- Bipolar Disorder
- Therapy Center
- When To See a Therapist
- Types of Therapy
- Best Online Therapy
- Best Couples Therapy
- Best Family Therapy
- Managing Stress
- Sleep and Dreaming
- Understanding Emotions
- Self-Improvement
- Healthy Relationships
- Student Resources
- Personality Types
- Guided Meditations
- Verywell Mind Insights
- 2023 Verywell Mind 25
- Mental Health in the Classroom
- Editorial Process
- Meet Our Review Board
- Crisis Support
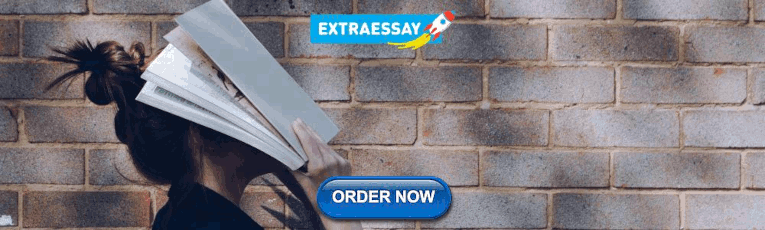
How to Write a Great Hypothesis
Hypothesis Format, Examples, and Tips
Kendra Cherry, MS, is a psychosocial rehabilitation specialist, psychology educator, and author of the "Everything Psychology Book."
:max_bytes(150000):strip_icc():format(webp)/IMG_9791-89504ab694d54b66bbd72cb84ffb860e.jpg)
Amy Morin, LCSW, is a psychotherapist and international bestselling author. Her books, including "13 Things Mentally Strong People Don't Do," have been translated into more than 40 languages. Her TEDx talk, "The Secret of Becoming Mentally Strong," is one of the most viewed talks of all time.
:max_bytes(150000):strip_icc():format(webp)/VW-MIND-Amy-2b338105f1ee493f94d7e333e410fa76.jpg)
Verywell / Alex Dos Diaz
- The Scientific Method
Hypothesis Format
Falsifiability of a hypothesis, operational definitions, types of hypotheses, hypotheses examples.
- Collecting Data
Frequently Asked Questions
A hypothesis is a tentative statement about the relationship between two or more variables. It is a specific, testable prediction about what you expect to happen in a study.
One hypothesis example would be a study designed to look at the relationship between sleep deprivation and test performance might have a hypothesis that states: "This study is designed to assess the hypothesis that sleep-deprived people will perform worse on a test than individuals who are not sleep-deprived."
This article explores how a hypothesis is used in psychology research, how to write a good hypothesis, and the different types of hypotheses you might use.
The Hypothesis in the Scientific Method
In the scientific method , whether it involves research in psychology, biology, or some other area, a hypothesis represents what the researchers think will happen in an experiment. The scientific method involves the following steps:
- Forming a question
- Performing background research
- Creating a hypothesis
- Designing an experiment
- Collecting data
- Analyzing the results
- Drawing conclusions
- Communicating the results
The hypothesis is a prediction, but it involves more than a guess. Most of the time, the hypothesis begins with a question which is then explored through background research. It is only at this point that researchers begin to develop a testable hypothesis. Unless you are creating an exploratory study, your hypothesis should always explain what you expect to happen.
In a study exploring the effects of a particular drug, the hypothesis might be that researchers expect the drug to have some type of effect on the symptoms of a specific illness. In psychology, the hypothesis might focus on how a certain aspect of the environment might influence a particular behavior.
Remember, a hypothesis does not have to be correct. While the hypothesis predicts what the researchers expect to see, the goal of the research is to determine whether this guess is right or wrong. When conducting an experiment, researchers might explore a number of factors to determine which ones might contribute to the ultimate outcome.
In many cases, researchers may find that the results of an experiment do not support the original hypothesis. When writing up these results, the researchers might suggest other options that should be explored in future studies.
In many cases, researchers might draw a hypothesis from a specific theory or build on previous research. For example, prior research has shown that stress can impact the immune system. So a researcher might hypothesize: "People with high-stress levels will be more likely to contract a common cold after being exposed to the virus than people who have low-stress levels."
In other instances, researchers might look at commonly held beliefs or folk wisdom. "Birds of a feather flock together" is one example of folk wisdom that a psychologist might try to investigate. The researcher might pose a specific hypothesis that "People tend to select romantic partners who are similar to them in interests and educational level."
Elements of a Good Hypothesis
So how do you write a good hypothesis? When trying to come up with a hypothesis for your research or experiments, ask yourself the following questions:
- Is your hypothesis based on your research on a topic?
- Can your hypothesis be tested?
- Does your hypothesis include independent and dependent variables?
Before you come up with a specific hypothesis, spend some time doing background research. Once you have completed a literature review, start thinking about potential questions you still have. Pay attention to the discussion section in the journal articles you read . Many authors will suggest questions that still need to be explored.
To form a hypothesis, you should take these steps:
- Collect as many observations about a topic or problem as you can.
- Evaluate these observations and look for possible causes of the problem.
- Create a list of possible explanations that you might want to explore.
- After you have developed some possible hypotheses, think of ways that you could confirm or disprove each hypothesis through experimentation. This is known as falsifiability.
In the scientific method , falsifiability is an important part of any valid hypothesis. In order to test a claim scientifically, it must be possible that the claim could be proven false.
Students sometimes confuse the idea of falsifiability with the idea that it means that something is false, which is not the case. What falsifiability means is that if something was false, then it is possible to demonstrate that it is false.
One of the hallmarks of pseudoscience is that it makes claims that cannot be refuted or proven false.
A variable is a factor or element that can be changed and manipulated in ways that are observable and measurable. However, the researcher must also define how the variable will be manipulated and measured in the study.
For example, a researcher might operationally define the variable " test anxiety " as the results of a self-report measure of anxiety experienced during an exam. A "study habits" variable might be defined by the amount of studying that actually occurs as measured by time.
These precise descriptions are important because many things can be measured in a number of different ways. One of the basic principles of any type of scientific research is that the results must be replicable. By clearly detailing the specifics of how the variables were measured and manipulated, other researchers can better understand the results and repeat the study if needed.
Some variables are more difficult than others to define. How would you operationally define a variable such as aggression ? For obvious ethical reasons, researchers cannot create a situation in which a person behaves aggressively toward others.
In order to measure this variable, the researcher must devise a measurement that assesses aggressive behavior without harming other people. In this situation, the researcher might utilize a simulated task to measure aggressiveness.
Hypothesis Checklist
- Does your hypothesis focus on something that you can actually test?
- Does your hypothesis include both an independent and dependent variable?
- Can you manipulate the variables?
- Can your hypothesis be tested without violating ethical standards?
The hypothesis you use will depend on what you are investigating and hoping to find. Some of the main types of hypotheses that you might use include:
- Simple hypothesis : This type of hypothesis suggests that there is a relationship between one independent variable and one dependent variable.
- Complex hypothesis : This type of hypothesis suggests a relationship between three or more variables, such as two independent variables and a dependent variable.
- Null hypothesis : This hypothesis suggests no relationship exists between two or more variables.
- Alternative hypothesis : This hypothesis states the opposite of the null hypothesis.
- Statistical hypothesis : This hypothesis uses statistical analysis to evaluate a representative sample of the population and then generalizes the findings to the larger group.
- Logical hypothesis : This hypothesis assumes a relationship between variables without collecting data or evidence.
A hypothesis often follows a basic format of "If {this happens} then {this will happen}." One way to structure your hypothesis is to describe what will happen to the dependent variable if you change the independent variable .
The basic format might be: "If {these changes are made to a certain independent variable}, then we will observe {a change in a specific dependent variable}."
A few examples of simple hypotheses:
- "Students who eat breakfast will perform better on a math exam than students who do not eat breakfast."
- Complex hypothesis: "Students who experience test anxiety before an English exam will get lower scores than students who do not experience test anxiety."
- "Motorists who talk on the phone while driving will be more likely to make errors on a driving course than those who do not talk on the phone."
Examples of a complex hypothesis include:
- "People with high-sugar diets and sedentary activity levels are more likely to develop depression."
- "Younger people who are regularly exposed to green, outdoor areas have better subjective well-being than older adults who have limited exposure to green spaces."
Examples of a null hypothesis include:
- "Children who receive a new reading intervention will have scores different than students who do not receive the intervention."
- "There will be no difference in scores on a memory recall task between children and adults."
Examples of an alternative hypothesis:
- "Children who receive a new reading intervention will perform better than students who did not receive the intervention."
- "Adults will perform better on a memory task than children."
Collecting Data on Your Hypothesis
Once a researcher has formed a testable hypothesis, the next step is to select a research design and start collecting data. The research method depends largely on exactly what they are studying. There are two basic types of research methods: descriptive research and experimental research.
Descriptive Research Methods
Descriptive research such as case studies , naturalistic observations , and surveys are often used when it would be impossible or difficult to conduct an experiment . These methods are best used to describe different aspects of a behavior or psychological phenomenon.
Once a researcher has collected data using descriptive methods, a correlational study can then be used to look at how the variables are related. This type of research method might be used to investigate a hypothesis that is difficult to test experimentally.
Experimental Research Methods
Experimental methods are used to demonstrate causal relationships between variables. In an experiment, the researcher systematically manipulates a variable of interest (known as the independent variable) and measures the effect on another variable (known as the dependent variable).
Unlike correlational studies, which can only be used to determine if there is a relationship between two variables, experimental methods can be used to determine the actual nature of the relationship—whether changes in one variable actually cause another to change.
A Word From Verywell
The hypothesis is a critical part of any scientific exploration. It represents what researchers expect to find in a study or experiment. In situations where the hypothesis is unsupported by the research, the research still has value. Such research helps us better understand how different aspects of the natural world relate to one another. It also helps us develop new hypotheses that can then be tested in the future.
Some examples of how to write a hypothesis include:
- "Staying up late will lead to worse test performance the next day."
- "People who consume one apple each day will visit the doctor fewer times each year."
- "Breaking study sessions up into three 20-minute sessions will lead to better test results than a single 60-minute study session."
The four parts of a hypothesis are:
- The research question
- The independent variable (IV)
- The dependent variable (DV)
- The proposed relationship between the IV and DV
Castillo M. The scientific method: a need for something better? . AJNR Am J Neuroradiol. 2013;34(9):1669-71. doi:10.3174/ajnr.A3401
Nevid J. Psychology: Concepts and Applications. Wadworth, 2013.
By Kendra Cherry, MSEd Kendra Cherry, MS, is a psychosocial rehabilitation specialist, psychology educator, and author of the "Everything Psychology Book."

Want to create or adapt books like this? Learn more about how Pressbooks supports open publishing practices.
Overview of the Scientific Method
Learning Objectives
- Distinguish between a theory and a hypothesis.
- Discover how theories are used to generate hypotheses and how the results of studies can be used to further inform theories.
- Understand the characteristics of a good hypothesis.
Theories and Hypotheses
Before describing how to develop a hypothesis, it is important to distinguish between a theory and a hypothesis. A theory is a coherent explanation or interpretation of one or more phenomena. Although theories can take a variety of forms, one thing they have in common is that they go beyond the phenomena they explain by including variables, structures, processes, functions, or organizing principles that have not been observed directly. Consider, for example, Zajonc’s theory of social facilitation and social inhibition (1965) [1] . He proposed that being watched by others while performing a task creates a general state of physiological arousal, which increases the likelihood of the dominant (most likely) response. So for highly practiced tasks, being watched increases the tendency to make correct responses, but for relatively unpracticed tasks, being watched increases the tendency to make incorrect responses. Notice that this theory—which has come to be called drive theory—provides an explanation of both social facilitation and social inhibition that goes beyond the phenomena themselves by including concepts such as “arousal” and “dominant response,” along with processes such as the effect of arousal on the dominant response.
Outside of science, referring to an idea as a theory often implies that it is untested—perhaps no more than a wild guess. In science, however, the term theory has no such implication. A theory is simply an explanation or interpretation of a set of phenomena. It can be untested, but it can also be extensively tested, well supported, and accepted as an accurate description of the world by the scientific community. The theory of evolution by natural selection, for example, is a theory because it is an explanation of the diversity of life on earth—not because it is untested or unsupported by scientific research. On the contrary, the evidence for this theory is overwhelmingly positive and nearly all scientists accept its basic assumptions as accurate. Similarly, the “germ theory” of disease is a theory because it is an explanation of the origin of various diseases, not because there is any doubt that many diseases are caused by microorganisms that infect the body.
A hypothesis , on the other hand, is a specific prediction about a new phenomenon that should be observed if a particular theory is accurate. It is an explanation that relies on just a few key concepts. Hypotheses are often specific predictions about what will happen in a particular study. They are developed by considering existing evidence and using reasoning to infer what will happen in the specific context of interest. Hypotheses are often but not always derived from theories. So a hypothesis is often a prediction based on a theory but some hypotheses are a-theoretical and only after a set of observations have been made, is a theory developed. This is because theories are broad in nature and they explain larger bodies of data. So if our research question is really original then we may need to collect some data and make some observations before we can develop a broader theory.
Theories and hypotheses always have this if-then relationship. “ If drive theory is correct, then cockroaches should run through a straight runway faster, and a branching runway more slowly, when other cockroaches are present.” Although hypotheses are usually expressed as statements, they can always be rephrased as questions. “Do cockroaches run through a straight runway faster when other cockroaches are present?” Thus deriving hypotheses from theories is an excellent way of generating interesting research questions.
But how do researchers derive hypotheses from theories? One way is to generate a research question using the techniques discussed in this chapter and then ask whether any theory implies an answer to that question. For example, you might wonder whether expressive writing about positive experiences improves health as much as expressive writing about traumatic experiences. Although this question is an interesting one on its own, you might then ask whether the habituation theory—the idea that expressive writing causes people to habituate to negative thoughts and feelings—implies an answer. In this case, it seems clear that if the habituation theory is correct, then expressive writing about positive experiences should not be effective because it would not cause people to habituate to negative thoughts and feelings. A second way to derive hypotheses from theories is to focus on some component of the theory that has not yet been directly observed. For example, a researcher could focus on the process of habituation—perhaps hypothesizing that people should show fewer signs of emotional distress with each new writing session.
Among the very best hypotheses are those that distinguish between competing theories. For example, Norbert Schwarz and his colleagues considered two theories of how people make judgments about themselves, such as how assertive they are (Schwarz et al., 1991) [2] . Both theories held that such judgments are based on relevant examples that people bring to mind. However, one theory was that people base their judgments on the number of examples they bring to mind and the other was that people base their judgments on how easily they bring those examples to mind. To test these theories, the researchers asked people to recall either six times when they were assertive (which is easy for most people) or 12 times (which is difficult for most people). Then they asked them to judge their own assertiveness. Note that the number-of-examples theory implies that people who recalled 12 examples should judge themselves to be more assertive because they recalled more examples, but the ease-of-examples theory implies that participants who recalled six examples should judge themselves as more assertive because recalling the examples was easier. Thus the two theories made opposite predictions so that only one of the predictions could be confirmed. The surprising result was that participants who recalled fewer examples judged themselves to be more assertive—providing particularly convincing evidence in favor of the ease-of-retrieval theory over the number-of-examples theory.
Theory Testing
The primary way that scientific researchers use theories is sometimes called the hypothetico-deductive method (although this term is much more likely to be used by philosophers of science than by scientists themselves). Researchers begin with a set of phenomena and either construct a theory to explain or interpret them or choose an existing theory to work with. They then make a prediction about some new phenomenon that should be observed if the theory is correct. Again, this prediction is called a hypothesis. The researchers then conduct an empirical study to test the hypothesis. Finally, they reevaluate the theory in light of the new results and revise it if necessary. This process is usually conceptualized as a cycle because the researchers can then derive a new hypothesis from the revised theory, conduct a new empirical study to test the hypothesis, and so on. As Figure 2.3 shows, this approach meshes nicely with the model of scientific research in psychology presented earlier in the textbook—creating a more detailed model of “theoretically motivated” or “theory-driven” research.
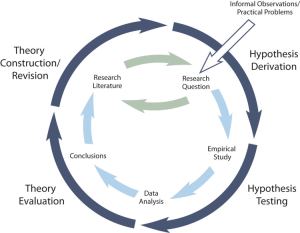
As an example, let us consider Zajonc’s research on social facilitation and inhibition. He started with a somewhat contradictory pattern of results from the research literature. He then constructed his drive theory, according to which being watched by others while performing a task causes physiological arousal, which increases an organism’s tendency to make the dominant response. This theory predicts social facilitation for well-learned tasks and social inhibition for poorly learned tasks. He now had a theory that organized previous results in a meaningful way—but he still needed to test it. He hypothesized that if his theory was correct, he should observe that the presence of others improves performance in a simple laboratory task but inhibits performance in a difficult version of the very same laboratory task. To test this hypothesis, one of the studies he conducted used cockroaches as subjects (Zajonc, Heingartner, & Herman, 1969) [3] . The cockroaches ran either down a straight runway (an easy task for a cockroach) or through a cross-shaped maze (a difficult task for a cockroach) to escape into a dark chamber when a light was shined on them. They did this either while alone or in the presence of other cockroaches in clear plastic “audience boxes.” Zajonc found that cockroaches in the straight runway reached their goal more quickly in the presence of other cockroaches, but cockroaches in the cross-shaped maze reached their goal more slowly when they were in the presence of other cockroaches. Thus he confirmed his hypothesis and provided support for his drive theory. (Zajonc also showed that drive theory existed in humans [Zajonc & Sales, 1966] [4] in many other studies afterward).
Incorporating Theory into Your Research
When you write your research report or plan your presentation, be aware that there are two basic ways that researchers usually include theory. The first is to raise a research question, answer that question by conducting a new study, and then offer one or more theories (usually more) to explain or interpret the results. This format works well for applied research questions and for research questions that existing theories do not address. The second way is to describe one or more existing theories, derive a hypothesis from one of those theories, test the hypothesis in a new study, and finally reevaluate the theory. This format works well when there is an existing theory that addresses the research question—especially if the resulting hypothesis is surprising or conflicts with a hypothesis derived from a different theory.
To use theories in your research will not only give you guidance in coming up with experiment ideas and possible projects, but it lends legitimacy to your work. Psychologists have been interested in a variety of human behaviors and have developed many theories along the way. Using established theories will help you break new ground as a researcher, not limit you from developing your own ideas.
Characteristics of a Good Hypothesis
There are three general characteristics of a good hypothesis. First, a good hypothesis must be testable and falsifiable . We must be able to test the hypothesis using the methods of science and if you’ll recall Popper’s falsifiability criterion, it must be possible to gather evidence that will disconfirm the hypothesis if it is indeed false. Second, a good hypothesis must be logical. As described above, hypotheses are more than just a random guess. Hypotheses should be informed by previous theories or observations and logical reasoning. Typically, we begin with a broad and general theory and use deductive reasoning to generate a more specific hypothesis to test based on that theory. Occasionally, however, when there is no theory to inform our hypothesis, we use inductive reasoning which involves using specific observations or research findings to form a more general hypothesis. Finally, the hypothesis should be positive. That is, the hypothesis should make a positive statement about the existence of a relationship or effect, rather than a statement that a relationship or effect does not exist. As scientists, we don’t set out to show that relationships do not exist or that effects do not occur so our hypotheses should not be worded in a way to suggest that an effect or relationship does not exist. The nature of science is to assume that something does not exist and then seek to find evidence to prove this wrong, to show that it really does exist. That may seem backward to you but that is the nature of the scientific method. The underlying reason for this is beyond the scope of this chapter but it has to do with statistical theory.
- Zajonc, R. B. (1965). Social facilitation. Science, 149 , 269–274 ↵
- Schwarz, N., Bless, H., Strack, F., Klumpp, G., Rittenauer-Schatka, H., & Simons, A. (1991). Ease of retrieval as information: Another look at the availability heuristic. Journal of Personality and Social Psychology, 61 , 195–202. ↵
- Zajonc, R. B., Heingartner, A., & Herman, E. M. (1969). Social enhancement and impairment of performance in the cockroach. Journal of Personality and Social Psychology, 13 , 83–92. ↵
- Zajonc, R.B. & Sales, S.M. (1966). Social facilitation of dominant and subordinate responses. Journal of Experimental Social Psychology, 2 , 160-168. ↵
A coherent explanation or interpretation of one or more phenomena.
A specific prediction about a new phenomenon that should be observed if a particular theory is accurate.
A cyclical process of theory development, starting with an observed phenomenon, then developing or using a theory to make a specific prediction of what should happen if that theory is correct, testing that prediction, refining the theory in light of the findings, and using that refined theory to develop new hypotheses, and so on.
The ability to test the hypothesis using the methods of science and the possibility to gather evidence that will disconfirm the hypothesis if it is indeed false.
Research Methods in Psychology Copyright © 2019 by Rajiv S. Jhangiani, I-Chant A. Chiang, Carrie Cuttler, & Dana C. Leighton is licensed under a Creative Commons Attribution-NonCommercial-ShareAlike 4.0 International License , except where otherwise noted.
Share This Book
Chapter 2: Psychological Research
The scientific method.
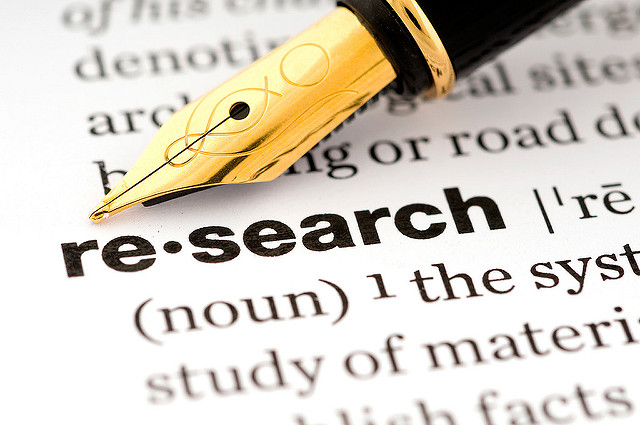
Scientists are engaged in explaining and understanding how the world around them works, and they are able to do so by coming up with theories that generate hypotheses that are testable and falsifiable. Theories that stand up to their tests are retained and refined, while those that do not are discarded or modified. In this way, research enables scientists to separate fact from simple opinion. Having good information generated from research aids in making wise decisions both in public policy and in our personal lives. In this section, you’ll see how psychologists use the scientific method to study and understand behavior.
Scientific research is a critical tool for successfully navigating our complex world. Without it, we would be forced to rely solely on intuition, other people’s authority, and blind luck. While many of us feel confident in our abilities to decipher and interact with the world around us, history is filled with examples of how very wrong we can be when we fail to recognize the need for evidence in supporting claims. At various times in history, we would have been certain that the sun revolved around a flat earth, that the earth’s continents did not move, and that mental illness was caused by possession (Figure 1). It is through systematic scientific research that we divest ourselves of our preconceived notions and superstitions and gain an objective understanding of ourselves and our world.
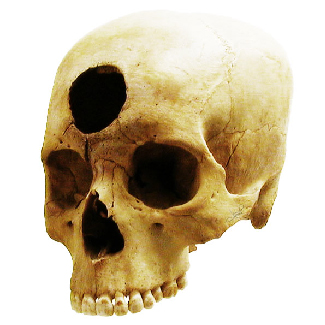
Figure 1 . Some of our ancestors, believed that trephination—the practice of making a hole in the skull—allowed evil spirits to leave the body, thus curing mental illness.
The goal of all scientists is to better understand the world around them. Psychologists focus their attention on understanding behavior, as well as the cognitive (mental) and physiological (body) processes that underlie behavior. In contrast to other methods that people use to understand the behavior of others, such as intuition and personal experience, the hallmark of scientific research is that there is evidence to support a claim. Scientific knowledge is empirical : It is grounded in objective, tangible evidence that can be observed time and time again, regardless of who is observing.
While behavior is observable, the mind is not. If someone is crying, we can see behavior. However, the reason for the behavior is more difficult to determine. Is the person crying due to being sad, in pain, or happy? Sometimes we can learn the reason for someone’s behavior by simply asking a question, like “Why are you crying?” However, there are situations in which an individual is either uncomfortable or unwilling to answer the question honestly, or is incapable of answering. For example, infants would not be able to explain why they are crying. In such circumstances, the psychologist must be creative in finding ways to better understand behavior. This module explores how scientific knowledge is generated, and how important that knowledge is in informing decisions in our personal lives and in the public domain.
The Process of Scientific Research
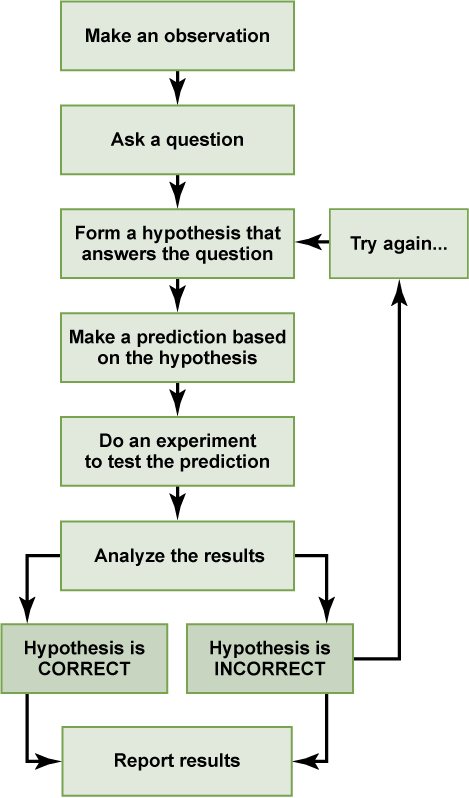
Figure 2 . The scientific method is a process for gathering data and processing information. It provides well-defined steps to standardize how scientific knowledge is gathered through a logical, rational problem-solving method.
Scientific knowledge is advanced through a process known as the scientific method. Basically, ideas (in the form of theories and hypotheses) are tested against the real world (in the form of empirical observations), and those empirical observations lead to more ideas that are tested against the real world, and so on.
The basic steps in the scientific method are:
- Observe a natural phenomenon and define a question about it
- Make a hypothesis, or potential solution to the question
- Test the hypothesis
- If the hypothesis is true, find more evidence or find counter-evidence
- If the hypothesis is false, create a new hypothesis or try again
- Draw conclusions and repeat–the scientific method is never-ending, and no result is ever considered perfect
In order to ask an important question that may improve our understanding of the world, a researcher must first observe natural phenomena. By making observations, a researcher can define a useful question. After finding a question to answer, the researcher can then make a prediction (a hypothesis) about what he or she thinks the answer will be. This prediction is usually a statement about the relationship between two or more variables. After making a hypothesis, the researcher will then design an experiment to test his or her hypothesis and evaluate the data gathered. These data will either support or refute the hypothesis. Based on the conclusions drawn from the data, the researcher will then find more evidence to support the hypothesis, look for counter-evidence to further strengthen the hypothesis, revise the hypothesis and create a new experiment, or continue to incorporate the information gathered to answer the research question.
Video 1. The Scientific Method explains the basic steps taken for most scientific inquiry.
The Basic Principles of the Scientific Method
Two key concepts in the scientific approach are theory and hypothesis. A theory is a well-developed set of ideas that propose an explanation for observed phenomena that can be used to make predictions about future observations. A hypothesis is a testable prediction that is arrived at logically from a theory. It is often worded as an if-then statement (e.g., if I study all night, I will get a passing grade on the test). The hypothesis is extremely important because it bridges the gap between the realm of ideas and the real world. As specific hypotheses are tested, theories are modified and refined to reflect and incorporate the result of these tests (Figure 3).
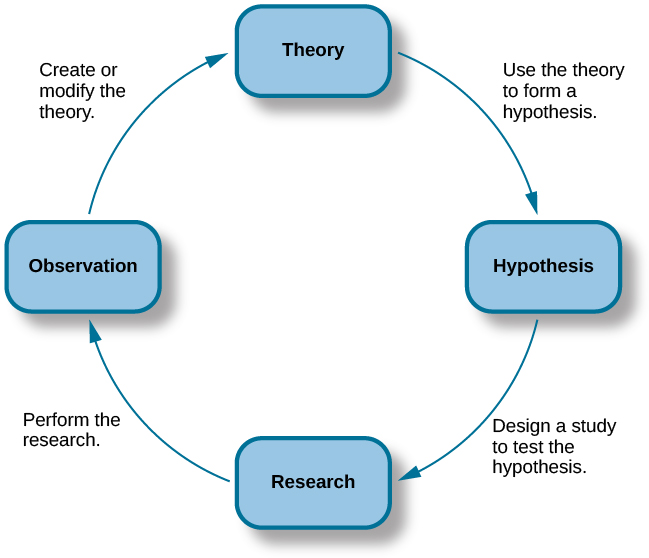
Figure 3 . The scientific method of research includes proposing hypotheses, conducting research, and creating or modifying theories based on results.
Other key components in following the scientific method include verifiability, predictability, falsifiability, and fairness. Verifiability means that an experiment must be replicable by another researcher. To achieve verifiability, researchers must make sure to document their methods and clearly explain how their experiment is structured and why it produces certain results.
Predictability in a scientific theory implies that the theory should enable us to make predictions about future events. The precision of these predictions is a measure of the strength of the theory.
Falsifiability refers to whether a hypothesis can be disproved. For a hypothesis to be falsifiable, it must be logically possible to make an observation or do a physical experiment that would show that there is no support for the hypothesis. Even when a hypothesis cannot be shown to be false, that does not necessarily mean it is not valid. Future testing may disprove the hypothesis. This does not mean that a hypothesis has to be shown to be false, just that it can be tested.
To determine whether a hypothesis is supported or not supported, psychological researchers must conduct hypothesis testing using statistics. Hypothesis testing is a type of statistics that determines the probability of a hypothesis being true or false. If hypothesis testing reveals that results were “statistically significant,” this means that there was support for the hypothesis and that the researchers can be reasonably confident that their result was not due to random chance. If the results are not statistically significant, this means that the researchers’ hypothesis was not supported.
Fairness implies that all data must be considered when evaluating a hypothesis. A researcher cannot pick and choose what data to keep and what to discard or focus specifically on data that support or do not support a particular hypothesis. All data must be accounted for, even if they invalidate the hypothesis.
Applying the Scientific Method
To see how this process works, let’s consider a specific theory and a hypothesis that might be generated from that theory. As you’ll learn in a later module, the James-Lange theory of emotion asserts that emotional experience relies on the physiological arousal associated with the emotional state. If you walked out of your home and discovered a very aggressive snake waiting on your doorstep, your heart would begin to race, and your stomach churn. According to the James-Lange theory, these physiological changes would result in your feeling of fear. A hypothesis that could be derived from this theory might be that a person who is unaware of the physiological arousal that the sight of the snake elicits will not feel fear.
Remember that a good scientific hypothesis is falsifiable, or capable of being shown to be incorrect. Recall from the introductory module that Sigmund Freud had lots of interesting ideas to explain various human behaviors (Figure 4). However, a major criticism of Freud’s theories is that many of his ideas are not falsifiable; for example, it is impossible to imagine empirical observations that would disprove the existence of the id, the ego, and the superego—the three elements of personality described in Freud’s theories. Despite this, Freud’s theories are widely taught in introductory psychology texts because of their historical significance for personality psychology and psychotherapy, and these remain the root of all modern forms of therapy.
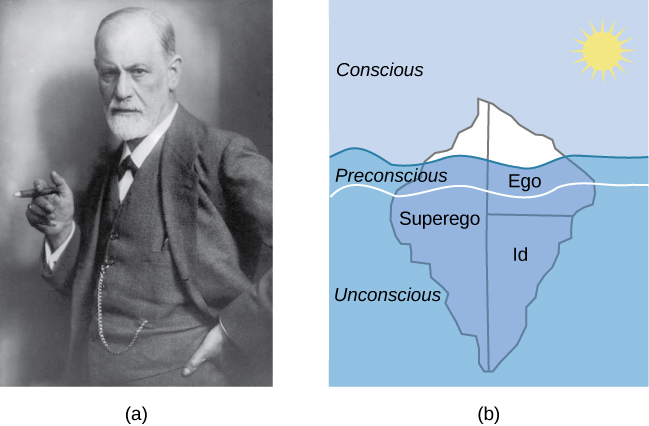
Figure 4 . Many of the specifics of (a) Freud’s theories, such as (b) his division of the mind into id, ego, and superego, have fallen out of favor in recent decades because they are not falsifiable. In broader strokes, his views set the stage for much of psychological thinking today, such as the unconscious nature of the majority of psychological processes.
In contrast, the James-Lange theory does generate falsifiable hypotheses, such as the one described above. Some individuals who suffer significant injuries to their spinal columns are unable to feel the bodily changes that often accompany emotional experiences. Therefore, we could test the hypothesis by determining how emotional experiences differ between individuals who have the ability to detect these changes in their physiological arousal and those who do not. In fact, this research has been conducted and while the emotional experiences of people deprived of an awareness of their physiological arousal may be less intense, they still experience emotion (Chwalisz, Diener, & Gallagher, 1988).
Link to Learning
Want to participate in a study? Visit this Psychological Research on the Net website and click on a link that sounds interesting to you in order to participate in online research.
Why the Scientific Method Is Important for Psychology
The use of the scientific method is one of the main features that separates modern psychology from earlier philosophical inquiries about the mind. Compared to chemistry, physics, and other “natural sciences,” psychology has long been considered one of the “social sciences” because of the subjective nature of the things it seeks to study. Many of the concepts that psychologists are interested in—such as aspects of the human mind, behavior, and emotions—are subjective and cannot be directly measured. Psychologists often rely instead on behavioral observations and self-reported data, which are considered by some to be illegitimate or lacking in methodological rigor. Applying the scientific method to psychology, therefore, helps to standardize the approach to understanding its very different types of information.
The scientific method allows psychological data to be replicated and confirmed in many instances, under different circumstances, and by a variety of researchers. Through replication of experiments, new generations of psychologists can reduce errors and broaden the applicability of theories. It also allows theories to be tested and validated instead of simply being conjectures that could never be verified or falsified. All of this allows psychologists to gain a stronger understanding of how the human mind works.
Scientific articles published in journals and psychology papers written in the style of the American Psychological Association (i.e., in “APA style”) are structured around the scientific method. These papers include an Introduction, which introduces the background information and outlines the hypotheses; a Methods section, which outlines the specifics of how the experiment was conducted to test the hypothesis; a Results section, which includes the statistics that tested the hypothesis and state whether it was supported or not supported, and a Discussion and Conclusion, which state the implications of finding support for, or no support for, the hypothesis. Writing articles and papers that adhere to the scientific method makes it easy for future researchers to repeat the study and attempt to replicate the results.
Today, scientists agree that good research is ethical in nature and is guided by a basic respect for human dignity and safety. However, as you will read in the Tuskegee Syphilis Study, this has not always been the case. Modern researchers must demonstrate that the research they perform is ethically sound. This section presents how ethical considerations affect the design and implementation of research conducted today.
Research Involving Human Participants
Any experiment involving the participation of human subjects is governed by extensive, strict guidelines designed to ensure that the experiment does not result in harm. Any research institution that receives federal support for research involving human participants must have access to an institutional review board (IRB) . The IRB is a committee of individuals often made up of members of the institution’s administration, scientists, and community members (Figure 1). The purpose of the IRB is to review proposals for research that involves human participants. The IRB reviews these proposals with the principles mentioned above in mind, and generally, approval from the IRB is required in order for the experiment to proceed.
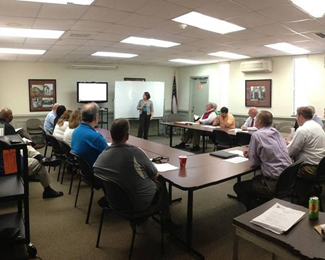
Figure 5 . An institution’s IRB meets regularly to review experimental proposals that involve human participants. (credit: modification of work by Lowndes Area Knowledge Exchange (LAKE)/Flickr)
An institution’s IRB requires several components in any experiment it approves. For one, each participant must sign an informed consent form before they can participate in the experiment. An informed consent form provides a written description of what participants can expect during the experiment, including potential risks and implications of the research. It also lets participants know that their involvement is completely voluntary and can be discontinued without penalty at any time. Furthermore, informed consent guarantees that any data collected in the experiment will remain completely confidential. In cases where research participants are under the age of 18, the parents or legal guardians are required to sign the informed consent form.
While the informed consent form should be as honest as possible in describing exactly what participants will be doing, sometimes deception is necessary to prevent participants’ knowledge of the exact research question from affecting the results of the study. Deception involves purposely misleading experiment participants in order to maintain the integrity of the experiment, but not to the point where the deception could be considered harmful. For example, if we are interested in how our opinion of someone is affected by their attire, we might use deception in describing the experiment to prevent that knowledge from affecting participants’ responses. In cases where deception is involved, participants must receive a full debriefing upon conclusion of the study—complete, honest information about the purpose of the experiment, how the data collected will be used, the reasons why deception was necessary, and information about how to obtain additional information about the study.
Dig Deeper: Ethics and the Tuskegee Syphilis Study
Unfortunately, the ethical guidelines that exist for research today were not always applied in the past. In 1932, poor, rural, black, male sharecroppers from Tuskegee, Alabama, were recruited to participate in an experiment conducted by the U.S. Public Health Service, with the aim of studying syphilis in black men (Figure 6). In exchange for free medical care, meals, and burial insurance, 600 men agreed to participate in the study. A little more than half of the men tested positive for syphilis, and they served as the experimental group (given that the researchers could not randomly assign participants to groups, this represents a quasi-experiment). The remaining syphilis-free individuals served as the control group. However, those individuals that tested positive for syphilis were never informed that they had the disease.
While there was no treatment for syphilis when the study began, by 1947 penicillin was recognized as an effective treatment for the disease. Despite this, no penicillin was administered to the participants in this study, and the participants were not allowed to seek treatment at any other facilities if they continued in the study. Over the course of 40 years, many of the participants unknowingly spread syphilis to their wives (and subsequently their children born from their wives) and eventually died because they never received treatment for the disease. This study was discontinued in 1972 when the experiment was discovered by the national press (Tuskegee University, n.d.). The resulting outrage over the experiment led directly to the National Research Act of 1974 and the strict ethical guidelines for research on humans described in this chapter. Why is this study unethical? How were the men who participated and their families harmed as a function of this research?
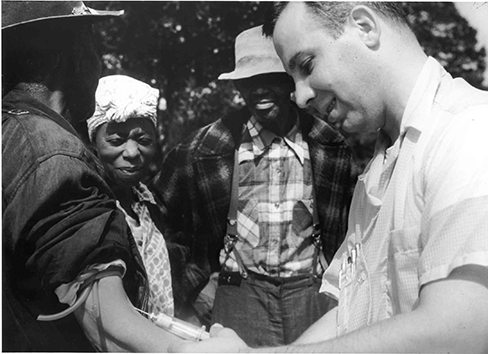
Figure 6 . A participant in the Tuskegee Syphilis Study receives an injection.
Visit this CDC website to learn more about the Tuskegee Syphilis Study.
Research Involving Animal Subjects
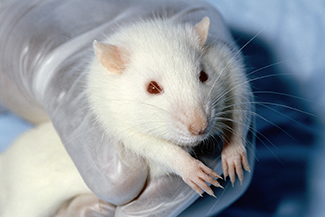
Figure 7 . Rats, like the one shown here, often serve as the subjects of animal research.
This does not mean that animal researchers are immune to ethical concerns. Indeed, the humane and ethical treatment of animal research subjects is a critical aspect of this type of research. Researchers must design their experiments to minimize any pain or distress experienced by animals serving as research subjects.
Whereas IRBs review research proposals that involve human participants, animal experimental proposals are reviewed by an Institutional Animal Care and Use Committee (IACUC) . An IACUC consists of institutional administrators, scientists, veterinarians, and community members. This committee is charged with ensuring that all experimental proposals require the humane treatment of animal research subjects. It also conducts semi-annual inspections of all animal facilities to ensure that the research protocols are being followed. No animal research project can proceed without the committee’s approval.
- Modification and adaptation. Provided by : Lumen Learning. License : CC BY-SA: Attribution-ShareAlike
- Psychology and the Scientific Method: From Theory to Conclusion, content on the scientific method principles. Provided by : Boundless. Located at : https://courses.lumenlearning.com/boundless-psychology/ . License : CC BY-SA: Attribution-ShareAlike
- Introduction to Psychological Research, Why is Research Important?, Ethics. Authored by : OpenStax College. Located at : http://cnx.org/contents/[email protected]:Hp5zMFYB@9/Why-Is-Research-Important . License : CC BY: Attribution . License Terms : Download for free at http://cnx.org/contents/[email protected]
- Research picture. Authored by : Mediterranean Center of Medical Sciences. Provided by : Flickr. Located at : https://www.flickr.com/photos/mcmscience/17664002728 . License : CC BY: Attribution
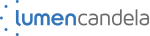
Privacy Policy
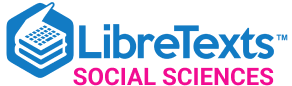
- school Campus Bookshelves
- menu_book Bookshelves
- perm_media Learning Objects
- login Login
- how_to_reg Request Instructor Account
- hub Instructor Commons
- Download Page (PDF)
- Download Full Book (PDF)
- Periodic Table
- Physics Constants
- Scientific Calculator
- Reference & Cite
- Tools expand_more
- Readability
selected template will load here
This action is not available.
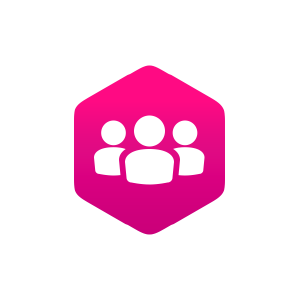
1.3: Psychologists Use the Scientific Method to Guide Their Research
- Last updated
- Save as PDF
- Page ID 107147

Learning Objectives
- Describe the principles of the scientific method and explain its importance in conducting and interpreting research.
- Differentiate laws from theories and explain how research hypotheses are developed and tested.
- Discuss the procedures that researchers use to ensure that their research with humans and with animals is ethical.
Psychologists aren’t the only people who seek to understand human behavior and solve social problems. Philosophers, religious leaders, and politicians, among others, also strive to provide explanations for human behavior. But psychologists believe that research is the best tool for understanding human beings and their relationships with others. Rather than accepting the claim of a philosopher that people do (or do not) have free will, a psychologist would collect data to empirically test whether or not people are able to actively control their own behavior. Rather than accepting a politician’s contention that creating (or abandoning) a new center for mental health will improve the lives of individuals in the inner city, a psychologist would empirically assess the effects of receiving mental health treatment on the quality of life of the recipients. The statements made by psychologists are empirical, which means they are based on systematic collection and analysis of data .
The Scientific Method
All scientists (whether they are physicists, chemists, biologists, sociologists, or psychologists) are engaged in the basic processes of collecting data and drawing conclusions about those data. The methods used by scientists have developed over many years and provide a common framework for developing, organizing, and sharing information. The scientific method is the set of assumptions, rules, and procedures scientists use to conduct research .
In addition to requiring that science be empirical, the scientific method demands that the procedures used be objective, or free from the personal bias or emotions of the scientist . The scientific method proscribes how scientists collect and analyze data, how they draw conclusions from data, and how they share data with others. These rules increase objectivity by placing data under the scrutiny of other scientists and even the public at large. Because data are reported objectively, other scientists know exactly how the scientist collected and analyzed the data. This means that they do not have to rely only on the scientist’s own interpretation of the data; they may draw their own, potentially different, conclusions.
Most new research is designed to replicate —that is, to repeat, add to, or modify—previous research findings. The scientific method therefore results in an accumulation of scientific knowledge through the reporting of research and the addition to and modifications of these reported findings by other scientists.
Laws and Theories as Organizing Principles
One goal of research is to organize information into meaningful statements that can be applied in many situations. Principles that are so general as to apply to all situations in a given domain of inquiry are known as laws. There are well-known laws in the physical sciences, such as the law of gravity and the laws of thermodynamics, and there are some universally accepted laws in psychology, such as the law of effect and Weber’s law. But because laws are very general principles and their validity has already been well established, they are themselves rarely directly subjected to scientific test.
The next step down from laws in the hierarchy of organizing principles is theory. A theory is an integrated set of principles that explains and predicts many, but not all, observed relationships within a given domain of inquiry . One example of an important theory in psychology is the stage theory of cognitive development proposed by the Swiss psychologist Jean Piaget. The theory states that children pass through a series of cognitive stages as they grow, each of which must be mastered in succession before movement to the next cognitive stage can occur. This is an extremely useful theory in human development because it can be applied to many different content areas and can be tested in many different ways.
Good theories have four important characteristics. First, good theories are general , meaning they summarize many different outcomes. Second, they are parsimonious , meaning they provide the simplest possible account of those outcomes. The stage theory of cognitive development meets both of these requirements. It can account for developmental changes in behavior across a wide variety of domains, and yet it does so parsimoniously—by hypothesizing a simple set of cognitive stages. Third, good theories provide ideas for future research . The stage theory of cognitive development has been applied not only to learning about cognitive skills, but also to the study of children’s moral (Kohlberg, 1966) and gender (Ruble & Martin, 1998) development.
Finally, good theories are falsifiable (Popper, 1959), which means the variables of interest can be adequately measured and the relationships between the variables that are predicted by the theory can be shown through research to be incorrect . The stage theory of cognitive development is falsifiable because the stages of cognitive reasoning can be measured and because if research discovers, for instance, that children learn new tasks before they have reached the cognitive stage hypothesized to be required for that task, then the theory will be shown to be incorrect.
No single theory is able to account for all behavior in all cases. Rather, theories are each limited in that they make accurate predictions in some situations or for some people but not in other situations or for other people. As a result, there is a constant exchange between theory and data: Existing theories are modified on the basis of collected data, and the new modified theories then make new predictions that are tested by new data, and so forth. When a better theory is found, it will replace the old one. This is part of the accumulation of scientific knowledge.
The Research Hypothesis
Theories are usually framed too broadly to be tested in a single experiment. Therefore, scientists use a more precise statement of the presumed relationship among specific parts of a theory—a research hypothesis—as the basis for their research. A research hypothesis is a specific and falsifiable prediction about the relationship between or among two or more variables , where a variable is any attribute that can assume different values among different people or across different times or places . The research hypothesis states the existence of a relationship between the variables of interest and the specific direction of that relationship. For instance, the research hypothesis “Using marijuana will reduce learning” predicts that there is a relationship between a variable “using marijuana” and another variable called “learning.” Similarly, in the research hypothesis “Participating in psychotherapy will reduce anxiety,” the variables that are expected to be related are “participating in psychotherapy” and “level of anxiety.”
When stated in an abstract manner, the ideas that form the basis of a research hypothesis are known as conceptual variables. Conceptual variables are abstract ideas that form the basis of research hypotheses . Sometimes the conceptual variables are rather simple—for instance, “age,” “gender,” or “weight.” In other cases the conceptual variables represent more complex ideas, such as “anxiety,” “cognitive development,” “learning,” self-esteem,” or “sexism.”
The first step in testing a research hypothesis involves turning the conceptual variables into measured variables, which are variables consisting of numbers that represent the conceptual variables . For instance, the conceptual variable “participating in psychotherapy” could be represented as the measured variable “number of psychotherapy hours the patient has accrued” and the conceptual variable “using marijuana” could be assessed by having the research participants rate, on a scale from 1 to 10, how often they use marijuana or by administering a blood test that measures the presence of the chemicals in marijuana.
Psychologists use the term operational definition to refer to a precise statement of how a conceptual variable is turned into a measured variable . The relationship between conceptual and measured variables in a research hypothesis is diagrammed in Figure \(\PageIndex{1}\). The conceptual variables are represented within circles at the top of the figure, and the measured variables are represented within squares at the bottom. The two vertical arrows, which lead from the conceptual variables to the measured variables, represent the operational definitions of the two variables. The arrows indicate the expectation that changes in the conceptual variables (psychotherapy and anxiety in this example) will cause changes in the corresponding measured variables. The measured variables are then used to draw inferences about the conceptual variables.
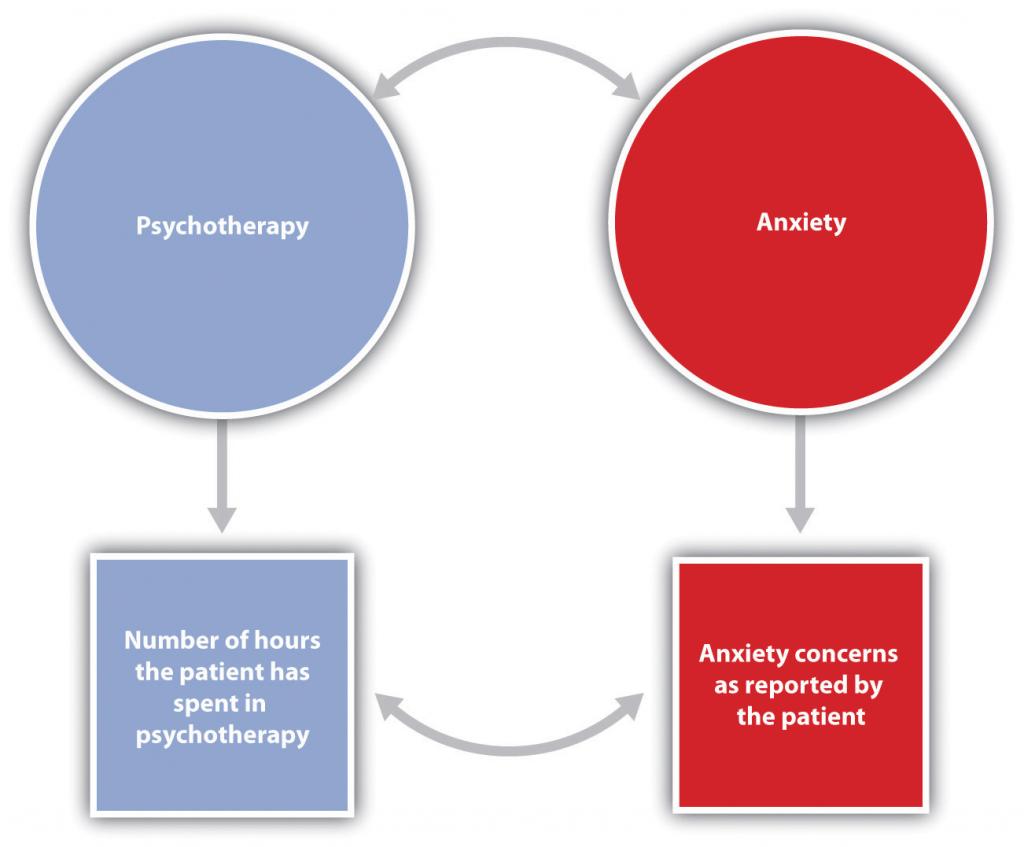
Table \(\PageIndex{1}\) lists some potential operational definitions of conceptual variables that have been used in psychological research. As you read through this list, note that in contrast to the abstract conceptual variables, the measured variables are very specific. This specificity is important for two reasons. First, more specific definitions mean that there is less danger that the collected data will be misunderstood by others. Second, specific definitions will enable future researchers to replicate the research.
Conducting Ethical Research
One of the questions that all scientists must address concerns the ethics of their research. Physicists are concerned about the potentially harmful outcomes of their experiments with nuclear materials. Biologists worry about the potential outcomes of creating genetically engineered human babies. Medical researchers agonize over the ethics of withholding potentially beneficial drugs from control groups in clinical trials. Likewise, psychologists are continually considering the ethics of their research.
Research in psychology may cause some stress, harm, or inconvenience for the people who participate in that research. For instance, researchers may require introductory psychology students to participate in research projects and then deceive these students, at least temporarily, about the nature of the research. Psychologists may induce stress, anxiety, or negative moods in their participants, expose them to weak electrical shocks, or convince them to behave in ways that violate their moral standards. And researchers may sometimes use animals in their research, potentially harming them in the process.
Decisions about whether research is ethical are made using established ethical codes developed by scientific organizations, such as the American Psychological Association, and federal governments. In the United States, the Department of Health and Human Services provides the guidelines for ethical standards in research. Some research, such as the research conducted by the Nazis on prisoners during World War II, is perceived as immoral by almost everyone. Other procedures, such as the use of animals in research testing the effectiveness of drugs, are more controversial.
Scientific research has provided information that has improved the lives of many people. Therefore, it is unreasonable to argue that because scientific research has costs, no research should be conducted. This argument fails to consider the fact that there are significant costs to not doing research and that these costs may be greater than the potential costs of conducting the research (Rosenthal, 1994). In each case, before beginning to conduct the research, scientists have attempted to determine the potential risks and benefits of the research and have come to the conclusion that the potential benefits of conducting the research outweigh the potential costs to the research participants.
Characteristics of an Ethical Research Project Using Human Participants
- Trust and positive rapport are created between the researcher and the participant.
- The rights of both the experimenter and participant are considered, and the relationship between them is mutually beneficial.
- The experimenter treats the participant with concern and respect and attempts to make the research experience a pleasant and informative one.
- Before the research begins, the participant is given all information relevant to his or her decision to participate, including any possibilities of physical danger or psychological stress.
- The participant is given a chance to have questions about the procedure answered, thus guaranteeing his or her free choice about participating.
- After the experiment is over, any deception that has been used is made public, and the necessity for it is explained.
- The experimenter carefully debriefs the participant, explaining the underlying research hypothesis and the purpose of the experimental procedure in detail and answering any questions.
- The experimenter provides information about how he or she can be contacted and offers to provide information about the results of the research if the participant is interested in receiving it. (Stangor, 2011)
This list presents some of the most important factors that psychologists take into consideration when designing their research. The most direct ethical concern of the scientist is to prevent harm to the research participants. One example is the well-known research of Stanley Milgram (1974) investigating obedience to authority. In these studies, participants were induced by an experimenter to administer electric shocks to another person so that Milgram could study the extent to which they would obey the demands of an authority figure. Most participants evidenced high levels of stress resulting from the psychological conflict they experienced between engaging in aggressive and dangerous behavior and following the instructions of the experimenter. Studies such as those by Milgram are no longer conducted because the scientific community is now much more sensitized to the potential of such procedures to create emotional discomfort or harm.
Another goal of ethical research is to guarantee that participants have free choice regarding whether they wish to participate in research. Students in psychology classes may be allowed, or even required, to participate in research, but they are also always given an option to choose a different study to be in, or to perform other activities instead. And once an experiment begins, the research participant is always free to leave the experiment if he or she wishes to. Concerns with free choice also occur in institutional settings, such as in schools, hospitals, corporations, and prisons, when individuals are required by the institutions to take certain tests, or when employees are told or asked to participate in research.
Researchers must also protect the privacy of the research participants. In some cases data can be kept anonymous by not having the respondents put any identifying information on their questionnaires. In other cases the data cannot be anonymous because the researcher needs to keep track of which respondent contributed the data. In this case one technique is to have each participant use a unique code number to identify his or her data, such as the last four digits of the student ID number. In this way the researcher can keep track of which person completed which questionnaire, but no one will be able to connect the data with the individual who contributed them.
Perhaps the most widespread ethical concern to the participants in behavioral research is the extent to which researchers employ deception. Deception occurs whenever research participants are not completely and fully informed about the nature of the research project before participating in it . Deception may occur in an active way, such as when the researcher tells the participants that he or she is studying learning when in fact the experiment really concerns obedience to authority. In other cases the deception is more passive, such as when participants are not told about the hypothesis being studied or the potential use of the data being collected.
Some researchers have argued that no deception should ever be used in any research (Baumrind, 1985). They argue that participants should always be told the complete truth about the nature of the research they are in, and that when participants are deceived there will be negative consequences, such as the possibility that participants may arrive at other studies already expecting to be deceived. Other psychologists defend the use of deception on the grounds that it is needed to get participants to act naturally and to enable the study of psychological phenomena that might not otherwise get investigated. They argue that it would be impossible to study topics such as altruism, aggression, obedience, and stereotyping without using deception because if participants were informed ahead of time what the study involved, this knowledge would certainly change their behavior. The codes of ethics of the American Psychological Association and other organizations allow researchers to use deception, but these codes also require them to explicitly consider how their research might be conducted without the use of deception.
Ensuring That Research Is Ethical
Making decisions about the ethics of research involves weighing the costs and benefits of conducting versus not conducting a given research project. The costs involve potential harm to the research participants and to the field, whereas the benefits include the potential for advancing knowledge about human behavior and offering various advantages, some educational, to the individual participants. Most generally, the ethics of a given research project are determined through a cost-benefit analysis , in which the costs are compared to the benefits. If the potential costs of the research appear to outweigh any potential benefits that might come from it, then the research should not proceed.
Arriving at a cost-benefit ratio is not simple. For one thing, there is no way to know ahead of time what the effects of a given procedure will be on every person or animal who participates or what benefit to society the research is likely to produce. In addition, what is ethical is defined by the current state of thinking within society, and thus perceived costs and benefits change over time. The U.S. Department of Health and Human Services regulations require that all universities receiving funds from the department set up an Institutional Review Board (IRB) to determine whether proposed research meets department regulations. The Institutional Review Board (IRB) is a committee of at least five members whose goal it is to determine the cost-benefit ratio of research conducted within an institution . The IRB approves the procedures of all the research conducted at the institution before the research can begin. The board may suggest modifications to the procedures, or (in rare cases) it may inform the scientist that the research violates Department of Health and Human Services guidelines and thus cannot be conducted at all.
One important tool for ensuring that research is ethical is the use of informed consent . A sample informed consent form is shown in Figure \(\PageIndex{2}\). Informed consent, conducted before a participant begins a research session, is designed to explain the research procedures and inform the participant of his or her rights during the investigation . The informed consent explains as much as possible about the true nature of the study, particularly everything that might be expected to influence willingness to participate, but it may in some cases withhold some information that allows the study to work.
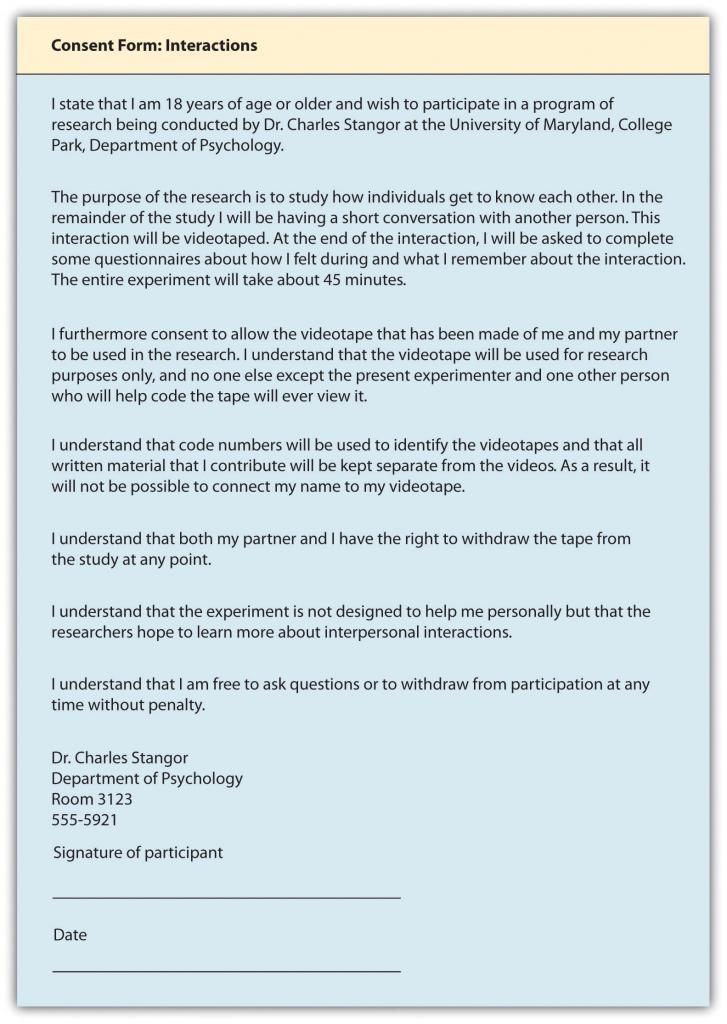
Because participating in research has the potential for producing long-term changes in the research participants, all participants should be fully debriefed immediately after their participation. The debriefing is a procedure designed to fully explain the purposes and procedures of the research and remove any harmful aftereffects of participation .
Research With Animals
Because animals make up an important part of the natural world, and because some research cannot be conducted using humans, animals are also participants in psychological research. Most psychological research using animals is now conducted with rats, mice, and birds, and the use of other animals in research is declining (Thomas & Blackman, 1992). As with ethical decisions involving human participants, a set of basic principles has been developed that helps researchers make informed decisions about such research; a summary is shown below.
APA Guidelines on Humane Care and Use of Animals in Research
The following are some of the most important ethical principles from the American Psychological Association’s guidelines on research with animals.
- Psychologists acquire, care for, use, and dispose of animals in compliance with current federal, state, and local laws and regulations, and with professional standards.
- Psychologists trained in research methods and experienced in the care of laboratory animals supervise all procedures involving animals and are responsible for ensuring appropriate consideration of their comfort, health, and humane treatment.
- Psychologists ensure that all individuals under their supervision who are using animals have received instruction in research methods and in the care, maintenance, and handling of the species being used, to the extent appropriate to their role.
- Psychologists make reasonable efforts to minimize the discomfort, infection, illness, and pain of animal subjects.
- Psychologists use a procedure subjecting animals to pain, stress, or privation only when an alternative procedure is unavailable and the goal is justified by its prospective scientific, educational, or applied value.
- Psychologists perform surgical procedures under appropriate anesthesia and follow techniques to avoid infection and minimize pain during and after surgery.
- When it is appropriate that an animal’s life be terminated, psychologists proceed rapidly, with an effort to minimize pain and in accordance with accepted procedures. (American Psychological Association, 2002)
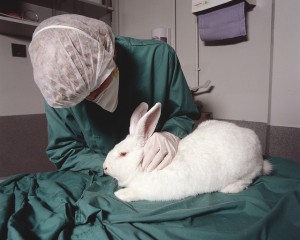
Because the use of animals in research involves a personal value, people naturally disagree about this practice. Although many people accept the value of such research (Plous, 1996), a minority of people, including animal-rights activists, believes that it is ethically wrong to conduct research on animals. This argument is based on the assumption that because animals are living creatures just as humans are, no harm should ever be done to them.
Most scientists, however, reject this view. They argue that such beliefs ignore the potential benefits that have and continue to come from research with animals. For instance, drugs that can reduce the incidence of cancer or AIDS may first be tested on animals, and surgery that can save human lives may first be practiced on animals. Research on animals has also led to a better understanding of the physiological causes of depression, phobias, and stress, among other illnesses. In contrast to animal-rights activists, then, scientists believe that because there are many benefits that accrue from animal research, such research can and should continue as long as the humane treatment of the animals used in the research is guaranteed.
Key Takeaways
- Psychologists use the scientific method to generate, accumulate, and report scientific knowledge.
- Basic research, which answers questions about behavior, and applied research, which finds solutions to everyday problems, inform each other and work together to advance science.
- Research reports describing scientific studies are published in scientific journals so that other scientists and laypersons may review the empirical findings.
- Organizing principles, including laws, theories and research hypotheses, give structure and uniformity to scientific methods.
- Concerns for conducting ethical research are paramount. Researchers assure that participants are given free choice to participate and that their privacy is protected. Informed consent and debriefing help provide humane treatment of participants.
- A cost-benefit analysis is used to determine what research should and should not be allowed to proceed.
Exercises and Critical Thinking
- Give an example from personal experience of how you or someone you know have benefited from the results of scientific research.
- Find and discuss a research project that in your opinion has ethical concerns. Explain why you find these concerns to be troubling.
- Indicate your personal feelings about the use of animals in research. When should and should not animals be used? What principles have you used to come to these conclusions?
American Psychological Association. (2002). Ethical principles of psychologists. American Psychologist, 57 , 1060–1073.
Baumrind, D. (1985). Research using intentional deception: Ethical issues revisited. American Psychologist, 40 , 165–174.
Kohlberg, L. (1966). A cognitive-developmental analysis of children’s sex-role concepts and attitudes. In E. E. Maccoby (Ed.), The development of sex differences . Stanford, CA: Stanford University Press.
Milgram, S. (1974). Obedience to authority: An experimental view . New York, NY: Harper and Row.
Plous, S. (1996). Attitudes toward the use of animals in psychological research and education. Psychological Science, 7 , 352–358.
Popper, K. R. (1959). The logic of scientific discovery . New York, NY: Basic Books.
Rosenthal, R. (1994). Science and ethics in conducting, analyzing, and reporting psychological research. Psychological Science, 5 , 127–134.
Ruble, D., & Martin, C. (1998). Gender development. In W. Damon (Ed.), Handbook of child psychology (5th ed., pp. 933–1016). New York, NY: John Wiley & Sons.
Stangor, C. (2011). Research methods for the behavioral sciences (4th ed.). Mountain View, CA: Cengage.
Thomas, G., & Blackman, D. (1992). The future of animal studies in psychology. American Psychologist, 47 , 1678.
2.4 Developing a Hypothesis
Learning objectives.
- Distinguish between a theory and a hypothesis.
- Discover how theories are used to generate hypotheses and how the results of studies can be used to further inform theories.
- Understand the characteristics of a good hypothesis.
Theories and Hypotheses
Before describing how to develop a hypothesis it is imporant to distinguish betwee a theory and a hypothesis. A theory is a coherent explanation or interpretation of one or more phenomena. Although theories can take a variety of forms, one thing they have in common is that they go beyond the phenomena they explain by including variables, structures, processes, functions, or organizing principles that have not been observed directly. Consider, for example, Zajonc’s theory of social facilitation and social inhibition. He proposed that being watched by others while performing a task creates a general state of physiological arousal, which increases the likelihood of the dominant (most likely) response. So for highly practiced tasks, being watched increases the tendency to make correct responses, but for relatively unpracticed tasks, being watched increases the tendency to make incorrect responses. Notice that this theory—which has come to be called drive theory—provides an explanation of both social facilitation and social inhibition that goes beyond the phenomena themselves by including concepts such as “arousal” and “dominant response,” along with processes such as the effect of arousal on the dominant response.
Outside of science, referring to an idea as a theory often implies that it is untested—perhaps no more than a wild guess. In science, however, the term theory has no such implication. A theory is simply an explanation or interpretation of a set of phenomena. It can be untested, but it can also be extensively tested, well supported, and accepted as an accurate description of the world by the scientific community. The theory of evolution by natural selection, for example, is a theory because it is an explanation of the diversity of life on earth—not because it is untested or unsupported by scientific research. On the contrary, the evidence for this theory is overwhelmingly positive and nearly all scientists accept its basic assumptions as accurate. Similarly, the “germ theory” of disease is a theory because it is an explanation of the origin of various diseases, not because there is any doubt that many diseases are caused by microorganisms that infect the body.
A hypothesis , on the other hand, is a specific prediction about a new phenomenon that should be observed if a particular theory is accurate. It is an explanation that relies on just a few key concepts. Hypotheses are often specific predictions about what will happen in a particular study. They are developed by considering existing evidence and using reasoning to infer what will happen in the specific context of interest. Hypotheses are often but not always derived from theories. So a hypothesis is often a prediction based on a theory but some hypotheses are a-theoretical and only after a set of observations have been made, is a theory developed. This is because theories are broad in nature and they explain larger bodies of data. So if our research question is really original then we may need to collect some data and make some observation before we can develop a broader theory.
Theories and hypotheses always have this if-then relationship. “ If drive theory is correct, then cockroaches should run through a straight runway faster, and a branching runway more slowly, when other cockroaches are present.” Although hypotheses are usually expressed as statements, they can always be rephrased as questions. “Do cockroaches run through a straight runway faster when other cockroaches are present?” Thus deriving hypotheses from theories is an excellent way of generating interesting research questions.
But how do researchers derive hypotheses from theories? One way is to generate a research question using the techniques discussed in this chapter and then ask whether any theory implies an answer to that question. For example, you might wonder whether expressive writing about positive experiences improves health as much as expressive writing about traumatic experiences. Although this question is an interesting one on its own, you might then ask whether the habituation theory—the idea that expressive writing causes people to habituate to negative thoughts and feelings—implies an answer. In this case, it seems clear that if the habituation theory is correct, then expressive writing about positive experiences should not be effective because it would not cause people to habituate to negative thoughts and feelings. A second way to derive hypotheses from theories is to focus on some component of the theory that has not yet been directly observed. For example, a researcher could focus on the process of habituation—perhaps hypothesizing that people should show fewer signs of emotional distress with each new writing session.
Among the very best hypotheses are those that distinguish between competing theories. For example, Norbert Schwarz and his colleagues considered two theories of how people make judgments about themselves, such as how assertive they are (Schwarz et al., 1991) [1] . Both theories held that such judgments are based on relevant examples that people bring to mind. However, one theory was that people base their judgments on the number of examples they bring to mind and the other was that people base their judgments on how easily they bring those examples to mind. To test these theories, the researchers asked people to recall either six times when they were assertive (which is easy for most people) or 12 times (which is difficult for most people). Then they asked them to judge their own assertiveness. Note that the number-of-examples theory implies that people who recalled 12 examples should judge themselves to be more assertive because they recalled more examples, but the ease-of-examples theory implies that participants who recalled six examples should judge themselves as more assertive because recalling the examples was easier. Thus the two theories made opposite predictions so that only one of the predictions could be confirmed. The surprising result was that participants who recalled fewer examples judged themselves to be more assertive—providing particularly convincing evidence in favor of the ease-of-retrieval theory over the number-of-examples theory.
Theory Testing
The primary way that scientific researchers use theories is sometimes called the hypothetico-deductive method (although this term is much more likely to be used by philosophers of science than by scientists themselves). A researcher begins with a set of phenomena and either constructs a theory to explain or interpret them or chooses an existing theory to work with. He or she then makes a prediction about some new phenomenon that should be observed if the theory is correct. Again, this prediction is called a hypothesis. The researcher then conducts an empirical study to test the hypothesis. Finally, he or she reevaluates the theory in light of the new results and revises it if necessary. This process is usually conceptualized as a cycle because the researcher can then derive a new hypothesis from the revised theory, conduct a new empirical study to test the hypothesis, and so on. As Figure 2.2 shows, this approach meshes nicely with the model of scientific research in psychology presented earlier in the textbook—creating a more detailed model of “theoretically motivated” or “theory-driven” research.

Figure 2.2 Hypothetico-Deductive Method Combined With the General Model of Scientific Research in Psychology Together they form a model of theoretically motivated research.
As an example, let us consider Zajonc’s research on social facilitation and inhibition. He started with a somewhat contradictory pattern of results from the research literature. He then constructed his drive theory, according to which being watched by others while performing a task causes physiological arousal, which increases an organism’s tendency to make the dominant response. This theory predicts social facilitation for well-learned tasks and social inhibition for poorly learned tasks. He now had a theory that organized previous results in a meaningful way—but he still needed to test it. He hypothesized that if his theory was correct, he should observe that the presence of others improves performance in a simple laboratory task but inhibits performance in a difficult version of the very same laboratory task. To test this hypothesis, one of the studies he conducted used cockroaches as subjects (Zajonc, Heingartner, & Herman, 1969) [2] . The cockroaches ran either down a straight runway (an easy task for a cockroach) or through a cross-shaped maze (a difficult task for a cockroach) to escape into a dark chamber when a light was shined on them. They did this either while alone or in the presence of other cockroaches in clear plastic “audience boxes.” Zajonc found that cockroaches in the straight runway reached their goal more quickly in the presence of other cockroaches, but cockroaches in the cross-shaped maze reached their goal more slowly when they were in the presence of other cockroaches. Thus he confirmed his hypothesis and provided support for his drive theory. (Zajonc also showed that drive theory existed in humans (Zajonc & Sales, 1966) [3] in many other studies afterward).
Incorporating Theory into Your Research
When you write your research report or plan your presentation, be aware that there are two basic ways that researchers usually include theory. The first is to raise a research question, answer that question by conducting a new study, and then offer one or more theories (usually more) to explain or interpret the results. This format works well for applied research questions and for research questions that existing theories do not address. The second way is to describe one or more existing theories, derive a hypothesis from one of those theories, test the hypothesis in a new study, and finally reevaluate the theory. This format works well when there is an existing theory that addresses the research question—especially if the resulting hypothesis is surprising or conflicts with a hypothesis derived from a different theory.
To use theories in your research will not only give you guidance in coming up with experiment ideas and possible projects, but it lends legitimacy to your work. Psychologists have been interested in a variety of human behaviors and have developed many theories along the way. Using established theories will help you break new ground as a researcher, not limit you from developing your own ideas.
Characteristics of a Good Hypothesis
There are three general characteristics of a good hypothesis. First, a good hypothesis must be testable and falsifiable . We must be able to test the hypothesis using the methods of science and if you’ll recall Popper’s falsifiability criterion, it must be possible to gather evidence that will disconfirm the hypothesis if it is indeed false. Second, a good hypothesis must be logical. As described above, hypotheses are more than just a random guess. Hypotheses should be informed by previous theories or observations and logical reasoning. Typically, we begin with a broad and general theory and use deductive reasoning to generate a more specific hypothesis to test based on that theory. Occasionally, however, when there is no theory to inform our hypothesis, we use inductive reasoning which involves using specific observations or research findings to form a more general hypothesis. Finally, the hypothesis should be positive. That is, the hypothesis should make a positive statement about the existence of a relationship or effect, rather than a statement that a relationship or effect does not exist. As scientists, we don’t set out to show that relationships do not exist or that effects do not occur so our hypotheses should not be worded in a way to suggest that an effect or relationship does not exist. The nature of science is to assume that something does not exist and then seek to find evidence to prove this wrong, to show that really it does exist. That may seem backward to you but that is the nature of the scientific method. The underlying reason for this is beyond the scope of this chapter but it has to do with statistical theory.
Key Takeaways
- A theory is broad in nature and explains larger bodies of data. A hypothesis is more specific and makes a prediction about the outcome of a particular study.
- Working with theories is not “icing on the cake.” It is a basic ingredient of psychological research.
- Like other scientists, psychologists use the hypothetico-deductive method. They construct theories to explain or interpret phenomena (or work with existing theories), derive hypotheses from their theories, test the hypotheses, and then reevaluate the theories in light of the new results.
- Practice: Find a recent empirical research report in a professional journal. Read the introduction and highlight in different colors descriptions of theories and hypotheses.
- Schwarz, N., Bless, H., Strack, F., Klumpp, G., Rittenauer-Schatka, H., & Simons, A. (1991). Ease of retrieval as information: Another look at the availability heuristic. Journal of Personality and Social Psychology, 61 , 195–202. ↵
- Zajonc, R. B., Heingartner, A., & Herman, E. M. (1969). Social enhancement and impairment of performance in the cockroach. Journal of Personality and Social Psychology, 13 , 83–92. ↵
- Zajonc, R.B. & Sales, S.M. (1966). Social facilitation of dominant and subordinate responses. Journal of Experimental Social Psychology, 2 , 160-168. ↵

Share This Book
- Increase Font Size
- Increase Font Size
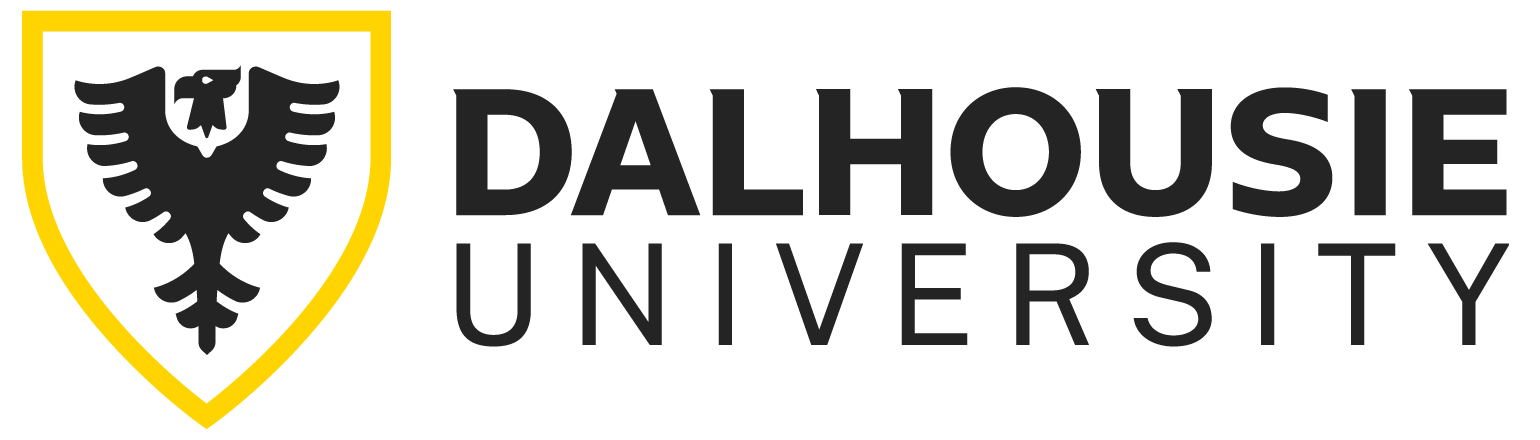
Want to create or adapt books like this? Learn more about how Pressbooks supports open publishing practices.
3 Chapter 3: From Theory to Hypothesis
From theory to hypothesis, 3.1 phenomena and theories.
A phenomenon (plural, phenomena) is a general result that has been observed reliably in systematic empirical research. In essence, it is an established answer to a research question. Some phenomena we have encountered in this book are that expressive writing improves health, women do not talk more than men, and cell phone usage impairs driving ability. Some others are that dissociative identity disorder (formerly called multiple personality disorder) increased greatly in prevalence during the late 20th century, people perform better on easy tasks when they are being watched by others (and worse on difficult tasks), and people recall items presented at the beginning and end of a list better than items presented in the middle.
Some Famous Psychological Phenomena
Phenomena are often given names by their discoverers or other researchers, and these names can catch on and become widely known. The following list is a small sample of famous phenomena in psychology.
· Blindsight. People with damage to their visual cortex are often able to respond to visual stimuli that they do not consciously see.
· Bystander effect. The more people who are present at an emergency situation, the less likely it is that any one of them will help.
· Fundamental attribution error. People tend to explain others’ behavior in terms of their personal characteristics as opposed to the situation they are in.
· McGurk effect. When audio of a basic speech sound is combined with video of a person making mouth movements for a different speech sound, people often perceive a sound that is intermediate between the two.
· Own-race effect. People recognize faces of people of their own race more accurately than faces of people of other races.
· Placebo effect. Placebos (fake psychological or medical treatments) often lead to improvements in people’s symptoms and functioning.
· Mere exposure effect. The more often people have been exposed to a stimulus, the more they like it—even when the stimulus is presented subliminally.
· Serial position effect. Stimuli presented near the beginning and end of a list are remembered better than stimuli presented in the middle.
· Spontaneous recovery. A conditioned response that has been extinguished often returns with no further training after the passage of time.
Although an empirical result might be referred to as a phenomenon after being observed only once, this term is more likely to be used for results that have been replicated. Replication means conducting a study again—either exactly as it was originally conducted or with modifications—to be sure that it produces the same results. Individual researchers usually replicate their own studies before publishing them. Many empirical research reports include an initial study and then one or more follow-up studies that replicate the initial study with minor modifications. Particularly interesting results come to the attention of other researchers who conduct their own replications. The positive effect of expressive writing on health and the negative effect of cell phone usage on driving ability are examples of phenomena that have been replicated many times by many different researchers.
Sometimes a replication of a study produces results that differ from the results of the initial study. This could mean that the results of the initial study or the results of the replication were a fluke—they occurred by chance and do not reflect something that is generally true. In either case, additional replications would be likely to resolve this. A failure to produce the same results could also mean that the replication differed in some important way from the initial study. For example, early studies showed that people performed a variety of tasks better and faster when they were watched by others than when they were alone. Some later replications, however, showed that people performed worse when they were watched by others. Eventually researcher Robert Zajonc identified a key difference between the two types of studies. People seemed to perform better when being watched on highly practiced tasks but worse when being watched on relatively unpracticed tasks (Zajonc, 1965). These two phenomena have now come to be called social facilitation and social inhibition.
What Is a Theory?
A theory is a coherent explanation or interpretation of one or more phenomena. Although theories can take a variety of forms, one thing they have in common is that they go beyond the phenomena they explain by including variables, structures, processes, functions, or organizing principles that have not been observed directly. Consider, for example, Zajonc’s theory of social facilitation and social inhibition. He proposed that being watched by others while performing a task creates a general state of physiological arousal, which increases the likelihood of the dominant (most likely) response. So for highly practiced tasks, being watched increases the tendency to make correct responses, but for relatively unpracticed tasks, being watched increases the tendency to make incorrect responses. Notice that this theory—which has come to be called drive theory—provides an explanation of both social facilitation and social inhibition that goes beyond the phenomena themselves by including concepts such as “arousal” and “dominant response,” along with processes such as the effect of arousal on the dominant response.
Outside of science, referring to an idea as a theory often implies that it is untested—perhaps no more than a wild guess. In science, however, the term theory has no such implication. A theory is simply an explanation or interpretation of a set of phenomena. It can be untested, but it can also be extensively tested, well supported, and accepted as an accurate description of the world by the scientific community. The theory of evolution by natural selection, for example, is a theory because it is an explanation of the diversity of life on earth—not because it is untested or unsupported by scientific research. On the contrary, the evidence for this theory is overwhelmingly positive and nearly all scientists accept its basic assumptions as accurate. Similarly, the “germ theory” of disease is a theory because it is an explanation of the origin of various diseases, not because there is any doubt that many diseases are caused by microorganisms that infect the body.
In addition to theory, researchers in psychology use several related terms to refer to their explanations and interpretations of phenomena. A perspective is a broad approach—more general than a theory—to explaining and interpreting phenomena. For example, researchers who take a biological perspective tend to explain phenomena in terms of genetics or nervous and endocrine system structures and processes, while researchers who take a behavioral perspective tend to explain phenomena in terms of reinforcement, punishment, and other external events. A model is a precise explanation or interpretation of a specific phenomenon—often expressed in terms of equations, computer programs, or biological structures and processes. A hypothesis can be an explanation that relies on just a few key concepts—although this term more commonly refers to a prediction about a new phenomenon based on a theory. Adding to the confusion is the fact that researchers often use these terms interchangeably. It would not be considered wrong to refer to the drive theory as the drive model or even the drive hypothesis. And the biopsychosocial model of health psychology—the general idea that health is determined by an interaction of biological, psychological, and social factors—is really more like a perspective as defined here. Keep in mind, however, that the most important distinction remains that between observations and interpretations.
What Are Theories For?
Of course, scientific theories are meant to provide accurate explanations or interpretations of phenomena. But there must be more to it than this. Consider that a theory can be accurate without being very useful. To say that expressive writing helps people “deal with their emotions” might be accurate as far as it goes, but it seems too vague to be of much use. Consider also that a theory can be useful without being entirely accurate.
3.2 Additional Purposes of Theories
Here we look at three additional purposes of theories: the organization of known phenomena, the prediction of outcomes in new situations, and the generation of new research.
Organization
One important purpose of scientific theories is to organize phenomena in ways that help people think about them clearly and efficiently. The drive theory of social facilitation and social inhibition, for example, helps to organize and make sense of a large number of seemingly contradictory results. The multistore model of human memory efficiently summarizes many important phenomena: the limited capacity and short retention time of information that is attended to but not rehearsed, the importance of rehearsing information for long-term retention, the serial-position effect, and so on.
Thus theories are good or useful to the extent that they organize more phenomena with greater clarity and efficiency. Scientists generally follow the principle of parsimony, which holds that a theory should include only as many concepts as are necessary to explain or interpret the phenomena of interest. Simpler, more parsimonious theories organize phenomena more efficiently than more complex, less parsimonious theories.
A second purpose of theories is to allow researchers and others to make predictions about what will happen in new situations. For example, a gymnastics coach might wonder whether a student’s performance is likely to be better or worse during a competition than when practicing alone. Even if this particular question has never been studied empirically, Zajonc’s drive theory suggests an answer. If the student generally performs with no mistakes, she is likely to perform better during competition. If she generally performs with many mistakes, she is likely to perform worse.
In clinical psychology, treatment decisions are often guided by theories. Consider, for example, dissociative identity disorder (formerly called multiple personality disorder). The prevailing scientific theory of dissociative identity disorder is that people develop multiple personalities (also called alters) because they are familiar with this idea from popular portrayals (e.g., the movie Sybil) and because they are unintentionally encouraged to do so by their clinicians (e.g., by asking to “meet” an alter). This theory implies that rather than encouraging patients to act out multiple personalities, treatment should involve discouraging them from doing this (Lilienfeld & Lynn, 2003).
Generation of New Research
A third purpose of theories is to generate new research by raising new questions. Consider, for example, the theory that people engage in self-injurious behavior such as cutting because it reduces negative emotions such as sadness, anxiety, and anger. This theory immediately suggests several new and interesting questions. Is there, in fact, a statistical relationship between cutting and the amount of negative emotions experienced? Is it causal? If so, what is it about cutting that has this effect? Is it the pain, the sight of the injury, or something else? Does cutting affect all negative emotions equally?
Notice that a theory does not have to be accurate to serve this purpose. Even an inaccurate theory can generate new and interesting research questions. Of course, if the theory is inaccurate, the answers to the new questions will tend to be inconsistent with the theory. This will lead researchers to reevaluate the theory and either revise it or abandon it for a new one. And this is how scientific theories become more detailed and accurate over time.
Multiple Theories
At any point in time, researchers are usually considering multiple theories for any set of phenomena. One reason is that because human behavior is extremely complex, it is always possible to look at it from different perspectives. For example, a biological theory of sexual orientation might focus on the role of sex hormones during critical periods of brain development, while a sociocultural theory might focus on cultural factors that influence how underlying biological tendencies are expressed. A second reason is that—even from the same perspective—there are usually different ways to “go beyond” the phenomena of interest. For example, in addition to the drive theory of social facilitation and social inhibition, there is another theory that explains them in terms of a construct called “evaluation apprehension”—anxiety about being evaluated by the audience. Both theories go beyond the phenomena to be interpreted, but they do so by proposing somewhat different underlying processes.
Different theories of the same set of phenomena can be complementary—with each one supplying one piece of a larger puzzle. A biological theory of sexual orientation and a sociocultural theory of sexual orientation might accurately describe different aspects of the same complex phenomenon. Similarly, social facilitation could be the result of both general physiological arousal and evaluation apprehension. But different theories of the same phenomena can also be competing in the sense that if one is accurate, the other is probably not. For example, an alternative theory of dissociative identity disorder—the posttraumatic theory—holds that alters are created unconsciously by the patient as a means of coping with sexual abuse or some other traumatic experience. Because the sociocognitive theory and the posttraumatic theories attribute dissociative identity disorder to fundamentally different processes, it seems unlikely that both can be accurate.
The fact that there are multiple theories for any set of phenomena does not mean that any theory is as good as any other or that it is impossible to know whether a theory provides an accurate explanation or interpretation. On the contrary, scientists are continually comparing theories in terms of their ability to organize phenomena, predict outcomes in new situations, and generate research. Those that fare poorly are assumed to be less accurate and are abandoned, while those that fare well are assumed to be more accurate and are retained and compared with newer—and hopefully better—theories. Although scientists generally do not believe that their theories ever provide perfectly accurate descriptions of the world, they do assume that this process produces theories that come closer and closer to that ideal.
Key Takeaways
· Scientists distinguish between phenomena, which are their systematic observations, and theories, which are their explanations or interpretations of phenomena.
· In addition to providing accurate explanations or interpretations, scientific theories have three basic purposes. They organize phenomena, allow people to predict what will happen in new situations, and help generate new research.
· Researchers generally consider multiple theories for any set of phenomena. Different theories of the same set of phenomena can be complementary or competing.
3.3 Using Theories in Psychological Research
We have now seen what theories are, what they are for, and the variety of forms that they take in psychological research. In this section we look more closely at how researchers actually use them. We begin with a general description of how researchers test and revise their theories, and we end with some practical advice for beginning researchers who want to incorporate theory into their research.
Theory Testing and Revision
The primary way that scientific researchers use theories is sometimes called the hypothetico-deductive method (although this term is much more likely to be used by philosophers of science than by scientists themselves). A researcher begins with a set of phenomena and either constructs a theory to explain or interpret them or chooses an existing theory to work with. He or she then makes a prediction about some new phenomenon that should be observed if the theory is correct. Again, this prediction is called a hypothesis. The researcher then conducts an empirical study to test the hypothesis. Finally, he or she reevaluates the theory in light of the new results and revises it if necessary. This process is usually conceptualized as a cycle because the researcher can then derive a new hypothesis from the revised theory, conduct a new empirical study to test the hypothesis, and so on. Together they form a model of theoretically motivated research.
As an example, let us return to Zajonc’s research on social facilitation and inhibition. He started with a somewhat contradictory pattern of results from the research literature. He then constructed his drive theory, according to which being watched by others while performing a task causes physiological arousal, which increases an organism’s tendency to make the dominant response. This leads to social facilitation for well-learned tasks and social inhibition for poorly learned tasks. He now had a theory that organized previous results in a meaningful way—but he still needed to test it. He hypothesized that if his theory was correct, he should observe that the presence of others improves performance in a simple laboratory task but inhibits performance in a difficult version of the very same laboratory task. To test this hypothesis, one of the studies he conducted used cockroaches as subjects (Zajonc, Heingartner, & Herman, 1969). The cockroaches ran either down a straight runway (an easy task for a cockroach) or through a cross-shaped maze (a difficult task for a cockroach) to escape into a dark chamber when a light was shined on them. They did this either while alone or in the presence of other cockroaches in clear plastic “audience boxes.” Zajonc found that cockroaches in the straight runway reached their goal more quickly in the presence of other cockroaches, but cockroaches in the cross-shaped maze reached their goal more slowly when they were in the presence of other cockroaches. Thus he confirmed his hypothesis and provided support for his drive theory.
Constructing or Choosing a Theory
Along with generating research questions, constructing theories is one of the more creative parts of scientific research. But as with all creative activities, success requires preparation and hard work more than anything else. To construct a good theory, a researcher must know in detail about the phenomena of interest and about any existing theories based on a thorough review of the literature. The new theory must provide a coherent explanation or interpretation of the phenomena of interest and have some advantage over existing theories. It could be more formal and therefore more precise, broader in scope, more parsimonious, or it could take a new perspective or theoretical approach. If there is no existing theory, then almost any theory can be a step in the right direction.
As we have seen, formality, scope, and theoretical approach are determined in part by the nature of the phenomena to be interpreted. But the researcher’s interests and abilities play a role too. For example, constructing a theory that specifies the neural structures and processes underlying a set of phenomena requires specialized knowledge and experience in neuroscience (which most professional researchers would acquire in college and then graduate school). But again, many theories in psychology are relatively informal, narrow in scope, and expressed in terms that even a beginning researcher can understand and even use to construct his or her own new theory.
It is probably more common, however, for a researcher to start with a theory that was originally constructed by someone else—giving due credit to the originator of the theory. This is another example of how researchers work collectively to advance scientific knowledge. Once they have identified an existing theory, they might derive a hypothesis from the theory and test it or modify the theory to account for some new phenomenon and then test the modified theory.
Deriving Hypotheses
Again, a hypothesis is a prediction about a new phenomenon that should be observed if a particular theory is accurate. Theories and hypotheses always have this if-then relationship. “If drive theory is correct, then cockroaches should run through a straight runway faster, and a branching runway more slowly, when other cockroaches are present.” Although hypotheses are usually expressed as statements, they can always be rephrased as questions. “Do cockroaches run through a straight runway faster when other cockroaches are present?” Thus deriving hypotheses from theories is an excellent way of generating interesting research questions.
But how do researchers derive hypotheses from theories? One way is to generate a research question using the techniques discussed in Chapter 2 and then ask whether any theory implies an answer to that question. For example, you might wonder whether expressive writing about positive experiences improves health as much as expressive writing about traumatic experiences. Although this is an interesting question on its own, you might then ask whether the habituation theory—the idea that expressive writing causes people to habituate to negative thoughts and feelings—implies an answer. In this case, it seems clear that if the habituation theory is correct, then expressive writing about positive experiences should not be effective because it would not cause people to habituate to negative thoughts and feelings. A second way to derive hypotheses from theories is to focus on some component of the theory that has not yet been directly observed. For example, a researcher could focus on the process of habituation—perhaps hypothesizing that people should show fewer signs of emotional distress with each new writing session.
Among the very best hypotheses are those that distinguish between competing theories. For example, Norbert Schwarz and his colleagues considered two theories of how people make judgments about themselves, such as how assertive they are (Schwarz et al., 1991). Both theories held that such judgments are based on relevant examples that people bring to mind. However, one theory was that people base their judgments on the number of examples they bring to mind and the other was that people base their judgments on how easily they bring those examples to mind. To test these theories, the researchers asked people to recall either six times when they were assertive (which is easy for most people) or 12 times (which is difficult for most people). Then they asked them to judge their own assertiveness. Note that the number-of-examples theory implies that people who recalled 12 examples should judge themselves to be more assertive because they recalled more examples, but the ease-of-examples theory implies that participants who recalled six examples should judge themselves as more assertive because recalling the examples was easier. Thus the two theories made opposite predictions so that only one of the predictions could be confirmed. The surprising result was that participants who recalled fewer examples judged themselves to be more assertive—providing particularly convincing evidence in favor of the ease-of-retrieval theory over the number-of-examples theory.
Evaluating and Revising Theories
If a hypothesis is confirmed in a systematic empirical study, then the theory has been strengthened. Not only did the theory make an accurate prediction, but there is now a new phenomenon that the theory accounts for. If a hypothesis is disconfirmed in a systematic empirical study, then the theory has been weakened. It made an inaccurate prediction, and there is now a new phenomenon that it does not account for.
Although this seems straightforward, there are some complications. First, confirming a hypothesis can strengthen a theory but it can never prove a theory. In fact, scientists tend to avoid the word “prove” when talking and writing about theories. One reason for this is that there may be other plausible theories that imply the same hypothesis, which means that confirming the hypothesis strengthens all those theories equally. A second reason is that it is always possible that another test of the hypothesis or a test of a new hypothesis derived from the theory will be disconfirmed. This is a version of the famous philosophical “problem of induction.” One cannot definitively prove a general principle (e.g., “All swans are white.”) just by observing confirming cases (e.g., white swans)—no matter how many. It is always possible that a disconfirming case (e.g., a black swan) will eventually come along. For these reasons, scientists tend to think of theories—even highly successful ones—as subject to revision based on new and unexpected observations.
A second complication has to do with what it means when a hypothesis is disconfirmed. According to the strictest version of the hypothetico-deductive method, disconfirming a hypothesis disproves the theory it was derived from. In formal logic, the premises “if A then B” and “not B” necessarily lead to the conclusion “not A.” If A is the theory and B is the hypothesis (“if A then B”), then disconfirming the hypothesis (“not B”) must mean that the theory is incorrect (“not A”). In practice, however, scientists do not give up on their theories so easily. One reason is that one disconfirmed hypothesis could be a fluke or it could be the result of a faulty research design. Perhaps the researcher did not successfully manipulate the independent variable or measure the dependent variable. A disconfirmed hypothesis could also mean that some unstated but relatively minor assumption of the theory was not met. For example, if Zajonc had failed to find social facilitation in cockroaches, he could have concluded that drive theory is still correct but it applies only to animals with sufficiently complex nervous systems.
This does not mean that researchers are free to ignore disconfirmations of their theories. If they cannot improve their research designs or modify their theories to account for repeated disconfirmations, then they eventually abandon their theories and replace them with ones that are more successful.
Incorporating Theory Into Your Research
It should be clear from this chapter that theories are not just “icing on the cake” of scientific research; they are a basic ingredient. If you can understand and use them, you will be much more successful at reading and understanding the research literature, generating interesting research questions, and writing and conversing about research. Of course, your ability to understand and use theories will improve with practice. But there are several things that you can do to incorporate theory into your research right from the start.
The first thing is to distinguish the phenomena you are interested in from any theories of those phenomena. Beware especially of the tendency to “fuse” a phenomenon to a commonsense theory of it. For example, it might be tempting to describe the negative effect of cell phone usage on driving ability by saying, “Cell phone usage distracts people from driving.” Or it might be tempting to describe the positive effect of expressive writing on health by saying, “Dealing with your emotions through writing makes you healthier.” In both of these examples, however, a vague commonsense explanation (distraction, “dealing with” emotions) has been fused to the phenomenon itself. The problem is that this gives the impression that the phenomenon has already been adequately explained and closes off further inquiry into precisely why or how it happens.
As another example, researcher Jerry Burger and his colleagues were interested in the phenomenon that people are more willing to comply with a simple request from someone with whom they are familiar (Burger, Soroka, Gonzago, Murphy, & Somervell, 1999). A beginning researcher who is asked to explain why this is the case might be at a complete loss or say something like, “Well, because they are familiar with them.” But digging just a bit deeper, Burger and his colleagues realized that there are several possible explanations. Among them are that complying with people we know creates positive feelings, that we anticipate needing something from them in the future, and that we like them more and follow an automatic rule that says to help people we like.
The next thing to do is turn to the research literature to identify existing theories of the phenomena you are interested in. Remember that there will usually be more than one plausible theory. Existing theories may be complementary or competing, but it is essential to know what they are. If there are no existing theories, you should come up with two or three of your own—even if they are informal and limited in scope. Then get in the habit of describing the phenomena you are interested in, followed by the two or three best theories of it. Do this whether you are speaking or writing about your research. When asked what their research was about, for example, Burger and his colleagues could have said something like the following:
It’s about the fact that we’re more likely to comply with requests from people we know [the phenomenon]. This is interesting because it could be because it makes us feel good [Theory 1], because we think we might get something in return [Theory 2], or because we like them more and have an automatic tendency to comply with people we like [Theory 3].
At this point, you may be able to derive a hypothesis from one of the theories. At the very least, for each research question you generate, you should ask what each plausible theory implies about the answer to that question. If one of them implies a particular answer, then you may have an interesting hypothesis to test. Burger and colleagues, for example, asked what would happen if a request came from a stranger whom participants had sat next to only briefly, did not interact with, and had no expectation of interacting with in the future. They reasoned that if familiarity created liking, and liking increased people’s tendency to comply (Theory 3), then this situation should still result in increased rates of compliance (which it did). If the question is interesting but no theory implies an answer to it, this might suggest that a new theory needs to be constructed or that existing theories need to be modified in some way. These would make excellent points of discussion in the introduction or discussion of an American Psychological Association (APA) style research report or research presentation.
When you do write your research report or plan your presentation, be aware that there are two basic ways that researchers usually include theory. The first is to raise a research question, answer that question by conducting a new study, and then offer one or more theories (usually more) to explain or interpret the results. This format works well for applied research questions and for research questions that existing theories do not address. The second way is to describe one or more existing theories, derive a hypothesis from one of those theories, test the hypothesis in a new study, and finally reevaluate the theory. This format works well when there is an existing theory that addresses the research question—especially if the resulting hypothesis is surprising or conflicts with a hypothesis derived from a different theory.
· Working with theories is not “icing on the cake.” It is a basic ingredient of psychological research.
· Like other scientists, psychologists use the hypothetico-deductive method. They construct theories to explain or interpret phenomena (or work with existing theories), derive hypotheses from their theories, test the hypotheses, and then reevaluate the theories in light of the new results.
· There are several things that even beginning researchers can do to incorporate theory into their research. These include clearly distinguishing phenomena from theories, knowing about existing theories, constructing one’s own simple theories, using theories to make predictions about the answers to research questions, and incorporating theories into one’s writing and speaking.
3.4 Understanding Null Hypothesis Testing
The Purpose of Null Hypothesis Testing
As we have seen, psychological research typically involves measuring one or more variables for a sample and computing descriptive statistics for that sample. In general, however, the researcher’s goal is not to draw conclusions about that sample but to draw conclusions about the population that the sample was selected from. Thus researchers must use sample statistics to draw conclusions about the corresponding values in the population. These corresponding values in the population are called parameters. Imagine, for example, that a researcher measures the number of depressive symptoms exhibited by each of 50 clinically depressed adults and computes the mean number of symptoms. The researcher probably wants to use this sample statistic (the mean number of symptoms for the sample) to draw conclusions about the corresponding population parameter (the mean number of symptoms for clinically depressed adults).
Unfortunately, sample statistics are not perfect estimates of their corresponding population parameters. This is because there is a certain amount of random variability in any statistic from sample to sample. This random variability in a statistic from sample to sample is called sampling error.
One implication of this is that when there is a statistical relationship in a sample, it is not always clear that there is a statistical relationship in the population. A small difference between two group means in a sample might indicate that there is a small difference between the two group means in the population. But it could also be that there is no difference between the means in the population and that the difference in the sample is just a matter of sampling error. Similarly, a Pearson’s r value of −.29 in a sample might mean that there is a negative relationship in the population. But it could also be that there is no relationship in the population and that the relationship in the sample is just a matter of sampling error.
In fact, any statistical relationship in a sample can be interpreted in two ways:
- There is a relationship in the population, and the relationship in the sample reflects this.
- There is no relationship in the population, and the relationship in the sample reflects only sampling error.
The purpose of null hypothesis testing is simply to help researchers decide between these two interpretations.
The Logic of Null Hypothesis Testing
Null hypothesis testing is a formal approach to deciding between two interpretations of a statistical relationship in a sample. One interpretation is called the null hypothesis (often symbolized H0 and read as “H-naught”). This is the idea that there is no relationship in the population and that the relationship in the sample reflects only sampling error. Informally, the null hypothesis is that the sample relationship “occurred by chance.” The other interpretation is called the alternative hypothesis (often symbolized as H1). This is the idea that there is a relationship in the population and that the relationship in the sample reflects this relationship in the population.
Again, every statistical relationship in a sample can be interpreted in either of these two ways: It might have occurred by chance, or it might reflect a relationship in the population. So researchers need a way to decide between them. Although there are many specific null hypothesis testing techniques, they are all based on the same general logic. The steps are as follows:
- Assume for the moment that the null hypothesis is true. There is no relationship between the variables in the population.
- Determine how likely the sample relationship would be if the null hypothesis were true.
- If the sample relationship would be extremely unlikely, then reject the null hypothesis in favor of the alternative hypothesis. If it would not be extremely unlikely, then retain the null hypothesis.
Following this logic, we can begin to understand why Mehl and his colleagues concluded that there is no difference in talkativeness between women and men in the population. In essence, they asked the following question: “If there were no difference in the population, how likely is it that we would find a small difference of d = 0.06 in our sample?” Their answer to this question was that this sample relationship would be fairly likely if the null hypothesis were true. Therefore, they retained the null hypothesis—concluding that there is no evidence of a sex difference in the population. We can also see why Kanner and his colleagues concluded that there is a correlation between hassles and symptoms in the population. They asked, “If the null hypothesis were true, how likely is it that we would find a strong correlation of +.60 in our sample?” Their answer to this question was that this sample relationship would be fairly unlikely if the null hypothesis were true. Therefore, they rejected the null hypothesis in favor of the alternative hypothesis—concluding that there is a positive correlation between these variables in the population.
A crucial step in null hypothesis testing is finding the likelihood of the sample result if the null hypothesis were true. This probability is called the p value. A low p value means that the sample result would be unlikely if the null hypothesis were true and leads to the rejection of the null hypothesis. A high p value means that the sample result would be likely if the null hypothesis were true and leads to the retention of the null hypothesis. But how low must the p value be before the sample result is considered unlikely enough to reject the null hypothesis? In null hypothesis testing, this criterion is called α (alpha) and is almost always set to .05. If there is less than a 5% chance of a result as extreme as the sample result if the null hypothesis were true, then the null hypothesis is rejected. When this happens, the result is said to be statistically significant. If there is greater than a 5% chance of a result as extreme as the sample result when the null hypothesis is true, then the null hypothesis is retained. This does not necessarily mean that the researcher accepts the null hypothesis as true—only that there is not currently enough evidence to conclude that it is true. Researchers often use the expression “fail to reject the null hypothesis” rather than “retain the null hypothesis,” but they never use the expression “accept the null hypothesis.”
The Misunderstood p Value
The p value is one of the most misunderstood quantities in psychological research (Cohen, 1994). Even professional researchers misinterpret it, and it is not unusual for such misinterpretations to appear in statistics textbooks!
The most common misinterpretation is that the p value is the probability that the null hypothesis is true—that the sample result occurred by chance. For example, a misguided researcher might say that because the p value is .02, there is only a 2% chance that the result is due to chance and a 98% chance that it reflects a real relationship in the population. But this is incorrect. The p value is really the probability of a result at least as extreme as the sample result if the null hypothesis were true. So a p value of .02 means that if the null hypothesis were true, a sample result this extreme would occur only 2% of the time.
You can avoid this misunderstanding by remembering that the p value is not the probability that any particular hypothesis is true or false. Instead, it is the probability of obtaining the sample result if the null hypothesis were true.
Role of Sample Size and Relationship Strength
Recall that null hypothesis testing involves answering the question, “If the null hypothesis were true, what is the probability of a sample result as extreme as this one?” In other words, “What is the p value?” It can be helpful to see that the answer to this question depends on just two considerations: the strength of the relationship and the size of the sample. Specifically, the stronger the sample relationship and the larger the sample, the less likely the result would be if the null hypothesis were true. That is, the lower the p value. This should make sense. Imagine a study in which a sample of 500 women is compared with a sample of 500 men in terms of some psychological characteristic, and Cohen’s d is a strong 0.50. If there were really no sex difference in the population, then a result this strong based on such a large sample should seem highly unlikely. Now imagine a similar study in which a sample of three women is compared with a sample of three men, and Cohen’s d is a weak 0.10. If there were no sex difference in the population, then a relationship this weak based on such a small sample should seem likely. And this is precisely why the null hypothesis would be rejected in the first example and retained in the second.
Of course, sometimes the result can be weak and the sample large, or the result can be strong and the sample small. In these cases, the two considerations trade off against each other so that a weak result can be statistically significant if the sample is large enough and a strong relationship can be statistically significant even if the sample is small. Weak relationships based on medium or small samples are never statistically significant and that strong relationships based on medium or larger samples are always statistically significant. If you keep this in mind, you will often know whether a result is statistically significant based on the descriptive statistics alone. It is extremely useful to be able to develop this kind of intuitive judgment. One reason is that it allows you to develop expectations about how your formal null hypothesis tests are going to come out, which in turn allows you to detect problems in your analyses. For example, if your sample relationship is strong and your sample is medium, then you would expect to reject the null hypothesis. If for some reason your formal null hypothesis test indicates otherwise, then you need to double-check your computations and interpretations. A second reason is that the ability to make this kind of intuitive judgment is an indication that you understand the basic logic of this approach in addition to being able to do the computations.
Statistical Significance Versus Practical Significance
A statistically significant result is not necessarily a strong one. Even a very weak result can be statistically significant if it is based on a large enough sample. This is closely related to Janet Shibley Hyde’s argument about sex differences (Hyde, 2007). The differences between women and men in mathematical problem solving and leadership ability are statistically significant. But the word significant can cause people to interpret these differences as strong and important—perhaps even important enough to influence the college courses they take or even who they vote for. As we have seen, however, these statistically significant differences are actually quite weak—perhaps even “trivial.”
This is why it is important to distinguish between the statistical significance of a result and the practical significance of that result. Practical significance refers to the importance or usefulness of the result in some real-world context. Many sex differences are statistically significant—and may even be interesting for purely scientific reasons—but they are not practically significant. In clinical practice, this same concept is often referred to as “clinical significance.” For example, a study on a new treatment for social phobia might show that it produces a statistically significant positive effect. Yet this effect still might not be strong enough to justify the time, effort, and other costs of putting it into practice—especially if easier and cheaper treatments that work almost as well already exist. Although statistically significant, this result would be said to lack practical or clinical significance.
· Null hypothesis testing is a formal approach to deciding whether a statistical relationship in a sample reflects a real relationship in the population or is just due to chance.
· The logic of null hypothesis testing involves assuming that the null hypothesis is true, finding how likely the sample result would be if this assumption were correct, and then making a decision. If the sample result would be unlikely if the null hypothesis were true, then it is rejected in favor of the alternative hypothesis. If it would not be unlikely, then the null hypothesis is retained.
· The probability of obtaining the sample result if the null hypothesis were true (the p value) is based on two considerations: relationship strength and sample size. Reasonable judgments about whether a sample relationship is statistically significant can often be made by quickly considering these two factors.
· Statistical significance is not the same as relationship strength or importance. Even weak relationships can be statistically significant if the sample size is large enough. It is important to consider relationship strength and the practical significance of a result in addition to its statistical significance.
References from Chapter 3
Burger, J. M., Soroka, S., Gonzago, K., Murphy, E., Somervell, E. (1999). The effect of fleeting attraction on compliance to requests. Personality and Social Psychology Bulletin, 27, 1578–1586.
Cohen, J. (1994). The world is round: p .05. American Psychologist, 49, 997–1003.
Hyde, J. S. (2007). New directions in the study of gender similarities and differences. Current Directions in Psychological Science, 16, 259–263.
Izawa, C. (Ed.) (1999). On human memory: Evolution, progress, and reflections on the 30th anniversary of the Atkinson-Shiffrin model. Mahwah, NJ: Erlbaum.
Lilienfeld, S. O., Lynn, S. J. (2003). Dissociative identity disorder: Multiplepersonalities, multiple controversies. In S. O. Lilienfeld, S. J. Lynn, J. M. Lohr (Eds.), Science and pseudoscience in clinical psychology (pp. 109–142). New York, NY: Guilford Press.
Neisser, U., Boodoo, G., Bouchard, T. J., Boykin, A. W., Brody, N., Ceci,…Urbina, S. (1996). Intelligence: Knowns and unknowns. American Psychologist, 51, 77–101.
Schwarz, N., Bless, H., Strack, F., Klumpp, G., Rittenauer-Schatka, H., Simons, A. (1991). Ease of retrieval as information: Another look at the availability heuristic. Journal of Personality and Social Psychology, 61, 195–202.
Zajonc, R. B. (1965). Social facilitation. Science, 149, 269–274.
Zajonc, R. B., Heingartner, A., Herman, E. M. (1969). Social enhancement and impairment of performance in the cockroach. Journal of Personality and Social Psychology, 13, 83–92.
Research Methods in Psychology & Neuroscience Copyright © by Dalhousie University Introduction to Psychology and Neuroscience Team. All Rights Reserved.
Share This Book
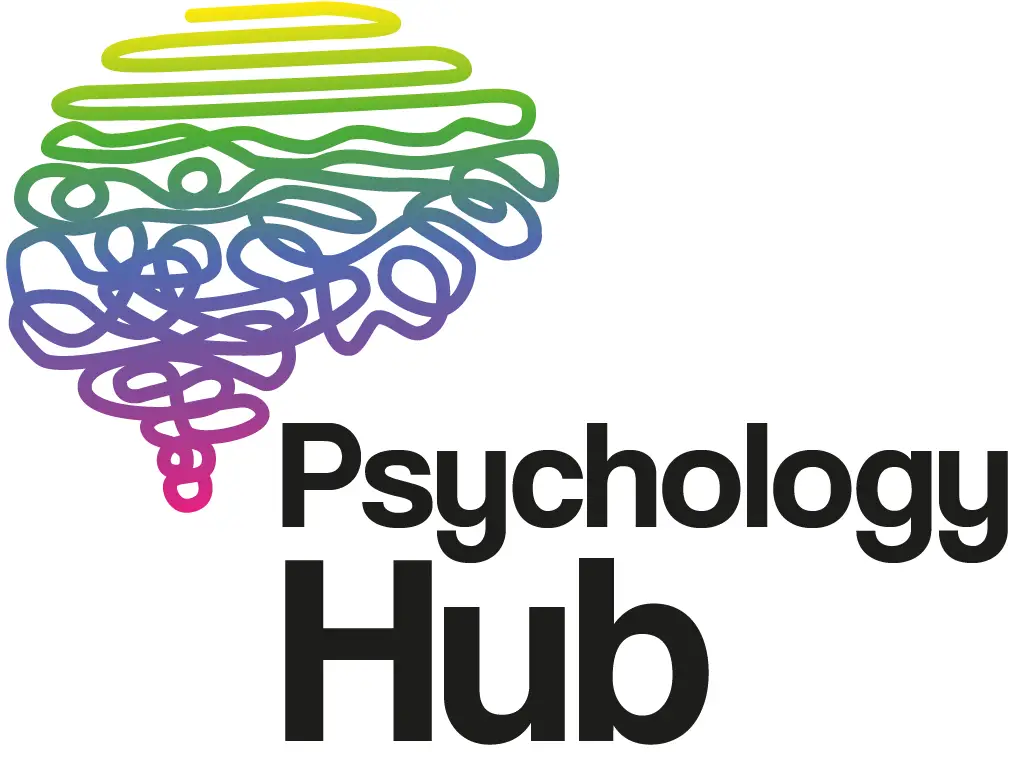
Aims And Hypotheses, Directional And Non-Directional
March 7, 2021 - paper 2 psychology in context | research methods.
- Back to Paper 2 - Research Methods
In Psychology, hypotheses are predictions made by the researcher about the outcome of a study. The research can chose to make a specific prediction about what they feel will happen in their research (a directional hypothesis) or they can make a ‘general,’ ‘less specific’ prediction about the outcome of their research (a non-directional hypothesis). The type of prediction that a researcher makes is usually dependent on whether or not any previous research has also investigated their research aim.
Variables Recap:
The independent variable (IV) is the variable that psychologists manipulate/change to see if changing this variable has an effect on the depen dent variable (DV).
The dependent variable (DV) is the variable that the psychologists measures (to see if the IV has had an effect).
It is important that the only variable that is changed in research is the independent variable (IV), all other variables have to be kept constant across the control condition and the experimental conditions. Only then will researchers be able to observe the true effects of just the independent variable (IV) on the dependent variable (DV).
Research/Experimental Aim(S):

An aim is a clear and precise statement of the purpose of the study. It is a statement of why a research study is taking place. This should include what is being studied and what the study is trying to achieve. (e.g. “This study aims to investigate the effects of alcohol on reaction times”.
It is important that aims created in research are realistic and ethical.
Hypotheses:
This is a testable statement that predicts what the researcher expects to happen in their research. The research study itself is therefore a means of testing whether or not the hypothesis is supported by the findings. If the findings do support the hypothesis then the hypothesis can be retained (i.e., accepted), but if not, then it must be rejected.
Three Different Hypotheses:

We're not around right now. But you can send us an email and we'll get back to you, asap.
Start typing and press Enter to search
Cookie Policy - Terms and Conditions - Privacy Policy
2.1 Why Is Research Important?
Learning objectives.
By the end of this section, you will be able to:
- Explain how scientific research addresses questions about behavior
- Discuss how scientific research guides public policy
- Appreciate how scientific research can be important in making personal decisions
Scientific research is a critical tool for successfully navigating our complex world. Without it, we would be forced to rely solely on intuition, other people’s authority, and blind luck. While many of us feel confident in our abilities to decipher and interact with the world around us, history is filled with examples of how very wrong we can be when we fail to recognize the need for evidence in supporting claims. At various times in history, we would have been certain that the sun revolved around a flat earth, that the earth’s continents did not move, and that mental illness was caused by possession ( Figure 2.2 ). It is through systematic scientific research that we divest ourselves of our preconceived notions and superstitions and gain an objective understanding of ourselves and our world.
The goal of all scientists is to better understand the world around them. Psychologists focus their attention on understanding behavior, as well as the cognitive (mental) and physiological (body) processes that underlie behavior. In contrast to other methods that people use to understand the behavior of others, such as intuition and personal experience, the hallmark of scientific research is that there is evidence to support a claim. Scientific knowledge is empirical : It is grounded in objective, tangible evidence that can be observed time and time again, regardless of who is observing.
While behavior is observable, the mind is not. If someone is crying, we can see behavior. However, the reason for the behavior is more difficult to determine. Is the person crying due to being sad, in pain, or happy? Sometimes we can learn the reason for someone’s behavior by simply asking a question, like “Why are you crying?” However, there are situations in which an individual is either uncomfortable or unwilling to answer the question honestly, or is incapable of answering. For example, infants would not be able to explain why they are crying. In such circumstances, the psychologist must be creative in finding ways to better understand behavior. This chapter explores how scientific knowledge is generated, and how important that knowledge is in forming decisions in our personal lives and in the public domain.
Use of Research Information
Trying to determine which theories are and are not accepted by the scientific community can be difficult, especially in an area of research as broad as psychology. More than ever before, we have an incredible amount of information at our fingertips, and a simple internet search on any given research topic might result in a number of contradictory studies. In these cases, we are witnessing the scientific community going through the process of reaching a consensus, and it could be quite some time before a consensus emerges. For example, the explosion in our use of technology has led researchers to question whether this ultimately helps or hinders us. The use and implementation of technology in educational settings has become widespread over the last few decades. Researchers are coming to different conclusions regarding the use of technology. To illustrate this point, a study investigating a smartphone app targeting surgery residents (graduate students in surgery training) found that the use of this app can increase student engagement and raise test scores (Shaw & Tan, 2015). Conversely, another study found that the use of technology in undergraduate student populations had negative impacts on sleep, communication, and time management skills (Massimini & Peterson, 2009). Until sufficient amounts of research have been conducted, there will be no clear consensus on the effects that technology has on a student's acquisition of knowledge, study skills, and mental health.
In the meantime, we should strive to think critically about the information we encounter by exercising a degree of healthy skepticism. When someone makes a claim, we should examine the claim from a number of different perspectives: what is the expertise of the person making the claim, what might they gain if the claim is valid, does the claim seem justified given the evidence, and what do other researchers think of the claim? This is especially important when we consider how much information in advertising campaigns and on the internet claims to be based on “scientific evidence” when in actuality it is a belief or perspective of just a few individuals trying to sell a product or draw attention to their perspectives.
We should be informed consumers of the information made available to us because decisions based on this information have significant consequences. One such consequence can be seen in politics and public policy. Imagine that you have been elected as the governor of your state. One of your responsibilities is to manage the state budget and determine how to best spend your constituents’ tax dollars. As the new governor, you need to decide whether to continue funding early intervention programs. These programs are designed to help children who come from low-income backgrounds, have special needs, or face other disadvantages. These programs may involve providing a wide variety of services to maximize the children's development and position them for optimal levels of success in school and later in life (Blann, 2005). While such programs sound appealing, you would want to be sure that they also proved effective before investing additional money in these programs. Fortunately, psychologists and other scientists have conducted vast amounts of research on such programs and, in general, the programs are found to be effective (Neil & Christensen, 2009; Peters-Scheffer, Didden, Korzilius, & Sturmey, 2011). While not all programs are equally effective, and the short-term effects of many such programs are more pronounced, there is reason to believe that many of these programs produce long-term benefits for participants (Barnett, 2011). If you are committed to being a good steward of taxpayer money, you would want to look at research. Which programs are most effective? What characteristics of these programs make them effective? Which programs promote the best outcomes? After examining the research, you would be best equipped to make decisions about which programs to fund.
Link to Learning
Watch this video about early childhood program effectiveness to learn how scientists evaluate effectiveness and how best to invest money into programs that are most effective.
Ultimately, it is not just politicians who can benefit from using research in guiding their decisions. We all might look to research from time to time when making decisions in our lives. Imagine that your sister, Maria, expresses concern about her two-year-old child, Umberto. Umberto does not speak as much or as clearly as the other children in his daycare or others in the family. Umberto's pediatrician undertakes some screening and recommends an evaluation by a speech pathologist, but does not refer Maria to any other specialists. Maria is concerned that Umberto's speech delays are signs of a developmental disorder, but Umberto's pediatrician does not; she sees indications of differences in Umberto's jaw and facial muscles. Hearing this, you do some internet searches, but you are overwhelmed by the breadth of information and the wide array of sources. You see blog posts, top-ten lists, advertisements from healthcare providers, and recommendations from several advocacy organizations. Why are there so many sites? Which are based in research, and which are not?
In the end, research is what makes the difference between facts and opinions. Facts are observable realities, and opinions are personal judgments, conclusions, or attitudes that may or may not be accurate. In the scientific community, facts can be established only using evidence collected through empirical research.
NOTABLE RESEARCHERS
Psychological research has a long history involving important figures from diverse backgrounds. While the introductory chapter discussed several researchers who made significant contributions to the discipline, there are many more individuals who deserve attention in considering how psychology has advanced as a science through their work ( Figure 2.3 ). For instance, Margaret Floy Washburn (1871–1939) was the first woman to earn a PhD in psychology. Her research focused on animal behavior and cognition (Margaret Floy Washburn, PhD, n.d.). Mary Whiton Calkins (1863–1930) was a preeminent first-generation American psychologist who opposed the behaviorist movement, conducted significant research into memory, and established one of the earliest experimental psychology labs in the United States (Mary Whiton Calkins, n.d.).
Francis Sumner (1895–1954) was the first African American to receive a PhD in psychology in 1920. His dissertation focused on issues related to psychoanalysis. Sumner also had research interests in racial bias and educational justice. Sumner was one of the founders of Howard University’s department of psychology, and because of his accomplishments, he is sometimes referred to as the “Father of Black Psychology.” Thirteen years later, Inez Beverly Prosser (1895–1934) became the first African American woman to receive a PhD in psychology. Prosser’s research highlighted issues related to education in segregated versus integrated schools, and ultimately, her work was very influential in the hallmark Brown v. Board of Education Supreme Court ruling that segregation of public schools was unconstitutional (Ethnicity and Health in America Series: Featured Psychologists, n.d.).
Although the establishment of psychology’s scientific roots occurred first in Europe and the United States, it did not take much time until researchers from around the world began to establish their own laboratories and research programs. For example, some of the first experimental psychology laboratories in South America were founded by Horatio Piñero (1869–1919) at two institutions in Buenos Aires, Argentina (Godoy & Brussino, 2010). In India, Gunamudian David Boaz (1908–1965) and Narendra Nath Sen Gupta (1889–1944) established the first independent departments of psychology at the University of Madras and the University of Calcutta, respectively. These developments provided an opportunity for Indian researchers to make important contributions to the field (Gunamudian David Boaz, n.d.; Narendra Nath Sen Gupta, n.d.).
When the American Psychological Association (APA) was first founded in 1892, all of the members were White males (Women and Minorities in Psychology, n.d.). However, by 1905, Mary Whiton Calkins was elected as the first female president of the APA, and by 1946, nearly one-quarter of American psychologists were female. Psychology became a popular degree option for students enrolled in the nation’s historically Black higher education institutions, increasing the number of Black Americans who went on to become psychologists. Given demographic shifts occurring in the United States and increased access to higher educational opportunities among historically underrepresented populations, there is reason to hope that the diversity of the field will increasingly match the larger population, and that the research contributions made by the psychologists of the future will better serve people of all backgrounds (Women and Minorities in Psychology, n.d.).
The Process of Scientific Research
Scientific knowledge is advanced through a process known as the scientific method . Basically, ideas (in the form of theories and hypotheses) are tested against the real world (in the form of empirical observations), and those empirical observations lead to more ideas that are tested against the real world, and so on. In this sense, the scientific process is circular. The types of reasoning within the circle are called deductive and inductive. In deductive reasoning , ideas are tested in the real world; in inductive reasoning , real-world observations lead to new ideas ( Figure 2.4 ). These processes are inseparable, like inhaling and exhaling, but different research approaches place different emphasis on the deductive and inductive aspects.
In the scientific context, deductive reasoning begins with a generalization—one hypothesis—that is then used to reach logical conclusions about the real world. If the hypothesis is correct, then the logical conclusions reached through deductive reasoning should also be correct. A deductive reasoning argument might go something like this: All living things require energy to survive (this would be your hypothesis). Ducks are living things. Therefore, ducks require energy to survive (logical conclusion). In this example, the hypothesis is correct; therefore, the conclusion is correct as well. Sometimes, however, an incorrect hypothesis may lead to a logical but incorrect conclusion. Consider this argument: all ducks are born with the ability to see. Quackers is a duck. Therefore, Quackers was born with the ability to see. Scientists use deductive reasoning to empirically test their hypotheses. Returning to the example of the ducks, researchers might design a study to test the hypothesis that if all living things require energy to survive, then ducks will be found to require energy to survive.
Deductive reasoning starts with a generalization that is tested against real-world observations; however, inductive reasoning moves in the opposite direction. Inductive reasoning uses empirical observations to construct broad generalizations. Unlike deductive reasoning, conclusions drawn from inductive reasoning may or may not be correct, regardless of the observations on which they are based. For instance, you may notice that your favorite fruits—apples, bananas, and oranges—all grow on trees; therefore, you assume that all fruit must grow on trees. This would be an example of inductive reasoning, and, clearly, the existence of strawberries, blueberries, and kiwi demonstrate that this generalization is not correct despite it being based on a number of direct observations. Scientists use inductive reasoning to formulate theories, which in turn generate hypotheses that are tested with deductive reasoning. In the end, science involves both deductive and inductive processes.
For example, case studies, which you will read about in the next section, are heavily weighted on the side of empirical observations. Thus, case studies are closely associated with inductive processes as researchers gather massive amounts of observations and seek interesting patterns (new ideas) in the data. Experimental research, on the other hand, puts great emphasis on deductive reasoning.
We’ve stated that theories and hypotheses are ideas, but what sort of ideas are they, exactly? A theory is a well-developed set of ideas that propose an explanation for observed phenomena. Theories are repeatedly checked against the world, but they tend to be too complex to be tested all at once; instead, researchers create hypotheses to test specific aspects of a theory.
A hypothesis is a testable prediction about how the world will behave if our idea is correct, and it is often worded as an if-then statement (e.g., if I study all night, I will get a passing grade on the test). The hypothesis is extremely important because it bridges the gap between the realm of ideas and the real world. As specific hypotheses are tested, theories are modified and refined to reflect and incorporate the result of these tests Figure 2.5 .
To see how this process works, let’s consider a specific theory and a hypothesis that might be generated from that theory. As you’ll learn in a later chapter, the James-Lange theory of emotion asserts that emotional experience relies on the physiological arousal associated with the emotional state. If you walked out of your home and discovered a very aggressive snake waiting on your doorstep, your heart would begin to race and your stomach churn. According to the James-Lange theory, these physiological changes would result in your feeling of fear. A hypothesis that could be derived from this theory might be that a person who is unaware of the physiological arousal that the sight of the snake elicits will not feel fear.
A scientific hypothesis is also falsifiable , or capable of being shown to be incorrect. Recall from the introductory chapter that Sigmund Freud had lots of interesting ideas to explain various human behaviors ( Figure 2.6 ). However, a major criticism of Freud’s theories is that many of his ideas are not falsifiable; for example, it is impossible to imagine empirical observations that would disprove the existence of the id, the ego, and the superego—the three elements of personality described in Freud’s theories. Despite this, Freud’s theories are widely taught in introductory psychology texts because of their historical significance for personality psychology and psychotherapy, and these remain the root of all modern forms of therapy.
In contrast, the James-Lange theory does generate falsifiable hypotheses, such as the one described above. Some individuals who suffer significant injuries to their spinal columns are unable to feel the bodily changes that often accompany emotional experiences. Therefore, we could test the hypothesis by determining how emotional experiences differ between individuals who have the ability to detect these changes in their physiological arousal and those who do not. In fact, this research has been conducted and while the emotional experiences of people deprived of an awareness of their physiological arousal may be less intense, they still experience emotion (Chwalisz, Diener, & Gallagher, 1988).
Scientific research’s dependence on falsifiability allows for great confidence in the information that it produces. Typically, by the time information is accepted by the scientific community, it has been tested repeatedly.
As an Amazon Associate we earn from qualifying purchases.
This book may not be used in the training of large language models or otherwise be ingested into large language models or generative AI offerings without OpenStax's permission.
Want to cite, share, or modify this book? This book uses the Creative Commons Attribution License and you must attribute OpenStax.
Access for free at https://openstax.org/books/psychology-2e/pages/1-introduction
- Authors: Rose M. Spielman, William J. Jenkins, Marilyn D. Lovett
- Publisher/website: OpenStax
- Book title: Psychology 2e
- Publication date: Apr 22, 2020
- Location: Houston, Texas
- Book URL: https://openstax.org/books/psychology-2e/pages/1-introduction
- Section URL: https://openstax.org/books/psychology-2e/pages/2-1-why-is-research-important
© Jan 6, 2024 OpenStax. Textbook content produced by OpenStax is licensed under a Creative Commons Attribution License . The OpenStax name, OpenStax logo, OpenStax book covers, OpenStax CNX name, and OpenStax CNX logo are not subject to the Creative Commons license and may not be reproduced without the prior and express written consent of Rice University.
- Privacy Policy
Buy Me a Coffee

Home » What is a Hypothesis – Types, Examples and Writing Guide
What is a Hypothesis – Types, Examples and Writing Guide
Table of Contents

Definition:
Hypothesis is an educated guess or proposed explanation for a phenomenon, based on some initial observations or data. It is a tentative statement that can be tested and potentially proven or disproven through further investigation and experimentation.
Hypothesis is often used in scientific research to guide the design of experiments and the collection and analysis of data. It is an essential element of the scientific method, as it allows researchers to make predictions about the outcome of their experiments and to test those predictions to determine their accuracy.
Types of Hypothesis
Types of Hypothesis are as follows:
Research Hypothesis
A research hypothesis is a statement that predicts a relationship between variables. It is usually formulated as a specific statement that can be tested through research, and it is often used in scientific research to guide the design of experiments.
Null Hypothesis
The null hypothesis is a statement that assumes there is no significant difference or relationship between variables. It is often used as a starting point for testing the research hypothesis, and if the results of the study reject the null hypothesis, it suggests that there is a significant difference or relationship between variables.
Alternative Hypothesis
An alternative hypothesis is a statement that assumes there is a significant difference or relationship between variables. It is often used as an alternative to the null hypothesis and is tested against the null hypothesis to determine which statement is more accurate.
Directional Hypothesis
A directional hypothesis is a statement that predicts the direction of the relationship between variables. For example, a researcher might predict that increasing the amount of exercise will result in a decrease in body weight.
Non-directional Hypothesis
A non-directional hypothesis is a statement that predicts the relationship between variables but does not specify the direction. For example, a researcher might predict that there is a relationship between the amount of exercise and body weight, but they do not specify whether increasing or decreasing exercise will affect body weight.
Statistical Hypothesis
A statistical hypothesis is a statement that assumes a particular statistical model or distribution for the data. It is often used in statistical analysis to test the significance of a particular result.
Composite Hypothesis
A composite hypothesis is a statement that assumes more than one condition or outcome. It can be divided into several sub-hypotheses, each of which represents a different possible outcome.
Empirical Hypothesis
An empirical hypothesis is a statement that is based on observed phenomena or data. It is often used in scientific research to develop theories or models that explain the observed phenomena.
Simple Hypothesis
A simple hypothesis is a statement that assumes only one outcome or condition. It is often used in scientific research to test a single variable or factor.
Complex Hypothesis
A complex hypothesis is a statement that assumes multiple outcomes or conditions. It is often used in scientific research to test the effects of multiple variables or factors on a particular outcome.
Applications of Hypothesis
Hypotheses are used in various fields to guide research and make predictions about the outcomes of experiments or observations. Here are some examples of how hypotheses are applied in different fields:
- Science : In scientific research, hypotheses are used to test the validity of theories and models that explain natural phenomena. For example, a hypothesis might be formulated to test the effects of a particular variable on a natural system, such as the effects of climate change on an ecosystem.
- Medicine : In medical research, hypotheses are used to test the effectiveness of treatments and therapies for specific conditions. For example, a hypothesis might be formulated to test the effects of a new drug on a particular disease.
- Psychology : In psychology, hypotheses are used to test theories and models of human behavior and cognition. For example, a hypothesis might be formulated to test the effects of a particular stimulus on the brain or behavior.
- Sociology : In sociology, hypotheses are used to test theories and models of social phenomena, such as the effects of social structures or institutions on human behavior. For example, a hypothesis might be formulated to test the effects of income inequality on crime rates.
- Business : In business research, hypotheses are used to test the validity of theories and models that explain business phenomena, such as consumer behavior or market trends. For example, a hypothesis might be formulated to test the effects of a new marketing campaign on consumer buying behavior.
- Engineering : In engineering, hypotheses are used to test the effectiveness of new technologies or designs. For example, a hypothesis might be formulated to test the efficiency of a new solar panel design.
How to write a Hypothesis
Here are the steps to follow when writing a hypothesis:
Identify the Research Question
The first step is to identify the research question that you want to answer through your study. This question should be clear, specific, and focused. It should be something that can be investigated empirically and that has some relevance or significance in the field.
Conduct a Literature Review
Before writing your hypothesis, it’s essential to conduct a thorough literature review to understand what is already known about the topic. This will help you to identify the research gap and formulate a hypothesis that builds on existing knowledge.
Determine the Variables
The next step is to identify the variables involved in the research question. A variable is any characteristic or factor that can vary or change. There are two types of variables: independent and dependent. The independent variable is the one that is manipulated or changed by the researcher, while the dependent variable is the one that is measured or observed as a result of the independent variable.
Formulate the Hypothesis
Based on the research question and the variables involved, you can now formulate your hypothesis. A hypothesis should be a clear and concise statement that predicts the relationship between the variables. It should be testable through empirical research and based on existing theory or evidence.
Write the Null Hypothesis
The null hypothesis is the opposite of the alternative hypothesis, which is the hypothesis that you are testing. The null hypothesis states that there is no significant difference or relationship between the variables. It is important to write the null hypothesis because it allows you to compare your results with what would be expected by chance.
Refine the Hypothesis
After formulating the hypothesis, it’s important to refine it and make it more precise. This may involve clarifying the variables, specifying the direction of the relationship, or making the hypothesis more testable.
Examples of Hypothesis
Here are a few examples of hypotheses in different fields:
- Psychology : “Increased exposure to violent video games leads to increased aggressive behavior in adolescents.”
- Biology : “Higher levels of carbon dioxide in the atmosphere will lead to increased plant growth.”
- Sociology : “Individuals who grow up in households with higher socioeconomic status will have higher levels of education and income as adults.”
- Education : “Implementing a new teaching method will result in higher student achievement scores.”
- Marketing : “Customers who receive a personalized email will be more likely to make a purchase than those who receive a generic email.”
- Physics : “An increase in temperature will cause an increase in the volume of a gas, assuming all other variables remain constant.”
- Medicine : “Consuming a diet high in saturated fats will increase the risk of developing heart disease.”
Purpose of Hypothesis
The purpose of a hypothesis is to provide a testable explanation for an observed phenomenon or a prediction of a future outcome based on existing knowledge or theories. A hypothesis is an essential part of the scientific method and helps to guide the research process by providing a clear focus for investigation. It enables scientists to design experiments or studies to gather evidence and data that can support or refute the proposed explanation or prediction.
The formulation of a hypothesis is based on existing knowledge, observations, and theories, and it should be specific, testable, and falsifiable. A specific hypothesis helps to define the research question, which is important in the research process as it guides the selection of an appropriate research design and methodology. Testability of the hypothesis means that it can be proven or disproven through empirical data collection and analysis. Falsifiability means that the hypothesis should be formulated in such a way that it can be proven wrong if it is incorrect.
In addition to guiding the research process, the testing of hypotheses can lead to new discoveries and advancements in scientific knowledge. When a hypothesis is supported by the data, it can be used to develop new theories or models to explain the observed phenomenon. When a hypothesis is not supported by the data, it can help to refine existing theories or prompt the development of new hypotheses to explain the phenomenon.
When to use Hypothesis
Here are some common situations in which hypotheses are used:
- In scientific research , hypotheses are used to guide the design of experiments and to help researchers make predictions about the outcomes of those experiments.
- In social science research , hypotheses are used to test theories about human behavior, social relationships, and other phenomena.
- I n business , hypotheses can be used to guide decisions about marketing, product development, and other areas. For example, a hypothesis might be that a new product will sell well in a particular market, and this hypothesis can be tested through market research.
Characteristics of Hypothesis
Here are some common characteristics of a hypothesis:
- Testable : A hypothesis must be able to be tested through observation or experimentation. This means that it must be possible to collect data that will either support or refute the hypothesis.
- Falsifiable : A hypothesis must be able to be proven false if it is not supported by the data. If a hypothesis cannot be falsified, then it is not a scientific hypothesis.
- Clear and concise : A hypothesis should be stated in a clear and concise manner so that it can be easily understood and tested.
- Based on existing knowledge : A hypothesis should be based on existing knowledge and research in the field. It should not be based on personal beliefs or opinions.
- Specific : A hypothesis should be specific in terms of the variables being tested and the predicted outcome. This will help to ensure that the research is focused and well-designed.
- Tentative: A hypothesis is a tentative statement or assumption that requires further testing and evidence to be confirmed or refuted. It is not a final conclusion or assertion.
- Relevant : A hypothesis should be relevant to the research question or problem being studied. It should address a gap in knowledge or provide a new perspective on the issue.
Advantages of Hypothesis
Hypotheses have several advantages in scientific research and experimentation:
- Guides research: A hypothesis provides a clear and specific direction for research. It helps to focus the research question, select appropriate methods and variables, and interpret the results.
- Predictive powe r: A hypothesis makes predictions about the outcome of research, which can be tested through experimentation. This allows researchers to evaluate the validity of the hypothesis and make new discoveries.
- Facilitates communication: A hypothesis provides a common language and framework for scientists to communicate with one another about their research. This helps to facilitate the exchange of ideas and promotes collaboration.
- Efficient use of resources: A hypothesis helps researchers to use their time, resources, and funding efficiently by directing them towards specific research questions and methods that are most likely to yield results.
- Provides a basis for further research: A hypothesis that is supported by data provides a basis for further research and exploration. It can lead to new hypotheses, theories, and discoveries.
- Increases objectivity: A hypothesis can help to increase objectivity in research by providing a clear and specific framework for testing and interpreting results. This can reduce bias and increase the reliability of research findings.
Limitations of Hypothesis
Some Limitations of the Hypothesis are as follows:
- Limited to observable phenomena: Hypotheses are limited to observable phenomena and cannot account for unobservable or intangible factors. This means that some research questions may not be amenable to hypothesis testing.
- May be inaccurate or incomplete: Hypotheses are based on existing knowledge and research, which may be incomplete or inaccurate. This can lead to flawed hypotheses and erroneous conclusions.
- May be biased: Hypotheses may be biased by the researcher’s own beliefs, values, or assumptions. This can lead to selective interpretation of data and a lack of objectivity in research.
- Cannot prove causation: A hypothesis can only show a correlation between variables, but it cannot prove causation. This requires further experimentation and analysis.
- Limited to specific contexts: Hypotheses are limited to specific contexts and may not be generalizable to other situations or populations. This means that results may not be applicable in other contexts or may require further testing.
- May be affected by chance : Hypotheses may be affected by chance or random variation, which can obscure or distort the true relationship between variables.
About the author
Muhammad Hassan
Researcher, Academic Writer, Web developer
You may also like

Data Collection – Methods Types and Examples

Delimitations in Research – Types, Examples and...

Research Process – Steps, Examples and Tips

Research Design – Types, Methods and Examples

Institutional Review Board – Application Sample...

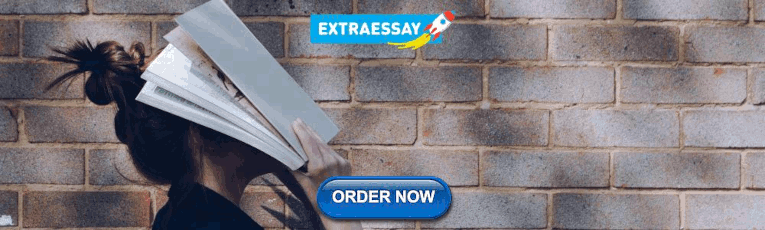
Evaluating Research – Process, Examples and...
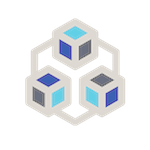
Want to create or adapt books like this? Learn more about how Pressbooks supports open publishing practices.
Psychological Research
Why Is Research Important?
OpenStaxCollege
[latexpage]
Learning Objectives
By the end of this section, you will be able to:
- Explain how scientific research addresses questions about behavior
- Discuss how scientific research guides public policy
- Appreciate how scientific research can be important in making personal decisions
Scientific research is a critical tool for successfully navigating our complex world. Without it, we would be forced to rely solely on intuition, other people’s authority, and blind luck. While many of us feel confident in our abilities to decipher and interact with the world around us, history is filled with examples of how very wrong we can be when we fail to recognize the need for evidence in supporting claims. At various times in history, we would have been certain that the sun revolved around a flat earth, that the earth’s continents did not move, and that mental illness was caused by possession ( [link] ). It is through systematic scientific research that we divest ourselves of our preconceived notions and superstitions and gain an objective understanding of ourselves and our world.
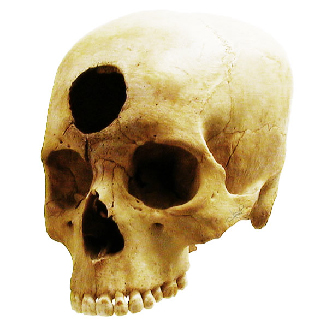
The goal of all scientists is to better understand the world around them. Psychologists focus their attention on understanding behavior, as well as the cognitive (mental) and physiological (body) processes that underlie behavior. In contrast to other methods that people use to understand the behavior of others, such as intuition and personal experience, the hallmark of scientific research is that there is evidence to support a claim. Scientific knowledge is empirical : It is grounded in objective, tangible evidence that can be observed time and time again, regardless of who is observing.
While behavior is observable, the mind is not. If someone is crying, we can see behavior. However, the reason for the behavior is more difficult to determine. Is the person crying due to being sad, in pain, or happy? Sometimes we can learn the reason for someone’s behavior by simply asking a question, like “Why are you crying?” However, there are situations in which an individual is either uncomfortable or unwilling to answer the question honestly, or is incapable of answering. For example, infants would not be able to explain why they are crying. In such circumstances, the psychologist must be creative in finding ways to better understand behavior. This chapter explores how scientific knowledge is generated, and how important that knowledge is in forming decisions in our personal lives and in the public domain.
USE OF RESEARCH INFORMATION
Trying to determine which theories are and are not accepted by the scientific community can be difficult, especially in an area of research as broad as psychology. More than ever before, we have an incredible amount of information at our fingertips, and a simple internet search on any given research topic might result in a number of contradictory studies. In these cases, we are witnessing the scientific community going through the process of reaching a consensus, and it could be quite some time before a consensus emerges. For example, the hypothesized link between exposure to media violence and subsequent aggression has been debated in the scientific community for roughly 60 years. Even today, we will find detractors, but a consensus is building. Several professional organizations view media violence exposure as a risk factor for actual violence, including the American Medical Association, the American Psychiatric Association, and the American Psychological Association (American Academy of Pediatrics, American Academy of Child & Adolescent Psychiatry, American Psychological Association, American Medical Association, American Academy of Family Physicians, American Psychiatric Association, 2000).
In the meantime, we should strive to think critically about the information we encounter by exercising a degree of healthy skepticism. When someone makes a claim, we should examine the claim from a number of different perspectives: what is the expertise of the person making the claim, what might they gain if the claim is valid, does the claim seem justified given the evidence, and what do other researchers think of the claim? This is especially important when we consider how much information in advertising campaigns and on the internet claims to be based on “scientific evidence” when in actuality it is a belief or perspective of just a few individuals trying to sell a product or draw attention to their perspectives.
We should be informed consumers of the information made available to us because decisions based on this information have significant consequences. One such consequence can be seen in politics and public policy. Imagine that you have been elected as the governor of your state. One of your responsibilities is to manage the state budget and determine how to best spend your constituents’ tax dollars. As the new governor, you need to decide whether to continue funding the D.A.R.E. (Drug Abuse Resistance Education) program in public schools ( [link] ). This program typically involves police officers coming into the classroom to educate students about the dangers of becoming involved with alcohol and other drugs. According to the D.A.R.E. website (www.dare.org), this program has been very popular since its inception in 1983, and it is currently operating in 75% of school districts in the United States and in more than 40 countries worldwide. Sounds like an easy decision, right? However, on closer review, you discover that the vast majority of research into this program consistently suggests that participation has little, if any, effect on whether or not someone uses alcohol or other drugs (Clayton, Cattarello, & Johnstone, 1996; Ennett, Tobler, Ringwalt, & Flewelling, 1994; Lynam et al., 1999; Ringwalt, Ennett, & Holt, 1991). If you are committed to being a good steward of taxpayer money, will you fund this particular program, or will you try to find other programs that research has consistently demonstrated to be effective?
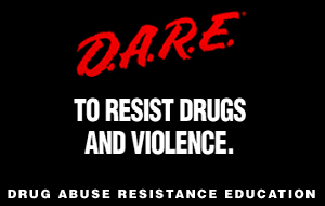
Watch this news report to learn more about some of the controversial issues surrounding the D.A.R.E. program.
Ultimately, it is not just politicians who can benefit from using research in guiding their decisions. We all might look to research from time to time when making decisions in our lives. Imagine you just found out that a close friend has breast cancer or that one of your young relatives has recently been diagnosed with autism. In either case, you want to know which treatment options are most successful with the fewest side effects. How would you find that out? You would probably talk with your doctor and personally review the research that has been done on various treatment options—always with a critical eye to ensure that you are as informed as possible.
In the end, research is what makes the difference between facts and opinions. Facts are observable realities, and opinions are personal judgments, conclusions, or attitudes that may or may not be accurate. In the scientific community, facts can be established only using evidence collected through empirical research.
THE PROCESS OF SCIENTIFIC RESEARCH
Scientific knowledge is advanced through a process known as the scientific method . Basically, ideas (in the form of theories and hypotheses) are tested against the real world (in the form of empirical observations), and those empirical observations lead to more ideas that are tested against the real world, and so on. In this sense, the scientific process is circular. The types of reasoning within the circle are called deductive and inductive. In deductive reasoning , ideas are tested against the empirical world; in inductive reasoning , empirical observations lead to new ideas ( [link] ). These processes are inseparable, like inhaling and exhaling, but different research approaches place different emphasis on the deductive and inductive aspects.
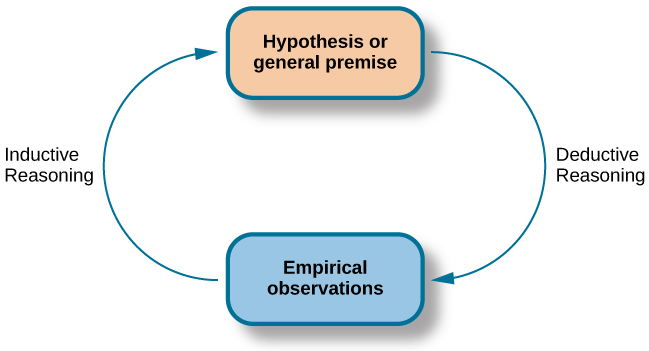
In the scientific context, deductive reasoning begins with a generalization—one hypothesis—that is then used to reach logical conclusions about the real world. If the hypothesis is correct, then the logical conclusions reached through deductive reasoning should also be correct. A deductive reasoning argument might go something like this: All living things require energy to survive (this would be your hypothesis). Ducks are living things. Therefore, ducks require energy to survive (logical conclusion). In this example, the hypothesis is correct; therefore, the conclusion is correct as well. Sometimes, however, an incorrect hypothesis may lead to a logical but incorrect conclusion. Consider this argument: all ducks are born with the ability to see. Quackers is a duck. Therefore, Quackers was born with the ability to see. Scientists use deductive reasoning to empirically test their hypotheses. Returning to the example of the ducks, researchers might design a study to test the hypothesis that if all living things require energy to survive, then ducks will be found to require energy to survive.
Deductive reasoning starts with a generalization that is tested against real-world observations; however, inductive reasoning moves in the opposite direction. Inductive reasoning uses empirical observations to construct broad generalizations. Unlike deductive reasoning, conclusions drawn from inductive reasoning may or may not be correct, regardless of the observations on which they are based. For instance, you may notice that your favorite fruits—apples, bananas, and oranges—all grow on trees; therefore, you assume that all fruit must grow on trees. This would be an example of inductive reasoning, and, clearly, the existence of strawberries, blueberries, and kiwi demonstrate that this generalization is not correct despite it being based on a number of direct observations. Scientists use inductive reasoning to formulate theories, which in turn generate hypotheses that are tested with deductive reasoning. In the end, science involves both deductive and inductive processes.
For example, case studies, which you will read about in the next section, are heavily weighted on the side of empirical observations. Thus, case studies are closely associated with inductive processes as researchers gather massive amounts of observations and seek interesting patterns (new ideas) in the data. Experimental research, on the other hand, puts great emphasis on deductive reasoning.
Play this “Deal Me In” interactive card game to practice using inductive reasoning.
We’ve stated that theories and hypotheses are ideas, but what sort of ideas are they, exactly? A theory is a well-developed set of ideas that propose an explanation for observed phenomena. Theories are repeatedly checked against the world, but they tend to be too complex to be tested all at once; instead, researchers create hypotheses to test specific aspects of a theory.
A hypothesis is a testable prediction about how the world will behave if our idea is correct, and it is often worded as an if-then statement (e.g., if I study all night, I will get a passing grade on the test). The hypothesis is extremely important because it bridges the gap between the realm of ideas and the real world. As specific hypotheses are tested, theories are modified and refined to reflect and incorporate the result of these tests [link] .
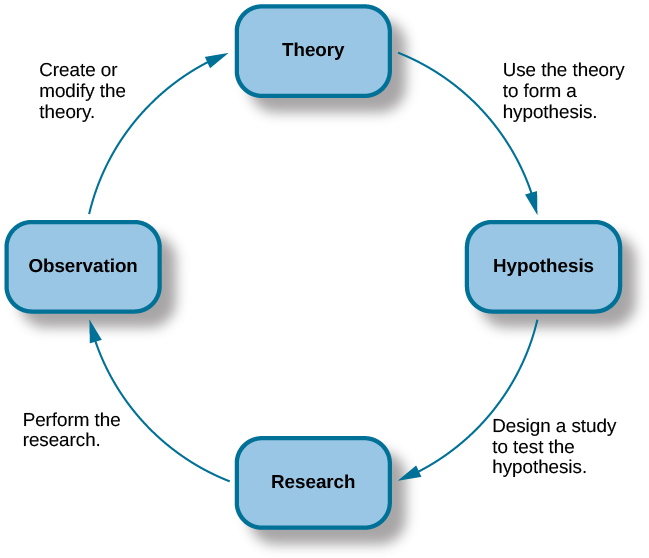
To see how this process works, let’s consider a specific theory and a hypothesis that might be generated from that theory. As you’ll learn in a later chapter, the James-Lange theory of emotion asserts that emotional experience relies on the physiological arousal associated with the emotional state. If you walked out of your home and discovered a very aggressive snake waiting on your doorstep, your heart would begin to race and your stomach churn. According to the James-Lange theory, these physiological changes would result in your feeling of fear. A hypothesis that could be derived from this theory might be that a person who is unaware of the physiological arousal that the sight of the snake elicits will not feel fear.
A scientific hypothesis is also falsifiable , or capable of being shown to be incorrect. Recall from the introductory chapter that Sigmund Freud had lots of interesting ideas to explain various human behaviors ( [link] ). However, a major criticism of Freud’s theories is that many of his ideas are not falsifiable; for example, it is impossible to imagine empirical observations that would disprove the existence of the id, the ego, and the superego—the three elements of personality described in Freud’s theories. Despite this, Freud’s theories are widely taught in introductory psychology texts because of their historical significance for personality psychology and psychotherapy, and these remain the root of all modern forms of therapy.
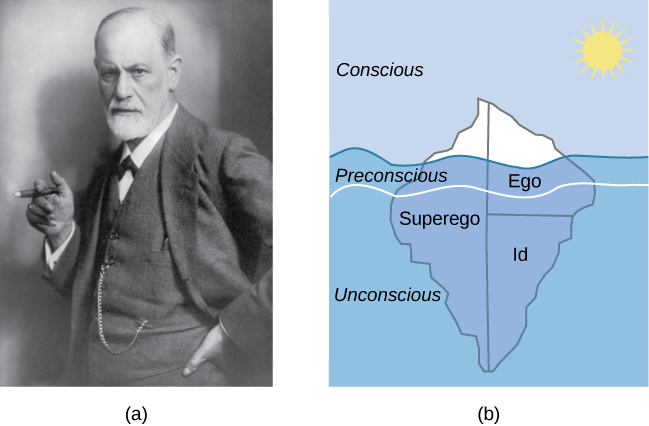
In contrast, the James-Lange theory does generate falsifiable hypotheses, such as the one described above. Some individuals who suffer significant injuries to their spinal columns are unable to feel the bodily changes that often accompany emotional experiences. Therefore, we could test the hypothesis by determining how emotional experiences differ between individuals who have the ability to detect these changes in their physiological arousal and those who do not. In fact, this research has been conducted and while the emotional experiences of people deprived of an awareness of their physiological arousal may be less intense, they still experience emotion (Chwalisz, Diener, & Gallagher, 1988).
Scientific research’s dependence on falsifiability allows for great confidence in the information that it produces. Typically, by the time information is accepted by the scientific community, it has been tested repeatedly.
Visit this website to apply the scientific method and practice its steps by using them to solve a murder mystery, determine why a student is in trouble, and design an experiment to test house paint.
Scientists are engaged in explaining and understanding how the world around them works, and they are able to do so by coming up with theories that generate hypotheses that are testable and falsifiable. Theories that stand up to their tests are retained and refined, while those that do not are discarded or modified. In this way, research enables scientists to separate fact from simple opinion. Having good information generated from research aids in making wise decisions both in public policy and in our personal lives.
Review Questions
Scientific hypotheses are ________ and falsifiable.
________ are defined as observable realities.
Scientific knowledge is ________.
A major criticism of Freud’s early theories involves the fact that his theories ________.
- were too limited in scope
- were too outrageous
- were too broad
- were not testable
Critical Thinking Questions
In this section, the D.A.R.E. program was described as an incredibly popular program in schools across the United States despite the fact that research consistently suggests that this program is largely ineffective. How might one explain this discrepancy?
There is probably tremendous political pressure to appear to be hard on drugs. Therefore, even though D.A.R.E. might be ineffective, it is a well-known program with which voters are familiar.
The scientific method is often described as self-correcting and cyclical. Briefly describe your understanding of the scientific method with regard to these concepts.
This cyclical, self-correcting process is primarily a function of the empirical nature of science. Theories are generated as explanations of real-world phenomena. From theories, specific hypotheses are developed and tested. As a function of this testing, theories will be revisited and modified or refined to generate new hypotheses that are again tested. This cyclical process ultimately allows for more and more precise (and presumably accurate) information to be collected.
Personal Application Questions
Healthcare professionals cite an enormous number of health problems related to obesity, and many people have an understandable desire to attain a healthy weight. There are many diet programs, services, and products on the market to aid those who wish to lose weight. If a close friend was considering purchasing or participating in one of these products, programs, or services, how would you make sure your friend was fully aware of the potential consequences of this decision? What sort of information would you want to review before making such an investment or lifestyle change yourself?
Why Is Research Important? Copyright © 2014 by OpenStaxCollege is licensed under a Creative Commons Attribution 4.0 International License , except where otherwise noted.

Want to create or adapt books like this? Learn more about how Pressbooks supports open publishing practices.
Developing a Hypothesis
Rajiv S. Jhangiani; I-Chant A. Chiang; Carrie Cuttler; and Dana C. Leighton
Learning Objectives
- Distinguish between a theory and a hypothesis.
- Discover how theories are used to generate hypotheses and how the results of studies can be used to further inform theories.
- Understand the characteristics of a good hypothesis.
Theories and Hypotheses
Before describing how to develop a hypothesis, it is important to distinguish between a theory and a hypothesis. A theory is a coherent explanation or interpretation of one or more phenomena. Although theories can take a variety of forms, one thing they have in common is that they go beyond the phenomena they explain by including variables, structures, processes, functions, or organizing principles that have not been observed directly. Consider, for example, Zajonc’s theory of social facilitation and social inhibition (1965) [1] . He proposed that being watched by others while performing a task creates a general state of physiological arousal, which increases the likelihood of the dominant (most likely) response. So for highly practiced tasks, being watched increases the tendency to make correct responses, but for relatively unpracticed tasks, being watched increases the tendency to make incorrect responses. Notice that this theory—which has come to be called drive theory—provides an explanation of both social facilitation and social inhibition that goes beyond the phenomena themselves by including concepts such as “arousal” and “dominant response,” along with processes such as the effect of arousal on the dominant response.
Outside of science, referring to an idea as a theory often implies that it is untested—perhaps no more than a wild guess. In science, however, the term theory has no such implication. A theory is simply an explanation or interpretation of a set of phenomena. It can be untested, but it can also be extensively tested, well supported, and accepted as an accurate description of the world by the scientific community. The theory of evolution by natural selection, for example, is a theory because it is an explanation of the diversity of life on earth—not because it is untested or unsupported by scientific research. On the contrary, the evidence for this theory is overwhelmingly positive and nearly all scientists accept its basic assumptions as accurate. Similarly, the “germ theory” of disease is a theory because it is an explanation of the origin of various diseases, not because there is any doubt that many diseases are caused by microorganisms that infect the body.
A hypothesis , on the other hand, is a specific prediction about a new phenomenon that should be observed if a particular theory is accurate. It is an explanation that relies on just a few key concepts. Hypotheses are often specific predictions about what will happen in a particular study. They are developed by considering existing evidence and using reasoning to infer what will happen in the specific context of interest. Hypotheses are often but not always derived from theories. So a hypothesis is often a prediction based on a theory but some hypotheses are a-theoretical and only after a set of observations have been made, is a theory developed. This is because theories are broad in nature and they explain larger bodies of data. So if our research question is really original then we may need to collect some data and make some observations before we can develop a broader theory.
Theories and hypotheses always have this if-then relationship. “ If drive theory is correct, then cockroaches should run through a straight runway faster, and a branching runway more slowly, when other cockroaches are present.” Although hypotheses are usually expressed as statements, they can always be rephrased as questions. “Do cockroaches run through a straight runway faster when other cockroaches are present?” Thus deriving hypotheses from theories is an excellent way of generating interesting research questions.
But how do researchers derive hypotheses from theories? One way is to generate a research question using the techniques discussed in this chapter and then ask whether any theory implies an answer to that question. For example, you might wonder whether expressive writing about positive experiences improves health as much as expressive writing about traumatic experiences. Although this question is an interesting one on its own, you might then ask whether the habituation theory—the idea that expressive writing causes people to habituate to negative thoughts and feelings—implies an answer. In this case, it seems clear that if the habituation theory is correct, then expressive writing about positive experiences should not be effective because it would not cause people to habituate to negative thoughts and feelings. A second way to derive hypotheses from theories is to focus on some component of the theory that has not yet been directly observed. For example, a researcher could focus on the process of habituation—perhaps hypothesizing that people should show fewer signs of emotional distress with each new writing session.
Among the very best hypotheses are those that distinguish between competing theories. For example, Norbert Schwarz and his colleagues considered two theories of how people make judgments about themselves, such as how assertive they are (Schwarz et al., 1991) [2] . Both theories held that such judgments are based on relevant examples that people bring to mind. However, one theory was that people base their judgments on the number of examples they bring to mind and the other was that people base their judgments on how easily they bring those examples to mind. To test these theories, the researchers asked people to recall either six times when they were assertive (which is easy for most people) or 12 times (which is difficult for most people). Then they asked them to judge their own assertiveness. Note that the number-of-examples theory implies that people who recalled 12 examples should judge themselves to be more assertive because they recalled more examples, but the ease-of-examples theory implies that participants who recalled six examples should judge themselves as more assertive because recalling the examples was easier. Thus the two theories made opposite predictions so that only one of the predictions could be confirmed. The surprising result was that participants who recalled fewer examples judged themselves to be more assertive—providing particularly convincing evidence in favor of the ease-of-retrieval theory over the number-of-examples theory.
Theory Testing
The primary way that scientific researchers use theories is sometimes called the hypothetico-deductive method (although this term is much more likely to be used by philosophers of science than by scientists themselves). Researchers begin with a set of phenomena and either construct a theory to explain or interpret them or choose an existing theory to work with. They then make a prediction about some new phenomenon that should be observed if the theory is correct. Again, this prediction is called a hypothesis. The researchers then conduct an empirical study to test the hypothesis. Finally, they reevaluate the theory in light of the new results and revise it if necessary. This process is usually conceptualized as a cycle because the researchers can then derive a new hypothesis from the revised theory, conduct a new empirical study to test the hypothesis, and so on. As Figure 2.3 shows, this approach meshes nicely with the model of scientific research in psychology presented earlier in the textbook—creating a more detailed model of “theoretically motivated” or “theory-driven” research.
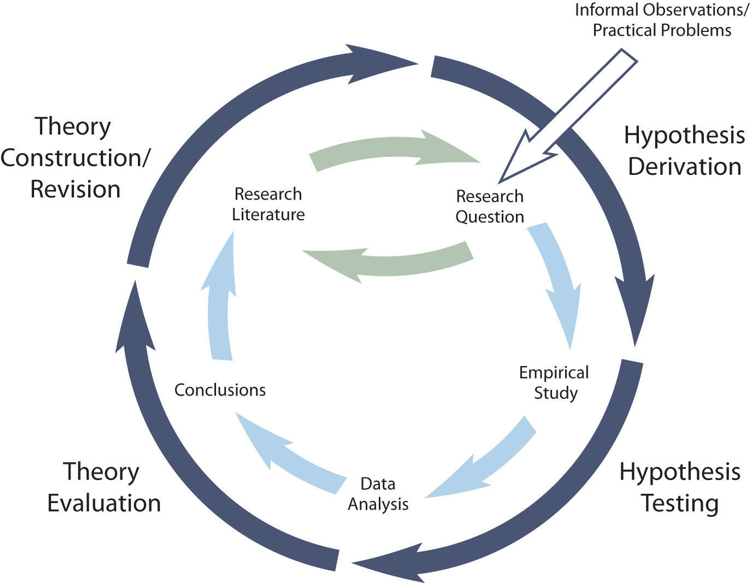
As an example, let us consider Zajonc’s research on social facilitation and inhibition. He started with a somewhat contradictory pattern of results from the research literature. He then constructed his drive theory, according to which being watched by others while performing a task causes physiological arousal, which increases an organism’s tendency to make the dominant response. This theory predicts social facilitation for well-learned tasks and social inhibition for poorly learned tasks. He now had a theory that organized previous results in a meaningful way—but he still needed to test it. He hypothesized that if his theory was correct, he should observe that the presence of others improves performance in a simple laboratory task but inhibits performance in a difficult version of the very same laboratory task. To test this hypothesis, one of the studies he conducted used cockroaches as subjects (Zajonc, Heingartner, & Herman, 1969) [3] . The cockroaches ran either down a straight runway (an easy task for a cockroach) or through a cross-shaped maze (a difficult task for a cockroach) to escape into a dark chamber when a light was shined on them. They did this either while alone or in the presence of other cockroaches in clear plastic “audience boxes.” Zajonc found that cockroaches in the straight runway reached their goal more quickly in the presence of other cockroaches, but cockroaches in the cross-shaped maze reached their goal more slowly when they were in the presence of other cockroaches. Thus he confirmed his hypothesis and provided support for his drive theory. (Zajonc also showed that drive theory existed in humans [Zajonc & Sales, 1966] [4] in many other studies afterward).
Incorporating Theory into Your Research
When you write your research report or plan your presentation, be aware that there are two basic ways that researchers usually include theory. The first is to raise a research question, answer that question by conducting a new study, and then offer one or more theories (usually more) to explain or interpret the results. This format works well for applied research questions and for research questions that existing theories do not address. The second way is to describe one or more existing theories, derive a hypothesis from one of those theories, test the hypothesis in a new study, and finally reevaluate the theory. This format works well when there is an existing theory that addresses the research question—especially if the resulting hypothesis is surprising or conflicts with a hypothesis derived from a different theory.
To use theories in your research will not only give you guidance in coming up with experiment ideas and possible projects, but it lends legitimacy to your work. Psychologists have been interested in a variety of human behaviors and have developed many theories along the way. Using established theories will help you break new ground as a researcher, not limit you from developing your own ideas.
Characteristics of a Good Hypothesis
There are three general characteristics of a good hypothesis. First, a good hypothesis must be testable and falsifiable . We must be able to test the hypothesis using the methods of science and if you’ll recall Popper’s falsifiability criterion, it must be possible to gather evidence that will disconfirm the hypothesis if it is indeed false. Second, a good hypothesis must be logical. As described above, hypotheses are more than just a random guess. Hypotheses should be informed by previous theories or observations and logical reasoning. Typically, we begin with a broad and general theory and use deductive reasoning to generate a more specific hypothesis to test based on that theory. Occasionally, however, when there is no theory to inform our hypothesis, we use inductive reasoning which involves using specific observations or research findings to form a more general hypothesis. Finally, the hypothesis should be positive. That is, the hypothesis should make a positive statement about the existence of a relationship or effect, rather than a statement that a relationship or effect does not exist. As scientists, we don’t set out to show that relationships do not exist or that effects do not occur so our hypotheses should not be worded in a way to suggest that an effect or relationship does not exist. The nature of science is to assume that something does not exist and then seek to find evidence to prove this wrong, to show that it really does exist. That may seem backward to you but that is the nature of the scientific method. The underlying reason for this is beyond the scope of this chapter but it has to do with statistical theory.
- Zajonc, R. B. (1965). Social facilitation. Science, 149 , 269–274 ↵
- Schwarz, N., Bless, H., Strack, F., Klumpp, G., Rittenauer-Schatka, H., & Simons, A. (1991). Ease of retrieval as information: Another look at the availability heuristic. Journal of Personality and Social Psychology, 61 , 195–202. ↵
- Zajonc, R. B., Heingartner, A., & Herman, E. M. (1969). Social enhancement and impairment of performance in the cockroach. Journal of Personality and Social Psychology, 13 , 83–92. ↵
- Zajonc, R.B. & Sales, S.M. (1966). Social facilitation of dominant and subordinate responses. Journal of Experimental Social Psychology, 2 , 160-168. ↵
A coherent explanation or interpretation of one or more phenomena.
A specific prediction about a new phenomenon that should be observed if a particular theory is accurate.
A cyclical process of theory development, starting with an observed phenomenon, then developing or using a theory to make a specific prediction of what should happen if that theory is correct, testing that prediction, refining the theory in light of the findings, and using that refined theory to develop new hypotheses, and so on.
The ability to test the hypothesis using the methods of science and the possibility to gather evidence that will disconfirm the hypothesis if it is indeed false.
Developing a Hypothesis Copyright © 2022 by Rajiv S. Jhangiani; I-Chant A. Chiang; Carrie Cuttler; and Dana C. Leighton is licensed under a Creative Commons Attribution-NonCommercial-ShareAlike 4.0 International License , except where otherwise noted.
Share This Book
Final dates! Join the tutor2u subject teams in London for a day of exam technique and revision at the cinema. Learn more →
Reference Library
Collections
- See what's new
- All Resources
- Student Resources
- Assessment Resources
- Teaching Resources
- CPD Courses
- Livestreams
Study notes, videos, interactive activities and more!
Psychology news, insights and enrichment
Currated collections of free resources
Browse resources by topic
- All Psychology Resources
Resource Selections
Currated lists of resources
- Study Notes
Aims and Hypotheses
Last updated 22 Mar 2021
- Share on Facebook
- Share on Twitter
- Share by Email
Observations of events or behaviour in our surroundings provoke questions as to why they occur. In turn, one or multiple theories might attempt to explain a phenomenon, and investigations are consequently conducted to test them. One observation could be that athletes tend to perform better when they have a training partner, and a theory might propose that this is because athletes are more motivated with peers around them.
The aim of an investigation, driven by a theory to explain a given observation, states the intent of the study in general terms. Continuing the above example, the consequent aim might be “to investigate the effect of having a training partner on athletes’ motivation levels”.
The theory attempting to explain an observation will help to inform hypotheses - predictions of an investigation’s outcome that make specific reference to the independent variables (IVs) manipulated and dependent variables (DVs) measured by the researchers.
There are two types of hypothesis:
- - H 1 – Research hypothesis
- - H 0 – Null hypothesis
H 1 – The Research Hypothesis
This predicts a statistically significant effect of an IV on a DV (i.e. an experiment), or a significant relationship between variables (i.e. a correlation study), e.g.
- In an experiment: “Athletes who have a training partner are likely to score higher on a questionnaire measuring motivation levels than athletes who train alone.”
- In a correlation study: ‘There will be a significant positive correlation between athletes’ motivation questionnaire scores and the number of partners athletes train with.”
The research hypothesis will be directional (one-tailed) if theory or existing evidence argues a particular ‘direction’ of the predicted results, as demonstrated in the two hypothesis examples above.
Non-directional (two-tailed) research hypotheses do not predict a direction, so here would simply predict “a significant difference” between questionnaire scores in athletes who train alone and with a training partner (in an experiment), or “a significant relationship” between questionnaire scores and number of training partners (in a correlation study).
H 0 – The Null Hypothesis
This predicts that a statistically significant effect or relationship will not be found, e.g.
- In an experiment: “There will be no significant difference in motivation questionnaire scores between athletes who train with and without a training partner.”
- In a correlation study: “There will be no significant relationship between motivation questionnaire scores and the number of partners athletes train with.”
When the investigation concludes, analysis of results will suggest that either the research hypothesis or null hypothesis can be retained, with the other rejected. Ultimately this will either provide evidence to support of refute the theory driving a hypothesis, and may lead to further research in the field.
You might also like
A level psychology topic quiz - research methods.
Quizzes & Activities
Research Methods: MCQ Revision Test 1 for AQA A Level Psychology
Topic Videos
Example Answers for Research Methods: A Level Psychology, Paper 2, June 2018 (AQA)
Exam Support
Our subjects
- › Criminology
- › Economics
- › Geography
- › Health & Social Care
- › Psychology
- › Sociology
- › Teaching & learning resources
- › Student revision workshops
- › Online student courses
- › CPD for teachers
- › Livestreams
- › Teaching jobs
Boston House, 214 High Street, Boston Spa, West Yorkshire, LS23 6AD Tel: 01937 848885
- › Contact us
- › Terms of use
- › Privacy & cookies
© 2002-2024 Tutor2u Limited. Company Reg no: 04489574. VAT reg no 816865400.
Thank you for visiting nature.com. You are using a browser version with limited support for CSS. To obtain the best experience, we recommend you use a more up to date browser (or turn off compatibility mode in Internet Explorer). In the meantime, to ensure continued support, we are displaying the site without styles and JavaScript.
- View all journals
- Explore content
- About the journal
- Publish with us
- Sign up for alerts
- Perspective
- Published: 29 November 2022
The fundamental importance of method to theory
- Rick Dale ORCID: orcid.org/0000-0001-7865-474X 1 ,
- Anne S. Warlaumont ORCID: orcid.org/0000-0001-9450-1372 1 &
- Kerri L. Johnson ORCID: orcid.org/0000-0002-1458-2019 1 , 2
Nature Reviews Psychology volume 2 , pages 55–66 ( 2023 ) Cite this article
721 Accesses
2 Citations
15 Altmetric
Metrics details
- Communication
- Human behaviour
Many domains of inquiry in psychology are concerned with rich and complex phenomena. At the same time, the field of psychology is grappling with how to improve research practices to address concerns with the scientific enterprise. In this Perspective, we argue that both of these challenges can be addressed by adopting a principle of methodological variety. According to this principle, developing a variety of methodological tools should be regarded as a scientific goal in itself, one that is critical for advancing scientific theory. To illustrate, we show how the study of language and communication requires varied methodologies, and that theory development proceeds, in part, by integrating disparate tools and designs. We argue that the importance of methodological variation and innovation runs deep, travelling alongside theory development to the core of the scientific enterprise. Finally, we highlight ongoing research agendas that might help to specify, quantify and model methodological variety and its implications.
This is a preview of subscription content, access via your institution
Access options
Subscribe to this journal
Receive 12 digital issues and online access to articles
$59.00 per year
only $4.92 per issue
Rent or buy this article
Prices vary by article type
Prices may be subject to local taxes which are calculated during checkout
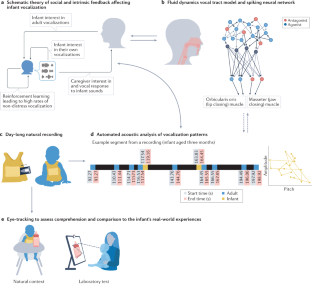
Similar content being viewed by others
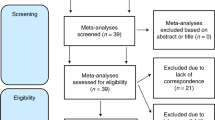
Comparing meta-analyses and preregistered multiple-laboratory replication projects
Amanda Kvarven, Eirik Strømland & Magnus Johannesson
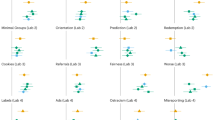
High replicability of newly discovered social-behavioural findings is achievable
John Protzko, Jon Krosnick, … Jonathan W. Schooler
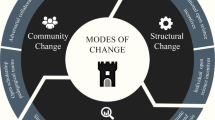
The replication crisis has led to positive structural, procedural, and community changes
Max Korbmacher, Flavio Azevedo, … Thomas Evans
Hacking, I. Representing and Intervening: Introductory Topics in The Philosophy of Natural Science (Cambridge Univ. Press, 1983).
Mayo, D. G. in PSA: Proceedings of the Biennial Meeting of the Philosophy of Science Association Vol. 1994, 270–279 (Cambridge Univ. Press, 1994).
Ackermann, R. The new experimentalism. Brit. J. Philos. Sci. 40 , 185–190 (1989).
Article Google Scholar
Simons, M. & Vagelli, M. Were experiments ever neglected? Ian Hacking and the history of philosophy of experiment. Phil. Inq. 9 , 167–188 (2021).
Google Scholar
Nosek, B. A. et al. Promoting an open research culture. Science 348 , 1422–1425 (2015).
Richardson, D. C., Dale, R. & Tomlinson, J. M. Conversation, gaze coordination, and beliefs about visual context. Cogn. Sci. 33 , 1468–1482 (2009).
Laidlaw, K. E., Foulsham, T., Kuhn, G. & Kingstone, A. Potential social interactions are important to social attention. Proc. Natl Acad. Sci. USA 108 , 5548–5553 (2011).
Richardson, D. C. et al. Joint perception: gaze and social context. Front. Hum. Neurosci. 6 , 194 (2012).
Risko, E. F., Richardson, D. C. & Kingstone, A. Breaking the fourth wall of cognitive science: real-world social attention and the dual function of gaze. Curr. Dir. Psychol. Sci. 25 , 70–74 (2016).
Levin, I. P., Schneider, S. L. & Gaeth, G. J. All frames are not created equal: a typology and critical analysis of framing effects. Organ. Behav. Hum. Decis. Process. 76 , 149–188 (1998).
Pothos, E. M. & Busemeyer, J. R. A quantum probability explanation for violations of ‘rational’ decision theory. Proc. R. Soc. B 276 , 2171–2178 (2009).
Pärnamets, P. et al. Biasing moral decisions by exploiting the dynamics of eye gaze. Proc. Natl Acad. Sci. USA 112 , 4170–4175 (2015).
Vinson, D. W., Dale, R. & Jones, M. N. Decision contamination in the wild: sequential dependencies in online review ratings. Behav. Res. Methods 51 , 1477–1484 (2019).
Longino, H. E. Gender, politics, and the theoretical virtues. Synthese 104 , 383–397 (1995).
Hansson, S. O. in Stanford Encyclopedia of Philosophy (ed. Zalta, E. N.) https://plato.stanford.edu/archives/fall2021/entries/pseudo-science/ (Stanford Univ., 2015).
Dupré, J. The Disorder of Things: Metaphysical Foundations of the Disunity of Science (Harvard Univ. Press, 1993).
Cartwright, N. The Dappled World: A Study of the Boundaries of Science (Cambridge Univ. Press, 1999).
McCauley, R. N. & Bechtel, W. Explanatory pluralism and heuristic identity theory. Theory Psychol. 11 , 736–760 (2001).
Mitchell, S. D. Integrative pluralism. Biol. Phil. 17 , 55–70 (2002).
Abrahamsen, A. & Bechtel, W. in Contemporary Debates in Cognitive Science (ed. Stainton, R.) 159–187 (Blackwell, 2006).
Kellert, S. H., Longino, H. E. & Waters, C. K. Scientific Pluralism (Univ. Minnesota Press, 2006).
Dale, R., Dietrich, E. & Chemero, A. Explanatory pluralism in cognitive science. Cogn. Sci. 33 , 739–742 (2009).
Weisberg, M. & Muldoon, R. Epistemic landscapes and the division of cognitive labor. Phil. Sci. 76 , 225–252 (2009).
Zollman, K. J. S. The epistemic benefit of transient diversity. Erkenntnis 72 , 17–35 (2010).
Horst, S. Beyond reduction: from naturalism to cognitive pluralism. Mind Matter 12 , 197–244 (2014).
Open Science Collaboration. Estimating the reproducibility of psychological science. Science 349 , 943 (2015).
Lin, H., Werner, K. M. & Inzlicht, M. Promises and perils of experimentation: the mutual-internal-validity problem. Perspect. Psychol. Sci. 16 , 854–863 (2021).
van Rooij, I. & Baggio, G. Theory before the test: how to build high-verisimilitude explanatory theories in psychological science. Perspect. Psychol. Sci. 16 , 682–697 (2021).
MacLeod, C. M. The Stroop task: the gold standard of attentional measures. J. Exp. Psychol. Gen. 121 , 12–14 (1992).
Eriksen, C. W. The flankers task and response competition: a useful tool for investigating a variety of cognitive problems. Vis. Cogn. 2 , 101–118 (1995).
Dickter, C. L. & Bartholow, B. D. Ingroup categorization and response conflict: interactive effects of target race, flanker compatibility, and infrequency on N2 amplitude. Psychophysiology 47 , 596–601 (2010).
Parris, B. A., Hasshim, N., Wadsley, M., Augustinova, M. & Ferrand, L. The loci of Stroop effects: a critical review of methods and evidence for levels of processing contributing to color-word Stroop effects and the implications for the loci of attentional selection. Psychol. Res. 86 , 1029–1053 (2022).
Barzykowski, K., Wereszczyski, M., Hajdas, S. & Radel, R. Cognitive inhibition behavioral tasks in online and laboratory settings: data from Stroop, SART and Eriksen Flanker tasks. Data Brief. 43 , 108398 (2022).
Gobel, M. S., Kim, H. S. & Richardson, D. C. The dual function of social gaze. Cognition 136 , 359–364 (2015).
Gallup, A. C., Chong, A. & Couzin, I. D. The directional flow of visual information transfer between pedestrians. Biol. Lett. 8 , 520–522 (2012).
Lick, D. J. & Johnson, K. L. Straight until proven gay: a systematic bias toward straight categorizations in sexual orientation judgments. J. Pers. Soc. Psychol. 110 , 801 (2016).
Alt, N. P., Lick, D. J. & Johnson, K. L. The straight categorization bias: a motivated and altruistic reasoning account. J. Pers. Soc. Psychol. https://doi.org/10.1037/pspi0000232 (2020).
Popper, K. Conjectures and Refutations: The Growth of Scientific Knowledge (Routledge, 2002).
Heit, E. & Hahn, U. Diversity-based reasoning in children. Cogn. Psychol. 43 , 243–273 (2001).
Heit, E., Hahn, U. & Feeney, A. in Categorization Inside and Outside the Laboratory: Essays in Honor of Douglas L. Medin 87–99 (American Psychological Association, 2005).
MacWhinney, B. in The Handbook of Linguistics 2nd edn (eds. Aronoff, M. & Rees-Miller, J.) 397–413 (Wiley, 2017).
Stivers, T. et al. Universals and cultural variation in turn-taking in conversation. Proc. Natl Acad. Sci. USA 106 , 10587–10592 (2009).
Louwerse, M. M., Dale, R., Bard, E. G. & Jeuniaux, P. Behavior matching in multimodal communication is synchronized. Cogn. Sci. 36 , 1404–1426 (2012).
Fusaroli, R., Bjørndahl, J. S., Roepstorff, A. & Tylén, K. A heart for interaction: shared physiological dynamics and behavioral coordination in a collective, creative construction task. J. Exp. Psychol. Hum. Percept. Perform. 42 , 1297 (2016).
Rasenberg, M., Özyürek, A. & Dingemanse, M. Alignment in multimodal interaction: an integrative framework. Cogn. Sci. 44 , e12911 (2020).
Dunn, M., Greenhill, S. J., Levinson, S. C. & Gray, R. D. Evolved structure of language shows lineage-specific trends in word-order universals. Nature 473 , 79–82 (2011).
Hua, X., Greenhill, S. J., Cardillo, M., Schneemann, H. & Bromham, L. The ecological drivers of variation in global language diversity. Nat. Commun. 10 , 1–10 (2019).
Christiansen, M. H. & Chater, N. The now-or-never bottleneck: a fundamental constraint on language. Behav. Brain Sci. 39 , e62 (2016).
Fitch, W. T., De Boer, B., Mathur, N. & Ghazanfar, A. A. Monkey vocal tracts are speech-ready. Sci. Adv. 2 , e1600723 (2016).
Hauser, M. D., Chomsky, N. & Fitch, W. T. The faculty of language: what is it, who has it, and how did it evolve? Science 298 , 1569–1579 (2002).
Cowley, S. J. Distributed Language (John Benjamins, 2011).
Samuels, R. Nativism in cognitive science. Mind Lang. 17 , 233–265 (2002).
Behme, C. & Deacon, S. H. Language learning in infancy: does the empirical evidence support a domain specific language acquisition device? Phil. Psychol. 21 , 641–671 (2008).
Chomsky, N. 4. A Review Of BF Skinner’s Verbal Behavior (Harvard Univ. Press, 2013).
Vihman, M. M. Phonological Development: The Origins of Language in the Child (Blackwell, 1996).
Oller, D. K. The Emergence of the Speech Capacity (Psychology Press, 2000).
Clark, E. V. & Casillas, M. First Language Acquisition (Routledge, 2015).
Goldstein, M. H., King, A. P. & West, M. J. Social interaction shapes babbling: testing parallels between birdsong and speech. Proc. Natl Acad. Sci. USA 100 , 8030–8035 (2003).
Warlaumont, A. S. Modeling the emergence of syllabic structure. J. Phonet. 53 , 61–65 (2015).
VanDam, M. et al. HomeBank: An online repository of daylong child-centered audio recordings. Semin. Speech Lang. 37 , 128–142 (2016).
Elmlinger, S. L., Schwade, J. A. & Goldstein, M. H. The ecology of prelinguistic vocal learning: parents simplify the structure of their speech in response to babbling. J. Child Lang. 46 , 998–1011 (2019).
Roy, B. C., Frank, M. C., DeCamp, P., Miller, M. & Roy, D. Predicting the birth of a spoken word. Proc. Natl Acad. Sci. USA 112 , 12663–12668 (2015).
McClelland, J. L. The place of modeling in cognitive science. Top. Cogn. Sci. 1 , 11–38 (2009).
Smaldino, P. E. in Computational Social Psychology (eds Vallacher’, R., Read, S. J. & Nowak, A.) 311–331 (Routledge, 2017).
Guest, O. & Martin, A. E. How computational modeling can force theory building in psychological science. Perspect. Psychol. Sci. 16 , 789–802 (2021).
Elman, J. L., Bates, E. A. & Johnson, M. H. Rethinking Innateness: A Connectionist Perspective on Development Vol. 10 (MIT Press, 1996).
Warlaumont, A. S., Westermann, G., Buder, E. H. & Oller, D. K. Prespeech motor learning in a neural network using reinforcement. Neural Netw. 38 , 64–75 (2013).
Warlaumont, A. S. & Finnegan, M. K. Learning to produce syllabic speech sounds via reward-modulated neural plasticity. PLoS One 11 , e0145096 (2016).
MacWhinney, B. & Snow, C. The child language data exchange system: an update. J. Child. Lang. 17 , 457–472 (1990).
MacWhinney, B. The CHILDES Project: Tools for Analyzing Talk 3rd edn (Psychology Press, 2014).
Kachergis, G., Marchman, V. A. & Frank, M. C. Toward a “standard model” of early language learning. Curr. Dir. Psychol. Sci. 31 , 20–27 (2022).
Lewis, J. D. & Elman, J. L. A connectionist investigation of linguistic arguments from the poverty of the stimulus: learning the unlearnable. In Proc. Annual Meeting of the Cognitive Science Society Vol. 23, 552–557 (eds. Moore, J. D. & Stenning, K.) (2001).
Regier, T. & Gahl, S. Learning the unlearnable: the role of missing evidence. Cognition 93 , 147–155 (2004).
Reali, F. & Christiansen, M. H. Uncovering the richness of the stimulus: structure dependence and indirect statistical evidence. Cogn. Sci. 29 , 1007–1028 (2005).
Foraker, S., Regier, T., Khetarpal, N., Perfors, A. & Tenenbaum, J. Indirect evidence and the poverty of the stimulus: the case of anaphoric one . Cognit. Sci. 33 , 287–300 (2009).
Saffran, J. R., Aslin, R. N. & Newport, E. L. Statistical learning by 8-month-old infants. Science 274 , 1926–1928 (1996).
McMurray, B. & Hollich, G. Core computational principles of language acquisition: can statistical learning do the job? Dev. Sci. 12 , 365–368 (2009).
Frost, R., Armstrong, B. C., Siegelman, N. & Christiansen, M. H. Domain generality versus modality specificity: the paradox of statistical learning. Trends Cogn. Sci. 19 , 117–125 (2015).
Isbilen, E. S., Frost, R. L. A., Monaghan, P. & Christiansen, M. H. Statistically based chunking of nonadjacent dependencies. J. Exp. Psychol. Gen. https://doi.org/10.1037/xge0001207 (2022).
Ruba, A. L., Pollak, S. D. & Saffran, J. R. Acquiring complex communicative systems: Statistical learning of language and emotion. Top. Cogn. Sci. https://doi.org/10.1111/tops.12612 (2022).
Abney, D. H., Warlaumont, A. S., Oller, D. K., Wallot, S. & Kello, C. T. Multiple coordination patterns in infant and adult vocalizations. Infancy 22 , 514–539 (2017).
Mendoza, J. K. & Fausey, C. M. Everyday music in infancy. Dev. Sci. 24 , e13122 (2019).
Ritwika, V. et al. Exploratory dynamics of vocal foraging during infant-caregiver communication. Sci. Rep. 10 , 10469 (2020).
Mendoza, J. K. & Fausey, C. M. Quantifying everyday ecologies: principles for manual annotation of many hours of infants’ lives. Front. Psychol. 12 , 710636 (2021).
Fernald, A., Zangl, R., Portillo, A. L. & Marchman, V. A. in Developmental Psycholinguistics: On-line Methods in Children’s Language Processing (eds. Sekerina, I. A., Fernández, E. M. & Clahsen, H.) Vol. 44, 97 (John Benjamins, 2008).
Weisleder, A. & Fernald, A. Talking to children matters: early language experience strengthens processing and builds vocabulary. Psychol. Sci. 24 , 2143–2152 (2013).
Bergelson, E. & Aslin, R. N. Nature and origins of the lexicon in 6-mo-olds. Proc. Natl Acad. Sci. USA 114 , 12916–12921 (2017).
Brennan, S. E., Galati, A. & Kuhlen, A. K. in Psychology of Learning and Motivation (ed. Ross, B. H.) Vol. 53, 301–344 (Elsevier, 2010).
Streeck, J., Goodwin, C. & LeBaron, C. Embodied Interaction: Language and Body in the Material World (Cambridge Univ. Press, 2011).
Goodwin, C. Co-operative Action (Cambridge Univ. Press, 2017).
Dale, R., Spivey, M. J. in Eye-Tracking In Interaction. Studies On The Role Of Eye Gaze In Dialogue (eds. Oben, B. and Brône, G.) 67–90 (John Benjamins, 2018).
Richardson, D. C. & Spivey, M. J. in Encyclopedia of Biomaterials and Biomedical Engineering 573–582 (CRC Press, 2004).
Tanenhaus, M. K., Spivey-Knowlton, M. J., Eberhard, K. M. & Sedivy, J. C. Integration of visual and linguistic information in spoken language comprehension. Science 268 , 1632–1634 (1995).
Spivey, M. J., Tanenhaus, M. K., Eberhard, K. M. & Sedivy, J. C. Eye movements and spoken language comprehension: effects of visual context on syntactic ambiguity resolution. Cogn. Psychol. 45 , 447–481 (2002).
Richardson, D. C., Dale, R. & Spivey, M. J. Eye movements in language and cognition. Methods Cogn. Linguist. 18 , 323–344 (2007).
Ferreira, F. & Clifton, C. Jr The independence of syntactic processing. J. Mem. Lang. 25 , 348–368 (1986).
Kamide, Y., Altmann, G. T. & Haywood, S. L. The time-course of prediction in incremental sentence processing: evidence from anticipatory eye movements. J. Mem. Lang. 49 , 133–156 (2003).
Coco, M. I., Keller, F. & Malcolm, G. L. Anticipation in real‐world scenes: the role of visual context and visual memory. Cogn. Sci. 40 , 1995–2024 (2016).
Coco, M. I. & Keller, F. Scan patterns predict sentence production in the cross‐modal processing of visual scenes. Cogn. Sci. 36 , 1204–1223 (2012).
Kieslich, P. J., Henninger, F., Wulff, D. U., Haslbeck, J. M. & Schulte-Mecklenbeck, M. in A Handbook of Process Tracing Methods 111–130 (Routledge, 2019).
Spivey, M. J., Grosjean, M. & Knoblich, G. Continuous attraction toward phonological competitors. Proc. Natl Acad. Sci. USA 102 , 10393–10398 (2005).
Freeman, J., Dale, R. & Farmer, T. Hand in motion reveals mind in motion. Front. Psychol. 2 , 59 (2011).
Freeman, J. B. & Johnson, K. L. More than meets the eye: split-second social perception. Trends Cogn. Sci. 20 , 362–374 (2016).
Goodale, B. M., Alt, N. P., Lick, D. J. & Johnson, K. L. Groups at a glance: perceivers infer social belonging in a group based on perceptual summaries of sex ratio. J. Exp. Psychol. Gen. 147 , 1660–1676 (2018).
Sneller, B. & Roberts, G. Why some behaviors spread while others don’t: a laboratory simulation of dialect contact. Cognition 170 , 298–311 (2018).
Atkinson, M., Mills, G. J. & Smith, K. Social group effects on the emergence of communicative conventions and language complexity. J. Lang. Evol. 4 , 1–18 (2019).
Raviv, L., Meyer, A. & Lev‐Ari, S. The role of social network structure in the emergence of linguistic structure. Cogn. Sci. 44 , e12876 (2020).
Lupyan, G. & Dale, R. Language structure is partly determined by social structure. PLoS One 5 , e8559 (2010).
Lupyan, G. & Dale, R. Why are there different languages? The role of adaptation in linguistic diversity. Trends Cogn. Sci. 20 , 649–660 (2016).
Wu, L., Waber, B. N., Aral, S., Brynjolfsson, E. & Pentland, A. Mining face-to-face interaction networks using sociometric badges: predicting productivity in an IT configuration task. Inf. Syst. Behav. Soc. Methods https://doi.org/10.2139/ssrn.1130251 (2008).
Paxton, A. & Dale, R. Argument disrupts interpersonal synchrony. Q. J. Exp. Psychol. 66 , 2092–2102 (2013).
Alviar, C., Dale, R. & Galati, A. Complex communication dynamics: exploring the structure of an academic talk. Cogn. Sci. 43 , e12718 (2019).
Joo, J., Bucy, E. P. & Seidel, C. Automated coding of televised leader displays: detecting nonverbal political behavior with computer vision and deep learning. Int. J. Commun. 13 , 4044–4066 (2019).
Metallinou, A. et al. The USC CreativeIT database of multimodal dyadic interactions: from speech and full body motion capture to continuous emotional annotations. Lang. Res. Eval. 50 , 497–521 (2016).
Pouw, W., Paxton, A., Harrison, S. J. & Dixon, J. A. Acoustic information about upper limb movement in voicing. Proc. Natl Acad. Sci. USA 117 , 11364–11367 (2020).
Enfield, N., Levinson, S. C., De Ruiter, J. P. & Stivers, T. in Field Manual Vol. 10, 96–99 (ed. Majid, A.) (Max Planck Institute for Psycholinguistics, 2007).
Enfield, N. & Sidnell, J. On the concept of action in the study of interaction. Discourse Stud. 19 , 515–535 (2017).
Duran, N. D., Paxton, A. & Fusaroli, R. ALIGN: analyzing linguistic interactions with generalizable techNiques — a Python library. Psychol. Methods 24 , 419 (2019).
Brennan, S. E. & Clark, H. H. Conceptual pacts and lexical choice in conversation. J. Exp. Psychol. Learn. Mem. Cogn. 22 , 1482–1493 (1996).
Hasson, U., Nir, Y., Levy, I., Fuhrmann, G. & Malach, R. Intersubject synchronization of cortical activity during natural vision. Science 303 , 1634–1640 (2004).
Huth, A. G., De Heer, W. A., Griffiths, T. L., Theunissen, F. E. & Gallant, J. L. Natural speech reveals the semantic maps that tile human cerebral cortex. Nature 532 , 453–458 (2016).
Shain, C. et al. Robust effects of working memory demand during naturalistic language comprehension in language-selective cortex. J. Neurosci. 42 , 7412–7430 (2022).
Fedorenko, E., Blank, I. A., Siegelman, M. & Mineroff, Z. Lack of selectivity for syntax relative to word meanings throughout the language network. Cognition 203 , 104348 (2020).
Stephens, G. J., Silbert, L. J. & Hasson, U. Speaker–listener neural coupling underlies successful communication. Proc. Natl Acad. Sci. USA 107 , 14425–14430 (2010).
Schilbach, L. et al. Toward a second-person neuroscience. Behav. Brain Sci. 36 , 393–414 (2013).
Redcay, E. & Schilbach, L. Using second-person neuroscience to elucidate the mechanisms of social interaction. Nat. Rev. Neurosci. 20 , 495–505 (2019).
Riley, M. A., Richardson, M., Shockley, K. & Ramenzoni, V. C. Interpersonal synergies. Front. Psychol. 2 , 38 (2011).
Dale, R., Fusaroli, R., Duran, N. D. & Richardson, D. C. in Psychology of Learning and Motivation (ed. Ross, B. H.) Vol. 59, 43–95 (Elsevier, 2013).
Fusaroli, R., Rączaszek-Leonardi, J. & Tylén, K. Dialog as interpersonal synergy. N. Ideas Psychol. 32 , 147–157 (2014).
Hadley, L. V., Naylor, G. & Hamilton, A. F. D. C. A review of theories and methods in the science of face-to-face social interaction. Nat. Rev. Psychol. 1 , 42–54 (2022).
Cornejo, C., Cuadros, Z., Morales, R. & Paredes, J. Interpersonal coordination: methods achievements and challenges. Front. Psychol. https://doi.org/10.3389/fpsyg.2017.01685 (2017).
Smaldino, P. E. in Computational Social Psychology 311–331 (Routledge, 2017).
Devezer, B., Nardin, L. G., Baumgaertner, B. & Buzbas, E. O. Scientific discovery in a model-centric framework: reproducibility, innovation, and epistemic diversity. PLoS One 14 , e0216125 (2019).
Sulik, J., Bahrami, B. & Deroy, O. The diversity gap: when diversity matters for knowledge. Perspect. Psychol. Sci. 17 , 752–767 (2022).
O’Connor, C. & Bruner, J. Dynamics and diversity in epistemic communities. Erkenntnis 84 , 101–119 (2019).
Longino, H. in The Stanford Encyclopedia of Philosophy (ed. Zalta, E. N.) https://plato.stanford.edu/archives/sum2019/entries/scientific-knowledge-social/ (Stanford Univ., 2019).
Van Rooij, I. The tractable cognition thesis. Cogn. Sci. 32 , 939–984 (2008).
Kwisthout, J., Wareham, T. & Van Rooij, I. Bayesian intractability is not an ailment that approximation can cure. Cogn. Sci. 35 , 779–784 (2011).
Contreras Kallens, P. & Dale, R. Exploratory mapping of theoretical landscapes through word use in abstracts. Scientometrics 116 , 1641–1674 (2018).
Methods for methods’ sake. Nat. Methods https://doi.org/10.1038/nmeth1004-1 (2004).
Oberauer, K. & Lewandowsky, S. Addressing the theory crisis in psychology. Psychon. Bull. Rev. 26 , 1596–1618 (2019).
Meehl, P. E. Theory-testing in psychology and physics: a methodological paradox. Phil. Sci. 34 , 103–115 (1967).
Klein, O. et al. A practical guide for transparency in psychological science. Collabra Psychol. 4 , 20 (2018).
Muthukrishna, M. & Henrich, J. A problem in theory. Nat. Hum. Behav. 3 , 221–229 (2019).
Eronen, M. I. & Bringmann, L. F. The theory crisis in psychology: how to move forward. Perspect. Psychol. Sci. https://doi.org/10.1177/1745691620970586 (2021).
Borsboom, D., van der Maas, H. L. J., Dalege, J., Kievit, R. A. & Haig, B. D. Theory construction methodology: a practical framework for building theories in psychology. Perspect. Psychol. Sci. https://doi.org/10.1177/1745691620969647 (2021).
Kyvik, S. & Reymert, I. Research collaboration in groups and networks: differences across academic fields. Scientometrics 113 , 951–967 (2017).
Tebes, J. K. & Thai, N. D. Interdisciplinary team science and the public: steps toward a participatory team science. Am. Psychol. 73 , 549 (2018).
Falk-Krzesinski, H. J. et al. Mapping a research agenda for the science of team science. Res. Eval. 20 , 145–158 (2011).
da Silva, J. A. T. The Matthew effect impacts science and academic publishing by preferentially amplifying citations, metrics and status. Scientometrics 126 , 5373–5377 (2021).
Scheel, A. M., Tiokhin, L., Isager, P. M. & Lakens, D. Why hypothesis testers should spend less time testing hypotheses. Perspect. Psychol. Sci. 16 , 744–755 (2020).
Jones, M. N. Big Data in Cognitive Science (Psychology Press, 2016).
Paxton, A. & Griffiths, T. L. Finding the traces of behavioral and cognitive processes in big data and naturally occurring datasets. Behav. Res. Methods 49 , 1630–1638 (2017).
Lupyan, G. & Goldstone, R. L. Beyond the lab: using big data to discover principles of cognition. Behav Res. Methods , 51 , 1554–3528 (2019).
Haspelmath, M., Dryer, M. S., Gil, D. & Comrie, B. (eds) The World Atlas of Language Structures (Max Planck Digital Library, 2013).
Eberhard, D. M., Simons, G. F. & Fennig, C. D. Ethnologue: Languages of the World (SIL International, 2021).
MacCorquodale, K. & Meehl, P. E. On a distinction between hypothetical constructs and intervening variables. Psychol. Rev. 55 , 95–107 (1948).
van Rooij, I. & Baggio, G. Theory before the test: how to build high-verisimilitude explanatory theories in psychological science. Perspect. Psychol. Sci . https://doi.org/10.1177/1745691620970604 (2021).
Christiansen, M. H. & Chater, N. Creating Language: Integrating Evolution, Acquisition, and Processing (MIT Press, 2016).
Berwick, R. C. & Chomsky, N. Why Only Us: Language and Evolution (MIT Press, 2016).
Gopnik, A. Scientific thinking in young children: theoretical advances, empirical research, and policy implications. Science 337 , 1623–1627 (2012).
Pereira, A. F., James, K. H., Jones, S. S. & Smith, L. B. Early biases and developmental changes in self-generated object views. J. Vis. 10 , 22–22 (2010).
Fagan, M. K. & Iverson, J. M. The influence of mouthing on infant vocalization. Infancy 11 , 191–202 (2007).
Martin, J., Ruthven, M., Boubertakh, R. & Miquel, M. E. Realistic dynamic numerical phantom for MRI of the upper vocal tract. J. Imaging 6 , 86 (2020).
Spivey, M. J. & Dale, R. Continuous dynamics in real-time cognition. Curr. Dir. Psychol. Sci. 15 , 207–211 (2006).
Publication Manual of the American Psychological Association 3rd edn (American Psychological Association, 1983).
Publication Manual of the American Psychological Association 6th edn (American Psychological Association, 2010).
Ashby, W. R. An Introduction to Cybernetics (Martino, 1956).
de Raadt, J. D. R. Ashby’s law of requisite variety: an empirical study. Cybern. Syst. 18 , 517–536 (1987).
Ward, L. M. Dynamical Cognitive Science (MIT Press, 2002).
Regier, T., Carstensen, A. & Kemp, C. Languages support efficient communication about the environment: words for snow revisited. PLoS One 11 , e0151138 (2016).
Newell, A. Unified Theories of Cognition (Harvard Univ. Press, 1990).
Rich, P., de Haan, R., Wareham, T. & van Rooij, I. in Proc. Annual Meeting of the Cognitive Science Society Vol. 43, 3034–3040 (eds. Fitch, T., Lamm, C., Leder, H., and Teßmar-Raible, K.) (2021).
Potochnik, A. & Sanches de Oliveira, G. Patterns in cognitive phenomena and pluralism of explanatory styles. Top. Cogn. Sci. 12 , 1306–1320 (2020).
Leydesdorff, L. & Schank, T. Dynamic animations of journal maps: indicators of structural changes and interdisciplinary developments. J. Am. Soc. Inf. Sci. Technol. 59 , 1810–1818 (2008).
Leydesdorff, L. & Goldstone, R. L. Interdisciplinarity at the journal and specialty level: the changing knowledge bases of the journal Cognitive Science . J. Assoc. Inf. Sci. Technol. 65 , 164–177 (2014).
DeStefano, I., Oey, L. A., Brockbank, E. & Vul, E. Integration by parts: collaboration and topic structure in the CogSci community. Top. Cogn. Sci. 13 , 399–413 (2021).
Cummins, R. in Explanation And Cognition (eds Keil, F. C. & Wilson, R.) 117–144 (MIT Press, 2000).
Boyd, N. M. & Bogen, J. in Stanford Encyclopedia of Philosophy (ed. Zalta, E. N.) https://plato.stanford.edu/archives/win2021/entries/science-theory-observation/ (Stanford Univ., 2021).
Smaldino, P. E. How to build a strong theoretical foundation. Psychol. Inq. 31 , 297–301 (2020).
Chang, H. Inventing Temperature: Measurement and Scientific Progress (Oxford Univ. Press, 2004).
Download references
Acknowledgements
A.S.W. was supported by the National Science Foundation (grants 1529127 and 1539129/1827744) and by the James S. McDonnell Foundation ( https://doi.org/10.37717/220020507 ). K.L.J. was supported by the National Science Foundation (grant 2017245).
Author information
Authors and affiliations.
Department of Communication, University of California, Los Angeles, Los Angeles, CA, USA
Rick Dale, Anne S. Warlaumont & Kerri L. Johnson
Department of Psychology, University of California, Los Angeles, Los Angeles, CA, USA
Kerri L. Johnson
You can also search for this author in PubMed Google Scholar
Contributions
R.D. discussed the submission theme with the editor and wrote the first draft. A.S.W. and K.L.J. refined and added to this plan and contributed major new sections of writing and revision. All authors contributed to developing the figures.
Corresponding author
Correspondence to Rick Dale .
Ethics declarations
Competing interests.
The authors declare no competing interests.
Peer review
Peer review information.
Nature Reviews Psychology thanks Berna Devezer; Michael Frank, who co-reviewed with Anjie Cao; and Justin Sulik for their contribution to the peer review of this work.
Additional information
Publisher’s note Springer Nature remains neutral with regard to jurisdictional claims in published maps and institutional affiliations.
Rights and permissions
Springer Nature or its licensor holds exclusive rights to this article under a publishing agreement with the author(s) or other rightsholder(s); author self-archiving of the accepted manuscript version of this article is solely governed by the terms of such publishing agreement and applicable law.
Reprints and permissions
About this article
Cite this article.
Dale, R., Warlaumont, A.S. & Johnson, K.L. The fundamental importance of method to theory. Nat Rev Psychol 2 , 55–66 (2023). https://doi.org/10.1038/s44159-022-00120-5
Download citation
Accepted : 22 September 2022
Published : 29 November 2022
Issue Date : January 2023
DOI : https://doi.org/10.1038/s44159-022-00120-5
Share this article
Anyone you share the following link with will be able to read this content:
Sorry, a shareable link is not currently available for this article.
Provided by the Springer Nature SharedIt content-sharing initiative
Quick links
- Explore articles by subject
- Guide to authors
- Editorial policies
Sign up for the Nature Briefing newsletter — what matters in science, free to your inbox daily.

The Role of Hypotheses in Psychological Research Expository Essay
Introduction, body of knowledge, reference list.
Psychological research is often done by developing and testing theories. A theory on the other hand is a well established, principle which has been developed to give a reasonable explanation to some worldly phenomenon. Most theories arise out of repeated observations, testing and other scientific facts, laws and tested hypotheses.
In most cases, deductive approach is used to generate specified predictions from other general theories. The process through which detailed predictions are drawn concerning the validity of a theory is the testing of hypothesis.
A hypothesis is a specified concept about a certain concept which can be tested about the anticipation of the outcome in the study (DeCarvalho, 1991, 48). For instance, a research developed to study the relationship between study habits among students and examination anxiety could have a hypothesis that states, “The study is developed to evaluate the hypothesis that students who studied better experienced less anxiety for examinations”.
The hypothesis explains what the researcher is expecting as the outcome unless it is explorative (Coon & Mitterer, 2011, p. 23). Therefore the first role of hypothesis is presenting the premise on which the psychological study is based on.
A well developed hypothesis in psychology will hence be able to give the researcher the direction of the experiment or investigation. The investigator will know which kind of data to collect, the methods to evaluate and measure the data, how to interpret and draw conclusions.
Therefore the hypothesis in psychological expereiemns has explanatory power. They also suggest to the researcher the expected association between the variables under investigation (Coon & Mitterer, 2011, p. 29). The hypothesis are required to be testable and aligned to the current pool of knowledge about certain concepts and factors.
The hypothesis also gives the reader a prelude of an experiment and therefore good hypothesis for psychological study should be simple and concise.
A hypothesis provides the psychologist with an uncertain explanation of an observable fact therefore sparking a feeling of curiosity and therefore inspires and facilitates expansion of knowledge in that sector. A hypothesis also gives the investigator a relational statement which can be tested by scientific methods and therefore helps to determine the type of study method that will be used for investigations.
As the method determines the data collected, hypothesis also guides the reporting of the findings and making conclusions (DeCarvalho, 1991, 48). This is because, the researcher seeks to accept or reject the hypothesis. And this is often the goal of the research.
In psychological studies, hypotheses are normally drawn from the existing knowledge and theories. As such hypotheses in this field function as the working instrument of certain theories that need to be proven or need more knowledge in terms of literature or findings (DeCarvalho, 1991, 48).
Psychology is described as the science of mental wellbeing and deals with both the status and the functioning. As a science, psychology is constituted by two essential principles (Hand 2004, p. 34). The first is that there has to be measurement. Hypothesis states the variables to be measured as a second role.
This role is very important because in science, before any phenomenon has been subjected to measurement it cannot attain a standing and dignity as a scientific fact (Fiske et al, 2010, p. 45). The second principle is that, psychological research should inform and be informed by a number of theories and hypotheses. By having a testable hypothesis, psychological research is made valid as it can be examined (Hand 2004, p. 36).
In psychology, there are two major empirical models of doing study and two types of hypotheses that can be tested. The different approaches deal with differ hypotheses. There is the causal hypothesis and the associative hypothesis. Sometimes the two can be examined together (Coon & Mitterer, 2011, p. 28).
Causal hypothesis helps a psychologist or a researcher to observe how manipulating some factors affects the outcomes of other variables in future. Associative hypothesis on the other hand investigates the frequency at which certain events happen concurrently (Coon & Mitterer, 2011, p. 29). Causal hypothesis therefore uses random allocation experimental methods of study. Causal uses random sampling.
In general, psychological researches revolve around generation of hypothesis and testing them (Fiske et al, 2010, p. 45). As hypotheses are speculation and testing them helps to develop other theories with integrity. Many studies focus on the methods of hoe these hypotheses are tested and dwell less on their generation strategies. Design of hypothesis determines the methods to be used for testing.
The more specific the hypothesis in a research is, the more intuitive the research will be and therefore the results will be more actionable. Normally, psychological research hypotheses are developed by a number of means. The common one is inductive reasoning and in this strategy, the researcher makes some observations which lead to him or her to develop a presumption (Fiske et al, 2010, p. 45).
To conduct the study, the researcher then employs larger battery of deductive methods to create a hypothesis that is falsifiable and practical.
Hypotheses help the research to build on the current knowledge in that they form precursors to theories and research problem which in most cases are questions in a research. For instance asking why student experience anxiety when examinations are going on. The problem question could be framed as ‘why do student experience anxiety during examination period?’ this is a very broad statement which is not testable by research method.
However it can draw literature views (Fiske et al, 2010, p. 45). To look into it, the researcher will have to conduct a literature review and use previously acquired knowledge and intuition to answer it. Intuition is not scientific.
A research hypothesis pairs down the problem to form a concept that is testable and falsifiable. From the above example, the investigator could the anxiety could be because student do not prepare well for the examinations (Coon & Mitterer, 2011, p. 29).
A psychologist must create a realistic hypothesis where the experiment will be designed. The researcher will then keep on referencing to the hypothesis for thought process and development of solutions to the research problem.
Psychologists regularly suggest theories to explain how human beings behave. On a more informal approach, people judge others, their intentions, the motivations and the cause of certain actions. These subjective and anecdotal opinions are subject to tests.
Hypotheses can be developed where these assumptions or perception can be tested by scientific methods in an object and systematic approach. It is pertinent to note that then hypothesis cannot be proved nor disapproved, rather the investigator accepts or rejects the hypothesis. When the hypothesis is rejected, it leads to development of another hypothesis which can also be tested.
Coon, D & Mitterer, J., 2011, Psychology: Modules for Active Learning with Concept Modules with Note-Taking and Practice Exams, 11 th Ed., Cengage Learning, New York.
DeCarvalho, R.J., 1991, The Growth Hypothesis in Psychology: The Humanistic Psychology of Abraham Maslow and Carl Rogers , EMText, San Francisco.
Fiske, S.T., Gilbert, D.T., & Lindzey, G., 2010, Handbook of Social Psychology , Volume 2, John Wiley and Sons, West Sussex.
Hand, D. J. 2004, Measurement Theory and Practice: The World through Quantification, Edward Arnold, London.
- Chicago (A-D)
- Chicago (N-B)
IvyPanda. (2024, March 27). The Role of Hypotheses in Psychological Research. https://ivypanda.com/essays/the-role-of-hypotheses-in-psychological-research/
"The Role of Hypotheses in Psychological Research." IvyPanda , 27 Mar. 2024, ivypanda.com/essays/the-role-of-hypotheses-in-psychological-research/.
IvyPanda . (2024) 'The Role of Hypotheses in Psychological Research'. 27 March.
IvyPanda . 2024. "The Role of Hypotheses in Psychological Research." March 27, 2024. https://ivypanda.com/essays/the-role-of-hypotheses-in-psychological-research/.
1. IvyPanda . "The Role of Hypotheses in Psychological Research." March 27, 2024. https://ivypanda.com/essays/the-role-of-hypotheses-in-psychological-research/.
Bibliography
IvyPanda . "The Role of Hypotheses in Psychological Research." March 27, 2024. https://ivypanda.com/essays/the-role-of-hypotheses-in-psychological-research/.
- The Cosmic Dance of Siva
- Social Research Conduction
- Experimental Research and Design Development: Perception
- Scientific Method: Role and Importance
- Psychological Properties of Colors
- Characteristics of a Good Hypothesis
- Public Administration Study: Research Methodology
- Science Meets Real Life: Studying Student Absence
- Variables Differentiation of the United States Army
- The Impact of Salts on Grass Growth
- Family Versus Individual Therapy
- Critical Thinking and What Really Constitutes Critical Thinking
- Critical Thinking Method vs. The Elements of Thought Questions: Finding Out Which Method Is More Effective
- Psychodynamic Approach Survey
- Objective Personality Tests
Public Health Notes
Your partner for better health, hypothesis in research: definition, types and importance .
April 21, 2020 Kusum Wagle Epidemiology 0
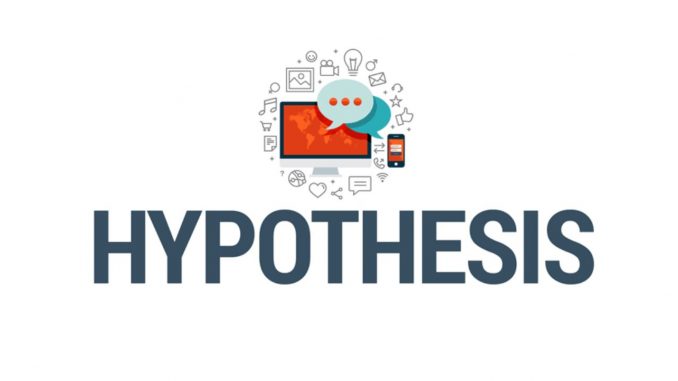
Table of Contents
What is Hypothesis?
- Hypothesis is a logical prediction of certain occurrences without the support of empirical confirmation or evidence.
- In scientific terms, it is a tentative theory or testable statement about the relationship between two or more variables i.e. independent and dependent variable.
Different Types of Hypothesis:
1. Simple Hypothesis:
- A Simple hypothesis is also known as composite hypothesis.
- In simple hypothesis all parameters of the distribution are specified.
- It predicts relationship between two variables i.e. the dependent and the independent variable
2. Complex Hypothesis:
- A Complex hypothesis examines relationship between two or more independent variables and two or more dependent variables.
3. Working or Research Hypothesis:
- A research hypothesis is a specific, clear prediction about the possible outcome of a scientific research study based on specific factors of the population.
4. Null Hypothesis:
- A null hypothesis is a general statement which states no relationship between two variables or two phenomena. It is usually denoted by H 0 .
5. Alternative Hypothesis:
- An alternative hypothesis is a statement which states some statistical significance between two phenomena. It is usually denoted by H 1 or H A .
6. Logical Hypothesis:
- A logical hypothesis is a planned explanation holding limited evidence.
7. Statistical Hypothesis:
- A statistical hypothesis, sometimes called confirmatory data analysis, is an assumption about a population parameter.
Although there are different types of hypothesis, the most commonly and used hypothesis are Null hypothesis and alternate hypothesis . So, what is the difference between null hypothesis and alternate hypothesis? Let’s have a look:
Major Differences Between Null Hypothesis and Alternative Hypothesis:
Importance of hypothesis:.
- It ensures the entire research methodologies are scientific and valid.
- It helps to assume the probability of research failure and progress.
- It helps to provide link to the underlying theory and specific research question.
- It helps in data analysis and measure the validity and reliability of the research.
- It provides a basis or evidence to prove the validity of the research.
- It helps to describe research study in concrete terms rather than theoretical terms.
Characteristics of Good Hypothesis:
- Should be simple.
- Should be specific.
- Should be stated in advance.
References and For More Information:
https://ocw.jhsph.edu/courses/StatisticalReasoning1/PDFs/2009/BiostatisticsLecture4.pdf
https://keydifferences.com/difference-between-type-i-and-type-ii-errors.html
https://www.khanacademy.org/math/ap-statistics/tests-significance-ap/error-probabilities-power/a/consequences-errors-significance
https://stattrek.com/hypothesis-test/hypothesis-testing.aspx
http://davidmlane.com/hyperstat/A2917.html
https://study.com/academy/lesson/what-is-a-hypothesis-definition-lesson-quiz.html
https://keydifferences.com/difference-between-null-and-alternative-hypothesis.html
https://blog.minitab.com/blog/adventures-in-statistics-2/understanding-hypothesis-tests-why-we-need-to-use-hypothesis-tests-in-statistics
- Characteristics of Good Hypothesis
- complex hypothesis
- example of alternative hypothesis
- example of null hypothesis
- how is null hypothesis different to alternative hypothesis
- Importance of Hypothesis
- null hypothesis vs alternate hypothesis
- simple hypothesis
- Types of Hypotheses
- what is alternate hypothesis
- what is alternative hypothesis
- what is hypothesis?
- what is logical hypothesis
- what is null hypothesis
- what is research hypothesis
- what is statistical hypothesis
- why is hypothesis necessary
Copyright © 2024 | WordPress Theme by MH Themes

An official website of the United States government
The .gov means it’s official. Federal government websites often end in .gov or .mil. Before sharing sensitive information, make sure you’re on a federal government site.
The site is secure. The https:// ensures that you are connecting to the official website and that any information you provide is encrypted and transmitted securely.
- Publications
- Account settings
Preview improvements coming to the PMC website in October 2024. Learn More or Try it out now .
- Advanced Search
- Journal List

Why we habitually engage in null-hypothesis significance testing: A qualitative study
Jonah stunt.
1 Department of Health Sciences, Section of Methodology and Applied Statistics, Vrije Universiteit, Amsterdam, The Netherlands
2 Department of Radiation Oncology, Erasmus Medical Center, Rotterdam, The Netherlands
Leonie van Grootel
3 Rathenau Institute, The Hague, The Netherlands
4 Department of Philosophy, Vrije Universiteit, Amsterdam, The Netherlands
5 Department of Epidemiology and Data Science, Amsterdam University Medical Centers, Amsterdam, The Netherlands
David Trafimow
6 Psychology Department, New Mexico State University, Las Cruces, New Mexico, United States of America
Trynke Hoekstra
Michiel de boer.
7 Department of General Practice and Elderly Care, University Medical Center Groningen, Groningen, The Netherlands
Associated Data
A full study protocol, including a detailed data analysis plan, was preregistered ( https://osf.io/4qg38/ ). At the start of this study, preregistration forms for qualitative studies were not developed yet. Therefore, preregistration for this study is based on an outdated form. Presently, there is a preregistration form available for qualitative studies. Information about data collection, data management, data sharing and data storage is described in a Data Management Plan. Sensitive data is stored in Darkstor, an offline archive for storing sensitive information or data (information that involves i.e., privacy or copyright). As the recordings and transcripts of the interviews and focus groups contain privacy-sensitive data, these files are archived in Darkstor and can be accessed only on request by authorized individuals (i.e., the original researcher or a research coordinator)1. Non-sensitive data is stored in DANS ( https://doi.org/10.17026/dans-2at-nzfs ) (Data Archiving and Networked Services; the Netherlands institute for permanent access to digital research resources). 1. Data requests can be send to ln.uv@mdr .
Null Hypothesis Significance Testing (NHST) is the most familiar statistical procedure for making inferences about population effects. Important problems associated with this method have been addressed and various alternatives that overcome these problems have been developed. Despite its many well-documented drawbacks, NHST remains the prevailing method for drawing conclusions from data. Reasons for this have been insufficiently investigated. Therefore, the aim of our study was to explore the perceived barriers and facilitators related to the use of NHST and alternative statistical procedures among relevant stakeholders in the scientific system.
Individual semi-structured interviews and focus groups were conducted with junior and senior researchers, lecturers in statistics, editors of scientific journals and program leaders of funding agencies. During the focus groups, important themes that emerged from the interviews were discussed. Data analysis was performed using the constant comparison method, allowing emerging (sub)themes to be fully explored. A theory substantiating the prevailing use of NHST was developed based on the main themes and subthemes we identified.
Twenty-nine interviews and six focus groups were conducted. Several interrelated facilitators and barriers associated with the use of NHST and alternative statistical procedures were identified. These factors were subsumed under three main themes: the scientific climate, scientific duty, and reactivity. As a result of the factors, most participants feel dependent in their actions upon others, have become reactive, and await action and initiatives from others. This may explain why NHST is still the standard and ubiquitously used by almost everyone involved.
Our findings demonstrate how perceived barriers to shift away from NHST set a high threshold for actual behavioral change and create a circle of interdependency between stakeholders. By taking small steps it should be possible to decrease the scientific community’s strong dependence on NHST and p-values.
Introduction
Empirical studies often start from the idea that there might be an association between a specific factor and a certain outcome within a population. This idea is referred to as the alternative hypothesis (H1). Its complement, the null hypothesis (H0), typically assumes no association or effect (although it is possible to test other effect sizes than no effect with the null hypothesis). At the stage of data-analysis, the probability of obtaining the observed, or a more extreme, association is calculated under the assumption of no effect in the population (H0) and a number of inferential assumptions [ 1 ]. The probability of obtaining the observed, or more extreme, data is known as ‘the p-value’. The p-value demonstrates the compatibility between the observed data and the expected data under the null hypothesis, where 0 is complete incompatibility and 1 is perfect compatibility [ 2 ]. When the p-value is smaller than a prespecified value (labelled as alpha, usually set at 5% (0.05)), results are generally declared to be statistically significant. At this point, researchers commonly reject the null hypothesis and accept the alternative hypothesis [ 2 ]. Assessing statistical significance by means of contrasting the data with the null hypothesis is called Null Hypothesis Significance Testing (NHST). NHST is the best known and most widely used statistical procedure for making inferences about population effects. The procedure has become the prevailing paradigm in empirical science [ 3 ], and reaching and being able to report statistically significant results has become the ultimate goal for many researchers.
Despite its widespread use, NHST and the p-value have been criticized since its inception. Numerous publications have addressed problems associated with NHST and p-values. Arguably the most important drawback is the fact that NHST is a form of indirect or inverse inference: researchers usually want to know if the null or alternative hypothesis can be accepted and use NHST to conclude either way. But with NHST, the probability of a finding, or more extreme findings, given the null hypothesis is calculated [ 4 ]. Ergo, NHST doesn’t tell us what we want to know. In fact, p-values were never meant to serve as a basis to draw conclusions, but as a continuous measure of incompatibility between empirical findings and a statistical model [ 2 ]. Moreover, the procedure promotes a dichotomous way of thinking, by using the outcome of a significance test as a dichotomous indicator for an effect (p<0.05: effect, p>0.05: no effect). Reducing empirical findings to two categories also results in a great loss of information. Further, a significant outcome is often unjustly interpreted as relevant, but a p-value does not convey any information about the strength or importance of the association. Worse yet, the p-values on which NHST is based confound effect size and sample size. A trivial effect size may nevertheless result in statistical significance provided a sufficiently large sample size. Or an important effect size may fail to result in statistical significance if the sample size is too small. P-values do not validly index the size, relevance, or precision of an effect [ 5 ]. Furthermore, statistical models include not only null hypotheses, but additional assumptions, some of which are wrong, such as the ubiquitous assumption of random and independent sampling from a defined population [ 1 ]. Therefore, although p-values validly index the incompatibility of data with models, p-values do not validly index incompatibility of data with hypotheses that are embedded in wrong models. These are important drawbacks rendering NHST unsuitable as the default procedure for drawing conclusions from empirical data [ 2 , 3 , 5 – 13 ].
A number of alternatives have been developed that overcome these pitfalls, such as Bayesian inference methods [ 7 , 11 , 14 , 15 ], informative hypothesis testing [ 9 , 16 ] and a priori inferential statistics [ 4 , 17 ]. These alternatives build on the idea that research usually starts with a more informed research-question than one merely assuming the null hypothesis of no effect. These methods overcome the problem of inverse inference, although the first two might still lead to dichotomous thinking with the use of thresholds. Despite the availability of alternatives, statistical behavior in the research community has hardly changed. Researchers have been slow to adopt alternative methods and NHST is still the prevailing paradigm for making inferences about population effects [ 3 ].
Until now, reasons for the continuous and ubiquitous use of NHST and the p-value have scarcely been investigated. One explanation is that NHST provides a very simple means for drawing conclusions from empirical data, usually based on the 5% cut-off. Secondly, most researchers are unaware of the pitfalls of NHST; it has been shown that NHST and the p-value are often misunderstood and misinterpreted [ 2 , 3 , 8 , 11 , 18 , 19 ]. Thirdly, NHST has a central role in most methods and statistics courses in higher education. Courses on alternative methods are increasingly being offered but are usually not mandatory. To our knowledge, there is a lack of in depth, empirical research, aimed at elucidating why NHST nevertheless remains the dominant approach, or what actions can be taken to shift the sciences away from NHST. Therefore, the aim of our study was to explore the perceived barriers and facilitators, as well as behavioral intentions related to the use of NHST and alternatives statistical procedures, among all relevant stakeholders in the scientific system.
Theoretical framework
In designing our study, we used two theories. Firstly, we used the ‘diffusion of innovation theory’ of Rogers [ 20 ]. This theory describes the dissemination of an innovation as a process consisting of four elements: 1) an innovation is 2) communicated through certain channels 3) over time 4) among the members of a social system [ 20 ]. In the current study, the innovation consists of the idea that we should stop with the default use of NHST and instead consider using alternative methods for drawing conclusions from empirical data. The science system forms the social structure in which the innovation should take place. The most important members, and potential adopters of the innovation, we identified are researchers, lecturers, editors of scientific journals and representatives of funding agencies. Rogers describes phases in the adoption process, which coincide with characteristics of the (potential) adopters of the idea: 1) innovators, 2) early adopters, 3) early majority adopters, 4) late majority adopters and 5) laggards. Innovators are the first to adopt an innovation. There are few innovators but these few are very important for bringing in new ideas. Early adopters form the second group to adopt an innovation. This group includes opinion leaders and role models for other stakeholders. The largest group consists of the early and late majority who follow the early adopters, and then there is a smaller group of laggards who resist the innovation until they are certain the innovation will not fail. The process of innovation adoption by individuals is described as a normal distribution ( Fig 1 ). For these five groups, the adoption of a new idea is influenced by the following five characteristics of the innovative idea and 1) its relative advantage, 2) its compatibility with current experiences, 3) its complexity, 4) its flexibility, and 5) its visibility [ 20 ]. Members of all four stakeholder groups could play an important role in the diffusion of the innovation of replacing NHST by its alternatives.

The innovativeness dimension, measured by the time at which an individual from an adopter category adopts an innovation. Each category is one of more standard deviations removed from the average time of adoption [ 20 ].
Another important theory for our study is the ‘theory of planned behavior’, that was developed in the 1960s [ 21 ]. This theory describes how human behavior in a certain context can be predicted and explained. The theory was updated in 2010, under the name ‘the reasoned action approach’ [ 22 ]. A central factor in this theory is the intention to perform a certain behavior, in this case, to change the default use of NHST. According to the theory, people’s intentions determine their behaviors. An intention indexes to what extent someone is motivated to perform the behavior. Intentions are determined by three independent determinants: the person’s attitudes toward the behavior—the degree to which a person sees the behavior as favorable or unfavorable, perceived subjective norms regarding the behavior—the perceived social pressure to perform the behavior or not, and perceptions of control regarding the behavior—the perceived ease or difficulty of performing the behavior. Underlying (i.e. responsible for) these three constructs are corresponding behavioral, normative, and control beliefs [ 21 , 22 ] (see Fig 2 ).

Both theories have served as a lens for both data collection and analysis. We used sensitizing concepts [ 23 ] within the framework of the grounded theory approach [ 24 ] from both theories as a starting point for this qualitative study, and more specifically, for the topic list for the interviews and focus groups, providing direction and guidance for the data collection and data analysis.
Many of the concepts of Rogers’ and Fishbein and Ajzen’s theory can be seen as facilitators and barriers for embracing and implementing innovation in the scientific system.
A qualitative study among stakeholders using semi-structured interviews and focus groups was performed. Data collection and analysis were guided by the principle of constant comparison traditional to the grounded theory approach we followed [ 24 ]. The grounded theory is a methodology that uses inductive reasoning, and aims to construct a theory through the collection and analysis of data. Constant comparison is the iterative process whereby each part of the data that emerges from the data analysis is compared with other parts of the data to thoroughly explore and validate the data. Concepts that have been extracted from the data are tagged with codes that are grouped into categories. These categories constitute themes, which (may) become the basis for a new theory. Data collection and analysis were continued until no new information was gained and data saturation had likely occurred within the identified themes.
The target population consisted of stakeholders relevant to our topic: junior and senior researchers, lecturers in statistics, editors of scientific journals and program leaders of funding agencies (see Tables Tables1 1 and and2). 2 ). We approached participants in the field of medical sciences, health- and life sciences and psychology. In line with the grounded theory approach, theoretical sampling was used to identify and recruit eligible participants. Theoretical sampling is a form of purposive sampling. This means that we aimed to purposefully select participants, based on their characteristics that fit the parameters of the research questions [ 25 ]. Recruitment took place by approaching persons in our professional networks and or the networks of the approached persons.
*The numbers between brackets represents the number of participants that were also interviewed.
Data collection
We conducted individual semi-structured interviews followed by focus groups. The aim of the interviews was to gain insight into the views of participants on the use of NHST and alternative methods and to examine potential barriers and facilitators related to these methods. The aim of the focus groups was to validate and further explore interview findings and to develop a comprehensive understanding of participants’ views and beliefs.
For the semi-structured interviews, we used a topic list (see Appendix 1 in S1 Appendix ). Questions addressed participants’ knowledge and beliefs about the concept of NHST, their familiarity with NHST, perceived attractiveness and drawbacks of the use of NHST, knowledge of the current NHST debate, knowledge of and views on alternative procedures and their views on the future of NHST. The topic list was slightly adjusted based on the interviews with editors and representatives from funding agencies (compared to the topic list for interviews with researchers and lecturers). Questions particularly focused on research and education were replaced by questions focused on policy (see Appendix 1 in S1 Appendix ).
The interviews were conducted between October 2017 and June 2018 by two researchers (L.v.G. and J.S.), both trained in qualitative research methods. Interviews lasted about one hour (range 31–86 minutes) and were voice-recorded. One interview was conducted by telephone; all others were face to face and took place at a location convenient for the participants, in most cases the participants’ work location.
Focus groups
During the focus groups, important themes that emerged from the interviews were discussed and explored. These include perceptions on NHST and alternatives and essential conditions to shift away from the default use of NHST.
Five focus groups included representatives from the different stakeholder groups. One focus group was homogenous, including solely lecturers. The focus groups consisted of ‘old’ as well as ‘new’ participants, that is, some of the participants of the focus groups were also in the interview sample. We also selected persons that were open for further contribution to the NHST debate and were willing to help think about (implementing) alternatives for NHST.
The focus groups were conducted between September and December 2018 by three researchers (L.v.G., J.S. and A.d.K.), all trained in qualitative research methods. The focus groups lasted about one-and-a-half hours (range 86–100 minutes).
Data analysis
All interviews and focus groups were transcribed verbatim. Atlas.ti 8.0 software was used for data management and analysis. All transcripts were read thoroughly several times to identify meaningful and relevant text fragments and analyzed by two researchers (J.S. and L.v.G.). Deductive predefined themes and theoretical concepts were used to guide the development of the topic list for the semi-structured interviews and focus groups, and were used as sensitizing concepts [ 23 ] in data collection and data analysis. Inductive themes were identified during the interview process and analysis of the data [ 26 ].
Transcripts were open-, axial- and selectively coded by two researchers (J.S. and L.v.G.). Open coding is the first step in the data-analysis, whereby phenomena found in the text are identified and named (coded). With axial coding, connections between codes are drawn. Selective coding is the process of selecting one central category and relating all other categories to that category, capturing the essence of the research. The constant comparison method [ 27 ] was applied allowing emerging (sub)themes to be fully explored. First, the two researchers independently developed a set of initial codes. Subsequently, findings were discussed until consensus was reached. Codes were then grouped into categories that were covered under subthemes, belonging to main themes. Finally, a theory substantiating the prevailing use of NHST was developed based on the main themes and subthemes.
Ethical issues
This research was conducted in accordance with the Dutch "General Data Protection Regulation" and the “Netherland’s code of conduct for research integrity”. The research protocol had been submitted for review and approved by the ethical review committee of the VU Faculty of Behavioral and Movement Sciences. In addition, the project had been submitted to the Medical Ethics Committee (METC) of the Amsterdam University Medical Centre who decided that the project is not subject to the Medical Research (Human Subjects) Act ( WMO). At the start of data collection, all participants signed an informed consent form.
A full study protocol, including a detailed data analysis plan, was preregistered ( https://osf.io/4qg38/ ). At the start of this study, preregistration forms for qualitative studies were not developed yet. Therefore, preregistration for this study is based on an outdated form. Presently, there is a preregistration form available for qualitative studies [ 28 ]. Information about data collection, data management, data sharing and data storage is described in a Data Management Plan. Sensitive data is stored in Darkstor, an offline archive for storing sensitive information or data (information that involves i.e., privacy or copyright). As the recordings and transcripts of the interviews and focus groups contain privacy-sensitive data, these files are archived in Darkstor and can be accessed only on request by authorized individuals (i.e., the original researcher or a research coordinator) (Data requests can be send to ln.uv@mdr ). Non-sensitive data is stored in DANS ( https://doi.org/10.17026/dans-2at-nzfs ) (Data Archiving and Networked Services; the Netherlands institute for permanent access to digital research resources).
Participant characteristics
Twenty-nine individual interviews and six focus groups were conducted. The focus groups included four to six participants per session. A total of 47 participants were included in the study (13 researchers, 15 lecturers, 11 editors of scientific journals and 8 representatives of funding agencies). Twenty-nine participants were interviewed. Twenty-seven participants took part in the focus group. Nine of the twenty-seven participants were both interviewed and took part in the focus groups. Some participants had multiple roles (i.e., editor and researcher, editor and lecturer or lecturer and researcher) but were classified based on their primary role (assistant professors were classified as lecturers). The lecturers in statistics in our sample were not statisticians themselves. Although they all received training in statistics, they were primarily trained as psychologists, medical doctors, or health scientists. Some lecturers in our sample taught an applied subject, with statistics as part of it. Other lectures taught Methodology and Statistics courses. Statistical skills and knowledge among lecturers varied from modest to quite advanced. Statistical skills and knowledge among participants from the other stakeholder groups varied from poor to quite advanced. All participants were working in the Netherlands. A general overview of the participants is presented in Table 1 . Participant characteristics split up by interviews and focus groups are presented in Table 2 .
Three main themes with sub-themes and categories emerged ( Fig 3 ): the green-colored compartments hold the three main themes: The scientific climate , The scientific duty and Reactivity . Each of these three main themes consists of subthemes, depicted by the yellow-colored compartments. In turn, some (but not all) of the 9 subthemes also have categories. These ‘lower level’ findings are not included in the figure but will be mentioned in the elaboration on the findings and are depicted in Appendix 2 in S1 Appendix . Fig 3 shows how the themes are related to each other. The blue arrows indicate that the themes are interrelated; factors influence each other. The scientific climate affects the way stakeholders perceive and fulfil their scientific duty, the way stakeholders give substance to their scientific duty shapes and maintain the scientific climate. The scientific duty and the scientific climate cause a state of reactivity. Many participants have adopted a ’wait and see’ attitude regarding behavioral changes with respect to statistical methods. They feel dependent on someone else’s action. This leads to a reactive (instead of a proactive) attitude and a low sense of responsibility. ‘Reactivity’ is the core theme, explaining the most critical problem with respect to the continuous and ubiquitous use of NHST.

Main themes and subthemes are numbered. Categories are mentioned in the body of the text in bold. ‘P’ stands for participant; ‘I’ stands for interviewer.
1. The scientific climate
The theme, ‘the scientific climate’, represents researchers’ (Dutch) perceptions of the many written and unwritten rules they face in the research environment. This theme concerns the opportunities and challenges participants encounter when working in the science system. Dutch academics feel pressured to publish fast and regularly, and to follow conventions and directions of those on whom they depend. They feel this comes at the expense of the quality of their work. Thus, the scientific climate in the Netherlands has a strong influence on the behavior of participants regarding how they set their priorities and control the quality of their work.
1 . 1 Quality control . Monitoring the quality of research is considered very important. Researchers, funding agencies and editors indicate they rely on their own knowledge, expertise, and insight, and those of their colleagues, to guarantee this quality. However, editors or funding agencies are often left with little choice when it comes to compiling an evaluation committee or a review panel. The choice is often like-knows-like-based. Given the limited choice, they are forced to trust the opinion of their consultants, but the question is whether this is justified.
I: “The ones who evaluate the statistics, do they have sufficient statistical knowledge?” P: “Ehhr, no, I don’t think so.” I: “Okay, interesting. So, there are manuscripts published of which you afterwards might think….” P: “Yes yes.” (Interview 18; Professor/editor, Medical Sciences)
1 . 2 Convention . The scientific system is built on mores and conventions, as this participant describes:
P: “There is science, and there is the sociology of science, that is, how we talk to each other, what we believe, how we connect. And at some point, it was agreed upon that we would talk to each other in this way.” (Interview 28, researcher, Medical Sciences)
And to these conventions, one (naturally) conforms. Stakeholders copy behavior and actions of others within their discipline, thereby causing particular behaviors and values to become conventional or normative. One of those conventions is the use of NHST and p-values. Everyone is trained with NHST and is used to applying this method. Another convention is the fact that significant results mean ‘success’, in the sense of successful research and being a successful researcher. Everyone is aware that ‘p is smaller than 0.05’ means the desired results are achieved and that publication and citation chances are increased.
P: “You want to find a significant result so badly. (…) Because people constantly think: I must find a significant result, otherwise my study is worthless.” (Focus group 4, lecturer, Medical Sciences)
Stakeholders rigidly hold on to the above-mentioned conventions and are not inclined to deviate from existing norms; they are, in other words, quite conservative . ‘We don’t know any better’ has been brought up as a valid argument by participants from various stakeholder groups to stick to current rules and conventions. Consequently, the status quo in the scientific system is being maintained.
P: “People hold on to….” I: ‘Everyone maintains the system?’ P: ‘Yes, we kind of hang to the conservative manner. This is what we know, what someone, everyone, accepts.” (Interview 17, researcher, Health Sciences)
Everyone is trained with NHST and considers it an accessible and easy to interpret method. The familiarity and perceived simplicity of NHST, user-friendly software such as SPSS and the clear cut-off value for significance are important facilitators for the use of NHST and at the same time barriers to start using alternative methods. Applied researchers stressed the importance of the accessibility of NHST as a method to test hypotheses and draw conclusions. This accessibility also justifies the use of NHST when researchers want to communicate their study results and messages in understandable ways to their readership.
P: “It is harder, also to explain, to use an alternative. So, I think, but maybe I’m overstepping, but if you want to go in that direction [alternative methods] it needs to be better facilitated for researchers. Because at the moment… I did some research, but, you know, there are those uncommon statistical packages.” (Interview 16, researcher/editor, Medical Sciences)
1 . 3 Publication pressure . Most researchers mentioned that they perceive publication pressure. This motivates them to use NHST and hope for significant results, as ‘significant p-values’ increase publication chances. They perceive a high workload and the way the scientific reward system is constructed as barriers for behavioral change pertaining to the use of statistical methods; potential negative consequences for publication and career chances prevent researchers from deviating from (un)written rules.
P: “I would like to learn it [alternative methods], but it might very well be that I will not be able to apply it, because I will not get my paper published. I find that quite tricky.” (Interview 1, Assistant Professor, Health Sciences)
2. The scientific duty
Throughout the interviews, participants reported a sense of duty in several variations. “What does it mean to be a scientific researcher?” seemed to be a question that was reflected upon during rather than prior to the interview, suggesting that many scientists had not really thought about the moral and professional obligations of being a scientist in general—let alone what that would mean for their use of NHST. Once they had given it some thought, the opinions concerning what constitutes the scientific duty varied to a large extent. Some participants attached great importance to issues such as reproducibility and transparency in scientific research and continuing education and training for researchers. For others, these topics seemed to play a less important role. A distinction was made between moral and professional obligations that participants described concerning their scientific duty.
2 . 1 Moral obligation . The moral obligation concerns issues such as doing research in a thorough and honest way, refraining from questionable research practices (QRPs) and investing in better research. It concerns tasks and activities that are not often rewarded or acknowledged.
Throughout the interviews and the focus groups, participants very frequently touched upon the responsibility they felt for doing ‘the right thing’ and making the right choice in doing research and using NHST, in particular. The extent to which they felt responsible varied among participants. When it comes to choices during doing research—for example, drawing conclusions from data—participants felt a strong sense of responsibility to do this correctly. However, when it comes to innovation and new practices, and feeling responsible for your own research, let alone improving scientific practice in general, opinions differed. This quotation from one of the focus groups illustrates that:
P1: “If you people [statisticians, methodologists] want me to improve the statistics I use in my research, then you have to hand it to me. I am not going to make any effort to improve that myself. “P3: “No. It is your responsibility as an academic to keep growing and learning and so, also to start familiarizing yourself when you notice that your statistics might need improvement.” (Focus group 2, participant 1 (PhD researcher, Medical Sciences) and 3 (Associate Professor, Health Sciences)
The sense of responsibility for improving research practices regarding the use of NHST was strongly felt and emphasized by a small group of participants. They emphasized the responsibility of the researcher to think, interpret and be critical when interpreting the p -value in NHST. It was felt that you cannot leave that up to the reader. Moreover, scrutinizing and reflecting upon research results was considered a primary responsibility of a scientist, and failing to do so, as not living up to what your job demands you to do:
P: “Yes, and if I want to be very provocative—and I often want that, because then people tend to wake up and react: then I say that hiding behind alpha.05 is just scientific laziness. Actually, it is worse: it is scientific cowardice. I would even say it is ‘relieving yourself from your duty’, but that may sound a bit harsh…” (Interview 2, Professor, Health Sciences)
These participants were convinced that scientists have a duty to keep scientific practice in general at the highest level possible.
The avoidance of questionable research practices (QRPs) was considered a means or a way to keep scientific practices high level and was often touched upon during the interviews and focus groups as being part of the scientific duty. Statisticians saw NHST as directly facilitating QRPs and providing ample examples of how the use of NHST leads to QRPs, whereas most applied researchers perceived NHST as the common way of doing research and were not aware of the risks related to QRPs. Participants did mention the violation of assumptions underlying NHST as being a QRP. Then, too, participants considered overinterpreting results as a QRP, including exaggerating the degree of significance. Although participants stated they were careful about interpreting and reporting p-values, they ‘admitted’ that statistical significance was a starting point for them. Most researchers indicated they search for information that could get their study published, which usually includes a low p-value (this also relates to the theme ‘Scientific climate’).
P: “We all know that a lot of weight is given to the p-value. So, if it is not significant, then that’s the end of it. If it ís significant, it just begins.” (Interview 5, lecturer, Psychology)
The term ‘sloppy science’ was mentioned in relation to efforts by researchers to reduce the p -value (a.k.a. p-hacking, data-dredging, and HARKing. HARKing is an acronym that refers to the questionable research question of Hypothesizing After the Results are Known. It occurs when researchers formulate a hypothesis after the data have been collected and analyzed, but make it look like it is an a priori hypothesis [ 29 ]). Preregistration and replication were mentioned as being promising solutions for some of the problems caused by NHST.
2 . 2 . Professional obligation . The theme professional obligation reflects participants’ expressions about what methodological knowledge scientists should have about NHST. In contrast moral obligations, there appeared to be some consensus about scientists’ professional obligations. Participants considered critical evaluation of research results a core professional obligation. Also, within all the stakeholder groups, participants agreed that sufficient statistical knowledge is required for using NHST, but they varied in their insights in the principles, potential and limitations of NHST. This also applied to the extent to which participants were aware of the current debate about NHST.
Participants considered critical thinking as a requirement for fulfilling their professional obligation. It specifically refers to the process of interpreting outcomes and taking all relevant contextual information into consideration. Critical thinking was not only literally referred to by participants, but also emerged by interpreting text fragments on the emphasis within their research. Researchers differed quite strongly in where the emphasis of their research outcomes should be put and what kind of information is required when reporting study results. Participants mentioned the proven effectiveness of a particular treatment, giving a summary of the research results, effect sizes, clinical relevance, p-values, or whether you have made a considerable contribution to science or society.
P: “I come back to the point where I said that people find it arbitrary to state that two points difference on a particular scale is relevant. They prefer to hide behind an alpha of 0.05, as if it is a God given truth, that it counts for one and for all. But it is just as well an invented concept and an invented guideline, an invented cut-off value, that isn’t more objective than other methods?” (Interview 2, Professor, Health Sciences)
For some participants, especially those representing funding agencies, critical thinking was primarily seen as a prerequisite for the utility of the research. The focus, when formulating the research question and interpreting the results, should be on practical relevance and the contribution the research makes to society.
The term ‘ignorance’ arose in the context of the participants’ concern regarding the level of statistical knowledge scientists and other stakeholders have versus what knowledge they should have to adequately apply statistical analysis in their research. The more statistically competent respondents in the sample felt quite strongly about how problematic the lack of knowledge about NHST is among those who regularly use it in their research, let alone the lack of knowledge about alternative methods. They felt that regularly retraining yourself in research methods is an essential part of the professional obligation one has. Applied researchers in the sample agreed that a certain level of background knowledge on NHST was required to apply it properly to research and acknowledged their own ignorance. However, they had different opinions about what level of knowledge is required. Moreover, not all of them regarded it as part of their scientific duty to be informed about all ins and outs of NHST. Some saw it as the responsibility of statisticians to actively inform them (see also the subtheme periphery). Some participants were not aware of their ignorance or stated that some of their colleagues are not aware of their ignorance, i.e., that they are unconsciously incompetent and without realizing it, poorly understood what the p-value and associated outcome measures actually mean.
P: “The worst, and I honestly think that this is the most common, is unconsciously incompetent, people don’t even understand that…” I: “Ignorance.” P: “Yes, but worse, ignorant and not even knowing you are ignorant.” (Interview 2, Professor, Health Sciences)
The lack of proper knowledge about statistical procedures was especially prevalent in the medical sciences. Participants working in or with the medical sciences all confirmed that there is little room for proper statistical training for medical students and that the level of knowledge is fairly low. NHST is often used because of its simplicity. It is especially attractive for medical PhD students because they need their PhD to get ahead in their medical career instead of pursuing a scientific career.
P: “I am not familiar with other ways of doing research. I would really like to learn, but I do not know where I could go. And I do not know whether there are better ways. So sometimes I do read studies of which I think: ‘this is something I could investigate with a completely different test. Apparently, this is also possible, but I don’t know how.’ Yes, there are courses, but I do not know what they are. And here in the medical center, a lot of research is done by medical doctors and these people have hardly been taught any statistics. Maybe they will get one or two statistics courses, they know how to do a t-test and that is about it. (…) And the courses have a very low level of statistics, so to say.” (Interview 1, Assistant Professor, Health Sciences)
Also, the term ‘ awareness ’ arose. Firstly, it refers to being conscious about the limitations of NHST. Secondly, it refers to the awareness of the ongoing discussions about NHST and more broadly, about the replication crisis. The statisticians in the sample emphasized the importance of knowing that NHST has limitations and that it cannot be considered the holy grail of data analysis. They also emphasized the importance of being aware of the debate. A certain level of awareness was considered a necessary requirement for critical thinking. There was variation in that awareness. Some participants were quite informed and were also fairly engaged in the discussion whereas others were very new to the discussion and larger contextual factors, such as the replication crisis.
I: “Are you aware of the debate going on in academia on this topic [NHST]? P: “No, I occasionally see some article sent by a colleague passing by. I have the idea that something is going on, but I do not know how the debate is conducted and how advanced it is. (Interview 6, lecturer, Psychology)
With respect to the theme, ‘the scientific duty’, participants differed to what extent they felt responsible for better and open science, for pioneering, for reviewing, and for growing and learning as a scientist. Participants had one commonality: although they strived for adherence to the norms of good research, the rampant feeling is that this is very difficult, due to the scientific climate. Consequently, participants perceive an internal conflict : a discrepancy between what they want or believe , and what they do . Participants often found themselves struggling with the responsibility they felt they had. Making the scientifically most solid choice was often difficult due to feasibility, time constraints, or certain expectations from supervisors (this is also directly related to the themes ‘Scientific climate’ and ‘Reactivity’). Thus, the scientific climate strongly influences the behavior of scientists regarding how they set their priorities and fulfill their scientific duties. The strong sense of scientific duty was perceived by some participants as a facilitator and by others as a barrier for the use of alternative methods.
3. Reactivity
A consequence of the foregoing factors is that most stakeholders have adopted a reactive attitude and behave accordingly. People are disinclined to take responsibility and await external signals and initiatives of others. This might explain why NHST is being continuously used and remains the default procedure to make inferences about population effects.
The core theme ‘reactivity’ can be explained by the following subthemes and categories:
3 . 1 Periphery . The NHST-problem resides in the periphery in several ways. First, it is a subject that is not given much priority. Secondly, some applied researchers and editors believe that methodological knowledge, as it is not their field of expertise, should not be part of their job requirement. This also applies to the NHST debate. Thirdly, and partly related to the second point, there is a lack of cooperation within and between disciplines.
The term ‘ priority’ was mentioned often when participants were asked to what extent the topic of NHST was subject of discussion in their working environment. Participants indicated that (too) little priority is given to statistics and the problems related to the subject. There is simply a lot going on in their research field and daily work, so there are always more important or urgent issues on the agenda.
P: “Discussions take place in the periphery; many people find it complicated. Or are just a little too busy.” (Interview 5, lecturer, Psychology)
As the NHST debate is not prioritized, initiatives with respect to this issue are not forthcoming. Moreover, researchers and lecturers claim there is neither time nor money available for training in statistics in general or acquiring more insight and skills with respect to (the use of) alternative methods. Busy working schedules were mentioned as an important barrier for improving statistical knowledge and skills.
P: “Well you can use your time once, so it is an issue low on the priority list.” (Focus group 5, researcher, Medical Sciences)
The NHST debate is perceived as the domain of statisticians and methodologists. Also, cooperation between different domains and domain-specific experts is perceived as complicated, as different perceptions and ways of thinking can clash. Therefore, some participants feel that separate worlds should be kept separate; put another way: stick to what you know!
P: “This part is not our job. The editorial staff, we have the assignment to ensure that it is properly written down. But the discussion about that [alternatives], that is outside our territory.” (Interview 26, editor, Medical Sciences)
Within disciplines, individuals tend to act on their own, not being aware that others are working on the same subject and that it would be worthwhile to join forces. The interviews and focus groups exposed that a modest number of participants actively try to change the current situation, but in doing that, feel like lone voices in the wilderness.
P1: “I mean, you become a lone voice in the wilderness.” P2: “Indeed, you don’t want that.” P1: “I get it, but no one listens. There is no audience.” (Focus Group 3, P1: MD, lecturer, medical Sciences, P2: editor, Medical Sciences)
To succeed at positive change, participants emphasized that it is essential that people (interdisciplinary) cooperate and join forces, rather than operate on individual levels, focusing solely on their own working environment.
The caution people show with respect to taking initiative is reenforced by the fear of encountering resistance from their working environment when one voices that change regarding the use of NHST is needed. A condition that was mentioned as essential to bring about change was tactical implementation , that is, taking very small steps. As everyone is still using NHST, taking big steps brings the risk of losing especially the more conservative people along the way. Also, the adjustment of policy, guidelines and educational programs are processes for which we need to provide time and scope.
P: “Everyone still uses it, so I think we have to be more critical, and I think we have to look at some kind of culture change, that means that we are going to let go of it (NHST) more and we will also use other tests, that in the long term will overthrow NHST. I: and what about alternatives? P: I think you should never be too fanatic in those discussion, because then you will provoke resistance. (…) That is not how it works in communication. You will touch them on a sore spot, and they will think: ‘and who are you?’ I: “and what works?” P: “well, gradualness. Tell them to use NHST, do not burn it to the ground, you do not want to touch peoples work, because it is close to their hearts. Instead, you say: ‘try to do another test next to NHST’. Be a pioneer yourself.” (Interview 5, lecturer, Psychology)
3 . 2 . Efficacy . Most participants stated they feel they are not in the position to initiate change. On the one hand, this feeling is related to their hierarchical positions within their working environments. On the other hand, the feeling is caused by the fact that statistics is perceived as a very complex field of expertise and people feel they lack sufficient knowledge and skills, especially about alternative methods.
Many participants stated they felt little sense of empowerment, or self-efficacy. The academic system is perceived as hierarchical, having an unequal balance of power. Most participants believe that it is not in their power to take a lead in innovative actions or to stand up against establishment, and think that this responsibility lies with other stakeholders, that have more status .
P: “Ideally, there would be a kind of an emergency letter from several people whose names open up doors, in which they indicate that in the medical sciences we are throwing away money because research is not being interpreted properly. Well, if these people that we listen to send such an emergency letter to the board of The Netherlands Organization for Health Research and Development [the largest Dutch funding agency for innovation and research in healthcare], I can imagine that this will initiate a discussion.” (…) I: “and with a big name you mean someone from within the science system? P: well, you know, ideally a chairman, or chairmen of the academic medical center. At that level. If they would put a letter together. Yes, that of course would have way more impact. Or some prominent medical doctors, yes, that would have more impact, than if some other person would send a letter yes.” (Interview 19, representative from funding agency, Physical Sciences)
Some participants indicated that they did try to make a difference but encountered too much resistance and therefore gave up their efforts. PhD students feel they have insufficient power to choose their own directions and make their own choices.
P: I am dependent on funding agencies and professors. In the end, I will write a grant application in that direction that gives me the greatest chance of eventually receiving that grant. Not primarily research that I think is the most optimal (…) If I know that reviewers believe the p-value is very important, well, of course I write down a method in which the p-value is central.” (Focus group 2, PhD-student, Medical Sciences)
With a sense of imperturbability, most participants accept that they cannot really change anything.
Lastly, the complexity of the subject is an obstacle for behavioral change. Statistics is perceived as a difficult subject. Participants indicate that they have a lack of knowledge and skills and that they are unsure about their own abilities. This applies to the ‘standard’ statistical methods (NHST), but to a greater extent to alternative methods. Many participants feel that they do not have the capacity to pursue a true understanding of (alternative) statistical methods.
P: “Statistics is just very hard. Time and again, research demonstrates that scientists, even the smartest, have a hard time with statistics.” (Focus group 3, PhD researcher, Psychology)
3 . 3 . Interdependency . As mentioned, participants feel they are not in a sufficiently strong position to take initiative or to behave in an anti-establishment manner. Therefore, they await external signals from people within the scientific system with more status, power, or knowledge. This can be people within their own stakeholder group, or from other stakeholder groups. As a consequence of this attitude, a situation arises in which peoples’ actions largely depend on others. That is, a complex state of interdependency evolves: scientists argue that if the reward system does not change, they are not able to alter their statistical behavior. According to researchers, editors and funding agencies are still very much focused on NHST and especially (significant) p-values, and thus, scientists wait for editors and funders to adjust their policy regarding statistics:
P: “I wrote an article and submitted it to an internal medicine journal. I only mentioned confidence intervals. Then I was asked to also write down the p-values. So, I had to do that. This is how they [editors] can use their power. They decide.” (Interview 1, Assistant Professor, Health Sciences)
Editors and funders in their turn claim they do not maintain a strict policy. Their main position is that scientists should reach consensus about the best statistical procedure, and they will then adjust their policy and guidelines.
P: “We actually believe that the research field itself should direct the quality of its research, and thus, also the discussions.” (Interview 22, representative from funding agency, Neurosciences)
Lecturers, for their part, argue that they cannot revise their educational programs due to the academic system, and university policies are adapted to NHST and p-values.
As most participants seem not to be aware of this process, a circle of interdependency arises that is difficult to break.
P: “Yes, the stupid thing about this perpetual circle is that you are educating people, let’s say in the department of cardiology. They must of course grow, and so they need to publish. If you want to publish you must meet the norms and values of the cardiology journals, so they will write down all those p-values. These people are trained and in twenty years they are on the editorial board of those journals, and then you never get rid of it [the p-value].” (Interview 18, Professor, editor, Medical Sciences)
3 . 4 . Degree of eagerness . Exerting certain behavior or behavioral change is (partly) determined by the extent to which people want to employ particular behavior, their behavioral intention [ 22 ]. Some participants indicated they are willing to change their behavior regarding the use of statistical methods, but only if it is absolutely necessary, imposed or if they think that the current conventions have too many negative consequences. Thus, true, intrinsic will-power to change behavior is lacking among these participants. Instead, they have a rather opportunistic attitude, meaning that their behavior is mostly driven by circumstances, not by principles.
P: “If tomorrow an alternative is offered by people that make that call, than I will move along. But I am not the one calling the shots on this issue.” (Interview 26, editor, Medical Sciences)
In addition, pragmatism often outweighs the perceived urgency to change. Participants argue they ‘just want to do their jobs’ and consider the practical consequences mainly in their actions. This attitude creates a certain degree of inertia. Although participants claim they are willing to change their behavior, this would contain much more than ‘doing their jobs, and thus, in the end, the NHST-debate is subject to ‘coffee talk’. People are open to discussion, but when it comes to taking action (and motivating others to do so), no one takes action.
P: “The endless analysis of your data to get something with a p-value less than 0.05… There are people that are more critical about that, and there are people that are less critical. But that is a subject for during the coffee break.” (Interview 18, professor, editor, Medical Sciences)
The goal of our study was to acquire in-depth insight into reasons why so many stakeholders from the scientific system keep using NHST as the default method to draw conclusions, despite its many well-documented drawbacks. Furthermore, we wanted to gain insight into the reasons for their reluctance to apply alternative methods. Using a theoretical framework [ 20 , 21 ], several interrelated facilitators and barriers associated with the use of NHST and alternative methods were identified. The identified factors are subsumed under three main themes: the scientific climate, the scientific duty and reactivity. The scientific climate is dominated by conventions, behavioral rules, and beliefs, of which the use of NHST and p-values is part. At the same time, stakeholders feel they have a (moral or professional) duty. For many participants, these two sides of the same coin are incompatible, leading to internal conflicts. There is a discrepancy between what participants want and what they do . As a result of these factors, the majority feels dependent on others and have thereby become reactive. Most participants are not inclined to take responsibility themselves but await action and initiatives from others. This may explain why NHST is still the standard and used by almost everyone involved.
The current study is closely related to the longstanding debate regarding NHST which recently increased to a level not seen before. In 2015, the editors of the journal ‘Basic and Applied Social Psychology’ (BASP) prohibited the use of NHST (and p-values and confidence intervals) [ 30 ]. Subsequently, in 2016, the American Statistical Association published the so-called ‘Statement on p-values’ in the American Statistician. This statement consists of critical standpoints regarding the use of NHST and p-values and warns against the abuse of the procedure. In 2019, the American Statistician devoted an entire edition to the implementation of reforms regarding the use of NHST; in more than forty articles, scientists debated statistical significance, advocated to embrace uncertainty, and suggested alternatives such as the use of s-values, False Positive Risks, reporting results as effect sizes and confidence intervals and more holistic approaches to p-values and outcome measures [ 31 ]. In addition, in the same year, several articles appeared in which an appeal was made to stop using statistical significance testing [ 32 , 33 ]. A number of counter-reactions were published [ 34 – 36 ], stating (i.e.) that banning statistical significance and, with that, abandoning clear rules for statistical analyses may create new problems with regard to statistical interpretation, study interpretations and objectivity. Also, some methodologists expressed the view that under certain circumstances the use of NHST and p-values is not problematic and can in fact provide useful answers [ 37 ]. Until recently, the NHST-debate was limited to mainly methodologists and statisticians. However, a growing number of scientists are getting involved in this lively debate and believe that a paradigm shift is desirable or even necessary.
The aforementioned publications have constructively contributed to this debate. In fact, since the publication of the special edition of the American Statistician, numerous scientific journals published editorials or revised, to a greater or lesser extent, their author guidelines [ 38 – 45 ]. Furthermore, following the American Statistical Association (ASA), the National Institute of Statistical Sciences (NISS) in the United States has also taken up the reform issue. However, real changes are still barely visible. It takes a long time before these kinds of initiatives translate into behavioral changes, and the widespread adoption by most of the scientific community is still far from accomplished. Debate alone will not lead to real changes, and therefore, our efforts to elucidate behavioral barriers and facilitators could provide a framework for potential effective initiatives that could be taken to reduce the default use of NHST. In fact, the debate could counteract behavioral change. If there is no consensus among statisticians and methodologists (the innovators), changing behavior cannot be expected from stakeholders with less statistical and methodological expertise. In other words, without agreement among innovators, early adopters might be reluctant to adopt the innovation.
Research has recently been conducted to explore the potential of behavioral change to improve Open Science behaviors. The adoption of open science behavior has increased in the last years, but uptake has been slow, due to firm barriers such as a lack of awareness about the subject, concerns about constrainment of the creative process, worries about being “scooped” and holding on to existing working practices [ 46 ]. The development regarding open science practices and the parallels these lines of research shows with the current study, might be of benefit to subserve behavioral change regarding the use of statistical methods.
The described obstacles to change behavior are related to features of both the ‘innovative idea’ and the potential adopters of the idea. First, there are characteristics of ‘the innovation’ that form barriers. The first barrier is the complexity of the innovation: most participants perceive alternative methods as difficult to understand and to use. A second barrier concerns the feasibility of trying the innovation; most people do not feel flexible about trying out or experimenting with the new idea. There is a lack of time and monetary resources to get acquainted with alternative methods (for example, by following a course). Also, the possible negative consequences of the use of alternatives (lower publications chances, the chance that the statistical method and message is too complicated for one’s readership) is holding people back from experimenting with these alternatives. And lastly, it is unclear for most participants what the visibility of the results of the new idea are. Up until now, the debate has mainly taken place among a small group of statisticians and methodologists. Many researchers are still not aware of the NHST debate and the idea to shift away from NHST and use alternative methods instead. Therefore, the question is how easily the benefits of the innovation can be made visible for a larger part of the scientific community. Thus, our study shows that, although the compatibility of the innovation is largely consistent with existing values (participants are critical about (the use of) NHST and the p-value and believe that there are better alternatives to NHST), important attributes of the innovative idea negatively affect the rate of adoption and consequently the diffusion of the innovation.
Due to the barriers mentioned above, most stakeholders do not have the intention to change their behavior and adopt the innovative idea. From the theory of planned behavior [ 21 ], it is known that behavioral intentions directly relate to performances of behaviors. The strength of the intention is shaped by attitudes, subjective norms, and perceived power. If people evaluate the suggested behavior as positive (attitude), and if they think others want them to perform the behavior (subjective norm), this leads to a stronger intention to perform that behavior. When an individual also perceives they have enough control over the behavior, they are likely to perform it. Although most participants have a positive attitude towards the behavior, or the innovative idea at stake, many participants think that others in their working environment believe that they should not perform the behavior—i.e., they do not approve of the use of alternative methods (social normative pressure). This is expressed, for example, in lower publication chances, negative judgements by supervisors or failing the requirements that are imposed by funding agencies. Thus, the perception about a particular behavior—the use of alternative methods—is negatively influenced by the (perceived) judgment of others. Moreover, we found that many participants have a low self-efficacy, meaning that there is a perceived lack of behavioral control, i.e., their perceived ability to engage in the behavior at issue is low. Also, participants feel a lack of authority (in the sense of knowledge and skills, but also power) to initiate behavioral change. The existing subjective norms and perceived behavioral control, and the negative attitudes towards performing the behavior, lead to a lower behavioral intention, and, ultimately, a lower chance of the performance of the actual behavior.
Several participants mentioned there is a need for people of stature (belonging to the group of early adopters) to take the lead and break down perceived barriers. Early adopters serve as role models and have opinion leadership, and form the next group (after the innovators, in this case statisticians and methodologists) to adopt an innovative idea [ 20 ] ( Fig 2 ). If early adopters would stand up, conveying a positive attitude towards the innovation, breaking down the described perceived barriers and facilitating the use of alternatives (for example by adjusting policy, guidelines and educational programs and making available financial resources for further training), this could positively affect the perceived social norms and self-efficacy of the early and late majority and ultimately laggards, which could ultimately lead to behavioral change among all stakeholders within the scientific community.
A strength of our study is that it is the first empirical study on views on the use of NHST, its alternatives and reasons for the prevailing use of NHST. Another strength is the method of coding which corresponds to the thematic approach from Braun & Clarke [ 47 ], which allows the researcher to move beyond just categorizing and coding the data, but also analyze how the codes are related to each other [ 47 ]. It provides a rich description of what is studied, linked to theory, but also generating new hypotheses. Moreover, two independent researchers coded all transcripts, which adds to the credibility of the study. All findings and the coding scheme were discussed by the two researchers, until consensus was reached. Also, interview results were further explored, enriched and validated by means of (mixed) focus groups. Important themes that emanated from the interviews, such as interdependency, perceptions on the scientific duty, perceived disadvantages of alternatives or the consequences of the current scientific climate, served as starting points and main subjects of the focus groups. This set-up provided more data, and more insight about the data and validation of the data. Lastly, the use of a theoretical framework [ 20 , 21 ] to develop the topic list, guide the interviews and focus groups, and guide their analysis is a strength as it provides structure to the analysis and substantiation of the results.
A limitation of this study is its sampling method. By using the network of members of the project group, and the fact that a relatively high proportion of those invited to participate refused because they thought they knew too little about the subject to be able to contribute, our sample was biased towards participants that are (somewhat) aware of the NHST debate. Our sample may also consist of people that are relatively critical towards the use of NHST, compared to the total population of researchers. It was not easy to include participants who were indifferent about or who were pro-NHST, as those were presumably less willing to make time and participate in this study. Even in our sample we found that the majority of our participants solely used NHST and perceived it as difficult if not impossible to change their behavior. These perceptions are thus probably even stronger in the target population. Another limitation, that is inherent to qualitative research, is the risk of interviewer bias. Respondents are unable, unwilling, or afraid to answer questions in good conscience, and instead provide socially desirable answers. In the context of our research, people are aware that, especially as a scientist, it does not look good to be conservative, complacent, or ignorant, or not to be open to innovation and new ideas. Therefore, some participants might have given a too favorable view of themselves. The interviewer bias can also take the other direction when values and expectations of the interviewer consciously or unconsciously influence the answers of the respondents. Although we have tried to be as neutral and objective as possible in asking questions and interpreting answers, we cannot rule out the chance that our views and opinions on the use of NHST have at times steered the respondents somewhat, potentially leading to the foregoing desirable answers.
Generalizability is a topic that is often debated in qualitative research methodology. Many researchers do not consider generalizability the purpose of qualitative research, but rather finding in-depth insights and explanations. However, this is an unjustified simplification, as generalizing of findings from qualitative research is possible. Three types of generalization in qualitative research are described: representational generalization (whether what is found in a sample can be generalized to the parent population of the sample), inferential generalization (whether findings from the study can be generalized to other settings), and theoretical generalization (where one draws theoretical statements from the findings of the study for more general application) [ 48 ]. The extent to which our results are generalizable is uncertain, as we used a theoretical sampling method, and our study was conducted exclusively in the Netherlands. We expect that the generic themes (reactivity, the scientific duty and the scientific climate) are applicable to academia in many countries across the world (inferential generalization). However, some elements, such as the Dutch educational system, will differ to a more or lesser extent from other countries (and thus can only be representationally generalized). In the Netherlands there is, for example, only one educational route after secondary school that has an academic orientation (scientific education, equivalent to the US university level education). This route consists of a bachelor’s program (typically 3 years), and a master’s program (typically 1, 2 or 3 years). Not every study program contains (compulsory) statistical courses, and statistical courses differ in depth and difficulty levels depending on the study program. Thus, not all the results will hold for other parts of the world, and further investigation is required.
Our findings demonstrate how perceived barriers to shift away from NHST set a high threshold for behavioral change and create a circle of interdependency. Behavioral change is a complex process. As ‘the stronger the intention to engage in a behavior, the more likely should be its performance’[ 21 ], further research on this subject should focus on how to influence the intention of behavior; i.e. which perceived barriers for the use of alternatives are most promising to break down in order to increase the intention for behavioral change. The present study shows that negative normative beliefs and a lack of perceived behavioral control regarding the innovation among individuals in the scientific system is a substantial problem. When social norms change in favor of the innovation, and control over the behavior increases, then the behavioral intention becomes a sufficient predictor of behavior [ 49 ]. An important follow-up question will therefore be: how can people be enthused and empowered, to ultimately take up the use of alternative methods instead of NHST? Answering this question can, in the long run, lead to the diffusion of the innovation through the scientific system as a whole.
NHST has been the leading paradigm for many decades and is deeply rooted in our science system, despite longstanding criticism. The aim of this study was to gain insight as to why we continue to use NHST. Our findings have demonstrated how perceived barriers to make a shift away from NHST set a high threshold for actual behavioral change and create a circle of interdependency between stakeholders in the scientific system. Consequently, people find themselves in a state of reactivity, which limits behavioral change with respect to the use of NHST. The next step would be to get more insight into ways to effectively remove barriers and thereby increase the intention to take a step back from NHST. A paradigm shift within a couple of years is not realistic. However, we believe that by taking small steps, one at a time, it is possible to decrease the scientific community’s strong dependence on NHST and p-values.
Supporting information
S1 appendix, acknowledgments.
The authors are grateful to Anja de Kruif for her contribution to the design of the study and for moderating one of the focus groups.
Funding Statement
This research was funded by the NWO (Nederlandse Organisatie voor Wetenschappelijk Onderzoek; Dutch Organization for Scientific Research) ( https://www.nwo.nl/ ) The funders had no role in study design, data collection and analysis, decision to publish, or preparation of the manuscript.
Data Availability
ORIGINAL RESEARCH article
Servant leadership, brand love, and work ethic: important predictors of general health in workers in the education sector.

- 1 UPG de Ciencias Empresariales, Escuela de Posgrado, Universidad Peruana Unión, Lima, Perú
- 2 EP de Administración, Facultad de Ciencias Empresariales, Universidad Peruana Unión, Juliaca, Perú
- 3 EP de Administración, Facultad de Ciencias Empresariales, Universidad Peruana Unión, Lima, Perú
Background: Building a path aimed at the wellbeing of workers in the education sector is the fundamental basis to encourage quality education. To fill the gap in knowledge and address this aspect by understanding the behavior of the study population, it was proposed as with the objective of determining if servant leadership, brand love and work ethic predict the general health in workers.
Methods: A non-probability sampling was applied for convenience. For this purpose, a sample of 509 workers from Peru was submitted to study, who completed a questionnaire consisting of: scale of servant leadership, work ethic, GHQ-12 and brand love. By applying a quantitative method using a structural equation modeling partial least squares approach.
Results: The present study demonstrated that the three constructs (servant leadership, brand love, and work ethic) predict the general health of workers in a positive and significant way, in a sample of Peruvian workers in the education sector. Furthermore, the results suggest that these factors can be used to improve the health of employees in educational institutions in Peru and possibly in other contexts as well.
Conclusion: Given these results and after knowing the solidity of the predictions, the importance of promoting general health in workers in the education sector.
Introduction
Currently, many companies have seen the great need to predict future changes in the management of human talent, therefore, one of their priority tasks is to redefine traditional roles and responsibilities, which allow increasing the internal health of the organization through the overall employee health ( Pino et al., 2020 ; Wang et al., 2020 ; Lunde et al., 2022 ; Tinella et al., 2022 ; Bezuidenhout et al., 2023 ); to achieve this end, organizations must have the ability to discover competent people with leadership qualities that promote better results in their work environment, as well as the use of strategies and mechanisms to develop more love ( Tijjang et al., 2023 ), commitment ( Grabowski et al., 2019 ; Tacadena and Muico, 2022 ; Mitonga-Monga et al., 2023 ) and ethics ( Grabowski et al., 2019 ; Sakr et al., 2022 ; Tacadena and Muico, 2022 ), from employees to your organization.
Today, there are few studies carried out to predict the general health of employees within the workplace ( Shi et al., 2022 ), in a post-COVID context where various jobs are carried out from the homes of employees; and it is here where there is a greater need to evaluate the changes in the general health of workers for the education sector. On the other hand, important scientific evidence shows that the practice of the leadership style of bosses/directors/managers has a significant impact on the health of workers ( Sakr et al., 2022 ; Tinella et al., 2022 ; McKimm et al., 2023 ). Although it is true, the transformational leadership is an affective and high-profile leadership style, which is why many institutions disseminate and practice it ( Miao and Cao, 2019 ), however, servant leadership has become one of the most studied, admired, disseminated and useful in various business sectors, due to the variety of benefits it produces ( Kaltiainen and Hakanen, 2022 ). In recent years, researchers have given greater consideration to the study of servant leadership, and how this construct behaves in the various dimensions of the organization ( der Kinderen et al., 2020 ), since not only favors collaborators, managers, senior managers, but its sphere of influence reaches all stakeholders ( Newman et al., 2017 ; Iqbal et al., 2020 ; Meuser and Smallfield, 2023 ).
Taking into consideration the current challenges in the educational environment, where the general health of academic staff is vital for the achievement of the purposes that it pursues, allowing anticipation of the changes and challenges posed in this very relevant field of life itself, it is important consider the factors that have been the reason for this study. Therefore, servant leadership is one that is recognized as a style of leadership focused on serving its followers and satisfying their needs, which in turn produces a shared spirit of purpose, trust, commitment, desire for wisdom, and effort in the community organization ( Gocen and Sen, 2021 ). For another on, brand love is an important factor in the recognition of institutional identity, it is the positive emotional connection with a brand, coming to manifest their love for it, allowing them to be more likely to commit and be loyal to it ( Larregui-Candelaria et al., 2019 ). And in turn, studies on work ethics have been validated in various business sectors, considering it as a set of norms and values that serve as a guideline for the activities of a job, taking the contribution of Sharma and Rai (2015) , who carried out the operationalization of this variable in three parameters from the point of view of the collaborator; work as a central interest in life, moral attitude toward work and intrinsic work motivation.
And in this context, general health has an important meaning in the lives of workers in the education sector, where this construct has currently been linked to many others: such as coping strategies ( Tinella et al., 2022 ), depression ( Gladstone et al., 2018 ), job insecurity ( Setati et al., 2015 ), psychological discomfort ( Jakubiec et al., 2014 ), machine learning ( Hoekstra et al., 2023 ), working capacity ( Kisiel et al., 2023 ), quality of life ( Bezuidenhout et al., 2023 ), among other. Understanding general health as the mental, emotional and physical wellbeing that allows us to face challenges ( Lunde et al., 2022 ) and acquire greater concentration in the activities that are carried out ( Chavez-Espinoza et al., 2022 ).
Previous studies have concentrated on some elements as predictors of general health ( Ebert et al., 2002 ; Mirsaleh et al., 2011 ; Nadi et al., 2020 ; Malakoutikhah et al., 2022 ), however, there is still a need for a scientific precedent where the usefulness of general health as a contextual factor to explore the behavior between servant leadership, brand love, and work ethic of educational workers ( Dahleez and Aboramadan, 2022 ).
In that sense, after a diligent review of the aforementioned background, there has been a growing interest in continuing to study these topics, both on the part of academics and professionals in the business and health sectors. Although scientific evidence validates that among the study topics, the one that has caused the greatest interest is general health focused on various contexts. On the other hand, bibliometric indicators reveal the 10 countries that most disseminate their scientific results on these topics, among which are: United States ( Meriac et al., 2023 ), United Kingdom ( Wang W. et al., 2022 ), Iran ( Malakoutikhah et al., 2022 ), China ( Yuan et al., 2020 ), Australia ( Sajtos et al., 2021 ), India ( Sharma and Rai, 2015 ), Pakistan ( Iqbal et al., 2020 ), Netherlands ( Fernstrand et al., 2017 ), Spain ( Ruiz-Palomino et al., 2021 ), and Germany ( Moll and Kretzschmar, 2017 ). The same ones who have applied their study to various areas, sectors and populations, such as: medicine ( Ebert et al., 2002 ), business ( Suryani et al., 2022 ), social sciences ( Mustafa et al., 2022 ), psychology ( Jakubiec et al., 2014 ), economics ( Peng et al., 2022 ), humanities ( Raja et al., 2020 ), among others. When discerning scientific dissemination by country, it has been found that the studies carried out in the Peruvian population ( Caycho-Rodríguez et al., 2020 ; Pino et al., 2020 ; Chavez-Espinoza et al., 2022 ; Rocha-Vallejos et al., 2022 ; Alipio et al., 2023 ) are very limited, that is, there is very little scientific literature that can provide support and guidance for future studies, and that can provide relevant information to develop communication strategies, health promotion, improving wellbeing and general health in the Peruvian context. Given the prevalence of diseases and the current situation of occupational health, this research aims to fill the knowledge gap and provide a valuable contribution to the academic community and professionals of the sectors involved. Based on existing evidence, the objective was determining whether servant leadership, brand love, and work ethics predict the general health of educational workers.
Literature review
Servant leadership.
Among the leadership approaches that focus on the collaborator is the transformational leadership approach that seeks to influence those they lead in order to achieve business objectives ( Charbonnier-Voirin et al., 2010 ; Miao and Cao, 2019 ; Purwanto, 2020 ; Sjamsoeddin et al., 2023 ), unlike the servant leadership approach that is oriented to the wellbeing of those led ( van Dierendonck and Nuijten, 2011 ). In this sense, a prudent review of the literature on this last topic will refer to the founder of the servant leadership movement, Robert Kiefner Greenleaf, a notable researcher who developed the theoretical foundation of this construct and published his famous work in 1970 called, “The Servant as Leader,” where he describes servant leaders as those who lead through service, but always with the aim of satisfying the needs of collaborators, providing them with learning opportunities and improving their self-management skills ( Eva et al., 2019 ); equally helps and guides the group by showing compassion, healing, awareness, persuasion, management, and commitment to its growth ( Wang W. et al., 2022 ).
Likewise, it encourages employees to reveal their doubts, take on challenges and reward this attitude by providing resources to feel this freedom ( Page and Wong, 2000 ; Olesia et al., 2014 ; Gandolfi and Stone, 2018 ; Lusiani et al., 2020 ; Pino et al., 2020 ; Gocen and Sen, 2021 ). Recent studies provide new evidence on how organizations, through servant leadership behaviors, help employees not only perform better in their tasks, but also to optimally manage work stress ( Quick and Henderson, 2016 ; Zetterberg et al., 2023 ), to reduce job exhaustion that is therefore associated with the psychological and physiological health of the employee ( Kaltiainen and Hakanen, 2022 ).
Approximately since 1990, the relationship between the company and its brand has been investigated ( Batra et al., 2012 ; Bagozzi et al., 2017 ; Na et al., 2023 ), in terms of loyalty, trust and commitment toward organizations, for this reason the study highlights the theme concerning the relationship and emotional connection that is established through trust, commitment, and loyalty ( Larregui-Candelaria et al., 2019 ). In the research by Shi et al. (2022) , refers that the positive connection between trust in the brand and love for the brand has been recently established. In the same way, brand trust is closely linked to attitudinal loyalty due to its ability to provoke positive emotions in customers and workers, in addition to developing a sense of belonging and commitment. There is also a relationship between the company and the employee, which means that employees can commit themselves and be loyal to the brand of the company where they work, considering the few studies that address the issue, some of them refer to the connection between the commitment of staff that goes beyond performing their professional tasks ( Wang and Binti, 2023 ), is also translated as that emotional connection that employees have with the company and how this can influence their health and wellbeing.
The work ethic is considered a construct that indicates how much a person values work in their life. It began as the Protestant work ethic, being a classic work by Max Weber around 1958, being the subject of many studies and discussions for being considered a religiously oriented work, however, over time it was called general ethics from work ( Sharma and Rai, 2015 ). Work ethic is the set of attitudes and behaviors at work, as a motivational construction reflected in behavior ( Sakr et al., 2022 ). In addition, in recent years, a number of investigations show that ethics is a predictor of important results at work, some theorists associate its dimensions with the individual’s job performance ( Woehr et al., 2023 ), job satisfaction and stress ( Meriac et al., 2023 ), therefore, it could be shown that it is somehow related to the health of the worker. Work ethic focuses on how ethical practices in the workplace can improve the health and wellbeing of employees. Recent studies highlight the need to support workers in maintaining ethical behavior in their workplace, even from other non-work settings ( Alfano, 2022 ).
General health
World Health Organization (2004) in its definition of health, it includes the three most important dimensions of life, referring to a complete state of physical, mental, and social wellbeing, and not only the absence of conditions or diseases. The definition of general health encompasses a series of components that define it as a continuous process of satisfaction that enables people to develop their abilities and potential naturally ( Chavez-Espinoza et al., 2022 ). General health reflects a person’s perception of physical symptoms, sleep disorders, anxiety symptoms, depressive symptoms, and social functioning ( Tinella et al., 2022 ), reflecting both positive and negative aspects of health ( Nadi et al., 2020 ).
Furthermore, previous studies have shown that general health reflects not only physical health but also mental health ( Bezuidenhout et al., 2023 ), because general health is a broader outcome than physical and mental health ( Shi et al., 2022 ). A wide range of factors are known to be associated with health status ( Hoekstra et al., 2023 ), such as lifestyle ( Isola, 2020 ), socioeconomic status ( Nadi et al., 2020 ), wellbeing at work ( de Ceballos and Santos, 2015 ), anxiety ( Malakoutikhah et al., 2022 ), perceived disease burden ( Nielsen et al., 2015 ), sociodemographic factors ( Tinella et al., 2022 ), work facilitation ( Shi et al., 2022 ), and work ability ( Kisiel et al., 2023 ). By conducting a thorough review, studies show other multidimensional models, however, it is believed that for this non-clinical study it is better suited using the GHQ-12 ( Montazeri et al., 2003 ; Hystad and Johnsen, 2020 ; Mayhew et al., 2021 ; Lütke et al., 2022 ).
Hypothesis development
Servant leadership predicts general health.
Servant leadership has emerged as an alternative to mitigate some of the stress experienced by professionals in their fields of work; in this context, the application of the PERMA model is proposed, a model that establishes the way in which each individual chooses to carry out activities that make them happy, contributing these activities to generate a feeling of wellbeing, this feeling being what allows the individual to have a positive experience ( Turner, 2022 ). The service leader brings together certain qualities of honesty, righteousness and selflessness that make his collaborators feel good, as they are immersed in an atmosphere of harmony, thus opening the way to a greater likelihood of greater wellbeing for both the collaborators and the leader ( Siu et al., 2015 ; Zeng et al., 2022 ). And servant leadership, beyond being an admirable quality, has a special recognition for fostering positive behavioral outcomes and active participation that contributes to the wellbeing of workers ( Yuan et al., 2020 ).
From another perspective ( Dollard and Bakker, 2010 ; Montano et al., 2017 ), the role of the leader is important for this purpose; thus, the leadership of someone who chooses to give priority to others is required, someone who supports his followers to develop their maximum potential, thus contributing to the mental and psychological health of the workers; that is, in this scenario, the active participation of a service leader who contributes, by his way of acting and proceeding, to the wellbeing of the workers is required; this is a key point in the construction of a healthy work environment ( Cottey and McKimm, 2019 ). This context reinforces the idea that a leader can develop a proactive approach to employee health, since his function, beyond managing human resources, is also based on generating a long-term vision that recognizes how important employee health is to the sustainable success of the company. Based on the above, the following study hypothesis is proposed:
H1: Servant leadership predicts general health in workers in the education sector.
Brand love predicts general health
The researchers ( Ahuvia et al., 2022 ) establish that a consumer who has a deep affinity for a product or service significantly values the brand, this fact denotes interpersonal connections that transcends or goes beyond the commercial transaction and according to the literature a brand has the ability to create a strong lasting emotional bond that impacts the consumer’s overall wellbeing. Furthermore, considering that brand love is associated with the consumer experience, scholars are convinced that from a holistic approach, brand love independently to support any marketing outcome, can also be part of the positive impact on the overall health of individuals and is that according to evidence, when a consumer develops brand love, it tends to create feelings of belonging and identity, thus releasing a positive impact on the welfare of consumers, their mental and physical health ( Junaid et al., 2020 ; Attiq et al., 2022 ; Rodrigues et al., 2022 ); in the context of this study, it is specified that a worker who loves a certain brand in the educational sector, can experience positive feelings of motivation and satisfaction that can boost their contribution to the objectives of the brand, thus maintaining a positive feeling of general health and increase the feeling of commitment to their educational work. In this way, a special emphasis is made that establishes that the investigations that study consumer behavior have begun to focus on consumer welfare that extends to the health of the same, as it is addressed, in some way, that emotional and psychological needs can be addressed within the environment where the brand and the consumer interact ( Bairrada et al., 2019 ). Based on the above, the following study hypothesis is proposed:
H2: Brand love predicts general health in workers in the education sector.
Work ethic predicts general health
Work ethic has been seen as a positive resource that fosters a sense of psychological wellbeing and general health in workers ( Maaz and Farroq, 2017 ; Raja et al., 2020 ). From this concept lies how important it is to preserve a positive state of those who make up an organization, thus having a vision of work ethics that extends to form part of the sustainable development of a company and when workers perceive that work ethics are valued within an organization, healthy working environments are promoted ( Chukwuma et al., 2023 ). In this context, special emphasis is given to human resource management systems where, independently of constantly striving for greater competitiveness, it can also improve work ethics practices ( Tadesse Bogale and Ayenew Birbirsa, 2023 ), since according to Bazzy (2018) , work ethics allows to break the paradigm that establishes that hard work, effort, and other sacrifices are indispensable to achieve success, this is how work ethics fulfills an important function of interceding for the wellbeing and health of workers, maintaining a healthy balance between the work and personal life of the worker. Based on the above, the following study hypothesis is proposed:
H3: Work ethic predicts general health .
This research has focused on determining whether servant leadership, brand love, and work ethics predict the general health of educational workers, and in this way provide relevant information to interest entities that seek to have employees who enjoy greater wellbeing in their work environments; thus, awakening a greater need in leaders to design strategies that seek a healthy balance in their institutions ( Figure 1 ).
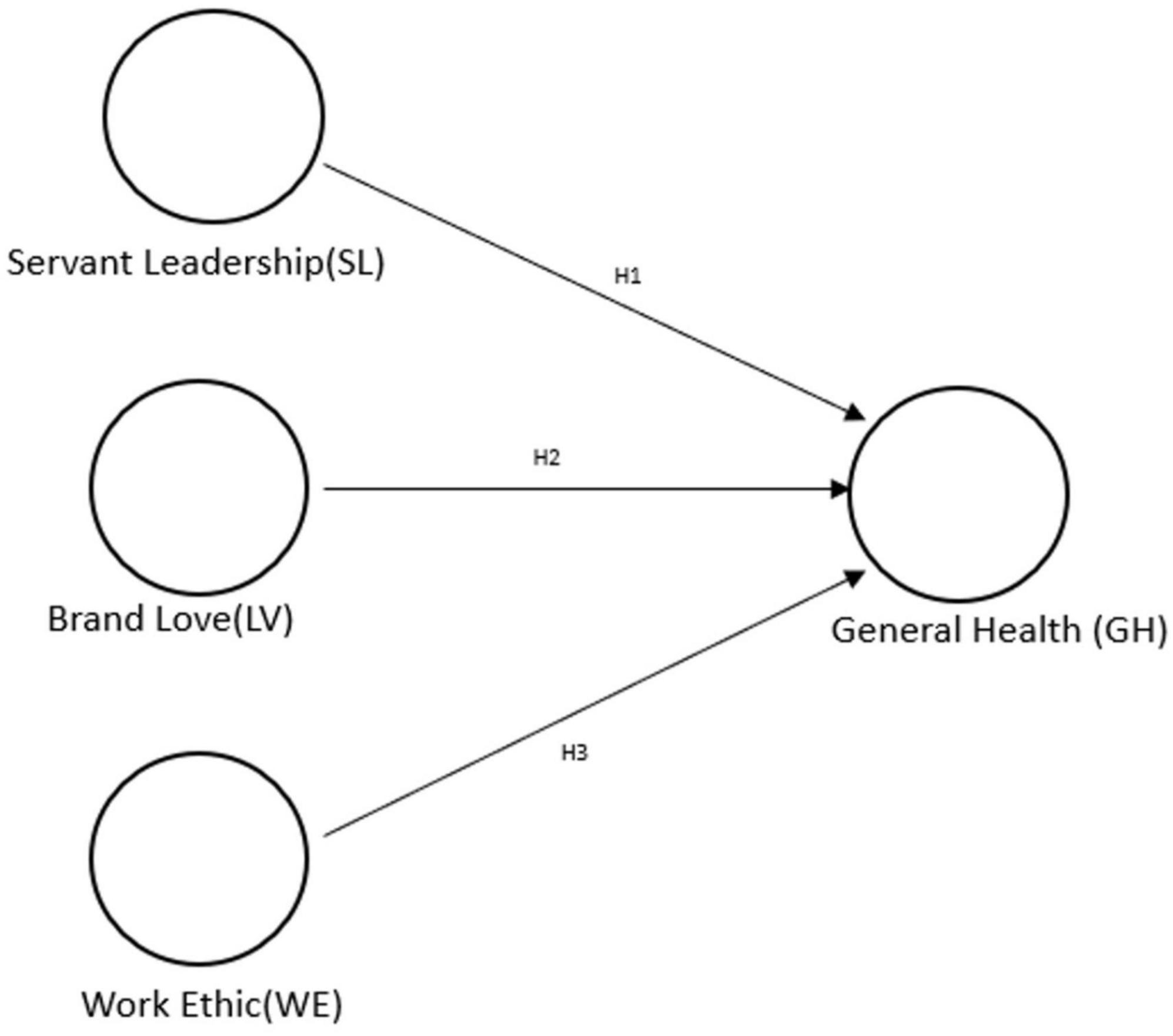
Figure 1. Theoretical model proposed. SL, servant leadership; WE, work ethic; LV, brand love; GH, general health.
Materials and methods
Design, procedure, and participants.
Cross-sectional explanatory study ( Ato et al., 2013 ).
In the survey, participation was voluntary for the sample of workers from regular basic education (56%), institutes (5%), and universities (40%). The sample comes from Peru. A non-probability sampling was applied for convenience. To be included in this study, participants had to meet the following inclusion criteria: work as a teacher or administrator in the public sector or private from a regular basic institution, institute, or university, have a minimum of 6 months working in the institution regardless of the type of work. Those who did not meet the inclusion criteria were excluded. The data was collected through an online survey platform (Google Forms). A total of 509 questionnaires were considered. From the descriptive analysis of the demographic information ( Table 1 ), 223 were men and 286 women, 43% of the respondents were between 31 and 43 years old and the majority of the subjects (55%) were married, and 36% of them were single, whilst the rest of the participants stated that they were cohabiting, divorced, and widowed. Workers from the coast and mountains predominate (39% each region). The greatest instruction that stood out was higher education (85%). The public sector stands out with 79%. Most of the participants declared less than 14 years of service at their institution (70%). The study was approved by the Ethics Committee of the EPG of the Universidad Peruana Unión (2022-CE-EPG-0000167). Informed consent and assent were obtained from the institutions participating in the study.
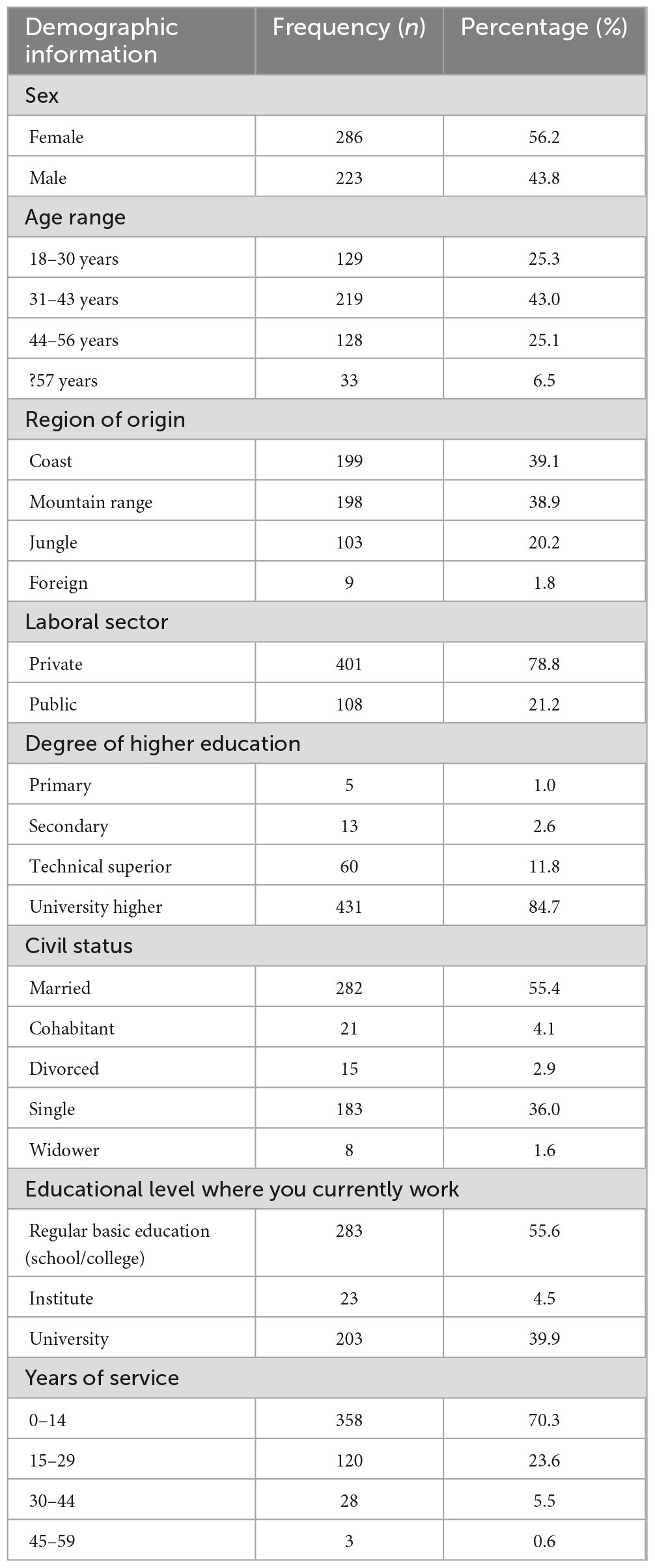
Table 1. Sociodemographic characteristics of the sample.
On the other hand, the surveys were self-administered by each participant. Regarding the servant leadership and work ethic scale, its original language was English, and it went through the pretest process. The survey techniques were in English, so they were translated by two native specialists who speak English and Spanish. Likewise, for the application of the four scales (servant leadership, work ethic, general health, and brand love), validation was carried out by expert judgment (six teachers with a master’s degree and doctorate) who evaluated clarity, objectivity, topicality, organization, sufficiency, intentionality, consistency, coherence, methodology, and relevance, resulting in an Aiken’s V indicator of 90.5%, 91.6%, 90.9%, and 90.7%, respectively. Additionally, a focus group session was held which allowed for sematic modifications of the work context, made up of workers from the education sector: two from regular basic education, two from institutes, and two from university. The questionnaire is ready to be applied to these three groups of educational workers. Regarding data collection, the questionnaire was hosted in the Google Form application, which was shared via WhatsApp, Messenger, and Instagram in order to have a greater reach to the study population. Its application lasted 31 weeks, from October 26, 2022 to June 4, 2023.
Outcome measures
For the purposes of this study, it was estimated to use four scales collected from articles housed in high-impact journals. The data collection instrument was built considering the four scales of the study, finally adding a section of questions to identify the educational level where the participant worked, as well as the institutional region, the labor sector, and other sociodemographic questions. The questionnaire consisted of 32 items: the first 7 items on leadership servant of Gocen and Sen (2021) . For work ethics, the scale of Sharma and Rai (2015) that used 10 items. For general health, the scale known as GHQ-12 applied to the Peruvian context by Chavez-Espinoza et al. (2022) , this instrument in question was adapted taking into account the questions that were adequate for the realization of the present study, of the 12 items only 6 factorially adequate were used. On the other hand, these first three variables were valued on a Likert-type response scale from 1 to 5, where 1 is “Strongly disagree” and 5 is “Strongly agree.” However, to measure love for the brand, 3 items established by Larregui-Candelaria et al. (2019) , the latter was assessed in a 5-point Likert-type response format, ranging from 1: “Completely disagree” to 5: “Completely agree.” Unlike the original instruments, these have been translated and adapted to the Peruvian educational context, considering the particularity of each sector (public and private).
Data analysis
In the data analysis, the partial least square structural equation modeling (PLS-SEM) was used to test the hypotheses. The PLS-SEM is a comprehensive approach to multivariate statistical analysis that includes measurement and structural components to simultaneously examine the relationships between each of the variables in a conceptual model, which has the characteristic of multivariate analysis, that is, it involves a quantity of variables ≥3 ( Hair et al., 2010 ). In addition, the PLS-SEM was used in the present study because it facilitates the construction of theory ( Hair et al., 2011 ). WarpPLS (Version 8.0) was used to perform the PLS-SEM analysis. This software was used because according to Kock (2014) , the WarpPLS provides options to use different algorithms for the external and internal models in the calculation of the scores of the latent variables, such as the path coefficient and the parameters associated with the p -value, identifying and taking into account non-linear relationships in the model structural ( Kock, 2011 ).
The evaluation of a model using PLS-SEM is a two-step process that involves the evaluation of the measurement and structural models ( Chin, 2010 ; Hair et al., 2011 ; Magno et al., 2022 ; Mustafa et al., 2022 ; Peng et al., 2022 ; Zada M. et al., 2022 ; Zada S. et al., 2022 ; Guenther et al., 2023 ).
Evaluation of the measurement model
To assess the quality of the reflective constructs, the convergent validity and reliability of the construct must be assessed, that is, internal consistency ( Chin, 2010 ; Hair et al., 2011 ; Kock, 2015 ). And the following indicators must be met ( Table 2 ):
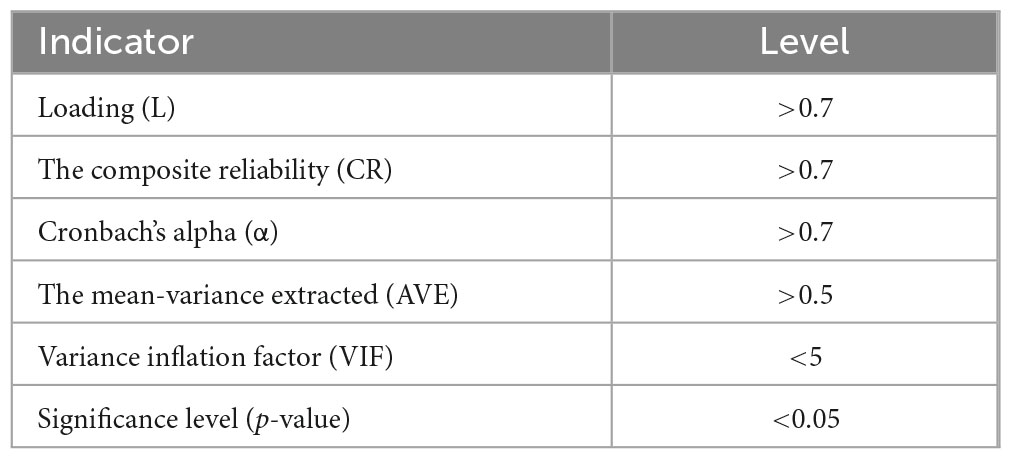
Table 2. Indicators to assess convergent validity and reliability of the constructs.
Table 3 shows that all the indicators are met. All loadings comply with being greater than 0.7 except for the items SL7 and WE7 whose values are 0.694 and 0.646, respectively, nevertheless, these items have been retained because the reliability indicators as a whole represent a good indicator; the Cronbach’s alpha and CR are greater than 0.7. Likewise, AVE also complies since they are all greater than 0.5. Also the full collinearity VIFs complies since all the values are less than 2.351 which is in the required range; under these terms ( Conforti et al., 2014 ) establish that tolerance lower than 0.2 or an indicator higher than 5 of VIFs represents a multicollinearity problem, for the case of this study the values oscillate between 1.116 and 2.351; this means that, the dispersion of the variables does not have a high correlation between them, this represents a high robustness in the results and estimated coefficient. According to the skewness and kurtosis, it is observed that the data do not have a normal distribution, however, one of the characteristics of the PLS-SEM approach is that it is relatively robust to deviations from normality ( Ramírez et al., 2014 )and requires assumptions less demanding about the distribution of data ( Hair et al., 2013 ). Since all the indicators comply, we proceed to the discriminant assessment.
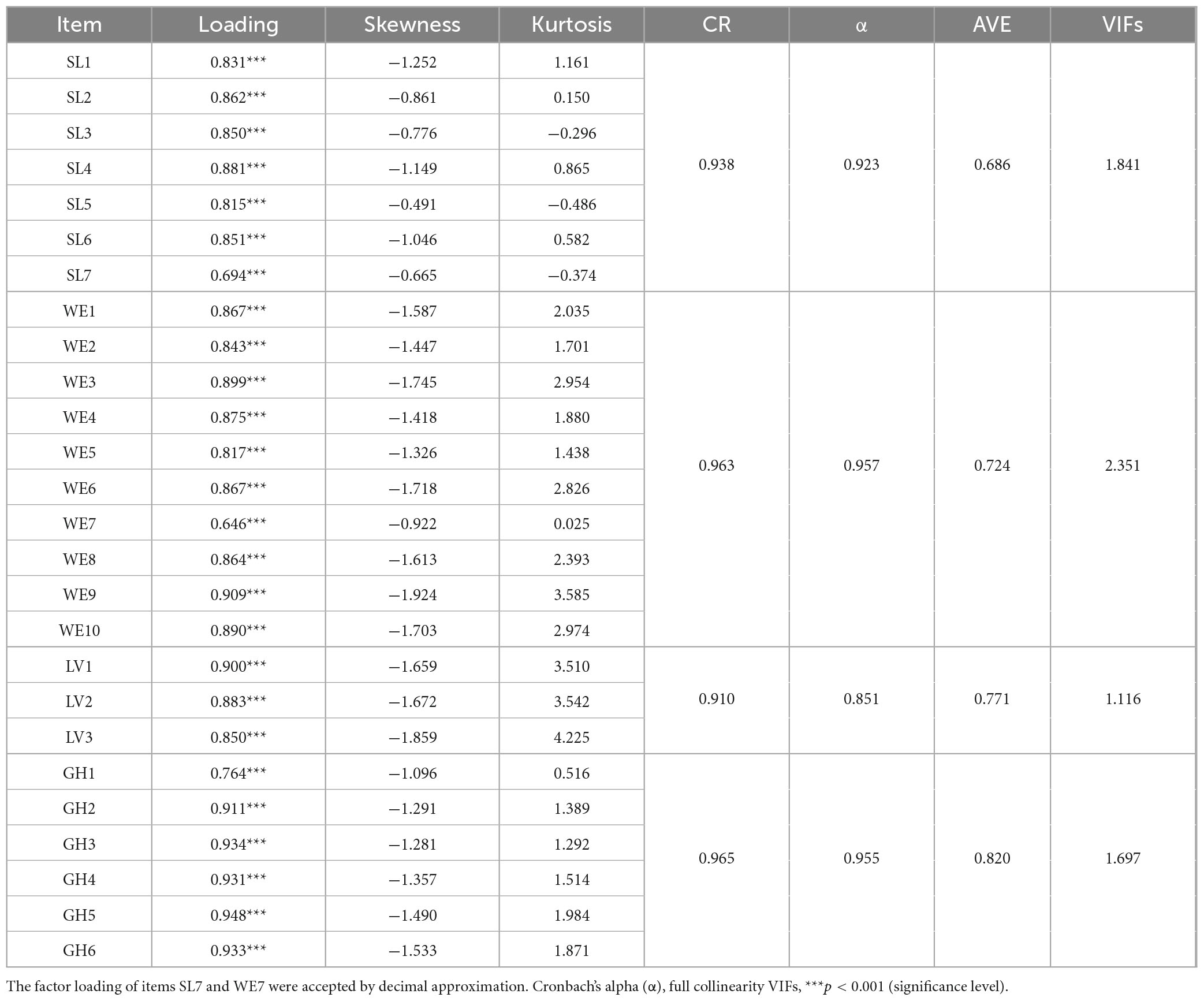
Table 3. Results of the evaluation of the measurement model.
Discriminant validity provides an indication of the extent to which each construct is different from other constructs in the model ( Chin, 2010 ). To meet discriminant validity, the square root of the AVE for each construct must be greater than the highest correlation between the construct and other constructs in the model ( Chin, 2010 ; Hair et al., 2011 ; Kock, 2014 ). Table 4 shows that the square root of the AVEs for all the constructs is greater than the correlation with the other constructs, indicating that the model has acceptable discriminant validity.
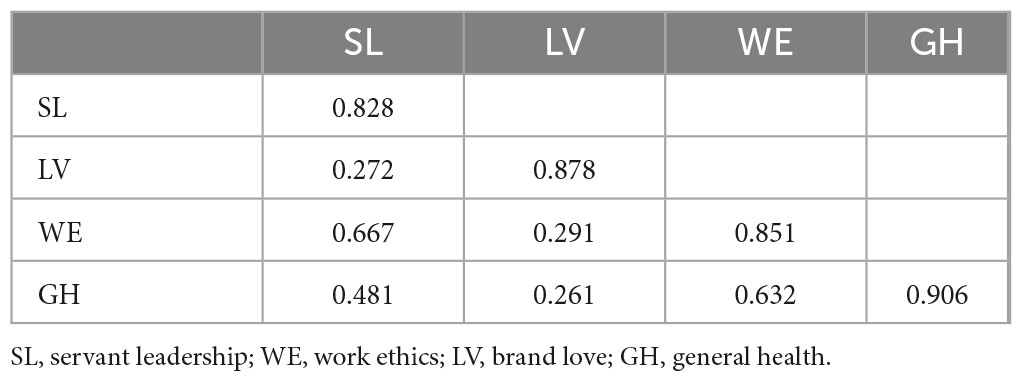
Table 4. Discriminant validity.
Goodness of fit of the structural model
Evaluating the fit of the statistical model to the study data involves evaluating the goodness of fit of the structural model. Table 5 shows the six goodness of fit indices that have been considered ( Kock, 2014 ), with a confidence level of 95%. In the case of the present study, the six fit indices suggested that the model fit was more than acceptable. The predictive validity of a construct can be confirmed when the value of its associated R 2 coefficient is greater than zero. This was the case for all values of the endogenous variables in the model, suggesting acceptable predictive validity across the model.
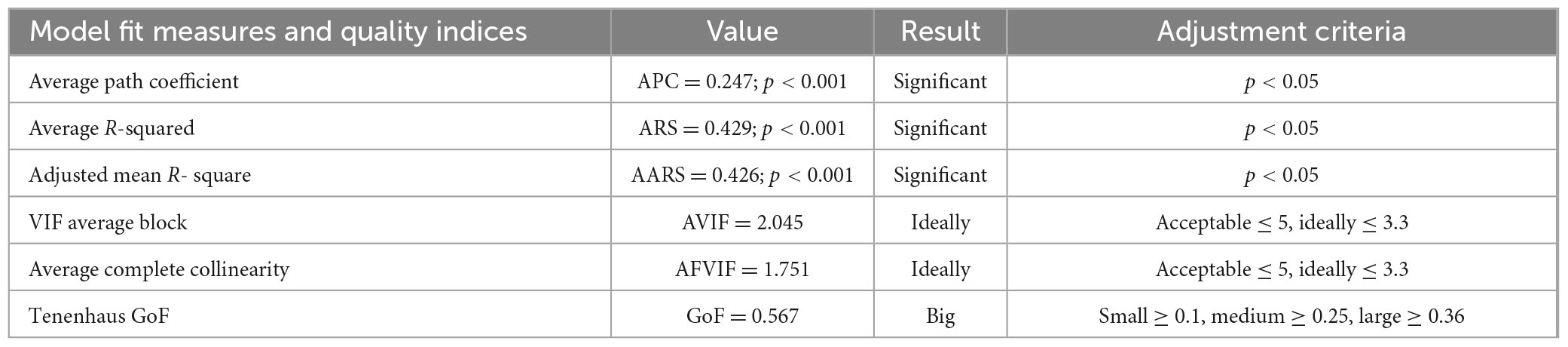
Table 5. Model fit and quality indices.
Structural model evaluation
To evaluate the structural model, two preliminary criteria must be verified and reported: the importance of the path coefficients and the coefficient value of R 2 for endogenous constructs. Each hypothesis is associated with a causal link in the structural model, which represents the relationships between a pair of constructs. Path coefficients have been calculated for each relationship in the model, as well as their corresponding p -values. Although the path coefficients must be significant, the value of the R 2 coefficient is highly dependent on the research area. Chin (1998) suggests values of 0.67, 0.33, and 0.19 as, respectively, substantial, moderate, and weak measures of R . In behavioral studies, a value of 0.2 for R 2 is generally considered acceptable ( Kock, 2013 ; Hair et al., 2014 ).
In the present study, the R 2 coefficient for GH was 0.43; this means that the proposed model explains 43% of the observed variability ( James et al., 2023 ); that is, servant leadership, love of the brand and work ethic predict the general health of workers by 43%. Therefore, this value has a high and acceptable level. Table 6 and Figure 2 show the results of the hypothesis tests and the evaluation of the path coefficients. The results show a positive and significant predictive value of SL on GH (H1), of LV on GH (H2), and WE on GH (H3).
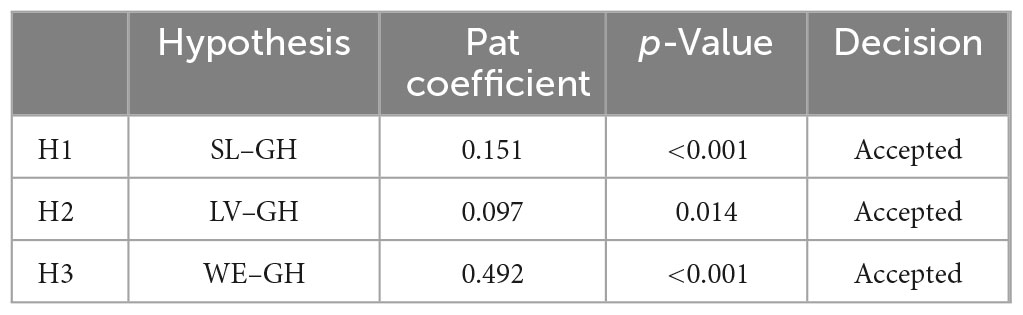
Table 6. Hypothesis test results.
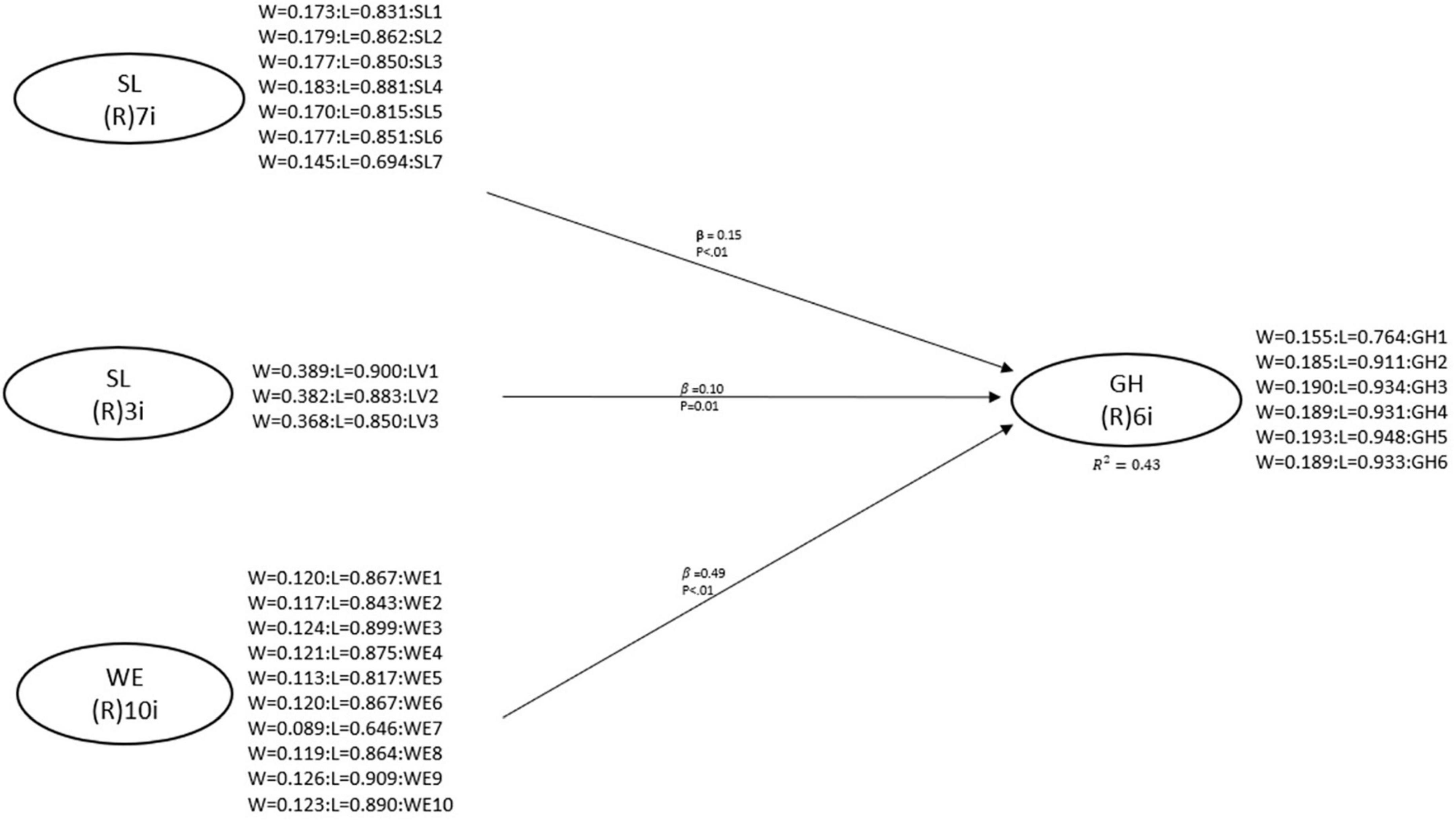
Figure 2. Results of the structural model.
For the fit index of the global model, the six indices of goodness of fit have been considered ( Kock, 2014 ), with a confidence level of 95%, the efficiency indices are the following:
• Average Path Coefficient (APC) and p < 0.05
• Average R -squared (ARS) and p < 0.05
• Adjusted mean R -square (AARS) > 0.02 and p > 0.05
• Average block VIF (AVIF), acceptable if ≤5, ideally ≤3.3
• Average complete collinearity (AFVIF), acceptable if ≤5, ideally ≤3.3
• Tenenhaus GoF, small ≥0.1, medium ≥0.25, large ≥0.36
In the case of the present study, the six fit indices suggested that the model fit was more than acceptable: APC = 0.247, p < 0.001; ARS = 0.429, p < 0.001; AARS = 0.426, p < 0.001; AVIF = 2,045 (acceptable if ≤5, ideally ≤3.3); AFVIF = 1.751 (acceptable if ≤5, ideally ≤3.3); and Tenenhaus GoF = 0.567 (small ≥0.1, medium ≥0.25, large ≥0.36). The predictive validity of a construct can be confirmed when the value of its associated to the coefficient R 2 is greater than zero. This was the case for all values of the endogenous variables in the model, which suggests an acceptable predictive validity in the whole model. This means that the fit indices submitted for evaluation support the robustness of the model, which affirms that servant leadership predicts general health by 15%; likewise, love for the brand also predicts general health by 10%, while work ethic predicts it by 49%; thus, it stands out that work ethic is a strong predictor of the variability of workers’ general health, this information provides an accurate picture to have a better understanding of the factors that predict general health.
The general health of workers has become an innate need in the work environment, especially in the educational sector, where teaching and administrative staff play a fundamental role in the development of students. Thus, it is important to promote the general health of workers in the education sector, since this act not only has benefits for them individually, but also has a positive impact on the work environment and on the quality of the education provided; in this context, this study proposed as its first objective to determine if servant leadership predicts the general health of employees. According to the findings, servant leadership predicts the general health of employees. This statement is supported by various scientists ( Hoch et al., 2016 ; Wang W. et al., 2022 ; Meuser and Smallfield, 2023 ), servant leadership has increased significantly among business leaders as it is considered a positive quality that is aimed at making them a role model due to their ethical behavior that contributes to the wellbeing of people; about, Wang Z. et al. (2022) and Iqbal et al. (2020) they show that the servant leader has a high capacity to promote the general health of employees through work motivation and empowerment; In this way, it is argued that promoting servant leadership is an assertive decision that opens the fulfillment of common objectives, thus generating a positive state in workers ( Xanthopoulou et al., 2012 ; Miao and Cao, 2019 ).
Additionally, a significant index of the brand love has been detected as a predictor of the general health of employees, this behavior is very recurrent, according to Junaid et al. (2020) the east arises as a result of positive feelings and satisfaction in different areas of life. About, Attiq et al. (2022) they highlight that the happiness, attachment and good relationship of people in the same environment can predict general health; specifically in the case of workers in the education sector, Strauss and Daniels (2013) establish that because teachers are exposed to extreme pressures due to daily work with students, parents and educational policies, their general wellbeing is at risk, attributing this fact to unhappiness in the workplace. work, for which they suggest healthy working conditions, which supposes a positive feeling in the school–teacher relationship, this statement is consistent with Li and Miao (2022) and Li et al. (2019) , who specify that positive emotions are vital issues in the teaching exercise, so the general health of workers is the result of their attachment or love that they maintain with their institution. And it is that every prosperous social connection established over time generates a special feeling called love, the same one that has the capacity to generate a feeling of wellbeing ( Oravecz et al., 2020 ).
Another of the findings guarantees the prediction of work ethics toward the general health of employees; about, Suryani et al. (2022) they place special emphasis on workers developing positive attitudes, including work ethic, which generates a feeling of wellbeing and health that can be moderated by the role assumed by the leader. In addition, another study linked to these results establishes that moral values are highly related to the prosperity of workers; that is, a healthy work environment promotes wellbeing and it is that according to research, workers value working conditions to a great extent, even when there are positive and negative aspects, they remain firm in their work ethic in order to achieve greater wellbeing ( King et al., 2011 ; Tacadena and Muico, 2022 ). Other studies that support the results of this research address work ethics as the construction of attitudes that derive from work-oriented values; in this way, a work environment governed by ethics and morality has a high potential to increase the general health of workers ( Sakr et al., 2022 ; Zúñiga et al., 2022 ); in this context, it is highlighted that promoting ethical principles creates a work environment where employees feel valued and motivated, and that the connection between work ethics and general health contains a solid approach that fosters an ideal work environment for personal development and professional of an individual.
Theoretical implications
This study leaves theoretical evidence of the connection of general health promoted as a result of servant leadership, brand love, and work ethic. The antecedents that were reviewed highlight that promoting servant leadership contributes to the general health of workers, so there is a need to create an adequate management culture in educational institutions, this implies maintaining a work environment where the leader promotes a climate of positive work that allows the wellbeing and health of workers. In addition, other research is highlighted that describes work ethics and love for the brand as a key element for the general health of workers, so a work environment where good practices exist will undoubtedly be a space where the worker express your wellbeing and general health, this being additionally supported by the emotional attachment you feel toward the institution where you work.
Practical implications
A concrete measure to take into account in the study population is to train leaders with a focus on servant leadership, this through training programs for leaders in order to develop the necessary skills that allow them to efficiently guide the work group. Likewise, it is necessary to foster a work environment that promotes for the brand love, establishing strategies and policies that strengthen the sense of belonging and pride of workers toward their institution. Finally, it is important to also prioritize work ethics, for which clear policies must be established in favor of integrity, equity and mutual respect. Therefore, all actions together could significantly improve working conditions, generating a virtuous circle where workers feel valued, encouraging them to contribute to quality education and the achievement of common institutional objectives.
Limitations and future research
Like previous studies, this research has some limitations that should be taken into account in future research. First, only one leadership style, servant leadership, was tested in this study. Therefore, future research should consider the use of other leadership styles (transformational, ethical, compassionate, charismatic, etc.) so that their behavior can be compared to a similar sample. Secondly, given that the study filtered out educational workers who had worked at the institution for a minimum of 6 months, it was a limitation for the research team, because in some institutions the worker was new staff, because the data began to be collected in the first 2 months of the academic year, in this sense, it is recommended to collect data after the half academic year, so that the leadership evaluation is relevant, unless an inclusion criterion unrelated to this is considered.
Third, the sociodemographic data from this study were not used in the hypothesis analysis. In future research, some sociodemographic data could be used as moderator variables, and consider expanding the sample. Fourth, this research only uses one leadership style, future research is expected to use charismatic leadership styles, transformational leadership, transactional leadership, business leadership, and e-leadership. And fifth, it is believed that it could be a great contribution to evaluate these constructs together with other associates, such as: environmental factors, job insecurity, labor ergonomics, among other important topics, as well as comparing the results in a longitudinal study.
And finally, based on the findings of this study, it is suggested that educational institutions promote the servant leadership style among their leaders and managers, including training in servant leadership skills and reinforcing programs that promote their application. In the same way, they should establish strategies that allow employees to foster love and loyalty toward their workplace, promoting the development of specialized programs that promote a healthy attachment to the brand. In addition to promoting a solid work ethic among leaders and employees, which allows favoring the organizational ethical climate. Knowing that this will also generate a better corporate reputation. In short, the results of this study have managed to achieve the proposed objectives and the hypotheses addressed at the beginning of the research have been tested, however, we believe that in future research the correlated construct could be evaluated in other economic sectors, as well as compare results over time.
The present study demonstrated that the three constructs (servant leadership, brand love, and work ethic) predict the general health of workers in a positive and significant way, in a sample of Peruvian workers in the education sector. Furthermore, the results suggest that these factors can be used to improve the health of employees in educational institutions in Peru and possibly in other contexts as well. The nature of the factors has shown that servant leadership skills, rather than a theoretical definition, is a significant factor shaping the health and wellbeing of an educational business community. Better leadership fosters professional attitudes, encourages ethical behavior, and improves worker health.
Data availability statement
The original contributions presented in this study are included in this article/supplementary material, further inquiries can be directed to the corresponding author.
Ethics statement
The studies involving human participants were reviewed and approved by the Ethics Committee of the Universidad Peruana Unión (2022-CE-EPG-0000167). The participants provided their written informed consent to participate in this study.
Author contributions
EL-A: Conceptualization, Data curation, Writing – original draft, Writing – review & editing. MV-G: Conceptualization, Investigation, Methodology, Project administration, Resources, Writing – original draft, Writing – review & editing. DM-L: Data curation, Formal analysis, Funding acquisition, Investigation, Resources, Software, Supervision, Validation, Visualization, Writing – review & editing.
The author(s) declare that no financial support was received for the research, authorship, and/or publication of this article.
Conflict of interest
The authors declare that the research was conducted in the absence of any commercial or financial relationships that could be construed as a potential conflict of interest.
Publisher’s note
All claims expressed in this article are solely those of the authors and do not necessarily represent those of their affiliated organizations, or those of the publisher, the editors and the reviewers. Any product that may be evaluated in this article, or claim that may be made by its manufacturer, is not guaranteed or endorsed by the publisher.
Ahuvia, A., Izberk-Bilgin, E., and Lee, K. (2022). Towards a theory of brand love in services: The power of identity and social relationships. J. Ser. Manag. 33, 453–464. doi: 10.1108/JOSM-06-2021-0221
Crossref Full Text | Google Scholar
Alfano, V. (2022). Work ethics, stay-at-home measures and COVID-19 diffusion: How is the pandemic affected by the way people perceive work? Eur. J. Health Econ. 23, 893–901. doi: 10.1007/s10198-021-01402-0
PubMed Abstract | Crossref Full Text | Google Scholar
Alipio, R., Avecillas, D., Santivañez, G., Mendoza, W., and Benites, E. (2023). Servant leadership and organizational performance: Mediating role of organizational culture. Problems Perspect. Manag. 21, 333–346. doi: 10.21511/ppm.21(4).2023.26
Ato, M., López, J. J., and Benavente, A. (2013). A classification system for research designs in psychology. Ann. Psychol. 29, 1038–1059. doi: 10.6018/analesps.29.3.178511
Attiq, S., Abdul, A., Khokhar, M., Shah, H., and Shahzad, A. (2022). ‘Wow! It’s cool’: How brand coolness affects the customer psychological well-being through brand love and brand engagement. Front. Psychol. 13:923870. doi: 10.3389/fpsyg.2022.923870
Bagozzi, R., Batra, R., and Ahuvia, A. (2017). Brand love: Development and validation of a practical scale. Market. Lett. 28, 1–14. doi: 10.1007/s11002-016-9406-1
Bairrada, C., Coelho, A., and Lizanets, V. (2019). The impact of brand personality on consumer behavior: The role of brand love. J. Fashion Market. Manag. Int. J. 23, 30–47. doi: 10.1108/JFMM-07-2018-0091
Batra, R., Ahuvia, A., and Bagozzi, R. P. (2012). Brand love. J. Market. 76, 1–16. doi: 10.1509/jm.09.0339
Bazzy, J. (2018). Work ethic dimensions as predictors of ego depletion. Curr. Psychol. 37, 198–206. doi: 10.1007/s12144-016-9503-6
Bezuidenhout, L., Rhoda, A., Conradsson, D., Mothabeng, J., and Joseph, C. (2023). The role of environmental factors on health conditions, general health and quality of life in persons with spinal cord injuries in South Africa. Int. J. Environ. Res. Public Health 20, 1–12. doi: 10.3390/ijerph20095709
Caycho-Rodríguez, T., Carbajal-León, C., and Noé-Grijalva, M. (2020). Loneliness, sex and general health perception in Peruvian older adults. Rev. Esp. Geriatría Gerontol. 55, 187–188. doi: 10.1016/j.regg.2020.02.005
Charbonnier-Voirin, A., El Akremi, A., and Vandenberghe, C. (2010). A multilevel model of transformational leadership and adaptive performance and the moderating role of climate for innovation. Group Organ. Manag. 35, 699–726. doi: 10.1177/1059601110390833
Chavez-Espinoza, J., Castillo-Romero, P. C., Ruiz-Reyes, R., Reyes-Mejía, M., and Hernández-López, M. Á (2022). Adaptación y validación peruana del cuestionario de salud general (ghq-12) en trabajadores de salud. Iatreia Rev. Méd. Univers. Antioq. 36, 1–22. doi: 10.17533/udea.iatreia.177
Chin, W. (1998). The partial least squares approach to structural formula modeling. Adv. Hosp. Leisure 8, 295–336.
Google Scholar
Chin, W. W. (2010). “How to write up and report PLS analyses,” in Handbook of partial least squares , eds V. Esposito Vinzi, W. Chin, J. Henseler, and H. Wang (Berlin: Springer), 655–690. doi: 10.1007/978-3-540-32827-8
Chukwuma, J., Areji, A., Obumse, N., Eze, E., Amadi, K., Agbo, C., et al. (2023). Impact of exposure to rational career reflective training on work-related ethics among student-philosophers. Medicine 102, 1–15. doi: 10.1097/MD.0000000000035608
Conforti, M., Pascale, S., Robustelli, G., and Sdao, F. (2014). Evaluation of prediction capability of the artificial neural networks for mapping landslide susceptibility in the turbolo river catchment (Northern Calabria, Italy). Catena 113, 236–250. doi: 10.1016/j.catena.2013.08.006
Cottey, L., and McKimm, J. (2019). Putting service back into health care through servant leadership. Br. J. Hosp. Med. 80, 220–224. doi: 10.12968/hmed.2019.80.4.220
Dahleez, K., and Aboramadan, M. (2022). Servant leadership and job satisfaction in higher education: The mediating roles of organizational justice and organizational trust. Int. J. Leadersh. Educ. 6, 1–22. doi: 10.1080/13603124.2022.2052753
de Ceballos, A. G., and Santos, G. B. (2015). Factors associated with musculoskeletal pain among teachers: Sociodemographics aspects, general health and well-being at work. Rev. Bras. Epidemiol. 18, 702–715. doi: 10.1590/1980-5497201500030015
der Kinderen, S., Valk, A., Khapova, S. N., and Tims, M. (2020). Facilitating eudaimonic well-being in mental health care organizations: The role of servant leadership and workplace civility climate. Int. J. Environ. Res. Public Health 17:1173. doi: 10.3390/ijerph17041173
Dollard, M., and Bakker, A. (2010). Psychosocial safety climate as a precursor to conducive work environments, psychological health problems, and employee engagement. J. Occup. Organ. Psychol. 83, 579–599. doi: 10.1348/096317909X470690
Ebert, S., Tucker, D. C., and Roth, D. L. (2002). Psychological resistance factors as predictors of general health status and physical symptom reporting. Psychol. Health Med. 7, 363–375. doi: 10.1080/13548500220139449
Eva, N., Robin, M., Sendjaya, S., van Dierendonck, D., and Liden, R. C. (2019). Servant leadership: A systematic review and call for future research. Leadersh. Q. 30, 111–132. doi: 10.1016/j.leaqua.2018.07.004
Fernstrand, A., Bury, D., Garssen, J., and Verster, J. C. (2017). Dietary intake of fibers: Differential effects in men and women on perceived general health and immune functioning. Food Nutr. Res. 61:1297053. doi: 10.1080/16546628.2017.1297053
Gandolfi, F., and Stone, S. (2018). Leadership, leadership styles, and servant leadership. J. Manag. Res. 18, 261–269.
Gladstone, T., Terrizzi, D. A., Paulson, A., Nidetz, J., Canel, J., Ching, E., et al. (2018). Effect of internet-based cognitive behavioral humanistic and interpersonal training vs internet-based general health education on adolescent depression in primary care: A randomized clinical trial. JAMA Netw. Open 1:e184278. doi: 10.1001/jamanetworkopen.2018.4278
Gocen, A., and Sen, S. (2021). A validation of servant leadership scale on multinational sample. Psychol. Rep. 124, 752–770. doi: 10.1177/0033294120957246
Grabowski, D., Chudzicka-Czupała, A., Chrupała-Pniak, M., Rachwaniec-Szczecińska, Z., Stasiła-Sieradzka, M., and Wojciechowska, W. (2019). Work ethic, organizational commitment and burnout. Med. Pracy 70, 305–316. doi: 10.13075/mp.5893.00800
Guenther, P., Guenther, M., Ringle, C. M., Zaefarian, G., and Cartwright, S. (2023). Improving PLS-SEM use for business marketing research. Ind. Market. Manag. 111, 127–142. doi: 10.1016/j.indmarman.2023.03.010
Hair, J. F., Anderson, R. E., Babin, B. J., and Black, W. C. (2010). Multivariate data analysis , 7th Edn, Vol. 8. Boston, MA: Cengage.
Hair, J. F., Ringle, C. M., and Sarstedt, M. (2013). Partial least squares structural equation modeling: Rigorous applications, better results and higher acceptance. Long Range Plann. 46, 1–12. doi: 10.1016/j.lrp.2013.01.001
Hair, J., Ringle, C. M., and Sarstedt, M. (2011). PLS-SEM: Indeed a silver bullet. J. Market. Theory Pract. 19, 139–152. doi: 10.2753/MTP1069-6679190202
Hair, J., Sarstedt, M., Hopkins, L., and Kuppelwieser, V. G. (2014). Partial least squares structural equation modeling (PLS-SEM): An emerging tool in business research. Eur. Bus. Rev. 26, 106–121. doi: 10.1108/EBR-10-2013-0128
Hoch, J., Bommer, W., and Wu, D. (2016). Do ethical, authentic, and servant leadership explain variance above and beyond transformational leadership? A meta-analysis. J. Manag. 44, 501–529. doi: 10.1177/0149206316665461
Hoekstra, J., Lenssen, E. S., Wong, A., Loef, B., Herber, G. C. M., Boshuizen, H. C., et al. (2023). Predicting self-perceived general health status using machine learning: An external exposome study. BMC Public Health 23:1027. doi: 10.1186/s12889-023-15962-8
Hystad, S., and Johnsen, B. H. (2020). The dimensionality of the 12-item general health questionnaire (GHQ-12): Comparisons of factor structures and invariance across samples and time. Front. Psychol. 11:1300. doi: 10.3389/fpsyg.2020.01300
Iqbal, A., Latif, K., and Ahmad, M. (2020). Servant leadership and employee innovative behaviour: Exploring psychological pathways. Leadersh. Organ. Dev. J. 41, 813–827. doi: 10.1108/LODJ-11-2019-0474
Isola, G. (2020). Oral health and related factors associated with general health and quality of life. Appl. Sci. 10:5. doi: 10.3390/app10134663
Jakubiec, D., Jagielska, K., Karmowski, M., Kubicka, K., Karmowski, A., and Sobiech, K. (2014). Effect of attending childbirth education classes on psychological distress in pregnant women measured by means of the general health questionnaire. Adv. Clin. Exp. Med. 23, 953–957. doi: 10.17219/acem/37351
James, G., Witten, D., Hastie, T., and Tibshirani, R. (2023). An introduction to statistical learning with applications in R , 2nd Edn. New York, NY: Springer.
Junaid, M., Hussain, K., Asghar, M., Javed, M., and Hou, F. (2020). “An investigation of the diners’ brand love in the value co-creation process. J. Hosp. Tour. Manag. 45, 172–181. doi: 10.1016/j.jhtm.2020.08.008
Kaltiainen, J., and Hakanen, J. (2022). Fostering task and adaptive performance through employee well-being: The role of servant leadership. BRQ Bus. Res. Q. 25, 28–43. doi: 10.1177/2340944420981599
King, E., Dawson, J., West, M., Gilrane, V., Peddie, C., and Bastin, L. (2011). Why organizational and community diversity matter: Representativeness and the emergence of incivility and organizational performance. Acad. Manag. 54, 1113–1118. doi: 10.5465/amj.2010.0016
Kisiel, M., Lee, S., Malmquist, S., Rykatkin, O., Holgert, S., Janols, H., et al. (2023). Clustering analysis identified three long COVID phenotypes and their association with general health status and working ability. J. Clin. Med. 12, 1–17. doi: 10.3390/jcm12113617
Kock, N. (2011). Using WarpPLS in E-collaboration studies: Descriptive statistics, settings, and key analysis results. Int. J. E Collab. 7, 1–18. doi: 10.4018/jec.2011040101
Kock, N. (2013). WarpPLS 4.0 user manual. Laredo, TX: ScriptWarp Systems.
Kock, N. (2014). Advanced mediating effects tests, multi-group analyses, and measurement model assessments in PLS-based SEM. Int. J. E Collab. 10, 1–13. doi: 10.4018/ijec.2014010101
Kock, N. (2015). A note on how to conduct a factor-based PLS-SEM analysis. Int. J. E Collab. 11, 1–9. doi: 10.4018/ijec.2015070101
Larregui-Candelaria, G., Sosa-Varela, J. C., and Ortíz-Soto, M. (2019). Amor hacia la marca: Una perspectiva de relación continua. Estud. Gerenciales 35, 271–282.
Li, B., and Miao, G. (2022). “On the role of Chinese English as a foreign language: Teachers’ well-being and loving pedagogy in their academic engagement. Front. Psychol. 13:941226. doi: 10.3389/fpsyg.2022.941226
Li, G., Sun, Z., and Jee, Y. (2019). The more technology the better? A comparison of teacher-student interaction in high and low technology use elementary EFL classrooms in China. System 84, 24–40. doi: 10.1016/j.system.2019.05.003
Lunde, L., Fløvik, L., Christensen, J., Johannessen, H. A., Finne, L., Jørgensen, I., et al. (2022). The relationship between telework from home and employee health: A systematic review. BMC Public Health 22:47. doi: 10.1186/s12889-021-12481-2
Lusiani, M., Abidin, Z., Fitrianingsih, D., Yusnita, E., Adiwinata, D., Rachmaniah, D., et al. (2020). Effect of servant, digital and green leadership toward business performance: Evidence from Indonesian manufacturing. Syst. Rev. Pharm. 11, 1351–1361. doi: 10.31838/SRP.2020.11.192
Lütke, S., Pfeifer, R., Lahmann, C., and Wünsch, A. (2022). How to measure the mental health of teachers? Psychometric properties of the GHQ-12 in a large sample of german teachers. International J. Environ. Res. Public Health 19, 1–17. doi: 10.3390/ijerph19159708
Maaz, U., and Farroq, S. (2017). Effect of Islamic work ethics on employee well-being, job stress and turnover intention. Sarhad J. Manag. Sci . 2, 1–8. doi: 10.31529/sjms.2016.2.2.5
Magno, F., Cassia, F., and Ringle, C. M. M. (2022). A brief review of partial least squares structural equation modeling (PLS-SEM) use in quality management studies. TQM J. 1–15. doi: 10.1108/TQM-06-2022-0197
Malakoutikhah, A., Zakeri, M., Derakhtanjani, A., and Dehghan, M. (2022). Anxiety, anger, and mindfulness as predictors of general health in the general population during COVID-19 outbreak: A survey in Southeast Iran. J. Commun. Psychol. 50, 916–927. doi: 10.1002/jcop.22690
Mayhew, E., Stuttard, L., and Beresford, B. (2021). An assessment of the psychometric properties of the GHQ-12 in an English population of autistic adults without learning difficulties. J. Autism Dev. Disord. 51, 1093–1106. doi: 10.1007/s10803-020-04604-2
McKimm, J., Ramani, S., Forrest, K., Bishop, J., Findyartini, A., Mills, C., et al. (2023). Adaptive leadership during challenging times: Effective strategies for health professions educators: AMEE guide no. 148. Med. Teach. 45, 128–138. doi: 10.1080/0142159X.2022.2057288
Meriac, J., Rasmussen, K. E., and Pang, J. (2023). Work ethic and grit: Explaining responses to dissatisfaction at work. Pers. Individ. Differ. 203:112037. doi: 10.1016/j.paid.2022.112037
Meuser, J., and Smallfield, J. (2023). Servant leadership: The missing community component. Bus. Horiz. 66, 251–264. doi: 10.1016/j.bushor.2022.07.002
Miao, R., and Cao, Y. (2019). High-performance work system, work well-being, and employee creativity: Cross-level moderating role of transformational leadership. Int. J. Environ. Res. Public Health 16:9. doi: 10.3390/ijerph16091640
Mirsaleh, Y. R., Rezai, H., Khabaz, M., Ardekani, I. A., and Abdi, K. (2011). Personality dimensions, religious tendencies and coping strategies as predictors of general health in iranian mothers of children with intellectual disability: A comparison with mothers of typically developing children. J. Appl. Res. Intell. Disabil. 24, 573–582. doi: 10.1111/j.1468-3148.2011.00639.x
Mitonga-Monga, J., Mokhethi, M., Keswa, B., Lekoma, B., Mathebula, L., and Mbatha, L. (2023). Ethical leadership in relation to employee commitment in a South African manufacturing company. Acta Comm. Independ. Res. J. Manag. Sci. 23, 1–9. doi: 10.4102/ac.v23i1.1046
Moll, J., and Kretzschmar, L. (2017). An investigation of the suitability of a servant leadership model for academic group leaders at german universities. J. Leadersh. Educ. 16, 166–182. doi: 10.12806/v16/i2/t1
Montano, D., Reeske, A., Franke, F., and Hüffmeier, J. (2017). “Leadership, followers’ mental health and job performance in organizations: A comprehensive meta-analysis from an occupational health perspective. J. Organ. Behav. 38, 327–350. doi: 10.1002/job.2124
Montazeri, A., Harirchi, A., Shariati, M., Garmaroudi, G., Ebadi, M., and Fateh, A. (2003). The 12-item general health questionnaire (GHQ-12): Translation and validation study of the Iranian version. Health Q. Life Outcomes 1:66. doi: 10.1186/1477-7525-1-66
Mustafa, K., Ahmad, F., Qaisar, M., Zada, S., Jamil, S., Anwer, N., et al. (2022). Brand love: Role of online customer experience, value co-creation, and relationship quality. Front. Psychol. 13:897933. doi: 10.3389/fpsyg.2022.897933
Na, M., Rong, L., Ali, M., Alam, S., Masukujjaman, M., and Ali, K. (2023). The mediating role of brand trust and brand love between brand experience and loyalty: A study on smartphones in China. Behav. Sci. 13:502. doi: 10.3390/bs13060502
Nadi, T., Poorolajal, J., and Doosti-Irani, A. (2020). Socioeconomic status and health literacy as the important predictors of general health in Iran: A structural equation modeling approach. Epidemiol. Biostat. Public Health 17, 1–11. doi: 10.2427/13312
Newman, A., Schwarz, G., Cooper, B., and Sendjaya, S. (2017). How servant leadership influences organizational citizenship behavior: The roles of LMX, empowerment, and proactive personality. J. Bus. Ethics 145, 49–62. doi: 10.1007/s10551-015-2827-6
Nielsen, A., Jensen, P., Gannik, D., Reventlow, S., Hollnagel, H., and De Fine Olivarius, N. (2015). Change in self-rated general health is associated with perceived illness burden: A 1-year follow up of patients newly diagnosed with type 2 diabetes disease epidemiology – chronic. BMC Public Health 15:439. doi: 10.1186/s12889-015-1790-6
Olesia, W., Namusonge, G., and Iravo, M. (2014). Servant leadership: The exemplifying behaviours. IOSR J. Hum. Soc. Sci. 19, 75–80.
Oravecz, Z., Dirsmith, J., Heshmati, S., Vandekerckhove, J., and Brick, T. (2020). Psychological well-being and personality traits are associated with experiencing love in everyday life. Pers. Individ. Differ. 153:109620. doi: 10.1016/j.paid.2019.109620
Page, D., and Wong, P. (2000). “A conceptual framework for measuring servant-leadership,” in The human factor in shaping the course of history and development , ed. S. Adjibolosoo (Boston, MA: University Press of America).
Peng, J., Samad, S., Comite, U., Ahmad, N., Han, H., Ariza-Montes, A., et al. (2022). Environmentally specific servant leadership and employees’ energy-specific pro-environmental behavior: Evidence from healthcare sector of a developing economy. Int. J. Environ. Res. Public Health 19, 1–16. doi: 10.3390/ijerph19137641
Pino, R., Arévalo-Avecillas, D., and Padilla-Lozano, C. (2020). Servant leadership and job satisfaction in master of business administration students. Form. Univers. 13, 205–216. doi: 10.4067/S0718-50062020000600205
Purwanto, A. (2020). The relationship of transformational leadership, organizational justice and organizational commitment: A mediation effect of job satisfaction. J. Crit. Rev. 7, 89–108.
Quick, J., and Henderson, D. F. (2016). Occupational stress: Preventing suffering, enhancing wellbeing. Int. J. Environ. Res. Public Health 13:459. doi: 10.3390/ijerph13050459
Raja, U., Haq, I. U., De Clercq, D., and Azeem, M. U. (2020). When ethics create misfit: Combined effects of despotic leadership and Islamic work ethic on job performance, job satisfaction, and psychological well-being. Int. J. Psychol. 55, 332–341. doi: 10.1002/ijop.12606
Ramírez, P. E., Mariano, A. M., and Salazar, E. A. (2014). Propuesta metodológica para aplicar modelos de ecuaciones estructurales Con PLS: El caso del uso de las bases de datos científicas en estudiantes universitarios. Rev. ADMPG 7, 133–139.
Rocha-Vallejos, K., Rabanal-Altamirano, S., Robles-Fabian, D., and Cordova-Buiza, F. (2022). Does brand love strictly increase purchase intention? The case of linio. Innov. Market. 18, 36–47. doi: 10.21511/im.18(4).2022.04
Rodrigues, P., Pinto, A., and Sousa, A. (2022). Authenticity as an antecedent of brand image in a positive emotional consumer relationship: The case of craft beer brands. Euromed J. Bus. 17, 634–651. doi: 10.1108/EMJB-03-2021-0041
Ruiz-Palomino, P., Linuesa-Langreo, J., and Elche, D. (2021). Team-level servant leadership and team performance: The mediating roles of organizational citizenship behavior and internal social capital. Bus. Ethics Environ. Respons. 32, 127–144. doi: 10.1111/BEER.12390
Sajtos, L., Cao, J. T., Espinosa, J. A., Phau, I., Rossi, P., Sung, B., et al. (2021). Brand love: Corroborating evidence across four continents. J. Bus. Res. 126, 591–604. doi: 10.1016/j.jbusres.2020.02.040
Sakr, F., Haddad, C., Zeenny, R., Sacre, H., Akel, M., Iskandar, K., et al. (2022). Work ethics and ethical attitudes among healthcare professionals: The role of leadership skills in determining ethics construct and professional behaviors. Healthcare 10:1399. doi: 10.3390/healthcare10081399
Setati, S., Stander, M., and Ukpere, W. (2015). Job insecurity, sense of coherence and the general health of employees at a higher education institution in South Africa. Corp. Ownersh. Control 12, 660–670. doi: 10.22495/cocv12i4c6p6
Sharma, B., and Rai, S. (2015). A study to develop an instrument to measure work ethic. Glob. Bus. Rev. 16, 244–257. doi: 10.1177/0972150914564417
Shi, Y., Li, D., Zhang, N., Jiang, P., Yuling, D., Xie, J., et al. (2022). “Job crafting and employees’ general health: The role of work–nonwork facilitation and perceived boundary control. BMC Public Health 22:1196. doi: 10.1186/s12889-022-13569-z
Siu, O., Cheung, F., and Lui, S. (2015). Linking positive emotions to work well-being and turnover intention among Hong Kong police officers: The role of psychological capital. J. Happiness Stud. 16, 367–380. doi: 10.1007/s10902-014-9513-8
Sjamsoeddin, S., Yusgiantoro, P., Saragih, H., and Soepandji, B. (2023). Transformational bureaucratic leadership model to support national defense policy in Indonesia. J. Ilmu Sos. Ilmu Politik 26, 227–239. doi: 10.22146/jsp.70204
Strauss, E., and Daniels, D. (2013). ‘Dis‘n tydbom. die skip gaan sink’: Emosionele welsyn van hoërskool-opvoeders in die Helderberg-area van die wes-kaap. Tydskrif Geesteswetenskappe 53, 391–403.
Suryani, S., Sudrajat, B., Hendryadi, H., Saihu, M., Amalia, E., and Fathoni, M. (2022). Development of thriving at work and organizational citizenship behavior through Islamic work ethics and humble leadership. Asian J. Bus. Ethics 12, 1–23. doi: 10.1007/s13520-022-00160-3
Tacadena, J., and Muico, E. (2022). A teacher’s work ethic: Exploring organizational behaviour, quality work life and commitment. J. Women Empower. Stud. 25, 36–41. doi: 10.55529/jwes.25.36.41
Tadesse Bogale, A., and Ayenew Birbirsa, Z. (2023). HR system and work ethics: A systematic review. Cogent Bus. Manag. 10:2278848. doi: 10.1080/23311975.2023.2278848
Tijjang, B., Junaidi, J., Nurfadhilah, N., and Putra, P. (2023). The role of brand love, trust, and commitment in fostering consumer satisfaction and loyalty. FWU J. Soc. Sci. 17, 110–126. doi: 10.51709/19951272/Spring
Tinella, L., Tinterri, A., Dipace, A., Ladogana, M., Loiodice, I., and Bosco, A. (2022). Sociodemographic and digital education factors are associated to general health, coping strategies, and academic outcomes of undergraduate students during the post-pandemic period. Eur. J. Invest. Health Psychol. Educ. 12, 1334–1348. doi: 10.3390/ejihpe12090093
Turner, K. (2022). Servant leadership to support wellbeing in higher education teaching. J. Further High. Educ. 46, 947–958. doi: 10.1080/0309877X.2021.2023733
van Dierendonck, D., and Nuijten, I. (2011). The servant leadership survey: Development and validation of a multidimensional measure. J. Bus. Psychol. 26, 249–267. doi: 10.1007/s10869-010-9194-1
Wang, C., Liu, J., Pu, R., Li, Z., Guo, W., Feng, Z., et al. (2020). Determinants of subjective health, happiness, and life satisfaction among young adults (18-24 Years) in Guyana. Biomed Res. Int. 2020:9063808. doi: 10.1155/2020/9063808
Wang, W., Kang, S., and Choi, S. (2022). Servant leadership and creativity: A study of the sequential mediating roles of psychological safety and employee well-being. Front. Psychol. 12:807070. doi: 10.3389/fpsyg.2021.807070
Wang, X., and Binti, N. A. (2023). Nexus between brand love, loyalty, affective commitment and positive word of mouth: In the context of social identity theory. Sustainability 15:3813. doi: 10.3390/su15043813
Wang, Z., Panaccio, A., Raja, U., Donia, M., Landry, G., Pereira, M., et al. (2022). Servant leadership and employee wellbeing: A crosscultural investigation of the moderated path model in Canada, Pakistan, China, the US, and Brazil. Int. J. Cross Cult. Manag. 22, 301–325. doi: 10.1177/14705958221112859
Woehr, D., Arciniega, L. M., González, L., and Stanley, L. J. (2023). Live to work, work to live, and work as a necessary evil: An examination of the structure and stability of work ethic profiles. Group Organ. Manag. 1–39. doi: 10.1177/10596011221146363
World Health Organization (2004). Completed report of the department of mental health and substance abuse of the World Health Organization . Available online at: http://www.asmi.es/arc/doc/promocion_de_la_salud_mental.pdf
Xanthopoulou, D., Bakker, A., and Ilies, R. (2012). Everyday working life: Explaining within-person fluctuations in employee well-being. Hum. Relat. 65, 1051–1069. doi: 10.1177/0018726712451283
Yuan, M., Cai, W., Gao, X., and Fu, J. (2020). How servant leadership leads to employees’ customer-oriented behavior in the service industry? A dual-mechanism model. Int. J. Environ. Res. Public Health 17:2296. doi: 10.3390/ijerph17072296
Zada, M., Zada, S., Khan, J., Saeed, I., Zhang, Y., Vega-Muñoz, A., et al. (2022). Does servant leadership control psychological distress in crisis? Moderation and mediation mechanism. Psychol. Res. Behav. Manag. 15, 607–622. doi: 10.2147/PRBM.S354093
Zada, S., Khan, J., Saeed, I., Yong Jun, Z., Vega-Muñoz, A., and Contreras-Barraza, N. (2022). Servant leadership behavior at workplace and knowledge hoarding: A moderation mediation examination. Front. Psychol. 13:888761. doi: 10.3389/fpsyg.2022.888761
Zeng, J., Lai, J., and Liu, X. (2022). How servant leadership motivates young university teachers’ workplace well-being: The role of occupational commitment and risk perception. Front. Psychol. 13:996497. doi: 10.3389/fpsyg.2022.996497
Zetterberg, H., Owiredua, C., Åsenlöf, P., Lennartsson, R., Jansen, G., Boersma, K., et al. (2023). Preventing pain and stress-related ill-health in employees: A 6-months follow-up of a psychosocial program in a cluster randomized controlled trial. J. Occup. Rehabil. 33, 316–328. doi: 10.1007/s10926-022-10074-3
Zúñiga, C., Aguado, D., and Cabrera-Tenecela, P. (2022). Examining work ethic across Latam populations: Differences between Ecuadorian and Chilean workers. J. Glob. Competitiv. Governabil. 16, 53–69. doi: 10.3232/GCG.2022.V16.N3.02
Keywords : servant leadership, brand love, work ethic, general health, education sector, Peru
Citation: Laura-Arias E, Villar-Guevara M and Millones-Liza DY (2024) Servant leadership, brand love, and work ethic: important predictors of general health in workers in the education sector. Front. Psychol. 15:1274965. doi: 10.3389/fpsyg.2024.1274965
Received: 16 August 2023; Accepted: 01 March 2024; Published: 05 April 2024.
Reviewed by:
Copyright © 2024 Laura-Arias, Villar-Guevara and Millones-Liza. This is an open-access article distributed under the terms of the Creative Commons Attribution License (CC BY) . The use, distribution or reproduction in other forums is permitted, provided the original author(s) and the copyright owner(s) are credited and that the original publication in this journal is cited, in accordance with accepted academic practice. No use, distribution or reproduction is permitted which does not comply with these terms.
*Correspondence: Miluska Villar-Guevara, [email protected]
Disclaimer: All claims expressed in this article are solely those of the authors and do not necessarily represent those of their affiliated organizations, or those of the publisher, the editors and the reviewers. Any product that may be evaluated in this article or claim that may be made by its manufacturer is not guaranteed or endorsed by the publisher.
What is The Null Hypothesis & When Do You Reject The Null Hypothesis
Julia Simkus
Editor at Simply Psychology
BA (Hons) Psychology, Princeton University
Julia Simkus is a graduate of Princeton University with a Bachelor of Arts in Psychology. She is currently studying for a Master's Degree in Counseling for Mental Health and Wellness in September 2023. Julia's research has been published in peer reviewed journals.
Learn about our Editorial Process
Saul Mcleod, PhD
Editor-in-Chief for Simply Psychology
BSc (Hons) Psychology, MRes, PhD, University of Manchester
Saul Mcleod, PhD., is a qualified psychology teacher with over 18 years of experience in further and higher education. He has been published in peer-reviewed journals, including the Journal of Clinical Psychology.
Olivia Guy-Evans, MSc
Associate Editor for Simply Psychology
BSc (Hons) Psychology, MSc Psychology of Education
Olivia Guy-Evans is a writer and associate editor for Simply Psychology. She has previously worked in healthcare and educational sectors.
On This Page:
A null hypothesis is a statistical concept suggesting no significant difference or relationship between measured variables. It’s the default assumption unless empirical evidence proves otherwise.
The null hypothesis states no relationship exists between the two variables being studied (i.e., one variable does not affect the other).
The null hypothesis is the statement that a researcher or an investigator wants to disprove.
Testing the null hypothesis can tell you whether your results are due to the effects of manipulating the dependent variable or due to random chance.
How to Write a Null Hypothesis
Null hypotheses (H0) start as research questions that the investigator rephrases as statements indicating no effect or relationship between the independent and dependent variables.
It is a default position that your research aims to challenge or confirm.
For example, if studying the impact of exercise on weight loss, your null hypothesis might be:
There is no significant difference in weight loss between individuals who exercise daily and those who do not.
Examples of Null Hypotheses
When do we reject the null hypothesis .
We reject the null hypothesis when the data provide strong enough evidence to conclude that it is likely incorrect. This often occurs when the p-value (probability of observing the data given the null hypothesis is true) is below a predetermined significance level.
If the collected data does not meet the expectation of the null hypothesis, a researcher can conclude that the data lacks sufficient evidence to back up the null hypothesis, and thus the null hypothesis is rejected.
Rejecting the null hypothesis means that a relationship does exist between a set of variables and the effect is statistically significant ( p > 0.05).
If the data collected from the random sample is not statistically significance , then the null hypothesis will be accepted, and the researchers can conclude that there is no relationship between the variables.
You need to perform a statistical test on your data in order to evaluate how consistent it is with the null hypothesis. A p-value is one statistical measurement used to validate a hypothesis against observed data.
Calculating the p-value is a critical part of null-hypothesis significance testing because it quantifies how strongly the sample data contradicts the null hypothesis.
The level of statistical significance is often expressed as a p -value between 0 and 1. The smaller the p-value, the stronger the evidence that you should reject the null hypothesis.
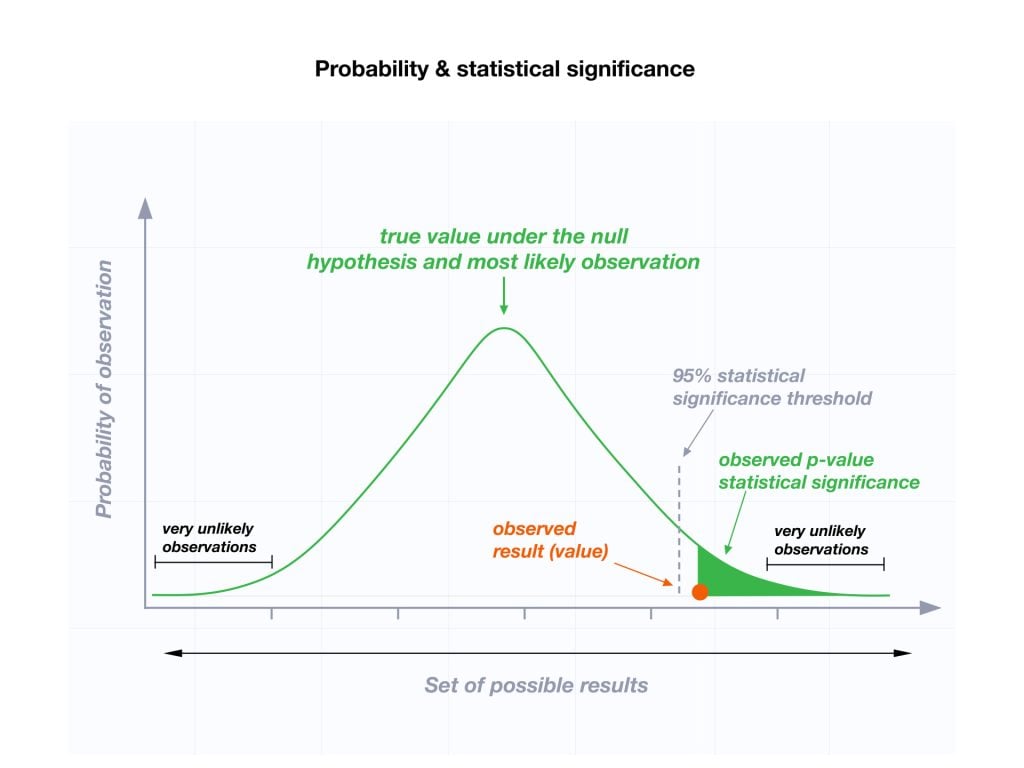
Usually, a researcher uses a confidence level of 95% or 99% (p-value of 0.05 or 0.01) as general guidelines to decide if you should reject or keep the null.
When your p-value is less than or equal to your significance level, you reject the null hypothesis.
In other words, smaller p-values are taken as stronger evidence against the null hypothesis. Conversely, when the p-value is greater than your significance level, you fail to reject the null hypothesis.
In this case, the sample data provides insufficient data to conclude that the effect exists in the population.
Because you can never know with complete certainty whether there is an effect in the population, your inferences about a population will sometimes be incorrect.
When you incorrectly reject the null hypothesis, it’s called a type I error. When you incorrectly fail to reject it, it’s called a type II error.
Why Do We Never Accept The Null Hypothesis?
The reason we do not say “accept the null” is because we are always assuming the null hypothesis is true and then conducting a study to see if there is evidence against it. And, even if we don’t find evidence against it, a null hypothesis is not accepted.
A lack of evidence only means that you haven’t proven that something exists. It does not prove that something doesn’t exist.
It is risky to conclude that the null hypothesis is true merely because we did not find evidence to reject it. It is always possible that researchers elsewhere have disproved the null hypothesis, so we cannot accept it as true, but instead, we state that we failed to reject the null.
One can either reject the null hypothesis, or fail to reject it, but can never accept it.
Why Do We Use The Null Hypothesis?
We can never prove with 100% certainty that a hypothesis is true; We can only collect evidence that supports a theory. However, testing a hypothesis can set the stage for rejecting or accepting this hypothesis within a certain confidence level.
The null hypothesis is useful because it can tell us whether the results of our study are due to random chance or the manipulation of a variable (with a certain level of confidence).
A null hypothesis is rejected if the measured data is significantly unlikely to have occurred and a null hypothesis is accepted if the observed outcome is consistent with the position held by the null hypothesis.
Rejecting the null hypothesis sets the stage for further experimentation to see if a relationship between two variables exists.
Hypothesis testing is a critical part of the scientific method as it helps decide whether the results of a research study support a particular theory about a given population. Hypothesis testing is a systematic way of backing up researchers’ predictions with statistical analysis.
It helps provide sufficient statistical evidence that either favors or rejects a certain hypothesis about the population parameter.
Purpose of a Null Hypothesis
- The primary purpose of the null hypothesis is to disprove an assumption.
- Whether rejected or accepted, the null hypothesis can help further progress a theory in many scientific cases.
- A null hypothesis can be used to ascertain how consistent the outcomes of multiple studies are.
Do you always need both a Null Hypothesis and an Alternative Hypothesis?
The null (H0) and alternative (Ha or H1) hypotheses are two competing claims that describe the effect of the independent variable on the dependent variable. They are mutually exclusive, which means that only one of the two hypotheses can be true.
While the null hypothesis states that there is no effect in the population, an alternative hypothesis states that there is statistical significance between two variables.
The goal of hypothesis testing is to make inferences about a population based on a sample. In order to undertake hypothesis testing, you must express your research hypothesis as a null and alternative hypothesis. Both hypotheses are required to cover every possible outcome of the study.
What is the difference between a null hypothesis and an alternative hypothesis?
The alternative hypothesis is the complement to the null hypothesis. The null hypothesis states that there is no effect or no relationship between variables, while the alternative hypothesis claims that there is an effect or relationship in the population.
It is the claim that you expect or hope will be true. The null hypothesis and the alternative hypothesis are always mutually exclusive, meaning that only one can be true at a time.
What are some problems with the null hypothesis?
One major problem with the null hypothesis is that researchers typically will assume that accepting the null is a failure of the experiment. However, accepting or rejecting any hypothesis is a positive result. Even if the null is not refuted, the researchers will still learn something new.
Why can a null hypothesis not be accepted?
We can either reject or fail to reject a null hypothesis, but never accept it. If your test fails to detect an effect, this is not proof that the effect doesn’t exist. It just means that your sample did not have enough evidence to conclude that it exists.
We can’t accept a null hypothesis because a lack of evidence does not prove something that does not exist. Instead, we fail to reject it.
Failing to reject the null indicates that the sample did not provide sufficient enough evidence to conclude that an effect exists.
If the p-value is greater than the significance level, then you fail to reject the null hypothesis.
Is a null hypothesis directional or non-directional?
A hypothesis test can either contain an alternative directional hypothesis or a non-directional alternative hypothesis. A directional hypothesis is one that contains the less than (“<“) or greater than (“>”) sign.
A nondirectional hypothesis contains the not equal sign (“≠”). However, a null hypothesis is neither directional nor non-directional.
A null hypothesis is a prediction that there will be no change, relationship, or difference between two variables.
The directional hypothesis or nondirectional hypothesis would then be considered alternative hypotheses to the null hypothesis.
Gill, J. (1999). The insignificance of null hypothesis significance testing. Political research quarterly , 52 (3), 647-674.
Krueger, J. (2001). Null hypothesis significance testing: On the survival of a flawed method. American Psychologist , 56 (1), 16.
Masson, M. E. (2011). A tutorial on a practical Bayesian alternative to null-hypothesis significance testing. Behavior research methods , 43 , 679-690.
Nickerson, R. S. (2000). Null hypothesis significance testing: a review of an old and continuing controversy. Psychological methods , 5 (2), 241.
Rozeboom, W. W. (1960). The fallacy of the null-hypothesis significance test. Psychological bulletin , 57 (5), 416.

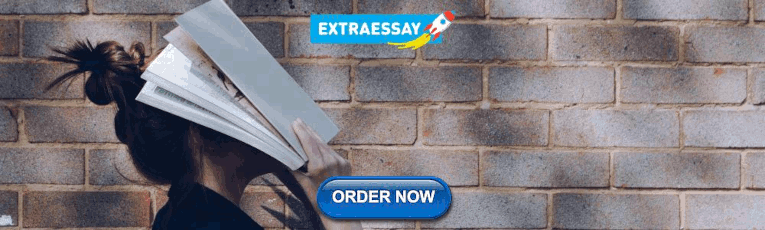
IMAGES
VIDEO
COMMENTS
Examples. A research hypothesis, in its plural form "hypotheses," is a specific, testable prediction about the anticipated results of a study, established at its outset. It is a key component of the scientific method. Hypotheses connect theory to data and guide the research process towards expanding scientific understanding.
In psychology, the hypothesis might focus on how a certain aspect of the environment might influence a particular behavior. Remember, a hypothesis does not have to be correct. ... These precise descriptions are important because many things can be measured in a number of different ways. One of the basic principles of any type of scientific ...
Theories and Hypotheses. Before describing how to develop a hypothesis, it is important to distinguish between a theory and a hypothesis. A theory is a coherent explanation or interpretation of one or more phenomena. Although theories can take a variety of forms, one thing they have in common is that they go beyond the phenomena they explain by including variables, structures, processes ...
The hypothesis is extremely important because it bridges the gap between the realm of ideas and the real world. As specific hypotheses are tested, theories are modified and refined to reflect and incorporate the result of these tests (Figure 3). Figure 3. The scientific method of research includes proposing hypotheses, conducting research, and ...
The research hypothesis states the existence of a relationship between the variables of interest and the specific direction of that relationship. For instance, the research hypothesis "Using marijuana will reduce learning" predicts that there is a relationship between a variable "using marijuana" and another variable called "learning."
Theories and Hypotheses. Before describing how to develop a hypothesis it is imporant to distinguish betwee a theory and a hypothesis. A theory is a coherent explanation or interpretation of one or more phenomena.Although theories can take a variety of forms, one thing they have in common is that they go beyond the phenomena they explain by including variables, structures, processes, functions ...
Hypotheses. A hypothesis (plural hypotheses) is a precise, testable statement of what the researchers predict will be the outcome of the study. This usually involves proposing a possible relationship between two variables: the independent variable (what the researcher changes) and the dependant variable (what the research measures).
A researcher begins with a set of phenomena and either constructs a theory to explain or interpret them or chooses an existing theory to work with. He or she then makes a prediction about some new phenomenon that should be observed if the theory is correct. Again, this prediction is called a hypothesis.
Three Different Hypotheses: (1) Directional Hypothesis: states that the IV will have an effect on the DV and what that effect will be (the direction of results). For example, eating smarties will significantly improve an individual's dancing ability. When writing a directional hypothesis, it is important that you state exactly how the IV will ...
A hypothesis is a testable prediction about how the world will behave if our idea is correct, and it is often worded as an if-then statement (e.g., if I study all night, I will get a passing grade on the test). The hypothesis is extremely important because it bridges the gap between the realm of ideas and the real world.
Research problems and hypotheses are important means for attaining valuable knowledge. They are pointers or guides to such knowledge, or as formulated by Kerlinger ( 1986, p. 19): " … they direct investigation.". There are many kinds of problems and hypotheses, and they may play various roles in knowledge construction.
Psychology: In psychology, hypotheses are used to test theories and models of human behavior and cognition. For example, a hypothesis might be formulated to test the effects of a particular stimulus on the brain or behavior. ... It is important to write the null hypothesis because it allows you to compare your results with what would be ...
A hypothesis is a testable prediction about how the world will behave if our idea is correct, and it is often worded as an if-then statement (e.g., if I study all night, I will get a passing grade on the test). The hypothesis is extremely important because it bridges the gap between the realm of ideas and the real world.
Theories and Hypotheses. Before describing how to develop a hypothesis, it is important to distinguish between a theory and a hypothesis. A theory is a coherent explanation or interpretation of one or more phenomena. Although theories can take a variety of forms, one thing they have in common is that they go beyond the phenomena they explain by including variables, structures, processes ...
The theory attempting to explain an observation will help to inform hypotheses - predictions of an investigation's outcome that make specific reference to the independent variables (IVs) manipulated and dependent variables (DVs) measured by the researchers. There are two types of hypothesis: H1 - The Research Hypothesis.
Here we have argued that the importance of methods runs deep, travelling alongside theory development to the core of the scientific enterprise. Methods are so critical to scientific progress that ...
Psychology is described as the science of mental wellbeing and deals with both the status and the functioning. As a science, psychology is constituted by two essential principles (Hand 2004, p. 34). The first is that there has to be measurement. Hypothesis states the variables to be measured as a second role.
The argument given in Table 1 suggests that a null hypothesis—in this case, that a person is an American—should be rejected whenever the observed results are unlikely under that hypothesis. NHST requires more than that, however. Implicitly, in order to reject a null hypothesis, NHST requires that the observed results must be more likely under an alternative hypothesis than under the null.
2. Complex Hypothesis: A Complex hypothesis examines relationship between two or more independent variables and two or more dependent variables. 3. Working or Research Hypothesis: A research hypothesis is a specific, clear prediction about the possible outcome of a scientific research study based on specific factors of the population. 4.
A p-value, or probability value, is a number describing how likely it is that your data would have occurred by random chance (i.e., that the null hypothesis is true). The level of statistical significance is often expressed as a p-value between 0 and 1. The smaller the p -value, the less likely the results occurred by random chance, and the ...
Assessing statistical significance by means of contrasting the data with the null hypothesis is called Null Hypothesis Significance Testing (NHST). NHST is the best known and most widely used statistical procedure for making inferences about population effects. The procedure has become the prevailing paradigm in empirical science [ 3 ], and ...
To evaluate the structural model, two preliminary criteria must be verified and reported: the importance of the path coefficients and the coefficient value of R 2 for endogenous constructs. Each hypothesis is associated with a causal link in the structural model, which represents the relationships between a pair of constructs.
When your p-value is less than or equal to your significance level, you reject the null hypothesis. In other words, smaller p-values are taken as stronger evidence against the null hypothesis. Conversely, when the p-value is greater than your significance level, you fail to reject the null hypothesis. In this case, the sample data provides ...