
- Create new account
- Reset your password
- Translation
- Alternative Standpoint
- HT Parekh Finance Column
- Law and Society
- Strategic Affairs
- Perspectives
- Special Articles
- The Economic Weekly (1949–1965)
- Economic and Political Weekly
- Open Access
- Notes for contributors
- Style Sheet
- Track Your Submission

- Debate Kits
- Discussion Maps
- Interventions
- Research Radio

Advanced Search
A + | A | A -
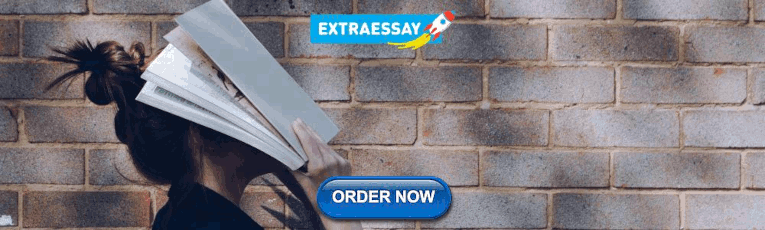
Impact of COVID-19 on the Indian Banking Sector
This article investigates the impact of COVID-19 on the banking sector through the trend analysis of return on assets and return on equity of the scheduled commercial banks. It covers both the pre- and post-lockdown periods. The results suggest that the governmental measures are helping ameliorate situations of bad quality loans and will improve future prospects of the banking industry.
The author is grateful to K B L Mathur, former economic advisor in the Ministry of Finance, Government of India, New Delhi for his useful comments and suggestions on the initial draft of this article. The usual disclaimers apply.
As on 31 August 2020, customers accounting for 40% of the outstanding bank loans availed the benefit of moratorium allowed by the Reserve Bank of India (RBI) for borrowers affected by COVID-19. Most sectors reported lower outstanding loans under the moratorium in August 2020 as compared to April 2020; however, micro, small and medium enterprises (MSMEs) registered a marginal increase, and the number of MSME customers availing moratorium increased to 78% in August 2020, reflecting the stress in this sector. The distribution of moratorium sought in MSME loans indicates that urban cooperative banks (UCBs) bore the brunt of the incipient stress, followed by PSBs and non-banking financial companies (NBFCs). In the case of the moratorium availed for outstanding individual loans, the share of small finance banks (SFBs) is the highest, followed by UCBs, and NBFCs. Nearly two-thirds of the total customers of PSBs and half of the total customers of PVBs exercised the option to defer payments in April 2020 (RBI 2020a). As on 31 August 2020, this was reversed, with PVBs accounting for a larger customer base under moratorium than the other categories of lenders, mainly due to a fourfold increase in their MSME customers availing the benefit, and with a sizeable customer base across categories (majorly individuals) opting out of moratorium, in the case of PSBs (RBI 2020b).
Large borrowal accounts (exposure of `5 crore and above) constituted 79.8% of the NPAs and 53.7% of the total loans at the end September 2020. During 201920, PSBs gross non-performing assets (GNPAs) ratio, as well as the ratio of restructured standard assets to total funded amounts, emanating from larger borrowal accounts, showed a downward trend. On the contrary, PVBs experienced an increasing share of NPAs in such accounts. The share of special mention accounts (SMA-0) witnessed a sharp rise in September 2020. This may be an initial sign of stress after the lifting of the moratorium on 31 August 2020. However, the share of other categories of SMAs, that is, SMA-1 and SMA-2 remained at a relatively lower level (RBI 2020c).1, 2

Dear Reader,
To continue reading, become a subscriber.
Explore our attractive subscription offers.
To gain instant access to this article (download).
(Readers in India)
(Readers outside India)
Your Support will ensure EPW’s financial viability and sustainability.
The EPW produces independent and public-spirited scholarship and analyses of contemporary affairs every week. EPW is one of the few publications that keep alive the spirit of intellectual inquiry in the Indian media.
Often described as a publication with a “social conscience,” EPW has never shied away from taking strong editorial positions. Our publication is free from political pressure, or commercial interests. Our editorial independence is our pride.
We rely on your support to continue the endeavour of highlighting the challenges faced by the disadvantaged, writings from the margins, and scholarship on the most pertinent issues that concern contemporary Indian society.
Every contribution is valuable for our future.
- About Engage
- For Contributors
- About Open Access
- Opportunities
Term & Policy
- Terms and Conditions
- Privacy Policy
Circulation
- Refund and Cancellation
- User Registration
- Delivery Policy
Advertisement
- Why Advertise in EPW?
- Advertisement Tariffs
Connect with us

320-322, A to Z Industrial Estate, Ganpatrao Kadam Marg, Lower Parel, Mumbai, India 400 013
Phone: +91-22-40638282 | Email: Editorial - [email protected] | Subscription - [email protected] | Advertisement - [email protected]
Designed, developed and maintained by Yodasoft Technologies Pvt. Ltd.
COVID-19 and its Impact on India’s banking sector
By Sonali Kulkarni
COVID-19 is undoubtedly one of the biggest global events of our lifetimes, presenting unprecedented challenges to many industries, governments and people all over the world. The pandemic remains a health and humanitarian crisis, and the business and economic impact has been deep and far reaching. Financial services firms, in particular, have the opportunity to help consumers and businesses weather the economic downturn and navigate the current storm.
A lot has been done already. Banks in India have focused on maintaining critical staff at branches and have temporarily redeployed staff to manage online or phone enquiries from customers. They’ve also deployed mobile ATMs and implemented doorstep banking for senior citizens and other customers that need additional attention. We expect financial firms to implement video collaboration tools, new chat and messaging software and other fintech innovations to continue live interactions with customers who have been coping with social distancing norms, with some already making use of common consumer apps to that end.
Several banks have made investments in technology and digital transformation over the past couple of years. A lot of them, however, are still heavily reliant on face-to-face interactions, supported by paper processes. So, we expect to see renewed vigor in the Indian financial services industry with banks making a concerted effort to up their digital game. This will be critical as COVID-19 is likely to have a prolonged impact, and banking touches every part of our economy.
That said, most banks have addressed the immediate challenges of COVID-19, related to protecting staff and providing much needed services to customers. They now have the chance to be active participants to help mitigate this crisis, and there are four key areas they can focus on to help navigate the current situation:
- Customer Service and Advice : As a result of social distancing, an increasing number of consumers are using online banking channels to manage their money. This is likely to result in a more permanent shift in customer preferences to digital channels and an increased demand for digital services. It’s important for banks to be accessible to all consumers, including the elderly or those not familiar with digital banking, providing education on how to use digital tools, keeping ATMs stocked and operational. As customers seek help and advice on short-term cash management and re-planning their future, banks would need to prioritize live interactions through video collaboration tools. This increase in digital customer engagement must go hand in hand with a ramp-up of cybersecurity and fraud-protection tools to protect customers.
- Credit Management: Even with the Indian government’s stimulus packages and Reserve Bank of India’s (RBI) liquidity measures, banks can expect an increase in loan defaults as borrowers across customer groups struggle to make payments in the face of an economic crisis resulting from lost business and jobs. Besides the moratorium facility announced by the RBI for all term loans, as part of the COVID-19 package, lenders should consider proactively restructuring loans to reduce the cashflow burden in the near term, thus reducing defaults in the immediate future. The industry must work together to make the financial relief process quick and easy to deploy. Banks should proactively initiate credit forbearance and modification programs using a data-driven approach to understand which customers need help and then rapidly reach out with tailored, relevant solutions. Even with these programs in place, some customers may still not be able to make their next payments. So, banks should prepare for losses and build capacity to deal with an increase in delinquent loans . As consumer demand picks up, albeit gradually, post lockdown, banks will need to repurpose their go-to-market and customer acquisition model, keeping in mind changing consumer behavior post COVID-19, as well as focus on digitally native journeys and re-look at underwriting norms for better risk discovery.
- Revenue Compression : Revenue from retail and commercial banking is falling sharply, as underlying consumption and transactions have seen an exponential dip. While central banks around the world slash interest rates, banks are reducing yields to generate business, thus significantly reducing net interest margins. Income from payments and other fee-based services are hit by a general decline in economic activity. With measures like moratorium periods provided on loans, banks’ cashflow have also taken a hit. We expect an overall drop of up to 10% in banks’ payments revenues, which means a USD 150 billion top-line decline for the industry globally, as demand in sectors like retail and entertainment falls sharply or moves to online channels, while activity in areas such as tourism and travel evaporates. There is little that banks can to do to stop the overall drop in revenue, but they can focus on making payments safer by increasing limits on contactless payment channels and educating consumers on digital wallets. Banks can also focus on cashback and loyalty rewards to encourage spending in sectors that need it the most.
- Operating Model Adjustments, Cost Elasticity and Innovation : Over the next few quarters, the banking sector will face a misalignment between short-term costs and revenues due to the economic impact of COVID-19. Banks would need to review and prioritize current projects to ensure allocation of resources to the most pressing needs. Banks should also focus on investing in areas that will outlive the current pandemic, including projects and initiatives that maintain or improve the customer experience such as a paperless utility, end-to-end digital advisory and lending capabilities, increased fraud and cybersecurity analysis and detection, etc. These new digital tools will make banks more efficient and resilient to future changes. Banks that haven’t focused on remote working and virtual collaboration in the past should explore establishing elastic operations. This will insure banks against such unprecedented lockdowns and perhaps better manage cost overheads.
COVID-19 will have long-lasting impact on many industries including banks. Post crisis, digital maturity and COVID-19 resiliency will determine strategy of banking players with three segments emerging: banks that are already future-ready with truly digital banking capabilities and cost elasticity, banks that are digital laggards and that need to evolve and renew due to sub-par COVID-19 resiliency, and lastly banks that will struggle to survive as a result of being digital laggards with sub-par financial and operational resiliency.
COVID-19 will change our behaviors as customers, citizens and employees in India and around the world. As people become more focused on their well-being, businesses will also need to understand how they can be part of a new health ecosystem that is likely to dominate customer thinking going forward. The idea that “every business is a health business” is already emerging in many corners of financial services, and that is perhaps one of the few positive lasting impacts to result from COVID-19.
(The author is Client Group Lead – India, Financial Services, Accenture and the views expressed in the article are her own)
Leave a Response Cancel reply
Save my name, email, and website in this browser for the next time I comment.
You Might Also Like
Is ai hurtling towards model collapse , google rolls out more ai features, zero-day exploits get costlier, a work-from-home reality check.
Useful Links
Information
Copyright © 2024 Trivone. All Rights Reserved.

The Economic Times daily newspaper is available online now.
View: the pivotal role of banking in india's covid fight.
One of the lessons learnt during the growth slowdown that was increasingly manifest during H2 FY2020 even before the Covid-19 lockdown was the importance -- even centrality -- of banks in facilitating sustained and robust growth.
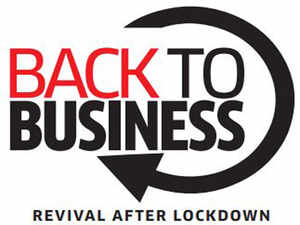
Read More News on
Download The Economic Times News App to get Daily Market Updates & Live Business News.
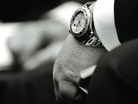
Better returns than stock, gold? Rolex, the new asset class for investments that transcends time.
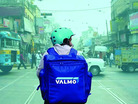
Meesho is taking a road less travelled to keep up in its fight with Amazon, Flipkart
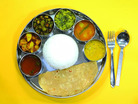
India is abandoning this healthy habit in droves
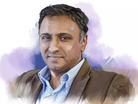
Where is Flipkart’s long-in-the-making IPO?
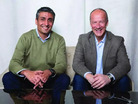
Turnaround Trouble: Why Thierry Delaporte is the third CEO to fail at turning around Wipro
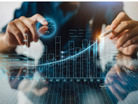
Weekly Largecap Top Picks: These stocks scored 10 on 10 on Stock Reports Plus

Find this comment offensive?
Choose your reason below and click on the Report button. This will alert our moderators to take action
Reason for reporting:
Your Reason has been Reported to the admin.
To post this comment you must
Log In/Connect with:
Fill in your details:
Will be displayed
Will not be displayed
Share this Comment:
Uh-oh this is an exclusive story available for selected readers only..
Worry not. You’re just a step away.
Prime Account Detected!
It seems like you're already an ETPrime member with
Login using your ET Prime credentials to enjoy all member benefits
Log out of your current logged-in account and log in again using your ET Prime credentials to enjoy all member benefits.
To read full story, subscribe to ET Prime
₹34 per week
Billed annually at ₹2499 ₹1749
Super Saver Sale - Flat 30% Off
On ET Prime Membership
Unlock this story and enjoy all members-only benefits.
Offer Exclusively For You
Save up to Rs. 700/-
ON ET PRIME MEMBERSHIP
Get 1 Year Free
With 1 and 2-Year ET prime membership
Get Flat 40% Off
Then ₹ 1749 for 1 year
ET Prime at ₹ 49 for 1 month
Stay Ahead in the New Financial Year
Get flat 20% off on ETPrime
90 Days Prime access worth Rs999 unlocked for you
Exclusive Economic Times Stories, Editorials & Expert opinion across 20+ sectors
Stock analysis. Market Research. Industry Trends on 4000+ Stocks
Get 1 Year Complimentary Subscription of TOI+ worth Rs.799/-
Stories you might be interested in

- Business and Finance /
- Retail Banking
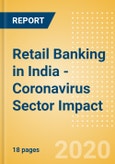
Retail Banking in India - Coronavirus (COVID-19) Sector Impact

- Region: India
- ID: 5031059
- Description
Table of Contents
Related topics, related reports.
- Purchase Options
- Ask a Question
- Recently Viewed Products
- Indian banks face pressure to increase their earnings and asset quality as a result of uncertainty surrounding the severity and duration of the pandemic, as well as the associated effects on Indian banks due to restrictions on economic activity.
- A drop in interest rates has been unsuccessful in boosting banks’ net interest margins as widespread retrenchment has adversely affected the repayment abilities of households.
- Fee income will fall, driven by decreased retail spending, while non-performing loan ratios will increase - particularly across SMEs. However, support measures should alleviate some of the asset-quality pressure that will emerge from this downturn.
- This will work in the short term and hold down the impaired loan ratio for the first half of 2020, but pressure will be on lenders in the months ahead.
- Make strategic decisions using top-level revised forecast data on the Indian retail lending and deposit industry.
- Understand the key market trends, challenges, and opportunities in the Indian retail lending and deposit industry.
- Receive a comprehensive insight into the total consumer loans in India, including mortgages, personal and credit card loans as well as retail deposits balances.
- COVID-19 Update
- Impact Assessment
- Retail Deposits
- Total Consumer Loans
- Mortgage Loans
- Credit Card Loans
- Other Consumer Loans
- Job Analysis
- Supplementary Data
- Definitions
- Methodology
- About the Publisher

Retail Banking Market - Global Industry Size, Share, Trends, Opportunity, and Forecast, 2018-2028F
- Report
- November 2023

Retail Banking Market Size, Share & Trends Analysis Report By Type (Public Sector Banks, Private Sector Banks, Foreign Banks), By Service (Saving & Checking Accounts, Credit & Debit Cards), By Region, And Segment Forecasts, 2023 - 2030

Asia-Pacific (APAC) Regional Retail Banking Analysis by Country, Consumer Credit, Retail Deposits and Residential Mortgages, 2023
- Asia Pacific

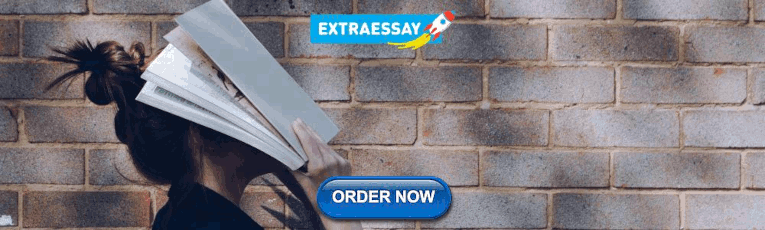
Retail Banking Sector Scorecard - Thematic Intelligence

Retail Banking Market By Type, By Function, By End User: Global Opportunity Analysis and Industry Forecast, 2023-2032
- October 2023
ASK A QUESTION
We request your telephone number so we can contact you in the event we have difficulty reaching you via email. We aim to respond to all questions on the same business day.
Request a Quote
YOUR ADDRESS
YOUR DETAILS
PRODUCT FORMAT
DOWNLOAD SAMPLE
Please fill in the information below to download the requested sample.
The impact of COVID-19 and the policy response in India
Subscribe to global connection, maurice kugler and maurice kugler professor of public policy, schar school of policy and government - george mason university @kugler_maurice shakti sinha shakti sinha senior fellow - world resources international (wri india).
July 13, 2020
Much has been written about how COVID-19 is affecting people in rich countries but less has been reported on what is happening in poor countries. Paradoxically, the first images of COVID-19 that India associates with are not ventilators or medical professionals in ICUs but of migrant laborers trudging back to their villages hundreds of miles away, lugging their belongings. With most of the economy shut down, the fragility of India’s labor market was patent. It is estimated that in the first wave, almost 10 million people returned to their villages, half a million of them walking or bicycling. After the economic stoppage, the International Labor Organization has projected that 400 million people in India risk falling into poverty .
Agriculture is the largest employer, at 42 percent of the workforce, but produces just 18 percent of GDP. Over 86 percent of all agricultural holdings have inefficient scale (below 2 hectares). Suppressed incomes due to low agricultural productivity prompt rural-urban migration. Migration is circular, as workers return for some seasons, such as harvesting.
Evidence of Indian labor market segmentation is widely available—with a small percentage of workers being employed formally, while the lion’s share of households relies on income from self-employment or precarious jobs without recourse to rights stipulated by labor regulations. Only about 10 percent of the workforce is formal with safe working conditions and social security. Perversely, modern-sector employment is becoming “informalized,” through outsourcing or hiring without direct contracts. The share of formal employment in the modern sector fell from 52 percent in 2005 to 45 percent in 2012. During this period, formal employment went up from 33.41 million to 38.56 million (about 15 percent), while nonagricultural informal employment increased from 160.83 million to 204.03 million (about 25 percent) .
Most informal workers labor for micro, small, and medium-sized enterprises (MSMEs) that emerged as intermediate inputs and services suppliers to the modern sector. However, workers struggle to get paid, which the government identifies as great challenge. Payroll and other taxes, as well as limited access to subsidized credit for large firms, are disincentives to MSME growth. Although over half of India has smartphone access, relatively few can telework. Retail and manufacturing jobs require physical presence involving direct client interaction. Indeed, income for families unable to telework has fallen faster.
Related Books
Geoffrey Kemp
May 3, 2010
June 14, 2012
Sebastian Edwards, Nora Claudia Lustig
September 1, 1997
The government’s crisis response has mitigated damage, with a fiscal stimulus of 20 trillion rupees , almost 10 percent of GDP. Also, the Reserve Bank of India enacted decisive expansionary monetary policy . Yet, banks accessed only 520 billion rupees out of the emergency guaranteed credit window of 3 trillion rupees. In fact, corporate credit in June is lower than June last year by a wide margin after bank lending’s fall. S&P has estimated the nonperforming loans would increase by 14 percent this fiscal year . Corporations have deleveraged retiring old debts and hoarding cash, as have households. Recovery through investment and consumption has stalled . These trends are exacerbated due to the pandemic. The manufacturing Purchasing Managers Index (PMI) recovered 50 percent since May but at 47.2 it remains in negative territory. Services contribute over half of GDP but its PMI, even after bouncing back , remains low at 33.7 in June. Consumption of electricity, petrol, and diesel have regained from the lockdown lows but are still 10-18 percent below June 2019 levels . Agriculture has been the bright spot, with 50 percent higher monsoon crop sowing and fertilizer consumption up 100 percent. Unemployment levels had spiked to 23.5 percent but with a mid-June recovery to 8.5 percent—and then crept up again marginally.
The National Rural Employment Guarantee Scheme (MNREGA) and supply of subsidized food grains have acted as useful buffers keeping unemployment down and ensuring social stability. Thirty-six million people sought work in May 2020 (25 million in May 2019). This went up to 40 million in June 2020 (average of 23.6 million during 2013-2019 period). The government has ramped up allocation to the highest level ever, totaling 1 trillion rupees. Similarly, in addition to a heavily subsidized supply of rice and wheat, a special scheme of free supply of 5 kilograms of wheat/rice per person for three months was started and since extended by another three months, covering 800 million people. There have also been cash transfers of 500 billion rupees to women and farmers .
However, MNREGA has an upper bound of 100 days guaranteed employment and it also does not cover urban areas. Agriculture cannot absorb more labor, with massive underlying disguised unemployment. A post-pandemic survey shows that the MSME sector expects earnings to fall up to 50 percent this year. Critically, the larger firms are perceived healthier. However, small and micro enterprises, who have minimal access to formal credit, constitute 99.2 percent of all MSMEs . These are the largest source of employment outside agriculture. Their inability to bounce back could see India face further economic and also social tensions. The economy is withstanding both supply and demand shocks, with the wholesale prices index declining sharply .
We identified labor market pressures toward increased poverty, both in the extensive margin (headcount) and intensive margin (deprivation depth). India needs to ramp up MNREGA, introduce a guaranteed urban employment scheme, and boost further cash transfers to poor households. Government efforts have been enormous in macroeconomic policy (fiscal stimulus and monetary loosening) to mitigate adversity but fiscal space is narrowing, requiring the World Bank and other international financial institutions to step up and help avert even greater hardship. Also, ongoing advances towards structural economic policy reforms have to continue.
Related Content
Ipchita Bharali, Preeti Kumar, Sakthivel Selvaraj
July 2, 2020
Urvashi Sahni
May 14, 2020
Stuti Khemani
April 30, 2020
Global Economy and Development
India South Asia
Homi Kharas, Charlotte Rivard
April 11, 2024
Brahima Sangafowa Coulibaly, Hafez Ghanem, Wafa Abedin
Robin Brooks

An official website of the United States government
The .gov means it’s official. Federal government websites often end in .gov or .mil. Before sharing sensitive information, make sure you’re on a federal government site.
The site is secure. The https:// ensures that you are connecting to the official website and that any information you provide is encrypted and transmitted securely.
- Publications
- Account settings
Preview improvements coming to the PMC website in October 2024. Learn More or Try it out now .
- Advanced Search
- Journal List
- Elsevier - PMC COVID-19 Collection

Banking sector performance during the COVID-19 crisis
This paper examines the impact of financial sector policy announcements on bank stocks around the world during the onset of the COVID-19 crisis. Overall, we find that liquidity support, borrower assistance programs and monetary easing moderated the adverse impact from the crisis, but their impact varied considerably across banks and countries. By contrast, countercyclical prudential measures led to negative abnormal returns in bank stocks, suggesting that markets price the downside risks associated with these policies.
1. Introduction
To reduce the spread of the novel COVID-19, governments enacted mitigation strategies based on social distancing, national quarantines, and shutdown of non-essential businesses. The halt to the economy represented a large shock to the corporate sector, which had to scramble for cash to cover operating costs as a result of the revenue shortfall. The financial sector, and banks in particular, are expected to play a key role absorbing the shock, by supplying much needed funding ( Acharya and Steffen, 2020 ; Borio, 2020 ). 1 Under these unprecedented circumstances, central banks and governments enacted a wide range of policy interventions. While some measures were aimed to reduce the sharp tightening of financial conditions in the short term, others sought to support the flow of credit to firms, either by direct intervention of credit markets (e.g., government-sponsored credit lines and liability guarantees), or by relaxing banks’ constraints on the use of capital buffers.
While credit institutions are being called to play an important countercyclical role to support the real sector, these actions also have a series of implications for the future resilience of the banking sector. For instance, as lenders exhaust their existing buffers, they might also experience deterioration of asset quality, threatening the systems’ stability. As the crisis is expected to continue, even after the lockdowns are lifted and economies start to reopen, the net effect of these policy measures on the banking sector is largely unknown.
The contribution of this paper is twofold. First, we use bank stock prices around the world to assess the impact of the pandemic on the banking sector. Second, we combine bank stock prices with a global database on financial sector policy responses during the pandemic. Using an event study methodology, we examine the stock market response to announcements of different policy initiatives. To better understand the impact of the measures implemented by monetary and supervisory authorities, we exploit the cross-sectional variation in the stock price of banks. In other words, we are interested in both the aggregate response of bank stocks to a particular announcement, as well as the differential effect across banks with different characteristics, such as size, liquidity, ownership, and others.
We use bank data including stock prices, balance sheets, and ownership, for 52 countries covering 896 commercial banks. We first document a systematic underperformance of bank stocks at the onset of the COVID-19 crisis, between March and April of 2020. More precisely, for most countries, bank stocks underperform relative to other publicly traded companies in their home country, and relative to non-bank financial institutions. While bank underperformance during downturns is common given their high betas, we show that early in the pandemic, banks’ stock returns were abnormally low when calculated relative to an asset pricing model with mixed factors, that is, global and domestic market returns as in Bekaert et al. (2014) . In the U.S., Acharya et al. (2021) also report a risk premium on bank stocks following the COVID-19 shock. We show that negative abnormal returns among banks are ubiquoutus to developed and developing countries during our observation period and are robust to multiple model specifications (e.g., Fama and French, 2015 ). Furthermore, we show that banks with lower liquidity buffers experienced larger than normal price drops.
To study the stock market reaction to different policy measures, we identify financial sector initiatives by government authorities from February 2 to April 17. The data was compiled and made publicly available by the World Bank ( Feyen et al., 2021 ). Our final sample contains 389 financial sector policy announcements in 45 countries (17 developed and 28 developing). We classify approved measures that target the banking sector into four categories. Liquidity support are measures used by monetary authorities to expand bank short-term funding in domestic and foreign currency. Prudential measures deal with the temporary relaxation of regulatory and supervisory requirements, including capital buffers. Borrower assistance include government-sponsored credit lines or liability guarantees to promote the flow of credit to households and firms. Finally, monetary policy includes policy rate cuts and quantitative easing (i.e., asset purchases). Our empirical methodology consists of estimating banks’ abnormal returns around the announcement day. Our findings can be summarized as follows:
- • Borrower assistance announcements appeared to have the strongest immediate impact on bank stock prices, both on aggregate and in the cross-section. Banks experienced large abnormal returns following the announcement of these policies. Additionally, larger banks seem to benefit more compared to smaller banks. This is consistent with the observation that new government credit lines, interest rate subsidies, and liability guarantees are more likely to be used by large banks. 2 Borrower assistance initiatives, which typically include the introduction of government guarantees, automatically transfer risks from banks’ balance sheets to the sovereign. In turn, these policies require significant fiscal commitments. Relatedly, we find that the positive association between excess stock returns and borrower assistance measures is exclusive to developed countries. In developing countries, where there is less room for fiscal expansion, announcements of borrower support had no effect on stock prices. The market response seems to suggest that the extent of borrower assistance measures is limited in such settings.
- • Liquidity support initiatives have a favorable impact in the reduction of the liquidity premium – stocks of banks with less liquid assets respond more strongly to these announcements. Also, smaller banks experienced large abnormal returns when liquidity support measures were announced.
- • In contrast, countercyclical prudential measures are associated with negative abnormal returns in bank stocks. Prudential policies allow banks to run down some of their buffers. They also send a strong signal of the willingness of policymakers to lessen the economic impact from the pandemic. However, the fact that bank stock prices drop following the announcements of these policies suggests that markets are also pricing the downside risk from the depletion of capital buffers, as well as the additional expansion of riskier loans in the balance sheets of banks.
- • Results for monetary policy announcements are more mixed. While such announcements were not associated with aggregate bank stock price increases, policy rate cuts did reduce the liquidity premium – banks with lower liquidity displayed higher stock returns around the announcement window. This result confirms that the interest rate policy remained a key tool at the onset of the crisis.
The most important threat to our identification strategy is the endogeneity of policy actions, which could bias our estimates. While the COVID-19 was exogenous, the adoption of a policy mix by financial authorities is not random. We conduct three exercises to mitigate this concern and confirm most of our findings. First, we focus on large cross-border banks in our sample and examine the reaction of their stock prices when policies are announced in countries where they have subsidiaries. Second, we examine the extent to which external rather than domestic factors influence the announcement of financial sector policies across jurisdictions. 3 Third, we examine bank stock prices in Euro area countries following broad cross-country policy announcements from the European Central Bank (i.e., a setting where policies are presumably less driven by within country characteristics). 4
Our paper is related to a growing literature that studies the economic impact of the pandemic using stock market data (e.g., Borio, 2020 ; Gormsen and Koijen, 2020 ; Landier and Thesmar, 2020 ; Ramelli and Wagner, 2020 ). Given the extraordinary scale and unprecedented nature of the crisis, it is difficult to quantify the effect of the shock versus the impact of the ensuing economic policies. We add to this literature by examining the effects of the pandemic on stock returns of banks based on an event study methodology.
We also contribute to a longstanding literature that examines the role of policy initiatives during financial crises ( Claessens et al., 2005 ; Reinhart and Rogoff, 2009 ; Taylor and Williams, 2009 ). Ait-Shalia et al. (2012) examine the effects of policy announcements on liquidity risk premia and interbank credit during the Global Financial Crisis (GFC). While we also study the market response to policy initiatives, there are noticeable differences between the COVID-19 shock versus previous events of financial and economic stress that grant further analysis. First, since the shock is truly exogenous to the financial sector, the overwhelming response from policymakers has been to relax regulatory requirements and use capital buffers: During our sample, 91% of countries used prudential measures. There is, however, no prior analysis of such a coordinated type of response, and no clear evidence about the medium- and long-term effects of these policies. To the best of our knowledge, our paper represents the first global analysis examining the market response to financial policy measures during the pandemic.
We use data from Refinitiv on all publicly traded banks across 52 countries between May 2, 2018 and May 12,020. The data set includes information on the daily stock prices, quarterly financial statements, and state ownership. We choose only stocks traded on major exchanges. Of the 1590 bank stocks in the data, we drop 242 stocks that traded less than 30% of the business days in each country throughout the sample period. We further drop 244 stocks that are not common stocks or are stocks with special features, such as depository receipts, real estate investment trusts, and preferred stocks. Finally, we drop from the dataset 208 banks that are owned by corporate groups whose core business is different from banking.
For several countries in our sample (i.e., oil-exporter countries) the contraction in the economy resulting from the COVID-19 shock was further exacerbated by a sharp contraction in oil prices resulting from the Russia-Saudi Arabia oil price war in early March. To control for the impact from the oil shock on banks, we use daily Brent crude oil prices and measure the degree to which individual banks were exposed to the oil sector prior to the COVID-19 pandemic. Specifically, we define oil sector exposure as the slope coefficient of an OLS regression of bank's stock returns on a constant, the market return where the bank is domiciled, and the rate of return of oil prices estimated using weekly data between May 2018 and December 2019.
We also obtained daily data on the total number of confirmed COVID-19 cases for every country since the beginning of the pandemic. 5 This information helps us measure the intensity of the pandemic over time and across countries.
Our final sample comprises 896 commercial banks. Table A1 in the Appendix presents the distribution of banks across the countries in our sample. Table 1 displays the summary statistics of the variables of interest. As Table 1 shows, the daily return of bank stocks from January to early May 2020 was on average -0.4%, with the median bank obtaining returns of -0.3%.
Summary statistics of banks.
Notes: The table presents the summary statistics of the 896 banks in the sample. Stock returns for each bank are averaged over the period January 1, 2020 to May 5, 2020. Liquidity ratio is defined as the ratio of liquid assets (cash & due from banks) to total assets averaged over the 2019Q1-2019Q4 period. Oil exposure corresponds to the slope coefficient of an OLS regression of bank's stock returns on a constant, the market return where the bank is domiciled, and the rate of return of oil prices using weekly data between May 2018 and December 2019. Size is calculated as the 2019Q1-2019Q4 average total assets for each bank and is reported in logs. Public bank is an indicator variable that equals one for banks with a non-zero equity participation from the domestic government and zero otherwise. Capital ratio corresponds to the 2019Q1-2019Q4 average ratio of Tier 1 + Tier 2 capital to total assets. COVID corresponds to the average number of confirmed COVID-19 cases per million citizens in the country of banks during the sample period.
For each bank in the sample, we calculate the average liquidity ratio in the year prior to the COVID-19 pandemic. The ex-ante liquidity ratio corresponds to the ratio of liquid assets to total assets averaged over the 2019Q1-2019Q4 period. Our measure of liquid assets comprises cash on hand and due from banks, which include receivables from, or short-term loans to, other banks and/or financial institutions, which usually bear minor interest earnings. The liquidity ratio of the median bank in the sample is 7%, with banks in the 25th percentile holding as little as 2% of cash and due from banks relative to their total assets. 6
Regarding the ex-ante exposure of banks to the oil sector, there is large heterogeneity around this measure, with the returns of banks in the 75th percentile experiencing a correlation with oil price returns of 0.23, compared to a correlation of -0.22 among banks in the 25th percentile. Bank size is calculated as the 2019Q1-2019Q4 average of the total assets for each bank and is reported in logs. The average log size of banks in the sample is 23.3, which corresponds to 13.5 billion dollars. In our sample, twelve percent of the banks are classified as public owned. 7 However, as Table A2 in the Appendix shows, there is large heterogeneity in the share of public banks across regions. Whereas state-owned banks in India represent 53% of all publicly traded banks in the country, in high-income countries they constitute just 2%. Regulatory capital ratios of banks (i.e., the sum of Tier 1 and Tier 2 capital relative to total assets) are averaged over the 2019Q1-2019Q4 period. The average bank in the sample entered the COVID-19 crisis with a regulatory capital ratio of 16.5%. There is also great variation on the number of COVID-19 cases per million citizens across countries in our sample. While in countries such as Nigeria or Vietnam there was on average less than 1 reported case per million from January to April, in countries such as Turkey or France the average reported cases per million exceeded 400.
3. Banks risk premium during the crisis
Following the large crash of global equity prices at the onset of the COVID-19 pandemic, banks’ share prices around the world underperformed relative to their local stock markets and relative to domestic non-financial firms ( Fig. 1 ). 8 Declines of bank stock prices are expected during sharp economic downturns or in crisis periods due to their exposure to market risk, often measured by their high equity betas. In this section, we show banks’ stock price underperformance is not explained by commonly used asset pricing models. Since we are using bank returns worldwide and banks with substantial differences in size, it is quite possible that the right asset pricing model differs across countries, or even across banks within a country.

Average stock returns of banks vs firms and non-bank financial companies
Notes: The figures plot average daily stock market returns of banks, firms and non-bank financials in the sample normalized to January 1, 2020. The average returns of firms in the first figure are equally weighted across countries and are net from bank returns. To calculate them, we exclude the returns of banks from the returns of each country's stock market using the index weights of banks. The average returns of banks are weighted by the contribution of each bank to the total bank assets of each region. The regional average bank returns are then equally weighted across regions. The same approach is used to obtain the average return of non-bank financials.
To examine the performance of bank stocks, we use an international factor model with two factors following Bekaert et al. (2014) –a global factor and a domestic factor [ R t G , R t , D ]. The two factors are value-weighted market indexes, so that the model potentially embeds a global CAPM, a domestic CAPM, and the mixed case when both global and domestic markets affect the pricing of a particular bank stock. To avoid spurrios correlation, the domestic market factor is value-weighted within the country, but excludes the returns of bank b itself, R t , D / b ( Table A1 presents the list of country indices used in the analysis). The model is:
where all the returns R are measured in U.S. dollars in excess of the three-month U.S. T-Bill rate. We estimate Eq. (1) for each bank in the sample using weekly data between May 2018 and December 2019. We then calculate weekly abnormal returns between January and April 2020 as the difference between the realized and the model implied returns: A R e t b , t = R b , t − α ^ b − β ^ G R t G − β U R ^ t , D / b .
The empirical analysis, outlined in Eq. (2 ), consists of a panel regression that relates the weekly abnormal returns of banks to a series of bank characteristics. The set of covariates includes the bank's ex-ante ratio of liquid assets, L i q u i d i t y r a t i o b , its ex-ante exposure to the oil sector, O i l e x p o s u r e b , its ex-ante size, S i z e b , an indicator variable that equals one for government-owned banks and zero otherwise, P u b l i c b a n k b , as well the bank's ex-ante regulatory capital ratio, C a p i t a l r a t i o b . We also control for the incidence of the pandemic using the percentage change of COVID-19 cases per million during the week C o v i d c , t . 9 The coefficients γ c and δ t are country and week fixed effects respectively, and u b , c , t is an error term clustered at the country level. Our final specification is as follows:
The results are summarized in Table 2 . In columns (1)–(3), we estimate abnormal returns for weeks during January and February of 2020, using alternative definitions of the liquidity ratio. The constant α 0 is economically small and statistically indistinguishable from zero, which suggests that banks’ stock returns during the first two months of the year are broadly explained by the two-factor model. On the contrary, during March and April (columns 4–6), bank stock returns are abnormally low; according to the most conservative estimate, weekly abnormal returns were on average -1.04% points. We further confirm our findings after calculating abnormal returns relative to a six-factor model ( Fama and French, 2015 ), where, in addition to the global and domestic markets, we add size, book to market, profitability, and investment risk factors from the Kenneth French website. Alternatively, we use a domestic CAPM where returns are estimated in local currency as a function of the domestic market returns (results for these alternative models are presented in Tables A3 and andA4 A4 in the Appendix).
Risk factors during the COVID-19 crisis.
Notes: The table presents OLS estimates of bank-week panel regressions. The dependent variable is abnormal returns, calculated as the difference between a bank stock returns during a week and the expected returns implied by a two-factor model with domestic and global factors. Returns are calculated in U.S. dollars. The definition of liquidity ratio, averaged over the 2019Q1-2019Q4 period, corresponds to: Liquid assets A/total assets, where liquid assets A include cash & due from banks (columns 1 and 4); liquid assets B/total deposits, where liquid assets B include cash & due from banks plus federal funds sold and securities purchased under resale agreements (columns 2 and 5); liquid assets C/total assets, where liquid assets C include cash & due from banks, federal funds sold and securities purchased under resale agreements, trading account assets, other short-term investments and total investment securities (columns 3 and 6). Oil exposure corresponds to the slope coefficient of an OLS regression of bank's stock returns on a constant, the domestic markets, and the percentage change of oil prices using weekly data between May 2018 and December 2019. Size is calculated as the 2019Q1-2019Q4 average total assets for each bank and is reported in logs. Public bank is an indicator variable that equals one for banks with non-zero equity participation from the domestic government and zero otherwise. Capital ratio corresponds to the 2019Q1-2019Q4 average ratio of Tier 1 + Tier 2 capital to total assets. COVID corresponds to the percentage change in number of confirmed COVID-19 cases of a country per million citizens during each week. All control variables are standardized with mean 0 and standard deviation 1. All specifications include country and week fixed effects. Standard errors are clustered at the country level.
The evidence also indicates that banks with lower liquidity ratios displayed the largest price drop during March and April. The coefficient for the liquidity ratio is positive and statistically significant for alternative definitions of liquid assets. For instance, a bank with a one-standard deviation liquidity ratio below the average underperformed other banks by 0.15% points per week. Other bank characteristics do not seem to correlate with the underperformance during the period. Overall, during March and April, markets were signaling an increased risk for the banking sector with an additional premium for less liquid banks.
Globally, banks entered the COVID-19 crisis better positioned to support the lending needs of the real economy. As documented by the BIS, the capital and liquidity buffers of banks at the onset of the crisis were substantially stronger than compared to the GFC ( Borio, 2020 ; Lewrick et al., 2020). Through aggressive interventions in the financial markets, governments actively encouraged banks to continue providing credit, allowing for buffers to draw down. In the next section, we study the immediate market response to these policy announcements.
4. Measuring the market response to financial sector distress policy initiatives
What impact did the different financial sector policy measures have on the banking sector? To answer this question, in this section we evaluate the market response to different financial sector policy initiatives.
4.1. Policy interventions
We use data on the dates and types of major policy initiatives to support the financial sector and address the impact of the COVID-19 emergency. The data were compiled and made publicly available by the World Bank ( Feyen et al., 2021 ). It covers policy measures in low, middle, and high-income countries. Information on each policy measure was collected from national authorities and international organizations. For each policy initiative, the dataset reports details on the announcement, and the day (and not the hour or minute) of the announcement which restricts our analysis to daily frequency. We classify approved measures that target the banking sector into the following categories: (i) liquidity support, (ii) prudential measures, (iii) borrower assistance, and (iv) monetary policy ( Table 3 ).
Policy announcements.
Liquidity support deals with potential shortages in funding, either because precautionary demand for liquidity has increased or because of lack of access to funding (e.g., wholesale funding markets have dried up). Measures included are provisions of domestic currency liquidity through broadened access to central bank refinancing, extended collateral framework, such as those in the short-term and long-term repo market, and available foreign currency liquidity through swap agreements between central banks.
Prudential measures include temporary relaxation of certain key regulatory and supervisory requirements, including changes in capital requirements, limits on exposure, concentration, loan-to-value ratios, minimum reserve requirements, and cancelation of stress tests. By slowing down the decline in banks’ regulatory capital ratios, these measures reduce the rate at which banks draw buffers down, spreading the recognition of losses and allowing a given amount of equity to support a larger lending volume.
Borrower assistance include measures to supply funds to firms and households due to the loss of revenue/income from the extended lockdowns. These include government liability guarantees for newly issued or existing wholesale financing, direct credit lines to strategic sectors, state support for interest-free loans and fully replenishable working capital financing. Other measures include enhancement of deposit protection schemes and simplified programs of loan restructuring.
Monetary policy are interest rate decisions (e.g., benchmark rate, repo rates, etc.), central bank purchases of government securities ( Quantitative Easing ), and credit easing, which typically consist of purchases of private sector debt in secondary markets. Given the different nature of conventional and unconventional monetary policy measures, we further split this category into Policy Rate announcements and Asset Purchases (both public and private bond buying programs and purchases of asset-backed securities).
Our final sample contains policies in 17 developed and 28 developing countries, for which we have stock market and policy data ( Table 4 ). Some European countries such as, Austria, Finland, and Netherlands, did not have domestic financial policy initiatives. We include banks of these countries in days of announcements by the European Central Bank. Other Euro countries, for example, France, Germany, Italy, and Spain have both financial support policies taken at the country level in addition to those taken by the monetary union authorities. 10 In total, the database includes 389 announcements between February 1 and April 17. 11 Prudential measures accounted for the largest share of policy initiatives (36%), followed by borrower support, liquidity provision measures, monetary policy and asset purchases (23%, 21%, 12% and 8%, respectively). However, the type of policies adopted differed substantially across countries (see Table A3 in the Appendix). While monetary policy announcements were frequent in developing countries (representing 22% of all measures), they only accounted for 4% in developed ones. In contrast, prudential measures in developed countries constituted 43% of all announcements, compared to 26% in developing countries.
Number of days with policy announcements.
Notes: Data obtained from the World Bank (2020) covering the period February 1 to April 17 2020.
Fig. 2 presents the timing of policy announcements. Early in February, financial markets and financial authorities had little reaction to the original outbreak in China and the ensuing lockdown of Wuhan. On February 20, when Italy announced the quarantine of eleven municipalities in the northern region, and once it was apparent that the outbreak had also spread to South Korea, and Iran, stock markets declined sharply. By this date, only five countries had taken financial sector policy interventions. Among these, Russia, Thailand, China and Indonesia lowered their short-term interest rates and Japan launched a crisis loan package to small businesses.

Timeline of policy announcements
Notes: Number of countries with at least one policy announcement (by category).
Following a myriad of national quarantines starting with Italy on March 10, travel bans, including the U.S. decision on March 12 to severely restrict travel from the EU, stock markets around the world declined more than 30% from their peak. Interestingly, at this stage of the global crisis, most financial authorities were only taking conventional monetary policy actions, reducing short-term rates. For example, between February 20 and March 18, seventeen countries in our sample had announced policy rate cuts, and only a few had either taken measures to assist borrowers or announced prudential measures (e.g., Italy, Germany, U.K., and South Korea).
In response to the unprecedented, and rapidly evolving situation, policymakers increased the scope and number of measures to support the financial system. After March 18, most government and monetary authorities included liquidity support measures and borrower assistance. For instance, by April 1735 countries had taken some measure of direct borrower support, in most cases, dealing with SME financing. The use of countercyclical prudential rules also became ubiquitous; by the end of our sample, 40 countries had introduced prudential actions, typically through a temporary relaxation of certain key regulatory and supervisory requirements.
A large number of interventions were announced simultaneously with other financial sector policies. For example, prudential measures in developed countries were often announced on the same day of borrower support program announcements ( Table A3 in the Appendix). However, as Tables A3 shows, there were also multiple single policy announcements for each of the policy categories we study. In developed countries, 42% of policies were announced on days with no other financial sector program announcements. This share is higher among developing countries, where 51% of policies were made on single announcement days.
4.2. Empirical methodology
We use an event study technique ( Brown and Warner, 1996 ; Kothari and Warner, 2007 ) to test the effect of policy announcements on the stock returns of banks. More specifically, we want to assess whether different policy initiatives convey new information about the ability of banks to properly operate during and after the crisis. Measuring bank-level stock returns instead of an aggregate index of bank stock prices (or interbank credit and liquidity risk premia), allows us to exploit variation across banks to better understand the market response to different types of policies. 12 For example, the cross-sectional analysis allows us to answer whether a specific type of government support measure favored a particular group of banks. Our focus is on the reaction of stock prices, as opposed to bond yields, because stocks are more frequently traded than bonds. Also, we do not use other bank-specific risk measures such as credit default swaps spreads, because these securities are not widely available for the majority of banks in developing countries.
To assess the stock market reaction to a policy announcement, we compute the accumulated abnormal stock returns of banks during the event. To be precise, we calculate accumulated abnormal returns over an event window n , which is the variation in the stock price one day prior to the announcement on day t , to n days after in excess to the expected returns implied by the mixed model with domestic and global factors ( A R e t b , t n ).
After calculating bank-level abnormal returns, we test whether policy announcements have significant effects on bank stock returns. In our benchmark specification, we test this hypothesis by estimating the following equation:
where A R e t b , c , t n represents the abnormal return of bank b located in country c in day t , measured over the [-1, n ] window (in days). As in the previous section, the covariates include a bank's ex-ante: liquidity ratio (measured as the ratio of cash & due from banks to total assets), oil exposure, size, and regulatory capital ratio; as well as an indicator variable for state-owned banks, and the percentage change in the number of COVID-19 cases per million on day t in each country. Finally, γ c and γ t are country and announcement date fixed effects. Standard errors are clustered at the country level.
We estimate Eq. (3) separately for each policy category. Since the variables are standardized (except for P u b l i c b a n k b ), the constant α 0 , n captures the abnormal stock returns of the average private bank for a type of announcement in the [-1, n ] window. The coefficients α 1 , n , α 2 , n , α 3 , n , α 4 , n and α 5 , n capture cross-sectional differences in the response of bank stock prices. For example, α 1 , n > 0 would suggest that banks with a larger share of liquid assets have higher accumulated abnormal returns during the event window for a specific type of announcement.
There are several challenges to our identification. First, owing to the high degree of global financial integration, there is extensive evidence of international spillovers from policy announcement by systemically important countries ( Ait-Sahalia et al., 2012 ; Morais et al., 2019 ). To avoid contaminating the analysis from the effects of financial policy initiatives taken by core countries, we exclude data from days when the U.S. or European authorities made major announcements covering the same broad policy type category. For example, on a day when the U.S. authorities announced liquidity support measures, we keep data from stock returns of U.S. banks in the sample but exclude banks in countries that issue liquidity announcement on the same day.
Second, due to the nature and scale of the crisis, in some cases, national government authorities announced multiple financial measures during the same day. In such situations, and without intraday time stamp for each announcement category, it is difficult to disentangle the effect of each policy on bank stock prices. To deal with these confounding factors, we further condition our sample to country-dates where only policies of a particular category were announced, and report results for this restricted sample.
Finally, selecting the length of the event window has important tradeoffs. Limiting the size of the window helps to avoid contaminating the analysis of a given announcement's effect with other news; particularly in a period when pandemic-related news was heightened. If the event window is too narrow, there might not be enough time for market participants to internalize the context and implications of complex policy announcements. This is likely to be more pronounced in developing countries, where trading volume in secondary markets is small and the speed of transactions is slower (e.g., the pace of business at which risk is transferred across market participants is longer). In turn, we use a five-day window, evaluating the change in stock prices from one day before the announcement to three days after, that is, n = {0,1,2,3}. We report accumulated abnormal returns for every day during this time window.
4.3. Impact of policy announcements
As a benchmark to gauge the magnitude of the stock market effects, we first plot the average abnormal returns during the event window for each policy category, pooling banks across all countries. Abnormal returns are obtained from estimating Eq. (3) on a constant, removing all other covariates and using day and country fixed effects. The results are displayed in Fig. 3 for the full sample (Panel A) and the restricted sample (Panel B) – when announcements of a particular category do not overlap with other initiatives. During our sample period, borrower assistance initiatives were strongly associated with large increases in the abnormal returns of bank stocks during the announcement day; 146 bps and 199 bps in the full and restricted sample respectively. We find that these large excess returns are present up to three days after the announcement. Borrower assistance initiatives typically include the introduction of government guarantees, which automatically transfer risks from banks’ balance sheets to the sovereign.

Abnormal returns of bank stocks around the announcement window
Notes: The variable plotted on the vertical axis shows the accumulated abnormal returns in percentage points within the event window of one day before the event and three days after the event, scaled to zero on the day before the announcement. Accumulated abnormal returns are averaged across banks for each policy category. The horizontal axis shows days within the event window, with "0" corresponding to the day of the announcement.
On days when prudential measures and policy rate reductions were announced, bank stocks display positive but small abnormal returns in the full sample (45bps and 39bps, respectively). These returns are quickly reversed within two days after the policy initiatives were announced. Notably, when we restrict the sample to single policy announcements, prudential measures seem to be accompanied by immediate price drops in bank stocks; 128 bps during the announcement day. 13 Prudential policies allow banks to run down some of their buffers to absorb the shock and send a strong signal about the resolve of policymakers. However, the fact that bank stock returns are negative might suggest that markets are also pricing the downside risk from the depletion of capital buffers.
Finally, we do not find evidence that liquidity support announcements had any aggregate short-term effects on bank stock prices. Although bank stocks seem to display positive abnormal returns when liquidity assistance measures are combined with other policies (Panel A in Fig. 4 ), in the restricted sample, abnormal returns are small and statistically indistinguishable from zero.

Cross-sectional impact of policy announcements (in percentage points)
Notes: Estimated slope coefficients of OLS regressions estimating daily accumulated abnormal returns on bank characteristics. Liquidity ratio, Oil exposure, Size and Capital ratio are standardized. “Public banks” is a dummy variable equal to 1 if the bank is state-owned and zero otherwise. The horizontal axis shows days within the event window, with "0" corresponding to the day of the announcement.
The results on the cross-sectional impact of financial sector policies are presented in Table 5 and Fig. 4. 14 While we present findings for the full and restricted sample, our preferred specification and discussion focuses on single policy initiatives. The constant in each model represents the average abnormal returns of private banks in the sample. The results confirm our previous finding that borrower assistance measures are strongly associated with banks’ stock price response. Other policy initiatives seem to have smaller or even negative aggregate effects on bank stocks.
Impact of policy initiatives.
Notes: The table presents OLS estimates of cross-sectional regressions of the impact of financial sector policies on the abnormal returns of banks on announcement days ( n = 0) and three days after the announcement ( n = 3). Panel A includes all announcements of a particular category in the sample. Panel B restricts the sample to announcements of a particular category that do not overlap with announcements of other financial sector initiatives. Abnormal returns are calculated as the difference between realized returns and the expected returns implied by a market model. Liquidity ratio is defined as the ratio of liquid assets (cash & due from banks) to total assets averaged over the 2019Q1-2019Q4 period. Oil exposure corresponds to the slope coefficient of an OLS regression of bank's stock returns on a constant, the market return where the bank is domiciled, and the rate of return of oil prices using weekly data between May 2018 and December 2019. Size is calculated as the 2019Q1-2019Q4 average total assets for each bank and is reported in logs. Public bank is an indicator variable that equals one for banks with a non-zero equity participation from the domestic government and zero otherwise. Capital ratio corresponds to the 2019Q1-2019Q4 average ratio of Tier 1 + Tier 2 capital to total assets. All control variables are standardized with mean 0 and standard deviation 1. All specifications include day and country fixed effects and control for the daily number
4.3.1. Liquidity support
Announcements of liquidity support are associated with a reduction in the liquidity premium. That is, stocks of banks with a smaller share of liquid assets overperform after policymakers announce liquidity and funding measures. The coefficient for the liquidity ratio can be read as follows: a bank with a liquidity ratio measure of one-standard deviation lower than the average, experienced an additional 210 bps of abnormal returns up to three days after the government announced liquidity support policies. The result is important because it confirms that central banks’ actions helped alleviate the sharp tightening of financial conditions at the onset of the crisis. During March, due to the overwhelming volatility in securities and FX markets, many financial entities reported having difficulties in accessing funding, which is consistent with the sharp increase in the liquidity premium documented in Section 3 . Overall, policies targeting funding availability (whether in domestic or foreign currency) appear to have a favorable impact in the reduction of the liquidity premium. We also see that stock prices of public banks and those of smaller banks benefit more from liquidity measures, potentially due to greater reductions in uncertainty these policies provide to such banks.
4.3.2. Prudential measures
In addition to having a negative impact on aggregate bank stock prices, prudential measures do not seem to be associated with clear reductions in the liquidity premium. The use of buffers, facilitation of loan restructuring, and other measures that require regulatory forbearance (e.g., loan moratoria and different treatment of non-performing loans), might help support credit flow throughout the lockdowns, but they entail large risks in the medium term, threatening financial stability. It appears that markets are pricing these risks, since abnormal returns are negative around the announcement of countercyclical prudential measures.
4.3.3. Borrower assistance
Announcements of borrower assistance were associated with large increases in abnormal returns of bank stocks. Recognizing the severity of the shock, especially to small non-essential businesses that would be unable to cover operating costs during extended lockdowns, many countries enhanced their public liability guarantees programs, with government guarantees up to 90% of loan values. Fiscal resources were also committed to subsidize interest-free loans and directly fund strategic sector during the pandemic. These initiatives generate large fiscal costs in the short term, while transferring the credit risks from banks to the government. It appears that markets internalize this information as good news for the banking industry, which could now offload part of the burden of the shock to the sovereign.
Announcements of borrower support programs had a greater impact among larger banks. For instance, a bank that is one-standard deviation above the mean in size, experiences an additional 109bps of abnormal returns up to three days after the announcement. Ornelas et al. (2019) document that during the GFC, large banks disproportionally benefited from allocating government-sponsored credit. While loans that are funded with government resources typically have interest rate ceilings, banks offset the lower revenue from these loans by extracting rents in other lending products to the recipients of government support. Smaller banks and those that operate in niche markets are less able to take advantage of cross-selling and enhanced sales in other products.
Consistent with the view that credit guarantees, and other state-funded lending programs reduce bank risk, we find that announcements of borrower assistance benefit banks with a higher share of lending portfolios prior to the COVID-19 crisis, as measured by their 2019Q1-2019Q4 average loan-to-asset ratios. 15
When we split the sample into developed and developing countries, we find that the positive association between abnormal stock returns and borrower support initiatives is exclusive to developed countries (see Table 6 and Fig. 5 ). As noted above, borrower assistance requires potentially large fiscal commitments. In developing countries, authorities have lower fiscal space to operate, and markets might anticipate that the extent of the borrower assistance measures in such settings is limited. 16 On the contrary, fiscal authorities in developed countries can pledge significant resources to help distressed households and firms, which in turn reduce bank risk.
Heterogeneity in the impact of policy initiatives (developed vs. developing countries).
Notes: The table presents OLS estimates of cross-sectional regressions of the impact of financial sector policies on the abnormal returns of banks on announcement days ( n = 0) and three days after the announcement ( n = 3) for the restricted sample of announcements of a particular category that do not overlap with announcements of other financial sector initiatives. Panel A restricts the sample to developed countries. Panel B restricts the sample to developing countries. Abnormal returns are calculated as the difference between realized returns and the expected returns implied by a market model. Liquidity ratio is defined as the ratio of liquid assets (cash & due from banks) to total assets averaged over the 2019Q1-2019Q4 period. Oil exposure corresponds to the slope coefficient of an OLS regression of bank's stock returns on a constant, the market return where the bank is domiciled, and the rate of return of oil prices using weekly data between May 2018 and December 2019. Size is calculated as the 2019Q1-2019Q4 average total assets for each bank and is reported in logs. Public bank is an indicator variable that equals one for banks with a non-zero equity participation from the domestic government and zero otherwise. Capital ratio corresponds to the 2019Q1-2019Q4 average ratio of Tier 1 + Tier 2 capital to total assets. All control variables are standardized with mean 0 and standard deviation 1. All specifications include day and country fixed effects and control for the daily number of COVID-19 cases in a country. Standard errors are clustered at the country level.

Abnormal returns of bank stocks after borrower assistance announcements: developed vs. developing countries
Notes: The variable plotted on the vertical axis shows the accumulated abnormal returns in percentage points for borrower assistance policies within the event window of one day before the event and three days after the event, scaled to zero on the day before the announcement. Accumulated abnormal returns are calculated by estimating Eq. (3) on a constant removing all other covariates and including country and announcement day fixed effects. The sample is split between developed and developing countries. The horizontal axis shows days within the event window, with "0" corresponding to the day of the announcement.
4.3.4. Monetary policy
Interest rate cuts were associated with significant declines in the liquidity premium. To be precise, during days of policy rate cuts, banks with lower liquidity experienced higher abnormal returns than more liquid banks (full and restricted sample in Table 5 ). The significant relative increase in abnormal stock returns among banks with lower liquidity ratios may have reflected markets’ expectation that lower interest rates would increase liquidity in the financial system, thereby benefiting banks with larger funding risks. The result also confirms that the interest rate policy remained a key policy tool at the onset of the crisis, since markets are familiar with conventional monetary policy.
Banks with more exposure to the oil shock seem to benefit less from interest rate actions, as evidenced in both the full and restricted sample. The result highlights the key difference between liquidity and solvency risks. Banks that are heavily exposed to the oil sector are more susceptible to losses due to the likelihood of defaults in this industry. To the extent that markets internalize that the profitability of these banks will be lower, interest rate cuts would have small effects. On the other hand, banks with short-term liquidity constraints, but with less exposed portfolios, stand to benefit more from the ease in funding conditions.
Announcement concerning asset purchases also appear to be followed by increases in the abnormal returns of less liquid banks, albeit the coefficient is statistically indistinguishable from zero in both the full and restricted sample. We interpret this finding as complementary evidence that unconventional monetary policy also played a role in reducing the liquidity premium.
Since announcements should have a greater impact if they are unexpected than if they are expected, we further restrict our sample to days with monetary policy surprises. That is, we restrict our sample to days when the monetary authority made policy rate announcements after emergency meetings, or when the domestic policy rate cut was larger than the expected by market analysts. 17 We further confirm earlier findings with the sample of unexpected monetary policy announcements. Although surprises of interest rate cuts were not associated with significant increases in bank stock prices, such announcements did appear to have differential effect across banks. For instance, monetary policy surprises were associated with declines in the liquidity premium and seemed to be more beneficial for banks with lower exposure to the oil sector ( Table A6 in the Appendix).
5. Endogeneity
Notwithstanding the robustness of the results, possible endogeneity issues remain a key challenge for our identification strategy. In particular, while the COVID-19 shock is exogenous, the policy mix adopted in a country is not random. To the extent that selected policies are precisely those that the financial authorities deemed more efficient for their jurisdictions, taking into account local economic conditions, banks’ characteristics and banks’ stock performance, our estimates would be biased. In this section, we present three exercises to address such endogeneity concerns. First, we examine the reaction of stock prices of large cross-border banks from policy announcements in countries where their subsidiaries make a significant share of their balance sheet. Second, we examine the extent to which domestic and external factors influence the announcement of financial sector policies. Third, we analyze the stock price response of banks in the euro area to policy announcements made by the ECB.
5.1. Cross-border banks
We focus on large cross-border banks headquartered outside a given jurisdiction but with exposure through a locally operating subsidiary. We examine the stock price reaction of these banks on days when fiscal and regulatory authorities at the country of the subsidiary introduce financial sector measures. Since local regulators are less likely to be influenced by the performance of individual banks with operations abroad, endogeneity concerns should be reduced.
We use data from Factset Revere Geographic Revenue (GeoRev). GeoRev provides annual information of companies’ revenue by geographic region and country. We use the last annual report for each bank in our sample prior to March 2021. We identify 150 banks that derived at least 1% of their total revenue from international subsidiaries. For example, according to GeoRev, Banco Santander, domiciled in Spain, generated 24% of its total revenue from Brazil, 17% from the United States, 11% from the United Kingdom, 8% from Mexico, and 5% from Chile during 2020. Table A7 presents the summary statistics of the 150 cross-border banks in our sample. As the table shows, subsidiaries abroad represent 5.9% of the total revenue of the average cross-border bank, with banks in the 25th percentile generating 1.6% of their revenues in their subsidiaries and banks in the 75th percentile making 6.8%. In terms of geographical penetration, the median cross-border bank in the sample operates in 6 countries, while banks in the 75th percentile operate in 12 countries. Of the 150 cross-border banks in the sample, 130 report revenues in subsidiaries located in developed countries, compared to 87 in developing countries.
We modify our event study methodology from Eq. (3) to examine the abnormal returns of a cross-border bank b in its country of domicile c on days when authorities in the country of its subsidiary s made a policy announcement, A R e t b , c , s , t n . To account for contemporaneous news that affect the banking sector at the country of domicile, we control for the average abnormal returns of banks in country c , excluding bank b : A R e t − b , c , t n . We also control for the incidence of the pandemic in both the location of the subsidiary and the bank's domicile using the percentage change of COVID-19 cases per million during the week, C o v i d s , t and C o v i d c , t respectively. We estimate the following equation:
where γ b and γ t are bank and day fixed effects and errors are clustered at the bank level. In Eq. (4) , the constant α 0 , n represents the average abnormal returns of cross-border banks in the time window [-1, n ] when a policy category is announced at the country of their subsidiary. To be precise, the abnormal returns of a bank around t are included in Eq. (4) anytime a policy is announced in a country where the bank operates a subsidiary. Following the analysis in Section 4 , we estimate Eq. (4) separately for each policy category, for announcements in developed and developing countries and focus on days with single policy announcements at the location of the subsidiary.
Table 7 presents our results. We examine the abnormal returns of cross-border banks for announcements in countries where the subsidiary represents at least 1 and 5% of the bank's yearly revenue. The results confirm our previous findings. In developed countries, countercyclical prudential measures are followed by negative abnormal stock returns up to three days after the announcement. Conversely, when developed countries announced borrower assistance programs, foreign banks with significant exposure to the country experience large and positive abnormal returns in their stock prices. Also, consistent with previous findings, borrowing assistance measures in developing countries seem to have no effect on bank stock prices.
Impact of policy initiatives on cross-border banks.
Notes: The table presents impact estimates of financial sector policies on the abnormal returns of cross-border banks with subsidiaries generating at least 1% of the banks' revenues and located in the country of the policy announcement. The estimates correspond to the abnormal returns of cross-border banks on the announcement day ( n = 0) and three days after the announcement ( n = 3). The sample of announcements is restricted to announcements of a particular category that do not overlap with announcements of other financial sector initiatives. Panel A restricts the sample to announcements in developed countries. Panel B restricts the sample to announcements in developing countries. Abnormal returns are calculated as the difference between realized returns and the expected returns implied by a market model. Controls include the average abnormal returns of banks in the country where the cross-border bank is domiciled, and the percentage change of COVID-19 cases per million during the week, both in the location of the subsidiary and in the country where the cross-border bank is domiciled. All specifications include day and bank fixed effects. Standard errors are clustered at the bank level.
To verify that our findings are not driven by spurious correlations in the stock prices of global banks at the onset of the pandemic, we estimate Eq. (4) for announcements in bank-country pairs where the revenue exposure is less than 1%. In this placebo test, we confirm that for all policy categories, the estimated α 0 , n is small and indistinguishable from zero. That is, the stock returns of large cross-border banks do not seem to be driven by announcements in countries where the subsidiary only represents a small fraction of total revenue.
5.2. Drivers of policy adoption
As an additional exercise, we examine the drivers behind the announcement of financial sector policies across jurisdictions. More precisely, we examine the extent to which domestic factors, such as the banking sector characteristics of a jurisdiction, and external factors, such as the adoption of similar policies in neighboring countries, predict the adoption of certain policies in a jurisdiction. Concerns about the endogeneity of policy measures would be mitigated if policy adoption is driven by external factors, for instance, due to policy contagion, rather than by domestic factors. Given the pace and uncertainty from the unprecedented global shock, local authorities might implement policies following the announcements in other countries. Indeed, Mistur et al. (2020) show that the actions of geographical neighbors and peer countries significantly influenced the decision to implement social distancing rules, close non-essential businesses and institute travel bans. Earlier work has also documented financial sector policy diffusion across borders in the adoption of international banking standards ( Jones and Zeitz, 2019 ) and in the implementation of deposit insurance ( Demirguc-Kunt et al., 2006 ).
To investigate the factors associated with the adoption of financial sector measures in a jurisdiction between the period of February to April, we run a series of OLS regressions at the country-day level that are summarized in Eq. (4 ). 18
The dependent variable of Eq. (4 ) equals one if country c announced a policy intervention type p on day t and zero otherwise. Since policies in our sample are present from the announcement date to the end of our observation period, we drop all post-announcement days. 19 The vector of internal factors I N T c includes the set of covariates that capture ex-ante banking sector characteristics of a jurisdiction: liquidity ratio, oil exposure, size, state ownership and capital ratio, averaged for all publicly traded banks in each country. 20 C o v i d c , t − 1 corresponds to the percentage change in the number of COVID-19 cases per million on day t-1 in country c . Time varying market factors include the accumulated returns of the domestic and global equity markets, as well as the banks’ stock returns in the country.
The vector of external factors E X T p c , t − 1 includes two variables that capture policy contagion: (i) The fraction of peer countries that by day t-1 have adopted the same type of policy p ( S a m e t − 1 , p ), and (ii) the fraction of peer countries that by day t-1 have announced financial sector policies other than p ( O t h e r t , p ). To evaluate contagion, we define peers as countries in the same geographical region within a particular income group as classified by the World Bank and calculate the share of countries within a region announcing a policy type at each day ( World Bank, 2019 ). 21 , 22 Appendix Table A8 presents summary statistics for the variables used in this section. 23
In Eq. (4) , γ w corresponds to week-fixed effects to control for unobserved common elements that make a particular financial policy initiative more desirable in countries over time. In some specifications, we further include fixed effects at the country*week level to isolate the role of policy contagion from all other time-varying developments within a country that may influence policy decisions of regulators. Errors are clustered at the country level and all covariates are standardized with mean 0 and standard deviation 1.
Table 8 and and8a presents 8a presents our main findings. Estimates for each policy announcement are displayed across three columns. In the first column of each policy measure, our results indicate that observable bank sector characteristics of a jurisdiction prior to the COVID-19 shock had little and in most cases no effect on the type of policy that was implemented in each country. One exception is the share of public banks, which is positively associated with an increased likelihood of announcing different measures. According to our results, a one-standard-deviation increase in a country's share of state-owned banks is linked to an increase in the likelihood of announcing prudential measures of 0.9% points and of 1.7% points in the likelihood of monetary policy announcements. Similarly, we find that the adoption of financial sector policies of a country is not driven by domestic market factors, measured by the lagged stock returns of banks in the country and the lagged accumulated returns of the domestic equity market. While global market factors have a statistically significant association with the adoption of certain measures, their economic magnitude is small. More precisely, a one-standard-deviation increase in the lagged accumulated returns of global equity markets leads to a 1.1% point increase in the probability of a liquidity measure announcement. The lack of explanatory power of ex-ante banking sector characteristics is not driven by the inclusion of lagged domestic and global factors in the regressions. That is, the coefficients and standard errors of the banking sector variables remain unaffected if we drop from the regressions the lagged accumulated returns of domestic and global equity markets and the lagged bank stock returns.
Impact of policy contagion on policy announcements.
Notes: The table presents the estimates of OLS regressions at the country-day level on the probability of announcing a financial sector policy from February 1 to April 17, 2020. The dependent variable corresponds to an indicator variable that equals 1 on days that a country announces the policy in each panel and zero otherwise. Post-announcement observations are dropped from the sample. For each country, Liquidity ratio (cash & due from banks/total assets), Oil exposure, Size (log of assets) and Capital ratio (Tier 1 + Tier 2 capital over total assets) are averaged over the period 2019Q1-2019Q4 across banks in the sample. Public bank is the country share of state-owned banks in the sample. COVID corresponds to the lagged daily percentage change of confirmed COVID-19 cases per million citizens. Domestic returns correspond to the one-day lagged accumulated domestic market returns. Bank returns measures the one-day lagged accumulated bank returns averaged for each country. World returns are the one-day lagged accumulated global market returns. Same corresponds to the regional one-day-lagged share of countries that announce the policy in each panel. Other corresponds to the regional one-day-lagged share of countries that announce other financial sector policies different from the policy in each panel. All control variables are standardized with mean 0 and standard deviation 1. All specifications include week fixed effects. Standard errors are clustered at the country level.
The next two columns of each panel show the role of country contagion on the likelihood of policy announcements. We find that past announcements of a specific policy type by regional peer countries have a statistically significant and economically meaningful effect on the policy adoption decision (the coefficient of S a m e t − 1 , p is positive). After controlling for time-varying factors within a country (captured by the country*week fixed effects), we find that a one-standard deviation increase in the share of countries announcing prudential measures in the previous day, is associated with a 23.4% point increase in the probability that a local authority implements a similar measure. This is a sizable increase, from 1.2% – the average likelihood of introducing a prudential measure on any given day – to 24.6% after peer countries implement prudential policies. Notably, past announcements of other policy types by regional peer countries do not increase the likelihood that a jurisdiction announces a specific policy (with the coefficient O t h e r t − 1 , p being either negative or in most cases indistinguishable from zero). We find similar results when using a Probit model instead of an OLS (reported in Appendix Table A9 ), although with the inclusion of week fixed effects we lose about half of the observations in most specifications and the inclusion of week*country fixed effects is prohibitive in this case.
Our evidence thus suggests that even after controlling for time-varying unobserved domestic factors, external factors, and in particular, policy contagion, are major drivers of policy adoption. That is, as more countries adopt a specific financial sector policy initiative, other countries become more likely to follow their lead. We interpret these findings as evidence that conditional on other countries' actions, regulators randomly employed financial sector policies in their jurisdictions. Moreover, given that our analysis throughout the paper includes country-fixed effects, we effectively are comparing how the announcement of a particular policy impacted banks with different characteristics within the country.
5.3. Cross-country announcements
As a final exercise to explore whether our results are biased by the endogeneity of policy actions, we examine broad cross-country policy measures in the euro area. Arguably, interventions from the European Central Bank are less likely to be driven by within country considerations. We estimate abnormal returns in bank stocks around policy announcements in Euro area countries. 24 We focus on countercyclical prudential measures because these offer the widest set of announcements to perform multiple robustness tests. For instance, prudential measures are announced both by local authorities, as is the case in France, Germany, Italy, and Spain, and at the ECB level. There are also days when only prudential measures were undertaken (liquidity, borrower support, and asset purchases were exclusively announced with other initiatives for these set of countries). 25
Columns (1) and (2) of Table A10 present the estimates of the accumulated abnormal returns from the announcement day to three days after the announcement. These results include days when prudential measures are introduced by domestic authorities or by the ECB. In line with previous findings, bank stocks display negative returns after prudential measures are announced. Larger banks within the EU display the lowest returns following prudential measures, although the coefficient is not statistically significant three days after the announcement. The results are robust to excluding domestic announcements (columns 3 and 4) which suggests that supranational prudential measures result in declines in bank stock prices. Our findings provide further credence to the interpretation that markets are pricing the additional risk to banks from the adoption of countercyclical measures. Finally, we restrict our sample to days when only prudential measures were announced (excluding days with multiple policy announcements in columns 5 and 6). Although the constant is not statistically significant in this case due to the reduced sample size, it appears that on average, bank stock prices have large declines up to three days following the announcement.
6. Conclusions
The spread of COVID-19 represents an unpresented global shock, with the disease itself and mitigation efforts –such as social distancing measures and partial and national lockdown measures– both having a significant impact on the economy. In the immediate aftermath, the financial sector, particularly banks, were expected to play an important role in absorbing the shock by supplying vital credit to the corporate sector and households. In an effort to facilitate this, central banks and governments around the world enacted a wide range of policy measures to provide greater liquidity and support the flow of credit. An important policy question is the potential impact of these countercyclical lending policies on the future stability of the banking systems and to what extent their strengthened capital positions since the global financial crisis will allow them to absorb this shock without undermining their resilience.
In this paper, we use daily stock prices and other balance sheet information for a sample of banks in 53 countries to take a first look at this issue. Our contribution is twofold. We first assess the impact of the pandemic on the banking sector and investigate whether the shock had a differential impact on banks versus corporates, as well as those banks with different characteristics. Second, using a global database of financial sector policy responses and an event study methodology, we investigate the role of different policy initiatives on addressing bank stress as perceived by markets, in the aggregate, as well as across different banks.
Our results suggest that the adverse impact of the COVID-19 shock on banks was much more pronounced and long-lasting than on the corporates as well as other non-bank financial institutions, revealing the expectation that banks are to absorb at least part of the shock to the corporate sector. Furthermore, larger banks and public banks suffered greater reductions in their stock returns, reflecting their greater anticipated role in dealing with the crisis. Banks with lower pre-crisis liquidity also suffered greater reduction in returns, consistent with their greater vulnerability to such a shock.
Investigating close to 400 policy announcements between February and April 2020, we next evaluate the impact of liquidity support, prudential measures, borrower assistance and monetary policy measures on bank abnormal returns. Our results suggest liquidity support and borrower assistance measures had the greatest positive impact on bank abnormal returns. Less liquid banks benefited most from liquidity support, whereas larger banks saw increased abnormal returns with the announcement of borrower assistance policies. However, since they rely on fiscal expenditures, these policies did not result in a positive impact on bank stock prices in developing countries where there is less room for fiscal expansion. Prudential measures appeared to have a negative impact on bank returns, suggesting that markets price the downside risk from depletion of capital buffers. Finally, policy rate cuts mostly benefited less liquid banks, confirming that monetary policy again played a key tool during this crisis.
Overall, our results suggest that the crisis and the countercyclical lending role they are expected to play has put banking systems around the world under stress, having a differential impact depending on their characteristics and pre-crisis vulnerabilities. While some policy measures such as liquidity support, borrower assistance and monetary easing moderated this adverse impact for some banks, this is not true for all banks or in all circumstances. For example, borrower assistance measures exacerbated the stress for banks that operate in countries with little fiscal space. These vulnerabilities will need to be carefully monitored as the pandemic continues to take its toll on the world economies.
CRediT authorship contribution statement
Asli Demirgüç-Kunt: Conceptualization, Supervision, Writing – original draft, Writing – review & editing. Alvaro Pedraza: Methodology, Investigation, Writing – original draft, Writing – review & editing. Claudia Ruiz-Ortega: Data curation, Investigation, Writing – original draft, Writing – review & editing.
Declaration of Competing Interest
1 Using U.S. data, Acharya and Steffen (2020) provide evidence of the transmission mechanism, whereby firms convert commited credit lines from banks into cash, exerting significant pressure on lenders.
3 We find that the adoption of financial sector policy measures is not associated with ex-ante characteristics of the banking sector in the country, or with time-varying market factors. In contrast, policy adoption appears largely explained by the actions of peer countries, namely, by policy contagion.
4 One remaining concern is whether our impact estimates capture investors’ reaction to information about the shock revealed through the policy announcement. However, this mechanism does not explain why bank returns are negative (positive) when prudential (borrower support) measures are announced, nor why markets only respond to borrower support measures announced in developed countries. Thus, our preferred interpretation is that our findings capture the impact of the policies rather than the information they carry.
5 The data on COVID-19 cases was retrieved from 'https://ourworldindata.org/coronavirus'.
6 While our liquidity measure omits other types of liquid assets held by banks, cash and due from banks are easier to observe and monitor, and their value is not subject to risk shifting ( Bonner et al., 2015 ; Calomiris et al., 2015 ; Calomiris, 2012 ), an issue particularly relevant in less developed financial markets ( Elliott, 2014 ). However, as a robustness check, we calculate two alternative definitions of bank liquidity. The first definition is the ratio of liquid assets to total deposits, where liquid assets includes cash and due from banks, federal funds sold, and securities purchased under resale agreements. The second measure consists of the ratio of liquid assets to total assets, where liquid assets include cash and due from banks, federal funds sold, securities purchased under resale agreements, trading account assets, other short-term investments, and total investment securities.
7 We define public banks as those with a non-zero equity participation from the domestic government. Our findings are robust to alternative definitions of public banks where the minimum threshold of state ownership is set to 10% and 30%.
8 Average returns of firms in Panel A of Fig. 1 are equally weighted across countries and are net from bank returns. To calculate these, we exclude the returns of banks from the returns of each country's stock market using the index weights of banks. The average returns of banks are weighted by the contribution of each bank to the total bank assets of each region. The regional average bank returns are then equally weighted across regions. For Panel B, we obtain data on all publicly traded non-bank financial companies across the 52 countries in our sample.
9 All covariates are standardized with mean 0 and standard deviation 1.
10 We also include eight policy announcements that were made during weekends. For these cases, we study the stock response using the first business day when the domestic stock market opened.
11 In most cases and for each policy category, the first time that a country announced the adoption of a financial sector measure was prior to April 17; 94% of first-time announcements in the sample (between Jan and Sep 2020).
12 Correa et al. (2014) also use bank stock prices to evaluate another kind of shock – the downgrade of the sovereign credit risk.
13 As Table A5 shows, in developed countries, 52 of the 99 prudential policy measures were jointly announced with borrower assistance initiatives. This might explain why in the full sample the net effect of prudential policies is higher than in the restricted sample.
14 To present all the policies in a single table, we only include same-day estimates ( n = 0) and three days after the announcement ( n = 3). However, the figures display estimates during the entire event window.
15 These unreported results can be shared upon request.
16 Fiscal expansion in developing economies is more costly, as these countries have a more difficult time raising money in the market ( Kose et al., 2017 ) and the debt threshold at which they experience growth rate decline is lower ( Reinhart and Rogoff, 2010 ).
17 We use the Reuters forecast based on the one-week analyst survey of benchmark rates. Some examples of monetary policy surprises include Thailand's 25bps cut in the benchmark rate (February 5), Canada's repo rate cut of 50bps (March 4), and the United Kingdom 50bps repo rate cut during the emergency meeting of March 11. The only case in which the rate cut was smaller than expected was in Thailand on March 20. We drop this date from the sample of monetary policy surprises.
18 While OLS regressions ease the interpretation of the coefficients, we also include the results from a Probit model.
19 The exercise resembles a hazard model of policy adoption.
20 To compute X c , we average the characteristics of banks in our sample using equal weights for each bank. As a robustness check, we compute X c using weighted averages, where the weight of a given bank is calculated using the assets (in logs) of the bank in 2019. We confirm that our results remain unchanged with this alternative measure of X c .
21 High-income countries are classified in two groups: (i) High-income OECD countries, (ii) High-income non-OECD countries. All other countries are classified in six regions: (i) East Asia & Pacific, (ii) Europe and Central Asia, (iii) Latin America & the Caribbean, (iv) Middle East & North Africa, (v) South Asia, (vi) Sub-Saharan Africa.
22 Career concerns among regulators might also promote policy contagion ( Gadinis, 2015 ).
23 In results not reported, we alternatively calculate contagion using the fraction of countries in the world that have announced a policy at each point in time. This definition deals with the possibility that some policy diffusion might occur from core to periphery countries, or even in different networks that form through cross-border financial linkages (e.g., Basel committee membership). The role of policy contagion remains similar when using this definition of peer countries.
24 In our sample, these are: Austria, Finland, France, Germany, Italy, the Netherlands, Portugal, and Spain.
25 There is no interest rate policy announcement in the sample of EU countries.
2 Using detailed loan-level data Ornelas et al. (2019) find that larger banks disproportionally allocated government-sponsored credit during the GFC.
Table A1 , Table A2 , Table A3 , Table A4 , Table A5 , Table A6 , Table A7 , Table A8 , Table A9 , Table A10
Number of banks by country.
Notes: The table lists the number of banks by country along with the sum of their weights (based on assets) within each region and their domestic market index.
Summary statistics of banks by region.
Risk factors during the COVID-19 crisis (implied by six-factor model).
Notes: The table presents OLS estimates of bank-week panel regressions. The dependent variable is abnormal returns, calculated as the difference between a bank stock returns during a week and the expected returns implied by a six-factor model: domestic market, global market, size, book to market, profitability, and investment risk. Returns are calculated in local U.S. dollars. The definition of liquidity ratio, averaged over the 2019Q1-2019Q4 period, corresponds to: Liquid assets A/total assets, where liquid assets A include cash & due from banks (columns 1 and 4); liquid assets B/total deposits, where liquid assets B include cash & due from banks plus federal funds sold and securities purchased under resale agreements (columns 2 and 5); liquid assets C/total assets, where liquid assets C include cash & due from banks, federal funds sold and securities purchased under resale agreements, trading account assets, other short-term investments and total investment securities (columns 3 and 6). Oil exposure corresponds to the slope coefficient of an OLS regression of bank's stock returns on a constant, the domestic markets, and the percentage change of oil prices using weekly data between May 2018 and December 2019. Size is calculated as the 2019Q1-2019Q4 average total assets for each bank and is reported in logs. Public bank is an indicator variable that equals one for banks with non-zero equity participation from the domestic government and zero otherwise. Capital ratio corresponds to the 2019Q1-2019Q4 average ratio of Tier 1 + Tier 2 capital to total assets. COVID corresponds to the percentage change in number of confirmed COVID-19 cases of a country per million citizens during each week. All control variables are standardized with mean 0 and standard deviation 1. All specifications include country and week fixed effects. Standard errors are clustered at the country level.
Risk factors during the COVID-19 crisis (implied by domestic market model).
Notes: The table presents OLS estimates of bank-week panel regressions. The dependent variable is abnormal returns, calculated as the difference between a bank stock returns during a week and the expected returns implied by a domestic market model. Returns are calculated in local currency. The definition of liquidity ratio, averaged over the 2019Q1-2019Q4 period, corresponds to: Liquid assets A/total assets, where liquid assets A include cash & due from banks (columns 1 and 4); liquid assets B/total deposits, where liquid assets B include cash & due from banks plus federal funds sold and securities purchased under resale agreements (columns 2 and 5); liquid assets C/total assets, where liquid assets C include cash & due from banks, federal funds sold and securities purchased under resale agreements, trading account assets, other short-term investments and total investment securities (columns 3 and 6). Oil exposure corresponds to the slope coefficient of an OLS regression of bank's stock returns on a constant, the domestic markets, and the percentage change of oil prices using weekly data between May 2018 and December 2019. Size is calculated as the 2019Q1-2019Q4 average total assets for each bank and is reported in logs. Public bank is an indicator variable that equals one for banks with non-zero equity participation from the domestic government and zero otherwise. Capital ratio corresponds to the 2019Q1-2019Q4 average ratio of Tier 1 + Tier 2 capital to total assets. COVID corresponds to the percentage change in number of confirmed COVID-19 cases of a country per million citizens during each week. All control variables are standardized with mean 0 and standard deviation 1. All specifications include country and week fixed effects. Standard errors are clustered at the country level.
Policy announcements in developed and developing countries.
Notes: Data obtained from the World Bank ( Feyen et al., 2021 ) covering the period February 1 to April 17 2020.
Impact of unexpected monetary policy rate announcements.
Notes: The table presents OLS estimates of cross-sectional regressions of the impact of financial sector policies on the abnormal returns of banks on announcement days ( n = 0) and three days after the announcement ( n = 3). Abnormal returns are calculated as the difference between realized returns and the expected returns implied by a market model. Liquidity ratio is defined as the ratio of liquid assets (cash & due from banks) to total assets averaged over the 2019Q1-2019Q4 period. Oil exposure corresponds to the slope coefficient of an OLS regression of bank's stock returns on a constant, the market return where the bank is domiciled, and the rate of return of oil prices using weekly data between May 2018 and December 2019. Size is calculated as the 2019Q1-2019Q4 average total assets for each bank and is reported in logs. Public bank is an indicator variable that equals one for banks with a non-zero equity participation from the domestic government and zero otherwise. Capital ratio corresponds to the 2019Q1-2019Q4 average ratio of Tier 1 + Tier 2 capital to total assets. All control variables are standardized with mean 0 and standard deviation 1. All specifications include day and country fixed effects and control for the daily number of COVID-19 cases in a country. Standard errors are clustered at the country level.
Summary statistics of cross-border banks.
Notes: The table presents summary statistics for our sample of 150 cross-border banks that derived at least 1% of their total revenue from international subsidiaries. The first panel presents statistics for all cross-border banks. The second and third panels present statistics for cross-border banks with subsidiaries in developed and developing countries, respectively. Revenue (%) corresponds to the share of total revenue generated by the subsidiaries of cross-border banks. Number of countries with subsidiaries per bank show the number of countries with subsidiaries of cross-border banks representing at least 1% of their total revenue.
Summary statistics of country-day level variables.
Notes: The table presents country-day level summary statistics from February 1 to April 17, 2020 for the variables used in the analysis of drivers of policy announcements. Post-announcement observations of the policy in each panel are dropped from the sample. For each country, Liquidity ratio (cash & due from banks/total assets), Oil exposure, Size (log of assets) and Capital ratio (Tier 1 + Tier 2 capital over total assets) are averaged over the period 2019Q1-2019Q4 across banks in the sample. Public bank is the country share of state-owned banks in the sample. COVID corresponds to the daily percentage change of confirmed COVID-19 cases per million citizens. Same corresponds to the regional one-day-lagged share of countries that announce the policy in each panel. Other corresponds to the regional one-day-lagged share of countries that announce other financial sector policies different from the policy in each panel.
Impact of policy contagion on policy announcements (Probit model).
Notes: The table presents the estimates of Probit regressions at the country-date level on the probability of announcing a financial sector policy from February 1 to April 17, 2020. The dependent variable corresponds to an indicator variable that equals 1 on dates that a country announces the policy in each panel and zero otherwise. Post-announcement observations are dropped from the sample. For each country, Liquidity ratio (cash & due from banks/total assets), Oil exposure, Size (log of assets) and Capital ratio (Tier 1 + Tier 2 capital over total assets) are averaged over the period 2019Q1-2019Q4 across banks in the sample. Public bank is the country share of state-owned banks in the sample. COVID corresponds to the lagged daily percentage change confirmed COVID-19 cases per million citizens. Same corresponds to the regional one-day-lagged share of countries that announce the policy in each panel. Other corresponds to the regional one-day-lagged share of countries that announce other financial sector policies different from the policy in each panel. Additional controls include one-day lagged accumulated bank returns averaged for each country as well as the one-day lagged accumulated domestic and world market returns. All control variables are standardized with mean 0 and standard deviation 1. All specifications include week fixed effects. Standard errors are clustered at the country level.
Impact of ECB policy announcements across Euro Area countries.
Notes: The table presents OLS estimates of cross-sectional regressions of the impact of financial sector policies on the abnormal returns of banks on announcement days ( n = 0) and three days after the announcement ( n = 3). Columns 1 and 2 include days when prudential measures are introduced by domestic authorities or by the ECB. Columns 3 and 4 exclude domestic policy announcements. Columns 5 and 6 exclude days with multiple policy announcements. Abnormal returns are calculated as the difference between realized returns and the expected returns implied by a market model. liquidity ratio is defined as the liquidity ratio of a bank averaged over the 2019Q1-2019Q4 period, calculated as the ratio of cash & due from banks to total assets. Oil exposure corresponds to the slope coefficient of an OLS regression of bank's stock returns on a constant, the market return where the bank is domiciled, and the rate of return of oil prices using weekly data between May 2018 and December 2019. Size is calculated as the 2019Q1-2019Q4 average total assets for each bank and is reported in logs. Public bank is an indicator variable that equals one for banks with a non-zero equity participation from the domestic government and zero otherwise. Capital ratio corresponds to the 2019Q1-2019Q4 average ratio of Tier 1 + Tier 2 capital to total assets. All control variables are standardized with mean 0 and standard deviation 1. All specifications include day and country fixed effects and control for the daily number of COVID-19 cases in a country. Standard errors are clustered at the country level.
- Acharya, V., Engle, R. & Steffen, S., 2021. Why did bank stocks crash during COVID-19?
- Acharya V., Steffen S. The risk of being a fallen angel and the corporate dash for cash in the midst of COVID. Rev. Corp. Finance Stud. 2020; 10 :430–471. [ Google Scholar ]
- Ait-Sahalia Y., et al. Market response to policy initiatives during the global financial crisis. J. Int. Econ. 2012; 87 :162–177. [ Google Scholar ]
- Bekaert G., Ehrmann M., Fratzscher M., Mehl A. The global crisis and equity market contagion. J. Finance. 2014; 69 (6):2587–2649. [ Google Scholar ]
- Bonner C., Van Lelyveld I., Zymek R. Banks’ liquidity buffers and the role of liquidity regulation. J. Financ. Serv. Res. 2015; 48 (3):215–234. [ Google Scholar ]
- Borio C. Bank of International Settlements; 2020. The Prudential Response to the COVID-19 Crisis. s.l. [ Google Scholar ]
- Brown S.J., Warner J.B. Using daily stock returns: the case of event studies. J. Financ. Econ. 1996; 14 :3–31. [ Google Scholar ]
- Calomiris C. Getting the right mix of capital and cash requirements in prudential bank regulation. J. Appl. Corp. Finance. 2012; 24 (1):33–41. [ Google Scholar ]
- Calomiris C., Heider F., Hoerova M. A theory of bank liquidity requirements. Columbia Bus. Sch. Res. Pap. 2015;(2):10. [ Google Scholar ]
- Claessens S., Klingebiel D., Laeven L. Systemic Financial Distress: Containment and Resolution. Cambridge University Press; Cambridge: 2005. Ciris resolution, policies, and institutions: empirical evidence. [ Google Scholar ]
- Correa R., Lee K.H., Sapriza H., Suarez G. Sovereign credit risk, banks' government support, and bank stock returns around the world. J Money Credit Bank. 2014; 46 (1):93–121. [ Google Scholar ]
- Demirguc-Kunt A., Kane E., Laeven L. The World Bank; 2006. Determinants of Deposit-Insurance Adoption and Design. [ Google Scholar ]
- Elliott D. The Brookings Institution; 2014. Bank Liquidity Requirements: An Introduction and Overview. s.l. [ Google Scholar ]
- Fama E., French K. A five-factor asset pricing model. J. Financ. Econ. 2015; 116 (1):1–22. [ Google Scholar ]
- Feyen E., Gispert T.A., Kliatskova T., Mare D.S. Financial Sector Policy Response to COVID-19 in Emerging Markets and Developing Economies. Journal of Banking & Finance. 2021:106–184. [ PMC free article ] [ PubMed ] [ Google Scholar ]
- Gadinis S. Three pathways to global standards: private, regulator, and ministry networks. Am. J. Int. Law. 2015; 109 (1):1–57. [ Google Scholar ]
- Gormsen N., Koijen R.S. Coronavirus: impact on stock prices and growth expectations. Rev. Asset Pricing Stud. 2020; 10 (4):574–597. [ Google Scholar ]
- Jones E., Zeitz A. Regulatory convergence in the financial periphery. Int. Stud. Q. 2019; 63 (4):908–922. [ Google Scholar ]
- Kose M., Kurlat S., Ohnsorge F., Sugawara N. The World Bank; 2017. A Cross-Country Database of Fiscal Space. s.l. [ Google Scholar ]
- Kothari S.P., Warner J.B. Handbook of Empirical Corporate Finance. Elsevier; 2007. Econometrics of event studies; pp. 3–36. s.l. [ Google Scholar ]
- Lewrick U., Schmieder C., Sobrun J., Takats E. Bank for International Settlements; 2020. Releasing bank buffers to cushion the crisis-a quantitative assessment (No. 11) [ Google Scholar ]
- Mistur, E., Givens, J.W. & Matisoff, D., 2020. Policy contagion during a pandemic. SSRN 3662444.
- Morais B., Peydro J.L., Roldan Peña J.R.O.C. The international bank lending channel of monetary policy. J. Finance. 2019; 74 (1):55–90. [ Google Scholar ]
- Ornelas J.R., Pedraza A., Ruiz-Ortega C., Silva T. World Bank; 2019. Winners and Losers when Private Banks Distribute Government Loans. [ Google Scholar ]
- Ramelli S., Wagner A. Feverish stock price reactions to COVID-19. Rev. Corp. Finance Stud. 2020; 9 (3):622–655. [ Google Scholar ]
- Reinhart C.M., Rogoff K.S. Princeton University Press; 2009. This time is Different: Eight Centuries of Financial Folly. s.l. [ Google Scholar ]
- Reinhart C., Rogoff K. Growth in a time of debt. Am. Econ. Rev. 2010; 100 (2):573–578. [ Google Scholar ]
- Taylor J., Williams J. A black swan in the money market. Am. Econ. J. Macroecon. 2009; 1 (1):58–83. [ Google Scholar ]
- World Bank . World Bank; Washington, D.C.: 2019. Global Financial Development Report 2019/2020: Bank Regulation and Supervision a Decade after the Global Financial Crisis. [ Google Scholar ]
- World Bank . World Bank; 2020. COVID-19 Finance Sector Related Policy Responses. https://datacatalog.worldbank.org/dataset/COVID-19-finance-sector-related-policy-responses [Online] Available at: [ Google Scholar ]
Covid19-IBO: A Covid-19 Impact on Indian Banking Ontology Along with an Efficient Schema Matching Approach
Affiliations.
- 1 Department of Software Engineering, School of Computing and Information Technology, Eastern International University, Binh Duong New City, Vietnam.
- 2 Office of DGA (Energy) Comptroller and Auditor General (CAG), Delhi, India.
- 3 Department of Computer Applications, National Institute of Technology, Kurukshetra, Haryana India.
- PMID: 34667368
- PMCID: PMC8517947
- DOI: 10.1007/s00354-021-00136-0
The exponential spread of Covid-19 is not only a serious concern for public health but has also severely affected the global economy. India is not an exception. The banking sector must plan innovatively in a wide range of scenarios focusing upon Covid-19 specific requirements. It becomes essential to examine the impact of Covid-19 on the performance of the Indian banking sector and take focused initiatives at both the tactical and the strategic levels. This paper offers the Covid-19 Impact on Banking Ontology (Covid19-IBO) that provides semantic information about the impact of Covid-19 on the banking sector of India. The developed ontology has been verified and validated and has been made available on the Linked Open Data cloud. It can be utilized to annotate the related data to provide meaningful insights. The Covid-19 ontologies already available have some overlapping information that causes redundancy. Unified integration of these ontologies is required to operate upon them unambiguously. It becomes reasonable to develop a matching approach to link all these ontologies semantically. We, therefore, also provide a schema matching approach with reasonable results to map the Covid-19 ontologies.
Keywords: Big data; Covid-19; Evaluation; Indian banking; Ontology; Schema matching.
© Ohmsha, Ltd. and Springer Japan KK, part of Springer Nature 2021.
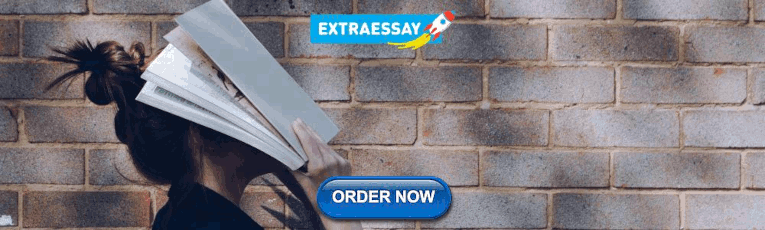
IMAGES
VIDEO
COMMENTS
Abstract. Since February 2020, the world is facing a global crisis, namely, the COVID-19 pandemic. This pandemic has adversely affected the banking system around the world. At the end of 2019, in ...
IMPACT OF COVID-19 ON THE INDIAN BANKING SECTOR: Global economic activity has come to a near standstill as Covid-19 related lockdowns areimposed across a widening swathe of affected countries. Financial markets have been facing highvolatility due to panic sell-offs resulting in destruction of equity markets. Financial institutionshave started ...
This article investigates the impact of COVID-19 on the banking sector through the trend analysis of return on assets and return on equity of the scheduled commercial banks. It covers both the pre- and post-lockdown periods. The results suggest that the governmental measures are helping ameliorate situations of bad quality loans and will improve future prospects of the banking
The findings of the study reveal that the impact of the COVID-19 pandemic on consumers' lifestyles has a direct and beneficial impact on the expansion of internet and mobile banking services. Expand. PDF. Decreased productivity and lockdowns have already started to take a toll on the financials of the corporate sector.
COVID-19 will have long-lasting impact on many industries including banks. Post crisis, digital maturity and COVID-19 resiliency will determine strategy of banking players with three segments ...
The COVID-19 outbreak has challenged Indian Banking sector adversely. The pandemic is impacting the Banking sector in multiple ways — from business continuity issues and operational considerations to the overall financial outlook.Indian Banks reported Increase in NPAs, demand of credit and in turn impacted profitability. Because of the shutdown, there were no source of Revenue and expenses ...
The research found that Indian Banking system has added various measures due to COVID-19 Pandemic to make Banking system smooth and effective. Most of the Indian Banks were facing the problem of NPA, Non recovery of loan, customer frauds, Bad Loans etc. and to add on to it COVID-19 has expedited the collapse of Indian Banking business.
It becomes essential to examine the impact of Covid-19 on the performance of the Indian banking sector and take focused initiatives at both the tactical and the strategic levels. This paper offers the Covid-19 Impact on Banking Ontology (Covid19-IBO) that provides semantic information about the impact of Covid-19 on the banking sector of India.
During this critical phase, banking services will be in place and geared up to support the pent-up demand for funds to aid a smooth economic recovery. GoI as well as state governments have responded to the Covid-19 pandemic with progressively heightened remedial stimulus steps, predominantly fiscal to counter the adverse impacts of this lockdown.
India now has the third-highest number of total COVID-19 cases in the world (behind the US and Brazil). This report focuses on the impact of the Coronavirus outbreak on the economy and the retail banking industry in India. Based on our proprietary datasets, the snap shot provides a detailed comparison between pre-COVID-19 forecasts and revised ...
The government's crisis response has mitigated damage, with a fiscal stimulus of 20 trillion rupees, almost 10 percent of GDP. Also, the Reserve Bank of India enacted decisive expansionary ...
Introduction. COVID-19 has emerged as the "Black Swan" event of the century for the global financial system, with disastrous consequences for India [17, 37, 63, 77, 78]. 1 COVID-19's exponentially negative spread has contributed to a huge drop in major financial system indicators in India, while having a small influence on the banking system's soundness [11, 14, 15, 47].
future if any of the pandemic impacts like Covid-19. This paper offers the Covid19 impact on Banking ontology (Covid19-IBO) that provides semantic information about the impact of the Covid-19 on the banking sector of India. The major contributions of the paper are listed below: • Development of Covid19 Impact on Banking ontology (Covid19-IBO)
The repercussions of the Covid-19 in the performance of the Indian banking sector is highlighted by creating and evaluating the largest comprehensive knowledge base called ontology (Covid19-IBO) in order to get semantic information and addresses few important research questions with respect to Indian economy. The COVID-19 pandemic adversely impacted various industrial sectors of India as well ...
Thirdly, it attempts to quantify the extent of economic losses in India using an Input-Output framework. Finally, it critically assesses the eco - nomic response of the Indian government in a comparative framework. The impact of the Covid-19 pandemic on the global economy has been profound. It was on a fragile global economy that the pandemic
Examination depends on impact of COVID - 19 in Indian Banking Sector. As all out world is experiencing the most noticeably awful circumstance. Indian Banking likewise influenced. ... Indian Government alongside Reserve Bank of India is constantly during the time spent growing new strategy, which assists with lessening effect of COVID-19. Along ...
We use data on the dates and types of major policy initiatives to support the financial sector and address the impact of the COVID-19 emergency. ... India: 20-Mar: 17-Apr: 5: 2: 1: 0: 2: Indonesia: 20-Feb: 14-Apr: 2: 1: 0: 1: 2: Ireland: 12-Mar: 16-Apr ... We first assess the impact of the pandemic on the banking sector and investigate whether ...
A Study on Impact of COVID-19 on Banking Sector: An Indian Perspective - Paripex - Indian Journal Of Research(PIJR) PIJR is a double reviewed monthly print journal that accepts research works
DOI: 10.1057/s41264-023-00244-6 Corpus ID: 261265071; The impact of COVID-19 on the banking sector's efficiency and growth trajectory @article{Jaiyeoba2023TheIO, title={The impact of COVID-19 on the banking sector's efficiency and growth trajectory}, author={Haruna Babatunde Jaiyeoba and Nafiu Olaniyi Oladokun and Razali Haron and Mohammed Ahmed Suleman}, journal={Journal of Financial ...
digital banking services which include internet banking mobile banking etc. and is increasing during this pandemic. Haq & Awan (2020): The study aimed to explore the service quality of e-banking and its impact on e-banking loyalty. The survey was undertaken with the help of the structured questionnaire from that it was
This paper offers the Covid-19 Impact on Banking Ontology (Covid19-IBO) that provides semantic information about the impact of Covid-19 on the banking sector of India. The developed ontology has been verified and validated and has been made available on the Linked Open Data cloud. It can be utilized to annotate the related data to provide ...
COVID-19 is in the first place, a pandemic with potential serious for people's health. It is an unprecedented challenge for our modern societies and health The consequences of the pandemic for our globaleconomy and financial are unpredictable. Economists are convinced that we are heading for a significant economic downturn; however, responses ...
This study is about Technologically Improved banking products adopted in the banking sector of India due to the implications of Covid-19 pandemic. ... Semantic Scholar's Logo. Search 217,645,333 papers from all fields of science. Search. Sign ... A Study on the Impact of COVID-19 Pandemic in the Adoption of Tech-Driven Banking in India @article ...