Student Engagement and School Dropout: Theories, Evidence, and Future Directions
- First Online: 20 October 2022
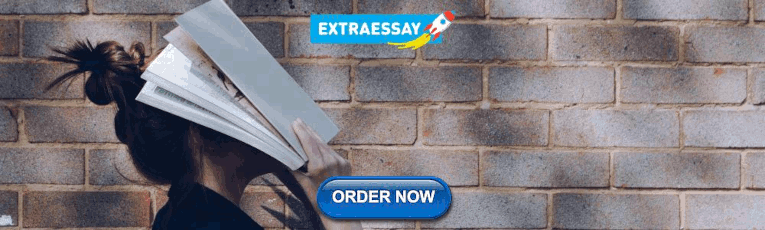
Cite this chapter
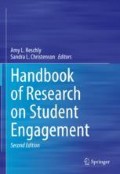
- Isabelle Archambault 3 ,
- Michel Janosz 3 ,
- Elizabeth Olivier 3 &
- Véronique Dupéré 3
3182 Accesses
13 Citations
School dropout is a major preoccupation in all countries. Several factors contribute to this outcome, but research suggests that dropouts mostly have gone through a process of disengaging from school. This chapter aims to present a synthesis of this process according to the major theories in the field and review empirical research linking student disengagement and school dropout. This chapter also presents the common risk and protective factors associated with these two issues, the profiles of students who drop out as well as the disengagement trajectories they follow and leading to their decision to quit school. Finally, it highlights the main challenges as well as the future directions that research should prioritize in the study of student engagement and school dropout.
This is a preview of subscription content, log in via an institution to check access.
Access this chapter
- Available as PDF
- Read on any device
- Instant download
- Own it forever
- Available as EPUB and PDF
- Compact, lightweight edition
- Dispatched in 3 to 5 business days
- Free shipping worldwide - see info
- Durable hardcover edition
Tax calculation will be finalised at checkout
Purchases are for personal use only
Institutional subscriptions
Afia, K., Dion, E., Dupéré, V., Archambault, I., & Toste, J. (2019). Parenting practices during middle adolescence and high school dropout. Journal of Adolescence, 76 , 55–64. https://doi.org/10.1016/j.adolescence.2019.08.012
Article PubMed Google Scholar
Agirdag, O., Van Houtte, M., & Van Avermaet, P. (2013). School segregation and self-fulfilling prophecies as determinants of academic achievement in Flanders. In S. De Groof & M. Elchardus (Eds.), Early school leaving and youth unemployment (pp. 46e74) . Amsterdam University Press.
Google Scholar
Alexander, K. L., Entwisle, D. R., & Kabbani, N. S. (2001). The dropout process in life course perspective: Early risk factors at home and school. Teachers College Record, 103 (5), 760–822. https://doi.org/10.1111/0161-4681.00134
Article Google Scholar
Alliance for Excellent Education. (2013). Saving futures, saving dollars: The impact of education on crime reduction and earnings . Retrived from https://mk0all4edorgjxiy8xf9.kinstacdn.com/wp-content/uploads/2013/09/SavingFutures.pdf .
Appleton, J. J., Christenson, S. L., Kim, D., & Reschly, A. L. (2006). Measuring cognitive and psychological engagement: Validation of the student engagement instrument. Journal of School Psychology, 44 (5), 427–445. https://doi.org/10.1016/j.jsp.2006.04.002
Archambault, I., Pascal, S., Tardif-Grenier, K., Dupéré, V., Janosz, M., Parent, S., & Pagani, L. (2021). The contribution of teacher structure, involvement, and autonomy support on student engagement in low-income elementary schools. Teachers and Teaching, 26 (5–6), 428–445. https://doi.org/10.1080/13540602.2020.1863208
Archambault, I., & Dupéré, V. (2017). Joint trajectories of behavioral, affective, and cognitive engagement in elementary school. The Journal of Educational Research, 110 (2), 188–198. https://doi.org/10.1080/00220671.2015.1060931
Archambault, I., Janosz, M., Dupéré, V., Brault, M.-C., & Andrew, M. M. (2017). Individual, social, and family factors associated with high school dropout among low- SES youth: Differential effects as a function of immigrant status. British Journal of Educational Psychology, 87 (3), 456–477. https://doi.org/10.1111/bjep.12159
Archambault, I., Janosz, M., Fallu, J.-S., & Pagani, L. S. (2009a). Student engagement and its relationship with early high school dropout. Journal of Adolescence, 32 (3), 651–670. https://doi.org/10.1016/j.adolescence.2008.06.007
Archambault, I., Janosz, M., Morizot, J., & Pagani, L. (2009b). Adolescent behavioral, affective, and cognitive engagement in school: Relationship to dropout. Journal of School Health, 79 (9), 408–415. https://doi.org/10.1111/j.1746-1561.2009.00428.x
Basharpoor, S., Issazadegan, A., Zahed, A., & Ahmadian, L. (2013). Comparing academic self-concept and engagement to school between students with learning disabilities and normal. The Journal of Education and Learning Studies, 5 , 47–64.
Bingham, G. E., & Okagaki, L. (2012). Ethnicity and student engagement. In S. L. Christenson, A. L. Reschly, & C. Wylie (Eds.), Handbook of research on student engagement (pp. 65–95). Springer Science + Business Media). https://doi.org/10.1007/978-1-4614-2018-7_4
Chapter Google Scholar
Björklund, A., & Salvanes, K. G. (2011). Education and family background: Mechanisms and policies. In E. A. Hanushek, S. Machin, & L. Woessmann (Eds.), Handbook in economics of education (Vol. 3, pp. 201–247). Elsevier.
Blondal, K. S., & Adalbjarnardottir, S. (2014). Parenting in relation to school dropout through student engagement: A longitudinal study. Journal of Marriage and Family, 76 (4), 778–795. https://doi.org/10.1111/jomf.12125
Bowers, A. J., & Sprott, R. (2012). Why tenth graders fail to finish high school: A dropout typology latent class analysis. Journal of Education for Students Placed at Risk, 17 (3), 129–148. https://doi.org/10.1080/10824669.2012.692071
Brault, M.-C., Janosz, M., & Archambault, I. (2014). Effects of school composition and school climate on teacher expectations of students: A multilevel analysis. Teaching and Teacher Education, 44 , 148–159. https://doi.org/10.1016/j.tate.2014.08.008
Brière, F. N., Pascal, S., Dupéré, V., Castellanos-Ryan, N., Allard, F., Yale-Soulière, G., & Janosz, M. (2017). Depressive and anxious symptoms and the risk of secondary school non-completion. The British Journal of Psychiatry, 211 , 163–168. https://doi.org/10.1192/bjp.bp.117.201418
Brooks-Gunn, J., & Duncan, G. J. (1997). The effects of poverty on children. The Future of Children: Children and Poverty, 7 (2), 55–71. https://doi.org/10.2307/1602387
Brozo, W. G., Sulkunen, S., Shiel, G., Garbe, C., Pandian, A., & Valtin, R. (2014). Reading, gender, and engagement. Journal of Adolescent & Adult Literacy, 57 (7), 584–593. https://doi.org/10.1002/jaal.291
Buhs, E. S., Koziol, N. A., Rudasill, K. M., & Crockett, L. J. (2018). Early temperament and middle school engagement: School social relationships as mediating processes. Journal of Educational Psychology, 110 (3), 338–354. https://doi.org/10.1037/edu0000224
Buhs, E. S. (2005). Peer rejection, negative peer treatment, and school adjustment: Self-concept and classroom engagement as mediating processes. Journal of School Psychology, 43 (5), 407–424. https://doi.org/10.1016/j.jsp.2005.09.001
Cappella, E., Kim, H. Y., Neal, J. W., & Jackson, D. R. (2013). Classroom peer relationships and behavioral engagement in elementary school: The role of social network equity. American Journal of Community Psychology, 52 (3–4), 367–379. https://doi.org/10.1007/s10464-013-9603-5
Article PubMed PubMed Central Google Scholar
Caraway, K., Tucker, C. M., Reinke, W. M., & Hall, C. (2003). Self-efficacy, goal orientation and fear of failure as predictors of school engagement in high school students. Psychology in the Schools, 40 (4), 417–427. https://doi.org/10.1002/pits.10092
Carmona-Halty, M., Salanova, M., Llorens, S., & Schaufeli, W. B. (2019). Linking positive emotions and academic performance: The mediated role of academic psychological capital and academic engagement. Current Psychology , 1–10. https://doi.org/10.1007/s12144-019-00227-8
Chen, J., Huebner, E., & Tian, L. (2020). Longitudinal relations between hope and academic achievement in elementary school students: Behavioral engagement as a mediator. Learning and Individual Differences, 78 , 101824. https://doi.org/10.1016/j.lindif.2020.101824
Cicchetti, D., & Rogosch, F. A. (1996). Equifinality and multifinality in developmental psychopathology. Development and Psychopathology, 8 , 597–600. https://doi.org/10.1017/S0954579400007318
Chiefs Assembly on Education. (2012). A portrait of first nations and education. Retrived from https://www.afn.ca/uploads/files/events/fact_sheet-ccoe-3.pdf
Christenson, S. L., & Thurlow, M. L. (2004). School dropouts: Prevention considerations, interventions, and challenges. Current Directions in Psychological Science, 13 (1), 36–39. https://doi.org/10.1111/j.0963-7214.2004.01301010.x
Christle, C. A., Jolivette, K., & Nelson, M. (2007). School characteristics related to high school dropout rates. Remedial and Special Education, 28 (6), 325–339. https://doi.org/10.1177/07419325070280060201
Cleary, T. J., et al. (2021). Linking student self-regulated learning profiles to achievement and engagement in mathematics. Psychology in the Schools, 58 (3), 443–457. https://doi.org/10.1002/pits.22456
Cornell, D., Gregory, A., Huang, F., & Fan, X. (2013). Perceived prevalence of teasing and bullying predicts high school dropout rates. Journal of Educational Psychology, 105 (1), 138–149. https://doi.org/10.1037/a0030416
Crosnoe, R., & Johnson, M. K. (2011). Research on adolescence in the twenty-first century. Annual Review of Sociology, 37 , 439–460. https://doi.org/10.1146/annurev-soc-081309-150008
Crosnoe, R., & Turley, R. N. (2011). K-12 educational outcomes of immigrant youth. The Future of Children, 21 (1), 129–152. https://doi.org/10.1353/foc.2011.0008
Crowder, K. D., & South, S. J. (2003). Neighborhood distress and school dropout: The variable significance of community context. Social Science Research, 32 , 659–698. https://doi.org/10.1016/S0049-089X(03)00035-8
Crul, M., & Mollenkopf, J. (2012). The changing face of world cities: Young adult children of immigrants in Europe and the United States (pp. 3–25). Russell Sage Foundation. https://www.jstor.org/stable/10.7758/9781610447911
Curhan, A. L., Rabinowitz, J. A., Pas, E. T., & Bradshaw, C. P. (2020). Informant discrepancies in internalizing and externalizing symptoms in an at-risk sample: The role of parenting and school engagement. Journal of Youth and Adolescence, 49 (1), 311–322. https://doi.org/10.1007/s10964-019-01107-x
Danneel, S., Colpin, H., Goossens, L., Engels, M., Van Leeuwen, K., Van Den Noortgate, W., & Verschueren, K. (2019). Emotional school engagement and global self-esteem in adolescents: Genetic susceptibility to peer acceptance and rejection. Merrill-Palmer Quarterly, 65 (2), 158–182. https://doi.org/10.13110/merrpalmquar1982.65.2.0158
Datu, J. A. D., & King, R. B. (2018). Subjective Well-being is reciprocally associated with academic engagement: A short-term longitudinal study. Journal of School Psychology, 69 , 100–110. https://doi.org/10.1016/j.jsp.2018.05.007
DePaoli, J. L., Hornig Fox, J., Ingram, E. S., Maushard, M., Bridgeland, J. M., & Balfanz, R. (2015). Building a grad nation: Progress and challenge in ending the high school dropout epidemic.
De Witte, K., Cabus, S., Thyssen, G., Groot, W., & van Den Brink, H. M. (2013). A critical review of the literature on school dropout. Educational Research Review, 10 , 13–28. https://doi.org/10.1016/j.edurev.2013.05.002
Dierendonck, C., Milmeister, P., Kerger, S., & Poncelet, D. (2020). Examining the measure of student engagement in the classroom using the bifactor model: Increased validity when predicting misconduct at school. International Journal of Behavioral Development, 44 (3), 279–286. https://doi.org/10.1177/0165025419876360
Dupéré, V., Dion, E., Cantin, S., Archambault, I., & Lacourse, E. (2020). Social contagion and high school dropout: The role of friends, romantic partners, and siblings. Journal of Education Psychology, 113 (3), 572–584. https://doi.org/10.1037/edu0000484
Dupéré, V., Dion, E., Leventhal, T., Crosnoe, R., Archambault, A., & Goulet, M. (2019). Circumstances preceding dropout among rural high schoolers: A comparison with urban peers. Journal of Research in Rural Education, 35 , 1–20. https://doi.org/10.1037/edu0000484
Dupéré, V., Dion, E., Leventhal, T., Archambault, I., Crosnoe, R., & Janosz, M. (2018). High school dropout in proximal context: The triggering role of stressful life events. Child Development, 89 (2), e107–e122. https://doi.org/10.1111/cdev.12792
Dupéré, V., Leventhal, T., Dion, E., Crosnoe, R., Archambault, I., & Janosz, M. (2015). Stressors and turning points in high school and dropout: A stress process, life course framework. Review of Educational Research, 859 (4), 591–629. https://doi.org/10.3102/0034654314559845
Duchesne, S., Larose, S., & Feng, B. (2019). Achievement goals and engagement with academic work in early high school: Does seeking help from teachers matter? The Journal of Early Adolescence, 39 (2), 222–252. https://doi.org/10.1177/0272431617737626
Duckworth, A. (2015). OECD report of skills for social progress: The power of social emotional skills (Peer Commentary on IECD report). Retrieved from https://www.oecd.org/edu/ceri/seminarandlaunchofthereportskillsforsocialprogressthepowerofsocialandemotionalskills.htm
Duncan, G. J., & Murnane, R. J. (2011). Whither opportunity? Rising inequality, schools, and children’s life chances . Russel Sage Foundation.
Eurostats. (2017). Decrease in “early school leavers” in the EU. Retrived from https://ec.europa.eu/eurostat/en/web/products-eurostat-news/-/edn-20170908-1 .
Fan, W., & Wolters, C. A. (2014). School motivation and high school dropout: The mediating role of educational expectations. British Journal of Educational Psychology, 84 (1), 22–39. https://doi.org/10.1111/bjep.12002
Farrell, E. (1990). Hanging in and dropping out: Voices of at-risk students . Teachers College Press.
Finn, J. D. (1989). Withdrawing from school. Review of Educational Research, 59 (2), 117–142. https://doi.org/10.3102/00346543059002117
Finn, J. D., & Zimmer, K. S. (2012). Student engagement: What is it? Why does it matter? In S. L. Christenson, A. L. Reschly, & C. Wylie (Eds.), Handbook of research on student engagement (pp. 97–131). Springer.
Fortin, L., Royer, É., Potvin, P., Marcotte, D., & Yergeau, É. (2004). La prediction du risque de decrochage scolaire au secondaire : Facteurs personnels, familiaux et scolaires [Prediction of risk for secondary school dropout: Personal, family and school factors]. Canadian Journal of Behavioural Science, 36 (3), 219–231. https://doi.org/10.1037/h0087232
Fraysier, K., Reschly, A., & Appleton, J. (2020). Predicting postsecondary enrollment with secondary student engagement data. Journal of Psychoeducational Assessment, 38 (7), 882–899. https://doi.org/10.1177/0734282920903168
Fredricks, J. A., Hofkens, T., Wang, M.-T., Mortenson, E., & Scott, P. (2018). Supporting girls’ and boys’ engagement in math and science learning: A mixed methods study. Journal of Research in Science Teaching, 55 (2), 271–298. https://doi.org/10.1002/tea.21419
Fredricks, J. A., Ye, F., Wang, M., & Brauer, S. (2019). Profiles of school disengagement: Not all disengaged students are alike. In J. A. Fredricks, A. L. Reschly, & S. L. Christenson (Eds.), Handbook of student engagement interventions (pp. 31–43). Academic Press.
Fredricks, J. A., Wang, M., Schall, J., Hokfkens, T., Snug, H., Parr, A., & Allerton, J. (2016). Using qualitative methods to develop a survey of math and science engagement. Learning and Instruction, 43 , 5–15. https://doi.org/10.1016/j.learninstruc.2016.01.009
Fredricks, J. A., Blumenfeld, P. C., & Paris, A. H. (2004). School engagement: Potential of the concept, state of the evidence. Review of Educational Research, 74 (1), 59–109. https://doi.org/10.3102/00346543074001059
French, D. C., & Conrad, J. (2001). School dropout as predicted by peer rejection and antisocial behavior. Journal of Research on Adolescence, 11 (3), 225–244. https://doi.org/10.1111/1532-7795.00011
García Coll, C. G., & Marks, A. K. (Eds.). (2012). The immigrant paradox in children and adolescents: Is becoming American a developmental risk? American Psychological Association.
Garrett-Peters, P. T., Mokrova, I. L., Carr, R. C., Vernon-Feagans, L., & Family Life Project Key Investigators. (2019). Early student (dis)engagement: Contributions of household chaos, parenting, and self-regulatory skills. Developmental Psychology, 55 (7), 1480–1492. https://doi.org/10.1037/dev0000720
Georgiades, K., Boyle, M. H., & Duku, E. (2007). Contextual influences on children’s mental health and school performance: The moderating effects of family immigrant status. Child Development, 78 (5), 1572–1591. https://doi.org/10.1111/j.1467-8624.2007.01084.x
Gonzales, N. A., Wong, J. J., Toomey, R. B., Millsap, R., Dumka, L. E., & Mauricio, A. M. (2014). School engagement mediates long-term prevention effects for Mexican American adolescents. Prevention Science, 15 (6), 929–939. https://doi.org/10.1007/s11121-013-0454-y
Goulet, M., Clément, M.-E., Helie, S., & Villatte, A. (2020). Longitudinal associations between risk profiles, school dropout risk, and substance abuse in adolescence. Child & Youth Care Forum, 49 , 687–706.
Gubbels, J., van der Put, C. E., & Assink, M. (2019). Risk factors for school absenteeism and dropout: A meta-analytic review. Journal of Youth and Adolescence, 48 (9), 1637–1667. https://doi.org/10.1007/s10964-019-01072-5
Henry, K. L., Knight, K. E., & Thornberry, T. P. (2012). School disengagement as a predictor of dropout, delinquency, and problem substance use during adolescence and early adulthood. Journal of Youth and Adolescence , 41 (2), 156–166. https://doi.org/10.1007/s10964-011-9665-3 .
Hoff, E., Laursen, B., & Tardiff, T. (2002). Socioeconomic status and parenting. In P. M. Greenfield & R. R. Cocking (Eds.), Cross-cultural roots of minority children development (pp. 285–313). Lawrence Erlbaum Associates.
Holen, S., Waaktaar, T., & Sagatun, Å. (2018). A chance lost in the prevention of school dropout? Teacher-student relationships mediate the effect of mental health problems on noncompletion of upper-secondary school. Scandinavian Journal of Educational Research, 62 (5), 737–753. https://doi.org/10.1080/00313831.2017.1306801
Hong, W., Zhen, R., Liu, R.-D., Wang, M.-T., Ding, Y., & Wang, J. (2020). The longitudinal linkages among Chinese children’s behavioral, cognitive, and emotional engagement within a mathematics context. Educational Psychology, 40 (6), 666–680. https://doi.org/10.1080/01443410.2020.1719981
Hosan, N. E., & Hoglund, W. (2017). Do teacher–child relationship and friendship quality matter for children’s school engagement and academic skills? School Psychology Review, 46 (2), 201–218. https://doi.org/10.17105/SPR-2017-0043.V46-2
Hospel, V., & Galand, B. (2016). Are both classroom autonomy support and structure equally important for students’ engagement? A multilevel analysis. Learning and Instruction, 41 , 1–10. https://doi.org/10.1016/j.learninstruc.2015.09.001
Hunt, J., Eisenberg, D., & Kilbourne, A. M. (2010). Consequences of receipt of a psychiatric diagnosis for completion of college. Psychiatric Services (Washington, D.C.), 61 (4), 399–404. https://doi.org/10.1176/ps.2010.61.4.399
Hymel, S., Comfort, C., Schonert-Reichl, K., & McDougall, P. (1996). Academic failure and school dropout: The influence of peers. In J. Juvonen & K. R. Wentzel (Eds.), Cambridge studies in social and emotional development. Social motivation: Understanding children’s school adjustment (pp. 313–345). Cambridge University Press. https://doi.org/10.1017/CBO9780511571190.015
Jang, H., Kim, E. J., & Reeve, J. (2012). Longitudinal test of self-determination theory’s motivation mediation model in a naturally occurring classroom context. Journal of Educational Psychology, 104 (4), 1175–1188. https://doi.org/10.1037/a0028089
Janosz, M., Archambault, I., Morizot, J., & Pagani, L. S. (2008a). School engagement trajectories and their differential predictive relations to dropout. Journal of Social Issues, 64 (1), 21–40. https://doi.org/10.1111/j.1540-4560.2008.00546.x
Janosz, M., Archambault, I., Pagani, L. S., Pascal, S., Morin, A. J., & Bowen, F. (2008b). Are there detrimental effects of witnessing school violence in early adolescence? The Journal of Adolescent Health : Official Publication of the Society for Adolescent Medicine, 43 (6), 600–608. https://doi.org/10.1016/j.jadohealth.2008.04.011
Janosz, M., Le Blanc, M., Boulerice, B., & Tremblay, R. E. (2000). Predicting different types of school dropouts: A typological approach with two longitudinal samples. Journal of Educational Psychology, 92 (1), 171–190. https://doi.org/10.1037/0022-0663.92.1.171
Janosz, M., Le Blanc, M., Boulerice, B., & Tremblay, R. E. (1997). Disentangling the weight of school dropout predictors: A test on two longitudinal samples. Journal of Youth and Adolescence, 26 (6), 733–762. https://doi.org/10.1023/A:1022300826371
Jiang, S., & Dong, L. (2020). The effects of teacher discrimination on depression among migrant adolescents: Mediated by school engagement and moderated by poverty status. Journal of Affective Disorders, 275 , 260–267. https://doi.org/10.1016/j.jad.2020.07.029
Jimerson, S. R., Campos, E., & Greif, J. L. (2003). Towards an understanding of definitions and measures of school engagement and related terms. The California School Psychologist, 8 , 7e28. https://doi.org/10.1016/S0022-4405(00)00051-0
Jimerson, S. R., Egeland, B., Sroufe, L. A., & Carlson, B. (2000). A prospective longitudinal study of high school dropouts: Examining multiple predictors across development. Journal of School Psychology, 38 (6), 525–549. https://doi.org/10.1016/S0022-4405(00)00051-0
Jordan, W. J., McPartland, J. M., & Lara, J. (1999). Rethinking the causes of high school dropout. The Prevention Researcher, 6 , 1–4.
Krauss, S. E., Kornbluh, M., & Zeldin, S. (2017). Community predictors of school engagement: The role of families and youth-adult partnership in Malaysia. Children and Youth Services Review, 73 , 328–337. https://doi.org/10.1016/j.childyouth.2017.01.009
Korhonen, J., Linnanmäki, K., & Aunio, P. (2014). Learning difficulties, academic well-being and educational dropout: A person-centered approach. Learning & Individual Differences, 31 , 1–10. https://doi.org/10.1016/j.lindif.2013.12.011
Kurdi, V., & Archambault, I. (2020). Self-perceptions and engagement in low socio-economic elementary school students: The moderating effects of immigration status and anxiety. School Mental Health, 12 , 400–416. https://doi.org/10.1007/s12310-020-09360-3
Ladd, G. W., & Dinella, L. M. (2009). Continuity and change in early school engagement: Predictive of children’s achievement trajectories from first to eighth grade? Journal of Educational Psychology, 101 (1), 190–206. https://doi.org/10.1037/a0013153
Ladd, G. W., Kochenderfer, B. J., & Coleman, C. C. (1997). Classroom peer acceptance, friendship, and victimization: Distinct relational systems that contribute uniquely to children’s school adjustment? Child Development, 68 (6), 1181–1197. https://www.jstor.org/stable/1132300
PubMed Google Scholar
Landis, R. N., & Reschly, A. L. (2013). Reexamining gifted underachievement and dropout through the lens of student engagement. Journal for the Education of the Gifted, 36 (2), 220–249. https://doi.org/10.1177/0162353213480864
Lansford, J. E., Dodge, K. A., Pettit, G. S., & Bates, J. E. (2016). A public health perspective on school dropout and adult outcomes: A prospective study of risk and protective factors from age 5 to 27 years. Journal of Adolescent Health, 58 (6), 652–658. https://doi.org/10.1016/2Fj.jadohealth.2016.01.014
Lavoie, L., Dupéré, V., Dion, E., Crosnoe, R., Lacourse, É., & Archambault, I. (2019). Gender differences in adolescents’ exposure to stressful life events and differential links to impaired school functioning. Journal of Abnormal Child Psychology, 47 (6), 1053–1064. https://doi.org/10.1007/s10802-018-00511-4
Lawson, M. A., & Lawson, H. A. (2013). New conceptual frameworks for student engagement research, policy, and practice. Review of Educational Research, 83 (3), 432–479. https://doi.org/10.3102/0034654313480891
Leventhal, T., & Dupéré, V. (2019). Neighborhood effects on youth development in experimental and nonexperimental research. Annual Review of Developmental Psychology, 1 , 149–176. https://doi.org/10.1146/annurev-devpsych-121318-085221
Lewis, A. D., Huebner, E. S., Malone, P. S., & Valois, R. F. (2011). Life satisfaction and student engagement in adolescents. Journal of Youth and Adolescence, 40 (3), 249–262. https://doi.org/10.1007/s10964-010-9517-6
Li, Y., & Lerner, R. M. (2013). Interrelations of behavioral, emotional, and cognitive school engagement in high school students. Journal of Youth and Adolescence, 42 (1), 20–32. https://doi.org/10.1007/s10964-012-9857-5
Li, Y., & Lerner, R. M. (2011). Trajectories of school engagement during adolescence:Implications for grades, depression, delinquency, and substance use. Developmental Psychology, 47 (1), 233–247. https://doi.org/10.1037/a0021307
Li, Y., Lerner, J. V., & Lerner, R. M. (2010). Personal and ecological assets and academic competence in early adolescence: The mediating role of school engagement. Journal of Youth and Adolescence, 39 , 801–815. https://doi.org/10.1007/s10964-010-9535-4
Liu, R.-D., Zhen, R., Ding, Y., Liu, Y., Wang, J., Jiang, R., & Xu, L. (2018). Teacher support and math engagement: Roles of academic self-efficacy and positive emotions. Educational Psychology, 38 (1), 3–16. https://doi.org/10.1080/01443410.2017.1359238
Lovelace, M. D., Reschly, M. L., & Appleton, J. J. (2018). Beyond school records: The value of cognitive and affective engagement in predicting dropout and on-time graduation. Professional School Counseling, 21 (1), 70–84. https://doi.org/10.5330/1096-2409-21.1.70
Luo, W., Hughes, J. N., Liew, J., & Kwok, O. (2009). Classifying academically at-risk first graders into engagement types: Association with long-term achievement trajectories. The Elementary School Journal, 109 (4), 380–405. https://doi.org/10.1086/593939
Mahuteau, S., Karmel, T., Mavromaras, K., & Zhu, R. (2015). Educational outcomes of young Indigenous Australians . National Centre for Student Equity in Higher Education, Curtin University, Bentley, viewed 7 February 2017. https://www.ncsehe.edu.au/educationaloutcomes-of-young-indigenous-australians/
Marsh, H. W., & Kleitman, S. (2005). Consequences of employment during high school: Character building, subversion of academic goals, or a threshold? American Educational Research Journal, 42 (2), 331–369. https://doi.org/10.3102/00028312042002331
Martin, A. J. (2007). Examining a multidimensional model of student motivation and engagement using a construct validation approach. British Journal of Educational Psychology, 77 , 413–440. https://doi.org/10.1348/000709906X118036
McDermott, E. R., Donlan, A. E., & Zaff, J. F. (2019). Why do students drop out? Turning points and long-term experiences. The Journal of Educational Research, 112 , 270–282. https://doi.org/10.1080/00220671.2018.1517296
McDermott, E. R., Anderson, S., & Zaff, J. (2017). Dropout typologies: Relating profiles of risk and support to later educational re-engagement. Applied Developmental Science, 22 , 217–232. https://doi.org/10.1080/10888691.2016.1270764
Melkevik, O., Nilsen, W., Evensen, M., Reneflot, A., & Mykletun, A. (2016). Internalizing disorders as risk factors for early school leaving: A systematic review. Adolescent Research Review, 1 (3), 245–255. https://doi.org/10.1007/s40894-016-0024-1
Mojtabai, R., Stuart, E. A., Hwang, I., Eaton, W. W., Sampson, N., & Kessler, R. C. (2015). Long-term effects of mental disorders on educational attainment in the National Comorbidity Survey ten-year follow-up. Social Psychiatry and Psychiatric Epidemiology, 50 (10), 1577–1591. https://doi.org/10.1007/s00127-015-1083-5
Organisation for Economic Co-operation and Development (OECD). (2018). Equity in education: Breaking down barriers to social mobility . PISA, OECD.
Olivier, E., Galand, B., Morin, A. J. S., & Hospel, V. (2020a). Need-supportive teaching and student engagement : Comparing the additive, synergistic, and balanced contributions. Learning and Instruction, 71 , 1–18. https://doi.org/10.1016/j.learninstruc.2020.101389
Olivier, E., Morin, A. J. S., Langlois, J., Tardif-Grenier, K., & Archambault, I. (2020b). Internalizing and externalizing behavior problems and student engagement in elementary and secondary school. Journal of Youth and Adolescence, 49 , 2327–2346. https://doi.org/10.1007/s10964-020-01295-x
Olivier, E., Archambault, I., & Dupéré, V. (2018). Boys’ and girls’ latent profiles of behavior and social adjustment in school: Longitudinal links with later student behavioral engagement and academic achievement? Journal of School Psychology, 69 , 28–44. https://doi.org/10.1016/j.jsp.2018.05.006
Organisation for Economic Co-operation and Development (OECD). (2015). Helping immigrant students to succeed at school – and beyond . OECD Publishing. https://www.oecd.org/education/Helping-immigrant-students-to-succeed-at-school-and-beyond.pdf
Pagani, L. S., Fitzpatrick, C., & Parent, S. (2012). Relating kindergarten attention to subsequent developmental pathways of classroom engagement in elementary school. Journal of Abnormal Child Psychology, 40 (5), 715–725. https://doi.org/10.1007/s10802-011-9605-4
Perry, J. C. (2008). School engagement among urban youth of color: Criterion pattern effects of vocational exploration and racial identity. Journal of Career Development, 34 (4), 397–422. https://doi.org/10.1177/0894845308316293
Pintrich, P. R. (2004). Conceptual framework for assessing motivation and self-regulated learning in college students. Psychological Bulletin, 16 , 385–407.
Reeve, J. (2009). Why teachers adopt a controlling motivating style toward students and how they can become more autonomy supportive. Educational Psychologist, 44 (3), 159–175. https://doi.org/10.1080/00461520903028990
Réseau Eurydice. (2010). Différences entre les genres en matière de réussite scolaire: étude sur les mesures prises et la situation actuelle en Europe.
Reschly, A. L., & Christenson, S. L. (2012). Jingle, jangle, and conceptual haziness: Evolution and future directions of the engagement construct. In S. L. Christenson, A. L. Reschly, & C. Wylie (Eds.), Handbook of research on student engagement (pp. 3–19). Springer Science + Business Media). https://doi.org/10.1007/978-1-4614-2018-7_1
Reschly, A., & Christenson, S. L. (2006a). Promoting school completion. In G. Bear & K. Minke (Eds.), Children’s needs III: Understanding and addressing the developmental needs of children . Bethesda.
Reschly, A. L., & Christenson, S. L. (2006b). Prediction of dropout among students with mild disabilities: A case for the inclusion of student engagement variables. Remedial and Special Education, 27 (5), 276–292. https://doi.org/10.1177/07419325060270050301
Rocque, M., & Snellings, Q. (2018). The new disciplinology: Research, theory, and remaining puzzles on the school-to-prison pipeline. Journal of Criminal Justice, 59 , 3–11.
Rosenthal, B. S. (1998). Non-school correlates of dropout: An integrative review of the literature. Children and Youth Services Review, 20 (5), 413–433. https://doi.org/10.1016/S0190-7409(98)00015-2
Rumberger, R. W. (2011). Dropping out: Why students drop out of high school and what can be done about it . Harvard University Press. https://doi.org/10.4159/harvard.9780674063167
Book Google Scholar
Rumberger, R. W., & Larson, K. A. (1998). Student mobility and the increased risk of high school dropout. American Journal of Education, 107 (1), 1–35. https://doi.org/10.1086/444201
Rumberger, R. W. (1987). High school dropouts: A review of issues and evidence. Review of Educational Research, 57 (2), 101–121. https://doi.org/10.3102/00346543057002101
Samuel, R., & Burger, K. (2020). Negative life events, self-efficacy, and social support: Risk and protective factors for school dropout intentions and dropout. Journal of Educational Psychology, 112 (5), 973–986. https://doi.org/10.1037/edu0000406
Skinner, E. A., & Pitzer, J. R. (2012). Developmental dynamics of student engagement, coping, and everyday resilience. In S. L. Christenson, A. L. Reschly, & C. Wylie (Eds.), Handbook of research on student engagement (pp. 21–44). Springer Science + Business Media). https://doi.org/10.1007/978-1-4614-2018-7_2
Skinner, E. A., Kindermann, T. A., & Furrer, C. J. (2009). A motivational perspective on engagement and disaffection: Conceptualization and assessment of children’s behavioral and emotional participation in academic activities in the classroom. Educational and Psychological Measurement, 69 (3), 493–525. https://doi.org/10.1177/0013164408323233
Skinner, E., Furrer, C., Marchand, G., & Kindermann, T. (2008). Engagement and disaffection in the classroom: Part of a larger motivational dynamic? Journal of Educational Psychology, 100 (4), 765–781. https://doi.org/10.1037/a0012840
Skinner, E. A., & Belmont, M. J. (1993). Motivation in the classroom: Reciprocal effects of teacher behavior and student engagement across the school year. Journal of Educational Psychology, 85 (4), 571–581. https://doi.org/10.1037/0022-0663.85.4.571
Staffs, J., & Kreager, D. A. (2008). Too cool for school? Violence, peer status and high school dropout. Social Forces, 87 (1), 445–471. https://doi.org/10.1353/sof.0.0068
Statistics Canada (2017). Insights on Canadian society young men and women without a high school diploma. Retrived from https://www150.statcan.gc.ca/n1/pub/75-006-x/2017001/article/14824-fra.htm
Suárez-Orozco, C., Rhodes, J., & Milburn, M. (2009). Unraveling the immigrant paradox: Academic engagement and disengagement among recently arrived immigrant youth. Journal of Education for Students Placed at Risk, 6 , 7–25. https://doi.org/10.1177/0044118X09333647
Strand, S. (2014). School effects and ethnic, gender and socio-economic gaps in educational achievement at age 11. Oxford Review of Education, 40 (2), 223–245. https://doi.org/10.1080/03054985.2014.891980
Taylor, G., Lekes, N., Gagnon, H., Kwan, L., & Koestner, R. (2012). Need satisfaction, work-school interference and school dropout: An application of self-determination theory. The British Journal of Educational Psychology, 82 (4), 622–646. https://doi.org/10.1111/j.2044-8279.2011.02050.x
Teese, R., Lamb, S., & Duru-Bellat, M. (2007). International studies in education inequality, theory and policy . Springer.
Tinto, V. (1975). Dropout from higher education: A theoretical synthesis of recent research. Review of Educational Research, 45 (1), 89–125. https://doi.org/10.2307/1170024
Tuominen-Soini, H., & Salmela-Aro, K. (2014). Schoolwork engagement and burnout among Finnish high school students and young adults: Profiles, progressions, and educational outcomes. Developmental Psychology, 50 (3), 649–662. https://doi.org/10.1037/a0033898
Tyler, J., & Lofstrom, M. (2009). Finishing high school: Alternative pathways and dropout recovery. The Future of Children, 19 (1), 77–103. https://doi.org/10.1353/foc.0.0019
UNESCO. (2020). World inequality database on education . UNESCO Institute for Statistics.
U.S. Department of Commerce. (2017). Census bureau, current population survey (CPS), selected years, October 1977 through 2017 . Table 2.5. Retrived from https://nces.ed.gov/programs/dropout/ind_02.asp .
U.S. Department of Education. (2016). Digest of Education Statistics 2016, table 219.70. Retrived from https://nces.ed.gov/pubs2017/2017094.pdf
Van Uden, J. M., Ritzen, H., & Pieters, J. M. (2016). Enhancing student engagement in pre-vocational and vocational education: a learning history. Teachers and Teaching, 22 (8), 983–999. https://doi.org/10.1080/13540602.2016.1200545
Véronneau, M.-H., Vitaro, F., Pedersen, S., & Tremblay, R. E. (2008). Do peers contribute to the likelihood of secondary school graduation among disadvantaged boys? Journal of Educational Psychology, 100 (2), 429–442. https://doi.org/10.1037/0022-0663.100.2.429
Wang, M. T., Fredricks, J., Ye, F., Hofkens, T., & Linn, J. S. (2019). Conceptualization and assessment of adolescents’ engagement and disengagement in school. European Journal of Psychological Assessment, 35 (4), 592–606. https://doi.org/10.1027/1015-5759/a000431
Wang, M.-T., Kiuru, N., Degol, J. L., & Salmela-Aro, K. (2018). Friends, academic achievement, and school engagement during adolescence: A social network approach to peer influence and selection effects. Learning and Instruction, 58 , 148–160. https://doi.org/10.1016/j.learninstruc.2018.06.003
Wang, M. T., Fredricks, J. A., Ye, F., Hofkens, T. L., & Linn, J. S. (2016). The math and science engagement scales: Scale development, validation, and psychometric properties. Learning and Instruction, 43 , 16–26. https://doi.org/10.1016/j.learninstruc.2016.01.008
Wang, M. T., & Degol, J. (2014). Motivational pathways to STEM career choices: Using expectancy-value perspective to understand individual and gender differences in STEM fields. Developmental Review, 33 , 304e340. https://doi.org/10.1016/j.dr.2013.08.001
Wang, M.-T., & Fredricks, J. A. (2014). The reciprocal links between school engagement, youth problem behaviors, and school dropout during adolescence. Child Development, 85 (2), 722–737. https://doi.org/10.1111/2Fcdev.12138
Wang, M. T., & Peck, S. C. (2013). Adolescent educational success and mental health vary across school engagement profiles. Developmental Psychology, 49 (7), 1266–1276. https://doi.org/10.1037/a0030028
Wang, M.-T., Willett, J. B., & Eccles, J. S. (2011). The assessment of school engagement: Examining dimensionality and measurement invariance by gender and race/ethnicity. Journal of School Psychology, 49 (4), 465–480. https://doi.org/10.1016/j.jsp.2011.04.001
Wang, M.-T., & Eccles, J. S. (2012). Adolescent behavioral, emotional, and cognitive engagement trajectories in school and their differential relations to educational success. Journal of Research on Adolescence, 22 (1), 31–39. https://doi.org/10.1111/j.1532-7795.2011.00753.x
Wehlage, G. G., Rutter, R. A., Smith, G. A., Lesko, N., & Fernandez, R. R. (1989). Reducing the risk: Schools as communities of support . The Falmer Press.
Wentzel, K. R., Jablansky, S., & Scalise, N. R. (2020). Peer social acceptance and academic achievement: A meta-analytic study. Journal of Educational Psychology, 113 (1), 157–180. https://doi.org/10.1037/edu0000468
Zhou, Q., Main, A., & Wang, Y. (2010). The relations of temperamental effortful control and anger/frustration to Chinese children’s academic achievement and social adjustment: A longitudinal study. Journal of Educational Psychology, 102 (1), 180–196. https://doi.org/10.1037/a001590
Download references
Author information
Authors and affiliations.
University of Montreal, Montreal, QC, Canada
Isabelle Archambault, Michel Janosz, Elizabeth Olivier & Véronique Dupéré
You can also search for this author in PubMed Google Scholar
Corresponding author
Correspondence to Isabelle Archambault .
Editor information
Editors and affiliations.
University of Georgia, Athens, GA, USA
Amy L. Reschly
University of Minnesota, Minneapolis, MN, USA
Sandra L. Christenson
Rights and permissions
Reprints and permissions
Copyright information
© 2022 The Author(s), under exclusive license to Springer Nature Switzerland AG
About this chapter
Archambault, I., Janosz, M., Olivier, E., Dupéré, V. (2022). Student Engagement and School Dropout: Theories, Evidence, and Future Directions. In: Reschly, A.L., Christenson, S.L. (eds) Handbook of Research on Student Engagement. Springer, Cham. https://doi.org/10.1007/978-3-031-07853-8_16
Download citation
DOI : https://doi.org/10.1007/978-3-031-07853-8_16
Published : 20 October 2022
Publisher Name : Springer, Cham
Print ISBN : 978-3-031-07852-1
Online ISBN : 978-3-031-07853-8
eBook Packages : Behavioral Science and Psychology Behavioral Science and Psychology (R0)
Share this chapter
Anyone you share the following link with will be able to read this content:
Sorry, a shareable link is not currently available for this article.
Provided by the Springer Nature SharedIt content-sharing initiative
- Publish with us
Policies and ethics
- Find a journal
- Track your research

An official website of the United States government
The .gov means it’s official. Federal government websites often end in .gov or .mil. Before sharing sensitive information, make sure you’re on a federal government site.
The site is secure. The https:// ensures that you are connecting to the official website and that any information you provide is encrypted and transmitted securely.
- Publications
- Account settings
Preview improvements coming to the PMC website in October 2024. Learn More or Try it out now .
- Advanced Search
- Journal List
- Eur J Investig Health Psychol Educ
- PMC10217510

Student Dropout as a Never-Ending Evergreen Phenomenon of Online Distance Education
Associated data.
The datasets used and/or analyzed during the current study are available from the corresponding author on reasonable request.
The research on student dropout demonstrates that there is no consensus on its definition and scope. Although there is an expanding collection of research on the topic, student dropout remains a significant issue, characterized by numerous uncertainties and ambiguous aspects. The primary aim of this investigation is to assess the research trends of student dropout within the distance education literature by employing data mining and analytic approaches. To identify these patterns, a total of 164 publications were examined by applying text mining and social network analysis. The study revealed some intriguing facts, such as the misinterpretation of the term “dropout” in different settings and the inadequacy of nonhuman analytics to explain the phenomenon, and promising implications on how to lessen dropout rates in open and distance learning environments. Based on the findings of the study, this article proposes possible directions for future research, including the need to provide a precise definition of the term “dropout” in the context of distance learning, to develop ethical principles, policies, and frameworks for the use of algorithmic approaches to predict student dropout, and finally, to adopt a human-centered approach aimed at fostering learners’ motivation, satisfaction, and independence to reduce the rate of dropout in distance education.
1. Introduction
Education has always been viewed as the key to the growth, development, and well-being of a society, and it has become even more of a prerequisite for citizens since the world is continuously evolving and the global challenges are getting more and more difficult to handle in everyday life. Recent advances in technology have made continued education and lifelong learning easier by allowing institutions to reach millions of students in a quicker and more resourceful way. This notion is also articulated in the Sustainable Development Goals, Number 4: Quality Education to provide “quality education for all is fundamental to creating a peaceful and prosperous world” [ 1 ]. Consequently, educational institutions globally have begun providing distance education courses to address this demand. Distance education has revolutionized learning by filling the geographical gap between institutions and learners [ 2 ] and, thanks to its flexible nature, offered people who have job or family commitments the opportunity to achieve their educational goals since its emergence [ 3 ].
However, despite the growing demand for distance learning, institutions suffer low retention rates within their distance learning environments [ 4 ], and it is not uncommon for learners to drop out, whether willingly or unwillingly, from these programs at some point. Furthermore, there is no single agreed-upon definition of the term “dropout”. For instance, Tinto [ 5 ] defines “dropout” as any person leaving their institution, while Kaplan et al. [ 6 ] state that dropouts are those who leave their departments voluntarily after the payment of the tuition fee is completed and/or the add/drop period is over. Conversely, Levy [ 7 ] presents a differing perspective, positing that students who choose to withdraw from a course within the “add/drop period” should not be classified as dropout students. This is due to the fact that they either receive a full refund for their tuition or do not face any financial repercussions for discontinuing the course during this specific time.
Although dropouts pose a major concern for all types of education, the dropout rate in distance education is much higher than that in traditional education, as Moore and Kearsley [ 8 ] have made clear. This, however, comes as no surprise when the spatial, temporal, and transactional distances separating the common target learner populations of distance education are considered. Providing educational opportunities to a relatively higher number of learners from any social, academic, or economic background, then, can become a downside, as high rates of dropout can be linked to a variety of issues in such a diverse context, which might prevent institutions from fully understanding and addressing the causes of dropout. Within the above-mentioned context, the overall purpose of this study is to examine the research trends and patterns of student dropout in distance education systems and to identify the emerging thematic research patterns.
Despite student dropout being a central concern for educational stakeholders since the inception of formal education systems [ 9 ], it was not until the early 1970s that theoretical frameworks began to emerge. The initial research aimed at understanding student dropout primarily centered on students’ physiological characteristics, including aspects such as personality, capabilities, and motivation [ 9 ], as well as personal deficiencies [ 9 , 10 , 11 ]. These investigations, categorized as psychological studies [ 9 , 12 ], were succeeded by additional theoretical models and research efforts that sought to elucidate the phenomenon from various angles, including psychological, environmental, sociological, economic, and organizational perspectives [ 9 , 10 ].
Since 1970, the phenomenon has been widely investigated, and various conceptual and theoretical models [ 5 , 11 , 13 , 14 , 15 , 16 , 17 , 18 ] have been put forward to explain the issue. These models aimed to help institutions identify at-risk students and present some implications for early interventions that practitioners and curriculum designers should be mindful of. Except for these models, there have been many other preliminary studies, most of which focus primarily on factors affecting student dropout.
In an attempt to reveal what has been said about the issue at a glance and provide a robust interpretation of all the relevant empirical evidence, there have also been several literature review studies. The first systematic review of dropout studies in the context of online learning was conducted by Lee and Choi [ 19 ]. In their seminal study, Lee and Choi [ 19 ] conducted a comprehensive analysis of 35 empirical research articles, exclusively from peer-reviewed academic publications, spanning the decade between 2000 and 2010. In order to find related studies, Lee and Choi [ 19 ] both searched for the keywords that might relate to relevant studies in three major educational databases: Education Research Complete, ERIC, and PsycINFO, and employed a snowball method. In their investigation, Lee and Choi [ 19 ] utilized the research classification frameworks established by Creswell, [ 20 ] and initially presented the authors, publication years, study samples, contexts, and dropout definitions pertaining to each individual study. Later Lee and Choi [ 19 ] focused on the factors affecting dropout and explained the phenomenon under categories such as student factors (academic background, relevant experiences, skills, and psychological attributes), course/program factors (course design, institutional support and interactions), and environment factors (work commitments and supportive environments).
Hart [ 21 ] presented a pioneering literature review that examined the factors potentially influencing students’ persistence in online courses. To select the relevant articles, Hart [ 21 ] employed the following criteria: (a) publication after 1999, (b) appearance in a peer-reviewed journal, and (c) the discussion of student factors promoting persistence. After evaluating 11 papers, Hart [ 21 ] offered a deeper understanding of persistence as a phenomenon, as well as identifying factors that encourage persistence and obstacles that hinder it.
In a more contemporary examination of the subject, Muljana and Luo [ 22 ] conducted a comprehensive review of the existing literature. Their investigation involved an analysis of 40 empirical studies published between 2010 and 2018, utilizing extensive database searches, abstract screenings, full-text evaluations, and synthesis procedures. Acknowledging the multifaceted and intricate nature of student dropout, Muljana and Luo [ 22 ] identified a plethora of contributing factors, such as institutional support, program difficulty, fostering a sense of belonging, enhancing learning, course structure, student behavior traits, demographic aspects, and other personal variables. Additionally, they found that prior academic performance, age, gender, personal circumstances, and abilities in online and self-directed learning emerged as the most prominent determinants of online dropout [ 22 ].
Pant et al. [ 23 ] were among those who aimed to provide a comprehensive picture of the literature on student dropout in online learning environments. However, Pant et al. [ 23 ] examined studies that focused on persistence specifically in MOOCs and were published between the years 2011 and 2020. In their analysis, Pant et al. [ 23 ] selected 50 research papers that investigated factors increasing student motivation and thus contributing to their persistence in MOOCs. The findings of this study demonstrated that learner-related factors, classified as personal, social, educational, and industrial, played a vital role in determining student enrollment in MOOCs.
Although not exactly the focus of this study, dropout has become more evident as an important problem in higher education during the COVID-19 pandemic. During the emergency remote teaching and learning practices implemented during the pandemic [ 24 , 25 ], it was observed that learners chose to drop out due to psychological, pedagogical, and socio-economic reasons. Among these reasons, psychological issues such as engagement and motivation were salient [ 26 , 27 ].
As can be seen in the literature, there have been many attempts to investigate students’ dropping out from online learning environments. Some of these studies were systematic reviews and contributed greatly to the existing literature since they delivered a rigorous summary and comparison of several primary studies and, therefore, addressed research questions better than a single study might do. Nevertheless, a key problem with much of the literature on the phenomenon is that none of the studies that tend to review the past literature have applied data mining and analytics methods. Employing such methods would help a study identify patterns and relationships in large volumes of data extracted from a great number of sources and represent them in a more meaningful way.
When conducting research in any discipline, it is important to identify the gaps and trends, determine the scholarly ground, and act accordingly. As reported in the literature review section, the existing research indicates that the concept of dropout in distance education is extensive, multifaceted, and multidimensional. Consequently, it is vital to comprehend the dropout phenomenon in distance education by exploring what has been previously discussed in order to gain deeper insights into potential new perspectives. In light of these considerations, the objective of the current study is to scrutinize dropout trends in the realm of distance education research. To achieve this, the study aims to address the subsequent research inquiry:
- What are the thematic research patterns for dropout studies in the field of distance education?
2. Materials and Methods
2.1. research design.
This study employed data mining and analytics approaches [ 28 ] to examine the articles on dropout from the perspective of distance education. In addition to descriptive statistics, tSNE analysis [ 29 ], social network analysis (SNA) [ 30 ], and text mining approaches [ 31 ] were used. Titles of the articles were analyzed using t-distributed stochastic neighbor embedding (t-SNE) to visualize “high-dimensional data by giving each datapoint [words in titles and abstracts] a location in a two [sic] or three-dimensional map” [ 29 ]. SNA [ 30 ] of the keywords was performed to better identify thematic clusters and significant nodes with strategic positions in the keyword network. In this analysis, each keyword identified by the authors of the article was considered a node, and its co-occurrences were considered relationships. By adopting text mining [ 31 ] through the lexical analysis of the titles and abstracts, the researchers were able to visualize a thematic concept map and identify major themes emerging from the research corpus. By applying different data mining and analytics approaches, the data could be triangulated, thereby increasing the validity and reliability of the study [ 32 ].
2.2. Inclusion Criteria and Sampling
In order to obtain a broad view, Scopus, which is the largest scholarly database, was selected for the sampling of the articles and proceedings. The research corpus was created by sampling publications that met the following inclusion criteria: (1) indexed in the Scopus database, (2) written in English or translated into English, and (3) presence of search query items in the title of the articles and proceedings ( Table 1 ). The search yielded a total of 164 publications (80 articles and 84 proceedings).
Search queries used for the inclusion criteria.
Throughout the sampling and research corpus creation processes, this study employed the PRISMA protocol, which pertains to the preferred reporting items for systematic reviews and meta-analyses ( Figure 1 ) [ 33 ]. Ultimately, a total of 164 publications were encompassed in the final phase.

The PRISMA Protocol.
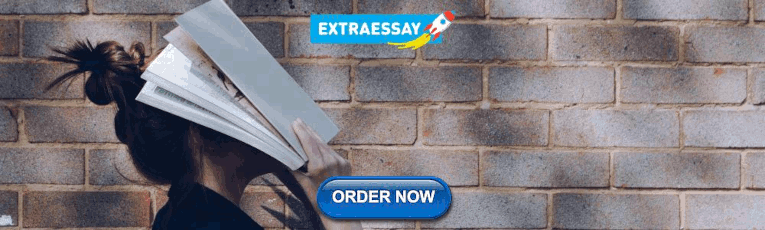
2.3. Data Collection Tools and Data Analysis Procedures
The data were crawled from the Scopus database, analyzed, and visualized by applying different data visualization tools. The study included a total of four phases. In the first phase of the study, time trends of the sampled studies were identified using descriptive statistics. In the second phase, the patterns in the titles were visualized by applying tSNE analysis [ 29 ]. In the third phase, the abstracts of the studies were analyzed using SNA [ 30 ] and text mining approaches [ 31 ], followed by identification of patterns and visualization of these patterns on a thematic concept map. In the final phase, using SNA [ 30 ], the keywords were analyzed based on their centrality metrics and then visualized on a network graphic.
2.4. Strengths and Limitations of the Study
The main strength of this study is its ability to use different data mining analytics approaches, which allowed for the visualization of the findings and identification of the research trends and patterns in an easily observable manner to facilitate analysis of the large volume of textual data. On the other hand, the study also has some limitations. First, the study examines publications that are only indexed in the Scopus database. However, publications not indexed in Scopus could have provided further insights into the research in question. Second, the study only examines peer-reviewed articles and proceedings, under the assumption that these provide the most robust and reliable findings. However, different types of publications (e.g., books, book chapters, reports, etc.) could have provided invaluable complementary findings. Third, while data mining techniques are considered a strength, they also have some limitations, and we acknowledge that our findings can only provide a partial view.
In the subsequent section, the findings from various analyses are presented, including time trend examination, tSNE evaluation of the titles, text mining of abstracts, and social network analysis (SNA) of the keywords.
3.1. Time Trend
When the time trends of the publications are examined, it can be seen that, while there is a slow but steady increase by the first decade of the 2000s, the number of the publications increases from 2010 onward and reaches its peak by 2020 ( Figure 2 ).

Time trends of the publications on dropout in distance education.
3.2. tSNE Analysis of the Titles
To identify the general focal point of the publications on dropout in distance education, a tSNE analysis, an unsupervised “nonlinear dimensionality reduction technique that aims to preserve the local structure of data” [ 29 ], was conducted. The analysis indicated that the publications distinctly focused on dropout in MOOCs (see the lower left corner in Figure 3 ), with a specific emphasis on predicting dropouts using different machine-based statistical models. The initial findings that emerged in tSNE analysis align with the insights gained from the time trends analysis.

tSNE analysis of the titles in the publications on dropout in distance education.
3.3. Text Mining of the Abstracts and SNA of the Keywords
In order to discern the research trends within the 164 publications addressing dropout in distance education, text mining techniques were employed to reveal patterns in the abstracts (see Figure 4 ), and SNA was utilized to uncover patterns in the keywords (see Figure 5 ). As a result, three overarching themes were identified through the triangulation of text mining and SNA findings. In this section, each theme was presented with evidence from text mining and SNA, and the entitled themes manifested themselves. Following that, we have explained these themes and discussed them in the next section by comparing and contrasting the related literature.

Thematic concept map of the abstracts in the publications on dropout in distance education.

Network graph of the keywords in the publications on dropout in distance education.
Theme 1: On defining dropout in MOOCs (see path in Figure 4 : flexibility, MOOC, problem, completion, rate, massive open challenges, attrition, online, and massive; see nodes in Figure 5 : lifelong learning, dropout rates, and MOOCs).
Theme 2: Non-human analytical data mining approaches to predict dropout (see path in Figure 4 : learner, MOOC, behavior, prediction, data, model, and methods; see nodes in Figure 5 : distance education, open and distance learning, dropout, learning analytics, educational data mining, machine learning, predictive analytics, data mining, and student dropout prediction).
Theme 3: Interaction, satisfaction, engagement, and personalization to reduce dropout rates (see path in Figure 4 : design, interaction, dropout, learning and support, student, distance, academic, e-learning, persistence and approach, learning, dropout, and at-risk; see nodes in Figure 5 : success, personalization, intention, design, academic locus of control, students satisfaction, e-learning, online learning, instructional design, students attrition, engagement, online education, distance learning, and higher education).
4. Discussion
4.1. time trend.
The first paper in the research corpus, written by Thompson [ 34 ] and published in 1984, addressed dropout in distance education from the perspective of the cognitive style of field dependence. Other earlier papers also used an exploratory research design. For instance, Sweet [ 35 ] examined dropout to validate Tinto’s model [ 5 ] for adult distance education students. The third paper in the research corpus, which was written by Garrison [ 36 ], noted that the field needs to go beyond simply descriptive studies and focus more on comprehensive and advanced research to better understand dropout in distance education. The first publications were explorative, and they highlighted the significance of the dropout phenomenon in distance education. In the 1990s, research on dropout began to attract some attention [ 37 ], which continued at a slow but steady pace until 2010. After 2013, there was a pronounced increase in the number of studies on dropout, and by 2017, the amount of research on dropout in distance education doubled before reaching its peak in 2020. Examination of the research corpus further showed that following the emergence of the first generation cMOOCs, the second generation of xMOOCs gained a lot of popularity [ 38 ], with the high dropout rates in MOOCs turning the spotlight on MOOC research [ 39 ]. For instance, publications on MOOCs and dropout [ 40 , 41 ] that focused on predictions using educational data mining [ 42 , 43 , 44 ] and publications that specifically focused on dropouts in online distance education [ 45 , 46 ] drew much attention. The findings indicate that the emergence of MOOCs and their popularity along with the emergence of online distance learning as a frequently used model in mainstream education further triggered the research on dropouts from the perspective of distance education. Another interesting finding is that most of the publications focused on predicting dropout behavior using educational data mining.
4.2. Research Patterns
This section provides a discussion of the research patterns. More specifically, it identifies the research themes through text mining of the abstracts and SNA of the keywords.
4.2.1. On Defining Dropout in MOOCs
A major flaw in the studies conducted to explore the reasons for students’ dropout from online environments is the lack of a proper definition of the term dropout. Previous attempts to come up with a precise definition have fallen short in many cases. In their comprehensive review, Lee and Choi [ 19 ] noted that many of the studies examined provided no general agreement on the definition of dropout from online courses, which made it quite challenging to compare dropout factors across different learning environments. Thus far, dropout students have been characterized in a variety of ways, including those who did the following:
- Departed from their institution for some reason [ 5 ];
- Voluntarily left their departments after finalizing tuition fee payments and the conclusion of the drop/add period [ 6 ];
- Did not register following three consecutive terms of non-enrollment [ 47 ];
- Earned a grade of F or formally withdrew from the course [ 48 ];
- Enrolled in a minimum of one module but failed to submit a single project [ 49 ];
- Were unable to complete a course during a semester [ 50 ];
- Went through the official withdrawal procedure [ 51 ];
- Opted to withdraw from e-learning, incurring financial penalties [ 7 ];
- Either withdrew or were dismissed from the program [ 52 ];
- Failed to meet the program requirement of completing two courses per year [ 53 ].
As for MOOCs, which globally offer open and flexible learning experiences for a large body of learners [ 54 ], the challenge to answer the question of whether every dropout is an actual dropout becomes more prominent [ 55 ]. According to Zheng et al. [ 56 ], some MOOC learners may only be interested in understanding particular concepts or some content rather than passing exams or achieving certificates, which may result in their leaving after acquiring the corresponding knowledge. Hence, previous attempts to define dropout seem to be inadequate and inconsistent due to the variety of ways that students join and leave MOOCs [ 57 ]. Furthermore, as Astin [ 58 ] stated, drawing a sharp distinction between dropouts and non-dropouts could be problematic in many ways because as long as the students in question are alive, they may return to college.
4.2.2. Non-Human Analytical Data Mining Approaches to Predict Dropout
As MOOCs have grown to include a massive number of students, effective machine learning models of complex student behavior patterns that identify at-risk students are needed [ 59 ]. The benefits of a diagnosis at an early stage include the ability to provide immediate intervention for at-risk students and determine in advance whether at-risk students lack interest in the teacher, the course, or MOOC itself [ 60 ]. Existing studies have placed great emphasis on supervised learning algorithms to build discriminative models that are capable of predicting dropout [ 61 , 62 ]. As Henrie et al. [ 63 ] suggested, the log data of learning behaviors collected by MOOC platforms where viewing is the basic learning behavior have been used to analyze dropout rates. Nonetheless, research predominantly utilizing clickstream data to construct a flow network model of collective attention in order to examine dropout learning patterns prompts numerous inquiries concerning the sufficiency of non-human analytics for exclusively explaining this phenomenon.
The literature reveals that the reasons for student dropout may vary. Previous studies explaining dropout reasons on personal grounds have highlighted the significance of psychological attributes, such as motivation [ 52 , 64 , 65 , 66 , 67 ], self-efficacy [ 52 , 67 ], and satisfaction [ 7 , 48 ]. Moreover, Perry et al. [ 53 ] point out that unexpected life events, such as health problems encountered by the student or a family member, death of a family member, or unplanned financial pressures, may also lead to dropout. In such unanticipated circumstances, misleading or inadequate data can emerge when learning algorithms rely too heavily on learner logs.
4.2.3. Interaction, Satisfaction, Engagement, and Personalization to Reduce Dropout Rates
The results emphatically indicate the need for implementing strategies to minimize learning obstacles and tackle the potential causes for withdrawal. The creation of supportive environments and provision of encouragement are deemed essential factors [ 4 ], irrespective of their origin. Support from various sources, such as family or friends [ 18 , 21 , 52 , 65 , 67 ] or the institution itself [ 64 , 68 ], considerably impacts students’ perseverance. Another crucial element is course satisfaction, as evidenced by multiple studies [ 7 , 48 ]. The level of satisfaction derived from e-learning plays a pivotal role in students’ decisions to either complete or withdraw from online courses [ 7 ]. Although benefitting from its most prominent characteristic of no time and space boundaries [ 69 ], distance education suffers from the negative impacts it has on transactional distance. Transactional distance, described as the degree of psychological distance between the learner and teacher by Moore [ 70 ], needs to be considered to improve learner satisfaction. Another dimension to consider is motivation. Motivated, goal-oriented, and engaged students tend to show better persistence in online learning [ 52 , 65 , 66 , 71 ]. Furthermore, previous studies have shown that the decision behind dropout in some cases lies in students’ locus of control [ 51 , 66 , 72 , 73 ]. However, there is no single factor on its own that can be held responsible for student dropout. Rather, it is a combination of different factors in different settings that results in non-persistence. Therefore, this brings attention to the significance of instructional design as an aspect of early intervention. According to Chyung et al. [ 74 ], an effective online learning environment, where student participation is high, involves well-designed curriculum and course materials that meet expectations and contribute to student satisfaction and motivation, accordingly. When the course is tailored to specific career goals and individual learning styles, as Perry et al. [ 53 ] suggest, the students will be less likely to drop out.
In all, the focal point of the aforementioned three themes is that dropout is a multidimensional and multilayered issue that has contextualized traits ranging from the way it is defined, the learning design (learner agency and personalized learning), the learning needs (e.g., formal learning requirements as in the higher education or lifelong learning needs as in the case of MOOCs), and socio-economic background (e.g., time needed, working status, family responsibilities, etc.) along with psychological status (e.g., satisfaction, motivation, engagement, etc.). This implies that the dropout equation has many variables and, to identify these variables and provide possible solution scenarios, future research can approach the research in question from different perspectives.
5. Conclusions and Suggestions
The issue of student dropout in distance education systems presents significant challenges for educators, administrators, and policy-making communities. By exploring the research trends and patterns of dropout in distance education systems using data mining and analytics methodologies, this study offers valuable theoretical and practical insights to enhance the current understanding of learner retention in online networked learning environments.
Dropout is a relative term, interpreted differently by different researchers, institutions, and educational systems. In most cases, intervention strategies that work for one system will not necessarily be applicable for another. Likewise, due to the sui generis feature of each online learning system, the reasons given for dropout may be unique to the program. Therefore, in order to avoid misinterpretation and to be able to come up with a proper diagnosis, a precise definition of the term “dropout” in the context of distance education is needed.
Another thing to consider is the excessive emphasis on supervised learning algorithms in building discriminative models to predict dropout. Considering the massive target populations of distance education programs, which include learners from any social, academic, or economic background, prediction models based only on learning algorithms may fall short because of their neglect of unanticipated circumstances of human nature. What is more, these models may lead to the exclusion of learners believed to be prone to dropout and leave them with no choice but failure. Thus, there is a need to develop ethical principles, policies, and frameworks regarding the use of algorithms to predict dropout in distance education. It should also be kept in mind that there may be other psychological and sociological reasons for dropping out of school, and in this context, it should be highlighted that algorithmic solutions cannot identify such reasons.
The findings of this study correlate fairly well with previous works and further support the idea that dropout from distance learning may arise from various factors. What seems to be fundamental to note here is that neither a single factor can be held responsible for student dropout, nor can a single formula ensure persistence. Rather, educators, institutions, and designers of online programs should adopt a human-centered approach that can foster learners’ motivation, satisfaction, and independence. In the end, learning is supposed to be a social process that requires giving more agency to learners and supporting them socially and academically to minimize the dropout rates.
Motivation can be introduced as an umbrella term for many factors, as it can be linked to many other factors to some extent. When learners are more motivated, it can eliminate both environmental- and student-related factors, since the first would better help them balance their family, work, and study life, and the latter would assist them in gaining self-regulation and self-efficacy skills. In order to increase motivation, learners should receive a considerable amount of encouragement, support, user-friendly learning instructions/interface, relevant content, hands-on experience, and guidance. Lastly, one of the most outstanding features of distance education, “learning at one’s own pace”, should be exploited to improve learner independence and autonomy. By doing so, learners can master their time management and workload.
Funding Statement
This research was funded by Anadolu University, grant number 2208E119 and 2207E099.
Author Contributions
Conceptualization, S.E. and A.B.; methodology, A.B.; software, A.B.; formal analysis, A.B.; investigation, S.E. and A.B.; data curation, A.B.; writing—original draft preparation, S.E. and A.B.; writing—review and editing, S.E. and A.B.; visualization, A.B.; supervision, A.B.; project administration, A.B.; funding acquisition, S.E. and A.B. All authors have read and agreed to the published version of the manuscript.
Institutional Review Board Statement
Not applicable.
Informed Consent Statement
Data availability statement, conflicts of interest.
The authors declare no conflict of interest.
Disclaimer/Publisher’s Note: The statements, opinions and data contained in all publications are solely those of the individual author(s) and contributor(s) and not of MDPI and/or the editor(s). MDPI and/or the editor(s) disclaim responsibility for any injury to people or property resulting from any ideas, methods, instructions or products referred to in the content.
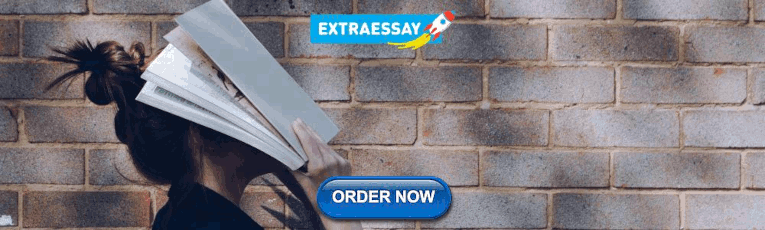
IMAGES
VIDEO
COMMENTS
Research on school dropout extends from early 20th-century pioneers until now, marking trends of causes and prevention. However, specific dropout causes reported by students from several nationally representative studies have never been examined together, which, if done, could lead to a better understanding of the dropout problem.
show that 3.4% of students dropped out that year. Alternatively, status dropout is the percentage. of 16- to 24-year-olds who are not enrolled in school and have not earned a high school ...
Why Students Drop Out of School: ... dropping out, a vast empirical research literature has examined numerous predictors of high school dropout and graduation. The empirical research comes from a number of social science ... high school dropout and graduation. The paper first reviews the theoretical literature on student
2018, the dropout rate for students was 3 %of the total stu-. dents, with 245,810 student dropouts, and in 2019 was 8 %, with the number of dropout students 698,261. In 2019, the. highest number ...
Different research studies conducted on the dropout rate in high schools have taken it as a complex process, resulting from several alterable and unalterable factors, responsible for influencing the students to leave the institution before graduation (Christenson et al., 2001).
dropping out, this study seeks to enrich the research base for engagement and dropout literature and to identify factors that can be implemented in future dropout prevention initiatives. Keywords: dropout, student engagement, alternative programs, small learning communities, blended learning, learning facilitator
Multiple factors in elementary or middle school may influence stu-dents' attitudes, behaviors, and performance in high school prior to drop-ping out. To better understand the underlying causes behind students' decisions for dropping out, we reviewed the past 25 years of research on dropouts. The review was based on 203 published studies ...
School dropout is a major concern in many societies. In Western countries in particular, a large proportion of youth quit school before obtaining a high school diploma (Eurostat, 2017; Statistics Canada, 2017; U.S. Department of Commerce, 2017).Many youth who drop out face important setbacks upon entering adulthood: compared to high school graduates, they rely more on social assistance ...
correlate highly with dropout status. Dropout rates were highest for students with late tuition fees, with approx-imately 94 percent of such students dropping out. 76 percent of debtors were dropouts. On the other hand, 86 percent of scholarship holders became graduates, which may be due to a higher desire to succeed and confirms
This report highlights key factors that lead students to drop out, with the major cause being socioeconomic conditions. Namoun and Alshanqiti [ 5 ] performed an exhaustive search that found 62 papers published in peer-reviewed journals between 2010 and 2020, which present intelligent models to predict student performance.
for dropping out, we reviewed the past 25 years of research on dropouts. The review was based on 203 published studies that analyzed a variety of. national, state, and local data to identify ...
the most important findings of our research into dropout so far in this paper. After giving the methodological background, we elaborate on the differences between students who dropped out, those who are at risk of dropout, and those who are persistent. We used multivariate statistical methods to determine the effects of the chief explanatory ...
These students had a 45% chance of dropping out if they had reached ninth grade, a 34% chance if they had reached tenth grade, a 23% chance if they had reached eleventh grade, and a 16% chance if they had reached twelfth grade (Neild & Balfanz, 2006). " Dropping out is a process that begins well before high school, and students exhibit ...
Globally, a large number of students drop out of school every year [21, 22]. While, a significant number of them are found living in poverty or receiving public assistance, imprisoned, unhealthy, ... There are a good number of research papers on school dropout in India, but very few focuses on adolescent population using longitudinal data. ...
Students with disabilities drop out of high school at a higher rate than typically learning students, impacting their short and long-term educational and employment opportunities and making long-term financial stability less likely. ... Dr. Majeika supports a portfolio of research on special education and multi-tiered systems of support (MTSS ...
Students who dropped out of the HRE Online master's degree program at the University of Illinois at Urbana-Champaign were the focus of this study. Students were admitted to the HRE Online degree program in cohorts of approximately 30 students. Data were collected from the first three cohorts of students.
Furthermore, predicting whether a student will drop out is not enough to diagnose and prevent the problem as it is also necessary to provide potential justifications for the dropout. This paper proposes an approach for creating and enriching a dataset for dropout prediction, which has been applied for dropout prediction using data from 19 ...
2.1. Research Design. This study employed data mining and analytics approaches [] to examine the articles on dropout from the perspective of distance education.In addition to descriptive statistics, tSNE analysis [], social network analysis (SNA) [], and text mining approaches [] were used.Titles of the articles were analyzed using t-distributed stochastic neighbor embedding (t-SNE) to ...
April 2013. Abstract. This paper reviews the growing literature on early school leaving. We clarify what is at stake. with early school leaving, and touch upon underlying pro blems and ...
The purpose of this paper is to establish what sociodemographic and institutional factors cause students to drop out, become uncertain about their intentions to obtain a degree, or confidently advance towards the fulfilment of their ambitions. Our analysis is based on the combined databases of large-sample questionnaire surveys carried out among former students who dropped out from higher ...
Over the ensuing years most law schools recovered, albeit with significant trimming, and for the most part rebalanced their faculty and student body in a sustainable fashion. On today's horizon, however, is a greater and more sustained threat. A shrunken student population pipeline has hit colleges and will soon reach law schools.
university. Method: In the present qualitative study, 15 students with a dropout experience were recruited. throughout a purposive sampling. The experie nces of participants were categorized into ...
In a report published by the Philippine Department of Education, on the average, within th e sc hool years 2005 to. 2013, 26% of el ementary school students drop out b efore the sixth grad e, and ...