- Daily Crossword
- Word Puzzle
- Word Finder
- Word of the Day
- Synonym of the Day
- Word of the Year
- Language stories
- All featured
- Gender and sexuality
- All pop culture
- Grammar Coach ™
- Writing hub
- Grammar essentials
- Commonly confused
- All writing tips
- Pop culture
- Writing tips
- representation
the act of representing.
the state of being represented.
the expression or designation by some term, character, symbol, or the like.
action or speech on behalf of a person, group, business house, state, or the like by an agent, deputy, or representative.
the state or fact of being so represented: to demand representation on a board of directors.
Government . the state, fact, or right of being represented by delegates having a voice in legislation or government.
the body or number of representatives, as of a constituency.
Diplomacy .
the act of speaking or negotiating on behalf of a state.
an utterance on behalf of a state.
presentation to the mind, as of an idea or image.
a mental image or idea so presented; concept.
the act of portrayal, picturing, or other rendering in visible form.
a picture, figure, statue, etc.
the production or a performance of a play or the like, as on the stage.
Often representations. a description or statement, as of things true or alleged.
a statement of facts, reasons, etc., made in appealing or protesting; a protest or remonstrance.
Law . an implication or statement of fact to which legal liability may attach if material: a representation of authority.
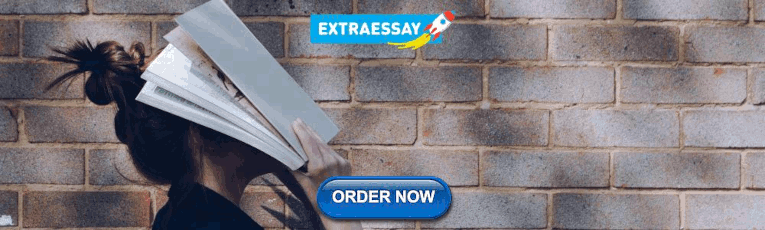
Origin of representation
Other words from representation.
- non·rep·re·sen·ta·tion, noun
- o·ver·rep·re·sen·ta·tion, noun
- pre·rep·re·sen·ta·tion, noun
- self-rep·re·sen·ta·tion, noun
- un·der·rep·re·sen·ta·tion, noun
Words Nearby representation
- reprehensible
- reprehension
- representational
- representationalism
- representative
- representatives
Dictionary.com Unabridged Based on the Random House Unabridged Dictionary, © Random House, Inc. 2024
How to use representation in a sentence
It was a metaphorical statement of giving and withdrawing consent for a show rooted in a literal representation of Coel being assaulted.
The mathematically manipulated results are passed on and augmented through the stages, finally producing an integrated representation of a face.
I hope this list—a representation of the most consequential changes taking places in our world—is similarly useful for you.
“Given the moment we are in, I can only hope our institutions really understand what this failure of representation means to our city,” he said.
The voters don’t want to have an elected city attorney on the, and representation said, that’s fine.
With all that said, representation of each of these respective communities has increased in the new Congress.
As this excellent piece in Mother Jones describes, however, Holsey had outrageously poor representation during his trial.
During that time days, Livvix went through court hearings without legal representation .
What do you think prompted the change in comic book representation of LGBTQ characters?
Barbie is an unrealistic, unhealthy, insulting representation of female appearance.
With less intelligent children traces of this tendency to take pictorial representation for reality may appear as late as four.
As observation widens and grows finer, the first bald representation becomes fuller and more life-like.
The child now aims at constructing a particular linear representation , that of a man, a horse, or what not.
He had heard it hinted that allowing the colonies representation in Parliament would be a simple plan for making taxes legal.
But sufficient can be discerned for the grasping of the idea, which seems to be a representation of the Nativity.
British Dictionary definitions for representation
/ ( ˌrɛprɪzɛnˈteɪʃən ) /
the act or an instance of representing or the state of being represented
anything that represents, such as a verbal or pictorial portrait
anything that is represented, such as an image brought clearly to mind
the principle by which delegates act for a constituency
a body of representatives
contract law a statement of fact made by one party to induce another to enter into a contract
an instance of acting for another, on his authority, in a particular capacity, such as executor or administrator
a dramatic production or performance
(often plural) a statement of facts, true or alleged, esp one set forth by way of remonstrance or expostulation
linguistics an analysis of a word, sentence, etc, into its constituents : phonetic representation
Collins English Dictionary - Complete & Unabridged 2012 Digital Edition © William Collins Sons & Co. Ltd. 1979, 1986 © HarperCollins Publishers 1998, 2000, 2003, 2005, 2006, 2007, 2009, 2012

Definition of 'representation'
- representation

Video: pronunciation of representation
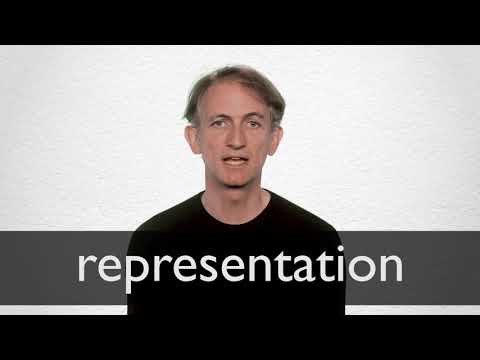
representation in British English
Representation in american english, examples of 'representation' in a sentence representation, cobuild collocations representation, trends of representation.
View usage for: All Years Last 10 years Last 50 years Last 100 years Last 300 years
In other languages representation
- American English : representation / rɛprɪzɛnˈteɪʃən /
- Brazilian Portuguese : representação
- Chinese : 代表
- European Spanish : representación
- French : représentation
- German : Vertretung
- Italian : rappresentanza
- Japanese : 代表
- Korean : 대표
- European Portuguese : representação
- Latin American Spanish : representación
- Thai : การมีตัวแทน
Browse alphabetically representation
- represent value
- representamen
- representant
- representation of reality
- representational
- representational painting
- All ENGLISH words that begin with 'R'
Related terms of representation
- ensure representation
- equal representation
- exact representation
- fair representation
- false representation
- View more related words
Quick word challenge
Quiz Review
Score: 0 / 5

Wordle Helper

Scrabble Tools

Words and phrases
Personal account.
- Access or purchase personal subscriptions
- Get our newsletter
- Save searches
- Set display preferences
Institutional access
Sign in with library card
Sign in with username / password
Recommend to your librarian
Institutional account management
Sign in as administrator on Oxford Academic
representation noun 1
- Hide all quotations
What does the noun representation mean?
There are 19 meanings listed in OED's entry for the noun representation , three of which are labelled obsolete. See ‘Meaning & use’ for definitions, usage, and quotation evidence.
representation has developed meanings and uses in subjects including
How common is the noun representation ?
How is the noun representation pronounced, british english, u.s. english, where does the noun representation come from.
Earliest known use
Middle English
The earliest known use of the noun representation is in the Middle English period (1150—1500).
OED's earliest evidence for representation is from around 1450, in St. Elizabeth of Spalbeck .
representation is of multiple origins. Either (i) a borrowing from French. Or (ii) a borrowing from Latin.
Etymons: French representation ; Latin repraesentātiōn- , repraesentātiō .
Nearby entries
- reprehensory, adj. 1576–1825
- repremiation, n. 1611
- represent, n. a1500–1635
- represent, v.¹ c1390–
- re-present, v.² 1564–
- representable, adj. & n. 1630–
- representamen, n. 1677–
- representance, n. 1565–
- representant, n. 1622–
- representant, adj. 1851–82
- representation, n.¹ c1450–
- re-presentation, n.² 1805–
- representational, adj. 1850–
- representationalism, n. 1846–
- representationalist, adj. & n. 1846–
- representationary, adj. 1856–
- representationism, n. 1842–
- representationist, n. & adj. 1842–
- representation theory, n. 1928–
- representative, adj. & n. a1475–
- representative fraction, n. 1860–
Thank you for visiting Oxford English Dictionary
To continue reading, please sign in below or purchase a subscription. After purchasing, please sign in below to access the content.
Meaning & use
Pronunciation, compounds & derived words, entry history for representation, n.¹.
representation, n.¹ was revised in December 2009.
representation, n.¹ was last modified in March 2024.
oed.com is a living text, updated every three months. Modifications may include:
- further revisions to definitions, pronunciation, etymology, headwords, variant spellings, quotations, and dates;
- new senses, phrases, and quotations.
Revisions and additions of this kind were last incorporated into representation, n.¹ in March 2024.
Earlier versions of this entry were published in:
OED First Edition (1906)
- Find out more
OED Second Edition (1989)
- View representation in OED Second Edition
Please submit your feedback for representation, n.¹
Please include your email address if you are happy to be contacted about your feedback. OUP will not use this email address for any other purpose.
Citation details
Factsheet for representation, n.¹, browse entry.
- Subscriber Services
- For Authors
- Publications
- Archaeology
- Art & Architecture
- Bilingual dictionaries
- Classical studies
- Encyclopedias
- English Dictionaries and Thesauri
- Language reference
- Linguistics
- Media studies
- Medicine and health
- Names studies
- Performing arts
- Science and technology
- Social sciences
- Society and culture
- Overview Pages
- Subject Reference
- English Dictionaries
- Bilingual Dictionaries
Recently viewed (0)
- Save Search
- Share This Facebook LinkedIn Twitter
Related Content
Related overviews.
descriptions
See all related overviews in Oxford Reference »
More Like This
Show all results sharing this subject:
representation
Quick reference.
[Latin repraesentare ‘to make present or manifest’]
1. Depicting or ‘making present’ something which is absent (e.g. people, places, events, or abstractions) in a different form: as in paintings, photographs, films, or language, rather than as a replica . See also description; compare absent presence.
2. The function of a sign or symbol of ‘standing for’ that to which it refers (its referent).
3. The various processes of production involved in generating representational texts in any medium, including the mass media (e.g. the filming, editing, and broadcasting of a television documentary). Such framings of the concept privilege authorial intention. See also auteur theory; authorial determinism; sender-oriented communication.
4. A text (in any medium) which is the product of such processes, usually regarded as amenable to textual analysis (‘a representation’).
5. What is explicitly or literally described, depicted, or denoted in a sign, text, or discourse in any medium as distinct from its symbolic meaning, metaphoric meaning, or connotations: its manifest referential content, as in ‘a representation of…’ See also mimesis; naturalism; referentiality.
6. How (in what ways) something is depicted. However ‘realistic’ texts may seem to be, they involve some form of transformation. Representations are unavoidably selective (none can ever ‘show the whole picture’), and within a limited frame, some things are foregrounded and others backgrounded: see also framing; generic representation; selective representation; stylization. In factual genres in the mass media, critics understandably focus on issues such as truth, accuracy, bias, and distortion ( see also reflectionism), or on whose realities are being represented and whose are being denied. See also dominant ideology; manipulative model; stereotyping; symbolic erasure.
7. The relation of a sign or text in any medium to its referent. In reflectionist framings, the transparent re- presentation, reflection, recording, transcription, or reproduction of a pre-existing reality ( see also imaginary signifier; mimesis; realism). In constructionist framings, the transformation of particular social realities, subjectivities, or identities in processes which are ostensibly merely re- presentations ( see also constitutive models; interpellation; reality construction). Some postmodern theorists avoid the term representation completely because the epistemological assumptions of realism seem to be embedded within it.
8. A cycle of processes of textual and meaning production and reception situated in a particular sociohistorical context ( see also circuit of communication; circuit of culture). This includes the active processes in which audiences engage in the interpretation of texts ( see also active audience theory; beholder's share; picture perception). Semiotics highlights representational codes which need to be decoded ( see also encoding/decoding model; photographic codes; pictorial codes; realism), and related to a relevant context ( see also Jakobson's model).
9. (narratology) Showing as distinct from telling (narration).
10. (mental representation) The process and product of encoding perceptual experience in the mind: see dual coding theory; gestalt laws; mental representation; perceptual codes; selective perception; selective retention.
11. A relationship in which one person (a representative) acting on behalf of another (as in law), or a political principle in which one person acts, in some sense, on behalf of a group of people, normally having been chosen by them to do so (as in representative democracies).
From: representation in A Dictionary of Media and Communication »
Subjects: Media studies
Related content in Oxford Reference
Reference entries, representation, problems.
View all reference entries »
View all related items in Oxford Reference »
Search for: 'representation' in Oxford Reference »
- Oxford University Press
PRINTED FROM OXFORD REFERENCE (www.oxfordreference.com). (c) Copyright Oxford University Press, 2023. All Rights Reserved. Under the terms of the licence agreement, an individual user may print out a PDF of a single entry from a reference work in OR for personal use (for details see Privacy Policy and Legal Notice ).
date: 04 April 2024
- Cookie Policy
- Privacy Policy
- Legal Notice
- Accessibility
- [66.249.64.20|185.80.150.64]
- 185.80.150.64
Character limit 500 /500
- More from M-W
- To save this word, you'll need to log in. Log In
representative
Definition of representative
(Entry 1 of 2)
Definition of representative (Entry 2 of 2)
- archetypical
- characteristic
Examples of representative in a Sentence
These examples are programmatically compiled from various online sources to illustrate current usage of the word 'representative.' Any opinions expressed in the examples do not represent those of Merriam-Webster or its editors. Send us feedback about these examples.
Word History
14th century, in the meaning defined at sense 1
1635, in the meaning defined at sense 1
Articles Related to representative
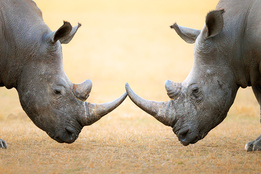
9 Words with Unnecessary Syllables
'Argumentative', 'interpretate', and more
Dictionary Entries Near representative
representationist
representative art
Cite this Entry
“Representative.” Merriam-Webster.com Dictionary , Merriam-Webster, https://www.merriam-webster.com/dictionary/representative. Accessed 4 Apr. 2024.
Kids Definition
Kids definition of representative.
Kids Definition of representative (Entry 2 of 2)
Legal Definition
Legal definition of representative.
Legal Definition of representative (Entry 2 of 2)
More from Merriam-Webster on representative
Nglish: Translation of representative for Spanish Speakers
Britannica English: Translation of representative for Arabic Speakers
Britannica.com: Encyclopedia article about representative
Subscribe to America's largest dictionary and get thousands more definitions and advanced search—ad free!
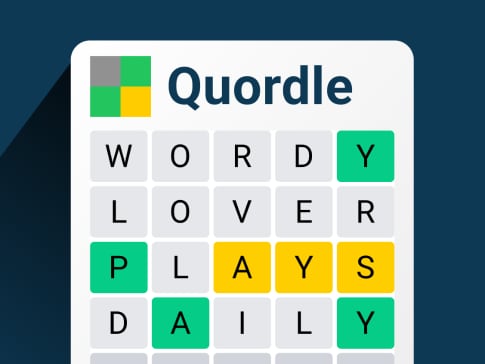
Can you solve 4 words at once?
Word of the day.
See Definitions and Examples »
Get Word of the Day daily email!
Popular in Grammar & Usage
The tangled history of 'it's' and 'its', more commonly misspelled words, why does english have so many silent letters, your vs. you're: how to use them correctly, every letter is silent, sometimes: a-z list of examples, popular in wordplay, the words of the week - mar. 29, 10 scrabble words without any vowels, 12 more bird names that sound like insults (and sometimes are), 8 uncommon words related to love, 9 superb owl words, games & quizzes.
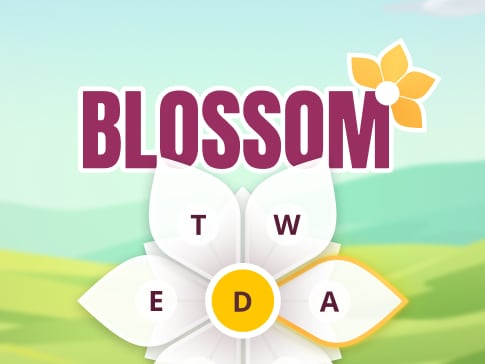

- Table of Contents
- Random Entry
- Chronological
- Editorial Information
- About the SEP
- Editorial Board
- How to Cite the SEP
- Special Characters
- Advanced Tools
- Support the SEP
- PDFs for SEP Friends
- Make a Donation
- SEPIA for Libraries
- Entry Contents
Bibliography
Academic tools.
- Friends PDF Preview
- Author and Citation Info
- Back to Top
Political Representation
The concept of political representation is misleadingly simple: everyone seems to know what it is, yet few can agree on any particular definition. In fact, there is an extensive literature that offers many different definitions of this elusive concept. [Classic treatments of the concept of political representations within this literature include Pennock and Chapman 1968; Pitkin, 1967 and Schwartz, 1988.] Hanna Pitkin (1967) provides, perhaps, one of the most straightforward definitions: to represent is simply to “make present again.” On this definition, political representation is the activity of making citizens’ voices, opinions, and perspectives “present” in public policy making processes. Political representation occurs when political actors speak, advocate, symbolize, and act on the behalf of others in the political arena. In short, political representation is a kind of political assistance. This seemingly straightforward definition, however, is not adequate as it stands. For it leaves the concept of political representation underspecified. Indeed, as we will see, the concept of political representation has multiple and competing dimensions: our common understanding of political representation is one that contains different, and conflicting, conceptions of how political representatives should represent and so holds representatives to standards that are mutually incompatible. In leaving these dimensions underspecified, this definition fails to capture this paradoxical character of the concept.
This encyclopedia entry has three main goals. The first is to provide a general overview of the meaning of political representation, identifying the key components of this concept. The second is to highlight several important advances that have been made by the contemporary literature on political representation. These advances point to new forms of political representation, ones that are not limited to the relationship between formal representatives and their constituents. The third goal is to reveal several persistent problems with theories of political representation and thereby to propose some future areas of research.
1.1 Delegate vs. Trustee
1.2 pitkin’s four views of representation, 2. changing political realities and changing concepts of political representation, 3. contemporary advances, 4. future areas of study, a. general discussions of representation, b. arguments against representation, c. non-electoral forms of representation, d. representation and electoral design, e. representation and accountability, f. descriptive representation, other internet resources, related entries, 1. key components of political representation.
Political representation, on almost any account, will exhibit the following five components:
- some party that is representing (the representative, an organization, movement, state agency, etc.);
- some party that is being represented (the constituents, the clients, etc.);
- something that is being represented (opinions, perspectives, interests, discourses, etc.); and
- a setting within which the activity of representation is taking place (the political context).
- something that is being left out (the opinions, interests, and perspectives not voiced).
Theories of political representation often begin by specifying the terms for the first four components. For instance, democratic theorists often limit the types of representatives being discussed to formal representatives — that is, to representatives who hold elected offices. One reason that the concept of representation remains elusive is that theories of representation often apply only to particular kinds of political actors within a particular context. How individuals represent an electoral district is treated as distinct from how social movements, judicial bodies, or informal organizations represent. Consequently, it is unclear how different forms of representation relate to each other. Andrew Rehfeld (2006) has offered a general theory of representation which simply identifies representation by reference to a relevant audience accepting a person as its representative. One consequence of Rehfeld’s general approach to representation is that it allows for undemocratic cases of representation.
However, Rehfeld’s general theory of representation does not specify what representative do or should do in order to be recognized as a representative. And what exactly representatives do has been a hotly contested issue. In particular, a controversy has raged over whether representatives should act as delegates or trustees .
Historically, the theoretical literature on political representation has focused on whether representatives should act as delegates or as trustees . Representatives who are delegates simply follow the expressed preferences of their constituents. James Madison (1787–8) describes representative government as “the delegation of the government...to a small number of citizens elected by the rest.” Madison recognized that “Enlightened statesmen will not always be at the helm.” Consequently, Madison suggests having a diverse and large population as a way to decrease the problems with bad representation. In other words, the preferences of the represented can partially safeguard against the problems of faction.
In contrast, trustees are representatives who follow their own understanding of the best action to pursue. Edmund Burke (1790) is famous for arguing that
Parliament is not a congress of ambassadors from different and hostile interests, which interest each must maintain, as an agent and advocate, against other agents and advocates; but Parliament is a deliberative assembly of one nation, with one interest, that of the whole… You choose a member, indeed; but when you have chosen him he is not a member of Bristol, but he is a member of Parliament (115).
The delegate and the trustee conception of political representation place competing and contradictory demands on the behavior of representatives. [For a discussion of the similarities and differences between Madison’s and Burke’s conception of representation, see Pitkin 1967, 191–192.] Delegate conceptions of representation require representatives to follow their constituents’ preferences, while trustee conceptions require representatives to follow their own judgment about the proper course of action. Any adequate theory of representation must grapple with these contradictory demands.
Famously, Hanna Pitkin argues that theorists should not try to reconcile the paradoxical nature of the concept of representation. Rather, they should aim to preserve this paradox by recommending that citizens safeguard the autonomy of both the representative and of those being represented. The autonomy of the representative is preserved by allowing them to make decisions based on his or her understanding of the represented’s interests (the trustee conception of representation). The autonomy of those being represented is preserved by having the preferences of the represented influence evaluations of representatives (the delegate conception of representation). Representatives must act in ways that safeguard the capacity of the represented to authorize and to hold their representatives accountable and uphold the capacity of the representative to act independently of the wishes of the represented.
Objective interests are the key for determining whether the autonomy of representative and the autonomy of the represented have been breached. However, Pitkin never adequately specifies how we are to identify constituents’ objective interests. At points, she implies that constituents should have some say in what are their objective interests, but ultimately she merely shifts her focus away from this paradox to the recommendation that representatives should be evaluated on the basis of the reasons they give for disobeying the preferences of their constituents. For Pitkin, assessments about representatives will depend on the issue at hand and the political environment in which a representative acts. To understand the multiple and conflicting standards within the concept of representation is to reveal the futility of holding all representatives to some fixed set of guidelines. In this way, Pitkin concludes that standards for evaluating representatives defy generalizations. Moreover, individuals, especially democratic citizens, are likely to disagree deeply about what representatives should be doing.
Pitkin offers one of the most comprehensive discussions of the concept of political representation, attending to its contradictory character in her The Concept of Representation . This classic discussion of the concept of representation is one of the most influential and oft-cited works in the literature on political representation. (For a discussion of her influence, see Dovi 2016). Adopting a Wittgensteinian approach to language, Pitkin maintains that in order to understand the concept of political representation, one must consider the different ways in which the term is used. Each of these different uses of the term provides a different view of the concept. Pitkin compares the concept of representation to “ a rather complicated, convoluted, three–dimensional structure in the middle of a dark enclosure.” Political theorists provide “flash-bulb photographs of the structure taken from different angles” [1967, 10]. More specifically, political theorists have provided four main views of the concept of representation. Unfortunately, Pitkin never explains how these different views of political representation fit together. At times, she implies that the concept of representation is unified. At other times, she emphasizes the conflicts between these different views, e.g. how descriptive representation is opposed to accountability. Drawing on her flash-bulb metaphor, Pitkin argues that one must know the context in which the concept of representation is placed in order to determine its meaning. For Pitkin, the contemporary usage of the term “representation” can signficantly change its meaning.
For Pitkin, disagreements about representation can be partially reconciled by clarifying which view of representation is being invoked. Pitkin identifies at least four different views of representation: formalistic representation, descriptive representation, symbolic representation, and substantive representation. (For a brief description of each of these views, see chart below.) Each view provides a different approach for examining representation. The different views of representation can also provide different standards for assessing representatives. So disagreements about what representatives ought to be doing are aggravated by the fact that people adopt the wrong view of representation or misapply the standards of representation. Pitkin has in many ways set the terms of contemporary discussions about representation by providing this schematic overview of the concept of political representation.
1. Formalistic Representation : Brief Description . The institutional arrangements that precede and initiate representation. Formal representation has two dimensions: authorization and accountability. Main Research Question . What is the institutional position of a representative? Implicit Standards for Evaluating Representatives . None. ( Authorization ): Brief Description . The means by which a representative obtains his or her standing, status, position or office. Main Research Questions . What is the process by which a representative gains power (e.g., elections) and what are the ways in which a representative can enforce his or her decisions? Implicit Standards for Evaluating Representatives . No standards for assessing how well a representative behaves. One can merely assess whether a representative legitimately holds his or her position. pdf include--> ( Accountability ): Brief Description . The ability of constituents to punish their representative for failing to act in accordance with their wishes (e.g. voting an elected official out of office) or the responsiveness of the representative to the constituents. Main Research Question . What are the sanctioning mechanisms available to constituents? Is the representative responsive towards his or her constituents’ preferences? Implicit Standards for Evaluating Representatives . No standards for assessing how well a representative behaves. One can merely determine whether a representative can be sanctioned or has been responsive.
Brief Description . The ways that a representative “stands for” the represented — that is, the meaning that a representative has for those being represented.
Main Research Question . What kind of response is invoked by the representative in those being represented?
Implicit Standards for Evaluating Representatives . Representatives are assessed by the degree of acceptance that the representative has among the represented.
Brief Description . The extent to which a representative resembles those being represented.
Main Research Question . Does the representative look like, have common interests with, or share certain experiences with the represented?
Implicit Standards for Evaluating Representatives . Assess the representative by the accuracy of the resemblance between the representative and the represented.
Brief Description . The activity of representatives—that is, the actions taken on behalf of, in the interest of, as an agent of, and as a substitute for the represented.
Main Research Question . Does the representative advance the policy preferences that serve the interests of the represented?
Implicit Standards for Evaluating Representatives . Assess a representative by the extent to which policy outcomes advanced by a representative serve “the best interests” of their constituents.
One cannot overestimate the extent to which Pitkin has shaped contemporary understandings of political representation, especially among political scientists. For example, her claim that descriptive representation opposes accountability is often the starting point for contemporary discussions about whether marginalized groups need representatives from their groups.
Similarly, Pitkin’s conclusions about the paradoxical nature of political representation support the tendency among contemporary theorists and political scientists to focus on formal procedures of authorization and accountability (formalistic representation). In particular, there has been a lot of theoretical attention paid to the proper design of representative institutions (e.g. Amy 1996; Barber, 2001; Christiano 1996; Guinier 1994). This focus is certainly understandable, since one way to resolve the disputes about what representatives should be doing is to “let the people decide.” In other words, establishing fair procedures for reconciling conflicts provides democratic citizens one way to settle conflicts about the proper behavior of representatives. In this way, theoretical discussions of political representation tend to depict political representation as primarily a principal-agent relationship. The emphasis on elections also explains why discussions about the concept of political representation frequently collapse into discussions of democracy. Political representation is understood as a way of 1) establishing the legitimacy of democratic institutions and 2) creating institutional incentives for governments to be responsive to citizens.
David Plotke (1997) has noted that this emphasis on mechanisms of authorization and accountability was especially useful in the context of the Cold War. For this understanding of political representation (specifically, its demarcation from participatory democracy) was useful for distinguishing Western democracies from Communist countries. Those political systems that held competitive elections were considered to be democratic (Schumpeter 1976). Plotke questions whether such a distinction continues to be useful. Plotke recommends that we broaden the scope of our understanding of political representation to encompass interest representation and thereby return to debating what is the proper activity of representatives. Plotke’s insight into why traditional understandings of political representation resonated prior to the end of the Cold War suggests that modern understandings of political representation are to some extent contingent on political realities. For this reason, those who attempt to define political representation should recognize how changing political realities can affect contemporary understandings of political representation. Again, following Pitkin, ideas about political representation appear contingent on existing political practices of representation. Our understandings of representation are inextricably shaped by the manner in which people are currently being represented. For an informative discussion of the history of representation, see Monica Brito Vieira and David Runican’s Representation .
As mentioned earlier, theoretical discussions of political representation have focused mainly on the formal procedures of authorization and accountability within nation states, that is, on what Pitkin called formalistic representation. However, such a focus is no longer satisfactory due to international and domestic political transformations. [For an extensive discussion of international and domestic transformations, see Mark Warren and Dario Castioglione (2004).] Increasingly international, transnational and non-governmental actors play an important role in advancing public policies on behalf of democratic citizens—that is, acting as representatives for those citizens. Such actors “speak for,” “act for” and can even “stand for” individuals within a nation-state. It is no longer desirable to limit one’s understanding of political representation to elected officials within the nation-state. After all, increasingly state “contract out” important responsibilities to non-state actors, e.g. environmental regulation. As a result, elected officials do not necessarily possess “the capacity to act,” the capacity that Pitkin uses to identify who is a representative. So, as the powers of nation-state have been disseminated to international and transnational actors, elected representatives are not necessarily the agents who determine how policies are implemented. Given these changes, the traditional focus of political representation, that is, on elections within nation-states, is insufficient for understanding how public policies are being made and implemented. The complexity of modern representative processes and the multiple locations of political power suggest that contemporary notions of accountability are inadequate. Grant and Keohane (2005) have recently updated notions of accountability, suggesting that the scope of political representation needs to be expanded in order to reflect contemporary realities in the international arena. Michael Saward (2009) has proposed an innovative type of criteria that should be used for evaluating non-elective representative claims. John Dryzek and Simon Niemayer (2008) has proposed an alternative conception of representation, what he calls discursive representation, to reflect the fact that transnational actors represent discourses, not real people. By discourses, they mean “a set of categories and concepts embodying specific assumptions, judgments, contentions, dispositions, and capabilities.” The concept of discursive representation can potentially redeem the promise of deliberative democracy when the deliberative participation of all affected by a collective decision is infeasible.
Domestic transformations also reveal the need to update contemporary understandings of political representation. Associational life — social movements, interest groups, and civic associations—is increasingly recognized as important for the survival of representative democracies. The extent to which interest groups write public policies or play a central role in implementing and regulating policies is the extent to which the division between formal and informal representation has been blurred. The fluid relationship between the career paths of formal and informal representatives also suggests that contemporary realities do not justify focusing mainly on formal representatives. Mark Warren’s concept of citizen representatives (2008) opens up a theoretical framework for exploring how citizens represent themselves and serve in representative capacities.
Given these changes, it is necessary to revisit our conceptual understanding of political representation, specifically of democratic representation. For as Jane Mansbridge has recently noted, normative understandings of representation have not kept up with recent empirical research and contemporary democratic practices. In her important article “Rethinking Representation” Mansbridge identifies four forms of representation in modern democracies: promissory, anticipatory, gyroscopic and surrogacy. Promissory representation is a form of representation in which representatives are to be evaluated by the promises they make to constituents during campaigns. Promissory representation strongly resembles Pitkin’s discussion of formalistic representation. For both are primarily concerned with the ways that constituents give their consent to the authority of a representative. Drawing on recent empirical work, Mansbridge argues for the existence of three additional forms of representation. In anticipatory representation, representatives focus on what they think their constituents will reward in the next election and not on what they promised during the campaign of the previous election. Thus, anticipatory representation challenges those who understand accountability as primarily a retrospective activity. In gyroscopic representation, representatives “look within” to derive from their own experience conceptions of interest and principles to serve as a basis for their action. Finally, surrogate representation occurs when a legislator represents constituents outside of their districts. For Mansbridge, each of these different forms of representation generates a different normative criterion by which representatives should be assessed. All four forms of representation, then, are ways that democratic citizens can be legitimately represented within a democratic regime. Yet none of the latter three forms representation operates through the formal mechanisms of authorization and accountability. Recently, Mansbridge (2009) has gone further by suggesting that political science has focused too much on the sanctions model of accountability and that another model, what she calls the selection model, can be more effective at soliciting the desired behavior from representatives. According to Mansbridge, a sanction model of accountability presumes that the representative has different interests from the represented and that the represented should not only monitor but reward the good representative and punish the bad. In contrast, the selection model of accountability presumes that representatives have self-motivated and exogenous reasons for carrying out the represented’s wishes. In this way, Mansbridge broadens our understanding of accountability to allow for good representation to occur outside of formal sanctioning mechanisms.
Mansbridge’s rethinking of the meaning of representation holds an important insight for contemporary discussions of democratic representation. By specifying the different forms of representation within a democratic polity, Mansbridge teaches us that we should refer to the multiple forms of democratic representation. Democratic representation should not be conceived as a monolithic concept. Moreover, what is abundantly clear is that democratic representation should no longer be treated as consisting simply in a relationship between elected officials and constituents within her voting district. Political representation should no longer be understood as a simple principal-agent relationship. Andrew Rehfeld has gone farther, maintaining that political representation should no longer be territorially based. In other words, Rehfeld (2005) argues that constituencies, e.g. electoral districts, should not be constructed based on where citizens live.
Lisa Disch (2011) also complicates our understanding of democratic representation as a principal-agent relationship by uncovering a dilemma that arises between expectations of democratic responsiveness to constituents and recent empirical findings regarding the context dependency of individual constituents’ preferences. In response to this dilemma, Disch proposes a mobilization conception of political representation and develops a systemic understanding of reflexivity as the measure of its legitimacy.
By far, one of the most important shifts in the literature on representation has been the “constructivist turn.” Constructivist approaches to representation emphasize the representative’s role in creating and framing the identities and claims of the represented. Here Michael Saward’s The Representative Claim is exemplary. For Saward, representation entails a series of relationships: “A maker of representations (M) puts forward a subject (S) which stands for an object (O) which is related to a referent (R) and is offered to an audience (A)” (2006, 302). Instead of presuming a pre-existing set of interests of the represented that representatives “bring into” the political arena, Saward stresses how representative claim-making is a “deeply culturally inflected practice.” Saward explicitly denies that theorists can know what are the interests of the represented. For this reason, the represented should have the ultimate say in judging the claims of the representative. The task of the representative is to create claims that will resonate with appropriate audiences.
Saward therefore does not evaluate representatives by the extent to which they advance the preferences or interests of the represented. Instead he focuses on the institutional and collective conditions in which claim-making takes place. The constructivist turn examines the conditions for claim-making, not the activities of particular representatives.
Saward’s “constructivist turn” has generated a new research direction for both political theorists and empirical scientists. For example, Lisa Disch (2015) considers whether the constructivist turn is a “normative dead” end, that is, whether the epistemological commitments of constructivism that deny the ability to identify interests will undermine the normative commitments to democratic politics. Disch offers an alternative approach, what she calls “the citizen standpoint”. This standpoint does not mean taking at face value whomever or whatever citizens regard as representing them. Rather, it is “an epistemological and political achievement that does not exist spontaneously but develops out of the activism of political movements together with the critical theories and transformative empirical research to which they give rise” (2015, 493). (For other critical engagements with Saward’s work, see Schaap et al, 2012 and Nässtrom, 2011).
There have been a number of important advances in theorizing the concept of political representation. In particular, these advances call into question the traditional way of thinking of political representation as a principal-agent relationship. Most notably, Melissa Williams’ recent work has recommended reenvisioning the activity of representation in light of the experiences of historically disadvantaged groups. In particular, she recommends understanding representation as “mediation.” In particular, Williams (1998, 8) identifies three different dimensions of political life that representatives must “mediate:” the dynamics of legislative decision-making, the nature of legislator-constituent relations, and the basis for aggregating citizens into representable constituencies. She explains each aspect by using a corresponding theme (voice, trust, and memory) and by drawing on the experiences of marginalized groups in the United States. For example, drawing on the experiences of American women trying to gain equal citizenship, Williams argues that historically disadvantaged groups need a “voice” in legislative decision-making. The “heavily deliberative” quality of legislative institutions requires the presence of individuals who have direct access to historically excluded perspectives.
In addition, Williams explains how representatives need to mediate the representative-constituent relationship in order to build “trust.” For Williams, trust is the cornerstone for democratic accountability. Relying on the experiences of African-Americans, Williams shows the consistent patterns of betrayal of African-Americans by privileged white citizens that give them good reason for distrusting white representatives and the institutions themselves. For Williams, relationships of distrust can be “at least partially mended if the disadvantaged group is represented by its own members”(1998, 14). Finally, representation involves mediating how groups are defined. The boundaries of groups according to Williams are partially established by past experiences — what Williams calls “memory.” Having certain shared patterns of marginalization justifies certain institutional mechanisms to guarantee presence.
Williams offers her understanding of representation as mediation as a supplement to what she regards as the traditional conception of liberal representation. Williams identifies two strands in liberal representation. The first strand she describes as the “ideal of fair representation as an outcome of free and open elections in which every citizen has an equally weighted vote” (1998, 57). The second strand is interest-group pluralism, which Williams describes as the “theory of the organization of shared social interests with the purpose of securing the equitable representation … of those groups in public policies” ( ibid .). Together, the two strands provide a coherent approach for achieving fair representation, but the traditional conception of liberal representation as made up of simply these two strands is inadequate. In particular, Williams criticizes the traditional conception of liberal representation for failing to take into account the injustices experienced by marginalized groups in the United States. Thus, Williams expands accounts of political representation beyond the question of institutional design and thus, in effect, challenges those who understand representation as simply a matter of formal procedures of authorization and accountability.
Another way of reenvisioning representation was offered by Nadia Urbinati (2000, 2002). Urbinati argues for understanding representation as advocacy. For Urbinati, the point of representation should not be the aggregation of interests, but the preservation of disagreements necessary for preserving liberty. Urbinati identifies two main features of advocacy: 1) the representative’s passionate link to the electors’ cause and 2) the representative’s relative autonomy of judgment. Urbinati emphasizes the importance of the former for motivating representatives to deliberate with each other and their constituents. For Urbinati the benefit of conceptualizing representation as advocacy is that it improves our understanding of deliberative democracy. In particular, it avoids a common mistake made by many contemporary deliberative democrats: focusing on the formal procedures of deliberation at the expense of examining the sources of inequality within civil society, e.g. the family. One benefit of Urbinati’s understanding of representation is its emphasis on the importance of opinion and consent formation. In particular, her agonistic conception of representation highlights the importance of disagreements and rhetoric to the procedures, practices, and ethos of democracy. Her account expands the scope of theoretical discussions of representation away from formal procedures of authorization to the deliberative and expressive dimensions of representative institutions. In this way, her agonistic understanding of representation provides a theoretical tool to those who wish to explain how non-state actors “represent.”
Other conceptual advancements have helped clarify the meaning of particular aspects of representation. For instance, Andrew Rehfeld (2009) has argued that we need to disaggregate the delegate/trustee distinction. Rehfeld highlights how representatives can be delegates and trustees in at least three different ways. For this reason, we should replace the traditional delegate/trustee distinction with three distinctions (aims, source of judgment, and responsiveness). By collapsing these three different ways of being delegates and trustees, political theorists and political scientists overlook the ways in which representatives are often partial delegates and partial trustees.
Other political theorists have asked us to rethink central aspects of our understanding of democratic representation. In Inclusion and Democracy Iris Marion Young asks us to rethink the importance of descriptive representation. Young stresses that attempts to include more voices in the political arena can suppress other voices. She illustrates this point using the example of a Latino representative who might inadvertently represent straight Latinos at the expense of gay and lesbian Latinos (1986, 350). For Young, the suppression of differences is a problem for all representation (1986, 351). Representatives of large districts or of small communities must negotiate the difficulty of one person representing many. Because such a difficulty is constitutive of representation, it is unreasonable to assume that representation should be characterized by a “relationship of identity.” The legitimacy of a representative is not primarily a function of his or her similarities to the represented. For Young, the representative should not be treated as a substitute for the represented. Consequently, Young recommends reconceptualizing representation as a differentiated relationship (2000, 125–127; 1986, 357). There are two main benefits of Young’s understanding of representation. First, her understanding of representation encourages us to recognize the diversity of those being represented. Second, her analysis of representation emphasizes the importance of recognizing how representative institutions include as well as they exclude. Democratic citizens need to remain vigilant about the ways in which providing representation for some groups comes at the expense of excluding others. Building on Young’s insight, Suzanne Dovi (2009) has argued that we should not conceptualize representation simply in terms of how we bring marginalized groups into democratic politics; rather, democratic representation can require limiting the influence of overrepresented privileged groups.
Moreover, based on this way of understanding political representation, Young provides an alterative account of democratic representation. Specifically, she envisions democratic representation as a dynamic process, one that moves between moments of authorization and moments of accountability (2000, 129). It is the movement between these moments that makes the process “democratic.” This fluidity allows citizens to authorize their representatives and for traces of that authorization to be evident in what the representatives do and how representatives are held accountable. The appropriateness of any given representative is therefore partially dependent on future behavior as well as on his or her past relationships. For this reason, Young maintains that evaluation of this process must be continuously “deferred.” We must assess representation dynamically, that is, assess the whole ongoing processes of authorization and accountability of representatives. Young’s discussion of the dynamic of representation emphasizes the ways in which evaluations of representatives are incomplete, needing to incorporate the extent to which democratic citizens need to suspend their evaluations of representatives and the extent to which representatives can face unanticipated issues.
Another insight about democratic representation that comes from the literature on descriptive representation is the importance of contingencies. Here the work of Jane Mansbridge on descriptive representation has been particularly influential. Mansbridge recommends that we evaluate descriptive representatives by contexts and certain functions. More specifically, Mansbridge (1999, 628) focuses on four functions and their related contexts in which disadvantaged groups would want to be represented by someone who belongs to their group. Those four functions are “(1) adequate communication in contexts of mistrust, (2) innovative thinking in contexts of uncrystallized, not fully articulated, interests, … (3) creating a social meaning of ‘ability to rule’ for members of a group in historical contexts where the ability has been seriously questioned and (4) increasing the polity’s de facto legitimacy in contexts of past discrimination.” For Mansbridge, descriptive representatives are needed when marginalized groups distrust members of relatively more privileged groups and when marginalized groups possess political preferences that have not been fully formed. The need for descriptive representation is contingent on certain functions.
Mansbridge’s insight about the contingency of descriptive representation suggests that at some point descriptive representatives might not be necessary. However, she doesn’t specify how we are to know if interests have become crystallized or trust has formed to the point that the need for descriptive representation would be obsolete. Thus, Mansbridge’s discussion of descriptive representation suggests that standards for evaluating representatives are fluid and flexible. For an interesting discussion of the problems with unified or fixed standards for evaluating Latino representatives, see Christina Beltran’s The Trouble with Unity .
Mansbridge’s discussion of descriptive representation points to another trend within the literature on political representation — namely, the trend to derive normative accounts of representation from the representative’s function. Russell Hardin (2004) captured this trend most clearly in his position that “if we wish to assess the morality of elected officials, we must understand their function as our representatives and then infer how they can fulfill this function.” For Hardin, only an empirical explanation of the role of a representative is necessary for determining what a representative should be doing. Following Hardin, Suzanne Dovi (2007) identifies three democratic standards for evaluating the performance of representatives: those of fair-mindedness, critical trust building, and good gate-keeping. In Ruling Passions , Andrew Sabl (2002) links the proper behavior of representatives to their particular office. In particular, Sabl focuses on three offices: senator, organizer and activist. He argues that the same standards should not be used to evaluate these different offices. Rather, each office is responsible for promoting democratic constancy, what Sabl understands as “the effective pursuit of interest.” Sabl (2002) and Hardin (2004) exemplify the trend to tie the standards for evaluating political representatives to the activity and office of those representatives.
There are three persistent problems associated with political representation. Each of these problems identifies a future area of investigation. The first problem is the proper institutional design for representative institutions within democratic polities. The theoretical literature on political representation has paid a lot of attention to the institutional design of democracies. More specifically, political theorists have recommended everything from proportional representation (e.g. Guinier, 1994 and Christiano, 1996) to citizen juries (Fishkin, 1995). However, with the growing number of democratic states, we are likely to witness more variation among the different forms of political representation. In particular, it is important to be aware of how non-democratic and hybrid regimes can adopt representative institutions to consolidate their power over their citizens. There is likely to be much debate about the advantages and disadvantages of adopting representative institutions.
This leads to a second future line of inquiry — ways in which democratic citizens can be marginalized by representative institutions. This problem is articulated most clearly by Young’s discussion of the difficulties arising from one person representing many. Young suggests that representative institutions can include the opinions, perspectives and interests of some citizens at the expense of marginalizing the opinions, perspectives and interests of others. Hence, a problem with institutional reforms aimed at increasing the representation of historically disadvantaged groups is that such reforms can and often do decrease the responsiveness of representatives. For instance, the creation of black districts has created safe zones for black elected officials so that they are less accountable to their constituents. Any decrease in accountability is especially worrisome given the ways citizens are vulnerable to their representatives. Thus, one future line of research is examining the ways that representative institutions marginalize the interests, opinions and perspectives of democratic citizens.
In particular, it is necessary for to acknowledge the biases of representative institutions. While E. E. Schattschneider (1960) has long noted the class bias of representative institutions, there is little discussion of how to improve the political representation of the disaffected — that is, the political representation of those citizens who do not have the will, the time, or political resources to participate in politics. The absence of such a discussion is particularly apparent in the literature on descriptive representation, the area that is most concerned with disadvantaged citizens. Anne Phillips (1995) raises the problems with the representation of the poor, e.g. the inability to define class, however, she argues for issues of class to be integrated into a politics of presence. Few theorists have taken up Phillip’s gauntlet and articulated how this integration of class and a politics of presence is to be done. Of course, some have recognized the ways in which interest groups, associations, and individual representatives can betray the least well off (e.g. Strolovitch, 2004). And some (Dovi, 2003) have argued that descriptive representatives need to be selected based on their relationship to citizens who have been unjustly excluded and marginalized by democratic politics. However, it is unclear how to counteract the class bias that pervades domestic and international representative institutions. It is necessary to specify the conditions under which certain groups within a democratic polity require enhanced representation. Recent empirical literature has suggested that the benefits of having descriptive representatives is by no means straightforward (Gay, 2002).
A third and final area of research involves the relationship between representation and democracy. Historically, representation was considered to be in opposition with democracy [See Dahl (1989) for a historical overview of the concept of representation]. When compared to the direct forms of democracy found in the ancient city-states, notably Athens, representative institutions appear to be poor substitutes for the ways that citizens actively ruled themselves. Barber (1984) has famously argued that representative institutions were opposed to strong democracy. In contrast, almost everyone now agrees that democratic political institutions are representative ones.
Bernard Manin (1997)reminds us that the Athenian Assembly, which often exemplifies direct forms of democracy, had only limited powers. According to Manin, the practice of selecting magistrates by lottery is what separates representative democracies from so-called direct democracies. Consequently, Manin argues that the methods of selecting public officials are crucial to understanding what makes representative governments democratic. He identifies four principles distinctive of representative government: 1) Those who govern are appointed by election at regular intervals; 2) The decision-making of those who govern retains a degree of independence from the wishes of the electorate; 3) Those who are governed may give expression to their opinions and political wishes without these being subject to the control of those who govern; and 4) Public decisions undergo the trial of debate (6). For Manin, historical democratic practices hold important lessons for determining whether representative institutions are democratic.
While it is clear that representative institutions are vital institutional components of democratic institutions, much more needs to be said about the meaning of democratic representation. In particular, it is important not to presume that all acts of representation are equally democratic. After all, not all acts of representation within a representative democracy are necessarily instances of democratic representation. Henry Richardson (2002) has explored the undemocratic ways that members of the bureaucracy can represent citizens. [For a more detailed discussion of non-democratic forms of representation, see Apter (1968). Michael Saward (2008) also discusses how existing systems of political representation do not necessarily serve democracy.] Similarly, it is unclear whether a representative who actively seeks to dismantle democratic institutions is representing democratically. Does democratic representation require representatives to advance the preferences of democratic citizens or does it require a commitment to democratic institutions? At this point, answers to such questions are unclear. What is certain is that democratic citizens are likely to disagree about what constitutes democratic representation.
One popular approach to addressing the different and conflicting standards used to evaluate representatives within democratic polities, is to simply equate multiple standards with democratic ones. More specifically, it is argued that democratic standards are pluralistic, accommodating the different standards possessed and used by democratic citizens. Theorists who adopt this approach fail to specify the proper relationship among these standards. For instance, it is unclear how the standards that Mansbridge identifies in the four different forms of representation should relate to each other. Does it matter if promissory forms of representation are replaced by surrogate forms of representation? A similar omission can be found in Pitkin: although Pitkin specifies there is a unified relationship among the different views of representation, she never describes how the different views interact. This omission reflects the lacunae in the literature about how formalistic representation relates to descriptive and substantive representation. Without such a specification, it is not apparent how citizens can determine if they have adequate powers of authorization and accountability.
Currently, it is not clear exactly what makes any given form of representation consistent, let alone consonant, with democratic representation. Is it the synergy among different forms or should we examine descriptive representation in isolation to determine the ways that it can undermine or enhance democratic representation? One tendency is to equate democratic representation simply with the existence of fluid and multiple standards. While it is true that the fact of pluralism provides justification for democratic institutions as Christiano (1996) has argued, it should no longer presumed that all forms of representation are democratic since the actions of representatives can be used to dissolve or weaken democratic institutions. The final research area is to articulate the relationship between different forms of representation and ways that these forms can undermine democratic representation.
- Andeweg, Rudy B., and Jacques J.A. Thomassen, 2005. “Modes of Political Representation: Toward a new typology,” Legislative Studies Quarterly , 30(4): 507–528.
- Ankersmit, Franklin Rudolph, 2002. Political Representation , Stanford: Stanford University Press.
- Alcoff, Linda, 1991. “The Problem of Speaking for Others,” Cultural Critique , Winter: 5–32.
- Alonso, Sonia, John Keane, and Wolfgang Merkel (eds.), 2011. The Future of Representative Democracy , Cambridge: Cambridge University Press.
- Beitz, Charles, 1989. Political Equality , Princeton, NJ: Princeton University Press. [Chapter 6 is on ‘Representation’]
- Burke, Edmund, 1790 [1968]. Reflections on the Revolution in France , London: Penguin Books.
- Dahl, Robert A., 1989. Democracy and Its Critics , New Haven: Yale University.
- Disch, Lisa, 2015. “The Constructivist Turn in Democratic Representation: A Normative Dead-End?,” Constellations , 22(4): 487–499.
- Dovi, Suzanne, 2007. The Good Representative , New York: Wiley-Blackwell Publishing.
- Downs, Anthony, 1957. An Economic Theory of Democracy , New York: Harper.
- Dryzek, John and Simon Niemeyer, 2008. “Discursive Representation,” American Political Science Review , 102(4): 481–493.
- Hardin, Russell, 2004. “Representing Ignorance,” Social Philosophy and Policy , 21: 76–99.
- Lublin, David, 1999. The Paradox of Representation: Racial Gerrymandering and Minority Interests in Congress , Princeton: Princeton University Press.
- Madison, James, Alexander Hamilton and John Jay, 1787–8 [1987]. The Federalist Papers , Isaac Kramnick (ed.), Harmondsworth: Penguin.
- Mansbridge, Jane, 2003. “Rethinking Representation,” American Political Science Review , 97(4): 515–28.
- Manin, Bernard, 1997. The Principles of Representative Government , Cambridge: Cambridge University Press.
- Nässtrom, Sofia, 2011. “Where is the representative turn going?” European journal of political theory, , 10(4): 501–510.
- Pennock, J. Roland and John Chapman (eds.), 1968. Representation , New York: Atherton Press.
- Pitkin, Hanna Fenichel, 1967. The Concept of Representation , Berkeley: University of California.
- Plotke, David, 1997. “Representation is Democracy,” Constellations , 4: 19–34.
- Rehfeld, Andrew, 2005. The Concept of Constituency: Political Representation, Democratic Legitimacy and Institutional Design , Cambridge: Cambridge University Press.
- –––, 2006. “Towards a General Theory of Political Representation,” The Journal of Politics , 68: 1–21.
- Rosenstone, Steven and John Hansen, 1993. Mobilization, Participation, and Democracy in America , New York: MacMillian Publishing Company.
- Runciman, David, 2007. “The Paradox of Political Representation,” Journal of Political Philosophy , 15: 93–114.
- Saward, Michael, 2014. “Shape-shifting representation”. American Political Science Review , 108(4): 723–736.
- –––, 2010. The Representative Claim , Oxford: Oxford University Press.
- –––, 2008. “Representation and democracy: revisions and possibilities,” Sociology compass , 2(3): 1000–1013.
- –––, 2006. “The representative claim”. Contemporary political theory , 5(3): 297–318.
- Sabl, Andrew, 2002. Ruling Passions: Political Offices and Democratic Ethics , Princeton: Princeton University Press.
- Schaap, Andrew, Thompson, Simon, Disch, Lisa, Castiglione, Dario and Saward, Michael, 2012. “Critical exchange on Michael Saward’s The Representative Claim,” Contemporary Political Theory , 11(1): 109–127.
- Schattschneider, E. E., 1960. The Semisovereign People , New York: Holt, Rinehart, and Winston.
- Schumpeter, Joseph, 1976. Capitalism, Socialism, and Democracy , London: Allen and Unwin.
- Schwartz, Nancy, 1988. The Blue Guitar: Political Representation and Community , Chicago: University of Chicago Press.
- Shapiro, Ian, Susan C. Stokes, Elisabeth Jean Wood and Alexander S. Kirshner (eds.), 2009. Political Representation , Cambridge: Cambridge University Press.
- Urbinati, Nadia, 2000. “Representation as Advocacy: A Study of Democratic Deliberation,” Political Theory , 28: 258–786.
- Urbinati, Nadia and Mark Warren, 2008. “The Concept of Representation in Contemporary Democratic Theory,” Annual Review of Political Science , 11: 387–412
- Vieira, Monica and David Runciman, 2008. Representation , Cambridge: Polity Press.
- Vieira, Monica (ed.), 2017. Reclaiming Representation: Contemporary Advances in the Theory of Political Representation , New York: Routledge Press.
- Warren, Mark and Dario Castiglione, 2004. “The Transformation of Democratic Representation,” Democracy and Society , 2(1): 5–22.
- Barber, Benjamin, 1984. Strong Democracy , Los Angeles, CA: University of California Press.
- Dryzek, John, 1996. “Political Inclusion and the Dynamics of Democratization,” American Political Science Review , 90 (September): 475–487.
- Pateman, Carole, 1970. Participation and Democratic Theory , Cambridge: Cambridge University Press.
- Rousseau, Jean Jacques, 1762, The Social Contract , Judith Masters and Roger Masters (trans.), New York: St. Martins Press, 1978.
- Saward, Michael, 2008. “Representation and Democracy: Revisions and Possibilities,” Sociology Compass , 2: 1000–1013.
- Apter, David, 1968. “Notes for a Theory of Nondemocratic Representation,” in Nomos X , Chapter 19, pp. 278–317.
- Brown, Mark, 2006. “Survey Article: Citizen Panels and the Concept of Representation,” Journal of Political Philosophy , 14: 203–225.
- Cohen, Joshua and Joel Rogers, 1995. Associations and Democracy (The Real Utopias Project: Volume 1), Erik Olin Wright (ed.), London: Verso.
- Dalton, Russell J., and Martin P. Wattenberg (eds.), 2002. Parties without partisans: Political change in advanced industrial democracies , Oxford: Oxford University Press.
- Montanaro, L., 2012. “The Democratic Legitimacy of Self-appointed Representatives,” The Journal of Politics , 74(4): 1094–1107.
- Ryden, David K., 1996. Representation in Crisis: The Constitution, Interest Groups, and Political Parties , Albany: State University of New York Press.
- Truman, David, 1951. The Governmental Process , New York: Knopf.
- Saward, Michael, 2009. “ Authorisation and Authenticity: Representation and the Unelected,” Journal of Political Philosophy , 17: 1–22.
- Steunenberg, Bernard and J. J. A.Thomassen, 2002. The European Parliament : Moving Toward Democracy in the EU , Oxford: Rowman & Littlefield.
- Schmitter, Philippe, 2000. “Representation,” in How to democratize the European Union and Why Bother? , Lanham, MD: Rowman and Littlefield, Ch. 3. pp. 53–74.
- Street, John, 2004. “Celebrity politicians: popular culture and political representation,” The British Journal of Politics and International Relations , 6(4): 435–452.
- Strolovitch, Dara Z., 2007. Affirmative Advocacy: Race, Class, and Gender in Interest Group Politics , Chicago: Chicago University Press.
- Tormey, S., 2012. “Occupy Wall Street: From representation to post-representation,” Journal of Critical Globalisation Studies , 5: 132–137.
- Richardson, Henry, 2002. “Representative government,” in Democratic Autonomy , Oxford: Oxford University Press, Ch. 14, pp. 193–202
- Runciman, David, 2010. “Hobbes’s Theory of Representation: anti-democratic or protodemoratic,” in Political Representation , Ian Shapiro, Susan C. Stokes, Elisabeth Jean Wood, and Alexander Kirshner (eds.), Cambridge: Cambridge University Press.
- Warren, Mark, 2001. Democracy and Association , Princeton, NJ: Princeton University Press.
- –––, 2008. “Citizen Representatives,” in Designing Deliberative Democracy: The British ColumbiaCitizens’ Assembly , Mark Warren and Hilary Pearse (eds.), Cambridge: Cambridge University Press, 50–69.
- Warren, Mark and Dario Castiglione, 2004. “The Transformation of Democratic Representation,” Democracy and Society , 2(I): 5, 20–22.
- Amy, Douglas, 1996. Real Choices/New Voices: The Case for Proportional Elections in the United States , New York: Columbia University Press.
- Barber, Kathleen, 2001. A Right to Representation: Proportional Election Systems for the 21 st Century , Columbia: Ohio University Press.
- Canon, David, 1999. Race, Redistricting, and Representation: The Unintended Consequences of Black Majority Districts , Chicago: University of Chicago Press.
- Christiano, Thomas, 1996. The Rule of the Many , Boulder: Westview Press.
- Cotta, Maurizio and Heinrich Best (eds.), 2007. Democratic Representation in Europe Diversity, Change, and Convergence , Oxford: Oxford University Press.
- Guinier, Lani, 1994. The Tyranny of the Majority: Fundamental Fairness in Representative Democracy , New York: Free Press.
- Przworksi, Adam, Susan C. Stokes, and Bernard Manin (eds.), 1999. Democracy, Accountability, and Representation , Cambridge: Cambridge University Press.
- Thompson, Dennis, 2002. Just Elections , Chicago: University of Chicago Press.
- Jacobs, Lawrence R. and Robert Y. Shapiro, 2000. Politicians Don’t Pander: Political Manipulation and the Loss of Democratic Responsiveness , Chicago: University of Chicago Press.
- Grant, Ruth and Robert O. Keohane, 2005. “Accountability and Abuses of Power in World Politics,” American Political Science Review , 99 (February): 29–44.
- Mansbridge, Jane, 2004. “Representation Revisited: Introduction to the Case Against Electoral Accountability,” Democracy and Society , 2(I): 12–13.
- –––, 2009. “A Selection Model of Representation,” Journal of Political Philosophy , 17(4): 369–398.
- Pettit, Philip, 2010. “Representation, Responsive and Indicative,” Constellations , 17(3): 426–434.
- Fishkin, John, 1995. The Voice of the People: Public Opinion and Democracy , New Haven, CT: Yale University Press.
- Gutmann, Amy and Dennis Thompson, 2004. Why Deliberative Democracy? , Princeton: Princeton University Press.
- Hibbing, John and Elizabeth Theiss-Morse, 2002. Stealth Democracy , Cambridge: Cambridge University Press.
- Saward, Michael (ed.), 2000. Democratic Innovation: Deliberation, Representation and Association , London: Routledge.
- Severs, E., 2010. “Representation As Claims-Making. Quid Responsiveness?” Representation , 46(4): 411–423.
- Williams, Melissa, 2000. “The Uneasy Alliance of Group Representation and Deliberative Democracy,” in Citizenship in Diverse Societies , W. Kymlicka and Wayne Norman (eds.), Oxford: Oxford University Press, Ch 5. pp. 124–153.
- Young, Iris Marion, 1999. “Justice, Inclusion, and Deliberative Democracy” in Deliberative Politics , Stephen Macedo (ed.), Oxford: Oxford University.
- Bentran, Cristina, 2010. The Trouble with Unity: Latino Politics and the Creation of Identity , Oxford: Oxford University Press.
- Celis, Karen, Sarah Childs, Johanna Kantola and Mona Lena Krook, 2008, “Rethinking Women’s Substantive Representation,” Representation , 44(2): 99–110.
- Childs Sarah, 2008. Women and British Party Politics: Descriptive, Substantive and Symbolic Representation , London: Routledge.
- Dovi, Suzanne, 2002. “Preferable Descriptive Representatives: Or Will Just Any Woman, Black, or Latino Do?,” American Political Science Review , 96: 745–754.
- –––, 2007. “Theorizing Women’s Representation in the United States?,” Politics and Gender , 3(3): 297–319. doi: 10.1017/S1743923X07000281
- –––, 2009. “In Praise of Exclusion,” Journal of Politics , 71 (3): 1172–1186.
- –––, 2016. “Measuring Representation: Rethinking the Role of Exclusion” Political Representation , Marc Bühlmann and Jan Fivaz (eds.), London: Routledge.
- Fenno, Richard F., 2003. Going Home: Black Representatives and Their Constituents , Chicago, IL: University of Chicago Press.
- Gay, Claudine, 2002. “Spirals of Trust?,” American Journal of Political Science , 4: 717–32.
- Gould, Carol, 1996. “Diversity and Democracy: Representing Differences,” in Democracy and Difference: Contesting the Boundaries of the Political , Seyla Benhabib (ed.), Princeton: Princeton University, pp. 171–186.
- Htun, Mala, 2004. “Is Gender like Ethnicity? The Political Representation of Identity Groups,” Perspectives on Politics , 2: 439–458.
- Mansbridge, Jane, 1999. “Should Blacks Represent Blacks and Women Represent Women? A Contingent ‘Yes’,” The Journal of Politics , 61: 628–57.
- –––, 2003. “Rethinking Representation,” American Political Science Review , 97: 515–528.
- Phillips, Anne, 1995. Politics of Presence , New York: Clarendon.
- –––, 1998. “Democracy and Representation: Or, Why Should It Matter Who Our Representatives Are?,” in Feminism and Politics , Oxford: Oxford University. pp. 224–240.
- Pitkin, Hanna, 1967. The Concept of Representation , Los Angeles: University of Press.
- Sapiro, Virginia, 1981. “When are Interests Interesting?,” American Political Science Review , 75 (September): 701–721.
- Strolovitch, Dara Z., 2004. “Affirmative Representation,” Democracy and Society , 2: 3–5.
- Swain, Carol M., 1993. Black Faces, Black Interests: The Representation of African Americans in Congress , Cambridge, MA: Harvard University.
- Thomas, Sue, 1991. “The Impact of Women on State Legislative Policies,” Journal of Politics , 53 (November): 958–976.
- –––, 1994. How Women Legislate , New York: Oxford University Press.
- Weldon, S. Laurel, 2002. “Beyond Bodies: Institutional Sources of Representation for Women in Democratic Policymaking,” Journal of Politics , 64(4): 1153–1174.
- Williams, Melissa, 1998. Voice, Trust, and Memory: Marginalized Groups and the Failings of Liberal Representation , Princeton, NJ: Princeton University.
- Young, Iris Marion, 1986. “Deferring Group Representation,” Nomos: Group Rights , Will Kymlicka and Ian Shapiro (eds.), New York: New York University Press, pp. 349–376.
- –––, 1990. Justice and the Politics of Difference , Princeton, NJ: Princeton University
- –––, 2000. Inclusion and Democracy , Oxford: Oxford University Press.
G. Democratic Representation
- Castiglione, D., 2015. “Trajectories and Transformations of the Democratic Representative System”. Global Policy , 6(S1): 8–16.
- Disch, Lisa, 2011. “Toward a Mobilization Conception of Democratic Representation,” American Political Science Review , 105(1): 100–114.
- –––, 2012. “Democratic representation and the constituency paradox,” Perspectives on Politics , 10(3): 599–616.
- –––, 2016. “Hanna Pitkin, The Concept of Representation,” The Oxford Handbook of Classics in Contemporary Political Theory , Jacob Levy (ed.), Oxford: Oxford University Press. doi: 10.1093/oxfordhb/9780198717133.013.24
- Mansbridge, Jane, 2003. “Rethinking Representation,” American Political Science Review , 97: 515–528.
- Näsström, Sofia, 2006. “Representative democracy as tautology: Ankersmit and Lefort on representation,” European Journal of Political Theory , 5(3): 321–342.
- Urbinati, Nadia, 2011. “Political Representation as Democratic Process,” Redescriptions (Yearbook of Political Thought and Conceptual History: Volume 10), Kari Palonen (ed.), Helsinki: Transaction Publishers.
How to cite this entry . Preview the PDF version of this entry at the Friends of the SEP Society . Look up topics and thinkers related to this entry at the Internet Philosophy Ontology Project (InPhO). Enhanced bibliography for this entry at PhilPapers , with links to its database.
- FairVote Program for Representative Government
- Proportional Representation Library , provides readings proportional representation elections created by Prof. Douglas J. Amy, Dept. of Politics, Mount Holyoke College
- Representation , an essay by Ann Marie Baldonado on the Postcolonial Studies website at Emory University.
- Representation: John Locke, Second Treatise, §§ 157–58 , in The Founders’ Constitution at the University of Chicago Press
- Popular Basis of Political Authority: David Hume, Of the Original Contract , in The Founders’ Constitution at the University of Chicago Press
Burke, Edmund | democracy
Copyright © 2018 by Suzanne Dovi < sdovi @ email . arizona . edu >
- Accessibility
Support SEP
Mirror sites.
View this site from another server:
- Info about mirror sites
The Stanford Encyclopedia of Philosophy is copyright © 2023 by The Metaphysics Research Lab , Department of Philosophy, Stanford University
Library of Congress Catalog Data: ISSN 1095-5054
- Dictionaries home
- American English
- Collocations
- German-English
- Grammar home
- Practical English Usage
- Learn & Practise Grammar (Beta)
- Word Lists home
- My Word Lists
- Recent additions
- Resources home
- Text Checker
Definition of represent verb from the Oxford Advanced American Dictionary
Definitions on the go
Look up any word in the dictionary offline, anytime, anywhere with the Oxford Advanced Learner’s Dictionary app.

Want to create or adapt books like this? Learn more about how Pressbooks supports open publishing practices.
1 Article 1 Set: What is Representation in Media?
There are THREE (3) article options in this set.
What Exactly is Media Representation Anyway?
Tawil, Y. (n.d.). What exactly is media representation anyway? Arab Film & Media Institute. https://arabfilminstitute.org/what-exactly-is-media-representation-anyway/
The importance of representation in media has been a prominent topic as of late. From the 2015 Oscars “so white” to the heavy discourse surrounding this year’s remake of Ghostbusters with an all-woman leading cast, we’ve finally begun to see a shift in attitudes towards the representation of underserved demographics in a variety of media. Even this year’s Olympics in Rio has been a huge force for representation, especially for women of color from all over the world. It is important to understand representation, though truly understanding the depth of a seemingly simple concept can be tough. We’re going to take you through some of the basic concepts of media representation and its importance, both to underserved communities, and to our society at large.

2016 Summer Olympics.
What does representation in the media really mean?
The basic definition of representation in the media is simply how media, such as television, film and books, portray certain types of people or communities. There are a number of groups who are underrepresented in most Western media. They include women, people of color, LBGTQA+ people, people with a range of body shapes and types, people of non-Christian religions, and differently-abled people. There has been a steady increase of diversity in media, but progress has been long and slow.

Ghostbusters (2016) image – Sony Pictures
But I see diversity in media all the time…
There are a few major ways producers of media can misrepresent a community, under the guise of creating diversity. The first is tokenism. Tokenism is when the inclusion of a minority or other underrepresented group is no more than a symbolic effort to make a story or environment seem equal or diverse. This can be seen in many different aspects of life, from the “diversity hires” at work, to the gay best friend in a television show. Another way to misrepresent people is through stereotyping and typecasting , which are two concepts that are very closely related. Stereotyping can be seen in media through the assumptions of how a certain type of person is supposed to be due to their racial, ethnic, gender, sexual or religious identity. Stereotypes, even those that seem harmless or even positive, can have a profound effect on society as they create unrealistic, and often negative, expectations and assumptions. Common stereotypes of Arabs in Western media include the Arab man as a terrorist or an oil-hungry sheikh. Typecasting, which generally refers specifically to actors, is when a person is repeatedly assigned the same type of role due to success with that role in the past or because they “look like” a specific stereotype. This can happen to all types of people, but underrepresented people tend to find themselves pigeonholed into the same roles again and again. Actor and comedian Aziz Ansari wrote a piece in the New York Times about the struggles he’s experienced as an Indian actor, and he touched specifically on typecasting. He wrote, “Even though I’ve sold out Madison Square Garden as a standup comedian and have appeared in several films and TV series, when my phone rings, the roles I’m offered are often defined by ethnicity and often require accents.”

Film still from Raiders of the Lost Ark (1981) showcasing a stereotypical Arab character.
What’s the importance of representation?
Strong and positive representation can help fight and break down stereotypes that can be detrimental to individuals and limiting to society . When a group of people is only ever represented in negative ways, it adversely affects the way others see them, as well as the way they see themselves. Which means, on the flip side, positive representation can build self-confidence in individuals. It offers them role models to look up to and people and characters to be inspired by and reinforces that they are not lesser than. They can be the superhero, the doctor, the actor or whoever they want to be. The benefits of better representation are also not limited to the people represented. We all benefit from learning about different experiences and expanding our notion of what is “normal”, isn’t this one of the fundamental purposes of art?
More diverse representation also opens up new and better opportunities. For example, there is a specific lack of acting opportunities available to non-white actors, simply because scripts insist on white characters. More diverse scripts, create more diverse roles which create more opportunities for non-white actors. And when this diversity goes a step further, beyond tokenism, it also opens the door for more interesting and complex roles for these actors. All of this ultimately leads to richer stories, new voices and new perspectives in our media.

Film still from Hidden Figures (2017)
Well, media companies are just doing what makes them the most money…right?
Not so true. The 2016 Hollywood Diversity Report from the Ralph J.Bunche Center for African American Studies at UCLA, found that in the United States “increasingly diverse audiences prefer diverse film and television content”. More specifically, the report found that films with more diverse cast had the highest median global box office receipts and the highest median return on investment. It also showed that social media engagement peaked for scripted broadcast and cable television shows that also had more diverse casts. So…that theory’s pretty much dead.

Still from Orange is the New Black, a show known for it’s diverse cast.
Want to learn more about diversity and representation in the media? Check out our blog post on diversity reports .
About the Author: Yasmina Tawil
Article ii:
Racial and cultural diversity in entertainment media.
MediaSmarts. (n.d.). Racial and cultural diversity in entertainment media. https://mediasmarts.ca/digital-media-literacy/media-issues/diversity-media/visible-minorities/racial-cultural-diversity-entertainment-media
In much the same way that racialized groups are under- or misrepresented in news media, they are also not accurately portrayed in entertainment media, which tends to reinforce themes that are conveyed in the news. Although positive change is occurring, it is important that media content more accurately and fairly reflect the reality of Canadian multiculturalism.
There is no question that entertainment media can have a profound effect on how young people see themselves and others. In a 2021 study, children aged nine to 12 who were asked how they would cast various roles were more than twice as likely to cast a White actor as the hero (52 percent, compared to 19 percent who would cast a Black actor and 12 percent who would cast an Asian actor) and more than twice as likely to cast a Black actor as poor. This held true no matter the race of the child: for example, only 16 percent of Black children cast a Black actor as the hero. [1]
Racialized groups in television
Dr. Minelle Mahtani, of the Institute for Social Justice at the University of British Columbia, argues that Canadian entertainment media shares many problems with American programming, exhibiting a similar tendency to under-represent and misrepresent racialized groups. According to Mahtani, this under-representation is “suggestive of [these groups’] unimportance or their non-existence.” [2] More recent research suggests that this hasn’t changed much. A 2018 study which analyzed 780 films from 1970 to 2018 found that “white actors are just over three and a half times more likely to speak than their population size would predict, leading to the underrepresentation of all other groups.” [3]
The recent international success of Canadian TV programs such as Anne with an E and Schitt’s Creek echoes this trend. Despite improved diversity in other areas, these shows feature predominantly White actors. [4] While racialized people make up 16.3 percent of speaking roles in Canadian television shows overall, [5] it wasn’t until 2019 that a Black Canadian actor was cast in a lead role for a primetime Canadian television program (Vinessa Antoine, in Diggstown. ) [6] Even now, the launch or cancellation of a single show, such as Kim’s Convenience [7] or The Porter, [8] has a significant impact on the number of non-White roles onscreen.
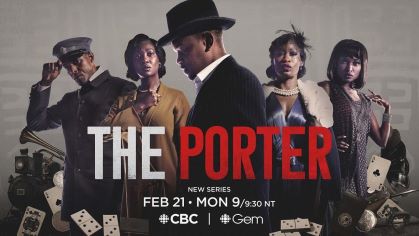
Until recently, it was still fairly common for White actors to play non-White characters in animated TV shows such as The Simpsons , Family Guy and Bojack Horseman. This has begun to change, however: most notably, Hank Azaria, who voiced Apu on The Simpsons – possibly the most widely-seen South Asian character in North American TV over the last three decades – decided in 2021 to stop playing the role, saying “if it’s an Indian character, or a Latinx character, or a Black character, please, let’s have that person voice the character. It’s more authentic, they’ll bring their experience to it. Let’s not take jobs away from people who don’t have enough.” [9]
How racialized characters are portrayed can be an issue, as well. Though negative stereotypes are still common [10] – for example, immigrants on American TV shows are often portrayed as being less educated and more likely to commit crimes than they are in reality [11] – an increasing trend is towards aggressively colour-blind casting. This can occur either in shows that simply pretend that race and ethnicity are no longer relevant to young people’s lives, like the CW’s Riverdale , or ones that imagine alternate worlds where the concepts are meaningless such as Prime’s Wheel of Time . While colour-blind casting provides more roles for non-White characters, treating colour-blindness as a positive value can, paradoxically, make viewers less sympathetic to the actual challenges faced by diverse communities: “Color-blindness is not just about showing and adding color to television; it is about assigning no meaning to color, positioning all ethnoracial groups in the same playing field.” [12]
Similarly, shows about law enforcement and crime typically offer a “colour-blind” vision of the justice system, rarely portraying things commonly experienced by racialized groups such as racial bias and racially-motivated police misconduct. [13]
While colour-blind casting may be a valuable starting point, a better standard would be shows that allow their characters to experience “mainstream” stories while recognizing and addressing their characters’ specific identities and the challenges associated with them, such as Netflix’s Never Have I Ever and CBC’s Sort Of . Chris Van Dusen, creator and show-runner of the Netflix show Bridgerton , took an approach he describes as “not color-blind [but] color-conscious” [14] in which the producers had “freedom to give people from diverse backgrounds a role but where a character’s race can still play a part in their story.” [15]
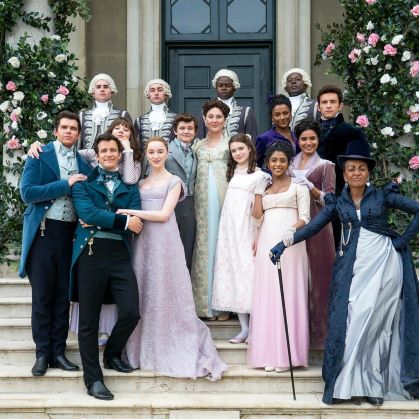
The cast of the second season of Netflix’s Bridgerton
Representation behind the screen is an issue, as well. A 2017 survey found that 91 percent of writers’ rooms in American TV were led by White showrunners, [16] and 64 percent of diverse writers in television had experienced bias, discrimination and harassment while working. [17]
The advent of streaming platforms such as Netflix and Disney Plus has resulted in more diversity both onscreen and off, [18] possibly because these services are better able to “narrowcast” to smaller audiences than traditional television. [19] While streaming platforms do make it easier to access international content, such as the South Korean hit Squid Game , because they feature little Canadian content they may provide a portrait of diversity just as inaccurate to Canadian audiences as American broadcast television.
Racialized groups in film
Film has a long history of racism and stereotyping. The first “blockbuster,” Birth of a Nation , not only valorized the Ku Klux Klan but led to its revival (and inspired a number of activities, such as cross-burning, which the original incarnation of the group had not practiced.) [20] It is true that there has been significant progress in the representation of racialized groups in film: compare, for instance, the original West Side Story, in which all but one of the Puerto Rican characters were played by White actors, to the 2021 remake, in which all are played by Latinx actors, and their Spanish dialogue is neither translated nor subtitled. [21] Nevertheless, significant issues and challenges remain.
Thanks to a number of factors, including the small size of Canada’s film industry and the prominence of the National Film Board (NFB), Canadian film has a fairly good history of racially and culturally diverse participation, both in front of and behind the camera. Canadian feature films such as White Elephant and Night of the Kings feature realistic and responsible fictional portrayals of racially and culturally diverse experience while the NFB has produced dozens of films on everything from hot-button multiculturalism issues to filmmakers’ family histories.
Still, these films make up a tiny portion of the movies watched by Canadian audiences, and too few to prevent actors from being lured away to the United States. As Fabienne Colas, founder of the Toronto Black Film Festival, put it, “in the U.S.A., you do have those roles for black people… we don’t have those roles; they don’t really exist.” [22] As well, the smaller size of the Canadian film industry increases the power of a small number of gatekeepers who have been, for most of its history, primarily White. [23]
There continues to be a fairly narrow range of roles available to racialized actors in both the Canadian and American film industries. A study of the 1,300 top-grossing films released between 2007 and 2019, for instance, found just 44 – or 3.4 percent – featured an Asian actor in a leading role. [24] (7.1 percent of the U.S. population identifies as “Asian or Pacific Islander”; [25] 14 percent of the Canadian population identifies as South Asian, Chinese, Filipino, Southeast Asian, Korean or Japanese.) [26] Of those 44 roles, just 13 percent were considered three-dimensional – not being defined as a foreigner, sidekick or villain – by the study’s authors. [27]
This may be because while a small number of racially and culturally diverse actors, such as Viola Davis and Dwayne Johnson, are among Hollywood’s biggest box-office draws, nearly all of the faces behind the camera are White. The Writers Guild of America’s Inclusion Report 2020 detailed that racialized people make up 20 percent of screenwriters in the United States even though they make up 40 percent of the population. [28] Racially and culturally diverse actors are also often only considered for parts specifically written as racialized, while parts of unspecified ethnicity are White by default. Kelly Edwards, vice-president of talent development and corporate diversity at NBC Universal, has said that casting directors and producers also tend to turn to actors with whom they’re already familiar, which often results in less diversity onscreen. [29]
Racialized groups in music
Canadian music has traditionally been a way through which minorities have been able to gain visibility, and this continues to the present day, with Ethiopian-Canadian artist The Weeknd and Drake, a biracial artist with a Black American father and Jewish Canadian mother, finding success both in Canada and internationally.
Popular music frequently displays certain racial themes. Toby Jenkins, professor of Integrative Studies and Higher Education, notes that rap and hip hop music express the realities of institutional racism and find their roots in the 1960s civil rights movement and integration of Black students into White society, giving these marginalized communities a way to express their thoughts and struggles at a time when they were often silenced and ignored in classrooms or social life. As Jenkins argues, “hip hop music tells the story of what it is like to be Black in America… hip hop music is one of the few cultural spaces where African Americans can voice their discontent with American power structures that make it difficult for Blacks to be successful.” [30]
Like American hip hop, much of Canadian hip hop is concerned with matters of race, culture and identity – but those of Black Canadians, whose origins, experiences and history are quite different from those of Black Americans. While it originated in close imitation of American hip hop, Canadian artists such as Kardinal Offishall and Boogat produce music which is much more influenced by the traditions of the Caribbean (two-thirds of African Canadians are of Caribbean origin) while first-generation immigrants such as K’naan express their personal and cultural experiences in producing their music and francophone hip hop performers such as Alaclair Ensemble draw on traditional Québécois folk music.
Other racially and culturally diverse groups have begun to make their presence known on the music scene, as well. The increasing prominence of South Asian culture, most visibly represented by “Bollywood” movies, has resulted in a greater visibility for groups like Delhi 2 Dublin which plays songs with lyrics in Punjabi and music played on instruments such as the sitar, dhol and tabla to mostly White audiences, with their 2019 album having more English lyrics. As with Canadian hip hop, this new wave of South Asian music isn’t just a transplant of traditional forms but a blend of influences – in this case South Asian banghra, Celtic folk and reggae. Raghav Mathur, whose music mashes Bollywood beats with hip hop and whose lyrics are equal parts English and Hindi, compares South Asian music in Canada to Latin artists such as Shakira and Camila Cabello, who’ve found success with both diverse and mainstream audiences.
Unfortunately, when it comes to diversity the move to streaming platforms has not had the same positive effects for diverse music as it has for film and television. This may be because users are more likely to allow the recommendation algorithm to make choices for them on music streaming platforms. This can result in a “rich get richer” cycle, which gives preference to mainstream acts even more than traditional media did. [31] TikTok, which for many young people is the main means of discovering new music, [32] has a history of preferentially boosting White creators who are performing Black performers’ music. [33] At the same time, unlike in traditional media, diverse acts can be found on Spotify and similar platforms – though the need to find and curate them is a significant barrier. In some cases, those same algorithms have also promoted music that would never have been widely heard on traditional radio, such as gay and Black artist Lil Nas X’s Old Town Road (which went on to win a Country Music Association Award.)
Racialized groups in advertising
Advertising has historically been a medium particularly prone to stereotyping. [34] Ads, which are by definition unwanted by their audience, have to make a strong impression in a brief exposure, [35] and stereotypes provoke the emotional reactions that drive brand loyalty and purchase intention. [36] While Aunt Jemima – the syrup mascot whose roots are in Nineteenth Century minstrel shows – has been retired, [37] research from 2021 found that half of people from historically under-represented communities have seen ads that stereotype them. [38]
Advertisers were slow to directly appeal to racialized groups. For instance, in 1963 Pepsi became one of the first large companies to advertise directly to Black audiences. [39] More recently, however, a growing number of advertisers have realized the value of appealing to racialized groups [40] as well as to young audiences who expect the brands they support to reflect their values. [41] As Allen Adamson, co-founder of marketing strategy firm Metaforce, explained, “it’s a cost-benefit thing. Most marketers have come to realize that no matter what they do, a certain segment is going to be offended. But the upside — seeming inclusive — outweighs the risk of ruffling feathers.” [42]
Ads do still draw on racial stereotypes, even from brands that have a long history with those communities. For example, in 2017, a Dove ad featured a Black woman transforming into a White woman and a Pepsi ad appeared to make light of Black Lives Matter protests. [43] Online, Black influencers routinely earn less per post than their White counterparts, [44] while the social networks that rely on their content also allow advertisers to target them with ads in ways that can be discriminatory [45] and even illegal. [46]
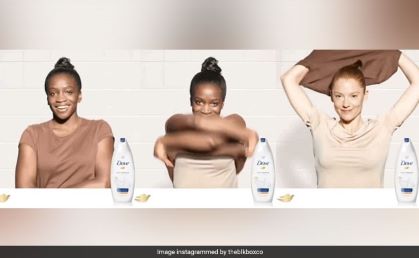
Dove’s body wash ad, which was withdrawn in response to protests.
Racialized groups in video games
Unlike other media, there is no domestic Canadian video game industry. While a number of extremely successful video game publishers, such as BioWare and Ubisoft, are based in Canada, their work is created for the American market. As a result, racially and culturally diverse representation in video games can only be considered in an American context.
Video games have perhaps the worst record of racially and culturally diverse representation. While 87 percent of Black teenagers play video games – more than any other teen demographic [47] – just two percent of game developers are Black. [48] Most Black video game characters are given background roles or “roles that enforce racist stereotypes,” [49] and when games do offer diverse characters they’re often reserved for downloadable add-ons sold separately. [50]
Violence, of course, is a frequent occurrence in video games, but while White characters are more likely to be shown engaging in fantasy violence – using swords or laser guns against monsters or aliens – racially and culturally diverse characters are much more likely to be part of realistic violent acts such as drive-by shootings, violent muggings and gang fights. [51] Asian characters are stereotyped even further, as they are almost exclusively shown engaging in martial arts. Many racialized groups – such as South Asians or Hispanics – are nearly or entirely absent. [52] Similarly, video games are, like animation, one of the few media where it is still common for White actors to voice non-White characters. [53]
Perhaps because the video game industry is overwhelmingly White, [54] it’s often proven to be insensitive on racial topics. For instance, it took Nintendo two decades to make it possible to change a character’s skin tone in the popular game Animal Crossing; the character Alloy in Horizon: Zero Dawn sports “appropriative and gross” [55] dreadlocks; World of Warcraft features characters called “Pandarens” with stereotypically Chinese clothing and mannerisms; [56] and Fortnite has reproduced dance moves created by Black artists without crediting or compensating them “despite being gaming’s biggest phenomenon.” [57] Ian Sundstrom, an independent video game developer, comments on these instances saying, “when it comes to the bigger AAA games with huge budgets, there’s really not an excuse to not be hiring black artists and designers to work on your game…[the] bare minimum [is] spending the extra time with the people you do have to add those different options and let people embody a character that looks like them.” [58]
Unlike other media such as television and film, adding onscreen diversity to video games can be a technical challenge – though this can sometimes be overused as an excuse. Ion Hazzikostas, director of the World of Warcraft expansion Shadowlands , explained why the game hadn’t previously offered a diverse range of facial features and hairstyles: “Some of it was technical constraints, going back to the way things were built, and the number of different textures that could be mapped onto a single model from the engine 15 years ago. But those are lines of code that can be changed. And yes, the real question is why didn’t we do it sooner? It’s a good question. We should’ve done this sooner, honestly.” [59]
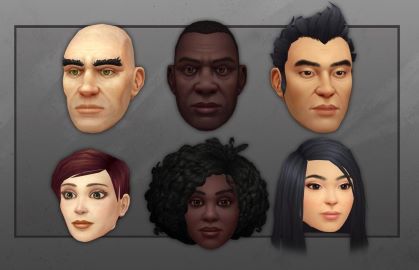
New character creation options in World of Warcraft: Shadowlands
Racialized groups in social media
Because of their networked nature , social media have had a mixed impact on racialized groups. On the one hand, the ability to publish content more or less directly to audiences has allowed racialized communities to bypass the gatekeepers associated with traditional media. Black communities use Twitter as a news source and [60] a resource for finding congenial businesses [61] and both Black and Asian communities use social media to demonstrate to skeptical White audiences the reality of racism in Canada [62] and elsewhere. [63]
But social networks are by no means free of racism themselves. There are many examples of White users making racist posts [64] or participating in “digital Blackface,” either by appropriating other cultures or using filters to literally make themselves look Black or Asian. [65] Other apps promote colourism by encouraging users to virtually lighten their skin. [66] Some platforms’ recommendation algorithms downrank posts about racial justice issues such as Black Lives Matter, [67] while many of those that allow users to earn revenue from advertising prevent them from monetizing those posts as a “brand safety” measure designed to keep ads from appearing alongside “controversial content. [68]
As with other media industries, this occurs in part because racialized communities are under-represented: just six percent of Twitter’s workforce, and four percent of Facebook’s, is Black. [69] Among decision-makers, the numbers are even lower. A 2016 study of 177 large US technology companies found that just 1.4 percent of executives and senior managers were Black. [70] Other forms of racial and ethnic prejudice can also limit participation and representation in the tech industry. While South Asians are well-represented in Silicon Valley, for example, workers from lower castes often experience caste-based discrimination and harassment and feel pressure to conceal their caste origins where possible. [71]
For more information on how to deal with hate directed towards racialized groups and other diverse communities, see our section on online hate .
Article iii:
Representation of diversity in media – overview.
MediaSmarts. (n.d.). Representation of diversity in media – Overview. https://mediasmarts.ca/digital-media-literacy/media-issues/diversity-media/representation-diversity-media-overview
Media representation issues
What we see – and don’t see – in media affects how we view reality. Media works can be imagined either as mirrors that reflect an audience’s own experience, windows that give them access to experiences they otherwise wouldn’t have known, or in some cases both. Rosemary Truglio, Senior Vice President of Sesame Workshop, described the diverse cast of Sesame Street as giving children “a mirror for them to see themselves, and (…) a window for them to learn about others.” [1]
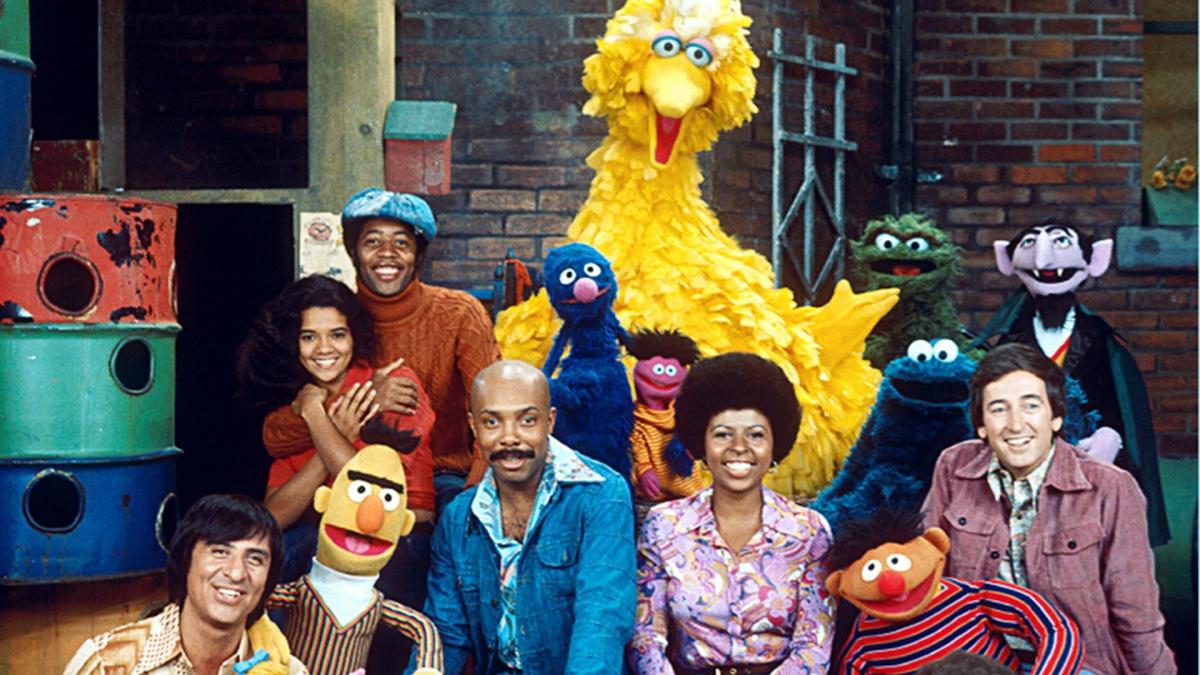
Sesame Street was a milestone in representation of race and disability in children’s media.
Media portrayals may provide different audiences with mirrors but not windows, or vice-versa, and a lack of either can have a negative impact. For members of historically under-represented groups, “when you have never seen yourself in books or movies or music, the first time you do is stunning.” [2] Similarly, “for children from dominant groups, window moments in stories come when the children realize they hold a powerful place in society and that there is something unjust about this.” [3] Unfortunately, fewer than half of Canadians feel that “Canadian media is a mirror in which all Canadians can see themselves.” [4]
In mass media, these issues typically play out in three ways:
Under-representation : Many groups have historically been under-represented in media. Even today, we are less likely to encounter many forms of diversity in mass media than we are in real life – and diverse communities are typically even less well-represented behind the scenes than onscreen. Under-representation can also make other representation issues worse because less representation means fewer opportunities for authentic representations of diversity within a group.
Besides being simply under-represented, groups may also be de-centred. That means making them or their culture a backdrop for more “mainstream” (e.g. White, abled, cisgender, etc.) protagonists. In some cases this may take the form of having a White character that excels in skills associated with a non-White culture, such as martial arts; stories where characters from under-represented groups need a White or other majority-culture character to “save” them; [5] and cases where aspects of an under-represented culture literally act as a prop. [6]
Stereotyping : This means portraying members of a particular group in just one or a small number of roles. This is particularly worrying when the stereotype is a negative one, but stereotyping can also do harm by only portraying a group in a narrow way. Even so-called “positive stereotypes” can have a negative effect because they limit how we see members of that group, as well as how we see ourselves. For example, if you belong to a group that is stereotyped as being good at sports, but are not particularly athletic, you may feel inadequate for being bad at something you’re “supposed” to be good at.
Another form of stereotyping is exoticizing , emphasizing the ways in which a character or culture are different from the (presumed) audience’s: for instance by overemphasizing aspects of a culture that mainstream audiences are most likely to find strange or disturbing, or by relying on things like accents or stereotyped characteristics for humour. Its most extreme form is othering , in which groups are shown as being fundamentally different from the audience and, in some cases, even as not being fully human.
Stereotyping can also happen when diverse identities always play the same role in the story. Author Corinne Duyvis identifies three ways that a character’s identity may be part of a work: “issue” stories where the identity and the challenges that come with it are what the story is about; “incidental” stories where a character’s identity is apparent but not relevant to the story, such as the main character’s sexual orientation in the Disney film Strange World, which provides a romantic subplot but is never specifically commented on; and “middle ground” stories where the identity is not the focus of the story but is recognized as always being relevant . As Duyvis puts it, “ableism, homophobia, and racism influence countless aspects of people’s everyday lives.” None of these is necessarily better than the other: what is most important is that audiences see all three kinds of stories, so that while marginalized communities’ specific issues and challenges are reflected in media they are also allowed to simply be . [7]
Whitewashing : While it has become rare for White actors to play Black or Asian characters, it remains common for disabled people, 2SLGBTQ+ people and other groups to be played by actors from outside those communities. Similarly, when works are adapted from one medium to another – such as when a book or comic is made into a movie or TV show – it is still fairly common for diverse characters to be changed into White ones, or for characters’ sexual orientation or disabilities to be downplayed or altered.
It’s important to point out that whitewashing only occurs when a character from a historically under-represented group is changed or recast so they are no longer part of that group, leading to reduced representation for that group and less diversity overall. For instance, the casting of a White actor to play an Asian character in the film Doctor Strange would count as whitewashing, while the casting of a Black actor to play a White character in the same film would not. [8]
These three issues are related, of course. Whitewashing contributes to both under-representation and stereotyping, as there are both fewer representations of historically under-represented groups in general and, in particular, fewer authentic representations. Similarly, under-representation contributes to and increases the impact of stereotypes because having fewer characters representing a particular group means fewer opportunities to show members of that group playing different roles in stories and in society.
For more examples of how these apply to different communities, see the specific articles on how each group is represented.
Impacts of media representation
All of these representation issues can have significant effects, both on audiences who are members of historically under-represented groups and those who aren’t. Seeing one’s own group stereotyped can lead to stress, negative self-image [9] and impaired academic achievement, [10] while being exposed to stereotyped portrayals of others can contribute to implicit or explicit prejudice. [11] Even more than changing individual attitudes, media portrayals – because they are seen as representing how others view a group – can have an impact on broader social attitudes towards different groups. [12] At the same time, exposure to authentic portrayals of oneself can improve self-esteem and promote a more positive view of one’s identity [13] or even improve academic performance, [14] while seeing authentic portrayals of other groups – which do not have to be uniformly positive ones [15] – can actually reduce prejudice. [16]
“Works of art are the only silver bullet we have against racism and sexism and hatred […] Art engenders empathy in a way that politics doesn’t, and in a way that nothing else really does. Art creates change in people’s hearts. But it happens slowly.” [17] Lin-Manuel Miranda
There can be significant impacts if different groups are not represented behind the scenes, as well. In mass media, under-representation behind the scenes generally results in under-representation on the screen, but it can also contribute to stereotyping as the portrayals are less likely to be authentic. [18] In digital media, not having historically under-represented groups involved at the design and management levels can lead to their experiences and concerns being ignored or treated as afterthoughts.
For more examples of how these affect different communities, see the specific articles on how each group is represented.
Intersectionality
While the other articles in this section address media portrayals of different groups separately, it’s important to point out that for many people they are not experienced that way. Many people identify with more than one historically marginalized or under-represented group, particularly when gender is added to the equation. Legal scholar Kimberlé Williams Crenshaw coined the term “intersectionality” to conceptualize “the way that different identity markers, such as race, gender, sexuality, and class, interact and affect each other.” [19]
Intersectionality does not mean that the impacts of different identities (including stereotyping) simply add on to one another, but that they transform and sometimes conflict with one another. East Asian women are frequently hypersexualized in media, [20] for example, while for East Asian men the stereotype is often the reverse. [21] However, audiences tend to consider just one aspect of intersectional identities. [22] When the stereotypes associated with two identities conflict with one another, people who identify with both may face confusion [23] or even hostility [24] from others.
It’s important to consider intersectionality both when making media and when critiquing it. For media makers, “shows and movies that attempt to lift up marginalized communities without thinking about intersectionality are only perpetuating different systems of prejudice and oppression.” [25] As well, some intersections may be an easier “sell” than others, both to audiences and the media industry. While the title character of House M.D. (2004-2012) both had a physical disability and was an outspoken atheist, he was also White; conversely, the showrunner of the currently running (2022) series Abbott Elementary said of one character’s canonical but unseen agnosticism “I honestly don’t know if we would be able to present that on ABC. It may not seem a big deal, but for a Black girl in Philadelphia — there are very few agnostic people.” [26]
When critiquing media, we should consider not just whether individual characters are stereotyped but whether a broad range of diversity, including intersecting identities, is represented. [27] As well, we should make a point of recognizing authentic portrayals of intersectionality in media, such as Reservation Dogs and Hawkeye. Finally, taking an intersectional approach to media education means considering other digital media issues – from cyberbullying to advertising to digital access and privacy – through an intersectional lens: not assuming, for example, that only White youth suffer from body image issues, and giving all young people a chance to confront the distinct ways that those issues affect them. [28]
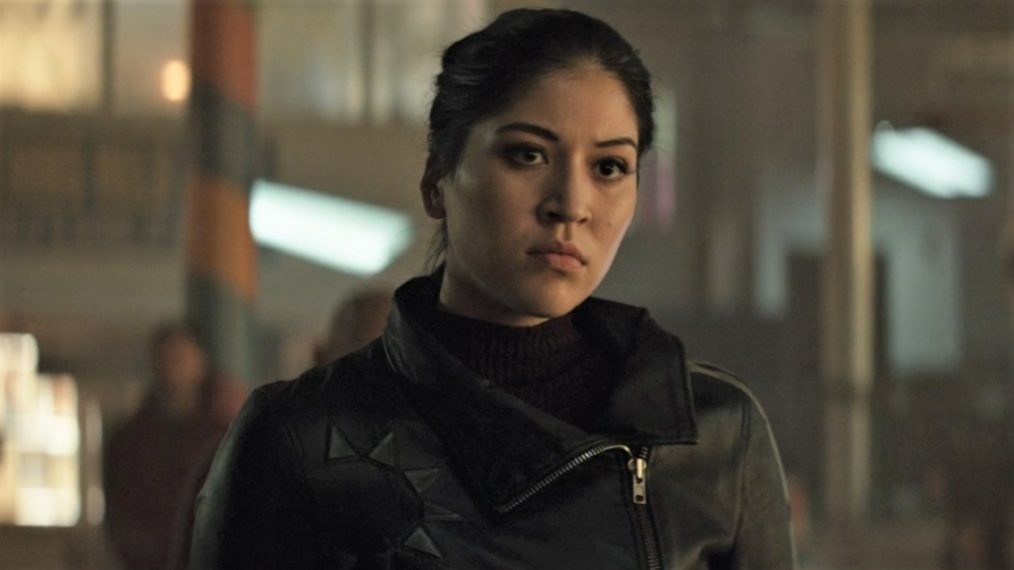
Maya Lopez from the Disney Plus series Hawkeye is Deaf and Indigenous, as is the actor who plays her, Alaqua Cox.
The importance of media education
Young people’s attitudes towards media representation changes over time. Children under nine don’t generally question whether what they see in media reflects their reality unless they are prompted to by parents or teachers. Tween and teens typically begin to become aware of media representation issues, especially if they are members of under-represented or stereotyped groups. By their later teens many actively seek out works with better representation. [29]
Media education can help young people put current images and messages into perspective by helping them understand how the media work, why stereotyping exists, how decisions are made and why it matters who is involved in making media works. Digital media literacy, especially if it explicitly addresses stereotyping and other media representation issues, can correct misperceptions of and prejudices towards other groups. [30] For young people who see stereotyped depictions of themselves in media works, media literacy can also mitigate negative effects on their self-esteem. [31]
Media education has also been shown to be an effective way of approaching issues like racism, providing a way of discussing difficult topics that feels safer while still challenging students’ assumptions and preconceptions [32] and can also help students affected by stereotyping deal with its effects. [33] Talking about media portrayals of diversity, especially positive ones, can also be a way of affirming students’ identities and encouraging them to create works that reflect those identities.
Positive portrayals don’t just avoid stereotyping, under-representation and the other issues identified above. They also:
- take the extra step of authentically portraying the challenges that members of under-represented communities face, such as racism or accessibility issues;
- tell stories of characters’ accommodations, resilience and agency in the face of those challenges; and
- show characters in the context of, and connected to, their communities. [34]
Media education is not about learning the right answers; it’s about consuming media images with an active, critical mind and asking the right questions.
Here are a few examples of the types of questions that could lead to a better understanding of how different groups are represented depicted in media:
Who selected or created these images and stories? Why does it matter who made these selections?
The first principle in media education is that nothing is objective—each and every media production is created with a viewpoint and for a purpose. The “reality” depicted in film or television productions is the result of many choices and each of these choices is based on the experience, knowledge and bias of the producers involved. More important than any conscious choices are the questions media makers don’t ask – the things they believe they already know. When members of historically marginalized groups are not involved in making shows, movies, news coverage or other media featuring them, it shows.
It’s also important to understand that media can have very different meanings depending on who made them, and that marginalized groups may “reclaim” stereotyped portrayals for their own purposes.
Whose voices are being heard? Whose voices are absent? Why?
Who is interviewed on a current affairs program? Which “experts” are chosen for sound bites on an issue? Whose perspectives are ignored completely? If characters or cultures representing a historically marginalized group are represented in a media text, have the creators of that text made significant efforts to consult with those communities, as Disney did when making Frozen II and Moana ? [35]
The question of whose voices are heard isn’t just important in mass media. While digital technology has made it easier than ever for people to make and share their own media, the online platforms where they share their work – whose ownership and workforce remain overwhelmingly White [36] – do not provide sufficient moderation and tools to push back against hate speech, they may fall silent in the face of online harassment. [37]
Why are certain stories selected or privileged and others not? Are some groups only represented in a small number of frames or contexts? Are characters representing diverse communities shown as real human beings in media, or are they defined exclusively by their identity? Do depictions respect differences and diversity within these communities?
Media producers, especially those in Hollywood, have used members of historically marginalized groups to tell mainstream cultures’ stories for generations. Rarely are diverse characters given complex personalities or autonomous roles. Rarely do they rely on their own values and judgements, or act upon their own motivations. Although efforts have been made to undo this tradition, old stereotypes die hard. [38]
This question highlights why it’s important not to look just as specific media works, but at the bigger picture. Each individual game, movie, or TV show with a White, non-disabled, cisgender, heterosexual, non-denominational Christian protagonist does not necessarily matter by itself, but when all of these are seen as the default identity for a main character it sends a powerful message about who can be the “main character” and who cannot.
How do commercial considerations, including the “conventional wisdom” in the industry, lead to issues around stereotyping and representation?
Commercial considerations are often given as a reason for excluding members of historically under-represented communities, whether explicitly (such as the assumption that White audiences won’t see movies with non-White leads) [39] or implicitly (by saying, for instance, that a movie needs a “big-name” lead to be successful – without saying out loud that most of those big names are White, non-disabled, heterosexual and cisgender). [40] While this industry conventional wisdom has been proven to be false, it’s still widely held. [41]
Characteristics of different media industries, in different countries, can also have an impact on whether diversity is represented. The Canadian television industry is often described as highly risk-averse, with licensing American shows seen as a safer bet than developing Canadian ones. As a result, when diversity does appear on private Canadian channels such as Global and CTV, it more often reflects the population of the United States than Canada’s. When private broadcasters do make original programming, they tend to play it safe – which usually means making shows aimed at White audiences. [42] As the report Deciding on Diversity puts it, “Risk narratives about equity-seeking stories and storytellers persist to preserve the status quo.” [43] Nathalie Younglai, founder of BIPOC TV and Film, paraphrases TV executives’ attitudes more bluntly: “How is this Canadian? How does someone in Saskatchewan relate to this?” [44]
Similarly, digital technology companies claim to be motivated by market pressures in deciding things like which languages digital assistants should be able to speak; this, too, often fails to hold true in the light of accurate data – Apple’s Siri, for instance, is offered in Finnish (which has about five million native speakers) but not Swahili (which has nearly a hundred million). [45]
How can different audiences “read against” or negotiate the meaning of a work with representation issues?
Some audiences, especially those from groups that have traditionally been marginalized in media industries, may engage in “resistant reading,” interpreting works in ways that are directly contrary to the generally received meaning. Nevertheless, it is true that, as bell hooks put it, “While audiences are clearly not passive and are able to pick and choose, it is simultaneously true that there are certain ‘received’ messages that are rarely mediated by the will of the audience.” [46]
In other words, while we don’t automatically accept the surface meaning of media works, most of us will take away a meaning that is fairly close to it. Only a small number of people, mostly those whose identity or experience lead them to a resistant reading, will have a significantly different interpretation. Until members of these groups have more meaningful participation in the media industries, however, neither the portrayals nor the mainstream audience’s interpretation of them are likely to change.
Resistant reading is also easier in some media than others: in most video games, for instance, ‘resistant play’ – choosing actions other than the ones the designers assume you will take – will prevent you from progressing very far in the game. [47]
How may the codes and conventions of the medium and genre perpetuate stereotyping and representation issues?
Both different media (such as TV, film or video games) and different genres (science fiction, advertising, animation, et cetera) have their own codes and conventions that may lead media makers to fall into stereotyping or under-representation, often unconsciously. For example, both advertising and news (especially headlines) have to grab the audience’s attention right away and communicate information in a small amount of time. As a result they often use stereotypes as a kind of “shorthand” that allows the audience to fill in what they already know (or think they know). Similarly, animation and comics – and works in other media that are based on comic or cartoon characters – often have characters whose racist origins are still apparent, or for whom traits like facial scarring, prosthetic limbs or stereotypically Jewish features serve as visual markers of villainy.
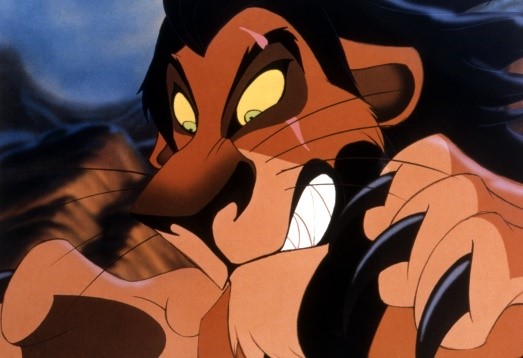
Even when media works try to confront racism, homophobia and other issues, there may be fundamental features of certain media, like the episodic nature of news and the focus in fictional media on individual characters, that lead them to portray these primarily as something perpetrated by individuals and downplay their systemic qualities. [48]
How can digital tools and platforms give voice to historically marginalized communities? How may they contribute to marginalization?
Unlike traditional media, there are no one-way connections in digital media. You can share content with other people as easily as a producer or distributor shares it with you. As a result, the barriers to participation are much lower than in traditional media and anyone can publish content and find an audience. But while power in networks is not hierarchical, neither is it evenly distributed: it rests in the nodes with the most links. This means that those who had gatekeeping power in the old media environment have had their influence reduced, but not eliminated.
For instance, while online publishing has made it possible for historically under-represented groups to “restory themselves” by making versions of popular culture works that include and even centre their own experiences, [49] online platforms also have tremendous power to either promote or suppress the same voices through the algorithms that determine what is shown or recommended to users. [50] As the historian of science Melvin Kranzberg put it, different technologies are neither inherently good nor inherently bad, but neither are they neutral: [51] like mass media, they reflect the beliefs, unconscious biases and unquestioned assumptions of their creators.
As a result, the impacts that networked technology have had on historically under-represented groups are complex. Online spaces can provide diverse communities, especially those that are geographically far-flung, with an ‘ecosystem’ that would not be possible with traditional media; [52] at the same time, content moderation systems can apply censorship that is more absolute than was ever found in film and television, limiting the ability of marginalized youth to access relevant health information, [53] to monetize content that reflects their community [54] and even to speak the name of their identity. [55]
Technical tools have an impact on how we use them not just through their affordances (what can be done with them) but also their defaults (what we are expected to do with them). For example, one study of video games found that while 23 percent had affordances that allowed players to choose their character’s race, 60 percent of those defaulted to a White character unless the player actively changed it. [56] Whether or not members of diverse communities were involved in the design of those affordances and defaults may determine whether they work successfully when used in or by those communities: a review of facial algorithms found that they were at least ten times as likely to mis-identify a Black or East Asian face as a White one, [57] for example, and many digital assistants such as Siri and Alexa routinely misunderstand Black users. [58]
Networked media can also make it possible, or easier, for marginalized groups to experience harms that were not possible or less likely with traditional media. One of these is amplification: [59] for example, the frictionless quality of networked media allows hate groups to broadcast their messages more widely and to tailor them to potential audiences at different levels of the “radicalization pyramid.” Amplification does not only apply to intentional acts, though. It can also reflect a harm that already exists and make it more widespread by embedding it in the operation of a networked tool – for example, for many years Google searches for terms such as “Asian girls” and “Black girls” returned primarily pornographic results, reflecting how they were most often used in the wider internet, while “White girls” did not. [60] Questions as simple as who appears in an image search for “doctor” or “happy family” can have a huge impact on how different groups are perceived. [61]
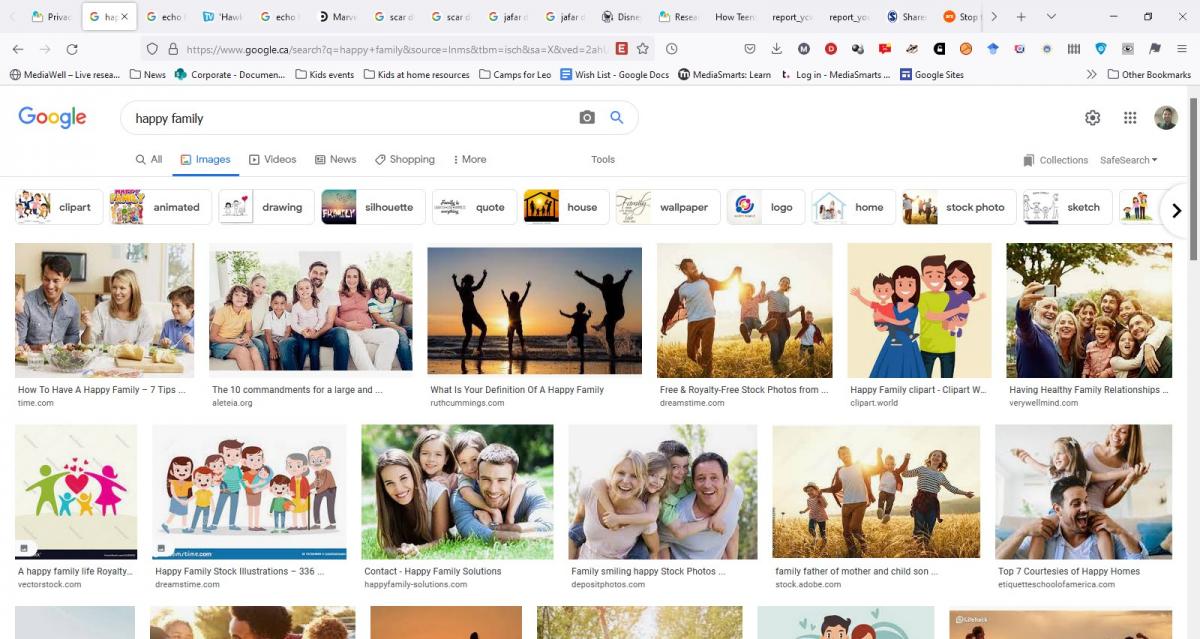
A Google image search for “happy family” conducted in March 2022.
As with the other search terms mentioned above, Google has taken some positive steps in this regard as a result of consumer pressure. [62] This demonstrates why a key part of media education is empowering young people to make their voices heard through making and publishing their own media, as well as to push back against stereotypes and other misrepresentations in media and to use digital tools to make a difference in their online and offline communities.
- MediaSmarts lessons that teach students to make their voices heard through media making include Representing Ourselves Online , Avatars and Body Image , Bias in News Sources , First Person and Art Exchange .
- MediaSmarts’ guide Talk Back! How to Take Action on Media Issues explains the rules and codes that apply to different media industries on issues such as stereotyping and representation and includes some advice on how to use social networks to speak out on a media issue.
- Speak Up! Your Guide to Changing the World, Online and Off explains how to use digital tools like social networks to share your views and organize others in making change.
How can educators limit resistance and backlash when addressing diversity in media?
Two of the most common risks of addressing diversity representation in media are resistance – in which students challenge the validity of media education as practice, such as by dismissing the work under study as “just an ad” or suggesting that the teacher is reading meaning into a work that isn’t there – and backlash , in which students feel the teacher is pushing their own views or interpretations, rather than encouraging students to articulate and argue their own.
One way of minimizing these is having young people explore questions, such as the ones listed above, rather than leading them towards a pre-ordained conclusion. While it is important to make them aware of the facts of representation in media, conclusions about the implications of those facts – and appropriate responses – should emerge from critical thinking and discussion.
Another important approach is to help students understand the key concept that all media have social and political implications – and that when they appear not to, it’s because they reinforce how you already see the world. Similarly, while we may be tempted to dismiss the importance of entertainment media relative to things like news, we are actually more likely to be persuaded by works that “transport” us and bypass our critical minds. [63]
As well, highlight to students that it is possible for a media work to be problematic in some aspects of its portrayal of diversity but successful in others. The 2016 film Doctor Strange , for instance, had many problematic elements in its portrayal of cultural diversity but also a fairly nuanced representation of the main character’s disability and his efforts to accommodate it. [64]
Perhaps most importantly, it’s important to teach students from early on that critiquing a part of something doesn’t mean you don’t like it, nor does critiquing a work mean that you’re criticizing anyone who likes it. Criticizing our children’s media choices can easily make them feel we’re criticizing them. There is a difference between a media work that was motivated by racism or sexism and one where it’s the result of the media-maker not questioning their assumptions or the “conventional wisdom” of their industry. Most of the time, the messages in the things they make aren’t on purpose but because of things they assumed or questions they didn’t think to ask. (It’s important to understand that the people who make media aren’t necessarily media literate in the critical sense.)
As Turner Classic Movies host Jacqueline Stewart points out, this is a distinction that people in historically under-represented groups often learn early. Describing a childhood viewing of Gone With the Wind, Stewart notes that “Black audiences have always juggled the pleasures and problems of mainstream media. I was learning that you can enjoy a film even as you are critiquing it.” [65] Of course, we also have to make a habit of studying accurate representations as well as critiquing negative ones – and recognize that a work may be positive in some aspects but problematic in others.
There may also be backlash from students relating specifically to the topic of diversity representation. This can be a result of a belief in the value of colour-blindness; though generally well-meaning, this attitude has been shown to contribute to prejudice, rather than reducing it, because it denies the identities and experiences of historically under-represented groups and prevents us from addressing the challenges and injustices they face. [66] Instead, stereotypes need to be acknowledged and faced head-on. As Jeffrey Adam Smith, author of Are We Born Racist? , puts it, “When we encounter a ‘slant-eyed, Oriental mastermind’ (to quote one old comic of mine), I stop, close the book, and tell [my son] that image is a product of prejudice, and that I think prejudice is wrong. I try to answer any questions he has. Then I re-open the book… and keep reading.” [67]
Young people may also want to distance themselves from a sense that they are accused of being prejudiced, or that they benefit from a prejudiced system. To explore different ways of addressing this, see our article on Privilege in the Media and our guide to Complicated Conversations in the Classroom .
For more tips on how to approach digital media literacy, see our article on Digital Media Literacy Fundamentals .
CS 050: College Writing Media Theme Copyright © by Confederation College Communications Department and Paterson Library Commons is licensed under a Creative Commons Attribution-NonCommercial-ShareAlike 4.0 International License , except where otherwise noted.
Share This Book
Thank you for visiting nature.com. You are using a browser version with limited support for CSS. To obtain the best experience, we recommend you use a more up to date browser (or turn off compatibility mode in Internet Explorer). In the meantime, to ensure continued support, we are displaying the site without styles and JavaScript.
- View all journals
- My Account Login
- Explore content
- About the journal
- Publish with us
- Sign up for alerts
- Open access
- Published: 02 April 2024
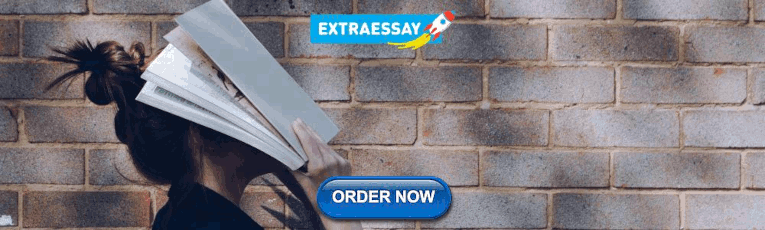
The covariance environment defines cellular niches for spatial inference
- Doron Haviv 1 , 2 ,
- Ján Remšík ORCID: orcid.org/0000-0002-3228-2896 3 ,
- Mohamed Gatie ORCID: orcid.org/0000-0001-8500-5609 4 ,
- Catherine Snopkowski 1 ,
- Meril Takizawa 1 ,
- Nathan Pereira 5 ,
- John Bashkin 5 ,
- Stevan Jovanovich ORCID: orcid.org/0000-0002-3285-1091 5 ,
- Tal Nawy ORCID: orcid.org/0000-0003-3720-3699 1 ,
- Ronan Chaligne ORCID: orcid.org/0000-0003-4332-3291 1 ,
- Adrienne Boire 3 , 6 , 7 ,
- Anna-Katerina Hadjantonakis 4 &
- Dana Pe’er ORCID: orcid.org/0000-0002-9259-8817 1 , 8
Nature Biotechnology ( 2024 ) Cite this article
1141 Accesses
31 Altmetric
Metrics details
- Computational models
A key challenge of analyzing data from high-resolution spatial profiling technologies is to suitably represent the features of cellular neighborhoods or niches. Here we introduce the covariance environment (COVET), a representation that leverages the gene–gene covariate structure across cells in the niche to capture the multivariate nature of cellular interactions within it. We define a principled optimal transport-based distance metric between COVET niches that scales to millions of cells. Using COVET to encode spatial context, we developed environmental variational inference (ENVI), a conditional variational autoencoder that jointly embeds spatial and single-cell RNA sequencing data into a latent space. ENVI includes two decoders: one to impute gene expression across the spatial modality and a second to project spatial information onto single-cell data. ENVI can confer spatial context to genomics data from single dissociated cells and outperforms alternatives for imputing gene expression on diverse spatial datasets.
Similar content being viewed by others
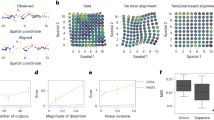
Alignment of spatial genomics data using deep Gaussian processes
Andrew Jones, F. William Townes, … Barbara E. Engelhardt
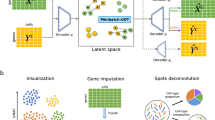
A unified computational framework for single-cell data integration with optimal transport
Kai Cao, Qiyu Gong, … Lin Wan
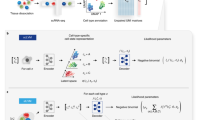
DestVI identifies continuums of cell types in spatial transcriptomics data
Romain Lopez, Baoguo Li, … Nir Yosef
Intense interest in cellular interactions and tissue context has spurred the growth of multiplexed spatial transcriptomics and antibody-based technologies, sparking the need for computational approaches to identify biological patterns within tissues 1 , 2 , 3 , 4 , 5 . The local neighborhood, or niche, of a cell is a useful resolution for defining cell interactions; it may represent functional anatomical subunits (such as stem cell niches) and is a basis for identifying larger spatial patterns. However, efficient representations of the cellular microenvironment that retain the full richness of the data and can be used to effectively compare niches are lacking 6 . At the same time, there is a need to address the limited molecular plexity of high-resolution spatial profiling technologies 7 .
Most methods for analyzing spatial data characterize each niche by tabulating discrete cell types within a given region 8 , 9 , 10 , 11 . Although these have generated important discoveries 2 , 8 , they were developed for low-plex antibody-based imaging methods that devote most markers to cell typing. Spatial transcriptomics methods, including commercial platforms, can now profile hundreds of genes 12 , 13 , 14 , 15 , 16 , 17 , 18 , meaning that analysis at the cell type level leads to substantial information loss. In single-cell genomics, the switch from discrete cell typing to continuous approaches, such as diffusion maps 19 and pseudotime 20 , 21 , has driven remarkable discovery. Moreover, setting thresholds for continuous cellular phenotypes is subjective and invokes problems of instability and bias. Even within highly discrete cell types, vast and meaningful variation often exists, such as the spectrum of activated and metabolic states within immune cell types 22 , 23 , 24 .
Thus, a niche representation is needed that considers the full measured expression and its continuous nature and that enables robust, efficient comparisons. We propose a representation that goes beyond cell typing and preserves complex patterns of gene expression, including covariation in genes across cell states. Specifically, we developed the covariance environment (COVET), a compact representation of a cell’s niche that assumes that interactions between the cell and its environment create biologically meaningful covariate structure in gene expression between cells of the niche. We developed a corresponding distance metric that unlocks the ability to compare and analyze niches using the full toolkit of approaches currently employed for cellular phenotypes, including dimensionality reduction, spatial gradient analysis and clustering.
Imaging-based spatial transcriptomics technologies face issues that practically limit quantification to hundreds of genes. Some methods can impute spatial information for genes not measured in the spatial modality, by integrating matched single-cell RNA sequencing (scRNA-seq) data 9 , 25 , 26 . However, integration methods do not explicitly model cellular microenvironment context from the spatial data, thereby limiting inference power.
To achieve transcriptome-wide spatial inference, we developed environmental variational inference (ENVI), a conditional variational autoencoder (CVAE) 27 , 28 , that simultaneously incorporates scRNA-seq and spatial data into a single embedding. ENVI leverages the covariate structure of COVET as a representation of cell microenvironment and achieves total integration by encoding both genome-wide expression and spatial context (the ability to reconstruct COVET matrices) into its latent embedding. Our approach is effective on data from a variety of multiplexed spatial technologies and outperforms other methods in accurately imputing the expression of genes in diverse developmental contexts. ENVI can also be used to project valuable spatial information onto dissociated scRNA-seq data and can capture continuous variation along spatial axes across large complex tissue regions.
COVET defines spatial neighborhoods
To move beyond cell type fraction and to characterize niches in a manner that leverages measured genes and enables quantitative comparison, we developed the COVET framework. Our core assumption is that a cell affects—and is also affected by—cells in its vicinity, generating covarying patterns of expression among the interacting cells. Our framework includes three components: (1) COVET, a robust per-cell representation of neighborhood information based on a modified formulation of gene–gene covariance among niche cells; (2) a distance metric that is essential for comparing and interpreting niches; and (3) an algorithm to efficiently compute this distance metric. Unlike mean expression, gene–gene covariance captures the relationships among genes and cell states that are shaped by cellular interplay within the niche. These relationships are rich, stable and enriched for biological signal; moreover, they contain substantial hidden information from unmeasured genes, providing an advantage for imputation tasks.
To calculate COVET, we first define the niche of each cell in a dataset by the k spatial nearest neighbors of that cell and then compute each niche’s gene–gene shifted covariance matrix (Fig. 1a and Methods ). Shifted covariance modifies the classic covariance formulation by using mean expression across the entire dataset rather than local mean expression as a reference. This constructs each cell’s covariance matrix relative to the entire population and critically enables direct comparison between niches, highlighting their shared and unique features. Gene–gene covariance provides the additional benefit of being more robust to technical artifacts 23 , facilitating integration across technologies.
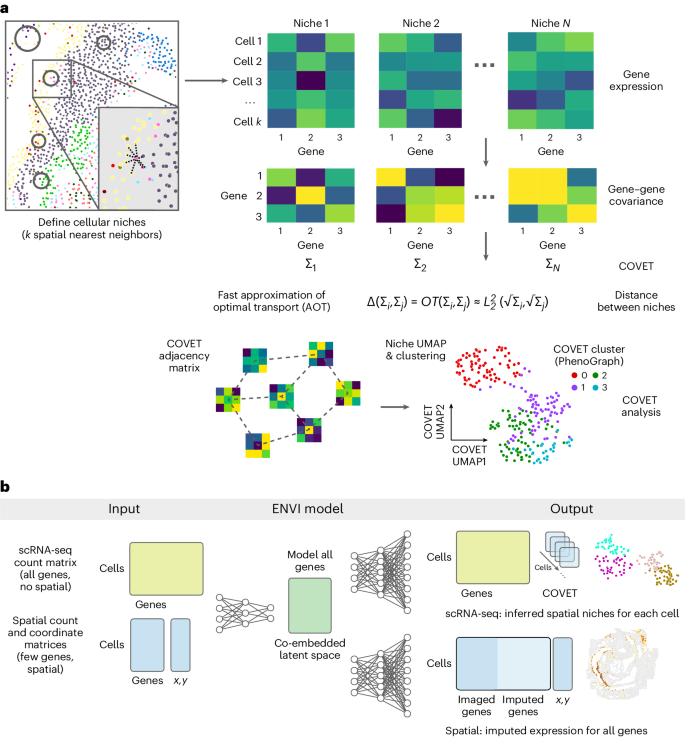
a , Schematics indicate steps in spatial covariance computation and ENVI operation. Each COVET matrix characterizes a cell’s niche, comprising k nearest spatial neighbors, based on the shifted covariance of gene expression within the niche. Shifted covariance is calculated relative to mean expression in the sample, enabling meaningful comparison of niches. Distance between niches is determined by an efficient approximation of optimal transport. The COVET adjacency matrix, based on AOT as the spatial similarity metric, can be used directly for other downstream spatial analyses, such as dimensionality reduction and clustering, where cells are grouped together by similar environment rather than by expression. b , ENVI is a conditional autoencoder that simultaneously embeds scRNA-seq and multiplexed spatial transcriptomic data into a unified latent embedding. ENVI models all genes (including those not imaged with spatial transcriptomics) and uses the COVET framework to represent information about cellular environment. An environment decoder allows ENVI to project spatial information onto single-cell data, and an expression decoder, which also includes genes captured only in the single-cell data, enables imputation of spatial expression transcriptome wide.
Despite being a compact and powerful representation of the niche, COVET requires a metric for comparison. Niche similarity cannot be determined by simply subtracting the cell-by-gene expression of matrices of two niches, because the result depends on cell order, which is set arbitrarily (it will change if an image is rotated, for example). We, thus, seek to quantify niche similarity in a permutation-invariant manner, for which the Fréchet distance provides a closed-form solution 29 . However, calculating Fréchet distance is computationally intractable, so we developed an approximation (approximate optimal transport (AOT)) that reduces runtime by over an order of magnitude and is substantially faster than another common metric, the Bhattacharyya distance 30 (Extended Data Fig. 1a ). AOT yields similar results to true optimal transport, and a GPU implementation takes under 1 min to compute the cell–cell AOT distance matrix of 100,000 cells (Extended Data Fig. 1b–d ).
As AOT can be computed via Euclidean distance, which underlies many standard single-cell analyses, such as clustering 31 , diffusion components 32 and uniform manifold approximation and projection (UMAP) 33 , niches can now be analyzed with the same algorithms designed to analyze phenotypes. Clustering niches can characterize canonical environments; visualization can be used to observe their relationships; and trajectory analysis can capture continuous trends, enabling facile interpretation. COVET thus provides a rich, robust and computationally efficient representation of cellular niches, derived from a mathematically principled formulation based on optimal transport.
The ENVI algorithm
ENVI employs a conditional variational autoencoder to infer spatial context in scRNA-seq data and impute missing genes in spatial data, by mapping both modalities to a common embedding (Fig. 1b and Methods ). Unlike other CVAEs used for spatial inference 25 , 34 , 35 , which only model genes measured in both modalities, ENVI explicitly models spatial information and gene expression genome wide. More importantly, it uses the COVET matrix to represent spatial information and simultaneously trains on samples from both spatial and single-cell datasets, optimizing a single latent space to decode the full transcriptome and spatial context for both modalities.
ENVI architecture includes a single encoder for both spatial and single-cell genomics data and two decoder networks: one for the full transcriptome and the second for the COVET matrix, providing spatial context. The requisite for decoding the spatial niche (and the use of a second decoder) is a unique aspect of ENVI. Intuitively, ENVI uses gene expression in the cell paired with its niche information (COVET) to learn an ‘environment’ regression model, which infers spatial context from gene expression input, and, simultaneously, an ‘imputation’ regression model trained to reproduce the full scRNA-seq dataset from the gene subset profiled by spatial transcriptomics. The nonlinear network architecture can capture complex dependencies between the variables.
Sequencing and spatial technologies measure different parameters and produce different data distributions and dynamic ranges (Extended Data Fig. 2a ). ENVI takes this into account by marginalizing technology-specific effects on expression, augmenting the standard variational autoencoder (VAE) by adding an auxiliary binary neuron to the input layers of encoding and decoding networks for each modality. Moreover, ENVI parameterizes each modality with different probabilistic distributions, modeling single-cell data with a negative binomial by default to account for dropout 36 , and spatial data with a Poisson by default to reflect the high capture rate of fluorescence in situ hybridization (FISH)-based technologies 3 . ENVI thus integrates, imputes and reconstructs spatial context with a single end-to-end model, using deep learning for high-dimensional regression and variational inference for optimal integration of scRNA-seq and spatial data. The method scales to atlas-size datasets including millions of cells with constant time computational complexity (Extended Data Fig. 2b ) while being robust to technology-specific artifacts, such as data sparsity (Extended Data Fig. 2c,d and Methods ).
ENVI imputes spatial patterns underlying gastrulation
We used ENVI to analyze a 350-gene sequential FISH (seqFISH) 37 of mouse organogenesis at embryonic day 8.75 (E8.75) and matched scRNA-seq dataset 38 at E8.5 (Fig. 2a ). Unlike the discrete layers of adult brain tissue 3 , 39 , 40 that dominate current spatial transcriptomic datasets, cells in developing embryos undergo rapid proliferation, differentiation and movement to create complex patterns and spatial gradients, presenting a challenging context for performance assessment. The most basic evaluation of any embedding-based data integration method is how well data across technologies co-embed, because this is critical for successful information transfer between modalities. The embedding learned by ENVI correctly maps major cell types to the combined latent space (Fig. 2b ), as measured by average batch silhouette score 41 ( Methods ).
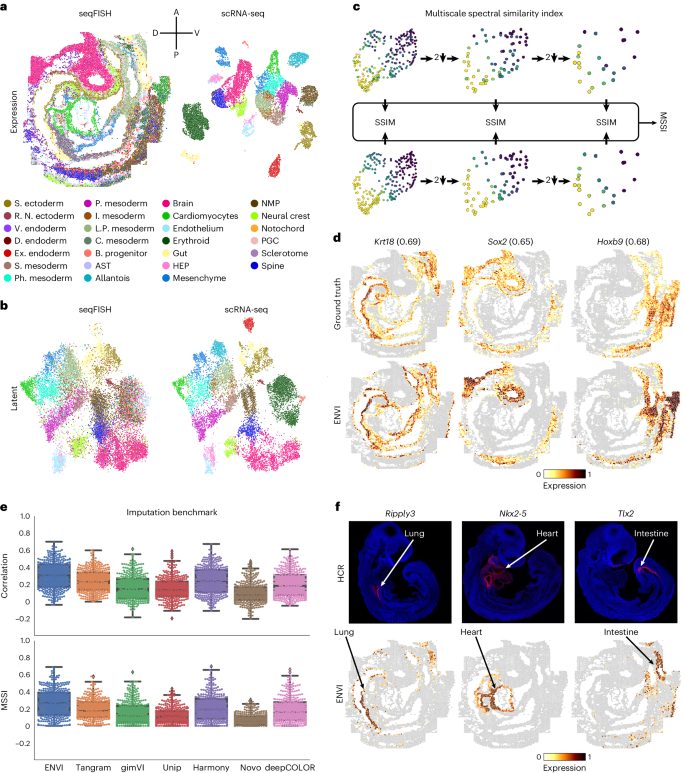
a , seqFISH 37 image of an E8.75 mouse embryo sagittal section (left) and UMAP embedding of matched scRNA-seq data 38 at E8.5 (right), both colored by major cell type compartment. b , UMAPs of ENVI latent embedding learned from mouse embryo data. Cells from seqFISH (left) and scRNA-seq (right) data are colored as in a . Average batch silhouette score bASW = 0.86 ( Methods ). c , Schematic of MSSI computation ( Methods ) for comparing two spatial expression profiles. Each profile is iteratively downsampled using spectral pooling on the cell proximity graph, and the SSIM is computed at each scale. MSSI is a weighted geometric mean of the SSIM computed at five scales, providing a spatially aware similarity metric on a scale from 0 to 1. d , seqFISH measurement in log counts (ground truth) and ENVI imputation for three withheld genes, marking endoderm ( Krt18 ), neural stem ( Sox2 ) and posterior section ( Hoxb9 ) cells. MSSI values for each gene appear in parentheses. e , Pearson correlation and MSSI scores between log of seqFISH and imputed expression across all genes predicted from five-fold cross-validation, comparing four algorithms run with default parameters ( Methods ). Novo, NovoSpaRc; Tg, Tangram; Unip; uniPort. Boxes and lines represent interquartile range (IQR) and median, respectively; whiskers represent ±1.5× IQR. In order, MSSI\Pearson correlation P values (one-sided t -test, n = 351) are: \(4.45\cdot {10}^{-11}\) \(\backslash 4.75\cdot {10}^{-9}\) , \(3.45\cdot {10}^{-58}\) \(\backslash \,3.02\cdot {10}^{-94}\) , \(1.44\cdot {10}^{-76}\backslash 6.68\cdot {10}^{-93},\) \(\,4.83\cdot {10}^{-18}\backslash 3.44\cdot {10}^{-50}\) , \(4.12\cdot {10}^{-79}\) \(\backslash 15.47\cdot {10}^{-85}\) and \(6.48\cdot {10}^{-32}\) \(\backslash 4.51\cdot {10}^{-69}\) . f , ENVI imputation (bottom) of organ marker genes not profiled by seqFISH: Ripply3 (lung), Nkx2-5 (heart) and Tlx2 (intestine). Imputed expression matches associated organs and is validated by whole-mount HCR in situ (top). AST, anterior somitic tissue; B., blood; C, caudal; D., definitive; Ex., extraembryonic; HEP, hematoendothelial progenitor; I., intermediate; L.P., lateral plate; Novo, NovoSpaRc; P., paraxial; PGC, primordial germ cell; Ph., pharyngeal; R.N., rostral neuro; S. Ectoderm, surface ectoderm; S. Mesoderm, somitic mesoderm; Unip, uniPort; V., visceral.
Current FISH-based technologies only quantify the expression of hundreds of genes 12 , 37 , 40 , prompting the development of algorithms to impute the spatial patterns of unmeasured genes 9 , 25 , 26 , 42 , 43 . Previous studies 9 , 25 , 44 used Pearson correlation and mean squared error between imputed and ground truth expression to evaluate the quality of imputation. However, both metrics are computed on a per-cell basis and ignore spatial context. To evaluate concordance between spatial patterns, we developed the multiscale spectral similarity index (MSSI), a metric that can capture similarity between spatial patterns by taking cell–cell proximity into account (Fig. 2c and Methods ). MSSI borrows from the multiscale structure similarity index measure (MS-SSIM) 45 , a spatial pattern similarity metric widely used in computer vision that iteratively subsamples an image and assesses similarity at multiple resolutions. Our MSSI metric uses a cell–cell neighbor graph based on spatial proximity to generate a series of images at progressively lower resolutions by aggregating proximal cells and then applies SSIM to compare similarity at each resolution. MSSI is, thus, a spatially aware similarity metric that uses full count matrices and incorporates patterning at the cellular rather than pixel level and has multiple use cases, such as comparing the similarity of different gene expression patterns 46 .
We used five-fold cross-validation ( Methods ) to compare ENVI imputation with measurements of held-out genes using both MSSI and Pearson correlation. The imputed expression of representative genes with clear spatial expression in endoderm ( Krt18 ), neural stem ( Sox2 ) and posterior section ( Hoxb9 ) was visually similar to ground truth (Fig. 2d ) and expressed in the correct organ. We found that some genes with correctly predicted organ-specific expression have high MSSI score but low Pearson correlation, supporting the importance of a spatially aware metric (Extended Data Fig. 3a ).
We compared ENVI against Tangram 9 , gimVI 25 and uniPort 47 , which were recently shown to outperform other integration methods 44 ; NovoSpaRc 26 , because it uses fused optimal transport to explicitly model spatial context; deepCOLOR 48 , because it uses a deep generative model; and Harmony 49 , for its widespread use as a batch correction method 50 . ENVI significantly outperforms all other methods based on both MSSI and Pearson correlation (Fig. 2e ).
Finally, we evaluated ENVI’s ability to impute genes beyond the 350-gene panel by assessing canonical markers of the developing lung ( Ripply3 ) 51 , heart ( Nkx2-5 ) 52 and intestine ( Tlx2 ) 53 . The expression of all three genes was validated as organ specific at E8.75 (before organ formation) by in situ hybridization chain reaction (HCR) imaging 54 and was correctly imputed by ENVI (Fig. 2f ). By contrast, Tangram and gimVI predicted weaker expression in the relevant region and anomalous expression beyond the organ (Extended Data Fig. 3b ).
ENVI ascribes spatial patterns to single-cell genomics data
In addition to gene imputation, ENVI can uniquely project spatial information onto dissociated cells profiled by scRNA-seq, by using its second decoder to reconstruct COVET matrices from the latent space. This approach can use limited spatial profiling data to confer spatial context onto the millions of cells in single-cell atlases. COVET represents gene–gene covariation between neighboring cells; thus, beyond deducing the cell type of neighbors, it can also infer their gene expression.
To demonstrate this ability, we used the mouse embryo dataset and focused on the gut tube, which generates the thymus, thyroid, lung, liver, pancreas, small intestine and colon in a stereotypical anterior-to-posterior sequence. Although E8.75 gut tube cells are anatomically indistinguishable, spatially delimited expression reveals that precursors are poised for their organ fates 55 . We computed COVET matrices for (measured) seqFISH data and used ENVI to infer COVET matrices for scRNA-seq data and then applied the AOT metric to co-embed matrices from both modalities (Fig. 3a ). The rich transcriptional information in scRNA-seq data facilitated the assignment of endodermal organ identity to these cells 55 , and ENVI’s highly concordant co-embedding allowed for label transfer to those cells measured with seqFISH, as confirmed by anatomical localization; thymus and thyroid cells fall into the most anterior ventral gut tube, followed by dorsal and ventral lung clusters and, finally, intestine (Fig. 3b ).
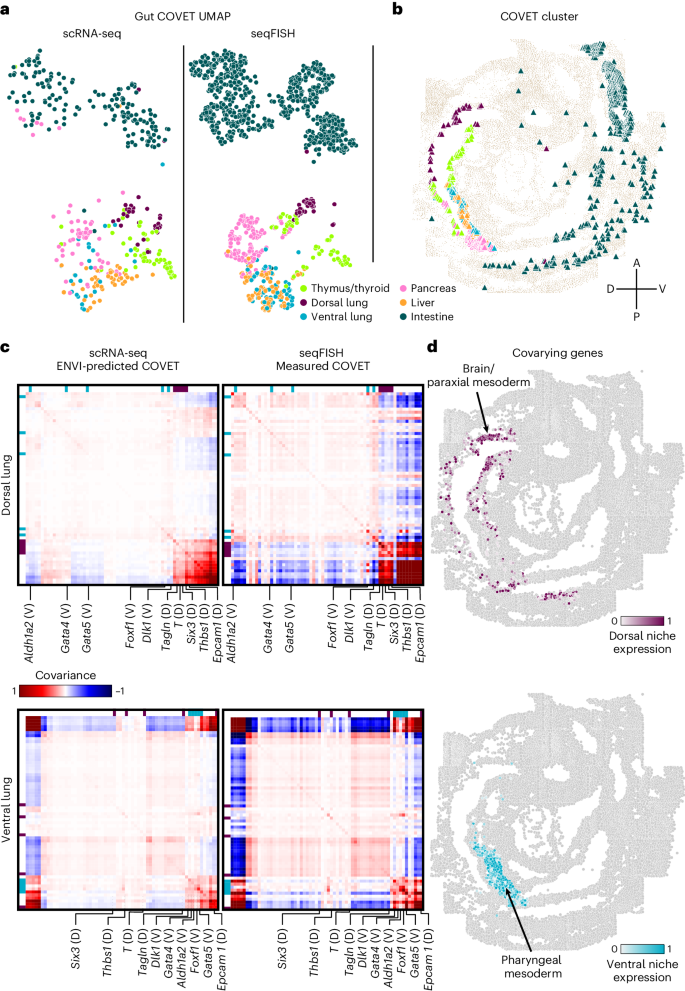
a , COVET UMAP of gut tube cells. Each cell is represented by its directly measured (seqFISH) or ENVI-inferred (scRNA-seq) COVET, embedded in 2D coordinates by UMAP using the AOT metric. Cells are colored by emerging organ. b , seqFISH endoderm cells, colored by organ (based on label transfer from scRNA-seq), delineating spatial regions of the gut tube and showing coherence of organ assignments in distinct spatial niches. c , Average ENVI-imputed (scRNA-seq, left) or empirically measured (seqFISH, right) COVET matrices of dorsal (top) and ventral (bottom) lung cells from scRNA-seq (left) and seqFISH (right) datasets. Labeled gene modules (top row of each matrix) are uniquely present in either dorsal (purple) or ventral (cyan) lung COVET representations. d , Mean expression of the uniquely covarying genes in either dorsal (top) or ventral (bottom) lung COVET matrices in c . Only mesoderm cells surrounding the endoderm gut tube (but not part of it) are colored.
Using only endodermal scRNA-seq data, we plotted the average COVET matrices of dorsal and ventral lung and observed that these closely match empirical matrices computed from the seqFISH data (Fig. 3c ). These COVET matrices infer modules of covarying genes in the niche environment and notably include genes expressed by adjacent mesodermal cells, which are known to provide spatial patterning cues to the endoderm 56 . To validate these inferred gene modules, we used the seqFISH data from mesodermal cells proximal to the gut tube (ignoring endodermal cells) and found that average ventral COVET gene expression is enriched in the ventral pharyngeal mesoderm, whereas average dorsal COVET gene expression is enriched in the dorsal brain and paraxial mesoderm (Fig. 3d ). Our observations validate the predicted dorsal and ventral subdomains within the gut tube and demonstrate that ENVI can identify biologically important signaling originating from cells that were not sampled directly.
Using spatial covariance can also markedly improve the simpler task of labeling organ identity for cells from the spatial modality, as seqFISH measures fewer genes and cells than scRNA-seq and is, thus, more difficult to label. Labeling scRNA-seq cells from the gut tube with organ-specific gene sets 55 ( Methods ) revealed an almost one-to-one matching between organ precursors and COVET clusters, whereas ENVI without COVET failed to generate accurate labels, and alternative approaches were even less accurate (Extended Data Fig. 4 ). ENVI-based label transfer is also robust to variation in neighborhood size when computing COVET (Extended Data Fig. 5 ).
ENVI learns spatial gradients from single-cell data
Although the gut tube is defined by relatively discrete primordial organs, many processes—such as the specification of spinal cord cells and their precursors, the neuromesodermal progenitors (NMPs), along the anteroposterior (AP) axis—are organized by continuous spatial gradients 57 . To highlight ENVI’s ability to model gradients, we co-embedded empirical seqFISH COVET matrices with ENVI-inferred scRNA-seq COVET matrices for NMPs and spine cells using a force-directed layout (FDL) 58 and calculated their diffusion components (DCs) (Fig. 4a ). The first DC is highly congruent with the AP axis (Pearson correlation = 0.86), demonstrating that COVET can capture gradual spatial trends (Fig. 4a,b and Extended Data Fig. 6a ). As the COVET DC is calculated from both seqFISH and scRNA-seq datasets, we can use it to assign AP pseudo-coordinates to NMPs and spine cells from scRNA-seq data.
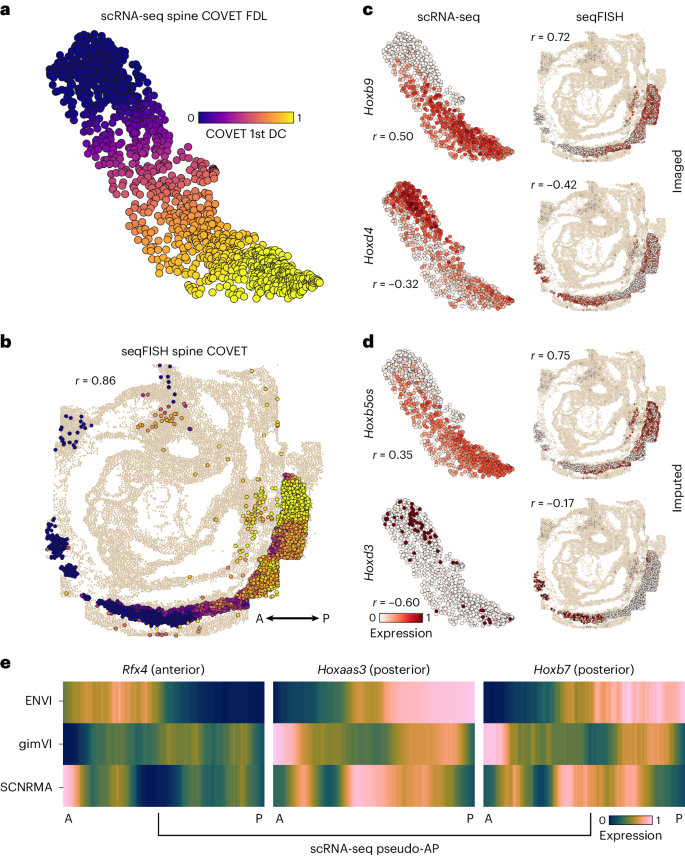
a , FDL based on ENVI-predicted COVET matrices of scRNA-seq spinal cord cells and their NMP precursors, colored by the first COVET diffusion component (pseudo-AP). FDL and DCs were calculated using both the seqFISH and scRNA-seq COVET matrices. b , seqFISH NMPs and spine cells colored by the first COVET DC, which traverses the AP axis. Pearson correlation ( r ) is calculated between pseudo-AP and true AP. c , Expression of Hoxd4 (anterior) and Hoxb9 (posterior) markers in NMPs and spine cells from scRNA-seq and seqFISH data, recapitulating expected AP localization. d , Same as c , colored by ENVI-imputed Hoxb5os (posterior) and Hoxd3 (anterior) expression. These markers were not imaged. e , scRNA-seq expression of canonical AP axis markers in NMPs and spine cells ordered along pseudo-AP axis according to different integration methods. SCNRMA, Scanorama.
The first COVET DC correctly reveals that scRNA-seq cells are enriched for Hoxd4 (refs. 55 , 59 ) (anterior) and Hoxb9 (ref. 60 ) (posterior) markers in their respective domains, consistent with seqFISH expression in NMPs and spine cells (Fig. 4c ). Furthermore, ENVI correctly mapped high expression of Hoxd3 (ref. 59 ) (anterior) and Hoxb5os 61 (posterior) markers to scRNA-seq cells in their corresponding AP domains, demonstrating that ENVI spatial modeling extends to genes that are not imaged (Fig. 4d ). Conversely, ENVI-imputed Hoxb5os and Hoxd3 expression for the seqFISH data mirrors the predicted spatial context of the scRNA-seq data.
We found that the major axis of variation (first DC) between the COVET matrices that model the niche reflects the spatial organization of the tissue; ordering NMPs and spine cells along DC 1 recovers a pseudo-AP axis that can be used to visualize predicted expression trends 57 (Fig. 4e ). Similar analysis using the gimVI latent space and Scanorama 62 integration ( Methods ) led to inferior alignment with the true AP axis (Extended Data Fig. 6b ), despite selecting the gimVI and Scanorama DCs most correlated ( r = 0.76 and r = 0.7070, respectively) with true AP polarity. This slightly lower correlation with the AP axis propagates into more pronounced inaccuracies in expression patterns; only ENVI correctly derived expected AP trends for Rfx4 (ref. 63 ), Hoxaas3 (ref. 61 ) and Hoxb7 (ref. 64 ) (Fig. 4e ). More generally, both anterior and posterior canonical markers are more correlated (or anti-correlated) with ENVI COVET pseudo-AP than with axes defined by gimVI and Scanorama (Extended Data Fig. 6c ). ENVI can, thus, correctly uncover AP polarity within single-cell NMPs and spine cells and correctly place them along this spatial axis.
ENVI delineates tissue-scale patterning in the motor cortex
Although data integration is typically evaluated on abundant neural cell types that dominate spatial regions, we challenged ENVI to recover rare cell types. Somatostatin ( Sst) -expressing interneurons are a cardinal class of inhibitory neurons in the cortex 65 that are implicated in Alzheimer’s disease and depression 66 and encompass substantial diversity 67 , 68 , 69 . Although we know that Sst interneurons influence their environment, their localization and its relationship to function and transcriptional states have not been fully explored.
To localize Sst interneurons, we analyzed the scRNA-seq (71,183-cell) and 252-gene MERFISH (276,556-cell) atlases of the motor cortex of the Brain Initiative Cell Census Network (BICCN) 40 , 70 . ENVI outperformed all other tested methods in both speed (training on this large atlas in minutes) and imputation (Extended Data Figs. 2b and 7 ), and it successfully co-embedded the 22 BICCN-annotated coarse cell types (Fig. 5a ). Notably, only scRNA-seq data can distinguish the nine distinct Sst subpopulations, as the MERFISH panel lacks requisite marker genes (Fig. 5b and Methods ).
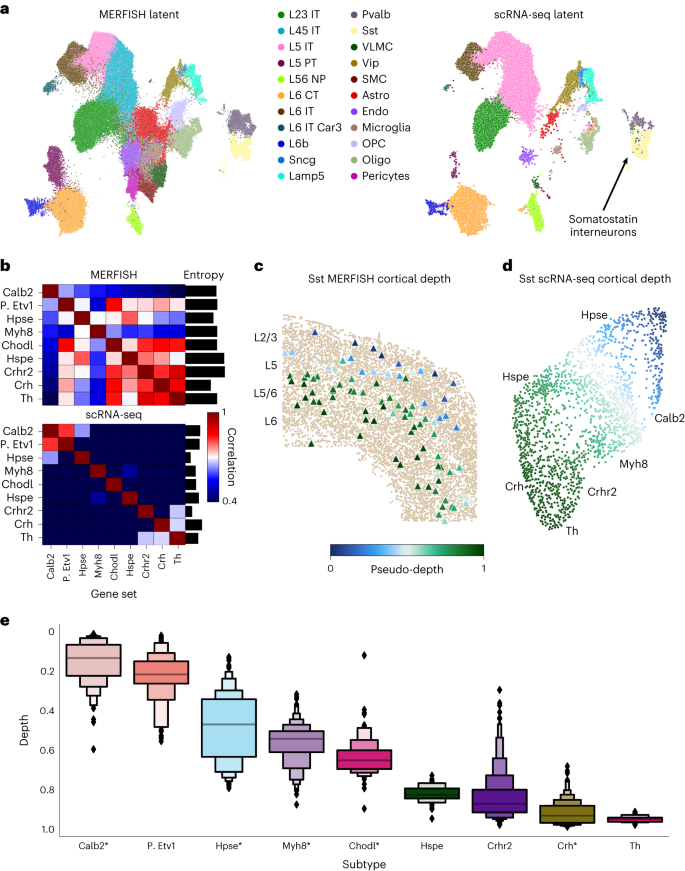
a , ENVI latent embedding of motor cortex MERFISH (left) and scRNA-seq (right) data, labeled by cell types from Zhang et al. 40 and Yao et al. 70 , and highlighting Sst interneurons. Latent integration bASW = 0.78. OPC, oligodendrocyte precursor cell. b , Correlation between subtype-specific gene sets (Supplementary Table 3 ) in Sst interneurons from the MERFISH and scRNA-seq datasets. Bar plots mark the entropy of overlap for each subgroup (higher values denote poorer delineation into distinct subtypes). c , Sst interneurons from MERFISH data, colored by first COVET DC representing pseudodepth. L2/3 to L6 indicate approximate location of cortical layers. d , FDL of scRNA-seq COVET for all Sst interneurons, colored by first COVET DC. COVET FDL and DCs are calculated based on both scRNA-seq and MERFISH datasets. Specific subtypes, labeled by Yao et al. 70 , are marked. e , COVET DC-predicted cortical depth of scRNA-seq Sst interneurons, grouped by labeled subtype. *, cell types imaged in Wu et al. 71 ; all empirical depths match ENVI predictions. In the box plot, line is median; first boxes include the 50th percentile; and each successive pair of boxes outward contain half of the remaining data.
Using ENVI-imputed COVET matrices, we mapped Sst interneurons labeled in the scRNA-seq dataset to their location within the cortex. We found that—despite being interspersed throughout the cortex, where cell types, such as excitatory neurons, dominate—the first DC of COVET matrices is highly correlated with cortical depth, thus defining a ‘pseudodepth’ axis (Fig. 5c,d ), and that Sst subtypes are predicted to stratify by depth (Fig. 5e ). Molecular imaging by genetic strategies targeting Sst subtypes 71 validates a number of our predictions, including the localization of Calb2 interneurons to the L2/3 layers and Crh interneurons to L6. Beyond these, ENVI predicted the cortical depth of many subtypes identified in the scRNA-seq atlas with unknown localization. For example, it placed Sst interneurons expressing high levels of the neurotransmitter metabolism gene Tyrosine hydroxylase in the deep L6 layer, as might be expected, suggesting that ENVI can articulate the interplay between transcriptional state and microenvironment.
ENVI can also capture spatial patterns within the cortex from datasets that include only a few imaged genes. Applied to a 33-gene osmFISH and matched scRNA-seq dataset of the somatosensory cortex 3 , ENVI successfully integrated the small datasets (fewer than 10,000 cells combined) into a unified embedding (Extended Data Fig. 8a,b ) and outperformed alternative methods in cell type resolution and spatial gene imputation (Extended Data Fig. 8c–e ). To determine whether ENVI can impute unimaged genes, we leveraged Allen Brain Atlas ground truth data for mouse brain cortex ( https://mouse.brain-map.org/ ) and confirmed that ENVI correctly imputes layer-specific spatial expression for Dti4l , Rprm and Ntst4 in the L2/3, L5/6 and CA1 regions, respectively (Extended Data Fig. 8f ).
ENVI integrates Xenium data on brain metastasis
Leptomeningeal metastasis (LM) is a lethal condition in which distant tumor cells spread into the fluid-filled space surrounding the central nervous system 72 , 73 . The poor understanding of interactions among tumor, immune and underlying brain parenchyma cells limits the discovery of therapeutics. We used the Xenium platform (10x Genomics) 16 to perform in situ hybridization (ISH) of 243 genes in a mouse model of melanoma LM 74 and also sequenced cells from an adjacent section using a custom single-nucleus RNA sequencing (snRNA-seq) protocol ( Methods ) that we developed by optimizing RNA extraction from formalin-fixed paraffin-embedded (FFPE) samples, followed by 10x Genomics Flex probe-based library preparation. We separately clustered and annotated the spatial and single-cell samples into major cell types based on marker genes (Fig. 6a and Extended Data Fig. 9a ). Even in this pathological context, ENVI performance with default parameters matches or exceeds competing methods on gene imputation (Extended Data Fig. 9b ) and harmonizes the two datasets into a unified latent space (Fig. 6b and Extended Data Fig. 9c ).
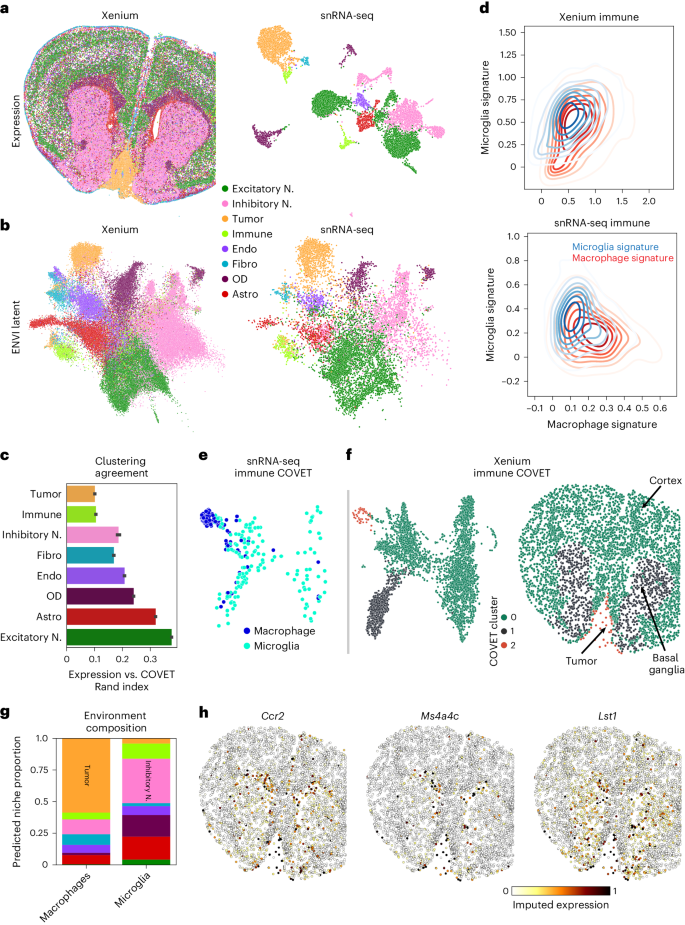
a , Xenium image and UMAP embedding of snRNA-seq data from mouse brain bearing a melanoma metastasis, colored by major cell type. b , UMAP embeddings of ENVI latent space showing cells from the spatial (left) and snRNA-seq (right) datasets. Similar cell types, including malignant cells, co-embed across modalities. bASW = 0.87. c , Average concordance of technical replicates between the expression of each cell type and its environment in the Xenium data, measured as ARI between k -means clusters of gene expression and COVET representation. Error bars, 95% confidence interval. d , Density plots of microglia and macrophage cell signature expression in immune-labeled cells from Xenium (top) and snRNA-seq (bottom) datasets. Only snRNA-seq data measure enough genes to separate cell types. e , UMAP embedding of the ENVI-predicted COVET representation of snRNA-seq immune cells, colored by subtype. f , COVET UMAP (left) and spatial coordinates (right) of Xenium immune cells, colored by COVET clusters representing major immune cell microenvironments: cortex (C0), basal ganglia (C1) and tumor (C2). COVET UMAP and clusters are calculated from both snRNA-seq and Xenium datasets. The vast majority (85%) of snRNA-seq macrophages were assigned to C2, predicting their localization to the tumor. g , Cell type fractions of ENVI-predicted environments for snRNA-seq macrophages and microglia, colored as in a , highlighting their predominant tumor and inhibitory neuron environments, respectively. h , ENVI-imputed macrophage infiltration marker genes on Xenium immune cell types are distinctly enriched within the metastasized tumor. Astro, astrocyte; Endo, endoderm; Fibro, fibroblast; OD, oligodendrocyte; N., neuron.
Our approach provides two representations of the Xenium data; we can visualize and cluster each cell based either on its gene expression or on its COVET matrix (representing the local niche). Measuring the agreement between clustering of the two representations reveals that, as expected, excitatory neuron expression depends strongly on spatial context, due to the association between distinct cortical layers and molecular markers 70 , whereas tumor and immune cell types show little concordance between expression and environmental context (Fig. 6c ).
Melanoma LM interacts with two key immune populations: tissue-resident brain macrophages, known as microglia, and monocyte-derived macrophages that are recruited to the tumor lesion and colonize it from the periphery 74 . The snRNA-seq data clearly distinguish these myeloid subtypes based on curated gene sets 75 , whereas the Xenium brain panel lacks the markers to distinguish macrophages and microglia (Fig. 6d ). To resolve where the subtypes localize, we co-embedded ENVI-imputed snRNA-seq COVET matrices with observed Xenium COVET matrices and clustered the data, revealing three distinct immune microenvironments consisting of cortical, basal ganglia or tumor cells (Fig. 6e,f ). The snRNA-seq data enabled the labeling of cluster 2 as non-resident macrophage, and the Xenium data allowed us to visualize the localization of cells from this COVET cluster. Confirming known patterns of neuroimmune cell types 76 , 77 , 78 , most microglia were assigned to the basal ganglia and cortex, whereas most macrophages were localized to the tumor and its boundary. COVET allowed us to infer the niche composition for each immune cell in the snRNA-seq data, which corroborates that macrophages are found mainly near tumor cells, whereas microglia are found mainly near neurons and other glial cells (Fig. 6g ).
Beyond localizing macrophages and microglia, ENVI can distinguish the transcriptional patterns of tumor-infiltrating macrophages from those on the boundary by imputing gene expression in the Xenium data (Fig. 6h ). For instance, imputation of Ccr2 , a chemokine receptor that recruits monocytes to the tumor and promotes their differentiation into tumor-associated macrophages 78 , was enriched in immune cells within the tumor and its vicinity. In contrast, clustering-based analysis of the gimVI latent on the immune cells does not clearly assign macrophages to a malignant microenvironment, and its gene imputation is also inaccurate, predicting that tumor infiltration genes are broadly expressed across the brain (Extended Data Fig. 9d,e ). Harmony and gimVI also fail to localize infiltration marker expression to immune cells within the tumor (Extended Data Fig. 10 ).
ENVI robustly integrates scRNA-seq and spatial transcriptomics data, overcoming technical biases while retaining biological information. The algorithm provides superior performance for imputing missing gene expression in spatial modalities; it scales to millions of cells; and it has the distinctive ability to infer the spatial context of dissociated cells, even across multiple cell types in complex tissues.
ENVI’s capabilities rely on COVET as a representation of spatial niches. Although most spatial representations are based on discrete cell typing, COVET takes full advantage of the quantitative nature of gene expression data. The COVET matrix captures covariation between markers in a cell’s niche and uses optimal transport to derive a principled and quantitative model of cellular neighborhoods. COVET powers a shift from discrete cell type to continuous cell state paradigms and the discovery of continuous trends in spatial microenvironments.
ENVI performance is primarily driven by three factors: (1) deep Bayesian inference to regress out modality-related confounders while learning nonlinear relationships between genes and niches; (2) explicit modeling of the entire transcriptome from scRNA-seq data; and (3) direct incorporation of spatial context via COVET. Whereas current methods only learn the genes that overlap between scRNA-seq and spatial datasets, ENVI models all available information and does not rely on post hoc inference. This proves invaluable, as the ENVI model is imbued with both spatial context and full transcriptome information, allowing for reliable transfer of information between modalities.
The ENVI COVET space can correctly predict primordial organ niches from seqFISH and scRNA-seq data of mouse gastrulation, and COVET-based DC analysis can highlight continuous AP trends of both expression and environment in the developing spine. ENVI’s critical ability to confer spatial context onto dissociated single cells drives the inference of circuits of Sst interneuron subtypes in the motor cortex. Moreover, it provides an accurate representation of both discrete and diffuse signals in healthy and pathological tissue contexts, enabling spatial reasoning along the full transcriptome, including the spatial distinction of subtly different tumor and non-tumor-associated macrophage cell states in metastatic tissue.
One caveat is that the range of spatial factors can vary, whereas COVET is currently defined at a single scale set by the neighborhood size, k . Although COVET is relatively robust to small changes of k , larger differences may lead to different outcomes, and its value should be tuned to the spatial questions of interest.
Computational methods
When comparing the spatial distribution of genes or markers across a tissue, it is imperative to have a robust metric that takes spatial structure into account. Although ubiquitous metrics, such as Pearson correlation, SSIM and root mean square error, can provide some insight, they lack spatial context (for example, cell–cell proximity or spatial patterns) and measure only per-cell discrepancy.
To devise a metric for spatial data, we borrowed the MS-SSIM 45 , a ubiquitous metric for the quality of image reconstruction, from computer vision. Given two images, MS-SSIM iteratively downsamples each image, creating an image pyramid 79 —a multiscale signal representation consisting of the same image at multiple resolutions. MS-SSIM returns a weighted geometric average of the standard SSIM scores between the two images at each scale of the pyramid. Standard SSIM for two images, x and y , is
M represents the maximum values between x and y ; μ x and μ y are their average values; σ x and σ y measure how each varies; and σ xy represents how much they covary. l ( x , y ), c ( x , y ) and s ( x , y ) are measures of ‘luminance’ (signal brightness), contrast and structure, respectively. Although SSIM is meant for images, it can also be calculated between any two vectors of similar sizes.
We introduce the MSSI as an adaptation of MS-SSIM to spatial transcriptomics that compares count matrices from segmented cells, rather than pixels, using a neighbor graph of spatially neighboring cells to capture structure. Intuitively, MSSI is a spectral analog of MS-SSIM; by rephrasing image coarsening to its graph-based counterpart, we can apply it to segmented cells and produce a multiscale, spatially driven score of expression reconstruction quality.
MSSI compares the expression profiles of two genes from a multiplexed image: \(X={\{{x}_{i}\}}_{i=1}^{N}\) and \(Y={\{{y}_{i}\}}_{i=1}^{N}\) , where the index i enumerates segmented cells, and x and y can be either (1) two different genes or (2) a ground truth gene and its imputed value. In addition, the spatial coordinate of each cell is \(D={\{{D}_{i}\}}_{i=1}^{N}\) . We first compute the k nearest neighbor (kNN) graph G 1 of segmented cells from \({\{{D}_{i}\}}_{i=1}^{N}\) . To generate a subsampled version of the kNN graph, we use a graph coarsening algorithm 80 , which pools nodes together based on their connectivity pattern, similarly to how image downsampling groups pixels together (Fig. 2c ). We iteratively coarsen and blur the graph four times by a factor of 2 and produce the expression of every gene at each scale.
Mathematically, each coarsening step produces a pooled version of the graph \({\{{G}^{s}\}}_{s=2}^{5}\) and a coarsening operator \({\{{C}^{s}\}}_{s=2}^{5}\) , which is the mapping between nodes at one scale to nodes at the next and allows us to generate pooled versions of the gene expression signals:
After MS-SSIM, we compute the MSSI between the expression profiles at each scale and return their weighted geometric mean. In detail, we compute the l , c and s SSIM-related values at each scale and derive MSSI based on their weighted product, as for the MS-SSIM:
where the weights are equivalent to those in MS-SSIM 45 :
When X i ,Y i are anti-correlated ( \({\sigma }_{xy} < 0\) ), s is negative, which prevents computing the weighted geometric mean; we, thus, clip negative values to 0. This implies that if, at any scale, X s , Y s are anti-correlated, the MSSI will be 0, its lowest possible value. We also normalize the original-scale gene expression to be between 0 and 1 but do not re-normalize at each coarsening scale.
Spatial covariance representation
Our spatial covariance framework includes three components: the COVET statistic, a similarity metric and an algorithm to robustly and efficiently compute the COVET metric. The COVET framework assumes that the interplay between the cell and its environment creates covarying patterns of expression between the cell and its niche, which can be formulated via the gene–gene covariance matrix of niche cells. The COVET statistic constructs a shifted covariance matrix (which preserves algebraic properties of the covariance matrix) and, thus, enables the use of any measure of statistical divergence between covariances to define a principled quantitative similarity metric to compare niches. The key is to build the COVET statistic in such a manner that two COVET matrices are comparable and to design a computationally efficient algorithm to quantify the statistical divergence between them.
The inputs to COVET are (1) the gene expression matrices ( \(X\in {R}^{n\times g}\) ), where n is the number of cells and g is the number of genes profiled; (2) the location of each cell in situ; and (3) a parameter ( k ) that defines the number of nearest cells to be included in the niche. For each cell, we identify its k nearest cells (excluding the cell itself) based on their spatial proximity and construct a niche matrix \({E}_{i}=\left\{{Y}_{ij}\in\right.\) \(\left.{R}^{g}|\,j\in kNN(i)\right\}\) , which represents the gene expression vector for each of those nearest neighbors. This produces an n × k × g tensor \(\varOmega =\,\left\{{E}_{i}\in\right.\) \(\left.{R}^{k\times g}|i=1,\ldots ,n\right\}\) , which combines the niche matrices of every cell.
The fundamental goal of COVET is to transform those niche matrices into effective representations of a cell’s niche. To this end, we calculate the ‘shifted’ gene–gene covariance matrix between cells in each niche matrix, where, instead of using the classical formulation
we swap the niche mean expression \({{\overline{E}}_{i}}\) with the total expression average \({\overline{X}}\) (computing the mean over the entire dataset). This enables direct comparison between covariance matrices, as they are constructed relative to the same reference:
This creates a representation relative to the entire population, which can better highlight the features that are unique to each niche while also holding the same algebraic properties that the standard covariance matrix holds, namely being positive semi-definite (PSD). Therefore, we can harness measures of statistical divergence to derive a metric on the COVET matrices and quantify differences and similarities between niches. Although we can conceptually use any statistical divergence measure, metrics such as Kullback–Leibler (KL) divergence and Bhattacharyya 30 distance are too computationally intensive and lack interpretability.
Distance between COVET matrices
To meaningfully compare between niches, we cannot simply use the sum difference between two niche matrices E i and E j , as changing the cells’ order would change the result (whereas there is no meaning to any given order). An intuitive way to quantify niche similarity is by finding the best matching of cells between niche matrices by solving the assignment problem 81 . Optimal transport (OT) 82 is a relaxed version of the assignment problem, where, instead of matching cells one to one, OT finds the best ‘soft assignment’ between cells. However, because this approach has no closed-form solution and does not scale to large datasets, we can use the closed-form solution of OT between covariance matrices, known as the Fréchet distance 29 , instead:
The Fréchet distance has time complexity of O ( k 3 ) and is, thus, computationally intractable for large-scale datasets, which would require billions of pairwise computations between all niches. To speed up computation, we swap the matrix square root (MSQR) and product operation in the last term of the Fréchet distance and define the AOT distance as:
If Σ i and Σ j are commutative, this is no longer an approximation and Δ AOT = Δ Fréchet . Both the approximate and true Fréchet distance require O ( k 3 ) operations between each pair of niches and O ( n 2 k 3 ) to compute the full distance matrix; however, using the identity that for symmetric matrices, \({\mathrm{Tr}}(AB)=\,\sum _{\gamma ,\delta }{A}_{\gamma \delta }\cdot {B}_{\gamma \delta }\) , we arrive at:
Therefore, when working in square root space, we do not require any computationally extraneous matrix multiplication and many calculations of MSQR. Instead, we first calculate the MSQR of each COVET matrix, which is \(O(n{k}^{3}\) , and then simply calculate pairwise (squared) Euclidean distance for a total time complexity of \(O(n{k}^{3}+{n}^{2}{k}^{2})\) , which is substantially more efficient than \(O({n}^{2}{k}^{3})\) for large n . For a given PSD matrix A , there could be many possible solutions B that fulfill the equation B 2 = A . Although this underdetermination is problematic, there is a unique symmetric PSD solution for the MSQR 83 . This solution can be found via spectral decomposition and reconstructing with standard square root of the matrix eigenvalues:
where λ i , v i are the eigenvalues/vector of A.
Because AOT can be formalized as the \({L}_{2}^{2}\) between MSQR of COVET matrices, it allows for direct use of any algorithm that is based on the squared Euclidean distance, such as UMAP, tSNE 84 and FDL 58 , clustering 31 and DC 32 analysis. We can simply compute MSQR of the COVET matrices, flatten the resulting matrices into one-dimensional (1D) vectors and apply the default implementations of all the mentioned algorithms. We can further leverage the squared Euclidean distance representation of the AOT metric and use computational accelerators designed to compute classical pairwise distances for additional speed gains.
We demonstrate that AOT is a good approximation by benchmarking against the true Fréchet distance and the Bhattacharyya distance, another common metric for distances between covariance matrices. Across various sizes of random sets of 64 × 64 covariance matrices, we test the runtime to compute the 10 nearest neighbors matrix in covariance space. As covariance matrices are PSD, to randomly generate n covariance matrices of 64 × 64 elements, we first sample n random 64 × 64 matrices (using the standard normal) and multiply each by its transpose, as a matrix Gramian is always PSD:
We find that, whereas AOT produces accurate similarities, its runtime is at least an order of magnitude smaller than that of other metrics, with Fréchet and Bhattacharyya failing on sample sizes larger than 3,000 matrices due to out-of-memory error. Using a GPU implementation of kNN distance built for the Euclidean metric, which can be easily adapted for AOT, the spatial covariance metric is indeed scalable to massive datasets, taking less than 1 min to compute the kNN matrix between 100,000 samples (Extended Data Fig. 1a ).
We observe accurate approximation on real COVET matrices, calculated from the eight nearest neighbors of the pharyngeal mesoderm cells from the seqFISH assay 37 , using the 64 most variable genes among the 350 imaged. Despite its efficiency, AOT does not sacrifice accuracy and concurs highly with Fréchet. We calculate the pairwise distance between the pharyngeal mesoderm COVET matrices according to Fréchet, AOT, Bhattacharyya and naive L 2 between matrices. For each pharyngeal mesoderm cell, we find its k nearest neighbors for every metric and compute their Jaccard index with the Fréchet nearest neighbors. Across a wide range of k , AOT-based kNN is highly congruent with Fréchet kNN, whereas Bhattacharyya and naive L 2 distances are not (Extended Data Fig. 1b ). Qualitatively, using Fréchet, AOT and Bhattacharyya pairwise distances to compute two-dimensional (2D) embeddings and PhenoGraph clusters for the COVET matrices returned similar results (Extended Data Fig. 1c,d ).
Choice of k
By default, we select k = 8 neighbors to construct COVET, which usually captures the immediate niche of a cell, but the exact choice of k should reflect the data. For all datasets analyzed in this study, we kept the value of k at the default, demonstrating how finding the optimal k is not required to gain insights from ENVI and COVET. Still, given the computational efficiency of both algorithms, we recommend that users attempt a range of k values at different scales, such as 8, 20 and 50. Users can visualize the ENVI-learned COVET representations with AOT and choose the most appropriate scale for their biological question. We also implemented an option for COVET to be computed on all cells within a given radius, rather than constant number of neighbors, to account for differences in cell density within a tissue.
ENVI algorithm
The ENVI algorithm integrates scRNA-seq and spatial transcriptomics data into a common latent embedding, in a manner that can infer spatial context for scRNA-seq and missing genes for spatial data. The core assumption of ENVI is that the interplay between a cell’s phenotype and its microenvironment, as captured by the COVET matrix, empowers better data integration.
ENVI is grounded on autoencoder variational inference but diverges from previous work 9 , 25 , 47 . Although current methods only model the expression of genes included in both single-cell and spatial datasets, ENVI explicitly incorporates both microenvironment context for spatial data and expression of the full transcriptome for scRNA-seq data. In addition, ENVI contains two decoders: one for expression, which includes additional neurons that learn gene expression only from scRNA-seq data, and one to predict spatial context. Using these decoders, ENVI trains the VAE 27 to reconstruct both full transcriptome expression and spatial context from partial transcriptome samples.
To integrate scRNA-seq and spatial data, ENVI learns a common latent space for both data modalities by marginalizing the technology-specific effect on expression via a CVAE 28 . It achieves this by augmenting the standard VAE with an auxiliary binary neuron in the input layers to the encoding and decoding networks representing each data modality. Integration is crucial, as each modality harbors technology-specific artifacts (Extended Data Fig. 2a ). ENVI takes as input the scRNA-seq count matrix X sc with n sc cells and their full transcriptome of g sc genes as well as counts of segmented cells from spatial transcriptomics matrix X st from n st cells and g st imaged genes. The algorithm is agnostic to the method used to segment cells before input. It uses the spatial data to compute the COVET matrix for each cell and their MSQR to align with the AOT distance formulation.
Next, ENVI’s conditional autoencoder builds a shared latent space for both data modalities. As the combined embedding must incorporate spatial context and full transcriptome information and must remove confounders relating to modality, we set the latent dimension to 512, substantially larger than standard VAEs in single-cell genomics, which usually contain around 10 neurons 25 , 34 , 35 . As input to the encoder, ENVI takes either spatial or scRNA-seq samples (the latter reduced to the subset of genes that have been imaged), along with the auxiliary neuron c having value 0 for the spatial data and 1 for scRNA-seq. The expression profile along with the auxiliary neuron are transformed into the latent variable l using the same encoding neural network, regardless of data modality:
where the encoder returns two vectors, μ l and σ l , which parameterize a Gaussian with diagonal covariance describing the posterior distribution of the latent. To calculate gradients through random samples, we use the reparameterization trick, which involves generating a sample from the standard normal ε ~ N (0,1) and describing the latent through a function of ε , μ l and σ l and treating ε as a constant:
Through the training process, our goal is to have the latent encode not only gene expression but also information about the spatial context of a given cell while removing confounding effects to allow transfer learning between modalities. This is achieved by optimizing a single latent space to accurately decode both the full transcriptome and COVET matrix for both data modalities, each missing one of these components. The requisite that the latent space be capable of decoding the spatial niche imbues sufficient spatial information into the latent space during training.
The latent of either modality, along with the appropriate auxiliary neurons, is fed into the ‘expression’ decoder network Dec Exp . The loss function, calculated by comparing the activations in the output layer to the true expression profiles, needs to reflect the underlying distribution of each data modality. We use the negative binomial distribution to model scRNA-seq data, similarly to previous work 25 , 36 , as it suffers from overdispersion and dropout. During training, the scRNA-seq data provide transcriptome-wide expression; therefore, we can include genes whose expression was not provided to the encoder in the loss function, allowing our encoder to model genome-wide expression.
The negative binomial has two parameters per gene: the number of failures, r , and success probability, p . Thus, the output layer of the decoder consists of \(2\cdot {g}_{\mathrm{sc}}\) neurons, where the first g sc neurons are the parameter r and the latter g sc neurons are p , using the ‘softplus’ nonlinearity for r and the sigmoid function for p to keep it a valid probability:
We use the Poisson distribution to model FISH-based multiplexed imaging data due to its high molecular capture rate 3 and have the first g st neurons in the output layer parameterize the per-gene rate parameter λ using ‘softplus’ nonlinearity to ensure that it is a valid rate value:
A standard CVAE, in which all neural parameters are shared aside from the auxiliary neurons, is sufficient to simply integrate between scRNA-seq batches, as demonstrated in scArches 34 . However, to successfully integrate scRNA-seq and multiplexed FISH-based technologies, a single auxiliary neuron is not sufficient to regress out all biases. In ENVI, only the first g st neurons of the output layer are shared by the two data modalities, whereas the rest are solely trained on the scRNA-seq data. These additional technology-specific parameters improve the ability of ENVI to regress out confounders from the latent embedding, beyond the auxiliary neuron.
Finally, ENVI includes an additional ‘environment’ decoder network Dec Env whose role is to reconstruct the COVET from the latent, which can be trained from the spatial data. The output layer of the environment decoder has \(\frac{{g}_{\mathrm{spatial}}\cdot ({g}_{\mathrm{spatial}}+1)}{2}\) output neurons parameterizing the lower triangular Cholesky factor. The Gramian matrix of the output layer is the mean parameter of a standard normal, reflecting our AOT distance, as the log likelihood of the standard normal is the \({L}_{2}^{2}\) distance.
where \(L={\mathrm{Dec}}_{\mathrm{Env}}(l)\) .
The output of the environment decoder is the MSQR of the COVET matrix, which is trained to minimize the \({L}_{2}^{2}\) error with the MSQR of the true COVET matrix. Using the AOT metric in this manner involves computing the MSQR of the COVET samples during training, which can be computationally prohibitive. Instead, we first calculate the MSQR of all COVET matrices, which ENVI is directly trained to reconstruct.
We train ENVI simultaneously on samples from both spatial and single-cell datasets, using mini-batch gradient descent on the variational inference loss. With the learned ENVI model, we impute missing genes for the spatial data by treating the latent embedding of the spatial data as if it were from the single-cell data, using the single-cell auxiliary variable and parameterizing as a negative binomial instead of a Poisson. Conversely, we reconstruct spatial context for the single-cell data by applying the ‘environment’ decoder on its latent, as if it was the latent of the spatial data.
In more detail, we train ENVI to optimize the evidence lower bound (ELBO) with a standard normal prior on the latent, with the goal of increasing the likelihood of the observed data \(\{{X}_{\mathrm{sc}},\,{X}_{\mathrm{st}},{\varSigma }_{\mathrm{st}}\}\) for the parameterization of their decoded distributions \(\{{\hat{X}}_{\mathrm{sc}},\,{\hat{X}}_{\mathrm{st}},{\hat{\varSigma }}_{\mathrm{st}}\}\) while minimizing the KL divergence between the latent distribution and \(N(0,1)\) :
To train ENVI to impute missing genes for the spatial data, we generate the latent embedding l st by passing X st through the encoder, and run the latent layer through the ‘expression’ decoder, but with the inverse auxiliary neuron, as if the embedding came from scRNA-seq data:
Similarly, we reconstruct the spatial context for dissociated scRNA-seq samples by passing the scRNA-seq latent embedding l sc through the ‘environment’ decoder:
To allow flexibility in modeling technologies with different count distributions and molecular capture rates, we implemented the normal, Poisson, negative binomial and zero-inflated negative binomial (ZINB) 85 distributions, which can be chosen for either modality to reflect pre-processing steps or varying levels of noise or dropout. The rate or mean parameters ( λ for Poisson, r for NB and ZINB and μ for normal) must all be defined per cell and per gene and shared across the single-cell and spatial data. However, all other parameters can be chosen to be either per cell and per gene or simply per gene and can be either shared between technologies or made distinct.
By default, the encoder and two decoder networks consist of three hidden layers, each with 1,024 neurons. The latent embedding consists of 512 neurons, and the prior coefficient is set to β = 0.3. For small datasets whose total samples size is fewer than 10,000 cells, we recommend increasing the reliance on the prior and set β = 1.0. We train ENVI for two 14 gradient descent steps with the ADAM optimizer 86 with learning rate 10 −3 (lowered to 10 −4 during the last quarter of training steps) and a batch consisting of 1,024 samples, half taken from scRNA-seq and the other half taken from spatial data. To reduce computational complexity, we subset the scRNA-seq dataset to the union of the 2,048 highly variable genes and all genes included in the spatial dataset rather than the full transcriptome.
ENVI training is constant in both time and memory, whereas methods such as Tangram and NovoSpaRc scale quadratically with dataset size and cannot be GPU accelerated on datasets above a few thousand cells. We benchmarked the run times of ENVI, Tangram 9 , NovoSpaRc 26 , gimVI 25 , uniPort 47 , deepCOLOR 48 and Harmony 49 on scRNA-seq datasets of various sizes and on osmFISH, seqFISH, Xenium and MERFISH datasets. All models were trained with their default parameters using a single 12 GB GeForce RTX 2080 GPU, except Tangram, which produced an out-of-memory error above 10,000 cells and was trained with a CPU instead. Model training was stopped prematurely if it exceeded 5 h.
As expected, ENVI’s training time was consistently around 10 min regardless of dataset size (Extended Data Fig. 2b ), and Harmony was also constant in time. gimVI runtime grew linearly with dataset size (the model trained for a predefined number of epochs over the datasets), and NovoSpaRc and Tangram were prohibitively slow on larger spatial and scRNA-seq datasets (they learn a cell-to-cell mapping between the spatial and single-cell datasets). We found that GPU acceleration is not possible for Tangram. deepCOLOR and uniPort were also substantially slower than ENVI at larger cell numbers.
Evaluation of integration quality
Batch average silhouette width (bASW), introduced in a recent benchmarking of batch integration methods for scRNA-seq atlases 41 , evaluates latent integration based on mixing between batches and co-localization of similar cell types within the latent. In brief, bASW computes, for each cell type, how well-mixed batch labels are using the silhouette coefficient and returns the average across all cell types. By treating each modality as a different batch, we could use the bASW score to measure the quality of ENVI’s learned latent. The latent of ENVI is large, consisting of 512 neurons; because silhouette coefficient is affected by the curse of dimensionality, we first compressed the ENVI latent to the top 10 principal components and computed bASW on them.
Benchmarking imputation
We benchmarked ENVI gene imputation following previous approaches 25 , 44 that generate a test set of held-out genes using cross-validation and compared imputed and true expression using Pearson correlation and our spatially aware MSSI metric. We evaluated log expression and imputation profiles, with pseudocount 0.1.
Many algorithms use scRNA-seq data to impute missing genes in spatial transcriptomics data 42 , 43 , 87 , 88 , 89 . We compared ENVI to gimVI, Tangram and uniPort for their competitive performance 44 , 47 , NovoSpaRc for its use of spatial context and optimal transport for data integration, deepCOLOR 48 for its use of a deep generative model and Harmony 49 for its prevalence as a batch correction method 50 .
On the osmFISH dataset, which includes only 33 genes, we performed a full leave-one-out cross-validation by hiding every gene in the imaging panel individually and predicting its expression. On the seqFISH, MERFISH and Xenium datasets, which assay hundreds of genes, we used five-fold cross-validation, whereby the imaged gene set was divided into five random groups, and each model was tested on one withheld group after training on four. To appraise performance, we used a ‘relative’ one-sided t -test, as scores are paired across genes.
We benchmarked all models using their default parameters and instructions on all datasets:
gimVI: We trained for 200 epochs with a batch size of 128 and latent dimension, per author recommendations ( https://docs.scvi-tools.org/en/stable/ ), and parameterized spatial and scRNA-seq datasets with NB and ZINB distributions, respectively. To impute genes with the trained model, we followed manuscript instructions and trained a kNN regression model on the scRNA-seq latent and full transcriptome expression, setting k as 5% of cells in the single-cell dataset. We then applied the regression model on the spatial data latent to predict the expression of unimaged genes.
Tangram: We trained for 1,000 epochs using default parameters ( https://github.com/broadinstitute/Tangram ). For osmFISH, seqFISH and Xenium datasets, we used the default ‘cells’ mode, and, for the much larger MERFISH atlas, we used the ‘cell-type’ mode, per the tutorial. We set the density prior to be uniform, as our spatial benchmark datasets are single-cell resolution. With the learned mapping, we used the ‘project_genes’ function to impute genes from scRNA-seq onto the spatial dataset.
NovoSpaRc: We followed the repository instructions ( https://github.com/rajewsky-lab/novosparc ), using an ‘alpha’ coefficient on a spatial location prior of 0.25 and smoothness parameter ‘epsilon’ of 0.005. To compute the scRNA-seq pairwise distance matrix, we used the union of the 2,048 most variable genes and all genes in the spatial dataset. For spatial datasets consisting of multiple samples, we trained a different model on each sample. Because NovoSpaRc does not scale well to large datasets, we reduced the MERFISH-related scRNA-seq dataset to a tenth of its size, sampling uniformly across each cell type. We applied the learned mapping to impute missing genes using the ‘tissue.sdge’ function.
uniPort: We replicated tutorial instructions for integrating spatial and single-cell datasets ( https://uniport.readthedocs.io/ ) by normalizing each dataset according to library size, log transforming counts, executing the ‘batch_scale’ function, training the model for 30,000 iterations with a ‘lambda_kl’ value of 5.0 and, finally, predicting the expression of hidden genes using the ‘predict’ function.
deepCOLOR: We trained for 500 epochs using default parameters from the tutorial ( https://github.com/kojikoji/deepcolor ). deepCOLOR does not directly impute unimaged genes, so we multiplied the resulting mapping matrix with the scRNA-seq expression of the hidden genes to predict their expression.
Harmony: We treated spatial and single-cell datasets as separate batches and integrated them using the default Harmony implementation in scanpy 90 ( https://scanpy.readthedocs.io/ ). We only included genes from the scRNA-seq data that were also in the spatial data (and removed test genes) to produce Harmony embeddings from the principal components of the concatenated dataset. Mirroring gimVI’s imputation procedure, we performed kNN regression on the Harmony embeddings to reconstruct expression of the manually hidden genes.
Impact of data sparsity on ENVI
To validate ENVI’s robustness to single-cell or count data sparsity, which can affect integration 9 , we benchmarked the full embryogenesis seqFISH and scRNA-seq data against random subsampling to 90% or 80% of counts according to the binomial distribution. For all three datasets, we performed five-fold cross-validation (see the ‘Benchmarking imputation’ subsection for details), finding that removing even 20% of counts does not greatly impact ENVI performance, which still surpasses Tangram on the full dataset (Extended Data Fig. 2c ). Using a kNN ( k = 5) classifier trained to predict cell type from the scRNA-seq latent space, we assigned labels to the seqFISH data and measured balanced accuracy compared to the original assignment, finding that the ENVI latent space remains reliable upon downsampling; datasets with higher sparsity are only slightly less accurate (Extended Data Fig. 2d ).
FDL and DCs
FDL 58 and DCs 32 capture and visualize continuous trends in single-cell data 19 . We calculated FDL following the implementation in Van Dijk et al. 91 , by computing a kNN matrix (using default k = 30), converting to an affinity matrix using an adaptive Gaussian kernel with width = 30 and k = 10, symmetrizing the matrix and using the ForceAtlas 92 function ‘force_directed_layout’ for visualization. DC computation followed a similar process to compute a data affinity matrix, except that we multiplied the affinity matrix by the inverse of its degree matrix to compute the normalized Laplacian. The eigenvectors of the Laplacian matrix, in order of eigenvalue magnitude, are the DCs.
Applying ENVI to seqFISH embryogenesis data
We started with pre-processed data from the E8.75 mouse gastrulation study 37 , which included 351 genes measured with seqFISH (57,536 imaged cells), and paired it with E8.5 scRNA-seq data (12,995 cells) from a second study 38 . We further processed the scRNA-seq data by removing mitochondrial genes, genes expressed in less than 1% of cells, cells with library size greater than 33,000 (set manually to match the knee point) and cells annotated as ‘nan’ or representing doublets. To avoid confounding batch effects 50 , we used only the largest scRNA-seq batch (labeled ‘3’). For the seqFISH dataset, we used only the first of three imaged embryos (‘embryo1’), removed cells with abnormally high total expression (threshold set manually to 600) and removed the gene Cavin3 , which did not appear in the scRNA-seq dataset. For both datasets, we used cell type annotations provided by the authors and visualized the seqFISH data using spatial coordinates and the scRNA-seq data using a UMAP embedding (Fig. 2a ). We also renamed several cell types to resolve nomenclature differences, including changing presomitic mesoderm to somitic mesoderm and splanchnic mesoderm to pharyngeal mesoderm.
We trained ENVI on the union of the 2,048 most variable genes in the scRNA-seq data, all seqFISH-measured genes, all HOX genes and several organ markers (Supplementary Table 1 ) using default parameters. We visualized the learned latent posterior of the seqFISH and scRNA-seq datasets using UMAP and found that cell types tend to co-embed regardless of modality (Fig. 2b ).
To test the imputation of unimaged canonical organ markers Ripply3 (ref. 51 ) (lung), Nkx2-5 (ref. 52 ) (heart) and intestine Tlx2 (ref. 53 ) (intestine), we visualized their imputed z-scored, logged expression and thresholded values lower than 2, finding almost exclusive expression in the correct organ (Fig. 2f ). To confirm that expression in the correct location at E8.75, before organ formation, we imaged each marker gene using whole-mount HCR (Fig. 2f ; see the ‘Whole-mount HCR’ subsection). HCR produces per-gene three-dimensional (3D) images, which we oriented coronally to match the seqFISH data. We similarly trained gimVI and Tangram on the complete scRNA-seq and seqFISH datasets to impute Ripply3 , Nkx2-5 and Tlx2 and visualized as for ENVI imputation, finding that ENVI imputation more closely matches the experimental data (Extended Data Fig. 3b ).
Spatial organization of emerging organs
At E8.5, scRNA-seq cell clusters correspond to primordial endodermal organs, ordered by where they will later emerge along the gut tube 55 . We identified organ-specific gene sets (Supplementary Table 2 ) by using the ‘rank_genes’ function in scanpy 91 to apply a Wilcoxon test for differentially expressed genes in each organ in a reference scRNA-seq endodermal atlas 55 . Thymus and thyroid are not well delineated at this stage, so we collapsed them into a single thymus/thyroid label, and we assigned small intestine and colon cells to a single ‘intestine’ label to avoid inconsistencies, as the seqFISH tissue section does not include colon 37 .
We used PhenoGraph to cluster the scRNA-seq gut tube cells into 12 clusters and labeled clusters by best matching organ based on z-scored and logged expression of each gene set, averaged across all cells in that cluster. Most clusters are highly distinct, whereas some co-express several programs. We labeled clusters for which the (z-scored) ratio between the highest and second-highest expressed gene set is above 1.5 with the most highly expressed organ. To assign ambiguous clusters with ratios below 1.5, we inspected marker expression individually:
Cluster5: Thymus/thyroid gene set expression is highest, but because lung marker Ripply3 (ref. 51 ) and Irx1 (ref. 93 ) expression is high (average z-score logged expression, 0.90) while thymus/thyroid marker Nkx2-1 (refs. 52 , 94 ) is low (−0.15), we labeled Cluster5 as ‘dorsal lung’ (second-highest expressing organ).
Cluster6: Dorsal lung gene set expression is highest, with pancreas a close second. Because the cluster has minimal Ripply3 and Irx1 expression (0.18) but is enriched for pancreas marker Pdx1 (ref. 95 ) expression (0.43), we labeled Cluster6 cells as pancreas.
Cluster7: Pancreas and liver gene set expression is highest and second highest, respectively. Due to high Pdx1 expression (0.99) and low liver marker Ppy 96 expression (−0.12), we kept the pancreas label for this cluster.
We inferred COVET representations for the scRNA-seq gut tube cells using the trained ENVI model and then measured pairwise AOT distances between the conjoined set of seqFISH and scRNA-seq COVET matrices to generate UMAP embeddings and PhenoGraph clusters. The data generated seven COVET clusters (CC0–CC7), which are highly congruent with emerging organs in the scRNA-seq data, indicating their spatial delineation (Extended Data Fig. 4a ): thymus/thyroid cells were assigned to CC0 (75%) or the spatially proximal CC1 (17%); dorsal lung cells were assigned to CC1 (52%) or CC0 (36%); ventral lung cells were assigned to CC2 (62%) or the highly related clusters CC1 (12%) or CC3 (19%); liver cells were assigned to CC2 (94%); pancreas cells were assigned to CC3 (58%) or the related cluster CC2 (26%); and intestine cells were assigned almost entirely to CC4–CC7, with only 1% assigned to CC3.
Gut tube cells in the seqFISH data were assigned organ labels via their COVET representations. We fit an AOT metric kNN classifier ( k = 5) on the scRNA-seq ENVI COVET matrices and their organ labels and used the classifier to assign budding organ labels to seqFISH COVET (Fig. 3a ). Projecting labels back onto their seqFISH coordinates reveals the spatial pattern of organogenesis, from thymus/thyroid to the lung compartments, liver, pancreas and intestine and colon from anterior to posterior (Fig. 3b ).
To calculate average COVET matrices predicted by ENVI (scRNA-seq organs) or measured directly (seqFISH), we compute the AOT average for the matrix set by calculating the matrix square of mean of their MSQR:
Mean COVET matrices are highly congruent between the two datasets for both dorsal and ventral lung cells, although the scRNA-seq COVET matrices are slightly smoother as they were inferred by ENVI rather than measured (Fig. 3c ). To find gene groupings, we performed hierarchal clustering on the 64 genes in each mean COVET matrix, finding that Dlk1 , Gata4 , Gata5 , Aldh1a2 and Foxf1 covary in the ventral lung COVET but not in the dorsal lung, whereas Tagln , Six3 , Thbs1 , T and Epcam1 exhibit the opposite pattern.
We generated clusters of each COVET matrix by plotting their average expression in cells near the anterior gut tube (fewer than 50 distance units away), but not the gut tube itself, and found that ventral niche genes are enriched in the pharyngeal mesoderm, whereas dorsal niche genes localize to brain and cranial mesoderm (Fig. 3d ). As pharyngeal mesoderm is ventral to the gut, and brain and cranium are dorsal, the uniquely covarying genes in the COVET matrices allow us to reconstruct each lung compartment’s spatial context.
We also assigned budding organs using integration methods that do not model spatial context (gimVI and Tangram) and computed ENVI without COVET to highlight the importance of explicit modeling of microenvironment:
gimVI: We trained gimVI on the full embryogenesis scRNA-seq and seqFISH datasets using defaults in the ‘Benchmarking imputation’ subsection (10 latent dimension, 200 epochs, NB for spatial and ZINB for single cell). We took the subset of gut tube cells in each modality from the learned latent embedding of scRNA-seq and seqFISH data and similarly learned a kNN classifier ( k = 5) from the single-cell latent and organ assignment, using it to predict labels on the spatial latent.
Tangram: Using parameters in the ‘Benchmarking imputation’ subsection (1,000 epochs, uniform density prior, ‘cells’ mode), we trained Tangram to learn a mapping matrix from scRNA-seq to spatial data. We subset the Tangram matrix to the mapping from scRNA-seq gut tube to seqFISH gut tube cells and re-normalized the columns to sum to 1. We transferred organ labels using Tangram’s ‘ project_cell_annotations’ function, which uses the subsetted mapping matrix to calculate the probability of each organ being assigned to each seqFISH gut tube cell, and we labeled according to the most probable organ.
ENVI without COVET: We retrained ENVI to solely reconstruct gene expression profiles, excluding any COVET-related information. A kNN classifier ( k = 5) on the learned latent was used to transfer organ labels from the scRNA-seq gut tube onto seqFISH cells.
Due to the lack of gene vocabulary and small number of gut tube cells, other methods could not assign labels as reliably as ENVI: gimVI failed to delineate dorsal lung from thymus/thyroid cells and missed almost all liver cells, and Tangram’s labeling lacked coherent spatial structure (Extended Data Fig. 4b ). Without COVET, ENVI was unable to distinguish between ventral lung and liver, although its results most closely resembled the COVET-based assignment and known organ organization.
ENVI robustness to neighborhood size
The optimal number of neighbors used to construct COVET depends on dataset features and desired analysis (see the ‘Spatial covariance representation’ subsection), but ENVI is nevertheless robust to variations of this parameter. For the seqFISH dataset, we calculated COVET matrices with k = 6, 8, 10 or 12 nearest neighbors (original, k = 8) and retrained ENVI on each representation. For each of the four ENVI models, we assigned organ labels onto the seqFISH gut tube cells, again using a kNN classifier on COVET matrices in AOT space. Despite doubling neighborhood size and inherent stochasticity in training deep learning models with batch gradient descent, all versions reliably assigned cells to spatial context (Extended Data Fig. 5a ). Although there are some differences, even the worst-performing mode ( k = 6), which mislabeled many dorsal lung cells as thymus/thyroid, is more accurate than competing methods (Extended Data Fig. 4b ).
AP polarity of developing spine and NMP cells
Spinal cord cells and their NMP precursors in the seqFISH data (total, 2,830 cells) span the embryo AP axis and make up a substantial fraction of cells in the scRNA-seq data (1,289 cells, 10% of total). To gauge whether ENVI can correctly map these cells and spatial trends along the AP axis, we first combined empirical seqFISH and ENVI-inferred scRNA-seq COVET matrices from spine and NMP cells and computed DCs via eigendecomposition of the Laplacian of the AOT kNN ( k = 30) graph in COVET space. We then compared to DCs of spatial coordinates of seqFISH spine and NMP cells, calculated using a kNN ( k = 30) graph with standard Euclidean distance, finding that pseudo-AP coordinates based on COVET DC are highly congruent with true AP coordinates based on seqFISH DCs (Fig. 4b and Extended Data Fig. 6a ) and (logged) expression of known posterior and anterior genes (Fig. 4c,d ).
We attempted to reconstruct pseudo-AP axes for gimVI and Scanorama. For gimVI, we used the model trained on the complete embryogenesis datasets and subset the learned gimVI combined latent to only the spine and NMP cells from the spatial and scRNA-seq datasets. We calculated the top three DCs from the latent embeddings and found that DC 2 was most correlated with true AP polarity (seqFISH spine and NMP cells), r = 0.76. Scanorama is designed for batch integration and uses mutual nearest neighbors to directly correct the gene expression count matrix and remove batch effect. Following scanpy instructions ( https://scanpy.readthedocs.io/en/stable/ ), we applied Scanorama to produce integrated count matrices of the seqFISH and scRNA-seq spine and NMP cells. We computed DCs from the combined Scanorama-corrected scRNA-seq and spatial datasets and found that DC 3 is most correlated with true AP, r = 0.70. Unlike ENVI, both of these methods produced spine and NMP cells in the posterior with low DC values (Extended Data Fig. 6b ). We note that, because DC order is arbitrary, we reversed any DC negatively correlated with the true AP. Tangram was excluded from this analysis as it does not calculate a combined embedding from which we can recover a pseudo-AP axis.
To assess the accuracy of pseudo-AP mapping, we ordered scRNA-seq spinal cells by pseudo-AP value and examined expression of canonical markers Rfx4 (ref. 63 ) (anterior), Hoxaas3 (ref. 61 ) (posterior) and Hoxb7 (ref. 64 ) (posterior) (Fig. 4e ). Gene expression values were logged and z-scored, and ordered profiles were smoothed with a first-order Savitzky–Golay filter with window size 128 for visual clarity.
To determine the quality of the pseudo-AP axis predicted for scRNA-seq spinal cells by each method, we calculated its correlation with the logged expression of known posterior genes Hoxaas3 , Hoxb5os 61 , Hoxb9 (ref. 60 ), Hoxb7 and Tlx2 (ref. 97 ) and anterior genes Foxa3 (refs. 98 , 99 ), Hoxd3 (ref. 59 ), Hoxa2 (refs. 100 , 101 , 102 ), Rfx4 and Hoxd4 (Extended Data Fig. 6c ), providing a quantitative recapitulation of pseudo-AP-ordered expression (Fig. 4e ).
Inferring Sst neuron cortical depth with MERFISH
We used the BICCN’s 252-gene MERFISH primary motor cortex atlas 40 and its matching scRNA-seq reference 70 to demonstrate ENVI in a tissue-wide context. For the single-cell data, we removed cells lacking cell type annotations or labeled as doublets or low quality, leaving 71,183 cells across three samples, and removed genes that both (1) appear in less than 5% of cells and (2) are not in the MERFISH panel. For the MERFISH data, we included all 12 samples, for a total of 276,556 cells from 64 motor cortex slices, and we removed cells lacking a cell type label and the genes Crispld2 and Igf2 , as they were absent from the scRNA-seq data, but avoided any additional pre-processing. Both spatial and scRNA-seq datasets were labeled into neuronal and non-neuronal cell types. For brevity and consistency between datasets, we relabeled the MERFISH GABAergic neurons from ‘Sst-chodl’ to ‘Sst’ and collapsed the ‘PVM’, ‘macrophage’ and ‘microglia’ labels to ‘microglia’.
We used five-fold cross-validation to benchmark ENVI imputation against Tangram, gimVI, NovoSpaRc, uniPort, Harmony and deepCOLOR with default parameters (see the ‘Benchmarking imputation’ subsection), except that we applied Tangram with ‘cell-type’ mode, which averages single-cell data per cell type, and ran NovoSpaRc independently for each slice, subsampling scRNA-seq data to 10% of each original size, because these methods do not otherwise scale to these data. ENVI MSSI and Pearson correlations were significantly higher than other methods (Extended Data Fig. 7a ), and ENVI imputation of unimaged genes matches ISH from the Allen Brain Atlas (Extended Data Fig. 7b ).
The full transcriptome information in scRNA-seq data allowed finer subtyping than the 22 cell types in the MERFISH dataset. Specifically, we further divided the Sst interneurons into nine subtypes and extracted gene sets for each subtype using the scanpy ‘rank_genes_group’ function. For the subset of MERFISH genes present in each gene set, we calculated average expression in every MERFISH Sst cell. We measured the pairwise correlation between gene sets within each modality and found that each subtype was delineated much more specifically in the single-cell data (Fig. 5b ). We quantified this by computing the per-gene-set entropy across the pairwise correlation matrix, after normalizing with ‘softmax’. The entropy for each gene set was higher in the MERFISH data, demonstrating the lack of distinction between subtypes.
To map the labeled scRNA-seq Sst interneurons to their cortical depth, we embedded ENVI-imputed scRNA-seq COVET matrices and MERFISH COVET matrices into DCs and FDL via a kNN graph ( k = 100) on AOT distance. The first COVET DC corresponds to pseudodepth and matches the cortical depth of MERFISH cells visualized on a single slice (Fig. 5c ) and aligns with the primary axis of the COVET FDL (Fig. 5d ). For each scRNA-seq Sst neuron, we predicted cortical depth using the pseudodepth axis (COVET DC 1), grouped the results by subtype and plotted their distribution (Fig. 5e ).
osmFISH imaging of somatosensory cortex
We applied ENVI to a 33-gene osmFISH dataset (4,530 cells, one sample) and complementary scRNA-seq dataset (30,005 cells) of the somatosensory cortex 3 , using the authors’ cell type annotations and no additional processing besides removing genes expressed in less than 1% of cells in the scRNA-seq data (Extended Data Fig. 8a ). As osmFISH data are more dispersed than MERFISH and seqFISH (Extended Data Fig. 2a ), we modeled them with the negative binomial instead of the Poisson distribution. Due to the limited size of the scRNA-seq dataset, we changed its parameterizing distribution from NB to ZINB. Because the total sample size is small (fewer than 10,000 cells), we also increased the reliance on the prior latent distribution and increased the regularization to β = 1.0, which is common practice in Bayesian modeling.
We visualized and compared the ENVI and gimVI learned latent spaces with a UMAP embedding labeled by cell type annotations from the osmFISH and scRNA-seq datasets. The ENVI embedding separates distinct cell types, with similar labels from the two data modalities occupying similar spaces (Extended Data Fig. 8b ), whereas gimVI confuses oligodendrocytes and pyramidal neurons and cannot accurately co-embed osmFISH and scRNA-seq endothelial cells (Extended Data Fig. 8c ).
We quantified integration quality and calculated the average center-of-mass embedding for each cell type, from both seqFISH and MERFISH datasets, in the gimVI and ENVI embedding spaces. ENVI and gimVI latent dimensions are vastly different in size (512 for ENVI compared to only 10 for gimVI), so we normalized each column in the pairwise distance to a maximum value of 1. In the ENVI latent, the center of mass for each osmFISH cell type is distinctly closer to its counterpart in the scRNA-seq data compared to other cell types, whereas cell types are less well separated in the gimVI latent (Extended Data Fig. 8d ). For each cell in the scRNA-seq data, we quantified this as the ratio of its five osmFISH nearest neighbors in the latent space that share its cell type and averaged across the six cell types. The latent cell type agreement was 0.58 for ENVI and 0.38 for gimVI.
Using leave-one-out cross-validation, ENVI outperformed alternative methods on spatial imputation (Extended Data Fig. 8e ). We further imputed the expression of three unimaged genes onto the osmFISH dataset using the full ENVI model (Extended Data Fig. 8f ) and validated by comparing them to Allen Brain Atlas ISH images of the somatosensory cortex. ENVI imputation and ISH images both specify Dti4l , Rprm and Ndst expression in the L2/3, L5–L6 and CA1 regions, respectively. The Allen Brain Atlas provides both raw ISH images and processed, cell-segmented expression profiles. Because each view is difficult to interpret on its own, we overlaid the processed profiles on top of the raw ISH images for clarity.
Xenium data analysis of LM
We assayed a slice of mouse brain bearing a LM of melanoma using snRNA-seq and Xenium (see the ‘Generation of mouse melanoma LM FFPE-snRNA-seq and Xenium datasets’ subsection). Raw Xenium imaging data were processed using the default pipeline provided by 10x Genomics 16 to produce a segmented cell-by-gene count matrix. In brief, nuclear segmentation was applied on DAPI stains, and all RNA molecules in each segmented mask and within a 15-μm dilation were assigned to cells to compose a count matrix.
We further filtered the Xenium data by removing cells with library size less than 10 and more than 300 and kept only genes that were in the snRNA-seq data. For the snRNA-seq data, we only kept cells with library size less than 10,000 and removed mitochondrial genes and any gene expressed in less than 5% of cells, unless it was in the Xenium panel. Finally, we removed any doublets predicted by DoubletDetection 103 from either dataset, followed by median library size normalization on the snRNA-seq data. This process resulted in 243 genes captured in 74,132 cells in the Xenium dataset and 9,230 genes sequenced in 9,870 cells by snRNA-seq.
To assign cells to cell types, we independently clustered each dataset with PhenoGraph and searched for per-cluster marker genes using the scanpy ‘rank_genes_groups’ function. We first labeled Xenium data by neuron, endothelium, oligodendrocyte, tumor, astrocyte and immune/fibroblast groups. We then reclustered neurons and annotated into excitatory and inhibitory compartments according to expression of Slc17a7 and Gad1 and separated immune/fibroblast into immune cells and fibroblasts. The snRNA-seq data followed a similar hierarchical process, except that fibroblasts and immune cells were distinguished in the first round of clustering. According to an independently curated set of genes for each group 75 , our cell typing matched known transcriptional markers (Extended Data Fig. 9a ). We benchmarked ENVI imputation against competing methods as for other methods (see the ‘Benchmarking imputation’ subsection), finding that ENVI outperforms all methods except for Harmony according to Pearson correlation but does equally well according to MSSI (Extended Data Fig. 9b ).
To evaluate cell type label transfer from snRNA-seq to Xenium data for ENVI, gimVI, Harmony, deepCOLOR and uniPort, we fitted a kNN ( k = 5) classifier on the snRNA-seq latent to predict cell type labels and used it to assign labels to the Xenium data. Tangram does not use a latent, so we used its ‘project_cell_annotations’ function and labeled each Xenium cell according to the most probable snRNA-seq cell type mapped to it. NovoSpaRc does not assign cell type labels and was not compared. We measured transfer accuracy with balanced accuracy, the per-cell-type arithmetic mean of precision and recall, averaged across cell types (Extended Data Fig. 9c ). ENVI transferred information as accurately as Tangram, uniPort and Harmony and was only slightly superseded by gimVI, validating our cell type annotation label transfer.
To uncover the relationship between phenotype and environment for each cell type in the Xenium dataset, we measured the agreement between clusters derived from expression (phenotype) and COVET representations (environment). Because non-parametric methods are biased by sample size, for each cell type we performed k -means ( k = 5) clustering on the logged expression of its cells and separately on its COVET representations. Each clustering was performed 10 times with random starting points. For each cell type, pairwise adjusted Rand index (ARI) was computed between each expression and COVET clustering, for a total of 100 values, and we reported their mean (Fig. 6c ).
Unlike excitatory neurons, whose localization pattern is mirrored in their transcriptional profiles, the niche of immune cells in the Xenium dataset (canonically either brain-resident microglia or tumor-colonizing macrophages 75 ) was not reflected in their gene expression. We attempted to divide the immune cells into macrophages or microglia (Supplementary Table 4 ) by computing the average logged expression of each cell type marker gene set in PanglaoDB 75 for every immune cell in the snRNA-seq and Xenium datasets, using only the subset of genes present in the Xenium assay (Fig. 6d ). The high degree of overlap between macrophage and microglia genes in the spatial data may explain why, unlike the snRNA-seq data, expression and microenvironment corresponded poorly for immune cells.
We mapped annotated snRNA-seq immune cells to spatial context using the COVET predictions from ENVI. PhenoGraph clustering of snRNA-seq and Xenium immune COVET representations revealed major microenvironment clusters C0, representing immune cells in the cortex surrounded by excitatory neurons, with 80% of snRNA-seq cells annotated as microglia; C1, representing immune cells in the basal ganglia, dominated by inhibitory neuron environments, with 80% of snRNA-seq cells annotated as microglia; and C2, representing cells in and around the tumor, with 90% of snRNA-seq cells annotated as macrophages. These strong associations predict that macrophages are localized to the tumor and its boundary, whereas microglia localize mainly to basal ganglia and cortex, recapitulating the known tendency for brain tumors to recruit bone-marrow-derived macrophages 76 , 77 .
For further interpretability, ENVI can also invoke the inferred COVET representations and explicitly predict the microenvironment composition of each snRNA-seq cell. For each cell in the Xenium dataset, we counted the instance of each cell type within its k = 8 nearest neighbor microenvironment, resulting in a n Xenium by | C | matrix titled M , where | C | = 8 is the number of distinct cell types. We then fit a kNN ( k = 5) regression model to predict M from COVET representations of the Xenium data. The trained model was applied to the COVET matrices that ENVI predicted for the snRNA-seq data to infer the distribution of cell types in each cell’s niche. As for COVET-based clusters, macrophage niches predicted from the snRNA-seq data were highly enriched for tumor cells, whereas microglia niches contained more inhibitory neurons and oligodendrocytes (Fig. 6g ).
ENVI can also be extended to identify markers of different macrophage types. Remsik et al. 75 identified Ccr2 , Ms4a4c and Lst1 as infiltrating monocyte markers based on cellular indexing of transcriptomes and epitopes by sequencing (CITE-seq) analysis. ENVI imputation of these genes on Xenium immune cells is indeed specific to cells within the tumor (Fig. 6h ).
Despite accurately transferring cell type information and imputing missing genes (Extended Data Fig. 9b,c ), the absence of direct spatial modeling prevents gimVI and Harmony from reliably inferring subtype-specific microenvironments. We clustered gimVI embeddings of snRNA-seq and Xenium immune cells and found no obvious tumor-related cluster; 90% of snRNA-seq macrophages and 68% of microglia were assigned to gimVI cluster C1, prohibiting clear assignment of subtype to microenvironment (Extended Data Fig. 9d,e ). Similarly, gimVI imputation of tumor infiltration genes did not distinctly enrich for immune cells within the tumor, and, despite outperforming ENVI according to Pearson correlation on imaged genes, Harmony also failed to accurately impute the expression of tumor-infiltrating markers (Extended Data Fig. 10 ).
Experimental methods
Whole-mount hcr.
Whole-mount HCR mRNA in situ was performed as described previously 54 , with minor modifications 104 . Mid-gestation embryos at E8.75 were treated with 10 µg ml −1 proteinase K for 5 min at room temperature, followed by washing and post-fixation in 4% paraformaldehyde (PFA) for 20 min. Embryos were incubated in hybridization buffer supplemented with 2 pmol of each probe ( Ripply3 , Nkx2-5 or Tlx2 ) overnight at 37 °C, followed by an amplification step with 60 pmol of each fluorophore-conjugated hairpin for 12–16 h at room temperature. Embryos were then stained with 0.5 µg ml −1 DAPI (Thermo Fisher Scientific) and cleared using a modified Ce3D+ clearing protocol 105 for 24–48 h. Images were acquired on a Nikon A1R laser scanning confocal microscope with a ×10 objective and 3.0-µm z -step size. Image rendering and optical sections were generated using IMARIS (version 9.9.0, BitPlane). All probes, hairpins and buffers were designed by and purchased from Molecular Instruments.
Generation of mouse melanoma LM FFPE-snRNA-seq and Xenium datasets
Animal studies were approved by the Memorial Sloan Kettering Cancer Center Institutional Animal Care and Use Committee under protocol 18-01-002. Mice were housed in specific pathogen-free conditions, in an environment with controlled temperature and humidity, on a 12-h light/dark cycle (lights on/off at 6:00/18:00), and with access to regular chow and sterilized tap water ad libitum. For this study, an 8-week-old female C57Bl/6- Tyr c-2 mouse (The Jackson Laboratory, 000058, albino C57Bl/6) was injected with 500 B16 LeptoM cells intracisternally, as described in Remsik et al. 74 . Two weeks after the injection, the mouse was deeply anaesthetized and transcardially perfused with PBS (MSK Media Core). Tissues, including the brain, were dissected and immediately placed into a tube containing histology-grade PFA (4%; Sigma-Aldrich, HT501128). After overnight incubation, tissue was rinsed with water and submerged in 70% ethanol. The brain was cut coronally into four 2–3-mm-thick sections, placed into a tissue cartridge and embedded in formalin using routine, automated procedure. The embedded tissue was stored at room temperature.
For snRNA-seq-FFPE, a 100-μm-thick section of tissue was pre-processed on a prototype Singulator system. The sample was automatically processed in a NIC+ cartridge (S2 Genomics, 100-215-389) by three 10-min deparaffinization steps (CitriSolv, VWR), rehydrated by successive 1-ml washes of 100%, 100%, 70%, 50% and 30% ethanol and followed by two washes of PBS. The sample was then spun at 1,000 g for 3 min and resuspended in 0.5 ml of Nuclei Isolation Reagent (NIR, S2 Genomics, 100-063-396) with 0.1 U ml −1 RNase inhibitor (Protector, MilliporeSigma, 3335399001); all subsequent solutions had RNase inhibitor. The sample was dissociated to single nuclei in a second NIC+ cartridge with 2 ml of NIR for 10 min, followed by a 2-ml wash with Nuclei Storage Reagent (NSR, S2 Genomics, 100-063-405). The single-nucleus suspension was spun at 500 g for 5 min, resuspended in NSR and counted, and then snRNA-seq was performed on the Chromium instrument (10x Genomics) following the user guide manual for Chromium Fixed RNA Kit, Mouse Transcriptome (SinglePlex). Final libraries were sequenced on an Illumina NovaSeq S4 (R1: 28 cycles; i7: eight cycles; R2: 90 cycles).
To perform Xenium spatial profiling, FFPE mouse brain tissue adjacent to that used for snRNA-seq was sectioned into 5-μm-thick slices with a microtome and placed onto the sample area of a Xenium slide (10x Genomics). Profiling was conducted following the 10x Genomics User Guide ( CG000578 , CG000580 and CG000582 ). In brief, tissue slices were baked at 42 °C for 3 h and stored overnight in a desiccating chamber. The tissue was then deparaffinized, serially rehydrated and de-crosslinked, before overnight hybridization with gene-specific padlock probes (Mouse Brain Panel, 10x Genomics). After this, the probes were ligated and amplified to generate the rolling circle amplification (RCA) product, which was then prepared for imaging with the Xenium. Before imaging, tissue autofluorescence was suppressed, and DAPI was applied as counterstain. The Xenium was loaded with the necessary reagents for decoding the RCA products, in conjunction with the selection of regions of interest for imaging based on the DAPI images captured by the Xenium.
Reporting summary
Further information on research design is available in the Nature Portfolio Reporting Summary linked to this article.
Data availability
Raw sequencing data and processed count matrices for snRNA-seq from brain tissue bearing a leptomeningeal metastasis are publicly available in the Gene Expression Omnibus ( https://www.ncbi.nlm.nih.gov/geo/ ) under accession number GSE246395 . Segmented and processed Xenium data are publicly available through Zenodo ( https://zenodo.org/ ) under accession number 10712720 .
Code availability
ENVI and COVET are available as Python packages at https://github.com/dpeerlab/ENVI and can be directly installed via ‘pip’ with the command ‘pip install scENVI’. A Jupyter notebook with an ENVI tutorial that reproduces motor cortex MERFISH results is available at github.com/dpeerlab/ENVI/blob/main/MOp_MERFISH_tutorial.ipynb .
Moffitt, J. R., Lundberg, E. & Heyn, H. The emerging landscape of spatial profiling technologies. Nat. Rev. Genet. 23 , 741–759 (2022).
Article CAS PubMed Google Scholar
Keren, L. et al. A structured tumor-immune microenvironment in triple negative breast cancer revealed by multiplexed ion beam imaging. Cell 174 , 1373–1387 (2018).
Article CAS PubMed PubMed Central Google Scholar
Codeluppi, S. et al. Spatial organization of the somatosensory cortex revealed by osmFISH. Nat. Methods 15 , 932–935 (2018).
Moffitt, J. R. et al. High-throughput single-cell gene-expression profiling with multiplexed error-robust fluorescence in situ hybridization. Proc. Natl Acad. Sci. USA 113 , 11046–11051 (2016).
Shah, S. et al. Dynamics and spatial genomics of the nascent transcriptome by intron seqFISH. Cell 174 , 363–376 (2018).
Atta, L. & Fan, J. Computational challenges and opportunities in spatially resolved transcriptomic data analysis. Nat. Commun. 12 , 5283 (2021).
Fang, S. et al. Computational approaches and challenges in spatial transcriptomics. Genomics Proteomics Bioinformatics 21 , 24–47 (2023).
Article PubMed Google Scholar
Goltsev, Y. et al. Deep profiling of mouse splenic architecture with CODEX multiplexed imaging. Cell 174 , 968–981 (2018).
Biancalani, T. et al. Deep learning and alignment of spatially resolved single-cell transcriptomes with Tangram. Nat. Methods 18 , 1352–1362 (2021).
Article PubMed PubMed Central Google Scholar
Wu, Z. et al. Graph deep learning for the characterization of tumour microenvironments from spatial protein profiles in tissue specimens. Nat. Biomed. Eng. 6 , 1435–1448 (2022).
Fischer, D. S., Schaar, A. C. & Theis, F. J. Modeling intercellular communication in tissues using spatial graphs of cells. Nat. Biotechnol. 41 , 332–336 (2023).
Moffitt, J. R. et al. Molecular, spatial, and functional single-cell profiling of the hypothalamic preoptic region. Science 362 , eaau5324 (2018).
Shah, S., Lubeck, E., Zhou, W. & Cai, L. In situ transcription profiling of single cells reveals spatial organization of cells in the mouse hippocampus. Neuron 92 , 342–357 (2016).
Merritt, C. R. et al. Multiplex digital spatial profiling of proteins and RNA in fixed tissue. Nat. Biotechnol. 38 , 586–599 (2020).
Liu, J. et al. Concordance of MERFISH spatial transcriptomics with bulk and single-cell RNA sequencing. Life Sci. Alliance 6 , e202201701 (2023).
Janesick, A. et al. High resolution mapping of the tumor microenvironment using integrated single-cell, spatial and in situ analysis. Nat. Commun. 14 , 8353 (2023).
Lee, J. H. et al. Fluorescent in situ sequencing (FISSEQ) of RNA for gene expression profiling in intact cells and tissues. Nat. Protoc. 10 , 442–458 (2015).
Eng, C.-H. L. et al. Transcriptome-scale super-resolved imaging in tissues by RNA seqFISH. Nature 568 , 235–239 (2019).
Haghverdi, L., Büttner, M., Wolf, F. A., Buettner, F. & Theis, F. J. Diffusion pseudotime robustly reconstructs lineage branching. Nat. Methods 13 , 845–848 (2016).
Bendall, S. C. et al. Single-cell trajectory detection uncovers progression and regulatory coordination in human B cell development. Cell 157 , 714–725 (2014).
Setty, M. et al. Characterization of cell fate probabilities in single-cell data with Palantir. Nat. Biotechnol. 37 , 451–460 (2019).
Gaublomme, J. T. et al. Single-cell genomics unveils critical regulators of Th17 cell pathogenicity. Cell 163 , 1400–1412 (2015).
Azizi, E. et al. Single-cell map of diverse immune phenotypes in the breast tumor microenvironment. Cell 174 , 1293–1308 (2018).
Geltink, R. I. K., Kyle, R. L. & Pearce, E. L. Unraveling the complex interplay between T cell metabolism and function. Annu. Rev. Immunol. 36 , 461–488 (2018).
Lopez, R. et al. A joint model of unpaired data from scRNA-seq and spatial transcriptomics for imputing missing gene expression measurements. Preprint at arXiv https://doi.org/10.48550/arXiv.1905.02269 (2019).
Nitzan, M., Karaiskos, N., Friedman, N. & Rajewsky, N. Gene expression cartography. Nature 576 , 132–137 (2019).
Kingma, D. P. & Welling, M. Auto-encoding variational Bayes. Preprint at arXiv https://doi.org/10.48550/arXiv.1312.6114 (2013).
Sohn, K., Yan, X. & Lee, H. Learning structured output representation using deep conditional generative models. In Proc. of the 28th International Conference on Neural Information Processing Systems 3483–3491 (MIT Press, 2015).
Dowson, D. C. & Landau, B. V. The Fréchet distance between multivariate normal distributions. J. Multivar. Anal. 12 , 450–455 (1982).
Article Google Scholar
Choi, E. & Lee, C. Feature extraction based on the Bhattacharyya distance. Pattern Recognit. 36 , 1703–1709 (2003).
Levine, J. H. et al. Data-driven phenotypic dissection of AML reveals progenitor-like cells that correlate with prognosis. Cell 162 , 184–197 (2015).
Coifman, R. R. Special issue on diffusion maps. Appl. Comput. Harmon. Anal. 21 , 3 (2006).
McInnes, L., Healy, L., Saul, N. & Großberger, L. UMAP: Uniform Manifold Approximation and Projection. J. Open Source Soft. 3 , 861 (2018).
Lotfollahi, M. et al. Mapping single-cell data to reference atlases by transfer learning. Nat. Biotechnol. 40 , 121–130 (2022).
Lopez, R., Regier, J., Cole, M. B., Jordan, M. I. & Yosef, N. Deep generative modeling for single-cell transcriptomics. Nat. Methods 15 , 1053–1058 (2018).
Hafemeister, C. & Satija, R. Normalization and variance stabilization of single-cell RNA-seq data using regularized negative binomial regression. Genome Biol. 20 , 296 (2019).
Lohoff, T. et al. Integration of spatial and single-cell transcriptomic data elucidates mouse organogenesis. Nat. Biotechnol. 40 , 74–85 (2022).
Pijuan-Sala, B. et al. A single-cell molecular map of mouse gastrulation and early organogenesis. Nature 566 , 490–495 (2019).
Alon, S. et al. Expansion sequencing: spatially precise in situ transcriptomics in intact biological systems. Science 371 , eaax2656 (2021).
Zhang, M. et al. Spatially resolved cell atlas of the mouse primary motor cortex by MERFISH. Nature 598 , 137–143 (2021).
Luecken, M. D. et al. Benchmarking atlas-level data integration in single-cell genomics. Nat. Methods 19 , 41–50 (2022).
Abdelaal, T., Mourragui, S., Mahfouz, A. & Reinders, M. J. T. SpaGE: spatial gene enhancement using scRNA-seq. Nucleic Acids Res. 48 , e107 (2020).
Liu, J. et al. Jointly defining cell types from multiple single-cell datasets using LIGER. Nat. Protoc. 15 , 3632–3662 (2020).
Li, B. et al. Benchmarking spatial and single-cell transcriptomics integration methods for transcript distribution prediction and cell type deconvolution. Nat. Methods 19 , 662–670 (2022).
Wang, Z., Simoncelli, E. P. & Bovik, A. C. Multiscale structural similarity for image quality assessment. In Thirty-Seventh Asilomar Conference on Signals, Systems & Computers 1398–1402 (IEEE, 2003).
DeTomaso, D. & Yosef, N. Hotspot identifies informative gene modules across modalities of single-cell genomics. Cell Syst. 12 , 446–456 (2021).
Cao, K., Gong, Q., Hong, Y. & Wan, L. A unified computational framework for single-cell data integration with optimal transport. Nat. Commun. 13 , 7419 (2022).
Kojima, Y. et al. Single-cell colocalization analysis using a deep generative model. Cell Syst. 15 , 180–192.e7 (2024).
Korsunsky, I. et al. Fast, sensitive and accurate integration of single-cell data with Harmony. Nat. Methods 16 , 1289–1296 (2019).
Tran, H. T. N. et al. A benchmark of batch-effect correction methods for single-cell RNA sequencing data. Genome Biol. 21 , 12 (2020).
Okubo, T. et al. Ripply3, a Tbx1 repressor, is required for development of the pharyngeal apparatus and its derivatives in mice. Development 138 , 339–348 (2011).
Lyons, I. et al. Myogenic and morphogenetic defects in the heart tubes of murine embryos lacking the homeo box gene Nkx2-5 . Genes Dev. 9 , 1654–1666 (1995).
Shirasawa, S. et al. Enx (Hox11L1) -deficient mice develop myenteric neuronal hyperplasia and megacolon. Nat. Med. 3 , 646–650 (1997).
Choi, H. M. T. et al. Third-generationin situhybridization chain reaction: multiplexed, quantitative, sensitive, versatile, robust. Development. 145 , dev165753 (2018).
Nowotschin, S. et al. The emergent landscape of the mouse gut endoderm at single-cell resolution. Nature 569 , 361–367 (2019).
Han, L. et al. Single cell transcriptomics identifies a signaling network coordinating endoderm and mesoderm diversification during foregut organogenesis. Nat. Commun. 11 , 4158 (2020).
Carpenter, E. M. Hox genes and spinal cord development. Dev. Neurosci. 24 , 24–34 (2002).
Fruchterman, T. M. J. & Reingold, E. M. Graph drawing by force-directed placement. Softw. Pract. Exp. 21 , 1129–1164 (1991).
López-Delgado, A. C., Delgado, I., Cadenas, V., Sánchez-Cabo, F. & Torres, M. Axial skeleton anterior-posterior patterning is regulated through feedback regulation between Meis transcription factors and retinoic acid. Development 148 , dev193813 (2021).
Chen, F. & Capecchi, M. R. Targeted mutations in Hoxa-9 and Hoxb-9 reveal synergistic interactions. Dev. Biol. 181 , 186–196 (1997).
Degani, N., Lubelsky, Y., Perry, R. B.-T., Ainbinder, E. & Ulitsky, I. Highly conserved and cis -acting lncRNAs produced from paralogous regions in the center of HOXA and HOXB clusters in the endoderm lineage. PLoS Genet. 17 , e1009681 (2021).
Hie, B., Bryson, B. & Berger, B. Efficient integration of heterogeneous single-cell transcriptomes using Scanorama. Nat. Biotechnol. 37 , 685–691 (2019).
Ashique, A. M. et al. The Rfx4 transcription factor modulates Shh signaling by regional control of ciliogenesis. Sci. Signal. 2 , ra70 (2009).
Chen, F., Greer, J. & Capecchi, M. R. Analysis of Hoxa7 / Hoxb7 mutants suggests periodicity in the generation of the different sets of vertebrae. Mech. Dev. 77 , 49–57 (1998).
Yao, Z. et al. A taxonomy of transcriptomic cell types across the isocortex and hippocampal formation. Cell 184 , 3222–3241 (2021).
Song, Y.-H., Yoon, J. & Lee, S.-H. The role of neuropeptide somatostatin in the brain and its application in treating neurological disorders. Exp. Mol. Med. 53 , 328–338 (2021).
Muñoz, W., Tremblay, R., Levenstein, D. & Rudy, B. Layer-specific modulation of neocortical dendritic inhibition during active wakefulness. Science 355 , 954–959 (2017).
Ma, Y., Hu, H., Berrebi, A. S., Mathers, P. H. & Agmon, A. Distinct subtypes of somatostatin-containing neocortical interneurons revealed in transgenic mice. J. Neurosci. 26 , 5069–5082 (2006).
Nigro, M. J., Hashikawa-Yamasaki, Y. & Rudy, B. Diversity and connectivity of layer 5 somatostatin-expressing interneurons in the mouse barrel cortex. J. Neurosci. 38 , 1622–1633 (2018).
Yao, Z. et al. A transcriptomic and epigenomic cell atlas of the mouse primary motor cortex. Nature 598 , 103–110 (2021).
Wu, S. J. et al. Cortical somatostatin interneuron subtypes form cell-type-specific circuits. Neuron 111 , 2675–2692 (2023).
Chamberlain, M. C. Leptomeningeal metastasis. Curr. Opin. Oncol. 22 , 627–635 (2010).
Wilcox, J. A., Li, M. J. & Boire, A. A. Leptomeningeal metastases: new opportunities in the modern era. Neurotherapeutics 19 , 1782–1798 (2022).
Remsik, J. et al. Leptomeningeal anti-tumor immunity follows unique signaling principles. Preprint at bioRxiv https://doi.org/10.1101/2023.03.17.533041 (2023).
Franzén, O., Gan, L.-M. & Björkegren, J. L. M. PanglaoDB: a web server for exploration of mouse and human single-cell RNA sequencing data. Database 2019 , baz046 (2019).
Guadagno, E. et al. Role of macrophages in brain tumor growth and progression. Int. J. Mol. Sci. 19 , 1005 (2018).
Goswami, S. et al. Immune profiling of human tumors identifies CD73 as a combinatorial target in glioblastoma. Nat. Med. 26 , 39–46 (2020).
Noy, R. & Pollard, J. W. Tumor-associated macrophages: from mechanisms to therapy. Immunity 41 , 49–61 (2014).
Andelson, E. H., Anderson, C. H., Bergen, J. R., Burt, P. J. & Ogden, J. M. Pyramid methods in image processing. RCA Engineer https://persci.mit.edu/pub_pdfs/RCA84.pdf (1984).
Loukas, A. Graph reduction with spectral and cut guarantees. J. Mach. Learn. Res. 20 , 1–42 (2018).
Google Scholar
Kuhn, H. W. The Hungarian method for the assignment problem. Nav. Res. Logist. Q. 2 , 83–97 (1955).
Villani, C. Optimal Transport (Springer, 2009).
Horn, R. A. & Johnson, C. R. Matrix Analysis (Cambridge Univ. Press, 2012).
Van Der Maaten, L., Courville, A., Fergus, R. & Manning, C. Accelerating t-SNE using tree-based algorithms. https://www.jmlr.org/papers/volume15/vandermaaten14a/vandermaaten14a.pdf (2014).
Risso, D., Perraudeau, F., Gribkova, S., Dudoit, S. & Vert, J.-P. A general and flexible method for signal extraction from single-cell RNA-seq data. Nat. Commun. 9 , 284 (2018).
Kingma, D. P. & Ba, J. Adam: a method for stochastic optimization. In Proc. 3rd International Conference on Learning Representations (ICLR) (ICLR, 2015).
Satija, R., Farrell, J. A., Gennert, D., Schier, A. F. & Regev, A. Spatial reconstruction of single-cell gene expression data. Nat. Biotechnol. 33 , 495–502 (2015).
Cang, Z. & Nie, Q. Inferring spatial and signaling relationships between cells from single cell transcriptomic data. Nat. Commun. 11 , 2084 (2020).
Shengquan, C., Boheng, Z., Xiaoyang, C., Xuegong, Z. & Rui, J. stPlus: a reference-based method for the accurate enhancement of spatial transcriptomics. Bioinformatics 37 , i299–i307 (2021).
Wolf, F. A., Angerer, P. & Theis, F. J. SCANPY: large-scale single-cell gene expression data analysis. Genome Biol. 19 , 15 (2018).
van Dijk, D. et al. Recovering gene interactions from single-cell data using data diffusion. Cell 174 , 716–729 (2018).
Jacomy, M., Venturini, T., Heymann, S. & Bastian, M. ForceAtlas2, a continuous graph layout algorithm for handy network visualization designed for the Gephi software. PLoS ONE 9 , e98679 (2014).
Becker, M. B., Zülch, A., Bosse, A. & Gruss, P. Irx1 and Irx2 expression in early lung development. Mech. Dev. 106 , 155–158 (2001).
Tanaka, M., Chen, Z., Bartunkova, S., Yamasaki, N. & Izumo, S. The cardiac homeobox gene Csx/Nkx2.5 lies genetically upstream of multiple genes essential for heart development. Development 126 , 1269–1280 (1999).
Offield, M. F. et al. PDX-1 is required for pancreatic outgrowth and differentiation of the rostral duodenum. Development 122 , 983–995 (1996).
Yang, Y., Akinci, E., Dutton, J. R., Banga, A. & Slack, J. M. W. Stage specific reprogramming of mouse embryo liver cells to a beta cell-like phenotype. Mech. Dev. 130 , 602–612 (2013).
Tang, S. J. et al. The Tlx-2 homeobox gene is a downstream target of BMP signalling and is required for mouse mesoderm development. Development 125 , 1877–1887 (1998).
Monaghan, A. P., Kaestner, K. H., Grau, E. & Schütz, G. Postimplantation expression patterns indicate a role for the mouse forkhead /HNF-3 α, β and γ genes in determination of the definitive endoderm, chordamesoderm and neuroectoderm. Development 119 , 567–578 (1993).
Kaestner, K. H., Hiemisch, H., Luckow, B. & Schütz, G. The HNF-3 gene family of transcription factors in mice: gene structure, cDNA sequence, and mRNA distribution. Genomics 20 , 377–385 (1994).
Gendron-Maguire, M., Mallo, M., Zhang, M. & Gridley, T. Hoxa-2 mutant mice exhibit homeotic transformation of skeletal elements derived from cranial neural crest. Cell 75 , 1317–1331 (1993).
Rijli, F. M. et al. A homeotic transformation is generated in the rostral branchial region of the head by disruption of Hoxa-2 , which acts as a selector gene. Cell 75 , 1333–1349 (1993).
Barrow, J. R. & Capecchi, M. R. Compensatory defects associated with mutations in Hoxa1 restore normal palatogenesis to Hoxa2 mutants. Development 126 , 5011–5026 (1999).
Shor, J. DoubletDetection: doublet detection in single-cell RNA-seq data. https://github.com/JonathanShor/DoubletDetection (2022).
Anderson, M. J., Magidson, V., Kageyama, R. & Lewandoski, M. Fgf4 maintains Hes7 levels critical for normal somite segmentation clock function. eLife. 9 , e55608 (2020).
Li, W., Germain, R. N. & Gerner, M. Y. Multiplex, quantitative cellular analysis in large tissue volumes with clearing-enhanced 3D microscopy (Ce3D). Proc. Natl Acad. Sci. USA 114 , E7321–E7330 (2017).
CAS PubMed PubMed Central Google Scholar
Download references
Acknowledgements
We thank C. Burdziak and E. Wershof for their insightful comments and the Single Cell Analytics Innovation Lab at Memorial Sloan Kettering Cancer Center for sample processing and protocol development related to brain metastasis data generation. This work was supported by National Cancer Institute (NCI) Cancer Center Support Grant P30 CA08748, NCI grant U54 CA209975, NCI grant R01 DK127821 (M.G.), NCI Human Tumor Atlas Network grant U2C CA233284 (D.P.), the Robert J. Kleberg, Jr. and Helen C. Kleberg Foundation (D.P.) and the Alan and Sandra Gerry Metastasis and Tumor Ecosystems Center at Memorial Sloan Kettering Cancer Center. D.P. is a Howard Hughes Medical Institute investigator.
Author information
Authors and affiliations.
Computational and Systems Biology Program, Sloan Kettering Institute, Memorial Sloan Kettering Cancer Center, New York, NY, USA
Doron Haviv, Catherine Snopkowski, Meril Takizawa, Tal Nawy, Ronan Chaligne & Dana Pe’er
Tri-Institutional Training Program in Computational Biology and Medicine, Weill Cornell Medicine, New York, NY, USA
Doron Haviv
Human Oncology & Pathogenesis Program, Memorial Sloan Kettering Cancer Center, New York, NY, USA
Ján Remšík & Adrienne Boire
Developmental Biology Program, Sloan Kettering Institute, Memorial Sloan Kettering Cancer Center, New York, NY, USA
Mohamed Gatie & Anna-Katerina Hadjantonakis
S2 Genomics, Livermore, CA, USA
Nathan Pereira, John Bashkin & Stevan Jovanovich
Department of Neurology, Memorial Sloan Kettering Cancer Center, New York, NY, USA
Adrienne Boire
Brain Tumor Center, Memorial Sloan Kettering Cancer Center, New York, NY, USA
Howard Hughes Medical Institute, New York, NY, USA
You can also search for this author in PubMed Google Scholar
Contributions
D.H. and D.P. conceived the study and algorithm design. D.H. implemented all algorithms used in this study and carried out testing, application and data analysis. D.H., M.G. and A.-K.H. carried out data analysis and interpretation of the embryogenesis data. M.G. collected the mouse embryogenesis HCR imaging data. D.H., J.R. and A.B. analyzed the leptomeningeal metastasis data. J.R. generated metastatic cell lines and performed animal experiments. N.P., J.B. and S.J. developed and optimized the automated nuclei extraction protocol from FFPE samples. C.N. and M.T. collected the Xenium and snRNA-seq data under the supervision of R.C. D.H., T.N. and D.P. wrote the paper. D.P. supervised the study.
Corresponding author
Correspondence to Dana Pe’er .
Ethics declarations
Competing interests.
D.P. is a member of the scientific advisory board of and has equity in Insitro. A.B. holds an unpaid position on the scientific advisory board of Evren Scientific and is an inventor on patents 62/258,044, 10/413,522 and 63/052,139 filed by Memorial Sloan Kettering Cancer Center. A.B. and J.R. are inventors of provisional patent applications 63/449,817 and 63/449,823 filed by Memorial Sloan Kettering Cancer Center. J.B., S.J. and N.P. were employees of S2 Genomics during this work and own company stock. The other authors declare no competing interests.
Peer review
Peer review information.
Nature Biotechnology thanks Tommaso Biancalani and the other, anonymous, reviewer(s) for their contribution to the peer review of this work.
Additional information
Publisher’s note Springer Nature remains neutral with regard to jurisdictional claims in published maps and institutional affiliations.
Extended data
Extended data fig. 1 approximate optimal transport (aot) yields similar results to optimal transport and bhattacharyya distance, but more efficiently..
a , Run times for computing the kNN graph between sets of randomly generated covariance matrices at various sample sizes. Both axes are in log scale. Fréchet and Bhattacharyya run times are not shown for samples larger than 4,000 cells due to out-of-memory error on a 768-GB, 64-core computing cluster. b , Agreement between the true Fréchet and AOT, Bhattacharyya and standard L2 kNN graphs, expressed as Jaccard Index values and computed on COVET matrices of pharyngeal mesoderm cells in the seqFISH embryogenesis dataset. c , COVET UMAP embeddings and PhenoGraph clustering of seqFISH pharyngeal mesoderm by different metrics, colored by PhenoGraph clusters of each. d , seqFISH data from pharyngeal mesoderm, colored by PhenoGraph clustering of COVET matrices according to each distance metric. Bhat, Bhattacharyya.
Extended Data Fig. 2 Modality-specific data features, run times and impact of sparsity on data integration.
a , Examples of three genes that exhibit very different expression distributions between four spatial datasets and their matching scRNA-seq data. b , Run time of spatial and single-cell integration methods on real datasets of different sizes. Programs were manually terminated at 5 h (18,000 s). c , Benchmarking ENVI imputation of the full embryogenesis seqFISH and scRNA-seq dataset against 80% and 90% subsampled versions, as well as Tangram (Tg) on the full dataset as reference. Boxes and lines represent interquartile range (IQR) and median, respectively; whiskers represent ±1.5 x IQR. d , Ability of ENVI to transfer cell-type label information from scRNA-seq to spatial data (datasets as in c ). ENVI retains cell-type information in the integrated latent even starting from sparser data.
Extended Data Fig. 3 ENVI accurately infers embryogenesis genes missing from seqFISH data.
a , Imputed expression of withheld genes from the seqFISH embryogenesis dataset 37 (bottom) compared to true (measured) expression (top), with corresponding MSSI and Pearson correlation reconstruction (Corr) scores. b , HCR images of Ripply3 , Nkx2-5 and Tlx2 and their imputation values according to ENVI, Tangram and gimVI. Organs marked by each gene are noted on the HCR and seqFISH images.
Extended Data Fig. 4 The use of COVET spatial covariance improves cell-type assignment.
a , Proportion of scRNA-seq gut tube cells in each organ (row) that fall into each COVET cluster (column), arranged from anterior to posterior. b , Assignment of developing organs to seqFISH gut tube cells via ENVI COVET space, latent space of ENVI when trained without COVET, gimVI latent space and Tangram cell-type mapping.
Extended Data Fig. 5 ENVI is robust to variation in COVET neighborhood size.
Transfer of organ labels onto seqFISH gut tube cells according to independent instances of ENVI, each trained according to COVET representations based on a different number of nearest spatial neighbors ( k ). Spatial context predictions remain robust across k values.
Extended Data Fig. 6 ENVI reliably recovers the AP axis during spine development.
a , Spine and NMP cells from seqFISH data, colored by AP polarity calculated from the first DC of their spatial coordinates. b , Pseudo-AP of seqFISH spine and NMP cells from DC analysis of gimVI and Scanorama. Values denote Pearson correlation with the true AP axis. c , Pearson correlation of ENVI COVET, gimVI and Scanorama pseudo-AP of spine and NMP scRNA-seq cells, for five canonical posterior markers (higher is better) and anterior markers (lower is better). Pseudo-AP axis is based on the DC best aligned with true depth (DC 1, 2 and 3 for ENVI, gimVI and Scanorama, respectively).
Extended Data Fig. 7 ENVI extends cortical tissue gene expression to the entire genome.
a , Five-fold cross-validation of imputation based on a 252-gene MERFISH dataset from the primary motor cortex 40 . Boxes and lines represent IQR and median, respectively; whiskers represent ±1.5 x IQR. Comparison of MSSI\Pearson correlations between ENVI and other methods (one-sided t-test, n = 252) generates p-values, from left (Tangram) to right (deepC), of \(4.67\cdot {10}^{-29}\backslash 1.80\cdot {10}^{-19}\) , \(1.62\cdot {10}^{-72}\backslash 7.29\cdot {10}^{-66}\) , \(4.70\cdot {10}^{-36}\backslash 1.72\cdot {10}^{-50}\) , \(5.25\cdot {10}^{-28}\backslash 4.10\cdot {10}^{-41}\) , \(5.23\cdot {10}^{-72}\backslash 1.35\cdot {10}^{-86}\) , \(1.87\cdot {10}^{-64}\backslash 2.38\cdot {10}^{-66}\) . b , ENVI imputation of genes selected due to their clear in situ hybridization profiles in the Allen Brain Atlas (mouse.brain-map.org), projected onto the MERFISH data, with corresponding ISH expression in the motor cortex. Novo, NovoSpaRc; deepC, deepCOLOR.
Extended Data Fig. 8 ENVI integrates osmFISH and scRNA-seq data from the somatosensory cortex.
a , osmFISH with segmented cells and UMAP visualization of scRNA-seq datasets of the mouse somatosensory cortex, colored by cell types as annotated in Codeluppi et al. 3 . b , UMAP visualizations of the ENVI integrated latent embedding of the osmFISH and scRNA-seq modalities, colored by cell types as in a . Latent integration score; bASW = 0.62. c , Same as b , but with latent embeddings from gimVI. d , Normalized distance between the center-of-mass of each cell type according to the ENVI and gimVI latent embeddings. e , Benchmarking of imputation based on leave-one-out training, evaluated by Pearson correlation and MSSI on a 33-gene osmFISH dataset of the somatosensory cortex 3 . Boxes and lines represent IQR and median, respectively; whiskers represent ±1.5 x IQR. In order, MSSI\Pearson correlation p-values (one-sided t-test, n = 33) are: \(1.41\cdot {10}^{-4}\backslash 6.69\cdot {10}^{-10}\) , \(9.03\cdot {10}^{-4}\backslash 2.64\cdot {10}^{-6}\) , \(5.25\cdot {10}^{-9}\backslash 2.03\cdot {10}^{-10}\) , \(1.21\cdot {10}^{-3}\backslash 1.62\cdot {10}^{-5}\) , \(4.34\cdot {10}^{-10}\backslash 1.43\cdot {10}^{-9}\) , \(7.11\cdot {10}^{-4}\backslash 9.08\cdot {10}^{-6}\) . f , ENVI-imputed expression of unimaged cortical markers Ddit4l (L2/3), Rprm (L5/6) and Ndst (Hippocampus, CA1) (top) and corresponding expression in the Allen Brain Atlas (mouse.brain-map.org) (bottom). Tg, Tangram; Hy, Harmony; Novo, NovoSpaRc; DC, deepCOLOR.
Extended Data Fig. 9 Cell type validation and extended benchmarking of ENVI on Xenium data.
a , Expression of marker genes for each annotated cell type in the snRNA-seq and Xenium data. b , Extended imputation benchmarking of ENVI against uniPort (Unip), Harmony and deepCOLOR. Boxes and lines represent IQR and median, respectively; whiskers represent ±1.5 x IQR. In order, MSSI\Pearson correlation p-values (one-sided t-test, n = 243) are: \(1.21\cdot {10}^{-11}\backslash 1.88\cdot {10}^{-3}\) , \(6.62\cdot {10}^{-16}\backslash 3.43\cdot {10}^{-13}\) , \(1.20\cdot {10}^{-33}\backslash 5.82\cdot {10}^{-7}\) , \(3.93\cdot {10}^{-1}\backslash 9.99\cdot {10}^{-1}\) , \(2.66\cdot {10}^{-45}\backslash 4.89\cdot {10}^{-19}\) , \(6.92\cdot {10}^{-12}\backslash 1.13\cdot {10}^{-4}\) . c , Balanced accuracy for annotating Xenium cell types from snRNA-seq labels. ENVI, Tangram (Tg), gimVI, uniPort (Unip) and Harmony all perform similarly. d , UMAP of gimVI latent space of snRNA-seq immune cells, colored by subtype. e , gimVI latent UMAP and PhenoGraph clusters of Xenium immune cells. UMAP and clusters are calculated using both Xenium and snRNA-seq immune cells. Most microglia (68%) and macrophages (90%) are assigned to cluster C1, preventing clear association between subtype and microenvironment.
Extended Data Fig. 10 Imputation of tumor-infiltrating macrophage markers at the tumor-immune boundary.
ENVI, gimVI and Harmony-based imputation of three tumor-infiltrating macrophage markers onto Xenium immune cells. Only ENVI correctly predicts the expected pattern of expression, showing enrichment in immune cells inside the tumor region.
Supplementary information
Reporting summary, supplementary table.
All tables, each in a dedicated Excel sheet
Rights and permissions
Open Access This article is licensed under a Creative Commons Attribution 4.0 International License, which permits use, sharing, adaptation, distribution and reproduction in any medium or format, as long as you give appropriate credit to the original author(s) and the source, provide a link to the Creative Commons licence, and indicate if changes were made. The images or other third party material in this article are included in the article’s Creative Commons licence, unless indicated otherwise in a credit line to the material. If material is not included in the article’s Creative Commons licence and your intended use is not permitted by statutory regulation or exceeds the permitted use, you will need to obtain permission directly from the copyright holder. To view a copy of this licence, visit http://creativecommons.org/licenses/by/4.0/ .
Reprints and permissions
About this article
Cite this article.
Haviv, D., Remšík, J., Gatie, M. et al. The covariance environment defines cellular niches for spatial inference. Nat Biotechnol (2024). https://doi.org/10.1038/s41587-024-02193-4
Download citation
Received : 18 April 2023
Accepted : 28 February 2024
Published : 02 April 2024
DOI : https://doi.org/10.1038/s41587-024-02193-4
Share this article
Anyone you share the following link with will be able to read this content:
Sorry, a shareable link is not currently available for this article.
Provided by the Springer Nature SharedIt content-sharing initiative
Quick links
- Explore articles by subject
- Guide to authors
- Editorial policies
Sign up for the Nature Briefing newsletter — what matters in science, free to your inbox daily.


- Cambridge Dictionary +Plus
Definition of representation – Learner’s Dictionary
Your browser doesn't support HTML5 audio
representation noun ( SPEAKING FOR )
Representation noun ( showing ).
(Definition of representation from the Cambridge Learner's Dictionary © Cambridge University Press)
Translations of representation
Get a quick, free translation!
Word of the Day
cloak-and-dagger
used to describe an exciting story involving secrets and mystery, often about spies, or something that makes you think of this

Shoots, blooms and blossom: talking about plants

Learn more with +Plus
- Recent and Recommended {{#preferredDictionaries}} {{name}} {{/preferredDictionaries}}
- Definitions Clear explanations of natural written and spoken English English Learner’s Dictionary Essential British English Essential American English
- Grammar and thesaurus Usage explanations of natural written and spoken English Grammar Thesaurus
- Pronunciation British and American pronunciations with audio English Pronunciation
- English–Chinese (Simplified) Chinese (Simplified)–English
- English–Chinese (Traditional) Chinese (Traditional)–English
- English–Dutch Dutch–English
- English–French French–English
- English–German German–English
- English–Indonesian Indonesian–English
- English–Italian Italian–English
- English–Japanese Japanese–English
- English–Norwegian Norwegian–English
- English–Polish Polish–English
- English–Portuguese Portuguese–English
- English–Spanish Spanish–English
- English–Swedish Swedish–English
- Dictionary +Plus Word Lists
- representation (SPEAKING FOR)
- representation (SHOWING)
- Translations
- All translations
Add representation to one of your lists below, or create a new one.
{{message}}
Something went wrong.
There was a problem sending your report.
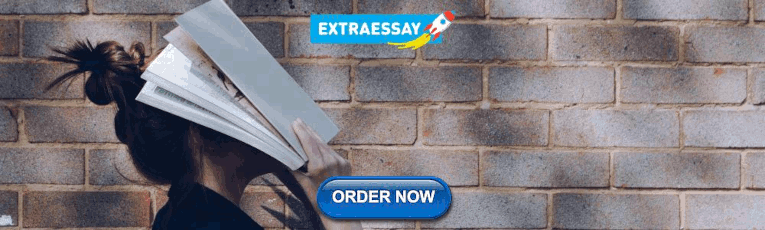
IMAGES
VIDEO
COMMENTS
representation: [noun] one that represents: such as. an artistic likeness or image. a statement or account made to influence opinion or action. an incidental or collateral statement of fact on the faith of which a contract is entered into. a dramatic production or performance. a usually formal statement made against something or to effect a ...
REPRESENTATION definition: 1. a person or organization that speaks, acts, or is present officially for someone else: 2. the…. Learn more.
REPRESENTATION meaning: 1. a person or organization that speaks, acts, or is present officially for someone else: 2. the…. Learn more.
Representation definition: . See examples of REPRESENTATION used in a sentence.
A representation acts or serves on behalf or in place of something. A lawyer provides legal representation for his client. A caricature is an exaggerated representation or likeness of a person.
representation by a lawyer; direct representation in Parliament; Whether guilty or innocent, we are still entitled to legal representation. They had a strong representation in government. The task force had broad representation with members drawn from different departments. The party has increased its representation in Parliament.
10 meanings: 1. the act or an instance of representing or the state of being represented 2. anything that represents, such as a.... Click for more definitions.
1 [uncountable, countable] the act of presenting someone or something in a particular way; something that shows or describes something synonym portrayal the negative representation of single mothers in the media The snake swallowing its tail is a representation of infinity.
There has been a decline in union representation in the auto industry. → proportional representation 2 [ countable] a painting, sign, description etc that shows something representation of The clock in the painting is a symbolic representation of the passage of time. 3 [ uncountable] the act of representing someone or something representation ...
REPRESENTATION definition: 1. speaking or doing something officially for another person: 2. the way someone or something is…. Learn more.
a : a statement made to influence the opinions or actions of others. Her representation of the situation was very confusing. He was accused of making false representations. b chiefly British : a formal and official complaint about something. Our ambassador has made representations to their government.
The meaning of REPRESENT is to bring clearly before the mind : present. How to use represent in a sentence.
There are 19 meanings listed in OED's entry for the noun representation, three of which are labelled obsolete. See 'Meaning & use' for definitions, usage, and quotation evidence. representation has developed meanings and uses in subjects including. visual arts (Middle English) theatre (late 1500s) philosophy (early 1600s) law (early 1600s ...
Depicting or 'making present' something which is absent (e.g. people, places, events, or abstractions) in a different form: as in paintings, photographs, films, or language, rather than as a replica. See also description; compare absent presence.2. The function of a sign or symbol of 'standing for' that to which it refers (its referent).3.
represent somebody/something to act or speak officially for somebody and defend their interests. The union represents over 200 000 teachers. The association was formed to represent the interests of women artists.; Ms Dale is representing the defendant (= is his/her lawyer) in the case.; As an MP, it is my duty to represent my constituents.
Representation is the use of signs that stand in for and take the place of something else. It is through representation that people organize the world and reality through the act of naming its elements. Signs are arranged in order to form semantic constructions and express relations.. Bust of Aristotle, Greek philosopher. For many philosophers, both ancient and modern, man is regarded as the ...
representative: [noun] one that represents another or others: such as. one that represents a constituency as a member of a legislative body. a member of the house of representatives of the U.S. Congress or a state legislature. one that represents another as agent, deputy, substitute, or delegate usually being invested with the authority of the ...
Political representation occurs when political actors speak, advocate, symbolize, and act on the behalf of others in the political arena. In short, political representation is a kind of political assistance. This seemingly straightforward definition, however, is not adequate as it stands. For it leaves the concept of political representation ...
representation, in government, method or process of enabling the citizenry, or some of them, to participate in the shaping of legislation and governmental policy through deputies chosen by them.. The rationale of representative government is that in large modern countries the people cannot all assemble, as they did in the marketplace of democratic Athens or Rome; and if, therefore, the people ...
act/speak for someone; 1 [often passive] represent somebody/something to be a member of a group of people and act or speak on their behalf at an event, a meeting, etc. The competition attracted over 500 contestants representing 8 different countries. Local businesses are well represented on the committee (= there are a lot of people from them on the committee).
The basic definition of representation in the media is simply how media, such as television, film and books, portray certain types of people or communities. There are a number of groups who are underrepresented in most Western media. They include women, people of color, LBGTQA+ people, people with a range of body shapes and types, people of non ...
REPRESENT definition: 1. to speak, act, or be present officially for another person or people: 2. to be the Member of…. Learn more.
We define a principled optimal transport-based distance metric between COVET niches that scales to millions of cells. ... a representation that leverages the gene-gene covariate structure across ...
Customer service is a team that helps customers solve problems with a business's products or services. As a customer service representative, you may allow a customer before or after they complete a purchase. Some duties may include: Asking and answering a customer's questions. Suggesting products or services to customers. Finding solutions ...
REPRESENTATION meaning: 1. speaking or doing something officially for another person: 2. the way someone or something is…. Learn more.