Get science-backed answers as you write with Paperpal's Research feature
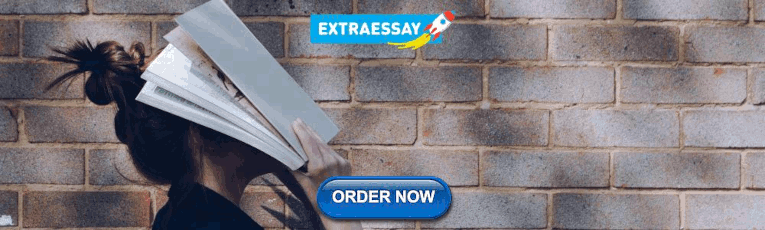
How to Write a Conclusion for Research Papers (with Examples)
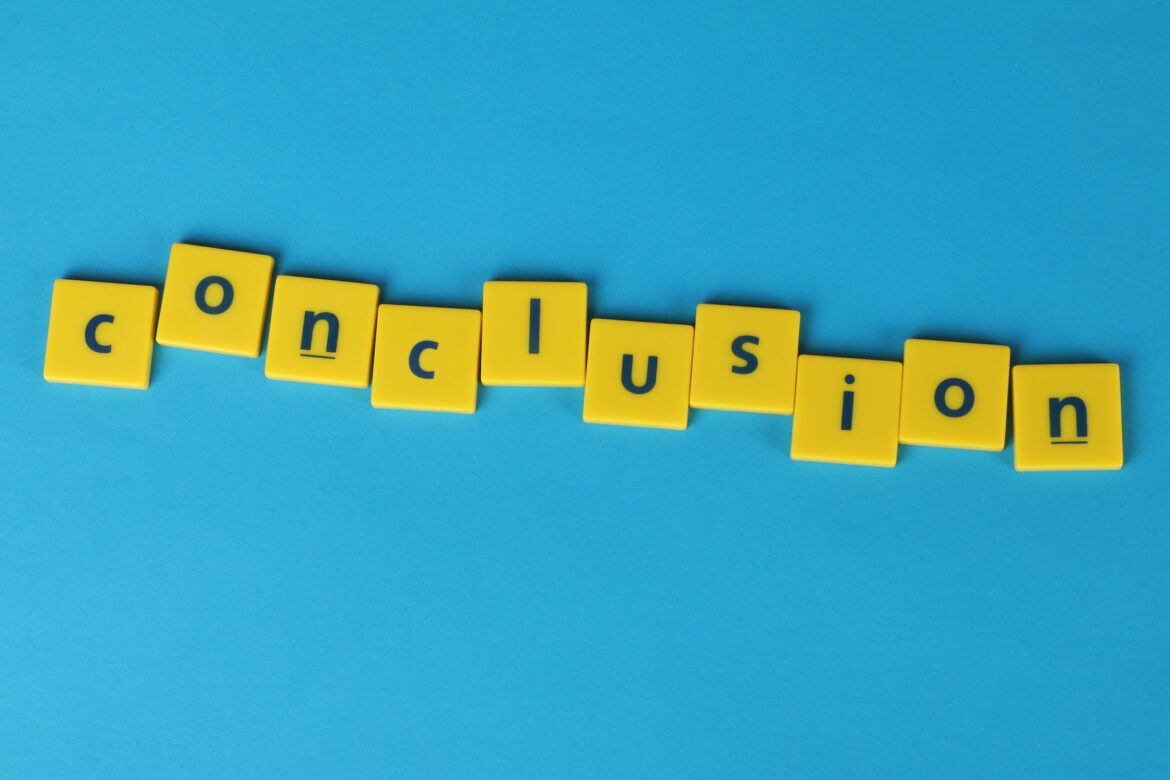
The conclusion of a research paper is a crucial section that plays a significant role in the overall impact and effectiveness of your research paper. However, this is also the section that typically receives less attention compared to the introduction and the body of the paper. The conclusion serves to provide a concise summary of the key findings, their significance, their implications, and a sense of closure to the study. Discussing how can the findings be applied in real-world scenarios or inform policy, practice, or decision-making is especially valuable to practitioners and policymakers. The research paper conclusion also provides researchers with clear insights and valuable information for their own work, which they can then build on and contribute to the advancement of knowledge in the field.
The research paper conclusion should explain the significance of your findings within the broader context of your field. It restates how your results contribute to the existing body of knowledge and whether they confirm or challenge existing theories or hypotheses. Also, by identifying unanswered questions or areas requiring further investigation, your awareness of the broader research landscape can be demonstrated.
Remember to tailor the research paper conclusion to the specific needs and interests of your intended audience, which may include researchers, practitioners, policymakers, or a combination of these.
Table of Contents
What is a conclusion in a research paper, summarizing conclusion, editorial conclusion, externalizing conclusion, importance of a good research paper conclusion, how to write a conclusion for your research paper, research paper conclusion examples.
- How to write a research paper conclusion with Paperpal?
Frequently Asked Questions
A conclusion in a research paper is the final section where you summarize and wrap up your research, presenting the key findings and insights derived from your study. The research paper conclusion is not the place to introduce new information or data that was not discussed in the main body of the paper. When working on how to conclude a research paper, remember to stick to summarizing and interpreting existing content. The research paper conclusion serves the following purposes: 1
- Warn readers of the possible consequences of not attending to the problem.
- Recommend specific course(s) of action.
- Restate key ideas to drive home the ultimate point of your research paper.
- Provide a “take-home” message that you want the readers to remember about your study.
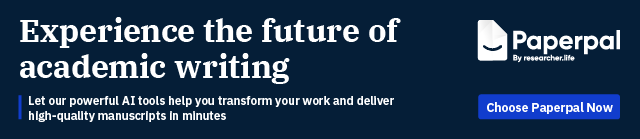
Types of conclusions for research papers
In research papers, the conclusion provides closure to the reader. The type of research paper conclusion you choose depends on the nature of your study, your goals, and your target audience. I provide you with three common types of conclusions:
A summarizing conclusion is the most common type of conclusion in research papers. It involves summarizing the main points, reiterating the research question, and restating the significance of the findings. This common type of research paper conclusion is used across different disciplines.
An editorial conclusion is less common but can be used in research papers that are focused on proposing or advocating for a particular viewpoint or policy. It involves presenting a strong editorial or opinion based on the research findings and offering recommendations or calls to action.
An externalizing conclusion is a type of conclusion that extends the research beyond the scope of the paper by suggesting potential future research directions or discussing the broader implications of the findings. This type of conclusion is often used in more theoretical or exploratory research papers.
Align your conclusion’s tone with the rest of your research paper. Start Writing with Paperpal Now!
The conclusion in a research paper serves several important purposes:
- Offers Implications and Recommendations : Your research paper conclusion is an excellent place to discuss the broader implications of your research and suggest potential areas for further study. It’s also an opportunity to offer practical recommendations based on your findings.
- Provides Closure : A good research paper conclusion provides a sense of closure to your paper. It should leave the reader with a feeling that they have reached the end of a well-structured and thought-provoking research project.
- Leaves a Lasting Impression : Writing a well-crafted research paper conclusion leaves a lasting impression on your readers. It’s your final opportunity to leave them with a new idea, a call to action, or a memorable quote.
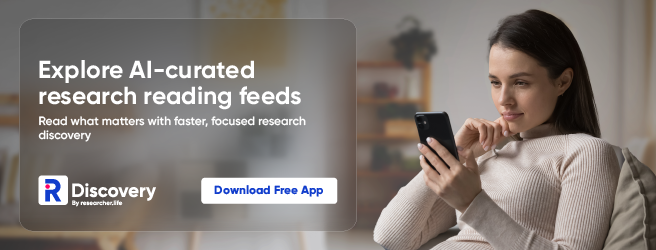
Writing a strong conclusion for your research paper is essential to leave a lasting impression on your readers. Here’s a step-by-step process to help you create and know what to put in the conclusion of a research paper: 2
- Research Statement : Begin your research paper conclusion by restating your research statement. This reminds the reader of the main point you’ve been trying to prove throughout your paper. Keep it concise and clear.
- Key Points : Summarize the main arguments and key points you’ve made in your paper. Avoid introducing new information in the research paper conclusion. Instead, provide a concise overview of what you’ve discussed in the body of your paper.
- Address the Research Questions : If your research paper is based on specific research questions or hypotheses, briefly address whether you’ve answered them or achieved your research goals. Discuss the significance of your findings in this context.
- Significance : Highlight the importance of your research and its relevance in the broader context. Explain why your findings matter and how they contribute to the existing knowledge in your field.
- Implications : Explore the practical or theoretical implications of your research. How might your findings impact future research, policy, or real-world applications? Consider the “so what?” question.
- Future Research : Offer suggestions for future research in your area. What questions or aspects remain unanswered or warrant further investigation? This shows that your work opens the door for future exploration.
- Closing Thought : Conclude your research paper conclusion with a thought-provoking or memorable statement. This can leave a lasting impression on your readers and wrap up your paper effectively. Avoid introducing new information or arguments here.
- Proofread and Revise : Carefully proofread your conclusion for grammar, spelling, and clarity. Ensure that your ideas flow smoothly and that your conclusion is coherent and well-structured.
Write your research paper conclusion 2x faster with Paperpal. Try it now!
Remember that a well-crafted research paper conclusion is a reflection of the strength of your research and your ability to communicate its significance effectively. It should leave a lasting impression on your readers and tie together all the threads of your paper. Now you know how to start the conclusion of a research paper and what elements to include to make it impactful, let’s look at a research paper conclusion sample.
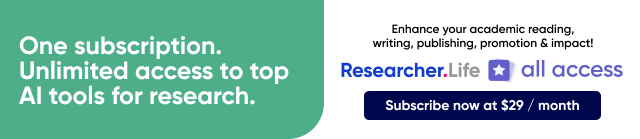
How to write a research paper conclusion with Paperpal?
A research paper conclusion is not just a summary of your study, but a synthesis of the key findings that ties the research together and places it in a broader context. A research paper conclusion should be concise, typically around one paragraph in length. However, some complex topics may require a longer conclusion to ensure the reader is left with a clear understanding of the study’s significance. Paperpal, an AI writing assistant trusted by over 800,000 academics globally, can help you write a well-structured conclusion for your research paper.
- Sign Up or Log In: Create a new Paperpal account or login with your details.
- Navigate to Features : Once logged in, head over to the features’ side navigation pane. Click on Templates and you’ll find a suite of generative AI features to help you write better, faster.
- Generate an outline: Under Templates, select ‘Outlines’. Choose ‘Research article’ as your document type.
- Select your section: Since you’re focusing on the conclusion, select this section when prompted.
- Choose your field of study: Identifying your field of study allows Paperpal to provide more targeted suggestions, ensuring the relevance of your conclusion to your specific area of research.
- Provide a brief description of your study: Enter details about your research topic and findings. This information helps Paperpal generate a tailored outline that aligns with your paper’s content.
- Generate the conclusion outline: After entering all necessary details, click on ‘generate’. Paperpal will then create a structured outline for your conclusion, to help you start writing and build upon the outline.
- Write your conclusion: Use the generated outline to build your conclusion. The outline serves as a guide, ensuring you cover all critical aspects of a strong conclusion, from summarizing key findings to highlighting the research’s implications.
- Refine and enhance: Paperpal’s ‘Make Academic’ feature can be particularly useful in the final stages. Select any paragraph of your conclusion and use this feature to elevate the academic tone, ensuring your writing is aligned to the academic journal standards.
By following these steps, Paperpal not only simplifies the process of writing a research paper conclusion but also ensures it is impactful, concise, and aligned with academic standards. Sign up with Paperpal today and write your research paper conclusion 2x faster .
The research paper conclusion is a crucial part of your paper as it provides the final opportunity to leave a strong impression on your readers. In the research paper conclusion, summarize the main points of your research paper by restating your research statement, highlighting the most important findings, addressing the research questions or objectives, explaining the broader context of the study, discussing the significance of your findings, providing recommendations if applicable, and emphasizing the takeaway message. The main purpose of the conclusion is to remind the reader of the main point or argument of your paper and to provide a clear and concise summary of the key findings and their implications. All these elements should feature on your list of what to put in the conclusion of a research paper to create a strong final statement for your work.
A strong conclusion is a critical component of a research paper, as it provides an opportunity to wrap up your arguments, reiterate your main points, and leave a lasting impression on your readers. Here are the key elements of a strong research paper conclusion: 1. Conciseness : A research paper conclusion should be concise and to the point. It should not introduce new information or ideas that were not discussed in the body of the paper. 2. Summarization : The research paper conclusion should be comprehensive enough to give the reader a clear understanding of the research’s main contributions. 3 . Relevance : Ensure that the information included in the research paper conclusion is directly relevant to the research paper’s main topic and objectives; avoid unnecessary details. 4 . Connection to the Introduction : A well-structured research paper conclusion often revisits the key points made in the introduction and shows how the research has addressed the initial questions or objectives. 5. Emphasis : Highlight the significance and implications of your research. Why is your study important? What are the broader implications or applications of your findings? 6 . Call to Action : Include a call to action or a recommendation for future research or action based on your findings.
The length of a research paper conclusion can vary depending on several factors, including the overall length of the paper, the complexity of the research, and the specific journal requirements. While there is no strict rule for the length of a conclusion, but it’s generally advisable to keep it relatively short. A typical research paper conclusion might be around 5-10% of the paper’s total length. For example, if your paper is 10 pages long, the conclusion might be roughly half a page to one page in length.
In general, you do not need to include citations in the research paper conclusion. Citations are typically reserved for the body of the paper to support your arguments and provide evidence for your claims. However, there may be some exceptions to this rule: 1. If you are drawing a direct quote or paraphrasing a specific source in your research paper conclusion, you should include a citation to give proper credit to the original author. 2. If your conclusion refers to or discusses specific research, data, or sources that are crucial to the overall argument, citations can be included to reinforce your conclusion’s validity.
The conclusion of a research paper serves several important purposes: 1. Summarize the Key Points 2. Reinforce the Main Argument 3. Provide Closure 4. Offer Insights or Implications 5. Engage the Reader. 6. Reflect on Limitations
Remember that the primary purpose of the research paper conclusion is to leave a lasting impression on the reader, reinforcing the key points and providing closure to your research. It’s often the last part of the paper that the reader will see, so it should be strong and well-crafted.
- Makar, G., Foltz, C., Lendner, M., & Vaccaro, A. R. (2018). How to write effective discussion and conclusion sections. Clinical spine surgery, 31(8), 345-346.
- Bunton, D. (2005). The structure of PhD conclusion chapters. Journal of English for academic purposes , 4 (3), 207-224.
Paperpal is a comprehensive AI writing toolkit that helps students and researchers achieve 2x the writing in half the time. It leverages 21+ years of STM experience and insights from millions of research articles to provide in-depth academic writing, language editing, and submission readiness support to help you write better, faster.
Get accurate academic translations, rewriting support, grammar checks, vocabulary suggestions, and generative AI assistance that delivers human precision at machine speed. Try for free or upgrade to Paperpal Prime starting at US$19 a month to access premium features, including consistency, plagiarism, and 30+ submission readiness checks to help you succeed.
Experience the future of academic writing – Sign up to Paperpal and start writing for free!
Related Reads:
- 5 Reasons for Rejection After Peer Review
- Ethical Research Practices For Research with Human Subjects
7 Ways to Improve Your Academic Writing Process
- Paraphrasing in Academic Writing: Answering Top Author Queries
Preflight For Editorial Desk: The Perfect Hybrid (AI + Human) Assistance Against Compromised Manuscripts
You may also like, ai in education: it’s time to change the..., is it ethical to use ai-generated abstracts without..., what are journal guidelines on using generative ai..., quillbot review: features, pricing, and free alternatives, what is an academic paper types and elements , should you use ai tools like chatgpt for..., publish research papers: 9 steps for successful publications , what are the different types of research papers, how to make translating academic papers less challenging, self-plagiarism in research: what it is and how....
- USC Libraries
- Research Guides
Organizing Your Social Sciences Research Paper
- 9. The Conclusion
- Purpose of Guide
- Design Flaws to Avoid
- Independent and Dependent Variables
- Glossary of Research Terms
- Reading Research Effectively
- Narrowing a Topic Idea
- Broadening a Topic Idea
- Extending the Timeliness of a Topic Idea
- Academic Writing Style
- Applying Critical Thinking
- Choosing a Title
- Making an Outline
- Paragraph Development
- Research Process Video Series
- Executive Summary
- The C.A.R.S. Model
- Background Information
- The Research Problem/Question
- Theoretical Framework
- Citation Tracking
- Content Alert Services
- Evaluating Sources
- Primary Sources
- Secondary Sources
- Tiertiary Sources
- Scholarly vs. Popular Publications
- Qualitative Methods
- Quantitative Methods
- Insiderness
- Using Non-Textual Elements
- Limitations of the Study
- Common Grammar Mistakes
- Writing Concisely
- Avoiding Plagiarism
- Footnotes or Endnotes?
- Further Readings
- Generative AI and Writing
- USC Libraries Tutorials and Other Guides
- Bibliography
The conclusion is intended to help the reader understand why your research should matter to them after they have finished reading the paper. A conclusion is not merely a summary of the main topics covered or a re-statement of your research problem, but a synthesis of key points derived from the findings of your study and, if applicable, where you recommend new areas for future research. For most college-level research papers, two or three well-developed paragraphs is sufficient for a conclusion, although in some cases, more paragraphs may be required in describing the key findings and their significance.
Conclusions. The Writing Center. University of North Carolina; Conclusions. The Writing Lab and The OWL. Purdue University.
Importance of a Good Conclusion
A well-written conclusion provides you with important opportunities to demonstrate to the reader your understanding of the research problem. These include:
- Presenting the last word on the issues you raised in your paper . Just as the introduction gives a first impression to your reader, the conclusion offers a chance to leave a lasting impression. Do this, for example, by highlighting key findings in your analysis that advance new understanding about the research problem, that are unusual or unexpected, or that have important implications applied to practice.
- Summarizing your thoughts and conveying the larger significance of your study . The conclusion is an opportunity to succinctly re-emphasize your answer to the "So What?" question by placing the study within the context of how your research advances past research about the topic.
- Identifying how a gap in the literature has been addressed . The conclusion can be where you describe how a previously identified gap in the literature [first identified in your literature review section] has been addressed by your research and why this contribution is significant.
- Demonstrating the importance of your ideas . Don't be shy. The conclusion offers an opportunity to elaborate on the impact and significance of your findings. This is particularly important if your study approached examining the research problem from an unusual or innovative perspective.
- Introducing possible new or expanded ways of thinking about the research problem . This does not refer to introducing new information [which should be avoided], but to offer new insight and creative approaches for framing or contextualizing the research problem based on the results of your study.
Bunton, David. “The Structure of PhD Conclusion Chapters.” Journal of English for Academic Purposes 4 (July 2005): 207–224; Conclusions. The Writing Center. University of North Carolina; Kretchmer, Paul. Twelve Steps to Writing an Effective Conclusion. San Francisco Edit, 2003-2008; Conclusions. The Writing Lab and The OWL. Purdue University; Assan, Joseph. "Writing the Conclusion Chapter: The Good, the Bad and the Missing." Liverpool: Development Studies Association (2009): 1-8.
Structure and Writing Style
I. General Rules
The general function of your paper's conclusion is to restate the main argument . It reminds the reader of the strengths of your main argument(s) and reiterates the most important evidence supporting those argument(s). Do this by clearly summarizing the context, background, and necessity of pursuing the research problem you investigated in relation to an issue, controversy, or a gap found in the literature. However, make sure that your conclusion is not simply a repetitive summary of the findings. This reduces the impact of the argument(s) you have developed in your paper.
When writing the conclusion to your paper, follow these general rules:
- Present your conclusions in clear, concise language. Re-state the purpose of your study, then describe how your findings differ or support those of other studies and why [i.e., what were the unique, new, or crucial contributions your study made to the overall research about your topic?].
- Do not simply reiterate your findings or the discussion of your results. Provide a synthesis of arguments presented in the paper to show how these converge to address the research problem and the overall objectives of your study.
- Indicate opportunities for future research if you haven't already done so in the discussion section of your paper. Highlighting the need for further research provides the reader with evidence that you have an in-depth awareness of the research problem but that further investigations should take place beyond the scope of your investigation.
Consider the following points to help ensure your conclusion is presented well:
- If the argument or purpose of your paper is complex, you may need to summarize the argument for your reader.
- If, prior to your conclusion, you have not yet explained the significance of your findings or if you are proceeding inductively, use the end of your paper to describe your main points and explain their significance.
- Move from a detailed to a general level of consideration that returns the topic to the context provided by the introduction or within a new context that emerges from the data [this is opposite of the introduction, which begins with general discussion of the context and ends with a detailed description of the research problem].
The conclusion also provides a place for you to persuasively and succinctly restate the research problem, given that the reader has now been presented with all the information about the topic . Depending on the discipline you are writing in, the concluding paragraph may contain your reflections on the evidence presented. However, the nature of being introspective about the research you have conducted will depend on the topic and whether your professor wants you to express your observations in this way. If asked to think introspectively about the topics, do not delve into idle speculation. Being introspective means looking within yourself as an author to try and understand an issue more deeply, not to guess at possible outcomes or make up scenarios not supported by the evidence.
II. Developing a Compelling Conclusion
Although an effective conclusion needs to be clear and succinct, it does not need to be written passively or lack a compelling narrative. Strategies to help you move beyond merely summarizing the key points of your research paper may include any of the following:
- If your essay deals with a critical, contemporary problem, warn readers of the possible consequences of not attending to the problem proactively.
- Recommend a specific course or courses of action that, if adopted, could address a specific problem in practice or in the development of new knowledge leading to positive change.
- Cite a relevant quotation or expert opinion already noted in your paper in order to lend authority and support to the conclusion(s) you have reached [a good source would be from your literature review].
- Explain the consequences of your research in a way that elicits action or demonstrates urgency in seeking change.
- Restate a key statistic, fact, or visual image to emphasize the most important finding of your paper.
- If your discipline encourages personal reflection, illustrate your concluding point by drawing from your own life experiences.
- Return to an anecdote, an example, or a quotation that you presented in your introduction, but add further insight derived from the findings of your study; use your interpretation of results from your study to recast it in new or important ways.
- Provide a "take-home" message in the form of a succinct, declarative statement that you want the reader to remember about your study.
III. Problems to Avoid
Failure to be concise Your conclusion section should be concise and to the point. Conclusions that are too lengthy often have unnecessary information in them. The conclusion is not the place for details about your methodology or results. Although you should give a summary of what was learned from your research, this summary should be relatively brief, since the emphasis in the conclusion is on the implications, evaluations, insights, and other forms of analysis that you make. Strategies for writing concisely can be found here .
Failure to comment on larger, more significant issues In the introduction, your task was to move from the general [the field of study] to the specific [the research problem]. However, in the conclusion, your task is to move from a specific discussion [your research problem] back to a general discussion framed around the implications and significance of your findings [i.e., how your research contributes new understanding or fills an important gap in the literature]. In short, the conclusion is where you should place your research within a larger context [visualize your paper as an hourglass--start with a broad introduction and review of the literature, move to the specific analysis and discussion, conclude with a broad summary of the study's implications and significance].
Failure to reveal problems and negative results Negative aspects of the research process should never be ignored. These are problems, deficiencies, or challenges encountered during your study. They should be summarized as a way of qualifying your overall conclusions. If you encountered negative or unintended results [i.e., findings that are validated outside the research context in which they were generated], you must report them in the results section and discuss their implications in the discussion section of your paper. In the conclusion, use negative results as an opportunity to explain their possible significance and/or how they may form the basis for future research.
Failure to provide a clear summary of what was learned In order to be able to discuss how your research fits within your field of study [and possibly the world at large], you need to summarize briefly and succinctly how it contributes to new knowledge or a new understanding about the research problem. This element of your conclusion may be only a few sentences long.
Failure to match the objectives of your research Often research objectives in the social and behavioral sciences change while the research is being carried out. This is not a problem unless you forget to go back and refine the original objectives in your introduction. As these changes emerge they must be documented so that they accurately reflect what you were trying to accomplish in your research [not what you thought you might accomplish when you began].
Resist the urge to apologize If you've immersed yourself in studying the research problem, you presumably should know a good deal about it [perhaps even more than your professor!]. Nevertheless, by the time you have finished writing, you may be having some doubts about what you have produced. Repress those doubts! Don't undermine your authority as a researcher by saying something like, "This is just one approach to examining this problem; there may be other, much better approaches that...." The overall tone of your conclusion should convey confidence to the reader about the study's validity and realiability.
Assan, Joseph. "Writing the Conclusion Chapter: The Good, the Bad and the Missing." Liverpool: Development Studies Association (2009): 1-8; Concluding Paragraphs. College Writing Center at Meramec. St. Louis Community College; Conclusions. The Writing Center. University of North Carolina; Conclusions. The Writing Lab and The OWL. Purdue University; Freedman, Leora and Jerry Plotnick. Introductions and Conclusions. The Lab Report. University College Writing Centre. University of Toronto; Leibensperger, Summer. Draft Your Conclusion. Academic Center, the University of Houston-Victoria, 2003; Make Your Last Words Count. The Writer’s Handbook. Writing Center. University of Wisconsin Madison; Miquel, Fuster-Marquez and Carmen Gregori-Signes. “Chapter Six: ‘Last but Not Least:’ Writing the Conclusion of Your Paper.” In Writing an Applied Linguistics Thesis or Dissertation: A Guide to Presenting Empirical Research . John Bitchener, editor. (Basingstoke,UK: Palgrave Macmillan, 2010), pp. 93-105; Tips for Writing a Good Conclusion. Writing@CSU. Colorado State University; Kretchmer, Paul. Twelve Steps to Writing an Effective Conclusion. San Francisco Edit, 2003-2008; Writing Conclusions. Writing Tutorial Services, Center for Innovative Teaching and Learning. Indiana University; Writing: Considering Structure and Organization. Institute for Writing Rhetoric. Dartmouth College.
Writing Tip
Don't Belabor the Obvious!
Avoid phrases like "in conclusion...," "in summary...," or "in closing...." These phrases can be useful, even welcome, in oral presentations. But readers can see by the tell-tale section heading and number of pages remaining that they are reaching the end of your paper. You'll irritate your readers if you belabor the obvious.
Assan, Joseph. "Writing the Conclusion Chapter: The Good, the Bad and the Missing." Liverpool: Development Studies Association (2009): 1-8.
Another Writing Tip
New Insight, Not New Information!
Don't surprise the reader with new information in your conclusion that was never referenced anywhere else in the paper. This why the conclusion rarely has citations to sources. If you have new information to present, add it to the discussion or other appropriate section of the paper. Note that, although no new information is introduced, the conclusion, along with the discussion section, is where you offer your most "original" contributions in the paper; the conclusion is where you describe the value of your research, demonstrate that you understand the material that you’ve presented, and position your findings within the larger context of scholarship on the topic, including describing how your research contributes new insights to that scholarship.
Assan, Joseph. "Writing the Conclusion Chapter: The Good, the Bad and the Missing." Liverpool: Development Studies Association (2009): 1-8; Conclusions. The Writing Center. University of North Carolina.
- << Previous: Limitations of the Study
- Next: Appendices >>
- Last Updated: Apr 11, 2024 1:27 PM
- URL: https://libguides.usc.edu/writingguide
In a short paper—even a research paper—you don’t need to provide an exhaustive summary as part of your conclusion. But you do need to make some kind of transition between your final body paragraph and your concluding paragraph. This may come in the form of a few sentences of summary. Or it may come in the form of a sentence that brings your readers back to your thesis or main idea and reminds your readers where you began and how far you have traveled.
So, for example, in a paper about the relationship between ADHD and rejection sensitivity, Vanessa Roser begins by introducing readers to the fact that researchers have studied the relationship between the two conditions and then provides her explanation of that relationship. Here’s her thesis: “While socialization may indeed be an important factor in RS, I argue that individuals with ADHD may also possess a neurological predisposition to RS that is exacerbated by the differing executive and emotional regulation characteristic of ADHD.”
In her final paragraph, Roser reminds us of where she started by echoing her thesis: “This literature demonstrates that, as with many other conditions, ADHD and RS share a delicately intertwined pattern of neurological similarities that is rooted in the innate biology of an individual’s mind, a connection that cannot be explained in full by the behavioral mediation hypothesis.”
Highlight the “so what”
At the beginning of your paper, you explain to your readers what’s at stake—why they should care about the argument you’re making. In your conclusion, you can bring readers back to those stakes by reminding them why your argument is important in the first place. You can also draft a few sentences that put those stakes into a new or broader context.
In the conclusion to her paper about ADHD and RS, Roser echoes the stakes she established in her introduction—that research into connections between ADHD and RS has led to contradictory results, raising questions about the “behavioral mediation hypothesis.”
She writes, “as with many other conditions, ADHD and RS share a delicately intertwined pattern of neurological similarities that is rooted in the innate biology of an individual’s mind, a connection that cannot be explained in full by the behavioral mediation hypothesis.”
Leave your readers with the “now what”
After the “what” and the “so what,” you should leave your reader with some final thoughts. If you have written a strong introduction, your readers will know why you have been arguing what you have been arguing—and why they should care. And if you’ve made a good case for your thesis, then your readers should be in a position to see things in a new way, understand new questions, or be ready for something that they weren’t ready for before they read your paper.
In her conclusion, Roser offers two “now what” statements. First, she explains that it is important to recognize that the flawed behavioral mediation hypothesis “seems to place a degree of fault on the individual. It implies that individuals with ADHD must have elicited such frequent or intense rejection by virtue of their inadequate social skills, erasing the possibility that they may simply possess a natural sensitivity to emotion.” She then highlights the broader implications for treatment of people with ADHD, noting that recognizing the actual connection between rejection sensitivity and ADHD “has profound implications for understanding how individuals with ADHD might best be treated in educational settings, by counselors, family, peers, or even society as a whole.”
To find your own “now what” for your essay’s conclusion, try asking yourself these questions:
- What can my readers now understand, see in a new light, or grapple with that they would not have understood in the same way before reading my paper? Are we a step closer to understanding a larger phenomenon or to understanding why what was at stake is so important?
- What questions can I now raise that would not have made sense at the beginning of my paper? Questions for further research? Other ways that this topic could be approached?
- Are there other applications for my research? Could my questions be asked about different data in a different context? Could I use my methods to answer a different question?
- What action should be taken in light of this argument? What action do I predict will be taken or could lead to a solution?
- What larger context might my argument be a part of?
What to avoid in your conclusion
- a complete restatement of all that you have said in your paper.
- a substantial counterargument that you do not have space to refute; you should introduce counterarguments before your conclusion.
- an apology for what you have not said. If you need to explain the scope of your paper, you should do this sooner—but don’t apologize for what you have not discussed in your paper.
- fake transitions like “in conclusion” that are followed by sentences that aren’t actually conclusions. (“In conclusion, I have now demonstrated that my thesis is correct.”)
- picture_as_pdf Conclusions

Conclusions
What this handout is about.
This handout will explain the functions of conclusions, offer strategies for writing effective ones, help you evaluate conclusions you’ve drafted, and suggest approaches to avoid.
About conclusions
Introductions and conclusions can be difficult to write, but they’re worth investing time in. They can have a significant influence on a reader’s experience of your paper.
Just as your introduction acts as a bridge that transports your readers from their own lives into the “place” of your analysis, your conclusion can provide a bridge to help your readers make the transition back to their daily lives. Such a conclusion will help them see why all your analysis and information should matter to them after they put the paper down.
Your conclusion is your chance to have the last word on the subject. The conclusion allows you to have the final say on the issues you have raised in your paper, to synthesize your thoughts, to demonstrate the importance of your ideas, and to propel your reader to a new view of the subject. It is also your opportunity to make a good final impression and to end on a positive note.
Your conclusion can go beyond the confines of the assignment. The conclusion pushes beyond the boundaries of the prompt and allows you to consider broader issues, make new connections, and elaborate on the significance of your findings.
Your conclusion should make your readers glad they read your paper. Your conclusion gives your reader something to take away that will help them see things differently or appreciate your topic in personally relevant ways. It can suggest broader implications that will not only interest your reader, but also enrich your reader’s life in some way. It is your gift to the reader.
Strategies for writing an effective conclusion
One or more of the following strategies may help you write an effective conclusion:
- Play the “So What” Game. If you’re stuck and feel like your conclusion isn’t saying anything new or interesting, ask a friend to read it with you. Whenever you make a statement from your conclusion, ask the friend to say, “So what?” or “Why should anybody care?” Then ponder that question and answer it. Here’s how it might go: You: Basically, I’m just saying that education was important to Douglass. Friend: So what? You: Well, it was important because it was a key to him feeling like a free and equal citizen. Friend: Why should anybody care? You: That’s important because plantation owners tried to keep slaves from being educated so that they could maintain control. When Douglass obtained an education, he undermined that control personally. You can also use this strategy on your own, asking yourself “So What?” as you develop your ideas or your draft.
- Return to the theme or themes in the introduction. This strategy brings the reader full circle. For example, if you begin by describing a scenario, you can end with the same scenario as proof that your essay is helpful in creating a new understanding. You may also refer to the introductory paragraph by using key words or parallel concepts and images that you also used in the introduction.
- Synthesize, don’t summarize. Include a brief summary of the paper’s main points, but don’t simply repeat things that were in your paper. Instead, show your reader how the points you made and the support and examples you used fit together. Pull it all together.
- Include a provocative insight or quotation from the research or reading you did for your paper.
- Propose a course of action, a solution to an issue, or questions for further study. This can redirect your reader’s thought process and help them to apply your info and ideas to their own life or to see the broader implications.
- Point to broader implications. For example, if your paper examines the Greensboro sit-ins or another event in the Civil Rights Movement, you could point out its impact on the Civil Rights Movement as a whole. A paper about the style of writer Virginia Woolf could point to her influence on other writers or on later feminists.
Strategies to avoid
- Beginning with an unnecessary, overused phrase such as “in conclusion,” “in summary,” or “in closing.” Although these phrases can work in speeches, they come across as wooden and trite in writing.
- Stating the thesis for the very first time in the conclusion.
- Introducing a new idea or subtopic in your conclusion.
- Ending with a rephrased thesis statement without any substantive changes.
- Making sentimental, emotional appeals that are out of character with the rest of an analytical paper.
- Including evidence (quotations, statistics, etc.) that should be in the body of the paper.
Four kinds of ineffective conclusions
- The “That’s My Story and I’m Sticking to It” Conclusion. This conclusion just restates the thesis and is usually painfully short. It does not push the ideas forward. People write this kind of conclusion when they can’t think of anything else to say. Example: In conclusion, Frederick Douglass was, as we have seen, a pioneer in American education, proving that education was a major force for social change with regard to slavery.
- The “Sherlock Holmes” Conclusion. Sometimes writers will state the thesis for the very first time in the conclusion. You might be tempted to use this strategy if you don’t want to give everything away too early in your paper. You may think it would be more dramatic to keep the reader in the dark until the end and then “wow” them with your main idea, as in a Sherlock Holmes mystery. The reader, however, does not expect a mystery, but an analytical discussion of your topic in an academic style, with the main argument (thesis) stated up front. Example: (After a paper that lists numerous incidents from the book but never says what these incidents reveal about Douglass and his views on education): So, as the evidence above demonstrates, Douglass saw education as a way to undermine the slaveholders’ power and also an important step toward freedom.
- The “America the Beautiful”/”I Am Woman”/”We Shall Overcome” Conclusion. This kind of conclusion usually draws on emotion to make its appeal, but while this emotion and even sentimentality may be very heartfelt, it is usually out of character with the rest of an analytical paper. A more sophisticated commentary, rather than emotional praise, would be a more fitting tribute to the topic. Example: Because of the efforts of fine Americans like Frederick Douglass, countless others have seen the shining beacon of light that is education. His example was a torch that lit the way for others. Frederick Douglass was truly an American hero.
- The “Grab Bag” Conclusion. This kind of conclusion includes extra information that the writer found or thought of but couldn’t integrate into the main paper. You may find it hard to leave out details that you discovered after hours of research and thought, but adding random facts and bits of evidence at the end of an otherwise-well-organized essay can just create confusion. Example: In addition to being an educational pioneer, Frederick Douglass provides an interesting case study for masculinity in the American South. He also offers historians an interesting glimpse into slave resistance when he confronts Covey, the overseer. His relationships with female relatives reveal the importance of family in the slave community.
Works consulted
We consulted these works while writing this handout. This is not a comprehensive list of resources on the handout’s topic, and we encourage you to do your own research to find additional publications. Please do not use this list as a model for the format of your own reference list, as it may not match the citation style you are using. For guidance on formatting citations, please see the UNC Libraries citation tutorial . We revise these tips periodically and welcome feedback.
Douglass, Frederick. 1995. Narrative of the Life of Frederick Douglass, an American Slave, Written by Himself. New York: Dover.
Hamilton College. n.d. “Conclusions.” Writing Center. Accessed June 14, 2019. https://www.hamilton.edu//academics/centers/writing/writing-resources/conclusions .
Holewa, Randa. 2004. “Strategies for Writing a Conclusion.” LEO: Literacy Education Online. Last updated February 19, 2004. https://leo.stcloudstate.edu/acadwrite/conclude.html.
You may reproduce it for non-commercial use if you use the entire handout and attribute the source: The Writing Center, University of North Carolina at Chapel Hill
Make a Gift
- Affiliate Program

- UNITED STATES
- 台灣 (TAIWAN)
- TÜRKIYE (TURKEY)
- Academic Editing Services
- - Research Paper
- - Journal Manuscript
- - Dissertation
- - College & University Assignments
- Admissions Editing Services
- - Application Essay
- - Personal Statement
- - Recommendation Letter
- - Cover Letter
- - CV/Resume
- Business Editing Services
- - Business Documents
- - Report & Brochure
- - Website & Blog
- Writer Editing Services
- - Script & Screenplay
- Our Editors
- Client Reviews
- Editing & Proofreading Prices
- Wordvice Points
- Partner Discount
- Plagiarism Checker
- APA Citation Generator
- MLA Citation Generator
- Chicago Citation Generator
- Vancouver Citation Generator
- - APA Style
- - MLA Style
- - Chicago Style
- - Vancouver Style
- Writing & Editing Guide
- Academic Resources
- Admissions Resources
How to Write a Research Paper Conclusion Section
What is a conclusion in a research paper?
The conclusion in a research paper is the final paragraph or two in a research paper. In scientific papers, the conclusion usually follows the Discussion section , summarizing the importance of the findings and reminding the reader why the work presented in the paper is relevant.
However, it can be a bit confusing to distinguish the conclusion section/paragraph from a summary or a repetition of your findings, your own opinion, or the statement of the implications of your work. In fact, the conclusion should contain a bit of all of these other parts but go beyond it—but not too far beyond!
The structure and content of the conclusion section can also vary depending on whether you are writing a research manuscript or an essay. This article will explain how to write a good conclusion section, what exactly it should (and should not) contain, how it should be structured, and what you should avoid when writing it.
Table of Contents:
What does a good conclusion section do, what to include in a research paper conclusion.
- Conclusion in an Essay
- Research Paper Conclusion
- Conclusion Paragraph Outline and Example
- What Not to Do When Writing a Conclusion
The conclusion of a research paper has several key objectives. It should:
- Restate your research problem addressed in the introduction section
- Summarize your main arguments, important findings, and broader implications
- Synthesize key takeaways from your study
The specific content in the conclusion depends on whether your paper presents the results of original scientific research or constructs an argument through engagement with previously published sources.
You presented your general field of study to the reader in the introduction section, by moving from general information (the background of your work, often combined with a literature review ) to the rationale of your study and then to the specific problem or topic you addressed, formulated in the form of the statement of the problem in research or the thesis statement in an essay.
In the conclusion section, in contrast, your task is to move from your specific findings or arguments back to a more general depiction of how your research contributes to the readers’ understanding of a certain concept or helps solve a practical problem, or fills an important gap in the literature. The content of your conclusion section depends on the type of research you are doing and what type of paper you are writing. But whatever the outcome of your work is, the conclusion is where you briefly summarize it and place it within a larger context. It could be called the “take-home message” of the entire paper.
What to summarize in the conclusion
Your conclusion section needs to contain a very brief summary of your work , a very brief summary of the main findings of your work, and a mention of anything else that seems relevant when you now look at your work from a bigger perspective, even if it was not initially listed as one of your main research questions. This could be a limitation, for example, a problem with the design of your experiment that either needs to be considered when drawing any conclusions or that led you to ask a different question and therefore draw different conclusions at the end of your study (compared to when you started out).
Once you have reminded the reader of what you did and what you found, you need to go beyond that and also provide either your own opinion on why your work is relevant (and for whom, and how) or theoretical or practical implications of the study , or make a specific call for action if there is one to be made.
How to Write an Essay Conclusion
Academic essays follow quite different structures than their counterparts in STEM and the natural sciences. Humanities papers often have conclusion sections that are much longer and contain more detail than scientific papers. There are three main types of academic essay conclusions.
Summarizing conclusion
The most typical conclusion at the end of an analytical/explanatory/argumentative essay is a summarizing conclusion . This is, as the name suggests, a clear summary of the main points of your topic and thesis. Since you might have gone through a number of different arguments or subtopics in the main part of your essay, you need to remind the reader again what those were, how they fit into each other, and how they helped you develop or corroborate your hypothesis.
For an essay that analyzes how recruiters can hire the best candidates in the shortest time or on “how starving yourself will increase your lifespan, according to science”, a summary of all the points you discussed might be all you need. Note that you should not exactly repeat what you said earlier, but rather highlight the essential details and present those to your reader in a different way.
Externalizing conclusion
If you think that just reminding the reader of your main points is not enough, you can opt for an externalizing conclusion instead, that presents new points that were not presented in the paper so far. These new points can be additional facts and information or they can be ideas that are relevant to the topic and have not been mentioned before.
Such a conclusion can stimulate your readers to think about your topic or the implications of your analysis in a whole new way. For example, at the end of a historical analysis of a specific event or development, you could direct your reader’s attention to some current events that were not the topic of your essay but that provide a different context for your findings.
Editorial conclusion
In an editorial conclusion , another common type of conclusion that you will find at the end of papers and essays, you do not add new information but instead present your own experiences or opinions on the topic to round everything up. What makes this type of conclusion interesting is that you can choose to agree or disagree with the information you presented in your paper so far. For example, if you have collected and analyzed information on how a specific diet helps people lose weight, you can nevertheless have your doubts on the sustainability of that diet or its practicability in real life—if such arguments were not included in your original thesis and have therefore not been covered in the main part of your paper, the conclusion section is the place where you can get your opinion across.
How to Conclude an Empirical Research Paper
An empirical research paper is usually more concise and succinct than an essay, because, if it is written well, it focuses on one specific question, describes the method that was used to answer that one question, describes and explains the results, and guides the reader in a logical way from the introduction to the discussion without going on tangents or digging into not absolutely relevant topics.
Summarize the findings
In a scientific paper, you should include a summary of the findings. Don’t go into great detail here (you will have presented your in-depth results and discussion already), but do clearly express the answers to the research questions you investigated.
Describe your main findings, even if they weren’t necessarily the ones anticipated, and explain the conclusion they led you to. Explain these findings in as few words as possible.
Instead of beginning with “ In conclusion, in this study, we investigated the effect of stress on the brain using fMRI …”, you should try to find a way to incorporate the repetition of the essential (and only the essential) details into the summary of the key points. “ The findings of this fMRI study on the effect of stress on the brain suggest that …” or “ While it has been known for a long time that stress has an effect on the brain, the findings of this fMRI study show that, surprisingly… ” would be better ways to start a conclusion.
You should also not bring up new ideas or present new facts in the conclusion of a research paper, but stick to the background information you have presented earlier, to the findings you have already discussed, and the limitations and implications you have already described. The one thing you can add here is a practical recommendation that you haven’t clearly stated before—but even that one needs to follow logically from everything you have already discussed in the discussion section.
Discuss the implications
After summing up your key arguments or findings, conclude the paper by stating the broader implications of the research , whether in methods , approach, or findings. Express practical or theoretical takeaways from your paper. This often looks like a “call to action” or a final “sales pitch” that puts an exclamation point on your paper.
If your research topic is more theoretical in nature, your closing statement should express the significance of your argument—for example, in proposing a new understanding of a topic or laying the groundwork for future research.
Future research example
Future research into education standards should focus on establishing a more detailed picture of how novel pedagogical approaches impact young people’s ability to absorb new and difficult concepts. Moreover, observational studies are needed to gain more insight into how specific teaching models affect the retention of relationships and facts—for instance, how inquiry-based learning and its emphasis on lateral thinking can be used as a jumping-off point for more holistic classroom approaches.
Research Conclusion Example and Outline
Let’s revisit the study on the effect of stress on the brain we mentioned before and see what the common structure for a conclusion paragraph looks like, in three steps. Following these simple steps will make it easy for you to wrap everything up in one short paragraph that contains all the essential information:
One: Short summary of what you did, but integrated into the summary of your findings:
While it has been known for a long time that stress has an effect on the brain, the findings of this fMRI study in 25 university students going through mid-term exams show that, surprisingly, one’s attitude to the experienced stress significantly modulates the brain’s response to it.
Note that you don’t need to repeat any methodological or technical details here—the reader has been presented with all of these before, they have read your results section and the discussion of your results, and even (hopefully!) a discussion of the limitations and strengths of your paper. The only thing you need to remind them of here is the essential outcome of your work.
Two: Add implications, and don’t forget to specify who this might be relevant for:
Students could be considered a specific subsample of the general population, but earlier research shows that the effect that exam stress has on their physical and mental health is comparable to the effects of other types of stress on individuals of other ages and occupations. Further research into practical ways of modulating not only one’s mental stress response but potentially also one’s brain activity (e.g., via neurofeedback training) are warranted.
This is a “research implication”, and it is nicely combined with a mention of a potential limitation of the study (the student sample) that turns out not to be a limitation after all (because earlier research suggests we can generalize to other populations). If there already is a lot of research on neurofeedback for stress control, by the way, then this should have been discussed in your discussion section earlier and you wouldn’t say such studies are “warranted” here but rather specify how your findings could inspire specific future experiments or how they should be implemented in existing applications.
Three: The most important thing is that your conclusion paragraph accurately reflects the content of your paper. Compare it to your research paper title , your research paper abstract , and to your journal submission cover letter , in case you already have one—if these do not all tell the same story, then you need to go back to your paper, start again from the introduction section, and find out where you lost the logical thread. As always, consistency is key.
Problems to Avoid When Writing a Conclusion
- Do not suddenly introduce new information that has never been mentioned before (unless you are writing an essay and opting for an externalizing conclusion, see above). The conclusion section is not where you want to surprise your readers, but the take-home message of what you have already presented.
- Do not simply copy your abstract, the conclusion section of your abstract, or the first sentence of your introduction, and put it at the end of the discussion section. Even if these parts of your paper cover the same points, they should not be identical.
- Do not start the conclusion with “In conclusion”. If it has its own section heading, that is redundant, and if it is the last paragraph of the discussion section, it is inelegant and also not really necessary. The reader expects you to wrap your work up in the last paragraph, so you don’t have to announce that. Just look at the above example to see how to start a conclusion in a natural way.
- Do not forget what your research objectives were and how you initially formulated the statement of the problem in your introduction section. If your story/approach/conclusions changed because of methodological issues or information you were not aware of when you started, then make sure you go back to the beginning and adapt your entire story (not just the ending).
Consider Receiving Academic Editing Services
When you have arrived at the conclusion of your paper, you might want to head over to Wordvice AI’s AI Writing Assistant to receive a free grammar check for any academic content.
After drafting, you can also receive English editing and proofreading services , including paper editing services for your journal manuscript. If you need advice on how to write the other parts of your research paper , or on how to make a research paper outline if you are struggling with putting everything you did together, then head over to the Wordvice academic resources pages , where we have a lot more articles and videos for you.

- Walden University
- Faculty Portal
Writing a Paper: Conclusions
Writing a conclusion.
A conclusion is an important part of the paper; it provides closure for the reader while reminding the reader of the contents and importance of the paper. It accomplishes this by stepping back from the specifics in order to view the bigger picture of the document. In other words, it is reminding the reader of the main argument. For most course papers, it is usually one paragraph that simply and succinctly restates the main ideas and arguments, pulling everything together to help clarify the thesis of the paper. A conclusion does not introduce new ideas; instead, it should clarify the intent and importance of the paper. It can also suggest possible future research on the topic.
An Easy Checklist for Writing a Conclusion
It is important to remind the reader of the thesis of the paper so he is reminded of the argument and solutions you proposed.
Think of the main points as puzzle pieces, and the conclusion is where they all fit together to create a bigger picture. The reader should walk away with the bigger picture in mind.
Make sure that the paper places its findings in the context of real social change.
Make sure the reader has a distinct sense that the paper has come to an end. It is important to not leave the reader hanging. (You don’t want her to have flip-the-page syndrome, where the reader turns the page, expecting the paper to continue. The paper should naturally come to an end.)
No new ideas should be introduced in the conclusion. It is simply a review of the material that is already present in the paper. The only new idea would be the suggesting of a direction for future research.
Conclusion Example
As addressed in my analysis of recent research, the advantages of a later starting time for high school students significantly outweigh the disadvantages. A later starting time would allow teens more time to sleep--something that is important for their physical and mental health--and ultimately improve their academic performance and behavior. The added transportation costs that result from this change can be absorbed through energy savings. The beneficial effects on the students’ academic performance and behavior validate this decision, but its effect on student motivation is still unknown. I would encourage an in-depth look at the reactions of students to such a change. This sort of study would help determine the actual effects of a later start time on the time management and sleep habits of students.
Related Webinar

Didn't find what you need? Email us at [email protected] .
- Previous Page: Thesis Statements
- Next Page: Writer's Block
- Office of Student Disability Services
Walden Resources
Departments.
- Academic Residencies
- Academic Skills
- Career Planning and Development
- Customer Care Team
- Field Experience
- Military Services
- Student Success Advising
- Writing Skills
Centers and Offices
- Center for Social Change
- Office of Academic Support and Instructional Services
- Office of Degree Acceleration
- Office of Research and Doctoral Services
- Office of Student Affairs
Student Resources
- Doctoral Writing Assessment
- Form & Style Review
- Quick Answers
- ScholarWorks
- SKIL Courses and Workshops
- Walden Bookstore
- Walden Catalog & Student Handbook
- Student Safety/Title IX
- Legal & Consumer Information
- Website Terms and Conditions
- Cookie Policy
- Accessibility
- Accreditation
- State Authorization
- Net Price Calculator
- Contact Walden
Walden University is a member of Adtalem Global Education, Inc. www.adtalem.com Walden University is certified to operate by SCHEV © 2024 Walden University LLC. All rights reserved.
How to Write a Conclusion for a Research Paper
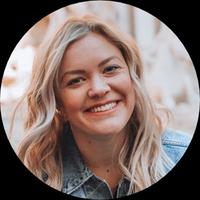
When you're wrapping up a research paper, the conclusion is like the grand finale of a fireworks show – it's your chance to leave a lasting impression. In this article, we'll break down the steps to help you write a winning research paper conclusion that not only recaps your main points but also ties everything together. Consider it the "So what?" moment – why should people care about your research? Our professional essay writers will guide you through making your conclusion strong, clear, and something that sticks with your readers long after they've put down your paper. So, let's dive in and ensure your research ends on a high note!
What Is a Conclusion in a Research Paper
In a research paper, the conclusion serves as the final segment, where you summarize the main points and findings of your study. It's not just a repetition of what you've already said but rather a chance to tie everything together and highlight the significance of your research. As you learn how to start a research paper , a good conclusion also often discusses the implications of your findings, suggests potential areas for further research, and leaves the reader with a lasting impression of the importance and relevance of your work in the broader context of the field. Essentially, it's your last opportunity to make a strong impact and leave your readers with a clear understanding of the significance of your research. Here’s a research paper conclusion example:
In conclusion, this research paper has navigated the intricacies of sustainable urban development, shedding light on the pivotal role of community engagement and innovative planning strategies. Through applying qualitative and quantitative research methods, we've uncovered valuable insights into the challenges and opportunities inherent in fostering environmentally friendly urban spaces. The implications of these findings extend beyond the confines of this study, emphasizing the imperative for continued exploration in the realms of urban planning and environmental sustainability. By emphasizing both the practical applications and theoretical contributions, this research underscores the significance of community involvement and forward-thinking strategies in shaping the future of urban landscapes. As cities evolve, incorporating these insights into planning and development practices will create resilient and harmonious urban environments.
Conclusion Outline for Research Paper
This outline for a research paper conclusion provides a structured framework to ensure that your ending effectively summarizes the key elements of your research paper and leaves a lasting impression on your readers. Adjust the content based on the specific requirements and focus of your research.
Restate the Thesis Statement
- Briefly restate the main thesis or research question.
- Emphasize the core objective or purpose of the study.
Summarize Key Findings
- Recap the main points and key findings from each section of the paper.
- Provide a concise overview of the research journey.
Discuss Implications
- Explore the broader implications of the research findings.
- Discuss how the results contribute to the existing body of knowledge in the field.
Address Limitations
- Acknowledge any limitations or constraints encountered during the research process.
- Explain how these limitations may impact the interpretation of the findings.
Suggest Areas for Future Research
- Propose potential directions for future studies related to the topic.
- Identify gaps in the current research that warrant further exploration.
Reaffirm Significance
- Reaffirm the importance and relevance of the research in the broader context.
- Highlight the practical applications or real-world implications of the study.
Concluding Statement
- Craft a strong, memorable closing statement that leaves a lasting impression.
- Sum up the overall impact of the research and its potential contribution to the field.
Study the full guide on how to make a research paper outline here, which will also specify the conclusion writing specifics to improve your general prowess.
Tips on How to Make a Conclusion in Research
Here are key considerations regarding a conclusion for research paper to not only recap the primary ideas in your work but also delve deeper to earn a higher grade:
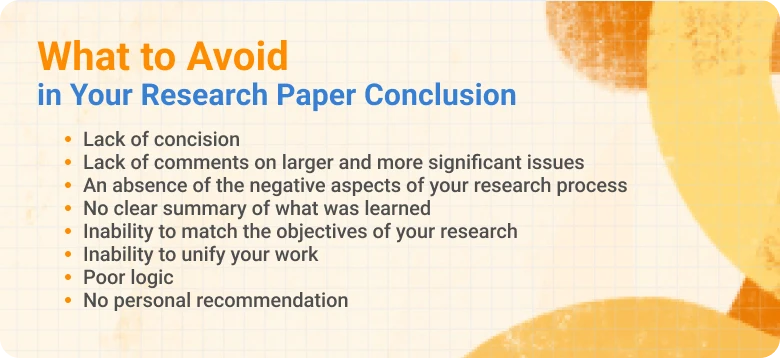
- Provide a concise recap of your main research outcomes.
- Remind readers of your research goals and their accomplishments.
- Stick to summarizing existing content; refrain from adding new details.
- Emphasize why your research matters and its broader implications.
- Clearly explain the practical or theoretical impact of your findings.
- Prompt readers to reflect on how your research influences their perspective.
- Briefly discuss the robustness of your research methods.
- End with a suggestion for future research or a practical application.
- Transparently address any constraints or biases in your study.
- End on a powerful note, leaving a memorable impression on your readers.
.webp)
For your inspiration, we’ve also prepared this research proposal example APA , which dwells on another important aspect of research writing.
How to Write a Research Paper Conclusion
As you finish your research paper, the conclusion takes center stage. In this section, we've got five practical tips for writing a conclusion for a research paper. We'll guide you through summarizing your key findings, revisiting your research goals, discussing the bigger picture, addressing any limitations, and ending on a powerful note. Think of it as your roadmap to creating a conclusion that not only wraps up your research but also leaves a lasting impact on your readers. Let's dive in and make sure your conclusion stands out for all the right reasons!
.webp)
Synthesize Core Discoveries. Initiate your conclusion by synthesizing the essential discoveries of your research. Offer a succinct recapitulation of the primary points and outcomes you have elucidated in your paper. This aids in reinforcing the gravity of your work and reiterates the pivotal information you have presented.
Revisit Research Objectives. Revisit the research objectives or questions you outlined at the beginning of your paper. Assess whether you have successfully addressed these objectives and if your findings align with the initial goals of your research. This reflection helps tie your conclusion back to the purpose of your study.
Discuss Implications and Contributions. Discuss the broader implications of your research and its potential contributions to the field. Consider how your findings might impact future research, applications, or understanding of the subject matter. This demonstrates the significance of your work and places it within a larger context.
Address Limitations and Future Research. Acknowledge any limitations in your study, such as constraints in data collection or potential biases. Briefly discuss how these limitations might have affected your results. Additionally, suggest areas for future research that could build upon your work, addressing any unanswered questions or unexplored aspects. This demonstrates a thoughtful approach to your research.
End with a Strong Conclusion Statement. Conclude your research paper with a strong and memorable statement that reinforces the key message you want readers to take away. This could be a call to action, a proposal for further investigation, or a reflection on the broader significance of your findings. Leave your readers with a lasting impression that emphasizes the importance of your research. Remember that you can buy a research paper anytime if you lack time or get stuck in writer’s block.
Want to Enjoy the Benefits of Academic Freedom?
With our top-notch writers and 24/7 support, you can relax and focus on what really matters
Stylistic Devices to Use in a Conclusion
Discover distinctive stylistic insights that you can apply when writing a conclusion for a research paper:
- Rhetorical Questions. When using rhetorical questions, strategically place them to engage readers' minds. For instance, you might pose a question that prompts reflection on the broader implications of your findings, leaving your audience with something to ponder.
- Powerful Language. Incorporate strong language to convey a sense of conviction and importance. Choose words that resonate with the overall tone of your research and amplify the significance of your conclusions. This adds weight to your key messages.
- Repetitions. Repetitions can be employed to reinforce essential ideas. Reiterate key phrases or concepts in a way that emphasizes their importance without sounding redundant. This technique serves to drive home your main points.
- Anecdotes. Integrating anecdotes into your conclusion can provide a human touch. Share a brief and relevant story that connects with your research, making the information more relatable and memorable for your audience.
- Vivid Imagery. Lastly, use vivid imagery to paint a picture in the minds of your readers. Appeal to their senses by describing scenarios or outcomes related to your research. This creates a more immersive and lasting impression.
If you have a larger paper to write, for example a thesis, use our custom dissertation writing can help you in no time.
How to Make a Conclusion Logically Appealing
Knowing how to write a conclusion for a research paper that is logically appealing is important for leaving a lasting impression on your readers. Here are some tips to achieve this:
Logical Sequencing
- Present your conclusion in a structured manner, following the natural flow of your paper. Readers should effortlessly follow your thought process, making your conclusion more accessible and persuasive.
Reinforce Main Arguments
- Emphasize the core arguments and findings from your research. By reinforcing key points, you solidify your stance and provide a logical culmination to your paper.
Address Counterarguments
- Acknowledge and address potential counterarguments or limitations in your research. Demonstrate intellectual honesty and strengthen your conclusion by preemptively addressing potential doubts.
Connect with Introduction
- Revisit themes or concepts introduced in your introduction to create a cohesive narrative, allowing readers to trace the logical progression of your research from start to finish.
Propose Actionable Insights
- Suggest practical applications or recommendations based on your findings. This will add a forward-looking dimension, making your conclusion more relevant and compelling.
Highlight Significance
- Clearly articulate the broader implications of your research to convey the importance of your work and its potential impact on the field, making your conclusion logically compelling.
Are you ready to produce an A-grade assignment? If not, opt for a custom research paper from our skilled writers across various disciplines.
Avoid These Things When Writing a Research Paper Conclusion
As you write your conclusion of research paper, there’s a list of things professional writers don’t recommend doing. Consider these issues carefully:
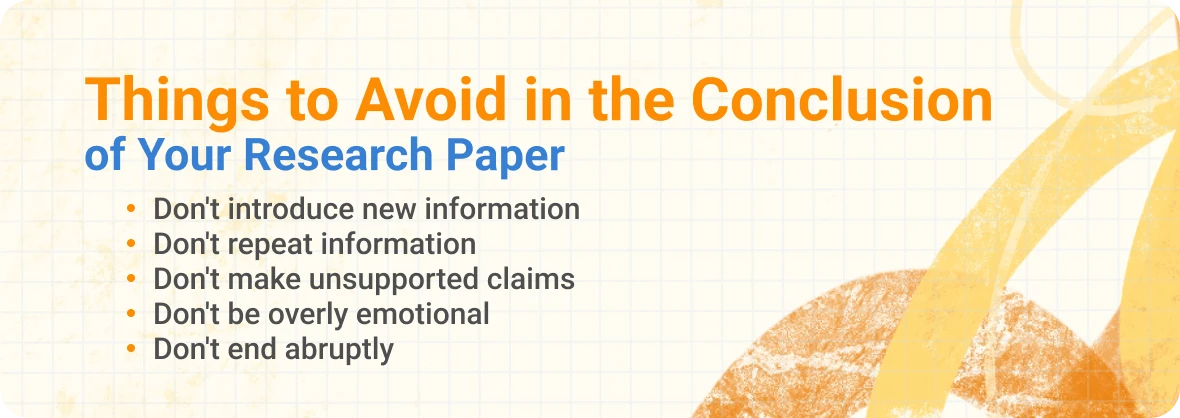
- Repetition of Exact Phrases
- Repetitively using the same phrases or sentences from the main body. Repetition can make your conclusion seem redundant and less engaging.
- Overly Lengthy Summaries
- Providing excessively detailed summaries of each section of your paper. Readers may lose interest if the conclusion becomes too long and detailed.
- Unclear Connection to the Introduction
- Failing to connect the conclusion back to the introduction. A lack of continuity may make the paper feel disjointed.
- Adding New Arguments or Ideas
- Introducing new arguments or ideas that were not addressed in the body. This can confuse the reader and disrupt the coherence of your paper.
- Overuse of Complex Jargon
- Using excessively complex or technical language without clarification. Clear communication is essential in the conclusion, ensuring broad understanding.
- Apologizing or Undermining Confidence
- Apologizing for limitations or expressing doubt about your work. Maintain a confident tone; if limitations exist, present them objectively without undermining your research.
- Sweeping Generalizations
- Making overly broad or unsupported generalizations. Such statements can weaken the credibility of your conclusion.
- Neglecting the Significance
- Failing to emphasize the broader significance of your research. Readers need to understand why your findings matter in a larger context.
- Abrupt Endings
- Concluding abruptly without a strong closing statement. A powerful ending leaves a lasting impression; avoid a sudden or weak conclusion.
Research Paper Conclusion Example
That covers the essential aspects of summarizing a research paper. The only remaining step is to review the conclusion examples for research paper provided by our team.
Like our examples? Order us to write paper according to your instructions to avoid plagiarizing and to keep your academic integrity strong.
Final Thoughts
In conclusion, the knowledge of how to write the conclusion of a research paper is pivotal for presenting your findings and leaving a lasting impression on your readers. By summarizing the key points, reiterating the significance of your research, and offering avenues for future exploration, you can create a conclusion that not only reinforces the value of your study but also encourages further academic discourse. Remember to balance brevity and completeness, ensuring your conclusion is concise yet comprehensive. Emphasizing the practical implications of your research and connecting it to the broader academic landscape will help solidify the impact of your work. Pay someone to write a research paper if you are having a hard time finishing your coursework on time.
Tired of Stressing Over Endless Essays and Deadlines?
Let us take the load off your shoulders! Order your custom research paper today and experience the relief of knowing that your assignment is in expert hands
Related Articles
%20(2).webp)
- Privacy Policy
Buy Me a Coffee

Home » Research Paper – Structure, Examples and Writing Guide
Research Paper – Structure, Examples and Writing Guide
Table of Contents

Research Paper
Definition:
Research Paper is a written document that presents the author’s original research, analysis, and interpretation of a specific topic or issue.
It is typically based on Empirical Evidence, and may involve qualitative or quantitative research methods, or a combination of both. The purpose of a research paper is to contribute new knowledge or insights to a particular field of study, and to demonstrate the author’s understanding of the existing literature and theories related to the topic.
Structure of Research Paper
The structure of a research paper typically follows a standard format, consisting of several sections that convey specific information about the research study. The following is a detailed explanation of the structure of a research paper:
The title page contains the title of the paper, the name(s) of the author(s), and the affiliation(s) of the author(s). It also includes the date of submission and possibly, the name of the journal or conference where the paper is to be published.
The abstract is a brief summary of the research paper, typically ranging from 100 to 250 words. It should include the research question, the methods used, the key findings, and the implications of the results. The abstract should be written in a concise and clear manner to allow readers to quickly grasp the essence of the research.
Introduction
The introduction section of a research paper provides background information about the research problem, the research question, and the research objectives. It also outlines the significance of the research, the research gap that it aims to fill, and the approach taken to address the research question. Finally, the introduction section ends with a clear statement of the research hypothesis or research question.
Literature Review
The literature review section of a research paper provides an overview of the existing literature on the topic of study. It includes a critical analysis and synthesis of the literature, highlighting the key concepts, themes, and debates. The literature review should also demonstrate the research gap and how the current study seeks to address it.
The methods section of a research paper describes the research design, the sample selection, the data collection and analysis procedures, and the statistical methods used to analyze the data. This section should provide sufficient detail for other researchers to replicate the study.
The results section presents the findings of the research, using tables, graphs, and figures to illustrate the data. The findings should be presented in a clear and concise manner, with reference to the research question and hypothesis.
The discussion section of a research paper interprets the findings and discusses their implications for the research question, the literature review, and the field of study. It should also address the limitations of the study and suggest future research directions.
The conclusion section summarizes the main findings of the study, restates the research question and hypothesis, and provides a final reflection on the significance of the research.
The references section provides a list of all the sources cited in the paper, following a specific citation style such as APA, MLA or Chicago.
How to Write Research Paper
You can write Research Paper by the following guide:
- Choose a Topic: The first step is to select a topic that interests you and is relevant to your field of study. Brainstorm ideas and narrow down to a research question that is specific and researchable.
- Conduct a Literature Review: The literature review helps you identify the gap in the existing research and provides a basis for your research question. It also helps you to develop a theoretical framework and research hypothesis.
- Develop a Thesis Statement : The thesis statement is the main argument of your research paper. It should be clear, concise and specific to your research question.
- Plan your Research: Develop a research plan that outlines the methods, data sources, and data analysis procedures. This will help you to collect and analyze data effectively.
- Collect and Analyze Data: Collect data using various methods such as surveys, interviews, observations, or experiments. Analyze data using statistical tools or other qualitative methods.
- Organize your Paper : Organize your paper into sections such as Introduction, Literature Review, Methods, Results, Discussion, and Conclusion. Ensure that each section is coherent and follows a logical flow.
- Write your Paper : Start by writing the introduction, followed by the literature review, methods, results, discussion, and conclusion. Ensure that your writing is clear, concise, and follows the required formatting and citation styles.
- Edit and Proofread your Paper: Review your paper for grammar and spelling errors, and ensure that it is well-structured and easy to read. Ask someone else to review your paper to get feedback and suggestions for improvement.
- Cite your Sources: Ensure that you properly cite all sources used in your research paper. This is essential for giving credit to the original authors and avoiding plagiarism.
Research Paper Example
Note : The below example research paper is for illustrative purposes only and is not an actual research paper. Actual research papers may have different structures, contents, and formats depending on the field of study, research question, data collection and analysis methods, and other factors. Students should always consult with their professors or supervisors for specific guidelines and expectations for their research papers.
Research Paper Example sample for Students:
Title: The Impact of Social Media on Mental Health among Young Adults
Abstract: This study aims to investigate the impact of social media use on the mental health of young adults. A literature review was conducted to examine the existing research on the topic. A survey was then administered to 200 university students to collect data on their social media use, mental health status, and perceived impact of social media on their mental health. The results showed that social media use is positively associated with depression, anxiety, and stress. The study also found that social comparison, cyberbullying, and FOMO (Fear of Missing Out) are significant predictors of mental health problems among young adults.
Introduction: Social media has become an integral part of modern life, particularly among young adults. While social media has many benefits, including increased communication and social connectivity, it has also been associated with negative outcomes, such as addiction, cyberbullying, and mental health problems. This study aims to investigate the impact of social media use on the mental health of young adults.
Literature Review: The literature review highlights the existing research on the impact of social media use on mental health. The review shows that social media use is associated with depression, anxiety, stress, and other mental health problems. The review also identifies the factors that contribute to the negative impact of social media, including social comparison, cyberbullying, and FOMO.
Methods : A survey was administered to 200 university students to collect data on their social media use, mental health status, and perceived impact of social media on their mental health. The survey included questions on social media use, mental health status (measured using the DASS-21), and perceived impact of social media on their mental health. Data were analyzed using descriptive statistics and regression analysis.
Results : The results showed that social media use is positively associated with depression, anxiety, and stress. The study also found that social comparison, cyberbullying, and FOMO are significant predictors of mental health problems among young adults.
Discussion : The study’s findings suggest that social media use has a negative impact on the mental health of young adults. The study highlights the need for interventions that address the factors contributing to the negative impact of social media, such as social comparison, cyberbullying, and FOMO.
Conclusion : In conclusion, social media use has a significant impact on the mental health of young adults. The study’s findings underscore the need for interventions that promote healthy social media use and address the negative outcomes associated with social media use. Future research can explore the effectiveness of interventions aimed at reducing the negative impact of social media on mental health. Additionally, longitudinal studies can investigate the long-term effects of social media use on mental health.
Limitations : The study has some limitations, including the use of self-report measures and a cross-sectional design. The use of self-report measures may result in biased responses, and a cross-sectional design limits the ability to establish causality.
Implications: The study’s findings have implications for mental health professionals, educators, and policymakers. Mental health professionals can use the findings to develop interventions that address the negative impact of social media use on mental health. Educators can incorporate social media literacy into their curriculum to promote healthy social media use among young adults. Policymakers can use the findings to develop policies that protect young adults from the negative outcomes associated with social media use.
References :
- Twenge, J. M., & Campbell, W. K. (2019). Associations between screen time and lower psychological well-being among children and adolescents: Evidence from a population-based study. Preventive medicine reports, 15, 100918.
- Primack, B. A., Shensa, A., Escobar-Viera, C. G., Barrett, E. L., Sidani, J. E., Colditz, J. B., … & James, A. E. (2017). Use of multiple social media platforms and symptoms of depression and anxiety: A nationally-representative study among US young adults. Computers in Human Behavior, 69, 1-9.
- Van der Meer, T. G., & Verhoeven, J. W. (2017). Social media and its impact on academic performance of students. Journal of Information Technology Education: Research, 16, 383-398.
Appendix : The survey used in this study is provided below.
Social Media and Mental Health Survey
- How often do you use social media per day?
- Less than 30 minutes
- 30 minutes to 1 hour
- 1 to 2 hours
- 2 to 4 hours
- More than 4 hours
- Which social media platforms do you use?
- Others (Please specify)
- How often do you experience the following on social media?
- Social comparison (comparing yourself to others)
- Cyberbullying
- Fear of Missing Out (FOMO)
- Have you ever experienced any of the following mental health problems in the past month?
- Do you think social media use has a positive or negative impact on your mental health?
- Very positive
- Somewhat positive
- Somewhat negative
- Very negative
- In your opinion, which factors contribute to the negative impact of social media on mental health?
- Social comparison
- In your opinion, what interventions could be effective in reducing the negative impact of social media on mental health?
- Education on healthy social media use
- Counseling for mental health problems caused by social media
- Social media detox programs
- Regulation of social media use
Thank you for your participation!
Applications of Research Paper
Research papers have several applications in various fields, including:
- Advancing knowledge: Research papers contribute to the advancement of knowledge by generating new insights, theories, and findings that can inform future research and practice. They help to answer important questions, clarify existing knowledge, and identify areas that require further investigation.
- Informing policy: Research papers can inform policy decisions by providing evidence-based recommendations for policymakers. They can help to identify gaps in current policies, evaluate the effectiveness of interventions, and inform the development of new policies and regulations.
- Improving practice: Research papers can improve practice by providing evidence-based guidance for professionals in various fields, including medicine, education, business, and psychology. They can inform the development of best practices, guidelines, and standards of care that can improve outcomes for individuals and organizations.
- Educating students : Research papers are often used as teaching tools in universities and colleges to educate students about research methods, data analysis, and academic writing. They help students to develop critical thinking skills, research skills, and communication skills that are essential for success in many careers.
- Fostering collaboration: Research papers can foster collaboration among researchers, practitioners, and policymakers by providing a platform for sharing knowledge and ideas. They can facilitate interdisciplinary collaborations and partnerships that can lead to innovative solutions to complex problems.
When to Write Research Paper
Research papers are typically written when a person has completed a research project or when they have conducted a study and have obtained data or findings that they want to share with the academic or professional community. Research papers are usually written in academic settings, such as universities, but they can also be written in professional settings, such as research organizations, government agencies, or private companies.
Here are some common situations where a person might need to write a research paper:
- For academic purposes: Students in universities and colleges are often required to write research papers as part of their coursework, particularly in the social sciences, natural sciences, and humanities. Writing research papers helps students to develop research skills, critical thinking skills, and academic writing skills.
- For publication: Researchers often write research papers to publish their findings in academic journals or to present their work at academic conferences. Publishing research papers is an important way to disseminate research findings to the academic community and to establish oneself as an expert in a particular field.
- To inform policy or practice : Researchers may write research papers to inform policy decisions or to improve practice in various fields. Research findings can be used to inform the development of policies, guidelines, and best practices that can improve outcomes for individuals and organizations.
- To share new insights or ideas: Researchers may write research papers to share new insights or ideas with the academic or professional community. They may present new theories, propose new research methods, or challenge existing paradigms in their field.
Purpose of Research Paper
The purpose of a research paper is to present the results of a study or investigation in a clear, concise, and structured manner. Research papers are written to communicate new knowledge, ideas, or findings to a specific audience, such as researchers, scholars, practitioners, or policymakers. The primary purposes of a research paper are:
- To contribute to the body of knowledge : Research papers aim to add new knowledge or insights to a particular field or discipline. They do this by reporting the results of empirical studies, reviewing and synthesizing existing literature, proposing new theories, or providing new perspectives on a topic.
- To inform or persuade: Research papers are written to inform or persuade the reader about a particular issue, topic, or phenomenon. They present evidence and arguments to support their claims and seek to persuade the reader of the validity of their findings or recommendations.
- To advance the field: Research papers seek to advance the field or discipline by identifying gaps in knowledge, proposing new research questions or approaches, or challenging existing assumptions or paradigms. They aim to contribute to ongoing debates and discussions within a field and to stimulate further research and inquiry.
- To demonstrate research skills: Research papers demonstrate the author’s research skills, including their ability to design and conduct a study, collect and analyze data, and interpret and communicate findings. They also demonstrate the author’s ability to critically evaluate existing literature, synthesize information from multiple sources, and write in a clear and structured manner.
Characteristics of Research Paper
Research papers have several characteristics that distinguish them from other forms of academic or professional writing. Here are some common characteristics of research papers:
- Evidence-based: Research papers are based on empirical evidence, which is collected through rigorous research methods such as experiments, surveys, observations, or interviews. They rely on objective data and facts to support their claims and conclusions.
- Structured and organized: Research papers have a clear and logical structure, with sections such as introduction, literature review, methods, results, discussion, and conclusion. They are organized in a way that helps the reader to follow the argument and understand the findings.
- Formal and objective: Research papers are written in a formal and objective tone, with an emphasis on clarity, precision, and accuracy. They avoid subjective language or personal opinions and instead rely on objective data and analysis to support their arguments.
- Citations and references: Research papers include citations and references to acknowledge the sources of information and ideas used in the paper. They use a specific citation style, such as APA, MLA, or Chicago, to ensure consistency and accuracy.
- Peer-reviewed: Research papers are often peer-reviewed, which means they are evaluated by other experts in the field before they are published. Peer-review ensures that the research is of high quality, meets ethical standards, and contributes to the advancement of knowledge in the field.
- Objective and unbiased: Research papers strive to be objective and unbiased in their presentation of the findings. They avoid personal biases or preconceptions and instead rely on the data and analysis to draw conclusions.
Advantages of Research Paper
Research papers have many advantages, both for the individual researcher and for the broader academic and professional community. Here are some advantages of research papers:
- Contribution to knowledge: Research papers contribute to the body of knowledge in a particular field or discipline. They add new information, insights, and perspectives to existing literature and help advance the understanding of a particular phenomenon or issue.
- Opportunity for intellectual growth: Research papers provide an opportunity for intellectual growth for the researcher. They require critical thinking, problem-solving, and creativity, which can help develop the researcher’s skills and knowledge.
- Career advancement: Research papers can help advance the researcher’s career by demonstrating their expertise and contributions to the field. They can also lead to new research opportunities, collaborations, and funding.
- Academic recognition: Research papers can lead to academic recognition in the form of awards, grants, or invitations to speak at conferences or events. They can also contribute to the researcher’s reputation and standing in the field.
- Impact on policy and practice: Research papers can have a significant impact on policy and practice. They can inform policy decisions, guide practice, and lead to changes in laws, regulations, or procedures.
- Advancement of society: Research papers can contribute to the advancement of society by addressing important issues, identifying solutions to problems, and promoting social justice and equality.
Limitations of Research Paper
Research papers also have some limitations that should be considered when interpreting their findings or implications. Here are some common limitations of research papers:
- Limited generalizability: Research findings may not be generalizable to other populations, settings, or contexts. Studies often use specific samples or conditions that may not reflect the broader population or real-world situations.
- Potential for bias : Research papers may be biased due to factors such as sample selection, measurement errors, or researcher biases. It is important to evaluate the quality of the research design and methods used to ensure that the findings are valid and reliable.
- Ethical concerns: Research papers may raise ethical concerns, such as the use of vulnerable populations or invasive procedures. Researchers must adhere to ethical guidelines and obtain informed consent from participants to ensure that the research is conducted in a responsible and respectful manner.
- Limitations of methodology: Research papers may be limited by the methodology used to collect and analyze data. For example, certain research methods may not capture the complexity or nuance of a particular phenomenon, or may not be appropriate for certain research questions.
- Publication bias: Research papers may be subject to publication bias, where positive or significant findings are more likely to be published than negative or non-significant findings. This can skew the overall findings of a particular area of research.
- Time and resource constraints: Research papers may be limited by time and resource constraints, which can affect the quality and scope of the research. Researchers may not have access to certain data or resources, or may be unable to conduct long-term studies due to practical limitations.
About the author
Muhammad Hassan
Researcher, Academic Writer, Web developer
You may also like

How to Cite Research Paper – All Formats and...

Data Collection – Methods Types and Examples

Delimitations in Research – Types, Examples and...

Research Paper Format – Types, Examples and...

Research Process – Steps, Examples and Tips

Research Design – Types, Methods and Examples
Research Objectives: The Compass of Your Study

Table of contents
- 1 Definition and Purpose of Setting Clear Research Objectives
- 2 How Research Objectives Fit into the Overall Research Framework
- 3 Types of Research Objectives
- 4 Aligning Objectives with Research Questions and Hypotheses
- 5 Role of Research Objectives in Various Research Phases
- 6.1 Key characteristics of well-defined research objectives
- 6.2 Step-by-Step Guide on How to Formulate Both General and Specific Research Objectives
- 6.3 How to Know When Your Objectives Need Refinement
- 7 Research Objectives Examples in Different Fields
- 8 Conclusion
Embarking on a research journey without clear objectives is like navigating the sea without a compass. This article delves into the essence of establishing precise research objectives, serving as the guiding star for your scholarly exploration.
We will unfold the layers of how the objective of study not only defines the scope of your research but also directs every phase of the research process, from formulating research questions to interpreting research findings. By bridging theory with practical examples, we aim to illuminate the path to crafting effective research objectives that are both ambitious and attainable. Let’s chart the course to a successful research voyage, exploring the significance, types, and formulation of research paper objectives.
Definition and Purpose of Setting Clear Research Objectives
Defining the research objectives includes which two tasks? Research objectives are clear and concise statements that outline what you aim to achieve through your study. They are the foundation for determining your research scope, guiding your data collection methods, and shaping your analysis. The purpose of research proposal and setting clear objectives in it is to ensure that your research efforts are focused and efficient, and to provide a roadmap that keeps your study aligned with its intended outcomes.
To define the research objective at the outset, researchers can avoid the pitfalls of scope creep, where the study’s focus gradually broadens beyond its initial boundaries, leading to wasted resources and time. Clear objectives facilitate communication with stakeholders, such as funding bodies, academic supervisors, and the broader academic community, by succinctly conveying the study’s goals and significance. Furthermore, they help in the formulation of precise research questions and hypotheses, making the research process more systematic and organized. Yet, it is not always easy. For this reason, PapersOwl is always ready to help. Lastly, clear research objectives enable the researcher to critically assess the study’s progress and outcomes against predefined benchmarks, ensuring the research stays on track and delivers meaningful results.
How Research Objectives Fit into the Overall Research Framework
Research objectives are integral to the research framework as the nexus between the research problem, questions, and hypotheses. They translate the broad goals of your study into actionable steps, ensuring every aspect of your research is purposefully aligned towards addressing the research problem. This alignment helps in structuring the research design and methodology, ensuring that each component of the study is geared towards answering the core questions derived from the objectives. Creating such a difficult piece may take a lot of time. If you need it to be accurate yet fast delivered, consider getting professional research paper writing help whenever the time comes. It also aids in the identification and justification of the research methods and tools used for data collection and analysis, aligning them with the objectives to enhance the validity and reliability of the findings.
Furthermore, by setting clear objectives, researchers can more effectively evaluate the impact and significance of their work in contributing to existing knowledge. Additionally, research objectives guide literature review, enabling researchers to focus their examination on relevant studies and theoretical frameworks that directly inform their research goals.
Types of Research Objectives
In the landscape of research, setting objectives is akin to laying down the tracks for a train’s journey, guiding it towards its destination. Constructing these tracks involves defining two main types of objectives: general and specific. Each serves a unique purpose in guiding the research towards its ultimate goals, with general objectives providing the broad vision and specific objectives outlining the concrete steps needed to fulfill that vision. Together, they form a cohesive blueprint that directs the focus of the study, ensuring that every effort contributes meaningfully to the overarching research aims.
- General objectives articulate the overarching goals of your study. They are broad, setting the direction for your research without delving into specifics. These objectives capture what you wish to explore or contribute to existing knowledge.
- Specific objectives break down the general objectives into measurable outcomes. They are precise, detailing the steps needed to achieve the broader goals of your study. They often correspond to different aspects of your research question , ensuring a comprehensive approach to your study.
To illustrate, consider a research project on the impact of digital marketing on consumer behavior. A general objective might be “to explore the influence of digital marketing on consumer purchasing decisions.” Specific objectives could include “to assess the effectiveness of social media advertising in enhancing brand awareness” and “to evaluate the impact of email marketing on customer loyalty.”
Aligning Objectives with Research Questions and Hypotheses
The harmony between what research objectives should be, questions, and hypotheses is critical. Objectives define what you aim to achieve; research questions specify what you seek to understand, and hypotheses predict the expected outcomes.
This alignment ensures a coherent and focused research endeavor. Achieving it necessitates a thoughtful consideration of how each component interrelates, ensuring that the objectives are not only ambitious but also directly answerable through the research questions and testable via the hypotheses. This interconnectedness facilitates a streamlined approach to the research process, enabling researchers to systematically address each aspect of their study in a logical sequence. Moreover, it enhances the clarity and precision of the research, making it easier for peers and stakeholders to grasp the study’s direction and potential contributions.
Role of Research Objectives in Various Research Phases
Throughout the research process, objectives guide your choices and strategies – from selecting the appropriate research design and methods to analyzing data and interpreting results. They are the criteria against which you measure the success of your study. In the initial stages, research objectives inform the selection of a topic, helping to narrow down a broad area of interest into a focused question that can be explored in depth. During the methodology phase, they dictate the type of data needed and the best methods for obtaining that data, ensuring that every step taken is purposeful and aligned with the study’s goals. As the research progresses, objectives provide a framework for analyzing the collected data, guiding the researcher in identifying patterns, drawing conclusions, and making informed decisions.
Crafting Effective Research Objectives

The effective objective of research is pivotal in laying the groundwork for a successful investigation. These objectives clarify the focus of your study and determine its direction and scope. Ensuring that your objectives are well-defined and aligned with the SMART criteria is crucial for setting a strong foundation for your research.
Key characteristics of well-defined research objectives
Well-defined research objectives are characterized by the SMART criteria – Specific, Measurable, Achievable, Relevant, and Time-bound. Specific objectives clearly define what you plan to achieve, eliminating any ambiguity. Measurable objectives allow you to track progress and assess the outcome. Achievable objectives are realistic, considering the research sources and time available. Relevant objectives align with the broader goals of your field or research question. Finally, Time-bound objectives have a clear timeline for completion, adding urgency and a schedule to your work.
Step-by-Step Guide on How to Formulate Both General and Specific Research Objectives
So lets get to the part, how to write research objectives properly?
- Understand the issue or gap in existing knowledge your study aims to address.
- Gain insights into how similar challenges have been approached to refine your objectives.
- Articulate the broad goal of research based on your understanding of the problem.
- Detail the specific aspects of your research, ensuring they are actionable and measurable.
How to Know When Your Objectives Need Refinement
Your objectives of research may require refinement if they lack clarity, feasibility, or alignment with the research problem. If you find yourself struggling to design experiments or methods that directly address your objectives, or if the objectives seem too broad or not directly related to your research question, it’s likely time for refinement. Additionally, objectives in research proposal that do not facilitate a clear measurement of success indicate a need for a more precise definition. Refinement involves ensuring that each objective is specific, measurable, achievable, relevant, and time-bound, enhancing your research’s overall focus and impact.
Research Objectives Examples in Different Fields
The application of research objectives spans various academic disciplines, each with its unique focus and methodologies. To illustrate how the objectives of the study guide a research paper across different fields, here are some research objective examples:
- In Health Sciences , a research aim may be to “determine the efficacy of a new vaccine in reducing the incidence of a specific disease among a target population within one year.” This objective is specific (efficacy of a new vaccine), measurable (reduction in disease incidence), achievable (with the right study design and sample size), relevant (to public health), and time-bound (within one year).
- In Environmental Studies , the study objectives could be “to assess the impact of air pollution on urban biodiversity over a decade.” This reflects a commitment to understanding the long-term effects of human activities on urban ecosystems, emphasizing the need for sustainable urban planning.
- In Economics , an example objective of a study might be “to analyze the relationship between fiscal policies and unemployment rates in developing countries over the past twenty years.” This seeks to explore macroeconomic trends and inform policymaking, highlighting the role of economic research study in societal development.
These examples of research objectives describe the versatility and significance of research objectives in guiding scholarly inquiry across different domains. By setting clear, well-defined objectives, researchers can ensure their studies are focused and impactful and contribute valuable knowledge to their respective fields.
Defining research studies objectives and problem statement is not just a preliminary step, but a continuous guiding force throughout the research journey. These goals of research illuminate the path forward and ensure that every stride taken is meaningful and aligned with the ultimate goals of the inquiry. Whether through the meticulous application of the SMART criteria or the strategic alignment with research questions and hypotheses, the rigor in crafting and refining these objectives underscores the integrity and relevance of the research. As scholars venture into the vast terrains of knowledge, the clarity, and precision of their objectives serve as beacons of light, steering their explorations toward discoveries that advance academic discourse and resonate with the broader societal needs.
Readers also enjoyed

WHY WAIT? PLACE AN ORDER RIGHT NOW!
Just fill out the form, press the button, and have no worries!
We use cookies to give you the best experience possible. By continuing we’ll assume you board with our cookie policy.
Conclusion | Definition, Types, Objectives, Characteristics, Structure and Examples
We explain what a conclusion is, what its objectives are and the types that exist. In addition, its characteristics, structure and some examples.
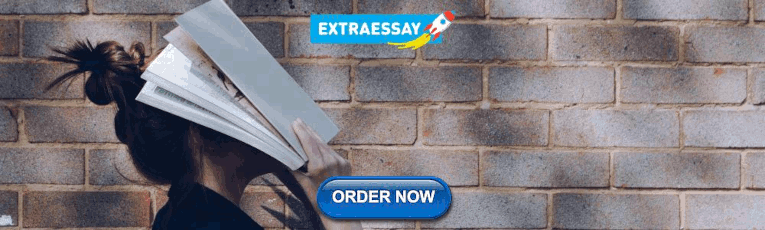
What is a conclusion?
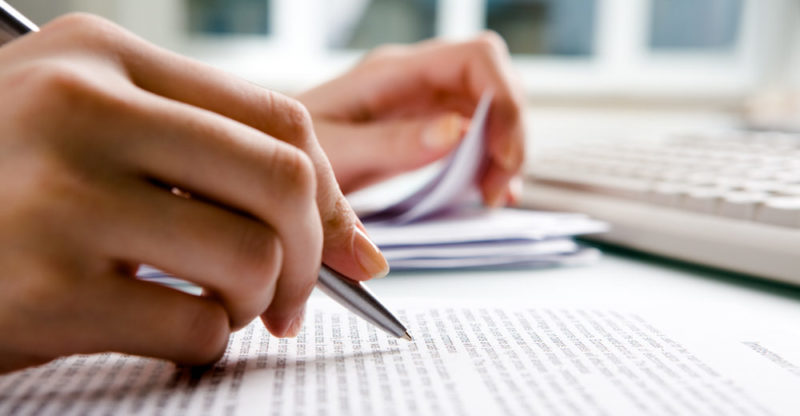
Objectives of a conclusion
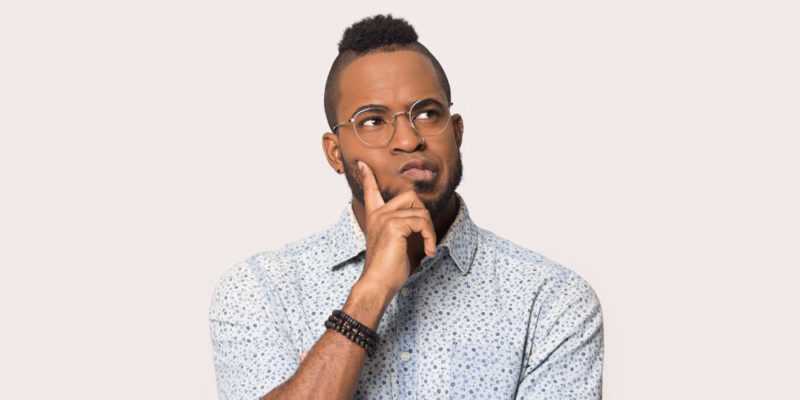
- Provide new, final, relevant information on the subject.
- Demonstrate the learning produced in the consideration of the premises.
- Reflect on the relevance of the problem established in the argument.
- Provide considerations regarding the appropriate way of thinking about the problem.
- Transmit the personal experience of the person who argues and their approach to the premises.
Types of conclusions
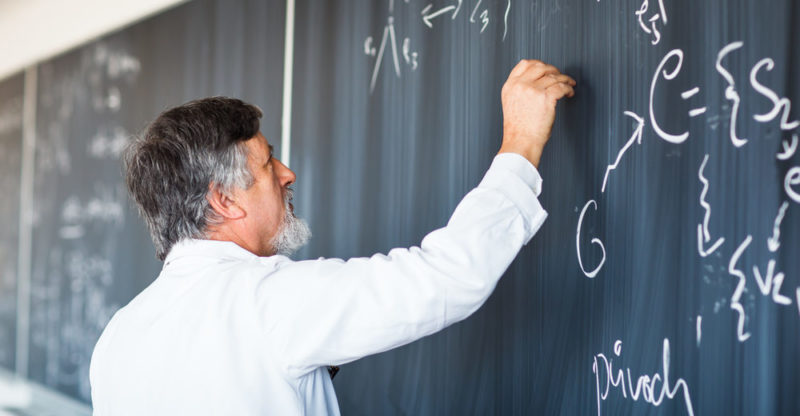
- Logical or formal: That which follows from its premises and is verifiable by any of the classical methods of formal reasoning, such as the syllogism or deduction.
- Personal: A conclusion rooted in the subjectivity of the person who formulates it, but that is not for that reason equivalent to an opinion. Personal conclusions must be valid, verifiable, even if they arise from individual experience.
- Theoretical: Those that propose new possible knowledge on which to build new research or reflections on the subject in the future .
- Summary: Summary conclusions are those that condense or round off what has been seen or argued previously, offering a kind of final recapitulation before adding final ideas.
- Recommendations: Those that reflect on the way in which the argumentation or investigation was carried out and offer clues to the future researcher from it
Why are the conclusions important?
A good conclusion.
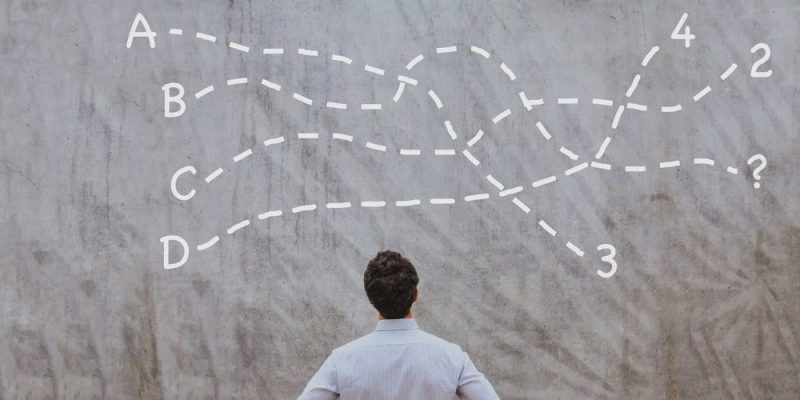
- Relevant: They have to do with the matter addressed in the premises and with the general approach of reasoning.
- Concise: They express something definite or determined, and not vagueness or repetitions of what has already been said in the body of the premises.
- Valid: The reader must be able to understand how these conclusions were reached, that is, they cannot be gratuitous or magical, pulled by the hair.
How are the conclusions drawn?
- Review and understand the premises: A valid conclusion cannot be reached from an unknown or half-known topic. It is convenient to review what has already been said and write down the most important points separately to make a kind of summary or synthesis.
- Round up or take up the problem: From the ideas in the synthesis, we can compare them with what was said in the introduction or the initial statement of the problem, to know how our vision of the matter has changed and what new ideas we have, what new knowledge was produced.
- Write the conclusions: Once we understand what was obtained from the research, we can write a text in which we explain what is the relevance that this has for the field of studies, human life or knowledge in general. Or, we can explain what knowledge in this regard deserves to occupy the place of closing the work, that is, with what final element on the subject we want the reader to keep.
Structure of the conclusion
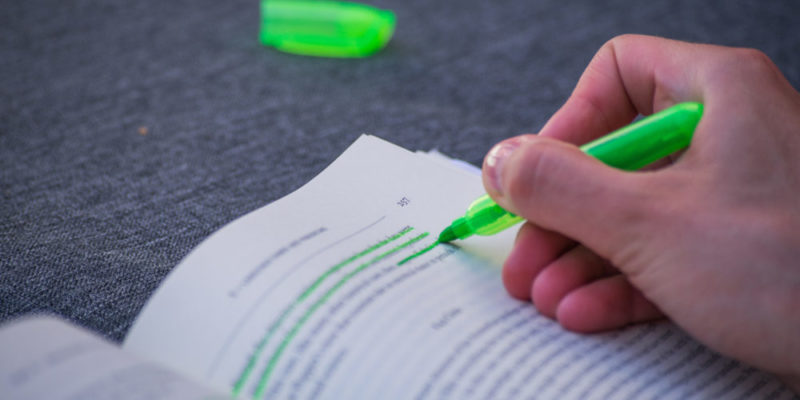
- A summary of what has been read that provides a new general perspective on the subject.
- A series of final ideas that give closure to the subject by providing new directions to it.
- An emphasis on the importance of what has been learned and its practical, theoretical or methodological utility.
- A series of recommendations for future researchers or difficulties encountered throughout the process.
Conclusions in academic works
Difference between conclusions and opinions.
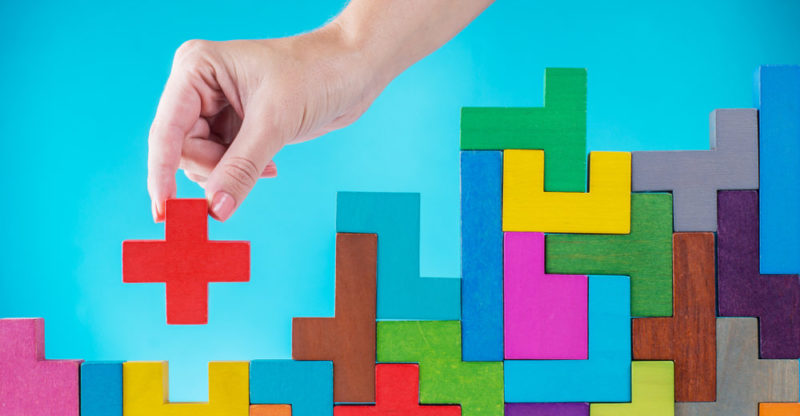
How to draw conclusions?
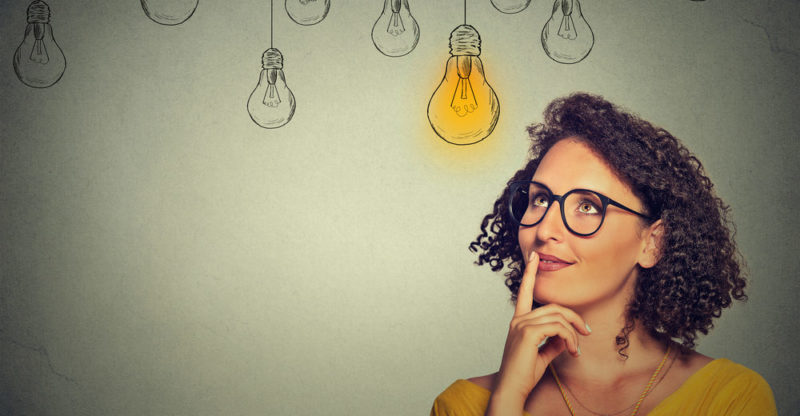
Examples of conclusions
A formal conclusion (syllogism) :.
All mammals are warm-blooded.
Since humans are mammals,
Conclusion : The human being has warm blood .
A written conclusion (in a medical diagnosis) :
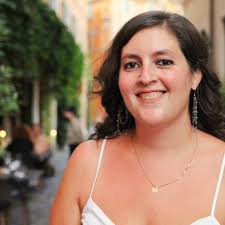
Amputee Ibi
She has pursued her studies in The United States, where she has graduated in Business and Economics and is currently finishing her Master studies in International Economics and Finance. Miss. Amputee is fluent in three languages: English, Spanish and Russian and has elementary knowledge of French and Italian. She love exploring how Collaborative Research Group can become the best tool to achieve the (necessary) educational change. .
Leave a reply
Social media, entertainment, recent post.
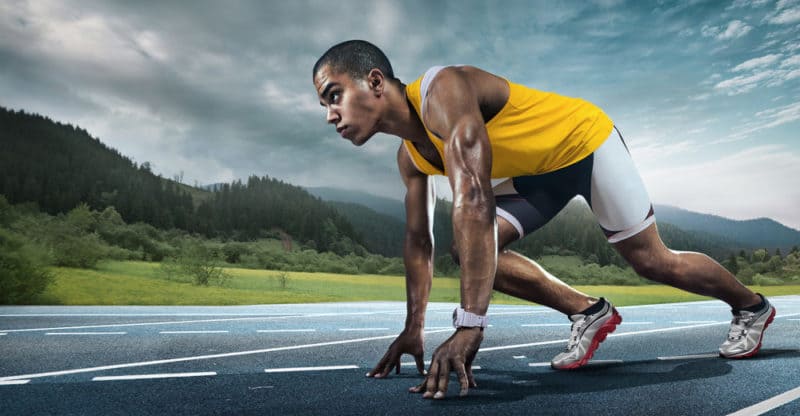
Sport: What Is It, Types, Risks, Features, Characteristics and Examples
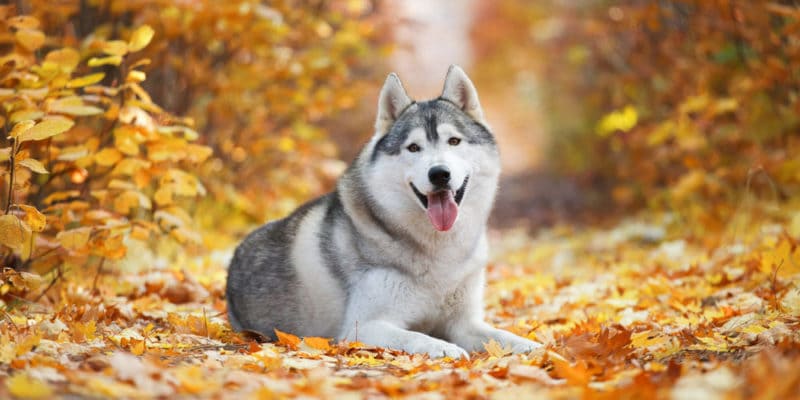
Dogs: Emergence, Features, Characteristics, Feeding and Breeds
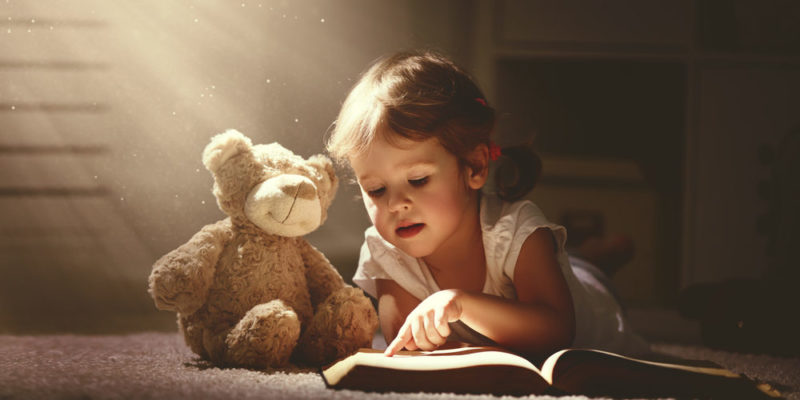
Story: Definition, Elements, Structure, Features and Characteristics
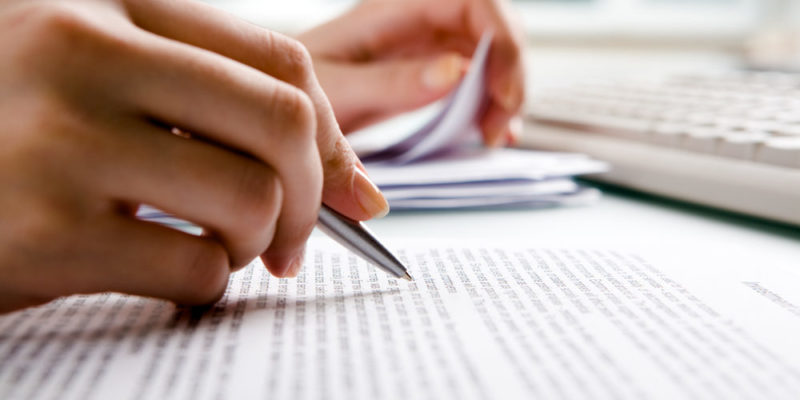
Essay: Definition, Structure, Features, Characteristics, How to Do It
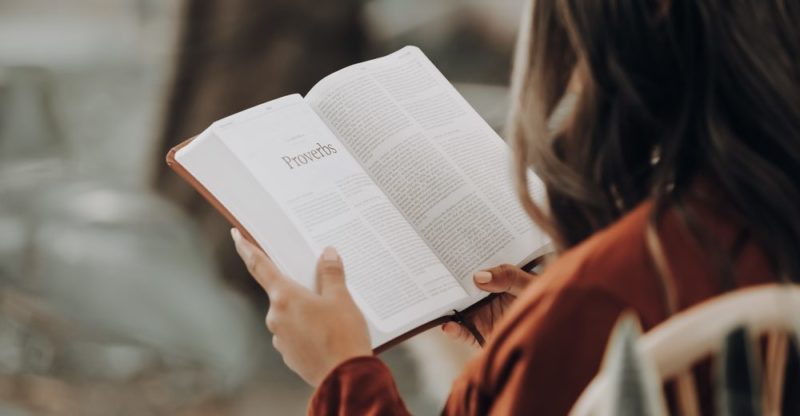
Narrative Text: What It Is, Structure, Features, Characteristics and Examples
Have a language expert improve your writing
Run a free plagiarism check in 10 minutes, generate accurate citations for free.
- Knowledge Base
Methodology
Research Methods | Definitions, Types, Examples
Research methods are specific procedures for collecting and analyzing data. Developing your research methods is an integral part of your research design . When planning your methods, there are two key decisions you will make.
First, decide how you will collect data . Your methods depend on what type of data you need to answer your research question :
- Qualitative vs. quantitative : Will your data take the form of words or numbers?
- Primary vs. secondary : Will you collect original data yourself, or will you use data that has already been collected by someone else?
- Descriptive vs. experimental : Will you take measurements of something as it is, or will you perform an experiment?
Second, decide how you will analyze the data .
- For quantitative data, you can use statistical analysis methods to test relationships between variables.
- For qualitative data, you can use methods such as thematic analysis to interpret patterns and meanings in the data.
Table of contents
Methods for collecting data, examples of data collection methods, methods for analyzing data, examples of data analysis methods, other interesting articles, frequently asked questions about research methods.
Data is the information that you collect for the purposes of answering your research question . The type of data you need depends on the aims of your research.
Qualitative vs. quantitative data
Your choice of qualitative or quantitative data collection depends on the type of knowledge you want to develop.
For questions about ideas, experiences and meanings, or to study something that can’t be described numerically, collect qualitative data .
If you want to develop a more mechanistic understanding of a topic, or your research involves hypothesis testing , collect quantitative data .
You can also take a mixed methods approach , where you use both qualitative and quantitative research methods.
Primary vs. secondary research
Primary research is any original data that you collect yourself for the purposes of answering your research question (e.g. through surveys , observations and experiments ). Secondary research is data that has already been collected by other researchers (e.g. in a government census or previous scientific studies).
If you are exploring a novel research question, you’ll probably need to collect primary data . But if you want to synthesize existing knowledge, analyze historical trends, or identify patterns on a large scale, secondary data might be a better choice.
Descriptive vs. experimental data
In descriptive research , you collect data about your study subject without intervening. The validity of your research will depend on your sampling method .
In experimental research , you systematically intervene in a process and measure the outcome. The validity of your research will depend on your experimental design .
To conduct an experiment, you need to be able to vary your independent variable , precisely measure your dependent variable, and control for confounding variables . If it’s practically and ethically possible, this method is the best choice for answering questions about cause and effect.
Prevent plagiarism. Run a free check.
Your data analysis methods will depend on the type of data you collect and how you prepare it for analysis.
Data can often be analyzed both quantitatively and qualitatively. For example, survey responses could be analyzed qualitatively by studying the meanings of responses or quantitatively by studying the frequencies of responses.
Qualitative analysis methods
Qualitative analysis is used to understand words, ideas, and experiences. You can use it to interpret data that was collected:
- From open-ended surveys and interviews , literature reviews , case studies , ethnographies , and other sources that use text rather than numbers.
- Using non-probability sampling methods .
Qualitative analysis tends to be quite flexible and relies on the researcher’s judgement, so you have to reflect carefully on your choices and assumptions and be careful to avoid research bias .
Quantitative analysis methods
Quantitative analysis uses numbers and statistics to understand frequencies, averages and correlations (in descriptive studies) or cause-and-effect relationships (in experiments).
You can use quantitative analysis to interpret data that was collected either:
- During an experiment .
- Using probability sampling methods .
Because the data is collected and analyzed in a statistically valid way, the results of quantitative analysis can be easily standardized and shared among researchers.
Receive feedback on language, structure, and formatting
Professional editors proofread and edit your paper by focusing on:
- Academic style
- Vague sentences
- Style consistency
See an example
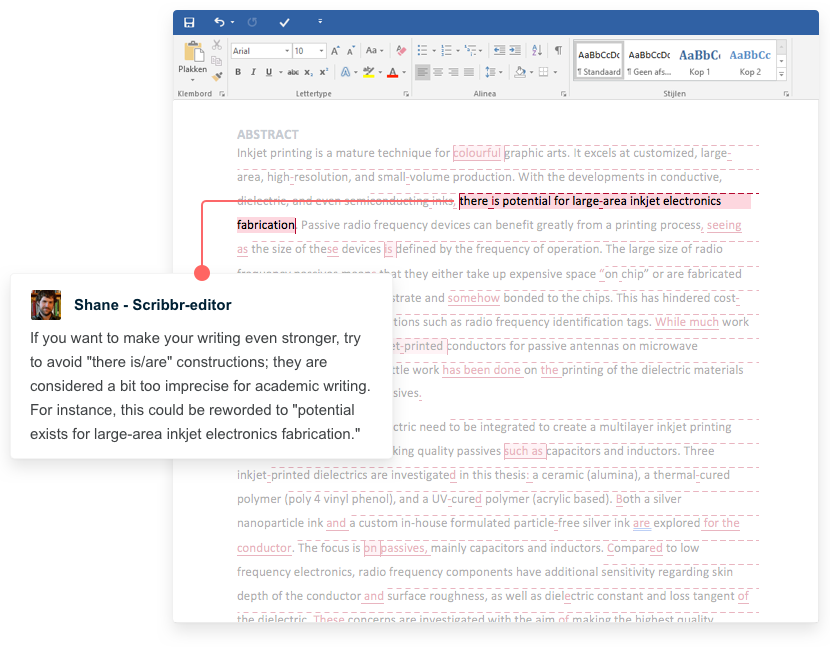
If you want to know more about statistics , methodology , or research bias , make sure to check out some of our other articles with explanations and examples.
- Chi square test of independence
- Statistical power
- Descriptive statistics
- Degrees of freedom
- Pearson correlation
- Null hypothesis
- Double-blind study
- Case-control study
- Research ethics
- Data collection
- Hypothesis testing
- Structured interviews
Research bias
- Hawthorne effect
- Unconscious bias
- Recall bias
- Halo effect
- Self-serving bias
- Information bias
Quantitative research deals with numbers and statistics, while qualitative research deals with words and meanings.
Quantitative methods allow you to systematically measure variables and test hypotheses . Qualitative methods allow you to explore concepts and experiences in more detail.
In mixed methods research , you use both qualitative and quantitative data collection and analysis methods to answer your research question .
A sample is a subset of individuals from a larger population . Sampling means selecting the group that you will actually collect data from in your research. For example, if you are researching the opinions of students in your university, you could survey a sample of 100 students.
In statistics, sampling allows you to test a hypothesis about the characteristics of a population.
The research methods you use depend on the type of data you need to answer your research question .
- If you want to measure something or test a hypothesis , use quantitative methods . If you want to explore ideas, thoughts and meanings, use qualitative methods .
- If you want to analyze a large amount of readily-available data, use secondary data. If you want data specific to your purposes with control over how it is generated, collect primary data.
- If you want to establish cause-and-effect relationships between variables , use experimental methods. If you want to understand the characteristics of a research subject, use descriptive methods.
Methodology refers to the overarching strategy and rationale of your research project . It involves studying the methods used in your field and the theories or principles behind them, in order to develop an approach that matches your objectives.
Methods are the specific tools and procedures you use to collect and analyze data (for example, experiments, surveys , and statistical tests ).
In shorter scientific papers, where the aim is to report the findings of a specific study, you might simply describe what you did in a methods section .
In a longer or more complex research project, such as a thesis or dissertation , you will probably include a methodology section , where you explain your approach to answering the research questions and cite relevant sources to support your choice of methods.
Is this article helpful?
Other students also liked, writing strong research questions | criteria & examples.
- What Is a Research Design | Types, Guide & Examples
- Data Collection | Definition, Methods & Examples
More interesting articles
- Between-Subjects Design | Examples, Pros, & Cons
- Cluster Sampling | A Simple Step-by-Step Guide with Examples
- Confounding Variables | Definition, Examples & Controls
- Construct Validity | Definition, Types, & Examples
- Content Analysis | Guide, Methods & Examples
- Control Groups and Treatment Groups | Uses & Examples
- Control Variables | What Are They & Why Do They Matter?
- Correlation vs. Causation | Difference, Designs & Examples
- Correlational Research | When & How to Use
- Critical Discourse Analysis | Definition, Guide & Examples
- Cross-Sectional Study | Definition, Uses & Examples
- Descriptive Research | Definition, Types, Methods & Examples
- Ethical Considerations in Research | Types & Examples
- Explanatory and Response Variables | Definitions & Examples
- Explanatory Research | Definition, Guide, & Examples
- Exploratory Research | Definition, Guide, & Examples
- External Validity | Definition, Types, Threats & Examples
- Extraneous Variables | Examples, Types & Controls
- Guide to Experimental Design | Overview, Steps, & Examples
- How Do You Incorporate an Interview into a Dissertation? | Tips
- How to Do Thematic Analysis | Step-by-Step Guide & Examples
- How to Write a Literature Review | Guide, Examples, & Templates
- How to Write a Strong Hypothesis | Steps & Examples
- Inclusion and Exclusion Criteria | Examples & Definition
- Independent vs. Dependent Variables | Definition & Examples
- Inductive Reasoning | Types, Examples, Explanation
- Inductive vs. Deductive Research Approach | Steps & Examples
- Internal Validity in Research | Definition, Threats, & Examples
- Internal vs. External Validity | Understanding Differences & Threats
- Longitudinal Study | Definition, Approaches & Examples
- Mediator vs. Moderator Variables | Differences & Examples
- Mixed Methods Research | Definition, Guide & Examples
- Multistage Sampling | Introductory Guide & Examples
- Naturalistic Observation | Definition, Guide & Examples
- Operationalization | A Guide with Examples, Pros & Cons
- Population vs. Sample | Definitions, Differences & Examples
- Primary Research | Definition, Types, & Examples
- Qualitative vs. Quantitative Research | Differences, Examples & Methods
- Quasi-Experimental Design | Definition, Types & Examples
- Questionnaire Design | Methods, Question Types & Examples
- Random Assignment in Experiments | Introduction & Examples
- Random vs. Systematic Error | Definition & Examples
- Reliability vs. Validity in Research | Difference, Types and Examples
- Reproducibility vs Replicability | Difference & Examples
- Reproducibility vs. Replicability | Difference & Examples
- Sampling Methods | Types, Techniques & Examples
- Semi-Structured Interview | Definition, Guide & Examples
- Simple Random Sampling | Definition, Steps & Examples
- Single, Double, & Triple Blind Study | Definition & Examples
- Stratified Sampling | Definition, Guide & Examples
- Structured Interview | Definition, Guide & Examples
- Survey Research | Definition, Examples & Methods
- Systematic Review | Definition, Example, & Guide
- Systematic Sampling | A Step-by-Step Guide with Examples
- Textual Analysis | Guide, 3 Approaches & Examples
- The 4 Types of Reliability in Research | Definitions & Examples
- The 4 Types of Validity in Research | Definitions & Examples
- Transcribing an Interview | 5 Steps & Transcription Software
- Triangulation in Research | Guide, Types, Examples
- Types of Interviews in Research | Guide & Examples
- Types of Research Designs Compared | Guide & Examples
- Types of Variables in Research & Statistics | Examples
- Unstructured Interview | Definition, Guide & Examples
- What Is a Case Study? | Definition, Examples & Methods
- What Is a Case-Control Study? | Definition & Examples
- What Is a Cohort Study? | Definition & Examples
- What Is a Conceptual Framework? | Tips & Examples
- What Is a Controlled Experiment? | Definitions & Examples
- What Is a Double-Barreled Question?
- What Is a Focus Group? | Step-by-Step Guide & Examples
- What Is a Likert Scale? | Guide & Examples
- What Is a Prospective Cohort Study? | Definition & Examples
- What Is a Retrospective Cohort Study? | Definition & Examples
- What Is Action Research? | Definition & Examples
- What Is an Observational Study? | Guide & Examples
- What Is Concurrent Validity? | Definition & Examples
- What Is Content Validity? | Definition & Examples
- What Is Convenience Sampling? | Definition & Examples
- What Is Convergent Validity? | Definition & Examples
- What Is Criterion Validity? | Definition & Examples
- What Is Data Cleansing? | Definition, Guide & Examples
- What Is Deductive Reasoning? | Explanation & Examples
- What Is Discriminant Validity? | Definition & Example
- What Is Ecological Validity? | Definition & Examples
- What Is Ethnography? | Definition, Guide & Examples
- What Is Face Validity? | Guide, Definition & Examples
- What Is Non-Probability Sampling? | Types & Examples
- What Is Participant Observation? | Definition & Examples
- What Is Peer Review? | Types & Examples
- What Is Predictive Validity? | Examples & Definition
- What Is Probability Sampling? | Types & Examples
- What Is Purposive Sampling? | Definition & Examples
- What Is Qualitative Observation? | Definition & Examples
- What Is Qualitative Research? | Methods & Examples
- What Is Quantitative Observation? | Definition & Examples
- What Is Quantitative Research? | Definition, Uses & Methods
"I thought AI Proofreading was useless but.."
I've been using Scribbr for years now and I know it's a service that won't disappoint. It does a good job spotting mistakes”
Short-Question
Practical answers to complex questions
What are the characteristics of a good conclusion in research?
Table of Contents
- 1 What are the characteristics of a good conclusion in research?
- 2 What is the characteristics of conclusion of an essay?
- 3 What are the characteristics of a well written thesis statement?
- 4 What makes a good conclusion in an essay?
- 5 What are the steps to write a conclusion?
- 6 Which should a conclusion do?
A good conclusion section should include: an analysis of compliance with the research objectives, a global response to the problem statement, a contrast between the results and the theoretical framework, areas for further research, and an acceptance or rejection of the established hypothesis (Allen 1973).
What is the characteristics of conclusion of an essay?
Features. A well-written conclusion should do several things for the essay. It should sum up an essay’s main points and restate the essay’s central argument, or thesis statement, in such a conclusive way as to illustrate to the reader that the essay has supported this argument.
What are the characteristics of a strong conclusion for an academic essay?
Key aspects to remember: A strong essay conclusion restates, not rewrites your thesis from the introduction. A strong essay conclusion consists of three sentences minimum. It concludes thoughts, not presents new ideas.
What does a conclusion consist of?
The conclusion paragraph should restate your thesis, summarize the key supporting ideas you discussed throughout the work, and offer your final impression on the central idea. This final summation should also contain the moral of your story or a revelation of a deeper truth.
What are the characteristics of a well written thesis statement?
A strong thesis statement contains the following qualities.
- Specificity. A thesis statement must concentrate on a specific area of a general topic.
- Ability to be argued.
- Ability to be demonstrated.
- Forcefulness.
- Confidence.
What makes a good conclusion in an essay?
A conclusion is the final piece of writing in a research paper, essay, or article that summarizes the entire work. The conclusion paragraph should restate your thesis, summarize the key supporting ideas you discussed throughout the work, and offer your final impression on the central idea.
What should you have in a conclusion?
What are all the things needed in a conclusion?
What are the steps to write a conclusion?
Which should a conclusion do.
Share this post
Privacy overview.
- Open access
- Published: 03 June 2022
Characteristics of the cancer stem cell niche and therapeutic strategies
- Feng Ju 1 na1 ,
- Manar M. Atyah 2 , 3 na1 ,
- Nellie Horstmann 1 ,
- Sheraz Gul 4 , 5 ,
- Razi Vago 6 ,
- Christiane J. Bruns 1 ,
- Yue Zhao ORCID: orcid.org/0000-0002-6790-3402 1 ,
- Qiong-Zhu Dong 7 , 8 &
- Ning Ren 2 , 3 , 7 , 8
Stem Cell Research & Therapy volume 13 , Article number: 233 ( 2022 ) Cite this article
7250 Accesses
43 Citations
11 Altmetric
Metrics details
Distinct regions harboring cancer stem cells (CSCs) have been identified within the microenvironment of various tumors, and as in the case of their healthy counterparts, these anatomical regions are termed “niche.” Thus far, a large volume of studies have shown that CSC niches take part in the maintenance, regulation of renewal, differentiation and plasticity of CSCs. In this review, we summarize and discuss the latest findings regarding CSC niche morphology, physical terrain, main signaling pathways and interactions within them. The cellular and molecular components of CSCs also involve genetic and epigenetic modulations that mediate and support their maintenance, ultimately leading to cancer progression. It suggests that the crosstalk between CSCs and their niche plays an important role regarding therapy resistance and recurrence. In addition, we updated diverse therapeutic strategies in different cancers in basic research and clinical trials in this review. Understanding the complex heterogeneity of CSC niches is a necessary pre-requisite for designing superior therapeutic strategies to target CSC-specific factors and/or components of the CSC niche.
Definitions and background
Stem cells are undifferentiated cells that have the capacity to self-renew and proliferate for longer than non-stem cells as well as having the ability to generate multiple types of cells in the body [ 1 , 2 ]. Regardless of their proliferation potential, stem cells are usually quiescent, remaining in an inactive dormant state (G1, G0) and are protected from cellular damage or mutations [ 3 , 4 ].
In general, stem cells exhibit various levels of differentiation potential, starting with totipotency (greatest differentiation potential), pluripotency, multipotency, oligopotency and finally unipotency/monopotency (stem cells can generate only one cell type) [ 4 ]. The survival of stem cells is ensured at two levels: cellular asymmetrical division and population asymmetrical renewal. With asymmetrical division, one stem cell gives rise to a stem cell and one differentiating cell. With population asymmetry, one stem cell produces two stem cells or two differentiating cells. As the frequency of these two processes are similar, both result in a comparable amount of stem cells and differentiating cells [ 5 ].
Some types of tissues have higher turnover and renewal rates than others (such as gastric and intestinal tissues and bone marrow as compared to brain or liver tissues), making the capability of stem cell self-renewal essential for their replenishment and providing an explanation for their broad presence in such tissues [ 6 ]. Many studies have explored whether the stemness of these cells is a result of an independent mechanism or the interaction with other cells and their surrounding environment and it has been established that these interactions are vital for the survival of stem cells [ 7 ].
Due to the inherent nature of stem cells, their use in regenerative medicine and stem cell-based therapy has been widely explored. However, to date, clinical applications of such therapeutic approaches are only available in hematopoietic malignancies and limited immune deficiencies [ 4 ].
Similar to normal stem cells, Cancer stem cells (CSCs) are also able to self-renew and differentiate into tumor cells [ 8 ]. With the multipotent capacity of CSCs, heterogeneous lineages of different kinds of cancer cells can be generated [ 9 ]. The ability of CSCs to initiate and reconstitute tumor lesions together with features such as differentiation and chemo/radiotherapy resistance has been widely investigated [ 10 ]. Although many features of CSCs are similar to normal stem cells, an important difference is that normal stem cells are usually dormant during adulthood until their regeneration ability is required, whereas this ability of CSCs is active. This leads to the possibility of detecting CSC based on markers found specifically in active stem cells but not dormant ones, such as PSF1 which has been identified in upregulated hematopoietic stem cells (HSCs) [ 7 ]. Many technologies and assays have been proposed for the identification of CSCs including microsphere assay, serial dilution assay, side population assay and aldehyde dehydrogenase activity assay, the gold standard for the CSC identification is the sphere colony formation assay and in vivo xeno transplantation of tumor cells into immunodeficient mouse models. Although each of these methods has limitations, they assist in identifying CSCs in several tumors such as breast cancer, brain cancer, liver cancer, stomach and colon cancer [ 10 ].
The fundamental mechanism by which CSCs function in cancer progression is unclear. A number of proposals have been made with regards to this, such as CSCs derived from normal somatic cells regain stemness due to cancerous changes and acquired genetic/epigenetic mutations [ 11 ]. For this, two models prevail, namely the hierarchical and stochastic models. In the hierarchical model, tumor initiation begins with stem cells that escape normal growth control and regulation. Therefore, they can transform into CSCs which give rise to a distinct population of cells forming the biological basis of tumors. This model has been validated clinically and would explain why only a full elimination of CSCs can prevent the relapse of cancers. Nevertheless, this model is unable to explain the interaction between CSCs and other differentiated cancer cells. In contrast, the stochastic model suggests that under suitable conditions all tumor cells (differentiated or not) can initiate further lesions. However, this model has limitations in explaining the relation between tumor heterogeneity and the capacity of initiating tumors. These findings have led to the concept of cellular plasticity, where both models are merged. Based on the genetic/epigenetic and microenvironmental signals, it is suggested that cancer cells have the ability to shift between stemness and differentiation states [ 12 ].
The microenvironment of CSCs is essential for their function and due to their complex nature and interactions with other components and factors, is referred to as the CSC niche. The CSC niche usually includes niche cells, such as cancer cells, stromal and endothelial cells extracellular matrix (ECM), signaling molecules, intrinsic factors, blood vessels and other cellular and acellular components such as exosomes. The components and structure of the niche can vary among organisms and different types of tissues in order to provide distinct functions in response to the needs of the tumor (Fig. 1 ) [ 13 , 14 , 15 , 16 ]. CSC niches have been identified in many locations including intestinal, tissues and neural tissues, aiding the regulation and maintenance of stem cell renewal and differentiation [ 6 , 17 ].
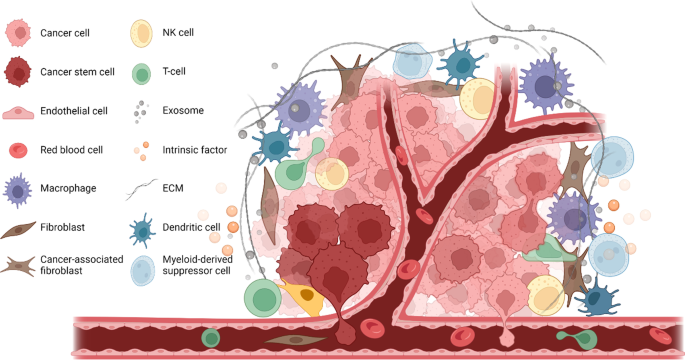
CSC niche in solid tumor. CSC niche has a complex microenvironment, it usually includes cancer cells, cancer stem cells, stromal cells, endothelial cells, fibroblasts, cancer-associated fibroblasts, ECM, exosomes and intrinsic factors. These components together contribute to the CSC renewal and maintain tumor malignancy
Due to the rarity of stem cells (compared to non-stem cells), it is challenging to clearly elucidate the mechanisms in the CSC niche [ 1 ]; however, some advances have been made with regards to this. The regulation mechanisms in the niche includes intrinsic mechanisms (associated with transcription factors expressed by cells), and extrinsic mechanisms (based on the signaling of the microenvironment and the connection to ECM). Disruption or interference in these mechanisms can lead to phenotypic changes that alter homeostasis of the niche [ 18 ]. Since the niche can contain more than one type of stem cells, competition between different stem cells within the niche is considered to control its function by factors such as E-cadherin [ 19 ], where the strength of the stem cell-niche connection can directly affect the fate of stem cells [ 14 ]. Even when only one type of stem cells are present in a niche, they still may lose their connection and, therefore, be replaced due to limited space or competition for occupancy [ 5 ] and this concept is crucial with regards to CSC niches.
Another important CSC feature is their ability to change and modify nearby stroma by CSC secreted proteins and molecular components, such as ECM proteins. The mechanism of ECM regulation has already been identified in different mammalian stem cells. In return, the niche can effectively regulate the biochemical status of keeping CSC in dormant. This is essential for the fate and plasticity of CSC and plays a role in their resistance to conventional therapies [ 15 ]. In order to better understand the characteristics of the CSC niche, we summarized the molecular features and mechanisms of the niche and reviewed the different therapeutic strategies targeting the CSC niche in various cancers from both basic and clinical aspects.
Conditions of the CSC niche
Hypoxia and its role in cscs.
Hypoxia is known for its role in the maintenance of the undifferentiated status of CSCs. The location of CSCs in hypoxic niches allows them to easily keep their slow cell cycling status with reduced proliferation, compared to a cycle with normal levels of oxygen. It is suggested that the rapid expansion of tumor mass and its vascular supply system might be the reason for tumor hypoxia that is responsible for the activation of several signaling pathways required in CSC functions. In solid tumors, the rapid growth of tumor tissues leads to a decrease in the quality of vascularization which affects the perfusion of blood and diffusion of substances it carries. That can be a cause for the poor outcome and the resistance of hypoxic niches against chemotherapy [ 20 ]. The hypoxic state of tumors is also linked to therapeutic resistance and local invasion [ 21 , 22 ]. It has also been shown that HSCs under hypoxic conditions can enhance the stemness of HSCs and shift cells from a quiescence to renewal phase [ 23 ]. Low levels of oxygen can also protect cells from DNA damage caused by oxidative stress which are common in aerobic metabolism. Studies have shown that rates of cellular damage are significantly higher at 20% O 2 relative to 3% O 2 . Low oxygen tension of 1% has also been shown to maintain the pluripotency of stem cells; therefore, hypoxia is essential for maintaining CSC characteristics [ 16 ].
In contrast to aerobic environments, hypoxic tissues use anaerobic glycolysis to metabolize glucose into lactate which produces much less ATP than in normal metabolism. This explains why cancer cells use higher rates of glucose compared to normal cells in aerobic conditions. However, reduced mitochondrial function is required, which is one way in which metabolic needs are down regulated in cancer cells and hypoxic niches. Such a difference between cancer cells and normal cells is usually controlled by the changes of oncogenes and tumor suppressor mutation profiles [ 20 ].
Many elements and factors participate in the control of hypoxic niches and related pathways such as mammalian target of rapamycin (mTOR) and the endoplasmic reticulum (ER) stress response. Examples of important transcription factors involved in the niche mechanisms are hypoxia inducible transcription factors (HIFs). Belonging to bHLH–PAS family of transcriptional factors, HIFs regulate many genes and play a role in oxygen homeostasis, glucose and iron metabolism and erythropoiesis [ 24 , 25 , 26 ]. These factors are also considered to be involved in several mechanisms such as the survival of cells under hypoxic conditions, cellular transcriptional responses, and activation of several signaling pathways. For example, expression of HIF-1α, CSC can promote tumor progression and metastasis. This transcription factor also affects genes involved in the regulation of the Notch and Oct4 pathways, as well as genes responsible for angiogenesis such as VEGF and GLUT-1 [ 1 ] by binding to HIF-1β and translocating to the nucleus where it can activate gene transcription. HIF signaling pathway can activate the angiogenic switch during tumor progression which is needed for the maintenance of oxygen homeostasis during the growth of tumor mass [ 27 ] (Fig. 2 ).
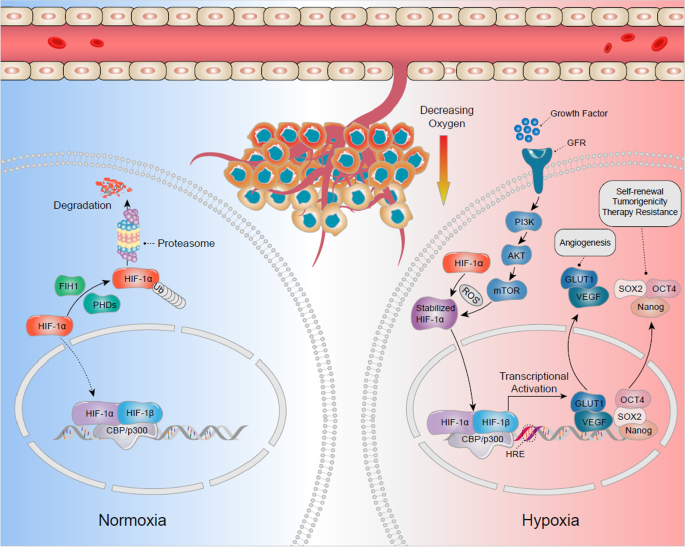
The hypoxic cancer stem cells (CSC) niches on CSC regulation. HIF-1α plays an important role in hypoxic process. Under normoxic conditions, HIF-1α is hydroxylated and inactivated by the function of poly1 hydroxylase domain-containing enzymes (PHDs) and Factor Inhibiting HIF (FIH1), and finally results in degradation. Under hypoxic conditions, both PHDs and FIH1 are inactivated, and HIF-1α stabilizes with the function of Reactive Oxygen Spices (ROS). HIF-1α could also be activated via activation of the PI-3 kinase/Akt-signaling pathway, which is induced by growth factor signaling. The stabilized HIF-1α translocates from cytoplasm to nucleus, where it dimerizes with HIF-1β and bind to Hypoxia Response Element (HRE). The heterodimer activates the downstream target genes transcription at these sites upon cofactor (CBP/p300) recruitment. These target genes in hypoxic niche, including Vascular Endothelial Growth Factor (VEGF), Glucose Transporter 1 (GLUT1), SOX2, Nanog and Octamer-binding Transcription Factor 4 (OCT4), may determine the fate of CSCs such as angiogenesis, self-renewal, tumorigenicity and induced therapy resistance
HIFs also play a role in regulating CSC proliferation, self-renewal, and tumorigenicity. The surface markers of CSC such as CD133 and CD44 show increased expression in hypoxic conditions [ 28 , 29 ]. With regards to therapy response, radiation has been found to double HIF-1 activity within 24–48 h following exposure, imposing further limitations on the outcome of such therapeutic approaches in cancer patients. Even after HIF activity is lost (usually occurring within minutes of activation), the targets may still show certain activities [ 20 ].
Metastasis of the CSC
Metastasis of CSCs was originally studied in hematopoietic malignancies followed by solid tumors which exhibited a similar phenomenon in brain, colon, breast, skin and pancreas cancers [ 30 , 31 , 32 , 33 , 34 ]. Most of these cancers are associated with specific metastasis-related genes as well as epigenetic amplification of cellular survival and renewal mechanisms than depend on driver mutations. Although migration occurs at an early stage of tumor progression, metastasis appears to take longer, due to the fact that not every migrated cell can initiate a tumor in distant locations and form a metastatic colony. Many conditions and mechanisms are required in both the migrated cells and the novel home tissues for this to occur, for example migrated cells must overcome many obstacles including lethal signals from reactive stroma that can downregulate the anti-apoptotic pathways in CSCs during the journey to the new environment [ 35 ]. CSCs must also survive the lack of growth factors and other environmental factors that are used to support their stemness and regulate their proliferation in the original niche [ 12 , 36 , 37 ]. Finally, after reaching the new site, the survivor CSCs need to induce specific niche factors to modulate the new niche according to their requirements.
It has also been found that one of the important stromal factors is POSTN (periostin) that normally plays a role in extracellular matrix formation and the development and function of teeth, bones, and heart tissues. In primary lesions, fibroblasts are responsible for POSTN expression; therefore, migrated tumor cells have to induce this factor in secondary lesions in order to successfully infiltrate into the new organs. POSTN is therefore a potential target for anti-metastasis therapies as it is considered to play a role in cancer stem cell maintenance. However, its deficiency only affects metastasis of tumors, but not normal cells nor primary lesions. Therefore, combination therapies may be necessary, but targeting POSTN alone may still prevent metastasis [ 38 ].
Following invasion of a new site, CSCs form a new niche to ensure their survival in the new environment where they face an aggressive immune response and a lack of survival signals and supportive stroma. Although most organs and tissues fend off CSC metastasis, some of these target tissues may be more susceptible than others. This creates an opportunity for CSCs to modulate the surrounding environment and build the metastatic niche (a novel niche in the new site that contains CSCs, niche components produced by CSCs themselves, and components produced by surrounding stromal cells) [ 12 , 35 ].
Epithelial to mesenchymal transition
During the development of tissues, some epithelial cells exhibit changes in their characteristics (such as a loss of adhesion contact with other cells and cellular polarity) and transform into a mesenchymal type with improved ability to relocate into different tissues. This phenomenon is termed the epithelial to mesenchymal transition (EMT) [ 1 ]. In cancer niches, transcription factors (e.g., Snail, Twist, Six1, Slug, Cripto, Zeb 1–2, E12-46, and others) can affect the connection of cells by activating EMT and downregulating important molecules such as E-cadherin and catenins. These molecules play an essential role in cellular adherens junctions (AJs) [ 9 ]. EMT is important during the development of organisms due to its impact on tissue morphogenesis; however, it can become a cause of concern in cancer tissues due to the risk of metastasis. In addition, cells having undergone EMT are associated with stronger invasiveness and resistance against apoptosis. They usually acquire features similar to CSCs and recent studies to suggest that the CSC itself may be a result of EMT (since all signals and conditions needed to initiate EMT can come from tumor microenvironment) [ 15 ]. It has also been reported that the de-differentiation of cancer cells into CSCs occurs after those cells have gone through EMT and migrate to other locations, thus indicating the important role of EMT in this process [ 39 ]. The interactions within the niche between microenvironment cells and components can also initiate EMT, with participation of cytokines and growth factors. Such interactions were also found to play a role in maintaining stemness of CSCs in several cancers such as breast and oral squamous cancers [ 15 ] (Fig. 3 ).
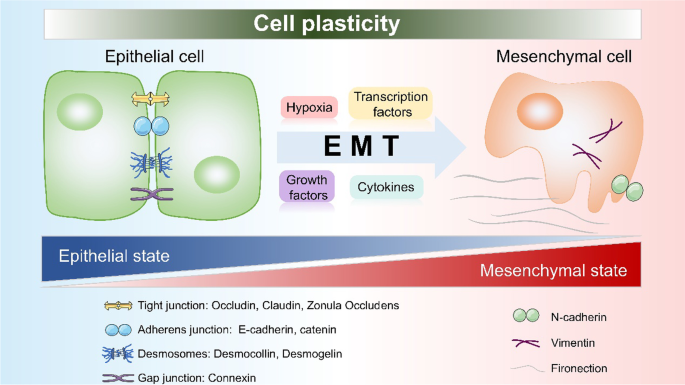
EMT and CSC niche. The crucial events in EMT are the dissolution of the epithelial cell–cell junctions, which include tight junction, adherens junction, desmosomes and gap junction. In CSC niche, those junctions start to break down via the induction of hypoxia, transcription factors, growth factors and cytokines. Then, the cells switch to a spindle-shaped mesenchymal morphology, as well as express markers (N-cadherin, vimentin and fibronectin) which would help to maintain the mesenchymal cell state
Additional studies found that EMT indeed strongly correlates with hypoxia, with HIF-1α activating several EMT-related transcription factors such as Snail and Twist. Hypoxia can also elevate the CSC self-renewal genes expression levels and activate related signaling pathways such as Wnt and Notch [ 40 , 41 ]. By activating EMT, hypoxic niches begin a chain of steps that eventually lead to metastasis, which also includes extracellular matrix modulation, intravasation, circulation, extravasation, homing, pre-metastatic niche formation, and mesenchymal epithelial transition (MET) [ 20 ].
Molecular mechanisms in the CSC niche
Essential molecules in the niche.
The homeostasis of the CSC niche is based on the interaction between different cells and components of the microenvironment. In order to maintain this homeostasis, many complicated mechanisms and molecules are required. Some of these molecules have been mentioned already in this review. In this section, we discuss other important molecules in the CSC niche modulation such as chemokines, cytokines and adhesion molecules.
Chemokines are common components in niche interactions, with one of the important chemokines discovered being SDF-1a and its receptor CXCR4. Studies in HSC found CXCR4 as essential for the retention, maintenance, and homing of tumor cells. The receptor is also important for the regulation of the CSCs’ renewal capacity. Mesenchymal stem cells (MSCs) are considered as a major source of the SDF-1 production whose expression is linked to metastasis in solid tumors (e.g., breast, liver, and lung cancers). Therefore, this chemokine has been suggested to play a contributing role in the changes that migrated CSCs apply on the surrounding environment during the initiation of metastasis colonization [ 1 , 6 , 15 ]. Another important component is reactive oxygen species (ROS) which usually affects the hypoxic conditions of the cancer niche in a dynamic manner. This can lead to DNA damage and changes in protein expression which are required in DNA repair [ 20 ]. Altering ROS levels in the cell also affects cellular stress responses which may favor tumor cells over normal ones. By increasing the levels of several factors (e.g., HIF-1α, HGF, EGF, TGF-β, TNF-α, TPA, MMP-3, and even micro-RNA), ROS can enhance and help the EMT and promote the metastasis of tumor cells [ 42 ].
Another family of molecules required for CSC metastasis is the cytokine family. CSCs, together with other tumor cells, can secrete different cytokines in the niche. Such cytokines and chemokines can further recruit several types of immune cells to create immunosuppression (e.g., tumor-associated neutrophils (TAN), tumor-associated macrophages (TAM), and myeloid-derived suppressor cells (MDSC)). Other cells involved in the niche region and are affected by CSCs include cancer-associated fibroblasts (CAFs), which are known to increase proliferation, enhance ECM production and secret essential factors such as CXCL12, VEGF, PDGF, and HGF [ 12 ].
A crucial ability of stem cell niches is the self-renewal of cells and cell–cell adhesion. Such adhesion relies on several molecular mechanisms and factors expressed in both the niche cells and stem cells. E-cadherin is a well-known example of such a cell adhesion molecule. In general, cadherins can modulate cell adhesion by interacting with intra- and extracellular domains, linking cells to cytoskeleton-associated proteins and nearby cells [ 43 ]. In doing so, cadherins can form strong AJs between cells. Cadherins have been found in stem cells in several types of tissues including brain stem cells and HSCs. Some cadherins can even regulate gene expression and signaling pathways by interacting with certain proteins and receptors. For example, N-cadherin can regulate the signaling by interacting with FGF receptors and also affect HSCs into quiescence by a similar mechanism.
In addition to cadherins, stem cells can also express integrins which are transmembrane molecules that modulate ECM interactions. Integrins can bind to ECM through certain proteins (e.g., collagen and laminin) and bind to surface adhesion molecules (e.g., CD54) and vascular cell adhesion molecules (e.g., CD106). Due to their ability to modulate cell adhesions, both cadherins and integrins are essential for spindle orientation of certain tissues (e.g., skin and brain) where the spindle of stem cells determines the type of division (symmetric or asymmetric division). It has been shown that the removal of such molecules can easily translate into randomized orientations. Other cellular mechanisms such as cell polarity, maintenance and signaling pathways can also be affected by adhesion molecules; therefore, it is difficult to draw a clear link between cellular adhesion and other cellular events. Other molecules that play a role in stem cells adhesion include Connexin 43 (a junction protein that support HSCs in stroma and affect CXCL12 secretion) and Vcam 1 [ 14 ].
Cancer-induced inflammation is a hallmark in solid tumor progression and considered to be essential in modifying their microenvironment. Although chemokines and cytokines, tumor surrounding cells (e.g., endothelial, mesenchymal, pericytes and fibroblast cells) can communicate directly with immune cells such as macrophages, neutrophiles, NK cells, T and B lymphocytes and other immune cells. Such interactions and the level of activity of each type of cell determine the resulting immune responses (pro or anti-tumor). For example, difference with tumor-induced inflammation is that it takes the profile of chronic inflammatory responses and uses cytokines and chemokines not only to communicate but also to recruit immune elements in order to resemble an unhealed wound. Consequently, the niche would remain in tumor favoring conditions such as hypoxia and EMT to enhance the survival and functioning of cells [ 9 ].
A complicated network of molecules is therefore responsible for the inflammatory response in the niche, and some of these are also involved in other pathways and functions. IL-6, IL-8, TGF-β, NFκ and TNF-α are some of the elements shown to play a role in the tumor-induced inflammatory environment. IL-6 is an important cytokine linked to proliferation, differentiation, and maturity of cells, making this molecule a promising target for many anti-tumor therapies. In CSCs, IL-6 has been found to play a role in Notch-3 regulation that leads to the malignant behavior of cells in human ductal breast carcinoma. The role of IL-6 in regulating CSCs was also studied in other types of human cancer (e.g., glioblastoma), where targeting it led to cellular apoptosis and reduction in tumor growth. NFκB pathway has also been found to be activated by inflammatory cytokines in the CSC niche. This pathway plays a role in modulating EMT factors Twist, Snail, and Slug. Therefore, NFκB is important for CSC migration and invasion in human cancers (e.g., pancreas, skin, and ovarian cancer) [ 1 ].
Current CSC-associated studies focus on their molecular features and differences and discovered novel markers including CD44, CD133, and CD24 [ 28 , 29 , 44 ]. However, markers specificity is still a challenge, since many are shared with normal stem cells and progenitors [ 3 ]. Several markers have already been linked to certain types of CSCs (breast cancer: CD44 + /CD24 − /ALDH + ; leukemia: CD34 + /CD8 − ; liver: CD90 + ; pancreas: CD44 + /CD24 + /ESA + ; epidermal surface antigen) [ 11 ]. Therefore, more efforts are required to identify CSC markers as there are contradicting reports regarding the exact role of markers in CSCs [ 6 ].
Genetics and epigenetic modulations in the CSC niche
The genetic diversity and heterogeneity of cancers has led to exploration of their genetic background and importance in CSC niches. Differences in genetic features or mutations among CSC clones can determine their phenotype (such as the level of activeness or dormancy, which can directly affect the survival of CSCs against chemotherapies) [ 45 ]. With the bidirectional relation between CSCs and their niches, genetic alterations on both sides can affect or determine the fate of CSCs or their link to the niche. This shows the advantage of the slow cycling of CSCs which helps avoid genetic mutations (which might not fit with the niche requirements) [ 3 , 46 ]. So far, two models prevail in explaining the intratumor heterogeneity, namely the hierarchical model which proposes that CSCs are originally organized in their niches in a cellular hierarchy similar to normal niches and the clonal evolution model which suggests that different somatic mutations and epigenetic alterations are acquired by different sub-clones of CSCs during tumor evolution, leading to molecular and biological differences among clones [ 8 ]. CSC niche conditions also influence the DNA profile and stability; for example, severe hypoxia leads to DNA replication arrests, which introduces different biological actions within the cells and adds to the intratumor heterogeneity [ 47 , 48 ]. As mentioned earlier, several transcriptional factors and signaling pathways can also affect the genetic profile of CSCs [ 49 ].
DNA methylation is a type of common epigenetic modification, and evidence is accumulating, suggesting that DNA methylation is a critical epigenetic reprogramming mechanism that may play a crucial role in CSCs biology [ 50 ]. BEX1, under the regulation of DNA methyltransferases 1 (DNMT1), was shown to have differential expression levels in Hepatoblastoma, CSC-hepatocellular carcinoma (HCC), and non-CSC HCC patients. This is essential for the self-renewal and maintenance of liver CSCs through activation of Wnt/β-catenin signaling [ 51 ]. Huang W et al. performed whole-genome bisulfite sequencing on tumor-repopulating cells (TRC), which are cancer stem cell (CSC)-like cells with highly tumorigenic and self-renewing abilities, their results showed that CSCs markers were biased toward altering their methylation in non-CG methylation and enriched in the gene body region, indicating non-CG DNA methylation plays a vital role in TRC selection [ 52 ].
Ubiquitination is also an important post-translational modification for CSCs self-renewal, maintenance, differentiation and tumorigenesis [ 53 ]. A recent study showed that targeting MYH9 could block HBX-induced GSK3β ubiquitination to activate the β-catenin destruction complex and then further suppress cancer stemness and EMT in hepatocellular carcinoma [ 54 ].
Besides, miRNAs and lncRNAs are also important modulating elements in the renewal and differentiation of CSCs. It has been shown that the loss of some miRNAs or lncRNAs can lead to the failure of the CSCs’ functioning (including the ability of CSCs to regulate stem cell markers, transcriptional factors, and binding proteins). Such changes affect the stemness and, therefore, the identity of CSCs. Interference with miRNAs or lncRNAs affects the role of cytokines and chemokines in CSCs, risks the whole balance of the CSC niche and influences the fate of those cells [ 11 , 55 , 56 , 57 , 58 ].
Signaling pathways regulating the CSC niche
The CSC niche contains many components that could maintain CSCs in a quiescence state and regulate the cell plasticity and quiescence by induction of several signaling pathways. Such signaling pathways in the CSC niche are the map or the network of all the interactions among cells and components in the niche. The importance of signaling pathways covers most characteristics of CSCs (e.g., self-renewal, proliferation, differentiation, metastasis, cell cycling, angiogenesis, and tumorigenesis) [ 15 , 59 ]. It has already been shown that several pathways in various types of niches such as Wnt, TGFβ, BMP, JAK-STAT, PI3K, and cell cycle pathways [ 60 ]. Signaling pathways in the CSC niche depend on the activation of other niche components (such as growth factors). In addition, many of these pathways act in the short term, thus requiring the CSCs to stay in the niche in order to benefit from the signaling [ 14 ]. Here, we highlight the common pathways often observed in the CSC niche (Fig. 4 ).
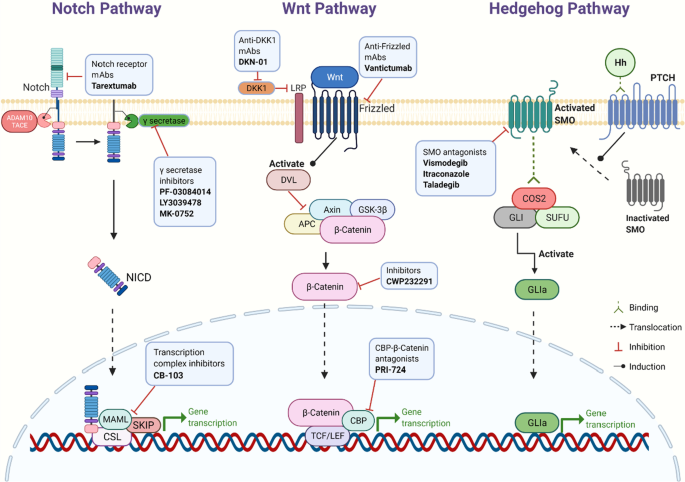
Key signaling pathways and related targeted inhibitors in the CSC niche. Notch pathway: After twice cleavages by ADAM10/TACE and γ-secretase, respectively, Notch intracellular domain (NICD) is finally released. NICD could translocate into the nucleus and interact with the transcription complex, promoting Notch target gene expression. Wnt pathway: When Wnt proteins bind to the Frizzled family receptors, dishevelled protein (DVL) is activated. DVL would inactivate the multiprotein destruction complex (including APC, Axin, and GSK-3β), which could lead β-catenin to degradation. With the DVL activation, β-catenin starts to accumulate in the cytoplasm. Part of β-catenin enters the nucleus to interact with TCF/LEF and CBP, promoting the expression of target genes. Hedgehog pathway: Binding PTCH with Hh would translocate smoothened (SMO) to the cell membrane and activate it. The activated SMO could easily bind to the COS2/GLI/SUFU complex, then GLI releases from the complex, referred to as GLIa. GLIa activates the transcription of downstream target genes
The Notch pathway is a well-known example in the interactions within the CSC niche. In this pathway, Notch binds to certain ligands that eventually translocate to the nucleus to activate cycling inhibitors (e.g., p21) [ 9 ]. Although the Notch pathway is responsible for the development of normal cells and maintenance of cellular proliferation and apoptosis, it is altered in several cancers (e.g., HCC) and can activate tumor initiation and progression. It has been shown that the Notch pathway is regulated by several genes including the tumor suppressor gene RUNX3, which explains the relation between Notch and tumor growth [ 59 ]. The Notch pathway is involved in the adhesion of CSCs in the niche, making it difficult to draw a clear line between the role of Notch in signaling and adhesion, especially in niches such as those in skin tissues [ 61 , 62 ]. All these functions of the Notch pathway make it a promising target of anti-tumor therapeutic approaches, regardless of its non-specific CSC nature. For example, blocking Notch-4 in breast cancer affected the ability of new cells formation [ 15 ].
The Wnt is another pathway and is similar to Notch as it is involved in the development and a functioning regulation of normal stem cells and therefore strongly correlates with CSCs. The hyper activation of Wnt has been shown to initiate cancer in the pituitary gland in animal models and increases the CSCs’ symmetrical division [ 63 , 64 , 65 ]. In HCC in particular, the Wnt/β-catenin pathway activation increases CSC activity and cells proliferation. Such an activation is controlled by PTEN, which is a tumor suppressor gene. Studies have shown that controlling or inhibiting the Wnt/β-catenin pathway can elevate the efficiency of drugs and other therapeutic approaches [ 66 , 67 , 68 ]. This pathway is also a potential target for future molecular therapies, since it depends on the concentration of β-catenin within the cytoplasm [ 69 ]. Together with Notch, Wnt is also involved in stemness stimulation in CSCs, which is essential for the identity of these cells [ 37 , 70 ].
The Hedgehog (Hh) pathway also plays a pivotal role in the CSC niche. In addition to its involvement in the development of cancer, it plays a role in determining the invasiveness and histological differentiation in tumors. Studies found that the simultaneous activation of the Hh pathway and EMT may be a reason for the chemoresistance that HCC cells usually exhibit [ 59 ]. Many drugs have been found to target Hh pathway (e.g., vismodegib, itraconazole, cyclopamine, and PTCN antibodies) to regulate CSC functions and inhibit tumor growth [ 11 , 15 ].
Another CSC controlling pathway is TGF-β. A recent study showed that tumor-initiating cells play a crucial role in creating a CSC niche microenvironment, which is required for cancer progression and therapy resistance [ 37 ]. Tumor cells release the interleukin-33 cytokine, which promotes the differentiation of the myeloid cells into macrophages. In turn, these macrophages further send TGF-β signals to CSCs, promoting their malignant progression and therapy resistance [ 71 ]. Other important pathways include TF pathway [ 72 ], Hippo pathway [ 73 ], and cell cycle pathways [ 74 ].
The CSC niche in different cancers
CSC niches have already been identified in several types of human cancers including brain, liver, breast, lung, head and neck, prostate, melanoma, gastric, pancreatic, renal, ovarian, esophageal and colorectal cancers [ 75 , 76 , 77 , 78 , 79 , 80 , 81 , 82 , 83 , 84 , 85 , 86 , 87 ]. Various studies have focused on the similarities and differences among the niches in different cancers and how cancer specific microenvironments can affect the formation and the development of CSC niches [ 4 , 6 , 88 , 89 , 90 ]. Here, we review some of the important examples of CSC niches in major cancers in order to further understand the interaction between tumors and CSC niches, and how the previously mentioned molecules and mechanisms participate in different tumors.
In general, the complexity of hepatic cancers posed a challenge for researchers since different tissues showed different sets of CSC markers and expression patterns [ 91 , 92 ]. To initiate a tumor in the liver, a CSC needs to survive the journey to the liver through the hepatic microvesiculation where it faces several intravascular death risks. CSCs therefore have to inhibit the liver immune responses to successfully complete clinical metastasis. In order to succeed, CSCs require several mechanisms and changes in the liver microenvironment, such as the inhibition of proteases by TIMP-1 which enables the formation of pre-metastatic niches and the expansion of metastasis. Hypoxia and HIF-1 can also activate HGF and its receptor Met which play a role in the development of liver metastasis. It has also been found that Kupffer cells and neutrophils in the niche increase the production of TNF-α, IL-1, and MMP-9 [ 68 ]. EGFR, VEGF, PDGF, TNF, SDF1, and other angiogenic factors enhance the growth and survival of hepatic cancer stem cells (HCSCs) and their resistance to radio- and chemotherapies [ 93 , 94 , 95 , 96 ].
So far, several HCSCs markers have been proposed (e.g., CD133, CD90, CD24, CD13, EpCAM, ALDH, and OV6) and current research efforts aim to link different combinations of these markers in HCSCs to the differences in phenotypes among CSC populations. For example, CD90 + cells have been found to upregulate genes related to inflammation and drug resistance while CD133 + cells have Akt/PKB pathway activation which translate into suppression of apoptosis and resistance to radiotherapy [ 59 ].
Studies showed that post surgery, any remaining HCSCs may be re-activated and even invade the vascular system leading to recurrence and metastasis of the primary tumor [ 97 ]. As for chemotherapy, HCSCs are more resistant to anti-tumor drugs including doxorubicin and 5-flouracil (5-FU). Such drugs can also alter the expression of certain genes (e.g., ABCG2) which increases the proliferation rate of HCSCs. Many agents are being developed to target certain molecules and markers of HCSCs, including AKT1 inhibitors, CD44 neutralizing antibodies, exogenous BMP4, pimozide, cylopamine, and other agents. Markers including CD133 and EpCAM also show great potential in the creation of specific targeted therapies as well as novel strategies targeting the crosstalk between the stroma and the tumor [ 59 ].
Gastric cancer is another type of malignancy with increasing incidence and mortality. Gastric cancer stem cells (GCSCs) have already been identified in different cell lines with markers including CD44, CD54, CD24, and CD71. Interestingly, many reports indicate the interaction between Helicobacter Pylori (HP) infection and GCSCs, such infection leads to modulation of stem cells into GCSCs in animal models [ 88 , 98 ]. The chronic inflammation caused by such an infection is also helpful for the GCSC niche. Since treatments such as surgery, chemotherapy, radiation, and immunotherapy are often challenged with the problem of tumor relapse, GCSCs have been proposed as potential targets for drugs that inhibit their stemness and functions. For example, Trastuzumab targets CD90 + GCSC and decreases the growth of gastric cancer tissues (when used with chemotherapy) [ 99 ]; Salinomycin targets ALDH + GCSC (a cell population which is resistant to chemotherapy drugs such as 5-FU and CDDP) [ 100 ].
In the case of breast cancer, it has been found that different populations of breast CSCs (BCSCs) can generate different lines of tumor cells. Yet, the genotype of the new cells may not completely resemble the original CSC genetic profile, which may indicate a number of mutations along the way [ 8 ]. It has been shown that BRCA1 expression (well-known tumor suppressor gene in breast cancer) is decreased by transcription factors such as Slug, while at the same time Slug stability is reduced by BRCA1 gene expression. This negative feedback-based balance is a key element in maintaining a normal growth of tumor and determining the level of stemness of niche cells [ 101 , 102 ]. Pluripotency factors including SOX are also over expressed in BCSC niches and other than their role in EMT and metastasis, the SOX family upregulates the expression of EZH2 which is important for histone methylation for several genes and can activate Raf1-β-catenin pathway [ 103 , 104 ]. With the identification of drugs that can specifically target BCSCs, essential pathways including Wnt can be inhibited. BCSCs resistance to radiotherapy can also be solved by advanced approaches including gold Nano-shell-mediated hyperthermia therapy [ 105 ].
In hematopoietic malignancies, HCSCs (also known as leukemia stem cells, LSCs) have been identified in acute myeloid leukemia (AML) in the 1990s, with specific markers such as CD34 + /CD38 − [ 45 , 106 ]. Although the interaction and communication mechanism between LSCs and their niche is not as clear as in solid tumors, targeting the niche may still be useful as it can affect the mobility of LSCs before escaping the original niche. This theory is supported by the expression of certain chemokines and receptors in the niche such as CXCR4 and CXCL12 [ 107 ]. In order to prevent relapse of primary cancer, targeting the niche might be of major therapeutical importance, as it provides a sanctuary for LSCs against conventional therapies [ 108 ].
The chemotherapeutic drugs cytarabine and daunorubicin have been the standard choices for many years, despite the risks of relapse or therapeutic resistance. As a result, there is an urgent need for novel therapies addressing the potential role of LSCs. In animal models, G-CSF treatment has shown promising results as it stimulates dormant LSCs and activated their cell cycle, leading to increased sensitivity to chemotherapy. Such treatments still require more development, but they certainly create new hope in this field [ 107 ].
In other examples of cancer, malignant bone tumors usually include osteosarcoma, multiple myeloma, and solid tumor metastasis. Bone metastasis pose a significant challenge as it is in general incurable and is associated with resistance to chemotherapy. Other than migrated tumor cells, the bones are also the source of HSCs. It has been shown that these two different populations of cells compete for the same niches in the bones, suggesting that stem cell niches may hold a great potential in the future as a target of treating metastatic tumors [ 109 ]. Although tumor stem cells can alter the microenvironment of the bone and the existing hematopoietic niches, the niche can still distinguish a normal stem cell from a CSC. Consequently, modulating the niche in favor of normal SC compared to CSC can prevent the invasion of migrated cells [ 110 ].
Therapeutic strategies targeting the CSC niche
Therapy resistance and tumor relapse are two major obstacles in the clinical management of solid cancers [ 111 ]. There is evidence that many residual tumors are enriched with CSCs after therapy [ 69 , 112 ]. Thus far, this phenomenon has been explained by the theory that even after a radical resection of tumor lesions ( R 0) by surgery and applying chemotherapy and radiotherapy, some CSCs still manage to escape and survive due to their therapy resistance [ 11 , 113 ]. CSCs are usually quiescent and slow cycling and have an active anti-apoptotic machinery [ 114 ], efficient DNA repairing systems (DNA checkpoint kinases) and stabilized stemness features, all of which may contribute to their resistance [ 115 ]. To date, many chemoresistance mechanisms have been found across distinct cancer types such as hypoxia, EMT and the signaling pathways which regulate CSC function, for instance Notch, Wnt and Hedgehog signaling. The recent clinical trials targeting CSCs or CSC-associated pathways are shown in Table 1 .
As described before, EMT is closely associated with the biological features of the CSC, including stemness, immune escape and resistance to chemotherapy [ 116 ]. Recent studies have shown that EMT plays a major role in therapy resistance [ 117 , 118 , 119 ]. Diverse factors and cytokines are involved in regulation of EMT, complicating targeted therapy approaches. For instance, responses to chemotherapy in a large group of breast cancer patients revealed a close association between chemoresistance and increased stromal gene expression, and this transcriptional upregulation appeared to be caused by activation of the EMT, which suggested anti-stromal agents may offer a new strategy to overcome therapy resistance [ 120 ]. A Phase I/II clinic trial showed that Plerixafor, as an anti-stromal agent which is an important inhibitor of CRCX4, combined with bortezomib restored the chemosensitivity in relapsed multiple myeloma, which suggested that the novel therapy may target the tumor microenvironment and overcome resistance to therapy [ 121 ]. In addition, Kari et al. established an EMT lineage-tracking system in breast-to-lung metastasis mice models, using a mesenchymal-specific Cre-mediated fluorescent marker switch system. They found out that EMT cells significantly contribute to recurrent lung metastasis formation after chemotherapy [ 122 ]. According to these capacities of the EMT, creating more effective treatment strategies to directly target the EMT program is promising and urgently needed.
Moreover, TGFβ signaling is one of the characterized pathways involved in EMT induction, as enhanced TGFβ signaling in cancer accelerates EMT program and maintains a highly proliferative phenotype [ 123 ]. Accumulating research studies have demonstrated that TGFβ-mediated EMT may initiate and facilitate therapy resistance, which is inhibited by blockade of TGFβ [ 124 , 125 , 126 ]. Fresolimumab, a TGFβ blocking antibody, showed an effective clinical application which was demonstrated in many clinical trials [ 127 , 128 , 129 ]. Furthermore, two doses of fresolimumab were explored in metastatic breast cancer in a prospective randomized trial, patients receiving the higher fresolimumab dose had a better systemic immune response and longer median overall survival compared to the lower dose group [ 130 ]. Therefore, TGFβ blockade might be a feasible and promising strategy for a CSC targeted therapy.
Additionally, another potential therapeutic target for preventing the induction of EMT is hepatocyte growth factor (HGF)-HGR receptor (HGFR, MET) signaling, which contributes to cancer pathogenesis, as exemplified by its frequent activation, often by point mutation or amplification of the MET, in many cancer types [ 131 ]. Therefore, targeting HGR or HGR-MET signaling is a promising therapeutic method which may also regulate CSCs. Recently, many studies show that anti-MET antibodies prevent HGF binding to MET and, subsequently, inhibit cancer progression and restore the chemoresistance, as well as improve the therapeutic efficiency [ 132 , 133 , 134 ].
In addition, hypoxia contributes to therapy resistance via a number of mechanisms, including maintaining CSC stemness, function of HIFs, drug transporters and affecting EMT [ 135 ]. Targeting these key factors is considered as a promising strategy for anti-CSC therapy. Acriflavine, a potent inhibitor of HIF-1α dimerization, could disturb glucose metabolism in melanoma regardless of the hypoxic condition [ 136 ]. Chetomin, another inhibitor of HIF-1α, could suppress the transcriptional activity via targeting p300 recruitment [ 137 ]. Furthermore, other agents, such as wortmannin, temsirolimus and camptothecin, have been proved to inhibit HIF-1α protein translation by targeting corresponding genes [ 138 ]. In addition, hypoxia can also mediate chemoresistance of CSCs by drug efflux through drug transporters [ATP-binding cassette (ABC) family membrane transporters]. ABCB1 and ABCG2 (BCRP), as the well-known ABC family transporters, have been reported to be induced in hypoxia [ 139 , 140 ]. A recent study showed that vatalanib, a multitargeted tyrosine kinase inhibitor for all isoforms of VEGFR, PDGFR and c-Kit, makes ABCB1 and ABCG2-overexpressing multidrug-resistant colon cancer cells sensitive to chemotherapy in hypoxic microenvironment. Utilizing the combination of vatalanib with conventional anti-tumor drugs might be a promising tool to restore the chemosensitivity in colon cancer treatment [ 141 ]. Similar mechanism is found in ovarian cancer stem cells: Hypoxia-induced HIF-2α overexpression endows ovarian cancer stem cells with resistance to adriamycin by promoting BCRP expression and adriamycin efflux [ 139 ].
We have described the Notch, Wnt and Hedgehog signaling pathways in CSC regulations before, which are widely explored in current CSC target therapies (Fig. 4 ). γ-secretase inhibitor (GSI), as a major clinical method used to inhibit Notch signaling, has been proved in many clinical trials. Combining trastuzumab plus a GSI has been reported to reduce recurrence rate for ErbB-2-positive breast tumors [ 142 ]. Another clinical trial shows that pharmacologic inhibition of the Notch pathway can reduce CSCs in breast cancer models [ 143 ]. A phase I study shows that ipafricept (OMP-54F28, a Decoy Receptor for Wnt Ligands) can be safely administered with manageable toxicities [ 144 ]. In addition, HH ligands, SMO and GLI transcription factors are three main targets of Hh pathway antagonists [ 60 ], whose effectiveness in basal cell carcinoma and prostate cancer was already shown in many trials [ 145 , 146 , 147 ].
In the past few years, CSC-directed immunotherapy has emerged. Besides the antibodies targeting CSC-related signaling pathways mentioned above, novel anti-CSC immunotherapeutic approaches, such as cancer vaccines, checkpoint inhibitors and chimeric antigen receptor T-cell (CAR-T cell) therapies, have been developed [ 148 , 149 ]. One personalized neoantigen dendritic cell vaccine was chosen to treat metastatic lung cancer (NCT02956551). In this trial, the disease control rate was 75% and the median overall survival was 7.9 months; the combination therapy of immune checkpoint inhibitors and the vaccines had a better progression free survival (2.2 vs 12.2 months) and overall survival (7.6 vs 11.2 months) trend; this study provided new evidence for cancer vaccine therapy and promising therapeutic opportunities for lung cancer treatment [ 150 ]. In addition, as a novel therapeutic strategy, CAR-T cell therapy is widely used in many types of cancer, especially against hematologic malignancies. CD19/CD22/CD33, been detected in leukemia patients, is a noteworthy target for immune-cellular therapy against AML [ 151 , 152 , 153 , 154 ]. The ongoing clinical trials of CAR-T cell therapy are listed in Table 2 .
The current studies have confirmed that CSCs are considered as the root of cancer relapse, metastasis and therapy resistance, while further research is required for the development of more effective strategies to eradicate CSCs.
Recent studies have expanded our knowledge regarding CSCs and their niche. The novel markers and mechanisms in CSC niches as greatly improved our understanding of cancer and its microenvironment. It also changed the proposition that metastasis is a late-stage complication of cancer and explains the long-standing dilemma of why a tumor may relapse even after a complete surgical removal, chemotherapy or radiotherapy. Regardless to the improvements in this field, we still need more efforts to annotate CSC niches regarding molecular interactions and mechanisms controlling their stemness capacity and relationship with dormancy and chemo-immuno resistance. More challenges are ahead before we can move forward from basic to translational research and further clinical applications; nonetheless, such findings may bring a great benefit for millions of patients and guide future anti-cancer therapeutic strategies.
Availability of data and materials
Not applicable.
Abbreviations
ATP-binding cassette sub-family G member 2
A disintegrin and metalloproteinase domain-containing protein 10
Adherens junctions
RAC-alpha serine/threonine-protein kinase
Aldehyde dehydrogenate
Acute myeloid leukemia
Adenomatous polyposis coli
Adenosine triphosphate
Axis inhibition protein
B-cell acute lymphoblastic leukemia
Breast cancer stem cells
Basic helix loop helix Per Arnt Sim
Bone morphogenetic proteins
BReast-CAncer susceptibility gene 1
Cancer-associated fibroblasts
CREB-binding protein
Cluster of differentiation
- Cancer stem cells
Colony stimulating factor 1
C-X-C motif chemokine 12 also known as SDF1
C-X-C chemokine receptor type 4
Dishevelled protein
Extracellular matrix
Epidermal growth factor
Epithelial cell adhesion molecule
Endoplasmic reticulum
Epidermal surface antigen
Enhancer of zeste homolog 2
Fibroblast growth factor
5-Flouracil
Gastric cancer stem cells
Glioma-associated oncogene
Glucose transporter 1
Glycogen synthase kinase 3 beta
Hepatocellular carcinoma
Hepatic cancer stem cells
Hepatocyte growth factor
Hedgehog pathway
Hypoxia inducible transcription factor
Helicobacter pylori
Hematopoietic stem cells
Insulin-like growth factor-1
Interleukin-6
Janus kinases
Leukemia stem cells
Myeloid-derived suppressor cell
Mesenchymal epithelial transition
Hepatocyte growth factor receptor
Matrix metalloproteinase 3
Mesenchymal stem cell
Mammalian target of rapamycin
Nuclear factor Kappa B
Notch intracellular domain
Natural killer cell
Octamer-binding transcription factor 4
Platelet derived growth factor
Phosphoinositide 3-kinase
Protein patched homolog
Partner of SLD five 1
Phosphatase and tensin homolog
Reactive oxygen species
Runt-related transcription factor 3
Sal-like protein 4
Stromal cell-derived factor-1
Sineoculis homeobox homolog 1
Zinc finger protein SNAI1
Signal transducer and activator of transcription proteins
Tumor necrosis factor-α converting enzyme
Tumor-associated macrophages
Tumor-associated neutrophils
T cell factor/lymphoid enhancer factor
Tissue factor
Transforming growth factor-beta
Tissue inhibitor of metalloproteinases 1
Tumor necrosis factor alpha
Tissue polypeptide antigen
Vascular cell adhesion molecule 1
Vascular endothelial growth factor
Zinc finger E-box binding homeobox
Cabarcas SM, Mathews LA, Farrar WL. The cancer stem cell niche–there goes the neighborhood? Int J Cancer. 2011;129(10):2315–27.
Article CAS PubMed PubMed Central Google Scholar
Kordes C, Häussinger D. Hepatic stem cell niches. J Clin Invest. 2013;123(5):1874–80.
Sottocornola R, Lo CC. Dormancy in the stem cell niche. Stem Cell Res Ther. 2012;3(2):10.
Lacina L, Plzak J, Kodet O, Szabo P, Chovanec M, Dvorankova B, Smetana K Jr. Cancer microenvironment: what can we learn from the stem cell niche. Int J Mol Sci. 2015;16(10):24094–110.
Article PubMed PubMed Central Google Scholar
Stine RR, Matunis EL. Stem cell competition: finding balance in the niche. Trends Cell Biol. 2013;23(8):357–64.
Ribatti D. Cancer stem cells and tumor angiogenesis. Cancer Lett. 2012;321(1):13–7.
Article CAS PubMed Google Scholar
Takakura N. Formation and regulation of the cancer stem cell niche. Cancer Sci. 2012;103(7):1177–81.
Guo W. Concise review: breast cancer stem cells: regulatory networks, stem cell niches, and disease relevance. Stem Cells Transl Med. 2014;3(8):942–8.
Shigdar S, Li Y, Bhattacharya S, O’Connor M, Pu C, Lin J, Wang T, Xiang D, Kong L, Wei MQ, et al. Inflammation and cancer stem cells. Cancer Lett. 2014;345(2):271–8.
Singh SR. Cancer stem cells: recent developments and future prospects. Cancer Lett. 2013;338(1):1–2.
Yu Z, Pestell TG, Lisanti MP, Pestell RG. Cancer stem cells. Int J Biochem Cell Biol. 2012;44(12):2144–51.
Plaks V, Kong N, Werb Z. The cancer stem cell niche: how essential is the niche in regulating stemness of tumor cells? Cell Stem Cell. 2015;16(3):225–38.
Batlle E, Clevers H. Cancer stem cells revisited. Nat Med. 2017;23(10):1124–34.
Chen S, Lewallen M, Xie T. Adhesion in the stem cell niche: biological roles and regulation. Development. 2013;140(2):255–65.
Yi SY, Hao YB, Nan KJ, Fan TL. Cancer stem cells niche: a target for novel cancer therapeutics. Cancer Treat Rev. 2013;39(3):290–6.
Mohyeldin A, Garzón-Muvdi T, Quiñones-Hinojosa A. Oxygen in stem cell biology: a critical component of the stem cell niche. Cell Stem Cell. 2010;7(2):150–61.
Liu L, Michowski W, Kolodziejczyk A, Sicinski P. The cell cycle in stem cell proliferation, pluripotency and differentiation. Nat Cell Biol. 2019;21(9):1060–7.
Watt FM, Huck WT. Role of the extracellular matrix in regulating stem cell fate. Nat Rev Mol Cell Biol. 2013;14(8):467–73.
Janiszewska M, Primi MC, Izard T. Cell adhesion in cancer: beyond the migration of single cells. J Biol Chem. 2020;295(8):2495–505.
Peitzsch C, Perrin R, Hill RP, Dubrovska A, Kurth I. Hypoxia as a biomarker for radioresistant cancer stem cells. Int J Radiat Biol. 2014;90(8):636–52.
Jing X, Yang F, Shao C, Wei K, Xie M, Shen H, Shu Y. Role of hypoxia in cancer therapy by regulating the tumor microenvironment. Mol Cancer. 2019;18(1):157.
Alsaab HO, Sau S, Alzhrani RM, Cheriyan VT, Polin LA, Vaishampayan U, Rishi AK, Iyer AK. Tumor hypoxia directed multimodal nanotherapy for overcoming drug resistance in renal cell carcinoma and reprogramming macrophages. Biomaterials. 2018;183:280–94.
Rovida E, Peppicelli S, Bono S, Bianchini F, Tusa I, Cheloni G, Marzi I, Cipolleschi MG, Calorini L, Sbarba PD. The metabolically-modulated stem cell niche: a dynamic scenario regulating cancer cell phenotype and resistance to therapy. Cell Cycle. 2014;13(20):3169–75.
Choudhry H, Harris AL. Advances in hypoxia-inducible factor biology. Cell Metab. 2018;27(2):281–98.
Ai Z, Lu Y, Qiu S, Fan Z. Overcoming cisplatin resistance of ovarian cancer cells by targeting HIF-1-regulated cancer metabolism. Cancer Lett. 2016;373(1):36–44.
Haase VH. Regulation of erythropoiesis by hypoxia-inducible factors. Blood Rev. 2013;27(1):41–53.
Schito L, Semenza GL. Hypoxia-inducible factors: master regulators of cancer progression. Trends Cancer. 2016;2(12):758–70.
Article PubMed Google Scholar
Won C, Kim BH, Yi EH, Choi KJ, Kim EK, Jeong JM, Lee JH, Jang JJ, Yoon JH, Jeong WI, et al. Signal transducer and activator of transcription 3-mediated CD133 up-regulation contributes to promotion of hepatocellular carcinoma. Hepatology. 2015;62(4):1160–73.
Bai J, Chen WB, Zhang XY, Kang XN, Jin LJ, Zhang H, Wang ZY. HIF-2α regulates CD44 to promote cancer stem cell activation in triple-negative breast cancer via PI3K/AKT/mTOR signaling. World J Stem Cells. 2020;12(1):87–99.
Lah TT, Novak M, Breznik B. Brain malignancies: glioblastoma and brain metastases. Semin Cancer Biol. 2020;60:262–73.
de Sousa e Melo F, Kurtova AV, Harnoss JM, Kljavin N, Hoeck JD, Hung J, Anderson JE, Storm EE, Modrusan Z, Koeppen H, et al. A distinct role for Lgr5(+) stem cells in primary and metastatic colon cancer. Nature. 2017;543(7647):676–80.
Article PubMed CAS Google Scholar
Carvalho R, Paredes J, Ribeiro AS. Impact of breast cancer cells’ secretome on the brain metastatic niche remodeling. Semin Cancer Biol. 2020;60:294–301.
Marzagalli M, Raimondi M, Fontana F, Montagnani Marelli M, Moretti RM, Limonta P. Cellular and molecular biology of cancer stem cells in melanoma: possible therapeutic implications. Semin Cancer Biol. 2019;59:221–35.
Lytle NK, Ferguson LP, Rajbhandari N, Gilroy K, Fox RG, Deshpande A, Schürch CM, Hamilton M, Robertson N, Lin W, et al. A Multiscale map of the stem cell state in pancreatic adenocarcinoma. Cell. 2019;177(3):572-86.e22.
Oskarsson T, Batlle E, Massagué J. Metastatic stem cells: sources, niches, and vital pathways. Cell Stem Cell. 2014;14(3):306–21.
Barbato L, Bocchetti M, Di Biase A, Regad T. Cancer stem cells and targeting strategies. Cells. 2019;8(8):926.
Article CAS PubMed Central Google Scholar
Oshimori N. Cancer stem cells and their niche in the progression of squamous cell carcinoma. Cancer Sci. 2020;111(11):3985–92.
Malanchi I, Santamaria-Martínez A, Susanto E, Peng H, Lehr HA, Delaloye JF, Huelsken J. Interactions between cancer stem cells and their niche govern metastatic colonization. Nature. 2011;481(7379):85–9.
Mani SA, Guo W, Liao MJ, Eaton EN, Ayyanan A, Zhou AY, Brooks M, Reinhard F, Zhang CC, Shipitsin M, et al. The epithelial-mesenchymal transition generates cells with properties of stem cells. Cell. 2008;133(4):704–15.
Zhang Z, Han H, Rong Y, Zhu K, Zhu Z, Tang Z, Xiong C, Tao J. Hypoxia potentiates gemcitabine-induced stemness in pancreatic cancer cells through AKT/Notch1 signaling. J Exp Clin Cancer Res. 2018;37(1):291.
Article PubMed PubMed Central CAS Google Scholar
Jiang N, Zou C, Zhu Y, Luo Y, Chen L, Lei Y, Tang K, Sun Y, Zhang W, Li S, et al. HIF-1ɑ-regulated miR-1275 maintains stem cell-like phenotypes and promotes the progression of LUAD by simultaneously activating Wnt/β-catenin and Notch signaling. Theranostics. 2020;10(6):2553–70.
Wang Z, Li Y, Sarkar FH. Signaling mechanism(s) of reactive oxygen species in epithelial–mesenchymal transition reminiscent of cancer stem cells in tumor progression. Curr Stem Cell Res Ther. 2010;5(1):74–80.
Kaszak I, Witkowska-Piłaszewicz O, Niewiadomska Z, Dworecka-Kaszak B, Ngosa Toka F, Jurka P. Role of cadherins in cancer-a review. Int J Mol Sci. 2020;21(20):7624.
Ooki A, VandenBussche CJ, Kates M, Hahn NM, Matoso A, McConkey DJ, Bivalacqua TJ, Hoque MO. CD24 regulates cancer stem cell (CSC)-like traits and a panel of CSC-related molecules serves as a non-invasive urinary biomarker for the detection of bladder cancer. Br J Cancer. 2018;119(8):961–70.
Minami Y. Overview: cancer stem cell and tumor environment. Oncology. 2015;89(Suppl 1):22–4.
Scadden DT. Nice neighborhood: emerging concepts of the stem cell niche. Cell. 2014;157(1):41–50.
Bristow RG, Hill RP. Hypoxia and metabolism. Hypoxia, DNA repair and genetic instability. Nat Rev Cancer. 2008;8(3):180–92.
Pires IM, Bencokova Z, Milani M, Folkes LK, Li JL, Stratford MR, Harris AL, Hammond EM. Effects of acute versus chronic hypoxia on DNA damage responses and genomic instability. Cancer Res. 2010;70(3):925–35.
Ruiz-Vela A, Aguilar-Gallardo C, Simón C. Building a framework for embryonic microenvironments and cancer stem cells. Stem Cell Rev Rep. 2009;5(4):319–27.
French R, Pauklin S. Epigenetic regulation of cancer stem cell formation and maintenance. Int J Cancer. 2021;148(12):2884–97.
Wang Q, Liang N, Yang T, Li Y, Li J, Huang Q, Wu C, Sun L, Zhou X, Cheng X, et al. DNMT1-mediated methylation of BEX1 regulates stemness and tumorigenicity in liver cancer. J Hepatol. 2021;75(5):1142–53.
Huang W, Hu H, Zhang Q, Wang N, Yang X, Guo AY. Genome-wide DNA Methylation enhances stemness in the mechanical selection of tumor-repopulating cells. Front Bioeng Biotechnol. 2020;8:88.
Deng L, Meng T, Chen L, Wei W, Wang P. The role of ubiquitination in tumorigenesis and targeted drug discovery. Signal Transduct Target Ther. 2020;5(1):11.
Lin X, Li AM, Li YH, Luo RC, Zou YJ, Liu YY, Liu C, Xie YY, Zuo S, Liu Z, et al. Silencing MYH9 blocks HBx-induced GSK3β ubiquitination and degradation to inhibit tumor stemness in hepatocellular carcinoma. Signal Transduct Target Ther. 2020;5(1):13.
Yang Q, Zhao S, Shi Z, Cao L, Liu J, Pan T, Zhou D, Zhang J. Chemotherapy-elicited exosomal miR-378a-3p and miR-378d promote breast cancer stemness and chemoresistance via the activation of EZH2/STAT3 signaling. J Exp Clin Cancer Res. 2021;40(1):120.
McCabe EM, Rasmussen TP. lncRNA involvement in cancer stem cell function and epithelial-mesenchymal transitions. Semin Cancer Biol. 2021;75:38–48.
He Y, Jiang X, Duan L, Xiong Q, Yuan Y, Liu P, Jiang L, Shen Q, Zhao S, Yang C, et al. LncRNA PKMYT1AR promotes cancer stem cell maintenance in non-small cell lung cancer via activating Wnt signaling pathway. Mol Cancer. 2021;20(1):156.
Ni H, Qin H, Sun C, Liu Y, Ruan G, Guo Q, Xi T, Xing Y, Zheng L. MiR-375 reduces the stemness of gastric cancer cells through triggering ferroptosis. Stem Cell Res Ther. 2021;12(1):325.
Sukowati CH, Tiribelli C. The biological implication of cancer stem cells in hepatocellular carcinoma: a possible target for future therapy. Expert Rev Gastroenterol Hepatol. 2013;7(8):749–57.
Clara JA, Monge C, Yang Y, Takebe N. Targeting signalling pathways and the immune microenvironment of cancer stem cells: a clinical update. Nat Rev Clin Oncol. 2020;17(4):204–32.
Totaro A, Castellan M, Battilana G, Zanconato F, Azzolin L, Giulitti S, Cordenonsi M, Piccolo S. YAP/TAZ link cell mechanics to Notch signalling to control epidermal stem cell fate. Nat Commun. 2017;8:15206.
Totaro A, Castellan M, Di Biagio D, Piccolo S. Crosstalk between YAP/TAZ and notch signaling. Trends Cell Biol. 2018;28(7):560–73.
Kim JH, Park SY, Jun Y, Kim JY, Nam JS. Roles of wnt target genes in the journey of cancer stem cells. Int J Mol Sci. 2017;18(8):1604.
Article PubMed Central CAS Google Scholar
Gaston-Massuet C, Andoniadou CL, Signore M, Jayakody SA, Charolidi N, Kyeyune R, Vernay B, Jacques TS, Taketo MM, Le Tissier P, et al. Increased Wingless (Wnt) signaling in pituitary progenitor/stem cells gives rise to pituitary tumors in mice and humans. Proc Natl Acad Sci U S A. 2011;108(28):11482–7.
Le Grand F, Jones AE, Seale V, Scimè A, Rudnicki MA. Wnt7a activates the planar cell polarity pathway to drive the symmetric expansion of satellite stem cells. Cell Stem Cell. 2009;4(6):535–47.
Katoh M. Canonical and non-canonical WNT signaling in cancer stem cells and their niches: cellular heterogeneity, omics reprogramming, targeted therapy and tumor plasticity (Review). Int J Oncol. 2017;51(5):1357–69.
Eyre R, Alférez DG, Santiago-Gómez A, Spence K, McConnell JC, Hart C, Simões BM, Lefley D, Tulotta C, Storer J, et al. Microenvironmental IL1β promotes breast cancer metastatic colonisation in the bone via activation of Wnt signalling. Nat Commun. 2019;10(1):5016.
Yang L, Shi P, Zhao G, Xu J, Peng W, Zhang J, Zhang G, Wang X, Dong Z, Chen F, et al. Targeting cancer stem cell pathways for cancer therapy. Signal Transduct Target Ther. 2020;5(1):8.
Najafi M, Farhood B, Mortezaee K. Cancer stem cells (CSCs) in cancer progression and therapy. J Cell Physiol. 2019;234(6):8381–95.
Zhan T, Rindtorff N, Boutros M. Wnt signaling in cancer. Oncogene. 2017;36(11):1461–73.
Taniguchi S, Elhance A, Van Duzer A, Kumar S, Leitenberger JJ, Oshimori N. Tumor-initiating cells establish an IL-33-TGF-β niche signaling loop to promote cancer progression. Science. 2020;369(6501):eaay1813.
Markopoulos GS, Roupakia E, Marcu KB, Kolettas E. Epigenetic regulation of inflammatory cytokine-induced epithelial-to-mesenchymal cell transition and cancer stem cell generation. Cells. 2019;8(10):1143.
Zhao B, Li L, Guan KL. Hippo signaling at a glance. J Cell Sci. 2010;123(Pt 23):4001–6.
Talukdar S, Bhoopathi P, Emdad L, Das S, Sarkar D, Fisher PB. Dormancy and cancer stem cells: an enigma for cancer therapeutic targeting. Adv Cancer Res. 2019;141:43–84.
Gimple RC, Bhargava S, Dixit D, Rich JN. Glioblastoma stem cells: lessons from the tumor hierarchy in a lethal cancer. Genes Dev. 2019;33(11–12):591–609.
Li Y, Tang T, Lee HJ, Song K. Selective anti-cancer effects of plasma-activated medium and its high efficacy with cisplatin on hepatocellular carcinoma with cancer stem cell characteristics. Int J Mol Sci. 2021;22(8):3956.
Lin X, Chen W, Wei F, Xie X. TV-circRGPD6 Nanoparticle suppresses breast cancer stem cell-mediated metastasis via the miR-26b/YAF2 axis. Mol Ther. 2021;29(1):244–62.
Raniszewska A, Vroman H, Dumoulin D, Cornelissen R, Aerts J, Domagała-Kulawik J. PD-L1(+) lung cancer stem cells modify the metastatic lymph-node immunomicroenvironment in nsclc patients. Cancer Immunol Immunother. 2021;70(2):453–61.
Liu C, Billet S, Choudhury D, Cheng R, Haldar S, Fernandez A, Biondi S, Liu Z, Zhou H, Bhowmick NA. Bone marrow mesenchymal stem cells interact with head and neck squamous cell carcinoma cells to promote cancer progression and drug resistance. Neoplasia. 2021;23(1):118–28.
Hagiwara M, Yasumizu Y, Yamashita N, Rajabi H, Fushimi A, Long MD, Li W, Bhattacharya A, Ahmad R, Oya M, et al. MUC1-C activates the BAF (mSWI/SNF) complex in prostate cancer stem cells. Cancer Res. 2021;81(4):1111–22.
Hsu MY, Yang MH, Schnegg CI, Hwang S, Ryu B, Alani RM. Notch3 signaling-mediated melanoma-endothelial crosstalk regulates melanoma stem-like cell homeostasis and niche morphogenesis. Lab Invest. 2017;97(6):725–36.
Hayakawa Y, Ariyama H, Stancikova J, Sakitani K, Asfaha S, Renz BW, Dubeykovskaya ZA, Shibata W, Wang H, Westphalen CB, et al. Mist1 expressing gastric stem cells maintain the normal and neoplastic gastric epithelium and are supported by a perivascular stem cell niche. Cancer Cell. 2015;28(6):800–14.
Nimmakayala RK, Leon F, Rachagani S, Rauth S, Nallasamy P, Marimuthu S, Shailendra GK, Chhonker YS, Chugh S, Chirravuri R, et al. Metabolic programming of distinct cancer stem cells promotes metastasis of pancreatic ductal adenocarcinoma. Oncogene. 2021;40(1):215–31.
Fendler A, Bauer D, Busch J, Jung K, Wulf-Goldenberg A, Kunz S, Song K, Myszczyszyn A, Elezkurtaj S, Erguen B, et al. Inhibiting WNT and NOTCH in renal cancer stem cells and the implications for human patients. Nat Commun. 2020;11(1):929.
Jain S, Annett SL, Morgan MP, Robson T. The cancer stem cell niche in ovarian cancer and its impact on immune surveillance. Int J Mol Sci. 2021;22(8):4091.
Yuan Y, Wang L, Ge D, Tan L, Cao B, Fan H, Xue L. Exosomal O-GlcNAc transferase from esophageal carcinoma stem cell promotes cancer immunosuppression through up-regulation of PD-1 in CD8(+) T cells. Cancer Lett. 2021;500:98–106.
Sphyris N, Hodder MC, Sansom OJ. Subversion of niche-signalling pathways in colorectal cancer: what makes and breaks the intestinal stem cell. Cancers (Basel). 2021;13(5):1000.
Singh SR. Gastric cancer stem cells: a novel therapeutic target. Cancer Lett. 2013;338(1):110–9.
Zhou C, Fan N, Liu F, Fang N, Plum PS, Thieme R, Gockel I, Gromnitza S, Hillmer AM, Chon SH, et al. Linking cancer stem cell plasticity to therapeutic resistance-mechanism and novel therapeutic strategies in esophageal cancer. Cells. 2020;9(6):1481.
Pinho S, Frenette PS. Haematopoietic stem cell activity and interactions with the niche. Nat Rev Mol Cell Biol. 2019;20(5):303–20.
Colombo F, Baldan F, Mazzucchelli S, Martin-Padura I, Marighetti P, Cattaneo A, Foglieni B, Spreafico M, Guerneri S, Baccarin M, et al. Evidence of distinct tumour-propagating cell populations with different properties in primary human hepatocellular carcinoma. PLoS ONE. 2011;6(6):e21369.
Wilson GS, Hu Z, Duan W, Tian A, Wang XM, McLeod D, Lam V, George J, Qiao L. Efficacy of using cancer stem cell markers in isolating and characterizing liver cancer stem cells. Stem Cells Dev. 2013;22(19):2655–64.
Nakamura T, Sakai K, Nakamura T, Matsumoto K. Hepatocyte growth factor twenty years on: much more than a growth factor. J Gastroenterol Hepatol. 2011;26(Suppl 1):188–202.
Lau CK, Yang ZF, Ho DW, Ng MN, Yeoh GC, Poon RT, Fan ST. An Akt/hypoxia-inducible factor-1alpha/platelet-derived growth factor-BB autocrine loop mediates hypoxia-induced chemoresistance in liver cancer cells and tumorigenic hepatic progenitor cells. Clin Cancer Res. 2009;15(10):3462–71.
Wang X, Sun W, Shen W, Xia M, Chen C, Xiang D, Ning B, Cui X, Li H, Li X, et al. Long non-coding RNA DILC regulates liver cancer stem cells via IL-6/STAT3 axis. J Hepatol. 2016;64(6):1283–94.
Fangmann L, Teller S, Stupakov P, Friess H, Ceyhan GO, Demir IE. 3D cancer migration assay with schwann cells. Methods Mol Biol. 2018;1739:317–25.
Dong HH, Xiang S, Liang HF, Li CH, Zhang ZW, Chen XP. The niche of hepatic cancer stem cell and cancer recurrence. Med Hypotheses. 2013;80(5):666–8.
Houghton J, Stoicov C, Nomura S, Rogers AB, Carlson J, Li H, Cai X, Fox JG, Goldenring JR, Wang TC. Gastric cancer originating from bone marrow-derived cells. Science. 2004;306(5701):1568–71.
Jiang J, Zhang Y, Chuai S, Wang Z, Zheng D, Xu F, Zhang Y, Li C, Liang Y, Chen Z. Trastuzumab (herceptin) targets gastric cancer stem cells characterized by CD90 phenotype. Oncogene. 2012;31(6):671–82.
Zhi QM, Chen XH, Ji J, Zhang JN, Li JF, Cai Q, Liu BY, Gu QL, Zhu ZG, Yu YY. Salinomycin can effectively kill ALDH(high) stem-like cells on gastric cancer. Biomed Pharmacother. 2011;65(7):509–15.
Proia TA, Keller PJ, Gupta PB, Klebba I, Jones AD, Sedic M, Gilmore H, Tung N, Naber SP, Schnitt S, et al. Genetic predisposition directs breast cancer phenotype by dictating progenitor cell fate. Cell Stem Cell. 2011;8(2):149–63.
Wu ZQ, Li XY, Hu CY, Ford M, Kleer CG, Weiss SJ. Canonical Wnt signaling regulates slug activity and links epithelial-mesenchymal transition with epigenetic breast Cancer 1, early onset (BRCA1) repression. Proc Natl Acad Sci USA. 2012;109(41):16654–9.
Tiwari N, Tiwari VK, Waldmeier L, Balwierz PJ, Arnold P, Pachkov M, Meyer-Schaller N, Schübeler D, van Nimwegen E, Christofori G. Sox4 is a master regulator of epithelial-mesenchymal transition by controlling Ezh2 expression and epigenetic reprogramming. Cancer Cell. 2013;23(6):768–83.
Chang CJ, Yang JY, Xia W, Chen CT, Xie X, Chao CH, Woodward WA, Hsu JM, Hortobagyi GN, Hung MC. EZH2 promotes expansion of breast tumor initiating cells through activation of RAF1-β-catenin signaling. Cancer Cell. 2011;19(1):86–100.
Atkinson RL, Zhang M, Diagaradjane P, Peddibhotla S, Contreras A, Hilsenbeck SG, Woodward WA, Krishnan S, Chang JC, Rosen JM. Thermal enhancement with optically activated gold nanoshells sensitizes breast cancer stem cells to radiation therapy. Sci Transl Med. 2010;2(55):55ra79.
Lapidot T, Sirard C, Vormoor J, Murdoch B, Hoang T, Caceres-Cortes J, Minden M, Paterson B, Caligiuri MA, Dick JE. A cell initiating human acute myeloid leukaemia after transplantation into SCID mice. Nature. 1994;367(6464):645–8.
Park D, Sykes DB, Scadden DT. The hematopoietic stem cell niche. Front Biosci (Landmark Ed). 2012;17:30–9.
Article CAS Google Scholar
Krause DS, Scadden DT, Preffer FI. The hematopoietic stem cell niche–home for friend and foe? Cytom B Clin Cytom. 2013;84(1):7–20.
Colmone A, Amorim M, Pontier AL, Wang S, Jablonski E, Sipkins DA. Leukemic cells create bone marrow niches that disrupt the behavior of normal hematopoietic progenitor cells. Science. 2008;322(5909):1861–5.
Chirgwin JM. The stem cell niche as a pharmaceutical target for prevention of skeletal metastases. Anticancer Agents Med Chem. 2012;12(3):187–93.
Idowu T, Samadder P, Arthur G, Schweizer F. Amphiphilic modulation of glycosylated antitumor ether lipids results in a potent triamino scaffold against epithelial cancer Cell lines and BT474 cancer stem cells. J Med Chem. 2017;60(23):9724–38.
Cazet AS, Hui MN, Elsworth BL, Wu SZ, Roden D, Chan CL, Skhinas JN, Collot R, Yang J, Harvey K, et al. Targeting stromal remodeling and cancer stem cell plasticity overcomes chemoresistance in triple negative breast cancer. Nat Commun. 2018;9(1):2897.
O’Leary DP, O’Leary E, Foley N, Cotter TG, Wang JH, Redmond HP. Effects of surgery on the cancer stem cell niche. Eur J Surg Oncol. 2016;42(3):319–25.
Lee KM, Giltnane JM, Balko JM, Schwarz LJ, Guerrero-Zotano AL, Hutchinson KE, Nixon MJ, Estrada MV, Sánchez V, Sanders ME, et al. MYC and MCL1 cooperatively promote chemotherapy-resistant breast cancer stem cells via regulation of mitochondrial oxidative phosphorylation. Cell Metab. 2017;26(4):633-47.e7.
Steinbichler TB, Dudás J, Skvortsov S, Ganswindt U, Riechelmann H, Skvortsova II. Therapy resistance mediated by cancer stem cells. Semin Cancer Biol. 2018;53:156–67.
Najafi M, Mortezaee K, Majidpoor J. Cancer stem cell (CSC) resistance drivers. Life Sci. 2019;234:116781.
Galle E, Thienpont B, Cappuyns S, Venken T, Busschaert P, Van Haele M, Van Cutsem E, Roskams T, van Pelt J, Verslype C, et al. DNA methylation-driven EMT is a common mechanism of resistance to various therapeutic agents in cancer. Clin Epigenetics. 2020;12(1):27.
Xu J, Liu D, Niu H, Zhu G, Xu Y, Ye D, Li J, Zhang Q. Resveratrol reverses doxorubicin resistance by inhibiting epithelial-mesenchymal transition (EMT) through modulating PTEN/Akt signaling pathway in gastric cancer. J Exp Clin Cancer Res. 2017;36(1):19.
Jin H, He Y, Zhao P, Hu Y, Tao J, Chen J, Huang Y. Targeting lipid metabolism to overcome EMT-associated drug resistance via integrin β3/FAK pathway and tumor-associated macrophage repolarization using legumain-activatable delivery. Theranostics. 2019;9(1):265–78.
Farmer P, Bonnefoi H, Anderle P, Cameron D, Wirapati P, Becette V, André S, Piccart M, Campone M, Brain E, et al. A stroma-related gene signature predicts resistance to neoadjuvant chemotherapy in breast cancer. Nat Med. 2009;15(1):68–74.
Ghobrial IM, Liu CJ, Zavidij O, Azab AK, Baz R, Laubach JP, Mishima Y, Armand P, Munshi NC, Basile F, et al. Phase I/II trial of the CXCR4 inhibitor plerixafor in combination with bortezomib as a chemosensitization strategy in relapsed/refractory multiple myeloma. Am J Hematol. 2019;94(11):1244–53.
Fischer KR, Durrans A, Lee S, Sheng J, Li F, Wong ST, Choi H, El Rayes T, Ryu S, Troeger J, et al. Epithelial-to-mesenchymal transition is not required for lung metastasis but contributes to chemoresistance. Nature. 2015;527(7579):472–6.
David CJ, Huang YH, Chen M, Su J, Zou Y, Bardeesy N, Iacobuzio-Donahue CA, Massagué J. TGF-β tumor suppression through a lethal EMT. Cell. 2016;164(5):1015–30.
Hua W, Ten Dijke P, Kostidis S, Giera M, Hornsveld M. TGFβ-induced metabolic reprogramming during epithelial-to-mesenchymal transition in cancer. Cell Mol Life Sci. 2020;77(11):2103–23.
Calon A, Espinet E, Palomo-Ponce S, Tauriello DV, Iglesias M, Céspedes MV, Sevillano M, Nadal C, Jung P, Zhang XH, et al. Dependency of colorectal cancer on a TGF-β-driven program in stromal cells for metastasis initiation. Cancer Cell. 2012;22(5):571–84.
Ahmadi A, Najafi M, Farhood B, Mortezaee K. Transforming growth factor-β signaling: tumorigenesis and targeting for cancer therapy. J Cell Physiol. 2019;234(8):12173–87.
den Hollander MW, Bensch F, Glaudemans AW, Oude Munnink TH, Enting RH, den Dunnen WF, Heesters MA, Kruyt FA, Lub-de Hooge MN, Cees de Groot J, et al. TGF-β antibody uptake in recurrent high-grade glioma imaged with 89Zr-fresolimumab PET. J Nucl Med. 2015;56(9):1310–4.
Trachtman H, Fervenza FC, Gipson DS, Heering P, Jayne DR, Peters H, Rota S, Remuzzi G, Rump LC, Sellin LK, et al. A phase 1, single-dose study of fresolimumab, an anti-TGF-β antibody, in treatment-resistant primary focal segmental glomerulosclerosis. Kidney Int. 2011;79(11):1236–43.
Lacouture ME, Morris JC, Lawrence DP, Tan AR, Olencki TE, Shapiro GI, Dezube BJ, Berzofsky JA, Hsu FJ, Guitart J. Cutaneous keratoacanthomas/squamous cell carcinomas associated with neutralization of transforming growth factor β by the monoclonal antibody fresolimumab (GC1008). Cancer Immunol Immunother. 2015;64(4):437–46.
Formenti SC, Hawtin RE, Dixit N, Evensen E, Lee P, Goldberg JD, Li X, Vanpouille-Box C, Schaue D, McBride WH, et al. Baseline T cell dysfunction by single cell network profiling in metastatic breast cancer patients. J Immunother Cancer. 2019;7(1):177.
Shibue T, Weinberg RA. EMT, CSCs, and drug resistance: the mechanistic link and clinical implications. Nat Rev Clin Oncol. 2017;14(10):611–29.
Huang X, Gan G, Wang X, Xu T, Xie W. The HGF-MET axis coordinates liver cancer metabolism and autophagy for chemotherapeutic resistance. Autophagy. 2019;15(7):1258–79.
Singh A, Settleman J. EMT, cancer stem cells and drug resistance: an emerging axis of evil in the war on cancer. Oncogene. 2010;29(34):4741–51.
Armstrong AJ, Freedland SJ, Garcia-Blanco M. Epithelial-mesenchymal transition in prostate cancer: providing new targets for therapy. Asian J Androl. 2011;13(2):179–80.
Sun X, Lv X, Yan Y, Zhao Y, Ma R, He M, Wei M. Hypoxia-mediated cancer stem cell resistance and targeted therapy. Biomed Pharmacother. 2020;130:110623.
Martí-Díaz R, Montenegro MF, Cabezas-Herrera J, Goding CR, Rodríguez-López JN, Sánchez-Del-Campo L. Acriflavine, a potent Inhibitor of HIF-1α, disturbs glucose metabolism and suppresses ATF4-protective pathways in melanoma under non-hypoxic conditions. Cancers (Basel). 2020;13(1):102.
Min S, Wang X, Du Q, Gong H, Yang Y, Wang T, Wu N, Liu X, Li W, Zhao C, et al. Chetomin, a Hsp90/HIF1α pathway inhibitor, effectively targets lung cancer stem cells and non-stem cells. Cancer Biol Ther. 2020;21(8):698–708.
Soni S, Padwad YS. HIF-1 in cancer therapy: two decade long story of a transcription factor. Acta Oncol. 2017;56(4):503–15.
He M, Wu H, Jiang Q, Liu Y, Han L, Yan Y, Wei B, Liu F, Deng X, Chen H, et al. Hypoxia-inducible factor-2α directly promotes BCRP expression and mediates the resistance of ovarian cancer stem cells to adriamycin. Mol Oncol. 2019;13(2):403–21.
Pinzón-Daza ML, Cuellar-Saenz Y, Nualart F, Ondo-Mendez A, Del Riesgo L, Castillo-Rivera F, Garzón R. Oxidative stress promotes doxorubicin-induced Pgp and BCRP expression in colon cancer cells under hypoxic conditions. J Cell Biochem. 2017;118(7):1868–78.
To KK, Poon DC, Wei Y, Wang F, Lin G, Fu LW. Vatalanib sensitizes ABCB1 and ABCG2-overexpressing multidrug resistant colon cancer cells to chemotherapy under hypoxia. Biochem Pharmacol. 2015;97(1):27–37.
Pandya K, Meeke K, Clementz AG, Rogowski A, Roberts J, Miele L, Albain KS, Osipo C. Targeting both Notch and ErbB-2 signalling pathways is required for prevention of ErbB-2-positive breast tumour recurrence. Br J Cancer. 2011;105(6):796–806.
Schott AF, Landis MD, Dontu G, Griffith KA, Layman RM, Krop I, Paskett LA, Wong H, Dobrolecki LE, Lewis MT, et al. Preclinical and clinical studies of gamma secretase inhibitors with docetaxel on human breast tumors. Clin Cancer Res. 2013;19(6):1512–24.
Jimeno A, Gordon M, Chugh R, Messersmith W, Mendelson D, Dupont J, Stagg R, Kapoun AM, Xu L, Uttamsingh S, et al. A first-in-human phase I study of the anticancer stem cell agent ipafricept (OMP-54F28), a decoy receptor for wnt ligands, in patients with advanced solid tumors. Clin Cancer Res. 2017;23(24):7490–7.
Basset-Séguin N, Hauschild A, Kunstfeld R, Grob J, Dréno B, Mortier L, Ascierto PA, Licitra L, Dutriaux C, Thomas L, et al. Vismodegib in patients with advanced basal cell carcinoma: primary analysis of STEVIE, an international, open-label trial. Eur J Cancer. 2017;86:334–48.
Antonarakis ES, Heath EI, Smith DC, Rathkopf D, Blackford AL, Danila DC, King S, Frost A, Ajiboye AS, Zhao M, et al. Repurposing itraconazole as a treatment for advanced prostate cancer: a noncomparative randomized phase II trial in men with metastatic castration-resistant prostate cancer. Oncologist. 2013;18(2):163–73.
Bendell J, Andre V, Ho A, Kudchadkar R, Migden M, Infante J, Tiu RV, Pitou C, Tucker T, Brail L, et al. Phase I study of LY2940680, a smo antagonist, in patients with advanced cancer including treatment-naïve and previously treated basal cell carcinoma. Clin Cancer Res. 2018;24(9):2082–91.
Dianat-Moghadam H, Mahari A, Salahlou R, Khalili M, Azizi M, Sadeghzadeh H. Immune evader cancer stem cells direct the perspective approaches to cancer immunotherapy. Stem Cell Res Ther. 2022;13(1):150.
Walcher L, Kistenmacher AK, Suo H, Kitte R, Dluczek S, Strauß A, Blaudszun AR, Yevsa T, Fricke S, Kossatz-Boehlert U. Cancer stem cells-origins and biomarkers: perspectives for targeted personalized therapies. Front Immunol. 2020;11:1280.
Ding Z, Li Q, Zhang R, Xie L, Shu Y, Gao S, Wang P, Su X, Qin Y, Wang Y, et al. Personalized neoantigen pulsed dendritic cell vaccine for advanced lung cancer. Signal Transduct Target Ther. 2021;6(1):26.
Ying Z, Huang XF, Xiang X, Liu Y, Kang X, Song Y, Guo X, Liu H, Ding N, Zhang T, et al. A safe and potent anti-CD19 CAR T cell therapy. Nat Med. 2019;25(6):947–53.
Singh N, Frey NV, Engels B, Barrett DM, Shestova O, Ravikumar P, Cummins KD, Lee YG, Pajarillo R, Chun I, et al. Antigen-independent activation enhances the efficacy of 4–1BB-costimulated CD22 CAR T cells. Nat Med. 2021;27(5):842–50.
Fathi E, Farahzadi R, Sheervalilou R, Sanaat Z, Vietor I. A general view of CD33(+) leukemic stem cells and CAR-T cells as interesting targets in acute myeloblatsic leukemia therapy. Blood Res. 2020;55(1):10–6.
Wang QS, Wang Y, Lv HY, Han QW, Fan H, Guo B, Wang LL, Han WD. Treatment of CD33-directed chimeric antigen receptor-modified T cells in one patient with relapsed and refractory acute myeloid leukemia. Mol Ther. 2015;23(1):184–91.
Download references
Acknowledgements
Figures were composed with the assistance of Servier Medical Art ( https://smart.servier.com/ ) and Biorender ( https://biorender.com/ ).
Open Access funding enabled and organized by Projekt DEAL. This work was supported by grants from the National Natural Science Foundation of China (81472672), International Science and Technology Cooperation Project of Shanghai (18410721900, 21490713700), Leading Talent Program of Minhang District (2017), the National Key Research and Development Program of China (2017YFC1308604), Köln Fortune Program/Faculty of Medicine, University of Cologne (457/2020), Medical Engineering Project of Fudan University (yg2021-017) and the Program of Shanghai Academic Research Leader (20XD1400900).
Author information
Feng Ju and Manar M. Atyah contributed equally to this work
Authors and Affiliations
General, Visceral and Cancer Surgery, University Hospital of Cologne, Kerpener Straße 62, Cologne, Germany
Feng Ju, Nellie Horstmann, Christiane J. Bruns & Yue Zhao
Department of Liver Surgery and Transplantation, Liver Cancer Institute, Zhongshan Hospital, Fudan University, No. 180 Fenglin Road, Shanghai, 200032, China
Manar M. Atyah & Ning Ren
Key Laboratory of Carcinogenesis and Cancer Invasion of Ministry of Education, Shanghai, China
Fraunhofer Institute for Translational Medicine and Pharmacology ITMP, 22525, Hamburg, Germany
Fraunhofer Cluster of Excellence for Immune-Mediated Diseases CIMD, 22525, Hamburg, Germany
Avram and Stella Goldstein-Goren, Department of Biotechnology Engineering, Ben-Gurion University of the Negev, Beer-Sheva, Israel
Institute of Fudan-Minhang Academic Health System, Minhang Hospital, Fudan University, Shanghai, 201199, China
Qiong-Zhu Dong & Ning Ren
Key Laboratory of Whole-Period Monitoring and Precise Intervention of Digestive Cancer of Shanghai Municipal Health Commission, Shanghai, 201199, China
You can also search for this author in PubMed Google Scholar
Contributions
Mr. FJ contributed to the writing and figure of the manuscript. Dr. MM. A participated in the reviewing of the literature and contributed to the writing and editing of the manuscript. Ms. NH participated in the reviewing and editing of the manuscript. Dr. SG and Prof. RV participated in the reviewing and editing of the manuscript. Prof. Dr. CJ Burns contributed to the reviewing and editing of the manuscript. Prof. Q-ZD, Dr. YZ and Prof. NR contributed to the construction of the manuscript and supervised the completion of the review. All authors read and approved the final manuscript.
Corresponding authors
Correspondence to Yue Zhao or Ning Ren .
Ethics declarations
Ethics approval and consent to participate, consent for publication.
All the authors have read and approved the paper and declare no potential conflicts of interest in the paper.
Competing interests
The authors declare that they have no competing interests.
Additional information
Publisher's note.
Springer Nature remains neutral with regard to jurisdictional claims in published maps and institutional affiliations.
Rights and permissions
Open Access This article is licensed under a Creative Commons Attribution 4.0 International License, which permits use, sharing, adaptation, distribution and reproduction in any medium or format, as long as you give appropriate credit to the original author(s) and the source, provide a link to the Creative Commons licence, and indicate if changes were made. The images or other third party material in this article are included in the article's Creative Commons licence, unless indicated otherwise in a credit line to the material. If material is not included in the article's Creative Commons licence and your intended use is not permitted by statutory regulation or exceeds the permitted use, you will need to obtain permission directly from the copyright holder. To view a copy of this licence, visit http://creativecommons.org/licenses/by/4.0/ . The Creative Commons Public Domain Dedication waiver ( http://creativecommons.org/publicdomain/zero/1.0/ ) applies to the data made available in this article, unless otherwise stated in a credit line to the data.
Reprints and permissions
About this article
Cite this article.
Ju, F., Atyah, M.M., Horstmann, N. et al. Characteristics of the cancer stem cell niche and therapeutic strategies. Stem Cell Res Ther 13 , 233 (2022). https://doi.org/10.1186/s13287-022-02904-1
Download citation
Received : 30 July 2021
Accepted : 16 May 2022
Published : 03 June 2022
DOI : https://doi.org/10.1186/s13287-022-02904-1
Share this article
Anyone you share the following link with will be able to read this content:
Sorry, a shareable link is not currently available for this article.
Provided by the Springer Nature SharedIt content-sharing initiative
- Niche/microenvironment
Stem Cell Research & Therapy
ISSN: 1757-6512
- Submission enquiries: Access here and click Contact Us
- General enquiries: [email protected]
Thank you for visiting nature.com. You are using a browser version with limited support for CSS. To obtain the best experience, we recommend you use a more up to date browser (or turn off compatibility mode in Internet Explorer). In the meantime, to ensure continued support, we are displaying the site without styles and JavaScript.
- View all journals
- My Account Login
- Explore content
- About the journal
- Publish with us
- Sign up for alerts
- Open access
- Published: 07 April 2023
Using machine learning to predict student retention from socio-demographic characteristics and app-based engagement metrics
- Sandra C. Matz 1 ,
- Christina S. Bukow 2 ,
- Heinrich Peters 1 ,
- Christine Deacons 3 ,
- Alice Dinu 5 na1 &
- Clemens Stachl 4
Scientific Reports volume 13 , Article number: 5705 ( 2023 ) Cite this article
7804 Accesses
2 Citations
35 Altmetric
Metrics details
- Human behaviour
An Author Correction to this article was published on 21 June 2023
This article has been updated
Student attrition poses a major challenge to academic institutions, funding bodies and students. With the rise of Big Data and predictive analytics, a growing body of work in higher education research has demonstrated the feasibility of predicting student dropout from readily available macro-level (e.g., socio-demographics or early performance metrics) and micro-level data (e.g., logins to learning management systems). Yet, the existing work has largely overlooked a critical meso-level element of student success known to drive retention: students’ experience at university and their social embeddedness within their cohort. In partnership with a mobile application that facilitates communication between students and universities, we collected both (1) institutional macro-level data and (2) behavioral micro and meso-level engagement data (e.g., the quantity and quality of interactions with university services and events as well as with other students) to predict dropout after the first semester. Analyzing the records of 50,095 students from four US universities and community colleges, we demonstrate that the combined macro and meso-level data can predict dropout with high levels of predictive performance (average AUC across linear and non-linear models = 78%; max AUC = 88%). Behavioral engagement variables representing students’ experience at university (e.g., network centrality, app engagement, event ratings) were found to add incremental predictive power beyond institutional variables (e.g., GPA or ethnicity). Finally, we highlight the generalizability of our results by showing that models trained on one university can predict retention at another university with reasonably high levels of predictive performance.
Similar content being viewed by others
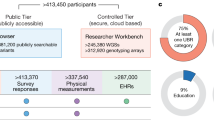
Genomic data in the All of Us Research Program
The All of Us Research Program Genomics Investigators
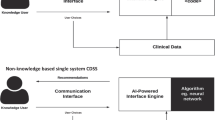
An overview of clinical decision support systems: benefits, risks, and strategies for success
Reed T. Sutton, David Pincock, … Karen I. Kroeker
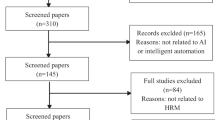
Ethics and discrimination in artificial intelligence-enabled recruitment practices
Zhisheng Chen
Introduction
In the US, only about 60% of full-time students graduate from their program 1 , 2 with the majority of those who discontinue their studies dropping out during their first year 3 These high attrition rates pose major challenges to students, universities, and funding bodies alike 4 , 5 .
Dropping out of university without a degree negatively impacts students’ finances and mental health. Over 65% of US undergraduate students receive student loans to help pay for college, causing them to incur heavy debts over the course of their studies 6 . According to the U.S. Department of Education, students who take out a loan but never graduate are three times more likely to default on loan repayment than students who graduate 7 . This is hardly surprising, given that students who drop out of university without a degree, earn 66% less than university graduates with a bachelor's degree and are far more likely to be unemployed 2 . In addition to financial losses, the feeling of failure often adversely impacts students’ well-being and mental health 8 .
At the same time, student attrition negatively impacts universities and federal funding bodies. For universities, student attrition results in an average annual revenue reduction of approximately $16.5 billion per year through the loss of tuition fees 9 , 10 . Similarly, student attrition wastes valuable resources provided by states and federal governments. For example, the US Department of Education Integrated Postsecondary Education Data System (IPEDS) shows that between 2003 and 2008, state and federal governments together provided more than $9 billion in grants and subsidies to students who did not return to the institution where they were enrolled for a second year 11 .
Given the high costs of attrition, the ability to predict at-risk students – and to provide them with additional support – is critical 12 , 13 . As most dropouts occur during the first year 14 , such predictions are most valuable if they can identify at-risk students as early as possible 13 , 15 , 16 . The earlier one can identify students who might struggle, the better the chances that interventions aimed at protecting them from gradually falling behind – and eventually discontinuing their studies – will be effective 17 , 18 .
Indicators of student retention
Previous research has identified various predictors of student retention, including previous academic performance, demographic and socio-economic factors, and the social embeddedness of a student in their home institution 19 , 20 , 21 , 22 , 23 .
Prior academic performance (e.g., high school GPA, SAT and ACT scores or college GPA) has been identified as one of the most consistent predictors of student retention: Students who are more successful academically are less likely to drop out 17 , 21 , 24 , 25 , 26 , 27 , 28 , 29 . Similarly, research has highlighted the role of demographic and socio-economic variables, including age, gender, and ethnicity 12 , 19 , 25 , 27 , 30 as well as socio-economic status 31 in predicting a students’ likelihood of persisting. For example, women are more likely to continue their studies than men 12 , 30 , 32 , 33 while White and Asian students are more likely to persist than students from other ethnic groups 19 , 27 , 30 . Moreover, a student’s socio-economic status and immediate financial situation have been shown to impact retention. Students are more likely to discontinue their studies if they are first generation students 34 , 35 , 36 or experience high levels of financial distress, (e.g., due to student loans or working nearly full time to cover college expenses) 37 , 38 . In contrast, students who receive financial support that does not have to be repaid post-graduation are more likely to complete their degree 39 , 40 .
While most of the outlined predictors of student retention are relatively stable intrapersonal characteristics and oftentimes difficult or costly to change, research also points to a more malleable pillar of retention: the students’ experience at university. In particular, the extent to which they are successfully integrated and socialized into the institution 16 , 22 , 41 , 42 . As Bean (2005) notes, “few would deny that the social lives of students in college and their exchanges with others inside and outside the institution are important in retention decisions” (p. 227) 41 . The extent to which a student is socially integrated and embedded into their institution has been studied in a number of ways, relating retention to the development of friendships with fellow students 43 , the student’s position in the social networks 16 , 29 , the experience of social connectedness 44 and a sense of belonging 42 , 45 , 46 . Taken together, these studies suggest that interactions with peers as well as faculty and staff – for example through participation in campus activities, membership of organizations, and the pursuit of extracurricular activities – help students better integrate into university life 44 , 47 . In contrast, a lack of social integration resulting from commuting (i.e., not living on campus with other students) has shown to negatively impact a student’s chances of completing their degree 48 , 49 , 50 , 51 . In short, the stronger a student is embedded and feels integrated into the university community – particularly in their first year – the less likely the student will drop out 42 , 52 .
Predicting retention using machine learning
A large proportion of research on student attrition has focused on understanding and explaining drivers of student retention. However, alongside the rise of computational methods and predictive modeling in the social sciences 53 , 54 , 55 , educational researchers and practitioners have started exploring the feasibility and value of data-driven approaches in supporting institutional decision making and educational effectiveness (for excellent overviews of the growing field see 56 , 57 ). In line with this broader trend, a growing body of work has shown the potential of predicting student dropout with the help of machine learning. In contrast to traditional inferential approaches, machine learning approaches are predominantly concerned with predictive performance (i.e., the ability to accurately forecast behavior that has not yet occurred) 54 . In the context of student retention this means: How accurately can we predict whether a student is going to complete or discontinue their studies (in the future) by analyzing their demographic and socio-economic characteristics, their past and current academic performance, as well as their current embeddedness in the university system and culture?
Echoing the National Academy of Education’s statement (2017) that “in the educational context, big data typically take the form of administrative data and learning process data, with each offering their own promise for educational research” (p.4) 58 , the vast majority of existing studies have focused on the prediction of student retention from demographic and socio-economic characteristics as well as students’ academic history and current performance 13 , 59 , 60 , 61 , 62 , 63 , 64 , 65 , 66 . In a recent study, Aulck and colleagues trained a model on the administrative data of over 66,000 first-year students enrolled in a public US university (e.g., race, gender, highschool GPA, entrance exam scores and early college performance/transcript data) to predict whether they would re-enroll in the second year and eventually graduate 59 . Specifically, they used a range of linear and non-linear machine learning models (e.g., regularized logistic regression, k-nearest neighbor, random forest, support vector machine, and gradient boosted trees) to predict retention out-of-sample using a standard cross-validation procedures. Their model was able to predict dropouts with an accuracy of 88% and graduation with an accuracy of 81% (where 50% is chance).
While the existing body of work provides robust evidence for the potential of predictive models for identifying at-risk students, it is based on similar sets of macro-level data (e.g., institutional data, academic performance) or micro-level data (e.g., click-stream data). Almost entirely absent from this research is data on students’ daily experience and engagement with both other students and the university itself (meso-level). Although there have been a small number of studies trying to capture part of this experience by inferring social networks from smart card transactions that were made by students in the same time and place 16 or engagement metrics with an open online course 67 , none of the existing work has offered a more holistic and comprehensive view on students’ daily experience. One potential explanation for this gap is that information about students’ social interactions with classmates or their day-to-day engagement with university services and events is difficult to track. While universities often have access to demographic or socio-economic variables through their Student Information Systems (SISs), and can easily track their academic performance, most universities do not have an easy way of capturing student’s deeper engagement with the system.
Research overview
In this research, we partner with an educational software company – READY Education – that offers a virtual one-stop interaction platform in the form of a smartphone application to facilitate communication between students, faculty, and staff. Students receive relevant information and announcements, can manage their university activities, and interact with fellow students in various ways. For example, the app offers a social media experience like Facebook, including private messaging, groups, public walls, and friendships. In addition, it captures students’ engagement with the university asking them to check into events (e.g., orientation, campus events, and student services) using QR code functionality and prompting them to rate their experience afterwards (see Methods for more details on the features we extracted from this data). As a result, the READY Education app allows us to observe a comprehensive set of information about students that include both (i) institutional data (i.e., demographic, and socio-economic characteristics as well as academic performance), and (ii) their idiosyncratic experience at university captured by their daily interactions with other students and the university services/events. Combining the two data sources captures a student’s profile more holistically and makes it possible to consider potential interactions between the variable sets. For example, being tightly embedded in a social support network of friends might be more important for retention among first-generation students who might not receive the same level of academic support or learn about implicit academic norms and rules from their parents.
Building on this unique dataset, we use machine learning models to predict student retention (i.e., dropout) from both institutional and behavioral engagement data. Given the desire to identify at-risk students as early as possible, we only use information gathered in the students’ first semester to predict whether the student dropped out at any point in time during their program. To thoroughly validate and scrutinize our analytical approach, generate insights for potential interventions, and probe the generalizability of our predictive models across different universities, we investigate the following three research questions:
How accurately can we predict a student's likelihood of discontinuing their studies using information from the first term of their studies (i.e., institutional data, behavioral engagement data, and a combination of both)?
Which features are the most predictive of student retention?
How well do the predictive models generalize across universities (i.e., how well can we predict student retention of students from one university if we use the model trained on data from another university and vice versa)?
Participants
We analyze de-identified data from four institutions with a total of 50,095 students (min = 476, max = 45,062). All students provided informed consent to the use of the anonymized data by READY Education and research partners. All experimental protocols were approved by the Columbia University Ethics Board, and all methods carried out were in accordance with the Board’s guidelines and regulations. The data stem from two sources: (a) Institutional data and (b) behavioral engagement data. The institutional data collected by the universities contain socio-demographics (e.g., gender, ethnicity), general study information (e.g., term of admission, study program), financial information (e.g., pell eligibility), students’ academic achievement scores (e.g., GPA, ACT) as well as the retention status. The latter indicated whether students continued or dropped out and serves as the outcome variable. As different universities collect different information about their students, the scope of institutional data varied between universities. Table 1 provides a descriptive overview of the most important sociodemographic characteristics for each of the four universities. In addition, it provides a descriptive overview of the app usage, including the average number of logs per student, the total number of sessions and logs, as well as the percentage of students in a cohort using the app (i.e., coverage). The broad coverage of students using the app, ranging between 70 and 98%, results in a largely representative sample of the student populations at the respective universities.
Notably, Universities 1–3 are traditional university campuses, while University 4 is a combination of 16 different community colleges. Given that there is considerable heterogeneity across campuses, the predictive accuracies for University 4 are a-priori expected to be lower than those observed for universities 1–3 (and partly speak to the generalizability of findings already). The decision to include University 4 as a single entity was based on the fact that separating out the 16 colleges would have resulted in an over-representation of community colleges that all share similar characteristics thereby artificially inflating the observed cross-university accuracies. Given these limitations (and the fact that the University itself collapsed the college campuses for many of their internal reports), we decided to analyze it as a single unit, acknowledging that this approach brings its own limitations.
The behavioral engagement data were generated through the app (see Table 1 for the specific data collection windows at each University). Behavioral engagement data were available in the form of time-stamped event-logs (i.e., each row in the raw data represented a registered event such as a tab clicked, a comment posted, a message sent). Each log could be assigned to a particular student via an anonymized, unique identifier. Across all four universities, the engagement data contained 7,477,630 sessions (Mean = 1,869,408, SD = 3,329,852) and 17,032,633 logs (Mean = 4,258,158, SD = 6,963,613) across all universities. For complete overview of all behavioral engagement metrics including a description see Table S1 in the Supplementary Materials.
Pre-processing and feature extraction
As a first step, we cleaned both the institutional and app data. For the institutional data, we excluded students who did not use the app and therefore could not be assigned a unique identifier. In addition, we excluded students without a term of admission to guarantee that we are only observing the students’ first semester. Lastly, we removed duplicate entries resulting from dual enrollment in different programs. For the app usage data, we visually inspected the variables in our data set for outliers that might stem from technical issues. We pre-processed data that reflected clicking through the app, named “clicked_[…]” and “viewed_[…]” (see Table S1 in the Supplementary Materials). A small number of observations showed unrealistically high numbers of clicks on the same tab in a very short period, which is likely a reflection of a student repeatedly clicking on a tab due to long loading time or other technical issues. To avoid oversampling these behaviors, we removed all clicks of the same type which were made by the same person less than one minute apart.
We extracted up to 462 features for each university across two broad categories: (i) institutional features and (ii) engagement features, using evidence from previous research as a reference point (see Table S2 in the Supplementary Materials for a comprehensive overview of all features and their availability for each of the universities). Institutional features contain students’ demographic, socio-economic and academic information. The engagement features represent the students’ behavior during their first term of studies. They can be further divided into app engagement and community engagement. The app engagement features represent the students’ behavior related to app usage, such as whether the students used the app before the start of the semester, how often they clicked on notifications or the community tabs, or whether their app use increased over the course of the semester. The community involvement features reflect social behavior and interaction with others, e.g., the number of messages sent, posts and comments made, events visited or a student’s position in the network as inferred from friendships and direct messages. Importantly, many of the features in our dataset will be inter-correlated. For example, living in college accommodation could signal higher levels of socio-economic status, but also make it more likely that students attend campus events and connect with other students living on campus. While intercorrelations among predictors is a challenge with standard inferential statistical techniques such as regression analyses, the methods we apply in this paper can account for a large number of correlated predictors.
Institutional features were directly derived from the data recorded by the institutions. As noted above, not all features were available for all universities, resulting in slightly different feature sets across universities. The engagement features were extracted from the app usage data. As we focused on an early prediction of drop-out, we restricted the data to event-logs that were recorded in the respective students' first term. Notably, the data captures students’ engagement as a time-stamped series of events, offering fine-grained insights into their daily experience. For reasons of simplicity and interpretability (see research question 2), we collapse the data into a single entry for each student. Specifically, we describe a student’s overall experience during the first semester, by calculating distribution measures for each student such as the arithmetic mean, standard deviation, kurtosis, skewness, and sum values. For example, we calculate how many daily messages a particular student sent or received during their first semester, or how many campus events they attended in total. However, we also account for changes in a student’s behavior over time by calculating more complex features such as entropy (e.g., the extent to which a person has frequent contact with few people or the same degree of contact with many people) and the development of specific behaviors over time measured by the slope of regression analyses, as well as features representing the regularity of behavior (e.g., the deviation of time between sending messages). Overall, the feature set was aimed at describing a student’s overall engagement with campus resources and other students during the first semester as well as changed in engagement over time. Finally, we extracted some of the features separately for weekdays and weekends to account for differences and similarities in students’ activities during the week and the weekend. For example, little social interaction on weekdays might predict retention differently than little social interaction on the weekend.
We further cleaned the data by discarding participants for whom the retention status was missing and those in which 95% or more of the values were zero or missing. Furthermore, features were removed if they showed little or no variance across participants, which makes them essentially meaningless in a prediction task. Specifically, we excluded numerical features which showed the same values for more than 90% of observations and categorical features which showed the same value for all observations.
In addition to these general pre-processing procedures, we integrated additional pre-processing steps into the resampling prior to training the models to avoid an overestimation of model performance 68 . To prevent problems with categorical features that occur when there are fewer levels in the test than in the training data, we first removed categories that did not occur in the training data. Second, we removed constant categorical features containing a single value only (and therefore no variation). Third, we imputed missing values using the following procedures: Categorical features were imputed with the mode. Following commonly used approaches to dealing with missing data, the imputation of numeric features varied between the learners. For the elastic net, we imputed those features with the median. For the random forest, we used twice the maximum to give missing values a distinct meaning that would allow the model to leverage this information. Lastly, we used the "Synthetic Minority Oversampling Technique" (SMOTE) to create artificial examples for the minority class in the training data 69 . The only exception was University 4 which followed a different procedure due to the large sample size and estimated computing power for implementing SMOTE. Instead of oversampling minority cases, we downsampled majority cases such that the positive and negative class were balanced. This was done to address the class imbalance caused by most students continuing their studies rather than dropping out 12 .
Predictive modeling approach
We predicted the retention status (1 = dropped out, 0 = continued) in a binary prediction task, with three sets of features: (1) institutional features (2) engagement features, and (3) a combined set of all features. To ensure the robustness of our predictions and to identify the model which is best suited for the current prediction context 54 , we compared a linear classifier ( elastic net; implemented in glmnet 4.1–4) 70 , 71 and a nonlinear classifier ( random forest ; implemented in randomForest 4.7–1) 72 , 73 . Both models are particularly well suited for our prediction context and are common choices in computational social science. That is, simple linear or logistic regression models are not suitable to work with datasets that have many inter-correlated predictors (in our case, a total of 462 predictors many of which are highly correlated) due to a high risk of overfitting. Both the elastic net and the random forest algorithm can effectively utilize large feature sets while reducing the risk of overfitting. We evaluate the performance of our six models for each school (2 algorithms and 3 feature sets), using out-of-sample benchmark experiments that estimate predictive performance and compare it against a common non-informative baseline model. The baseline represents a null-model that does not include any features, but instead always predicts the majority class, which in our samples means “continued.” 74 Below, we provide more details about the specific algorithms (i.e., elastic net and random forest), the cross-validation procedure, and the performance metrics we used for model evaluation.
Elastic net model
The elastic net is a regularized regression approach that combines advantages of ridge regression 75 with those of the LASSO 76 and is motivated by the need to handle large feature sets. The elastic net shrinks the beta-coefficients of features that add little predictive value (e.g., intercorrelated, little variance). Additionally, the elastic net can effectively remove variables from the model by reducing the respective beta coefficients to zero 70 . Unlike classical regression models, the elastic net does not aim to optimize the sum of least squares, but includes two penalty terms (L1, L2) that incentivize the model to reduce the estimated beta value of features that do not add information to the model. Combining the L1 (the sum of absolute values of the coefficients) and L2 (the sum of the squared values of the coefficients) penalties, elastic net addresses the limitations of alternative linear models such as LASSO regression (not capable of handling multi-collinearity) and Ridge Regression (may not produce sparse-enough solutions) 70 .
Formally, following Hastie & Qian (2016) the model equation of elastic net for binary classification problems can be written as follows 77 . Suppose the response variable takes values in G = {0,1}, y i denoted as I(g i = 1), the model formula is written as
After applying the log-odds transformation, the model formula can be written as
The objective function for logistic regression is the penalized negative binomial log-likelihood
where λ is the regularization parameter that controls the overall strength of the regularization, α is the mixing parameter that controls the balance between L1 and L2 regularization with α values closer to zero to result in sparser models (lasso regression α = 1, ridge regression α = 0). β represents coefficients of the regression model, ||β|| 1 is the is the L1 norm of the coefficients (the sum of absolute values of the coefficients), ||β|| 2 is the L2 norm of the coefficients (the sum of the squared values of the coefficients).
The regularized regression approach is especially relevant for our model because many of the app-based engagement features are highly correlated (e.g., the number of clicks is related to the number of activities registered in the app). In addition, we favored the elastic net algorithm over more complex alternatives, because the regularized beta coefficients can be interpreted as feature importance, allowing insights into which predictors are most informative of college dropout 78 , 79 .
Random forest model
Random forest models are a widely used ensemble learning method that grows many bagged and decorrelated decision trees to come up with a “collective” prediction of the outcome (i.e., the outcome that is chosen by most trees in a classification problem) 72 . Individual decision trees recursively split the feature space (rules to distinguish classes) with the goal to separate the different classes of the criterion (drop out vs. remain in our case). For a detailed description of how individual decision trees operate and translate to a random forest see Pargent, Schoedel & Stachl 80 .
Unlike the elastic net, random forest models can account for nonlinear associations between features and criterion and automatically include multi-dimensional interactions between features. Each decision tree in a random forest considers a random subset of bootstrapped cases and features, thereby increasing the variance of predictions across trees and the robustness of the overall prediction. For the splitting in each node of each tree, a random subset of features (mtry hyperparameter that we optimize in our models) are used by randomly drawing from the total set. For each split, all combinations of split variables and split points are compared, with the model choosing the splits that optimize the separation between classes 72 .
The random forest algorithm can be formally described as follows (verbatim from Hastie et al., 2016, p. 588):
For b = 1 to B:
Draw a bootstrap sample of size N from the training data.
Grow a decision tree to the bootstrapped data, by recursively repeating the following steps for each terminal node of the tree, until the minimum node size is reached.
Select m variables at random from the p variables.
Pick the best variable/split-point among the m according to the loss function (in our case Gini-impurity decrease)
Split the node into two daughter nodes.
Output the ensemble of trees
New predictions can then be made by generating a prediction for each tree and aggregating the results using majority vote.
The aggregation of predictions across trees in random forests improves the prediction performance compared to individual decision trees, as it can benefit from the trees’ variance and greatly reduces it to arrive at a single prediction 72 , 81 .
(Nested) Cross-validation: Out-of-sample model evaluation
We evaluate the performance of our predictive models using an out-of-sample validation approach. The idea behind out-of-sample validation is to increase the likelihood that a model will accurately predict student dropout on new data (e.g. new students) by using different datasets when training and evaluating the model. A commonly used, efficient technique for out-of-sample validation is to repeatedly fit (cf. training) and evaluate (cf. testing) models on non-overlapping parts of the same datasets and to combine the individual estimates across multiple iterations. This procedure – known as cross-validation – can also be used for model optimization (e.g., hyperparameter-tuning, pre-processing, variable selection), by repeatedly evaluating different settings for optimal predictive performance. When both approaches are combined, evaluation and optimization steps need to be performed in a nested fashion to ensure a strict separation of training and test data for a realistic out-of-sample performance estimation. The general idea is to emulate all modeling steps in each fold of the resampling as if it were a single in-sample model. Here, we use nested cross-validation to estimate the predictive performance of our models, to optimize model hyperparameters, and to pre-process data. We illustrate the procedure in Fig. 1 .
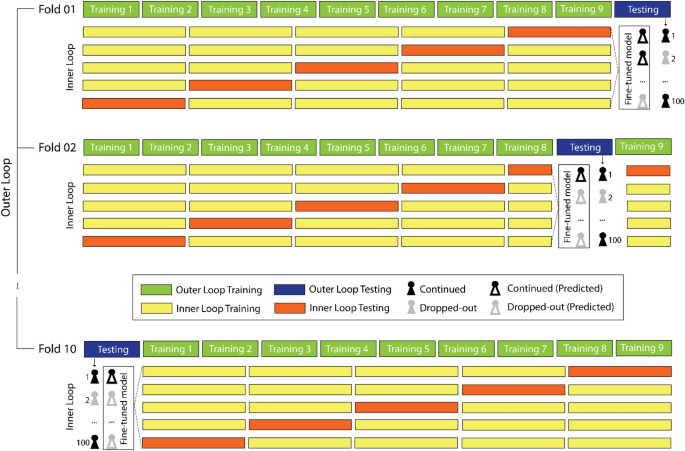
Schematic cross-validation procedure for out-of-sample predictions. The figure shows a tenfold cross-validation in the outer loop which is used to estimate the overall performance of the model by comparing the predicted outcomes for each student in the previously unseen test set with their actual outcomes. Within each of the 10 outer loops, a fivefold cross-validation in the inner loop is used to finetune model hyperparameters by evaluating different model settings.
The cross-validation procedure works as follows: Say we have a dataset with 1,000 students. In a first step, the dataset is split into ten different subsamples, each containing data from 100 students. In the first round, nine of these subsamples are used for training (i.e., fitting the model to estimate parameters, green boxes). That means, the data from the first 900 students will be included in training the model to relate the different features to the retention outcome. Once training is completed, the model’s performance can be evaluated on the data of the remaining 100 students (i.e., test dataset, blue boxes). For each student, the actual outcome (retained or discontinued, grey and black figures) is compared to the predicted outcome (retained or discontinued, grey and black figures). This comparison allows for the calculation of various performance metrics (see “ Performance metrics ” section below for more details). In contrast to the application of traditional inferential statistics, the evaluation process in predictive models separates the data used to train a model from the data used to evaluate these associations. Hence any overfitting that occurs at the training stage (e.g., using researcher degrees of freedom or due to the model learning relationships that are unique to the training data), hurts the predictive performance in the testing stage. To further increase the robustness of findings and leverage the entire dataset, this process is repeated for all 10 subsamples, such that each subsample is used nine times for training and once for testing. Finally, the obtained estimates from those ten iterations are aggregated to arrive at a cross-validated estimate of model performance. This tenfold cross validation procedure is referred to as the “outer loop”.
In addition to the outer loop, our models also contain an “inner loop”. The inner loop consists of an additional cross-validation procedure that is used to identify ideal hyperparameter settings (see “ Hyperparameter tuning ” section below). That is, in each of the ten iterations of the outer loop, the training sample is further divided into a training and test set to identify the best parameter constellations before model evaluation in the outer loop. We used fivefold cross-validation in the inner loop. All analyses scripts for the pre-processing and modeling steps are available on OSF ( https://osf.io/bhaqp/?view_only=629696d6b2854aa9834d5745425cdbbc ).
Performance metrics
We evaluate model performance based on four different metrics. Our main metric for model performance is AUC (area under the received operating characteristics curve). AUC is commonly used to assess the performance of a model over a 50%-chance baseline, and can range anywhere between 0 and 1. The AUC metric captures the area under the receiver operating characteristic (ROC) curve, which plots the true positive rate (TPR or recall; i.e. the percentage of correctly classified dropouts among all students who actually dropped out), against the false positive rate (FPR; i.e. the percentage of students erroneously classified as dropouts among all the students who actually continued). When the AUC is 0.5, the model’s predictive performance is equal to chance or a coin flip. The closer to 1, the higher the model’s predictive performance in distinguishing between students who continued and those who dropped out.
In addition, we report the F1 score, which ranges between 0 and 1 82 . The F1 score is based on the model’s positive predictive value (or precision, i.e., the percentage of correctly classified dropouts among all students predicted to have dropped out) as well as the model's TPR. A high F1 score hence indicates that there are both few false positives and few false negatives.
Given the specific context, we also report the TPR and the true negative rates (TNR, i.e. the percentage of students predicted to continue among all students who actually continued). Depending on their objective, universities might place a stronger emphasis on optimizing the TPR to make sure no student who is at risk of dropping out gets overlooked or on optimizing the TNR to save resources and assure that students do not get overly burdened. Notably, in most cases, universities are likely to strive for a balance between the two, which is reflected in our main AUC measure. All reported performance metrics represent the mean predictive performance across the 10 cross-validation folds of the outer loop 54 .
Hyperparameter tuning
We used a randomized search with 50 iterations and fivefold cross-validation for hyperparameter tuning in the inner loop of our cross-validation. The randomized search algorithm fits models with hyperparameter configurations randomly selected from a previously defined hyperparameter space and then picks the model that shows the best generalized performance averaged over the five cross-validation folds. The best hyperparamter configuration is used for training in the outer resampling loop to evaluate model performance.
For the elastic net classifier, we tuned the regularization parameter lambda, the decision rule used to choose lambda, and the L1-ratio parameter. The search space for lambda encompassed the 100 glmnet default values 71 . The space of decision rules for lambda included lambda.min which chooses the value of lambda that results in the minimum mean cross-validation error, and lambda.1se which chooses the value of lambda that results in the most regularized model such that the cross-validation error remains within one standard error of the minimum. The search space for the L1-ratio parameter included the range of values between 0 (ridge) to 1 (lasso). For the random forest classifier, we tuned the number of features selected for each split within a decision tree (mtry) and the minimum node size (i.e., how many cases are required to be left in the resulting end-nodes of the tree). The search space for the number of input features per decision tree was set to a range of 1 to p, where p represents the dimensionality of the feature space. The search space for minimum node size was set to a range of 1 to 5. Additionally, for both models, we tuned the oversampling rate and the number or neighbors used to generate new samples utilized by the SMOTE algorithm. The oversampling rate was set to a range of 2 to 15 and the number of nearest neighbors was set to a range of 1 to 10.
RQ1: How accurately can we predict a student's likelihood of discontinuing their studies using information from the first term of their studies?
Figure 2 displays AUC scores (Y-axis) across the different universities (rows), separated by the different feature sets (colors) and predictive algorithms (X-axis labels). The figure displays the distribution of AUC accuracies across the 10 cross-validation folds, alongside their mean and standard deviation. Independent t-tests using Holm corrections for multiple comparisons indicate statistical differences in the predictive performance across the different models and feature sets within each university. Table 2 provides the predictive performance across all four metrics.

AUC performance across the four universities for different feature sets and model. Inst. = Institutional data. Engag. = Engagement data. (EN) = Elastic Net. (RF) = Random Forest.
Overall, our models showed high levels of predictive accuracies across universities, models, feature sets and performance metrics, significantly outperforming the baseline in all instances. The main performance metric AUC reached an average of 73% (where 50% is chance), with a maximum of 88% for the random forest model and the full feature set in University 1. Both institutional features and engagement features significantly contributed to predictive performance, highlighting the fact that a student’s likelihood to drop out is both a function of their more stable socio-demographic characteristics as well as their experience of campus life. In most cases, the joint model (i.e., the combination of institutional and engagement features) performed better than each of the individual models alone. Finally, the random forest models produced higher levels of predictive performance than the elastic net in most cases (average AUC elastic net = 70%, AUC random forest = 75%), suggesting that the features are likely to interact with one another in predicting student retention and might not always be linearly related to the outcome.
RQ2: Which features are the most predictive of student retention?
To provide insights into the underlying relationships between student retention and socio-demographic as well as behavioral features, we examined two indicators of feature importance that both offer unique insights. First, we calculated the zero-order correlations between the features and the outcome for each of the four universities. We chose zero-order correlations over elastic net coefficients as they represent the relationships unaltered by the model’s regularization procedure (i.e., the relationship between a feature and the outcome is shown independently of the importance of the other features in the model). To improve the robustness of our findings, we only included the variables that passed the threshold for data inclusion in our models and had less than 50% of the data imputed. The top third of Table 3 displays the 10 most important features (i.e., highest absolute correlation with retention). The sign in brackets indicates the direction of the effects with ( +) indicating a protective factor and (−) indicating a risk factor. Features that showed up in the top 10 for more than 1 university are highlighted in bold.
Second, we calculated permutation variable importance scores for the elastic net and random forest models. For the elastic net model, feature importance is reported as the model coefficient after shrinking the coefficients according to their incremental predictive power. Compared to the zero-order correlation, the elastic net coefficients hence identify the features that have the strongest unique variance. For the random forest models, feature importance is reported as a model-agnostic metric that estimates the importance of a feature by observing the drop in model predictive performance when the actual association between the feature and the outcome is broken by randomly shuffling observations 72 , 83 . A feature is considered important if shuffling its values increases the model error (and therefore decreases the model’s predictive performance). In contrast to the coefficients from the elastic net model, the permutation feature importance scores are undirected and do not provide insights into the specific nature of the relationship between the feature and the outcome. However, they account for the fact that some features might not be predictive themselves but could still prove valuable in the overall model performance because they moderate the impact of other features. For example, minority or first-generation students might benefit more from being embedded in a strong social network than majority students who do not face the same barriers and are likely to have a stronger external support network. The bottom of Table 3 displays the 10 most important features in the elastic net and random forest models (i.e., highest permutation variable importance).
Supporting the findings reported in RQ1, the zero-order correlations confirm that both institutional and behavioral engagement features play an important role in predicting student retention. Aligned with prior work, students’ performance (measured by GPA or ACT) repeatedly appeared as one of the most important predictors across universities and models. In addition, many of the engagement features (e.g., services attended, chat messages network centrality) are related to social activities or network features, supporting the notion that a student’s social connections and support play a critical role in student retention. In addition, the extent to which students are positively engaged with their institutions (e.g., by attending events and rating them highly) appears to play a critical role in preventing dropout.
RQ3: How well do the predictive models generalize across universities?
To test the generalizability of our models across universities, we used the predictive model trained on one university (e.g., University 1) to predict retention of the remaining three universities (e.g., Universities 2–4). Figures 3 A,B display the AUCs across all possible pairs, indicating which university was used for training (X-axis) and which was used for testing (Y-axis, see Figure S1 in the SI for graphs illustrating the findings for F1, TNR and TPR).
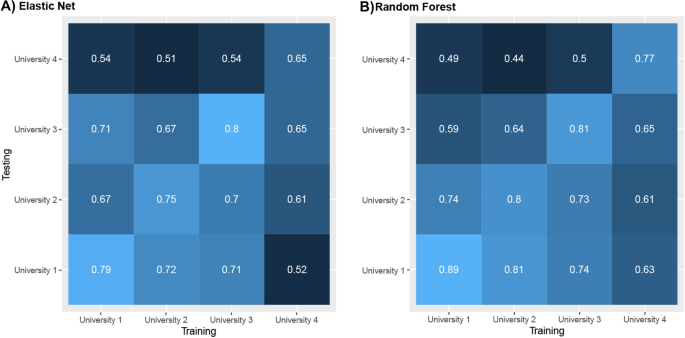
Performance (average AUC) of cross-university predictions.
Overall, we observed reasonably high levels of predictive performance when applying a model trained on one university to the data of another. The average AUC observed was 63% (for both the elastic net and the random forest), with the highest predictive performance reaching 74% (trained on University 1, predicting University 2), just 1%-point short of the predictive performance observed for the prediction from the universities own model (trained on University 2, predicting University 2). Contrary to the findings in RQ1, the random forest models did not perform better than the elastic net when making predictions for other universities. This suggests that the benefits afforded by the random forest models capture complex interaction patterns that are somewhat unique to each university but might not generalize well to new contexts. The main outlier in generalizability was University 4, where none of the other models reached accuracies much better than chance, and whose model produced relatively low levels of accuracies when predicting student retention across universities 1–2. This is likely a result of the fact that University 4 was qualitatively different from the other universities in several ways, including the fact that University 4 was a community college and consisted of 16 different campuses that were merged for the purpose of this analysis (see Methods for more details).
We show that student retention can be predicted from institutional data, behavioral engagement data, and their combination. Using data from over 50,000 students across four Universities, our predictive models achieve out-of-sample accuracies of up to 88% (where 50% is chance). Notably, while both institutional data and behavioral engagement data significantly predict retention, the combination of the two performs best in most instances. This finding is further supported by our feature importance analyses which suggest that both institutional and behavioral engagement features are among the most important predictors of student retention. Specifically, academic performance as measured by GPA and behavioral metrics associated with campus engagement (e.g., event attendances or ratings) or a student’s position in the network (e.g., closeness or centrality) were shown to consistently act as protective factors. Finally, we highlight the generalizability of our models across universities. Models trained on one university were able to predict student retention at another with reasonably high levels of predictive performance. As one might expect, the generalizability across universities heavily depends on the extent to which the universities are similar on important structural dimensions, with prediction accuracies dropping radically in cases where similarity is low (see low cross-generalization for University 4).
Contributions to the scientific literature
Our findings contribute to the existing literature in several ways. First, they respond to recent calls for more predictive research in psychology 54 , 55 as well as the use of Big Data analytics in education research 56 , 57 . Not only do our models consider socio-demographic characteristics that are collected by universities, but they also capture students’ daily experience and university engagement by tracking behaviors via the READY Education app. Our findings suggest, these more psychological predictors of student retention can improve the performance of predictive models above and beyond socio-demographic variables. This is consistent with previous findings suggesting that the inclusion of engagement metrics improves the performance of predictive models 16 , 84 , 85 . Overall, our models showed superior accuracies to models of former studies that were trained only on demographics and transcript records 15 , 25 or less comprehensive behavioral features 16 and provided results comparable to those reported in studies that additionally included a wide range of socio-economic variables 12 . Given that the READY Education app captures only a fraction of the students' actual experience, the high predictive accuracies make an even stronger case for the importance of student engagement in college retention.
Second, our findings provide insights into the features that are most important in predicting whether a student is going to drop out or not. By doing so they complement our predictive approach with layers of understanding that are conducive to not only validating our models but also generating insights into potential protective and risk factors. Most importantly, our findings highlight the relevance of the behavioral engagement metrics for predicting student retention. Most features identified as being important in the prediction were related to app and community engagement. In line with previous research, features indicative of early and deep social integration, such as interactions with peers and faculty or the development of friendships and social networks, were found to be highly predictive 16 , 41 . For example, it is reasonable to assume that a short time between app registration and the first visit of a campus event (one of the features identified as important) has a positive impact on retention, because campus events offer ideal opportunities for students to socialize 86 . Early participation in a campus event implies early integration and networking with others, protecting students from perceived stress 87 and providing better social and emotional support 88 . In contrast, a student who never attends an event or does so very late in the semester may be less connected to the campus life and the student community which in turn increases the likelihood of dropping out. This interpretation is strengthened by the fact that a high proportion of positive event ratings was identified as an important predictor of a student continuing their studies. Students who enjoy an event are likely to feel more comfortable, be embedded in the university life, make more connections, and build stronger connections. This might result in a virtuous cycle in which students continue attending events and over time create a strong social connection to their peers. As in most previous work, a high GPA score was consistently related to a higher likelihood of continuing one’s studies 21 , 24 . Although their importance varied across universities, ethnicity was also found to play a major role for retention, with consistent inequalities replicating in our predictive models 12 , 19 , 47 . For example, Black students were on average more likely to drop-out, suggesting that universities should dedicate additional resources to protect this group. Importantly, all qualitative interpretations are post-hoc. While many of the findings are intuitive and align with previous research on the topic, future studies should validate our results and investigate the causality underlying the effects in experimental or longitudinal within-person designs 54 , 78 .
Finally, our findings are the first to explore the extent to which the relationships between certain socio-demographic and behavioral characteristics might be idiosyncratic and unique to a specific university. By being able to compare the models across four different universities, we were able to show that many of the insights gained from one university can be leveraged to predict student retention at another. However, our findings also point to important boundary conditions: The more dissimilar universities are in their organizational structures and student experience, the more idiosyncratic the patterns between certain socio-demographic and behavioral features with student retention will be and the harder it is to merely translate general insights to the specific university campus.
Practical contributions
Our findings also have important practical implications. In the US, student attrition results in an average annual revenue loss of approximately $16.5 billion per year 9 , 10 and over $9 billion wasted in federal and state grants and subsidies that are awarded to students who do not finish their degree 11 . Hence, it is critical to predict potential dropouts as early and as accurately as possible to be able to offer dedicated support and allocate resources where they are needed the most. Our models rely exclusively on data collected in the first semester at university and are therefore an ideal “early warning” system for universities who want to predict whether their students will likely continue their studies or drop out at some point. Depending on the university’s resources and goals, the predictive models can be optimized for different performance measures. Indeed, a university might decide to focus on the true positive rate to capture as many dropouts as possible. While this would mean erroneously classifying “healthy “ students as potential dropouts, universities might decide that the burden of providing “unnecessary “ support to these healthy students is worth the reduced risk of missing a dropout. Importantly, our models go beyond mere socio-demographic variables and allow for a more nuanced, personal model that considers not just “who someone is” but also what their experience on campus looks like. As such, our models make it possible to acknowledge individuality rather than using over-generalized assessments of entire socio-demographic segments.
Importantly, however, it is critical to subject these models to continuous quality assurance. While predictive models could allow universities to flag at-risk students early, they could also perpetuate biases that get calcified in the predictive models themselves. For example, students who are traditionally less likely to discontinue their studies might have to pass a much higher level of dysfunctional engagement behavior before their file gets flagged as “at-risk”. Similarly, a person from a traditionally underrepresented group might receive an unnecessarily high volume of additional check-ins even though they are generally flourishing in their day-to-day experience. Given that being labeled as “at-risk” can be associated with stigma that could reinforce stigmas around historically marginalized groups, it will be critical to monitor both the performance of the model over time as well as the perception of its helpfulness among administrators, faculty, and students.
Limitations and future research
Our study has several limitations and highlights avenues for future research. First, our sample consisted of four US universities. Thus, our results are not necessarily generalizable to countries with more collectivistic cultures and other education systems such as Asia, where the reasons for dropping out might be different 89 , 90 , or Europe where most students work part-time jobs and live off-campus. Future research should investigate the extent to which our models can generalize to other cultural contexts and identify the features of student retention that are universally valid across contexts.
Second, our predictive models relied on app usage data. Therefore, our predictive approach could only be applied to students who decided to use the app. This selection, in and by itself, is likely to introduce a sampling bias, as students who decide to use the app might be more likely to retain in the first place, restricting the variance in observations, and excluding students for whom app usage data was not available. However, as our findings suggest, the institutional data alone provide predictive performance independent of the app features, making this a viable alternative for students who do not use the app.
Third, our predictive models rely on cross-sectional predictions. That is, we observe a students’ behavior over the course of an entire semester and based on the patterns observed in other students we predict whether that student is likely to drop out or not. Future research could try to improve both the predictive performance of the model and its usefulness for applied contexts by modeling within-person trends dynamically. Given enough data, the model could observe a person’s baseline behavior and identify changes from that baseline as potentially problematic. In fact, more social contact with other students might be considered a protective factor in our cross-sectional model. However, there are substantial individual differences in how much social contact individuals seek out and enjoy 91 . Hence, sending 10 chat messages a week might be considered a lot for one person, but very little for another. Future research should hence investigate whether the behavioral engagement features allow for a more dynamic within-person model that makes it possible to take base rates into account and provide a dynamic, momentary assessment of a student’s likelihood to drop out.
Fourth, although the engagement data was captured as a longitudinal time series with time-stamped events, we collapsed the data into a single set of cross-sectional features for each student. Although some of these features captures variation in behaviors over time (e.g., entropy and linear trends), future research should try to implement more advanced machine learning models to account for this time series data directly. For example, long short-term memory models (LSTMs) 92 – a type of recurrent neural network – are capable of learning patterns in longitudinal, sequential data like ours.
Fifth, even though the current research provides initial insights into the workings of the models by highlighting the importance of certain features, the conclusions that can be drawn from these analyses are limited as the importance metrics are calculated for the overall population. Future research could aim to calculate the importance of certain features at the individual level to test whether their importance varies across certain socio-demographic features. Estimating the importance of a person’s position in the social network on an individual level, for example, would make it possible to see whether the importance is correlated with institutional data such as minority or first-generation status.
Finally, our results lay the foundation for developing interventions that foster retention through shaping students’ experience at university 93 . Interventions which have been shown to have a positive effect on retention, include orientation programs and academic advising 94 , student support services like mentoring and coaching as well as need-based grants 95 . However, to date, the first-year experience programs meant to strengthen social integration of first year students, do not seem to have yielded positive results 96 , 97 . Our findings could support the development of interventions aimed at improving and maintaining student integration on campus. On a high level, the insights into the most important features provide an empirical path for developing relevant interventions that target the most important levers of student retention. For example, the fact that the time between registration and the first event attendance has such a big impact on student retention means that universities should do everything they can to get students to attend events as early as possible. Similarly, they could develop interventions that lead to more cohesive networks among cohorts and make sure that all students connect to their community. On a deeper, more sophisticated level, new approaches to model explainability could allow universities to tailor their intervention to each student 98 , 99 . For example, explainable AI makes it possible to derive decision rules for each student, indicating which features were critical in predicting the students’ outcome. While student A might be predicted to drop out because they are disconnected from the network, student B might be predicted to drop out because they don’t access the right information on the app. Given this information, universities would be able to personalize their offerings to the specific needs of the student. While student A might be encouraged to spend more time socializing with other students, student B might be reminded to check out important course information. Hence, predictive models could not only be used to identify students at risk but also provide an automated path to offering personalized guidance and support.
For every study that is discontinued, an educational dream shatters. And every shattered dream has a negative long-term impact both on the student and the university the student attended. In this study we introduce an approach to accurately predicting student retention after the first term. Our results show that student retention can be predicted with relatively high levels of predictive performance when considering institutional data, behavioral engagement data, or a combination of the two. By combining socio-demographic characteristics with passively observed behavioral traces reflecting a student’s daily activities, our models offer a holistic picture of students' university experiences and its relation to retention. Overall, such predictive models have great potential both for the early identification of at-risk students and for enabling timely, evidence-based interventions.
Data availability
Raw data are not publicly available due to their proprietary nature and the risks associated with de-anonymization, but they are available from the corresponding author on reasonable request. The pre-processed data and all analyses codes are available on OSF ( https://osf.io/bhaqp/ ) to facilitate reproducibility of our work. Data were analyzed using R, version 4.0.0 (R Core Team, 2020; see subsections for specific packages and versions used). The study’s design relies on secondary data and the analyses were not preregistered.
Change history
21 june 2023.
A Correction to this paper has been published: https://doi.org/10.1038/s41598-023-36579-2
Ginder, S. A., Kelly-Reid, J. E. & Mann, F. B. Graduation Rates for Selected Cohorts, 2009–14; Outcome Measures for Cohort Year 2009–10; Student Financial Aid, Academic Year 2016–17; and Admissions in Postsecondary Institutions, Fall 2017. First Look (Provisional Data). NCES 2018–151. National Center for Education Statistics (2018).
Snyder, T. D., de Brey, C. & Dillow, S. A. Digest of Education Statistics 2017 NCES 2018-070. Natl. Cent. Educ. Stat. (2019).
NSC Research Center. Persistence & Retention – 2019. NSC Research Center https://nscresearchcenter.org/snapshotreport35-first-year-persistence-and-retention/ (2019).
Bound, J., Lovenheim, M. F. & Turner, S. Why have college completion rates declined? An analysis of changing student preparation and collegiate resources. Am. Econ. J. Appl. Econ. 2 , 129–157 (2010).
Article PubMed PubMed Central Google Scholar
Bowen, W. G., Chingos, M. M. & McPherson, M. S. Crossing the finish line. in Crossing the Finish Line (Princeton University Press, 2009).
McFarland, J. et al. The Condition of Education 2019. NCES 2019-144. Natl. Cent. Educ. Stat. (2019).
Education, U. S. D. of. Fact sheet: Focusing higher education on student success. [Fact Sheet] (2015).
Freudenberg, N. & Ruglis, J. Peer reviewed: Reframing school dropout as a public health issue. Prev. Chronic Dis. 4 , 4 (2007).
Google Scholar
Raisman, N. The cost of college attrition at four-year colleges & universities-an analysis of 1669 US institutions. Policy Perspect. (2013).
Wellman, J., Johnson, N. & Steele, P. Measuring (and Managing) the Invisible Costs of Postsecondary Attrition. Policy brief. Delta Cost Proj. Am. Instit. Res. (2012).
Schneider, M. Finishing the first lap: The cost of first year student attrition in America’s four year colleges and universities (American Institutes for Research, 2010).
Delen, D. A comparative analysis of machine learning techniques for student retention management. Decis. Support Syst. 49 , 498–506 (2010).
Article Google Scholar
Yu, R., Lee, H. & Kizilcec, R. F. Should College Dropout Prediction Models Include Protected Attributes? in Proceedings of the Eighth ACM Conference on Learning@ Scale 91–100 (2021).
Tinto, V. Reconstructing the first year of college. Plan. High. Educ. 25 , 1–6 (1996).
Ortiz-Lozano, J. M., Rua-Vieites, A., Bilbao-Calabuig, P. & Casadesús-Fa, M. University student retention: Best time and data to identify undergraduate students at risk of dropout. Innov. Educ. Teach. Int. 57 , 74–85 (2020).
Ram, S., Wang, Y., Currim, F. & Currim, S. Using big data for predicting freshmen retention. in 2015 international conference on information systems: Exploring the information frontier, ICIS 2015 (Association for Information Systems, 2015).
Levitz, R. S., Noel, L. & Richter, B. J. Strategic moves for retention success. N. Dir. High. Educ. 1999 , 31–49 (1999).
Veenstra, C. P. A strategy for improving freshman college retention. J. Qual. Particip. 31 , 19–23 (2009).
Astin, A. W. How, “good” is your institution’s retention rate?. Res. High. Educ. 38 , 647–658 (1997).
Coleman, J. S. Social capital in the creation of human capital. Am. J. Sociol. 94 , S95–S120 (1988).
Reason, R. D. Student variables that predict retention: Recent research and new developments. J. Stud. Aff. Res. Pract. 40 , 704–723 (2003).
Tinto, V. Dropout from higher education: A theoretical synthesis of recent research. Rev Educ Res 45 , 89–125 (1975).
Tinto, V. Completing college: Rethinking institutional action (University of Chicago Press, 2012).
Book Google Scholar
Astin, A. Retaining and Satisfying Students. Educ. Rec. 68 , 36–42 (1987).
Aulck, L., Velagapudi, N., Blumenstock, J. & West, J. Predicting student dropout in higher education. arXiv preprint arXiv:1606.06364 (2016).
Bogard, M., Helbig, T., Huff, G. & James, C. A comparison of empirical models for predicting student retention (Western Kentucky University, 2011).
Murtaugh, P. A., Burns, L. D. & Schuster, J. Predicting the retention of university students. Res. High. Educ. 40 , 355–371 (1999).
Porter, K. B. Current trends in student retention: A literature review. Teach. Learn. Nurs. 3 , 3–5 (2008).
Thomas, S. L. Ties that bind: A social network approach to understanding student integration and persistence. J. High. Educ. 71 , 591–615 (2000).
Peltier, G. L., Laden, R. & Matranga, M. Student persistence in college: A review of research. J. Coll. Stud. Ret. 1 , 357–375 (2000).
Nandeshwar, A., Menzies, T. & Nelson, A. Learning patterns of university student retention. Expert Syst. Appl. 38 , 14984–14996 (2011).
Boero, G., Laureti, T. & Naylor, R. An econometric analysis of student withdrawal and progression in post-reform Italian universities. (2005).
Tinto, V. Leaving college: Rethinking the causes and cures of student attrition (ERIC, 1987).
Choy, S. Students whose parents did not go to college: Postsecondary access, persistence, and attainment. Findings from the condition of education, 2001. (2001).
Ishitani, T. T. Studying attrition and degree completion behavior among first-generation college students in the United States. J. High. Educ. 77 , 861–885 (2006).
Thayer, P. B. Retention of students from first generation and low income backgrounds. (2000).
Britt, S. L., Ammerman, D. A., Barrett, S. F. & Jones, S. Student loans, financial stress, and college student retention. J. Stud. Financ. Aid 47 , 3 (2017).
McKinney, L. & Burridge, A. B. Helping or hindering? The effects of loans on community college student persistence. Res. High Educ. 56 , 299–324 (2015).
Hochstein, S. K. & Butler, R. R. The effects of the composition of a financial aids package on student retention. J. Stud. Financ. Aid 13 , 21–26 (1983).
Singell, L. D. Jr. Come and stay a while: Does financial aid effect retention conditioned on enrollment at a large public university?. Econ. Educ. Rev. 23 , 459–471 (2004).
Bean, J. P. Nine themes of college student. Coll. Stud. Retent. Formula Stud. Success 215 , 243 (2005).
Tinto, V. Through the eyes of students. J. Coll. Stud. Ret. 19 , 254–269 (2017).
Cabrera, A. F., Nora, A. & Castaneda, M. B. College persistence: Structural equations modeling test of an integrated model of student retention. J. High. Educ. 64 , 123–139 (1993).
Roberts, J. & Styron, R. Student satisfaction and persistence: Factors vital to student retention. Res. High. Educ. J. 6 , 1 (2010).
Gopalan, M. & Brady, S. T. College students’ sense of belonging: A national perspective. Educ. Res. 49 , 134–137 (2020).
Hoffman, M., Richmond, J., Morrow, J. & Salomone, K. Investigating, “sense of belonging” in first-year college students. J. Coll. Stud. Ret. 4 , 227–256 (2002).
Terenzini, P. T. & Pascarella, E. T. Toward the validation of Tinto’s model of college student attrition: A review of recent studies. Res. High Educ. 12 , 271–282 (1980).
Astin, A. W. The impact of dormitory living on students. Educational record (1973).
Astin, A. W. Student involvement: A developmental theory for higher education. J. Coll. Stud. Pers. 25 , 297–308 (1984).
Terenzini, P. T. & Pascarella, E. T. Studying college students in the 21st century: Meeting new challenges. Rev. High Ed. 21 , 151–165 (1998).
Thompson, J., Samiratedu, V. & Rafter, J. The effects of on-campus residence on first-time college students. NASPA J. 31 , 41–47 (1993).
Tinto, V. Research and practice of student retention: What next?. J. Coll. Stud. Ret. 8 , 1–19 (2006).
Lazer, D. et al. Computational social science. Science 1979 (323), 721–723 (2009).
Yarkoni, T. & Westfall, J. Choosing prediction over explanation in psychology: Lessons from machine learning. Perspect. Psychol. Sci. 12 , 1100–1122 (2017).
Peters, H., Marrero, Z. & Gosling, S. D. The Big Data toolkit for psychologists: Data sources and methodologies. in The psychology of technology: Social science research in the age of Big Data. 87–124 (American Psychological Association, 2022). doi: https://doi.org/10.1037/0000290-004 .
Fischer, C. et al. Mining big data in education: Affordances and challenges. Rev. Res. Educ. 44 , 130–160 (2020).
Hilbert, S. et al. Machine learning for the educational sciences. Rev. Educ. 9 , e3310 (2021).
National Academy of Education. Big data in education: Balancing the benefits of educational research and student privacy . (2017).
Aulck, L., Nambi, D., Velagapudi, N., Blumenstock, J. & West, J. Mining university registrar records to predict first-year undergraduate attrition. Int. Educ. Data Min. Soc. (2019).
Beaulac, C. & Rosenthal, J. S. Predicting university students’ academic success and major using random forests. Res. High Educ. 60 , 1048–1064 (2019).
Berens, J., Schneider, K., Görtz, S., Oster, S. & Burghoff, J. Early detection of students at risk–predicting student dropouts using administrative student data and machine learning methods. Available at SSRN 3275433 (2018).
Dawson, S., Jovanovic, J., Gašević, D. & Pardo, A. From prediction to impact: Evaluation of a learning analytics retention program. in Proceedings of the seventh international learning analytics & knowledge conference 474–478 (2017).
Dekker, G. W., Pechenizkiy, M. & Vleeshouwers, J. M. Predicting students drop Out: A case study. Int. Work. Group Educ. Data Min. (2009).
del Bonifro, F., Gabbrielli, M., Lisanti, G. & Zingaro, S. P. Student dropout prediction. in International Conference on Artificial Intelligence in Education 129–140 (Springer, 2020).
Hutt, S., Gardner, M., Duckworth, A. L. & D’Mello, S. K. Evaluating fairness and generalizability in models predicting on-time graduation from college applications. Int. Educ. Data Min. Soc. (2019).
Jayaprakash, S. M., Moody, E. W., Lauría, E. J. M., Regan, J. R. & Baron, J. D. Early alert of academically at-risk students: An open source analytics initiative. J. Learn. Anal. 1 , 6–47 (2014).
Balakrishnan, G. & Coetzee, D. Predicting student retention in massive open online courses using hidden markov models. Elect. Eng. Comput. Sci. Univ. Calif. Berkeley 53 , 57–58 (2013).
Hastie, T., Tibshirani, R. & Friedman, J. The elements of statistical learning (Springer series in statistics, New York, NY, USA, 2001).
Book MATH Google Scholar
Chawla, N. V., Bowyer, K. W., Hall, L. O. & Kegelmeyer, W. P. SMOTE: Synthetic minority over-sampling technique. J. Artif. Intell. Res. 16 , 321–357 (2002).
Article MATH Google Scholar
Zou, H. & Hastie, T. Regularization and variable selection via the elastic net. J. R. Stat. Soc. Seri. B Stat. Methodol. 67 , 301–320 (2005).
Article MathSciNet MATH Google Scholar
Friedman, J., Hastie, T. & Tibshirani, R. Regularization paths for generalized linear models via coordinate descent. J. Stat. Softw. 33 , 1 (2010).
Breiman, L. Random forests. Mach. Learn. 45 , 5–32 (2001).
Liaw, A. & Wiener, M. Classification and regression by randomForest. R News 2 , 18–22 (2002).
Pargent, F., Schoedel, R. & Stachl, C. An introduction to machine learning for psychologists in R. Psyarxiv (2022).
Hoerl, A. E. & Kennard, R. W. Ridge Regression. in Encyclopedia of Statistical Sciences vol. 8 129–136 (John Wiley & Sons, Inc., 2004).
Tibshirani, R. Regression shrinkage and selection via the Lasso. J. R. Stat. Soc. Ser. B (Methodol.) 58 , 267–288 (1996).
MathSciNet MATH Google Scholar
Hastie, T. & Qian, J. Glmnet vignette. vol. 9 1–42 https://hastie.su.domains/Papers/Glmnet_Vignette.pdf (2016).
Orrù, G., Monaro, M., Conversano, C., Gemignani, A. & Sartori, G. Machine learning in psychometrics and psychological research. Front. Psychol. 10 , 2970 (2020).
Pargent, F. & Albert-von der Gönna, J. Predictive modeling with psychological panel data. Z Psychol (2019).
Pargent, F., Schoedel, R. & Stachl, C. Best practices in supervised machine learning: A tutorial for psychologists. Doi: https://doi.org/10.31234/osf.io/89snd (2023).
Friedman, J., Hastie, T. & Tibshirani, R. The elements of statistical learning Vol. 1 (Springer series in statistics, 2001).
MATH Google Scholar
Rijsbergen, V. & Joost, C. K. Information Retrieval Butterworths London. Google Scholar Google Scholar Digital Library Digital Library (1979).
Molnar, C. Interpretable machine learning . (Lulu. com, 2020).
Aguiar, E., Ambrose, G. A., Chawla, N. v, Goodrich, V. & Brockman, J. Engagement vs Performance: Using Electronic Portfolios to Predict First Semester Engineering Student Persistence . Journal of Learning Analytics vol. 1 (2014).
Chai, K. E. K. & Gibson, D. Predicting the risk of attrition for undergraduate students with time based modelling. Int. Assoc. Dev. Inf. Soc. (2015).
Saenz, T., Marcoulides, G. A., Junn, E. & Young, R. The relationship between college experience and academic performance among minority students. Int. J. Educ. Manag (1999).
Pidgeon, A. M., Coast, G., Coast, G. & Coast, G. Psychosocial moderators of perceived stress, anxiety and depression in university students: An international study. Open J. Soc. Sci. 2 , 23 (2014).
Wilcox, P., Winn, S. & Fyvie-Gauld, M. ‘It was nothing to do with the university, it was just the people’: The role of social support in the first-year experience of higher education. Stud. High. Educ. 30 , 707–722 (2005).
Guiffrida, D. A. Toward a cultural advancement of Tinto’s theory. Rev. High Ed. 29 , 451–472 (2006).
Triandis, H. C., McCusker, C. & Hui, C. H. Multimethod probes of individualism and collectivism. J. Pers. Soc. Psychol. 59 , 1006 (1990).
Watson, D. & Clark, L. A. Extraversion and its positive emotional core. in Handbook of personality psychology 767–793 (Elsevier, 1997).
Greff, K., Srivastava, R. K., Koutník, J., Steunebrink, B. R. & Schmidhuber, J. LSTM: A search space odyssey. IEEE Trans. Neural Netw. Learn. Syst. 28 , 2222–2232 (2017).
Article MathSciNet PubMed Google Scholar
Arnold, K. E. & Pistilli, M. D. Course signals at Purdue: Using learning analytics to increase student success. in Proceedings of the 2nd international conference on learning analytics and knowledge 267–270 (2012).
Braxton, J. M. & McClendon, S. A. The fostering of social integration and retention through institutional practice. J. Coll. Stud. Ret. 3 , 57–71 (2001).
Sneyers, E. & de Witte, K. Interventions in higher education and their effect on student success: A meta-analysis. Educ. Rev. (Birm) 70 , 208–228 (2018).
Jamelske, E. Measuring the impact of a university first-year experience program on student GPA and retention. High Educ. (Dordr) 57 , 373–391 (2009).
Purdie, J. R. & Rosser, V. J. Examining the academic performance and retention of first-year students in living-learning communities and first-year experience courses. Coll. Stud. Aff. J. 29 , 95 (2011).
Lundberg, S. M. et al. From local explanations to global understanding with explainable AI for trees. Nat. Mach. Intell. 2 , 56–67 (2020).
Ramon, Y., Farrokhnia, R. A., Matz, S. C. & Martens, D. Explainable AI for psychological profiling from behavioral data: An application to big five personality predictions from financial transaction records. Information 12 , 518 (2021).
Download references
Author information
Alice Dinu is an Independent Researcher.
Authors and Affiliations
Columbia University, New York, USA
Sandra C. Matz & Heinrich Peters
Ludwig Maximilian University of Munich, Munich, Germany
Christina S. Bukow
Ready Education, Montreal, Canada
Christine Deacons
University of St. Gallen, St. Gallen, Switzerland
Clemens Stachl
Montreal, Canada
You can also search for this author in PubMed Google Scholar
Contributions
S.C.M., C.B, A.D., H.P., and C.S. designed the research. C.D. and A.D. provided the data. S.C.M, C.B. and H.P. analyzed the data. S.C.M and C.B. wrote the manuscript. All authors reviewed the manuscript. Earlier versions of thi research were part of the C.B.’s masters thesis which was supervised by S.C.M. and C.S.
Corresponding author
Correspondence to Sandra C. Matz .
Ethics declarations
Competing interests.
C.D. is a former employee of Ready Education. None of the other authors have conflict of interests related to this submission.
Additional information
Publisher's note.
Springer Nature remains neutral with regard to jurisdictional claims in published maps and institutional affiliations.
The original online version of this Article was revised: Alice Dinu was omitted from the author list in the original version of this Article. The Author Contributions section now reads: “S.C.M., C.B, A.D., H.P., and C.S. designed the research. C.D. and A.D. provided the data. S.C.M, C.B. and H.P. analyzed the data. S.C.M and C.B. wrote the manuscript. All authors reviewed the manuscript. Earlier versions of this research were part of the C.B.’s masters thesis which was supervised by S.C.M. and C.S.” Additionally, the Article contained an error in Data Availability section and the legend of Figure 2 was incomplete.
Supplementary Information
Supplementary information., rights and permissions.
Open Access This article is licensed under a Creative Commons Attribution 4.0 International License, which permits use, sharing, adaptation, distribution and reproduction in any medium or format, as long as you give appropriate credit to the original author(s) and the source, provide a link to the Creative Commons licence, and indicate if changes were made. The images or other third party material in this article are included in the article's Creative Commons licence, unless indicated otherwise in a credit line to the material. If material is not included in the article's Creative Commons licence and your intended use is not permitted by statutory regulation or exceeds the permitted use, you will need to obtain permission directly from the copyright holder. To view a copy of this licence, visit http://creativecommons.org/licenses/by/4.0/ .
Reprints and permissions
About this article
Cite this article.
Matz, S.C., Bukow, C.S., Peters, H. et al. Using machine learning to predict student retention from socio-demographic characteristics and app-based engagement metrics. Sci Rep 13 , 5705 (2023). https://doi.org/10.1038/s41598-023-32484-w
Download citation
Received : 09 August 2022
Accepted : 28 March 2023
Published : 07 April 2023
DOI : https://doi.org/10.1038/s41598-023-32484-w
Share this article
Anyone you share the following link with will be able to read this content:
Sorry, a shareable link is not currently available for this article.
Provided by the Springer Nature SharedIt content-sharing initiative
By submitting a comment you agree to abide by our Terms and Community Guidelines . If you find something abusive or that does not comply with our terms or guidelines please flag it as inappropriate.
Quick links
- Explore articles by subject
- Guide to authors
- Editorial policies
Sign up for the Nature Briefing newsletter — what matters in science, free to your inbox daily.

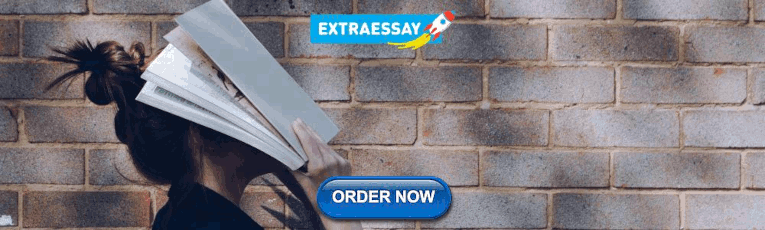
IMAGES
VIDEO
COMMENTS
Table of contents. Step 1: Restate the problem. Step 2: Sum up the paper. Step 3: Discuss the implications. Research paper conclusion examples. Frequently asked questions about research paper conclusions.
The characteristics of a research paper conclusion include: Clear and concise: The conclusion should be written in a clear and concise manner, summarizing the key findings and their significance. Comprehensive: The conclusion should address all of the main points of the research paper, including the research question or problem, the methodology ...
Provide a brief description of your study: Enter details about your research topic and findings. This information helps Paperpal generate a tailored outline that aligns with your paper's content. Generate the conclusion outline: After entering all necessary details, click on 'generate'.
6 Conciseness. Above all, every research paper conclusion should be written with conciseness. In general, conclusions should be short, so keep an eye on your word count as you write and aim to be as succinct as possible. You can expound on your topic in the body of your paper, but the conclusion is more for summarizing and recapping.
The conclusion is intended to help the reader understand why your research should matter to them after they have finished reading the paper. A conclusion is not merely a summary of the main topics covered or a re-statement of your research problem, but a synthesis of key points derived from the findings of your study and, if applicable, where you recommend new areas for future research.
Highlight the "so what". At the beginning of your paper, you explain to your readers what's at stake—why they should care about the argument you're making. In your conclusion, you can bring readers back to those stakes by reminding them why your argument is important in the first place. You can also draft a few sentences that put ...
Step 2: Summarize and reflect on your research. Step 3: Make future recommendations. Step 4: Emphasize your contributions to your field. Step 5: Wrap up your thesis or dissertation. Full conclusion example. Conclusion checklist. Other interesting articles. Frequently asked questions about conclusion sections.
The conclusion is where you describe the consequences of your arguments by justifying to your readers why your arguments matter (Hamilton College, 2014). Derntl (2014) also describes conclusion as the counterpart of the introduction. Using the Hourglass Model (Swales, 1993) as a visual reference, Derntl describes conclusion as the part of the ...
Conclusion Section for Research Papers. Conclusions are often the last section your audience reads, so they are just as important as introductions in research papers. They are your final opportunity to leave a good impression on the reader. Some academic readers will even jump to read the conclusion to help them decide if they should read the ...
The conclusion pushes beyond the boundaries of the prompt and allows you to consider broader issues, make new connections, and elaborate on the significance of your findings. Your conclusion should make your readers glad they read your paper. Your conclusion gives your reader something to take away that will help them see things differently or ...
Research paper conclusion examples. Below, we've created basic templates showing the key parts of a research paper conclusion. Keep in mind that the length of your conclusion will depend on the length of your paper. The order of the parts may vary, too; these templates only demonstrate how to tie them together. 1. Empirical research paper ...
The conclusion of a research paper has several key objectives. It should: Restate your research problem addressed in the introduction section. Summarize your main arguments, important findings, and broader implications. Synthesize key takeaways from your study. The specific content in the conclusion depends on whether your paper presents the ...
Writing a Conclusion. A conclusion is an important part of the paper; it provides closure for the reader while reminding the reader of the contents and importance of the paper. It accomplishes this by stepping back from the specifics in order to view the bigger picture of the document. In other words, it is reminding the reader of the main ...
Present your conclusion in a structured manner, following the natural flow of your paper. Readers should effortlessly follow your thought process, making your conclusion more accessible and persuasive. Reinforce Main Arguments. Emphasize the core arguments and findings from your research.
1. Restate the thesis. An effective conclusion brings the reader back to the main point, reminding the reader of the purpose of the essay. However, avoid repeating the thesis verbatim. Paraphrase your argument slightly while still preserving the primary point. 2. Reiterate supporting points.
Here are some common characteristics of research papers: Evidence-based: Research papers are based on empirical evidence, which is collected through rigorous research methods such as experiments, surveys, observations, or interviews. They rely on objective data and facts to support their claims and conclusions.
The primary goal or purpose of research in any field of inquiry; is to add to what is known about the phenomenon under investigation by applying scientific methods. Though each research has its own specific goals, we may enumerate the following 4 broad goals of scientific research: Exploration and Explorative Research.
Key characteristics of well-defined research objectives. Well-defined research objectives are characterized by the SMART criteria - Specific, Measurable, Achievable, Relevant, and Time-bound. ... Conclusion. Defining research studies objectives and problem statement is not just a preliminary step, but a continuous guiding force throughout the ...
We explain what a conclusion is, what its objectives are and the types that exist. In addition, its characteristics, structure and some examples. What is a conclusion? In logic, the final proposition of an argument is called a conclusion . Its content follows in one way or another from the previous premises (as long as it is a valid argument).
Research methods are specific procedures for collecting and analyzing data. Developing your research methods is an integral part of your research design. When planning your methods, there are two key decisions you will make. First, decide how you will collect data. Your methods depend on what type of data you need to answer your research question:
This work seeks to help students in improving their first research reports, based on natural language processing techniques. We present a Conclusion model that includes three schemes: Goal Connectedness, Judgment and Speculation. These subsystems try to account for the main expected attributes in conclusions, specifically the Connectedness with the general objective of the research, the ...
6 Simple Steps To Write A Good Conclusion For Your Essay 1. Start with an opening sentence 2. Summarize your main arguments 3. Answer the question "So what.". 4. Provoke the Reader 5. Align it with your introduction 6. Build a connection with the audience.
will outline and ex plain in detail the. characteristics of quality research, attributes of go od rese arch, research. ethics, step- by -step g uide to writing a. scientific article. This guide ...
Conclusion. Research is a process of investigating a particular topic with defined objectives. To conduct good research, it becomes important to understand the objective of the research and its characteristics. Good research generates new ideas and concepts, which further help develop new techniques and materials.
Distinct regions harboring cancer stem cells (CSCs) have been identified within the microenvironment of various tumors, and as in the case of their healthy counterparts, these anatomical regions are termed "niche." Thus far, a large volume of studies have shown that CSC niches take part in the maintenance, regulation of renewal, differentiation and plasticity of CSCs. In this review, we ...
Individual characteristics and professional inequalities in healthcare may be potential modifiable factors for policy makers seeking to mitigate risks for healthcare providers. ... Conclusion. Nurses and doctors working in ICU during the COVID-19 pandemic sustained psychological impacts, manifesting as stress, anxiety, and for some, symptoms of ...
Conclusion The findings spotlight the influence of maternal characteristics and infant feeding on infant growth. This asserts the need for evidence-based programmes targeting mothers to promote optimal feeding practices and foster healthy child growth.
Fifth, even though the current research provides initial insights into the workings of the models by highlighting the importance of certain features, the conclusions that can be drawn from these ...
Conclusions from this study were limited by the small number of patients, variable prior therapies, and the retrospective nature of the study. AFFIRM-AL is an ongoing confirmatory global Phase 3 clinical trial of birtamimab + SoC in newly diagnosed treatment-naïve patients with Mayo Stage IV AL amyloidosis (NCT04973137) [ Citation 87 ...