
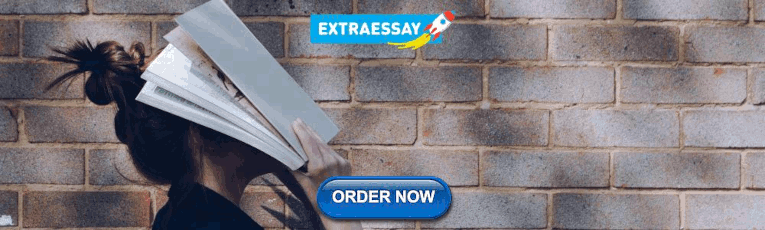
Quantitative and Qualitative Research
- I NEED TO . . .
What is Quantitative Research?
- What is Qualitative Research?
- Quantitative vs Qualitative
- Step 1: Accessing CINAHL
- Step 2: Create a Keyword Search
- Step 3: Create a Subject Heading Search
- Step 4: Repeat Steps 1-3 for Second Concept
- Step 5: Repeat Steps 1-3 for Quantitative Terms
- Step 6: Combining All Searches
- Step 7: Adding Limiters
- Step 8: Save Your Search!
- What Kind of Article is This?
- More Research Help This link opens in a new window
Quantitative methodology is the dominant research framework in the social sciences. It refers to a set of strategies, techniques and assumptions used to study psychological, social and economic processes through the exploration of numeric patterns . Quantitative research gathers a range of numeric data. Some of the numeric data is intrinsically quantitative (e.g. personal income), while in other cases the numeric structure is imposed (e.g. ‘On a scale from 1 to 10, how depressed did you feel last week?’). The collection of quantitative information allows researchers to conduct simple to extremely sophisticated statistical analyses that aggregate the data (e.g. averages, percentages), show relationships among the data (e.g. ‘Students with lower grade point averages tend to score lower on a depression scale’) or compare across aggregated data (e.g. the USA has a higher gross domestic product than Spain). Quantitative research includes methodologies such as questionnaires, structured observations or experiments and stands in contrast to qualitative research. Qualitative research involves the collection and analysis of narratives and/or open-ended observations through methodologies such as interviews, focus groups or ethnographies.
Coghlan, D., Brydon-Miller, M. (2014). The SAGE encyclopedia of action research (Vols. 1-2). London, : SAGE Publications Ltd doi: 10.4135/9781446294406
What is the purpose of quantitative research?
The purpose of quantitative research is to generate knowledge and create understanding about the social world. Quantitative research is used by social scientists, including communication researchers, to observe phenomena or occurrences affecting individuals. Social scientists are concerned with the study of people. Quantitative research is a way to learn about a particular group of people, known as a sample population. Using scientific inquiry, quantitative research relies on data that are observed or measured to examine questions about the sample population.
Allen, M. (2017). The SAGE encyclopedia of communication research methods (Vols. 1-4). Thousand Oaks, CA: SAGE Publications, Inc doi: 10.4135/9781483381411
How do I know if the study is a quantitative design? What type of quantitative study is it?
Quantitative Research Designs: Descriptive non-experimental, Quasi-experimental or Experimental?
Studies do not always explicitly state what kind of research design is being used. You will need to know how to decipher which design type is used. The following video will help you determine the quantitative design type.
- << Previous: I NEED TO . . .
- Next: What is Qualitative Research? >>
- Last Updated: May 3, 2024 2:09 PM
- URL: https://libguides.uta.edu/quantitative_and_qualitative_research
University of Texas Arlington Libraries 702 Planetarium Place · Arlington, TX 76019 · 817-272-3000
- Internet Privacy
- Accessibility
- Problems with a guide? Contact Us.

What is Quantitative Research? Definition, Examples, Key Advantages, Methods and Best Practices
By Nick Jain
Published on: May 17, 2023
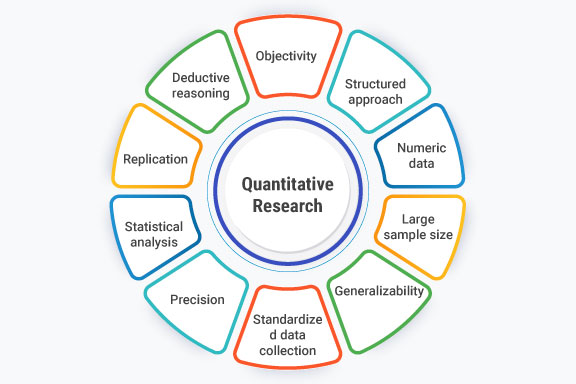
Table of Contents
What is Quantitative Research?
Quantitative research examples, quantitative research: key advantages, quantitative research methodology, 7 best practices to conduct quantitative research.
Quantitative research stands as a powerful research methodology dedicated to the systematic collection and analysis of measurable data. Through rigorous statistical and mathematical techniques, this method extracts insights from structured surveys, controlled experiments, or other defined data-gathering methods.
The primary objective of quantitative research is to measure and quantify variables, relationships, and patterns within the dataset. By testing hypotheses, making predictions, and drawing generalizable conclusions, it plays a crucial role in fields such as psychology, sociology, economics, and education. This approach often involves significant sample sizes, ensuring robust results.
Explore the depth of quantitative research with this comprehensive guide, offering practical examples and applications to demonstrate its real-world impact. Stay updated with the latest trends and developments in quantitative research as we continually refine our insights to provide you with the most relevant and cutting-edge information.
Quantitative Research: Key Characteristics
Below are the key characteristics of quantitative research:
- Objectivity: Quantitative research is grounded in the principles of objectivity and empiricism, which means that the research is focused on observable and measurable phenomena, rather than personal opinions or experiences.
- Structured approach: Quantitative research follows a structured and systematic approach to data collection and analysis, using clearly defined variables, hypotheses, and research questions.
- Numeric data: Quantitative research uses numerical data to describe and analyze the phenomena under study, such as statistical analysis, surveys, and experiments.
- Large sample size: Quantitative research often involves large sample sizes to ensure statistical significance and to generalize findings to a larger population.
- Standardized data collection: Quantitative research typically involves standardized data collection methods, such as surveys or experiments, to minimize potential sources of bias and increase reliability.
- Deductive reasoning: Quantitative research uses deductive reasoning, where the researcher tests a specific hypothesis based on prior knowledge and theory.
- Replication: Quantitative research emphasizes the importance of replication, where other researchers can reproduce the study’s methods and obtain similar results.
- Statistical analysis: Quantitative research involves statistical analysis to analyze the data and test the research hypotheses, often using software programs to assist with data analysis.
- Precision: Quantitative research aims to be precise in its measurement and analysis of data. It seeks to quantify and measure the specific aspects of a phenomenon being studied.
- Generalizability: Quantitative research aims to generalize findings from a sample to a larger population. It seeks to draw conclusions that apply to a broader group beyond the specific sample being studied.
Below are 3 examples of quantitative research:
Example 1: Boosting Employee Performance with Innovative Training Programs
In this quantitative study, we delve into the transformative impact of a cutting-edge training program on employee productivity within corporate environments. Employing a quasi-experimental framework, we meticulously analyze the outcomes of a cohort undergoing innovative training against a control group.
Through advanced statistical methodologies, we unveil actionable insights into performance enhancements, arming organizations with data-driven strategies for workforce development and competitive advantage.
Example 2: Unveiling the Power of Physical Exercise on Mental Well-being
Unlocking the correlation between physical exercise and mental health, this quantitative inquiry stands at the forefront of holistic wellness research.
Through meticulous data collection and rigorous statistical analyses, we dissect the nuanced relationship between exercise regimens and mental well-being indicators.
Our findings not only underscore the profound impact of exercise on psychological resilience but also provide actionable insights for healthcare professionals and individuals striving for optimal mental health.
Example 3: Revolutionizing Education with Innovative Teaching Methodologies
In this groundbreaking study, we embark on a quantitative exploration of the transformative potential of innovative teaching methods on student learning outcomes. Utilizing a quasi-experimental design, we meticulously evaluate the efficacy of novel pedagogical approaches against conventional teaching methodologies.
Through rigorous statistical analyses of pre-test and post-test data, we unearth compelling evidence of enhanced academic performance, paving the way for educational institutions to embrace innovation and elevate learning experiences.
Example 4: Assessing the Impact of Social Media Usage on Academic Performance
In this quantitative research endeavor, we delve into the relationship between social media usage patterns and academic achievement among college students. Employing a correlational research design, we collect data on students’ social media habits and their corresponding GPA scores.
Through regression analysis and other statistical techniques, we uncover insights into how factors such as frequency of social media use, types of platforms utilized, and time spent online correlate with academic performance. These findings provide valuable insights for educators, policymakers, and students themselves to optimize study habits and achieve academic success in the digital age.
Example 5: Analyzing the Effects of Financial Literacy Programs on Saving Behavior
This quantitative study investigates the efficacy of financial literacy programs in promoting responsible saving behavior among young adults. Using a randomized controlled trial methodology, we administer financial education interventions to a sample population and compare their saving habits to a control group over a specified period.
Through statistical analysis of savings rates, expenditure patterns, and financial decision-making, we discern the impact of financial literacy interventions on participants’ saving behaviors. These findings offer valuable implications for financial institutions, policymakers, and educators seeking to empower individuals with the knowledge and skills to make sound financial decisions and achieve long-term financial well-being.
Learn more: What is Quantitative Market Research?
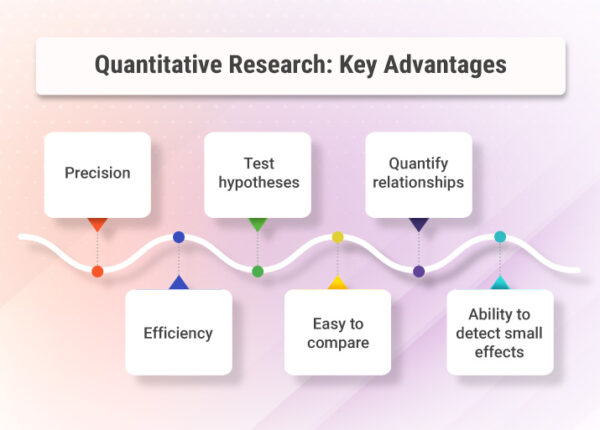
The advantages of quantitative research make it a valuable research method in a variety of fields, particularly in fields that require precise measurement and testing of hypotheses.
- Precision: Quantitative research aims to be precise in its measurement and analysis of data. This can increase the accuracy of the results and enable researchers to make more precise predictions.
- Test hypotheses: Quantitative research is well-suited for testing specific hypotheses or research questions, allowing researchers to draw clear conclusions and make predictions based on the data.
- Quantify relationships: Quantitative research enables researchers to quantify and measure relationships between variables, allowing for more precise and quantitative comparisons.
- Efficiency: Quantitative research often involves the use of standardized procedures and data collection methods, which can make the research process more efficient and reduce the amount of time and resources required.
- Easy to compare: Quantitative research often involves the use of standardized measures and scales, which makes it easier to compare results across different studies or populations.
- Ability to detect small effects: Quantitative research is often able to detect small effects that may not be observable through qualitative research methods, due to the use of statistical analysis and large sample sizes.
Quantitative research is a type of research that focuses on collecting and analyzing numerical data to answer research questions. There are two main methods used to conduct quantitative research:
1. Primary Method
There are several methods of primary quantitative research, each with its own strengths and limitations.
Surveys: Surveys are a common method of quantitative research and involve collecting data from a sample of individuals using standardized questionnaires or interviews. Surveys can be conducted in various ways, such as online, by mail, by phone, or in person. Surveys can be used to study attitudes, behaviors, opinions, and demographics.
One of the main advantages of surveys is that they can be conducted on a large scale, making it possible to obtain representative data from a population. However, surveys can suffer from issues such as response bias, where participants may not provide accurate or truthful answers, and nonresponse bias, where certain groups may be less likely to participate in the survey.
Experiments: Experiments involve manipulating one or more variables to determine their effects on an outcome of interest. Experiments can be carried out in controlled laboratory settings or in real-world field environments. Experiments can be used to test causal relationships between variables and to establish cause-and-effect relationships.
One of the main advantages of experiments is that they provide a high level of control over the variables being studied, which can increase the internal validity of the study. However, experiments can suffer from issues such as artificiality, where the experimental setting may not accurately reflect real-world situations, and demand characteristics, where participants may change their behavior due to the experimental setting.
Observational studies: Observational studies involve observing and recording data without manipulating any variables. Observational studies can be conducted in various settings, such as naturalistic environments or controlled laboratory settings. Observational studies can be used to study behaviors, interactions, and phenomena that cannot be manipulated experimentally.
One of the main advantages of observational studies is that they can provide rich and detailed data about real-world phenomena. However, observational studies can suffer from issues such as observer bias, where the observer may interpret the data in a subjective manner, and reactivity, where the presence of the observer may change the behavior being observed.
Content analysis: Content analysis involves analyzing media or communication content, such as text, images, or videos, to identify patterns or trends. Content analysis can be used to study media representations of social issues or to identify patterns in social media data.
One of the main advantages of content analysis is that it can provide insights into the cultural and social values reflected in media content. However, content analysis can suffer from issues such as the subjectivity of the coding process and the potential for errors or bias in the data collection process.
Psychometrics: Psychometrics involves the development and validation of standardized tests or measures, such as personality tests or intelligence tests. Psychometrics can be used to study individual differences in psychological traits and to assess the validity and reliability of psychological measures.
One of the main advantages of psychometrics is that it can provide a standardized and objective way to measure psychological constructs. However, psychometrics can suffer from issues such as the cultural specificity of the measures and the potential for response bias in self-report measures.
2. Secondary Method
Secondary quantitative research methods involve analyzing existing data that was collected for other purposes. This can include data from government records, public opinion polls, or market research studies. Secondary research is often quicker and less expensive than primary research, but it may not provide data that is as specific to the research question.
One of the main advantages of secondary data analysis is that it can be a cost-effective way to obtain large amounts of data. However, secondary data analysis can suffer from issues such as the quality and relevance of the data, and the potential for missing or incomplete data.
Learn more: What is Quantitative Observation?
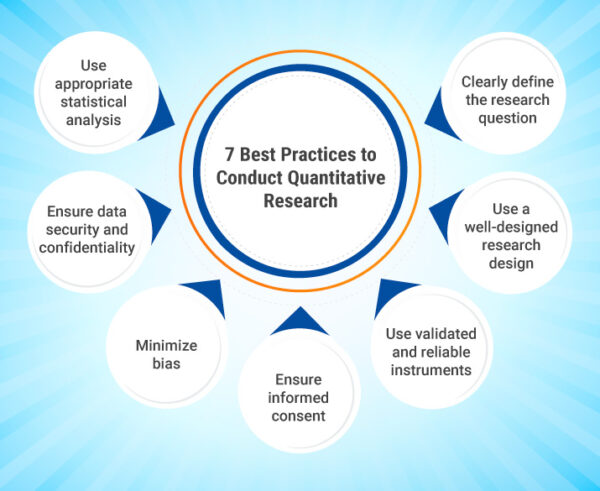
Here are the key best practices that should be followed when conducting quantitative research:
1. Clearly define the research question: The research question should be specific, measurable, and focused on a clear problem or issue.
2. Use a well-designed research design: The research design should be appropriate for the research question, and should include a clear sampling strategy, data collection methods, and statistical analysis plan.
3. Use validated and reliable instruments: The instruments used to collect data should be validated and reliable to ensure that the data collected is accurate and consistent.
4. Ensure informed consent: Participants should be fully informed about the purpose of the research, their rights, and how their data will be used. Informed consent should be obtained before data collection begins.
5. Minimize bias: Researchers should take steps to minimize bias in all stages of the research process, including study design, data collection, and data analysis.
6. Ensure data security and confidentiality: Data should be kept secure and confidential to protect the privacy of participants and prevent unauthorized access.
7. Use appropriate statistical analysis: Statistical analysis should be appropriate for the research question and the data collected. Accurate and clear reporting of results is imperative in quantitative research.
Learn more: What is Qualitative Research?
Enhance Your Research
Collect feedback and conduct research with IdeaScale’s award-winning software
Elevate Research And Feedback With Your IdeaScale Community!
IdeaScale is an innovation management solution that inspires people to take action on their ideas. Your community’s ideas can change lives, your business and the world. Connect to the ideas that matter and start co-creating the future.
Copyright © 2024 IdeaScale
Privacy Overview
Have a language expert improve your writing
Run a free plagiarism check in 10 minutes, automatically generate references for free.
- Knowledge Base
- Methodology
- What Is Quantitative Research? | Definition & Methods
What Is Quantitative Research? | Definition & Methods
Published on 4 April 2022 by Pritha Bhandari . Revised on 10 October 2022.
Quantitative research is the process of collecting and analysing numerical data. It can be used to find patterns and averages, make predictions, test causal relationships, and generalise results to wider populations.
Quantitative research is the opposite of qualitative research , which involves collecting and analysing non-numerical data (e.g. text, video, or audio).
Quantitative research is widely used in the natural and social sciences: biology, chemistry, psychology, economics, sociology, marketing, etc.
- What is the demographic makeup of Singapore in 2020?
- How has the average temperature changed globally over the last century?
- Does environmental pollution affect the prevalence of honey bees?
- Does working from home increase productivity for people with long commutes?
Table of contents
Quantitative research methods, quantitative data analysis, advantages of quantitative research, disadvantages of quantitative research, frequently asked questions about quantitative research.
You can use quantitative research methods for descriptive, correlational or experimental research.
- In descriptive research , you simply seek an overall summary of your study variables.
- In correlational research , you investigate relationships between your study variables.
- In experimental research , you systematically examine whether there is a cause-and-effect relationship between variables.
Correlational and experimental research can both be used to formally test hypotheses , or predictions, using statistics. The results may be generalised to broader populations based on the sampling method used.
To collect quantitative data, you will often need to use operational definitions that translate abstract concepts (e.g., mood) into observable and quantifiable measures (e.g., self-ratings of feelings and energy levels).
Prevent plagiarism, run a free check.
Once data is collected, you may need to process it before it can be analysed. For example, survey and test data may need to be transformed from words to numbers. Then, you can use statistical analysis to answer your research questions .
Descriptive statistics will give you a summary of your data and include measures of averages and variability. You can also use graphs, scatter plots and frequency tables to visualise your data and check for any trends or outliers.
Using inferential statistics , you can make predictions or generalisations based on your data. You can test your hypothesis or use your sample data to estimate the population parameter .
You can also assess the reliability and validity of your data collection methods to indicate how consistently and accurately your methods actually measured what you wanted them to.
Quantitative research is often used to standardise data collection and generalise findings . Strengths of this approach include:
- Replication
Repeating the study is possible because of standardised data collection protocols and tangible definitions of abstract concepts.
- Direct comparisons of results
The study can be reproduced in other cultural settings, times or with different groups of participants. Results can be compared statistically.
- Large samples
Data from large samples can be processed and analysed using reliable and consistent procedures through quantitative data analysis.
- Hypothesis testing
Using formalised and established hypothesis testing procedures means that you have to carefully consider and report your research variables, predictions, data collection and testing methods before coming to a conclusion.
Despite the benefits of quantitative research, it is sometimes inadequate in explaining complex research topics. Its limitations include:
- Superficiality
Using precise and restrictive operational definitions may inadequately represent complex concepts. For example, the concept of mood may be represented with just a number in quantitative research, but explained with elaboration in qualitative research.
- Narrow focus
Predetermined variables and measurement procedures can mean that you ignore other relevant observations.
- Structural bias
Despite standardised procedures, structural biases can still affect quantitative research. Missing data , imprecise measurements or inappropriate sampling methods are biases that can lead to the wrong conclusions.
- Lack of context
Quantitative research often uses unnatural settings like laboratories or fails to consider historical and cultural contexts that may affect data collection and results.
Quantitative research deals with numbers and statistics, while qualitative research deals with words and meanings.
Quantitative methods allow you to test a hypothesis by systematically collecting and analysing data, while qualitative methods allow you to explore ideas and experiences in depth.
In mixed methods research , you use both qualitative and quantitative data collection and analysis methods to answer your research question .
Data collection is the systematic process by which observations or measurements are gathered in research. It is used in many different contexts by academics, governments, businesses, and other organisations.
Operationalisation means turning abstract conceptual ideas into measurable observations.
For example, the concept of social anxiety isn’t directly observable, but it can be operationally defined in terms of self-rating scores, behavioural avoidance of crowded places, or physical anxiety symptoms in social situations.
Before collecting data , it’s important to consider how you will operationalise the variables that you want to measure.
Reliability and validity are both about how well a method measures something:
- Reliability refers to the consistency of a measure (whether the results can be reproduced under the same conditions).
- Validity refers to the accuracy of a measure (whether the results really do represent what they are supposed to measure).
If you are doing experimental research , you also have to consider the internal and external validity of your experiment.
Hypothesis testing is a formal procedure for investigating our ideas about the world using statistics. It is used by scientists to test specific predictions, called hypotheses , by calculating how likely it is that a pattern or relationship between variables could have arisen by chance.
Cite this Scribbr article
If you want to cite this source, you can copy and paste the citation or click the ‘Cite this Scribbr article’ button to automatically add the citation to our free Reference Generator.
Bhandari, P. (2022, October 10). What Is Quantitative Research? | Definition & Methods. Scribbr. Retrieved 6 May 2024, from https://www.scribbr.co.uk/research-methods/introduction-to-quantitative-research/
Is this article helpful?
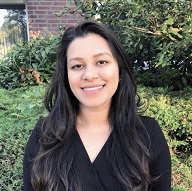
Pritha Bhandari
- Reviews / Why join our community?
- For companies
- Frequently asked questions
Quantitative Research
What is Quantitative Research?
Quantitative research is the methodology which researchers use to test theories about people’s attitudes and behaviors based on numerical and statistical evidence. Researchers sample a large number of users (e.g., through surveys) to indirectly obtain measurable, bias-free data about users in relevant situations.
“Quantification clarifies issues which qualitative analysis leaves fuzzy. It is more readily contestable and likely to be contested. It sharpens scholarly discussion, sparks off rival hypotheses, and contributes to the dynamics of the research process.” — Angus Maddison, Notable scholar of quantitative macro-economic history
- Transcript loading…
See how quantitative research helps reveal cold, hard facts about users which you can interpret and use to improve your designs.
Use Quantitative Research to Find Mathematical Facts about Users
Quantitative research is a subset of user experience (UX) research . Unlike its softer, more individual-oriented “counterpart”, qualitative research , quantitative research means you collect statistical/numerical data to draw generalized conclusions about users’ attitudes and behaviors . Compare and contrast quantitative with qualitative research, below:
Qualitative Research
You Aim to Determine
The “what”, “where” & “when” of the users’ needs & problems – to help keep your project’s focus on track during development
The “why” – to get behind how users approach their problems in their world
Highly structured (e.g., surveys) – to gather data about what users do & find patterns in large user groups
Loosely structured (e.g., contextual inquiries) – to learn why users behave how they do & explore their opinions
Number of Representative Users
Ideally 30+
Often around 5
Level of Contact with Users
Less direct & more remote (e.g., analytics)
More direct & less remote (e.g., usability testing to examine users’ stress levels when they use your design)
Statistically
Reliable – if you have enough test users
Less reliable, with need for great care with handling non-numerical data (e.g., opinions), as your own opinions might influence findings
Quantitative research is often best done from early on in projects since it helps teams to optimally direct product development and avoid costly design mistakes later. As you typically get user data from a distance—i.e., without close physical contact with users—also applying qualitative research will help you investigate why users think and feel the ways they do. Indeed, in an iterative design process quantitative research helps you test the assumptions you and your design team develop from your qualitative research. Regardless of the method you use, with proper care you can gather objective and unbiased data – information which you can complement with qualitative approaches to build a fuller understanding of your target users. From there, you can work towards firmer conclusions and drive your design process towards a more realistic picture of how target users will ultimately receive your product.
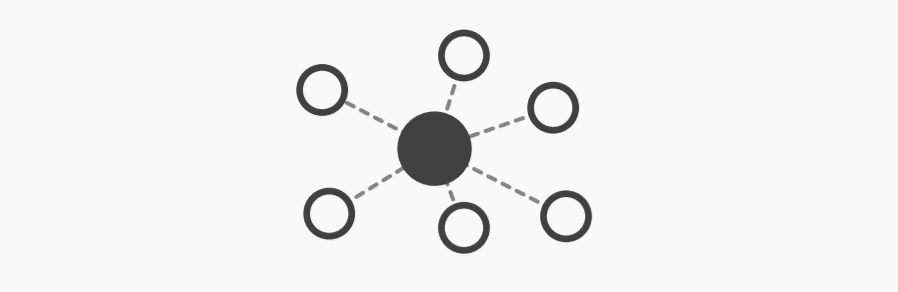
Quantitative analysis helps you test your assumptions and establish clearer views of your users in their various contexts.
Quantitative Research Methods You Can Use to Guide Optimal Designs
There are many quantitative research methods, and they help uncover different types of information on users. Some methods, such as A/B testing, are typically done on finished products, while others such as surveys could be done throughout a project’s design process. Here are some of the most helpful methods:
A/B testing – You test two or more versions of your design on users to find the most effective. Each variation differs by just one feature and may or may not affect how users respond. A/B testing is especially valuable for testing assumptions you’ve drawn from qualitative research. The only potential concerns here are scale—in that you’ll typically need to conduct it on thousands of users—and arguably more complexity in terms of considering the statistical significance involved.
Analytics – With tools such as Google Analytics, you measure metrics (e.g., page views, click-through rates) to build a picture (e.g., “How many users take how long to complete a task?”).
Desirability Studies – You measure an aspect of your product (e.g., aesthetic appeal) by typically showing it to participants and asking them to select from a menu of descriptive words. Their responses can reveal powerful insights (e.g., 78% associate the product/brand with “fashionable”).
Surveys and Questionnaires – When you ask for many users’ opinions, you will gain massive amounts of information. Keep in mind that you’ll have data about what users say they do, as opposed to insights into what they do . You can get more reliable results if you incentivize your participants well and use the right format.
Tree Testing – You remove the user interface so users must navigate the site and complete tasks using links alone. This helps you see if an issue is related to the user interface or information architecture.
Another powerful benefit of conducting quantitative research is that you can keep your stakeholders’ support with hard facts and statistics about your design’s performance—which can show what works well and what needs improvement—and prove a good return on investment. You can also produce reports to check statistics against different versions of your product and your competitors’ products.
Most quantitative research methods are relatively cheap. Since no single research method can help you answer all your questions, it’s vital to judge which method suits your project at the time/stage. Remember, it’s best to spend appropriately on a combination of quantitative and qualitative research from early on in development. Design improvements can be costly, and so you can estimate the value of implementing changes when you get the statistics to suggest that these changes will improve usability. Overall, you want to gather measurements objectively, where your personality, presence and theories won’t create bias.
Learn More about Quantitative Research
Take our User Research course to see how to get the most from quantitative research.
See how quantitative research methods fit into your design research landscape .
This insightful piece shows the value of pairing quantitative with qualitative research .
Find helpful tips on combining quantitative research methods in mixed methods research .
Questions related to Quantitative Research
Qualitative and quantitative research differ primarily in the data they produce. Quantitative research yields numerical data to test hypotheses and quantify patterns. It's precise and generalizable. Qualitative research, on the other hand, generates non-numerical data and explores meanings, interpretations, and deeper insights. Watch our video featuring Professor Alan Dix on different types of research methods.
This video elucidates the nuances and applications of both research types in the design field.
In quantitative research, determining a good sample size is crucial for the reliability of the results. William Hudson, CEO of Syntagm, emphasizes the importance of statistical significance with an example in our video.
He illustrates that even with varying results between design choices, we need to discern whether the differences are statistically significant or products of chance. This ensures the validity of the results, allowing for more accurate interpretations. Statistical tools like chi-square tests can aid in analyzing the results effectively. To delve deeper into these concepts, take William Hudson’s Data-Driven Design: Quantitative UX Research Course .
Quantitative research is crucial as it provides precise, numerical data that allows for high levels of statistical inference. Our video from William Hudson, CEO of Syntagm, highlights the importance of analytics in examining existing solutions.
Quantitative methods, like analytics and A/B testing, are pivotal for identifying areas for improvement, understanding user behaviors, and optimizing user experiences based on solid, empirical evidence. This empirical nature ensures that the insights derived are reliable, allowing for practical improvements and innovations. Perhaps most importantly, numerical data is useful to secure stakeholder buy-in and defend design decisions and proposals. Explore this approach in our Data-Driven Design: Quantitative Research for UX Research course and learn from William Hudson’s detailed explanations of when and why to use analytics in the research process.
After establishing initial requirements, statistical data is crucial for informed decisions through quantitative research. William Hudson, CEO of Syntagm, sheds light on the role of quantitative research throughout a typical project lifecycle in this video:
During the analysis and design phases, quantitative research helps validate user requirements and understand user behaviors. Surveys and analytics are standard tools, offering insights into user preferences and design efficacy. Quantitative research can also be used in early design testing, allowing for optimal design modifications based on user interactions and feedback, and it’s fundamental for A/B and multivariate testing once live solutions are available.
To write a compelling quantitative research question:
Create clear, concise, and unambiguous questions that address one aspect at a time.
Use common, short terms and provide explanations for unusual words.
Avoid leading, compound, and overlapping queries and ensure that questions are not vague or broad.
According to our video by William Hudson, CEO of Syntagm, quality and respondent understanding are vital in forming good questions.
He emphasizes the importance of addressing specific aspects and avoiding intimidating and confusing elements, such as extensive question grids or ranking questions, to ensure participant engagement and accurate responses. For more insights, see the article Writing Good Questions for Surveys .
Survey research is typically quantitative, collecting numerical data and statistical analysis to make generalizable conclusions. However, it can also have qualitative elements, mainly when it includes open-ended questions, allowing for expressive responses. Our video featuring the CEO of Syntagm, William Hudson, provides in-depth insights into when and how to effectively utilize surveys in the product or service lifecycle, focusing on user satisfaction and potential improvements.
He emphasizes the importance of surveys in triangulating data to back up qualitative research findings, ensuring we have a complete understanding of the user's requirements and preferences.
Descriptive research focuses on describing the subject being studied and getting answers to questions like what, where, when, and who of the research question. However, it doesn’t include the answers to the underlying reasons, or the “why” behind the answers obtained from the research. We can use both f qualitative and quantitative methods to conduct descriptive research. Descriptive research does not describe the methods, but rather the data gathered through the research (regardless of the methods used).
When we use quantitative research and gather numerical data, we can use statistical analysis to understand relationships between different variables. Here’s William Hudson, CEO of Syntagm with more on correlation and how we can apply tests such as Pearson’s r and Spearman Rank Coefficient to our data.
This helps interpret phenomena such as user experience by analyzing session lengths and conversion values, revealing whether variables like time spent on a page affect checkout values, for example.
Random Sampling: Each individual in the population has an equitable opportunity to be chosen, which minimizes biases and simplifies analysis.
Systematic Sampling: Selecting every k-th item from a list after a random start. It's simpler and faster than random sampling when dealing with large populations.
Stratified Sampling: Segregate the population into subgroups or strata according to comparable characteristics. Then, samples are taken randomly from each stratum.
Cluster Sampling: Divide the population into clusters and choose a random sample.
Multistage Sampling: Various sampling techniques are used at different stages to collect detailed information from diverse populations.
Convenience Sampling: The researcher selects the sample based on availability and willingness to participate, which may only represent part of the population.
Quota Sampling: Segment the population into subgroups, and samples are non-randomly selected to fulfill a predetermined quota from each subset.
These are just a few techniques, and choosing the right one depends on your research question, discipline, resource availability, and the level of accuracy required. In quantitative research, there isn't a one-size-fits-all sampling technique; choosing a method that aligns with your research goals and population is critical. However, a well-planned strategy is essential to avoid wasting resources and time, as highlighted in our video featuring William Hudson, CEO of Syntagm.
He emphasizes the importance of recruiting participants meticulously, ensuring their engagement and the quality of their responses. Accurate and thoughtful participant responses are crucial for obtaining reliable results. William also sheds light on dealing with failing participants and scrutinizing response quality to refine the outcomes.
The 4 types of quantitative research are Descriptive, Correlational, Causal-Comparative/Quasi-Experimental, and Experimental Research. Descriptive research aims to depict ‘what exists’ clearly and precisely. Correlational research examines relationships between variables. Causal-comparative research investigates the cause-effect relationship between variables. Experimental research explores causal relationships by manipulating independent variables. To gain deeper insights into quantitative research methods in UX, consider enrolling in our Data-Driven Design: Quantitative Research for UX course.
The strength of quantitative research is its ability to provide precise numerical data for analyzing target variables.This allows for generalized conclusions and predictions about future occurrences, proving invaluable in various fields, including user experience. William Hudson, CEO of Syntagm, discusses the role of surveys, analytics, and testing in providing objective insights in our video on quantitative research methods, highlighting the significance of structured methodologies in eliciting reliable results.
To master quantitative research methods, enroll in our comprehensive course, Data-Driven Design: Quantitative Research for UX .
This course empowers you to leverage quantitative data to make informed design decisions, providing a deep dive into methods like surveys and analytics. Whether you’re a novice or a seasoned professional, this course at Interaction Design Foundation offers valuable insights and practical knowledge, ensuring you acquire the skills necessary to excel in user experience research. Explore our diverse topics to elevate your understanding of quantitative research methods.
Answer a Short Quiz to Earn a Gift
What is the primary goal of quantitative research in design?
- To analyze numerical data and identify patterns
- To explore abstract design concepts for implementation
- To understand people's subjective experiences and opinions
Which of the following methods is an example of quantitative research?
- Conduct a focus groups to collect detailed user feedback
- Participate in open-ended interviews to explore user experiences
- Run usability tests and measure task completion times
What is one key advantage of quantitative research?
- It allows participants to express their opinions in a flexible manner.
- It provides researchers with detailed narratives of user experiences and perspectives.
- It produces standardized, comparable data that researchers can statistically analyze.
What is a significant challenge of quantitative research?
- It lacks objectivity which makes its results difficult to reproduce.
- It may oversimplify complex user behaviors into numbers and miss contextual insights.
- It often results in biased or misleading conclusions.
How can designers effectively combine qualitative and quantitative research?
- They can collect quantitative data first, followed by qualitative insights to explain the findings.
- They can completely replace quantitative methods with qualitative approaches.
- They can treat them as interchangeable methods to gather similar data.
Better luck next time!
Do you want to improve your UX / UI Design skills? Join us now
Congratulations! You did amazing
You earned your gift with a perfect score! Let us send it to you.
Check Your Inbox
We’ve emailed your gift to [email protected] .
Literature on Quantitative Research
Here’s the entire UX literature on Quantitative Research by the Interaction Design Foundation, collated in one place:
Learn more about Quantitative Research
Take a deep dive into Quantitative Research with our course User Research – Methods and Best Practices .
How do you plan to design a product or service that your users will love , if you don't know what they want in the first place? As a user experience designer, you shouldn't leave it to chance to design something outstanding; you should make the effort to understand your users and build on that knowledge from the outset. User research is the way to do this, and it can therefore be thought of as the largest part of user experience design .
In fact, user research is often the first step of a UX design process—after all, you cannot begin to design a product or service without first understanding what your users want! As you gain the skills required, and learn about the best practices in user research, you’ll get first-hand knowledge of your users and be able to design the optimal product—one that’s truly relevant for your users and, subsequently, outperforms your competitors’ .
This course will give you insights into the most essential qualitative research methods around and will teach you how to put them into practice in your design work. You’ll also have the opportunity to embark on three practical projects where you can apply what you’ve learned to carry out user research in the real world . You’ll learn details about how to plan user research projects and fit them into your own work processes in a way that maximizes the impact your research can have on your designs. On top of that, you’ll gain practice with different methods that will help you analyze the results of your research and communicate your findings to your clients and stakeholders—workshops, user journeys and personas, just to name a few!
By the end of the course, you’ll have not only a Course Certificate but also three case studies to add to your portfolio. And remember, a portfolio with engaging case studies is invaluable if you are looking to break into a career in UX design or user research!
We believe you should learn from the best, so we’ve gathered a team of experts to help teach this course alongside our own course instructors. That means you’ll meet a new instructor in each of the lessons on research methods who is an expert in their field—we hope you enjoy what they have in store for you!
All open-source articles on Quantitative Research
Best practices for qualitative user research.
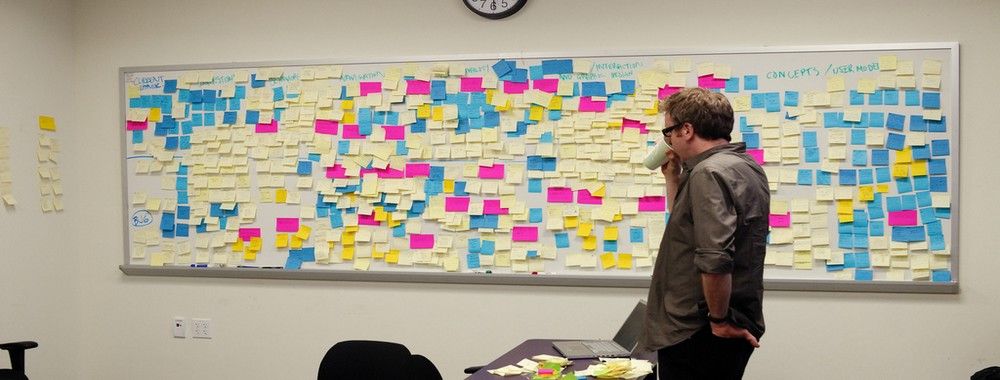
- 3 years ago
Card Sorting
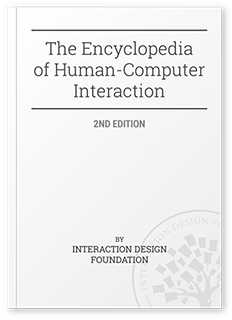
Understand the User’s Perspective through Research for Mobile UX
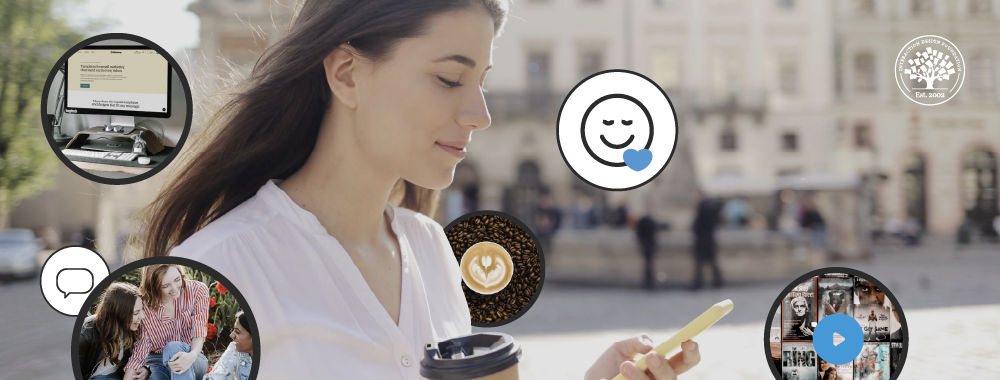
- 11 mths ago
7 Simple Ways to Get Better Results From Ethnographic Research
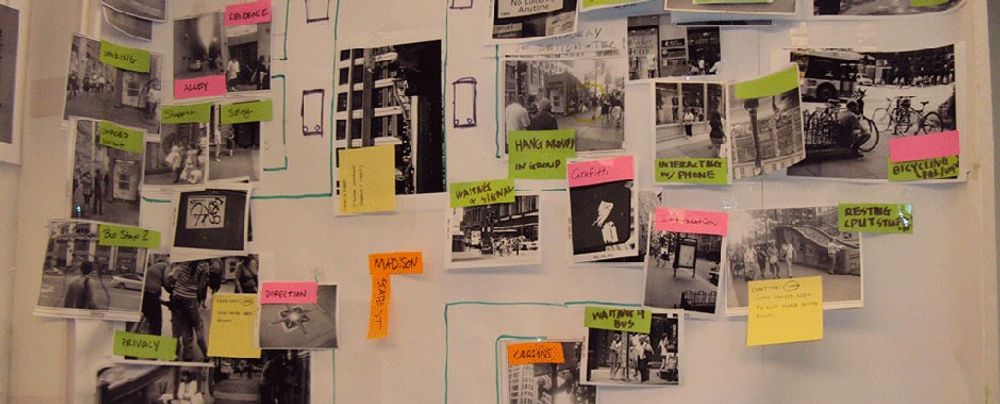
Question Everything
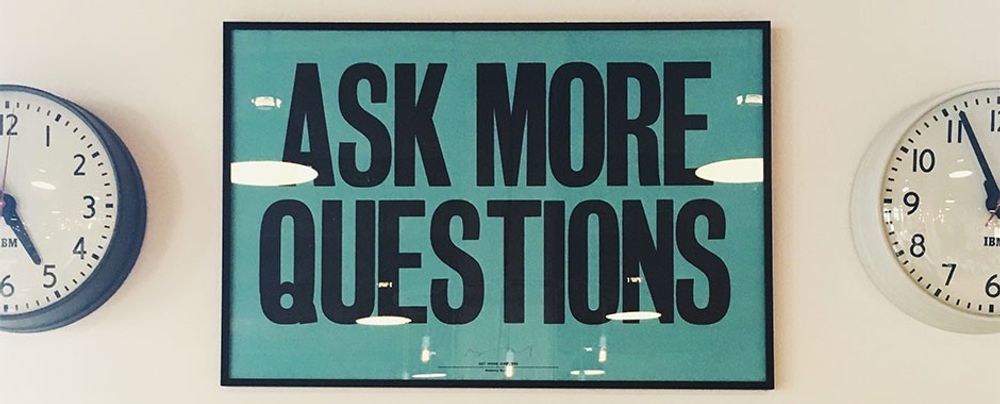
Tree Testing
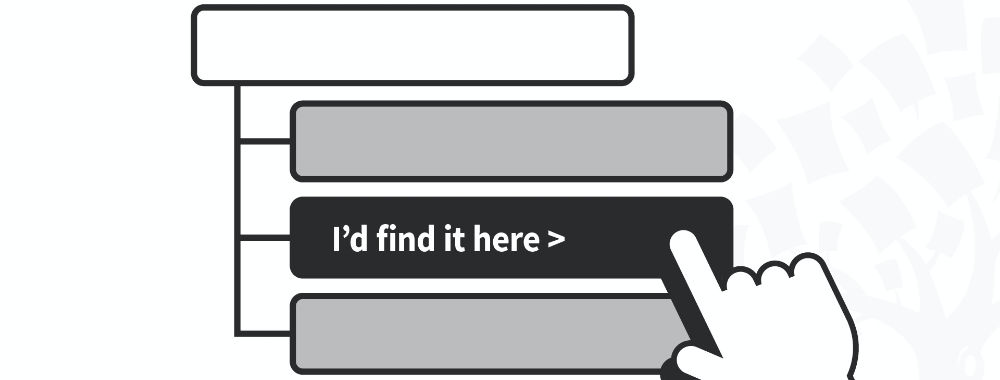
Adding Quality to Your Design Research with an SSQS Checklist
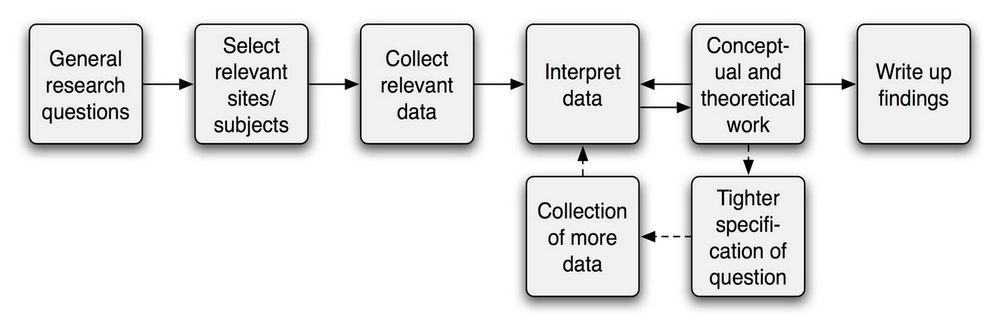
- 8 years ago
How to Fit Quantitative Research into the Project Lifecycle
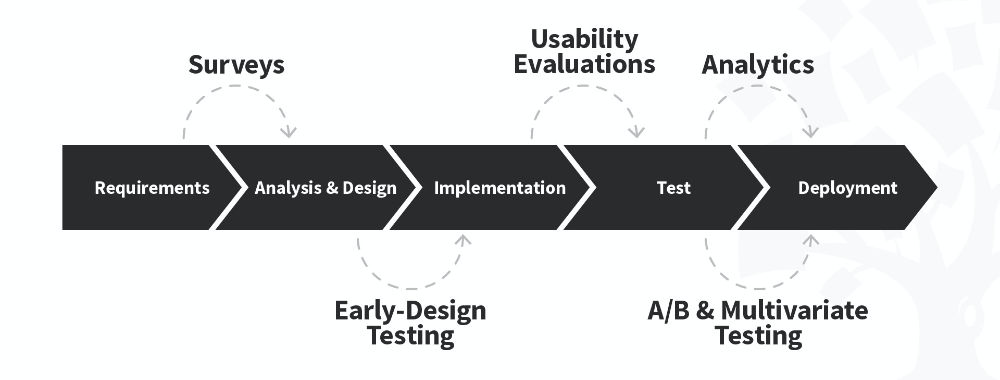
Why and When to Use Surveys
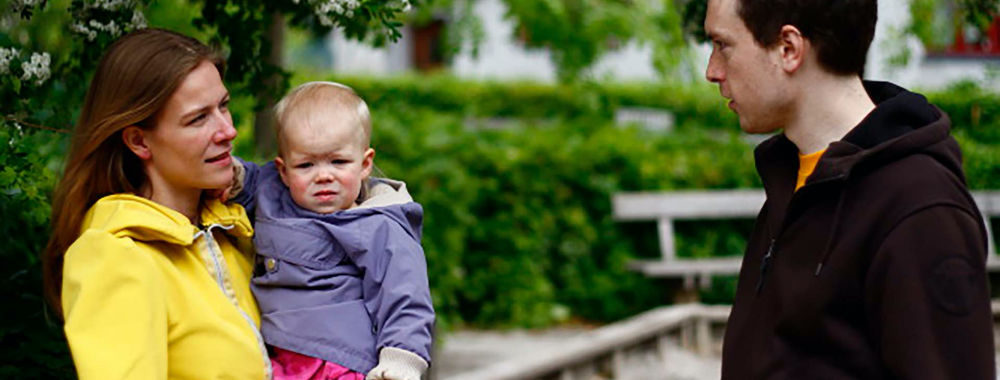
Correlation in User Experience
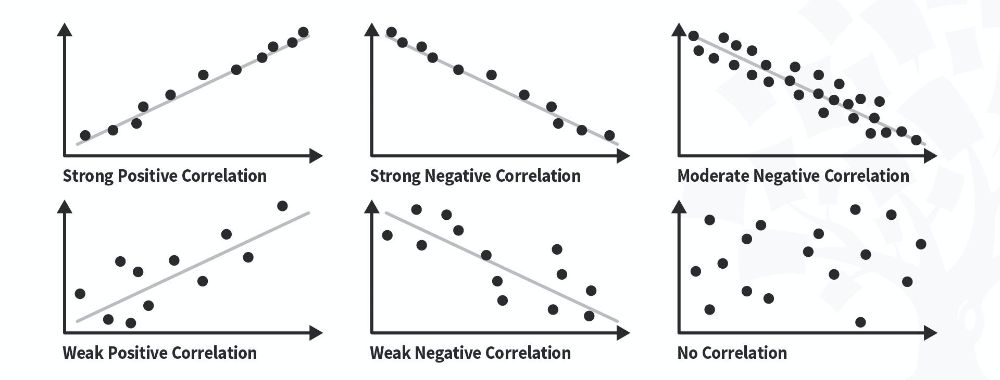
First-Click Testing
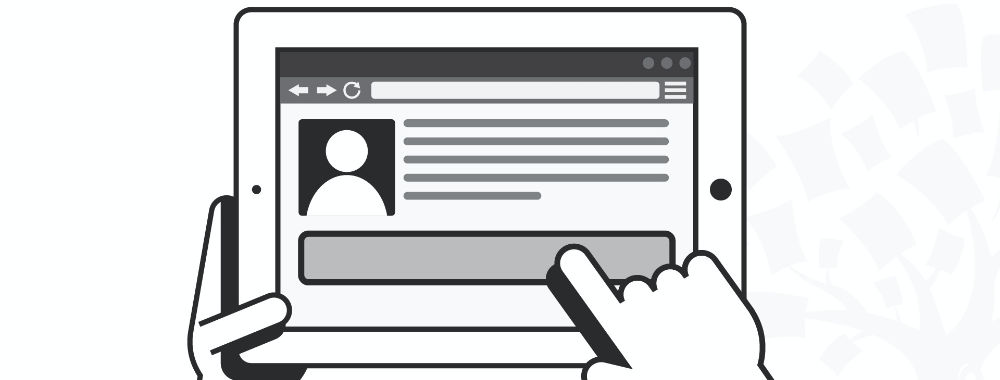
Rating Scales in UX Research: The Ultimate Guide
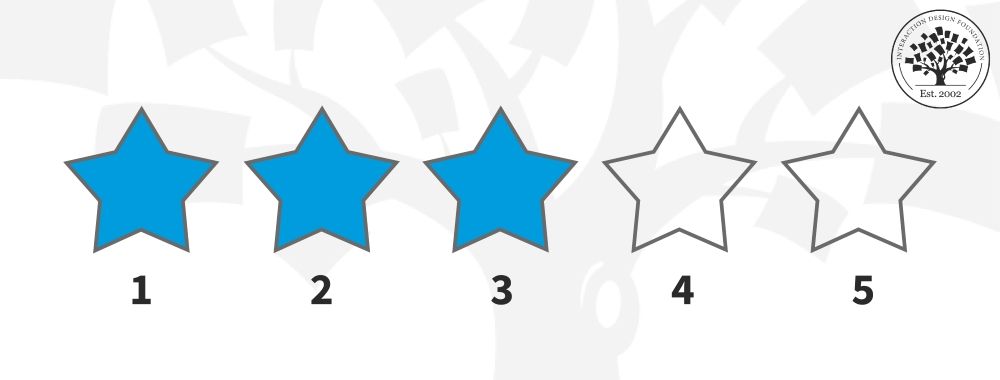
What to Test
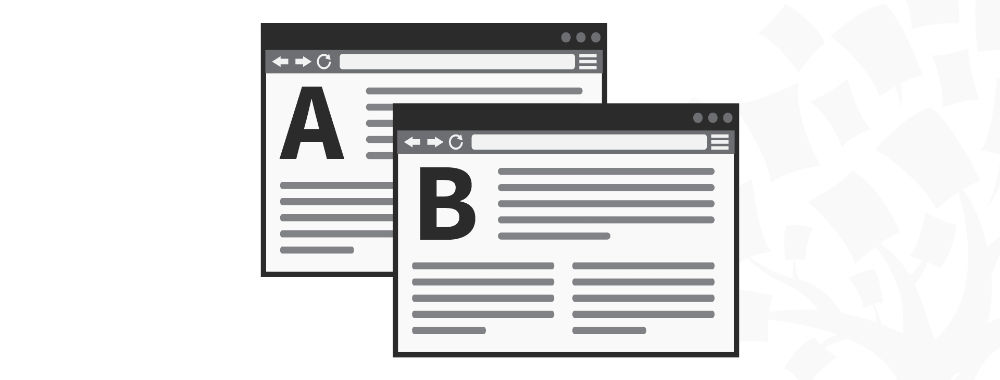
Open Access—Link to us!
We believe in Open Access and the democratization of knowledge . Unfortunately, world-class educational materials such as this page are normally hidden behind paywalls or in expensive textbooks.
If you want this to change , cite this page , link to us, or join us to help us democratize design knowledge !
Privacy Settings
Our digital services use necessary tracking technologies, including third-party cookies, for security, functionality, and to uphold user rights. Optional cookies offer enhanced features, and analytics.
Experience the full potential of our site that remembers your preferences and supports secure sign-in.
Governs the storage of data necessary for maintaining website security, user authentication, and fraud prevention mechanisms.
Enhanced Functionality
Saves your settings and preferences, like your location, for a more personalized experience.
Referral Program
We use cookies to enable our referral program, giving you and your friends discounts.
Error Reporting
We share user ID with Bugsnag and NewRelic to help us track errors and fix issues.
Optimize your experience by allowing us to monitor site usage. You’ll enjoy a smoother, more personalized journey without compromising your privacy.
Analytics Storage
Collects anonymous data on how you navigate and interact, helping us make informed improvements.
Differentiates real visitors from automated bots, ensuring accurate usage data and improving your website experience.
Lets us tailor your digital ads to match your interests, making them more relevant and useful to you.
Advertising Storage
Stores information for better-targeted advertising, enhancing your online ad experience.
Personalization Storage
Permits storing data to personalize content and ads across Google services based on user behavior, enhancing overall user experience.
Advertising Personalization
Allows for content and ad personalization across Google services based on user behavior. This consent enhances user experiences.
Enables personalizing ads based on user data and interactions, allowing for more relevant advertising experiences across Google services.
Receive more relevant advertisements by sharing your interests and behavior with our trusted advertising partners.
Enables better ad targeting and measurement on Meta platforms, making ads you see more relevant.
Allows for improved ad effectiveness and measurement through Meta’s Conversions API, ensuring privacy-compliant data sharing.
LinkedIn Insights
Tracks conversions, retargeting, and web analytics for LinkedIn ad campaigns, enhancing ad relevance and performance.
LinkedIn CAPI
Enhances LinkedIn advertising through server-side event tracking, offering more accurate measurement and personalization.
Google Ads Tag
Tracks ad performance and user engagement, helping deliver ads that are most useful to you.
Share Knowledge, Get Respect!
or copy link
Cite according to academic standards
Simply copy and paste the text below into your bibliographic reference list, onto your blog, or anywhere else. You can also just hyperlink to this page.
New to UX Design? We’re Giving You a Free ebook!
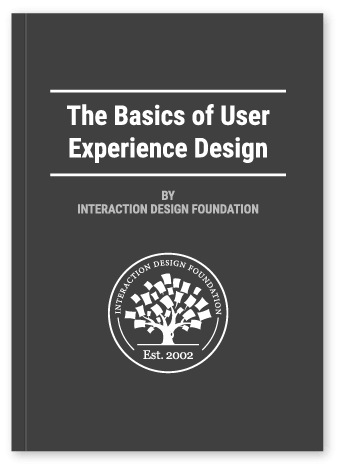
Download our free ebook The Basics of User Experience Design to learn about core concepts of UX design.
In 9 chapters, we’ll cover: conducting user interviews, design thinking, interaction design, mobile UX design, usability, UX research, and many more!
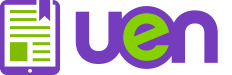
Key Concepts in Quantitative Research
In this module, we are going to explore the nuances of quantitative research, including the main types of quantitative research, more exploration into variables (including confounding and extraneous variables), and causation.
Content includes:
- Flaws, “Proof”, and Rigor
- The Steps of Quantitative Methodology
- Major Classes of Quantitative Research
- Experimental versus Non-Experimental Research
- Types of Experimental Research
- Types of Non-Experimental Research
- Research Variables
- Confounding/Extraneous Variables
- Causation versus correlation/association
Objectives:
- Discuss the flaws, proof, and rigor in research.
- Describe the differences between independent variables and dependent variables.
- Describe the steps in quantitative research methodology.
- Describe experimental, quasi-experimental, and non-experimental research studies
- Describe confounding and extraneous variables.
- Differentiate cause-and-effect (causality) versus association/correlation
Flaws, Proof, and Rigor in Research
One of the biggest hurdles that students and seasoned researchers alike struggle to grasp, is that research cannot “ prove ” nor “ disprove ”. Research can only support a hypothesis with reasonable, statistically significant evidence.
Indeed. You’ve heard it incorrectly your entire life. You will hear professors, scientists, radio ads, podcasts, and even researchers comment something to the effect of, “It has been proven that…” or “Research proves that…” or “Finally! There is proof that…”
We have been duped. Consider the “ prove ” word a very bad word in this course. The forbidden “P” word. Do not say it, write it, allude to it, or repeat it. And, for the love of avocados and all things fluffy, do not include the “P” word on your EBP poster. You will be deducted some major points.
We can only conclude with reasonable certainty through statistical analyses that there is a high probability that something did not happen by chance but instead happened due to the intervention that the researcher tested. Got that? We will come back to that concept but for now know that it is called “statistical significance”.
All research has flaws. We might not know what those flaws are, but we will be learning about confounding and extraneous variables later on in this module to help explain how flaws can happen.
Remember this: Sometimes, the researcher might not even know that there was a flaw that occurred. No research project is perfect. There is no 100% awesome. This is a major reason why it is so important to be able to duplicate a research project and obtain similar results. The more we can duplicate research with the same exact methodology and protocols, the more certainty we have in the results and we can start accounting for flaws that may have sneaked in.
Finally, not all research is equal. Some research is done very sloppily, and other research has a very high standard of rigor. How do we know which is which when reading an article? Well, within this module, we will start learning about some things to look for in a published research article to help determine rigor. We do not want lazy research to determine our actions as nurses, right? We want the strongest, most reliable, most valid, most rigorous research evidence possible so that we can take those results and embed them into patient care. Who wants shoddy evidence determining the actions we take with your grandmother’s heart surgery?
Independent Variables and Dependent Variables
As we were already introduced to, there are measures called “variables” in research. This will be a bit of a review but it is important to bring up again, as it is a hallmark of quantitative research. In quantitative studies, the concepts being measured are called variables (AKA: something that varies). Variables are something that can change – either by manipulation or from something causing a change. In the article snapshots that we have looked at, researchers are trying to find causes for phenomena. Does a nursing intervention cause an improvement in patient outcomes? Does the cholesterol medication cause a decrease in cholesterol level? Does smoking cause cancer?
The presumed cause is called the independent variable. The presumed effect is called the dependent variable. The dependent variable is “dependent” on something causing it to change. The dependent variable is the outcome that a researcher is trying to understand, explain, or predict.
Think back to our PICO questions. You can think of the intervention (I) as the independent variable and the outcome (O) as the dependent variable.
The independent variable is manipulated by the researcher or can be variants of influence. Whereas the dependent variable is never manipulated.
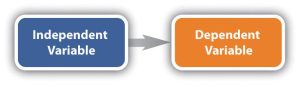
Variables do not always measure cause-and-effect. They can also measure a direction of influence.
Here is an example of that: If we compared levels of depression among men and women diagnosed with pancreatic cancer and found men to be more depressed, we cannot conclude that depression was caused by gender. However, we can note that the direction of influence clearly runs from gender to depression. It makes no sense to suggest the depression influenced their gender.
In the above example, what is the independent variable (IV) and what is the dependent variable (DV)? If you guessed gender as the IV and depression as the DV, you are correct! Important to note in this case that the researcher did not manipulate the IV, but the IV is manipulated on its own (male or female).
Researchers do not always have just one IV. In some cases, more than one IV may be measured. Take, for instance, a study that wants to measure the factors that influence one’s study habits. Independent variables of gender, sleep habits, and hours of work may be considered. Likewise, multiple DVs can be measured. For example, perhaps we want to measure weight and abdominal girth on a plant-based diet (IV).
Now, some studies do not have an intervention. We will come back to that when we talk about non-experimental research.
The point of variables is so that researchers have a very specific measurement that they seek to study.
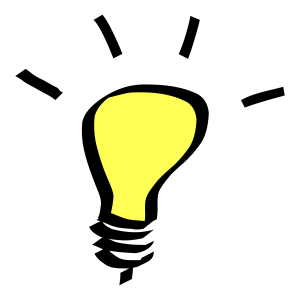
Let’s look at a couple of examples:
Now you try! Identify the IVs and DVs:
IV and DV Case Studies (Leibold, 2020)
Case Three: Independent variable: Healthy Lifestyle education with a focus on physical activity; Dependent variable: Physical activity rate before and after education intervention, Heart rate before and after education intervention, Blood pressures before and after education intervention.
Case Four: Independent variable: Playing classical music; Dependent variable: Grade point averages post classical music, compared to pre-classical music.
Case Five: Independent variable: No independent variable as there is no intervention. Dependent variable: The themes that emerge from the qualitative data.
The Steps in Quantitative Research Methodology
Now, as we learned in the last module, quantitative research is completely objective. There is no subjectivity to it. Why is this? Well, as we have learned, the purpose of quantitative research is to make an inference about the results in order to generalize these results to the population.
In quantitative studies, there is a very systematic approach that moves from the beginning point of the study (writing a research question) to the end point (obtaining an answer). This is a very linear and purposeful flow across the study, and all quantitative research should follow the same sequence.
- Identifying a problem and formulating a research question . Quantitative research begins with a theory . As in, “something is wrong and we want to fix it or improve it”. Think back to when we discussed research problems and formulating a research question. Here we are! That is the first step in formulating a quantitative research plan.
- Formulate a hypothesis . This step is key. Researchers need to know exactly what they are testing so that testing the hypothesis can be achieved through specific statistical analyses.
- A thorough literature review . At this step, researchers strive to understand what is already known about a topic and what evidence already exists.
- Identifying a framework . When an appropriate framework is identified, the findings of a study may have broader significance and utility (Polit & Beck, 2021).
- Choosing a study design . The research design will determine exactly how the researcher will obtain the answers to the research question(s). The entire design needs to be structured and controlled, with the overarching goal of minimizing bias and errors. The design determines what data will be collected and how, how often data will be collected, what types of comparisons will be made. You can think of the study design as the architectural backbone of the entire study.
- Sampling . The researcher needs to determine a subset of the population that is to be studied. We will come back to the sampling concept in the next module. However, the goal of sampling is to choose a subset of the population that adequate reflects the population of interest.
- I nstruments to be used to collect data (with reliability and validity as a priority). Researchers must find a way to measure the research variables (intervention and outcome) accurately. The task of measuring is complex and challenging, as data needs to be collected reliably (measuring consistently each time) and valid. Reliability and validity are both about how well a method measures something. The next module will cover this in detail.
- Obtaining approval for ethical/legal human rights procedures . As we will learn in an upcoming module, there needs to be methods in place to safeguard human rights.
- Data collection . The fun part! Finally, after everything has been organized and planned, the researcher(s) begin to collect data. The pre-established plan (methodology) determines when data collection begins, how to accomplish it, how data collection staff will be trained, and how data will be recorded.
- Data analysis . Here comes the statistical analyses. The next module will dive into this.
- Discussion . After all the analyses have been complete, the researcher then needs to interpret the results and examine the implications. Researchers attempt to explain the findings in light of the theoretical framework, prior evidence, theory, clinical experience, and any limitations in the study now that it has been completed. Often, the researcher discusses not just the statistical significance, but also the clinical significance, as it is common to have one without the other.
- Summary/references . Part of the final steps of any research project is to disseminate (AKA: share) the findings. This may be in a published article, conference, poster session, etc. The point of this step is to communicate to others the information found through the study. All references are collected so that the researchers can give credit to others.
- Budget and funding . As a last mention in the overall steps, budget and funding for research is a consideration. Research can be expensive. Often, researchers can obtain a grant or other funding to help offset the costs.
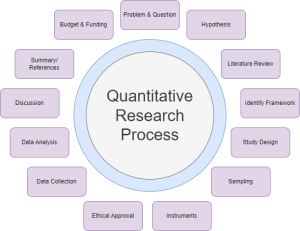
Edit: Steps in Quantitative Research video. Step 12 should say “Dissemination” (sharing the results).
Experimental, Quasi-Experimental, and Non-Experimental Studies
To start this section, please watch this wonderful video by Jenny Barrow, MSN, RN, CNE, that explains experimental versus nonexperimental research.
(Jenny Barrow, 2019)
Now that you have that overview, continue reading this module.
Experimental Research : In experimental research, the researcher is seeking to draw a conclusion between an independent variable and a dependent variable. This design attempts to establish cause-effect relationships among the variables. You could think of experimental research as experimenting with “something” to see if it caused “something else”.
A true experiment is called a Randomized Controlled Trial (or RCT). An RCT is at the top of the echelon as far as quantitative experimental research. It’s the gold standard of scientific research. An RCT, a true experimental design, must have 3 features:
- An intervention : The experiment does something to the participants by the option of manipulating the independent variable.
- Control : Some participants in the study receive either the standard care, or no intervention at all. This is also called the counterfactual – meaning, it shows what would happen if no intervention was introduced.
- Randomization : Randomization happens when the researcher makes sure that it is completely random who receives the intervention and who receives the control. The purpose is to make the groups equal regarding all other factors except receipt of the intervention.
Note: There is a lot of confusion with students (and even some researchers!) when they refer to “ random assignment ” versus “ random sampling ”. Random assignment is a signature of a true experiment. This means that if participants are not truly randomly assigned to intervention groups, then it is not a true experiment. We will talk more about random sampling in the next module.
One very common method for RCT’s is called a pretest-posttest design . This is when the researcher measures the outcome before and after the intervention. For example, if the researcher had an IV (intervention/treatment) of a pain medication, the DV (pain) would be measured before the intervention is given and after it is given. The control group may just receive a placebo. This design permits the researcher to see if the change in pain was caused by the pain medication because only some people received it (Polit & Beck, 2021).
Another experimental design is called a crossover design . This type of design involves exposing participants to more than one treatment. For example, subject 1 first receives treatment A, then treatment B, then treatment C. Subject 2 might first receive treatment B, then treatment A, and then treatment C. In this type of study, the three conditions for an experiment are met: Intervention, randomization, and control – with the subjects serving as their own control group.
Control group conditions can be done in 4 ways:
- No intervention is used; control group gets no treatment at all
- “Usual care” or standard of care or normal procedures used
- An alternative intervention is uses (e.g. auditory versus visual stimulation)
- A placebo or pseudo-intervention, presumed to have no therapeutic value, is used
Quasi-Experimental Research : Quasi-experiments involve an experiment just like true experimental research. However, they lack randomization and some even lack a control group. Therefore, there is implementation and testing of an intervention, but there is an absence of randomization.
For example, perhaps we wanted to measure the effect of yoga for nursing students. The IV (intervention of yoga) is being offered to all nursing students and therefore randomization is not possible. For comparison, we could measure quality of life data on nursing students at a different university. Data is collected from both groups at baseline and then again after the yoga classes. Note, that in quasi-experiments, the phrase “comparison group” is sometimes used instead of “control group” against which outcome measures are collected.
Sometimes there is no comparison group either. This would be called a one-group pretest-posttest design .
Non-Experimental Research : Sometimes, cause-problem research questions cannot be answered with an experimental or quasi-experimental design because the IV cannot be manipulated. For example, if we want to measure what impact prerequisite grades have on student success in nursing programs, we obviously cannot manipulate the prerequisite grades. In another example, if we wanted to investigate how low birth weight impacts developmental progression in children, we cannot manipulate the birth weight. Often, you will see the word “observational” in lieu of non-experimental researcher. This does not mean the researcher is just standing and watching people, but instead it refers to the method of observing data that has already been established without manipulation.
There are various types of non-experimental research:
Correlational research : A correlational research design investigates relationships between two variables (or more) without the researcher controlling or manipulating any of them. In the example of prerequisites and nursing program success, that is a correlational design. Consider hypothetically, a researcher is studying a correlation between cancer and marriage. In this study, there are two variables: disease and marriage. Let us say marriage has a negative association with cancer. This means that married people are less likely to develop cancer.
Cohort design (also called a prospective design) : In a cohort study, the participants do not have the outcome of interest to begin with. They are selected based on the exposure status of the individual. They are then followed over time to evaluate for the occurrence of the outcome of interest. Cohorts may be divided into exposure categories once baseline measurements of a defined population are made. For example, the Framingham Cardiovascular Disease Study (CVD) used baseline measurements to divide the population into categories of CVD risk factors. Another example: An example of a cohort study is comparing the test scores of one group of people who underwent extensive tutoring and a special curriculum and those who did not receive any extra help. The group could be studied for years to assess whether their scores improve over time and at what rate.
Retrospective design : In retrospective studies, the outcome of interest has already occurred (or not occurred – e.g., in controls) in each individual by the time s/he is enrolled, and the data are collected either from records or by asking participants to recall exposures. There is no follow-up of participants. For example, a researcher might examine the medical histories of 1000 elderly women to identify the causes of health problems.
Case-control design : A study that compares two groups of people: those with the disease or condition under study (cases) and a very similar group of people who do not have the condition. For example, investigators conducted a case-control study to determine if there is an association between colon cancer and a high fat diet. Cases were all confirmed colon cancer cases in North Carolina in 2010. Controls were a sample of North Carolina residents without colon cancer.
Descriptive research : Descriptive research design is a type of research design that aims to obtain information to systematically describe a phenomenon, situation, or population. More specifically, it helps answer the what, when, where, and how questions regarding the research problem, rather than the why. For example, the researcher might wish to discover the percentage of motorists who tailgate – the prevalence of a certain behavior.
There are two other designs to mention, which are both on a time continuum basis.
Cross-sectional design : All data are collected at a single point in time. Retrospective studies are usually cross-sectional. The IV usually concerns events or behaviors occurring in the past. One cross-sectional study example in medicine is a data collection of smoking habits and lung cancer incidence in a given population. A cross-sectional study like this cannot solely determine that smoking habits cause lung cancer, but it can suggest a relationship that merits further investigation. Cross-sectional studies serve many purposes, and the cross-sectional design is the most relevant design when assessing the prevalence of disease, attitudes and knowledge among patients and health personnel, in validation studies comparing, for example, different measurement instruments, and in reliability studies.
Longitudinal design : Data are collected two or more times over an extended period. Longitudinal designs are better at showing patterns of change and at clarifying whether a cause occurred before an effect (outcome). A challenge in longitudinal studies is attrition or the loss of participants over time. In a longitudinal study subjects are followed over time with continuous or repeated monitoring of risk factors or health outcomes, or both. Such investigations vary enormously in their size and complexity. At one extreme a large population may be studied over decades. An example of a longitudinal design is a multiyear comparative study of the same children in an urban and a suburban school to record their cognitive development in depth.
Confounding and Extraneous Variables
Confounding variables are a type of extraneous variable that occur which interfere with or influence the relationship between the independent and dependent variables. In research that investigates a potential cause-and-effect relationship, a confounding variable is an unmeasured third variable that influences both the supposed cause and the supposed effect.
It’s important to consider potential confounding variables and account for them in research designs to ensure results are valid. You can imagine that if something sneaks in to influence the measured variables, it can really muck up the study!
Here is an example:
You collect data on sunburns and ice cream consumption. You find that higher ice cream consumption is associated with a higher probability of sunburn. Does that mean ice cream consumption causes sunburn?
Here, the confounding variable is temperature: hot temperatures cause people to both eat more ice cream and spend more time outdoors under the sun, resulting in more sunburns.
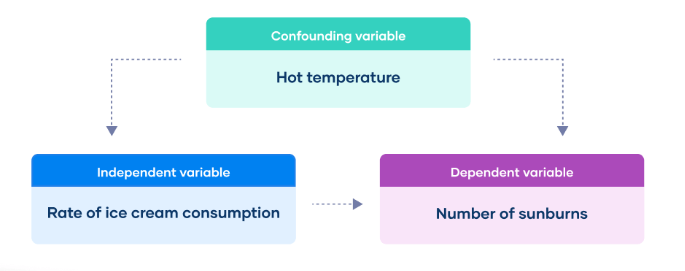
To ensure the internal validity of research, the researcher must account for confounding variables. If he/she fails to do so, the results may not reflect the actual relationship between the variables that they are interested in.
For instance, they may find a cause-and-effect relationship that does not actually exist, because the effect they measure is caused by the confounding variable (and not by the independent variable).
Here is another example:
The researcher finds that babies born to mothers who smoked during their pregnancies weigh significantly less than those born to non-smoking mothers. However, if the researcher does not account for the fact that smokers are more likely to engage in other unhealthy behaviors, such as drinking or eating less healthy foods, then he/she might overestimate the relationship between smoking and low birth weight.
Extraneous variables are any variables that the researcher is not investigating that can potentially affect the outcomes of the research study. If left uncontrolled, extraneous variables can lead to inaccurate conclusions about the relationship between IVs and DVs.
Extraneous variables can threaten the internal validity of a study by providing alternative explanations for the results. In an experiment, the researcher manipulates an independent variable to study its effects on a dependent variable.
In a study on mental performance, the researcher tests whether wearing a white lab coat, the independent variable (IV), improves scientific reasoning, the dependent variable (DV).
Students from a university are recruited to participate in the study. The researcher manipulates the independent variable by splitting participants into two groups:
- Participants in the experimental group are asked to wear a lab coat during the study.
- Participants in the control group are asked to wear a casual coat during the study.
All participants are given a scientific knowledge quiz, and their scores are compared between groups.
When extraneous variables are uncontrolled, it’s hard to determine the exact effects of the independent variable on the dependent variable, because the effects of extraneous variables may mask them.
Uncontrolled extraneous variables can also make it seem as though there is a true effect of the independent variable in an experiment when there’s actually none.
In the above experiment example, these extraneous variables can affect the science knowledge scores:
- Participant’s major (e.g., STEM or humanities)
- Participant’s interest in science
- Demographic variables such as gender or educational background
- Time of day of testing
- Experiment environment or setting
If these variables systematically differ between the groups, you can’t be sure whether your results come from your independent variable manipulation or from the extraneous variables.
In summary, an extraneous variable is anything that could influence the dependent variable. A confounding variable influences the dependent variable, and also correlates with or causally affects the independent variable.
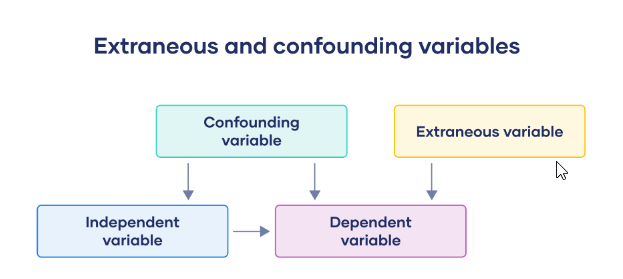
Cause-and-Effect (Causality) Versus Association/Correlation
A very important concept to understand is cause-and-effect, also known as causality, versus correlation. Let’s look at these two concepts in very simplified statements. Causation means that one thing caused another thing to happen. Correlation means there is some association between the two thing we are measuring.
It would be nice if it were as simple as that. These two concepts can indeed by confused by many. Let’s dive deeper.
Two or more variables are considered to be related or associated, in a statistical context, if their values change so that as the value of one variable increases or decreases so does the value of the other variable (or the opposite direction).
For example, for the two variables of “hours worked” and “income earned”, there is a relationship between the two if the increase in hours is associated with an increase in income earned.
However, correlation is a statistical measure that describes the size and direction of a relationship between two or more variables. A correlation does not automatically mean that the change in one variable caused the change in value in the other variable.
Theoretically, the difference between the two types of relationships is easy to identify — an action or occurrence can cause another (e.g. smoking causes an increase in the risk of developing lung cancer), or it can correlate with another (e.g. smoking is correlated with alcoholism, but it does not cause alcoholism). In practice, however, it remains difficult to clearly establish cause and effect, compared with establishing correlation.
Simplified in this image, we can say that hot and sunny weather causes an increase in ice cream consumption. Similarly, we can demise that hot and sunny weather increases the incidence of sunburns. However, we cannot say that ice cream caused a sunburn (or that a sunburn increases consumption of ice cream). It is purely coincidental. In this example, it is pretty easy to anecdotally surmise correlation versus causation. However, in research, we have statistical tests that help researchers differentiate via specialized analyses.
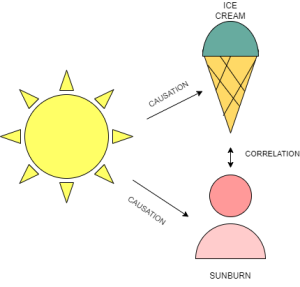
Here is a great Khan Academy video of about 5 minutes that shows a worked example of correlation versus causation with regard to sledding accidents and frostbite cases:
https://www.khanacademy.org/test-prep/praxis-math/praxis-math-lessons/gtp–praxis-math–lessons–statistics-and-probability/v/gtp–praxis-math–video–correlation-and-causation
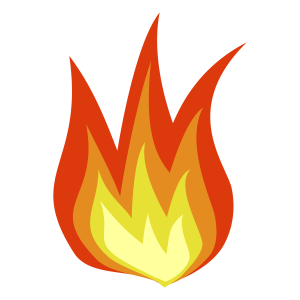
References & Attribution
“ Light bulb doodle ” by rawpixel licensed CC0 .
“ Magnifying glass ” by rawpixel licensed CC0
“ Orange flame ” by rawpixel licensed CC0 .
Jenny Barrow. (2019). Experimental versus nonexperimental research. https://www.youtube.com/watch?v=FJo8xyXHAlE
Leibold, N. (2020). Research variables. Measures and Concepts Commonly Encountered in EBP. Creative Commons License: BY NC
Polit, D. & Beck, C. (2021). Lippincott CoursePoint Enhanced for Polit’s Essentials of Nursing Research (10th ed.). Wolters Kluwer Health.
Evidence-Based Practice & Research Methodologies Copyright © by Tracy Fawns is licensed under a Creative Commons Attribution-NonCommercial-ShareAlike 4.0 International License , except where otherwise noted.
Share This Book
Quantitative research
Affiliation.
- 1 Faculty of Health and Social Care, University of Hull, Hull, England.
- PMID: 25828021
- DOI: 10.7748/ns.29.31.44.e8681
This article describes the basic tenets of quantitative research. The concepts of dependent and independent variables are addressed and the concept of measurement and its associated issues, such as error, reliability and validity, are explored. Experiments and surveys – the principal research designs in quantitative research – are described and key features explained. The importance of the double-blind randomised controlled trial is emphasised, alongside the importance of longitudinal surveys, as opposed to cross-sectional surveys. Essential features of data storage are covered, with an emphasis on safe, anonymous storage. Finally, the article explores the analysis of quantitative data, considering what may be analysed and the main uses of statistics in analysis.
Keywords: Experiments; measurement; nursing research; quantitative research; reliability; surveys; validity.
- Biomedical Research / methods*
- Double-Blind Method
- Evaluation Studies as Topic
- Longitudinal Studies
- Randomized Controlled Trials as Topic
- United Kingdom
Educational resources and simple solutions for your research journey
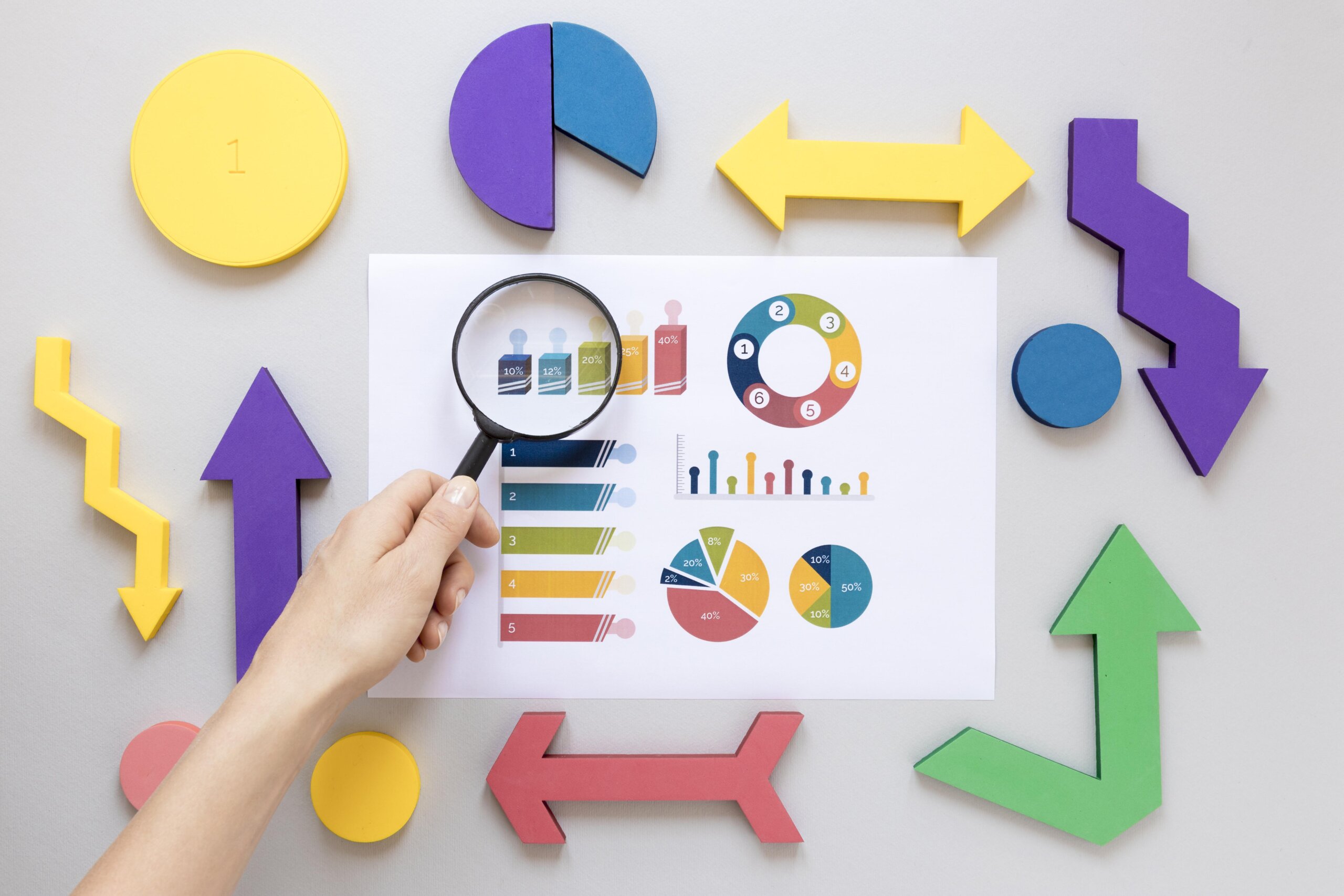
What is Quantitative Research? Definition, Methods, Types, and Examples

If you’re wondering what is quantitative research and whether this methodology works for your research study, you’re not alone. If you want a simple quantitative research definition , then it’s enough to say that this is a method undertaken by researchers based on their study requirements. However, to select the most appropriate research for their study type, researchers should know all the methods available.
Selecting the right research method depends on a few important criteria, such as the research question, study type, time, costs, data availability, and availability of respondents. There are two main types of research methods— quantitative research and qualitative research. The purpose of quantitative research is to validate or test a theory or hypothesis and that of qualitative research is to understand a subject or event or identify reasons for observed patterns.
Quantitative research methods are used to observe events that affect a particular group of individuals, which is the sample population. In this type of research, diverse numerical data are collected through various methods and then statistically analyzed to aggregate the data, compare them, or show relationships among the data. Quantitative research methods broadly include questionnaires, structured observations, and experiments.
Here are two quantitative research examples:
- Satisfaction surveys sent out by a company regarding their revamped customer service initiatives. Customers are asked to rate their experience on a rating scale of 1 (poor) to 5 (excellent).
- A school has introduced a new after-school program for children, and a few months after commencement, the school sends out feedback questionnaires to the parents of the enrolled children. Such questionnaires usually include close-ended questions that require either definite answers or a Yes/No option. This helps in a quick, overall assessment of the program’s outreach and success.
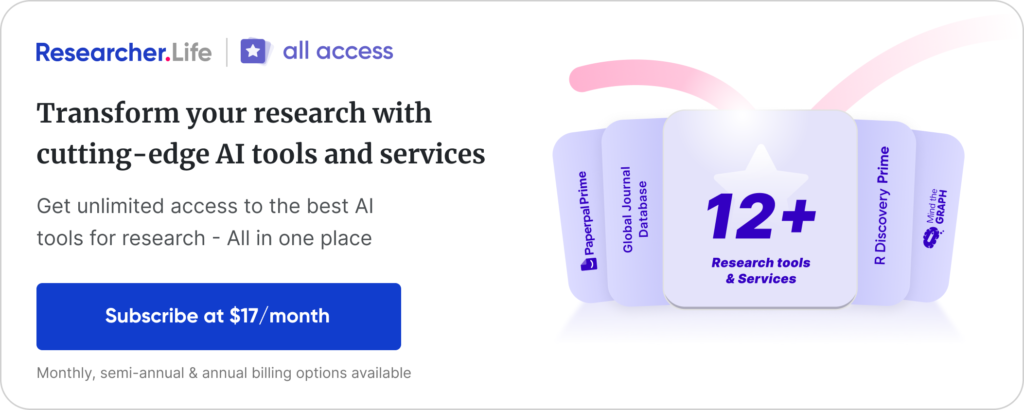
Table of Contents
What is quantitative research ? 1,2

The steps shown in the figure can be grouped into the following broad steps:
- Theory : Define the problem area or area of interest and create a research question.
- Hypothesis : Develop a hypothesis based on the research question. This hypothesis will be tested in the remaining steps.
- Research design : In this step, the most appropriate quantitative research design will be selected, including deciding on the sample size, selecting respondents, identifying research sites, if any, etc.
- Data collection : This process could be extensive based on your research objective and sample size.
- Data analysis : Statistical analysis is used to analyze the data collected. The results from the analysis help in either supporting or rejecting your hypothesis.
- Present results : Based on the data analysis, conclusions are drawn, and results are presented as accurately as possible.
Quantitative research characteristics 4
- Large sample size : This ensures reliability because this sample represents the target population or market. Due to the large sample size, the outcomes can be generalized to the entire population as well, making this one of the important characteristics of quantitative research .
- Structured data and measurable variables: The data are numeric and can be analyzed easily. Quantitative research involves the use of measurable variables such as age, salary range, highest education, etc.
- Easy-to-use data collection methods : The methods include experiments, controlled observations, and questionnaires and surveys with a rating scale or close-ended questions, which require simple and to-the-point answers; are not bound by geographical regions; and are easy to administer.
- Data analysis : Structured and accurate statistical analysis methods using software applications such as Excel, SPSS, R. The analysis is fast, accurate, and less effort intensive.
- Reliable : The respondents answer close-ended questions, their responses are direct without ambiguity and yield numeric outcomes, which are therefore highly reliable.
- Reusable outcomes : This is one of the key characteristics – outcomes of one research can be used and replicated in other research as well and is not exclusive to only one study.
Quantitative research methods 5
Quantitative research methods are classified into two types—primary and secondary.
Primary quantitative research method:
In this type of quantitative research , data are directly collected by the researchers using the following methods.
– Survey research : Surveys are the easiest and most commonly used quantitative research method . They are of two types— cross-sectional and longitudinal.
->Cross-sectional surveys are specifically conducted on a target population for a specified period, that is, these surveys have a specific starting and ending time and researchers study the events during this period to arrive at conclusions. The main purpose of these surveys is to describe and assess the characteristics of a population. There is one independent variable in this study, which is a common factor applicable to all participants in the population, for example, living in a specific city, diagnosed with a specific disease, of a certain age group, etc. An example of a cross-sectional survey is a study to understand why individuals residing in houses built before 1979 in the US are more susceptible to lead contamination.
->Longitudinal surveys are conducted at different time durations. These surveys involve observing the interactions among different variables in the target population, exposing them to various causal factors, and understanding their effects across a longer period. These studies are helpful to analyze a problem in the long term. An example of a longitudinal study is the study of the relationship between smoking and lung cancer over a long period.
– Descriptive research : Explains the current status of an identified and measurable variable. Unlike other types of quantitative research , a hypothesis is not needed at the beginning of the study and can be developed even after data collection. This type of quantitative research describes the characteristics of a problem and answers the what, when, where of a problem. However, it doesn’t answer the why of the problem and doesn’t explore cause-and-effect relationships between variables. Data from this research could be used as preliminary data for another study. Example: A researcher undertakes a study to examine the growth strategy of a company. This sample data can be used by other companies to determine their own growth strategy.

– Correlational research : This quantitative research method is used to establish a relationship between two variables using statistical analysis and analyze how one affects the other. The research is non-experimental because the researcher doesn’t control or manipulate any of the variables. At least two separate sample groups are needed for this research. Example: Researchers studying a correlation between regular exercise and diabetes.
– Causal-comparative research : This type of quantitative research examines the cause-effect relationships in retrospect between a dependent and independent variable and determines the causes of the already existing differences between groups of people. This is not a true experiment because it doesn’t assign participants to groups randomly. Example: To study the wage differences between men and women in the same role. For this, already existing wage information is analyzed to understand the relationship.
– Experimental research : This quantitative research method uses true experiments or scientific methods for determining a cause-effect relation between variables. It involves testing a hypothesis through experiments, in which one or more independent variables are manipulated and then their effect on dependent variables are studied. Example: A researcher studies the importance of a drug in treating a disease by administering the drug in few patients and not administering in a few.
The following data collection methods are commonly used in primary quantitative research :
- Sampling : The most common type is probability sampling, in which a sample is chosen from a larger population using some form of random selection, that is, every member of the population has an equal chance of being selected. The different types of probability sampling are—simple random, systematic, stratified, and cluster sampling.
- Interviews : These are commonly telephonic or face-to-face.
- Observations : Structured observations are most commonly used in quantitative research . In this method, researchers make observations about specific behaviors of individuals in a structured setting.
- Document review : Reviewing existing research or documents to collect evidence for supporting the quantitative research .
- Surveys and questionnaires : Surveys can be administered both online and offline depending on the requirement and sample size.
The data collected can be analyzed in several ways in quantitative research , as listed below:
- Cross-tabulation —Uses a tabular format to draw inferences among collected data
- MaxDiff analysis —Gauges the preferences of the respondents
- TURF analysis —Total Unduplicated Reach and Frequency Analysis; helps in determining the market strategy for a business
- Gap analysis —Identify gaps in attaining the desired results
- SWOT analysis —Helps identify strengths, weaknesses, opportunities, and threats of a product, service, or organization
- Text analysis —Used for interpreting unstructured data
Secondary quantitative research methods :
This method involves conducting research using already existing or secondary data. This method is less effort intensive and requires lesser time. However, researchers should verify the authenticity and recency of the sources being used and ensure their accuracy.
The main sources of secondary data are:
- The Internet
- Government and non-government sources
- Public libraries
- Educational institutions
- Commercial information sources such as newspapers, journals, radio, TV
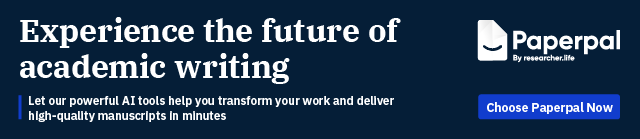
When to use quantitative research 6
Here are some simple ways to decide when to use quantitative research . Use quantitative research to:
- recommend a final course of action
- find whether a consensus exists regarding a particular subject
- generalize results to a larger population
- determine a cause-and-effect relationship between variables
- describe characteristics of specific groups of people
- test hypotheses and examine specific relationships
- identify and establish size of market segments
A research case study to understand when to use quantitative research 7
Context: A study was undertaken to evaluate a major innovation in a hospital’s design, in terms of workforce implications and impact on patient and staff experiences of all single-room hospital accommodations. The researchers undertook a mixed methods approach to answer their research questions. Here, we focus on the quantitative research aspect.
Research questions : What are the advantages and disadvantages for the staff as a result of the hospital’s move to the new design with all single-room accommodations? Did the move affect staff experience and well-being and improve their ability to deliver high-quality care?
Method: The researchers obtained quantitative data from three sources:
- Staff activity (task time distribution): Each staff member was shadowed by a researcher who observed each task undertaken by the staff, and logged the time spent on each activity.
- Staff travel distances : The staff were requested to wear pedometers, which recorded the distances covered.
- Staff experience surveys : Staff were surveyed before and after the move to the new hospital design.
Results of quantitative research : The following observations were made based on quantitative data analysis:
- The move to the new design did not result in a significant change in the proportion of time spent on different activities.
- Staff activity events observed per session were higher after the move, and direct care and professional communication events per hour decreased significantly, suggesting fewer interruptions and less fragmented care.
- A significant increase in medication tasks among the recorded events suggests that medication administration was integrated into patient care activities.
- Travel distances increased for all staff, with highest increases for staff in the older people’s ward and surgical wards.
- Ratings for staff toilet facilities, locker facilities, and space at staff bases were higher but those for social interaction and natural light were lower.
Advantages of quantitative research 1,2
When choosing the right research methodology, also consider the advantages of quantitative research and how it can impact your study.
- Quantitative research methods are more scientific and rational. They use quantifiable data leading to objectivity in the results and avoid any chances of ambiguity.
- This type of research uses numeric data so analysis is relatively easier .
- In most cases, a hypothesis is already developed and quantitative research helps in testing and validatin g these constructed theories based on which researchers can make an informed decision about accepting or rejecting their theory.
- The use of statistical analysis software ensures quick analysis of large volumes of data and is less effort intensive.
- Higher levels of control can be applied to the research so the chances of bias can be reduced.
- Quantitative research is based on measured value s, facts, and verifiable information so it can be easily checked or replicated by other researchers leading to continuity in scientific research.
Disadvantages of quantitative research 1,2
Quantitative research may also be limiting; take a look at the disadvantages of quantitative research.
- Experiments are conducted in controlled settings instead of natural settings and it is possible for researchers to either intentionally or unintentionally manipulate the experiment settings to suit the results they desire.
- Participants must necessarily give objective answers (either one- or two-word, or yes or no answers) and the reasons for their selection or the context are not considered.
- Inadequate knowledge of statistical analysis methods may affect the results and their interpretation.
- Although statistical analysis indicates the trends or patterns among variables, the reasons for these observed patterns cannot be interpreted and the research may not give a complete picture.
- Large sample sizes are needed for more accurate and generalizable analysis .
- Quantitative research cannot be used to address complex issues.
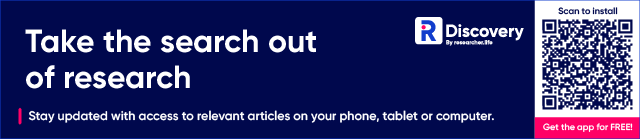
Frequently asked questions on quantitative research
Q: What is the difference between quantitative research and qualitative research? 1
A: The following table lists the key differences between quantitative research and qualitative research, some of which may have been mentioned earlier in the article.
Q: What is the difference between reliability and validity? 8,9
A: The term reliability refers to the consistency of a research study. For instance, if a food-measuring weighing scale gives different readings every time the same quantity of food is measured then that weighing scale is not reliable. If the findings in a research study are consistent every time a measurement is made, then the study is considered reliable. However, it is usually unlikely to obtain the exact same results every time because some contributing variables may change. In such cases, a correlation coefficient is used to assess the degree of reliability. A strong positive correlation between the results indicates reliability.
Validity can be defined as the degree to which a tool actually measures what it claims to measure. It helps confirm the credibility of your research and suggests that the results may be generalizable. In other words, it measures the accuracy of the research.
The following table gives the key differences between reliability and validity.
Q: What is mixed methods research? 10
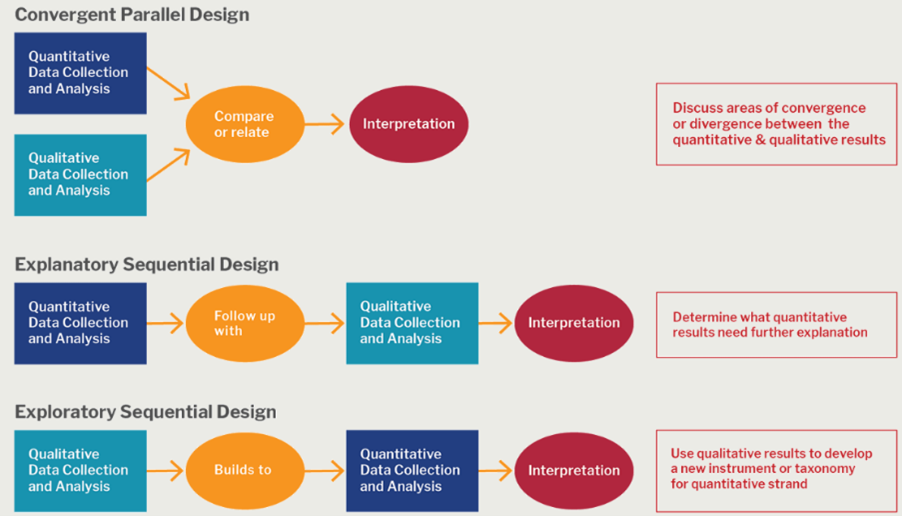
A: A mixed methods approach combines the characteristics of both quantitative research and qualitative research in the same study. This method allows researchers to validate their findings, verify if the results observed using both methods are complementary, and explain any unexpected results obtained from one method by using the other method. A mixed methods research design is useful in case of research questions that cannot be answered by either quantitative research or qualitative research alone. However, this method could be more effort- and cost-intensive because of the requirement of more resources. The figure 3 shows some basic mixed methods research designs that could be used.
Thus, quantitative research is the appropriate method for testing your hypotheses and can be used either alone or in combination with qualitative research per your study requirements. We hope this article has provided an insight into the various facets of quantitative research , including its different characteristics, advantages, and disadvantages, and a few tips to quickly understand when to use this research method.
References
- Qualitative vs quantitative research: Differences, examples, & methods. Simply Psychology. Accessed Feb 28, 2023. https://simplypsychology.org/qualitative-quantitative.html#Quantitative-Research
- Your ultimate guide to quantitative research. Qualtrics. Accessed February 28, 2023. https://www.qualtrics.com/uk/experience-management/research/quantitative-research/
- The steps of quantitative research. Revise Sociology. Accessed March 1, 2023. https://revisesociology.com/2017/11/26/the-steps-of-quantitative-research/
- What are the characteristics of quantitative research? Marketing91. Accessed March 1, 2023. https://www.marketing91.com/characteristics-of-quantitative-research/
- Quantitative research: Types, characteristics, methods, & examples. ProProfs Survey Maker. Accessed February 28, 2023. https://www.proprofssurvey.com/blog/quantitative-research/#Characteristics_of_Quantitative_Research
- Qualitative research isn’t as scientific as quantitative methods. Kmusial blog. Accessed March 5, 2023. https://kmusial.wordpress.com/2011/11/25/qualitative-research-isnt-as-scientific-as-quantitative-methods/
- Maben J, Griffiths P, Penfold C, et al. Evaluating a major innovation in hospital design: workforce implications and impact on patient and staff experiences of all single room hospital accommodation. Southampton (UK): NIHR Journals Library; 2015 Feb. (Health Services and Delivery Research, No. 3.3.) Chapter 5, Case study quantitative data findings. Accessed March 6, 2023. https://www.ncbi.nlm.nih.gov/books/NBK274429/
- McLeod, S. A. (2007). What is reliability? Simply Psychology. www.simplypsychology.org/reliability.html
- Reliability vs validity: Differences & examples. Accessed March 5, 2023. https://statisticsbyjim.com/basics/reliability-vs-validity/
- Mixed methods research. Community Engagement Program. Harvard Catalyst. Accessed February 28, 2023. https://catalyst.harvard.edu/community-engagement/mmr
Researcher.Life is a subscription-based platform that unifies the best AI tools and services designed to speed up, simplify, and streamline every step of a researcher’s journey. The Researcher.Life All Access Pack is a one-of-a-kind subscription that unlocks full access to an AI writing assistant, literature recommender, journal finder, scientific illustration tool, and exclusive discounts on professional publication services from Editage.
Based on 21+ years of experience in academia, Researcher.Life All Access empowers researchers to put their best research forward and move closer to success. Explore our top AI Tools pack, AI Tools + Publication Services pack, or Build Your Own Plan. Find everything a researcher needs to succeed, all in one place – Get All Access now starting at just $17 a month !
Related Posts
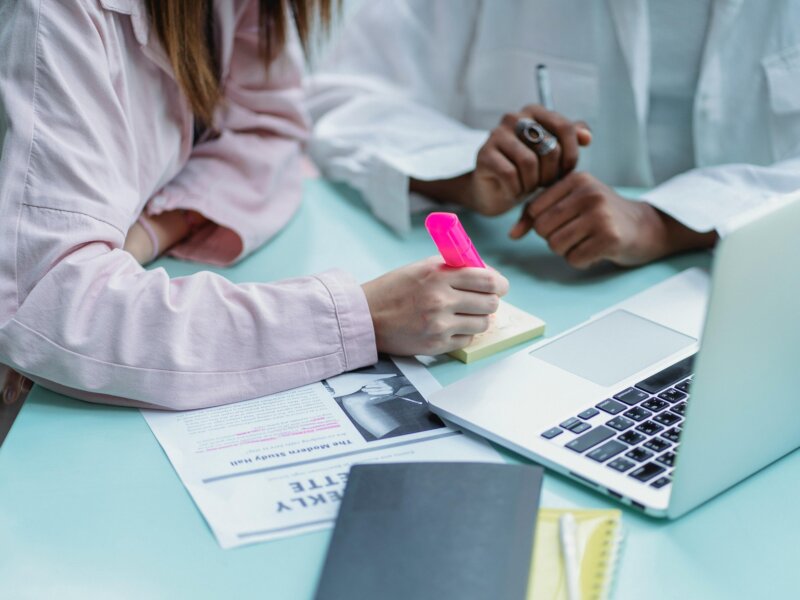
Research Process Steps: What Are They and How to Follow Them?
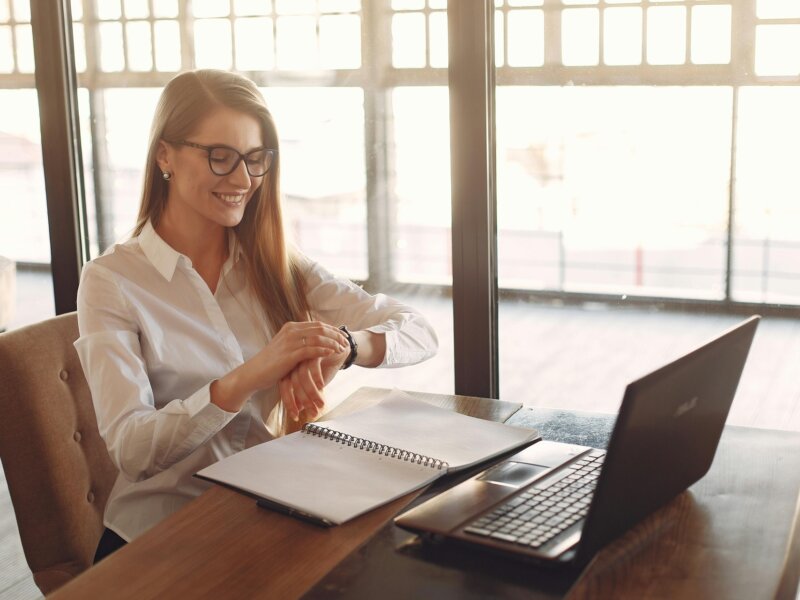
Academic Publishing Speed: Researcher.Life Adds Time to Publish Metric to Enhance Journal Selection
- Privacy Policy

Home » Quantitative Research – Methods, Types and Analysis
Quantitative Research – Methods, Types and Analysis
Table of Contents

Quantitative Research
Quantitative research is a type of research that collects and analyzes numerical data to test hypotheses and answer research questions . This research typically involves a large sample size and uses statistical analysis to make inferences about a population based on the data collected. It often involves the use of surveys, experiments, or other structured data collection methods to gather quantitative data.
Quantitative Research Methods

Quantitative Research Methods are as follows:
Descriptive Research Design
Descriptive research design is used to describe the characteristics of a population or phenomenon being studied. This research method is used to answer the questions of what, where, when, and how. Descriptive research designs use a variety of methods such as observation, case studies, and surveys to collect data. The data is then analyzed using statistical tools to identify patterns and relationships.
Correlational Research Design
Correlational research design is used to investigate the relationship between two or more variables. Researchers use correlational research to determine whether a relationship exists between variables and to what extent they are related. This research method involves collecting data from a sample and analyzing it using statistical tools such as correlation coefficients.
Quasi-experimental Research Design
Quasi-experimental research design is used to investigate cause-and-effect relationships between variables. This research method is similar to experimental research design, but it lacks full control over the independent variable. Researchers use quasi-experimental research designs when it is not feasible or ethical to manipulate the independent variable.
Experimental Research Design
Experimental research design is used to investigate cause-and-effect relationships between variables. This research method involves manipulating the independent variable and observing the effects on the dependent variable. Researchers use experimental research designs to test hypotheses and establish cause-and-effect relationships.
Survey Research
Survey research involves collecting data from a sample of individuals using a standardized questionnaire. This research method is used to gather information on attitudes, beliefs, and behaviors of individuals. Researchers use survey research to collect data quickly and efficiently from a large sample size. Survey research can be conducted through various methods such as online, phone, mail, or in-person interviews.
Quantitative Research Analysis Methods
Here are some commonly used quantitative research analysis methods:
Statistical Analysis
Statistical analysis is the most common quantitative research analysis method. It involves using statistical tools and techniques to analyze the numerical data collected during the research process. Statistical analysis can be used to identify patterns, trends, and relationships between variables, and to test hypotheses and theories.
Regression Analysis
Regression analysis is a statistical technique used to analyze the relationship between one dependent variable and one or more independent variables. Researchers use regression analysis to identify and quantify the impact of independent variables on the dependent variable.
Factor Analysis
Factor analysis is a statistical technique used to identify underlying factors that explain the correlations among a set of variables. Researchers use factor analysis to reduce a large number of variables to a smaller set of factors that capture the most important information.
Structural Equation Modeling
Structural equation modeling is a statistical technique used to test complex relationships between variables. It involves specifying a model that includes both observed and unobserved variables, and then using statistical methods to test the fit of the model to the data.
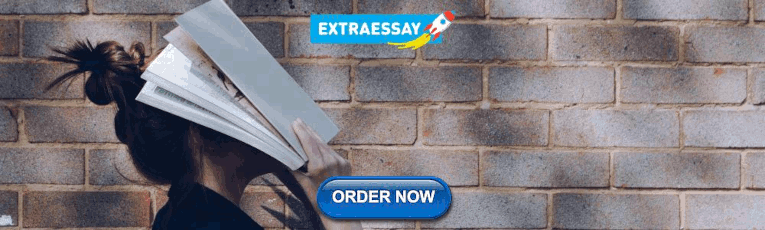
Time Series Analysis
Time series analysis is a statistical technique used to analyze data that is collected over time. It involves identifying patterns and trends in the data, as well as any seasonal or cyclical variations.
Multilevel Modeling
Multilevel modeling is a statistical technique used to analyze data that is nested within multiple levels. For example, researchers might use multilevel modeling to analyze data that is collected from individuals who are nested within groups, such as students nested within schools.
Applications of Quantitative Research
Quantitative research has many applications across a wide range of fields. Here are some common examples:
- Market Research : Quantitative research is used extensively in market research to understand consumer behavior, preferences, and trends. Researchers use surveys, experiments, and other quantitative methods to collect data that can inform marketing strategies, product development, and pricing decisions.
- Health Research: Quantitative research is used in health research to study the effectiveness of medical treatments, identify risk factors for diseases, and track health outcomes over time. Researchers use statistical methods to analyze data from clinical trials, surveys, and other sources to inform medical practice and policy.
- Social Science Research: Quantitative research is used in social science research to study human behavior, attitudes, and social structures. Researchers use surveys, experiments, and other quantitative methods to collect data that can inform social policies, educational programs, and community interventions.
- Education Research: Quantitative research is used in education research to study the effectiveness of teaching methods, assess student learning outcomes, and identify factors that influence student success. Researchers use experimental and quasi-experimental designs, as well as surveys and other quantitative methods, to collect and analyze data.
- Environmental Research: Quantitative research is used in environmental research to study the impact of human activities on the environment, assess the effectiveness of conservation strategies, and identify ways to reduce environmental risks. Researchers use statistical methods to analyze data from field studies, experiments, and other sources.
Characteristics of Quantitative Research
Here are some key characteristics of quantitative research:
- Numerical data : Quantitative research involves collecting numerical data through standardized methods such as surveys, experiments, and observational studies. This data is analyzed using statistical methods to identify patterns and relationships.
- Large sample size: Quantitative research often involves collecting data from a large sample of individuals or groups in order to increase the reliability and generalizability of the findings.
- Objective approach: Quantitative research aims to be objective and impartial in its approach, focusing on the collection and analysis of data rather than personal beliefs, opinions, or experiences.
- Control over variables: Quantitative research often involves manipulating variables to test hypotheses and establish cause-and-effect relationships. Researchers aim to control for extraneous variables that may impact the results.
- Replicable : Quantitative research aims to be replicable, meaning that other researchers should be able to conduct similar studies and obtain similar results using the same methods.
- Statistical analysis: Quantitative research involves using statistical tools and techniques to analyze the numerical data collected during the research process. Statistical analysis allows researchers to identify patterns, trends, and relationships between variables, and to test hypotheses and theories.
- Generalizability: Quantitative research aims to produce findings that can be generalized to larger populations beyond the specific sample studied. This is achieved through the use of random sampling methods and statistical inference.
Examples of Quantitative Research
Here are some examples of quantitative research in different fields:
- Market Research: A company conducts a survey of 1000 consumers to determine their brand awareness and preferences. The data is analyzed using statistical methods to identify trends and patterns that can inform marketing strategies.
- Health Research : A researcher conducts a randomized controlled trial to test the effectiveness of a new drug for treating a particular medical condition. The study involves collecting data from a large sample of patients and analyzing the results using statistical methods.
- Social Science Research : A sociologist conducts a survey of 500 people to study attitudes toward immigration in a particular country. The data is analyzed using statistical methods to identify factors that influence these attitudes.
- Education Research: A researcher conducts an experiment to compare the effectiveness of two different teaching methods for improving student learning outcomes. The study involves randomly assigning students to different groups and collecting data on their performance on standardized tests.
- Environmental Research : A team of researchers conduct a study to investigate the impact of climate change on the distribution and abundance of a particular species of plant or animal. The study involves collecting data on environmental factors and population sizes over time and analyzing the results using statistical methods.
- Psychology : A researcher conducts a survey of 500 college students to investigate the relationship between social media use and mental health. The data is analyzed using statistical methods to identify correlations and potential causal relationships.
- Political Science: A team of researchers conducts a study to investigate voter behavior during an election. They use survey methods to collect data on voting patterns, demographics, and political attitudes, and analyze the results using statistical methods.
How to Conduct Quantitative Research
Here is a general overview of how to conduct quantitative research:
- Develop a research question: The first step in conducting quantitative research is to develop a clear and specific research question. This question should be based on a gap in existing knowledge, and should be answerable using quantitative methods.
- Develop a research design: Once you have a research question, you will need to develop a research design. This involves deciding on the appropriate methods to collect data, such as surveys, experiments, or observational studies. You will also need to determine the appropriate sample size, data collection instruments, and data analysis techniques.
- Collect data: The next step is to collect data. This may involve administering surveys or questionnaires, conducting experiments, or gathering data from existing sources. It is important to use standardized methods to ensure that the data is reliable and valid.
- Analyze data : Once the data has been collected, it is time to analyze it. This involves using statistical methods to identify patterns, trends, and relationships between variables. Common statistical techniques include correlation analysis, regression analysis, and hypothesis testing.
- Interpret results: After analyzing the data, you will need to interpret the results. This involves identifying the key findings, determining their significance, and drawing conclusions based on the data.
- Communicate findings: Finally, you will need to communicate your findings. This may involve writing a research report, presenting at a conference, or publishing in a peer-reviewed journal. It is important to clearly communicate the research question, methods, results, and conclusions to ensure that others can understand and replicate your research.
When to use Quantitative Research
Here are some situations when quantitative research can be appropriate:
- To test a hypothesis: Quantitative research is often used to test a hypothesis or a theory. It involves collecting numerical data and using statistical analysis to determine if the data supports or refutes the hypothesis.
- To generalize findings: If you want to generalize the findings of your study to a larger population, quantitative research can be useful. This is because it allows you to collect numerical data from a representative sample of the population and use statistical analysis to make inferences about the population as a whole.
- To measure relationships between variables: If you want to measure the relationship between two or more variables, such as the relationship between age and income, or between education level and job satisfaction, quantitative research can be useful. It allows you to collect numerical data on both variables and use statistical analysis to determine the strength and direction of the relationship.
- To identify patterns or trends: Quantitative research can be useful for identifying patterns or trends in data. For example, you can use quantitative research to identify trends in consumer behavior or to identify patterns in stock market data.
- To quantify attitudes or opinions : If you want to measure attitudes or opinions on a particular topic, quantitative research can be useful. It allows you to collect numerical data using surveys or questionnaires and analyze the data using statistical methods to determine the prevalence of certain attitudes or opinions.
Purpose of Quantitative Research
The purpose of quantitative research is to systematically investigate and measure the relationships between variables or phenomena using numerical data and statistical analysis. The main objectives of quantitative research include:
- Description : To provide a detailed and accurate description of a particular phenomenon or population.
- Explanation : To explain the reasons for the occurrence of a particular phenomenon, such as identifying the factors that influence a behavior or attitude.
- Prediction : To predict future trends or behaviors based on past patterns and relationships between variables.
- Control : To identify the best strategies for controlling or influencing a particular outcome or behavior.
Quantitative research is used in many different fields, including social sciences, business, engineering, and health sciences. It can be used to investigate a wide range of phenomena, from human behavior and attitudes to physical and biological processes. The purpose of quantitative research is to provide reliable and valid data that can be used to inform decision-making and improve understanding of the world around us.
Advantages of Quantitative Research
There are several advantages of quantitative research, including:
- Objectivity : Quantitative research is based on objective data and statistical analysis, which reduces the potential for bias or subjectivity in the research process.
- Reproducibility : Because quantitative research involves standardized methods and measurements, it is more likely to be reproducible and reliable.
- Generalizability : Quantitative research allows for generalizations to be made about a population based on a representative sample, which can inform decision-making and policy development.
- Precision : Quantitative research allows for precise measurement and analysis of data, which can provide a more accurate understanding of phenomena and relationships between variables.
- Efficiency : Quantitative research can be conducted relatively quickly and efficiently, especially when compared to qualitative research, which may involve lengthy data collection and analysis.
- Large sample sizes : Quantitative research can accommodate large sample sizes, which can increase the representativeness and generalizability of the results.
Limitations of Quantitative Research
There are several limitations of quantitative research, including:
- Limited understanding of context: Quantitative research typically focuses on numerical data and statistical analysis, which may not provide a comprehensive understanding of the context or underlying factors that influence a phenomenon.
- Simplification of complex phenomena: Quantitative research often involves simplifying complex phenomena into measurable variables, which may not capture the full complexity of the phenomenon being studied.
- Potential for researcher bias: Although quantitative research aims to be objective, there is still the potential for researcher bias in areas such as sampling, data collection, and data analysis.
- Limited ability to explore new ideas: Quantitative research is often based on pre-determined research questions and hypotheses, which may limit the ability to explore new ideas or unexpected findings.
- Limited ability to capture subjective experiences : Quantitative research is typically focused on objective data and may not capture the subjective experiences of individuals or groups being studied.
- Ethical concerns : Quantitative research may raise ethical concerns, such as invasion of privacy or the potential for harm to participants.
About the author
Muhammad Hassan
Researcher, Academic Writer, Web developer
You may also like

Questionnaire – Definition, Types, and Examples

Case Study – Methods, Examples and Guide

Observational Research – Methods and Guide

Qualitative Research Methods

Explanatory Research – Types, Methods, Guide

Survey Research – Types, Methods, Examples
Home • Knowledge hub • What is quantitative research?
What is quantitative research?
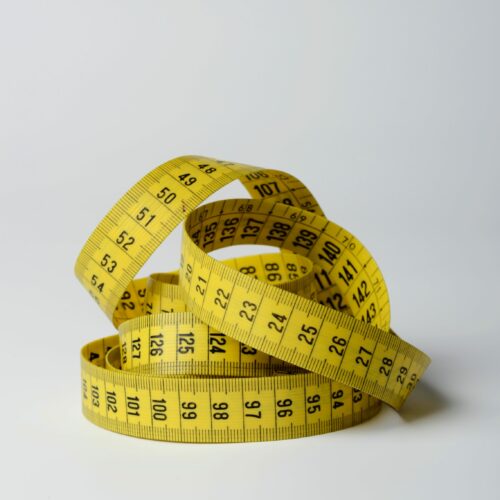
Quantitative research is an important part of market research that relies on hard facts and numerical data to gain as objective a picture of people’s opinions as possible.
It’s different from qualitative research in a number of important ways and is a highly useful tool for researchers.
Quantitative research is a systematic empirical approach used in the social sciences and various other fields to gather, analyze, and interpret numerical data. It focuses on obtaining measurable data and applying statistical methods to generalize findings to a larger population.
Researchers use structured instruments such as surveys, questionnaires, or experiments to collect data from a representative sample in quantitative research. The data collected is typically numerical values or categorical responses that can be analyzed using statistical techniques. These statistical analyses help researchers identify patterns, relationships, trends, or associations among variables.
Quantitative research aims to generate objective and reliable information about a particular phenomenon, population, or group. It aims to better understand the subject under investigation by employing statistical measures such as means, percentages, correlations, or regression analyses.
Quantitative research provides:
- A quantitative understanding of social phenomena.
- Allowing researchers to make generalizations.
- Predictions.
- Comparisons based on numerical data.
It is widely used in psychology, sociology, economics, marketing, and many other disciplines to explore and gain insights into various research questions.
In this article, we’ll take a deep dive into quantitative research, why it’s important, and how to use it effectively.
How is quantitative research different from qualitative research?
Although they’re both extremely useful, there are a number of key differences between quantitative and qualitative market research strategies. A solid market research strategy will make use of both qualitative and quantitative research.
- Quantitative research relies on gathering numerical data points. Qualitative research on the other hand, as the name suggests, seeks to gather qualitative data by speaking to people in individual or group settings.
- Quantitative research normally uses closed questions, while qualitative research uses open questions more frequently.
- Quantitative research is great for establishing trends and patterns of behavior, whereas qualitative methods are great for explaining the “why” behind them.
Why is quantitative research useful?
Quantitative research has a crucial role to play in any market research strategy for a range of reasons:
- It enables you to conduct research at scale
- When quantitative research is conducted in a representative way, it can reveal insights about broader groups of people or the population as a whole
- It enables us to easily compare different groups (e.g. by age, gender or market) to understand similarities or differences
- It can help businesses understand the size of a new opportunity
- It can be helpful for reducing a complex problem or topic to a limited number of variables
Get regular insights
Keep up to date with the latest insights from our research as well as all our company news in our free monthly newsletter.
- First Name *
- Last Name *
- Business Email *
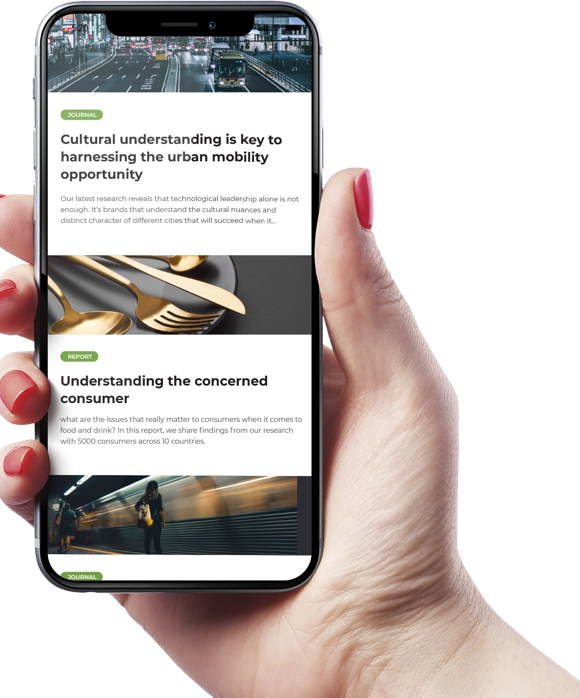
Quantitative Research Design
Quantitative research design refers to the overall plan and structure that guides the collection, analysis, and interpretation of numerical data in a quantitative research study. It outlines the specific steps, procedures, and techniques used to address research questions or test hypotheses systematically and rigorously. A well-designed quantitative research study ensures that the data collected is reliable, valid, and capable of answering the research objectives.
There are several key components involved in designing a quantitative research study:
- Research Questions or Hypotheses: The research design begins with clearly defined research questions or hypotheses articulating the study’s objectives. These questions guide the selection of variables and the development of research instruments.
- Sampling: A critical aspect of quantitative research design is selecting a representative sample from the target population. The sample should be carefully chosen to ensure it adequately represents the population of interest, allowing for the generalizability of the findings.
- Variables and Operationalization: Quantitative research involves the measurement of variables. In the research design phase, researchers identify the variables they will study and determine how to operationalize them into measurable and observable forms. This includes defining the indicators or measures used to assess each variable.
- Data Collection Methods: Quantitative research typically involves collecting data through structured instruments, such as surveys, questionnaires, or tests. The research design specifies the data collection methods, including the procedures for administering the instruments, the timing of data collection, and the strategies for maximizing response rates.
- Data Analysis: Quantitative research design includes decisions about the statistical techniques and analyses applied to the collected data. This may involve descriptive statistics (e.g., means, percentages) and inferential statistics (e.g., t-tests, regression analyses) to examine variables’ relationships, differences, or associations.
- Validity and Reliability: Ensuring the validity and reliability of the data is a crucial consideration in quantitative research design. Validity refers to the extent to which a measurement instrument or procedure accurately measures what it intends to measure. Reliability refers to the consistency and stability of the measurement over time and across different conditions. Researchers employ pilot testing, validity checks, and statistical measures to enhance validity and reliability.
- Ethical Considerations: Quantitative research design also includes ethical considerations, such as obtaining informed consent from participants, protecting their privacy and confidentiality, and ensuring the study adheres to ethical guidelines and regulations.
By carefully designing a quantitative research study, researchers can ensure their investigations are methodologically sound, reliable, and valid.
Well-designed research provides a solid foundation for collecting and analyzing numerical data, allowing researchers to draw meaningful conclusions and contribute to the body of knowledge in their respective fields.
Quantitative research data collection methods
When collecting and analyzing the data you need for quantitative research, you have a number of possibilities available to you. Each has its own pros and cons, and it might be best to use a mix. Here are some of the main research methods:
Survey research
This involves sending out surveys to your target audience to collect information before statistically analyzing the results to draw conclusions and insights. It’s a great way to better understand your target customers or explore a new market and can be turned around quickly.
There are a number of different ways of conducting surveys, such as:
- Email — this is a quick way of reaching a large number of people and can be more affordable than the other methods described below.
- Phone — not everyone has access to the internet so if you’re looking to reach a particular demographic that may struggle to engage in this way (e.g. older consumers) telephone can be a better approach. That said, it can be expensive and time-consuming.
- Post or Mail — as with the phone, you can reach a wide segment of the population, but it’s expensive and takes a long time. As organizations look to identify and react to changes in consumer behavior at speed, postal surveys have become somewhat outdated.
- In-person — in some instances it makes sense to conduct quantitative research in person. Examples of this include intercepts where you need to collect quantitative data about the customer experience in the moment or taste tests or central location tests , where you need consumers to physically interact with a product to provide useful feedback. Conducting research in this way can be expensive and logistically challenging to organize and carry out.
Survey questions for quantitative research usually include closed-ended questions rather than the open-ended questions used in qualitative research. For example, instead of asking
“How do you feel about our delivery policy?”
You might ask…
“How satisfied are you with our delivery policy? “Very satisfied / Satisfied / Don’t Know / Dissatisfied / Very Dissatisfied”
This way, you’ll gain data that can be categorized and analyzed in a quantitative, numbers-based way.
Correlational Research
Correlational research is a specific type of quantitative research that examines the relationship between two or more variables. It focuses on determining whether there is a statistical association or correlation between variables without establishing causality. In other words, correlational research helps to understand how changes in one variable correspond to changes in another.
One of the critical features of correlational research is that it allows researchers to analyze data from existing sources or collect data through surveys or questionnaires. By measuring the variables of interest, researchers can calculate a correlation coefficient, such as Pearson’s, to quantify the strength and direction of the relationship. The correlation coefficient ranges from -1 to +1, where a positive value indicates a positive relationship, a negative value indicates a negative relationship and a value close to zero suggests no significant relationship. Correlational research is valuable in various fields, such as psychology, sociology, and economics, as it helps researchers explore connections between variables that may not be feasible to manipulate in an experimental setting. For example, a psychologist might use correlational research to investigate the relationship between sleep duration and student academic performance. By collecting data on these variables, they can determine whether there is a correlation between the two factors and to what extent they are related. It is important to note that correlational research does not imply causation. While a correlation suggests an association between variables, it does not provide evidence for a cause-and-effect relationship. Other factors, known as confounding variables, may be influencing the observed relationship. Therefore, researchers must exercise caution in interpreting correlational findings and consider additional research methods, such as experimental studies, to establish causality. Correlational research is vital in quantitative research and analysis by investigating relationships between variables. It provides valuable insights into the strength and direction of associations and helps researchers generate hypotheses for further investigation. By understanding the limitations of correlational research, researchers can use this method effectively to explore connections between variables in various disciplines.
Experimental Research
Experimental research is a fundamental approach within quantitative research that aims to establish cause-and-effect relationships between variables. It involves the manipulation of an independent variable and measuring its effects on a dependent variable while controlling for potential confounding variables. Experimental research is highly regarded for its ability to provide rigorous evidence and draw conclusions about causal relationships. The hallmark of experimental research is the presence of at least two groups: the experimental and control groups. The experimental group receives the manipulated variable, the independent variable, while the control group does not. By comparing the outcomes or responses of the two groups, researchers can attribute any differences observed to the effects of the independent variable. Several key components are employed to ensure the reliability and validity of experimental research. Random assignment is a crucial step that involves assigning participants to either the experimental or control group in a random and unbiased manner. This minimizes the potential for pre-existing differences between groups and strengthens the study’s internal validity. Another essential feature of experimental research is the ability to control extraneous variables. By carefully designing the study environment and procedures, researchers can minimize the influence of factors other than the independent variable on the dependent variable. This control enhances the ability to isolate the manipulated variable’s effects and increases the study’s internal validity. Quantitative data is typically collected in experimental research through objective and standardized measurements. Researchers use instruments such as surveys, tests, observations, or physiological measurements to gather numerical data that can be analyzed statistically. This allows for applying various statistical techniques, such as t-tests or analysis of variance (ANOVA), to determine the significance of the observed effects and draw conclusions about the relationship between variables. Experimental research is widely used across psychology, medicine, education, and the natural sciences. It enables researchers to test hypotheses, evaluate interventions or treatments, and provide evidence-based recommendations. Experimental research offers valuable insights into the effectiveness or impact of specific variables, interventions, or strategies by establishing cause-and-effect relationships. Despite its strengths, experimental research also has limitations. The artificial nature of laboratory settings and the need for control may reduce the generalizability of findings to real-world contexts. Ethical considerations also play a crucial role in experimental research, as researchers must ensure participants’ well-being and informed consent. Experimental research is a powerful tool in the quantitative research arsenal. It enables researchers to establish cause-and-effect relationships, control extraneous variables, and gather objective numerical data. Experimental research contributes to evidence-based decision-making and advances knowledge in various fields by employing rigorous methods.
Analyzing results
Once you have your results, the next step — and one of the most important overall — is to categorize and analyze them.
There are many ways to do this. One powerful method is cross-tabulation, where you separate your results into categories based on demographic subgroups. For example, of the people who answered ‘yes’ to a question, how many of them were business leaders and how many were entry-level employees?
You’ll also need to take time to clean the data (for example removing people who sped through the survey, selecting the same answer) to make sure you can confidently draw conclusions. This can all be taken care of by the right team of experts.
The importance of quantitative research
Quantitative research is a powerful tool for anyone looking to learn more about their market and customers. It allows you to gain reliable, objective insights from data and clearly understand trends and patterns.
Where quantitative research falls short is in explaining the ‘why’. This is where you need to turn to other methods, like qualitative research, where you’ll actually talk to your audience and delve into the more subjective factors driving their decision-making.
At Kadence, it’s our job to help you with every aspect of your research strategy. We’ve done this with countless businesses, and we’d love to do it with you. To find out more, get in touch with us .
Helping brands uncover valuable insights
We’ve been working with Kadence on a couple of strategic projects, which influenced our product roadmap roll-out within the region. Their work has been exceptional in providing me the insights that I need. Senior Marketing Executive Arla Foods
Kadence’s reports give us the insight, conclusion and recommended execution needed to give us a different perspective, which provided us with an opportunity to relook at our go to market strategy in a different direction which we are now reaping the benefits from. Sales & Marketing Bridgestone
Kadence helped us not only conduct a thorough and insightful piece of research, its interpretation of the data provided many useful and unexpected good-news stories that we were able to use in our communications and interactions with government bodies. General Manager PR -Internal Communications & Government Affairs Mitsubishi
Kadence team is more like a partner to us. We have run a number of projects together and … the pro-activeness, out of the box thinking and delivering in spite of tight deadlines are some of the key reasons we always reach out to them. Vital Strategies
Kadence were an excellent partner on this project; they took time to really understand our business challenges, and developed a research approach that would tackle the exam question from all directions. The impact of the work is still being felt now, several years later. Customer Intelligence Director Wall Street Journal
Get In Touch
" (Required) " indicates required fields
Privacy Overview
Qualitative vs Quantitative Research Methods & Data Analysis
Saul Mcleod, PhD
Editor-in-Chief for Simply Psychology
BSc (Hons) Psychology, MRes, PhD, University of Manchester
Saul Mcleod, PhD., is a qualified psychology teacher with over 18 years of experience in further and higher education. He has been published in peer-reviewed journals, including the Journal of Clinical Psychology.
Learn about our Editorial Process
Olivia Guy-Evans, MSc
Associate Editor for Simply Psychology
BSc (Hons) Psychology, MSc Psychology of Education
Olivia Guy-Evans is a writer and associate editor for Simply Psychology. She has previously worked in healthcare and educational sectors.
On This Page:
What is the difference between quantitative and qualitative?
The main difference between quantitative and qualitative research is the type of data they collect and analyze.
Quantitative research collects numerical data and analyzes it using statistical methods. The aim is to produce objective, empirical data that can be measured and expressed in numerical terms. Quantitative research is often used to test hypotheses, identify patterns, and make predictions.
Qualitative research , on the other hand, collects non-numerical data such as words, images, and sounds. The focus is on exploring subjective experiences, opinions, and attitudes, often through observation and interviews.
Qualitative research aims to produce rich and detailed descriptions of the phenomenon being studied, and to uncover new insights and meanings.
Quantitative data is information about quantities, and therefore numbers, and qualitative data is descriptive, and regards phenomenon which can be observed but not measured, such as language.
What Is Qualitative Research?
Qualitative research is the process of collecting, analyzing, and interpreting non-numerical data, such as language. Qualitative research can be used to understand how an individual subjectively perceives and gives meaning to their social reality.
Qualitative data is non-numerical data, such as text, video, photographs, or audio recordings. This type of data can be collected using diary accounts or in-depth interviews and analyzed using grounded theory or thematic analysis.
Qualitative research is multimethod in focus, involving an interpretive, naturalistic approach to its subject matter. This means that qualitative researchers study things in their natural settings, attempting to make sense of, or interpret, phenomena in terms of the meanings people bring to them. Denzin and Lincoln (1994, p. 2)
Interest in qualitative data came about as the result of the dissatisfaction of some psychologists (e.g., Carl Rogers) with the scientific study of psychologists such as behaviorists (e.g., Skinner ).
Since psychologists study people, the traditional approach to science is not seen as an appropriate way of carrying out research since it fails to capture the totality of human experience and the essence of being human. Exploring participants’ experiences is known as a phenomenological approach (re: Humanism ).
Qualitative research is primarily concerned with meaning, subjectivity, and lived experience. The goal is to understand the quality and texture of people’s experiences, how they make sense of them, and the implications for their lives.
Qualitative research aims to understand the social reality of individuals, groups, and cultures as nearly as possible as participants feel or live it. Thus, people and groups are studied in their natural setting.
Some examples of qualitative research questions are provided, such as what an experience feels like, how people talk about something, how they make sense of an experience, and how events unfold for people.
Research following a qualitative approach is exploratory and seeks to explain ‘how’ and ‘why’ a particular phenomenon, or behavior, operates as it does in a particular context. It can be used to generate hypotheses and theories from the data.
Qualitative Methods
There are different types of qualitative research methods, including diary accounts, in-depth interviews , documents, focus groups , case study research , and ethnography.
The results of qualitative methods provide a deep understanding of how people perceive their social realities and in consequence, how they act within the social world.
The researcher has several methods for collecting empirical materials, ranging from the interview to direct observation, to the analysis of artifacts, documents, and cultural records, to the use of visual materials or personal experience. Denzin and Lincoln (1994, p. 14)
Here are some examples of qualitative data:
Interview transcripts : Verbatim records of what participants said during an interview or focus group. They allow researchers to identify common themes and patterns, and draw conclusions based on the data. Interview transcripts can also be useful in providing direct quotes and examples to support research findings.
Observations : The researcher typically takes detailed notes on what they observe, including any contextual information, nonverbal cues, or other relevant details. The resulting observational data can be analyzed to gain insights into social phenomena, such as human behavior, social interactions, and cultural practices.
Unstructured interviews : generate qualitative data through the use of open questions. This allows the respondent to talk in some depth, choosing their own words. This helps the researcher develop a real sense of a person’s understanding of a situation.
Diaries or journals : Written accounts of personal experiences or reflections.
Notice that qualitative data could be much more than just words or text. Photographs, videos, sound recordings, and so on, can be considered qualitative data. Visual data can be used to understand behaviors, environments, and social interactions.
Qualitative Data Analysis
Qualitative research is endlessly creative and interpretive. The researcher does not just leave the field with mountains of empirical data and then easily write up his or her findings.
Qualitative interpretations are constructed, and various techniques can be used to make sense of the data, such as content analysis, grounded theory (Glaser & Strauss, 1967), thematic analysis (Braun & Clarke, 2006), or discourse analysis.
For example, thematic analysis is a qualitative approach that involves identifying implicit or explicit ideas within the data. Themes will often emerge once the data has been coded.
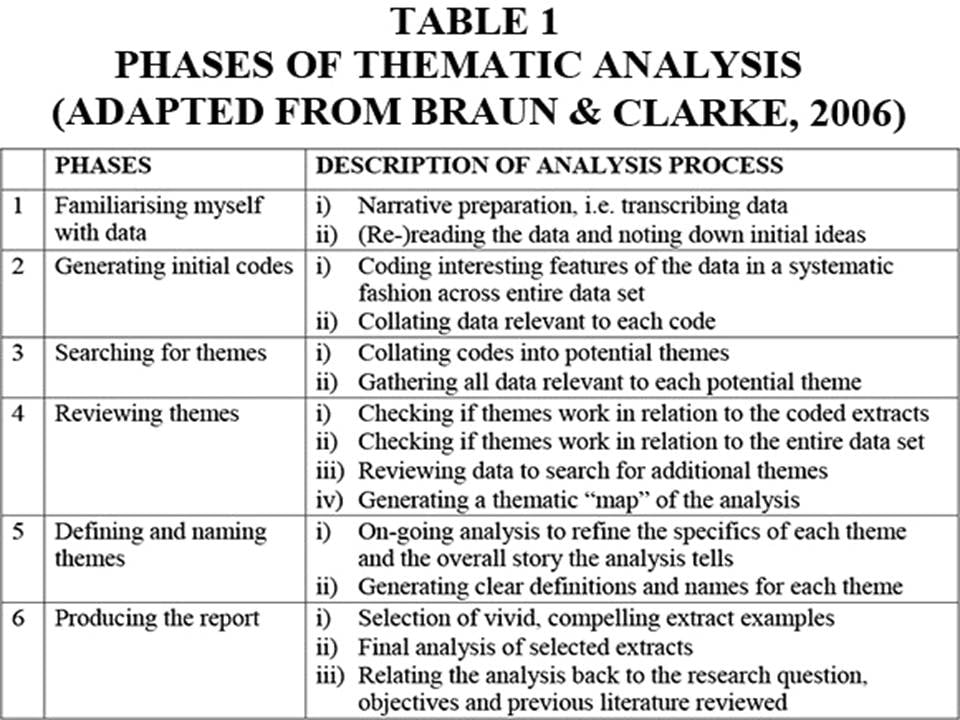
Key Features
- Events can be understood adequately only if they are seen in context. Therefore, a qualitative researcher immerses her/himself in the field, in natural surroundings. The contexts of inquiry are not contrived; they are natural. Nothing is predefined or taken for granted.
- Qualitative researchers want those who are studied to speak for themselves, to provide their perspectives in words and other actions. Therefore, qualitative research is an interactive process in which the persons studied teach the researcher about their lives.
- The qualitative researcher is an integral part of the data; without the active participation of the researcher, no data exists.
- The study’s design evolves during the research and can be adjusted or changed as it progresses. For the qualitative researcher, there is no single reality. It is subjective and exists only in reference to the observer.
- The theory is data-driven and emerges as part of the research process, evolving from the data as they are collected.
Limitations of Qualitative Research
- Because of the time and costs involved, qualitative designs do not generally draw samples from large-scale data sets.
- The problem of adequate validity or reliability is a major criticism. Because of the subjective nature of qualitative data and its origin in single contexts, it is difficult to apply conventional standards of reliability and validity. For example, because of the central role played by the researcher in the generation of data, it is not possible to replicate qualitative studies.
- Also, contexts, situations, events, conditions, and interactions cannot be replicated to any extent, nor can generalizations be made to a wider context than the one studied with confidence.
- The time required for data collection, analysis, and interpretation is lengthy. Analysis of qualitative data is difficult, and expert knowledge of an area is necessary to interpret qualitative data. Great care must be taken when doing so, for example, looking for mental illness symptoms.
Advantages of Qualitative Research
- Because of close researcher involvement, the researcher gains an insider’s view of the field. This allows the researcher to find issues that are often missed (such as subtleties and complexities) by the scientific, more positivistic inquiries.
- Qualitative descriptions can be important in suggesting possible relationships, causes, effects, and dynamic processes.
- Qualitative analysis allows for ambiguities/contradictions in the data, which reflect social reality (Denscombe, 2010).
- Qualitative research uses a descriptive, narrative style; this research might be of particular benefit to the practitioner as she or he could turn to qualitative reports to examine forms of knowledge that might otherwise be unavailable, thereby gaining new insight.
What Is Quantitative Research?
Quantitative research involves the process of objectively collecting and analyzing numerical data to describe, predict, or control variables of interest.
The goals of quantitative research are to test causal relationships between variables , make predictions, and generalize results to wider populations.
Quantitative researchers aim to establish general laws of behavior and phenomenon across different settings/contexts. Research is used to test a theory and ultimately support or reject it.
Quantitative Methods
Experiments typically yield quantitative data, as they are concerned with measuring things. However, other research methods, such as controlled observations and questionnaires , can produce both quantitative information.
For example, a rating scale or closed questions on a questionnaire would generate quantitative data as these produce either numerical data or data that can be put into categories (e.g., “yes,” “no” answers).
Experimental methods limit how research participants react to and express appropriate social behavior.
Findings are, therefore, likely to be context-bound and simply a reflection of the assumptions that the researcher brings to the investigation.
There are numerous examples of quantitative data in psychological research, including mental health. Here are a few examples:
Another example is the Experience in Close Relationships Scale (ECR), a self-report questionnaire widely used to assess adult attachment styles .
The ECR provides quantitative data that can be used to assess attachment styles and predict relationship outcomes.
Neuroimaging data : Neuroimaging techniques, such as MRI and fMRI, provide quantitative data on brain structure and function.
This data can be analyzed to identify brain regions involved in specific mental processes or disorders.
For example, the Beck Depression Inventory (BDI) is a clinician-administered questionnaire widely used to assess the severity of depressive symptoms in individuals.
The BDI consists of 21 questions, each scored on a scale of 0 to 3, with higher scores indicating more severe depressive symptoms.
Quantitative Data Analysis
Statistics help us turn quantitative data into useful information to help with decision-making. We can use statistics to summarize our data, describing patterns, relationships, and connections. Statistics can be descriptive or inferential.
Descriptive statistics help us to summarize our data. In contrast, inferential statistics are used to identify statistically significant differences between groups of data (such as intervention and control groups in a randomized control study).
- Quantitative researchers try to control extraneous variables by conducting their studies in the lab.
- The research aims for objectivity (i.e., without bias) and is separated from the data.
- The design of the study is determined before it begins.
- For the quantitative researcher, the reality is objective, exists separately from the researcher, and can be seen by anyone.
- Research is used to test a theory and ultimately support or reject it.
Limitations of Quantitative Research
- Context: Quantitative experiments do not take place in natural settings. In addition, they do not allow participants to explain their choices or the meaning of the questions they may have for those participants (Carr, 1994).
- Researcher expertise: Poor knowledge of the application of statistical analysis may negatively affect analysis and subsequent interpretation (Black, 1999).
- Variability of data quantity: Large sample sizes are needed for more accurate analysis. Small-scale quantitative studies may be less reliable because of the low quantity of data (Denscombe, 2010). This also affects the ability to generalize study findings to wider populations.
- Confirmation bias: The researcher might miss observing phenomena because of focus on theory or hypothesis testing rather than on the theory of hypothesis generation.
Advantages of Quantitative Research
- Scientific objectivity: Quantitative data can be interpreted with statistical analysis, and since statistics are based on the principles of mathematics, the quantitative approach is viewed as scientifically objective and rational (Carr, 1994; Denscombe, 2010).
- Useful for testing and validating already constructed theories.
- Rapid analysis: Sophisticated software removes much of the need for prolonged data analysis, especially with large volumes of data involved (Antonius, 2003).
- Replication: Quantitative data is based on measured values and can be checked by others because numerical data is less open to ambiguities of interpretation.
- Hypotheses can also be tested because of statistical analysis (Antonius, 2003).
Antonius, R. (2003). Interpreting quantitative data with SPSS . Sage.
Black, T. R. (1999). Doing quantitative research in the social sciences: An integrated approach to research design, measurement and statistics . Sage.
Braun, V. & Clarke, V. (2006). Using thematic analysis in psychology . Qualitative Research in Psychology , 3, 77–101.
Carr, L. T. (1994). The strengths and weaknesses of quantitative and qualitative research : what method for nursing? Journal of advanced nursing, 20(4) , 716-721.
Denscombe, M. (2010). The Good Research Guide: for small-scale social research. McGraw Hill.
Denzin, N., & Lincoln. Y. (1994). Handbook of Qualitative Research. Thousand Oaks, CA, US: Sage Publications Inc.
Glaser, B. G., Strauss, A. L., & Strutzel, E. (1968). The discovery of grounded theory; strategies for qualitative research. Nursing research, 17(4) , 364.
Minichiello, V. (1990). In-Depth Interviewing: Researching People. Longman Cheshire.
Punch, K. (1998). Introduction to Social Research: Quantitative and Qualitative Approaches. London: Sage
Further Information
- Designing qualitative research
- Methods of data collection and analysis
- Introduction to quantitative and qualitative research
- Checklists for improving rigour in qualitative research: a case of the tail wagging the dog?
- Qualitative research in health care: Analysing qualitative data
- Qualitative data analysis: the framework approach
- Using the framework method for the analysis of
- Qualitative data in multi-disciplinary health research
- Content Analysis
- Grounded Theory
- Thematic Analysis

- Skip to main content
- Skip to primary sidebar
- Skip to footer
- QuestionPro

- Solutions Industries Gaming Automotive Sports and events Education Government Travel & Hospitality Financial Services Healthcare Cannabis Technology Use Case NPS+ Communities Audience Contactless surveys Mobile LivePolls Member Experience GDPR Positive People Science 360 Feedback Surveys
- Resources Blog eBooks Survey Templates Case Studies Training Help center

Home Market Research
Quantitative Analysis: Definition, Importance + Types
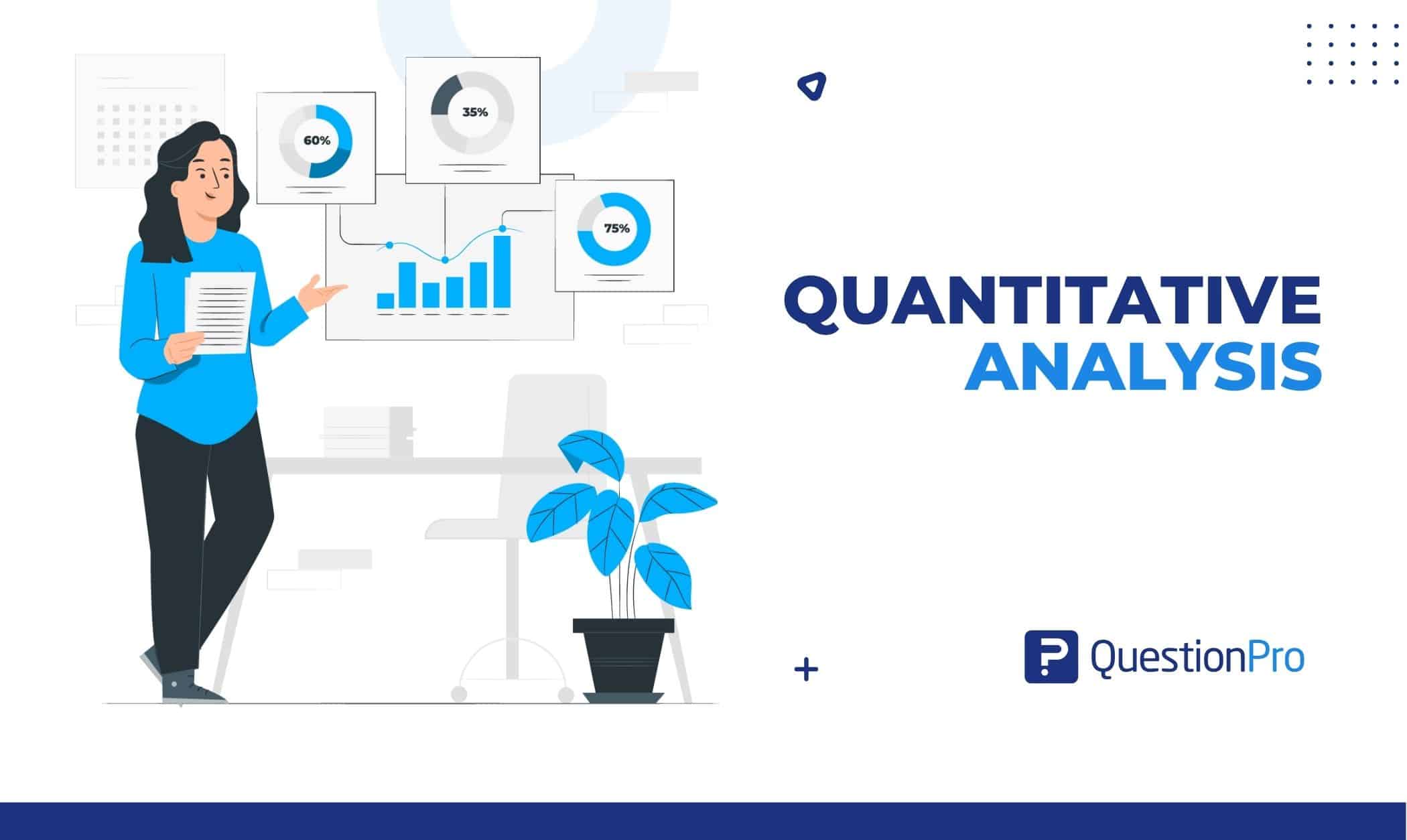
Quantitative data is what we talk about when we ask questions like “How many?” or “How often?” or “How much?” Mathematical approaches can be utilized to check and evaluate the accuracy of this information in a time-efficient manner. Quantitative analysis analyzes and interprets quantitative data using numerical and statistical methods. This analysis seeks to identify data patterns, trends, and linkages to inform decisions and predictions.
Quantitative data analysis uses statistics and math to solve problems in business, finance, and risk management problems. It is an important technique that helps financial analysts, scientists, and researchers understand challenging ideas and issues.
This blog discusses quantitative analysis, its types, and techniques used in business.
What is quantitative analysis?
Quantitative analysis is a way to figure out how good an investment or asset is by using numbers and statistics. It involves using mathematical and statistical models to look at data and make decisions about investments, business operations, or other complex systems.
Quantitative analysis aims to make better informed and more logical decisions by using data and objective analysis instead of relying on subjective judgment or intuition.
Quantitative analysts, also called “quants,” use tools and methods like statistical analysis , econometric modeling, machine learning, and computer programming to look at large amounts of data.
They could use this analysis to help them make financial decisions, like which trading strategies to use, how to handle risks, and how to divide up their assets. Quantitative analysis can be used in many fields, such as finance, economics, marketing, and political science.
Quantitative analysis can be used to answer some of the following types of questions:
- How does the performance of an investment or portfolio compare to a benchmark or another benchmark?
- How does a certain asset or security price change when the market or other things change?
- How likely is it that a certain event will happen, and how might it affect how well an investment or business operation does?
This analysis uses data and objective analysis to make more informed and logical decisions rather than relying on subjective judgment or intuition.
LEARN ABOUT: Research Process Steps
Importance of quantitative analysis
Quantitative analysis plays a crucial role in various fields because it provides objective, numerical insights and supports informed decision-making. Here are some key reasons why quantitative analysis is important:
- Objective Decision-Making
Quantitative analysis relies on data and mathematical/statistical methods, which help minimize subjectivity and bias in decision-making. This objectivity is particularly valuable when dealing with complex issues that require evidence-based conclusions.
- Data-driven Insights
It allows researchers and analysts to extract meaningful insights from large datasets. Patterns, trends, relationships, and anomalies that might not be apparent through qualitative methods can be uncovered using quantitative research techniques.
- Comparison and Benchmarking
Quantitative analysis deals with the comparison of different variables, scenarios, or strategies in a systematic and measurable way. This aids in identifying the most effective or efficient approach among options.
- Risk Assessment and Management
It assesses and quantifies risks in various contexts, from financial markets to engineering projects. This helps understand the potential impact of different risk factors and make informed decisions to mitigate them.
- Predictive Modeling
Many quantitative techniques, such as regression analysis and time series analysis, are used to build predictive models. These models help forecast future outcomes, allowing businesses and organizations to plan ahead and make proactive decisions.
- Resource Allocation
Quantitative analysis assists in optimizing the allocation of resources, whether it’s allocating budgets, manpower, or time. Organizations can make efficient use of their resources by understanding the relationships between variables.
- Performance Evaluation
Evaluating the performance of investments, projects, or products in fields like business and finance is crucial. It provides a structured way to assess whether goals and targets are being met.
- Evidence in Research
Quantitative analysis provides empirical evidence to support or refute hypotheses in scientific research. It helps establish causation and correlation relationships by analyzing data objectively.
- Quality Control and Assurance
Quantitative methods are often used to monitor and control quality in manufacturing and production processes. Statistical process control helps detect deviations from expected norms and ensures consistent product quality.
- Policy Formulation
Quantitative models inform policy decisions by providing data-driven insights into the potential impact of different policy options. This is essential in areas such as economics, public health, and social sciences.
- Market Research and Consumer Behavior
In marketing, quantitative analysis helps understand consumer behavior, preferences, and trends. It assists businesses in tailoring their products and marketing strategies to target audiences effectively.
- Validation and Verification
In engineering and computer science fields, quantitative analysis is used to validate and verify designs, simulations, and software systems. This ensures that products and systems meet predefined specifications and standards.
LEARN ABOUT: Data Asset Management
Types of quantitative analysis
Despite the fact that quantitative analysis involves numbers, there are various approaches to this type of analysis. This analysis comes in a variety of types, including:
1. Regression Analysis
Regression analysis is a typical form used by statisticians, economists, company owners, and other professionals. It entails making predictions or estimating the effects of one variable on another using statistical equations.
For example, it can reveal how interest rates impact customers’ asset investment decisions. Establishing the impact of education and work experience on employees’ annual salaries is another essential use of regression analysis.
Business owners can use regression analysis to ascertain how advertising costs affect revenue. This method allows a business owner to determine whether there is a positive or negative correlation between two factors.
2. Linear programming
Most businesses occasionally have a scarcity of resources, including office space, equipment for production, and manpower. Company managers must devise strategies to deploy resources wisely in such circumstances.
A quantitative research approach that specifies how to arrive at such an ideal solution is linear programming. It is also used to assess, under a set of limitations, such as labor, how a business may maximize earnings and cut expenditures.
3. Data Mining
Data mining combines statistical techniques with computer programming knowledge. As the variety and size of available data sets increase, data mining’s popularity also rises.
Many data are analyzed using mining techniques to look for hidden patterns or relationships.
LEARN ABOUT: Data Mining Techniques
Difference between quantitative analysis and qualitative analysis?
The choice between quantitative and qualitative analysis depends on the research question, objectives, available data, and desired insights. The key differences between qualitative and quantitative analysis:
Quantitative analysis is figuring out how well a business is doing using math and statistics. Before this analysis was invented, many company directors made decisions based on their experience and gut feelings.
Business owners can now use quantitative analysis techniques to predict trends, decide how to use resources, and manage projects. Quantitative analysis types and methods are also used to evaluate investments. This way, organizations can determine which assets to invest in and when to do it.
The QuestionPro Research Suite is a set of tools that can help with quantitative analysis by giving a place to run and look at surveys. It lets you make and send out surveys to get information from many people. It also gives you various tools for analyzing data and making reports.
If you want to see a demo or find out more, you can get a free trial from QuestionPro.
FREE TRIAL LEARN MORE
MORE LIKE THIS
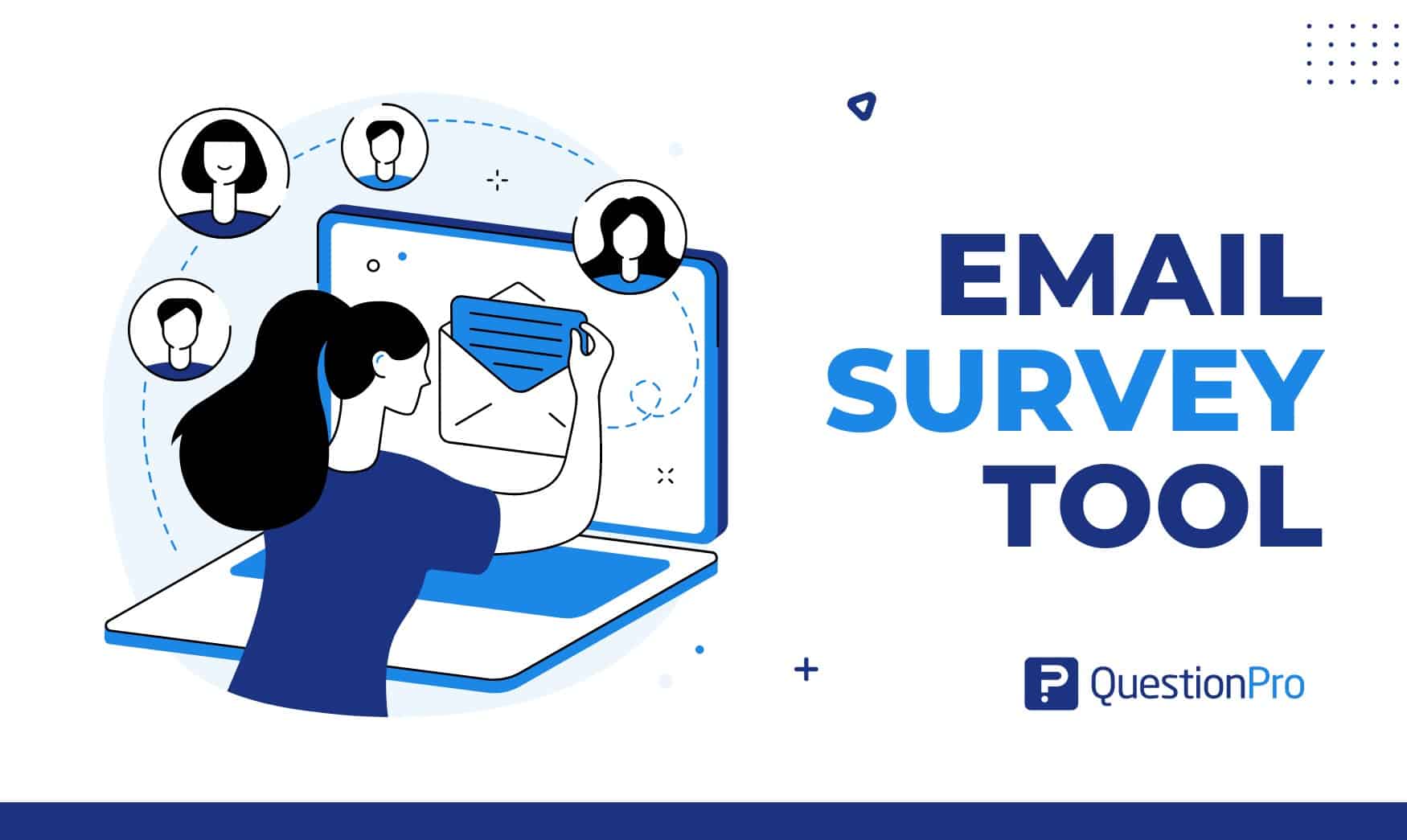
The Best Email Survey Tool to Boost Your Feedback Game
May 7, 2024
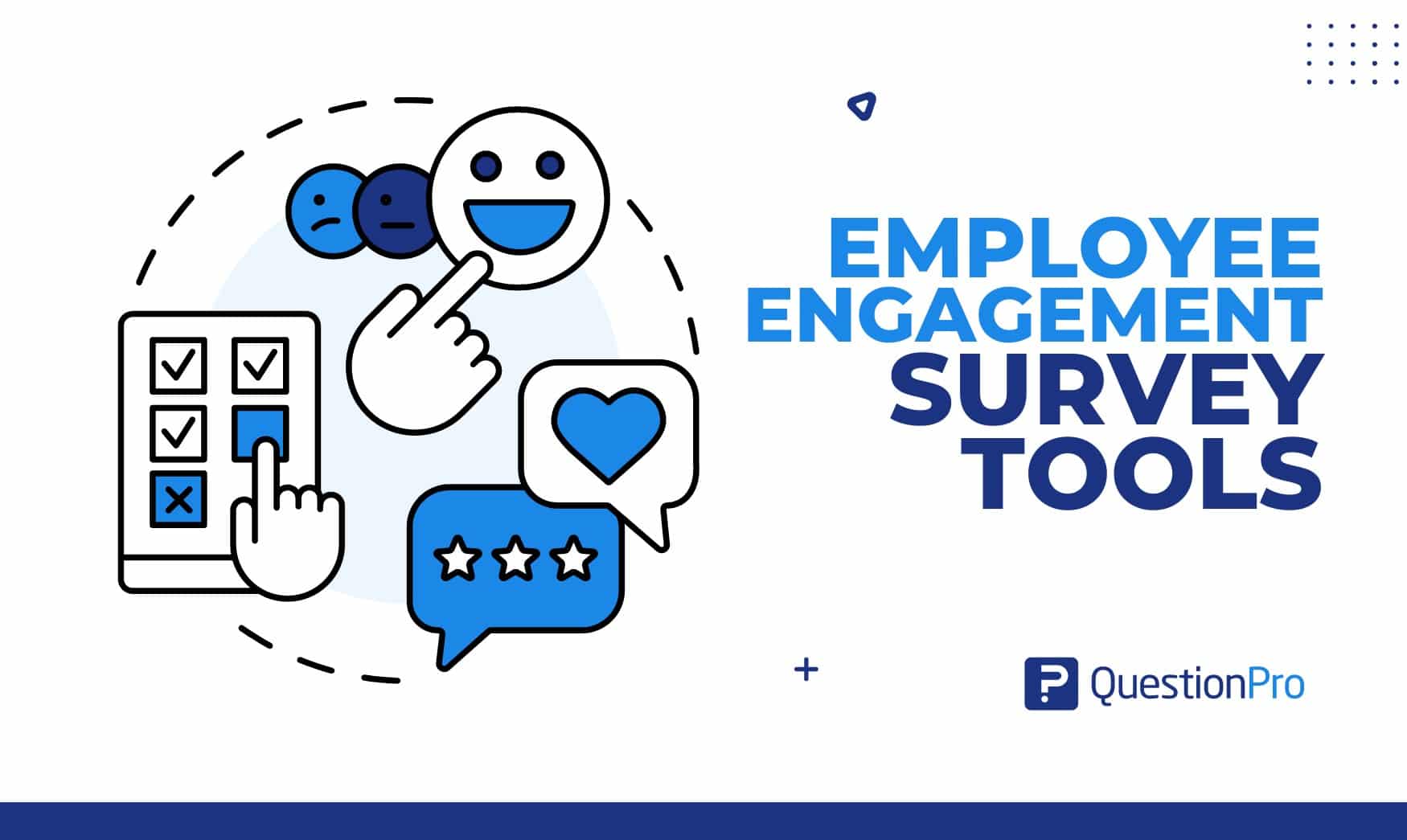
Top 10 Employee Engagement Survey Tools
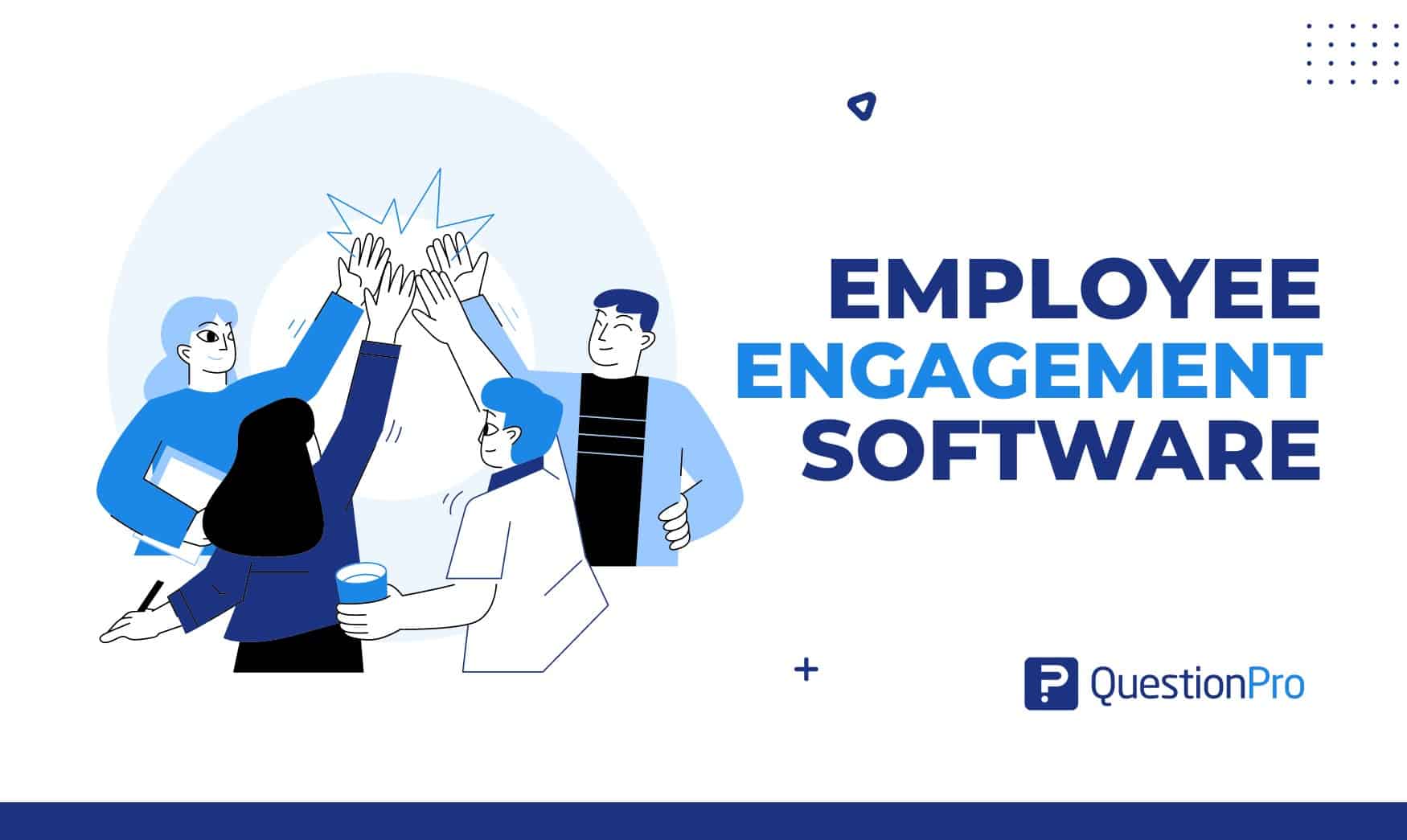
Top 20 Employee Engagement Software Solutions
May 3, 2024
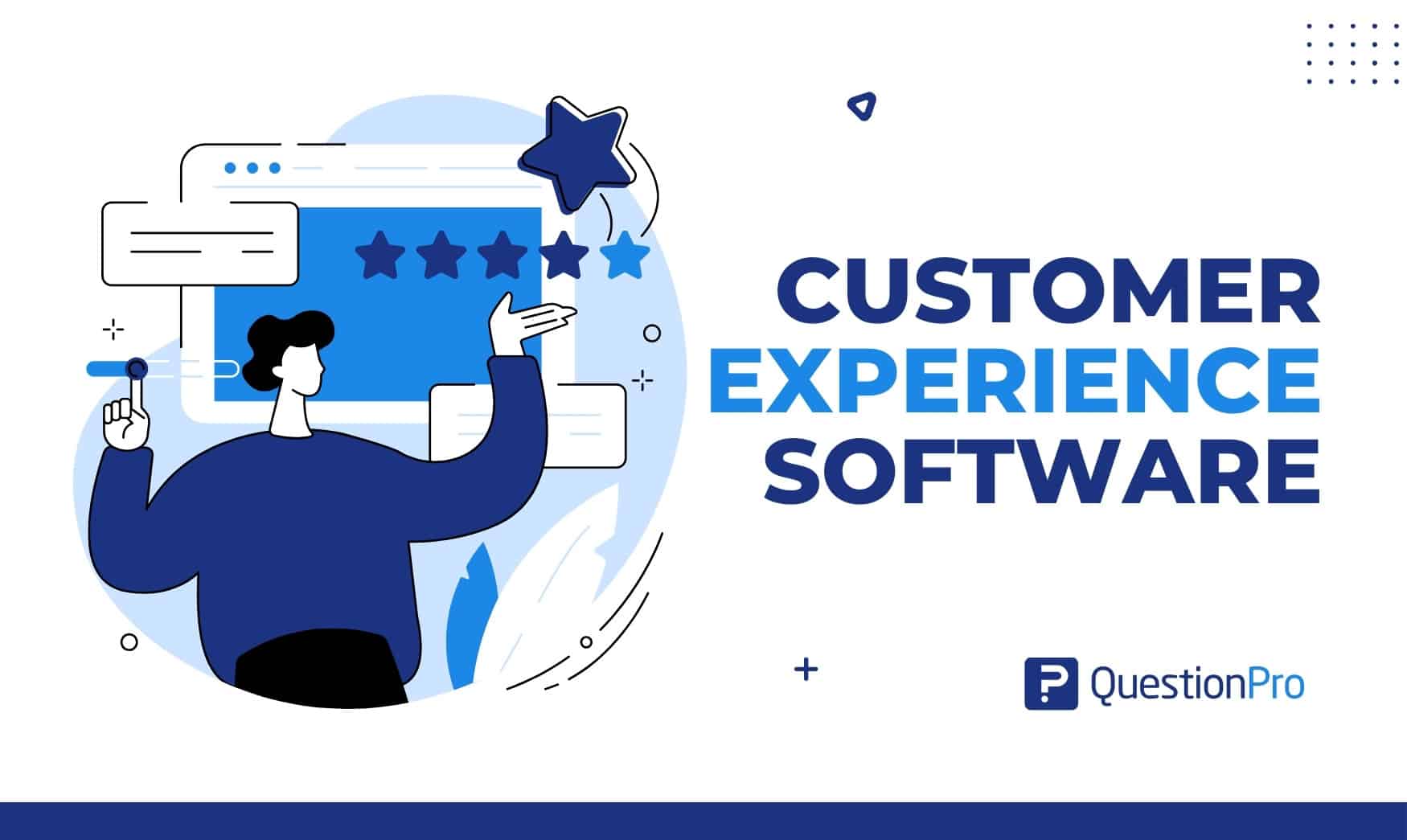
15 Best Customer Experience Software of 2024
May 2, 2024
Other categories
- Academic Research
- Artificial Intelligence
- Assessments
- Brand Awareness
- Case Studies
- Communities
- Consumer Insights
- Customer effort score
- Customer Engagement
- Customer Experience
- Customer Loyalty
- Customer Research
- Customer Satisfaction
- Employee Benefits
- Employee Engagement
- Employee Retention
- Friday Five
- General Data Protection Regulation
- Insights Hub
- Life@QuestionPro
- Market Research
- Mobile diaries
- Mobile Surveys
- New Features
- Online Communities
- Question Types
- Questionnaire
- QuestionPro Products
- Release Notes
- Research Tools and Apps
- Revenue at Risk
- Survey Templates
- Training Tips
- Uncategorized
- Video Learning Series
- What’s Coming Up
- Workforce Intelligence
What Is Research, and Why Do People Do It?
- Open Access
- First Online: 03 December 2022
Cite this chapter
You have full access to this open access chapter
- James Hiebert 6 ,
- Jinfa Cai 7 ,
- Stephen Hwang 7 ,
- Anne K Morris 6 &
- Charles Hohensee 6
Part of the book series: Research in Mathematics Education ((RME))
17k Accesses
Abstractspiepr Abs1
Every day people do research as they gather information to learn about something of interest. In the scientific world, however, research means something different than simply gathering information. Scientific research is characterized by its careful planning and observing, by its relentless efforts to understand and explain, and by its commitment to learn from everyone else seriously engaged in research. We call this kind of research scientific inquiry and define it as “formulating, testing, and revising hypotheses.” By “hypotheses” we do not mean the hypotheses you encounter in statistics courses. We mean predictions about what you expect to find and rationales for why you made these predictions. Throughout this and the remaining chapters we make clear that the process of scientific inquiry applies to all kinds of research studies and data, both qualitative and quantitative.
You have full access to this open access chapter, Download chapter PDF
Part I. What Is Research?
Have you ever studied something carefully because you wanted to know more about it? Maybe you wanted to know more about your grandmother’s life when she was younger so you asked her to tell you stories from her childhood, or maybe you wanted to know more about a fertilizer you were about to use in your garden so you read the ingredients on the package and looked them up online. According to the dictionary definition, you were doing research.
Recall your high school assignments asking you to “research” a topic. The assignment likely included consulting a variety of sources that discussed the topic, perhaps including some “original” sources. Often, the teacher referred to your product as a “research paper.”
Were you conducting research when you interviewed your grandmother or wrote high school papers reviewing a particular topic? Our view is that you were engaged in part of the research process, but only a small part. In this book, we reserve the word “research” for what it means in the scientific world, that is, for scientific research or, more pointedly, for scientific inquiry .
Exercise 1.1
Before you read any further, write a definition of what you think scientific inquiry is. Keep it short—Two to three sentences. You will periodically update this definition as you read this chapter and the remainder of the book.
This book is about scientific inquiry—what it is and how to do it. For starters, scientific inquiry is a process, a particular way of finding out about something that involves a number of phases. Each phase of the process constitutes one aspect of scientific inquiry. You are doing scientific inquiry as you engage in each phase, but you have not done scientific inquiry until you complete the full process. Each phase is necessary but not sufficient.
In this chapter, we set the stage by defining scientific inquiry—describing what it is and what it is not—and by discussing what it is good for and why people do it. The remaining chapters build directly on the ideas presented in this chapter.
A first thing to know is that scientific inquiry is not all or nothing. “Scientificness” is a continuum. Inquiries can be more scientific or less scientific. What makes an inquiry more scientific? You might be surprised there is no universally agreed upon answer to this question. None of the descriptors we know of are sufficient by themselves to define scientific inquiry. But all of them give you a way of thinking about some aspects of the process of scientific inquiry. Each one gives you different insights.
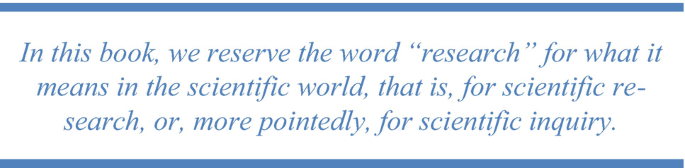
Exercise 1.2
As you read about each descriptor below, think about what would make an inquiry more or less scientific. If you think a descriptor is important, use it to revise your definition of scientific inquiry.
Creating an Image of Scientific Inquiry
We will present three descriptors of scientific inquiry. Each provides a different perspective and emphasizes a different aspect of scientific inquiry. We will draw on all three descriptors to compose our definition of scientific inquiry.
Descriptor 1. Experience Carefully Planned in Advance
Sir Ronald Fisher, often called the father of modern statistical design, once referred to research as “experience carefully planned in advance” (1935, p. 8). He said that humans are always learning from experience, from interacting with the world around them. Usually, this learning is haphazard rather than the result of a deliberate process carried out over an extended period of time. Research, Fisher said, was learning from experience, but experience carefully planned in advance.
This phrase can be fully appreciated by looking at each word. The fact that scientific inquiry is based on experience means that it is based on interacting with the world. These interactions could be thought of as the stuff of scientific inquiry. In addition, it is not just any experience that counts. The experience must be carefully planned . The interactions with the world must be conducted with an explicit, describable purpose, and steps must be taken to make the intended learning as likely as possible. This planning is an integral part of scientific inquiry; it is not just a preparation phase. It is one of the things that distinguishes scientific inquiry from many everyday learning experiences. Finally, these steps must be taken beforehand and the purpose of the inquiry must be articulated in advance of the experience. Clearly, scientific inquiry does not happen by accident, by just stumbling into something. Stumbling into something unexpected and interesting can happen while engaged in scientific inquiry, but learning does not depend on it and serendipity does not make the inquiry scientific.
Descriptor 2. Observing Something and Trying to Explain Why It Is the Way It Is
When we were writing this chapter and googled “scientific inquiry,” the first entry was: “Scientific inquiry refers to the diverse ways in which scientists study the natural world and propose explanations based on the evidence derived from their work.” The emphasis is on studying, or observing, and then explaining . This descriptor takes the image of scientific inquiry beyond carefully planned experience and includes explaining what was experienced.
According to the Merriam-Webster dictionary, “explain” means “(a) to make known, (b) to make plain or understandable, (c) to give the reason or cause of, and (d) to show the logical development or relations of” (Merriam-Webster, n.d. ). We will use all these definitions. Taken together, they suggest that to explain an observation means to understand it by finding reasons (or causes) for why it is as it is. In this sense of scientific inquiry, the following are synonyms: explaining why, understanding why, and reasoning about causes and effects. Our image of scientific inquiry now includes planning, observing, and explaining why.

We need to add a final note about this descriptor. We have phrased it in a way that suggests “observing something” means you are observing something in real time—observing the way things are or the way things are changing. This is often true. But, observing could mean observing data that already have been collected, maybe by someone else making the original observations (e.g., secondary analysis of NAEP data or analysis of existing video recordings of classroom instruction). We will address secondary analyses more fully in Chap. 4 . For now, what is important is that the process requires explaining why the data look like they do.
We must note that for us, the term “data” is not limited to numerical or quantitative data such as test scores. Data can also take many nonquantitative forms, including written survey responses, interview transcripts, journal entries, video recordings of students, teachers, and classrooms, text messages, and so forth.
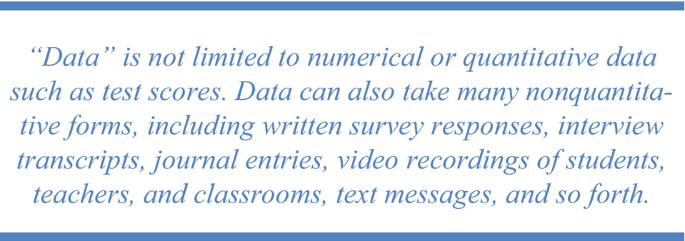
Exercise 1.3
What are the implications of the statement that just “observing” is not enough to count as scientific inquiry? Does this mean that a detailed description of a phenomenon is not scientific inquiry?
Find sources that define research in education that differ with our position, that say description alone, without explanation, counts as scientific research. Identify the precise points where the opinions differ. What are the best arguments for each of the positions? Which do you prefer? Why?
Descriptor 3. Updating Everyone’s Thinking in Response to More and Better Information
This descriptor focuses on a third aspect of scientific inquiry: updating and advancing the field’s understanding of phenomena that are investigated. This descriptor foregrounds a powerful characteristic of scientific inquiry: the reliability (or trustworthiness) of what is learned and the ultimate inevitability of this learning to advance human understanding of phenomena. Humans might choose not to learn from scientific inquiry, but history suggests that scientific inquiry always has the potential to advance understanding and that, eventually, humans take advantage of these new understandings.
Before exploring these bold claims a bit further, note that this descriptor uses “information” in the same way the previous two descriptors used “experience” and “observations.” These are the stuff of scientific inquiry and we will use them often, sometimes interchangeably. Frequently, we will use the term “data” to stand for all these terms.
An overriding goal of scientific inquiry is for everyone to learn from what one scientist does. Much of this book is about the methods you need to use so others have faith in what you report and can learn the same things you learned. This aspect of scientific inquiry has many implications.
One implication is that scientific inquiry is not a private practice. It is a public practice available for others to see and learn from. Notice how different this is from everyday learning. When you happen to learn something from your everyday experience, often only you gain from the experience. The fact that research is a public practice means it is also a social one. It is best conducted by interacting with others along the way: soliciting feedback at each phase, taking opportunities to present work-in-progress, and benefitting from the advice of others.
A second implication is that you, as the researcher, must be committed to sharing what you are doing and what you are learning in an open and transparent way. This allows all phases of your work to be scrutinized and critiqued. This is what gives your work credibility. The reliability or trustworthiness of your findings depends on your colleagues recognizing that you have used all appropriate methods to maximize the chances that your claims are justified by the data.
A third implication of viewing scientific inquiry as a collective enterprise is the reverse of the second—you must be committed to receiving comments from others. You must treat your colleagues as fair and honest critics even though it might sometimes feel otherwise. You must appreciate their job, which is to remain skeptical while scrutinizing what you have done in considerable detail. To provide the best help to you, they must remain skeptical about your conclusions (when, for example, the data are difficult for them to interpret) until you offer a convincing logical argument based on the information you share. A rather harsh but good-to-remember statement of the role of your friendly critics was voiced by Karl Popper, a well-known twentieth century philosopher of science: “. . . if you are interested in the problem which I tried to solve by my tentative assertion, you may help me by criticizing it as severely as you can” (Popper, 1968, p. 27).
A final implication of this third descriptor is that, as someone engaged in scientific inquiry, you have no choice but to update your thinking when the data support a different conclusion. This applies to your own data as well as to those of others. When data clearly point to a specific claim, even one that is quite different than you expected, you must reconsider your position. If the outcome is replicated multiple times, you need to adjust your thinking accordingly. Scientific inquiry does not let you pick and choose which data to believe; it mandates that everyone update their thinking when the data warrant an update.
Doing Scientific Inquiry
We define scientific inquiry in an operational sense—what does it mean to do scientific inquiry? What kind of process would satisfy all three descriptors: carefully planning an experience in advance; observing and trying to explain what you see; and, contributing to updating everyone’s thinking about an important phenomenon?
We define scientific inquiry as formulating , testing , and revising hypotheses about phenomena of interest.
Of course, we are not the only ones who define it in this way. The definition for the scientific method posted by the editors of Britannica is: “a researcher develops a hypothesis, tests it through various means, and then modifies the hypothesis on the basis of the outcome of the tests and experiments” (Britannica, n.d. ).

Notice how defining scientific inquiry this way satisfies each of the descriptors. “Carefully planning an experience in advance” is exactly what happens when formulating a hypothesis about a phenomenon of interest and thinking about how to test it. “ Observing a phenomenon” occurs when testing a hypothesis, and “ explaining ” what is found is required when revising a hypothesis based on the data. Finally, “updating everyone’s thinking” comes from comparing publicly the original with the revised hypothesis.
Doing scientific inquiry, as we have defined it, underscores the value of accumulating knowledge rather than generating random bits of knowledge. Formulating, testing, and revising hypotheses is an ongoing process, with each revised hypothesis begging for another test, whether by the same researcher or by new researchers. The editors of Britannica signaled this cyclic process by adding the following phrase to their definition of the scientific method: “The modified hypothesis is then retested, further modified, and tested again.” Scientific inquiry creates a process that encourages each study to build on the studies that have gone before. Through collective engagement in this process of building study on top of study, the scientific community works together to update its thinking.
Before exploring more fully the meaning of “formulating, testing, and revising hypotheses,” we need to acknowledge that this is not the only way researchers define research. Some researchers prefer a less formal definition, one that includes more serendipity, less planning, less explanation. You might have come across more open definitions such as “research is finding out about something.” We prefer the tighter hypothesis formulation, testing, and revision definition because we believe it provides a single, coherent map for conducting research that addresses many of the thorny problems educational researchers encounter. We believe it is the most useful orientation toward research and the most helpful to learn as a beginning researcher.
A final clarification of our definition is that it applies equally to qualitative and quantitative research. This is a familiar distinction in education that has generated much discussion. You might think our definition favors quantitative methods over qualitative methods because the language of hypothesis formulation and testing is often associated with quantitative methods. In fact, we do not favor one method over another. In Chap. 4 , we will illustrate how our definition fits research using a range of quantitative and qualitative methods.
Exercise 1.4
Look for ways to extend what the field knows in an area that has already received attention by other researchers. Specifically, you can search for a program of research carried out by more experienced researchers that has some revised hypotheses that remain untested. Identify a revised hypothesis that you might like to test.
Unpacking the Terms Formulating, Testing, and Revising Hypotheses
To get a full sense of the definition of scientific inquiry we will use throughout this book, it is helpful to spend a little time with each of the key terms.
We first want to make clear that we use the term “hypothesis” as it is defined in most dictionaries and as it used in many scientific fields rather than as it is usually defined in educational statistics courses. By “hypothesis,” we do not mean a null hypothesis that is accepted or rejected by statistical analysis. Rather, we use “hypothesis” in the sense conveyed by the following definitions: “An idea or explanation for something that is based on known facts but has not yet been proved” (Cambridge University Press, n.d. ), and “An unproved theory, proposition, or supposition, tentatively accepted to explain certain facts and to provide a basis for further investigation or argument” (Agnes & Guralnik, 2008 ).
We distinguish two parts to “hypotheses.” Hypotheses consist of predictions and rationales . Predictions are statements about what you expect to find when you inquire about something. Rationales are explanations for why you made the predictions you did, why you believe your predictions are correct. So, for us “formulating hypotheses” means making explicit predictions and developing rationales for the predictions.
“Testing hypotheses” means making observations that allow you to assess in what ways your predictions were correct and in what ways they were incorrect. In education research, it is rarely useful to think of your predictions as either right or wrong. Because of the complexity of most issues you will investigate, most predictions will be right in some ways and wrong in others.
By studying the observations you make (data you collect) to test your hypotheses, you can revise your hypotheses to better align with the observations. This means revising your predictions plus revising your rationales to justify your adjusted predictions. Even though you might not run another test, formulating revised hypotheses is an essential part of conducting a research study. Comparing your original and revised hypotheses informs everyone of what you learned by conducting your study. In addition, a revised hypothesis sets the stage for you or someone else to extend your study and accumulate more knowledge of the phenomenon.
We should note that not everyone makes a clear distinction between predictions and rationales as two aspects of hypotheses. In fact, common, non-scientific uses of the word “hypothesis” may limit it to only a prediction or only an explanation (or rationale). We choose to explicitly include both prediction and rationale in our definition of hypothesis, not because we assert this should be the universal definition, but because we want to foreground the importance of both parts acting in concert. Using “hypothesis” to represent both prediction and rationale could hide the two aspects, but we make them explicit because they provide different kinds of information. It is usually easier to make predictions than develop rationales because predictions can be guesses, hunches, or gut feelings about which you have little confidence. Developing a compelling rationale requires careful thought plus reading what other researchers have found plus talking with your colleagues. Often, while you are developing your rationale you will find good reasons to change your predictions. Developing good rationales is the engine that drives scientific inquiry. Rationales are essentially descriptions of how much you know about the phenomenon you are studying. Throughout this guide, we will elaborate on how developing good rationales drives scientific inquiry. For now, we simply note that it can sharpen your predictions and help you to interpret your data as you test your hypotheses.
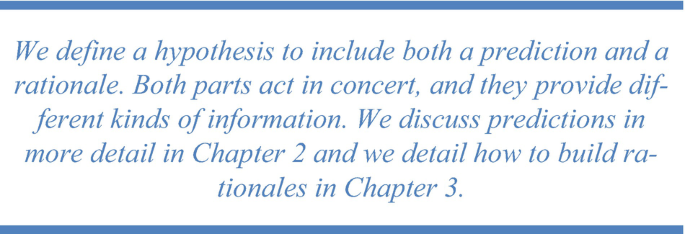
Hypotheses in education research take a variety of forms or types. This is because there are a variety of phenomena that can be investigated. Investigating educational phenomena is sometimes best done using qualitative methods, sometimes using quantitative methods, and most often using mixed methods (e.g., Hay, 2016 ; Weis et al. 2019a ; Weisner, 2005 ). This means that, given our definition, hypotheses are equally applicable to qualitative and quantitative investigations.
Hypotheses take different forms when they are used to investigate different kinds of phenomena. Two very different activities in education could be labeled conducting experiments and descriptions. In an experiment, a hypothesis makes a prediction about anticipated changes, say the changes that occur when a treatment or intervention is applied. You might investigate how students’ thinking changes during a particular kind of instruction.
A second type of hypothesis, relevant for descriptive research, makes a prediction about what you will find when you investigate and describe the nature of a situation. The goal is to understand a situation as it exists rather than to understand a change from one situation to another. In this case, your prediction is what you expect to observe. Your rationale is the set of reasons for making this prediction; it is your current explanation for why the situation will look like it does.
You will probably read, if you have not already, that some researchers say you do not need a prediction to conduct a descriptive study. We will discuss this point of view in Chap. 2 . For now, we simply claim that scientific inquiry, as we have defined it, applies to all kinds of research studies. Descriptive studies, like others, not only benefit from formulating, testing, and revising hypotheses, but also need hypothesis formulating, testing, and revising.
One reason we define research as formulating, testing, and revising hypotheses is that if you think of research in this way you are less likely to go wrong. It is a useful guide for the entire process, as we will describe in detail in the chapters ahead. For example, as you build the rationale for your predictions, you are constructing the theoretical framework for your study (Chap. 3 ). As you work out the methods you will use to test your hypothesis, every decision you make will be based on asking, “Will this help me formulate or test or revise my hypothesis?” (Chap. 4 ). As you interpret the results of testing your predictions, you will compare them to what you predicted and examine the differences, focusing on how you must revise your hypotheses (Chap. 5 ). By anchoring the process to formulating, testing, and revising hypotheses, you will make smart decisions that yield a coherent and well-designed study.
Exercise 1.5
Compare the concept of formulating, testing, and revising hypotheses with the descriptions of scientific inquiry contained in Scientific Research in Education (NRC, 2002 ). How are they similar or different?
Exercise 1.6
Provide an example to illustrate and emphasize the differences between everyday learning/thinking and scientific inquiry.
Learning from Doing Scientific Inquiry
We noted earlier that a measure of what you have learned by conducting a research study is found in the differences between your original hypothesis and your revised hypothesis based on the data you collected to test your hypothesis. We will elaborate this statement in later chapters, but we preview our argument here.
Even before collecting data, scientific inquiry requires cycles of making a prediction, developing a rationale, refining your predictions, reading and studying more to strengthen your rationale, refining your predictions again, and so forth. And, even if you have run through several such cycles, you still will likely find that when you test your prediction you will be partly right and partly wrong. The results will support some parts of your predictions but not others, or the results will “kind of” support your predictions. A critical part of scientific inquiry is making sense of your results by interpreting them against your predictions. Carefully describing what aspects of your data supported your predictions, what aspects did not, and what data fell outside of any predictions is not an easy task, but you cannot learn from your study without doing this analysis.
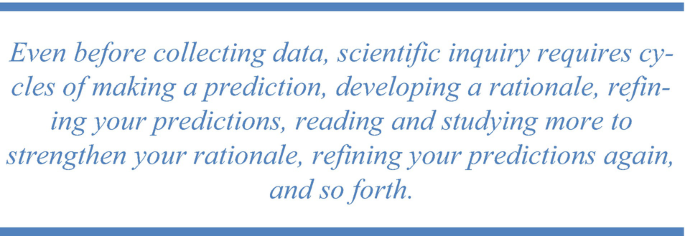
Analyzing the matches and mismatches between your predictions and your data allows you to formulate different rationales that would have accounted for more of the data. The best revised rationale is the one that accounts for the most data. Once you have revised your rationales, you can think about the predictions they best justify or explain. It is by comparing your original rationales to your new rationales that you can sort out what you learned from your study.
Suppose your study was an experiment. Maybe you were investigating the effects of a new instructional intervention on students’ learning. Your original rationale was your explanation for why the intervention would change the learning outcomes in a particular way. Your revised rationale explained why the changes that you observed occurred like they did and why your revised predictions are better. Maybe your original rationale focused on the potential of the activities if they were implemented in ideal ways and your revised rationale included the factors that are likely to affect how teachers implement them. By comparing the before and after rationales, you are describing what you learned—what you can explain now that you could not before. Another way of saying this is that you are describing how much more you understand now than before you conducted your study.
Revised predictions based on carefully planned and collected data usually exhibit some of the following features compared with the originals: more precision, more completeness, and broader scope. Revised rationales have more explanatory power and become more complete, more aligned with the new predictions, sharper, and overall more convincing.
Part II. Why Do Educators Do Research?
Doing scientific inquiry is a lot of work. Each phase of the process takes time, and you will often cycle back to improve earlier phases as you engage in later phases. Because of the significant effort required, you should make sure your study is worth it. So, from the beginning, you should think about the purpose of your study. Why do you want to do it? And, because research is a social practice, you should also think about whether the results of your study are likely to be important and significant to the education community.
If you are doing research in the way we have described—as scientific inquiry—then one purpose of your study is to understand , not just to describe or evaluate or report. As we noted earlier, when you formulate hypotheses, you are developing rationales that explain why things might be like they are. In our view, trying to understand and explain is what separates research from other kinds of activities, like evaluating or describing.
One reason understanding is so important is that it allows researchers to see how or why something works like it does. When you see how something works, you are better able to predict how it might work in other contexts, under other conditions. And, because conditions, or contextual factors, matter a lot in education, gaining insights into applying your findings to other contexts increases the contributions of your work and its importance to the broader education community.
Consequently, the purposes of research studies in education often include the more specific aim of identifying and understanding the conditions under which the phenomena being studied work like the observations suggest. A classic example of this kind of study in mathematics education was reported by William Brownell and Harold Moser in 1949 . They were trying to establish which method of subtracting whole numbers could be taught most effectively—the regrouping method or the equal additions method. However, they realized that effectiveness might depend on the conditions under which the methods were taught—“meaningfully” versus “mechanically.” So, they designed a study that crossed the two instructional approaches with the two different methods (regrouping and equal additions). Among other results, they found that these conditions did matter. The regrouping method was more effective under the meaningful condition than the mechanical condition, but the same was not true for the equal additions algorithm.
What do education researchers want to understand? In our view, the ultimate goal of education is to offer all students the best possible learning opportunities. So, we believe the ultimate purpose of scientific inquiry in education is to develop understanding that supports the improvement of learning opportunities for all students. We say “ultimate” because there are lots of issues that must be understood to improve learning opportunities for all students. Hypotheses about many aspects of education are connected, ultimately, to students’ learning. For example, formulating and testing a hypothesis that preservice teachers need to engage in particular kinds of activities in their coursework in order to teach particular topics well is, ultimately, connected to improving students’ learning opportunities. So is hypothesizing that school districts often devote relatively few resources to instructional leadership training or hypothesizing that positioning mathematics as a tool students can use to combat social injustice can help students see the relevance of mathematics to their lives.
We do not exclude the importance of research on educational issues more removed from improving students’ learning opportunities, but we do think the argument for their importance will be more difficult to make. If there is no way to imagine a connection between your hypothesis and improving learning opportunities for students, even a distant connection, we recommend you reconsider whether it is an important hypothesis within the education community.
Notice that we said the ultimate goal of education is to offer all students the best possible learning opportunities. For too long, educators have been satisfied with a goal of offering rich learning opportunities for lots of students, sometimes even for just the majority of students, but not necessarily for all students. Evaluations of success often are based on outcomes that show high averages. In other words, if many students have learned something, or even a smaller number have learned a lot, educators may have been satisfied. The problem is that there is usually a pattern in the groups of students who receive lower quality opportunities—students of color and students who live in poor areas, urban and rural. This is not acceptable. Consequently, we emphasize the premise that the purpose of education research is to offer rich learning opportunities to all students.
One way to make sure you will be able to convince others of the importance of your study is to consider investigating some aspect of teachers’ shared instructional problems. Historically, researchers in education have set their own research agendas, regardless of the problems teachers are facing in schools. It is increasingly recognized that teachers have had trouble applying to their own classrooms what researchers find. To address this problem, a researcher could partner with a teacher—better yet, a small group of teachers—and talk with them about instructional problems they all share. These discussions can create a rich pool of problems researchers can consider. If researchers pursued one of these problems (preferably alongside teachers), the connection to improving learning opportunities for all students could be direct and immediate. “Grounding a research question in instructional problems that are experienced across multiple teachers’ classrooms helps to ensure that the answer to the question will be of sufficient scope to be relevant and significant beyond the local context” (Cai et al., 2019b , p. 115).
As a beginning researcher, determining the relevance and importance of a research problem is especially challenging. We recommend talking with advisors, other experienced researchers, and peers to test the educational importance of possible research problems and topics of study. You will also learn much more about the issue of research importance when you read Chap. 5 .
Exercise 1.7
Identify a problem in education that is closely connected to improving learning opportunities and a problem that has a less close connection. For each problem, write a brief argument (like a logical sequence of if-then statements) that connects the problem to all students’ learning opportunities.
Part III. Conducting Research as a Practice of Failing Productively
Scientific inquiry involves formulating hypotheses about phenomena that are not fully understood—by you or anyone else. Even if you are able to inform your hypotheses with lots of knowledge that has already been accumulated, you are likely to find that your prediction is not entirely accurate. This is normal. Remember, scientific inquiry is a process of constantly updating your thinking. More and better information means revising your thinking, again, and again, and again. Because you never fully understand a complicated phenomenon and your hypotheses never produce completely accurate predictions, it is easy to believe you are somehow failing.
The trick is to fail upward, to fail to predict accurately in ways that inform your next hypothesis so you can make a better prediction. Some of the best-known researchers in education have been open and honest about the many times their predictions were wrong and, based on the results of their studies and those of others, they continuously updated their thinking and changed their hypotheses.
A striking example of publicly revising (actually reversing) hypotheses due to incorrect predictions is found in the work of Lee J. Cronbach, one of the most distinguished educational psychologists of the twentieth century. In 1955, Cronbach delivered his presidential address to the American Psychological Association. Titling it “Two Disciplines of Scientific Psychology,” Cronbach proposed a rapprochement between two research approaches—correlational studies that focused on individual differences and experimental studies that focused on instructional treatments controlling for individual differences. (We will examine different research approaches in Chap. 4 ). If these approaches could be brought together, reasoned Cronbach ( 1957 ), researchers could find interactions between individual characteristics and treatments (aptitude-treatment interactions or ATIs), fitting the best treatments to different individuals.
In 1975, after years of research by many researchers looking for ATIs, Cronbach acknowledged the evidence for simple, useful ATIs had not been found. Even when trying to find interactions between a few variables that could provide instructional guidance, the analysis, said Cronbach, creates “a hall of mirrors that extends to infinity, tormenting even the boldest investigators and defeating even ambitious designs” (Cronbach, 1975 , p. 119).
As he was reflecting back on his work, Cronbach ( 1986 ) recommended moving away from documenting instructional effects through statistical inference (an approach he had championed for much of his career) and toward approaches that probe the reasons for these effects, approaches that provide a “full account of events in a time, place, and context” (Cronbach, 1986 , p. 104). This is a remarkable change in hypotheses, a change based on data and made fully transparent. Cronbach understood the value of failing productively.
Closer to home, in a less dramatic example, one of us began a line of scientific inquiry into how to prepare elementary preservice teachers to teach early algebra. Teaching early algebra meant engaging elementary students in early forms of algebraic reasoning. Such reasoning should help them transition from arithmetic to algebra. To begin this line of inquiry, a set of activities for preservice teachers were developed. Even though the activities were based on well-supported hypotheses, they largely failed to engage preservice teachers as predicted because of unanticipated challenges the preservice teachers faced. To capitalize on this failure, follow-up studies were conducted, first to better understand elementary preservice teachers’ challenges with preparing to teach early algebra, and then to better support preservice teachers in navigating these challenges. In this example, the initial failure was a necessary step in the researchers’ scientific inquiry and furthered the researchers’ understanding of this issue.
We present another example of failing productively in Chap. 2 . That example emerges from recounting the history of a well-known research program in mathematics education.
Making mistakes is an inherent part of doing scientific research. Conducting a study is rarely a smooth path from beginning to end. We recommend that you keep the following things in mind as you begin a career of conducting research in education.
First, do not get discouraged when you make mistakes; do not fall into the trap of feeling like you are not capable of doing research because you make too many errors.
Second, learn from your mistakes. Do not ignore your mistakes or treat them as errors that you simply need to forget and move past. Mistakes are rich sites for learning—in research just as in other fields of study.
Third, by reflecting on your mistakes, you can learn to make better mistakes, mistakes that inform you about a productive next step. You will not be able to eliminate your mistakes, but you can set a goal of making better and better mistakes.
Exercise 1.8
How does scientific inquiry differ from everyday learning in giving you the tools to fail upward? You may find helpful perspectives on this question in other resources on science and scientific inquiry (e.g., Failure: Why Science is So Successful by Firestein, 2015).
Exercise 1.9
Use what you have learned in this chapter to write a new definition of scientific inquiry. Compare this definition with the one you wrote before reading this chapter. If you are reading this book as part of a course, compare your definition with your colleagues’ definitions. Develop a consensus definition with everyone in the course.
Part IV. Preview of Chap. 2
Now that you have a good idea of what research is, at least of what we believe research is, the next step is to think about how to actually begin doing research. This means how to begin formulating, testing, and revising hypotheses. As for all phases of scientific inquiry, there are lots of things to think about. Because it is critical to start well, we devote Chap. 2 to getting started with formulating hypotheses.
Agnes, M., & Guralnik, D. B. (Eds.). (2008). Hypothesis. In Webster’s new world college dictionary (4th ed.). Wiley.
Google Scholar
Britannica. (n.d.). Scientific method. In Encyclopaedia Britannica . Retrieved July 15, 2022 from https://www.britannica.com/science/scientific-method
Brownell, W. A., & Moser, H. E. (1949). Meaningful vs. mechanical learning: A study in grade III subtraction . Duke University Press..
Cai, J., Morris, A., Hohensee, C., Hwang, S., Robison, V., Cirillo, M., Kramer, S. L., & Hiebert, J. (2019b). Posing significant research questions. Journal for Research in Mathematics Education, 50 (2), 114–120. https://doi.org/10.5951/jresematheduc.50.2.0114
Article Google Scholar
Cambridge University Press. (n.d.). Hypothesis. In Cambridge dictionary . Retrieved July 15, 2022 from https://dictionary.cambridge.org/us/dictionary/english/hypothesis
Cronbach, J. L. (1957). The two disciplines of scientific psychology. American Psychologist, 12 , 671–684.
Cronbach, L. J. (1975). Beyond the two disciplines of scientific psychology. American Psychologist, 30 , 116–127.
Cronbach, L. J. (1986). Social inquiry by and for earthlings. In D. W. Fiske & R. A. Shweder (Eds.), Metatheory in social science: Pluralisms and subjectivities (pp. 83–107). University of Chicago Press.
Hay, C. M. (Ed.). (2016). Methods that matter: Integrating mixed methods for more effective social science research . University of Chicago Press.
Merriam-Webster. (n.d.). Explain. In Merriam-Webster.com dictionary . Retrieved July 15, 2022, from https://www.merriam-webster.com/dictionary/explain
National Research Council. (2002). Scientific research in education . National Academy Press.
Weis, L., Eisenhart, M., Duncan, G. J., Albro, E., Bueschel, A. C., Cobb, P., Eccles, J., Mendenhall, R., Moss, P., Penuel, W., Ream, R. K., Rumbaut, R. G., Sloane, F., Weisner, T. S., & Wilson, J. (2019a). Mixed methods for studies that address broad and enduring issues in education research. Teachers College Record, 121 , 100307.
Weisner, T. S. (Ed.). (2005). Discovering successful pathways in children’s development: Mixed methods in the study of childhood and family life . University of Chicago Press.
Download references
Author information
Authors and affiliations.
School of Education, University of Delaware, Newark, DE, USA
James Hiebert, Anne K Morris & Charles Hohensee
Department of Mathematical Sciences, University of Delaware, Newark, DE, USA
Jinfa Cai & Stephen Hwang
You can also search for this author in PubMed Google Scholar
Rights and permissions
Open Access This chapter is licensed under the terms of the Creative Commons Attribution 4.0 International License ( http://creativecommons.org/licenses/by/4.0/ ), which permits use, sharing, adaptation, distribution and reproduction in any medium or format, as long as you give appropriate credit to the original author(s) and the source, provide a link to the Creative Commons license and indicate if changes were made.
The images or other third party material in this chapter are included in the chapter's Creative Commons license, unless indicated otherwise in a credit line to the material. If material is not included in the chapter's Creative Commons license and your intended use is not permitted by statutory regulation or exceeds the permitted use, you will need to obtain permission directly from the copyright holder.
Reprints and permissions
Copyright information
© 2023 The Author(s)
About this chapter
Hiebert, J., Cai, J., Hwang, S., Morris, A.K., Hohensee, C. (2023). What Is Research, and Why Do People Do It?. In: Doing Research: A New Researcher’s Guide. Research in Mathematics Education. Springer, Cham. https://doi.org/10.1007/978-3-031-19078-0_1
Download citation
DOI : https://doi.org/10.1007/978-3-031-19078-0_1
Published : 03 December 2022
Publisher Name : Springer, Cham
Print ISBN : 978-3-031-19077-3
Online ISBN : 978-3-031-19078-0
eBook Packages : Education Education (R0)
Share this chapter
Anyone you share the following link with will be able to read this content:
Sorry, a shareable link is not currently available for this article.
Provided by the Springer Nature SharedIt content-sharing initiative
- Publish with us
Policies and ethics
- Find a journal
- Track your research
The University of Tennessee, Knoxville
Educational leadership and policy studies, educational leadership and policy studies college of education, health, and human sciences.

How Do I Critically Consume Quantitative Research?
By Austin Boyd
Every measurement, evaluation, statistics, and assessment (MESA) professional, whether they are established educators and practitioners or aspiring students, engages with academic literature in some capacity. Sometimes for work, other times for pleasure, but always in the pursuit of new knowledge. But how do we as consumers of research determine whether the quantitative research we engage with is high quality?

- What is the purpose of the article?
The first question to keep in mind when reading an article is , “ W hat is its purpose?” Articles may state these in the form of research questions or even in the title by using words such as “empirical”, “validation”, and “meta-analysis”. While the purpose of an article has no bearing on its quality, it does impact the type of information a reader should expect to obtain from it. Do the research questions indicate that the article will be presenting new exploratory research on a new phenomenon or attempting to validate previous research findings ? Remaining aware of the article’s purpose allows you to determine if the information is relevant and in the scope of what it should be providing.
- What information is provided about obtaining participants and about the participants themselves?
The backbone of quantitative research is data. In order to have any data, participants or cases must be found and measured for the phenomena of interest. These participants are all unique, and it is this uniqueness that needs to be disclosed to the reader. Information on the population of interest, how the selected participants were recruited, who they are, and why their results were or were not included in the analysis is essential for understanding the c ontext of the research. Beyond the article itself, the demographics of the participants are also important for planning future research. While research participants are largely Western, educated, industrialized, rich, and democratic societies ( WEIRD; Henrich et al., 2010), it should not be assumed that this is the case for all research. The author(s) of an article should disclose demographic information of the participants , so the readers understand the context of the data and the generalizability of the results, and so that researchers can accurately replicate or expand the research to ne w contexts.
- Do the analyses used make sense for the data and proposed research question(s)?
In order to obtain results from the quantitative data collected, some form of analysis must be conducted. The most basic methods of exploring quantitative data are called statistics ( Sheard, 2018 ). T he selected statistical analysis should align with the variables presented in the article and answer the research question(s) guiding the project. There is a level of subjectivity as to which statistical analysis should be used to analyze data. Variables measured on a nominal scale should not be used as the outcome variable when conducting analyses that look at the differences between group means, such as t-tests and ANOVAs, while ratio scale variables should not be used to conduct analyses dealing with frequency distributions, such as chi-square tests. However, there are analyses which require the same variable types, making them seemingly interchangeable. For example, t-tests, logistic regressions, and point biserial analyses all use two variables, one continuous and one binary. However, each of these analyses addresses different research questions such as “Is there a difference between groups?”, “Can we predict an outcome?”, and “Is there a relationship between variables?”. While there is a level of subjectivity as to which statistical analysis can be used to analyze data, there are objectively incorrect analyses based on both the overarching research questions and the scale of measurement of the available variables in the data.
- What results are provided?
While a seemingly straightforward question, there is a lot of information that can be provided about a given analysis. The most basic, and least informative, is a blanket statement about the statistical significance. Even if there is no statistically significant result to report, a blanket statement is not sufficient information about the analyses with all the different values that can be reported for each analysis. For example, a t-test has a t value, degrees of freedom, p value, confidence interval, power level, and effect size, all of which provide valuable information about the results. While having some of these values does allow the reader to calculate the missing ones, the onus should not be put on the reader to do so (Cohen, 1990). Additionally, depending on the type of statistical analysis chosen, additional tests of the data must be conducted to determine if the data meets the assumptions necessary for the analysis. The results of these tests of assumptions and the decisions made based on them should be reported and supported by the existing literature.
- Is there any discussion of limitations?
Almost every article has limitations in some form or other, which should be made known to the reader. If an article didn’t have any limitations, the author would make a point to state as much. Limitations include limits to the generalizability of the findings, confounding variables, or simply time constraints. While these might seem negative, they are not immediate reasons to discredit an article entirely. As was the case for the demographics, the limitations provide further context about the research. They can even be useful in providing direction for follow-up studies in the same way a future research section would.
- Do you find yourself still having questions after finishing the article?
The final question to keep in mind once you have finished reading an article is “Do you still have questions?” At the end of an article, you shouldn’t find yourself needing more information about the study. You might want to know more about the topic or similar research, but you shouldn’t be left wondering about pieces of the research design or other methodological aspects of the study. High-quality research deserves an equally high-quality article, which includes ample information about every aspect of the study.
While not an exhaustive list, these six questions are designed to provide a starting point for determining if research with quantitative data is of high quality. Not all research is peer-reviewed, including conference presentations, blog posts, and white papers, and simply being peer-reviewed does not make a publication infallible. It is important to understand how to critically consume research in order to successfully navigate the ever-expanding body of scientific research.
Additional Resources:
https://blogs.lse.ac.uk/impactofsocialsciences/2016/05/09/how-to-read-and-understand-a-scientific-paper-a-guide-for-non-scientists/
https://statmodeling.stat.columbia.edu/2021/06/16/wow-just-wow-if-you-think-psychological-science-as-bad-in-the-2010-2015-era-you-cant-imagine-how-bad-it-was-back-in-1999/
https://totalinternalreflectionblog.com/2018/05/21/check-the-technique-a-short-guide-to-critical-reading-of-scientific-papers/
https://undsci.berkeley.edu/understanding-science-101/how-science-works/scrutinizing-science-peer-review/
https://www.linkedin.com/pulse/critical-consumers-scientific-literature-researchers-patients-savitz/
References:
Cohen, J. (1990). Things I have learned (So Far). The American Psychologist , 45 (12), 1304–1312. DOI: 10.1037/0003-066X.45.12.1304
Henrich, J., Heine, S., & Norenzayan, A. (2010). The weirdest people in the world? Behavioral and Brain Sciences, 33 (2-3), 61-83 DOI: 10.1017/S0140525X0999152X
Sheard, J. (2018). Chapter 18 – Quantitative data analysis. In K. Williamson & G. Johanson (Eds.), Research Methods (2nd ed., pp. 429-452). Chandos Publishing. DOI: 10.1016/B978-0-08-102220-7.00018-2
The flagship campus of the University of Tennessee System and partner in the Tennessee Transfer Pathway .
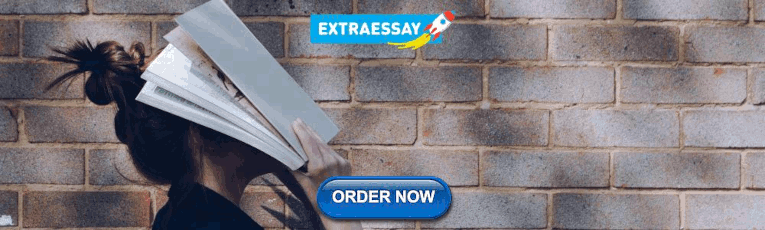
IMAGES
VIDEO
COMMENTS
Revised on June 22, 2023. Quantitative research is the process of collecting and analyzing numerical data. It can be used to find patterns and averages, make predictions, test causal relationships, and generalize results to wider populations. Quantitative research is the opposite of qualitative research, which involves collecting and analyzing ...
Unlike research questions in quantitative research, research questions in qualitative research are usually continuously reviewed and reformulated. ... To construct effective research questions and hypotheses, it is very important to 1) clarify the background and 2) identify the research problem at the outset of the research, within a specific ...
What is Quantitative Research? Quantitative methodology is the dominant research framework in the social sciences. It refers to a set of strategies, techniques and assumptions used to study psychological, social and economic processes through the exploration of numeric patterns.Quantitative research gathers a range of numeric data.
Advantages of Quantitative Research. Quantitative researchers aim to create a general understanding of behavior and other phenomena across different settings and populations. Quantitative studies are often fast, focused, scientific and relatable. 4. The speed and efficiency of the quantitative method are attractive to many researchers.
Quantitative research is a type of research that focuses on collecting and analyzing numerical data to answer research questions. There are two main methods used to conduct quantitative research: 1. Primary Method. There are several methods of primary quantitative research, each with its own strengths and limitations.
Quantitative research is a research strategy that focuses on quantifying the collection and analysis of data. It is formed from a deductive approach where emphasis is placed on the testing of theory, shaped by empiricist and positivist philosophies.. Associated with the natural, applied, formal, and social sciences this research strategy promotes the objective empirical investigation of ...
Revised on 10 October 2022. Quantitative research is the process of collecting and analysing numerical data. It can be used to find patterns and averages, make predictions, test causal relationships, and generalise results to wider populations. Quantitative research is the opposite of qualitative research, which involves collecting and ...
The importance of quantitative research. Quantitative research is a powerful tool for anyone looking to learn more about their market and customers. It allows you to gain reliable, objective insights from data and clearly understand trends and patterns. Where quantitative research falls short is in explaining the 'why'.
Mixed-methods research is a flexible approach, where the research design is determined by what we want to find out rather than by any predetermined epistemological position. In mixed-methods research, qualitative or quantitative components can predominate, or both can have equal status. 1.4. Units and variables.
Quantitative research methods are concerned with the planning, design, and implementation of strategies to collect and analyze data. Descartes, the seventeenth-century philosopher, suggested that how the results are achieved is often more important than the results themselves, as the journey taken along the research path is a journey of discovery. . High-quality quantitative research is ...
Quantitative research is the methodology which researchers use to test theories about people's attitudes and behaviors based on numerical and statistical evidence. Researchers sample a large number of users (e.g., through surveys) to indirectly obtain measurable, bias-free data about users in relevant situations.
Key Concepts in Quantitative Research In this module, we are going to explore the nuances of quantitative research, including the main types of quantitative research, more exploration into variables (including confounding and extraneous variables), and causation. ... This is a major reason why it is so important to be able to duplicate a ...
Quantitative Research. Quantitative research is an objective, formal, systematic process in which numerical data are used to quantify or measure social phenomena and produce findings. ... and with important implications for policy. Among all the problems mentioned by the survey respondents, which included for instance employment and debt, it ...
Abstract. This article describes the basic tenets of quantitative research. The concepts of dependent and independent variables are addressed and the concept of measurement and its associated issues, such as error, reliability and validity, are explored. Experiments and surveys - the principal research designs in quantitative research - are ...
Quantitative research is the process of collecting and analyzing numerical data to describe, predict, or control variables of interest. This type of research helps in testing the causal relationships between variables, making predictions, and generalizing results to wider populations. The purpose of quantitative research is to test a predefined ...
Quantitative research involves analyzing and gathering numerical data to uncover trends, calculate averages, evaluate relationships, and derive overarching insights. It's used in various fields, including the natural and social sciences. Quantitative data analysis employs statistical techniques for processing and interpreting numeric data.
Quantitative research is a method of inquiry that uses numbers and mathematical operations to explore questions about reality. About us; Disclaimer; ... It is important to clearly communicate the research question, methods, results, and conclusions to ensure that others can understand and replicate your research. When to use Quantitative Research.
Quantitative research is an important part of market research that relies on hard facts and numerical data to gain as objective a picture of people's opinions as possible. It's different from qualitative research in a number of important ways and is a highly useful tool for researchers. Quantitative research is a systematic empirical ...
Qualitative research aims to produce rich and detailed descriptions of the phenomenon being studied, and to uncover new insights and meanings. Quantitative data is information about quantities, and therefore numbers, and qualitative data is descriptive, and regards phenomenon which can be observed but not measured, such as language.
This analysis seeks to identify data patterns, trends, and linkages to inform decisions and predictions. Quantitative data analysis uses statistics and math to solve problems in business, finance, and risk management problems. It is an important technique that helps financial analysts, scientists, and researchers understand challenging ideas ...
Quantitative research generates factual, reliable outcome data that are usually generalizable to some larger populations, and qualitative research produces rich, detailed and valid process data based on the participant's, rather than the investigator's, perspectives and interpretations ( 1 ). Quantitative research is usually deductive ...
The phrase "research" refers to seeking knowledge. It is a scholarly and systematic search for relevant knowledge on a specified subject. The Oxford Learner's Dictionaries defines "Research ...
Abstractspiepr Abs1. Every day people do research as they gather information to learn about something of interest. In the scientific world, however, research means something different than simply gathering information. Scientific research is characterized by its careful planning and observing, by its relentless efforts to understand and explain ...
Not all research is peer-reviewed, including conference presentations, blog posts, and white papers, and simply being peer-reviewed does not make a publication infallible. It is important to understand how to critically consume research in order to successfully navigate the ever-expanding body of scientific research.