Have a language expert improve your writing
Run a free plagiarism check in 10 minutes, automatically generate references for free.
- Knowledge Base
- Methodology
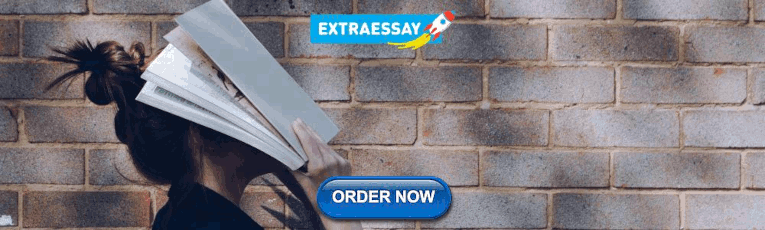
Research Design | Step-by-Step Guide with Examples
Published on 5 May 2022 by Shona McCombes . Revised on 20 March 2023.
A research design is a strategy for answering your research question using empirical data. Creating a research design means making decisions about:
- Your overall aims and approach
- The type of research design you’ll use
- Your sampling methods or criteria for selecting subjects
- Your data collection methods
- The procedures you’ll follow to collect data
- Your data analysis methods
A well-planned research design helps ensure that your methods match your research aims and that you use the right kind of analysis for your data.
Table of contents
Step 1: consider your aims and approach, step 2: choose a type of research design, step 3: identify your population and sampling method, step 4: choose your data collection methods, step 5: plan your data collection procedures, step 6: decide on your data analysis strategies, frequently asked questions.
- Introduction
Before you can start designing your research, you should already have a clear idea of the research question you want to investigate.
There are many different ways you could go about answering this question. Your research design choices should be driven by your aims and priorities – start by thinking carefully about what you want to achieve.
The first choice you need to make is whether you’ll take a qualitative or quantitative approach.
Qualitative research designs tend to be more flexible and inductive , allowing you to adjust your approach based on what you find throughout the research process.
Quantitative research designs tend to be more fixed and deductive , with variables and hypotheses clearly defined in advance of data collection.
It’s also possible to use a mixed methods design that integrates aspects of both approaches. By combining qualitative and quantitative insights, you can gain a more complete picture of the problem you’re studying and strengthen the credibility of your conclusions.
Practical and ethical considerations when designing research
As well as scientific considerations, you need to think practically when designing your research. If your research involves people or animals, you also need to consider research ethics .
- How much time do you have to collect data and write up the research?
- Will you be able to gain access to the data you need (e.g., by travelling to a specific location or contacting specific people)?
- Do you have the necessary research skills (e.g., statistical analysis or interview techniques)?
- Will you need ethical approval ?
At each stage of the research design process, make sure that your choices are practically feasible.
Prevent plagiarism, run a free check.
Within both qualitative and quantitative approaches, there are several types of research design to choose from. Each type provides a framework for the overall shape of your research.
Types of quantitative research designs
Quantitative designs can be split into four main types. Experimental and quasi-experimental designs allow you to test cause-and-effect relationships, while descriptive and correlational designs allow you to measure variables and describe relationships between them.
With descriptive and correlational designs, you can get a clear picture of characteristics, trends, and relationships as they exist in the real world. However, you can’t draw conclusions about cause and effect (because correlation doesn’t imply causation ).
Experiments are the strongest way to test cause-and-effect relationships without the risk of other variables influencing the results. However, their controlled conditions may not always reflect how things work in the real world. They’re often also more difficult and expensive to implement.
Types of qualitative research designs
Qualitative designs are less strictly defined. This approach is about gaining a rich, detailed understanding of a specific context or phenomenon, and you can often be more creative and flexible in designing your research.
The table below shows some common types of qualitative design. They often have similar approaches in terms of data collection, but focus on different aspects when analysing the data.
Your research design should clearly define who or what your research will focus on, and how you’ll go about choosing your participants or subjects.
In research, a population is the entire group that you want to draw conclusions about, while a sample is the smaller group of individuals you’ll actually collect data from.
Defining the population
A population can be made up of anything you want to study – plants, animals, organisations, texts, countries, etc. In the social sciences, it most often refers to a group of people.
For example, will you focus on people from a specific demographic, region, or background? Are you interested in people with a certain job or medical condition, or users of a particular product?
The more precisely you define your population, the easier it will be to gather a representative sample.
Sampling methods
Even with a narrowly defined population, it’s rarely possible to collect data from every individual. Instead, you’ll collect data from a sample.
To select a sample, there are two main approaches: probability sampling and non-probability sampling . The sampling method you use affects how confidently you can generalise your results to the population as a whole.
Probability sampling is the most statistically valid option, but it’s often difficult to achieve unless you’re dealing with a very small and accessible population.
For practical reasons, many studies use non-probability sampling, but it’s important to be aware of the limitations and carefully consider potential biases. You should always make an effort to gather a sample that’s as representative as possible of the population.
Case selection in qualitative research
In some types of qualitative designs, sampling may not be relevant.
For example, in an ethnography or a case study, your aim is to deeply understand a specific context, not to generalise to a population. Instead of sampling, you may simply aim to collect as much data as possible about the context you are studying.
In these types of design, you still have to carefully consider your choice of case or community. You should have a clear rationale for why this particular case is suitable for answering your research question.
For example, you might choose a case study that reveals an unusual or neglected aspect of your research problem, or you might choose several very similar or very different cases in order to compare them.
Data collection methods are ways of directly measuring variables and gathering information. They allow you to gain first-hand knowledge and original insights into your research problem.
You can choose just one data collection method, or use several methods in the same study.
Survey methods
Surveys allow you to collect data about opinions, behaviours, experiences, and characteristics by asking people directly. There are two main survey methods to choose from: questionnaires and interviews.
Observation methods
Observations allow you to collect data unobtrusively, observing characteristics, behaviours, or social interactions without relying on self-reporting.
Observations may be conducted in real time, taking notes as you observe, or you might make audiovisual recordings for later analysis. They can be qualitative or quantitative.
Other methods of data collection
There are many other ways you might collect data depending on your field and topic.
If you’re not sure which methods will work best for your research design, try reading some papers in your field to see what data collection methods they used.
Secondary data
If you don’t have the time or resources to collect data from the population you’re interested in, you can also choose to use secondary data that other researchers already collected – for example, datasets from government surveys or previous studies on your topic.
With this raw data, you can do your own analysis to answer new research questions that weren’t addressed by the original study.
Using secondary data can expand the scope of your research, as you may be able to access much larger and more varied samples than you could collect yourself.
However, it also means you don’t have any control over which variables to measure or how to measure them, so the conclusions you can draw may be limited.
As well as deciding on your methods, you need to plan exactly how you’ll use these methods to collect data that’s consistent, accurate, and unbiased.
Planning systematic procedures is especially important in quantitative research, where you need to precisely define your variables and ensure your measurements are reliable and valid.
Operationalisation
Some variables, like height or age, are easily measured. But often you’ll be dealing with more abstract concepts, like satisfaction, anxiety, or competence. Operationalisation means turning these fuzzy ideas into measurable indicators.
If you’re using observations , which events or actions will you count?
If you’re using surveys , which questions will you ask and what range of responses will be offered?
You may also choose to use or adapt existing materials designed to measure the concept you’re interested in – for example, questionnaires or inventories whose reliability and validity has already been established.
Reliability and validity
Reliability means your results can be consistently reproduced , while validity means that you’re actually measuring the concept you’re interested in.
For valid and reliable results, your measurement materials should be thoroughly researched and carefully designed. Plan your procedures to make sure you carry out the same steps in the same way for each participant.
If you’re developing a new questionnaire or other instrument to measure a specific concept, running a pilot study allows you to check its validity and reliability in advance.
Sampling procedures
As well as choosing an appropriate sampling method, you need a concrete plan for how you’ll actually contact and recruit your selected sample.
That means making decisions about things like:
- How many participants do you need for an adequate sample size?
- What inclusion and exclusion criteria will you use to identify eligible participants?
- How will you contact your sample – by mail, online, by phone, or in person?
If you’re using a probability sampling method, it’s important that everyone who is randomly selected actually participates in the study. How will you ensure a high response rate?
If you’re using a non-probability method, how will you avoid bias and ensure a representative sample?
Data management
It’s also important to create a data management plan for organising and storing your data.
Will you need to transcribe interviews or perform data entry for observations? You should anonymise and safeguard any sensitive data, and make sure it’s backed up regularly.
Keeping your data well organised will save time when it comes to analysing them. It can also help other researchers validate and add to your findings.
On their own, raw data can’t answer your research question. The last step of designing your research is planning how you’ll analyse the data.
Quantitative data analysis
In quantitative research, you’ll most likely use some form of statistical analysis . With statistics, you can summarise your sample data, make estimates, and test hypotheses.
Using descriptive statistics , you can summarise your sample data in terms of:
- The distribution of the data (e.g., the frequency of each score on a test)
- The central tendency of the data (e.g., the mean to describe the average score)
- The variability of the data (e.g., the standard deviation to describe how spread out the scores are)
The specific calculations you can do depend on the level of measurement of your variables.
Using inferential statistics , you can:
- Make estimates about the population based on your sample data.
- Test hypotheses about a relationship between variables.
Regression and correlation tests look for associations between two or more variables, while comparison tests (such as t tests and ANOVAs ) look for differences in the outcomes of different groups.
Your choice of statistical test depends on various aspects of your research design, including the types of variables you’re dealing with and the distribution of your data.
Qualitative data analysis
In qualitative research, your data will usually be very dense with information and ideas. Instead of summing it up in numbers, you’ll need to comb through the data in detail, interpret its meanings, identify patterns, and extract the parts that are most relevant to your research question.
Two of the most common approaches to doing this are thematic analysis and discourse analysis .
There are many other ways of analysing qualitative data depending on the aims of your research. To get a sense of potential approaches, try reading some qualitative research papers in your field.
A sample is a subset of individuals from a larger population. Sampling means selecting the group that you will actually collect data from in your research.
For example, if you are researching the opinions of students in your university, you could survey a sample of 100 students.
Statistical sampling allows you to test a hypothesis about the characteristics of a population. There are various sampling methods you can use to ensure that your sample is representative of the population as a whole.
Operationalisation means turning abstract conceptual ideas into measurable observations.
For example, the concept of social anxiety isn’t directly observable, but it can be operationally defined in terms of self-rating scores, behavioural avoidance of crowded places, or physical anxiety symptoms in social situations.
Before collecting data , it’s important to consider how you will operationalise the variables that you want to measure.
The research methods you use depend on the type of data you need to answer your research question .
- If you want to measure something or test a hypothesis , use quantitative methods . If you want to explore ideas, thoughts, and meanings, use qualitative methods .
- If you want to analyse a large amount of readily available data, use secondary data. If you want data specific to your purposes with control over how they are generated, collect primary data.
- If you want to establish cause-and-effect relationships between variables , use experimental methods. If you want to understand the characteristics of a research subject, use descriptive methods.
Cite this Scribbr article
If you want to cite this source, you can copy and paste the citation or click the ‘Cite this Scribbr article’ button to automatically add the citation to our free Reference Generator.
McCombes, S. (2023, March 20). Research Design | Step-by-Step Guide with Examples. Scribbr. Retrieved 22 April 2024, from https://www.scribbr.co.uk/research-methods/research-design/
Is this article helpful?
Shona McCombes

Want to create or adapt books like this? Learn more about how Pressbooks supports open publishing practices.
Research Components
12 Research Design
The story continues….
The next morning, as Harry and Physicus finished the last cold slice of George’s pizza, they had also finished collecting their literature to conduct a review. The friends concluded their theory about the number of mice influencing Pickles’ behavior had considerable support in the research literature. After writing the Literature Review, their research questions will be fully grounded, justified, and worthwhile to be answered.
“So, how do we go about answering our research questions?” asked Harry.
Physicus explained that they will have to analyze their research questions to see what types of answers are required. Knowing this will guide their decisions about how to design the project to answer their questions.
“There are two basic types of answers to research questions, quantitative and qualitative. The types of answers the research questions require tell us what type of research design we need,” said Physicus.
“I guess if I ask how we decide which type of research design we should choose, you will say, ‘It depends?'” uttered Harry.
Physicus’ face brightened as he blurted out, “Absolutely not! Negative!” Physicus continued, “If the research questions are stated well, there will only be two ways in which they can be answered. The research questions are king; they make all the decisions.”
“How come?” Harry appeared confused.
“Well, let us see. Think about our first question. How many mice will Pickles attack at one time? What type of answer does this question require? It requires a numeric answer, correct?” Physicus asked.
“Yes, that is correct,” Harry said.
Physicus continued, “Good. So, does our second question also require a numeric answer?”
“The second question is also answered with a number,” replied Harry
Physicus blurted, “Correct! This means we need to use a quantitative research design!”
Physicus continued, “Now if we had research questions that could not be answered with numbers, we would need to use a qualitative research design to answer our questions with words or phrases instead.”
Harry now appeared relieved, “I get it. So in designing a research project, we simply look for a way to answer the research questions. That’s easy!”
“Well, it depends,” answered Physicus smiling.
Interpreting the Story
There are qualitative, quantitative, mixed methods, and applied research designs. Based on the research questions, the research design will be obvious. Physicus led Harry in determining their study would need a quantitative design, because they only needed numerical data to answer their research questions. If Harry’s questions could only be answered with words or phrases, then a qualitative design would be needed. If the friends had questions needing to be answered with numbers and phrases, then either a mixed methods or an applied research design would have been the choice.
Research Design
The Research Design explains what type of research is being conducted. The writing in this heading also explains why this type of research is needed to obtain the answers to the research or guiding questions for the project. The design provides a blueprint for the methodology. Articulating the nature of the research design is critical for explaining the Methodology (see the next chapter).
There are four categories of research designs used in educational research and a variety of specific research designs in each category. The first step in determining which category to use is to identify what type of data will answer the research questions. As in our story, Harry and Physicus had research questions that required quantitative answers, so the category of their research design is quantitative.
The next step in finding the specific research design is to consider the purpose (goal) of the research project. The research design must support the purpose. In our story, Harry and Physicus need a quantitative research design that supports their goal of determining the effect of the number of mice Pickles encounters at one time on his behavior. A causal-comparative or quasi-experimental research design is the best choice for the friends because these are specific quantitative designs used to find a cause-and-effect relationship.
Quantitative Research Designs
Quantitative research designs seek results based on statistical analyses of the collected numerical data. The primary quantitative designs used in educational research include descriptive, correlational, causal-comparative, and quasi-experimental designs. Numerical data are collected and analyzed using statistical calculations appropriate for the design. For example, analyses like mean, median, mode, range, etc. are used to describe or explain a phenomenon observed in a descriptive research design. A correlational research design uses statistics, such as correlation coefficient or regression analyses to explain how two phenomena are related. Causal-comparative and quasi-experimental designs use analyses needed to establish causal relationships, such as pre-post testing, or behavior change (like in our story).
The use of numerical data guides both the methodology and the analysis protocols. The design also guides and limits how the results are interpreted. Examples of quantitative data found in educational research include test scores, grade point averages, and dropout rates.
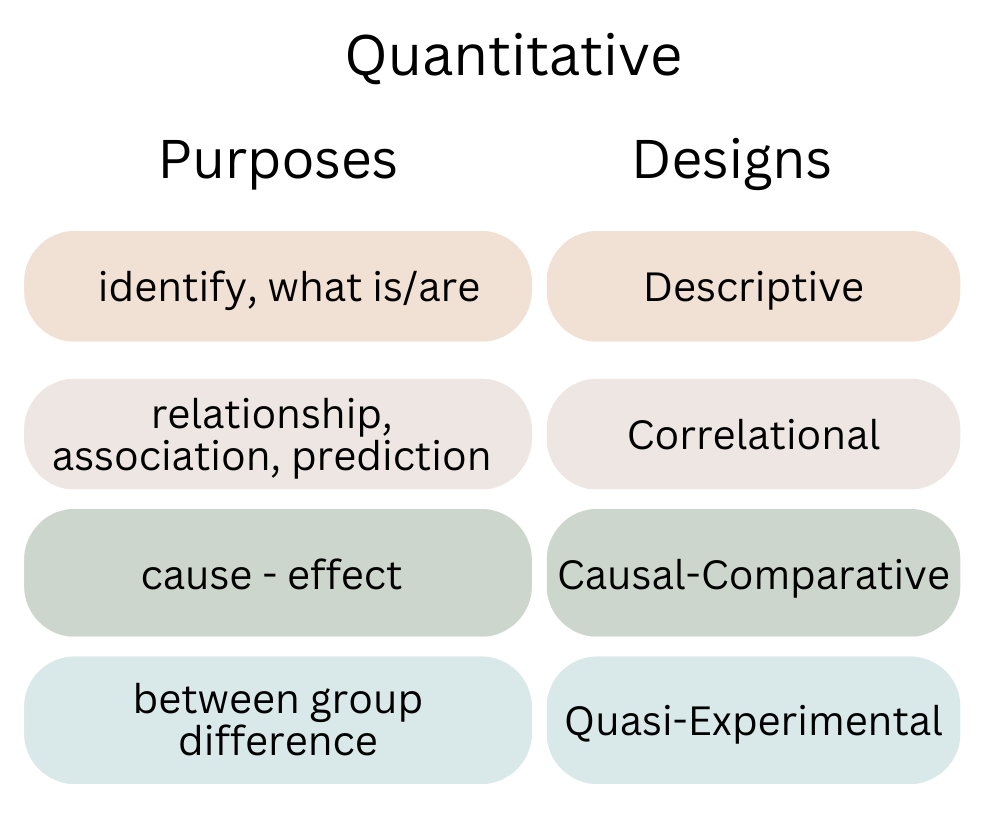
Qualitative Research Designs
Qualitative research designs involve obtaining verbal, perspective, and/or visual results using code-based analyses of collected data. Typical qualitative designs used in educational research include the case study, phenomenological, grounded theory, and ethnography. These designs involve exploring behaviors, perceptions/feelings, and social/cultural phenomena found in educational settings.
Qualitative designs result in a written description of the findings. Data collection strategies include observations, interviews, focus groups, surveys, and documentation reviews. The data are recorded as words, phrases, sentences, and paragraphs. Data are then grouped together to form themes. The process of grouping data to form themes is called coding. The labeled themes become the “code” used to interpret the data. The coding can be determined ahead of time before data are collected, or the coding emerges from the collected data. Data collection strategies often include media such as video and audio recordings. These recordings are transcribed into words to allow for the coding analysis.
The use of qualitative data guides both the methodology and the analysis protocols. The “squishy” nature of qualitative data (words vs. numbers) and the data coding analysis limits the interpretation and conclusions made from the results. It is important to explain the coding analysis used to provide clear reasoning for the themes and how these relate to the research questions.
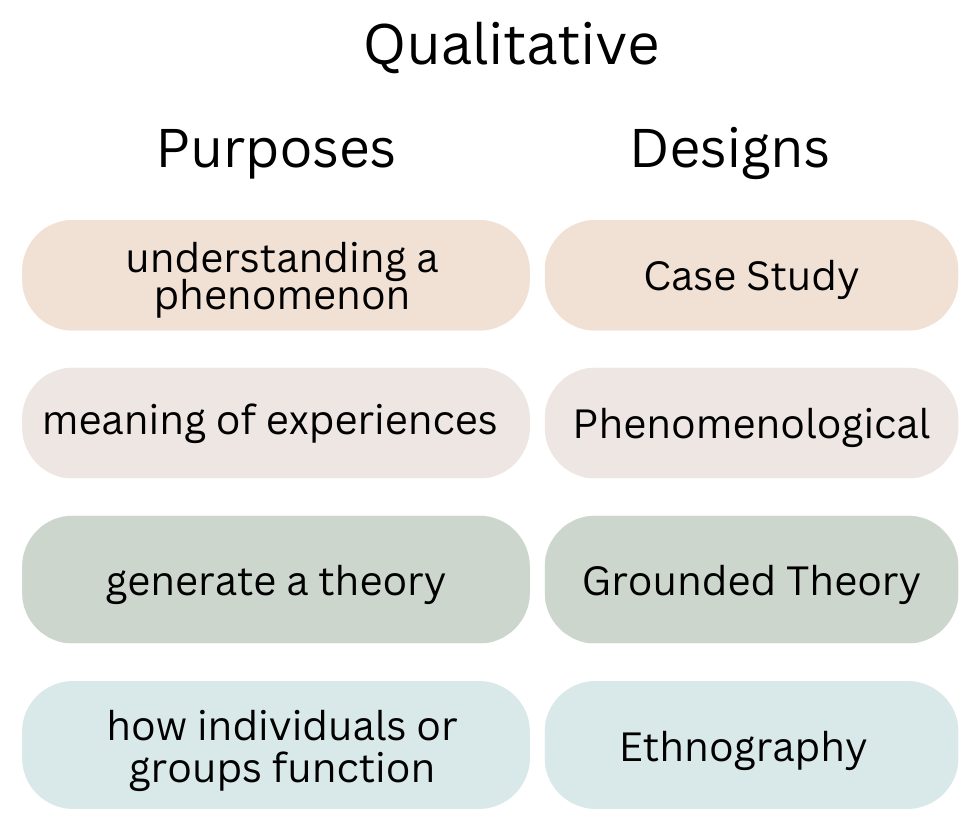
Mixed Method Designs
Mixed Methods research designs are used when the research questions must be answered with results that are both quantitative and qualitative. These designs integrate the data results to arrive at conclusions. A mixed method design is used when there are greater benefits to using multiple data types, sources, and analyses. Examples of typical mixed methods design approaches in education include convergent, explanatory, exploratory, and embedded designs. Using mixed methods approaches in educational research allows the researcher to triangulate, complement, or expand understanding using multiple types of data.
The use of mixed methods data guides the methodology, analysis, and interpretation of the results. Using both qualitative (quant) and quantitative (qual) data analyses provides a clearer or more balanced picture of the results. Data are analyzed sequentially or concurrently depending on the design. While the quantitative and qualitative data are analyzed independently, the results are interpreted integratively. The findings are a synthesis of the quantitative and qualitative analyses.
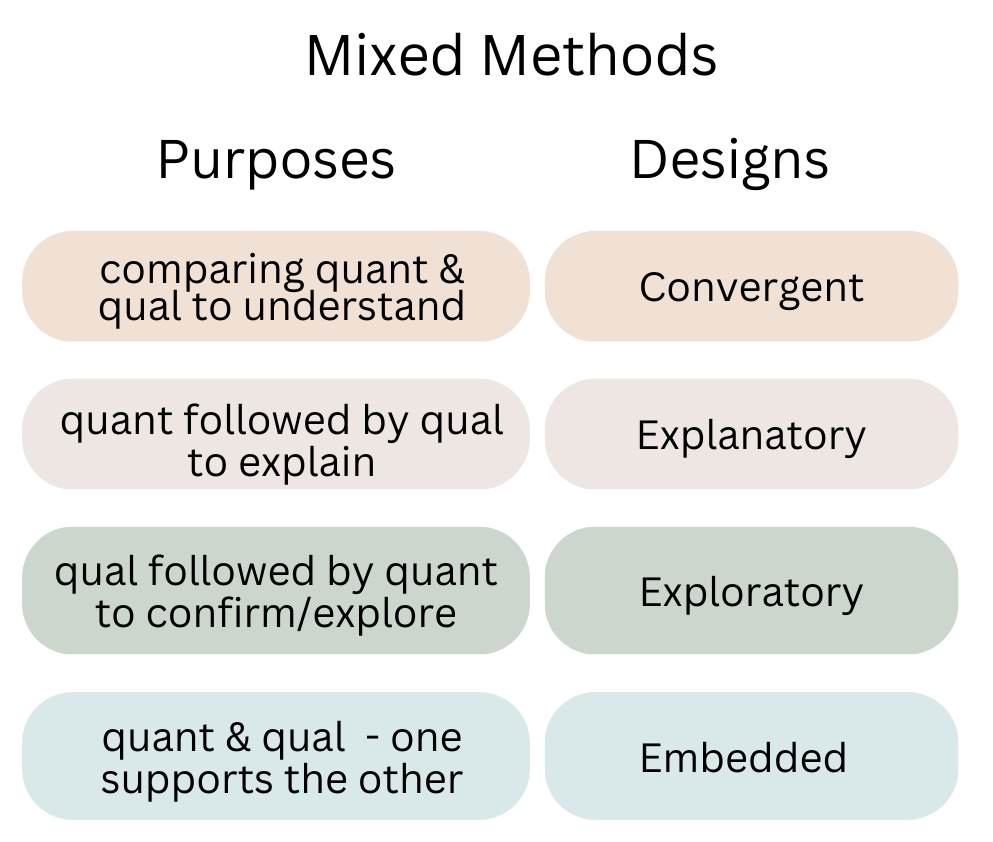
Applied Research Designs
Applied research designs seek both quantitative and qualitative results to address issues of educational practice. Applied research designs include evaluation, design and development, and action research. The purposes of applied research are to identify best practices, to innovate or improve current practices or policies, to test pedagogy, and to evaluate effectiveness. The results of applied research designs provide practical solutions to problems in educational practice.
Applied designs use both theoretical and empirical data. Theoretical data are collected from published theories or other research. Empirical data are obtained by conducting a needs assessment or other data collection methods. Data analyses include both quantitative and qualitative procedures. The findings are interpreted integratively as in mixed methods approaches, and then “applied” to the problem to form a solution.
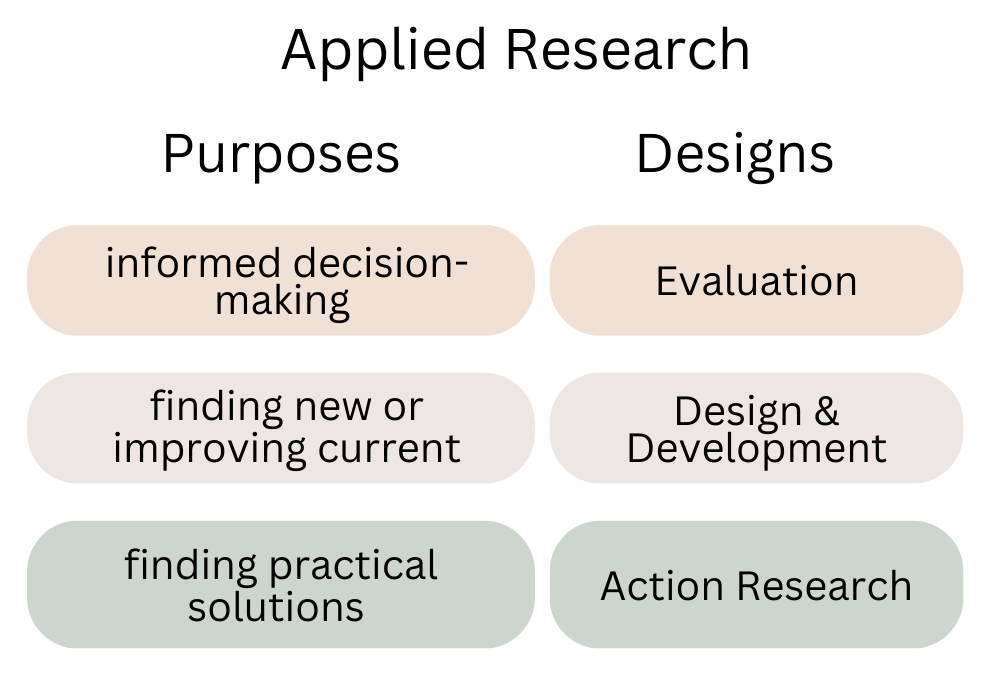
Telling the research story
The Research Design in a research project tells the story of what direction the plot of the story will take. The writing in this heading sets the stage for the rising action of the plot in the research story. The Research Design describes the journey that is about to take place. It functions to guide the reader in understanding the type of path the story will follow. The Research Design is the overall direction of the research story and is determined before deciding on the specific steps to take in obtaining and analyzing the data.
The Research Design heading appears in Chapter 3 of the thesis and dissertation projects under the Methodology heading. A literature review project does not have this heading.
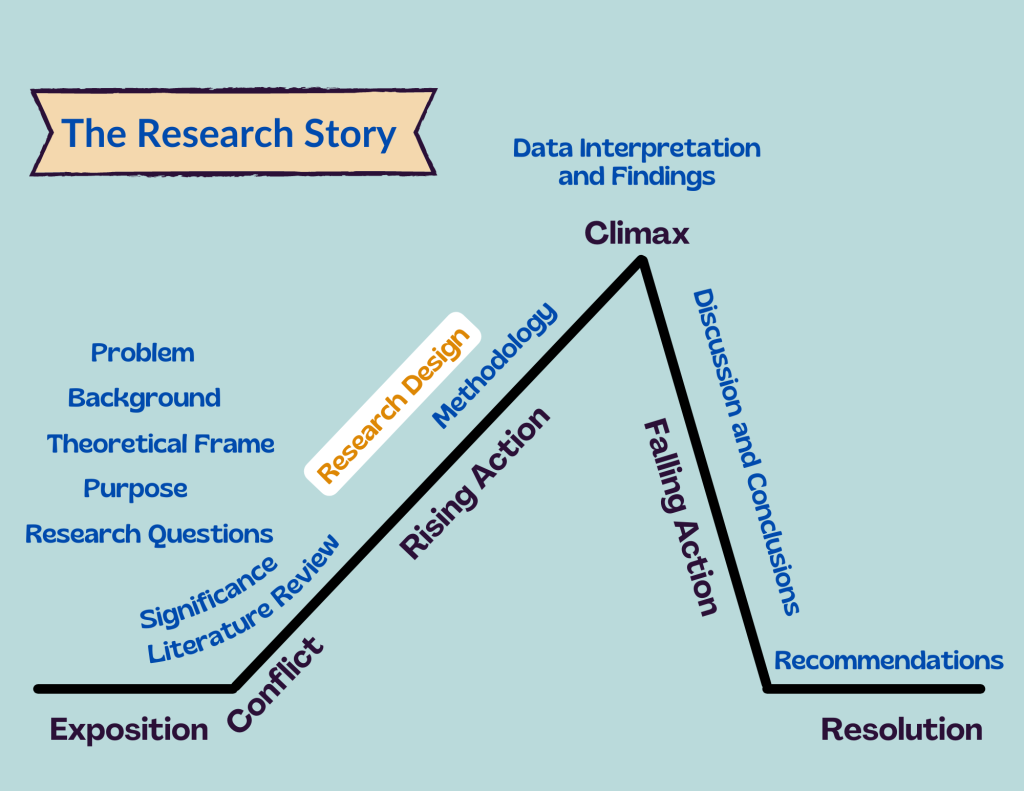
Graduate Research in Education: Learning the Research Story Copyright © 2022 by Kimberly Chappell and Greg I. Voykhansky is licensed under a Creative Commons Attribution-NonCommercial-ShareAlike 4.0 International License , except where otherwise noted.
- Subject List
- Take a Tour
- For Authors
- Subscriber Services
- Publications
- African American Studies
- African Studies
- American Literature
- Anthropology
- Architecture Planning and Preservation
- Art History
- Atlantic History
- Biblical Studies
- British and Irish Literature
- Childhood Studies
- Chinese Studies
- Cinema and Media Studies
- Communication
- Criminology
- Environmental Science
- Evolutionary Biology
- International Law
- International Relations
- Islamic Studies
- Jewish Studies
- Latin American Studies
- Latino Studies
- Linguistics
- Literary and Critical Theory
- Medieval Studies
- Military History
- Political Science
- Public Health
- Renaissance and Reformation
- Social Work
- Urban Studies
- Victorian Literature
- Browse All Subjects
How to Subscribe
- Free Trials
In This Article Expand or collapse the "in this article" section Quantitative Research Designs in Educational Research
Introduction, general overviews.
- Survey Research Designs
- Correlational Designs
- Other Nonexperimental Designs
- Randomized Experimental Designs
- Quasi-Experimental Designs
- Single-Case Designs
- Single-Case Analyses
Related Articles Expand or collapse the "related articles" section about
About related articles close popup.
Lorem Ipsum Sit Dolor Amet
Vestibulum ante ipsum primis in faucibus orci luctus et ultrices posuere cubilia Curae; Aliquam ligula odio, euismod ut aliquam et, vestibulum nec risus. Nulla viverra, arcu et iaculis consequat, justo diam ornare tellus, semper ultrices tellus nunc eu tellus.
- Methodologies for Conducting Education Research
- Mixed Methods Research
- Multivariate Research Methodology
- Qualitative Data Analysis Techniques
- Qualitative, Quantitative, and Mixed Methods Research Sampling Strategies
- Researcher Development and Skills Training within the Context of Postgraduate Programs
- Single-Subject Research Design
- Social Network Analysis
- Statistical Assumptions
Other Subject Areas
Forthcoming articles expand or collapse the "forthcoming articles" section.
- English as an International Language for Academic Publishing
- Girls' Education in the Developing World
- History of Education in Europe
- Find more forthcoming articles...
- Export Citations
- Share This Facebook LinkedIn Twitter
Quantitative Research Designs in Educational Research by James H. McMillan , Richard S. Mohn , Micol V. Hammack LAST REVIEWED: 24 July 2013 LAST MODIFIED: 24 July 2013 DOI: 10.1093/obo/9780199756810-0113
The field of education has embraced quantitative research designs since early in the 20th century. The foundation for these designs was based primarily in the psychological literature, and psychology and the social sciences more generally continued to have a strong influence on quantitative designs until the assimilation of qualitative designs in the 1970s and 1980s. More recently, a renewed emphasis on quasi-experimental and nonexperimental quantitative designs to infer causal conclusions has resulted in many newer sources specifically targeting these approaches to the field of education. This bibliography begins with a discussion of general introductions to all quantitative designs in the educational literature. The sources in this section tend to be textbooks or well-known sources written many years ago, though still very relevant and helpful. It should be noted that there are many other sources in the social sciences more generally that contain principles of quantitative designs that are applicable to education. This article then classifies quantitative designs primarily as either nonexperimental or experimental but also emphasizes the use of nonexperimental designs for making causal inferences. Among experimental designs the article distinguishes between those that include random assignment of subjects, those that are quasi-experimental (with no random assignment), and those that are single-case (single-subject) designs. Quasi-experimental and nonexperimental designs used for making causal inferences are becoming more popular in education given the practical difficulties and expense in conducting well-controlled experiments, particularly with the use of structural equation modeling (SEM). There have also been recent developments in statistical analyses that allow stronger causal inferences. Historically, quantitative designs have been tied closely to sampling, measurement, and statistics. In this bibliography there are important sources for newer statistical procedures that are needed for particular designs, especially single-case designs, but relatively little attention to sampling or measurement. The literature on quantitative designs in education is not well focused or comprehensively addressed in very many sources, except in general overview textbooks. Those sources that do include the range of designs are introductory in nature; more advanced designs and statistical analyses tend to be found in journal articles and other individual documents, with a couple exceptions. Another new trend in educational research designs is the use of mixed-method designs (both quantitative and qualitative), though this article does not emphasize these designs.
For many years there have been textbooks that present the range of quantitative research designs, both in education and the social sciences more broadly. Indeed, most of the quantitative design research principles are much the same for education, psychology, and other social sciences. These sources provide an introduction to basic designs that are used within the broader context of other educational research methodologies such as qualitative and mixed-method. Examples of these textbooks written specifically for education include Johnson and Christensen 2012 ; Mertens 2010 ; Arthur, et al. 2012 ; and Creswell 2012 . An example of a similar text written for the social sciences, including education that is dedicated only to quantitative research, is Gliner, et al. 2009 . In these texts separate chapters are devoted to different types of quantitative designs. For example, Creswell 2012 contains three quantitative design chapters—experimental, which includes both randomized and quasi-experimental designs; correlational (nonexperimental); and survey (also nonexperimental). Johnson and Christensen 2012 also includes three quantitative design chapters, with greater emphasis on quasi-experimental and single-subject research. Mertens 2010 includes a chapter on causal-comparative designs (nonexperimental). Often survey research is addressed as a distinct type of quantitative research with an emphasis on sampling and measurement (how to design surveys). Green, et al. 2006 also presents introductory chapters on different types of quantitative designs, but each of the chapters has different authors. In this book chapters extend basic designs by examining in greater detail nonexperimental methodologies structured for causal inferences and scaled-up experiments. Two additional sources are noted because they represent the types of publications for the social sciences more broadly that discuss many of the same principles of quantitative design among other types of designs. Bickman and Rog 2009 uses different chapter authors to cover topics such as statistical power for designs, sampling, randomized controlled trials, and quasi-experiments, and educational researchers will find this information helpful in designing their studies. Little 2012 provides a comprehensive coverage of topics related to quantitative methods in the social, behavioral, and education fields.
Arthur, James, Michael Waring, Robert Coe, and Larry V. Hedges, eds. 2012. Research methods & methodologies in education . Thousand Oaks, CA: SAGE.
Readers will find this book more of a handbook than a textbook. Different individuals author each of the chapters, representing quantitative, qualitative, and mixed-method designs. The quantitative chapters are on the treatment of advanced statistical applications, including analysis of variance, regression, and multilevel analysis.
Bickman, Leonard, and Debra J. Rog, eds. 2009. The SAGE handbook of applied social research methods . 2d ed. Thousand Oaks, CA: SAGE.
This handbook includes quantitative design chapters that are written for the social sciences broadly. There are relatively advanced treatments of statistical power, randomized controlled trials, and sampling in quantitative designs, though the coverage of additional topics is not as complete as other sources in this section.
Creswell, John W. 2012. Educational research: Planning, conducting, and evaluating quantitative and qualitative research . 4th ed. Boston: Pearson.
Creswell presents an introduction to all major types of research designs. Three chapters cover quantitative designs—experimental, correlational, and survey research. Both the correlational and survey research chapters focus on nonexperimental designs. Overall the introductions are complete and helpful to those beginning their study of quantitative research designs.
Gliner, Jeffrey A., George A. Morgan, and Nancy L. Leech. 2009. Research methods in applied settings: An integrated approach to design and analysis . 2d ed. New York: Routledge.
This text, unlike others in this section, is devoted solely to quantitative research. As such, all aspects of quantitative designs are covered. There are separate chapters on experimental, nonexperimental, and single-subject designs and on internal validity, sampling, and data-collection techniques for quantitative studies. The content of the book is somewhat more advanced than others listed in this section and is unique in its quantitative focus.
Green, Judith L., Gregory Camilli, and Patricia B. Elmore, eds. 2006. Handbook of complementary methods in education research . Mahwah, NJ: Lawrence Erlbaum.
Green, Camilli, and Elmore edited forty-six chapters that represent many contemporary issues and topics related to quantitative designs. Written by noted researchers, the chapters cover design experiments, quasi-experimentation, randomized experiments, and survey methods. Other chapters include statistical topics that have relevance for quantitative designs.
Johnson, Burke, and Larry B. Christensen. 2012. Educational research: Quantitative, qualitative, and mixed approaches . 4th ed. Thousand Oaks, CA: SAGE.
This comprehensive textbook of educational research methods includes extensive coverage of qualitative and mixed-method designs along with quantitative designs. Three of twenty chapters focus on quantitative designs (experimental, quasi-experimental, and single-case) and nonexperimental, including longitudinal and retrospective, designs. The level of material is relatively high, and there are introductory chapters on sampling and quantitative analyses.
Little, Todd D., ed. 2012. The Oxford handbook of quantitative methods . Vol. 1, Foundations . New York: Oxford Univ. Press.
This handbook is a relatively advanced treatment of quantitative design and statistical analyses. Multiple authors are used to address strengths and weaknesses of many different issues and methods, including advanced statistical tools.
Mertens, Donna M. 2010. Research and evaluation in education and psychology: Integrating diversity with quantitative, qualitative, and mixed methods . 3d ed. Thousand Oaks, CA: SAGE.
This textbook is an introduction to all types of educational designs and includes four chapters devoted to quantitative research—experimental and quasi-experimental, causal comparative and correlational, survey, and single-case research. The author’s treatment of some topics is somewhat more advanced than texts such as Creswell 2012 , with extensive attention to threats to internal validity for some of the designs.
back to top
Users without a subscription are not able to see the full content on this page. Please subscribe or login .
Oxford Bibliographies Online is available by subscription and perpetual access to institutions. For more information or to contact an Oxford Sales Representative click here .
- About Education »
- Meet the Editorial Board »
- Academic Achievement
- Academic Audit for Universities
- Academic Freedom and Tenure in the United States
- Action Research in Education
- Adjuncts in Higher Education in the United States
- Administrator Preparation
- Adolescence
- Advanced Placement and International Baccalaureate Courses
- Advocacy and Activism in Early Childhood
- African American Racial Identity and Learning
- Alaska Native Education
- Alternative Certification Programs for Educators
- Alternative Schools
- American Indian Education
- Animals in Environmental Education
- Art Education
- Artificial Intelligence and Learning
- Assessing School Leader Effectiveness
- Assessment, Behavioral
- Assessment, Educational
- Assessment in Early Childhood Education
- Assistive Technology
- Augmented Reality in Education
- Beginning-Teacher Induction
- Bilingual Education and Bilingualism
- Black Undergraduate Women: Critical Race and Gender Perspe...
- Blended Learning
- Case Study in Education Research
- Changing Professional and Academic Identities
- Character Education
- Children’s and Young Adult Literature
- Children's Beliefs about Intelligence
- Children's Rights in Early Childhood Education
- Citizenship Education
- Civic and Social Engagement of Higher Education
- Classroom Learning Environments: Assessing and Investigati...
- Classroom Management
- Coherent Instructional Systems at the School and School Sy...
- College Admissions in the United States
- College Athletics in the United States
- Community Relations
- Comparative Education
- Computer-Assisted Language Learning
- Computer-Based Testing
- Conceptualizing, Measuring, and Evaluating Improvement Net...
- Continuous Improvement and "High Leverage" Educational Pro...
- Counseling in Schools
- Critical Approaches to Gender in Higher Education
- Critical Perspectives on Educational Innovation and Improv...
- Critical Race Theory
- Crossborder and Transnational Higher Education
- Cross-National Research on Continuous Improvement
- Cross-Sector Research on Continuous Learning and Improveme...
- Cultural Diversity in Early Childhood Education
- Culturally Responsive Leadership
- Culturally Responsive Pedagogies
- Culturally Responsive Teacher Education in the United Stat...
- Curriculum Design
- Data Collection in Educational Research
- Data-driven Decision Making in the United States
- Deaf Education
- Desegregation and Integration
- Design Thinking and the Learning Sciences: Theoretical, Pr...
- Development, Moral
- Dialogic Pedagogy
- Digital Age Teacher, The
- Digital Citizenship
- Digital Divides
- Disabilities
- Distance Learning
- Distributed Leadership
- Doctoral Education and Training
- Early Childhood Education and Care (ECEC) in Denmark
- Early Childhood Education and Development in Mexico
- Early Childhood Education in Aotearoa New Zealand
- Early Childhood Education in Australia
- Early Childhood Education in China
- Early Childhood Education in Europe
- Early Childhood Education in Sub-Saharan Africa
- Early Childhood Education in Sweden
- Early Childhood Education Pedagogy
- Early Childhood Education Policy
- Early Childhood Education, The Arts in
- Early Childhood Mathematics
- Early Childhood Science
- Early Childhood Teacher Education
- Early Childhood Teachers in Aotearoa New Zealand
- Early Years Professionalism and Professionalization Polici...
- Economics of Education
- Education For Children with Autism
- Education for Sustainable Development
- Education Leadership, Empirical Perspectives in
- Education of Native Hawaiian Students
- Education Reform and School Change
- Educational Statistics for Longitudinal Research
- Educator Partnerships with Parents and Families with a Foc...
- Emotional and Affective Issues in Environmental and Sustai...
- Emotional and Behavioral Disorders
- Environmental and Science Education: Overlaps and Issues
- Environmental Education
- Environmental Education in Brazil
- Epistemic Beliefs
- Equity and Improvement: Engaging Communities in Educationa...
- Equity, Ethnicity, Diversity, and Excellence in Education
- Ethical Research with Young Children
- Ethics and Education
- Ethics of Teaching
- Ethnic Studies
- Evidence-Based Communication Assessment and Intervention
- Family and Community Partnerships in Education
- Family Day Care
- Federal Government Programs and Issues
- Feminization of Labor in Academia
- Finance, Education
- Financial Aid
- Formative Assessment
- Future-Focused Education
- Gender and Achievement
- Gender and Alternative Education
- Gender, Power and Politics in the Academy
- Gender-Based Violence on University Campuses
- Gifted Education
- Global Mindedness and Global Citizenship Education
- Global University Rankings
- Governance, Education
- Grounded Theory
- Growth of Effective Mental Health Services in Schools in t...
- Higher Education and Globalization
- Higher Education and the Developing World
- Higher Education Faculty Characteristics and Trends in the...
- Higher Education Finance
- Higher Education Governance
- Higher Education Graduate Outcomes and Destinations
- Higher Education in Africa
- Higher Education in China
- Higher Education in Latin America
- Higher Education in the United States, Historical Evolutio...
- Higher Education, International Issues in
- Higher Education Management
- Higher Education Policy
- Higher Education Research
- Higher Education Student Assessment
- High-stakes Testing
- History of Early Childhood Education in the United States
- History of Education in the United States
- History of Technology Integration in Education
- Homeschooling
- Inclusion in Early Childhood: Difference, Disability, and ...
- Inclusive Education
- Indigenous Education in a Global Context
- Indigenous Learning Environments
- Indigenous Students in Higher Education in the United Stat...
- Infant and Toddler Pedagogy
- Inservice Teacher Education
- Integrating Art across the Curriculum
- Intelligence
- Intensive Interventions for Children and Adolescents with ...
- International Perspectives on Academic Freedom
- Intersectionality and Education
- Knowledge Development in Early Childhood
- Leadership Development, Coaching and Feedback for
- Leadership in Early Childhood Education
- Leadership Training with an Emphasis on the United States
- Learning Analytics in Higher Education
- Learning Difficulties
- Learning, Lifelong
- Learning, Multimedia
- Learning Strategies
- Legal Matters and Education Law
- LGBT Youth in Schools
- Linguistic Diversity
- Linguistically Inclusive Pedagogy
- Literacy Development and Language Acquisition
- Literature Reviews
- Mathematics Identity
- Mathematics Instruction and Interventions for Students wit...
- Mathematics Teacher Education
- Measurement for Improvement in Education
- Measurement in Education in the United States
- Meta-Analysis and Research Synthesis in Education
- Methodological Approaches for Impact Evaluation in Educati...
- Mindfulness, Learning, and Education
- Motherscholars
- Multiliteracies in Early Childhood Education
- Multiple Documents Literacy: Theory, Research, and Applica...
- Museums, Education, and Curriculum
- Music Education
- Narrative Research in Education
- Native American Studies
- Nonformal and Informal Environmental Education
- Note-Taking
- Numeracy Education
- One-to-One Technology in the K-12 Classroom
- Online Education
- Open Education
- Organizing for Continuous Improvement in Education
- Organizing Schools for the Inclusion of Students with Disa...
- Outdoor Play and Learning
- Outdoor Play and Learning in Early Childhood Education
- Pedagogical Leadership
- Pedagogy of Teacher Education, A
- Performance Objectives and Measurement
- Performance-based Research Assessment in Higher Education
- Performance-based Research Funding
- Phenomenology in Educational Research
- Philosophy of Education
- Physical Education
- Podcasts in Education
- Policy Context of United States Educational Innovation and...
- Politics of Education
- Portable Technology Use in Special Education Programs and ...
- Post-humanism and Environmental Education
- Pre-Service Teacher Education
- Problem Solving
- Productivity and Higher Education
- Professional Development
- Professional Learning Communities
- Program Evaluation
- Programs and Services for Students with Emotional or Behav...
- Psychology Learning and Teaching
- Psychometric Issues in the Assessment of English Language ...
- Qualitative, Quantitative, and Mixed Methods Research Samp...
- Qualitative Research Design
- Quantitative Research Designs in Educational Research
- Queering the English Language Arts (ELA) Writing Classroom
- Race and Affirmative Action in Higher Education
- Reading Education
- Refugee and New Immigrant Learners
- Relational and Developmental Trauma and Schools
- Relational Pedagogies in Early Childhood Education
- Reliability in Educational Assessments
- Religion in Elementary and Secondary Education in the Unit...
- Researcher Development and Skills Training within the Cont...
- Research-Practice Partnerships in Education within the Uni...
- Response to Intervention
- Restorative Practices
- Risky Play in Early Childhood Education
- Scale and Sustainability of Education Innovation and Impro...
- Scaling Up Research-based Educational Practices
- School Accreditation
- School Choice
- School Culture
- School District Budgeting and Financial Management in the ...
- School Improvement through Inclusive Education
- School Reform
- Schools, Private and Independent
- School-Wide Positive Behavior Support
- Science Education
- Secondary to Postsecondary Transition Issues
- Self-Regulated Learning
- Self-Study of Teacher Education Practices
- Service-Learning
- Severe Disabilities
- Single Salary Schedule
- Single-sex Education
- Social Context of Education
- Social Justice
- Social Pedagogy
- Social Science and Education Research
- Social Studies Education
- Sociology of Education
- Standards-Based Education
- Student Access, Equity, and Diversity in Higher Education
- Student Assignment Policy
- Student Engagement in Tertiary Education
- Student Learning, Development, Engagement, and Motivation ...
- Student Participation
- Student Voice in Teacher Development
- Sustainability Education in Early Childhood Education
- Sustainability in Early Childhood Education
- Sustainability in Higher Education
- Teacher Beliefs and Epistemologies
- Teacher Collaboration in School Improvement
- Teacher Evaluation and Teacher Effectiveness
- Teacher Preparation
- Teacher Training and Development
- Teacher Unions and Associations
- Teacher-Student Relationships
- Teaching Critical Thinking
- Technologies, Teaching, and Learning in Higher Education
- Technology Education in Early Childhood
- Technology, Educational
- Technology-based Assessment
- The Bologna Process
- The Regulation of Standards in Higher Education
- Theories of Educational Leadership
- Three Conceptions of Literacy: Media, Narrative, and Gamin...
- Tracking and Detracking
- Traditions of Quality Improvement in Education
- Transformative Learning
- Transitions in Early Childhood Education
- Tribally Controlled Colleges and Universities in the Unite...
- Understanding the Psycho-Social Dimensions of Schools and ...
- University Faculty Roles and Responsibilities in the Unite...
- Using Ethnography in Educational Research
- Value of Higher Education for Students and Other Stakehold...
- Virtual Learning Environments
- Vocational and Technical Education
- Wellness and Well-Being in Education
- Women's and Gender Studies
- Young Children and Spirituality
- Young Children's Learning Dispositions
- Young Children's Working Theories
- Privacy Policy
- Cookie Policy
- Legal Notice
- Accessibility
Powered by:
- [66.249.64.20|81.177.182.159]
- 81.177.182.159
Educational Design Research
- First Online: 01 January 2013
Cite this chapter
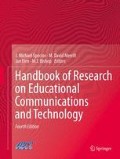
- Susan McKenney 5 &
- Thomas C. Reeves 6
32k Accesses
78 Citations
3 Altmetric
Educational design research is a genre of research in which the iterative development of solutions to practical and complex educational problems provides the setting for scientific inquiry. The solutions can be educational products, processes, programs, or policies. Educational design research not only targets solving significant problems facing educational practitioners but at the same time seeks to discover new knowledge that can inform the work of others facing similar problems. Working systematically and simultaneously toward these dual goals is perhaps the most defining feature of educational design research. This chapter seeks to clarify the nature of educational design research by distinguishing it from other types of inquiry conducted in the field of educational communications and technology. Examples of design research conducted by different researchers working in the field of educational communications and technology are described. The chapter concludes with a discussion of several important issues facing educational design researchers as they pursue future work using this innovative research approach.
This is a preview of subscription content, log in via an institution to check access.
Access this chapter
- Available as PDF
- Read on any device
- Instant download
- Own it forever
- Available as EPUB and PDF
Tax calculation will be finalised at checkout
Purchases are for personal use only
Institutional subscriptions
Anderson, T., & Shattuck, J. (2012). Design-based research: A decade of progress in education research? Educational Researcher, 41 (1), 16–25.
Google Scholar
Bannan-Ritland, B., & Baek, J. (2008). Teacher design research: An emerging paradigm for teachers’ professional development. In A. Kelly, R. Lesh, & J. Baek (Eds.), Handbook of design research methods in education: Innovations in science, technology, engineering, and mathematics learning and teaching . London: Routledge.
Barab, S., & Squire, K. (2004). Design-based research: Putting a stake in the ground. The Journal of the Learning Sciences, 13 (1), 1–14.
Article Google Scholar
Basham, J. D., Meyer, H., & Perry, E. (2010). The design and application of the digital backpack. Journal of Research on Technology in Education, 42 (4), 339–359.
Bell, P. (2004). On the theoretical breadth of design-based research in education. Educational Psychologist, 39 (4), 243–253.
Bell, P., Hoadley, C., & Linn, M. (2004). Design-based research in education. In M. Linn, E. Davis, & P. Bell (Eds.), Internet environments of science education (pp. 73–85). Mahwah, NJ: Lawrence Earlbaum Associates.
Bereiter, C. (2002). Design research for sustained innovation. Cognitive Studies, 9 (3), 321–327.
Boote, D. N., & Beile, P. (2005). Scholars before researchers: On the centrality of the dissertation literature review in research preparation. Educational Researcher, 34 (6), 3–15.
Brar, R. (2010). The design and study of a learning environment to support growth and change in students’ knowledge of fraction multiplication . Unpublished doctoral dissertation, The University of California, Berkeley.
*Brown, A. L. (1992). Design experiments: Theoretical and methodological challenges in creating complex interventions in classroom settings. Journal of the Learning Sciences, 2 (2), 141–178.
Burkhardt, H. (2006). From design research to large-scale impact: Engineering research in education. In J. van den Akker, K. Gravemeijer, S. McKenney, & N. Nieveen (Eds.), Educational design research . London: Routledge.
Burkhardt, H. (2009). On strategic design. Educational Designer: Journal of the International Society for Design and Development in Education, 1 (3). Available online at: http://www.educationaldesigner.org/ed/volume1/issue3/article9/index.htm
Carter, C. A., Ruhe, M. C., Weyer, S. M., Litaker, D., Fry, R. E., & Stange, K. C. (2007). An appreciative inquiry approach to practice improvement and transformative change in health care settings. Quality Management in Health Care, 16 (3), 194–204.
Clarke, A. (1999). Evaluation research: An introduction to principles, methods and practice . London: Sage.
*Collins, A. (1992). Toward a design science of education. In E. Lagemann & L. Shulman (Eds.), Issues in education research: problems and possibilities (pp. 15–22). San Francisco, CA: Jossey-Bass.
Dede, C. (2004). If design-based research is the answer, what is the question? Journal of the Learning Sciences, 13 (1), 105–114.
Design-Based Research Collective. (2003). Design-based research: An emerging paradigm for educational inquiry. Educational Researcher, 32 (1), 5–8.
Dewey, J. (1900). Psychology and social practice. Psychological Review, 7 , 105–124.
Drexler, W. (2010). The networked student: A design-based research case study of student constructed personal learning environments in a middle school science course . Unpublished doctoral dissertation, The University of Florida.
Edelson, D. (2002). Design research: What we learn when we engage in design. The Journal of the Learning Sciences, 11 (1), 105–121.
Ejersbo, L., Engelhardt, R., Frølunde, L., Hanghøj, T., Magnussen, R., & Misfeldt, M. (2008). Balancing product design and theoretical insight. In A. Kelly, R. Lesh, & J. Baek (Eds.), The handbook of design research methods in education (pp. 149–163). Mahwah, NJ: Lawrence Erlbaum Associates.
*Firestone, W. A. (1993). Alternative arguments for generalizing from data as applied to qualitative research. Educational Researcher, 22 (4), 16–23.
Fullan, M., & Pomfret, A. (1977). Research on curriculum and instruction implementation. Review of Educational Research, 47 (2), 335–397.
Glaser, R. (1976). Components of a psychology of instruction: Toward a science of design. Review of Educational Research, 46 (1), 29–39.
Gravemeijer, K., & Cobb, P. (2006). Outline of a method for design research in mathematics education. In J. V. D. Akker, K. Gravemeijer, S. McKenney, & N. Nieveen (Eds.), Educational design research . London: Routledge.
Hall, G. E., Wallace, R. C., & Dossett, W. A. (1973). A developmental conceptualization of the adoption process within educational institutions . Austin, TX: The Research and Development Center for Teacher Education.
Havelock, R. (1971). Planning for innovation through dissemination and utilization of knowledge . Ann Arbor, MI: Center for Research on Utilization of Scientific Knowledge.
Kaestle, C. F. (1993). The awful reputation of education research. Educational Researcher, 22 (1), 23, 26–31.
Kali, Y. (2008). The design principles database as means for promoting design-based research. In A. Kelly, R. Lesh, & J. Baek (Eds.), Handbook of design research methods in education (pp. 423–438). London: Routledge.
Kelly, A. (2003). Research as design. Educational Researcher, 32 (1), 3–4.
*Kelly, A., Lesh, R., & Baek, J. (Eds.). (2008). Handbook of design research methods in education . New York, NY: Routledge.
Kim, H., & Hannafin, M. (2008). Grounded design of web-enhanced case-based activity. Educational Technology Research and Development, 56 , 161–179.
Klopfer, E., & Squire, K. (2008). Environmental detectives—The development of an augmented reality platform for environmental simulations. Educational Technology Research and Development, 56 (2), 203–228.
Labaree, D. F. (2006). The trouble with ed schools . New Haven, CT: Yale University Press.
Lagemann, E. (2002). An elusive science: The troubling history of education research . Chicago: University of Chicago Press.
Laurel, B. (2003). Design research: Methods and perspectives . Cambridge, MA: MIT Press.
Lee, J. J. (2009). Understanding how identity supportive games can impact ethnic minority possible selves and learning: A design-based research study . Unpublished dissertation study, The Pennsylvania State University.
*Lincoln, Y. S., & Guba, E. G. (1985). Naturalistic inquiry. Newbury Park, CA: Sage Publications.
Linn, M., & Eylon, B. (2006). Science education: Integrating views of learning and instruction. In P. Alexander & P. Winne (Eds.), Handbook of educational psychology (2nd ed., pp. 511–544). Mahwah, NJ: Lawrence Erlbaum Associates.
McKenney, S. E., & Reeves, T. C. (2013). Systematic review of design-based research progress: Is a little knowledge a dangerous thing? Educational Researcher, 42 (2), 97–100.
*McKenney, S. E., & Reeves, T. C. (2012). Conducting educational design research . London: Routledge.
McKenney, S., van den Akker, J., & Nieveen, N. (2006). Design research from the curriculum perspective. In J. Van den Akker, K. Gravemeijer, S. McKenney, & N. Nieveen (Eds.), Educational design research (pp. 67–90). London: Routledge.
Mills, G. E. (2002). Action research: A guide for the teacher researcher (2nd ed.). Englewood Cliffs, NJ: Prentice-Hall.
Mishra, P., & Koehler, M. (2006). Technological pedagogical content knowledge: A framework for teacher knowledge. Teachers College Record, 108 (6), 1017–1054.
Münsterberg, H. (1899). Psychology and life . Boston, MA: Houghton Mifflin.
Book Google Scholar
Newman, D. (1990). Opportunities for research on the organizational impact of school computers. Educational Researcher, 19 (3), 8–13.
Oh, E. (2011). Collaborative group work in an online learning environment: A design research study . Unpublished doctoral dissertation, The University of Georgia.
Oh, E., & Reeves, T. (2010). The implications of the differences between design research and instructional systems design for educational technology researchers and practitioners. Educational Media International, 47 (4), 263–275.
*Penuel, W., Fishman, B., Cheng, B., & Sabelli, N. (2011). Organizing research and development at the intersection of learning, implementation and design. Educational Researcher, 40 (7), 331–337.
Plomp, T., & Nieveen, N. (Eds.). (2009). An introduction to educational design research: Proceedings of the seminar conducted at the East China Normal University, Shanghai . Enschede, The Netherlands: SLO—Netherlands Institute for Curriculum Institute.
Quintana, C., Reiser, B., Davis, E., Krajcik, J., Fretz, E., Duncan, R. G. et al. (2004). A scaffolding design framework for software to support science inquiry. The Journal of the Learning Sciences, 13 (3), 337–386.
Raval, H. (2010). Supporting para-teachers in an Indian NGO: The plan-enact-reflect cycle . Unpublished doctoral dissertation, Twente University.
Reeves, T. C. (2011). Can educational research be both rigorous and relevant? Educational Designer: Journal of the International Society for Design and Development in Education , 1 (4). Available online at: http://www.educationaldesigner.org/ed/volume1/issue4/
Reeves, T. C. (2006). Design research from the technology perspective. In J. V. Akker, K. Gravemeijer, S. McKenney, & N. Nieveen (Eds.), Educational design research (pp. 86–109). London: Routledge.
*Reinking, D., & Bradley, B. (2008). Formative and design experiments: Approaches to language and literacy research . New York, NY: Teachers College Press.
Reynolds, R., & Caperton, I. (2011). Contrasts in student engagement, meaning-making, dislikes, and challenges in a discovery-based program of game design learning. Educational Technology Research and Development, 59 (2), 267–289.
Richey, R., & Klein, J. (2007). Design and development research . Mahwah, NJ: Lawrence Erlbaum Associates.
Schoenfeld, A. H. (2009). Bridging the cultures of educational research and design. Educational Designer: Journal of the International Society for Design and Development in Education, 1 (2). Available online at: http://www.educationaldesigner.org/ed/volume1/issue2/article5/index.htm
Schwarz, B. B., & Asterhan, C. S. (2011). E-moderation of synchronous discussions in educational settings: A nascent practice. The Journal of the Learning Sciences, 20 (3), 395–442.
Shavelson, R., Phillips, D., Towne, L., & Feuer, M. (2003). On the science of education design studies. Educational Researcher, 32 (1), 25–28.
Stokes, D. (1997). Pasteurs quadrant: Basic science and technological innovation . Washington, DC: Brookings Institution Press.
Thomas, M. K., Barab, S. A., & Tuzun, H. (2009). Developing critical implementations of technology-rich innovations: A cross-case study of the implementation of Quest Atlantis. Journal of Educational Computing Research, 41 (2), 125–153.
van Aken, X. (2004). Management research based on the paradigm of the design sciences: The quest for field-tested and grounded technological rules. Journal of Management Studies, 41 (2), 219–246.
*van den Akker, J. (1999). Principles and methods of development research. In J. van den Akker, R. Branch, K. Gustafson, N. Nieveen, & T. Plomp (Eds.), Design approaches and tools in education and training (pp. 1–14). Dordrecht: Kluwer Academic Publishers.
van den Akker, J., Gravemeijer, K., McKenney, S., & Nieveen, N. (Eds.). (2006a). Educational design research . London: Routledge.
van den Akker, J., Gravemeijer, K., McKenney, S., & Nieveen, N. (2006b). Introducing educational design research. In J. Van den Akker, K. Gravemeijer, S. McKenney, & N. Nieveen (Eds.), Educational design research (pp. 3–7). London: Routledge.
Wang, F., & Hannafin, M. (2005). Design-based research and technology-enhanced learning environments. Educational Technology Research and Development, 53 (4), 5–23.
Xie, Y., & Sharma, P. (2011). Exploring evidence of reflective thinking in student artifacts of blogging-mapping tool: A design-based research approach. Instructional Science, 39 (5), 695–719.
Yin, R. (1989). Case study research: Design and methods . Newbury Park, CA: Sage Publishing.
Download references
Author information
Authors and affiliations.
Open University of the Netherlands & University of Twente, OWK/GW/UTwente, 217, Enschede, 7500 AE, The Netherlands
Susan McKenney
College of Education, The University of Georgia, 325C Aderhold Hall, Athens, GA, 30602-7144, USA
Thomas C. Reeves
You can also search for this author in PubMed Google Scholar
Corresponding author
Correspondence to Susan McKenney .
Editor information
Editors and affiliations.
, Department of Learning Technologies, C, University of North Texas, North Elm 3940, Denton, 76207-7102, Texas, USA
J. Michael Spector
W. Sunset Blvd. 1812, St. George, 84770, Utah, USA
M. David Merrill
, Centr. Instructiepsychol.&-technologie, K.U. Leuven, Andreas Vesaliusstraat 2, Leuven, 3000, Belgium
Research Drive, Iacocca A109 111, Bethlehem, 18015, Pennsylvania, USA
M. J. Bishop
Rights and permissions
Reprints and permissions
Copyright information
© 2014 Springer Science+Business Media New York
About this chapter
McKenney, S., Reeves, T.C. (2014). Educational Design Research. In: Spector, J., Merrill, M., Elen, J., Bishop, M. (eds) Handbook of Research on Educational Communications and Technology. Springer, New York, NY. https://doi.org/10.1007/978-1-4614-3185-5_11
Download citation
DOI : https://doi.org/10.1007/978-1-4614-3185-5_11
Published : 22 May 2013
Publisher Name : Springer, New York, NY
Print ISBN : 978-1-4614-3184-8
Online ISBN : 978-1-4614-3185-5
eBook Packages : Humanities, Social Sciences and Law Education (R0)
Share this chapter
Anyone you share the following link with will be able to read this content:
Sorry, a shareable link is not currently available for this article.
Provided by the Springer Nature SharedIt content-sharing initiative
- Publish with us
Policies and ethics
- Find a journal
- Track your research
- Tools and Resources
- Customer Services
- Original Language Spotlight
- Alternative and Non-formal Education
- Cognition, Emotion, and Learning
- Curriculum and Pedagogy
- Education and Society
- Education, Change, and Development
- Education, Cultures, and Ethnicities
- Education, Gender, and Sexualities
- Education, Health, and Social Services
- Educational Administration and Leadership
- Educational History
- Educational Politics and Policy
- Educational Purposes and Ideals
- Educational Systems
- Educational Theories and Philosophies
- Globalization, Economics, and Education
- Languages and Literacies
- Professional Learning and Development
- Research and Assessment Methods
- Technology and Education
- Share This Facebook LinkedIn Twitter
Article contents
Qualitative design research methods.
- Michael Domínguez Michael Domínguez San Diego State University
- https://doi.org/10.1093/acrefore/9780190264093.013.170
- Published online: 19 December 2017
Emerging in the learning sciences field in the early 1990s, qualitative design-based research (DBR) is a relatively new methodological approach to social science and education research. As its name implies, DBR is focused on the design of educational innovations, and the testing of these innovations in the complex and interconnected venue of naturalistic settings. As such, DBR is an explicitly interventionist approach to conducting research, situating the researcher as a part of the complex ecology in which learning and educational innovation takes place.
With this in mind, DBR is distinct from more traditional methodologies, including laboratory experiments, ethnographic research, and large-scale implementation. Rather, the goal of DBR is not to prove the merits of any particular intervention, or to reflect passively on a context in which learning occurs, but to examine the practical application of theories of learning themselves in specific, situated contexts. By designing purposeful, naturalistic, and sustainable educational ecologies, researchers can test, extend, or modify their theories and innovations based on their pragmatic viability. This process offers the prospect of generating theory-developing, contextualized knowledge claims that can complement the claims produced by other forms of research.
Because of this interventionist, naturalistic stance, DBR has also been the subject of ongoing debate concerning the rigor of its methodology. In many ways, these debates obscure the varied ways DBR has been practiced, the varied types of questions being asked, and the theoretical breadth of researchers who practice DBR. With this in mind, DBR research may involve a diverse range of methods as researchers from a variety of intellectual traditions within the learning sciences and education research design pragmatic innovations based on their theories of learning, and document these complex ecologies using the methodologies and tools most applicable to their questions, focuses, and academic communities.
DBR has gained increasing interest in recent years. While it remains a popular methodology for developmental and cognitive learning scientists seeking to explore theory in naturalistic settings, it has also grown in importance to cultural psychology and cultural studies researchers as a methodological approach that aligns in important ways with the participatory commitments of liberatory research. As such, internal tension within the DBR field has also emerged. Yet, though approaches vary, and have distinct genealogies and commitments, DBR might be seen as the broad methodological genre in which Change Laboratory, design-based implementation research (DBIR), social design-based experiments (SDBE), participatory design research (PDR), and research-practice partnerships might be categorized. These critically oriented iterations of DBR have important implications for educational research and educational innovation in historically marginalized settings and the Global South.
- design-based research
- learning sciences
- social-design experiment
- qualitative research
- research methods
Educational research, perhaps more than many other disciplines, is a situated field of study. Learning happens around us every day, at all times, in both formal and informal settings. Our worlds are replete with complex, dynamic, diverse communities, contexts, and institutions, many of which are actively seeking guidance and support in the endless quest for educational innovation. Educational researchers—as a source of potential expertise—are necessarily implicated in this complexity, linked to the communities and institutions through their very presence in spaces of learning, poised to contribute with possible solutions, yet often positioned as separate from the activities they observe, creating dilemmas of responsibility and engagement.
So what are educational scholars and researchers to do? These tensions invite a unique methodological challenge for the contextually invested researcher, begging them to not just produce knowledge about learning, but to participate in the ecology, collaborating on innovations in the complex contexts in which learning is taking place. In short, for many educational researchers, our backgrounds as educators, our connections to community partners, and our sociopolitical commitments to the process of educational innovation push us to ensure that our work is generative, and that our theories and ideas—our expertise—about learning and education are made pragmatic, actionable, and sustainable. We want to test what we know outside of laboratories, designing, supporting, and guiding educational innovation to see if our theories of learning are accurate, and useful to the challenges faced in schools and communities where learning is messy, collaborative, and contested. Through such a process, we learn, and can modify our theories to better serve the real needs of communities. It is from this impulse that qualitative design-based research (DBR) emerged as a new methodological paradigm for education research.
Qualitative design-based research will be examined, documenting its origins, the major tenets of the genre, implementation considerations, and methodological issues, as well as variance within the paradigm. As a relatively new methodology, much tension remains in what constitutes DBR, and what design should mean, and for whom. These tensions and questions, as well as broad perspectives and emergent iterations of the methodology, will be discussed, and considerations for researchers looking toward the future of this paradigm will be considered.
The Origins of Design-Based Research
Qualitative design-based research (DBR) first emerged in the learning sciences field among a group of scholars in the early 1990s, with the first articulation of DBR as a distinct methodological construct appearing in the work of Ann Brown ( 1992 ) and Allan Collins ( 1992 ). For learning scientists in the 1970s and 1980s, the traditional methodologies of laboratory experiments, ethnographies, and large-scale educational interventions were the only methods available. During these decades, a growing community of learning science and educational researchers (e.g., Bereiter & Scardamalia, 1989 ; Brown, Campione, Webber, & McGilley, 1992 ; Cobb & Steffe, 1983 ; Cole, 1995 ; Scardamalia & Bereiter, 1991 ; Schoenfeld, 1982 , 1985 ; Scribner & Cole, 1978 ) interested in educational innovation and classroom interventions in situated contexts began to find the prevailing methodologies insufficient for the types of learning they wished to document, the roles they wished to play in research, and the kinds of knowledge claims they wished to explore. The laboratory, or laboratory-like settings, where research on learning was at the time happening, was divorced from the complexity of real life, and necessarily limiting. Alternatively, most ethnographic research, while more attuned to capturing these complexities and dynamics, regularly assumed a passive stance 1 and avoided interceding in the learning process, or allowing researchers to see what possibility for innovation existed from enacting nascent learning theories. Finally, large-scale interventions could test innovations in practice but lost sight of the nuance of development and implementation in local contexts (Brown, 1992 ; Collins, Joseph, & Bielaczyc, 2004 ).
Dissatisfied with these options, and recognizing that in order to study and understand learning in the messiness of socially, culturally, and historically situated settings, new methods were required, Brown ( 1992 ) proposed an alternative: Why not involve ourselves in the messiness of the process, taking an active, grounded role in disseminating our theories and expertise by becoming designers and implementers of educational innovations? Rather than observing from afar, DBR researchers could trace their own iterative processes of design, implementation, tinkering, redesign, and evaluation, as it unfolded in shared work with teachers, students, learners, and other partners in lived contexts. This premise, initially articulated as “design experiments” (Brown, 1992 ), would be variously discussed over the next decade as “design research,” (Edelson, 2002 ) “developmental research,” (Gravemeijer, 1994 ), and “design-based research,” (Design-Based Research Collective, 2003 ), all of which reflect the original, interventionist, design-oriented concept. The latter term, “design-based research” (DBR), is used here, recognizing this as the prevailing terminology used to refer to this research approach at present. 2
Regardless of the evolving moniker, the prospects of such a methodology were extremely attractive to researchers. Learning scientists acutely aware of various aspects of situated context, and interested in studying the applied outcomes of learning theories—a task of inquiry into situated learning for which canonical methods were rather insufficient—found DBR a welcome development (Bell, 2004 ). As Barab and Squire ( 2004 ) explain: “learning scientists . . . found that they must develop technological tools, curriculum, and especially theories that help them systematically understand and predict how learning occurs” (p. 2), and DBR methodologies allowed them to do this in proactive, hands-on ways. Thus, rather than emerging as a strict alternative to more traditional methodologies, DBR was proposed to fill a niche that other methodologies were ill-equipped to cover.
Effectively, while its development is indeed linked to an inherent critique of previous research paradigms, neither Brown nor Collins saw DBR in opposition to other forms of research. Rather, by providing a bridge from the laboratory to the real world, where learning theories and proposed innovations could interact and be implemented in the complexity of lived socio-ecological contexts (Hoadley, 2004 ), new possibilities emerged. Learning researchers might “trace the evolution of learning in complex, messy classrooms and schools, test and build theories of teaching and learning, and produce instructional tools that survive the challenges of everyday practice” (Shavelson, Phillips, Towne, & Feuer, 2003 , p. 25). Thus, DBR could complement the findings of laboratory, ethnographic, and large-scale studies, answering important questions about the implementation, sustainability, limitations, and usefulness of theories, interventions, and learning when introduced as innovative designs into situated contexts of learning. Moreover, while studies involving these traditional methodologies often concluded by pointing toward implications—insights subsequent studies would need to take up—DBR allowed researchers to address implications iteratively and directly. No subsequent research was necessary, as emerging implications could be reflexively explored in the context of the initial design, offering considerable insight into how research is translated into theory and practice.
Since its emergence in 1992 , DBR as a methodological approach to educational and learning research has quickly grown and evolved, used by researchers from a variety of intellectual traditions in the learning sciences, including developmental and cognitive psychology (e.g., Brown & Campione, 1996 , 1998 ; diSessa & Minstrell, 1998 ), cultural psychology (e.g., Cole, 1996 , 2007 ; Newman, Griffin, & Cole, 1989 ; Gutiérrez, Bien, Selland, & Pierce, 2011 ), cultural anthropology (e.g., Barab, Kinster, Moore, Cunningham, & the ILF Design Team, 2001 ; Polman, 2000 ; Stevens, 2000 ; Suchman, 1995 ), and cultural-historical activity theory (e.g., Engeström, 2011 ; Espinoza, 2009 ; Espinoza & Vossoughi, 2014 ; Gutiérrez, 2008 ; Sannino, 2011 ). Given this plurality of epistemological and theoretical fields that employ DBR, it might best be understood as a broad methodology of educational research, realized in many different, contested, heterogeneous, and distinct iterations, and engaging a variety of qualitative tools and methods (Bell, 2004 ). Despite tensions among these iterations, and substantial and important variances in the ways they employ design-as-research in community settings, there are several common, methodological threads that unite the broad array of research that might be classified as DBR under a shared, though pluralistic, paradigmatic umbrella.
The Tenets of Design-Based Research
Why design-based research.
As we turn to the core tenets of the design-based research (DBR) paradigm, it is worth considering an obvious question: Why use DBR as a methodology for educational research? To answer this, it is helpful to reflect on the original intentions for DBR, particularly, that it is not simply the study of a particular, isolated intervention. Rather, DBR methodologies were conceived of as the complete, iterative process of designing, modifying, and assessing the impact of an educational innovation in a contextual, situated learning environment (Barab & Kirshner, 2001 ; Brown, 1992 ; Cole & Engeström, 2007 ). The design process itself—inclusive of the theory of learning employed, the relationships among participants, contextual factors and constraints, the pedagogical approach, any particular intervention, as well as any changes made to various aspects of this broad design as it proceeds—is what is under study.
Considering this, DBR offers a compelling framework for the researcher interested in having an active and collaborative hand in designing for educational innovation, and interested in creating knowledge about how particular theories of learning, pedagogical or learning practices, or social arrangements function in a context of learning. It is a methodology that can put the researcher in the position of engineer , actively experimenting with aspects of learning and sociopolitical ecologies to arrive at new knowledge and productive outcomes, as Cobb, Confrey, diSessa, Lehrer, and Schauble ( 2003 ) explain:
Prototypically, design experiments entail both “engineering” particular forms of learning and systematically studying those forms of learning within the context defined by the means of supporting them. This designed context is subject to test and revision, and the successive iterations that result play a role similar to that of systematic variation in experiment. (p. 9)
This being said, how directive the engineering role the researcher takes on varies considerably among iterations of DBR. Indeed, recent approaches have argued strongly for researchers to take on more egalitarian positionalities with respect to the community partners with whom they work (e.g., Zavala, 2016 ), acting as collaborative designers, rather than authoritative engineers.
Method and Methodology in Design-Based Research
Now, having established why we might use DBR, a recurring question that has faced the DBR paradigm is whether DBR is a methodology at all. Given the variety of intellectual and ontological traditions that employ it, and thus the pluralism of methods used in DBR to enact the “engineering” role (whatever shape that may take) that the researcher assumes, it has been argued that DBR is not, in actuality a methodology at all (Kelly, 2004 ). The proliferation and diversity of approaches, methods, and types of analysis purporting to be DBR have been described as a lack of coherence that shows there is no “argumentative grammar” or methodology present in DBR (Kelly, 2004 ).
Now, the conclusions one will eventually draw in this debate will depend on one’s orientations and commitments, but it is useful to note that these demands for “coherence” emerge from previous paradigms in which methodology was largely marked by a shared, coherent toolkit for data collection and data analysis. These previous paradigmatic rules make for an odd fit when considering DBR. Yet, even if we proceed—within the qualitative tradition from which DBR emerges—defining methodology as an approach to research that is shaped by the ontological and epistemological commitments of the particular researcher, and methods as the tools for research, data collection, and analysis that are chosen by the researcher with respect to said commitments (Gutiérrez, Engeström, & Sannino, 2016 ), then a compelling case for DBR as a methodology can be made (Bell, 2004 ).
Effectively, despite the considerable variation in how DBR has been and is employed, and tensions within the DBR field, we might point to considerable, shared epistemic common ground among DBR researchers, all of whom are invested in an approach to research that involves engaging actively and iteratively in the design and exploration of learning theory in situated, natural contexts. This common epistemic ground, even in the face of pluralistic ideologies and choices of methods, invites in a new type of methodological coherence, marked by “intersubjectivity without agreement” (Matusov, 1996 ), that links DBR from traditional developmental and cognitive psychology models of DBR (e.g., Brown, 1992 ; Brown & Campione, 1998 ; Collins, 1992 ), to more recent critical and sociocultural manifestations (e.g., Bang & Vossoughi, 2016 ; Engeström, 2011 ; Gutiérrez, 2016 ), and everything in between.
Put in other terms, even as DBR researchers may choose heterogeneous methods for data collection, data analysis, and reporting results complementary to the ideological and sociopolitical commitments of the particular researcher and the types of research questions that are under examination (Bell, 2004 ), a shared epistemic commitment gives the methodology shape. Indeed, the common commitment toward design innovation emerges clearly across examples of DBR methodological studies ranging in method from ethnographic analyses (Salvador, Bell, & Anderson, 1999 ) to studies of critical discourse within a design (Kärkkäinen, 1999 ), to focused examinations of metacognition of individual learners (White & Frederiksen, 1998 ), and beyond. Rather than indicating a lack of methodology, or methodological weakness, the use of varying qualitative methods for framing data collection and retrospective analyses within DBR, and the tensions within the epistemic common ground itself, simply reflects the scope of its utility. Learning in context is complex, contested, and messy, and the plurality of methods present across DBR allow researchers to dynamically respond to context as needed, employing the tools that fit best to consider the questions that are present, or may arise.
All this being the case, it is useful to look toward the coherent elements—the “argumentative grammar” of DBR, if you will—that can be identified across the varied iterations of DBR. Understanding these shared features, in the context and terms of the methodology itself, help us to appreciate what is involved in developing robust and thorough DBR research, and how DBR seeks to make strong, meaningful claims around the types of research questions it takes up.
Coherent Features of Design-Based Research
Several scholars have provided comprehensive overviews and listings of what they see as the cross-cutting features of DBR, both in the context of more traditional models of DBR (e.g., Cobb et al., 2003 ; Design-Based Research Collective, 2003 ), and in regards to newer iterations (e.g., Gutiérrez & Jurow, 2016 ; Bang & Vossoughi, 2016 ). Rather than try to offer an overview of each of these increasingly pluralistic classifications, the intent here is to attend to three broad elements that are shared across articulations of DBR and reflect the essential elements that constitute the methodological approach DBR offers to educational researchers.
Design research is concerned with the development, testing, and evolution of learning theory in situated contexts
This first element is perhaps most central to what DBR of all types is, anchored in what Brown ( 1992 ) was initially most interested in: testing the pragmatic validity of theories of learning by designing interventions that engaged with, or proposed, entire, naturalistic, ecologies of learning. Put another way, while DBR studies may have various units of analysis, focuses, and variables, and may organize learning in many different ways, it is the theoretically informed design for educational innovation that is most centrally under evaluation. DBR actively and centrally exists as a paradigm that is engaged in the development of theory, not just the evaluation of aspects of its usage (Bell, 2004 ; Design-Based Research Collective, 2003 ; Lesh & Kelly, 2000 ; van den Akker, 1999 ).
Effectively, where DBR is taking place, theory as a lived possibility is under examination. Specifically, in most DBR, this means a focus on “intermediate-level” theories of learning, rather than “grand” ones. In essence, DBR does not contend directly with “grand” learning theories (such as developmental or sociocultural theory writ large) (diSessa, 1991 ). Rather, DBR seeks to offer constructive insights by directly engaging with particular learning processes that flow from these theories on a “grounded,” “intermediate” level. This is not, however, to say DBR is limited in what knowledge it can produce; rather, tinkering in this “intermediate” realm can produce knowledge that informs the “grand” theory (Gravemeijer, 1994 ). For example, while cognitive and motivational psychology provide “grand” theoretical frames, interest-driven learning (IDL) is an “intermediate” theory that flows from these and can be explored in DBR to both inform the development of IDL designs in practice and inform cognitive and motivational psychology more broadly (Joseph, 2004 ).
Crucially, however, DBR entails putting the theory in question under intense scrutiny, or, “into harm’s way” (Cobb et al., 2003 ). This is an especially core element to DBR, and one that distinguishes it from the proliferation of educational-reform or educational-entrepreneurship efforts that similarly take up the discourse of “design” and “innovation.” Not only is the reflexive, often participatory element of DBR absent from such efforts—that is, questioning and modifying the design to suit the learning needs of the context and partners—but the theory driving these efforts is never in question, and in many cases, may be actively obscured. Indeed, it is more common to see educational-entrepreneur design innovations seek to modify a context—such as the way charter schools engage in selective pupil recruitment and intensive disciplinary practices (e.g., Carnoy et al., 2005 ; Ravitch, 2010 ; Saltman, 2007 )—rather than modify their design itself, and thus allow for humility in their theory. Such “innovations” and “design” efforts are distinct from DBR, which must, in the spirit of scientific inquiry, be willing to see the learning theory flail and struggle, be modified, and evolve.
This growth and evolution of theory and knowledge is of course central to DBR as a rigorous research paradigm; moving it beyond simply the design of local educational programs, interventions, or innovations. As Barab and Squire ( 2004 ) explain:
Design-based research requires more than simply showing a particular design works but demands that the researcher (move beyond a particular design exemplar to) generate evidence-based claims about learning that address contemporary theoretical issues and further the theoretical knowledge of the field. (pp. 5–6)
DBR as a research paradigm offers a design process through which theories of learning can be tested; they can be modified, and by allowing them to operate with humility in situated conditions, new insights and knowledge, even new theories, may emerge that might inform the field, as well as the efforts and directions of other types of research inquiry. These productive, theory-developing outcomes, or “ontological innovations” (diSessa & Cobb, 2004 ), represent the culmination of an effective program of DBR—the production of new ways to understand, conceptualize, and enact learning as a lived, contextual process.
Design research works to understand learning processes, and the design that supports them in situated contexts
As a research methodology that operates by tinkering with “grounded” learning theories, DBR is itself grounded, and seeks to develop its knowledge claims and designs in naturalistic, situated contexts (Brown, 1992 ). This is, again, a distinguishing element of DBR—setting it apart from laboratory research efforts involving design and interventions in closed, controlled environments. Rather than attempting to focus on singular variables, and isolate these from others, DBR is concerned with the multitude of variables that naturally occur across entire learning ecologies, and present themselves in distinct ways across multiple planes of possible examination (Rogoff, 1995 ; Collins, Joseph, & Bielaczyc, 2004 ). Certainly, specific variables may be identified as dependent, focal units of analysis, but identifying (while not controlling for) the variables beyond these, and analyzing their impact on the design and learning outcomes, is an equally important process in DBR (Collins et al., 2004 ; Barab & Kirshner, 2001 ). In practice, this of course varies across iterations in its depth and breadth. Traditional models of developmental or cognitive DBR may look to account for the complexity and nuance of a setting’s social, developmental, institutional, and intellectual characteristics (e.g., Brown, 1992 ; Cobb et al., 2003 ), while more recent, critical iterations will give increased attention to how historicity, power, intersubjectivity, and culture, among other things, influence and shape a setting, and the learning that occurs within it (e.g., Gutiérrez, 2016 ; Vakil, de Royston, Nasir, & Kirshner, 2016 ).
Beyond these variations, what counts as “design” in DBR varies widely, and so too will what counts as a naturalistic setting. It has been well documented that learning occurs all the time, every day, and in every space imaginable, both formal and informal (Leander, Phillips, & Taylor, 2010 ), and in ways that span strictly defined setting boundaries (Engeström, Engeström, & Kärkkäinen, 1995 ). DBR may take place in any number of contexts, based on the types of questions asked, and the learning theories and processes that a researcher may be interested in exploring. DBR may involve one-to-one tutoring and learning settings, single classrooms, community spaces, entire institutions, or even holistically designed ecologies (Design-Based Research Collective, 2003 ; Engeström, 2008 ; Virkkunen & Newnham, 2013 ). In all these cases, even the most completely designed experimental ecology, the setting remains naturalistic and situated because DBR actively embraces the uncontrollable variables that participants bring with them to the learning process for and from their situated worlds, lives, and experiences—no effort is made to control for these complicated influences of life, simply to understand how they operate in a given ecology as innovation is attempted. Thus, the extent of the design reflects a broader range of qualitative and theoretical study, rather than an attempt to control or isolate some particular learning process from outside influence.
While there is much variety in what design may entail, where DBR takes place, what types of learning ecologies are under examination, and what methods are used, situated ecologies are always the setting of this work. In this way, conscious of naturalistic variables, and the influences that culture, historicity, participation, and context have on learning, researchers can use DBR to build on prior research, and extend knowledge around the learning that occurs in the complexity of situated contexts and lived practices (Collins et al., 2004 ).
Design based research is iterative; it changes, grows, and evolves to meet the needs and emergent questions of the context, and this tinkering process is part of the research
The final shared element undergirding models of DBR is that it is an iterative, active, and interventionist process, interested in and focused on producing educational innovation by actually and actively putting design innovations into practice (Brown, 1992 , Collins, 1992 ; Gutiérrez, 2008 ). Given this interventionist, active stance, tinkering with the design and the theory of learning informing the design is as much a part of the research process as the outcome of the intervention or innovation itself—we learn what impacts learning as much, if not more, than we learn what was learned. In this sense, DBR involves a focus on analyzing the theory-driven design itself, and its implementation as an object of study (Edelson, 2002 ; Penuel, Fishman, Cheng, & Sabelli, 2011 ), and is ultimately interested in the improvement of the design—of how it unfolds, how it shifts, how it is modified, and made to function productively for participants in their contexts and given their needs (Kirshner & Polman, 2013 ).
While DBR is iterative and contextual as a foundational methodological principle, what this means varies across conceptions of DBR. For instance, in more traditional models, Brown and Campione ( 1996 ) pointed out the dangers of “lethal mutation” in which a design, introduced into a context, may become so warped by the influence, pressures, incomplete implementation, or misunderstanding of participants in the local context, that it no longer reflects or tests the theory under study. In short, a theory-driven intervention may be put in place, and then subsumed to such a degree by participants based on their understanding and needs, that it remains the original innovative design in name alone. The assertion here is that in these cases, the research ceases to be DBR in the sense that the design is no longer central, actively shaping learning. We cannot, they argue, analyze a design—and the theory it was meant to reflect—as an object of study when it has been “mutated,” and it is merely a banner under which participants are enacting their idiosyncratic, pragmatic needs.
While the ways in which settings and individuals might disrupt designs intended to produce robust learning is certainly a tension to be cautious of in DBR, it is also worth noting that in many critical approaches to DBR, such mutations—whether “lethal” to the original design or not—are seen as compelling and important moments. Here, where collaboration and community input is more central to the design process, iterative is understood differently. Thus, a “mutation” becomes a point where reflexivity, tension, and contradiction might open the door for change, for new designs, for reconsiderations of researcher and collaborative partner positionalities, or for ethnographic exploration into how a context takes up, shapes, and ultimately engages innovations in a particular sociocultural setting. In short, accounting for and documenting changes in design is a vital part of the DBR process, allowing researchers to respond to context in a variety of ways, always striving for their theories and designs to act with humility, and in the interest of usefulness .
With this in mind, the iterative nature of DBR means that the relationships researchers have with other design partners (educators and learners) in the ecology are incredibly important, and vital to consider (Bang et al., 2016 ; Engeström, 2007 ; Engeström, Sannino, & Virkkunen, 2014 ). Different iterations of DBR might occur in ways in which the researcher is more or less intimately involved in the design and implementation process, both in terms of actual presence and intellectual ownership of the design. Regarding the former, in some cases, a researcher may hand a design off to others to implement, periodically studying and modifying it, while in other contexts or designs, the researcher may be actively involved, tinkering in every detail of the implementation and enactment of the design. With regard to the latter, DBR might similarly range from a somewhat prescribed model, in which the researcher is responsible for the original design, and any modifications that may occur based on their analyses, without significant input from participants (e.g., Collins et al., 2004 ), to incredibly participatory models, in which all parties (researchers, educators, learners) are part of each step of the design-creation, modification, and research process (e.g., Bang, Faber, Gurneau, Marin, & Soto, 2016 ; Kirshner, 2015 ).
Considering the wide range of ideological approaches and models for DBR, we might acknowledge that DBR can be gainfully conducted through many iterations of “openness” to the design process. However, the strength of the research—focused on analyzing the design itself as a unit of study reflective of learning theory—will be bolstered by thoughtfully accounting for how involved the researcher will be, and how open to participation the modification process is. These answers should match the types of questions, and conceptual or ideological framing, with which researchers approach DBR, allowing them to tinker with the process of learning as they build on prior research to extend knowledge and test theory (Barab & Kirshner, 2001 ), while thoughtfully documenting these changes in the design as they go.
Implementation and Research Design
As with the overarching principles of design-based research (DBR), even amid the pluralism of conceptual frameworks of DBR researchers, it is possible, and useful, to trace the shared contours in how DBR research design is implemented. Though texts provide particular road maps for undertaking various iterations of DBR consistent with the specific goals, types of questions, and ideological orientations of these scholarly communities (e.g., Cole & Engeström, 2007 ; Collins, Joseph, & Bielaczyc, 2004 ; Fishman, Penuel, Allen, Cheng, & Sabelli, 2013 ; Gutiérrez & Jurow, 2016 ; Virkkunen & Newnham, 2013 ), certain elements, realized differently, can be found across all of these models, and may be encapsulated in five broad methodological phases.
Considering the Design Focus
DBR begins by considering what the focus of the design, the situated context, and the units of analysis for research will be. Prospective DBR researchers will need to consider broader research in regard to the “grand” theory of learning with which they work to determine what theoretical questions they have, or identify “intermediate” aspects of the theories that might be studied and strengthened by a design process in situated contexts, and what planes of analysis (Rogoff, 1995 ) will be most suitable for examination. This process allows for the identification of the critical theoretical elements of a design, and articulation of initial research questions.
Given the conceptual framework, theoretical and research questions, and sociopolitical interests at play, researchers may undertake this, and subsequent steps in the process, on their own, or in close collaboration with the communities and individuals in the situated contexts in which the design will unfold. As such, across iterations of DBR, and with respect to the ways DBR researchers choose to engage with communities, the origin of the design will vary, and might begin in some cases with theoretical questions, or arise in others as a problem of practice (Coburn & Penuel, 2016 ), though as has been noted, in either case, theory and practice are necessarily linked in the research.
Creating and Implementing a Designed Innovation
From the consideration and identification of the critical elements, planned units of analysis, and research questions that will drive a design, researchers can then actively create (either on their own or in conjunction with potential design partners) a designed intervention reflecting these critical elements, and the overarching theory.
Here, the DBR researcher should consider what partners exist in the process and what ownership exists around these partnerships, determine exactly what the pragmatic features of the intervention/design will be and who will be responsible for them, and consider when checkpoints for modification and evaluation will be undertaken, and by whom. Additionally, researchers should at this stage consider questions of timeline and of recruiting participants, as well as what research materials will be needed to adequately document the design, its implementation, and its outcomes, and how and where collected data will be stored.
Once a design (the planned, theory-informed innovative intervention) has been produced, the DBR researcher and partners can begin the implementation process, putting the design into place and beginning data collection and documentation.
Assessing the Impact of the Design on the Learning Ecology
Chronologically, the next two methodological steps happen recursively in the iterative process of DBR. The researcher must assess the impact of the design, and then, make modifications as necessary, before continuing to assess the impact of these modifications. In short, these next two steps are a cycle that continues across the life and length of the research design.
Once a design has been created and implemented, the researcher begins to observe and document the learning, the ecology, and the design itself. Guided by and in conversation with the theory and critical elements, the researcher should periodically engage in ongoing data analysis, assessing the success of the design, and of learning, paying equal attention to the design itself, and how its implementation is working in the situated ecology.
Within the realm of qualitative research, measuring or assessing variables of learning and assessing the design may look vastly different, require vastly different data-collection and data-analysis tools, and involve vastly different research methods among different researchers.
Modifying the Design
Modification, based on ongoing assessment of the design, is what makes DBR iterative, helping the researcher extend the field’s knowledge about the theory, design, learning, and the context under examination.
Modification of the design can take many forms, from complete changes in approach or curriculum, to introducing an additional tool or mediating artifact into a learning ecology. Moreover, how modification unfolds involves careful reflection from the researcher and any co-designing participants, deciding whether modification will be an ongoing, reflexive, tinkering process, or if it will occur only at predefined checkpoints, after formal evaluation and assessment. Questions of ownership, issues of resource availability, technical support, feasibility, and communication are all central to the work of design modification, and answers will vary given the research questions, design parameters, and researchers’ epistemic commitments.
Each moment of modification indicates a new phase in a DBR project, and a new round of assessing—through data analysis—the impact of the design on the learning ecology, either to guide continued or further modification, report the results of the design, or in some cases, both.
Reporting the Results of the Design
The final step in DBR methodology is to report on the results of the designed intervention, how it contributed to understandings of theory, and how it impacted the local learning ecology or context. The format, genre, and final data analysis methods used in reporting data and research results will vary across iterations of DBR. However, it is largely understood that to avoid methodological confusion, DBR researchers should clearly situate themselves in the DBR paradigm by clearly describing and detailing the design itself; articulating the theory, central elements, and units of analysis under scrutiny, what modifications occurred and what precipitated these changes, and what local effects were observed; and exploring any potential contributions to learning theory, while accounting for the context and their interventionist role and positionality in the design. As such, careful documentation of pragmatic and design decisions for retrospective data analysis, as well as research findings, should be done at each stage of this implementation process.
Methodological Issues in the Design-Based Research Paradigm
Because of its pluralistic nature, its interventionist, nontraditional stance, and the fact that it remains in its conceptual infancy, design-based research (DBR) is replete with ongoing methodological questions and challenges, both from external and internal sources. While there are many more that may exist, addressed will be several of the most pressing the prospective DBR researcher may encounter, or want to consider in understanding the paradigm and beginning a research design.
Challenges to Rigor and Validity
Perhaps the place to begin this reflection on tensions in the DBR paradigm is the recurrent and ongoing challenge to the rigor and validity of DBR, which has asked: Is DBR research at all? Given the interventionist and activist way in which DBR invites the researcher to participate, and the shift in orientation from long-accepted research paradigms, such critiques are hardly surprising, and fall in line with broader challenges to the rigor and objectivity of qualitative social science research in general. Historically, such complaints about DBR are linked to decades of critique of any research that does not adhere to the post-positivist approach set out as the U.S. Department of Education began to prioritize laboratory and large-scale randomized control-trial experimentation as the “gold standard” of research design (e.g., Mosteller & Boruch, 2002 ).
From the outset, DBR, as an interventionist, local, situated, non-laboratory methodology, was bound to run afoul of such conservative trends. While some researchers involved in (particularly traditional developmental and cognitive) DBR have found broader acceptance within these constraints, the rigor of DBR remains contested. It has been suggested that DBR is under-theorized and over-methologized, a haphazard way for researchers to do activist work without engaging in the development of robust knowledge claims about learning (Dede, 2004 ), and an approach lacking in coherence that sheltered interventionist projects of little impact to developing learning theory and allowed researchers to make subjective, pet claims through selective analysis of large bodies of collected data (Kelly, 2003 , 2004 ).
These critiques, however, impose an external set of criteria on DBR, desiring it to fit into the molds of rigor and coherence as defined by canonical methodologies. Bell ( 2004 ) and Bang and Vossoughi ( 2016 ) have made compelling cases for the wide variety of methods and approaches present in DBR not as a fracturing, but as a generative proliferation of different iterations that can offer powerful insights around the different types of questions that exist about learning in the infinitely diverse settings in which it occurs. Essentially, researchers have argued that within the DBR paradigm, and indeed within educational research more generally, the practical impact of research on learning, context, and practices should be a necessary component of rigor (Gutiérrez & Penuel, 2014 ), and the pluralism of methods and approaches available in DBR ensures that the practical impacts and needs of the varied contexts in which the research takes place will always drive the design and research tools.
These moves are emblematic of the way in which DBR is innovating and pushing on paradigms of rigor in educational research altogether, reflecting how DBR fills a complementary niche with respect to other methodologies and attends to elements and challenges of learning in lived, real environments that other types of research have consistently and historically missed. Beyond this, Brown ( 1992 ) was conscious of the concerns around data collection, validity, rigor, and objectivity from the outset, identifying this dilemma—the likelihood of having an incredible amount of data collected in a design only a small fraction of which can be reported and shared, thus leading potentially to selective data analysis and use—as the Bartlett Effect (Brown, 1992 ). Since that time, DBR researchers have been aware of this challenge, actively seeking ways to mitigate this threat to validity by making data sets broadly available, documenting their design, tinkering, and modification processes, clearly situating and describing disconfirming evidence and their own position in the research, and otherwise presenting the broad scope of human and learning activity that occurs within designs in large learning ecologies as comprehensively as possible.
Ultimately, however, these responses are likely to always be insufficient as evidence of rigor to some, for the root dilemma is around what “counts” as education science. While researchers interested and engaged in DBR ought rightly to continue to push themselves to ensure the methodological rigor of their work and chosen methods, it is also worth noting that DBR should seek to hold itself to its own criteria of assessment. This reflects broader trends in qualitative educational research that push back on narrow constructions of what “counts” as science, recognizing the ways in which new methodologies and approaches to research can help us examine aspects of learning, culture, and equity that have continued to be blind spots for traditional education research; invite new voices and perspectives into the process of achieving rigor and validity (Erickson & Gutiérrez, 2002 ); bolster objectivity by bringing it into conversation with the positionality of the researcher (Harding, 1993 ); and perhaps most important, engage in axiological innovation (Bang, Faber, Gurneau, Marin, & Soto, 2016 ), or the exploration of and design for what is, “good right, true, and beautiful . . . in cultural ecologies” (p. 2).
Questions of Generalizability and Usefulness
The generalizability of research results in DBR has been an ongoing and contentious issue in the development of the paradigm. Indeed, by the standards of canonical methods (e.g., laboratory experimentation, ethnography), these local, situated interventions should lack generalizability. While there is reason to discuss and question the merit of generalizability as a goal of qualitative research at all, researchers in the DBR paradigm have long been conscious of this issue. Understanding the question of generalizability around DBR, and how the paradigm has responded to it, can be done in two ways.
First, by distinguishing questions specific to a particular design from the generalizability of the theory. Cole’s (Cole & Underwood, 2013 ) 5th Dimension work, and the nationwide network of linked, theoretically similar sites, operating nationwide with vastly different designs, is a powerful example of this approach to generalizability. Rather than focus on a single, unitary, potentially generalizable design, the project is more interested in variability and sustainability of designs across local contexts (e.g., Cole, 1995 ; Gutiérrez, Bien, Selland, & Pierce, 2011 ; Jurow, Tracy, Hotchkiss, & Kirshner, 2012 ). Through attention to sustainable, locally effective innovations, conscious of the wide variation in culture and context that accompanies any and all learning processes, 5th Dimension sites each derive their idiosyncratic structures from sociocultural theory, sharing some elements, but varying others, while seeking their own “ontological innovations” based on the affordances of their contexts. This pattern reflects a key element of much of the DBR paradigm: that questions of generalizability in DBR may be about the generalizability of the theory of learning, and the variability of learning and design in distinct contexts, rather than the particular design itself.
A second means of addressing generalizability in DBR has been to embrace the pragmatic impacts of designing innovations. This response stems from Messick ( 1992 ) and Schoenfeld’s ( 1992 ) arguments early on in the development of DBR that the consequentialness and validity of DBR efforts as potentially generalizable research depend on the “ usefulness ” of the theories and designs that emerge. Effectively, because DBR is the examination of situated theory, a design must be able to show pragmatic impact—it must succeed at showing the theory to be useful . If there is evidence of usefulness to both the context in which it takes place, and the field of educational research more broadly, then the DBR researcher can stake some broader knowledge claims that might be generalizable. As a result, the DBR paradigm tends to “treat changes in [local] contexts as necessary evidence for the viability of a theory” (Barab & Squire, 2004 , p. 6). This of course does not mean that DBR is only interested in successful efforts. A design that fails or struggles can provide important information and knowledge to the field. Ultimately, though, DBR tends to privilege work that proves the usefulness of designs, whose pragmatic or theoretical findings can then be generalized within the learning science and education research fields.
With this said, the question of usefulness is not always straightforward, and is hardly unitary. While many DBR efforts—particularly those situated in developmental and cognitive learning science traditions—are interested in the generalizability of their useful educational designs (Barab & Squire, 2004 ; Cobb, Confrey, diSessa, Lehrer, & Schauble, 2003 ; Joseph, 2004 ; Steffe & Thompson, 2000 ), not all are. Critical DBR researchers have noted that if usefulness remains situated in the extant sociopolitical and sociocultural power-structures—dominant conceptual and popular definitions of what useful educational outcomes are—the result will be a bar for research merit that inexorably bends toward the positivist spectrum (Booker & Goldman, 2016 ; Dominguez, 2015 ; Zavala, 2016 ). This could potentially, and likely, result in excluding the non-normative interventions and innovations that are vital for historically marginalized communities, but which might have vastly different-looking outcomes, that are nonetheless useful in the sociopolitical context they occur in. Alternative framings to this idea of usefulness push on and extend the intention, and seek to involve the perspectives and agency of situated community partners and their practices in what “counts” as generative and rigorous research outcomes (Gutiérrez & Penuel, 2014 ). An example in this regard is the idea of consequential knowledge (Hall & Jurow, 2015 ; Jurow & Shea, 2015 ), which suggests outcomes that are consequential will be taken up by participants in and across their networks, and over-time—thus a goal of consequential knowledge certainly meets the standard of being useful , but it also implicates the needs and agency of communities in determining the success and merit of a design or research endeavor in important ways that strict usefulness may miss.
Thus, the bar of usefulness that characterizes the DBR paradigm should not be approached without critical reflection. Certainly designs that accomplish little for local contexts should be subject to intense questioning and critique, but considering the sociopolitical and systemic factors that might influence what “counts” as useful in local contexts and education science more generally, should be kept firmly in mind when designing, choosing methods, and evaluating impacts (Zavala, 2016 ). Researchers should think deeply about their goals, whether they are reaching for generalizability at all, and in what ways they are constructing contextual definitions of success, and be clear about these ideologically influenced answers in their work, such that generalizability and the usefulness of designs can be adjudicated based on and in conversation with the intentions and conceptual framework of the research and researcher.
Ethical Concerns of Sustainability, Participation, and Telos
While there are many external challenges to rigor and validity of DBR, another set of tensions comes from within the DBR paradigm itself. Rather than concerns about rigor or validity, these internal critiques are not unrelated to the earlier question of the contested definition of usefulness , and more accurately reflect questions of research ethics and grow from ideological concerns with how an intentional, interventionist stance is taken up in research as it interacts with situated communities.
Given that the nature of DBR is to design and implement some form of educational innovation, the DBR researcher will in some way be engaging with an individual or community, becoming part of a situated learning ecology, complete with a sociopolitical and cultural history. As with any research that involves providing an intervention or support, the question of what happens when the research ends is as much an ethical as a methodological one. Concerns then arise given how traditional models of DBR seem intensely focused on creating and implementing a “complete” cycle of design, but giving little attention to what happens to the community and context afterward (Engeström, 2011 ). In contrast to this privileging of “completeness,” sociocultural and critical approaches to DBR have suggested that if research is actually happening in naturalistic, situated contexts that authentically recognize and allow social and cultural dimensions to function (i.e., avoid laboratory-type controls to mitigate independent variables), there can never be such a thing as “complete,” for the design will, and should, live on as part of the ecology of the space (Cole, 2007 ; Engeström, 2000 ). Essentially, these internal critiques push DBR to consider sustainability, and sustainable scale, as equally important concerns to the completeness of an innovation. Not only are ethical questions involved, but accounting for the unbounded and ongoing nature of learning as a social and cultural activity can help strengthen the viability of knowledge claims made, and what degree of generalizability is reasonably justified.
Related to this question of sustainability are internal concerns regarding the nature and ethics of participation in DBR, whether partners in a design are being adequately invited to engage in the design and modification processes that will unfold in their situated contexts and lived communities (Bang et al., 2016 ; Engeström, 2011 ). DBR has actively sought to examine multiple planes of analysis in learning that might be occurring in a learning ecology but has rarely attended to the subject-subject dynamics (Bang et al., 2016 ), or “relational equity” (DiGiacomo & Gutiérrez, 2015 ) that exists between researchers and participants as a point of focus. Participatory design research (PDR) (Bang & Vossoughi, 2016 ) models have recently emerged as a way to better attend to these important dimensions of collective participation (Engeström, 2007 ), power (Vakil et al., 2016 ), positionality (Kirshner, 2015 ), and relational agency (Edwards, 2007 , 2009 ; Sannino & Engeström, 2016 ) as they unfold in DBR.
Both of these ethical questions—around sustainability and participation—reflect challenges to what we might call the telos —or direction—that DBR takes to innovation and research. These are questions related to whose voices are privileged, in what ways, for what purposes, and toward what ends. While DBR, like many other forms of educational research, has involved work with historically marginalized communities, it has, like many other forms of educational research, not always done so in humanizing ways. Put another way, there are ethical and political questions surrounding whether the designs, goals, and standards of usefulness we apply to DBR efforts should be purposefully activist, and have explicitly liberatory ends. To this point, critical and decolonial perspectives have pushed on the DBR paradigm, suggesting that DBR should situate itself as being a space of liberatory innovation and potential, in which communities and participants can become designers and innovators of their own futures (Gutiérrez, 2005 ). This perspective is reflected in the social design experiment (SDE) approach to DBR (Gutiérrez, 2005 , 2008 ; Gutierréz & Vossoughi, 2010 ; Gutiérrez, 2016 ; Gutiérrez & Jurow, 2016 ), which begins in participatory fashion, engaging a community in identifying its own challenges and desires, and reflecting on the historicity of learning practices, before proleptic design efforts are undertaken that ensure that research is done with , not on , communities of color (Arzubiaga, Artiles, King, & Harris-Murri, 2008 ), and intentionally focused on liberatory goals.
Global Perspectives and Unique Iterations
While design-based research (DBR) has been a methodology principally associated with educational research in the United States, its development is hardly limited to the U.S. context. Rather, while DBR emerged in U.S. settings, similar methods of situated, interventionist research focused on design and innovation were emerging in parallel in European contexts (e.g., Gravemeijer, 1994 ), most significantly in the work of Vygotskian scholars both in Europe and the United States (Cole, 1995 ; Cole & Engeström, 1993 , 2007 ; Engeström, 1987 ).
Particularly, where DBR began in the epistemic and ontological terrain of developmental and cognitive psychology, this vein of design-based research work began deeply grounded in cultural-historical activity theory (CHAT). This ontological and epistemic grounding meant that the approach to design that was taken was more intensively conscious of context, historicity, hybridity, and relational factors, and framed around understanding learning as a complex, collective activity system that, through design, could be modified and transformed (Cole & Engeström, 2007 ). The models of DBR that emerged in this context abroad were the formative intervention (Engeström, 2011 ; Engeström, Sannino, & Virkkunen, 2014 ), which relies heavily on Vygotskian double-stimulation to approach learning in nonlinear, unbounded ways, accounting for the role of learner, educator, and researcher in a collective process, shifting and evolving and tinkering with the design as the context needs and demands; and the Change Laboratory (Engeström, 2008 ; Virkkunen & Newnham, 2013 ), which similarly relies on the principle of double stimulation, while presenting holistic way to approach transforming—or changing—entire learning activity systems in fundamental ways through designs that encourage collective “expansive learning” (Engeström, 2001 ), through which participants can produce wholly new activity systems as the object of learning itself.
Elsewhere in the United States, still parallel to the developmental- or cognitive-oriented DBR work that was occurring, American researchers employing CHAT began to leverage the tools and aims of expansive learning in conversation with the tensions and complexity of the U.S. context (Cole, 1995 ; Gutiérrez, 2005 ; Gutiérrez & Rogoff, 2003 ). Like the CHAT design research of the European context, there was a focus on activity systems, historicity, nonlinear and unbounded learning, and collective learning processes and outcomes. Rather than a simple replication, however, these researchers put further attention on questions of equity, diversity, and justice in this work, as Gutiérrez, Engeström, and Sannino ( 2016 ) note:
The American contribution to a cultural historical activity theoretic perspective has been its attention to diversity, including how we theorize, examine, and represent individuals and their communities. (p. 276)
Effectively, CHAT scholars in parts of the United States brought critical and decolonial perspectives to bear on their design-focused research, focusing explicitly on the complex cultural, racial, and ethnic terrain in which they worked, and ensuring that diversity, equity, justice, and non-dominant perspectives would become central principles to the types of design research conducted. The result was the emergence of the aforementioned social design experiments (e.g., Gutiérrez, 2005 , 2016 ), and participatory design research (Bang & Vossoughi, 2016 ) models, which attend intentionally to historicity and relational equity, tailor their methods to the liberation of historically marginalized communities, aim intentionally for liberatory outcomes as key elements of their design processes, and seek to produce outcomes in which communities of learners become designers of new community futures (Gutiérrez, 2016 ). While these approaches emerged in the United States, their origins reflect ontological and ideological perspectives quite distinct from more traditional learning science models of DBR, and dominant U.S. ontologies in general. Indeed, these iterations of DBR are linked genealogically to the ontologies, ideologies, and concerns of peoples in the Global South, offering some promise for the method in those regions, though DBR has yet to broadly take hold among researchers beyond the United States and Europe.
There is, of course, much more nuance to these models, and each of these models (formative interventions, Change Laboratories, social design experiments, and participatory design research) might itself merit independent exploration and review well beyond the scope here. Indeed, there is some question as to whether all adherents of these CHAT design-based methodologies, with their unique genealogies and histories, would even consider themselves under the umbrella of DBR. Yet, despite significant ontological divergences, these iterations share many of the same foundational tenets of the traditional models (though realized differently), and it is reasonable to argue that they do indeed share the same, broad methodological paradigm (DBR), or at the very least, are so intimately related that any discussion of DBR, particularly one with a global view, should consider the contributions CHAT iterations have made to the DBR methodology in the course of their somewhat distinct, but parallel, development.
Possibilities and Potentials for Design-Based Research
Since its emergence in 1992 , the DBR methodology for educational research has continued to grow in popularity, ubiquity, and significance. Its use has begun to expand beyond the confines of the learning sciences, taken up by researchers in a variety of disciplines, and across a breadth of theoretical and intellectual traditions. While still not as widely recognized as more traditional and well-established research methodologies, DBR as a methodology for rigorous research is unquestionably here to stay.
With this in mind, the field ought to still be cautious of the ways in which the discourse of design is used. Not all design is DBR, and preserving the integrity, rigor, and research ethics of the paradigm (on its own terms) will continue to require thoughtful reflection as its pluralistic parameters come into clearer focus. Yet the proliferation of methods in the DBR paradigm should be seen as a positive. There are far too many theories of learning and ideological perspectives that have meaningful contributions to make to our knowledge of the world, communities, and learning to limit ourselves to a unitary approach to DBR, or set of methods. The paradigm has shown itself to have some core methodological principles, but there is no reason not to expect these to grow, expand, and evolve over time.
In an increasingly globalized, culturally diverse, and dynamic world, there is tremendous potential for innovation couched in this proliferation of DBR. Particularly in historically marginalized communities and across the Global South, we will need to know how learning theories can be lived out in productive ways in communities that have been understudied, and under-engaged. The DBR paradigm generally, and critical and CHAT iterations particularly, can fill an important need for participatory, theory-developing research in these contexts that simultaneously creates lived impacts. Participatory design research (PDR), social design experiments (SDE), and Change Laboratory models of DBR should be of particular interest and attention moving forward, as current trends toward culturally sustaining pedagogies and learning will need to be explored in depth and in close collaboration with communities, as participatory design partners, in the press toward liberatory educational innovations.
Bibliography
The following special issues of journals are encouraged starting points for engaging more deeply with current and past trends in design-based research.
- Bang, M. , & Vossoughi, S. (Eds.). (2016). Participatory design research and educational justice: Studying learning and relations within social change making [Special issue]. Cognition and Instruction , 34 (3).
- Barab, S. (Ed.). (2004). Design-based research [Special issue]. Journal of the Learning Sciences , 13 (1).
- Cole, M. , & The Distributed Literacy Consortium. (2006). The Fifth Dimension: An after-school program built on diversity . New York, NY: Russell Sage Foundation.
- Kelly, A. E. (Ed.). (2003). Special issue on the role of design in educational research [Special issue]. Educational Researcher , 32 (1).
- Arzubiaga, A. , Artiles, A. , King, K. , & Harris-Murri, N. (2008). Beyond research on cultural minorities: Challenges and implications of research as situated cultural practice. Exceptional Children , 74 (3), 309–327.
- Bang, M. , Faber, L. , Gurneau, J. , Marin, A. , & Soto, C. (2016). Community-based design research: Learning across generations and strategic transformations of institutional relations toward axiological innovations. Mind, Culture, and Activity , 23 (1), 28–41.
- Bang, M. , & Vossoughi, S. (2016). Participatory design research and educational justice: Studying learning and relations within social change making. Cognition and Instruction , 34 (3), 173–193.
- Barab, S. , Kinster, J. G. , Moore, J. , Cunningham, D. , & The ILF Design Team. (2001). Designing and building an online community: The struggle to support sociability in the Inquiry Learning Forum. Educational Technology Research and Development , 49 (4), 71–96.
- Barab, S. , & Squire, K. (2004). Design-based research: Putting a stake in the ground. Journal of the Learning Sciences , 13 (1), 1–14.
- Barab, S. A. , & Kirshner, D. (2001). Methodologies for capturing learner practices occurring as part of dynamic learning environments. Journal of the Learning Sciences , 10 (1–2), 5–15.
- Bell, P. (2004). On the theoretical breadth of design-based research in education. Educational Psychologist , 39 (4), 243–253.
- Bereiter, C. , & Scardamalia, M. (1989). Intentional learning as a goal of instruction. In L. B. Resnick (Ed.), Knowing, learning, and instruction: Essays in honor of Robert Glaser (pp. 361–392). Hillsdale, NJ: Lawrence Erlbaum.
- Booker, A. , & Goldman, S. (2016). Participatory design research as a practice for systemic repair: Doing hand-in-hand math research with families. Cognition and Instruction , 34 (3), 222–235.
- Brown, A. L. (1992). Design experiments: Theoretical and methodological challenges in creating complex interventions in classroom settings. Journal of the Learning Sciences , 2 (2), 141–178.
- Brown, A. , & Campione, J. C. (1996). Psychological theory and the design of innovative learning environments: On procedures, principles, and systems. In L. Schauble & R. Glaser (Eds.), Innovations in learning: New environments for education (pp. 289–325). Mahwah, NJ: Lawrence Erlbaum.
- Brown, A. L. , & Campione, J. C. (1998). Designing a community of young learners: Theoretical and practical lessons. In N. M. Lambert & B. L. McCombs (Eds.), How students learn: Reforming schools through learner-centered education (pp. 153–186). Washington, DC: American Psychological Association.
- Brown, A. , Campione, J. , Webber, L. , & McGilley, K. (1992). Interactive learning environments—A new look at learning and assessment. In B. R. Gifford & M. C. O’Connor (Eds.), Future assessment: Changing views of aptitude, achievement, and instruction (pp. 121–211). Boston, MA: Academic Press.
- Carnoy, M. , Jacobsen, R. , Mishel, L. , & Rothstein, R. (2005). The charter school dust-up: Examining the evidence on enrollment and achievement . Washington, DC: Economic Policy Institute.
- Carspecken, P. (1996). Critical ethnography in educational research . New York, NY: Routledge.
- Cobb, P. , Confrey, J. , diSessa, A. , Lehrer, R. , & Schauble, L. (2003). Design experiments in educational research. Educational Researcher , 32 (1), 9–13.
- Cobb, P. , & Steffe, L. P. (1983). The constructivist researcher as teacher and model builder. Journal for Research in Mathematics Education , 14 , 83–94.
- Coburn, C. , & Penuel, W. (2016). Research-practice partnerships in education: Outcomes, dynamics, and open questions. Educational Researcher , 45 (1), 48–54.
- Cole, M. (1995). From Moscow to the Fifth Dimension: An exploration in romantic science. In M. Cole & J. Wertsch (Eds.), Contemporary implications of Vygotsky and Luria (pp. 1–38). Worcester, MA: Clark University Press.
- Cole, M. (1996). Cultural psychology: A once and future discipline . Cambridge, MA: Harvard University Press.
- Cole, M. (2007). Sustaining model systems of educational activity: Designing for the long haul. In J. Campione , K. Metz , & A. S. Palinscar (Eds.), Children’s learning in and out of school: Essays in honor of Ann Brown (pp. 71–89). New York, NY: Routledge.
- Cole, M. , & Engeström, Y. (1993). A cultural historical approach to distributed cognition. In G. Saloman (Ed.), Distributed cognitions: Psychological and educational considerations (pp. 1–46). Cambridge, U.K.: Cambridge University Press.
- Cole, M. , & Engeström, Y. (2007). Cultural-historical approaches to designing for development. In J. Valsiner & A. Rosa (Eds.), The Cambridge handbook of sociocultural psychology , Cambridge, U.K.: Cambridge University Press.
- Cole, M. , & Underwood, C. (2013). The evolution of the 5th Dimension. In The Story of the Laboratory of Comparative Human Cognition: A polyphonic autobiography . https://lchcautobio.ucsd.edu/polyphonic-autobiography/section-5/chapter-12-the-later-life-of-the-5th-dimension-and-its-direct-progeny/ .
- Collins, A. (1992). Toward a design science of education. In E. Scanlon & T. O’Shea (Eds.), New directions in educational technology (pp. 15–22). New York, NY: Springer-Verlag.
- Collins, A. , Joseph, D. , & Bielaczyc, K. (2004). Design research: Theoretical and methodological issues. Journal of the Learning Sciences , 13 (1), 15–42.
- Dede, C. (2004). If design-based research is the answer, what is the question? A commentary on Collins, Joseph, and Bielaczyc; DiSessa and Cobb; and Fishman, Marx, Blumenthal, Krajcik, and Soloway in the JLS special issue on design-based research. Journal of the Learning Sciences , 13 (1), 105–114.
- Design-Based Research Collective . (2003). Design-based research: An emerging paradigm for educational inquiry. Educational Researcher , 32 (1), 5–8.
- DiGiacomo, D. , & Gutiérrez, K. D. (2015). Relational equity as a design tool within making and tinkering activities. Mind, Culture, and Activity , 22 (3), 1–15.
- diSessa, A. A. (1991). Local sciences: Viewing the design of human-computer systems as cognitive science. In J. M. Carroll (Ed.), Designing interaction: Psychology at the human-computer interface (pp. 162–202). Cambridge, U.K.: Cambridge University Press.
- diSessa, A. A. , & Cobb, P. (2004). Ontological innovation and the role of theory in design experiments. Journal of the Learning Sciences , 13 (1), 77–103.
- diSessa, A. A. , & Minstrell, J. (1998). Cultivating conceptual change with benchmark lessons. In J. G. Greeno & S. Goldman (Eds.), Thinking practices (pp. 155–187). Mahwah, NJ: Lawrence Erlbaum.
- Dominguez, M. (2015). Decolonizing teacher education: Explorations of expansive learning and culturally sustaining pedagogy in a social design experiment (Doctoral dissertation). University of Colorado, Boulder.
- Edelson, D. (2002). Design research: What we learn when we engage in design. Journal of the Learning Sciences , 11 (1), 105–121.
- Edwards, A. (2007). Relational agency in professional practice: A CHAT analysis. Actio: An International Journal of Human Activity Theory , 1 , 1–17.
- Edwards, A. (2009). Agency and activity theory: From the systemic to the relational. In A. Sannino , H. Daniels , & K. Gutiérrez (Eds.), Learning and expanding with activity theory (pp. 197–211). Cambridge, U.K.: Cambridge University Press.
- Engeström, Y. (1987). Learning by expanding . Helsinki, Finland: University of Helsinki, Department of Education.
- Engeström, Y. (2000). Can people learn to master their future? Journal of the Learning Sciences , 9 , 525–534.
- Engeström, Y. (2001). Expansive learning at work: Toward an activity theoretical reconceptualization. Journal of Education and Work , 14 (1), 133–156.
- Engeström, Y. (2007). Enriching the theory of expansive learning: Lessons from journeys toward co-configuration. Mind, Culture, and Activity , 14 (1–2), 23–39.
- Engeström, Y. (2008). Putting Vygotksy to work: The Change Laboratory as an application of double stimulation. In H. Daniels , M. Cole , & J. Wertsch (Eds.), Cambridge companion to Vygotsky (pp. 363–382). New York, NY: Cambridge University Press.
- Engeström, Y. (2011). From design experiments to formative interventions. Theory & Psychology , 21 (5), 598–628.
- Engeström, Y. , Engeström, R. , & Kärkkäinen, M. (1995). Polycontextuality and boundary crossing in expert cognition: Learning and problem solving in complex work activities. Learning and Instruction , 5 (4), 319–336.
- Engeström, Y. , & Sannino, A. (2010). Studies of expansive learning: Foundations, findings and future challenges. Educational Research Review , 5 (1), 1–24.
- Engeström, Y. , & Sannino, A. (2011). Discursive manifestations of contradictions in organizational change efforts: A methodological framework. Journal of Organizational Change Management , 24 (3), 368–387.
- Engeström, Y. , Sannino, A. , & Virkkunen, J. (2014). On the methodological demands of formative interventions. Mind, Culture, and Activity , 2 (2), 118–128.
- Erickson, F. , & Gutiérrez, K. (2002). Culture, rigor, and science in educational research. Educational Researcher , 31 (8), 21–24.
- Espinoza, M. (2009). A case study of the production of educational sanctuary in one migrant classroom. Pedagogies: An International Journal , 4 (1), 44–62.
- Espinoza, M. L. , & Vossoughi, S. (2014). Perceiving learning anew: Social interaction, dignity, and educational rights. Harvard Educational Review , 84 (3), 285–313.
- Fine, M. (1994). Dis-tance and other stances: Negotiations of power inside feminist research. In A. Gitlin (Ed.), Power and method (pp. 13–25). New York, NY: Routledge.
- Fishman, B. , Penuel, W. , Allen, A. , Cheng, B. , & Sabelli, N. (2013). Design-based implementation research: An emerging model for transforming the relationship of research and practice. National Society for the Study of Education , 112 (2), 136–156.
- Gravemeijer, K. (1994). Educational development and developmental research in mathematics education. Journal for Research in Mathematics Education , 25 (5), 443–471.
- Gutiérrez, K. (2005). Intersubjectivity and grammar in the third space . Scribner Award Lecture.
- Gutiérrez, K. (2008). Developing a sociocritical literacy in the third space. Reading Research Quarterly , 43 (2), 148–164.
- Gutiérrez, K. (2016). Designing resilient ecologies: Social design experiments and a new social imagination. Educational Researcher , 45 (3), 187–196.
- Gutiérrez, K. , Bien, A. , Selland, M. , & Pierce, D. M. (2011). Polylingual and polycultural learning ecologies: Mediating emergent academic literacies for dual language learners. Journal of Early Childhood Literacy , 11 (2), 232–261.
- Gutiérrez, K. , Engeström, Y. , & Sannino, A. (2016). Expanding educational research and interventionist methodologies. Cognition and Instruction , 34 (2), 275–284.
- Gutiérrez, K. , & Jurow, A. S. (2016). Social design experiments: Toward equity by design. Journal of Learning Sciences , 25 (4), 565–598.
- Gutiérrez, K. , & Penuel, W. R. (2014). Relevance to practice as a criterion for rigor. Educational Researcher , 43 (1), 19–23.
- Gutiérrez, K. , & Rogoff, B. (2003). Cultural ways of learning: Individual traits or repertoires of practice. Educational Researcher , 32 (5), 19–25.
- Gutierréz, K. , & Vossoughi, S. (2010). Lifting off the ground to return anew: Mediated praxis, transformative learning, and social design experiments. Journal of Teacher Education , 61 (1–2), 100–117.
- Hall, R. , & Jurow, A. S. (2015). Changing concepts in activity: Descriptive and design studies of consequential learning in conceptual practices. Educational Psychologist , 50 (3), 173–189.
- Harding, S. (1993). Rethinking standpoint epistemology: What is “strong objectivity”? In L. Alcoff & E. Potter (Eds.), Feminist epistemologies (pp. 49–82). New York, NY: Routledge.
- Hoadley, C. (2002). Creating context: Design-based research in creating and understanding CSCL. In G. Stahl (Ed.), Computer support for collaborative learning 2002 (pp. 453–462). Mahwah, NJ: Lawrence Erlbaum.
- Hoadley, C. (2004). Methodological alignment in design-based research. Educational Psychologist , 39 (4), 203–212.
- Joseph, D. (2004). The practice of design-based research: Uncovering the interplay between design, research, and the real-world context. Educational Psychologist , 39 (4), 235–242.
- Jurow, A. S. , & Shea, M. V. (2015). Learning in equity-oriented scale-making projects. Journal of the Learning Sciences , 24 (2), 286–307.
- Jurow, S. , Tracy, R. , Hotchkiss, J. , & Kirshner, B. (2012). Designing for the future: How the learning sciences can inform the trajectories of preservice teachers. Journal of Teacher Education , 63 (2), 147–60.
- Kärkkäinen, M. (1999). Teams as breakers of traditional work practices: A longitudinal study of planning and implementing curriculum units in elementary school teacher teams . Helsinki, Finland: University of Helsinki, Department of Education.
- Kelly, A. (2004). Design research in education: Yes, but is it methodological? Journal of the Learning Sciences , 13 (1), 115–128.
- Kelly, A. E. , & Sloane, F. C. (2003). Educational research and the problems of practice. Irish Educational Studies , 22 , 29–40.
- Kirshner, B. (2015). Youth activism in an era of education inequality . New York: New York University Press.
- Kirshner, B. , & Polman, J. L. (2013). Adaptation by design: A context-sensitive, dialogic approach to interventions. National Society for the Study of Education Yearbook , 112 (2), 215–236.
- Leander, K. M. , Phillips, N. C. , & Taylor, K. H. (2010). The changing social spaces of learning: Mapping new mobilities. Review of Research in Education , 34 , 329–394.
- Lesh, R. A. , & Kelly, A. E. (2000). Multi-tiered teaching experiments. In A. E. Kelly & R. A. Lesh (Eds.), Handbook of research design in mathematics and science education (pp. 197–230). Mahwah, NJ: Lawrence Erlbaum.
- Matusov, E. (1996). Intersubjectivty without agreement. Mind, Culture, and Activity , 3 (1), 29–45.
- Messick, S. (1992). The interplay of evidence and consequences in the validation of performance assessments. Educational Researcher , 23 (2), 13–23.
- Mosteller, F. , & Boruch, R. F. (Eds.). (2002). Evidence matters: Randomized trials in education research . Washington, DC: Brookings Institution Press.
- Newman, D. , Griffin, P. , & Cole, M. (1989). The construction zone: Working for cognitive change in school . London, U.K.: Cambridge University Press.
- Penuel, W. R. , Fishman, B. J. , Cheng, B. H. , & Sabelli, N. (2011). Organizing research and development at the intersection of learning, implementation, and design. Educational Researcher , 40 (7), 331–337.
- Polman, J. L. (2000). Designing project-based science: Connecting learners through guided inquiry . New York, NY: Teachers College Press.
- Ravitch, D. (2010). The death and life of the great American school system: How testing and choice are undermining education . New York, NY: Basic Books.
- Rogoff, B. (1990). Apprenticeship in thinking: Cognitive development in social context . New York, NY: Oxford University Press.
- Rogoff, B. (1995). Observing sociocultural activity on three planes: Participatory appropriation, guided participation, and apprenticeship. In J. V. Wertsch , P. D. Rio , & A. Alvarez (Eds.), Sociocultural studies of mind (pp. 139–164). Cambridge U.K.: Cambridge University Press.
- Saltman, K. J. (2007). Capitalizing on disaster: Taking and breaking public schools . Boulder, CO: Paradigm.
- Salvador, T. , Bell, G. , & Anderson, K. (1999). Design ethnography. Design Management Journal , 10 (4), 35–41.
- Sannino, A. (2011). Activity theory as an activist and interventionist theory. Theory & Psychology , 21 (5), 571–597.
- Sannino, A. , & Engeström, Y. (2016). Relational agency, double stimulation and the object of activity: An intervention study in a primary school. In A. Edwards (Ed.), Working relationally in and across practices: Cultural-historical approaches to collaboration (pp. 58–77). Cambridge, U.K.: Cambridge University Press.
- Scardamalia, M. , & Bereiter, C. (1991). Higher levels of agency for children in knowledge building: A challenge for the design of new knowledge media. Journal of the Learning Sciences , 1 , 37–68.
- Schoenfeld, A. H. (1982). Measures of problem solving performance and of problem solving instruction. Journal for Research in Mathematics Education , 13 , 31–49.
- Schoenfeld, A. H. (1985). Mathematical problem solving . Orlando, FL: Academic Press.
- Schoenfeld, A. H. (1992). On paradigms and methods: What do you do when the ones you know don’t do what you want them to? Issues in the analysis of data in the form of videotapes. Journal of the Learning Sciences , 2 (2), 179–214.
- Scribner, S. , & Cole, M. (1978). Literacy without schooling: Testing for intellectual effects. Harvard Educational Review , 48 (4), 448–461.
- Shavelson, R. J. , Phillips, D. C. , Towne, L. , & Feuer, M. J. (2003). On the science of education design studies. Educational Researcher , 32 (1), 25–28.
- Steffe, L. P. , & Thompson, P. W. (2000). Teaching experiment methodology: Underlying principles and essential elements. In A. Kelly & R. Lesh (Eds.), Handbook of research design in mathematics and science education (pp. 267–307). Mahwah, NJ: Erlbaum.
- Stevens, R. (2000). Divisions of labor in school and in the workplace: Comparing computer and paper-supported activities across settings. Journal of the Learning Sciences , 9 (4), 373–401.
- Suchman, L. (1995). Making work visible. Communications of the ACM , 38 (9), 57–64.
- Vakil, S. , de Royston, M. M. , Nasir, N. , & Kirshner, B. (2016). Rethinking race and power in design-based research: Reflections from the field. Cognition and Instruction , 34 (3), 194–209.
- van den Akker, J. (1999). Principles and methods of development research. In J. van den Akker , R. M. Branch , K. Gustafson , N. Nieveen , & T. Plomp (Eds.), Design approaches and tools in education and training (pp. 1–14). Boston, MA: Kluwer Academic.
- Virkkunen, J. , & Newnham, D. (2013). The Change Laboratory: A tool for collaborative development of work and education . Rotterdam, The Netherlands: Sense.
- White, B. Y. , & Frederiksen, J. R. (1998). Inquiry, modeling, and metacognition: Making science accessible to all students. Cognition and Instruction , 16 , 3–118.
- Zavala, M. (2016). Design, participation, and social change: What design in grassroots spaces can teach learning scientists. Cognition and Instruction , 34 (3), 236–249.
1. The reader should note the emergence of critical ethnography (e.g., Carspecken, 1996 ; Fine, 1994 ), and other more participatory models of ethnography that deviated from this traditional paradigm during this same time period. These new forms of ethnography comprised part of the genealogy of the more critical approaches to DBR, described later in this article.
2. The reader will also note that the adjective “qualitative” largely drops away from the acronym “DBR.” This is largely because, as described, DBR, as an exploration of naturalistic ecologies with multitudes of variables, and social and learning dynamics, necessarily demands a move beyond what can be captured by quantitative measurement alone. The qualitative nature of the research is thus implied and embedded as part of what makes DBR a unique and distinct methodology.
Related Articles
- Qualitative Data Analysis
- The Entanglements of Ethnography and Participatory Action Research (PAR) in Educational Research in North America
- Writing Educational Ethnography
- Qualitative Data Analysis and the Use of Theory
- Comparative Case Study Research
- Use of Qualitative Methods in Evaluation Studies
- Writing Qualitative Dissertations
- Ethnography in Early Childhood Education
- A History of Qualitative Research in Education in China
- Qualitative Research in the Field of Popular Education
- Qualitative Methodological Considerations for Studying Undocumented Students in the United States
- Culturally Responsive Evaluation as a Form of Critical Qualitative Inquiry
- Participatory Action Research in Education
- Complexity Theory as a Guide to Qualitative Methodology in Teacher Education
- Observing Schools and Classrooms
Printed from Oxford Research Encyclopedias, Education. Under the terms of the licence agreement, an individual user may print out a single article for personal use (for details see Privacy Policy and Legal Notice).
date: 23 April 2024
- Cookie Policy
- Privacy Policy
- Legal Notice
- Accessibility
- [66.249.64.20|81.177.182.159]
- 81.177.182.159
Character limit 500 /500
- USC Libraries
- Research Guides
Organizing Your Social Sciences Research Paper
- Types of Research Designs
- Purpose of Guide
- Design Flaws to Avoid
- Independent and Dependent Variables
- Glossary of Research Terms
- Reading Research Effectively
- Narrowing a Topic Idea
- Broadening a Topic Idea
- Extending the Timeliness of a Topic Idea
- Academic Writing Style
- Applying Critical Thinking
- Choosing a Title
- Making an Outline
- Paragraph Development
- Research Process Video Series
- Executive Summary
- The C.A.R.S. Model
- Background Information
- The Research Problem/Question
- Theoretical Framework
- Citation Tracking
- Content Alert Services
- Evaluating Sources
- Primary Sources
- Secondary Sources
- Tiertiary Sources
- Scholarly vs. Popular Publications
- Qualitative Methods
- Quantitative Methods
- Insiderness
- Using Non-Textual Elements
- Limitations of the Study
- Common Grammar Mistakes
- Writing Concisely
- Avoiding Plagiarism
- Footnotes or Endnotes?
- Further Readings
- Generative AI and Writing
- USC Libraries Tutorials and Other Guides
- Bibliography
Introduction
Before beginning your paper, you need to decide how you plan to design the study .
The research design refers to the overall strategy and analytical approach that you have chosen in order to integrate, in a coherent and logical way, the different components of the study, thus ensuring that the research problem will be thoroughly investigated. It constitutes the blueprint for the collection, measurement, and interpretation of information and data. Note that the research problem determines the type of design you choose, not the other way around!
De Vaus, D. A. Research Design in Social Research . London: SAGE, 2001; Trochim, William M.K. Research Methods Knowledge Base. 2006.
General Structure and Writing Style
The function of a research design is to ensure that the evidence obtained enables you to effectively address the research problem logically and as unambiguously as possible . In social sciences research, obtaining information relevant to the research problem generally entails specifying the type of evidence needed to test the underlying assumptions of a theory, to evaluate a program, or to accurately describe and assess meaning related to an observable phenomenon.
With this in mind, a common mistake made by researchers is that they begin their investigations before they have thought critically about what information is required to address the research problem. Without attending to these design issues beforehand, the overall research problem will not be adequately addressed and any conclusions drawn will run the risk of being weak and unconvincing. As a consequence, the overall validity of the study will be undermined.
The length and complexity of describing the research design in your paper can vary considerably, but any well-developed description will achieve the following :
- Identify the research problem clearly and justify its selection, particularly in relation to any valid alternative designs that could have been used,
- Review and synthesize previously published literature associated with the research problem,
- Clearly and explicitly specify hypotheses [i.e., research questions] central to the problem,
- Effectively describe the information and/or data which will be necessary for an adequate testing of the hypotheses and explain how such information and/or data will be obtained, and
- Describe the methods of analysis to be applied to the data in determining whether or not the hypotheses are true or false.
The research design is usually incorporated into the introduction of your paper . You can obtain an overall sense of what to do by reviewing studies that have utilized the same research design [e.g., using a case study approach]. This can help you develop an outline to follow for your own paper.
NOTE : Use the SAGE Research Methods Online and Cases and the SAGE Research Methods Videos databases to search for scholarly resources on how to apply specific research designs and methods . The Research Methods Online database contains links to more than 175,000 pages of SAGE publisher's book, journal, and reference content on quantitative, qualitative, and mixed research methodologies. Also included is a collection of case studies of social research projects that can be used to help you better understand abstract or complex methodological concepts. The Research Methods Videos database contains hours of tutorials, interviews, video case studies, and mini-documentaries covering the entire research process.
Creswell, John W. and J. David Creswell. Research Design: Qualitative, Quantitative, and Mixed Methods Approaches . 5th edition. Thousand Oaks, CA: Sage, 2018; De Vaus, D. A. Research Design in Social Research . London: SAGE, 2001; Gorard, Stephen. Research Design: Creating Robust Approaches for the Social Sciences . Thousand Oaks, CA: Sage, 2013; Leedy, Paul D. and Jeanne Ellis Ormrod. Practical Research: Planning and Design . Tenth edition. Boston, MA: Pearson, 2013; Vogt, W. Paul, Dianna C. Gardner, and Lynne M. Haeffele. When to Use What Research Design . New York: Guilford, 2012.
Action Research Design
Definition and Purpose
The essentials of action research design follow a characteristic cycle whereby initially an exploratory stance is adopted, where an understanding of a problem is developed and plans are made for some form of interventionary strategy. Then the intervention is carried out [the "action" in action research] during which time, pertinent observations are collected in various forms. The new interventional strategies are carried out, and this cyclic process repeats, continuing until a sufficient understanding of [or a valid implementation solution for] the problem is achieved. The protocol is iterative or cyclical in nature and is intended to foster deeper understanding of a given situation, starting with conceptualizing and particularizing the problem and moving through several interventions and evaluations.
What do these studies tell you ?
- This is a collaborative and adaptive research design that lends itself to use in work or community situations.
- Design focuses on pragmatic and solution-driven research outcomes rather than testing theories.
- When practitioners use action research, it has the potential to increase the amount they learn consciously from their experience; the action research cycle can be regarded as a learning cycle.
- Action research studies often have direct and obvious relevance to improving practice and advocating for change.
- There are no hidden controls or preemption of direction by the researcher.
What these studies don't tell you ?
- It is harder to do than conducting conventional research because the researcher takes on responsibilities of advocating for change as well as for researching the topic.
- Action research is much harder to write up because it is less likely that you can use a standard format to report your findings effectively [i.e., data is often in the form of stories or observation].
- Personal over-involvement of the researcher may bias research results.
- The cyclic nature of action research to achieve its twin outcomes of action [e.g. change] and research [e.g. understanding] is time-consuming and complex to conduct.
- Advocating for change usually requires buy-in from study participants.
Coghlan, David and Mary Brydon-Miller. The Sage Encyclopedia of Action Research . Thousand Oaks, CA: Sage, 2014; Efron, Sara Efrat and Ruth Ravid. Action Research in Education: A Practical Guide . New York: Guilford, 2013; Gall, Meredith. Educational Research: An Introduction . Chapter 18, Action Research. 8th ed. Boston, MA: Pearson/Allyn and Bacon, 2007; Gorard, Stephen. Research Design: Creating Robust Approaches for the Social Sciences . Thousand Oaks, CA: Sage, 2013; Kemmis, Stephen and Robin McTaggart. “Participatory Action Research.” In Handbook of Qualitative Research . Norman Denzin and Yvonna S. Lincoln, eds. 2nd ed. (Thousand Oaks, CA: SAGE, 2000), pp. 567-605; McNiff, Jean. Writing and Doing Action Research . London: Sage, 2014; Reason, Peter and Hilary Bradbury. Handbook of Action Research: Participative Inquiry and Practice . Thousand Oaks, CA: SAGE, 2001.
Case Study Design
A case study is an in-depth study of a particular research problem rather than a sweeping statistical survey or comprehensive comparative inquiry. It is often used to narrow down a very broad field of research into one or a few easily researchable examples. The case study research design is also useful for testing whether a specific theory and model actually applies to phenomena in the real world. It is a useful design when not much is known about an issue or phenomenon.
- Approach excels at bringing us to an understanding of a complex issue through detailed contextual analysis of a limited number of events or conditions and their relationships.
- A researcher using a case study design can apply a variety of methodologies and rely on a variety of sources to investigate a research problem.
- Design can extend experience or add strength to what is already known through previous research.
- Social scientists, in particular, make wide use of this research design to examine contemporary real-life situations and provide the basis for the application of concepts and theories and the extension of methodologies.
- The design can provide detailed descriptions of specific and rare cases.
- A single or small number of cases offers little basis for establishing reliability or to generalize the findings to a wider population of people, places, or things.
- Intense exposure to the study of a case may bias a researcher's interpretation of the findings.
- Design does not facilitate assessment of cause and effect relationships.
- Vital information may be missing, making the case hard to interpret.
- The case may not be representative or typical of the larger problem being investigated.
- If the criteria for selecting a case is because it represents a very unusual or unique phenomenon or problem for study, then your interpretation of the findings can only apply to that particular case.
Case Studies. Writing@CSU. Colorado State University; Anastas, Jeane W. Research Design for Social Work and the Human Services . Chapter 4, Flexible Methods: Case Study Design. 2nd ed. New York: Columbia University Press, 1999; Gerring, John. “What Is a Case Study and What Is It Good for?” American Political Science Review 98 (May 2004): 341-354; Greenhalgh, Trisha, editor. Case Study Evaluation: Past, Present and Future Challenges . Bingley, UK: Emerald Group Publishing, 2015; Mills, Albert J. , Gabrielle Durepos, and Eiden Wiebe, editors. Encyclopedia of Case Study Research . Thousand Oaks, CA: SAGE Publications, 2010; Stake, Robert E. The Art of Case Study Research . Thousand Oaks, CA: SAGE, 1995; Yin, Robert K. Case Study Research: Design and Theory . Applied Social Research Methods Series, no. 5. 3rd ed. Thousand Oaks, CA: SAGE, 2003.
Causal Design
Causality studies may be thought of as understanding a phenomenon in terms of conditional statements in the form, “If X, then Y.” This type of research is used to measure what impact a specific change will have on existing norms and assumptions. Most social scientists seek causal explanations that reflect tests of hypotheses. Causal effect (nomothetic perspective) occurs when variation in one phenomenon, an independent variable, leads to or results, on average, in variation in another phenomenon, the dependent variable.
Conditions necessary for determining causality:
- Empirical association -- a valid conclusion is based on finding an association between the independent variable and the dependent variable.
- Appropriate time order -- to conclude that causation was involved, one must see that cases were exposed to variation in the independent variable before variation in the dependent variable.
- Nonspuriousness -- a relationship between two variables that is not due to variation in a third variable.
- Causality research designs assist researchers in understanding why the world works the way it does through the process of proving a causal link between variables and by the process of eliminating other possibilities.
- Replication is possible.
- There is greater confidence the study has internal validity due to the systematic subject selection and equity of groups being compared.
- Not all relationships are causal! The possibility always exists that, by sheer coincidence, two unrelated events appear to be related [e.g., Punxatawney Phil could accurately predict the duration of Winter for five consecutive years but, the fact remains, he's just a big, furry rodent].
- Conclusions about causal relationships are difficult to determine due to a variety of extraneous and confounding variables that exist in a social environment. This means causality can only be inferred, never proven.
- If two variables are correlated, the cause must come before the effect. However, even though two variables might be causally related, it can sometimes be difficult to determine which variable comes first and, therefore, to establish which variable is the actual cause and which is the actual effect.
Beach, Derek and Rasmus Brun Pedersen. Causal Case Study Methods: Foundations and Guidelines for Comparing, Matching, and Tracing . Ann Arbor, MI: University of Michigan Press, 2016; Bachman, Ronet. The Practice of Research in Criminology and Criminal Justice . Chapter 5, Causation and Research Designs. 3rd ed. Thousand Oaks, CA: Pine Forge Press, 2007; Brewer, Ernest W. and Jennifer Kubn. “Causal-Comparative Design.” In Encyclopedia of Research Design . Neil J. Salkind, editor. (Thousand Oaks, CA: Sage, 2010), pp. 125-132; Causal Research Design: Experimentation. Anonymous SlideShare Presentation; Gall, Meredith. Educational Research: An Introduction . Chapter 11, Nonexperimental Research: Correlational Designs. 8th ed. Boston, MA: Pearson/Allyn and Bacon, 2007; Trochim, William M.K. Research Methods Knowledge Base. 2006.
Cohort Design
Often used in the medical sciences, but also found in the applied social sciences, a cohort study generally refers to a study conducted over a period of time involving members of a population which the subject or representative member comes from, and who are united by some commonality or similarity. Using a quantitative framework, a cohort study makes note of statistical occurrence within a specialized subgroup, united by same or similar characteristics that are relevant to the research problem being investigated, rather than studying statistical occurrence within the general population. Using a qualitative framework, cohort studies generally gather data using methods of observation. Cohorts can be either "open" or "closed."
- Open Cohort Studies [dynamic populations, such as the population of Los Angeles] involve a population that is defined just by the state of being a part of the study in question (and being monitored for the outcome). Date of entry and exit from the study is individually defined, therefore, the size of the study population is not constant. In open cohort studies, researchers can only calculate rate based data, such as, incidence rates and variants thereof.
- Closed Cohort Studies [static populations, such as patients entered into a clinical trial] involve participants who enter into the study at one defining point in time and where it is presumed that no new participants can enter the cohort. Given this, the number of study participants remains constant (or can only decrease).
- The use of cohorts is often mandatory because a randomized control study may be unethical. For example, you cannot deliberately expose people to asbestos, you can only study its effects on those who have already been exposed. Research that measures risk factors often relies upon cohort designs.
- Because cohort studies measure potential causes before the outcome has occurred, they can demonstrate that these “causes” preceded the outcome, thereby avoiding the debate as to which is the cause and which is the effect.
- Cohort analysis is highly flexible and can provide insight into effects over time and related to a variety of different types of changes [e.g., social, cultural, political, economic, etc.].
- Either original data or secondary data can be used in this design.
- In cases where a comparative analysis of two cohorts is made [e.g., studying the effects of one group exposed to asbestos and one that has not], a researcher cannot control for all other factors that might differ between the two groups. These factors are known as confounding variables.
- Cohort studies can end up taking a long time to complete if the researcher must wait for the conditions of interest to develop within the group. This also increases the chance that key variables change during the course of the study, potentially impacting the validity of the findings.
- Due to the lack of randominization in the cohort design, its external validity is lower than that of study designs where the researcher randomly assigns participants.
Healy P, Devane D. “Methodological Considerations in Cohort Study Designs.” Nurse Researcher 18 (2011): 32-36; Glenn, Norval D, editor. Cohort Analysis . 2nd edition. Thousand Oaks, CA: Sage, 2005; Levin, Kate Ann. Study Design IV: Cohort Studies. Evidence-Based Dentistry 7 (2003): 51–52; Payne, Geoff. “Cohort Study.” In The SAGE Dictionary of Social Research Methods . Victor Jupp, editor. (Thousand Oaks, CA: Sage, 2006), pp. 31-33; Study Design 101. Himmelfarb Health Sciences Library. George Washington University, November 2011; Cohort Study. Wikipedia.
Cross-Sectional Design
Cross-sectional research designs have three distinctive features: no time dimension; a reliance on existing differences rather than change following intervention; and, groups are selected based on existing differences rather than random allocation. The cross-sectional design can only measure differences between or from among a variety of people, subjects, or phenomena rather than a process of change. As such, researchers using this design can only employ a relatively passive approach to making causal inferences based on findings.
- Cross-sectional studies provide a clear 'snapshot' of the outcome and the characteristics associated with it, at a specific point in time.
- Unlike an experimental design, where there is an active intervention by the researcher to produce and measure change or to create differences, cross-sectional designs focus on studying and drawing inferences from existing differences between people, subjects, or phenomena.
- Entails collecting data at and concerning one point in time. While longitudinal studies involve taking multiple measures over an extended period of time, cross-sectional research is focused on finding relationships between variables at one moment in time.
- Groups identified for study are purposely selected based upon existing differences in the sample rather than seeking random sampling.
- Cross-section studies are capable of using data from a large number of subjects and, unlike observational studies, is not geographically bound.
- Can estimate prevalence of an outcome of interest because the sample is usually taken from the whole population.
- Because cross-sectional designs generally use survey techniques to gather data, they are relatively inexpensive and take up little time to conduct.
- Finding people, subjects, or phenomena to study that are very similar except in one specific variable can be difficult.
- Results are static and time bound and, therefore, give no indication of a sequence of events or reveal historical or temporal contexts.
- Studies cannot be utilized to establish cause and effect relationships.
- This design only provides a snapshot of analysis so there is always the possibility that a study could have differing results if another time-frame had been chosen.
- There is no follow up to the findings.
Bethlehem, Jelke. "7: Cross-sectional Research." In Research Methodology in the Social, Behavioural and Life Sciences . Herman J Adèr and Gideon J Mellenbergh, editors. (London, England: Sage, 1999), pp. 110-43; Bourque, Linda B. “Cross-Sectional Design.” In The SAGE Encyclopedia of Social Science Research Methods . Michael S. Lewis-Beck, Alan Bryman, and Tim Futing Liao. (Thousand Oaks, CA: 2004), pp. 230-231; Hall, John. “Cross-Sectional Survey Design.” In Encyclopedia of Survey Research Methods . Paul J. Lavrakas, ed. (Thousand Oaks, CA: Sage, 2008), pp. 173-174; Helen Barratt, Maria Kirwan. Cross-Sectional Studies: Design Application, Strengths and Weaknesses of Cross-Sectional Studies. Healthknowledge, 2009. Cross-Sectional Study. Wikipedia.
Descriptive Design
Descriptive research designs help provide answers to the questions of who, what, when, where, and how associated with a particular research problem; a descriptive study cannot conclusively ascertain answers to why. Descriptive research is used to obtain information concerning the current status of the phenomena and to describe "what exists" with respect to variables or conditions in a situation.
- The subject is being observed in a completely natural and unchanged natural environment. True experiments, whilst giving analyzable data, often adversely influence the normal behavior of the subject [a.k.a., the Heisenberg effect whereby measurements of certain systems cannot be made without affecting the systems].
- Descriptive research is often used as a pre-cursor to more quantitative research designs with the general overview giving some valuable pointers as to what variables are worth testing quantitatively.
- If the limitations are understood, they can be a useful tool in developing a more focused study.
- Descriptive studies can yield rich data that lead to important recommendations in practice.
- Appoach collects a large amount of data for detailed analysis.
- The results from a descriptive research cannot be used to discover a definitive answer or to disprove a hypothesis.
- Because descriptive designs often utilize observational methods [as opposed to quantitative methods], the results cannot be replicated.
- The descriptive function of research is heavily dependent on instrumentation for measurement and observation.
Anastas, Jeane W. Research Design for Social Work and the Human Services . Chapter 5, Flexible Methods: Descriptive Research. 2nd ed. New York: Columbia University Press, 1999; Given, Lisa M. "Descriptive Research." In Encyclopedia of Measurement and Statistics . Neil J. Salkind and Kristin Rasmussen, editors. (Thousand Oaks, CA: Sage, 2007), pp. 251-254; McNabb, Connie. Descriptive Research Methodologies. Powerpoint Presentation; Shuttleworth, Martyn. Descriptive Research Design, September 26, 2008; Erickson, G. Scott. "Descriptive Research Design." In New Methods of Market Research and Analysis . (Northampton, MA: Edward Elgar Publishing, 2017), pp. 51-77; Sahin, Sagufta, and Jayanta Mete. "A Brief Study on Descriptive Research: Its Nature and Application in Social Science." International Journal of Research and Analysis in Humanities 1 (2021): 11; K. Swatzell and P. Jennings. “Descriptive Research: The Nuts and Bolts.” Journal of the American Academy of Physician Assistants 20 (2007), pp. 55-56; Kane, E. Doing Your Own Research: Basic Descriptive Research in the Social Sciences and Humanities . London: Marion Boyars, 1985.
Experimental Design
A blueprint of the procedure that enables the researcher to maintain control over all factors that may affect the result of an experiment. In doing this, the researcher attempts to determine or predict what may occur. Experimental research is often used where there is time priority in a causal relationship (cause precedes effect), there is consistency in a causal relationship (a cause will always lead to the same effect), and the magnitude of the correlation is great. The classic experimental design specifies an experimental group and a control group. The independent variable is administered to the experimental group and not to the control group, and both groups are measured on the same dependent variable. Subsequent experimental designs have used more groups and more measurements over longer periods. True experiments must have control, randomization, and manipulation.
- Experimental research allows the researcher to control the situation. In so doing, it allows researchers to answer the question, “What causes something to occur?”
- Permits the researcher to identify cause and effect relationships between variables and to distinguish placebo effects from treatment effects.
- Experimental research designs support the ability to limit alternative explanations and to infer direct causal relationships in the study.
- Approach provides the highest level of evidence for single studies.
- The design is artificial, and results may not generalize well to the real world.
- The artificial settings of experiments may alter the behaviors or responses of participants.
- Experimental designs can be costly if special equipment or facilities are needed.
- Some research problems cannot be studied using an experiment because of ethical or technical reasons.
- Difficult to apply ethnographic and other qualitative methods to experimentally designed studies.
Anastas, Jeane W. Research Design for Social Work and the Human Services . Chapter 7, Flexible Methods: Experimental Research. 2nd ed. New York: Columbia University Press, 1999; Chapter 2: Research Design, Experimental Designs. School of Psychology, University of New England, 2000; Chow, Siu L. "Experimental Design." In Encyclopedia of Research Design . Neil J. Salkind, editor. (Thousand Oaks, CA: Sage, 2010), pp. 448-453; "Experimental Design." In Social Research Methods . Nicholas Walliman, editor. (London, England: Sage, 2006), pp, 101-110; Experimental Research. Research Methods by Dummies. Department of Psychology. California State University, Fresno, 2006; Kirk, Roger E. Experimental Design: Procedures for the Behavioral Sciences . 4th edition. Thousand Oaks, CA: Sage, 2013; Trochim, William M.K. Experimental Design. Research Methods Knowledge Base. 2006; Rasool, Shafqat. Experimental Research. Slideshare presentation.
Exploratory Design
An exploratory design is conducted about a research problem when there are few or no earlier studies to refer to or rely upon to predict an outcome . The focus is on gaining insights and familiarity for later investigation or undertaken when research problems are in a preliminary stage of investigation. Exploratory designs are often used to establish an understanding of how best to proceed in studying an issue or what methodology would effectively apply to gathering information about the issue.
The goals of exploratory research are intended to produce the following possible insights:
- Familiarity with basic details, settings, and concerns.
- Well grounded picture of the situation being developed.
- Generation of new ideas and assumptions.
- Development of tentative theories or hypotheses.
- Determination about whether a study is feasible in the future.
- Issues get refined for more systematic investigation and formulation of new research questions.
- Direction for future research and techniques get developed.
- Design is a useful approach for gaining background information on a particular topic.
- Exploratory research is flexible and can address research questions of all types (what, why, how).
- Provides an opportunity to define new terms and clarify existing concepts.
- Exploratory research is often used to generate formal hypotheses and develop more precise research problems.
- In the policy arena or applied to practice, exploratory studies help establish research priorities and where resources should be allocated.
- Exploratory research generally utilizes small sample sizes and, thus, findings are typically not generalizable to the population at large.
- The exploratory nature of the research inhibits an ability to make definitive conclusions about the findings. They provide insight but not definitive conclusions.
- The research process underpinning exploratory studies is flexible but often unstructured, leading to only tentative results that have limited value to decision-makers.
- Design lacks rigorous standards applied to methods of data gathering and analysis because one of the areas for exploration could be to determine what method or methodologies could best fit the research problem.
Cuthill, Michael. “Exploratory Research: Citizen Participation, Local Government, and Sustainable Development in Australia.” Sustainable Development 10 (2002): 79-89; Streb, Christoph K. "Exploratory Case Study." In Encyclopedia of Case Study Research . Albert J. Mills, Gabrielle Durepos and Eiden Wiebe, editors. (Thousand Oaks, CA: Sage, 2010), pp. 372-374; Taylor, P. J., G. Catalano, and D.R.F. Walker. “Exploratory Analysis of the World City Network.” Urban Studies 39 (December 2002): 2377-2394; Exploratory Research. Wikipedia.
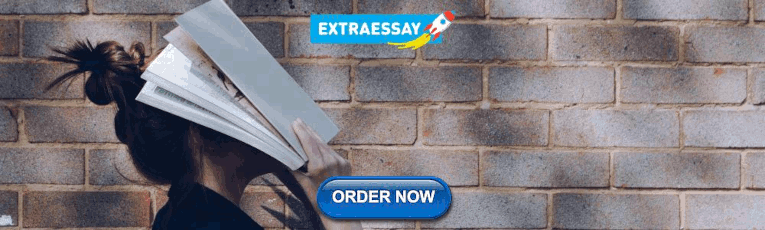
Field Research Design
Sometimes referred to as ethnography or participant observation, designs around field research encompass a variety of interpretative procedures [e.g., observation and interviews] rooted in qualitative approaches to studying people individually or in groups while inhabiting their natural environment as opposed to using survey instruments or other forms of impersonal methods of data gathering. Information acquired from observational research takes the form of “ field notes ” that involves documenting what the researcher actually sees and hears while in the field. Findings do not consist of conclusive statements derived from numbers and statistics because field research involves analysis of words and observations of behavior. Conclusions, therefore, are developed from an interpretation of findings that reveal overriding themes, concepts, and ideas. More information can be found HERE .
- Field research is often necessary to fill gaps in understanding the research problem applied to local conditions or to specific groups of people that cannot be ascertained from existing data.
- The research helps contextualize already known information about a research problem, thereby facilitating ways to assess the origins, scope, and scale of a problem and to gage the causes, consequences, and means to resolve an issue based on deliberate interaction with people in their natural inhabited spaces.
- Enables the researcher to corroborate or confirm data by gathering additional information that supports or refutes findings reported in prior studies of the topic.
- Because the researcher in embedded in the field, they are better able to make observations or ask questions that reflect the specific cultural context of the setting being investigated.
- Observing the local reality offers the opportunity to gain new perspectives or obtain unique data that challenges existing theoretical propositions or long-standing assumptions found in the literature.
What these studies don't tell you
- A field research study requires extensive time and resources to carry out the multiple steps involved with preparing for the gathering of information, including for example, examining background information about the study site, obtaining permission to access the study site, and building trust and rapport with subjects.
- Requires a commitment to staying engaged in the field to ensure that you can adequately document events and behaviors as they unfold.
- The unpredictable nature of fieldwork means that researchers can never fully control the process of data gathering. They must maintain a flexible approach to studying the setting because events and circumstances can change quickly or unexpectedly.
- Findings can be difficult to interpret and verify without access to documents and other source materials that help to enhance the credibility of information obtained from the field [i.e., the act of triangulating the data].
- Linking the research problem to the selection of study participants inhabiting their natural environment is critical. However, this specificity limits the ability to generalize findings to different situations or in other contexts or to infer courses of action applied to other settings or groups of people.
- The reporting of findings must take into account how the researcher themselves may have inadvertently affected respondents and their behaviors.
Historical Design
The purpose of a historical research design is to collect, verify, and synthesize evidence from the past to establish facts that defend or refute a hypothesis. It uses secondary sources and a variety of primary documentary evidence, such as, diaries, official records, reports, archives, and non-textual information [maps, pictures, audio and visual recordings]. The limitation is that the sources must be both authentic and valid.
- The historical research design is unobtrusive; the act of research does not affect the results of the study.
- The historical approach is well suited for trend analysis.
- Historical records can add important contextual background required to more fully understand and interpret a research problem.
- There is often no possibility of researcher-subject interaction that could affect the findings.
- Historical sources can be used over and over to study different research problems or to replicate a previous study.
- The ability to fulfill the aims of your research are directly related to the amount and quality of documentation available to understand the research problem.
- Since historical research relies on data from the past, there is no way to manipulate it to control for contemporary contexts.
- Interpreting historical sources can be very time consuming.
- The sources of historical materials must be archived consistently to ensure access. This may especially challenging for digital or online-only sources.
- Original authors bring their own perspectives and biases to the interpretation of past events and these biases are more difficult to ascertain in historical resources.
- Due to the lack of control over external variables, historical research is very weak with regard to the demands of internal validity.
- It is rare that the entirety of historical documentation needed to fully address a research problem is available for interpretation, therefore, gaps need to be acknowledged.
Howell, Martha C. and Walter Prevenier. From Reliable Sources: An Introduction to Historical Methods . Ithaca, NY: Cornell University Press, 2001; Lundy, Karen Saucier. "Historical Research." In The Sage Encyclopedia of Qualitative Research Methods . Lisa M. Given, editor. (Thousand Oaks, CA: Sage, 2008), pp. 396-400; Marius, Richard. and Melvin E. Page. A Short Guide to Writing about History . 9th edition. Boston, MA: Pearson, 2015; Savitt, Ronald. “Historical Research in Marketing.” Journal of Marketing 44 (Autumn, 1980): 52-58; Gall, Meredith. Educational Research: An Introduction . Chapter 16, Historical Research. 8th ed. Boston, MA: Pearson/Allyn and Bacon, 2007.
Longitudinal Design
A longitudinal study follows the same sample over time and makes repeated observations. For example, with longitudinal surveys, the same group of people is interviewed at regular intervals, enabling researchers to track changes over time and to relate them to variables that might explain why the changes occur. Longitudinal research designs describe patterns of change and help establish the direction and magnitude of causal relationships. Measurements are taken on each variable over two or more distinct time periods. This allows the researcher to measure change in variables over time. It is a type of observational study sometimes referred to as a panel study.
- Longitudinal data facilitate the analysis of the duration of a particular phenomenon.
- Enables survey researchers to get close to the kinds of causal explanations usually attainable only with experiments.
- The design permits the measurement of differences or change in a variable from one period to another [i.e., the description of patterns of change over time].
- Longitudinal studies facilitate the prediction of future outcomes based upon earlier factors.
- The data collection method may change over time.
- Maintaining the integrity of the original sample can be difficult over an extended period of time.
- It can be difficult to show more than one variable at a time.
- This design often needs qualitative research data to explain fluctuations in the results.
- A longitudinal research design assumes present trends will continue unchanged.
- It can take a long period of time to gather results.
- There is a need to have a large sample size and accurate sampling to reach representativness.
Anastas, Jeane W. Research Design for Social Work and the Human Services . Chapter 6, Flexible Methods: Relational and Longitudinal Research. 2nd ed. New York: Columbia University Press, 1999; Forgues, Bernard, and Isabelle Vandangeon-Derumez. "Longitudinal Analyses." In Doing Management Research . Raymond-Alain Thiétart and Samantha Wauchope, editors. (London, England: Sage, 2001), pp. 332-351; Kalaian, Sema A. and Rafa M. Kasim. "Longitudinal Studies." In Encyclopedia of Survey Research Methods . Paul J. Lavrakas, ed. (Thousand Oaks, CA: Sage, 2008), pp. 440-441; Menard, Scott, editor. Longitudinal Research . Thousand Oaks, CA: Sage, 2002; Ployhart, Robert E. and Robert J. Vandenberg. "Longitudinal Research: The Theory, Design, and Analysis of Change.” Journal of Management 36 (January 2010): 94-120; Longitudinal Study. Wikipedia.
Meta-Analysis Design
Meta-analysis is an analytical methodology designed to systematically evaluate and summarize the results from a number of individual studies, thereby, increasing the overall sample size and the ability of the researcher to study effects of interest. The purpose is to not simply summarize existing knowledge, but to develop a new understanding of a research problem using synoptic reasoning. The main objectives of meta-analysis include analyzing differences in the results among studies and increasing the precision by which effects are estimated. A well-designed meta-analysis depends upon strict adherence to the criteria used for selecting studies and the availability of information in each study to properly analyze their findings. Lack of information can severely limit the type of analyzes and conclusions that can be reached. In addition, the more dissimilarity there is in the results among individual studies [heterogeneity], the more difficult it is to justify interpretations that govern a valid synopsis of results. A meta-analysis needs to fulfill the following requirements to ensure the validity of your findings:
- Clearly defined description of objectives, including precise definitions of the variables and outcomes that are being evaluated;
- A well-reasoned and well-documented justification for identification and selection of the studies;
- Assessment and explicit acknowledgment of any researcher bias in the identification and selection of those studies;
- Description and evaluation of the degree of heterogeneity among the sample size of studies reviewed; and,
- Justification of the techniques used to evaluate the studies.
- Can be an effective strategy for determining gaps in the literature.
- Provides a means of reviewing research published about a particular topic over an extended period of time and from a variety of sources.
- Is useful in clarifying what policy or programmatic actions can be justified on the basis of analyzing research results from multiple studies.
- Provides a method for overcoming small sample sizes in individual studies that previously may have had little relationship to each other.
- Can be used to generate new hypotheses or highlight research problems for future studies.
- Small violations in defining the criteria used for content analysis can lead to difficult to interpret and/or meaningless findings.
- A large sample size can yield reliable, but not necessarily valid, results.
- A lack of uniformity regarding, for example, the type of literature reviewed, how methods are applied, and how findings are measured within the sample of studies you are analyzing, can make the process of synthesis difficult to perform.
- Depending on the sample size, the process of reviewing and synthesizing multiple studies can be very time consuming.
Beck, Lewis W. "The Synoptic Method." The Journal of Philosophy 36 (1939): 337-345; Cooper, Harris, Larry V. Hedges, and Jeffrey C. Valentine, eds. The Handbook of Research Synthesis and Meta-Analysis . 2nd edition. New York: Russell Sage Foundation, 2009; Guzzo, Richard A., Susan E. Jackson and Raymond A. Katzell. “Meta-Analysis Analysis.” In Research in Organizational Behavior , Volume 9. (Greenwich, CT: JAI Press, 1987), pp 407-442; Lipsey, Mark W. and David B. Wilson. Practical Meta-Analysis . Thousand Oaks, CA: Sage Publications, 2001; Study Design 101. Meta-Analysis. The Himmelfarb Health Sciences Library, George Washington University; Timulak, Ladislav. “Qualitative Meta-Analysis.” In The SAGE Handbook of Qualitative Data Analysis . Uwe Flick, editor. (Los Angeles, CA: Sage, 2013), pp. 481-495; Walker, Esteban, Adrian V. Hernandez, and Micheal W. Kattan. "Meta-Analysis: It's Strengths and Limitations." Cleveland Clinic Journal of Medicine 75 (June 2008): 431-439.
Mixed-Method Design
- Narrative and non-textual information can add meaning to numeric data, while numeric data can add precision to narrative and non-textual information.
- Can utilize existing data while at the same time generating and testing a grounded theory approach to describe and explain the phenomenon under study.
- A broader, more complex research problem can be investigated because the researcher is not constrained by using only one method.
- The strengths of one method can be used to overcome the inherent weaknesses of another method.
- Can provide stronger, more robust evidence to support a conclusion or set of recommendations.
- May generate new knowledge new insights or uncover hidden insights, patterns, or relationships that a single methodological approach might not reveal.
- Produces more complete knowledge and understanding of the research problem that can be used to increase the generalizability of findings applied to theory or practice.
- A researcher must be proficient in understanding how to apply multiple methods to investigating a research problem as well as be proficient in optimizing how to design a study that coherently melds them together.
- Can increase the likelihood of conflicting results or ambiguous findings that inhibit drawing a valid conclusion or setting forth a recommended course of action [e.g., sample interview responses do not support existing statistical data].
- Because the research design can be very complex, reporting the findings requires a well-organized narrative, clear writing style, and precise word choice.
- Design invites collaboration among experts. However, merging different investigative approaches and writing styles requires more attention to the overall research process than studies conducted using only one methodological paradigm.
- Concurrent merging of quantitative and qualitative research requires greater attention to having adequate sample sizes, using comparable samples, and applying a consistent unit of analysis. For sequential designs where one phase of qualitative research builds on the quantitative phase or vice versa, decisions about what results from the first phase to use in the next phase, the choice of samples and estimating reasonable sample sizes for both phases, and the interpretation of results from both phases can be difficult.
- Due to multiple forms of data being collected and analyzed, this design requires extensive time and resources to carry out the multiple steps involved in data gathering and interpretation.
Burch, Patricia and Carolyn J. Heinrich. Mixed Methods for Policy Research and Program Evaluation . Thousand Oaks, CA: Sage, 2016; Creswell, John w. et al. Best Practices for Mixed Methods Research in the Health Sciences . Bethesda, MD: Office of Behavioral and Social Sciences Research, National Institutes of Health, 2010Creswell, John W. Research Design: Qualitative, Quantitative, and Mixed Methods Approaches . 4th edition. Thousand Oaks, CA: Sage Publications, 2014; Domínguez, Silvia, editor. Mixed Methods Social Networks Research . Cambridge, UK: Cambridge University Press, 2014; Hesse-Biber, Sharlene Nagy. Mixed Methods Research: Merging Theory with Practice . New York: Guilford Press, 2010; Niglas, Katrin. “How the Novice Researcher Can Make Sense of Mixed Methods Designs.” International Journal of Multiple Research Approaches 3 (2009): 34-46; Onwuegbuzie, Anthony J. and Nancy L. Leech. “Linking Research Questions to Mixed Methods Data Analysis Procedures.” The Qualitative Report 11 (September 2006): 474-498; Tashakorri, Abbas and John W. Creswell. “The New Era of Mixed Methods.” Journal of Mixed Methods Research 1 (January 2007): 3-7; Zhanga, Wanqing. “Mixed Methods Application in Health Intervention Research: A Multiple Case Study.” International Journal of Multiple Research Approaches 8 (2014): 24-35 .
Observational Design
This type of research design draws a conclusion by comparing subjects against a control group, in cases where the researcher has no control over the experiment. There are two general types of observational designs. In direct observations, people know that you are watching them. Unobtrusive measures involve any method for studying behavior where individuals do not know they are being observed. An observational study allows a useful insight into a phenomenon and avoids the ethical and practical difficulties of setting up a large and cumbersome research project.
- Observational studies are usually flexible and do not necessarily need to be structured around a hypothesis about what you expect to observe [data is emergent rather than pre-existing].
- The researcher is able to collect in-depth information about a particular behavior.
- Can reveal interrelationships among multifaceted dimensions of group interactions.
- You can generalize your results to real life situations.
- Observational research is useful for discovering what variables may be important before applying other methods like experiments.
- Observation research designs account for the complexity of group behaviors.
- Reliability of data is low because seeing behaviors occur over and over again may be a time consuming task and are difficult to replicate.
- In observational research, findings may only reflect a unique sample population and, thus, cannot be generalized to other groups.
- There can be problems with bias as the researcher may only "see what they want to see."
- There is no possibility to determine "cause and effect" relationships since nothing is manipulated.
- Sources or subjects may not all be equally credible.
- Any group that is knowingly studied is altered to some degree by the presence of the researcher, therefore, potentially skewing any data collected.
Atkinson, Paul and Martyn Hammersley. “Ethnography and Participant Observation.” In Handbook of Qualitative Research . Norman K. Denzin and Yvonna S. Lincoln, eds. (Thousand Oaks, CA: Sage, 1994), pp. 248-261; Observational Research. Research Methods by Dummies. Department of Psychology. California State University, Fresno, 2006; Patton Michael Quinn. Qualitiative Research and Evaluation Methods . Chapter 6, Fieldwork Strategies and Observational Methods. 3rd ed. Thousand Oaks, CA: Sage, 2002; Payne, Geoff and Judy Payne. "Observation." In Key Concepts in Social Research . The SAGE Key Concepts series. (London, England: Sage, 2004), pp. 158-162; Rosenbaum, Paul R. Design of Observational Studies . New York: Springer, 2010;Williams, J. Patrick. "Nonparticipant Observation." In The Sage Encyclopedia of Qualitative Research Methods . Lisa M. Given, editor.(Thousand Oaks, CA: Sage, 2008), pp. 562-563.
Philosophical Design
Understood more as an broad approach to examining a research problem than a methodological design, philosophical analysis and argumentation is intended to challenge deeply embedded, often intractable, assumptions underpinning an area of study. This approach uses the tools of argumentation derived from philosophical traditions, concepts, models, and theories to critically explore and challenge, for example, the relevance of logic and evidence in academic debates, to analyze arguments about fundamental issues, or to discuss the root of existing discourse about a research problem. These overarching tools of analysis can be framed in three ways:
- Ontology -- the study that describes the nature of reality; for example, what is real and what is not, what is fundamental and what is derivative?
- Epistemology -- the study that explores the nature of knowledge; for example, by what means does knowledge and understanding depend upon and how can we be certain of what we know?
- Axiology -- the study of values; for example, what values does an individual or group hold and why? How are values related to interest, desire, will, experience, and means-to-end? And, what is the difference between a matter of fact and a matter of value?
- Can provide a basis for applying ethical decision-making to practice.
- Functions as a means of gaining greater self-understanding and self-knowledge about the purposes of research.
- Brings clarity to general guiding practices and principles of an individual or group.
- Philosophy informs methodology.
- Refine concepts and theories that are invoked in relatively unreflective modes of thought and discourse.
- Beyond methodology, philosophy also informs critical thinking about epistemology and the structure of reality (metaphysics).
- Offers clarity and definition to the practical and theoretical uses of terms, concepts, and ideas.
- Limited application to specific research problems [answering the "So What?" question in social science research].
- Analysis can be abstract, argumentative, and limited in its practical application to real-life issues.
- While a philosophical analysis may render problematic that which was once simple or taken-for-granted, the writing can be dense and subject to unnecessary jargon, overstatement, and/or excessive quotation and documentation.
- There are limitations in the use of metaphor as a vehicle of philosophical analysis.
- There can be analytical difficulties in moving from philosophy to advocacy and between abstract thought and application to the phenomenal world.
Burton, Dawn. "Part I, Philosophy of the Social Sciences." In Research Training for Social Scientists . (London, England: Sage, 2000), pp. 1-5; Chapter 4, Research Methodology and Design. Unisa Institutional Repository (UnisaIR), University of South Africa; Jarvie, Ian C., and Jesús Zamora-Bonilla, editors. The SAGE Handbook of the Philosophy of Social Sciences . London: Sage, 2011; Labaree, Robert V. and Ross Scimeca. “The Philosophical Problem of Truth in Librarianship.” The Library Quarterly 78 (January 2008): 43-70; Maykut, Pamela S. Beginning Qualitative Research: A Philosophic and Practical Guide . Washington, DC: Falmer Press, 1994; McLaughlin, Hugh. "The Philosophy of Social Research." In Understanding Social Work Research . 2nd edition. (London: SAGE Publications Ltd., 2012), pp. 24-47; Stanford Encyclopedia of Philosophy . Metaphysics Research Lab, CSLI, Stanford University, 2013.
Sequential Design
- The researcher has a limitless option when it comes to sample size and the sampling schedule.
- Due to the repetitive nature of this research design, minor changes and adjustments can be done during the initial parts of the study to correct and hone the research method.
- This is a useful design for exploratory studies.
- There is very little effort on the part of the researcher when performing this technique. It is generally not expensive, time consuming, or workforce intensive.
- Because the study is conducted serially, the results of one sample are known before the next sample is taken and analyzed. This provides opportunities for continuous improvement of sampling and methods of analysis.
- The sampling method is not representative of the entire population. The only possibility of approaching representativeness is when the researcher chooses to use a very large sample size significant enough to represent a significant portion of the entire population. In this case, moving on to study a second or more specific sample can be difficult.
- The design cannot be used to create conclusions and interpretations that pertain to an entire population because the sampling technique is not randomized. Generalizability from findings is, therefore, limited.
- Difficult to account for and interpret variation from one sample to another over time, particularly when using qualitative methods of data collection.
Betensky, Rebecca. Harvard University, Course Lecture Note slides; Bovaird, James A. and Kevin A. Kupzyk. "Sequential Design." In Encyclopedia of Research Design . Neil J. Salkind, editor. (Thousand Oaks, CA: Sage, 2010), pp. 1347-1352; Cresswell, John W. Et al. “Advanced Mixed-Methods Research Designs.” In Handbook of Mixed Methods in Social and Behavioral Research . Abbas Tashakkori and Charles Teddle, eds. (Thousand Oaks, CA: Sage, 2003), pp. 209-240; Henry, Gary T. "Sequential Sampling." In The SAGE Encyclopedia of Social Science Research Methods . Michael S. Lewis-Beck, Alan Bryman and Tim Futing Liao, editors. (Thousand Oaks, CA: Sage, 2004), pp. 1027-1028; Nataliya V. Ivankova. “Using Mixed-Methods Sequential Explanatory Design: From Theory to Practice.” Field Methods 18 (February 2006): 3-20; Bovaird, James A. and Kevin A. Kupzyk. “Sequential Design.” In Encyclopedia of Research Design . Neil J. Salkind, ed. Thousand Oaks, CA: Sage, 2010; Sequential Analysis. Wikipedia.
Systematic Review
- A systematic review synthesizes the findings of multiple studies related to each other by incorporating strategies of analysis and interpretation intended to reduce biases and random errors.
- The application of critical exploration, evaluation, and synthesis methods separates insignificant, unsound, or redundant research from the most salient and relevant studies worthy of reflection.
- They can be use to identify, justify, and refine hypotheses, recognize and avoid hidden problems in prior studies, and explain data inconsistencies and conflicts in data.
- Systematic reviews can be used to help policy makers formulate evidence-based guidelines and regulations.
- The use of strict, explicit, and pre-determined methods of synthesis, when applied appropriately, provide reliable estimates about the effects of interventions, evaluations, and effects related to the overarching research problem investigated by each study under review.
- Systematic reviews illuminate where knowledge or thorough understanding of a research problem is lacking and, therefore, can then be used to guide future research.
- The accepted inclusion of unpublished studies [i.e., grey literature] ensures the broadest possible way to analyze and interpret research on a topic.
- Results of the synthesis can be generalized and the findings extrapolated into the general population with more validity than most other types of studies .
- Systematic reviews do not create new knowledge per se; they are a method for synthesizing existing studies about a research problem in order to gain new insights and determine gaps in the literature.
- The way researchers have carried out their investigations [e.g., the period of time covered, number of participants, sources of data analyzed, etc.] can make it difficult to effectively synthesize studies.
- The inclusion of unpublished studies can introduce bias into the review because they may not have undergone a rigorous peer-review process prior to publication. Examples may include conference presentations or proceedings, publications from government agencies, white papers, working papers, and internal documents from organizations, and doctoral dissertations and Master's theses.
Denyer, David and David Tranfield. "Producing a Systematic Review." In The Sage Handbook of Organizational Research Methods . David A. Buchanan and Alan Bryman, editors. ( Thousand Oaks, CA: Sage Publications, 2009), pp. 671-689; Foster, Margaret J. and Sarah T. Jewell, editors. Assembling the Pieces of a Systematic Review: A Guide for Librarians . Lanham, MD: Rowman and Littlefield, 2017; Gough, David, Sandy Oliver, James Thomas, editors. Introduction to Systematic Reviews . 2nd edition. Los Angeles, CA: Sage Publications, 2017; Gopalakrishnan, S. and P. Ganeshkumar. “Systematic Reviews and Meta-analysis: Understanding the Best Evidence in Primary Healthcare.” Journal of Family Medicine and Primary Care 2 (2013): 9-14; Gough, David, James Thomas, and Sandy Oliver. "Clarifying Differences between Review Designs and Methods." Systematic Reviews 1 (2012): 1-9; Khan, Khalid S., Regina Kunz, Jos Kleijnen, and Gerd Antes. “Five Steps to Conducting a Systematic Review.” Journal of the Royal Society of Medicine 96 (2003): 118-121; Mulrow, C. D. “Systematic Reviews: Rationale for Systematic Reviews.” BMJ 309:597 (September 1994); O'Dwyer, Linda C., and Q. Eileen Wafford. "Addressing Challenges with Systematic Review Teams through Effective Communication: A Case Report." Journal of the Medical Library Association 109 (October 2021): 643-647; Okoli, Chitu, and Kira Schabram. "A Guide to Conducting a Systematic Literature Review of Information Systems Research." Sprouts: Working Papers on Information Systems 10 (2010); Siddaway, Andy P., Alex M. Wood, and Larry V. Hedges. "How to Do a Systematic Review: A Best Practice Guide for Conducting and Reporting Narrative Reviews, Meta-analyses, and Meta-syntheses." Annual Review of Psychology 70 (2019): 747-770; Torgerson, Carole J. “Publication Bias: The Achilles’ Heel of Systematic Reviews?” British Journal of Educational Studies 54 (March 2006): 89-102; Torgerson, Carole. Systematic Reviews . New York: Continuum, 2003.
- << Previous: Purpose of Guide
- Next: Design Flaws to Avoid >>
- Last Updated: Apr 22, 2024 9:12 AM
- URL: https://libguides.usc.edu/writingguide
- Privacy Policy
Buy Me a Coffee

Home » Research Design – Types, Methods and Examples
Research Design – Types, Methods and Examples
Table of Contents

Research Design
Definition:
Research design refers to the overall strategy or plan for conducting a research study. It outlines the methods and procedures that will be used to collect and analyze data, as well as the goals and objectives of the study. Research design is important because it guides the entire research process and ensures that the study is conducted in a systematic and rigorous manner.
Types of Research Design
Types of Research Design are as follows:
Descriptive Research Design
This type of research design is used to describe a phenomenon or situation. It involves collecting data through surveys, questionnaires, interviews, and observations. The aim of descriptive research is to provide an accurate and detailed portrayal of a particular group, event, or situation. It can be useful in identifying patterns, trends, and relationships in the data.
Correlational Research Design
Correlational research design is used to determine if there is a relationship between two or more variables. This type of research design involves collecting data from participants and analyzing the relationship between the variables using statistical methods. The aim of correlational research is to identify the strength and direction of the relationship between the variables.
Experimental Research Design
Experimental research design is used to investigate cause-and-effect relationships between variables. This type of research design involves manipulating one variable and measuring the effect on another variable. It usually involves randomly assigning participants to groups and manipulating an independent variable to determine its effect on a dependent variable. The aim of experimental research is to establish causality.
Quasi-experimental Research Design
Quasi-experimental research design is similar to experimental research design, but it lacks one or more of the features of a true experiment. For example, there may not be random assignment to groups or a control group. This type of research design is used when it is not feasible or ethical to conduct a true experiment.
Case Study Research Design
Case study research design is used to investigate a single case or a small number of cases in depth. It involves collecting data through various methods, such as interviews, observations, and document analysis. The aim of case study research is to provide an in-depth understanding of a particular case or situation.
Longitudinal Research Design
Longitudinal research design is used to study changes in a particular phenomenon over time. It involves collecting data at multiple time points and analyzing the changes that occur. The aim of longitudinal research is to provide insights into the development, growth, or decline of a particular phenomenon over time.
Structure of Research Design
The format of a research design typically includes the following sections:
- Introduction : This section provides an overview of the research problem, the research questions, and the importance of the study. It also includes a brief literature review that summarizes previous research on the topic and identifies gaps in the existing knowledge.
- Research Questions or Hypotheses: This section identifies the specific research questions or hypotheses that the study will address. These questions should be clear, specific, and testable.
- Research Methods : This section describes the methods that will be used to collect and analyze data. It includes details about the study design, the sampling strategy, the data collection instruments, and the data analysis techniques.
- Data Collection: This section describes how the data will be collected, including the sample size, data collection procedures, and any ethical considerations.
- Data Analysis: This section describes how the data will be analyzed, including the statistical techniques that will be used to test the research questions or hypotheses.
- Results : This section presents the findings of the study, including descriptive statistics and statistical tests.
- Discussion and Conclusion : This section summarizes the key findings of the study, interprets the results, and discusses the implications of the findings. It also includes recommendations for future research.
- References : This section lists the sources cited in the research design.
Example of Research Design
An Example of Research Design could be:
Research question: Does the use of social media affect the academic performance of high school students?
Research design:
- Research approach : The research approach will be quantitative as it involves collecting numerical data to test the hypothesis.
- Research design : The research design will be a quasi-experimental design, with a pretest-posttest control group design.
- Sample : The sample will be 200 high school students from two schools, with 100 students in the experimental group and 100 students in the control group.
- Data collection : The data will be collected through surveys administered to the students at the beginning and end of the academic year. The surveys will include questions about their social media usage and academic performance.
- Data analysis : The data collected will be analyzed using statistical software. The mean scores of the experimental and control groups will be compared to determine whether there is a significant difference in academic performance between the two groups.
- Limitations : The limitations of the study will be acknowledged, including the fact that social media usage can vary greatly among individuals, and the study only focuses on two schools, which may not be representative of the entire population.
- Ethical considerations: Ethical considerations will be taken into account, such as obtaining informed consent from the participants and ensuring their anonymity and confidentiality.
How to Write Research Design
Writing a research design involves planning and outlining the methodology and approach that will be used to answer a research question or hypothesis. Here are some steps to help you write a research design:
- Define the research question or hypothesis : Before beginning your research design, you should clearly define your research question or hypothesis. This will guide your research design and help you select appropriate methods.
- Select a research design: There are many different research designs to choose from, including experimental, survey, case study, and qualitative designs. Choose a design that best fits your research question and objectives.
- Develop a sampling plan : If your research involves collecting data from a sample, you will need to develop a sampling plan. This should outline how you will select participants and how many participants you will include.
- Define variables: Clearly define the variables you will be measuring or manipulating in your study. This will help ensure that your results are meaningful and relevant to your research question.
- Choose data collection methods : Decide on the data collection methods you will use to gather information. This may include surveys, interviews, observations, experiments, or secondary data sources.
- Create a data analysis plan: Develop a plan for analyzing your data, including the statistical or qualitative techniques you will use.
- Consider ethical concerns : Finally, be sure to consider any ethical concerns related to your research, such as participant confidentiality or potential harm.
When to Write Research Design
Research design should be written before conducting any research study. It is an important planning phase that outlines the research methodology, data collection methods, and data analysis techniques that will be used to investigate a research question or problem. The research design helps to ensure that the research is conducted in a systematic and logical manner, and that the data collected is relevant and reliable.
Ideally, the research design should be developed as early as possible in the research process, before any data is collected. This allows the researcher to carefully consider the research question, identify the most appropriate research methodology, and plan the data collection and analysis procedures in advance. By doing so, the research can be conducted in a more efficient and effective manner, and the results are more likely to be valid and reliable.
Purpose of Research Design
The purpose of research design is to plan and structure a research study in a way that enables the researcher to achieve the desired research goals with accuracy, validity, and reliability. Research design is the blueprint or the framework for conducting a study that outlines the methods, procedures, techniques, and tools for data collection and analysis.
Some of the key purposes of research design include:
- Providing a clear and concise plan of action for the research study.
- Ensuring that the research is conducted ethically and with rigor.
- Maximizing the accuracy and reliability of the research findings.
- Minimizing the possibility of errors, biases, or confounding variables.
- Ensuring that the research is feasible, practical, and cost-effective.
- Determining the appropriate research methodology to answer the research question(s).
- Identifying the sample size, sampling method, and data collection techniques.
- Determining the data analysis method and statistical tests to be used.
- Facilitating the replication of the study by other researchers.
- Enhancing the validity and generalizability of the research findings.
Applications of Research Design
There are numerous applications of research design in various fields, some of which are:
- Social sciences: In fields such as psychology, sociology, and anthropology, research design is used to investigate human behavior and social phenomena. Researchers use various research designs, such as experimental, quasi-experimental, and correlational designs, to study different aspects of social behavior.
- Education : Research design is essential in the field of education to investigate the effectiveness of different teaching methods and learning strategies. Researchers use various designs such as experimental, quasi-experimental, and case study designs to understand how students learn and how to improve teaching practices.
- Health sciences : In the health sciences, research design is used to investigate the causes, prevention, and treatment of diseases. Researchers use various designs, such as randomized controlled trials, cohort studies, and case-control studies, to study different aspects of health and healthcare.
- Business : Research design is used in the field of business to investigate consumer behavior, marketing strategies, and the impact of different business practices. Researchers use various designs, such as survey research, experimental research, and case studies, to study different aspects of the business world.
- Engineering : In the field of engineering, research design is used to investigate the development and implementation of new technologies. Researchers use various designs, such as experimental research and case studies, to study the effectiveness of new technologies and to identify areas for improvement.
Advantages of Research Design
Here are some advantages of research design:
- Systematic and organized approach : A well-designed research plan ensures that the research is conducted in a systematic and organized manner, which makes it easier to manage and analyze the data.
- Clear objectives: The research design helps to clarify the objectives of the study, which makes it easier to identify the variables that need to be measured, and the methods that need to be used to collect and analyze data.
- Minimizes bias: A well-designed research plan minimizes the chances of bias, by ensuring that the data is collected and analyzed objectively, and that the results are not influenced by the researcher’s personal biases or preferences.
- Efficient use of resources: A well-designed research plan helps to ensure that the resources (time, money, and personnel) are used efficiently and effectively, by focusing on the most important variables and methods.
- Replicability: A well-designed research plan makes it easier for other researchers to replicate the study, which enhances the credibility and reliability of the findings.
- Validity: A well-designed research plan helps to ensure that the findings are valid, by ensuring that the methods used to collect and analyze data are appropriate for the research question.
- Generalizability : A well-designed research plan helps to ensure that the findings can be generalized to other populations, settings, or situations, which increases the external validity of the study.
Research Design Vs Research Methodology
About the author.
Muhammad Hassan
Researcher, Academic Writer, Web developer
You may also like

How to Cite Research Paper – All Formats and...

Data Collection – Methods Types and Examples

Delimitations in Research – Types, Examples and...

Research Paper Format – Types, Examples and...

Research Process – Steps, Examples and Tips

Institutional Review Board – Application Sample...
Leave a comment x.
Save my name, email, and website in this browser for the next time I comment.
Fernando Rodriguez Jr.
EDUC 10: Educational Research Design
This course teaches students important concepts of education research design. The course covers the development of research questions, participant selection, educational measurement and data collection, experimental and correlational research designs, and quantitative and qualitative research methodologies.
The learning goals for the course are as follows.
- Understand the nature of knowledge (where knowledge comes from, perspectives about truth, how knowledge is justified), and how education research informs what we know about teaching and learning.
- Understand how to develop a research question and plan a research study.
- Understand important research design concepts (sample, independent vs. dependent variables, survey design, research design)
- Understand concepts of validity in education research (e.g., internal, external, construct validity)
- Understand qualitative and quantitative approaches to collecting data
- Critically evaluate educational research studies with the above concepts in mind.
- Design a study to answer a research question relevant to education in an ethical and rigorous way.
Unique about this course is that students gain hands-on experience with the research process by developing a set of research questions and administering surveys. As a COIL Initiative course, students also survey their peers from International Christian University (ICU) and Aoyama Gakuin University. Students then present their results to one another and engage in discussions on Discord and Zoom.


Scientific Research in Education (2002)
Chapter: 5 designs for the conduct of scientific research in education, 5 designs for the conduct of scientific research in education.
The salient features of education delineated in Chapter 4 and the guiding principles of scientific research laid out in Chapter 3 set boundaries for the design and conduct of scientific education research. Thus, the design of a study (e.g., randomized experiment, ethnography, multiwave survey) does not itself make it scientific. However, if the design directly addresses a question that can be addressed empirically, is linked to prior research and relevant theory, is competently implemented in context, logically links the findings to interpretation ruling out counterinterpretations, and is made accessible to scientific scrutiny, it could then be considered scientific. That is: Is there a clear set of questions underlying the design? Are the methods appropriate to answer the questions and rule out competing answers? Does the study take previous research into account? Is there a conceptual basis? Are data collected in light of local conditions and analyzed systematically? Is the study clearly described and made available for criticism? The more closely aligned it is with these principles, the higher the quality of the scientific study. And the particular features of education require that the research process be explicitly designed to anticipate the implications of these features and to model and plan accordingly.
RESEARCH DESIGN
Our scientific principles include research design—the subject of this chapter—as but one aspect of a larger process of rigorous inquiry. How-
ever, research design (and corresponding scientific methods) is a crucial aspect of science. It is also the subject of much debate in many fields, including education. In this chapter, we describe some of the most frequently used and trusted designs for scientifically addressing broad classes of research questions in education.
In doing so, we develop three related themes. First, as we posit earlier, a variety of legitimate scientific approaches exist in education research. Therefore, the description of methods discussed in this chapter is illustrative of a range of trusted approaches; it should not be taken as an authoritative list of tools to the exclusion of any others. 1 As we stress in earlier chapters, the history of science has shown that research designs evolve, as do the questions they address, the theories they inform, and the overall state of knowledge.
Second, we extend the argument we make in Chapter 3 that designs and methods must be carefully selected and implemented to best address the question at hand. Some methods are better than others for particular purposes, and scientific inferences are constrained by the type of design employed. Methods that may be appropriate for estimating the effect of an educational intervention, for example, would rarely be appropriate for use in estimating dropout rates. While researchers—in education or any other field—may overstate the conclusions from an inquiry, the strength of scientific inference must be judged in terms of the design used to address the question under investigation. A comprehensive explication of a hierarchy of appropriate designs and analytic approaches under various conditions would require a depth of treatment found in research methods textbooks. This is not our objective. Rather, our goal is to illustrate that among available techniques, certain designs are better suited to address particular kinds of questions under particular conditions than others.
Third, in order to generate a rich source of scientific knowledge in education that is refined and revised over time, different types of inquiries and methods are required. At any time, the types of questions and methods depend in large part on an accurate assessment of the overall state of knowl-
edge and professional judgment about how a particular line of inquiry could advance understanding. In areas with little prior knowledge, for example, research will generally need to involve careful description to formulate initial ideas. In such situations, descriptive studies might be undertaken to help bring education problems or trends into sharper relief or to generate plausible theories about the underlying structure of behavior or learning. If the effects of education programs that have been implemented on a large scale are to be understood, however, investigations must be designed to test a set of causal hypotheses. Thus, while we treat the topic of design in this chapter as applying to individual studies, research design has a broader quality as it relates to lines of inquiry that develop over time.
While a full development of these notions goes considerably beyond our charge, we offer this brief overview to place the discussion of methods that follows into perspective. Also, in the concluding section of this chapter, we make a few targeted suggestions for the kinds of work we believe are most needed in education research to make further progress toward robust knowledge.
TYPES OF RESEARCH QUESTIONS
In discussing design, we have to be true to our admonition that the research question drives the design, not vice versa. To simplify matters, the committee recognized that a great number of education research questions fall into three (interrelated) types: description—What is happening? cause—Is there a systematic effect? and process or mechanism—Why or how is it happening?
The first question—What is happening?—invites description of various kinds, so as to properly characterize a population of students, understand the scope and severity of a problem, develop a theory or conjecture, or identify changes over time among different educational indicators—for example, achievement, spending, or teacher qualifications. Description also can include associations among variables, such as the characteristics of schools (e.g., size, location, economic base) that are related to (say) the provision of music and art instruction. The second question is focused on establishing causal effects: Does x cause y ? The search for cause, for example,
can include seeking to understand the effect of teaching strategies on student learning or state policy changes on district resource decisions. The third question confronts the need to understand the mechanism or process by which x causes y . Studies that seek to model how various parts of a complex system—like U.S. education—fit together help explain the conditions that facilitate or impede change in teaching, learning, and schooling. Within each type of question, we separate the discussion into subsections that show the use of different methods given more fine-grained goals and conditions of an inquiry.
Although for ease of discussion we treat these types of questions separately, in practice they are closely related. As our examples show, within particular studies, several kinds of queries can be addressed. Furthermore, various genres of scientific education research often address more than one of these types of questions. Evaluation research—the rigorous and systematic evaluation of an education program or policy—exemplifies the use of multiple questions and corresponding designs. As applied in education, this type of scientific research is distinguished from other scientific research by its purpose: to contribute to program improvement (Weiss, 1998a). Evaluation often entails an assessment of whether the program caused improvements in the outcome or outcomes of interest (Is there a systematic effect?). It also can involve detailed descriptions of the way the program is implemented in practice and in what contexts ( What is happening? ) and the ways that program services influence outcomes (How is it happening?).
Throughout the discussion, we provide several examples of scientific education research, connecting them to scientific principles ( Chapter 3 ) and the features of education ( Chapter 4 ). We have chosen these studies because they align closely with several of the scientific principles. These examples include studies that generate hypotheses or conjectures as well as those that test them. Both tasks are essential to science, but as a general rule they cannot be accomplished simultaneously.
Moreover, just as we argue that the design of a study does not itself make it scientific, an investigation that seeks to address one of these questions is not necessarily scientific either. For example, many descriptive studies—however useful they may be—bear little resemblance to careful scientific study. They might record observations without any clear conceptual viewpoint, without reproducible protocols for recording data, and so
forth. Again, studies may be considered scientific by assessing the rigor with which they meet scientific principles and are designed to account for the context of the study.
Finally, we have tended to speak of research in terms of a simple dichotomy— scientific or not scientific—but the reality is more complicated. Individual research projects may adhere to each of the principles in varying degrees, and the extent to which they meet these goals goes a long way toward defining the scientific quality of a study. For example, while all scientific studies must pose clear questions that can be investigated empirically and be grounded in existing knowledge, more rigorous studies will begin with more precise statements of the underlying theory driving the inquiry and will generally have a well-specified hypothesis before the data collection and testing phase is begun. Studies that do not start with clear conceptual frameworks and hypotheses may still be scientific, although they are obviously at a more rudimentary level and will generally require follow-on study to contribute significantly to scientific knowledge.
Similarly, lines of research encompassing collections of studies may be more or less productive and useful in advancing knowledge. An area of research that, for example, does not advance beyond the descriptive phase toward more precise scientific investigation of causal effects and mechanisms for a long period of time is clearly not contributing as much to knowledge as one that builds on prior work and moves toward more complete understanding of the causal structure. This is not to say that descriptive work cannot generate important breakthroughs. However, the rate of progress should—as we discuss at the end of this chapter—enter into consideration of the support for advanced lines of inquiry. The three classes of questions we discuss in the remainder of this chapter are ordered in a way that reflects the sequence that research studies tend to follow as well as their interconnected nature.
WHAT IS HAPPENING?
Answers to “What is happening?” questions can be found by following Yogi Berra’s counsel in a systematic way: if you want to know what’s going on, you have to go out and look at what is going on. Such inquiries are descriptive. They are intended to provide a range of information from
documenting trends and issues in a range of geopolitical jurisdictions, populations, and institutions to rich descriptions of the complexities of educational practice in a particular locality, to relationships among such elements as socioeconomic status, teacher qualifications, and achievement.
Estimates of Population Characteristics
Descriptive scientific research in education can make generalizable statements about the national scope of a problem, student achievement levels across the states, or the demographics of children, teachers, or schools. Methods that enable the collection of data from a randomly selected sample of the population provide the best way of addressing such questions. Questionnaires and telephone interviews are common survey instruments developed to gather information from a representative sample of some population of interest. Policy makers at the national, state, and sometimes district levels depend on this method to paint a picture of the educational landscape. Aggregate estimates of the academic achievement level of children at the national level (e.g., National Center for Education Statistics [NCES], National Assessment of Educational Progress [NAEP]), the supply, demand, and turnover of teachers (e.g., NCES Schools and Staffing Survey), the nation’s dropout rates (e.g., NCES Common Core of Data), how U.S. children fare on tests of mathematics and science achievement relative to children in other nations (e.g., Third International Mathematics and Science Study) and the distribution of doctorate degrees across the nation (e.g., National Science Foundation’s Science and Engineering Indicators) are all based on surveys from populations of school children, teachers, and schools.
To yield credible results, such data collection usually depends on a random sample (alternatively called a probability sample) of the target population. If every observation (e.g., person, school) has a known chance of being selected into the study, researchers can make estimates of the larger population of interest based on statistical technology and theory. The validity of inferences about population characteristics based on sample data depends heavily on response rates, that is, the percentage of those randomly selected for whom data are collected. The measures used must have known reliability—that is, the extent to which they reproduce results. Finally, the value of a data collection instrument hinges not only on the
sampling method, participation rate, and reliability, but also on their validity: that the questionnaire or survey items measure what they are supposed to measure.
The NAEP survey tracks national trends in student achievement across several subject domains and collects a range of data on school, student, and teacher characteristics (see Box 5-1 ). This rich source of information enables several kinds of descriptive work. For example, researchers can estimate the average score of eighth graders on the mathematics assessment (i.e., measures of central tendency) and compare that performance to prior years. Part of the study we feature (see below) about college women’s career choices featured a similar estimation of population characteristics. In that study, the researchers developed a survey to collect data from a representative sample of women at the two universities to aid them in assessing the generalizability of their findings from the in-depth studies of the 23 women.
Simple Relationships
The NAEP survey also illustrates how researchers can describe patterns of relationships between variables. For example, NCES reports that in 2000, eighth graders whose teachers majored in mathematics or mathematics education scored higher, on average, than did students whose teachers did not major in these fields (U.S. Department of Education, 2000). This finding is the result of descriptive work that explores the correlation between variables: in this case, the relationship between student mathematics performance and their teachers’ undergraduate major.
Such associations cannot be used to infer cause. However, there is a common tendency to make unsubstantiated jumps from establishing a relationship to concluding cause. As committee member Paul Holland quipped during the committee’s deliberations, “Casual comparisons inevitably invite careless causal conclusions.” To illustrate the problem with drawing causal inferences from simple correlations, we use an example from work that compares Catholic schools to public schools. We feature this study later in the chapter as one that competently examines causal mechanisms. Before addressing questions of mechanism, foundational work involved simple correlational results that compared the performance of Catholic high school students on standardized mathematics tests with their
counterparts in public schools. These simple correlations revealed that average mathematics achievement was considerably higher for Catholic school students than for public school students (Bryk, Lee, and Holland, 1993). However, the researchers were careful not to conclude from this analysis that attending a Catholic school causes better student outcomes, because there are a host of potential explanations (other than attending a Catholic school) for this relationship between school type and achievement. For example, since Catholic schools can screen children for aptitude, they may have a more able student population than public schools at the outset. (This is an example of the classic selectivity bias that commonly threatens the validity of causal claims in nonrandomized studies; we return to this issue in the next section.) In short, there are other hypotheses that could explain the observed differences in achievement between students in different sectors that must be considered systematically in assessing the potential causal relationship between Catholic schooling and student outcomes.
Descriptions of Localized Educational Settings
In some cases, scientists are interested in the fine details (rather than the distribution or central tendency) of what is happening in a particular organization, group of people, or setting. This type of work is especially important when good information about the group or setting is non-existent or scant. In this type of research, then, it is important to obtain first-hand, in-depth information from the particular focal group or site. For such purposes, selecting a random sample from the population of interest may not be the proper method of choice; rather, samples may be purposively selected to illuminate phenomena in depth. 2 For example, to better understand a high-achieving school in an urban setting with children of predominantly low socioeconomic status, a researcher might conduct a detailed case study or an ethnographic study (a case study with a focus on culture) of such a school (Yin and White, 1986; Miles and Huberman,
1994). This type of scientific description can provide rich depictions of the policies, procedures, and contexts in which the school operates and generate plausible hypotheses about what might account for its success. Researchers often spend long periods of time in the setting or group in order to understand what decisions are made, what beliefs and attitudes are formed, what relationships are developed, and what forms of success are celebrated. These descriptions, when used in conjunction with causal methods, are often critical to understand such educational outcomes as student achievement because they illuminate key contextual factors.
Box 5-2 provides an example of a study that described in detail (and also modeled several possible mechanisms; see later discussion) a small group of women, half who began their college careers in science and half in what were considered more traditional majors for women. This descriptive part of the inquiry involved an ethnographic study of the lives of 23 first-year women enrolled in two large universities.
Scientific description of this type can generate systematic observations about the focal group or site, and patterns in results may be generalizable to other similar groups or sites or for the future. As with any other method, a scientifically rigorous case study has to be designed to address the research question it addresses. That is, the investigator has to choose sites, occasions, respondents, and times with a clear research purpose in mind and be sensitive to his or her own expectations and biases (Maxwell, 1996; Silverman, 1993). Data should typically be collected from varied sources, by varied methods, and corroborated by other investigators. Furthermore, the account of the case needs to draw on original evidence and provide enough detail so that the reader can make judgments about the validity of the conclusions (Yin, 2000).
Results may also be used as the basis for new theoretical developments, new experiments, or improved measures on surveys that indicate the extent of generalizability. In the work done by Holland and Eisenhart (1990), for example (see Box 5-2 ), a number of theoretical models were developed and tested to explain how women decide to pursue or abandon nontraditional careers in the fields they had studied in college. Their finding that commitment to college life—not fear of competing with men or other hypotheses that had previously been set forth—best explained these decisions was new knowledge. It has been shown in subsequent studies to
generalize somewhat to similar schools, though additional models seem to exist at some schools (Seymour and Hewitt, 1997).
Although such purposively selected samples may not be scientifically generalizable to other locations or people, these vivid descriptions often appeal to practitioners. Scientifically rigorous case studies have strengths and weaknesses for such use. They can, for example, help local decision makers by providing them with ideas and strategies that have promise in their educational setting. They cannot (unless combined with other methods) provide estimates of the likelihood that an educational approach might work under other conditions or that they have identified the right underlying causes. As we argue throughout this volume, research designs can often be strengthened considerably by using multiple methods— integrating the use of both quantitative estimates of population characteristics and qualitative studies of localized context.
Other descriptive designs may involve interviews with respondents or document reviews in a fairly large number of cases, such as 30 school districts or 60 colleges. Cases are often selected to represent a variety of conditions (e.g., urban/rural; east/west; affluent/poor). Such descriptive studies can be longitudinal, returning to the same cases over several years to see how conditions change.
These examples of descriptive work meet the principles of science, and have clearly contributed important insights to the base of scientific knowledge. If research is to be used to answer questions about “what works,” however, it must advance to other levels of scientific investigation such as those considered next.
IS THERE A SYSTEMATIC EFFECT?
Research designs that attempt to identify systematic effects have at their root an intent to establish a cause-and-effect relationship. Causal work is built on both theory and descriptive studies. In other words, the search for causal effects cannot be conducted in a vacuum: ideally, a strong theoretical base as well as extensive descriptive information are in place to provide the intellectual foundation for understanding causal relationships.
The simple question of “does x cause y ?” typically involves several different kinds of studies undertaken sequentially (Holland, 1993). In basic
terms, several conditions must be met to establish cause. Usually, a relationship or correlation between the variables is first identified. 3 Researchers also confirm that x preceded y in time (temporal sequence) and, crucially, that all presently conceivable rival explanations for the observed relationship have been “ruled out.” As alternative explanations are eliminated, confidence increases that it was indeed x that caused y . “Ruling out” competing explanations is a central metaphor in medical research, diagnosis, and other fields, including education, and it is the key element of causal queries (Campbell and Stanley 1963; Cook and Campbell 1979, 1986).
The use of multiple qualitative methods, especially in conjunction with a comparative study of the kind we describe in this section, can be particularly helpful in ruling out alternative explanations for the results observed (Yin, 2000; Weiss, in press). Such investigative tools can enable stronger causal inferences by enhancing the analysis of whether competing explanations can account for patterns in the data (e.g., unreliable measures or contamination of the comparison group). Similarly, qualitative methods can examine possible explanations for observed effects that arise outside of the purview of the study. For example, while an intervention was in progress, another program or policy may have offered participants opportunities similar to, and reinforcing of, those that the intervention provided. Thus, the “effects” that the study observed may have been due to the other program (“history” as the counterinterpretation; see Chapter 3 ). When all plausible rival explanations are identified and various forms of data can be used as evidence to rule them out, the causal claim that the intervention caused the observed effects is strengthened. In education, research that explores students’ and teachers’ in-depth experiences, observes their actions, and documents the constraints that affect their day-to-day activities provides a key source of generating plausible causal hypotheses.
We have organized the remainder of this section into two parts. The first treats randomized field trials, an ideal method when entities being examined can be randomly assigned to groups. Experiments are especially well-suited to situations in which the causal hypothesis is relatively simple. The second describes situations in which randomized field trials are not
feasible or desirable, and showcases a study that employed causal modeling techniques to address a complex causal question. We have distinguished randomized studies from others primarily to signal the difference in the strength with which causal claims can typically be made from them. The key difference between randomized field trials and other methods with respect to making causal claims is the extent to which the assumptions that underlie them are testable. By this simple criterion, nonrandomized studies are weaker in their ability to establish causation than randomized field trials, in large part because the role of other factors in influencing the outcome of interest is more difficult to gauge in nonrandomized studies. Other conditions that affect the choice of method are discussed in the course of the section.
Causal Relationships When Randomization Is Feasible
A fundamental scientific concept in making causal claims—that is, inferring that x caused y —is comparison. Comparing outcomes (e.g., student achievement) between two groups that are similar except for the causal variable (e.g., the educational intervention) helps to isolate the effect of that causal agent on the outcome of interest. 4 As we discuss in Chapter 4 , it is sometimes difficult to retain the sharpness of a comparison in education due to proximity (e.g., a design that features students in one classroom assigned to different interventions is subject to “spillover” effects) or human volition (e.g., teacher, parent, or student decisions to switch to another condition threaten the integrity of the randomly formed groups). Yet, from a scientific perspective, randomized trials (we also use the term “experiment” to refer to causal studies that feature random assignment) are the ideal for establishing whether one or more factors caused change in an outcome because of their strong ability to enable fair comparisons (Campbell and Stanley, 1963; Boruch, 1997; Cook and Payne, in press). Random allocation of students, classrooms, schools—whatever the unit of comparison may be—to different treatment groups assures that these comparison groups are, roughly speaking, equivalent at the time an intervention is introduced (that is, they do not differ systematically on account of hidden
influences) and chance differences between the groups can be taken into account statistically. As a result, the independent effect of the intervention on the outcome of interest can be isolated. In addition, these studies enable legitimate statistical statements of confidence in the results.
The Tennessee STAR experiment (see Chapter 3 ) on class-size reduction is a good example of the use of randomization to assess cause in an education study; in particular, this tool was used to gauge the effectiveness of an intervention. Some policy makers and scientists were unwilling to accept earlier, largely nonexperimental studies on class-size reduction as a basis for major policy decisions in the state. Those studies could not guarantee a fair comparison of children in small versus large classes because the comparisons relied on statistical adjustment rather than on actual construction of statistically equivalent groups. In Tennessee, statistical equivalence was achieved by randomly assigning eligible children and teachers to classrooms of different size. If the trial was properly carried out, 5 this randomization would lead to an unbiased estimate of the relative effect of class-size reduction and a statistical statement of confidence in the results.
Randomized trials are used frequently in the medical sciences and certain areas of the behavioral and social sciences, including prevention studies of mental health disorders (e.g., Beardslee, Wright, Salt, and Drezner, 1997), behavioral approaches to smoking cessation (e.g., Pieterse, Seydel, DeVries, Mudde, and Kok, 2001), and drug abuse prevention (e.g., Cook, Lawrence, Morse, and Roehl, 1984). It would not be ethical to assign individuals randomly to smoke and drink, and thus much of the evidence regarding the harmful effects of nicotine and alcohol comes from descriptive and correlational studies. However, randomized trials that show reductions in health detriments and improved social and behavioral functioning strengthen the causal links that have been established between drug use and adverse health and behavioral outcomes (Moses, 1995; Mosteller, Gilbert, and McPeek, 1980). In medical research, the relative effectiveness of the Salk vaccine (see Lambert and Markel, 2000) and streptomycin (Medical Research Council, 1948) was demonstrated through such trials. We have also learned about which drugs and surgical treatments are useless by depending on randomized controlled experiments (e.g., Schulte et al.,
2001; Gorman et al., 2001; Paradise et al., 1999). Randomized controlled trials are also used in industrial, market, and agricultural research.
Such trials are not frequently conducted in education research (Boruch, De Moya, and Snyder, in press). Nonetheless, it is not difficult to identify good examples in a variety of education areas that demonstrate their feasibility (see Boruch, 1997; Orr, 1999; and Cook and Payne, in press). For example, among the education programs whose effectiveness have been evaluated in randomized trials are the Sesame Street television series (Bogatz and Ball, 1972), peer-assisted learning and tutoring for young children with reading problems (Fuchs, Fuchs, and Kazdan, 1999), and Upward Bound (Myers and Schirm, 1999). And many of these trials have been successfully implemented on a large scale, randomizing entire classrooms or schools to intervention conditions. For numerous examples of trials in which schools, work places, and other entities are the units of random allocation and analysis, see Murray (1998), Donner and Klar (2000), Boruch and Foley (2000), and the Campbell Collaboration register of trials at http://campbell.gse.upenn.edu .
Causal Relationships When Randomization Is Not Feasible
In this section we discuss the conditions under which randomization is not feasible nor desirable, highlight alternative methods for addressing causal questions, and provide an illustrative example. Many nonexperimental methods and analytic approaches are commonly classified under the blanket rubric “quasi-experiment” because they attempt to approximate the underlying logic of the experiment without random assignment (Campbell and Stanley, 1963; Caporaso and Roos, 1973). These designs were developed because social science researchers recognized that in some social contexts (e.g., schools), researchers do not have the control afforded in laboratory settings and thus cannot always randomly assign units (e.g., classrooms).
Quasi-experiments (alternatively called observational studies), 6 for example, sometimes compare groups of interest that exist naturally (e.g.,
existing classes varying in size) rather than assigning them randomly to different conditions (e.g., assigning students to small, medium, or large class size). These studies must attempt to ensure fair comparisons through means other than randomization, such as by using statistical techniques to adjust for background variables that may account for differences in the outcome of interest. For example, researchers might come across schools that vary in the size of their classes and compare the achievement of students in large and small classes, adjusting for other differences among schools and children. If the class size conjecture holds after this adjustment is made, the researchers would expect students in smaller classes to have higher achievement scores than students in larger size classes. If indeed this difference is observed, the causal effect is more plausible.
The plausibility of the researchers’ causal interpretation, however, depends on some strong assumptions. They must assume that their attempts to equate schools and children were, indeed, successful. Yet, there is always the possibility that some unmeasured, prior existing difference among schools and children caused the effect, not the reduced class size. Or, there is the possibility that teachers with reduced classes were actively involved in school reform and that their increased effort and motivation (which might wane over time) caused the effect, not the smaller classes themselves. In short, these designs are less effective at eliminating competing plausible hypotheses with the same authority as a true experiment.
The major weakness of nonrandomized designs is selectivity bias—the counter-interpretation that the treatment did not cause the difference in outcomes but, rather, unmeasured prior existing differences (differential selectivity) between the groups did. 7 For example, a comparison of early literacy skills among low-income children who participated in a local preschool program and those who did not may be confounded by selectivity bias. That is, the parents of the children who were enrolled in preschool may be more motivated than other parents to provide reading experiences to their children at home, thus making it difficult to disentangle the several potential causes (e.g., preschool program or home reading experiences) for early reading success.
It is critical in such studies, then, to be aware of potential sources of bias and to measure them so their influence can be accounted for in relation to the outcome of interest. 8 It is when these biases are not known that quasi-experiments may yield misleading results. Thus, the scientific principle of making assumptions explicit and carefully attending to ruling out competing hypotheses about what caused a difference takes on heightened importance.
In some settings, well-controlled quasi-experiments may have greater “external validity”—generalizability to other people, times, and settings— than experiments with completely random assignment (Cronbach et al., 1980; Weiss, 1998a). It may be useful to take advantage of the experience and investment of a school with a particular program and try to design a quasi-experiment that compares the school that has a good implementation of the program to a similar school without the program (or with a different program). In such cases, there is less risk of poor implementation, more investment of the implementers in the program, and potentially greater impact. The findings may be more generalizable than in a randomized experiment because the latter may be externally mandated (i.e., by the researcher) and thus may not be feasible to implement in the “real-life” practice of education settings. The results may also have stronger external validity because if a school or district uses a single program, the possible contamination of different programs because teachers or administrators talk and interact will be reduced. Random assignment within a school at the level of the classroom or child often carries the risk of dilution or blending the programs. If assignment is truly random, such threats to internal validity will not bias the comparison of programs—just the estimation of the strength of the effects.
In the section above ( What Is Happening? ), we note that some kinds of correlational work make important contributions to understanding broad patterns of relationships among educational phenomena; here, we highlight a correlational design that allows causal inferences about the relationship between two or more variables. When correlational methods use what are called “model-fitting” techniques based on a theoretically gener-
ated system of variables, they permit stronger, albeit still tentative, causal inferences.
In Chapter 3 , we offer an example that illustrates the use of model-fitting techniques from the geophysical sciences that tested alternative hypotheses about the causes of glaciation. In Box 5-3 , we provide an example of causal modeling that shows the value of such techniques in education. This work examined the potential causal connection between teacher compensation and student dropout rates. Exploring this relationship is quite relevant to education policy, but it cannot be studied through a randomized field trail: teacher salaries, of course, cannot be randomly assigned nor can students be randomly assigned to those teachers. Because important questions like these often cannot be examined experimentally, statisticians have developed sophisticated model-fitting techniques to statistically rule out potential alternative explanations and deal with the problem of selection bias.
The key difference between simple correlational work and model-fitting is that the latter enhances causal attribution. In the study examining teacher compensation and dropout rates, for example, researchers introduced a conceptual model for the relationship between student outcomes and teacher salary, set forth an explicit hypothesis to test about the nature of that relationship, and assessed competing models of interpretation. By empirically rejecting competing theoretical models, confidence is increased in the explanatory power of the remaining model(s) (although other alternative models may also exist that provide a comparable fit to the data).
The study highlighted in Box 5-3 tested different models in this way. Loeb and Page (2000) took a fresh look at a question that had a good bit of history, addressing what appeared to be converging evidence that there was no causal relationship between teacher salaries and student outcomes. They reasoned that one possible explanation for these results was that the usual “production-function” model for the effects of salary on student outcomes was inadequately specified. Specifically, they hypothesized that nonpecuniary job characteristics and alternative wage opportunities that previous models had not accounted for may be relevant in understanding the relationship between teacher compensation and student outcomes. After incorporating these opportunity costs in their model and finding a sophisticated way to control the fact that wealthier parents are likely to send their
children to schools that pay teachers more, Loeb and Page found that raising teacher wages by 10 percent reduced high school dropout rates by 3 to 4 percent.
WHY OR HOW IS IT HAPPENING?
In many situations, finding that a causal agent ( x ) leads to the outcome ( y ) is not sufficient. Important questions remain about how x causes y . Questions about how things work demand attention to the processes and mechanisms by which the causes produce their effects. However, scientific research can also legitimately proceed in the opposite direction: that is, the search for mechanism can come before an effect has been established. For example, if the process by which an intervention influences student outcomes is established, researchers can often predict its effectiveness with known probability. In either case, the processes and mechanisms should be linked to theories so as to form an explanation for the phenomena of interest.
The search for causal mechanisms, especially once a causal effect has garnered strong empirical support, can use all of the designs we have discussed. In Chapter 2 , we trace a sequence of investigations in molecular biology that investigated how genes are turned on and off. Very different techniques, but ones that share the same basic intellectual approach to casual analysis reflected in these genetic studies, have yielded understandings in education. Consider, for example, the Tennessee class-size experiment (see discussion in Chapter 3 ). In addition to examining whether reduced class size produced achievement benefits, especially for minority students, a research team and others in the field asked (see, e.g., Grissmer, 1999) what might explain the Tennessee and other class-size effects. That is, what was the causal mechanism through which reduced class size affected achievement? To this end, researchers (Bohrnstedt and Stecher, 1999) used classroom observations and interviews to compare teaching in different class sizes. They conducted ethnographic studies in search of mechanism. They correlated measures of teaching behavior with student achievement scores. These questions are important because they enhance understanding of the foundational processes at work when class size is reduced and thus
improve the capacity to implement these reforms effectively in different times, places, and contexts.
Exploring Mechanism When Theory Is Fairly Well Established
A well-known study of Catholic schools provides another example of a rigorous attempt to understand mechanism (see Box 5-4 ). Previous and highly controversial work on Catholic schools (e.g., Coleman, Hoffer, and
Kilgore, 1982) had examined the relative benefits to students of Catholic and public schools. Drawing on these studies, as well as a fairly substantial literature related to effective schools, Bryk and his colleagues (Byrk, Lee, and Holland, 1993) focused on the mechanism by which Catholic schools seemed to achieve success relative to public schools. A series of models were developed (sector effects only, compositional effects, and school effects) and tested to explain the mechanism by which Catholic schools successfully achieve an equitable social distribution of academic achievement. The
researchers’ analyses suggested that aspects of school life that enhance a sense of community within Catholic schools most effectively explained the differences in student outcomes between Catholic and public schools.
Exploring Mechanism When Theory Is Weak
When the theoretical basis for addressing questions related to mechanism is weak, contested, or poorly understood, other types of methods may be more appropriate. These queries often have strong descriptive components and derive their strength from in-depth study that can illuminate unforeseen relationships and generate new insights. We provide two examples in this section of such approaches: the first is the ethnographic study of college women (see Box 5-2 ) and the second is a “design study” that resulted in a theoretical model for how young children learn the mathematical concepts of ratio and proportion.
After generating a rich description of women’s lives in their universities based on extensive analysis of ethnographic and survey data, the researchers turned to the question of why women who majored in nontraditional majors typically did not pursue those fields as careers (see Box 5-2 ). Was it because women were not well prepared before college? Were they discriminated against? Did they not want to compete with men? To address these questions, the researchers developed several theoretical models depicting commitment to schoolwork to describe how the women participated in college life. Extrapolating from the models, the researchers predicted what each woman would do after completing college, and in all cases, the models’ predictions were confirmed.
A second example highlights another analytic approach for examining mechanism that begins with theoretical ideas that are tested through the design, implementation, and systematic study of educational tools (curriculum, teaching methods, computer applets) that embody the initial conjectured mechanism. The studies go by different names; perhaps the two most popular names are “design studies” (Brown, 1992) and “teaching experiments” (Lesh and Kelly, 2000; Schoenfeld, in press).
Box 5-5 illustrates a design study whose aim was to develop and elaborate the theoretical mechanism by which ratio reasoning develops in young children and to build and modify appropriate tasks and assessments that
incorporate the models of learning developed through observation and interaction in the classroom. The work was linked to substantial existing literature in the field about the theoretical nature of ratio and proportion as mathematical ideas and teaching approaches to convey them (e.g., Behr, Lesh, Post, and Silver, 1983; Harel and Confrey, 1994; Mack, 1990, 1995). The initial model was tested and refined as careful distinctions and extensions were noted, explained, and considered as alternative explanations as the work progressed over a 3-year period, studying one classroom intensively. The design experiment methodology was selected because, unlike laboratory or other highly controlled approaches, it involved research within the complex interactions of teachers and students and allowed the everyday demands and opportunities of schooling to affect the investigation.
Like many such design studies, there were two main products of this work. First, through a theory-driven process of designing—and a data-driven process of refining—instructional strategies for teaching ratio and proportion, researchers produced an elaborated explanatory model of how young children come to understand these core mathematical concepts. Second, the instructional strategies developed in the course of the work itself hold promise because they were crafted based on a number of relevant research literatures. Through comparisons of achievement outcomes between children who received the new instruction and students in other classrooms and schools, the researchers provided preliminary evidence that the intervention designed to embody this theoretical mechanism is effective. The intervention would require further development, testing, and comparisons of the kind we describe in the previous section before it could be reasonably scaled up for widespread curriculum use.
Steffe and Thompson (2000) are careful to point out that design studies and teaching experiments must be conducted scientifically. In their words:
We use experiment in “teaching experiment” in a scientific sense…. What is important is that the teaching experiments are done to test hypotheses as well as to generate them. One does not embark on the intensive work of a teaching experiment without having major research hypotheses to test (p. 277).
This genre of method and approach is a relative newcomer to the field of education research and is not nearly as accepted as many of the other
methods described in this chapter. We highlight it here as an illustrative example of the creative development of new methods to embed the complex instructional settings that typify U.S. education in the research process. We echo Steffe and Thompson’s (2000) call to ensure a careful application of the scientific principles we describe in this report in the conduct of such research. 9
CONCLUDING COMMENTS
This chapter, building on the scientific principles outlined in Chapter 3 and the features of education that influence their application in education presented in Chapter 4 , illustrates that a wide range of methods can legitimately be employed in scientific education research and that some methods are better than others for particular purposes. As John Dewey put it:
We know that some methods of inquiry are better than others in just the same way in which we know that some methods of surgery, arming, road-making, navigating, or what-not are better than others. It does not follow in any of these cases that the “better” methods are ideally perfect…We ascertain how and why certain means and agencies have provided warrantably assertible conclusions, while others have not and cannot do so (Dewey, 1938, p. 104, italics in original).
The chapter also makes clear that knowledge is generated through a sequence of interrelated descriptive and causal studies, through a constant process of refining theory and knowledge. These lines of inquiry typically require a range of methods and approaches to subject theories and conjectures to scrutiny from several perspectives.
We conclude this chapter with several observations and suggestions about the current state of education research that we believe warrant attention if scientific understanding is to advance beyond its current state. We do not provide a comprehensive agenda for the nation. Rather, we
wish to offer constructive guidance by pointing to issues we have identified throughout our deliberations as key to future improvements.
First, there are a number of areas in education practice and policy in which basic theoretical understanding is weak. For example, very little is known about how young children learn ratio and proportion—mathematical concepts that play a key role in developing mathematical proficiency. The study we highlight in this chapter generated an initial theoretical model that must undergo sustained development and testing. In such areas, we believe priority should be given to descriptive and theory-building studies of the sort we highlight in this chapter. Scientific description is an essential part of any scientific endeavor, and education is no different. These studies are often extremely valuable in themselves, and they also provide the critical theoretical grounding needed to conduct causal studies. We believe that attention to the development and systematic testing of theories and conjectures across multiple studies and using multiple methods—a key scientific principle that threads throughout all of the questions and designs we have discussed—is currently undervalued in education relative to other scientific fields. The physical sciences have made progress by continuously developing and testing theories; something of that nature has not been done systematically in education. And while it is not clear that grand, unifying theories exist in the social world, conceptual understanding forms the foundation for scientific understanding and progresses—as we showed in Chapter 2 —through the systematic assessment and refinement of theory.
Second, while large-scale education policies and programs are constantly undertaken, we reiterate our belief that they are typically launched without an adequate evidentiary base to inform their development, implementation, or refinement over time (Campbell, 1969; President’s Committee of Advisors on Science and Technology, 1997). The “demand” for education research in general, and education program evaluation in particular, is very difficult to quantify, but we believe it tends to be low from educators, policy makers, and the public. There are encouraging signs that public attitudes toward the use of objective evidence to guide decisions is improving (e.g., statutory requirements to set aside a percentage of annual appropriations to conduct evaluations of federal programs, the Government Performance and Results Act, and common rhetoric about “evidence-based” and “research-based” policy and practice). However, we believe stronger
scientific knowledge is needed about educational interventions to promote its use in decision making.
In order to generate a rich store of scientific evidence that could enhance effective decision making about education programs, it will be necessary to strengthen a few related strands of work. First, systematic study is needed about the ways that programs are implemented in diverse educational settings. We view implementation research—the genre of research that examines the ways that the structural elements of school settings interact with efforts to improve instruction—as a critical, underfunded, and underappreciated form of education research. We also believe that understanding how to “scale up” (Elmore, 1996) educational interventions that have promise in a small number of cases will depend critically on a deep understanding of how policies and practices are adopted and sustained (Rogers, 1995) in the complex U.S. education system. 10
In all of this work, more knowledge is needed about causal relationships. In estimating the effects of programs, we urge the expanded use of random assignment. Randomized experiments are not perfect. Indeed, the merits of their use in education have been seriously questioned (Cronbach et al., 1980; Cronbach, 1982; Guba and Lincoln, 1981). For instance, they typically cannot test complex causal hypotheses, they may lack generalizability to other settings, and they can be expensive. However, we believe that these and other issues do not generate a compelling rationale against their use in education research and that issues related to ethical concerns, political obstacles, and other potential barriers often can be resolved. We believe that the credible objections to their use that have been raised have clarified the purposes, strengths, limitations, and uses of randomized experiments as well as other research methods in education. Establishing cause is often exceedingly important—for example, in the large-scale deployment of interventions—and the ambiguity of correlational studies or quasi-experiments can be undesirable for practical purposes.
In keeping with our arguments throughout this report, we also urge that randomized field trials be supplemented with other methods, including in-depth qualitative approaches that can illuminate important nuances,
identify potential counterhypotheses, and provide additional sources of evidence for supporting causal claims in complex educational settings.
In sum, theory building and rigorous studies of implementations and interventions are two broad-based areas that we believe deserve attention. Within the framework of a comprehensive research agenda, targeting these aspects of research will build on the successes of the enterprise we highlight throughout this report.
Researchers, historians, and philosophers of science have debated the nature of scientific research in education for more than 100 years. Recent enthusiasm for "evidence-based" policy and practice in education—now codified in the federal law that authorizes the bulk of elementary and secondary education programs—have brought a new sense of urgency to understanding the ways in which the basic tenets of science manifest in the study of teaching, learning, and schooling.
Scientific Research in Education describes the similarities and differences between scientific inquiry in education and scientific inquiry in other fields and disciplines and provides a number of examples to illustrate these ideas. Its main argument is that all scientific endeavors share a common set of principles, and that each field—including education research—develops a specialization that accounts for the particulars of what is being studied. The book also provides suggestions for how the federal government can best support high-quality scientific research in education.
READ FREE ONLINE
Welcome to OpenBook!
You're looking at OpenBook, NAP.edu's online reading room since 1999. Based on feedback from you, our users, we've made some improvements that make it easier than ever to read thousands of publications on our website.
Do you want to take a quick tour of the OpenBook's features?
Show this book's table of contents , where you can jump to any chapter by name.
...or use these buttons to go back to the previous chapter or skip to the next one.
Jump up to the previous page or down to the next one. Also, you can type in a page number and press Enter to go directly to that page in the book.
Switch between the Original Pages , where you can read the report as it appeared in print, and Text Pages for the web version, where you can highlight and search the text.
To search the entire text of this book, type in your search term here and press Enter .
Share a link to this book page on your preferred social network or via email.
View our suggested citation for this chapter.
Ready to take your reading offline? Click here to buy this book in print or download it as a free PDF, if available.
Get Email Updates
Do you enjoy reading reports from the Academies online for free ? Sign up for email notifications and we'll let you know about new publications in your areas of interest when they're released.

An official website of the United States government
The .gov means it’s official. Federal government websites often end in .gov or .mil. Before sharing sensitive information, make sure you’re on a federal government site.
The site is secure. The https:// ensures that you are connecting to the official website and that any information you provide is encrypted and transmitted securely.
- Publications
- Account settings
Preview improvements coming to the PMC website in October 2024. Learn More or Try it out now .
- Advanced Search
- Journal List
- J Grad Med Educ
- v.8(1); 2016 Feb
Research Design Considerations
Associated data.
Editor's Note: The online version of this article contains references and resources for further reading and the authors' professional information.
The Challenge
“I'd really like to do a survey” or “Let's conduct some interviews” might sound like reasonable starting points for a research project. However, it is crucial that researchers examine their philosophical assumptions and those underpinning their research questions before selecting data collection methods. Philosophical assumptions relate to ontology, or the nature of reality, and epistemology, the nature of knowledge. Alignment of the researcher's worldview (ie, ontology and epistemology) with methodology (research approach) and methods (specific data collection, analysis, and interpretation tools) is key to quality research design. This Rip Out will explain philosophical differences between quantitative and qualitative research designs and how they affect definitions of rigorous research.
What Is Known
Worldviews offer different beliefs about what can be known and how it can be known, thereby shaping the types of research questions that are asked, the research approach taken, and ultimately, the data collection and analytic methods used. Ontology refers to the question of “What can we know?” Ontological viewpoints can be placed on a continuum: researchers at one end believe that an observable reality exists independent of our knowledge of it, while at the other end, researchers believe that reality is subjective and constructed, with no universal “truth” to be discovered. 1,2 Epistemology refers to the question of “How can we know?” 3 Epistemological positions also can be placed on a continuum, influenced by the researcher's ontological viewpoint. For example, the positivist worldview is based on belief in an objective reality and a truth to be discovered. Therefore, knowledge is produced through objective measurements and the quantitative relationships between variables. 4 This might include measuring the difference in examination scores between groups of learners who have been exposed to 2 different teaching formats, in order to determine whether a particular teaching format influenced the resulting examination scores.
In contrast, subjectivists (also referred to as constructionists or constructivists ) are at the opposite end of the continuum, and believe there are multiple or situated realities that are constructed in particular social, cultural, institutional, and historical contexts. According to this view, knowledge is created through the exploration of beliefs, perceptions, and experiences of the world, often captured and interpreted through observation, interviews, and focus groups. A researcher with this worldview might be interested in exploring the perceptions of students exposed to the 2 teaching formats, to better understand how learning is experienced in the 2 settings. It is crucial that there is alignment between ontology (what can we know?), epistemology (how can we know it?), methodology (what approach should be used?), and data collection and analysis methods (what specific tools should be used?). 5
Key Differences in Qualitative and Quantitative Approaches
Use of theory.
Quantitative approaches generally test theory, while qualitative approaches either use theory as a lens that shapes the research design or generate new theories inductively from their data. 4
Use of Logic
Quantitative approaches often involve deductive logic, starting off with general arguments of theories and concepts that result in data points. 4 Qualitative approaches often use inductive logic or both inductive and deductive logic, start with the data, and build up to a description, theory, or explanatory model. 4
Purpose of Results
Quantitative approaches attempt to generalize findings; qualitative approaches pay specific attention to particular individuals, groups, contexts, or cultures to provide a deep understanding of a phenomenon in local context. 4
Establishing Rigor
Quantitative researchers must collect evidence of validity and reliability. Some qualitative researchers also aim to establish validity and reliability. They seek to be as objective as possible through techniques, including cross-checking and cross-validating sources during observations. 6 Other qualitative researchers have developed specific frameworks, terminology, and criteria on which qualitative research should be evaluated. 6,7 For example, the use of credibility, transferability, dependability, and confirmability as criteria for rigor seek to establish the accuracy, trustworthiness, and believability of the research, rather than its validity and reliability. 8 Thus, the framework of rigor you choose will depend on your chosen methodology (see “Choosing a Qualitative Research Approach” Rip Out).
View of Objectivity
A goal of quantitative research is to maintain objectivity, in other words, to reduce the influence of the researcher on data collection as much as possible. Some qualitative researchers also attempt to reduce their own influence on the research. However, others suggest that these approaches subscribe to positivistic ideals, which are inappropriate for qualitative research, 6,9,10 as researchers should not seek to eliminate the effects of their influence on the study but to understand them through reflexivity . 11 Reflexivity is an acknowledgement that, to make sense of the social world, a researcher will inevitably draw on his or her own values, norms, and concepts, which prevent a totally objective view of the social world. 12
Sampling Strategies
Quantitative research favors using large, randomly generated samples, especially if the intent of the research is to generalize to other populations. 6 Instead, qualitative research often focuses on participants who are likely to provide rich information about the study questions, known as purposive sampling . 6
How You Can Start TODAY
- Consider how you can best address your research problem and what philosophical assumptions you are making.
- Consider your ontological and epistemological stance by asking yourself: What can I know about the phenomenon of interest? How can I know what I want to know? W hat approach should I use and why? Answers to these questions might be relatively fixed but should be flexible enough to guide methodological choices that best suit different research problems under study. 5
- Select an appropriate sampling strategy. Purposive sampling is often used in qualitative research, with a goal of finding information-rich cases, not to generalize. 6
- Be reflexive: Examine the ways in which your history, education, experiences, and worldviews have affected the research questions you have selected and your data collection methods, analyses, and writing. 13
How You Can Start TODAY—An Example
Let's assume that you want to know about resident learning on a particular clinical rotation. Your initial thought is to use end-of-rotation assessment scores as a way to measure learning. However, these assessments cannot tell you how or why residents are learning. While you cannot know for sure that residents are learning, consider what you can know—resident perceptions of their learning experiences on this rotation.
Next, you consider how to go about collecting this data—you could ask residents about their experiences in interviews or watch them in their natural settings. Since you would like to develop a theory of resident learning in clinical settings, you decide to use grounded theory as a methodology, as you believe asking residents about their experience using in-depth interviews is the best way for you to elicit the information you are seeking. You should also do more research on grounded theory by consulting related resources, and you will discover that grounded theory requires theoretical sampling. 14,15 You also decide to use the end-of-rotation assessment scores to help select your sample.
Since you want to know how and why students learn, you decide to sample extreme cases of students who have performed well and poorly on the end-of-rotation assessments. You think about how your background influences your standpoint about the research question: Were you ever a resident? How did you score on your end-of-rotation assessments? Did you feel this was an accurate representation of your learning? Are you a clinical faculty member now? Did your rotations prepare you well for this role? How does your history shape the way you view the problem? Seek to challenge, elaborate, and refine your assumptions throughout the research.
As you proceed with the interviews, they trigger further questions, and you then decide to conduct interviews with faculty members to get a more complete picture of the process of learning in this particular resident clinical rotation.
What You Can Do LONG TERM
- Familiarize yourself with published guides on conducting and evaluating qualitative research. 5,16–18 There is no one-size-fits-all formula for qualitative research. However, there are techniques for conducting your research in a way that stays true to the traditions of qualitative research.
- Consider the reporting style of your results. For some research approaches, it would be inappropriate to quantify results through frequency or numerical counts. 19 In this case, instead of saying “5 respondents reported X,” you might consider “respondents who reported X described Y.”
- Review the conventions and writing styles of articles published with a methodological approach similar to the one you are considering. If appropriate, consider using a reflexive writing style to demonstrate understanding of your own role in shaping the research. 6
Supplementary Material
REDLAB: Research in Education and Design

Contact Us:
» Follow us on Twitter: @stanfordREDlab
» REDlab on Facebook
- Stanford University
- School of Education
- 485 Lasuen Mall
- Stanford, CA 94305-3096

- publications and resources
- news and events
Research in Education & Design
©Stanford University. Stanford, CA 94305 (650) 723-2300
Help | Advanced Search
Physics > Physics Education
Title: research in teaching and learning sequence design. to what extent do designers theoretical orientations about learning and the nature of science shape design decisions.
Abstract: Over the last three decades, various didactic proposals have been published in an attempt to connect theory and research findings with the design of Teaching-Learning Sequences (TLS) in various contexts. Many studies have analysed the process of designing teaching-learning sequences as a research activity. This line of research aims to increase the impact and transferability of educational practice. However, the information usually provided about the relation between the theory and research findings with the design of the TLS is insufficiently detailed to provide the basis for a critique. Furthermore, not all TLS proposals include evaluation in terms of learning outcomes and very rarely are these learning outcomes specifically related to the design process. This lack of detailed information on the design and evaluation of proposed TLS makes it difficult to properly assess their potential effectiveness or to systematically discuss and improve their design. In this chapter we want to contribute to make the rationale for design decisions explicit. The aim of this paper is to describe in detail how the theoretical orientations of designers of teaching materials towards cognition and learning can shape the structure and pedagogical strategies of the resulting TLS. We will analyse the relationship of two design tools (Epistemological analysis and Learning demands) to theoretical assumptions about learning and the nature of science. We want to highlight the benefits of reflecting on and discussing theoretical elements and their links to design decisions, which makes TLS design more productive on a practical level to broaden the teaching and learning perspectives of TLS. Finally, we will explore the question to what extent the theoretical orientations of curriculum designers towards cognition and learning can influence the structure and pedagogical strategies of the resulting TLS
Submission history
Access paper:.
- Other Formats

References & Citations
- Google Scholar
- Semantic Scholar
BibTeX formatted citation

Bibliographic and Citation Tools
Code, data and media associated with this article, recommenders and search tools.
- Institution
arXivLabs: experimental projects with community collaborators
arXivLabs is a framework that allows collaborators to develop and share new arXiv features directly on our website.
Both individuals and organizations that work with arXivLabs have embraced and accepted our values of openness, community, excellence, and user data privacy. arXiv is committed to these values and only works with partners that adhere to them.
Have an idea for a project that will add value for arXiv's community? Learn more about arXivLabs .
An official website of the United States government
Here's how you know
Official websites use .gov A .gov website belongs to an official government organization in the United States.
Secure .gov websites use HTTPS. A lock ( Lock Locked padlock ) or https:// means you've safely connected to the .gov website. Share sensitive information only on official, secure websites.
NRT research and innovation: Advancing the understanding of complex living systems
Discoveries in biology allow the exploration of the very foundations of life, from the smallest molecules and simplest cells to entire civilizations and complex ecosystems. Biological research that bridges diverse scientific disciplines is vital to deepening the understanding of dynamic living systems and how to help them thrive. It can lead to technological breakthroughs around the most critical societal challenges — from developing innovative medicines that cure disease to improving the environmental resilience of plants for global food security.
The U.S. National Science Foundation Research Traineeship Program (NRT) is committed to supporting researchers across the country who are advancing the biological sciences. By funding innovative science, technology, engineering and mathematics graduate programs focused on cutting-edge, convergent biological research, NRT is building a new generation of biologists prepared to become leaders in a wide range of fields, including botany, ecology, neuroscience, genetics and beyond. Read about three institutions leveraging NRT to support breakthroughs in the biological sciences.
Plants3D — Discover, Design, Deploy at the University of California, Riverside
Harnessing the power and wisdom of plants holds great promise in addressing some of the greatest challenges in agriculture and biotechnology. With the planet's population rapidly expanding, biologists are seeking new ways to improve the environmental resilience of plants to increase crop production and ensure global food security. At the University of California, Riverside, the Plants3D — Discover, Design, Deploy NRT based at the Center for Plant Cell Biology has set out to provide a new generation of STEM graduate students with "the knowledge and skills to combine plant and microbial biology with engineering technologies to discover, design and deploy plant-inspired solutions" to our food security challenges.
Offering highly convergent plant science training related to food security and human health, Plants3D connects faculty and graduate students from various disciplines and departments, such as botany, genetics, biochemistry, plant pathology, engineering, computer science and beyond. In addition to in-depth, interdisciplinary coursework, the program provides the resources for trainees to pursue cutting-edge team-based research. This work fosters entrepreneurialism by encouraging trainees to translate their research discoveries into practical applications. Plants3D is committed to changing the fact that only 3% of the biotechnology workforce is Hispanic or Black by significantly expanding opportunities for minority and first-generation STEM students. This work prepares a new generation of biologists to take on careers in industry, academia and government agencies and lead crucial discoveries in agricultural biotechnology.
Synthetic Biology PhD Training Program at Northwestern University
The field of synthetic biology, which focuses on transforming existing biological organisms and redesigning them for new purposes, is ripe with possibility. Synthetic biologists are making vital research breakthroughs that touch nearly every aspect of daily life, from creating new foods and fuels using sustainable materials to inventing "smart medicines" to treat disease and improve health.
The Synthetic Biology PhD Training Program (SynBAS) NRT at Northwestern University has developed a STEM graduate program allowing trainees to explore "how biology becomes technology" and harness the power of synthetic biology. Trainees explore the principles of living systems — such as molecules, cells, organisms and communities — while drawing on lessons from chemistry, physics, biology, engineering, mathematics, business and more. This convergent approach to synthetic biology training provides unique insight into how synthesizing diverse phenomena can lead to powerful technological breakthroughs. Beyond innovative coursework, SynBAS provides trainees with career mentoring and networking connections to academia and industry, preparing them to become leaders in the synthetic biological sciences.
Data Driven Biology at the University of California, Santa Barbara
In 2021, the University of California, Santa Barbara launched the Data Driven Biology (DDB) NRT with the goal of training "a new generation of biological scientists and engineers who are able to work across disciplines to advance fundamental research in quantitative biology and bioengineering." DDB builds connections among trainees working in a wide range of fields, from electrical, computer and mechanical engineering to molecular, cellular and developmental biology. Through coursework, mentoring and hands-on "in vivo research," the program builds trainees' fluency in data analytics and experimental and modeling methods to engage in data-dense quantitative biology experiments.
ORIGINAL RESEARCH article
Increasing phd student self-awareness and self-confidence through strengths-based professional development submission type: research article provisionally accepted.
- 1 Department of Physiology, Pharmacology, and Toxicology, School of Medicine, West Virginia University, United States
- 2 Health Sciences Center, West Virginia University, United States
The final, formatted version of the article will be published soon.
Strengths-based programs have emerged as asset-based approaches to professional development that promote positive student engagement and success. This paper shares the outcomes of a strengths-based professional development program provided to biomedical and health sciences graduate doctoral students within an academic health center. Program outcomes and changes in participants’ perceived confidence when identifying and applying their strengths in different contexts were evaluated through a mixed methods design that included a Likert-based survey and thematic analysis of qualitative responses. Findings strongly suggest that most participants lacked the self-confidence and/or self-awareness to recognize their own strengths prior to the program. Themes that emerged upon implementation of the program point to the following outcomes: participants gained an increased understanding of their strengths, confidence that the knowledge gained about their strengths would help them learn more effectively in laboratory settings, an increased belief that they possess natural talents and skills that make them good scientists and strong members of their research team, and confidence that applying their strengths will help them to overcome both personal and professional challenges. This program shows promise to strengthen graduate student self-awareness and self-confidence. Further studies are needed to understand and measure how asset-based programs such as this can impact graduate student resilience, science identity, and overall student success.
Keywords: graduate, Strengths, Professional Development, biomedical, Health Sciences, Science identity, stem
Received: 31 Jan 2024; Accepted: 22 Apr 2024.
Copyright: © 2024 Lockman and Ferguson. This is an open-access article distributed under the terms of the Creative Commons Attribution License (CC BY) . The use, distribution or reproduction in other forums is permitted, provided the original author(s) or licensor are credited and that the original publication in this journal is cited, in accordance with accepted academic practice. No use, distribution or reproduction is permitted which does not comply with these terms.
* Correspondence: Dr. Julie A. Lockman, West Virginia University, Department of Physiology, Pharmacology, and Toxicology, School of Medicine, Morgantown, United States
People also looked at
College of Education Faculty Receive Design Award at AERA Conference in Philadelphia

College of Education faculty members Torrey Trust and Robert W. Maloy , along with learning, media, and technology master’s program alum, Viacheslav Yurchenkov, received the 2024 Outstanding Design Case from the Design and Technology Special Interest Group (SIG) at the American Educational Research Association (AERA) Conference held in Philadelphia earlier this month.
The award recognizes the writing and publishing of their open online eBook, “ Building Democracy for All: Interactive Explorations of Government and Civic Life ,” available through online free textbook and journal publisher edtechbooks.org .
First published in 2023 by Indiana University’s International Journal for Designs for Learning , the design case showcases the development of an interactive, student-centered, multimodal, multicultural open-access eBook that promotes civic learning among upper elementary, middle and high school students.
In their design case, Trust, Maloy and Yurchenkov highlight the steps they took to redesign a traditional print-based textbook into an open access, interactive, multicultural and multimodal learning format that can be used both for in-person and online classroom learning. “Building Democracy for All” has separate chapters that address each of the 50 eighth grade learning standards in the statewide Massachusetts history and science curriculum.
The book encourages students and teachers to investigate, uncover and engage civics and history content while utilizing eBook features including live links to key online resources, embedded video and audio materials, interactive glossary and search functions and more.
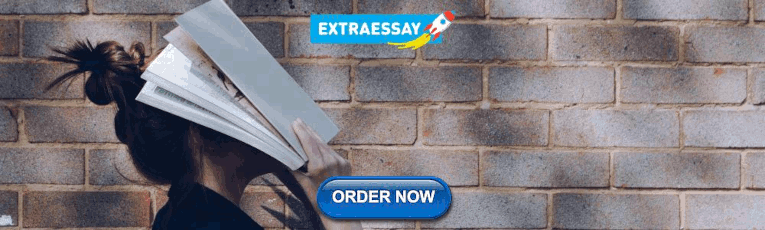
IMAGES
VIDEO
COMMENTS
A research design is a strategy for answering your research question using empirical data. Creating a research design means making decisions about: Your overall research objectives and approach. Whether you'll rely on primary research or secondary research. Your sampling methods or criteria for selecting subjects. Your data collection methods.
While many books and articles guide various qualitative research methods and analyses, there is currently no concise resource that explains and differentiates among the most common qualitative approaches. We believe novice qualitative researchers, students planning the design of a qualitative study or taking an introductory qualitative research course, and faculty teaching such courses can ...
Step 1: Consider your aims and approach. Step 2: Choose a type of research design. Step 3: Identify your population and sampling method. Step 4: Choose your data collection methods. Step 5: Plan your data collection procedures. Step 6: Decide on your data analysis strategies. Frequently asked questions.
Examples of typical mixed methods design approaches in education include convergent, explanatory, exploratory, and embedded designs. Using mixed methods approaches in educational research allows the researcher to triangulate, complement, or expand understanding using multiple types of data. ... The Research Design is the overall direction of ...
Thesis. Educational design research (EDR) is a genre of research that features the gaining of in‐depth understanding of a problem before any prototype solution is designed and tested. It is different from other forms of scientific inquiry because it is committed to the simultaneous development of both theoretical insights and practical ...
Presents an overview of qualitative, quantitative and mixed-methods research designs, including how to choose the design based on the research question. This book is particularly helpful for those who want to design mixed-methods studies. Green, J. L., G. Camilli, and P. B. Elmore. 2006. Handbook of complementary methods for research in education.
This exploratory paper seeks to shed light on the methodological challenges of education systems research. There is growing consensus that interventions to improve learning outcomes must be designed and studied as part of a broader system of education, and that learning outcomes are affected by a complex web of dynamics involving different inputs, actors, processes and socio-political contexts.
The first, Design-Based Implementation Research or DBIR (Penuel et al. Citation 2011) may be thought of as an offshoot from DBR when applied to problems of practice that relate to systems of education, tightly tied to the notion of Research-Practice Partnerships (Penuel et al., Citation 2015). DBR and DBIR share a contextualized, emergent ...
Introduction. The field of education has embraced quantitative research designs since early in the 20th century. The foundation for these designs was based primarily in the psychological literature, and psychology and the social sciences more generally continued to have a strong influence on quantitative designs until the assimilation of qualitative designs in the 1970s and 1980s.
Conducting Educational Design Research, 2 nd Edition has been written to support graduate students as well as experienced researchers who are new to this approach. Part I describes the origins, outcomes, and generic approach. Part II discusses the core processes of the generic approach in detail. Part III recommends how to propose, report, and ...
Educational design research is a genre of research in which the iterative development of solutions to practical and complex educational problems provides the setting for scientific inquiry. The solutions can be educational products, processes, programs, or policies. Educational design research not only targets solving significant problems ...
Summary. Emerging in the learning sciences field in the early 1990s, qualitative design-based research (DBR) is a relatively new methodological approach to social science and education research. As its name implies, DBR is focused on the design of educational innovations, and the testing of these innovations in the complex and interconnected ...
The research design is usually incorporated into the introduction of your paper. You can obtain an overall sense of what to do by reviewing studies that have utilized the same research design [e.g., using a case study approach]. ... Action Research in Education: A Practical Guide. New York: Guilford, 2013; Gall, Meredith.
Educational design research is a genre of research in which the iterative development of solutions to practical and complex educational problems provides the setting for scientific inquiry. The ...
Education: Research design is essential in the field of education to investigate the effectiveness of different teaching methods and learning strategies. Researchers use various designs such as experimental, quasi-experimental, and case study designs to understand how students learn and how to improve teaching practices.
Research in Education provides a space for fully peer-reviewed, critical, trans-disciplinary, debates on theory, policy and practice in relation to Education. International in scope, we publish challenging, well-written and theoretically innovative contributions that question and explore the concept, practice and institution of Education as an object of study.
Research contribute Type seek to test, to improved. #1: Foundational of systems or learning processes. Research. in methodologies and/o technologies of teaching provides or education the fundamental that will influence and infor outcomes. and may knowledge development in contexts. research and innovations of.
This course teaches students important concepts of education research design. The course covers the development of research questions, participant selection, educational measurement and data collection, experimental and correlational research designs, and quantitative and qualitative research methodologies. The learning goals for the course are ...
The salient features of education delineated in Chapter 4 and the guiding principles of scientific research laid out in Chapter 3 set boundaries for the design and conduct of scientific education research. Thus, the design of a study (e.g., randomized experiment, ethnography, multiwave survey) does not itself make it scientific.
2. Survey and Correlational Research Design. A nonexperimental research design used to describe an individual or a group by having participants complete a survey or questionnaire. A correlational design uses data to determine if two or more factors are related/correlated. 3. Qualitative Research. Process of collecting, analyzing, and ...
Purposive sampling is often used in qualitative research, with a goal of finding information-rich cases, not to generalize. 6. Be reflexive: Examine the ways in which your history, education, experiences, and worldviews have affected the research questions you have selected and your data collection methods, analyses, and writing. 13. Go to:
Research in Education & Design. REDlab's mission is to conduct research to inform our understanding of design thinking in K-12, undergraduate and graduate educational settings. Design thinking focuses on needfinding, challenging assumptions, generating a range of possibilities, and learning through targeted stages of iterative prototyping. A ...
Using a randomized control trial research design, teachers from 44 physical education classes and their 1185 secondary-grade students either did or did not participate in an autonomy-supportive teaching workshop. ... This research was supported by the College of Education, Korea University Grant in 2023 and by the Ministry of Education of the ...
Over the last three decades, various didactic proposals have been published in an attempt to connect theory and research findings with the design of Teaching-Learning Sequences (TLS) in various contexts. Many studies have analysed the process of designing teaching-learning sequences as a research activity. This line of research aims to increase the impact and transferability of educational ...
Patients participating in an earlier study, with self-experience of COPD as a special competence, were involved as research partners at the design of this study. Methods. Eleven individual and two group interviews with five participants in each group were conducted. Results. Group-based self-management education with a digital website supports ...
Special education policy and scholarship have increasingly emphasized the use of research that encompasses interdependent methodological approaches designed to address specific questions. Consequen...
At the University of California, Riverside, the Plants3D — Discover, Design, Deploy NRT based at the Center for Plant Cell Biology has set out to provide a new generation of STEM graduate students with "the knowledge and skills to combine plant and microbial biology with engineering technologies to discover, design and deploy plant-inspired ...
Strengths-based programs have emerged as asset-based approaches to professional development that promote positive student engagement and success. This paper shares the outcomes of a strengths-based professional development program provided to biomedical and health sciences graduate doctoral students within an academic health center.Program outcomes and changes in participants' perceived ...
Share This. College of Education faculty members Torrey Trust and Robert W. Maloy, along with learning, media, and technology master's program alum, Viacheslav Yurchenkov, received the 2024 Outstanding Design Case from the Design and Technology Special Interest Group (SIG) at the American Educational Research Association (AERA) Conference ...
Eric R. Felix is an associate professor at San Diego State University where he leads the CCHALES Research Collective examining the systems, structures, and practices within higher education that hinder racial equity. His research explores the ways policymakers craft higher education reform and how institutional leaders implement them, highlighting the possibilities of policy to improve racial ...