Secondary Menu
- Job Market Paper
The job market paper is a piece of original research, usually included as one of three chapters in the dissertation, that best demonstrates a job market candidate's skills, training, and aptitude as an economist. A job market paper also should contribute to the extant literature by providing novel answers and insights regarding a well-defined research question.
- Location & Directions
- Our Newsletter
- EcoTeach Center
- Standards of Conduct and Values
- Commencement 2024
- What Economists Do
- Career Paths
- B.S. Concentration in Financial Economics
- B.A. in Economics
- Economics Minor
- Financial Economics Minor
- Differences between the B.A. and B.S. Degrees
- Major Declaration & Registration
- Independent Study
- Co-Curricular Finance Programs
- Duke Economic Analytics Laboratory (DEAL)
- Duke Journal of Economics
- Economics Student Union
- Work-Study Jobs, Internships & Funding
- Commonly Used Forms
- Tutoring & Support Services
- Mentors for Majors
- Instructions, Dates, Resources & Templates
- Showcases, Awards & Past Theses
- Paths to Honors and Suggested Courses
- Trinity Ambassadors
- M.A. Economics
- M.A. Analytical Political Economy
- M.S. Economics & Computation
- M.S. Quantitative Financial Economics
- Info for Prospective Applicants
- Resources for Current Students
- Master's Alumni Advisory Board
- Master's Program Alumni Spotlights
- Master's Program News
- Fields of Study
- Year-by-Year Overview
- Advising and Mentoring of Ph.D. Students
- Application and Admission Information
- Financial Support
- Job Market Placements
- Graduate Life
- Graduate Mentorship Program
- Pre-Graduate Fellows Program
- Year-By-Year Overview
- Preliminary Exam
- Dissertation
- Modules, Seminars & Workshops
- Research & Travel Funding
- Teaching Assistant Resources
- Student Grievance Process
- Job Market Candidates
- All Courses
- Core Undergrad Economics Courses
- Ph.D. Modules, Seminars & Workshops
- Regular Rank Faculty
- Secondary Faculty
- Instructional Faculty
- Emeritus Faculty
- PhD Students
- Doctoral Candidates
- Development
- Econometrics
- Energy & Environment
- History of Political Economy
- Industrial Organization
- International Economics & Trade
- Labor & Health
- Macroeconomics & Finance
- Microeconomic Theory
- Public & Political Economy
- Conferences, Jamborees, & Lectures
- Visitors Program Speakers
- Working Paper Series
- Journals & Editorships
- Featured Publications
- Selected Faculty Books
- Center for the History of Political Economy
- Duke Financial Economics Center
- About the DEAL
- DEAL Events
- Woodman Scholars
- Woodman Scholar Accomplishments
- Learning Resources
- Duke Help Resources
- For Our Students
- Assisting Duke Students
- Washington State University
- I Want to Give
- Economics Degrees
- Advising Sheets
- Online B.S. Economics
- Academic Advising
- Transfer Information
- Scholarships & Internship Opportunities
- Econ Lab - Tutoring
- Pre-Master Program
- Master of Applied Economics
- Math Refresher
- Courses and Learning Objectives
- Benefits of our Masters program
- MSE Courses
- PhD-bound option
- Frequently Asked Questions
- PhD Courses
- Graduate Assistantships
- PhD Field Requirements
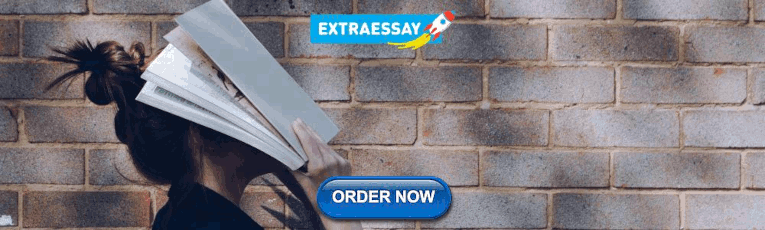
Job Market Guide
- Job Placement
- Graduate Student Resources
- School of Economic Sciences Graduate Student Association
- Research Guidelines
- PhD-Students
- MS Students
- Working Paper Series
- Community and Regional Economics
- Health Economics
- Specialty Crops Economics
- Wheat & Small Grains
- Livestock Economics
- Tree Fruit Economics
- Environmental & Natural Resources
- Crop Enterprise Budgets
- Michael Brady
- Randy Fortenbery
- Karina Gallardo
- Bidisha Mandal
- Shannon Neibergs
- IMPACT Center
- Publications
- Freight Policy Transportation Institute
- Freight Data Warehouse
- Our Mission
- Research Project Videos
- Friday’s SES Seminar Series
- Student Seminar Series
- Leigh Lecture
- Alumni & Friends Banquet & Auction
- Active Shooter Resources
- History of the School of Economic Sciences
- Living in Pullman
This guide provides basic information about the process of job market preparation and participation for WSU School of Economic Sciences doctoral students. It is not a comprehensive road map; it serves only to provide a platform for communication with faculty advisors. Most of the discussion assumes that a doctoral student is seeking an academic position after completing a four year course of doctoral study. Adjustments for timing and job market targets should be expected and discussed with advisors .
You should also look at “ A Guide and Advice for Economists on the U.S. Junior Academic Job Market (pdf) ” on the JOE website. It provides valuable additional information, especially with some details about the formal economics job market. It is imperative for students to recognize that job market preparation and initiation of faculty support in the process is the responsibility of the student .
Harvard Business School NOM Unit Working Paper No. 19-027
Job Market Meetings During the Year
Spring Semester Meeting
During the Spring Semester, a job market meeting for all PhD students will be conducted to provide students with an overview of how the job market works. The main beneficiaries will be second and third year students since the discussion will focus mainly on how to build your portfolio to become competitive in the job market when the time comes. ( Slides from meeting (pdf) )
First Fall Semester Meeting
There are two meetings during Fall. The first Fall meeting focuses on 4th and 5th year students who are thinking of entering the job market. We discuss in detail the timing of events, the application packet and important deadlines. ( Fall 2023 Slides from meeting (pdf)
Second Fall Semester Meeting
The second Fall meeting is exclusively for students who enter the job market. We discuss tips on interviews and scheduling.
Where to Find Jobs
The main listing for job openings for economists is housed in the AEA webpage. However, there are other links for specialized fields or International universities or agencies.
- aeaweb JOE Listings – August 1, 2018 – January 31, 2019
- Career Center Jobs – Agricultural & Applied Economics Association
- Econ Job Market
- Academic Economist Jobs Economist Jobs by Econ-Jobs.com
- Discover Asst. Prof. / Lecturer Jobs at INOMICS
- Job Market Open Positions EAERE
- Economic Openings at HigherEdJobs
- Agricultural Economics and Agribusiness Openings at HigherEdJobs
- Chronicle of Higher Education – Economics Jobs
- Academic Positions in the European Union – Economics
- Researchers in Motion – European Economics Jobs
- The Chinese Economists Society
Some of these postings may coincide with those in AEA, but some are European universities that only post their ads in either of the above websites. Note that many of the universities posting jobs in the Econjobmarket.org website only conduct interviews at the Spanish Economic Association Meeting (even if the university is not Spanish) in mid-December. You can find more information here: SAEe 2018 – Madrid .
Timeline for Job Market Preparation
Preliminary job interviews for all fields are held at the ASSA conferences in early January of every year. For those students writing in agricultural, resource and environmental fields, interviews are also conducted at the AAEA meetings in late July or early August, although increasingly, many agricultural and applied economics departments are recruiting at the ASSA meetings. Students must have a polished paper for inclusion in their job applications, which are sent out up to three months in advance of the meetings. Most institutions looking to hire at the ASSA expect you to be finished by August of the following year. Thus, besides one polished paper you need to be able to demonstrate that you have made substantial progress on your entire dissertation. You need your paper for the ASSA meetings ready by November 1.
After the meetings, students must be prepared for multi-day on-site interviews with employers on short notice (within days of the interviews at the meetings, with as short as one-week notice for the visit). Therefore, preparation for research presentations must begin early (you will be grilled about your research at the conference interviews as well as when you do campus visits). Students should schedule at least one practice presentation in SES at least one week prior to the campus interview. You should also plan on doing a mock interview with SES faculty before the ASSA or AAEA meetings. The following schedule puts you on track to be ready for the job market by the beginning of your fourth year of study:
- Year 1 : In addition to coursework, explore research interests, meet faculty, and discuss dissertation ideas.
- Year 2 : Begin serious exploratory work on a dissertation. Visit professors, kick around ideas, and delve into the literature of your area of interest. By fall of the third year, a solid idea for a dissertation should be in mind and preliminary work underway.
- Keep track of job postings in Job Openings for Economists (JOE) and the AAEA website early in the year, but especially June onward.
- Send applications out for any possibilities
- Coordinate brief interviews at the AAEA meetings through the employment center.
- Attend AAEA meetings in late July, early August.
- All year, but especially October onward, regularly check employment opportunities in JOE. The most important issue is October, followed closely by the November, and then residual job announcements in the December issue.
- Schedule a seminar in SES for September to get broad feedback on your job market paper.
- Have job market paper polished by early November for ASSA applications.
- Early November onward: submit applications for possible ASSA interviews.
- Mid December: Finalize job market presentations and give practice seminars. Organize practice interviews with the assistance of faculty.
- Early January. Interview for jobs at ASSA Meetings.
- If still on the Job market, revisit the AAEA meetings (discussed under year 3).
- Finish dissertation by May or August of year 4.
Compiling Application Packets for Academic Jobs
Job opening announcements usually request all or most of the following:
These materials should be the most carefully crafted documents you have ever created. A misspelled word or bad grammar in the cover letter or anywhere else in your application can send your application to the no-hire stack immediately. Proofread your research papers and other documents, and arrange with other students on the market to do an exchange–you proofread their papers and they can proofread your papers.
Cover Letter
For academic positions, the cover letter should be tailored to each specific job. It should include a brief description of why you are interested in this particular job and why you think the search committee should be interested in you for this specific job. You can very briefly summarize your research but do not put an entire abstract in the letter. For the most part, your vita and supporting material will sell you. The point of the letter is to get the reader to look further at your materials.
Curriculum Vita
Curriculum vitae (CV) includes information about education, experience, teaching, research, grant-getting and other related experience and productivity. Compared to a standard private sector resume a CV tends to be longer and more inclusive. A template for a CV for a doctoral student from this school is provide at LateX.
For the .tex file please contact the main office.
Letters of Reference
Some announcements request letters of reference to be sent for the initial application [usually under separate cover directly from references]; others request a list of references with contact information in order to request letters after initial review. References and contact information should be listed on your CV even if letters are not requested in the initial announcement. Choose your references carefully, and ask prospective letter writers if they are willing to write strong letters on your behalf. Provide your reference writers with easy access to your CV, the job announcement, and any other information that you think would help them write a strong letter for you, including but not limited to job market papers and a summary of where you stand in your dissertation progress. When choosing your references, try to choose a set of people that can speak to each important aspect of your background and experience (e.g., someone who knows you as a student in their class, your dissertation advisor and, if you worked as a TA, your TA advisor.)
Do not ask too many people to provide references. While three is the minimum, usually four, and most definitely five, is the maximum. Three strong letters are better than three strong letters and one tepid letter. Usually, any letters above three should be addressing something the other recommenders cannot adequately address, for example, your superior teaching skills.
Official Transcripts
Some positions require that you have official transcripts mailed to them. Others require e-mailed transcripts. If you need assistance in scanning your official transcript, check with the main office.
One or Two Samples of Research Work
A statement of teaching philosophy.
If you do not have experience teaching you will need to come up with a statement about your teaching philosophy.
Preparation for Interviews
Job market paper.
You should select one component of your research, one paper from your dissertation, to use as your job market paper. This paper will be sent in your application packet (perhaps along with other papers), and it will be the basis for your seminar. It should be a paper that you either have or expect to submit for review at a peer-reviewed journal. The choice of your job market paper is an important one. Of the research you might be working on, the job market paper is that which provides the best illustration of your skills, interests, and capacity to perform independent, interesting, and cutting-edge research.
Further, this job market paper must be very polished by the time you send it off in an application, which means that you should have it completed by the middle of your fall semester of your fourth academic year. This also means that you may have to plan your dissertation work accordingly such that you finish your job market paper earlier, leaving other parts of your dissertation for later completion. But keep in mind that employers expect you to be done with your degree by August at the latest. If all you have is one paper, you are not ready to go on the market.
Practice Presentations
It is beyond the scope of this document to provide guidance for presentation preparation, except to reiterate the importance of giving your job talk seminar at least once prior to any interview, in addition to their dissertation oral exams. As a student, you will have ample opportunity to do so. A student preparing for the job market should, early in the Fall semester, schedule one of the regular Friday seminar slots to present his or her work. If these seminar slots are taken, then schedule for another day and advertise to get good attendance. Be sure to realize that a seminar in your home department is as good as it gets: the audience will be rooting for you, they will be very willing to provide advice, and the more people that are there, the more advice you are likely to receive. This will undoubtedly increase the likelihood of performing well during a job interview.
Practice Interviews
Holding a practice interview is an excellent way of getting a feel for what kinds of questions and experiences to expect during a job interview. Sometime prior to attending a conference interview or any type of interview, you should ask your committee and perhaps others to participate in one on your behalf. At a minimum, this practice interview should be a half-hour session in which faculty asks the candidate the type of questions that a candidate would get during a real interview. Preferably, this question and answer session would be followed up by a half-hour debriefing, with advice and further questions about the interview process for the given type of interview that the student is facing.
Student Responsibilities and the Role of the School
As the doctoral candidate facing the job market, all responsibility to prepare for the job market is incumbent upon the student. However, the faculty of the School of Economic Sciences will facilitate you in several ways. Throughout the process, keep in mind the goal is to find the right job for you, not necessarily the job at the highest ranked department, etc. It is your life, and your preference and responsibilities are what will determine whether or not the job market results in a good outcome for you.
Your dissertation advisors should be the primary source of advice regarding the job market and your goals in it. However, it is up to the student to initiate discussions and ask questions when necessary. It is also the student’s responsibility to develop a personal timeline for completion and for job market preparation and participation. Faculty advisors will provide guidance to facilitate the completion of these goals given student initiative. In addition with the guidance on dissertation writing, faculty advisors and committee members will facilitate and participate, whenever possible, in practice seminars, practice interview sessions, and will write letters of recommendation if asked.
If a student is planning to enter the job market in January via the ASSA meetings, he or she should have a serious planning discussion about completion of a job market paper, a dissertation, the student’s job market preferences and aspirations, and any other necessary issues by the end of the previous Spring semester. The Placement Coordinator is not a substitute for your committee chair and committee members. However, the placement coordinator is available to answer questions that you might have about the job market, to help facilitate some aspects of preparation, and to provide additional opinion regarding job market preparation.
Conference Interviews
For academic positions, it is common for competitive job candidates to have two interviews for a given position: the first at a conference employment program for about a half hour to an hour, and if successful, an on-campus interview that may last up to two full days. Descriptions of the conference interview process are provided below, followed by a description of the on-site interview process. To begin, it is important to realize that personal interactions are crucial. It is important to show interest in your own work, the work that you would be expected to pursue, and the department in which you would be working. The primary way of conveying your interest is by being informed about the position, department, and people, so you can ask and answer questions effectively. So, below are a set of questions you will likely face and questions you ought to ask in interviews:
10 Questions to be Ready to Answer:
- What is your dissertation (job market paper) about? (2 minute and 5 minute answers)
- Why is your research important?
- Where do you expect to publish your work?
- What will be the focus of your research over the next 5 years (research agenda)?
- What do you have to offer our department? (Mention 3 things: research, teaching, and team player or collegial)
- Who on this faculty might you collaborate with in research?
- Why this person?
- What two classes would you most like to teach? Why?
- What is your teaching philosophy?
- What do you like about this department/town/region?
7 Questions to Ask Each (or almost each) Faculty Member:
- What do you like most about working in this department?
- What do you like least about working in this department?
- What do you think the person who takes this position will be expected to focus on?
- In what ways do the faculty formally and informally support junior faculty?
- What is the tenure process like?
- How are junior faculty evaluated?
- Ask something specific about the research that the faculty member is doing.
You will be busy during interviews. Here is a list of 10 things you should have and use at conference and on-campus interviews:
- Mouthwash/breath mints
- Deodorant/antiperspirant (important regardless of whether you use these products normally)
- Men: extra tie
- Women: Extra stockings
- Watch or timepiece (DO NOT be late)
- Pen and pad of paper
- Copies of cv
- Copies of job paper
- [perhaps a ONE page front and back cv and summary of job paper combination]
For doctoral students in our program, there are two primary conference-based employment interview opportunities, one at the ASSA meetings in early January (of which the American Economics Association meetings are a part), and at the American Agricultural Economics Association meetings in late July and/or early August. The ASSA meetings are larger and apply to a broader set of job openings. The employment center at the AAEA meetings are of interest primarily to those specializing in agricultural, natural resources, and environmental economics, but may also cover other areas of interest as well.
ASSA Interview Process
The ASSA meetings in early January are the primary forum for preliminary interviews for the academic economics market. You should submit job applications in November or at the vary latest early December. Interested employers start contacting candidates for appointments at the meetings in early or mid-December, so you need to have your applications out. Moreover, you need to be available. A cell phone is a very valuable investment, and you should put your cell number on your vita and in your letter of application. Keep your cell phone turned on, and answer it. The point is, right now you want to be very accessible.
ASSA interviews typically range from thirty minutes to one hour long. Job candidates with multiple interviews will be going from interview room to interview room, and will often involve walking to and from multiple hotels in the vicinity to get to their interviews, so try to schedule interviews to allow you time in between. Ask the interviewers what hotel they expect to be at, and try to congregate your interviews accordingly. Sometimes the hotels are not nearly as close as they appear, and 15 minutes may not be sufficient time between interviews. Wear formal employment attire to the ASSA interviews. Men should wear suits, although you can probably get by with a sport coat and tie. Women should wear normal business attire.
When you set up the interview, ask who will be interviewing you. If you are given the names, spend some time on the Internet getting to know a little bit about the people in the room. Find out their fields, and maybe their most recent research. Take some brief notes you can refer to just before the interview. Also learn a little bit generally about the people at the institution working in or near your field.
A central goal for you as an interviewee is to convince the potential employer that they should want to ask you out for an on-site campus interview (a fly-out). In general, this entails convincing the interviewers that
- you are a talented scholar/teacher/researcher that will contribute the productivity and standing of the department,
- your expertise fits the job requirements well,
- you would be an engaged and valued colleague to other department members, and
- you are very interested in the position responsibilities and opportunities.
Keep these goals in mind as you prepare and engage in the interview.
The ASSA interview will usually go something like this. After initial introductions, you will usually be asked to give a brief summary (~5 minutes) about your research. Your summary should be right on the tip of your tongue; well rehearsed, concise, and clear, but try very hard to sound extemporaneous. If you come off sounding bored or rote, the interviewers will lose interest quickly. You will probably be interrupted with questions throughout the summary. You will also be asked about ideas for future research beyond your dissertation (remember, the employer wants to know what you will do after they hire you; what you have done to date is only an imperfect predictor of that). If the position entails teaching, you will be asked about your teaching experience, skills, and perhaps your perspectives and philosophy on teaching.
The answers to all of the above questions should be provided with points (a), (b), and (c) in mind. Always be ready to answer tough questions as well as possible; be willing to say I don’t know sometimes; and accept the fact that if you bluff about your knowledge of a topic, it will be noticed. Your answers to these questions should also integrate your knowledge of the members of your potential employer’s department. (See the point above about doing some research before the interview.) Be ready to ask and answer questions about how your research relates to that of members of the department, and vice versa. This type of background research on your part will be interpreted as an indicator of interest in this position and a willingness to investment in the possibility of taking that job, and will almost always makes an interview more engaging and interesting.
Although you are an expert on your dissertation, don’t get into arguments about your research. You can disagree, but do not try to convince your interviewer that he or she is wrong-just make your points and defend them. Nothing can kill an interview faster than saying something disparaging about someone’s work, and then finding out they are a friend, coauthor or colleague of the person interviewing you, or the person themselves, so try not to disparage other work in your interview. Remember, you don’t sell yourself by tearing down others. Also keep in mind that any given job may not be ideal from your perspective in any of a number of ways. During your interview your responses should be made with both the position description and your own preferences in mind. You should not promise anything you are unwilling to do, and for your own sake, provide through your responses a clear and balanced picture of your preferences. Your goal is to get the right job for you, not just any job, and not necessarily a job at the highest ranked program.
You will also be asked if you have questions about the position or department. From a strategic perspective, this question should be responded to in a balanced way. You should show interest by asking questions that will inform you regarding whether or not you are interested in an on-campus interview. Do not ask questions that a little bit of work on your part, usually through the web, would have answered. For example, you should know if the department is in a business school or liberal arts college. Time constraints in these interviews are usually such that you should withhold most of them, with a comment that they could wait until an on-campus interview.
AAEA Interview Process
The AAEA employment center meeting process is somewhat different than the ASSA meetings. As a job candidate, you should treat these interviews with the same formality as the ASSA meetings. A description of the AAEA employment center and how it works can be found at the annual meeting site. Job listings maintained by the AAEA can be found on their website. As a job candidate, you should begin keeping an eye on these job listings early and regularly; certainly by March to know when application deadlines are for the summer job postings. The AAEA employment center is different in one important respect from the ASSA meetings. It is generally more acceptable for students to participate earlier in their Ph.D. program (one-half to even one full year earlier) than at the ASSA meetings. So, for those students who are interested in AAEA-type job openings should plan on interviewing at the AAEA meetings the summer before interviewing at the ASSA meetings.
On-Site interviews
Timing of on-site interviews.
After the ASSA meetings, employers will rank all candidates who are considered worth hiring for their position and then, within usually a week or two of the ASSA meetings, they contact the top 2-4 for on-site interviews. In some cases employers will send letters immediately to those who do not suit the position at all, but if you are neither in the top set of finalists nor in the immediate no-hire group, you will not hear from the employer for several weeks or even a month or two. In the meantime, they will proceed with all on-site interviews, one interviewee at a time, make job offers, wait up to a week or more for a response to a job offer, and then either come to closure on the position or repeat the process, ultimately perhaps even with a second set of fly-outs if the first-round is unsuccessful. If you are not one of these second round candidates, you have to wait longer still to get a response. The point is that a quick response is either good or bad. A long wait is not a reason to lose hope.
Interview Schedule
If you are invited for an on-campus interview, the employer will generally arrange your travel with your input. You may be asked to fly out within just a couple of days of being contacted. This is why you should be very ready for an on-campus interview before the ASSA meetings, and have made at least one presentation of your research to SES before the meetings. On-site visits last at least a day, and often two. You should do substantial pre-visit research (usually online) to better know the interests of the people you will be meeting. Never put your seminar materials in checked luggage, in fact always try to carry on your luggage. At the very least, carry on your interview clothes. You always want to be prepared for the worst, so you can make your best presentation. The purpose of the on-site interview is for both the employer and candidate to gather additional information about regarding the position. You will almost always be asked to give a research seminar, and occasionally you will be asked to present a teaching seminar as well. You will be scheduled for meetings with faculty and administrators (department heads, deans, and others) or managers. You will have breakfast, lunch, dinner, and usually a reception with department members. It will be exhausting, but it should also be interesting, exhilarating, and hopefully fun.
The meetings with faculty will be similar to the ASSA meeting. Be prepared to describe your work, ask and answer the same questions, multiple times. Try not to sound bored, even if you’ve said the same thing 50 times. Beyond that you will be asked many questions. Again, do the best you can answering questions, ask lots of questions, and very importantly, use your knowledge about the individuals in the department to engage them and develop connections between you. This last point is hard to overstate.
The seminar is likely to be the single most important event in the interview process. It will likely last 1.5 hours or so, including questions. Usually you should have 45 minutes or more of (questionless) material prepared, and then be prepared to adapt to interruptions and questions. Get details about time requirements before the interview so you have time to adjust your presentation.
Almost all seminars should utilize a slide presentation. Find out beforehand if you need your own computer or if they will provide one, and you only need your thumb-drive and make sure a computer-linked projector is available. Have backups of your presentation available, so if you computer dies enroute, you are still prepared. Have one presentation on the web that you can download if needed.
It is hard to characterize exactly how the seminar will go. Some places adhere to very strict rules about no questions, except brief clarifying questions, until the end of the formal presentations. Other places start interrogating you from almost the first moment. At some departments graduate students are expected to challenge you, and the faculty will be silent. The point is, it is hard to judge how the seminar is going from the reaction and interaction of the audience, so don’t read too much into it as it is on-going. In all cases, be ready and willing to adapt to the questioning style graciously. If you come to an impasse with a questioner, agree to disagree, and move on. Keep control of the seminar, after all, it is your seminar, and also feel free to (occasionally) admit you do not know the answer to a question.
The goal of your presentation is to provide an interesting and engaging experience for your audience while convincing them of the value of your work and the care and knowledge with which you have created it. Therefore, again, know your audience, and present your seminar accordingly. If it is an economics department, then speak to a general audience of economists. This would be a substantially different presentation than if you were speaking mainly to econometricians in an analysis arm of a large firm or agency.
After the Interview
While it will not likely influence decisions, proper etiquette is to send a thank you to the chair, committee members and other people you had significant interaction with during your interview. Doing this by email is fine, even though Emily Post would disagree.
After all of the interviewees have come and gone, the faculty meets and discusses the candidates. After this discussion, the faculty usually votes. This vote often amounts to a recommendation to the department head (or the hiring committee). The department head then makes a recommendation to the dean, and the dean may then make a recommendation to the provost. The point is to keep in mind that virtually everyone you meet will have a voice, if not the ultimate responsibility, of deciding whether you are offered a job.
Thank you for visiting nature.com. You are using a browser version with limited support for CSS. To obtain the best experience, we recommend you use a more up to date browser (or turn off compatibility mode in Internet Explorer). In the meantime, to ensure continued support, we are displaying the site without styles and JavaScript.
- View all journals
- My Account Login
- Explore content
- About the journal
- Publish with us
- Sign up for alerts
- Open access
- Published: 18 January 2024
The impact of artificial intelligence on employment: the role of virtual agglomeration
- Yang Shen ORCID: orcid.org/0000-0002-6781-6915 1 &
- Xiuwu Zhang 1
Humanities and Social Sciences Communications volume 11 , Article number: 122 ( 2024 ) Cite this article
45k Accesses
3 Citations
16 Altmetric
Metrics details
- Development studies
Sustainable Development Goal 8 proposes the promotion of full and productive employment for all. Intelligent production factors, such as robots, the Internet of Things, and extensive data analysis, are reshaping the dynamics of labour supply and demand. In China, which is a developing country with a large population and labour force, analysing the impact of artificial intelligence technology on the labour market is of particular importance. Based on panel data from 30 provinces in China from 2006 to 2020, a two-way fixed-effect model and the two-stage least squares method are used to analyse the impact of AI on employment and to assess its heterogeneity. The introduction and installation of artificial intelligence technology as represented by industrial robots in Chinese enterprises has increased the number of jobs. The results of some mechanism studies show that the increase of labour productivity, the deepening of capital and the refinement of the division of labour that has been introduced into industrial enterprises through the introduction of robotics have successfully mitigated the damaging impact of the adoption of robot technology on employment. Rather than the traditional perceptions of robotics crowding out labour jobs, the overall impact on the labour market has exerted a promotional effect. The positive effect of artificial intelligence on employment exhibits an inevitable heterogeneity, and it serves to relatively improves the job share of women and workers in labour-intensive industries. Mechanism research has shown that virtual agglomeration, which evolved from traditional industrial agglomeration in the era of the digital economy, is an important channel for increasing employment. The findings of this study contribute to the understanding of the impact of modern digital technologies on the well-being of people in developing countries. To give full play to the positive role of artificial intelligence technology in employment, we should improve the social security system, accelerate the process of developing high-end domestic robots and deepen the reform of the education and training system.
Similar content being viewed by others
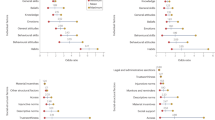
Determinants of behaviour and their efficacy as targets of behavioural change interventions
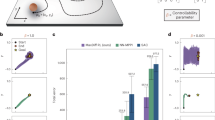
Maximum diffusion reinforcement learning
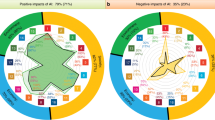
The role of artificial intelligence in achieving the Sustainable Development Goals
Introduction.
Ensuring people’s livelihood requires diligence, but diligence is not scarce. Diversification, technological upgrading, and innovation all contribute to achieving the Sustainable Development Goal of full and productive employment for all (SDGs 8). Since the outbreak of the industrial revolution, human society has undergone four rounds of technological revolution, and each technological change can be regarded as the deepening of automation technology. The conflict and subsequent rebalancing of efficiency and employment are constantly being repeated in the process of replacing people with machines (Liu 2018 ; Morgan 2019 ). When people realize the new wave of human economic and social development that is created by advanced technological innovation, they must also accept the “creative destruction” brought by the iterative renewal of new technologies (Michau 2013 ; Josifidis and Supic 2018 ; Forsythe et al. 2022 ). The questions of where technology will eventually lead humanity, to what extent artificial intelligence will change the relationship between humans and work, and whether advanced productivity will lead to large-scale structural unemployment have been hotly debated. China has entered a new stage of deep integration and development of the “new technology cluster” that is represented by the internet and the real economy. Physical space, cyberspace, and biological space have become fully integrated, and new industries, new models, and new forms of business continue to emerge. In the process of the vigorous development of digital technology, its characteristics in terms of employment, such as strong absorption capacity, flexible form, and diversified job demands are more prominent, and many new occupations have emerged. The new practice of digital survival that is represented by the platform economy, sharing economy, full-time economy, and gig economy, while adapting to, leading to, and innovating the transformation and development of the economy, has also led to significant changes in employment carriers, employment forms, and occupational skill requirements (Dunn 2020 ; Wong et al. 2020 ; Li et al. 2022 ).
Artificial intelligence (AI) is one of the core areas of the fourth industrial revolution, along with the transformation of the mechanical technology, electric power technology, and information technology, and it serves to promote the transformation and upgrading of the digital economy industry. Indeed, the rapid iteration and cross-border integration of general information technology in the era of the digital economy has made a significant contribution to the stabilization of employment and the promotion of growth, but this is due only to the “employment effect” caused by the ongoing development of the times and technological progress in the field of social production. Digital technology will inevitably replace some of the tasks that were once performed by human labour. In recent years, due to the influence of China’s labour market and employment structure, some enterprises have needed help in recruiting workers. Driven by the rapid development of artificial intelligence technology, some enterprises have accelerated the pace of “machine replacement,” resulting in repetitive and standardized jobs being performed by robots. Deep learning and AI enable machines and operating systems to perform more complex tasks, and the employment prospects of enterprise employees face new challenges in the digital age. According to the Future of Jobs 2020 report released by the World Economic Forum, the recession caused by the COVID-19 pandemic and the rapid development of automation technology are changing the job market much faster than expected, and automation and the new division of labour between humans and machines will disrupt 85 million jobs in 15 industries worldwide over the next five years. The demand for skilled jobs, such as data entry, accounting, and administrative services, has been hard hit. Thanks to the wave of industrial upgrading and the vigorous development of digitalization, the recruitment demand for AI, big data, and manufacturing industries in China has maintained high growth year-on-year under the premise of macroenvironmental uncertainty during the period ranging from 2019 to 2022, and the average annual growth rate of new jobs was close to 30%. However, this growth has also aggravated the sense of occupational crisis among white-collar workers. The research shows that the agriculture, forestry, animal husbandry, fishery, mining, manufacturing, and construction industries, which are expected to adopt a high level of intelligence, face a high risk of occupational substitution, and older and less educated workers are faced with a very high risk of substitution (Wang et al. 2022 ). Whether AI, big data, and intelligent manufacturing technology, as brand-new forms of digital productivity, will lead to significant changes in the organic composition of capital and effectively decrease labour employment has yet to reach consensus. As the “pearl at the top of the manufacturing crown,” a robot is an essential carrier of intelligent manufacturing and AI technology as materialized in machinery and equipment, and it is also an important indicator for measuring a country’s high-end manufacturing industry. Due to the large number of manufacturing employees in China, the challenge of “machine substitution” to the labour market is more severe than that in other countries, and the use of AI through robots is poised to exert a substantial impact on the job market (Xie et al. 2022 ). In essence, the primary purpose of the digital transformation of industrial enterprises is to improve quality and efficiency, but the relationship between machines and workers has been distorted in the actual application of digital technology. Industrial companies use robots as an entry point, and the study delves into the impact of AI on the labour market to provide experience and policy suggestions on the best ways of coordinating the relationship between enterprise intelligent transformation and labour participation and to help realize Chinese-style modernization.
As a new general technology, AI technology represents remarkable progress in productivity. Objectively analysing the dual effects of substitution and employment creation in the era of artificial intelligence to actively integrate change and adapt to development is essential to enhancing comprehensive competitiveness and better qualifying workers for current and future work. This research is organized according to a research framework from the published literature (Luo et al. 2023 ). In this study, we used data published by the International Federation of Robotics (IFR) and take the installed density of industrial robots in China as the main indicator of AI. Based on panel data from 30 provinces in China covering the period from 2006–2020, the impact of AI technology on employment in a developing country with a large population size is empirically examined. The issues that need to be solved in this study include the following: The first goal is to examine the impact of AI on China’s labour market from the perspective of the economic behaviour of those enterprises that have adopted the use of industrial robots in production. The realistic question we expect to answer is whether the automated processing of daily tasks has led to unemployment in China during the past fifteen years. The second goal is to answer the question of how AI will continue to affect the employment market by increasing labour productivity, changing the technical composition of capital, and deepening the division of labour. The third goal is to examine how the transformation of industrial organization types in the digital economy era affects employment through digital industrial clusters or virtual clusters. The fourth goal is to test the role of AI in eliminating gender discrimination, especially in regard to whether it can improve the employment opportunities of female employees. Then, whether workers face different employment difficulties in different industry attributes is considered. The final goal is to provide some policy insights into how a developing country can achieve full employment in the face a new technological revolution in the context of a large population and many low-skilled workers.
The remainder of the paper is organized as follows. In Section Literature Review, we summarize the literature on the impact of AI on the labour market and employment and classify it from three perspectives: pessimistic, negative, and neutral. Based on a literature review, we then summarize the marginal contribution of this study. In Section Theoretical mechanism and research hypothesis, we provide a theoretical analysis of AI’s promotion of employment and present the research hypotheses to be tested. In Section Study design and data sources, we describe the data source, variable setting and econometric model. In Section Empirical analysis, we test Hypothesis 1 and conduct a robustness test and the causal identification of the conclusion. In Section Extensibility analysis, we test Hypothesis 2 and Hypothesis 3, as well as testing the heterogeneity of the baseline regression results. The heterogeneity test employee gender and industry attributes increase the relevance of the conclusions. Finally, Section Conclusions and policy implications concludes.
Literature review
The social effect of technological progress has the unique characteristics of the times and progresses through various stages, and there is variation in our understanding of its development and internal mechanism. A classic argument of labour sociology and labour economics is that technological upgrading objectively causes workers to lose their jobs, but the actual historical experience since the industrial revolution tells us that it does not cause large-scale structural unemployment (Zhang 2023a ). While neoclassical liberals such as Adam Smith claimed that technological progress would not lead to unemployment, other scholars such as Sismondi were adamant that it would. David Ricardo endorsed the “Luddite fear” in his book On Machinery, and Marx argued that technological progress can increase labour productivity while also excluding labour participation, thus leaving workers in poverty. The worker being turned ‘into a crippled monstrosity’ by modern machinery. Technology is not used to reduce working hours and improve the quality of work, rather, it is used to extend working hours and speed up work (Spencer 2023 ). According to Schumpeter’s innovation theory, within a unified complex system, the essence of technological innovation forms from the unity of positive and negative feedback and the oneness of opposites such as “revolutionary” and “destructive.” Even a tiny technological impact can cause drastic consequences. The impact of AI on employment is different from the that of previous industrial revolutions, and it is exceptional in that “machines” are no longer straightforward mechanical tools but have assumed more of a “worker” role, just as people who can learn and think tend to do (Boyd and Holton 2018 ). AI-related technologies continue to advance, the industrialization and commercialization process continues to accelerate, and the industry continues to explore the application of AI across multiple fields. Since AI was first proposed at the Dartmouth Conference in 1956, discussions about “AI replacing human labor” and “AI defeating humans” have endlessly emerged. This dynamic has increased in intensity since the emergence of ChatGPT, which has aroused people’s concerns about technology replacing the workforce. Summarizing the literature, we can find three main arguments concerning the relationship between AI and employment:
First, AI has the effect of creating and filling jobs. The intelligent manufacturing industry paradigm characterized by AI technology will assist in forming a high-quality “human‒machine cooperation” employment mode. In an enlightened society, the social state of shared prosperity benefits the lowest class of people precisely because of the advanced productive forces and higher labour efficiency created through the refinement of the division of labour. By improving production efficiency, reducing the sales price of final products, and stimulating social consumption, technological progress exerts both price effects and income effects, which in turn drive related enterprises to expand their production scale, which, in turn, increases the demand for labour (Li et al. 2021 ; Ndubuisi et al. 2021 ; Yang 2022 ; Sharma and Mishra 2023 ; Li et al. 2022 ). People habitually regard robots as competitors for human beings, but this view only represents the materialistic view of traditional machinery. The coexistence of man and machine is not a zero-sum game. When the task evolves from “cooperation for all” to “cooperation between man and machine,” it results in fewer production constraints and maximizes total factor productivity, thus creating more jobs and generating novel collaborative tasks (Balsmeier and Woerter 2019 ; Duan et al. 2023 ). At the same time, materialized AI technology can improve the total factor production efficiency in ways that are suitable for its factor endowment structure and improve the production efficiency between upstream and downstream enterprises in the industrial chain and the value chain. This increase in the efficiency of the entire market will subsequently drive the expansion of the production scale of enterprises and promote reproduction, and its synergy will promote the synchronous growth of the labour demand involving various skills, thus resulting in a creative effect (Liu et al. 2022 ). As an essential force in the fourth industrial revolution, AI inevitably affects the social status of humans and changes the structure of the labour force (Chen 2023 ). AI and machines increase labour productivity by automating routine tasks while expanding employee skills and increasing the value of work. As a result, in a machine-for-machine employment model, low-skilled jobs will disappear, while new and currently unrealized job roles will emerge (Polak 2021 ). We can even argue that digital technology, artificial intelligence, and robot encounters are helping to train skilled robots and raise their relative wages (Yoon 2023 ).
Second, AI has both a destructive effect and a substitution effect on employment. As soon as machines emerged as the means of labour, they immediately began to compete with the workers themselves. As a modern new technology, artificial intelligence is essentially humanly intelligent labour that condenses complex labour. Like the disruptive general-purpose technologies of early industrialization, automation technologies such as AI offer both promise and fear in regard to “machine replacement.” Technological progress leads to an increase in the organic composition of capital and the relative surplus population. The additional capital formed in capital accumulation comes to absorb fewer and fewer workers compared to its quantity. At the same time, old capital, which is periodically reproduced according to the new composition, will begin to increasingly exclude the workers it previously employed, resulting in severe “technological unemployment.” The development of productivity creates more free time, especially in industries such as health care, transportation, and production environment control, which have seen significant benefits from AI. In recent years, however, some industrialized countries have faced the dilemma of declining income from labour and the slow growth of total labour productivity while applying AI on a large scale (Autor 2019 ). Low-skilled and incapacitated workers enjoy a high probability of being replaced by automation (Ramos et al. 2022 ; Jetha et al. 2023 ). It is worth noting that with the in-depth development of digital technologies, such as deep learning and big data analysis, some complex, cognitive, and creative jobs that are currently considered irreplaceable in the traditional view will also be replaced by AI, which indicates that automation technology is not only a substitute for low-skilled labour (Zhao and Zhao 2017 ; Dixon et al. 2021 ; Novella et al. 2023 ; Nikitas et al. 2021 ). Among factors, AI and robotics exert a particularly significant impact on the manufacturing job market, and industry-related jobs will face a severe unemployment problem due to the disruptive effect of AI and robotics (Zhou and Chen 2022 ; Sun and Liu 2023 ). At this stage, most of the world’s economies are facing the deep integration of the digital wave in their national economy, and any work, including high-level tasks, is being affected by digitalization and AI (Gardberg et al. 2020 ). The power of AI models is growing exponentially rather than linearly, and the rapid development and rapid diffusion of technology will undoubtedly have a devastating effect on knowledge workers, as did the industrial revolution (Liu and Peng 2023 ). In particular, the development and improvement of AI-generated content in recent years poses a more significant threat to higher-level workers, such as researchers, data analysts, and product managers, than to physical labourers. White collar workers are facing unprecedented anxiety and unease (Nam 2019 ; Fossen and Sorgner 2022 ; Wang et al. 2023 ). A classic study suggests that AI could replace 47% of the 702 job types in the United States within 20 years (Frey and Osborne 2017 ). Since the 2020 epidemic, digitization has accelerated, and online and digital resources have become a must for enterprises. Many occupations are gradually moving away from humans (Wu and Yang 2022 ; Männasoo et al. 2023 ). It is obvious that the intelligent robot arm on the factory assembly line is poised to allow factory assembly line workers to exit the stage and move into history. Career guides are being replaced by mobile phone navigation software.
Third, the effect of AI on employment is uncertain, and its impact on human work does not fall into a simple “utopian” or “dystopian” scene, but rather leads to a combination of “utopia” and “dystopia” (Kolade and Owoseni 2022 ). The job-creation effects of robotics and the emergence of new jobs that result from technological change coexist at the enterprise level (Ni and Obashi 2021 ). Adopting a suitable AI operation mode can adjust for the misallocation of resources by the market, enterprises, and individuals to labour-intensive tasks, reverse the nondirectional allocation of robots in the labour sector, and promote their reallocation in the manufacturing and service industries. The size of the impact on employment through the whole society is uncertain (Fabo et al. 2017 ; Huang and Rust 2018 ; Berkers et al. 2020 ; Tschang and Almirall 2021 ; Reljic et al. 2021 ). For example, Oschinski and Wyonch ( 2017 ) claimed that those jobs that are easily replaced by AI technology in Canada account for only 1.7% of the total labour market, and they have yet to find evidence that automation technology will cause mass unemployment in the short term. Wang et al. ( 2022 ) posited that the impact of industrial robots on labour demand in the short term is mainly negative, but in the long run, its impact on employment is mainly that of job creation. Kirov and Malamin ( 2022 ) claimed that the pessimism underlying the idea that AI will destroy the jobs and quality of language workers on a large scale is unjustified. Although some jobs will be eliminated as such technology evolves, many more will be created in the long run.
In the view that modern information technology and digital technology increase employment, the literature holds that foreign direct investment (Fokam et al. 2023 ), economic systems (Bouattour et al. 2023 ), labour skills and structure (Yang 2022 ), industrial technological intensity (Graf and Mohamed 2024 ), and the easing of information friction (Jin et al. 2023 ) are important mechanisms. The research on whether AI technology crowds out jobs is voluminous, but the conclusions are inconsistent (Filippi et al. 2023 ). This paper is focused on the influence of AI on the employment scale of the manufacturing industry, examines the job creation effect of technological progress from the perspectives of capital deepening, labour refinement, and labour productivity, and systematically examines the heterogeneous impact of the adoption of industrial robots on employment demand, structure, and different industries. The marginal contributions of this paper are as follows: first, the installation density of industrial robots is used as an indicator to measure AI, and the question of whether AI has had negative effects on employment in the manufacturing sector from the perspective of machine replacement is examined. The second contribution is the analysis of the heterogeneity of AI’s employment creation effect from the perspective of gender and industry attributes and the claim that women and the employees of labour-intensive enterprises are more able to obtain additional work benefits in the digital era. Most importantly, in contrast to the literature, this paper innovatively introduces virtual agglomeration into the path mechanism of the effect of robots on employment and holds that information technologies such as the internet, big data, and the industrial Internet of Things, which rely upon AI, have reshaped the management mode and organizational structure of enterprises. Online and offline integration work together, and information, knowledge, and technology are interconnected. In the past, the job matching mode of one person, one post, and specific individuals has changed into a multiple faceted set of tasks involving one person, many posts, and many types of people. The internet platform spawned by digital technology frees the employment mode of enterprises from being limited to single enterprises and specific gathering areas. Traditional industrial geographical agglomeration has gradually evolved into virtual agglomeration, which geometrically enlarges the agglomeration effect and mechanism and enhances the spillover effect. In the online world, individual practitioners and entrepreneurs can obtain orders, receive training, connect resources and employment needs more widely and efficiently, and they can achieve higher-quality self-employment. Virtual agglomeration has become a new path by which AI affects employment. Another literature contribution is that this study used the linear regression model of the machine learning model in the robustness test part, which verified the employment creation effect of AI from the perspective of positive contribution proportion. In causal identification, this study innovatively uses the industrial feed-in price as a tool variable to analyse the causal path of AI promoting employment.
Theoretical mechanism and research hypothesis
The direct influence of ai on employment.
With advances in machine learning, big data, artificial intelligence, and other technologies, a new generation of intelligent robots that can perform routine, repetitive, and regular production tasks requiring human judgement, problem-solving, and analytical skills has emerged. Robotic process automation technology can learn and imitate the way that workers perform repeated new tasks regarding the collecting of data, running of reports, copying of data, checking of data integrity, reading, processing, and the sending of emails, and it can play an essential role in processing large amounts of data (Alan 2023 ). In the context of an informatics- and technology-oriented economy, companies are asking employees to transition into creative jobs. According to the theory of the combined task framework, the most significant advantage of the productivity effect produced by intelligent technology is creation of new demands, that is, the creation of new tasks (Acemoglu and Restrepo 2018 ). These new task packages update the existing tasks and create new task combinations with more complex technical difficulties. Although intelligent technology is widely used in various industries, it may have a substitution effect on workers and lead to technical unemployment. However, with the rise of a new round of technological innovation and revolution, high efficiency leads to the development and growth of a series of emerging industries and exerts job creation effects. Technological progress has the effect of creating new jobs. That is, such progress creates new jobs that are more in line with the needs of social development and thus increases the demand for labour (Borland and Coelli 2017 ). Therefore, the intelligent development of enterprises will come to replace their initial programmed tasks and produce more complex new tasks, and human workers in nonprogrammed positions, such as technology and knowledge, will have more comparative advantages.
Generally, the “new technology-economy” paradigm that is derived from automation machine and AI technology is affecting the breadth and depth of employment, which is manifested as follows:
It reduces the demand for coded jobs in enterprises while increasing the demand for nonprogrammed complex labour.
The development of digital technology has deepened and refined the division of labour, accelerated the service trend of the manufacturing industry, increased the employment share of the modern service industry and created many emerging jobs.
Advanced productive forces give workers higher autonomy and increased efficiency in their work, improving their job satisfaction and employment quality. As described in Das Kapital, “Although machines actually crowd out and potentially replace a large number of workers, with the development of machines themselves (which is manifested by the increase in the number of the same kind of factories or the expansion of the scale of existing factories), the number of factory workers may eventually be more than the number of handicraft workers in the workshops or handicrafts that they crowd out… It can be seen that the relative reduction and absolute increase of employed workers go hand in hand” (Li and Zhang 2022 ).
Internet information technology reduces the distance between countries in both time and space, promotes the transnational flow of production factors, and deepens the international division of labour. The emergence of AI technology leads to the decline of a country’s traditional industries and departments. Under the new changes to the division of labour, these industries and departments may develop in late-developing countries and serve to increase their employment through international labour export.
From a long-term perspective, AI will create more jobs through the continuous expansion of the social production scale, the continuous improvement of production efficiency, and the more detailed industrial categories that it engenders. With the accumulation of human capital under the internet era, practitioners are gradually becoming liberated from heavy and dangerous work, and workers’ skills and job adaptability will undergo continuous improvement. The employment creation and compensation effects caused by technological and industrial changes are more significant than the substitution effects (Han et al. 2022 ). Accordingly, the article proposes the following two research hypotheses:
Hypothesis 1 (H1): AI increases employment .
Hypothesis 2 (H2): AI promotes employment by improving labour productivity, deepening capital, and refining the division of labour .
Role of virtual agglomeration
The research on economic geography and “new” economic geography agglomeration theory focuses on industrial agglomeration in the traditional sense. This model is a geographical agglomeration model that depends on spatial proximity from a geographical perspective. Assessing the role of externalities requires a particular geographical scope, as it has both physical and scope limitations. Virtual agglomeration transcends Marshall’s theory of economies of scale, which is not limited to geographical agglomeration from the perspective of natural territory but rather takes on more complex and multidimensional forms (such as virtual clusters, high-tech industrial clusters, and virtual business circles). Under the influence of a new generation of digital technology that is characterized by big data, the Internet of Things, and the industrial internet, the digital, intelligent, and platform transformation trend is prominent in some industries and enterprises, and industrial digitalization and digital industrialization jointly promote industrial upgrading. The innovation of information technology leads to “distance death” (Schultz 1998 ). With the further materialization of digital and networked services of enterprises, the trading mode of digital knowledge and services, such as professional knowledge, information combination, cultural products, and consulting services, has transitioned from offline to digital trade, and the original geographical space gathering mode between enterprises has gradually evolved into a virtual network gathering that places the real-time exchange of data and information as its core (Wang et al. 2018 ). Tan and Xia ( 2022 ) stated that virtual agglomeration geometrically magnifies the social impact of industrial agglomeration mechanisms and agglomeration effects, and enterprises in the same industry and their upstream and downstream affiliated enterprises can realize low-cost long-distance transactions, services, and collaborative production through digital trade, resulting in large-scale zero-distance agglomeration along with neighbourhood-style production, service, circulation, and consumption. First, the knowledge and information underlying the production, design, research and development, organization, and trading of all kinds of enterprises are increasingly being completed by digital technology. The tacit knowledge that used to require face-to-face communication has become codable, transmissible, and reproducible under digital technology. Tacit knowledge has gradually become explicit, and knowledge spillover and technology diffusion have become more pronounced, which further leads to an increase in the demand for unconventional task labour (Zhang and Li 2022 ). Second, the cloud platform causes the labour pool effect of traditional geographical agglomeration to evolve into the labour “conservation land” of virtual agglomeration, and employment is no longer limited to the internal organization or constrained within a particular regional scope. Digital technology allows enterprises to hire “ghost workers” for lower wages to compensate for the possibility of AI’s “last mile.” Information technology and network platforms seek connections with all social nodes, promoting the time and space for work in a way that transcends standardized fixed frameworks. At the same time, joining or quitting work tasks, indirectly increasing the temporary and transitional nature of work and forming a decentralized management organization model of supplementary cooperation, social networks, industry experts, and skilled labour all become more convenient for workers (Wen and Liu 2021 ). With a mobile phone and a computer, labourers worldwide can create value for enterprises or customers, and the forms of labour are becoming more flexible and diverse. Workers can provide digital real-time services to employers far away from their residence, and they can also obtain flexible employment information and improve their digital skills through the leveraging of digital resources, resulting in the odd-job economy, crowdsourcing economy, sharing economy, and other economic forms. Finally, the network virtual space can accommodate almost unlimited enterprises simultaneously. In the commercial background of digital trade, while any enterprise can obtain any intermediate supply in the online market, its final product output can instantly become the intermediate input of other enterprises. Therefore, enterprises’ raw material supply and product sales rely on the whole market. At this time, the market scale effect of intermediate inputs can be infinitely amplified, as it is no longer confined to the limited space of geographical agglomeration (Duan and Zhang 2023 ). Accordingly, the following research hypothesis is proposed:
Hypothesis 3 (H3): AI promotes employment by improving the VA of enterprises .
Study design and data sources
Variable setting, explained variable.
Employment scale (ES). Compared with the agriculture and service industry, the industrial sector accommodates more labour, and robot technology is mainly applied in the industrial sector, which has the greatest demand shock effect on manufacturing jobs. In this paper, we select the number of employees in manufacturing cities and towns as the proxy variable for employment scale.
Core explanatory variable
Artificial intelligence (AI). Emerging technologies endow industrial robots with more complete technical attributes, which increases their ability to act as human beings in many work projects, enabling them to either independently complete production tasks or to assist humans in completing such tasks. This represents an important form of AI technology embedded into machinery and equipment. In this paper, the installation density of industrial robots is selected as the proxy variable for AI. Robot data mainly come from the number of robots installed in various industries at various national levels as published by the International Federation of Robotics (IFR). Because the dataset published by the IFR provides the dataset at the national-industry level and its industry classification standards are significantly different from those in China, the first lessons for this paper are drawn from the practices of Yan et al. ( 2020 ), who matches the 14 manufacturing categories published by the IFR with the subsectors in China’s manufacturing sector, and then uses the mobile share method to merge and sort out the employment numbers of various industries in various provinces. First, the national subsector data provided by the IFR are matched with the second National Economic Census data. Next, the share of employment in different industries to the total employment in the province is used to develop weights and decompose the industry-level robot data into the local “provincial-level industry” level. Finally, the application of robots in various industries at the provincial level is summarized. The Bartik shift-share instrumental variable is now widely used to measure robot installation density at the city (province) level (Wu 2023 ; Yang and Shen, 2023 ; Shen and Yang 2023 ). The calculation process is as follows:
In Eq. ( 1 ), N is a collection of manufacturing industries, Robot it is the robot installation density of province i in year t, \({{{\mathrm{employ}}}}_{{{{\mathrm{ij}}}},{{{\mathrm{t}}}} = 2006}\) is the number of employees in industry j of province i in 2006, \({{{\mathrm{employ}}}}_{{{{\mathrm{i}}}},{{{\mathrm{t}}}} = 2006}\) is the total number of employees in province i in 2006, and \({{{\mathrm{Robot}}}}_{{{{\mathrm{jt}}}}}{{{\mathrm{/employ}}}}_{{{{\mathrm{i}}}},{{{\mathrm{t}}}} = 2006}\) represents the robot installation density of each year and industry level.
Mediating variables
Labour productivity (LP). According to the definition and measurement method proposed by Marx’s labour theory of value, labour productivity is measured by the balance of the total social product minus the intermediate goods and the amount of labour consumed by the pure production sector. The specific calculation process is \(AL = Y - k/l\) , where Y represents GDP, l represents employment, k represents capital depreciation, and AL represents labour productivity. Capital deepening (CD). The per capita fixed capital stock of industrial enterprises above a designated size is used in this study as a proxy variable for capital deepening. The division of labour refinement (DLR) is refined and measured by the number of employees in producer services. Virtual agglomeration (VA) is mainly a continuation of the location entropy method in the traditional industrial agglomeration measurement idea, and weights are assigned according to the proportion of the number of internet access ports in the country. Because of the dependence of virtual agglomeration on digital technology and network information platforms, the industrial agglomeration degree of each region is first calculated in this paper by using the number of information transmissions, computer services, and software practitioners and then multiplying that number by the internet port weight. The specific expression is \(Agg_{it} = \left( {M_{it}/M_t} \right)/\left( {E_{it}/E_t} \right) \times \left( {Net_{it}/Net_t} \right)\) , where \(M_{it}\) represents the number of information transmissions, computer services and software practitioners in region i in year t, \(M_t\) represents the total number of national employees in this industry, \(E_{it}\) represents the total number of employees in region i, \(E_t\) represents the total number of national employees, \(Net_{it}\) represents the number of internet broadband access ports in region i, and \(Net_t\) represents the total number of internet broadband access ports in the country. VA represents the degree of virtual agglomeration.
Control variables
To avoid endogeneity problems caused by unobserved variables and to obtain more accurate estimation results, seven control variables were also selected. Road accessibility (RA) is measured by the actual road area at the end of the year. Industrial structure (IS) is measured by the proportion of the tertiary industry’s added value and the secondary industry’s added value. The full-time equivalent of R&D personnel is used to measure R&D investment (RD). Wage cost (WC) is calculated using city average salary as a proxy variable; Marketization (MK) is determined using Fan Gang marketization index as a proxy variable; Urbanization (UR) is measured by the proportion of the urban population to the total population at the end of the year; and the proportion of general budget expenditure to GDP is used to measure Macrocontrol (MC).
Econometric model
To investigate the impact of AI on employment, based on the selection and definition of the variables detailed above and by mapping the research ideas to an empirical model, the following linear regression model is constructed:
In Eq. ( 2 ), ES represents the scale of manufacturing employment, AI represents artificial intelligence, and subscripts t, i and m represent time t, individual i and the m th control variable, respectively. \(\mu _i\) , \(\nu _t\) and \(\varepsilon _{it}\) represent the individual effect, time effect and random disturbance terms, respectively. \(\delta _0\) is the constant term, a is the parameter to be fitted, and Control represents a series of control variables. To further test whether there is a mediating effect of mechanism variables in the process of AI affecting employment, only the influence of AI on mechanism variables is tested in the empirical part according to the modelling process and operational suggestions of the intermediary effects as proposed by Jiang ( 2022 ) to overcome the inherent defects of the intermediary effects. On the basis of Eq. ( 2 ), the following econometric model is constructed:
In Eq. ( 3 ), Media represents the mechanism variable. β 1 represents the degree of influence of AI on mechanism variables, and its significance and symbolic direction still need to be emphasized. The meanings of the remaining symbols are consistent with those of Eq. ( 2 ).
Data sources
Following the principle of data availability, the panel data of 30 provinces (municipalities and autonomous regions) in China from 2006 to 2020 (samples from Tibet and Hong Kong, Macao, and Taiwan were excluded due to data availability) were used as statistical investigation samples. The raw data on the installed density of industrial robots and the number of workers in the manufacturing industry come from the International Federation of Robotics and the China Labour Statistics Yearbook. The original data for the remaining indicators came from the China Statistical Yearbook, China Population and Employment Statistical Yearbook, China’s Marketization Index Report by Province (2021), the provincial and municipal Bureau of Statistics, and the global statistical data analysis platform of the Economy Prediction System (EPS). The few missing values are supplemented through linear interpolation. It should be noted that although the IFR has yet to release the number of robots installed at the country-industry level in 2020, it has published the overall growth rate of new robot installations, which is used to calculate the robot stock in 2020 for this study. The descriptive statistical analysis of relevant variables is shown in Table 1 .
Empirical analysis
To reduce the volatility of the data and address the possible heteroscedasticity problem, all the variables are located. The results of the Hausmann test and F test both reject the null hypothesis at the 1% level, indicating that the fixed effect model is the best-fitting model. Table 2 reports the fitting results of the baseline regression.
As shown in Table 2 , the results of the two-way fixed-effect (TWFE) model displayed in Column (5) show that the fitting coefficient of AI on employment is 0.989 and is significant at the 1% level. At the same time, the fitting results of other models show that the impact of AI on employment is significantly positive. The results confirm that the effect of AI on employment is positive and the effect of job creation is greater than the effect of destruction, and these conclusions are robust, thus verifying the employment creation mechanism of technological progress. Research Hypothesis 1 (H1) is supported. The new round of scientific and technological revolution represented by artificial intelligence involves the upgrading of traditional industries, the promotion of major changes in the economy and society, the driving of rapid development of the “unmanned economy,” the spawning a large number of new products, new technologies, new formats, and new models, and the provision of more possibilities for promoting greater and higher quality employment. Classical and neoclassical economics view the market mechanism as a process of automatic correction that can offset the job losses caused by labour-saving technological innovation. Under the premise of the “employment compensation” theory, the new products, new models, and new industrial sectors created by the progress of AI technology can directly promote employment. At the same time, the scale effect caused by advanced productivity results in lower product prices and higher worker incomes, which drives increased demand and economic growth, increasing output growth and employment (Ge and Zhao 2023 ). In conjunction with the empirical results of this paper, we have reason to believe that enterprises adopt the strategy of “machine replacement” to replace procedural and repetitive labour positions in the pursuit of high efficiency and high profits. However, AI improves not only enterprises’ production efficiency but also their production capacity and scale economy. To occupy a favourable share of market competition, enterprises expand the scale of reproduction. At this point, new and more complex tasks continue to emerge, eventually leading companies to hire more labour. At this stage, robot technology and application in developing countries are still in their infancy. Whether regarding the application scenario or the application scope of robots, the automation technology represented by industrial robots has not yet been widely promoted, which increases the time required for the automation technology to completely replace manual tasks, so the destruction effect of automation technology on jobs is not apparent. The fundamental market situation of the low cost of China’s labour market drives enterprises to pay more attention to technology upgrading and efficiency improvement when introducing industrial robots. The implementation of the machine replacement strategy is mainly caused by the labour shortage driven by high work intensity, high risk, simple process repetition, and poor working conditions. The intelligent transformation of enterprises points to more than the simple saving of labour costs (Dixon et al. 2021 ).
Robustness test
The above results show that the effect of AI on job creation is greater than the effect of substitution and the overall promotion of enterprises for the enhancement of employment demand. To verify the robustness of the benchmark results, the following three means of verifying the results are adopted in this study. First, we replace the explained variables. In addition to industrial manufacturing, robots are widely used in service industries, such as medical care, finance, catering, and education. To reflect the dynamic change relationship between the employment share of the manufacturing sector and the employment number of all sectors, the absolute number of manufacturing employees is replaced by the ratio of the manufacturing industry to all employment numbers. The second means is increasing the missing variables. Since many factors affect employment, this paper considers the living cots, human capital, population density, and union power in the basic regression model. The impact of these variables on employment is noticeable; for example, the existence of trade unions improves employee welfare and the working environment but raises the entry barrier for workers in the external market. The new missing variables are the average selling price of commercial and residential buildings, urban population density (person/square kilometre), nominal human capital stock, and the number of grassroots trade union organizations in the China Human Capital Report 2021 issued by Central University of Finance and Economics, which are used as proxy variables. The third means involves the use of linear regression (the gradient descent method) in machine learning regression to calculate the importance of AI to the increase in employment size. The machine learning model has a higher goodness of fit and fitting effect on the predicted data, and its mean square error and mean absolute error are more minor (Wang Y et al. 2022 ).
As seen from the robustness part of Table 3 , the results of Method 1 show that AI exerts a positive impact on the employment share in the manufacturing industry; that is, AI can increase the proportion of employment in the manufacturing industry, the use of AI creates more derivative jobs for the manufacturing industry, and the demand for the labour force of enterprises further increases. The results of method 2 show that after increasing the number of control variables, the influence of robots on employment remains significantly positive, indicating no social phenomenon of “machine replacement.” The results of method 3 show that the weight of AI is 84.3%, indicating that AI can explain most of the increase in the manufacturing employment scale and has a positive promoting effect. The above three methods confirm the robustness of the baseline regression results.
Endogenous problem
Although further control variables are used to alleviate the endogeneity problem caused by missing variables to the greatest extent possible, the bidirectional causal relationship between labour demand and robot installation (for example, enterprises tend to passively adopt the machine replacement strategy in the case of labour shortages and recruitment difficulties) still threatens the accuracy of the statistical inference results in this paper. To eliminate the potential endogeneity problem of the model, the two-stage least squares method (2SLS) was applied. In general, the cost factor that enterprises need to consider when introducing industrial robots is not only the comparative advantage between the efficiency cost of machinery and the costs of equipment and labour wages but also the cost of electricity to maintain the efficient operation of machinery and equipment. Changes in industrial electricity prices indicate that the dynamic conditions between installing robots and hiring workers have changed, and decision-makers need to reweigh the costs and profits of intelligent transformation. Changes in industrial electricity prices can impact the demand for labour by enterprises; this path does not directly affect the labour market but is rather based on the power consumption, work efficiency, and equipment prices of robots. Therefore, industrial electricity prices are exogenous relative to employment, and the demand for robots is correlated.
Electricity production and operation can be divided into power generation, transmission, distribution, and sales. China has realized the integration of exports and distribution, so there are two critical prices in practice: on-grid and sales tariffs (Yu and Liu 2017 ). The government determines the on-grid tariff according to different cost-plus models, and its regulatory policy has roughly proceeded from that of principal and interest repayment, through operating period pricing, to benchmark pricing. The sales price (also known as the catalogue price) is the price of electric energy sold by power grid operators to end users, and its price structure is formed based on the “electric heating price” that was implemented in 1976. There is differentiated pricing between industrial and agricultural electricity. Generally, government departments formulate on-grid tariffs, integrating the interests of power plants, grid enterprises, and end users. As China’s thermal power installed capacity accounts for more than 70% of the installed capacity of generators, the price of coal becomes an essential factor affecting the price of industrial internet access. The pricing strategy for electricity sales is not determined by market-oriented transmission and distribution electricity price, on-grid electricity price, or tax but rather by the goal of “stable growth and ensuring people’s livelihood” (Tang and Yang 2014 ). The externality of the feed-in price is more robust, so the paper chooses the feed-in price as an instrumental variable.
It can be seen from Table 3 that the instrumental variables in the first stage positively affect the robot installation density at the level of 1%. Meanwhile, the results of the validity test of the instrumental variables show that there are no weak instrumental variables or unidentifiable problems with this variable, thus satisfying the principle of correlation and exclusivity. The second-stage results show that robots still positively affect the demand for labour at the 1% level, but the fitting coefficient is smaller than that of the benchmark regression model. In summary, the results of fitting the calculation with the causal inference paradigm still support the conclusion that robots create more jobs and increase the labour demand of enterprises.
Extensibility analysis
Robot adoption and gender bias.
The quantity and quality of labour needed by various industries in the manufacturing sector vary greatly, and labour-intensive and capital-intensive industries have different labour needs. Over the past few decades, the demand for female employees has grown. Female employees obtain more job opportunities and better salaries today (Zhang et al. 2023 ). Female employees may benefit from reducing the content of manual labour jobs, meaning that further study of AI heterogeneity from the perspective of gender bias may be needed. As seen from Table 4 , AI has a significant positive impact on the employment of both male and female practitioners, indicating that AI technology does not have a heterogeneous effect on the dynamic gender structure. By comparing the coefficients of the two (the estimated results for men and those for women), it can be found that robots have a more significant promotion effect on female employees. AI has significantly improved the working environment of front-line workers, reduced the level of labour intensity, enabled people to free themselves of dirty and heavy work tasks, and indirectly improved the job adaptability of female workers. Intellectualization increases the flexibility of the time, place, and manner of work for workers, correspondingly improves the working freedom of female workers, and alleviates the imbalance in the choice between family and career for women to a certain extent (Lu et al. 2023 ). At the same time, women are born with the comparative advantage of cognitive skills that allow them to pay more nuanced attention to work details. By introducing automated technology, companies are increasing the demand for cognitive skills such as mental labour and sentiment analysis, thus increasing the benefits for female workers (Wang and Zhang 2022 ). Flexible employment forms, such as online car hailing, community e-commerce, and online live broadcasting, provide a broader stage for women’s entrepreneurship and employment. According to the “Didi Digital Platform and Female Ecology Research Report”, the number of newly registered female online taxi drivers in China has exceeded 265,000 since 2020, and approximately 60 percent of the heads of the e-commerce platform, Orange Heart, are women.
Industry heterogeneity
Given the significant differences in the combination of factors across the different industries in China’s manufacturing sector, there is also a significant gap in the installation density of robots; even compared to AI density, in industries with different production characteristics, indicating that there may be an opposite employment phenomenon at play. According to the number of employees and their salary level, capital stock, R&D investment, and patent technology, the manufacturing industry is divided into labour-intensive (LI), capital-intensive (CI), and technology-intensive (TI) industries.
As seen from the industry-specific test results displayed in Table 4 , the impact of AI on employment in the three attribute industries is significantly positive, which is consistent with the results of Beier et al. ( 2022 ). In contrast, labour-intensive industries can absorb more workers, and industry practitioners are better able to share digital dividends from these new workers, which is generally in line with expectations (in the labour-intensive case, the regression coefficient of AI on employment is 0.054, which is significantly larger than the regression coefficient of the other two industries). This conclusion shows that enterprises use AI to replace the labour force of procedural and process-based positions in pursuit of cost-effective performance. However, the scale effect generated by improving enterprise production efficiency leads to increased labour demand, namely, productivity and compensation effects. For example, AGV-handling robots are used to replace porters in monotonous and repetitive high-intensity work, thus realizing the uncrewed operation of storage links and the automatic handling of goods, semifinished products, and raw materials in the production process. This reduces the cost of goods storage while improving the efficiency of logistics handling, increasing the capital investment of enterprises in the expansion of market share and extension of the industrial chain.
Mechanism test
To reveal the path mechanism through which AI affects employment, in combination with H2 and H3 and the intermediary effect model constructed with Eq. ( 3 ), the TWFE model was used to fit the results shown in Table 5 .
It can be seen from Table 5 that the fitting coefficients of AI for capital deepening, labour productivity, and division of labour are 0.052, 0.071, and 0.302, respectively, and are all significant at the 1% level, indicating that AI can promote employment through the above three mechanisms, and thus research Hypothesis 2 (H2) is supported. Compared with the workshop and handicraft industry, machine production has driven incomparably broad development in the social division of labour. Intelligent transformation helps to open up the internal and external data chain, improve the combination of production factors, reduce costs and increase efficiency to enable the high-quality development of enterprises. At the macro level, the impact of robotics on social productivity, industrial structure, and product prices affects the labour demand of enterprises. At the micro level, robot technology changes the employment carrier, skill requirements, and employment form of labour and impacts the matching of labour supply and demand. The combination of the price and income effects can drive the impact of technological progress on employment creation. While improving labour productivity, AI technology reduces product production costs. In the case of constant nominal income, the market increases the demand for the product, which in turn drives the expansion of the industrial scale and increases output, resulting in an increase in the demand for labour. At the same time, the emergence of robotics has refined the division of labour. Most importantly, the development of AI technology results in productivity improvements that cannot be matched by pure labour input, which not only enables 24 h automation but also reduces error rates, improves precision, and accelerates production speeds.
Table 5 also shows that the fitting coefficient of AI to virtual agglomeration is 0.141 and significant at the 5% level, indicating that AI and digital technology can promote employment by promoting the agglomeration degree of enterprises in the cloud and network. Research Hypothesis 3 is thus supported. Industrial internet, AI, collaborative robots, and optical fidelity information transmission technology are necessary for the future of the manufacturing industry, and smart factories will become the ultimate direction of manufacturing. Under the intelligent manufacturing model, by leveraging cloud links, industrial robots, and the technological depth needed to achieve autonomous management, the proximity advantage of geographic spatial agglomeration gradually begins to fade. The panconnective features of digital technology break through the situational constraints of work, reshaping the static, linear, and demarcated organizational structure and management modes of the industrial era and increasingly facilitates dynamic, network-based, borderless organizational forms, despite the fact that traditional work tasks can be carried out on a broader network platform employing online office platforms and online meetings. While promoting cost reduction and efficiency increase, such connectivity also creates new occupations that rely on this network to achieve efficient virtual agglomeration. On the other hand, robot technology has also broken the fixed connection between people and jobs, and the previous post matching mode of one person and one specific individual has gradually evolved into an organizational structure involving multiple posts and multiple people, thus providing more diverse and inclusive jobs for different groups.
Conclusions and policy implications
Research conclusion.
The decisive impact of digitization and automation on the functioning of all society’s social subsystems is indisputable. Technological progress alone does not impart any purpose to technology, and its value (consciousness) can only be defined by its application in the social context in which it emerges (Rakowski et al. 2021 ). The recent launch of the intelligent chatbot ChatGPT by the US artificial intelligence company OpenAI, with its powerful word processing capabilities and human-computer interaction, has once again sparked global concerns about its potential impact on employment in related industries. Automation technology represented by intelligent manufacturing profoundly affects the labour supply and demand map and significantly impacts economic and social development. The application of industrial robots is a concrete reflection of the integration of AI technology and industry, and its widespread promotion and popularization in the manufacturing field have resulted in changes in production methods and exerted impacts on the labour market. In this paper, the internal mechanism of AI’s impact on employment is first delineated and then empirical tests based on panel data from 30 provinces (municipalities and autonomous regions, excluding Hong Kong, Macao, Taiwan, and Xizang) in China from 2006 to 2020 are subsequently conducted. As mentioned in relation to the theory of “employment compensation,” the research described in this paper shows that the overall impact of AI on employment is positive, revealing a pronounced job creation effect, and the impact of automation technology on the labour market is mainly positively manifested as “icing on the cake.” Our conclusion is consistent with the literature (Sharma and Mishra 2023 ; Feng et al. 2024 ). This conclusion remains after replacing variables, adding missing variables, and controlling for endogeneity problems. The positive role of AI in promoting employment does not have exert opposite effects resulting from gender and industry differences. However, it brings greater digital welfare to female practitioners and workers in labour-intensive industries while relatively reducing the overall proportion of male practitioners in the manufacturing industry. Mechanism analysis shows that AI drives employment through mechanisms that promote capital deepening, the division of labour, and increased labour productivity. The digital trade derived from digital technology and internet platforms has promoted the transformation of traditional industrial agglomeration into virtual agglomeration, the constructed network flow space system is more prone to the free spillover of knowledge, technology, and creativity, and the agglomeration effect and agglomeration mechanism are amplified by geometric multiples. Industrial virtual agglomeration has become a new mechanism and an essential channel through which AI promotes employment, which helps to enhance labour autonomy, improve job suitability and encourage enterprises to share the welfare of labour among “cultivation areas.”
Policy implications
Technology is neutral, and its key lies in its use. Artificial intelligence technology, as an open new general technology, represents significant progress in productivity and is an essential driving force with the potential to boost economic development. However, it also inevitably poses many potential risks and social problems. This study helps to clarify the argument that technology replaces jobs by revealing the impact of automation technology on China’s labour market at the present stage, and its findings alleviate the social anxiety caused by the fear of machine replacement. According to the above research conclusions, the following valuable implications can be obtained.
Investment in AI research and development should be increased, and the high-end development of domestic robots should be accelerated. The development of AI has not only resulted in the improvement of production efficiency but has also triggered a change in industrial structure and labour structure, and it has also generated new jobs as it has replaced human labour. Currently, the impact of AI on employment in China is positive and helps to stabilize employment. Speeding up the development of the information infrastructure, accelerating the intelligent upgrade of the traditional physical infrastructure, and realizing the inclusive promotion of intelligent infrastructure are necessary to ensure efficient development. 5G technology and the development dividend of the digital economy can be used to increase the level of investment in new infrastructure such as cloud computing, the Internet of Things, blockchain, and the industrial internet and to improve the level of intelligent application across the industry. We need to implement the intelligent transformation of old infrastructure, upgrade traditional old infrastructure to smart new infrastructure, and digitally transform traditional forms of infrastructure such as power, reservoirs, rivers, and urban sewer pipes through the employment of sensors and access algorithms to solve infrastructure problems more intelligently. Second, the diversification and agglomeration of industrial lines are facilitated through the transformation of industrial intelligence and automation. At the same time, it is necessary to speed up the process of industrial intelligence and cultivate the prospects of emerging industries and employment carriers, particularly in regard to the development and growth of emerging producer services. The development of domestic robots should be task-oriented and application-oriented, should adhere to the effective transformation of scientific and technological achievements under the guidance of the development of the service economy. A “1 + 2 + N” collaborative innovation ecosystem should be constructed with a focus on cultivating, incubating, and supporting critical technological innovation in each subindustry of the manufacturing industry, optimizing the layout, and forming a matrix multilevel achievement transformation service. We need to improve the mechanisms used for complementing research and production, such as technology investment and authorization. To move beyond standard robot system development technology, the research and development of bionic perception and knowledge, as well as other cutting-edge technologies need to be developed to overcome the core technology “bottleneck” problem.
It is suggested that government departments improve the social security system and stabilize employment through multiple channels. The first channel is the evaluation and monitoring of the potential destruction of the low-end labour force by AI, enabled through the cooperation of the government and enterprises, to build relevant information platforms, improve the transparency of the labour market information, and reasonably anticipate structural unemployment. Big data should be fully leveraged, a sound national employment information monitoring platform should be built, real-time monitoring of the dynamic changes in employment in critical regions, fundamental groups, and key positions should be implemented, employment status information should be released, and employment early warning, forecasting, and prediction should be provided. Second, the backstop role of public service, including human resources departments and social security departments at all levels, should improve the relevant social security system in a timely manner. A mixed-guarantee model can be adopted for the potential unemployed and laws and regulations to protect the legitimate rights and interests of entrepreneurs and temporary employees should be improved. We can gradually expand the coverage of unemployment insurance and basic living allowances. For the extremely poor, unemployed or extreme labour shortage groups, public welfare jobs or special subsidies can be used to stabilize their basic lifestyles. The second is to understand the working conditions of the bottom workers at the grassroots level in greater depth, strengthen the statistical investigation and professional evaluation of AI technology and related jobs, provide skills training, employment assistance, and unemployment subsidies for workers who are unemployed due to the use of AI, and encourage unemployed groups to participate in vocational skills training to improve their applicable skillsets. Workers should be encouraged to use their fragmented time to participate in the gig and sharing economies and achieve flexible employment according to dominant conditions. Finally, a focus should be established on the impact of AI on the changing demand for jobs in specific industries, especially transportation equipment manufacturing and communications equipment, computers, and other electronic equipment manufacturing.
It is suggested that education departments promote the reform of the education and training system and deepen the coordinated development of industry-university research. Big data, the Internet of Things, and AI, as new digital production factors, have penetrated daily economic activities, driving industrial changes and changes in the supply and demand dynamics of the job market. Heterogeneity analysis results confirmed that AI imparts a high level of digital welfare for women and workers in labour-intensive industrial enterprises, but to stimulate the spillover of technology dividends in the whole society, it is necessary to dynamically optimize human capital and improve the adaptability of man-machine collaborative work; otherwise, the disruptive effect of intelligent technology on low-end, routine and programmable work will be obscured. AI has a creativity promoting effect on irregular, creative, and stylized technical positions. Hence, the contradiction between supply and demand in the labour market and the slow transformation of the labour skill structure requires attention. The relevant administrative departments of the state should take the lead in increasing investment in basic research and forming a scientific research division system in which enterprises increase their levels of investment in experimental development and multiple subjects participate in R&D. Relevant departments should clarify the urgent need for talent in the digital economy era, deepen the reform of the education system as a guide, encourage all kinds of colleges and universities to add related majors around AI and big data analysis, accelerate the research on the skill needs of new careers and jobs, and establish a lifelong learning and employment training system that meets the needs of the innovative economy and intelligent society. We need to strengthen the training of innovative, technical, and professional technical personnel, focus on cultivating interdisciplinary talent and AI-related professionals to improve worker adaptability to new industries and technologies, deepen the adjustment of the educational structure, increase the skills and knowledge of perceptual, creative, and social abilities of the workforce, and cultivate the skills needed to perform complex jobs in the future that are difficult to replace by AI. The lifelong education and training system should be improved, and enterprise employees should be encouraged to participate in vocational skills training and cultural knowledge learning through activities such as vocational and technical schools, enterprise universities, and personnel exchanges.
Research limitations
The study used panel data from 30 provinces in China from 2006 to 2020 to examine the impact of AI on employment using econometric models. Therefore, the conclusions obtained in this study are only applicable to the economic reality in China during the sample period. There are three shortcomings in this study. First, only the effect and mechanism of AI in promoting employment from a macro level are investigated in this study, which is limited by the large data particles and small sample data that are factors that reduce the reliability and validity of statistical inference. The digital economy has grown rapidly in the wake of the COVID-19 pandemic, and the related industrial structures and job types have been affected by sudden public events. An examination of the impact of AI on employment based on nearly three years of micro-data (particularly the data obtained from field research) is urgent. When conducting empirical analysis, combining case studies of enterprises that are undergoing digital transformation is very helpful. Second, although the two-way fixed effect model and instrumental variable method can reveal conclusions regarding causality to a certain extent, these conclusions are not causal inference in the strict sense. Due to the lack of good policy pilots regarding industrial robots and digital parks, the topic cannot be thoroughly evaluated for determining policy and calculating resident welfare. In future research, researchers can look for policies and systems such as big data pilot zones, intelligent industrial parks, and digital economy demonstration zones to perform policy evaluations through quasinatural experiments. The use of difference in differences (DID), regression discontinuity (RD), and synthetic control method (SCM) to perform regression is beneficial. In addition, the diffusion effect caused by introducing and installing industrial robots leads to the flow of labour between regions, resulting in a potential spatial spillover effect. Although the spatial econometric model is used above, it is mainly used as a robustness test, and the direct effect is considered. This paper has yet to discuss the spatial effect from the perspective of the spatial spillover effect. Last, it is important to note that the digital infrastructure, workforce, and industrial structure differ from country to country. The study focused on a sample of data from China, making the findings only partially applicable to other countries. Therefore, the sample size of countries should be expanded in future studies, and the possible heterogeneity of AI should be explored and compared by classifying different countries according to their stage of development.
Data availability
The data generated during and/or analyzed during the current study are provided in Supplementary File “database”.
Acemoglu D, Restrepo P (2018) Low-Skill and High-Skill Automation. J Hum Cap 12(2):204–232. https://doi.org/10.1086/697242
Article Google Scholar
Alan H (2023) A systematic bibliometric analysis on the current digital human resources management studies and directions for future research. J Chin Hum Resour Manag 14(1):38–59. https://doi.org/10.47297/wspchrmWSP2040-800502.20231401
Autor D (2019) Work of the past, work of the future. AEA Pap Proc 109(4):1–32. https://doi.org/10.1257/pandp.20191110
Balsmeier B, Woerter M (2019) Is this time different? How digitalization influences job creation and destruction. Res Policy 48(8):103765. https://doi.org/10.1016/j.respol.2019.03.010
Beier G, Matthess M, Shuttleworth L, Guan T, Grudzien DIDP, Xue B et al. (2022) Implications of Industry 4.0 on industrial employment: A comparative survey from Brazilian, Chinese, and German practitioners. Technol Soc 70:102028. https://doi.org/10.1016/j.techsoc.2022.102028
Berkers H, Smids J, Nyholm SR, Le Blanc PM (2020) Robotization and meaningful work in logistic warehouses: threats and opportunities. Gedrag Organisatie 33(4):324–347
Google Scholar
Borland J, Coelli M (2017) Are robots taking our jobs? Aust Economic Rev 50(4):377–397. https://doi.org/10.1111/1467-8462.12245
Bouattour A, Kalai M, Helali K (2023) The nonlinear impact of technologies import on industrial employment: A panel threshold regression approach. Heliyon 9(10):e20266. https://doi.org/10.1016/j.heliyon.2023.e20266
Article PubMed PubMed Central Google Scholar
Boyd R, Holton RJ (2018) Technology, innovation, employment and power: Does robotics and artificial intelligence really mean social transformation? J Sociol 54(3):331–345. https://doi.org/10.1177/1440783317726591
Chen Z (2023) Ethics and discrimination in artificial intelligence-enabled recruitment practices. Humanities Soc Sci Commun 10:567. https://doi.org/10.1057/s41599-023-02079-x
Dixon J, Hong B, Wu L (2021) The robot revolution: Managerial and employment consequences for firms. Manag Sci 67(9):5586–5605. https://doi.org/10.1287/mnsc.2020.3812
Duan SX, Deng H, Wibowo S (2023) Exploring the impact of digital work on work-life balance and job performance: a technology affordance perspective. Inf Technol People 36(5):2009–2029. https://doi.org/10.1108/ITP-01-2021-0013
Duan X, Zhang Q (2023) Industrial digitization, virtual agglomeration and total factor productivity. J Northwest Norm Univ(Soc Sci) 60(1):135–144. https://doi.org/10.16783/j.cnki.nwnus.2023.01.016
Dunn M (2020) Making gigs work: digital platforms, job quality and worker motivations. N. Technol Work Employ 35(2):232–249. https://doi.org/10.1111/ntwe.12167
Fabo B, Karanovic J, Dukova K (2017) In search of an adequate European policy response to the platform economy. Transf: Eur Rev Labour Res 23(2):163–175. https://doi.org/10.1177/1024258916688861
Feng R, Shen C, Guo Y (2024) Digital finance and labor demand of manufacturing enterprises: Theoretical mechanism and heterogeneity analysis. Int Rev Econ Financ 89(Part A):17–32. https://doi.org/10.1016/j.iref.2023.07.065
Filippi E, Bannò M, Trento S (2023) Automation technologies and their impact on employment: A review, synthesis and future research agenda. Technol Forecast Soc Change 191:122448. https://doi.org/10.1016/j.techfore.2023.122448
Fokam DNDT, Kamga BF, Nchofoung TN (2023) Information and communication technologies and employment in developing countries: Effects and transmission channels. Telecommun Policy 47(8):102597. https://doi.org/10.1016/j.telpol.2023.102597
Forsythe E, Kahn LB, Lange F, Wiczer D (2022) Where have all the workers gone? Recalls, retirements, and reallocation in the COVID recovery. Labour Econ 78:102251. https://doi.org/10.1016/j.labeco.2022.102251
Fossen FM, Sorgner A (2022) New digital technologies and heterogeneous wage and employment dynamics in the United States: Evidence from individual-level data. Technol Forecast Soc Change 175:121381. https://doi.org/10.1016/j.techfore.2021.121381
Frey CB, Osborne MA (2017) The future of employment: How susceptible are jobs to computerisation? Technol Forecast Soc Change 114:254–280. https://doi.org/10.1016/j.techfore.2016.08.019
Gardberg M, Heyman F, Norbäck P, Persson L (2020) Digitization-based automation and occupational dynamics. Econ Lett 189:109032. https://doi.org/10.1016/j.econlet.2020.109032
Ge P, Zhao Z (2023) The rise of robots and employment change: 2009–2017. J Renmin Univ China 37(1):102–115
Graf H, Mohamed H (2024) Robotization and employment dynamics in German manufacturing value chains. Struct Change Economic Dyn 68:133–147. https://doi.org/10.1016/j.strueco.2023.10.014
Han J, Yan X, Wei N (2022) Study on regional differences of the impact of artificial intelligence on China’s employment skill structure. Northwest Popul J 43(3):45–57. https://doi.org/10.15884/j.cnki.issn.1007-0672.2022.03.004
Article CAS Google Scholar
Huang M, Rust RT (2018) Artificial intelligence in service. J Serv Res 21(2):155–172. https://doi.org/10.1177/1094670517752459
Jiang T (2022) Mediating effects and moderating effects in causal inference. China Ind Econ 410(5):100–120. https://doi.org/10.19581/j.cnki.ciejournal.2022.05.005
Article MathSciNet Google Scholar
Jin X, Ma B, Zhang H (2023) Impact of fast internet access on employment: Evidence from a broadband expansion in China. China Econ Rev 81:102038. https://doi.org/10.1016/j.chieco.2023.102038
Jetha A, Bonaccio S, Shamaee A, Banks CG, Bültmann U, Smith PM et al. (2023) Divided in a digital economy: Understanding disability employment inequities stemming from the application of advanced workplace technologies. SSM - Qual Res Health 3:100293. https://doi.org/10.1016/j.ssmqr.2023.100293
Josifidis K, Supic N (2018) Income polarization of the US working class: An institutionalist view. J Econ Issues 52(2):498–508. https://doi.org/10.1080/00213624.2018.1469929
Kirov V, Malamin B (2022) Are translators afraid of artificial intelligence? Societies 12(2):70. https://doi.org/10.3390/soc12020070
Kolade O, Owoseni A (2022) Employment 5.0: The work of the future and the future of work. Technol Soc 71:102086. https://doi.org/10.1016/j.techsoc.2022.102086
Li L, Mo Y, Zhou G (2022) Platform economy and China’ s labor market: structural transformation and policy challenges. China Econ J 15(2):139–152. https://doi.org/10.1080/17538963.2022.2067685
Li Q, Zhang R (2022) Study on the challenges and countermeasures of coordinated development of quantity and quality of employment under the new technology-economy paradigm. J Xiangtan Univ(Philos Soc Sci) 46(5):42–45+58. https://doi.org/10.13715/j.cnki.jxupss.2022.05.019
Li Z, Hong Y, Zhang Z (2021) The empowering and competition effects of the platform-based sharing economy on the supply and demand sides of the labor market. J Manag Inf Syst 38(1):140–165. https://doi.org/10.1080/07421222.2021.1870387
Liu L (2018) Occupational therapy in the fourth industrial revolution. Can J Occup Ther 85(4):272–283. https://doi.org/10.1177/0008417418815179
Article ADS Google Scholar
Liu N, Gu X, Lei CK (2022) The equilibrium effects of digital technology on banking, production, and employment. Financ Res Lett 49:103196. https://doi.org/10.1016/j.frl.2022.103196
Liu Y, Peng J (2023) The impact of “AI unemployment” on contemporary youth and its countermeasures. Youth Exploration 241(1):43–51. https://doi.org/10.13583/j.cnki.issn1004-3780.2023.01.004
Lu J, Xiao Q, Wang T (2023) Does the digital economy generate a gender dividend for female employment? Evidence from China. Telecommun Policy 47(6):102545. https://doi.org/10.1016/j.telpol.2023.102545
Luo J, Zhuo W, Xu B (2023). The bigger, the better? Optimal NGO size of human resources and governance quality of entrepreneurship in circular economy. Management Decision ahead-of-print. https://doi.org/10.1108/MD-03-2023-0325
Männasoo K, Pareliussen JK, Saia A (2023) Digital capacity and employment outcomes: Microdata evidence from pre- and post-COVID-19 Europe. Telemat Inform 83:102024. https://doi.org/10.1016/j.tele.2023.102024
Michau JB (2013) Creative destruction with on-the-job search. Rev Econ Dyn 16(4):691–707. https://doi.org/10.1016/j.red.2012.10.011
Morgan J (2019) Will we work in twenty-first century capitalism? A critique of the fourth industrial revolution literature. Econ Soc 48(3):371–398. https://doi.org/10.1080/03085147.2019.1620027
Nam T (2019) Technology usage, expected job sustainability, and perceived job insecurity. Technol Forecast Soc Change 138:155–165. https://doi.org/10.1016/j.techfore.2018.08.017
Ndubuisi G, Otioma C, Tetteh GK (2021) Digital infrastructure and employment in services: Evidence from Sub-Saharan African countries. Telecommun Policy 45(8):102153. https://doi.org/10.1016/j.telpol.2021.102153
Ni B, Obashi A (2021) Robotics technology and firm-level employment adjustment in Japan. Jpn World Econ 57:101054. https://doi.org/10.1016/j.japwor.2021.101054
Nikitas A, Vitel AE, Cotet C (2021) Autonomous vehicles and employment: An urban futures revolution or catastrophe? Cities 114:103203. https://doi.org/10.1016/j.cities.2021.103203
Novella R, Rosas-Shady D, Alvarado A (2023) Are we nearly there yet? New technology adoption and labor demand in Peru. Sci Public Policy 50(4):565–578. https://doi.org/10.1093/scipol/scad007
Oschinski A, Wyonch R (2017). Future shock?: the impact of automation on Canada’s labour market.C.D. Howe Institute Commentary working paper
Polak P (2021) Welcome to the digital era—the impact of AI on business and society. Society 58:177–178. https://doi.org/10.1007/s12115-021-00588-6
Rakowski R, Polak P, Kowalikova P (2021) Ethical aspects of the impact of AI: the status of humans in the era of artificial intelligence. Society 58:196–203. https://doi.org/10.1007/s12115-021-00586-8
Ramos ME, Garza-Rodríguez J, Gibaja-Romero DE (2022) Automation of employment in the presence of industry 4.0: The case of Mexico. Technol Soc 68:101837. https://doi.org/10.1016/j.techsoc.2021.101837
Reljic J, Evangelista R, Pianta M (2021). Digital technologies, employment, and skills. Industrial and Corporate Change dtab059. https://doi.org/10.1093/icc/dtab059
Schultz DE (1998) The death of distance—How the communications revolution will change our lives. Int Mark Rev 15(4):309–311. https://doi.org/10.1108/imr.1998.15.4.309.1
Sharma C, Mishra RK (2023) Imports, technology, and employment: Job creation or creative destruction. Manag Decis Econ 44(1):152–170. https://doi.org/10.1002/mde.3671
Shen Y, Yang Z (2023) Chasing green: The synergistic effect of industrial intelligence on pollution control and carbon reduction and its mechanisms. Sustainability 15(8):6401. https://doi.org/10.3390/su15086401
Spencer DA (2023) Technology and work: Past lessons and future directions. Technol Soc 74:102294. https://doi.org/10.1016/j.techsoc.2023.102294
Sun W, Liu Y (2023) Research on the influence mechanism of artificial intelligence on labor market. East China Econ Manag 37(3):1–9. https://doi.org/10.19629/j.cnki.34-1014/f.220706008
Tan H, Xia C (2022) Digital trade reshapes the theory and model of industrial agglomeration — From geographic agglomeration to online agglomeration. Res Financial Econ Issues 443(6):43–52. https://doi.org/10.19654/j.cnki.cjwtyj.2022.06.004
Tang J, Yang J (2014) Research on the economic impact of the hidden subsidy of sales price and reform. China Ind Econ 321(12):5–17. https://doi.org/10.19581/j.cnki.ciejournal.2014.12.001
Tschang FT, Almirall E (2021) Artificial intelligence as augmenting automation: Implications for employment. Acad Manag Perspect 35(4):642–659. https://doi.org/10.5465/amp.2019.0062
Wang PX, Kim S, Kim M (2023) Robot anthropomorphism and job insecurity: The role of social comparison. J Bus Res 164:114003. https://doi.org/10.1016/j.jbusres.2023.114003
Wang L, Hu S, Dong Z (2022) Artificial intelligence technology, Task attribute and occupational substitutable risk: Empirical evidence from the micro-level. J Manag World 38(7):60–79. https://doi.org/10.19744/j.cnki.11-1235/f.2022.0094
Wang R, Liang Q, Li G (2018) Virtual agglomeration: a new form of spatial organization with the deep integration of new generation information technology and real economy. J Manag World 34(2):13–21. https://doi.org/10.19744/j.cnki.11-1235/f.2018.02.002
Wang X, Zhu X, Wang Y (2022) TheImpactofRobotApplicationonManufacturingEmployment. J Quant Technol Econ 39(4):88–106. https://doi.org/10.13653/j.cnki.jqte.2022.04.002
Wang Y, Zhang Y (2022) Dual employment effect of digital economy and higher quality employment development. Expanding Horiz 231(3):43–50
Wang Y, Zhang Y, Liu J (2022) Digital finance and carbon emissions: an empirical test based on micro data and machine learning model. China Popul,Resour Environ 32(6):1–11
Wen J, Liu Y (2021) Uncertainty of new employment form: Digital labor in platform capital space and the reflection on it. J Zhejiang Gongshang Univ 171(6):92–106. https://doi.org/10.14134/j.cnki.cn33-1337/c.2021.06.009
Wong SI, Fieseler C, Kost D (2020) Digital labourers’ proactivity and the venture for meaningful work: Fruitful or fruitless? J-of-Occup-and-Organ-Psychol 93(4):887–911. https://doi.org/10.1111/joop.12317
Wu B, Yang W (2022) Empirical test of the impact of the digital economy on China’s employment structure. Financ Res Lett 49:103047. https://doi.org/10.1016/j.frl.2022.103047
Wu Q (2023) Sustainable growth through industrial robot diffusion: Quasi-experimental evidence from a Bartik shift-share design. Economics of Transition and Institutional Change Early Access https://doi.org/10.1111/ecot.12367
Xie M, Dong L, Xia Y, Guo J, Pan J, Wang H (2022) Does artificial intelligence affect the pattern of skill demand? Evidence from Chinese manufacturing firms. Econ Model 96:295–309. https://doi.org/10.1016/j.econmod.2021.01.009
Yan X, Zhu K, Ma C (2020) Employment under robot Impact: Evidence from China manufacturing. Stat Res 37(1):74–87. https://doi.org/10.19343/j.cnki.11-1302/c.2020.01.006
Yang CH (2022) How artificial intelligence technology affects productivity and employment: Firm-level evidence from Taiwan. Res Policy 51(6):104536. https://doi.org/10.1016/j.respol.2022.104536
Yang Z, Shen Y (2023) The impact of intelligent manufacturing on industrial green total factor productivity and its multiple mechanisms. Front Environ Sci 10:1058664. https://doi.org/10.3389/fenvs.2022.1058664
Yoon C (2023) Technology adoption and jobs: The effects of self-service kiosks in restaurants on labor outcomes. Technol Soc 74:102336. https://doi.org/10.1016/j.techsoc.2023.102336
Yu L, Liu Y (2017) Consumers’ welfare in China’s electric power industry competition. Res Econ Manag 38(8):55–64. https://doi.org/10.13502/j.cnki.issn1000-7636.2017.08.006
Zhang Q, Zhang F, Mai Q (2023) Robot adoption and labor demand: A new interpretation from external competition. Technol Soc 74:102310. https://doi.org/10.1016/j.techsoc.2023.102310
Zhang Y, Li X (2022) The new digital infrastructure, gig employment and spatial spillover effect. China Bus Mark 36(11):103–117. https://doi.org/10.14089/j.cnki.cn11-3664/f.2022.11.010
Article MathSciNet CAS Google Scholar
Zhang Z (2023a) The impact of the artificial intelligence industry on the number and structure of employments in the digital economy environment. Technol Forecast Soc Change 197:122881. https://doi.org/10.1016/j.techfore.2023.122881
Zhao L, Zhao X (2017) Is AI endangering human job opportunities?—From a perspective of marxism. J Hebei Univ Econ Bus 38(6):17–22. https://doi.org/10.14178/j.cnki.issn1007-2101.2017.06.004
Zhou S, Chen B (2022) Robots and industrial employment: Based on the perspective of subtask model. Stat Decis 38(23):85–89. https://doi.org/10.13546/j.cnki.tjyjc.2022.23.016
Download references
Acknowledgements
This work was financially supported by the Natural Science Foundation of Fujian Province (Grant No. 2022J01320).
Author information
Authors and affiliations.
Institute of Quantitative Economics, Huaqiao University, Xiamen, 361021, China
Yang Shen & Xiuwu Zhang
You can also search for this author in PubMed Google Scholar
Contributions
YS: Data analysis, Writing – original draft, Software, Methodology, Formal analysis; XZ: Data collection; Supervision, Project administration, Writing – review & editing, Funding acquisition. All authors substantially contributed to the article and accepted the published version of the manuscript.
Corresponding author
Correspondence to Yang Shen .
Ethics declarations
Competing interests.
The authors declare no competing interests.
Ethical approval
This article does not contain any studies featuring human participants performed by any of the authors.
Informed consent
This study does not contain any study with human participants performed by any of the authors.
Additional information
Publisher’s note Springer Nature remains neutral with regard to jurisdictional claims in published maps and institutional affiliations.
Supplementary information
Rights and permissions.
Open Access This article is licensed under a Creative Commons Attribution 4.0 International License, which permits use, sharing, adaptation, distribution and reproduction in any medium or format, as long as you give appropriate credit to the original author(s) and the source, provide a link to the Creative Commons license, and indicate if changes were made. The images or other third party material in this article are included in the article’s Creative Commons license, unless indicated otherwise in a credit line to the material. If material is not included in the article’s Creative Commons license and your intended use is not permitted by statutory regulation or exceeds the permitted use, you will need to obtain permission directly from the copyright holder. To view a copy of this license, visit http://creativecommons.org/licenses/by/4.0/ .
Reprints and permissions
About this article
Cite this article.
Shen, Y., Zhang, X. The impact of artificial intelligence on employment: the role of virtual agglomeration. Humanit Soc Sci Commun 11 , 122 (2024). https://doi.org/10.1057/s41599-024-02647-9
Download citation
Received : 23 August 2023
Accepted : 09 January 2024
Published : 18 January 2024
DOI : https://doi.org/10.1057/s41599-024-02647-9
Share this article
Anyone you share the following link with will be able to read this content:
Sorry, a shareable link is not currently available for this article.
Provided by the Springer Nature SharedIt content-sharing initiative
Quick links
- Explore articles by subject
- Guide to authors
- Editorial policies

- Open access
- Published: 19 March 2022
Evaluation of the trends in jobs and skill-sets using data analytics: a case study
- Armin Alibasic ORCID: orcid.org/0000-0001-8747-2593 1 ,
- Himanshu Upadhyay 1 ,
- Mecit Can Emre Simsekler 1 ,
- Thomas Kurfess 2 ,
- Wei Lee Woon 3 &
- Mohammed Atif Omar 1
Journal of Big Data volume 9 , Article number: 32 ( 2022 ) Cite this article
7319 Accesses
6 Citations
Metrics details
Introduction
Fast-emerging technologies are making the job market dynamic, causing desirable skills to evolve continuously. It is therefore important to understand the transitions in the job market to proactively identify skill sets required.
Case description
A novel data-driven approach is developed to identify trending jobs through a case study in the oil and gas industry. The proposed approach leverages a range of data analytics tools, including Latent Semantic Indexing (LSI), Latent Dirichlet Allocation (LDA), Factor Analysis and Non-Negative Matrix Factorization (NMF), to study changes in the market. Further, our approach is capable of identifying disparities between skills that are covered by the educational system, and the skills that are required in the job market.
Discussion and evaluation
The results of the case study show that, while the jobs most likely to be replaced are generally low-skilled, some high-skilled jobs may also be at risk. In addition, mismatches are identified between skills that are imparted by the education system and the skills required in the job market.
Conclusions
This study presents how job market and skills required evolved over time, which can help decision-makers to prepare the workforce for highly demanding jobs and skills. Our findings are in line with the concerns that automation is decreasing the demand for certain skills. On the other hand, we also identify the new skills that are required to strengthen the need for collaboration between minds and machines.
Emerging technologies, such as Artificial Intelligence (AI), Machine Learning (ML) and robotics have accelerated the march towards widespread automation in many industries and domains. This trend has become increasingly apparent over the past decade, where the performance of AI systems have exceeded that of humans in a growing list of applications [ 1 ]. Such disruptive technologies started transforming our lives in many ways. The impact on the nature of work and on the skills that are required in the job market, is particularly notable. Even “knowledge-based” jobs are not exempt, as is evidenced by a recent study where an automated medical diagnosis system was shown to be as accurate as the best physicians [ 2 ]. Accordingly, in this study, we analyze the oil and gas industry where cognitive skills may have more value when compared to manual routine skills.
Earlier studies have concluded that low skilled jobs requiring manual labour are being replaced by machines and automation [ 3 , 4 , 5 , 6 , 7 ]. Due to this reason, there has been a significant drop in the demand for such skills over the last decade. On the other hand, the same studies have discovered that interpersonal skills and social activities which consist of skills like emotional intelligence, caring for others, creativity are seeing increasing demand.
The benefits of automation are not limited to manual work; AI agents can now act as negotiators in e-commerce [ 8 ], while automation has greatly enhanced assistive technologies for old people [ 9 ]. There has been a dramatic increase in the demand for AI skills over 2010-2019 in the US economy across most industries [ 10 ]. Indeed, computers are supplanting human labour in an increasing variety of cognitive tasks [ 11 ]. Due to these reasons, the ability to analyze and anticipate changes in the skill sets required for future jobs is critically important, and will have major implications on public and HR policies and even the educational system. This will also help in creating general awareness about the impact of automation and AI on the demand for certain skills in the job market.
It seems clear that insights about job market trends can be extremely valuable to job-seekers, employers and policymakers, particularly that during challenging eocnomic times in the economy [ 12 ]. By analyzing how skills change over time [ 13 ], researchers focus on variations in the skills composition of various jobs, widely know as intensive analysis . However, studying the extensive margin, i.e. variations in the demand and distribution of jobs, is also of equal importance. For example, the study of extensive margin changes can be very helpful for Human Resource (HR) employees for getting relevant insights about hiring, and will ultimately help a company in tackling its turnover issues. This will eventually lead to an improvement in the retention rate of the company and hence it may attract more of the talent which is very significant for the company’s growth in the long run. This is particularly important in the oil and gas industry as this industry boasts of being among the top ten sectors when it comes to the Talent Turnover Rate [ 14 ].
The studies mentioned above have extensively discussed the problem, however, there is still a lack of any comprehensive study which focuses on the mismatch between the skills required in the job market and the skills which are imparted by the current education system. Besides, there is a deficit of studies which specify the change magnitude of such skills and what the actual demand is from the job market [ 15 ]. Due to these reasons, it becomes very crucial to evaluate jobs and workforce to find out the mismatch between the skill sets endowed by education and the demand in the job market. If we focus on these questions, we will be better off in predicting the skills which will be in higher demand.
The objective of this research paper is to fill the gaps mentioned earlier with the help of a model which uses multiple data science techniques to develop a framework that can provide significant insights for strategic decision-making. This will further help in enhancing the capability of a policymaker or an HR to establish a match between jobs and the corresponding skill sets. To achieve the aforementioned, this research endeavors to answer the following research questions along with using data science techniques for oil & gas industry.
What are the skill factors most affected by a rapid advancement of technology, i.e. AI advancement?
What will be the new emerging skills if the current skill sets will lose relevance?
If such changes occur, what will be the corresponding impact on the job market in the oil & gas industry? Hence, what could be the role of the Federal government to tackle these challenges?
This section presents the research problem and the literature review, the rest of the paper is structured as mentioned. Section " Case description " talks about the applied methodology and walks us through the development of the framework. Section " Results " presents the study results. Section " Discussion and evaluation " provides a comprehensive discussion of the results. Section " Conclusion " talks about the limitations of the study. The same section contains the conclusion while providing recommendations for further studies in the future.
In this study, data mining techniques were used to analyze the changes in the skill sets required and job market demand in the oil & gas sector. These techniques are discussed and explained further in the subsequent sections.
- Data mining
To analyze our research questions, we collected unstructured textual data. Then, we made use of Natural Language Processing (NLP) to derive all the necessary information from the extracted text. This process is commonly known as “Text Mining”. Text mining methods have many fields of application. Some of them are mining of electronic health records [ 16 ], text mining of online news to perform sentimental analysis [ 17 ], predicting component failures [ 18 ], etc.
Data collection
We used scraping, which is a data mining technique for the collection of data. Publicly accessible data from the internet can be collected using the scraping technique. First of all, we explored and decided to choose the best websites with job postings. Then to ensure that the mined data is diversified, we collected data from various website sources. This guarantee that the collected data approximately represents the overall job market in the sector we are targeting to study. In our study, ‘demand’ represents the employers who are in search of employees with a particular skill set. We also studied the ‘supply’ side, which corresponds to the comprehensive analysis of various faculty courses i.e. the skill sets imparted into students till graduation. So by the help of data analytics, we will be finding the gap existing between the skills sets imparted into students by faculty courses and the skill sets which are in demand in the market. Later, these observations after the analysis can be very useful for the future knowledge-based policymakers in making informed decisions for education and training.
The data collection procedure started with extracting specific information from the chosen websites, so to achieve this goal we used the python libraries Beautiful Soap and Regular Expression. Job postings available on these websites provide a rich source of real-time and detailed data on the qualifications and skills sought by employers across industries, occupations and locations [ 19 ]. Each job consists of many features such as salary, company name, required skill set, etc. but for our study, we focused on the title and description of the job. Utilizing the above-mentioned libraries we were able to get refined and relevant data according to our requirements for performing the analysis.
Job postings that we collected belong to a period from July 2015 to June 2018. The data collected were for oil & gas sector in the GCC countries consisting of United Arab Emirates (UAE), Saudi Arabia, Oman, Qatar, Bahrain and Kuwait and USA (Texas, California, Louisiana, Oklahoma, and Pennsylvania). For the same period, corresponding data to the syllabuses of the academic courses were collected. The data was collected from the recognized educational institutions in the United Arab Emirates for the oil & gas sector.
The next step was to standardize the data which was collected from various websites. In order to achieve this, we used the Occupational Information Network (O*NET) Footnote 1 . O*NET is one of the primary sources of occupational information for researches involving job analysis. The O*NET database provides comprehensive information on over 1110 occupations, and for each of such occupations, there are hundreds of standardized and occupation-specific descriptors [ 15 ]. To identify the change of skills during years, abilities, task statements, and work activities were used from the ONET. Finally, using this full description information, it was possible to match jobs gathered from the Web with the O*NET occupations.
The choice of oil & gas industry and data from academia for oil & gas sector was primarily due to the reason that this sector holds immense importance in the GCC countries. In addition, gathering the data from GCC and USA countries ensured that the developed models are robust—this will further ensure that the models are independent of the geographical location where the data is collected. Also, it is well known that the O*NET database is a US-based program. To assure that the O*NET database is a good representation of not only US data but also GCC job market data is critical. Table 1 shows the exact number of job postings data we collected. It also mentions the data obtained from the academia about courses syllabuses in the oil & gas sector.
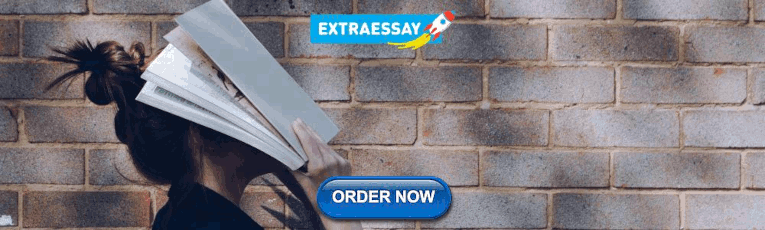
Data preprocessing
The conventional input in text mining is a document—term matrix. For the data we used, a document is synonymous to a job, and terms are words from the job description. Word frequency, which is the number of occurrences of a word in the document, signifies the importance of a word. Some words like English stop words generally have high frequency but they don’t have any additional information value. Hence we don’t include these in terms.
We followed the state of the art text mining techniques in order to pre-process our data [ 20 , 21 ]. We removed the common words and expressions. This helped in reducing the size and noise. The pre-processing steps followed are described as follows:
Tokenize Data
We first performed Tokenization. This refers to a process by which the text is split into significant tokens. Using the ideas from the reviewed literature [ 22 ], we did the tokenization step on our data. We converted our data into single word tokens and counted the frequencies of the words. O*NET data corpus produced a 1110 × 12964 matrix. The rows (records) represent the occupations and columns (features) represent unique words called tokens. The matrix thus produced was a sparse matrix. Few words were outliers and this was resolved as demonstrated in the next step.
Removal of English Stop-Words
The most frequently occurring words which were mainly the English Stop-Words such as and, or, to, as, appeared almost in every record. Thus these words seemingly created no value. Hence these words were removed. This helped in reducing the matrix size too.
Additional Filtering step
To avoid the issues of overfitting and underfitting, we removed all words that appeared in more than 90% of the occupations. This step can be termed as additional filtering. We tried different threshold percentages but 90% was the best in order to create a balance between underfitting and overfitting. The words that are too rare do not contribute much information about document similarity [ 23 ], hence we removed those who appear only in 1% of the documents. This step leads to further improvement in the generalization of the model and helps in significantly compressing the size of the matrix.
The process of removing morphological affixes from words such that only the word stem is left, is called stemming. For example, a Stemming algorithm reduces the words ‘working’, ‘works’, and ‘worker’ to the root word, ‘work’. So we deployed the stemming step and this resulted in the final matrix size of \((1110 \times 4655)\) . The matrix density increased to 2.35%. The total number of unique words reduced to 4655 words. The word ‘equip’ which is stemmed from the word ‘equipment’ was the most frequent word after the preprocessing completion. It had a count of 2412 across all documents.
The preprocessing step mentioned above was described on the O*NET data in this paper, but the same pre-processing steps were also applied to the jobs data collected from the web and the academic data.
Models for intensive change analysis
The intensive change refers to changes in the task content within an occupation. If we analyze the variations in the composition of the jobs, we will be further able to investigate if they were being replaced by automation.
Factor analysis (FA)
From the O*NET database db_20_0, we collected 1110 occupations. The three job descriptors we used were Skills, Abilities, and Work Activities. We got \(954 \times 128\) matrix after consolidating based on the importance level from the descriptors mentioned earlier. Factor Analysis (FA) method is very helpful in describing the variability, called factors [ 24 , 25 ]. In our data, we had 128 features. So by FA, we aim to find only several most informative unobserved or underlying features which can explain the entire features in our data. These underlying features are called latent or hidden features. This is very similar to Principal Component Analysis (PCA) [ 26 ] but the FA method also has independent and Gaussian distributed error terms associated with each input variable.
The importance of a skill n at time t as a function of the importance of all skill’s involved at a prior point of time \(t-1\) is being defined by the following equation:
This method has a drawback when some of the skills are found in many of the occupations. This may lead to a phenomenon called split loadings [ 27 ]. As a result, the correlation of such skills with multiple factors becomes very low. So it becomes hard to put such a skill into a specific factor group. These shortcomings can be overcome by Non-negative Matrix Factorization (NMF). So we deployed it and then compared the results with the FA model.
Non-negative matrix factorization (NMF)
By using NMF, sparse matrix V can be written as a multiplication of the two denser matrices W and H as given below:
All these three matrices have no negative elements which makes them easy to handle and that is one of the advantages of NMF over FA. NMF can handle polysemy which is the issue of a single word having multiple meanings [ 28 ].
Here V is row vectors matrix which contains the input data, W represents the transformed data set and H represents the basis set which defines a linear combination of columns of W .
The main differences between NMF and FA are summarized in Table 2 .
Extensive change analysis
The study of changes across occupations is referred to as extensive margin analysis, and can only be fully understood by analyzing the distribution of demand for the different occupation classes. However, a priori definitions of the combinations of skills and activities which constitute specific jobs can bias our analyses [ 6 ]. Instead, we take an empirical approach to analyzing the occupational changes, where the definition of specific occupations are extracted using observed patterns and distributions. Data for the targeted job markets were gathered for the period between 2015 and 2018. For the same job title in the standardized O*NET database, there will be multiple job postings from the web advertisements in totally different structure in regards to the title and description of the job. If we are about to simply count the jobs from the web, there will be a huge number of job titles and descriptions for the same one standardized job as described in the ONET. Hence, for data analysis, we applied Latent Semantic Indexing (LSI) that resolve some of the polysemy and synonymy issues. In LSI, we map documents and terms into a latent semantic space via a singular value decomposition SVD method. In addition, Latent Dirichlet Allocation (LDA) model is applied for further extensive analysis. These two models are further described in detail in the following subsections " Latent Semantic Indexing (LSI) ", " Latent Dirichlet Allocation (LDA) ".
Latent Semantic Indexing (LSI)
Existing machine learning techniques for text classification usually require hand-labeled training data to effectively classify new sets of data. As such, the amount of available training data is often insufficient, which negatively affects model performance. Unsupervised methods like Latent Semantic Indexing (LSI) (Dumais et al. [ 29 ]) can help to overcome this problem by extracting semantic relationships present in unlabeled text corpora. This semantic space is termed as the latent space and the relationship between words and documents can be better elucidated in this space [ 30 ].
To achieve the above, we made use of the Gensim python library [ 31 ], which automatically extracts semantic topics from the unstructured text. Gensim supports two different topic modeling algorithms, Latent Semantic Analysis (LSA) and Latent Dirichlet Allocation (LDA), both of which are unsupervised algorithms which require no manual labeling of training data. The algorithms search for co-occurrence patterns of the words within a corpus which can be used to decompose the documents into their underlying semantic representations or topics . Using this approach, unstructured documents collected from the Web can be compared with occupations listed in O*NET by comparing the corresponding topics. LSI also deals with the issues of synonymy , in which multiple words share the same meaning, and polysemy , where individual words may have more than one meaning.
We transformed O*NET occupation descriptions into vector space where each element in the vector represents a word. The textual data is then represented as a word-document matrix X ( n × m ). n represents the size of the terms, and m represents O*NET occupation descriptions size. Any given element \(x_{t,d}\) gives us the number of occurrences of the term t in the document d . We then assigned weights to the terms with the help of Term Frequency-Inverse Document Frequency (TF-IDF) formula weighting scheme. This helps in moderating the weights of frequently occurring terms and increasing the weights of rare terms [ 32 ], but we did not use it in the pre-processing steps because it sets the TF-IDF value to zero if the word of interest appears in all documents. After this, we performed Singular Value Decomposition (SVD). The SVD of matrix X is given by:
Here L represents the left singular value vector, R represents the right singular value vector and S represents the diagonal matrix of singular values which are ordered by magnitude. If the dimensions of matrix X is ( m × n ) then respective dimensions for L, S, and R will be ( m × k ), ( k × k ) and ( k × n ) respectively. Due to this order, the matrices can be simplified by setting the smallest optimal value of k for the matrix S . We then set the value of k as 300 according to already existing literature [ 31 , 33 ]. So to summarize, in our case, after applying TDF-IF we got our matrix “X” with dimensions ( 1110 × 4655 ). Then when we applied LSI, we got matrices L (Documents by topics), S (Singular Values) and R (Topics by words) with dimensions ( 1110 × 300 ), ( 300 × 300 ) and ( 300 × 4655 ) respectively. Moving forward, we identified the similarities. We projected each job posting to a query vector \(Q_{k}\) . The weights for a single job posting are the entries of the query vector \(Q_{k}\) , where \(Q_{k}\) is given explicitly by the following displayed formula. As described shortly, we will then normalize these weights across all p job postings and finally sum the normalized weights across the p job postings to obtain weights corresponding to each occupation. The semantic space is of k dimensions. The following formula was used for this purpose:
Here \(S^{-1}\) represents the inverse of matrix S defined earlier, \(L^T\) represents the transpose of the matrix L , and Q represents the term vector. We performed numerous similarity tests but Cosine similarity turned out to be the most appropriate in our case. The Cosine similarity for two vectors \(u_{a}\) and \(u_{b}\) is given by:
where \(u_{a}\) and \(u_{b}\) are n-dimensional vectors over the term set \(U = u_{1},...,u_{n}\) .
For normalization we used z-score normalization given below:
where \(\mu\) represents the mean of feature x and \(\sigma\) represents the standard deviation of feature x.
In the end z-scores are fed into the p vectors whose values correspond to each job postings. Then, p vectors are summed together to form a single vector W . Each element of W is a demand weight for each occupation in the O*NET database. Later, all these weights were being used to perform intensive and extensive analysis. The results of the analyses are discussed in the section " Results ". LSI turned out to be a very robust model as it can be applied to different job markets. Further, LSI can be applied irrespective of geographical location and still produce accurate and reliable results.
Latent Dirichlet Allocation (LDA)
Latent Dirichlet Allocation (LDA) is a generative probabilistic model for collections of discrete data, such as text corpora [ 34 ]. Schematic diagram of the applied LDA methodology is given in Fig. 1 . LDA uses Dirichlet distribution [ 35 ] which supposes that all occupations cover only a small set of topics, and the topics are represented with a small set of words. Implementing LDA is usually done with two approaches: Using an Expectation Maximization (EM)-like procedure called variational inference and Using a randomized algorithm called Gibbs sampling. In our case, we want to discover the topics (combination of the O*NET jobs) looking at only words that are occurring in the web jobs descriptions. LDA observes all the words in a given document, and hence tells us which topics are present in it. Thus this generates a topic distribution. Figure 2 depicts the LDA Model where:
Nodes are random variables,
Edges denote possible dependence,
Observed variables are shaded,
Plates denote replicated structure,
M denotes the number of documents,
N is the number of words in a document,
\(\alpha\) is the parameter of the Dirichlet prior on the per-document topic distributions,
\(\beta\) is the parameter of the Dirichlet prior on the per-topic word distribution,
\(\theta _{m}\) is the topic distribution for document m ,
\(\varphi _{k}\) is the word distribution for topic k ,
\(z_{mn}\) is the topic for the n-th word in a document m ,
\(w_{mn}\) is the observed word in a document m
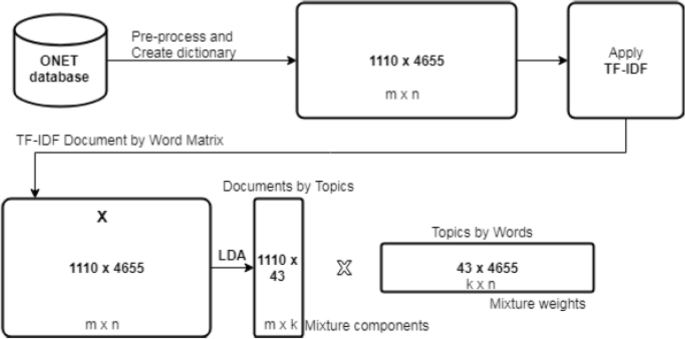
Schematic diagram of the applied Latent Dirichlet Allocation (LDA) model
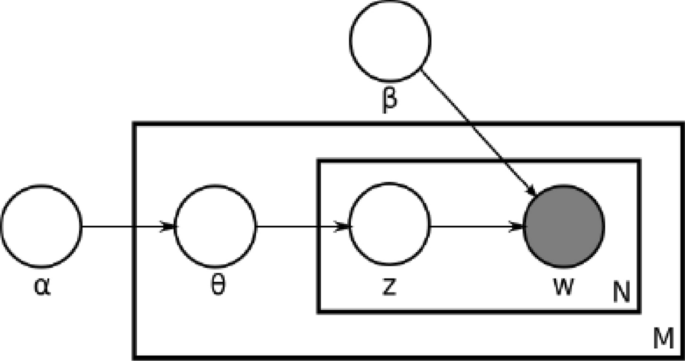
Latent Dirichlet Allocation (LDA) model
Adjusting the hyperparameters \(\alpha\) and \(\beta\) controls the learning of the model. Setting \(\alpha\) to a higher value will make every document a mixture of many topics. On the contrary, setting \(\alpha\) to lower value will mean that the document is represented by only a few topics. In our case, we want \(\alpha\) to be lower value because our occupations are represented with only a few topics where a topic is simply a probability distribution over words. Subsequent, modifying \(\beta\) to a higher value will mean that each topic is a mixture of many words. Conversely, changing \(\beta\) to lower value will mean that the topic is a mixture of just a few words. We want topics to be as distinct as possible, although there will be words appearing in several topics due to overlapping skills in a different type of jobs hence, \(\beta\) is also set to a lower value. The lower values of hyperparameters \(\alpha\) and \(\beta\) will result in more decisive topic associations [ 36 ]. The model‘s only observed variable is words, while the other unobserved (latent) variable is topics which will be inferred from the observed variable words.
The joint distribution of a topic mixture \(\theta\) , a set of N topics z , and a set of N words, w is given by:
here \(p(z_n|\theta )\) represents \(\theta _i\) for a unique value of i such that it satisfies \(z_n^i=1\) . Also the parameters \(\alpha\) and \(\beta\) are given. The marginal distribution of a document is obtained by integrating over \(\theta\) and summing over z . It can be described as follows:
Next, we need to choose the value of k , which represents the number of topics, and which can significantly impact the final outputs of the model. Unfortunately, the choice of k is somewhat subjective and most approaches tend to be iterative [ 37 ]. In this paper, we used two techniques to decide the value of k :
The number of topics can be approximately given by \((m \times n) / t\) . Here t is the number of entries in D which are non-zero [ 38 ]. D in the formula represents the matrix of size m by n formed by a collection of documents. We plugged the values from our data into the formula and get the value of k to be \((1110 \times 4655) / 121472 \approx 43\) .
The other technique we used is the Coherence score. For a topic to be more intuitive, its coherence score should be higher. Coherence is given by the Formula 11 which was given by Mimno et al. [ 39 ].
Here D ( v ) denotes the document frequency of word type v and D ( v , w ) denotes the co-document frequency of word types v and w . Also, \(V(t) = (v_{1}(t),v_{2}(t),.....v_{M}(t))\) is the list of M most probable words in topic t .
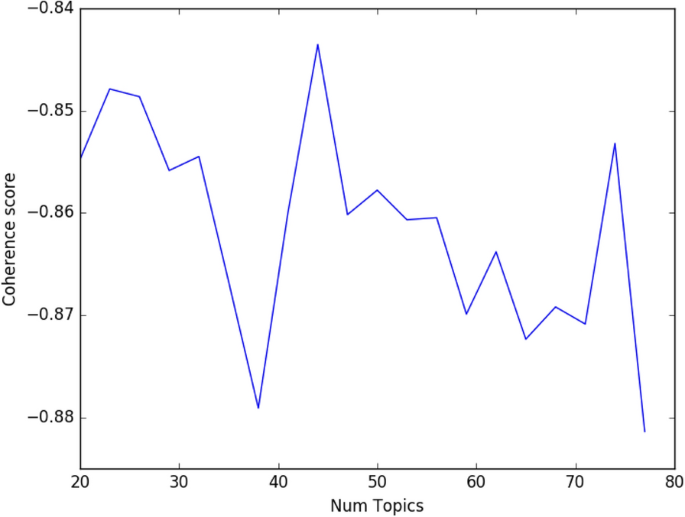
Coherence vs number of topics
We tried to find a perfect balance between overfitting and underfitting. As can be seen in Fig. 3 , the highest coherence score was 43 topics. This is the same as we got from approximation in our first technique. So we set the value k to be 43.
We then validated the number of topics suggested by the coherence measure with the help of visualization. To facilitate visualization, we deployed pyLDAvis library. Figure 4 shows that the topics are distinguished as one can easily identify that topic 1 is about oil & gas (distinctive words—process, research, develop, etc.). The bigger the size of the circle, the more that topic is present in the data. We can also get significant information about the estimated term frequency within a topic that is selected (red color) when compared to the overall frequency of that particular term (blue color).
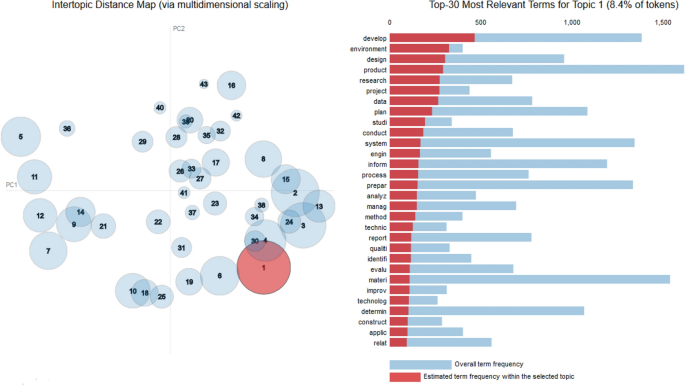
Topic 1—oil & gas
In the next step, we calculated the similarity weights. For this purpose, Hellinger distance [ 40 ] method was used. If there ate two probability distributions given by \(P = (p_1,...p_k)\) and \(Q = (q_1,...q_k)\) , Hellinger distance can be calculated using the Formula 12 .
The higher the value of Hellinger measure, the lower is the similarity between two given probabilities. In order to make the given equation consistent with the cosine similarity, we changed the formula as follows:
As a final step, we performed the intensive and extensive analysis by using the generated weights from the LDA model. It is important to mention that neither LSI nor LDA necessarily yields an accurate ground truth, however, LSI proved to be more robust than LDA which is in accordance to the findings of other researchers as well [ 41 ]. The results of the analyses can be seen in detail in section " Results ".
Job analysis framework tool
Since this study is limited only to oil & gas sector, we developed a job analysis system that anybody can utilize for further analysis. Job Analysis Framework is designed to evaluate job data for extensive and intensive changes. The ultimate goal of the framework is to enable users to analyze their data for any job market or industrial sector with only one click and without any programming knowledge.
For these purposes, https://www.kudsom.org/ website is developed which employs all developed models in Python programming language. Every website contains two main parts for development: front-end, and back-end. In simple words, the front-end is what users see on the web page when they open the website. On the other hand, the back-end is what regular user does not see. Behind the scenes, back-end developers are involved with databases, servers, and application. The following steps briefly explain applied tools to build the job analysis framework website:
Flask—back-end framework
Bootstrap—front-end framework
PythonAnywhere—web hosting service
1. Flask Footnote 2 is a web framework which allows website development using Python programming language. This allows users of the website to easily upload their data for the jobs, and run a comprehensive analysis with only one click.
2. As important as the back-end, is the front-end development. It is very important to provide the user with a friendly interface website. To allow this, Bootstrap Footnote 3 open-source toolkit is deployed.
3. Finally, to deploy developed site on the server, we used PythonAnywhere Footnote 4 free web hosting service.
After deploying the LDA and LSI models on our dataset, we came up with a matrix of size \(1110 \times 2\) . This matrix contains the title of the occupation from the O*NET database and the corresponding weights. Then we sorted the occupations in descending order depending upon the weights. We updated the complete data on our job analysis website provided earlier. In this paper, for illustrative purposes, we are providing the top 10 occupations only in the form of tables in the results section.
LSI model results—job market data
The top 10 occupations with the highest weights in oil & gas industry of GCC and USA are being displayed in Table 3 .
We can see that the occupation ‘Mechanical Engineers’ has a higher weighting than ‘Civil Engineers’. This shows that more Mechanical Engineer than Civil Engineer positions are available in the GCC oil and gas industry, which results in the relevant skills being in greater demand. As might be expected, both the oil & gas industries in the USA and the GCC have some overlapping jobs that are in high demand like ‘Mechanical Engineer’ and ‘Electrical Engineer’. However, it is interesting to notice that the proposed model also exposed notable differences between the two job markets, where engineers tend to be in greater demand in the GCC, while Managerial and Human Resources (HR) positions are relatively more prevalent in the USA. A possible explanation for this was presented in [ 42 ], which postulated that HR is seen as a ‘cost’ to the employer in GCC countries and not a valued resource to be invested in.
We further observe that the LSI model which we generated came up with very relevant top occupations from the oil & gas industry.
LDA oil & gas job market data
Table 4 shows the top 10 occupations in the GCC (left) and USA (right) oil & gas industry sorted by generated weights where the highest position corresponds to the greatest demand. For example, we can see that the occupation ‘Intelligence Analysts’ has a higher demand than for example ‘Anthropologists’ from which it can be inferred that more Intelligence Analysts than Anthropologists positions are wanted in the GCC oil & gas industry, and hence that the corresponding skills would be in greater demand. However, what is unique about LDA is that it bring some interesting results that LSI model did not find, for example, positions like ‘GeoScientists’ are in the top demand for GCC oil & gas industry. In addition, it is encouraging to see demanding positions for ‘Sustainability’ in oil & gas Sector. There are also some occupations that on the first look does not seem to be related to oil & gas industry like ‘Curators’ and ‘Statisticians’ but indeed if we look at the description of these jobs, they have related words with some of the description jobs from oil & gas industry. As LDA is a probabilistic model determining which topics are present in any given document by observing all the words this leads it to conclusions that these occupations are part of oil & gas industry which can be considered as a shortcoming of the LDA model. For example, in the ‘Curators’ occupation we find sentences like “Plan and organize the acquisition, storage, and exhibition of collections and related materials, including the selection of exhibition themes and designs, and develop or install exhibit materials” or “Plan and conduct special research projects in the area of interest or expertise. Confer with the board of directors to formulate and interpret policies, to determine budget requirements, and to plan overall operations.” while in the ‘Statisticians’ description we can find related sentences: “Develop or apply mathematical or statistical theory and methods to collect, organize, interpret, and summarize numerical data to provide usable information.” or “Adapt statistical methods to solve specific problems in many fields, such as economics, biology, and engineering.”
For the USA and GCC oil & gas data, both regions have some overlapping jobs that are in high demand like ‘Chief Sustainability Officers’. In LSI results for USA oil & gas data, we had a trend for Managers and HR positions. LDA shows this trend even more explicit where the majority of the top 10 demanded positions are related with Managers. Thus, we can conclude that USA and GCC job markets are quite different within the same oil & gas industry.
LSI and LDA model results—academic data
In this paper, to get a complete picture of the changes in the job market, we not only analyzed the demand side but also analyzed the supply side to provide a complete picture. For doing the supply-side analysis, we collected academic data from various top-rated educational institutes in the UAE for oil & gas related courses. We collected course syllabuses from these institutions. The top 10 occupations based on the supply-side data of the oil & gas industry are being shown in Table 5 . The one at the top represents the occupation with the highest supply and vice versa.
As can be seen from Table 5 , all the jobs located at the top are very closely related to oil & gas industry, but this should not be surprising that majority of job demands are associated to ‘teachers’. This can be attributed to the fact that in the corpus of collected academic data, there have been a high frequency of words like ‘course’, ‘research’ and ’students’.
When we observe the top oil & gas industry jobs from the supply side, we get an indication that the faculty in the UAE can provide appropriate general knowledge to the students, but for jobs like ‘Civil Engineers’ and ’Petroleum Engineers’, very specific and specialized knowledge is required. In order to better understand the mismatch between the supply side and the demand side, we exploited the heatmap visualizations " LSI and LDA weights visualization using ". This will help us in finding out if the courses taught are imparting right skills into the students to make them ready for the job market.
For the oil & gas academic data, the LDA model also provided insightful results. Interestingly, in oil & gas academic data, we find more positions that are related to general knowledge from this industry, rather than specific occupations. Hence, this can be a possible indicator that faculty related to oil & gas industry needs to focus more at teaching specific high skill knowledge like for example we see in demand ‘Petroleum Engineers’. Although we see some specific knowledge like for example in academic data we have ‘Geospatial Information Scientists and Technologists’ which can correspond to demand position ‘Geoscientists’.
LSI and LDA results validation
We used SurveyMonkey platform to circulate a questionnaire which asked the participants to select either “Relevant” or “Irrelevant” for each of the job titles in oil & gas industry based on data analysis of job titles. For example, for all job titles that are related to oil & gas industry, the participant will select “Relevant”, otherwise, if the job title is not relevant to the industry, the user will select “Irrelevant”.
As can be inferred from the Fig. 5 LSI and LDA models provided very relevant top 10 occupations in the oil & gas industry.
It was observed that the LSI model was providing better results when compared to the LDA model. There were a significant number of occupations for which 100% of the participants agreed that the given occupation is relevant to the oil & gas industry. To see all the results of the survey, follow this link: https://www.surveymonkey.com/results/SM-FVTF6PJXL/ .
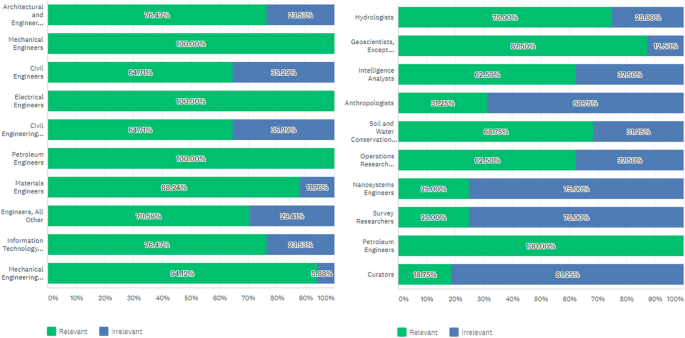
Results for LSI (left) and LDA (right) models for the oil & gas industry
LSI and LDA weights visualization using Heatmaps
We visualized the job demands with the help of heatmaps to make the results more comprehensible. But it’s difficult for humans to comprehend more than 3-d graphs, and hence it becomes difficult to intuitively visualize our high-dimensional matrix. So we deployed Principal Components Analysis (PCA) on the occupations-skills matrix. PCA simply applies linear transformations and finds out the maximum variance direction in multi-dimensional data. These directions are referred to as principal components. Later on, when required, we can retrieve the original data with a very small loss in information. In order to make it simplified, we extracted only the first two components of the skills and occupations matrix. These components has been represented as the x and y axes in the graphs presented in this paper.
Then we estimated the contours from weights with the help of k-nearest neighbors (k-NN) algorithm [ 43 ]. After this, we overlayed these contours on the two-dimensional space generated by Principal Component Analysis described earlier.
The color coding legend can be seen in Fig. 6 . Dark blue represents high demand while light green signifies low demand. All the intermediate demands are represented with intermediate shades between dark blue and light green. As you can see on the legend, only 6 occupation titles are being represented to make visibility clearer. Each of these occupations are from different weight groups. The corresponding representations are marked with shapes like square and circle as can be seen in the legend.
The six occupations which are being chosen are chosen randomly from the 6 different demand categories. Hence the results can be deemed reliable. As we aimed to present all the low demand and high demand occupation scenarios, there was a need of categorization because otherwise all the six chosen occupations might have turned out to be from one specific area (Low demand or high demand). We also randomly selected few occupations from very low demand to ensure that these jobs with very low demand are not from the oil & gas industry. For example, if we see “Petroleum engineers” in the low demand area, then we can get an idea that our model has something wrong with it.
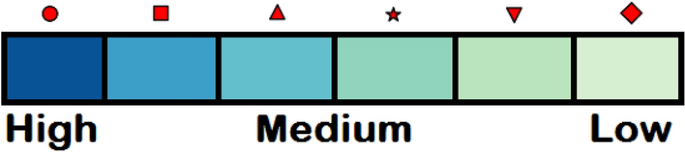
Heatmaps scale based on weights
Heatmaps generated from the LSI weights
The heatmap for the GCC (left) and USA (right) oil & gas industry is shown in Fig. 7 . As can be seen in the heatmaps, our heatmap visualizations are in alignment with the top 10 occupations which came out from the LSI model. There is a similarity between job market data of the US and GCC. This confirms our model is reliable no matter what geographical location it is applied on. As can be seen, heatmap 7 confirm that there appears to be a greater managerial and/or HR jobs for USA market in oil and gas companies, while demand for the same industry operating in the GCC region seems to be focused more on technical engineering jobs as expected.
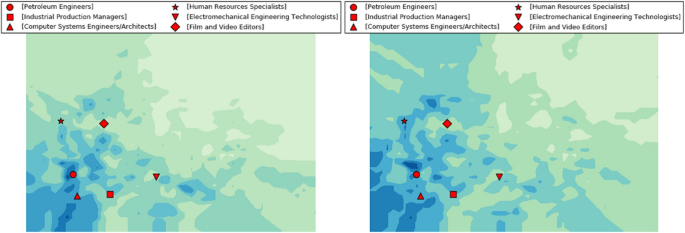
LSI Heatmap for GCC (left) and USA (right) oil & gas
Figure 8 shows oil & gas industry demand (left) and supply (right) heatmaps for the GCC region. We can see that occupation ‘Petroleum Engineers’ has both high demand and supply; however, “teaching” occupations that have a high supply like ‘Environmental Science Teachers’, does not match the same intensity of demand on the job market. In addition, interdisciplinary jobs like ‘Sales Managers’ that have high demand in the job market, does not match the same supply which can indicate that interdisciplinary skills are lacking with students. One possible reason for this mismatch is the different use of terminology between academia and industry. For instance “course” and “students” are common in academia compared to industrial sector [ 15 ]. Other reason could be the mismatch between the faculty courses and the specific skills requirements of the job market.
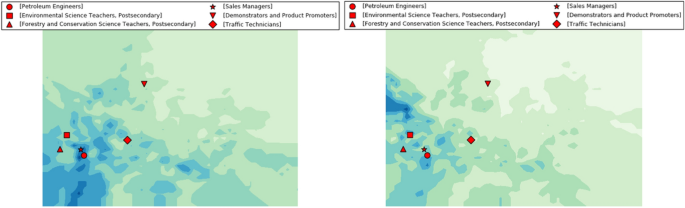
LSI Heatmap for GCC oil & gas Demand (left) and Supply (right)
Heatmaps generated from the LDA weights
The LDA heatmaps also turn to be in agreement with our top 10 occupations findings using the LDA model. There is agreement between oil & gas job market data and academic data; or oil & gas industry with different geographical location. This confirms the LDA model is also robust no matter which geographical location it is implemented on.
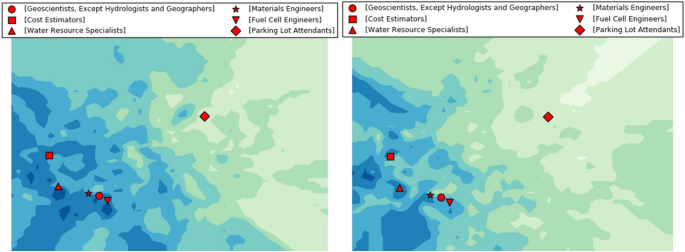
LDA Heatmap for GCC (left) and USA (right) oil & gas
Figure 9 shows oil & gas industry heatmaps for GCC (left) and USA (right) regions. As expected, both regions USA and GCC for oil & gas industry have some overlapping jobs that are in high demand like ‘Water Resource Specialist’, ‘Cost Estimators’. LDA shows slightly higher demand in GCC for positions ‘GeoScientists’, Except ’Hydrologists and Geographers’, and ‘Fuel Cell Engineers’. In addition, it can be seen that there is an overall difference between two regions, which is in assent to the previous conclusion that USA and GCC job markets are considerably different within the same Oil and Gas industry.
Same conclusions can be made from Fig. 10 GCC Demand (left) and UAE Supply (right) oil & gas where it can be inferred that demand is covering many more occupations comparing to what is provided with supply. Since we cover only six occupations in heatmaps visualizations all occupations cannot be shown. However, one interesting occupation that heatmap discovered is ‘Marine Engineers’ which can be found in high demand but low supply weight. Once again, seems that interdisciplinary knowledge is lacking on the supply side.
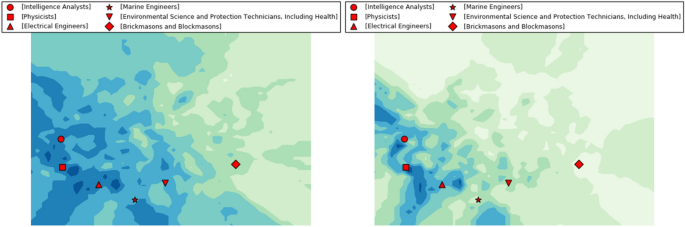
LDA Heatmap for GCC demand (left) and UAE supply (right) oil & gas
Results of the factor analysis (FA) and non-negative matrix (NMF) factorization
After the extensive analysis, we applied the generated weights for analyzing the intensive margin changes. Negative values in Fig. 11 represent that average occupational requirements are lower for that skill factor in 2015 than in 2008. This figure was generated solely based on the O*NET database, that is why there are no any weights on it.
NMF model extracted six O*NET Factors or we can call them skill sets. Their description can be seen in Table 6 . Those factors are comprehensively explained below:
Management Performing Administrative Activities, Documenting Information, Processing Information, and similar skills are in decreasing because they are replaced by new technology. For example, to document and record information we do not need to hold a collection of physical documents records. Instead, we have electronic versions and store them in the cloud or some similar online platform.
Quality Control Inspecting products, services, or processes to control quality and/or performance is similar like Equipment factor. However, here we focus more on software and end products rather than on machines itself. As expected, these skills are in more demand.
Analytics This factor has a quite low standard deviation, so it is almost unchanged for these 7 years because even computers are great in dealing with numbers we still need mathematical skills for new algorithms and various kinds of optimization problems.
Equipment Equipment skills are showing positive value which means that average occupational requirements are higher for this skill factor in 2015 than in 2008 which is normal due to the fact that now we have so many machines and automated processes which require humans to look after these.
Manual We see that manual is most affected which is expected because these kinds of jobs consist of the routine manual tasks which can be easily automated and replaced with machines. Hence, manual skills’ importance within jobs has decreased over time.
Cognitive This factor is showing negative value without weights and slightly negative with weights. This can be due to skills within it that can be shared with many other occupations.
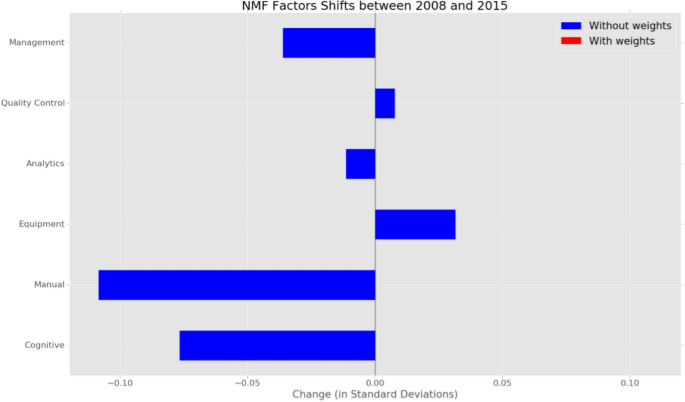
NMF factors shifts between 2008 and 2015
As was anticipated, depending on the data from which weights are applied, these factors can prevail on a different scale of demand—from lower to higher or vice versa. An interesting example is the one from weights related to academia. From Fig. 12 it can be seen that both LSI (left) and LDA (right) weights caused factor ‘Analytics’ predominate from lower to a higher demand. Two more factors that changed demand, but this time from higher to lower are ‘Equipment’ and ‘Quality Control’. Acknowledging the data weights are from academia, this is not a surprise because these two factors are more related to the industry.
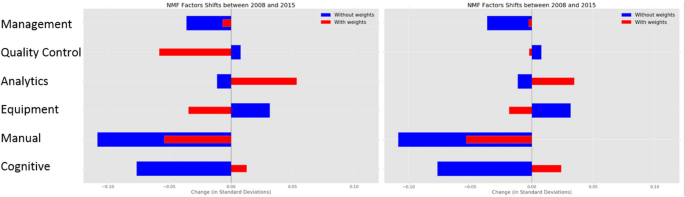
NMF GCC oil& gas courses LSI (left) and LDA (right)
FA model extracted five O*NET factors as shown in Table 7 .
FA model results for five O*NET Factors as described below:
Supervision This includes skills related to scheduling, work coordination, guiding, etc. In past years, technology has enabled workers to conduct their work without supervisory intervention. For instance, online resources, such as email exchanges and e-meetings, enable people to collaborate without requesting resources or scheduling meetings through supervisors. An even better example is a customer that now summon Uber car with one click on the application so no need to interact with a human dispatcher who in this example will be the supervisor.
Mathematics This factor was also extracted with NMF technique, so this again confirms the consistency of these two techniques. We get almost the same results with subtle standard deviation for mathematical skills which shows that these skills were almost unchanged in demand comparing 2008 and 2015 year.
Perception The importance of its skills are also decreased over time. This is because nowadays computers comprehend speech in ways they could never do before. Also, computer vision capabilities have advanced rapidly because of sensors so now computers can recognize various type of objects, comprehend writing, identify production defects on assembly lines, etc.
Equipment This factor was also extracted with NMF technique so this confirms the consistency of these two techniques. FA here shows an even stronger demand for these skills than NMF model. Interestingly, weights from the LSI model are showing even more importance to Equipment skill sets.
Problem Solving Obviously, these are the skills where a computer is still underperforming compared to the human brain. However, with fast-improving on the machine learning field, especially deep learning area, these are also threatened in the future to be replaced by the machine.
When the weights from LSI and LDA models were introduced to these five factors produced by FA model, there were no big changes in terms of changing the sides of demand, only the intensity of demand was strengthened. Nonetheless, in GCC and USA oil & gas industry there seems to be a slight discrepancy between LSI and LDA model as Figs. 13 , 14 , suggest. Videlicet, for both USA and GCC oil & gas industry, LDA model shows slightly more demand for Mathematical skill sets in 2015 compared to the 2008 year; while the LSI model shows imperceptibly less demand for the same skill-sets. The aforementioned disagreement can be neglected as the changes in standard deviations for this skill-sets are very small.
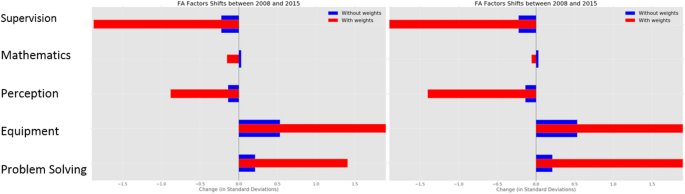
FA GCC (left) and USA (right) oil & gas LSI
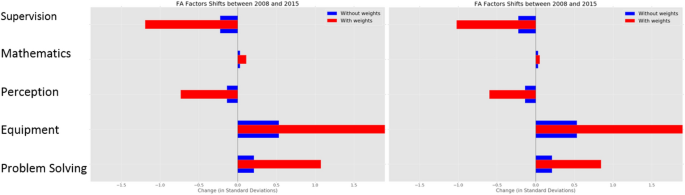
FA GCC (left) and USA (right) oil & gas LDA
The study aims to utilize data science techniques to come up with useful insights into the changes happening in the demand for certain jobs and skills in the oil & gas industry. To get a wholesome analysis, we did extensive as well as intensive analysis of the changes in jobs fro two geographical locations, USA and the GCC. There is extensive literature already existing on this topic but we are filling the gap of measuring the extent or magnitude of the changes in demand and supply of oil & gas industry jobs. The most important findings of this research are being discussed further:
Intensive Analysis The NMF model discovered that Quality Control Analysis factor is also in higher demand. By analyzing the skills from this factor, we see the importance of skills such as troubleshooting, programming, etc. One more factor that is in higher demand and which is identified by the FA model is Problem Solving. Indeed, this is also expected since today’s problems are not routine (the routine problems are easily solved with machines) but rather need critical thinking to be solved. Factors whose demand is lower in the year 2015 in comparison to the year 2008 are manual, cognitive, management, perception, and supervision. Due to continuous improvements in the capability of machines, they nowadays can understand speech, writing and can distinguish between different objects. Hence we can expect the factors like manual, perception and cognitive to get lower in demand over the years which is what our model tells us. But it comes as a surprise to see factors like supervision and management getting lower in terms of demand in oil & gas job market. When we examine the skills related to these two factors, we will also notice that they have been replaced with new technologies over time, as a result of which, the demand of these skills has been getting lower. A good example of the management skill “document and record information” we do not need any more to hold a collection of physical documents records. Instead, we have electronic versions and store them in the cloud or some similar online platform. Example for supervision factor can be a customer that now summon Uber car with one click on the application so no need to interact with a human dispatcher who in this example will be the supervisor. Nevertheless, one interesting skill where computers miserably fail and yet humans do it with ease is common sense.
One can easily observe from our findings, that in the oil & gas industry, the skills that are disappearing from demand in the job market are mostly those where machines can replace humans as a better alternative. But not only routine and manual skills are getting their demand lowered over time, few cognitive skills like management and supervision are also seeing a significant decline in their demand. We can also see examples where computers are able to generate artistic images [ 44 ] or music [ 45 ]. In addition, by applying weights from extensive analysis prior to factor extraction, we discovered that in academia, Mathematical and Analytical skill-sets are in higher demand in the year 2018 in comparison to the year 2008. The conclusion is that we should not ‘fight’ against the machines, rather we should cooperate, which as a result will have the best combination of skills in which performance humans and machines are the best. Furthermore, this will let humans focus more on the skills which computers still couldn’t perform well e.g. critical thinking and social skills like motivation and leadership. In particular, firms have become increasingly more likely to demand Social skills [ 46 ].
Extensive Analysis The extensive analysis for the GCC and USA oil & gas industry showed us that both regions have some overlapping jobs that are in high demand like ‘Petroleum Engineers’, ‘Mechanical Engineers’, ‘Civil Engineers’. However, more interestingly the results expose the different type of job markets between USA and GCC, where GCC is in more demand for engineers, while in the USA we can find high demand jobs for Manager and Human Resources (HR) positions. The question for future research is will the UAE job market for oil & gas industry lean more towards similar jobs as we can find now in the same industry in the USA. For the academic data of the oil & gas sector based on the observed results, the conclusion can be that the faculty is not providing the students with the specific skills which are required to perform a job in the oil & gas industry. They are imparting only some adequate general knowledge into the students. This can be compensated by additional training series organized by companies related to the industry. Through these series, by educating the students, companies will provide them with the required skills to work in the fast-developing oil & gas industry. In addition, students will need to explore beyond their field, to find interdisciplinary jobs that can be related to their field of expertise, so they can acquire new skills in order to ease the transfer into new interdisciplinary jobs that require the fusion of their old and newly acquired skills.
Recommendations based on findings in this article (In the context of the UAE):
The Job Analysis Framework Tool provided by us can be very helpful to policy decision-makers. Through this tool, they will be able to find the mismatch which exists between the skills which are imparted by educational institutions into the students and the skills which are required to perform specific jobs in the oil & gas industry. This may lead a way for the policymakers to enforce collaborations between several educational institutions with the required infrastructure and the companies in oil & gas sector. As a result of which, the educational institutions will be walking hand in hand with the industry to design the course curriculum and projects that can have a real impact on the industry. An example of such collaboration can be a University and its founded projects with private sectors. Aforementioned will help students to perform applied research and by doing so gain the experience needed in the real job market. Further, private sectors can suggest universities to add practice and help educational entities to design adequate courses to match skills sets with those that are in-demand at the job market. Hence when the students will graduate, they will have the required skills to immediately take up a job in one of those companies. This scenario would cause a win-win situation for both companies and educational institutions.
Due to the saturation of the public sector, UAE nationals should increase their involvement in the private sector. UAE is among the leading countries when it comes to automation of public sector processes. This is a major factor in the reduction in the number of jobs in the UAE public sector. Hence acquiring of right skills and proper guidance and support becomes very important. Our analysis tool provides intrinsic analysis which will help the UAE nationals to ultimately know the required skills and attain them. This will make life easy for the students in order to get a job in the private sector. Furthermore, policymakers should highly recommend educational systems to study more about problems that are highly related to the UAE like water desalinization, increasing demand for energy, food production, etc. which should inspire Emiratis to start their own private companies that should tackle these or similar issues and help increase the creative solutions developed inside the UAE.
When we have a look at our Extrinsic analysis, we find out that there has been an increase in the importance of certain occupations that are interdisciplinary in nature. Thus, Interdisciplinary knowledge, entrepreneurship, and innovation must be promoted in the students and the youth. This will ultimately lead to the flourishing of the startup ecosystem and new companies will be born.
Although in this article we only discussed about oil & gas industry in GCC and USA, it should be noted that the job analysis website https://www.kudsom.org/ can serve as an open resource for further research. It can be used for validating and verifying the results for any industry in any geographical location. Finally, the main objective of the research is attained, and that is to provide a comprehensive data analytics method to enhance the process of studying the actual market behavior and future needs based on real data acquired.
While the displacement of manual labor for repetitive and low skilled jobs has been ongoing for some time, the automation of high skilled, knowledge intensive tasks is a recent trend, and has profoundly affected almost all sectors of the oil & gas industry. Understanding and predicting the future impact of these changes is vital if appropriate actions are to be taken and effective policies formulated in response to these trends. Of equal importance is the need to create public awareness about the extent to which automation and other disruptive technologies will affect existing occupations in the near future. This should help to mitigate the impact on society and allow individuals to make informed decisions about their career paths.
The factorization techniques presented in this paper can be used to study the changes in demand for skills over specific industries and market. As a demonstration of this, the use of FA and NMF were demonstrated using occupational data from the years 2008 and 2015, and later 2008 and 2018. These valuable findings confirmed the concerns that automation is decreasing the certain skills demand. On the other hand, we also identified the new emerging skills that are required to strengthen the need for collaboration between minds and machines. LSI and LDA models for extrinsic analysis also proved to be incredibly useful for analyzing changes in job demands. Not only this analysis provided summarized jobs ordered by demand in a standardized view, but it also helps identify the differences between UAE and USA markets job markets. Furthermore, it helped to get insights into differences between skills that academia is equipping students, and skills that industry is requiring on the field.
Nevertheless, the analysis and decision making with regards to the HR industry to predict needed skills for the future of jobs shall be accompanied by an analytical tool. Therefore, a state of art approach has been developed and implemented in this study to further support decision-makers to make informed decisions by leveraging the use of data available in the job market.
It can be concluded that in the oil & gas industry, there are skills required which can not be completely replaced by machines but some of the skills can be replaced. If humans compete against machines in the skills where machines can perform better, then we would face the issue of under productivity. Hence humans should learn to work along with the machines and complement each other to get maximum benefits. In the era of new invasive emerging technologies, such as AI and ML, the future of work will be very much controlled by how we humans develop a symbiotic relationship with these technologies.
Availability of data and materials
The datasets generated and/or analysed during the current study are available in the following GitHub link: https://github.com/ArminMne/JobAnalysisFramework
https://www.onetcenter.org/ .
Flask: http://flask.pocoo.org/ .
Bootstrap: https://getbootstrap.com/ .
PythonAnywhere: https://www.pythonanywhere.com/ .
Rossetti MD, Felder RA, Kumar A. Simulation of robotic courier deliveries in hospital distribution services. Health Care Manag Sci. 2000;3(3):201–13.
Article Google Scholar
Halal W, Kolber J, Davies O, Global T. Forecasts of AI and future jobs in 2030: muddling through likely, with two alternative scenarios. J Future Stud. 2017;21(2):83–96.
Google Scholar
Moravec H. Mind children: the future of robot and human intelligence. Cambridge: Harvard University Press; 1988.
Brynjolfsson E, McAfee A. Race against the machine: how the digital revolution is accelerating innovation, driving productivity, and irreversibly transforming employment and the economy. Brynjolfsson and McAfee; 2012.
Brynjolfsson E, McAfee A. The second machine age: work, progress, and prosperity in a time of brilliant technologies. New York: WW Norton & Company; 2014.
MacCrory F, Westerman G, Alhammadi Y, Brynjolfsson E. Racing with and against the machine: changes in occupational skill composition in an era of rapid technological advance (2014)
Illéssy M, Makó C. Automation and creativity in work: Which jobs are at risk of automation? Intersections. East Eur J Soc Polit. 2020;6(2).
Kowalczyk R. Fuzzy e-negotiation agents. Soft Comput. 2002;6(5):337–47.
Broadbent E, Stafford R, MacDonald B. Acceptance of healthcare robots for the older population: review and future directions. Int J Soc Robot. 2009;1(4):319.
Alekseeva L, Azar J, Gine M, Samila S, Taska B. The demand for AI skills in the labor market. Labour Econ. 2021;71:102002.
Lee C-S, Wang M-H, Wu M-J, Nakagawa Y, Tsuji H, Yamazaki Y, Hirota K. Soft-computing-based emotional expression mechanism for game of computer go. Soft Comput. 2013;17(7):1263–82.
Karakatsanis I, AlKhader W, MacCrory F, Alibasic A, Omar MA, Aung Z, Woon WL. Data mining approach to monitoring the requirements of the job market: a case study. Inf Syst. 2017;65:1–6.
Woon, W.L., Aung, Z., AlKhader, W., Svetinovic, D., Omar, M.A.: Changes in occupational skills-a case study using non-negative matrix factorization. In: International Conference on Neural Information Processing, pp. 627–634. Springer (2015)
Booz M. These 3 industries have the highest talent turnover rates. LinkedIn Talent Blog. Business LinkedIn. 2018.
Alibasic A, Simsekler MCE, Kurfess T, Woon WL, Omar MA. Utilizing data science techniques to analyze skill and demand changes in healthcare occupations: case study on USA and UAE healthcare sector. Soft Comput. 2020;24(7):4959–76.
Jensen PB, Jensen LJ, Brunak S. Mining electronic health records: towards better research applications and clinical care. Nat Rev Genet. 2012;13(6):395.
Li J, Fong S, Zhuang Y, Khoury R. Hierarchical classification in text mining for sentiment analysis of online news. Soft Comput. 2016;20(9):3411–20.
Rajpathak D, De S. A data-and ontology-driven text mining-based construction of reliability model to analyze and predict component failures. Knowl Inf Syst. 2016;46(1):87–113.
Brüning, N., Mangeol, P.: What skills do employers seek in graduates?: Using online job posting data to support policy and practice in higher education (2020)
Olson DL, Delen D. Advanced data mining techniques. Berlin: Springer; 2008.
MATH Google Scholar
Han J, Pei J, Kamber M. Data mining: concepts and techniques. Amsterdam: Elsevier; 2011.
Sarkar D. Processing and understanding text. In: Text Analytics with Python, Springer. 2016, p. 107–165.
Denny MJ, Spirling A. Text preprocessing for unsupervised learning: why it matters, when it misleads, and what to do about it. Polit Anal. 2018;26(2):168–89.
Horst P. Factor analysis of data matrices. New York: Holt, Rinehart and Winston; 1965.
Lawley DN, Maxwell AE. Factor analysis as a statistical method, vol. 18. Hoboken: Wiley Online Library; 1971.
Jolliffe I. Principal component analysis. Encyclopedia of statistics in behavioral science (2005)
Yong AG, Pearce S. A beginner’s guide to factor analysis: focusing on exploratory factor analysis. Tutor Quant Methods Psychol. 2013;9(2):79–94.
Lee DD, Seung HS. Learning the parts of objects by non-negative matrix factorization. Nature. 1999;401(6755):788.
Dumais ST, Furnas GW, Landauer TK, Deerwester S, Harshman R: Using latent semantic analysis to improve access to textual information. In: Proceedings of the SIGCHI Conference on Human Factors in Computing Systems, pp. 281–285 (1988). ACM
Deerwester S, Dumais ST, Furnas GW, Landauer TK, Harshman R. Indexing by latent semantic analysis. J Am Soc Inf Sci. 1990;41(6):391.
Rehurek R, Sojka P. Software framework for topic modelling with large corpora. In: Proceedings of the LREC 2010 Workshop on New Challenges for NLP Frameworks, pp. 45–50. ELRA, Valletta, Malta. 2010.
Manning CD, Raghavan P, Schütze H, et al. Introduction to information retrieval, vol. 1. Cambridge: Cambridge University Press Cambridge; 2008.
Book Google Scholar
Bradford, R.B.: An empirical study of required dimensionality for large-scale latent semantic indexing applications. In: Proceedings of the 17th ACM Conference on Information and Knowledge Management, pp. 153–162 (2008). ACM.
Blei DM, Ng AY, Jordan MI. Latent Dirichlet Allocation. J Mach Learn Res. 2003;3:993–1022.
Ma Z, Lai Y, Xie J, Meng D, Kleijn WB, Guo J, Yu J. Dirichlet process mixture of generalized inverted dirichlet distributions for positive vector data with extended variational inference. IEEE Trans Neural Netw Learn Syst. 2021. https://doi.org/10.1109/TNNLS.2021.3072209 .
Gregor H. Parameter estimation for text analysis. Technical report (2005)
Zhao W, Chen JJ, Perkins R, Liu Z, Ge W, Ding Y, Zou W. A heuristic approach to determine an appropriate number of topics in topic modeling. BMC Bioinform. 2015;16:8.
Can F, Ozkarahan EA. Concepts and effectiveness of the cover-coefficient-based clustering methodology for text databases. ACM Trans Database Systems (TODS). 1990;15(4):483–517.
Mimno D, Wallach HM, Talley E, Leenders M, McCallum A. Optimizing semantic coherence in topic models. In: Proceedings of the Conference on Empirical Methods in Natural Language Processing, pp. 262–272 (2011). Association for Computational Linguistics.
Hellinger E. Neue begründung der theorie quadratischer formen von unendlichvielen veränderlichen. Journal für die reine und angewandte Mathematik. 1909;136:210–71.
Article MathSciNet Google Scholar
Anaya LH. Comparing Latent Dirichlet Allocation and latent semantic analysis as classifiers. Ohio: ERIC; 2011.
Harry W. Employment creation and localization: the crucial human resource issues for the gcc. Int J Hum Resour Manag. 2007;18(1):132–46.
Larose DT. k-nearest neighbor algorithm. Discovering knowledge in data: An introduction to data mining, 90–106 (2005)
Gatys LA, Ecker AS, Bethge M. A neural algorithm of artistic style. arXiv preprint arXiv:1508.06576 ; 2015.
Gautam S, Soni S. Artificial intelligence techniques for music composition. Int J Sci Res Comput Sci Eng Inform Technol. 2018;3(3):385–9.
Hansen S, Ramdas T, Sadun R, Fuller J. The demand for executive skills. National Bureau of Economic Research: Technical report; 2021.
Download references
Acknowledgements
Not applicable.
This publication is based upon work supported by the Khalifa University of Science and Technology under Award No. RCII-2019-002, Center for Digital Supply Chain and Operations Management.
Author information
Authors and affiliations.
Department of Industrial and Systems Engineering, Khalifa University of Science and Technology, 127788, Abu Dhabi, UAE
Armin Alibasic, Himanshu Upadhyay, Mecit Can Emre Simsekler & Mohammed Atif Omar
George W. Woodruff School of Mechanical Engineering, Georgia Insitute of Technology, Atlanta, GA, 30332, US
Thomas Kurfess
Department of Marketplaces and Yield Data Science, Expedia Group, Seattle, WA, 98119, US
Wei Lee Woon
You can also search for this author in PubMed Google Scholar
Contributions
AA: Conceptualization, Methodology, Software, Data curation, Writing- Original draft preparation. HU: Writing- Reviewing and Editing. MCES: Writing- Reviewing and Editing TK: Writing- Reviewing and Editing. WLW: Writing- Reviewing and Editing. MAO: Writing- Reviewing and Editing. All authors read and approved the final manuscript.
Corresponding author
Correspondence to Armin Alibasic .
Ethics declarations
Ethics approval and consent to participate.
This article does not contain any studies with human participants or animals performed by any of the authors.
Competing interests
The authors declare that they have no competing interests.
Additional information
Publisher's note.
Springer Nature remains neutral with regard to jurisdictional claims in published maps and institutional affiliations.
Rights and permissions
Open Access This article is licensed under a Creative Commons Attribution 4.0 International License, which permits use, sharing, adaptation, distribution and reproduction in any medium or format, as long as you give appropriate credit to the original author(s) and the source, provide a link to the Creative Commons licence, and indicate if changes were made. The images or other third party material in this article are included in the article's Creative Commons licence, unless indicated otherwise in a credit line to the material. If material is not included in the article's Creative Commons licence and your intended use is not permitted by statutory regulation or exceeds the permitted use, you will need to obtain permission directly from the copyright holder. To view a copy of this licence, visit http://creativecommons.org/licenses/by/4.0/ .
Reprints and permissions
About this article
Cite this article.
Alibasic, A., Upadhyay, H., Simsekler, M.C.E. et al. Evaluation of the trends in jobs and skill-sets using data analytics: a case study. J Big Data 9 , 32 (2022). https://doi.org/10.1186/s40537-022-00576-5
Download citation
Received : 09 September 2021
Accepted : 06 February 2022
Published : 19 March 2022
DOI : https://doi.org/10.1186/s40537-022-00576-5
Share this article
Anyone you share the following link with will be able to read this content:
Sorry, a shareable link is not currently available for this article.
Provided by the Springer Nature SharedIt content-sharing initiative
- Future of work
- Jobs and skills analysis
- Data analytics
- Natural language processing
- Publications
- Key Findings
- Economy, industry, region and skill profiles
- Infographics
- Full report
The Future of Jobs Report 2023
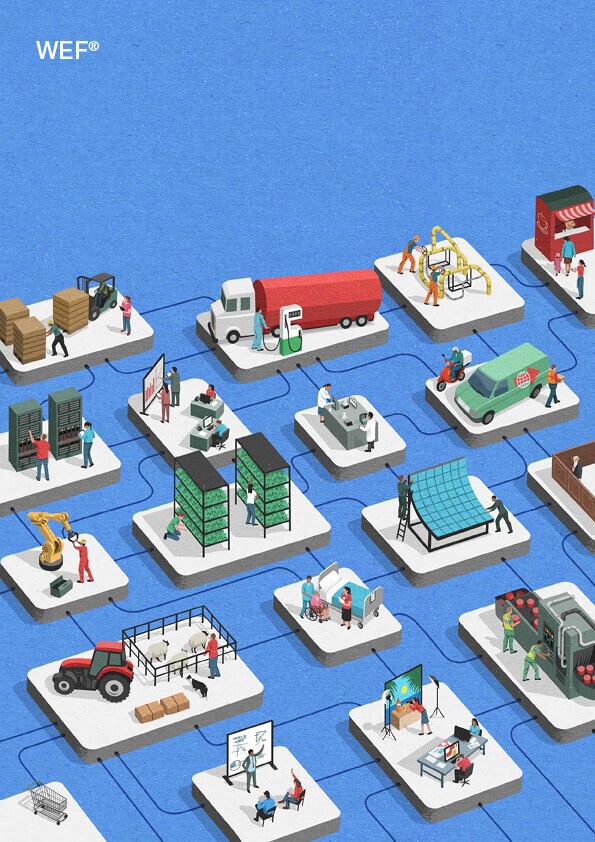
1. Introduction: the global labour market landscape in 2023
The past three years have been shaped by a challenging combination of health, economic and geopolitical volatility combined with growing social and environmental pressures. These accelerating transformations have and continue to reconfigure the world’s labour markets and shape the demand for jobs and skills of tomorrow, driving divergent economic trajectories within and across countries, in developing and developed economies alike. The Fourth Industrial Revolution, changing worker and consumer expectations, and the urgent need for a green and energy transition are also reconfiguring the sectoral composition of the workforce and stimulating demand for new occupations and skills. Global supply chains must also quickly adapt to the challenges of increasing geopolitical volatility, economic uncertainty, rising inflation and increasing commodity prices.
Like previous editions, The Future of Jobs Report 2023 offers insights into these transformations and unpacks how businesses are expecting to navigate these labour-market changes from 2023 to 2027, leveraging a unique cross-sectoral and global survey of Chief Human Resources, Chief Learning Officers and Chief Executive Officers of leading global employers and their peers.
This report is structured as follows: Chapter 1 reviews the global labour-market landscape at the beginning of 2023. Chapter 2 explores how key macrotrends are expected to transform this landscape over the 2023–2027 period. Chapters 3 and 4 then discuss the resulting global outlooks for jobs and skills over the 2023–2027 period. Chapter 5 reviews emerging workforce and talent strategies in response to these trends. The report’s appendices provide an overview of the report’s survey methodology and detailed sectoral breakdowns of the five-year outlook for macrotrends, technology adoption and skills.
In addition, The Future of Jobs Report 2023 features a comprehensive set of Economy, Industry, and – for the first time – Skill Profiles. User Guides are provided for each of these profiles, to support their use as practical, standalone tools.
As a foundation for analysing respondents’ expectations of the future of jobs and skills in the next five years, this chapter now assesses the current state of the global labour-market at the beginning of 2023.
Diverging labour-market outcomes between low-, middle- and high-income countries
The intertwined economic and geopolitical crises of the past three years created an uncertain and divergent outlook for labour markets, widening disparities between developed and emerging economies and among workers. Even as a growing number of economies have begun to recover from the COVID-19 pandemic and its associated lockdowns, low- and lower-middle-income countries continue to face elevated unemployment, while high-income countries are generally experiencing tight labour markets.
At the time of publication, the latest unemployment rates stand below pre-pandemic rates in three quarters of OECD countries, 1 and across a majority of G20 economies (Figure 1.1). At 4.9%, the 2022 unemployment rate across the OECD area is at its lowest level since 2001. 2
By contrast, many developing economies have experienced a comparatively slow labour-market recovery from the disruptions induced by the COVID-19 pandemic. In South Africa, for example, the formal unemployment rate has climbed to 30%, five percentage points higher than it was pre-pandemic (Figure 1.1). Developing economies, especially those reliant on the sectors hardest hit by recurring lockdowns, such as hospitality and tourism, still exhibit slow labour-market recoveries.
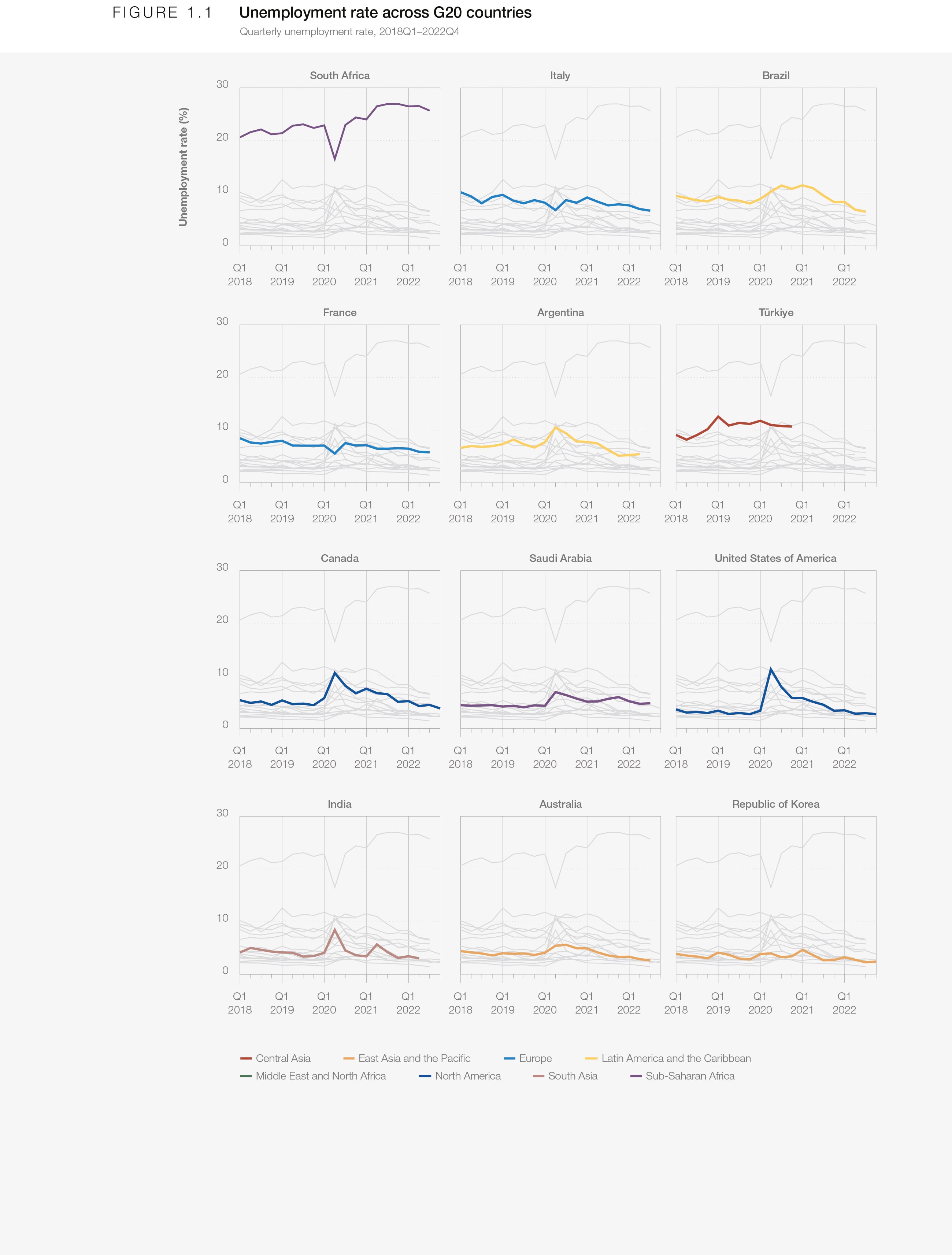
The asymmetry of the recovery is exacerbated by countries’ varying capacities to maintain policy measures to protect the most vulnerable and maintain employment levels. While advanced economies were able to adopt far-reaching measures, emerging economies have provided less support to the most vulnerable firms and workers due to their limited fiscal space. 3,4
In 2022, various employment indicators pointed towards a strong labour-market recovery for high-income countries, with many sectors experiencing labour shortages. In Europe, for example, almost three in 10 manufacturing and service firms reported production constraints in the second quarter of 2022 due to a lack of workers. 5 Nursing professionals, plumbers and pipefitters, software developers, systems analysts, welders and flame cutters, bricklayers and related workers, and heavy truck and lorry drivers were among the most needed professions (Figure 1.2).
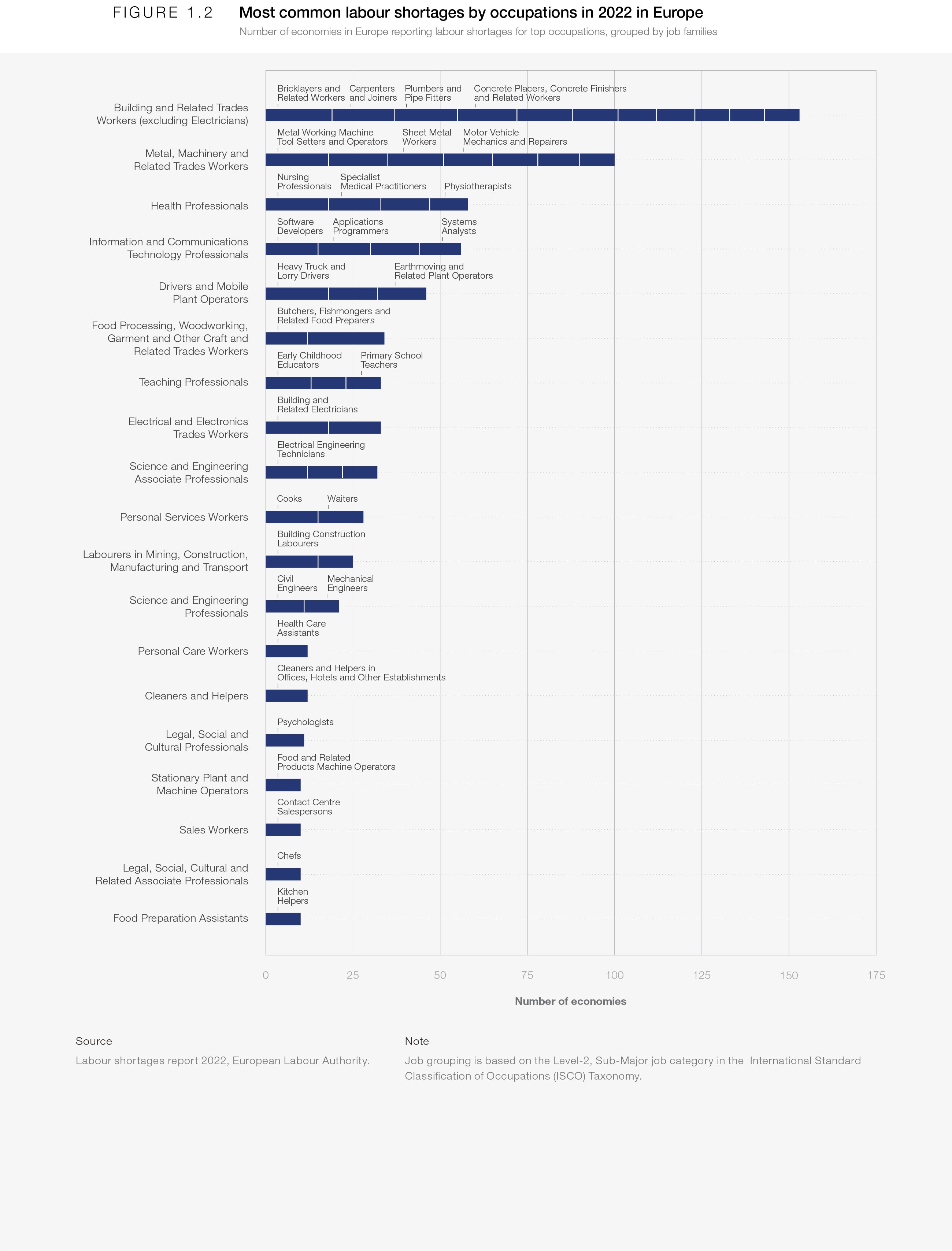
In the United States, businesses in Retail and Wholesale of Consumer Goods reported close to 70% of job openings remaining unfilled, with close to 55% of roles unfilled in manufacturing and 45% in leisure and hospitality. 6 Businesses also reported difficulties in retaining workers. According to a global survey conducted in late 2022 across 44 countries, one in five employees reported they intend to switch employers in the coming year. 7
Diverging employment levels by gender, age and education level
Women experienced greater employment loss than men during the pandemic 8 , and according to the World Economic Forum’s Global Gender Gap Report 2022 9 , gender parity in the labour force stands at 62.9% – the lowest level registered since the index was first compiled. The global pandemic also disproportionately impacted young workers, with less than half of the global youth employment deficit projected to have recovered by the end of 2022. 10 As highlighted in Figure 1.3, the youth employment deficit relative to 2019 is largest in Southern Asia, Latin America, Northern Africa and Eastern Europe, with only Europe and North America likely to have fully recovered at the time of publication.
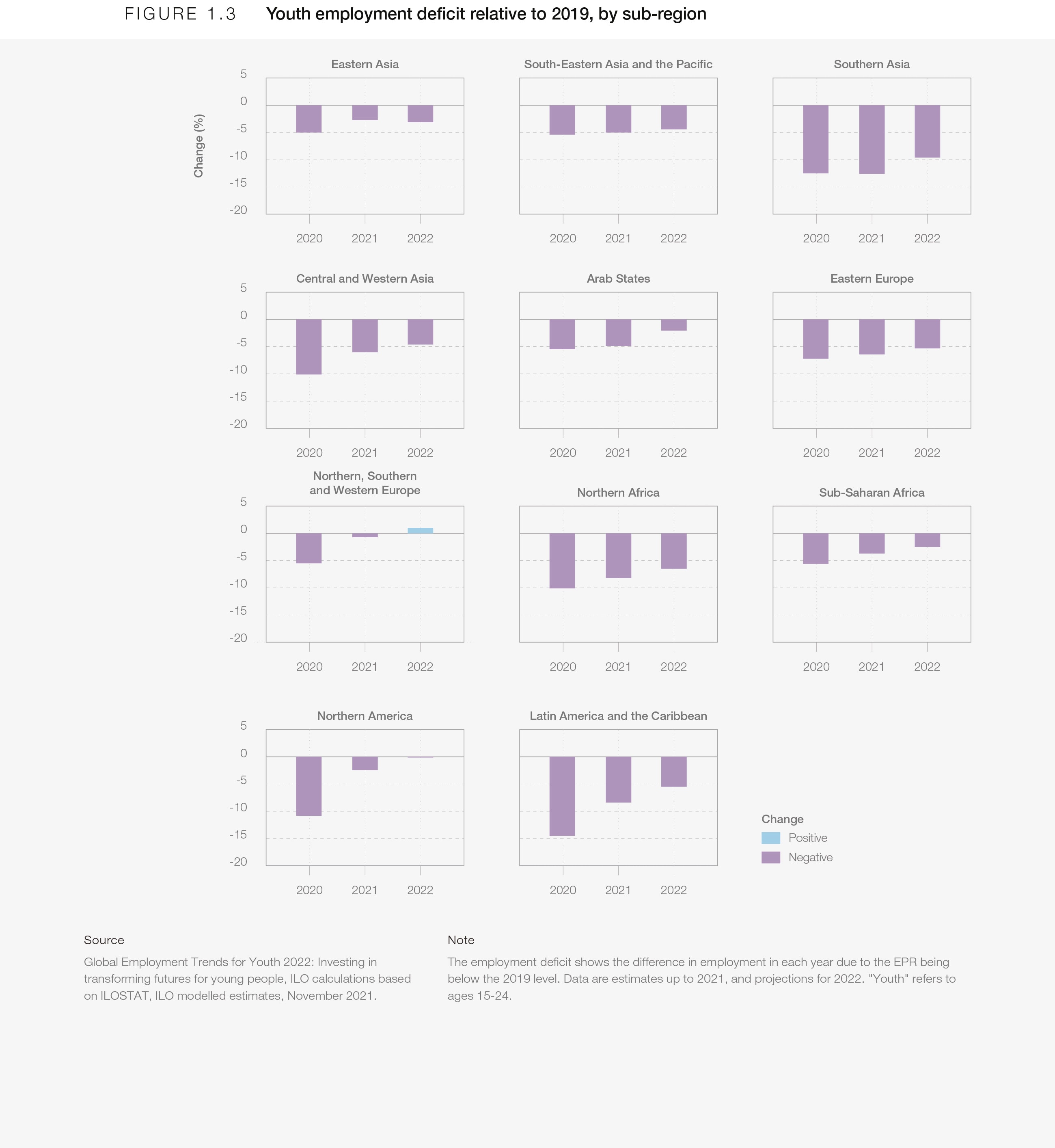
Workers with a basic education were also hardest hit in 2020, and slower to recover their prior participation in the labour market. In many countries the increase in unemployment from 2019 to 2021 of workers with a basic education level was more than twice as large as the impact on workers with advanced education (Figure 1.4).
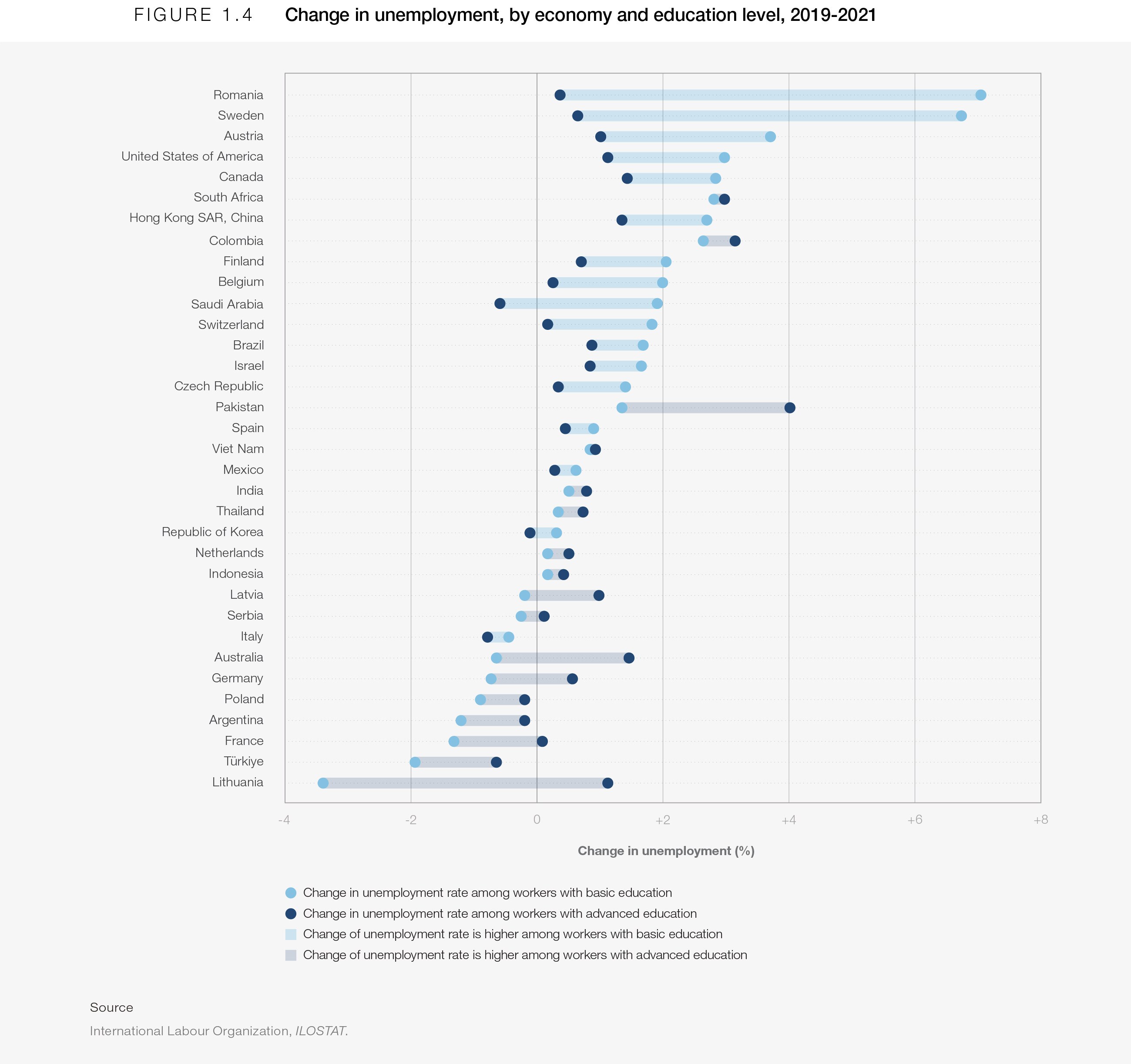
Access to social protection
From January 2020 to January 2022, almost 3,900 social-protection measures were implemented across 223 economies to support the labour force impacted by COVID-19. 11 These measures are estimated to have reached close to 1.2 billion people globally. Wage subsidies, cash transfers, training measures and extending unemployment-benefit coverage have all been crucial tools to protect the most vulnerable during the pandemic. Most such short-term support measures are now being phased out, 12 and targeted medium to long-term investments will be needed to alleviate the long-term effects of recurring economic shocks on firms and workers.
Yet, there remains an urgent need to provide adequate social protection to those not covered by full-time employment contracts (Figure 1.5). Nearly 2 billion workers globally are in informal employment, representing close to 70% of workers in developing and low-income countries, as well as 18% in high income ones. 13 Given their susceptibility to economic shocks and working poverty, informal workers represent a crucial labour-market cohort and need better representation in data, broad-based income support in the short term and a longer term shift towards formalization.
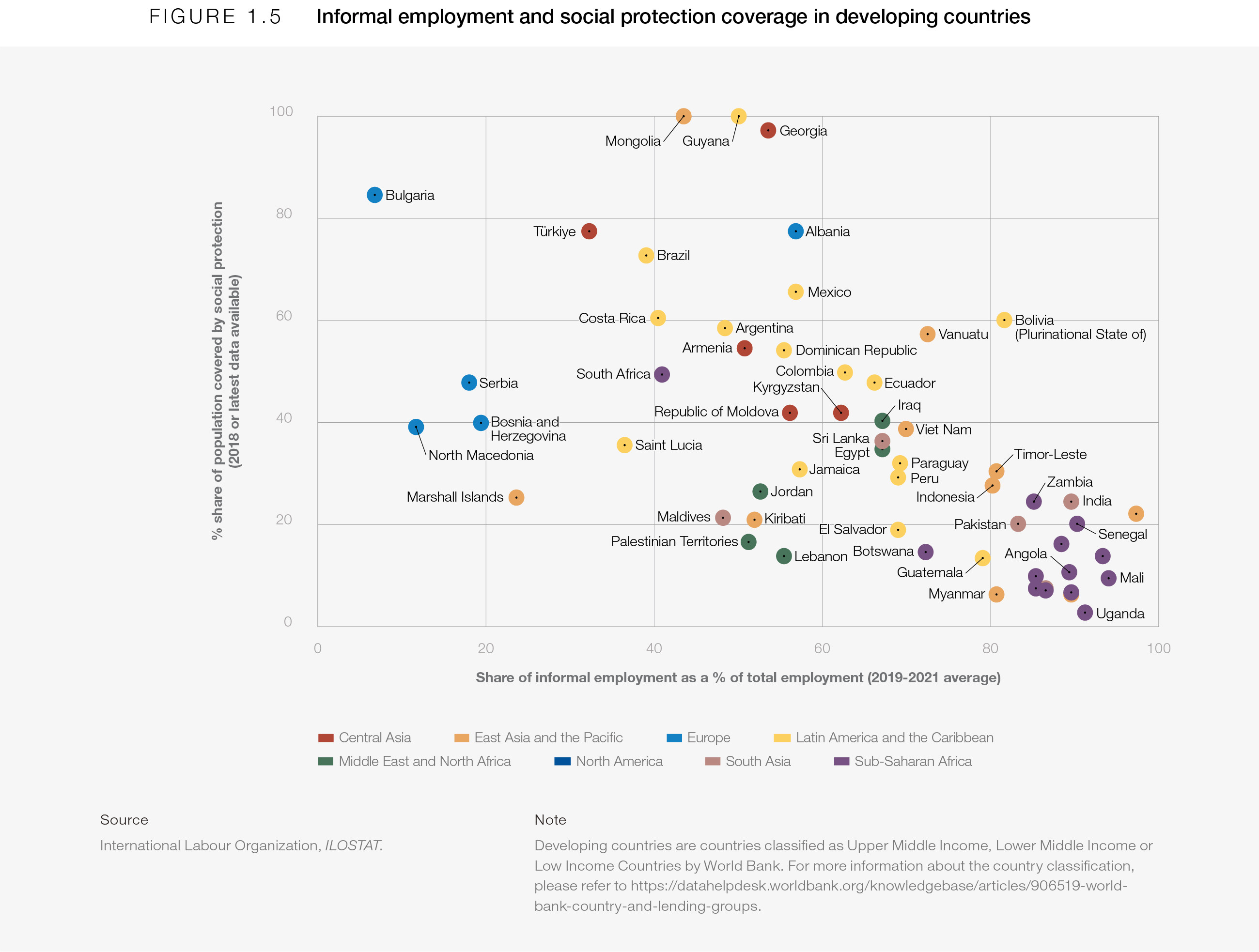
Real wages and cost of living
According to the International Labour Organization (ILO), labour income in many developing countries remains below pre-pandemic levels. 14 In 2020, the global economy started experiencing inflation levels not seen in almost 40 years. 15 With high inflation, the global cost-of-living crisis has hit the most vulnerable hardest. 16 According to the ILO, for the first time over the last 15 years, workers’ real wages have declined – by 0.9% in the first half of 2022. 17
Across regions, real wage growth was most affected in Northern, Southern and Western Europe; Latin America; Asia Pacific; and North America. 18 In Africa, real wages saw a 10.5% drop in 2020 due to the global pandemic. 19 However, real wages have continued to increase in 2022 across Asia Pacific, Central and Western Asia and Arab states. 20
In line with rising inflation, purchasing power has declined for the most vulnerable, given the higher weight of energy and food in expenditures of the lowest-income households. 21 According to recent research by the United Nations Development Programme (UNDP), rising food and energy prices could push up to 71 million people into poverty, with hot spots in Sub-Saharan Africa, the Balkans and the Caspian Basin. 22 This cost-of-living crisis highlights the importance of designing permanent models of social protection for non-standard employment and the informal economy that provide security and support resilience. 23
Worker preferences
In this context of diverging labour-market outcomes, issues around the quality of work have come to the fore. This section reviews some of the latest worker preference research to analyse which job attributes are of most importance to workers currently. As a starting point, data shows workers, openness to changing employer. Data on worker preferences from CultureAmp 24 and Adecco 25 find that more than a quarter (33% and 27% of workers, respectively) do not see themselves at their current company of employment in two years’ time. In line with this, a little under half of workers (42% and 45%, according to CultureAmp and Adecco, respectively) actively explore opportunities at different companies.
Worker surveys at both CultureAmp 26 and Randstad 27 suggest that salary levels are the main reason workers decide to change their job. 52% of Randstad respondents say they worry about the impact of economic uncertainty on their employment and 61% of respondents to Adecco’s worker-preference survey worry that their salary is not high enough to keep pace with the cost of living given rising rates of inflation. 28
Additional data explores the protection and flexibility of employment: 92% of respondents to Randstad’s employee survey 29 say job security is important and more than half of these respondents wouldn’t accept a job that didn’t give assurances regarding job security. 83% prioritize flexible hours and 71% prioritize flexible locations.
A fourth theme identified by workers is work-life balance and burnout: 35% of CultureAmp respondents indicate that work-life balance and burnout would be the primary reason to leave their employer. Workers responding to Randstad’s employee survey 30 value salary and work-life balance equally, with a 94% share identifying both aspects of employment as important to choosing to work in a particular role.
Data also suggests that diversity, equity and inclusion (DEI) at work is particularly important to young workers. According to Manpower, 31 68% of Gen Z workers are not satisfied with their organization’s progress in creating a diverse and inclusive work environment, and 56% of Gen Z workers would not accept a role without diverse leadership. Meanwhile, data suggests that fewer women than men are trained.
Lastly, workers across age ranges indicate dissatisfaction about training opportunities. Manpower data 32 show that 57% of surveyed employees are pursuing training outside of work, because company training programmes do not teach them relevant skills, advance their career development or help them stay competitive in the labour market. Respondents to Adecco’s survey criticize companies for focusing their efforts too much on managers’ development, skills and rewards. Only 36% of non-managers who responded to Adecco’s survey said that their company is investing effectively in developing their skills, compared to 64% of managers.
Employment shifts across sectors
The past two years have witnessed a volatility in the demand and supply of goods and services resulting from lockdowns and supply-chain disruptions. The global economic rebound has reconfigured the sectoral distribution of employment across industries. Figure 6 presents OECD data demonstrating that, while Information Technology and Digital Communications experienced a strong rebound in most countries, the Accommodation, Food and Leisure; Manufacturing and Consumer; and Wholesale and Consumer Goods sectors are experiencing a slower rate of recovery. Since the first quarter of 2019, a majority of countries have experienced employment growth in Professional Services, Education and Training, Health and Healthcare, and Government and Public Sector, but employment in the Supply Chain and Transportation and Media, Entertainment and Sports sectors lags behind 2019 levels.
In addition to the pandemic-induced employment shifts we have seen across sectors during the last few years, generative AI models are likely to continue shaping sectoral shifts in employment. While AI applications are shown to be effective general-purpose technologies, 33 the development of general-purpose technologies have previously been hard to predict, which is why regulation needs to be both prompt and adaptable as institutions learn how these technologies can be used.
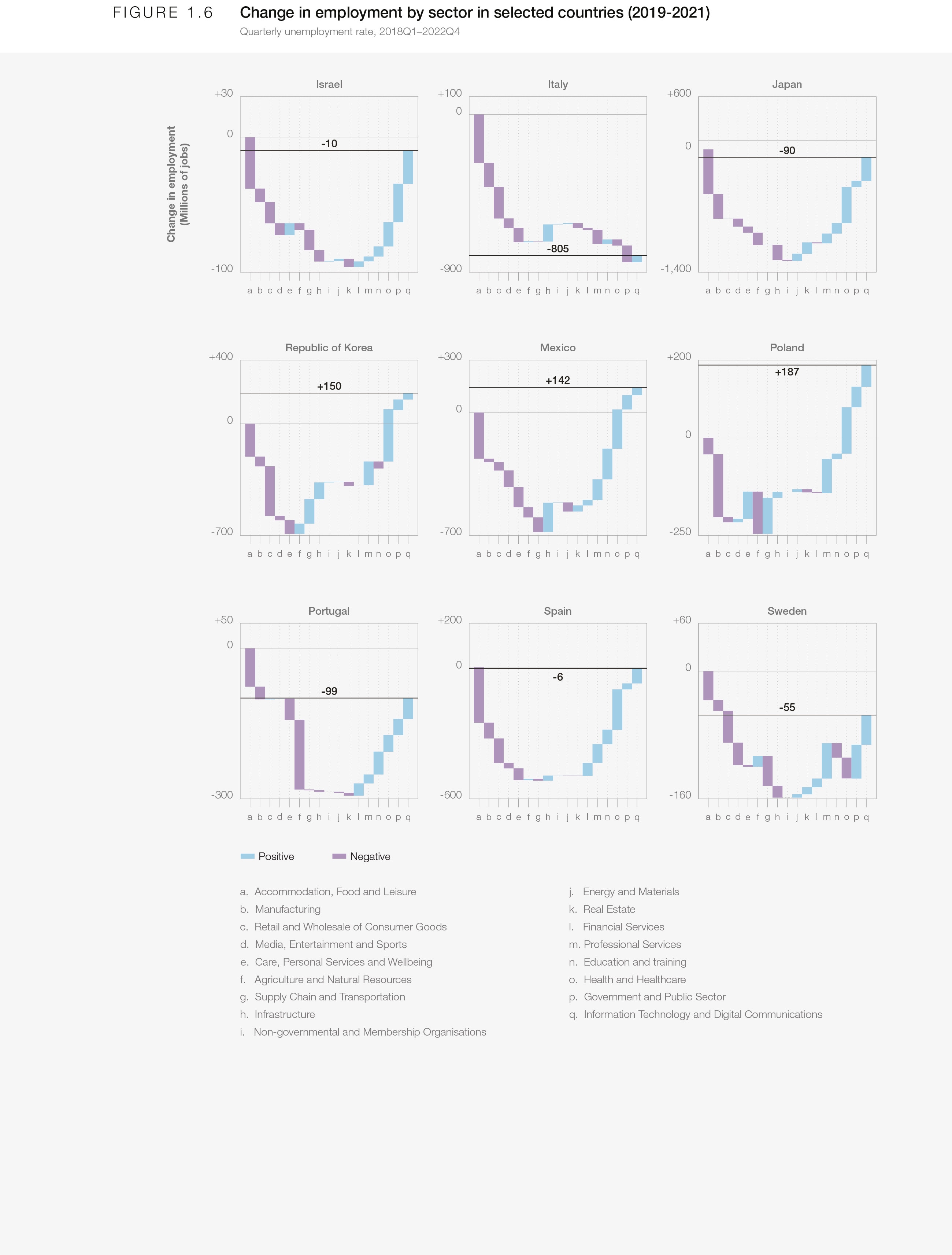
Through research conducted for the Future of Jobs Report, LinkedIn has identified the fastest growing roles globally over the past four years, shedding further light on the types of jobs employers have been seeking (Box 1.1).
The transformations that labour markets are experiencing have also increased the need for swifter and more efficient job reallocation mechanisms within and across different firms and sectors. The coming years represent a generational opportunity for businesses and policy-makers to embrace a future of work which fosters economic inclusion and opportunity, sets in place policies which will influence not only the rate of growth but its direction, and contribute to shaping more inclusive, sustainable and resilient economies and societies.
The green transition, technological change, supply-chain transformations and changing consumer expectations are all generating demand for new jobs across industries and regions. However, these positive drivers are offset by growing geoeconomic tensions and a cost-of-living crisis. 34
The Future of Jobs Survey was conducted in late 2022 and early 2023 bringing together the perspective of 803 companies – collectively employing more than 11.3 million workers – across 27 industry clusters and 45 economies from all world regions. The Survey covers questions of macrotrends and technology trends, their impact on jobs, their impact on skills, and the workforce transformation strategies businesses plan to use.
This chapter analyses findings from the World Economic Forum’s Future of Jobs Survey to explore how businesses expect macrotrends and technology adoption to drive industry transformation and employment.
BOX 1.1 The fastest-growing jobs support sales growth and customer engagement, the search for talent, and technology/IT
In collaboration with LinkedIn
Research conducted by LinkedIn for the Future of Jobs Report 2023 describes the 100 roles that have grown fastest, consistently and globally, over the last four years – known as the “Jobs on the Rise”. While ILO and OECD data show which sectors are employing more people, Jobs on the Rise data identifies the specific job types that have experienced significant growth. Figure B.1 organizes the 100 Jobs on the Rise into broad types.
In line with ILO and OECD data on the growth of roles in the Information Technology and Digital Communication sector, Technology and IT related roles make up 16 of the top 100 Jobs on the Rise, the third-highest of all job groupings. Jobs related to Sales Growth and Customer Engagement top the list, with 22 of the 100 roles. With roles such as Sales Development Representatives, Director of Growth, and Customer Success Engineer featuring in this group, this may suggest an increasing focus on broadening customer groups and growth models in a world with increasing digital access and rapid technological advancement (more detail on how increasing digital access and adoption of frontier technologies could transform demand for specific job types is available in Chapter 3). Human Resources and Talent Acquisition roles are the second-most popular roles, and most of these relate to Talent Acquisition and Recruitment, including a specific role for Information Technology Recruitment – perhaps illustrating the increasing difficulty and importance of accessing talent in a generally strong labour market.
Of the groups further down the list, Sustainability and Environment related roles are notable for all being in the top 40, including three of the top 10 roles (Figure B.2). This might suggest the green transition is both a significant and developing labour-market trend, where roles have titles such as “Sustainability Analyst”. Chapter 3 further examines the outlook for roles related to a green transition.
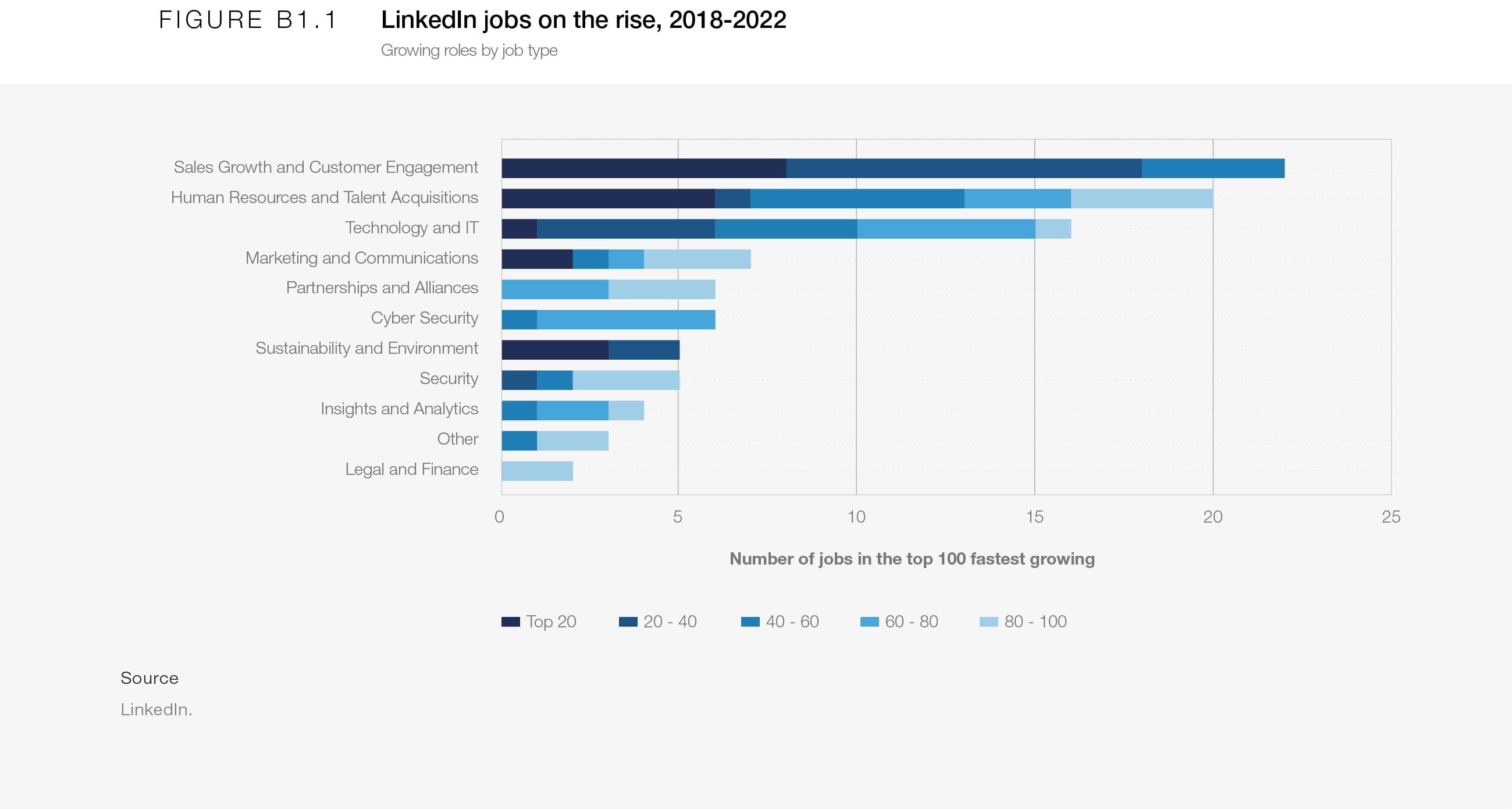
Job Market Paper
“does mobility make a nice person theory and evidence from across the globe”.
In much of modern life, cooperation takes the form of people engaging in costly behavior that helps another. It is easy to understand how cooperation might be achieved in small communities where members interact repeatedly. However, in our modern world, there is a high degree of relational mobility – where individuals can easily change locations and/or social groups. How does this affect cooperative behavior? I examine this question from both theoretical and empirical perspectives. I first develop a model of repeated prisoner’s dilemma. Individuals are matched but have some ability to leave the relationship. This is sufficient to show that, perhaps surprisingly, greater relational mobility actually leads to more cooperation in equilibrium, and the model predicts a stronger effect when players are more patient. I take these predictions to the data by first conducting a meta-analysis of twelve prisoner’s dilemma experiments that varied the amount of mobility in and out of relationships. I also examine the predictions of the model using data from the World Values Survey and Gallup World Poll. I find that looking across individuals, more relational mobility in the region is associated with greater cooperation and that this relationship is stronger when individuals are more patient. Both the experimental and the observational evidence are consistent with the theoretical mechanism.
- Original Article
- Open access
- Published: 02 November 2023
Job market polarization and American poverty
- Abu Bakkar Siddique ORCID: orcid.org/0000-0002-9964-7511 1
Journal for Labour Market Research volume 57 , Article number: 30 ( 2023 ) Cite this article
2318 Accesses
1 Citations
2 Altmetric
Metrics details
The article posits that the puzzles of stagnating poverty rates amidst high growth and declining unemployment in the United States can be substantially explained by polarized job markets characterized by job quality and job distribution. In recent decades, there has been an increased number of poor-quality jobs and an unequal distribution of jobs in the developed world, particularly in the United States. I have calculated measures of uneven job distribution indices that account for the distribution of jobs across households. A higher value of the uneven job distribution indices implies that there are relatively large numbers of households with multiple employed people and households with no employed people. Similarly, poor-quality jobs are those jobs that do not offer full-time work. Two-way fixed-effect models estimate that higher uneven job distribution across households worsens aggregated poverty at the state level. Similarly, good-quality jobs help households escape poverty, whereas poor-quality jobs do not. This paper suggests that eradicating poverty requires the government to direct labor market policies to be tailored more toward distributing jobs from individuals to households and altering bad jobs into good jobs, rather than merely creating more jobs in the economy. This paper contributes by elaborating on relations of employment and poverty, addressing employment quality and distribution, and providing empirical evidence.
1 Introduction
Poverty in the United States is measured by a threshold, and people fall into and escape poverty for many reasons. Many consider employment as the primary policy solution to all forms of poverty, accounting for both falling into and out of poverty (Middleton and Loumidis 2002 ; Saunders 2006 ; Krishna 2007 ). Footnote 1 In the recent pre-pandemic time, the United States reached its highest level of employment in 50 years, with low unemployment. By many metrics, the job market was doing well (Kelly 2019 ). However, poverty in America has remained stagnant for many decades (Desmond 2018 ). What kind of jobs are available? Do they not pay enough to live on? Are these jobs not equally distributed? Footnote 2
Although it may seem logical that higher employment rates would reduce poverty, as the income and consumption of poor people largely come from their work, the relationship between employment and poverty is not straightforward. While some scholars assume that increasing employment reduces poverty (Cantillon et al. 2003 ), others believe that creating more non-subsidized jobs may lead to a higher number of low-paid jobs, leaving more working people in poverty (Kalleberg 2009 , 2011 ). In other words, when employment growth occurs at the cost of wage reduction it does not eradicate poverty (Sloane and Theodossiou 1996 ; Gardiner and Millar 2006 ). Moreover, poverty reduction may not be realized if employment growth occurs in sectors that do not accommodate many poor people (Ravallion and Datt 2002 ; Satchi and Temple 2009 ) or in industries that require higher levels of skills (Loayza and Raddatz 2010 ) and capital (Siddique 2016 ). Furthermore, poor people cannot always afford to be unemployed, and they are not necessarily unemployed people (Visaria 1981 ; Saunders 2002 ). Despite this complexity, there is little research exploring the relationship between employment and poverty, leading to a lack of evidence-based policy actions in this area. Specifically, there is a need for scientific empirical evidence on the relationship between job distribution, job quality, and poverty, and this paper makes an important contribution in these fields.
Despite strong economic performances, poverty rates in the United States have remained stubbornly high, and past attempts to explain this phenomenon have fallen short (Hoover et al. 2004 ; Hoynes et al. 2006 ; Edelman 2013 ; Meyers 2014 ; Pacas and Rothwell 2020 ). In this paper, I argue that job market polarization is a major factor that perpetuates poverty, even during periods of high employment and low unemployment. When jobs are distributed unequally across households and are of poor quality, with no full-time work available, the benefits of job growth fail to reach those who need them the most. This means that the “trickle-down” economy may not work for majority of poor Americans, who face both poor job quality and uneven job distribution. As a result, job growth in a polarized job market does not benefit jobless households, and poverty remains stagnant. Recent data shows that employment-rich households are experiencing faster employment growth than employment-poor households (England 2017 ), exacerbating the problem. In a more equitable, non-polarized job market, the unemployment rate would be the same for both individuals and households (Gregg et al. 2010 ).
The United States has not also succeeded in reducing poverty despite high employment rates due to the poor-quality of available jobs. The job market in the United States has become increasingly bifurcated, which has significant implications for poverty reduction strategies and outcomes. This trend started in the 1980s as the service sector began to replace the manufacturing sector and technology started playing major roles in the labor market (Autor and Dorn 2013 ; Goos et al. 2014 ; Salvatori 2018 ). Since then, Americans have faced an increase in low-paying jobs with few hours, little job security, and no entitled benefits. These poor-quality jobs are prevalent across multiple industries and have impacted the daily lives of millions of Americans. Several studies have documented this trend, including Herzenberg et al. ( 2000 ), Desmond and Gershenson ( 2016 ), Kelly ( 2019 ), Newman ( 2009 ), and Kalleberg ( 2009 , 2011 ). Contrastingly, while some industries like technological offer attractive salaries, promotions, benefits, and even equity in the company, they struggle to find a sufficient pool of skilled applicants to fill their job openings (Goos and Manning 2003 ; Salvatori 2018 ; Kelly 2019 ), contributing to job market polarization. Therefore, economic prosperity alone cannot effectively reduce poverty if it does not generate an adequate number of good-quality jobs (Odhiambo 2011 ; Page and Shimeles 2015 ). Unfortunately, the majority of recently available jobs are low-paying, part-time contract positions that does not provide healthcare or other benefits. The rise of these poor-quality jobs contributes to the growth of the working poor and inequality, Footnote 3 which could be a potential explanation for the persistent high levels of poverty in the United States.
In this research paper, the impact of uneven job distribution and poor-quality jobs on poverty rates in the United States is examined. A longitudinal dataset was constructed by aggregating household-level data to the state-level to match with state-level variables, and a two-way fixed effect model was applied to estimate their effects. The results indicate that the eradication of poverty in the United States may depend on redistributing jobs from individuals to households and on improving quality of jobs. Rather than solely focusing on creating more jobs, policymakers should prioritize transforming existing poor-quality jobs into good-quality ones and ensuring their equitable distribution. It is important to note that creating more jobs is not discouraged, only the creation of poor-quality jobs. Moreover, the findings suggest that a high-growth economy and high employment rates are unlikely to alleviate poverty.
This paper makes a significant contribution by expanding our understanding about the intricate relationship between employment and poverty and presenting novel empirical evidence. Notably, this analysis introduces a fresh perspective by focusing on the United States context, which distinguishes it from previous research that primarily examined the United Kingdom and other European economies. In addition, it opens up avenues for further research into the potential implications of issues related to job distribution and job quality, not only on poverty but also on various aspects of socio-economic well-being.
The following section will delve into the intricate relationships between family dynamics, employment, and poverty. Data, measuring key variables, and empirical strategy will provide detailed insights into data, the metrics used for key variables of interest, and chosen empirical methodologies. Results: two-way fixed effect model will unveil the findings, and, finally, in Sect. 5, I will wrap up this paper with a conclusion.
2 Job distribution, job quality, and poverty
2.1 family is central to avoid poverty.
In most societies, the risk of poverty is unevenly distributed, affecting specific groups such as ethnic minorities, single parents, and people with disabilities more significantly than others. Unexpected events, such as illness, can also lead to poverty (Flaherty et al. 2004 ; Gardiner and Millar 2006 ). Vulnerable individuals often employ strategies like living with family members or relying on state transfer benefits and tax credits to prevent falling into poverty. Gardiner and Millar ( 2006 ) conducted a study in British society and found that over 30% of low-paid workers can escape poverty by depending on the income of other family members. More than 60% rely on the income of their partners and other adults in the household. Approximately 8% of low-paid workers manage to avoid poverty by working long hours to compensate for their low earnings, while around 13% achieve this by combining incomes from state transfers. Living with other individuals plays a crucial role in helping many low-income individuals mitigate the impact of poverty. Pooling together all sources of income is effective, as even a family member with relatively low earnings can improve the overall financial well-being of the household. It's important to note that a single individual earning a decent income has the potential to lift the entire family out of poverty.
Over the past four to five decades, the family structure in the United States has undergone significant transformations, marked by a notable decrease in marriage rates, a decline in the proportion of children born within marriages, and a rise in the number of children born outside of wedlock (Cancian and Haskins 2014 ; Thiede et al. 2017 ). Simultaneously, there has been a substantial increase in women entering the labor market, while many men, particularly those with lower levels of education, have faced diminishing employment opportunities (Cancian and Haskins 2014 ; Binder and Bound 2019 ). These shifts in family structure have had a considerable impact on poverty through their influence on labor market opportunities. Increased participation of women in the workforce may have a poverty-reducing effect if they entered the labor market to compensate for limited family income. Data from the United States Census and the American Community Survey show that families headed by single females with children have consistently experienced an average poverty rate of around 40% over the past four to five decades. In contrast, families headed by married couples with children have maintained a poverty rate of less than 8% during the same period. Moreover, the poverty rate for married couples without children has been even lower, averaging less than 4%. Conversely, families headed by single males and single females have experienced poverty rates higher than 15% on average (Cancian and Haskins 2014 ). Thus, families play a crucial role in shielding many individuals from falling into poverty.
When a single female gets married, the household's needs are likely to increase. However, this marriage also introduces a second earning adult to the household, potentially reducing the risk of poverty. Similarly, if she joins a joint family with another earner, similar benefits can arise. This phenomenon is known as economies of scale, where each additional person added to a household results in less than proportional increases in needs (Cancian and Haskins 2014 ; Reyes 2020 ). The current state of individual and household employment is influenced by various factors, including modernization, the prevalence of nuclear families, the feminization of labor markets, and an increasing number of individuals pursuing tertiary education (Corluy and Vandenbroucke 2017 ; Thiede et al. 2017 ). Considering these trends in family structure, I hypothesize that the unequal distribution of jobs among households may explain the persistent high levels of poverty in the United States. Surprisingly, there have been no studies to date that have specifically explored the distribution of employment across households and its associated consequences on poverty in the country.
2.2 Jobs quality, labor market policies, and poverty
The gap between good and bad jobs is widening in the United States, representing another dimension of job market polarization. The availability of good jobs that offer fringe benefits is declining, while the number of bad jobs without such benefits is increasing. In non-regulated and non-competitive labor markets, both good jobs and bad jobs can coexist. Acemoglu ( 2001 ) suggests that in a laissez-faire equilibrium, the labor market is biased towards poor-quality jobs due to a phenomenon known as "hold-up." According to his search model (Acemoglu 2001 ), the presence of diverse job creation costs results in differentiated compensation for similar workers. In this market, employers and employees share rents, thus breaking the relationship between wages and marginal productivity. Additionally, employers fail to internalize the externalities arising from rent-sharing, which could otherwise be addressed through market allocation. Capital-intensive firms that have made substantial sunk investments are compelled to negotiate and create significant positive pecuniary externalities for workers. Consequently, these firms tend to create a scarcity of good jobs while simultaneously generating an excess number of bad jobs.
There are also several policy factors that play a crucial role in determining the quality of jobs in the market. These factors may include but not limited to inadequate social security programs, such as unemployment benefits, and the absence of minimum wage laws. Both policies can potentially incentivize workers to wait for better job opportunities, consequently reducing firms' profits from creating low-quality jobs and shifting overall job compositions (Carter 1995 ; Acemoglu 1996 ). In the United States, due to the limited prevalence of social security programs, most workers cannot afford to remain unemployed while waiting for better job offers. This results in an oversupply of labor in the market, prompting firms to shift towards a higher proportion of poor-quality jobs. On the contrary, if workers have the protection of unemployment insurance, the cost of waiting for a better job would be less burdensome. Therefore, social protection programs can potentially reduce the labor supply by increasing reservation wages (Marinescu and Skandalis 2021 ).
Similarly, the absence of a minimum wage requirement is also contributing to the growth of poor-quality jobs. Firms find it more profitable to offer poor-quality jobs when there are no higher minimum wage laws in place. Conversely, setting a higher minimum wage in the economy would have compelled firms to pay higher wages for poor-quality jobs, making them less economically profitable and encouraging firms to improve their job compositions (Bulow and Summers 1986 ; Carter 1998 ).
Poor-quality work may not alleviate poverty but rather perpetuate it. To comprehend the implications for poverty, in addition to the economic theories discussed above, it is necessary to examine the policy changes that have promoted a flexible labor market over the last five decades. The Regulatory Flexibility Act (RFA) of 1980 was adopted on a bipartisan basis, reflecting liberal economic principles aimed at deregulating laws and enhancing business power with an aim to improve innovation and production. These flexible labor policies have encouraged the creation of low-quality jobs while reducing the number of high-quality jobs. Sectors that offer low-quality jobs experience high employee turnover (Albrecht and Vroman 1992 ; Carter 1998 ). During economic downturns, these positions can easily be terminated without incurring costs, only to be rehired when production needs increase (Kalleberg 2000 ; Van Arsdale 2013 ), offer no job security to these workers. In other words, the underlying logic of creating low-quality jobs is to pay only for the units of work performed by these positions, and thus, this policy change has direct implications for poverty.
Within these flexible labor law regimes, new third-party staffing firms (e.g., Kelly Services Inc., Robert Half, Toptal, etc.) have emerged to manage this flexible workforce, creating a triangular relationship and operating their businesses at the expense of labor wages (Van Arsdale 2013 ). Major corporations like Amazon, Microsoft, and Sheraton often outsource numerous positions to independent staffing companies, predominantly offering jobs with unpredictable schedules. Surprisingly, approximately 40% of hourly employees receive their work schedules only a week or less in advance (Desmond 2018 ). It is common to see cleaners, reception assistants, and security officers working at prominent corporations, yet these workers are not directly employed by these companies. Instead, they are engaged through independent contractors who take a share of their wages. These intermediary companies not only deduct a portion of the employees' wages offered by the host companies but also deny workers any opportunity for career advancement within the host company, regardless of their hard work. Consequently, many workers under these contracts do not even receive their full wages. By outsourcing these positions to independent contracting agencies, large organizations evade their responsibilities to provide healthcare and other security benefits.
In this triangular relationship, staffing firms negotiate wage and work conditions between employing firms and employees, often leaving employees with little to no voice. Consequently, employees frequently reject job offers, resulting in an increased pool of unemployed individuals, further enhancing labor market flexibility, and boosting company profit margins. The consequence of a flexible labor market is increased poverty, as a large pool of flexible labor enables staffing companies to exploit employees, leading to more individuals earning poverty-level wages and a disproportionate transfer of resources to businesses. The decline in regular employment by host companies and the growth in employment through these staffing firms are strategic policies employed by businesses to avoid the costs associated with adding people to their payrolls, such as health insurance, bonuses, and other human resource expenses, particularly during economic recessions (Van Arsdale 2013 ). The poverty implications of this changing employment era have not been thoroughly studied yet.
The growth of poor-quality jobs is also linked to various recent developments, including technological advancements, changes in work arrangements, the expansion of service sectors, and the decline of industrial employment. It is further associated with shifts in corporate governance and employer strategies (Kalleberg 2011 ). Moreover, it is intertwined with emerging trends such as privatization, marketization, and individualization (Keune 2013 ), along with the declining influence of trade unions (Farber and Levy 2000 ). Consequently, poverty reduction efforts have been stalled.
3 Data, measuring key variables, and empirical strategy
I utilized publicly available data in this study, primarily drawing from the American Community Survey (ACS). The ACS provides individual-level data with household and geographic codes, enabling precise estimates at the local administrative unit level and tracking long-term trends. This dataset is collected by the Census Bureau and is representative of the entire USA population, based on a 1% sample. To align with state-level macro-variables, the data was initially aggregated to the household level and subsequently to the state level. The household-level employment status data is derived from the ACS, while individual unemployment data at the state level is sourced from the Department of Labor and Training. Furthermore, the ACS data was instrumental in measuring households with good-quality jobs and poor-quality jobs. The ACS survey questionnaire includes multiple questions that inquire about the employment status of household members, facilitating the classification of households into full-time and part-time employment categories.
Other macroeconomic variables, such as GDP per capita and the estimated Theil inequality index, were acquired from the US Bureau of Economic Analysis (BEA). Data related to government expenditure and revenue were obtained from the National Association of State Budget Officers (NASBO) community. Human capital and educational attainment data were sourced from the US Census Bureau. Regarding poverty measures, I employed the official poverty measurement utilized by the Federal Government, which is based on the US Census Bureau's poverty threshold of $20,212 for a family consisting of two adults and one child in 2018. This poverty threshold has been adjusted for the number of children, meaning that households with more than one child have a higher poverty threshold. Table 1 in the paper presents the descriptive statistics of the data after aggregating it to the state level.
I aggregated individual and household-level data to the state level for several compelling reasons. Firstly, income is a flow variable that is commonly used to measure poverty, while employment / unemployment status represents the labor market conditions at a specific point in time, rendering it a stock variable. Consequently, if an individual is interviewed during a period of unemployment but remains employed for the rest of the year, their annual income may surpass the poverty line (Saunders 2002 ). Moreover, income is typically reported annually, whereas employment status is not regularly reported on an annual basis. Therefore, analyzing the impact of employment status on poverty without aggregation can lead to misleading results.
Secondly, employment status is usually analyzed at the individual level, whereas poverty measures consider the combined income of the entire family to determine the poverty level. As discussed earlier in this paper, an individual may have low or zero income but not meet the criteria for poverty according to Federal guidelines, as other family members may have higher earnings. Income within a household is shared, and it is the collective income that determines whether the individual or family qualifies for welfare benefits. Hence, measures of poverty and employment should ideally be aggregated at the family level over the entire year.
Thirdly, microdata sources like ACS exhibit variation over time but do not follow a panel format, which means they do not observe the same individual or household over an extended period. Without panel data, it is not possible to apply fixed-effect (FE) models, which allow for controlling observable and unobservable characteristics that remain constant over time (more details of empirical strategies are in Empirical strategies ). The two-way FE model is more suitable for statistical inference than the pooled cross-section model (Lechner et al. 2016 ). Lastly, public welfare expenditure is a crucial determinant of poverty. However, individual or household-level records in the ACS do not provide information about the benefits received from public welfare programs, such as cash assistance. Aggregating the data at the state level is necessary because states are the primary authorities responsible for disbursing these types of payments. Additionally, states play a crucial role in decision-making regarding most poverty reduction programs.
3.2 Measuring the index of uneven job distribution
The index of uneven job distribution indicates an increasing concentration of jobs at the individual level and a decreasing concentration of jobs at the household level. This index essentially reflects unequal job distribution between individuals and households, as opposed to the usual measures of job polarization in occupation, pay, and sector, as explained elsewhere (Goos and Manning 2003 ; Salvatori 2018 ; Jaimovich and Siu 2020 ). Footnote 4 In this paper, the index of uneven job distribution is measured using the method of Gregg & Wadsworth ( 2008 ), which discerns the discrepancy between individual-level joblessness and household-level joblessness. When a state's population consists of individuals 'i', the total number of households ‘H’ consists of individual household ‘h’, and person 'k' resides within household 'h', the total population 'P' can be expressed as:
When an individual person living in a household has no job, a binary outcome value of 1 ( \({n}_{ih}=1\) ) is assigned, and when an individual person living in a household has a job, a binary outcome value of 0 ( \({n}_{ih}=0\) ) is set. Therefore, the individual-level joblessness rate in the population will be:
Let's now consider household-level joblessness, where households can host both jobless individuals and individuals with a job. Such joblessness can now be grouped by households. When a household does not have any people with a job, a binary outcome value of 1 ( \({m}_{h}=1\) ) is assigned, and when a household has at least one person with a job, a binary value of 0 ( \({m}_{h}=0\) ) is set. Therefore, the household-level joblessness will be:
Here, 'm' essentially reflects the number of households with a value of 1 for \({m}_{h}\) weighted by the total number of households ' H '. A simple example will be highly useful here to understand the distinction between joblessness at the individual level and at the household level, and why this distinction should matter for poverty prevalence. Consider a small economy with only two households, each consisting of two people, for a total of four people. The economy offers only two jobs, leaving only two possible scenarios. In the first scenario, one person from each household is unemployed, denoted by \(N=[\left\{\mathrm{1,0}\right\}, \left\{\mathrm{1,0}\right\}]\) , with an associated joblessness rate of 50%. In the second scenario, both working individuals belong to the same household, leaving the other household with no employed individuals, denoted by \(N=[\left\{\mathrm{1,1}\right\}, \left\{\mathrm{0,0}\right\}]\) , with an associated joblessness rate of 50%. Despite both scenarios having the same unemployment rate, they have different rates of joblessness at the household level. In the first scenario, no households are jobless, whereas in the second scenario, 50% of households are jobless.
This illustrates how aggregated individual-level job statistics fail to reflect the actual distribution of jobs across households, much like how typical income distribution measures fail to capture the necessary details of income distribution. To address this, we can measure the degree of uneven job distribution across households by calculating the difference between the actual rate of jobless households and the rate predicted under a counterfactual scenario in which jobs are randomly distributed among the working-age population. This difference can offer insight into the extent to which households experience joblessness beyond what would be expected under random job distribution.
Assuming that all other factors remain constant, it is more likely for fewer individuals to be present in a household where all occupants are employed if the family structure is nuclear, as opposed to extended. This is because households are categorized based solely on their size, and as family sizes decrease, the number of households without any employed individuals is expected to increase. Consequently, the size of workless households can be attributed to a combination of the individual-level unemployment rate and the number of individuals in the household.
where \({uneven job distribution}_{it}\) represents the extent of uneven job distribution in state i for year t . Meanwhile, \({JL}_{it}\) denotes the fraction of households that do not have any employed individuals in state i for year t , regardless their size of households. \({JL}_{it}^{e}\) represents the expected rate of jobless households in state i for year t if jobs were randomly distributed. We can present a counterfactual rate of households without any employed individuals, denoted as \({\hat{J} }_{k}\) for households with k as follows:
Assuming that joblessness is randomly distributed, every individual adult in a household \(k\) has an equal chance of being unemployed. Consequently, the probability of observing an adult in a jobless household (counterfactual rate) should be the same as the observed unemployment rate at the individual level. Similarly, the likelihood of a household with two adults being jobless would be twice the individual unemployment rate (Gregg and Wadsworth 2008 ). To benchmark the probabilities of equal job distribution, we can consider it as having an intuitive appeal similar to the criterion of income equality used in the Lorenz curve. Finally, we can aggregate the counterfactual households with no employed individuals and weigh them by the population, ( \({s}_{k}\) ), of households of size k :
So, the uneven job distribution is the distinction between observed and counterfactual jobless households.
The measure of uneven job distribution discussed here does not have any normative implications. A higher index of uneven job distribution indicates a larger proportion of households are jobless and greater job distribution inequality. Since job numbers are generally limited, positive values in the index can be considered as reflecting ‘Matthew effects,’ wherein additional jobs are concentrated among households who already have employment, rather than being spread out more evenly across population, particularly households with all members are jobless. Footnote 5 Conversely, negative value of uneven job distribution index suggests that there are fewer jobless households than would be expected if jobs were randomly distributed, which can be seen as a form of solidarity. If the expected and observed rates of jobless households are identical, the index of uneven job distribution should have a value of zero.
We never know the counterfactual jobless household rate. While the individual unemployment rate can be a reasonable proxy for the counterfactual jobless households, this will still be an extremely conservative measure of uneven job distribution. This is because there are more jobs available than the number of households in the country. Consider a state with a population of 1 million; there will be a million households if each household only has one person. If the state's unemployment rate is 5%, then both the individual unemployment rate and household joblessness rate will also be 5%. Since job numbers are generally fixed at a point in time, a negative or positive uneven job distribution index will only emerge when two or more individuals form a family, and some of them are employed while others are not. If one household has multiple jobholders, it comes at the expense of another family that has no employed individuals. The value of the index of uneven job distribution increases as more households have no employed individuals while others have multiple jobholders.
3.3 Description of uneven job distribution measures and poverty with data from United States
Let’s consider the data provided by the United States Census Bureau for 2018. According to their report, the United States had a population of 326.68 million people, with 60% of them falling within the working-age bracket, totaling approximately 196.01 million individuals. With an average family size of 2.53, we can estimate that there were around 129.13 million households in the country. In comparison to the number of households, there were approximately 197.31 million available jobs in the country for the year 2018. If these jobs were randomly distributed, every household should ideally have at least one employed member (as shown in Table 2 ). Therefore, the percentage of workless households can serve as a measure of uneven job distribution, which, while still conservative, is less so than the measure expressed in Eq. ( 3 ). In this paper, I will examine the association between both uneven job distribution measures and the poverty rate. The less conservative uneven job distribution measure will be referred to as Uneven Job Distribution Index 1 (UJD-1), while the more conservative measure mentioned in Eq. ( 3 ) will be known as Uneven Job Distribution Index 2 (UJD-2).
With an average family size of 2.53, we can estimate that there were around 129.13 million households in the country. In comparison to the number of households, there were approximately 197.31 million available jobs in the country for the year 2018. If these jobs were randomly distributed, every household should ideally have at least one employed member (as shown in Table 2 ). Therefore, the percentage of workless households can serve as a measure of uneven job distribution, which, while still conservative, is less so than the measure expressed in Eq. ( 3 ).
In this paper, I will examine the association between both measures of uneven job distribution and the poverty rate. The less conservative measure of uneven job distribution will be referred to as Uneven Job Distribution Index 1 (UJD-1), while the more conservative measure mentioned in Eq. ( 3 ) will be known as Uneven Job Distribution Index 2 (UJD-2). Figures 1 and 2 provide valuable insights into job distribution measures at the national level as well as within and across states. Figure 1 demonstrates a noticeable divergence between the unemployment rate at the individual level and the prevalence of jobless households from 2008 to 2018. The state level heterogeneities in the same divergence trend are presented in the Appendix A.
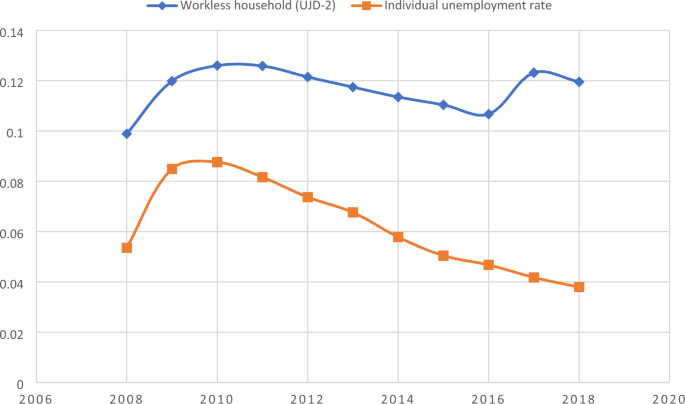
Workless households and individual unemployment rate in the United States. This was calculated using American Community Survey (ACS) and Bureau of Labor Statistics (BLS) data
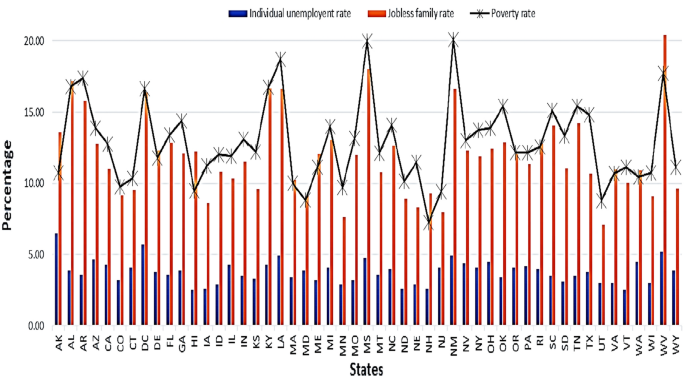
Employment and poverty across states for the year 2018. The data sources are ACS and BLS
The main concern highlighted by these illustrations is the increasing rate of workless households (UJD-2) in the United States despite a stable decrease in the individual-level unemployment rate. Consequently, there has been a growing disparity between the rates of unemployment for individuals and jobless households (UJD-2) in the country. This divergence indicates that job growth in the United States over the past few decades has not benefitted workless households. States with higher employment growth have primarily witnessed benefits for individuals or households with already employed members, resulting in a lack of improvement in job distributions at the household level and, in fact, worsening the situation. Similar trends of employment growth predominantly benefiting households with employed individuals have been observed not only in the United States but also in the UK, Netherlands, and other developed economies (Gregg and Wadsworth 1994 , 2003 ; Beer 2001 ; Cantillon et al. 2003 ; Corluy and Vandenbroucke 2017 ).
While the growing gap in employment distribution between households and individuals may pose various issues concerning labor market performance in the country, the rising rate of workless households (even if it is a stagnant one) carries significant implications for poverty reduction strategies. As mentioned earlier, in an ideal scenario with a normative world of random employment distribution, the unemployment rate at the individual level and the rate of joblessness in households should be identical, and more importantly, with zero joblessness at the household level in the United States. Therefore, the values presented in Fig. 1 represent the extent of uneven job distribution in the United States. Despite positive job market outcomes at the individual level, the situation at the household level has been deteriorating. In this paper, I propose the hypothesis that this higher index of uneven job distribution may be responsible for the increased poverty levels in the country.
Figure 2 showcases the percentage of workless families and individual unemployment rates in relation to the poverty rate across states for the year of 2018. It also highlights the disparity between individual-level unemployment and workless households across different states. Notably, the individual-level unemployment rate is significantly lower than the rate of workless households. Several states, including West Virginia, Mississippi, Alabama, Kentucky, New Mexico, Louisiana, and others, exhibit considerably higher index of uneven job distribution, indicating a pronounced discrepancy between individual-level unemployment and workless households. Intriguingly, these states with higher rates of jobless families are also characterized by higher poverty rates, as depicted in Fig. 2 . In contrast, the states of Utah, Minnesota, New Jersey, Nebraska, Iowa, North Dakota, Wisconsin, Maryland, Colorado, New Hampshire, Connecticut, and others show the lowest index of uneven job distribution. These states demonstrate a more balanced distribution of job opportunities, with a smaller gap between individual-level unemployment and workless households, and lower poverty rate.
3.4 Measure of job quality
Measuring job quality, particularly when identifying households with poor-quality jobs, presents complex challenges. While it's true that low-paying jobs are often categorized as poor-quality jobs, as conventionally defined in economics (Acemoglu 2001 ), this criterion alone doesn't provide the most comprehensive measure of job quality. Job quality is multifaceted (Findlay et al. 2013 ), and the International Labor Organization (2019) offers a more comprehensive definition of a high-quality job, encompassing factors such as higher pay, job security, safety, work-life balance, fairness in employment, social protections, and various other socio-economic considerations. Additionally, scholars like Stecy-Hildebrandt et al. ( 2019 ) and Adamson and Roper ( 2019 ) emphasize additional indicators, including fringe benefits, job security, and favorable income trajectories as characteristics of high-quality jobs. It's important to recognize that job quality is also subjective and influenced by individual perceptions (Clarke 2015 ). In some cases, even a low-paying job can be viewed as a high-quality job if it includes in-work benefits, job security, full-time employment, and other favorable aspects, particularly if it is perceived as such. Many employees prioritize factors like job security, as it can have a more substantial positive impact on their overall well-being than wages alone.
This paper defines a 'good job' as one held by a household with at least one full-time worker who commits to 35 h or more of work per week, and I refer to such households as having 'good jobs.' Conversely, it defines a 'bad job' when a household has only part-time worker(s) putting in less than 35 h of work per week and has no full-time worker, and I refer to such households as having 'bad jobs.' This classification of full-time and part-time employment status at the household level can serve as proxies for assessing job quality at the household level. Moreover, it is expected that part-time and full-time work status is associated with other indicators of job quality such as wages, and it provides suitable quantitative measurement for regression analysis in this paper. While it can be argued that some women and students voluntarily choose part-time positions to allocate more time to family responsibilities (Walsh 1999 ; Hill et al. 2004 ; Pech et al. 2021 ) and education, it's crucial to recognize here that the measurement used in this paper operates at the household level, where it is reasonable to expect that at least one member of the household should be willing to take on a full-time role, unless the household is a single-parent household or its member(s) require special care (e.g., disability).
Furthermore, there is strong evidence to suggest that a significant proportion of part-time jobs are involuntary, with individuals preferring full-time contracts if they were given the opportunity (Tilly 1991 , 2010 ; Kalleberg 2009 ; Kauhanen and Nätti 2015 ). Involuntary part-time jobs represent a form of underemployment, as these part-time workers are actively seeking full-time positions but have not been able to secure them. Notably, part-time workers constitute more than one-fifth of the total workforce in the United States, and their presence in the labor market has been steadily increasing since 1970 (Tilly 1991 ; Fullerton et al. 2020 ). This growth in involuntary part-time employment is primarily driven by employer demands for flexible scheduling, cost minimization, and their predictability of available labor forces, rather than worker’s preferences (Tilly 1991 , 2010 ; Kalleberg 2009 ).
Two significant theories, neoclassical and institutionalist, can potentially elucidate the issue of job composition in terms of part-time and full-time employment, particularly regarding involuntary part-time positions. The neoclassical approach can be extended in two ways—building a microeconomic foundation to analyze market constraints and addressing the lack of aggregate demand in the macroeconomic environment. Bulow and Summers ( 1986 ), who developed the "efficiency wage" model based on a microeconomic foundation, suggest that firms pay full-time workers higher wages than their marginal product, while part-time workers receive lower wages at the market rate. The higher pay for full-time workers is efficient because it disincentivizes them from shirking, leading to lower employee turnover and increased productivity. In contrast, part-time employees, who face low wages and high turnover, become less productive even though they may be identical to full-time employees in all other aspects. In such situations, involuntary part-timers may consider the loss of their job and associated income to be significantly more detrimental than accepting lower wages. Full-timers are also treated as insiders, while part-timers are seen as outsiders (Lindbeck and Snower 1986 ). Insiders have the power to threaten to leave, leading to higher costs for firms related to hiring and training new employees, but part-timers lack such bargaining power.
In macroeconomic contexts, involuntary part-time employment or underemployment emerges as a natural consequence. During economic downturns, part-time employment may be the preferred choice for employers, enabling them to retain skilled employees at reduced wages while reducing the workforce. For instance, the recent COVID-19 pandemic vividly exemplified that, as part-time workers were more susceptible to job loss, full-time workers were better equipped to retain their employment. In such economic downturns, workers often find that accepting lower wages is a more viable option than losing their jobs, particularly in the absence of unemployment insurance.
The institutionalist approach, while acknowledging the logic of neoclassical perspectives, underscores that part-time employment is distinguished not only by hours worked but also by various other attributes. Institutional theories contend that a fundamental shift in the organizational environment, including elements such as labor unions and policies, is necessary to transition between different job compositions rather than merely adjusting to economic variables within the same framework (Woodbury 1987 ; Tilly 2010 ). In contrast to the neoclassical approach, which assumes that market actors act rationally and that labor market outcomes are determined by the standard demand–supply model, the institutional approach recognizes that a range of labor market outcomes is possible and that these outcomes are shaped by socially imposed norms and traditions. Given the tradition of strong capitalism and a history of limited policy regulations in place in the United States, employers enjoy greater flexibility in crafting job configurations that maximize their profit margins compared to other welfare-oriented nations.
These part-time positions consistently come with a wage penalty (Hirsch 2005 ; Baffoe-Bonnie and Gyapong 2018 ; Gallego-Granados 2019 ) and provide limited opportunities for career advancement, resulting in high turnover rates (Tilly 1991 ; Sloane and Theodossiou 1996 ). Part-time work status also implies limited access to social protections due to shorter lengths of service (Stecy-Hildebrandt et al. 2019 ) and is generally associated with lower rates of unionization (Anderson et al. 2006 ). Research has also demonstrated that the prevalence of part-time jobs, primarily driven by neoliberal market restructuring, contributes to wage disparities across race (Wilson and Roscigno 2016 ) and gender (Fuller 2005 ). In addition to the reduced income potential associated with part-time positions, studies by McDonald et al. ( 2009 ) have raised several other concerns, including limitations on career progression, restricted access to high-status roles, increased workloads, challenging work environments, and related issues. For these various reasons, part-time positions are a fair representation of poor-quality jobs, while full-time positions signify good-quality jobs.
Hence, it is reasserted here that economic prosperity alone may not effectively alleviate poverty unless it results in a sufficient number of good-quality jobs with full-time contracts for households. An increase in the number of families with good-quality jobs is expected to play a pivotal role in poverty eradication in the United States. The combined remuneration package associated with full-time employment has the potential to lift a household above the poverty threshold. Conversely, households relying solely on part-time jobs are likely to contribute to an increase in poverty rates across the states. These household-level indicators of job quality are anticipated to have a direct and significant impact on the economic status of people living in poverty, surpassing the influence of broader macro-level determinants, such as overall economic growth and employment levels.
3.5 Empirical strategies
The identification strategy of this paper is to estimate Eqs. 4 and 5 using the two-way fixed effect (TWFE) method for a strong balanced panel data that spans from 2008 to 2018. With a large number of independent clusters of observations (i.e. states), the coefficient of our variable of interests can be consistently estimated using a TWFE regression specification, and by clustering standard errors the conclusion will be an asymptotically valid inference (Roth et al. 2023 ).
Where \({Y}_{it}\) represents the poverty rate, \({UJD}_{it}\) represents either the uneven job distribution index-1 or index-2, and \({JQ}_{it}\) represents either the measure of good-quality jobs or poor-quality jobs in state i for year t . \({X}_{it}\) is a vector of characteristics that vary over time at the state level, including GDP per capita, GDP growth rate, state population size, regional inequality, per capita tax revenue, intergovernmental transfers, population demographics (such as age groups, immigration/citizenship status, and human capital), and others. \({\varepsilon }_{it}\) is the random error term. By applying the two-way fixed effect method, I examine the within-state variation to estimate the impact of polarized job distribution and job quality on the poverty rate.
However, it is important to note that, given our observational data, this analysis does not intend to interpret the \(\beta\) coefficient as a strictly causal relationship between employment variables and the poverty rate outcome. The state fixed effect, \({\delta }_{i}\) , estimate accounts for both observable and unobservable time-invariant factors that may impact poverty rates, such as colonial history and geographic locations. The year fixed effect, \({\mu }_{t}\) , captures any unusual time trends, such as financial crises, that may also influence the poverty rate. However, the two-way fixed-effect model cannot address problems that may arise if employment distribution and quality and poverty are simultaneously related. For instance, it may not account for scenarios where higher poverty leads to either higher or lower uneven job distribution or job quality, thus failing to discern any such reverse effect. To tackle this issue, we would require an instrument that is strongly correlated with poverty but has no association with our employment variables, and then apply an instrumental variables regression approach. Unfortunately, our data lacks such an instrumental variable that fulfills both of these conditions for a good instrument. This concern regarding internal validity remains unaddressed in this paper.
Furthermore, while the two-way fixed-effect model accounts for observed and unobserved time-invariant factors, it does not consider omitted time-varying factors, such as union and firm density in the local area. These factors could not be controlled for due to the absence of long-panel data used in this paper. Similarly, measurement errors pose potential threats to estimating unbiased coefficients. For instance, using part-time and full-time working status at the household level as proxies for job quality may not accurately capture the true measures of job quality and may introduce bias into the estimates. If alternative measures of job quality in terms of pay and benefits were available, the results might differ from those presented in this paper, thus posing a threat to the external validity of these results in this paper.
4 Results: two-way fixed effect model
Figure 3 presents the scatterplot matrix depicting the relationships among the key variables of interest. Both the less conservative and more conservative measures of uneven job distribution are positively associated with the poverty rate. Conversely, there exists a strong negative relationship between poverty and good-quality jobs (households with full-time workers), while the relationship between poverty and poor-quality jobs (families with only part-time workers) is positive. All of these relationships confirm our earlier predictions in this paper that an unequal job distribution across households is a significant predictor of persistent poverty in the United States. Similarly, bad jobs also serve as a predictor of higher poverty, whereas good jobs act as a predictor of lower poverty in the country. While these scatterplots provide a visual representation of the basic strength, direction, and nature of the relationship between poverty and variables related to job distribution and quality, the following sections delve into the empirical findings obtained through the application of two-way fixed effect methods.
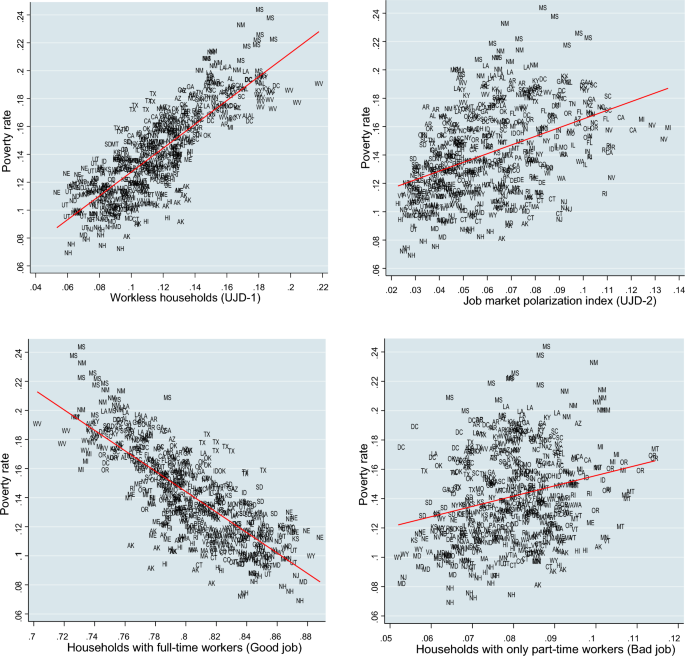
Scatterplot of uneven job distribution, job quality, and poverty
4.1 Uneven job distributions and poverty
Table 3 presents the two-way fixed effect estimates of the effect of uneven job distribution on the poverty rate. The poverty rate is measured as the average poverty across all races, based on the Federal poverty guideline at 100%. Uneven job distribution consists of two measures: the more conservative index-2 from Eq. ( 3 ) and the less conservative index-1, which represents the share of jobless households as discussed in section III. The estimates in Table 3 are presented as percentage point estimates for both job distribution indices. The results strongly support the prediction made earlier in this paper that higher job market polarization in the form of job distribution leads to a higher poverty.
Notably, the uneven job distribution index-1 has a significantly larger impact on the poverty rate compared to uneven job distribution index-2. The interpretation of these coefficients is straightforward. A one percentage point increase in uneven job distribution index-1 will result in approximately a 0.50 percentage point increase in the poverty rate. On the other hand, a one percentage point increase in uneven job distribution index-2 will lead to an approximately 0.25 percentage point increase in the poverty rate. This disparity in magnitudes between index-1 and index-2 can be attributed to the subtraction of unequal job distribution from index-1 to obtain index-2. This difference reinforces the hypothesis that as the level of uneven job distribution increases, the poverty rate also increases. In other words, this provides strong evidence that equal distribution of jobs is a necessary condition to reduce poverty in the United States. Furthermore, these results indicate that the higher employment levels in the United States did not benefit all families, especially low-income families. The findings persist in terms of both effect size and significance level across all model specifications.
The most vulnerable group of people in every society comprises those living in families where no one is employed (Gallie et al. 2000 ; Cantillon et al. 2003 ). Our results in this paper align with the findings from earlier literature. Even after controlling for a comprehensive set of variables as presented in Table 3 , the effect size remains large and statistically significant which is an alarming issue for the country. Förster (2000) reported raw figures indicating that the average poverty rate among households with a working-age head but only one employed member is approximately 36% across 16 OECD countries. In contrast, the corresponding figures are only about 13% for families with one employed household member and merely 3% for households with two employed members. It is important to note that the coefficient presented in this paper cannot be directly compared to the raw numbers reported in Förster's (2000) work. Nonetheless, our coefficients suggest that a ten-percentage point increase in jobless families (uneven job distribution index-1) leads to a substantial five-percentage point increase in poverty.
While factors such as the share of children, elderly individuals, non-white population, and non-professional occupations also contribute to a higher overall poverty rate in the USA, their magnitudes are considerably smaller compared to the job distribution measures. Moreover, variables negatively associated with poverty rates, such as GDP per capita, human capital, and public expenditures, also exhibit relatively small effects. Previous studies that excluded these job distribution measures in their analyses of poverty had less predictive power as a result. Appendix B presents a comparison of aggregated household-level employment (such as job distribution index) versus aggregated individual-level unemployment rate. The uneven job distribution index at the household level exhibits more than three times the predictive power to explain poverty compared to the individual unemployment rate.
A higher percentage of the non-white population and individuals outside the working age (such as children and older people) is positively associated with the poverty rate, which aligns with earlier literature (Bradbury et al. 2001 ; Hoover et al. 2004 ). This result suggests that a portion of children and individuals outside the working age lack the support of working-age individuals within their families. It is worth noting that the fraction of immigrants and non-white individuals has been increasing over the past few decades. Data indicates that recent immigrants, on average, have lower levels of education and fewer skillsets compared to native individuals, resulting in a higher proportion of immigrants earning lower incomes and living in poverty (Hoynes et al. 2006 ; Siddique et al. 2022 ). Alternatively, the influx of immigrants may reduce job market opportunities for native individuals. Consequently, if this argument holds, the overall association between the share of immigrants and the poverty rate will be positive, although the evidence in the literature is mixed (Llull 2017 ). Moreover, the increasing share of non-white and immigrant populations leads to greater ethnic diversity within states, which in turn contributes less to public income and resources (Siddique 2021 , 2022 ). This indirect effect may further hinder poverty reduction efforts.
On average, historically non-white populations face relative disadvantages in this country. Therefore, an increasing fraction of the non-white population can be seen as a predictor of higher poverty, indicating the ongoing disadvantages faced by non-white individuals in society (Siddique 2022 ). However, the statistical association between immigration status (citizenship) and poverty is not significant, except in model 3.2. This is because although recent immigrants experience higher poverty rates compared to earlier immigrant cohorts, their share in the overall population of the United States is relatively small (the non-citizen population is 4.91% as shown in Table 1 ) to significantly impact the state-level poverty rate. The growth rate among non-white children surpasses that of white children, and non-white children are more likely to experience poverty due to their parents' lower economic resources (Mordechay and Orfield 2017 ; Siddique 2022 ). Therefore, a higher share of non-white children may contribute to an overall increase in poverty. However, the share of non-white children is not statistically significant, suggesting that the effects may have been absorbed by the share of the non-white population as a whole.
Spatial variations and economic development can potentially play a role in determining poverty and inequality (Glasmeier 2002 ; Khan and Siddique 2021 ). As the spatial differences between states are likely to be fixed during our study period and are accounted for by fixed effects estimates, I also control for within-state spatial economic inequality, measured by Theil regional inequality, along with GDP per capita. The results show that higher GDP per capita is negatively associated with poverty and is statistically significant, while the Theil local inequality index is not statistically significant. The insignificant coefficient for regional inequality is small because regional inequality within states has remained relatively stable throughout the study period (Khan and Siddique 2021 ). Although a higher GDP per capita is negatively associated with the poverty rate, the relationship between the two variables is weak in terms of effect size. This finding is consistent with earlier literature in the United States and across countries (Adams 2004 ; Hoynes et al. 2006 ).
The proportion of non-management/professional jobs also serves as a predictor of higher poverty, although its impact is less consistent. On average, 62% of employment falls within this occupational category. In addition, I have included controls for various measures of human capital or educational attainment and government expenditure. Human capital has always played a crucial role in explaining economic growth and poverty. It directly influences employment and growth patterns by providing the skills necessary for the growth process, thereby impacting poverty (Gutierrez 2007). Controlling for human capital takes into account the reverse impact of poverty on employment. Limited human capital may prevent many low-income families from accessing good job opportunities. By including measures of human capital or educational attainment, we can capture the impact of poverty on employment, if any. Most educational achievements are negatively associated with the poverty rate, with the exception of those who have completed less than the 9th grade and the share of high school graduates or higher. Education below the 9th grade is likely insufficient in terms of human capital to prevent poverty. Increasing the percentage of high school graduates among individuals aged 25 years and above (who are likely to be part of the workforce) helps in poverty prevention, whereas the share of high school graduates among the overall population does not have the same effect, as many individuals in this group are not part of the labor force. Those who are not in the labor force do not utilize their human capital to earn income. Overall, the relationship between educational attainment and poverty aligns with the existing literature, which suggests that higher levels of education, such as a bachelor's degree or associate degree, help people escape poverty (Assari 2018 ).
Government taxes and transfers play a vital role as income sources for the poor. While a higher share of public expenditure is negatively associated with the poverty rate, a higher percentage of public revenues does not show the same relationship (models 3.5–6). The link between public spending and poverty is well-established in the literature (Hidalgo-Hidalgo and Iturbe-Ormaetxe 2018 ); however, there are debates regarding which types of public expenditures effectively help the poor escape poverty (Fan et al. 2000 ; Jung et al. 2009 ). Furthermore, I have included controls for two different measures related to welfare expenditures: the Temporary Assistance to Needy Families (TANF) program and other cash assistance. The TANF program does not have a significant impact on poverty reduction, whereas the other cash assistance program shows a significant negative effect on the poverty rate. Government transfers can have both direct and indirect consequences on family earnings. The immediate impact is that government transfers provide households with cash and other benefits, which directly affect income and poverty. However, there is an indirect effect as well, where households may adjust their behavior due to the availability of government transfers, potentially reducing their incentive to work and resulting in lower incomes (Schoeni and Blank 2000 ). Thus, the indirect impact may offset the direct effect. Moreover, estimating the immediate impact of government benefits on poverty can be challenging due to the various types of benefits and the definition of the poverty level used in this study. The TANF program, for example, provides cash benefits to low-income households with children. Assuming no behavioral changes due to TANF, it should directly increase the incomes of poor families. However, its impact on poverty reduction may be limited since TANF transfers phase out at income levels around the poverty line (Hoynes et al. 2006 ). Therefore, these estimates do not show any effects of TANF on the poverty rate.
4.2 Job quality and poverty
Table 4 presents the impact of job quality measures on the overall poverty rate at the state level. Good-quality jobs have a significant effect on poverty reduction, while poor-quality jobs have a significant opposite effect on poverty (increasing effect) across all model specifications in Table 4 . As previously defined, "good-quality jobs" refer to households with at least one full-time employee, while "poor-quality jobs" encompass households with only part-time employment. The model specifications in Table 4 are identical to those in Table 3 , except that here, I replaced the uneven job distribution index-1 and index-2 with measures of good-quality jobs and poor-quality jobs.
The interpretation of this result is simple: a one percentage point increase in the share of households with good-quality jobs reduces the poverty rate by 0.42 to 0.44 percentage points. On the other hand, a one percentage point increase in the share of households with poor-quality jobs increases the poverty rate by 0.35 to 0.38 percentage points. This evidence demonstrates that job quality matters for poverty reduction. The existence of a substantial share of poor-quality jobs in the economy, concentrated in households that have no other good jobs, is responsible for the higher persistent poverty rate in the country. In addition to uneven job distribution, as we have seen in the earlier section, job quality is another factor that can explain the persistent level of poverty in the United States. The significance level and size of coefficients are consistent, and the estimated model has high goodness of fit measures (R-square = 0.81 and 0.76 in models 4.5–6). After controlling for both state and year fixed effects and gradually including control variables, no inconsistencies in terms of the size of the coefficients and their significance levels have been noticed. These findings provide robust support for partial causal evidence that job quality plays a critical role in determining the poverty rate in the country: bad jobs increase poverty, and good jobs reduce poverty.
When people are working but still living below the poverty line, it is referred to as "poverty in work," as we have observed in the case of households with poor-quality jobs, which aligns with earlier evidence (Burkhauser and Finegan 1989 ). Due to the lack of extensive unemployment insurance, minimum wage protections, and the diminishing presence of trade unions in the USA, most poor individuals cannot afford to remain unemployed and wait for offers of good jobs. Consequently, they are compelled to accept these bad jobs regardless of their quality and level of compensation (Berry and Sabot 1978 ; Visaria 1981 ; Acemoglu 2001 ; Saunders 2002 ). The existence of bad jobs stems from employers being able to find an adequate labor supply to fill these positions. Therefore, the presence of bad jobs and higher poverty rates are closely intertwined. If these bad jobs persist in the labor market, it is unlikely that poverty will disappear from the economy.
Previous literature attempted to explain why the poverty rate remained persistent in the United States despite positive economic indicators (Hoover et al. 2004 ; Hoynes et al. 2006 ). However, they failed to adequately consider the role of employment quality and employment distribution, which are the primary sources of income for the poor. If jobs are not evenly distributed and predominantly consist of poor-quality positions, a rapidly growing economy is unlikely to help poor individuals escape poverty. A $1,000 increase in GDP per capita only reduces the poverty rate by 0.001 percentage points, which is minimal compared to the impact of uneven job distributions and job quality on poverty, as demonstrated in Tables 3 and 4 . These results suggest that the "trickle-down" economy does not effectively benefit the poor if employment, their main source of earnings, does not prioritize their well-being. In the United States, the role of uneven job distributions and job quality in determining poverty is more significant compared to other developed economies, as alternative means of support for the poor, such as transfers and redistribution, are relatively limited in this country (Gilens 2009 ; Garfinkel et al. 2010 ). Therefore, without sufficient redistribution programs, higher levels of uneven job distributions and the prevalence of low-quality jobs may explain why the income poverty rate remains high and persistent in the country, even during periods of robust economic growth and low unemployment.
5 Conclusions
The poverty rate in the United States has persistently remained high, posing a challenge to understand the underlying reasons. This paper aims to explore whether higher levels of uneven job distribution and the prevalence of poor-quality jobs contribute significantly to poverty in the country. The findings reveal that both uneven job distribution and poor-quality jobs play an important role in explaining poverty in the United States.
An increase of one percentage point in the uneven job distribution index—1, which represents workless households, leads to an almost 0.48 percentage point increase in the poverty rate. This suggests that for a state with an average poverty rate of 14 percent, a 1 percent increase in workless households will result in a corresponding rise of approximately 3.43 percent in the poverty rate. Similarly, a one percentage point increase in the share of households with poor-quality jobs results in a 0.35 percentage point increase in the poverty rate. This implies that in a state where the average poverty rate stands at 14 percent, a mere one percentage point rise in households with only part-time workers can potentially contribute to an increase in poverty by approximately 2.5 percent. In contrast, a mere one percentage point increment in the proportion of households with good-quality jobs results in a poverty reduction of 0.42 percentage points. Consequently, in a state with an average poverty rate of 14 percent, this translates to a significant decline in poverty by approximately 3 percent.
In comparison to other commonly studied variables in the literature, such as individual unemployment rate, GDP per capita, public expenditure, and human capital, which are expected to impact the poverty rate, the role of job distribution and job quality is significantly prominent and noteworthy. The evidence presented in this paper can serve as a valuable resource for public policy debates aimed at reducing poverty in developed economies. It sheds light on the reasons behind the stagnation in poverty reduction efforts in the United States. Both uneven job distribution and poor-quality jobs are structural problems that hinder progress in achieving poverty reduction targets. This is not to say that safety-net programs and other measures do not alleviate poverty—they likely lift millions of households above the poverty line each year. However, I find that the most effective anti-poverty solutions lie in the availability of good-quality jobs and their equitable distribution among households.
In the absence of policy guidance, the issue of uneven job distribution is likely to worsen in the future, potentially hindering the achievement of poverty reduction goals. Traditionally, when the first person from a family enters the labor market, it is more likely to be a male, unless the family is headed by a single mother. Subsequently, the second person from a family to enter the labor market is more likely to be a woman. When a woman becomes the second earner due to financial hardship, the family's economic situation improves, increasing their chances of surpassing the poverty threshold (Blackburn and Bloom 1994 ). However, in recent decades, a significant portion of the female labor force, particularly those married to well-paid men, has joined the workforce (Stier and Lewin 2002 ; Averett et al. 2021 ). There is a positive correlation between husbands' and wives' incomes (Averett et al. 2021 ). As female labor market opportunities expand, high-earning women tend to marry high-earning men, resulting in a rise in households with two high-earning individuals. Such structural forces play a role in determining employment opportunities, and as a result, the likelihood of both spouses in some families being unemployed may increase (De Graaf and Ultee 2000 ; Stier and Lewin 2002 ).
Therefore, it is crucial to establish public policy guidelines for the recruitment process that prioritize the common benefits over private benefits in order to reduce poverty in our society. One effective policy approach could be implementing a preference system similar to the veteran preference policy, where job applicants from workless families are entitled to preferences over applicants from households with already employed individuals in recruitment from competitive lists. Similar strategies should also be followed during firm’s downsizing times where employee retention offer should prioritize individual whose household does not have a second earning individual. This preference system can be implemented in the job market without sacrificing efficiency since candidates must still meet the minimum qualifications. It can be further strengthened by restricting job offers to employees' spouses, a practice that some institutions such as universities have recently adopted, although it overlooks the overall societal benefits. While achieving comprehensive job distribution efforts may pose challenges in the short term, even limited success would yield significant societal equity gains in the long-term.
Similarly, in the absence of a minimum wage, unemployment insurance, and trade unions, the proportion of poor-quality jobs will continue to rise and coexist with good-quality jobs. As a result, a high poverty rate will also persist. There is a significant disconnect between the booming labor market and the well-being of the people, particularly those at the bottom. The labor market is trapped in a cycle of bad jobs. The continuous rise of employment in gig economies may make the employment rate appear impressive, but without proper regulation and policies, the economy will keep producing more poor-quality jobs. This growth in poor-quality jobs is a byproduct of the massive scale development of the service sector, such as healthcare, leisure, hospitality, and restaurants, which predominantly hire people on a part-time basis and pay low wages. This trend also coincides with the declining manufacturing sector.
To reduce poverty, it is not only important to stop creating new poor-quality jobs, but also to replace the current poor-quality jobs with good-quality ones. Both direct and indirect policy guidance is necessary. The direct approach may include policy guidance by setting minimum work conditions and wages for all jobs in the market. Setting higher standards and a higher minimum wage would not only directly regulate job quality but also reduce incentives for firms to create more poor-quality jobs. Poor-quality jobs would be less beneficial for them compared to creating more good-quality jobs.
The indirect method should consist of increasing the coverage of unemployment insurance, investing in education to ensure equitable access to higher education for all, and allowing trade unions to function within each institution. Unemployment insurance should enable people to wait for better job offers instead of immediately accepting poor-quality jobs. It would also reduce the labor supply in the market, which would further push firms to raise pay and improve job quality. Similarly, increasing access to higher education is another way to create demand for good-quality jobs and reduce the supply of recipients of poor-quality jobs. This would leave firms with no choice but to produce more good-quality jobs. Highly skilled workers typically demand higher job quality than low-skilled workers (Cortés and Tessada 2011 ). Historically, unions have played a significant role in protecting workers' interests, and strengthening workers' unions can extend institutional regulations to represent worker interests and generate collective pressure to improve job quality (Simms 2017 ).
There are more full-time, good-quality jobs in the United States than the total number of households, as demonstrated in this paper. Therefore, their equitable distribution among families can play a significant role in eradicating poverty. While achieving a completely even distribution of jobs across households may not be immediately feasible due to structural constraints, combined and simultaneous efforts to allocate jobs from individuals to households and implement policies to enhance job quality would help alleviate poverty. Future research should explore methods to improve the distribution of jobs among households and transition from low-quality to high-quality jobs without sacrificing efficiency, as this paper did not adequately address these aspects, which fall beyond its scope.
Data are from the US Census Bureau, American Community Survey (ACS), World Development Indicators, and the Department of Labor.
Availability of data and materials
The associated data are publicly available, and the Stata code can be requested at [email protected] for reproducing and replicating the results.
Standard literature on poverty identified a wide range of factors that are responsible for poverty such as lack of education (Hofmarcher 2021 ), industrialization (Kimura and Chang 2017 ), technology adoption (Comin et al. 2010 ), redistribution (Jouini et al. 2018 ), and others. Read Ravallion ( 2012 ), McMillan ( 2016 ), and Rosenzweig ( 2012 ) for a more standard explanation of poverty.
In economics, this is called the productivity-pay gap when economic expansion does not broaden social uplift.
According to the Bureau of Labor Statistics, in 2016, there were about 7.6 million “working poor” who spent at least half the year either working or looking for employment.
For example, job polarization in occupation means higher growth of job in the highest-wage and lowest-wage occupations while wiping out the mid-waged occupational jobs (Goos and Manning 2003 ).
The Matthew effect is related to a statement from the Gospel of St Matthew—“For to all those who have, more will be given.” In recent times, this concept is used to present form of self-reinforcing inequality in income, wealth, political power, prestige, and others (Rigney 2010 ).
Acemoglu, D.: Good jobs versus bad jobs. J. Labor Econ. 19 , 1–21 (2001)
Article Google Scholar
Acemoglu D. (1996) Good jobs versus bad jobs: Theory and some evidence
Adams, R.H.: Economic growth, inequality and poverty: estimating the growth elasticity of poverty. World Dev. 32 , 1989–2014 (2004). https://doi.org/10.1016/j.worlddev.2004.08.006
Adamson, M., Roper, I.: ‘Good’ jobs and ‘bad’ jobs: contemplating job quality in different contexts. Work Employ Soc. 33 , 551–559 (2019). https://doi.org/10.1177/0950017019855510
Albrecht, J.W., Vroman, S.B.: Dual labor markets, efficiency wages, and search. J. Labor Econ. 10 , 438–461 (1992). https://doi.org/10.1086/298295
Anderson, J., Beaton, J., Laxer, K.: The union dimension: Mitigating precarious employment? precarious employ underst labour mark insecurity can. McGill-Queen’s University Press, Montreal (2006)
Google Scholar
Assari, S.: Parental education better helps white than black families escape poverty: national survey of children’s health. Economies 6 , 30 (2018). https://doi.org/10.3390/economies6020030
Autor, D.H., Dorn, D.: The growth of low-skill service jobs and the polarization of the US labor market. Am. Econ. Rev. 103 , 1553–1597 (2013)
Averett, S.L., Bansak, C., Smith, J.K.: Behind every high earning man is a conscientious woman: the impact of spousal personality on earnings and marriage. J. Fam. Econ. Issues 42 , 29–46 (2021). https://doi.org/10.1007/s10834-020-09692-x
Baffoe-Bonnie, J., Gyapong, A.O.: Definition of full-time and part-time employment, and distributional assumptions: the implications for the estimated full-time and part-time wage equations. Int. Rev. Appl. Econ. 32 , 259–279 (2018). https://doi.org/10.1080/02692171.2017.1340436
Beer PT (2001) Over werken in de postindustriële samenleving. SCP, Sociaal en Cultureel Planbureau
Berry, A., Sabot, R.H.: Labour market performance in developing countries: a survey. World Dev. 6 , 1199–1242 (1978). https://doi.org/10.1016/0305-750X(78)90011-6
Binder, A.J., Bound, J.: The declining labor market prospects of less-educated men. J. Econ. Perspect. 33 , 163–190 (2019). https://doi.org/10.1257/jep.33.2.163
Blackburn ML, Bloom DE (1994) Changes in the structure of family income inequality in the US and other industrialized nations during the 1980s. LIS Working Paper Series
Bradbury, B., Jenkins, S.P., Micklewright, J.: The dynamics of child poverty in industrialised countries. Cambridge University Press (2001)
Book Google Scholar
Bulow, J.I., Summers, L.H.: A theory of dual labor markets with application to industrial policy, discrimination, and keynesian unemployment. J. Labor Econ. 4 , 376–414 (1986). https://doi.org/10.1086/298116
Burkhauser, R.V., Finegan, T.A.: The minimum wage and the poor: the end of a relationship. J. Policy Anal. Manage. 8 , 53–71 (1989)
Cancian, M., Haskins, R.: Changes in family composition: implications for income, poverty, and public policy. Ann. Am. Acad. Pol. Soc. Sci. 654 , 31–47 (2014). https://doi.org/10.1177/0002716214525322
Cantillon, B., Marx, I., Van Den Bosch, K.: The puzzle of egalitarianism. the relationship between employment, wage inequality, social expenditure and poverty. Eur. J. Soc. Secur. 5 , 108–127 (2003). https://doi.org/10.1177/138826270300500203
Carter, T.J.: Efficiency wages: employment versus welfare. South. Econ. J. 62 (1), 116–125 (1995)
Carter, T.J.: Policy in a two-sector efficiency wage model: substituting good jobs for bad. J. Post. Keynes. Econ. 20 , 445–461 (1998). https://doi.org/10.1080/01603477.1998.11490163
Choodari-Oskooei, B., Royston, P., Parmar, M.K.B.: A simulation study of predictive ability measures in a survival model I: explained variation measures. Stat. Med. 31 , 2627–2643 (2012). https://doi.org/10.1002/sim.4242
Clarke, M.: To what extent a “bad” job? employee perceptions of job quality in community aged care. Empl. Relat. 37 (2), 192–208 (2015)
Comin, D., Easterly, W., Gong, E.: Was the wealth of nations determined in 1000 BC? Am. Econ. J. Macroecon. 2 , 65–97 (2010)
Corluy V, Vandenbroucke F (2017) Individual employment, household employment and risk of poverty in the EU. A decomposition analysis. Monit Soc Incl Eur Stat Books Eurostat Luxemb Publ Off Eur Union 2017
Cortés, P., Tessada, J.: Low-skilled immigration and the labor supply of highly skilled women. Am. Econ. J. Appl. Econ. 3 , 88–123 (2011). https://doi.org/10.1257/app.3.3.88
Desmond, M.: Americans want to believe jobs are the solution to poverty. They’re not. N. y. times. 11 , 11 (2018)
Desmond, M., Gershenson, C.: Housing and employment insecurity among the working poor. Soc. Probl. 63 , 46–67 (2016). https://doi.org/10.1093/socpro/spv025
Edelman, P.: So rich, so poor: why it’s so hard to end poverty in America. New Press, The (2013)
England, P.: Households, employment, and gender: a social, economic, and demographic view. Routledge (2017)
Fan, S., Hazell, P., Thorat, S.K.: Impact of public expenditure on poverty in rural India. Econ. Polit. Wkly. 35 , 3581–3588 (2000)
Farber, H.S., Levy, H.: Recent trends in employer-sponsored health insurance coverage: are bad jobs getting worse? J. Health Econ. 19 , 93–119 (2000). https://doi.org/10.1016/S0167-6296(99)00027-2
Findlay, P., Kalleberg, A.L., Warhurst, C.: The challenge of job quality. Hum. Relat. 66 , 441–451 (2013)
Flaherty, J., Veit-Wilson, J., Dornan, P.: Poverty: the Facts. CPAG, London (2004)
Förster MF, Pellizzari M (2000) Trends and driving factors in income distribution and poverty in the OECD area
Foster, F.D., Smith, T., Whaley, R.E.: Assessing goodness-of-fit of asset pricing models: the distribution of the maximal R2. J Finance 52 , 591–607 (1997). https://doi.org/10.1111/j.1540-6261.1997.tb04814.x
Fuller, S.: Public sector employment and gender wage inequalities in British Columbia: Assessing the effects of a shrinking public sector. Can. J. Sociol Can. Sociol. 1 , 405–439 (2005)
Fullerton, A.S., Dixon, J.C., McCollum, D.B.: The institutionalization of part-time work: cross-national differences in the relationship between part-time work and perceived insecurity. Soc. Sci. Res. 87 , 102402 (2020). https://doi.org/10.1016/j.ssresearch.2019.102402
Gallego-Granados, P.: The part-time wage gap across the wage distribution. Social Science Research Network, Rochester, NY (2019)
Gallie, D., Jacobs, S., Paugam, S.: Poverty and financial hardship among the unemployed. Welf Regimes Exp Unempl Eur. 2000 , 47–68 (2000)
Gardiner, K., Millar, J.: How low-paid employees avoid poverty: an analysis by family type and household structure. J. Soc. Policy 35 , 351–369 (2006)
Garfinkel, I., Rainwater, L., Smeeding, T.: Wealth and welfare states: Is America a laggard or leader? Oxford University Press (2010)
Gilens, M.: Why Americans hate welfare: race, media, and the politics of antipoverty policy. University of Chicago Press (2009)
Glasmeier, A.K.: One nation, pulling apart: the basis of persistent poverty in the USA. Prog. Hum. Geogr. 26 , 155–173 (2002)
Goos, M., Manning, A.: McJobs and MacJobs: the growing polarisation of jobs in the UK. Labour Mark New Labour State Work Br 2003 , 70–85 (2003)
Goos, M., Manning, A., Salomons, A.: Explaining job polarization: routine-biased technological change and offshoring. Am. Econ. Rev. 104 , 2509–2526 (2014)
De Graaf PM, Ultee WC (2000) United in employment, united in unemployment?: Employment and unemployment of couples in the European Union in 1994. In: Welfare regimes and the experience of unemployment in Europe. Oxford University Press, pp 265–285
Gregg P, Wadsworth J. (1994) More work in fewer households? National Institute of Economic and Social Research London
Gregg, P., Wadsworth, J.: Workless Households and the Recovery. In: Dickens, R., Gregg, P., Wadsworth, J. (eds.) The labour market under new labour: the state of working Britain 2003, pp. 32–39. Palgrave Macmillan UK, London (2003)
Chapter Google Scholar
Gregg, P., Wadsworth, J.: Two sides to every story: measuring polarization and inequality in the distribution of work. J. R. Stat. Soc. Ser. A Stat. Soc. 171 , 857–875 (2008)
Gregg, P., Scutella, R., Wadsworth, J.: Reconciling workless measures at the individual and household level. Theory and evidence from the United States, Britain, Germany. Spain and Australia. J Popul Econ 23 , 139–167 (2010)
Carlo, G.C.O., Paci, P.S., Pieter,: Does employment generation really matter for poverty reduction ? The World Bank, Washington, DC (2007)
Hagerty, M.R., Srinivasan, V.: Comparing the predictive powers of alternative multiple regression models. Psychometrika 56 , 77–85 (1991). https://doi.org/10.1007/BF02294587
Herzenberg, S.A., Alic, J.A., Wial, H.: New rules for a new economy: employment and opportunity in postindustrial America. Cornell University Press, New York (2000)
Hidalgo-Hidalgo, M., Iturbe-Ormaetxe, I.: Long-run effects of public expenditure on poverty. J. Econ. Inequal 16 , 1–22 (2018). https://doi.org/10.1007/s10888-017-9360-z
Hill, E.J., Märtinson, V.K., Ferris, M., Baker, R.Z.: Beyond the mommy track: the influence of new-concept part-time work for professional women on work and family. J. Fam. Econ. Issues 25 , 121–136 (2004). https://doi.org/10.1023/B:JEEI.0000016726.06264.91
Hirsch, B.T.: Why do part-time workers earn less? the role of worker and job skills. ILR Rev. 58 , 525–551 (2005). https://doi.org/10.1177/001979390505800401
Hofmarcher, T.: The effect of education on poverty: a European perspective. Econ. Educ. Rev. 83 , 102124 (2021). https://doi.org/10.1016/j.econedurev.2021.102124
Hoover, G.A., Formby, J.P., Kim, H.: Poverty, non-white poverty, and the sen index. Rev. Income Wealth 50 , 543–559 (2004). https://doi.org/10.1111/j.0034-6586.2004.00139.x
Hoynes, H.W., Page, M.E., Stevens, A.H.: Poverty in America: trends and explanations. J. Econ. Perspect. 20 , 47–68 (2006)
ILO (2019) Work for a Brighter Future: The Global Commission for the Future of Work. International Labour Organisation (ILO)
Jaimovich, N., Siu, H.E.: Job polarization and jobless recoveries. Rev. Econ. Stat. 102 , 129–147 (2020)
Jouini, N., Lustig, N., Moummi, A., Shimeles, A.: Fiscal policy, income redistribution, and poverty reduction: evidence from Tunisia. Rev. Income Wealth 64 , S225–S248 (2018). https://doi.org/10.1111/roiw.12372
Jung S, Cho S-H, Roberts RK (2009) Public Expenditure and Poverty Reduction in the Southern United States. In: AgEcon Search. https://ageconsearch.umn.edu/record/47145 . Accessed 1 Oct 2019
Kalleberg, A.L.: Nonstandard employment relations: Part-time, temporary and contract work. Annu. Rev. Sociol. 26 , 341–365 (2000)
Kalleberg, A.L.: Precarious work, insecure workers: employment relations in transition. Am. Sociol. Rev. 74 , 1–22 (2009). https://doi.org/10.1177/000312240907400101
Kalleberg, A.L.: Good jobs, bad jobs: the rise of polarized and precarious employment systems in the United States, 1970s–2000s. Russell Sage Foundation (2011)
Kauhanen, M., Nätti, J.: Involuntary temporary and part-time work, job quality and well-being at work. Soc. Indic. Res. 120 , 783–799 (2015). https://doi.org/10.1007/s11205-014-0617-7
Kelly J (2019) The Frightening Rise In Low-Quality, Low-Paying Jobs: Is This Really A Strong Job Market? Forbes
Keune, M.: Trade union responses to precarious work in seven European countries. Int. J. Labour Res. 5 , 59 (2013)
Khan, M.S., Siddique, A.B.: Spatial analysis of regional and income inequality in the United States. Economies 9 , 159 (2021). https://doi.org/10.3390/economies9040159
Kimura, F., Chang, M.S.: Industrialization and poverty reduction in East Asia: internal labor movements matter. J. Asian Econ. 48 , 23–37 (2017). https://doi.org/10.1016/j.asieco.2016.10.005
Krishna, A.: For reducing poverty faster: target reasons before people. World Dev. 35 , 1947–1960 (2007). https://doi.org/10.1016/j.worlddev.2006.12.003
Lechner, M., Rodriguez-Planas, N., Kranz, D.F.: Difference-in-difference estimation by FE and OLS when there is panel non-response. J. Appl. Stat. 43 , 2044–2052 (2016). https://doi.org/10.1080/02664763.2015.1126240
Lindbeck, A., Snower, D.J.: Wage setting, unemployment, and insider-outsider relations. Am. Econ. Rev. 76 , 235–239 (1986)
Llull, J.: The effect of immigration on wages: exploiting exogenous variation at the national level. J. Hum. Res. (2017). https://doi.org/10.3368/jhr.53.3.0315-7032R2
Loayza, N.V., Raddatz, C.: The composition of growth matters for poverty alleviation. J. Dev. Econ. 93 , 137–151 (2010)
Marinescu, I., Skandalis, D.: Unemployment insurance and job search behavior. Q. J. Econ. 136 , 887–931 (2021)
McDonald, P., Bradley, L., Brown, K.: ‘Full-time is a Given Here’: part-time versus full-time job quality*. Br. J. Manag. 20 , 143–157 (2009). https://doi.org/10.1111/j.1467-8551.2008.00560.x
McMillan, M.: Understanding African poverty over the Longue Durée: a review of Africa’s development in historical perspective. J Econ Lit 54 , 893–905 (2016). https://doi.org/10.1257/jel.20151293
Meyers, M.K.: Are we asking the right questions about poverty in America? Soc. Serv. Rev. 88 , 728–744 (2014). https://doi.org/10.1086/679331
Middleton S, Loumidis J (2002) Young people, poverty and part-time work. In: Hidden Hands. Routledge, pp 40–52
Mordechay, K., Orfield, G.: Demographic transformation in a policy vacuum: the changing face of u.s. metropolitan society and challenges for public schools. Educ Forum 81 , 193–203 (2017). https://doi.org/10.1080/00131725.2017.1280758
Newman, K.S.: No shame in my game: the working poor in the inner city. Vintage, New York (2009)
Odhiambo, N.M.: Growth, employment and poverty in south africa. in search of a trickle-down effect. J. Income Distrib. 20 , 49–62 (2011)
Pacas, J.D., Rothwell, D.W.: Why is poverty higher in rural America according to the supplemental poverty measure? an investigation of the geographic adjustment. Popul. Res. Policy Rev. 39 , 941–975 (2020). https://doi.org/10.1007/s11113-020-09612-9
Page, J., Shimeles, A.: Aid, employment and poverty reduction in Africa. Afr. Dev. Rev. 27 , 17–30 (2015). https://doi.org/10.1111/1467-8268.12136
Pech, C., Klainot-Hess, E., Norris, D.: Part-time by gender, not choice: the gender gap in involuntary part-time work. Sociol. Perspect. 64 , 280–300 (2021)
Ravallion, M.: Fighting poverty one experiment at a time: <em>poor economics: a radical rethinking of the way to fight global poverty</em>: review essay. J Econ Lit 50 , 103–114 (2012). https://doi.org/10.1257/jel.50.1.103
Ravallion, M., Datt, G.: Why has economic growth been more pro-poor in some states of India than others? J. Dev. Econ. 68 , 381–400 (2002)
Reyes, A.M.: Mitigating poverty through the formation of extended family households: race and ethnic differences. Soc. Probl. 67 , 782–799 (2020). https://doi.org/10.1093/socpro/spz046
Rigney D (2010) The Matthew Effect: How Advantage Begets Further Advantage. Columbia University Press
Rosenzweig, M.R.: Thinking small: <em>poor economics: a radical rethinking of the way to fight global poverty</em>: review essay. J. Econ. Lit. 50 , 115–127 (2012). https://doi.org/10.1257/jel.50.1.115
Roth, J., Sant’Anna PHC, Bilinski A, Poe J,: What’s trending in difference-in-differences? a synthesis of the recent econometrics literature. J. Econom. 235 , 2218–2244 (2023). https://doi.org/10.1016/j.jeconom.2023.03.008
Salvatori, A.: The anatomy of job polarisation in the UK. J. Labour Mark Res. 52 , 8 (2018). https://doi.org/10.1186/s12651-018-0242-z
Satchi, M., Temple, J.: Labor markets and productivity in developing countries. Rev. Econ. Dyn. 12 , 183–204 (2009). https://doi.org/10.1016/j.red.2008.09.001
Saunders, P.: The direct and indirect effects of unemployment on poverty and inequality. Aust. J. Labour Econ. 5 , 507 (2002)
Saunders P (2006) A perennial problem: Employment, joblessness and poverty. Social Policy Research Centre Sydney
Schoeni RF, Blank RM (2000) What has welfare reform accomplished? Impacts on welfare participation, employment, income, poverty, and family structure. National bureau of economic research
Siddique, A.B.: Comparative advantage defying development strategy and cross country poverty incidence. J. Econ. Dev. 41 , 45–78 (2016). https://doi.org/10.35866/caujed.2016.41.4.003
Siddique, A.B.: Does ethnic diversity hurt fiscal sustainability? Econ. Bull. 41 , 532–543 (2021)
Siddique AB (2022) White prototypicality threats, inequality in diversity, and collective goods provisions
Siddique, A.B., Haynes, K.E., Kulkarni, R., Li, M.-H.: Regional poverty and infection disease: early exploratory evidence from the COVID-19 pandemic. Ann. Reg. Sci. (2022). https://doi.org/10.1007/s00168-022-01109-x
Simms, M.: Unions and job quality in the UK: Extending interest representation within regulation institutions. Work. Occup. 44 , 47–67 (2017)
Sloane, P.J., Theodossiou, I.: Earnings mobility, family income and low pay. Econ. J. 106 , 657–666 (1996). https://doi.org/10.2307/2235573
Stecy-Hildebrandt, N., Fuller, S., Burns, A.: ‘Bad’jobs in a ‘Good’sector: examining the employment outcomes of temporary work in the Canadian public sector. Work Employ Soc. 33 , 560–579 (2019)
Stier, H., Lewin, A.C.: Does women’s employment reduce poverty? evidence from Israel. Work Employ Soc. 16 , 211–230 (2002). https://doi.org/10.1177/095001702400426811
Thiede, B.C., Kim, H., Slack, T.: Marriage, work, and racial inequalities in poverty: evidence from the United States. J. Marriage Fam. 79 , 1241–1257 (2017). https://doi.org/10.1111/jomf.12427
Tilly, C.: Reasons for the continuing growth of part-time employment. Mon. Labor Rev. 114 , 10–18 (1991)
Tilly, C.: Half a job: bad and good part-time jobs in a changing labor market. Temple University Press, Philadelphia (2010)
Van Arsdale, D.G.: The temporary work revolution: the shift from jobs that solve poverty to jobs that make poverty. Workingusa 16 , 87–112 (2013)
Visaria, P.: Poverty and unemployment in India: An analysis of recent evidence. World Dev. 9 , 277–300 (1981). https://doi.org/10.1016/0305-750X(81)90031-0
Walsh, J.: Myths and counter-myths: an analysis of part-time female employees and their orientations to work and working hours. Work Employ Soc. 13 , 179–203 (1999)
Wilson, G., Roscigno, V.J.: Public sector reform and racial occupational mobility. Work. Occup. 43 , 259–293 (2016)
Woodbury, S.A.: Power in the labor market: institutionalist approaches to labor problems. J. Econ. Issues 21 , 1781–1807 (1987). https://doi.org/10.1080/00213624.1987.11504722
Download references
Acknowledgements
I would like to express my gratitude to Drs. M. Kugler, K. Reinert, N. Koizumi, and S. Slavov for their invaluable guidance. Additionally, I extend my thanks to the two anonymous reviewers for their insightful and thorough referee reports. Special thanks to Prof. Christian Pfeifer, the Associate Editor of JLMR, for overseeing and managing the peer-review process for this manuscript and feedbacks. Your collective contributions have significantly enriched the quality of this work.
This research did not receive any financial support from any sources.
Author information
Authors and affiliations.
School of Public Administration, Florida Atlantic University, 777 Glades Road, Boca Raton, FL, 33431, USA
Abu Bakkar Siddique
You can also search for this author in PubMed Google Scholar
Contributions
All analysis and manuscript writing were done by ABS.
Corresponding author
Correspondence to Abu Bakkar Siddique .
Ethics declarations
Conflict of interests.
There are no conflicts of interest.
Additional information
Publisher's note.
Springer Nature remains neutral with regard to jurisdictional claims in published maps and institutional affiliations.
1.1 Appendix A
See Fig. 4 .
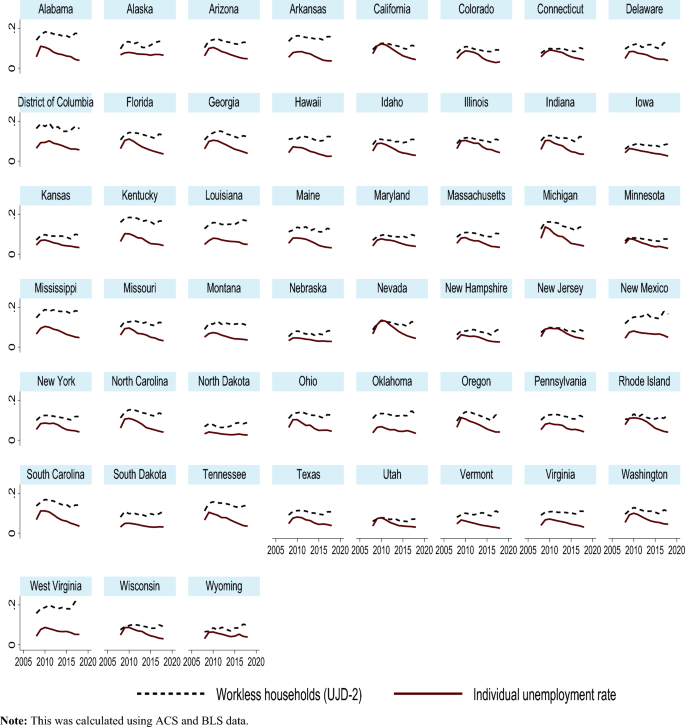
Workless households and individual unemployment rate by states. This was calculated using ACS and BLS data
1.2 Appendix B
Household-level versus aggregate-level measures.
This paper highlights an important issue regarding the significance of household-level employment measures compared to aggregate-level employment measures. To support this argument, a comparison is made between the predictive abilities of the job distribution index, job quality measure, and individual-level unemployment rate. Since the predictive power of two-way fixed effect estimates is largely driven by state and year fixed effects, I employ an ordinary least square model and compare their predictive power to offer suggestive evidence. In determining the statistical model's explanatory power, R-square is widely used and has been employed here (Hagerty and Srinivasan 1991 ; Foster et al. 1997 ; Choodari-Oskooei et al. 2012 ). Table 5 presents various estimates using the uneven job distribution index, job quality measures, and individual unemployment rate separately, as well as their estimates jointly. Remarkably, the uneven job distribution index, measured at the household level, exhibits more than three times the predictive power for poverty compared to the individual unemployment rate (R-square of 0.66 versus 0.19 in columns 5.1 to 5.2). Interestingly, including the individual unemployment rate alongside the uneven job distribution does not improve the R-square value. Additionally, it is important to note that good-quality jobs demonstrate higher explanatory power (R-square 0.60) as well, while poor-quality jobs have limited explanatory power. Therefore, the key takeaway from this analysis is that household-level employment distribution holds significantly greater explanatory power in understanding poverty than the commonly used economic indicator of the unemployment rate.
Rights and permissions
Open Access This article is licensed under a Creative Commons Attribution 4.0 International License, which permits use, sharing, adaptation, distribution and reproduction in any medium or format, as long as you give appropriate credit to the original author(s) and the source, provide a link to the Creative Commons licence, and indicate if changes were made. The images or other third party material in this article are included in the article's Creative Commons licence, unless indicated otherwise in a credit line to the material. If material is not included in the article's Creative Commons licence and your intended use is not permitted by statutory regulation or exceeds the permitted use, you will need to obtain permission directly from the copyright holder. To view a copy of this licence, visit http://creativecommons.org/licenses/by/4.0/ .
Reprints and permissions
About this article
Cite this article.
Siddique, A.B. Job market polarization and American poverty. J Labour Market Res 57 , 30 (2023). https://doi.org/10.1186/s12651-023-00356-5
Download citation
Received : 18 October 2022
Accepted : 16 October 2023
Published : 02 November 2023
DOI : https://doi.org/10.1186/s12651-023-00356-5
Share this article
Anyone you share the following link with will be able to read this content:
Sorry, a shareable link is not currently available for this article.
Provided by the Springer Nature SharedIt content-sharing initiative
- Job market polarization
- Job quality
- Job distribution
JEL Classification
- Harvard Business School →
- Faculty & Research →
- Research Summary
Job Market Paper
Description.
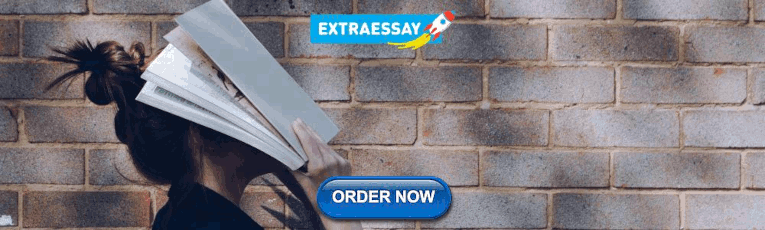
IMAGES
VIDEO
COMMENTS
The job market paper is a piece of original research, usually included as one of three chapters in the dissertation, that best demonstrates a job market candidate's skills, training, and aptitude as an economist. A job market paper also should contribute to the extant literature by providing novel answers and insights regarding a well-defined research question.
The Ten Most Important Rules of Writing Your Job Market Paper. Rule #1: You will probably not have a Nobel Prize winning idea. Theorem #1: It is always possible to transform a good idea into a great paper and a superb presentation. Theorem #2: Even if your idea is Nobel-worthy, you can always make it into a poorly written paper and a lousy ...
Elaboration on Above Comment. In economics and certain business school fields, a major part of junior academic hiring is the job market paper (JMP). The JMP is an original piece of research by the applicant that constitutes their best research to date. It is meant to serve as a signal of the candidate's potential for becoming a good researcher.
In this paper, we provide a thorough review of existing research on data science techniques used to analyze the job market, focusing on publications from the last seven years. The review examines more than 101 studies, describing the process of analyzing the job market and categorizing the different data sources and approaches applied in every ...
Of the research you might be working on, the job market paper is that which provides the best illustration of your skills, interests, and capacity to perform independent, interesting, and cutting-edge research. Further, this job market paper must be very polished by the time you send it off in an application, which means that you should have it ...
improving your paper, your interview skills, and your job talk (your 90-minute seminar based on the job market paper). During your search, keep in mind two basic facts about the job market for new economics Ph.D.s. The first is trickle down. Each year, almost every economics Ph.D. program produces more Ph.D.s than it can hire.
A: Having more than one presentable paper is a plus, but it is not necessary. In most cases, you will want to identify as your job market paper the one that best displays your skills and is the best forecast of your research agenda. In special cases you can identify a job market paper for one audience (e.g., theory) and one for another
Dr. Karen's Five-Paragraph Model. Paragraph 1: A brief sketch of the main themes and topics of your research as well as how it relates to your field. Paragraph 2: A summary of your dissertation research. This may be similar to the paragraph on the dissertation in your cover letter, but it must have more detail about the methods, the ...
The impact of artificial intelligence (AI) on the job market continues to be a topic of debate. While AI has been perceived as a potential threat to employment, it also presents opportunities. for ...
Prepare your interview presentation: you will need one-line, 1-min, and 30-min versions of your description of your job market paper. Focus on why your job market paper is important and interesting and why anyone should care. Also, get a good haircut before the interviews. 3.10 At the first-round interviews. All interviews are more-or-less the ...
This paper is focused on the influence of AI on the employment scale of the manufacturing industry, examines the job creation effect of technological progress from the perspectives of capital ...
Introduction Fast-emerging technologies are making the job market dynamic, causing desirable skills to evolve continuously. It is therefore important to understand the transitions in the job market to proactively identify skill sets required. Case description A novel data-driven approach is developed to identify trending jobs through a case study in the oil and gas industry. The proposed ...
Job Search and Employment Success: A Quantitative Review and Future Research Agenda. July 2020. Journal of Applied Psychology. DOI: 10.1037/apl0000675. Authors: Edwin van Hooft. University of ...
Job Market Paper. The Economic Geography of Lifecycle Human Capital Accumulation: The Competing Effects of Labor Markets and Childhood Environments. Ben Sprung-Keyser and Sonya Porter. Abstract. We examine how place shapes the production of human capital across the lifecycle. We ask: do those places that most effectively produce human capital ...
Like previous editions, The Future of Jobs Report 2023 offers insights into these transformations and unpacks how businesses are expecting to navigate these labour-market changes from 2023 to 2027, leveraging a unique cross-sectoral and global survey of Chief Human Resources, Chief Learning Officers and Chief Executive Officers of leading global employers and their peers.
The paper analyzes the job market trends from 2019 to 2023 based on the latest research and reports, and discusses the implications of AI for the workforce and policymakers. The paper concludes ...
The Journal for Labour Market Research is a journal in the interdisciplinary field of labour market research. As of 2016 the Journal publishes open access. The journal follows international research standards and strives for international visibility. With its empirical and multidisciplinary orientation, the journal publishes papers in English ...
Finally, we survey work on job search interventions and conclude with an overview of pressing job search issues in need of future research. You can access this article via the following e-print ...
Job Market Paper. "Does Mobility Make a Nice Person? Theory and. Evidence from Across the Globe". In much of modern life, cooperation takes the form of people engaging in costly behavior that helps another. It is easy to understand how cooperation might be achieved in small communities where members interact repeatedly.
In this paper, I argue that job market polarization is a major factor that perpetuates poverty, even during periods of high employment and low unemployment. When jobs are distributed unequally across households and are of poor quality, with no full-time work available, the benefits of job growth fail to reach those who need them the most.
Abstract and Figures. Artificial intelligence (AI) is rapidly transforming the way we live and work, affecting a wide range of industries and job markets. In this research paper, the focus will be ...
Description. Much of the prior research on CEO successions focuses on differences between CEOs appointed from within the firm and those appointed from outside; however, this dichotomy neglects significant heterogeneity in CEOs' career trajectories. In this study, I examine the environmental antecedents and performance consequences of ...
that by 2022, AI and ML will create 133 million new jobs while displac ing 75 million (WEF, 2020). Artificial. intelligence (AI) and machine learning (ML) are rapidly changing the way businesses ...