Statistical Analysis in Climate Research
About this ebook, about the author.
Hans von Storch is Director of the Institute of Hydrophysics of the GKSS Research Centre in Geesthacht, Germany and a Professor at the Meteorological Institute of the University of Hamburg.
Francis W. Zwiers is Chief of the Canadian Centre for Climate Modelling and Analysis, Atmospheric Environment Service, Victoria, Canada, and an Adjunct Professor at the Department of Mathematicw and Statistics of the University of Victoria.
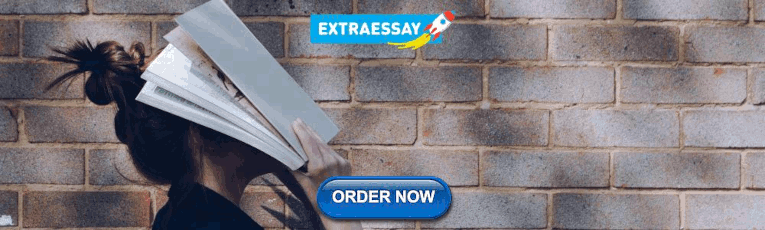
Rate this ebook
Reading information, more by hans von storch.
Similar ebooks
Academia.edu no longer supports Internet Explorer.
To browse Academia.edu and the wider internet faster and more securely, please take a few seconds to upgrade your browser .
Enter the email address you signed up with and we'll email you a reset link.
- We're Hiring!
- Help Center
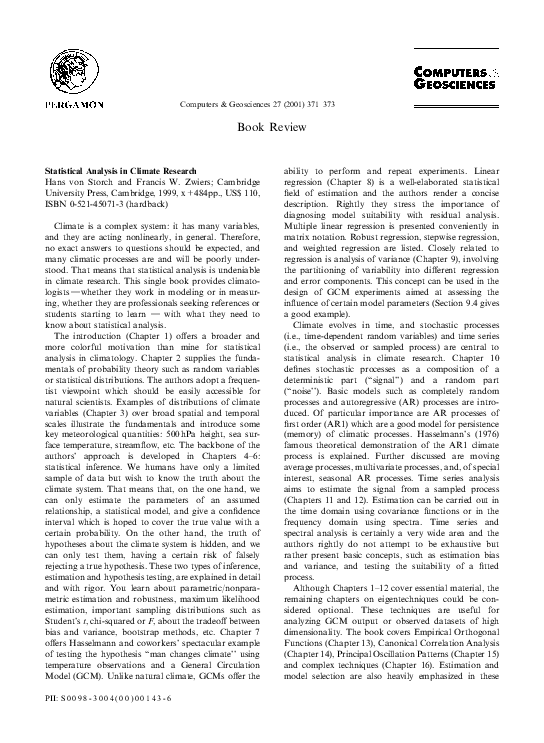
Statistical Analysis in Climate Research

The purpose of this book is to help the climatologist understand the basic precepts of the statistician's art and to provide some of the background needed to apply statistical methodology correctly and usefully. The book is self contained: introductory material, standard advanced techniques, and the specialized techniques used specifically by climatologists are all contained within this one source. There are a wealth of real-world examples drawn from the climate literature to demonstrate the need, power and pitfalls of statistical analysis in climate research.
Related Papers
Intern. J Climatol. 24: 665–680
Hans von Storch
We review the role of statistical analysis in the climate sciences. Special emphasis is given to attempts to construct dynamical knowledge from limited observational evidence, and to the ongoing task of drawing detailed and reliable information on the state, and change, of climate that is needed, for example, for short-term and seasonal forecasting. We conclude with recommendations of how to improve the practice of statistical analysis in the climate sciences by drawing more efficiently on relevant developments in statistical mathematics.
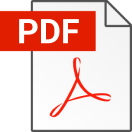
Peter Guttorp
Norman Dreier
Zenodo (CERN European Organization for Nuclear Research)
Eben Nornormey
American Journal of Climate Change
Comptes Rendus Geoscience
Caspar Ammann
wasana Jayawardena
RELATED PAPERS
Monthly Notices of the Royal Astronomical Society
Jorge Garcíarojas
Fire Science and Engineering
Gwon Hyun Ko
Management & Marketing
Simona Vasilache
Experimental Dermatology
Roslyn Isseroff
Rolf Saalfrank
Dini Wahyuni
Annals of Translational Medicine
Angela McCall
Journal of tropical medicine
Nagib A Elmarzugi
Traffic Injury Prevention
Paul Begeman
Applied Sciences
Young-Ji Byon
Global Change Biology
Dominic Hodgson
European Journal of Cancer Prevention
Christophe Depuydt
Journal of Economics and Sustainable Development
Carol Munyao
Proceedings of the National Academy of Sciences
sudipta chatterjee
ana stephan
Acta bioquímica clínica latinoamericana
medi falahati
mehdi falahati
Camillo La Mesa
World Applied Sciences Journal
Ali Ahmed Bhutto
Studi Francesi
michel arrous
SIAM Journal on Numerical Analysis
Raytcho Lazarov
Social Science Research Network
Amber Conner
Journal of Krishi Vigyan vol 12 issue 1
Manoj Sharma
Frontiers in Medicine
Wolfram Weckwerth
RELATED TOPICS
- We're Hiring!
- Help Center
- Find new research papers in:
- Health Sciences
- Earth Sciences
- Cognitive Science
- Mathematics
- Computer Science
- Academia ©2024
Climate Data Analysis Tools & Methods
View all of our climate data analysis tools & methods or use the list below to jump to a certain group.
Climate Model Evaluation
Climate data processing & visualization, climate data formats, statistical methods, evaluation of interannual-to-decadal climate variability in earth system models, model configurations, overview: climate model intercomparison project (cmip), common spectral model grid resolutions, overview: climate data processing, web based visualization and processing for climate and weather, climate data processing software, regridding overview, common climate data formats: overview, text (ascii) files, binary (data format), netcdf overview, statistical & diagnostic methods overview, empirical orthogonal function (eof) analysis and rotated eof analysis, taylor diagrams, trend analysis.
Thank you for visiting nature.com. You are using a browser version with limited support for CSS. To obtain the best experience, we recommend you use a more up to date browser (or turn off compatibility mode in Internet Explorer). In the meantime, to ensure continued support, we are displaying the site without styles and JavaScript.
- View all journals
- My Account Login
- Explore content
- About the journal
- Publish with us
- Sign up for alerts
- Open access
- Published: 17 April 2024
Climate damage projections beyond annual temperature
- Paul Waidelich ORCID: orcid.org/0000-0002-5081-776X 1 ,
- Fulden Batibeniz ORCID: orcid.org/0000-0002-1751-3385 2 , 3 , 4 ,
- James Rising ORCID: orcid.org/0000-0001-8514-4748 5 ,
- Jarmo S. Kikstra ORCID: orcid.org/0000-0001-9405-1228 6 , 7 , 8 &
- Sonia I. Seneviratne ORCID: orcid.org/0000-0001-9528-2917 2
Nature Climate Change ( 2024 ) Cite this article
4461 Accesses
105 Altmetric
Metrics details
- Climate-change impacts
- Environmental economics
- Projection and prediction
Estimates of global economic damage from climate change assess the effect of annual temperature changes. However, the roles of precipitation, temperature variability and extreme events are not yet known. Here, by combining projections of climate models with empirical dose–response functions translating shifts in temperature means and variability, rainfall patterns and extreme precipitation into economic damage, we show that at +3 ° C global average losses reach 10% of gross domestic product, with worst effects (up to 17%) in poorer, low-latitude countries. Relative to annual temperature damage, the additional impacts of projecting variability and extremes are smaller and dominated by interannual variability, especially at lower latitudes. However, accounting for variability and extremes when estimating the temperature dose–response function raises global economic losses by nearly two percentage points and exacerbates economic tail risks. These results call for region-specific risk assessments and the integration of other climate variables for a better understanding of climate change impacts.
Similar content being viewed by others
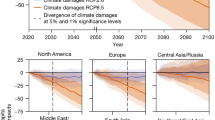
The economic commitment of climate change
Maximilian Kotz, Anders Levermann & Leonie Wenz
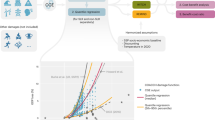
New damage curves and multimodel analysis suggest lower optimal temperature
Kaj-Ivar van der Wijst, Francesco Bosello, … Detlef van Vuuren
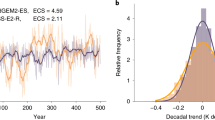
Decadal global temperature variability increases strongly with climate sensitivity
Femke J. M. M. Nijsse, Peter M. Cox, … Mark S. Williamson
Projections of economic damage from climate change are key for evaluating climate mitigation benefits, identifying effects on vulnerable communities and informing discussions around adaptation needs, as well as loss and damage financing. On a global or country level, such assessments have focused on how projected changes in annual mean temperatures affect gross domestic product (GDP) 1 , 2 , 3 , 4 . However, the widespread losses in recent years driven by flooding and drought suggest that precipitation variability and extremes are similarly important 5 , 6 . Anthropogenic forcing is increasing the frequency and intensity of precipitation extremes and variability on multiple scales, altering daily temperature patterns and driving an overall increase in precipitation over land 7 , 8 . Continued global warming is expected to exacerbate these trends, potentially with uneven impacts across regions 5 , 9 , 10 . Therefore, including precipitation, variability and extremes can improve the precision, comprehensiveness and interpretability of climate change damage estimations 11 .
Economic damage from climate change can be assessed either bottom-up by quantifying, valuating and aggregating specific impacts (for example, crop failures or labour supply changes) or top-down by identifying the statistical relationship between observed climatic shifts and economic growth. While both approaches have advantages and limitations, top-down approaches usually neglect climatic shifts beyond annual temperature changes 12 . To address this shortcoming, recent studies have estimated the relationship between macrolevel income and a wider range of climatic indicators, such as total precipitation 13 , 14 , 15 , temperature variability 16 , 17 or temperature and precipitation extremes and anomalies 14 , 18 , 19 . However, these studies do not investigate how much the inclusion of these climate indicators alters previous economic assessments of climate change, which is highly relevant for policy-making and future adaptation. A notable exception is ref. 15 , which projects the effects of annual precipitation and temperature shifts on inequality. A comprehensive assessment of the projected economic impacts of intense periods of precipitation and temperature anomalies, however, is still missing.
In this study, we draw upon recent advances in estimating dose–response functions, which relate shifts in various climate indicators (total precipitation, temperature variability, precipitation anomalies and extremes) to GDP changes 14 . Combining these functions with projections from 33 models of Coupled Model Intercomparison Project Phase 6 (CMIP6), including two large ensembles, we investigate how including these indicators affects the understanding of future economic impacts at different global warming levels. Variability and extremes introduce substantial climatic and associated economic uncertainties and we conduct a variance decomposition to determine the main uncertainty drivers. Furthermore, we explore how including variability and extremes in empirical regressions alters the dose–response function for annual mean temperature, which the extant literature has estimated controlling only for annual precipitation 1 , 2 , 4 , 20 , 21 .
Projecting GDP impacts for six climate indicators
Compared to annual temperature, future changes in precipitation and temperature variability under climate change are subject to high uncertainties 8 , 22 , 23 . To capture these uncertainties, we use projections from various CMIP6 models 10 to analyse four climate indicators besides annual mean temperature and annual precipitation: (1) day-to-day temperature variability (how much daily temperatures deviate from monthly means); (2) extreme precipitation (the annual sum of precipitation on days with exceptionally high precipitation exceeding the historical 99.9th percentile); (3) monthly precipitation deviation (how much monthly precipitation deviates from historical means throughout the year); and (4) the number of ‘wet days’ with precipitation above 1 mm d −1 . These indicators have been linked to forcing from GHGs 24 , 25 as well as to income growth using a global sample 14 , 16 . Considering all indicators in one coherent estimation framework is crucial because variability and extremes correlate strongly with annual temperature, total precipitation and each other (Supplementary Fig. 3 ). Therefore, combining dose–response functions from different estimations risks double-counting impacts. Notably, our coherent estimation framework 14 does not model damage from droughts and heat waves. Therefore, we include heat in a complementary analysis, whereas we find no significant statistical link to economic growth for droughts, potentially due to limited spatial and temporal resolution and impact heterogeneity across regions (Supplementary Appendix F ).
We illustrate our approach for the example of extreme precipitation impacts on economic output for New York State at +3°C of global warming (Fig. 1 ). On the basis of how a given CMIP6 model and scenario project the respective climate indicator (Fig. 1a ), we compare the GDP impacts in a given year against the average impacts if the climate were to remain constant at levels of a recent baseline period (Fig. 1b ) 2 , 15 . For each model and scenario, the baseline period is the 41-year period during which global warming equals the historical warming between 1979 and 2019 (+0.84 °C), which is the climatic baseline used for estimating the dose–response functions used here ( Methods ) 14 . We then repeat this procedure for different CMIP6 models and potential damage parameter estimates based on statistical uncertainty and aggregate results to the national level. This yields a distribution of GDP impacts for each country featuring all years in each model and scenario associated with the same global warming level (Fig. 1c ). Therefore, the main sources of uncertainty in our GDP impact distribution for a given global warming level and territory are (1) internal variability for the same CMIP6 model because the magnitude of extremes can vary strongly from year to year and, for large ensembles, across model runs, (2) statistical uncertainty in the dose–response functions and (3) projection differences between CMIP6 models.
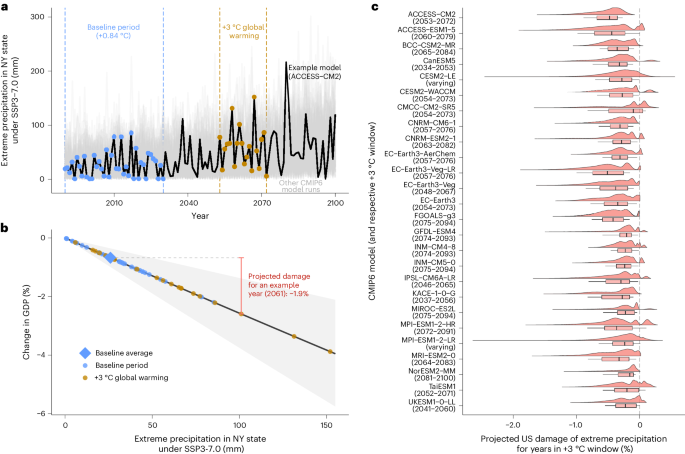
a , Projected extreme precipitation under SSP3-7.0 for an example model run (ACCESS-CM2, black) and other CMIP6 model runs (grey). Vertical lines denote the baseline period (blue) and the +3 °C global warming window (brown). b , Dose–response function for extreme precipitation (black line) and 95% confidence interval (grey area). Coloured dots and the blue diamond represent extreme precipitation levels from a and the baseline period average. The red error bar illustrates the difference between the dose–response function for an example year (2061) and the baseline average, which equals the projected damage for this year. Dose–response function values are transformed from the original logarithmic changes to percentage of GDP by exponentiating and subtracting one ( Methods ). c , Distribution of projected extreme precipitation damage at +3 °C by CMIP6 model. Boxplot centre, hinges and whiskers denote median, upper/lower quartiles and upper/lower deciles, respectively. For the CESM2-LE and MPI-ESM1-2-LR large ensembles, the +3 °C global warming-level window varies across ensemble members.
Source data
Global results.
We examine the impact on global GDP for all indicators combined, as well as the separate impacts from annual temperature, annual precipitation and the four variability and extremes indicators (Fig. 2a ). Global GDP is estimated to be 3.2% lower (lower/upper decile: 1.2–5.4%) at +1.5 °C of global warming, compared to a world with no further climate change beyond recent levels. At +3 °C, global GDP decreases by 10.0% (5.1–14.9%). When disaggregated by climate indicator, global impacts are strongly determined by the annual mean temperature changes, which account for a GDP reduction of 10.0% at +3 °C. This estimate is consistent with recent top-down studies focusing exclusively on damage from annual temperature changes and projecting impacts of 7–14% of GDP per capita loss by the end of the century at global warming levels of more than +4 °C (refs. 4 , 8 , 20 ); with other top-down studies estimating damage even higher 2 as a result of their assumption that temperature changes impact growth trajectories persistently 12 , 26 , 27 . For context, a 10% reduction exceeds the GDP loss of the COVID-19 pandemic when global growth plummeted from +2.6% in 2019 to −3.1% in 2020 or the effect of the global financial crisis in 2009 when global output shrunk by −1.3% (ref. 28 ). Importantly, recent research suggests that damage attributed to annual temperature covers heat wave impacts at least partially 18 . Indeed, when disentangling the two, we find that almost half of annual temperature damage can be attributed to heat extremes (Supplementary Appendix F ).
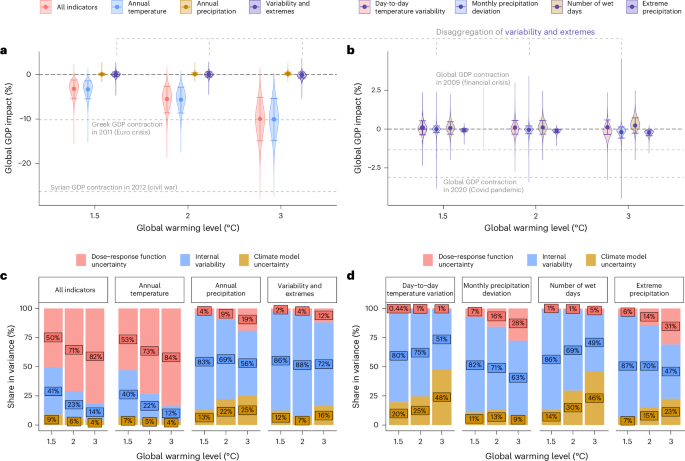
a , Points and the error bars centred around them denote the mean and upper-to-lower-decile range, respectively. ‘Variability and extremes’ comprises the four indicators in b . Labelled grey horizontal lines denote example growth rates in real GDP 28 . b , Same as a , with ‘variability and extremes’ impacts disaggregated by indicator. c , Variance decomposition for the combined GDP impacts of all climate indicators and for indicator-specific impacts. Indicator-specific decompositions are feasible because impacts in the underlying regression model are additive and hence can be projected separately. For absolute variances and coefficients of variation, see Supplementary Figs. 8 and 9 . d , Same as c , with ‘variability and extremes’ disaggregated by indicator.
By contrast, increases in annual precipitation in many areas have a small positive impact on global GDP (0.2% at +3 ° C warming) and the combined impact of the variability and extremes indicators remains centred around zero. While this suggests a lack of signal, disaggregating projections by individual indicators reveals otherwise (Fig. 2b ). At +3 °C, extreme precipitation reduces global GDP by 0.2% (0.1–0.4%), with 99% of our impact distribution indicating economic losses as extreme precipitation increases around the globe (Supplementary Fig. 5 ). Notably, these impacts are much lower than annual temperature damage. This is somewhat expected because extremes have a lower temporal and spatial correlation. Therefore, aggregation from daily, location-specific events to annual indicators and country-level projections reduces signals more strongly compared to annual mean temperature 13 , 14 . However, a 0.2% GDP loss due to extreme precipitation globally for an average year still represents a tenth of the damage caused by the catastrophic 2022 floods in Pakistan, estimated at 2.2% of GDP 29 . Global GDP losses from extreme precipitation are compensated, on average, by temperature variability reductions in higher latitudes (+0.1% of global GDP at +3 °C) 24 , 30 and fewer wet days (+0.2%). However, only 63% and 74% of the impact distribution imply global economic gains for these indicators, respectively. Monthly precipitation deviations, on average, add to global GDP losses (0.2% at +3 °C), but uncertainty ranges remain centred around zero.
To explore uncertainty drivers, we decompose the variance in GDP impacts from each climate indicator into statistical dose–response function uncertainty, climate model uncertainty and internal variability (Fig. 2c ). For annual temperature damage, uncertainty is primarily driven by the dose–response function, particularly at higher global warming levels. By contrast, impact uncertainty for annual precipitation and variability and extremes is dominated by internal variability. Additional analyses focusing on the two large ensembles in our sample suggest that this stems primarily from interannual variation within model runs rather than differences across ensemble members (Supplementary Figs. 15 and 16 ). Moreover, disagreement between CMIP6 models plays either a comparable or a more substantial role than dose–response function uncertainty for these additional indicators (except for monthly precipitation deviation) and is particularly pronounced for day-to-day temperature variability and the number of wet days (Fig. 2d ). Notably, the share of climate model uncertainty decreases in global warming for annual temperature impacts but not for variability and extremes because even for a stronger global warming signal, GDP impact projections do not converge between models.
Country-level results
Because global aggregates risk masking heterogeneities across regions, we further investigate the combined country-level GDP impacts from all six climate indicators at +3 ° C of warming (Fig. 3a ). All countries face GDP losses, in line with recent evidence that climate change might not benefit cooler countries economically 20 . Impacts are more severe in the Global South and highest in Africa and the Middle East, where higher initial temperatures make countries particularly vulnerable to additional warming. Considering the combined GDP impact of all four variability and extremes indicators reveals a clear North–South divide (Fig. 3b ). While for higher latitudes, the decrease in temperature variability and, for some countries, wet days (Supplementary Fig. 5) mitigates overall GDP damage, variability and extremes exacerbate GDP losses in most parts of the Global South. However, these effects vary substantially across the full distribution of projected impacts for each country.
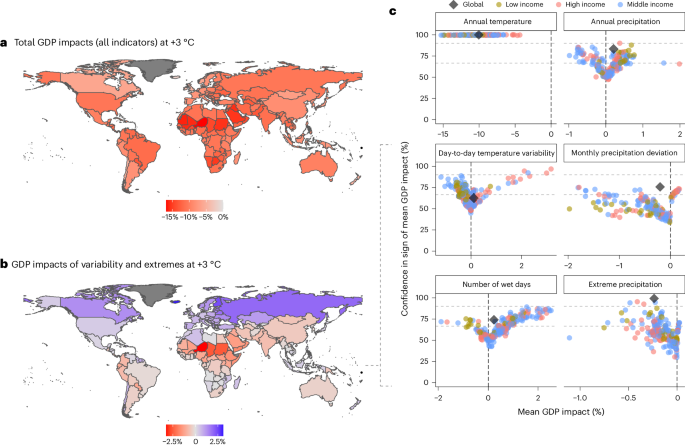
a , Mean GDP impact at +3 °C of global warming for sovereign countries (other territories in dark grey) considering all six indicators in c , using shapefiles from ref. 42 . b , Same as a but only considering the bottom four ‘variability and extremes’ indicators in c . c , Mean GDP impact ( x axis) and share of the impact distribution agreeing with the sign of the mean ( y axis) for sovereign countries by World Bank income group (colour) and the global economy (grey diamond) at +3 °C. Middle income comprises lower- and upper-middle-income countries for conciseness. Dashed horizontal lines denote thresholds for 66% and 90% likelihood following IPCC terminology 8 .
Annual temperature is the only indicator where negative impacts arise for at least 90% of our impact distribution for all countries (upper dashed line in Fig. 3c ), including substantial impacts in several colder countries partially because the temperature dose-response function deployed here implicitly features damages from higher inter-annual temperature variability as well (Supplementary Appendix C). Annual precipitation increases benefit most countries on average, but for many countries, less than two-thirds of the distribution supports the sign of expected impacts (lower dashed line). For day-to-day temperature variability, we find a clear divide between relatively certain gains for a few high-income countries and less certain, smaller losses for many lower-income countries as a result of variability increases in lower latitudes 24 . While extreme precipitation increases in most regions, projected damages are highest and least uncertain for middle- and high-income countries in higher latitudes such as China and the United States 31 . In contrast, low-income countries are more likely to face losses from shifts in precipitation deviation and wet days, but high uncertainties limit the conclusions that can be drawn.
Overall impact of including variability and extremes
The results in the previous sections seemingly suggest that including variability and extremes in GDP impact projections exacerbates disparities between higher- and lower-income countries (Fig. 3 ) but does not substantially alter the implications of climate change for global GDP (Fig. 2 ), particularly since annual temperature damages capture heat wave impacts to some extent already. However, providing an apples-to-apples comparison with the recent climate economics literature requires calculating damage on the basis of the current ‘status quo’ approach, which (1) projects only damage from annual temperature changes and (2) estimates the relationship between income growth and annual temperature controlling only for annual precipitation 1 , 2 , 4 , 20 , 21 . When comparing the resulting global GDP impacts following this status quo methodology to our approach, which (1) projects damage for all six indicators and (2) controls for all our climate indicators when estimating the temperature dose–response function (Fig. 4a ), we find that including variability and extremes increases global damage at +3 °C of global warming by 1.8 percentage points (10.0% instead of 8.2%).
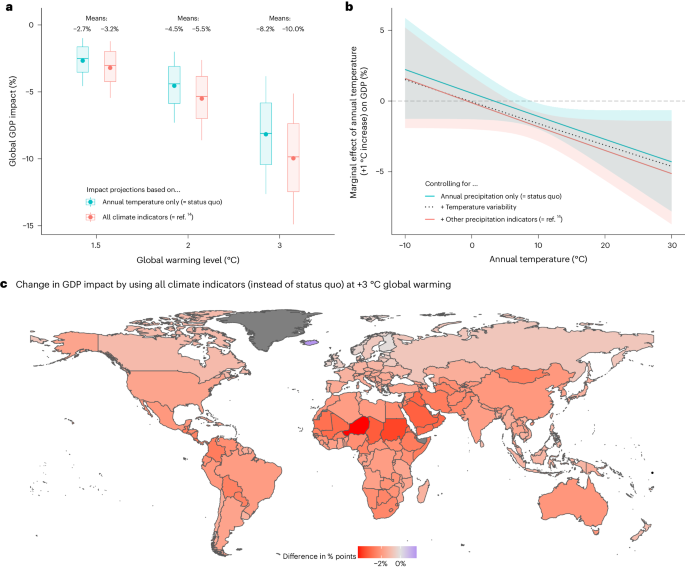
a , Dots represent mean values of the GDP impact distribution, while boxplot centre, hinges and whiskers denote median, upper/lower quartiles and upper/lower deciles, respectively. b , GDP impact of a +1 °C increase in the annual temperature of a territory for different initial temperature levels ( x axis) using mean marginal effects (lines) with 95% confidence intervals around them (shaded area). Estimated using Supplementary equation ( 22 ) and the regression models in Supplementary Table 7 (columns 1, 2 and 5). No confidence interval shown for ‘+ Temperature variability’ for visual conciseness. c , Difference in mean GDP impacts between our main approach and the status quo approach at +3 °C for sovereign countries (other territories marked in dark grey), using shapefiles from ref. 42 .
The main reason for this increase is that controlling for variability and extremes, instead of only for annual precipitation, increases the estimated effect of mean temperature changes (Fig. 4b ). The marginal GDP impact of a +1 °C rise in annual temperature increases by more than 0.5 percentage points irrespective of the initial temperature level when all climate indicators are included as control variables (red line). Most of this effect is driven by including temperature variability (dotted line), which leads to higher impacts, particularly for colder regions. Therefore, the positive impacts of temperature variability in Fig. 3 obscure that, in fact, including this parameter leads to higher global damage since it disentangles potential benefits of reduced variability from the negative effects of temperature increases. As a result, including all climate indicators exacerbates GDP impacts across the globe (Fig. 4c ).
Exposure to tail risks
Aside from average impacts and the uncertainty around them, prudent risk management by policy-makers also requires information about tail risks. Therefore, we examine the percentage of the present global population living in countries that have a non-negligible chance (at least 5%) of suffering from damage exceeding different thresholds at different global warming levels (Fig. 5a ), both for our main approach (solid line) and the status quo approach (dotted line). Even at +1.5 °C, tail risks are substantial, with 99% of the global population living in countries with a non-negligible risk of suffering GDP damage of 5% or higher if all climate indicators are included. Notably, including variability and extremes increases tail risks considerably (Fig. 5b ). While under the status quo, 54% of the global population is projected to face damage of at least 15% with a likelihood of at least 5% at +3 ° C of warming, this increases to 68% of the population when variability and extremes are included. The share of the global population facing catastrophic impacts of 20% or higher with a 5% chance rises from 4% to 17%. However, disaggregating these results by individual climate indicators (Supplementary Fig. 6 ) highlights that the increase in global exposure to catastrophic climate change damage is primarily driven by higher temperature damage if underlying regression models control for more climate indicators than just annual precipitation and less by the direct impacts of these indicators on global GDP.
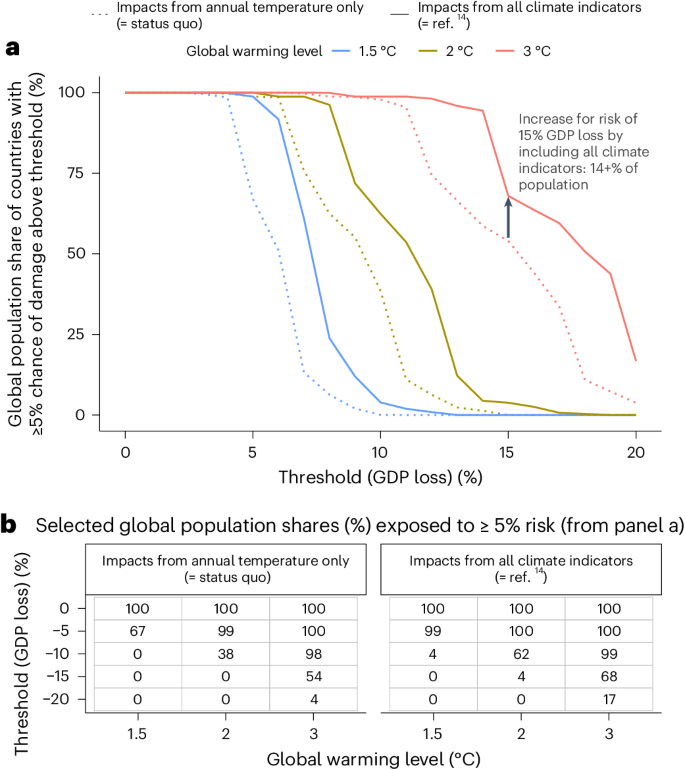
a , Share of the current global population living in countries whose projected GDP impacts for a given warming level (colour) exceeds the respective threshold ( x axis) for at least 5% of the GDP impact distribution, based on the status quo approach (dotted lines) and our main approach using all climate indicators (solid lines), respectively. The grey arrow and text annotation provide a reading example. b , Selected values from a .
Taken together, our results illustrate that projecting top-down damage of variability and extremes exacerbates global disparities further. Aggregate GDP loss projections, however, remain primarily driven by the impacts of mean temperature changes, which seem to cover economic losses due to heat waves at least partially 18 —an important finding for climate–economy modelling that complementary assessments of economic damage should corroborate to disentangle different impact channels better. As a result, overall uncertainty in GDP losses is dominated by the temperature dose–response function. However, substantial climatic uncertainties still limit the understanding of direct impacts by variability and extremes, particularly for low-income countries, which are expected to suffer the most but exhibit the largest uncertainties.
For scholars studying the economic effects of climate change, our results suggest a potential downward bias in temperature damage estimates by not disentangling the impacts of changes in temperature means and temperature variability. Future studies estimating temperature dose–response functions should test how including variability and extremes indicators linked to economic growth alters their findings. Notably, such biases could also be caused by other climate indicators not explicitly considered here and their direction and magnitude are likely to vary by location 32 . Furthermore, since the signal clarity is highest for extreme precipitation, this indicator seems most suitable to be included in climate–economy calculations, such as the social cost of carbon.
While our results rest on strong empirical foundations and a wide range of state-of-the-art climate models, there are several reasons why actual GDP impacts may exceed our projections. First, while the temperature dose–response function seems to include heat impacts at least partially, the dose–response functions used here do not explicitly cover important climate extremes, most notably droughts 33 . Second, to be conservative, we abstract from the possibility that climatic shifts do not only change GDP growth in a given year but alter a country’s long-run growth trajectory persistently. While such persistence in GDP losses remains empirically debated 1 , 2 , 14 , 21 , 34 , it would increase damage estimates substantially 26 , 27 . Third, aggregation across time and space is more likely to reduce signals in precipitation patterns because of their lower spatial and temporal correlation compared to annual mean temperature 13 , 14 . For these reasons, our results should be seen as an important first step, but they certainly do not exclude the possibility of larger GDP losses. Furthermore, econometric-based dose–response functions such as the ones used here have several limitations; for example, the risk of conflating weather impacts with climatic shifts or the extrapolation of impacts to warming levels that go far beyond historical observations, with the implicit assumption that adaptation remains at historically observed levels 35 , 36 . In addition, specification questions can further exacerbate socioeconomic uncertainties 21 and uniform dose–response functions for aggregate GDP can mask heterogeneities between countries, sectors and income segments 15 . Moreover, considering impacts in percentage of GDP implicitly assigns lower weights to poorer regions within countries that are disproportionately exposed to climate change risks 37 . Lastly, the distributions presented here might underestimate true climatic uncertainties for at least three reasons: (1) measurement imperfections in the reanalysis data underlying the dose–response functions, particularly for precipitation and lower-income regions 32 , 38 ; (2) using single runs for most CMIP6 models may underestimate tail risk events (Supplementary Appendix E ); and (3) not all CMIP6 models, despite representing the current frontier of global climatic projections, fully capture future changes in temperature variability and precipitation 24 , 25 , 39 , 40 , 41 .
Nevertheless, our study represents a key improvement in top-down damage projections, highlights the risks of omitting climate indicators beyond annual temperature, either as impact channels or control variables, and identifies the most promising fields for additional research. Building on our work, researchers could integrate further climate indicators, such as droughts, into a comprehensive assessment of climate change impacts. Aside from improvements in climate modelling, particularly for developing countries, this would also require more empirical studies to robustly identify the link between economic growth and different climatic extremes, ideally combined with an improved temporal or spatial resolution 17 . In addition, future research should assess the impact of controlling for these extremes on temperature dose–response functions and enhance the understanding of potential adaptation mechanisms and the persistence of GDP losses, ideally by consistently estimating and implementing persistence for each climate indicator under consideration.
Climatic data
Daily temperature and precipitation projections are taken from 33 CMIP6 models under two low-emission scenarios (Shared Socioeconomic Pathways SSP1-1.9 and SSP1-2.6) and one high-emission scenario (SSP3-7.0) to calculate bias-corrected, annual climate indicators for the 1850–2100 period. Owing to computational constraints, we use only one realization for most model–scenario pairs. However, to explore the role of intra-ensemble variation, we include 30 realizations of MPI-ESM1-2-LR and all 100 realizations of the CESM2-LE large ensemble under SSP3-7.0, which provides us with a total of 199 model-realization–scenario pairings (Supplementary Tables 1–3 ). Time series switch between historical scenarios and the respective Representative Concentration Pathway (RCP)–SSP pair in 2015 and are regridded onto a common 2.5° × 2.5° longitude–latitude grid using conservative remapping 43 .
Consistent with our source of empirically calibrated dose–response functions, which relies on ERA5 data 14 , we calculate annual average temperature T , annual total precipitation RA as well as four climate indicators using the equations listed below before downscaling and regridding the annual indicators from 2.5° to 0.25° (the grid resolution of ERA5). Notably, the indicators used here have been motivated and subjected to various robustness checks by previous studies 14 , 16 .
Day-to-day temperature variability:
where T x ,d,a,t is the temperature for grid cell x of day d of month a in year t and D a ∈ {28, 30, 31} is the number of days in the respective month a . \({\bar{T}}_{\rm{x,a,t}}\) denotes the mean temperature in month a of year t for the respective grid cell.
Extreme precipitation:
where R x,d,t is the precipitation of grid cell x on day d of year t , I () is an indicator function and R x,99p9,base denotes the 99.9th percentile of daily precipitation in grid cell x over a historical baseline period.
Number of wet days with precipitation exceeding 1 mm d −1 :
Grid-cell-level annual climate indicators are then aggregated to the subnational region level (ADM1) using the geospatial data from the Database of Global Administrative Areas (GADM, v.3.6) and area weighting.
Monthly precipitation deviation, which we calculate only at the ADM1 level and not at the grid-cell level, consistent with ref. 14 :
where R i, a ,t denotes precipitation totals in month a of year t for a given ADM1-level region i . Variables denoted by a bar represent averages across the baseline period, either for the full year or for a specific month, while σ i,a,base denotes the month-specific standard deviation across the baseline period for region i . As for all other climate indicators, region-level monthly precipitation R i,a,t is derived from grid-cell-level values based on area weighting.
For the baseline-dependent climate indicators \(\hat{\rm{RD}}\) and RM, our source of dose–response functions 14 uses 1979–2019 as the historical baseline period, during which global warming relative to pre-industrial levels over 1850–1900 averaged +0.84 °C according to Berkeley Earth data (the best estimate for the observed warming and, in a previous version, used in the IPCC AR6; ref. 8 ). However, the 1979–2019 time period can differ climatically across CMIP6 models, which warm at very different paces 44 . To maintain consistency and ensure that all climate indicators are based on the same baseline in terms of global warming, we, therefore, identify the corresponding 41-year window during which global warming relative to pre-industrial levels over 1850–1900 averages +0.84 °C for each climate model-realization and scenario. Then, we use the +0.84 °C window to calculate all values with a ‘base’ subscript in equations ( 2 ) and ( 4 ). Warming-level windows for each model-realization–scenario pairing are calculated following the approach by ref. 10 and shown in Supplementary Tables 1–3 . However, percentile-based indicators, such as our extreme precipitation measure, can exhibit artificial jumps at the end of the baseline period, causing potential frequency increases and discontinuities outside this period 10 , 45 , 46 . To correct this, we use the bootstrap resampling procedure developed by ref. 46 , estimating the percentile applied to each year in the baseline period on the basis of the remaining 40 years in the baseline period and then using the average across these percentiles for all years outside the baseline period. Mathematical expressions for the bootstrap resampling procedure and the calculation of global warming levels, as well as more information on the suitability of CMIP6 and ERA5 data to assess variability and extremes, are provided in Supplementary Appendix A .
Bias correction
We bias-correct annual climate indicators using the change factor method 47 , which adds model-projected changes compared to a reference period to the corresponding reference period average of an observational dataset. For any climate indicator C out of the six indicators considered here, bias-corrected values are obtained as follows:
where C x,t,m,r,s represents the raw climate indicator output of climate model m ’s realization r under scenario s in year t for grid cell x . \({\bar{C}}{\,\!}_{\rm{x},{\rm{ref}}}^{\rm{ERA5}}\) and \({\bar{C}}_{\rm{x},{\rm{ref}},m,r,s}\) represent the reference period average in ERA5 and for the climate model run, respectively. As a reference period, we use 1950–1990, during which global warming averaged +0.38 °C according to Berkeley Earth. Therefore, \({\bar{C}}_{\rm{x},{\rm{ref}},\rm{m,r,s}}\) is calculated for the 41-year global warming-level window corresponding to +0.38 °C (for the specific global warming-level windows, see Supplementary Tables 1–3 ). We bias-correct each annual indicator separately and, for the monthly precipitation deviation, apply the change factor method to the underlying monthly precipitation amounts. As a reference period, we use 1950–1990 because of its low influence of anthropogenic forcing and, to avoid impossible values, further impose zero lower bounds for all non-negative climate indicators and an upper bound of 365 for the number of wet days. In addition, the bias-corrected monthly precipitation deviation in some selected cases yields values that are one or two orders of magnitude above the maximum in our raw CMIP6 data. To address these outliers, we cap bias-corrected monthly precipitation deviation on the basis of the highest values observed for the raw CMIP6 data for up to +3 °C of global warming (~11.6; Supplementary Table 4 ), which affects only observations beyond the 99.993th percentile of our distribution.
Bias-correcting annual climate indicators ensures the highest consistency for each indicator with the ERA5 data used to estimate dose–response functions by ref. 14 (Supplementary Figs. 1–3 ). However, it can lead to inconsistencies between the different climate indicators derived from the same daily temperature or precipitation and, as outlined above, to outlier values in a few cases. As a robustness check, we apply the change factor method to the underlying daily temperature and precipitation values of an example model run instead, which increases the computational burden of bias correction considerably but leaves our conclusions unchanged (Supplementary Fig. 4 ).
GDP impacts
Dose–response functions for subnational economic growth are taken from ref. 14 , which jointly estimates the impact of all six indicators on income per capita growth. The resulting dose–response functions for each climate indicator are shown in Supplementary Fig. 7 and the underlying regression is reproduced in Supplementary Table 7 , column 5. Importantly, this regression model has been subjected to comprehensive robustness checks, such as using alternative datasets and variable definitions, controlling for region-specific time trends or assessing seasonal heterogeneities 14 . Mathematically, the specification of the regression can be summarized as
while the full model is written out in Supplementary equation ( 5 ). Here, g i,t denotes the economic growth of ADM1-level region i in year t , measured as the first difference of the log-transformed gross regional product per capita 48 . α i , δ t and ϵ i,t denote fixed effects and the error term and h C is the estimated dose–response function specific to climate indicator C i,t where \(C\in \{T,{\rm{RA}},\widetilde{T},\hat{{\rm{RD}}},{\rm{RD}},{\rm{RM}}\}\) . For instance, for annual precipitation RA, the relationship with economic growth is estimated as a quadratic relationship such that
where \({\beta }_{1}^{{\rm{RA}}}\) and \({\beta }_{2}^{{\rm{RA}}}\) are the respective regression coefficients.
To calculate the impacts of climate change, we compare annual economic impacts against the average impact during the historical baseline period for the same model–realization–scenario pairing, such that our impacts represent changes from a hypothetical scenario in which climate remains constant, following previous studies 2 , 15 . As a baseline period for GDP impacts, we again use the +0.84 ° C global warming-level window for a given realization r of climate model m and RCP–SSP pair s for consistency with the calculation of our climate indicators. Therefore, annual impacts, in percentage of GDP, of all climate indicators combined due to shifts relative to the baseline period are calculated as follows
where K is the 41-year model–realization–scenario-specific baseline period corresponding to +0.84 °C of global warming. Note that we exponentiate and subtract one to convert logarithmic changes to percentage of GDP, but impacts of different indicators and years are added and averaged in log scale. Individual GDP impacts of each climate indicator are obtained by using only the respective individual dose–response function in equation ( 8 ), instead of the sum across dose–response functions ∑ C h C ( C i,k ). Similarly, the combined GDP impacts of variability and extremes are calculated by summing only the dose–response functions for \(\widetilde{T}\) , \(\hat{{\rm{RD}}}\) , RD and RM in equation ( 8 ). More detailed mathematical expressions for all steps in equation ( 8 ) are provided in Supplementary Appendix C .
Importantly, the model specification by ref. 14 features annual temperature in first-differences compared to previous years and not in absolute levels:
To translate these regression coefficients into impact projections, we calculate cumulative impacts following ref. 4 , such that the dose–response function for annual temperature used in equation ( 8 ) reads as follows:
where k 0 denotes the first year in the baseline period K . As we discuss in Supplementary Appendix C , this procedure implicitly includes impacts of inter-annual temperature variability that persist over time.
For extreme precipitation \(\hat{{\rm{RD}}}\) , the dose–response function estimated by ref. 14 interacts extreme rainfall with the annual mean temperature T because the marginal impact of extreme precipitation is lower in warmer climates. Projecting this out under climate change, however, would make the strong assumption that global warming increases the resilience of countries to extreme precipitation worldwide. Because there is no evidence supporting such a positive feedback of warming and because the heterogeneity of extreme rainfall effects in ref. 14 is equally well-explained by the latitude of a country (see R2 and Adjusted R2 in Supplementary Table 4 of ref. 14 ), which is time-constant, we hold temperature in the interaction constant at the average level during the baseline period such that
When projecting damage of climate change, a core methodological choice is whether to assume that impacts affect GDP levels, such that the economy bounces back in the following year or whether to assume that a part of the damage persists and alters the long-run growth trajectory. Assuming persistence has a substantial impact on damage projections and the associated uncertainty 21 , 26 , 27 . Recent empirical analyses differ in methods and outcomes, with no consensus yet 1 , 2 , 12 , 21 , 34 . To be conservative, here we assume no persistence in implementing δ i,t , aside from any persistence implicit in the methodology by ref. 4 , and provide further mathematical expressions and discussions of damage persistence in Supplementary Appendix C . Furthermore, by holding historical dose–response functions and baseline periods for climate indicators constant, our approach rests on the common implicit assumption that future adaptation outcomes mirror historical ones 2 , 4 , 35 , in line with the mixed evidence on macro-economic adaptation so far 2 , 20 , 21 . In addition, we follow ref. 27 in equating relative GDP per capita impacts with relative GDP impacts (that is, assuming that any decrease in GDP per capita is caused by a climate change-induced reduction in economic output and not by an increase in population).
Spatial aggregation of GDP impacts
We aggregate the GDP impacts calculated via equation ( 8 ) from the subnational detail (ADM1) to the country level (ADM0) using GDP weighting. For GDP weights, we use 2010 GDP data downscaled to a 0.5° grid by ref. 49 . To deal with 105 outlier grid cells with raw GDP data exceeding US$10 20 , we apply a ceiling at $10 10 , which is the next highest grid-cell GDP value in the dataset. Note that we hold this intracountry income distribution constant across all years and SSPs. This simplification stems from the SSPs not directly informing spatial intracountry GDP per capita distributions and also prevents our results from being driven by changes in the weighting scheme over time rather than climatic changes, which is standard practice in the literature 2 . To calculate GDP impacts at the global level, we weigh each country i with its share in global GDP in year t as per the respective SSP. Since the SSP database does not provide GDP growth trajectories for a few sovereign countries, namely Andorra, Liechtenstein, Kosovo, Nauru, North Korea, San Marino, South Sudan and Tuvalu, these economies are not represented in our damage projections for the global economy.
GDP impact distribution
To capture dose–response function uncertainty, we draw 1,000 estimates for the dose–response function parameters \({\beta }_{1}^{{\rm{RA}}},{\beta }_{2}^{{\rm{RA}}},{\beta }_{1}^{\rm{T}},\ldots\) jointly from the multivariate Gaussian distribution estimated by ref. 14 (main specification, standard errors clustered at the country level). Applying each Monte Carlo draw to each of the 199 model–realization–scenario pairings provides us with 199,000 different impact projection pathways for each territory. For each model-realization–scenario pairing, we then identify the 20-year window corresponding to a global warming level of +1 °C, +1.5 °C, +2 °C, +3 °C and +4 °C, respectively, following the approach by ref. 10 (for the specific global warming-level windows, see Supplementary Tables 1–3 . This provides us with a conditional distribution of GDP impacts for a given territory and warming level. Aside from reducing the importance of individual RCP–SSP scenarios, conditioning results on global warming levels also reduces the need to omit or down-weight ‘hot models’ in CMIP6, which project too much warming 44 . Since not all models reach all warming levels and to prevent the two large ensembles from dominating our results, we weight models inversely such that each CMIP6 model has the same sampling probability for each warming level following ref. 18 . All summary statistics of the distribution (means, percentiles, variances and so on) are calculated using these CMIP6 model weights.
Variance decomposition
To decompose the observed variance in our conditional global GDP impact distribution for a given warming level, we adapt the approach by ref. 50 based on the partitioning method by ref. 51 . First, we carry out projections using point estimates for all dose–response function parameters (abstracting from dose–response function uncertainty) and calculate internal variability as the average across CMIP6 models of each model’s variance of global GDP impacts in a given global warming-level window. For CMIP6 models with only a single run in our analysis, this within-model variance stems from climatic differences between different scenario–years, whereas for the two large ensembles, it also includes differences between ensemble members. Correspondingly, we calculate climate model uncertainty as the variance between the mean global GDP impact of CMIP6 models for a given global warming level. Lastly, we calculate dose–response function uncertainty as the variance across dose–response function Monte Carlo draws of the mean GDP impact for each Monte Carlo draw (that is, an average across all CMIP6 models and scenario–years in the respective global warming-level window). Mathematical expressions for each variance component are provided in Supplementary Appendix D . Notably, this approach rests on the commonly made assumption that variance drivers are orthogonal, thus abstracting from interaction terms 52 . As a robustness check, we use an alternative approach by ref. 3 (Supplementary Appendix D ), which does not affect our conclusions.
Reporting summary
Further information on research design is available in the Nature Portfolio Reporting Summary linked to this article.
Data availability
CMIP6 temperature and precipitation indicators are available on the ETH Zurich CMIP6 repository 53 . CESM2 large ensemble outputs are available at https://www.earthsystemgrid.org/dataset/ucar.cgd.cesm2le.output.html . Tx5d and PDSI values from ref. 18 (used in Supplementary Appendix F ) are available at https://github.com/ccallahan45/CallahanMankin_ExtremeHeatEconomics_2022 (ref. 54 ). The historical climate data and the economic growth data to estimate the dose–response functions from ref. 14 are available from ref. 55 . ERA5 reanalysis data are available at https://www.ecmwf.int/en/forecasts/datasets/reanalysis-datasets/era5 . Source data are provided with this paper. All additional data are publicly available from ref. 56 .
Code availability
All scripts used to conduct the analysis and create the figures are publicly available from ref. 56 .
Dell, M., Jones, B. F. & Olken, B. A. Temperature shocks and economic growth: evidence from the last half century. Am. Econ. J. Macroecon. 4 , 66–95 (2012).
Article Google Scholar
Burke, M., Hsiang, S. M. & Miguel, E. Global non-linear effect of temperature on economic production. Nature 527 , 235–239 (2015).
Article CAS Google Scholar
Hsiang, S. et al. Estimating economic damage from climate change in the United States. Science 356 , 1362–1369 (2017).
Kalkuhl, M. & Wenz, L. The impact of climate conditions on economic production: evidence from a global panel of regions. J. Environ. Econ. Manag. 103 , 102360 (2020).
Seneviratne, S. I. et al. in Climate Change 2021: The Physical Science Basis (eds Masson-Delmotte, V. et al.) 1513–1766 (Cambridge Univ. Press, 2021).
Newman, R. & Noy, I. The global costs of extreme weather that are attributable to climate change. Nat. Commun. 14 , 6103 (2023).
Pendergrass, A. G., Knutti, R., Lehner, F., Deser, C. & Sanderson, B. M. Precipitation variability increases in a warmer climate. Sci. Rep. 7 , 17966 (2017).
Masson-Delmotte, V. et al. (eds) Climate Change 2021: The Physical Science Basis (Cambridge Univ. Press, 2021).
Bathiany, S., Dakos, V., Scheffer, M. & Lenton, T. M. Climate models predict increasing temperature variability in poor countries. Sci. Adv. 4 , eaar5809 (2018).
Batibeniz, F., Hauser, M. & Seneviratne, S. I. Countries most exposed to individual and concurrent extremes and near-permanent extreme conditions at different global warming levels. Earth Syst. Dynam. 14 , 485–505 (2023).
Burke, M. et al. Opportunities for advances in climate change economics. Science 352 , 292–293 (2016).
Piontek, F. et al. Integrated perspective on translating biophysical to economic impacts of climate change. Nat. Clim. Change 11 , 563–572 (2021).
Damania, R., Desbureaux, S. & Zaveri, E. Does rainfall matter for economic growth? Evidence from global sub-national data (1990–2014). J. Environ. Econ. Manag. 102 , 102335 (2020).
Kotz, M., Levermann, A. & Wenz, L. The effect of rainfall changes on economic production. Nature 601 , 223–227 (2022).
Palagi, E., Coronese, M., Lamperti, F. & Roventini, A. Climate change and the nonlinear impact of precipitation anomalies on income inequality. Proc. Natl Acad. Sci. USA 119 , e2203595119 (2022).
Kotz, M., Wenz, L., Stechemesser, A., Kalkuhl, M. & Levermann, A. Day-to-day temperature variability reduces economic growth. Nat. Clim. Change 11 , 319–325 (2021).
Linsenmeier, M. Temperature variability and long-run economic development. J. Environ. Econ. Manag. 121 , 102840 (2023).
Callahan, C. W. & Mankin, J. S. Globally unequal effect of extreme heat on economic growth. Sci. Adv. 8 , eadd3726 (2022).
Holtermann, L. Precipitation anomalies, economic production and the role of ‘first-nature’ and ‘second-nature’ geographies: a disaggregated analysis in high-income countries. Glob. Environ. Change 65 , 102167 (2020).
Kahn, M. E. et al. Long-term macroeconomic effects of climate change: a cross-country analysis. Energy Econ. 104 , 105624 (2021).
Newell, R. G., Prest, B. C. & Sexton, S. E. The GDP–temperature relationship: implications for climate change damages. J. Environ. Econ. Manag. 108 , 102445 (2021).
Orlowsky, B. & Seneviratne, S. I. Elusive drought: uncertainty in observed trends and short- and long-term CMIP5 projections. Hydrol. Earth Syst. Sci. 17 , 1765–1781 (2013).
Pfahl, S., O’Gorman, P. A. & Fischer, E. M. Understanding the regional pattern of projected future changes in extreme precipitation. Nat. Clim. Change 7 , 423–427 (2017).
Kotz, M., Wenz, L. & Levermann, A. Footprint of greenhouse forcing in daily temperature variability. Proc. Natl Acad. Sci. USA 118 , e2103294118 (2021).
Kotz, M., Lange, S., Wenz, L. & Levermann, A. Constraining the pattern and magnitude of projected extreme precipitation change in a multimodel ensemble. J. Clim. 37 , 97–111 (2024).
Moore, F. C. & Diaz, D. B. Temperature impacts on economic growth warrant stringent mitigation policy. Nat. Clim. Change 5 , 127–131 (2015).
Kikstra, J. S. et al. The social cost of carbon dioxide under climate–economy feedbacks and temperature variability. Environ. Res. Lett. 16 , 094037 (2021).
World Development Indicators: GDP (constant 2015 US$) (World Bank, accessed 3 May 2023); https://databank.worldbank.org/source/world-development-indicators
Pakistan Floods 2022: Post-Disaster Needs Assessment (Government of Pakistan, 2022); https://www.undp.org/pakistan/publications/pakistan-floods-2022-post-disaster-needs-assessment-pdna
Screen, J. A. Arctic amplification decreases temperature variance in northern mid- to high-latitudes. Nat. Clim. Change 4 , 577–582 (2014).
de Vries, I. E., Sippel, S., Pendergrass, A. G. & Knutti, R. Robust global detection of forced changes in mean and extreme precipitation despite observational disagreement on the magnitude of change. Earth Syst. Dynam. 14 , 81–100 (2023).
Auffhammer, M., Hsiang, S. M., Schlenker, W. & Sobel, A. Using weather data and climate model output in economic analyses of climate change. Rev. Environ. Econ. Policy 7 , 181–198 (2013).
Naumann, G., Cammalleri, C., Mentaschi, L. & Feyen, L. Increased economic drought impacts in Europe with anthropogenic warming. Nat. Clim. Change 11 , 485–491 (2021).
Bastien-Olvera, B. A., Granella, F. & Moore, F. C. Persistent effect of temperature on GDP identified from lower frequency temperature variability. Environ. Res. Lett. 17 , 084038 (2022).
Dell, M., Jones, B. F. & Olken, B. A. What do we learn from the weather? The new climate–economy literature. J. Econ. Lit. 52 , 740–798 (2014).
Auffhammer, M. Quantifying economic damages from climate change. J. Econ. Perspect. 32 , 33–52 (2018).
Byers, E. et al. Global exposure and vulnerability to multi-sector development and climate change hotspots. Environ. Res. Lett. 13 , 055012 (2018).
Dinku, T. in Extreme Hydrology and Climate Variability (eds Melesse, A. M. et al.) 71–80 (Elsevier, 2019).
Kornhuber, K. et al. Risks of synchronized low yields are underestimated in climate and crop model projections. Nat. Commun. 14 , 3528 (2023).
Schwarzwald, K., Goddard, L., Seager, R., Ting, M. & Marvel, K. Understanding CMIP6 biases in the representation of the Greater Horn of Africa long and short rains. Clim. Dynam. 61 , 1229–1255 (2023).
Ayugi, B. et al. Comparison of CMIP6 and CMIP5 models in simulating mean and extreme precipitation over East Africa. Int. J. Climatol. 41 , 6474–6496 (2021).
South, A. rnaturalearthdata: World vector map data from Natural Earth used in ’rnaturalearth’. R package version 0.1.0 https://github.com/ropenscilabs/rnaturalearthdata (2017).
Jones, P. First- and second-order conservative remapping schemes for grids in spherical coordinates. Mon. Weather Rev. 127 , 2204–2210 (1999).
Hausfather, Z., Marvel, K., Schmidt, G. A., Nielsen-Gammon, J. W. & Zelinka, M. Climate simulations: recognize the ‘hot model’ problem. Nature 605 , 26–29 (2022).
Zhang, X. et al. Indices for monitoring changes in extremes based on daily temperature and precipitation data. WIREs Clim. Change 2 , 851–870 (2011).
Zhang, X., Hegerl, G., Zwiers, F. W. & Kenyon, J. Avoiding inhomogeneity in percentile-based indices of temperature extremes. J. Clim. 18 , 1641–1651 (2005).
Tabor, K. & Williams, J. W. Globally downscaled climate projections for assessing the conservation impacts of climate change. Ecol. Appl. 20 , 554–565 (2010).
Wenz, L., Carr, R. D., Kögel, N., Kotz, M. & Kalkuhl, M. DOSE—global data set of reported sub-national economic output. Sci. Data 10 , 425 (2023).
Murakami, D., Yoshida, T. & Yamagata, Y. Gridded GDP projections compatible with the five SSPs (shared socioeconomic pathways). Front. Built Environ. 7 , 760306 (2021).
Schwarzwald, K. & Lenssen, N. The importance of internal climate variability in climate impact projections. Proc. Natl Acad. Sci. USA 119 , e2208095119 (2022).
Hawkins, E. & Sutton, R. The potential to narrow uncertainty in regional climate predictions. Bull. Am. Meteorol. Soc. 90 , 1095–1108 (2009).
Lehner, F. et al. Partitioning climate projection uncertainty with multiple large ensembles and CMIP5/6. Earth Syst. Dynam. 11 , 491–508 (2020).
Brunner, L., Hauser, M., Lorenz, R. & Beyerle, U. The ETH Zurich CMIP6 next generation archive: technical documentation. Zenodo https://zenodo.org/doi/10.5281/zenodo.3734127 (2020).
Callahan, C. W. & Mankin, J. S. Globally unequal effect of extreme heat on economic growth. GitHub https://github.com/ccallahan45/CallahanMankin_ExtremeHeatEconomics_2022 (2022).
Kotz, M., Levermann, A., & Wenz, L. Data and code for the publication ‘The effect of rainfall changes on economic production’. Zenodo https://zenodo.org/doi/10.5281/zenodo.5657456 (2021).
Waidelich, P., Batibeniz, F., Rising, J., Kikstra, J. S., & Seneviratne, S. Scripts and data for ‘Climate damage projections beyond annual temperature’. Zenodo https://zenodo.org/doi/10.5281/zenodo.10465253 (2024).
Download references
Acknowledgements
We thank M. Hauser, J. Krug and I. de Vries, as well as participants at the NAVIGATE-ENGAGE Summer School 2023 and the 16th IAMC Annual Meeting 2023 for valuable comments. This work was supported by the European Union’s Horizon 2020 research and innovation programme, European Research Council under grant agreement no. 948220, project no. GREENFIN (P.W.) and by the Natural Environment Research Council under grant agreement no. NE/S007415/1 (J.S.K.).
Open access funding provided by Swiss Federal Institute of Technology Zurich.
Author information
Authors and affiliations.
Climate Finance and Policy Group, ETH Zurich, Zurich, Switzerland
Paul Waidelich
Institute for Atmospheric and Climate Science, ETH Zurich, Zurich, Switzerland
Fulden Batibeniz & Sonia I. Seneviratne
Oeschger Center for Climate Change Research, University of Bern, Bern, Switzerland
Fulden Batibeniz
Climate and Environmental Physics, Physics Institute, University of Bern, Bern, Switzerland
School of Marine Science & Policy, University of Delaware, Newark, DE, US
James Rising
Centre for Environmental Policy, Imperial College London, London, UK
Jarmo S. Kikstra
Grantham Institute for Climate Change and the Environment, Imperial College London, London, UK
Energy, Climate and Environment (ECE) Program, International Institute for Applied Systems Analysis, Laxenburg, Austria
You can also search for this author in PubMed Google Scholar
Contributions
All authors conceived the study. F.B. prepared the underlying climate data and conducted the bias correction. P.W. and J.R. performed the data aggregation and developed the projection methodology. P.W. carried out the impact projections, analysed and visualized the results and wrote the manuscript. All authors reviewed the manuscript.
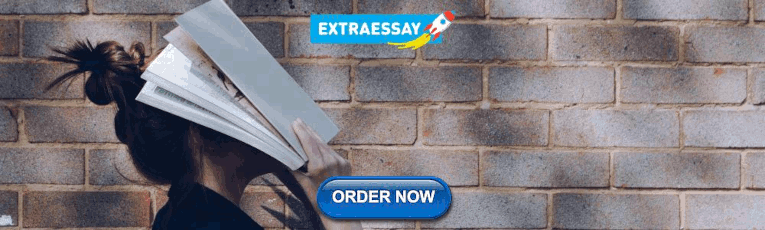
Corresponding author
Correspondence to Paul Waidelich .
Ethics declarations
Competing interests.
The authors declare no competing interests.
Peer review
Peer review information.
Nature Climate Change thanks Marshall Burke and the other, anonymous, reviewer(s) for their contribution to the peer review of this work.
Additional information
Publisher’s note Springer Nature remains neutral with regard to jurisdictional claims in published maps and institutional affiliations.
Supplementary information
Supplementary information.
Supplementary Discussion (Appendices A–F), Figs. 1–19 and Tables 1–10.
Reporting Summary
Source data fig. 1.
Statistical source data.
Source Data Fig. 2
Source data fig. 3, source data fig. 4, source data fig. 5, rights and permissions.
Open Access This article is licensed under a Creative Commons Attribution 4.0 International License, which permits use, sharing, adaptation, distribution and reproduction in any medium or format, as long as you give appropriate credit to the original author(s) and the source, provide a link to the Creative Commons licence, and indicate if changes were made. The images or other third party material in this article are included in the article’s Creative Commons licence, unless indicated otherwise in a credit line to the material. If material is not included in the article’s Creative Commons licence and your intended use is not permitted by statutory regulation or exceeds the permitted use, you will need to obtain permission directly from the copyright holder. To view a copy of this licence, visit http://creativecommons.org/licenses/by/4.0/ .
Reprints and permissions
About this article
Cite this article.
Waidelich, P., Batibeniz, F., Rising, J. et al. Climate damage projections beyond annual temperature. Nat. Clim. Chang. (2024). https://doi.org/10.1038/s41558-024-01990-8
Download citation
Received : 24 July 2023
Accepted : 20 March 2024
Published : 17 April 2024
DOI : https://doi.org/10.1038/s41558-024-01990-8
Share this article
Anyone you share the following link with will be able to read this content:
Sorry, a shareable link is not currently available for this article.
Provided by the Springer Nature SharedIt content-sharing initiative
Quick links
- Explore articles by subject
- Guide to authors
- Editorial policies
Sign up for the Nature Briefing newsletter — what matters in science, free to your inbox daily.


- Kindle Store
- Kindle eBooks
- Science & Math
Buy $128.00
Promotions apply when you purchase
These promotions will be applied to this item:
Some promotions may be combined; others are not eligible to be combined with other offers. For details, please see the Terms & Conditions associated with these promotions.
- Highlight, take notes, and search in the book
- In this edition, page numbers are just like the physical edition
- Create digital flashcards instantly
Rent $49.89
Today through selected date:
Rental price is determined by end date.
Buy for others
Buying and sending ebooks to others.
- Select quantity
- Buy and send eBooks
- Recipients can read on any device
These ebooks can only be redeemed by recipients in the US. Redemption links and eBooks cannot be resold.

Download the free Kindle app and start reading Kindle books instantly on your smartphone, tablet, or computer - no Kindle device required .
Read instantly on your browser with Kindle for Web.
Using your mobile phone camera - scan the code below and download the Kindle app.

Image Unavailable

- To view this video download Flash Player
Follow the authors

Statistical Analysis in Climate Research Kindle Edition
- ISBN-13 978-0521012300
- Sticky notes On Kindle Scribe
- Publisher Cambridge University Press
- Publication date February 21, 2002
- Language English
- File size 49877 KB
- See all details
- Kindle (5th Generation)
- Kindle Keyboard
- Kindle (2nd Generation)
- Kindle (1st Generation)
- Kindle Paperwhite
- Kindle Paperwhite (5th Generation)
- Kindle Touch
- Kindle Voyage
- Kindle Oasis
- Kindle Scribe (1st Generation)
- Kindle Fire HDX 8.9''
- Kindle Fire HDX
- Kindle Fire HD (3rd Generation)
- Fire HDX 8.9 Tablet
- Fire HD 7 Tablet
- Fire HD 6 Tablet
- Kindle Fire HD 8.9"
- Kindle Fire HD(1st Generation)
- Kindle Fire(2nd Generation)
- Kindle Fire(1st Generation)
- Kindle for Windows 8
- Kindle for Windows Phone
- Kindle for BlackBerry
- Kindle for Android Phones
- Kindle for Android Tablets
- Kindle for iPhone
- Kindle for iPod Touch
- Kindle for iPad
- Kindle for Mac
- Kindle for PC
- Kindle Cloud Reader
Customers who bought this item also bought

Editorial Reviews
About the author, product details.
- ASIN : B00CF0JKZE
- Publisher : Cambridge University Press (February 21, 2002)
- Publication date : February 21, 2002
- Language : English
- File size : 49877 KB
- Simultaneous device usage : Up to 4 simultaneous devices, per publisher limits
- Text-to-Speech : Enabled
- Screen Reader : Supported
- Enhanced typesetting : Enabled
- X-Ray : Not Enabled
- Word Wise : Not Enabled
- Sticky notes : On Kindle Scribe
- Print length : 500 pages
- Page numbers source ISBN : 0521012309
About the authors
H. v. storch.
Discover more of the author’s books, see similar authors, read author blogs and more
Hans von Storch
Customer reviews.
Customer Reviews, including Product Star Ratings help customers to learn more about the product and decide whether it is the right product for them.
To calculate the overall star rating and percentage breakdown by star, we don’t use a simple average. Instead, our system considers things like how recent a review is and if the reviewer bought the item on Amazon. It also analyzed reviews to verify trustworthiness.
- Sort reviews by Top reviews Most recent Top reviews
Top reviews from the United States
There was a problem filtering reviews right now. please try again later..

Top reviews from other countries

- Amazon Newsletter
- About Amazon
- Accessibility
- Sustainability
- Press Center
- Investor Relations
- Amazon Devices
- Amazon Science
- Sell on Amazon
- Sell apps on Amazon
- Supply to Amazon
- Protect & Build Your Brand
- Become an Affiliate
- Become a Delivery Driver
- Start a Package Delivery Business
- Advertise Your Products
- Self-Publish with Us
- Become an Amazon Hub Partner
- › See More Ways to Make Money
- Amazon Visa
- Amazon Store Card
- Amazon Secured Card
- Amazon Business Card
- Shop with Points
- Credit Card Marketplace
- Reload Your Balance
- Amazon Currency Converter
- Your Account
- Your Orders
- Shipping Rates & Policies
- Amazon Prime
- Returns & Replacements
- Manage Your Content and Devices
- Recalls and Product Safety Alerts
- Conditions of Use
- Privacy Notice
- Consumer Health Data Privacy Disclosure
- Your Ads Privacy Choices
Sandia LabNews
- Download This Issue (PDF)
New issues every two weeks. Unsubscribe at any time. Email addresses are never shared.
Subscribe Successful Thank you for subscribing!
Statistician seeks to understand climate change through variable relationships
BY SARAH JEWEL JOHNSON
THURSDAY, APRIL 18, 2024
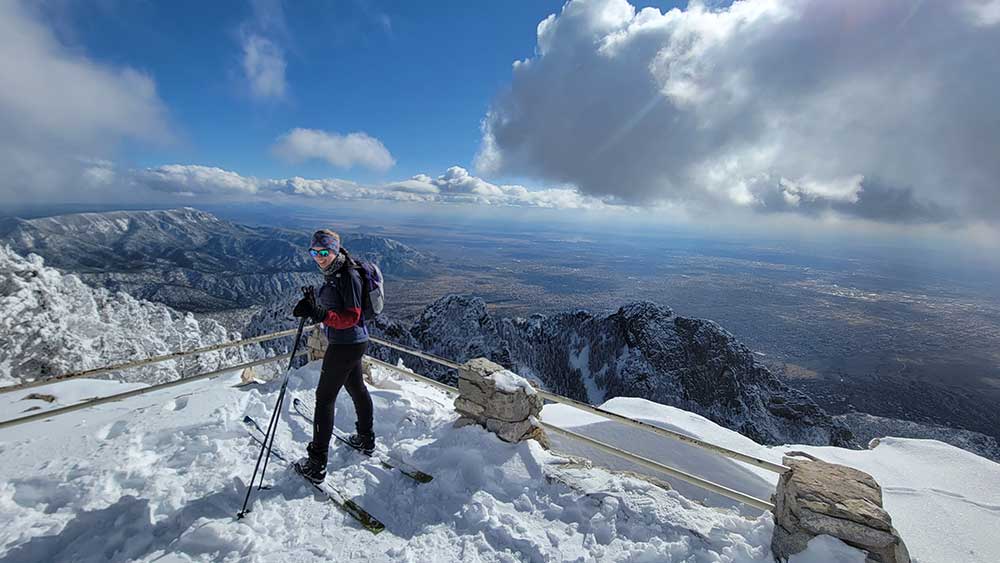
Climate change is a confusing maze of inputs and outputs. Katherine Goode spends her days trying make the variable chaos behave.
Katherine is a research and development statistician at Sandia currently supporting the CLDERA Grand Challenge . She is part of a large research team working to understand climate change mitigation strategies and their potential downstream effects. Katherine lends her statistical expertise to create novel algorithms that identify patterns and relation points in inputs and variables that climate scientists believe are related.
“I’m working with observational data collected by satellites to try to understand how different climate variables are related to some sort of climate event. It’s not an easy problem, but it’s a really interesting challenge trying to understand those relationships,” Katherine said.
Machine-learning models relate inputs and outputs to predict future outcomes. Katherine applies machine learning to better understand the negative impacts of climate change, causes and potential outcomes of future mitigation techniques — such as climate geoengineering to slow the rate of change.
“We have this really complex climate system, but we try to understand if a climate change mitigation strategy was taken, how one variable may affect another variable which will affect another variable,” Katherine said. “One of the mitigation strategies we are studying is stratospheric aerosol injections, where aerosols would be injected into the stratosphere with the intention of deflecting the sun’s rays. We would like to understand how a change in the amount of aerosols relates to temperature changes.”
Statistical relationships
For Katherine, the quest to explain variable relationships is nothing new. She graduated with a doctorate from Iowa State University in 2021 and started as a Sandia intern in 2019. Her dissertation explored the mathematical relationships between the inputs and the predictions that come out of black-box machine-learning models.
“Machine learning models are powerful tools for making predictions, but the mathematical complexity of the algorithms makes it difficult to understand how the inputs relate to the outputs. Hence the term ‘black-box models’. I work to develop techniques that provide transparency to these models. For example, if we build a model to predict temperature given the quantity of aerosols and other climate variables, how does the model make use of the aerosols for prediction,” Katherine says.
Katherine’s passion to mitigate climate change stems from her love of nature and her desire to prevent potentially catastrophic consequences in the future.
“People are developing possible mitigation strategies for dealing with climate change that could have negative downstream consequences that we still don’t fully understand. If one nation chooses to use a mitigation strategy, it could negatively affect another nation. At Sandia, we’re well positioned with individuals with varied backgrounds to solve complicated problems like this,” Katherine said.
Making connections
Because of the complicated and complex nature of both climate change and quantitative data, Katherine doesn’t hesitate to reach out to peers and constantly reads literature on diverse application spaces for statistical techniques. While she rarely has an opportunity to discuss her work with the public, Katherine takes time at conferences and events to network with researchers and fellow statisticians.
“I go to a lot of conferences, and I think that’s a place I can really talk about the application space,” shares Katherine. “There aren’t a lot of great techniques that already exist that can just be directly applied to the type of climate data that’s being worked with — which is huge amounts of data — or that can be used to address the questions that individuals have, but that’s what makes it interesting. You get to be really creative and think about how we could go about using existing tools and adapting them to work with this particular situation.”
So, what does the world look like in the future according to Katherine?
Twenty years from now, we could have a better understanding of climate change and be able to spend more time addressing other threats to national security. We could have algorithms to explain the unexplainable. But we will still need to adapt.
“I feel like we’re going to have to continue taking what we’ve learned from our current research process and I’m sure new issues will arise that we haven’t foreseen. Climate mitigation is such a meaningful place to be doing work, but it’s still a newer area of research. It’s a new application space. It’s challenging but we’ve got to overcome the hurdles,” Katherine said.

- Science serving others
- Carlsbad site welcomes new leader
- Battling an existential threat
Numbers, Facts and Trends Shaping Your World
Read our research on:
Full Topic List
Regions & Countries
- Publications
- Our Methods
- Short Reads
- Tools & Resources
Read Our Research On:
Key facts about Americans and guns
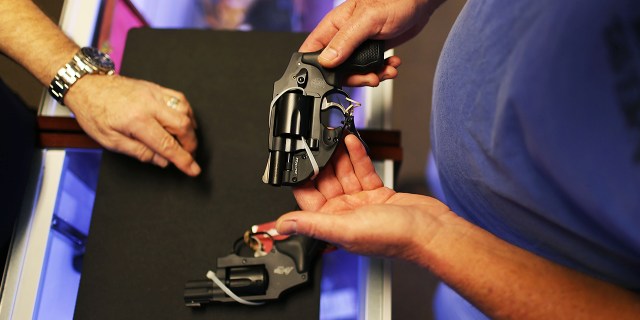
Guns are deeply ingrained in American society and the nation’s political debates.
The Second Amendment to the United States Constitution guarantees the right to bear arms, and about a third of U.S. adults say they personally own a gun. At the same time, in response to concerns such as rising gun death rates and mass shootings , President Joe Biden has proposed gun policy legislation that would expand on the bipartisan gun safety bill Congress passed last year.
Here are some key findings about Americans’ views of gun ownership, gun policy and other subjects, drawn primarily from a Pew Research Center survey conducted in June 2023 .
Pew Research Center conducted this analysis to summarize key facts about Americans and guns. We used data from recent Center surveys to provide insights into Americans’ views on gun policy and how those views have changed over time, as well as to examine the proportion of adults who own guns and their reasons for doing so.
The analysis draws primarily from a survey of 5,115 U.S. adults conducted from June 5 to June 11, 2023. Everyone who took part in the surveys cited is a member of the Center’s American Trends Panel (ATP), an online survey panel that is recruited through national, random sampling of residential addresses. This way nearly all U.S. adults have a chance of selection. The survey is weighted to be representative of the U.S. adult population by gender, race, ethnicity, partisan affiliation, education and other categories. Read more about the ATP’s methodology .
Here are the questions used for the analysis on gun ownership , the questions used for the analysis on gun policy , and the survey’s methodology .
Additional information about the fall 2022 survey of parents and its methodology can be found at the link in the text of this post.
Measuring gun ownership in the United States comes with unique challenges. Unlike many demographic measures, there is not a definitive data source from the government or elsewhere on how many American adults own guns.
The Pew Research Center survey conducted June 5-11, 2023, on the Center’s American Trends Panel, asks about gun ownership using two separate questions to measure personal and household ownership. About a third of adults (32%) say they own a gun, while another 10% say they do not personally own a gun but someone else in their household does. These shares have changed little from surveys conducted in 2021 and 2017 . In each of those surveys, 30% reported they owned a gun.
These numbers are largely consistent with rates of gun ownership reported by Gallup , but somewhat higher than those reported by NORC’s General Social Survey . Those surveys also find only modest changes in recent years.
The FBI maintains data on background checks on individuals attempting to purchase firearms in the United States. The FBI reported a surge in background checks in 2020 and 2021, during the coronavirus pandemic. The number of federal background checks declined in 2022 and through the first half of this year, according to FBI statistics .
About four-in-ten U.S. adults say they live in a household with a gun, including 32% who say they personally own one, according to an August report based on our June survey. These numbers are virtually unchanged since the last time we asked this question in 2021.
There are differences in gun ownership rates by political affiliation, gender, community type and other factors.
- Republicans and Republican-leaning independents are more than twice as likely as Democrats and Democratic leaners to say they personally own a gun (45% vs. 20%).
- 40% of men say they own a gun, compared with 25% of women.
- 47% of adults living in rural areas report personally owning a firearm, as do smaller shares of those who live in suburbs (30%) or urban areas (20%).
- 38% of White Americans own a gun, compared with smaller shares of Black (24%), Hispanic (20%) and Asian (10%) Americans.
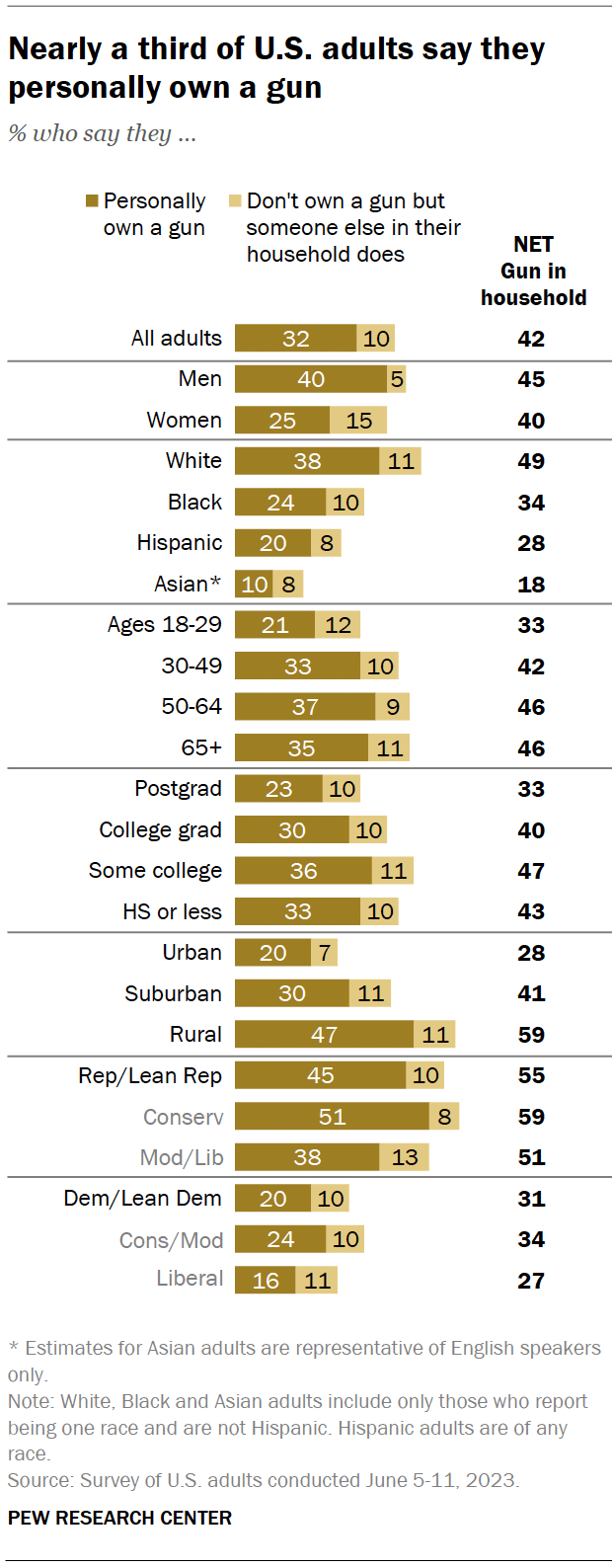
Personal protection tops the list of reasons gun owners give for owning a firearm. About three-quarters (72%) of gun owners say that protection is a major reason they own a gun. Considerably smaller shares say that a major reason they own a gun is for hunting (32%), for sport shooting (30%), as part of a gun collection (15%) or for their job (7%).
The reasons behind gun ownership have changed only modestly since our 2017 survey of attitudes toward gun ownership and gun policies. At that time, 67% of gun owners cited protection as a major reason they owned a firearm.
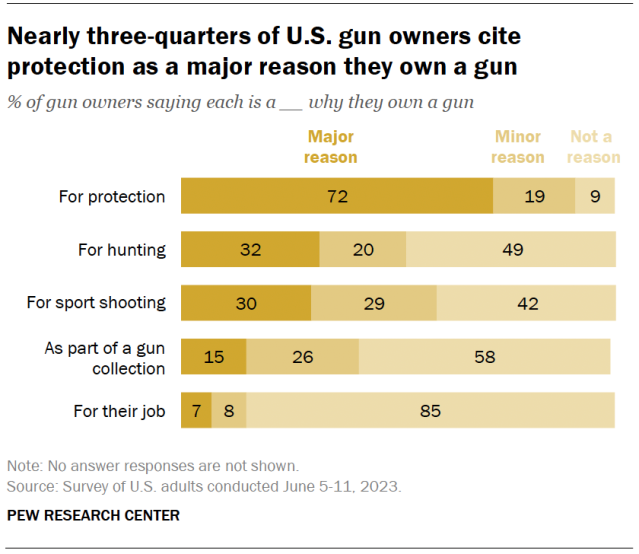
Gun owners tend to have much more positive feelings about having a gun in the house than non-owners who live with them. For instance, 71% of gun owners say they enjoy owning a gun – but far fewer non-gun owners in gun-owning households (31%) say they enjoy having one in the home. And while 81% of gun owners say owning a gun makes them feel safer, a narrower majority (57%) of non-owners in gun households say the same about having a firearm at home. Non-owners are also more likely than owners to worry about having a gun in the home (27% vs. 12%, respectively).
Feelings about gun ownership also differ by political affiliation, even among those who personally own firearms. Republican gun owners are more likely than Democratic owners to say owning a gun gives them feelings of safety and enjoyment, while Democratic owners are more likely to say they worry about having a gun in the home.
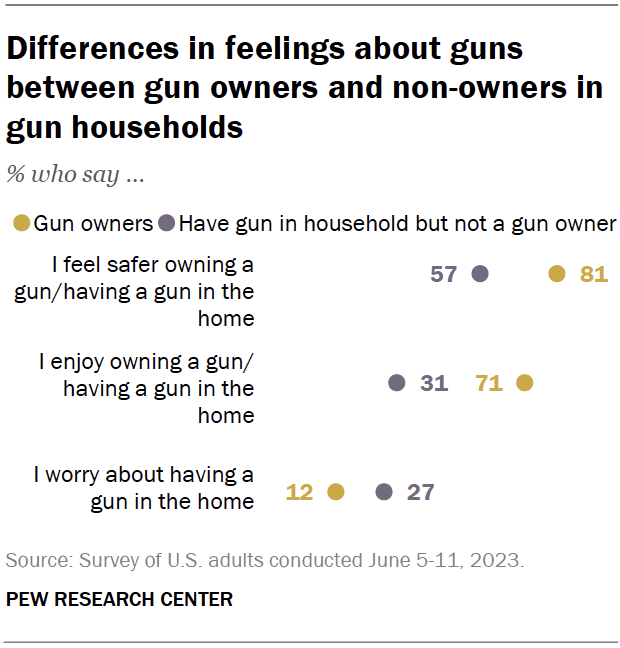
Non-gun owners are split on whether they see themselves owning a firearm in the future. About half (52%) of Americans who don’t own a gun say they could never see themselves owning one, while nearly as many (47%) could imagine themselves as gun owners in the future.
Among those who currently do not own a gun:
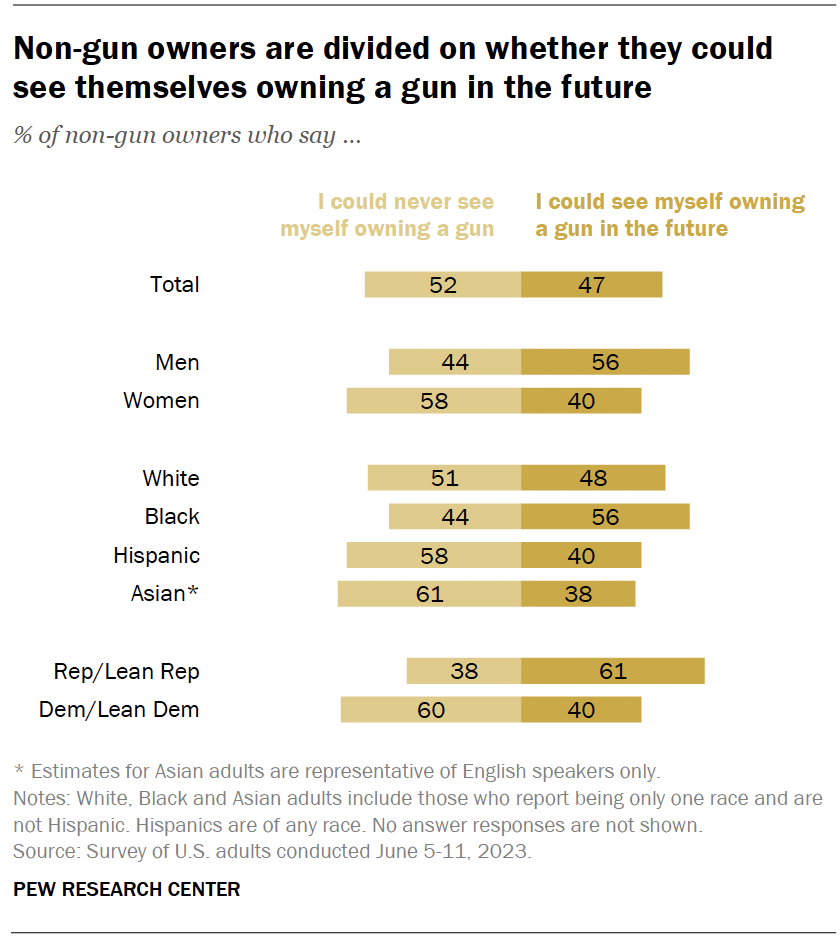
- 61% of Republicans and 40% of Democrats who don’t own a gun say they would consider owning one in the future.
- 56% of Black non-owners say they could see themselves owning a gun one day, compared with smaller shares of White (48%), Hispanic (40%) and Asian (38%) non-owners.
Americans are evenly split over whether gun ownership does more to increase or decrease safety. About half (49%) say it does more to increase safety by allowing law-abiding citizens to protect themselves, but an equal share say gun ownership does more to reduce safety by giving too many people access to firearms and increasing misuse.
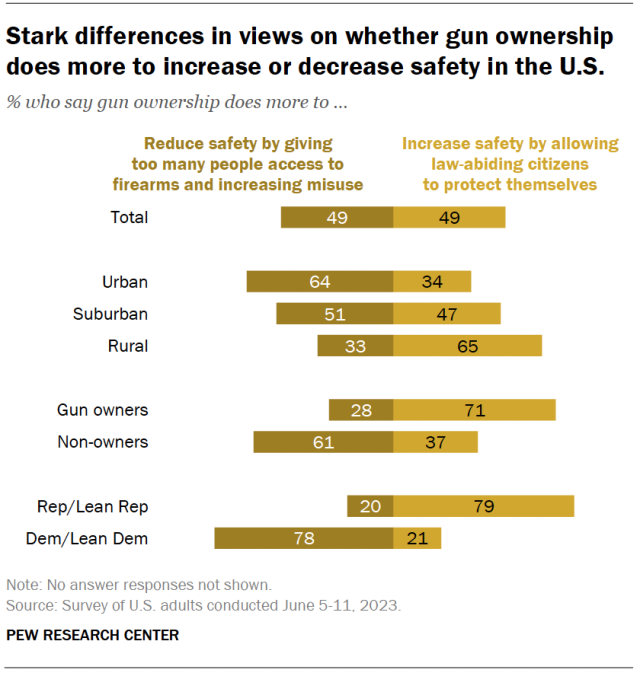
Republicans and Democrats differ on this question: 79% of Republicans say that gun ownership does more to increase safety, while a nearly identical share of Democrats (78%) say that it does more to reduce safety.
Urban and rural Americans also have starkly different views. Among adults who live in urban areas, 64% say gun ownership reduces safety, while 34% say it does more to increase safety. Among those who live in rural areas, 65% say gun ownership increases safety, compared with 33% who say it does more to reduce safety. Those living in the suburbs are about evenly split.
Americans increasingly say that gun violence is a major problem. Six-in-ten U.S. adults say gun violence is a very big problem in the country today, up 9 percentage points from spring 2022. In the survey conducted this June, 23% say gun violence is a moderately big problem, and about two-in-ten say it is either a small problem (13%) or not a problem at all (4%).
Looking ahead, 62% of Americans say they expect the level of gun violence to increase over the next five years. This is double the share who expect it to stay the same (31%). Just 7% expect the level of gun violence to decrease.
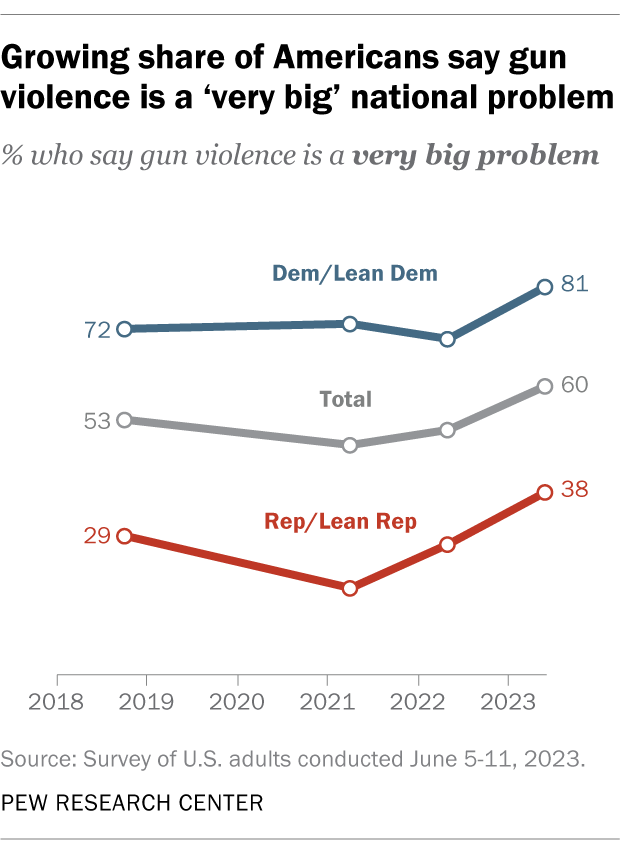
A majority of Americans (61%) say it is too easy to legally obtain a gun in this country. Another 30% say the ease of legally obtaining a gun is about right, and 9% say it is too hard to get a gun. Non-gun owners are nearly twice as likely as gun owners to say it is too easy to legally obtain a gun (73% vs. 38%). Meanwhile, gun owners are more than twice as likely as non-owners to say the ease of obtaining a gun is about right (48% vs. 20%).
Partisan and demographic differences also exist on this question. While 86% of Democrats say it is too easy to obtain a gun legally, 34% of Republicans say the same. Most urban (72%) and suburban (63%) dwellers say it’s too easy to legally obtain a gun. Rural residents are more divided: 47% say it is too easy, 41% say it is about right and 11% say it is too hard.
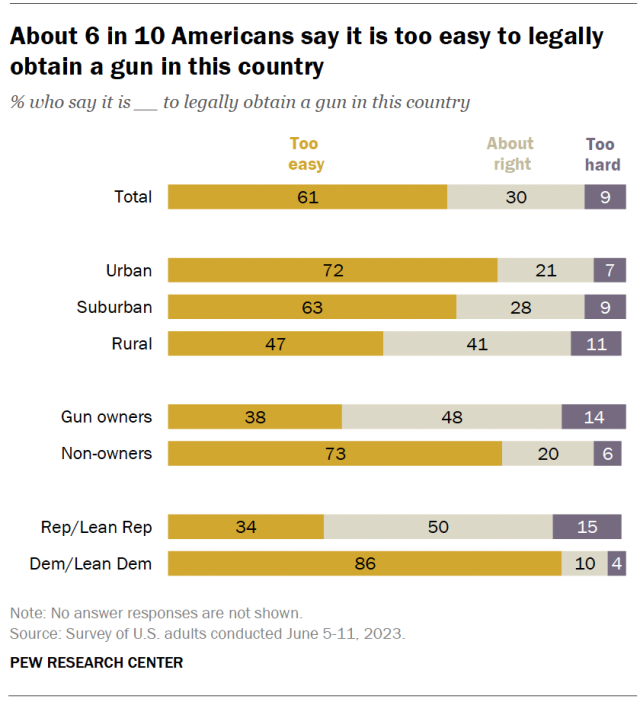
About six-in-ten U.S. adults (58%) favor stricter gun laws. Another 26% say that U.S. gun laws are about right, and 15% favor less strict gun laws. The percentage who say these laws should be stricter has fluctuated a bit in recent years. In 2021, 53% favored stricter gun laws, and in 2019, 60% said laws should be stricter.
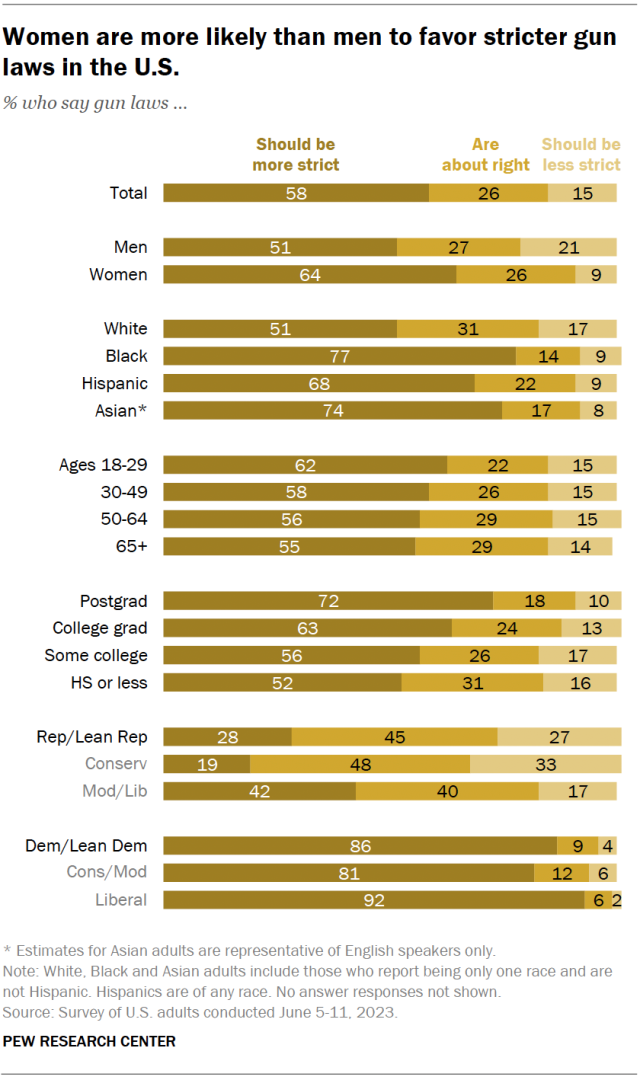
About a third (32%) of parents with K-12 students say they are very or extremely worried about a shooting ever happening at their children’s school, according to a fall 2022 Center survey of parents with at least one child younger than 18. A similar share of K-12 parents (31%) say they are not too or not at all worried about a shooting ever happening at their children’s school, while 37% of parents say they are somewhat worried.
Among all parents with children under 18, including those who are not in school, 63% see improving mental health screening and treatment as a very or extremely effective way to prevent school shootings. This is larger than the shares who say the same about having police officers or armed security in schools (49%), banning assault-style weapons (45%), or having metal detectors in schools (41%). Just 24% of parents say allowing teachers and school administrators to carry guns in school would be a very or extremely effective approach, while half say this would be not too or not at all effective.
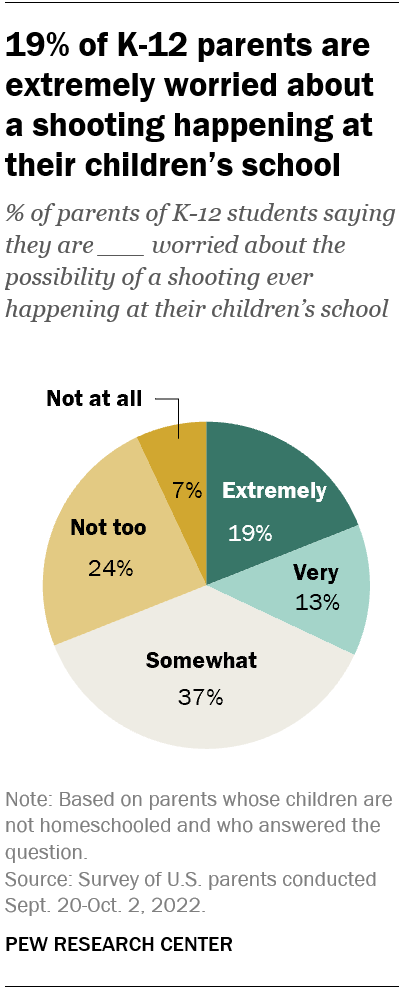
There is broad partisan agreement on some gun policy proposals, but most are politically divisive, the June 2023 survey found . Majorities of U.S. adults in both partisan coalitions somewhat or strongly favor two policies that would restrict gun access: preventing those with mental illnesses from purchasing guns (88% of Republicans and 89% of Democrats support this) and increasing the minimum age for buying guns to 21 years old (69% of Republicans, 90% of Democrats). Majorities in both parties also oppose allowing people to carry concealed firearms without a permit (60% of Republicans and 91% of Democrats oppose this).
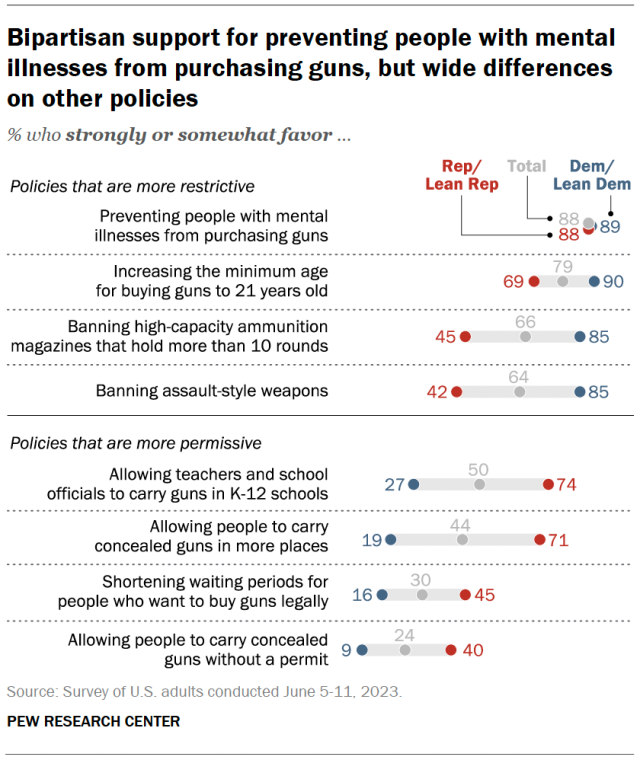
Republicans and Democrats differ on several other proposals. While 85% of Democrats favor banning both assault-style weapons and high-capacity ammunition magazines that hold more than 10 rounds, majorities of Republicans oppose these proposals (57% and 54%, respectively).
Most Republicans, on the other hand, support allowing teachers and school officials to carry guns in K-12 schools (74%) and allowing people to carry concealed guns in more places (71%). These proposals are supported by just 27% and 19% of Democrats, respectively.
Gun ownership is linked with views on gun policies. Americans who own guns are less likely than non-owners to favor restrictions on gun ownership, with a notable exception. Nearly identical majorities of gun owners (87%) and non-owners (89%) favor preventing mentally ill people from buying guns.
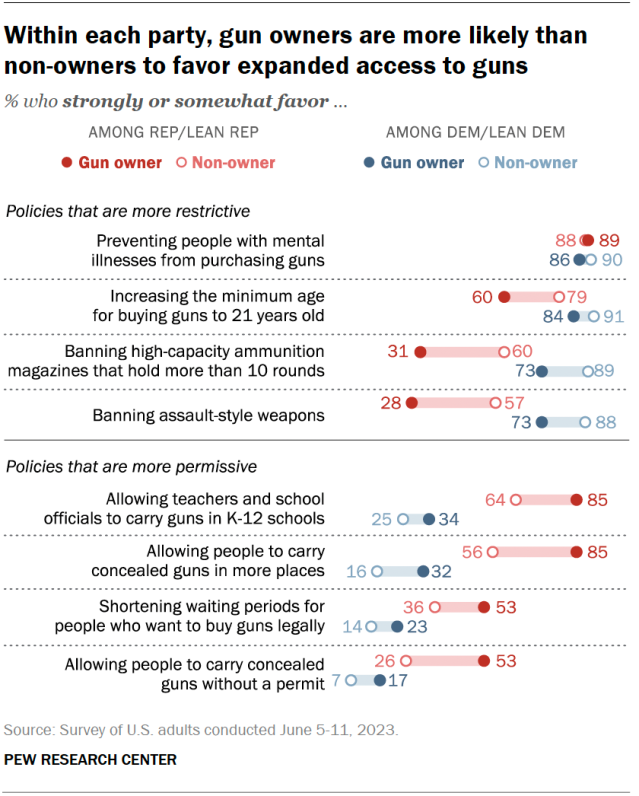
Within both parties, differences between gun owners and non-owners are evident – but they are especially stark among Republicans. For example, majorities of Republicans who do not own guns support banning high-capacity ammunition magazines and assault-style weapons, compared with about three-in-ten Republican gun owners.
Among Democrats, majorities of both gun owners and non-owners favor these two proposals, though support is greater among non-owners.
Note: This is an update of a post originally published on Jan. 5, 2016 .
- Partisanship & Issues
- Political Issues
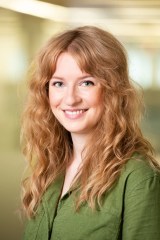
About 1 in 4 U.S. teachers say their school went into a gun-related lockdown in the last school year
Striking findings from 2023, for most u.s. gun owners, protection is the main reason they own a gun, gun violence widely viewed as a major – and growing – national problem, what the data says about gun deaths in the u.s., most popular.
1615 L St. NW, Suite 800 Washington, DC 20036 USA (+1) 202-419-4300 | Main (+1) 202-857-8562 | Fax (+1) 202-419-4372 | Media Inquiries
Research Topics
- Age & Generations
- Coronavirus (COVID-19)
- Economy & Work
- Family & Relationships
- Gender & LGBTQ
- Immigration & Migration
- International Affairs
- Internet & Technology
- Methodological Research
- News Habits & Media
- Non-U.S. Governments
- Other Topics
- Politics & Policy
- Race & Ethnicity
- Email Newsletters
ABOUT PEW RESEARCH CENTER Pew Research Center is a nonpartisan fact tank that informs the public about the issues, attitudes and trends shaping the world. It conducts public opinion polling, demographic research, media content analysis and other empirical social science research. Pew Research Center does not take policy positions. It is a subsidiary of The Pew Charitable Trusts .
Copyright 2024 Pew Research Center
Terms & Conditions
Privacy Policy
Cookie Settings
Reprints, Permissions & Use Policy
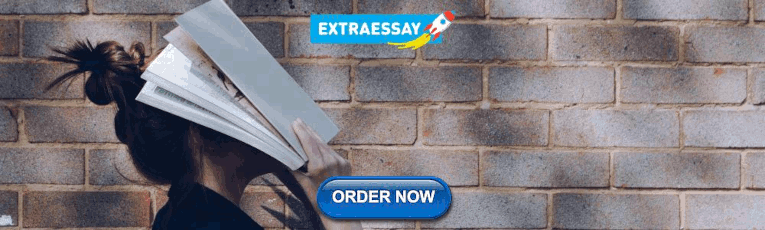
COMMENTS
There are a wealth of real-world examples drawn from the climate literature to demonstrate the need, power and pitfalls of statistical analysis in climate research. Suitable for graduate courses on statistics for climatic, atmospheric and oceanic science, this book will also be valuable as a reference source for researchers in climatology ...
Instead, we use probabilistic ideas and statistics to describe the 'climate' system. Four factors ensure that the climate system is amenable to statistical thinking. † The climate is controlled by innumerable factors. Only a small proportion of these factors can be considered, while the rest are necessarily interpreted as background noise.
Statistical Analysis in Climate Research. Climatology is, to a large degree, the study of the statistics of our climate. The powerful tools of mathematical statistics therefore find wide application in climatological research. The purpose of this book is to help the climatologist understand the basic precepts of the statistician's art and to ...
Includes both the mathematics and the intuition needed for climate data analysis. Professor Dennis L Hartmann, University of Washington. ... education, learning, and research at the highest international levels of excellence. www.cambridge.org ... 978-1-108-47241-8 — Statistical Methods for Climate Scientists Timothy DelSole , Michael Tippett ...
This chapter discusses statistical concepts in climate research, as well as time series and stochastic processes, and some of the techniques used to estimate covariance functions and spectra. 1. Introduction Part I. Fundamentals: 2. Probability theory 3. Distributions of climate variables 4. Concepts in statistical inference 5. Estimation Part II. Confirmation and Analysis: 6. The statistical ...
Climate Research, Vol. 89, Issue. , p. 61. CrossRef; Google Scholar; DelSole, Timothy and Tippett, Michael K. 2022. ... 'This text will be useful for teaching advanced undergraduates and graduate students about the applications of statistical methods to climate data analysis. It is filled with many relevant examples informed by the authors ...
Authors. Hans von Storch, Universität Hamburg Hans von Storch is Director of the Institute of Hydrophysics of the GKSS Research Centre in Geesthacht, Germany and a Professor at the Meteorological Institute of the University of Hamburg.. Francis W. Zwiers, University of Victoria, British Columbia Francis W. Zwiers is Chief of the Canadian Centre for Climate Modelling and Analysis, Atmospheric ...
The statistical analysis in climate research is universally compatible with any devices to read and is available in the digital library an online access to it is set as public so you can download it instantly. Thank you very much for reading statistical analysis in climate research. Maybe you have knowledge that, people have search numerous times for their chosen readings like this statistical ...
Statistical Analysis in Climate Research - Ebook written by Hans von Storch, Francis W. Zwiers. Read this book using Google Play Books app on your PC, android, iOS devices. Download for offline reading, highlight, bookmark or take notes while you read Statistical Analysis in Climate Research.
Chapter 2 supplies the funda- statistical analysis in climate research. Chapter 10 mentals of probability theory such as random variables defines stochastic processes as a composition of a or statistical distributions. The authors adopt a frequen- deterministic part (''signal'') and a random part tist viewpoint which should be easily ...
We review the role of statistical analysis in the climate sciences. Special emphasis is given to attempts to construct dynamical knowledge from limited observational evidence, and to the ongoing task of drawing detailed and reliable information on the state, and change, of climate that is needed, for example, for short-term and seasonal ...
Climate Data Analysis Tools & Methods. View all of our climate data analysis tools & methods or use the list below to jump to a certain group. Climate Model Evaluation. Climate Data Processing & Visualization. Climate Data Formats. Statistical Methods.
Recently, it has been widely argued that common climate variables (e.g., P) will be stationary if the mean remains constant over climatic (e.g., 30-y) timescales (for example, in ref. 13 and earlier in refs. 14 and 15).However, the formal definition of stationarity goes beyond the mean and involves higher-order statistics (variance and higher-order moments) as well as the autocovariance (or ...
"Statistical analysis in Climate Research" is the statistics book which has been most relevant for me that I have read until this time. The information density is very high in this book. It is necessary with some prior knowledge about statistics to read this book. It does not contain any proofs, but many references to other books.
Trend analysis of climate variables is the central process in assessing the state of the climate of a region and provides an overall estimate about the variations in the climate variables 16 ...
The paradigm of climate research evolved from the purely descriptive approach towards an understanding of the dynamics of climate with the advent of computers and the ability to simulate the climatic state and its variability. Statistics plays an important role in this new paradigm. The climate is a dynamical system influenced not only by ...
Statistical Analysis in Climate Research, by von Storch and Zwiers, Cambridge Univ Press. Applied Multivariate Statistical Analysis, by Johnson and Wichern. Software Homework computations are expected to be done using the package R. Class Schedule 08/29 field significance 09/05 NO CLASS: attend GMU seminar on August 28
Buy Statistical Analysis in Climate Research on Amazon.com FREE SHIPPING on qualified orders Statistical Analysis in Climate Research: Storch, Hans von, Zwiers, Francis W.: 9780521450713: Amazon.com: Books
However, our review of case studies shows that in climate research, big-data elements have been applied beyond small problems. Based on our considerations, this is justified when these elements ...
PUBLISHED BY THE PRESS SYNDICATE OF THE UNIVERSITY OF CAMBRIDGE The Pitt Building, Trumpington Street, Cambridge CB2 1RP, United Kingdom CAMBRIDGE UNIVERSITY PRESS
At +3 °C, global GDP decreases by 10.0% (5.1-14.9%). When disaggregated by climate indicator, global impacts are strongly determined by the annual mean temperature changes, which account for a ...
There are a wealth of real-world examples drawn from the climate literature to demonstrate the need, power and pitfalls of statistical analysis in climate research. Suitable for graduate courses on statistics for climatic, atmospheric and oceanic science, this book will also be valuable as a reference source for researchers in climatology ...
Statistical Analysis in Climate Research. Kindle Edition. Climatology is, to a large degree, the study of the statistics of our climate. The powerful tools of mathematical statistics therefore find wide application in climatological research. The purpose of this book is to help the climatologist understand the basic precepts of the statistician ...
Over the past two decades, the Gravity Recovery and Climate Experiment (GRACE) satellite mission and its successor, GRACE-follow on (GRACE-FO), have played a vital role in climate research. However, the absence of certain observations during and between these missions has presented a persistent challenge. Despite numerous studies attempting to address this issue with mathematical and ...
Climate change is a confusing maze of inputs and outputs. Katherine Goode spends her days trying make the variable chaos behave. Katherine is a research and development statistician at Sandia currently supporting the CLDERA Grand Challenge. She is part of a large research team working to understand climate change mitigation strategies and their potential downstream effects.
Our innovative products and services for learners, authors and customers are based on world-class research and are relevant, exciting and inspiring. Academic Research, Teaching and Learning English Language Learning
Coastal ecosystems are facing heightened risks due to human-induced climate change, including rising water levels and intensified storm events. Accurate bathymetry data is crucial for assessing the impacts of these threats. Traditional data collection methods can be cost-prohibitive. This study investigates the feasibility of using freely accessible Landsat and Sentinel satellite imagery to ...
Pew Research Center conducted this analysis to summarize key facts about Americans and guns. We used data from recent Center surveys to provide insights into Americans' views on gun policy and how those views have changed over time, as well as to examine the proportion of adults who own guns and their reasons for doing so. ... The analysis ...