
- SUGGESTED TOPICS
- The Magazine
- Newsletters
- Managing Yourself
- Managing Teams
- Work-life Balance
- The Big Idea
- Data & Visuals
- Reading Lists
- Case Selections
- HBR Learning
- Topic Feeds
- Account Settings
- Email Preferences
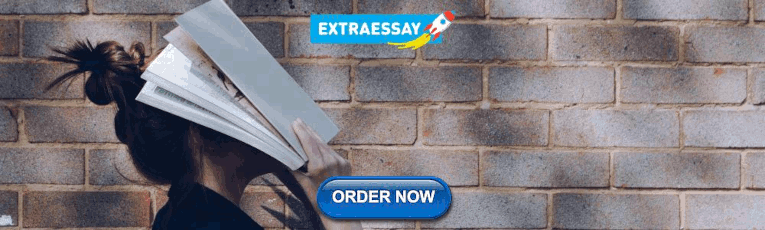
Present Your Data Like a Pro
- Joel Schwartzberg

Demystify the numbers. Your audience will thank you.
While a good presentation has data, data alone doesn’t guarantee a good presentation. It’s all about how that data is presented. The quickest way to confuse your audience is by sharing too many details at once. The only data points you should share are those that significantly support your point — and ideally, one point per chart. To avoid the debacle of sheepishly translating hard-to-see numbers and labels, rehearse your presentation with colleagues sitting as far away as the actual audience would. While you’ve been working with the same chart for weeks or months, your audience will be exposed to it for mere seconds. Give them the best chance of comprehending your data by using simple, clear, and complete language to identify X and Y axes, pie pieces, bars, and other diagrammatic elements. Try to avoid abbreviations that aren’t obvious, and don’t assume labeled components on one slide will be remembered on subsequent slides. Every valuable chart or pie graph has an “Aha!” zone — a number or range of data that reveals something crucial to your point. Make sure you visually highlight the “Aha!” zone, reinforcing the moment by explaining it to your audience.
With so many ways to spin and distort information these days, a presentation needs to do more than simply share great ideas — it needs to support those ideas with credible data. That’s true whether you’re an executive pitching new business clients, a vendor selling her services, or a CEO making a case for change.

- JS Joel Schwartzberg oversees executive communications for a major national nonprofit, is a professional presentation coach, and is the author of Get to the Point! Sharpen Your Message and Make Your Words Matter and The Language of Leadership: How to Engage and Inspire Your Team . You can find him on LinkedIn and X. TheJoelTruth
Partner Center
We use essential cookies to make Venngage work. By clicking “Accept All Cookies”, you agree to the storing of cookies on your device to enhance site navigation, analyze site usage, and assist in our marketing efforts.
Manage Cookies
Cookies and similar technologies collect certain information about how you’re using our website. Some of them are essential, and without them you wouldn’t be able to use Venngage. But others are optional, and you get to choose whether we use them or not.
Strictly Necessary Cookies
These cookies are always on, as they’re essential for making Venngage work, and making it safe. Without these cookies, services you’ve asked for can’t be provided.
Show cookie providers
- Google Login
Functionality Cookies
These cookies help us provide enhanced functionality and personalisation, and remember your settings. They may be set by us or by third party providers.
Performance Cookies
These cookies help us analyze how many people are using Venngage, where they come from and how they're using it. If you opt out of these cookies, we can’t get feedback to make Venngage better for you and all our users.
- Google Analytics
Targeting Cookies
These cookies are set by our advertising partners to track your activity and show you relevant Venngage ads on other sites as you browse the internet.
- Google Tag Manager
- Infographics
- Daily Infographics
- Template Lists
- Graphic Design
- Graphs and Charts
- Data Visualization
- Human Resources
- Beginner Guides
Blog Data Visualization
10 Data Presentation Examples For Strategic Communication
By Krystle Wong , Sep 28, 2023
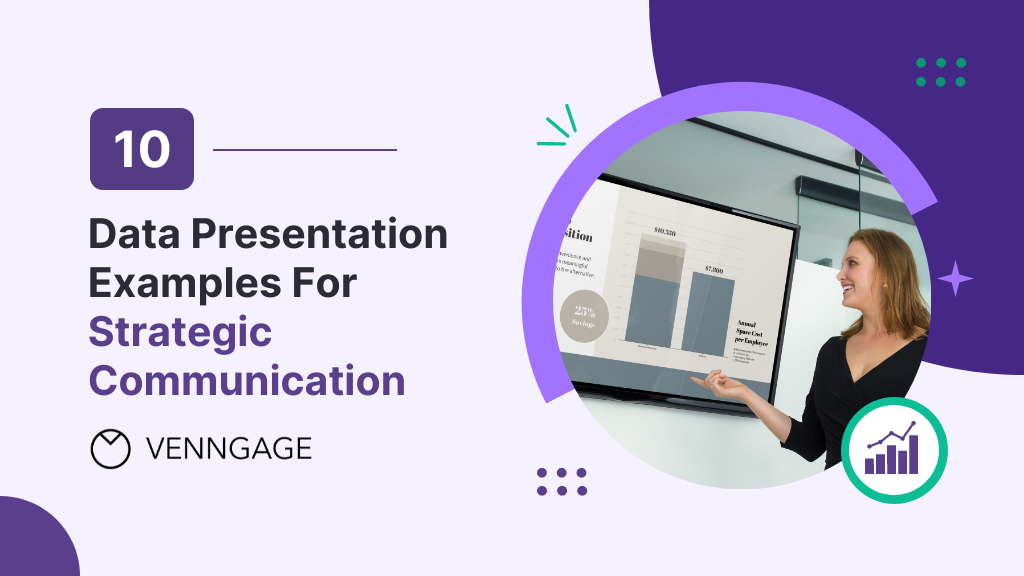
Knowing how to present data is like having a superpower.
Data presentation today is no longer just about numbers on a screen; it’s storytelling with a purpose. It’s about captivating your audience, making complex stuff look simple and inspiring action.
To help turn your data into stories that stick, influence decisions and make an impact, check out Venngage’s free chart maker or follow me on a tour into the world of data storytelling along with data presentation templates that work across different fields, from business boardrooms to the classroom and beyond. Keep scrolling to learn more!
Click to jump ahead:
10 Essential data presentation examples + methods you should know
What should be included in a data presentation, what are some common mistakes to avoid when presenting data, faqs on data presentation examples, transform your message with impactful data storytelling.
Data presentation is a vital skill in today’s information-driven world. Whether you’re in business, academia, or simply want to convey information effectively, knowing the different ways of presenting data is crucial. For impactful data storytelling, consider these essential data presentation methods:
1. Bar graph
Ideal for comparing data across categories or showing trends over time.
Bar graphs, also known as bar charts are workhorses of data presentation. They’re like the Swiss Army knives of visualization methods because they can be used to compare data in different categories or display data changes over time.
In a bar chart, categories are displayed on the x-axis and the corresponding values are represented by the height of the bars on the y-axis.
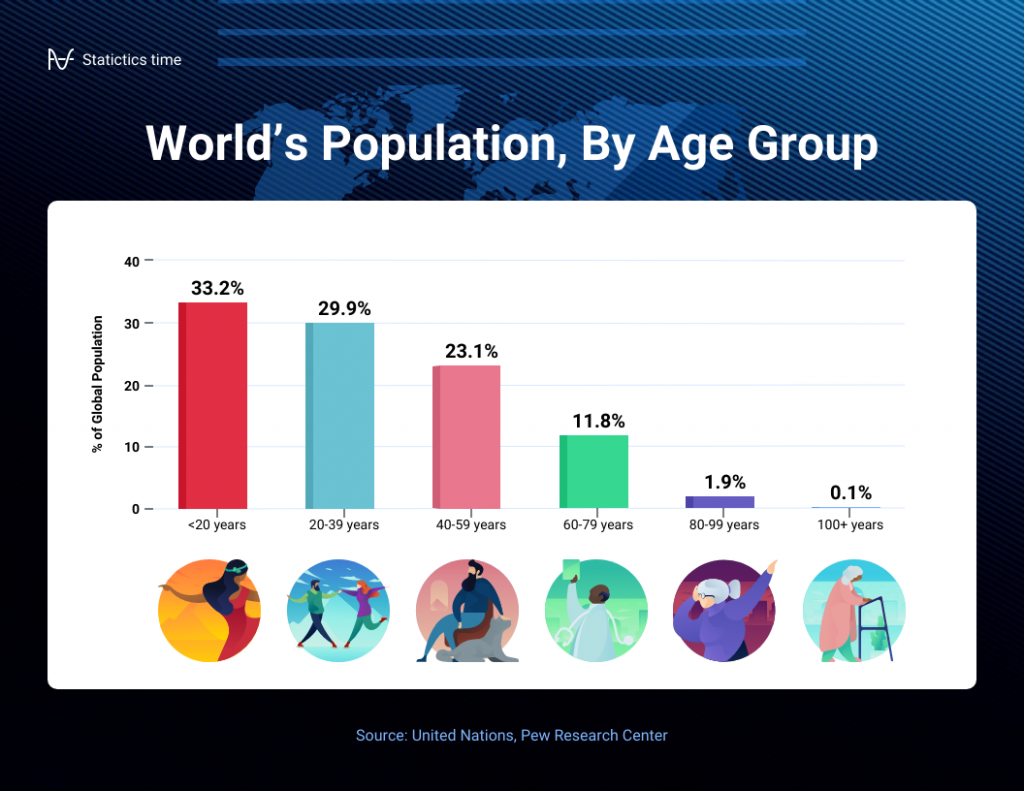
It’s a straightforward and effective way to showcase raw data, making it a staple in business reports, academic presentations and beyond.
Make sure your bar charts are concise with easy-to-read labels. Whether your bars go up or sideways, keep it simple by not overloading with too many categories.
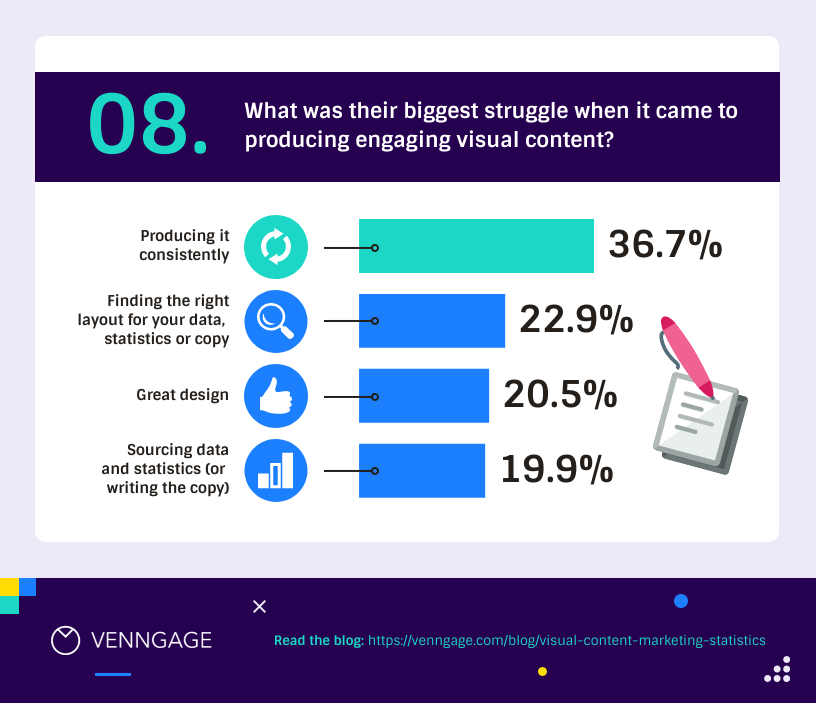
2. Line graph
Great for displaying trends and variations in data points over time or continuous variables.
Line charts or line graphs are your go-to when you want to visualize trends and variations in data sets over time.
One of the best quantitative data presentation examples, they work exceptionally well for showing continuous data, such as sales projections over the last couple of years or supply and demand fluctuations.
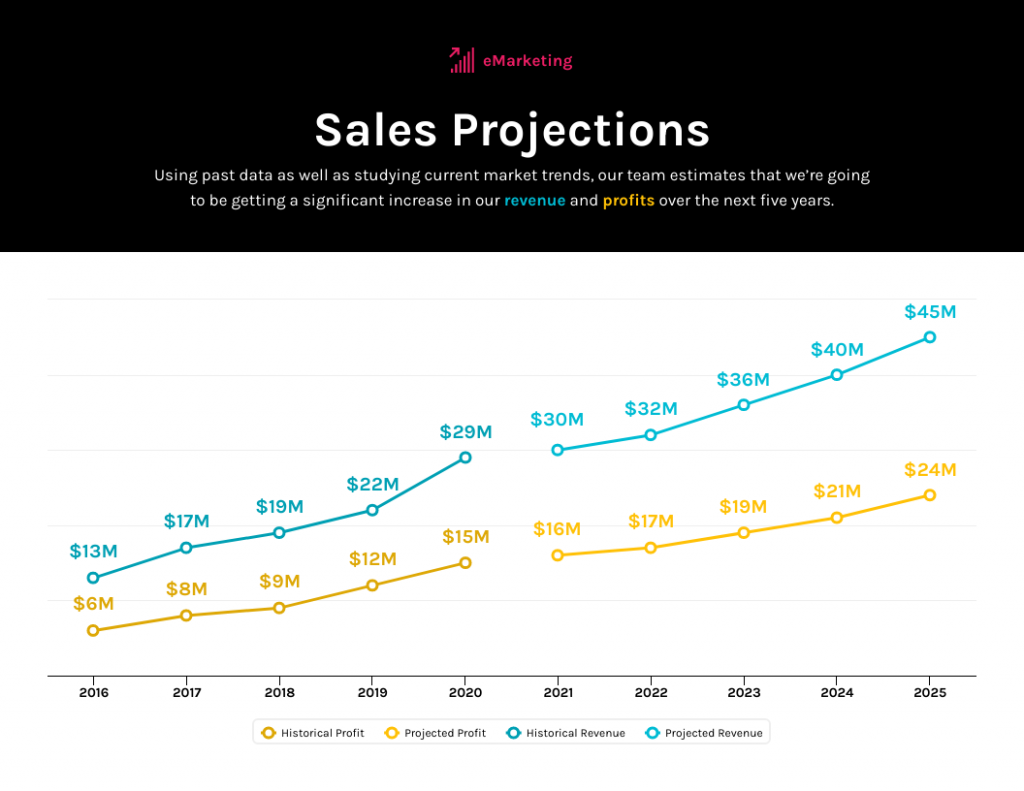
The x-axis represents time or a continuous variable and the y-axis represents the data values. By connecting the data points with lines, you can easily spot trends and fluctuations.
A tip when presenting data with line charts is to minimize the lines and not make it too crowded. Highlight the big changes, put on some labels and give it a catchy title.
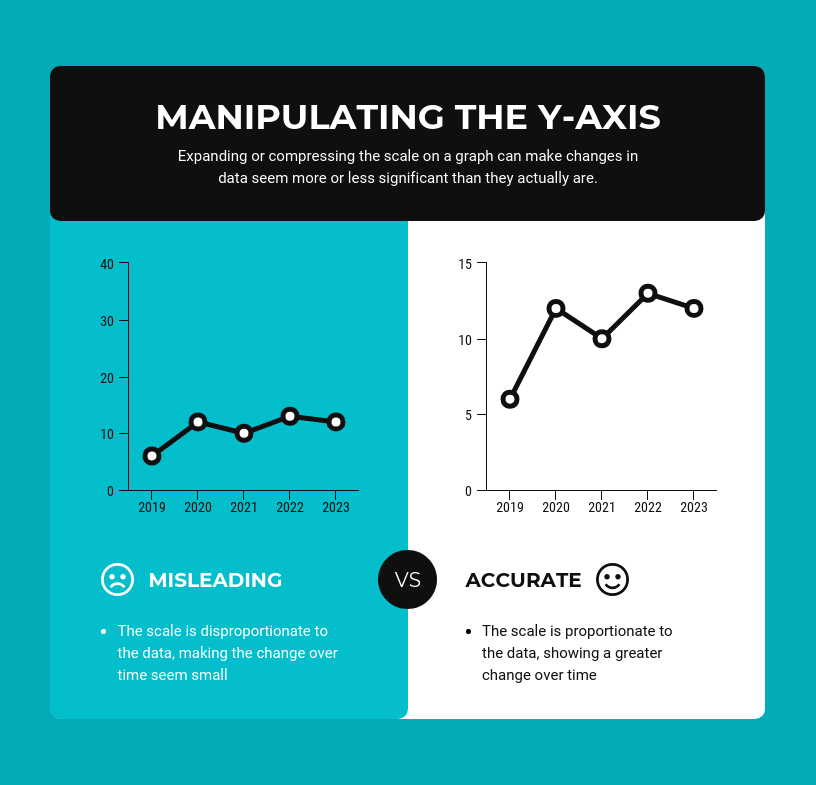
3. Pie chart
Useful for illustrating parts of a whole, such as percentages or proportions.
Pie charts are perfect for showing how a whole is divided into parts. They’re commonly used to represent percentages or proportions and are great for presenting survey results that involve demographic data.
Each “slice” of the pie represents a portion of the whole and the size of each slice corresponds to its share of the total.
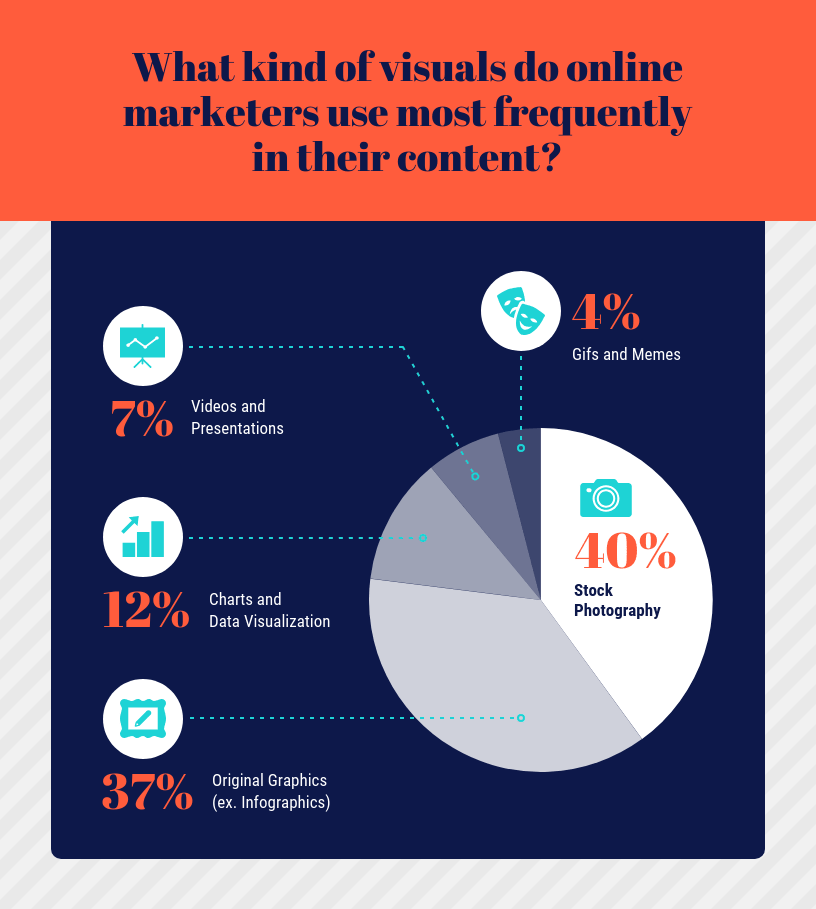
While pie charts are handy for illustrating simple distributions, they can become confusing when dealing with too many categories or when the differences in proportions are subtle.
Don’t get too carried away with slices — label those slices with percentages or values so people know what’s what and consider using a legend for more categories.
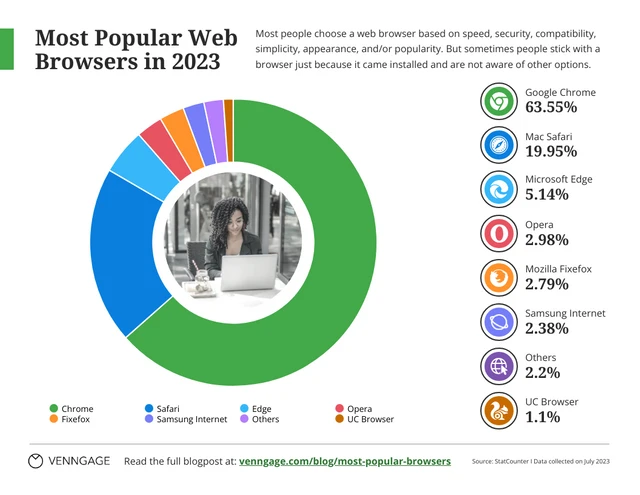
4. Scatter plot
Effective for showing the relationship between two variables and identifying correlations.
Scatter plots are all about exploring relationships between two variables. They’re great for uncovering correlations, trends or patterns in data.
In a scatter plot, every data point appears as a dot on the chart, with one variable marked on the horizontal x-axis and the other on the vertical y-axis.
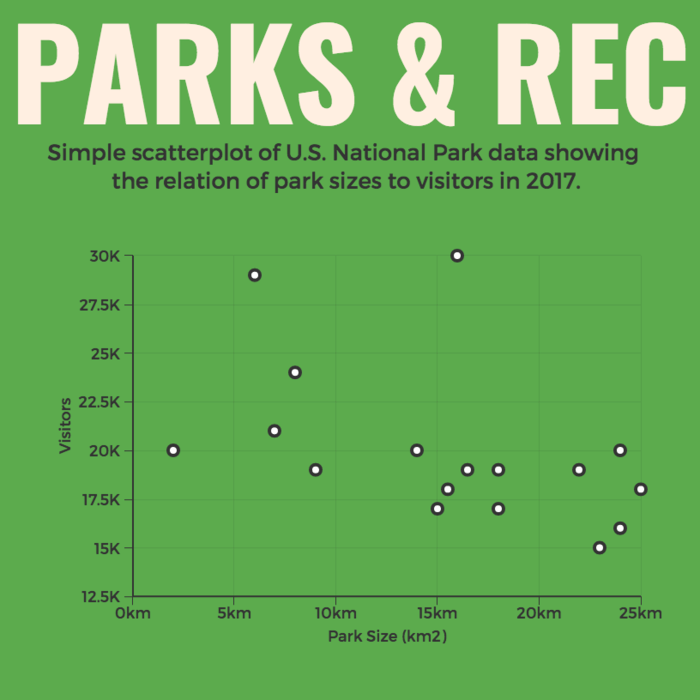
By examining the scatter of points, you can discern the nature of the relationship between the variables, whether it’s positive, negative or no correlation at all.
If you’re using scatter plots to reveal relationships between two variables, be sure to add trendlines or regression analysis when appropriate to clarify patterns. Label data points selectively or provide tooltips for detailed information.
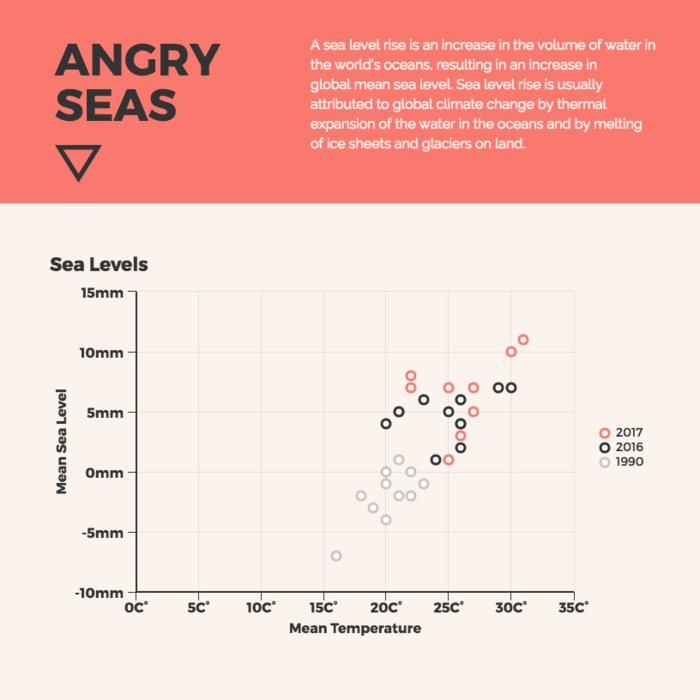
5. Histogram
Best for visualizing the distribution and frequency of a single variable.
Histograms are your choice when you want to understand the distribution and frequency of a single variable.
They divide the data into “bins” or intervals and the height of each bar represents the frequency or count of data points falling into that interval.
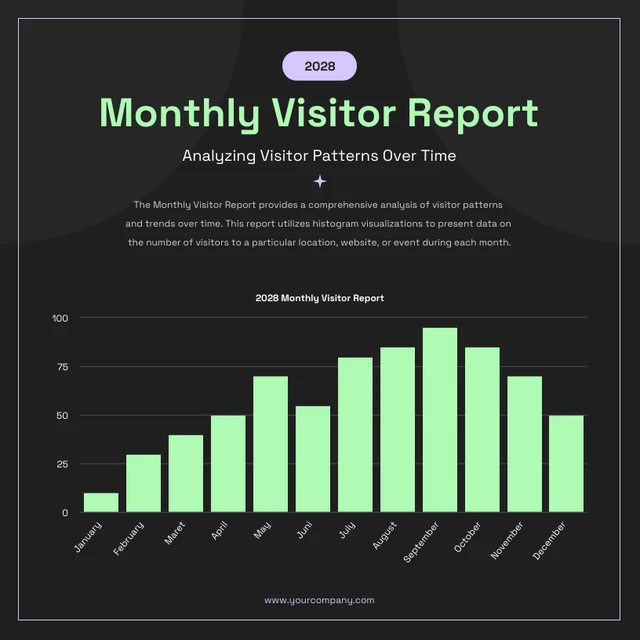
Histograms are excellent for helping to identify trends in data distributions, such as peaks, gaps or skewness.
Here’s something to take note of — ensure that your histogram bins are appropriately sized to capture meaningful data patterns. Using clear axis labels and titles can also help explain the distribution of the data effectively.
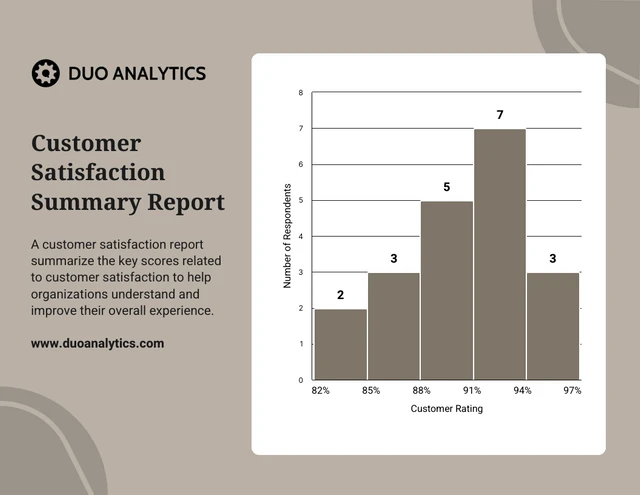
6. Stacked bar chart
Useful for showing how different components contribute to a whole over multiple categories.
Stacked bar charts are a handy choice when you want to illustrate how different components contribute to a whole across multiple categories.
Each bar represents a category and the bars are divided into segments to show the contribution of various components within each category.
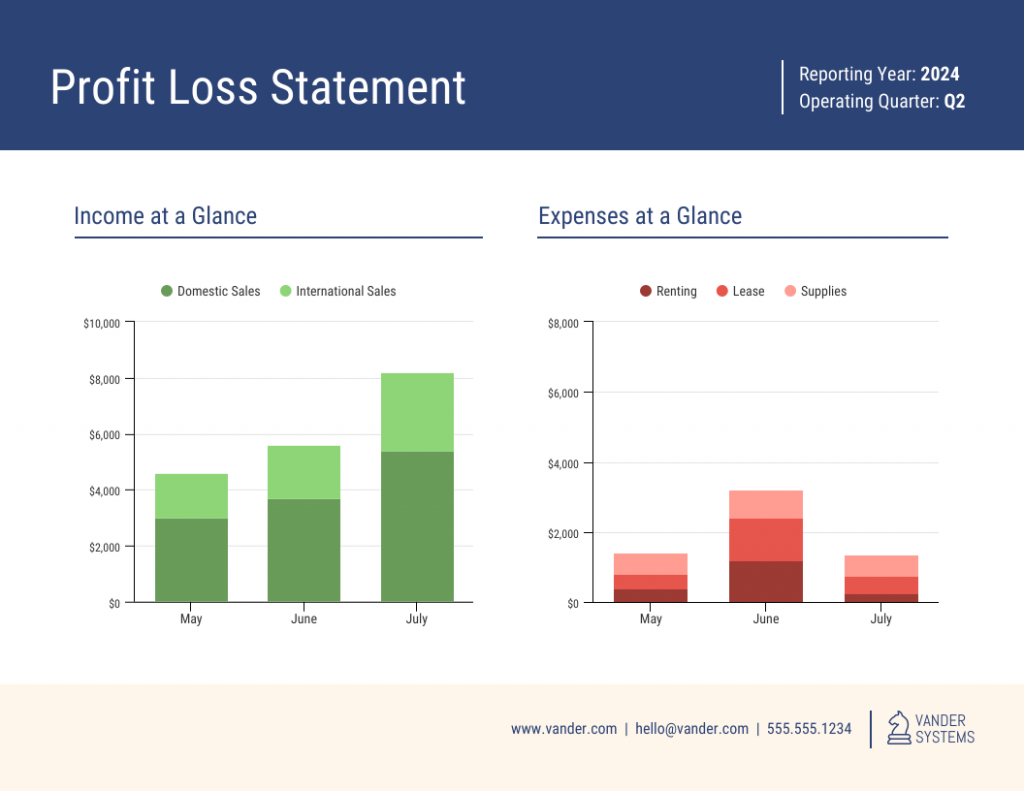
This method is ideal for highlighting both the individual and collective significance of each component, making it a valuable tool for comparative analysis.
Stacked bar charts are like data sandwiches—label each layer so people know what’s what. Keep the order logical and don’t forget the paintbrush for snazzy colors. Here’s a data analysis presentation example on writers’ productivity using stacked bar charts:
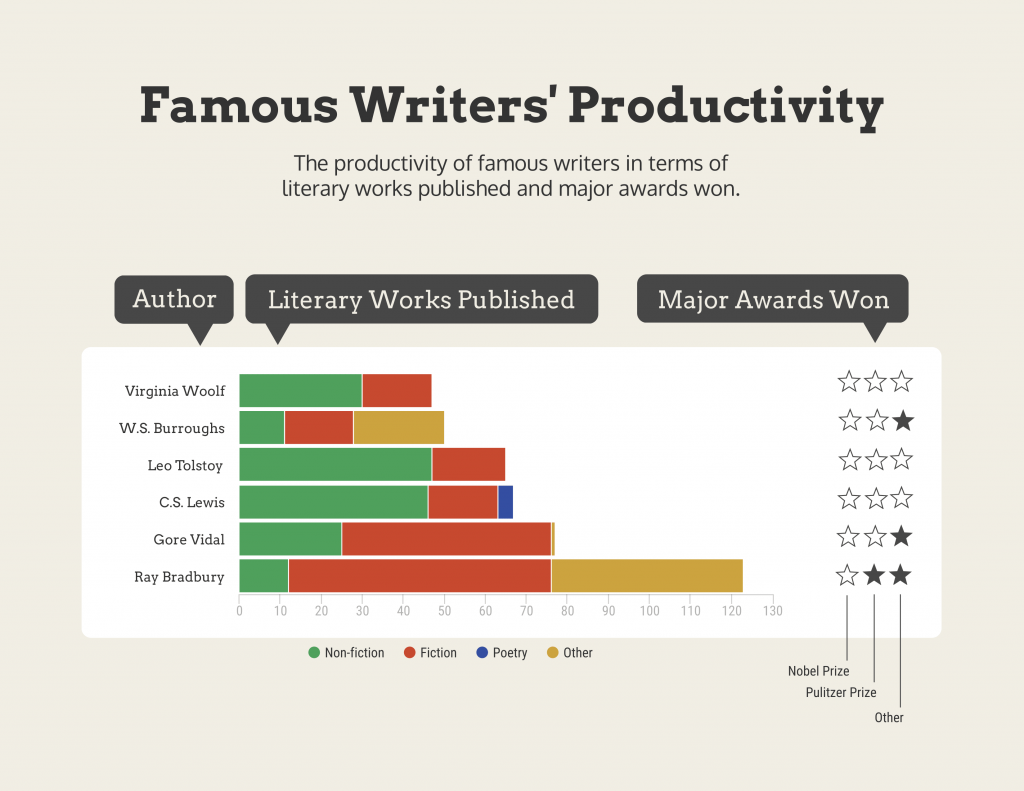
7. Area chart
Similar to line charts but with the area below the lines filled, making them suitable for showing cumulative data.
Area charts are close cousins of line charts but come with a twist.
Imagine plotting the sales of a product over several months. In an area chart, the space between the line and the x-axis is filled, providing a visual representation of the cumulative total.
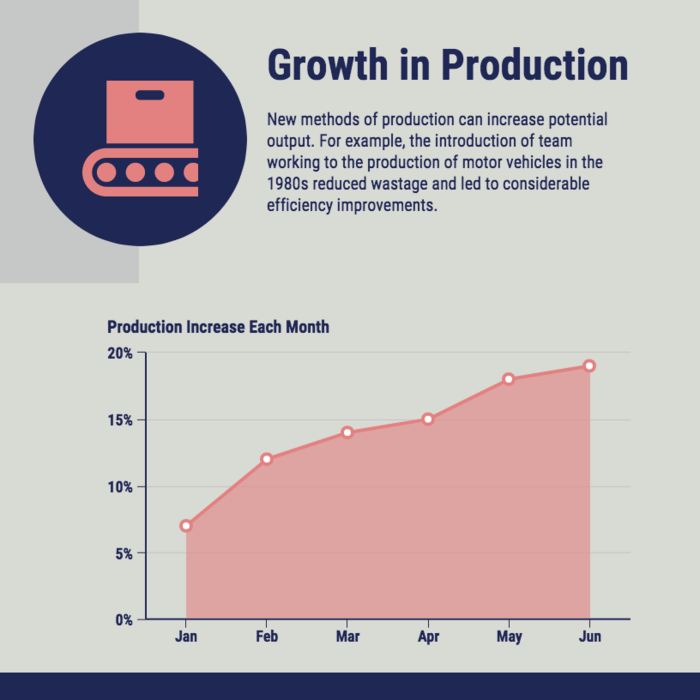
This makes it easy to see how values stack up over time, making area charts a valuable tool for tracking trends in data.
For area charts, use them to visualize cumulative data and trends, but avoid overcrowding the chart. Add labels, especially at significant points and make sure the area under the lines is filled with a visually appealing color gradient.
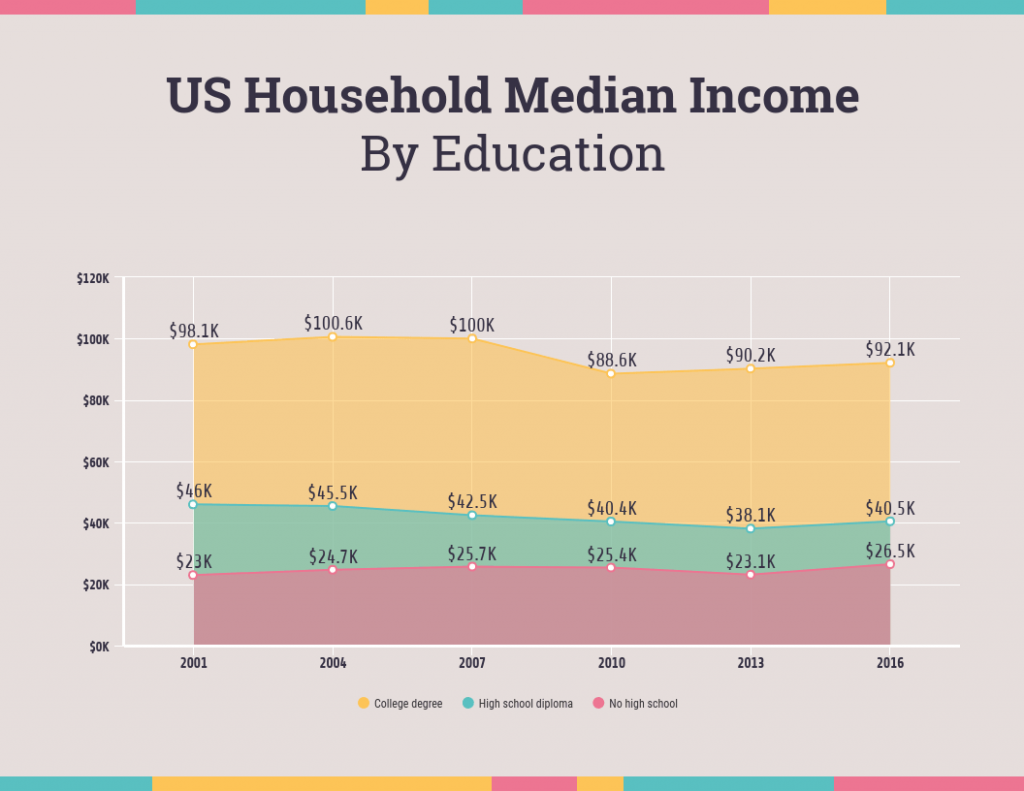
8. Tabular presentation
Presenting data in rows and columns, often used for precise data values and comparisons.
Tabular data presentation is all about clarity and precision. Think of it as presenting numerical data in a structured grid, with rows and columns clearly displaying individual data points.
A table is invaluable for showcasing detailed data, facilitating comparisons and presenting numerical information that needs to be exact. They’re commonly used in reports, spreadsheets and academic papers.
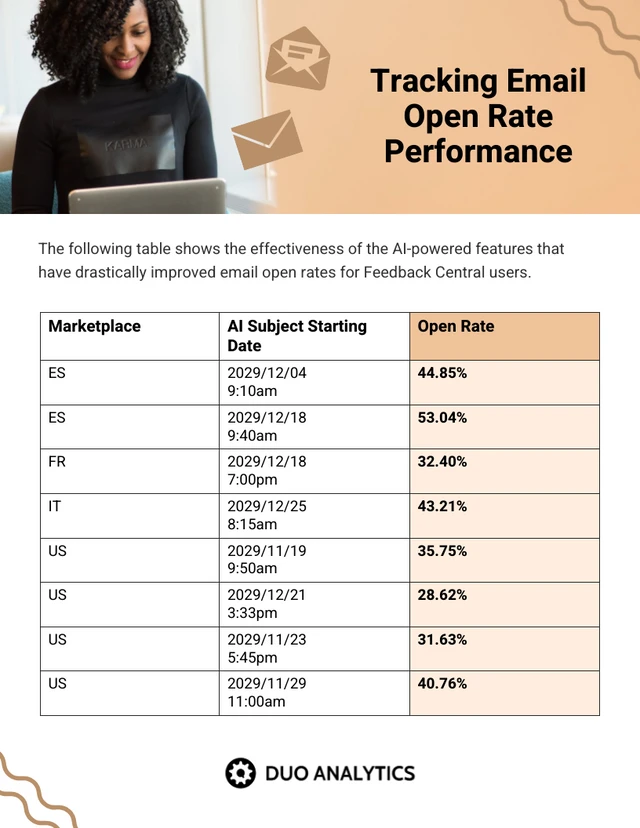
When presenting tabular data, organize it neatly with clear headers and appropriate column widths. Highlight important data points or patterns using shading or font formatting for better readability.
9. Textual data
Utilizing written or descriptive content to explain or complement data, such as annotations or explanatory text.
Textual data presentation may not involve charts or graphs, but it’s one of the most used qualitative data presentation examples.
It involves using written content to provide context, explanations or annotations alongside data visuals. Think of it as the narrative that guides your audience through the data.
Well-crafted textual data can make complex information more accessible and help your audience understand the significance of the numbers and visuals.
Textual data is your chance to tell a story. Break down complex information into bullet points or short paragraphs and use headings to guide the reader’s attention.
10. Pictogram
Using simple icons or images to represent data is especially useful for conveying information in a visually intuitive manner.
Pictograms are all about harnessing the power of images to convey data in an easy-to-understand way.
Instead of using numbers or complex graphs, you use simple icons or images to represent data points.
For instance, you could use a thumbs up emoji to illustrate customer satisfaction levels, where each face represents a different level of satisfaction.
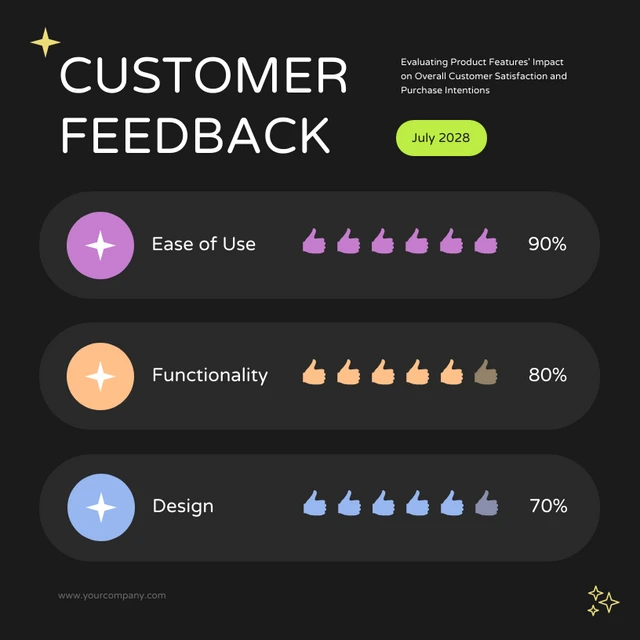
Pictograms are great for conveying data visually, so choose symbols that are easy to interpret and relevant to the data. Use consistent scaling and a legend to explain the symbols’ meanings, ensuring clarity in your presentation.
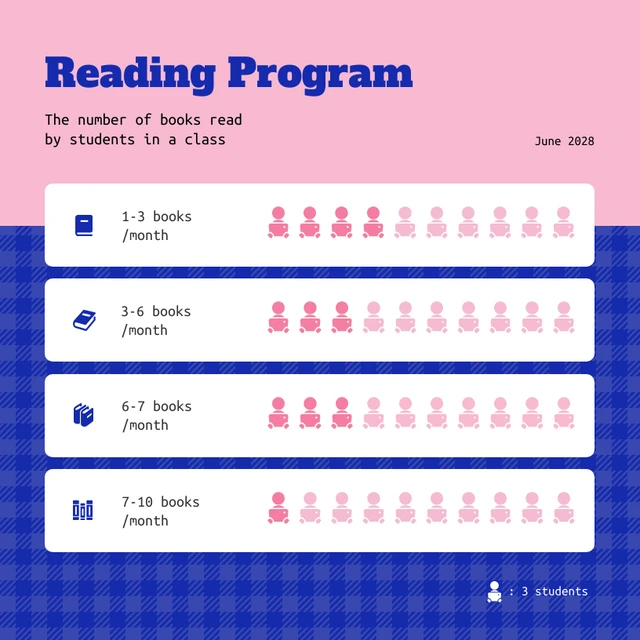
Looking for more data presentation ideas? Use the Venngage graph maker or browse through our gallery of chart templates to pick a template and get started!
A comprehensive data presentation should include several key elements to effectively convey information and insights to your audience. Here’s a list of what should be included in a data presentation:
1. Title and objective
- Begin with a clear and informative title that sets the context for your presentation.
- State the primary objective or purpose of the presentation to provide a clear focus.
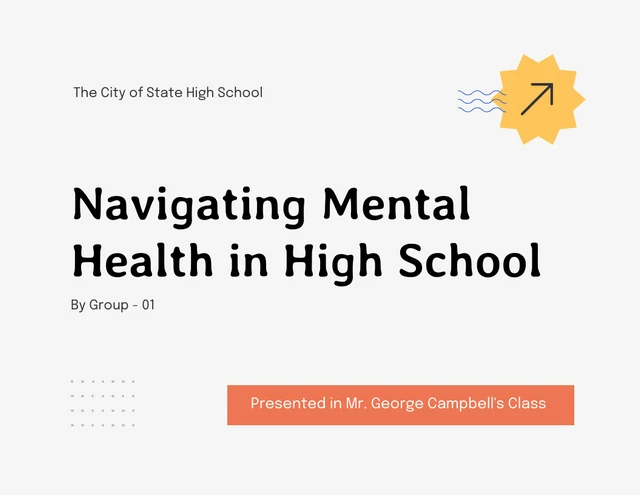
2. Key data points
- Present the most essential data points or findings that align with your objective.
- Use charts, graphical presentations or visuals to illustrate these key points for better comprehension.
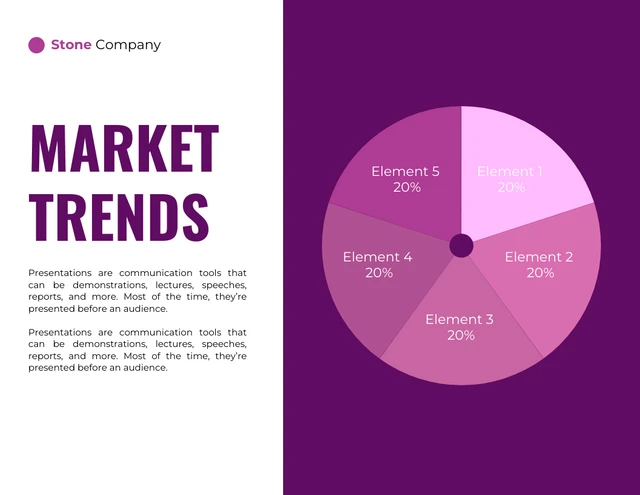
3. Context and significance
- Provide a brief overview of the context in which the data was collected and why it’s significant.
- Explain how the data relates to the larger picture or the problem you’re addressing.
4. Key takeaways
- Summarize the main insights or conclusions that can be drawn from the data.
- Highlight the key takeaways that the audience should remember.
5. Visuals and charts
- Use clear and appropriate visual aids to complement the data.
- Ensure that visuals are easy to understand and support your narrative.
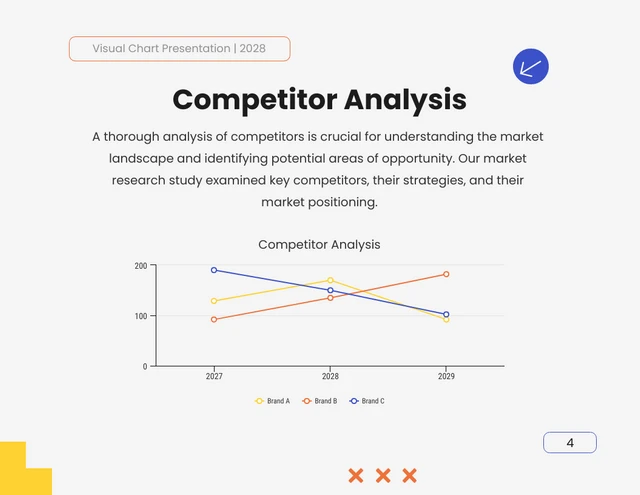
6. Implications or actions
- Discuss the practical implications of the data or any recommended actions.
- If applicable, outline next steps or decisions that should be taken based on the data.
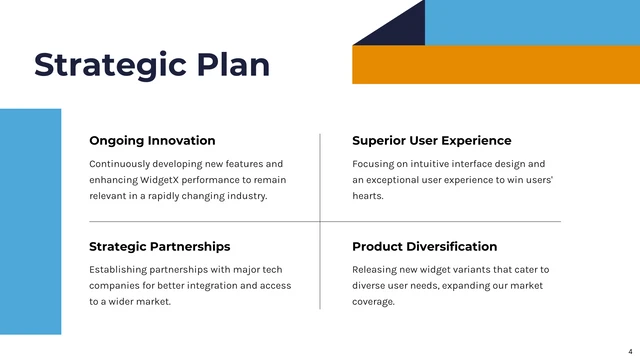
7. Q&A and discussion
- Allocate time for questions and open discussion to engage the audience.
- Address queries and provide additional insights or context as needed.
Presenting data is a crucial skill in various professional fields, from business to academia and beyond. To ensure your data presentations hit the mark, here are some common mistakes that you should steer clear of:
Overloading with data
Presenting too much data at once can overwhelm your audience. Focus on the key points and relevant information to keep the presentation concise and focused. Here are some free data visualization tools you can use to convey data in an engaging and impactful way.
Assuming everyone’s on the same page
It’s easy to assume that your audience understands as much about the topic as you do. But this can lead to either dumbing things down too much or diving into a bunch of jargon that leaves folks scratching their heads. Take a beat to figure out where your audience is coming from and tailor your presentation accordingly.
Misleading visuals
Using misleading visuals, such as distorted scales or inappropriate chart types can distort the data’s meaning. Pick the right data infographics and understandable charts to ensure that your visual representations accurately reflect the data.
Not providing context
Data without context is like a puzzle piece with no picture on it. Without proper context, data may be meaningless or misinterpreted. Explain the background, methodology and significance of the data.
Not citing sources properly
Neglecting to cite sources and provide citations for your data can erode its credibility. Always attribute data to its source and utilize reliable sources for your presentation.
Not telling a story
Avoid simply presenting numbers. If your presentation lacks a clear, engaging story that takes your audience on a journey from the beginning (setting the scene) through the middle (data analysis) to the end (the big insights and recommendations), you’re likely to lose their interest.
Infographics are great for storytelling because they mix cool visuals with short and sweet text to explain complicated stuff in a fun and easy way. Create one with Venngage’s free infographic maker to create a memorable story that your audience will remember.
Ignoring data quality
Presenting data without first checking its quality and accuracy can lead to misinformation. Validate and clean your data before presenting it.
Simplify your visuals
Fancy charts might look cool, but if they confuse people, what’s the point? Go for the simplest visual that gets your message across. Having a dilemma between presenting data with infographics v.s data design? This article on the difference between data design and infographics might help you out.
Missing the emotional connection
Data isn’t just about numbers; it’s about people and real-life situations. Don’t forget to sprinkle in some human touch, whether it’s through relatable stories, examples or showing how the data impacts real lives.
Skipping the actionable insights
At the end of the day, your audience wants to know what they should do with all the data. If you don’t wrap up with clear, actionable insights or recommendations, you’re leaving them hanging. Always finish up with practical takeaways and the next steps.
Can you provide some data presentation examples for business reports?
Business reports often benefit from data presentation through bar charts showing sales trends over time, pie charts displaying market share,or tables presenting financial performance metrics like revenue and profit margins.
What are some creative data presentation examples for academic presentations?
Creative data presentation ideas for academic presentations include using statistical infographics to illustrate research findings and statistical data, incorporating storytelling techniques to engage the audience or utilizing heat maps to visualize data patterns.
What are the key considerations when choosing the right data presentation format?
When choosing a chart format , consider factors like data complexity, audience expertise and the message you want to convey. Options include charts (e.g., bar, line, pie), tables, heat maps, data visualization infographics and interactive dashboards.
Knowing the type of data visualization that best serves your data is just half the battle. Here are some best practices for data visualization to make sure that the final output is optimized.
How can I choose the right data presentation method for my data?
To select the right data presentation method, start by defining your presentation’s purpose and audience. Then, match your data type (e.g., quantitative, qualitative) with suitable visualization techniques (e.g., histograms, word clouds) and choose an appropriate presentation format (e.g., slide deck, report, live demo).
For more presentation ideas , check out this guide on how to make a good presentation or use a presentation software to simplify the process.
How can I make my data presentations more engaging and informative?
To enhance data presentations, use compelling narratives, relatable examples and fun data infographics that simplify complex data. Encourage audience interaction, offer actionable insights and incorporate storytelling elements to engage and inform effectively.
The opening of your presentation holds immense power in setting the stage for your audience. To design a presentation and convey your data in an engaging and informative, try out Venngage’s free presentation maker to pick the right presentation design for your audience and topic.
What is the difference between data visualization and data presentation?
Data presentation typically involves conveying data reports and insights to an audience, often using visuals like charts and graphs. Data visualization , on the other hand, focuses on creating those visual representations of data to facilitate understanding and analysis.
Now that you’ve learned a thing or two about how to use these methods of data presentation to tell a compelling data story , it’s time to take these strategies and make them your own.
But here’s the deal: these aren’t just one-size-fits-all solutions. Remember that each example we’ve uncovered here is not a rigid template but a source of inspiration. It’s all about making your audience go, “Wow, I get it now!”
Think of your data presentations as your canvas – it’s where you paint your story, convey meaningful insights and make real change happen.
So, go forth, present your data with confidence and purpose and watch as your strategic influence grows, one compelling presentation at a time.
- Online Degree Explore Bachelor’s & Master’s degrees
- MasterTrack™ Earn credit towards a Master’s degree
- University Certificates Advance your career with graduate-level learning
- Top Courses
- Join for Free

Data Analysis and Presentation Skills: the PwC Approach Specialization
Make Smarter Business Decisions With Data Analysis. Understand data, apply data analytics tools and create effective business intelligence presentations
Taught in English
Some content may not be translated

Instructor: Alex Mannella
Financial aid available
157,406 already enrolled
Specialization - 5 course series
(9,839 reviews)
Skills you'll gain
- Data Analysis
- Microsoft Excel
- Data Visualization
- Presentation
Details to know

Add to your LinkedIn profile
See how employees at top companies are mastering in-demand skills
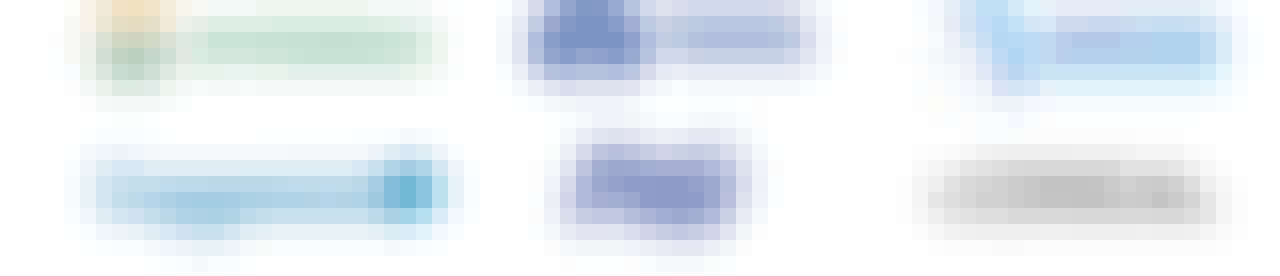
Advance your subject-matter expertise
- Learn in-demand skills from university and industry experts
- Master a subject or tool with hands-on projects
- Develop a deep understanding of key concepts
- Earn a career certificate from PwC
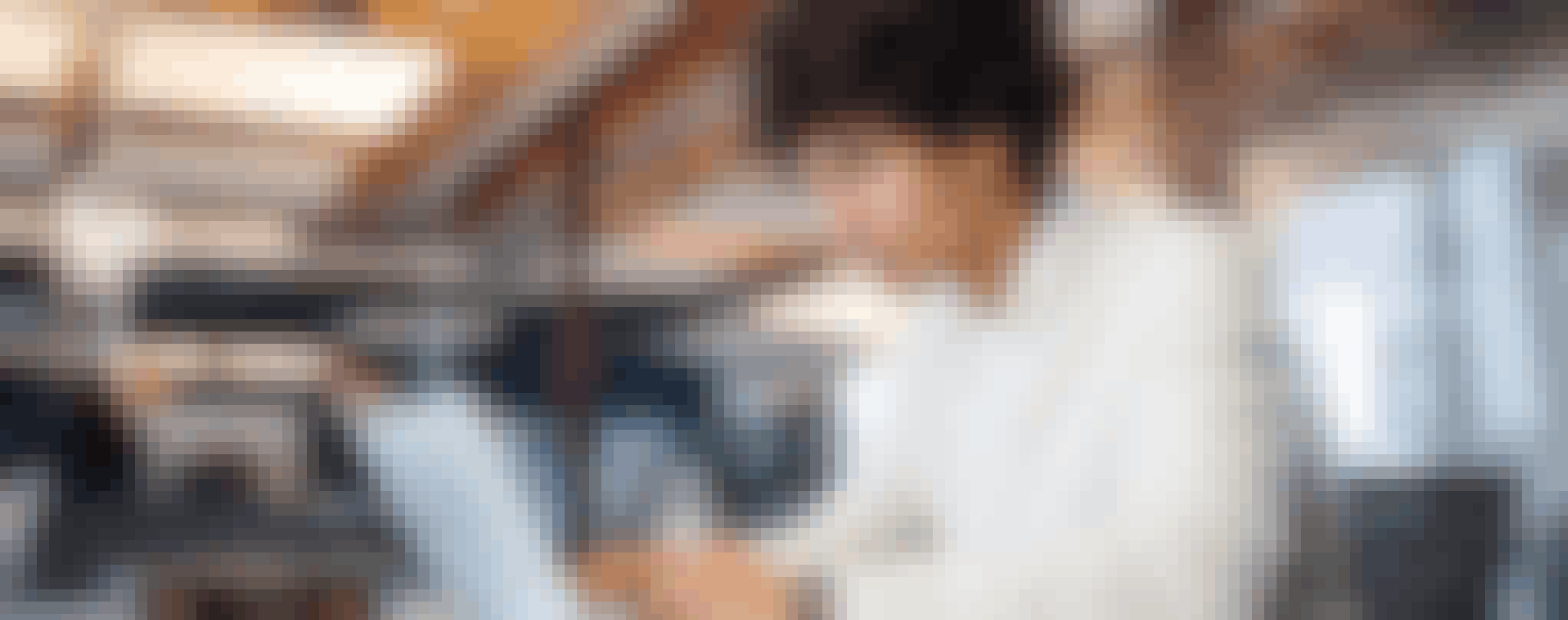
Earn a career certificate
Add this credential to your LinkedIn profile, resume, or CV
Share it on social media and in your performance review
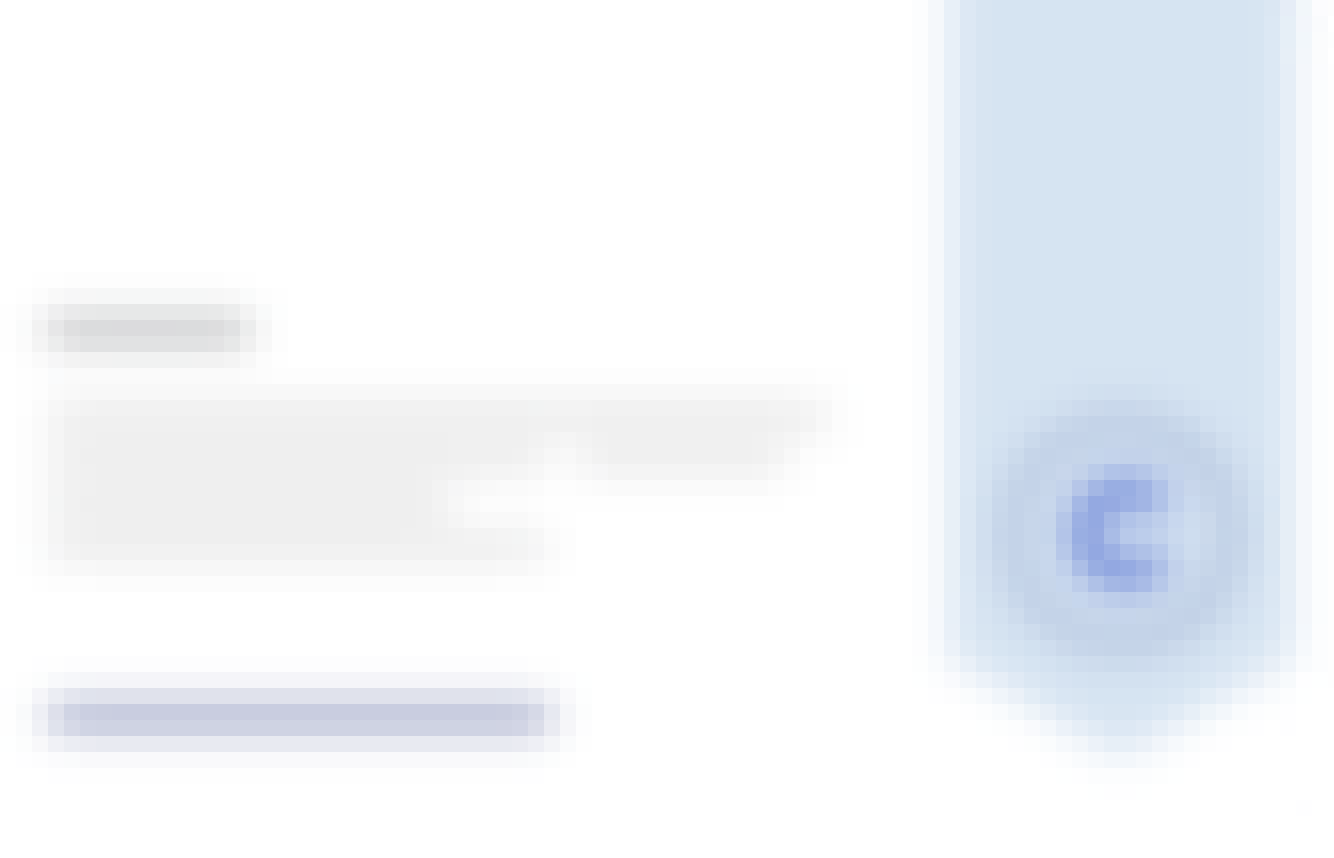
If you are a PwC Employee, gain access to the PwC Specialization and Courses for free using the instructions on Vantage.
This Specialization will help you get practical with data analysis, turning business intelligence into real-world outcomes. We'll explore how a combination of better understanding, filtering, and application of data can help you solve problems faster - leading to smarter and more effective decision-making. You’ll learn how to use Microsoft Excel, PowerPoint, and other common data analysis and communication tools, and perhaps most importantly, we'll help you to present data to others in a way that gets them engaged in your story and motivated to act.
Please note: If you'd like to audit the courses in this Specialization, you'll need to enroll in each course separately and then you will see the audit option.
This specialization was created by PricewaterhouseCoopers LLP with an address at 300 Madison Avenue, New York, New York, 10017.
Applied Learning Project
This specialization will include a project at the end of each module and a capstone project at the end of the specialization. Each project will provide you the chance to apply the skills of that lesson. In the first module you'll plan an analysis approach, in the second and third modules you will analyze sets of data using the Excel skills you learn. In the fourth module you will prepare a business presentation.
In the final Capstone Project, you'll apply the skills you’ve learned by working through a mock client business problem. You'll analyze a set of data, looking for the business insights. Then you'll create and visualize your findings, before recording a video to present your recommendations to the client.
Data-driven Decision Making
What you'll learn.
Welcome to Data-driven Decision Making. In this course, you'll get an introduction to Data Analytics and its role in business decisions. You'll learn why data is important and how it has evolved. You'll be introduced to “Big Data” and how it is used. You'll also be introduced to a framework for conducting Data Analysis and what tools and techniques are commonly used. Finally, you'll have a chance to put your knowledge to work in a simulated business setting.
This course was created by PricewaterhouseCoopers LLP with an address at 300 Madison Avenue, New York, New York, 10017.
Problem Solving with Excel
This course explores Excel as a tool for solving business problems. In this course you will learn the basic functions of excel through guided demonstration. Each week you will build on your excel skills and be provided an opportunity to practice what you’ve learned. Finally, you will have a chance to put your knowledge to work in a final project. Please note, the content in this course was developed using a Windows version of Excel 2013.
Data Visualization with Advanced Excel
In this course, you will get hands-on instruction of advanced Excel 2013 functions. You’ll learn to use PowerPivot to build databases and data models. We’ll show you how to perform different types of scenario and simulation analysis and you’ll have an opportunity to practice these skills by leveraging some of Excel's built in tools including, solver, data tables, scenario manager and goal seek. In the second half of the course, will cover how to visualize data, tell a story and explore data by reviewing core principles of data visualization and dashboarding. You’ll use Excel to build complex graphs and Power View reports and then start to combine them into dynamic dashboards.
Note: Learners will need PowerPivot to complete some of the exercises. Please use MS Excel 2013 version. If you have other MS Excel versions or a MAC you might not be able to complete all assignments. This course was created by PricewaterhouseCoopers LLP with an address at 300 Madison Avenue, New York, New York, 10017.
Effective Business Presentations with Powerpoint
This course is all about presenting the story of the data, using PowerPoint. You'll learn how to structure a presentation, to include insights and supporting data. You'll also learn some design principles for effective visuals and slides. You'll gain skills for client-facing communication - including public speaking, executive presence and compelling storytelling. Finally, you'll be given a client profile, a business problem, and a set of basic Excel charts, which you'll need to turn into a presentation - which you'll deliver with iterative peer feedback.
Data Analysis and Presentation Skills: the PwC Approach Final Project
In this Capstone Project, you'll bring together all the new skills and insights you've learned through the four courses. You'll be given a 'mock' client problem and a data set. You'll need to analyze the data to gain business insights, research the client's domain area, and create recommendations. You'll then need to visualize the data in a client-facing presentation. You'll bring it all together in a recorded video presentation.

With offices in 157 countries and more than 208,000 people, PwC is among the leading professional services networks in the world. Our purpose is to build trust in society and solve important problems. We help organisations and individuals create the value they’re looking for, by delivering quality in assurance, tax and advisory services.
Why people choose Coursera for their career

New to Business Essentials? Start here.

Open new doors with Coursera Plus
Unlimited access to 7,000+ world-class courses, hands-on projects, and job-ready certificate programs - all included in your subscription
Advance your career with an online degree
Earn a degree from world-class universities - 100% online
Join over 3,400 global companies that choose Coursera for Business
Upskill your employees to excel in the digital economy
Frequently asked questions
How long does it take to complete the specialization.
Exactly how long it takes will vary, depending on your schedule. Most learners complete the Specialization in five to six months.
What background knowledge is necessary?
You don't need any background knowledge. We've designed this Specialization for learners who are new to the field of data and analytics.
Do I need to take the courses in a specific order?
We recommend you take them in the order they appear on Coursera. Each course builds on the knowledge you learned in the last one.
Will I earn university credit for completing the Specialization?
Coursera courses and certificates don't carry university credit, though some universities may choose to accept Specialization Certificates for credit. You should check with your institution to find out more.
What will I be able to do upon completing the Specialization?
You'll be able to use the data and analytics framework to develop a plan to solve a business problem. You'll be able to use Excel to analyze data using formulas and present a series of visualizations with a summary recommendation to solve the business problem. You'll also be able to take data and create a dynamic data dashboard in Excel that accepts inputs and refreshes with new data. Finally, you'll be able to develop and deliver a presentation using PowerPoint and the results of your data analysis - so you can share your point of view on how to solve the business problem.
How do I audit the Specialization?
If you'd like to audit the courses in this Specialization, you'll need to enroll in each course separately and then you will see the audit option.
What tools do I need for this Specialization?
In the "Data Visualization and Advance Excel" course learners will need PowerPivot to complete some of the exercises. Please use MS Excel 2013 version. If you have other MS Excel versions or a MAC you might not be able to complete all assignments.
Is this course really 100% online? Do I need to attend any classes in person?
This course is completely online, so there’s no need to show up to a classroom in person. You can access your lectures, readings and assignments anytime and anywhere via the web or your mobile device.
What is the refund policy?
If you subscribed, you get a 7-day free trial during which you can cancel at no penalty. After that, we don’t give refunds, but you can cancel your subscription at any time. See our full refund policy Opens in a new tab .
Can I just enroll in a single course?
Yes! To get started, click the course card that interests you and enroll. You can enroll and complete the course to earn a shareable certificate, or you can audit it to view the course materials for free. When you subscribe to a course that is part of a Specialization, you’re automatically subscribed to the full Specialization. Visit your learner dashboard to track your progress.
Is financial aid available?
Yes. In select learning programs, you can apply for financial aid or a scholarship if you can’t afford the enrollment fee. If fin aid or scholarship is available for your learning program selection, you’ll find a link to apply on the description page.
Can I take the course for free?
When you enroll in the course, you get access to all of the courses in the Specialization, and you earn a certificate when you complete the work. If you only want to read and view the course content, you can audit the course for free. If you cannot afford the fee, you can apply for financial aid Opens in a new tab .
More questions
Your Modern Business Guide To Data Analysis Methods And Techniques

Table of Contents
1) What Is Data Analysis?
2) Why Is Data Analysis Important?
3) What Is The Data Analysis Process?
4) Types Of Data Analysis Methods
5) Top Data Analysis Techniques To Apply
6) Quality Criteria For Data Analysis
7) Data Analysis Limitations & Barriers
8) Data Analysis Skills
9) Data Analysis In The Big Data Environment
In our data-rich age, understanding how to analyze and extract true meaning from our business’s digital insights is one of the primary drivers of success.
Despite the colossal volume of data we create every day, a mere 0.5% is actually analyzed and used for data discovery , improvement, and intelligence. While that may not seem like much, considering the amount of digital information we have at our fingertips, half a percent still accounts for a vast amount of data.
With so much data and so little time, knowing how to collect, curate, organize, and make sense of all of this potentially business-boosting information can be a minefield – but online data analysis is the solution.
In science, data analysis uses a more complex approach with advanced techniques to explore and experiment with data. On the other hand, in a business context, data is used to make data-driven decisions that will enable the company to improve its overall performance. In this post, we will cover the analysis of data from an organizational point of view while still going through the scientific and statistical foundations that are fundamental to understanding the basics of data analysis.
To put all of that into perspective, we will answer a host of important analytical questions, explore analytical methods and techniques, while demonstrating how to perform analysis in the real world with a 17-step blueprint for success.
What Is Data Analysis?
Data analysis is the process of collecting, modeling, and analyzing data using various statistical and logical methods and techniques. Businesses rely on analytics processes and tools to extract insights that support strategic and operational decision-making.
All these various methods are largely based on two core areas: quantitative and qualitative research.
To explain the key differences between qualitative and quantitative research, here’s a video for your viewing pleasure:
Gaining a better understanding of different techniques and methods in quantitative research as well as qualitative insights will give your analyzing efforts a more clearly defined direction, so it’s worth taking the time to allow this particular knowledge to sink in. Additionally, you will be able to create a comprehensive analytical report that will skyrocket your analysis.
Apart from qualitative and quantitative categories, there are also other types of data that you should be aware of before dividing into complex data analysis processes. These categories include:
- Big data: Refers to massive data sets that need to be analyzed using advanced software to reveal patterns and trends. It is considered to be one of the best analytical assets as it provides larger volumes of data at a faster rate.
- Metadata: Putting it simply, metadata is data that provides insights about other data. It summarizes key information about specific data that makes it easier to find and reuse for later purposes.
- Real time data: As its name suggests, real time data is presented as soon as it is acquired. From an organizational perspective, this is the most valuable data as it can help you make important decisions based on the latest developments. Our guide on real time analytics will tell you more about the topic.
- Machine data: This is more complex data that is generated solely by a machine such as phones, computers, or even websites and embedded systems, without previous human interaction.
Why Is Data Analysis Important?
Before we go into detail about the categories of analysis along with its methods and techniques, you must understand the potential that analyzing data can bring to your organization.
- Informed decision-making : From a management perspective, you can benefit from analyzing your data as it helps you make decisions based on facts and not simple intuition. For instance, you can understand where to invest your capital, detect growth opportunities, predict your income, or tackle uncommon situations before they become problems. Through this, you can extract relevant insights from all areas in your organization, and with the help of dashboard software , present the data in a professional and interactive way to different stakeholders.
- Reduce costs : Another great benefit is to reduce costs. With the help of advanced technologies such as predictive analytics, businesses can spot improvement opportunities, trends, and patterns in their data and plan their strategies accordingly. In time, this will help you save money and resources on implementing the wrong strategies. And not just that, by predicting different scenarios such as sales and demand you can also anticipate production and supply.
- Target customers better : Customers are arguably the most crucial element in any business. By using analytics to get a 360° vision of all aspects related to your customers, you can understand which channels they use to communicate with you, their demographics, interests, habits, purchasing behaviors, and more. In the long run, it will drive success to your marketing strategies, allow you to identify new potential customers, and avoid wasting resources on targeting the wrong people or sending the wrong message. You can also track customer satisfaction by analyzing your client’s reviews or your customer service department’s performance.
What Is The Data Analysis Process?

When we talk about analyzing data there is an order to follow in order to extract the needed conclusions. The analysis process consists of 5 key stages. We will cover each of them more in detail later in the post, but to start providing the needed context to understand what is coming next, here is a rundown of the 5 essential steps of data analysis.
- Identify: Before you get your hands dirty with data, you first need to identify why you need it in the first place. The identification is the stage in which you establish the questions you will need to answer. For example, what is the customer's perception of our brand? Or what type of packaging is more engaging to our potential customers? Once the questions are outlined you are ready for the next step.
- Collect: As its name suggests, this is the stage where you start collecting the needed data. Here, you define which sources of data you will use and how you will use them. The collection of data can come in different forms such as internal or external sources, surveys, interviews, questionnaires, and focus groups, among others. An important note here is that the way you collect the data will be different in a quantitative and qualitative scenario.
- Clean: Once you have the necessary data it is time to clean it and leave it ready for analysis. Not all the data you collect will be useful, when collecting big amounts of data in different formats it is very likely that you will find yourself with duplicate or badly formatted data. To avoid this, before you start working with your data you need to make sure to erase any white spaces, duplicate records, or formatting errors. This way you avoid hurting your analysis with bad-quality data.
- Analyze : With the help of various techniques such as statistical analysis, regressions, neural networks, text analysis, and more, you can start analyzing and manipulating your data to extract relevant conclusions. At this stage, you find trends, correlations, variations, and patterns that can help you answer the questions you first thought of in the identify stage. Various technologies in the market assist researchers and average users with the management of their data. Some of them include business intelligence and visualization software, predictive analytics, and data mining, among others.
- Interpret: Last but not least you have one of the most important steps: it is time to interpret your results. This stage is where the researcher comes up with courses of action based on the findings. For example, here you would understand if your clients prefer packaging that is red or green, plastic or paper, etc. Additionally, at this stage, you can also find some limitations and work on them.
Now that you have a basic understanding of the key data analysis steps, let’s look at the top 17 essential methods.
17 Essential Types Of Data Analysis Methods
Before diving into the 17 essential types of methods, it is important that we go over really fast through the main analysis categories. Starting with the category of descriptive up to prescriptive analysis, the complexity and effort of data evaluation increases, but also the added value for the company.
a) Descriptive analysis - What happened.
The descriptive analysis method is the starting point for any analytic reflection, and it aims to answer the question of what happened? It does this by ordering, manipulating, and interpreting raw data from various sources to turn it into valuable insights for your organization.
Performing descriptive analysis is essential, as it enables us to present our insights in a meaningful way. Although it is relevant to mention that this analysis on its own will not allow you to predict future outcomes or tell you the answer to questions like why something happened, it will leave your data organized and ready to conduct further investigations.
b) Exploratory analysis - How to explore data relationships.
As its name suggests, the main aim of the exploratory analysis is to explore. Prior to it, there is still no notion of the relationship between the data and the variables. Once the data is investigated, exploratory analysis helps you to find connections and generate hypotheses and solutions for specific problems. A typical area of application for it is data mining.
c) Diagnostic analysis - Why it happened.
Diagnostic data analytics empowers analysts and executives by helping them gain a firm contextual understanding of why something happened. If you know why something happened as well as how it happened, you will be able to pinpoint the exact ways of tackling the issue or challenge.
Designed to provide direct and actionable answers to specific questions, this is one of the world’s most important methods in research, among its other key organizational functions such as retail analytics , e.g.
c) Predictive analysis - What will happen.
The predictive method allows you to look into the future to answer the question: what will happen? In order to do this, it uses the results of the previously mentioned descriptive, exploratory, and diagnostic analysis, in addition to machine learning (ML) and artificial intelligence (AI). Through this, you can uncover future trends, potential problems or inefficiencies, connections, and casualties in your data.
With predictive analysis, you can unfold and develop initiatives that will not only enhance your various operational processes but also help you gain an all-important edge over the competition. If you understand why a trend, pattern, or event happened through data, you will be able to develop an informed projection of how things may unfold in particular areas of the business.
e) Prescriptive analysis - How will it happen.
Another of the most effective types of analysis methods in research. Prescriptive data techniques cross over from predictive analysis in the way that it revolves around using patterns or trends to develop responsive, practical business strategies.
By drilling down into prescriptive analysis, you will play an active role in the data consumption process by taking well-arranged sets of visual data and using it as a powerful fix to emerging issues in a number of key areas, including marketing, sales, customer experience, HR, fulfillment, finance, logistics analytics , and others.

As mentioned at the beginning of the post, data analysis methods can be divided into two big categories: quantitative and qualitative. Each of these categories holds a powerful analytical value that changes depending on the scenario and type of data you are working with. Below, we will discuss 17 methods that are divided into qualitative and quantitative approaches.
Without further ado, here are the 17 essential types of data analysis methods with some use cases in the business world:
A. Quantitative Methods
To put it simply, quantitative analysis refers to all methods that use numerical data or data that can be turned into numbers (e.g. category variables like gender, age, etc.) to extract valuable insights. It is used to extract valuable conclusions about relationships, differences, and test hypotheses. Below we discuss some of the key quantitative methods.
1. Cluster analysis
The action of grouping a set of data elements in a way that said elements are more similar (in a particular sense) to each other than to those in other groups – hence the term ‘cluster.’ Since there is no target variable when clustering, the method is often used to find hidden patterns in the data. The approach is also used to provide additional context to a trend or dataset.
Let's look at it from an organizational perspective. In a perfect world, marketers would be able to analyze each customer separately and give them the best-personalized service, but let's face it, with a large customer base, it is timely impossible to do that. That's where clustering comes in. By grouping customers into clusters based on demographics, purchasing behaviors, monetary value, or any other factor that might be relevant for your company, you will be able to immediately optimize your efforts and give your customers the best experience based on their needs.
2. Cohort analysis
This type of data analysis approach uses historical data to examine and compare a determined segment of users' behavior, which can then be grouped with others with similar characteristics. By using this methodology, it's possible to gain a wealth of insight into consumer needs or a firm understanding of a broader target group.
Cohort analysis can be really useful for performing analysis in marketing as it will allow you to understand the impact of your campaigns on specific groups of customers. To exemplify, imagine you send an email campaign encouraging customers to sign up for your site. For this, you create two versions of the campaign with different designs, CTAs, and ad content. Later on, you can use cohort analysis to track the performance of the campaign for a longer period of time and understand which type of content is driving your customers to sign up, repurchase, or engage in other ways.
A useful tool to start performing cohort analysis method is Google Analytics. You can learn more about the benefits and limitations of using cohorts in GA in this useful guide . In the bottom image, you see an example of how you visualize a cohort in this tool. The segments (devices traffic) are divided into date cohorts (usage of devices) and then analyzed week by week to extract insights into performance.
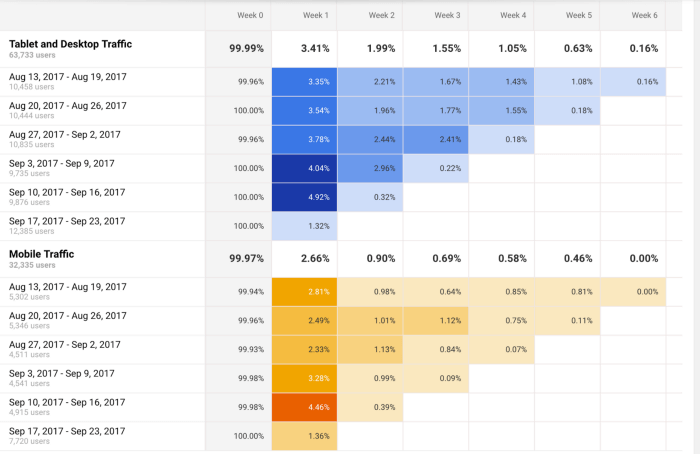
3. Regression analysis
Regression uses historical data to understand how a dependent variable's value is affected when one (linear regression) or more independent variables (multiple regression) change or stay the same. By understanding each variable's relationship and how it developed in the past, you can anticipate possible outcomes and make better decisions in the future.
Let's bring it down with an example. Imagine you did a regression analysis of your sales in 2019 and discovered that variables like product quality, store design, customer service, marketing campaigns, and sales channels affected the overall result. Now you want to use regression to analyze which of these variables changed or if any new ones appeared during 2020. For example, you couldn’t sell as much in your physical store due to COVID lockdowns. Therefore, your sales could’ve either dropped in general or increased in your online channels. Through this, you can understand which independent variables affected the overall performance of your dependent variable, annual sales.
If you want to go deeper into this type of analysis, check out this article and learn more about how you can benefit from regression.
4. Neural networks
The neural network forms the basis for the intelligent algorithms of machine learning. It is a form of analytics that attempts, with minimal intervention, to understand how the human brain would generate insights and predict values. Neural networks learn from each and every data transaction, meaning that they evolve and advance over time.
A typical area of application for neural networks is predictive analytics. There are BI reporting tools that have this feature implemented within them, such as the Predictive Analytics Tool from datapine. This tool enables users to quickly and easily generate all kinds of predictions. All you have to do is select the data to be processed based on your KPIs, and the software automatically calculates forecasts based on historical and current data. Thanks to its user-friendly interface, anyone in your organization can manage it; there’s no need to be an advanced scientist.
Here is an example of how you can use the predictive analysis tool from datapine:

**click to enlarge**
5. Factor analysis
The factor analysis also called “dimension reduction” is a type of data analysis used to describe variability among observed, correlated variables in terms of a potentially lower number of unobserved variables called factors. The aim here is to uncover independent latent variables, an ideal method for streamlining specific segments.
A good way to understand this data analysis method is a customer evaluation of a product. The initial assessment is based on different variables like color, shape, wearability, current trends, materials, comfort, the place where they bought the product, and frequency of usage. Like this, the list can be endless, depending on what you want to track. In this case, factor analysis comes into the picture by summarizing all of these variables into homogenous groups, for example, by grouping the variables color, materials, quality, and trends into a brother latent variable of design.
If you want to start analyzing data using factor analysis we recommend you take a look at this practical guide from UCLA.
6. Data mining
A method of data analysis that is the umbrella term for engineering metrics and insights for additional value, direction, and context. By using exploratory statistical evaluation, data mining aims to identify dependencies, relations, patterns, and trends to generate advanced knowledge. When considering how to analyze data, adopting a data mining mindset is essential to success - as such, it’s an area that is worth exploring in greater detail.
An excellent use case of data mining is datapine intelligent data alerts . With the help of artificial intelligence and machine learning, they provide automated signals based on particular commands or occurrences within a dataset. For example, if you’re monitoring supply chain KPIs , you could set an intelligent alarm to trigger when invalid or low-quality data appears. By doing so, you will be able to drill down deep into the issue and fix it swiftly and effectively.
In the following picture, you can see how the intelligent alarms from datapine work. By setting up ranges on daily orders, sessions, and revenues, the alarms will notify you if the goal was not completed or if it exceeded expectations.
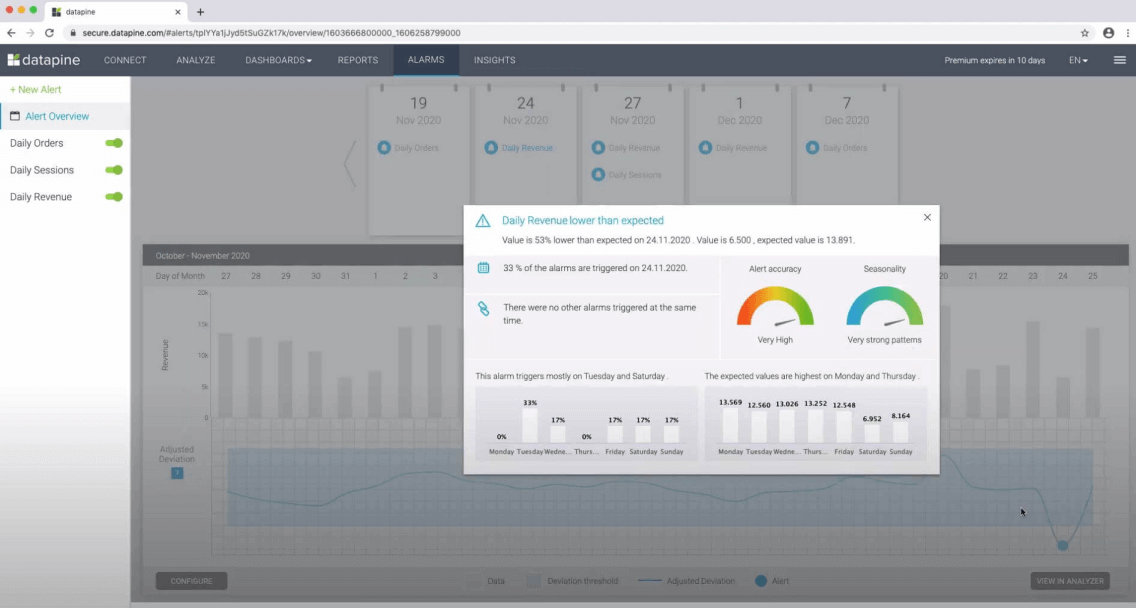
7. Time series analysis
As its name suggests, time series analysis is used to analyze a set of data points collected over a specified period of time. Although analysts use this method to monitor the data points in a specific interval of time rather than just monitoring them intermittently, the time series analysis is not uniquely used for the purpose of collecting data over time. Instead, it allows researchers to understand if variables changed during the duration of the study, how the different variables are dependent, and how did it reach the end result.
In a business context, this method is used to understand the causes of different trends and patterns to extract valuable insights. Another way of using this method is with the help of time series forecasting. Powered by predictive technologies, businesses can analyze various data sets over a period of time and forecast different future events.
A great use case to put time series analysis into perspective is seasonality effects on sales. By using time series forecasting to analyze sales data of a specific product over time, you can understand if sales rise over a specific period of time (e.g. swimwear during summertime, or candy during Halloween). These insights allow you to predict demand and prepare production accordingly.
8. Decision Trees
The decision tree analysis aims to act as a support tool to make smart and strategic decisions. By visually displaying potential outcomes, consequences, and costs in a tree-like model, researchers and company users can easily evaluate all factors involved and choose the best course of action. Decision trees are helpful to analyze quantitative data and they allow for an improved decision-making process by helping you spot improvement opportunities, reduce costs, and enhance operational efficiency and production.
But how does a decision tree actually works? This method works like a flowchart that starts with the main decision that you need to make and branches out based on the different outcomes and consequences of each decision. Each outcome will outline its own consequences, costs, and gains and, at the end of the analysis, you can compare each of them and make the smartest decision.
Businesses can use them to understand which project is more cost-effective and will bring more earnings in the long run. For example, imagine you need to decide if you want to update your software app or build a new app entirely. Here you would compare the total costs, the time needed to be invested, potential revenue, and any other factor that might affect your decision. In the end, you would be able to see which of these two options is more realistic and attainable for your company or research.
9. Conjoint analysis
Last but not least, we have the conjoint analysis. This approach is usually used in surveys to understand how individuals value different attributes of a product or service and it is one of the most effective methods to extract consumer preferences. When it comes to purchasing, some clients might be more price-focused, others more features-focused, and others might have a sustainable focus. Whatever your customer's preferences are, you can find them with conjoint analysis. Through this, companies can define pricing strategies, packaging options, subscription packages, and more.
A great example of conjoint analysis is in marketing and sales. For instance, a cupcake brand might use conjoint analysis and find that its clients prefer gluten-free options and cupcakes with healthier toppings over super sugary ones. Thus, the cupcake brand can turn these insights into advertisements and promotions to increase sales of this particular type of product. And not just that, conjoint analysis can also help businesses segment their customers based on their interests. This allows them to send different messaging that will bring value to each of the segments.
10. Correspondence Analysis
Also known as reciprocal averaging, correspondence analysis is a method used to analyze the relationship between categorical variables presented within a contingency table. A contingency table is a table that displays two (simple correspondence analysis) or more (multiple correspondence analysis) categorical variables across rows and columns that show the distribution of the data, which is usually answers to a survey or questionnaire on a specific topic.
This method starts by calculating an “expected value” which is done by multiplying row and column averages and dividing it by the overall original value of the specific table cell. The “expected value” is then subtracted from the original value resulting in a “residual number” which is what allows you to extract conclusions about relationships and distribution. The results of this analysis are later displayed using a map that represents the relationship between the different values. The closest two values are in the map, the bigger the relationship. Let’s put it into perspective with an example.
Imagine you are carrying out a market research analysis about outdoor clothing brands and how they are perceived by the public. For this analysis, you ask a group of people to match each brand with a certain attribute which can be durability, innovation, quality materials, etc. When calculating the residual numbers, you can see that brand A has a positive residual for innovation but a negative one for durability. This means that brand A is not positioned as a durable brand in the market, something that competitors could take advantage of.
11. Multidimensional Scaling (MDS)
MDS is a method used to observe the similarities or disparities between objects which can be colors, brands, people, geographical coordinates, and more. The objects are plotted using an “MDS map” that positions similar objects together and disparate ones far apart. The (dis) similarities between objects are represented using one or more dimensions that can be observed using a numerical scale. For example, if you want to know how people feel about the COVID-19 vaccine, you can use 1 for “don’t believe in the vaccine at all” and 10 for “firmly believe in the vaccine” and a scale of 2 to 9 for in between responses. When analyzing an MDS map the only thing that matters is the distance between the objects, the orientation of the dimensions is arbitrary and has no meaning at all.
Multidimensional scaling is a valuable technique for market research, especially when it comes to evaluating product or brand positioning. For instance, if a cupcake brand wants to know how they are positioned compared to competitors, it can define 2-3 dimensions such as taste, ingredients, shopping experience, or more, and do a multidimensional scaling analysis to find improvement opportunities as well as areas in which competitors are currently leading.
Another business example is in procurement when deciding on different suppliers. Decision makers can generate an MDS map to see how the different prices, delivery times, technical services, and more of the different suppliers differ and pick the one that suits their needs the best.
A final example proposed by a research paper on "An Improved Study of Multilevel Semantic Network Visualization for Analyzing Sentiment Word of Movie Review Data". Researchers picked a two-dimensional MDS map to display the distances and relationships between different sentiments in movie reviews. They used 36 sentiment words and distributed them based on their emotional distance as we can see in the image below where the words "outraged" and "sweet" are on opposite sides of the map, marking the distance between the two emotions very clearly.

Aside from being a valuable technique to analyze dissimilarities, MDS also serves as a dimension-reduction technique for large dimensional data.
B. Qualitative Methods
Qualitative data analysis methods are defined as the observation of non-numerical data that is gathered and produced using methods of observation such as interviews, focus groups, questionnaires, and more. As opposed to quantitative methods, qualitative data is more subjective and highly valuable in analyzing customer retention and product development.
12. Text analysis
Text analysis, also known in the industry as text mining, works by taking large sets of textual data and arranging them in a way that makes it easier to manage. By working through this cleansing process in stringent detail, you will be able to extract the data that is truly relevant to your organization and use it to develop actionable insights that will propel you forward.
Modern software accelerate the application of text analytics. Thanks to the combination of machine learning and intelligent algorithms, you can perform advanced analytical processes such as sentiment analysis. This technique allows you to understand the intentions and emotions of a text, for example, if it's positive, negative, or neutral, and then give it a score depending on certain factors and categories that are relevant to your brand. Sentiment analysis is often used to monitor brand and product reputation and to understand how successful your customer experience is. To learn more about the topic check out this insightful article .
By analyzing data from various word-based sources, including product reviews, articles, social media communications, and survey responses, you will gain invaluable insights into your audience, as well as their needs, preferences, and pain points. This will allow you to create campaigns, services, and communications that meet your prospects’ needs on a personal level, growing your audience while boosting customer retention. There are various other “sub-methods” that are an extension of text analysis. Each of them serves a more specific purpose and we will look at them in detail next.
13. Content Analysis
This is a straightforward and very popular method that examines the presence and frequency of certain words, concepts, and subjects in different content formats such as text, image, audio, or video. For example, the number of times the name of a celebrity is mentioned on social media or online tabloids. It does this by coding text data that is later categorized and tabulated in a way that can provide valuable insights, making it the perfect mix of quantitative and qualitative analysis.
There are two types of content analysis. The first one is the conceptual analysis which focuses on explicit data, for instance, the number of times a concept or word is mentioned in a piece of content. The second one is relational analysis, which focuses on the relationship between different concepts or words and how they are connected within a specific context.
Content analysis is often used by marketers to measure brand reputation and customer behavior. For example, by analyzing customer reviews. It can also be used to analyze customer interviews and find directions for new product development. It is also important to note, that in order to extract the maximum potential out of this analysis method, it is necessary to have a clearly defined research question.
14. Thematic Analysis
Very similar to content analysis, thematic analysis also helps in identifying and interpreting patterns in qualitative data with the main difference being that the first one can also be applied to quantitative analysis. The thematic method analyzes large pieces of text data such as focus group transcripts or interviews and groups them into themes or categories that come up frequently within the text. It is a great method when trying to figure out peoples view’s and opinions about a certain topic. For example, if you are a brand that cares about sustainability, you can do a survey of your customers to analyze their views and opinions about sustainability and how they apply it to their lives. You can also analyze customer service calls transcripts to find common issues and improve your service.
Thematic analysis is a very subjective technique that relies on the researcher’s judgment. Therefore, to avoid biases, it has 6 steps that include familiarization, coding, generating themes, reviewing themes, defining and naming themes, and writing up. It is also important to note that, because it is a flexible approach, the data can be interpreted in multiple ways and it can be hard to select what data is more important to emphasize.
15. Narrative Analysis
A bit more complex in nature than the two previous ones, narrative analysis is used to explore the meaning behind the stories that people tell and most importantly, how they tell them. By looking into the words that people use to describe a situation you can extract valuable conclusions about their perspective on a specific topic. Common sources for narrative data include autobiographies, family stories, opinion pieces, and testimonials, among others.
From a business perspective, narrative analysis can be useful to analyze customer behaviors and feelings towards a specific product, service, feature, or others. It provides unique and deep insights that can be extremely valuable. However, it has some drawbacks.
The biggest weakness of this method is that the sample sizes are usually very small due to the complexity and time-consuming nature of the collection of narrative data. Plus, the way a subject tells a story will be significantly influenced by his or her specific experiences, making it very hard to replicate in a subsequent study.
16. Discourse Analysis
Discourse analysis is used to understand the meaning behind any type of written, verbal, or symbolic discourse based on its political, social, or cultural context. It mixes the analysis of languages and situations together. This means that the way the content is constructed and the meaning behind it is significantly influenced by the culture and society it takes place in. For example, if you are analyzing political speeches you need to consider different context elements such as the politician's background, the current political context of the country, the audience to which the speech is directed, and so on.
From a business point of view, discourse analysis is a great market research tool. It allows marketers to understand how the norms and ideas of the specific market work and how their customers relate to those ideas. It can be very useful to build a brand mission or develop a unique tone of voice.
17. Grounded Theory Analysis
Traditionally, researchers decide on a method and hypothesis and start to collect the data to prove that hypothesis. The grounded theory is the only method that doesn’t require an initial research question or hypothesis as its value lies in the generation of new theories. With the grounded theory method, you can go into the analysis process with an open mind and explore the data to generate new theories through tests and revisions. In fact, it is not necessary to collect the data and then start to analyze it. Researchers usually start to find valuable insights as they are gathering the data.
All of these elements make grounded theory a very valuable method as theories are fully backed by data instead of initial assumptions. It is a great technique to analyze poorly researched topics or find the causes behind specific company outcomes. For example, product managers and marketers might use the grounded theory to find the causes of high levels of customer churn and look into customer surveys and reviews to develop new theories about the causes.
How To Analyze Data? Top 17 Data Analysis Techniques To Apply

Now that we’ve answered the questions “what is data analysis’”, why is it important, and covered the different data analysis types, it’s time to dig deeper into how to perform your analysis by working through these 17 essential techniques.
1. Collaborate your needs
Before you begin analyzing or drilling down into any techniques, it’s crucial to sit down collaboratively with all key stakeholders within your organization, decide on your primary campaign or strategic goals, and gain a fundamental understanding of the types of insights that will best benefit your progress or provide you with the level of vision you need to evolve your organization.
2. Establish your questions
Once you’ve outlined your core objectives, you should consider which questions will need answering to help you achieve your mission. This is one of the most important techniques as it will shape the very foundations of your success.
To help you ask the right things and ensure your data works for you, you have to ask the right data analysis questions .
3. Data democratization
After giving your data analytics methodology some real direction, and knowing which questions need answering to extract optimum value from the information available to your organization, you should continue with democratization.
Data democratization is an action that aims to connect data from various sources efficiently and quickly so that anyone in your organization can access it at any given moment. You can extract data in text, images, videos, numbers, or any other format. And then perform cross-database analysis to achieve more advanced insights to share with the rest of the company interactively.
Once you have decided on your most valuable sources, you need to take all of this into a structured format to start collecting your insights. For this purpose, datapine offers an easy all-in-one data connectors feature to integrate all your internal and external sources and manage them at your will. Additionally, datapine’s end-to-end solution automatically updates your data, allowing you to save time and focus on performing the right analysis to grow your company.
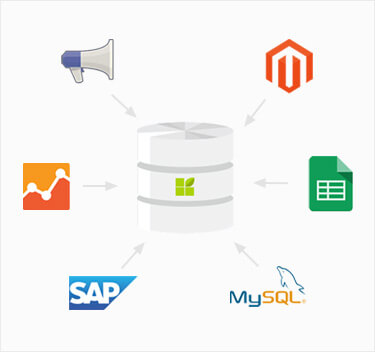
4. Think of governance
When collecting data in a business or research context you always need to think about security and privacy. With data breaches becoming a topic of concern for businesses, the need to protect your client's or subject’s sensitive information becomes critical.
To ensure that all this is taken care of, you need to think of a data governance strategy. According to Gartner , this concept refers to “ the specification of decision rights and an accountability framework to ensure the appropriate behavior in the valuation, creation, consumption, and control of data and analytics .” In simpler words, data governance is a collection of processes, roles, and policies, that ensure the efficient use of data while still achieving the main company goals. It ensures that clear roles are in place for who can access the information and how they can access it. In time, this not only ensures that sensitive information is protected but also allows for an efficient analysis as a whole.
5. Clean your data
After harvesting from so many sources you will be left with a vast amount of information that can be overwhelming to deal with. At the same time, you can be faced with incorrect data that can be misleading to your analysis. The smartest thing you can do to avoid dealing with this in the future is to clean the data. This is fundamental before visualizing it, as it will ensure that the insights you extract from it are correct.
There are many things that you need to look for in the cleaning process. The most important one is to eliminate any duplicate observations; this usually appears when using multiple internal and external sources of information. You can also add any missing codes, fix empty fields, and eliminate incorrectly formatted data.
Another usual form of cleaning is done with text data. As we mentioned earlier, most companies today analyze customer reviews, social media comments, questionnaires, and several other text inputs. In order for algorithms to detect patterns, text data needs to be revised to avoid invalid characters or any syntax or spelling errors.
Most importantly, the aim of cleaning is to prevent you from arriving at false conclusions that can damage your company in the long run. By using clean data, you will also help BI solutions to interact better with your information and create better reports for your organization.
6. Set your KPIs
Once you’ve set your sources, cleaned your data, and established clear-cut questions you want your insights to answer, you need to set a host of key performance indicators (KPIs) that will help you track, measure, and shape your progress in a number of key areas.
KPIs are critical to both qualitative and quantitative analysis research. This is one of the primary methods of data analysis you certainly shouldn’t overlook.
To help you set the best possible KPIs for your initiatives and activities, here is an example of a relevant logistics KPI : transportation-related costs. If you want to see more go explore our collection of key performance indicator examples .

7. Omit useless data
Having bestowed your data analysis tools and techniques with true purpose and defined your mission, you should explore the raw data you’ve collected from all sources and use your KPIs as a reference for chopping out any information you deem to be useless.
Trimming the informational fat is one of the most crucial methods of analysis as it will allow you to focus your analytical efforts and squeeze every drop of value from the remaining ‘lean’ information.
Any stats, facts, figures, or metrics that don’t align with your business goals or fit with your KPI management strategies should be eliminated from the equation.
8. Build a data management roadmap
While, at this point, this particular step is optional (you will have already gained a wealth of insight and formed a fairly sound strategy by now), creating a data governance roadmap will help your data analysis methods and techniques become successful on a more sustainable basis. These roadmaps, if developed properly, are also built so they can be tweaked and scaled over time.
Invest ample time in developing a roadmap that will help you store, manage, and handle your data internally, and you will make your analysis techniques all the more fluid and functional – one of the most powerful types of data analysis methods available today.
9. Integrate technology
There are many ways to analyze data, but one of the most vital aspects of analytical success in a business context is integrating the right decision support software and technology.
Robust analysis platforms will not only allow you to pull critical data from your most valuable sources while working with dynamic KPIs that will offer you actionable insights; it will also present them in a digestible, visual, interactive format from one central, live dashboard . A data methodology you can count on.
By integrating the right technology within your data analysis methodology, you’ll avoid fragmenting your insights, saving you time and effort while allowing you to enjoy the maximum value from your business’s most valuable insights.
For a look at the power of software for the purpose of analysis and to enhance your methods of analyzing, glance over our selection of dashboard examples .
10. Answer your questions
By considering each of the above efforts, working with the right technology, and fostering a cohesive internal culture where everyone buys into the different ways to analyze data as well as the power of digital intelligence, you will swiftly start to answer your most burning business questions. Arguably, the best way to make your data concepts accessible across the organization is through data visualization.
11. Visualize your data
Online data visualization is a powerful tool as it lets you tell a story with your metrics, allowing users across the organization to extract meaningful insights that aid business evolution – and it covers all the different ways to analyze data.
The purpose of analyzing is to make your entire organization more informed and intelligent, and with the right platform or dashboard, this is simpler than you think, as demonstrated by our marketing dashboard .

This visual, dynamic, and interactive online dashboard is a data analysis example designed to give Chief Marketing Officers (CMO) an overview of relevant metrics to help them understand if they achieved their monthly goals.
In detail, this example generated with a modern dashboard creator displays interactive charts for monthly revenues, costs, net income, and net income per customer; all of them are compared with the previous month so that you can understand how the data fluctuated. In addition, it shows a detailed summary of the number of users, customers, SQLs, and MQLs per month to visualize the whole picture and extract relevant insights or trends for your marketing reports .
The CMO dashboard is perfect for c-level management as it can help them monitor the strategic outcome of their marketing efforts and make data-driven decisions that can benefit the company exponentially.
12. Be careful with the interpretation
We already dedicated an entire post to data interpretation as it is a fundamental part of the process of data analysis. It gives meaning to the analytical information and aims to drive a concise conclusion from the analysis results. Since most of the time companies are dealing with data from many different sources, the interpretation stage needs to be done carefully and properly in order to avoid misinterpretations.
To help you through the process, here we list three common practices that you need to avoid at all costs when looking at your data:
- Correlation vs. causation: The human brain is formatted to find patterns. This behavior leads to one of the most common mistakes when performing interpretation: confusing correlation with causation. Although these two aspects can exist simultaneously, it is not correct to assume that because two things happened together, one provoked the other. A piece of advice to avoid falling into this mistake is never to trust just intuition, trust the data. If there is no objective evidence of causation, then always stick to correlation.
- Confirmation bias: This phenomenon describes the tendency to select and interpret only the data necessary to prove one hypothesis, often ignoring the elements that might disprove it. Even if it's not done on purpose, confirmation bias can represent a real problem, as excluding relevant information can lead to false conclusions and, therefore, bad business decisions. To avoid it, always try to disprove your hypothesis instead of proving it, share your analysis with other team members, and avoid drawing any conclusions before the entire analytical project is finalized.
- Statistical significance: To put it in short words, statistical significance helps analysts understand if a result is actually accurate or if it happened because of a sampling error or pure chance. The level of statistical significance needed might depend on the sample size and the industry being analyzed. In any case, ignoring the significance of a result when it might influence decision-making can be a huge mistake.
13. Build a narrative
Now, we’re going to look at how you can bring all of these elements together in a way that will benefit your business - starting with a little something called data storytelling.
The human brain responds incredibly well to strong stories or narratives. Once you’ve cleansed, shaped, and visualized your most invaluable data using various BI dashboard tools , you should strive to tell a story - one with a clear-cut beginning, middle, and end.
By doing so, you will make your analytical efforts more accessible, digestible, and universal, empowering more people within your organization to use your discoveries to their actionable advantage.
14. Consider autonomous technology
Autonomous technologies, such as artificial intelligence (AI) and machine learning (ML), play a significant role in the advancement of understanding how to analyze data more effectively.
Gartner predicts that by the end of this year, 80% of emerging technologies will be developed with AI foundations. This is a testament to the ever-growing power and value of autonomous technologies.
At the moment, these technologies are revolutionizing the analysis industry. Some examples that we mentioned earlier are neural networks, intelligent alarms, and sentiment analysis.
15. Share the load
If you work with the right tools and dashboards, you will be able to present your metrics in a digestible, value-driven format, allowing almost everyone in the organization to connect with and use relevant data to their advantage.
Modern dashboards consolidate data from various sources, providing access to a wealth of insights in one centralized location, no matter if you need to monitor recruitment metrics or generate reports that need to be sent across numerous departments. Moreover, these cutting-edge tools offer access to dashboards from a multitude of devices, meaning that everyone within the business can connect with practical insights remotely - and share the load.
Once everyone is able to work with a data-driven mindset, you will catalyze the success of your business in ways you never thought possible. And when it comes to knowing how to analyze data, this kind of collaborative approach is essential.
16. Data analysis tools
In order to perform high-quality analysis of data, it is fundamental to use tools and software that will ensure the best results. Here we leave you a small summary of four fundamental categories of data analysis tools for your organization.
- Business Intelligence: BI tools allow you to process significant amounts of data from several sources in any format. Through this, you can not only analyze and monitor your data to extract relevant insights but also create interactive reports and dashboards to visualize your KPIs and use them for your company's good. datapine is an amazing online BI software that is focused on delivering powerful online analysis features that are accessible to beginner and advanced users. Like this, it offers a full-service solution that includes cutting-edge analysis of data, KPIs visualization, live dashboards, reporting, and artificial intelligence technologies to predict trends and minimize risk.
- Statistical analysis: These tools are usually designed for scientists, statisticians, market researchers, and mathematicians, as they allow them to perform complex statistical analyses with methods like regression analysis, predictive analysis, and statistical modeling. A good tool to perform this type of analysis is R-Studio as it offers a powerful data modeling and hypothesis testing feature that can cover both academic and general data analysis. This tool is one of the favorite ones in the industry, due to its capability for data cleaning, data reduction, and performing advanced analysis with several statistical methods. Another relevant tool to mention is SPSS from IBM. The software offers advanced statistical analysis for users of all skill levels. Thanks to a vast library of machine learning algorithms, text analysis, and a hypothesis testing approach it can help your company find relevant insights to drive better decisions. SPSS also works as a cloud service that enables you to run it anywhere.
- SQL Consoles: SQL is a programming language often used to handle structured data in relational databases. Tools like these are popular among data scientists as they are extremely effective in unlocking these databases' value. Undoubtedly, one of the most used SQL software in the market is MySQL Workbench . This tool offers several features such as a visual tool for database modeling and monitoring, complete SQL optimization, administration tools, and visual performance dashboards to keep track of KPIs.
- Data Visualization: These tools are used to represent your data through charts, graphs, and maps that allow you to find patterns and trends in the data. datapine's already mentioned BI platform also offers a wealth of powerful online data visualization tools with several benefits. Some of them include: delivering compelling data-driven presentations to share with your entire company, the ability to see your data online with any device wherever you are, an interactive dashboard design feature that enables you to showcase your results in an interactive and understandable way, and to perform online self-service reports that can be used simultaneously with several other people to enhance team productivity.
17. Refine your process constantly
Last is a step that might seem obvious to some people, but it can be easily ignored if you think you are done. Once you have extracted the needed results, you should always take a retrospective look at your project and think about what you can improve. As you saw throughout this long list of techniques, data analysis is a complex process that requires constant refinement. For this reason, you should always go one step further and keep improving.
Quality Criteria For Data Analysis
So far we’ve covered a list of methods and techniques that should help you perform efficient data analysis. But how do you measure the quality and validity of your results? This is done with the help of some science quality criteria. Here we will go into a more theoretical area that is critical to understanding the fundamentals of statistical analysis in science. However, you should also be aware of these steps in a business context, as they will allow you to assess the quality of your results in the correct way. Let’s dig in.
- Internal validity: The results of a survey are internally valid if they measure what they are supposed to measure and thus provide credible results. In other words , internal validity measures the trustworthiness of the results and how they can be affected by factors such as the research design, operational definitions, how the variables are measured, and more. For instance, imagine you are doing an interview to ask people if they brush their teeth two times a day. While most of them will answer yes, you can still notice that their answers correspond to what is socially acceptable, which is to brush your teeth at least twice a day. In this case, you can’t be 100% sure if respondents actually brush their teeth twice a day or if they just say that they do, therefore, the internal validity of this interview is very low.
- External validity: Essentially, external validity refers to the extent to which the results of your research can be applied to a broader context. It basically aims to prove that the findings of a study can be applied in the real world. If the research can be applied to other settings, individuals, and times, then the external validity is high.
- Reliability : If your research is reliable, it means that it can be reproduced. If your measurement were repeated under the same conditions, it would produce similar results. This means that your measuring instrument consistently produces reliable results. For example, imagine a doctor building a symptoms questionnaire to detect a specific disease in a patient. Then, various other doctors use this questionnaire but end up diagnosing the same patient with a different condition. This means the questionnaire is not reliable in detecting the initial disease. Another important note here is that in order for your research to be reliable, it also needs to be objective. If the results of a study are the same, independent of who assesses them or interprets them, the study can be considered reliable. Let’s see the objectivity criteria in more detail now.
- Objectivity: In data science, objectivity means that the researcher needs to stay fully objective when it comes to its analysis. The results of a study need to be affected by objective criteria and not by the beliefs, personality, or values of the researcher. Objectivity needs to be ensured when you are gathering the data, for example, when interviewing individuals, the questions need to be asked in a way that doesn't influence the results. Paired with this, objectivity also needs to be thought of when interpreting the data. If different researchers reach the same conclusions, then the study is objective. For this last point, you can set predefined criteria to interpret the results to ensure all researchers follow the same steps.
The discussed quality criteria cover mostly potential influences in a quantitative context. Analysis in qualitative research has by default additional subjective influences that must be controlled in a different way. Therefore, there are other quality criteria for this kind of research such as credibility, transferability, dependability, and confirmability. You can see each of them more in detail on this resource .
Data Analysis Limitations & Barriers
Analyzing data is not an easy task. As you’ve seen throughout this post, there are many steps and techniques that you need to apply in order to extract useful information from your research. While a well-performed analysis can bring various benefits to your organization it doesn't come without limitations. In this section, we will discuss some of the main barriers you might encounter when conducting an analysis. Let’s see them more in detail.
- Lack of clear goals: No matter how good your data or analysis might be if you don’t have clear goals or a hypothesis the process might be worthless. While we mentioned some methods that don’t require a predefined hypothesis, it is always better to enter the analytical process with some clear guidelines of what you are expecting to get out of it, especially in a business context in which data is utilized to support important strategic decisions.
- Objectivity: Arguably one of the biggest barriers when it comes to data analysis in research is to stay objective. When trying to prove a hypothesis, researchers might find themselves, intentionally or unintentionally, directing the results toward an outcome that they want. To avoid this, always question your assumptions and avoid confusing facts with opinions. You can also show your findings to a research partner or external person to confirm that your results are objective.
- Data representation: A fundamental part of the analytical procedure is the way you represent your data. You can use various graphs and charts to represent your findings, but not all of them will work for all purposes. Choosing the wrong visual can not only damage your analysis but can mislead your audience, therefore, it is important to understand when to use each type of data depending on your analytical goals. Our complete guide on the types of graphs and charts lists 20 different visuals with examples of when to use them.
- Flawed correlation : Misleading statistics can significantly damage your research. We’ve already pointed out a few interpretation issues previously in the post, but it is an important barrier that we can't avoid addressing here as well. Flawed correlations occur when two variables appear related to each other but they are not. Confusing correlations with causation can lead to a wrong interpretation of results which can lead to building wrong strategies and loss of resources, therefore, it is very important to identify the different interpretation mistakes and avoid them.
- Sample size: A very common barrier to a reliable and efficient analysis process is the sample size. In order for the results to be trustworthy, the sample size should be representative of what you are analyzing. For example, imagine you have a company of 1000 employees and you ask the question “do you like working here?” to 50 employees of which 49 say yes, which means 95%. Now, imagine you ask the same question to the 1000 employees and 950 say yes, which also means 95%. Saying that 95% of employees like working in the company when the sample size was only 50 is not a representative or trustworthy conclusion. The significance of the results is way more accurate when surveying a bigger sample size.
- Privacy concerns: In some cases, data collection can be subjected to privacy regulations. Businesses gather all kinds of information from their customers from purchasing behaviors to addresses and phone numbers. If this falls into the wrong hands due to a breach, it can affect the security and confidentiality of your clients. To avoid this issue, you need to collect only the data that is needed for your research and, if you are using sensitive facts, make it anonymous so customers are protected. The misuse of customer data can severely damage a business's reputation, so it is important to keep an eye on privacy.
- Lack of communication between teams : When it comes to performing data analysis on a business level, it is very likely that each department and team will have different goals and strategies. However, they are all working for the same common goal of helping the business run smoothly and keep growing. When teams are not connected and communicating with each other, it can directly affect the way general strategies are built. To avoid these issues, tools such as data dashboards enable teams to stay connected through data in a visually appealing way.
- Innumeracy : Businesses are working with data more and more every day. While there are many BI tools available to perform effective analysis, data literacy is still a constant barrier. Not all employees know how to apply analysis techniques or extract insights from them. To prevent this from happening, you can implement different training opportunities that will prepare every relevant user to deal with data.
Key Data Analysis Skills
As you've learned throughout this lengthy guide, analyzing data is a complex task that requires a lot of knowledge and skills. That said, thanks to the rise of self-service tools the process is way more accessible and agile than it once was. Regardless, there are still some key skills that are valuable to have when working with data, we list the most important ones below.
- Critical and statistical thinking: To successfully analyze data you need to be creative and think out of the box. Yes, that might sound like a weird statement considering that data is often tight to facts. However, a great level of critical thinking is required to uncover connections, come up with a valuable hypothesis, and extract conclusions that go a step further from the surface. This, of course, needs to be complemented by statistical thinking and an understanding of numbers.
- Data cleaning: Anyone who has ever worked with data before will tell you that the cleaning and preparation process accounts for 80% of a data analyst's work, therefore, the skill is fundamental. But not just that, not cleaning the data adequately can also significantly damage the analysis which can lead to poor decision-making in a business scenario. While there are multiple tools that automate the cleaning process and eliminate the possibility of human error, it is still a valuable skill to dominate.
- Data visualization: Visuals make the information easier to understand and analyze, not only for professional users but especially for non-technical ones. Having the necessary skills to not only choose the right chart type but know when to apply it correctly is key. This also means being able to design visually compelling charts that make the data exploration process more efficient.
- SQL: The Structured Query Language or SQL is a programming language used to communicate with databases. It is fundamental knowledge as it enables you to update, manipulate, and organize data from relational databases which are the most common databases used by companies. It is fairly easy to learn and one of the most valuable skills when it comes to data analysis.
- Communication skills: This is a skill that is especially valuable in a business environment. Being able to clearly communicate analytical outcomes to colleagues is incredibly important, especially when the information you are trying to convey is complex for non-technical people. This applies to in-person communication as well as written format, for example, when generating a dashboard or report. While this might be considered a “soft” skill compared to the other ones we mentioned, it should not be ignored as you most likely will need to share analytical findings with others no matter the context.
Data Analysis In The Big Data Environment
Big data is invaluable to today’s businesses, and by using different methods for data analysis, it’s possible to view your data in a way that can help you turn insight into positive action.
To inspire your efforts and put the importance of big data into context, here are some insights that you should know:
- By 2026 the industry of big data is expected to be worth approximately $273.4 billion.
- 94% of enterprises say that analyzing data is important for their growth and digital transformation.
- Companies that exploit the full potential of their data can increase their operating margins by 60% .
- We already told you the benefits of Artificial Intelligence through this article. This industry's financial impact is expected to grow up to $40 billion by 2025.
Data analysis concepts may come in many forms, but fundamentally, any solid methodology will help to make your business more streamlined, cohesive, insightful, and successful than ever before.
Key Takeaways From Data Analysis
As we reach the end of our data analysis journey, we leave a small summary of the main methods and techniques to perform excellent analysis and grow your business.
17 Essential Types of Data Analysis Methods:
- Cluster analysis
- Cohort analysis
- Regression analysis
- Factor analysis
- Neural Networks
- Data Mining
- Text analysis
- Time series analysis
- Decision trees
- Conjoint analysis
- Correspondence Analysis
- Multidimensional Scaling
- Content analysis
- Thematic analysis
- Narrative analysis
- Grounded theory analysis
- Discourse analysis
Top 17 Data Analysis Techniques:
- Collaborate your needs
- Establish your questions
- Data democratization
- Think of data governance
- Clean your data
- Set your KPIs
- Omit useless data
- Build a data management roadmap
- Integrate technology
- Answer your questions
- Visualize your data
- Interpretation of data
- Consider autonomous technology
- Build a narrative
- Share the load
- Data Analysis tools
- Refine your process constantly
We’ve pondered the data analysis definition and drilled down into the practical applications of data-centric analytics, and one thing is clear: by taking measures to arrange your data and making your metrics work for you, it’s possible to transform raw information into action - the kind of that will push your business to the next level.
Yes, good data analytics techniques result in enhanced business intelligence (BI). To help you understand this notion in more detail, read our exploration of business intelligence reporting .
And, if you’re ready to perform your own analysis, drill down into your facts and figures while interacting with your data on astonishing visuals, you can try our software for a free, 14-day trial .

- Certifications

Data Analysis 101: How to Make Your Presentations Practical and Effective
- December 27, 2022
- 53 Comments
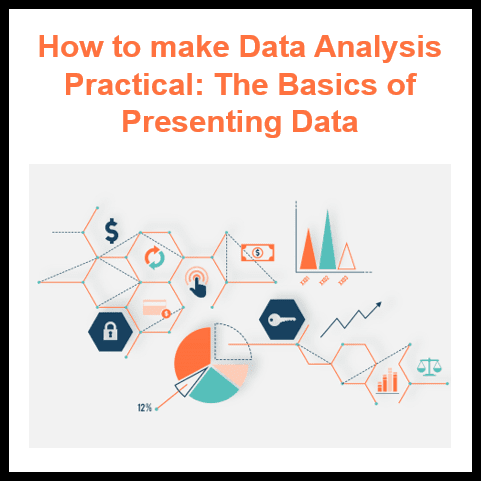
Understanding Importance of Data Analysis
The results of data analysis can give business the vital insights they need to turn in to successful and profitable ventures. It could be the difference between a successful business operation and a business operation that is in trouble.
Data analysis, though one of the most in-demand job roles globally, doesn’t require a degree in statistics or mathematics to do well, and employers from a wide variety of industries are very keen to recruit data analysts.
Businesses hire data analysts in the field of finance, marketing, administration, HR, IT and procurement, to name just a few. Understand the big picture and provide answers. By engaging in data analysis, you can actually delve deep and discover hidden truths that most business people would never be able to do.
What skills you should master to be a data analyst?
While Data Analyst roles are on the rise, there are certain skills that are vital for anyone who wants to become a data analyst . Before the job, a candidate needs to have either a degree in statistics, business or computer science or a related subject, or work experience in these areas.
If you’re interested in becoming a data analyst, you’ll need to know:
- Programming and algorithms
- Data Visualization
- Open-source and cloud technologies
- No coding experience is required.
How much is a data analyst worth? Data analysts earn an average salary of £32,403 per annum, according to jobs site Glassdoor. This pays for a salary, with benefits such as medical insurance and paid leave included in the starting salary. If you think you have the right skills, there are plenty of roles on offer.
What data analysis entails
Data analysis is an analytical process which involves recording and tabulating (recording and entering, entering and tabulating) the quantities of a product, such as numbers of units produced, costs of materials and expenses.
While data analyst can take different forms, for example in databases, in other structures such as spreadsheets, numbers are the main means of data entry. This involves entering and entering the required data in a data analysis system such as Excel.
For example, although a database doesn’t require a data analyst, it can still benefit from data analysis techniques such as binomial testing, ANOVA and Fisher’s exact tests. Where is the data analysis courses in IT? Given the ever-increasing reliance on technology in business, data analysis courses are vital skills.
What are the types of data analysis methods?
- Cluster analysis
The act of grouping a specific set of data in a manner that those elements are more similar to one another than to those in other groups – hence the term ‘cluster.’ Since there is no special target variable while doing clustering, the method is often used to find hidden patterns in the data. The approach is purposely used to offer additional context to a particular trend or dataset.
- Cohort analysis
This type of data analysis method uses historical data to examine and compare a determined segment of users’ behavior, which can then be grouped with others with similar characteristics. By using this data analysis methodology, it’s possible to gain a wealth of insight into consumer needs or a firm understanding of a broader target group.
A dependent variable is an element of a complex system that is assumed to have a single cause, but it’s affected by multiple factors, thus giving researchers an indication as to how a complex system function.
- Regression analysis
The regression analysis is used to predict how the value of a dependent variable changes when one or more independent variables change, stay the same or the dependent variable is not moved. Regression is a sophisticated statistical method that includes mathematical functions that are typically called “segmentation,” “distribution,” and “intercept” functions.
Regression is a type of regression analysis that only contains linear and quadratic functions. You can change the types of factors (or the independent variables) that are selected in regression analysis (it’s typically called “nonlinear regression analysis”) by changing the order in which the models are constructed.To begin, let’s explain how regression analysis works.
Examples in business world
The Oracle Corporation is one of the first multinational companies to adopt this type of analysis method, based on which the company was able to develop predictive modelling systems for marketing purposes.
In a more specific sense, a Regression analysis is a popular type of data analysis used for analyzing the likelihood that a random variable will move up or down a range of parameters in response to a change in a specific control variable.
Companies who use this type of analysis are looking for trends and patterned performance over time. For example, how a company may respond to a rising cost of labor and its effect on its business bottom line, a weather-related issue like an earthquake, a new advertising campaign, or even a surge in customer demand in some areas.
What are basic pointers to consider while presenting data
Recognize that presentation matters.
Too often, analysts make the mistake of presenting information in order to show an abstracted version of it. For instance, say a B2B company has 4 ways to improve their sales funnel:
- More Visually Engaging
- More Easily Transacted
- More Cost Effective
Then, “informative” would mean that a B2B company needs to optimize their sales funnel to each of these to be more “convenient, faster, easier, more visually engaging, or most cost effective.” Sure, it would be nice if they all improved – they would all provide a competitive advantage in some way. But that’s not what the data tells us.
Don’t scare people with numbers
When you’re presenting data, show as many as possible, in as many charts as possible. Then, try to talk through the implications of the data, rather than overwhelming people with an overwhelming amount of data.
Why? Research suggests that when a number is presented in a visual, people become more likely to process it and learn from it. I recommend using video, text, graphs, and pictures to represent your numbers. This creates a more visually appealing data set. The number of followers on Twitter is visually appealing. The number of followers on Facebook is visually appealing. But nobody looks at their Twitter followers. If you don’t know what your numbers mean, how will your audience? That doesn’t mean numbers aren’t important.
Maximize the data pixel ratio
The more data you show to a critical stakeholder, the more likely they are to get lost and distracted from what you’re actually trying to communicate. This is especially important in the case of people in the sales and marketing function.
Do you have a sales person out in the field who is trying to close a deal? It would be a shame if that person got lost in your Excel analytics and lost out on the sale. This problem also occurs on the web.
Consider how web visitors respond to large, colorful charts and graphs. If we’re talking about visualizations that depict web performance, a visual might be helpful. But how often do we see this done? Research shows that people respond better to web-based data in a simplified, less complex format.
Save 3-D for the movies
There are great stories in the universe. This is an oversimplification, but if you look at history, humans only understand stories. We are great storytellers. We develop, through trial and error, our own intuition about the “right” way to tell stories.
One of the most powerful and effective ways to present data is to go beyond the visual to the audible, that is, to tell stories in a way that people can relate to. Everything you hear about computers being a series of numbers is wrong. We visualize numbers in a precise, quantitative way. But the numbers are not a collection of isolated events. To understand them, we need to understand the broader context.
Friends don’t let friends use pie charts
Businesses and analysts have done this since pie charts first appeared on Microsoft Excel sheets. When presenting data, break down your pie chart into its component segments.
As opposed to an equal-sized circle for the average earnings for all the employees, share a pie chart where the percentages for each individual segment are different, with a link to the corresponding chart.
Pair with explanatory text, show their correlation, and make your choice based on your audience, not on whether you want to scare or “educate” them. The majority of audiences will see the same image, regardless of whether it’s presented in a bar chart, bar chart, line chart, or something else.
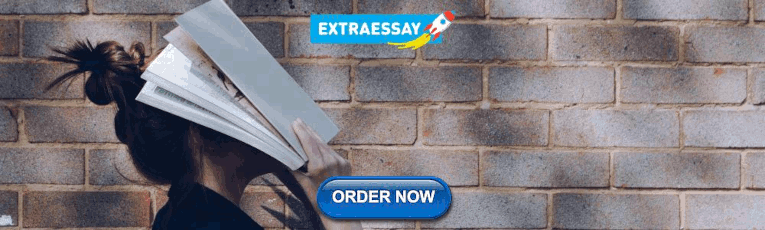
Choose the appropriate chart
Does the data make logical sense? Check your assumptions against the data. Are the graphs charting only part of the story? Include other variables in the graphs. Avoid using axis labels to mislead. Never rely on axes to infer, “logical” conclusions. Trust your eyes: you know what information your brain can process.
Think of numbers like music — they are pleasing, but not overwhelming. Save 3D for the movies. When everyone is enjoying 4K, 8K, and beyond, it’s hard to envision your audience without the new stuff. I remember the first time I got to see HDTV. At home, I sat behind a chair and kept turning around to watch the TV. But at the theatre, I didn’t need a chair. All I had to do was look up, and see the giant screen, the contrast, and the detail.
Don’t mix chart types for no reason
Excel chart s with colored areas help people focus. Arrows give us scale. Assume your audience doesn’t understand what you’re saying, even if they do. Nobody wants to open a recipe book to learn how to cook soup. Instead, we start with a recipe.
Use a formula to communicate your analysis with as few words as possible. Keep it simple. Resist the urge to over-complicate your presentation. A word cloud is not a word cloud. A bar chart is not a bar chart. If you use a word cloud to illustrate a chart, consider replacing a few words with a gif. A bar chart doesn’t need clouds. And a bar chart doesn’t need clouds. If there’s one thing that’s sure to confuse your audience, it’s bar charts.
Use color with intention
Use color with intention. It’s not about pretty. When it comes to presenting data clearly, “informative” is more important than “beautiful.”
However, visualizations like maps, axes, or snapshots can help visual communication to avoid this pitfall. If you are going to show a few locations on a map, make sure each location has a voice and uses a distinct color. Avoid repeating colors from the map or bottom bar in all the visuals. Be consistent with how you present the data . A pie chart is not very interesting if all it shows is a bunch of varying sizes of the pie.
Data analysis in the workplace, and how it will impact the future of business
Business leaders are taking note of the importance of data analysis skills in their organisation, as it can make an enormous impact on business.
Larger organisations such as Google, Amazon and Facebook employ huge teams of analysts to create their data and statistics. We are already seeing the rise of the next generation of big data analysts – those who can write code that analyses and visualizes the data and report back information to a company to help it improve efficiency and increase revenue.
The increasing need for high-level understanding of data analysis has already led to the role of data analyst becoming available at university level. It is no longer a mandatory business qualification but one that can enhance your CV.
By understanding the importance of each variable, you can improve your business by managing your time and creating more effective systems and processes for running your business. The focus shifts from just providing services to providing value to your customers, creating a better, more intuitive experience for them so they can work with your company for the long-term.
Adopting these small steps will allow you to be more effective in your business and go from being an employee to an entrepreneur.
Share This Post:
53 thoughts on “data analysis 101: how to make your presentations practical and effective”.
Buy Zyvox Online – Special offer: Save up to $498 – buy antibiotics online and get discount for all purchased!
Thanks again for the post.Thanks Again. Cool.
Thanks for great information. What trips can you recommend in 2024? Astro tourism, eco diving, home swapping, train stations are the new food destinations,sports tourism, coolcationing, gig tripping, private group travel?
Enjoyed every bit of your article.Really looking forward to read more. Great.
Really appreciate you sharing this post. Want more.
I am so grateful for your blog. Really Great.
Hey, thanks for the blog post.
Great, thanks for sharing this blog.Thanks Again. Will read on…
Thanks for sharing, this is a fantastic blog.Thanks Again. Great.
Thanks for sharing, this is a fantastic article.Really thank you! Fantastic.
Major thankies for the article post.Thanks Again. Will read on…
Hey, thanks for the blog post. Cool.
A round of applause for your blog post.Really looking forward to read more. Cool.
Appreciate you sharing, great article post. Great.
wow, awesome blog.Much thanks again. Cool.
Say, you got a nice blog.Really thank you! Cool.
Enjoyed every bit of your blog article.Much thanks again. Want more.
A round of applause for your blog post.Much thanks again. Cool.
I’m not sure where you’re getting your info, but good topic. I needs to spend some time learning more or understanding more. Thanks for wonderful info I was looking for this info for my mission.
Im thankful for the blog post.Much thanks again.
A big thank you for your article.Really thank you!
I truly appreciate this article post.Really looking forward to read more. Really Cool.
I really enjoy the article post.Much thanks again.
wow, awesome blog.Thanks Again. Will read on…
Awesome blog post.Much thanks again. Much obliged.
Im thankful for the blog.Much thanks again. Want more.
Thanks a lot for the post.Much thanks again. Want more.
I really liked your article.Really thank you! Really Cool.
A round of applause for your post.Thanks Again. Much obliged.
Say, you got a nice article.Really thank you! Fantastic.
I value the blog article. Really Cool.
A round of applause for your blog article.Really looking forward to read more. Great.
Really appreciate you sharing this blog article. Really Cool.
Really informative article. Really Great.
Fantastic article post.Really looking forward to read more. Really Great.
I really liked your post.Much thanks again. Much obliged.
Beneficial document helps make frequent advance, appreciate it write about, this pile-up connected with expertise is usually to hold finding out, focus is usually the beginning of money.
I really liked your blog article.Much thanks again. Really Great.
I really liked your article post.Really thank you! Fantastic.
Great, thanks for sharing this blog.Thanks Again. Awesome.
Enjoyed every bit of your blog article. Cool.
I really enjoy the blog. Want more.
Im grateful for the blog.Thanks Again. Awesome.
Very neat blog.Thanks Again. Cool.
Muchos Gracias for your blog article.
Im grateful for the post.Really looking forward to read more. Keep writing.
Great, thanks for sharing this post.Really looking forward to read more. Cool.
Thank you ever so for you article post.Really looking forward to read more. Will read on…
Great article post.Much thanks again. Great.
I really like and appreciate your blog post.Thanks Again. Awesome.
Really appreciate you sharing this blog. Really Cool.
Greetings! Incredibly helpful suggestions within this short article! It’s the very little adjustments that make the greatest modifications. Quite a few many thanks for sharing!
Awesome blog.Really looking forward to read more. Much obliged.
Add a Comment Cancel reply
Save my name, email, and website in this browser for the next time I comment.
Get A 5X Raise In Salary
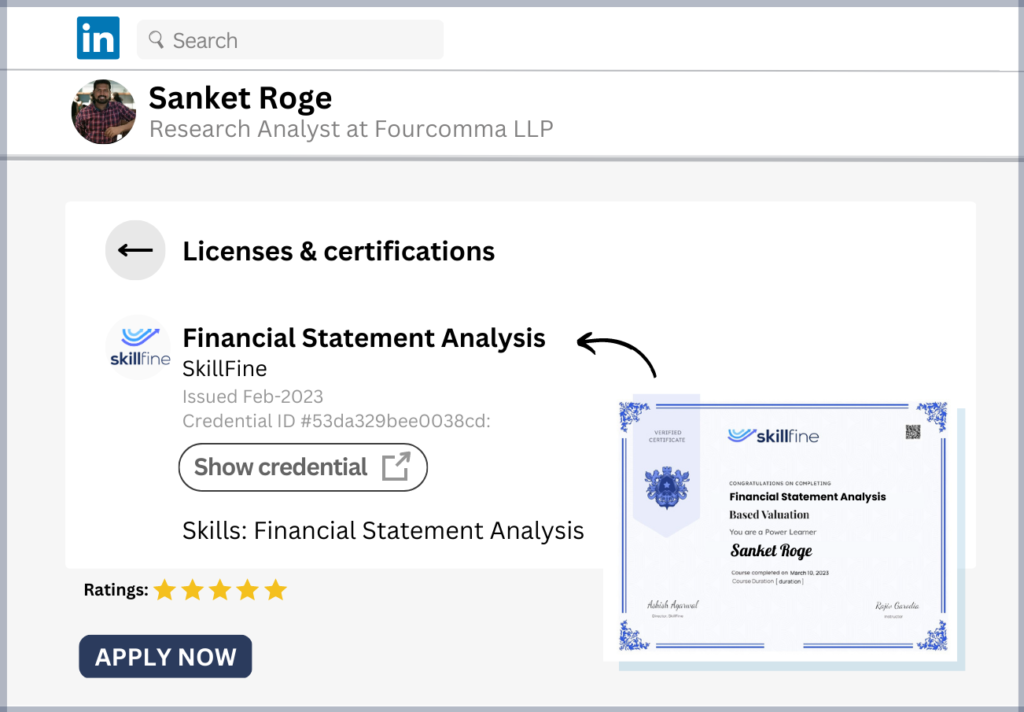
Reset Password
Insert/edit link.
Enter the destination URL
Or link to existing content
Call Us Today! +91 99907 48956 | [email protected]

It is the simplest form of data Presentation often used in schools or universities to provide a clearer picture to students, who are better able to capture the concepts effectively through a pictorial Presentation of simple data.
2. Column chart

It is a simplified version of the pictorial Presentation which involves the management of a larger amount of data being shared during the presentations and providing suitable clarity to the insights of the data.
3. Pie Charts

Pie charts provide a very descriptive & a 2D depiction of the data pertaining to comparisons or resemblance of data in two separate fields.
4. Bar charts

A bar chart that shows the accumulation of data with cuboid bars with different dimensions & lengths which are directly proportionate to the values they represent. The bars can be placed either vertically or horizontally depending on the data being represented.
5. Histograms
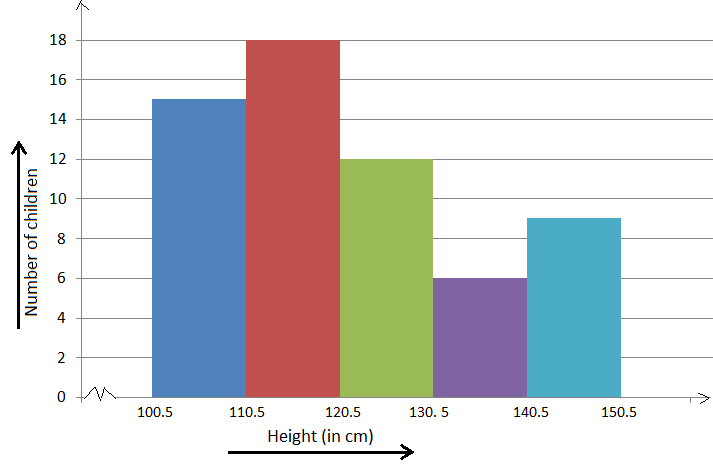
It is a perfect Presentation of the spread of numerical data. The main differentiation that separates data graphs and histograms are the gaps in the data graphs.
6. Box plots

Box plot or Box-plot is a way of representing groups of numerical data through quartiles. Data Presentation is easier with this style of graph dealing with the extraction of data to the minutes of difference.

Map Data graphs help you with data Presentation over an area to display the areas of concern. Map graphs are useful to make an exact depiction of data over a vast case scenario.
All these visual presentations share a common goal of creating meaningful insights and a platform to understand and manage the data in relation to the growth and expansion of one’s in-depth understanding of data & details to plan or execute future decisions or actions.
Importance of Data Presentation
Data Presentation could be both can be a deal maker or deal breaker based on the delivery of the content in the context of visual depiction.
Data Presentation tools are powerful communication tools that can simplify the data by making it easily understandable & readable at the same time while attracting & keeping the interest of its readers and effectively showcase large amounts of complex data in a simplified manner.
If the user can create an insightful presentation of the data in hand with the same sets of facts and figures, then the results promise to be impressive.
There have been situations where the user has had a great amount of data and vision for expansion but the presentation drowned his/her vision.
To impress the higher management and top brass of a firm, effective presentation of data is needed.
Data Presentation helps the clients or the audience to not spend time grasping the concept and the future alternatives of the business and to convince them to invest in the company & turn it profitable both for the investors & the company.
Although data presentation has a lot to offer, the following are some of the major reason behind the essence of an effective presentation:-
- Many consumers or higher authorities are interested in the interpretation of data, not the raw data itself. Therefore, after the analysis of the data, users should represent the data with a visual aspect for better understanding and knowledge.
- The user should not overwhelm the audience with a number of slides of the presentation and inject an ample amount of texts as pictures that will speak for themselves.
- Data presentation often happens in a nutshell with each department showcasing their achievements towards company growth through a graph or a histogram.
- Providing a brief description would help the user to attain attention in a small amount of time while informing the audience about the context of the presentation
- The inclusion of pictures, charts, graphs and tables in the presentation help for better understanding the potential outcomes.
- An effective presentation would allow the organization to determine the difference with the fellow organization and acknowledge its flaws. Comparison of data would assist them in decision making.
Recommended Courses

Data Visualization
Using powerbi &tableau.

Tableau for Data Analysis

MySQL Certification Program

The PowerBI Masterclass
Need help call our support team 7:00 am to 10:00 pm (ist) at (+91 999-074-8956 | 9650-308-956), keep in touch, email: [email protected].
WhatsApp us
Home PowerPoint Templates Data Analysis
Data Analysis PowerPoint Templates & Presentation Slides
Download 100% editable data analysis PowerPoint templates and backgrounds for presentations in Microsoft PowerPoint.
Featured Templates
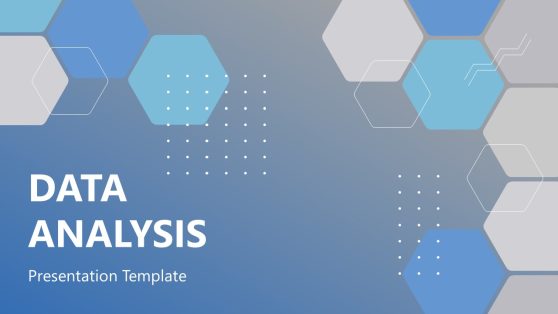
Data Analysis PowerPoint Template
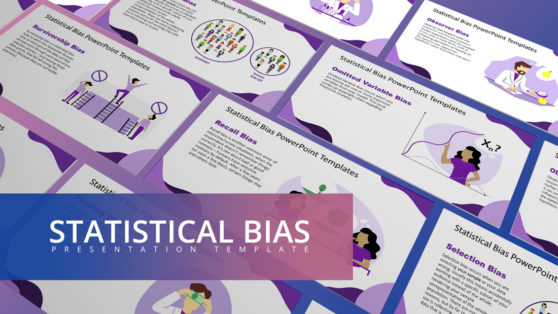
Statistical Bias PowerPoint Templates
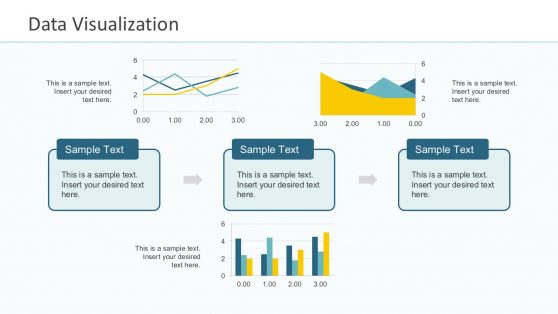
Data Visualization PowerPoint Template
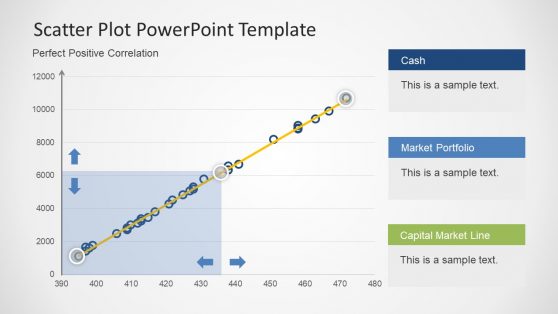
Scatter Plots Correlations PowerPoint Templates
Latest templates.
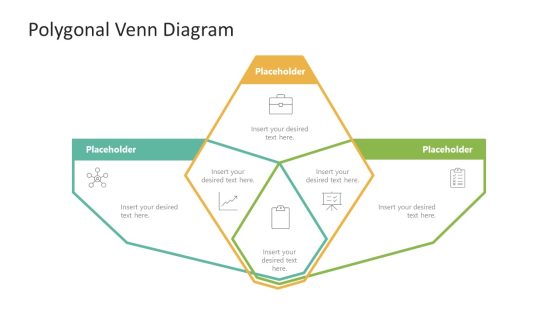
Polygonal Venn Diagram
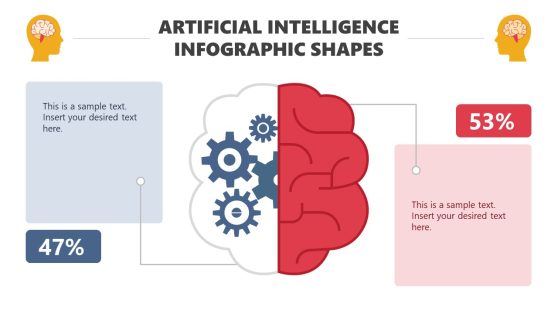
Artificial Intelligence Infographic Shapes for PowerPoint
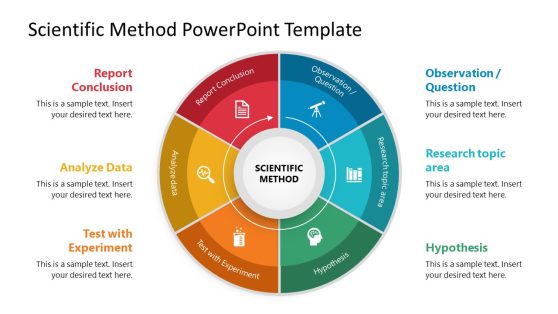
Scientific Method Diagram PowerPoint Template
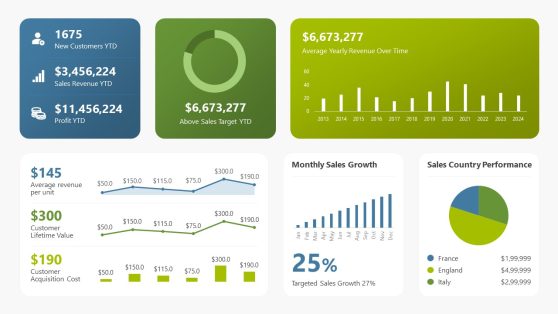
Sales Performance Dashboard PowerPoint Template
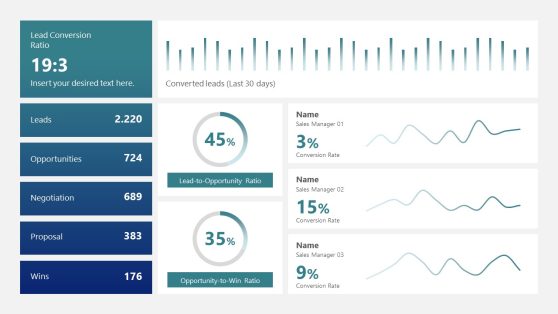
Sales Dashboard Template for PowerPoint
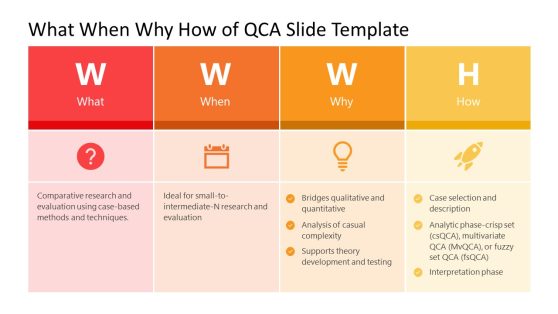
What When Why How of QCA Template for PowerPoint
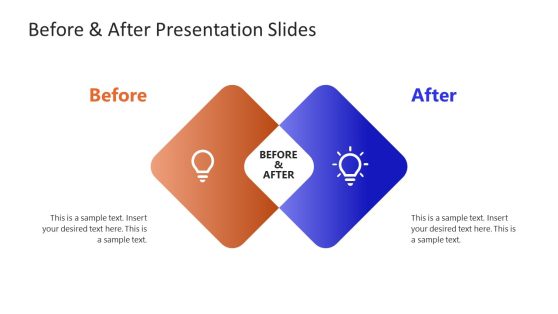
Before & After Presentation Slides
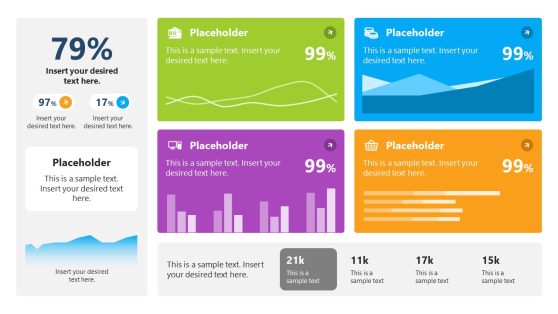
Executive Dashboard PowerPoint Template
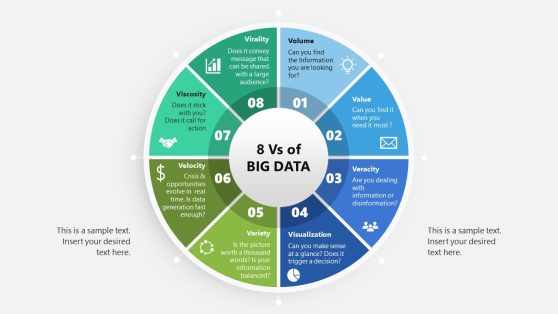
8Vs of Big Data PowerPoint Template
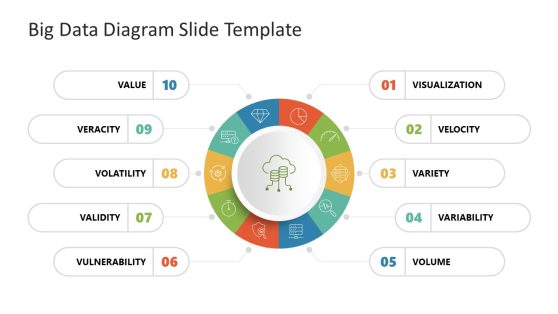
Big Data Diagram PowerPoint Template
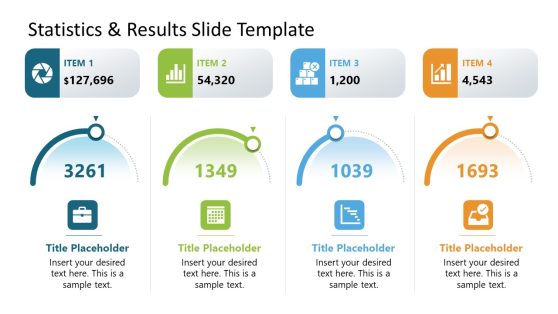
Statistics & Results PowerPoint Template
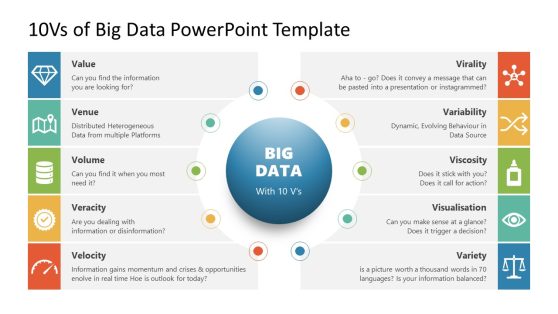
10Vs of Big Data PowerPoint Template
Data Analysis PowerPoint presentation templates are pre-designed slides that can be used for presenting results, insights, and conclusions derived from the analysis of various kinds of data. They often contain a variety of slide layouts, diagrams, charts, and other graphic elements that can effectively communicate complex data in a visually engaging and digestible manner.
Our editable data analysis presentation slides can help to prepare impeccable business reports and data analysis presentations with the help of editable & high-quality data analysis slide templates compatible with PowerPoint & Google Slides presentations.
Possible use cases, applications and presentation ideas for data analysis slide templates:
- Business Intelligence: A company might use data analysis templates to present results from its business intelligence efforts. This could include data about sales trends, customer demographics, and operational efficiency.
- Academic Research: Researchers can use data analysis presentation templates to present their research findings in conferences or seminars. They can showcase data about a variety of subjects, from social sciences to natural sciences.
- Marketing Campaign Analysis: Marketing professionals might use data analysis PowerPoint templates to present the results of a marketing campaign, analyzing data like audience engagement, conversion rates, and return on investment.
- SEO Strategy: A data analysis can also be used in a SEO-oriented presentation. This can help digital marketing teams, businesses, and SEO agencies to plan, implement, and report their SEO strategies effectively. The use of tools such as Google’s BigQuery can also demonstrate the ability to handle and analyze big data, which is increasingly important in today’s data-driven marketing landscape.
- Financial Analysis: Financial analysts could use slide templates on data analysis to present financial data such as revenue trends, cost analysis, budgeting, and forecasting.
- Healthcare Data Analysis: In the healthcare sector, data analysis templates can be used to present data on patient demographics, treatment effectiveness, and disease prevalence, for example.
- Consulting: Consultants and consulting firms often need to present data-driven insights to their clients. A data analysis PowerPoint template or presentation template for Google Slides would be suitable for this.
- Government & Public Policy: Government officials or policy analysts may use data analysis presentation templates to present data on social issues, economic trends, or the impact of certain policies.
These data analysis infographics and charts can help to prepare compelling data analysis presentation designs with charts and visually appealing graphics.
Download Unlimited Content
Our annual unlimited plan let you download unlimited content from slidemodel. save hours of manual work and use awesome slide designs in your next presentation..
Got any suggestions?
We want to hear from you! Send us a message and help improve Slidesgo
Top searches
Trending searches
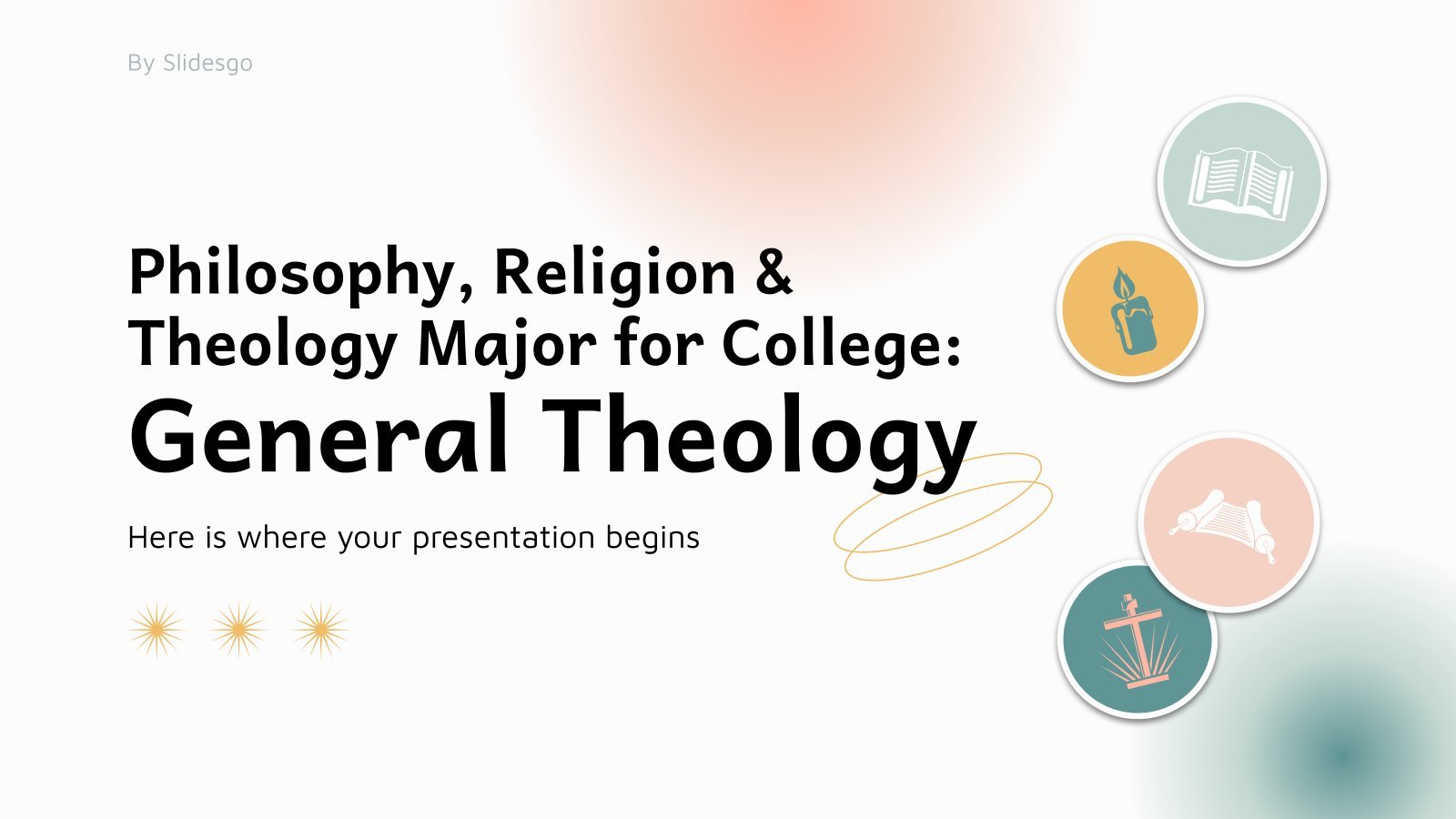
46 templates
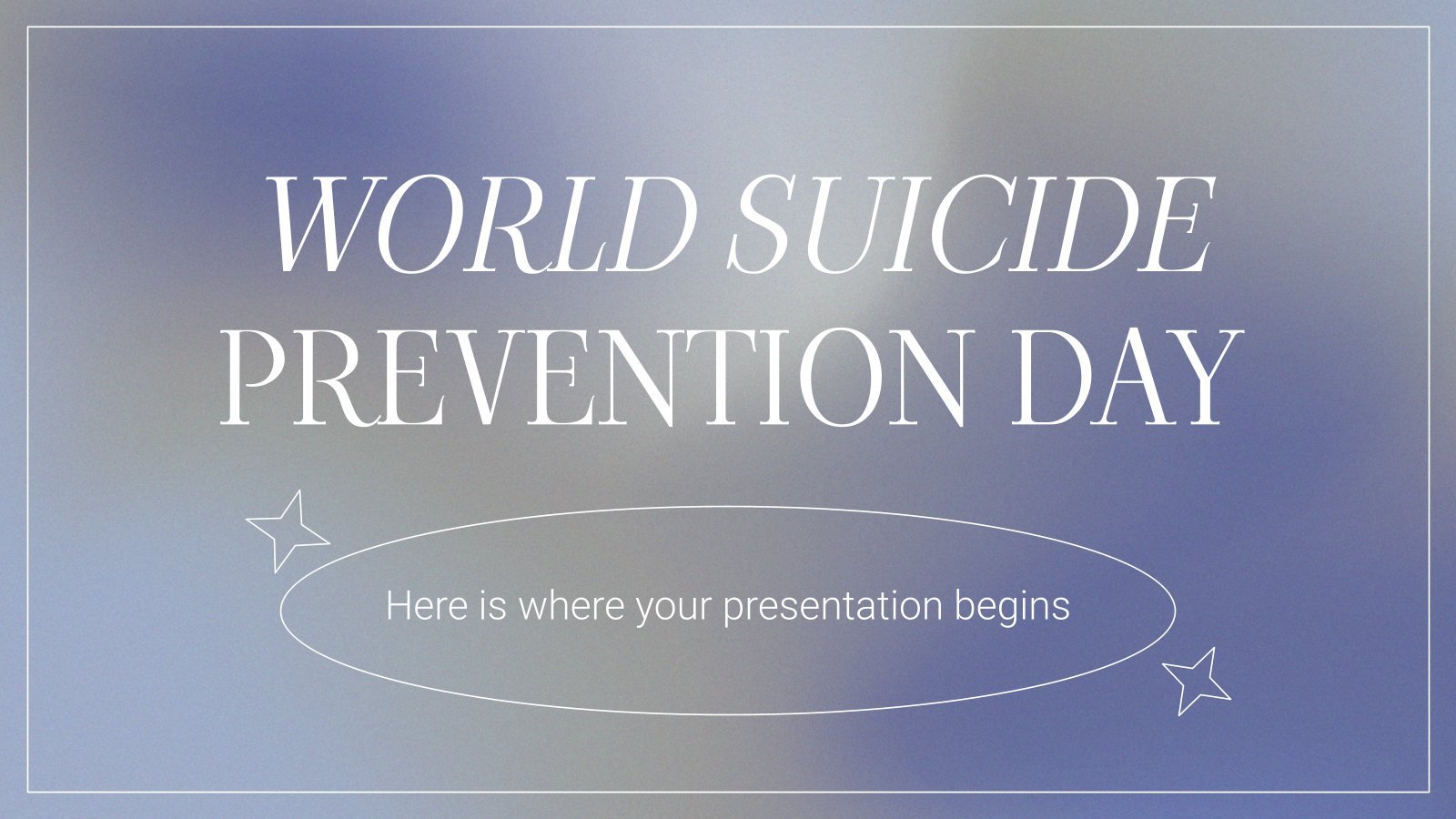
suicide prevention
8 templates
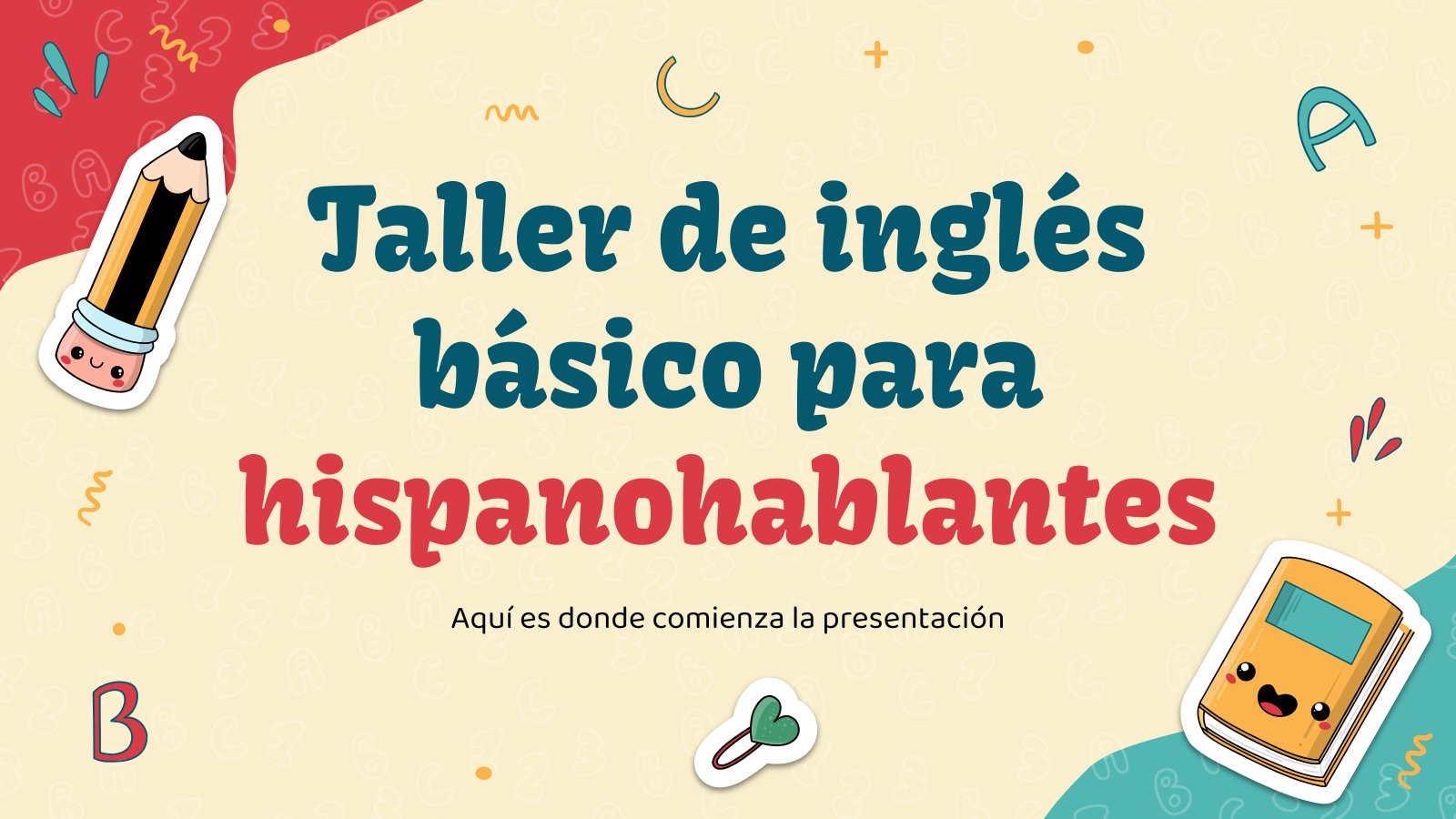
49 templates
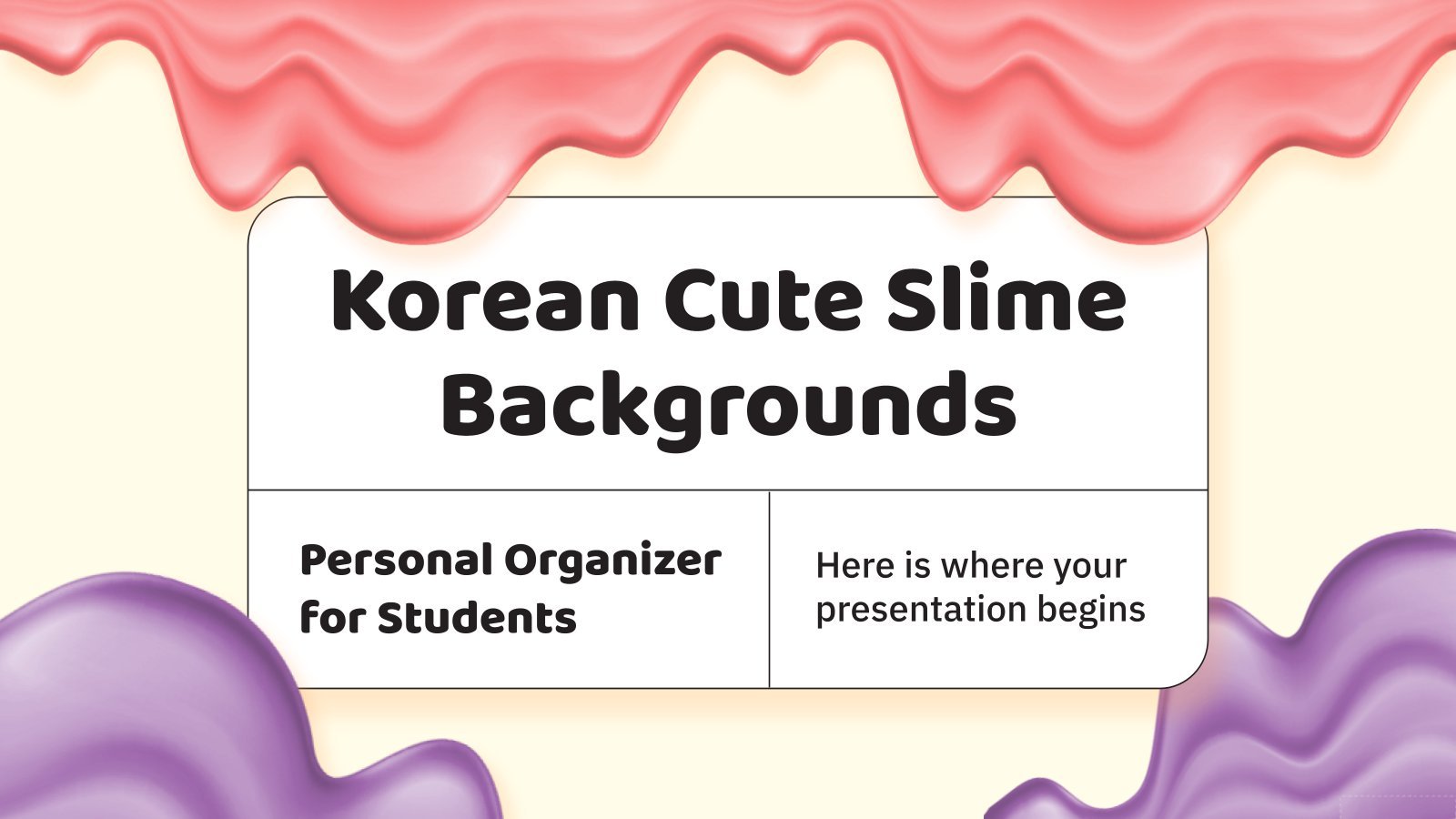
18 templates
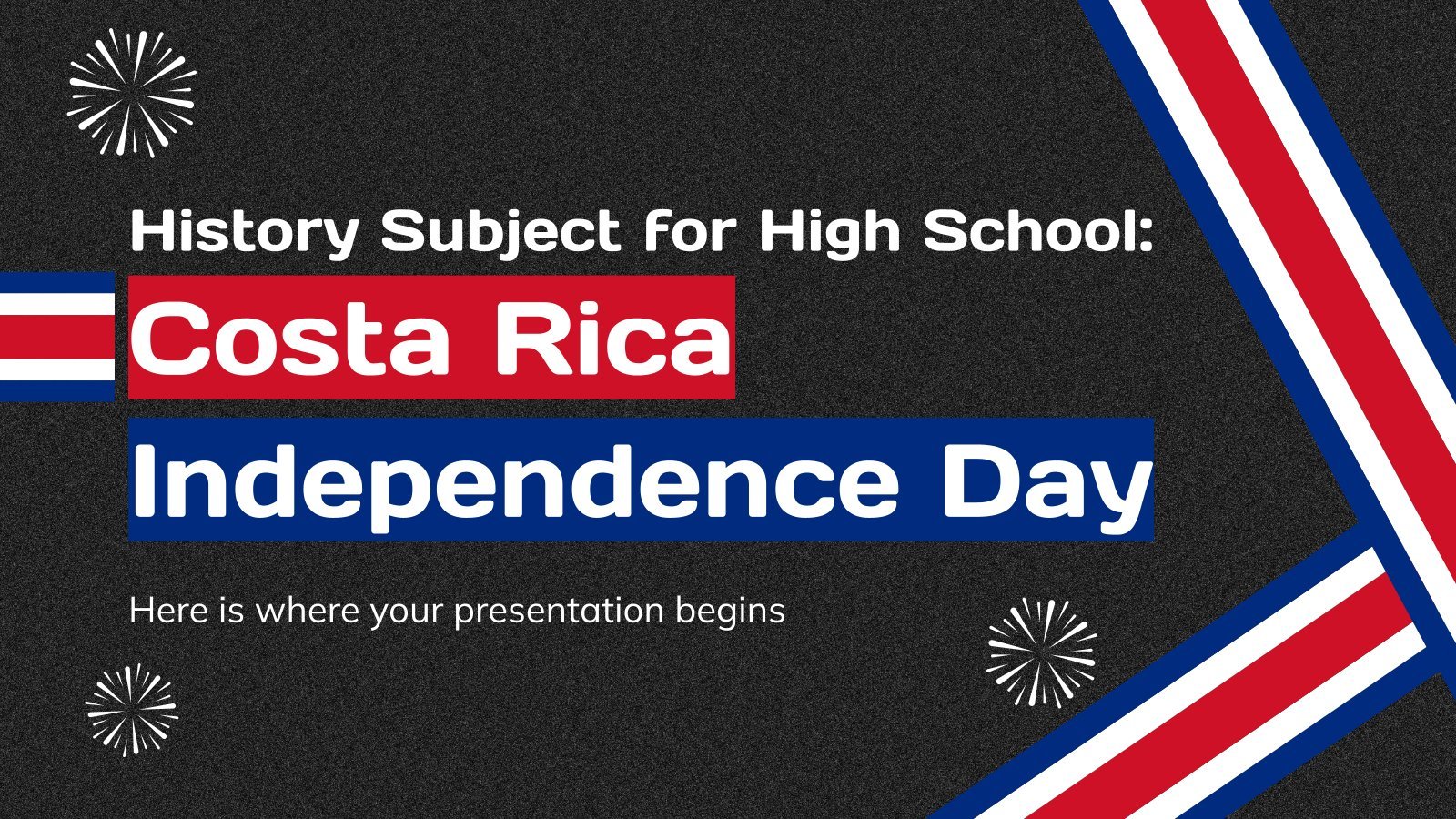
41 templates
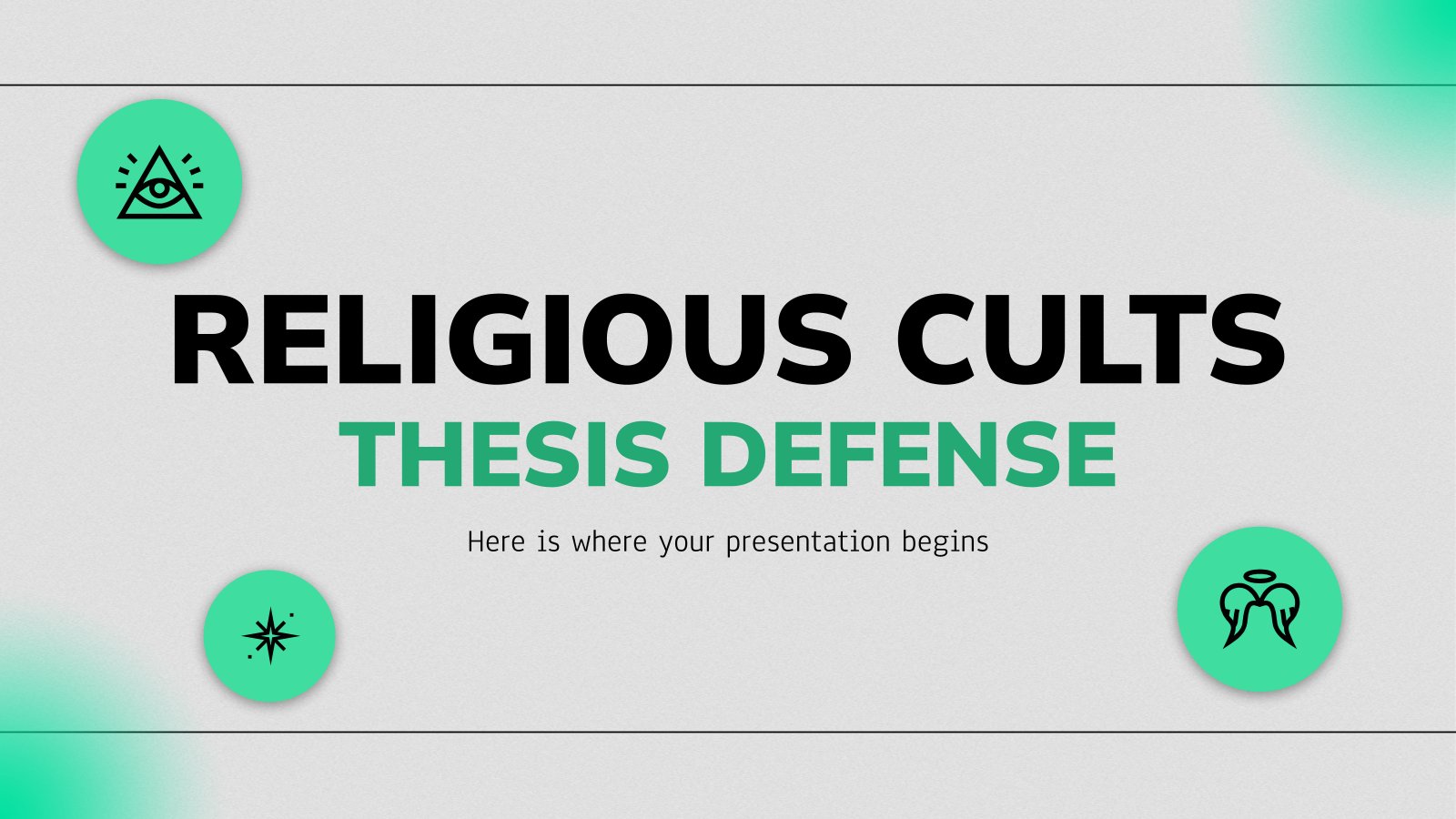
29 templates
Data Analysis for Business
Data analysis for business presentation, free google slides theme, powerpoint template, and canva presentation template.
What helps employees of a company know how the business is performing and recognize current problems that are to be solved? Data analysis laid out in a presentation, for example. Since we all want to do our best in our jobs, this template can come in handy for you. Its design has gradients, linear elements such as maps, icons or decorative shapes, and a menu at the top with text that can be clicked to jump to different sections. Analyze data with style!
Features of this template
- 100% editable and easy to modify
- 35 different slides to impress your audience
- Contains easy-to-edit graphics such as graphs, maps, tables, timelines and mockups
- Includes 500+ icons and Flaticon’s extension for customizing your slides
- Designed to be used in Google Slides, Canva, and Microsoft PowerPoint
- 16:9 widescreen format suitable for all types of screens
- Includes information about fonts, colors, and credits of the free resources used
How can I use the template?
Am I free to use the templates?
How to attribute?
Combines with:
This template can be combined with this other one to create the perfect presentation:
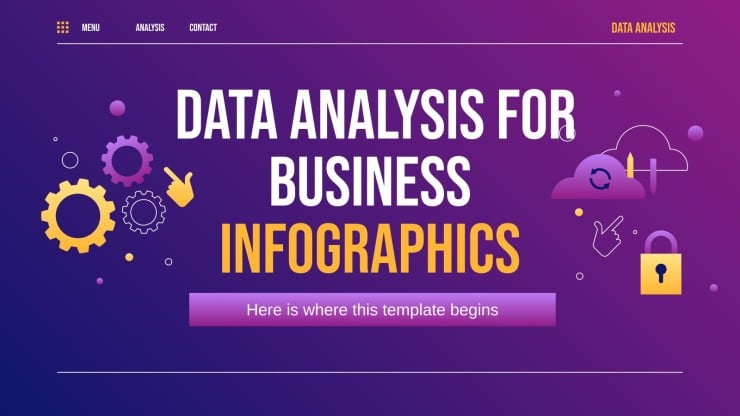
Attribution required If you are a free user, you must attribute Slidesgo by keeping the slide where the credits appear. How to attribute?
Related posts on our blog.
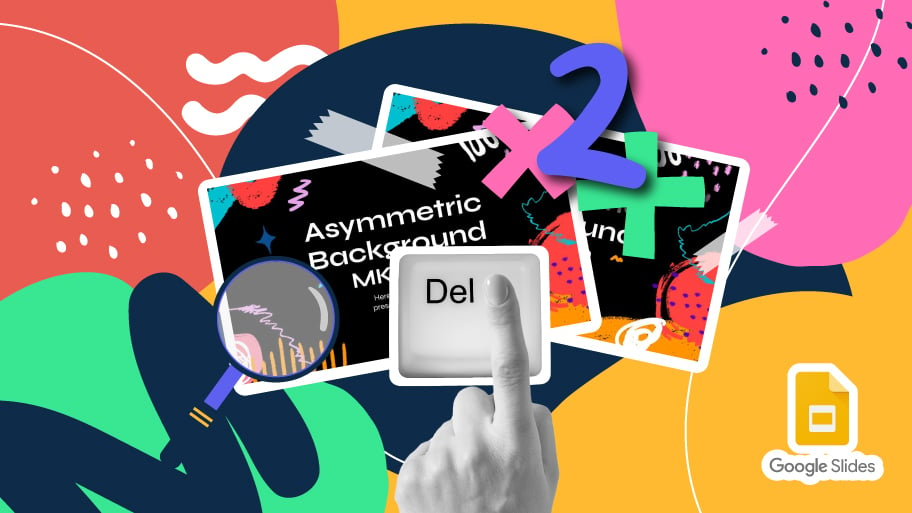
How to Add, Duplicate, Move, Delete or Hide Slides in Google Slides
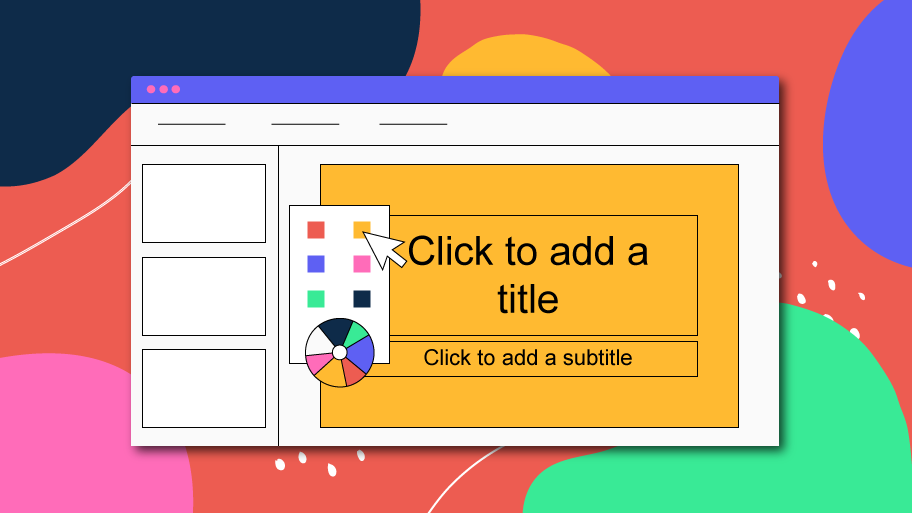
How to Change Layouts in PowerPoint
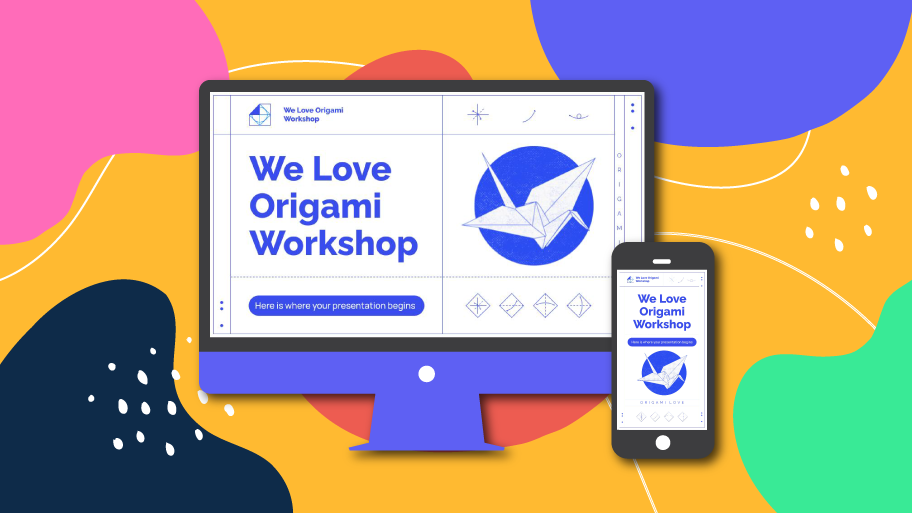
How to Change the Slide Size in Google Slides
Related presentations.
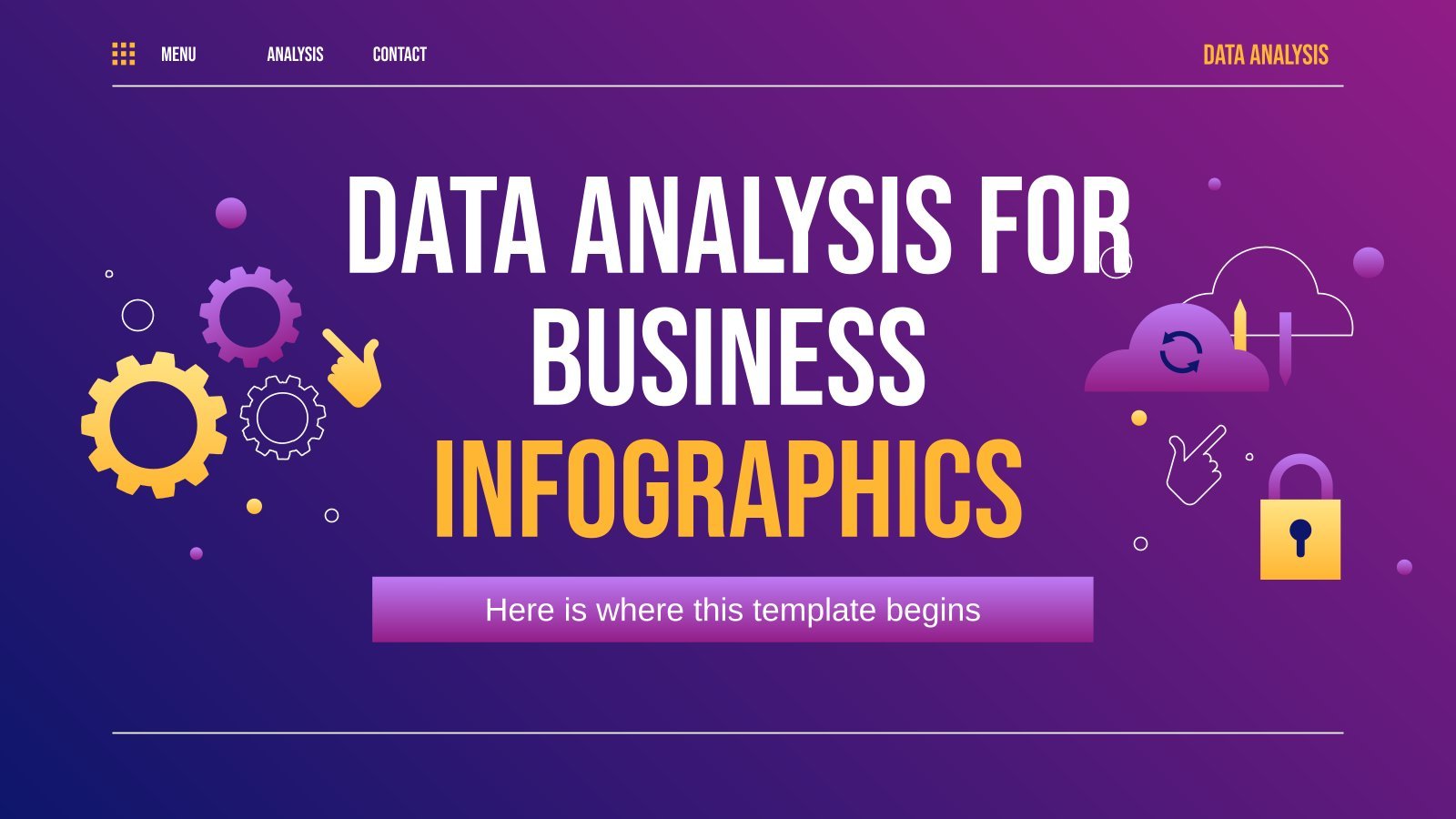
Premium template
Unlock this template and gain unlimited access
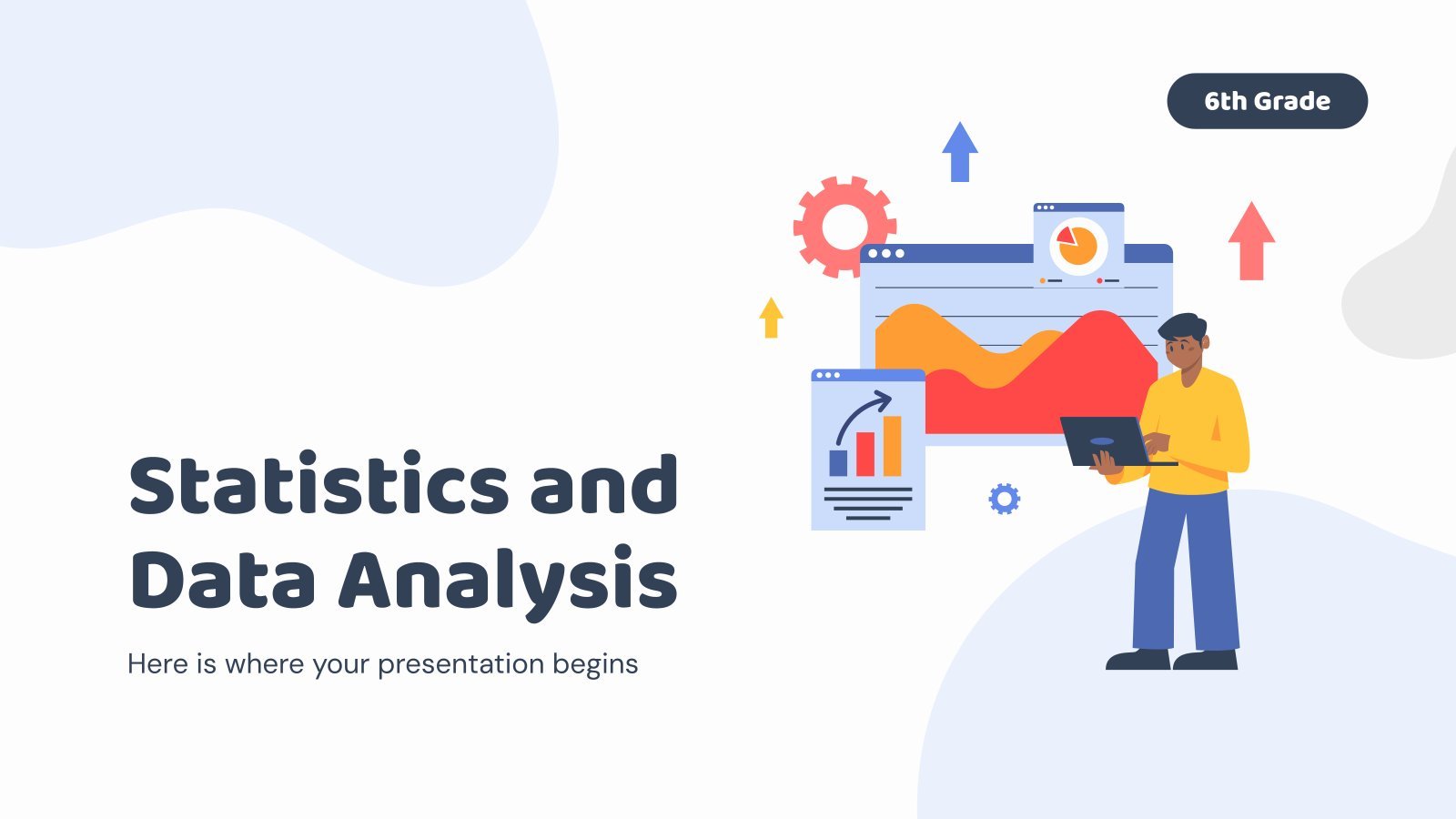
Register for free and start editing online
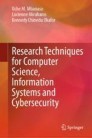
Research Techniques for Computer Science, Information Systems and Cybersecurity pp 115–138 Cite as
Data Collection, Presentation and Analysis
- Uche M. Mbanaso 4 ,
- Lucienne Abrahams 5 &
- Kennedy Chinedu Okafor 6
- First Online: 25 May 2023
506 Accesses
This chapter covers the topics of data collection, data presentation and data analysis. It gives attention to data collection for studies based on experiments, on data derived from existing published or unpublished data sets, on observation, on simulation and digital twins, on surveys, on interviews and on focus group discussions. One of the interesting features of this chapter is the section dealing with using measurement scales in quantitative research, including nominal scales, ordinal scales, interval scales and ratio scales. It explains key facets of qualitative research including ethical clearance requirements. The chapter discusses the importance of data visualization as key to effective presentation of data, including tabular forms, graphical forms and visual charts such as those generated by Atlas.ti analytical software.
This is a preview of subscription content, log in via an institution .
Buying options
- Available as PDF
- Read on any device
- Instant download
- Own it forever
- Available as EPUB and PDF
- Durable hardcover edition
- Dispatched in 3 to 5 business days
- Free shipping worldwide - see info
Tax calculation will be finalised at checkout
Purchases are for personal use only
Bibliography
Abdullah, M. F., & Ahmad, K. (2013). The mapping process of unstructured data to structured data. Proceedings of the 2013 International Conference on Research and Innovation in Information Systems (ICRIIS) , Malaysia , 151–155. https://doi.org/10.1109/ICRIIS.2013.6716700
Adnan, K., & Akbar, R. (2019). An analytical study of information extraction from unstructured and multidimensional big data. Journal of Big Data, 6 , 91. https://doi.org/10.1186/s40537-019-0254-8
Article Google Scholar
Alsheref, F. K., & Fattoh, I. E. (2020). Medical text annotation tool based on IBM Watson Platform. Proceedings of the 2020 6th international conference on advanced computing and communication systems (ICACCS) , India , 1312–1316. https://doi.org/10.1109/ICACCS48705.2020.9074309
Cinque, M., Cotroneo, D., Della Corte, R., & Pecchia, A. (2014). What logs should you look at when an application fails? Insights from an industrial case study. Proceedings of the 2014 44th Annual IEEE/IFIP International Conference on Dependable Systems and Networks , USA , 690–695. https://doi.org/10.1109/DSN.2014.69
Gideon, L. (Ed.). (2012). Handbook of survey methodology for the social sciences . Springer.
Google Scholar
Leedy, P., & Ormrod, J. (2015). Practical research planning and design (12th ed.). Pearson Education.
Madaan, A., Wang, X., Hall, W., & Tiropanis, T. (2018). Observing data in IoT worlds: What and how to observe? In Living in the Internet of Things: Cybersecurity of the IoT – 2018 (pp. 1–7). https://doi.org/10.1049/cp.2018.0032
Chapter Google Scholar
Mahajan, P., & Naik, C. (2019). Development of integrated IoT and machine learning based data collection and analysis system for the effective prediction of agricultural residue/biomass availability to regenerate clean energy. Proceedings of the 2019 9th International Conference on Emerging Trends in Engineering and Technology – Signal and Information Processing (ICETET-SIP-19) , India , 1–5. https://doi.org/10.1109/ICETET-SIP-1946815.2019.9092156 .
Mahmud, M. S., Huang, J. Z., Salloum, S., Emara, T. Z., & Sadatdiynov, K. (2020). A survey of data partitioning and sampling methods to support big data analysis. Big Data Mining and Analytics, 3 (2), 85–101. https://doi.org/10.26599/BDMA.2019.9020015
Miswar, S., & Kurniawan, N. B. (2018). A systematic literature review on survey data collection system. Proceedings of the 2018 International Conference on Information Technology Systems and Innovation (ICITSI) , Indonesia , 177–181. https://doi.org/10.1109/ICITSI.2018.8696036
Mosina, C. (2020). Understanding the diffusion of the internet: Redesigning the global diffusion of the internet framework (Research report, Master of Arts in ICT Policy and Regulation). LINK Centre, University of the Witwatersrand. https://hdl.handle.net/10539/30723
Nkamisa, S. (2021). Investigating the integration of drone management systems to create an enabling remote piloted aircraft regulatory environment in South Africa (Research report, Master of Arts in ICT Policy and Regulation). LINK Centre, University of the Witwatersrand. https://hdl.handle.net/10539/33883
QuestionPro. (2020). Survey research: Definition, examples and methods . https://www.questionpro.com/article/survey-research.html
Rajanikanth, J. & Kanth, T. V. R. (2017). An explorative data analysis on Bangalore City Weather with hybrid data mining techniques using R. Proceedings of the 2017 International Conference on Current Trends in Computer, Electrical, Electronics and Communication (CTCEEC) , India , 1121-1125. https://doi/10.1109/CTCEEC.2017.8455008
Rao, R. (2003). From unstructured data to actionable intelligence. IT Professional, 5 , 29–35. https://www.researchgate.net/publication/3426648_From_Unstructured_Data_to_Actionable_Intelligence
Schulze, P. (2009). Design of the research instrument. In P. Schulze (Ed.), Balancing exploitation and exploration: Organizational antecedents and performance effects of innovation strategies (pp. 116–141). Gabler. https://doi.org/10.1007/978-3-8349-8397-8_6
Usanov, A. (2015). Assessing cybersecurity: A meta-analysis of threats, trends and responses to cyber attacks . The Hague Centre for Strategic Studies. https://www.researchgate.net/publication/319677972_Assessing_Cyber_Security_A_Meta-analysis_of_Threats_Trends_and_Responses_to_Cyber_Attacks
Van de Kaa, G., De Vries, H. J., van Heck, E., & van den Ende, J. (2007). The emergence of standards: A meta-analysis. Proceedings of the 2007 40th Annual Hawaii International Conference on Systems Science (HICSS’07) , USA , 173a–173a. https://doi.org/10.1109/HICSS.2007.529
Download references
Author information
Authors and affiliations.
Centre for Cybersecurity Studies, Nasarawa State University, Keffi, Nigeria
Uche M. Mbanaso
LINK Centre, University of the Witwatersrand, Johannesburg, South Africa
Lucienne Abrahams
Department of Mechatronics Engineering, Federal University of Technology, Owerri, Nigeria
Kennedy Chinedu Okafor
You can also search for this author in PubMed Google Scholar
Rights and permissions
Reprints and permissions
Copyright information
© 2023 The Author(s), under exclusive license to Springer Nature Switzerland AG
About this chapter
Cite this chapter.
Mbanaso, U.M., Abrahams, L., Okafor, K.C. (2023). Data Collection, Presentation and Analysis. In: Research Techniques for Computer Science, Information Systems and Cybersecurity. Springer, Cham. https://doi.org/10.1007/978-3-031-30031-8_7
Download citation
DOI : https://doi.org/10.1007/978-3-031-30031-8_7
Published : 25 May 2023
Publisher Name : Springer, Cham
Print ISBN : 978-3-031-30030-1
Online ISBN : 978-3-031-30031-8
eBook Packages : Engineering Engineering (R0)
Share this chapter
Anyone you share the following link with will be able to read this content:
Sorry, a shareable link is not currently available for this article.
Provided by the Springer Nature SharedIt content-sharing initiative
- Publish with us
Policies and ethics
- Find a journal
- Track your research
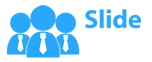
- Data Analytics
Powerpoint Templates
Icon Bundle
Kpi Dashboard
Professional
Business Plans
Swot Analysis
Gantt Chart
Business Proposal
Marketing Plan
Project Management
Business Case
Business Model
- Cyber Security
Business PPT
Digital Marketing
- Digital Transformation
Human Resources
Product Management
- Artificial Intelligence
Company Profile
Acknowledgement PPT
PPT Presentation
Reports Brochures
One Page Pitch
Interview PPT
All Categories

Best Data Analytics PPT Presentations, Templates & Slides
- Sub Categories
- 5G Technology
- Agile and Scrum
- Big Data Analytics
- Cloud Computing
- Cryptocurrency
- Green Energy
- Internet Of Things
- IT Certification
- Machine Learning
- Microprocessors
- Nanotechnology
- Robotic Process Automation
- Software Testing
- Technology Project Management
- Technology Quality Assurance
- Technology Stack
- Virtualization
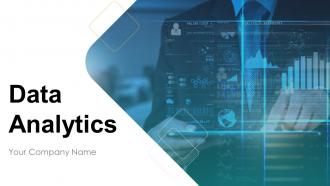
This complete deck is oriented to make sure you do not lag in your presentations. Our creatively crafted slides come with apt research and planning. This exclusive deck with twenty slides is here to help you to strategize, plan, analyze, or segment the topic with clear understanding and apprehension. Utilize ready to use presentation slides on Data Analytics Powerpoint Presentation Slides with all sorts of editable templates, charts and graphs, overviews, analysis templates. The presentation is readily available in both 4:3 and 16:9 aspect ratio. Alter the colors, fonts, font size, and font types of the template as per the requirements. It can be changed into formats like PDF, JPG, and PNG. It is usable for marking important decisions and covering critical issues. This presentation deck can be used by all professionals, managers, individuals, internal-external teams involved in any company organization.
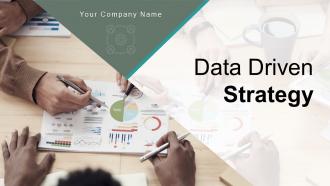
This complete deck can be used to present to your team. It has PPT slides on various topics highlighting all the core areas of your business needs. This complete deck focuses on Data Driven Strategy Analytics Technology Approach Corporate and has professionally designed templates with suitable visuals and appropriate content. This deck consists of total of thirteen slides. All the slides are completely customizable for your convenience. You can change the colour, text and font size of these templates. You can add or delete the content if needed. Get access to this professionally designed complete presentation by clicking the download button below.
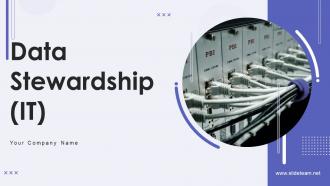
Enthrall your audience with this Data Stewardship IT Powerpoint Presentation Slides. Increase your presentation threshold by deploying this well-crafted template. It acts as a great communication tool due to its well-researched content. It also contains stylized icons, graphics, visuals etc, which make it an immediate attention-grabber. Comprising ninty slides, this complete deck is all you need to get noticed. All the slides and their content can be altered to suit your unique business setting. Not only that, other components and graphics can also be modified to add personal touches to this prefabricated set.
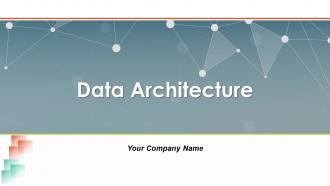
Sharing Data Architecture PowerPoint Presentation Slides. This PowerPoint complete deck includes 29 professional designs. Customers can edit the fonts, text, and color as slide are fully editable. You can easily download the presentation in widescreen and standard screen. The presentation is supported by Google Slides. PowerPoint templates can be converted into JPG or PDF format.
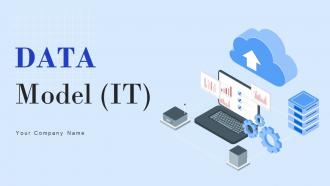
Deliver this complete deck to your team members and other collaborators. Encompassed with stylized slides presenting various concepts, this Data Model IT Powerpoint Presentation Slides is the best tool you can utilize. Personalize its content and graphics to make it unique and thought-provoking. All the fifty eight slides are editable and modifiable, so feel free to adjust them to your business setting. The font, color, and other components also come in an editable format making this PPT design the best choice for your next presentation. So, download now.
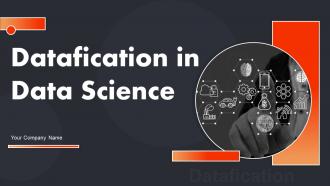
Enthrall your audience with this Datafication In Data Science Powerpoint Presentation Slides. Increase your presentation threshold by deploying this well-crafted template. It acts as a great communication tool due to its well-researched content. It also contains stylized icons, graphics, visuals etc, which make it an immediate attention-grabber. Comprising fifty six slides, this complete deck is all you need to get noticed. All the slides and their content can be altered to suit your unique business setting. Not only that, other components and graphics can also be modified to add personal touches to this prefabricated set.
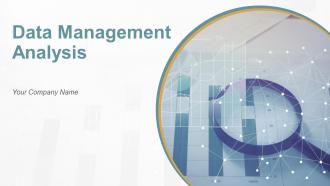
High-quality data management PowerPoint slides which are compatible with google sides. Easy conversion to your desired format and quick downloading. Widely used by business owners, marketers, investors, financial executives, professors and students for change and data management. You can modify and personalize these PPT's presentation by including the company name and logo by following the instructions given.
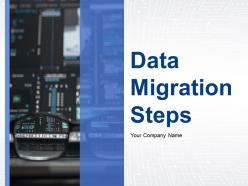
Presenting this set of slides with name - Data Migration Steps Powerpoint Presentation Slides. This PPT deck displays twenty-six slides with in-depth research. We provide a ready to use deck with all sorts of relevant topics subtopics templates, charts and graphs, overviews, analysis templates. When you download this deck by clicking the download button below, you get the presentation in both standard and widescreen format. All slides are fully editable. change the colors, font size, add or delete text if needed. The presentation is fully supported with Google Slides. It can be easily converted into JPG or PDF format.
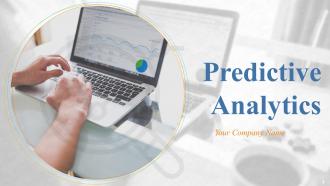
Well-Constructed PPT templates beneficial for different business professionals from diverse sectors, simply amendable shapes, patterns and subject matters, authentic and relevant PPT Images with pliable data options, smooth downloads, runs smoothly with all available software’s, high quality picture presentation graphics which remain unaffected when projected on wide screen, well adaptable on Google slides also.
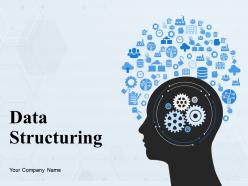
Presenting this set of slides with name - Data Structuring Powerpoint Presentation Slides. We bring to you to the point topic specific slides with apt research and understanding. Putting forth our PPT deck comprises of thirty one slides. Our tailor made Data Structuring Powerpoint Presentation Slides editable deck assists planners to segment and expound the topic with brevity. The advantageous slides on Data Structuring Powerpoint Presentation Slides is braced with multiple charts and graphs, overviews, analysis templates agenda slides etc. to help boost important aspects of your presentation. Highlight all sorts of related usable templates for important considerations. Our deck finds applicability amongst all kinds of professionals, managers, individuals, temporary permanent teams involved in any company organization from any field.
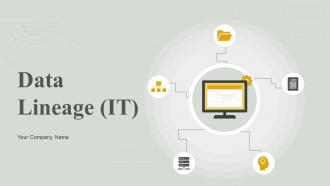
Deliver an informational PPT on various topics by using this Data Lineage IT Powerpoint Presentation Slides. This deck focuses and implements best industry practices, thus providing a birds-eye view of the topic. Encompassed with ninty slides, designed using high-quality visuals and graphics, this deck is a complete package to use and download. All the slides offered in this deck are subjective to innumerable alterations, thus making you a pro at delivering and educating. You can modify the color of the graphics, background, or anything else as per your needs and requirements. It suits every business vertical because of its adaptable layout.
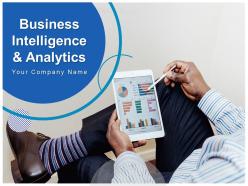
Presenting Business Intelligence And Analytics Powerpoint Presentation Slides. You can modify the font size, type, and color of the slide as per your requirements. This slide can be downloaded into formats like PDF, JPG, and PNG without any problem. It is Google Slides friendly which makes it accessible at once. This slide is available in both the standard(4:9) and the widescreen(16:9) aspect ratio.
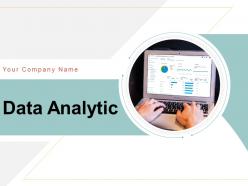
Grab our Data Analytic PowerPoint Presentation Slides PowerPoint Presentation Slides that are sure to impress executives, inspire team members, and other audience. This PPT is the most comprehensive presentation of intelligent data use you could have asked for your business. We have used beautiful PowerPoint graphics, templates, icons, and diagrams. The content has been well researched by our excellent team of researchers. You can change the colour, fonts, texts, images without any hassle to suit your business needs. Download the presentation, enter your content in the placeholders, and present it with confidence!
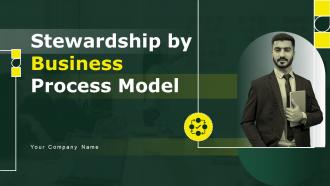
This complete deck covers various topics and highlights important concepts. It has PPT slides which cater to your business needs. This complete deck presentation emphasizes Stewardship By Business Process Model Powerpoint Presentation Slides and has templates with professional background images and relevant content. This deck consists of total of eighty two slides. Our designers have created customizable templates, keeping your convenience in mind. You can edit the color, text and font size with ease. Not just this, you can also add or delete the content if needed. Get access to this fully editable complete presentation by clicking the download button below.
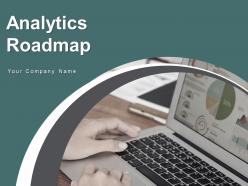
This complete deck can be used to present to your team. It has PPT slides on various topics highlighting all the core areas of your business needs. This complete deck focuses on Analytics Roadmap Developing Management Platform Automation Framework Technological Business and has professionally designed templates with suitable visuals and appropriate content. This deck consists of total of twelve slides. All the slides are completely customizable for your convenience. You can change the colour, text and font size of these templates. You can add or delete the content if needed. Get access to this professionally designed complete presentation by clicking the download button below.
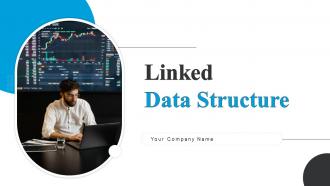
Enthrall your audience with this Linked Data Structure Powerpoint Presentation Slides. Increase your presentation threshold by deploying this well-crafted template. It acts as a great communication tool due to its well-researched content. It also contains stylized icons, graphics, visuals etc, which make it an immediate attention-grabber. Comprising fifty one slides, this complete deck is all you need to get noticed. All the slides and their content can be altered to suit your unique business setting. Not only that, other components and graphics can also be modified to add personal touches to this prefabricated set.
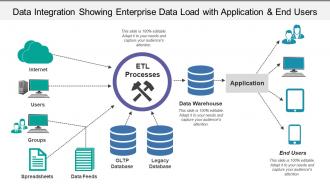
Presenting this set of slides with name - Data Integration Showing Enterprise Data Load With Application And End Users. This is a three stage process. The stages in this process are Data Integration, Data Management, Data Analysis.
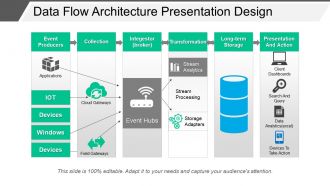
Presenting, the data flow architecture presentation PowerPoint deck. This data flow architecture PPT runs steadily and on various software. You can now steadily convert it into a JPG, PDF or even both formats for ease! Compatible with Google Slides and available in both standard 4:3 and widescreen format 16:9 after downloading. Widescreen projection without PPT graphics pixelation. Availability to insert company logo, name, and trademark for personalization has been taken care of. This is an entirely customizable PPT layout that includes font, text, color, and design. Download this design within a snap.
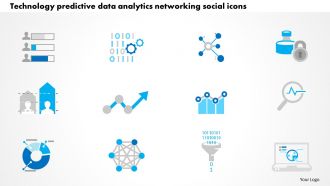
Fine quality, high resolution PPT icons. Bright coloured PPT graphics for a vibrant visual impact. Customisation of any icon as per need of the hour. Easy inclusion and exclusion of data possible. Hassle free conversion into varied formats. Compatible with multiple info graphic software. Beneficial for business owners, managers, investors, financers, marketers, entrepreneurs, teachers, students.
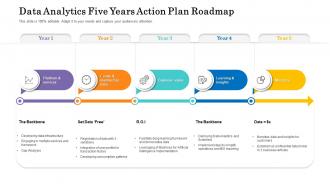
Presenting Data Analytics Five Years Action Plan Roadmap PowerPoint slide. This PPT theme is available in both 4,3 and 16,9 aspect ratios. This PowerPoint template is customizable so you can modify the font size, font type, color, and shapes as per your requirements. This PPT presentation is Google Slides compatible hence it is easily accessible. You can download and save this PowerPoint layout in different formats like PDF, PNG, and JPG.
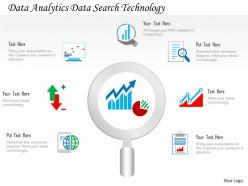
PPT slides are entirely compatible with Google slides. Standard and widescreen view display options available. 100% editable PowerPoint template design to enable customization. Simple to convert into JPEG and PDF document. Downloading is easy and can be insert in your presentation. Useful for every small and large scale organization. The stages in this process are big data analytics.
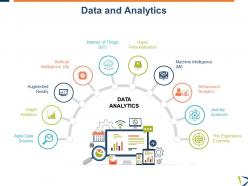
Presenting this set of slides with name Data And Analytics Artificial Intelligence Ppt Powerpoint Presentation Slides Icons. This is a ten stage process. The stages in this process are Machine Intelligence, Behavioural Analytics, Graph Analytics, Augmented Reality, Artificial Intelligence. This is a completely editable PowerPoint presentation and is available for immediate download. Download now and impress your audience.
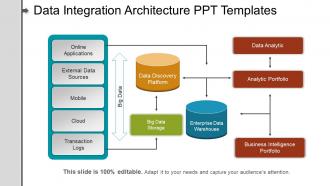
SlideTeam presents to you this data integration architecture PPT template. This slideshow is 100% editable so you can make a lot of changes related to the font, orientation, color, size, shape, etc. of various features and diagrammatic images used in the presentation. This PPT can be viewed in standard size display ratio of 4:3 or widescreen display ratio of 16:9. This template is Google slides friendly. The PowerPoint template can be saved in either JPG or PDF format.
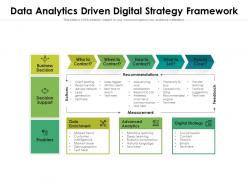
Presenting this set of slides with name Data Analytics Driven Digital Strategy Framework. The topics discussed in these slides are Business, Decision, Enablers. This is a completely editable PowerPoint presentation and is available for immediate download. Download now and impress your audience.
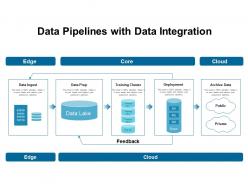
Presenting this set of slides with name Data Pipelines With Data Integration. This is a five stage process. The stages in this process are Data Ingest, Data Prep, Training Cluster, Deployment, Archive Data. This is a completely editable PowerPoint presentation and is available for immediate download. Download now and impress your audience.
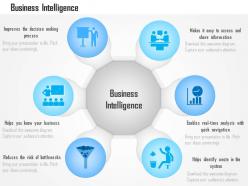
Fully modifiable PowerPoint design. High resolution presentation slide as can be anticipated on wide screen. Easily adjustable with maximum number of software i.e. JPG and PDF. Trouble free inclusion and omission of content as per industry need. Glorious quality of PowerPoint design. Presentation illustration download with different nodes and stages. Possible to download with standard and widescreen view.
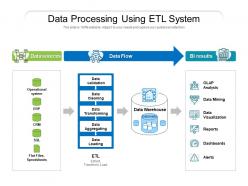
Presenting this set of slides with name Data Processing Using ETL System. This is a three stage process. The stages in this process are Operational system, Data validation, Data Cleaning, Data Aggregating, Data Transforming, Data Loading, Data Visualization, Dashboards, CRM, ERP. This is a completely editable PowerPoint presentation and is available for immediate download. Download now and impress your audience.
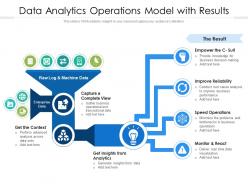
Presenting our set of slides with name Data Analytics Operations Model With Results. This exhibits information on four stages of the process. This is an easy to edit and innovatively designed PowerPoint template. So download immediately and highlight information on Raw Log And Machine Data, Capture A Complete View, Get Insights From Analytics.
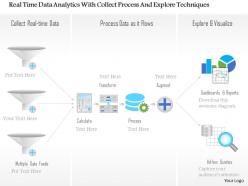
They are capable of providing up-to-date information about the enterprise. Better and quicker business decisions can be made using these layouts. Helps in forming a well organised business system. Compatible with Google slides. Modifiable by following simple instructions that come pre-defined with these PPT presentation Patterns.The stages in this process are networking, storage, big data analytics.
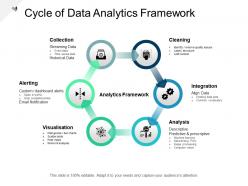
Presenting this set of slides with name - Cycle Of Data Analytics Framework. This is a six stages process. The stages in this process are Analytics Architecture, Analytics Framework, Data Analysis.
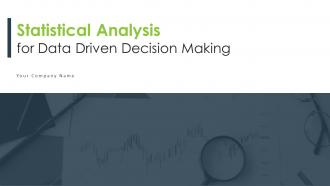
Deliver an informational PPT on various topics by using this Statistical Analysis For Data Driven Decision Making Powerpoint Presentation Slides. This deck focuses and implements best industry practices, thus providing a birds-eye view of the topic. Encompassed with seventy one slides, designed using high-quality visuals and graphics, this deck is a complete package to use and download. All the slides offered in this deck are subjective to innumerable alterations, thus making you a pro at delivering and educating. You can modify the color of the graphics, background, or anything else as per your needs and requirements. It suits every business vertical because of its adaptable layout.
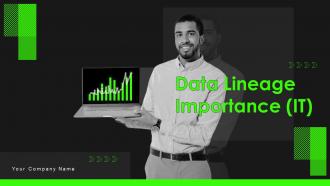
Deliver an informational PPT on various topics by using this Data Lineage Importance IT Powerpoint Presentation Slides. This deck focuses and implements best industry practices, thus providing a birds-eye view of the topic. Encompassed with ninety slides, designed using high-quality visuals and graphics, this deck is a complete package to use and download. All the slides offered in this deck are subjective to innumerable alterations, thus making you a pro at delivering and educating. You can modify the color of the graphics, background, or anything else as per your needs and requirements. It suits every business vertical because of its adaptable layout.
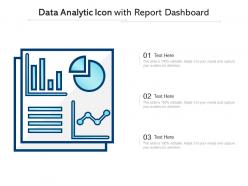
Presenting this set of slides with name Data Analytic Icon With Report Dashboard Snapshot. The topics discussed in these slide is Data Analytic Icon With Report Dashboard. This is a completely editable PowerPoint presentation and is available for immediate download. Download now and impress your audience.
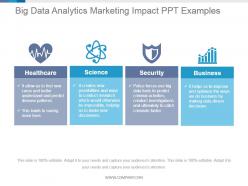
Presenting big data analytics marketing impact ppt examples. This is a big data analytics marketing impact ppt examples. This is a four stage process. The stages in this process are healthcare, science, security, business.
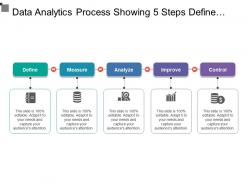
Presenting this set of slides with name - Data Analytics Process Showing 5 Steps Define Measure Improve Control. This is a five stage process. The stages in this process are Data Analytics Process, Data Analysis Cycle, Data Visualization Process.
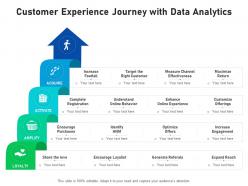
Presenting our set of slides with Customer Experience Journey With Data Analytics. This exhibits information on four stages of the process. This is an easy-to-edit and innovatively designed PowerPoint template. So download immediately and highlight information on Loyalty, Amplify, Activate, Acquire.
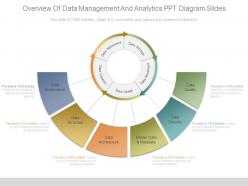
Presenting overview of data management and analytics ppt diagram slides. This is a overview of data management and analytics ppt diagram slides. This is a six stage process. The stages in this process are data retirement, data storage, data movement, data creation, data usage, data governance, data structure, data architecture, master data and metadata, data security, data quality.
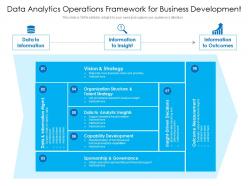
Introducing our Data Analytics Operations Framework For Business Development set of slides. The topics discussed in these slides are Organization Structure And Talent Strategy, Data To Analytic Insights, Capability Development. This is an immediately available PowerPoint presentation that can be conveniently customized. Download it and convince your audience.
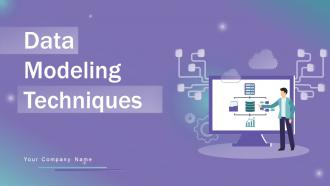
Deliver this complete deck to your team members and other collaborators. Encompassed with stylized slides presenting various concepts, this Data Modeling Techniques Powerpoint Presentation Slides is the best tool you can utilize. Personalize its content and graphics to make it unique and thought-provoking. All the fifty eight slides are editable and modifiable, so feel free to adjust them to your business setting. The font, color, and other components also come in an editable format making this PPT design the best choice for your next presentation. So, download now.
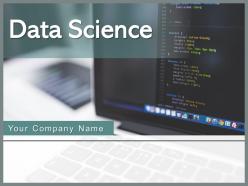
This complete presentation has PPT slides on wide range of topics highlighting the core areas of your business needs. It has professionally designed templates with relevant visuals and subject driven content. This presentation deck has total of eleven slides. Get access to the customizable templates. Our designers have created editable templates for your convenience. You can edit the colour, text and font size as per your need. You can add or delete the content if required. You are just a click to away to have this ready-made presentation. Click the download button now.
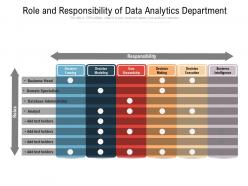
Presenting this set of slides with name Role And Responsibility Of Data Analytics Department. The topics discussed in these slides are Business, Responsibility, Decision Making, Business Intelligence, Decision Modeling. This is a completely editable PowerPoint presentation and is available for immediate download. Download now and impress your audience.
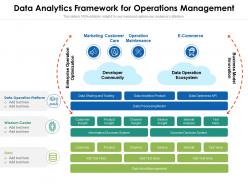
Introducing our Data Analytics Framework For Operations Management set of slides. The topics discussed in these slides are Data Sharing And Trading, Data Analytics Product, Information Discovery System. This is an immediately available PowerPoint presentation that can be conveniently customized. Download it and convince your audience.
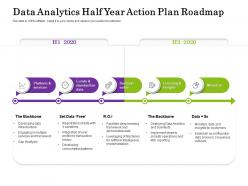
Presenting Data Analytics Half Year Action Plan Roadmap PowerPoint slide which is percent editable. You can change the color, font size, font type, and shapes of this PPT layout according to your needs. This PPT template is compatible with Google Slides and is available in both 4,3 and 16,9 aspect ratios. This ready to use PowerPoint presentation can be downloaded in various formats like PDF, JPG, and PNG.
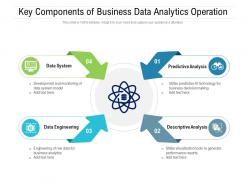
Presenting our set of slides with name Key Components Of Business Data Analytics Operation. This exhibits information on four stages of the process. This is an easy to edit and innovatively designed PowerPoint template. So download immediately and highlight information on Predictive Analysis, Descriptive Analysis, Data Engineering.
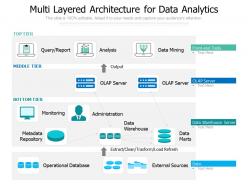
Presenting this set of slides with name Multi Layered Architecture For Data Analytics. The topics discussed in these slides are Analysis, Data Mining, Administration, Data Warehouse, Metadata Repository. This is a completely editable PowerPoint presentation and is available for immediate download. Download now and impress your audience.
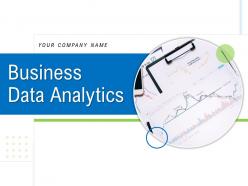
Enthrall your audience with this Business Data Analytics Powerpoint Presentation Slides. Increase your presentation threshold by deploying this well crafted template. It acts as a great communication tool due to its well researched content. It also contains stylized icons, graphics, visuals etc, which make it an immediate attention grabber. Comprising twenty nine slides, this complete deck is all you need to get noticed. All the slides and their content can be altered to suit your unique business setting. Not only that, other components and graphics can also be modified to add personal touches to this prefabricated set.
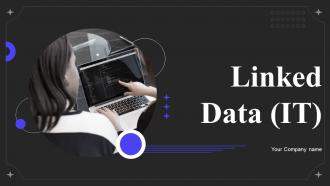
This complete presentation has PPT slides on wide range of topics highlighting the core areas of your business needs. It has professionally designed templates with relevant visuals and subject driven content. This presentation deck has total of fifty three slides. Get access to the customizable templates. Our designers have created editable templates for your convenience. You can edit the color, text and font size as per your need. You can add or delete the content if required. You are just a click to away to have this ready-made presentation. Click the download button now.

Deliver this complete deck to your team members and other collaborators. Encompassed with stylized slides presenting various concepts, this Data Lineage Types IT Powerpoint Presentation Slides is the best tool you can utilize. Personalize its content and graphics to make it unique and thought-provoking. All the ninty slides are editable and modifiable, so feel free to adjust them to your business setting. The font, color, and other components also come in an editable format making this PPT design the best choice for your next presentation. So, download now.
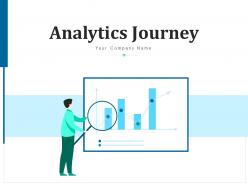
Deliver a credible and compelling presentation by deploying this Analytics Journey Data Exploration Operational Reporting Value Creation. Intensify your message with the right graphics, images, icons, etc. presented in this complete deck. This PPT template is a great starting point to convey your messages and build a good collaboration. The twelve slides added to this PowerPoint slideshow helps you present a thorough explanation of the topic. You can use it to study and present various kinds of information in the form of stats, figures, data charts, and many more. This Analytics Journey Data Exploration Operational Reporting Value Creation PPT slideshow is available for use in standard and widescreen aspects ratios. So, you can use it as per your convenience. Apart from this, it can be downloaded in PNG, JPG, and PDF formats, all completely editable and modifiable. The most profound feature of this PPT design is that it is fully compatible with Google Slides making it suitable for every industry and business domain.
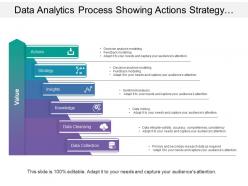
Presenting this set of slides with name - Data Analytics Process Showing Actions Strategy Insights And Data Collection. This is a six stage process. The stages in this process are Data Analytics Process, Data Analysis Cycle, Data Visualization Process.
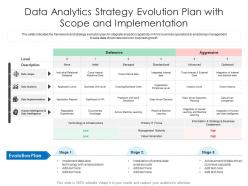
Introducing our Data Analytics Strategy Evolution Plan With Scope And Implementation set of slides. The topics discussed in these slides are Defensive, Aggressive, Evolution Plan. This is an immediately available PowerPoint presentation that can be conveniently customized. Download it and convince your audience.
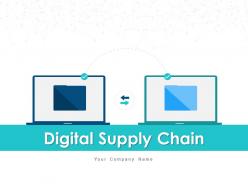
Introduce your topic and host expert discussion sessions with this Digital Supply Chain Data Analytics Demand Forecasting Channel Optimization. This template is designed using high-quality visuals, images, graphics, etc, that can be used to showcase your expertise. Different topics can be tackled using the twelve slides included in this template. You can present each topic on a different slide to help your audience interpret the information more effectively. Apart from this, this PPT slideshow is available in two screen sizes, standard and widescreen making its delivery more impactful. This will not only help in presenting a birds-eye view of the topic but also keep your audience engaged. Since this PPT slideshow utilizes well-researched content, it induces strategic thinking and helps you convey your message in the best possible manner. The biggest feature of this design is that it comes with a host of editable features like color, font, background, etc. So, grab it now to deliver a unique presentation every time.
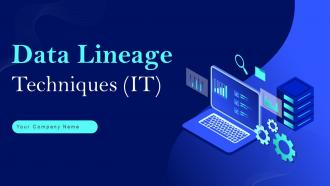
This complete presentation has PPT slides on wide range of topics highlighting the core areas of your business needs. It has professionally designed templates with relevant visuals and subject driven content. This presentation deck has total of ninty slides. Get access to the customizable templates. Our designers have created editable templates for your convenience. You can edit the color, text and font size as per your need. You can add or delete the content if required. You are just a click to away to have this ready-made presentation. Click the download button now.
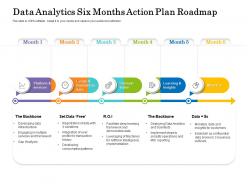
Presenting Data Analytics Six Months Action Plan Roadmap PowerPoint slide. This PPT presentation is Google Slides compatible hence it is easily accessible. This PPT theme is available in both 4,3 and 16,9 aspect ratios. This PowerPoint template is customizable so you can modify the font size, font type, color, and shapes as per your requirements. You can download and save this PowerPoint layout in different formats like PDF, PNG, and JPG.
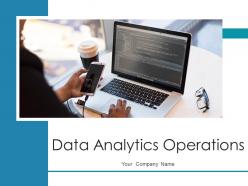
If you require a professional template with great design, then this Data Analytics Operations Business Marketing Sale Developer Community Ecosystem is an ideal fit for you. Deploy it to enthrall your audience and increase your presentation threshold with the right graphics, images, and structure. Portray your ideas and vision using eleven slides included in this complete deck. This template is suitable for expert discussion meetings presenting your views on the topic. With a variety of slides having the same thematic representation, this template can be regarded as a complete package. It employs some of the best design practices, so everything is well-structured. Not only this, it responds to all your needs and requirements by quickly adapting itself to the changes you make. This PPT slideshow is available for immediate download in PNG, JPG, and PDF formats, further enhancing its usability. Grab it by clicking the download button.
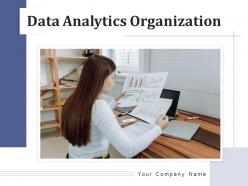
Engage buyer personas and boost brand awareness by pitching yourself using this prefabricated set. This Data Analytics Organization Environment Representing Financial Information is a great tool to connect with your audience as it contains high-quality content and graphics. This helps in conveying your thoughts in a well-structured manner. It also helps you attain a competitive advantage because of its unique design and aesthetics. In addition to this, you can use this PPT design to portray information and educate your audience on various topics. With twelve slides, this is a great design to use for your upcoming presentations. Not only is it cost-effective but also easily pliable depending on your needs and requirements. As such color, font, or any other design component can be altered. It is also available for immediate download in different formats such as PNG, JPG, etc. So, without any further ado, download it now.
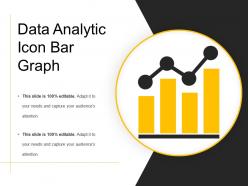
Presenting Data Analytic Icon Bar Graph template. The slide is compatible with Google Slides which makes it accessible at once. The slide is completely editable. It can be saved in various document formats such as JPEG, PNG, or PDF. Moreover, both standard screen(4:3) and widescreen(16:9) aspect ratios are supported. High-quality graphics ensure that distortion does not occur.
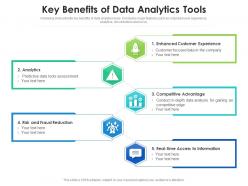
Following slide exhibits key benefits of data analytics tools. It includes major features such as- improved user experience, analytics, risk reduction and so on. Presenting our set of slides with Key Benefits Of Data Analytics Tools. This exhibits information on five stages of the process. This is an easy-to-edit and innovatively designed PowerPoint template. So download immediately and highlight information on Analytics, Information, Competitive Advantage, Customer Experience, Risk.
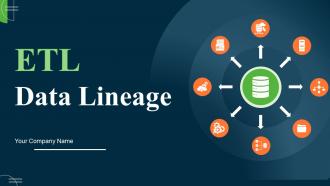
Enthrall your audience with this ETL Data Lineage Powerpoint Presentation Slides. Increase your presentation threshold by deploying this well-crafted template. It acts as a great communication tool due to its well-researched content. It also contains stylized icons, graphics, visuals etc, which make it an immediate attention-grabber. Comprising ninety slides, this complete deck is all you need to get noticed. All the slides and their content can be altered to suit your unique business setting. Not only that, other components and graphics can also be modified to add personal touches to this prefabricated set.
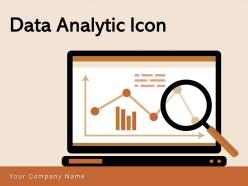
This complete deck can be used to present to your team. It has PPT slides on various topics highlighting all the core areas of your business needs. This complete deck focuses on Data Analytic Icon Business Growth Analysis Gear Magnifying Glass Dashboard and has professionally designed templates with suitable visuals and appropriate content. This deck consists of total of twelve slides. All the slides are completely customizable for your convenience. You can change the colour, text and font size of these templates. You can add or delete the content if needed. Get access to this professionally designed complete presentation by clicking the download button below.
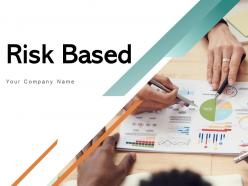
It covers all the important concepts and has relevant templates which cater to your business needs. This complete deck has PPT slides on Risk Based Approach Success Assurance Management Analytics Assessment with well suited graphics and subject driven content. This deck consists of total of twelve slides. All templates are completely editable for your convenience. You can change the colour, text and font size of these slides. You can add or delete the content as per your requirement. Get access to this professionally designed complete deck presentation by clicking the download button below.
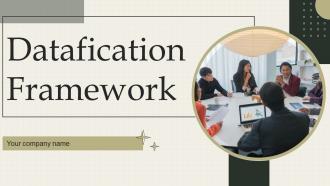
This complete presentation has PPT slides on wide range of topics highlighting the core areas of your business needs. It has professionally designed templates with relevant visuals and subject driven content. This presentation deck has total of fifty six slides. Get access to the customizable templates. Our designers have created editable templates for your convenience. You can edit the color, text and font size as per your need. You can add or delete the content if required. You are just a click to away to have this ready-made presentation. Click the download button now.
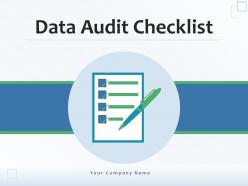
Deliver a lucid presentation by utilizing this Data Audit Checklist Analytics Organization Maintenance Management Business. Use it to present an overview of the topic with the right visuals, themes, shapes, and graphics. This is an expertly designed complete deck that reinforces positive thoughts and actions. Use it to provide visual cues to your audience and help them make informed decisions. A wide variety of discussion topics can be covered with this creative bundle such as Data Audit Checklist, Analytics, Organization, Maintenance, Management. All the twelve slides are available for immediate download and use. They can be edited and modified to add a personal touch to the presentation. This helps in creating a unique presentation every time. Not only that, with a host of editable features, this presentation can be used by any industry or business vertical depending on their needs and requirements. The compatibility with Google Slides is another feature to look out for in the PPT slideshow.
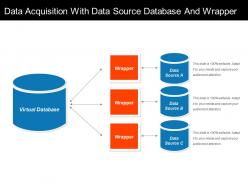
Presenting data acquisition with data source database and wrapper. This is a data acquisition with data source database and wrapper. This is a three stage process. The stages in this process are signal processing, data acquisition, signal acquisition.
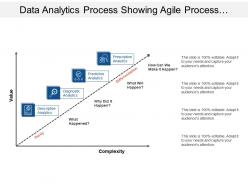
Presenting this set of slides with name - Data Analytics Process Showing Agile Process With Value And Complexity. This is a four stage process. The stages in this process are Data Analytics Process, Data Analysis Cycle, Data Visualization Process.
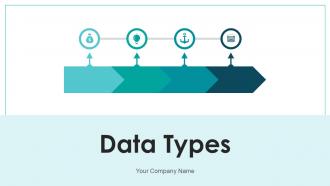
Engage buyer personas and boost brand awareness by pitching yourself using this prefabricated set. This Data Types Analytics Business Programming Financial Government Statistics is a great tool to connect with your audience as it contains high-quality content and graphics. This helps in conveying your thoughts in a well-structured manner. It also helps you attain a competitive advantage because of its unique design and aesthetics. In addition to this, you can use this PPT design to portray information and educate your audience on various topics. With twelve slides, this is a great design to use for your upcoming presentations. Not only is it cost-effective but also easily pliable depending on your needs and requirements. As such color, font, or any other design component can be altered. It is also available for immediate download in different formats such as PNG, JPG, etc. So, without any further ado, download it now.
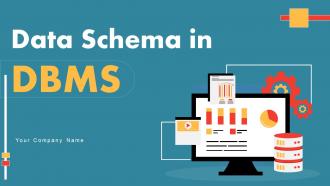
Deliver this complete deck to your team members and other collaborators. Encompassed with stylized slides presenting various concepts, this Data Schema In DBMS Powerpoint Presentation Slides is the best tool you can utilize. Personalize its content and graphics to make it unique and thought-provoking. All the fifty eight slides are editable and modifiable, so feel free to adjust them to your business setting. The font, color, and other components also come in an editable format making this PPT design the best choice for your next presentation. So, download now.
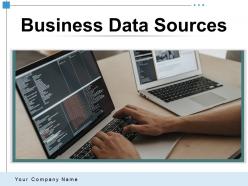
Introduce your topic and host expert discussion sessions with this Business Data Sources Product Customer Analytics Organization Generation. This template is designed using high-quality visuals, images, graphics, etc, that can be used to showcase your expertise. Different topics can be tackled using the twelve slides included in this template. You can present each topic on a different slide to help your audience interpret the information more effectively. Apart from this, this PPT slideshow is available in two screen sizes, standard and widescreen making its delivery more impactful. This will not only help in presenting a birds-eye view of the topic but also keep your audience engaged. Since this PPT slideshow utilizes well-researched content, it induces strategic thinking and helps you convey your message in the best possible manner. The biggest feature of this design is that it comes with a host of editable features like color, font, background, etc. So, grab it now to deliver a unique presentation every time.
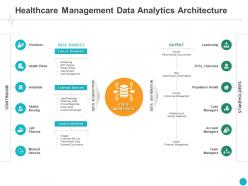
Presenting this set of slides with name Healthcare Management Data Analytics Architecture Leadership Ppt Powerpoint Presentation. This is a twelve stage process. The stages in this process are Management, Data, Analytics, Architecture, Leadership. This is a completely editable PowerPoint presentation and is available for immediate download. Download now and impress your audience.
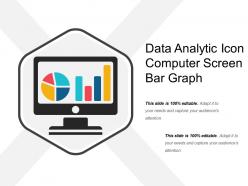
Presenting data analytic icon computer screen bar graph. This is a data analytic icon computer screen bar graph. This is a one stage process. The stages in this process are data analytics icons, information analytics icons, content analytics icons.
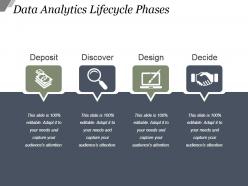
Presenting data analytics lifecycle phases powerpoint slide background designs. This is a data analytics lifecycle phases powerpoint slide background designs. This is a four stage process. The stages in this process are deposit, discover, design, decide.
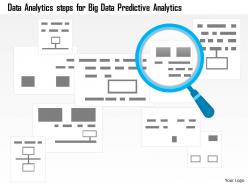
Versatile and dynamic PPT presentation layouts. Loaded with influential patterns and icons. Offers thousands of icons to alter the appearance. Integrated with subtle complementary colours to impress the audience. Can be viewed in big screens without affecting the quality of images. Easy to merge and to operate. Performs incredibly fast.
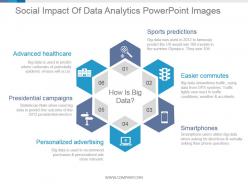
Presenting social impact of data analytics powerpoint images. This is a social impact of data analytics powerpoint images. This is a six stage process. The stages in this process are how is big data, sports predictions, easier commutes, smartphones, personalized advertising, presidential campaigns, advanced healthcare.
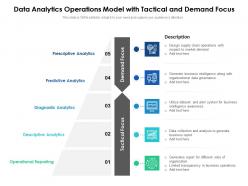
Introducing our premium set of slides with name Data Analytics Operations Model With Tactical And Demand Focus. Ellicudate the five stages and present information using this PPT slide. This is a completely adaptable PowerPoint template design that can be used to interpret topics like Prescriptive Analytics, Diagnostic Analytics, Operational Reporting. So download instantly and tailor it with your information.
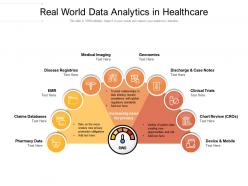
Presenting this set of slides with name Real World Data Analytics In Healthcare. This is a ten stage process. The stages in this process are Clinical Trials, Pharmacy Data, Device And Mobile. This is a completely editable PowerPoint presentation and is available for immediate download. Download now and impress your audience.
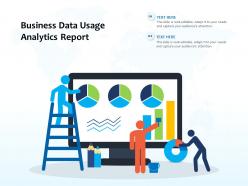
Introducing our Business Data Usage Analytics Report set of slides. The topics discussed in these slides are Business Data Usage Analytics Report. This is an immediately available PowerPoint presentation that can be conveniently customized. Download it and convince your audience.
Item 1 to 60 of 262 total items
- You're currently reading page 1

PCR/qPCR Data Analysis
A Technical Guide to PCR Technologies
- PCR/qPCR Qualitative Analysis
qPCR Data Analysis
- Deriving Accurate Cq Values
Setting the Threshold
Qpcr quantification strategies, standard curve quantification, relative/comparative quantification, normalization, reference gene selection, analysis of reference gene stability, alternative normalization methods, statistical analysis and data visualization, visualization techniques for univariate analysis, statistical tests, hierarchical clustering, principal component analysis, pcr/qpcr qualitative data analysis.
After a traditional PCR has been completed, the data are analyzed by resolution through an agarose gel or, more recently, through a capillary electrophoresis system. For some applications, a qPCR will be run with the end-point data used for analysis, such as for SNP genotyping. In each case, endpoint data provides a qualitative analysis after the PCR has reached plateau phase. In some cases, it may be possible to analyze end-point data to make a semi-quantitative analysis of the PCR yield, but quantitative measurements are more often made using qPCR and analysis of quantification cycle values (C q ) 1 values.
Throughout this guide, the factors that contribute to variations in the measurement of nucleic acid using PCR or qPCR have been highlighted. Each of these factors should be optimized to result in an assay that provides the closest possible value to the actual quantity of gene (target) in the reaction. The result of these processes is the generation of a set of C q values for each target in each sample. The process of deriving and analyzing those C q values to provide reliable data that represent the biological story is presented in this chapter.
Deriving Accurate C q Values
Baseline correction.
A C q value is determined for each target in each sample. Different analysis packages that are associated with different instruments, have alternative approaches for determining the C q (and also use alternative names, e.g., C t , C p , take off point). It is beyond the scope of this guide to delve into the fine details of all of these algorithms. However, qPCR measurements that are based on amplification curves are sensitive to background fluorescence. The background fluorescence may be caused by a range of factors, which include choice of plasticware, remaining probe fluorescence that is not quenched, light leaking into the sample well, and differences in the optical detection for a given microtiter plate well. In well-designed assays, the background is low when compared to the amplified signal. However, variation in background signal may hinder quantitative comparison of different samples. Therefore, it is important to correct for background fluorescence variations that cause differences in the baseline ( Figure 10.1 ).

Figure 10.1 The components of amplification plots. This graph shows the increase of fluorescence with the number of cycles for different samples. The threshold is set above the detection limit but well below the plateau phase during which the amplification rate slows down.
A common approach is to use the fluorescence intensity during early cycles, such as between cycles 5 to15, to identify a constant and linear component of the background fluorescence. This is then defined as the background or baseline for the amplification plot. Due to transient effects, it is advisable to avoid the first few cycles (e.g., cycles 1 to 5) for baseline definition because these often show reaction stabilizing artefacts. The more cycles that are used for the baseline correction, the better the potential accuracy of the linear component of the baseline variations. Many instrument software packages allow manual setting of the cycles to be considered for baseline definition. These functions should be explored by the user and the temptation to accept default settings strongly resisted.
An example of the effect of baseline setting is shown in Figure 10.1 . As can be seen, C q values and the apparent shape of the amplification plot are affected by accurate baseline setting. In the example, the baseline for the curve labeled C3 has been incorrectly adjusted manually so that the baseline cycles calculated from the data in cycles 5 to cycle 31. This causes the curve to dip blow the zero baseline level ( Figure 10.2A ) with a C q of 28.80. To correct this, the raw data, R, are viewed and the last cycle of the linear background (the last cycle before amplification) is identified. In Figure 10.2B , this can be seen to be cycle 22. The baseline is correctly set to be zero between cycle 5 and cycle 22 ( Figure 10.2C ), and the amplification plot is then corrected ( Figure 10.2D ). The corrected C q is 26.12. Therefore, note that there was a substantial difference between the C q values with the incorrect and correct baselines settings, demonstrating that setting the correct baseline is an important component of data analysis.

Figure 10.2A–B. A) Typical example of data dropping below the zero normalized fluorescence reading when the baseline setting is incorrect (blue amplification plot). B) Raw data of the same amplification plots showing the limit of the linear baseline and that the data are not at fault.

Figure 10.2C–D. C) The limits of the start and end of the baseline are defined using the appropriate software settings. D) Application of the corrected baseline setting results in good quality data
Although some researchers advocate mapping individual amplification plot to estimate amplification efficiency and target quantities in measured samples 2,3,4 , the original and most common approach to deriving the C q is to use a threshold. The wide adoption of this approach is likely to be due to the threshold method being a simple and effective quantification method.
The principle behind the threshold method is that; in order to visualize the associated fluorescent signal from the qPCR amplification, the signal must increase so that it is above the detection limit of the instrument (and therefore, the baseline; Figure 10.1 ). The number of cycles required for this to occur is proportional to the initial starting copy number of the target in the sample. Hence, more cycles are required for the signal to increase above the baseline if the original copy number is low and fewer cycles if the copy number is high. Since the baseline is set at the limit of detection for the system, measurements at the baseline would be very inaccurate. Therefore, rather than measuring to the intensity of minimum fluorescence that the system can detect, a higher fluorescence is selected and an artificial threshold is introduced.
The selection of the threshold intensity requires adherence to some fundamental principles. It is important that the threshold is set at a fixed intensity for a given target and for all samples that are to be compared. If there are too many samples to fit on a single plate, then an inter-plate calibration scheme must be adopted, e.g., inclusion of a replicated control that serves as an inter-plate control or a standard curve serial dilution. In theory, the threshold can be set anywhere on the log-linear phase of the amplification curve. However, in practice, the log-linear phase of the amplification may be disturbed by the background fluorescence baseline drifting, the plateau phase, or differences in assay efficiency and therefore amplification plot gradient at higher cycles. It is recommended that the threshold is set as follows:
- Sufficiently above the background fluorescence baseline to be confident of avoiding the amplification plot crossing the threshold prematurely due to background fluorescence.
- In the log phase of the amplification plot where it is unaffected by the plateau phase (this is most easily seen by viewing the amplification plots on a log view, Figure 10.3A ).
- At a position where the log phases of all amplification plots are parallel.
The process of threshold setting is demonstrated in Figure 10.3 . In Figure 10.3A , the amplification plots are viewed on a Y axis log scale, thus providing a visual expansion of the log phase of amplification and presenting this as a linear portion of the amplification plot. The threshold is set at the highest fluorescence intensity (refer to Y axis) that is within this log phase and where all amplification plots are parallel. The scale is then returned to the linear view ( Figure 10.3B ) showing the highest setting that fulfils the threshold setting requirements. Alternatively the threshold may be set at the lower end of this log phase ( Figures 10.3C and 10.3D ). As long as the log phase of the amplification plots are parallel, the ΔC q between samples is unaffected by the threshold setting.

Figure 10.3 The threshold setting influences the absolute Cq recorded and can influence ΔCq between samples. A). Using a log vs linear plot of the data, the threshold is set at the highest fluorescence intensity but where the amplification plots show parallel log phases. B). The threshold setting is maintained from A) and is displayed on the linear vs linear plot. C). Using a log vs linear plot of the data, the threshold is set at the lowest fluorescence intensity but where the amplification plots show parallel log phases. D). The threshold setting is maintained from C) and is displayed on the linear vs linear plot. In each case, the ΔCq values between samples are the same.
The requirement for a threshold setting at a position where the log-linear phases of the amplification plots are parallel becomes more pertinent when data at higher cycles are included in the analysis. The threshold setting procedure that was described for the data in Figure 10.3 was repeated on a data set of higher C q and the results presented in Figure 10.4 . The resulting C q data in Table 10.1 serve to illustrate the variability in the C q , and more importantly, the ΔC q values for three amplification plots with three threshold settings ( Figure 10.4 ). The ΔC q values and therefore the estimate of the relative quantity of target in each sample are highly dependent on the setting of the threshold ( Figure 10.4 ) because the amplification plots are not parallel.

Figure 10.4. The analysis that was performed and demonstrated in Figure 10.3 was repeated using a different data set. In this case, the amplification plots are not parallel due to a difference in efficiency of the reaction at high Cq. The lowest settings for A) and B) result in different ΔCq values than the highest settings for C) and D) (Summarized in Table 10.1).
Accurate baseline and threshold setting is imperative for reliable quantification. After setting each of these, a C q value is generated and this is used as the basis for quantification. The quantity of target in a given sample is then determined using either a standard curve or relative/comparative quantification.
As the name implies, standard curve quantification requires the use of a standard curve to determine quantities of targets in test samples. All quantities determined for samples are, therefore, relative to the quantity assigned to the standard curve. This requires running additional, external standards alongside every set of sample reactions. The choice of material for the standard curve is important for eliminating potential differences in quantification due to differences between assay efficiencies in the samples and in the standards. The primer binding sites of the external standards must be the same as those in the target, contain sequences that are the same as the target, have similar complexity and be handled in as similar a manner as possible. Therefore, when measuring the concentration of a target in cDNA, it is preferable to measure the same cDNA in a serial dilution of a control sample. However, for some studies there are practical reasons that prevent this, so it is important to reproduce the sample conditions as closely as possible, e.g., by adding gDNA from a species unrelated to the test species, to an artificial oligonucleotide standard or linearized plasmid carrying the standard sequence. Once a suitable construct or amplicon is identified, a standard curve of serial dilutions is generated. The C q for the target is determined for each of the standards and plotted against the concentration or relative concentration/dilution factor on a log scale. This results in a standard curve that is then used to determine the concentrations of test samples by comparison of the C q values derived from amplification of the unknown samples. When using a standard curve for quantification, the threshold setting must be kept constant for determination of C q for the standard and for the samples on the same plate. The threshold can differ between plates.
Relative or comparative quantification uses the difference in C q as a determinant of the differences in concentration of the target sequence in different samples. Rather than measuring quantities of target per sample as with the standard curve method, this leads to sets of data showing fold changes between samples.
In the original form of this approach 5 , the efficiency of all of the assays was assumed to be 100%, leading to the assumption that a C q difference of 1 (ΔC q = 1) was as the result of a 2-fold difference in target. To determine a fold change in the target or gene of interest (GOI), the data must also be referenced to a loading control (reference gene, ref; see the following for a discussion regarding data normalization).
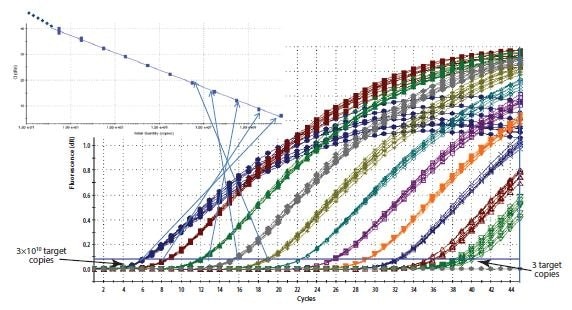
Figure 10.5. Construction of a Standard Curve. The Cq recorded for each sample of a dilution series is plotted on a log linear scale against the relative concentration.
In Equation 1 , the ratio of the GOI, after correction to the ref gene, in 2 samples (A relative to B) is measured as: 2 (assuming 100% efficient reactions) raised to the power of the differences in the C q values for the GOI divided by 2 raised to the power of the differences in the C q values for the ref gene

Equation 1. Original (Livak) Relative Quantification Model.
However, as illustrated in Assay Optimization and Validation , the efficiencies of reactions vary considerably and this can have a large impact on data. Therefore, the assumptions in Equation 1 were addressed ( Equation 2 ) 6 , so that the differences in reaction efficiencies could be incorporated into the analyses. In this case, the amplification factor 2 is replaced by the actual efficiency of the PCR (as determined by a standard curve analysis; see Assay Optimization and Validation ).

Equation 2. Efficiency Adapted (Pfaffl) Relative Quantification Model
As an example of using the efficiency adapted ( Equation 2 ) relative quantification model, a set of C q values are presented in Table 10.2 . The efficiency for the GOI is 1.8 and for the ref gene 1.94.
This is a very simple example of a study with the requirement to measure the fold difference between one gene in two samples and after normalization to a single reference gene. The ratio shows the fold change of the GOI in sample 2 relative to sample 1, after correction to the single Ref gene. However, it has become apparent that selection of a single, suitable reference gene is often impossible and, therefore, more sophisticated approaches for normalization have been suggested.
The major objective of most PCR-based experiments is to address the basic question of whether the target is present in the sample (unknown, UNK). At the very simplest level, this is answered by running a gel and examining the fragments for the presence or absence of the desired GOI. When the fragment is present, the confirmation of fragment size gives reassurance of a positive result. However, when absent, there is the potential of a false negative result. Therefore, it is critical to repeat the test assay and also perform at least one additional PCR to serve as a loading and positive PCR control. The universal, inhibition control assay, SPUD (see Sample Purification and Quality Assessment ), can be used to support confidence in a negative result. An alternative approach is to run an assay that is specific to a reference gene or genes. Traditionally, PCR assays detecting the reference genes, GAPDH, 18S ribosomal RNA, or β actin were run alongside those for the GOI and the resulting fragments visualized on a gel. GAPDH, 18S ribosomal RNA, and β actin are constitutively expressed and were therefore used as loading controls in semi-quantitative analyses. However, it soon became apparent that these genes are not ubiquitously expressed at the same concentration in all cells, regardless of experimental design. Therefore, the need arose for a stable reference when the objective was to measure relative nucleic acid concentrations, usually cDNA but also gDNA when, for example, examining the copy number variation of a gene.
Normalization is the process of correcting technical measurements to a stable reference in order to examine true biological variation. There are many methods for normalizing technical differences which means that the appropriate approach for the specific experiment must be selected and validated 7 . It is critical to recognize that adoption of inappropriate normalization techniques may be more detrimental to the overall analytical process than not normalizing at all 8 .
The Effect of Sample Quality On Assay Normalization
The effect of sample integrity and purity on target quantity measurements by qPCR and RT-qPCR was discussed at length ( Sample Purification and Quality Assessment , Sample Quality Control and Reverse Transcription , Reverse Transcription). It was demonstrated that inhibitors in the sample and RNA degradation have a differential effect on the measurement of a given target 9 . Inhibitors effect the measurement of any target but to a different degree, depending on the assay design. Degradation of total RNA effects the measurement of mRNA and miRNA 10 , again being highly dependent on the overall experimental design. Therefore, it is critical to consider the effect of template concentration on the RT reaction and the effect of the sample quality on data after normalization. Normalization will not counter the effect of low quality assays or samples (see Assay Optimization and Validation ).
Normalization Approaches
Ideally, normalization methods counteract variability that may be introduced during the multi-step process that is required to perform a qPCR analysis ( Figure 10.6 ). However, applying normalization at any one stage in the process may not control for technical error and/or bias that was, or will be, introduced at an earlier or later stage, respectively. Normalization methods are not mutually exclusive and so adopting a combination of controls is recommended 11 .

Figure 10.6. qPCR is a multistep process and each step must be controlled. Normalization must be considered within a series of controls.
The objective of normalization is to provide a stable reference point against which the measurements can be referred; therefore, the choice of normalization factor must be a measurement which is stable throughout the experiment. This may be stable reference gene(s), or one of the alternatives, such as cell number, tissue mass, RNA/DNA concentration, an external spike 12 , or a representative measure of the global expressed genes.
Reference genes are targets whose quantity does not change as a result of the experiment. When quantifying DNA copy number variation in which the number of copies of the sequence of interest may change, the measurement is simply normalized by targeting an alternative genomic region that is known not to change. An example of how this may be applied is when measuring Human Epidermal Growth Factor Receptor 2 (HER-2) genomic amplification 13 . HER-2 genomic instability is a prognostic indicator in breast cancer and accurate measurement of HER-2 amplification status is important in patient management. HER-2 status can be measured by qPCR by comparing the copies of HER-2 with another genomic target that is acting as a control.
When measuring gene expression, reference genes are targets with mRNA concentrations that do not change as a result of the experiment. An example study would be one in which the effect on the expression of gene X is being measured after addition of a mitogenic compound to a cell monolayer. A reference point is required in order to measure the change in gene X. Therefore, another gene (or genes) that are known not to be affected by the mitogen in question is also measured. This provides the researcher with the immediate challenge of finding a mRNA target that is not affected by the experimental procedure, before being able to study the GOI. This process of validation of reference genes is fundamental for an accurate measurement of the GOI. The most widely used approach to normalization is to ignore this process and normalize the gene expression data to a single, unvalidated reference gene. This practice is not recommended and is in direct opposition to the MIQE guidelines 1 . The quantification of mRNA by RT-qPCR has routinely been compromised by the incorrect choice of reference genes. It is not acceptable to follow the relatively common practices of using a reference gene because the primers are in the freezer already, it was used historically on Northern blots, it is used by a colleague, or used in another laboratory for a different experiment. Reference genes need to be validated under specific experimental scenarios to be assured that the reference gene in question is not affected by the experiment. If this validation is not carried out and the reference gene is affected by the experiment, the results could be incorrect and subsequent interpretations are likely to result in meaningless data 8 .
There is a range of scientific literature describing different methods for normalization 7-14 as well as a plethora of publications describing the protocols required to identify the most appropriate normalizer genes for a given experimental scenario. While in the past, a key question was whether to select single or multiple reference genes, lower running costs means that current best practices have moved towards measuring multiple reference genes.
Selection of stable reference genes requires the analyst to evaluate the stability of qPCR for a number (usually 10 to 20 genes) of candidate mRNA targets 7 on a subset of samples that represent the test and control mRNAs. A full protocol is provided in Appendix A , Protocols, of this guide and may be used in combination with different analytical methods using programs such as REST 15 , GeNorm 14 , Bestkeeper 16 , or NormFinder 17 . This procedure is described in more detail in the following section, Analysis of Reference Gene Stability.
The reference gene is literally the pivot point for qPCR relative quantification assays. It is therefore critical for the reliability of the entire assay that the reference gene is stable. If the reference gene expression varies between samples, the variation will be directly transferred to the quantification results and the added variability may obscure the desired observable biological effect or, even worse, may create an entirely artificial appearance of a biological effect, one that is unrelated to the actual gene of interest. For these reasons, it is strongly recommended that several safety measures are followed to render reference gene variability insignificant and make measures of biological effects as significant as possible.
Arguably, the most important safety measure is to use not only one, but two or more, reference genes. The expression of several reference genes can be averaged to reduce technical variability due to normalization. This can be useful to improve significance in measurements of small biological effects. However, more importantly, two or more reference genes provide mutual controls for maintained stability and control for unexpected occurrences that may influence the expression levels of one of the reference genes. With a single reference gene, there is a risk that unexpected influences of gene expression may be undetected in the assay.
Another safety measure is to use more than one method of identifying stable reference genes. The following is an example to illustrate several aspects of reference gene normalization, including a possible advantage of using both geNorm and NormFinder methods on the same data set.
Table 10.3 holds a list of reference gene candidates that were evaluated during a workshop we previously conducted with EMBL. Samples were collected from a human cell culture in two different treatment groups. This data set will be used to demonstrate aspects of reference gene validation.
Both the NormFinder and geNorm algorithms have been developed with the assumption that testing a multitude of reference gene candidates can be used to rank the stability of individual reference gene candidates. The assumption may be true if, for example, all reference gene candidates vary stochastically around stable expression levels. However, this may not necessarily be true in reality. To avoid misleading results, it is therefore prudent to avoid regulated and in particular co-regulated reference gene candidates.
The list of reference gene candidates shown in Table 10.3 was specifically chosen to select genes that belong to different functional classes, reducing the chance that the genes may be co-regulated. A notable exception is GAPDH, which is present here in two versions. Although this does not affect this analysis, it is best practice is to avoid multiple entries of genes that may be suspected of being co-regulated.
The first algorithm to be demonstrated is geNorm. This provides an evaluation of gene stabilities by calculating a gene stability measure called the M-value, which is based on pairwise comparisons between the analyzed reference gene candidate and all other reference gene candidates in the data set. It is performed in an iterative fashion, meaning that in this example, the procedure is first performed on all 15 reference gene candidates, the least stable is removed, the process is repeated on the remaining 14, the second least stable candidate is removed, and so on until two reference genes remain.
There may be times when identification of the most stable reference gene may be particularly challenging. One case may be when all reference gene candidates perform poorly. Another case may be if all reference gene candidates perform well. To distinguish between these two cases, a useful guideline is that reference genes with an M-value below 0.5 may be considered stably expressed.
The second algorithm to be demonstrated is NormFinder, which is a freely available reference gene analysis package (Appendix B, Additional Resources). The underlying algorithm takes a ANOVA-like approach to reference gene stability evaluation in that the whole and subgroups are analyzed for variations. One advantage of this is that the obtained measures are directly related to gene expression levels. A standard deviation of 0.20 in C q units therefore represents about 15% variation in copy number expression levels of the particular reference gene candidate.
For convenience, in this demonstration, both of these analysis packages are accessed using GenEx (MultiD) data analysis software, but they are also available as independent packages (Appendix B, Additional Resources).
The bar diagrams shown in Figure 10.7 illustrate reference genes ranked according to their respective stability measures using both algorithms. In addition, a graph showing the accumulated standard deviation from NormFinder indicates that a combination of up to the three best reference genes may yield stability improvements.

Figure 10.7. Bar diagrams showing stability measures: M-values for geNorm and standard deviations for NormFinder. In addition, a graph showing the accumulated standard deviation from NormFinder indicates that a combination of up to the three best reference genes may yield stability improvements. The data set was generated from assays designed for the reference gene candidates shown in Table 10.3 and measured on a human cell culture in two different treatment groups. Notice that, in this instance, the reference gene stability algorithms geNorm and NormFinder do not agree about the best reference genes.

Figure 10.8. Mean centered expression profile of the reference gene candidates of the two samples in each treatment group. Samples 1 and 2 belong to the first treatment group and samples 3 and 4 belong to the second treatment group. Expression profiles of SDHA and CANX are indicated in red. Expression profile of UBC is indicated in yellow. The table lists the measured Cq values in the data set.
Due to the deviating expression profiles, it is possible that SDHA and CANX are regulated by the different treatment alternatives and therefore, are not suitable as reference genes. Removing these from the data set and repeating the analysis results in agreement between both algorithms and that the best choice of reference genes is EIF4A2 and ATP53 ( Figure 10.9 ). In the NormFinder calculation of accumulated standard deviations, it is also apparent that the addition of more reference genes does not improve stability.

Figure 10.9. Inspection of the expression profiles and measured Cq values (Figure 10.8) raised concern that SDHA and CANX may be co-regulated in the applied assay. The co-regulation may disrupt reference gene stability algorithms. Bar diagrams showing stability measures: A) M-values for geNorm and B) standard deviations for NormFinder. The data set is the same as the one used in Figure 10.8 except that the data for SDHA and CANX have been removed. Notice that with this reduced data set the reference gene stability algorithms geNorm and NormFinder do agree about the best reference genes.
The analysis of data in this example serves to illustrate that using geNorm and NormFinder in parallel allows for identification of co-regulated reference gene candidates and that removing these genes from further studies provides a final identification of reference genes that can be adopted with more confidence than after using a single analysis. Identification and selection of stable reference genes leads to greater security of data analysis.
While normalization to reference genes is the most common method for assay normalization, there are situations where this approach is not suitable, such as when a large number of genes in a heterogeneous group of samples is to be compared, or when profiling miRNA. In these scenarios it is necessary to adopt an alternative strategy.
Normalization to Tissue Mass or Cell Number
Measurement of cell number or tissue mass to use as a normalization factor is not as simple as it may first appear. Cell culture experiments are relatively easy to normalize based on cell count. However, addition of a treatment might impact cell morphology, complicating the ratio of cell number to total RNA/genes expressed when compared with a control culture. The experimental treatment may result in the production of extra cellular matrix causing differences in nucleic acid extraction efficiencies.
Biological tissues can be highly heterogeneous within and between subjects, with more variation being apparent when healthy tissue is compared with diseased tissue. Even apparently less complex tissues, such as blood, can differ considerably in cell count and composition such that gene expression varies considerably between apparently healthy donors 18 .
Any delays in the processes used to purify nucleic acid will result in alterations in the measured RNA. For example, delays in processing peripheral blood mononuclear cells and extracting RNA from cells, results in considerable changes in gene expression 19 . The methods underlying the extraction procedures are also major sources of technical variation. Even the isolation process selected for sampling blood derived cells and RNA purification result in differences in apparent gene expression profiles 20 . Therefore, the first normalization consideration is to ensure that collection and processing is absolutely identical for all samples. It is then critical to perform sufficient quality control to be certain of the sample concentration, integrity, and purity ( Sample Purification and Quality Assessment and associated protocols in Appendix A ).
Normalization to RNA Concentration
As a minimum, an estimation of template concentration (DNA for qPCR or RNA for RT-qPCR) is important and, as mentioned in Sample Purification and Quality Assessment , it is critical to ensure that the same instrument is used for all measurements because the determination of nucleic acid concentration is also variable and technique dependent.
When measuring total RNA concentration, the vast majority of the sample is composed of rRNA, with only a small fraction consisting of the mRNA of interest when examining gene expression, or the sncRNA when examining gene expression regulation. This means that if the rRNA concentration increases a small amount but the mRNA remains constant, the total RNA concentration will increase. The mRNA concentration must increase a significant amount to cause an apparent increase in the total RNA concentration. Hence, rRNA concentration is an unreliable measure of the mRNA concentration, but for many protocols, equal RNA concentration is required to ensure accurate reverse transcription (see Reverse Transcription ).
Normalization to Global Gene Expression
When measuring large numbers of targets, the analyst can estimate the global mean of the total gene expression and identify regulated RNA sequences that deviate from this mean. This approach is conventionally used for normalization of gene expression arrays. It is a valuable alternative to using reference genes and may be preferable where many targets are being measured.
Another recently explored approach is the measurement of endogenously expressed repeat elements (ERE) that are present within many of the mRNAs. Many species contain these repeat elements (ALU in primates, B elements in mice), which can provide an estimation of the mRNA fraction. Measurement of these target sequences has been shown to perform as conventional normalizing systems 9 (Le Bert, et al., in preparation) and may offer a universal solution or an alternative for complex experiments where stable reference gene combinations are unavailable.
Normalization of miRNA Data
As yet there have been no reports of a miRNA universal reference gene. Therefore, the selection of normalization system is still rather empirical. When possible, stable invariant miRNAs may be identified from genome-wide approaches, i.e., microarrays. Small nucleolar RNAs (snoRNAs) have also been used as reference genes. Global gene expression is also a useful method of normalizing miRNA expression when a stable reference is unknown and several hundred targets have been analyzed 21,22,23 . This method is more appropriate for those using approaches resulting in capture of all miRNAs as cDNA in a multiplexed form, e.g., Exiqon and miQPCR systems (refer to Castoldi et al. in PCR Technologies, Current Innovations 24 ).
Biological and Technical Replicates
The purpose of normalization is to avoid systematic errors and to reduce data variability for the eventual statistical analysis. Another important aspect of setting up data for statistical analysis is the use of data replicates.
Biological replicates are absolutely necessary for statistical analysis. Statistical significance levels are often set at a 5% significance cut-off. For biological effects close to such a significance level, it may be necessary to have at least 20 biological replicates to determine the assays significance level (1:20 corresponding to 5%). In fact, it has been suggested that at least 50 times the number of observations are required to be recorded for an accurate estimate of significance 25 , i.e., on the order of a thousand biological samples. Naturally, practical limitations seldom allow for biological replicates at these levels. Furthermore, accurate estimates of the number of necessary biological replicates to meet a given significance level also depend on the level of variability of the data. Nevertheless, it is important to realize that a common mistake is to underestimate the necessary number of biological replicates to be able to arrive at reliable conclusions. It is recommended to perform an initial pilot study to evaluate the assay’s inherent variability and the potential size of the observable biological effect in order to have a good basis to estimate the necessary number of biological replicates 26 .
Technical replicates are not used directly for the statistical analysis. Instead, technical replicates are used to backup samples (in case some samples are lost in the technical handling process) and to improve assessment of data accuracy. Technical replicates can improve data accuracy if the assumption holds true that they vary stochastically around the accurate measurement at each stage of the technical handling process. The average of the technical replicates is closer to the accurate measurement. The effect of averaging technical replicates can be illustrated by noting the size of the confidence interval in a simulated data set with a predetermined variability, i.e., standard deviation set at one. As seen in Table 10.4 , the confidence interval becomes smaller with an increasing number of technical replicates (samples), indicating a more precise estimate of the accurate measurement. Furthermore, the narrowing of the confidence interval is most dramatic at the low number of technical replicates. Increasing the replicate number from 2–3 decreases the confidence interval from 8.99–2.48, i.e., a more than 3-fold improvement of the precision in the estimate of the accurate measurement. While additional replicates continue to improve the estimate of the accuracy of the measurement, the effect is at a decreasing magnitude. Therefore, it is apparent that in cases where technical handling variability is an issue, it may be a great advantage to use triplicates rather than duplicates.
Technical replicates can be collected at several stages throughout the sample handling process, including RNA extraction, reverse transcription and qPCR detection. If technical replicates are detected at several stages, a nested experimental design is generated. A pilot study that takes advantage of a nested experimental design may help to identify sample handling stages that contribute the most to technical handling errors and an optimal sampling plan can be calculated based on this information 27 .
Scientific analysis of biological data centers on the formulation and testing of hypotheses. The formulation of a hypothesis requires a detailed understanding of the conditions and variables of the assay. Successful testing of a hypothesis involves careful execution and an appropriate experimental design to maximize the desired observable signal while minimizing technical variability. In this context, it is useful to distinguish between exploratory and confirmatory studies ( Figure 10.10 ).

Figure 10.10. Flowchart illustrating operations involved in exploratory and confirmatory statistical analyses. The left-hand side of the figure, before the dashed arrow, shows operations in an exploratory statistical study. The right-hand side of the figure, after the dashed arrow, shows operations in a confirmatory statistical study.
The purpose of the exploratory study is to analyze data with one or several different techniques in order to substantiate a hypothesis. The data set may be redefined and/or different analysis techniques may be employed repeatedly in order to support one or several hypotheses. The exploratory study is thus very flexible to the specifics of any scientific question. However, the repeated probing of hypotheses testing on one data set may lead to issues that undermine statistical conclusions. This is due to multiple testing, which refers to the fact that a statistical test with several independent hypotheses is more likely to yield a positive significance and that the chances of this increases as additional hypotheses are tested, even if the underlying probability distributions are identical. To avoid misleading statistical results, the exploratory study is therefore often combined with a confirmatory study.
The requirements for a confirmatory study are based on much stricter statistical criteria. First, the hypothesis of study, including criteria for significance, needs to be defined before the collection of data and before the analysis. In addition, the data set for analysis needs to have been collected exclusively for this purpose. It is statistically incorrect to reuse the data set from the exploratory study in the confirmatory study since that data set would inherently favor the proposed hypothesis. The end result of the confirmatory study is a rejected or accepted hypothesis according to the pre-stated criteria.
For statistical testing, the likelihood that an observed phenomenon occurred by random chance is analyzed. This is called the Null hypothesis 28 . If the observed phenomenon is rare according to the Null hypothesis, the conclusion is that it is unlikely that the Null hypothesis is valid. The Null hypothesis is rejected and the likelihood of the alternative hypothesis as significant is accepted.
The estimated likelihood that the observed phenomenon occurred by random chance is called the p -value. The p -value is measured in a range from 0 to 1, or equivalently, in percentage units. The statistical criteria for a confirmatory study include an alpha cut-off under which calculated p -values would indicate significance for the observed phenomenon. An alpha cut-off of 5% is commonly used, although this must be adjusted to fit desired and necessary criteria that are specific to the subject of study.
Many algorithms have been developed for calculating p -values under various assumptions and for different purposes. A common algorithm is the Student’s t-test. The Student’s t-test is used to calculate a p -value based on the difference in the mean values between two groups of data. The main assumption of Student’s t-test is that the two groups of data are independent and conform to normal distributions. An advantage of the Student’s t-test is that it is powerful, compared to nonparametric statistical tests 29 . A non-parametric test that is equivalent to the Student’s t-test may be one of the most well-known non-parametric statistical tests; the Wilcoxon rank-sum test (sometimes called Mann-Whitney U test; not to be confused with Wilcoxon signed-rank test which is used to compare two paired groups). Non‑parametric statistical tests, such as the Wilcoxon ranksum test, have an advantage over parametric statistical tests, such as the Student’s t-test, in that they do not depend on prior assumptions of the data set distributions. A Kolmogorov- Smirnov’s test for normal distribution may be used to decide whether to apply the Student’s t-test or one of the nonparametric tests
In addition to the choice of algorithm for p -value calculation, data sets that are fed into the p -value calculation algorithm may be manipulated to facilitate observation of desired properties in the data set. The combination of raw data manipulation steps and choice of p -value calculation algorithm is part of building a hypothesis model.
There is a high level of freedom in building hypothesis models in the exploratory phase of a statistical analysis and this is an important part of scientific inquiry. However, a hypothesis is never proven using a scientific, statistical approach. A correct scientific approach is to formulate a Null hypothesis, use an independent (preferably a newly collected) data set, and accept or reject the Null hypothesis according to the confirmatory study flowchart ( Figure 10.10 ).
Just as there are many analysis methods available, there are also many data visualization techniques from which to choose. For univariate data analysis, a simple bar diagram with associated error bars is an appropriate visualization technique. Even though this is a common and simple visualization technique, there are issues that are worth emphasizing. First, error bars may illustrate different sources of variability; the inherent variability of the data (the standard deviation, SD) or the precision by which the mean value has been determined. Secondly, the precision by which the mean value has been determined can be illustrated in different ways, but it ultimately depends on a combination of the inherent variability of the data together with the number of samples (N) and in its raw form, it is called the standard error of the mean (SEM, Equation 1 ):

Equation 1. SEM
However, the SEM is not a very intuitive measure and it is not straight forward to compare SEMs from different experiments in a meaningful way. A more popular way of illustrating the precision of the estimated mean and indicating statistical significance in a graphical way, is the confidence interval (CI, Equation 2 ):

Equation 10-2. Cl
The presence of the SEM can be recognized in the equation for the confidence interval as the ratio between the standard deviation (SD) and the square root of the number of samples (N) and thus it is evident that the confidence interval is based upon the SEM. The lower limit of the confidence interval is constructed by subtracting the SEM multiplied by a percentile of a t-distribution from the mean. The upper limit of the confidence interval is constructed by adding the SEM multiplied by a percentile of a t-distribution from the mean. The confidence level of the confidence interval is set by the confidence level associated with the critical value t*; typically a 95% confidence level.
Figure 10.11 shows a bar graph with error bars denoting the 95% confidence interval within each experimental group, highlighting the uncertainty associated with the mean estimate for an example gene expression in samples from different organs after treatment with several drug doses. In addition, the t-test statistical significance p -values are shown for the difference in gene expression between the control samples and each of the three different samples from different drug dose responses, indicated by means of an asterisk notation. It is customary to have one asterisk correspond to a p -value below 0.05, two asterisks correspond to a p -value below 0.01 and three asterisks correspond to a p -value below 0.001.

Figure 10.11. Fold change (log2) expression of a gene of interest relative to a pair of reference genes, relative to the expression in the sample with lowest expression within each organ type. Bar heights indicate mean expression of the gene in several samples in groups of non-treated (Dose 0) samples or samples treated at one of three different drug doses (Dose 1, Dose 2, and Dose 3). Error bars indicate 95% confidence interval estimates of the mean expressions. One asterisk indicates statistically significant difference between the means of a treated sample set compared to the mean of the non-treated sample set to 5%; two asterisks indicate statistically significant difference to 1%; three asterisks indicate statistically significant difference to 0.1%.
Given that the asterisk notation hides the absolute value of p , it is often encouraged to include a table with the absolute values of p , as shown in the example in Table 10.5 . One reason behind this is that a p -value of for example 0.032 is only slightly more “significant” than a p -value of 0.055. Borderline cases like this can lead to some confusion when deciding precisely what cut-off to use when classifying data as significant. In realistic cases, a p -value of 0.051 could be just as significant as a p -value of 0.049, yet a strict (although fundamentally arbitrary) cut-off of 0.05 would classify one as significant and the other not.
However, there is a variant of the bar diagram visualization that takes advantage of the confidence interval of the difference between means to avoid many, if not all, of the disadvantages of traditional bar diagrams 24 . With the confidence interval of the difference between means, it is possible to estimate directly the statistical significance with associated error bars while at the same time highlight biological effect size and data variability. Figure 10.12 shows the variant with the confidence interval of the difference between means of the data used in Figure 10.11 . Notice that confidence intervals that do not encompass the zero difference between means correspond to significant results at the confidence level corresponding to the p -value cut-off (5% in Figure 10.11 and Table 10.5 ).

Figure 10.12. Bar diagram showing the difference between means of the nontreated sample set (Dose 0) and one of the treated sample sets (Dose 1, Dose 2 or Dose 3) in the data set from Figure 10.11. Error bars show the confidence interval of the difference between means. Error bars that do not cross the x-axis indicate the corresponding means comparison is statistically significant to 5% in a t-test. PCR Technology, Current Innovations-3rd ed. by Taylor and Francis Group LLC Books. Reproduced with permission of Taylor and Francis Group LLC Books in the format reuse in a book/e-book via Copyright Clearance Center.
Multivariate data are data collected on several variables for each sampling unit. The data used in Figures 10.11 and 10.12 are multivariate in that they depend on variables such as dose and organ type. However, the statistical analyses in Figures 10.11 and 10.12 are nevertheless univariate in that each representation (bar) only illustrates one variable, gene expression, relative to fixed measures of the other variables. For multivariate data analysis techniques, hierarchical clustering and principal component analysis are good options for data representation.
One of the easiest and useful methods to characterize data is by plotting the data in a scatterplot (for example plotting measured C q values of one gene against the corresponding C q values of another gene for a set of biological samples in a 2D plot). Plots in one or two dimensions are conveniently visualized by human eyes. Plots in three dimensions may also be possible with appropriate tools, but higher dimensional plots are significantly harder to visualize. However, for exploratory studies, the data set is inherently multidimensional and scatterplots of whole data sets may thus become impractical. From a qPCR data set, there may be, for example, several genes and/or several types of biological samples represented.
A popular, alternative way of characterizing and visualizing data from exploratory studies is to analyze measures of distances between data points in the scatterplot. Different distance measures exist, including Euclidean, Manhattan and Pearson correlations. With computational power, it is straightforward to calculate distances, even for multidimensional data of much higher dimensionality than three dimensions. For agglomerative hierarchical clustering, the following iterative process is performed: 1) Find the two closest objects and merge them into a cluster; 2) Define the new cluster as a new object through a clustering method; 3) Repeat from 1) until all objects have been combined into clusters 30 . Alternatives for clustering methods include Ward’s method, Single linkage and Average linkage 31 . A dendrogram is often used to visualize results from hierarchical clustering.
Interpretation of hierarchical clustering dendrograms of qPCR data often results in conclusions about gene expression profile similarities. In an exploratory study, these similarities may then be used to formulate hypotheses about gene expression coregulation, which may be accepted or rejected in subsequent confirmatory studies. The advantages of hierarchical clustering dendrograms include the clarity by which similarity relationships are visualized. On the other hand, the strong emphasis on similarity measures may be perceived as limiting with respect to formulating hypotheses, since similar expression profiles may be redundant attributes in hypotheses. It may be of higher value to identify sets of expression profiles that complement each other in a specific combination, to answer the desired hypothesis.
Another popular, alternative way to characterize and visualize data from exploratory studies is to take advantage of the information contained in the whole, multidimensional data set, select desired properties and project it to a lower dimensional scatterplot, such as a 2D or 3D plot. This can be achieved using principal components analysis (PCA) 32,33,34, 35 . Here, the original coordinate system of the data set (i.e., the expression profiles measured by qPCR) is transformed onto a new multidimensional space where new variables (principal components: PC or factors) are constructed. Each PC is a linear combination of the subjects in the original data set. By mathematical definition, the PC’s are extracted in successive order of importance. This means that the first PC explains most of the information (variance) present in the data, the second less and so forth. Therefore, the first two or three PC coordinates (termed scores) can be used to obtain a projection of the whole data set onto a conveniently small dimension, suitable for visualization in a 2D or 3D plot. By using the first two or three PCs for representation, the projection that accounts for the most variability in the data set is obtained. Variance from experimental design conditions is expected to be systematic, while confounding variance is expected to be random, so this representation may be desired under appropriate conditions.
As previously noted for hierarchical clustering, the interpretation of qPCR PCA often results in conclusions about gene expression profile similarities. Although PCA and hierarchical clustering may yield complementary insights into gene expression co-regulation patterns, both techniques focus on gene expression profile similarities. This places limitations on the types of hypotheses that can be found in exploratory studies using these techniques alone. To expand on the reach of generated hypotheses in exploratory studies, a hypothesisdriven approach to multivariate analysis was recently proposed 24 . Hypothesis-driven, custom-designed algorithms may identify biologically relevant hypotheses that may otherwise be missed by commonly used techniques for multivariate data analysis.
Network error: Failed to fetch
- PCR/qPCR/dPCR Assay Design
- PCR-based Assay Regulations and Validation
- Mgat4 May Play a Role in Increased Sialylation by Overexpressing Functional MGAT1 in Mgat1-Disrupted Chinese Hamster Ovary (CHO) Cells
- Complete Solutions for PCR Assay Development
- PCR Assay Optimization and Validation
- DNA Oligonucleotide Synthesis
- Locked Nucleic Acid
- Technical Guide to PCR Technologies
To continue reading please sign in or create an account.
Research. Development. Production.
We are a leading supplier to the global Life Science industry with solutions and services for research, biotechnology development and production, and pharmaceutical drug therapy development and production.

© 2024 Merck KGaA, Darmstadt, Germany and/or its affiliates. All Rights Reserved.
Reproduction of any materials from the site is strictly forbidden without permission.
- English - EN
- Español - ES
Create an account
Create a free IEA account to download our reports or subcribe to a paid service.
The Role of Critical Minerals in Clean Energy Transitions
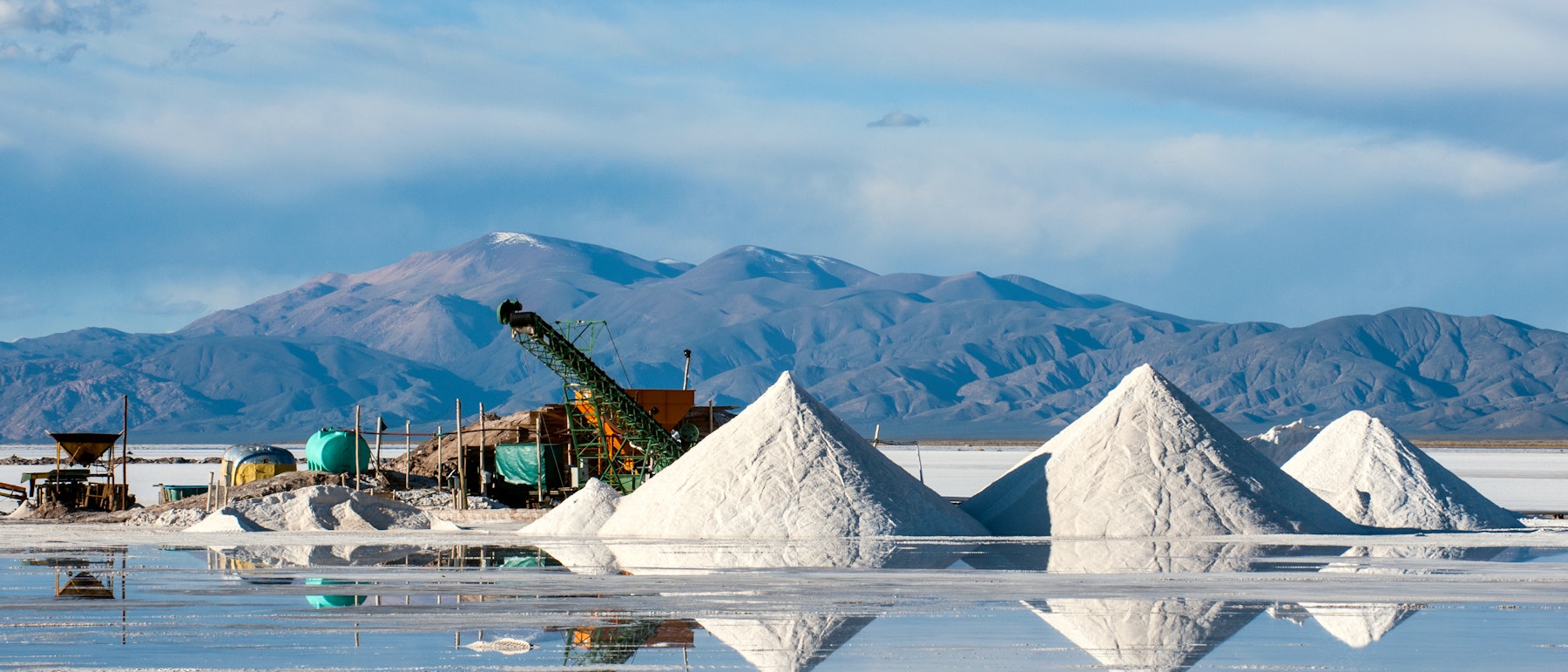
This report is part of World Energy Outlook
About this report
Minerals are essential components in many of today’s rapidly growing clean energy technologies – from wind turbines and electricity networks to electric vehicles. Demand for these minerals will grow quickly as clean energy transitions gather pace. This new World Energy Outlook Special Report provides the most comprehensive analysis to date of the complex links between these minerals and the prospects for a secure, rapid transformation of the energy sector.
Alongside a wealth of detail on mineral demand prospects under different technology and policy assumptions, it examines whether today’s mineral investments can meet the needs of a swiftly changing energy sector. It considers the task ahead to promote responsible and sustainable development of mineral resources, and offers vital insights for policy makers, including six key IEA recommendations for a new, comprehensive approach to mineral security.
Online table of contents
1.0 executive summary.
Read online
2.0 The state of play
3.0 mineral requirements for clean energy transitions, 4.0 reliable supply of minerals, 5.0 sustainable and responsible development of minerals, related files.
- Launch presentation Download "Launch presentation"
- Acknowledgements Download "Acknowledgements"
- References Download "References"
Cite report
IEA (2021), The Role of Critical Minerals in Clean Energy Transitions , IEA, Paris https://www.iea.org/reports/the-role-of-critical-minerals-in-clean-energy-transitions, Licence: CC BY 4.0
Share this report
- Share on Twitter Twitter
- Share on Facebook Facebook
- Share on LinkedIn LinkedIn
- Share on Email Email
- Share on Print Print
Subscription successful
Thank you for subscribing. You can unsubscribe at any time by clicking the link at the bottom of any IEA newsletter.
- Open access
- Published: 16 April 2024
Clinical presentation and management of methanol poisoning outbreaks in Riyadh, Saudi Arabia: a retrospective analysis
- Faisal Alhusain 1 , 2 ,
- Mohammed Alshalhoub 1 , 2 ,
- Moath Bin Homaid 3 ,
- Laila Carolina Abu Esba 2 , 4 ,
- Mohammad Alghafees 2 , 5 &
- Mohammad Al Deeb 1 , 2
BMC Emergency Medicine volume 24 , Article number: 64 ( 2024 ) Cite this article
154 Accesses
5 Altmetric
Metrics details
Acute methanol intoxication, whether unintentional or deliberate, necessitates prompt intervention to prevent severe morbidity and mortality. Homemade alcoholic beverages are a frequent source of such poisoning. This retrospective analysis examined two outbreaks of methanol intoxication in Saudi Arabia. It investigated the clinical presentation, implemented management strategies, and any lasting complications (sequelae) associated with these cases. The aim was to assess the potential impact of different treatment modalities and the timeliness of their initiation on patient outcomes.
This was a retrospective case series of methanol poisoning cases which presented to the adult emergency department (ED) at King Abdulaziz Medical City (KAMC) in Riyadh, Saudi Arabia. There were two separate outbreaks in the city, the first one was from September 1 to September 10, 2020 and the second one was from May 14 to May 20, 2021. Electronic charts were reviewed, and data were extracted to previously prepared data extraction sheets.
From the 22 patients who arrived in the ED alive, the most common complaints were nausea or vomiting followed by altered level of consciousness. About 9% from the patient were hypotensive, 36% were tachycardic, 41% were tachypneic and 4% were having SpO2 < 94%. Brain CT was abnormal in 6 patients. Vision impairment was the most common sequalae of methanol poisoning (7 out of 12 patients who were assessed by ophthalmologist, 58%). When the patients were divided based on severity (mild, moderate, severe), nausea or vomiting and loss of consciousness were the most common complaints among the moderate group while loss of consciousness predominated in the severe group. Two patients presented with low blood pressure and were in the sever group. The severe group had a mean Glasgow Coma Scale (GCS) of 8. Most of the patients in the severity groups underwent the same management apart from those who died or deposited. Eight patients in the severe group had to be intubated.
This study demonstrates the multifaceted clinical presentation of methanol poisoning, culminating in a 17.4% mortality rate. Notably, our findings emphasize the critical role of prompt diagnosis and swift initiation of combined fomepizole therapy and hemodialysis in mitigating mortality and minimizing the potential for chronic visual sequelae associated with methanol poisoning.
Peer Review reports
Introduction
Methanol is one of the poisonous alcohols frequently used as a solvent in automobiles, paint thinners and other industrial applications. Poisoning often arises from consumption of illicit or non-commercially produced alcoholic beverages, sometimes referred to as “moonshine.” These beverages inadvertently produce methanol during their synthesis [ 1 ]. Methanol poisoning, either accidental or intentional, is very harmful if not managed rapidly and may lead to significant morbidity and even mortality [ 2 ]. Methanol has a depressant effect on the central nervous system (CNS) when ingested or inhaled but the toxicity of methanol is attributed to its metabolite -formic acid- formed from the oxidation of methanol to formaldehyde and then to formic acid. Formic acid is toxic to the optic nerve, the CNS and the mitochondria and its concentration is directly related to the risk of morbidity and mortality [ 3 ]. Ingesting 50–100 milliliter of pure methanol can cause perpetual blindness and neurological deterioration resulting in death [ 4 ]. The clinical presentation of methanol poisoning varies according to the route of exposure, the amount ingested, and the elapsed time after ingestion. Early symptoms of methanol poisoning include nausea, vomiting, dizziness along with epigastric pain. Later -after a period of 12 to 48 h since ingestion- methanol poisoning can lead to neurologic dysfunction, blindness and even death. Metabolic acidosis with a high anion gap is the most prominent laboratory abnormality [ 5 ]. Various case studies have reported complications ranging from ischemia and necrosis to hypotension and coma [ 6 , 7 ].
Managing methanol toxicity depends on the extent of exposure and requires close monitoring of laboratory parameters. Therapy with an antidote and/or extracorporeal treatment is the mainstay of treatment [ 8 , 9 ]. The treatment approach is directed towards interrupting methanol breakdown to formic acid using a competitive alcohol dehydrogenase enzyme inhibitor, such as fomepizole or ethanol. In addition to directly eliminating the toxic metabolites through hemodialysis [ 4 ]. Administration of sodium bicarbonate is recommended to tackle metabolic acidosis and to reduce formic acid penetration into the CNS and optic nerve [ 3 ]. The use of folic acid is also recommended to accelerate the breakdown of formate [ 10 ]. Early administration of fomepizole has shown to reduce mortality and prevent the need for dialysis. In a multicenter prospective trial, fomepizole administration to 11 patients with methanol poisoning resulted in a fall in concentration of formic acid and an improvement in metabolic acidosis in all patients [ 11 ]. None of the 7 surviving patients that initially presented with visual abnormalities had any decrements in visual acuity at the end of the trial [ 11 ]. Dialysis is also required in severe cases to eliminate the toxic metabolite from the body, however a retrospective study reported a survival of 5 out of 15 patients (33.3%) who were treated with dialysis [ 9 , 12 ].
The global significance of methanol toxicity has been underscored during the COVID-19 pandemic. Some regions witnessed methanol poisoning surges due to sanitizer consumption or misconceptions about alcohol’s protective effects against the virus. Notably, the outbreaks we describe, while coinciding with the pandemic, were linked to the illegal distribution of adulterated alcohol [ 12 ]. Given the profound health implications, including coma and death, early diagnosis and intervention are paramount. This study aimed to describe the clinical presentation, treatment strategies, and outcomes of patients from two distinct methanol poisoning outbreaks in Riyadh, Saudi Arabia, thereby filling existing knowledge gaps and underscoring the importance of timely public health interventions.
Study design
This study was a single center retrospective case series of methanol poisoning cases. It focused on patients that presented to the adult emergency department (ED) at King Abdulaziz Medical City (KAMC), a tertiary care academic hospital in Riyadh, Saudi Arabia. KAMC provides services to a rapidly growing patient population and houses 1,973 beds. The ED at KAMC offers care for national guard employees, their families, and critically ill or injured individuals. The study period encompassed two outbreaks between September 2020 and June 2021.
Data collection
Data for this study were extracted from the electronic medical records at KAMC. The two documented outbreaks occurred from September 1 to September 10, 2020, and May 14 to May 20, 2021. Given the prohibition on the sale, purchase, and consumption of alcohol in Saudi Arabia [ 13 ]. As a result, some might resort to “illicit or non-commercially” alcohol produced illegally by local individuals in the country. For the scope of this study, 5 patients were considered from the first outbreak and 18 from the second. Diagnosis criteria depended on a positive methanol serum concentration exceeding 20 mg/dL. Details such as demographic information, symptoms upon arrival, initial vital signs, laboratory results, GCS, brain computed tomography (CT) findings, and treatment (encompassing fomepizole, sodium bicarbonate, dialysis, folate, and mechanical ventilation) were compiled. Additionally, assessments by ophthalmologist and/or neurologist were conducted for patients presenting with vision or neurological complaints.
Data analysis
The gathered data were subjected to analysis using the Statistical Package for the Social Sciences (IBM SPSS Statistics for Windows, Version 22.0). Demographic data and baseline characteristics were summarized and presented as frequencies and proportions.
Based on the severity and clinical presentation, patients were categorized into three groups. A brief rationale for the grouping is: methanol poisoning’s severity can be gauged through clinical manifestations such as coma or seizures and laboratory indicators like blood pH levels. A lower pH often indicates acidosis, a common consequence of methanol poisoning. The severity groups were defined as: Mild: patients not in a coma, no seizures, and an initial pH > 7.2, Moderate: patients not in a coma, no seizures, but an initial pH ranging from 7.0 to 7.2 and Severe: patients in a coma, had seizures, or their initial pH was below 7.0. This classification helped in understanding the clinical implications of varying severities of methanol poisoning and guided subsequent interventions and prognosis evaluation.
Patient demographics
A total of 23 patients presented to the ED over the two methanol toxicity outbreaks. The majority of patients were male (19/23, 83%) with a mean age of 29-years-old. Out of the 23 patients, one was pronounced dead on arrival, one died in the ED and the rest were discharged from the ED or were admitted for further management. Table 1 lists the initial presentation of the patients upon arrival to the ED. From the 22 patients who arrived alive to the ED, the most common symptoms were nausea or vomiting (17/22, 74%), altered level of consciousness (10/22, 44%), impaired vision (9/22, 39%) and abdominal pain (7/22, 30%). Only one patient presented asymptomatic with only a history of possible ingestion of methanol and positive methanol serum level. Two patients (9%) were hypotensive upon arrival, eight patients (36%) were initially tachycardic and nine patients were tachypneic (41%). All the patients (100%) had a normal initial temperature. Only eight patients from the total patients (35%) had brain CT and was abnormal in six patients, of which four showed brain edematous changes, and two had no brain perfusion and the rest were normal.
Patient outcomes
Table 2 characterizes those who presented to the ED with methanol poisoning according to severity. The groups were mild, moderate, and severe (3/23; 13%, 11/23; 48%, and 8/23; 35%, respectively). The mean age of the patients was 38 years old in the mild group, 27 years old in the moderate group and 28 years old in the severe group. All patients in the mild and moderate groups were males and only 50% of those in severe group were male. Nausea or vomiting and loss of consciousness were the most common complaints among the moderate group while loss of consciousness predominates in the severe group. Blood pressure readings were normal in the mild and moderate groups but was low (SBP < 100) in 25% of those in the severe group. Tachycardia (> 100 beats/min) among groups were as following: 33% in the mild group, 36% in the moderate group and 37% in the severe group. Tachypnea (> 20 breaths/min) was almost similar in the mild and moderate groups (33% and 27%, respectively). Tachypnea was almost doubled in the severe group (63%). Only 1 patient was having SpO2 < 94% and he was in the severe group. The mild and moderate groups showed an initial mean GCS of 14 and 15, respectively. The severe group, on the other hand, had a mean GCS of 8. VBG results showed a mean PH of 7.2 in the mild group, 7.1 in the moderate group and 6.8 in the severe group. HCO3 concentration had a mean of 15 mmol/l in the mild group, 9 mmol/l in the moderate group, and 14 mmol/l in the severe group. Mean methanol concentration was 136 mg/dl in the severe group, 177 mg/dl in the mild group and 113 mg/dl in the moderate group. White blood cells showed an upward trend among the groups: 9 cells per cubic millimeter in mild group, 14 cells per cubic millimeter in moderate group and 20 cells per cubic millimeter in severe group. In addition, creatinine was 139 µmol/L in the severe group while it was 78 µmol/L and 109 µmol/L in the mild and moderate groups, respectively. The mean anion gap (AGAP) and lactic acid were very high in the severe group (AGAP:25, Lactate:9 mmol/L). While the AGAP in the mild and moderate groups were 25 and 29 respectively and the Lactate were 1.84 mmom/L for mild group and 2.43 for the moderate group. Brain CT showed abnormal changes in certain patients in the severe group. Osmolality was 315 mOsm/kg in the mild group, 336 mOsm/kg in the moderate group and 362 mOsm/kg in the severe group.
The overall mortality rate was (4/23), 17.4%, three patients that died were in the severe group and one patient died up on arrival. Among those who were discharged from the hospital, vision impairment was the most common sequalae of methanol poisoning (7/12 who were assessed by ophthalmology, 58%), four patients (36%) in the moderate group and three patients (38%) in the severe group. Moreover, four patients (63%) in the severe group were diagnosed with brain death or edematous changes. Appendix 1 includes the full data for the patients.
Patient managements
ED management included fomepizole, dialysis, sodium bicarbonate and folate. Almost 91% of the alive cases was started on hemodialysis (20/22). One of those who was not started on dialysis was in mild group and was asymptomatic and the other died in the ED before initiation of the dialysis. For mild group, one case was dialyzed for one time and the other had two sessions of dialysis while in the moderate group, eight cases had only one time of dialysis and three cases needed two sessions. Two cases in the severe group had three sessions of dialysis and two case needed three times of dialysis. The majority of the patients (11/20, 55%), were dialyzed within five hours or less from arrival to the ED. Twenty-two patients (22/22, 100%) in this study were started on fomepizole. All patients in the severe group had to be intubated (8/8, 100%) compared to two from the moderate group (2/11, 18%) and none from the mild group (0/3, 0%).
The multifaceted presentation of methanol poisoning poses a substantial diagnostic challenge, often presenting with a heterogenous constellation of symptoms across patients, potentially delaying suspicion and contributing to its significant morbidity and mortality [ 14 ]. However, prompt recognition and swift therapeutic intervention can dramatically mitigate the severity of sequelae [ 15 ]. Therefore, rapid source identification, coupled with proactive communication and heightened awareness amongst potentially exposed individuals, presents a significant opportunity for improved clinical outcomes. In our healthcare facility, timely diagnosis was achieved on the initial presentation itself, underscoring the critical role of early recognition in combating this potentially devastating toxicologic entity. The initial presenting complaint in this outbreak differed from previous reports. While nausea and vomiting were the most common symptoms observed, consistent with two prior outbreaks [ 14 , 16 ], this contrasts with other studies where visual impairment was the dominant presentation [ 17 , 18 , 19 ]. The potential for ethanol co-ingestion, a less harmful alcohol, might explain this disparity, although further investigation is warranted. Upon emergency department presentation, a comprehensive laboratory evaluation including CBC, electrolytes, VBG, methanol, lactate, and osmolality was conducted based on clinical suspicion. Consistent with established literature [ 14 , 16 , 17 , 18 ], the group with severe presentations exhibited the lowest mean serum pH, alongside the highest mean levels of methanol, potassium, lactate, WBCs, and osmolality. These findings underscore the importance of considering diverse presenting features in methanol poisoning, while highlighting the consistent laboratory profile associated with disease severity. All patients in the severe group, with the exception of the individual who demised before ICU admission, necessitated intensive care support. Notably, the severe group exhibited signs of nephrotoxicity, as evidenced by elevated mean creatinine (139 µmol/L) and blood urea nitrogen (BUN) levels (5.7 mmol/L). This observation aligns with the known nephrotoxic potential of methanol’s direct cytotoxic metabolite. While previous research suggests hypotension as a potential contributor to methanol-induced kidney injury [ 16 ], it is noteworthy that all patients within the hypotensive subgroup in this study also presented with renal impairment. These findings warrant further investigation to elucidate the precise mechanisms underlying and the potential interplay between hypotension and the direct cytotoxic effects of methanol metabolites in the pathogenesis of methanol-associated kidney injury. Upon suspicion of methanol poisoning based on a combination of clinical history, presentation, and metabolic acidosis, immediate therapeutic interventions were initiated as per the established local protocol. This aggressive management employed fomepizole to competitively inhibit methanol metabolism, sodium bicarbonate to rapidly rectify severe acidemia, folate for enhanced formic acid clearance, and hemodialysis for expeditious toxin removal. Notably, the observed mortality rate of 17.4% fell significantly below the average reported in other outbreaks (28-48%). 14,16 This discrepancy can be primarily attributed to the swift diagnosis and prompt initiation of fomepizole and hemodialysis therapy, in contrast to delays or limited availability noted in previous reports. Notably, the majority of patients received fomepizole and dialysis within 3–5 h of emergency department arrival. While a slight delay in fomepizole administration for the initial case occurred, swift recognition of a potential influx of cases triggered a multi-faceted response. This led to operational collaboration and ensured timely fomepizole access for subsequent patients, prompting a comprehensive review of all antidote availability and distribution protocols, detailed elsewhere [ 20 ]. Several prior investigations have established a correlation between the severity of metabolic acidosis and mortality in methanol poisoning, aligning with our observations in this study. Patients who demised in the emergency department exhibited pH values below 7. Notably, four individuals within our cohort presented with similarly low pH (< 7), yet three experienced favorable outcomes and were discharged home, with one leaving against medical advice [ 14 , 16 , 17 , 18 ]. This apparent discrepancy may be attributed to the prompt administration of fomepizole, the swift initiation of hemodialysis, and the number of dialysis sessions undergone. These findings suggest that an aggressive combined therapeutic approach, targeting both metabolic acidosis correction and toxin elimination, may mitigate the adverse prognostic implications associated with severe acidosis in methanol poisoning. Further research is warranted to elucidate the precise interplay between acidosis severity, early intervention, and ultimate prognosis in this complex clinical entity.
Early fomepizole administration can be crucial in preventing death and disability from methanol poisoning, as highlighted in a previous case series from our region with nine cases [ 21 ]. This is particularly important in Saudi Arabia, where alcohol consumption is prohibited due to religious and health reasons. However, there have been multiple outbreaks of methanol poisoning, especially among young people, during the COVID-19 pandemic. The pandemic likely played a role in these outbreaks by disrupting access to regulated alcoholic beverages, potentially leading to increased consumption of unregulated and often methanol-contaminated alternatives. For healthcare systems, this emphasizes the importance of having readily available stocks of essential antidotes like fomepizole and hemodialysis equipment, which can be lifesaving in such cases. Additionally, ongoing education for healthcare providers on the clinical management of toxic alcohol ingestions and the potential for outbreaks, particularly during public health crises, is crucial. By taking these steps, we can be better prepared to respond to future methanol poisoning outbreaks and improve patient outcomes.
This study demonstrates the diverse clinical presentation of methanol poisoning, encompassing a spectrum of gastrointestinal, ophthalmic, and central nervous system manifestations. Notably, the observed low mortality and morbidity rate can be primarily attributed to the prompt diagnostic approach, swift initiation of fomepizole therapy, and rapid deployment of hemodialysis. These findings underscore the paramount importance of prioritizing early recognition and intervention in emergency departments during suspected methanol poisoning outbreaks. Establishing standardized protocols for expedited clinical assessment and laboratory testing, particularly in regions with a higher prevalence of unregulated alcohol consumption, holds crucial value in mitigating the potential morbidity and mortality associated with this toxicological entity.
Data availability
The datasets generated during the current study are available.
Pressman P, Clemens R, Sahu S, Hayes AW. A review of methanol poisoning: a crisis beyond ocular toxicology. 2020;39:173–9. https://doi.org/10.1080/15569527.2020.1768402 .
CAS Google Scholar
Korabathina K. Methanol Toxicity . 2015.
Barceloux DG, Krenzelok EP, Olson K, Watson W, Miller H. American academy of clinical toxicology practice guidelines on the treatment of ethylene glycol poisoning. J Toxicol - Clin Toxicol. 1999;37:537.
Article CAS PubMed Google Scholar
Kruse JA. Methanol and Ethylene Glycol Intoxication. Crit Care Clin. 2012;28:661–711.
Article PubMed Google Scholar
Aisa TM, Ballut OM. Methanol intoxication with cerebral hemorrhage. Neurosciences. 2016;21:275–7.
Article PubMed PubMed Central Google Scholar
Phang PT, Passerini L, Mielke B, Berendt R, King EG. Brain hemorrhage associated with methanol poisoning. Crit Care Med. 1988;16:137–40.
Ekins BR, Rollins DE, Duffy DP, Gregory MC. Standardized treatment of severe methanol poisoning with ethanol and hemodialysis. West J Med. 1985;142:337–40.
CAS PubMed PubMed Central Google Scholar
Chan APL, Chan TYK. Methanol as an unlisted ingredient in supposedly alcohol-based hand rub can pose serious health risk. Int J Environ Res Public Health. 2018;15. https://doi.org/10.3390/ijerph15071440 .
Ahmed F, Khan NU, Ali N, Feroze A. Methanol poisoning: 27 years’ experience at a tertiary care hospital. J Pak Med Assoc. 2017;67:1751–2.
PubMed Google Scholar
Becker CE. Methanol poisoning. J Emerg Med. 1983;1:51–8.
Effrey J, Rent B, Enneth MCM, Artin K, Cott S, Hillips P, Aron YA et al. The New Eng land Jour nal of Medicine FOMEPIZOLE FOR THE TREATMENT OF METHANOL POISONING Background Methanol poisoning may result in. 2001.
Sefidbakht S, Lotfi M, Jalli R, Moghadami M, Sabetian G, Iranpour P. Methanol toxicity outbreak: when fear of COVID-19 goes viral. Emerg Med J. 2020;37:416.
About Saudi - Visit Saudi Official Website. https://www.visitsaudi.com/en/understand (accessed 20 Nov2022).
Md Noor J, Hawari R, Mokhtar MF, Yussof SJ, Chew N, Norzan NA, et al. Methanol outbreak: a Malaysian tertiary hospital experience. Int J Emerg Med. 2020;13:1–7.
Article Google Scholar
Collister D, Duff G, Palatnick W, Komenda P, Tangri N, Hingwala J. A methanol intoxication outbreak from recreational ingestion of Fracking Fluid. Am J Kidney Dis. 2017;69:696–700.
Paasma R, Hovda KE, Tikkerberi A, Jacobsen D. Methanol mass poisoning in Estonia: outbreak in 154 patients. Clin Toxicol. 2007;45:152–7.
Article CAS Google Scholar
Liu JJ, Daya MR, Carrasquillo O, Kales SN. Prognostic factors in patients with methanol poisoning. J Toxicol Clin Toxicol. 1998;36:175–81.
Hovda KE, Hunderi OH, Tafjord AB, Dunlop O, Rudberg N, Jacobsen D. Methanol outbreak in Norway 2002–2004: epidemiology, clinical features and prognostic signs. J Intern Med. 2005;258:181–90.
Naraqi S, Dethlefs RF, Slobodniuk RA, Sairere JS. An outbreak of acute methyl alcohol intoxication. Aust N Z J Med. 1979;9:65–8.
Abu Esba LC, Mardawi G, Al Deeb M. Can’t find the antidote: a root cause analysis. Front Pharmacol. 2022;13. https://doi.org/10.3389/FPHAR.2022.895841 .
Eskandrani R, Almulhim K, Altamimi A, Alhaj A, Alnasser S, Alawi L, et al. Methanol poisoning outbreak in Saudi Arabia: a case series. J Med Case Rep. 2022;16:1–7.
Download references
Acknowledgements
We wholeheartedly acknowledge the invaluable contributions of King Abdulaziz Medical City (KAMC) and King Abdullah International Medical Research Center (KAIMRC) in the successful completion of this study. KAMC provided unwavering support on multiple fronts. By granting access to patient electronic records and fostering a conducive clinical environment for data collection, they laid the cornerstone for our research. Notably, KAMC’s emergency department served as the focal point for both patient presentation and management of the methanol poisoning cases, granting us an unparalleled opportunity for thorough retrospective analysis of the events.
Not applicable.
Author information
Authors and affiliations.
Emergency Medicine Department, Ministry of the National Guard - Health Affairs, Riyadh, Saudi Arabia
Faisal Alhusain, Mohammed Alshalhoub & Mohammad Al Deeb
King Abdullah International Medical Research Center, Riyadh, Saudi Arabia
Faisal Alhusain, Mohammed Alshalhoub, Laila Carolina Abu Esba, Mohammad Alghafees & Mohammad Al Deeb
Emergency Medicine Department, King Faisal Specialist Hospital and Research Center, Riyadh, Saudi Arabia
Moath Bin Homaid
Pharmaceutical Care Services, Ministry of the National Guard– Health Affairs, Riyadh, Saudi Arabia
Laila Carolina Abu Esba
Surgery Department, Ministry of the National Guard - Health Affairs, Riyadh, Saudi Arabia
Mohammad Alghafees
You can also search for this author in PubMed Google Scholar
Contributions
Faisal Alhusain: Literature review and manuscript writing Mohammed Alshalhoub: Literature review and manuscript writing Moath Bin Homaid: Literature review, data collection and manuscript review Laila Carolina Abu Esba: Literature review, data collection and data analysisMohammad Alghafees: Literature review and data collection Mohammad Al Deeb: Literature review, manuscript review and work supervision.
Corresponding author
Correspondence to Faisal Alhusain .
Ethics declarations
Ethics approval and consent to participate.
Ethical approval was granted by Institutional Review Board King Abdullah International Medical Research Centre via reference number NRC22R/348/07. The informed consent was waived by the same abovementioned ethics committee that approved the study.
Consent for publication
Competing interests.
The authors declare no competing interests.
Additional information
Publisher’s note.
Springer Nature remains neutral with regard to jurisdictional claims in published maps and institutional affiliations.
Electronic supplementary material
Below is the link to the electronic supplementary material.
Supplementary Material 1
Rights and permissions.
Open Access This article is licensed under a Creative Commons Attribution 4.0 International License, which permits use, sharing, adaptation, distribution and reproduction in any medium or format, as long as you give appropriate credit to the original author(s) and the source, provide a link to the Creative Commons licence, and indicate if changes were made. The images or other third party material in this article are included in the article’s Creative Commons licence, unless indicated otherwise in a credit line to the material. If material is not included in the article’s Creative Commons licence and your intended use is not permitted by statutory regulation or exceeds the permitted use, you will need to obtain permission directly from the copyright holder. To view a copy of this licence, visit http://creativecommons.org/licenses/by/4.0/ . The Creative Commons Public Domain Dedication waiver ( http://creativecommons.org/publicdomain/zero/1.0/ ) applies to the data made available in this article, unless otherwise stated in a credit line to the data.
Reprints and permissions
About this article
Cite this article.
Alhusain, F., Alshalhoub, M., Homaid, M.B. et al. Clinical presentation and management of methanol poisoning outbreaks in Riyadh, Saudi Arabia: a retrospective analysis. BMC Emerg Med 24 , 64 (2024). https://doi.org/10.1186/s12873-024-00976-1
Download citation
Received : 17 January 2023
Accepted : 27 March 2024
Published : 16 April 2024
DOI : https://doi.org/10.1186/s12873-024-00976-1
Share this article
Anyone you share the following link with will be able to read this content:
Sorry, a shareable link is not currently available for this article.
Provided by the Springer Nature SharedIt content-sharing initiative
- Methanol toxicity
- Methanol poisoning outbreak
- Saudi Arabia
BMC Emergency Medicine
ISSN: 1471-227X
- General enquiries: [email protected]
ORIGINAL RESEARCH article
Use of the canadian ct head rule for patients on anticoagulant/anti-platelet therapy presenting with mild traumatic brain injury: prospective observational study.
- 1 Emergency Department—EOC—Ospedale Regionale di Lugano, Lugano, Switzerland
- 2 Surgery Department—EOC—Ospedale Regionale di Lugano, Lugano, Switzerland
Background and importance: Mild traumatic brain injury (mTBI) is a frequent presentation in Emergency Department (ED). There are standardised guidelines, the Canadian CT Head Rule (CCHR), for CT scan in mTBI that rule out patients on either anticoagulant or anti-platelet therapy. All patients with these therapies undergo a CT scan irrespectively of other consideration.
Objective: To determine whether standard guidelines could be applied to patients on anticoagulants or anti-platelet drugs.
Design, settings, and participants: 1,015 patients with mTBI and Glasgow Coma Score (GCS) of 15 were prospectively recruited, 509 either on anticoagulant or anti-platelet therapy and 506 on neither. All patients on neither therapy underwent CT scan following guidelines. All patients with mTBI on either therapy underwent CT scan irrespective of the guidelines.
Outcome measure and analysis: Primary endpoint was the incidence of post-traumatic intracranial bleeding in patients either on anticoagulants or anti-platelet drugs and in patients who were not on these therapies. Bayesian statistical analysis with calculation of Confidence Intervals (CI) was then performed.
Main results: Sixty scans were positive for bleeding: 59 patients fulfilled the criteria and 1 did not. Amongst patients with haemorrhage, 24 were on either therapy and only one did not meet the guidelines but in this patient the CT scan was performed before 2 h from the mTBI. Patients on either therapy did not have higher bleeding rates than patients on neither. There were higher bleeding rates in patients on anti-platelet therapy who met the guidelines vs. patients who did not. These rates overlapped with patients on neither therapy, meeting CCHR.
Conclusion: The CCHR might be used for mTBI patients on either therapy. Anticoagulants and anti-platelet drugs should not be considered a risk factor for patients with mTBI and a GCS of 15. Multicentric studies are needed to confirm this result.
Introduction
Traumatic brain injury (TBI), defined as brain function impairment due to external forces ( 1 , 2 ) resulting in loss of consciousness, amnesia or disorientation ( 3 ) is one of the commonest occurrences at the Emergency Department (ED) worldwide ( 4 ).
TBI is classified in severe (GCS ≤ 8), moderate (GCS from 9 to 13) and mild (GCS ≥ 14) ( 5 ).
Whilst there is evidence about the need of a head CT scan for patients with a moderate or severe TBI, there is still discussion on when a patient with mild traumatic brain injury (mTBI) should undergo CT. Several guidelines exist, the most important of which is the Canadian CT Head Rule (CCHR) ( 6 , 7 ), to assist in deciding when further diagnostic investigation is required for mTBI ( 1 ). The CCHR for patients with mTBI is 99%–100% sensitive in detecting patients needing a neurosurgical intervention, but is lacking in specificity (39%–51%) ( 8 ). The incidence of intracranial bleeding in patients with GCS 15 who meet the criteria for the rule is about 5%–8% ( 9 ). The CCHR was derived excluding people on anticoagulant or anti-platelet medication assuming all those with any TBI symptoms (loss of consciousness, amnesia, etc.) would require CT imaging. There was no explicit comment on the need for CT imaging in the context of head injury without clear evidence of TBI in people with anticoagulant and anti-platelet medication. A recent systematic review could not identify robust empirical data to inform recommendations in this population ( 10 , 11 ).
Historically anticoagulant and anti-platelet drugs have been considered a risk factor in traumatic brain injury. The American College of Emergency Physicians (2008) states that the management of patients on anticoagulants is unclear and gives no specific recommendations ( 8 ). The latest update of the NICE Head Injury Guidelines ( 11 ) recommend a head CT for any medium risk patient (GCS 15 within 2 h of injury with history of loss of consciousness or amnesia) taking any anticoagulant or anti-platelet regime – excluding aspirin monotherapy. Where no loss of consciousness or amnesia has occurred shared decision making rather than a mandatory CT brain scan is recommended. The consequence is that every patient on either anticoagulants or anti-platelet drugs with a mTBI undergoes a CT scan.
The number of patients on anticoagulant or anti-platelet therapy is increasing. This is mainly due to the increase in the ageing population. The incidence of hospital presentation for mild TBI will also be a significant issue in an ageing population.
The total anticoagulant prescription nearly doubled from 2014 to 2019 in the UK (15.0 million doses vs. 33.0 million doses) ( 8 ). Around 43 million adults in the US (19.0%) took aspirin at least three times per week for more than 3 months in 2010. This was an increase of 57% in aspirin use compared with 2005 ( 12 ).
The resulting increasing number of patients on these therapies presenting with mTBI makes it necessary for the clinician to weigh the risk of haemorrhage and the risk of irradiating the brain which can lead to radiation-related damage, as well as the costs of performing unnecessary examinations ( 13 – 15 ).
Intracranial bleeding represents the most feared complication in patients under antithrombotic agents, since it is associated with high morbidity and mortality. There is, however, limited evidence of the role of these drugs on mortality after mTBI ( 4 , 16 , 17 ). Literature suggests that anti-platelet and anticoagulant therapy increase the risk for intracranial haematoma and its progression after mTBI ( 16 , 17 ), but these evidence is based on patients using vitamin K antagonists (VKAs) or anti-platelet drugs. In the last few years, direct oral anticoagulants (DOACs) diffusion has led to the knowledge that these drugs could be safer than VKAs also in the setting of mTBI ( 18 ).
The present study aims at determining whether there is a difference in intracerebral haemorrhage rates in patients with GCS 15 taking either antithrombotic or anticoagulant therapy in the mild TBI patients vs. those not on either therapy as an independent risk factor. If this is the case, CCHR could be applied to these patients, reducing the exposure to unneeded radiation. This will help define the management of mTBI in patients under anticoagulant or anti-platelet treatments.
Materials and methods
Study design and study period.
This is a mono-centre prospective cohort observational study, involving patients’ charts data collection from adults presenting with mTBI (that is head trauma resulting in loss of consciousness, amnesia or disorientation) to the Emergency Department from the 29th April 2021 to the 31st June 2022.
The study received the approval of the local Ethics Committee and the participants signed an informed consent. The study was conducted in accordance with the declaration of Helsinki.
Sample size calculation
A sample size calculation was undertaken. Null hypothesis was that there are no differences in bleeding between patients with mild brain injury and GCS 15 on anticoagulant/anti-platelet therapy vs. patients with mild brain injury and GCS 15 not on anticoagulant/anti-platelet therapy. To test this null hypothesis, we had to assume a difference between patients who were and were not on either therapy. We assumed 5%, as this is the incidence of intracranial bleeding in patients neither on anticoagulants nor on anti-platelet drugs meeting CCHR ( 5 ). The sample size was calculated to have 80% power, 95% confidence level and 2% margin of error. This sample size was 457 subjects for each group. Therefore, we planned to recruit at least 914 patients.
Participants description
We included all the adult patients presenting during the study period with mTBI and GCS 15, both on anticoagulants or anti-platelet drugs and neither meeting criteria for mild traumatic brain injury. Exclusion criteria were: medical cause of head trauma (e.g., syncope, epilepsy…), GCS 2 h after trauma of 14 or less, presence of seizures after injury, pregnancy, delayed presentation (>24 h), not having taken regular anti-platelet or anticoagulant therapy, absence of written consent.
The regularity of taking anticoagulant or anti-platelet therapy was ascertained by interviewing patients, family members and family physicians.
Patients not on therapy were included in the study only when meeting CCHR criteria for performing a head CT scan.
One month after the access to ED, enrolled patients received a follow-up phone call to find out their condition.
Outcome measures
Primary endpoint was the incidence of intracranial bleeding in patients either on anticoagulants or anti-platelet drugs and in patients who were not on these therapies.
Secondary endpoints were:
• the need for intervention after post traumatic head bleeding in the two groups;
• the reliability of CT Head Rule in patients on either anticoagulants or anti-platelet drugs;
• to assess whether anticoagulants and/or anti-platelet drugs are a risk factor for patients presenting with mTBI and GCS of 15; and
• to compare mortality and morbidity after mTBI in the two groups with a follow-up period of 1 month.
Data validation
Two months were selected randomly for data validation. An independent research collaborator was identified to determine the number of patients who should be included in the study within the data collection period and check if any were missed or added unrightfully. This collaborator was not involved in the initial data collection. No differences were detected.
Data analysis
Statistical analysis was performed using the open source packages “Pandas,” “NumPy,” “SciPy,” “Seaborn,” and “PyMC” for Mac Os X versions 1.4.1, 1.21.2, 1.7.3, 0.11.2, and 3.11.14, respectively. Statistical significance was considered achieved based on highly credible intervals of parameter estimates and p < 0.05. Confidence intervals (CI) were calculated at 95%.
Since we needed to compare proportions of haemorrhages in different sub-populations, we performed both a two-tail t-test computation based on classic proportion comparison using Fischer exact test, and a Bayesian estimate of the parameter distribution of a Bernoulli stochastic variable to model bleeding occurrences using a non-informative uniform prior distribution over the interval 0–1. The estimate was obtained by using the Metropolis-Hastings algorithm in a Markov-chain Monte Carlo (MCMC) model, with a burn-in of 5,000 iterations and runs lasting 40,000 iterations. Traces were inspected to verify convergence diagnostics (Geweke plots and Raftery-Lewis analysis). The posterior parameter distribution was then plotted in order to have a graphical overview and confidence intervals were estimated. Furthermore, by sampling the posterior distributions, we were able to estimate both probability that the parameters describing one population would be different from each other as well as the estimate confidence intervals for Relative Risk ( 19 ).
Between April 2021 and June 2022, 1,015 patients were enrolled, 509 on either anticoagulants or anti-platelet drugs and 506 on neither.
Personal data, causes of injury, met criteria for CCHR, presence or absence of haemorrhage at CT, need for surgical intervention, reason for anticoagulant/anti-platelet therapy, are summarised in Tables 1 , 2 .
INR values of 37 out of 52 patients anticoagulated with VKA were recorded. The mean value was 1.78 (range 1.2–6.6), 3 patients had a subtherapeutic value (≤1.5), 18 patients a therapeutic value (1.5 < INR < 2.5) and 16 patients an overtherapeutic value (≥2.5).
Of the 1,015 CT scans performed 60 resulted positive for haemorrhage (5.9%).
We considered a CT scan positive when there was any trace of blood, even the smallest (even one single petechia). Of these positive patients, 24 were patients on either anticoagulants or anti-platelets and 36 on neither. Amongst the 60 patients who resulted positive for haemorrhage at CT scan, only one seemed not to meet the criteria for the CCHR. This was a 74 year old patient on aspirin (in primary prevention) who accidentally fell from her height and hit the back of the head. She arrived at the hospital by ambulance 30 min after the accident and GCS assessment was performed at the arrival (GCS 15). CT scan was performed at 50 min from the fall and was positive for subdural haematoma. Thirty minutes after she became confused and did not recognise her son (GCS 12 E3 V3 M6). She underwent neurosurgical intervention as a consequence of the positive CT scan for subdural haematoma.
The remaining 59 positive patients met CCHR ( Table 3 ) criteria for head CT, had minimal bleeding, remained stable at the next CT check-up and did not require surgery. Anti-platelet and anticoagulant therapy was discontinued (with the exception of one patient on warfarin therapy for a mechanical mitral valve, who was anticoagulated with unfractionated heparin and closely monitored, with no progression of minimal subarachnoid haemorrhage detected on CT).
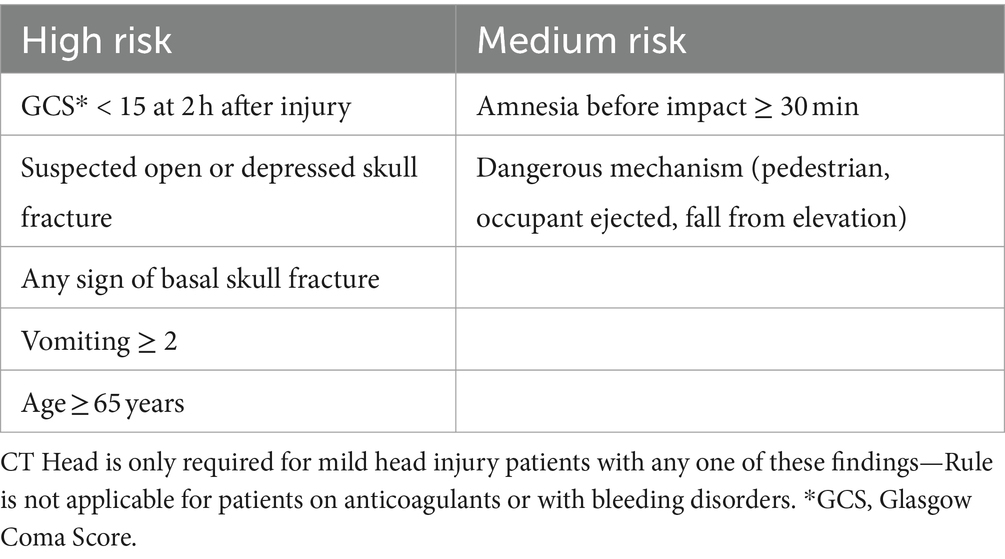
Table 3 . Canadian CT head rule criteria—from the work of Stiell et al. ( 6 ).
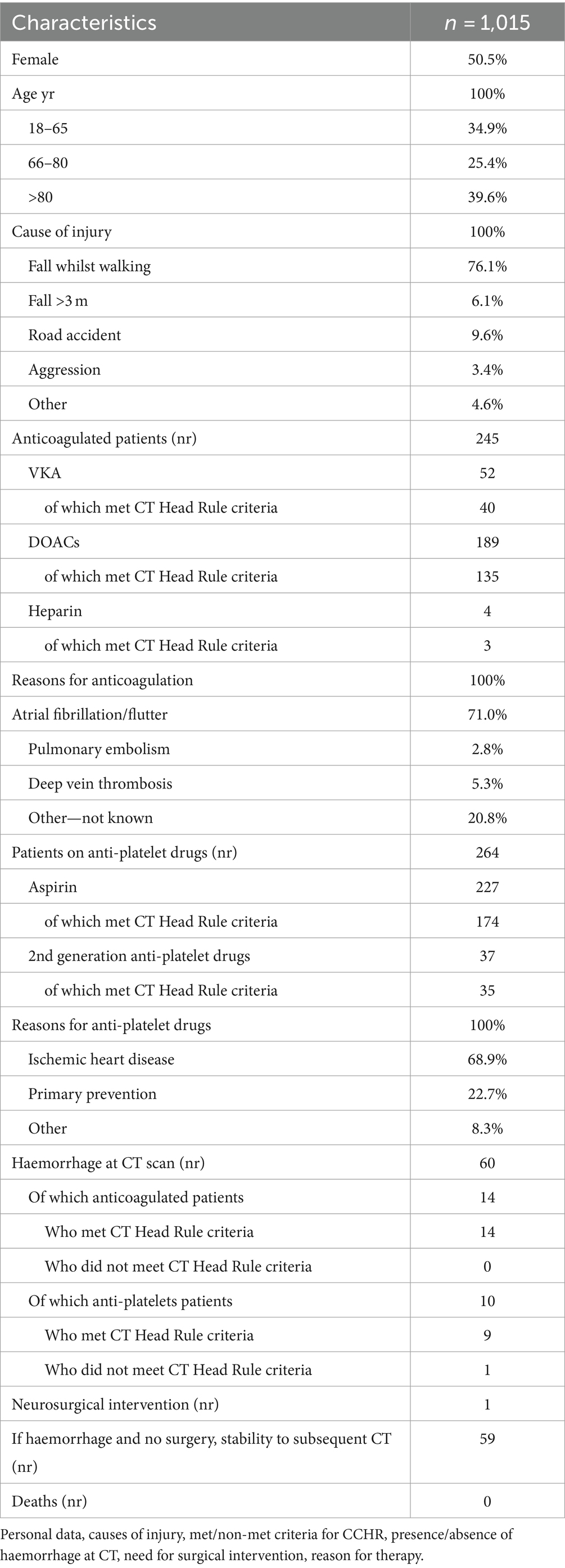
Table 1 . Description of sample.
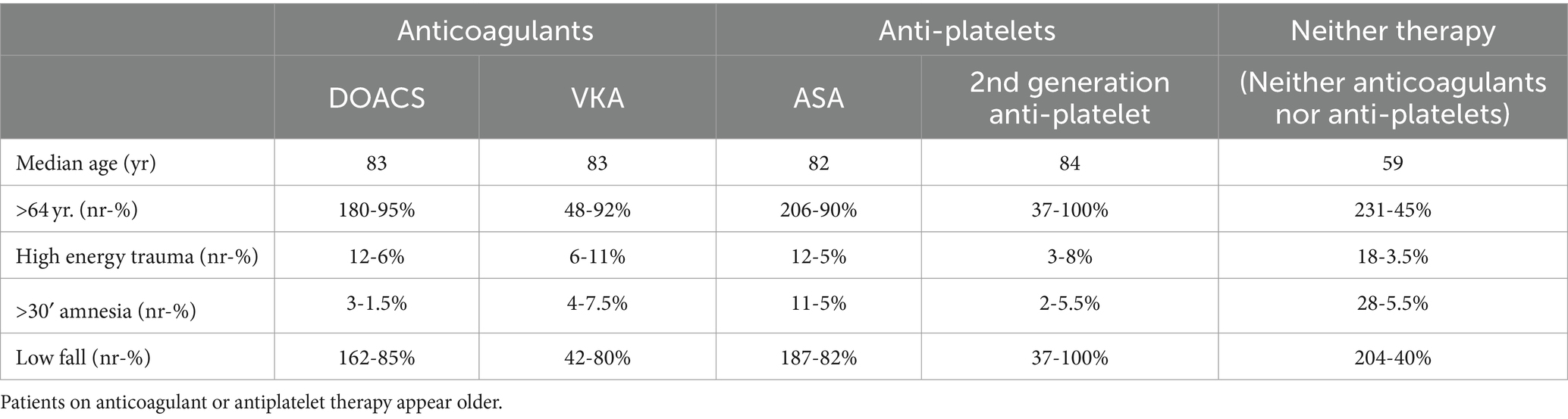
Table 2 . Description of sample by confounding factors.
No reversal agents were administered to any patient with a positive scan due to the scarcity of bleeding.
There was no difference in terms of bleeding in the two groups, on anticoagulant/anti-platelet therapy and patients on neither. The two CI greatly overlapped.
At 1 month follow-up we could reach all patients but 20 with a telephone call: 18 did not answer and for two patients phone number was missing. No patient had died or suffered complications following trauma. One patient on DOACs with negative CT scan had suspended rivaroxaban and suffered from ischemic stroke 3 days later. Two patients on neither therapy returned to the ED after 4 and 7 days, reporting headache and neck pain. Investigations revealed no complications and they were discharged home. One last patient on neither drug reported paraesthesias in all four limbs after trauma: an MRI of the spine ruled out major complications.
Of the 509 patients on anticoagulants/anti-platelets, 387 met inclusion criteria for CCHR.
The comparison of patients undergoing either therapy who did and did not fulfil the criteria of the CCHR was statistically significant, as patients who fulfilled the criteria had a higher probability of haemorrhage ( p = 0.023, CI 4.0%–9.1% for fulfilled and 1.0%–4.9% for unfulfilled criteria).
Amongst participants who met CCHR criteria, the comparison between patients who did take anticoagulants or anti-platelet drugs and the patients on neither was not statistically significant. The two groups overlapped.
When separating the categories of anti-platelets and anticoagulants, the difference in bleeding rate of those who did and did not meet the criteria for the Rule was statistically significant for anti-platelets ( p = 0.013 CI 4.8–13.1% for met criteria and 1.0–6.3% for non-meet criteria; Figure 1 ).
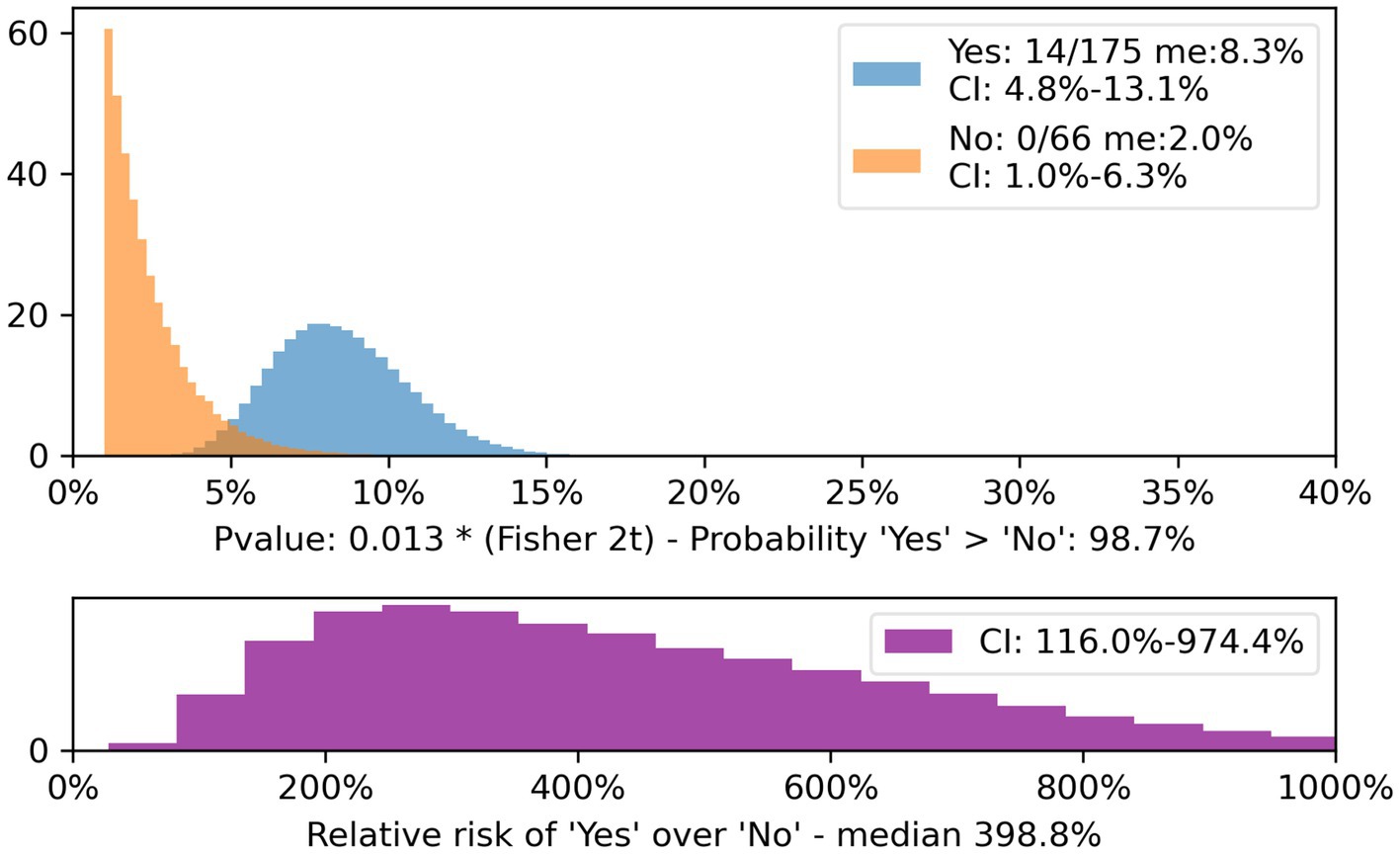
Figure 1 . Patients on anti-platelets meeting and non-meeting CT Head Rule, CI and relative risk. The population of patients on anti-platelet drugs who did not meet the CT Head Rule criteria (yellow) is compared with those who did (blue).
The population that met the CCHR criteria had a significantly higher rate of intracranial bleeding and not statistically significant for anticoagulants (CI 2.2%–8.0% for met criteria and 1.1%–6.2% for non-met criteria) with a trend towards more bleeding for patients on anticoagulants who met the criteria.
Comparing anticoagulated patients who met the Rule with patients on anti-platelets who met the Rule, the difference was not statistically significant, with a tendency towards more haemorrhages in patients on anticoagulant drugs.
The comparison between patients anticoagulated with vitamin K antagonists (VKA) and anticoagulated with direct oral anticoagulants (DOACs) was also not statistically significant: the two categories overlapped.
Data availability
Data from this study are available on https://datadryad.org/stash .
This study shows that anticoagulant or anti-platelet therapies are not an independent risk factor for brain haemorrhage in GCS 15 patients and that the CCHR might be used for patients with mTBI undergoing these treatments.
In the literature mTBI has so far been discussed under the assumption that all patients on anticoagulant or anti-platelet therapy were at high risk, even if they had a GCS of 15 ( 8 , 11 , 20 ). Many retrospective studies evaluated the incidence of bleeding in anticoagulated patients, whilst this paper is giving an answer in a prospective study ( 4 , 16 – 18 ).
The results reported are of high importance and likely to impact clinical practice in the ED.
The question is not about whether anticoagulants and anti-platelets are actually a risk factor for haemorrhage in brain injury, even when mild. Indeed, it seems quite clear (although the studies are mostly retrospective) that taking these therapies does carry with it a certain increased risk of developing intracranial bleeding after trauma ( 21 – 23 ).
With this regard, several authors emphasise that anti-platelets vs. anticoagulants, and amongst the latter, VKAs vs. DOACs, are at higher risk ( 4 , 13 , 22 , 24 – 26 ).
The question is whether patients with GCS of 15 within 2 h after trauma should be considered in the same way as other patients. The present work shows that this might be possible. If 2 h after trauma they have maintained an intact neurological state, they should be considered low-risk patients because it is unlikely to be severe damage inside the brain ( 27 ). In fact, with regard to patients on either therapy, in this study the probability of bleeding even when meeting the criteria remained comparable to that of patients on neither therapy meeting the same criteria. As for patients on anticoagulants, it appears that those with GCS 15 do not have a higher bleeding rate even when selected using the Rule criteria.
Considering possible confounding factors (median age, percentage of patients > 64 years old, percentage of high-energy incidents, amnesia > 30 min, percentage of low energy traumas), patients on anticoagulant or anti-platelet therapy appear older.
The two groups are also comparable in terms of low energy trauma (with a slight tendency to higher energy trauma for the anticoagulants/anti-platelet group) and amnesia.
We did not proceed with further calculations because, given their older age and similar energy of traumas, they are theoretically at an increased risk of ICH. Since the comparison between anticoagulants/anti-platelets and patients on neither therapy yielded similar results, this reinforces our findings.
In the entire study population, one patient needed surgery for evacuation of a subdural haematoma and she was discharged without neurological sequelae, resuming her normal activity in 1 month. Retrospective analysis of the emergency department management of this patient revealed that head GCS score was registered early. In fact, it had been performed upon the patient’s arrival in the emergency department. At 2 h after the trauma (the time pointed out by CCHR to assess GCS), the patient was no longer GCS 15 and thus theoretically should have been excluded from the study.
With regard to the other 58 patients whose CT was positive for haemorrhage, CT control was stable in all cases. All patients were discharged without neurological sequelae and resumed their normal activity.
Our results are giving an answer to the question whether CCHR is reliable also for patients on anticoagulant and anti-platelet treatment.
More than the immediate symptoms after a mTBI (amnesia, disorientation, transient loss of consciousness), a normal neurological state after 2 h is important, regardless of the treatment the patient is taking.
It is possible to speculate, that the vast majority of CTs performed on GCS 15 patients, even when they meet the Rule’s criteria, are unnecessary, with the exception of patients on anti-platelet therapy.
Limitations
Our study has some limitations:
• We were not able to measure antiXa activity in the vast majority of patients on DOACs, so we did not actually know their coagulation status, even though the patients we enrolled were regularly taking their therapy. Similarly, we did not assess the platelet function of patients on anti-platelet therapy. Adherence to therapy seemed to us a good surrogate as DOACs level measures are not routinely requested for these therapies. However, this needs further investigation in the context of traumatic intracranial haemorrhage.
• This is a single-centre study that needs confirmation on several sites.
• We were not able to contact 20 patients on follow-up. However, negative outcomes in these 20 individuals are really unlikely (control CT in hospital was stable) and could hardly have changed the outcome of the study.
• This study analysed a group of anticoagulant or anti-platelet medication users combined: bigger studies are needed that analyse anticoagulants alone and anti-platelet drugs alone.
The CCHR could possibly be used for mTBI patients on anticoagulant or anti-platelet therapy, although the number of diagnostic tests requested with the help of this Rule is probably still too high. Multicenter studies are needed to reinforce this opinion.
Anticoagulants and anti-platelet drugs should not be considered per se a risk factor for patients with mTBI and a GCS of 15; the need for CT scan should be weighed against the guidelines used for patients on neither therapy.
Data availability statement
Raw data from this study is in the supplementary material.
Ethics statement
The studies involving humans were approved by Comitato etico del Canton Ticino. The studies were conducted in accordance with the local legislation and institutional requirements. The participants provided their written informed consent to participate in this study.
Author contributions
LU: Conceptualization, Data curation, Formal analysis, Investigation, Methodology, Project administration, Supervision, Writing – original draft, Writing – review & editing. CR: Conceptualization, Investigation, Methodology, Writing – original draft. FP: Conceptualization, Validation, Writing – original draft. CB: Data curation, Methodology, Writing – review & editing. GU: Data curation, Formal analysis, Writing – review & editing. RP: Data curation, Formal analysis, Writing – review & editing. PM-H: Conceptualization, Project administration, Supervision, Writing – review & editing.
The author(s) declare that no financial support was received for the research, authorship, and/or publication of this article.
Acknowledgments
Thanks to Lorenzo Emilitri: without his unparalleled statistical expertise we would not be able to continue our work. Thanks also to Elena Porro whose support during the preparation of protocol was constant and precious.
Conflict of interest
The authors declare that the research was conducted in the absence of any commercial or financial relationships that could be construed as a potential conflict of interest.
Publisher’s note
All claims expressed in this article are solely those of the authors and do not necessarily represent those of their affiliated organizations, or those of the publisher, the editors and the reviewers. Any product that may be evaluated in this article, or claim that may be made by its manufacturer, is not guaranteed or endorsed by the publisher.
Supplementary material
The Supplementary material for this article can be found online at: https://www.frontiersin.org/articles/10.3389/fneur.2024.1327871/full#supplementary-material
1. Menon, DK, Schwab, K, Wright, DW, and Maas, AI. Demographics and clinical assessment working Group of the International and Interagency Initiative toward common data elements for research on traumatic brain injury and psychological health. Position statement: definition of traumatic brain injury. Arch Phys Med Rehabil . (2010) 91:1637–40. doi: 10.1016/j.apmr.2010.05.017
PubMed Abstract | Crossref Full Text | Google Scholar
2. Furlan, JC, Radan, MM, and Tator, CH. A scoping review of registered clinical studies on mild traumatic brain injury and concussion (2000 to 2019). Neurosurgery . (2020) 87:891–9. doi: 10.1093/neuros/nyaa151
3. Saadat, S, Ghodsi, SM, Naieni, KH, Firouznia, K, Hosseini, M, Kadkhodaie, HR, et al. Prediction of intracranial computed tomography findings in patients with minor head injury by using logistic regression. J Neurosurg . (2009) 111:688–94. doi: 10.3171/2009.2.JNS08909
Crossref Full Text | Google Scholar
4. Uccella, L, Zoia, C, Bongetta, D, Gaetani, P, Martig, F, Candrian, C, et al. Are antiplatelet and anticoagulants drugs a risk factor for bleeding in mild traumatic brain injury? World Neurosurg . (2018) 110:e339–45. doi: 10.1016/j.wneu.2017.10.173
5. Mena, JH, Sanchez, AI, Rubiano, AM, Peitzman, AB, Sperry, JL, Gutierrez, MI, et al. Effect of the modified Glasgow coma scale score criteria for mild traumatic brain injury on mortality prediction: comparing classic and modified Glasgow coma scale score model scores of 13. J Trauma . (2011) 71:1185–93. doi: 10.1097/TA.0b013e31823321f8
6. Stiell, IG, Wells, GA, Vandemheen, K, Clement, C, Lesiuk, H, Laupacis, A, et al. The Canadian CT head rule for patients with minor head injury. Lancet . (2001) 357:1391–6. doi: 10.1016/s0140-6736(00)04561-x
7. Kavalci, C, Aksel, G, Salt, O, Yilmaz, MS, Demir, A, Kavalci, G, et al. Comparison of the Canadian CT head rule and the New Orleans criteria in patients with minor head injury. World J Emerg Surg . (2014) 9:31. doi: 10.1186/1749-7922-9-31
8. Ho, KH, van Hove, M, and Leng, G. Trends in anticoagulant prescribing: a review of local policies in English primary care. BMC Health Serv Res . (2020) 20:279. doi: 10.1186/s12913-020-5058-1
9. Skandsen, T, Nilsen, TL, Einarsen, C, Normann, I, McDonagh, D, Haberg, AK, et al. Incidence of mild traumatic brain injury: a prospective hospital, emergency room and general practitioner-based study. Front Neurol . (2019) 10:638. doi: 10.3389/fneur.2019.00638
10. Jagoda, AS, Bazarian, JJ, Bruns, JJ Jr, Cantrill, SV, Gean, AD, Howard, PK, et al. American College of Emergency Physicians; Centers for Disease Control and Prevention. Clinical policy: neuroimaging and decisionmaking in adult mild traumatic brain injury in the acute setting. Ann Emerg Med . (2008) 52:714–48. doi: 10.1016/j.annemergmed.2008.08.021
11. NICE . Head injury: assessment and early management Clinical guideline (2023). Available at: https://www.nice.org.uk/guidance/ng232
Google Scholar
12. Zhou, Y, Boudreau, DM, and Freedman, AN. Trends in the use of aspirin and nonsteroidal anti-inflammatory drugs in the general U.S. population. Pharmacoepidemiol Drug Saf . (2014) 23:43–50. doi: 10.1002/pds.3463
13. Uccella, L, Zoia, C, Perlasca, F, Bongetta, D, Codecà, R, and Gaetani, P. Mild traumatic brain injury in patients on long-term anticoagulation therapy: do they really need repeated head CT scan? World Neurosurg . (2016) 93:100–3. doi: 10.1016/j.wneu.2016.05.061
14. Van den Brand, CL, Perotti, JR, van der Linden, MC, Tolido, T, and Jellema, K. Effect of the implementation of a new guideline for minor head injury on computed tomography-ratio and hospitalizations in the Netherlands. Eur J Emerg Med . (2020) 27:441–6. doi: 10.1097/MEJ.0000000000000714
15. Cheng, AHY, Campbell, S, Chartier, LB, Dowling, S, Goddard, T, Gosselin, S, et al. Choosing wisely Canada's emergency medicine recommendations: time for a revision. CJEM . (2019) 21:717–20. doi: 10.1017/cem.2019.405
16. Ganti, L, Stead, T, Daneshvar, Y, Bodhit, AN, Pulvino, C, Ayala, SW, et al. GCS 15: when mild TBI isn't so mild. Neurol Res Pract . (2019) 1:6. doi: 10.1186/s42466-018-0001-1
17. Cipriano, A, Park, N, Pecori, A, Bionda, A, Bardini, M, Frassi, F, et al. Predictors of post-traumatic complication of mild brain injury in anticoagulated patients: DOACs are safer than VKAs. Intern Emerg Med . (2021) 16:1061–70. doi: 10.1007/s11739-020-02576-w
18. Cipriano, A, Pecori, A, Bionda, AE, Bardini, M, Frassi, F, Leoli, F, et al. Intracranial hemorrhage in anticoagulated patients with mild traumatic brain injury: significant differences between direct oral anticoagulants and vitamin K antagonists. Intern Emerg Med . (2018) 13:1077–87. doi: 10.1007/s11739-018-1806-1
19. Kruschke, JK . Bayesian estimation supersedes the t-test. J Exp Psychol Gen . (2013) 142:573–603. doi: 10.1037/a0029146
20. Cohen, DB, Rinker, C, and Wilberger, JE. Traumatic brain injury in anticoagulated patients. J Trauma . (2006) 60:553–7. doi: 10.1097/01.ta.0000196542.54344.05
21. Uccella, L, Bongetta, D, Fumagalli, L, Raffa, G, and Zoia, C. Acute alcohol intoxication as a confounding factor for mild traumatic brain injury. Neurol Sci . (2020) 41:2127–34. doi: 10.1007/s10072-020-04313-9
22. Cheng, L, Cui, G, and Yang, R. The impact of preinjury use of antiplatelet drugs on outcomes of traumatic brain injury: a systematic review and Meta-analysis. Front Neurol . (2022) 13:724641. doi: 10.3389/fneur.2022.724641
23. Probst, MA, Gupta, M, Hendey, GW, Rodriguez, RM, Winkel, G, Loo, GT, et al. Prevalence of intracranial injury in adult patients with blunt head trauma with and without anticoagulant or antiplatelet use. Ann Emerg Med . (2020) 75:354–64. doi: 10.1016/j.annemergmed.2019.10.004
24. Alter, SM, Mazer, BA, Solano, JJ, Shih, RD, Hughes, MJ, Clayton, LM, et al. Antiplatelet therapy is associated with a high rate of intracranial hemorrhage in patients with head injuries. Trauma Surg Acute Care Open . (2020) 5:e000520. doi: 10.1136/tsaco-2020-000520
25. Van den Brand, CL, Tolido, T, Rambach, AH, Hunink, MG, Patka, P, and Jellema, K. Systematic review and Meta-analysis: is pre-injury antiplatelet therapy associated with traumatic intracranial hemorrhage? J Neurotrauma . (2017) 34:1–7. doi: 10.1089/neu.2015.4393
26. Turcato, G, Zannoni, M, Zaboli, A, Zorzi, E, Ricci, G, Pfeifer, N, et al. Direct Oral anticoagulant treatment and mild traumatic brain injury: risk of early and delayed bleeding and the severity of injuries compared with vitamin K antagonists. J Emerg Med . (2019) 57:817–24. doi: 10.1016/j.jemermed.2019.09.007
27. Weber, MW, Nie, JZ, Espinosa, JA, Delfino, KR, and Michael, AP. Assessing the efficacy of mild traumatic brain injury management. Clin Neurol Neurosurg . (2021) 202:106518. doi: 10.1016/j.clineuro.2021.106518
Keywords: anticoagulants, anti-platelet, brain concussion, brain injury, Canadian CT head rule, GCS 15, mild traumatic brain injury
Citation: Uccella L, Riboni C, Polinelli F, Biondi C, Uccheddu G, Petrino R and Majno-Hurst P (2024) Use of the Canadian CT head rule for patients on anticoagulant/anti-platelet therapy presenting with mild traumatic brain injury: prospective observational study. Front. Neurol . 15:1327871. doi: 10.3389/fneur.2024.1327871
Received: 26 October 2023; Accepted: 02 April 2024; Published: 18 April 2024.
Reviewed by:
Copyright © 2024 Uccella, Riboni, Polinelli, Biondi, Uccheddu, Petrino and Majno-Hurst. This is an open-access article distributed under the terms of the Creative Commons Attribution License (CC BY) . The use, distribution or reproduction in other forums is permitted, provided the original author(s) and the copyright owner(s) are credited and that the original publication in this journal is cited, in accordance with accepted academic practice. No use, distribution or reproduction is permitted which does not comply with these terms.
*Correspondence: Laura Uccella, [email protected]
Disclaimer: All claims expressed in this article are solely those of the authors and do not necessarily represent those of their affiliated organizations, or those of the publisher, the editors and the reviewers. Any product that may be evaluated in this article or claim that may be made by its manufacturer is not guaranteed or endorsed by the publisher.
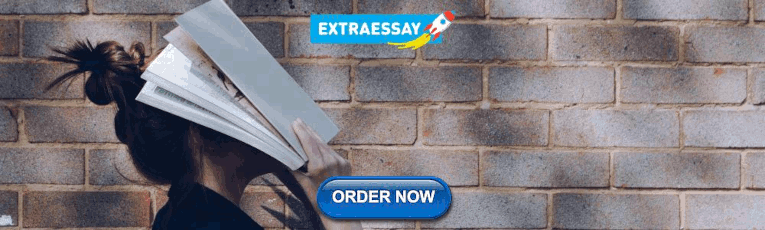
IMAGES
VIDEO
COMMENTS
TheJoelTruth. While a good presentation has data, data alone doesn't guarantee a good presentation. It's all about how that data is presented. The quickest way to confuse your audience is by ...
In the histogram data analysis presentation example, imagine an instructor analyzing a class's grades to identify the most common score range. A histogram could effectively display the distribution. It will show whether most students scored in the average range or if there are significant outliers. Step 1: Gather Data. He begins by gathering ...
Template 5: Data Management Analysis PPT Framework . For achieving business excellence, the quest for efficient and time-saving solutions is a universal endeavor. Recognizing your aspirations, we present the Data Management Analysis PowerPoint Presentation — an invaluable asset for seamless change management and effective data analysis. It ...
Here's my five-step routine to make and deliver your data presentation right where it is intended —. 1. Understand Your Data & Make It Seen. Data slides aren't really about data; they're about the meaning of that data. As data professionals, everyone approaches data differently.
Be the first to add your personal experience. 6. Deliver your presentation. 7. Here's what else to consider. Be the first to add your personal experience. Data analysis is a valuable skill, but ...
8. Tabular presentation. Presenting data in rows and columns, often used for precise data values and comparisons. Tabular data presentation is all about clarity and precision. Think of it as presenting numerical data in a structured grid, with rows and columns clearly displaying individual data points.
Here are 10 data presentation tips to effectively communicate with executives, senior managers, marketing managers, and other stakeholders. 1. Choose a Communication Style. Every data professional has a different way of presenting data to their audience. Some people like to tell stories with data, illustrating solutions to existing and ...
Presentation length. This is my formula to determine how many slides to include in my main presentation assuming I spend about five minutes per slide. (Presentation length in minutes-10 minutes for questions ) / 5 minutes per slide. For an hour presentation that comes out to ( 60-10 ) / 5 = 10 slides.
These 20 free PowerPoint and Google Slides templates for data presentations will help you cut down your preparation time significantly. You'll be able to focus on what matters most - ensuring the integrity of your data and its analysis. We'll take care of the design end for you!
In the first module you'll plan an analysis approach, in the second and third modules you will analyze sets of data using the Excel skills you learn. In the fourth module you will prepare a business presentation. In the final Capstone Project, you'll apply the skills you've learned by working through a mock client business problem.
Data Presentation. Tools for effective data presentation. Over 1.8 million professionals use CFI to learn accounting, financial analysis, modeling and more. Start with a free account to explore 20+ always-free courses and hundreds of finance templates and cheat sheets.
1. Collect your data. First things first, and that is to have all your information ready. Especially for long business presentations, there can be a lot of information to consider when working on your slides. Having it all organized and ready to use will make the whole process much easier to go through. 2.
A method of data analysis that is the umbrella term for engineering metrics and insights for additional value, direction, and context. By using exploratory statistical evaluation, data mining aims to identify dependencies, relations, patterns, and trends to generate advanced knowledge.
What data analysis entails. Data analysis is an analytical process which involves recording and tabulating (recording and entering, entering and tabulating) the quantities of a product, such as numbers of units produced, costs of materials and expenses. While data analyst can take different forms, for example in databases, in other structures ...
Data Analysis and Data Presentation have a practical implementation in every possible field. It can range from academic studies, commercial, industrial and marketing activities to professional practices. In its raw form, data can be extremely complicated to decipher and in order to extract meaningful insights from the data, data analysis is an important step towards breaking down data into ...
A data analysis PowerPoint template or presentation template for Google Slides would be suitable for this. Government & Public Policy: Government officials or policy analysts may use data analysis presentation templates to present data on social issues, economic trends, or the impact of certain policies.
See a slide-by-slide example of a presentation deck for a data analytics report. Connor, a Marketing Analytics Manager at Google Cloud, walks you through exa...
What Is Data Presentation? Data presentation is a process of comparing two or more data sets with visual aids, such as graphs. Using a graph, you can represent how the information relates to other data. This process follows data analysis and helps organise information by visualising and putting it into a more readable format.
Data Analysis for Business Presentation. Free Google Slides theme, PowerPoint template, and Canva presentation template. What helps employees of a company know how the business is performing and recognize current problems that are to be solved? Data analysis laid out in a presentation, for example. Since we all want to do our best in our jobs ...
Abstract. This chapter covers the topics of data collection, data presentation and data analysis. It gives attention to data collection for studies based on experiments, on data derived from existing published or unpublished data sets, on observation, on simulation and digital twins, on surveys, on interviews and on focus group discussions.
Utilize ready to use presentation slides on Data Analytics Powerpoint Presentation Slides with all sorts of editable templates, charts and graphs, overviews, analysis templates. The presentation is readily available in both 4:3 and 16:9 aspect ratio. Alter the colors, fonts, font size, and font types of the template as per the requirements.
DATA PRESENTATION, ANALYSIS AND INTERPRETATION. 4.0 Introduction. This chapter is concerned with data pres entation, of the findings obtained through the study. The. findings are presented in ...
1 of 38. Chapter 10-DATA ANALYSIS & PRESENTATION. 1. Data Analysis and Presentation. 2. PLANNING FOR DATA ANALYSIS. 3. Data Analysis The purpose To answer the research questions and to help determine the trends and relationships among the variables. 4.
qPCR Data Analysis. Throughout this guide, the factors that contribute to variations in the measurement of nucleic acid using PCR or qPCR have been highlighted. Each of these factors should be optimized to result in an assay that provides the closest possible value to the actual quantity of gene (target) in the reaction.
Minerals are essential components in many of today's rapidly growing clean energy technologies - from wind turbines and electricity networks to electric vehicles. Demand for these minerals will grow quickly as clean energy transitions gather pace. This new World Energy Outlook Special Report provides the most comprehensive analysis to date ...
Tennessee State Government - TN.gov
Association analysis of single cells with bulk data and acquisition of antigen presentation-related prognostic gene set results. Distribution of negative and positive cells in ovarian cancer single-cell data t-SNE diagram (A). The number of positive versus negative cells in each cell type is shown in a bar chart (B).
Data analysis. The gathered data were subjected to analysis using the Statistical Package for the Social Sciences (IBM SPSS Statistics for Windows, Version 22.0). Demographic data and baseline characteristics were summarized and presented as frequencies and proportions. Based on the severity and clinical presentation, patients were categorized ...
1 Emergency Department—EOC—Ospedale Regionale di Lugano, Lugano, Switzerland; 2 Surgery Department—EOC—Ospedale Regionale di Lugano, Lugano, Switzerland; Background and importance: Mild traumatic brain injury (mTBI) is a frequent presentation in Emergency Department (ED). There are standardised guidelines, the Canadian CT Head Rule (CCHR), for CT scan in mTBI that rule out patients on ...