- Login to Survey Tool Review Center
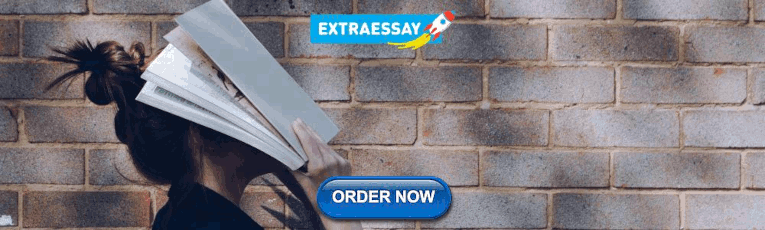
Secondary Research Advantages, Limitations, and Sources
Summary: secondary research should be a prerequisite to the collection of primary data, but it rarely provides all the answers you need. a thorough evaluation of the secondary data is needed to assess its relevance and accuracy..
5 minutes to read. By author Michaela Mora on January 25, 2022 Topics: Relevant Methods & Tips , Business Strategy , Market Research
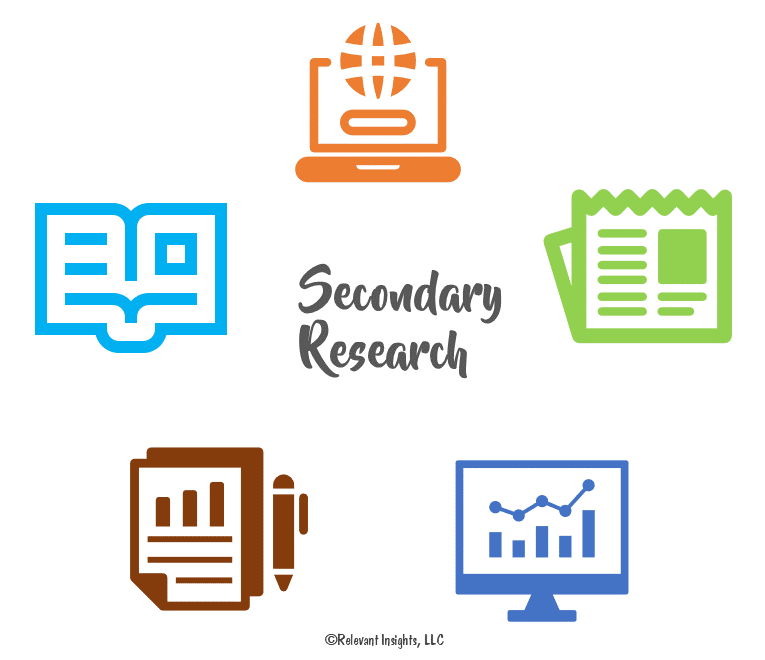
Secondary research is based on data already collected for purposes other than the specific problem you have. Secondary research is usually part of exploratory market research designs.
The connection between the specific purpose that originates the research is what differentiates secondary research from primary research. Primary research is designed to address specific problems. However, analysis of available secondary data should be a prerequisite to the collection of primary data.
Advantages of Secondary Research
Secondary data can be faster and cheaper to obtain, depending on the sources you use.
Secondary research can help to:
- Answer certain research questions and test some hypotheses.
- Formulate an appropriate research design (e.g., identify key variables).
- Interpret data from primary research as it can provide some insights into general trends in an industry or product category.
- Understand the competitive landscape.
Limitations of Secondary Research
The usefulness of secondary research tends to be limited often for two main reasons:
Lack of relevance
Secondary research rarely provides all the answers you need. The objectives and methodology used to collect the secondary data may not be appropriate for the problem at hand.
Given that it was designed to find answers to a different problem than yours, you will likely find gaps in answers to your problem. Furthermore, the data collection methods used may not provide the data type needed to support the business decisions you have to make (e.g., qualitative research methods are not appropriate for go/no-go decisions).
Lack of Accuracy
Secondary data may be incomplete and lack accuracy depending on;
- The research design (exploratory, descriptive, causal, primary vs. repackaged secondary data, the analytical plan, etc.)
- Sampling design and sources (target audiences, recruitment methods)
- Data collection method (qualitative and quantitative techniques)
- Analysis point of view (focus and omissions)
- Reporting stages (preliminary, final, peer-reviewed)
- Rate of change in the studied topic (slowly vs. rapidly evolving phenomenon, e.g., adoption of specific technologies).
- Lack of agreement between data sources.
Criteria for Evaluating Secondary Research Data
Before taking the information at face value, you should conduct a thorough evaluation of the secondary data you find using the following criteria:
- Purpose : Understanding why the data was collected and what questions it was trying to answer will tell us how relevant and useful it is since it may or may not be appropriate for your objectives.
- Methodology used to collect the data : Important to understand sources of bias.
- Accuracy of data: Sources of errors may include research design, sampling, data collection, analysis, and reporting.
- When the data was collected : Secondary data may not be current or updated frequently enough for the purpose that you need.
- Content of the data : Understanding the key variables, units of measurement, categories used and analyzed relationships may reveal how useful and relevant it is for your purposes.
- Source reputation : In the era of purposeful misinformation on the Internet, it is important to check the expertise, credibility, reputation, and trustworthiness of the data source.
Secondary Research Data Sources
Compared to primary research, the collection of secondary data can be faster and cheaper to obtain, depending on the sources you use.
Secondary data can come from internal or external sources.
Internal sources of secondary data include ready-to-use data or data that requires further processing available in internal management support systems your company may be using (e.g., invoices, sales transactions, Google Analytics for your website, etc.).
Prior primary qualitative and quantitative research conducted by the company are also common sources of secondary data. They often generate more questions and help formulate new primary research needed.
However, if there are no internal data collection systems yet or prior research, you probably won’t have much usable secondary data at your disposal.
External sources of secondary data include:
- Published materials
- External databases
- Syndicated services.
Published Materials
Published materials can be classified as:
- General business sources: Guides, directories, indexes, and statistical data.
- Government sources: Census data and other government publications.
External Databases
In many industries across a variety of topics, there are private and public databases that can bed accessed online or by downloading data for free, a fixed fee, or a subscription.
These databases can include bibliographic, numeric, full-text, directory, and special-purpose databases. Some public institutions make data collected through various methods, including surveys, available for others to analyze.
Syndicated Services
These services are offered by companies that collect and sell pools of data that have a commercial value and meet shared needs by a number of clients, even if the data is not collected for specific purposes those clients may have.
Syndicated services can be classified based on specific units of measurements (e.g., consumers, households, organizations, etc.).
The data collection methods for these data may include:
- Surveys (Psychographic and Lifestyle, advertising evaluations, general topics)
- Household panels (Purchase and media use)
- Electronic scanner services (volume tracking data, scanner panels, scanner panels with Cable TV)
- Audits (retailers, wholesalers)
- Direct inquiries to institutions
- Clipping services tracking PR for institutions
- Corporate reports
You can spend hours doing research on Google in search of external sources, but this is likely to yield limited insights. Books, articles journals, reports, blogs posts, and videos you may find online are usually analyses and summaries of data from a particular perspective. They may be useful and give you an indication of the type of data used, but they are not the actual data. Whenever possible, you should look at the actual raw data used to draw your own conclusion on its value for your research objectives. You should check professionally gathered secondary research.
Here are some external secondary data sources often used in market research that you may find useful as starting points in your research. Some are free, while others require payment.
- Pew Research Center : Reports about the issues, attitudes, and trends shaping the world. It conducts public opinion polling, demographic research, media content analysis, and other empirical social science research.
- Data.Census.gov : Data dissemination platform to access demographic and economic data from the U.S. Census Bureau.
- Data.gov : The US. government’s open data source with almost 200,00 datasets ranges in topics from health, agriculture, climate, ecosystems, public safety, finance, energy, manufacturing, education, and business.
- Google Scholar : A web search engine that indexes the full text or metadata of scholarly literature across an array of publishing formats and disciplines.
- Google Public Data Explorer : Makes large, public-interest datasets easy to explore, visualize and communicate.
- Google News Archive : Allows users to search historical newspapers and retrieve scanned images of their pages.
- Mckinsey & Company : Articles based on analyses of various industries.
- Statista : Business data platform with data across 170+ industries and 150+ countries.
- Claritas : Syndicated reports on various market segments.
- Mintel : Consumer reports combining exclusive consumer research with other market data and expert analysis.
- MarketResearch.com : Data aggregator with over 350 publishers covering every sector of the economy as well as emerging industries.
- Packaged Facts : Reports based on market research on consumer goods and services industries.
- Dun & Bradstreet : Company directory with business information.
Related Articles
- What Is Market Research?
- Step by Step Guide to the Market Research Process
- How to Leverage UX and Market Research To Understand Your Customers
- Why Your Business Needs Discovery Research
- Your Market Research Plan to Succeed As a Startup
- Top Reason Why Businesses Fail & What To Do About It
- What To Value In A Market Research Vendor
- Don’t Let The Budget Dictate Your Market Research Approach
- How To Use Research To Find High-Order Brand Benefits
- How To Prioritize What To Research
- Don’t Just Trust Your Gut — Do Research
- Understanding the Pros and Cons of Mixed-Mode Research
Subscribe to our newsletter to get notified about future articles
Subscribe and don’t miss anything!
Recent Articles
- Re: Design/Growth Podcast – Researching User Experiences for Business Growth
- Why You Need Positioning Concept Testing in New Product Development
- Why Conjoint Analysis Is Best for Price Research
- The Rise of UX
- Making the Case Against the Van Westendorp Price Sensitivity Meter
- How to Future-Proof Experience Management and Your Business
- When Using Focus Groups Makes Sense
- How to Make Segmentation Research Actionable
- How To Integrate Market Research and UX Research for Desired Business Outcomes
- How To Get Value Out of Your Research Budget
Popular Articles
- Which Rating Scales Should I Use?
- What To Consider in Survey Design
- 6 Decisions To Make When Designing Product Concept Tests
- Write Winning Product Concepts To Get Accurate Results In Concept Tests
- How to Use Qualitative and Quantitative Research in Product Development
- The Opportunity of UX Research Webinar
- Myths & Misunderstandings About UX – MR Realities Podcast
- 12 Research Techniques to Solve Choice Overload
- Concept Testing for UX Researchers
- UX Research Geeks Podcast – Using Market Research for Better Context in UX
- A Researcher’s Path – Data Stories Leaders At Work Podcast
- How To Improve Racial and Gender Inclusion in Survey Design
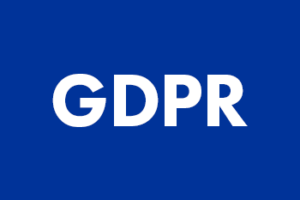
- Privacy Overview
- Strictly Necessary Cookies
This website uses cookies so that we can provide you with the best user experience possible. Cookie information is stored in your browser and performs functions such as recognising you when you return to our website and helping our team to understand which sections of the website you find most interesting and useful.
Strictly Necessary Cookie should be enabled at all times so that we can save your preferences for cookie settings.
If you disable this cookie, we will not be able to save your preferences. This means that every time you visit this website you will need to enable or disable cookies again.
- How it works
Disadvantages of Secondary Research – A Definitive Guide
Published by Jamie Walker at October 19th, 2021 , Revised On August 29, 2023
Secondary research is referred to as desk research because it implies collecting data from the Internet, journals, books, and public sources such as government repositories, or public and private libraries.
The study design of the research encompasses collecting a variety of different data sets and analysing them empirically in order to obtain research findings.
The aim is to evaluate pre-existing patterns from previous studies and adapt them to their own research setting. Although secondary research is quite useful, it has certain disadvantages.
Read more about the advantages of secondary research
Read more about the advantages of primary research
Read more about the disadvantages of primary research
Disadvantages of Secondary Research
Let’s take a look at the six most common disadvantages of secondary research mentioned below.
- Quality of the Secondary Data
- Out-dated Data
- Missing Information
- Availability of the Secondary Data
- Relevance of the Secondary Data
- Adequacy of Data
1. Quality of the Secondary Data
A study conducted with primary methods is monitored by the researchers themselves to a large extent. In contrast, this is not the case for data obtained from other sources (secondary sources). For this reason, the quality of secondary research should be carefully evaluated, as the origin of the information may be suspect.
Companies and researchers that rely on secondary data for decision-making need to closely examine the authenticity and reliability of the information by investigating the way the data was collected, analysed, and interpreted.
Secondary data may seem like a cheap and time-saving option, it is important to consider the source of the data and thus its authenticity and credibility. In other words, a disadvantage may be that the source is unreliable and calls into question the results of your own research.
Also Read: The Difference Between Primary and Secondary Research
2. Out-dated Data
It is important to be cautious while using secondary data that has been attained in the past. Out of date information can be of limited use to researchers who are conducting researches in the fast-changing markets and research areas.
3. Missing Information
A researcher often finds that an interesting study is simply a “teaser”. In such a case, only a limited part of the research is made public free of cost. It is quite obvious that the researcher will need to view the full report to obtain the missing information, but the publisher could charge a fee to provide access to the full report.
4. Availability of the Secondary Data
When taking into consideration the uses of secondary data, it is imperative to ascertain whether or not the data is available on your chosen topic, population , or variables . If secondary data is not in relevance to your requirements, primary data must be used despite its disadvantages.
5. Relevance of the Secondary Data
It is important to check the relevance of the data before using a secondary dataset. For instance, if the dimensional units do not match those required by the researcher for their study, or the ideas presented are somehow different from the ones that are required for the current study, then secondary data may be rendered as irrelevant for a new study. A serious downside of secondary data is the use of data that is not relevant.
6. Adequacy of Data
Secondary data may be alluring, but there is a risk that the amount and the relevance of data will not be sufficient to meet your research objectives. In other words, before you decide to do secondary research, you need to make sure that there is enough data on the topic at hand to meet your research questions .
While secondary research has some substantial disadvantages, if the data obtained are checked for their feasibility, reliability, and suitability for the research project at hand, they can still be of great use for your own research.
Need Help with Secondary Data Collection?
If you are a student, a researcher, or a business looking to collect secondary research for a report, a dissertation, an essay, or another type of project, feel free to get in touch with us. You can also read about our secondary data collection service here . Our experts include highly qualified academicians, doctors, and researchers who are sure to collect authentic, reliable, up to date and relevant sources for your research study.
Hire an Expert Researcher
Orders completed by our expert writers are
- Formally drafted in academic style
- 100% Plagiarism free & 100% Confidential
- Never resold
- Include unlimited free revisions
- Completed to match exact client requirements
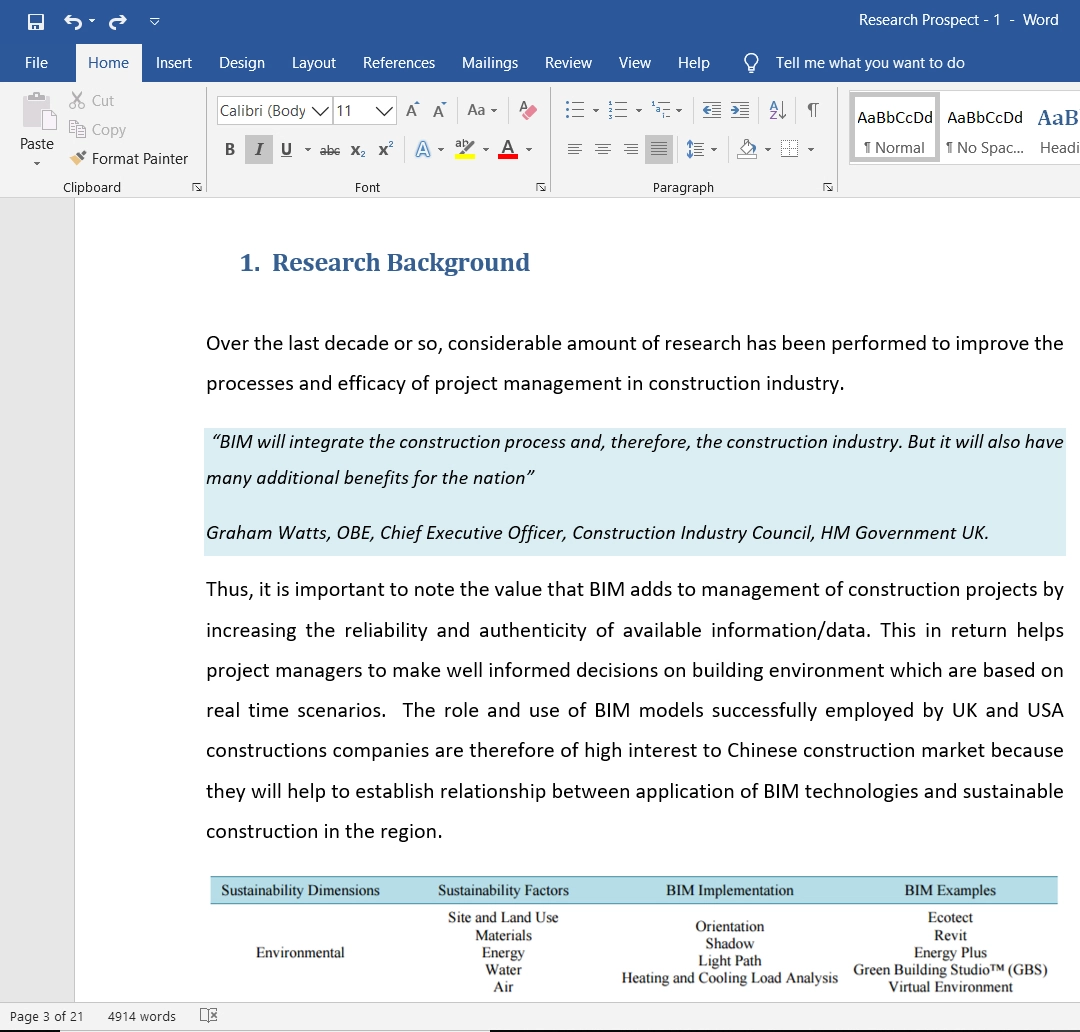
Frequently Asked Questions
How to perform secondary research.
To perform secondary research:
- Define research objectives.
- Collect existing data and sources.
- Analyze scholarly articles, books, and reports.
- Extract relevant information.
- Compare and synthesize findings.
- Properly cite sources used.
You May Also Like
A variable is a characteristic that can change and have more than one value, such as age, height, and weight. But what are the different types of variables?
Descriptive research is carried out to describe current issues, programs, and provides information about the issue through surveys and various fact-finding methods.
Content analysis is used to identify specific words, patterns, concepts, themes, phrases, or sentences within the content in the recorded communication.
USEFUL LINKS
LEARNING RESOURCES
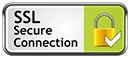
COMPANY DETAILS
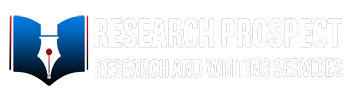
- How It Works
Have a language expert improve your writing
Run a free plagiarism check in 10 minutes, generate accurate citations for free.
- Knowledge Base
Methodology
- What is Secondary Research? | Definition, Types, & Examples
What is Secondary Research? | Definition, Types, & Examples
Published on January 20, 2023 by Tegan George . Revised on January 12, 2024.
Secondary research is a research method that uses data that was collected by someone else. In other words, whenever you conduct research using data that already exists, you are conducting secondary research. On the other hand, any type of research that you undertake yourself is called primary research .
Secondary research can be qualitative or quantitative in nature. It often uses data gathered from published peer-reviewed papers, meta-analyses, or government or private sector databases and datasets.
Table of contents
When to use secondary research, types of secondary research, examples of secondary research, advantages and disadvantages of secondary research, other interesting articles, frequently asked questions.
Secondary research is a very common research method, used in lieu of collecting your own primary data. It is often used in research designs or as a way to start your research process if you plan to conduct primary research later on.
Since it is often inexpensive or free to access, secondary research is a low-stakes way to determine if further primary research is needed, as gaps in secondary research are a strong indication that primary research is necessary. For this reason, while secondary research can theoretically be exploratory or explanatory in nature, it is usually explanatory: aiming to explain the causes and consequences of a well-defined problem.
Here's why students love Scribbr's proofreading services
Discover proofreading & editing
Secondary research can take many forms, but the most common types are:
Statistical analysis
Literature reviews, case studies, content analysis.
There is ample data available online from a variety of sources, often in the form of datasets. These datasets are often open-source or downloadable at a low cost, and are ideal for conducting statistical analyses such as hypothesis testing or regression analysis .
Credible sources for existing data include:
- The government
- Government agencies
- Non-governmental organizations
- Educational institutions
- Businesses or consultancies
- Libraries or archives
- Newspapers, academic journals, or magazines
A literature review is a survey of preexisting scholarly sources on your topic. It provides an overview of current knowledge, allowing you to identify relevant themes, debates, and gaps in the research you analyze. You can later apply these to your own work, or use them as a jumping-off point to conduct primary research of your own.
Structured much like a regular academic paper (with a clear introduction, body, and conclusion), a literature review is a great way to evaluate the current state of research and demonstrate your knowledge of the scholarly debates around your topic.
A case study is a detailed study of a specific subject. It is usually qualitative in nature and can focus on a person, group, place, event, organization, or phenomenon. A case study is a great way to utilize existing research to gain concrete, contextual, and in-depth knowledge about your real-world subject.
You can choose to focus on just one complex case, exploring a single subject in great detail, or examine multiple cases if you’d prefer to compare different aspects of your topic. Preexisting interviews , observational studies , or other sources of primary data make for great case studies.
Content analysis is a research method that studies patterns in recorded communication by utilizing existing texts. It can be either quantitative or qualitative in nature, depending on whether you choose to analyze countable or measurable patterns, or more interpretive ones. Content analysis is popular in communication studies, but it is also widely used in historical analysis, anthropology, and psychology to make more semantic qualitative inferences.
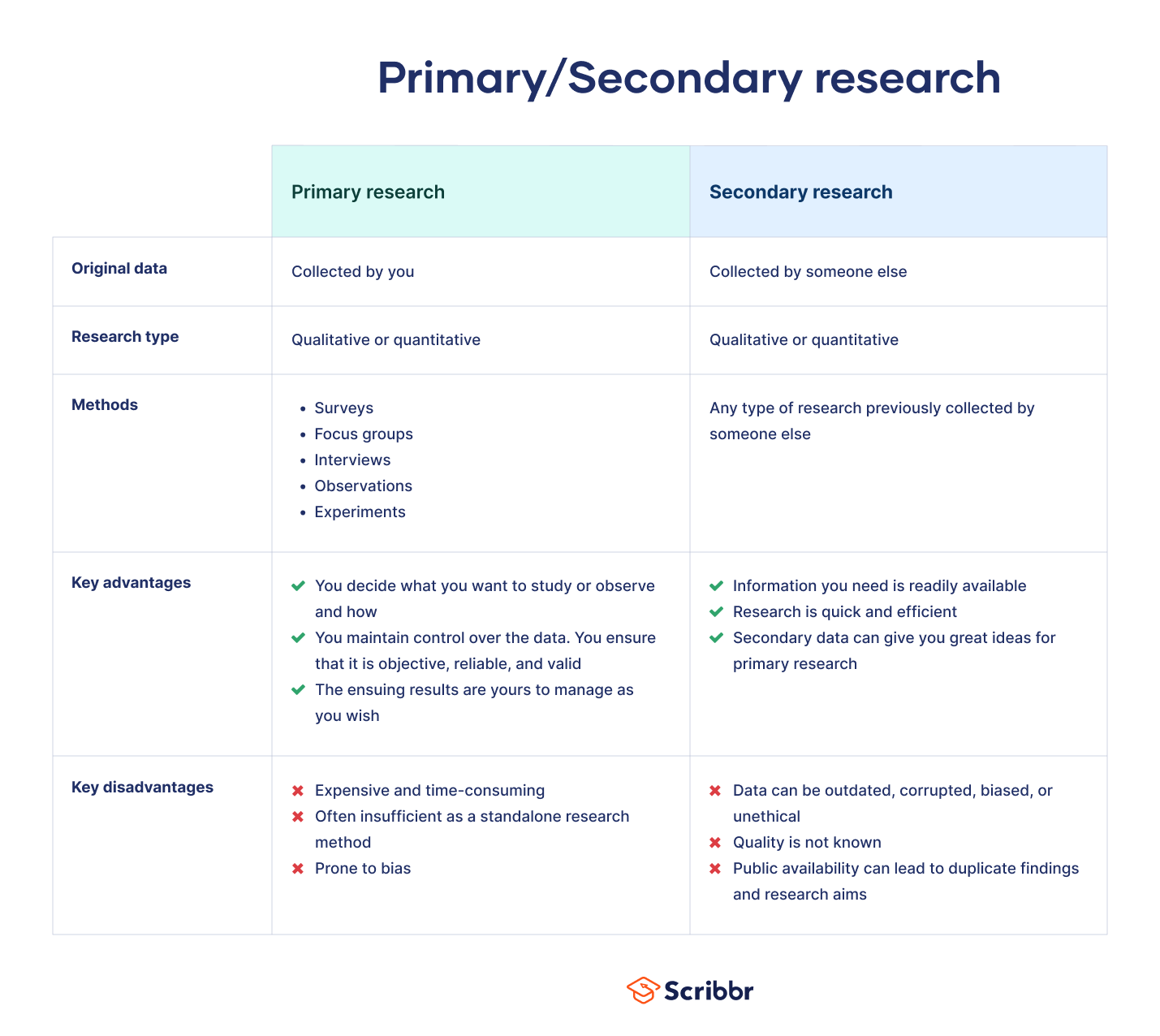
Secondary research is a broad research approach that can be pursued any way you’d like. Here are a few examples of different ways you can use secondary research to explore your research topic .
Secondary research is a very common research approach, but has distinct advantages and disadvantages.
Advantages of secondary research
Advantages include:
- Secondary data is very easy to source and readily available .
- It is also often free or accessible through your educational institution’s library or network, making it much cheaper to conduct than primary research .
- As you are relying on research that already exists, conducting secondary research is much less time consuming than primary research. Since your timeline is so much shorter, your research can be ready to publish sooner.
- Using data from others allows you to show reproducibility and replicability , bolstering prior research and situating your own work within your field.
Disadvantages of secondary research
Disadvantages include:
- Ease of access does not signify credibility . It’s important to be aware that secondary research is not always reliable , and can often be out of date. It’s critical to analyze any data you’re thinking of using prior to getting started, using a method like the CRAAP test .
- Secondary research often relies on primary research already conducted. If this original research is biased in any way, those research biases could creep into the secondary results.
Many researchers using the same secondary research to form similar conclusions can also take away from the uniqueness and reliability of your research. Many datasets become “kitchen-sink” models, where too many variables are added in an attempt to draw increasingly niche conclusions from overused data . Data cleansing may be necessary to test the quality of the research.
Receive feedback on language, structure, and formatting
Professional editors proofread and edit your paper by focusing on:
- Academic style
- Vague sentences
- Style consistency
See an example
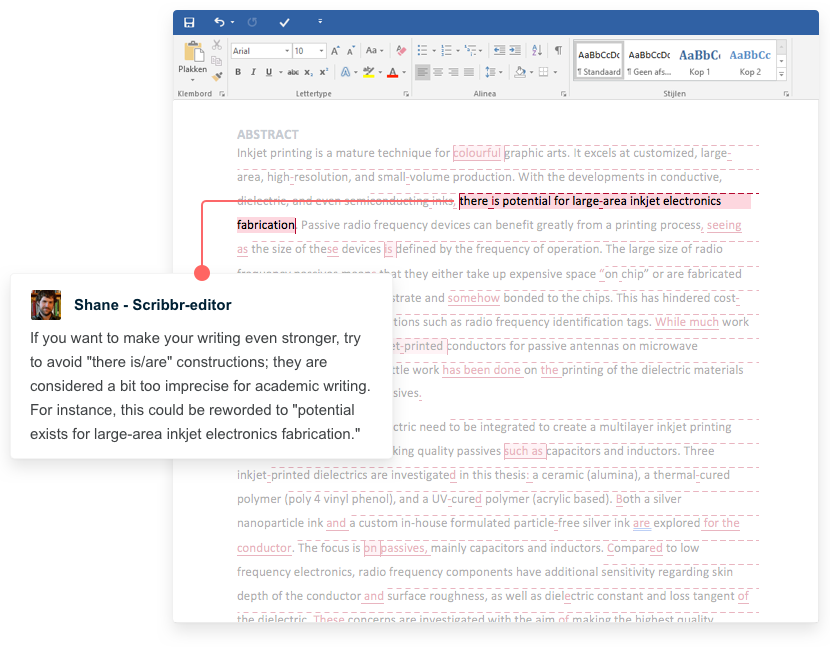
If you want to know more about statistics , methodology , or research bias , make sure to check out some of our other articles with explanations and examples.
- Normal distribution
- Degrees of freedom
- Null hypothesis
- Discourse analysis
- Control groups
- Mixed methods research
- Non-probability sampling
- Quantitative research
- Inclusion and exclusion criteria
Research bias
- Rosenthal effect
- Implicit bias
- Cognitive bias
- Selection bias
- Negativity bias
- Status quo bias
A systematic review is secondary research because it uses existing research. You don’t collect new data yourself.
The research methods you use depend on the type of data you need to answer your research question .
- If you want to measure something or test a hypothesis , use quantitative methods . If you want to explore ideas, thoughts and meanings, use qualitative methods .
- If you want to analyze a large amount of readily-available data, use secondary data. If you want data specific to your purposes with control over how it is generated, collect primary data.
- If you want to establish cause-and-effect relationships between variables , use experimental methods. If you want to understand the characteristics of a research subject, use descriptive methods.
Quantitative research deals with numbers and statistics, while qualitative research deals with words and meanings.
Quantitative methods allow you to systematically measure variables and test hypotheses . Qualitative methods allow you to explore concepts and experiences in more detail.
Sources in this article
We strongly encourage students to use sources in their work. You can cite our article (APA Style) or take a deep dive into the articles below.
George, T. (2024, January 12). What is Secondary Research? | Definition, Types, & Examples. Scribbr. Retrieved April 9, 2024, from https://www.scribbr.com/methodology/secondary-research/
Largan, C., & Morris, T. M. (2019). Qualitative Secondary Research: A Step-By-Step Guide (1st ed.). SAGE Publications Ltd.
Peloquin, D., DiMaio, M., Bierer, B., & Barnes, M. (2020). Disruptive and avoidable: GDPR challenges to secondary research uses of data. European Journal of Human Genetics , 28 (6), 697–705. https://doi.org/10.1038/s41431-020-0596-x
Is this article helpful?
Tegan George
Other students also liked, primary research | definition, types, & examples, how to write a literature review | guide, examples, & templates, what is a case study | definition, examples & methods, unlimited academic ai-proofreading.
✔ Document error-free in 5minutes ✔ Unlimited document corrections ✔ Specialized in correcting academic texts
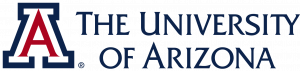
Want to create or adapt books like this? Learn more about how Pressbooks supports open publishing practices.
4 Chapter 5 Secondary Research
Learning Objectives
By the end of this chapter, students must be able to:
- Explain the concept of secondary research
- Highlight the key benefits and limitations of secondary research
- Evaluate different sources of secondary data
What is Secondary Research?
In situations where the researcher has not been involved in the data gathering process (primary research), one may have to rely on existing information and data to arrive at specific research conclusions or outcomes. Secondary research, also known as desk research, is a research method that involves the use of information previously collected for another research purpose.
In this chapter, we are going to explain what secondary research is, how it works, and share some examples of it in practice.
Marketing textbook © 2022 Western Sydney University taken by Sally Tsoutas Western Sydney University Photographer is licensed under an Attribution-NonCommercial-NoDerivatives 4.0 International
Sources of secondary data.
The two main sources of secondary data are:
- Internal sources
- External sources
Internal sources of secondary data exist within the organization. There could be reports, previous research findings, or old documents which may still be used to understand a particular phenomenon. This information may only be available to the organization’s members and could be a valuable asset.
External sources of secondary data lie outside the organization and refer to information held at the public library, government departments, council offices, various associations as well as in newspapers or journal articles.
Benefits of using Secondary Data
It is only logical for researchers to look for secondary information thoroughly before investing their time and resources in collecting primary data. In academic research, scholars are not permitted to move to the next stage till they demonstrate they have undertaken a review of all previous studies. Suppose a researcher would like to examine the characteristics of a migrant population in the Western Sydney region. The following pieces of information are already available in various reports generated from the Australian Bureau of Statistics’ census data:
- Birthplace of residents
- Language spoken at home by residents
- Family size
- Income levels
- Level of education
By accessing such readily available secondary data, the researcher is able to save time, money, and effort. When the data comes from a reputable source, it further adds to the researchers’ credibility of identifying a trustworthy source of information.
Evaluation of Secondary Data
[1] Assessing secondary data is important. It may not always be available free of cost. The following factors must be considered as these relate to the reliability and validity of research results, such as whether:
- the source is trusted
- the sample characteristics, time of collection, and response rate (if relevant) of the data are appropriate
- the methods of data collection are appropriate and acceptable in your discipline
- the data were collected in a consistent way
- any data coding or modification is appropriate and sufficient
- the documentation of the original study in which the data were collected is detailed enough for you to assess its quality
- there is enough information in the metadata or data to properly cite the original source.
In addition to the above-mentioned points, some practical issues which need to be evaluated include the cost of accessing and the time frame involved in getting access to the data is relevant.
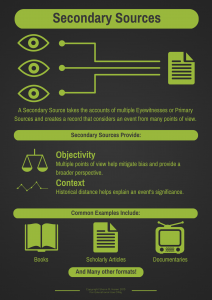
The infographic Secondary Sources created by Shonn M. Haren, 2015 is licensed under a Creative Commons Attribution 4.0 International Licence [2]
Table 2: differences between primary and secondary research.
- Griffith University n.d., Research data: get started, viewed 28 February 2022,<https://libraryguides.griffith.edu.au/finddata>. ↵
- Shonnmaren n.d., Secondary sources, viewed 28 February 2020, Wikimedia Commons, <https://commons.wikimedia.org/wiki/File:Secondary_Sources.png> ↵
- Qualtrics XM n.d., S econdary research: definition, methods and examples , viewed 28 February 2022, <https://www.qualtrics.com/au/experience-management/research/secondary-research/#:~:text=Unlike%20primary%20research%2C%20secondary%20research,secondary%20research%20have%20their%20places>. ↵
About the author
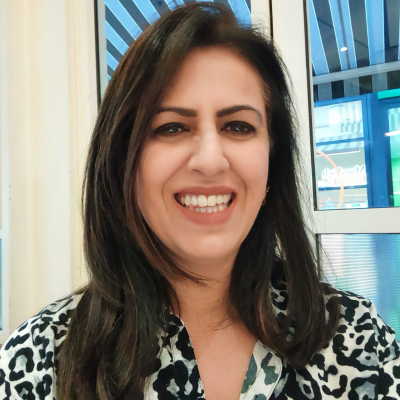
name: Aila Khan
institution: Western Sydney University
Chapter 5 Secondary Research Copyright © by Aila Khan is licensed under a Creative Commons Attribution-NonCommercial-ShareAlike 4.0 International License , except where otherwise noted.
Share This Book
- Skip to main content
- Skip to primary sidebar
- Skip to footer
- QuestionPro

- Solutions Industries Gaming Automotive Sports and events Education Government Travel & Hospitality Financial Services Healthcare Cannabis Technology Use Case NPS+ Communities Audience Contactless surveys Mobile LivePolls Member Experience GDPR Positive People Science 360 Feedback Surveys
- Resources Blog eBooks Survey Templates Case Studies Training Help center

Home Market Research
Secondary Research: Definition, Methods and Examples.
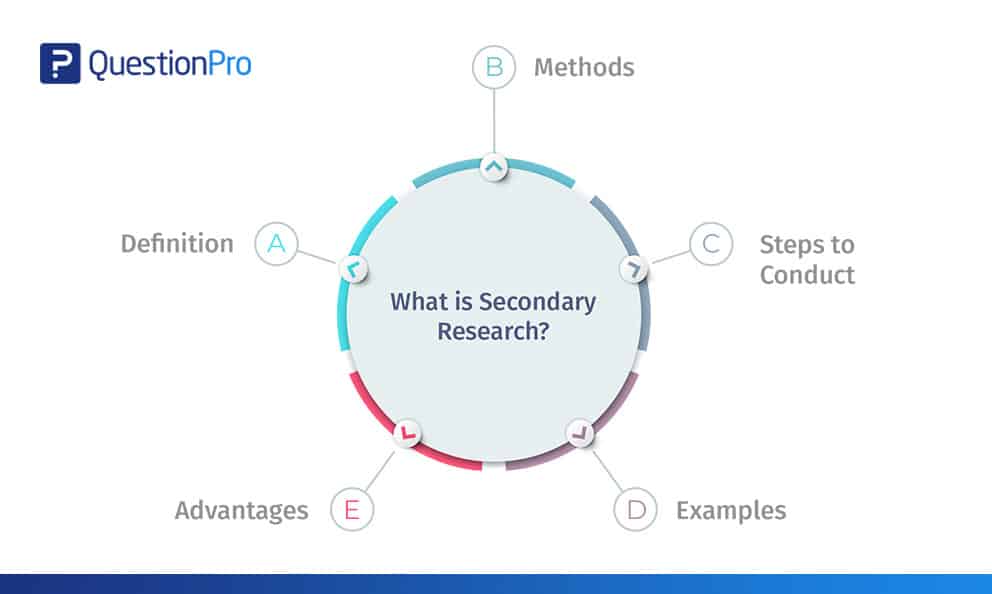
In the world of research, there are two main types of data sources: primary and secondary. While primary research involves collecting new data directly from individuals or sources, secondary research involves analyzing existing data already collected by someone else. Today we’ll discuss secondary research.
One common source of this research is published research reports and other documents. These materials can often be found in public libraries, on websites, or even as data extracted from previously conducted surveys. In addition, many government and non-government agencies maintain extensive data repositories that can be accessed for research purposes.
LEARN ABOUT: Research Process Steps
While secondary research may not offer the same level of control as primary research, it can be a highly valuable tool for gaining insights and identifying trends. Researchers can save time and resources by leveraging existing data sources while still uncovering important information.
What is Secondary Research: Definition
Secondary research is a research method that involves using already existing data. Existing data is summarized and collated to increase the overall effectiveness of the research.
One of the key advantages of secondary research is that it allows us to gain insights and draw conclusions without having to collect new data ourselves. This can save time and resources and also allow us to build upon existing knowledge and expertise.
When conducting secondary research, it’s important to be thorough and thoughtful in our approach. This means carefully selecting the sources and ensuring that the data we’re analyzing is reliable and relevant to the research question . It also means being critical and analytical in the analysis and recognizing any potential biases or limitations in the data.
LEARN ABOUT: Level of Analysis
Secondary research is much more cost-effective than primary research , as it uses already existing data, unlike primary research, where data is collected firsthand by organizations or businesses or they can employ a third party to collect data on their behalf.
LEARN ABOUT: Data Analytics Projects
Secondary Research Methods with Examples
Secondary research is cost-effective, one of the reasons it is a popular choice among many businesses and organizations. Not every organization is able to pay a huge sum of money to conduct research and gather data. So, rightly secondary research is also termed “ desk research ”, as data can be retrieved from sitting behind a desk.
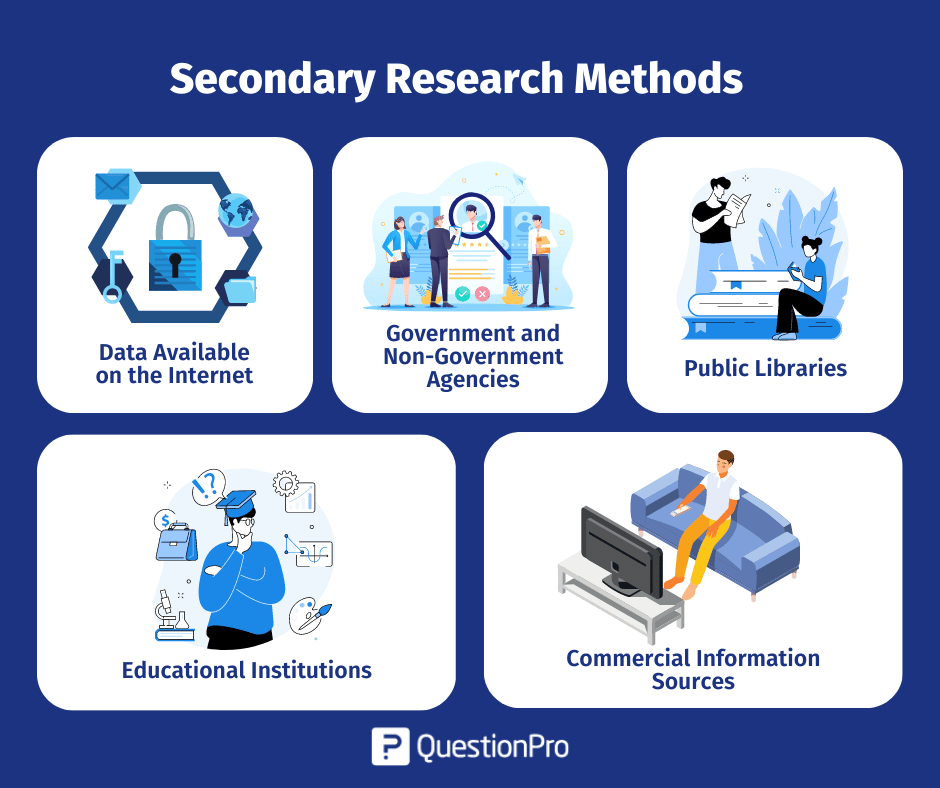
The following are popularly used secondary research methods and examples:
1. Data Available on The Internet
One of the most popular ways to collect secondary data is the internet. Data is readily available on the internet and can be downloaded at the click of a button.
This data is practically free of cost, or one may have to pay a negligible amount to download the already existing data. Websites have a lot of information that businesses or organizations can use to suit their research needs. However, organizations need to consider only authentic and trusted website to collect information.
2. Government and Non-Government Agencies
Data for secondary research can also be collected from some government and non-government agencies. For example, US Government Printing Office, US Census Bureau, and Small Business Development Centers have valuable and relevant data that businesses or organizations can use.
There is a certain cost applicable to download or use data available with these agencies. Data obtained from these agencies are authentic and trustworthy.
3. Public Libraries
Public libraries are another good source to search for data for this research. Public libraries have copies of important research that were conducted earlier. They are a storehouse of important information and documents from which information can be extracted.
The services provided in these public libraries vary from one library to another. More often, libraries have a huge collection of government publications with market statistics, large collection of business directories and newsletters.
4. Educational Institutions
Importance of collecting data from educational institutions for secondary research is often overlooked. However, more research is conducted in colleges and universities than any other business sector.
The data that is collected by universities is mainly for primary research. However, businesses or organizations can approach educational institutions and request for data from them.
5. Commercial Information Sources
Local newspapers, journals, magazines, radio and TV stations are a great source to obtain data for secondary research. These commercial information sources have first-hand information on economic developments, political agenda, market research, demographic segmentation and similar subjects.
Businesses or organizations can request to obtain data that is most relevant to their study. Businesses not only have the opportunity to identify their prospective clients but can also know about the avenues to promote their products or services through these sources as they have a wider reach.
Key Differences between Primary Research and Secondary Research
Understanding the distinction between primary research and secondary research is essential in determining which research method is best for your project. These are the two main types of research methods, each with advantages and disadvantages. In this section, we will explore the critical differences between the two and when it is appropriate to use them.
How to Conduct Secondary Research?
We have already learned about the differences between primary and secondary research. Now, let’s take a closer look at how to conduct it.
Secondary research is an important tool for gathering information already collected and analyzed by others. It can help us save time and money and allow us to gain insights into the subject we are researching. So, in this section, we will discuss some common methods and tips for conducting it effectively.
Here are the steps involved in conducting secondary research:
1. Identify the topic of research: Before beginning secondary research, identify the topic that needs research. Once that’s done, list down the research attributes and its purpose.
2. Identify research sources: Next, narrow down on the information sources that will provide most relevant data and information applicable to your research.
3. Collect existing data: Once the data collection sources are narrowed down, check for any previous data that is available which is closely related to the topic. Data related to research can be obtained from various sources like newspapers, public libraries, government and non-government agencies etc.
4. Combine and compare: Once data is collected, combine and compare the data for any duplication and assemble data into a usable format. Make sure to collect data from authentic sources. Incorrect data can hamper research severely.
4. Analyze data: Analyze collected data and identify if all questions are answered. If not, repeat the process if there is a need to dwell further into actionable insights.
Advantages of Secondary Research
Secondary research offers a number of advantages to researchers, including efficiency, the ability to build upon existing knowledge, and the ability to conduct research in situations where primary research may not be possible or ethical. By carefully selecting their sources and being thoughtful in their approach, researchers can leverage secondary research to drive impact and advance the field. Some key advantages are the following:
1. Most information in this research is readily available. There are many sources from which relevant data can be collected and used, unlike primary research, where data needs to collect from scratch.
2. This is a less expensive and less time-consuming process as data required is easily available and doesn’t cost much if extracted from authentic sources. A minimum expenditure is associated to obtain data.
3. The data that is collected through secondary research gives organizations or businesses an idea about the effectiveness of primary research. Hence, organizations or businesses can form a hypothesis and evaluate cost of conducting primary research.
4. Secondary research is quicker to conduct because of the availability of data. It can be completed within a few weeks depending on the objective of businesses or scale of data needed.
As we can see, this research is the process of analyzing data already collected by someone else, and it can offer a number of benefits to researchers.
Disadvantages of Secondary Research
On the other hand, we have some disadvantages that come with doing secondary research. Some of the most notorious are the following:
1. Although data is readily available, credibility evaluation must be performed to understand the authenticity of the information available.
2. Not all secondary data resources offer the latest reports and statistics. Even when the data is accurate, it may not be updated enough to accommodate recent timelines.
3. Secondary research derives its conclusion from collective primary research data. The success of your research will depend, to a greater extent, on the quality of research already conducted by primary research.
LEARN ABOUT: 12 Best Tools for Researchers
In conclusion, secondary research is an important tool for researchers exploring various topics. By leveraging existing data sources, researchers can save time and resources, build upon existing knowledge, and conduct research in situations where primary research may not be feasible.
There are a variety of methods and examples of secondary research, from analyzing public data sets to reviewing previously published research papers. As students and aspiring researchers, it’s important to understand the benefits and limitations of this research and to approach it thoughtfully and critically. By doing so, we can continue to advance our understanding of the world around us and contribute to meaningful research that positively impacts society.
QuestionPro can be a useful tool for conducting secondary research in a variety of ways. You can create online surveys that target a specific population, collecting data that can be analyzed to gain insights into consumer behavior, attitudes, and preferences; analyze existing data sets that you have obtained through other means or benchmark your organization against others in your industry or against industry standards. The software provides a range of benchmarking tools that can help you compare your performance on key metrics, such as customer satisfaction, with that of your peers.
Using QuestionPro thoughtfully and strategically allows you to gain valuable insights to inform decision-making and drive business success. Start today for free! No credit card is required.
LEARN MORE FREE TRIAL
MORE LIKE THIS
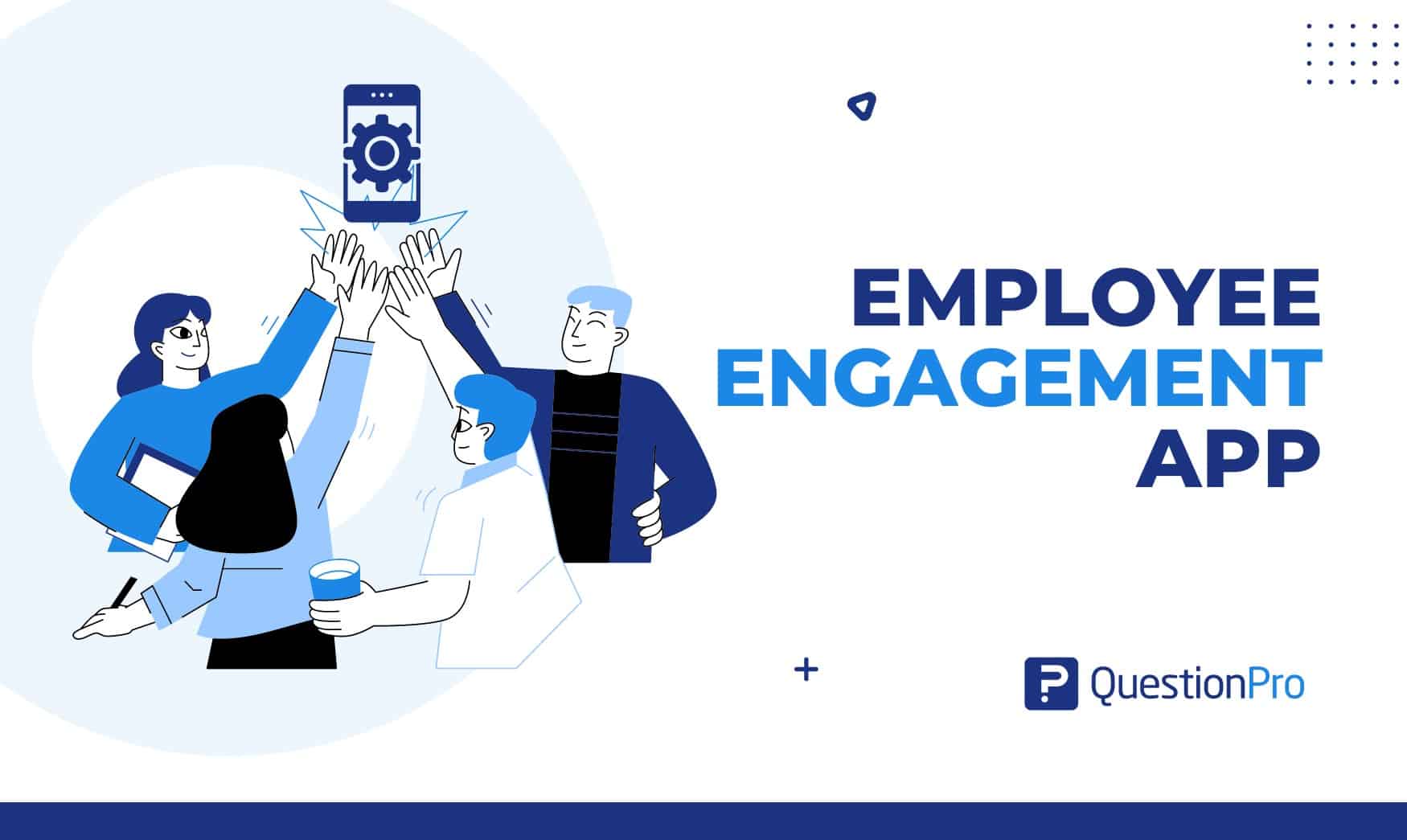
Employee Engagement App: Top 11 For Workforce Improvement
Apr 10, 2024
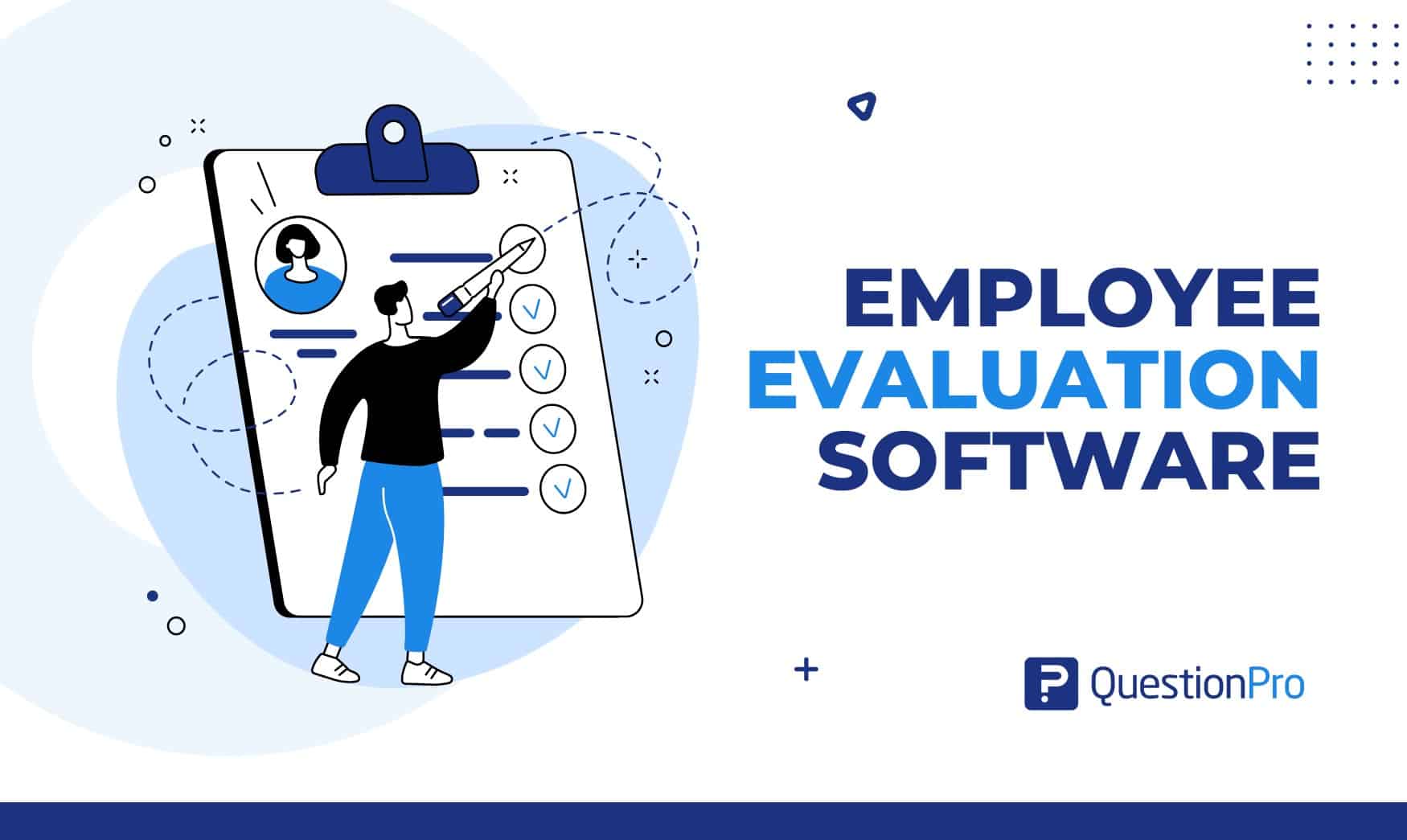
Top 15 Employee Evaluation Software to Enhance Performance
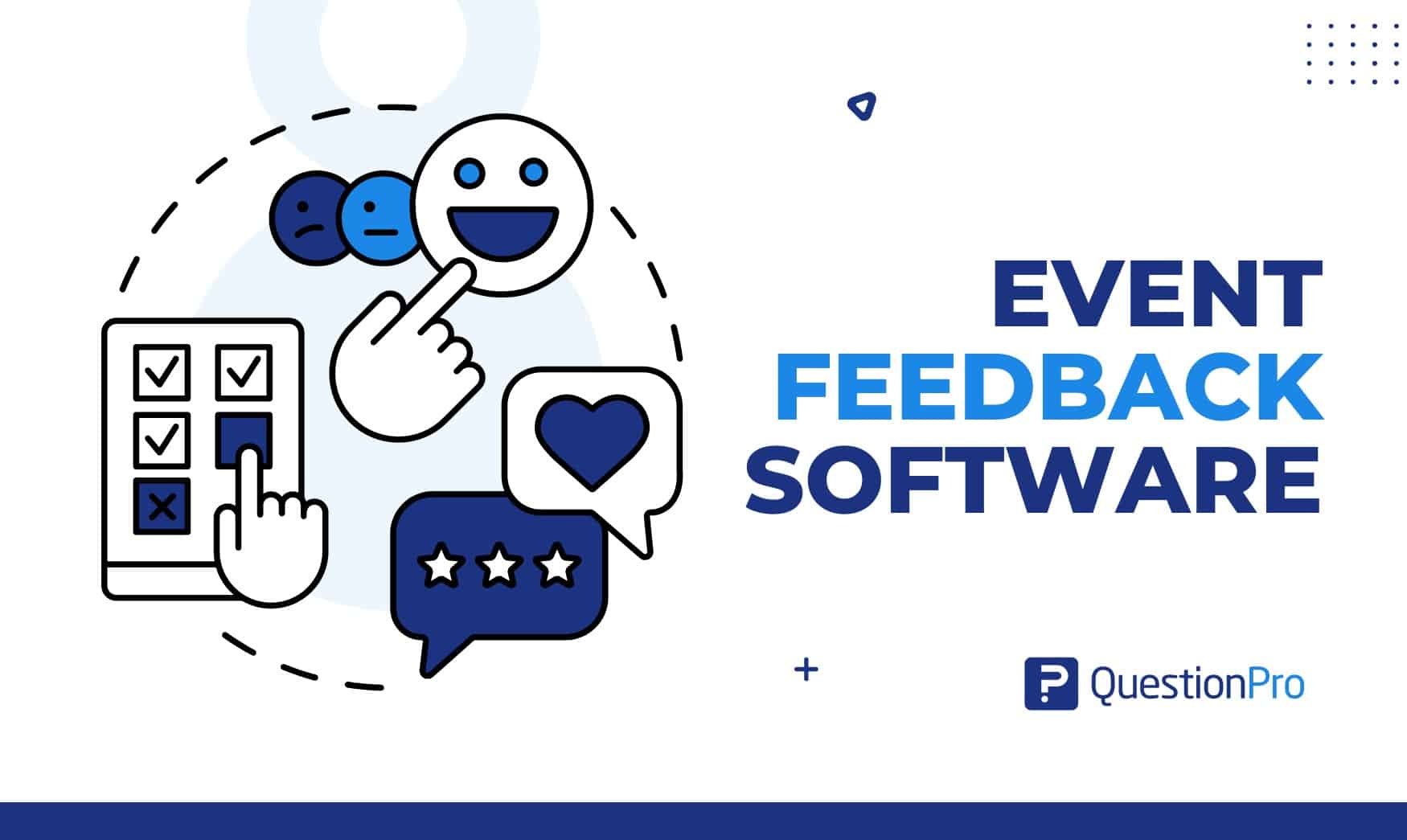
Event Feedback Software: Top 11 Best in 2024
Apr 9, 2024
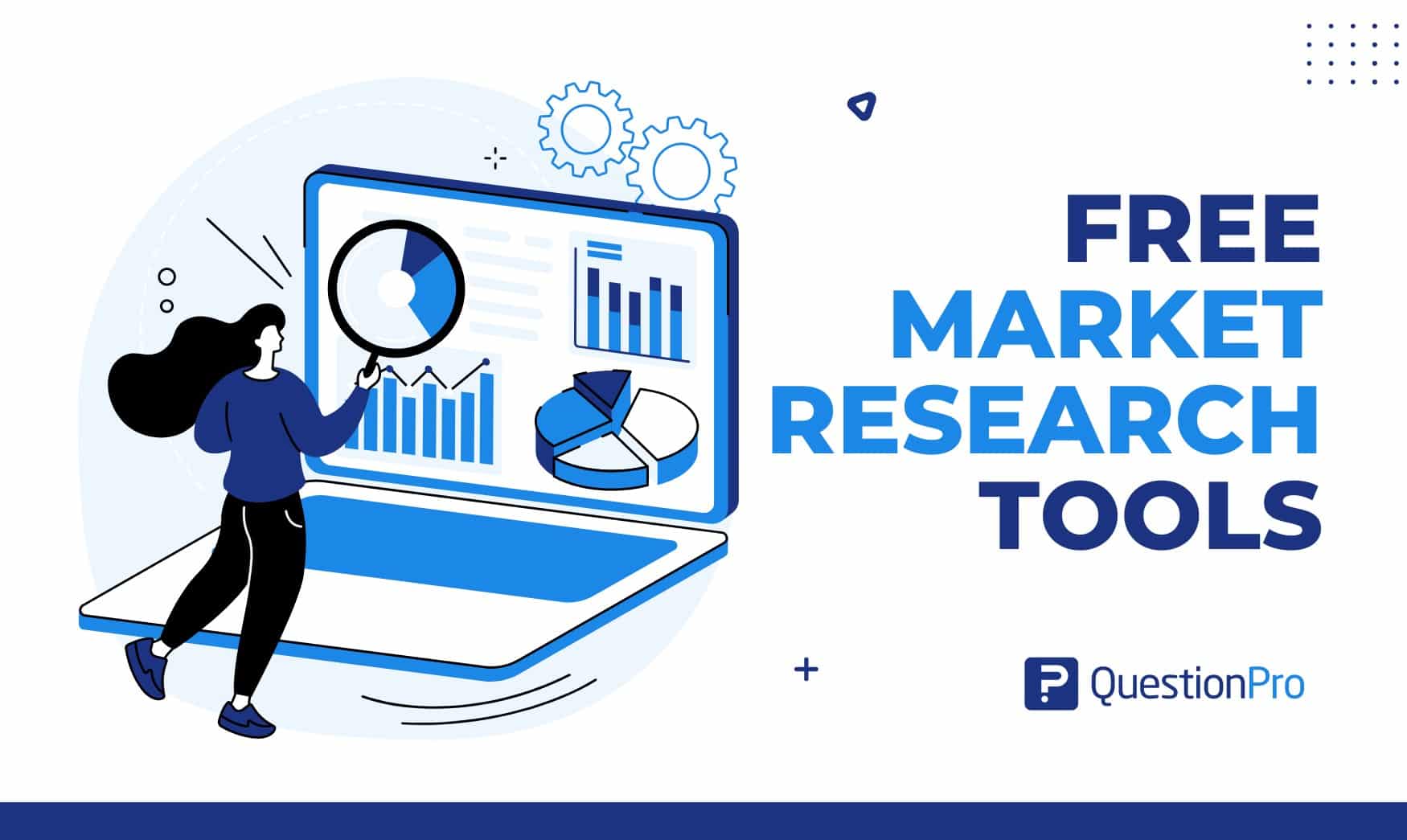
Top 10 Free Market Research Tools to Boost Your Business
Other categories.
- Academic Research
- Artificial Intelligence
- Assessments
- Brand Awareness
- Case Studies
- Communities
- Consumer Insights
- Customer effort score
- Customer Engagement
- Customer Experience
- Customer Loyalty
- Customer Research
- Customer Satisfaction
- Employee Benefits
- Employee Engagement
- Employee Retention
- Friday Five
- General Data Protection Regulation
- Insights Hub
- Life@QuestionPro
- Market Research
- Mobile diaries
- Mobile Surveys
- New Features
- Online Communities
- Question Types
- Questionnaire
- QuestionPro Products
- Release Notes
- Research Tools and Apps
- Revenue at Risk
- Survey Templates
- Training Tips
- Uncategorized
- Video Learning Series
- What’s Coming Up
- Workforce Intelligence
What is Secondary Research? Types, Methods, Examples
Appinio Research · 20.09.2023 · 13min read
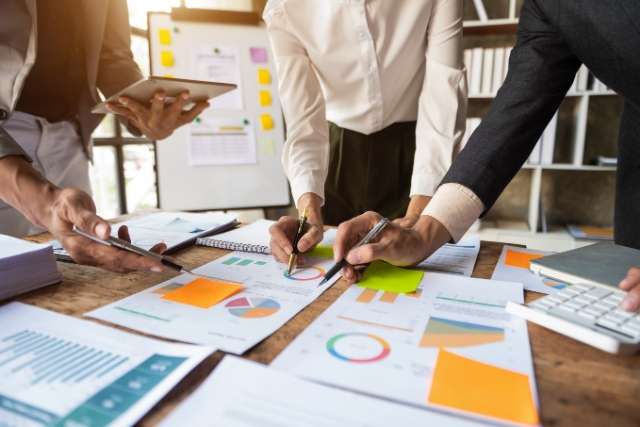
Have you ever wondered how researchers gather valuable insights without conducting new experiments or surveys? That's where secondary research steps in—a powerful approach that allows us to explore existing data and information others collect.
Whether you're a student, a professional, or someone seeking to make informed decisions, understanding the art of secondary research opens doors to a wealth of knowledge.
What is Secondary Research?
Secondary Research refers to the process of gathering and analyzing existing data, information, and knowledge that has been previously collected and compiled by others. This approach allows researchers to leverage available sources, such as articles, reports, and databases, to gain insights, validate hypotheses, and make informed decisions without collecting new data.
Benefits of Secondary Research
Secondary research offers a range of advantages that can significantly enhance your research process and the quality of your findings.
- Time and Cost Efficiency: Secondary research saves time and resources by utilizing existing data sources, eliminating the need for data collection from scratch.
- Wide Range of Data: Secondary research provides access to vast information from various sources, allowing for comprehensive analysis.
- Historical Perspective: Examining past research helps identify trends, changes, and long-term patterns that might not be immediately apparent.
- Reduced Bias: As data is collected by others, there's often less inherent bias than in conducting primary research, where biases might affect data collection.
- Support for Primary Research: Secondary research can lay the foundation for primary research by providing context and insights into gaps in existing knowledge.
- Comparative Analysis : By integrating data from multiple sources, you can conduct robust comparative analyses for more accurate conclusions.
- Benchmarking and Validation: Secondary research aids in benchmarking performance against industry standards and validating hypotheses.
Primary Research vs. Secondary Research
When it comes to research methodologies, primary and secondary research each have their distinct characteristics and advantages. Here's a brief comparison to help you understand the differences.
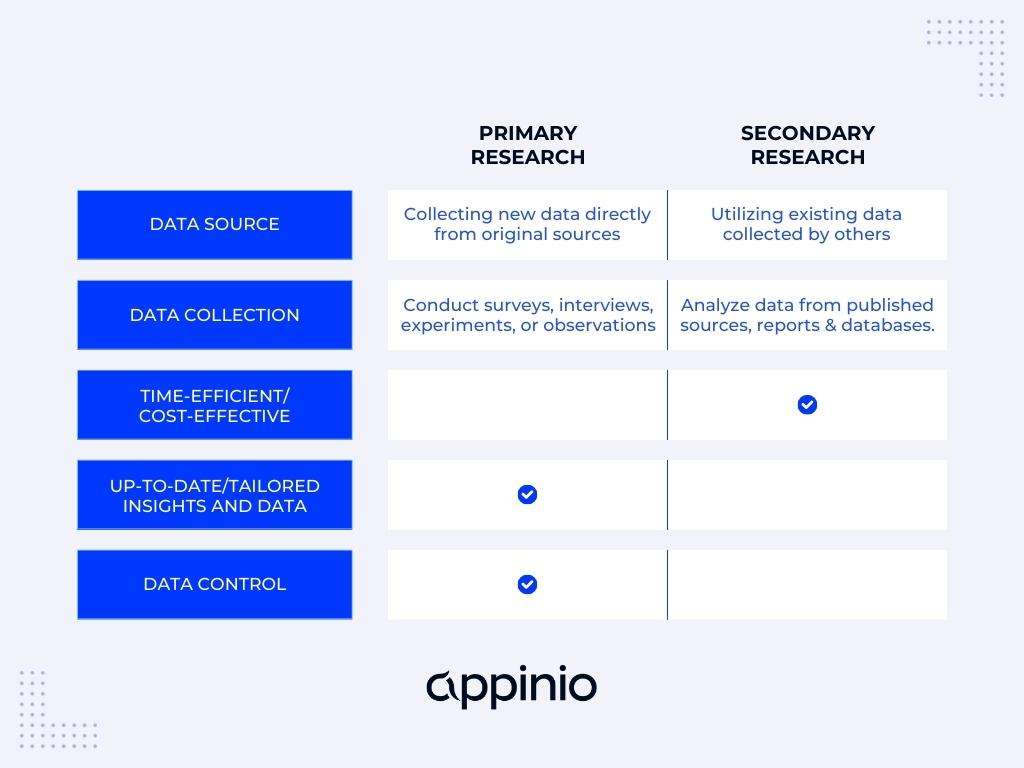
Primary Research
- Data Source: Involves collecting new data directly from original sources.
- Data Collection: Researchers design and conduct surveys, interviews, experiments, or observations.
- Time and Resources: Typically requires more time, effort, and resources due to data collection.
- Fresh Insights: Provides firsthand, up-to-date information tailored to specific research questions.
- Control: Researchers control the data collection process and can shape methodologies.
Secondary Research
- Data Source: Involves utilizing existing data and information collected by others.
- Data Collection: Researchers search, select, and analyze data from published sources, reports, and databases.
- Time and Resources: Generally more time-efficient and cost-effective as data is already available.
- Existing Knowledge: Utilizes data that has been previously compiled, often providing broader context.
- Less Control: Researchers have limited control over how data was collected originally, if any.
Choosing between primary and secondary research depends on your research objectives, available resources, and the depth of insights you require.
Types of Secondary Research
Secondary research encompasses various types of existing data sources that can provide valuable insights for your research endeavors. Understanding these types can help you choose the most relevant sources for your objectives.
Here are the primary types of secondary research:
Internal Sources
Internal sources consist of data generated within your organization or entity. These sources provide valuable insights into your own operations and performance.
- Company Records and Data: Internal reports, documents, and databases that house information about sales, operations, and customer interactions.
- Sales Reports and Customer Data: Analysis of past sales trends, customer demographics, and purchasing behavior.
- Financial Statements and Annual Reports: Financial data, such as balance sheets and income statements, offer insights into the organization's financial health.
External Sources
External sources encompass data collected and published by entities outside your organization.
These sources offer a broader perspective on various subjects.
- Published Literature and Journals: Scholarly articles, research papers, and academic studies available in journals or online databases.
- Market Research Reports: Reports from market research firms that provide insights into industry trends, consumer behavior, and market forecasts.
- Government and NGO Databases: Data collected and maintained by government agencies and non-governmental organizations, offering demographic, economic, and social information.
- Online Media and News Articles: News outlets and online publications that cover current events, trends, and societal developments.
Each type of secondary research source holds its value and relevance, depending on the nature of your research objectives. Combining these sources lets you understand the subject matter and make informed decisions.
How to Conduct Secondary Research?
Effective secondary research involves a thoughtful and systematic approach that enables you to extract valuable insights from existing data sources. Here's a step-by-step guide on how to navigate the process:
1. Define Your Research Objectives
Before delving into secondary research, clearly define what you aim to achieve. Identify the specific questions you want to answer, the insights you're seeking, and the scope of your research.
2. Identify Relevant Sources
Begin by identifying the most appropriate sources for your research. Consider the nature of your research objectives and the data type you require. Seek out sources such as academic journals, market research reports, official government databases, and reputable news outlets.
3. Evaluate Source Credibility
Ensuring the credibility of your sources is crucial. Evaluate the reliability of each source by assessing factors such as the author's expertise, the publication's reputation, and the objectivity of the information provided. Choose sources that align with your research goals and are free from bias.
4. Extract and Analyze Information
Once you've gathered your sources, carefully extract the relevant information. Take thorough notes, capturing key data points, insights, and any supporting evidence. As you accumulate information, start identifying patterns, trends, and connections across different sources.
5. Synthesize Findings
As you analyze the data, synthesize your findings to draw meaningful conclusions. Compare and contrast information from various sources to identify common themes and discrepancies. This synthesis process allows you to construct a coherent narrative that addresses your research objectives.
6. Address Limitations and Gaps
Acknowledge the limitations and potential gaps in your secondary research. Recognize that secondary data might have inherent biases or be outdated. Where necessary, address these limitations by cross-referencing information or finding additional sources to fill in gaps.
7. Contextualize Your Findings
Contextualization is crucial in deriving actionable insights from your secondary research. Consider the broader context within which the data was collected. How does the information relate to current trends, societal changes, or industry shifts? This contextual understanding enhances the relevance and applicability of your findings.
8. Cite Your Sources
Maintain academic integrity by properly citing the sources you've used for your secondary research. Accurate citations not only give credit to the original authors but also provide a clear trail for readers to access the information themselves.
9. Integrate Secondary and Primary Research (If Applicable)
In some cases, combining secondary and primary research can yield more robust insights. If you've also conducted primary research, consider integrating your secondary findings with your primary data to provide a well-rounded perspective on your research topic.
You can use a market research platform like Appinio to conduct primary research with real-time insights in minutes!
10. Communicate Your Findings
Finally, communicate your findings effectively. Whether it's in an academic paper, a business report, or any other format, present your insights clearly and concisely. Provide context for your conclusions and use visual aids like charts and graphs to enhance understanding.
Remember that conducting secondary research is not just about gathering information—it's about critically analyzing, interpreting, and deriving valuable insights from existing data. By following these steps, you'll navigate the process successfully and contribute to the body of knowledge in your field.
Secondary Research Examples
To better understand how secondary research is applied in various contexts, let's explore a few real-world examples that showcase its versatility and value.
Market Analysis and Trend Forecasting
Imagine you're a marketing strategist tasked with launching a new product in the smartphone industry. By conducting secondary research, you can:
- Access Market Reports: Utilize market research reports to understand consumer preferences, competitive landscape, and growth projections.
- Analyze Trends: Examine past sales data and industry reports to identify trends in smartphone features, design, and user preferences.
- Benchmark Competitors: Compare market share, customer satisfaction, and pricing strategies of key competitors to develop a strategic advantage.
- Forecast Demand: Use historical sales data and market growth predictions to estimate demand for your new product.
Academic Research and Literature Reviews
Suppose you're a student researching climate change's effects on marine ecosystems. Secondary research aids your academic endeavors by:
- Reviewing Existing Studies: Analyze peer-reviewed articles and scientific papers to understand the current state of knowledge on the topic.
- Identifying Knowledge Gaps: Identify areas where further research is needed based on what existing studies still need to cover.
- Comparing Methodologies: Compare research methodologies used by different studies to assess the strengths and limitations of their approaches.
- Synthesizing Insights: Synthesize findings from various studies to form a comprehensive overview of the topic's implications on marine life.
Competitive Landscape Assessment for Business Strategy
Consider you're a business owner looking to expand your restaurant chain to a new location. Secondary research aids your strategic decision-making by:
- Analyzing Demographics: Utilize demographic data from government databases to understand the local population's age, income, and preferences.
- Studying Local Trends: Examine restaurant industry reports to identify the types of cuisines and dining experiences currently popular in the area.
- Understanding Consumer Behavior: Analyze online reviews and social media discussions to gauge customer sentiment towards existing restaurants in the vicinity.
- Assessing Economic Conditions: Access economic reports to evaluate the local economy's stability and potential purchasing power.
These examples illustrate the practical applications of secondary research across various fields to provide a foundation for informed decision-making, deeper understanding, and innovation.
Secondary Research Limitations
While secondary research offers many benefits, it's essential to be aware of its limitations to ensure the validity and reliability of your findings.
- Data Quality and Validity: The accuracy and reliability of secondary data can vary, affecting the credibility of your research.
- Limited Contextual Information: Secondary sources might lack detailed contextual information, making it important to interpret findings within the appropriate context.
- Data Suitability: Existing data might not align perfectly with your research objectives, leading to compromises or incomplete insights.
- Outdated Information: Some sources might provide obsolete information that doesn't accurately reflect current trends or situations.
- Potential Bias: While secondary data is often less biased, biases might still exist in the original data sources, influencing your findings.
- Incompatibility of Data: Combining data from different sources might pose challenges due to variations in definitions, methodologies, or units of measurement.
- Lack of Control: Unlike primary research, you have no control over how data was collected or its quality, potentially affecting your analysis. Understanding these limitations will help you navigate secondary research effectively and make informed decisions based on a well-rounded understanding of its strengths and weaknesses.
Secondary research is a valuable tool that businesses can use to their advantage. By tapping into existing data and insights, companies can save time, resources, and effort that would otherwise be spent on primary research. This approach equips decision-makers with a broader understanding of market trends, consumer behaviors, and competitive landscapes. Additionally, benchmarking against industry standards and validating hypotheses empowers businesses to make informed choices that lead to growth and success.
As you navigate the world of secondary research, remember that it's not just about data retrieval—it's about strategic utilization. With a clear grasp of how to access, analyze, and interpret existing information, businesses can stay ahead of the curve, adapt to changing landscapes, and make decisions that are grounded in reliable knowledge.
How to Conduct Secondary Research in Minutes?
In the world of decision-making, having access to real-time consumer insights is no longer a luxury—it's a necessity. That's where Appinio comes in, revolutionizing how businesses gather valuable data for better decision-making. As a real-time market research platform, Appinio empowers companies to tap into the pulse of consumer opinions swiftly and seamlessly.
- Fast Insights: Say goodbye to lengthy research processes. With Appinio, you can transform questions into actionable insights in minutes.
- Data-Driven Decisions: Harness the power of real-time consumer insights to drive your business strategies, allowing you to make informed choices on the fly.
- Seamless Integration: Appinio handles the research and technical complexities, freeing you to focus on what truly matters: making rapid data-driven decisions that propel your business forward.
Join the loop 💌
Be the first to hear about new updates, product news, and data insights. We'll send it all straight to your inbox.
Get the latest market research news straight to your inbox! 💌
Wait, there's more
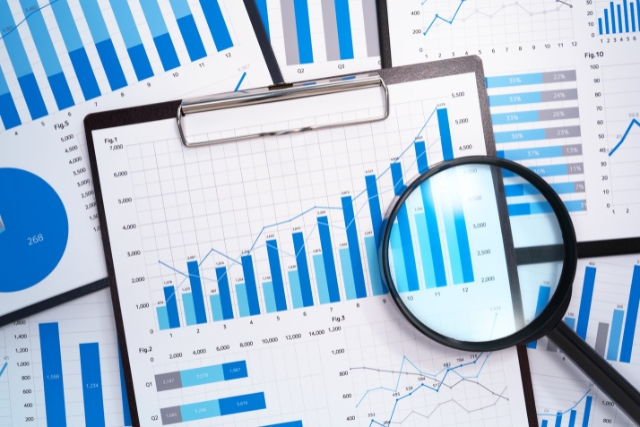
11.04.2024 | 34min read
What is Data Analysis? Definition, Tools, Examples
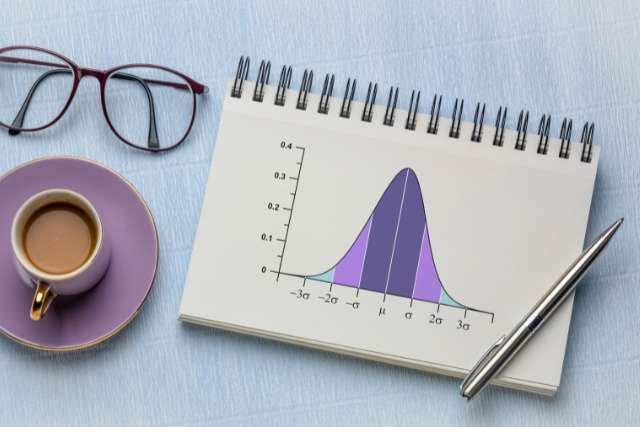
09.04.2024 | 29min read
What is a Confidence Interval and How to Calculate It?
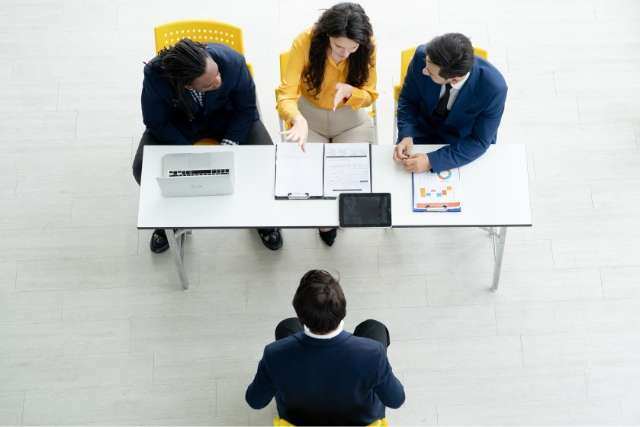
05.04.2024 | 28min read
What is Field Research? Definition, Types, Methods, Examples
What is secondary research?
Last updated
7 February 2023
Reviewed by
Cathy Heath
In this guide, we explain in detail what secondary research is, including the difference between this research method and primary research, the different sources for secondary research, and how you can benefit from this research method.
Analyze your secondary research
Bring your secondary research together inside Dovetail, tag PDFs, and uncover actionable insights
- Overview of secondary research
Secondary research is a method by which the researcher finds existing data, filters it to meet the context of their research question, analyzes it, and then summarizes it to come up with valid research conclusions.
This research method involves searching for information, often via the internet, using keywords or search terms relevant to the research question. The goal is to find data from internal and external sources that are up-to-date and authoritative, and that fully answer the question.
Secondary research reviews existing research and looks for patterns, trends, and insights, which helps determine what further research, if any, is needed.
- Secondary research methods
Secondary research is more economical than primary research, mainly because the methods for this type of research use existing data and do not require the data to be collected first-hand or by a third party that you have to pay.
Secondary research is referred to as ‘desk research’ or ‘desktop research,’ since the data can be retrieved from behind a desk instead of having to host a focus group and create the research from scratch.
Finding existing research is relatively easy since there are numerous accessible sources organizations can use to obtain the information they need. These include:
The internet: This data is either free or behind a paywall. Yet, while there are plenty of sites on the internet with information that can be used, businesses need to be careful to collect information from trusted and authentic websites to ensure the data is accurate.
Government agencies: Government agencies are typically known to provide valuable, trustworthy information that companies can use for their research.
The public library: This establishment holds paper-based and online sources of reliable information, including business databases, magazines, newspapers, and government publications. Be mindful of any copyright restrictions that may apply when using these sources.
Commercial information: This source provides first-hand information on politics, demographics, and economic developments through information aggregators, newspapers, magazines, radio, blogs, podcasts, and journals. This information may be free or behind a paywall.
Educational and scientific facilities: Universities, colleges, and specialized research facilities carry out significant amounts of research. As a result, they have data that may be available to the public and businesses for use.
- Key differences between primary research and secondary research
Both primary and secondary research methods provide researchers with vital, complementary information, despite some major differences between the two approaches.
Primary research involves gathering first-hand information by directly working with the target market, users, and interviewees. Researchers ask questions directly using surveys , interviews, and focus groups.
Through the primary research method, researchers obtain targeted responses and accurate results directly related to their overall research goals.
Secondary research uses existing data, such as published reports, that have already been completed through earlier primary and secondary research. Researchers can use this existing data to support their research goals and preliminary research findings.
Other notable differences between primary and secondary research include:
Relevance: Primary research uses raw data relevant to the investigation's goals. Secondary research may contain irrelevant data or may not neatly fit the parameters of the researcher's goals.
Time: Primary research takes a lot of time. Secondary research can be done relatively quickly.
Researcher bias: Primary research can be subject to researcher bias.
Cost: Primary research can be expensive. Secondary research can be more affordable because the data is often free. However, valuable data is often behind a paywall. The piece of secondary research you want may not exist or be very expensive, so you may have to turn to primary research to fill the information gap.
- When to conduct secondary research
Both primary and secondary research have roles to play in providing a holistic and accurate understanding of a topic. Generally, secondary research is done at the beginning of the research phase, especially if the topic is new.
Secondary research can provide context and critical background information to understand the issue at hand and identify any gaps, that could then be filled by primary research.
- How to conduct secondary research
Researchers usually follow several steps for secondary research.
1. Identify and define the research topic
Before starting either of these research methods, you first need to determine the following:
Topic to be researched
Purpose of this research
For instance, you may want to explore a question, determine why something happened, or confirm whether an issue is true.
At this stage, you also need to consider what search terms or keywords might be the most effective for this topic. You could do this by looking at what synonyms exist for your topic, the use of industry terms and acronyms, as well as the balance between statistical or quantitative data and contextual data to support your research topic.
It’s also essential to define what you don’t want to cover in your secondary research process. This might be choosing only to use recent information or only focusing on research based on a particular country or type of consumer. From there, once you know what you want to know and why you can decide whether you need to use both primary and secondary research to answer your questions.
2. Find research and existing data sources
Once you have determined your research topic , select the information sources that will provide you with the most appropriate and relevant data for your research. If you need secondary research, you want to determine where this information can likely be found, for example:
Trade associations
Government sources
Create a list of the relevant data sources , and other organizations or people that can help you find what you need.
3. Begin searching and collecting the existing data
Once you have narrowed down your sources, you will start gathering this information and putting it into an organized system. This often involves:
Checking the credibility of the source
Setting up meetings with research teams
Signing up for accounts to access certain websites or journals
One search result on the internet often leads to other pieces of helpful information, known as ‘pearl gathering’ or ‘pearl harvesting.’ This is usually a serendipitous activity, which can lead to valuable nuggets of information you may not have been aware of or considered.
4. Combine the data and compare the results
Once you have gathered all the data, start going through it by carefully examining all the information and comparing it to ensure the data is usable and that it isn’t duplicated or corrupted. Contradictory information is useful—just make sure you note the contradiction and the context. Be mindful of copyright and plagiarism when using secondary research and always cite your sources.
Once you have assessed everything, you will begin to look at what this information tells you by checking out the trends and comparing the different datasets. You will also investigate what this information means for your research, whether it helps your overall goal, and any gaps or deficiencies.
5. Analyze your data and explore further
In the final stage of conducting secondary research, you will analyze the data you have gathered and determine if it answers the questions you had before you started researching. Check that you understand the information, whether it fills in all your gaps, and whether it provides you with other insights or actions you should take next.
If you still need further data, repeat these steps to find additional information that can help you explore your topic more deeply. You may also need to supplement what you find with primary research to ensure that your data is complete, accurate, transparent, and credible.
- The advantages of secondary research
There are numerous advantages to performing secondary research. Some key benefits are:
Quicker than primary research: Because the data is already available, you can usually find the information you need fairly quickly. Not only will secondary research help you research faster, but you will also start optimizing the data more quickly.
Plenty of available data: There are countless sources for you to choose from, making research more accessible. This data may be already compiled and arranged, such as statistical information, so you can quickly make use of it.
Lower costs: Since you will not have to carry out the research from scratch, secondary research tends to be much more affordable than primary research.
Opens doors to further research: Existing research usually identifies whether more research needs to be done. This could mean follow-up surveys or telephone interviews with subject matter experts (SME) to add value to your own research.
- The disadvantages of secondary research
While there are plenty of benefits to secondary research are plenty, there are some issues you should be aware of. These include:
Credibility issues: It is important to verify the sources used. Some information may be biased and not reflect or hide, relevant issues or challenges. It could also be inaccurate.
No recent information: Even if data may seem accurate, it may not be up to date, so the information you gather may no longer be correct. Outdated research can distort your overall findings.
Poor quality: Because secondary research tends to make conclusions from primary research data, the success of secondary research will depend on the quality and context of the research that has already been completed. If the research you are using is of poor quality, this will bring down the quality of your own findings.
Research doesn’t exist or is not easily accessible, or is expensive: Sometimes the information you need is confidential or proprietary, such as sales or earnings figures. Many information-based businesses attach value to the information they hold or publish, so the costs to access this information can be prohibitive.
Should you complete secondary research or primary research first?
Due to the costs and time involved in primary research, it may be more beneficial to conduct secondary market research first. This will save you time and provide a picture of what issues you may come across in your research. This allows you to focus on using more expensive primary research to get the specific answers you want.
What should you ask yourself before using secondary research data?
Check the date of the research to make sure it is still relevant. Also, determine the data source so you can assess how credible and trustworthy it is likely to be. For example, data from known brands, professional organizations, and even government agencies are usually excellent sources to use in your secondary research, as it tends to be trustworthy.
Be careful when using some websites and personal blogs as they may be based on opinions rather than facts. However, these sources can be useful for determining sentiment about a product or service, and help direct any primary research.
Get started today
Go from raw data to valuable insights with a flexible research platform
Editor’s picks
Last updated: 21 December 2023
Last updated: 16 December 2023
Last updated: 6 October 2023
Last updated: 5 March 2024
Last updated: 25 November 2023
Last updated: 15 February 2024
Last updated: 11 March 2024
Last updated: 12 December 2023
Last updated: 6 March 2024
Last updated: 10 April 2023
Last updated: 20 December 2023
Latest articles
Related topics, log in or sign up.
Get started for free
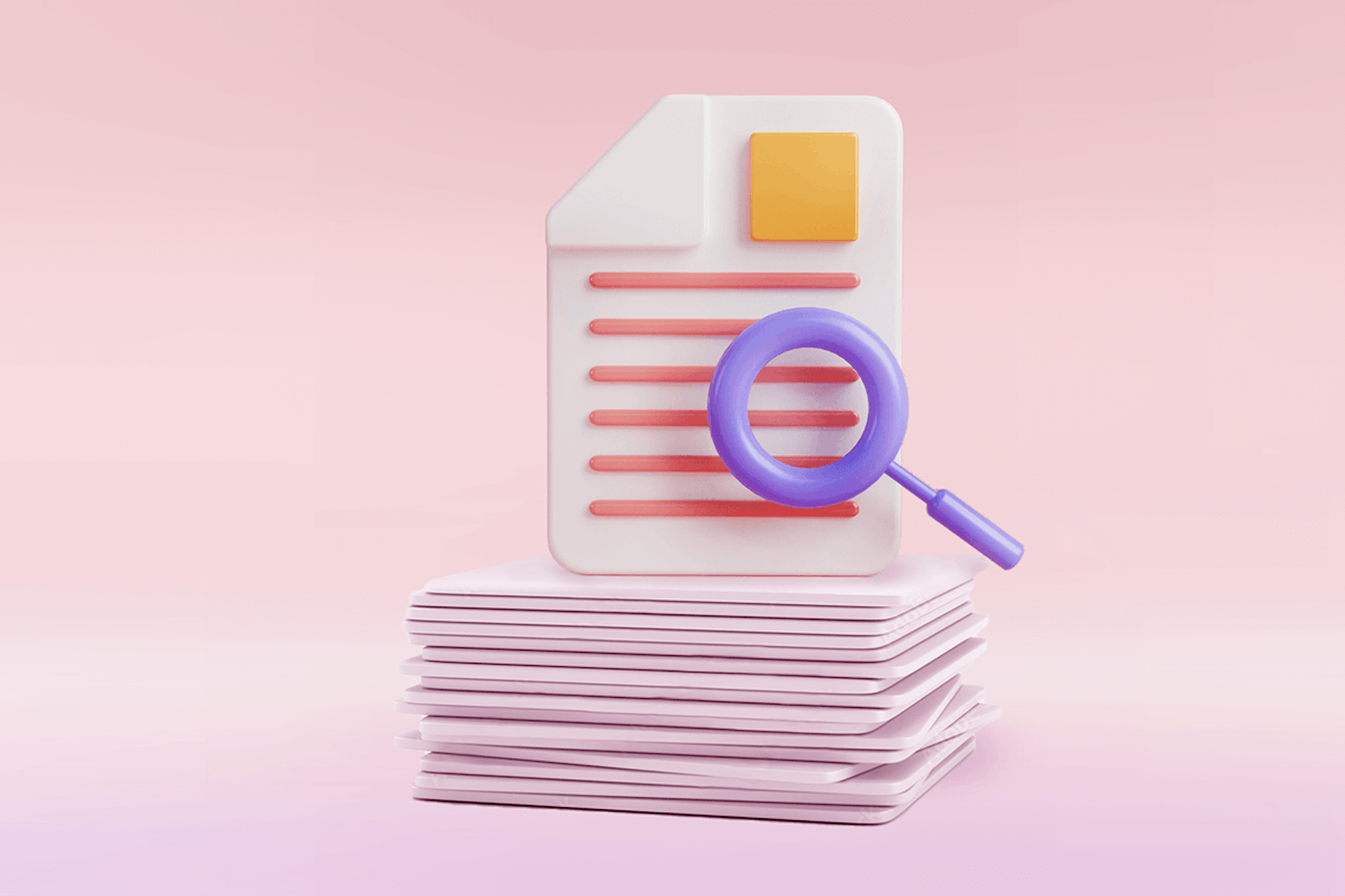
Secondary Research Guide: Definition, Methods, Examples
Apr 3, 2024
8 min. read
The internet has vastly expanded our access to information, allowing us to learn almost anything about everything. But not all market research is created equal , and this secondary research guide explains why.
There are two key ways to do research. One is to test your own ideas, make your own observations, and collect your own data to derive conclusions. The other is to use secondary research — where someone else has done most of the heavy lifting for you.
Here’s an overview of secondary research and the value it brings to data-driven businesses.
Secondary Research Definition: What Is Secondary Research?
Primary vs Secondary Market Research
What Are Secondary Research Methods?
Advantages of secondary research, disadvantages of secondary research, best practices for secondary research, how to conduct secondary research with meltwater.
Secondary research definition: The process of collecting information from existing sources and data that have already been analyzed by others.
Secondary research (aka desk research ) provides a foundation to help you understand a topic, with the goal of building on existing knowledge. They often cover the same information as primary sources, but they add a layer of analysis and explanation to them.
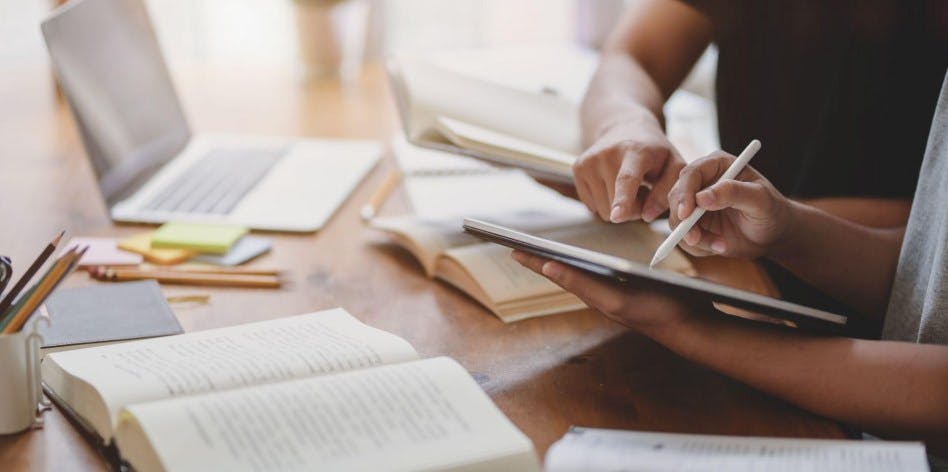
Users can choose from several secondary research types and sources, including:
- Journal articles
- Research papers
With secondary sources, users can draw insights, detect trends , and validate findings to jumpstart their research efforts.
Primary vs. Secondary Market Research
We’ve touched a little on primary research , but it’s essential to understand exactly how primary and secondary research are unique.
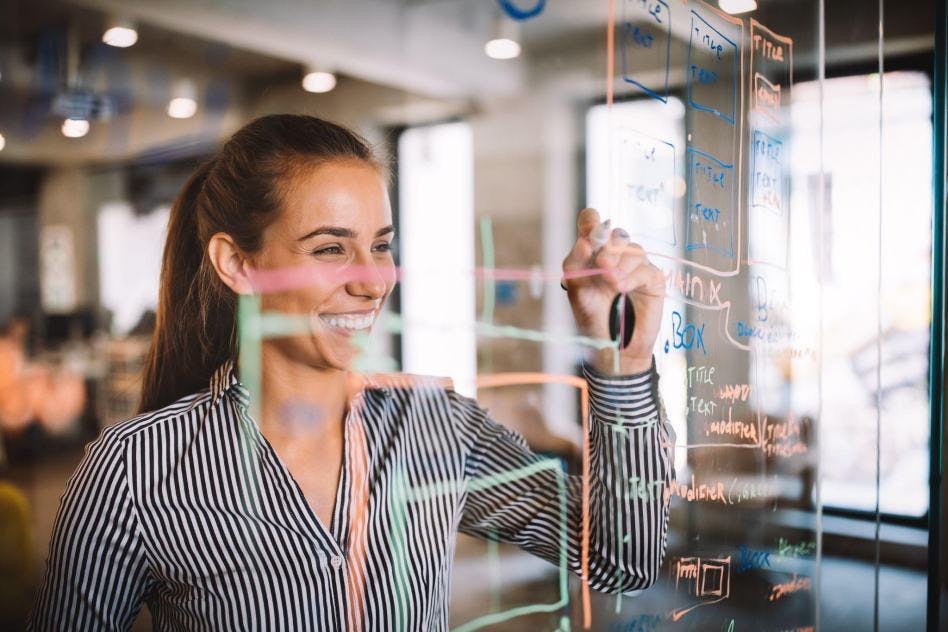
Think of primary research as the “thing” itself, and secondary research as the analysis of the “thing,” like these primary and secondary research examples:
- An expert gives an interview (primary research) and a marketer uses that interview to write an article (secondary research).
- A company conducts a consumer satisfaction survey (primary research) and a business analyst uses the survey data to write a market trend report (secondary research).
- A marketing team launches a new advertising campaign across various platforms (primary research) and a marketing research firm, like Meltwater for market research , compiles the campaign performance data to benchmark against industry standards (secondary research).
In other words, primary sources make original contributions to a topic or issue, while secondary sources analyze, synthesize, or interpret primary sources.
Both are necessary when optimizing a business, gaining a competitive edge , improving marketing, or understanding consumer trends that may impact your business.
Secondary research methods focus on analyzing existing data rather than collecting primary data . Common examples of secondary research methods include:
- Literature review . Researchers analyze and synthesize existing literature (e.g., white papers, research papers, articles) to find knowledge gaps and build on current findings.
- Content analysis . Researchers review media sources and published content to find meaningful patterns and trends.
- AI-powered secondary research . Platforms like Meltwater for market research analyze vast amounts of complex data and use AI technologies like natural language processing and machine learning to turn data into contextual insights.
Researchers today have access to more market research tools and technology than ever before, allowing them to streamline their efforts and improve their findings.
Want to see how Meltwater can complement your secondary market research efforts? Simply fill out the form at the bottom of this post, and we'll be in touch.
Conducting secondary research offers benefits in every job function and use case, from marketing to the C-suite. Here are a few advantages you can expect.
Cost and time efficiency
Using existing research saves you time and money compared to conducting primary research. Secondary data is readily available and easily accessible via libraries, free publications, or the Internet. This is particularly advantageous when you face time constraints or when a project requires a large amount of data and research.
Access to large datasets
Secondary data gives you access to larger data sets and sample sizes compared to what primary methods may produce. Larger sample sizes can improve the statistical power of the study and add more credibility to your findings.
Ability to analyze trends and patterns
Using larger sample sizes, researchers have more opportunities to find and analyze trends and patterns. The more data that supports a trend or pattern, the more trustworthy the trend becomes and the more useful for making decisions.
Historical context
Using a combination of older and recent data allows researchers to gain historical context about patterns and trends. Learning what’s happened before can help decision-makers gain a better current understanding and improve how they approach a problem or project.
Basis for further research
Ideally, you’ll use secondary research to further other efforts . Secondary sources help to identify knowledge gaps, highlight areas for improvement, or conduct deeper investigations.
Tip: Learn how to use Meltwater as a research tool and how Meltwater uses AI.
Secondary research comes with a few drawbacks, though these aren’t necessarily deal breakers when deciding to use secondary sources.
Reliability concerns
Researchers don’t always know where the data comes from or how it’s collected, which can lead to reliability concerns. They don’t control the initial process, nor do they always know the original purpose for collecting the data, both of which can lead to skewed results.
Potential bias
The original data collectors may have a specific agenda when doing their primary research, which may lead to biased findings. Evaluating the credibility and integrity of secondary data sources can prove difficult.
Outdated information
Secondary sources may contain outdated information, especially when dealing with rapidly evolving trends or fields. Using outdated information can lead to inaccurate conclusions and widen knowledge gaps.
Limitations in customization
Relying on secondary data means being at the mercy of what’s already published. It doesn’t consider your specific use cases, which limits you as to how you can customize and use the data.
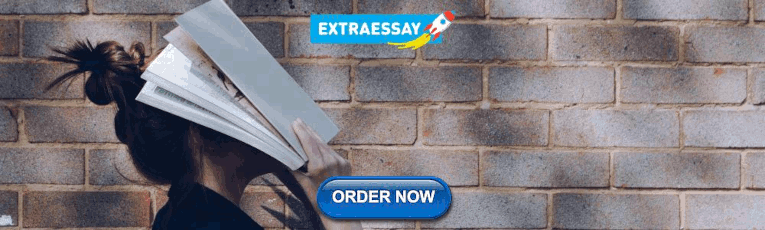
A lack of relevance
Secondary research rarely holds all the answers you need, at least from a single source. You typically need multiple secondary sources to piece together a narrative, and even then you might not find the specific information you need.
To make secondary market research your new best friend, you’ll need to think critically about its strengths and find ways to overcome its weaknesses. Let’s review some best practices to use secondary research to its fullest potential.
Identify credible sources for secondary research
To overcome the challenges of bias, accuracy, and reliability, choose secondary sources that have a demonstrated history of excellence . For example, an article published in a medical journal naturally has more credibility than a blog post on a little-known website.
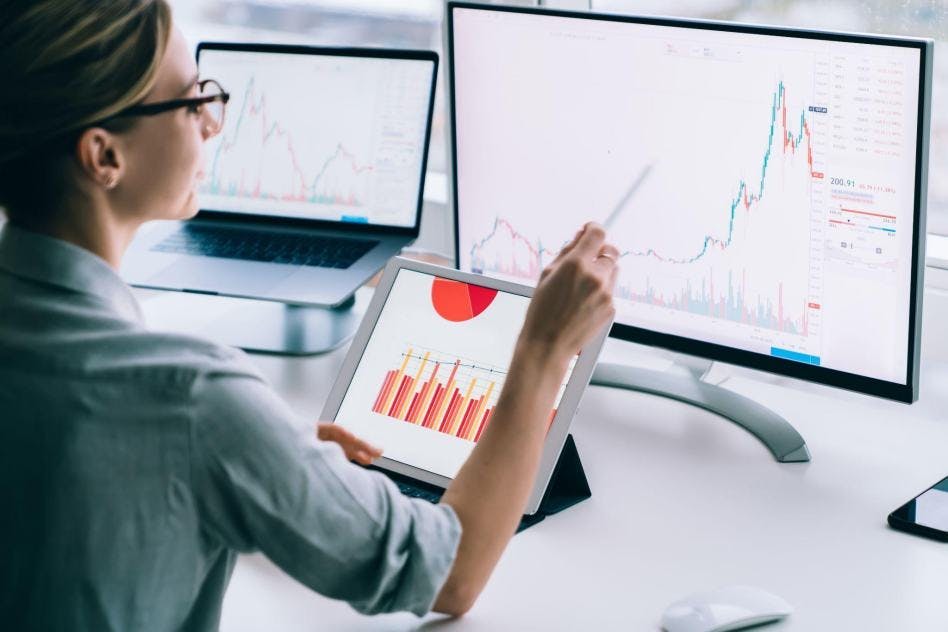
Assess credibility based on peer reviews, author expertise, sampling techniques, publication reputation, and data collection methodologies. Cross-reference the data with other sources to gain a general consensus of truth.
The more credibility “factors” a source has, the more confidently you can rely on it.
Evaluate the quality and relevance of secondary data
You can gauge the quality of the data by asking simple questions:
- How complete is the data?
- How old is the data?
- Is this data relevant to my needs?
- Does the data come from a known, trustworthy source?
It’s best to focus on data that aligns with your research objectives. Knowing the questions you want to answer and the outcomes you want to achieve ahead of time helps you focus only on data that offers meaningful insights.
Document your sources
If you’re sharing secondary data with others, it’s essential to document your sources to gain others’ trust. They don’t have the benefit of being “in the trenches” with you during your research, and sharing your sources can add credibility to your findings and gain instant buy-in.
Secondary market research offers an efficient, cost-effective way to learn more about a topic or trend, providing a comprehensive understanding of the customer journey . Compared to primary research, users can gain broader insights, analyze trends and patterns, and gain a solid foundation for further exploration by using secondary sources.
Meltwater for market research speeds up the time to value in using secondary research with AI-powered insights, enhancing your understanding of the customer journey. Using natural language processing, machine learning, and trusted data science processes, Meltwater helps you find relevant data and automatically surfaces insights to help you understand its significance. Our solution identifies hidden connections between data points you might not know to look for and spells out what the data means, allowing you to make better decisions based on accurate conclusions. Learn more about Meltwater's power as a secondary research solution when you request a demo by filling out the form below:
Continue Reading
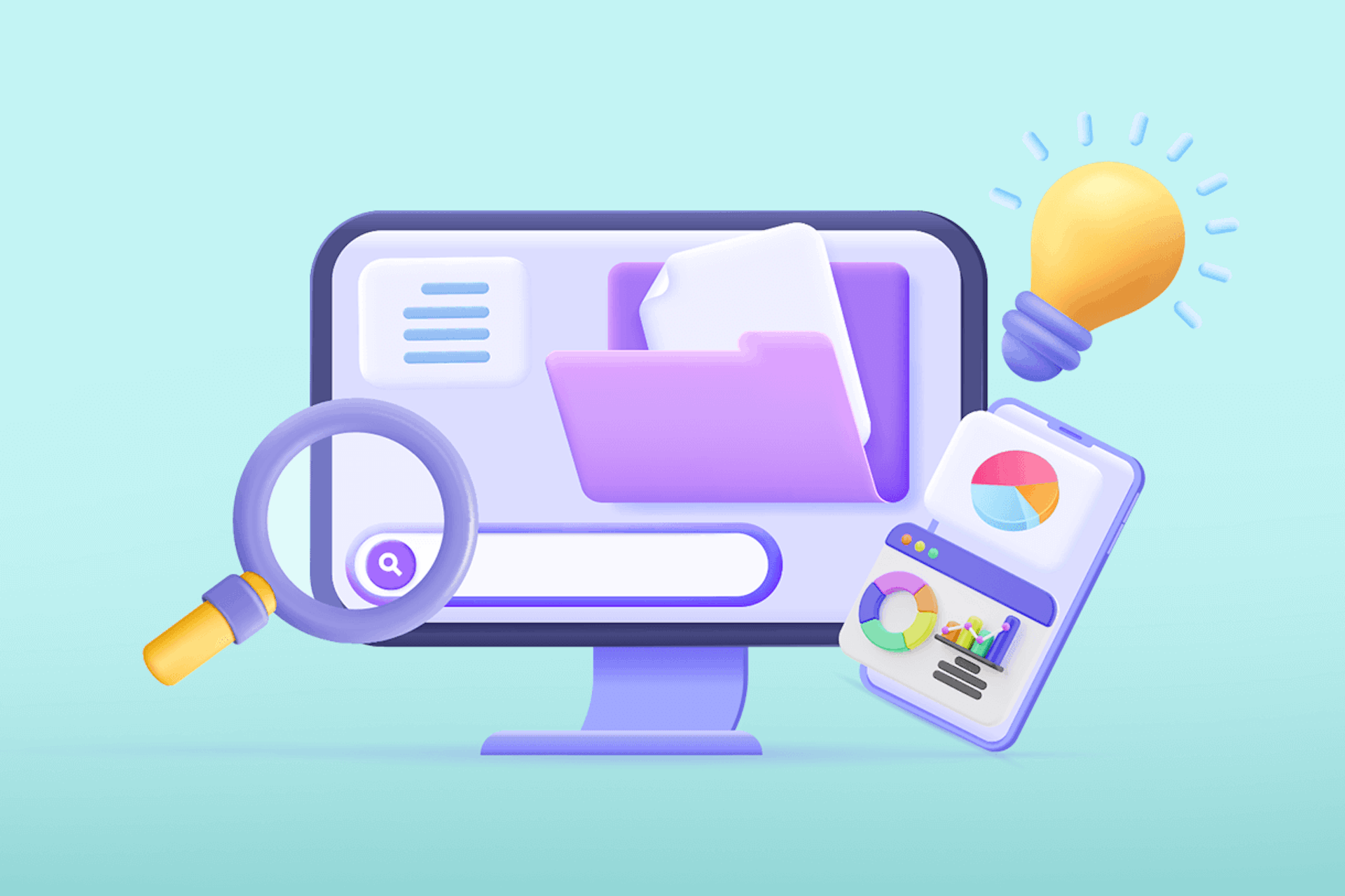
How To Do Market Research: Definition, Types, Methods
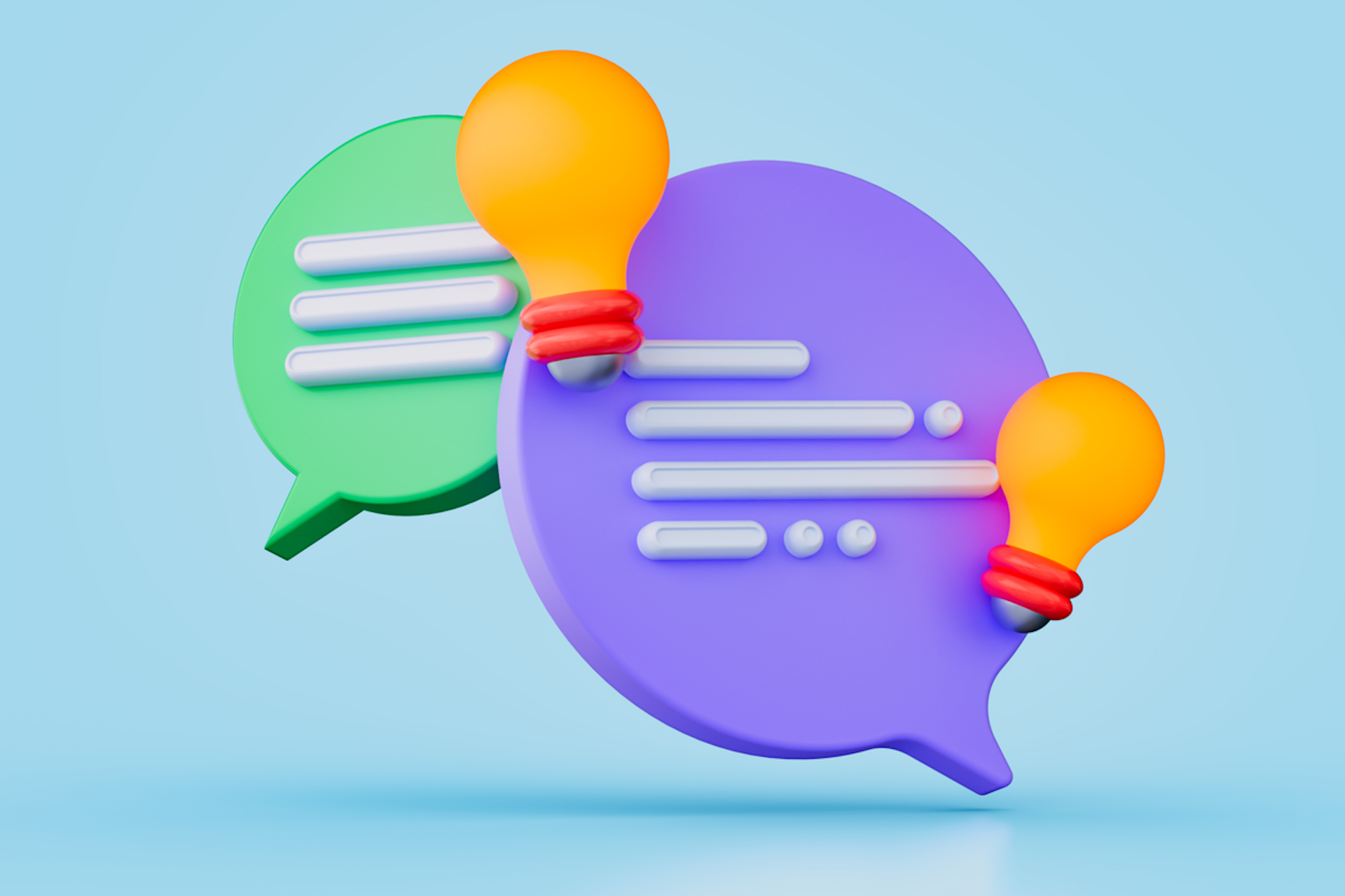
What Are Consumer Insights? Meaning, Examples, Strategy
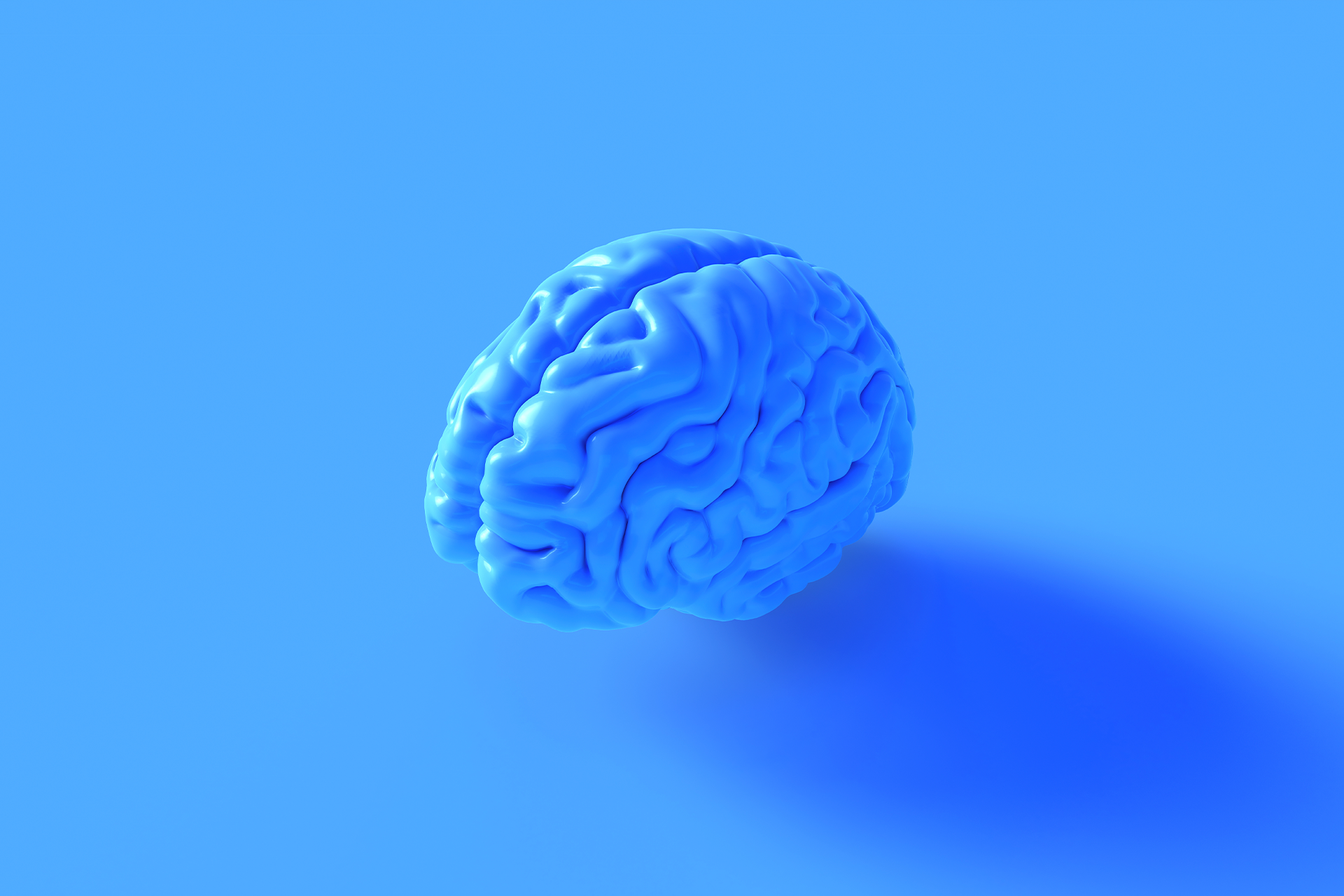
Market Intelligence 101: What It Is & How To Use It
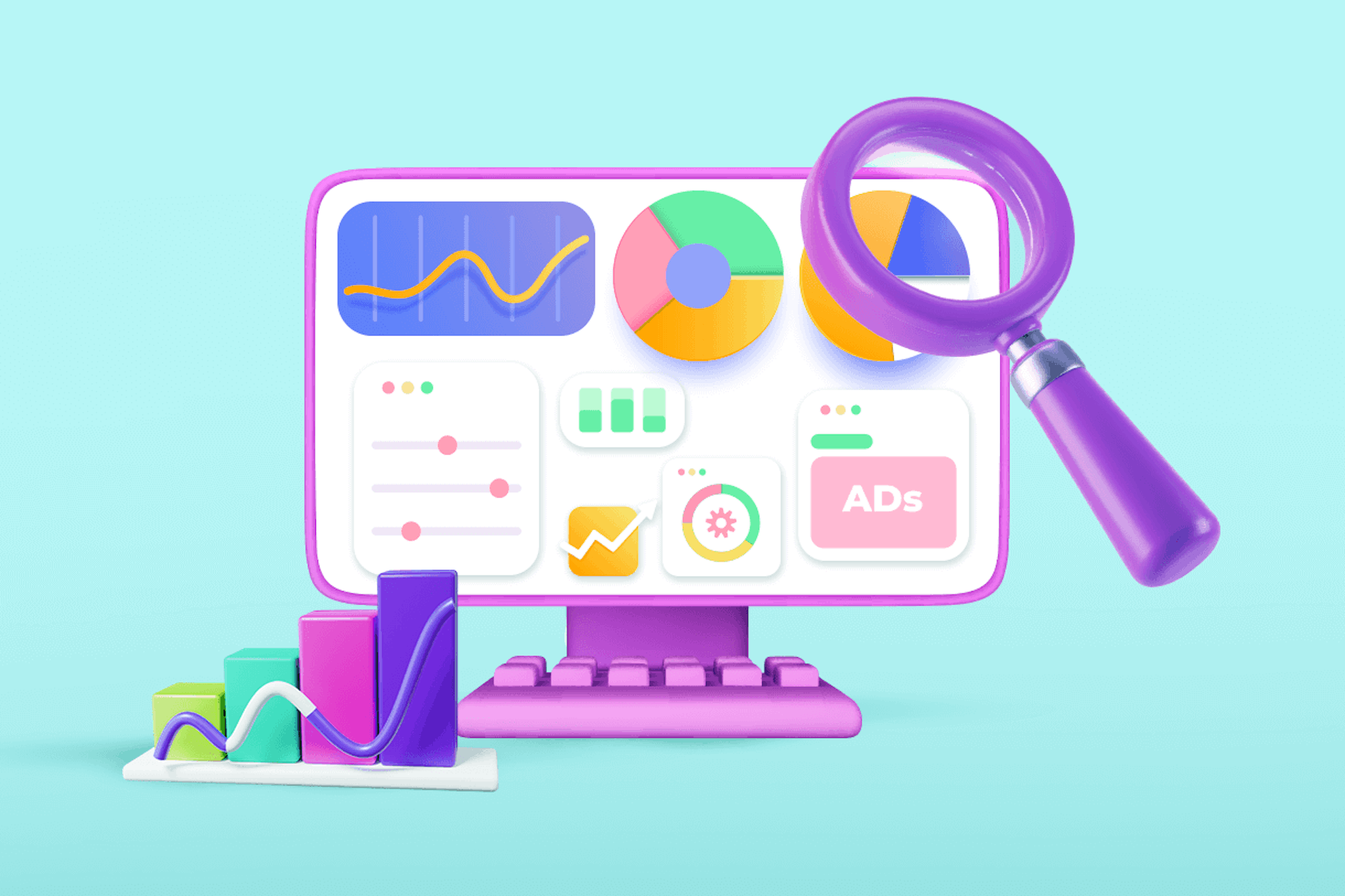
The 13 Best Market Research Tools
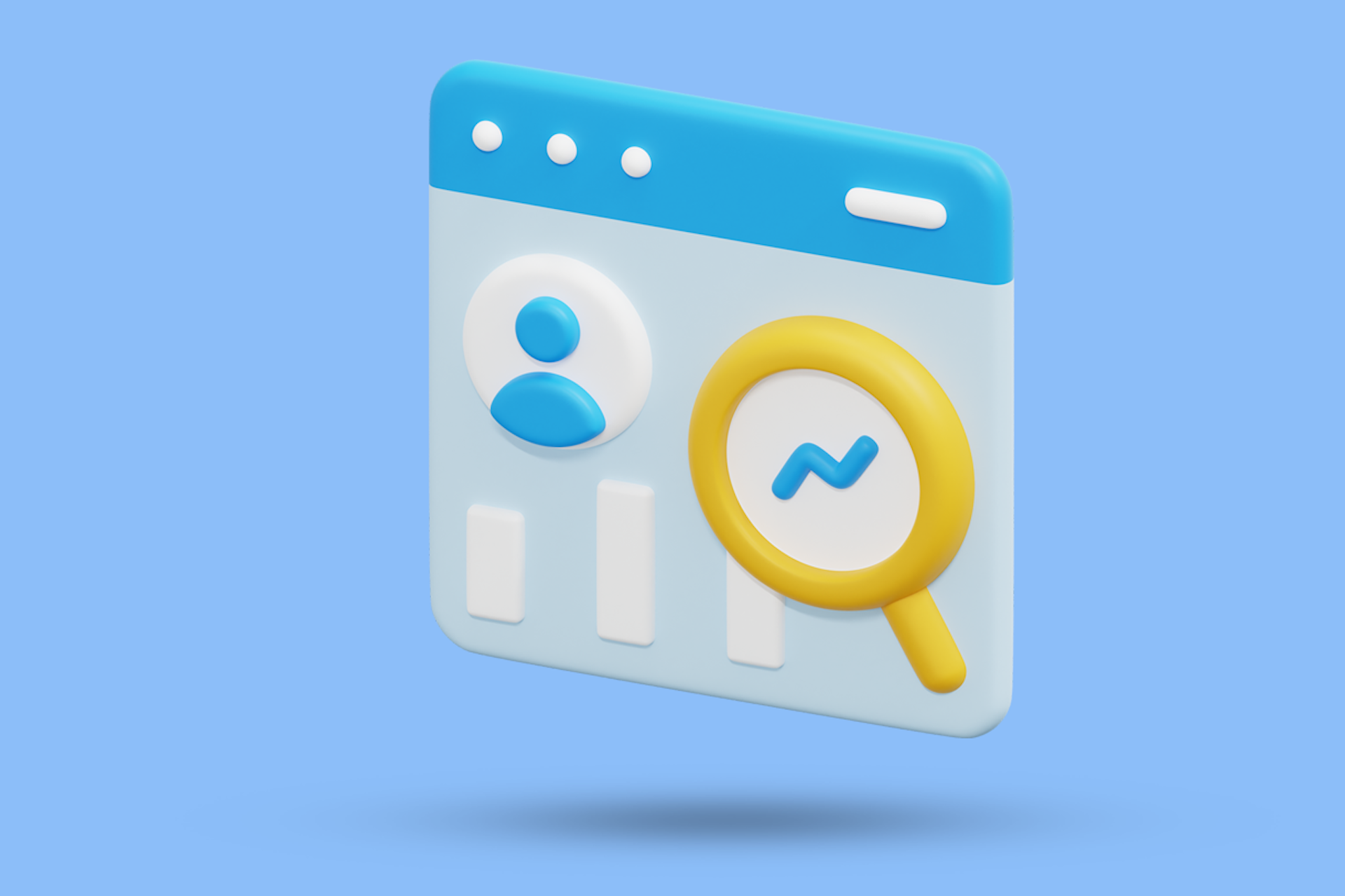
Consumer Intelligence: Definition & Examples
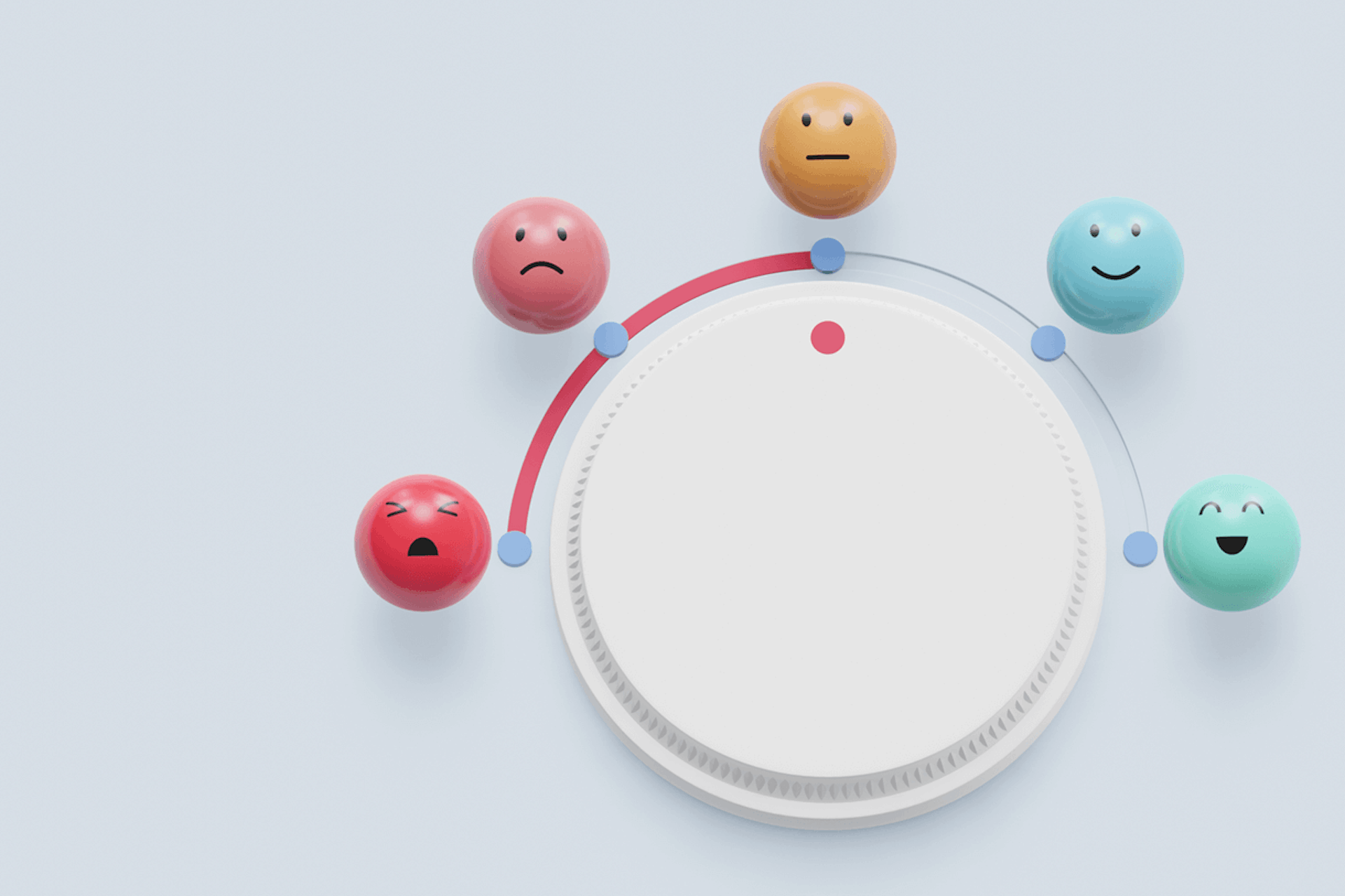
9 Top Consumer Insights Tools & Companies
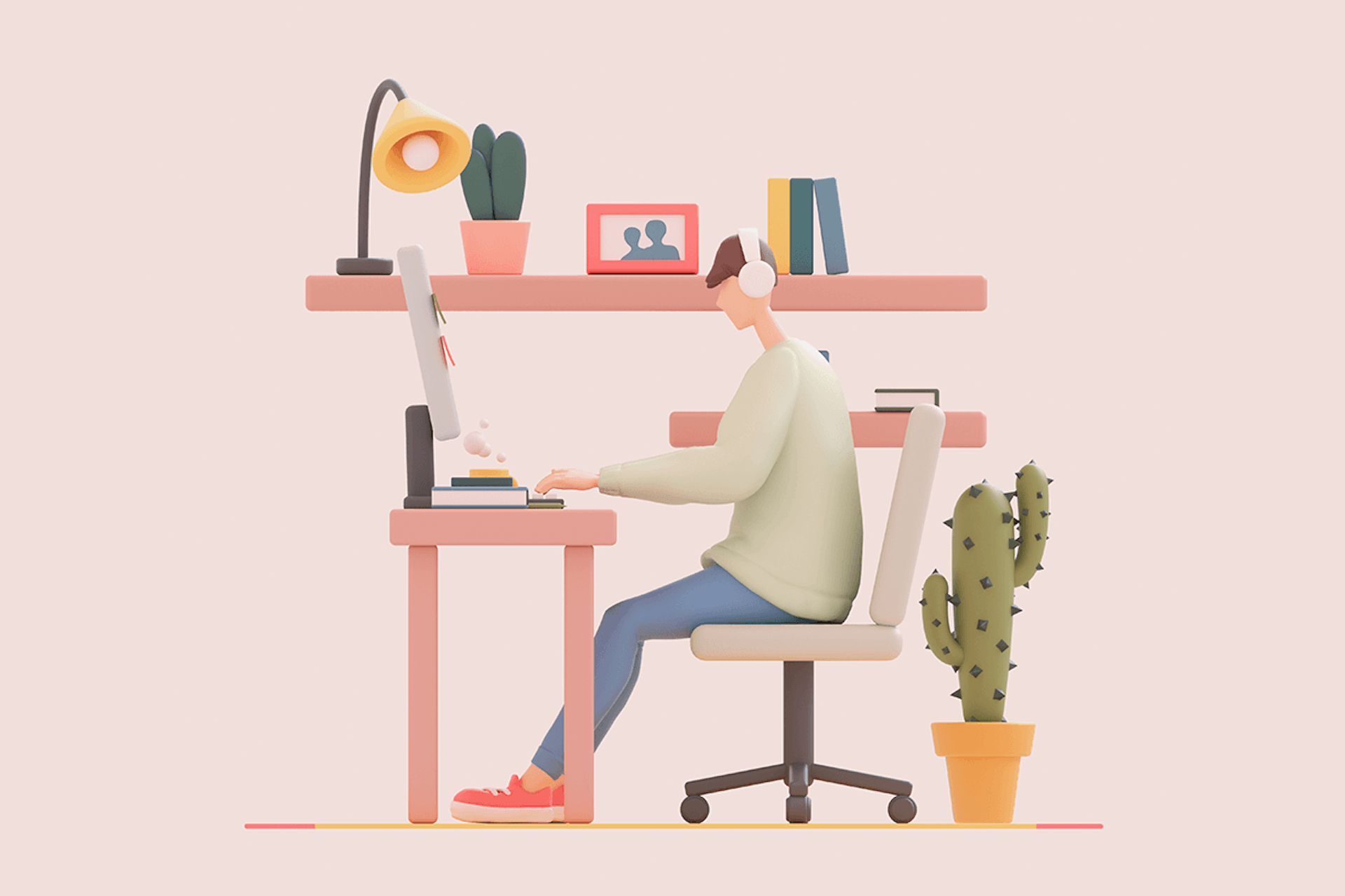
What Is Desk Research? Meaning, Methodology, Examples
12 Pros and Cons of Secondary Research
Last Updated on March 11, 2021 by Filip Poutintsev
Secondary research is the research method of collecting all the data and documents available from other sources. Some major companies or statistics written in some books or information gathered from some newspaper or thesis or individual research all these data are eligible to be secondary data.

It is a convenient and powerful tool for researchers looking to ask broad questions on a large scale. It benefits researchers as all the data are already taken down so it can be time-consuming but the area where it takes time might be if those data are ideal for the researcher’s goal or not.
A large amount of information can be gathered with a small effort and summarizing and relating it increases the effectiveness of research. Some pros and cons of secondary research are pointed out below.
Pros Of Secondary Research
1. accessibility.
A few years ago when you needed to collect some data then going to libraries or particular organizations was a must. And it was even impossible to gather such data by the public. The Internet has played a great role in accessing the data so easily in a single click.
The problem here will be your patience to search where it is, it’s accessed for free. Some market research or the poll by the organization or product or comment on some of the sites about the product or some news. Anything necessary for your analysis will be available you just need to search in the right place.
2. Low Cost
When the data already exists and is collected and summarized. The large sum of money is saved where you don’t need to pay the institution for the data or organize some workshops to know the people’s opinion, you can easily use social media platforms which saves you the manpower and its cost. Researchers are easily tempted by secondary data, which can be easily accessed and prepared in a short period of time without any investment.
3. Saves Time
The data are collected or documented already on the social platform in magazines or on the internet. Using internet large numbers of data are gathered by the researchers without their own effort.
The data are already been documented by the organization or the researchers which you can just collect directly and start analysis over it. This saves lots and lots of time for you where you can study the variables and ups and downs regarding the data.
4. May Help Clarify Research Question
Where primary research is most expensive because it requires both the effort and time. Secondary research tips lots of important questions that are needed while conducting primary research.
The data collected through secondary research gives an organization or the personnel an idea about the effectiveness and the overview of the issue without conducting the primary research. This saves lots of money and time here.
5. Government & Agencies
There are many database analysis performed by the government itself for the census, for health issue protocols and other general information about the citizens. This research are being carried out for a long period of time and covers almost the entire population.
Likewise, many NGO’s and INGO’s conduct such data collection during their campaign in some scarcity or spreading awareness. Including such information provided by the government publicly increases the authenticity and accuracy of your secondary research data.
6. Understand The Problem
The secondary researcher needs to analyze and examine the data they collect from the source. In this process, the researcher goes deep into the procedure of how and when were the data collected and the difficulties encountered while gathering the data.
Some reports of multinational companies while attempting the large market research already includes the obstacles faced like the people declining and people interested during research.
These data are useful to plan how’s your research feedback is going to be or how to conduct or what to change during the research to get the desired outcome or what area to cover to make our outcome more subtle or accurate.
7. New Conclusion Or Data
The data analyzed and collected are very vast varied and shows the perspective of lots of issues with different variables. This continuous and frequent analysis of these data may develop or give the statistical graph of the new variable.
For example, knowing how many hospitals are there and the number of aware citizens about healthcare gives us the data about how many doctors are needed to carry the campaign and how many connected district, city or province is going to need new hospitals and new technology.
This helps us come up with a new conclusion while verifying and confirming how the previous research was carried out.
Cons Of Secondary Research
1. quality of research.
As we know the secondary research is derived from the conclusion of the primary research, how hard we analyze it depends on the quality of the research conducted primarily.
If the originator is concerned about organizations or institutions those data might be false and may have been shown to attract clients or shareholders. Thus the validity of the data is necessary but reliability on other’s data prevents it.
2. May Not Fulfill Researcher’s Need
Secondary research data does not show exactly how or what the researcher was looking for. It is the collection of lots of data from lots of perspectives and people, some may be easy to ignore and some may be hard to validate and find its authenticity.
The researcher will be looking for data with some concern or with some particular question in mind but the data might not be collected regarding the particular issue or agenda. Meanwhile, all the data studied are not collected by the researcher they have no control over what the secondary data set may contain.
3. Incomplete Information
Not being able to get complete information about the data he/she wants to collect will affect the researcher’s study. As they are unable to know exactly how and when the procedure went wrong during execution.
It will not only be difficult to continue the research process but also confuses the researcher about where the issue is leading them.
4. Outdated Information
The most important thing one must consider while using secondary data is to note the date when the information was collected. They must be aware of how are those products and companies doing in the current situation.
It helps them to verify and ignore the achieved data. It is not possible to get all the updated reports or statistics of the data. One must be aware of not using the most outdated information in their research.
5. Lack Of Quality Data
The mindset of the researcher will be something else, they have to work on the data collected or data found in the research process. Since they are not able to carry out primary research, they should be depending on someone else’s data disregarding its quality.
As we know data are available in many forms and we are unable to know who performed the research we are forced to note down and analyze the data compromising its quality and validity.

Related Posts

10 Significant Benefits of Community College

8 Important Pros and Cons of Learning to Code

10 Benefits of Bilingual Education

10 Pros and Cons of School Uniform

10 Pros and Cons of a Community College

Advantages and Disadvantages of Electrical Engineering
- Online Business
- Entertainment
- Home Improvement
- Environment
Type above and press Enter to search. Press Esc to cancel.
Protecting against researcher bias in secondary data analysis: challenges and potential solutions
- Open access
- Published: 13 January 2022
- Volume 37 , pages 1–10, ( 2022 )
Cite this article
You have full access to this open access article
- Jessie R. Baldwin ORCID: orcid.org/0000-0002-5703-5058 1 , 2 ,
- Jean-Baptiste Pingault 1 , 2 ,
- Tabea Schoeler 1 ,
- Hannah M. Sallis 3 , 4 , 5 &
- Marcus R. Munafò 3 , 4 , 6
46k Accesses
31 Citations
182 Altmetric
Explore all metrics
Analysis of secondary data sources (such as cohort studies, survey data, and administrative records) has the potential to provide answers to science and society’s most pressing questions. However, researcher biases can lead to questionable research practices in secondary data analysis, which can distort the evidence base. While pre-registration can help to protect against researcher biases, it presents challenges for secondary data analysis. In this article, we describe these challenges and propose novel solutions and alternative approaches. Proposed solutions include approaches to (1) address bias linked to prior knowledge of the data, (2) enable pre-registration of non-hypothesis-driven research, (3) help ensure that pre-registered analyses will be appropriate for the data, and (4) address difficulties arising from reduced analytic flexibility in pre-registration. For each solution, we provide guidance on implementation for researchers and data guardians. The adoption of these practices can help to protect against researcher bias in secondary data analysis, to improve the robustness of research based on existing data.
Similar content being viewed by others
Reporting and Transparency in Big Data: The Nexus of Ethics and Methodology
Preregistration of Studies with Existing Data
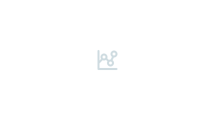
Secondary analysis of statutorily collected routine data
M. Trenner, H.-H. Eckstein, … A. Kühnl
Avoid common mistakes on your manuscript.
Introduction
Secondary data analysis has the potential to provide answers to science and society’s most pressing questions. An abundance of secondary data exists—cohort studies, surveys, administrative data (e.g., health records, crime records, census data), financial data, and environmental data—that can be analysed by researchers in academia, industry, third-sector organisations, and the government. However, secondary data analysis is vulnerable to questionable research practices (QRPs) which can distort the evidence base. These QRPs include p-hacking (i.e., exploiting analytic flexibility to obtain statistically significant results), selective reporting of statistically significant, novel, or “clean” results, and hypothesising after the results are known (HARK-ing [i.e., presenting unexpected results as if they were predicted]; [ 1 ]. Indeed, findings obtained from secondary data analysis are not always replicable [ 2 , 3 ], reproducible [ 4 ], or robust to analytic choices [ 5 , 6 ]. Preventing QRPs in research based on secondary data is therefore critical for scientific and societal progress.
A primary cause of QRPs is common cognitive biases that affect the analysis, reporting, and interpretation of data [ 7 – 10 ]. For example, apophenia (the tendency to see patterns in random data) and confirmation bias (the tendency to focus on evidence that is consistent with one’s beliefs) can lead to particular analytical choices and selective reporting of “publishable” results [ 11 – 13 ]. In addition, hindsight bias (the tendency to view past events as predictable) can lead to HARK-ing, so that observed results appear more compelling.
The scope for these biases to distort research outputs from secondary data analysis is perhaps particularly acute, for two reasons. First, researchers now have increasing access to high-dimensional datasets that offer a multitude of ways to analyse the same data [ 6 ]. Such analytic flexibility can lead to different conclusions depending on the analytical choices made [ 5 , 14 , 15 ]. Second, current incentive structures in science reward researchers for publishing statistically significant, novel, and/or surprising findings [ 16 ]. This combination of opportunity and incentive may lead researchers—consciously or unconsciously—to run multiple analyses and only report the most “publishable” findings.
One way to help protect against the effects of researcher bias is to pre-register research plans [ 17 , 18 ]. This can be achieved by pre-specifying the rationale, hypotheses, methods, and analysis plans, and submitting these to either a third-party registry (e.g., the Open Science Framework [OSF]; https://osf.io/ ), or a journal in the form of a Registered Report [ 19 ]. Because research plans and hypotheses are specified before the results are known, pre-registration reduces the potential for cognitive biases to lead to p-hacking, selective reporting, and HARK-ing [ 20 ]. While pre-registration is not necessarily a panacea for preventing QRPs (Table 1 ), meta-scientific evidence has found that pre-registered studies and Registered Reports are more likely to report null results [ 21 – 23 ], smaller effect sizes [ 24 ], and be replicated [ 25 ]. Pre-registration is increasingly being adopted in epidemiological research [ 26 , 27 ], and is even required for access to data from certain cohorts (e.g., the Twins Early Development Study [ 28 ]). However, pre-registration (and other open science practices; Table 2 ) can pose particular challenges to researchers conducting secondary data analysis [ 29 ], motivating the need for alternative approaches and solutions. Here we describe such challenges, before proposing potential solutions to protect against researcher bias in secondary data analysis (summarised in Fig. 1 ).
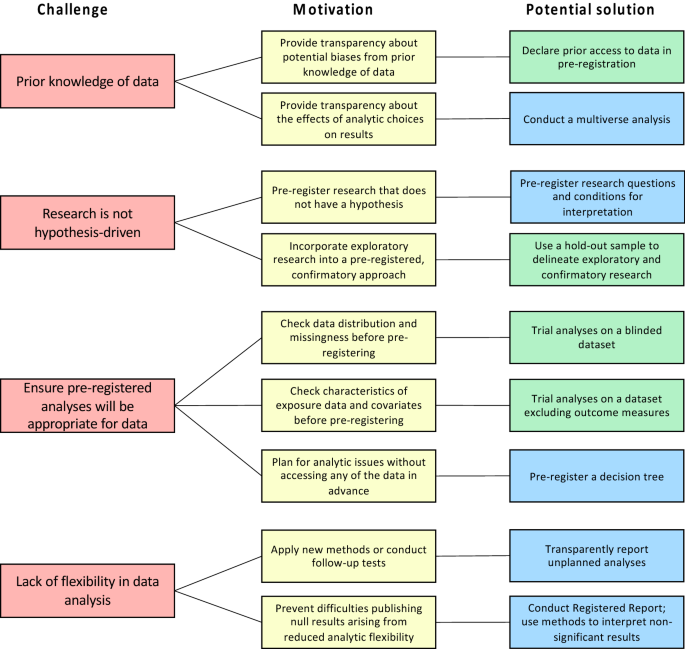
Challenges in pre-registering secondary data analysis and potential solutions (according to researcher motivations). Note : In the “Potential solution” column, blue boxes indicate solutions that are researcher-led; green boxes indicate solutions that should be facilitated by data guardians
Challenges of pre-registration for secondary data analysis
Prior knowledge of the data.
Researchers conducting secondary data analysis commonly analyse data from the same dataset multiple times throughout their careers. However, prior knowledge of the data increases risk of bias, as prior expectations about findings could motivate researchers to pursue certain analyses or questions. In the worst-case scenario, a researcher might perform multiple preliminary analyses, and only pursue those which lead to notable results (perhaps posting a pre-registration for these analyses, even though it is effectively post hoc). However, even if the researcher has not conducted specific analyses previously, they may be biased (either consciously or subconsciously) to pursue certain analyses after testing related questions with the same variables, or even by reading past studies on the dataset. As such, pre-registration cannot fully protect against researcher bias when researchers have previously accessed the data.
Research may not be hypothesis-driven
Pre-registration and Registered Reports are tailored towards hypothesis-driven, confirmatory research. For example, the OSF pre-registration template requires researchers to state “specific, concise, and testable hypotheses”, while Registered Reports do not permit purely exploratory research [ 30 ], although a new Exploratory Reports format now exists [ 31 ]. However, much research involving secondary data is not focused on hypothesis testing, but is exploratory, descriptive, or focused on estimation—in other words, examining the magnitude and robustness of an association as precisely as possible, rather than simply testing a point null. Furthermore, without a strong theoretical background, hypotheses will be arbitrary and could lead to unhelpful inferences [ 32 , 33 ], and so should be avoided in novel areas of research.
Pre-registered analyses are not appropriate for the data
With pre-registration, there is always a risk that the data will violate the assumptions of the pre-registered analyses [ 17 ]. For example, a researcher might pre-register a parametric test, only for the data to be non-normally distributed. However, in secondary data analysis, the extent to which the data shape the appropriate analysis can be considerable. First, longitudinal cohort studies are often subject to missing data and attrition. Approaches to deal with missing data (e.g., listwise deletion; multiple imputation) depend on the characteristics of missing data (e.g., the extent and patterns of missingness [ 34 ]), and so pre-specifying approaches to dealing with missingness may be difficult, or extremely complex. Second, certain analytical decisions depend on the nature of the observed data (e.g., the choice of covariates to include in a multiple regression might depend on the collinearity between the measures, or the degree of missingness of different measures that capture the same construct). Third, much secondary data (e.g., electronic health records and other administrative data) were never collected for research purposes, so can present several challenges that are impossible to predict in advance [ 35 ]. These issues can limit a researcher’s ability to pre-register a precise analytic plan prior to accessing secondary data.
Lack of flexibility in data analysis
Concerns have been raised that pre-registration limits flexibility in data analysis, including justifiable exploration [ 36 – 38 ]. For example, by requiring researchers to commit to a pre-registered analysis plan, pre-registration could prevent researchers from exploring novel questions (with a hypothesis-free approach), conducting follow-up analyses to investigate notable findings [ 39 ], or employing newly published methods with advantages over those pre-registered. While this concern is also likely to apply to primary data analysis, it is particularly relevant to certain fields involving secondary data analysis, such as genetic epidemiology, where new methods are rapidly being developed [ 40 ], and follow-up analyses are often required (e.g., in a genome-wide association study to further investigate the role of a genetic variant associated with a phenotype). However, this concern is perhaps over-stated – pre-registration does not preclude unplanned analyses; it simply makes it more transparent that these analyses are post hoc. Nevertheless, another understandable concern is that reduced analytic flexibility could lead to difficulties in publishing papers and accruing citations. For example, pre-registered studies are more likely to report null results [ 22 , 23 ], likely due to reduced analytic flexibility and selective reporting. While this is a positive outcome for research integrity, null results are less likely to be published [ 13 , 41 , 42 ] and cited [ 11 ], which could disadvantage researchers’ careers.
In this section, we describe potential solutions to address the challenges involved in pre-registering secondary data analysis, including approaches to (1) address bias linked to prior knowledge of the data, (2) enable pre-registration of non-hypothesis-driven research, (3) ensure that pre-planned analyses will be appropriate for the data, and (4) address potential difficulties arising from reduced analytic flexibility.
Challenge: Prior knowledge of the data
Declare prior access to data.
To increase transparency about potential biases arising from knowledge of the data, researchers could routinely report all prior data access in a pre-registration [ 29 ]. This would ideally include evidence from an independent gatekeeper (e.g., a data guardian of the study) stating whether data and relevant variables were accessed by each co-author. To facilitate this process, data guardians could set up a central “electronic checkout” system that records which researchers have accessed data, what data were accessed, and when [ 43 ]. The researcher or data guardian could then provide links to the checkout histories for all co-authors in the pre-registration, to verify their prior data access. If it is not feasible to provide such objective evidence, authors could self-certify their prior access to the dataset and where possible, relevant variables—preferably listing any publications and in-preparation studies based on the dataset [ 29 ]. Of course, self-certification relies on trust that researchers will accurately report prior data access, which could be challenging if the study involves a large number of authors, or authors who have been involved on many studies on the dataset. However, it is likely to be the most feasible option at present as many datasets do not have available electronic records of data access. For further guidance on self-certifying prior data access when pre-registering secondary data analysis studies on a third-party registry (e.g., the OSF), we recommend referring to the template by Van den Akker, Weston [ 29 ].
The extent to which prior access to data renders pre-registration invalid is debatable. On the one hand, even if data have been accessed previously, pre-registration is likely to reduce QRPs by encouraging researchers to commit to a pre-specified analytic strategy. On the other hand, pre-registration does not fully protect against researcher bias where data have already been accessed, and can lend added credibility to study claims, which may be unfounded. Reporting prior data access in a pre-registration is therefore important to make these potential biases transparent, so that readers and reviewers can judge the credibility of the findings accordingly. However, for a more rigorous solution which protects against researcher bias in the context of prior data access, researchers should consider adopting a multiverse approach.
Conduct a multiverse analysis
A multiverse analysis involves identifying all potential analytic choices that could justifiably be made to address a given research question (e.g., different ways to code a variable, combinations of covariates, and types of analytic model), implementing them all, and reporting the results [ 44 ]. Notably, this method differs from the traditional approach in which findings from only one analytic method are reported. It is conceptually similar to a sensitivity analysis, but it is far more comprehensive, as often hundreds or thousands of analytic choices are reported, rather than a handful. By showing the results from all defensible analytic approaches, multiverse analysis reduces scope for selective reporting and provides insight into the robustness of findings against analytical choices (for example, if there is a clear convergence of estimates, irrespective of most analytical choices). For causal questions in observational research, Directed Acyclic Graphs (DAGs) could be used to inform selection of covariates in multiverse approaches [ 45 ] (i.e., to ensure that confounders, rather than mediators or colliders, are controlled for).
Specification curve analysis [ 46 ] is a form of multiverse analysis that has been applied to examine the robustness of epidemiological findings to analytic choices [ 6 , 47 ]. Specification curve analysis involves three steps: (1) identifying all analytic choices – termed “specifications”, (2) displaying the results graphically with magnitude of effect size plotted against analytic choice, and (3) conducting joint inference across all results. When applied to the association between digital technology use and adolescent well-being [ 6 ], specification curve analysis showed that the (small, negative) association diminished after accounting for adequate control variables and recall bias – demonstrating the sensitivity of results to analytic choices.
Despite the benefits of the multiverse approach in addressing analytic flexibility, it is not without limitations. First, because each analytic choice is treated as equally valid, including less justifiable models could bias the results away from the truth. Second, the choice of specifications can be biased by prior knowledge (e.g., a researcher may choose to omit a covariate to obtain a particular result). Third, multiverse analysis may not entirely prevent selective reporting (e.g., if the full range of results are not reported), although pre-registering multiverse approaches (and specifying analytic choices) could mitigate this. Last, and perhaps most importantly, multiverse analysis is technically challenging (e.g., when there are hundreds or thousands of analytic choices) and can be impractical for complex analyses, very large datasets, or when computational resources are limited. However, this burden can be somewhat reduced by tutorials and packages which are being developed to standardise the procedure and reduce computational time [see 48 , 49 ].
Challenge: Research may not be hypothesis-driven
Pre-register research questions and conditions for interpreting findings.
Observational research arguably does not need to have a hypothesis to benefit from pre-registration. For studies that are descriptive or focused on estimation, we recommend pre-registering research questions, analysis plans, and criteria for interpretation. Analytic flexibility will be limited by pre-registering specific research questions and detailed analysis plans, while post hoc interpretation will be limited by pre-specifying criteria for interpretation [ 50 ]. The potential for HARK-ing will also be minimised because readers can compare the published study to the original pre-registration, where a-priori hypotheses were not specified.
Detailed guidance on how to pre-register research questions and analysis plans for secondary data is provided in Van den Akker’s [ 29 ] tutorial. To pre-specify conditions for interpretation, it is important to anticipate – as much as possible – all potential findings, and state how each would be interpreted. For example, suppose that a researcher aims to test a causal relationship between X and Y using a multivariate regression model with longitudinal data. Assuming that all potential confounders have been fully measured and controlled for (albeit a strong assumption) and statistical power is high, three broad sets of results and interpretations could be pre-specified. First, an association between X and Y that is similar in magnitude to the unadjusted association would be consistent with a causal relationship. Second, an association between X and Y that is attenuated after controlling for confounders would suggest that the relationship is partly causal and partly confounded. Third, a minimal, non-statistically significant adjusted association would suggest a lack of evidence for a causal effect of X on Y. Depending on the context of the study, criteria could also be provided on the threshold (or range of thresholds) at which the effect size would justify different interpretations [ 51 ], be considered practically meaningful, or the smallest effect size of interest for equivalence tests [ 52 ]. While researcher biases might still affect the pre-registered criteria for interpreting findings (e.g., toward over-interpreting a small effect size as meaningful), this bias will at least be transparent in the pre-registration.
Use a holdout sample to delineate exploratory and confirmatory research
Where researchers wish to integrate exploratory research into a pre-registered, confirmatory study, a holdout sample approach can be used [ 18 ]. Creating a holdout sample refers to the process of randomly splitting the dataset into two parts, often referred to as ‘training’ and ‘holdout’ datasets. To delineate exploratory and confirmatory research, researchers can first conduct exploratory data analysis on the training dataset (which should comprise a moderate fraction of the data, e.g., 35% [ 53 ]. Based on the results of the discovery process, researchers can pre-register hypotheses and analysis plans to formally test on the holdout dataset. This process has parallels with cross-validation in machine learning, in which the dataset is split and the model is developed on the training dataset, before being tested on the test dataset. The approach enables a flexible discovery process, before formally testing discoveries in a non-biased way.
When considering whether to use the holdout sample approach, three points should be noted. First, because the training dataset is not reusable, there will be a reduced sample size and loss of power relative to analysing the whole dataset. As such, the holdout sample approach will only be appropriate when the original dataset is large enough to provide sufficient power in the holdout dataset. Second, when the training dataset is used for exploration, subsequent confirmatory analyses on the holdout dataset may be overfitted (due to both datasets being drawn from the same sample), so replication in independent samples is recommended. Third, the holdout dataset should be created by an independent data manager or guardian, to ensure that the researcher does not have knowledge of the full dataset. However, it is straightforward to randomly split a dataset into a holdout and training sample and we provide example R code at: https://github.com/jr-baldwin/Researcher_Bias_Methods/blob/main/Holdout_script.md .
Challenge: Pre-registered analyses are not appropriate for the data
Use blinding to test proposed analyses.
One method to help ensure that pre-registered analyses will be appropriate for the data is to trial the analyses on a blinded dataset [ 54 ], before pre-registering. Data blinding involves obscuring the data values or labels prior to data analysis, so that the proposed analyses can be trialled on the data without observing the actual findings. Various types of blinding strategies exist [ 54 ], but one method that is appropriate for epidemiological data is “data scrambling” [ 55 ]. This involves randomly shuffling the data points so that any associations between variables are obscured, whilst the variable distributions (and amounts of missing data) remain the same. We provide a tutorial for how to implement this in R (see https://github.com/jr-baldwin/Researcher_Bias_Methods/blob/main/Data_scrambling_tutorial.md ). Ideally the data scrambling would be done by a data guardian who is independent of the research, to ensure that the main researcher does not access the data prior to pre-registering the analyses. Once the researcher is confident with the analyses, the study can be pre-registered, and the analyses conducted on the unscrambled dataset.
Blinded analysis offers several advantages for ensuring that pre-registered analyses are appropriate, with some limitations. First, blinded analysis allows researchers to directly check the distribution of variables and amounts of missingness, without having to make assumptions about the data that may not be met, or spend time planning contingencies for every possible scenario. Second, blinded analysis prevents researchers from gaining insight into the potential findings prior to pre-registration, because associations between variables are masked. However, because of this, blinded analysis does not enable researchers to check for collinearity, predictors of missing data, or other covariances that may be necessary for model specification. As such, blinded analysis will be most appropriate for researchers who wish to check the data distribution and amounts of missingness before pre-registering.
Trial analyses on a dataset excluding the outcome
Another method to help ensure that pre-registered analyses will be appropriate for the data is to trial analyses on a dataset excluding outcome data. For example, data managers could provide researchers with part of the dataset containing the exposure variable(s) plus any covariates and/or auxiliary variables. The researcher can then trial and refine the analyses ahead of pre-registering, without gaining insight into the main findings (which require the outcome data). This approach is used to mitigate bias in propensity score matching studies [ 26 , 56 ], as researchers use data on the exposure and covariates to create matched groups, prior to accessing any outcome data. Once the exposed and non-exposed groups have been matched effectively, researchers pre-register the protocol ahead of viewing the outcome data. Notably though, this approach could help researchers to identify and address other analytical challenges involving secondary data. For example, it could be used to check multivariable distributional characteristics, test for collinearity between multiple predictor variables, or identify predictors of missing data for multiple imputation.
This approach offers certain benefits for researchers keen to ensure that pre-registered analyses are appropriate for the observed data, with some limitations. Regarding benefits, researchers will be able to examine associations between variables (excluding the outcome), unlike the data scrambling approach described above. This would be helpful for checking certain assumptions (e.g., collinearity or characteristics of missing data such as whether it is missing at random). In addition, the approach is easy to implement, as the dataset can be initially created without the outcome variable, which can then be added after pre-registration, minimising burden on data guardians. Regarding limitations, it is possible that accessing variables in advance could provide some insight into the findings. For example, if a covariate is known to be highly correlated with the outcome, testing the association between the covariate and the exposure could give some indication of the relationship between the exposure and the outcome. To make this potential bias transparent, researchers should report the variables that they already accessed in the pre-registration. Another limitation is that researchers will not be able to identify analytical issues relating to the outcome data in advance of pre-registration. Therefore, this approach will be most appropriate where researchers wish to check various characteristics of the exposure variable(s) and covariates, rather than the outcome. However, a “mixed” approach could be applied in which outcome data is provided in scrambled format, to enable researchers to also assess distributional characteristics of the outcome. This would substantially reduce the number of potential challenges to be considered in pre-registered analytical pipelines.
Pre-register a decision tree
If it is not possible to access any of the data prior to pre-registering (e.g., to enable analyses to be trialled on a dataset that is blinded or missing outcome data), researchers could pre-register a decision tree. This defines the sequence of analyses and rules based on characteristics of the observed data [ 17 ]. For example, the decision tree could specify testing a normality assumption, and based on the results, whether to use a parametric or non-parametric test. Ideally, the decision tree should provide a contingency plan for each of the planned analyses, if assumptions are not fulfilled. Of course, it can be challenging and time consuming to anticipate every potential issue with the data and plan contingencies. However, investing time into pre-specifying a decision tree (or a set of contingency plans) could save time should issues arise during data analysis, and can reduce the likelihood of deviating from the pre-registration.
Challenge: Lack of flexibility in data analysis
Transparently report unplanned analyses.
Unplanned analyses (such as applying new methods or conducting follow-up tests to investigate an interesting or unexpected finding) are a natural and often important part of the scientific process. Despite common misconceptions, pre-registration does not permit such unplanned analyses from being included, as long as they are transparently reported as post-hoc. If there are methodological deviations, we recommend that researchers should (1) clearly state the reasons for using the new method, and (2) if possible, report results from both methods, to ideally show that the change in methods was not due to the results [ 57 ]. This information can either be provided in the manuscript or in an update to the original pre-registration (e.g., on the third-party registry such as the OSF), which can be useful when journal word limits are tight. Similarly, if researchers wish to include additional follow-up analyses to investigate an interesting or unexpected finding, this should be reported but labelled as “exploratory” or “post-hoc” in the manuscript.
Ensure a paper’s value does not depend on statistically significant results
Researchers may be concerned that reduced analytic flexibility from pre-registration could increase the likelihood of reporting null results [ 22 , 23 ], which are harder to publish [ 13 , 42 ]. To address this, we recommend taking steps to ensure that the value and success of a study does not depend on a significant p-value. First, methodologically strong research (e.g., with high statistical power, valid and reliable measures, robustness checks, and replication samples) will advance the field, whatever the findings. Second, methods can be applied to allow for the interpretation of statistically non-significant findings (e.g., Bayesian methods [ 58 ] or equivalence tests, which determine whether an observed effect is surprisingly small [ 52 , 59 , 60 ]. This means that the results will be informative whatever they show, in contrast to approaches relying solely on null hypothesis significance testing, where statistically non-significant findings cannot be interpreted as meaningful. Third, researchers can submit the proposed study as a Registered Report, where it will be evaluated before the results are available. This is arguably the strongest way to protect against publication bias, as in-principle study acceptance is granted without any knowledge of the results. In addition, Registered Reports can improve the methodology, as suggestions from expert reviewers can be incorporated into the pre-registered protocol.
Under a system that rewards novel and statistically significant findings, it is easy for subconscious human biases to lead to QRPs. However, researchers, along with data guardians, journals, funders, and institutions, have a responsibility to ensure that findings are reproducible and robust. While pre-registration can help to limit analytic flexibility and selective reporting, it involves several challenges for epidemiologists conducting secondary data analysis. The approaches described here aim to address these challenges (Fig. 1 ), to either improve the efficacy of pre-registration or provide an alternative approach to address analytic flexibility (e.g., a multiverse analysis). The responsibility in adopting these approaches should not only fall on researchers’ shoulders; data guardians also have an important role to play in recording and reporting access to data, providing blinded datasets and hold-out samples, and encouraging researchers to pre-register and adopt these solutions as part of their data request. Furthermore, wider stakeholders could incentivise these practices; for example, journals could provide a designated space for researchers to report deviations from the pre-registration, and funders could provide grants to establish best practice at the cohort level (e.g., data checkout systems, blinded datasets). Ease of adoption is key to ensure wide uptake, and we therefore encourage efforts to evaluate, simplify and improve these practices. Steps that could be taken to evaluate these practices are presented in Box 1.
More broadly, it is important to emphasise that researcher biases do not operate in isolation, but rather in the context of wider publication bias and a “publish or perish” culture. These incentive structures not only promote QRPs [ 61 ], but also discourage researchers from pre-registering and adopting other time-consuming reproducible methods. Therefore, in addition to targeting bias at the individual researcher level, wider initiatives from journals, funders, and institutions are required to address these institutional biases [ 7 ]. Systemic changes that reward rigorous and reproducible research will help researchers to provide unbiased answers to science and society’s most important questions.
Box 1. Evaluation of approaches
To evaluate, simplify and improve approaches to protect against researcher bias in secondary data analysis, the following steps could be taken.
Co-creation workshops to refine approaches
To obtain feedback on the approaches (including on any practical concerns or feasibility issues) co-creation workshops could be held with researchers, data managers, and wider stakeholders (e.g., journals, funders, and institutions).
Empirical research to evaluate efficacy of approaches
To evaluate the effectiveness of the approaches in preventing researcher bias and/or improving pre-registration, empirical research is needed. For example, to test the extent to which the multiverse analysis can reduce selective reporting, comparisons could be made between effect sizes from multiverse analyses versus effect sizes from meta-analyses (of non-pre-registered studies) addressing the same research question. If smaller effect sizes were found in multiverse analyses, it would suggest that the multiverse approach can reduce selective reporting. In addition, to test whether providing a blinded dataset or dataset missing outcome variables could help researchers develop an appropriate analytical protocol, researchers could be randomly assigned to receive such a dataset (or no dataset), prior to pre-registration. If researchers who received such a dataset had fewer eventual deviations from the pre-registered protocol (in the final study), it would suggest that this approach can help ensure that proposed analyses are appropriate for the data.
Pilot implementation of the measures
To assess the practical feasibility of the approaches, data managers could pilot measures for users of the dataset (e.g., required pre-registration for access to data, provision of datasets that are blinded or missing outcome variables). Feedback could then be collected from researchers and data managers via about the experience and ease of use.
Kerr NL. HARKing: Hypothesizing after the results are known. Pers Soc Psychol Rev. 1998;2(3):196–217.
CAS PubMed Google Scholar
Border R, Johnson EC, Evans LM, et al. No support for historical candidate gene or candidate gene-by-interaction hypotheses for major depression across multiple large samples. Am J Psychiatry. 2019;176(5):376–87.
PubMed PubMed Central Google Scholar
Duncan LE, Keller MC. A critical review of the first 10 years of candidate gene-by-environment interaction research in psychiatry. Am J Psychiatry. 2011;168(10):1041–9.
Seibold H, Czerny S, Decke S, et al. A computational reproducibility study of PLOS ONE articles featuring longitudinal data analyses. PLoS ONE. 2021;16(6):e0251194. https://doi.org/10.1371/journal.pone.0251194 .
Article CAS PubMed PubMed Central Google Scholar
Botvinik-Nezer R, Holzmeister F, Camerer CF, et al. Variability in the analysis of a single neuroimaging dataset by many teams. Nature. 2020;582:84–8.
CAS PubMed PubMed Central Google Scholar
Orben A, Przybylski AK. The association between adolescent well-being and digital technology use. Nat Hum Behav. 2019;3(2):173.
PubMed Google Scholar
Munafò MR, Nosek BA, Bishop DV, et al. A manifesto for reproducible science. Nat Hum Behav. 2017;1(1):0021.
Nuzzo R. How scientists fool themselves–and how they can stop. Nature News. 2015;526(7572):182.
CAS Google Scholar
Bishop DV. The psychology of experimental psychologists: Overcoming cognitive constraints to improve research: The 47th Sir Frederic Bartlett lecture. Q J Exp Psychol. 2020;73(1):1–19.
Google Scholar
Greenland S. Invited commentary: The need for cognitive science in methodology. Am J Epidemiol. 2017;186(6):639–45.
De Vries Y, Roest A, de Jonge P, Cuijpers P, Munafò M, Bastiaansen J. The cumulative effect of reporting and citation biases on the apparent efficacy of treatments: The case of depression. Psychol Med. 2018;48(15):2453–5.
Nickerson RS. Confirmation bias: A ubiquitous phenomenon in many guises. Rev Gen Psychol. 1998;2(2):175–220.
Franco A, Malhotra N, Simonovits G. Publication bias in the social sciences: Unlocking the file drawer. Science. 2014;345(6203):1502–5.
Silberzahn R, Uhlmann EL, Martin DP, et al. Many analysts, one data set: Making transparent how variations in analytic choices affect results. Adv Methods Pract Psychol Sci. 2018;1(3):337–56.
Simmons JP, Nelson LD, Simonsohn U. False-positive psychology: Undisclosed flexibility in data collection and analysis allows presenting anything as significant. Psychol Sci. 2011;22(11):1359–66.
Metcalfe J, Wheat, K., Munafo, M., Parry, J. Research integrity: A landscape study: UK Research and innovation 2020.
Nosek BA, Ebersole CR, DeHaven AC, Mellor DT. The preregistration revolution. Proc Natl Acad Sci. 2018;115(11):2600–6.
Wagenmakers E-J, Wetzels R, Borsboom D, van der Maas HL, Kievit RA. An agenda for purely confirmatory research. Perspect Psychol Sci. 2012;7(6):632–8.
Chambers CD. Registered reports: A new publishing initiative at Cortex. Cortex. 2013;49(3):609–10.
Nosek BA, Beck ED, Campbell L, et al. Preregistration is hard, and worthwhile. Trends Cogn Sci. 2019;23(10):815–8.
Kaplan RM, Irvin VL. Likelihood of null effects of large NHLBI clinical trials has increased over time. PLoS One. 2015;10(8):e0132382.
Allen C, Mehler DM. Open science challenges, benefits and tips in early career and beyond. PLoS Biol. 2019;17(5):e3000246.
Scheel AM, Schijen MR, Lakens D. An excess of positive results: Comparing the standard psychology literature with registered reports. Adv Methods Pract Psychol Sci. 2021;4(2):25152459211007468.
Schäfer T, Schwarz MA. The meaningfulness of effect sizes in psychological research: differences between sub-disciplines and the impact of potential biases. Front Psychol. 2019;10:813.
Protzko J, Krosnick J, Nelson LD, et al. High replicability of newly-discovered social-behavioral findings is achievable. PsyArXiv. 2020. doi: https://doi.org/10.31234/osf.io/n2a9x
Small DS, Firth D, Keele L, et al. Protocol for a study of the effect of surface mining in central appalachia on adverse birth outcomes. arXiv.org. 2020
Deshpande SK, Hasegawa RB, Weiss J, Small DS. Protocol for an observational study on the effects of playing football in adolescence on mental health in early adulthood. arXiv preprint 2018
Twins Early Development Study. TEDS Data Access Policy: 6. Pre-registration of analysis. https://www.teds.ac.uk/researchers/teds-data-access-policy#preregistration . Accessed 18 March 2021
Van den Akker O, Weston SJ, Campbell L, et al. Preregistration of secondary data analysis: a template and tutorial. PsyArXiv. 2019. doi: https://doi.org/10.31234/osf.io/hvfmr
Chambers C, Tzavella L. Registered reports: past, present and future. MetaArXiv. 2020. doi: https://doi.org/10.31222/osf.io/43298
McIntosh RD. Exploratory reports: A new article type for cortex. Cortex. 2017;96:A1–4.
Scheel AM, Tiokhin L, Isager PM, Lakens D. Why hypothesis testers should spend less time testing hypotheses. Perspect Psychol Sci. 2020;16(4):744–55.
Colhoun HM, McKeigue PM, Smith GD. Problems of reporting genetic associations with complex outcomes. Lancet. 2003;361(9360):865–72.
Hughes RA, Heron J, Sterne JAC, Tilling K. Accounting for missing data in statistical analyses: Multiple imputation is not always the answer. Int J Epidemiol. 2019;48(4):1294–304. https://doi.org/10.1093/ije/dyz032 .
Article PubMed PubMed Central Google Scholar
Goldstein BA. Five analytic challenges in working with electronic health records data to support clinical trials with some solutions. Clin Trials. 2020;17(4):370–6.
Goldin-Meadow S. Why preregistration makes me nervous. APS Observer. 2016;29(7).
Lash TL. Preregistration of study protocols is unlikely to improve the yield from our science, but other strategies might. Epidemiology. 2010;21(5):612–3. https://doi.org/10.1097/EDE.0b013e3181e9bba6 .
Article PubMed Google Scholar
Lawlor DA. Quality in epidemiological research: should we be submitting papers before we have the results and submitting more hypothesis-generating research? Int J Epidemiol. 2007;36(5):940–3.
Vandenbroucke JP. Preregistration of epidemiologic studies: An ill-founded mix of ideas. Epidemiology. 2010;21(5):619–20.
Pingault J-B, O’reilly PF, Schoeler T, Ploubidis GB, Rijsdijk F, Dudbridge F. Using genetic data to strengthen causal inference in observational research. Nat Rev Genet. 2018;19(9):566.
Fanelli D. Negative results are disappearing from most disciplines and countries. Scientometrics. 2012;90(3):891–904.
Greenwald AG. Consequences of prejudice against the null hypothesis. Psychol Bull. 1975;82(1):1.
Scott KM, Kline M. Enabling confirmatory secondary data analysis by logging data checkout. Adv Methods Pract Psychol Sci. 2019;2(1):45–54. https://doi.org/10.1177/2515245918815849 .
Article Google Scholar
Steegen S, Tuerlinckx F, Gelman A, Vanpaemel W. Increasing transparency through a multiverse analysis. Perspect Psychol Sci. 2016;11(5):702–12.
Del Giudice M, Gangestad SW. A traveler’s guide to the multiverse: Promises, pitfalls, and a framework for the evaluation of analytic decisions. Adv Methods Pract Psychol Sci. 2021;4(1):2515245920954925.
Simonsohn U, Simmons JP, Nelson LD. Specification curve: descriptive and inferential statistics on all reasonable specifications. SSRN. 2015. https://doi.org/10.2139/ssrn.2694998 .
Rohrer JM, Egloff B, Schmukle SC. Probing birth-order effects on narrow traits using specification-curve analysis. Psychol Sci. 2017;28(12):1821–32.
Masur P. How to do specification curve analyses in R: Introducing ‘specr’. 2020. https://philippmasur.de/2020/01/02/how-to-do-specification-curve-analyses-in-r-introducing-specr/ . Accessed 23rd July 2020.
Masur PK, Scharkow M. specr: Conducting and visualizing specification curve analyses: R package. (2020).
Kiyonaga A, Scimeca JM. Practical considerations for navigating registered reports. Trends Neurosci. 2019;42(9):568–72.
McPhetres J. What should a preregistration contain? PsyArXiv. (2020).
Lakens D. Equivalence tests: A practical primer for t tests, correlations, and meta-analyses. Soc Psychol Personal Sci. 2017;8(4):355–62.
Anderson ML, Magruder J. Split-sample strategies for avoiding false discoveries: National Bureau of Economic Research2017. Report No.: 0898-2937.
MacCoun R, Perlmutter S. Blind analysis: Hide results to seek the truth. Nature. 2015;526(7572):187–9.
MacCoun R, Perlmutter S. Blind analysis as a correction for confirmatory bias in physics and in psychology. Psychological science under scrutiny 2017. p. 295-322.
Rubin DB. The design versus the analysis of observational studies for causal effects: Parallels with the design of randomized trials. Stat Med. 2007;26(1):20–36.
Claesen A, Gomes SLBT, Tuerlinckx F, Vanpaemel W. Preregistration: Comparing dream to reality. 2019.
Schönbrodt FD, Wagenmakers E-J. Bayes factor design analysis: Planning for compelling evidence. Psychon Bull Rev. 2018;25(1):128–42.
Lakens D, Scheel AM, Isager PM. Equivalence testing for psychological research: A tutorial. Adv Methods Pract Psychol Sci. 2018;1(2):259–69.
Lakens D, McLatchie N, Isager PM, Scheel AM, Dienes Z. Improving inferences about null effects with Bayes factors and equivalence tests. J Gerontol Ser B. 2020;75(1):45–57.
Gopalakrishna G, ter Riet G, Vink G, Stoop I, Wicherts J, Bouter L. Prevalence of questionable research practices, research misconduct and their potential explanatory factors: a survey among academic researchers in The Netherlands. 2021.
Goldacre B, Drysdale, H., Powell-Smith, A., Dale, A., Milosevic, I., Slade, E., Hartley, H., Marston, C., Mahtani, K., Heneghan, C. The compare trials project. 2021. https://compare-trials.org . Accessed 23rd July 2020.
Mathieu S, Boutron I, Moher D, Altman DG, Ravaud P. Comparison of registered and published primary outcomes in randomized controlled trials. JAMA. 2009;302(9):977–84.
Rubin M. Does preregistration improve the credibility of research findings? arXiv preprint 2020.
Szollosi A, Kellen D, Navarro D, et al. Is preregistration worthwhile? Cell. 2019.
Quintana DS. A synthetic dataset primer for the biobehavioural sciences to promote reproducibility and hypothesis generation. Elife. 2020;9:e53275.
Weston SJ, Ritchie SJ, Rohrer JM, Przybylski AK. Recommendations for increasing the transparency of analysis of preexisting data sets. Adv Methods Pract Psychol Sci. 2019;2(3):214–27.
Thompson WH, Wright J, Bissett PG, Poldrack RA. Meta-research: dataset decay and the problem of sequential analyses on open datasets. Elife. 2020;9:e53498.
Download references
Acknowledgements
The authors are grateful to Professor George Davey for his helpful comments on this article.
J.R.B is funded by a Wellcome Trust Sir Henry Wellcome fellowship (grant 215917/Z/19/Z). J.B.P is a supported by the Medical Research Foundation 2018 Emerging Leaders 1 st Prize in Adolescent Mental Health (MRF-160–0002-ELP-PINGA). M.R.M and H.M.S work in a unit that receives funding from the University of Bristol and the UK Medical Research Council (MC_UU_00011/5, MC_UU_00011/7), and M.R.M is also supported by the National Institute for Health Research (NIHR) Biomedical Research Centre at the University Hospitals Bristol National Health Service Foundation Trust and the University of Bristol.
Author information
Authors and affiliations.
Department of Clinical, Educational and Health Psychology, Division of Psychology and Language Sciences, University College London, London, WC1H 0AP, UK
Jessie R. Baldwin, Jean-Baptiste Pingault & Tabea Schoeler
Social, Genetic and Developmental Psychiatry Centre, Institute of Psychiatry, Psychology and Neuroscience, King’s College London, London, UK
Jessie R. Baldwin & Jean-Baptiste Pingault
MRC Integrative Epidemiology Unit at the University of Bristol, Bristol Medical School, University of Bristol, Bristol, UK
Hannah M. Sallis & Marcus R. Munafò
School of Psychological Science, University of Bristol, Bristol, UK
Centre for Academic Mental Health, Population Health Sciences, University of Bristol, Bristol, UK
Hannah M. Sallis
NIHR Biomedical Research Centre, University Hospitals Bristol NHS Foundation Trust and University of Bristol, Bristol, UK
Marcus R. Munafò
You can also search for this author in PubMed Google Scholar
Contributions
JRB and MRM developed the idea for the article. The first draft of the manuscript was written by JRB, with support from MRM and all authors commented on previous versions of the manuscript. All authors read and approved the final manuscript.
Corresponding author
Correspondence to Jessie R. Baldwin .
Ethics declarations
Conflict of interest.
Author declares that they have no conflict of interest.
Additional information
Publisher's note.
Springer Nature remains neutral with regard to jurisdictional claims in published maps and institutional affiliations.
Rights and permissions
Open Access This article is licensed under a Creative Commons Attribution 4.0 International License, which permits use, sharing, adaptation, distribution and reproduction in any medium or format, as long as you give appropriate credit to the original author(s) and the source, provide a link to the Creative Commons licence, and indicate if changes were made. The images or other third party material in this article are included in the article's Creative Commons licence, unless indicated otherwise in a credit line to the material. If material is not included in the article's Creative Commons licence and your intended use is not permitted by statutory regulation or exceeds the permitted use, you will need to obtain permission directly from the copyright holder. To view a copy of this licence, visit http://creativecommons.org/licenses/by/4.0/ .
Reprints and permissions
About this article
Baldwin, J.R., Pingault, JB., Schoeler, T. et al. Protecting against researcher bias in secondary data analysis: challenges and potential solutions. Eur J Epidemiol 37 , 1–10 (2022). https://doi.org/10.1007/s10654-021-00839-0
Download citation
Received : 19 October 2021
Accepted : 28 December 2021
Published : 13 January 2022
Issue Date : January 2022
DOI : https://doi.org/10.1007/s10654-021-00839-0
Share this article
Anyone you share the following link with will be able to read this content:
Sorry, a shareable link is not currently available for this article.
Provided by the Springer Nature SharedIt content-sharing initiative
- Secondary data analysis
- Pre-registration
- Open science
- Researcher bias
Advertisement
- Find a journal
- Publish with us
- Track your research
Root out friction in every digital experience, super-charge conversion rates, and optimize digital self-service
Uncover insights from any interaction, deliver AI-powered agent coaching, and reduce cost to serve
Increase revenue and loyalty with real-time insights and recommendations delivered to teams on the ground
Know how your people feel and empower managers to improve employee engagement, productivity, and retention
Take action in the moments that matter most along the employee journey and drive bottom line growth
Whatever they’re are saying, wherever they’re saying it, know exactly what’s going on with your people
Get faster, richer insights with qual and quant tools that make powerful market research available to everyone
Run concept tests, pricing studies, prototyping + more with fast, powerful studies designed by UX research experts
Track your brand performance 24/7 and act quickly to respond to opportunities and challenges in your market
Explore the platform powering Experience Management
- Free Account
- For Digital
- For Customer Care
- For Human Resources
- For Researchers
- Financial Services
- All Industries
Popular Use Cases
- Customer Experience
- Employee Experience
- Employee Exit Interviews
- Net Promoter Score
- Voice of Customer
- Customer Success Hub
- Product Documentation
- Training & Certification
- XM Institute
- Popular Resources
- Customer Stories
Market Research
- Artificial Intelligence
- Partnerships
- Marketplace
The annual gathering of the experience leaders at the world’s iconic brands building breakthrough business results, live in Salt Lake City.
- English/AU & NZ
- Español/Europa
- Español/América Latina
- Português Brasileiro
- REQUEST DEMO
- Experience Management
- Primary vs Secondary Research
Try Qualtrics for free
Primary vs secondary research – what’s the difference.
14 min read Find out how primary and secondary research are different from each other, and how you can use them both in your own research program.
Primary vs secondary research: in a nutshell
The essential difference between primary and secondary research lies in who collects the data.
- Primary research definition
When you conduct primary research, you’re collecting data by doing your own surveys or observations.
- Secondary research definition:
In secondary research, you’re looking at existing data from other researchers, such as academic journals, government agencies or national statistics.
Free Ebook: The Qualtrics Handbook of Question Design
When to use primary vs secondary research
Primary research and secondary research both offer value in helping you gather information.
Each research method can be used alone to good effect. But when you combine the two research methods, you have the ingredients for a highly effective market research strategy. Most research combines some element of both primary methods and secondary source consultation.
So assuming you’re planning to do both primary and secondary research – which comes first? Counterintuitive as it sounds, it’s more usual to start your research process with secondary research, then move on to primary research.
Secondary research can prepare you for collecting your own data in a primary research project. It can give you a broad overview of your research area, identify influences and trends, and may give you ideas and avenues to explore that you hadn’t previously considered.
Given that secondary research can be done quickly and inexpensively, it makes sense to start your primary research process with some kind of secondary research. Even if you’re expecting to find out what you need to know from a survey of your target market, taking a small amount of time to gather information from secondary sources is worth doing.
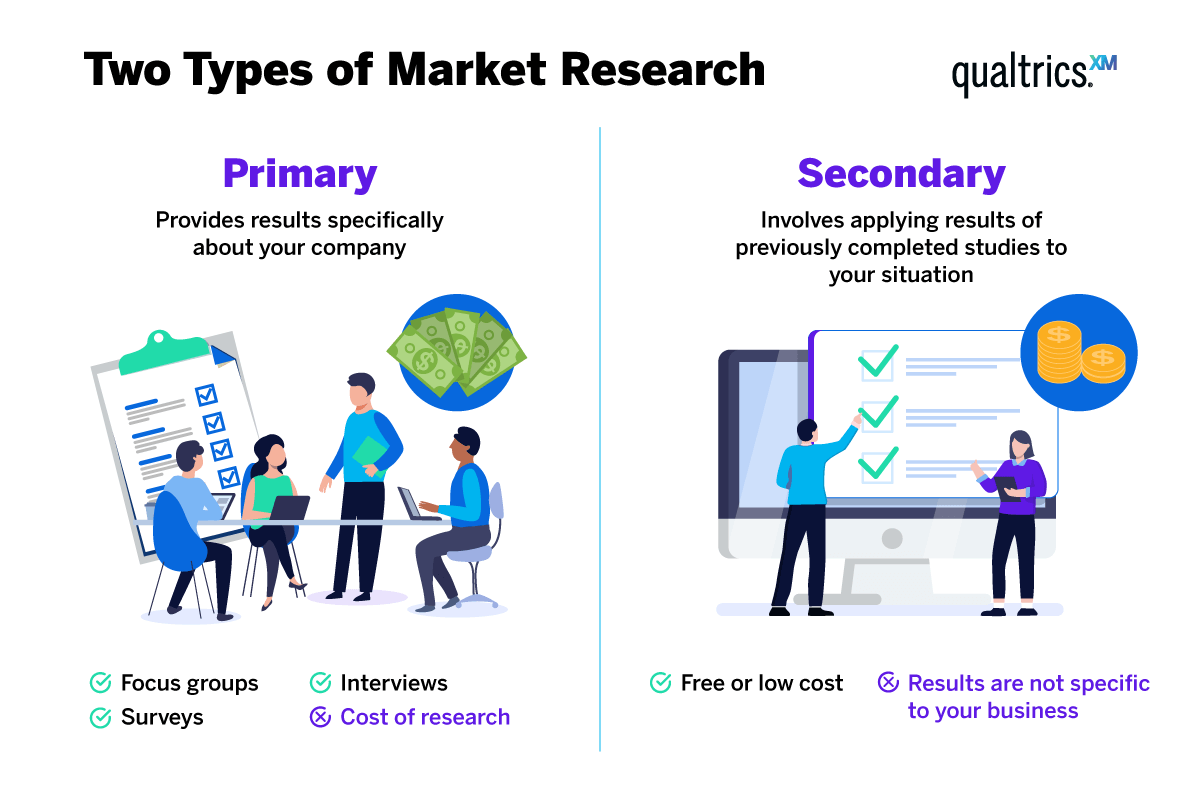
Primary research
Primary market research is original research carried out when a company needs timely, specific data about something that affects its success or potential longevity.
Primary research data collection might be carried out in-house by a business analyst or market research team within the company, or it may be outsourced to a specialist provider, such as an agency or consultancy. While outsourcing primary research involves a greater upfront expense, it’s less time consuming and can bring added benefits such as researcher expertise and a ‘fresh eyes’ perspective that avoids the risk of bias and partiality affecting the research data.
Primary research gives you recent data from known primary sources about the particular topic you care about, but it does take a little time to collect that data from scratch, rather than finding secondary data via an internet search or library visit.
Primary research involves two forms of data collection:
- Exploratory research This type of primary research is carried out to determine the nature of a problem that hasn’t yet been clearly defined. For example, a supermarket wants to improve its poor customer service and needs to understand the key drivers behind the customer experience issues. It might do this by interviewing employees and customers, or by running a survey program or focus groups.
- Conclusive research This form of primary research is carried out to solve a problem that the exploratory research – or other forms of primary data – has identified. For example, say the supermarket’s exploratory research found that employees weren’t happy. Conclusive research went deeper, revealing that the manager was rude, unreasonable, and difficult, making the employees unhappy and resulting in a poor employee experience which in turn led to less than excellent customer service. Thanks to the company’s choice to conduct primary research, a new manager was brought in, employees were happier and customer service improved.
Examples of primary research
All of the following are forms of primary research data.
- Customer satisfaction survey results
- Employee experience pulse survey results
- NPS rating scores from your customers
- A field researcher’s notes
- Data from weather stations in a local area
- Recordings made during focus groups
Primary research methods
There are a number of primary research methods to choose from, and they are already familiar to most people. The ones you choose will depend on your budget, your time constraints, your research goals and whether you’re looking for quantitative or qualitative data.
A survey can be carried out online, offline, face to face or via other media such as phone or SMS. It’s relatively cheap to do, since participants can self-administer the questionnaire in most cases. You can automate much of the process if you invest in good quality survey software.
Primary research interviews can be carried out face to face, over the phone or via video calling. They’re more time-consuming than surveys, and they require the time and expense of a skilled interviewer and a dedicated room, phone line or video calling setup. However, a personal interview can provide a very rich primary source of data based not only on the participant’s answers but also on the observations of the interviewer.
Focus groups
A focus group is an interview with multiple participants at the same time. It often takes the form of a discussion moderated by the researcher. As well as taking less time and resources than a series of one-to-one interviews, a focus group can benefit from the interactions between participants which bring out more ideas and opinions. However this can also lead to conversations going off on a tangent, which the moderator must be able to skilfully avoid by guiding the group back to the relevant topic.
Secondary research
Secondary research is research that has already been done by someone else prior to your own research study.
Secondary research is generally the best place to start any research project as it will reveal whether someone has already researched the same topic you’re interested in, or a similar topic that helps lay some of the groundwork for your research project.
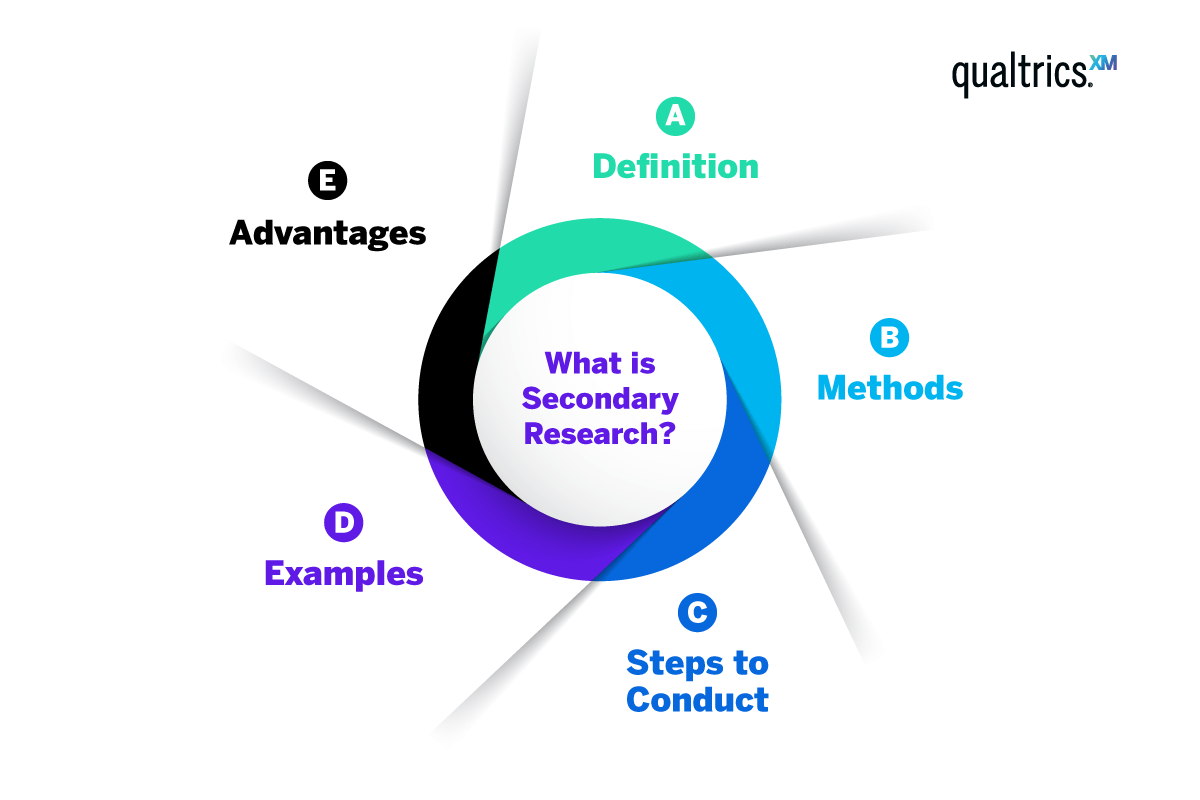
Even if your preliminary secondary research doesn’t turn up a study similar to your own research goals, it will still give you a stronger knowledge base that you can use to strengthen and refine your research hypothesis. You may even find some gaps in the market you didn’t know about before.
The scope of secondary research resources is extremely broad. Here are just a few of the places you might look for relevant information.
Books and magazines
A public library can turn up a wealth of data in the form of books and magazines – and it doesn’t cost a penny to consult them.
Market research reports
Secondary research from professional research agencies can be highly valuable, as you can be confident the data collection methods and data analysis will be sound
Scholarly journals, often available in reference libraries
Peer-reviewed journals have been examined by experts from the relevant educational institutions, meaning there has been an extra layer of oversight and careful consideration of the data points before publication.
Government reports and studies
Public domain data, such as census data, can provide relevant information for your research project, not least in choosing the appropriate research population for a primary research method. If the information you need isn’t readily available, try contacting the relevant government agencies.
White papers
Businesses often produce white papers as a means of showcasing their expertise and value in their field. White papers can be helpful in secondary research methods, although they may not be as carefully vetted as academic papers or public records.
Trade or industry associations
Associations may have secondary data that goes back a long way and offers a general overview of a particular industry. This data collected over time can be very helpful in laying the foundations of your particular research project.
Private company data
Some businesses may offer their company data to those conducting research in return for fees or with explicit permissions. However, if a business has data that’s closely relevant to yours, it’s likely they are a competitor and may flat out refuse your request.
Learn more about secondary research
Examples of secondary research data
These are all forms of secondary research data in action:
- A newspaper report quoting statistics sourced by a journalist
- Facts from primary research articles quoted during a debate club meeting
- A blog post discussing new national figures on the economy
- A company consulting previous research published by a competitor
Secondary research methods
Literature reviews.
A core part of the secondary research process, involving data collection and constructing an argument around multiple sources. A literature review involves gathering information from a wide range of secondary sources on one topic and summarizing them in a report or in the introduction to primary research data.
Content analysis
This systematic approach is widely used in social science disciplines. It uses codes for themes, tropes or key phrases which are tallied up according to how often they occur in the secondary data. The results help researchers to draw conclusions from qualitative data.
Data analysis using digital tools
You can analyze large volumes of data using software that can recognize and categorize natural language. More advanced tools will even be able to identify relationships and semantic connections within the secondary research materials.
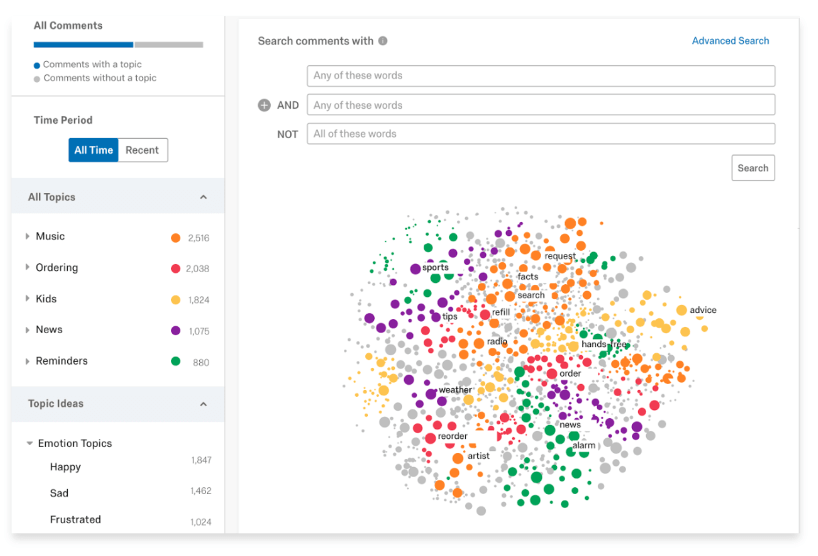
Comparing primary vs secondary research
We’ve established that both primary research and secondary research have benefits for your business, and that there are major differences in terms of the research process, the cost, the research skills involved and the types of data gathered. But is one of them better than the other?
The answer largely depends on your situation. Whether primary or secondary research wins out in your specific case depends on the particular topic you’re interested in and the resources you have available. The positive aspects of one method might be enough to sway you, or the drawbacks – such as a lack of credible evidence already published, as might be the case in very fast-moving industries – might make one method totally unsuitable.
Here’s an at-a-glance look at the features and characteristics of primary vs secondary research, illustrating some of the key differences between them.
What are the pros and cons of primary research?
Primary research provides original data and allows you to pinpoint the issues you’re interested in and collect data from your target market – with all the effort that entails.
Benefits of primary research:
- Tells you what you need to know, nothing irrelevant
- Yours exclusively – once acquired, you may be able to sell primary data or use it for marketing
- Teaches you more about your business
- Can help foster new working relationships and connections between silos
- Primary research methods can provide upskilling opportunities – employees gain new research skills
Limitations of primary research:
- Lacks context from other research on related subjects
- Can be expensive
- Results aren’t ready to use until the project is complete
- Any mistakes you make in in research design or implementation could compromise your data quality
- May not have lasting relevance – although it could fulfill a benchmarking function if things change
What are the pros and cons of secondary research?
Secondary research relies on secondary sources, which can be both an advantage and a drawback. After all, other people are doing the work, but they’re also setting the research parameters.
Benefits of secondary research:
- It’s often low cost or even free to access in the public domain
- Supplies a knowledge base for researchers to learn from
- Data is complete, has been analyzed and checked, saving you time and costs
- It’s ready to use as soon as you acquire it
Limitations of secondary research
- May not provide enough specific information
- Conducting a literature review in a well-researched subject area can become overwhelming
- No added value from publishing or re-selling your research data
- Results are inconclusive – you’ll only ever be interpreting data from another organization’s experience, not your own
- Details of the research methodology are unknown
- May be out of date – always check carefully the original research was conducted
Related resources
Business research methods 12 min read, qualitative research interviews 11 min read, market intelligence 10 min read, marketing insights 11 min read, ethnographic research 11 min read, qualitative vs quantitative research 13 min read, qualitative research questions 11 min read, request demo.
Ready to learn more about Qualtrics?

An official website of the United States government
The .gov means it’s official. Federal government websites often end in .gov or .mil. Before sharing sensitive information, make sure you’re on a federal government site.
The site is secure. The https:// ensures that you are connecting to the official website and that any information you provide is encrypted and transmitted securely.
- Publications
- Account settings
Preview improvements coming to the PMC website in October 2024. Learn More or Try it out now .
- Advanced Search
- Journal List

Protecting against researcher bias in secondary data analysis: challenges and potential solutions
Jessie r. baldwin.
1 Department of Clinical, Educational and Health Psychology, Division of Psychology and Language Sciences, University College London, London, WC1H 0AP UK
2 Social, Genetic and Developmental Psychiatry Centre, Institute of Psychiatry, Psychology and Neuroscience, King’s College London, London, UK
Jean-Baptiste Pingault
Tabea schoeler, hannah m. sallis.
3 MRC Integrative Epidemiology Unit at the University of Bristol, Bristol Medical School, University of Bristol, Bristol, UK
4 School of Psychological Science, University of Bristol, Bristol, UK
5 Centre for Academic Mental Health, Population Health Sciences, University of Bristol, Bristol, UK
Marcus R. Munafò
6 NIHR Biomedical Research Centre, University Hospitals Bristol NHS Foundation Trust and University of Bristol, Bristol, UK
Analysis of secondary data sources (such as cohort studies, survey data, and administrative records) has the potential to provide answers to science and society’s most pressing questions. However, researcher biases can lead to questionable research practices in secondary data analysis, which can distort the evidence base. While pre-registration can help to protect against researcher biases, it presents challenges for secondary data analysis. In this article, we describe these challenges and propose novel solutions and alternative approaches. Proposed solutions include approaches to (1) address bias linked to prior knowledge of the data, (2) enable pre-registration of non-hypothesis-driven research, (3) help ensure that pre-registered analyses will be appropriate for the data, and (4) address difficulties arising from reduced analytic flexibility in pre-registration. For each solution, we provide guidance on implementation for researchers and data guardians. The adoption of these practices can help to protect against researcher bias in secondary data analysis, to improve the robustness of research based on existing data.
Introduction
Secondary data analysis has the potential to provide answers to science and society’s most pressing questions. An abundance of secondary data exists—cohort studies, surveys, administrative data (e.g., health records, crime records, census data), financial data, and environmental data—that can be analysed by researchers in academia, industry, third-sector organisations, and the government. However, secondary data analysis is vulnerable to questionable research practices (QRPs) which can distort the evidence base. These QRPs include p-hacking (i.e., exploiting analytic flexibility to obtain statistically significant results), selective reporting of statistically significant, novel, or “clean” results, and hypothesising after the results are known (HARK-ing [i.e., presenting unexpected results as if they were predicted]; [ 1 ]. Indeed, findings obtained from secondary data analysis are not always replicable [ 2 , 3 ], reproducible [ 4 ], or robust to analytic choices [ 5 , 6 ]. Preventing QRPs in research based on secondary data is therefore critical for scientific and societal progress.
A primary cause of QRPs is common cognitive biases that affect the analysis, reporting, and interpretation of data [ 7 – 10 ]. For example, apophenia (the tendency to see patterns in random data) and confirmation bias (the tendency to focus on evidence that is consistent with one’s beliefs) can lead to particular analytical choices and selective reporting of “publishable” results [ 11 – 13 ]. In addition, hindsight bias (the tendency to view past events as predictable) can lead to HARK-ing, so that observed results appear more compelling.
The scope for these biases to distort research outputs from secondary data analysis is perhaps particularly acute, for two reasons. First, researchers now have increasing access to high-dimensional datasets that offer a multitude of ways to analyse the same data [ 6 ]. Such analytic flexibility can lead to different conclusions depending on the analytical choices made [ 5 , 14 , 15 ]. Second, current incentive structures in science reward researchers for publishing statistically significant, novel, and/or surprising findings [ 16 ]. This combination of opportunity and incentive may lead researchers—consciously or unconsciously—to run multiple analyses and only report the most “publishable” findings.
One way to help protect against the effects of researcher bias is to pre-register research plans [ 17 , 18 ]. This can be achieved by pre-specifying the rationale, hypotheses, methods, and analysis plans, and submitting these to either a third-party registry (e.g., the Open Science Framework [OSF]; https://osf.io/ ), or a journal in the form of a Registered Report [ 19 ]. Because research plans and hypotheses are specified before the results are known, pre-registration reduces the potential for cognitive biases to lead to p-hacking, selective reporting, and HARK-ing [ 20 ]. While pre-registration is not necessarily a panacea for preventing QRPs (Table (Table1), 1 ), meta-scientific evidence has found that pre-registered studies and Registered Reports are more likely to report null results [ 21 – 23 ], smaller effect sizes [ 24 ], and be replicated [ 25 ]. Pre-registration is increasingly being adopted in epidemiological research [ 26 , 27 ], and is even required for access to data from certain cohorts (e.g., the Twins Early Development Study [ 28 ]). However, pre-registration (and other open science practices; Table Table2) 2 ) can pose particular challenges to researchers conducting secondary data analysis [ 29 ], motivating the need for alternative approaches and solutions. Here we describe such challenges, before proposing potential solutions to protect against researcher bias in secondary data analysis (summarised in Fig. 1 ).
Limitations in the use of pre-registration to address QRPs
Challenges and potential solutions regarding sharing pre-existing data

Challenges in pre-registering secondary data analysis and potential solutions (according to researcher motivations). Note : In the “Potential solution” column, blue boxes indicate solutions that are researcher-led; green boxes indicate solutions that should be facilitated by data guardians
Challenges of pre-registration for secondary data analysis
Prior knowledge of the data.
Researchers conducting secondary data analysis commonly analyse data from the same dataset multiple times throughout their careers. However, prior knowledge of the data increases risk of bias, as prior expectations about findings could motivate researchers to pursue certain analyses or questions. In the worst-case scenario, a researcher might perform multiple preliminary analyses, and only pursue those which lead to notable results (perhaps posting a pre-registration for these analyses, even though it is effectively post hoc). However, even if the researcher has not conducted specific analyses previously, they may be biased (either consciously or subconsciously) to pursue certain analyses after testing related questions with the same variables, or even by reading past studies on the dataset. As such, pre-registration cannot fully protect against researcher bias when researchers have previously accessed the data.
Research may not be hypothesis-driven
Pre-registration and Registered Reports are tailored towards hypothesis-driven, confirmatory research. For example, the OSF pre-registration template requires researchers to state “specific, concise, and testable hypotheses”, while Registered Reports do not permit purely exploratory research [ 30 ], although a new Exploratory Reports format now exists [ 31 ]. However, much research involving secondary data is not focused on hypothesis testing, but is exploratory, descriptive, or focused on estimation—in other words, examining the magnitude and robustness of an association as precisely as possible, rather than simply testing a point null. Furthermore, without a strong theoretical background, hypotheses will be arbitrary and could lead to unhelpful inferences [ 32 , 33 ], and so should be avoided in novel areas of research.
Pre-registered analyses are not appropriate for the data
With pre-registration, there is always a risk that the data will violate the assumptions of the pre-registered analyses [ 17 ]. For example, a researcher might pre-register a parametric test, only for the data to be non-normally distributed. However, in secondary data analysis, the extent to which the data shape the appropriate analysis can be considerable. First, longitudinal cohort studies are often subject to missing data and attrition. Approaches to deal with missing data (e.g., listwise deletion; multiple imputation) depend on the characteristics of missing data (e.g., the extent and patterns of missingness [ 34 ]), and so pre-specifying approaches to dealing with missingness may be difficult, or extremely complex. Second, certain analytical decisions depend on the nature of the observed data (e.g., the choice of covariates to include in a multiple regression might depend on the collinearity between the measures, or the degree of missingness of different measures that capture the same construct). Third, much secondary data (e.g., electronic health records and other administrative data) were never collected for research purposes, so can present several challenges that are impossible to predict in advance [ 35 ]. These issues can limit a researcher’s ability to pre-register a precise analytic plan prior to accessing secondary data.
Lack of flexibility in data analysis
Concerns have been raised that pre-registration limits flexibility in data analysis, including justifiable exploration [ 36 – 38 ]. For example, by requiring researchers to commit to a pre-registered analysis plan, pre-registration could prevent researchers from exploring novel questions (with a hypothesis-free approach), conducting follow-up analyses to investigate notable findings [ 39 ], or employing newly published methods with advantages over those pre-registered. While this concern is also likely to apply to primary data analysis, it is particularly relevant to certain fields involving secondary data analysis, such as genetic epidemiology, where new methods are rapidly being developed [ 40 ], and follow-up analyses are often required (e.g., in a genome-wide association study to further investigate the role of a genetic variant associated with a phenotype). However, this concern is perhaps over-stated – pre-registration does not preclude unplanned analyses; it simply makes it more transparent that these analyses are post hoc. Nevertheless, another understandable concern is that reduced analytic flexibility could lead to difficulties in publishing papers and accruing citations. For example, pre-registered studies are more likely to report null results [ 22 , 23 ], likely due to reduced analytic flexibility and selective reporting. While this is a positive outcome for research integrity, null results are less likely to be published [ 13 , 41 , 42 ] and cited [ 11 ], which could disadvantage researchers’ careers.
In this section, we describe potential solutions to address the challenges involved in pre-registering secondary data analysis, including approaches to (1) address bias linked to prior knowledge of the data, (2) enable pre-registration of non-hypothesis-driven research, (3) ensure that pre-planned analyses will be appropriate for the data, and (4) address potential difficulties arising from reduced analytic flexibility.
Challenge: Prior knowledge of the data
Declare prior access to data.
To increase transparency about potential biases arising from knowledge of the data, researchers could routinely report all prior data access in a pre-registration [ 29 ]. This would ideally include evidence from an independent gatekeeper (e.g., a data guardian of the study) stating whether data and relevant variables were accessed by each co-author. To facilitate this process, data guardians could set up a central “electronic checkout” system that records which researchers have accessed data, what data were accessed, and when [ 43 ]. The researcher or data guardian could then provide links to the checkout histories for all co-authors in the pre-registration, to verify their prior data access. If it is not feasible to provide such objective evidence, authors could self-certify their prior access to the dataset and where possible, relevant variables—preferably listing any publications and in-preparation studies based on the dataset [ 29 ]. Of course, self-certification relies on trust that researchers will accurately report prior data access, which could be challenging if the study involves a large number of authors, or authors who have been involved on many studies on the dataset. However, it is likely to be the most feasible option at present as many datasets do not have available electronic records of data access. For further guidance on self-certifying prior data access when pre-registering secondary data analysis studies on a third-party registry (e.g., the OSF), we recommend referring to the template by Van den Akker, Weston [ 29 ].
The extent to which prior access to data renders pre-registration invalid is debatable. On the one hand, even if data have been accessed previously, pre-registration is likely to reduce QRPs by encouraging researchers to commit to a pre-specified analytic strategy. On the other hand, pre-registration does not fully protect against researcher bias where data have already been accessed, and can lend added credibility to study claims, which may be unfounded. Reporting prior data access in a pre-registration is therefore important to make these potential biases transparent, so that readers and reviewers can judge the credibility of the findings accordingly. However, for a more rigorous solution which protects against researcher bias in the context of prior data access, researchers should consider adopting a multiverse approach.
Conduct a multiverse analysis
A multiverse analysis involves identifying all potential analytic choices that could justifiably be made to address a given research question (e.g., different ways to code a variable, combinations of covariates, and types of analytic model), implementing them all, and reporting the results [ 44 ]. Notably, this method differs from the traditional approach in which findings from only one analytic method are reported. It is conceptually similar to a sensitivity analysis, but it is far more comprehensive, as often hundreds or thousands of analytic choices are reported, rather than a handful. By showing the results from all defensible analytic approaches, multiverse analysis reduces scope for selective reporting and provides insight into the robustness of findings against analytical choices (for example, if there is a clear convergence of estimates, irrespective of most analytical choices). For causal questions in observational research, Directed Acyclic Graphs (DAGs) could be used to inform selection of covariates in multiverse approaches [ 45 ] (i.e., to ensure that confounders, rather than mediators or colliders, are controlled for).
Specification curve analysis [ 46 ] is a form of multiverse analysis that has been applied to examine the robustness of epidemiological findings to analytic choices [ 6 , 47 ]. Specification curve analysis involves three steps: (1) identifying all analytic choices – termed “specifications”, (2) displaying the results graphically with magnitude of effect size plotted against analytic choice, and (3) conducting joint inference across all results. When applied to the association between digital technology use and adolescent well-being [ 6 ], specification curve analysis showed that the (small, negative) association diminished after accounting for adequate control variables and recall bias – demonstrating the sensitivity of results to analytic choices.
Despite the benefits of the multiverse approach in addressing analytic flexibility, it is not without limitations. First, because each analytic choice is treated as equally valid, including less justifiable models could bias the results away from the truth. Second, the choice of specifications can be biased by prior knowledge (e.g., a researcher may choose to omit a covariate to obtain a particular result). Third, multiverse analysis may not entirely prevent selective reporting (e.g., if the full range of results are not reported), although pre-registering multiverse approaches (and specifying analytic choices) could mitigate this. Last, and perhaps most importantly, multiverse analysis is technically challenging (e.g., when there are hundreds or thousands of analytic choices) and can be impractical for complex analyses, very large datasets, or when computational resources are limited. However, this burden can be somewhat reduced by tutorials and packages which are being developed to standardise the procedure and reduce computational time [see 48 , 49 ].
Challenge: Research may not be hypothesis-driven
Pre-register research questions and conditions for interpreting findings.
Observational research arguably does not need to have a hypothesis to benefit from pre-registration. For studies that are descriptive or focused on estimation, we recommend pre-registering research questions, analysis plans, and criteria for interpretation. Analytic flexibility will be limited by pre-registering specific research questions and detailed analysis plans, while post hoc interpretation will be limited by pre-specifying criteria for interpretation [ 50 ]. The potential for HARK-ing will also be minimised because readers can compare the published study to the original pre-registration, where a-priori hypotheses were not specified.
Detailed guidance on how to pre-register research questions and analysis plans for secondary data is provided in Van den Akker’s [ 29 ] tutorial. To pre-specify conditions for interpretation, it is important to anticipate – as much as possible – all potential findings, and state how each would be interpreted. For example, suppose that a researcher aims to test a causal relationship between X and Y using a multivariate regression model with longitudinal data. Assuming that all potential confounders have been fully measured and controlled for (albeit a strong assumption) and statistical power is high, three broad sets of results and interpretations could be pre-specified. First, an association between X and Y that is similar in magnitude to the unadjusted association would be consistent with a causal relationship. Second, an association between X and Y that is attenuated after controlling for confounders would suggest that the relationship is partly causal and partly confounded. Third, a minimal, non-statistically significant adjusted association would suggest a lack of evidence for a causal effect of X on Y. Depending on the context of the study, criteria could also be provided on the threshold (or range of thresholds) at which the effect size would justify different interpretations [ 51 ], be considered practically meaningful, or the smallest effect size of interest for equivalence tests [ 52 ]. While researcher biases might still affect the pre-registered criteria for interpreting findings (e.g., toward over-interpreting a small effect size as meaningful), this bias will at least be transparent in the pre-registration.
Use a holdout sample to delineate exploratory and confirmatory research
Where researchers wish to integrate exploratory research into a pre-registered, confirmatory study, a holdout sample approach can be used [ 18 ]. Creating a holdout sample refers to the process of randomly splitting the dataset into two parts, often referred to as ‘training’ and ‘holdout’ datasets. To delineate exploratory and confirmatory research, researchers can first conduct exploratory data analysis on the training dataset (which should comprise a moderate fraction of the data, e.g., 35% [ 53 ]. Based on the results of the discovery process, researchers can pre-register hypotheses and analysis plans to formally test on the holdout dataset. This process has parallels with cross-validation in machine learning, in which the dataset is split and the model is developed on the training dataset, before being tested on the test dataset. The approach enables a flexible discovery process, before formally testing discoveries in a non-biased way.
When considering whether to use the holdout sample approach, three points should be noted. First, because the training dataset is not reusable, there will be a reduced sample size and loss of power relative to analysing the whole dataset. As such, the holdout sample approach will only be appropriate when the original dataset is large enough to provide sufficient power in the holdout dataset. Second, when the training dataset is used for exploration, subsequent confirmatory analyses on the holdout dataset may be overfitted (due to both datasets being drawn from the same sample), so replication in independent samples is recommended. Third, the holdout dataset should be created by an independent data manager or guardian, to ensure that the researcher does not have knowledge of the full dataset. However, it is straightforward to randomly split a dataset into a holdout and training sample and we provide example R code at: https://github.com/jr-baldwin/Researcher_Bias_Methods/blob/main/Holdout_script.md .
Challenge: Pre-registered analyses are not appropriate for the data
Use blinding to test proposed analyses.
One method to help ensure that pre-registered analyses will be appropriate for the data is to trial the analyses on a blinded dataset [ 54 ], before pre-registering. Data blinding involves obscuring the data values or labels prior to data analysis, so that the proposed analyses can be trialled on the data without observing the actual findings. Various types of blinding strategies exist [ 54 ], but one method that is appropriate for epidemiological data is “data scrambling” [ 55 ]. This involves randomly shuffling the data points so that any associations between variables are obscured, whilst the variable distributions (and amounts of missing data) remain the same. We provide a tutorial for how to implement this in R (see https://github.com/jr-baldwin/Researcher_Bias_Methods/blob/main/Data_scrambling_tutorial.md ). Ideally the data scrambling would be done by a data guardian who is independent of the research, to ensure that the main researcher does not access the data prior to pre-registering the analyses. Once the researcher is confident with the analyses, the study can be pre-registered, and the analyses conducted on the unscrambled dataset.
Blinded analysis offers several advantages for ensuring that pre-registered analyses are appropriate, with some limitations. First, blinded analysis allows researchers to directly check the distribution of variables and amounts of missingness, without having to make assumptions about the data that may not be met, or spend time planning contingencies for every possible scenario. Second, blinded analysis prevents researchers from gaining insight into the potential findings prior to pre-registration, because associations between variables are masked. However, because of this, blinded analysis does not enable researchers to check for collinearity, predictors of missing data, or other covariances that may be necessary for model specification. As such, blinded analysis will be most appropriate for researchers who wish to check the data distribution and amounts of missingness before pre-registering.
Trial analyses on a dataset excluding the outcome
Another method to help ensure that pre-registered analyses will be appropriate for the data is to trial analyses on a dataset excluding outcome data. For example, data managers could provide researchers with part of the dataset containing the exposure variable(s) plus any covariates and/or auxiliary variables. The researcher can then trial and refine the analyses ahead of pre-registering, without gaining insight into the main findings (which require the outcome data). This approach is used to mitigate bias in propensity score matching studies [ 26 , 56 ], as researchers use data on the exposure and covariates to create matched groups, prior to accessing any outcome data. Once the exposed and non-exposed groups have been matched effectively, researchers pre-register the protocol ahead of viewing the outcome data. Notably though, this approach could help researchers to identify and address other analytical challenges involving secondary data. For example, it could be used to check multivariable distributional characteristics, test for collinearity between multiple predictor variables, or identify predictors of missing data for multiple imputation.
This approach offers certain benefits for researchers keen to ensure that pre-registered analyses are appropriate for the observed data, with some limitations. Regarding benefits, researchers will be able to examine associations between variables (excluding the outcome), unlike the data scrambling approach described above. This would be helpful for checking certain assumptions (e.g., collinearity or characteristics of missing data such as whether it is missing at random). In addition, the approach is easy to implement, as the dataset can be initially created without the outcome variable, which can then be added after pre-registration, minimising burden on data guardians. Regarding limitations, it is possible that accessing variables in advance could provide some insight into the findings. For example, if a covariate is known to be highly correlated with the outcome, testing the association between the covariate and the exposure could give some indication of the relationship between the exposure and the outcome. To make this potential bias transparent, researchers should report the variables that they already accessed in the pre-registration. Another limitation is that researchers will not be able to identify analytical issues relating to the outcome data in advance of pre-registration. Therefore, this approach will be most appropriate where researchers wish to check various characteristics of the exposure variable(s) and covariates, rather than the outcome. However, a “mixed” approach could be applied in which outcome data is provided in scrambled format, to enable researchers to also assess distributional characteristics of the outcome. This would substantially reduce the number of potential challenges to be considered in pre-registered analytical pipelines.
Pre-register a decision tree
If it is not possible to access any of the data prior to pre-registering (e.g., to enable analyses to be trialled on a dataset that is blinded or missing outcome data), researchers could pre-register a decision tree. This defines the sequence of analyses and rules based on characteristics of the observed data [ 17 ]. For example, the decision tree could specify testing a normality assumption, and based on the results, whether to use a parametric or non-parametric test. Ideally, the decision tree should provide a contingency plan for each of the planned analyses, if assumptions are not fulfilled. Of course, it can be challenging and time consuming to anticipate every potential issue with the data and plan contingencies. However, investing time into pre-specifying a decision tree (or a set of contingency plans) could save time should issues arise during data analysis, and can reduce the likelihood of deviating from the pre-registration.
Challenge: Lack of flexibility in data analysis
Transparently report unplanned analyses.
Unplanned analyses (such as applying new methods or conducting follow-up tests to investigate an interesting or unexpected finding) are a natural and often important part of the scientific process. Despite common misconceptions, pre-registration does not permit such unplanned analyses from being included, as long as they are transparently reported as post-hoc. If there are methodological deviations, we recommend that researchers should (1) clearly state the reasons for using the new method, and (2) if possible, report results from both methods, to ideally show that the change in methods was not due to the results [ 57 ]. This information can either be provided in the manuscript or in an update to the original pre-registration (e.g., on the third-party registry such as the OSF), which can be useful when journal word limits are tight. Similarly, if researchers wish to include additional follow-up analyses to investigate an interesting or unexpected finding, this should be reported but labelled as “exploratory” or “post-hoc” in the manuscript.
Ensure a paper’s value does not depend on statistically significant results
Researchers may be concerned that reduced analytic flexibility from pre-registration could increase the likelihood of reporting null results [ 22 , 23 ], which are harder to publish [ 13 , 42 ]. To address this, we recommend taking steps to ensure that the value and success of a study does not depend on a significant p-value. First, methodologically strong research (e.g., with high statistical power, valid and reliable measures, robustness checks, and replication samples) will advance the field, whatever the findings. Second, methods can be applied to allow for the interpretation of statistically non-significant findings (e.g., Bayesian methods [ 58 ] or equivalence tests, which determine whether an observed effect is surprisingly small [ 52 , 59 , 60 ]. This means that the results will be informative whatever they show, in contrast to approaches relying solely on null hypothesis significance testing, where statistically non-significant findings cannot be interpreted as meaningful. Third, researchers can submit the proposed study as a Registered Report, where it will be evaluated before the results are available. This is arguably the strongest way to protect against publication bias, as in-principle study acceptance is granted without any knowledge of the results. In addition, Registered Reports can improve the methodology, as suggestions from expert reviewers can be incorporated into the pre-registered protocol.
Under a system that rewards novel and statistically significant findings, it is easy for subconscious human biases to lead to QRPs. However, researchers, along with data guardians, journals, funders, and institutions, have a responsibility to ensure that findings are reproducible and robust. While pre-registration can help to limit analytic flexibility and selective reporting, it involves several challenges for epidemiologists conducting secondary data analysis. The approaches described here aim to address these challenges (Fig. 1 ), to either improve the efficacy of pre-registration or provide an alternative approach to address analytic flexibility (e.g., a multiverse analysis). The responsibility in adopting these approaches should not only fall on researchers’ shoulders; data guardians also have an important role to play in recording and reporting access to data, providing blinded datasets and hold-out samples, and encouraging researchers to pre-register and adopt these solutions as part of their data request. Furthermore, wider stakeholders could incentivise these practices; for example, journals could provide a designated space for researchers to report deviations from the pre-registration, and funders could provide grants to establish best practice at the cohort level (e.g., data checkout systems, blinded datasets). Ease of adoption is key to ensure wide uptake, and we therefore encourage efforts to evaluate, simplify and improve these practices. Steps that could be taken to evaluate these practices are presented in Box 1.
More broadly, it is important to emphasise that researcher biases do not operate in isolation, but rather in the context of wider publication bias and a “publish or perish” culture. These incentive structures not only promote QRPs [ 61 ], but also discourage researchers from pre-registering and adopting other time-consuming reproducible methods. Therefore, in addition to targeting bias at the individual researcher level, wider initiatives from journals, funders, and institutions are required to address these institutional biases [ 7 ]. Systemic changes that reward rigorous and reproducible research will help researchers to provide unbiased answers to science and society’s most important questions.
Box 1. Evaluation of approaches
To evaluate, simplify and improve approaches to protect against researcher bias in secondary data analysis, the following steps could be taken.
Co-creation workshops to refine approaches
To obtain feedback on the approaches (including on any practical concerns or feasibility issues) co-creation workshops could be held with researchers, data managers, and wider stakeholders (e.g., journals, funders, and institutions).
Empirical research to evaluate efficacy of approaches
To evaluate the effectiveness of the approaches in preventing researcher bias and/or improving pre-registration, empirical research is needed. For example, to test the extent to which the multiverse analysis can reduce selective reporting, comparisons could be made between effect sizes from multiverse analyses versus effect sizes from meta-analyses (of non-pre-registered studies) addressing the same research question. If smaller effect sizes were found in multiverse analyses, it would suggest that the multiverse approach can reduce selective reporting. In addition, to test whether providing a blinded dataset or dataset missing outcome variables could help researchers develop an appropriate analytical protocol, researchers could be randomly assigned to receive such a dataset (or no dataset), prior to pre-registration. If researchers who received such a dataset had fewer eventual deviations from the pre-registered protocol (in the final study), it would suggest that this approach can help ensure that proposed analyses are appropriate for the data.
Pilot implementation of the measures
To assess the practical feasibility of the approaches, data managers could pilot measures for users of the dataset (e.g., required pre-registration for access to data, provision of datasets that are blinded or missing outcome variables). Feedback could then be collected from researchers and data managers via about the experience and ease of use.
Acknowledgements
The authors are grateful to Professor George Davey for his helpful comments on this article.
Author contributions
JRB and MRM developed the idea for the article. The first draft of the manuscript was written by JRB, with support from MRM and all authors commented on previous versions of the manuscript. All authors read and approved the final manuscript.
J.R.B is funded by a Wellcome Trust Sir Henry Wellcome fellowship (grant 215917/Z/19/Z). J.B.P is a supported by the Medical Research Foundation 2018 Emerging Leaders 1 st Prize in Adolescent Mental Health (MRF-160–0002-ELP-PINGA). M.R.M and H.M.S work in a unit that receives funding from the University of Bristol and the UK Medical Research Council (MC_UU_00011/5, MC_UU_00011/7), and M.R.M is also supported by the National Institute for Health Research (NIHR) Biomedical Research Centre at the University Hospitals Bristol National Health Service Foundation Trust and the University of Bristol.
Declarations
Author declares that they have no conflict of interest.
Publisher's Note
Springer Nature remains neutral with regard to jurisdictional claims in published maps and institutional affiliations.
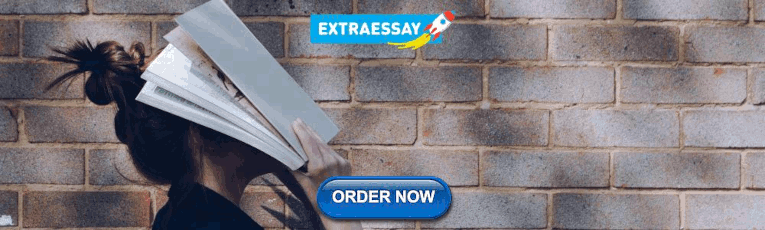
IMAGES
VIDEO
COMMENTS
Compared to primary research, the collection of secondary data can be faster and cheaper to obtain, depending on the sources you use. Secondary data can come from internal or external sources. Internal sources of secondary data include ready-to-use data or data that requires further processing available in internal management support systems ...
Let's take a look at the six most common disadvantages of secondary research mentioned below. Quality of the Secondary Data. Out-dated Data. Missing Information. Availability of the Secondary Data. Relevance of the Secondary Data. Adequacy of Data. 1. Quality of the Secondary Data.
Secondary research is a research method that uses data that was collected by someone else. In other words, whenever you conduct research using data that already exists, you are conducting secondary research. On the other hand, any type of research that you undertake yourself is called primary research. Example: Secondary research.
These disadvantages of secondary data, however, can be mitigated. Data collected for primary research with a particular question in mind may not fit or be appropriate for the secondary research question (Heaton, 2008; Sindin, 2017). Yet, if the data is relevant to the secondary research question although not in the ideal format, with ...
The disadvantages of secondary research are worth considering in advance of conducting research: Secondary research data can be out of date - Secondary sources can be updated regularly, but if you're exploring the data between two updates, the data can be out of date. Researchers will need to consider whether the data available provides the ...
Secondary analysis of data collected by another researcher for a different purpose, or SDA, is increasing in the medical and social sciences. This is not surprising, given the immense body of health care-related research performed worldwide and the potential beneficial clinical implications of the timely expansion of primary research (Johnston, 2014; Tripathy, 2013).
Secondary Research. First-hand research to collect data. May require a lot of time. The research collects existing, published data. Requires less time. Creates raw data that the researcher owns. The researcher has no control over data method or ownership. Relevant to the goals of the research. May not be relevant to the goals of the research.
Disadvantages of Secondary Research. On the other hand, we have some disadvantages that come with doing secondary research. Some of the most notorious are the following: 1. Although data is readily available, credibility evaluation must be performed to understand the authenticity of the information available. 2. Not all secondary data resources ...
Secondary Research. Data Source: Involves utilizing existing data and information collected by others. Data Collection: Researchers search, select, and analyze data from published sources, reports, and databases. Time and Resources: Generally more time-efficient and cost-effective as data is already available.
Secondary research is considered human subjects research that requires IRB review when the specimens/data are identifiable to the researchers and were collected for another purpose than the planned research. The following is an example of secondary research: An investigator learns of preliminary data from a study that suggests cigarette smoking leads to specific epigenetic changes that ...
Overview of secondary research. Secondary research is a method by which the researcher finds existing data, filters it to meet the context of their research question, analyzes it, and then summarizes it to come up with valid research conclusions. This research method involves searching for information, often via the internet, using keywords or ...
Secondary research methods focus on analyzing existing data rather than collecting primary data. Common examples of secondary research methods include: Literature review. Researchers analyze and synthesize existing literature (e.g., white papers, research papers, articles) to find knowledge gaps and build on current findings. Content analysis.
Conducting successful secondary research requires a systematic approach involving identifying the research question, searching for relevant data, evaluating the quality of data sources found, and assessing advantages & disadvantages. Secondary research is applicable in various fields such as marketing and social sciences.
This critical interpretive synthesis examined research articles (n = 71) published between 2006 and 2016 that involved qualitative secondary data analysis and assessed the context, purpose, and methodologies that were reported. ... Identification of limitations in secondary analysis. Most articles reported limitations in their studies that are ...
Though not without its limitations, Hinds et al. (1997) argue that it is a "respected, common, and cost-effective approach to maximizing the usefulness of collected data" (p. 408). ... Thorne S (1994) Secondary analysis in qualitative research: Issues and implications. In: More JM (ed.) ...
Secondary data analysis. Secondary analysis refers to the use of existing research data to find answer to a question that was different from the original work ( 2 ). Secondary data can be large scale surveys or data collected as part of personal research. Although there is general agreement about sharing the results of large scale surveys, but ...
Pros Of Secondary Research. 1. Accessibility. A few years ago when you needed to collect some data then going to libraries or particular organizations was a must. And it was even impossible to gather such data by the public. The Internet has played a great role in accessing the data so easily in a single click.
Analysis of secondary data sources (such as cohort studies, survey data, and administrative records) has the potential to provide answers to science and society's most pressing questions. However, researcher biases can lead to questionable research practices in secondary data analysis, which can distort the evidence base. While pre-registration can help to protect against researcher biases ...
Benefits of secondary research: It's often low cost or even free to access in the public domain; Supplies a knowledge base for researchers to learn from; Data is complete, has been analyzed and checked, saving you time and costs; It's ready to use as soon as you acquire it; Limitations of secondary research. May not provide enough specific ...
6 Cite and reference the data. The last step to overcome the limitations of secondary research is to cite and reference the data sources that you used. This is important to avoid plagiarism, to ...
This entry discusses resources for locating secondary data and considers secondary data's advantages and disadvantages in a research context. Discover the world's research 25+ million members
Secondary research is widely used in many fields of study and industries, such as legal research and market research. In the sciences, for instance, one of the most common methods of secondary research is a systematic review. In a systematic review, scientists review existing literature and studies on a certain topic through systematic methods ...
Introduction. Secondary data analysis has the potential to provide answers to science and society's most pressing questions. An abundance of secondary data exists—cohort studies, surveys, administrative data (e.g., health records, crime records, census data), financial data, and environmental data—that can be analysed by researchers in academia, industry, third-sector organisations, and ...