Quantitative Data Analysis: A Comprehensive Guide
By: Ofem Eteng | Published: May 18, 2022
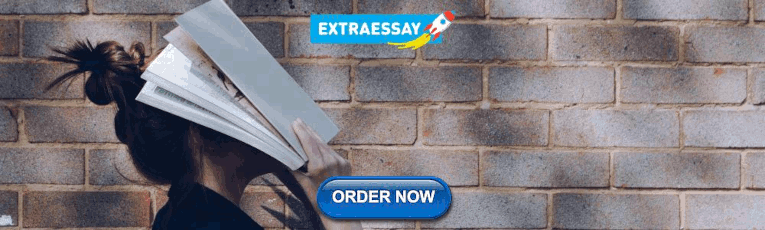
Related Articles
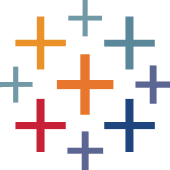
A healthcare giant successfully introduces the most effective drug dosage through rigorous statistical modeling, saving countless lives. A marketing team predicts consumer trends with uncanny accuracy, tailoring campaigns for maximum impact.
Table of Contents
These trends and dosages are not just any numbers but are a result of meticulous quantitative data analysis. Quantitative data analysis offers a robust framework for understanding complex phenomena, evaluating hypotheses, and predicting future outcomes.
In this blog, we’ll walk through the concept of quantitative data analysis, the steps required, its advantages, and the methods and techniques that are used in this analysis. Read on!
What is Quantitative Data Analysis?
Quantitative data analysis is a systematic process of examining, interpreting, and drawing meaningful conclusions from numerical data. It involves the application of statistical methods, mathematical models, and computational techniques to understand patterns, relationships, and trends within datasets.
Quantitative data analysis methods typically work with algorithms, mathematical analysis tools, and software to gain insights from the data, answering questions such as how many, how often, and how much. Data for quantitative data analysis is usually collected from close-ended surveys, questionnaires, polls, etc. The data can also be obtained from sales figures, email click-through rates, number of website visitors, and percentage revenue increase.
Quantitative Data Analysis vs Qualitative Data Analysis
When we talk about data, we directly think about the pattern, the relationship, and the connection between the datasets – analyzing the data in short. Therefore when it comes to data analysis, there are broadly two types – Quantitative Data Analysis and Qualitative Data Analysis.
Quantitative data analysis revolves around numerical data and statistics, which are suitable for functions that can be counted or measured. In contrast, qualitative data analysis includes description and subjective information – for things that can be observed but not measured.
Let us differentiate between Quantitative Data Analysis and Quantitative Data Analysis for a better understanding.
Data Preparation Steps for Quantitative Data Analysis
Quantitative data has to be gathered and cleaned before proceeding to the stage of analyzing it. Below are the steps to prepare a data before quantitative research analysis:
- Step 1: Data Collection
Before beginning the analysis process, you need data. Data can be collected through rigorous quantitative research, which includes methods such as interviews, focus groups, surveys, and questionnaires.
- Step 2: Data Cleaning
Once the data is collected, begin the data cleaning process by scanning through the entire data for duplicates, errors, and omissions. Keep a close eye for outliers (data points that are significantly different from the majority of the dataset) because they can skew your analysis results if they are not removed.
This data-cleaning process ensures data accuracy, consistency and relevancy before analysis.
- Step 3: Data Analysis and Interpretation
Now that you have collected and cleaned your data, it is now time to carry out the quantitative analysis. There are two methods of quantitative data analysis, which we will discuss in the next section.
However, if you have data from multiple sources, collecting and cleaning it can be a cumbersome task. This is where Hevo Data steps in. With Hevo, extracting, transforming, and loading data from source to destination becomes a seamless task, eliminating the need for manual coding. This not only saves valuable time but also enhances the overall efficiency of data analysis and visualization, empowering users to derive insights quickly and with precision
Hevo is the only real-time ELT No-code Data Pipeline platform that cost-effectively automates data pipelines that are flexible to your needs. With integration with 150+ Data Sources (40+ free sources), we help you not only export data from sources & load data to the destinations but also transform & enrich your data, & make it analysis-ready.
Start for free now!
Now that you are familiar with what quantitative data analysis is and how to prepare your data for analysis, the focus will shift to the purpose of this article, which is to describe the methods and techniques of quantitative data analysis.
Methods and Techniques of Quantitative Data Analysis
Quantitative data analysis employs two techniques to extract meaningful insights from datasets, broadly. The first method is descriptive statistics, which summarizes and portrays essential features of a dataset, such as mean, median, and standard deviation.
Inferential statistics, the second method, extrapolates insights and predictions from a sample dataset to make broader inferences about an entire population, such as hypothesis testing and regression analysis.
An in-depth explanation of both the methods is provided below:
- Descriptive Statistics
- Inferential Statistics
1) Descriptive Statistics
Descriptive statistics as the name implies is used to describe a dataset. It helps understand the details of your data by summarizing it and finding patterns from the specific data sample. They provide absolute numbers obtained from a sample but do not necessarily explain the rationale behind the numbers and are mostly used for analyzing single variables. The methods used in descriptive statistics include:
- Mean: This calculates the numerical average of a set of values.
- Median: This is used to get the midpoint of a set of values when the numbers are arranged in numerical order.
- Mode: This is used to find the most commonly occurring value in a dataset.
- Percentage: This is used to express how a value or group of respondents within the data relates to a larger group of respondents.
- Frequency: This indicates the number of times a value is found.
- Range: This shows the highest and lowest values in a dataset.
- Standard Deviation: This is used to indicate how dispersed a range of numbers is, meaning, it shows how close all the numbers are to the mean.
- Skewness: It indicates how symmetrical a range of numbers is, showing if they cluster into a smooth bell curve shape in the middle of the graph or if they skew towards the left or right.
2) Inferential Statistics
In quantitative analysis, the expectation is to turn raw numbers into meaningful insight using numerical values, and descriptive statistics is all about explaining details of a specific dataset using numbers, but it does not explain the motives behind the numbers; hence, a need for further analysis using inferential statistics.
Inferential statistics aim to make predictions or highlight possible outcomes from the analyzed data obtained from descriptive statistics. They are used to generalize results and make predictions between groups, show relationships that exist between multiple variables, and are used for hypothesis testing that predicts changes or differences.
There are various statistical analysis methods used within inferential statistics; a few are discussed below.
- Cross Tabulations: Cross tabulation or crosstab is used to show the relationship that exists between two variables and is often used to compare results by demographic groups. It uses a basic tabular form to draw inferences between different data sets and contains data that is mutually exclusive or has some connection with each other. Crosstabs help understand the nuances of a dataset and factors that may influence a data point.
- Regression Analysis: Regression analysis estimates the relationship between a set of variables. It shows the correlation between a dependent variable (the variable or outcome you want to measure or predict) and any number of independent variables (factors that may impact the dependent variable). Therefore, the purpose of the regression analysis is to estimate how one or more variables might affect a dependent variable to identify trends and patterns to make predictions and forecast possible future trends. There are many types of regression analysis, and the model you choose will be determined by the type of data you have for the dependent variable. The types of regression analysis include linear regression, non-linear regression, binary logistic regression, etc.
- Monte Carlo Simulation: Monte Carlo simulation, also known as the Monte Carlo method, is a computerized technique of generating models of possible outcomes and showing their probability distributions. It considers a range of possible outcomes and then tries to calculate how likely each outcome will occur. Data analysts use it to perform advanced risk analyses to help forecast future events and make decisions accordingly.
- Analysis of Variance (ANOVA): This is used to test the extent to which two or more groups differ from each other. It compares the mean of various groups and allows the analysis of multiple groups.
- Factor Analysis: A large number of variables can be reduced into a smaller number of factors using the factor analysis technique. It works on the principle that multiple separate observable variables correlate with each other because they are all associated with an underlying construct. It helps in reducing large datasets into smaller, more manageable samples.
- Cohort Analysis: Cohort analysis can be defined as a subset of behavioral analytics that operates from data taken from a given dataset. Rather than looking at all users as one unit, cohort analysis breaks down data into related groups for analysis, where these groups or cohorts usually have common characteristics or similarities within a defined period.
- MaxDiff Analysis: This is a quantitative data analysis method that is used to gauge customers’ preferences for purchase and what parameters rank higher than the others in the process.
- Cluster Analysis: Cluster analysis is a technique used to identify structures within a dataset. Cluster analysis aims to be able to sort different data points into groups that are internally similar and externally different; that is, data points within a cluster will look like each other and different from data points in other clusters.
- Time Series Analysis: This is a statistical analytic technique used to identify trends and cycles over time. It is simply the measurement of the same variables at different times, like weekly and monthly email sign-ups, to uncover trends, seasonality, and cyclic patterns. By doing this, the data analyst can forecast how variables of interest may fluctuate in the future.
- SWOT analysis: This is a quantitative data analysis method that assigns numerical values to indicate strengths, weaknesses, opportunities, and threats of an organization, product, or service to show a clearer picture of competition to foster better business strategies
How to Choose the Right Method for your Analysis?
Choosing between Descriptive Statistics or Inferential Statistics can be often confusing. You should consider the following factors before choosing the right method for your quantitative data analysis:
1. Type of Data
The first consideration in data analysis is understanding the type of data you have. Different statistical methods have specific requirements based on these data types, and using the wrong method can render results meaningless. The choice of statistical method should align with the nature and distribution of your data to ensure meaningful and accurate analysis.
2. Your Research Questions
When deciding on statistical methods, it’s crucial to align them with your specific research questions and hypotheses. The nature of your questions will influence whether descriptive statistics alone, which reveal sample attributes, are sufficient or if you need both descriptive and inferential statistics to understand group differences or relationships between variables and make population inferences.
Pros and Cons of Quantitative Data Analysis
1. Objectivity and Generalizability:
- Quantitative data analysis offers objective, numerical measurements, minimizing bias and personal interpretation.
- Results can often be generalized to larger populations, making them applicable to broader contexts.
Example: A study using quantitative data analysis to measure student test scores can objectively compare performance across different schools and demographics, leading to generalizable insights about educational strategies.
2. Precision and Efficiency:
- Statistical methods provide precise numerical results, allowing for accurate comparisons and prediction.
- Large datasets can be analyzed efficiently with the help of computer software, saving time and resources.
Example: A marketing team can use quantitative data analysis to precisely track click-through rates and conversion rates on different ad campaigns, quickly identifying the most effective strategies for maximizing customer engagement.
3. Identification of Patterns and Relationships:
- Statistical techniques reveal hidden patterns and relationships between variables that might not be apparent through observation alone.
- This can lead to new insights and understanding of complex phenomena.
Example: A medical researcher can use quantitative analysis to pinpoint correlations between lifestyle factors and disease risk, aiding in the development of prevention strategies.
1. Limited Scope:
- Quantitative analysis focuses on quantifiable aspects of a phenomenon , potentially overlooking important qualitative nuances, such as emotions, motivations, or cultural contexts.
Example: A survey measuring customer satisfaction with numerical ratings might miss key insights about the underlying reasons for their satisfaction or dissatisfaction, which could be better captured through open-ended feedback.
2. Oversimplification:
- Reducing complex phenomena to numerical data can lead to oversimplification and a loss of richness in understanding.
Example: Analyzing employee productivity solely through quantitative metrics like hours worked or tasks completed might not account for factors like creativity, collaboration, or problem-solving skills, which are crucial for overall performance.
3. Potential for Misinterpretation:
- Statistical results can be misinterpreted if not analyzed carefully and with appropriate expertise.
- The choice of statistical methods and assumptions can significantly influence results.
This blog discusses the steps, methods, and techniques of quantitative data analysis. It also gives insights into the methods of data collection, the type of data one should work with, and the pros and cons of such analysis.
Gain a better understanding of data analysis with these essential reads:
- Data Analysis and Modeling: 4 Critical Differences
- Exploratory Data Analysis Simplified 101
- 25 Best Data Analysis Tools in 2024
Carrying out successful data analysis requires prepping the data and making it analysis-ready. That is where Hevo steps in.
Want to give Hevo a try? Sign Up for a 14-day free trial and experience the feature-rich Hevo suite first hand. You may also have a look at the amazing Hevo price , which will assist you in selecting the best plan for your requirements.
Share your experience of understanding Quantitative Data Analysis in the comment section below! We would love to hear your thoughts.
Ofem is a freelance writer specializing in data-related topics, who has expertise in translating complex concepts. With a focus on data science, analytics, and emerging technologies.
No-code Data Pipeline for your Data Warehouse
- Data Analysis
- Data Warehouse
- Quantitative Data Analysis
Continue Reading
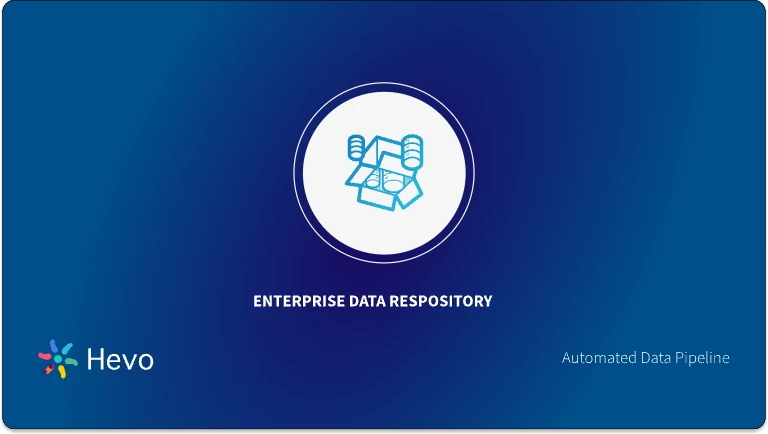
Enterprise Data Repository: Types, Benefits, & Best Practices
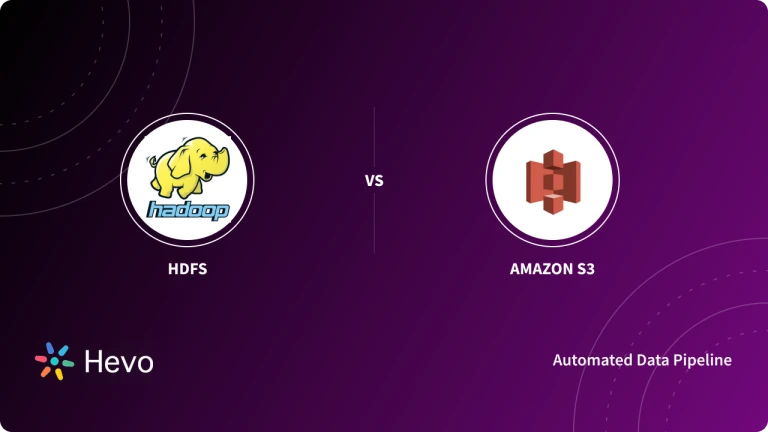
Aakash Raman
Hadoop S3 Comparison: 7 Critical Differences
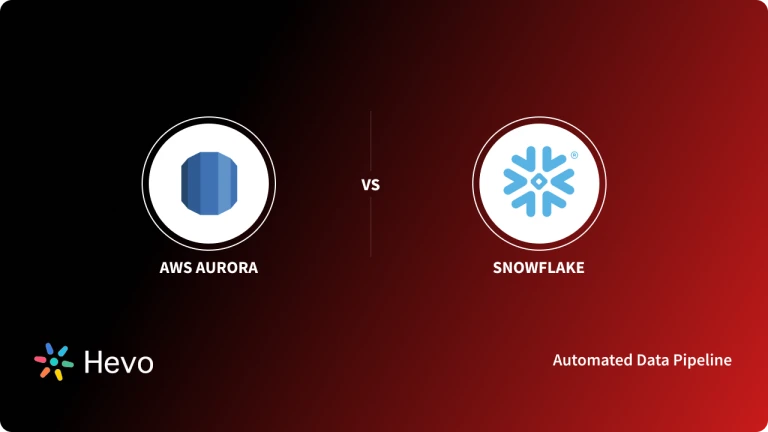
Manisha Jena
AWS Aurora vs Snowflake: 5 Critical Differences
I want to read this e-book.

Quantitative Data Analysis 101
The lingo, methods and techniques, explained simply.
By: Derek Jansen (MBA) and Kerryn Warren (PhD) | December 2020
Quantitative data analysis is one of those things that often strikes fear in students. It’s totally understandable – quantitative analysis is a complex topic, full of daunting lingo , like medians, modes, correlation and regression. Suddenly we’re all wishing we’d paid a little more attention in math class…
The good news is that while quantitative data analysis is a mammoth topic, gaining a working understanding of the basics isn’t that hard , even for those of us who avoid numbers and math . In this post, we’ll break quantitative analysis down into simple , bite-sized chunks so you can approach your research with confidence.
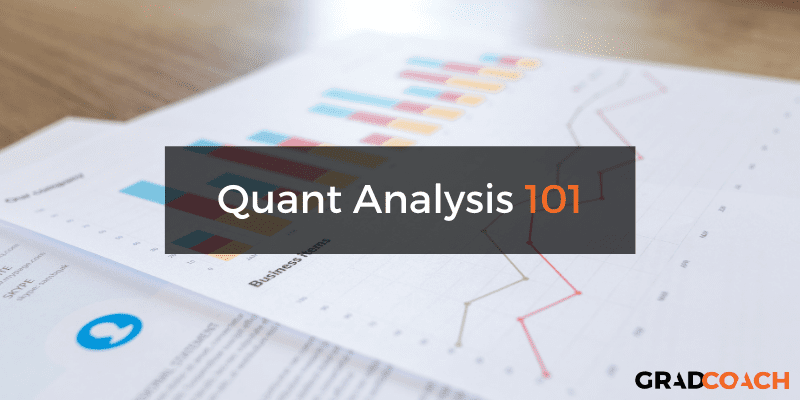
Overview: Quantitative Data Analysis 101
- What (exactly) is quantitative data analysis?
- When to use quantitative analysis
- How quantitative analysis works
The two “branches” of quantitative analysis
- Descriptive statistics 101
- Inferential statistics 101
- How to choose the right quantitative methods
- Recap & summary
What is quantitative data analysis?
Despite being a mouthful, quantitative data analysis simply means analysing data that is numbers-based – or data that can be easily “converted” into numbers without losing any meaning.
For example, category-based variables like gender, ethnicity, or native language could all be “converted” into numbers without losing meaning – for example, English could equal 1, French 2, etc.
This contrasts against qualitative data analysis, where the focus is on words, phrases and expressions that can’t be reduced to numbers. If you’re interested in learning about qualitative analysis, check out our post and video here .
What is quantitative analysis used for?
Quantitative analysis is generally used for three purposes.
- Firstly, it’s used to measure differences between groups . For example, the popularity of different clothing colours or brands.
- Secondly, it’s used to assess relationships between variables . For example, the relationship between weather temperature and voter turnout.
- And third, it’s used to test hypotheses in a scientifically rigorous way. For example, a hypothesis about the impact of a certain vaccine.
Again, this contrasts with qualitative analysis , which can be used to analyse people’s perceptions and feelings about an event or situation. In other words, things that can’t be reduced to numbers.
How does quantitative analysis work?
Well, since quantitative data analysis is all about analysing numbers , it’s no surprise that it involves statistics . Statistical analysis methods form the engine that powers quantitative analysis, and these methods can vary from pretty basic calculations (for example, averages and medians) to more sophisticated analyses (for example, correlations and regressions).
Sounds like gibberish? Don’t worry. We’ll explain all of that in this post. Importantly, you don’t need to be a statistician or math wiz to pull off a good quantitative analysis. We’ll break down all the technical mumbo jumbo in this post.
Need a helping hand?
As I mentioned, quantitative analysis is powered by statistical analysis methods . There are two main “branches” of statistical methods that are used – descriptive statistics and inferential statistics . In your research, you might only use descriptive statistics, or you might use a mix of both , depending on what you’re trying to figure out. In other words, depending on your research questions, aims and objectives . I’ll explain how to choose your methods later.
So, what are descriptive and inferential statistics?
Well, before I can explain that, we need to take a quick detour to explain some lingo. To understand the difference between these two branches of statistics, you need to understand two important words. These words are population and sample .
First up, population . In statistics, the population is the entire group of people (or animals or organisations or whatever) that you’re interested in researching. For example, if you were interested in researching Tesla owners in the US, then the population would be all Tesla owners in the US.
However, it’s extremely unlikely that you’re going to be able to interview or survey every single Tesla owner in the US. Realistically, you’ll likely only get access to a few hundred, or maybe a few thousand owners using an online survey. This smaller group of accessible people whose data you actually collect is called your sample .
So, to recap – the population is the entire group of people you’re interested in, and the sample is the subset of the population that you can actually get access to. In other words, the population is the full chocolate cake , whereas the sample is a slice of that cake.
So, why is this sample-population thing important?
Well, descriptive statistics focus on describing the sample , while inferential statistics aim to make predictions about the population, based on the findings within the sample. In other words, we use one group of statistical methods – descriptive statistics – to investigate the slice of cake, and another group of methods – inferential statistics – to draw conclusions about the entire cake. There I go with the cake analogy again…
With that out the way, let’s take a closer look at each of these branches in more detail.
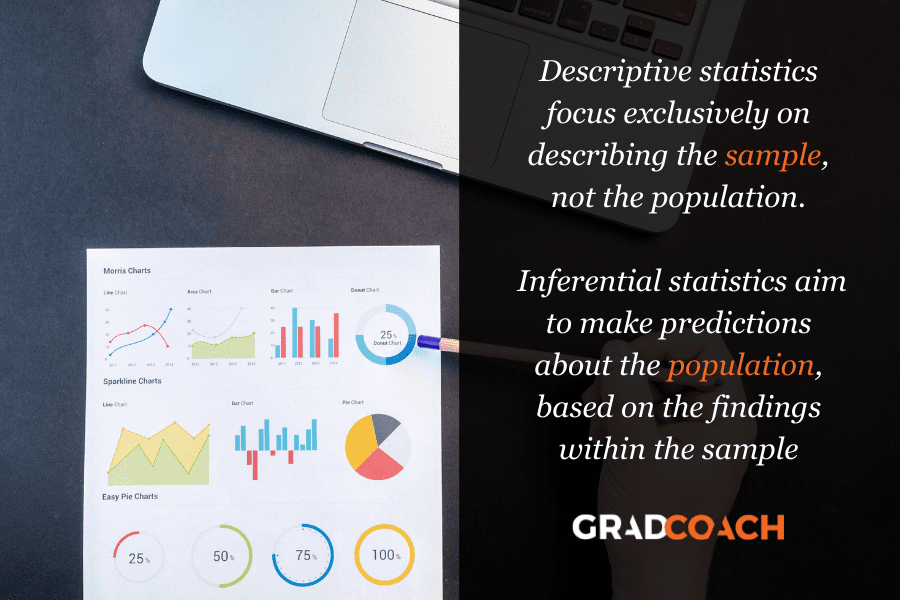
Branch 1: Descriptive Statistics
Descriptive statistics serve a simple but critically important role in your research – to describe your data set – hence the name. In other words, they help you understand the details of your sample . Unlike inferential statistics (which we’ll get to soon), descriptive statistics don’t aim to make inferences or predictions about the entire population – they’re purely interested in the details of your specific sample .
When you’re writing up your analysis, descriptive statistics are the first set of stats you’ll cover, before moving on to inferential statistics. But, that said, depending on your research objectives and research questions , they may be the only type of statistics you use. We’ll explore that a little later.
So, what kind of statistics are usually covered in this section?
Some common statistical tests used in this branch include the following:
- Mean – this is simply the mathematical average of a range of numbers.
- Median – this is the midpoint in a range of numbers when the numbers are arranged in numerical order. If the data set makes up an odd number, then the median is the number right in the middle of the set. If the data set makes up an even number, then the median is the midpoint between the two middle numbers.
- Mode – this is simply the most commonly occurring number in the data set.
- In cases where most of the numbers are quite close to the average, the standard deviation will be relatively low.
- Conversely, in cases where the numbers are scattered all over the place, the standard deviation will be relatively high.
- Skewness . As the name suggests, skewness indicates how symmetrical a range of numbers is. In other words, do they tend to cluster into a smooth bell curve shape in the middle of the graph, or do they skew to the left or right?
Feeling a bit confused? Let’s look at a practical example using a small data set.
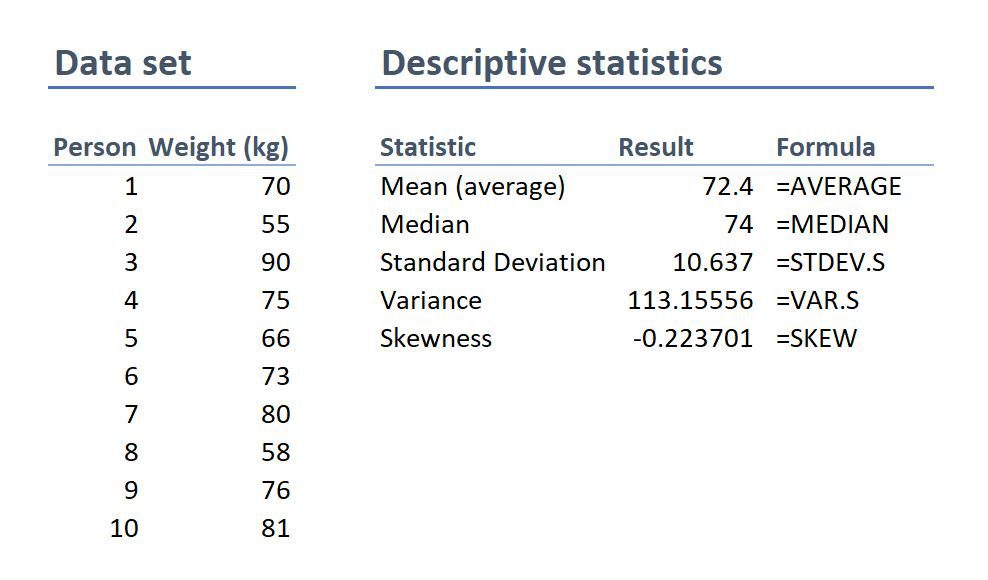
On the left-hand side is the data set. This details the bodyweight of a sample of 10 people. On the right-hand side, we have the descriptive statistics. Let’s take a look at each of them.
First, we can see that the mean weight is 72.4 kilograms. In other words, the average weight across the sample is 72.4 kilograms. Straightforward.
Next, we can see that the median is very similar to the mean (the average). This suggests that this data set has a reasonably symmetrical distribution (in other words, a relatively smooth, centred distribution of weights, clustered towards the centre).
In terms of the mode , there is no mode in this data set. This is because each number is present only once and so there cannot be a “most common number”. If there were two people who were both 65 kilograms, for example, then the mode would be 65.
Next up is the standard deviation . 10.6 indicates that there’s quite a wide spread of numbers. We can see this quite easily by looking at the numbers themselves, which range from 55 to 90, which is quite a stretch from the mean of 72.4.
And lastly, the skewness of -0.2 tells us that the data is very slightly negatively skewed. This makes sense since the mean and the median are slightly different.
As you can see, these descriptive statistics give us some useful insight into the data set. Of course, this is a very small data set (only 10 records), so we can’t read into these statistics too much. Also, keep in mind that this is not a list of all possible descriptive statistics – just the most common ones.
But why do all of these numbers matter?
While these descriptive statistics are all fairly basic, they’re important for a few reasons:
- Firstly, they help you get both a macro and micro-level view of your data. In other words, they help you understand both the big picture and the finer details.
- Secondly, they help you spot potential errors in the data – for example, if an average is way higher than you’d expect, or responses to a question are highly varied, this can act as a warning sign that you need to double-check the data.
- And lastly, these descriptive statistics help inform which inferential statistical techniques you can use, as those techniques depend on the skewness (in other words, the symmetry and normality) of the data.
Simply put, descriptive statistics are really important , even though the statistical techniques used are fairly basic. All too often at Grad Coach, we see students skimming over the descriptives in their eagerness to get to the more exciting inferential methods, and then landing up with some very flawed results.
Don’t be a sucker – give your descriptive statistics the love and attention they deserve!
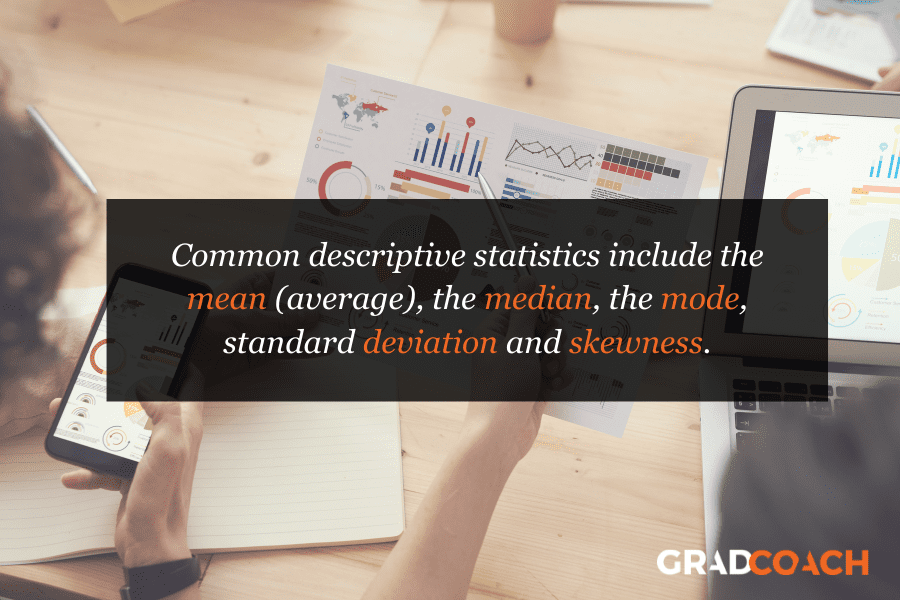
Branch 2: Inferential Statistics
As I mentioned, while descriptive statistics are all about the details of your specific data set – your sample – inferential statistics aim to make inferences about the population . In other words, you’ll use inferential statistics to make predictions about what you’d expect to find in the full population.
What kind of predictions, you ask? Well, there are two common types of predictions that researchers try to make using inferential stats:
- Firstly, predictions about differences between groups – for example, height differences between children grouped by their favourite meal or gender.
- And secondly, relationships between variables – for example, the relationship between body weight and the number of hours a week a person does yoga.
In other words, inferential statistics (when done correctly), allow you to connect the dots and make predictions about what you expect to see in the real world population, based on what you observe in your sample data. For this reason, inferential statistics are used for hypothesis testing – in other words, to test hypotheses that predict changes or differences.
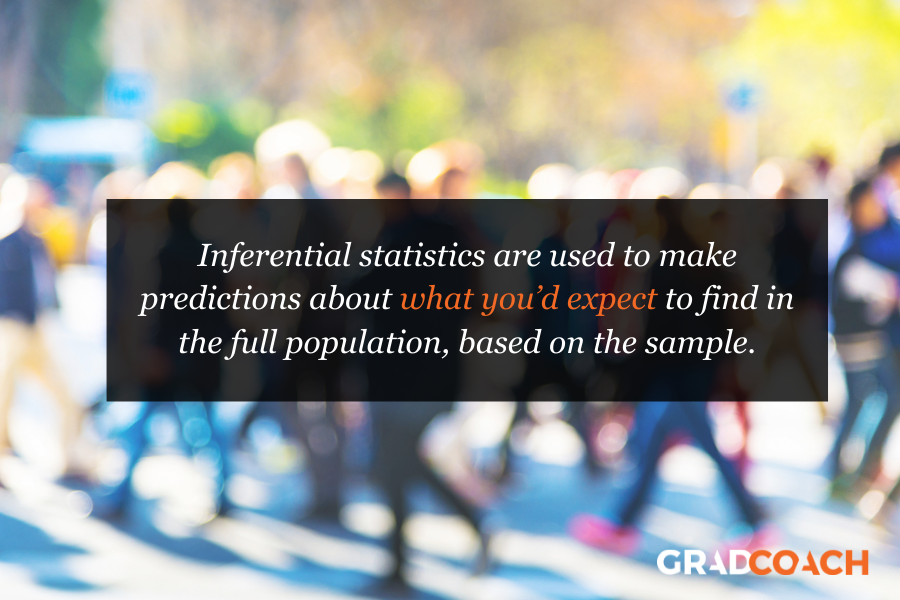
Of course, when you’re working with inferential statistics, the composition of your sample is really important. In other words, if your sample doesn’t accurately represent the population you’re researching, then your findings won’t necessarily be very useful.
For example, if your population of interest is a mix of 50% male and 50% female , but your sample is 80% male , you can’t make inferences about the population based on your sample, since it’s not representative. This area of statistics is called sampling, but we won’t go down that rabbit hole here (it’s a deep one!) – we’ll save that for another post .
What statistics are usually used in this branch?
There are many, many different statistical analysis methods within the inferential branch and it’d be impossible for us to discuss them all here. So we’ll just take a look at some of the most common inferential statistical methods so that you have a solid starting point.
First up are T-Tests . T-tests compare the means (the averages) of two groups of data to assess whether they’re statistically significantly different. In other words, do they have significantly different means, standard deviations and skewness.
This type of testing is very useful for understanding just how similar or different two groups of data are. For example, you might want to compare the mean blood pressure between two groups of people – one that has taken a new medication and one that hasn’t – to assess whether they are significantly different.
Kicking things up a level, we have ANOVA, which stands for “analysis of variance”. This test is similar to a T-test in that it compares the means of various groups, but ANOVA allows you to analyse multiple groups , not just two groups So it’s basically a t-test on steroids…
Next, we have correlation analysis . This type of analysis assesses the relationship between two variables. In other words, if one variable increases, does the other variable also increase, decrease or stay the same. For example, if the average temperature goes up, do average ice creams sales increase too? We’d expect some sort of relationship between these two variables intuitively , but correlation analysis allows us to measure that relationship scientifically .
Lastly, we have regression analysis – this is quite similar to correlation in that it assesses the relationship between variables, but it goes a step further to understand cause and effect between variables, not just whether they move together. In other words, does the one variable actually cause the other one to move, or do they just happen to move together naturally thanks to another force? Just because two variables correlate doesn’t necessarily mean that one causes the other.
Stats overload…
I hear you. To make this all a little more tangible, let’s take a look at an example of a correlation in action.
Here’s a scatter plot demonstrating the correlation (relationship) between weight and height. Intuitively, we’d expect there to be some relationship between these two variables, which is what we see in this scatter plot. In other words, the results tend to cluster together in a diagonal line from bottom left to top right.
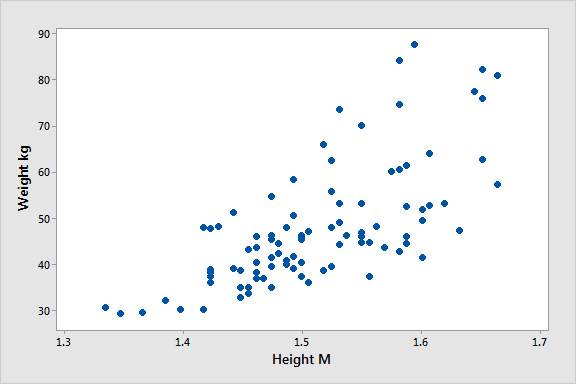
As I mentioned, these are are just a handful of inferential techniques – there are many, many more. Importantly, each statistical method has its own assumptions and limitations .
For example, some methods only work with normally distributed (parametric) data, while other methods are designed specifically for non-parametric data. And that’s exactly why descriptive statistics are so important – they’re the first step to knowing which inferential techniques you can and can’t use.
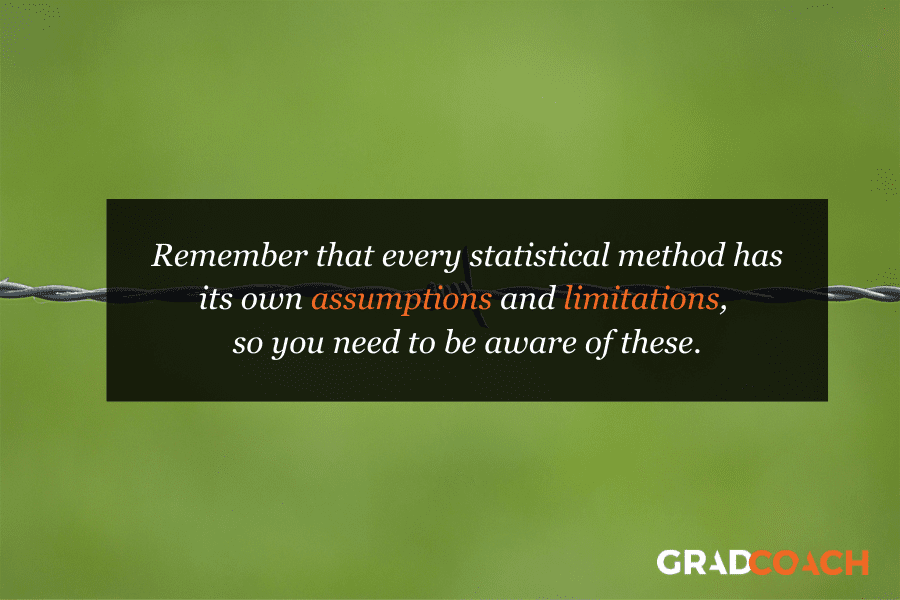
How to choose the right analysis method
To choose the right statistical methods, you need to think about two important factors :
- The type of quantitative data you have (specifically, level of measurement and the shape of the data). And,
- Your research questions and hypotheses
Let’s take a closer look at each of these.
Factor 1 – Data type
The first thing you need to consider is the type of data you’ve collected (or the type of data you will collect). By data types, I’m referring to the four levels of measurement – namely, nominal, ordinal, interval and ratio. If you’re not familiar with this lingo, check out the video below.
Why does this matter?
Well, because different statistical methods and techniques require different types of data. This is one of the “assumptions” I mentioned earlier – every method has its assumptions regarding the type of data.
For example, some techniques work with categorical data (for example, yes/no type questions, or gender or ethnicity), while others work with continuous numerical data (for example, age, weight or income) – and, of course, some work with multiple data types.
If you try to use a statistical method that doesn’t support the data type you have, your results will be largely meaningless . So, make sure that you have a clear understanding of what types of data you’ve collected (or will collect). Once you have this, you can then check which statistical methods would support your data types here .
If you haven’t collected your data yet, you can work in reverse and look at which statistical method would give you the most useful insights, and then design your data collection strategy to collect the correct data types.
Another important factor to consider is the shape of your data . Specifically, does it have a normal distribution (in other words, is it a bell-shaped curve, centred in the middle) or is it very skewed to the left or the right? Again, different statistical techniques work for different shapes of data – some are designed for symmetrical data while others are designed for skewed data.
This is another reminder of why descriptive statistics are so important – they tell you all about the shape of your data.
Factor 2: Your research questions
The next thing you need to consider is your specific research questions, as well as your hypotheses (if you have some). The nature of your research questions and research hypotheses will heavily influence which statistical methods and techniques you should use.
If you’re just interested in understanding the attributes of your sample (as opposed to the entire population), then descriptive statistics are probably all you need. For example, if you just want to assess the means (averages) and medians (centre points) of variables in a group of people.
On the other hand, if you aim to understand differences between groups or relationships between variables and to infer or predict outcomes in the population, then you’ll likely need both descriptive statistics and inferential statistics.
So, it’s really important to get very clear about your research aims and research questions, as well your hypotheses – before you start looking at which statistical techniques to use.
Never shoehorn a specific statistical technique into your research just because you like it or have some experience with it. Your choice of methods must align with all the factors we’ve covered here.
Time to recap…
You’re still with me? That’s impressive. We’ve covered a lot of ground here, so let’s recap on the key points:
- Quantitative data analysis is all about analysing number-based data (which includes categorical and numerical data) using various statistical techniques.
- The two main branches of statistics are descriptive statistics and inferential statistics . Descriptives describe your sample, whereas inferentials make predictions about what you’ll find in the population.
- Common descriptive statistical methods include mean (average), median , standard deviation and skewness .
- Common inferential statistical methods include t-tests , ANOVA , correlation and regression analysis.
- To choose the right statistical methods and techniques, you need to consider the type of data you’re working with , as well as your research questions and hypotheses.
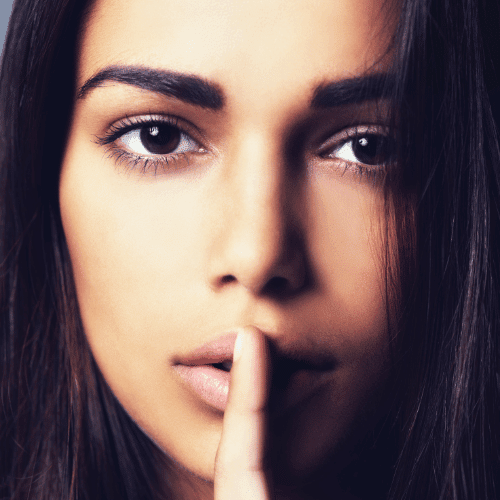
Psst... there’s more!
This post was based on one of our popular Research Bootcamps . If you're working on a research project, you'll definitely want to check this out ...
You Might Also Like:
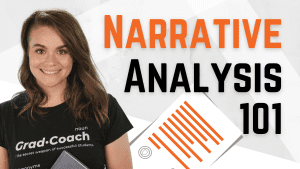
75 Comments
Hi, I have read your article. Such a brilliant post you have created.
Thank you for the feedback. Good luck with your quantitative analysis.
Thank you so much.
Thank you so much. I learnt much well. I love your summaries of the concepts. I had love you to explain how to input data using SPSS
Amazing and simple way of breaking down quantitative methods.
This is beautiful….especially for non-statisticians. I have skimmed through but I wish to read again. and please include me in other articles of the same nature when you do post. I am interested. I am sure, I could easily learn from you and get off the fear that I have had in the past. Thank you sincerely.
Send me every new information you might have.
i need every new information
Thank you for the blog. It is quite informative. Dr Peter Nemaenzhe PhD
It is wonderful. l’ve understood some of the concepts in a more compréhensive manner
Your article is so good! However, I am still a bit lost. I am doing a secondary research on Gun control in the US and increase in crime rates and I am not sure which analysis method I should use?
Based on the given learning points, this is inferential analysis, thus, use ‘t-tests, ANOVA, correlation and regression analysis’
Well explained notes. Am an MPH student and currently working on my thesis proposal, this has really helped me understand some of the things I didn’t know.
I like your page..helpful
wonderful i got my concept crystal clear. thankyou!!
This is really helpful , thank you
Thank you so much this helped
Wonderfully explained
thank u so much, it was so informative
THANKYOU, this was very informative and very helpful
This is great GRADACOACH I am not a statistician but I require more of this in my thesis
Include me in your posts.
This is so great and fully useful. I would like to thank you again and again.
Glad to read this article. I’ve read lot of articles but this article is clear on all concepts. Thanks for sharing.
Thank you so much. This is a very good foundation and intro into quantitative data analysis. Appreciate!
You have a very impressive, simple but concise explanation of data analysis for Quantitative Research here. This is a God-send link for me to appreciate research more. Thank you so much!
Avery good presentation followed by the write up. yes you simplified statistics to make sense even to a layman like me. Thank so much keep it up. The presenter did ell too. i would like more of this for Qualitative and exhaust more of the test example like the Anova.
This is a very helpful article, couldn’t have been clearer. Thank you.
Awesome and phenomenal information.Well done
The video with the accompanying article is super helpful to demystify this topic. Very well done. Thank you so much.
thank you so much, your presentation helped me a lot
I don’t know how should I express that ur article is saviour for me 🥺😍
It is well defined information and thanks for sharing. It helps me a lot in understanding the statistical data.
I gain a lot and thanks for sharing brilliant ideas, so wish to be linked on your email update.
Very helpful and clear .Thank you Gradcoach.
Thank for sharing this article, well organized and information presented are very clear.
VERY INTERESTING AND SUPPORTIVE TO NEW RESEARCHERS LIKE ME. AT LEAST SOME BASICS ABOUT QUANTITATIVE.
An outstanding, well explained and helpful article. This will help me so much with my data analysis for my research project. Thank you!
wow this has just simplified everything i was scared of how i am gonna analyse my data but thanks to you i will be able to do so
simple and constant direction to research. thanks
This is helpful
Great writing!! Comprehensive and very helpful.
Do you provide any assistance for other steps of research methodology like making research problem testing hypothesis report and thesis writing?
Thank you so much for such useful article!
Amazing article. So nicely explained. Wow
Very insightfull. Thanks
I am doing a quality improvement project to determine if the implementation of a protocol will change prescribing habits. Would this be a t-test?
The is a very helpful blog, however, I’m still not sure how to analyze my data collected. I’m doing a research on “Free Education at the University of Guyana”
tnx. fruitful blog!
So I am writing exams and would like to know how do establish which method of data analysis to use from the below research questions: I am a bit lost as to how I determine the data analysis method from the research questions.
Do female employees report higher job satisfaction than male employees with similar job descriptions across the South African telecommunications sector? – I though that maybe Chi Square could be used here. – Is there a gender difference in talented employees’ actual turnover decisions across the South African telecommunications sector? T-tests or Correlation in this one. – Is there a gender difference in the cost of actual turnover decisions across the South African telecommunications sector? T-tests or Correlation in this one. – What practical recommendations can be made to the management of South African telecommunications companies on leveraging gender to mitigate employee turnover decisions?
Your assistance will be appreciated if I could get a response as early as possible tomorrow
This was quite helpful. Thank you so much.
wow I got a lot from this article, thank you very much, keep it up
Thanks for yhe guidance. Can you send me this guidance on my email? To enable offline reading?
Thank you very much, this service is very helpful.
Every novice researcher needs to read this article as it puts things so clear and easy to follow. Its been very helpful.
Wonderful!!!! you explained everything in a way that anyone can learn. Thank you!!
I really enjoyed reading though this. Very easy to follow. Thank you
Many thanks for your useful lecture, I would be really appreciated if you could possibly share with me the PPT of presentation related to Data type?
Thank you very much for sharing, I got much from this article
This is a very informative write-up. Kindly include me in your latest posts.
Very interesting mostly for social scientists
Thank you so much, very helpfull
You’re welcome 🙂
woow, its great, its very informative and well understood because of your way of writing like teaching in front of me in simple languages.
I have been struggling to understand a lot of these concepts. Thank you for the informative piece which is written with outstanding clarity.
very informative article. Easy to understand
Beautiful read, much needed.
Always greet intro and summary. I learn so much from GradCoach
Quite informative. Simple and clear summary.
I thoroughly enjoyed reading your informative and inspiring piece. Your profound insights into this topic truly provide a better understanding of its complexity. I agree with the points you raised, especially when you delved into the specifics of the article. In my opinion, that aspect is often overlooked and deserves further attention.
Absolutely!!! Thank you
Thank you very much for this post. It made me to understand how to do my data analysis.
its nice work and excellent job ,you have made my work easier
Submit a Comment Cancel reply
Your email address will not be published. Required fields are marked *
Save my name, email, and website in this browser for the next time I comment.
- Print Friendly
Your Modern Business Guide To Data Analysis Methods And Techniques

Table of Contents
1) What Is Data Analysis?
2) Why Is Data Analysis Important?
3) What Is The Data Analysis Process?
4) Types Of Data Analysis Methods
5) Top Data Analysis Techniques To Apply
6) Quality Criteria For Data Analysis
7) Data Analysis Limitations & Barriers
8) Data Analysis Skills
9) Data Analysis In The Big Data Environment
In our data-rich age, understanding how to analyze and extract true meaning from our business’s digital insights is one of the primary drivers of success.
Despite the colossal volume of data we create every day, a mere 0.5% is actually analyzed and used for data discovery , improvement, and intelligence. While that may not seem like much, considering the amount of digital information we have at our fingertips, half a percent still accounts for a vast amount of data.
With so much data and so little time, knowing how to collect, curate, organize, and make sense of all of this potentially business-boosting information can be a minefield – but online data analysis is the solution.
In science, data analysis uses a more complex approach with advanced techniques to explore and experiment with data. On the other hand, in a business context, data is used to make data-driven decisions that will enable the company to improve its overall performance. In this post, we will cover the analysis of data from an organizational point of view while still going through the scientific and statistical foundations that are fundamental to understanding the basics of data analysis.
To put all of that into perspective, we will answer a host of important analytical questions, explore analytical methods and techniques, while demonstrating how to perform analysis in the real world with a 17-step blueprint for success.
What Is Data Analysis?
Data analysis is the process of collecting, modeling, and analyzing data using various statistical and logical methods and techniques. Businesses rely on analytics processes and tools to extract insights that support strategic and operational decision-making.
All these various methods are largely based on two core areas: quantitative and qualitative research.
To explain the key differences between qualitative and quantitative research, here’s a video for your viewing pleasure:
Gaining a better understanding of different techniques and methods in quantitative research as well as qualitative insights will give your analyzing efforts a more clearly defined direction, so it’s worth taking the time to allow this particular knowledge to sink in. Additionally, you will be able to create a comprehensive analytical report that will skyrocket your analysis.
Apart from qualitative and quantitative categories, there are also other types of data that you should be aware of before dividing into complex data analysis processes. These categories include:
- Big data: Refers to massive data sets that need to be analyzed using advanced software to reveal patterns and trends. It is considered to be one of the best analytical assets as it provides larger volumes of data at a faster rate.
- Metadata: Putting it simply, metadata is data that provides insights about other data. It summarizes key information about specific data that makes it easier to find and reuse for later purposes.
- Real time data: As its name suggests, real time data is presented as soon as it is acquired. From an organizational perspective, this is the most valuable data as it can help you make important decisions based on the latest developments. Our guide on real time analytics will tell you more about the topic.
- Machine data: This is more complex data that is generated solely by a machine such as phones, computers, or even websites and embedded systems, without previous human interaction.
Why Is Data Analysis Important?
Before we go into detail about the categories of analysis along with its methods and techniques, you must understand the potential that analyzing data can bring to your organization.
- Informed decision-making : From a management perspective, you can benefit from analyzing your data as it helps you make decisions based on facts and not simple intuition. For instance, you can understand where to invest your capital, detect growth opportunities, predict your income, or tackle uncommon situations before they become problems. Through this, you can extract relevant insights from all areas in your organization, and with the help of dashboard software , present the data in a professional and interactive way to different stakeholders.
- Reduce costs : Another great benefit is to reduce costs. With the help of advanced technologies such as predictive analytics, businesses can spot improvement opportunities, trends, and patterns in their data and plan their strategies accordingly. In time, this will help you save money and resources on implementing the wrong strategies. And not just that, by predicting different scenarios such as sales and demand you can also anticipate production and supply.
- Target customers better : Customers are arguably the most crucial element in any business. By using analytics to get a 360° vision of all aspects related to your customers, you can understand which channels they use to communicate with you, their demographics, interests, habits, purchasing behaviors, and more. In the long run, it will drive success to your marketing strategies, allow you to identify new potential customers, and avoid wasting resources on targeting the wrong people or sending the wrong message. You can also track customer satisfaction by analyzing your client’s reviews or your customer service department’s performance.
What Is The Data Analysis Process?

When we talk about analyzing data there is an order to follow in order to extract the needed conclusions. The analysis process consists of 5 key stages. We will cover each of them more in detail later in the post, but to start providing the needed context to understand what is coming next, here is a rundown of the 5 essential steps of data analysis.
- Identify: Before you get your hands dirty with data, you first need to identify why you need it in the first place. The identification is the stage in which you establish the questions you will need to answer. For example, what is the customer's perception of our brand? Or what type of packaging is more engaging to our potential customers? Once the questions are outlined you are ready for the next step.
- Collect: As its name suggests, this is the stage where you start collecting the needed data. Here, you define which sources of data you will use and how you will use them. The collection of data can come in different forms such as internal or external sources, surveys, interviews, questionnaires, and focus groups, among others. An important note here is that the way you collect the data will be different in a quantitative and qualitative scenario.
- Clean: Once you have the necessary data it is time to clean it and leave it ready for analysis. Not all the data you collect will be useful, when collecting big amounts of data in different formats it is very likely that you will find yourself with duplicate or badly formatted data. To avoid this, before you start working with your data you need to make sure to erase any white spaces, duplicate records, or formatting errors. This way you avoid hurting your analysis with bad-quality data.
- Analyze : With the help of various techniques such as statistical analysis, regressions, neural networks, text analysis, and more, you can start analyzing and manipulating your data to extract relevant conclusions. At this stage, you find trends, correlations, variations, and patterns that can help you answer the questions you first thought of in the identify stage. Various technologies in the market assist researchers and average users with the management of their data. Some of them include business intelligence and visualization software, predictive analytics, and data mining, among others.
- Interpret: Last but not least you have one of the most important steps: it is time to interpret your results. This stage is where the researcher comes up with courses of action based on the findings. For example, here you would understand if your clients prefer packaging that is red or green, plastic or paper, etc. Additionally, at this stage, you can also find some limitations and work on them.
Now that you have a basic understanding of the key data analysis steps, let’s look at the top 17 essential methods.
17 Essential Types Of Data Analysis Methods
Before diving into the 17 essential types of methods, it is important that we go over really fast through the main analysis categories. Starting with the category of descriptive up to prescriptive analysis, the complexity and effort of data evaluation increases, but also the added value for the company.
a) Descriptive analysis - What happened.
The descriptive analysis method is the starting point for any analytic reflection, and it aims to answer the question of what happened? It does this by ordering, manipulating, and interpreting raw data from various sources to turn it into valuable insights for your organization.
Performing descriptive analysis is essential, as it enables us to present our insights in a meaningful way. Although it is relevant to mention that this analysis on its own will not allow you to predict future outcomes or tell you the answer to questions like why something happened, it will leave your data organized and ready to conduct further investigations.
b) Exploratory analysis - How to explore data relationships.
As its name suggests, the main aim of the exploratory analysis is to explore. Prior to it, there is still no notion of the relationship between the data and the variables. Once the data is investigated, exploratory analysis helps you to find connections and generate hypotheses and solutions for specific problems. A typical area of application for it is data mining.
c) Diagnostic analysis - Why it happened.
Diagnostic data analytics empowers analysts and executives by helping them gain a firm contextual understanding of why something happened. If you know why something happened as well as how it happened, you will be able to pinpoint the exact ways of tackling the issue or challenge.
Designed to provide direct and actionable answers to specific questions, this is one of the world’s most important methods in research, among its other key organizational functions such as retail analytics , e.g.
c) Predictive analysis - What will happen.
The predictive method allows you to look into the future to answer the question: what will happen? In order to do this, it uses the results of the previously mentioned descriptive, exploratory, and diagnostic analysis, in addition to machine learning (ML) and artificial intelligence (AI). Through this, you can uncover future trends, potential problems or inefficiencies, connections, and casualties in your data.
With predictive analysis, you can unfold and develop initiatives that will not only enhance your various operational processes but also help you gain an all-important edge over the competition. If you understand why a trend, pattern, or event happened through data, you will be able to develop an informed projection of how things may unfold in particular areas of the business.
e) Prescriptive analysis - How will it happen.
Another of the most effective types of analysis methods in research. Prescriptive data techniques cross over from predictive analysis in the way that it revolves around using patterns or trends to develop responsive, practical business strategies.
By drilling down into prescriptive analysis, you will play an active role in the data consumption process by taking well-arranged sets of visual data and using it as a powerful fix to emerging issues in a number of key areas, including marketing, sales, customer experience, HR, fulfillment, finance, logistics analytics , and others.

As mentioned at the beginning of the post, data analysis methods can be divided into two big categories: quantitative and qualitative. Each of these categories holds a powerful analytical value that changes depending on the scenario and type of data you are working with. Below, we will discuss 17 methods that are divided into qualitative and quantitative approaches.
Without further ado, here are the 17 essential types of data analysis methods with some use cases in the business world:
A. Quantitative Methods
To put it simply, quantitative analysis refers to all methods that use numerical data or data that can be turned into numbers (e.g. category variables like gender, age, etc.) to extract valuable insights. It is used to extract valuable conclusions about relationships, differences, and test hypotheses. Below we discuss some of the key quantitative methods.
1. Cluster analysis
The action of grouping a set of data elements in a way that said elements are more similar (in a particular sense) to each other than to those in other groups – hence the term ‘cluster.’ Since there is no target variable when clustering, the method is often used to find hidden patterns in the data. The approach is also used to provide additional context to a trend or dataset.
Let's look at it from an organizational perspective. In a perfect world, marketers would be able to analyze each customer separately and give them the best-personalized service, but let's face it, with a large customer base, it is timely impossible to do that. That's where clustering comes in. By grouping customers into clusters based on demographics, purchasing behaviors, monetary value, or any other factor that might be relevant for your company, you will be able to immediately optimize your efforts and give your customers the best experience based on their needs.
2. Cohort analysis
This type of data analysis approach uses historical data to examine and compare a determined segment of users' behavior, which can then be grouped with others with similar characteristics. By using this methodology, it's possible to gain a wealth of insight into consumer needs or a firm understanding of a broader target group.
Cohort analysis can be really useful for performing analysis in marketing as it will allow you to understand the impact of your campaigns on specific groups of customers. To exemplify, imagine you send an email campaign encouraging customers to sign up for your site. For this, you create two versions of the campaign with different designs, CTAs, and ad content. Later on, you can use cohort analysis to track the performance of the campaign for a longer period of time and understand which type of content is driving your customers to sign up, repurchase, or engage in other ways.
A useful tool to start performing cohort analysis method is Google Analytics. You can learn more about the benefits and limitations of using cohorts in GA in this useful guide . In the bottom image, you see an example of how you visualize a cohort in this tool. The segments (devices traffic) are divided into date cohorts (usage of devices) and then analyzed week by week to extract insights into performance.
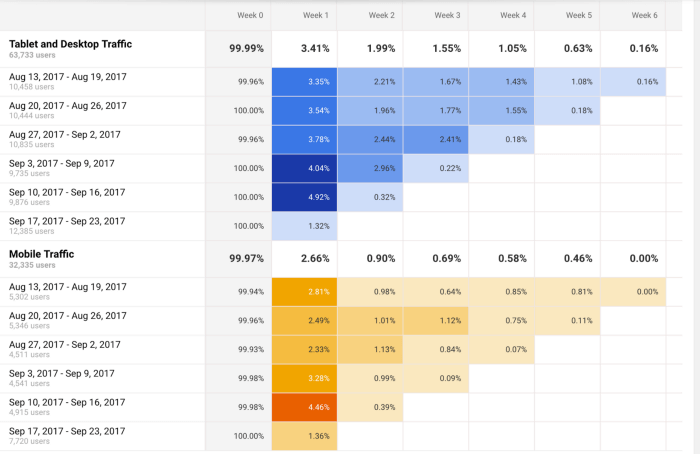
3. Regression analysis
Regression uses historical data to understand how a dependent variable's value is affected when one (linear regression) or more independent variables (multiple regression) change or stay the same. By understanding each variable's relationship and how it developed in the past, you can anticipate possible outcomes and make better decisions in the future.
Let's bring it down with an example. Imagine you did a regression analysis of your sales in 2019 and discovered that variables like product quality, store design, customer service, marketing campaigns, and sales channels affected the overall result. Now you want to use regression to analyze which of these variables changed or if any new ones appeared during 2020. For example, you couldn’t sell as much in your physical store due to COVID lockdowns. Therefore, your sales could’ve either dropped in general or increased in your online channels. Through this, you can understand which independent variables affected the overall performance of your dependent variable, annual sales.
If you want to go deeper into this type of analysis, check out this article and learn more about how you can benefit from regression.
4. Neural networks
The neural network forms the basis for the intelligent algorithms of machine learning. It is a form of analytics that attempts, with minimal intervention, to understand how the human brain would generate insights and predict values. Neural networks learn from each and every data transaction, meaning that they evolve and advance over time.
A typical area of application for neural networks is predictive analytics. There are BI reporting tools that have this feature implemented within them, such as the Predictive Analytics Tool from datapine. This tool enables users to quickly and easily generate all kinds of predictions. All you have to do is select the data to be processed based on your KPIs, and the software automatically calculates forecasts based on historical and current data. Thanks to its user-friendly interface, anyone in your organization can manage it; there’s no need to be an advanced scientist.
Here is an example of how you can use the predictive analysis tool from datapine:

**click to enlarge**
5. Factor analysis
The factor analysis also called “dimension reduction” is a type of data analysis used to describe variability among observed, correlated variables in terms of a potentially lower number of unobserved variables called factors. The aim here is to uncover independent latent variables, an ideal method for streamlining specific segments.
A good way to understand this data analysis method is a customer evaluation of a product. The initial assessment is based on different variables like color, shape, wearability, current trends, materials, comfort, the place where they bought the product, and frequency of usage. Like this, the list can be endless, depending on what you want to track. In this case, factor analysis comes into the picture by summarizing all of these variables into homogenous groups, for example, by grouping the variables color, materials, quality, and trends into a brother latent variable of design.
If you want to start analyzing data using factor analysis we recommend you take a look at this practical guide from UCLA.
6. Data mining
A method of data analysis that is the umbrella term for engineering metrics and insights for additional value, direction, and context. By using exploratory statistical evaluation, data mining aims to identify dependencies, relations, patterns, and trends to generate advanced knowledge. When considering how to analyze data, adopting a data mining mindset is essential to success - as such, it’s an area that is worth exploring in greater detail.
An excellent use case of data mining is datapine intelligent data alerts . With the help of artificial intelligence and machine learning, they provide automated signals based on particular commands or occurrences within a dataset. For example, if you’re monitoring supply chain KPIs , you could set an intelligent alarm to trigger when invalid or low-quality data appears. By doing so, you will be able to drill down deep into the issue and fix it swiftly and effectively.
In the following picture, you can see how the intelligent alarms from datapine work. By setting up ranges on daily orders, sessions, and revenues, the alarms will notify you if the goal was not completed or if it exceeded expectations.
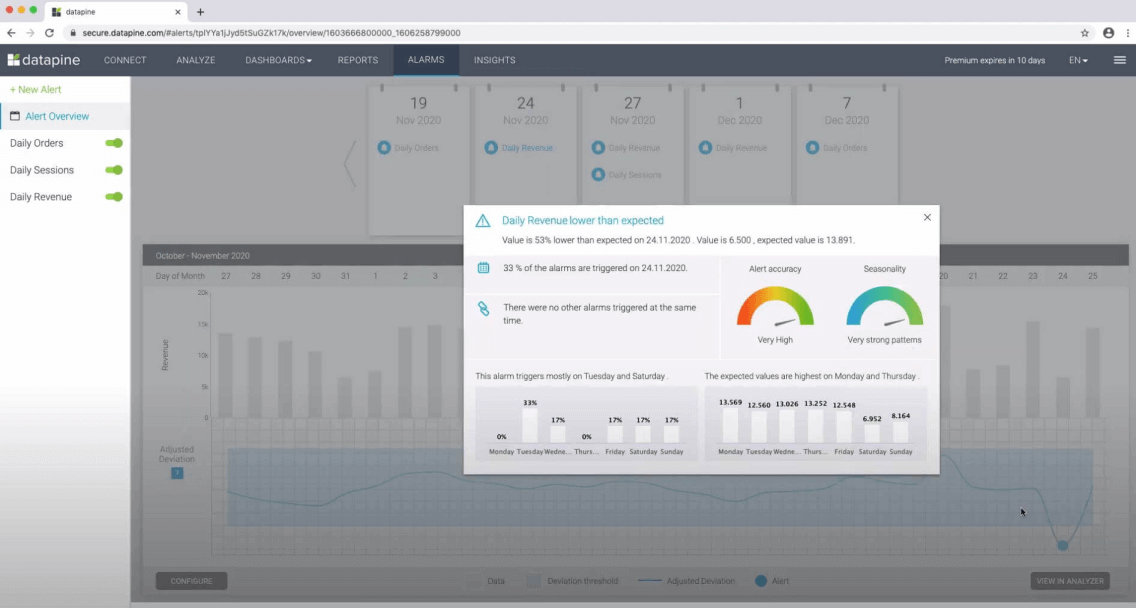
7. Time series analysis
As its name suggests, time series analysis is used to analyze a set of data points collected over a specified period of time. Although analysts use this method to monitor the data points in a specific interval of time rather than just monitoring them intermittently, the time series analysis is not uniquely used for the purpose of collecting data over time. Instead, it allows researchers to understand if variables changed during the duration of the study, how the different variables are dependent, and how did it reach the end result.
In a business context, this method is used to understand the causes of different trends and patterns to extract valuable insights. Another way of using this method is with the help of time series forecasting. Powered by predictive technologies, businesses can analyze various data sets over a period of time and forecast different future events.
A great use case to put time series analysis into perspective is seasonality effects on sales. By using time series forecasting to analyze sales data of a specific product over time, you can understand if sales rise over a specific period of time (e.g. swimwear during summertime, or candy during Halloween). These insights allow you to predict demand and prepare production accordingly.
8. Decision Trees
The decision tree analysis aims to act as a support tool to make smart and strategic decisions. By visually displaying potential outcomes, consequences, and costs in a tree-like model, researchers and company users can easily evaluate all factors involved and choose the best course of action. Decision trees are helpful to analyze quantitative data and they allow for an improved decision-making process by helping you spot improvement opportunities, reduce costs, and enhance operational efficiency and production.
But how does a decision tree actually works? This method works like a flowchart that starts with the main decision that you need to make and branches out based on the different outcomes and consequences of each decision. Each outcome will outline its own consequences, costs, and gains and, at the end of the analysis, you can compare each of them and make the smartest decision.
Businesses can use them to understand which project is more cost-effective and will bring more earnings in the long run. For example, imagine you need to decide if you want to update your software app or build a new app entirely. Here you would compare the total costs, the time needed to be invested, potential revenue, and any other factor that might affect your decision. In the end, you would be able to see which of these two options is more realistic and attainable for your company or research.
9. Conjoint analysis
Last but not least, we have the conjoint analysis. This approach is usually used in surveys to understand how individuals value different attributes of a product or service and it is one of the most effective methods to extract consumer preferences. When it comes to purchasing, some clients might be more price-focused, others more features-focused, and others might have a sustainable focus. Whatever your customer's preferences are, you can find them with conjoint analysis. Through this, companies can define pricing strategies, packaging options, subscription packages, and more.
A great example of conjoint analysis is in marketing and sales. For instance, a cupcake brand might use conjoint analysis and find that its clients prefer gluten-free options and cupcakes with healthier toppings over super sugary ones. Thus, the cupcake brand can turn these insights into advertisements and promotions to increase sales of this particular type of product. And not just that, conjoint analysis can also help businesses segment their customers based on their interests. This allows them to send different messaging that will bring value to each of the segments.
10. Correspondence Analysis
Also known as reciprocal averaging, correspondence analysis is a method used to analyze the relationship between categorical variables presented within a contingency table. A contingency table is a table that displays two (simple correspondence analysis) or more (multiple correspondence analysis) categorical variables across rows and columns that show the distribution of the data, which is usually answers to a survey or questionnaire on a specific topic.
This method starts by calculating an “expected value” which is done by multiplying row and column averages and dividing it by the overall original value of the specific table cell. The “expected value” is then subtracted from the original value resulting in a “residual number” which is what allows you to extract conclusions about relationships and distribution. The results of this analysis are later displayed using a map that represents the relationship between the different values. The closest two values are in the map, the bigger the relationship. Let’s put it into perspective with an example.
Imagine you are carrying out a market research analysis about outdoor clothing brands and how they are perceived by the public. For this analysis, you ask a group of people to match each brand with a certain attribute which can be durability, innovation, quality materials, etc. When calculating the residual numbers, you can see that brand A has a positive residual for innovation but a negative one for durability. This means that brand A is not positioned as a durable brand in the market, something that competitors could take advantage of.
11. Multidimensional Scaling (MDS)
MDS is a method used to observe the similarities or disparities between objects which can be colors, brands, people, geographical coordinates, and more. The objects are plotted using an “MDS map” that positions similar objects together and disparate ones far apart. The (dis) similarities between objects are represented using one or more dimensions that can be observed using a numerical scale. For example, if you want to know how people feel about the COVID-19 vaccine, you can use 1 for “don’t believe in the vaccine at all” and 10 for “firmly believe in the vaccine” and a scale of 2 to 9 for in between responses. When analyzing an MDS map the only thing that matters is the distance between the objects, the orientation of the dimensions is arbitrary and has no meaning at all.
Multidimensional scaling is a valuable technique for market research, especially when it comes to evaluating product or brand positioning. For instance, if a cupcake brand wants to know how they are positioned compared to competitors, it can define 2-3 dimensions such as taste, ingredients, shopping experience, or more, and do a multidimensional scaling analysis to find improvement opportunities as well as areas in which competitors are currently leading.
Another business example is in procurement when deciding on different suppliers. Decision makers can generate an MDS map to see how the different prices, delivery times, technical services, and more of the different suppliers differ and pick the one that suits their needs the best.
A final example proposed by a research paper on "An Improved Study of Multilevel Semantic Network Visualization for Analyzing Sentiment Word of Movie Review Data". Researchers picked a two-dimensional MDS map to display the distances and relationships between different sentiments in movie reviews. They used 36 sentiment words and distributed them based on their emotional distance as we can see in the image below where the words "outraged" and "sweet" are on opposite sides of the map, marking the distance between the two emotions very clearly.

Aside from being a valuable technique to analyze dissimilarities, MDS also serves as a dimension-reduction technique for large dimensional data.
B. Qualitative Methods
Qualitative data analysis methods are defined as the observation of non-numerical data that is gathered and produced using methods of observation such as interviews, focus groups, questionnaires, and more. As opposed to quantitative methods, qualitative data is more subjective and highly valuable in analyzing customer retention and product development.
12. Text analysis
Text analysis, also known in the industry as text mining, works by taking large sets of textual data and arranging them in a way that makes it easier to manage. By working through this cleansing process in stringent detail, you will be able to extract the data that is truly relevant to your organization and use it to develop actionable insights that will propel you forward.
Modern software accelerate the application of text analytics. Thanks to the combination of machine learning and intelligent algorithms, you can perform advanced analytical processes such as sentiment analysis. This technique allows you to understand the intentions and emotions of a text, for example, if it's positive, negative, or neutral, and then give it a score depending on certain factors and categories that are relevant to your brand. Sentiment analysis is often used to monitor brand and product reputation and to understand how successful your customer experience is. To learn more about the topic check out this insightful article .
By analyzing data from various word-based sources, including product reviews, articles, social media communications, and survey responses, you will gain invaluable insights into your audience, as well as their needs, preferences, and pain points. This will allow you to create campaigns, services, and communications that meet your prospects’ needs on a personal level, growing your audience while boosting customer retention. There are various other “sub-methods” that are an extension of text analysis. Each of them serves a more specific purpose and we will look at them in detail next.
13. Content Analysis
This is a straightforward and very popular method that examines the presence and frequency of certain words, concepts, and subjects in different content formats such as text, image, audio, or video. For example, the number of times the name of a celebrity is mentioned on social media or online tabloids. It does this by coding text data that is later categorized and tabulated in a way that can provide valuable insights, making it the perfect mix of quantitative and qualitative analysis.
There are two types of content analysis. The first one is the conceptual analysis which focuses on explicit data, for instance, the number of times a concept or word is mentioned in a piece of content. The second one is relational analysis, which focuses on the relationship between different concepts or words and how they are connected within a specific context.
Content analysis is often used by marketers to measure brand reputation and customer behavior. For example, by analyzing customer reviews. It can also be used to analyze customer interviews and find directions for new product development. It is also important to note, that in order to extract the maximum potential out of this analysis method, it is necessary to have a clearly defined research question.
14. Thematic Analysis
Very similar to content analysis, thematic analysis also helps in identifying and interpreting patterns in qualitative data with the main difference being that the first one can also be applied to quantitative analysis. The thematic method analyzes large pieces of text data such as focus group transcripts or interviews and groups them into themes or categories that come up frequently within the text. It is a great method when trying to figure out peoples view’s and opinions about a certain topic. For example, if you are a brand that cares about sustainability, you can do a survey of your customers to analyze their views and opinions about sustainability and how they apply it to their lives. You can also analyze customer service calls transcripts to find common issues and improve your service.
Thematic analysis is a very subjective technique that relies on the researcher’s judgment. Therefore, to avoid biases, it has 6 steps that include familiarization, coding, generating themes, reviewing themes, defining and naming themes, and writing up. It is also important to note that, because it is a flexible approach, the data can be interpreted in multiple ways and it can be hard to select what data is more important to emphasize.
15. Narrative Analysis
A bit more complex in nature than the two previous ones, narrative analysis is used to explore the meaning behind the stories that people tell and most importantly, how they tell them. By looking into the words that people use to describe a situation you can extract valuable conclusions about their perspective on a specific topic. Common sources for narrative data include autobiographies, family stories, opinion pieces, and testimonials, among others.
From a business perspective, narrative analysis can be useful to analyze customer behaviors and feelings towards a specific product, service, feature, or others. It provides unique and deep insights that can be extremely valuable. However, it has some drawbacks.
The biggest weakness of this method is that the sample sizes are usually very small due to the complexity and time-consuming nature of the collection of narrative data. Plus, the way a subject tells a story will be significantly influenced by his or her specific experiences, making it very hard to replicate in a subsequent study.
16. Discourse Analysis
Discourse analysis is used to understand the meaning behind any type of written, verbal, or symbolic discourse based on its political, social, or cultural context. It mixes the analysis of languages and situations together. This means that the way the content is constructed and the meaning behind it is significantly influenced by the culture and society it takes place in. For example, if you are analyzing political speeches you need to consider different context elements such as the politician's background, the current political context of the country, the audience to which the speech is directed, and so on.
From a business point of view, discourse analysis is a great market research tool. It allows marketers to understand how the norms and ideas of the specific market work and how their customers relate to those ideas. It can be very useful to build a brand mission or develop a unique tone of voice.
17. Grounded Theory Analysis
Traditionally, researchers decide on a method and hypothesis and start to collect the data to prove that hypothesis. The grounded theory is the only method that doesn’t require an initial research question or hypothesis as its value lies in the generation of new theories. With the grounded theory method, you can go into the analysis process with an open mind and explore the data to generate new theories through tests and revisions. In fact, it is not necessary to collect the data and then start to analyze it. Researchers usually start to find valuable insights as they are gathering the data.
All of these elements make grounded theory a very valuable method as theories are fully backed by data instead of initial assumptions. It is a great technique to analyze poorly researched topics or find the causes behind specific company outcomes. For example, product managers and marketers might use the grounded theory to find the causes of high levels of customer churn and look into customer surveys and reviews to develop new theories about the causes.
How To Analyze Data? Top 17 Data Analysis Techniques To Apply

Now that we’ve answered the questions “what is data analysis’”, why is it important, and covered the different data analysis types, it’s time to dig deeper into how to perform your analysis by working through these 17 essential techniques.
1. Collaborate your needs
Before you begin analyzing or drilling down into any techniques, it’s crucial to sit down collaboratively with all key stakeholders within your organization, decide on your primary campaign or strategic goals, and gain a fundamental understanding of the types of insights that will best benefit your progress or provide you with the level of vision you need to evolve your organization.
2. Establish your questions
Once you’ve outlined your core objectives, you should consider which questions will need answering to help you achieve your mission. This is one of the most important techniques as it will shape the very foundations of your success.
To help you ask the right things and ensure your data works for you, you have to ask the right data analysis questions .
3. Data democratization
After giving your data analytics methodology some real direction, and knowing which questions need answering to extract optimum value from the information available to your organization, you should continue with democratization.
Data democratization is an action that aims to connect data from various sources efficiently and quickly so that anyone in your organization can access it at any given moment. You can extract data in text, images, videos, numbers, or any other format. And then perform cross-database analysis to achieve more advanced insights to share with the rest of the company interactively.
Once you have decided on your most valuable sources, you need to take all of this into a structured format to start collecting your insights. For this purpose, datapine offers an easy all-in-one data connectors feature to integrate all your internal and external sources and manage them at your will. Additionally, datapine’s end-to-end solution automatically updates your data, allowing you to save time and focus on performing the right analysis to grow your company.
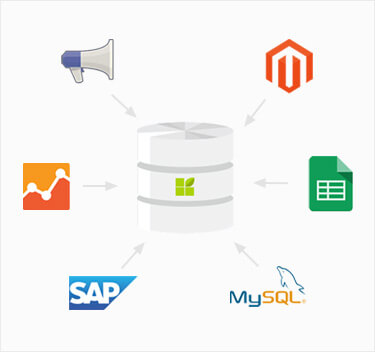
4. Think of governance
When collecting data in a business or research context you always need to think about security and privacy. With data breaches becoming a topic of concern for businesses, the need to protect your client's or subject’s sensitive information becomes critical.
To ensure that all this is taken care of, you need to think of a data governance strategy. According to Gartner , this concept refers to “ the specification of decision rights and an accountability framework to ensure the appropriate behavior in the valuation, creation, consumption, and control of data and analytics .” In simpler words, data governance is a collection of processes, roles, and policies, that ensure the efficient use of data while still achieving the main company goals. It ensures that clear roles are in place for who can access the information and how they can access it. In time, this not only ensures that sensitive information is protected but also allows for an efficient analysis as a whole.
5. Clean your data
After harvesting from so many sources you will be left with a vast amount of information that can be overwhelming to deal with. At the same time, you can be faced with incorrect data that can be misleading to your analysis. The smartest thing you can do to avoid dealing with this in the future is to clean the data. This is fundamental before visualizing it, as it will ensure that the insights you extract from it are correct.
There are many things that you need to look for in the cleaning process. The most important one is to eliminate any duplicate observations; this usually appears when using multiple internal and external sources of information. You can also add any missing codes, fix empty fields, and eliminate incorrectly formatted data.
Another usual form of cleaning is done with text data. As we mentioned earlier, most companies today analyze customer reviews, social media comments, questionnaires, and several other text inputs. In order for algorithms to detect patterns, text data needs to be revised to avoid invalid characters or any syntax or spelling errors.
Most importantly, the aim of cleaning is to prevent you from arriving at false conclusions that can damage your company in the long run. By using clean data, you will also help BI solutions to interact better with your information and create better reports for your organization.
6. Set your KPIs
Once you’ve set your sources, cleaned your data, and established clear-cut questions you want your insights to answer, you need to set a host of key performance indicators (KPIs) that will help you track, measure, and shape your progress in a number of key areas.
KPIs are critical to both qualitative and quantitative analysis research. This is one of the primary methods of data analysis you certainly shouldn’t overlook.
To help you set the best possible KPIs for your initiatives and activities, here is an example of a relevant logistics KPI : transportation-related costs. If you want to see more go explore our collection of key performance indicator examples .

7. Omit useless data
Having bestowed your data analysis tools and techniques with true purpose and defined your mission, you should explore the raw data you’ve collected from all sources and use your KPIs as a reference for chopping out any information you deem to be useless.
Trimming the informational fat is one of the most crucial methods of analysis as it will allow you to focus your analytical efforts and squeeze every drop of value from the remaining ‘lean’ information.
Any stats, facts, figures, or metrics that don’t align with your business goals or fit with your KPI management strategies should be eliminated from the equation.
8. Build a data management roadmap
While, at this point, this particular step is optional (you will have already gained a wealth of insight and formed a fairly sound strategy by now), creating a data governance roadmap will help your data analysis methods and techniques become successful on a more sustainable basis. These roadmaps, if developed properly, are also built so they can be tweaked and scaled over time.
Invest ample time in developing a roadmap that will help you store, manage, and handle your data internally, and you will make your analysis techniques all the more fluid and functional – one of the most powerful types of data analysis methods available today.
9. Integrate technology
There are many ways to analyze data, but one of the most vital aspects of analytical success in a business context is integrating the right decision support software and technology.
Robust analysis platforms will not only allow you to pull critical data from your most valuable sources while working with dynamic KPIs that will offer you actionable insights; it will also present them in a digestible, visual, interactive format from one central, live dashboard . A data methodology you can count on.
By integrating the right technology within your data analysis methodology, you’ll avoid fragmenting your insights, saving you time and effort while allowing you to enjoy the maximum value from your business’s most valuable insights.
For a look at the power of software for the purpose of analysis and to enhance your methods of analyzing, glance over our selection of dashboard examples .
10. Answer your questions
By considering each of the above efforts, working with the right technology, and fostering a cohesive internal culture where everyone buys into the different ways to analyze data as well as the power of digital intelligence, you will swiftly start to answer your most burning business questions. Arguably, the best way to make your data concepts accessible across the organization is through data visualization.
11. Visualize your data
Online data visualization is a powerful tool as it lets you tell a story with your metrics, allowing users across the organization to extract meaningful insights that aid business evolution – and it covers all the different ways to analyze data.
The purpose of analyzing is to make your entire organization more informed and intelligent, and with the right platform or dashboard, this is simpler than you think, as demonstrated by our marketing dashboard .

This visual, dynamic, and interactive online dashboard is a data analysis example designed to give Chief Marketing Officers (CMO) an overview of relevant metrics to help them understand if they achieved their monthly goals.
In detail, this example generated with a modern dashboard creator displays interactive charts for monthly revenues, costs, net income, and net income per customer; all of them are compared with the previous month so that you can understand how the data fluctuated. In addition, it shows a detailed summary of the number of users, customers, SQLs, and MQLs per month to visualize the whole picture and extract relevant insights or trends for your marketing reports .
The CMO dashboard is perfect for c-level management as it can help them monitor the strategic outcome of their marketing efforts and make data-driven decisions that can benefit the company exponentially.
12. Be careful with the interpretation
We already dedicated an entire post to data interpretation as it is a fundamental part of the process of data analysis. It gives meaning to the analytical information and aims to drive a concise conclusion from the analysis results. Since most of the time companies are dealing with data from many different sources, the interpretation stage needs to be done carefully and properly in order to avoid misinterpretations.
To help you through the process, here we list three common practices that you need to avoid at all costs when looking at your data:
- Correlation vs. causation: The human brain is formatted to find patterns. This behavior leads to one of the most common mistakes when performing interpretation: confusing correlation with causation. Although these two aspects can exist simultaneously, it is not correct to assume that because two things happened together, one provoked the other. A piece of advice to avoid falling into this mistake is never to trust just intuition, trust the data. If there is no objective evidence of causation, then always stick to correlation.
- Confirmation bias: This phenomenon describes the tendency to select and interpret only the data necessary to prove one hypothesis, often ignoring the elements that might disprove it. Even if it's not done on purpose, confirmation bias can represent a real problem, as excluding relevant information can lead to false conclusions and, therefore, bad business decisions. To avoid it, always try to disprove your hypothesis instead of proving it, share your analysis with other team members, and avoid drawing any conclusions before the entire analytical project is finalized.
- Statistical significance: To put it in short words, statistical significance helps analysts understand if a result is actually accurate or if it happened because of a sampling error or pure chance. The level of statistical significance needed might depend on the sample size and the industry being analyzed. In any case, ignoring the significance of a result when it might influence decision-making can be a huge mistake.
13. Build a narrative
Now, we’re going to look at how you can bring all of these elements together in a way that will benefit your business - starting with a little something called data storytelling.
The human brain responds incredibly well to strong stories or narratives. Once you’ve cleansed, shaped, and visualized your most invaluable data using various BI dashboard tools , you should strive to tell a story - one with a clear-cut beginning, middle, and end.
By doing so, you will make your analytical efforts more accessible, digestible, and universal, empowering more people within your organization to use your discoveries to their actionable advantage.
14. Consider autonomous technology
Autonomous technologies, such as artificial intelligence (AI) and machine learning (ML), play a significant role in the advancement of understanding how to analyze data more effectively.
Gartner predicts that by the end of this year, 80% of emerging technologies will be developed with AI foundations. This is a testament to the ever-growing power and value of autonomous technologies.
At the moment, these technologies are revolutionizing the analysis industry. Some examples that we mentioned earlier are neural networks, intelligent alarms, and sentiment analysis.
15. Share the load
If you work with the right tools and dashboards, you will be able to present your metrics in a digestible, value-driven format, allowing almost everyone in the organization to connect with and use relevant data to their advantage.
Modern dashboards consolidate data from various sources, providing access to a wealth of insights in one centralized location, no matter if you need to monitor recruitment metrics or generate reports that need to be sent across numerous departments. Moreover, these cutting-edge tools offer access to dashboards from a multitude of devices, meaning that everyone within the business can connect with practical insights remotely - and share the load.
Once everyone is able to work with a data-driven mindset, you will catalyze the success of your business in ways you never thought possible. And when it comes to knowing how to analyze data, this kind of collaborative approach is essential.
16. Data analysis tools
In order to perform high-quality analysis of data, it is fundamental to use tools and software that will ensure the best results. Here we leave you a small summary of four fundamental categories of data analysis tools for your organization.
- Business Intelligence: BI tools allow you to process significant amounts of data from several sources in any format. Through this, you can not only analyze and monitor your data to extract relevant insights but also create interactive reports and dashboards to visualize your KPIs and use them for your company's good. datapine is an amazing online BI software that is focused on delivering powerful online analysis features that are accessible to beginner and advanced users. Like this, it offers a full-service solution that includes cutting-edge analysis of data, KPIs visualization, live dashboards, reporting, and artificial intelligence technologies to predict trends and minimize risk.
- Statistical analysis: These tools are usually designed for scientists, statisticians, market researchers, and mathematicians, as they allow them to perform complex statistical analyses with methods like regression analysis, predictive analysis, and statistical modeling. A good tool to perform this type of analysis is R-Studio as it offers a powerful data modeling and hypothesis testing feature that can cover both academic and general data analysis. This tool is one of the favorite ones in the industry, due to its capability for data cleaning, data reduction, and performing advanced analysis with several statistical methods. Another relevant tool to mention is SPSS from IBM. The software offers advanced statistical analysis for users of all skill levels. Thanks to a vast library of machine learning algorithms, text analysis, and a hypothesis testing approach it can help your company find relevant insights to drive better decisions. SPSS also works as a cloud service that enables you to run it anywhere.
- SQL Consoles: SQL is a programming language often used to handle structured data in relational databases. Tools like these are popular among data scientists as they are extremely effective in unlocking these databases' value. Undoubtedly, one of the most used SQL software in the market is MySQL Workbench . This tool offers several features such as a visual tool for database modeling and monitoring, complete SQL optimization, administration tools, and visual performance dashboards to keep track of KPIs.
- Data Visualization: These tools are used to represent your data through charts, graphs, and maps that allow you to find patterns and trends in the data. datapine's already mentioned BI platform also offers a wealth of powerful online data visualization tools with several benefits. Some of them include: delivering compelling data-driven presentations to share with your entire company, the ability to see your data online with any device wherever you are, an interactive dashboard design feature that enables you to showcase your results in an interactive and understandable way, and to perform online self-service reports that can be used simultaneously with several other people to enhance team productivity.
17. Refine your process constantly
Last is a step that might seem obvious to some people, but it can be easily ignored if you think you are done. Once you have extracted the needed results, you should always take a retrospective look at your project and think about what you can improve. As you saw throughout this long list of techniques, data analysis is a complex process that requires constant refinement. For this reason, you should always go one step further and keep improving.
Quality Criteria For Data Analysis
So far we’ve covered a list of methods and techniques that should help you perform efficient data analysis. But how do you measure the quality and validity of your results? This is done with the help of some science quality criteria. Here we will go into a more theoretical area that is critical to understanding the fundamentals of statistical analysis in science. However, you should also be aware of these steps in a business context, as they will allow you to assess the quality of your results in the correct way. Let’s dig in.
- Internal validity: The results of a survey are internally valid if they measure what they are supposed to measure and thus provide credible results. In other words , internal validity measures the trustworthiness of the results and how they can be affected by factors such as the research design, operational definitions, how the variables are measured, and more. For instance, imagine you are doing an interview to ask people if they brush their teeth two times a day. While most of them will answer yes, you can still notice that their answers correspond to what is socially acceptable, which is to brush your teeth at least twice a day. In this case, you can’t be 100% sure if respondents actually brush their teeth twice a day or if they just say that they do, therefore, the internal validity of this interview is very low.
- External validity: Essentially, external validity refers to the extent to which the results of your research can be applied to a broader context. It basically aims to prove that the findings of a study can be applied in the real world. If the research can be applied to other settings, individuals, and times, then the external validity is high.
- Reliability : If your research is reliable, it means that it can be reproduced. If your measurement were repeated under the same conditions, it would produce similar results. This means that your measuring instrument consistently produces reliable results. For example, imagine a doctor building a symptoms questionnaire to detect a specific disease in a patient. Then, various other doctors use this questionnaire but end up diagnosing the same patient with a different condition. This means the questionnaire is not reliable in detecting the initial disease. Another important note here is that in order for your research to be reliable, it also needs to be objective. If the results of a study are the same, independent of who assesses them or interprets them, the study can be considered reliable. Let’s see the objectivity criteria in more detail now.
- Objectivity: In data science, objectivity means that the researcher needs to stay fully objective when it comes to its analysis. The results of a study need to be affected by objective criteria and not by the beliefs, personality, or values of the researcher. Objectivity needs to be ensured when you are gathering the data, for example, when interviewing individuals, the questions need to be asked in a way that doesn't influence the results. Paired with this, objectivity also needs to be thought of when interpreting the data. If different researchers reach the same conclusions, then the study is objective. For this last point, you can set predefined criteria to interpret the results to ensure all researchers follow the same steps.
The discussed quality criteria cover mostly potential influences in a quantitative context. Analysis in qualitative research has by default additional subjective influences that must be controlled in a different way. Therefore, there are other quality criteria for this kind of research such as credibility, transferability, dependability, and confirmability. You can see each of them more in detail on this resource .
Data Analysis Limitations & Barriers
Analyzing data is not an easy task. As you’ve seen throughout this post, there are many steps and techniques that you need to apply in order to extract useful information from your research. While a well-performed analysis can bring various benefits to your organization it doesn't come without limitations. In this section, we will discuss some of the main barriers you might encounter when conducting an analysis. Let’s see them more in detail.
- Lack of clear goals: No matter how good your data or analysis might be if you don’t have clear goals or a hypothesis the process might be worthless. While we mentioned some methods that don’t require a predefined hypothesis, it is always better to enter the analytical process with some clear guidelines of what you are expecting to get out of it, especially in a business context in which data is utilized to support important strategic decisions.
- Objectivity: Arguably one of the biggest barriers when it comes to data analysis in research is to stay objective. When trying to prove a hypothesis, researchers might find themselves, intentionally or unintentionally, directing the results toward an outcome that they want. To avoid this, always question your assumptions and avoid confusing facts with opinions. You can also show your findings to a research partner or external person to confirm that your results are objective.
- Data representation: A fundamental part of the analytical procedure is the way you represent your data. You can use various graphs and charts to represent your findings, but not all of them will work for all purposes. Choosing the wrong visual can not only damage your analysis but can mislead your audience, therefore, it is important to understand when to use each type of data depending on your analytical goals. Our complete guide on the types of graphs and charts lists 20 different visuals with examples of when to use them.
- Flawed correlation : Misleading statistics can significantly damage your research. We’ve already pointed out a few interpretation issues previously in the post, but it is an important barrier that we can't avoid addressing here as well. Flawed correlations occur when two variables appear related to each other but they are not. Confusing correlations with causation can lead to a wrong interpretation of results which can lead to building wrong strategies and loss of resources, therefore, it is very important to identify the different interpretation mistakes and avoid them.
- Sample size: A very common barrier to a reliable and efficient analysis process is the sample size. In order for the results to be trustworthy, the sample size should be representative of what you are analyzing. For example, imagine you have a company of 1000 employees and you ask the question “do you like working here?” to 50 employees of which 49 say yes, which means 95%. Now, imagine you ask the same question to the 1000 employees and 950 say yes, which also means 95%. Saying that 95% of employees like working in the company when the sample size was only 50 is not a representative or trustworthy conclusion. The significance of the results is way more accurate when surveying a bigger sample size.
- Privacy concerns: In some cases, data collection can be subjected to privacy regulations. Businesses gather all kinds of information from their customers from purchasing behaviors to addresses and phone numbers. If this falls into the wrong hands due to a breach, it can affect the security and confidentiality of your clients. To avoid this issue, you need to collect only the data that is needed for your research and, if you are using sensitive facts, make it anonymous so customers are protected. The misuse of customer data can severely damage a business's reputation, so it is important to keep an eye on privacy.
- Lack of communication between teams : When it comes to performing data analysis on a business level, it is very likely that each department and team will have different goals and strategies. However, they are all working for the same common goal of helping the business run smoothly and keep growing. When teams are not connected and communicating with each other, it can directly affect the way general strategies are built. To avoid these issues, tools such as data dashboards enable teams to stay connected through data in a visually appealing way.
- Innumeracy : Businesses are working with data more and more every day. While there are many BI tools available to perform effective analysis, data literacy is still a constant barrier. Not all employees know how to apply analysis techniques or extract insights from them. To prevent this from happening, you can implement different training opportunities that will prepare every relevant user to deal with data.
Key Data Analysis Skills
As you've learned throughout this lengthy guide, analyzing data is a complex task that requires a lot of knowledge and skills. That said, thanks to the rise of self-service tools the process is way more accessible and agile than it once was. Regardless, there are still some key skills that are valuable to have when working with data, we list the most important ones below.
- Critical and statistical thinking: To successfully analyze data you need to be creative and think out of the box. Yes, that might sound like a weird statement considering that data is often tight to facts. However, a great level of critical thinking is required to uncover connections, come up with a valuable hypothesis, and extract conclusions that go a step further from the surface. This, of course, needs to be complemented by statistical thinking and an understanding of numbers.
- Data cleaning: Anyone who has ever worked with data before will tell you that the cleaning and preparation process accounts for 80% of a data analyst's work, therefore, the skill is fundamental. But not just that, not cleaning the data adequately can also significantly damage the analysis which can lead to poor decision-making in a business scenario. While there are multiple tools that automate the cleaning process and eliminate the possibility of human error, it is still a valuable skill to dominate.
- Data visualization: Visuals make the information easier to understand and analyze, not only for professional users but especially for non-technical ones. Having the necessary skills to not only choose the right chart type but know when to apply it correctly is key. This also means being able to design visually compelling charts that make the data exploration process more efficient.
- SQL: The Structured Query Language or SQL is a programming language used to communicate with databases. It is fundamental knowledge as it enables you to update, manipulate, and organize data from relational databases which are the most common databases used by companies. It is fairly easy to learn and one of the most valuable skills when it comes to data analysis.
- Communication skills: This is a skill that is especially valuable in a business environment. Being able to clearly communicate analytical outcomes to colleagues is incredibly important, especially when the information you are trying to convey is complex for non-technical people. This applies to in-person communication as well as written format, for example, when generating a dashboard or report. While this might be considered a “soft” skill compared to the other ones we mentioned, it should not be ignored as you most likely will need to share analytical findings with others no matter the context.
Data Analysis In The Big Data Environment
Big data is invaluable to today’s businesses, and by using different methods for data analysis, it’s possible to view your data in a way that can help you turn insight into positive action.
To inspire your efforts and put the importance of big data into context, here are some insights that you should know:
- By 2026 the industry of big data is expected to be worth approximately $273.4 billion.
- 94% of enterprises say that analyzing data is important for their growth and digital transformation.
- Companies that exploit the full potential of their data can increase their operating margins by 60% .
- We already told you the benefits of Artificial Intelligence through this article. This industry's financial impact is expected to grow up to $40 billion by 2025.
Data analysis concepts may come in many forms, but fundamentally, any solid methodology will help to make your business more streamlined, cohesive, insightful, and successful than ever before.
Key Takeaways From Data Analysis
As we reach the end of our data analysis journey, we leave a small summary of the main methods and techniques to perform excellent analysis and grow your business.
17 Essential Types of Data Analysis Methods:
- Cluster analysis
- Cohort analysis
- Regression analysis
- Factor analysis
- Neural Networks
- Data Mining
- Text analysis
- Time series analysis
- Decision trees
- Conjoint analysis
- Correspondence Analysis
- Multidimensional Scaling
- Content analysis
- Thematic analysis
- Narrative analysis
- Grounded theory analysis
- Discourse analysis
Top 17 Data Analysis Techniques:
- Collaborate your needs
- Establish your questions
- Data democratization
- Think of data governance
- Clean your data
- Set your KPIs
- Omit useless data
- Build a data management roadmap
- Integrate technology
- Answer your questions
- Visualize your data
- Interpretation of data
- Consider autonomous technology
- Build a narrative
- Share the load
- Data Analysis tools
- Refine your process constantly
We’ve pondered the data analysis definition and drilled down into the practical applications of data-centric analytics, and one thing is clear: by taking measures to arrange your data and making your metrics work for you, it’s possible to transform raw information into action - the kind of that will push your business to the next level.
Yes, good data analytics techniques result in enhanced business intelligence (BI). To help you understand this notion in more detail, read our exploration of business intelligence reporting .
And, if you’re ready to perform your own analysis, drill down into your facts and figures while interacting with your data on astonishing visuals, you can try our software for a free, 14-day trial .
Quantitative Data Analysis
A Companion for Accounting and Information Systems Research
- © 2017
- Willem Mertens 0 ,
- Amedeo Pugliese 1 ,
- Jan Recker ORCID: https://orcid.org/0000-0002-2072-5792 2
QUT Business School, Queensland University of Technology, Brisbane, Australia
You can also search for this author in PubMed Google Scholar
Dept. of Economics and Management, University of Padova, Padova, Italy
School of accountancy, queensland university of technology, brisbane, australia.
- Offers a guide through the essential steps required in quantitative data analysis
- Helps in choosing the right method before starting the data collection process
- Presents statistics without the math!
- Offers numerous examples from various diciplines in accounting and information systems
- No need to invest in expensive and complex software packages
48k Accesses
24 Citations
13 Altmetric
This is a preview of subscription content, log in via an institution to check access.
Access this book
- Available as EPUB and PDF
- Read on any device
- Instant download
- Own it forever
- Compact, lightweight edition
- Dispatched in 3 to 5 business days
- Free shipping worldwide - see info
- Durable hardcover edition
Tax calculation will be finalised at checkout
Other ways to access
Licence this eBook for your library
Institutional subscriptions
Table of contents (9 chapters)
Front matter, introduction.
- Willem Mertens, Amedeo Pugliese, Jan Recker
Comparing Differences Across Groups
Assessing (innocuous) relationships, models with latent concepts and multiple relationships: structural equation modeling, nested data and multilevel models: hierarchical linear modeling, analyzing longitudinal and panel data, causality: endogeneity biases and possible remedies, how to start analyzing, test assumptions and deal with that pesky p -value, keeping track and staying sane, back matter.
- quantitative data analysis
- nested models
- quantitative data analysis method
- building data analysis skills
About this book
Authors and affiliations.
Willem Mertens
Amedeo Pugliese
About the authors
Willem Mertens is a Postdoctoral Research Fellow at Queensland University of Technology, Brisbane, Australia, and a Research Fellow of Vlerick Business School, Belgium. His main research interests lie in the areas of innovation, positive deviance and organizational behavior in general.
Amedeo Pugliese (PhD, University of Naples, Federico II) is currently Associate Professor of Financial Accounting and Governance at the University of Padova and Colin Brain Research Fellow in Corporate Governance and Ethics at Queensland University of Technology. His research interests span across boards of directors and the role of financial information and corporate disclosure on capital markets. Specifically he is studying how information risk faced by board members and its effects on the decision-making quality and monitoring in the boardroom.
Jan Recker is Alexander-von-Humboldt Fellow and tenured Full Professor of Information Systems at Queensland University of Technology. His research focuses on process-oriented systems analysis, Green Information Systems and IT-enabled innovation. He has written a textbook on scientific research in Information Systems that is used in many doctoral programs all over the world. He is Editor-in-Chief of the Communications of the Association for Information Systems, and Associate Editor for the MIS Quarterly.
Bibliographic Information
Book Title : Quantitative Data Analysis
Book Subtitle : A Companion for Accounting and Information Systems Research
Authors : Willem Mertens, Amedeo Pugliese, Jan Recker
DOI : https://doi.org/10.1007/978-3-319-42700-3
Publisher : Springer Cham
eBook Packages : Business and Management , Business and Management (R0)
Copyright Information : Springer International Publishing Switzerland 2017
Hardcover ISBN : 978-3-319-42699-0 Published: 10 October 2016
Softcover ISBN : 978-3-319-82640-0 Published: 14 June 2018
eBook ISBN : 978-3-319-42700-3 Published: 29 September 2016
Edition Number : 1
Number of Pages : X, 164
Number of Illustrations : 9 b/w illustrations, 20 illustrations in colour
Topics : Business Information Systems , Statistics for Business, Management, Economics, Finance, Insurance , Information Systems and Communication Service , Corporate Governance , Methodology of the Social Sciences
- Publish with us
Policies and ethics
- Find a journal
- Track your research
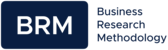
Quantitative Data Analysis
In quantitative data analysis you are expected to turn raw numbers into meaningful data through the application of rational and critical thinking. Quantitative data analysis may include the calculation of frequencies of variables and differences between variables. A quantitative approach is usually associated with finding evidence to either support or reject hypotheses you have formulated at the earlier stages of your research process .
The same figure within data set can be interpreted in many different ways; therefore it is important to apply fair and careful judgement.
For example, questionnaire findings of a research titled “A study into the impacts of informal management-employee communication on the levels of employee motivation: a case study of Agro Bravo Enterprise” may indicate that the majority 52% of respondents assess communication skills of their immediate supervisors as inadequate.
This specific piece of primary data findings needs to be critically analyzed and objectively interpreted through comparing it to other findings within the framework of the same research. For example, organizational culture of Agro Bravo Enterprise, leadership style, the levels of frequency of management-employee communications need to be taken into account during the data analysis.
Moreover, literature review findings conducted at the earlier stages of the research process need to be referred to in order to reflect the viewpoints of other authors regarding the causes of employee dissatisfaction with management communication. Also, secondary data needs to be integrated in data analysis in a logical and unbiased manner.
Let’s take another example. You are writing a dissertation exploring the impacts of foreign direct investment (FDI) on the levels of economic growth in Vietnam using correlation quantitative data analysis method . You have specified FDI and GDP as variables for your research and correlation tests produced correlation coefficient of 0.9.
In this case simply stating that there is a strong positive correlation between FDI and GDP would not suffice; you have to provide explanation about the manners in which the growth on the levels of FDI may contribute to the growth of GDP by referring to the findings of the literature review and applying your own critical and rational reasoning skills.
A set of analytical software can be used to assist with analysis of quantitative data. The following table illustrates the advantages and disadvantages of three popular quantitative data analysis software: Microsoft Excel, Microsoft Access and SPSS.
Advantages and disadvantages of popular quantitative analytical software
Quantitative data analysis with the application of statistical software consists of the following stages [1] :
- Preparing and checking the data. Input of data into computer.
- Selecting the most appropriate tables and diagrams to use according to your research objectives.
- Selecting the most appropriate statistics to describe your data.
- Selecting the most appropriate statistics to examine relationships and trends in your data.
It is important to note that while the application of various statistical software and programs are invaluable to avoid drawing charts by hand or undertake calculations manually, it is easy to use them incorrectly. In other words, quantitative data analysis is “a field where it is not at all difficult to carry out an analysis which is simply wrong, or inappropriate for your data or purposes. And the negative side of readily available specialist statistical software is that it becomes that much easier to generate elegantly presented rubbish” [2] .
Therefore, it is important for you to seek advice from your dissertation supervisor regarding statistical analyses in general and the choice and application of statistical software in particular.
My e-book, The Ultimate Guide to Writing a Dissertation in Business Studies: a step by step approach contains a detailed, yet simple explanation of quantitative data analysis methods . The e-book explains all stages of the research process starting from the selection of the research area to writing personal reflection. Important elements of dissertations such as research philosophy, research approach, research design, methods of data collection and data analysis are explained in simple words. John Dudovskiy
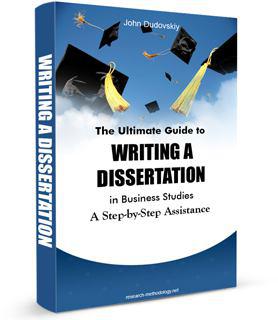
[1] Saunders, M., Lewis, P. & Thornhill, A. (2012) “Research Methods for Business Students” 6th edition, Pearson Education Limited.
[2] Robson, C. (2011) Real World Research: A Resource for Users of Social Research Methods in Applied Settings (3rd edn). Chichester: John Wiley.
- Privacy Policy

Home » Quantitative Research – Methods, Types and Analysis
Quantitative Research – Methods, Types and Analysis
Table of Contents

Quantitative Research
Quantitative research is a type of research that collects and analyzes numerical data to test hypotheses and answer research questions . This research typically involves a large sample size and uses statistical analysis to make inferences about a population based on the data collected. It often involves the use of surveys, experiments, or other structured data collection methods to gather quantitative data.
Quantitative Research Methods

Quantitative Research Methods are as follows:
Descriptive Research Design
Descriptive research design is used to describe the characteristics of a population or phenomenon being studied. This research method is used to answer the questions of what, where, when, and how. Descriptive research designs use a variety of methods such as observation, case studies, and surveys to collect data. The data is then analyzed using statistical tools to identify patterns and relationships.
Correlational Research Design
Correlational research design is used to investigate the relationship between two or more variables. Researchers use correlational research to determine whether a relationship exists between variables and to what extent they are related. This research method involves collecting data from a sample and analyzing it using statistical tools such as correlation coefficients.
Quasi-experimental Research Design
Quasi-experimental research design is used to investigate cause-and-effect relationships between variables. This research method is similar to experimental research design, but it lacks full control over the independent variable. Researchers use quasi-experimental research designs when it is not feasible or ethical to manipulate the independent variable.
Experimental Research Design
Experimental research design is used to investigate cause-and-effect relationships between variables. This research method involves manipulating the independent variable and observing the effects on the dependent variable. Researchers use experimental research designs to test hypotheses and establish cause-and-effect relationships.
Survey Research
Survey research involves collecting data from a sample of individuals using a standardized questionnaire. This research method is used to gather information on attitudes, beliefs, and behaviors of individuals. Researchers use survey research to collect data quickly and efficiently from a large sample size. Survey research can be conducted through various methods such as online, phone, mail, or in-person interviews.
Quantitative Research Analysis Methods
Here are some commonly used quantitative research analysis methods:
Statistical Analysis
Statistical analysis is the most common quantitative research analysis method. It involves using statistical tools and techniques to analyze the numerical data collected during the research process. Statistical analysis can be used to identify patterns, trends, and relationships between variables, and to test hypotheses and theories.
Regression Analysis
Regression analysis is a statistical technique used to analyze the relationship between one dependent variable and one or more independent variables. Researchers use regression analysis to identify and quantify the impact of independent variables on the dependent variable.
Factor Analysis
Factor analysis is a statistical technique used to identify underlying factors that explain the correlations among a set of variables. Researchers use factor analysis to reduce a large number of variables to a smaller set of factors that capture the most important information.
Structural Equation Modeling
Structural equation modeling is a statistical technique used to test complex relationships between variables. It involves specifying a model that includes both observed and unobserved variables, and then using statistical methods to test the fit of the model to the data.
Time Series Analysis
Time series analysis is a statistical technique used to analyze data that is collected over time. It involves identifying patterns and trends in the data, as well as any seasonal or cyclical variations.
Multilevel Modeling
Multilevel modeling is a statistical technique used to analyze data that is nested within multiple levels. For example, researchers might use multilevel modeling to analyze data that is collected from individuals who are nested within groups, such as students nested within schools.
Applications of Quantitative Research
Quantitative research has many applications across a wide range of fields. Here are some common examples:
- Market Research : Quantitative research is used extensively in market research to understand consumer behavior, preferences, and trends. Researchers use surveys, experiments, and other quantitative methods to collect data that can inform marketing strategies, product development, and pricing decisions.
- Health Research: Quantitative research is used in health research to study the effectiveness of medical treatments, identify risk factors for diseases, and track health outcomes over time. Researchers use statistical methods to analyze data from clinical trials, surveys, and other sources to inform medical practice and policy.
- Social Science Research: Quantitative research is used in social science research to study human behavior, attitudes, and social structures. Researchers use surveys, experiments, and other quantitative methods to collect data that can inform social policies, educational programs, and community interventions.
- Education Research: Quantitative research is used in education research to study the effectiveness of teaching methods, assess student learning outcomes, and identify factors that influence student success. Researchers use experimental and quasi-experimental designs, as well as surveys and other quantitative methods, to collect and analyze data.
- Environmental Research: Quantitative research is used in environmental research to study the impact of human activities on the environment, assess the effectiveness of conservation strategies, and identify ways to reduce environmental risks. Researchers use statistical methods to analyze data from field studies, experiments, and other sources.
Characteristics of Quantitative Research
Here are some key characteristics of quantitative research:
- Numerical data : Quantitative research involves collecting numerical data through standardized methods such as surveys, experiments, and observational studies. This data is analyzed using statistical methods to identify patterns and relationships.
- Large sample size: Quantitative research often involves collecting data from a large sample of individuals or groups in order to increase the reliability and generalizability of the findings.
- Objective approach: Quantitative research aims to be objective and impartial in its approach, focusing on the collection and analysis of data rather than personal beliefs, opinions, or experiences.
- Control over variables: Quantitative research often involves manipulating variables to test hypotheses and establish cause-and-effect relationships. Researchers aim to control for extraneous variables that may impact the results.
- Replicable : Quantitative research aims to be replicable, meaning that other researchers should be able to conduct similar studies and obtain similar results using the same methods.
- Statistical analysis: Quantitative research involves using statistical tools and techniques to analyze the numerical data collected during the research process. Statistical analysis allows researchers to identify patterns, trends, and relationships between variables, and to test hypotheses and theories.
- Generalizability: Quantitative research aims to produce findings that can be generalized to larger populations beyond the specific sample studied. This is achieved through the use of random sampling methods and statistical inference.
Examples of Quantitative Research
Here are some examples of quantitative research in different fields:
- Market Research: A company conducts a survey of 1000 consumers to determine their brand awareness and preferences. The data is analyzed using statistical methods to identify trends and patterns that can inform marketing strategies.
- Health Research : A researcher conducts a randomized controlled trial to test the effectiveness of a new drug for treating a particular medical condition. The study involves collecting data from a large sample of patients and analyzing the results using statistical methods.
- Social Science Research : A sociologist conducts a survey of 500 people to study attitudes toward immigration in a particular country. The data is analyzed using statistical methods to identify factors that influence these attitudes.
- Education Research: A researcher conducts an experiment to compare the effectiveness of two different teaching methods for improving student learning outcomes. The study involves randomly assigning students to different groups and collecting data on their performance on standardized tests.
- Environmental Research : A team of researchers conduct a study to investigate the impact of climate change on the distribution and abundance of a particular species of plant or animal. The study involves collecting data on environmental factors and population sizes over time and analyzing the results using statistical methods.
- Psychology : A researcher conducts a survey of 500 college students to investigate the relationship between social media use and mental health. The data is analyzed using statistical methods to identify correlations and potential causal relationships.
- Political Science: A team of researchers conducts a study to investigate voter behavior during an election. They use survey methods to collect data on voting patterns, demographics, and political attitudes, and analyze the results using statistical methods.
How to Conduct Quantitative Research
Here is a general overview of how to conduct quantitative research:
- Develop a research question: The first step in conducting quantitative research is to develop a clear and specific research question. This question should be based on a gap in existing knowledge, and should be answerable using quantitative methods.
- Develop a research design: Once you have a research question, you will need to develop a research design. This involves deciding on the appropriate methods to collect data, such as surveys, experiments, or observational studies. You will also need to determine the appropriate sample size, data collection instruments, and data analysis techniques.
- Collect data: The next step is to collect data. This may involve administering surveys or questionnaires, conducting experiments, or gathering data from existing sources. It is important to use standardized methods to ensure that the data is reliable and valid.
- Analyze data : Once the data has been collected, it is time to analyze it. This involves using statistical methods to identify patterns, trends, and relationships between variables. Common statistical techniques include correlation analysis, regression analysis, and hypothesis testing.
- Interpret results: After analyzing the data, you will need to interpret the results. This involves identifying the key findings, determining their significance, and drawing conclusions based on the data.
- Communicate findings: Finally, you will need to communicate your findings. This may involve writing a research report, presenting at a conference, or publishing in a peer-reviewed journal. It is important to clearly communicate the research question, methods, results, and conclusions to ensure that others can understand and replicate your research.
When to use Quantitative Research
Here are some situations when quantitative research can be appropriate:
- To test a hypothesis: Quantitative research is often used to test a hypothesis or a theory. It involves collecting numerical data and using statistical analysis to determine if the data supports or refutes the hypothesis.
- To generalize findings: If you want to generalize the findings of your study to a larger population, quantitative research can be useful. This is because it allows you to collect numerical data from a representative sample of the population and use statistical analysis to make inferences about the population as a whole.
- To measure relationships between variables: If you want to measure the relationship between two or more variables, such as the relationship between age and income, or between education level and job satisfaction, quantitative research can be useful. It allows you to collect numerical data on both variables and use statistical analysis to determine the strength and direction of the relationship.
- To identify patterns or trends: Quantitative research can be useful for identifying patterns or trends in data. For example, you can use quantitative research to identify trends in consumer behavior or to identify patterns in stock market data.
- To quantify attitudes or opinions : If you want to measure attitudes or opinions on a particular topic, quantitative research can be useful. It allows you to collect numerical data using surveys or questionnaires and analyze the data using statistical methods to determine the prevalence of certain attitudes or opinions.
Purpose of Quantitative Research
The purpose of quantitative research is to systematically investigate and measure the relationships between variables or phenomena using numerical data and statistical analysis. The main objectives of quantitative research include:
- Description : To provide a detailed and accurate description of a particular phenomenon or population.
- Explanation : To explain the reasons for the occurrence of a particular phenomenon, such as identifying the factors that influence a behavior or attitude.
- Prediction : To predict future trends or behaviors based on past patterns and relationships between variables.
- Control : To identify the best strategies for controlling or influencing a particular outcome or behavior.
Quantitative research is used in many different fields, including social sciences, business, engineering, and health sciences. It can be used to investigate a wide range of phenomena, from human behavior and attitudes to physical and biological processes. The purpose of quantitative research is to provide reliable and valid data that can be used to inform decision-making and improve understanding of the world around us.
Advantages of Quantitative Research
There are several advantages of quantitative research, including:
- Objectivity : Quantitative research is based on objective data and statistical analysis, which reduces the potential for bias or subjectivity in the research process.
- Reproducibility : Because quantitative research involves standardized methods and measurements, it is more likely to be reproducible and reliable.
- Generalizability : Quantitative research allows for generalizations to be made about a population based on a representative sample, which can inform decision-making and policy development.
- Precision : Quantitative research allows for precise measurement and analysis of data, which can provide a more accurate understanding of phenomena and relationships between variables.
- Efficiency : Quantitative research can be conducted relatively quickly and efficiently, especially when compared to qualitative research, which may involve lengthy data collection and analysis.
- Large sample sizes : Quantitative research can accommodate large sample sizes, which can increase the representativeness and generalizability of the results.
Limitations of Quantitative Research
There are several limitations of quantitative research, including:
- Limited understanding of context: Quantitative research typically focuses on numerical data and statistical analysis, which may not provide a comprehensive understanding of the context or underlying factors that influence a phenomenon.
- Simplification of complex phenomena: Quantitative research often involves simplifying complex phenomena into measurable variables, which may not capture the full complexity of the phenomenon being studied.
- Potential for researcher bias: Although quantitative research aims to be objective, there is still the potential for researcher bias in areas such as sampling, data collection, and data analysis.
- Limited ability to explore new ideas: Quantitative research is often based on pre-determined research questions and hypotheses, which may limit the ability to explore new ideas or unexpected findings.
- Limited ability to capture subjective experiences : Quantitative research is typically focused on objective data and may not capture the subjective experiences of individuals or groups being studied.
- Ethical concerns : Quantitative research may raise ethical concerns, such as invasion of privacy or the potential for harm to participants.
About the author
Muhammad Hassan
Researcher, Academic Writer, Web developer
You may also like

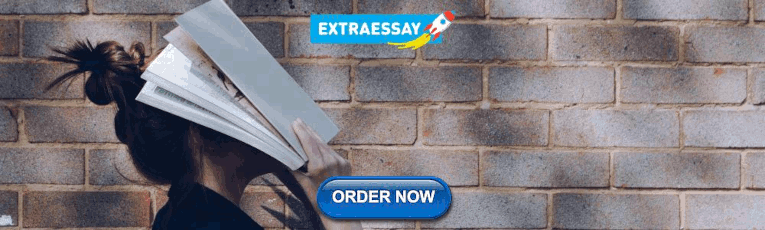
Questionnaire – Definition, Types, and Examples

Case Study – Methods, Examples and Guide

Observational Research – Methods and Guide

Qualitative Research Methods

Explanatory Research – Types, Methods, Guide

Survey Research – Types, Methods, Examples
- Skip to main content
- Skip to primary sidebar
- Skip to footer
- QuestionPro

- Solutions Industries Gaming Automotive Sports and events Education Government Travel & Hospitality Financial Services Healthcare Cannabis Technology Use Case NPS+ Communities Audience Contactless surveys Mobile LivePolls Member Experience GDPR Positive People Science 360 Feedback Surveys
- Resources Blog eBooks Survey Templates Case Studies Training Help center

Home Market Research
Data Analysis in Research: Types & Methods
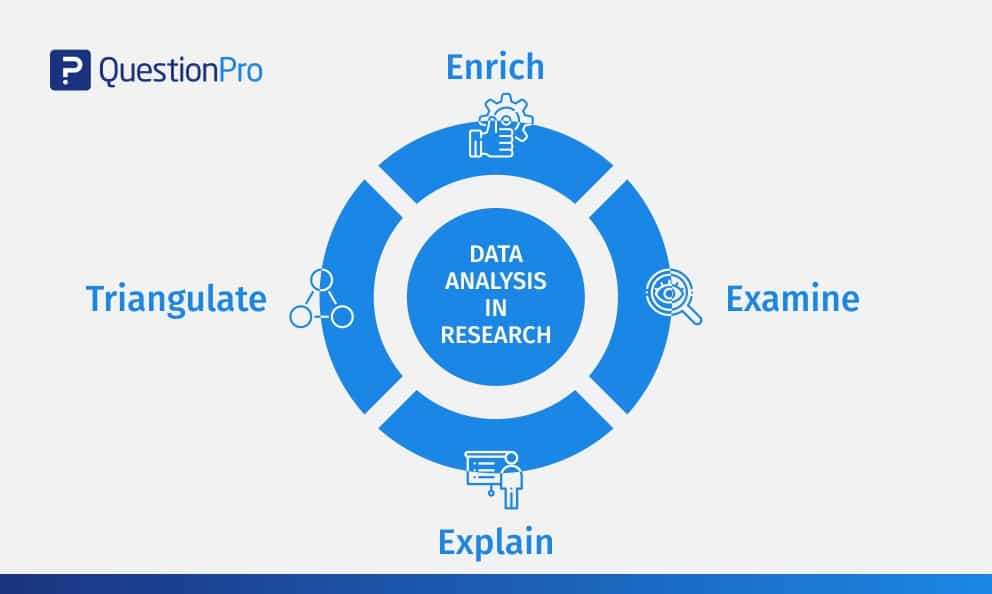
Content Index
Why analyze data in research?
Types of data in research, finding patterns in the qualitative data, methods used for data analysis in qualitative research, preparing data for analysis, methods used for data analysis in quantitative research, considerations in research data analysis, what is data analysis in research.
Definition of research in data analysis: According to LeCompte and Schensul, research data analysis is a process used by researchers to reduce data to a story and interpret it to derive insights. The data analysis process helps reduce a large chunk of data into smaller fragments, which makes sense.
Three essential things occur during the data analysis process — the first is data organization . Summarization and categorization together contribute to becoming the second known method used for data reduction. It helps find patterns and themes in the data for easy identification and linking. The third and last way is data analysis – researchers do it in both top-down and bottom-up fashion.
LEARN ABOUT: Research Process Steps
On the other hand, Marshall and Rossman describe data analysis as a messy, ambiguous, and time-consuming but creative and fascinating process through which a mass of collected data is brought to order, structure and meaning.
We can say that “the data analysis and data interpretation is a process representing the application of deductive and inductive logic to the research and data analysis.”
Researchers rely heavily on data as they have a story to tell or research problems to solve. It starts with a question, and data is nothing but an answer to that question. But, what if there is no question to ask? Well! It is possible to explore data even without a problem – we call it ‘Data Mining’, which often reveals some interesting patterns within the data that are worth exploring.
Irrelevant to the type of data researchers explore, their mission and audiences’ vision guide them to find the patterns to shape the story they want to tell. One of the essential things expected from researchers while analyzing data is to stay open and remain unbiased toward unexpected patterns, expressions, and results. Remember, sometimes, data analysis tells the most unforeseen yet exciting stories that were not expected when initiating data analysis. Therefore, rely on the data you have at hand and enjoy the journey of exploratory research.
Create a Free Account
Every kind of data has a rare quality of describing things after assigning a specific value to it. For analysis, you need to organize these values, processed and presented in a given context, to make it useful. Data can be in different forms; here are the primary data types.
- Qualitative data: When the data presented has words and descriptions, then we call it qualitative data . Although you can observe this data, it is subjective and harder to analyze data in research, especially for comparison. Example: Quality data represents everything describing taste, experience, texture, or an opinion that is considered quality data. This type of data is usually collected through focus groups, personal qualitative interviews , qualitative observation or using open-ended questions in surveys.
- Quantitative data: Any data expressed in numbers of numerical figures are called quantitative data . This type of data can be distinguished into categories, grouped, measured, calculated, or ranked. Example: questions such as age, rank, cost, length, weight, scores, etc. everything comes under this type of data. You can present such data in graphical format, charts, or apply statistical analysis methods to this data. The (Outcomes Measurement Systems) OMS questionnaires in surveys are a significant source of collecting numeric data.
- Categorical data: It is data presented in groups. However, an item included in the categorical data cannot belong to more than one group. Example: A person responding to a survey by telling his living style, marital status, smoking habit, or drinking habit comes under the categorical data. A chi-square test is a standard method used to analyze this data.
Learn More : Examples of Qualitative Data in Education
Data analysis in qualitative research
Data analysis and qualitative data research work a little differently from the numerical data as the quality data is made up of words, descriptions, images, objects, and sometimes symbols. Getting insight from such complicated information is a complicated process. Hence it is typically used for exploratory research and data analysis .
Although there are several ways to find patterns in the textual information, a word-based method is the most relied and widely used global technique for research and data analysis. Notably, the data analysis process in qualitative research is manual. Here the researchers usually read the available data and find repetitive or commonly used words.
For example, while studying data collected from African countries to understand the most pressing issues people face, researchers might find “food” and “hunger” are the most commonly used words and will highlight them for further analysis.
LEARN ABOUT: Level of Analysis
The keyword context is another widely used word-based technique. In this method, the researcher tries to understand the concept by analyzing the context in which the participants use a particular keyword.
For example , researchers conducting research and data analysis for studying the concept of ‘diabetes’ amongst respondents might analyze the context of when and how the respondent has used or referred to the word ‘diabetes.’
The scrutiny-based technique is also one of the highly recommended text analysis methods used to identify a quality data pattern. Compare and contrast is the widely used method under this technique to differentiate how a specific text is similar or different from each other.
For example: To find out the “importance of resident doctor in a company,” the collected data is divided into people who think it is necessary to hire a resident doctor and those who think it is unnecessary. Compare and contrast is the best method that can be used to analyze the polls having single-answer questions types .
Metaphors can be used to reduce the data pile and find patterns in it so that it becomes easier to connect data with theory.
Variable Partitioning is another technique used to split variables so that researchers can find more coherent descriptions and explanations from the enormous data.
LEARN ABOUT: Qualitative Research Questions and Questionnaires
There are several techniques to analyze the data in qualitative research, but here are some commonly used methods,
- Content Analysis: It is widely accepted and the most frequently employed technique for data analysis in research methodology. It can be used to analyze the documented information from text, images, and sometimes from the physical items. It depends on the research questions to predict when and where to use this method.
- Narrative Analysis: This method is used to analyze content gathered from various sources such as personal interviews, field observation, and surveys . The majority of times, stories, or opinions shared by people are focused on finding answers to the research questions.
- Discourse Analysis: Similar to narrative analysis, discourse analysis is used to analyze the interactions with people. Nevertheless, this particular method considers the social context under which or within which the communication between the researcher and respondent takes place. In addition to that, discourse analysis also focuses on the lifestyle and day-to-day environment while deriving any conclusion.
- Grounded Theory: When you want to explain why a particular phenomenon happened, then using grounded theory for analyzing quality data is the best resort. Grounded theory is applied to study data about the host of similar cases occurring in different settings. When researchers are using this method, they might alter explanations or produce new ones until they arrive at some conclusion.
LEARN ABOUT: 12 Best Tools for Researchers
Data analysis in quantitative research
The first stage in research and data analysis is to make it for the analysis so that the nominal data can be converted into something meaningful. Data preparation consists of the below phases.
Phase I: Data Validation
Data validation is done to understand if the collected data sample is per the pre-set standards, or it is a biased data sample again divided into four different stages
- Fraud: To ensure an actual human being records each response to the survey or the questionnaire
- Screening: To make sure each participant or respondent is selected or chosen in compliance with the research criteria
- Procedure: To ensure ethical standards were maintained while collecting the data sample
- Completeness: To ensure that the respondent has answered all the questions in an online survey. Else, the interviewer had asked all the questions devised in the questionnaire.
Phase II: Data Editing
More often, an extensive research data sample comes loaded with errors. Respondents sometimes fill in some fields incorrectly or sometimes skip them accidentally. Data editing is a process wherein the researchers have to confirm that the provided data is free of such errors. They need to conduct necessary checks and outlier checks to edit the raw edit and make it ready for analysis.
Phase III: Data Coding
Out of all three, this is the most critical phase of data preparation associated with grouping and assigning values to the survey responses . If a survey is completed with a 1000 sample size, the researcher will create an age bracket to distinguish the respondents based on their age. Thus, it becomes easier to analyze small data buckets rather than deal with the massive data pile.
LEARN ABOUT: Steps in Qualitative Research
After the data is prepared for analysis, researchers are open to using different research and data analysis methods to derive meaningful insights. For sure, statistical analysis plans are the most favored to analyze numerical data. In statistical analysis, distinguishing between categorical data and numerical data is essential, as categorical data involves distinct categories or labels, while numerical data consists of measurable quantities. The method is again classified into two groups. First, ‘Descriptive Statistics’ used to describe data. Second, ‘Inferential statistics’ that helps in comparing the data .
Descriptive statistics
This method is used to describe the basic features of versatile types of data in research. It presents the data in such a meaningful way that pattern in the data starts making sense. Nevertheless, the descriptive analysis does not go beyond making conclusions. The conclusions are again based on the hypothesis researchers have formulated so far. Here are a few major types of descriptive analysis methods.
Measures of Frequency
- Count, Percent, Frequency
- It is used to denote home often a particular event occurs.
- Researchers use it when they want to showcase how often a response is given.
Measures of Central Tendency
- Mean, Median, Mode
- The method is widely used to demonstrate distribution by various points.
- Researchers use this method when they want to showcase the most commonly or averagely indicated response.
Measures of Dispersion or Variation
- Range, Variance, Standard deviation
- Here the field equals high/low points.
- Variance standard deviation = difference between the observed score and mean
- It is used to identify the spread of scores by stating intervals.
- Researchers use this method to showcase data spread out. It helps them identify the depth until which the data is spread out that it directly affects the mean.
Measures of Position
- Percentile ranks, Quartile ranks
- It relies on standardized scores helping researchers to identify the relationship between different scores.
- It is often used when researchers want to compare scores with the average count.
For quantitative research use of descriptive analysis often give absolute numbers, but the in-depth analysis is never sufficient to demonstrate the rationale behind those numbers. Nevertheless, it is necessary to think of the best method for research and data analysis suiting your survey questionnaire and what story researchers want to tell. For example, the mean is the best way to demonstrate the students’ average scores in schools. It is better to rely on the descriptive statistics when the researchers intend to keep the research or outcome limited to the provided sample without generalizing it. For example, when you want to compare average voting done in two different cities, differential statistics are enough.
Descriptive analysis is also called a ‘univariate analysis’ since it is commonly used to analyze a single variable.
Inferential statistics
Inferential statistics are used to make predictions about a larger population after research and data analysis of the representing population’s collected sample. For example, you can ask some odd 100 audiences at a movie theater if they like the movie they are watching. Researchers then use inferential statistics on the collected sample to reason that about 80-90% of people like the movie.
Here are two significant areas of inferential statistics.
- Estimating parameters: It takes statistics from the sample research data and demonstrates something about the population parameter.
- Hypothesis test: I t’s about sampling research data to answer the survey research questions. For example, researchers might be interested to understand if the new shade of lipstick recently launched is good or not, or if the multivitamin capsules help children to perform better at games.
These are sophisticated analysis methods used to showcase the relationship between different variables instead of describing a single variable. It is often used when researchers want something beyond absolute numbers to understand the relationship between variables.
Here are some of the commonly used methods for data analysis in research.
- Correlation: When researchers are not conducting experimental research or quasi-experimental research wherein the researchers are interested to understand the relationship between two or more variables, they opt for correlational research methods.
- Cross-tabulation: Also called contingency tables, cross-tabulation is used to analyze the relationship between multiple variables. Suppose provided data has age and gender categories presented in rows and columns. A two-dimensional cross-tabulation helps for seamless data analysis and research by showing the number of males and females in each age category.
- Regression analysis: For understanding the strong relationship between two variables, researchers do not look beyond the primary and commonly used regression analysis method, which is also a type of predictive analysis used. In this method, you have an essential factor called the dependent variable. You also have multiple independent variables in regression analysis. You undertake efforts to find out the impact of independent variables on the dependent variable. The values of both independent and dependent variables are assumed as being ascertained in an error-free random manner.
- Frequency tables: The statistical procedure is used for testing the degree to which two or more vary or differ in an experiment. A considerable degree of variation means research findings were significant. In many contexts, ANOVA testing and variance analysis are similar.
- Analysis of variance: The statistical procedure is used for testing the degree to which two or more vary or differ in an experiment. A considerable degree of variation means research findings were significant. In many contexts, ANOVA testing and variance analysis are similar.
- Researchers must have the necessary research skills to analyze and manipulation the data , Getting trained to demonstrate a high standard of research practice. Ideally, researchers must possess more than a basic understanding of the rationale of selecting one statistical method over the other to obtain better data insights.
- Usually, research and data analytics projects differ by scientific discipline; therefore, getting statistical advice at the beginning of analysis helps design a survey questionnaire, select data collection methods , and choose samples.
LEARN ABOUT: Best Data Collection Tools
- The primary aim of data research and analysis is to derive ultimate insights that are unbiased. Any mistake in or keeping a biased mind to collect data, selecting an analysis method, or choosing audience sample il to draw a biased inference.
- Irrelevant to the sophistication used in research data and analysis is enough to rectify the poorly defined objective outcome measurements. It does not matter if the design is at fault or intentions are not clear, but lack of clarity might mislead readers, so avoid the practice.
- The motive behind data analysis in research is to present accurate and reliable data. As far as possible, avoid statistical errors, and find a way to deal with everyday challenges like outliers, missing data, data altering, data mining , or developing graphical representation.
LEARN MORE: Descriptive Research vs Correlational Research The sheer amount of data generated daily is frightening. Especially when data analysis has taken center stage. in 2018. In last year, the total data supply amounted to 2.8 trillion gigabytes. Hence, it is clear that the enterprises willing to survive in the hypercompetitive world must possess an excellent capability to analyze complex research data, derive actionable insights, and adapt to the new market needs.
LEARN ABOUT: Average Order Value
QuestionPro is an online survey platform that empowers organizations in data analysis and research and provides them a medium to collect data by creating appealing surveys.
MORE LIKE THIS
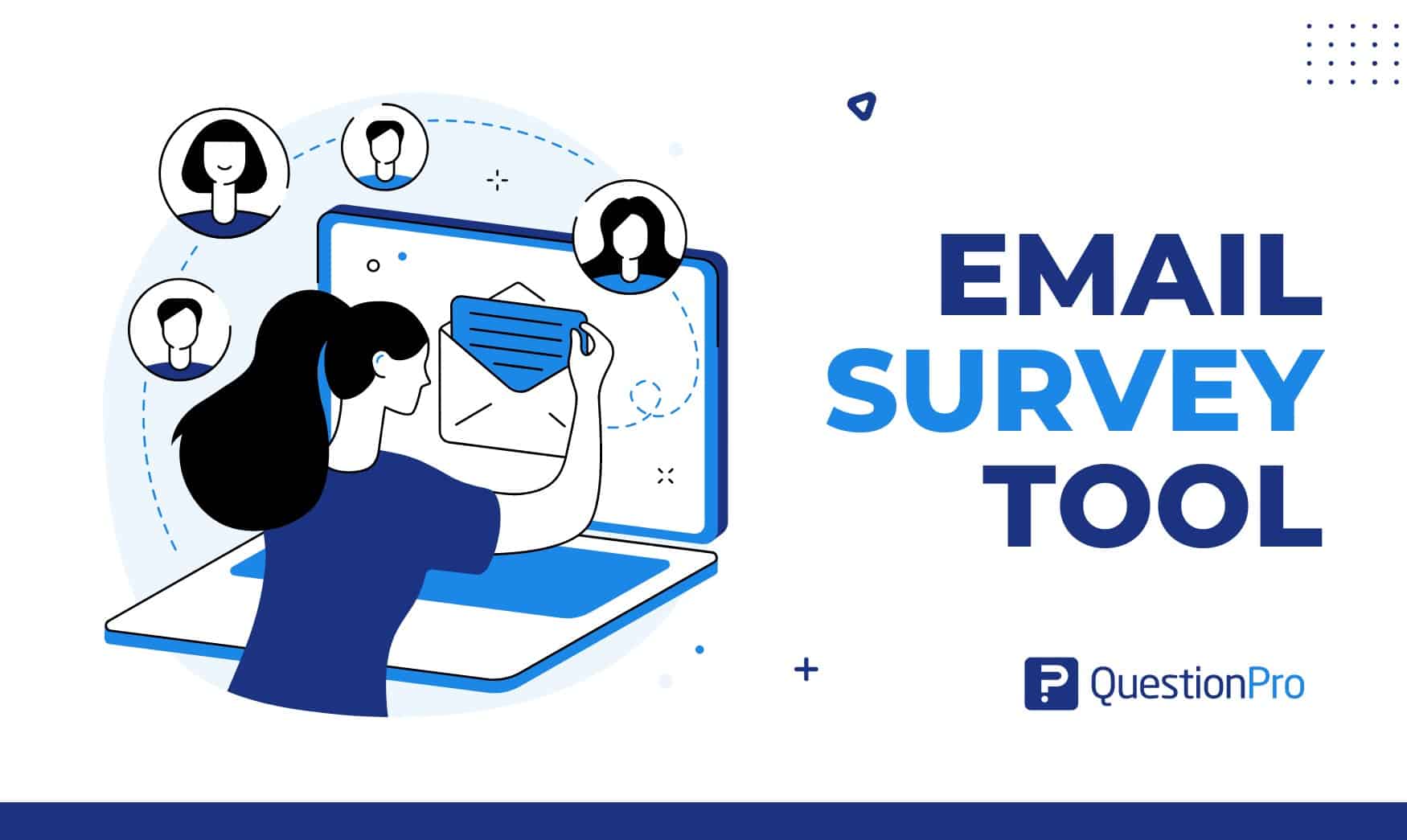
The Best Email Survey Tool to Boost Your Feedback Game
May 7, 2024
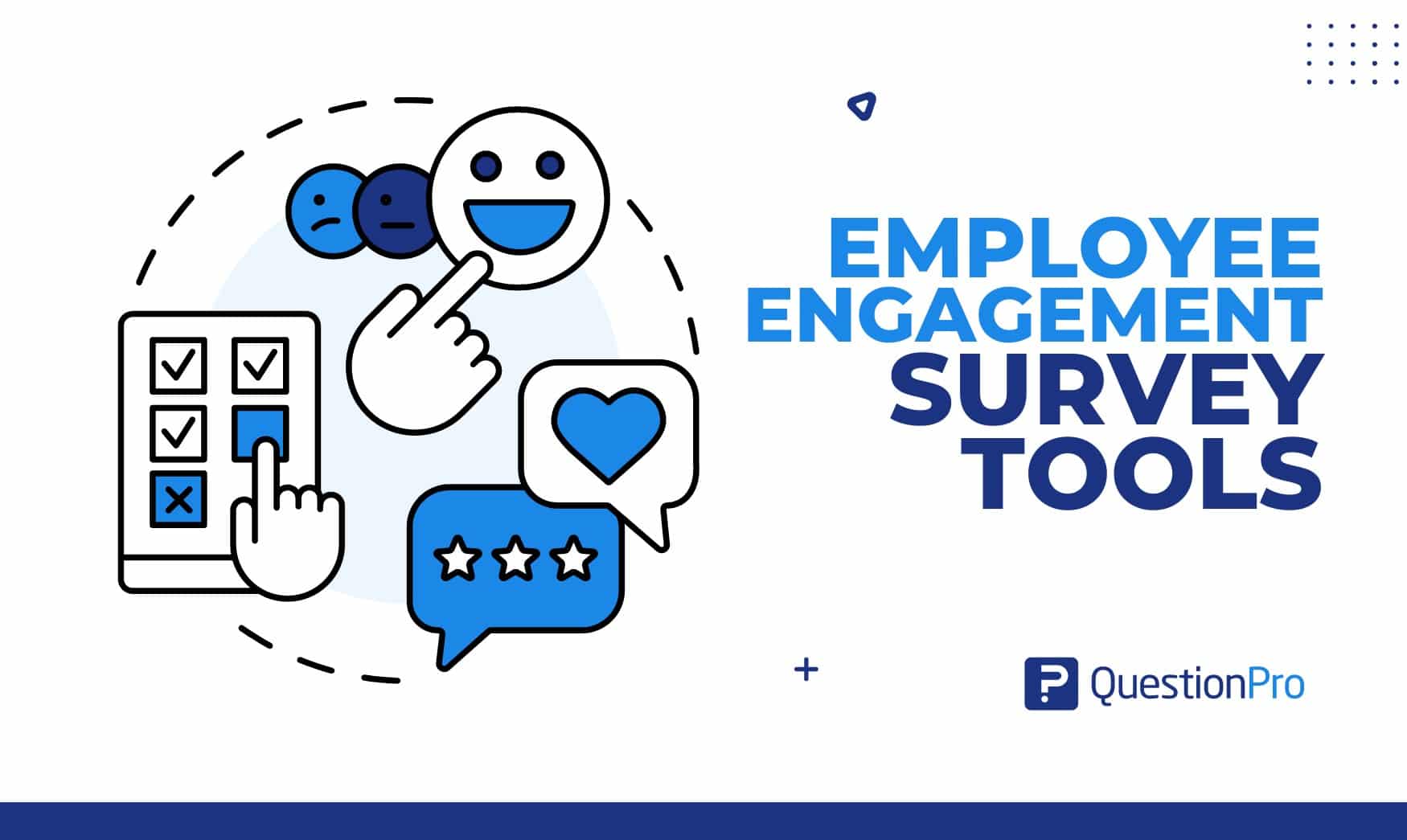
Top 10 Employee Engagement Survey Tools
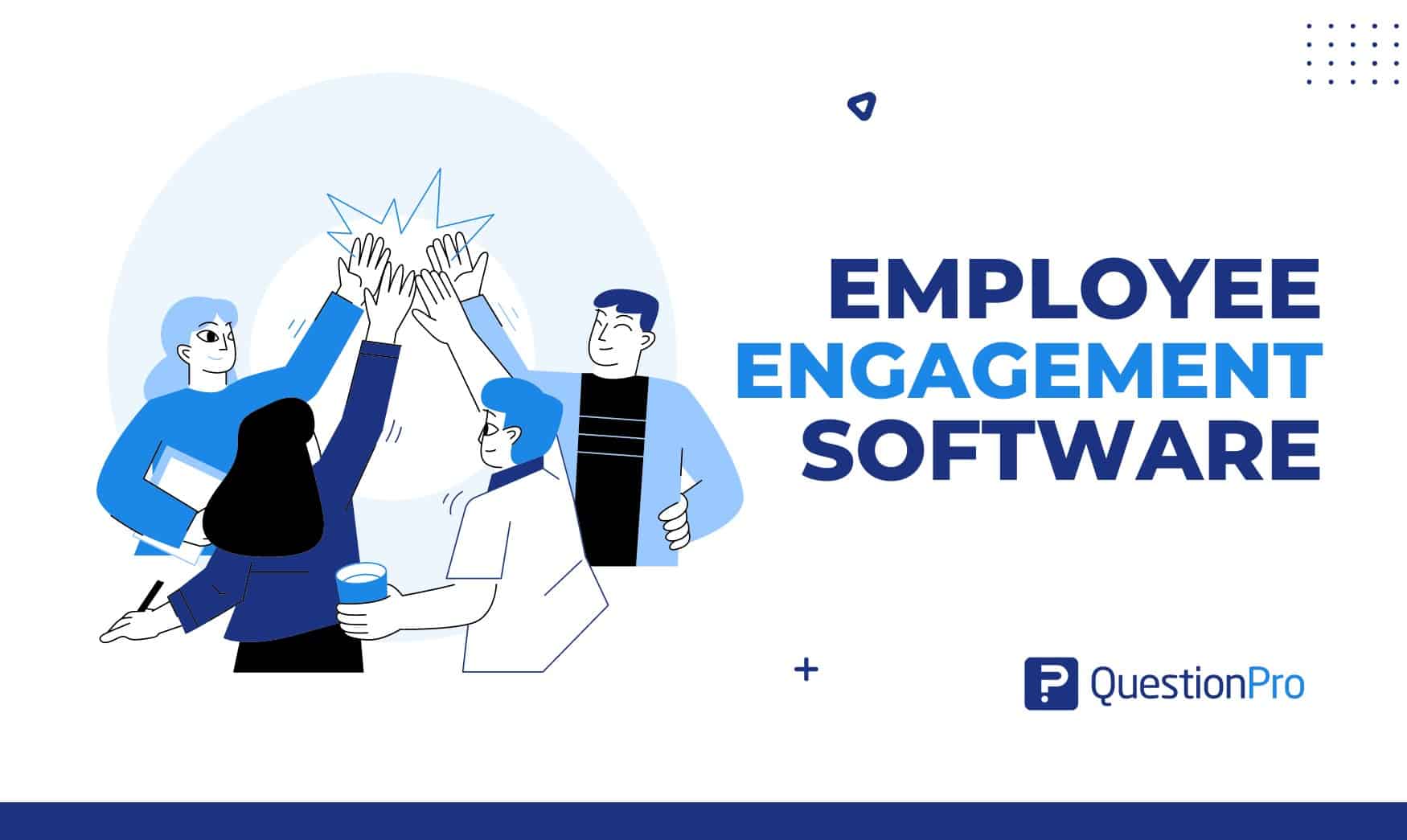
Top 20 Employee Engagement Software Solutions
May 3, 2024
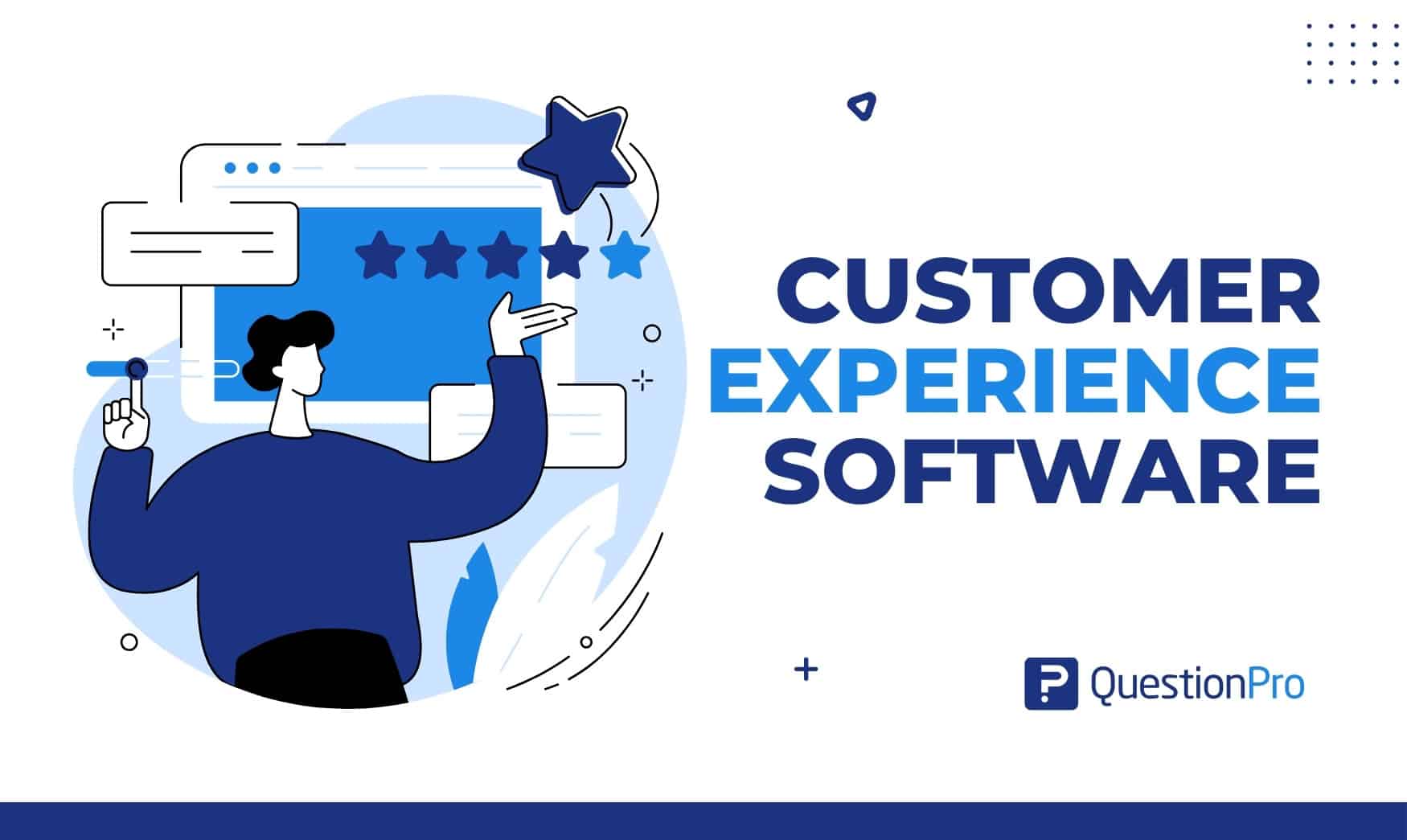
15 Best Customer Experience Software of 2024
May 2, 2024
Other categories
- Academic Research
- Artificial Intelligence
- Assessments
- Brand Awareness
- Case Studies
- Communities
- Consumer Insights
- Customer effort score
- Customer Engagement
- Customer Experience
- Customer Loyalty
- Customer Research
- Customer Satisfaction
- Employee Benefits
- Employee Engagement
- Employee Retention
- Friday Five
- General Data Protection Regulation
- Insights Hub
- Life@QuestionPro
- Market Research
- Mobile diaries
- Mobile Surveys
- New Features
- Online Communities
- Question Types
- Questionnaire
- QuestionPro Products
- Release Notes
- Research Tools and Apps
- Revenue at Risk
- Survey Templates
- Training Tips
- Uncategorized
- Video Learning Series
- What’s Coming Up
- Workforce Intelligence
- Search Menu
- Browse content in Arts and Humanities
- Browse content in Archaeology
- Anglo-Saxon and Medieval Archaeology
- Archaeological Methodology and Techniques
- Archaeology by Region
- Archaeology of Religion
- Archaeology of Trade and Exchange
- Biblical Archaeology
- Contemporary and Public Archaeology
- Environmental Archaeology
- Historical Archaeology
- History and Theory of Archaeology
- Industrial Archaeology
- Landscape Archaeology
- Mortuary Archaeology
- Prehistoric Archaeology
- Underwater Archaeology
- Urban Archaeology
- Zooarchaeology
- Browse content in Architecture
- Architectural Structure and Design
- History of Architecture
- Residential and Domestic Buildings
- Theory of Architecture
- Browse content in Art
- Art Subjects and Themes
- History of Art
- Industrial and Commercial Art
- Theory of Art
- Biographical Studies
- Byzantine Studies
- Browse content in Classical Studies
- Classical History
- Classical Philosophy
- Classical Mythology
- Classical Literature
- Classical Reception
- Classical Art and Architecture
- Classical Oratory and Rhetoric
- Greek and Roman Papyrology
- Greek and Roman Epigraphy
- Greek and Roman Law
- Greek and Roman Archaeology
- Late Antiquity
- Religion in the Ancient World
- Digital Humanities
- Browse content in History
- Colonialism and Imperialism
- Diplomatic History
- Environmental History
- Genealogy, Heraldry, Names, and Honours
- Genocide and Ethnic Cleansing
- Historical Geography
- History by Period
- History of Emotions
- History of Agriculture
- History of Education
- History of Gender and Sexuality
- Industrial History
- Intellectual History
- International History
- Labour History
- Legal and Constitutional History
- Local and Family History
- Maritime History
- Military History
- National Liberation and Post-Colonialism
- Oral History
- Political History
- Public History
- Regional and National History
- Revolutions and Rebellions
- Slavery and Abolition of Slavery
- Social and Cultural History
- Theory, Methods, and Historiography
- Urban History
- World History
- Browse content in Language Teaching and Learning
- Language Learning (Specific Skills)
- Language Teaching Theory and Methods
- Browse content in Linguistics
- Applied Linguistics
- Cognitive Linguistics
- Computational Linguistics
- Forensic Linguistics
- Grammar, Syntax and Morphology
- Historical and Diachronic Linguistics
- History of English
- Language Evolution
- Language Reference
- Language Acquisition
- Language Variation
- Language Families
- Lexicography
- Linguistic Anthropology
- Linguistic Theories
- Linguistic Typology
- Phonetics and Phonology
- Psycholinguistics
- Sociolinguistics
- Translation and Interpretation
- Writing Systems
- Browse content in Literature
- Bibliography
- Children's Literature Studies
- Literary Studies (Romanticism)
- Literary Studies (American)
- Literary Studies (Asian)
- Literary Studies (European)
- Literary Studies (Eco-criticism)
- Literary Studies (Modernism)
- Literary Studies - World
- Literary Studies (1500 to 1800)
- Literary Studies (19th Century)
- Literary Studies (20th Century onwards)
- Literary Studies (African American Literature)
- Literary Studies (British and Irish)
- Literary Studies (Early and Medieval)
- Literary Studies (Fiction, Novelists, and Prose Writers)
- Literary Studies (Gender Studies)
- Literary Studies (Graphic Novels)
- Literary Studies (History of the Book)
- Literary Studies (Plays and Playwrights)
- Literary Studies (Poetry and Poets)
- Literary Studies (Postcolonial Literature)
- Literary Studies (Queer Studies)
- Literary Studies (Science Fiction)
- Literary Studies (Travel Literature)
- Literary Studies (War Literature)
- Literary Studies (Women's Writing)
- Literary Theory and Cultural Studies
- Mythology and Folklore
- Shakespeare Studies and Criticism
- Browse content in Media Studies
- Browse content in Music
- Applied Music
- Dance and Music
- Ethics in Music
- Ethnomusicology
- Gender and Sexuality in Music
- Medicine and Music
- Music Cultures
- Music and Media
- Music and Religion
- Music and Culture
- Music Education and Pedagogy
- Music Theory and Analysis
- Musical Scores, Lyrics, and Libretti
- Musical Structures, Styles, and Techniques
- Musicology and Music History
- Performance Practice and Studies
- Race and Ethnicity in Music
- Sound Studies
- Browse content in Performing Arts
- Browse content in Philosophy
- Aesthetics and Philosophy of Art
- Epistemology
- Feminist Philosophy
- History of Western Philosophy
- Metaphysics
- Moral Philosophy
- Non-Western Philosophy
- Philosophy of Language
- Philosophy of Mind
- Philosophy of Perception
- Philosophy of Science
- Philosophy of Action
- Philosophy of Law
- Philosophy of Religion
- Philosophy of Mathematics and Logic
- Practical Ethics
- Social and Political Philosophy
- Browse content in Religion
- Biblical Studies
- Christianity
- East Asian Religions
- History of Religion
- Judaism and Jewish Studies
- Qumran Studies
- Religion and Education
- Religion and Health
- Religion and Politics
- Religion and Science
- Religion and Law
- Religion and Art, Literature, and Music
- Religious Studies
- Browse content in Society and Culture
- Cookery, Food, and Drink
- Cultural Studies
- Customs and Traditions
- Ethical Issues and Debates
- Hobbies, Games, Arts and Crafts
- Lifestyle, Home, and Garden
- Natural world, Country Life, and Pets
- Popular Beliefs and Controversial Knowledge
- Sports and Outdoor Recreation
- Technology and Society
- Travel and Holiday
- Visual Culture
- Browse content in Law
- Arbitration
- Browse content in Company and Commercial Law
- Commercial Law
- Company Law
- Browse content in Comparative Law
- Systems of Law
- Competition Law
- Browse content in Constitutional and Administrative Law
- Government Powers
- Judicial Review
- Local Government Law
- Military and Defence Law
- Parliamentary and Legislative Practice
- Construction Law
- Contract Law
- Browse content in Criminal Law
- Criminal Procedure
- Criminal Evidence Law
- Sentencing and Punishment
- Employment and Labour Law
- Environment and Energy Law
- Browse content in Financial Law
- Banking Law
- Insolvency Law
- History of Law
- Human Rights and Immigration
- Intellectual Property Law
- Browse content in International Law
- Private International Law and Conflict of Laws
- Public International Law
- IT and Communications Law
- Jurisprudence and Philosophy of Law
- Law and Politics
- Law and Society
- Browse content in Legal System and Practice
- Courts and Procedure
- Legal Skills and Practice
- Primary Sources of Law
- Regulation of Legal Profession
- Medical and Healthcare Law
- Browse content in Policing
- Criminal Investigation and Detection
- Police and Security Services
- Police Procedure and Law
- Police Regional Planning
- Browse content in Property Law
- Personal Property Law
- Study and Revision
- Terrorism and National Security Law
- Browse content in Trusts Law
- Wills and Probate or Succession
- Browse content in Medicine and Health
- Browse content in Allied Health Professions
- Arts Therapies
- Clinical Science
- Dietetics and Nutrition
- Occupational Therapy
- Operating Department Practice
- Physiotherapy
- Radiography
- Speech and Language Therapy
- Browse content in Anaesthetics
- General Anaesthesia
- Neuroanaesthesia
- Clinical Neuroscience
- Browse content in Clinical Medicine
- Acute Medicine
- Cardiovascular Medicine
- Clinical Genetics
- Clinical Pharmacology and Therapeutics
- Dermatology
- Endocrinology and Diabetes
- Gastroenterology
- Genito-urinary Medicine
- Geriatric Medicine
- Infectious Diseases
- Medical Toxicology
- Medical Oncology
- Pain Medicine
- Palliative Medicine
- Rehabilitation Medicine
- Respiratory Medicine and Pulmonology
- Rheumatology
- Sleep Medicine
- Sports and Exercise Medicine
- Community Medical Services
- Critical Care
- Emergency Medicine
- Forensic Medicine
- Haematology
- History of Medicine
- Browse content in Medical Skills
- Clinical Skills
- Communication Skills
- Nursing Skills
- Surgical Skills
- Browse content in Medical Dentistry
- Oral and Maxillofacial Surgery
- Paediatric Dentistry
- Restorative Dentistry and Orthodontics
- Surgical Dentistry
- Medical Ethics
- Medical Statistics and Methodology
- Browse content in Neurology
- Clinical Neurophysiology
- Neuropathology
- Nursing Studies
- Browse content in Obstetrics and Gynaecology
- Gynaecology
- Occupational Medicine
- Ophthalmology
- Otolaryngology (ENT)
- Browse content in Paediatrics
- Neonatology
- Browse content in Pathology
- Chemical Pathology
- Clinical Cytogenetics and Molecular Genetics
- Histopathology
- Medical Microbiology and Virology
- Patient Education and Information
- Browse content in Pharmacology
- Psychopharmacology
- Browse content in Popular Health
- Caring for Others
- Complementary and Alternative Medicine
- Self-help and Personal Development
- Browse content in Preclinical Medicine
- Cell Biology
- Molecular Biology and Genetics
- Reproduction, Growth and Development
- Primary Care
- Professional Development in Medicine
- Browse content in Psychiatry
- Addiction Medicine
- Child and Adolescent Psychiatry
- Forensic Psychiatry
- Learning Disabilities
- Old Age Psychiatry
- Psychotherapy
- Browse content in Public Health and Epidemiology
- Epidemiology
- Public Health
- Browse content in Radiology
- Clinical Radiology
- Interventional Radiology
- Nuclear Medicine
- Radiation Oncology
- Reproductive Medicine
- Browse content in Surgery
- Cardiothoracic Surgery
- Gastro-intestinal and Colorectal Surgery
- General Surgery
- Neurosurgery
- Paediatric Surgery
- Peri-operative Care
- Plastic and Reconstructive Surgery
- Surgical Oncology
- Transplant Surgery
- Trauma and Orthopaedic Surgery
- Vascular Surgery
- Browse content in Science and Mathematics
- Browse content in Biological Sciences
- Aquatic Biology
- Biochemistry
- Bioinformatics and Computational Biology
- Developmental Biology
- Ecology and Conservation
- Evolutionary Biology
- Genetics and Genomics
- Microbiology
- Molecular and Cell Biology
- Natural History
- Plant Sciences and Forestry
- Research Methods in Life Sciences
- Structural Biology
- Systems Biology
- Zoology and Animal Sciences
- Browse content in Chemistry
- Analytical Chemistry
- Computational Chemistry
- Crystallography
- Environmental Chemistry
- Industrial Chemistry
- Inorganic Chemistry
- Materials Chemistry
- Medicinal Chemistry
- Mineralogy and Gems
- Organic Chemistry
- Physical Chemistry
- Polymer Chemistry
- Study and Communication Skills in Chemistry
- Theoretical Chemistry
- Browse content in Computer Science
- Artificial Intelligence
- Computer Architecture and Logic Design
- Game Studies
- Human-Computer Interaction
- Mathematical Theory of Computation
- Programming Languages
- Software Engineering
- Systems Analysis and Design
- Virtual Reality
- Browse content in Computing
- Business Applications
- Computer Security
- Computer Games
- Computer Networking and Communications
- Digital Lifestyle
- Graphical and Digital Media Applications
- Operating Systems
- Browse content in Earth Sciences and Geography
- Atmospheric Sciences
- Environmental Geography
- Geology and the Lithosphere
- Maps and Map-making
- Meteorology and Climatology
- Oceanography and Hydrology
- Palaeontology
- Physical Geography and Topography
- Regional Geography
- Soil Science
- Urban Geography
- Browse content in Engineering and Technology
- Agriculture and Farming
- Biological Engineering
- Civil Engineering, Surveying, and Building
- Electronics and Communications Engineering
- Energy Technology
- Engineering (General)
- Environmental Science, Engineering, and Technology
- History of Engineering and Technology
- Mechanical Engineering and Materials
- Technology of Industrial Chemistry
- Transport Technology and Trades
- Browse content in Environmental Science
- Applied Ecology (Environmental Science)
- Conservation of the Environment (Environmental Science)
- Environmental Sustainability
- Environmentalist Thought and Ideology (Environmental Science)
- Management of Land and Natural Resources (Environmental Science)
- Natural Disasters (Environmental Science)
- Nuclear Issues (Environmental Science)
- Pollution and Threats to the Environment (Environmental Science)
- Social Impact of Environmental Issues (Environmental Science)
- History of Science and Technology
- Browse content in Materials Science
- Ceramics and Glasses
- Composite Materials
- Metals, Alloying, and Corrosion
- Nanotechnology
- Browse content in Mathematics
- Applied Mathematics
- Biomathematics and Statistics
- History of Mathematics
- Mathematical Education
- Mathematical Finance
- Mathematical Analysis
- Numerical and Computational Mathematics
- Probability and Statistics
- Pure Mathematics
- Browse content in Neuroscience
- Cognition and Behavioural Neuroscience
- Development of the Nervous System
- Disorders of the Nervous System
- History of Neuroscience
- Invertebrate Neurobiology
- Molecular and Cellular Systems
- Neuroendocrinology and Autonomic Nervous System
- Neuroscientific Techniques
- Sensory and Motor Systems
- Browse content in Physics
- Astronomy and Astrophysics
- Atomic, Molecular, and Optical Physics
- Biological and Medical Physics
- Classical Mechanics
- Computational Physics
- Condensed Matter Physics
- Electromagnetism, Optics, and Acoustics
- History of Physics
- Mathematical and Statistical Physics
- Measurement Science
- Nuclear Physics
- Particles and Fields
- Plasma Physics
- Quantum Physics
- Relativity and Gravitation
- Semiconductor and Mesoscopic Physics
- Browse content in Psychology
- Affective Sciences
- Clinical Psychology
- Cognitive Psychology
- Cognitive Neuroscience
- Criminal and Forensic Psychology
- Developmental Psychology
- Educational Psychology
- Evolutionary Psychology
- Health Psychology
- History and Systems in Psychology
- Music Psychology
- Neuropsychology
- Organizational Psychology
- Psychological Assessment and Testing
- Psychology of Human-Technology Interaction
- Psychology Professional Development and Training
- Research Methods in Psychology
- Social Psychology
- Browse content in Social Sciences
- Browse content in Anthropology
- Anthropology of Religion
- Human Evolution
- Medical Anthropology
- Physical Anthropology
- Regional Anthropology
- Social and Cultural Anthropology
- Theory and Practice of Anthropology
- Browse content in Business and Management
- Business Ethics
- Business Strategy
- Business History
- Business and Technology
- Business and Government
- Business and the Environment
- Comparative Management
- Corporate Governance
- Corporate Social Responsibility
- Entrepreneurship
- Health Management
- Human Resource Management
- Industrial and Employment Relations
- Industry Studies
- Information and Communication Technologies
- International Business
- Knowledge Management
- Management and Management Techniques
- Operations Management
- Organizational Theory and Behaviour
- Pensions and Pension Management
- Public and Nonprofit Management
- Strategic Management
- Supply Chain Management
- Browse content in Criminology and Criminal Justice
- Criminal Justice
- Criminology
- Forms of Crime
- International and Comparative Criminology
- Youth Violence and Juvenile Justice
- Development Studies
- Browse content in Economics
- Agricultural, Environmental, and Natural Resource Economics
- Asian Economics
- Behavioural Finance
- Behavioural Economics and Neuroeconomics
- Econometrics and Mathematical Economics
- Economic History
- Economic Systems
- Economic Methodology
- Economic Development and Growth
- Financial Markets
- Financial Institutions and Services
- General Economics and Teaching
- Health, Education, and Welfare
- History of Economic Thought
- International Economics
- Labour and Demographic Economics
- Law and Economics
- Macroeconomics and Monetary Economics
- Microeconomics
- Public Economics
- Urban, Rural, and Regional Economics
- Welfare Economics
- Browse content in Education
- Adult Education and Continuous Learning
- Care and Counselling of Students
- Early Childhood and Elementary Education
- Educational Equipment and Technology
- Educational Strategies and Policy
- Higher and Further Education
- Organization and Management of Education
- Philosophy and Theory of Education
- Schools Studies
- Secondary Education
- Teaching of a Specific Subject
- Teaching of Specific Groups and Special Educational Needs
- Teaching Skills and Techniques
- Browse content in Environment
- Applied Ecology (Social Science)
- Climate Change
- Conservation of the Environment (Social Science)
- Environmentalist Thought and Ideology (Social Science)
- Natural Disasters (Environment)
- Social Impact of Environmental Issues (Social Science)
- Browse content in Human Geography
- Cultural Geography
- Economic Geography
- Political Geography
- Browse content in Interdisciplinary Studies
- Communication Studies
- Museums, Libraries, and Information Sciences
- Browse content in Politics
- African Politics
- Asian Politics
- Chinese Politics
- Comparative Politics
- Conflict Politics
- Elections and Electoral Studies
- Environmental Politics
- European Union
- Foreign Policy
- Gender and Politics
- Human Rights and Politics
- Indian Politics
- International Relations
- International Organization (Politics)
- International Political Economy
- Irish Politics
- Latin American Politics
- Middle Eastern Politics
- Political Behaviour
- Political Economy
- Political Institutions
- Political Methodology
- Political Communication
- Political Philosophy
- Political Sociology
- Political Theory
- Politics and Law
- Public Policy
- Public Administration
- Quantitative Political Methodology
- Regional Political Studies
- Russian Politics
- Security Studies
- State and Local Government
- UK Politics
- US Politics
- Browse content in Regional and Area Studies
- African Studies
- Asian Studies
- East Asian Studies
- Japanese Studies
- Latin American Studies
- Middle Eastern Studies
- Native American Studies
- Scottish Studies
- Browse content in Research and Information
- Research Methods
- Browse content in Social Work
- Addictions and Substance Misuse
- Adoption and Fostering
- Care of the Elderly
- Child and Adolescent Social Work
- Couple and Family Social Work
- Developmental and Physical Disabilities Social Work
- Direct Practice and Clinical Social Work
- Emergency Services
- Human Behaviour and the Social Environment
- International and Global Issues in Social Work
- Mental and Behavioural Health
- Social Justice and Human Rights
- Social Policy and Advocacy
- Social Work and Crime and Justice
- Social Work Macro Practice
- Social Work Practice Settings
- Social Work Research and Evidence-based Practice
- Welfare and Benefit Systems
- Browse content in Sociology
- Childhood Studies
- Community Development
- Comparative and Historical Sociology
- Economic Sociology
- Gender and Sexuality
- Gerontology and Ageing
- Health, Illness, and Medicine
- Marriage and the Family
- Migration Studies
- Occupations, Professions, and Work
- Organizations
- Population and Demography
- Race and Ethnicity
- Social Theory
- Social Movements and Social Change
- Social Research and Statistics
- Social Stratification, Inequality, and Mobility
- Sociology of Religion
- Sociology of Education
- Sport and Leisure
- Urban and Rural Studies
- Browse content in Warfare and Defence
- Defence Strategy, Planning, and Research
- Land Forces and Warfare
- Military Administration
- Military Life and Institutions
- Naval Forces and Warfare
- Other Warfare and Defence Issues
- Peace Studies and Conflict Resolution
- Weapons and Equipment

- < Previous chapter
- Next chapter >
15 Data Analysis I: Overview of Data Analysis Strategies
Julia Brannen is Professor of Sociology of the Family, Thomas Coram Research Unit, Institute of Education, University of London. Her substantive research has focused on the family lives of parents, children and young people in Britain and Europe, working families and food, and intergenerational relations. She is an academician of the Academy of Social Science in the UK. She has a particular interest in methodology including mixed methods, biographical and narrative approaches and comparative research. Co-founder of The International Journal of Social Research Methodology, she coedited the journal for 17 years with Rosalind Edwards and she is an Associate editor of The Journal of Mixed Methods. An early exponent of MMR, in 1992 she edited Mixing Methods: Qualitative and Quantitative Research (London: Gower). She has written many books journal articles and contributions to methodological texts. Recent books include: The Handbook of Social Research Methods (Sage 2010) Work, Family and Organizations in Transition: A European Perspective (Policy 2009), Transitions to Parenthood in Europe: A comparative life course perspective (Policy 2012).
Rebecca O’Connell is a Senior Research Officer at the Thomas Coram Research Unit (TCRU), Institute of Education, University of London, UK. She is a social anthropologist whose research interests focus on the intersection of care and work, particularly foodwork and childcare. She is currently Principal Investigator on two studies: ‘Families, Food and Work: taking a long view’, a multi-methods longitudinal study funded by the Department of Health and the Economic and Social Research Council (ESRC); and ‘Families and Food in Hard Times, a subproject of the ESRC's National Centre for Research Methods ‘Novella’ node, which is led by Professor Ann Phoenix. Professor Julia Brannen is co-investigator on both studies. From May 2014 Rebecca leads a study of ‘Families and food poverty in three European countries in an age of austerity’, a five-year project funded by the European Research Council. Rebecca is also co-convenor of the British Sociological Association Food Study Group.
- Published: 19 January 2016
- Cite Icon Cite
- Permissions Icon Permissions
This chapter enables the reader to consider issues that are likely to affect the analysis of mixed method research (MMMR). It identifies the ways in which data from multimethod and mixed methods research can be integrated in principle and gives detailed examples of different strategies in practice. Furthermore, it examines a particular type of MMMR and discusses an exemplar study in which national survey data are analyzed alongside a longitudinal qualitative study whose sample is drawn from the same national survey. By working through three analytic issues, it shows the complexities and challenges involved in integrating qualitative and quantitative data: issues about linking data sets, similarity (or not) of units of analysis, and concepts and meaning. It draws some conclusions and sets out some future directions for MMMR.
Introduction
This chapter begins with a consideration of the conditions under which integration is possible (or not). A number of factors that need to be considered before a researcher can decide that integration is possible are briefly discussed. This discussion is followed by a consideration of Caracelli and Greene’s (1993) analysis strategies. Examples of mixed method studies that involve these strategies are described, including the ways they attempt to integrate different data, in particular data transformation, examine typologies and outlier cases, and merge data sets. It is shown that these strategies are not always standalone but can merge into each other. The chapter concludes with a discussion of an extended example of the ways in which a study we carried out called Families, Food and Work (2009–2014) sought to employ analysis of relevant questions from different large-scale data sets with data from a qualitative study of how working parents and children negotiate food and eating ( O’Connell & Brannen, 2015 ).
Issues to Consider Before Conducting Mixed Method Research and Analysis
The researcher should consider a number of issues that need to be revisited during the analysis of the data before embarking on multimethod and mixed methods research (MMMR).
The first concerns the ontological and epistemological assumptions underpinning the choice of methods used to generate the data. Working from the principle that the choice of method is not made in a philosophical void, the data should be thought about in relation to epistemological assumptions underpinning the aspect of the research problem/question being addressed (see, e.g., Barbour, 1999 ). Thus in terms of best practice, researchers may be well advised to consider what kind of knowledge they seek to generate. Most multimethod and mixed methods researchers, while not necessarily thinking of themselves as pragmatists in a philosophical sense, adopt a pragmatic approach ( Bryman, 2008 ). Pragmatism dominates in MMMR ( Omwuegbuzie & Leech, 2005 ), especially among those from more applied fields of the social sciences (in which MMMR has been most widespread). However, pragmatism in this context connotes its common-sense meaning, sidelining philosophical issues so that MMMR strategies are employed as a matter of pragmatics ( Bryman, 2008 ). Some might argue that if different questions are addressed in a study that require different types of knowledge, then the data cannot be integrated unproblematically in the analysis phase. However, it depends on what one means by “integration,” as we later discuss.
The second issue concerns the level of reality under study. Some research questions are about understanding social phenomena at the micro level while others are concerned with social phenomena at the macro level. Thus researchers in the former group emphasize the agency of those they study through an emphasis on studying individuals’ subjective interpretations and perspectives and have allegiances to interpretivist and postmodernist epistemologies. Those working at the macro level are concerned with identifying larger scale patterns and trends and seek to hypothesize or create structural explanations, which may call on realist epistemologies. However, all researchers aim to focus to some extent on the relation between individuals and society. If one is to transcend conceptually the micro and the macro levels, then methods must be developed to reflect this transcendence ( Kelle, 2001 ). For example, in qualitative research that focuses on individuals’ perspectives, it is important to set those perspectives in their social structural and historical contexts. Whether those who apply a paradigm of rationality will apply both qualitative and quantitative methods will depend on the extent to which they seek to produce different levels and types of explanation. This will mean interrogating the linkages between the data analyzes made at these levels.
The third issue relates to the kinds of human experience and social action that the study’s research questions are designed to address. For example, if one is interested in life experiences over long periods of time, researchers will employ life story or other narrative methods. In this case, they need to take into account the way stories are framed, and in particular how temporal perspectives, the purposes of the narrator, and the way stories are told influence the stories. The data the researchers will collect are therefore narrative data. Hence how these stories fit, for example, with quantitative data collected as part of a MMMR approach will require close interrogation in the analysis of the two data sets, taking into account both interpretive and realist historical approaches.
The fourth issue to consider is whether the data are primary or secondary and, in the latter case, whether they are subjected to secondary analysis. Secondary data are by definition collected by other people, although access to them may not be straightforward. If the data have already been coded and the original data are not available, the types of secondary analysis possible will be limited. Moreover, the preexistence of these data may influence the timetabling of the MMMR project and may also shape the questions that are framed in any subsequent qualitative phase and in the data analysis. Depending on the nature and characteristics of the data, one data set may prove intrinsically more interesting; thus more time and attention may be given to its analysis. A related issue therefore concerns the possibilities for operationalizing the concepts employed in relation to the different parts of the MMMR inquiry. Preexisting data, especially those of a quantitative type, may make it difficult to reconceptualize the problem. At a practical level, the questions asked in a survey may poorly relate to those that fit the MMMR inquiry, as we later illustrate. Since one does not know what one does not know, it may be only at later stages that researchers working across disciplines and methodologies may come to realize which questions cannot be addressed and which data are missing.
The fifth issue relates to the environments in which researchers are located. For example, are the research and the researcher operating within the same research setting, for example the same discipline, the same theoretical and methodological tradition, or the same policy social context? MMMR fits with the political currency accorded to “practical inquiry” that speaks to policy and policymakers and that informs practice, as distinct from scientific research ( Hammersley, 2000 ). However, with respect to policy, this has to be set in the context of the continued policy importance afforded to large-scale data but also the increased scale of these data sets and the growth in the availability of official administrative data. In turn, these trends have been matched by the increased capacity of computing power to manage and analyze these data ( Brannen & Moss, 2013 ) and the increased pressure on social scientists to specialize in high-level quantitative data analysis. As more such data accrue, the apparent demand for quantitative analysis increases ( Brannen & Moss, 2013 ). However, MMMR strategies are also often employed alongside such quantitative analysis, especially in policy-driven research. For example, in cross-national research, governmental organizations require comparative data to assess how countries are doing in a number of different fields, a process that has become an integral part of performance monitoring. But, equally, there is a requirement for policy analysis and inquiries into how policies work in particular local conditions. Such micro-level analysis will require methods like documentary analysis, discourse analysis, case study designs, and intensive research approaches. Furthermore, qualitative data are thought useful to “bring alive” research for policy and practitioner audiences ( O’Cathain, 2009 ).
Another aspect of environment relates to the sixth issue concerning the constitution of the research team and the extent to which it is inter or transdisciplinary . Research teams can be understood as “communities of practice” ( Denscombe, 2008 ). While paradigms are pervasive ways of dividing social science research, as Morgan (2007) argues, we need to think in terms of shared beliefs within communities of researchers. This requires an ethic of “precarity” to prevail ( Ettlinger, 2007 , p. 319), through which researchers are open to others’ ideas and can relinquish entrenched positions. However, the success of communities of practice will depend on the political context, their composition, and whether they are democratic ( Hammersley, 2005 ). Thus in the analysis of MMMR, it is important to be cognizant of the power relations with such communities of practice since they will influence the researcher’s room for maneuvering in determining directions and outputs of the data analysis. At the same time, these political issues affect analysis and dissemination in research teams in which members share disciplinary approaches.
Finally, there are the methodological preferences, skills, and specialisms of the researcher, all of which have implications for the quality of the data and the data analysis. MMMR offers the opportunity to learn about a range of methods and thus to be open to new ways of addressing research questions. Broadening one’s methodological repertoire mitigates against “trained incapacities” as Reiss (1968) termed the issue—the entrenchment of researchers in particular types of research paradigms, as well as questions, research methods, and types of analysis.
The Context of Inquiry: Research Questions and Research Design
The rationale for MMMR must be clear both in the phase of the project’s research design (the context of the inquiry) and in the analysis phase (the context of justification). At the research design phase, researchers wrestle with such fundamental methodological questions as to what kinds of knowledge they seek to generate, such as whether to describe and understand a social phenomenon or seek to explain it. Do we wish to do both, that is, to understand and explain? In the latter case, the research strategy will typically translate itself into employing a mix of qualitative and quantitative methods, which some argue is the defining characteristic of mixed method research (MMR) ( Tashakorri & Creswell, 2007 ).
If a MMR strategy is employed, this generally implies that a number of research questions will address a substantive issue. MMMR is also justified in terms of its capacity to address different aspects of a research question. This is turns leads researchers to consider how to frame their research questions and how these determine the methods chosen. Typically research questions are formulated in the research proposal. However, they should also be amenable to adaptation ( Harrits, 2011 , citing Dewey, 1991 ); adaptations may be necessary as researchers respond to the actual conditions of the inquiry. According to Law (2004) , research is an “assemblage,” that is, something not fixed in shape but incorporating tacit knowledge, research skills, resources, and political agenda that are “constructed” as they are woven together (p. 42). Methodology should be rebuilt during the research process in a way that responds to research needs and the conditions encountered—what Seltzer-Kelly, Westwood, and Pena-Guzman (2012) term “a constructivist stance at the methodological level” (p. 270). This can also happen at the phase when data are analyzed.
Developing a coherent methodology with a close link between the research question and the research strategy holds out the best hope for answering a project’s objectives and questions ( Woolley, 2009 , p. 8). Thus Yin (2006) would say that to carry out an MMMR analysis it is essential to have an integrated set of research questions. However, it is not easy to determine what constitutes coherence. For example, the research question concerning the link between the quality of children’s diet in the general population and whether mothers are in paid employment may be considered a very different and not necessarily complementary question to the research question about the conditions under which the children of working mothers are fed. Thus we have to consider here how tightly or loosely the research questions interconnect.
The framing of the research question influences the method chosen that, in turn, influences the choice of analytic method. Thus in our study of children’s food that examined the link between children’s diet and maternal employment, we examined a number of large-scale data sets and carried out statistical analyzes on these, while in studying the conditions under which children in working families get fed, we carried out qualitative case analysis on a subset of households selected from one of the large-scale data sets.
The Context of Justification: The Analysis Phase
In the analysis phase of MMMR, the framing of the research questions becomes critical, affecting when, to what extent, and in what ways data from different methods are integrated. So, for example, we have to consider the temporal ordering of methods. For example, quantitative data on a research topic may be available and the results already analyzed. This analysis may influence the questions to be posed in the qualitative phase of inquiry.
Thus it is also necessary to consider the compatibility between the units of analysis in the quantitative phase and the qualitative phase of the study, for example, between variables studied in a survey and the analytic units studied in a qualitative study. Are we seeking analytic units that are equivalent (but not similar), or are we seeking to analyze a different aspect of a social phenomenon? If the latter, how do the two analyses relate? This may become more critical if the same population is covered in both the qualitative and quantitative phases. What happens when a nested or integrated sampling strategy is employed, as in the case of a large-scale survey analysis and a qualitative analysis based on a subsample of the survey?
A number of frameworks have been suggested for integrating data produced by quantitative and qualitative methods ( Brannen, 1992 ; Caracelli & Greene, 1993 ; Greene, Caracelli, & Graham, 1989 ). While these may provide a guide to the variety of ways to integrate data, they should not be used as fixed templates. Indeed, they may provide a basis for reflection after the analysis has been completed.
Corroboration —in which one set of results based on one method are confirmed by those gained through the application of another method.
Elaboration or expansion —in which qualitative data analysis may exemplify how patterns based on quantitative data analysis apply in particular cases. Here the use of one type of data analysis adds to the understanding gained by another.
Initiation —in which the use of a first method sparks new hypotheses or research questions that can be pursued using a different method.
Complementarity —in which qualitative and quantitative results are regarded as different beasts but are meshed together so that each data analysis enhances the other ( Mason, 2006 ). The data analyses from the two methods are juxtaposed and generate complementary insights that together create a bigger picture.
Contradiction —in which qualitative data and quantitative findings conflict. Exploring contradictions between different types of data assumed to reflect the same phenomenon may lead to an interrogation of the methods and to discounting one method in favor of another (in terms of assessments of validity or reliability). Alternatively, the researcher may simply juxtapose the contradictions for others to explore in further research. More commonly, one type of data may be presented and assumed to be “better,” rather than seeking to explain the contradictions in relation to some ontological reality ( Denzin & Lincoln, 2005 ; Greene et al., 1989 ).
As Hammersley (2005) points out, all these ways of combining different data analyses to some extent make assumptions that there is some reality out there to be captured, despite the caveats expressed about how each method constructs the data differently. Thus, just as seeking to corroborate data may not lead us down the path of “validation,” so too the complementarity rationale for mixing methods may not complete the picture either. There may be no meeting point between epistemological positions. As Hammersley (2008) suggests, there is a need for a dialogue between them in the recognition that absolute certainty is never justified and that “we must treat knowledge claims as equally doubtful or that we should judge them on grounds other than their likely truth” (p. 51).
Multimethod and Mixed Methods Research Analysis Strategies: Examples of Studies
Caracelli and Greene (1993) suggest analysis strategies for integrating qualitative and quantitative data. In practice these strategies are not always standalone but blur into each other. Moreover, as Bryman (2008) has observed, it is relatively rare for mixed method researchers to give full rationales for MMMR designs. They can involve data transformation in which, for example, qualitative data are treated quantitatively. They may involve typology development in which cases are categorized in patterns and outlier cases are scrutinized. They may involve data merging in which both data sets are treated in similar ways, for instance, by creating similar variables or equivalent units of analysis across data sets. In this section, drawing on the categorization of Caracelli and Greene, we give some examples of studies in which qualitative and quantitative data are integrated in these different ways (Table 15.1 ). These are not intended to be exhaustive, nor are the studies pure examples of these strategies.
Qualitative Data Are Transformed into Quantitative Data or Vice Versa
In survey research, in order to test how respondents understand questions it is commonplace to transform qualitative data into quantitative data. This is termed cognitive testing . The aim here is to find a fit between responses given in both the survey and the qualitative testing. For example, most personality scales are based on prior clinical research. An example of data transformation on a larger scale is taken from a program of research on the wider benefits of adult learning ( Hammond, 2005 ). The rationale for the study was that the research area was underresearched and the research questions relatively unformulated (p. 241). Qualitative research was carried out to identify variables to test on an existing national longitudinal data set. The qualitative phase involved biographical interviews with adult learners. The quantitative data consisted of data from an existing UK cohort study (the 1958 National Child Development Study). A main justification for using these latter data concerned the further exploitation of data that are expensive to collect. The qualitative component was conceived as a “mapping” exercise carried out to inform the research design and the implementation of the quantitative phase, that is, the identification of variables for quantitative analysis ( Hammond, 2005 , p. 243). This approach has parallels with qualitative pilot work carried out as a prologue to a survey, although the qualitative material was also analyzed in its own right. However, while the qualitative data were used with the aim of finding common measures that fit with the quantitative inquiry, Hammond also insisted that the qualitative data not be used to explain quantitatively derived outcomes but to interrogate them further ( Hammond, 2005 , p. 244). Inevitably, contradictions between the respective findings arose. For example, Hammond reported that the effect of adult learning on life satisfaction (the transformed measure) found in the National Child Development Study cohort analysis was greater for men than for women, while women reported themselves in the biographical interview responses to be positive about the courses they had taken. On this issue, the biographical interviews were regarded as being “more sensitive” than the quantitative measure. Hammond also suggested that the interview data showed that an improved sense of well-being (another transformed measure) experienced by the respondents in the present was not necessarily incompatible with having a negative view of the future. The quantitative data conflated satisfaction with “life so far” and with “life in the future.” Contradictions were also explained in terms of the lack of representativeness of the qualitative study (the samples did not overlap). In addition, it is possible that priority was given by the researcher to the biographical interviews and may have placed more trust in this approach. Another possibly relevant factor was that the researcher had no stake in creating or shaping the quantitative data set. In any event, the biographical interviews were conducted before the quantitative analyses and were used to influence the decisions about which analyses to focus on in the quantitative analysis. Hence the qualitative data threw up hypotheses that the quantitative data were used to reject or support. What is interesting about using qualitative data to test on quantitative evidence is the opportunity it offers to pose or initiate new lines of questioning ( Greene et al., 1989 )—a result not necessarily anticipated at the outset of this research.
Typologies, Deviant, Negative, or Outlier Cases Are Subjected to Further Scrutiny Later or in Another Data Set
A longitudinal or multilayered design provides researchers with opportunities to examine the strength of the conclusions that can be drawn about the cases and the phenomena under study ( Nilsen & Brannen, 2010 ). For an example of this strategy, we turn to the classic study carried by Glueck and Glueck (1950 , 1968 ). The study Five Hundred Criminal Careers was based on longitudinal research of delinquents and nondelinquents (1949–1965). The Gluecks studied the two groups at three age points; 14, 25, and 32. The study had a remarkably high (92%) response rate when adjusted for mortality at the third wave. The Gluecks collected very rich data on a variety of dimensions and embedded open-ended questions within a survey framework. Interviews with respondents and their families, as well as key informants (social workers, school teachers, employers, and neighbors), were carried out, together with home observations and the study of official records and criminal histories. Some decades later, Laub and Sampson (1993 , 1998) reanalyzed these data longitudinally (the Gluecks’ original analyzes were cross-sectional).
Laub and Sampson (1998) note that the Gluecks’ material “represents the comparison, reconciliation and integration of these multiple sources of data” (p. 217) although the Gluecks did not treat the qualitative data in their own right. The original study was firmly grounded in a quantitative logic where the purpose was to arrive at causal explanations and the ability to predict criminal behavior . However, the Gluecks were carrying out their research in a pre-computer age, a fact that facilitated reanalysis of the material. When Laub and Sampson came to recode the raw data many years later, they rebuilt the Gluecks’ original data set and used their coding schemes to validate their original analyzes. Laub and Sampson then constructed the criminal histories of the sample, drawing on and integrating the different kinds of data available. This involved merging data.
Next they purposively selected a subset of cases for intensive qualitative analysis in order to explore consistencies and inconsistencies between the original findings and the original study’s predictions for the delinquents’ future criminal careers—what happened to them some decades later. They examined “off diagonal” and “negative cases” that did not fit the quantitative results and predictions. In particular, they selected individuals who, on the basis of their earlier careers, were expected to follow a life of crime but did not and those expected to cease criminality but did not.
Citing Jick (1979) , Laub and Sampson (1998) suggest how divergence can become an opportunity for enriching explanations (p. 223). By examining deviant cases on the basis of one data analysis and interrogating these in a second data analysis, they demonstrated complex processes of individual pathways into and out of crime, including identified pathways, that take place over long time periods ( Laub & Sampson, 1998 , p. 222). They argued that “without qualitative data, discussions of continuity often mask complex and rich qualitative processes” (Sampson & Laub, 1997, quoted in Laub & Sampson 1998 , p. 229).
In addition they supported a biographical approach that enables the researcher to interpret data in historical context, in this case to understand criminality in relation to the type and level of crime prevalent at the time. Laub and Sampson (1998) selected a further subsample of the original sample of delinquents, having managed to trace them after 50 years ( Laub & Sampson, 1993 ) and asked them to review their past lives. The researchers were particularly interested in identifying turning points to understand what had shaped the often unexpected discontinuities and continuities in the careers of these one-time delinquents.
This is an exemplar study of the analytic strategy of subjecting typologies to deeper scrutiny. It also afforded an opportunity to theorize about the conditions concerning cases that deviated from predicted trajectories.
Data Merging: The Same Set of Variables Is Created Across Quantitative and Qualitative Data Sets
Here assumptions are made that the phenomena under study are similar in both the qualitative and quantitative parts of an inquiry, a strategy exemplified in the following two studies. The treatment of the data in both parts of the study was seamless, so that one type of data is transformed into the other. In a longitudinal study, Blatchford (2005) examined the relationship between classroom size and pupils’ educational achievement. Blatchford justifies using a mixed method strategy in terms of the power of mixed methods to reconciling inconsistencies found in previous research. The rationale given for using qualitative methods was the need to assess the relationships between the same variables but in particular case studies. Blatchford notes that “priorities had to be set and some areas of investigation received more attention than others” (p. 204). The dominance of the quantitative analysis occurred despite the collection of “fine grained data on classroom processes” that could have lent themselves to other kinds of analysis, such as understanding how students learn in different classroom environments. The qualitative data were in fact put to limited use and were merged with the quantitative data.
Sammons et al. (2005) similarly employed a longitudinal quantitative design to explore the effects of preschool education on children’s attainment and development at entry to school. Using a purposive rationale, they selected a smaller number of early education centers from their original sample on the basis of their contrasting profiles. Sammons et al. coded the qualitative data in such a way that the “reduced data” (p. 219) were used to provide statistical explanations for the outcomes produced in the quantitative longitudinal study. Thus, again, the insights derived from the qualitative data analysis were merged with the quantitative variables, which were correlated with outcome variables on children’s attainment. The researchers in question could have drawn on both the qualitative and quantitative data for different insights, as is required in case study research ( Yin, 2006 ) and as suggested in their purposive choice of preschool centers.
Using Quantitative and Qualitative Data: A Longitudinal Study of Working Families and Food
In this final part of the chapter we take an example from our own work in which we faced a number of methodological issues in integrating and meshing different types of data. In this section we discuss some of the challenges involved in the collection and analysis of such data.
The study we carried out is an example of designing quantitative and qualitative constituent parts to address differently framed questions. Its questions were, and remain, currently highly topical in the Western world and concern the influences of health policy on healthy eating, including in childhood, and its implications for obesity. 1 Much of the health evidence is unable to explain why it is that families appear to ignore advice and continue to eat in unhealthy ways. The project arose in the context of some existing research that suggests an association between parental (maternal) employment and children’s (poor) diet ( Hawkins, Cole, & Law, 2009 ). We pursued these issues by framing the research phenomenon in different ways and through the analysis of different data sets.
The project was initiated in a policy context in which we tendered successfully for a project that enabled us to exploit a data set commissioned by government to examine the nation’s diet. Somewhat of a landmark study in the UK, the project is directly linked to the National Diet and Nutrition Survey (NDNS) funded by the UK’s Food Standards Agency and Department of Health, a study largely designed by those from public health and nutritionist perspectives. These data, from the first wave of the new rolling survey, were unavailable to others at that time. We were also facilitated in selecting a subsample of households with children from the NDNS that we subjected to a range of qualitative methods. The research team worked closely with the UK government to gain access to the data collected and managed by an independent research agency in the identification of a subsample to meet the research criteria and in seeking the consent of the survey subsample participants.
Applying anthropological and sociological lenses, the ethnographically trained researchers in the team sought to explore inductively parents’ experiences of negotiating the demands of “work” and “home” and domestic food provisioning in families. We therefore sought to understand the contextual and embodied meanings of food practices and their situatedness in different social contexts (inside and outside the home). We also assumed that children are agents in their own lives, and therefore we included children in the study and examined the ways in which children reported food practices and attributed meaning to food. The main research questions (RQ) for the study were:
What is the relationship between parental employment and the diets of children (aged 1.5 to 10 years)?
How does food fit into working family life and how do parents experience the demands of “work” and “home” in managing food provisioning?
How do parents and children negotiate food practices?
What foods do children of working parents eat in different contexts—home, childcare, and school—and how do children negotiate food practices?
The study not only employed a MMMR strategy but was also longitudinal, a design that is rarely discussed in the MMMR literature. We conducted a follow-up study (Wave 2) approximately two years later, which repeated some questions and additionally asked about social change, the division of food work, and the social practice of family meals. The first research question was to be addressed through the survey data while RQ 2, 3, and 4 were addressed through the qualitative study. In the qualitative study, a variety of ethnographic methods were to be deployed with both parents and children ages 2 to 10 years. The ethnographic methods included a range of interactive research tools, which were used flexibly with the children since their age span is wide: interviews, drawing methods, and, with some children, photo elicitation interviews in which children photographed foods and meals consumed within and outside the home and discussed these with the researcher at a later visit. Semistructured interviews were carried out with parents who defined themselves as the main food providers and sometimes with an additional parent or care-provider who was involved in food work and also wished to participate.
In the context of existing research that suggests an association between parental (maternal) employment and household income with children’s (poor) diet ( Hawkins et al., 2009 ) carried out on a different UK data set and also supported by some US research (e.g. Crepinsek & Burstein, 2004 ; McIntosh et al., 2008 ), it was important to investigate whether this association was born out elsewhere. In addition and in parallel, we therefore carried out secondary analysis on the NDNS Year 1 (2008/2009) data and on two other large-scale national surveys, the Health Survey for England (surveys, 2007, 2008) and the Avon Longitudinal Study of Parents and Children (otherwise known as “Children of the Nineties”) (data sweeps 1995/1996, 1996/1997, and 1997/1999) to examine the first research question. This part of the work was not straightforward. First we found that, contrary to a previous NDNS (1997) survey that had classified mothers’ working hours as full or part-time, neither mothers’ hours of work nor full/part-time status had been collected in the new rolling NDNS survey. Rather, this information was limited in most cases to whether a mother was or was not in paid employment. Thus it was not possible to disentangle the effects of mothers working full-time from those doing part-time hours on children’s diets. This was unfortunate since the NDNS provided very detailed data on children’s nutrition based on food diaries, unlike the Millennium Cohort Study, which collected only mothers’ reports of children’s snacking between meals at home ( Hawkins et al., 2009 ). While the Millennium Cohort Study analysis found a relationship between long hours of maternal employment and children’s dietary intake, no association between mothers’ employment and children’s dietary intake was found in the NDNS ( O’Connell, Brannen, Mooney, Knight, & Simon, 2011 ; Simon et al., forthcoming ). However, it is possible that a relationship might have been found if we had been able to disaggregate women’s employment by hours.
In the following we describe three instances of data analysis in this longitudinal MMMR study in relation to some of the key analytic issues set out in the research questions described previously (see Table 15.2 ).
Studying children’s diets in a MMMR design
Examining the division of household food work in a MMMR design
Making sense of family meals in a MMMR design
Linking Data in a Longitudinal Multimethod and Mixed Methods Research Design: Studying Children’s Diets
The research problem.
Together with drawing a sample for qualitative study from the national survey, we aimed to carry out secondary analysis on the NDNS data in order to generate patterns of “what” is eaten by children and parents and to explore associations with a range of independent variables, notably mothers’ employment. The NDNS diet data were based on four-day unweighed food diaries that recorded detailed information about quantities of foods and drinks consumed, as well as where, when, and with whom foods were eaten ( Bates, Lennox, Bates, & Swan, 2011 ). On behalf of the NDNS survey, the diaries were subjected by researchers at Human Nutrition Research, Cambridge University, to an analysis of nutrient intakes using specialist dietary recording and analysis software ( Bates et al., 2011 ; Data In Nutrients Out [DINO]).
Qualitative vs Quantitative Research Methods & Data Analysis
Saul Mcleod, PhD
Editor-in-Chief for Simply Psychology
BSc (Hons) Psychology, MRes, PhD, University of Manchester
Saul Mcleod, PhD., is a qualified psychology teacher with over 18 years of experience in further and higher education. He has been published in peer-reviewed journals, including the Journal of Clinical Psychology.
Learn about our Editorial Process
Olivia Guy-Evans, MSc
Associate Editor for Simply Psychology
BSc (Hons) Psychology, MSc Psychology of Education
Olivia Guy-Evans is a writer and associate editor for Simply Psychology. She has previously worked in healthcare and educational sectors.
On This Page:
What is the difference between quantitative and qualitative?
The main difference between quantitative and qualitative research is the type of data they collect and analyze.
Quantitative research collects numerical data and analyzes it using statistical methods. The aim is to produce objective, empirical data that can be measured and expressed in numerical terms. Quantitative research is often used to test hypotheses, identify patterns, and make predictions.
Qualitative research , on the other hand, collects non-numerical data such as words, images, and sounds. The focus is on exploring subjective experiences, opinions, and attitudes, often through observation and interviews.
Qualitative research aims to produce rich and detailed descriptions of the phenomenon being studied, and to uncover new insights and meanings.
Quantitative data is information about quantities, and therefore numbers, and qualitative data is descriptive, and regards phenomenon which can be observed but not measured, such as language.
What Is Qualitative Research?
Qualitative research is the process of collecting, analyzing, and interpreting non-numerical data, such as language. Qualitative research can be used to understand how an individual subjectively perceives and gives meaning to their social reality.
Qualitative data is non-numerical data, such as text, video, photographs, or audio recordings. This type of data can be collected using diary accounts or in-depth interviews and analyzed using grounded theory or thematic analysis.
Qualitative research is multimethod in focus, involving an interpretive, naturalistic approach to its subject matter. This means that qualitative researchers study things in their natural settings, attempting to make sense of, or interpret, phenomena in terms of the meanings people bring to them. Denzin and Lincoln (1994, p. 2)
Interest in qualitative data came about as the result of the dissatisfaction of some psychologists (e.g., Carl Rogers) with the scientific study of psychologists such as behaviorists (e.g., Skinner ).
Since psychologists study people, the traditional approach to science is not seen as an appropriate way of carrying out research since it fails to capture the totality of human experience and the essence of being human. Exploring participants’ experiences is known as a phenomenological approach (re: Humanism ).
Qualitative research is primarily concerned with meaning, subjectivity, and lived experience. The goal is to understand the quality and texture of people’s experiences, how they make sense of them, and the implications for their lives.
Qualitative research aims to understand the social reality of individuals, groups, and cultures as nearly as possible as participants feel or live it. Thus, people and groups are studied in their natural setting.
Some examples of qualitative research questions are provided, such as what an experience feels like, how people talk about something, how they make sense of an experience, and how events unfold for people.
Research following a qualitative approach is exploratory and seeks to explain ‘how’ and ‘why’ a particular phenomenon, or behavior, operates as it does in a particular context. It can be used to generate hypotheses and theories from the data.
Qualitative Methods
There are different types of qualitative research methods, including diary accounts, in-depth interviews , documents, focus groups , case study research , and ethnography.
The results of qualitative methods provide a deep understanding of how people perceive their social realities and in consequence, how they act within the social world.
The researcher has several methods for collecting empirical materials, ranging from the interview to direct observation, to the analysis of artifacts, documents, and cultural records, to the use of visual materials or personal experience. Denzin and Lincoln (1994, p. 14)
Here are some examples of qualitative data:
Interview transcripts : Verbatim records of what participants said during an interview or focus group. They allow researchers to identify common themes and patterns, and draw conclusions based on the data. Interview transcripts can also be useful in providing direct quotes and examples to support research findings.
Observations : The researcher typically takes detailed notes on what they observe, including any contextual information, nonverbal cues, or other relevant details. The resulting observational data can be analyzed to gain insights into social phenomena, such as human behavior, social interactions, and cultural practices.
Unstructured interviews : generate qualitative data through the use of open questions. This allows the respondent to talk in some depth, choosing their own words. This helps the researcher develop a real sense of a person’s understanding of a situation.
Diaries or journals : Written accounts of personal experiences or reflections.
Notice that qualitative data could be much more than just words or text. Photographs, videos, sound recordings, and so on, can be considered qualitative data. Visual data can be used to understand behaviors, environments, and social interactions.
Qualitative Data Analysis
Qualitative research is endlessly creative and interpretive. The researcher does not just leave the field with mountains of empirical data and then easily write up his or her findings.
Qualitative interpretations are constructed, and various techniques can be used to make sense of the data, such as content analysis, grounded theory (Glaser & Strauss, 1967), thematic analysis (Braun & Clarke, 2006), or discourse analysis.
For example, thematic analysis is a qualitative approach that involves identifying implicit or explicit ideas within the data. Themes will often emerge once the data has been coded.
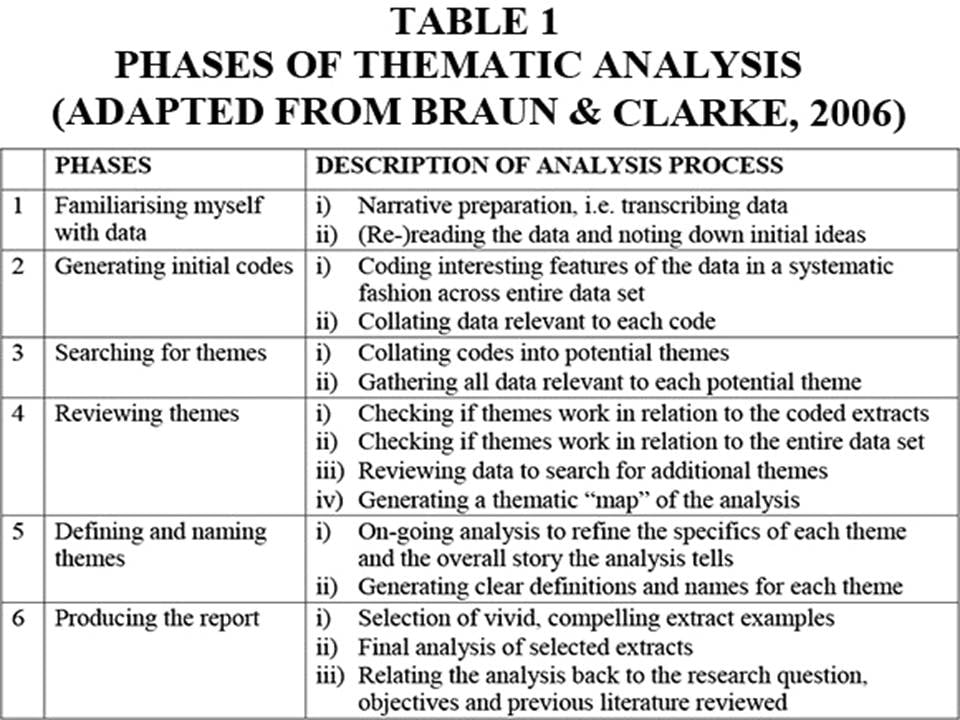
Key Features
- Events can be understood adequately only if they are seen in context. Therefore, a qualitative researcher immerses her/himself in the field, in natural surroundings. The contexts of inquiry are not contrived; they are natural. Nothing is predefined or taken for granted.
- Qualitative researchers want those who are studied to speak for themselves, to provide their perspectives in words and other actions. Therefore, qualitative research is an interactive process in which the persons studied teach the researcher about their lives.
- The qualitative researcher is an integral part of the data; without the active participation of the researcher, no data exists.
- The study’s design evolves during the research and can be adjusted or changed as it progresses. For the qualitative researcher, there is no single reality. It is subjective and exists only in reference to the observer.
- The theory is data-driven and emerges as part of the research process, evolving from the data as they are collected.
Limitations of Qualitative Research
- Because of the time and costs involved, qualitative designs do not generally draw samples from large-scale data sets.
- The problem of adequate validity or reliability is a major criticism. Because of the subjective nature of qualitative data and its origin in single contexts, it is difficult to apply conventional standards of reliability and validity. For example, because of the central role played by the researcher in the generation of data, it is not possible to replicate qualitative studies.
- Also, contexts, situations, events, conditions, and interactions cannot be replicated to any extent, nor can generalizations be made to a wider context than the one studied with confidence.
- The time required for data collection, analysis, and interpretation is lengthy. Analysis of qualitative data is difficult, and expert knowledge of an area is necessary to interpret qualitative data. Great care must be taken when doing so, for example, looking for mental illness symptoms.
Advantages of Qualitative Research
- Because of close researcher involvement, the researcher gains an insider’s view of the field. This allows the researcher to find issues that are often missed (such as subtleties and complexities) by the scientific, more positivistic inquiries.
- Qualitative descriptions can be important in suggesting possible relationships, causes, effects, and dynamic processes.
- Qualitative analysis allows for ambiguities/contradictions in the data, which reflect social reality (Denscombe, 2010).
- Qualitative research uses a descriptive, narrative style; this research might be of particular benefit to the practitioner as she or he could turn to qualitative reports to examine forms of knowledge that might otherwise be unavailable, thereby gaining new insight.
What Is Quantitative Research?
Quantitative research involves the process of objectively collecting and analyzing numerical data to describe, predict, or control variables of interest.
The goals of quantitative research are to test causal relationships between variables , make predictions, and generalize results to wider populations.
Quantitative researchers aim to establish general laws of behavior and phenomenon across different settings/contexts. Research is used to test a theory and ultimately support or reject it.
Quantitative Methods
Experiments typically yield quantitative data, as they are concerned with measuring things. However, other research methods, such as controlled observations and questionnaires , can produce both quantitative information.
For example, a rating scale or closed questions on a questionnaire would generate quantitative data as these produce either numerical data or data that can be put into categories (e.g., “yes,” “no” answers).
Experimental methods limit how research participants react to and express appropriate social behavior.
Findings are, therefore, likely to be context-bound and simply a reflection of the assumptions that the researcher brings to the investigation.
There are numerous examples of quantitative data in psychological research, including mental health. Here are a few examples:
Another example is the Experience in Close Relationships Scale (ECR), a self-report questionnaire widely used to assess adult attachment styles .
The ECR provides quantitative data that can be used to assess attachment styles and predict relationship outcomes.
Neuroimaging data : Neuroimaging techniques, such as MRI and fMRI, provide quantitative data on brain structure and function.
This data can be analyzed to identify brain regions involved in specific mental processes or disorders.
For example, the Beck Depression Inventory (BDI) is a clinician-administered questionnaire widely used to assess the severity of depressive symptoms in individuals.
The BDI consists of 21 questions, each scored on a scale of 0 to 3, with higher scores indicating more severe depressive symptoms.
Quantitative Data Analysis
Statistics help us turn quantitative data into useful information to help with decision-making. We can use statistics to summarize our data, describing patterns, relationships, and connections. Statistics can be descriptive or inferential.
Descriptive statistics help us to summarize our data. In contrast, inferential statistics are used to identify statistically significant differences between groups of data (such as intervention and control groups in a randomized control study).
- Quantitative researchers try to control extraneous variables by conducting their studies in the lab.
- The research aims for objectivity (i.e., without bias) and is separated from the data.
- The design of the study is determined before it begins.
- For the quantitative researcher, the reality is objective, exists separately from the researcher, and can be seen by anyone.
- Research is used to test a theory and ultimately support or reject it.
Limitations of Quantitative Research
- Context: Quantitative experiments do not take place in natural settings. In addition, they do not allow participants to explain their choices or the meaning of the questions they may have for those participants (Carr, 1994).
- Researcher expertise: Poor knowledge of the application of statistical analysis may negatively affect analysis and subsequent interpretation (Black, 1999).
- Variability of data quantity: Large sample sizes are needed for more accurate analysis. Small-scale quantitative studies may be less reliable because of the low quantity of data (Denscombe, 2010). This also affects the ability to generalize study findings to wider populations.
- Confirmation bias: The researcher might miss observing phenomena because of focus on theory or hypothesis testing rather than on the theory of hypothesis generation.
Advantages of Quantitative Research
- Scientific objectivity: Quantitative data can be interpreted with statistical analysis, and since statistics are based on the principles of mathematics, the quantitative approach is viewed as scientifically objective and rational (Carr, 1994; Denscombe, 2010).
- Useful for testing and validating already constructed theories.
- Rapid analysis: Sophisticated software removes much of the need for prolonged data analysis, especially with large volumes of data involved (Antonius, 2003).
- Replication: Quantitative data is based on measured values and can be checked by others because numerical data is less open to ambiguities of interpretation.
- Hypotheses can also be tested because of statistical analysis (Antonius, 2003).
Antonius, R. (2003). Interpreting quantitative data with SPSS . Sage.
Black, T. R. (1999). Doing quantitative research in the social sciences: An integrated approach to research design, measurement and statistics . Sage.
Braun, V. & Clarke, V. (2006). Using thematic analysis in psychology . Qualitative Research in Psychology , 3, 77–101.
Carr, L. T. (1994). The strengths and weaknesses of quantitative and qualitative research : what method for nursing? Journal of advanced nursing, 20(4) , 716-721.
Denscombe, M. (2010). The Good Research Guide: for small-scale social research. McGraw Hill.
Denzin, N., & Lincoln. Y. (1994). Handbook of Qualitative Research. Thousand Oaks, CA, US: Sage Publications Inc.
Glaser, B. G., Strauss, A. L., & Strutzel, E. (1968). The discovery of grounded theory; strategies for qualitative research. Nursing research, 17(4) , 364.
Minichiello, V. (1990). In-Depth Interviewing: Researching People. Longman Cheshire.
Punch, K. (1998). Introduction to Social Research: Quantitative and Qualitative Approaches. London: Sage
Further Information
- Designing qualitative research
- Methods of data collection and analysis
- Introduction to quantitative and qualitative research
- Checklists for improving rigour in qualitative research: a case of the tail wagging the dog?
- Qualitative research in health care: Analysing qualitative data
- Qualitative data analysis: the framework approach
- Using the framework method for the analysis of
- Qualitative data in multi-disciplinary health research
- Content Analysis
- Grounded Theory
- Thematic Analysis

Related Articles
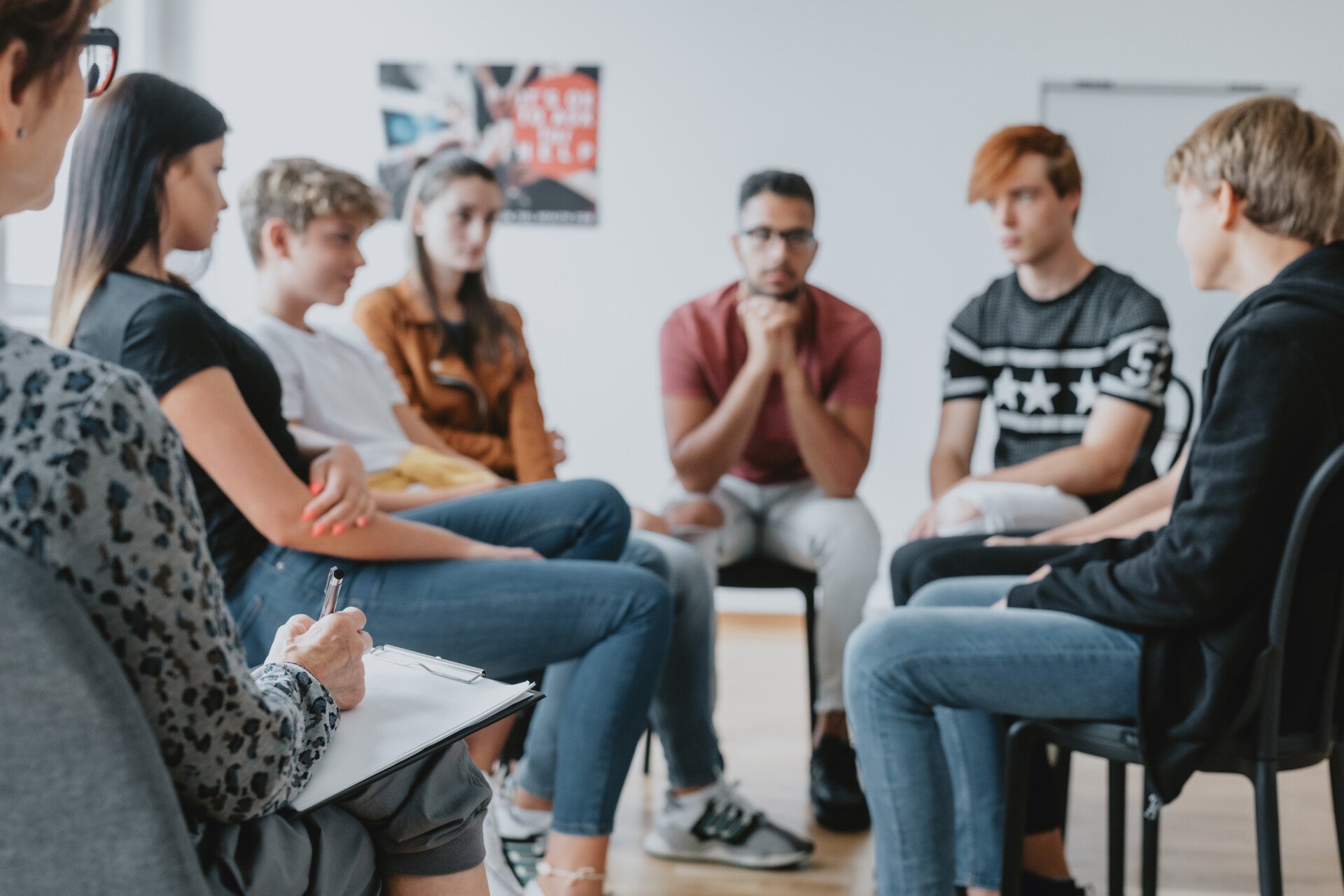
Research Methodology
What Is a Focus Group?
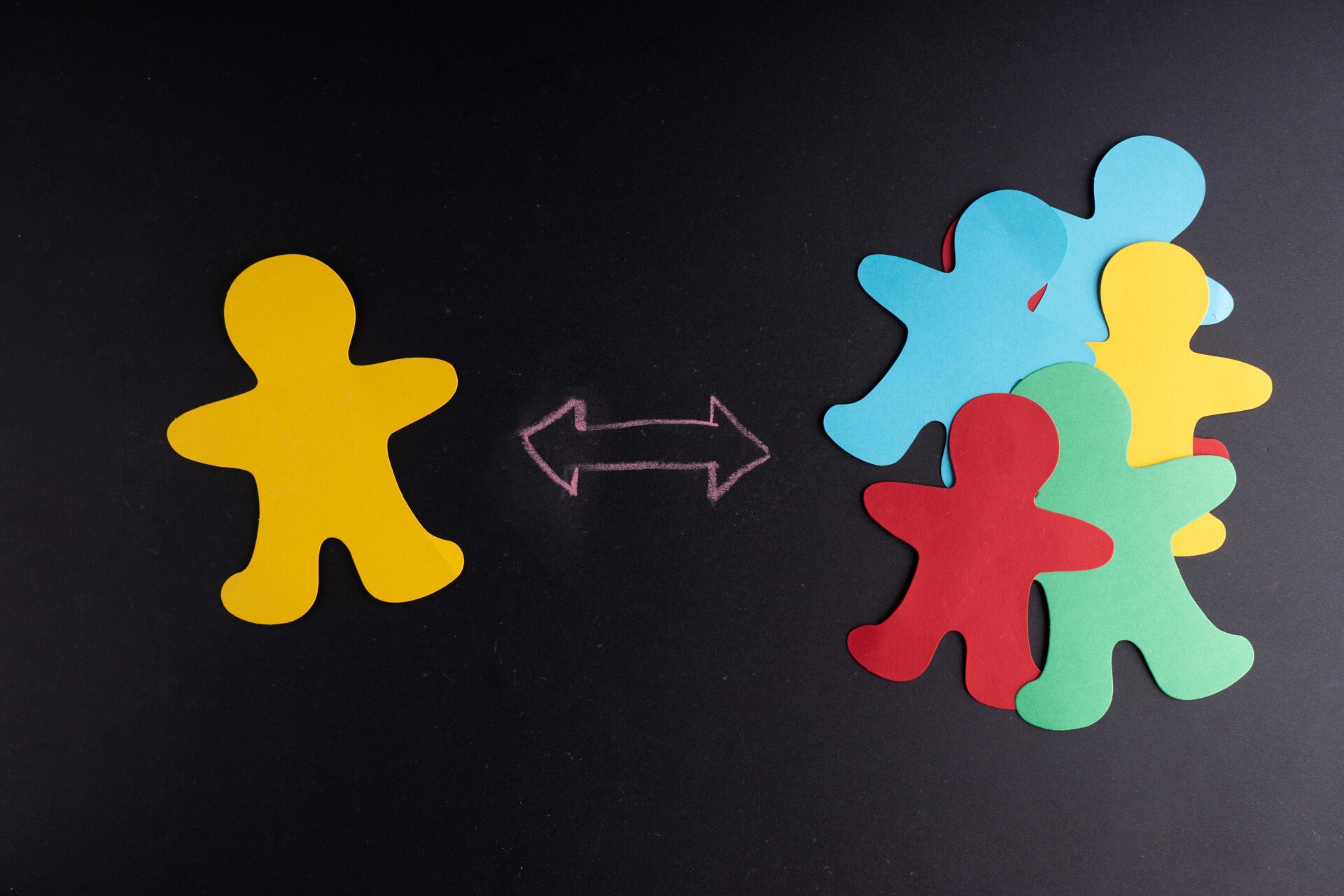
Cross-Cultural Research Methodology In Psychology
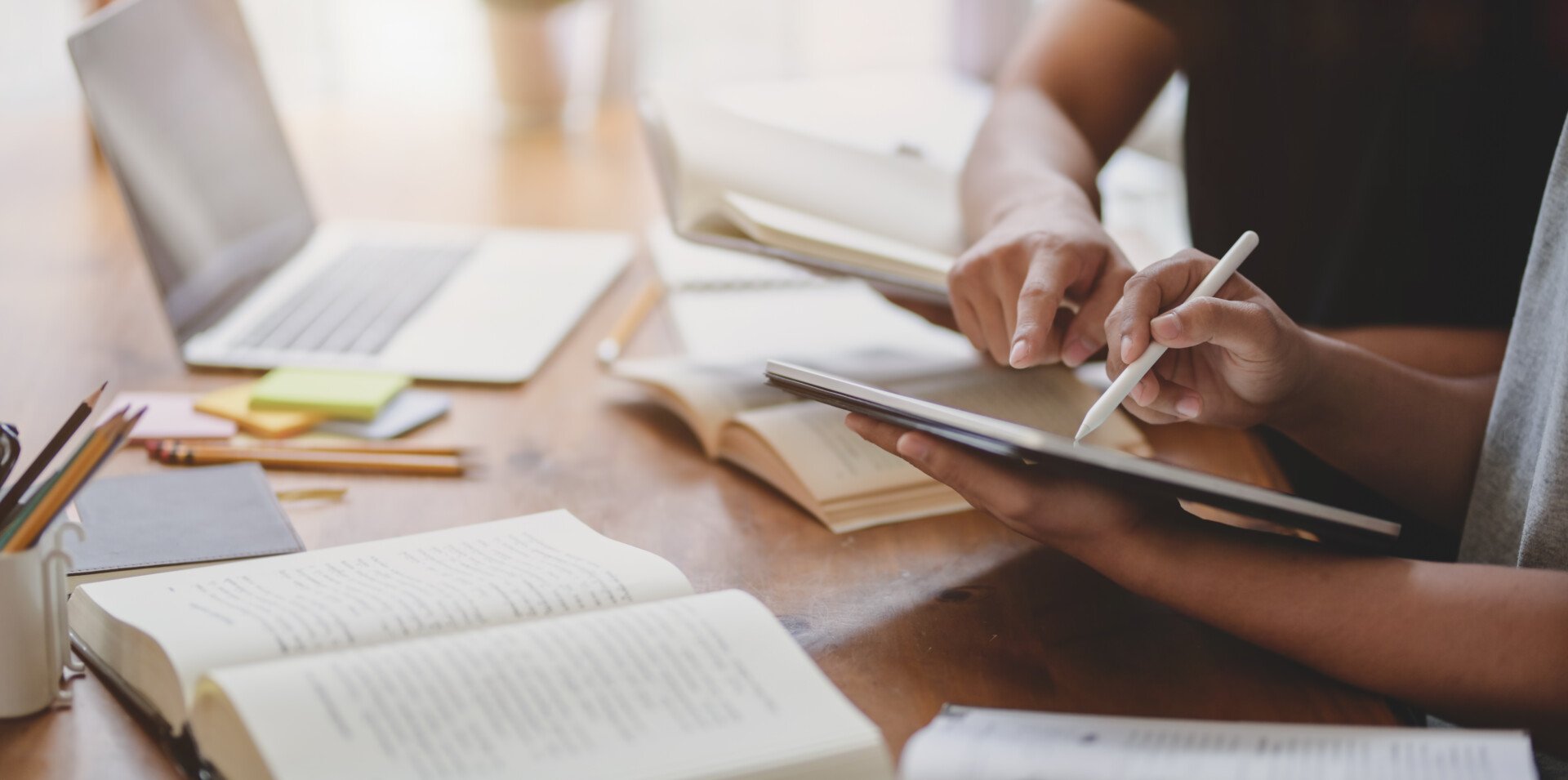
What Is Internal Validity In Research?
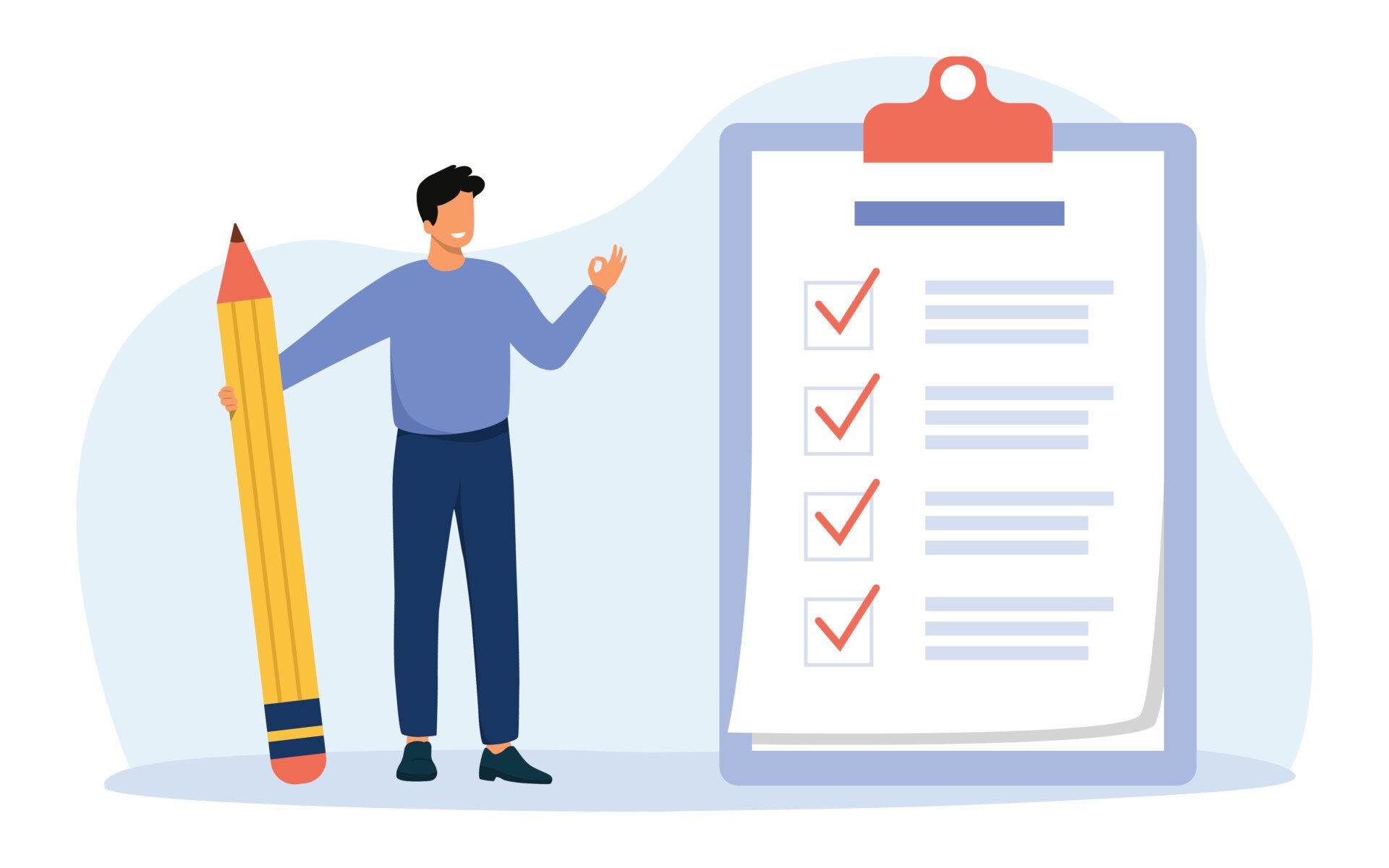
Research Methodology , Statistics
What Is Face Validity In Research? Importance & How To Measure
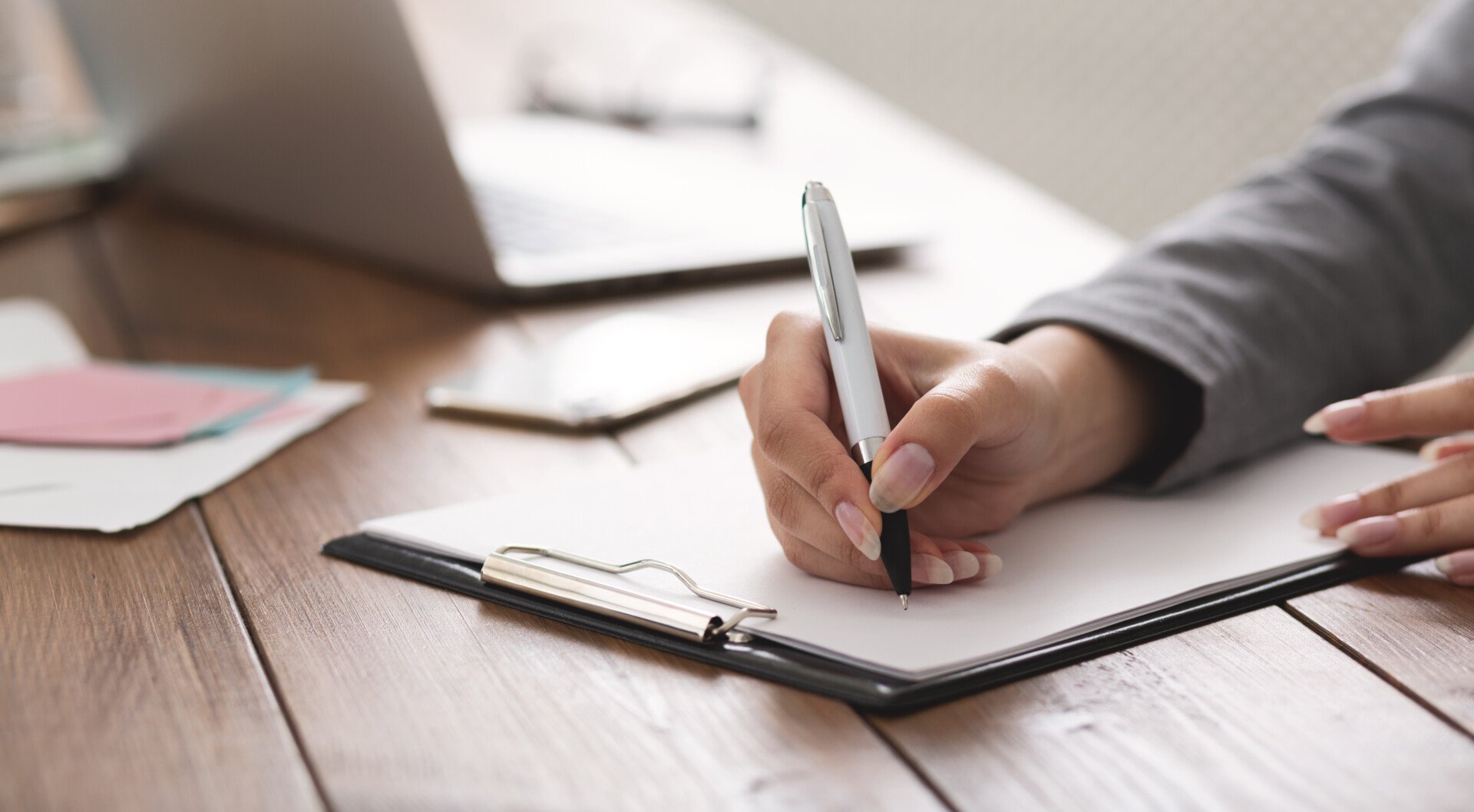
Criterion Validity: Definition & Examples
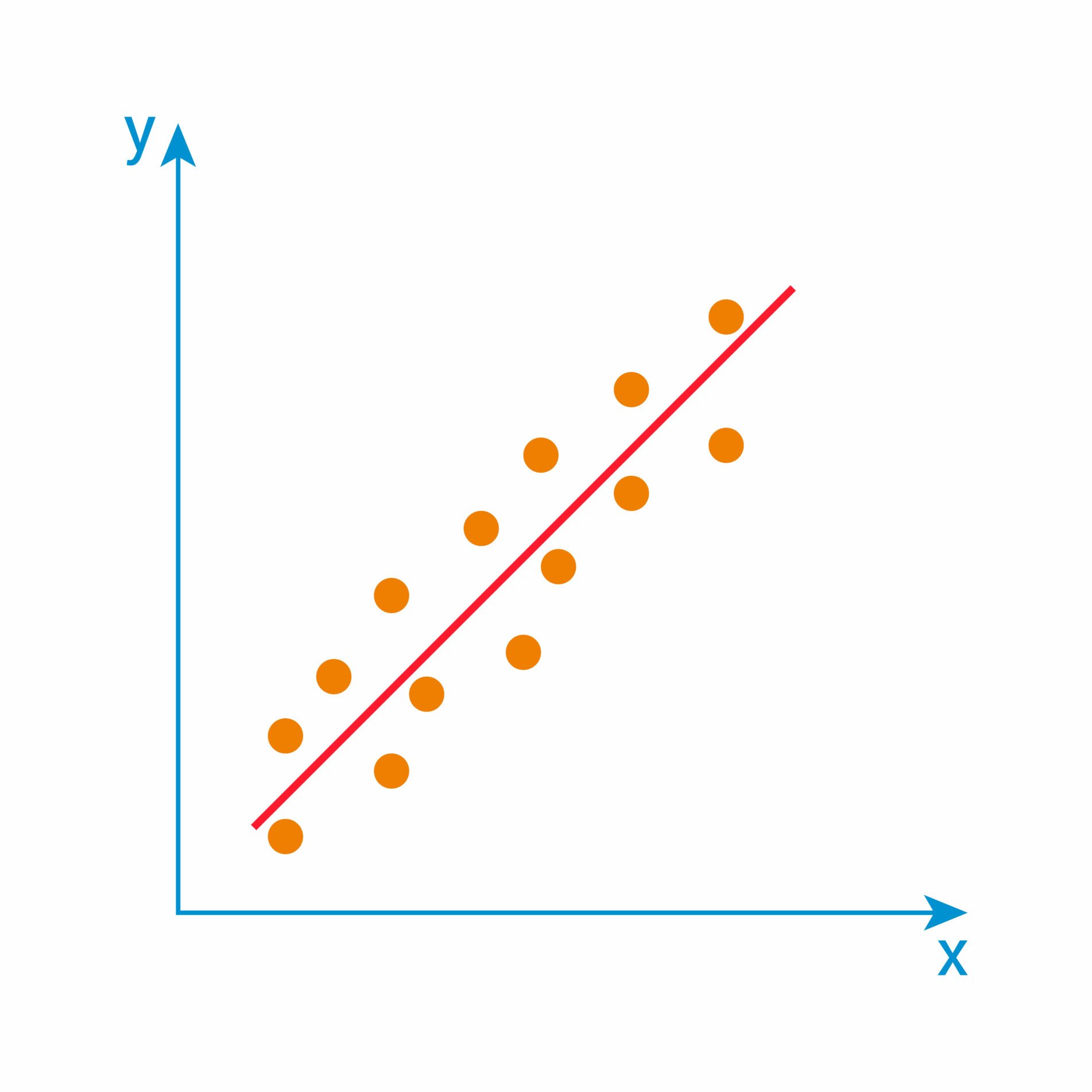
Convergent Validity: Definition and Examples
Understanding data analysis: A beginner's guide
Before data can be used to tell a story, it must go through a process that makes it usable. Explore the role of data analysis in decision-making.
What is data analysis?
Data analysis is the process of gathering, cleaning, and modeling data to reveal meaningful insights. This data is then crafted into reports that support the strategic decision-making process.
Types of data analysis
There are many different types of data analysis. Each type can be used to answer a different question.
Descriptive analytics
Descriptive analytics refers to the process of analyzing historical data to understand trends and patterns. For example, success or failure to achieve key performance indicators like return on investment.
An example of descriptive analytics is generating reports to provide an overview of an organization's sales and financial data, offering valuable insights into past activities and outcomes.
Predictive analytics
Predictive analytics uses historical data to help predict what might happen in the future, such as identifying past trends in data to determine if they’re likely to recur.
Methods include a range of statistical and machine learning techniques, including neural networks, decision trees, and regression analysis.
Diagnostic analytics
Diagnostic analytics helps answer questions about what caused certain events by looking at performance indicators. Diagnostic analytics techniques supplement basic descriptive analysis.
Generally, diagnostic analytics involves spotting anomalies in data (like an unexpected shift in a metric), gathering data related to these anomalies, and using statistical techniques to identify potential explanations.
Cognitive analytics
Cognitive analytics is a sophisticated form of data analysis that goes beyond traditional methods. This method uses machine learning and natural language processing to understand, reason, and learn from data in a way that resembles human thought processes.
The goal of cognitive analytics is to simulate human-like thinking to provide deeper insights, recognize patterns, and make predictions.
Prescriptive analytics
Prescriptive analytics helps answer questions about what needs to happen next to achieve a certain goal or target. By using insights from prescriptive analytics, organizations can make data-driven decisions in the face of uncertainty.
Data analysts performing prescriptive analysis often rely on machine learning to find patterns in large semantic models and estimate the likelihood of various outcomes.
analyticsText analytics
Text analytics is a way to teach computers to understand human language. It involves using algorithms and other techniques to extract information from large amounts of text data, such as social media posts or customer previews.
Text analytics helps data analysts make sense of what people are saying, find patterns, and gain insights that can be used to make better decisions in fields like business, marketing, and research.
The data analysis process
Compiling and interpreting data so it can be used in decision making is a detailed process and requires a systematic approach. Here are the steps that data analysts follow:
1. Define your objectives.
Clearly define the purpose of your analysis. What specific question are you trying to answer? What problem do you want to solve? Identify your core objectives. This will guide the entire process.
2. Collect and consolidate your data.
Gather your data from all relevant sources using data analysis software . Ensure that the data is representative and actually covers the variables you want to analyze.
3. Select your analytical methods.
Investigate the various data analysis methods and select the technique that best aligns with your objectives. Many free data analysis software solutions offer built-in algorithms and methods to facilitate this selection process.
4. Clean your data.
Scrutinize your data for errors, missing values, or inconsistencies using the cleansing features already built into your data analysis software. Cleaning the data ensures accuracy and reliability in your analysis and is an important part of data analytics.
5. Uncover valuable insights.
Delve into your data to uncover patterns, trends, and relationships. Use statistical methods, machine learning algorithms, or other analytical techniques that are aligned with your goals. This step transforms raw data into valuable insights.
6. Interpret and visualize the results.
Examine the results of your analyses to understand their implications. Connect these findings with your initial objectives. Then, leverage the visualization tools within free data analysis software to present your insights in a more digestible format.
7. Make an informed decision.
Use the insights gained from your analysis to inform your next steps. Think about how these findings can be utilized to enhance processes, optimize strategies, or improve overall performance.
By following these steps, analysts can systematically approach large sets of data, breaking down the complexities and ensuring the results are actionable for decision makers.
The importance of data analysis
Data analysis is critical because it helps business decision makers make sense of the information they collect in our increasingly data-driven world. Imagine you have a massive pile of puzzle pieces (data), and you want to see the bigger picture (insights). Data analysis is like putting those puzzle pieces together—turning that data into knowledge—to reveal what’s important.
Whether you’re a business decision maker trying to make sense of customer preferences or a scientist studying trends, data analysis is an important tool that helps us understand the world and make informed choices.
Primary data analysis methods
Quantitative analysis
Quantitative analysis deals with numbers and measurements (for example, looking at survey results captured through ratings). When performing quantitative analysis, you’ll use mathematical and statistical methods exclusively and answer questions like ‘how much’ or ‘how many.’
Qualitative analysis
Qualitative analysis is about understanding the subjective meaning behind non-numerical data. For example, analyzing interview responses or looking at pictures to understand emotions. Qualitative analysis looks for patterns, themes, or insights, and is mainly concerned with depth and detail.
Data analysis solutions and resources
Turn your data into actionable insights and visualize the results with ease.
Microsoft 365
Process data and turn ideas into reality with innovative apps, including Excel.
Importance of backing up data
Learn how to back up your data and devices for peace of mind—and added security.
Copilot in Excel
Go deeper with your data using Microsoft Copilot—your AI assistant.
Excel expense template
Organize and track your business expenses using Excel.
Excel templates
Boost your productivity with free, customizable Excel templates for all types of documents.
Chart designs
Enhance presentations, research, and other materials with customizable chart templates.
Follow Microsoft
- SVP Experience
- Ethical Business Decisions Playbook
- Silicon Valley Hiring Guide
- Tuition and Financial Aid
- GMAT/GRE Waiver
- Admissions Requirements
- Application Deadlines
- How to Apply
- Networking Opportunities
- Student Success
Quantitative Methods in Business Analytics
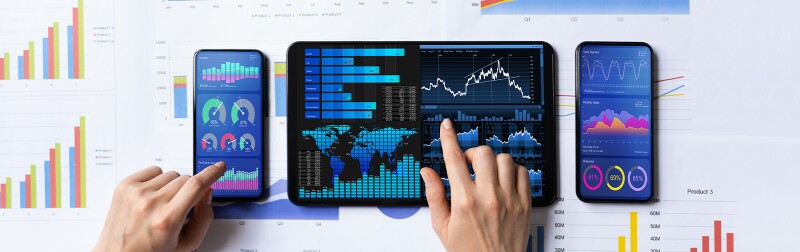
Quantitative data analysis for business intelligence (BI) examines business issues through statistical, mathematical, or computational techniques. Business analysts collect and examine numerical data to identify trends, patterns, and relationships that inform strategic business decisions. 1
Quantitative methods in BI drive decision-making by giving business leaders a solid foundation for making informed choices backed by data rather than intuition alone. Using quantitative analysis, leaders can forecast future trends, optimize operations, improve product offerings, and increase customer satisfaction. 1
This article will examine how quantitative methods in business intelligence support strategic decision-making and foster innovation and competitive advantage.
Descriptive Analytics
Descriptive analytics, a term for analytical models based on historical data, answers the question, “What happened?” These models provide insight into past business performance by analyzing historical records. Descriptive analytic models uncover meaningful patterns and relationships in data that can be displayed through summary statistics and data visualization techniques. They also serve as a starting point for more in-depth, advanced forms of analysis, such as predictive and prescriptive analytics. 2
Data visualization tools present complex datasets in visually appealing and easily understandable formats. Tools such as bar charts, line graphs, heat maps, and scatter plots allow analysts and business stakeholders to understand trends, outliers, and patterns intuitively, at a glance. Effective visualization acts as a powerful tool for communicating the story behind the data, enabling decision-makers to derive actionable insights quickly and efficiently. 3
Summary statistics provide a concise overview of data distributions. These metrics help business leaders understand the general behavior of data, highlighting key data points and identifying anomalies. 4
Data exploration is an introductory step in more complex data analysis. Analysts examine datasets to discover initial insights by cleaning data, identifying missing values, and understanding the basic structure of the dataset. Through data exploration, businesses can uncover hidden opportunities, gain a deeper understanding of consumer behavior , and make more informed decisions. 5
Predictive Analytics
Predictive analytics uses statistical techniques that analyze current and historical facts to make predictions about future or otherwise unknown events. It answers the question, “What will happen?” It includes different forecasting methods and predictive modeling techniques, including advanced machine learning algorithms and time series analysis to anticipate future trends, behaviors and activities. 6
Time series analysis is useful when the data is sequential and indexed by time. It analyzes time-ordered data points to help business leaders understand the underlying structure and function that produce the sequences. This analysis helps in forecasting future values based on past observations, which is particularly useful in domains such as finance, weather forecasting and inventory planning. 6
Forecasting methods apply mathematical models to historical data to predict future occurrences. Techniques range from simple moving averages to complex algorithms that adjust for seasonality, trends, and cyclical patterns. These methods help in planning and decision-making for businesses and organizations. 6
Predictive modeling techniques that use machine learning can learn from historical data and improve over time, making them exceptionally powerful for predicting future events. These models can handle complex interactions between variables and scale with data, so they have a wide variety of use cases, including marketing, finance, and healthcare. Through predictive analytics, business leaders can anticipate changes, optimize strategies, and mitigate risks effectively. 6
Prescriptive Analytics
Prescriptive analytics takes statistical analysis in business analytics further by recommending actions that can potentially lead to desired results. It answers the question, “How can we make something happen?” This advanced form of analytics uses tools and techniques such as optimization, simulation, and decision analysis, to advise on possible outcomes and guide decision-makers. 7
Optimization techniques, such as linear programming, find the best possible solution from a set of available alternatives under given constraints. These techniques are widely used in logistics, resource allocation, and scheduling to make sure that resources are used efficiently to maximize output or minimize costs. 8
Simulation methods allow organizations to model complex systems and scenarios to predict the outcomes of different decisions. By creating a virtual replica of a real-world process, simulations can explore a vast range of possibilities and their outcomes, making them invaluable in risk management and strategic planning. 9
Decision trees and decision analysis offer a structured approach to decision-making, breaking down complex decisions into simpler, smaller parts. This helps in visualizing the outcomes of different actions, weighing them against each other, and determining the path that leads to the best possible outcome. Together, these tools empower businesses to make informed, data-driven decisions that can significantly impact their success and growth. 10
Inferential Statistics
Inferential statistics is a mathematical model in business intelligence that allows businesses to make predictions or inferences about a population based on a sample of data drawn from that population. This methodology bridges the gap between the data businesses have and the conclusions they need to draw about a broader context. Hypothesis testing and confidence intervals allow analysts to make broader generalizations from sample data. 11
Hypothesis testing provides a framework for making decisions and drawing conclusions about population parameters. It starts by forming a null hypothesis, which is a statement of no effect or difference, and an alternative hypothesis, which is a statement indicating an effect. Through statistical tests, analysts can assess the strength of the evidence against the null hypothesis and determine whether it can be rejected in favor of the alternative hypothesis. 11
Confidence intervals offer another way to understand the uncertainty of an estimate. A confidence interval provides a range of values derived from the sample data that is likely to contain the true population parameter. The width of the interval gives an idea of the estimate's precision, with narrower intervals indicating more reliable estimates. 11
Regression analysis—another type of inferential statistics—is used to examine the relationship between two or more variables. It helps to understand how the dependent variable changes when any one of the independent variables is altered while the other independent variables stay the same. This analysis helps predict outcomes and test theories in various fields, from economics to social sciences. 12
Data-Driven Knowledge: Getting Ahead is Good Business
Stay ahead of the ongoing advancements in business by earning Santa Clara University’s Online Master of Science in Business Analytics . Mentored by our experienced faculty of industry experts , you’ll become proficient in business analytics, machine learning, and information technology, learning to use data-driven insights to lead innovation and foster organizational growth.
Balance your education with your employment and personal commitments. Our flexible online program is designed for working professionals looking to expand their networks and improve their career potential. It delivers the foundational business knowledge and principled leadership skills to propel your advancement, in Silicon Valley and beyond.
To learn more about how the Leavey School of Business can help you reach your goals, schedule a call with an admissions outreach advisor today.
- Retrieved on March 22, 2024, from linkedin.com/pulse/quantitative-techniques-understand-importance-business-ram-m/
- Retrieved on March 22, 2024, from investopedia.com/terms/d/descriptive-analytics.asp
- Retrieved on March 22, 2024, from aws.amazon.com/what-is/data-visualization/#
- Retrieved on March 22, 2024, from betterevaluation.org/methods-approaches/methods/summary-statistics#
- Retrieved on March 22, 2024, from spotfire.com/glossary/what-is-data-exploration#
- Retrieved on March 22, 2024, from ibm.com/topics/predictive-analytics
- Retrieved on March 22, 2024, from investopedia.com/terms/p/prescriptive-analytics.asp
- Retrieved on March 22, 2024, from mathworks.com/discovery/prescriptive-analytics.html#
- Retrieved on March 22, 2024, from baobabsoluciones.es/en/blog/2020/11/19/prescriptive-analytics-optimisation-and-simulation/
- Retrieved on March 22, 2024, from lumivero.com/resources/blog/the-analytics-pyramid-why-analytics-are-critical-for-defensible-objective-decision-making
- Retrieved on March 22, 2024, from corporatefinanceinstitute.com/resources/data-science/inferential-statistics/#
- Retrieved on March 22, 2024, from cuemath.com/data/inferential-statistics/
Return to Media
Santa Clara University has engaged Everspring , a leading provider of education and technology services, to support select aspects of program delivery.
Interested in one of our online programs? Receive a program brochure.
Artificial intelligence in strategy
Can machines automate strategy development? The short answer is no. However, there are numerous aspects of strategists’ work where AI and advanced analytics tools can already bring enormous value. Yuval Atsmon is a senior partner who leads the new McKinsey Center for Strategy Innovation, which studies ways new technologies can augment the timeless principles of strategy. In this episode of the Inside the Strategy Room podcast, he explains how artificial intelligence is already transforming strategy and what’s on the horizon. This is an edited transcript of the discussion. For more conversations on the strategy issues that matter, follow the series on your preferred podcast platform .
Joanna Pachner: What does artificial intelligence mean in the context of strategy?
Yuval Atsmon: When people talk about artificial intelligence, they include everything to do with analytics, automation, and data analysis. Marvin Minsky, the pioneer of artificial intelligence research in the 1960s, talked about AI as a “suitcase word”—a term into which you can stuff whatever you want—and that still seems to be the case. We are comfortable with that because we think companies should use all the capabilities of more traditional analysis while increasing automation in strategy that can free up management or analyst time and, gradually, introducing tools that can augment human thinking.
Joanna Pachner: AI has been embraced by many business functions, but strategy seems to be largely immune to its charms. Why do you think that is?
Subscribe to the Inside the Strategy Room podcast
Yuval Atsmon: You’re right about the limited adoption. Only 7 percent of respondents to our survey about the use of AI say they use it in strategy or even financial planning, whereas in areas like marketing, supply chain, and service operations, it’s 25 or 30 percent. One reason adoption is lagging is that strategy is one of the most integrative conceptual practices. When executives think about strategy automation, many are looking too far ahead—at AI capabilities that would decide, in place of the business leader, what the right strategy is. They are missing opportunities to use AI in the building blocks of strategy that could significantly improve outcomes.
I like to use the analogy to virtual assistants. Many of us use Alexa or Siri but very few people use these tools to do more than dictate a text message or shut off the lights. We don’t feel comfortable with the technology’s ability to understand the context in more sophisticated applications. AI in strategy is similar: it’s hard for AI to know everything an executive knows, but it can help executives with certain tasks.
When executives think about strategy automation, many are looking too far ahead—at AI deciding the right strategy. They are missing opportunities to use AI in the building blocks of strategy.
Joanna Pachner: What kind of tasks can AI help strategists execute today?
Yuval Atsmon: We talk about six stages of AI development. The earliest is simple analytics, which we refer to as descriptive intelligence. Companies use dashboards for competitive analysis or to study performance in different parts of the business that are automatically updated. Some have interactive capabilities for refinement and testing.
The second level is diagnostic intelligence, which is the ability to look backward at the business and understand root causes and drivers of performance. The level after that is predictive intelligence: being able to anticipate certain scenarios or options and the value of things in the future based on momentum from the past as well as signals picked in the market. Both diagnostics and prediction are areas that AI can greatly improve today. The tools can augment executives’ analysis and become areas where you develop capabilities. For example, on diagnostic intelligence, you can organize your portfolio into segments to understand granularly where performance is coming from and do it in a much more continuous way than analysts could. You can try 20 different ways in an hour versus deploying one hundred analysts to tackle the problem.
Predictive AI is both more difficult and more risky. Executives shouldn’t fully rely on predictive AI, but it provides another systematic viewpoint in the room. Because strategic decisions have significant consequences, a key consideration is to use AI transparently in the sense of understanding why it is making a certain prediction and what extrapolations it is making from which information. You can then assess if you trust the prediction or not. You can even use AI to track the evolution of the assumptions for that prediction.
Those are the levels available today. The next three levels will take time to develop. There are some early examples of AI advising actions for executives’ consideration that would be value-creating based on the analysis. From there, you go to delegating certain decision authority to AI, with constraints and supervision. Eventually, there is the point where fully autonomous AI analyzes and decides with no human interaction.
Because strategic decisions have significant consequences, you need to understand why AI is making a certain prediction and what extrapolations it’s making from which information.
Joanna Pachner: What kind of businesses or industries could gain the greatest benefits from embracing AI at its current level of sophistication?
Yuval Atsmon: Every business probably has some opportunity to use AI more than it does today. The first thing to look at is the availability of data. Do you have performance data that can be organized in a systematic way? Companies that have deep data on their portfolios down to business line, SKU, inventory, and raw ingredients have the biggest opportunities to use machines to gain granular insights that humans could not.
Companies whose strategies rely on a few big decisions with limited data would get less from AI. Likewise, those facing a lot of volatility and vulnerability to external events would benefit less than companies with controlled and systematic portfolios, although they could deploy AI to better predict those external events and identify what they can and cannot control.
Third, the velocity of decisions matters. Most companies develop strategies every three to five years, which then become annual budgets. If you think about strategy in that way, the role of AI is relatively limited other than potentially accelerating analyses that are inputs into the strategy. However, some companies regularly revisit big decisions they made based on assumptions about the world that may have since changed, affecting the projected ROI of initiatives. Such shifts would affect how you deploy talent and executive time, how you spend money and focus sales efforts, and AI can be valuable in guiding that. The value of AI is even bigger when you can make decisions close to the time of deploying resources, because AI can signal that your previous assumptions have changed from when you made your plan.
Joanna Pachner: Can you provide any examples of companies employing AI to address specific strategic challenges?
Yuval Atsmon: Some of the most innovative users of AI, not coincidentally, are AI- and digital-native companies. Some of these companies have seen massive benefits from AI and have increased its usage in other areas of the business. One mobility player adjusts its financial planning based on pricing patterns it observes in the market. Its business has relatively high flexibility to demand but less so to supply, so the company uses AI to continuously signal back when pricing dynamics are trending in a way that would affect profitability or where demand is rising. This allows the company to quickly react to create more capacity because its profitability is highly sensitive to keeping demand and supply in equilibrium.
Joanna Pachner: Given how quickly things change today, doesn’t AI seem to be more a tactical than a strategic tool, providing time-sensitive input on isolated elements of strategy?
Yuval Atsmon: It’s interesting that you make the distinction between strategic and tactical. Of course, every decision can be broken down into smaller ones, and where AI can be affordably used in strategy today is for building blocks of the strategy. It might feel tactical, but it can make a massive difference. One of the world’s leading investment firms, for example, has started to use AI to scan for certain patterns rather than scanning individual companies directly. AI looks for consumer mobile usage that suggests a company’s technology is catching on quickly, giving the firm an opportunity to invest in that company before others do. That created a significant strategic edge for them, even though the tool itself may be relatively tactical.
Joanna Pachner: McKinsey has written a lot about cognitive biases and social dynamics that can skew decision making. Can AI help with these challenges?
Yuval Atsmon: When we talk to executives about using AI in strategy development, the first reaction we get is, “Those are really big decisions; what if AI gets them wrong?” The first answer is that humans also get them wrong—a lot. [Amos] Tversky, [Daniel] Kahneman, and others have proven that some of those errors are systemic, observable, and predictable. The first thing AI can do is spot situations likely to give rise to biases. For example, imagine that AI is listening in on a strategy session where the CEO proposes something and everyone says “Aye” without debate and discussion. AI could inform the room, “We might have a sunflower bias here,” which could trigger more conversation and remind the CEO that it’s in their own interest to encourage some devil’s advocacy.
We also often see confirmation bias, where people focus their analysis on proving the wisdom of what they already want to do, as opposed to looking for a fact-based reality. Just having AI perform a default analysis that doesn’t aim to satisfy the boss is useful, and the team can then try to understand why that is different than the management hypothesis, triggering a much richer debate.
In terms of social dynamics, agency problems can create conflicts of interest. Every business unit [BU] leader thinks that their BU should get the most resources and will deliver the most value, or at least they feel they should advocate for their business. AI provides a neutral way based on systematic data to manage those debates. It’s also useful for executives with decision authority, since we all know that short-term pressures and the need to make the quarterly and annual numbers lead people to make different decisions on the 31st of December than they do on January 1st or October 1st. Like the story of Ulysses and the sirens, you can use AI to remind you that you wanted something different three months earlier. The CEO still decides; AI can just provide that extra nudge.
Joanna Pachner: It’s like you have Spock next to you, who is dispassionate and purely analytical.
Yuval Atsmon: That is not a bad analogy—for Star Trek fans anyway.
Joanna Pachner: Do you have a favorite application of AI in strategy?
Yuval Atsmon: I have worked a lot on resource allocation, and one of the challenges, which we call the hockey stick phenomenon, is that executives are always overly optimistic about what will happen. They know that resource allocation will inevitably be defined by what you believe about the future, not necessarily by past performance. AI can provide an objective prediction of performance starting from a default momentum case: based on everything that happened in the past and some indicators about the future, what is the forecast of performance if we do nothing? This is before we say, “But I will hire these people and develop this new product and improve my marketing”— things that every executive thinks will help them overdeliver relative to the past. The neutral momentum case, which AI can calculate in a cold, Spock-like manner, can change the dynamics of the resource allocation discussion. It’s a form of predictive intelligence accessible today and while it’s not meant to be definitive, it provides a basis for better decisions.
Joanna Pachner: Do you see access to technology talent as one of the obstacles to the adoption of AI in strategy, especially at large companies?
Yuval Atsmon: I would make a distinction. If you mean machine-learning and data science talent or software engineers who build the digital tools, they are definitely not easy to get. However, companies can increasingly use platforms that provide access to AI tools and require less from individual companies. Also, this domain of strategy is exciting—it’s cutting-edge, so it’s probably easier to get technology talent for that than it might be for manufacturing work.
The bigger challenge, ironically, is finding strategists or people with business expertise to contribute to the effort. You will not solve strategy problems with AI without the involvement of people who understand the customer experience and what you are trying to achieve. Those who know best, like senior executives, don’t have time to be product managers for the AI team. An even bigger constraint is that, in some cases, you are asking people to get involved in an initiative that may make their jobs less important. There could be plenty of opportunities for incorporating AI into existing jobs, but it’s something companies need to reflect on. The best approach may be to create a digital factory where a different team tests and builds AI applications, with oversight from senior stakeholders.
The big challenge is finding strategists to contribute to the AI effort. You are asking people to get involved in an initiative that may make their jobs less important.
Joanna Pachner: Do you think this worry about job security and the potential that AI will automate strategy is realistic?
Yuval Atsmon: The question of whether AI will replace human judgment and put humanity out of its job is a big one that I would leave for other experts.
The pertinent question is shorter-term automation. Because of its complexity, strategy would be one of the later domains to be affected by automation, but we are seeing it in many other domains. However, the trend for more than two hundred years has been that automation creates new jobs, although ones requiring different skills. That doesn’t take away the fear some people have of a machine exposing their mistakes or doing their job better than they do it.
Joanna Pachner: We recently published an article about strategic courage in an age of volatility that talked about three types of edge business leaders need to develop. One of them is an edge in insights. Do you think AI has a role to play in furnishing a proprietary insight edge?
Yuval Atsmon: One of the challenges most strategists face is the overwhelming complexity of the world we operate in—the number of unknowns, the information overload. At one level, it may seem that AI will provide another layer of complexity. In reality, it can be a sharp knife that cuts through some of the clutter. The question to ask is, Can AI simplify my life by giving me sharper, more timely insights more easily?
Joanna Pachner: You have been working in strategy for a long time. What sparked your interest in exploring this intersection of strategy and new technology?
Yuval Atsmon: I have always been intrigued by things at the boundaries of what seems possible. Science fiction writer Arthur C. Clarke’s second law is that to discover the limits of the possible, you have to venture a little past them into the impossible, and I find that particularly alluring in this arena.
AI in strategy is in very nascent stages but could be very consequential for companies and for the profession. For a top executive, strategic decisions are the biggest way to influence the business, other than maybe building the top team, and it is amazing how little technology is leveraged in that process today. It’s conceivable that competitive advantage will increasingly rest in having executives who know how to apply AI well. In some domains, like investment, that is already happening, and the difference in returns can be staggering. I find helping companies be part of that evolution very exciting.
Explore a career with us
Related articles.

Strategic courage in an age of volatility
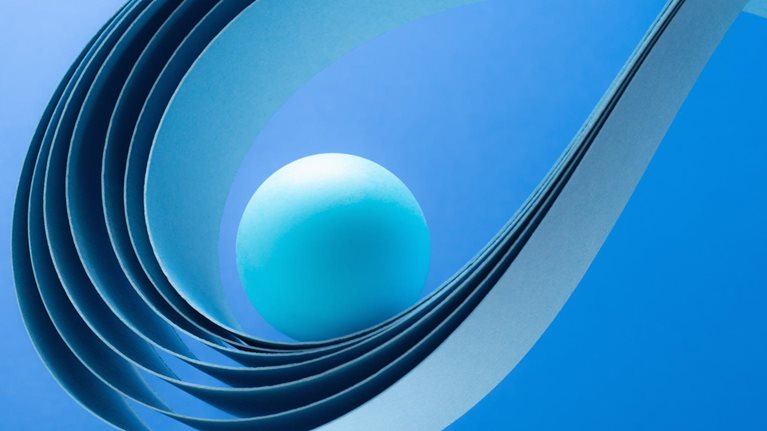
Bias Busters Collection
- Open access
- Published: 09 May 2024
Examining the feasibility of assisted index case testing for HIV case-finding: a qualitative analysis of barriers and facilitators to implementation in Malawi
- Caroline J. Meek 1 , 2 ,
- Tiwonge E. Mbeya Munkhondya 3 ,
- Mtisunge Mphande 4 ,
- Tapiwa A. Tembo 4 ,
- Mike Chitani 4 ,
- Milenka Jean-Baptiste 2 ,
- Dhrutika Vansia 4 ,
- Caroline Kumbuyo 4 ,
- Jiayu Wang 2 ,
- Katherine R. Simon 4 ,
- Sarah E. Rutstein 5 ,
- Clare Barrington 2 ,
- Maria H. Kim 4 ,
- Vivian F. Go 2 &
- Nora E. Rosenberg 2
BMC Health Services Research volume 24 , Article number: 606 ( 2024 ) Cite this article
102 Accesses
Metrics details
Assisted index case testing (ICT), in which health care workers take an active role in referring at-risk contacts of people living with HIV for HIV testing services, has been widely recognized as an evidence-based intervention with high potential to increase status awareness in people living with HIV. While the available evidence from eastern and southern Africa suggests that assisted ICT can be an effective, efficient, cost-effective, acceptable, and low-risk strategy to implement in the region, it reveals that feasibility barriers to implementation exist. This study aims to inform the design of implementation strategies to mitigate these feasibility barriers by examining “assisting” health care workers’ experiences of how barriers manifest throughout the assisted ICT process, as well as their perceptions of potential opportunities to facilitate feasibility.
In-depth interviews were conducted with 26 lay health care workers delivering assisted ICT in Malawian health facilities. Interviews explored health care workers’ experiences counseling index clients and tracing these clients’ contacts, aiming to inform development of a blended learning implementation package. Transcripts were inductively analyzed using Dedoose coding software to identify and describe key factors influencing feasibility of assisted ICT. Analysis included multiple rounds of coding and iteration with the data collection team.
Participants reported a variety of barriers to feasibility of assisted index case testing implementation, including sensitivities around discussing ICT with clients, privacy concerns, limited time for assisted index case testing amid high workloads, poor quality contact information, and logistical obstacles to tracing. Participants also reported several health care worker characteristics that facilitate feasibility (knowledge, interpersonal skills, non-stigmatizing attitudes and behaviors, and a sense of purpose), as well as identified process improvements with the potential to mitigate barriers.
Conclusions
Maximizing assisted ICT’s potential to increase status awareness in people living with HIV requires equipping health care workers with effective training and support to address and overcome the many feasibility barriers that they face in implementation. Findings demonstrate the need for, as well as inform the development of, implementation strategies to mitigate barriers and promote facilitators to feasibility of assisted ICT.
Trial registration
NCT05343390. Date of registration: April 25, 2022.
Peer Review reports
Introduction
To streamline progress towards its goal of ending AIDS as a public health threat by 2030, the Joint United Nations Programme on HIV/AIDS (UNAIDS) launched a set of HIV testing and treatment targets [ 1 ]. Adopted by United Nations member states in June 2021, the targets call for 95% of all people living with HIV (PLHIV) to know their HIV status, 95% of all PLHIV to be accessing sustained antiretroviral therapy (ART), and 95% of all people receiving ART to achieve viral suppression by 2025 [ 2 ]. Eastern and southern Africa has seen promising regional progress towards these targets in recent years, and the region is approaching the first target related to status awareness in PLHIV- in 2022, 92% of PLHIV in the region were aware of their status [ 3 ]. However, several countries in the region lag behind [ 4 ], and as 2025 approaches, it is critical to scale up adoption of evidence-based interventions to sustain and accelerate progress.
Index case testing (ICT), which targets provision of HIV testing services (HTS) for sexual partners, biological children, and other contacts of known PLHIV (“index clients”), is a widely recognized evidence-based intervention used to identify PLHIV by streamlining testing efforts to populations most at risk [ 5 , 6 , 7 ]. Traditional approaches to ICT rely on passive referral, in which index clients invite their contacts for testing [ 5 ]. However, the World Health Organization (WHO) and the President’s Emergency Plan for HIV/AIDS Relief (PEPFAR) have both recommended assisted approaches to ICT [ 6 , 8 , 9 , 10 ], in which health care workers (HCWs) take an active role in referral of at-risk contacts for testing, due to evidence of improved effectiveness in identifying PLHIV compared to passive approaches [ 10 , 11 , 12 , 13 , 14 ]. As a result, there have been several efforts to scale assisted ICT throughout eastern and southern Africa in recent years [ 15 , 16 , 17 , 18 , 19 , 20 ]. In addition to evidence indicating that assisted ICT can be effective in increasing HIV testing and case-finding [ 16 , 17 , 21 , 22 , 23 , 24 ], implementation evidence [ 25 ] from the region suggests that assisted ICT can be an efficient [ 14 ], acceptable [ 5 , 13 , 15 , 18 , 20 , 21 , 26 ], cost-effective [ 27 ], and low-risk [ 21 , 22 , 24 , 28 , 29 ] strategy to promote PLHIV status awareness. However, the few studies that focus on feasibility, or the extent to which HCWs can successfully carry out assisted ICT [ 25 ], suggest that barriers exist to feasibility of effective implementation [ 18 , 19 , 20 , 30 , 31 , 32 ]. Developing informed implementation strategies to mitigate these barriers requires more detailed examination of how these barriers manifest throughout the assisted ICT process, as well as of potential opportunities to facilitate feasibility, from the perspective of the HCWs who are doing the “assisting”.
This qualitative analysis addresses this need for further detail by exploring “assisting” HCWs’ perspectives of factors that influence the feasibility of assisted ICT, with a unique focus on informing development of effective implementation strategies to best support assisted ICT delivery in the context of an implementation science trial in Malawi.
This study was conducted in the Machinga and Balaka districts of Malawi. Malawi is a country in southeastern Africa in which 7.1% of the population lives with HIV and 94% of PLHIV know their status [ 4 ]. Machinga and Balaka are two relatively densely populated districts in the southern region of Malawi [ 33 ] with HIV prevalence rates similar to the national average [ 34 ]. We selected Machinga and Balaka because they are prototypical of districts in Malawi implementing Ministry of Health programs with support from an implementing partner.
Malawi has a long-established passive ICT program, and in 2019 the country also adopted an assisted component, known as voluntary assisted partner notification, as part of its national HIV testing policy [ 32 ]. In Malawi, ICT is conducted through the following four methods, voluntarily selected by the index client: 1) passive referral, in which HCWs encourage the index client to refer partners for voluntary HTS, 2) contract referral, in which HCWs establish an informal ‘contract’ with index clients that agrees upon a date that the HCW can contact the contact clients if they have not yet presented for HTS; 3) provider referral, in which HCWs contact and offer voluntary HTS to contact clients; and 3) dual referral, in which HCWs accompany and provide support to index clients in disclosing their status and offering HTS to their partners [ 8 ].
While Malawi has one of the lowest rates of qualified clinical HCWs globally (< 5 clinicians per 100,000 people) [ 35 ], the country has a strong track record of shifting HTS tasks to lay HCWs, who have been informally trained to perform certain health care delivery functions but do not have a formal professional/para-professional certification or tertiary education degree, in order to mitigate this limited medical workforce capacity [ 32 , 36 ]. In Malawi, lay HCW roles include HIV Diagnostic Assistants (who are primarily responsible for HIV testing and counseling, including index case counseling) and community health workers (who are responsible for a wider variety of tasks, including index case counseling and contact tracing) [ 32 ]. Non-governmental organization implementing partners, such as the Tingathe Program, play a critical role in harnessing Malawian lay HCW capacity to rapidly and efficiently scale up HTS, including assisted ICT [ 32 , 37 , 38 , 39 ].
Study design
Data for this analysis were collected as part of formative research for a two-arm cluster randomized control trial examining a blended learning implementation package as a strategy for building HCW capacity in assisted ICT [ 40 ]. Earlier work [ 32 ] established the theoretical basis for testing the blended learning implementation package, which combines individual asynchronous modules with synchronous small-group interactive sessions to enhance training and foster continuous quality improvement. The formative research presented in this paper aimed to further explore factors influencing feasibility of the assisted ICT from the perspective of HCWs in order to inform development of the blended learning implementation package.
Prior to the start of the trial (October-December 2021), the research team conducted 26 in-depth interviews (IDIs) with lay HCWs at 14 of the 34 facilities included in the parent trial. We purposively selected different types of facilities (hospitals, health centers, and dispensaries) in both districts and from both randomization arms, as this served as a qualitative baseline for a randomized trial. Within these facilities, we worked with facility supervisors to purposively select HCWs who were actively engaged in Malawi’s ICT program from the larger sample of HCWs eligible for the parent trial (had to be at least 18 years old, employed full-time at one of the health facilities included in the parent trial, and involved in counseling index clients and/or tracing their contacts). The parent trial enrolled 306 HCWs, who were primarily staff hired by Tingathe Program to support facilities implementing Malawi’s national HIV program.
Data collection
IDIs were conducted by three trained Malawian interviewers in a private setting using a semi-structured guide. IDIs were conducted over the phone when possible ( n = 18) or in-person at sites with limited phone service ( n = 8). The semi-structured guide was developed for this study through a series of rigorous, iterative discussions among the research team (Additional file 1 ). The questions used for this analysis were a subset of a larger interview. The interview guide questions for this analysis explored HCWs’ experiences with assisted ICT, including barriers and facilitators to implementation. Probing separately about the processes of counseling index clients and tracing their contacts, interviewers asked questions such as “What is the first thing that comes to mind when you think of counseling index clients/tracing contacts?”, “What aspects do you [like/not like] about…?” and “What do your colleagues say about…?”. When appropriate, interviewers probed further about how specific factors mentioned by the participant facilitate or impede the ICT implementation experience.
The IDIs lasted from 60–90 min and were conducted in Chichewa, a local language in Malawi. Eleven audio recordings were transcribed verbatim in Chichewa before being translated into English and 15 recordings were directly translated and transcribed into English. Interviewers summarized each IDI after it was completed, and these summaries were discussed with the research team routinely.
Data analysis
The research team first reviewed all of the interview summaries individually and then met multiple times to discuss initial observations, refining the research question and scope of analysis. A US-based analyst (CJM) with training in qualitative analysis used an inductive approach to develop a codebook, deriving broad codes from the implementation factors mentioned by participants throughout their interviews. Along with focused examination of the transcripts, she consulted team members who had conducted the IDIs with questions or clarifications. CJM regularly met with Malawian team members (TEMM, MM, TAT) who possess the contextual expertise necessary to verify and enhance meaning. She used the Dedoose (2019) web application to engage in multiple rounds of coding, starting with codes representing broad implementation factors and then further refining the codebook as needed to capture the nuanced manifestations of these barriers and facilitators. Throughout codebook development and refinement, the analyst engaged in memoing to track first impressions, thought processes, and coding decisions. The analyst presented the codebook and multiple rounds of draft results to the research team. All transcripts and applied codes were also reviewed in detail by additional team members (MJB, DV). Additional refinements to the codebook and results interpretations were iteratively made based on team feedback.
Ethical clearance
Ethical clearance was provided by UNC’s IRB, Malawi’s National Health Sciences Research Committee, and the Baylor College of Medicine IRB. Written informed consent was obtained from all participants in the main study and interviewers confirmed verbal consent before starting the IDIs.
Participant characteristics are described in Table 1 below.
Factors influencing feasibility of assisted ICT: barriers and facilitators
Participants described a variety of barriers and facilitators to feasibility of assisted ICT, manifesting across the index client counseling and contact client tracing phases of the implementation process. Identified barriers included sensitivities around discussing ICT with clients, privacy concerns, limited time for ICT amid high workloads, poor quality contact information, and logistical obstacles to tracing. In addition to these barriers, participants also described several HCW characteristics that facilitated feasibility: ICT knowledge, interpersonal skills, positive attitudes towards clients, and sense of purpose. Barriers and facilitators are mapped to the ICT process in Fig. 1 and described in greater detail in further sections.
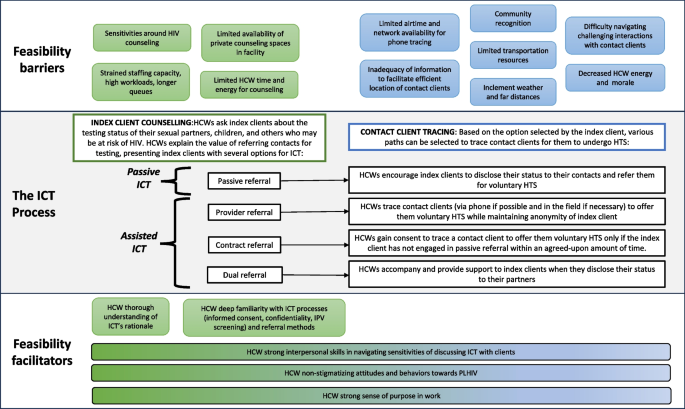
Conceptual diagram mapping feasibility barriers and facilitators to the ICT process
Feasibility barriers
Sensitivities around discussing ict with clients.
Participants described ICT as a highly sensitive topic to approach with clients. Many expressed a feeling of uncertainty around how open index clients will be to sharing information about their contacts, as well as how contacts will react when approached for HTS. When asked about difficult aspects of counseling index clients, many HCWs mentioned clients’ hesitance or declination to participate in assisted ICT and share their contacts. Further, several HCWs mentioned that some index clients would provide false contact information. These index client behaviors were often attributed to confidentiality concerns, fear of unwanted status disclosure, and fear of the resulting implications of status disclosure: “They behave that way because they think you will be telling other people about their status…they also think that since you know it means their life is done, you will be looking at them differently .” Populations commonly identified as particularly likely to hesitate, refuse, or provide false information included youth (described as “ shy ” “ thinking they know a lot ” and “ difficult to reveal their contacts ”) and newly diagnosed clients (“it may be hard for them to accept [their HIV diagnosis]” ). One participant suggested that efforts to pair index clients with same-sex HCWs could make them more comfortable to discuss their contacts.
When asked about the first things that come to mind when starting to trace contacts, many participants discussed wondering how they will be received by the contact and preparing themselves to approach the contact. When conducting provider or contract referral, HCWs described a variety of challenging reactions that can occur when they approach a contact for HTS- including delay or refusal of testing, excessive questioning about the identity of the index client who referred them for testing, and even anger or aggression. Particularly mentioned in the context of male clients, these kinds of reactions can lead to stress and uncertain next steps for HCWs: “I was very tensed up. I was wondering to myself what was going to happen…he was talking with anger.”
Participants also noted the unique sensitivities inherent in conducting dual referral and interacting with sexual partners of index clients, explaining that HIV disclosure can create acute conflict in couples due to perceived blame and assumptions of infidelity. They recounted these scenarios as particularly difficult to navigate, with high stakes that require high-quality counseling skills: “sometimes if you do not have good counseling the marriage happens to get to an end.” . Some participants discussed concern about index client risk of intimate partner violence (IPV) upon partner disclosure: “they think that if they go home and [disclose their HIV status], the marriage will end right there, or for some getting to a point of [being] beaten.”
Privacy concerns
Participants also reported that clients highly value privacy, which can be difficult to secure throughout the ICT process. In the facility, while participants largely indicated that counseling index clients was much more successful when conducted in a private area, many reported limited availability of private counseling space. One participant described this challenge: “ if I’m counseling an index client and people keep coming into the room…this compromises the whole thing because the client becomes uncomfortable in the end.” Some HCWs mentioned working around this issue through use of screens, “do-not-disturb” signs, outdoor spots, and tents.
Participants also noted maintaining privacy as a challenge when tracing contact clients in the field, as they sometimes find clients in a situation that is not conducive to private conversations. One participant described: “ we get to the house and find that there are 4, 5 people with our [contact client]…it doesn’t go well…That is a mission gone wrong. ” Participants also noted that HCWs are also often easily recognizable in the community due to their bikes and cars, which exacerbates the risk of compromising privacy. To address privacy challenges in the community, participants reported strategies to increase discretion, including dressing to blend in with the community, preparing an alternate reason to be looking for the client, and offering HTS to multiple people or households to avoid singling out one person.
Limited time for ICT amid high workloads
Some participants indicated that strained staffing capacity leads HCWs to have to perform multiple roles, expressing challenges in balancing their ICT work with their other tasks. As one participant described, “Sometimes it is found that you are assigned a task here at the hospital to screen anyone who comes for blood testing, but you are also supposed to follow up [with] the contacts the same day- so it becomes a problem…you fail to follow up [with] the contacts.” Some also described being the only, or one of few staff responsible for ICT: “You’re doing this work alone, so you can see that it is a big task to do it single-handedly.” The need to counsel each index client individually, as a result of confidentiality concerns, further increases workload for the limited staff assigned to this work. Further, HCWs often described contact tracing in the field as time-consuming and physically taxing, which leaves them less time and energy for counseling. Many HCWs noted the need to hire more staff dedicated to ICT work.
High workloads also resulted in shorter appointments and less time to counsel index clients, which participants reported limits the opportunity for rapport that facilitates openness or probes for detailed information about sexual partners. Participants emphasized the importance of having enough time to meaningfully engage with index clients: “For counseling you cannot have a limit to say, ‘I will talk to him for 5 min only.’ …That is not counseling then. You are supposed to stay up until…you feel that this [person] is fulfilled.” . In addition, high workload can reduce the capacity of HCWs to deliver quality counseling: “So you find that as you go along with the counseling, you can do better with the first three clients but the rest, you are tired and you do short cuts.”
High workloads also lead to longer queues, which may deter clients from coming into the clinic or cause them to leave before receiving services: “Sometimes because of shortage of staff, it happens that you have been assigned a certain task that you were supposed to do but at the same time there are clients who were supposed to be counseled. As a result, because you spent more time on the other task as a result you lose out some of the clients because you find that they have gone.” In response to long queues, several participants described ‘fast-tracking’ contact clients who come in for HTS in effort to maximize case-finding by prioritizing those who have been identified as at risk of HIV.
Poor quality contact information
Participants repeatedly discussed the importance of eliciting accurate information about a person’s sexual partners, including where, when, and how to best contact them. As one participant said, “ Once the index has given us the wrong information then everything cannot work, it becomes wrong…if he gives us full information [with] the right details then everything becomes successful and happens without a problem. ” Adequate information is a critical component of the ICT process, and incorrect or incomplete information delays or prevents communication with contact clients.
Inadequate information, which can include incorrect or incomplete names, phone numbers, physical addresses, and contextual details, can arise from a variety of scenarios. Most participants mentioned index clients providing incorrect information as a concern. This occurred either intentionally to avoid disclosure or unintentionally if information was not known. Poor quality contact information also results from insufficient probing and poor documentation, which is often exacerbated by aforementioned HCW time and energy constraints. In one participant’s words, “The person who has enlisted the contact…is the key person who can make sure that our tracing is made easy.” Participants noted the pivotal role of the original HCW who first interacts with the index client in not only eliciting correct locator information but also eliciting detailed contextual information. For example, details about a contact client’s profession are helpful to trace the client at a time when they will likely be at home. Other helpful information included nicknames, HIV testing history, and notes about confidentiality concerns.
Logistical obstacles to tracing
Some contact clients are reached by phone whereas others must be physically traced in the community. Some participants reported difficulty with tracing via phone, frequently citing network problems and lack of sufficient airtime allocated by the facility. Participants also reported that some clients were unreachable by phone, necessitating physical tracing. Physically tracing a contact client requires a larger investment of resources than phone tracing, especially when the client lives at a far distance from the clinic. Participants frequently discussed having to travel far distances to reach contact clients, an issue some saw as exacerbated by people who travel to clinics at far distances due to privacy concerns.
While most participants reported walking or biking to reach contact clients in the community, some mentioned using a motorcycle or Tingathe vehicle. However, access to vehicles is often limited and these transportation methods require additional expenses for fuel. Walking or biking was also reported to expose HCWs to inclement weather, including hot or rainy seasons, and potential safety risks such as violence.
Participants reported that traveling far distances can be physically taxing and time-consuming, sometimes rendering them too tired or busy to attend to other tasks. Frequent travel influenced HCW morale, particularly when a tracing effort did not result in successfully recruiting a contact client. Participants frequently described this perception of wasted time and energy as “ painful ”, with the level of distress often portrayed as increasing with the distance travelled. As one HCW said, “You [can] find out that he gave a false address. That is painful because it means you have done nothing for the person, you travelled for nothing.”
HCWs described multiple approaches used to strategically allocate limited resources for long distances. These approaches included waiting to physically trace until there are multiple clients in a particular area, reserving vehicle use for longer trips, and coordinating across HCWs to map out contact client locations. HCWs also mentioned provision of rain gear and sun protection to mitigate uncomfortable travel. Another approach involved allocating contact tracing to HCWs based in the same communities as the contact clients.
Feasibility facilitators
Hcw knowledge about ict.
Participants reported that HCWs with a thorough understanding of ICT’s rationale and purpose can facilitate client openness. Clients were more likely to engage with HCWs about assisted ICT if they understood the benefits to themselves and their loved ones. One HCW stated, “If the person understands why we need the information, they will give us accurate information.”
Participants also discussed the value of deep HCW familiarity with ICT procedures and processes, particularly regarding screening clients for IPV and choosing referral method. One participant described the importance of clearly explaining various referral methods to clients: “So…people come and choose the method they like…when you explain things clearly it is like the index client is free to choose a method which the contact can use for testing”. Thorough knowledge of available referral methods allows HCWs to actively engage with index clients to discuss strategies to refer contacts in a way that fits their unique confidentiality needs, which was framed as particularly important when IPV is identified as a concern. Multiple participants suggested the use of flipcharts or videos, saying these would save limited HCW time and energy, fill information gaps, and provide clients with a visual aid to supplement the counseling. Others suggested recurring opportunities for training, to continuously “refresh” their ICT knowledge in order to facilitate implementation.
HCW interpersonal skills
In addition, HCWs’ ability to navigate sensitive conversations about HIV was noted as a key facilitator of successful implementation. Interpersonal skills were mentioned as mitigating the role’s day-to-day uncertainty by preparing HCWs to engage with clients, especially newly diagnosed clients: “ I need to counsel them skillfully so that they understand what I mean regardless that they have just tested positive for HIV.”
When discussing strategies to build HCW skills in counseling index clients and tracing contact clients, participants suggested establishing regular opportunities to discuss challenges and share approaches to address these challenges: “ I think that there should be much effort on the [HCWs] doing [ICT]. For example, what do I mean, they should be having a meeting with the facility people to ask what challenges are you facing and how can we end them?”. Another participant further elaborated, saying “We should be able to share experiences with our [colleagues] so that we can all learn from one another. And also, there are other people who are really brilliant at their job. Those people ought to come visit us and see how we are doing. That is very motivating.”
HCW non-stigmatizing attitudes and behaviors
Participants also highlighted the role of empathy and non-judgement in building trust with clients: “ Put yourself in that other person’s shoes. In so doing, the counseling session goes well. Understanding that person, that what is happening to them can also happen to you. ”. Participants viewed trust-building as critical to facilitating client comfort and openness: “if they trust you enough, they will give you the right information.” Further, participants associated HCW assurance of confidentiality with promoting trust and greater information sharing: “ Also assuring them on the issue of confidentiality because confidentiality is a paramount. If there will not be confidentiality then the clients will not reveal.”
HCW sense of purpose
Lastly, several participants reported that a sense of purpose and desire to help people motivated them to overcome the challenges of delivering assisted ICT. One participant said, “ Some of these jobs are a ministry. Counseling is not easy. You just need to tell yourself that you are there to help that person. ” Many seemed to take comfort in the knowledge that their labors, however taxing, would ultimately allow people to know their status, take control of their health, and prevent the spread of HIV. Participants framed the sense of fulfillment from successful ICT implementation as a mitigating factor amidst challenges: “ If [the contact client] has accepted it then I feel that mostly I have achieved the aim of being in the health field…that is why it is appealing to me ”.
Participants described a variety of barriers to assisted ICT implementation, including sensitivities around discussing ICT with clients, privacy concerns, limited time for ICT amid high workloads, poor quality contact information, and logistical obstacles to tracing. These barriers manifested across each step of the process of counseling index clients and tracing contacts. However, participants also identified HCW characteristics and process improvements that can mitigate these barriers.
Further, participants’ descriptions of the assisted ICT process revealed the intimately interconnected nature of factors that influence feasibility of assisted ICT. Sensitivities around HIV, privacy limitations, time constraints, and HCW characteristics all contribute to the extent to which counseling index clients elicits adequate information to facilitate contact tracing. Information quality has implications for HCW capacity, as inadequate information can lead to wasted resources, including HCW time and energy, on contact tracing. The opportunity cost of wasted efforts, which increases as the distance from which the contact client lives from the clinic increases, depletes HCW morale. The resulting acceleration of burnout, which is already fueled by busy workloads and the inherent uncertainty of day-to-day ICT work, further impairs HCW capacity to effectively engage in quality counseling that elicits adequate information from index clients. This interconnectedness suggests that efforts to mitigate barriers at any step of the assisted ICT process may have the potential to ripple across the whole process.
Participants’ descriptions of client confidentiality and privacy concerns, as well as fear of consequences of disclosure, align with previous studies that emphasize stigma as a key barrier to assisted ICT [ 15 , 18 , 19 , 20 , 30 , 31 ] and the overall HIV testing and treatment cascade [ 41 ]. Our findings suggest that anticipated stigma, or the fear of discrimination upon disclosure [ 42 ], drives several key barriers to feasibility of assisted ICT implementation. Previous studies also highlight the key role of HCWs in mitigating barriers related to anticipated stigma; noting the key role of HCW ICT knowledge, interpersonal skills, and non-stigmatizing attitudes/behaviors in securing informed consent from clients for ICT, tailoring the referral strategy to minimize risk to client confidentiality and safety, building trust and rapport with the client, and eliciting accurate contact information from index clients to facilitate contact tracing [ 18 , 19 , 20 , 30 ].
Our findings also reflect previous evidence of logistical challenges related to limited time, space, and resources that can present barriers to feasibility for HCWs [ 18 , 19 , 20 , 30 , 31 ]. Participants in the current study described these logistical challenges as perpetuating HCW burnout, making it harder for them to engage in effective counseling. Cumulative evidence of barriers across different settings (further validated by this study) suggests that assisted ICT implementation may pose greater burden on HCWs than previously thought [ 7 ]. However, our findings also suggest that strategic investment in targeted implementation strategies has the potential to help overcome these feasibility barriers.
In our own work, these findings affirmed the rationale for and informed the development of the blended learning implementation package tested in our trial [ 40 , 43 ]. Findings indicated the need for evidence-based training and support to promote HCW capacity to foster facilitating characteristics. Participants discussed the value of "refresher" opportunities in building knowledge, as well as the value of learning from other’s experiences. The blended learning implementation package balances both needs by providing time for HCWs to master ICT knowledge and skills with a combination of asynchronous, digitally delivered content (which allows for continuous review as a "refresher") and in-person sessions (which allow for sharing, practicing, and feedback). Our findings also highlight the value of flexible referral methods that align with the client’s needs, so our training content includes a detailed description of each referral method process. Further, our training content emphasizes client-centered, non-judgmental counseling as our findings add to cumulative evidence of stigma as a key barrier to assisted ICT implementation [ 41 ].
In addition, participants frequently mentioned informal workarounds currently in use to mitigate barriers or offered up ideas for potential solutions to try. Our blended learning implementation package streamlines these problem-solving processes by offering monthly continuous quality improvement sessions at each facility in our enhanced arm. These sessions allow for structured time to discuss identified barriers, share ideas to mitigate barriers, and develop solutions for sustained process improvement tailored to their specific setting. Initial focus areas for continuous quality improvement discussions include use of space, staffing, allocation of airtime and vehicles, and documentation, which were identified as barriers to feasibility in the current study.
Our study provides a uniquely in-depth examination of HCWs’ experiences implementing assisted ICT, exploring how barriers can manifest and interact with each other at each step of the process to hinder successful implementation. Further, our study has a highly actionable focus on informing development of implementation strategies to support HCWs implementing assisted ICT. Our study also has limitations. Firstly, while our sole focus on HCWs allowed for deeper exploration of assisted ICT from the perspective of those actually implementing it on the ground, this meant that our analysis did not include perspectives of index or contact clients. In addition, we did not conduct sub-group analyses as interpretation of results would be limited by our small sample size.
Assisted ICT has been widely recognized as an evidence-based intervention with high promise to increase PLHIV status awareness [ 5 , 6 , 7 , 10 , 12 , 13 , 14 , 15 , 16 , 17 , 18 , 19 , 20 , 21 , 23 , 24 , 26 , 27 , 28 , 29 ], which is important as countries in eastern and southern Africa strive to reach global UNAIDS targets. Study findings support cumulative evidence that HCWs face a variety of feasibility barriers to assisted ICT implementation in the region; further, the study’s uniquely in-depth focus on the experiences of those doing the “assisting” enhances understanding of how these barriers manifest and informs the development of implementation strategies to mitigate these barriers. Maximizing assisted ICT’s potential to increase HIV testing requires equipping HCWs with effective training and support to address and overcome the many feasibility barriers they face in implementation. Findings demonstrate the need for, as well as inform the development of, implementation strategies to mitigate barriers and promote facilitators to feasibility of assisted ICT.
Availability of data and materials
Qualitative data on which this analysis is based, as well as data collection materials and codebooks, are available from the last author upon reasonable request. The interview guide is included as an additional file.
Abbreviations
Acquired Immunodeficiency Syndrome
Antiretroviral Therapy
Health Care Worker
Human Immunodeficiency Virus
HIV Testing Services
Index Case Testing
In-Depth Interview
Intimate Partner Violence
Institutional Review Board
President’s Emergency Plan for HIV/AIDS Relief
People Living With HIV
Joint United Nations Programme on HIV/AIDS
World Health Organization
UNAIDS. Prevailing against pandemics by putting people at the centre. Geneva: UNAIDS; 2020.
Google Scholar
Frescura L, Godfrey-Faussett P, Feizzadeh AA, El-Sadr W, Syarif O, Ghys PD, et al. Achieving the 95 95 95 targets for all: A pathway to ending AIDS. PLoS One. 2022;17(8):e0272405.
Article CAS PubMed PubMed Central Google Scholar
UNAIDS. UNAIDS global AIDS update 2023: The path that ends AIDS. New York: United Nations; 2023.
Book Google Scholar
UNAIDS. UNAIDS data 2023. Geneva: Joint United Nations Programme on HIV/AIDS; 2023.
Kahabuka C, Plotkin M, Christensen A, Brown C, Njozi M, Kisendi R, et al. Addressing the first 90: A highly effective partner notification approach reaches previously undiagnosed sexual partners in Tanzania. AIDS Behav. 2017;21(8):2551–60.
Article PubMed PubMed Central Google Scholar
Lasry A, Medley A, Behel S, Mujawar MI, Cain M, Diekman ST, et al. Scaling up testing for human immunodeficiency virus infection among contacts of index patients - 20 countries, 2016–2018. MMWR Morb Mortal Wkly Rep. 2019;68(21):474–7.
Onovo A, Kalaiwo A, Agweye A, Emmanuel G, Keiser O. Diagnosis and case finding according to key partner risk populations of people living with HIV in Nigeria: A retrospective analysis of community-led index partner testing services. EClinicalMedicine. 2022;43:101265.
World Health Organization (WHO). Guidelines on HIV self-testing and partner notification : supplement to Consolidated guidelines on HIV testing services. 2016. https://apps.who.int/iris/bitstream/handle/10665/251655/9789241549868-eng.pdf?sequence=1 . Accessed 19 Apr 2024.
Watts H. Why PEPFAR is going all in on partner notification services. 2019. https://programme.ias2019.org/PAGMaterial/PPT/1934_117/Why%20PEPFAR%20is%20all%20in%20for%20PNS%2007192019%20rev.pptx . Accessed 19 Apr 2024.
Dalal S, Johnson C, Fonner V, Kennedy CE, Siegfried N, Figueroa C, et al. Improving HIV test uptake and case finding with assisted partner notification services. AIDS. 2017;31(13):1867–76.
Article PubMed Google Scholar
Mathews C, Coetzee N, Zwarenstein M, Lombard C, Guttmacher S, Oxman A, et al. A systematic review of strategies for partner notification for sexually transmitted diseases, including HIV/AIDS. Int J STD AIDS. 2002;13(5):285–300.
Hogben M, McNally T, McPheeters M, Hutchinson AB. The effectiveness of HIV partner counseling and referral services in increasing identification of HIV-positive individuals a systematic review. Am J Prev Med. 2007;33(2 Suppl):S89-100.
Brown LB, Miller WC, Kamanga G, Nyirenda N, Mmodzi P, Pettifor A, et al. HIV partner notification is effective and feasible in sub-Saharan Africa: opportunities for HIV treatment and prevention. J Acquir Immune Defic Syndr. 2011;56(5):437–42.
Sharma M, Ying R, Tarr G, Barnabas R. Systematic review and meta-analysis of community and facility-based HIV testing to address linkage to care gaps in sub-Saharan Africa. Nature. 2015;528(7580):S77-85.
Edosa M, Merdassa E, Turi E. Acceptance of index case HIV testing and its associated factors among HIV/AIDS Clients on ART follow-up in West Ethiopia: A multi-centered facility-based cross-sectional study. HIV AIDS (Auckl). 2022;14:451–60.
PubMed Google Scholar
Williams D, MacKellar D, Dlamini M, Byrd J, Dube L, Mndzebele P, et al. HIV testing and ART initiation among partners, family members, and high-risk associates of index clients participating in the CommLink linkage case management program, Eswatini, 2016–2018. PLoS ONE. 2021;16(12):e0261605.
Remera E, Nsanzimana S, Chammartin F, Semakula M, Rwibasira GN, Malamba SS, et al. Brief report: Active HIV case finding in the city of Kigali, Rwanda: Assessment of voluntary assisted partner notification modalities to detect undiagnosed HIV infections. J Acquir Immune Defic Syndr. 2022;89(4):423–7.
Article CAS PubMed Google Scholar
Quinn C, Nakyanjo N, Ddaaki W, Burke VM, Hutchinson N, Kagaayi J, et al. HIV partner notification values and preferences among sex workers, fishermen, and mainland community members in Rakai, Uganda: A qualitative study. AIDS Behav. 2018;22(10):3407–16.
Monroe-Wise A, Maingi Mutiti P, Kimani H, Moraa H, Bukusi DE, Farquhar C. Assisted partner notification services for patients receiving HIV care and treatment in an HIV clinic in Nairobi, Kenya: a qualitative assessment of barriers and opportunities for scale-up. J Int AIDS Soc. 2019;22 Suppl 3(Suppl Suppl 3):e25315.
Liu W, Wamuti BM, Owuor M, Lagat H, Kariithi E, Obong’o C, et al. “It is a process” - a qualitative evaluation of provider acceptability of HIV assisted partner services in western Kenya: experiences, challenges, and facilitators. BMC Health Serv Res. 2022;22(1):616.
Myers RS, Feldacker C, Cesar F, Paredes Z, Augusto G, Muluana C, et al. Acceptability and effectiveness of assisted human immunodeficiency virus partner services in Mozambique: Results from a pilot program in a public. Urban Clinic Sex Transm Dis. 2016;43(11):690–5.
Rosenberg NE, Mtande TK, Saidi F, Stanley C, Jere E, Paile L, et al. Recruiting male partners for couple HIV testing and counselling in Malawi’s option B+ programme: an unblinded randomised controlled trial. Lancet HIV. 2015;2(11):e483–91.
Mahachi N, Muchedzi A, Tafuma TA, Mawora P, Kariuki L, Semo BW, et al. Sustained high HIV case-finding through index testing and partner notification services: experiences from three provinces in Zimbabwe. J Int AIDS Soc. 2019;22 Suppl 3(Suppl Suppl 3):e25321.
Cherutich P, Golden MR, Wamuti B, Richardson BA, Asbjornsdottir KH, Otieno FA, et al. Assisted partner services for HIV in Kenya: a cluster randomised controlled trial. Lancet HIV. 2017;4(2):e74–82.
Proctor E, Silmere H, Raghavan R, Hovmand P, Aarons G, Bunger A, et al. Outcomes for implementation research: conceptual distinctions, measurement challenges, and research agenda. Adm Policy Ment Health. 2011;38(2):65–76.
Kamanga G, Brown L, Jawati P, Chiwanda D, Nyirenda N. Maximizing HIV partner notification opportunities for index patients and their sexual partners in Malawi. Malawi Med J. 2015;27(4):140–4.
Rutstein SE, Brown LB, Biddle AK, Wheeler SB, Kamanga G, Mmodzi P, et al. Cost-effectiveness of provider-based HIV partner notification in urban Malawi. Health Policy Plan. 2014;29(1):115–26.
Wamuti BM, Welty T, Nambu W, Chimoun FT, Shields R, Golden MR, et al. Low risk of social harms in an HIV assisted partner services programme in Cameroon. J Int AIDS Soc. 2019;22 Suppl 3(Suppl Suppl 3):e25308.
Henley C, Forgwei G, Welty T, Golden M, Adimora A, Shields R, et al. Scale-up and case-finding effectiveness of an HIV partner services program in Cameroon: an innovative HIV prevention intervention for developing countries. Sex Transm Dis. 2013;40(12):909–14.
Klabbers RE, Muwonge TR, Ayikobua E, Izizinga D, Bassett IV, Kambugu A, et al. Health worker perspectives on barriers and facilitators of assisted partner notification for HIV for refugees and Ugandan nationals: A mixed methods study in West Nile Uganda. AIDS Behav. 2021;25(10):3206–22.
Mugisha N, Tirera F, Coulibaly-Kouyate N, Aguie W, He Y, Kemper K, et al. Implementation process and challenges of index testing in Cote d’Ivoire from healthcare workers’ perspectives. PLoS One. 2023;18(2):e0280623.
Rosenberg NE, Tembo TA, Simon KR, Mollan K, Rutstein SE, Mwapasa V, et al. Development of a Blended Learning Approach to Delivering HIV-Assisted Contact Tracing in Malawi: Applied Theory and Formative Research. JMIR Form Res. 2022;6(4):e32899.
Government of Malawi National Statistical Office. 2018 Malawi population and housing census : main report. 2019. https://malawi.unfpa.org/sites/default/files/resource-pdf/2018%20Malawi%20Population%20and%20Housing%20Census%20Main%20Report%20%281%29.pdf . Accessed 19 April 2024.
Wolock TM, Flaxman S, Chimpandule T, Mbiriyawanda S, Jahn A, Nyirenda R, et al. Subnational HIV incidence trends in Malawi: large, heterogeneous declines across space. medRxiv (PREPRINT). 2023. https://www.ncbi.nlm.nih.gov/pmc/articles/PMC9915821/ . Accessed 19 Apr 2024.
World Health Organization (WHO). Medical doctors (per 10,000). 2020. https://www.who.int/data/gho/data/indicators/indicator-details/GHO/medical-doctors-(per-10-000-population . Accessed 19 Apr 2024.
Flick RJ, Simon KR, Nyirenda R, Namachapa K, Hosseinipour MC, Schooley A, et al. The HIV diagnostic assistant: early findings from a novel HIV testing cadre in Malawi. AIDS. 2019;33(7):1215–24.
Kim MH, Ahmed S, Buck WC, Preidis GA, Hosseinipour MC, Bhalakia A, et al. The Tingathe programme: a pilot intervention using community health workers to create a continuum of care in the prevention of mother to child transmission of HIV (PMTCT) cascade of services in Malawi. J Int AIDS Soc. 2012;15(Suppl 2):17389.
Simon KR, Hartig M, Abrams EJ, Wetzel E, Ahmed S, Chester E, et al. The Tingathe Surge: a multi-strategy approach to accelerate HIV case finding in Malawi. Public Health Action. 2019;9(3):128–34.
Ahmed S, Kim MH, Dave AC, Sabelli R, Kanjelo K, Preidis GA, et al. Improved identification and enrolment into care of HIV-exposed and -infected infants and children following a community health worker intervention in Lilongwe, Malawi. J Int AIDS Soc. 2015;18(1):19305.
Tembo TA, Mollan K, Simon K, Rutstein S, Chitani MJ, Saha PT, et al. Does a blended learning implementation package enhance HIV index case testing in Malawi? A protocol for a cluster randomised controlled trial. BMJ Open. 2024;14(1):e077706.
Nyblade L, Mingkwan P, Stockton MA. Stigma reduction: an essential ingredient to ending AIDS by 2030. Lancet HIV. 2021;8(2):e106–13.
Nyblade L, Stockton M, Nyato D, Wamoyi J. Perceived, anticipated and experienced stigma: exploring manifestations and implications for young people’s sexual and reproductive health and access to care in North-Western Tanzania. Cult Health Sex. 2017;19(10):1092–107.
Tembo TA, Simon KR, Kim MH, Chikoti C, Huffstetler HE, Ahmed S, et al. Pilot-Testing a Blended Learning Package for Health Care Workers to Improve Index Testing Services in Southern Malawi: An Implementation Science Study. J Acquir Immune Defic Syndr. 2021;88(5):470–6.
Download references
Acknowledgements
We are grateful to the Malawian health care workers who shared their experiences through in-depth interviews, as well as to the study team members in Malawi and the United States for their contributions.
Research reported in this publication was funded by the National Institutes of Health (R01 MH124526) with support from the University of North Carolina at Chapel Hill Center for AIDS Research (P30 AI50410) and the Fogarty International Center of the National Institutes of Health (D43 TW010060 and R01 MH115793-04). The funders had no role in trial design, data collection and analysis, decision to publish or preparation of the manuscript.
Author information
Authors and affiliations.
RTI International, Research Triangle Park, NC, USA
Caroline J. Meek
Gillings School of Global Public Health, University of North Carolina at Chapel Hill, Chapel Hill, NC, USA
Caroline J. Meek, Milenka Jean-Baptiste, Jiayu Wang, Clare Barrington, Vivian F. Go & Nora E. Rosenberg
Kamuzu University of Health Sciences, Blantyre, Malawi
Tiwonge E. Mbeya Munkhondya
Baylor College of Medicine Children’s Foundation, Lilongwe, Malawi
Mtisunge Mphande, Tapiwa A. Tembo, Mike Chitani, Dhrutika Vansia, Caroline Kumbuyo, Katherine R. Simon & Maria H. Kim
Department of Medicine, Division of Infectious Diseases, University of North Carolina at Chapel Hill, Chapel Hill, NC, USA
Sarah E. Rutstein
You can also search for this author in PubMed Google Scholar
Contributions
TAT, KRS, SER, MHK, VFG, and NER contributed to overall study conceptualization, with CJM, CB, and NER leading conceptualization of the analysis presented in this study. Material preparation and data collection were performed by TEMM, MM, TAT, MC, and CK. Analysis was led by CJM with support from MJB and DV. The first draft of the manuscript was written by CJM with consultation from NER, TEMM, MM, TAT, MJB, and DV. JW provided quantitative analysis support for participant characteristics. All authors commented on previous versions of the manuscript. All authors read and approved the final manuscript.
Corresponding author
Correspondence to Caroline J. Meek .
Ethics declarations
Ethics approval and consent to participate.
Ethical clearance was provided by the Malawi National Health Science Research Committee (NHSRC; #20/06/2566), University of North Carolina Institution Review Board (UNC IRB; #20–1810) and the Baylor College of Medicine institutional review board (Baylor IRB; H-48800). The procedures used in this study adhere to the tenets of the Declaration of Helsinki. Written informed consent for participation was obtained from all study participants prior to enrollment in the parent study. Interviewers also engaged in informal verbal discussion of consent immediately ahead of in-depth interviews.
Consent for publication
Not applicable. No identifying information is included in the manuscript.
Competing interests
The authors declare no competing interests.
Additional information
Publisher’s note.
Springer Nature remains neutral with regard to jurisdictional claims in published maps and institutional affiliations.
Supplementary Information
Supplementary material 1., rights and permissions.
Open Access This article is licensed under a Creative Commons Attribution 4.0 International License, which permits use, sharing, adaptation, distribution and reproduction in any medium or format, as long as you give appropriate credit to the original author(s) and the source, provide a link to the Creative Commons licence, and indicate if changes were made. The images or other third party material in this article are included in the article's Creative Commons licence, unless indicated otherwise in a credit line to the material. If material is not included in the article's Creative Commons licence and your intended use is not permitted by statutory regulation or exceeds the permitted use, you will need to obtain permission directly from the copyright holder. To view a copy of this licence, visit http://creativecommons.org/licenses/by/4.0/ . The Creative Commons Public Domain Dedication waiver ( http://creativecommons.org/publicdomain/zero/1.0/ ) applies to the data made available in this article, unless otherwise stated in a credit line to the data.
Reprints and permissions
About this article
Cite this article.
Meek, C.J., Munkhondya, T.E.M., Mphande, M. et al. Examining the feasibility of assisted index case testing for HIV case-finding: a qualitative analysis of barriers and facilitators to implementation in Malawi. BMC Health Serv Res 24 , 606 (2024). https://doi.org/10.1186/s12913-024-10988-z
Download citation
Received : 31 August 2023
Accepted : 12 April 2024
Published : 09 May 2024
DOI : https://doi.org/10.1186/s12913-024-10988-z
Share this article
Anyone you share the following link with will be able to read this content:
Sorry, a shareable link is not currently available for this article.
Provided by the Springer Nature SharedIt content-sharing initiative
- HIV testing and counseling
- Index case testing
- Assisted partner notification services
- Implementation science
- Health care workers
BMC Health Services Research
ISSN: 1472-6963
- General enquiries: [email protected]
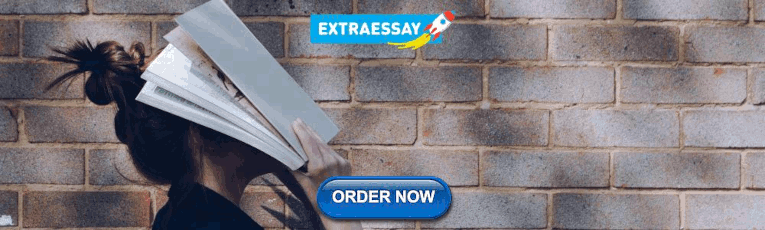
COMMENTS
Quantitative data has to be gathered and cleaned before proceeding to the stage of analyzing it. Below are the steps to prepare a data before quantitative research analysis: Step 1: Data Collection. Before beginning the analysis process, you need data. Data can be collected through rigorous quantitative research, which includes methods such as ...
Revised on June 22, 2023. Quantitative research is the process of collecting and analyzing numerical data. It can be used to find patterns and averages, make predictions, test causal relationships, and generalize results to wider populations. Quantitative research is the opposite of qualitative research, which involves collecting and analyzing ...
Quantitative data analysis is one of those things that often strikes fear in students. It's totally understandable - quantitative analysis is a complex topic, full of daunting lingo, like medians, modes, correlation and regression.Suddenly we're all wishing we'd paid a little more attention in math class…. The good news is that while quantitative data analysis is a mammoth topic ...
nominal. It is important to know w hat kind of data you are planning to collect or analyse as this w ill. affect your analysis method. A 12 step approach to quantitative data analysis. Step 1 ...
Quantitative Research: Focus: Quantitative research focuses on numerical data, seeking to quantify variables and examine relationships between them. It aims to provide statistical evidence and generalize findings to a larger population. Measurement: Quantitative research involves standardized measurement instruments, such as surveys or questionnaires, to collect data.
Abstract. Quantitative data analysis serves as part of an essential process of evidence-making in health and social sciences. It is adopted for any types of research question and design whether it is descriptive, explanatory, or causal. However, compared with qualitative counterpart, quantitative data analysis has less flexibility.
Without further ado, here are the 17 essential types of data analysis methods with some use cases in the business world: A. Quantitative Methods . To put it simply, quantitative analysis refers to all methods that use numerical data or data that can be turned into numbers (e.g. category variables like gender, age, etc.) to extract valuable ...
Abstract. This chapter describes the types of data analysis techniques in quantitative research and sampling strategies suitable for quantitative studies, particularly probability sampling, to produce credible and trustworthy explanations of a phenomenon. Initially, it briefly describes the measurement levels of variables.
Data analysis is a comprehensive method of inspecting, cleansing, transforming, and modeling data to discover useful information, draw conclusions, and support decision-making. It is a multifaceted process involving various techniques and methodologies to interpret data from various sources in different formats, both structured and unstructured.
Offers a guide through the essential steps required in quantitative data analysis; Helps in choosing the right method before starting the data collection process; ... executing and reporting appropriate data analysis methods to answer their research questions. It provides readers with a basic understanding of the steps that each method involves ...
Quantitative research is a research strategy that focuses on quantifying the collection and analysis of data. It is formed from a deductive approach where emphasis is placed on the testing of theory, shaped by empiricist and positivist philosophies.. Associated with the natural, applied, formal, and social sciences this research strategy promotes the objective empirical investigation of ...
This article is a practical guide to conducting data analysis in general literature reviews. The general literature review is a synthesis and analysis of published research on a relevant clinical issue, and is a common format for academic theses at the bachelor's and master's levels in nursing, physiotherapy, occupational therapy, public health and other related fields.
quantitative (numbers) and qualitative (words or images) data. The combination of. quantitative and qualitative research methods is called mixed methods. For example, first, numerical data are ...
Quantitative data analysis with the application of statistical software consists of the following stages [1]: Preparing and checking the data. Input of data into computer. Selecting the most appropriate tables and diagrams to use according to your research objectives. Selecting the most appropriate statistics to describe your data.
Education Research: Quantitative research is used in education research to study the effectiveness of teaching methods, assess student learning outcomes, and identify factors that influence student success. Researchers use experimental and quasi-experimental designs, as well as surveys and other quantitative methods, to collect and analyze data.
Definition of research in data analysis: According to LeCompte and Schensul, research data analysis is a process used by researchers to reduce data to a story and interpret it to derive insights. The data analysis process helps reduce a large chunk of data into smaller fragments, which makes sense. Three essential things occur during the data ...
The last step of designing your research is planning your data analysis strategies. In this video, we'll take a look at some common approaches for both quant...
Expand Multimethod and Mixed Methods Research Analysis Strategies: ... it shows the complexities and challenges involved in integrating qualitative and quantitative data: issues about linking data sets, similarity (or not) of units of analysis, and concepts and meaning. It draws some conclusions and sets out some future directions for MMMR.
INTRODUCTION. Scientific research is usually initiated by posing evidenced-based research questions which are then explicitly restated as hypotheses.1,2 The hypotheses provide directions to guide the study, solutions, explanations, and expected results.3,4 Both research questions and hypotheses are essentially formulated based on conventional theories and real-world processes, which allow the ...
When collecting and analyzing data, quantitative research deals with numbers and statistics, while qualitative research deals with words and meanings. Both are important for gaining different kinds of knowledge. Quantitative research. Quantitative research is expressed in numbers and graphs. It is used to test or confirm theories and assumptions.
Descriptive analysis is a quantitative data analysis approach that assists researchers in presenting data in an easily understood, quantitative format, assisting in the interpretation and ...
Qualitative research aims to produce rich and detailed descriptions of the phenomenon being studied, and to uncover new insights and meanings. Quantitative data is information about quantities, and therefore numbers, and qualitative data is descriptive, and regards phenomenon which can be observed but not measured, such as language.
Also from Sage. CQ Library Elevating debate opens in new tab; Sage Data Uncovering insight opens in new tab; Sage Business Cases Shaping futures opens in new tab; Sage Campus Unleashing potential opens in new tab; Sage Knowledge Multimedia learning resources opens in new tab; Sage Research Methods Supercharging research opens in new tab; Sage Video Streaming knowledge opens in new tab
Gather your data from all relevant sources using data analysis software. Ensure that the data is representative and actually covers the variables you want to analyze. 3. Select your analytical methods. Investigate the various data analysis methods and select the technique that best aligns with your objectives.
Quantitative data analysis for business intelligence (BI) examines business issues through statistical, mathematical, or computational techniques. Business analysts collect and examine numerical data to identify trends, patterns, and relationships that inform strategic business decisions. 1 Quantitative methods in BI drive decision-making by giving business leaders a solid foundation for ...
Yuval Atsmon: When people talk about artificial intelligence, they include everything to do with analytics, automation, and data analysis. Marvin Minsky, the pioneer of artificial intelligence research in the 1960s, talked about AI as a "suitcase word"—a term into which you can stuff whatever you want—and that still seems to be the case.
The research team first reviewed all of the interview summaries individually and then met multiple times to discuss initial observations, refining the research question and scope of analysis. A US-based analyst (CJM) with training in qualitative analysis used an inductive approach to develop a codebook, deriving broad codes from the ...
Follow. Dublin, May 13, 2024 (GLOBE NEWSWIRE) -- The "Kingfisher - Digital Transformation Strategies" company profile has been added to ResearchAndMarkets.com's offering. The report offers a ...
Contact Data CONTACT: ResearchAndMarkets.com Laura Wood,Senior Press Manager [email protected] For E.S.T Office Hours Call 1-917-300-0470 For U.S./ CAN Toll Free Call 1-800-526-8630 For ...
Dublin, May 13, 2024 (GLOBE NEWSWIRE) -- The . Air France Digital Transformation Strategy Analysis Report 2024: Accelerators, Incubators, Training and Innovation Programs