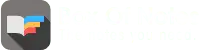
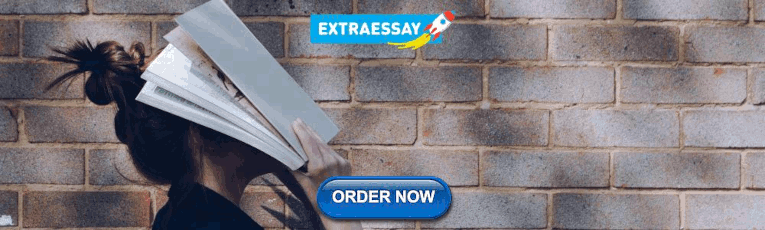
Problem Solving Agents in Artificial Intelligence
In this post, we will talk about Problem Solving agents in Artificial Intelligence, which are sort of goal-based agents. Because the straight mapping from states to actions of a basic reflex agent is too vast to retain for a complex environment, we utilize goal-based agents that may consider future actions and the desirability of outcomes.
You Will Learn
Problem Solving Agents
Problem Solving Agents decide what to do by finding a sequence of actions that leads to a desirable state or solution.
An agent may need to plan when the best course of action is not immediately visible. They may need to think through a series of moves that will lead them to their goal state. Such an agent is known as a problem solving agent , and the computation it does is known as a search .
The problem solving agent follows this four phase problem solving process:
- Goal Formulation: This is the first and most basic phase in problem solving. It arranges specific steps to establish a target/goal that demands some activity to reach it. AI agents are now used to formulate goals.
- Problem Formulation: It is one of the fundamental steps in problem-solving that determines what action should be taken to reach the goal.
- Search: After the Goal and Problem Formulation, the agent simulates sequences of actions and has to look for a sequence of actions that reaches the goal. This process is called search, and the sequence is called a solution . The agent might have to simulate multiple sequences that do not reach the goal, but eventually, it will find a solution, or it will find that no solution is possible. A search algorithm takes a problem as input and outputs a sequence of actions.
- Execution: After the search phase, the agent can now execute the actions that are recommended by the search algorithm, one at a time. This final stage is known as the execution phase.
Problems and Solution
Before we move into the problem formulation phase, we must first define a problem in terms of problem solving agents.
A formal definition of a problem consists of five components:
Initial State
Transition model.
It is the agent’s starting state or initial step towards its goal. For example, if a taxi agent needs to travel to a location(B), but the taxi is already at location(A), the problem’s initial state would be the location (A).
It is a description of the possible actions that the agent can take. Given a state s, Actions ( s ) returns the actions that can be executed in s. Each of these actions is said to be appropriate in s.
It describes what each action does. It is specified by a function Result ( s, a ) that returns the state that results from doing action an in state s.
The initial state, actions, and transition model together define the state space of a problem, a set of all states reachable from the initial state by any sequence of actions. The state space forms a graph in which the nodes are states, and the links between the nodes are actions.
It determines if the given state is a goal state. Sometimes there is an explicit list of potential goal states, and the test merely verifies whether the provided state is one of them. The goal is sometimes expressed via an abstract attribute rather than an explicitly enumerated set of conditions.
It assigns a numerical cost to each path that leads to the goal. The problem solving agents choose a cost function that matches its performance measure. Remember that the optimal solution has the lowest path cost of all the solutions .
Example Problems
The problem solving approach has been used in a wide range of work contexts. There are two kinds of problem approaches
- Standardized/ Toy Problem: Its purpose is to demonstrate or practice various problem solving techniques. It can be described concisely and precisely, making it appropriate as a benchmark for academics to compare the performance of algorithms.
- Real-world Problems: It is real-world problems that need solutions. It does not rely on descriptions, unlike a toy problem, yet we can have a basic description of the issue.
Some Standardized/Toy Problems
Vacuum world problem.
Let us take a vacuum cleaner agent and it can move left or right and its jump is to suck up the dirt from the floor.
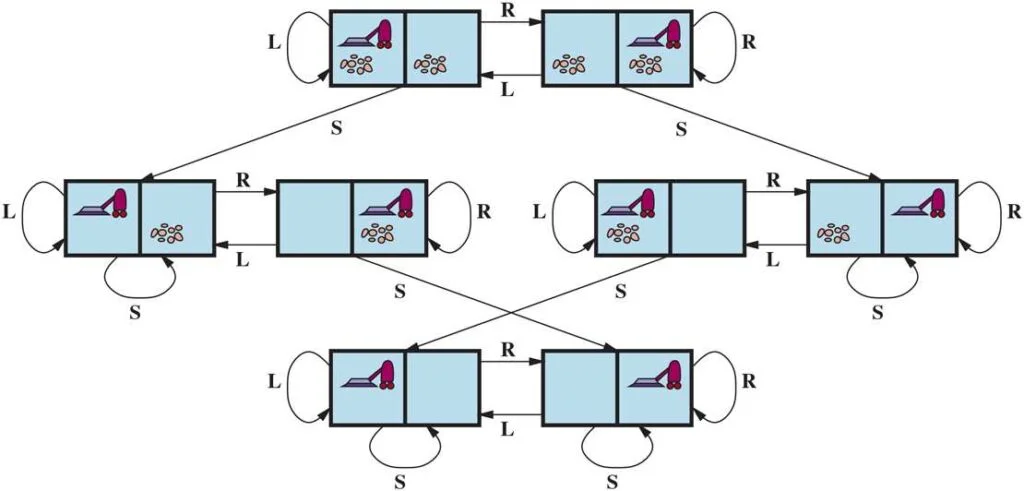
The vacuum world’s problem can be stated as follows:
States: A world state specifies which objects are housed in which cells. The objects in the vacuum world are the agent and any dirt. The agent can be in either of the two cells in the simple two-cell version, and each call can include dirt or not, therefore there are 2×2×2 = 8 states. A vacuum environment with n cells has n×2 n states in general.
Initial State: Any state can be specified as the starting point.
Actions: We defined three actions in the two-cell world: sucking, moving left, and moving right. More movement activities are required in a two-dimensional multi-cell world.
Transition Model: Suck cleans the agent’s cell of any filth; Forward moves the agent one cell forward in the direction it is facing unless it meets a wall, in which case the action has no effect. Backward moves the agent in the opposite direction, whilst TurnRight and TurnLeft rotate it by 90°.
Goal States: The states in which every cell is clean.
Action Cost: Each action costs 1.
8 Puzzle Problem
In a sliding-tile puzzle , a number of tiles (sometimes called blocks or pieces) are arranged in a grid with one or more blank spaces so that some of the tiles can slide into the blank space. One variant is the Rush Hour puzzle, in which cars and trucks slide around a 6 x 6 grid in an attempt to free a car from the traffic jam. Perhaps the best-known variant is the 8- puzzle (see Figure below ), which consists of a 3 x 3 grid with eight numbered tiles and one blank space, and the 15-puzzle on a 4 x 4 grid. The object is to reach a specified goal state, such as the one shown on the right of the figure. The standard formulation of the 8 puzzles is as follows:
STATES : A state description specifies the location of each of the tiles.
INITIAL STATE : Any state can be designated as the initial state. (Note that a parity property partitions the state space—any given goal can be reached from exactly half of the possible initial states.)
ACTIONS : While in the physical world it is a tile that slides, the simplest way of describing action is to think of the blank space moving Left , Right , Up , or Down . If the blank is at an edge or corner then not all actions will be applicable.
TRANSITION MODEL : Maps a state and action to a resulting state; for example, if we apply Left to the start state in the Figure below, the resulting state has the 5 and the blank switched.
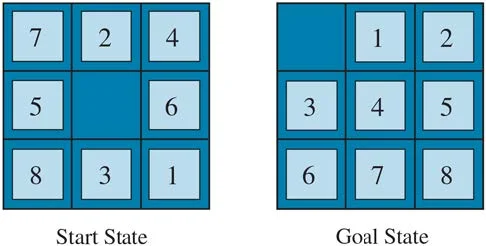
GOAL STATE : It identifies whether we have reached the correct goal state. Although any state could be the goal, we typically specify a state with the numbers in order, as in the Figure above.
ACTION COST : Each action costs 1.
You Might Like:
- Agents in Artificial Intelligence
Types of Environments in Artificial Intelligence
- Understanding PEAS in Artificial Intelligence
- River Crossing Puzzle | Farmer, Wolf, Goat and Cabbage
Share Article:
Digital image processing: all you need to know.
- Trending Now
- Foundational Courses
- Data Science
- Practice Problem
- Machine Learning
- System Design
- DevOps Tutorial
- Statistical Machine Translation of Languages in Artificial Intelligence
- Breadth-first Search is a special case of Uniform-cost search
- Artificial Intelligence - Boon or Bane
- Stochastic Games in Artificial Intelligence
- Resolution Algorithm in Artificial Intelligence
- Types of Environments in AI
- PEAS Description of Task Environment
- Optimal Decision Making in Multiplayer Games
- Game Theory in AI
- Emergence Of Artificial Intelligence
- Propositional Logic based Agent
- GPT-3 : Next AI Revolution
- Advantages and Disadvantage of Artificial Intelligence
- Understanding PEAS in Artificial Intelligence
- Sparse Rewards in Reinforcement Learning
- Propositional Logic Hybrid Agent and Logical State
- Prepositional Logic Inferences
- Linguistic variable And Linguistic hedges
- Knowledge based agents in AI
Problem Solving in Artificial Intelligence
The reflex agent of AI directly maps states into action. Whenever these agents fail to operate in an environment where the state of mapping is too large and not easily performed by the agent, then the stated problem dissolves and sent to a problem-solving domain which breaks the large stored problem into the smaller storage area and resolves one by one. The final integrated action will be the desired outcomes.
On the basis of the problem and their working domain, different types of problem-solving agent defined and use at an atomic level without any internal state visible with a problem-solving algorithm. The problem-solving agent performs precisely by defining problems and several solutions. So we can say that problem solving is a part of artificial intelligence that encompasses a number of techniques such as a tree, B-tree, heuristic algorithms to solve a problem.
We can also say that a problem-solving agent is a result-driven agent and always focuses on satisfying the goals.
There are basically three types of problem in artificial intelligence:
1. Ignorable: In which solution steps can be ignored.
2. Recoverable: In which solution steps can be undone.
3. Irrecoverable: Solution steps cannot be undo.
Steps problem-solving in AI: The problem of AI is directly associated with the nature of humans and their activities. So we need a number of finite steps to solve a problem which makes human easy works.
These are the following steps which require to solve a problem :
- Problem definition: Detailed specification of inputs and acceptable system solutions.
- Problem analysis: Analyse the problem thoroughly.
- Knowledge Representation: collect detailed information about the problem and define all possible techniques.
- Problem-solving: Selection of best techniques.
Components to formulate the associated problem:
- Initial State: This state requires an initial state for the problem which starts the AI agent towards a specified goal. In this state new methods also initialize problem domain solving by a specific class.
- Action: This stage of problem formulation works with function with a specific class taken from the initial state and all possible actions done in this stage.
- Transition: This stage of problem formulation integrates the actual action done by the previous action stage and collects the final stage to forward it to their next stage.
- Goal test: This stage determines that the specified goal achieved by the integrated transition model or not, whenever the goal achieves stop the action and forward into the next stage to determines the cost to achieve the goal.
- Path costing: This component of problem-solving numerical assigned what will be the cost to achieve the goal. It requires all hardware software and human working cost.
Please Login to comment...
Similar reads.
- Artificial Intelligence
- What are Tiktok AI Avatars?
- Poe Introduces A Price-per-message Revenue Model For AI Bot Creators
- Truecaller For Web Now Available For Android Users In India
- Google Introduces New AI-powered Vids App
- 30 OOPs Interview Questions and Answers (2024)
Improve your Coding Skills with Practice
What kind of Experience do you want to share?
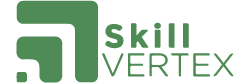
Problem-Solving Agents In Artificial Intelligence
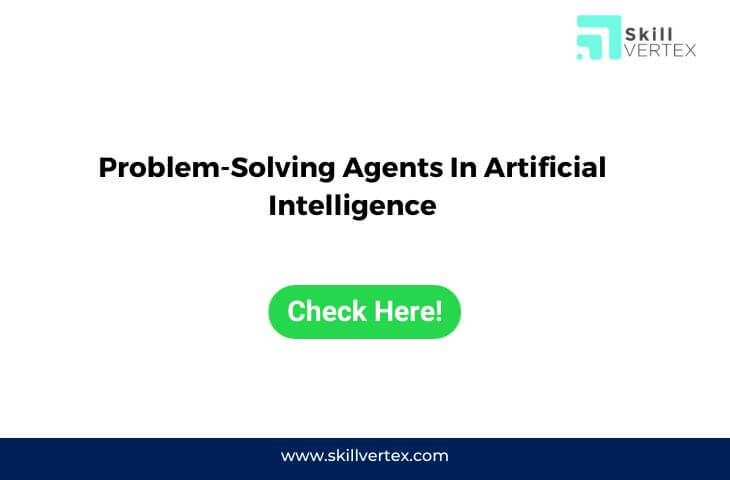
In artificial intelligence, a problem-solving agent refers to a type of intelligent agent designed to address and solve complex problems or tasks in its environment. These agents are a fundamental concept in AI and are used in various applications, from game-playing algorithms to robotics and decision-making systems. Here are some key characteristics and components of a problem-solving agent:
- Perception : Problem-solving agents typically have the ability to perceive or sense their environment. They can gather information about the current state of the world, often through sensors, cameras, or other data sources.
- Knowledge Base : These agents often possess some form of knowledge or representation of the problem domain. This knowledge can be encoded in various ways, such as rules, facts, or models, depending on the specific problem.
- Reasoning : Problem-solving agents employ reasoning mechanisms to make decisions and select actions based on their perception and knowledge. This involves processing information, making inferences, and selecting the best course of action.
- Planning : For many complex problems, problem-solving agents engage in planning. They consider different sequences of actions to achieve their goals and decide on the most suitable action plan.
- Actuation : After determining the best course of action, problem-solving agents take actions to interact with their environment. This can involve physical actions in the case of robotics or making decisions in more abstract problem-solving domains.
- Feedback : Problem-solving agents often receive feedback from their environment, which they use to adjust their actions and refine their problem-solving strategies. This feedback loop helps them adapt to changing conditions and improve their performance.
- Learning : Some problem-solving agents incorporate machine learning techniques to improve their performance over time. They can learn from experience, adapt their strategies, and become more efficient at solving similar problems in the future.
Problem-solving agents can vary greatly in complexity, from simple algorithms that solve straightforward puzzles to highly sophisticated AI systems that tackle complex, real-world problems. The design and implementation of problem-solving agents depend on the specific problem domain and the goals of the AI application.
Hello, I’m Hridhya Manoj. I’m passionate about technology and its ever-evolving landscape. With a deep love for writing and a curious mind, I enjoy translating complex concepts into understandable, engaging content. Let’s explore the world of tech together
Which Of The Following Is A Privilege In SQL Standard
Implicit Return Type Int In C
Leave a Comment Cancel reply
Save my name, email, and website in this browser for the next time I comment.
Reach Out to Us for Any Query
SkillVertex is an edtech organization that aims to provide upskilling and training to students as well as working professionals by delivering a diverse range of programs in accordance with their needs and future aspirations.
© 2024 Skill Vertex
Thank you for visiting nature.com. You are using a browser version with limited support for CSS. To obtain the best experience, we recommend you use a more up to date browser (or turn off compatibility mode in Internet Explorer). In the meantime, to ensure continued support, we are displaying the site without styles and JavaScript.
- View all journals
- Explore content
- About the journal
- Publish with us
- Sign up for alerts
- Perspective
- Published: 25 January 2022
Intelligent problem-solving as integrated hierarchical reinforcement learning
- Manfred Eppe ORCID: orcid.org/0000-0002-5473-3221 1 nAff4 ,
- Christian Gumbsch ORCID: orcid.org/0000-0003-2741-6551 2 , 3 ,
- Matthias Kerzel 1 ,
- Phuong D. H. Nguyen 1 ,
- Martin V. Butz ORCID: orcid.org/0000-0002-8120-8537 2 &
- Stefan Wermter 1
Nature Machine Intelligence volume 4 , pages 11–20 ( 2022 ) Cite this article
5200 Accesses
30 Citations
8 Altmetric
Metrics details
- Cognitive control
- Computational models
- Computer science
- Learning algorithms
- Problem solving
According to cognitive psychology and related disciplines, the development of complex problem-solving behaviour in biological agents depends on hierarchical cognitive mechanisms. Hierarchical reinforcement learning is a promising computational approach that may eventually yield comparable problem-solving behaviour in artificial agents and robots. However, so far, the problem-solving abilities of many human and non-human animals are clearly superior to those of artificial systems. Here we propose steps to integrate biologically inspired hierarchical mechanisms to enable advanced problem-solving skills in artificial agents. We first review the literature in cognitive psychology to highlight the importance of compositional abstraction and predictive processing. Then we relate the gained insights with contemporary hierarchical reinforcement learning methods. Interestingly, our results suggest that all identified cognitive mechanisms have been implemented individually in isolated computational architectures, raising the question of why there exists no single unifying architecture that integrates them. As our final contribution, we address this question by providing an integrative perspective on the computational challenges to develop such a unifying architecture. We expect our results to guide the development of more sophisticated cognitively inspired hierarchical machine learning architectures.
This is a preview of subscription content, access via your institution
Access options
Access Nature and 54 other Nature Portfolio journals
Get Nature+, our best-value online-access subscription
24,99 € / 30 days
cancel any time
Subscribe to this journal
Receive 12 digital issues and online access to articles
111,21 € per year
only 9,27 € per issue
Rent or buy this article
Prices vary by article type
Prices may be subject to local taxes which are calculated during checkout

Similar content being viewed by others
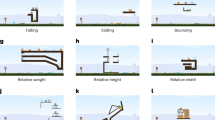
Phy-Q as a measure for physical reasoning intelligence
Cheng Xue, Vimukthini Pinto, … Jochen Renz
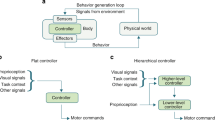
Hierarchical motor control in mammals and machines
Josh Merel, Matthew Botvinick & Greg Wayne
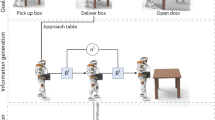
Hierarchical generative modelling for autonomous robots
Kai Yuan, Noor Sajid, … Zhibin Li
Gruber, R. et al. New Caledonian crows use mental representations to solve metatool problems. Curr. Biol. 29 , 686–692 (2019).
Article Google Scholar
Butz, M. V. & Kutter, E. F. How the Mind Comes into Being (Oxford Univ. Press, 2017).
Perkins, D. N. & Salomon, G. in International Encyclopedia of Education (eds. Husen T. & Postelwhite T. N.) 6452–6457 (Pergamon Press, 1992).
Botvinick, M. M., Niv, Y. & Barto, A. C. Hierarchically organized behavior and its neural foundations: a reinforcement learning perspective. Cognition 113 , 262–280 (2009).
Tomov, M. S., Yagati, S., Kumar, A., Yang, W. & Gershman, S. J. Discovery of hierarchical representations for efficient planning. PLoS Comput. Biol. 16 , e1007594 (2020).
Arulkumaran, K., Deisenroth, M. P., Brundage, M. & Bharath, A. A. Deep reinforcement learning: a brief survey. IEEE Signal Process. Mag. 34 , 26–38 (2017).
Li, Y. Deep reinforcement learning: an overview. Preprint at https://arxiv.org/abs/1701.07274 (2018).
Sutton, R. S. & Barto, A. G. Reinforcement Learning : An Introduction 2nd edn (MIT Press, 2018).
Neftci, E. O. & Averbeck, B. B. Reinforcement learning in artificial and biological systems. Nat. Mach. Intell. 1 , 133–143 (2019).
Eppe, M., Nguyen, P. D. H. & Wermter, S. From semantics to execution: integrating action planning with reinforcement learning for robotic causal problem-solving. Front. Robot. AI 6 , 123 (2019).
Oh, J., Singh, S., Lee, H. & Kohli, P. Zero-shot task generalization with multi-task deep reinforcement learning. In Proc. 34th International Conference on Machine Learning ( ICML ) (eds. Precup, D. & Teh, Y. W.) 2661–2670 (PMLR, 2017).
Sohn, S., Oh, J. & Lee, H. Hierarchical reinforcement learning for zero-shot generalization with subtask dependencies. In Proc. 32nd International Conference on Neural Information Processing Systems ( NeurIPS ) (eds Bengio S. et al.) Vol. 31, 7156–7166 (ACM, 2018).
Hegarty, M. Mechanical reasoning by mental simulation. Trends Cogn. Sci. 8 , 280–285 (2004).
Klauer, K. J. Teaching for analogical transfer as a means of improving problem-solving, thinking and learning. Instruct. Sci. 18 , 179–192 (1989).
Duncker, K. & Lees, L. S. On problem-solving. Psychol. Monographs 58, No.5 (whole No. 270), 85–101 https://doi.org/10.1037/h0093599 (1945).
Dayan, P. Goal-directed control and its antipodes. Neural Netw. 22 , 213–219 (2009).
Dolan, R. J. & Dayan, P. Goals and habits in the brain. Neuron 80 , 312–325 (2013).
O’Doherty, J. P., Cockburn, J. & Pauli, W. M. Learning, reward, and decision making. Annu. Rev. Psychol. 68 , 73–100 (2017).
Tolman, E. C. & Honzik, C. H. Introduction and removal of reward, and maze performance in rats. Univ. California Publ. Psychol. 4 , 257–275 (1930).
Google Scholar
Butz, M. V. & Hoffmann, J. Anticipations control behavior: animal behavior in an anticipatory learning classifier system. Adaptive Behav. 10 , 75–96 (2002).
Miller, G. A., Galanter, E. & Pribram, K. H. Plans and the Structure of Behavior (Holt, Rinehart & Winston, 1960).
Botvinick, M. & Weinstein, A. Model-based hierarchical reinforcement learning and human action control. Philos. Trans. R. Soc. B Biol. Sci. 369 , 20130480 (2014).
Wiener, J. M. & Mallot, H. A. ’Fine-to-coarse’ route planning and navigation in regionalized environments. Spatial Cogn. Comput. 3 , 331–358 (2003).
Stock, A. & Stock, C. A short history of ideo-motor action. Psychol. Res. 68 , 176–188 (2004).
Hommel, B., Müsseler, J., Aschersleben, G. & Prinz, W. The theory of event coding (TEC): a framework for perception and action planning. Behav. Brain Sci. 24 , 849–878 (2001).
Hoffmann, J. in Anticipatory Behavior in Adaptive Learning Systems : Foundations , Theories and Systems (eds Butz, M. V. et al.) 44–65 (Springer, 2003).
Kunde, W., Elsner, K. & Kiesel, A. No anticipation-no action: the role of anticipation in action and perception. Cogn. Process. 8 , 71–78 (2007).
Barsalou, L. W. Grounded cognition. Annu. Rev. Psychol. 59 , 617–645 (2008).
Butz, M. V. Toward a unified sub-symbolic computational theory of cognition. Front. Psychol. 7 , 925 (2016).
Pulvermüller, F. Brain embodiment of syntax and grammar: discrete combinatorial mechanisms spelt out in neuronal circuits. Brain Lang. 112 , 167–179 (2010).
Sutton, R. S., Precup, D. & Singh, S. Between MDPs and semi-MDPs: a framework for temporal abstraction in reinforcement learning. Artif. Intell. 112 , 181–211 (1999).
Article MathSciNet MATH Google Scholar
Flash, T. & Hochner, B. Motor primitives in vertebrates and invertebrates. Curr. Opin. Neurobiol. 15 , 660–666 (2005).
Schaal, S. in Adaptive Motion of Animals and Machines (eds. Kimura, H. et al.) 261–280 (Springer, 2006).
Feldman, J., Dodge, E. & Bryant, J. in The Oxford Handbook of Linguistic Analysis (eds Heine, B. & Narrog, H.) 111–138 (Oxford Univ. Press, 2009).
Fodor, J. A. Language, thought and compositionality. Mind Lang. 16 , 1–15 (2001).
Frankland, S. M. & Greene, J. D. Concepts and compositionality: in search of the brain’s language of thought. Annu. Rev. Psychol. 71 , 273–303 (2020).
Hummel, J. E. Getting symbols out of a neural architecture. Connection Sci. 23 , 109–118 (2011).
Haynes, J. D., Wisniewski, D., Gorgen, K., Momennejad, I. & Reverberi, C. FMRI decoding of intentions: compositionality, hierarchy and prospective memory. In Proc. 3rd International Winter Conference on Brain-Computer Interface ( BCI ), 1-3 (IEEE, 2015).
Gärdenfors, P. The Geometry of Meaning : Semantics Based on Conceptual Spaces (MIT Press, 2014).
Book MATH Google Scholar
Lakoff, G. & Johnson, M. Philosophy in the Flesh (Basic Books, 1999).
Eppe, M. et al. A computational framework for concept blending. Artif. Intell. 256 , 105–129 (2018).
Turner, M. The Origin of Ideas (Oxford Univ. Press, 2014).
Deci, E. L. & Ryan, R. M. Self-determination theory and the facilitation of intrinsic motivation. Am. Psychol. 55 , 68–78 (2000).
Friston, K. et al. Active inference and epistemic value. Cogn. Neurosci. 6 , 187–214 (2015).
Berlyne, D. E. Curiosity and exploration. Science 153 , 25–33 (1966).
Loewenstein, G. The psychology of curiosity: a review and reinterpretation. Psychol. Bull. 116 , 75–98 (1994).
Oudeyer, P.-Y., Kaplan, F. & Hafner, V. V. Intrinsic motivation systems for autonomous mental development. In IEEE Transactions on Evolutionary Computation (eds. Coello, C. A. C. et al.) Vol. 11, 265–286 (IEEE, 2007).
Pisula, W. Play and exploration in animals—a comparative analysis. Polish Psychol. Bull. 39 , 104–107 (2008).
Jeannerod, M. Mental imagery in the motor context. Neuropsychologia 33 , 1419–1432 (1995).
Kahnemann, D. & Tversky, A. in Judgement under Uncertainty : Heuristics and Biases (eds Kahneman, D. et al.) Ch. 14, 201–208 (Cambridge Univ. Press, 1982).
Wells, G. L. & Gavanski, I. Mental simulation of causality. J. Personal. Social Psychol. 56 , 161–169 (1989).
Taylor, S. E., Pham, L. B., Rivkin, I. D. & Armor, D. A. Harnessing the imagination: mental simulation, self-regulation and coping. Am. Psychol. 53 , 429–439 (1998).
Kaplan, F. & Oudeyer, P.-Y. in Embodied Artificial Intelligence , Lecture Notes in Computer Science Vol. 3139 (eds Iida, F. et al.) 259–270 (Springer, 2004).
Schmidhuber, J. Formal theory of creativity, fun, and intrinsic motivation. IEEE Trans. Auton. Mental Dev. 2 , 230–247 (2010).
Friston, K., Mattout, J. & Kilner, J. Action understanding and active inference. Biol. Cybern. 104 , 137–160 (2011).
Oudeyer, P.-Y. Computational theories of curiosity-driven learning. In The New Science of Curiosity (ed. Goren Gordon), 43-72 (Nova Science Publishers, 2018); https://arxiv.org/abs/1802.10546
Colombo, M. & Wright, C. First principles in the life sciences: the free-energy principle, organicism and mechanism. Synthese 198 , 3463–3488 (2021).
Article MathSciNet Google Scholar
Huang, Y. & Rao, R. P. Predictive coding. WIREs Cogn. Sci. 2 , 580–593 (2011).
Friston, K. The free-energy principle: a unified brain theory? Nat. Rev. Neurosci. 11 , 127–138 (2010).
Knill, D. C. & Pouget, A. The Bayesian brain: the role of uncertainty in neural coding and computation. Trends Neurosci. 27 , 712–719 (2004).
Clark, A. Whatever next? Predictive brains, situated agents, and the future of cognitive science. Behav. Brain Sci. 36 , 181–204 (2013).
Clark, A. Surfing Uncertainty : Prediction , Action and the Embodied Mind (Oxford Univ. Press, 2016).
Zacks, J. M., Speer, N. K., Swallow, K. M., Braver, T. S. & Reyonolds, J. R. Event perception: a mind/brain perspective. Psychol. Bull. 133 , 273–293 (2007).
Eysenbach, B., Ibarz, J., Gupta, A. & Levine, S. Diversity is all you need: learning skills without a reward function. In International Conference on Learning Representations (ICLR, 2019).
Frans, K., Ho, J., Chen, X., Abbeel, P. & Schulman, J. Meta learning shared hierarchies. In Proc. International Conference on Learning Representations https://openreview.net/pdf?id=SyX0IeWAW (ICLR, 2018).
Heess, N. et al. Learning and transfer of modulated locomotor controllers. Preprint at https://arxiv.org/abs/1610.05182 (2016).
Jiang, Y., Gu, S., Murphy, K. & Finn, C. Language as an abstraction for hierarchical deep reinforcement learning. In Neural Information Processing Systems ( NeurIPS ) (eds. Wallach, H. et al.) 9414–9426 (ACM, 2019).
Li, A. C., Florensa, C., Clavera, I. & Abbeel, P. Sub-policy adaptation for hierarchical reinforcement learning. In Proc. International Conference on Learning Representations https://openreview.net/forum?id=ByeWogStDS (ICLR, 2020).
Qureshi, A. H. et al. Composing task-agnostic policies with deep reinforcement learning. In Proc. International Conference on Learning Representations https://openreview.net/forum?id=H1ezFREtwH (ICLR, 2020).
Sharma, A., Gu, S., Levine, S., Kumar, V. & Hausman, K. Dynamics-aware unsupervised discovery of skills. In Proc. International Conference on Learning Representations https://openreview.net/forum?id=HJgLZR4KvH (ICLR, 2020).
Tessler, C., Givony, S., Zahavy, T., Mankowitz, D. J. & Mannor, S. A deep hierarchical approach to lifelong learning in minecraft. In Proc. 31st AAAI Conference on Artificial Intelligence 1553–1561 (AAAI, 2017).
Vezhnevets, A. et al. Strategic attentive writer for learning macro-actions. In Neural Information Processing Systems ( NIPS ) (eds. Lee, D. et al.) 3494–3502 (NIPS, 2016).
Devin, C., Gupta, A., Darrell, T., Abbeel, P. & Levine, S. Learning modular neural network policies for multi-task and multi-robot transfer. In Proc. International Conference on Robotics and Automation ( ICRA ) (eds. Okamura, A. et al.) 2169–2176 (IEEE, 2017).
Hejna, D. J., Abbeel, P. & Pinto, L. Hierarchically decoupled morphological transfer. In Proc. International Conference on Machine Learning ( ICML ) (eds. Daumé III, H. & Singh, A.) 11409–11420 (PMLR, 2020).
Hamrick, J. B. et al. On the role of planning in model-based deep reinforcement learning. In Proc. International Conference on Learning Representations https://openreview.net/pdf?id=IrM64DGB21 (ICLR, 2021).
Sutton, R. S. Integrated architectures for learning, planning, and reacting based on approximating dynamic programming. In Proc. 7th International Conference on Machine Learning ( ICML ) (eds. Porter, B. W. & Mooney, R. J.) 216–224 (Morgan Kaufmann, 1990).
Nau, D. et al. SHOP2: an HTN planning system. J. Artif. Intell. Res. 20 , 379–404 (2003).
Article MATH Google Scholar
Lyu, D., Yang, F., Liu, B. & Gustafson, S. SDRL: interpretable and data-efficient deep reinforcement learning leveraging symbolic planning. In Proc. AAAI Conference on Artificial Intelligence Vol. 33, 2970–2977 (AAAI, 2019).
Ma, A., Ouimet, M. & Cortés, J. Hierarchical reinforcement learning via dynamic subspace search for multi-agent planning. Auton. Robot. 44 , 485–503 (2020).
Bacon, P.-L., Harb, J. & Precup, D. The option-critic architecture. In Proc. 31st AAAI Conference on Artificial Intelligence 1726–1734 (AAAI, 2017).
Dietterich, T. G. State abstraction in MAXQ hierarchical reinforcement learning. In Advances in Neural Information Processing Systems ( NIPS ) (eds. Solla, S. et al.) Vol. 12, 994–1000 (NIPS, 1999).
Kulkarni, T. D., Narasimhan, K. R., Saeedi, A. & Tenenbaum, J. B. Hierarchical deep reinforcement learning: integrating temporal abstraction and intrinsic motivation. In Neural Information Processing Systems ( NIPS ) (eds. Lee, D. et al.) 3675–3683 (NIPS, 2016).
Shankar, T., Pinto, L., Tulsiani, S. & Gupta, A. Discovering motor programs by recomposing demonstrations. In Proc. International Conference on Learning Representations https://openreview.net/attachment?id=rkgHY0NYwr&name=original_pdf (ICLR, 2020).
Vezhnevets, A. S., Wu, Y. T., Eckstein, M., Leblond, R. & Leibo, J. Z. Options as responses: grounding behavioural hierarchies in multi-agent reinforcement learning. In Proc. International Conference on Machine Learning ( ICML ) (eds. Daumé III, H. & Singh, A.) 9733–9742 (PMLR, 2020).
Ghazanfari, B., Afghah, F. & Taylor, M. E. Sequential association rule mining for autonomously extracting hierarchical task structures in reinforcement learning. IEEE Access 8 , 11782–11799 (2020).
Levy, A., Konidaris, G., Platt, R. & Saenko, K. Learning multi-level hierarchies with hindsight. In Proc. International Conference on Learning Representations https://openreview.net/pdf?id=ryzECoAcY7 (ICLR, 2019).
Nachum, O., Gu, S., Lee, H. & Levine, S. Data-efficient hierarchical reinforcement learning. In Proc. 32nd International Conference on Neural Information Processing Systems (NIPS) (eds. Bengio, S. et al.) 3307–3317 (NIPS, 2018).
Rafati, J. & Noelle, D. C. Learning representations in model-free hierarchical reinforcement learning. In Proc. 33rd AAAI Conference on Artificial Intelligence 10009–10010 (AAAI, 2019).
Röder, F., Eppe, M., Nguyen, P. D. H. & Wermter, S. Curious hierarchical actor-critic reinforcement learning. In Proc. International Conference on Artificial Neural Networks ( ICANN ) (eds. Farkaš, I. et al.) 408–419 (Springer, 2020).
Zhang, T., Guo, S., Tan, T., Hu, X. & Chen, F. Generating adjacency-constrained subgoals in hierarchical reinforcement learning. In Neural Information Processing Systems ( NIPS ) (eds. Larochelle, H. et al.) 21579-21590 (NIPS, 2020).
Lample, G. & Chaplot, D. S. Playing FPS games with deep reinforcement learning. In Proc. 31st AAAI Conference on Artificial Intelligence 2140–2146 (AAAI, 2017).
Vezhnevets, A. S. et al. FeUdal networks for hierarchical reinforcement learning. In Proc. 34th International Conference on Machine Learning ( ICML ) (eds. Precup, D. & Teh, Y. W.) Vol. 70, 3540–3549 (PMLR, 2017).
Wulfmeier, M. et al. Compositional Transfer in Hierarchical Reinforcement Learning. In Robotics: Science and System XVI (RSS) (eds. Toussaint M. et al.) (Robotics: Science and Systems Foundation, 2020); https://arxiv.org/abs/1906.11228
Yang, Z., Merrick, K., Jin, L. & Abbass, H. A. Hierarchical deep reinforcement learning for continuous action control. IEEE Trans. Neural Netw. Learn. Syst. 29 , 5174–5184 (2018).
Toussaint, M., Allen, K. R., Smith, K. A. & Tenenbaum, J. B. Differentiable physics and stable modes for tool-use and manipulation planning. In Proc. Robotics : Science and Systems XIV ( RSS ) (eds. Kress-Gazit, H. et al.) https://ipvs.informatik.uni-stuttgart.de/mlr/papers/18-toussaint-RSS.pdf (Robotics: Science and Systems Foundation, 2018).
Akrour, R., Veiga, F., Peters, J. & Neumann, G. Regularizing reinforcement learning with state abstraction. In Proc. IEEE / RSJ International Conference on Intelligent Robots and Systems ( IROS ) 534–539 (IEEE, 2018).
Schaul, T. & Ring, M. Better generalization with forecasts. In Proc. 23rd International Joint Conference on Artificial Intelligence ( IJCAI ) (ed. Rossi, F.) 1656–1662 (AAAI, 2013).
Colas, C., Akakzia, A., Oudeyer, P.-Y., Chetouani, M. & Sigaud, O. Language-conditioned goal generation: a new approach to language grounding for RL. Preprint at https://arxiv.org/abs/2006.07043 (2020).
Blaes, S., Pogancic, M. V., Zhu, J. J. & Martius, G. Control what you can: intrinsically motivated task-planning agent. Neural Inf. Process. Syst. 32 , 12541–12552 (2019).
Haarnoja, T., Hartikainen, K., Abbeel, P. & Levine, S. Latent space policies for hierarchical reinforcement learning. In Proc. International Conference on Machine Learning ( ICML ) (eds. Dy, J. & Krause, A.) Vol. 4, 2965–2975 (PMLR, 2018).
Rasmussen, D., Voelker, A. & Eliasmith, C. A neural model of hierarchical reinforcement learning. PLoS ONE 12 , e0180234 (2017).
Riedmiller, M. et al. Learning by playing—solving sparse reward tasks from scratch. In Proc. International Conference on Machine Learning ( ICML ) (eds. Dy, J. & Krause, A.) Vol. 10, 6910–6919 (PMLR, 2018).
Yang, F., Lyu, D., Liu, B. & Gustafson, S. PEORL: integrating symbolic planning and hierarchical reinforcement learning for robust decision-making. In Proc. 27th International Joint Conference on Artificial Intelligence ( IJCAI ) (ed. Lang, J.) 4860–4866 (IJCAI, 2018).
Machado, M. C., Bellemare, M. G. & Bowling, M. A Laplacian framework for option discovery in reinforcement learning. In Proc. International Conference on Machine Learning (ICML) (eds. Precup, D. & Teh, Y. W.) Vol. 5, 3567–3582 (PMLR, 2017).
Pathak, D., Agrawal, P., Efros, A. A. & Darrell, T. Curiosity-driven exploration by self-supervised prediction. In Proc. 34th International Conference on Machine Learning ( ICML ) (eds. Precup, D. & Teh, Y. W.) 2778–2787 (PMLR, 2017).
Schillaci, G. et al. Intrinsic motivation and episodic memories for robot exploration of high-dimensional sensory spaces. Adaptive Behav. 29 549–566 (2020).
Colas, C., Fournier, P., Sigaud, O., Chetouani, M. & Oudeyer, P.-Y. CURIOUS: intrinsically motivated modular multi-goal reinforcement learning. In Proc. International Conference on Machine Learning ( ICML ) (eds. Chaudhuri, K. & Salakhutdinov, R.) 1331–1340 (PMLR, 2019).
Hafez, M. B., Weber, C., Kerzel, M. & Wermter, S. Improving robot dual-system motor learning with intrinsically motivated meta-control and latent-space experience imagination. Robot. Auton. Syst. 133 , 103630 (2020).
Yamamoto, K., Onishi, T. & Tsuruoka, Y. Hierarchical reinforcement learning with abductive planning. In Proc. ICML / IJCAI / AAMAS 2018 Workshop on Planning and Learning ( PAL-18 ) (2018).
Wu, B., Gupta, J. K. & Kochenderfer, M. J. Model primitive hierarchical lifelong reinforcement learning . In Proc. International Joint Conference on Autonomous Agents and Multiagent Systems ( AAMAS ) (eds. Agmon, N. et al.) Vol. 1, 34–42 (IFAAMAS, 2019).
Li, Z., Narayan, A. & Leong, T. Y. An efficient approach to model-based hierarchical reinforcement learning. In Proc. 31st AAAI Conference on Artificial Intelligence 3583–3589 (AAAI, 2017).
Hafner, D., Lillicrap, T. & Norouzi, M. Dream to control: learning behaviors by latent imagination. In Proc. International Conference on Learning Representations https://openreview.net/pdf?id=S1lOTC4tDS (ICLR, 2020).
Deisenroth, M. P., Rasmussen, C. E. & Fox, D. Learning to control a low-cost manipulator using data-efficient reinforcement learning. In Robotics : Science and Systems VII ( RSS ) (eds. Durrant-Whyte, H. et al.) 57–64 (Robotics: Science and Systems Foundation, 2011).
Ha, D. & Schmidhuber, J. Recurrent world models facilitate policy evolution. In Proc. 32nd International Conference on Neural Information Processing Systems (NeurIPS) (eds. Bengio, S. et al.) 2455–2467 (NIPS, 2018).
Battaglia, P. W. et al. Relational inductive biases, deep learning and graph networks. Preprint at https://arxiv.org/abs/1806.01261 (2018).
Andrychowicz, M. et al. Hindsight experience replay. In Proc. Neural Information Processing Systems ( NIPS ) (eds. Guyon I. et al.) 5048–5058 (NIPS, 2017); https://papers.nips.cc/paper/7090-hindsight-experience-replay.pdf
Schwartenbeck, P. et al. Computational mechanisms of curiosity and goal-directed exploration. eLife 8 , e41703 (2019).
Haarnoja, T., Zhou, A., Abbeel, P. & Levine, S. Soft actor-critic: off-policy maximum entropy deep reinforcement learning with a stochastic actor. In Proc. International Conference on Machine Learning ( ICML ) (eds. Dy, J. & Krause, A.) 1861–1870 (PMLR, 2018).
Yu, A. J. & Dayan, P. Uncertainty, neuromodulation and attention. Neuron 46 , 681–692 (2005).
Baldwin, D. A. & Kosie, J. E. How does the mind render streaming experience as events? Top. Cogn. Sci. 13 , 79–105 (2021).
Download references
Acknowledgements
We acknowledge funding from the DFG (projects IDEAS, LeCAREbot, TRR169, SPP 2134, RTG 1808 and EXC 2064/1), the Humboldt Foundation and Max Planck Research School IMPRS-IS.
Author information
Manfred Eppe
Present address: Hamburg University of Technology, Hamburg, Germany
Authors and Affiliations
Universität Hamburg, Hamburg, Germany
Manfred Eppe, Matthias Kerzel, Phuong D. H. Nguyen & Stefan Wermter
University of Tübingen, Tübingen, Germany
Christian Gumbsch & Martin V. Butz
Max Planck Institute for Intelligent Systems, Tübingen, Germany
Christian Gumbsch
You can also search for this author in PubMed Google Scholar
Corresponding author
Correspondence to Manfred Eppe .
Ethics declarations
Competing interests.
The authors declare no competing interests.
Additional information
Publisher’s note Springer Nature remains neutral with regard to jurisdictional claims in published maps and institutional affiliations.
Supplementary information
Supplementary information.
Supplementary Boxes 1–6 and Table 1.
Rights and permissions
Reprints and permissions
About this article
Cite this article.
Eppe, M., Gumbsch, C., Kerzel, M. et al. Intelligent problem-solving as integrated hierarchical reinforcement learning. Nat Mach Intell 4 , 11–20 (2022). https://doi.org/10.1038/s42256-021-00433-9
Download citation
Received : 18 December 2020
Accepted : 07 December 2021
Published : 25 January 2022
Issue Date : January 2022
DOI : https://doi.org/10.1038/s42256-021-00433-9
Share this article
Anyone you share the following link with will be able to read this content:
Sorry, a shareable link is not currently available for this article.
Provided by the Springer Nature SharedIt content-sharing initiative
This article is cited by
Efficient stacking and grasping in unstructured environments.
- Jinbiao Zhu
Journal of Intelligent & Robotic Systems (2024)
Four attributes of intelligence, a thousand questions
- Matthieu Bardal
- Eric Chalmers
Biological Cybernetics (2023)
An Alternative to Cognitivism: Computational Phenomenology for Deep Learning
- Pierre Beckmann
- Guillaume Köstner
- Inês Hipólito
Minds and Machines (2023)
Quick links
- Explore articles by subject
- Guide to authors
- Editorial policies
Sign up for the Nature Briefing newsletter — what matters in science, free to your inbox daily.

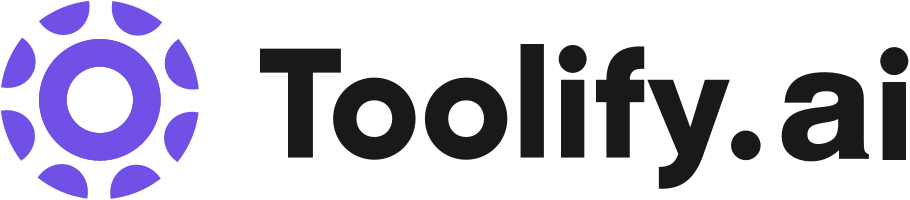
The Latest AIs, every day
AIs with the most favorites on Toolify
AIs with the highest website traffic (monthly visits)
AI Tools by browser extensions
AI Tools by Apps
Discover the Discord of AI
Top AI lists by month and monthly visits.
Top AI lists by category and monthly visits.
Top AI lists by region and monthly visits.
Top AI lists by source and monthly visits.
Top AI lists by revenue and real traffic.
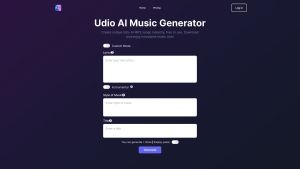
Unlocking the Power of Problem-Solving Agents in AI
Updated on Feb 19,2024
Table of Contents
Introduction, what is problem-solving agent, understanding the role of support in problem-solving, starting the problem-solving process, the efficiency of golf state in problem-solving, benefits of subscribing to problem-solving agents, incorporating quiz function in problem-solving, the resultant state and its significance, the use of problem-solving agent in medicine.
In today's world, problem-solving skills are highly valued, especially in exams where the likelihood of encountering questions related to this topic is significantly high. It is important to delve deeper into understanding what exactly a problem-solving agent entails. Essentially, a problem-solving agent refers to the support system that helps in identifying and resolving problems. Whether it is through chat or golf, the aim is to find the starting point to solve the problem efficiently. By subscribing to problem-solving agents, users gain access to their expertise and follow a systematic approach towards resolving issues. This article will explore the concept of problem-solving agents in detail, highlighting their benefits and how they can be effectively utilized in various domains.
A problem-solving agent serves as a crucial tool in addressing and resolving various issues. It acts as a support system that systematically identifies and solves problems. The initial step in utilizing a problem-solving agent is to start a chat, wherein the user describes the problem that needs to be solved. The agent then navigates through the problem-solving process, searching for the best Course of action to address the problem efficiently. This may involve accessing Relevant information, utilizing the golf state, or following a predefined set of steps. Ultimately, the problem-solving agent aims to provide solutions and guide users towards resolving their problems effectively.
Support plays a vital role in the problem-solving process. When a problem is presented to a problem-solving agent, it takes the responsibility of finding the most suitable approach to solve it. The agent starts by analyzing the problem, identifying its root causes, and devising an action plan accordingly. The support provided by the problem-solving agent is crucial as it guides users in determining Where To begin the problem-solving process. Whether it is through chat or accessing relevant resources, the support system ensures that users have a clear direction to follow in order to reach a solution.
The starting point of the problem-solving process is crucial in determining the efficiency and effectiveness of the overall solution. By initiating a chat, users can provide the necessary information about the problem at HAND . This allows the problem-solving agent to understand the context and formulate the most appropriate course of action. The initial chat sets the foundation for resolving the problem, ensuring that the subsequent steps are focused on finding the optimal solution. It serves as a starting point to Gather relevant insights and create a roadmap that leads towards problem resolution.
Golf state is an efficient approach in problem-solving that aids in finding the best solution. By exploring various options and evaluating their outcomes, the agent can navigate through different states to reach the most desirable outcome. The golf state allows the problem-solving agent to assess the potential outcomes of different actions and select the one that yields the desired results. This method of problem-solving guarantees efficiency and effectiveness as it considers the consequences of each step before proceeding further.
Subscribing to problem-solving agents offers numerous benefits in addressing various issues. Firstly, it provides access to expert support, ensuring that users receive guidance from professionals in the respective field. Moreover, problem-solving agents streamline the problem-solving process and enable users to follow a systematic approach towards finding a solution. Additionally, subscribing to these agents enhances efficiency by providing quick and accurate responses to problems. The utilization of problem-solving agents saves time and effort while delivering effective solutions.
Integrating a quiz function in problem-solving enhances the overall learning experience. By incorporating Quizzes , problem-solving agents can assess the user's understanding of the problem and recommend appropriate solutions. Quizzes serve as a knowledge check and help users gauge their expertise in problem-solving. Furthermore, they enable users to practice their problem-solving skills and enhance their competency in addressing various issues.
The resultant state in problem-solving signifies the successful resolution of the problem. It represents the endpoint where the problem-solving process has reached fruition, and a solution has been obtained. The resultant state is a crucial indicator of success and serves as a milestone in the problem-solving journey. Achieving the resultant state validates the effectiveness of the problem-solving agent and demonstrates the user's ability to overcome challenges.
Problem-solving agents have found application in various fields, including medicine. In the medical domain, problem-solving agents aid in diagnosing and resolving medical issues. They analyze symptoms, medical history, and test results to arrive at accurate diagnoses and suggest appropriate treatment plans. The utilization of problem-solving agents in medicine enhances efficiency and ensures accurate and Timely medical interventions.
Problem-solving agents play a significant role in addressing and resolving various issues efficiently. By subscribing to these agents, users gain access to expert support, which guides them through the problem-solving process. The integration of quizzes and the utilization of golf states further enhance the problem-solving experience. Whether in medicine or other domains, problem-solving agents offer valuable assistance in finding optimal solutions. By employing these agents, users can overcome challenges and achieve successful outcomes.
- Problem-solving agents act as support systems to address and resolve problems efficiently.
- The starting point in problem-solving is crucial in determining the effectiveness of the solution.
- Golf state is an efficient approach that evaluates potential outcomes to find the best solution.
- Subscribing to problem-solving agents provides expert guidance and streamlines the problem-solving process.
- Quizzes enhance the learning experience and help users improve their problem-solving skills.
- Problem-solving agents are utilized in various fields, including medicine, for accurate diagnoses and treatment plans.
The above is a brief introduction to Unlocking the Power of Problem-Solving Agents in AI
Let's move on to the first section of Unlocking the Power of Problem-Solving Agents in AI
Most people like
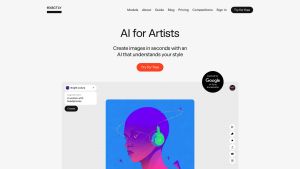
Find AI tools in Toolify
Join TOOLIFY to find the ai tools
Get started
- Discover Leanbe: Boost Your Customer Engagement and Product Development
- Unlock Your Productivity Potential with LeanBe
- Unleash Your Naval Power! Best Naval Civs in Civilization 5 - Part 7
- Master Algebra: Essential Guide for March SAT Math
- Let God Lead and Watch Your Life Transform | Inspirational Video
- Magewell XI204XE SD/HD Video Capture Card Review
- Discover Nepal's Ultimate Hiking Adventure
- Master the Art of Debugging with Our Step-by-Step Guide
- Maximize Customer Satisfaction with Leanbe's Feedback Tool
- Unleashing the Power of AI: A Closer Look
- Transform Your Images with Microsoft's BING and DALL-E 3
- Create Stunning Images with AI for Free!
- Unleash Your Creativity with Microsoft Bing AI Image Creator
- Create Unlimited AI Images for Free!
- Discover the Amazing Microsoft Bing Image Creator
- Create Stunning Images with Microsoft Image Creator
- AI Showdown: Stable Diffusion vs Dall E vs Bing Image Creator
- Create Stunning Images with Free Ai Text to Image Tool
- Unleashing Generative AI: Exploring Opportunities in QE&T
- Create a YouTube Channel with AI: ChatGPT, Bing Image Maker, Canva
- Google's AI Demo Scandal Sparks Stock Plunge
- Unveiling the Yoga Master: the Life of Tirumalai Krishnamacharya
- Hilarious Encounter: Jimmy's Unforgettable Moment with Robert Irwin
- Google's Incredible Gemini Demo: Unveiling the Future
- Say Goodbye to Under Eye Dark Circles - Simple Makeup Tips
- Discover Your Magical Soul Mate in ASMR Cosplay Role Play
- Boost Kidney Health with these Top Foods
- OpenAI's GEMINI 1.0 Under Scrutiny
- Unveiling the Mind-Blowing Gemini Ultra!
- Shocking AI News: Google's Deception Exposed!
- Unraveling Analog Devices Interview Puzzle
- Top 3 AMD Motherboards for RTX 4060
- Intel: The Trusted Foundation of the Cloud
- Unveiling Intel's Open Source Legacy
- Unlocking AI: Insights from Intel's AI Everywhere Head
- Unlocking Tech Insights: Edge Computing & Analytics Evolution
- Unlocking Intel's AI Strategy
- Intel Arc Desktop Lineup Leak: Big Xe vs. Big Ampere
- Unveiling Quantum Computing: A Journey into the Future
- Unveiling 12th Gen: Overclocking Intel's Powerhouse
Unraveling the Enigma: The SCP-079 Old AITable of Contents: Introduction SCP-079: The Old AI The Or
MR. ILLUSTRATED
The Potential of AI in Healthcare: Revolutionizing Holistic Patient CareTable of Contents Introduct
This Week Health
The Future of Battle Royale: Gaming and Movies ConvergeTable of Contents Introduction What is a Bat
Riccardo Silano
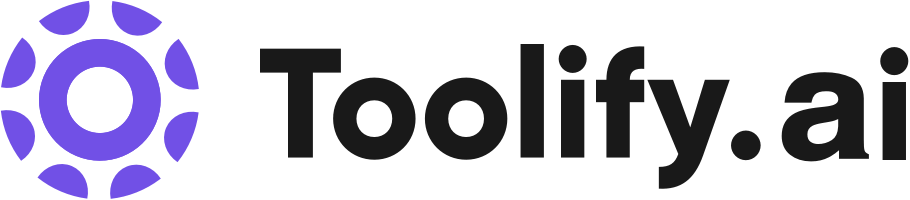
The Best AI Websites & AI Tools Directory
- Most Saved AIs
- Most Used AIs
- AI Browser Extensions
- Discord of AI
- Top AI By Monthly
- Top AI By Categories
- Top AI By Regions
- Top AI By Source
- Top AI by Revenue
- More Business
- Stable Video Diffusion
- Top AI Tools
- How to boost your SQL Coding Efficiency in a Multi-Database Environment
- Unleash Your Potential: Why ITIL Certification is the Smartest Investment for Your Future
- Navigating the Web Unseen: How Dolphin Anty Shields Your Digital Identity
- Human Writing vs. AI Writing: What to Choose for College Education Needs
- Privacy Policy
- [email protected]
- ai watermark remove
- ai voice noise removal
- ai voice background remover
- ai vocal remove
- ai video text remover
- ai video remove watermark
- ai content remover
- ai object removal
- ai text remover
- ai voice remover
Copyright ©2024 toolify
Towards Problem Solving Agents that Communicate and Learn
Anjali Narayan-Chen , Colin Graber , Mayukh Das , Md Rakibul Islam , Soham Dan , Sriraam Natarajan , Janardhan Rao Doppa , Julia Hockenmaier , Martha Palmer , Dan Roth
Export citation
- Preformatted
Markdown (Informal)
[Towards Problem Solving Agents that Communicate and Learn](https://aclanthology.org/W17-2812) (Narayan-Chen et al., RoboNLP 2017)
- Towards Problem Solving Agents that Communicate and Learn (Narayan-Chen et al., RoboNLP 2017)
- Anjali Narayan-Chen, Colin Graber, Mayukh Das, Md Rakibul Islam, Soham Dan, Sriraam Natarajan, Janardhan Rao Doppa, Julia Hockenmaier, Martha Palmer, and Dan Roth. 2017. Towards Problem Solving Agents that Communicate and Learn . In Proceedings of the First Workshop on Language Grounding for Robotics , pages 95–103, Vancouver, Canada. Association for Computational Linguistics.
- Speakers & Mentors
- AI services
Learn About Problem Solving Agents in Artificial Intelligence on Tutorialspoint
Artificial intelligence is a rapidly growing field that aims to develop computer systems capable of performing tasks that typically require human intelligence. One of the key areas in AI is problem solving, where agents are designed to find solutions to complex problems.
TutorialsPoint provides an in-depth tutorial on problem solving agents in artificial intelligence, covering various techniques and algorithms used to tackle different types of problems. Whether you are a beginner or an experienced AI practitioner, this tutorial will equip you with the knowledge and skills needed to build effective problem solving agents.
In this tutorial, you will learn about different problem solving frameworks, such as the goal-based approach, the utility-based approach, and the constraint satisfaction approach. You will also explore various search algorithms, including uninformed search algorithms like depth-first search and breadth-first search, as well as informed search algorithms like A* search and greedy search.
Problem solving agents in artificial intelligence play a crucial role in many real-world applications, ranging from robotics and automation to data analysis and decision-making systems. By mastering the concepts and techniques covered in this tutorial, you will be able to design and develop intelligent agents that can effectively solve complex problems.
What are Problem Solving Agents?
In the field of artificial intelligence, problem solving agents are intelligent systems that are designed to solve complex problems. These agents are equipped with the ability to analyze a given problem, search for possible solutions, and select the best solution based on a set of defined criteria.
Problem solving agents can be thought of as entities that can interact with their environment and take actions to achieve a desired goal. These agents are typically equipped with sensors to perceive the environment, an internal representation of the problem, and actuators to take actions.
One of the key challenges in designing problem solving agents is to define a suitable representation of the problem and its associated constraints. This representation allows the agent to reason about the problem and generate potential solutions. The agent can then evaluate these solutions and select the one that is most likely to achieve the desired goal.
Problem solving agents can be encountered in various domains, such as robotics, computer vision, natural language processing, and even in game playing. These agents can be implemented using different techniques, including search algorithms, constraint satisfaction algorithms, and machine learning algorithms.
In conclusion, problem solving agents are intelligent systems that are designed to solve complex problems by analyzing the environment, searching for solutions, and selecting the best solution based on a set of defined criteria. These agents can be encountered in various domains and can be implemented using different techniques.
Types of Problem Solving Agents
In the field of artificial intelligence, problem solving agents are designed to tackle a wide range of issues and tasks. These agents are built to analyze problems, explore potential solutions, and make decisions based on their findings. Here, we will explore some of the common types of problem solving agents.
Simple Reflex Agents
A simple reflex agent is a basic type of problem solving agent that relies on a set of predefined rules or conditions to make decisions. These rules are typically in the form of “if-then” statements, where the agent takes certain actions based on the current state of the problem. Simple reflex agents are often used in situations where the problem can be easily mapped to a small set of conditions.
Model-Based Reflex Agents
A model-based reflex agent goes beyond simple reflex agents by maintaining an internal model of the problem and its environment. This model allows the agent to have a better understanding of its current state and make more informed decisions. Model-based reflex agents use the current state and the model to determine the appropriate action to take. These agents are often used in more complex problem-solving scenarios.
Goal-Based Agents
A goal-based agent is designed to achieve a specific goal or set of goals. These agents analyze the current state of the problem and then determine a sequence of actions that will lead to the desired outcome. Goal-based agents often use search algorithms to explore the possible paths and make decisions based on their analysis. These agents are commonly used in planning and optimization problems.
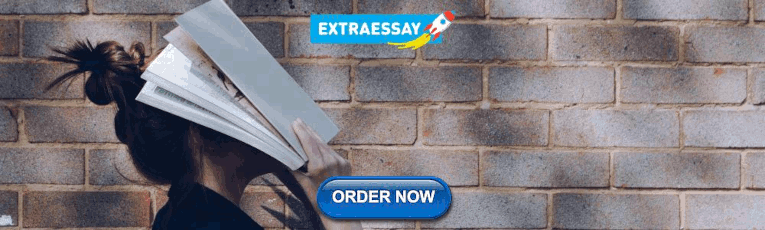
Utility-Based Agents
Utility-based agents make decisions based on a utility function or a measure of the desirability of different outcomes. These agents assign a value or utility to each possible action and choose the action that maximizes the overall utility. Utility-based agents are commonly used in decision-making problems where there are multiple possible outcomes with varying levels of desirability.
These are just a few examples of the types of problem solving agents that can be found in the field of artificial intelligence. Each type of agent has its own strengths and weaknesses and is suited to different problem-solving scenarios. By understanding the different types of agents, developers and researchers can choose the most appropriate agent for their specific problem and improve the efficiency and effectiveness of their problem-solving solutions.
Components of Problem Solving Agents
A problem-solving agent is a key concept in the field of artificial intelligence (AI). It is an agent that can analyze a given problem and take appropriate actions to solve it. The agents are designed using a set of components that work together to achieve their goals.
One of the main components of a problem-solving agent is the solving component. This component is responsible for applying different algorithms and techniques to find the best solution to a given problem. The solving component can use various approaches such as search algorithms, constraint satisfaction, optimization techniques, and machine learning.
TutorialsPoint is a popular online platform that offers a wealth of resources on various topics, including artificial intelligence and problem-solving agents. These tutorials provide step-by-step instructions and examples to help learners understand and implement different problem-solving techniques.
Another important component of a problem-solving agent is the knowledge component. This component stores the agent’s knowledge about the problem domain, including facts, rules, and constraints. The knowledge component is crucial for guiding the agent’s problem-solving process and making informed decisions.
The problem component is responsible for representing and defining the problem that the agent needs to solve. It includes information such as the initial state, goal state, and possible actions that the agent can take. The problem component provides the necessary context for the agent to analyze and solve the problem effectively.
Finally, the agents component is responsible for coordinating the activities of different components and controlling the overall behavior of the problem-solving agent. It receives inputs from the environment, communicates with the other components, and takes actions based on the current state of the problem. The agents component plays a crucial role in ensuring the problem-solving agent operates efficiently and effectively.
In conclusion, problem-solving agents in artificial intelligence are designed using various components such as solving, knowledge, problem, and agents. These components work together to analyze a problem, apply appropriate techniques, and find the best solution. TutorialsPoint is a valuable resource for learning about different problem-solving techniques and implementing them in practice.
Search Strategies for Problem Solving Agents
Intelligence is a complex and fascinating field that encompasses a wide range of topics and technologies. One area of focus in artificial intelligence is problem solving. Problem solving agents are designed to find solutions to specific problems by searching through a large space of possible solutions.
TutorialsPoint provides valuable resources and tutorials on problem solving agents in artificial intelligence. These tutorials cover various search strategies that can be employed by problem solving agents to efficiently find optimal solutions.
Search strategies play a crucial role in the efficiency and effectiveness of problem solving agents. Some common search strategies include:
These are just a few examples of search strategies that problem solving agents can utilize. The choice of search strategy depends on the specific problem at hand and the available resources.
TutorialsPoint’s comprehensive tutorials on problem solving agents in artificial intelligence provide in-depth explanations, examples, and implementation guides for each search strategy. By leveraging these tutorials, developers and researchers can enhance their understanding of problem solving agents and apply them to real-world scenarios.
Uninformed Search Algorithms
Uninformed search algorithms are a category of algorithms used by problem-solving agents in artificial intelligence. These algorithms do not have any information about the problem domain and make decisions solely based on the current state and possible actions.
Breadth-First Search
Breadth-first search is one of the basic uninformed search algorithms. It explores all the neighbor nodes at the present depth before moving on to nodes at the next depth level. It guarantees the shortest path to the goal state if there is one, but it can be inefficient for large search spaces.
Depth-First Search
Depth-first search is another uninformed search algorithm. It explores a path all the way to the deepest level before backtracking. It is often implemented using a stack data structure. Depth-first search is not guaranteed to find the shortest path to the goal state, but it can be more memory-efficient than breadth-first search.
Uninformed search algorithms are widely used in problem-solving scenarios where there is no additional information available about the problem domain. They are important tools in the field of artificial intelligence and are taught in many tutorials and courses, including the ones available on TutorialsPoint.
Breadth First Search (BFS)
Breadth First Search (BFS) is a fundamental algorithm used in artificial intelligence for problem solving. It is a graph traversal algorithm that explores all vertices of a graph in a breadthward motion, starting from a given source vertex.
BFS is commonly used to solve problems in AI, such as finding the shortest path between two nodes, determining if a graph is connected, or generating all possible solutions to a problem.
In BFS, the algorithm continuously explores the vertices adjacent to the current vertex before moving on to the next level of vertices. This approach ensures that all vertices at each level are visited before proceeding to the next level. The algorithm uses a queue to keep track of the vertices that need to be visited.
The main steps of the BFS algorithm are as follows:
- Choose a source vertex and mark it as visited.
- Enqueue the source vertex.
- While the queue is not empty, dequeue a vertex and visit it.
- Enqueue all the adjacent vertices of the visited vertex that have not been visited before.
- Mark the dequeued vertex as visited.
By following these steps, BFS explores the graph level by level, guaranteeing that the shortest path from the source vertex to any other vertex is found.
BFS has a time complexity of O(V + E), where V is the number of vertices and E is the number of edges in the graph. It is considered an efficient algorithm for solving problems in artificial intelligence.
Depth First Search (DFS)
Depth First Search (DFS) is a popular graph traversal algorithm commonly used in artificial intelligence and problem solving agents. It is also commonly used in tutorialspoint for teaching the concepts of graph traversal algorithms.
DFS starts at a given node of a graph and explores as far as possible along each branch before backtracking. It uses a stack data structure to keep track of the nodes to be visited. The algorithm visit nodes in a depthward motion, meaning that it explores the deepest paths in the graph first.
The DFS algorithm can be implemented as follows:
- Start at the given node and mark it as visited.
- If the node has unvisited neighbors, choose one and push it onto the stack.
- If there are no unvisited neighbors, backtrack by popping the stack.
- If the stack is empty, the algorithm terminates.
- Repeat steps 2-4 until all nodes are visited.
DFS is often used to solve problems that can be represented as graphs, such as finding solutions in a maze or searching for a path between two points. It can also be used to perform topological sorting and cycle detection in directed graphs.
DFS has a few advantages over other graph traversal algorithms. It requires less memory than breadth-first search (BFS) because it only needs to store the visited nodes in a stack. It can also be easily implemented recursively.
However, DFS may not find the shortest path between two nodes in a graph, as it explores deep paths first. It can also get stuck in infinite loops if the graph contains cycles.
Overall, DFS is a powerful algorithm that can be used to solve a wide range of problems in artificial intelligence and problem solving agents. By understanding how it works, developers can effectively apply it to various scenarios in their projects.
Iterative Deepening Depth First Search (IDDFS)
The Iterative Deepening Depth First Search (IDDFS) is a technique used in artificial intelligence to solve problems efficiently. It is a combination of depth-first search (DFS) and breadth-first search (BFS) algorithms.
The IDDFS algorithm starts with a depth limit of 0 and gradually increases the depth limit until the goal is found or all possibilities have been explored. It works by performing a depth-first search up to the current depth limit, and if the goal is not found, it resets the visited nodes and increases the depth limit by 1.
This approach allows the IDDFS algorithm to explore the search space more efficiently compared to standard depth-first search. It avoids the disadvantages of BFS, such as high memory usage, by only keeping track of the current path.
The IDDFS algorithm is particularly useful in situations where the search space is large and the goal is likely to be found at a relatively small depth. It guarantees that the algorithm will find the solution if it exists within the search space.
Overall, the IDDFS algorithm is a powerful tool in problem-solving agents in artificial intelligence. It combines the advantages of depth-first search and breadth-first search, making it an efficient and effective approach for solving complex problems.
Uniform Cost Search (UCS)
Uniform Cost Search (UCS) is a problem-solving algorithm used in the field of artificial intelligence. It is a variant of the general graph search algorithm, which aims to find the cheapest path from a starting node to a goal node in a weighted graph.
In UCS, each action in the problem domain has a cost associated with it. The algorithm expands the nodes in a graph in a cost-effective manner, always choosing the node with the lowest cost so far. This ensures that the optimal solution with the minimum total cost is found.
The UCS algorithm maintains a priority queue of nodes, where the priority is based on the accumulated cost to reach each node. Initially, the start node is inserted into the priority queue with a cost of zero. The algorithm then iteratively selects and expands the node with the lowest cost, updating the priority queue accordingly.
During the expansion process, the algorithm checks if the goal node has been reached. If not, it generates the successor nodes of the expanded node and adds them to the priority queue. The cost of each successor node is calculated by adding the cost of the action that led to that node to the total cost so far. This process continues until the goal node is reached.
Uniform Cost Search is considered to be complete and optimal, meaning it will always find a solution if one exists, and it will find the optimal solution with the minimum cost. However, it can be computationally expensive, especially in large graphs or graphs with high branching factors.
In summary, Uniform Cost Search is a powerful problem-solving algorithm used in artificial intelligence to find the cheapest path from a starting node to a goal node in a weighted graph. By prioritizing nodes based on their accumulated cost, UCS ensures that the optimal solution with the minimum total cost is found.
Informed Search Algorithms
In the field of Artificial Intelligence, informed search algorithms are a group of problem-solving agents that use knowledge or information about the problem domain to guide the search process. Unlike uninformed search algorithms, which do not have any additional information about the problem, informed search algorithms make use of heuristics or other measures of desirability to guide the search towards the goal state more efficiently.
One of the most well-known informed search algorithms is the A* algorithm. The A* algorithm uses a combination of the cost to reach a certain state and an estimate of the cost from that state to the goal state, known as the heuristic function. By selecting the states with the lowest total cost, the A* algorithm can efficiently navigate through the search space and find the optimal solution.
Another popular informed search algorithm is the greedy best-first search. Greedy best-first search evaluates each state based solely on the heuristic estimate of the cost to reach the goal state. It always chooses the state that appears to be the closest to the goal, without considering the overall cost. Although this algorithm is efficient for some problems, it can also get stuck in local optima and fail to find the optimal solution.
In addition to A* and greedy best-first search, there are various other informed search algorithms, such as the iterative deepening A* (IDA*) algorithm and the simulated annealing algorithm. Each algorithm has its strengths and weaknesses and is suited for different types of problems.
In conclusion, informed search algorithms play an important role in problem-solving in the field of Artificial Intelligence. They use additional knowledge or information about the problem domain to guide the search process and find optimal solutions more efficiently. By considering both the cost to reach a certain state and an estimate of the cost to the goal state, these algorithms can effectively navigate through the search space and find the best possible solution.
Heuristic Functions
In the field of artificial intelligence, heuristic functions play a crucial role in problem solving. A heuristic function is a function that estimates the cost or utility of reaching a goal state from a given state in a problem. It provides a way for agents to make informed decisions about their actions while navigating through a problem-solving process.
Heuristic functions are designed to guide the search process towards the most promising directions in order to find a solution efficiently. They provide a measure of how close a particular state is to the goal state, without having complete information about the problem domain. This allows the agent to prioritize its actions and choose the most promising ones at each step.
When designing a heuristic function, it is important to consider the specific problem at hand and the available knowledge about it. The function should be able to assess the cost or utility of reaching the goal state based on the available information and the characteristics of the problem. It should also be computationally efficient to ensure that it can be used in real-time problem-solving scenarios.
The effectiveness of a heuristic function depends on its ability to accurately estimate the cost or utility of reaching the goal state. A good heuristic function should provide a tight lower bound on the actual cost, meaning that it should never overestimate the distance to the goal. This allows the agent to efficiently explore the solution space and reach the goal state optimally.
In conclusion, heuristic functions are essential tools in artificial intelligence for problem solving. They enable agents to make informed decisions and guide the search process towards the most promising directions. By accurately estimating the cost or utility of reaching the goal state, heuristic functions help agents find optimal solutions efficiently.
A* Algorithm
The A* algorithm is a commonly used artificial intelligence technique for solving problems. It is particularly useful in problem-solving agents that aim to find the optimal path or solution. With the A* algorithm, an agent can navigate through a problem space, evaluating and selecting the most promising paths based on heuristic estimates and actual costs.
A* combines the advantages of both uniform cost search and best-first search algorithms. It uses a heuristic function to estimate the cost from the current state to the goal state, allowing the agent to prioritize its search and explore the most promising paths first. The heuristic function provides an estimation of the remaining cost, often referred to as the “H-cost”.
The algorithm maintains a priority queue, also known as an open list, that keeps track of the states or nodes to be expanded. At each step, the agent selects the state with the lowest combined cost, known as the “F-cost”, which is calculated as the sum of the actual cost from the starting state to the current state (known as the “G-cost”) and the heuristic cost.
The A* algorithm guarantees to find the optimal path if certain conditions are met. The heuristic function must be admissible, meaning that it never overestimates the actual cost. Additionally, the function should be consistent or monotonic, which ensures that the heuristic estimate is always less than or equal to the cost of reaching the goal state from the current state.
In conclusion, the A* algorithm is a powerful tool used in artificial intelligence for solving problems. It combines the benefits of both uniform cost search and best-first search algorithms, making it an efficient and effective choice for problem-solving agents. By prioritizing the most promising paths, as estimated by the heuristic function, the A* algorithm can find the optimal solution in a timely manner.
Greedy Best-first Search
The Greedy Best-first Search is a type of problem solving algorithm that is used in artificial intelligence. It is an informed search algorithm that uses heuristics to guide its search through a problem space.
The goal of the Greedy Best-first Search is to find a solution to a problem by always choosing the most promising path at each step. It does this by evaluating the estimated cost of each possible next step based on a heuristic function. The heuristic function provides an estimate of how close the current state is to the goal state.
This algorithm is called “greedy” because it always chooses the path that appears to be the best at the current moment, without considering the future consequences. It does not take into account the possibility that a different path might lead to a better solution in the long run.
Despite its simplicity, the Greedy Best-first Search can be quite effective in certain types of problems. However, it has some limitations. Since it only considers the local information at each step, it may overlook better paths that require more steps to reach the goal. In addition, it may get stuck in loops or infinite cycles if there are no better paths to follow.
Algorithm Steps:
- Initialize the search with the initial state.
- Choose the most promising state based on the heuristic function.
- Move to the chosen state.
- If the chosen state is the goal state, stop the search.
In conclusion, the Greedy Best-first Search is a simple yet effective problem solving algorithm in artificial intelligence. It can be a useful tool when solving certain types of problems, but it also has its limitations. It is important to understand the strengths and weaknesses of different search algorithms when applying them to real-world problems.
Hill Climbing
The Hill Climbing algorithm is a popular search algorithm used in artificial intelligence to solve problem-solving tasks. It is commonly used in optimization problems where the goal is to find the best possible solution among a set of alternatives.
In hill climbing, the agent starts with an initial solution and iteratively makes small improvements to the solution by locally exploring the neighboring states. The agent selects the next state to move to based on an evaluation function, which assigns a value to each state indicating its desirability. The agent moves to the state with the highest value and repeats the process until no better state can be found.
The hill climbing algorithm can be thought of as climbing a hill, where the agent starts at the bottom and tries to reach the highest point by taking steps uphill. However, hill climbing can get stuck at local optima, which are points that are higher than their immediate neighbors but lower than the global optimum. This means that the algorithm may fail to find the best solution if it gets trapped in a local maximum.
Types of Hill Climbing
There are different variations of the hill climbing algorithm that address its limitations:
Steepest Ascent Hill Climbing
The steepest ascent hill climbing algorithm is a variation of hill climbing where the agent considers all possible moves from the current state and selects the one that leads to the state with the highest value. This approach ensures that the agent moves uphill as much as possible in each iteration, but it can be computationally expensive as it needs to evaluate all possible moves.
First-Choice Hill Climbing
The first-choice hill climbing algorithm is another variation of hill climbing where the agent randomly selects one move from the current state and moves to the state with the highest value among the randomly selected moves. This approach can be more efficient than steepest ascent hill climbing as it does not need to evaluate all possible moves, but it may still get stuck in local optima.
In conclusion, hill climbing is a widely used algorithm in artificial intelligence for solving problem-solving tasks. Despite its limitations, it can be effective in finding good solutions for optimization problems. However, it is important to be aware of the possibility of getting stuck in local optima and consider using other algorithms or techniques to overcome this limitation.
Simulated Annealing
Simulated Annealing is a problem-solving algorithm used in artificial intelligence. It is a probabilistic method that is inspired by the annealing process used in metallurgy.
The goal of simulated annealing is to find a good solution to a problem, even when the search space is large and the problem is difficult to solve. It starts with an initial solution and gradually explores the search space, making small random changes to the solution. These changes may improve the solution or make it worse.
The algorithm uses a temperature parameter to control the probability of accepting a worse solution. At high temperatures, there is a high probability of accepting a worse solution, allowing the algorithm to escape local maxima. As the temperature decreases, the probability of accepting a worse solution decreases, leading to the convergence of the algorithm to a near-optimal solution.
Simulated annealing is particularly useful for solving problems where finding the optimal solution is difficult or time-consuming. It has been successfully applied to various problems such as the traveling salesman problem, the job shop scheduling problem, and the graph coloring problem.
In conclusion, simulated annealing is a powerful tool for problem-solving agents in artificial intelligence. It allows them to navigate complex search spaces and find near-optimal solutions. By using a probabilistic approach and gradually reducing the temperature, it can efficiently explore the solution space and overcome local maxima. This makes it an essential technique for solving challenging problems in artificial intelligence.
Genetic Algorithms
Genetic Algorithms (GAs) are a type of Artificial Intelligence (AI) technique used for problem-solving. They are commonly used in various fields, including optimization, machine learning, and data mining. GAs are inspired by the process of natural selection and evolution.
In a GA, a population of candidate solutions evolves over time to find the optimal solution to a given problem. Each candidate solution, also known as an individual, is represented as a set of chromosomes, which are strings of binary digits. The fitness of each individual is evaluated based on how well it solves the problem.
During the evolution process, individuals with higher fitness have a higher chance of being selected for reproduction. Genetic operators such as crossover and mutation are applied to the selected individuals to create new offspring. The new offspring then replace the less fit individuals in the population.
This process of selection, reproduction, and replacement continues over multiple generations until the population converges to a near-optimal solution. The convergence speed and quality of the solution depend on the parameters and characteristics of the GA, such as the selection method, crossover rate, mutation rate, and population size.
The use of GAs allows for the exploration of a large search space and can often find good solutions to complex problems. However, GAs do not guarantee finding the optimal solution, but rather give a good approximation.
TutorialsPoint is a great resource for learning about Artificial Intelligence, including Genetic Algorithms. They provide detailed tutorials and examples that help beginners understand the concepts and implementation of GAs. By following their tutorials, you can learn how to apply Genetic Algorithms to solve various problems in the field of AI.
Constraint Satisfaction Problems (CSPs)
In the field of artificial intelligence, problem solving agents often encounter situations where they need to fulfill a set of constraints in order to find a solution. These problems are known as Constraint Satisfaction Problems (CSPs). CSPs involve finding values for a set of variables that satisfy a given set of constraints.
A CSP can be defined as a triple (X, D, C), where:
- X is a set of variables, each with a domain of possible values.
- D is a set of domains, where each domain contains the possible values for its corresponding variable.
- C is a set of constraints, which define the relationship between the variables and their possible values.
Solving CSPs
Solving CSPs involves finding assignments of values to variables that satisfy all the given constraints. This can be done using various techniques, such as:
- Backtracking : This is a systematic search algorithm that explores the possible assignments of values to variables, backtracking when a conflict is encountered.
- Constraint propagation : This technique involves using the constraints to eliminate values from the domains of variables, reducing the search space.
- Constraint satisfaction algorithms : These algorithms use heuristics to guide the search for solutions, making it more efficient.
CSPs are used to solve a wide range of problems, such as scheduling, planning, resource allocation, and configuration. They provide a powerful framework for representing and solving problems in the field of artificial intelligence.
CSP Formulation
In the context of problem-solving agents, a CSP (Constraint Satisfaction Problem) is a formulation used to represent and solve problems in artificial intelligence. CSPs are widely used in various domains, such as scheduling, planning, and optimization.
In a CSP, the problem is represented as a set of variables, each with a domain of possible values, and a set of constraints that specify the relationships between variables. The goal is to find an assignment of values to variables that satisfies all the constraints.
Variables and Domains
Variables represent the unknowns in the problem, and each variable has a domain that defines the possible values it can take. For example, in a scheduling problem, the variables could represent tasks, and the domain of each variable could be the possible time slots for that task.
Constraints
Constraints define the relationships between variables. They specify the conditions that must be satisfied by the variable assignments. For example, in a scheduling problem, a constraint could be that two tasks cannot be scheduled at the same time.
Constraints can have different types, such as binary constraints that involve two variables, or unary constraints that involve only one variable. They can also have different degrees, such as constraints that involve more than two variables.
To solve a CSP, the agent searches for a consistent assignment of values to variables that satisfies all the constraints. This can be done using various search algorithms, such as backtracking or constraint propagation.
Let’s consider an example of a CSP formulation for a scheduling problem. We have three tasks to schedule, A, B, and C, and each task can be scheduled at two possible time slots, 1 and 2.
The variables are A, B, and C, and their domains are {1, 2}. The constraints are:
The goal is to find an assignment of values to variables that satisfies all the constraints. In this case, the valid assignments are:
- A = 1, B = 2, C = 1
- A = 2, B = 1, C = 2
These assignments satisfy all the constraints, as there are no conflicts between the tasks scheduled at the same time.
In conclusion, CSP formulation is a powerful technique used in problem-solving agents to represent and solve problems in artificial intelligence. It provides a flexible and efficient way to model and reason about complex problems.
Backtracking Algorithm
In the field of artificial intelligence, problem-solving agents are designed to find solutions to complex problems. One popular approach is the use of backtracking algorithms.
Backtracking is a systematic way of finding solutions by exploring all possible paths and discarding those that do not lead to a solution. It is often used when the problem can be represented as a search tree, where each node represents a partial solution and the edges represent possible choices.
The Backtracking Process
The backtracking algorithm starts by examining the first choice and moves forward along the path until a dead end is reached. At this point, it backtracks to the previous choice and explores the next option. This process continues until a solution is found or all possibilities have been exhausted.
During the backtracking process, the algorithm uses pruning techniques to optimize the search. Pruning involves eliminating portions of the search tree that are known to lead to dead ends, reducing the number of nodes that need to be explored.
Applications of Backtracking
The backtracking algorithm can be applied to a wide range of problem-solving tasks in artificial intelligence. Some common applications include:
- Constraint satisfaction problems: Backtracking can be used to find solutions that satisfy a set of constraints. For example, in a Sudoku puzzle, backtracking can be used to fill in the empty cells while ensuring that no number is repeated in a row, column, or block.
- Graph problems: Backtracking can be used to find paths in a graph that satisfy certain conditions. For example, in a maze, backtracking can be used to find a path from the starting point to the goal.
- Combinatorial optimization: Backtracking can be used to find the optimal solution among a set of possibilities. For example, in the traveling salesman problem, backtracking can be used to find the shortest possible route that visits all cities.
In summary, backtracking algorithms are a powerful tool for solving complex problems in artificial intelligence. They allow problem-solving agents to systematically explore all possible solutions and find the best one.
Forward Checking Algorithm
Forward Checking is an algorithm used in artificial intelligence to solve problems by systematically exploring the search space. It is particularly useful in constraint satisfaction problems where a set of constraints must be satisfied.
In the context of problem solving agents, the Forward Checking algorithm is used to efficiently eliminate values from the domains of variables during the search process. It works by propagating information about constraints from assigned variables to unassigned variables, reducing their domains and increasing the efficiency of the search.
The Forward Checking algorithm can be summarized in the following steps:
Step 1: Initialization
Initialize the domain of each variable with all possible values.
Step 2: Assign a Value
Select an unassigned variable and assign a value from its domain.
Step 3: Forward Checking
Update the domains of other unassigned variables based on the assigned value and the constraints.
In the table above, the domains of the variables are updated after assigning a value to one of the variables.
The Forward Checking algorithm continues by selecting the next unassigned variable with the smallest domain and repeating steps 2 and 3 until a solution is found or all variables have been assigned.
By efficiently propagating constraints, the Forward Checking algorithm can greatly reduce the search space and improve the efficiency of problem solving agents in artificial intelligence.
Constraint Propagation
Constraint propagation is one of the key techniques used by problem-solving agents in artificial intelligence. It is the process of using constraints to narrow down the search space and reduce the number of possible solutions.
In the context of artificial intelligence, a constraint represents a restriction or limitation on the values that certain variables can take. These constraints can be used to model real-world constraints or dependencies between variables. For example, in a scheduling problem, the constraint might state that two tasks cannot be scheduled at the same time.
Constraint propagation works by iteratively applying constraints to the problem domain and updating the values of variables based on the constraints. The goal is to eliminate values that are not consistent with the constraints, thus reducing the search space and making it easier to find a solution.
Types of Constraint Propagation
There are different types of constraint propagation techniques, including:
- Local Constraint Propagation: This technique applies constraints at a local level, focusing on individual variables or groups of variables. It updates the value of a variable based on the constraints without considering the global context of the problem.
- Global Constraint Propagation: This technique considers the global context of the problem and applies constraints across all variables simultaneously. It updates the values of variables based on the constraints and the implications of those constraints on other variables.
Constraint propagation can be a powerful technique for problem-solving agents as it allows them to prune the search space and focus on more promising solutions. It can help reduce the time and computational resources required to find a solution to a problem.
In conclusion, constraint propagation is a fundamental technique used by problem-solving agents in artificial intelligence. It leverages constraints to reduce the search space and find consistent values for variables. By applying constraints at a local or global level, agents can effectively narrow down the possibilities and improve the efficiency of their problem-solving process.
Local Search in CSPs
Local search is a common approach used in solving constraint satisfaction problems (CSPs) in artificial intelligence. CSPs involve finding solutions to a set of variables subject to a set of constraints. Local search algorithms focus on improving a current solution by making small modifications to it.
In the context of CSPs, local search algorithms explore the solution space by starting with an initial assignment of values to variables. They then iteratively improve this assignment by considering different neighborhoods of the current solution.
Local search algorithms aim to find the best possible assignment of values that satisfies all constraints in the problem. However, they do not guarantee finding the global optimal solution. Instead, they focus on finding a solution that is acceptable or meets certain criteria within a given time limit.
Tutorialspoint offers various tutorials and resources on local search algorithms in artificial intelligence. These tutorials provide in-depth explanations and implementations of different local search algorithms, such as hill climbing, simulated annealing, and genetic algorithms.
By learning and understanding local search algorithms, you can apply them to solve a wide range of problem-solving tasks in artificial intelligence. Whether it’s optimizing a complex scheduling problem or finding the best configuration for a system, local search algorithms provide practical solutions to real-world problems.
Tabu Search
Tabu Search is an artificial intelligence technique used for solving complex problem instances. It is a metaheuristic method that efficiently explores a problem space by keeping track of previously visited states, known as the tabu list, to avoid revisiting them. This allows the search algorithm to overcome local optima and find better solutions.
The main idea behind Tabu Search is to use memory to guide the search process. It maintains a short-term memory of recent moves and a long-term memory of the best solutions found so far. This allows the algorithm to make informed decisions and avoid getting stuck in suboptimal regions of the problem space.
During the search, Tabu Search uses different strategies to explore the problem space, such as generating and evaluating neighboring solutions, choosing the best move, and updating the tabu list. The tabu list contains forbidden moves that are temporarily avoided to prevent the search algorithm from going backward or cycling through the same solutions.
Tabu Search is particularly effective in solving optimization problems, combinatorial problems, and scheduling problems. It has been successfully applied in various domains, including operations research, computer science, engineering, and economics.
In conclusion, Tabu Search is a powerful technique used by problem-solving agents in artificial intelligence to tackle complex problem instances. It leverages memory to guide the search process and avoid revisiting previously explored states. By doing so, it is able to overcome local optima and find better solutions.+
Min-Conflict Algorithm
The Min-Conflict algorithm is a popular approach in the field of problem-solving intelligence. It is commonly used to solve constraint satisfaction problems.
This algorithm comes in handy when we need to find a solution that satisfies a set of constraints. It is especially useful when we encounter a problem where we have partial information or conflicting information.
The Min-Conflict algorithm works by iteratively adjusting the current solution to the problem until a feasible solution is found. It starts with an initial solution and then repeatedly selects a variable with a conflict and changes its value to minimize the number of conflicts. This process continues until either a solution with no conflicts is found or a predefined number of iterations is reached.
One of the advantages of the Min-Conflict algorithm is its ability to quickly find solutions to complex problems. It can handle large domains and a high number of constraints efficiently, making it a favored technique in artificial intelligence.
Implementing the Min-Conflict Algorithm
To implement the Min-Conflict algorithm, we need to follow these steps:
- Initialize the problem with an initial solution.
- While a solution with no conflicts is not found and the maximum number of iterations is not reached, repeat the following steps:
- Select a variable with conflicts.
- Choose a value for that variable that minimizes the number of conflicts.
- Update the assignments based on the new value.
- If a solution is found within the iterations limit, return it. Otherwise, return that no solution exists.
The Min-Conflict algorithm is a powerful approach for solving constraint satisfaction problems. Its iterative nature and ability to handle conflicts efficiently make it a preferred technique in artificial intelligence. By following a few simple steps, we can implement this algorithm to solve a wide range of complex problems.
Questions and answers
What are problem-solving agents in artificial intelligence.
Problem-solving agents in artificial intelligence are programs that are designed to find solutions to specific problems by searching through a set of possible actions and states.
How do problem-solving agents work?
Problem-solving agents work by starting with an initial state and applying a series of actions to reach a goal state. They use different search algorithms, such as depth-first search or breadth-first search, to explore the problem space and find the most optimal solution.
What are some examples of problem-solving agents?
Examples of problem-solving agents include route-planning systems, chess-playing programs, and automated theorem provers. These agents are designed to solve specific problems by searching for the best possible solution.
What are the advantages of problem-solving agents in artificial intelligence?
Problem-solving agents in artificial intelligence have several advantages. They can solve complex problems that would be difficult for humans to solve manually. They can also work quickly and efficiently, and they can explore a large number of possible solutions to find the best one.
What are some limitations of problem-solving agents in artificial intelligence?
Although problem-solving agents are powerful tools in artificial intelligence, they have some limitations. They require a well-defined problem and goal state, and they may not always find the optimal solution. Additionally, the search space can be very large, which can make finding a solution time-consuming or even infeasible.
Related posts:
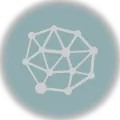
About the author
Cohere: Bridging Language and AI for a Smarter Future
Microsoft office 365 ai. microsoft copilot, understanding the distinctions between artificial intelligence and human intelligence, exploring the impact of artificial intelligence on discrimination in insurance pricing and underwriting.

- SUGGESTED TOPICS
- The Magazine
- Newsletters
- Managing Yourself
- Managing Teams
- Work-life Balance
- The Big Idea
- Data & Visuals
- Reading Lists
- Case Selections
- HBR Learning
- Topic Feeds
- Account Settings
- Email Preferences
Share Podcast

Do You Understand the Problem You’re Trying to Solve?
To solve tough problems at work, first ask these questions.
- Apple Podcasts
- Google Podcasts
Problem solving skills are invaluable in any job. But all too often, we jump to find solutions to a problem without taking time to really understand the dilemma we face, according to Thomas Wedell-Wedellsborg , an expert in innovation and the author of the book, What’s Your Problem?: To Solve Your Toughest Problems, Change the Problems You Solve .
In this episode, you’ll learn how to reframe tough problems by asking questions that reveal all the factors and assumptions that contribute to the situation. You’ll also learn why searching for just one root cause can be misleading.
Key episode topics include: leadership, decision making and problem solving, power and influence, business management.
HBR On Leadership curates the best case studies and conversations with the world’s top business and management experts, to help you unlock the best in those around you. New episodes every week.
- Listen to the original HBR IdeaCast episode: The Secret to Better Problem Solving (2016)
- Find more episodes of HBR IdeaCast
- Discover 100 years of Harvard Business Review articles, case studies, podcasts, and more at HBR.org .
HANNAH BATES: Welcome to HBR on Leadership , case studies and conversations with the world’s top business and management experts, hand-selected to help you unlock the best in those around you.
Problem solving skills are invaluable in any job. But even the most experienced among us can fall into the trap of solving the wrong problem.
Thomas Wedell-Wedellsborg says that all too often, we jump to find solutions to a problem – without taking time to really understand what we’re facing.
He’s an expert in innovation, and he’s the author of the book, What’s Your Problem?: To Solve Your Toughest Problems, Change the Problems You Solve .
In this episode, you’ll learn how to reframe tough problems, by asking questions that reveal all the factors and assumptions that contribute to the situation. You’ll also learn why searching for one root cause can be misleading. And you’ll learn how to use experimentation and rapid prototyping as problem-solving tools.
This episode originally aired on HBR IdeaCast in December 2016. Here it is.
SARAH GREEN CARMICHAEL: Welcome to the HBR IdeaCast from Harvard Business Review. I’m Sarah Green Carmichael.
Problem solving is popular. People put it on their resumes. Managers believe they excel at it. Companies count it as a key proficiency. We solve customers’ problems.
The problem is we often solve the wrong problems. Albert Einstein and Peter Drucker alike have discussed the difficulty of effective diagnosis. There are great frameworks for getting teams to attack true problems, but they’re often hard to do daily and on the fly. That’s where our guest comes in.
Thomas Wedell-Wedellsborg is a consultant who helps companies and managers reframe their problems so they can come up with an effective solution faster. He asks the question “Are You Solving The Right Problems?” in the January-February 2017 issue of Harvard Business Review. Thomas, thank you so much for coming on the HBR IdeaCast .
THOMAS WEDELL-WEDELLSBORG: Thanks for inviting me.
SARAH GREEN CARMICHAEL: So, I thought maybe we could start by talking about the problem of talking about problem reframing. What is that exactly?
THOMAS WEDELL-WEDELLSBORG: Basically, when people face a problem, they tend to jump into solution mode to rapidly, and very often that means that they don’t really understand, necessarily, the problem they’re trying to solve. And so, reframing is really a– at heart, it’s a method that helps you avoid that by taking a second to go in and ask two questions, basically saying, first of all, wait. What is the problem we’re trying to solve? And then crucially asking, is there a different way to think about what the problem actually is?
SARAH GREEN CARMICHAEL: So, I feel like so often when this comes up in meetings, you know, someone says that, and maybe they throw out the Einstein quote about you spend an hour of problem solving, you spend 55 minutes to find the problem. And then everyone else in the room kind of gets irritated. So, maybe just give us an example of maybe how this would work in practice in a way that would not, sort of, set people’s teeth on edge, like oh, here Sarah goes again, reframing the whole problem instead of just solving it.
THOMAS WEDELL-WEDELLSBORG: I mean, you’re bringing up something that’s, I think is crucial, which is to create legitimacy for the method. So, one of the reasons why I put out the article is to give people a tool to say actually, this thing is still important, and we need to do it. But I think the really critical thing in order to make this work in a meeting is actually to learn how to do it fast, because if you have the idea that you need to spend 30 minutes in a meeting delving deeply into the problem, I mean, that’s going to be uphill for most problems. So, the critical thing here is really to try to make it a practice you can implement very, very rapidly.
There’s an example that I would suggest memorizing. This is the example that I use to explain very rapidly what it is. And it’s basically, I call it the slow elevator problem. You imagine that you are the owner of an office building, and that your tenants are complaining that the elevator’s slow.
Now, if you take that problem framing for granted, you’re going to start thinking creatively around how do we make the elevator faster. Do we install a new motor? Do we have to buy a new lift somewhere?
The thing is, though, if you ask people who actually work with facilities management, well, they’re going to have a different solution for you, which is put up a mirror next to the elevator. That’s what happens is, of course, that people go oh, I’m busy. I’m busy. I’m– oh, a mirror. Oh, that’s beautiful.
And then they forget time. What’s interesting about that example is that the idea with a mirror is actually a solution to a different problem than the one you first proposed. And so, the whole idea here is once you get good at using reframing, you can quickly identify other aspects of the problem that might be much better to try to solve than the original one you found. It’s not necessarily that the first one is wrong. It’s just that there might be better problems out there to attack that we can, means we can do things much faster, cheaper, or better.
SARAH GREEN CARMICHAEL: So, in that example, I can understand how A, it’s probably expensive to make the elevator faster, so it’s much cheaper just to put up a mirror. And B, maybe the real problem people are actually feeling, even though they’re not articulating it right, is like, I hate waiting for the elevator. But if you let them sort of fix their hair or check their teeth, they’re suddenly distracted and don’t notice.
But if you have, this is sort of a pedestrian example, but say you have a roommate or a spouse who doesn’t clean up the kitchen. Facing that problem and not having your elegant solution already there to highlight the contrast between the perceived problem and the real problem, how would you take a problem like that and attack it using this method so that you can see what some of the other options might be?
THOMAS WEDELL-WEDELLSBORG: Right. So, I mean, let’s say it’s you who have that problem. I would go in and say, first of all, what would you say the problem is? Like, if you were to describe your view of the problem, what would that be?
SARAH GREEN CARMICHAEL: I hate cleaning the kitchen, and I want someone else to clean it up.
THOMAS WEDELL-WEDELLSBORG: OK. So, my first observation, you know, that somebody else might not necessarily be your spouse. So, already there, there’s an inbuilt assumption in your question around oh, it has to be my husband who does the cleaning. So, it might actually be worth, already there to say, is that really the only problem you have? That you hate cleaning the kitchen, and you want to avoid it? Or might there be something around, as well, getting a better relationship in terms of how you solve problems in general or establishing a better way to handle small problems when dealing with your spouse?
SARAH GREEN CARMICHAEL: Or maybe, now that I’m thinking that, maybe the problem is that you just can’t find the stuff in the kitchen when you need to find it.
THOMAS WEDELL-WEDELLSBORG: Right, and so that’s an example of a reframing, that actually why is it a problem that the kitchen is not clean? Is it only because you hate the act of cleaning, or does it actually mean that it just takes you a lot longer and gets a lot messier to actually use the kitchen, which is a different problem. The way you describe this problem now, is there anything that’s missing from that description?
SARAH GREEN CARMICHAEL: That is a really good question.
THOMAS WEDELL-WEDELLSBORG: Other, basically asking other factors that we are not talking about right now, and I say those because people tend to, when given a problem, they tend to delve deeper into the detail. What often is missing is actually an element outside of the initial description of the problem that might be really relevant to what’s going on. Like, why does the kitchen get messy in the first place? Is it something about the way you use it or your cooking habits? Is it because the neighbor’s kids, kind of, use it all the time?
There might, very often, there might be issues that you’re not really thinking about when you first describe the problem that actually has a big effect on it.
SARAH GREEN CARMICHAEL: I think at this point it would be helpful to maybe get another business example, and I’m wondering if you could tell us the story of the dog adoption problem.
THOMAS WEDELL-WEDELLSBORG: Yeah. This is a big problem in the US. If you work in the shelter industry, basically because dogs are so popular, more than 3 million dogs every year enter a shelter, and currently only about half of those actually find a new home and get adopted. And so, this is a problem that has persisted. It’s been, like, a structural problem for decades in this space. In the last three years, where people found new ways to address it.
So a woman called Lori Weise who runs a rescue organization in South LA, and she actually went in and challenged the very idea of what we were trying to do. She said, no, no. The problem we’re trying to solve is not about how to get more people to adopt dogs. It is about keeping the dogs with their first family so they never enter the shelter system in the first place.
In 2013, she started what’s called a Shelter Intervention Program that basically works like this. If a family comes and wants to hand over their dog, these are called owner surrenders. It’s about 30% of all dogs that come into a shelter. All they would do is go up and ask, if you could, would you like to keep your animal? And if they said yes, they would try to fix whatever helped them fix the problem, but that made them turn over this.
And sometimes that might be that they moved into a new building. The landlord required a deposit, and they simply didn’t have the money to put down a deposit. Or the dog might need a $10 rabies shot, but they didn’t know how to get access to a vet.
And so, by instigating that program, just in the first year, she took her, basically the amount of dollars they spent per animal they helped went from something like $85 down to around $60. Just an immediate impact, and her program now is being rolled out, is being supported by the ASPCA, which is one of the big animal welfare stations, and it’s being rolled out to various other places.
And I think what really struck me with that example was this was not dependent on having the internet. This was not, oh, we needed to have everybody mobile before we could come up with this. This, conceivably, we could have done 20 years ago. Only, it only happened when somebody, like in this case Lori, went in and actually rethought what the problem they were trying to solve was in the first place.
SARAH GREEN CARMICHAEL: So, what I also think is so interesting about that example is that when you talk about it, it doesn’t sound like the kind of thing that would have been thought of through other kinds of problem solving methods. There wasn’t necessarily an After Action Review or a 5 Whys exercise or a Six Sigma type intervention. I don’t want to throw those other methods under the bus, but how can you get such powerful results with such a very simple way of thinking about something?
THOMAS WEDELL-WEDELLSBORG: That was something that struck me as well. This, in a way, reframing and the idea of the problem diagnosis is important is something we’ve known for a long, long time. And we’ve actually have built some tools to help out. If you worked with us professionally, you are familiar with, like, Six Sigma, TRIZ, and so on. You mentioned 5 Whys. A root cause analysis is another one that a lot of people are familiar with.
Those are our good tools, and they’re definitely better than nothing. But what I notice when I work with the companies applying those was those tools tend to make you dig deeper into the first understanding of the problem we have. If it’s the elevator example, people start asking, well, is that the cable strength, or is the capacity of the elevator? That they kind of get caught by the details.
That, in a way, is a bad way to work on problems because it really assumes that there’s like a, you can almost hear it, a root cause. That you have to dig down and find the one true problem, and everything else was just symptoms. That’s a bad way to think about problems because problems tend to be multicausal.
There tend to be lots of causes or levers you can potentially press to address a problem. And if you think there’s only one, if that’s the right problem, that’s actually a dangerous way. And so I think that’s why, that this is a method I’ve worked with over the last five years, trying to basically refine how to make people better at this, and the key tends to be this thing about shifting out and saying, is there a totally different way of thinking about the problem versus getting too caught up in the mechanistic details of what happens.
SARAH GREEN CARMICHAEL: What about experimentation? Because that’s another method that’s become really popular with the rise of Lean Startup and lots of other innovation methodologies. Why wouldn’t it have worked to, say, experiment with many different types of fixing the dog adoption problem, and then just pick the one that works the best?
THOMAS WEDELL-WEDELLSBORG: You could say in the dog space, that’s what’s been going on. I mean, there is, in this industry and a lot of, it’s largely volunteer driven. People have experimented, and they found different ways of trying to cope. And that has definitely made the problem better. So, I wouldn’t say that experimentation is bad, quite the contrary. Rapid prototyping, quickly putting something out into the world and learning from it, that’s a fantastic way to learn more and to move forward.
My point is, though, that I feel we’ve come to rely too much on that. There’s like, if you look at the start up space, the wisdom is now just to put something quickly into the market, and then if it doesn’t work, pivot and just do more stuff. What reframing really is, I think of it as the cognitive counterpoint to prototyping. So, this is really a way of seeing very quickly, like not just working on the solution, but also working on our understanding of the problem and trying to see is there a different way to think about that.
If you only stick with experimentation, again, you tend to sometimes stay too much in the same space trying minute variations of something instead of taking a step back and saying, wait a minute. What is this telling us about what the real issue is?
SARAH GREEN CARMICHAEL: So, to go back to something that we touched on earlier, when we were talking about the completely hypothetical example of a spouse who does not clean the kitchen–
THOMAS WEDELL-WEDELLSBORG: Completely, completely hypothetical.
SARAH GREEN CARMICHAEL: Yes. For the record, my husband is a great kitchen cleaner.
You started asking me some questions that I could see immediately were helping me rethink that problem. Is that kind of the key, just having a checklist of questions to ask yourself? How do you really start to put this into practice?
THOMAS WEDELL-WEDELLSBORG: I think there are two steps in that. The first one is just to make yourself better at the method. Yes, you should kind of work with a checklist. In the article, I kind of outlined seven practices that you can use to do this.
But importantly, I would say you have to consider that as, basically, a set of training wheels. I think there’s a big, big danger in getting caught in a checklist. This is something I work with.
My co-author Paddy Miller, it’s one of his insights. That if you start giving people a checklist for things like this, they start following it. And that’s actually a problem, because what you really want them to do is start challenging their thinking.
So the way to handle this is to get some practice using it. Do use the checklist initially, but then try to step away from it and try to see if you can organically make– it’s almost a habit of mind. When you run into a colleague in the hallway and she has a problem and you have five minutes, like, delving in and just starting asking some of those questions and using your intuition to say, wait, how is she talking about this problem? And is there a question or two I can ask her about the problem that can help her rethink it?
SARAH GREEN CARMICHAEL: Well, that is also just a very different approach, because I think in that situation, most of us can’t go 30 seconds without jumping in and offering solutions.
THOMAS WEDELL-WEDELLSBORG: Very true. The drive toward solutions is very strong. And to be clear, I mean, there’s nothing wrong with that if the solutions work. So, many problems are just solved by oh, you know, oh, here’s the way to do that. Great.
But this is really a powerful method for those problems where either it’s something we’ve been banging our heads against tons of times without making progress, or when you need to come up with a really creative solution. When you’re facing a competitor with a much bigger budget, and you know, if you solve the same problem later, you’re not going to win. So, that basic idea of taking that approach to problems can often help you move forward in a different way than just like, oh, I have a solution.
I would say there’s also, there’s some interesting psychological stuff going on, right? Where you may have tried this, but if somebody tries to serve up a solution to a problem I have, I’m often resistant towards them. Kind if like, no, no, no, no, no, no. That solution is not going to work in my world. Whereas if you get them to discuss and analyze what the problem really is, you might actually dig something up.
Let’s go back to the kitchen example. One powerful question is just to say, what’s your own part in creating this problem? It’s very often, like, people, they describe problems as if it’s something that’s inflicted upon them from the external world, and they are innocent bystanders in that.
SARAH GREEN CARMICHAEL: Right, or crazy customers with unreasonable demands.
THOMAS WEDELL-WEDELLSBORG: Exactly, right. I don’t think I’ve ever met an agency or consultancy that didn’t, like, gossip about their customers. Oh, my god, they’re horrible. That, you know, classic thing, why don’t they want to take more risk? Well, risk is bad.
It’s their business that’s on the line, not the consultancy’s, right? So, absolutely, that’s one of the things when you step into a different mindset and kind of, wait. Oh yeah, maybe I actually am part of creating this problem in a sense, as well. That tends to open some new doors for you to move forward, in a way, with stuff that you may have been struggling with for years.
SARAH GREEN CARMICHAEL: So, we’ve surfaced a couple of questions that are useful. I’m curious to know, what are some of the other questions that you find yourself asking in these situations, given that you have made this sort of mental habit that you do? What are the questions that people seem to find really useful?
THOMAS WEDELL-WEDELLSBORG: One easy one is just to ask if there are any positive exceptions to the problem. So, was there day where your kitchen was actually spotlessly clean? And then asking, what was different about that day? Like, what happened there that didn’t happen the other days? That can very often point people towards a factor that they hadn’t considered previously.
SARAH GREEN CARMICHAEL: We got take-out.
THOMAS WEDELL-WEDELLSBORG: S,o that is your solution. Take-out from [INAUDIBLE]. That might have other problems.
Another good question, and this is a little bit more high level. It’s actually more making an observation about labeling how that person thinks about the problem. And what I mean with that is, we have problem categories in our head. So, if I say, let’s say that you describe a problem to me and say, well, we have a really great product and are, it’s much better than our previous product, but people aren’t buying it. I think we need to put more marketing dollars into this.
Now you can go in and say, that’s interesting. This sounds like you’re thinking of this as a communications problem. Is there a different way of thinking about that? Because you can almost tell how, when the second you say communications, there are some ideas about how do you solve a communications problem. Typically with more communication.
And what you might do is go in and suggest, well, have you considered that it might be, say, an incentive problem? Are there incentives on behalf of the purchasing manager at your clients that are obstructing you? Might there be incentive issues with your own sales force that makes them want to sell the old product instead of the new one?
So literally, just identifying what type of problem does this person think about, and is there different potential way of thinking about it? Might it be an emotional problem, a timing problem, an expectations management problem? Thinking about what label of what type of problem that person is kind of thinking as it of.
SARAH GREEN CARMICHAEL: That’s really interesting, too, because I think so many of us get requests for advice that we’re really not qualified to give. So, maybe the next time that happens, instead of muddying my way through, I will just ask some of those questions that we talked about instead.
THOMAS WEDELL-WEDELLSBORG: That sounds like a good idea.
SARAH GREEN CARMICHAEL: So, Thomas, this has really helped me reframe the way I think about a couple of problems in my own life, and I’m just wondering. I know you do this professionally, but is there a problem in your life that thinking this way has helped you solve?
THOMAS WEDELL-WEDELLSBORG: I’ve, of course, I’ve been swallowing my own medicine on this, too, and I think I have, well, maybe two different examples, and in one case somebody else did the reframing for me. But in one case, when I was younger, I often kind of struggled a little bit. I mean, this is my teenage years, kind of hanging out with my parents. I thought they were pretty annoying people. That’s not really fair, because they’re quite wonderful, but that’s what life is when you’re a teenager.
And one of the things that struck me, suddenly, and this was kind of the positive exception was, there was actually an evening where we really had a good time, and there wasn’t a conflict. And the core thing was, I wasn’t just seeing them in their old house where I grew up. It was, actually, we were at a restaurant. And it suddenly struck me that so much of the sometimes, kind of, a little bit, you love them but they’re annoying kind of dynamic, is tied to the place, is tied to the setting you are in.
And of course, if– you know, I live abroad now, if I visit my parents and I stay in my old bedroom, you know, my mother comes in and wants to wake me up in the morning. Stuff like that, right? And it just struck me so, so clearly that it’s– when I change this setting, if I go out and have dinner with them at a different place, that the dynamic, just that dynamic disappears.
SARAH GREEN CARMICHAEL: Well, Thomas, this has been really, really helpful. Thank you for talking with me today.
THOMAS WEDELL-WEDELLSBORG: Thank you, Sarah.
HANNAH BATES: That was Thomas Wedell-Wedellsborg in conversation with Sarah Green Carmichael on the HBR IdeaCast. He’s an expert in problem solving and innovation, and he’s the author of the book, What’s Your Problem?: To Solve Your Toughest Problems, Change the Problems You Solve .
We’ll be back next Wednesday with another hand-picked conversation about leadership from the Harvard Business Review. If you found this episode helpful, share it with your friends and colleagues, and follow our show on Apple Podcasts, Spotify, or wherever you get your podcasts. While you’re there, be sure to leave us a review.
We’re a production of Harvard Business Review. If you want more podcasts, articles, case studies, books, and videos like this, find it all at HBR dot org.
This episode was produced by Anne Saini, and me, Hannah Bates. Ian Fox is our editor. Music by Coma Media. Special thanks to Maureen Hoch, Adi Ignatius, Karen Player, Ramsey Khabbaz, Nicole Smith, Anne Bartholomew, and you – our listener.
See you next week.
- Subscribe On:
Latest in this series
This article is about leadership.
- Decision making and problem solving
- Power and influence
- Business management
Partner Center
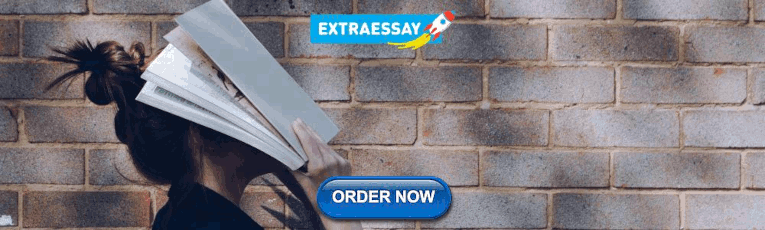
IMAGES
VIDEO
COMMENTS
The problem solving agent follows this four phase problem solving process: Goal Formulation: This is the first and most basic phase in problem solving. It arranges specific steps to establish a target/goal that demands some activity to reach it. AI agents are now used to formulate goals. Problem Formulation: It is one of the fundamental steps ...
Problem-solving agents can be used in a number of different ways in artificial intelligence. They can be used to help find solutions to specific problems or tasks, or they can be used to generalize a problem and find potential solutions. In either case, the problem-solving agent is able to understand complex instructions and carry out specific ...
The problem-solving agent performs precisely by defining problems and several solutions. So we can say that problem solving is a part of artificial intelligence that encompasses a number of techniques such as a tree, B-tree, heuristic algorithms to solve a problem. We can also say that a problem-solving agent is a result-driven agent and always ...
The problem solving agent chooses a cost function that reflects its own performance measure. The solution to the problem is an action sequence that leads from initial state to goal state and the ...
Problem-Solving Agents. goal formulation objectives that need to be achieved problem formulation actions and states to consider problem types single-/multiple state, contingency, exploration example problems toy problems real-world problems search strategies solution execution of the action sequence.
March 5, 2024. In artificial intelligence, a problem-solving agent refers to a type of intelligent agent designed to address and solve complex problems or tasks in its environment. These agents are a fundamental concept in AI and are used in various applications, from game-playing algorithms to robotics and decision-making systems.
Problem formulation ♦ Example problems ♦ Basic search algorithms Chapter 3 2 Problem-solving agents Restricted form of general agent: function Simple-Problem-Solving-Agent (percept) returns an action static: seq, an action sequence, initially empty state, some description of the current world state goal, a goal, initially null problem, a ...
Chapter3 1. Problem-solving agents. function Simple-Problem-Solving-Agent(percept) returns an action static: seq, an action sequence, initially empty state, some description of the current world state goal, a goal, initially null problem, a problem formulation. state←Update-State(state,percept)
After formulating a goal and a problem to solve, the agent calls a search procedure to solve it. It then uses the solution to guide its actions, doing whatever the solution recommends as 1 Notice that each of these "states" actually corresponds to a large set of world states, because a real world state specifies every aspect of reality.
Problem Solving Agents and Uninformed Search An intelligent agents act to increase their performance measure. Some do this by adopting a goal. Four general steps in problem solving: Goal formulation - deciding on what the goal states are - based on current situation and agent's performance measure - What are the successful world states
Problem-solving agents Restricted form of general agent: function Simple-Problem-Solving-Agent(percept) returns an action static: seq, an action sequence, initially empty state, some description of the current world state goal, a goal, initially null problem, a problem formulation state Update-State(state,percept) if seq is empty then
Problem Solving and Search Problem Solving • Agent knows world dynamics • World state is finite, small enough to enumerate • World is deterministic • Utility for a sequence of states is a sum over path The utility for sequences of states is a sum over the path of the utilities of the individual states.
Artificial Intelligence Series: Problem Solving Agents In this article we will be discussing about problem solving agents and how to formulate problems for the agents to solve. 5 min read · Oct ...
Figure 1: Creative Problem Solving (CPS) occurs when the initial conceptual space of the agent is insufficient to com-plete the task, and the agent needs to expand its conceptual space to achieve the task goal. Traditional planning or learn-ing in AI would return a failure in such scenarios.
00:00 - 3.1 Problem-solving agents (The Romania problem)02:10 - 3.1.1 Search problems and solutions 09:10 - 3.1.2 Formulating problems11:55 - 3.2.1 Standardi...
By utilizing heuristic information, Informed agents can affect more efficient and effective problem-solving. Informed agents are best suited for problems where the state space is large, complex, or infinite, and where heuristic information can significantly narrow down the search. These agents excel in scenarios where:
According to cognitive psychology and related disciplines, the development of complex problem-solving behaviour in biological agents depends on hierarchical cognitive mechanisms. Hierarchical ...
When a problem is presented to a problem-solving agent, it takes the responsibility of finding the most suitable approach to solve it. The agent starts by analyzing the problem, identifying its root causes, and devising an action plan accordingly. The support provided by the problem-solving agent is crucial as it guides users in determining ...
The problem-solving agent must assign a color to each region in such a way that no adjacent regions have the same color. CSPs can be solved using various algorithms, such as backtracking, constraint propagation, and local search. These algorithms iteratively explore the search space of possible variable assignments, while taking into account ...
Abstract Agents that communicate back and forth with humans to help them execute non-linguistic tasks are a long sought goal of AI. These agents need to translate between utterances and actionable meaning representations that can be interpreted by task-specific problem solvers in a context-dependent manner.
A problem-solving agent is a key concept in the field of artificial intelligence (AI). It is an agent that can analyze a given problem and take appropriate actions to solve it. The agents are designed using a set of components that work together to achieve their goals.
ROHINI COLLEGE OF ENGINEERING AND TECHNOLOGY CS34 91-ARTIFICIAL INTELLIGENCE AND MACHINE LEARNING Steps performed by Problem-solving agent Goal Formulation: It is the first and simplest step in problem-solving. It organizes the steps/sequence required to formulate one goal out of multiple goals as well as actions to
Finding a suitable solution for issues can be accomplished by following the basic four-step problem-solving process and methodology outlined below. Step. Characteristics. 1. Define the problem. Differentiate fact from opinion. Specify underlying causes. Consult each faction involved for information. State the problem specifically.
To solve tough problems at work, first ask these questions. Problem solving skills are invaluable in any job. But all too often, we jump to find solutions to a problem without taking time to ...
Human intelligence is characterized by our remarkable ability to solve complex problems. This involves planning a sequence of actions that leads us from an initial state to a desired goal state. Quantifying and comparing problem-solving capabilities across species and finding its evolutional roots is a fundamental challenge in cognitive science, and is critical for understanding how the brain ...
In this edition of Readers & Leaders, sharpen your business problem-solving skills and learn ways to overcome friction, strengthen teams, and enhance project management efforts. After studying more than 2,000 teams, Robert I. Sutton shares friction-fixing tips to streamline processes for greater efficiency and less frustration. Andrew McAfee ...