
- Last updated November 18, 2021
- In AI Origins & Evolution
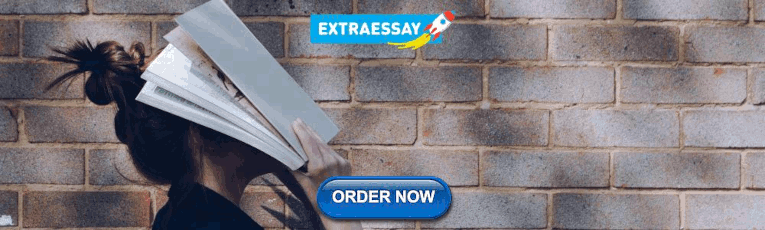
Top Machine Learning Research Papers Released In 2021
- Published on November 18, 2021
- by Dr. Nivash Jeevanandam
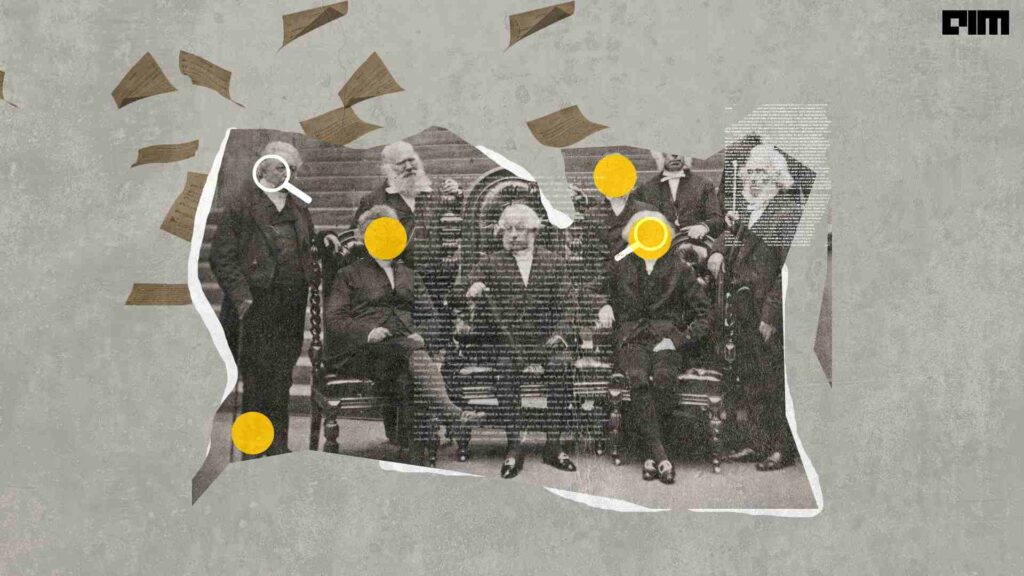
Advances in machine learning and deep learning research are reshaping our technology. Machine learning and deep learning have accomplished various astounding feats this year in 2021, and key research articles have resulted in technical advances used by billions of people. The research in this sector is advancing at a breakneck pace and assisting you to keep up. Here is a collection of the most important recent scientific study papers.
Rebooting ACGAN: Auxiliary Classifier GANs with Stable Training
The authors of this work examined why ACGAN training becomes unstable as the number of classes in the dataset grows. The researchers revealed that the unstable training occurs due to a gradient explosion problem caused by the unboundedness of the input feature vectors and the classifier’s poor classification capabilities during the early training stage. The researchers presented the Data-to-Data Cross-Entropy loss (D2D-CE) and the Rebooted Auxiliary Classifier Generative Adversarial Network to alleviate the instability and reinforce ACGAN (ReACGAN). Additionally, extensive tests of ReACGAN demonstrate that it is resistant to hyperparameter selection and is compatible with a variety of architectures and differentiable augmentations.
This article is ranked #1 on CIFAR-10 for Conditional Image Generation.
For the research paper, read here .
For code, see here .
Dense Unsupervised Learning for Video Segmentation
The authors presented a straightforward and computationally fast unsupervised strategy for learning dense spacetime representations from unlabeled films in this study. The approach demonstrates rapid convergence of training and a high degree of data efficiency. Furthermore, the researchers obtain VOS accuracy superior to previous results despite employing a fraction of the previously necessary training data. The researchers acknowledge that the research findings may be utilised maliciously, such as for unlawful surveillance, and that they are excited to investigate how this skill might be used to better learn a broader spectrum of invariances by exploiting larger temporal windows in movies with complex (ego-)motion, which is more prone to disocclusions.
This study is ranked #1 on DAVIS 2017 for Unsupervised Video Object Segmentation (val).
Temporally-Consistent Surface Reconstruction using Metrically-Consistent Atlases
The authors offer an atlas-based technique for producing unsupervised temporally consistent surface reconstructions by requiring a point on the canonical shape representation to translate to metrically consistent 3D locations on the reconstructed surfaces. Finally, the researchers envisage a plethora of potential applications for the method. For example, by substituting an image-based loss for the Chamfer distance, one may apply the method to RGB video sequences, which the researchers feel will spur development in video-based 3D reconstruction.
This article is ranked #1 on ANIM in the category of Surface Reconstruction.
EdgeFlow: Achieving Practical Interactive Segmentation with Edge-Guided Flow
The researchers propose a revolutionary interactive architecture called EdgeFlow that uses user interaction data without resorting to post-processing or iterative optimisation. The suggested technique achieves state-of-the-art performance on common benchmarks due to its coarse-to-fine network design. Additionally, the researchers create an effective interactive segmentation tool that enables the user to improve the segmentation result through flexible options incrementally.
This paper is ranked #1 on Interactive Segmentation on PASCAL VOC
Learning Transferable Visual Models From Natural Language Supervision
The authors of this work examined whether it is possible to transfer the success of task-agnostic web-scale pre-training in natural language processing to another domain. The findings indicate that adopting this formula resulted in the emergence of similar behaviours in the field of computer vision, and the authors examine the social ramifications of this line of research. CLIP models learn to accomplish a range of tasks during pre-training to optimise their training objective. Using natural language prompting, CLIP can then use this task learning to enable zero-shot transfer to many existing datasets. When applied at a large scale, this technique can compete with task-specific supervised models, while there is still much space for improvement.
This research is ranked #1 on Zero-Shot Transfer Image Classification on SUN
CoAtNet: Marrying Convolution and Attention for All Data Sizes
The researchers in this article conduct a thorough examination of the features of convolutions and transformers, resulting in a principled approach for combining them into a new family of models dubbed CoAtNet. Extensive experiments demonstrate that CoAtNet combines the advantages of ConvNets and Transformers, achieving state-of-the-art performance across a range of data sizes and compute budgets. Take note that this article is currently concentrating on ImageNet classification for model construction. However, the researchers believe their approach is relevant to a broader range of applications, such as object detection and semantic segmentation.
This paper is ranked #1 on Image Classification on ImageNet (using extra training data).
SwinIR: Image Restoration Using Swin Transformer
The authors of this article suggest the SwinIR image restoration model, which is based on the Swin Transformer . The model comprises three modules: shallow feature extraction, deep feature extraction, and human-recognition reconstruction. For deep feature extraction, the researchers employ a stack of residual Swin Transformer blocks (RSTB), each formed of Swin Transformer layers, a convolution layer, and a residual connection.
This research article is ranked #1 on Image Super-Resolution on Manga109 – 4x upscaling.
Access all our open Survey & Awards Nomination forms in one place >>
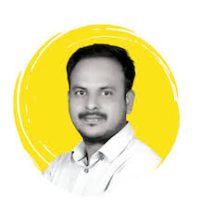
Dr. Nivash Jeevanandam
Download our mobile app.
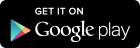
CORPORATE TRAINING PROGRAMS ON GENERATIVE AI
Generative ai skilling for enterprises, our customized corporate training program on generative ai provides a unique opportunity to empower, retain, and advance your talent., 3 ways to join our community, telegram group.
Discover special offers, top stories, upcoming events, and more.
Discord Server
Stay Connected with a larger ecosystem of data science and ML Professionals
Subscribe to our Daily newsletter
Get our daily awesome stories & videos in your inbox, recent stories.

Top 10 LMS Platforms for Enterprise AI Training and Development
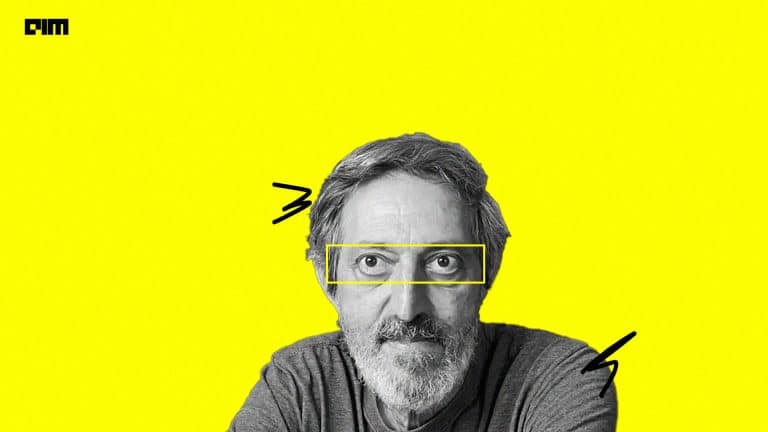
Father of Computational Theory Wins 2023 Turing Award
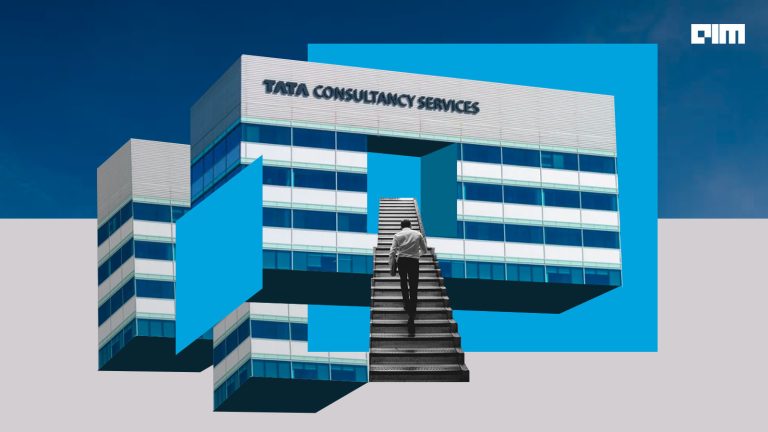
TCS Records $900 Million AI and GenAI Pipeline This Quarter
Generative AI has significantly enhanced TCS’ service offerings across cloud platforms, security, and enterprise solutions.
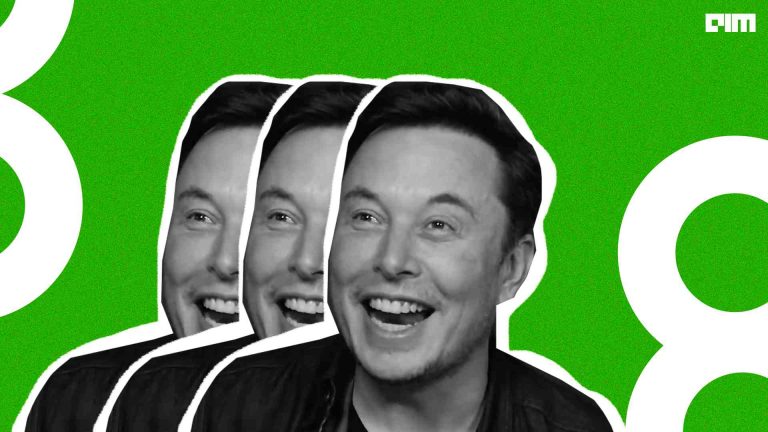
Elon Musk’s xAI Unveils Grok-1.5 Vision, Beats OpenAI’s GPT-4V

Ola Krutrim Makes History with In-House Cloud Infrastructure, Skips AWS and Azure
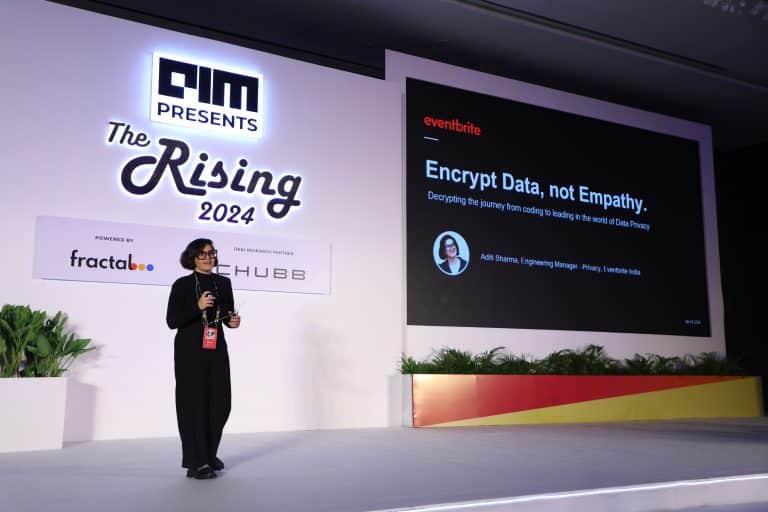
Eventbrite’s Data Privacy Manager, Aditi Sharma on ‘Encrypt Data, not Empathy’
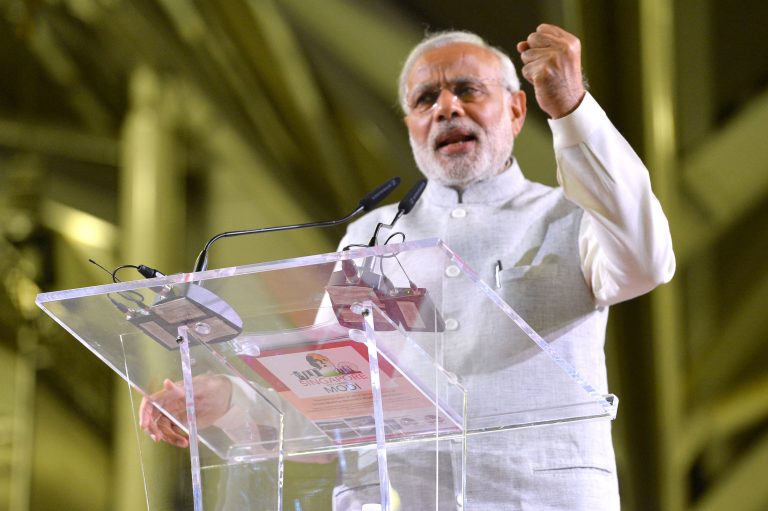
NaMo App Introduces AI Chatbot NaMo AI, Offers Quick Answers to Government Schemes
Users can inquire about initiatives like “Har Ghar Jal” and get insights.
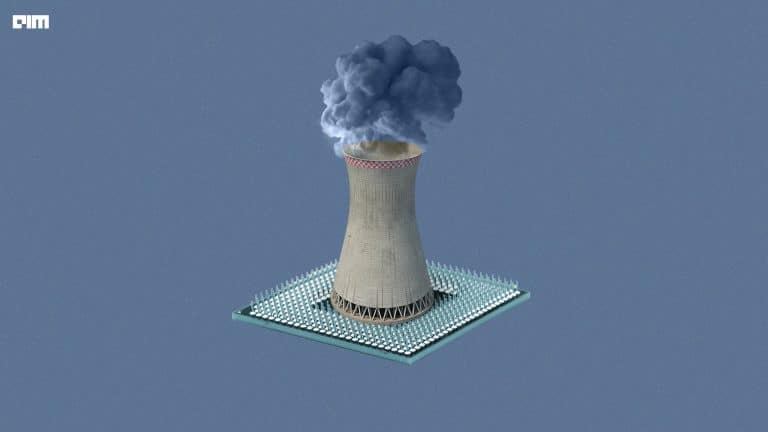
Data Centres’ Lean Towards Nuclear-Powered Future to Combat Energy Needs
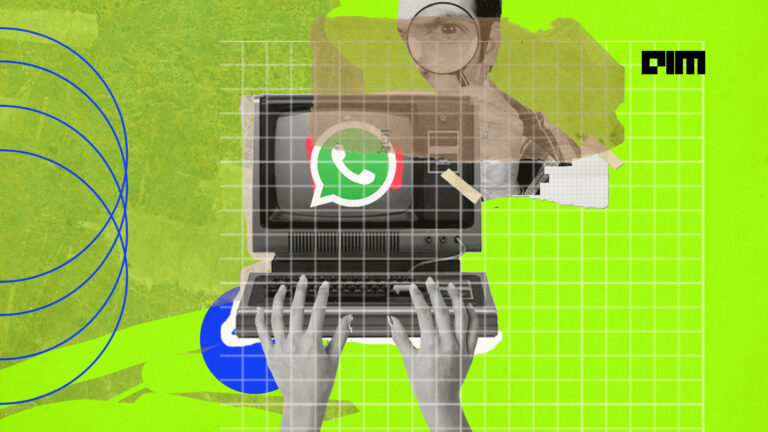
Meta Releases AI on WhatsApp, Looks Like Perplexity AI
Our mission is to bring about better-informed and more conscious decisions about technology through authoritative, influential, and trustworthy journalism., shape the future of ai.
© Analytics India Magazine Pvt Ltd & AIM Media House LLC 2024
- Terms of use
- Privacy Policy
Subscribe to Our Newsletter
The Belamy, our weekly Newsletter is a rage. Just enter your email below.
Thank you for visiting nature.com. You are using a browser version with limited support for CSS. To obtain the best experience, we recommend you use a more up to date browser (or turn off compatibility mode in Internet Explorer). In the meantime, to ensure continued support, we are displaying the site without styles and JavaScript.
- View all journals
Machine learning articles from across Nature Portfolio
Machine learning is the ability of a machine to improve its performance based on previous results. Machine learning methods enable computers to learn without being explicitly programmed and have multiple applications, for example, in the improvement of data mining algorithms.
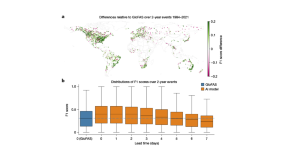
Artificial intelligence can provide accurate forecasts of extreme floods at global scale
Anthropogenic climate change is accelerating the hydrological cycle, causing an increase in the risk of flood-related disasters. A system that uses artificial intelligence allows the creation of reliable, global river flood forecasts, even in places where accurate local data are not available.
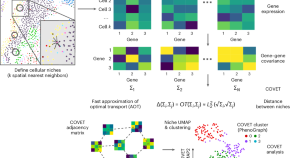
Capturing and modeling cellular niches from dissociated single-cell and spatial data
Cells interact with their local environment to enact global tissue function. By harnessing gene–gene covariation in cellular neighborhoods from spatial transcriptomics data, the covariance environment (COVET) niche representation and the environmental variational inference (ENVI) data integration method model phenotype–microenvironment interplay and reconstruct the spatial context of dissociated single-cell RNA sequencing datasets.
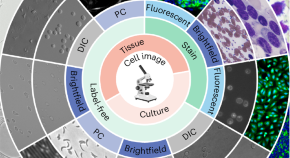
Creating a universal cell segmentation algorithm
Cell segmentation currently involves the use of various bespoke algorithms designed for specific cell types, tissues, staining methods and microscopy technologies. We present a universal algorithm that can segment all kinds of microscopy images and cell types across diverse imaging protocols.
Latest Research and Reviews
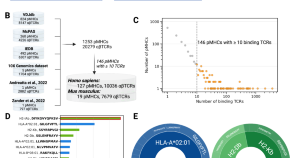
Deep learning predictions of TCR-epitope interactions reveal epitope-specific chains in dual alpha T cells
Prediction of the specificity of a T cell receptor from amino acid sequence has been performed using different methods and approaches. Here the authors use TCRab sequences with known specificity to develop a deep learning TCR-epitope interaction predictor and use this method to predict specificity of dual alpha chain TCRs and TCRs specific for different antigens.
- Giancarlo Croce
- Sara Bobisse
- David Gfeller
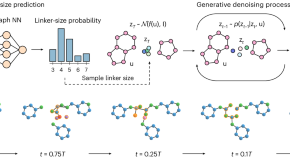
Equivariant 3D-conditional diffusion model for molecular linker design
Fragment-based molecular design uses chemical motifs and combines them into bio-active compounds. While this approach has grown in capability, molecular linker methods are restricted to linking fragments one by one, which makes the search for effective combinations harder. Igashov and colleagues use a conditional diffusion model to link multiple fragments in a one-shot generative process.
- Ilia Igashov
- Hannes Stärk
- Bruno Correia
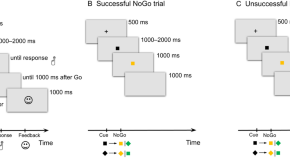
Machine learning reveals differential effects of depression and anxiety on reward and punishment processing
- Anna Grabowska
- Jakub Zabielski
- Magdalena Senderecka
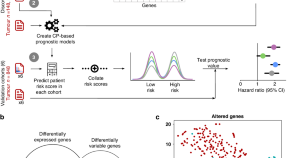
Pathway-based signatures predict patient outcome, chemotherapy benefit and synthetic lethal dependencies in invasive lobular breast cancer
- John Alexander
- Koen Schipper
- Syed Haider
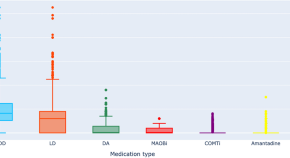
A decision support system based on recurrent neural networks to predict medication dosage for patients with Parkinson's disease
- Atiye Riasi
- Mehdi Delrobaei
- Mehri Salari
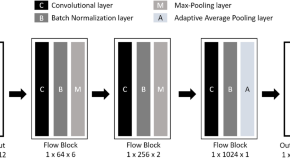
Deep learning assists in acute leukemia detection and cell classification via flow cytometry using the acute leukemia orientation tube
- Fu-Ming Cheng
- Shih-Chang Lo
- Kai-Cheng Hsu
News and Comment
Protein language models using convolutions.
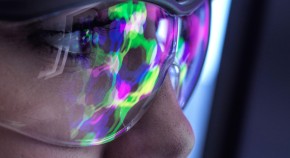
Designer antibiotics by generative AI
Researchers developed an AI model that designs novel, synthesizable antibiotic compounds — several of which showed potent in vitro activity against priority pathogens.
- Karen O’Leary
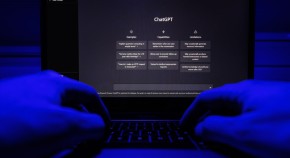
Is ChatGPT corrupting peer review? Telltale words hint at AI use
A study of review reports identifies dozens of adjectives that could indicate text written with the help of chatbots.
- Dalmeet Singh Chawla
How to break big tech’s stranglehold on AI in academia
- Michał Woźniak
- Paweł Ksieniewicz
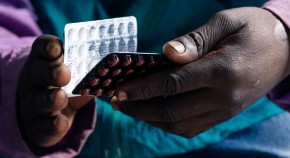
AI can help to tailor drugs for Africa — but Africans should lead the way
Computational models that require very little data could transform biomedical and drug development research in Africa, as long as infrastructure, trained staff and secure databases are available.
- Gemma Turon
- Mathew Njoroge
- Kelly Chibale
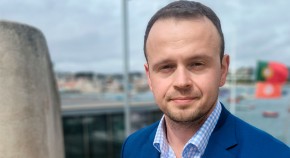
Three ways ChatGPT helps me in my academic writing
Generative AI can be a valuable aid in writing, editing and peer review – if you use it responsibly, says Dritjon Gruda.
- Dritjon Gruda
Quick links
- Explore articles by subject
- Guide to authors
- Editorial policies

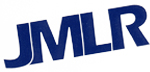
Frequently Asked Questions
Journal of Machine Learning Research
The Journal of Machine Learning Research (JMLR), established in 2000 , provides an international forum for the electronic and paper publication of high-quality scholarly articles in all areas of machine learning. All published papers are freely available online.
- 2024.02.18 : Volume 24 completed; Volume 25 began.
- 2023.01.20 : Volume 23 completed; Volume 24 began.
- 2022.07.20 : New special issue on climate change .
- 2022.02.18 : New blog post: Retrospectives from 20 Years of JMLR .
- 2022.01.25 : Volume 22 completed; Volume 23 began.
- 2021.12.02 : Message from outgoing co-EiC Bernhard Schölkopf .
- 2021.02.10 : Volume 21 completed; Volume 22 began.
- More news ...
Latest papers
More PAC-Bayes bounds: From bounded losses, to losses with general tail behaviors, to anytime validity Borja Rodríguez-Gálvez, Ragnar Thobaben, Mikael Skoglund , 2024. [ abs ][ pdf ][ bib ]
Neural Hilbert Ladders: Multi-Layer Neural Networks in Function Space Zhengdao Chen , 2024. [ abs ][ pdf ][ bib ]
QDax: A Library for Quality-Diversity and Population-based Algorithms with Hardware Acceleration Felix Chalumeau, Bryan Lim, Raphaël Boige, Maxime Allard, Luca Grillotti, Manon Flageat, Valentin Macé, Guillaume Richard, Arthur Flajolet, Thomas Pierrot, Antoine Cully , 2024. (Machine Learning Open Source Software Paper) [ abs ][ pdf ][ bib ] [ code ]
Random Forest Weighted Local Fréchet Regression with Random Objects Rui Qiu, Zhou Yu, Ruoqing Zhu , 2024. [ abs ][ pdf ][ bib ] [ code ]
PhAST: Physics-Aware, Scalable, and Task-Specific GNNs for Accelerated Catalyst Design Alexandre Duval, Victor Schmidt, Santiago Miret, Yoshua Bengio, Alex Hernández-García, David Rolnick , 2024. [ abs ][ pdf ][ bib ] [ code ]
Unsupervised Anomaly Detection Algorithms on Real-world Data: How Many Do We Need? Roel Bouman, Zaharah Bukhsh, Tom Heskes , 2024. [ abs ][ pdf ][ bib ] [ code ]
Multi-class Probabilistic Bounds for Majority Vote Classifiers with Partially Labeled Data Vasilii Feofanov, Emilie Devijver, Massih-Reza Amini , 2024. [ abs ][ pdf ][ bib ]
Information Processing Equalities and the Information–Risk Bridge Robert C. Williamson, Zac Cranko , 2024. [ abs ][ pdf ][ bib ]
Nonparametric Regression for 3D Point Cloud Learning Xinyi Li, Shan Yu, Yueying Wang, Guannan Wang, Li Wang, Ming-Jun Lai , 2024. [ abs ][ pdf ][ bib ] [ code ]
AMLB: an AutoML Benchmark Pieter Gijsbers, Marcos L. P. Bueno, Stefan Coors, Erin LeDell, Sébastien Poirier, Janek Thomas, Bernd Bischl, Joaquin Vanschoren , 2024. [ abs ][ pdf ][ bib ] [ code ]
Materials Discovery using Max K-Armed Bandit Nobuaki Kikkawa, Hiroshi Ohno , 2024. [ abs ][ pdf ][ bib ]
Semi-supervised Inference for Block-wise Missing Data without Imputation Shanshan Song, Yuanyuan Lin, Yong Zhou , 2024. [ abs ][ pdf ][ bib ]
Adaptivity and Non-stationarity: Problem-dependent Dynamic Regret for Online Convex Optimization Peng Zhao, Yu-Jie Zhang, Lijun Zhang, Zhi-Hua Zhou , 2024. [ abs ][ pdf ][ bib ]
Scaling Speech Technology to 1,000+ Languages Vineel Pratap, Andros Tjandra, Bowen Shi, Paden Tomasello, Arun Babu, Sayani Kundu, Ali Elkahky, Zhaoheng Ni, Apoorv Vyas, Maryam Fazel-Zarandi, Alexei Baevski, Yossi Adi, Xiaohui Zhang, Wei-Ning Hsu, Alexis Conneau, Michael Auli , 2024. [ abs ][ pdf ][ bib ] [ code ]
MAP- and MLE-Based Teaching Hans Ulrich Simon, Jan Arne Telle , 2024. [ abs ][ pdf ][ bib ]
A General Framework for the Analysis of Kernel-based Tests Tamara Fernández, Nicolás Rivera , 2024. [ abs ][ pdf ][ bib ]
Overparametrized Multi-layer Neural Networks: Uniform Concentration of Neural Tangent Kernel and Convergence of Stochastic Gradient Descent Jiaming Xu, Hanjing Zhu , 2024. [ abs ][ pdf ][ bib ]
Sparse Representer Theorems for Learning in Reproducing Kernel Banach Spaces Rui Wang, Yuesheng Xu, Mingsong Yan , 2024. [ abs ][ pdf ][ bib ]
Exploration of the Search Space of Gaussian Graphical Models for Paired Data Alberto Roverato, Dung Ngoc Nguyen , 2024. [ abs ][ pdf ][ bib ]
The good, the bad and the ugly sides of data augmentation: An implicit spectral regularization perspective Chi-Heng Lin, Chiraag Kaushik, Eva L. Dyer, Vidya Muthukumar , 2024. [ abs ][ pdf ][ bib ] [ code ]
Stochastic Approximation with Decision-Dependent Distributions: Asymptotic Normality and Optimality Joshua Cutler, Mateo Díaz, Dmitriy Drusvyatskiy , 2024. [ abs ][ pdf ][ bib ]
Minimax Rates for High-Dimensional Random Tessellation Forests Eliza O'Reilly, Ngoc Mai Tran , 2024. [ abs ][ pdf ][ bib ]
Nonparametric Estimation of Non-Crossing Quantile Regression Process with Deep ReQU Neural Networks Guohao Shen, Yuling Jiao, Yuanyuan Lin, Joel L. Horowitz, Jian Huang , 2024. [ abs ][ pdf ][ bib ]
Spatial meshing for general Bayesian multivariate models Michele Peruzzi, David B. Dunson , 2024. [ abs ][ pdf ][ bib ] [ code ]
A Semi-parametric Estimation of Personalized Dose-response Function Using Instrumental Variables Wei Luo, Yeying Zhu, Xuekui Zhang, Lin Lin , 2024. [ abs ][ pdf ][ bib ]
Learning Non-Gaussian Graphical Models via Hessian Scores and Triangular Transport Ricardo Baptista, Youssef Marzouk, Rebecca Morrison, Olivier Zahm , 2024. [ abs ][ pdf ][ bib ] [ code ]
On the Learnability of Out-of-distribution Detection Zhen Fang, Yixuan Li, Feng Liu, Bo Han, Jie Lu , 2024. [ abs ][ pdf ][ bib ]
Win: Weight-Decay-Integrated Nesterov Acceleration for Faster Network Training Pan Zhou, Xingyu Xie, Zhouchen Lin, Kim-Chuan Toh, Shuicheng Yan , 2024. [ abs ][ pdf ][ bib ] [ code ]
On the Eigenvalue Decay Rates of a Class of Neural-Network Related Kernel Functions Defined on General Domains Yicheng Li, Zixiong Yu, Guhan Chen, Qian Lin , 2024. [ abs ][ pdf ][ bib ]
Tight Convergence Rate Bounds for Optimization Under Power Law Spectral Conditions Maksim Velikanov, Dmitry Yarotsky , 2024. [ abs ][ pdf ][ bib ]
ptwt - The PyTorch Wavelet Toolbox Moritz Wolter, Felix Blanke, Jochen Garcke, Charles Tapley Hoyt , 2024. (Machine Learning Open Source Software Paper) [ abs ][ pdf ][ bib ] [ code ]
Choosing the Number of Topics in LDA Models – A Monte Carlo Comparison of Selection Criteria Victor Bystrov, Viktoriia Naboka-Krell, Anna Staszewska-Bystrova, Peter Winker , 2024. [ abs ][ pdf ][ bib ] [ code ]
Functional Directed Acyclic Graphs Kuang-Yao Lee, Lexin Li, Bing Li , 2024. [ abs ][ pdf ][ bib ]
Unlabeled Principal Component Analysis and Matrix Completion Yunzhen Yao, Liangzu Peng, Manolis C. Tsakiris , 2024. [ abs ][ pdf ][ bib ] [ code ]
Distributed Estimation on Semi-Supervised Generalized Linear Model Jiyuan Tu, Weidong Liu, Xiaojun Mao , 2024. [ abs ][ pdf ][ bib ]
Towards Explainable Evaluation Metrics for Machine Translation Christoph Leiter, Piyawat Lertvittayakumjorn, Marina Fomicheva, Wei Zhao, Yang Gao, Steffen Eger , 2024. [ abs ][ pdf ][ bib ]
Differentially private methods for managing model uncertainty in linear regression Víctor Peña, Andrés F. Barrientos , 2024. [ abs ][ pdf ][ bib ]
Data Summarization via Bilevel Optimization Zalán Borsos, Mojmír Mutný, Marco Tagliasacchi, Andreas Krause , 2024. [ abs ][ pdf ][ bib ]
Pareto Smoothed Importance Sampling Aki Vehtari, Daniel Simpson, Andrew Gelman, Yuling Yao, Jonah Gabry , 2024. [ abs ][ pdf ][ bib ] [ code ]
Policy Gradient Methods in the Presence of Symmetries and State Abstractions Prakash Panangaden, Sahand Rezaei-Shoshtari, Rosie Zhao, David Meger, Doina Precup , 2024. [ abs ][ pdf ][ bib ] [ code ]
Scaling Instruction-Finetuned Language Models Hyung Won Chung, Le Hou, Shayne Longpre, Barret Zoph, Yi Tay, William Fedus, Yunxuan Li, Xuezhi Wang, Mostafa Dehghani, Siddhartha Brahma, Albert Webson, Shixiang Shane Gu, Zhuyun Dai, Mirac Suzgun, Xinyun Chen, Aakanksha Chowdhery, Alex Castro-Ros, Marie Pellat, Kevin Robinson, Dasha Valter, Sharan Narang, Gaurav Mishra, Adams Yu, Vincent Zhao, Yanping Huang, Andrew Dai, Hongkun Yu, Slav Petrov, Ed H. Chi, Jeff Dean, Jacob Devlin, Adam Roberts, Denny Zhou, Quoc V. Le, Jason Wei , 2024. [ abs ][ pdf ][ bib ]
Tangential Wasserstein Projections Florian Gunsilius, Meng Hsuan Hsieh, Myung Jin Lee , 2024. [ abs ][ pdf ][ bib ] [ code ]
Learnability of Linear Port-Hamiltonian Systems Juan-Pablo Ortega, Daiying Yin , 2024. [ abs ][ pdf ][ bib ] [ code ]
Off-Policy Action Anticipation in Multi-Agent Reinforcement Learning Ariyan Bighashdel, Daan de Geus, Pavol Jancura, Gijs Dubbelman , 2024. [ abs ][ pdf ][ bib ] [ code ]
On Unbiased Estimation for Partially Observed Diffusions Jeremy Heng, Jeremie Houssineau, Ajay Jasra , 2024. (Machine Learning Open Source Software Paper) [ abs ][ pdf ][ bib ] [ code ]
Improving Lipschitz-Constrained Neural Networks by Learning Activation Functions Stanislas Ducotterd, Alexis Goujon, Pakshal Bohra, Dimitris Perdios, Sebastian Neumayer, Michael Unser , 2024. [ abs ][ pdf ][ bib ] [ code ]
Mathematical Framework for Online Social Media Auditing Wasim Huleihel, Yehonathan Refael , 2024. [ abs ][ pdf ][ bib ]
An Embedding Framework for the Design and Analysis of Consistent Polyhedral Surrogates Jessie Finocchiaro, Rafael M. Frongillo, Bo Waggoner , 2024. [ abs ][ pdf ][ bib ]
Low-rank Variational Bayes correction to the Laplace method Janet van Niekerk, Haavard Rue , 2024. [ abs ][ pdf ][ bib ] [ code ]
Scaling the Convex Barrier with Sparse Dual Algorithms Alessandro De Palma, Harkirat Singh Behl, Rudy Bunel, Philip H.S. Torr, M. Pawan Kumar , 2024. [ abs ][ pdf ][ bib ] [ code ]
Causal-learn: Causal Discovery in Python Yujia Zheng, Biwei Huang, Wei Chen, Joseph Ramsey, Mingming Gong, Ruichu Cai, Shohei Shimizu, Peter Spirtes, Kun Zhang , 2024. (Machine Learning Open Source Software Paper) [ abs ][ pdf ][ bib ] [ code ]
Decomposed Linear Dynamical Systems (dLDS) for learning the latent components of neural dynamics Noga Mudrik, Yenho Chen, Eva Yezerets, Christopher J. Rozell, Adam S. Charles , 2024. [ abs ][ pdf ][ bib ] [ code ]
Existence and Minimax Theorems for Adversarial Surrogate Risks in Binary Classification Natalie S. Frank, Jonathan Niles-Weed , 2024. [ abs ][ pdf ][ bib ]
Data Thinning for Convolution-Closed Distributions Anna Neufeld, Ameer Dharamshi, Lucy L. Gao, Daniela Witten , 2024. [ abs ][ pdf ][ bib ] [ code ]
A projected semismooth Newton method for a class of nonconvex composite programs with strong prox-regularity Jiang Hu, Kangkang Deng, Jiayuan Wu, Quanzheng Li , 2024. [ abs ][ pdf ][ bib ]
Revisiting RIP Guarantees for Sketching Operators on Mixture Models Ayoub Belhadji, Rémi Gribonval , 2024. [ abs ][ pdf ][ bib ]
Monotonic Risk Relationships under Distribution Shifts for Regularized Risk Minimization Daniel LeJeune, Jiayu Liu, Reinhard Heckel , 2024. [ abs ][ pdf ][ bib ] [ code ]
Polygonal Unadjusted Langevin Algorithms: Creating stable and efficient adaptive algorithms for neural networks Dong-Young Lim, Sotirios Sabanis , 2024. [ abs ][ pdf ][ bib ]
Axiomatic effect propagation in structural causal models Raghav Singal, George Michailidis , 2024. [ abs ][ pdf ][ bib ]
Optimal First-Order Algorithms as a Function of Inequalities Chanwoo Park, Ernest K. Ryu , 2024. [ abs ][ pdf ][ bib ] [ code ]
Resource-Efficient Neural Networks for Embedded Systems Wolfgang Roth, Günther Schindler, Bernhard Klein, Robert Peharz, Sebastian Tschiatschek, Holger Fröning, Franz Pernkopf, Zoubin Ghahramani , 2024. [ abs ][ pdf ][ bib ]
Trained Transformers Learn Linear Models In-Context Ruiqi Zhang, Spencer Frei, Peter L. Bartlett , 2024. [ abs ][ pdf ][ bib ]
Adam-family Methods for Nonsmooth Optimization with Convergence Guarantees Nachuan Xiao, Xiaoyin Hu, Xin Liu, Kim-Chuan Toh , 2024. [ abs ][ pdf ][ bib ]
Efficient Modality Selection in Multimodal Learning Yifei He, Runxiang Cheng, Gargi Balasubramaniam, Yao-Hung Hubert Tsai, Han Zhao , 2024. [ abs ][ pdf ][ bib ]
A Multilabel Classification Framework for Approximate Nearest Neighbor Search Ville Hyvönen, Elias Jääsaari, Teemu Roos , 2024. [ abs ][ pdf ][ bib ] [ code ]
Probabilistic Forecasting with Generative Networks via Scoring Rule Minimization Lorenzo Pacchiardi, Rilwan A. Adewoyin, Peter Dueben, Ritabrata Dutta , 2024. [ abs ][ pdf ][ bib ] [ code ]
Multiple Descent in the Multiple Random Feature Model Xuran Meng, Jianfeng Yao, Yuan Cao , 2024. [ abs ][ pdf ][ bib ]
Mean-Square Analysis of Discretized Itô Diffusions for Heavy-tailed Sampling Ye He, Tyler Farghly, Krishnakumar Balasubramanian, Murat A. Erdogdu , 2024. [ abs ][ pdf ][ bib ]
Invariant and Equivariant Reynolds Networks Akiyoshi Sannai, Makoto Kawano, Wataru Kumagai , 2024. (Machine Learning Open Source Software Paper) [ abs ][ pdf ][ bib ] [ code ]
Personalized PCA: Decoupling Shared and Unique Features Naichen Shi, Raed Al Kontar , 2024. [ abs ][ pdf ][ bib ] [ code ]
Survival Kernets: Scalable and Interpretable Deep Kernel Survival Analysis with an Accuracy Guarantee George H. Chen , 2024. [ abs ][ pdf ][ bib ] [ code ]
On the Sample Complexity and Metastability of Heavy-tailed Policy Search in Continuous Control Amrit Singh Bedi, Anjaly Parayil, Junyu Zhang, Mengdi Wang, Alec Koppel , 2024. [ abs ][ pdf ][ bib ]
Convergence for nonconvex ADMM, with applications to CT imaging Rina Foygel Barber, Emil Y. Sidky , 2024. [ abs ][ pdf ][ bib ] [ code ]
Distributed Gaussian Mean Estimation under Communication Constraints: Optimal Rates and Communication-Efficient Algorithms T. Tony Cai, Hongji Wei , 2024. [ abs ][ pdf ][ bib ]
Sparse NMF with Archetypal Regularization: Computational and Robustness Properties Kayhan Behdin, Rahul Mazumder , 2024. [ abs ][ pdf ][ bib ] [ code ]
Deep Network Approximation: Beyond ReLU to Diverse Activation Functions Shijun Zhang, Jianfeng Lu, Hongkai Zhao , 2024. [ abs ][ pdf ][ bib ]
Effect-Invariant Mechanisms for Policy Generalization Sorawit Saengkyongam, Niklas Pfister, Predrag Klasnja, Susan Murphy, Jonas Peters , 2024. [ abs ][ pdf ][ bib ]
Pygmtools: A Python Graph Matching Toolkit Runzhong Wang, Ziao Guo, Wenzheng Pan, Jiale Ma, Yikai Zhang, Nan Yang, Qi Liu, Longxuan Wei, Hanxue Zhang, Chang Liu, Zetian Jiang, Xiaokang Yang, Junchi Yan , 2024. (Machine Learning Open Source Software Paper) [ abs ][ pdf ][ bib ] [ code ]
Heterogeneous-Agent Reinforcement Learning Yifan Zhong, Jakub Grudzien Kuba, Xidong Feng, Siyi Hu, Jiaming Ji, Yaodong Yang , 2024. [ abs ][ pdf ][ bib ] [ code ]
Sample-efficient Adversarial Imitation Learning Dahuin Jung, Hyungyu Lee, Sungroh Yoon , 2024. [ abs ][ pdf ][ bib ]
Stochastic Modified Flows, Mean-Field Limits and Dynamics of Stochastic Gradient Descent Benjamin Gess, Sebastian Kassing, Vitalii Konarovskyi , 2024. [ abs ][ pdf ][ bib ]
Rates of convergence for density estimation with generative adversarial networks Nikita Puchkin, Sergey Samsonov, Denis Belomestny, Eric Moulines, Alexey Naumov , 2024. [ abs ][ pdf ][ bib ]
Additive smoothing error in backward variational inference for general state-space models Mathis Chagneux, Elisabeth Gassiat, Pierre Gloaguen, Sylvain Le Corff , 2024. [ abs ][ pdf ][ bib ]
Optimal Bump Functions for Shallow ReLU networks: Weight Decay, Depth Separation, Curse of Dimensionality Stephan Wojtowytsch , 2024. [ abs ][ pdf ][ bib ]
Numerically Stable Sparse Gaussian Processes via Minimum Separation using Cover Trees Alexander Terenin, David R. Burt, Artem Artemev, Seth Flaxman, Mark van der Wilk, Carl Edward Rasmussen, Hong Ge , 2024. [ abs ][ pdf ][ bib ] [ code ]
On Tail Decay Rate Estimation of Loss Function Distributions Etrit Haxholli, Marco Lorenzi , 2024. [ abs ][ pdf ][ bib ] [ code ]
Deep Nonparametric Estimation of Operators between Infinite Dimensional Spaces Hao Liu, Haizhao Yang, Minshuo Chen, Tuo Zhao, Wenjing Liao , 2024. [ abs ][ pdf ][ bib ]
Post-Regularization Confidence Bands for Ordinary Differential Equations Xiaowu Dai, Lexin Li , 2024. [ abs ][ pdf ][ bib ]
On the Generalization of Stochastic Gradient Descent with Momentum Ali Ramezani-Kebrya, Kimon Antonakopoulos, Volkan Cevher, Ashish Khisti, Ben Liang , 2024. [ abs ][ pdf ][ bib ]
Pursuit of the Cluster Structure of Network Lasso: Recovery Condition and Non-convex Extension Shotaro Yagishita, Jun-ya Gotoh , 2024. [ abs ][ pdf ][ bib ]
Iterate Averaging in the Quest for Best Test Error Diego Granziol, Nicholas P. Baskerville, Xingchen Wan, Samuel Albanie, Stephen Roberts , 2024. [ abs ][ pdf ][ bib ] [ code ]
Nonparametric Inference under B-bits Quantization Kexuan Li, Ruiqi Liu, Ganggang Xu, Zuofeng Shang , 2024. [ abs ][ pdf ][ bib ]
Black Box Variational Inference with a Deterministic Objective: Faster, More Accurate, and Even More Black Box Ryan Giordano, Martin Ingram, Tamara Broderick , 2024. [ abs ][ pdf ][ bib ] [ code ]
On Sufficient Graphical Models Bing Li, Kyongwon Kim , 2024. [ abs ][ pdf ][ bib ]
Localized Debiased Machine Learning: Efficient Inference on Quantile Treatment Effects and Beyond Nathan Kallus, Xiaojie Mao, Masatoshi Uehara , 2024. [ abs ][ pdf ][ bib ] [ code ]
On the Effect of Initialization: The Scaling Path of 2-Layer Neural Networks Sebastian Neumayer, Lénaïc Chizat, Michael Unser , 2024. [ abs ][ pdf ][ bib ]
Improving physics-informed neural networks with meta-learned optimization Alex Bihlo , 2024. [ abs ][ pdf ][ bib ]
A Comparison of Continuous-Time Approximations to Stochastic Gradient Descent Stefan Ankirchner, Stefan Perko , 2024. [ abs ][ pdf ][ bib ]
Critically Assessing the State of the Art in Neural Network Verification Matthias König, Annelot W. Bosman, Holger H. Hoos, Jan N. van Rijn , 2024. [ abs ][ pdf ][ bib ]
Estimating the Minimizer and the Minimum Value of a Regression Function under Passive Design Arya Akhavan, Davit Gogolashvili, Alexandre B. Tsybakov , 2024. [ abs ][ pdf ][ bib ]
Modeling Random Networks with Heterogeneous Reciprocity Daniel Cirkovic, Tiandong Wang , 2024. [ abs ][ pdf ][ bib ]
Exploration, Exploitation, and Engagement in Multi-Armed Bandits with Abandonment Zixian Yang, Xin Liu, Lei Ying , 2024. [ abs ][ pdf ][ bib ]
On Efficient and Scalable Computation of the Nonparametric Maximum Likelihood Estimator in Mixture Models Yangjing Zhang, Ying Cui, Bodhisattva Sen, Kim-Chuan Toh , 2024. [ abs ][ pdf ][ bib ] [ code ]
Decorrelated Variable Importance Isabella Verdinelli, Larry Wasserman , 2024. [ abs ][ pdf ][ bib ]
Model-Free Representation Learning and Exploration in Low-Rank MDPs Aditya Modi, Jinglin Chen, Akshay Krishnamurthy, Nan Jiang, Alekh Agarwal , 2024. [ abs ][ pdf ][ bib ]
Seeded Graph Matching for the Correlated Gaussian Wigner Model via the Projected Power Method Ernesto Araya, Guillaume Braun, Hemant Tyagi , 2024. [ abs ][ pdf ][ bib ] [ code ]
Fast Policy Extragradient Methods for Competitive Games with Entropy Regularization Shicong Cen, Yuting Wei, Yuejie Chi , 2024. [ abs ][ pdf ][ bib ]
Power of knockoff: The impact of ranking algorithm, augmented design, and symmetric statistic Zheng Tracy Ke, Jun S. Liu, Yucong Ma , 2024. [ abs ][ pdf ][ bib ]
Lower Complexity Bounds of Finite-Sum Optimization Problems: The Results and Construction Yuze Han, Guangzeng Xie, Zhihua Zhang , 2024. [ abs ][ pdf ][ bib ]
On Truthing Issues in Supervised Classification Jonathan K. Su , 2024. [ abs ][ pdf ][ bib ]
Subscribe to the PwC Newsletter
Join the community, natural language processing, representation learning.

Disentanglement
Graph representation learning, sentence embeddings.

Network Embedding
Classification.

Text Classification

Graph Classification

Audio Classification

Medical Image Classification
Language modelling.

Long-range modeling
Protein language model, sentence pair modeling, deep hashing, table retrieval, question answering.

Open-Ended Question Answering

Open-Domain Question Answering
Conversational question answering.

Answer Selection
Translation, image generation.

Image-to-Image Translation

Image Inpainting

Text-to-Image Generation

Conditional Image Generation
Data augmentation.

Image Augmentation

Text Augmentation
Machine translation.

Transliteration
Bilingual lexicon induction.

Multimodal Machine Translation

Unsupervised Machine Translation
Text generation.

Dialogue Generation

Data-to-Text Generation

Multi-Document Summarization
Text style transfer.

Topic Models

Document Classification

Sentence Classification

Emotion Classification
2d semantic segmentation, image segmentation.

Scene Parsing

Reflection Removal
Visual question answering (vqa).

Visual Question Answering

Machine Reading Comprehension

Chart Question Answering

Embodied Question Answering
Named entity recognition (ner).

Nested Named Entity Recognition
Chinese named entity recognition, few-shot ner, sentiment analysis.

Aspect-Based Sentiment Analysis (ABSA)

Multimodal Sentiment Analysis

Aspect Sentiment Triplet Extraction

Twitter Sentiment Analysis
Few-shot learning.

One-Shot Learning

Few-Shot Semantic Segmentation
Cross-domain few-shot.

Unsupervised Few-Shot Learning
Word embeddings.

Learning Word Embeddings

Multilingual Word Embeddings
Embeddings evaluation, contextualised word representations, optical character recognition (ocr).

Active Learning

Handwriting Recognition
Handwritten digit recognition, irregular text recognition, continual learning.

Class Incremental Learning
Continual named entity recognition, unsupervised class-incremental learning, text summarization.

Abstractive Text Summarization
Document summarization, opinion summarization, information retrieval.

Passage Retrieval
Cross-lingual information retrieval, table search, relation extraction.

Relation Classification
Document-level relation extraction, joint entity and relation extraction, temporal relation extraction, link prediction.

Inductive Link Prediction
Dynamic link prediction, hyperedge prediction, anchor link prediction, natural language inference.

Answer Generation

Visual Entailment
Cross-lingual natural language inference, reading comprehension.

Intent Recognition
Implicit relations, large language model, active object detection, emotion recognition.
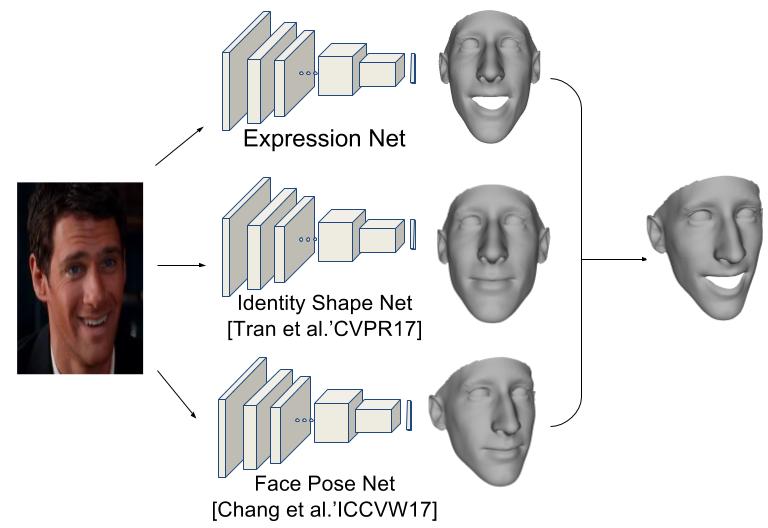
Speech Emotion Recognition

Emotion Recognition in Conversation

Multimodal Emotion Recognition
Emotion-cause pair extraction, natural language understanding, vietnamese social media text processing.

Emotional Dialogue Acts
Semantic textual similarity.

Paraphrase Identification

Cross-Lingual Semantic Textual Similarity
Image captioning.

3D dense captioning
Controllable image captioning, aesthetic image captioning.

Relational Captioning
Event extraction, event causality identification, zero-shot event extraction, dialogue state tracking, task-oriented dialogue systems.

Visual Dialog
Dialogue understanding, semantic parsing.

AMR Parsing
Semantic dependency parsing, drs parsing, ucca parsing, coreference resolution, coreference-resolution, cross document coreference resolution, in-context learning, semantic similarity, conformal prediction.

Text Simplification

Music Source Separation
Audio source separation.

Decision Making Under Uncertainty

Sentence Embedding
Sentence compression, joint multilingual sentence representations, sentence embeddings for biomedical texts, code generation.

Code Translation

Code Documentation Generation
Class-level code generation, library-oriented code generation, dependency parsing.

Transition-Based Dependency Parsing
Prepositional phrase attachment, unsupervised dependency parsing, cross-lingual zero-shot dependency parsing, specificity, information extraction, extractive summarization, temporal information extraction, low resource named entity recognition, cross-lingual, cross-lingual transfer, cross-lingual document classification.

Cross-Lingual Entity Linking
Cross-language text summarization, response generation, common sense reasoning.

Physical Commonsense Reasoning
Riddle sense, anachronisms, instruction following, visual instruction following, memorization, data integration.

Entity Alignment

Entity Resolution
Table annotation, entity linking.

Prompt Engineering

Visual Prompting
Question generation, poll generation.

Topic coverage
Dynamic topic modeling, part-of-speech tagging.

Unsupervised Part-Of-Speech Tagging
Abuse detection, hate speech detection, mathematical reasoning.

Math Word Problem Solving
Formal logic, geometry problem solving, abstract algebra, open information extraction.

Hope Speech Detection
Hate speech normalization, hate speech detection crisishatemm benchmark, data mining.

Argument Mining

Opinion Mining
Subgroup discovery, cognitive diagnosis, parallel corpus mining, word sense disambiguation.

Word Sense Induction
Language identification, dialect identification, native language identification, few-shot relation classification, implicit discourse relation classification, cause-effect relation classification, bias detection, selection bias, fake news detection, relational reasoning.

Semantic Role Labeling

Predicate Detection
Semantic role labeling (predicted predicates).

Textual Analogy Parsing
Slot filling.

Zero-shot Slot Filling
Extracting covid-19 events from twitter, grammatical error correction.

Grammatical Error Detection
Text matching, document text classification, learning with noisy labels, multi-label classification of biomedical texts, political salient issue orientation detection, pos tagging, deep clustering, trajectory clustering, deep nonparametric clustering, nonparametric deep clustering, stance detection, zero-shot stance detection, few-shot stance detection, stance detection (us election 2020 - biden), stance detection (us election 2020 - trump), spoken language understanding, dialogue safety prediction, multi-modal entity alignment, intent detection.

Open Intent Detection
Word similarity, text-to-speech synthesis.
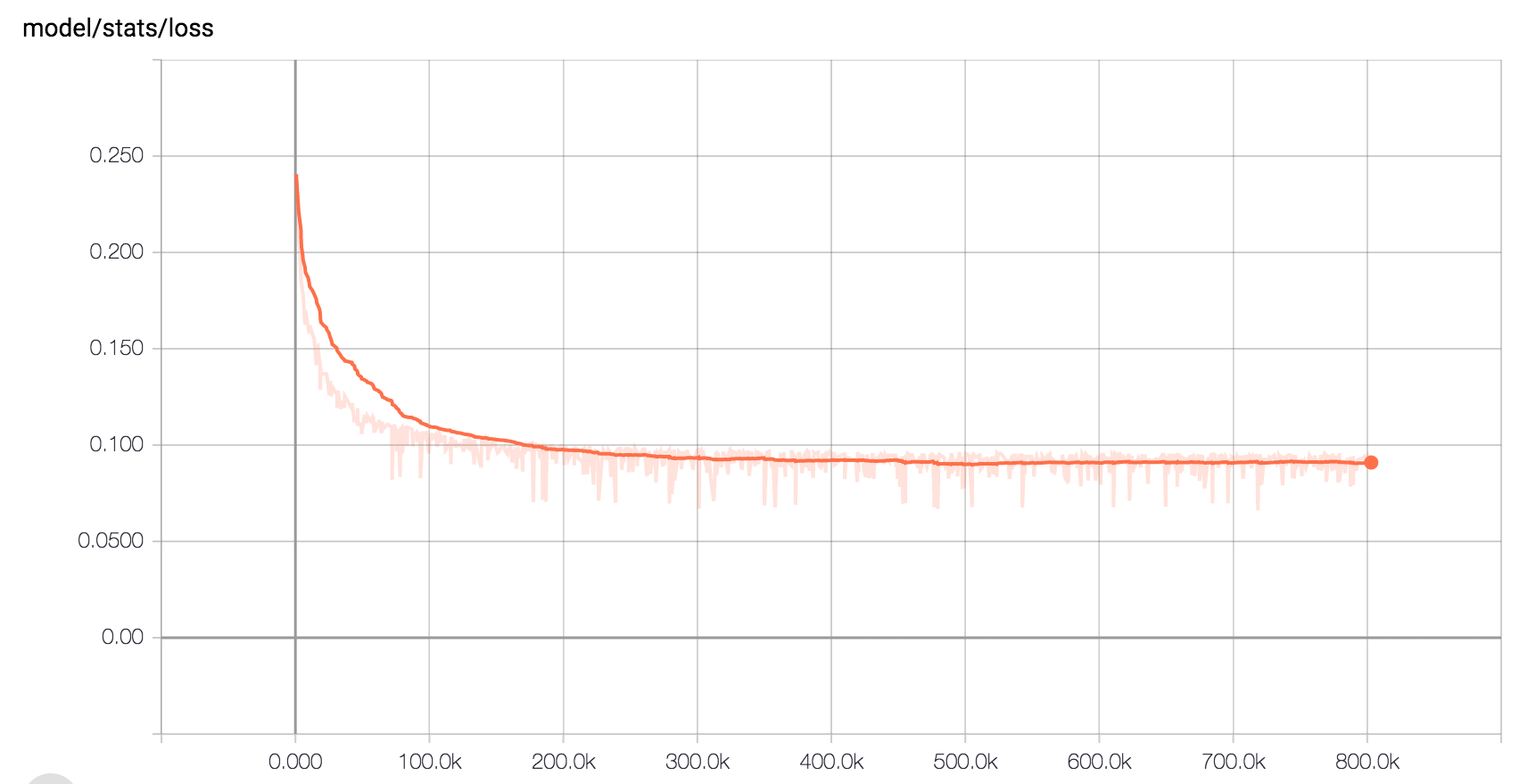
Prosody Prediction
Zero-shot multi-speaker tts, zero-shot cross-lingual transfer, cross-lingual ner, fact verification, intent classification.

Document AI
Document understanding, language acquisition, grounded language learning, constituency parsing.

Constituency Grammar Induction
Entity typing.

Entity Typing on DH-KGs
Self-learning, cross-modal retrieval, image-text matching, multilingual cross-modal retrieval.

Zero-shot Composed Person Retrieval
Cross-modal retrieval on rsitmd, ad-hoc information retrieval, document ranking.

Word Alignment
Open-domain dialog, dialogue evaluation, novelty detection, model editing, knowledge editing, multimodal deep learning, multimodal text and image classification, discourse parsing, discourse segmentation, connective detection, multi-label text classification.

Text-based Image Editing
Text-guided-image-editing.

Zero-Shot Text-to-Image Generation
Concept alignment, conditional text-to-image synthesis.

Shallow Syntax
Sarcasm detection.
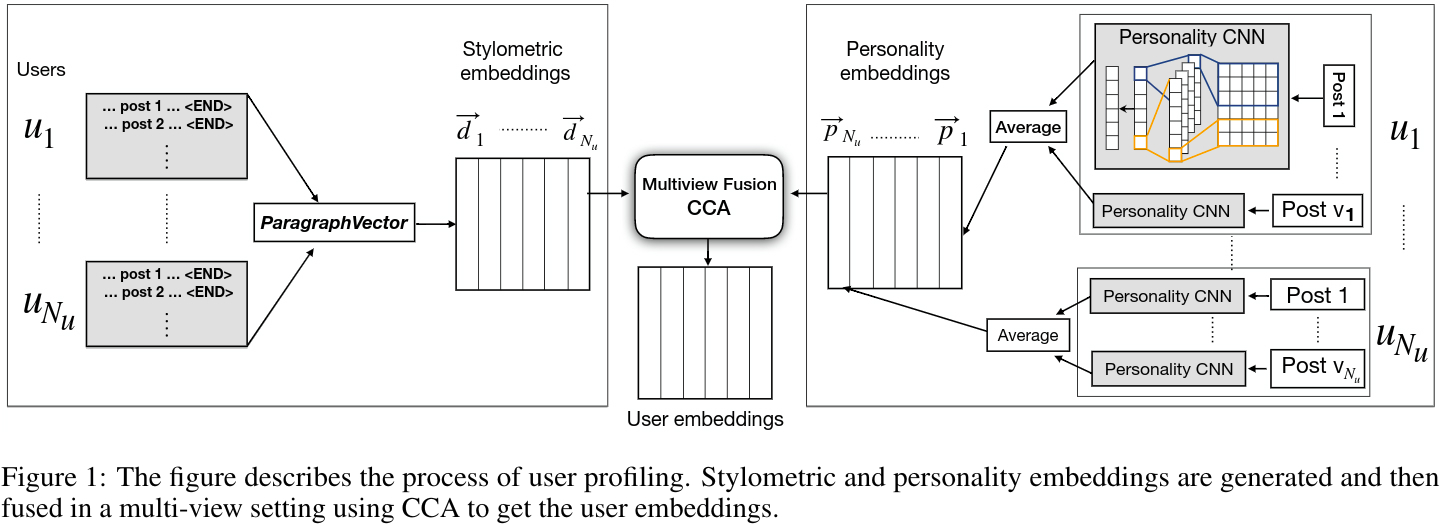
De-identification
Privacy preserving deep learning, explanation generation, lemmatization, morphological analysis.

Session Search

Aspect Extraction
Extract aspect, aspect category sentiment analysis.

Aspect-oriented Opinion Extraction

Aspect-Category-Opinion-Sentiment Quadruple Extraction
Molecular representation.

Chinese Word Segmentation
Handwritten chinese text recognition, chinese spelling error correction, chinese zero pronoun resolution, offline handwritten chinese character recognition, entity disambiguation, text-to-video generation, text-to-video editing, subject-driven video generation, conversational search, source code summarization, method name prediction, speech-to-text translation, simultaneous speech-to-text translation, text clustering.

Short Text Clustering

Open Intent Discovery
Authorship attribution, keyphrase extraction, linguistic acceptability.

Column Type Annotation
Cell entity annotation, columns property annotation, row annotation, abusive language.

Visual Storytelling

KG-to-Text Generation

Unsupervised KG-to-Text Generation
Few-shot text classification, zero-shot out-of-domain detection, term extraction, text2text generation, keyphrase generation, figurative language visualization, sketch-to-text generation, protein folding, phrase grounding, grounded open vocabulary acquisition, deep attention, morphological inflection, word translation, multilingual nlp, spam detection, context-specific spam detection, traditional spam detection, summarization, unsupervised extractive summarization, query-focused summarization.
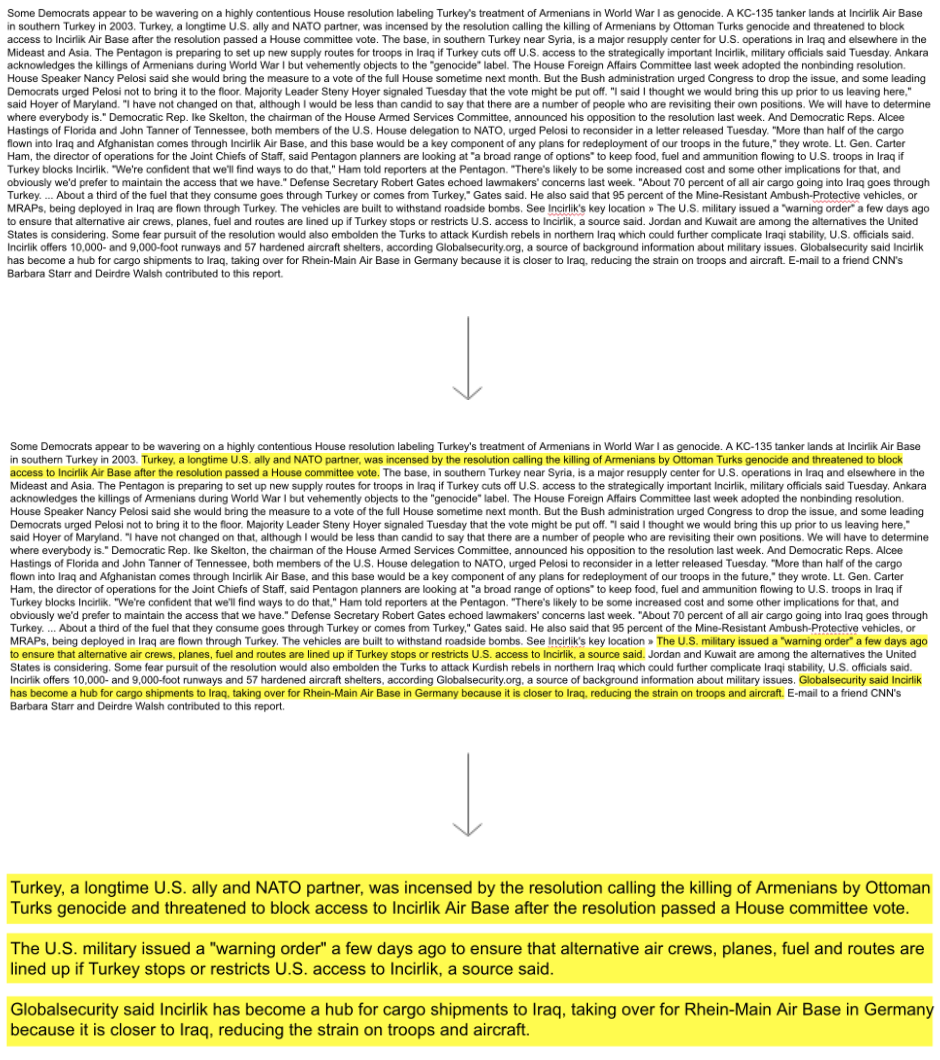
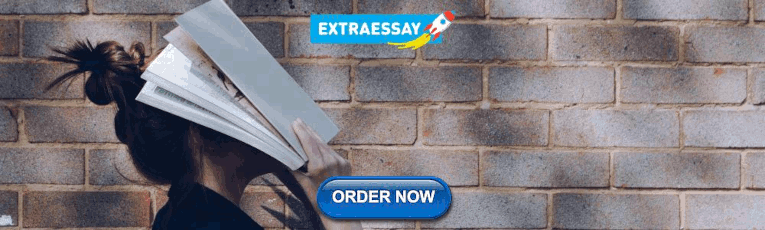
Knowledge Base Population
Natural language transduction, conversational response selection, cross-lingual word embeddings, text annotation, passage ranking, image-to-text retrieval, news classification, key information extraction, biomedical information retrieval.

SpO2 estimation
Graph-to-sequence, authorship verification.

Sentence Summarization
Unsupervised sentence summarization, automated essay scoring, keyword extraction, story generation, temporal processing, timex normalization, document dating, meme classification, hateful meme classification, multimodal association, multimodal generation, morphological tagging, nlg evaluation, key point matching, component classification, argument pair extraction (ape), claim extraction with stance classification (cesc), claim-evidence pair extraction (cepe), weakly supervised classification, weakly supervised data denoising, entity extraction using gan.

Rumour Detection
Semantic composition.

Sentence Ordering
Comment generation.

Lexical Simplification
Token classification, toxic spans detection.

Blackout Poetry Generation
Semantic retrieval, subjectivity analysis.

Taxonomy Learning
Taxonomy expansion, hypernym discovery, conversational response generation.

Personalized and Emotional Conversation
Passage re-ranking, review generation, sentence-pair classification, emotional intelligence, dark humor detection, lexical normalization, pronunciation dictionary creation, negation detection, negation scope resolution, question similarity, medical question pair similarity computation, goal-oriented dialog, user simulation, intent discovery, propaganda detection, propaganda span identification, propaganda technique identification, lexical analysis, lexical complexity prediction, question rewriting, punctuation restoration, reverse dictionary, humor detection.

Meeting Summarization
Table-based fact verification, legal reasoning, pretrained multilingual language models, formality style transfer, semi-supervised formality style transfer, word attribute transfer, attribute value extraction, diachronic word embeddings, persian sentiment analysis, clinical concept extraction.

Clinical Information Retreival
Constrained clustering.

Only Connect Walls Dataset Task 1 (Grouping)
Incremental constrained clustering, aspect category detection, dialog act classification, extreme summarization.

Hallucination Evaluation
Long-context understanding, recognizing emotion cause in conversations.

Causal Emotion Entailment

Nested Mention Recognition
Relationship extraction (distant supervised), binary classification, llm-generated text detection, cancer-no cancer per breast classification, cancer-no cancer per image classification, suspicous (birads 4,5)-no suspicous (birads 1,2,3) per image classification, cancer-no cancer per view classification, clickbait detection, decipherment, semantic entity labeling, text compression, handwriting verification, bangla spelling error correction, ccg supertagging, gender bias detection, linguistic steganography, probing language models, toponym resolution.

Timeline Summarization
Multimodal abstractive text summarization, reader-aware summarization, code repair, thai word segmentation, stock prediction, text-based stock prediction, event-driven trading, pair trading.

Face to Face Translation
Multimodal lexical translation, explanatory visual question answering, vietnamese visual question answering, aggression identification, arabic text diacritization, commonsense causal reasoning, fact selection, suggestion mining, temporal relation classification, vietnamese datasets, vietnamese word segmentation, arabic sentiment analysis, aspect category polarity, complex word identification, cross-lingual bitext mining, morphological disambiguation, scientific document summarization, lay summarization, text attribute transfer.

Image-guided Story Ending Generation
Speculation detection, speculation scope resolution, abstract argumentation, dialogue rewriting, logical reasoning reading comprehension.

Unsupervised Sentence Compression
Sign language production, stereotypical bias analysis, temporal tagging, anaphora resolution, bridging anaphora resolution.

Abstract Anaphora Resolution
Hope speech detection for english, hope speech detection for malayalam, hope speech detection for tamil, hidden aspect detection, latent aspect detection, chinese spell checking, cognate prediction, japanese word segmentation, memex question answering, multi-agent integration, polyphone disambiguation, spelling correction, table-to-text generation.

KB-to-Language Generation
Text anonymization, zero-shot sentiment classification, conditional text generation, contextualized literature-based discovery, multimedia generative script learning, image-sentence alignment, open-world social event classification, personality generation, personality alignment, action parsing, author attribution, binary condescension detection, conversational web navigation, croatian text diacritization, czech text diacritization, definition modelling, document-level re with incomplete labeling, domain labelling, french text diacritization, hungarian text diacritization, irish text diacritization, latvian text diacritization, misogynistic aggression identification, morpheme segmentaiton, multi-label condescension detection, news annotation, open relation modeling, personality recognition in conversation.

Reading Order Detection
Record linking, role-filler entity extraction, romanian text diacritization, slovak text diacritization, spanish text diacritization, syntax representation, text-to-video search, turkish text diacritization, turning point identification, twitter event detection.

Vietnamese Language Models
Vietnamese text diacritization, zero-shot machine translation.

Conversational Sentiment Quadruple Extraction
Attribute extraction, legal outcome extraction, automated writing evaluation, chemical indexing, clinical assertion status detection.

Coding Problem Tagging
Collaborative plan acquisition, commonsense reasoning for rl, context query reformulation.

Variable Disambiguation
Cross-lingual text-to-image generation, crowdsourced text aggregation.

Description-guided molecule generation

Multi-modal Dialogue Generation
Page stream segmentation.

Email Thread Summarization
Emergent communications on relations, emotion detection and trigger summarization, extractive tags summarization.

Hate Intensity Prediction
Hate span identification, job prediction, joint entity and relation extraction on scientific data, joint ner and classification, literature mining, math information retrieval, meme captioning, multi-grained named entity recognition, multilingual machine comprehension in english hindi, multimodal text prediction, negation and speculation cue detection, negation and speculation scope resolution, only connect walls dataset task 2 (connections), overlapping mention recognition, paraphrase generation, multilingual paraphrase generation, phrase ranking, phrase tagging, phrase vector embedding, poem meters classification, query wellformedness.

Question-Answer categorization
Readability optimization, reliable intelligence identification, sentence completion, hurtful sentence completion, speaker attribution in german parliamentary debates (germeval 2023, subtask 1), text effects transfer, text-variation, vietnamese aspect-based sentiment analysis, sentiment dependency learning, vietnamese natural language understanding, web page tagging, workflow discovery, incongruity detection, multi-word expression embedding, multi-word expression sememe prediction, trustable and focussed llm generated content, pcl detection, semeval-2022 task 4-1 (binary pcl detection), semeval-2022 task 4-2 (multi-label pcl detection), automatic writing, complaint comment classification, counterspeech detection, extractive text summarization, face selection, job classification, multi-lingual text-to-image generation, multlingual neural machine translation, optical charater recogntion, bangla text detection, question to declarative sentence, relation mention extraction.

Tweet-Reply Sentiment Analysis
Vietnamese parsing.
- Data Science
- Quantum Computing
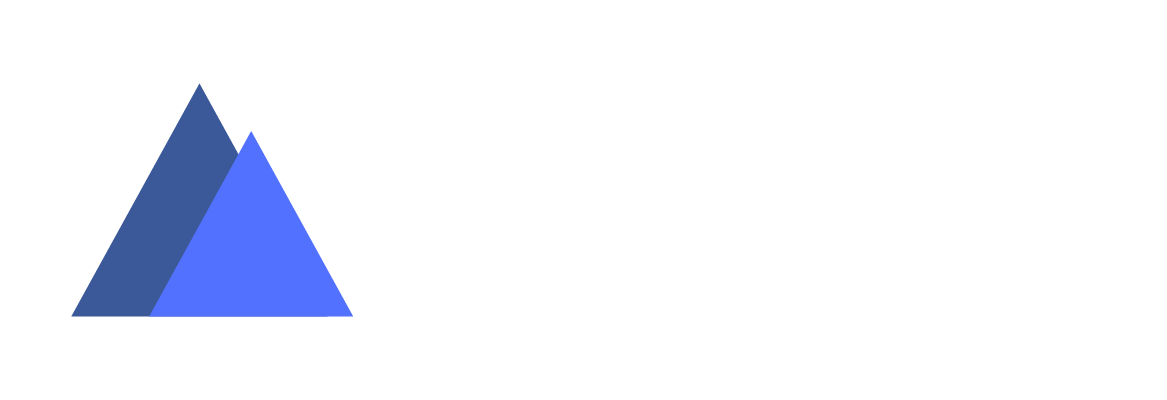
- Miscellaneous
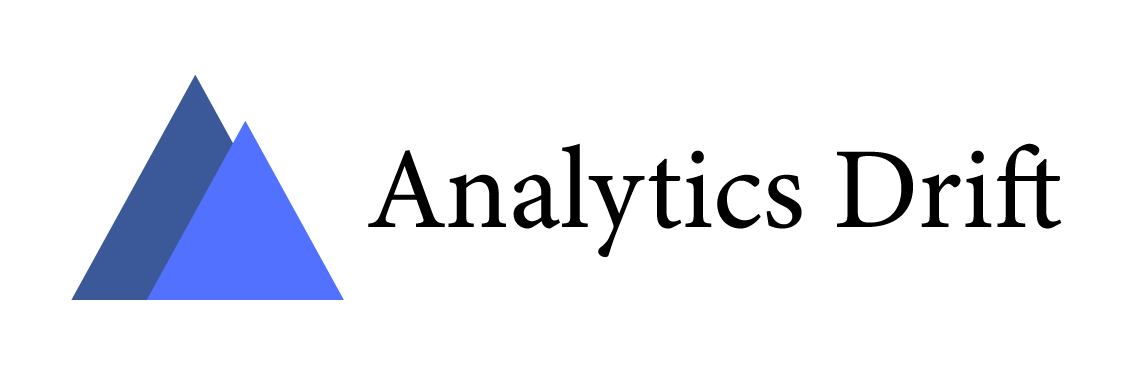
A Comprehensive Guide on RTMP Streaming
Blockchain booms, risks loom: the ai rescue mission in smart contract auditing, developing incident response plans for insider threats, weis wave: revolutionizing market analysis, top machine learning (ml) research papers released in 2022.
For every Machine Learning (ML) enthusiast, we bring you a curated list of the major breakthroughs in ML research in 2022.

Machine learning (ML) is gaining much traction in recent years owing to the disruption and development it brings in enhancing existing technologies. Every month, hundreds of ML papers from various organizations and universities get uploaded on the internet to share the latest breakthroughs in this domain. As the year ends, we bring you the Top 22 ML research papers of 2022 that created a huge impact in the industry. The following list does not reflect the ranking of the papers, and they have been selected on the basis of the recognitions and awards received at international conferences in machine learning.
- Bootstrapped Meta-Learning
Meta-learning is a promising field that investigates ways to enable machine learners or RL agents (which include hyperparameters) to learn how to learn in a quicker and more robust manner, and it is a crucial study area for enhancing the efficiency of AI agents.
This 2022 ML paper presents an algorithm that teaches the meta-learner how to overcome the meta-optimization challenge and myopic meta goals. The algorithm’s primary objective is meta-learning using gradients, which ensures improved performance. The research paper also examines the potential benefits due to bootstrapping. The authors highlight several interesting theoretical aspects of this algorithm, and the empirical results achieve new state-of-the-art (SOTA) on the ATARI ALE benchmark as well as increased efficiency in multitask learning.
- Competition-level code generation with AlphaCode
One of the exciting uses for deep learning and large language models is programming. The rising need for coders has sparked the race to build tools that can increase developer productivity and provide non-developers with tools to create software. However, these models still perform badly when put to the test on more challenging, unforeseen issues that need more than just converting instructions into code.
The popular ML paper of 2022 introduces AlphaCode, a code generation system that, in simulated assessments of programming contests on the Codeforces platform, averaged a rating in the top 54.3%. The paper describes the architecture, training, and testing of the deep-learning model.
- Restoring and attributing ancient texts using deep neural networks
The epigraphic evidence of the ancient Greek era — inscriptions created on durable materials such as stone and pottery — had already been broken when it was discovered, rendering the inscribed writings incomprehensible. Machine learning can help in restoring, and identifying chronological and geographical origins of damaged inscriptions to help us better understand our past.
This ML paper proposed a machine learning model built by DeepMind, Ithaca, for the textual restoration and geographical and chronological attribution of ancient Greek inscriptions. Ithaca was trained on a database of just under 80,000 inscriptions from the Packard Humanities Institute. It had a 62% accuracy rate compared to historians, who had a 25% accuracy rate on average. But when historians used Ithaca, they quickly achieved a 72% accuracy.
- Tensor Programs V: Tuning Large Neural Networks via Zero-Shot Hyperparameter Transfer
Large neural networks use more resources to train hyperparameters since each time, the network must estimate which hyperparameters to utilize. This groundbreaking ML paper of 2022 suggests a novel zero-shot hyperparameter tuning paradigm for more effectively tuning massive neural networks. The research, co-authored by Microsoft Research and OpenAI, describes a novel method called µTransfer that leverages µP to zero-shot transfer hyperparameters from small models and produces nearly perfect HPs on large models without explicitly tuning them.
This method has been found to reduce the amount of trial and error necessary in the costly process of training large neural networks. By drastically lowering the need to predict which training hyperparameters to use, this approach speeds up research on massive neural networks like GPT-3 and perhaps its successors in the future.
- PaLM: Scaling Language Modeling with Pathways
Large neural networks trained for language synthesis and recognition have demonstrated outstanding results in various tasks in recent years. This trending 2022 ML paper introduced Pathways Language Model (PaLM), a 780 billion high-quality text token, and 540 billion parameter-dense decoder-only autoregressive transformer.
Although PaLM just uses a decoder and makes changes like SwiGLU Activation, Parallel Layers, Multi-Query Attention, RoPE Embeddings, Shared Input-Output Embeddings, and No Biases and Vocabulary, it is based on a typical transformer model architecture. The paper describes the company’s latest flagship surpassing several human baselines while achieving state-of-the-art in numerous zero, one, and few-shot NLP tasks.
- Robust Speech Recognition via Large-Scale Weak Supervision
Machine learning developers have found it challenging to build speech-processing algorithms that are trained to predict a vast volume of audio transcripts on the internet. This year, OpenAI released Whisper , a new state-of-the-art (SotA) model in speech-to-text that can transcribe any audio to text and translate it into several languages. It has received 680,000 hours of training on a vast amount of voice data gathered from the internet. According to OpenAI, this model is robust to accents, background noise, and technical terminology. Additionally, it allows transcription into English from 99 different languages and translation into English from those languages.
The OpenAI ML paper mentions the author ensured that about one-third of the audio data is non-English. This helped the team outperform other supervised state-of-the-art models by maintaining a diversified dataset.
- OPT: Open Pre-trained Transformer Language Models
Large language models have demonstrated extraordinary performance f on numerous tasks (e.g., zero and few-shot learning). However, these models are difficult to duplicate without considerable funding due to their high computing costs. Even while the public can occasionally interact with these models through paid APIs, complete research access is still only available from a select group of well-funded labs. This limited access has hindered researchers’ ability to comprehend how and why these language models work, which has stalled progress on initiatives to improve their robustness and reduce ethical drawbacks like bias and toxicity.
The popular 2022 ML paper introduces Open Pre-trained Transformers (OPT), a suite of decoder-only pre-trained transformers with 125 million to 175 billion parameters that the authors want to share freely and responsibly with interested academics. The biggest OPT model, OPT-175B (it is not included in the code repository but is accessible upon request), which is impressively proven to perform similarly to GPT-3 (which also has 175 billion parameters) uses just 15% of GPT-3’s carbon footprint during development and training.
- A Path Towards Autonomous Machine Intelligence
Yann LeCun is a prominent and respectable researcher in the field of artificial intelligence and machine learning. In June, his much-anticipated paper “ A Path Towards Autonomous Machine Intelligence ” was published on OpenReview. LeCun offered a number of approaches and architectures in his paper that might be combined and used to create self-supervised autonomous machines.
He presented a modular architecture for autonomous machine intelligence that combines various models to operate as distinct elements of a machine’s brain and mirror the animal brain. Due to the differentiability of all the models, they are all interconnected to power certain brain-like activities, such as identification and environmental response. It incorporates ideas like a configurable predictive world model, behavior-driven through intrinsic motivation, and hierarchical joint embedding architectures trained with self-supervised learning.
- LaMDA: Language Models for Dialog Applications
Despite tremendous advances in text generation, many of the chatbots available are still rather irritating and unhelpful. This 2022 ML paper from Google describes the LaMDA — short for “Language Model for Dialogue Applications” — system, which caused the uproar this summer when a former Google engineer, Blake Lemoine, alleged that it is sentient. LaMDA is a family of large language models for dialog applications built on Google’s Transformer architecture, which is known for its efficiency and speed in language tasks such as translation. The model’s ability to be adjusted using data that has been human-annotated and the capability of consulting external sources are its most intriguing features.
The model, which has 137 billion parameters, was pre-trained using 1.56 trillon words from publicly accessible conversation data and online publications. The model is also adjusted based on the three parameters of quality, safety, and groundedness.
- Privacy for Free: How does Dataset Condensation Help Privacy?
One of the primary proposals in the award-winning ML paper is to use dataset condensation methods to retain data efficiency during model training while also providing membership privacy. The authors argue that dataset condensation, which was initially created to increase training effectiveness, is a better alternative to data generators for producing private data since it offers privacy for free.
Though existing data generators are used to produce differentially private data for model training to minimize unintended data leakage, they result in high training costs or subpar generalization performance for the sake of data privacy. This study was published by Sony AI and received the Outstanding Paper Award at ICML 2022.
- TranAD: Deep Transformer Networks for Anomaly Detection in Multivariate Time Series Data
The use of a model that converts time series into anomaly scores at each time step is essential in any system for detecting time series anomalies. Recognizing and diagnosing anomalies in multivariate time series data is critical for modern industrial applications. Unfortunately, developing a system capable of promptly and reliably identifying abnormal observations is challenging. This is attributed to a shortage of anomaly labels, excessive data volatility, and the expectations of modern applications for ultra-low inference times.
In this study , the authors present TranAD, a deep transformer network-based anomaly detection and diagnosis model that leverages attention-based sequence encoders to quickly execute inference while being aware of the more general temporal patterns in the data. TranAD employs adversarial training to achieve stability and focus score-based self-conditioning to enable robust multi-modal feature extraction. The paper mentions extensive empirical experiments on six publicly accessible datasets show that TranAD can perform better in detection and diagnosis than state-of-the-art baseline methods with data- and time-efficient training.
- Photorealistic Text-to-Image Diffusion Models with Deep Language Understanding
In the last few years, generative models called “diffusion models” have been increasingly popular. This year saw these models capture the excitement of AI enthusiasts around the world.
Going ahead of the current text to speech technology of recent times, this outstanding 2022 ML paper introduced the viral text-to-image diffusion model from Google, Imagen. This diffusion model achieves a new state-of-the-art FID score of 7.27 on the COCO dataset by combining the deep language understanding of transformer-based large language models with the photorealistic image-generating capabilities of diffusion models. A text-only frozen language model provides the text representation, and a diffusion model with two super-resolution upsampling stages, up to 1024×2014, produces the images. It employs several training approaches, including classifier-free guiding, to teach itself conditional and unconditional generation. Another important feature of Imagen is the use of dynamic thresholding, which stops the diffusion process from being saturated in specific areas of the picture, a behavior that reduces image quality, particularly when the weight placed on text conditional creation is large.
- No Language Left Behind: Scaling Human-Centered Machine Translation
This ML paper introduced the most popular Meta projects of the year 2022: NLLB-200. This paper talks about how Meta built and open-sourced this state-of-the-art AI model at FAIR, which is capable of translating 200 languages between each other. It covers every aspect of this technology: language analysis, moral issues, effect analysis, and benchmarking.
No matter what language a person speaks, accessibility via language ensures that everyone can benefit from the growth of technology. Meta claims that several languages that NLLB-200 translates, such as Kamba and Lao, are not currently supported by any translation systems in use. The tech behemoth also created a dataset called “FLORES-200” to evaluate the effectiveness of the NLLB-200 and show that accurate translations are offered. According to Meta, NLLB-200 offers an average of 44% higher-quality translations than its prior model.
- A Generalist Agent
AI pundits believe that multimodality will play a huge role in the future of Artificial General Intelligence (AGI). One of the most talked ML papers of 2022 by DeepMind introduces Gato – a generalist agent . This AGI agent is a multi-modal, multi-task, multi-embodiment network, which means that the same neural network (i.e. a single architecture with a single set of weights) can do all tasks while integrating inherently diverse types of inputs and outputs.
DeepMind claims that the general agent can be improved with new data to perform even better on a wider range of tasks. They argue that having a general-purpose agent reduces the need for hand-crafting policy models for each region, enhances the volume and diversity of training data, and enables continuous advances in the data, computing, and model scales. A general-purpose agent can also be viewed as the first step toward artificial general intelligence, which is the ultimate goal of AGI.
Gato demonstrates the versatility of transformer-based machine learning architectures by exhibiting their use in a variety of applications. Unlike previous neural network systems tailored for playing games, stack blocks with a real robot arm, read words, and caption images, Gato is versatile enough to perform all of these tasks on its own, using only a single set of weights and a relatively simple architecture.
- The Forward-Forward Algorithm: Some Preliminary Investigations
AI pioneer Geoffrey Hinton is known for writing paper on the first deep convolutional neural network and backpropagation. In his latest paper presented at NeurIPS 2022, Hinton proposed the “forward-forward algorithm,” a new learning algorithm for artificial neural networks based on our understanding of neural activations in the brain. This approach draws inspiration from Boltzmann machines (Hinton and Sejnowski, 1986) and noise contrast estimation (Gutmann and Hyvärinen, 2010). According to Hinton, forward-forward, which is still in its experimental stages, can substitute the forward and backward passes of backpropagation with two forward passes, one with positive data and the other with negative data that the network itself could generate. Further, the algorithm could simulate hardware more efficiently and provide a better explanation for the brain’s cortical learning process.
Without employing complicated regularizers, the algorithm obtained a 1.4 percent test error rate on the MNIST dataset in an empirical study, proving that it is just as effective as backpropagation.
The paper also suggests a novel “mortal computing” model that can enable the forward-forward algorithm and understand our brain’s energy-efficient processes.
- Focal Modulation Networks
In humans, the ciliary muscles alter the shape of the eye and hence the radius of the curvature lens to focus on near or distant objects. Changing the shape of the eye lens, changes the focal length of the lens. Mimicking this behavior of focal modulation in computer vision systems can be tricky.
This machine learning paper introduces FocalNet, an iterative information extraction technique that employs the premise of foveal attention to post-process Deep Neural Network (DNN) outputs by performing variable input/feature space sampling. Its attention-free design outperforms SoTA self-attention (SA) techniques in a wide range of visual benchmarks. According to the paper, focal modulation consists of three parts: According to the paper, focal modulation consists of three parts:
a. hierarchical contextualization, implemented using a stack of depth-wise convolutional layers, to encode visual contexts from close-up to a great distance;
b. gated aggregation to selectively gather contexts for each query token based on its content; and
c. element-wise modulation or affine modification to inject the gathered context into the query.
- Learning inverse folding from millions of predicted structures
The field of structural biology is being fundamentally changed by cutting-edge technologies in machine learning, protein structure prediction, and innovative ultrafast structural aligners. Time and money are no longer obstacles to obtaining precise protein models and extensively annotating their functionalities. However, determining a protein sequence from its backbone atom coordinates remained a challenge for scientists. To date, machine learning methods to this challenge have been constrained by the amount of empirically determined protein structures available.
In this ICML Outstanding Paper (Runner Up) , authors explain tackling this problem by increasing training data by almost three orders of magnitude by using AlphaFold2 to predict structures for 12 million protein sequences. With the use of this additional data, a sequence-to-sequence transformer with invariant geometric input processing layers is able to recover native sequence on structurally held-out backbones in 51% of cases while recovering buried residues in 72% of cases. This is an improvement of over 10% over previous techniques. In addition to designing protein complexes, partly masked structures, binding interfaces, and numerous states, the concept generalises to a range of other more difficult tasks.
- MineDojo: Building Open-Ended Embodied Agents with Internet-Scale Knowledge
Within the AI research community, using video games as a training medium for AI has gained popularity. These autonomous agents have had great success in Atari games, Starcraft, Dota, and Go. Although these developments have gained popularity in the field of artificial intelligence research, the agents do not generalize beyond a narrow range of activities, in contrast to humans, who continually learn from open-ended tasks.
This thought-provoking 2022 ML paper suggests MineDojo, a unique framework for embodied agent research based on the well-known game Minecraft. In addition to building an internet-scale information base with Minecraft videos, tutorials, wiki pages, and forum discussions, Minecraft provides a simulation suite with tens of thousands of open-ended activities. Using MineDojo data, the author proposes a unique agent learning methodology that employs massive pre-trained video-language models as a learnt reward function. Without requiring a dense shaping reward that has been explicitly created, MinoDojo autonomous agent can perform a wide range of open-ended tasks that are stated in free-form language.
- Is Out-of-Distribution Detection Learnable?
Machine learning (supervised ML) models are frequently trained using the closed-world assumption, which assumes that the distribution of the testing data will resemble that of the training data. This assumption doesn’t hold true when used in real-world activities, which causes a considerable decline in their performance. While this performance loss is acceptable for applications like product recommendations, developing an out-of-distribution (OOD) identification algorithm is crucial to preventing ML systems from making inaccurate predictions in situations where data distribution in real-world activities typically drifts over time (self-driving cars).
In this paper , authors explore the probably approximately correct (PAC) learning theory of OOD detection, which is proposed by researchers as an open problem, to study the applicability of OOD detection. They first focus on identifying a prerequisite for OOD detection’s learnability. Following that, they attempt to show a number of impossibility theorems regarding the learnability of OOD detection in a handful yet different scenarios.
- Gradient Descent: The Ultimate Optimizer
Gradient descent is a popular optimization approach for training machine learning models and neural networks. The ultimate aim of any machine learning (neural network) method is to optimize parameters, but selecting the ideal step size for an optimizer is difficult since it entails lengthy and error-prone manual work. Many strategies exist for automated hyperparameter optimization; however, they often incorporate additional hyperparameters to govern the hyperparameter optimization process. In this study , MIT CSAIL and Meta researchers offer a unique approach that allows gradient descent optimizers like SGD and Adam to tweak their hyperparameters automatically.
They propose learning the hyperparameters by self-using gradient descent, as well as learning the hyper-hyperparameters via gradient descent, and so on indefinitely. This paper describes an efficient approach for allowing gradient descent optimizers to autonomously adjust their own hyperparameters, which may be layered recursively to many levels. As these gradient-based optimizer towers expand in size, they become substantially less sensitive to the selection of top-level hyperparameters, reducing the load on the user to search for optimal values.
- ProcTHOR: Large-Scale Embodied AI Using Procedural Generation
Embodied AI is a developing study field that has been influenced by recent advancements in artificial intelligence, machine learning, and computer vision. This method of computer learning makes an effort to translate this connection to artificial systems. The paper proposes ProcTHOR, a framework for procedural generation of Embodied AI environments. ProcTHOR allows researchers to sample arbitrarily huge datasets of diverse, interactive, customisable, and performant virtual environments in order to train and assess embodied agents across navigation, interaction, and manipulation tasks.
According to the authors, models trained on ProcTHOR using only RGB images and without any explicit mapping or human task supervision achieve cutting-edge results in 6 embodied AI benchmarks for navigation, rearrangement, and arm manipulation, including the ongoing Habitat2022, AI2-THOR Rearrangement2022, and RoboTHOR challenges. The paper received the Outstanding Paper award at NeurIPS 2022.
- A Commonsense Knowledge Enhanced Network with Retrospective Loss for Emotion Recognition in Spoken Dialog
Emotion Recognition in Spoken Dialog (ERSD) has recently attracted a lot of attention due to the growth of open conversational data. This is due to the fact that excellent speech recognition algorithms have emerged as a result of the integration of emotional states in intelligent spoken human-computer interactions. Additionally, it has been demonstrated that recognizing emotions makes it possible to track the development of human-computer interactions, allowing for dynamic change of conversational strategies and impacting the result (e.g., customer feedback). But the volume of the current ERSD datasets restricts the model’s development.
This ML paper proposes a Commonsense Knowledge Enhanced Network (CKE-Net) with a retrospective loss to carry out dialog modeling, external knowledge integration, and historical state retrospect hierarchically.
Subscribe to our newsletter
Subscribe and never miss out on such trending AI-related articles.
Join our WhatsApp Channel and Discord Server to be a part of an engaging community.
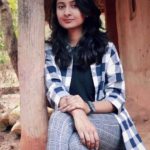
RELATED ARTICLES
Enhancing efficiency: the role of data storage in ai systems, from insight to impact: the power of data expanding your business, the ultimate guide to scrape websites for data using web scraping tools, leave a reply cancel reply.
Save my name, email, and website in this browser for the next time I comment.
Most Popular
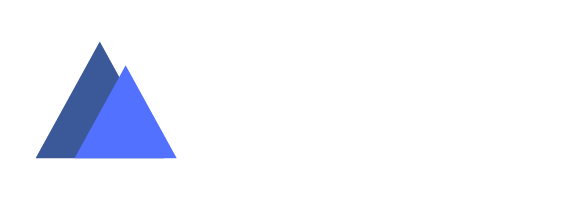
Analytics Drift strives to keep you updated with the latest technologies such as Artificial Intelligence, Data Science, Machine Learning, and Deep Learning. We are on a mission to build the largest data science community in the world by serving you with engaging content on our platform.
Contact us: [email protected]
Copyright © 2024 Analytics Drift Private Limited.
Machine Intelligence
Google is at the forefront of innovation in Machine Intelligence, with active research exploring virtually all aspects of machine learning, including deep learning and more classical algorithms. Exploring theory as well as application, much of our work on language, speech, translation, visual processing, ranking and prediction relies on Machine Intelligence. In all of those tasks and many others, we gather large volumes of direct or indirect evidence of relationships of interest, applying learning algorithms to understand and generalize.
Machine Intelligence at Google raises deep scientific and engineering challenges, allowing us to contribute to the broader academic research community through technical talks and publications in major conferences and journals. Contrary to much of current theory and practice, the statistics of the data we observe shifts rapidly, the features of interest change as well, and the volume of data often requires enormous computation capacity. When learning systems are placed at the core of interactive services in a fast changing and sometimes adversarial environment, combinations of techniques including deep learning and statistical models need to be combined with ideas from control and game theory.
Recent Publications
Some of our teams.
Algorithms & optimization
Applied science
Climate and sustainability
Graph mining
Learning theory
Market algorithms
Operations research
Security, privacy and abuse
System performance
We're always looking for more talented, passionate people.
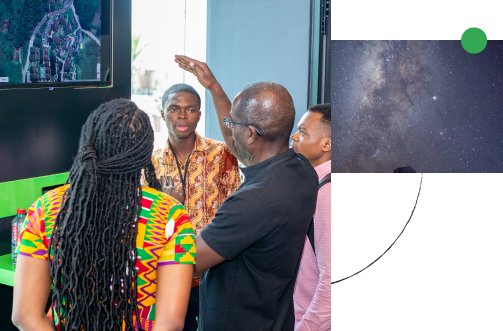
Advertisement
Artificial intelligence and machine learning research: towards digital transformation at a global scale
- Published: 17 April 2021
- Volume 13 , pages 3319–3321, ( 2022 )
Cite this article
- Akila Sarirete 1 ,
- Zain Balfagih 1 ,
- Tayeb Brahimi 1 ,
- Miltiadis D. Lytras 1 , 2 &
- Anna Visvizi 3 , 4
8107 Accesses
12 Citations
Explore all metrics
Avoid common mistakes on your manuscript.
Artificial intelligence (AI) is reshaping how we live, learn, and work. Until recently, AI used to be a fanciful concept, more closely associated with science fiction rather than with anything else. However, driven by unprecedented advances in sophisticated information and communication technology (ICT), AI today is synonymous technological progress already attained and the one yet to come in all spheres of our lives (Chui et al. 2018 ; Lytras et al. 2018 , 2019 ).
Considering that Machine Learning (ML) and AI are apt to reach unforeseen levels of accuracy and efficiency, this special issue sought to promote research on AI and ML seen as functions of data-driven innovation and digital transformation. The combination of expanding ICT-driven capabilities and capacities identifiable across our socio-economic systems along with growing consumer expectations vis-a-vis technology and its value-added for our societies, requires multidisciplinary research and research agenda on AI and ML (Lytras et al. 2021 ; Visvizi et al. 2020 ; Chui et al. 2020 ). Such a research agenda should oscilate around the following five defining issues (Fig. 1 ):
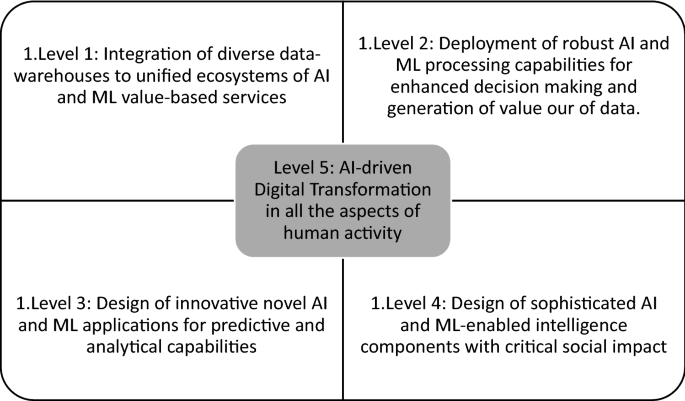
Source: The Authors
An AI-Driven Digital Transformation in all aspects of human activity/
Integration of diverse data-warehouses to unified ecosystems of AI and ML value-based services
Deployment of robust AI and ML processing capabilities for enhanced decision making and generation of value our of data.
Design of innovative novel AI and ML applications for predictive and analytical capabilities
Design of sophisticated AI and ML-enabled intelligence components with critical social impact
Promotion of the Digital Transformation in all the aspects of human activity including business, healthcare, government, commerce, social intelligence etc.
Such development will also have a critical impact on government, policies, regulations and initiatives aiming to interpret the value of the AI-driven digital transformation to the sustainable economic development of our planet. Additionally the disruptive character of AI and ML technology and research will required further research on business models and management of innovation capabilities.
This special issue is based on submissions invited from the 17th Annual Learning and Technology Conference 2019 that was held at Effat University and open call jointly. Several very good submissions were received. All of them were subjected a rigorous peer review process specific to the Ambient Intelligence and Humanized Computing Journal.
A variety of innovative topics are included in the agenda of the published papers in this special issue including topics such as:
Stock market Prediction using Machine learning
Detection of Apple Diseases and Pests based on Multi-Model LSTM-based Convolutional Neural Networks
ML for Searching
Machine Learning for Learning Automata
Entity recognition & Relation Extraction
Intelligent Surveillance Systems
Activity Recognition and K-Means Clustering
Distributed Mobility Management
Review Rating Prediction with Deep Learning
Cybersecurity: Botnet detection with Deep learning
Self-Training methods
Neuro-Fuzzy Inference systems
Fuzzy Controllers
Monarch Butterfly Optimized Control with Robustness Analysis
GMM methods for speaker age and gender classification
Regression methods for Permeability Prediction of Petroleum Reservoirs
Surface EMG Signal Classification
Pattern Mining
Human Activity Recognition in Smart Environments
Teaching–Learning based Optimization Algorithm
Big Data Analytics
Diagnosis based on Event-Driven Processing and Machine Learning for Mobile Healthcare
Over a decade ago, Effat University envisioned a timely platform that brings together educators, researchers and tech enthusiasts under one roof and functions as a fount for creativity and innovation. It was a dream that such platform bridges the existing gap and becomes a leading hub for innovators across disciplines to share their knowledge and exchange novel ideas. It was in 2003 that this dream was realized and the first Learning & Technology Conference was held. Up until today, the conference has covered a variety of cutting-edge themes such as Digital Literacy, Cyber Citizenship, Edutainment, Massive Open Online Courses, and many, many others. The conference has also attracted key, prominent figures in the fields of sciences and technology such as Farouq El Baz from NASA, Queen Rania Al-Abdullah of Jordan, and many others who addressed large, eager-to-learn audiences and inspired many with unique stories.
While emerging innovations, such as Artificial Intelligence technologies, are seen today as promising instruments that could pave our way to the future, these were also the focal points around which fruitful discussions have always taken place here at the L&T. The (AI) was selected for this conference due to its great impact. The Saudi government realized this impact of AI and already started actual steps to invest in AI. It is stated in the Kingdome Vision 2030: "In technology, we will increase our investments in, and lead, the digital economy." Dr. Ahmed Al Theneyan, Deputy Minister of Technology, Industry and Digital Capabilities, stated that: "The Government has invested around USD 3 billion in building the infrastructure so that the country is AI-ready and can become a leader in AI use." Vision 2030 programs also promote innovation in technologies. Another great step that our country made is establishing NEOM city (the model smart city).
Effat University realized this ambition and started working to make it a reality by offering academic programs that support the different sectors needed in such projects. For example, the master program in Energy Engineering was launched four years ago to support the energy sector. Also, the bachelor program of Computer Science has tracks in Artificial Intelligence and Cyber Security which was launched in Fall 2020 semester. Additionally, Energy & Technology and Smart Building Research Centers were established to support innovation in the technology and energy sectors. In general, Effat University works effectively in supporting the KSA to achieve its vision in this time of national transformation by graduating skilled citizen in different fields of technology.
The guest editors would like to take this opportunity to thank all the authors for the efforts they put in the preparation of their manuscripts and for their valuable contributions. We wish to express our deepest gratitude to the referees, who provided instrumental and constructive feedback to the authors. We also extend our sincere thanks and appreciation for the organizing team under the leadership of the Chair of L&T 2019 Conference Steering Committee, Dr. Haifa Jamal Al-Lail, University President, for her support and dedication.
Our sincere thanks go to the Editor-in-Chief for his kind help and support.
Chui KT, Lytras MD, Visvizi A (2018) Energy sustainability in smart cities: artificial intelligence, smart monitoring, and optimization of energy consumption. Energies 11(11):2869
Article Google Scholar
Chui KT, Fung DCL, Lytras MD, Lam TM (2020) Predicting at-risk university students in a virtual learning environment via a machine learning algorithm. Comput Human Behav 107:105584
Lytras MD, Visvizi A, Daniela L, Sarirete A, De Pablos PO (2018) Social networks research for sustainable smart education. Sustainability 10(9):2974
Lytras MD, Visvizi A, Sarirete A (2019) Clustering smart city services: perceptions, expectations, responses. Sustainability 11(6):1669
Lytras MD, Visvizi A, Chopdar PK, Sarirete A, Alhalabi W (2021) Information management in smart cities: turning end users’ views into multi-item scale development, validation, and policy-making recommendations. Int J Inf Manag 56:102146
Visvizi A, Jussila J, Lytras MD, Ijäs M (2020) Tweeting and mining OECD-related microcontent in the post-truth era: A cloud-based app. Comput Human Behav 107:105958
Download references
Author information
Authors and affiliations.
Effat College of Engineering, Effat Energy and Technology Research Center, Effat University, P.O. Box 34689, Jeddah, Saudi Arabia
Akila Sarirete, Zain Balfagih, Tayeb Brahimi & Miltiadis D. Lytras
King Abdulaziz University, Jeddah, 21589, Saudi Arabia
Miltiadis D. Lytras
Effat College of Business, Effat University, P.O. Box 34689, Jeddah, Saudi Arabia
Anna Visvizi
Institute of International Studies (ISM), SGH Warsaw School of Economics, Aleja Niepodległości 162, 02-554, Warsaw, Poland
You can also search for this author in PubMed Google Scholar
Corresponding author
Correspondence to Akila Sarirete .
Additional information
Publisher's note.
Springer Nature remains neutral with regard to jurisdictional claims in published maps and institutional affiliations.
Rights and permissions
Reprints and permissions
About this article
Sarirete, A., Balfagih, Z., Brahimi, T. et al. Artificial intelligence and machine learning research: towards digital transformation at a global scale. J Ambient Intell Human Comput 13 , 3319–3321 (2022). https://doi.org/10.1007/s12652-021-03168-y
Download citation
Published : 17 April 2021
Issue Date : July 2022
DOI : https://doi.org/10.1007/s12652-021-03168-y
Share this article
Anyone you share the following link with will be able to read this content:
Sorry, a shareable link is not currently available for this article.
Provided by the Springer Nature SharedIt content-sharing initiative
- Find a journal
- Publish with us
- Track your research
machine learning Recently Published Documents
Total documents.
- Latest Documents
- Most Cited Documents
- Contributed Authors
- Related Sources
- Related Keywords
An explainable machine learning model for identifying geographical origins of sea cucumber Apostichopus japonicus based on multi-element profile
A comparison of machine learning- and regression-based models for predicting ductility ratio of rc beam-column joints, alexa, is this a historical record.
Digital transformation in government has brought an increase in the scale, variety, and complexity of records and greater levels of disorganised data. Current practices for selecting records for transfer to The National Archives (TNA) were developed to deal with paper records and are struggling to deal with this shift. This article examines the background to the problem and outlines a project that TNA undertook to research the feasibility of using commercially available artificial intelligence tools to aid selection. The project AI for Selection evaluated a range of commercial solutions varying from off-the-shelf products to cloud-hosted machine learning platforms, as well as a benchmarking tool developed in-house. Suitability of tools depended on several factors, including requirements and skills of transferring bodies as well as the tools’ usability and configurability. This article also explores questions around trust and explainability of decisions made when using AI for sensitive tasks such as selection.
Automated Text Classification of Maintenance Data of Higher Education Buildings Using Text Mining and Machine Learning Techniques
Data-driven analysis and machine learning for energy prediction in distributed photovoltaic generation plants: a case study in queensland, australia, modeling nutrient removal by membrane bioreactor at a sewage treatment plant using machine learning models, big five personality prediction based in indonesian tweets using machine learning methods.
<span lang="EN-US">The popularity of social media has drawn the attention of researchers who have conducted cross-disciplinary studies examining the relationship between personality traits and behavior on social media. Most current work focuses on personality prediction analysis of English texts, but Indonesian has received scant attention. Therefore, this research aims to predict user’s personalities based on Indonesian text from social media using machine learning techniques. This paper evaluates several machine learning techniques, including <a name="_Hlk87278444"></a>naive Bayes (NB), K-nearest neighbors (KNN), and support vector machine (SVM), based on semantic features including emotion, sentiment, and publicly available Twitter profile. We predict the personality based on the big five personality model, the most appropriate model for predicting user personality in social media. We examine the relationships between the semantic features and the Big Five personality dimensions. The experimental results indicate that the Big Five personality exhibit distinct emotional, sentimental, and social characteristics and that SVM outperformed NB and KNN for Indonesian. In addition, we observe several terms in Indonesian that specifically refer to each personality type, each of which has distinct emotional, sentimental, and social features.</span>
Compressive strength of concrete with recycled aggregate; a machine learning-based evaluation
Temperature prediction of flat steel box girders of long-span bridges utilizing in situ environmental parameters and machine learning, computer-assisted cohort identification in practice.
The standard approach to expert-in-the-loop machine learning is active learning, where, repeatedly, an expert is asked to annotate one or more records and the machine finds a classifier that respects all annotations made until that point. We propose an alternative approach, IQRef , in which the expert iteratively designs a classifier and the machine helps him or her to determine how well it is performing and, importantly, when to stop, by reporting statistics on a fixed, hold-out sample of annotated records. We justify our approach based on prior work giving a theoretical model of how to re-use hold-out data. We compare the two approaches in the context of identifying a cohort of EHRs and examine their strengths and weaknesses through a case study arising from an optometric research problem. We conclude that both approaches are complementary, and we recommend that they both be employed in conjunction to address the problem of cohort identification in health research.
Export Citation Format
Share document.
How to Read Research Papers: A Pragmatic Approach for ML Practitioners
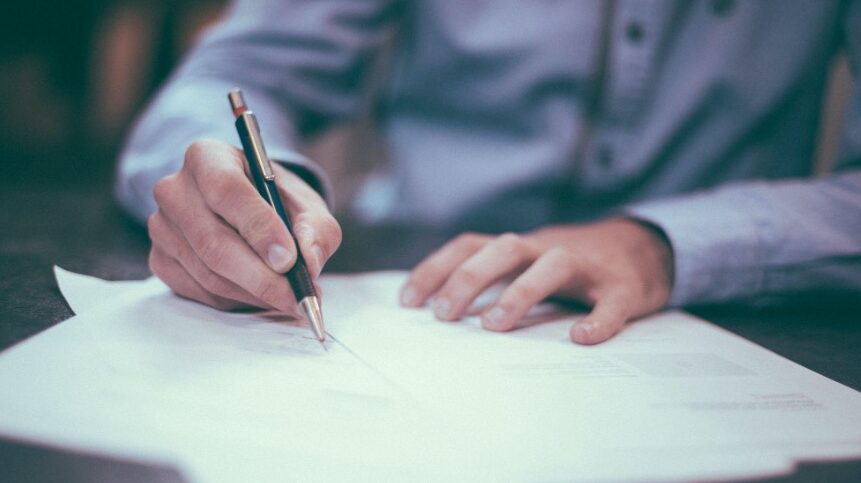
Is it necessary for data scientists or machine-learning experts to read research papers?
The short answer is yes. And don’t worry if you lack a formal academic background or have only obtained an undergraduate degree in the field of machine learning.
Reading academic research papers may be intimidating for individuals without an extensive educational background. However, a lack of academic reading experience should not prevent Data scientists from taking advantage of a valuable source of information and knowledge for machine learning and AI development .
This article provides a hands-on tutorial for data scientists of any skill level to read research papers published in academic journals such as NeurIPS , JMLR , ICML, and so on.
Before diving wholeheartedly into how to read research papers, the first phases of learning how to read research papers cover selecting relevant topics and research papers.
Step 1: Identify a topic
The domain of machine learning and data science is home to a plethora of subject areas that may be studied. But this does not necessarily imply that tackling each topic within machine learning is the best option.
Although generalization for entry-level practitioners is advised, I’m guessing that when it comes to long-term machine learning, career prospects, practitioners, and industry interest often shifts to specialization.
Identifying a niche topic to work on may be difficult, but good. Still, a rule of thumb is to select an ML field in which you are either interested in obtaining a professional position or already have experience.
Deep Learning is one of my interests, and I’m a Computer Vision Engineer that uses deep learning models in apps to solve computer vision problems professionally. As a result, I’m interested in topics like pose estimation, action classification, and gesture identification.
Based on roles, the following are examples of ML/DS occupations and related themes to consider.
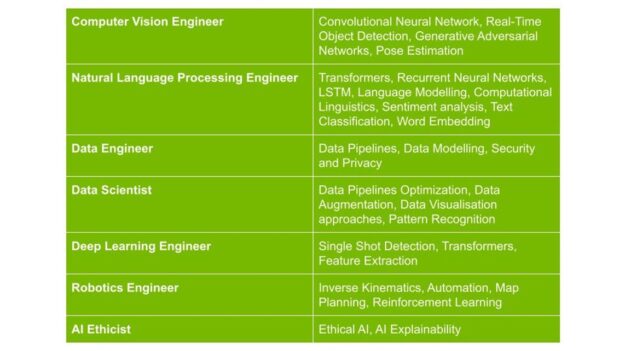
For this article, I’ll select the topic Pose Estimation to explore and choose associated research papers to study.
Step 2: Finding research papers
One of the most excellent tools to use while looking at machine learning-related research papers, datasets, code, and other related materials is PapersWithCode .
We use the search engine on the PapersWithCode website to get relevant research papers and content for our chosen topic, “Pose Estimation.” The following image shows you how it’s done.
The search results page contains a short explanation of the searched topic, followed by a table of associated datasets, models, papers, and code. Without going into too much detail, the area of interest for this use case is the “Greatest papers with code”. This section contains the relevant papers related to the task or topic. For the purpose of this article, I’ll select the DensePose: Dense Human Pose Estimation In The Wild .
Step 3: First pass (gaining context and understanding)
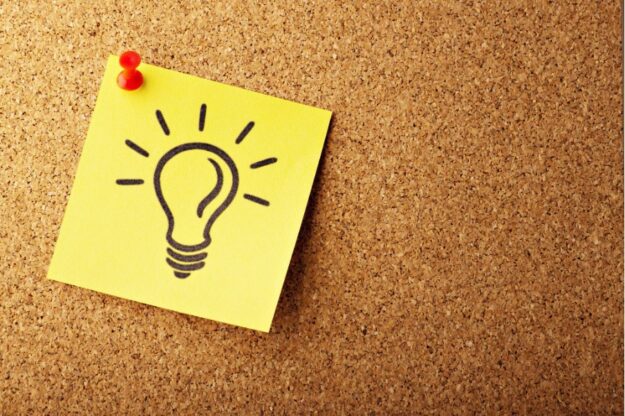
At this point, we’ve selected a research paper to study and are prepared to extract any valuable learnings and findings from its content.
It’s only natural that your first impulse is to start writing notes and reading the document from beginning to end, perhaps taking some rest in between. However, having a context for the content of a study paper is a more practical way to read it. The title, abstract, and conclusion are three key parts of any research paper to gain an understanding.
The goal of the first pass of your chosen paper is to achieve the following:
- Assure that the paper is relevant.
- Obtain a sense of the paper’s context by learning about its contents, methods, and findings.
- Recognize the author’s goals, methodology, and accomplishments.
The title is the first point of information sharing between the authors and the reader. Therefore, research papers titles are direct and composed in a manner that leaves no ambiguity.
The research paper title is the most telling aspect since it indicates the study’s relevance to your work. The importance of the title is to give a brief perception of the paper’s content.
In this situation, the title is “DensePose: Dense Human Pose Estimation in the Wild.” This gives a broad overview of the work and implies that it will look at how to provide pose estimations in environments with high levels of activity and realistic situations properly.
The abstract portion gives a summarized version of the paper. It’s a short section that contains 300-500 words and tells you what the paper is about in a nutshell. The abstract is a brief text that provides an overview of the article’s content, researchers’ objectives, methods, and techniques.
When reading an abstract of a machine-learning research paper, you’ll typically come across mentions of datasets, methods, algorithms, and other terms. Keywords relevant to the article’s content provide context. It may be helpful to take notes and keep track of all keywords at this point.
For the paper: “ DensePose: Dense Human Pose Estimation In The Wild “, I identified in the abstract the following keywords: pose estimation, COCO dataset, CNN, region-based models, real-time.
It’s not uncommon to experience fatigue when reading the paper from top to bottom at your first initial pass, especially for Data Scientists and practitioners with no prior advanced academic experience. Although extracting information from the later sections of a paper might seem tedious after a long study session, the conclusion sections are often short. Hence reading the conclusion section in the first pass is recommended.
The conclusion section is a brief compendium of the work’s author or authors and/or contributions and accomplishments and promises for future developments and limitations.
Before reading the main content of a research paper, read the conclusion section to see if the researcher’s contributions, problem domain, and outcomes match your needs.
Following this particular brief first pass step enables a sufficient understanding and overview of the research paper’s scope and objectives, as well as a context for its content. You’ll be able to get more detailed information out of its content by going through it again with laser attention.
Step 4: Second pass (content familiarization)
Content familiarization is a process that’s relevant to the initial steps. The systematic approach to reading the research paper presented in this article. The familiarity process is a step that involves the introduction section and figures within the research paper.
As previously mentioned, the urge to plunge straight into the core of the research paper is not required because knowledge acclimatization provides an easier and more comprehensive examination of the study in later passes.
Introduction
Introductory sections of research papers are written to provide an overview of the objective of the research efforts. This objective mentions and explains problem domains, research scope, prior research efforts, and methodologies.
It’s normal to find parallels to past research work in this area, using similar or distinct methods. Other papers’ citations provide the scope and breadth of the problem domain, which broadens the exploratory zone for the reader. Perhaps incorporating the procedure outlined in Step 3 is sufficient at this point.
Another aspect of the benefit provided by the introduction section is the presentation of requisite knowledge required to approach and understand the content of the research paper.
Graph, diagrams, figures
Illustrative materials within the research paper ensure that readers can comprehend factors that support problem definition or explanations of methods presented. Commonly, tables are used within research papers to provide information on the quantitative performances of novel techniques in comparison to similar approaches.
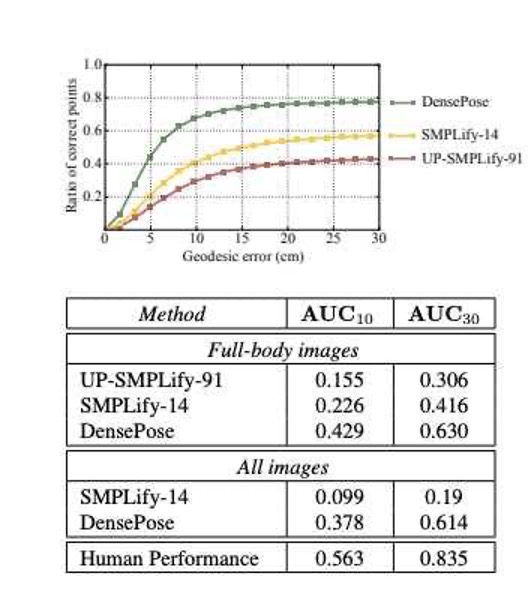
Generally, the visual representation of data and performance enables the development of an intuitive understanding of the paper’s context. In the Dense Pose paper mentioned earlier, illustrations are used to depict the performance of the author’s approach to pose estimation and create. An overall understanding of the steps involved in generating and annotating data samples.
In the realm of deep learning, it’s common to find topological illustrations depicting the structure of artificial neural networks. Again this adds to the creation of intuitive understanding for any reader. Through illustrations and figures, readers may interpret the information themselves and gain a fuller perspective of it without having any preconceived notions about what outcomes should be.
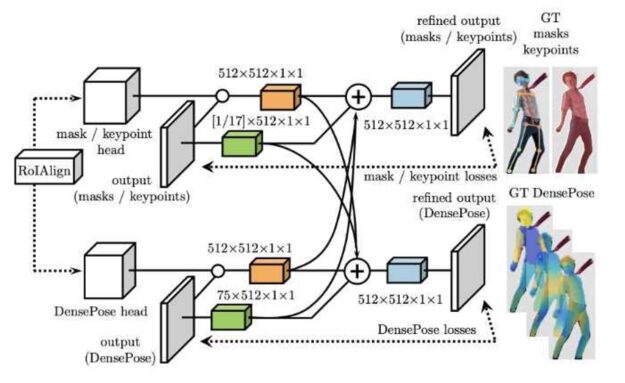
Step 5: Third pass (deep reading)
The third pass of the paper is similar to the second, though it covers a greater portion of the text. The most important thing about this pass is that you avoid any complex arithmetic or technique formulations that may be difficult for you. During this pass, you can also skip over any words and definitions that you don’t understand or aren’t familiar with. These unfamiliar terms, algorithms, or techniques should be noted to return to later.
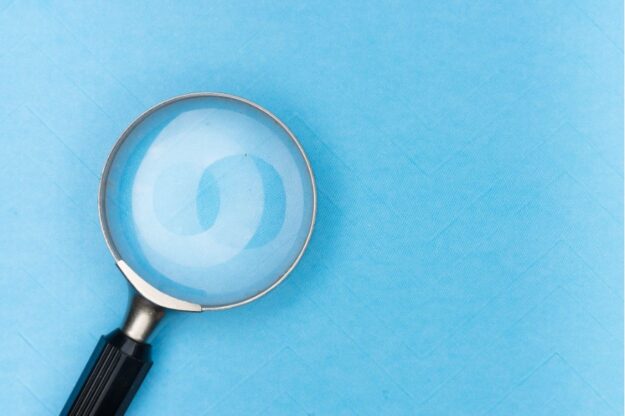
During this pass, your primary objective is to gain a broad understanding of what’s covered in the paper. Approach the paper, starting again from the abstract to the conclusion, but be sure to take intermediary breaks in between sections. Moreover, it’s recommended to have a notepad, where all key insights and takeaways are noted, alongside the unfamiliar terms and concepts.
The Pomodoro Technique is an effective method of managing time allocated to deep reading or study. Explained simply, the Pomodoro Technique involves the segmentation of the day into blocks of work, followed by short breaks.
What works for me is the 50/15 split, that is, 50 minutes studying and 15 minutes allocated to breaks. I tend to execute this split twice consecutively before taking a more extended break of 30 minutes. If you are unfamiliar with this time management technique, adopt a relatively easy division such as 25/5 and adjust the time split according to your focus and time capacity.
Step 6: Forth pass (final pass)
The final pass is typically one that involves an exertion of your mental and learning abilities, as it involves going through the unfamiliar terms, terminologies, concepts, and algorithms noted in the previous pass. This pass focuses on using external material to understand the recorded unfamiliar aspects of the paper.
In-depth studies of unfamiliar subjects have no specified time length, and at times efforts span into the days and weeks. The critical factor to a successful final pass is locating the appropriate sources for further exploration.
Unfortunately, there isn’t one source on the Internet that provides the wealth of information you require. Still, there are multiple sources that, when used in unison and appropriately, fill knowledge gaps. Below are a few of these resources.
- The Machine Learning Subreddit
- The Deep Learning Subreddit
- PapersWithCode
- Top conferences such as NIPS , ICML , ICLR
- Research Gate
- Machine Learning Apple
The Reference sections of research papers mention techniques and algorithms. Consequently, the current paper either draws inspiration from or builds upon, which is why the reference section is a useful source to use in your deep reading sessions.
Step 7: Summary (optional)
In almost a decade of academic and professional undertakings of technology-associated subjects and roles, the most effective method of ensuring any new information learned is retained in my long-term memory through the recapitulation of explored topics. By rewriting new information in my own words, either written or typed, I’m able to reinforce the presented ideas in an understandable and memorable manner.
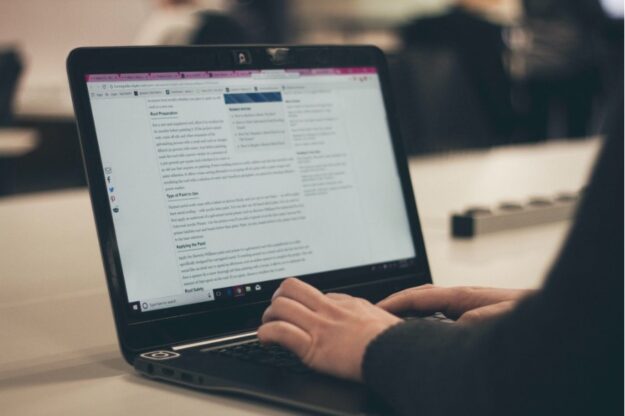
To take it one step further, it’s possible to publicize learning efforts and notes through the utilization of blogging platforms and social media. An attempt to explain the freshly explored concept to a broad audience, assuming a reader isn’t accustomed to the topic or subject, requires understanding topics in intrinsic details.
Undoubtedly, reading research papers for novice Data Scientists and ML practitioners can be daunting and challenging; even seasoned practitioners find it difficult to digest the content of research papers in a single pass successfully.
The nature of the Data Science profession is very practical and involved. Meaning, there’s a requirement for its practitioners to employ an academic mindset, more so as the Data Science domain is closely associated with AI, which is still a developing field.
To summarize, here are all of the steps you should follow to read a research paper:
- Identify A Topic.
- Finding associated Research Papers
- Read title, abstract, and conclusion to gain a vague understanding of the research effort aims and achievements.
- Familiarize yourself with the content by diving deeper into the introduction; including the exploration of figures and graphs presented in the paper.
- Use a deep reading session to digest the main content of the paper as you go through the paper from top to bottom.
- Explore unfamiliar terms, terminologies, concepts, and methods using external resources.
- Summarize in your own words essential takeaways, definitions, and algorithms.
Thanks for reading!
Related resources
- DLI course: Building Transformer-Based Natural Language Processing
- GTC session: Enterprise MLOps 101
- GTC session: Intro to Large Language Models: LLM Tutorial and Disease Diagnosis LLM Lab
- GTC session: Build AI Applications with GPU Vector Databases
- NGC Containers: MATLAB
- Webinar: The Impact of Large Language Models (LLMs) on Life Sciences
About the Authors
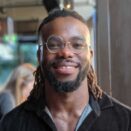
Related posts
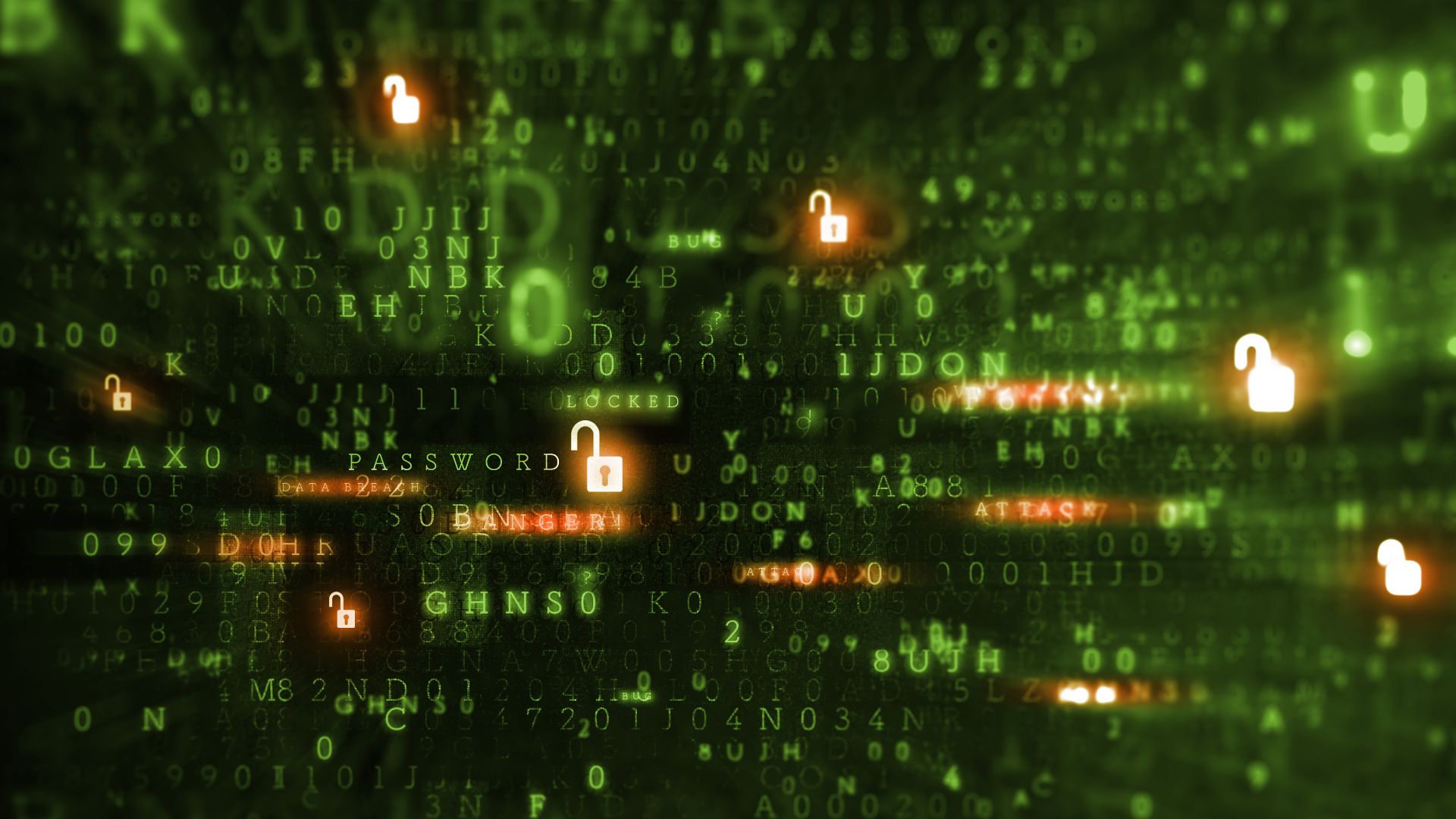
Improving Machine Learning Security Skills at a DEF CON Competition

Community Spotlight: Democratizing Computer Vision and Conversational AI in Kenya
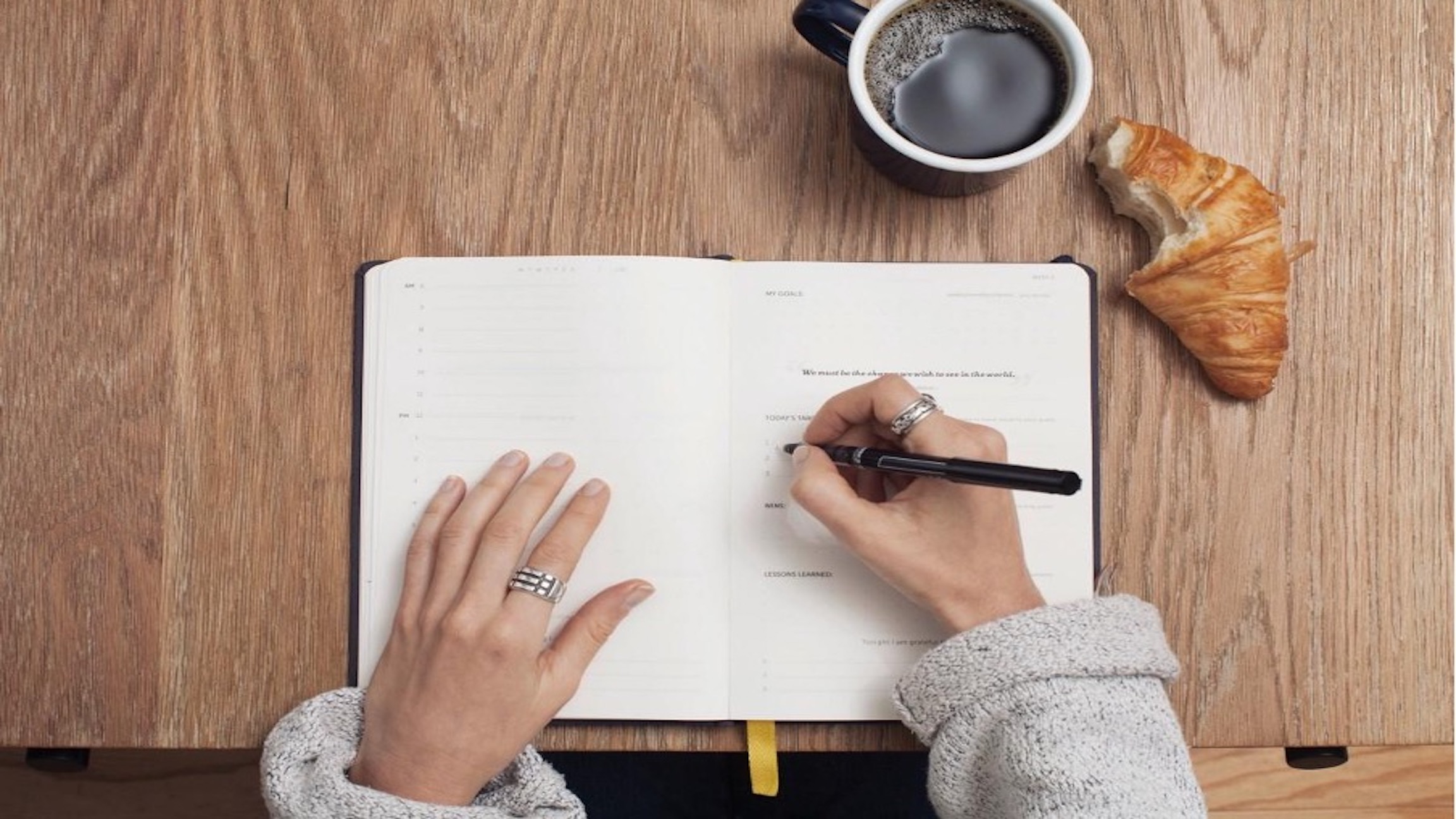
An Important Skill for Data Scientists and Machine Learning Practitioners
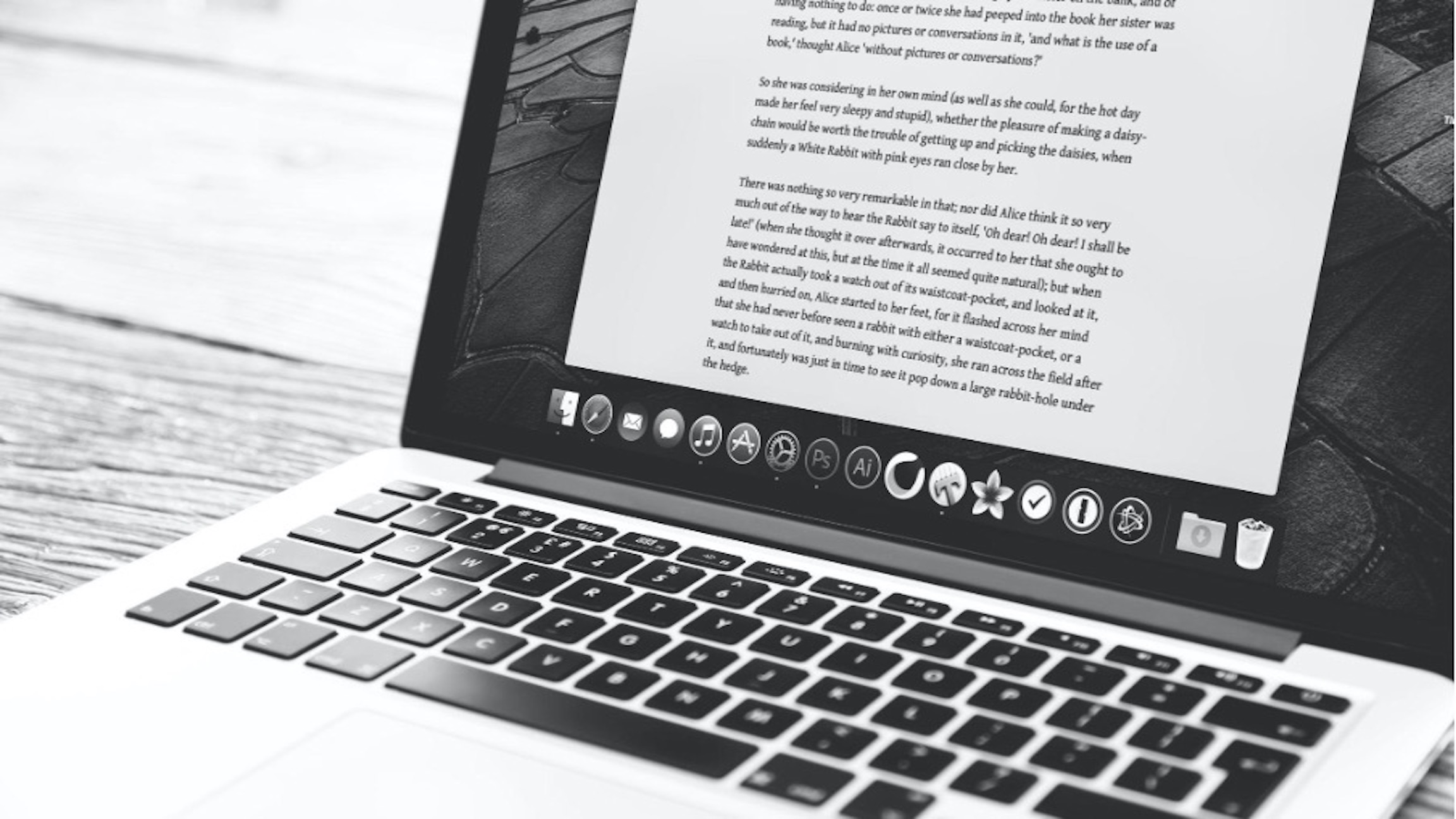
AI Pioneers Write So Should Data Scientists
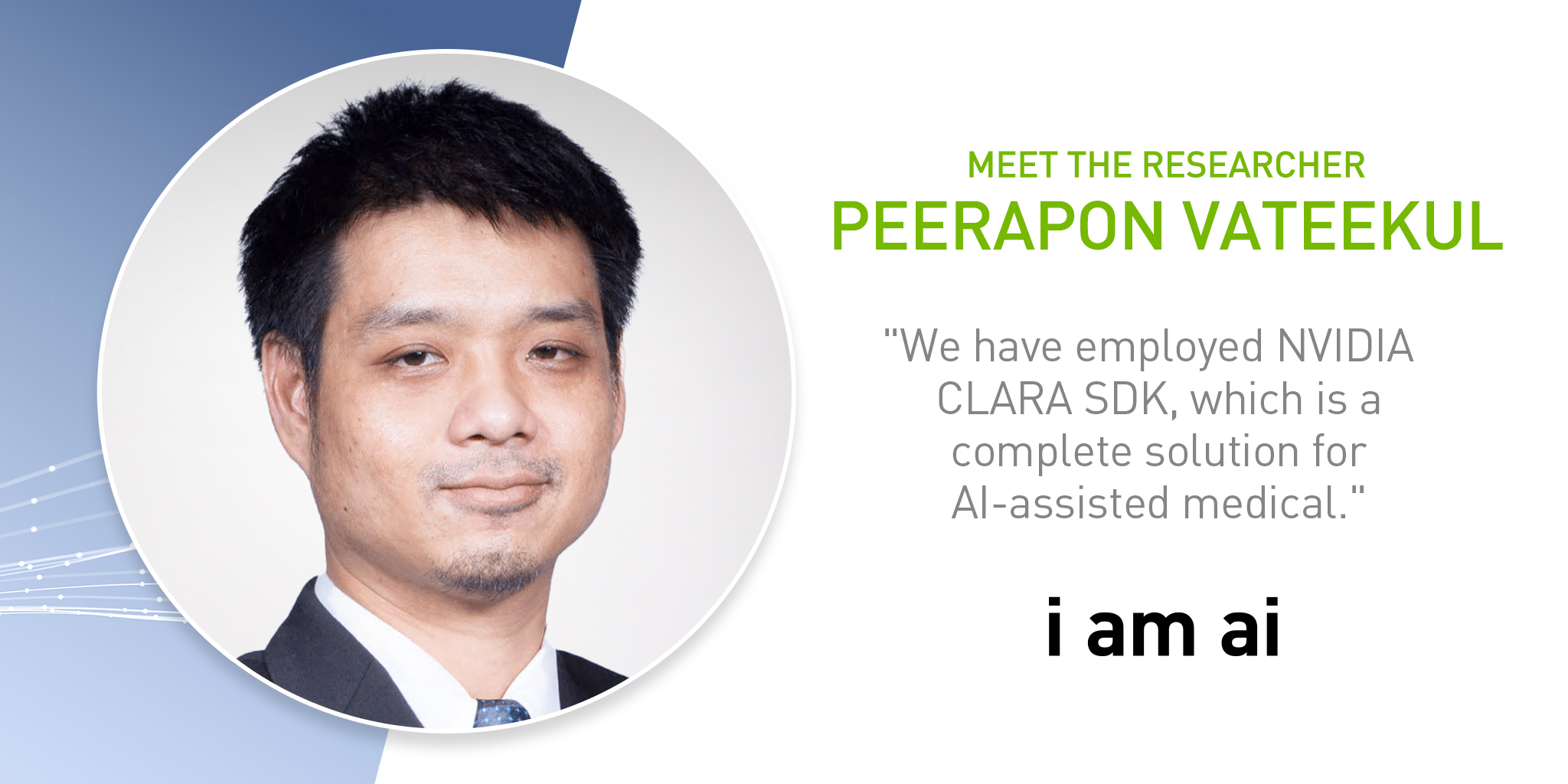
Meet the Researcher: Peerapon Vateekul, Deep Learning Solutions for Medical Diagnosis and NLP
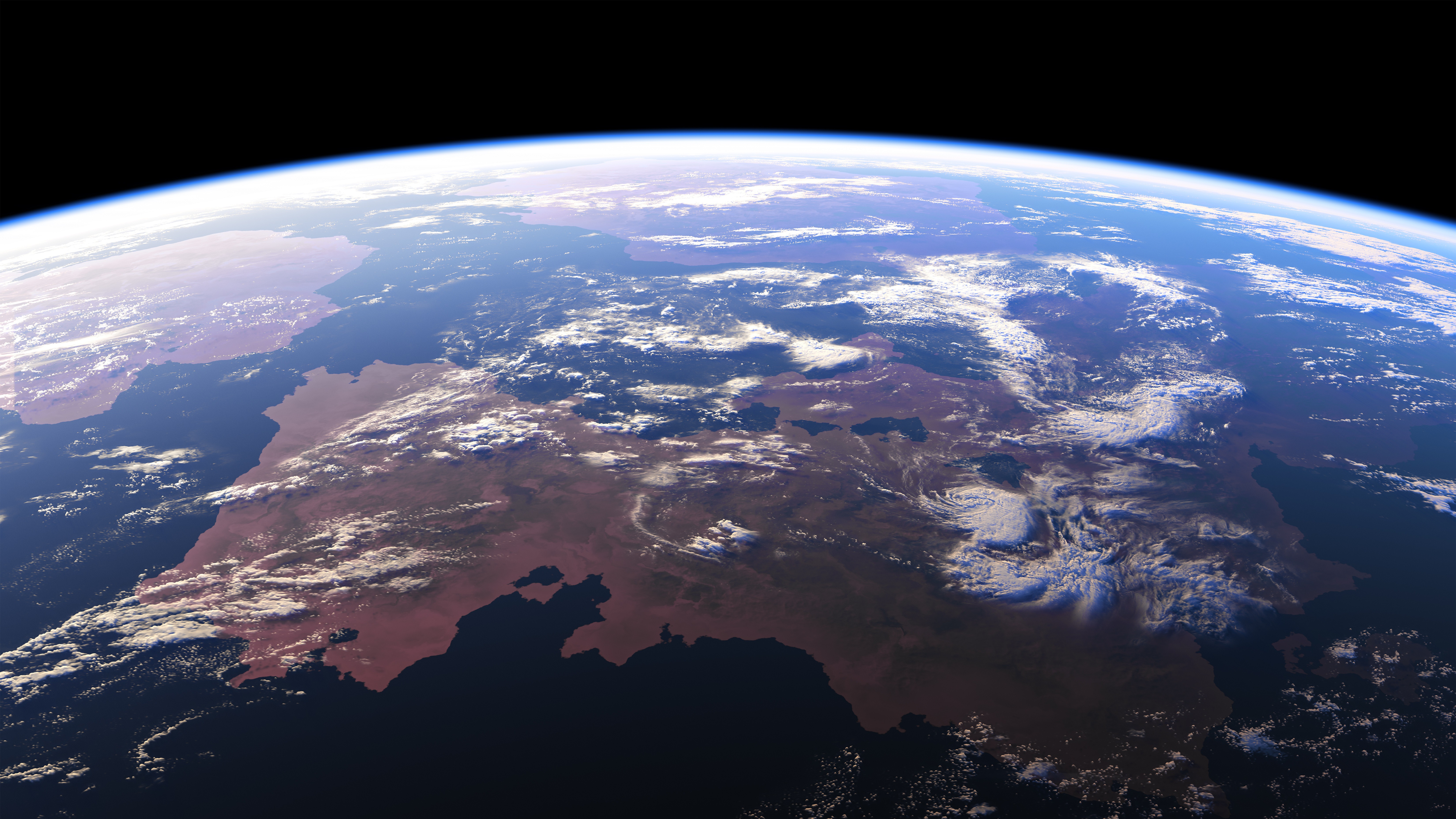
Next-Generation Seismic Monitoring with Neural Operators
Simulating realistic traffic behavior with a bi-level imitation learning ai model.
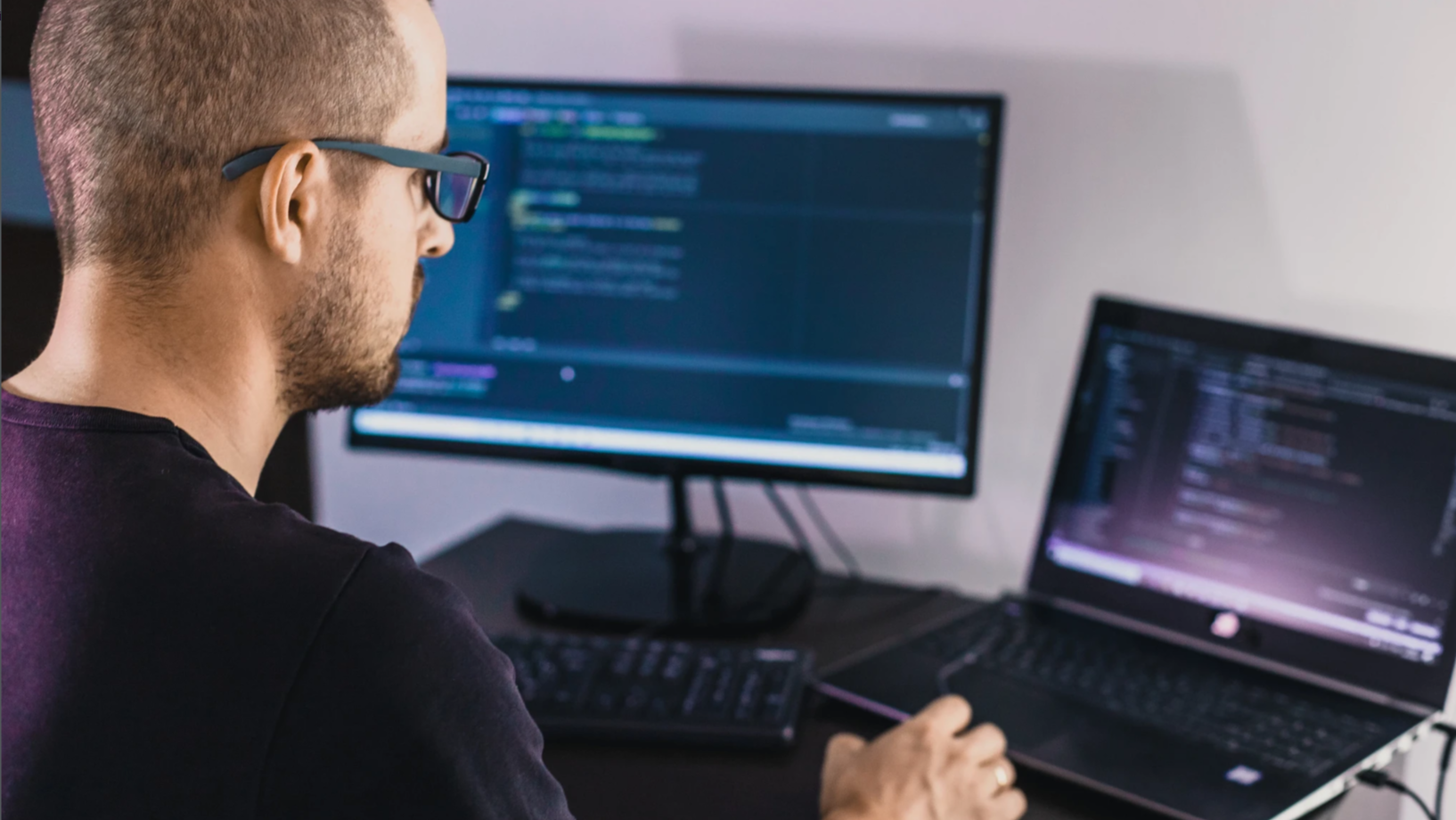
Analyzing the Security of Machine Learning Research Code
Research unveils breakthrough deep learning tool for understanding neural activity and movement control, generative ai research empowers creators with guided image structure control.

An official website of the United States government
The .gov means it’s official. Federal government websites often end in .gov or .mil. Before sharing sensitive information, make sure you’re on a federal government site.
The site is secure. The https:// ensures that you are connecting to the official website and that any information you provide is encrypted and transmitted securely.
- Publications
- Account settings
Preview improvements coming to the PMC website in October 2024. Learn More or Try it out now .
- Advanced Search
- Journal List
- Healthcare (Basel)

Machine-Learning-Based Disease Diagnosis: A Comprehensive Review
Md manjurul ahsan.
1 School of Industrial and Systems Engineering, University of Oklahoma, Norman, OK 73019, USA
Shahana Akter Luna
2 Medicine & Surgery, Dhaka Medical College & Hospital, Dhaka 1000, Bangladesh; [email protected]
Zahed Siddique
3 Department of Aerospace and Mechanical Engineering, University of Oklahoma, Norman, OK 73019, USA; ude.uo@euqiddisz
Globally, there is a substantial unmet need to diagnose various diseases effectively. The complexity of the different disease mechanisms and underlying symptoms of the patient population presents massive challenges in developing the early diagnosis tool and effective treatment. Machine learning (ML), an area of artificial intelligence (AI), enables researchers, physicians, and patients to solve some of these issues. Based on relevant research, this review explains how machine learning (ML) is being used to help in the early identification of numerous diseases. Initially, a bibliometric analysis of the publication is carried out using data from the Scopus and Web of Science (WOS) databases. The bibliometric study of 1216 publications was undertaken to determine the most prolific authors, nations, organizations, and most cited articles. The review then summarizes the most recent trends and approaches in machine-learning-based disease diagnosis (MLBDD), considering the following factors: algorithm, disease types, data type, application, and evaluation metrics. Finally, in this paper, we highlight key results and provides insight into future trends and opportunities in the MLBDD area.
1. Introduction
In medical domains, artificial intelligence (AI) primarily focuses on developing the algorithms and techniques to determine whether a system’s behavior is correct in disease diagnosis. Medical diagnosis identifies the disease or conditions that explain a person’s symptoms and signs. Typically, diagnostic information is gathered from the patient’s history and physical examination [ 1 ]. It is frequently difficult due to the fact that many indications and symptoms are ambiguous and can only be diagnosed by trained health experts. Therefore, countries that lack enough health professionals for their populations, such as developing countries like Bangladesh and India, face difficulty providing proper diagnostic procedures for their maximum population of patients [ 2 ]. Moreover, diagnosis procedures often require medical tests, which low-income people often find expensive and difficult to afford.
As humans are prone to error, it is not surprising that a patient may have overdiagnosis occur more often. If overdiagnosis, problems such as unnecessary treatment will arise, impacting individuals’ health and economy [ 3 ]. According to the National Academics of Science, Engineering, and Medicine report of 2015, the majority of people will encounter at least one diagnostic mistake during their lifespan [ 4 ]. Various factors may influence the misdiagnosis, which includes:
- lack of proper symptoms, which often unnoticeable
- the condition of rare disease
- the disease is omitted mistakenly from the consideration
Machine learning (ML) is used practically everywhere, from cutting-edge technology (such as mobile phones, computers, and robotics) to health care (i.e., disease diagnosis, safety). ML is gaining popularity in various fields, including disease diagnosis in health care. Many researchers and practitioners illustrate the promise of machine-learning-based disease diagnosis (MLBDD), which is inexpensive and time-efficient [ 5 ]. Traditional diagnosis processes are costly, time-consuming, and often require human intervention. While the individual’s ability restricts traditional diagnosis techniques, ML-based systems have no such limitations, and machines do not get exhausted as humans do. As a result, a method to diagnose disease with outnumbered patients’ unexpected presence in health care may be developed. To create MLBDD systems, health care data such as images (i.e., X-ray, MRI) and tabular data (i.e., patients’ conditions, age, and gender) are employed [ 6 ].
Machine learning (ML) is a subset of AI that uses data as an input resource [ 7 ]. The use of predetermined mathematical functions yields a result (classification or regression) that is frequently difficult for humans to accomplish. For example, using ML, locating malignant cells in a microscopic image is frequently simpler, which is typically challenging to conduct just by looking at the images. Furthermore, since advances in deep learning (a form of machine learning), the most current study shows MLBDD accuracy of above 90% [ 5 ]. Alzheimer’s disease, heart failure, breast cancer, and pneumonia are just a few of the diseases that may be identified with ML. The emergence of machine learning (ML) algorithms in disease diagnosis domains illustrates the technology’s utility in medical fields.
Recent breakthroughs in ML difficulties, such as imbalanced data, ML interpretation, and ML ethics in medical domains, are only a few of the many challenging fields to handle in a nutshell [ 8 ]. In this paper, we provide a review that highlights the novel uses of ML and DL in disease diagnosis and gives an overview of development in this field in order to shed some light on this current trend, approaches, and issues connected with ML in disease diagnosis. We begin by outlining several methods to machine learning and deep learning techniques and particular architecture for detecting and categorizing various forms of disease diagnosis.
The purpose of this review is to provide insights to recent and future researchers and practitioners regarding machine-learning-based disease diagnosis (MLBDD) that will aid and enable them to choose the most appropriate and superior machine learning/deep learning methods, thereby increasing the likelihood of rapid and reliable disease detection and classification in diagnosis. Additionally, the review aims to identify potential studies related to the MLBDD. In general, the scope of this study is to provide the proper explanation for the following questions:
- 1. What are some of the diseases that researchers and practitioners are particularly interested in when evaluating data-driven machine learning approaches?
- 2. Which MLBDD datasets are the most widely used?
- 3. Which machine learning and deep learning approaches are presently used in health care to classify various forms of disease?
- 4. Which architecture of convolutional neural networks (CNNs) is widely employed in disease diagnosis?
- 5. How is the model’s performance evaluated? Is that sufficient?
In this paper, we summarize the different machine learning (ML) and deep learning (DL) methods utilized in various disease diagnosis applications. The remainder of the paper is structured as follows. In Section 2 , we discuss the background and overview of ML and DL, whereas in Section 3 , we detail the article selection technique. Section 4 includes bibliometric analysis. In Section 5 , we discuss the use of machine learning in various disease diagnoses, and in Section 6 , we identify the most frequently utilized ML methods and datatypes based on the linked research. In Section 7 , we discuss the findings, anticipated trends, and problems. Finally, Section 9 concludes the article with a general conclusion.
2. Basics and Background
Machine learning (ML) is an approach that analyzes data samples to create main conclusions using mathematical and statistical approaches, allowing machines to learn without programming. Arthur Samuel presented machine learning in games and pattern recognition algorithms to learn from experience in 1959, which was the first time the important advancement was recognized. The core principle of ML is to learn from data in order to forecast or make decisions depending on the assigned task [ 9 ]. Thanks to machine learning (ML) technology, many time-consuming jobs may now be completed swiftly and with minimal effort. With the exponential expansion of computer power and data capacity, it is becoming simpler to train data-driven ML models to predict outcomes with near-perfect accuracy. Several papers offer various sorts of ML approaches [ 10 , 11 ].
The ML algorithms are generally classified into three categories such as supervised, unsupervised, and semisupervised [ 10 ]. However, ML algorithms can be divided into several subgroups based on different learning approaches, as shown in Figure 1 . Some of the popular ML algorithms include linear regression, logistic regression, support vector machines (SVM), random forest (RF), and naïve Bayes (NB) [ 10 ].

Different types of machine learning algorithms.
2.1. Machine Learning Algorithms
This section provides a comprehensive review of the most frequently used machine learning algorithms in disease diagnosis.
2.1.1. Decision Tree
The decision tree (DT) algorithm follows divide-and-conquer rules. In DT models, the attribute may take on various values known as classification trees; leaves indicate distinct classes, whereas branches reflect the combination of characteristics that result in those class labels. On the other hand, DT can take continuous variables called regression trees. C4.5 and EC4.5 are the two famous and most widely used DT algorithms [ 12 ]. DT is used extensively by following reference literature: [ 13 , 14 , 15 , 16 ].
2.1.2. Support Vector Machine
For classification and regression-related challenges, support vector machine (SVM) is a popular ML approach. SVM was introduced by Vapnik in the late twentieth century [ 17 ]. Apart from disease diagnosis, SVMs have been extensively employed in various other disciplines, including facial expression recognition, protein fold, distant homology discovery, speech recognition, and text classification. For unlabeled data, supervised ML algorithms are unable to perform. Using a hyperplane to find the clustering among the data, SVM can categorize unlabeled data. However, SVM output is not nonlinearly separable. To overcome such problems, selecting appropriate kernel and parameters is two key factors when applying SVM in data analysis [ 11 ].
2.1.3. K -Nearest Neighbor
K -nearest neighbor (KNN) classification is a nonparametric classification technique invented in 1951 by Evelyn Fix and Joseph Hodges. KNN is suitable for classification as well as regression analysis. The outcome of KNN classification is class membership. Voting mechanisms are used to classify the item. Euclidean distance techniques are utilized to determine the distance between two data samples. The projected value in regression analysis is the average of the values of the KNN [ 18 ].
2.1.4. Naïve Bayes
The naïve Bayes (NB) classifier is a Bayesian-based probabilistic classifier. Based on a given record or data point, it forecasts membership probability for each class. The most probable class is the one having the greatest probability. Instead of predictions, the NB classifier is used to project likelihood [ 11 ].
2.1.5. Logistic Regression
Logistic regression (LR) is an ML approach that is used to solve classification issues. The LR model has a probabilistic framework, with projected values ranging from 0 to 1. Examples of LR-based ML include spam email identification, online fraud transaction detection, and malignant tumor detection. The cost function, often known as the sigmoid function, is used by LR. The sigmoid function transforms every real number between 0 and 1 [ 19 ].
2.1.6. AdaBoost
Yoav Freund and Robert Schapire developed Adaptive Boosting, popularly known as AdaBoost. AdaBoost is a classifier that combines multiple weak classifiers into a single classifier. AdaBoost works by giving greater weight to samples that are harder to classify and less weight to those that are already well categorized. It may be used for categorization as well as regression analysis [ 20 ].
2.2. Deep Learning Overview
Deep learning (DL) is a subfield of machine learning (ML) that employs multiple layers to extract both higher and lower-level information from input (i.e., images, numerical value, categorical values). The majority of contemporary DL models are built on artificial neural networks (ANN), notably convolutional neural networks (CNN), which may be integrated with other DL models, including generative models, deep belief networks, and the Boltzmann machine. Deep learning may be classified into three types: supervised, semisupervised, and unsupervised. Deep neural networks (DNN), reinforcement learning, and recurrent neural networks (RNN) are some of the most prominent DL architectures (RNN) [ 21 ].
Each level in DL learns to convert its input data to the succeeding layers while learning distinct data attributes. For example, the raw input may be a pixel matrix in image recognition applications, and the first layers may detect the image’s edges. On the other hand, the second layer will construct and encode the nose and eyes, and the third layer may recognize the face by merging all of the information gathered from the previous two layers [ 6 ].
In medical fields, DL has enormous promise. Radiology and pathology are two well-known medical fields that have widely used DL in disease diagnosis over the years [ 22 ]. Furthermore, collecting valuable information from molecular state and determining disease progression or therapy sensitivity are practical uses of DL that are frequently unidentified by human investigations [ 23 ].
Convolutional Neural Network
Convolutional neural networks (CNNs) are a subclass of artificial neural networks (ANNs) that are extensively used in image processing. CNN is widely employed in face identification, text analysis, human organ localization, and biological image detection or recognition [ 24 ]. Since the initial development of CNN in 1989, a different type of CNN has been proposed that has performed exceptionally well in disease diagnosis over the last three decades. A CNN architecture comprises three parts: input layer, hidden layer, and output layer. The intermediate levels of any feedforward network are known as hidden layers, and the number of hidden layers varies depending on the type of architecture. Convolutions are performed in hidden layers, which contain dot products of the convolution kernel with the input matrix. Each convolutional layer provides feature maps used as input by the subsequent layers. Following the concealed layer are more layers, such as pooling and fully connected layers [ 21 ]. Several CNN models have been proposed throughout the years, and the most extensively used and popular CNN models are shown in Figure 2 .

Some of the most well-known CNN models, along with their development time frames.
In general, it may be considered that ML and DL have grown substantially throughout the years. The increased computational capability of computers and the enormous number of data available inspire academics and practitioners to employ ML/DL more efficiently. A schematic overview of machine learning and deep learning algorithms and their development chronology is shown in Figure 3 , which may be a helpful resource for future researchers and practitioner.

Illustration of machine learning and deep learning algorithms development timeline.
2.3. Performance Evaluations
This section describes the performance measures used in reference literature. Performance indicators, including accuracy, precision, recall, and F1 score, are widely employed in disease diagnosis. For example, lung cancer can be categorized as true positive ( T P ) or true-negative ( T N ) if individuals are diagnosed correctly, while it can be categorized into false positive ( F P ) or false negative ( F N ) if misdiagnosed. The most widely used metrics are described below [ 10 ].
Accuracy (Acc) : The accuracy denotes total correctly identifying instances among all of the instances. Accuracy can be calculated using following formulas:
Precision ( P n ): Precision is measured as the proportion of precisely predicted to all expected positive observations.
Recall ( R c ): The proportion of overall relevant results that the algorithm properly recognizes is referred to as recall.
Sensitivity ( S n ) : Sensitivity denotes only true positive measure considering total instances and can be measured as follows:
Specificity ( S p ): It identifies how many true negatives are appropriately identified and calculated as follows:
F-measure: The F1 score is the mean of accuracy and recall in a harmonic manner. The highest F score is 1, indicating perfect precision and recall score.
Area under curve (AUC): The area under the curve represents the models’ behaviors in different situations. The AUC can be calculated as follows:
where l p and l n denotes positive and negative data samples and R i is the rating of the i th positive samples.
3. Article Selection
3.1. identification.
The Scopus and Web of Science (WOS) databases are utilized to find original research publications. Due to their high quality and peer review paper index, Scopus and WOS are prominent databases for article searching, as many academics and scholars utilized them for systematic review [ 25 , 26 ]. Using keywords along with Boolean operators, the title search was carried out as follows:
“disease” AND (“diagnsois” OR “Supprot vector machine” OR “SVM” OR “KNN” OR “K-nearest neighbor” OR “logistic regression” OR “K-means clustering” OR “random forest” OR “RF” OR “adaboost” OR “XGBoost”, “decision tree” OR “neural network” OR “NN” OR “artificial neural network” OR “ANN” OR “convolutional neural network” OR “CNN” OR “deep neural network” OR “DNN” OR “machine learning" or “adversarial network” or “GAN”).
The initial search yielded 16,209 and 2129 items, respectively, from Scopus and Web of Science (WOS).
3.2. Screening
Once the search period was narrowed to 2012–2021 and only peer-reviewed English papers were evaluated, the total number of articles decreased to 9117 for Scopus and 1803 for WOS, respectively.
3.3. Eligibility and Inclusion
These publications were chosen for further examination if they are open access and are journal articles. There were 1216 full-text articles (724 from the Scopus database and 492 from WOS). Bibliographic analysis was performed on all 1216 publications. One investigator (Z.S.) imported the 1216 article information as excel CSV data for future analysis. Excel duplication functions were used to identify and eliminate duplicates. Two independent reviewers (M.A. and Z.S.) examined the titles and abstracts of 1192 publications. Disagreements were settled through conversation. We omitted studies that were not relevant to machine learning but were relevant to disease diagnosis or vice versa.
After screening the titles and abstracts, the complete text of 102 papers was examined, and all 102 articles satisfied all inclusion requirements. Factors that contributed to the article’s exclusion from the full-text screening includes:
- 1. Inaccessibility of the entire text
- 2. Nonhuman studies, book chapters, reviews
- 3. Incomplete information related to test result
Figure 4 shows the flow diagram of the systematic article selection procedure used in this study.

MLBDD article selection procedure used in this study.
4. Bibliometric Analysis
The bibliometric study in this section was carried out using reference literature gathered from the Scopus and WOS databases. The bibliometric study examines publications in terms of the subject area, co-occurrence network, year of publication, journal, citations, countries, and authors.
4.1. Subject Area
Many research disciplines have uncovered machine learning-based disease diagnostics throughout the years. Figure 5 depicts a schematic representation of machine learning-based disease detection spread across several research fields. According to the graph, computer science (40%) and engineering (31.2%) are two dominating fields that vigorously concentrated on MLBDD.

Distribution of articles by subject area.
4.2. Co-Occurrence Network
Co-occurrence of keywords provides an overview of how the keywords are interconnected or used by the researchers. Figure 6 displays the co-occurrence network of the article’s keywords and their connection, developed by VOSviewer software. The figure shows that some of the significant clusters include neural networks (NN), decision trees (DT), machine learning (ML), and logistic regression (LR). Each cluster is also connected with other keywords that fall under that category. For instance, the NN cluster contains support vector machine (SVM), Parkinson’s disease, and classification.

Bibliometric map representing co-occurrence analysis of keywords in network visualization.
4.3. Publication by Year
The exponential growth of journal publications is observed from 2017. Figure 7 displays the number of publications between 2012 to 2021 based on the Scopus and WOS data. Note that while the image may not accurately depict the MLBDD’s real contribution, it does illustrate the influence of MLBDD over time.

Publications of machine-learning-based disease diagnosis (MLBDD) by year.
4.4. Publication by Journal
We investigated the most prolific journals in MLBDD domains based on our referred literature.The top ten journals and the number of articles published in the last ten years are depicted in Figure 8 . IEEE Access and Scientific Reports are the most productive journals that published 171 and 133 MLBDD articles, respectively.

Publications by journals.
4.5. Publication by Citations
Citations are one of the primary indicators of an article’s effect. Here, we have identified the top ten cited articles using the R Studio tool. Table 1 summarizes the top articles that achieved the highest citation during the year between 2012 to 2021. Note that Google Scholar and other online databases may have various indexing procedures and times; therefore, the citations in this manuscript may differ from the number of citations shown in this study. The table shows that published articles by [ 27 ] earned the most citations (257), with 51.4 citations per year, followed by Gray [ 28 ]’s article, which obtained 218 citations. It is assumed that all the authors included in Table 1 are among those prominent authors that contributed to MLBDD.
Top ten cited papers published in MLBDD in between 2012–2021 based on Scopus and WOS database.
4.6. Publication by Countries
Figure 9 displayed that China published the most publications in MLBDD, total 259 articles. USA and India are placed 2nd and 3rd, respectively, as they published 139 and 103 papers related to MLBDD. Interestingly, four out of the top ten productive countries are from Asia: China, India, Korea, and Japan.

Top ten countries that contributed to MLBDD literature.
4.7. Publication by Author
According to Table 2 , author Kim J published the most publications (20 out of 1216). Wang Y and Li J Ranked 2nd and 3rd by publishing 19 and 18 articles, respectively. As shown in Table 2 , the number of papers produced by the top 10 authors ranges between 15–20.
Top ten authors based on total number of publications.
5. Machine Learning Techniques for Different Disease Diagnosis
Many academics and practitioners have used machine learning (ML) approaches in disease diagnosis. This section describes many types of machine-learning-based disease diagnosis (MLBDD) that have received much attention because of their importance and severity. For example, due to the global relevance of COVID-19, several studies concentrated on COVID-19 disease detection using ML from 2020 to the present, which also received greater priority in our study. Severe diseases such as heart disease, kidney disease, breast cancer, diabetes, Parkinson’s, Alzheimer’s, and COVID-19 are discussed briefly, while other diseases are covered briefly under the “other disease”.
5.1. Heart Disease
Most researchers and practitioners use machine learning (ML) approaches to identify cardiac disease [ 37 , 38 ]. Ansari et al. (2011), for example, offered an automated coronary heart disease diagnosis system based on neurofuzzy integrated systems that yield around 89% accuracy [ 37 ]. One of the study’s significant weaknesses is the lack of a clear explanation for how their proposed technique would work in various scenarios such as multiclass classification, big data analysis, and unbalanced class distribution. Furthermore, there is no explanation about the credibility of the model’s accuracy, which has lately been highly encouraged in medical domains, particularly to assist users who are not from the medical domains in understanding the approach.
Rubin et al. (2017) uses deep-convolutional-neural-network-based approaches to detect irregular cardiac sounds. The authors of this study adjusted the loss function to improve the training dataset’s sensitivity and specificity. Their suggested model was tested in the 2016 PhysioNet computing competition. They finished second in the competition, with a final prediction of 0.95 specificity and 0.73 sensitivity [ 39 ].
Aside from that, deep-learning (DL)-based algorithms have lately received attention in detecting cardiac disease. Miao and Miao et al. (2018), for example, offered a DL-based technique to diagnosing cardiotocographic fetal health based on a multiclass morphologic pattern. The created model is used to differentiate and categorize the morphologic pattern of individuals suffering from pregnancy complications. Their preliminary computational findings include accuracy of 88.02%, a precision of 85.01%, and an F-score of 0.85 [ 40 ]. During that study, they employed multiple dropout strategies to address overfitting problems, which finally increased training time, which they acknowledged as a tradeoff for higher accuracy.
Although ML applications have been widely employed in heart disease diagnosis, no research has been conducted that addressed the issues associated with unbalanced data with multiclass classification. Furthermore, the model’s explainability during final prediction is lacking in most cases. Table 3 summarizes some of the cited publications that employed ML and DL approaches in the diagnosis of cardiac disease. However, further information about machine-learning-based cardiac disease diagnosis can be found in [ 5 ].
Referenced literature that considered machine-learning-based heart disease diagnosis.
5.2. Kidney Disease
Kidney disease, often known as renal disease, refers to nephropathy or kidney damage. Patients with kidney disease have decreased kidney functional activity, which can lead to kidney failure if not treated promptly. According to the National Kidney Foundation, 10% of the world’s population has chronic kidney disease (CKD), and millions die each year due to insufficient treatment. The recent advancement of ML- and DL-based kidney disease diagnosis may provide a possibility for those countries that are unable to handle the kidney disease diagnostic-related tests [ 49 ]. For instance, Charleonnan et al. (2016) used publicly available datasets to evaluate four different ML algorithms: K -nearest neighbors (KNN), support vector machine (SVM), logistic regression (LR), and decision tree classifiers and received the accuracy of 98.1%, 98.3%, 96.55%, and 94.8%, respectively [ 50 ]. Aljaaf et al. (2018) conducted a similar study. The authors tested different ML algorithms, including RPART, SVM, LOGR, and MLP, using a comparable dataset, CKD, as used by [ 50 ], and found that MLP performed best (98.1 percent) in identifying chronic kidney disease [ 51 ]. To identify chronic kidney disease, Ma et al. (2020) utilizes a collection of datasets containing data from many sources [ 52 ]. Their suggested heterogeneous modified artificial neural network (HMANN) model obtained an accuracy of 87–99%.
Table 4 summarizes some of the cited publications that employed ML and DL approaches to diagnose kidney disease.
Referenced literature that considered machine-learning-based kidney disease diagnosis.
5.3. Breast Cancer
Many scholars in the medical field have proposed machine-learning (ML)-based breast cancer analysis as a potential solution to early-stage diagnosis. Miranda and Felipe (2015), for example, proposed fuzzy-logic-based computer-aided diagnosis systems for breast cancer categorization. The advantage of fuzzy logic over other classic ML techniques is that it can minimize computational complexity while simulating the expert radiologist’s reasoning and style. If the user inputs parameters such as contour, form, and density, the algorithm offers a cancer categorization based on their preferred method [ 57 ]. Miranda and Felipe (2015)’s proposed model had an accuracy of roughly 83.34%. The authors employed an approximately equal ratio of images for the experiment, which resulted in improved accuracy and unbiased performance. However, as the study did not examine the interpretation of their results in an explainable manner, it may be difficult to conclude that accuracy, in general, indicates true accuracy for both benign and malignant classifications. Furthermore, no confusion matrix is presented to demonstrate the models’ actual prediction for the each class.
Zheng et al. (2014) presented hybrid strategies for diagnosing breast cancer disease utilizing k -means clustering (KMC) and SVM. Their proposed model considerably decreased the dimensional difficulties and attained an accuracy of 97.38% using Wisconsin Diagnostic Breast Cancer (WDBC) dataset [ 58 ]. The dataset is normally distributed and has 32 features divided into 10 categories. It is difficult to conclude that their suggested model will outperform in a dataset with an unequal class ratio, which may contain missing value as well.
To determine the best ML models, Asri et al. (2016) applied various ML approaches such as SVM, DT (C4.5), NB, and KNN on the Wisconsin Breast Cancer (WBC) datasets. According to their findings, SVM outperformed all other ML algorithms, obtaining an accuracy of 97.13% [ 59 ]. However, if a same experiment is repeated in a different database, the results may differ. Furthermore, experimental results accompanied by ground truth values may provide a more precise estimate in determining which ML model is the best or not.
Mohammed et al. (2020) conducted a nearly identical study. The authors employ three ML algorithms to find the best ML methods: DT (J48), NB, and sequential minimal optimization (SMO), and the experiment was conducted on two popular datasets: WBC and breast cancer datasets. One of the interesting aspects of this research is that they focused on data imbalance issues and minimized the imbalance problem through the use of resampling data labeling procedures. Their findings showed that the SMO algorithms exceeded the other two classifiers, attaining more than 95% accuracy on both datasets [ 60 ]. However, in order to reduce the imbalance ratio, they used resampling procedures numerous times, potentially lowering the possibility of data diversity. As a result, the performance of those three ML methods may suffer on a dataset that is not normally distributed or imbalanced.
Assegie (2021) used the grid search approach to identify the best k -nearest neighbor (KNN) settings. Their investigation showed that parameter adjustment had a considerable impact on the model’s performance. They demonstrated that by fine-tuning the settings, it is feasible to get 94.35% accuracy, whereas the default KNN achieved around 90% accuracy [ 61 ].
To detect breast cancer, Bhattacherjee et al. (2020) employed a backpropagation neural network (BNN). The experiment was carried out in the WBC dataset with nine features, and they achieved 99.27% accuracy [ 62 ]. Alshayeji et al. (2021) used the WBCD and WDBI datasets to develop a shallow ANN model for classifying breast cancer tumors. The authors demonstrated that the suggested model could classify tumors up to 99.85% properly without selecting characteristics or tweaking the algorithms [ 63 ].
Sultana et al. (2021) detect breast cancer using a different ANN architecture on the WBC dataset. They employed a variety of NN architectures, including the multilayer perceptron (MLP) neural network, the Jordan/Elman NN, the modular neural network (MNN), the generalized feedforward neural network (GFFNN), the self-organizing feature map (SOFM), the SVM neural network, the probabilistic neural network (PNN), and the recurrent neural network (RNN). Their final computational result demonstrates that the PNN with 98.24% accuracy outperforms the other NN models utilized in that study [ 64 ]. However, this study lacks the interpretability as of many other investigations because it does not indicate which features are most important during the prediction phase.
Deep learning (DL) was also used by Ghosh et al. (2021). The WBC dataset was used by the authors to train seven deep learning (DL) models: ANN, CNN, GRU, LSTM, MLP, PNN, and RNN. Long short-term memory (LSTM) and gated recurrent unit (GRU) demonstrated the best performance among all DL models, achieving an accuracy of roughly 99% [ 65 ]. Table 5 summarizes some of the referenced literature that used ML and DL techniques in breast cancer diagnosis.
Referenced literature that considered machine-learning-based breast cancer disease diagnosis.
5.4. Diabetes
According to the International Diabetes Federation (IDF), there are currently over 382 million individuals worldwide who have diabetes, with that number anticipated to increase to 629 million by 2045 [ 71 ]. Numerous studies widely presented ML-based systems for diabetes patient detection. For example, Kandhasamy and Balamurali (2015) compared ML classifiers (J48 DT, KNN, RF, and SVM) for classifying patients with diabetes mellitus. The experiment was conducted on the UCI Diabetes dataset, and the KNN (K = 1) and RF classifiers obtained near-perfect accuracy [ 72 ]. However, one disadvantage of this work is that it used a simplified Diabetes dataset with only eight binary-classified parameters. As a result, getting 100% accuracy with a less difficult dataset is unsurprising. Furthermore, there is no discussion of how the algorithms influence the final prediction or how the result should be viewed from a nontechnical position in the experiment.
Yahyaoui et al. (2019) presented a Clinical Decision Support Systems (CDSS) to aid physicians or practitioners with Diabetes diagnosis. To reach this goal, the study utilized a variety of ML techniques, including SVM, RF, and deep convolutional neural network (CNN). RF outperformed all other algorithms in their computations, obtaining an accuracy of 83.67%, while DL and SVM scored 76.81% and 65.38% accuracy, respectively [ 73 ].
Naz and Ahuja (2020) employed a variety of ML techniques, including artificial neural networks (ANN), NB, DT, and DL, to analyze open-source PIMA Diabetes datasets. Their study indicates that DL is the most accurate method for detecting the development of diabetes, with an accuracy of approximately 98.07% [ 71 ]. The PIMA dataset is one of the most thoroughly investigated and primary datasets, making it easy to perform conventional and sophisticated ML-based algorithms. As a result, gaining greater accuracy with the PIMA Indian dataset is not surprising. Furthermore, the paper makes no mention of interpretability issues and how the model would perform with an unbalanced dataset or one with a significant number of missing variables. As is widely recognized in healthcare, several types of data can be created that are not always labeled, categorized, and preprocessed in the same way as the PIMA Indian dataset. As a result, it is critical to examine the algorithms’ fairness, unbiasedness, dependability, and interpretability while developing a CDSS, especially when a considerable amount of information is missing in a multiclass classification dataset.
Ashiquzzaman et al. (2017) developed a deep learning strategy to address the issue of overfitting in diabetes datasets. The experiment was carried out on the PIMA Indian dataset and yielded an accuracy of 88.41%. The authors claimed that performance improved significantly when dropout techniques were utilized and the overfitting problems were reduced [ 74 ]. Overuse of the dropout approach, on the other hand, lengthens overall training duration. As a result, as they did not address these concerns in their study, assessing whether their proposed model is optimum in terms of computational time is difficult.
Alhassan et al. (2018) introduced the King Abdullah International Research Center for Diabetes (KAIMRCD) dataset, which includes data from 14k people and is the world’s largest diabetic dataset. During that experiment, the author presented a CDSS architecture based on LSTM and GRU-based deep neural networks, which obtained up to 97% accuracy [ 75 ]. Table 6 highlights some of the relevant publications that employed ML and DL approaches in the diagnosis of diabetic disease.
Referenced literature that considered machine-learning-based diabetic disease diagnosis.
5.5. Parkinson’s Disease
Parkinson’s disease is one of the conditions that has received a great amount of attention in the ML literature. It is a slow-progressing chronic neurological disorder. When dopamine-producing neurons in certain parts of the brain are harmed or die, people have difficulty speaking, writing, walking, and doing other core activities [ 80 ]. There are several ML-based approaches have been proposed. For instance, Sriram et al. (2013) used KNN, SVM, NB, and RF algorithms to develop intelligent Parkinson’s disease diagnosis systems. Their computational result shows that, among all other algorithms, RF shows the best performance (90.26% accuracy), and NB demonstrate the worst performance (69.23% accuracy) [ 81 ].
Esmaeilzadeh et al. (2018) proposed a deep CNN-based model to diagnose Parkinson’s disease and achieved almost 100% accuracy on train and test set [ 82 ]. However, there was no mention of any overfitting difficulties in the trial. Furthermore, the experimental results do not provide a good interpretation of the final classification and regression, which is now widely expected, particularly in CDSS. Grover et al. (2018) also used DL-based approaches on UCI’s Parkinson’s telemonitoring voice dataset. Their experiment using DNN has achieved around 81.67% accuracy in diagnosing patients with Parkinson’s disease symptoms [ 80 ].
Warjurkar and Ridhorkar (2021) conducted a thorough study on the performance of the ML-based approach in decision support systems that can detect both brain tumors and diagnose Parkinson’s patients. Based on their findings, it was obvious that, when compared to other algorithms, boosted logistic regression surpassed all other models, attaining 97.15% accuracy in identifying Parkinson’s disease patients. In tumor segmentation, however, the Markov random technique performed best, obtaining an accuracy of 97.4% [ 83 ]. Parkinson’s disease diagnosis using ML and DL approaches is summarized in Table 7 , which includes a number of references to the relevant research.
Referenced literature that considered machine-learning-based Parkinson’s disease diagnosis.
5.6. COVID-19
The new severe acute respiratory syndrome coronavirus 2 (SARS-CoV-2), also known as COVID-19, pandemic has become humanity’s greatest challenge in contemporary history. Despite the fact that a vaccine had been advanced in distribution because to the global emergency, it was unavailable to the majority of people for the duration of the crisis [ 88 ]. Because of the new COVID-19 Omicron strain’s high transmission rates and vaccine-related resistance, there is an extra layer of concern. The gold standard for diagnosing COVID-19 infection is now Real-Time Reverse Transcription-Polymerase Chain Reaction (RT-PCR) [ 89 , 90 ]. Throughout the epidemic, the researcher advocated other technologies including as chest X-rays and Computed Tomography (CT) combined with Machine Learning and Artificial Intelligence to aid in the early detection of people who might be infected. For example, Chen et al. (2020) proposed a UNet++ model employing CT images from 51 COVID-19 and 82 non-COVID-19 patients and achieved an accuracy of 98.5% [ 91 ]. Ardakani et al. (2020) used a small dataset of 108 COVID-19 and 86 non-COVID-19 patients to evaluate ten different DL models and achieved a 99% overall accuracy [ 92 ]. Wang et al. (2020) built an inception-based model with a large dataset, containing 453 CT scan images, and achieved 73.1% accuracy. However, the model’s network activity and region of interest were poorly explained [ 93 ]. Li et al. (2020) suggested the COVNet model and obtain 96% accuracy utilizing a large dataset of 4356 chest CT images of Pneumonia patients, 1296 of which were verified COVID-19 cases [ 94 ].
Several studies investigated and advised screening COVID-19 patients utilizing chest X-ray images in parallel, with major contributions in [ 95 , 96 , 97 ]. For example, Hemdan et al. (2020) used a small dataset of only 50 images to identify COVID-19 patients from chest X-ray images with an accuracy of 90% and 95%, respectively, using VGG19 and ResNet50 models [ 95 ]. Using a dataset of 100 chest X-ray images, Narin et al. (2021) distinguished COVID-19 patients from those with Pneumonia with 86% accuracy [ 97 ].
In addition, in order to develop more robust and better screening systems, other studies considered larger datasets. For example, Brunese et al. (2020) employed 6505 images with a data ratio of 1:1.17, with 3003 images classified as COVID-19 symptoms and 3520 as “other patients” for the objectives of that study [ 98 ]. With a dataset of 5941 images, Ghoshal and Tucker (2020) achieved 92.9% accuracy [ 99 ]. However, neither study looked at how their proposed models would work with data that was severely unbalanced and had mismatched class ratios. Apostolopoulos and Mpesiana (2020) employed a CNN-based Xception model on an imbalanced dataset of 284 COVID-19 and 967 non-COVID-19 patient chest X-ray images and achieved 89.6% accuracy [ 100 ].
The following Table 8 summarizes some of the relevant literature that employed ML and DL approaches to diagnose COVID-19 disease.
Referenced literature that considered machine-learning-based COVID-19 disease diagnosis.
5.7. Alzheimer’s Disease
Alzheimer is a brain illness that often begins slowly but progresses over time, and it affects 60–70% of those who are diagnosed with dementia [ 103 ]. Alzheimer’s disease symptoms include language problems, confusion, mood changes, and other behavioral disorders. Body functions gradually deteriorated, and the usual life expectancy is three to nine years after diagnosis. Early diagnosis, on the other hand, may assist to avoid and take required actions to enter into suitable treatment as soon as possible, which will also raise the possibility of life expectancy. Machine learning and deep learning have shown promising outcomes in detecting Alzheimer’s disease patients throughout the years. For instance, Neelaveni and Devasana (2020) proposed a model that can detect Alzheimer patients using SVM and DT, and achieved an accuracy of 85% and 83% respectively [ 104 ]. Collij et al. (2016) also used SVM to detect single-subject Alzheimer’s disease and mild cognitive impairment (MCI) prediction and achieved an accuracy of 82% [ 105 ].
Multiple algorithms have been adopted and tested in developing ML based Alzheimer disease diagnosis. For example, Vidushi and Shrivastava (2019) experimented using Logistic Regression (LR), SVM, DT, ensemble Random Forest (RF), and Boosting Adaboost and achieved an accuracy of 78.95%, 81.58%, 81.58%, 84.21%, and 84.21% respectively [ 106 ]. Many of the study adopted CNN based approach to detect Alzheimer patients as CNN demonstrates robust results in image processing compared to other existing algorithms. As a consequence, Ahmed et al. (2020) proposed a CNN model for earlier diagnosis and classification of Alzheimer disease. Within the dataset consists of 6628 MRI images, the proposed model achieved 99% accuracy [ 107 ]. Nawaz et al. (2020) proposed deep feature-based models and achieved an accuracy of 99.12% [ 108 ]. Additionally, Studies conducted by Haft-Javaherian et al. (2019) [ 109 ] and Aderghal et al. (2017) [ 110 ] are some of the CNN based study that also demonstrates the robustness of CNN based approach in Alzheimer disease diagnosis. ML and DL approaches employed in the diagnosis of Alzheimer’s disease are summarized in Table 9 .
Referenced literature that considered Machine Learning-based Alzheimer disease diagnosis.
5.8. Other Diseases
Beyond the disease mentioned above, ML and DL have been used to identify various other diseases. Big data and increasing computer processing power are two key reasons for this increased use. For example, Mao et al. (2020) used Decision Tree (DT) and Random Forest (RF) to disease classification based on eye movement [ 114 ]. Nosseir and Shawky (2019) evaluated KNN and SVM to develop automatic skin disease classification systems, and the best performance was observed using KNN by achieving an accuracy of 98.22% [ 115 ]. Khan et al. (2020) employed CNN-based approaches such as VGG16 and VGG19 to classify multimodal Brain tumors. The experiment was carried out using publicly available three image datasets: BraTs2015, BraTs2017, and BraTs2018, and achieved 97.8%, 96.9%, and 92.5% accuracy, respectively [ 116 ]. Amin et al. (2018) conducted a similar experiment utilizing the RF classifier for tumor segmentation. The authors achieved 98.7%, 98.7%, 98.4%, 90.2%, and 90.2% accuracy using BRATS 2012, BRATS 2013, BRATS 2014, BRATS 2015, and ISLES 2015 dataset, respectively [ 117 ].
Dai et al. (2019) proposed a CNN-based model to develop an application to detect Skin cancer. The authors used a publicly available dataset, HAM10000, to experiment and achieved 75.2% accuracy [ 118 ]. Daghrir et al. (2020) evaluated KNN, SVM, CNN, Majority Voting using ISIC (International Skin Imaging Collaboration) dataset to detect Melanoma skin cancer. The best result was found using Majority Voting (88.4% accuracy) [ 119 ]. Table 10 summarizes some of the referenced literature that used ML and DL techniques in various disease diagnosis.
Referenced literature that considered Machine Learning on various disease diagnoses.
6. Algorithm and Dataset Analysis
Most of the referenced literature considered multiple algorithms in MLBDD approaches. Here we have addressed multiple algorithms as hybrid approaches. For instance, Sun et al. (2021) used hybrid approaches to predict coronary Heart disease using Gaussian Naïve Bayes, Bernoulli Naïve Bayes, and Random Forest (RF) algorithms [ 111 ]. Bemando et al. (2021) adopted CNN and SVM to automate the diagnosis of Alzheimer’s disease and mild cognitive impairment [ 41 ]. Saxena et al. (2019) used KNN and Decision Tree (DT) in Heart disease diagnosis [ 131 ]; Elsalamony (2018) employed Neural Networks (NN) and SVM in detecting Anaemia disease in human red blood cells [ 132 ]. One of the key benefits of using the hybrid technique is that it is more accurate than using single ML models.
According to the relevant literature, the most extensively utilized induvial algorithms in developing MLBDD models are CNN, SVM, and LR. For instance, Kalaiselvi et al. (2020) proposed CNN based approach in Brain tumor diagnosis [ 123 ]; Dai et al. (2019) used CNN in developing a device inference app for Skin cancer detection [ 118 ]; Fathi et al. (2020) used SVM to classify liver diseases [ 121 ]; Sing et al. (2019) used SVM to classify the patients with Heart disease symptoms [ 43 ]; and Basheer et al. (2019) used Logistic Regression to detect Heart disease [ 133 ].
Figure 10 depicts the most commonly used Machine Learning algorithms in disease diagnosis. The bolder and larger font emphasizes the importance and frequency with which the algorithms in MLBDD are used. Based on the Figure, we can observe that Neural Networks, CNN, SVM, and Logistic Regression are the most commonly employed algorithms by MLBDD researchers.

Word cloud for most frequently used ML algorithms in MLBDD publications.
Most MLBDD researchers utilize publically accessible datasets since they do not require permission and provide sufficient information to do the entire study. Manually gathering data from patients, on the other hand, is time-consuming; yet, numerous research utilized privately collected/owned data, either owing to their special necessity based on their experiment setup or to produce a result with actual data [ 46 , 55 , 56 , 68 , 70 ]. The Cleveland Heart disease dataset, PIMA dataset, and Parkinson dataset are the most often utilized datasets in disease diagnosis areas. Table 11 lists publicly available datasets and sources that may be useful to future academics and practitioners.
Most widely used disease diagnosis dataset URL along with the referenced literature (accessed on 16 December 2021).
7. Discussion
In the last 10 years, Machine Learning (ML) and Deep Learning (DL) have grown in prominence in disease diagnosis, which the annotated literature has strengthened in this study. The review began with specific research questions and attempted to answer them using the reference literature. According to the overall research, CNN is one of the most emerging algorithms, outperforming all other ML algorithms due to its solid performance with both image and tabular data [ 94 , 123 , 128 , 137 ]. Transfer learning is also gaining popularity since it does not necessitate constructing a CNN model from scratch and produces better results than typical ML methods [ 47 , 91 ]. Aside from CNN, the reference literature lists SVM, RF, and DT as some of the most common algorithms utilized widely in MLBDD. Furthermore, several researchers are emphasizing ensemble techniques in MLBDD [ 127 , 130 ]. Nonetheless, when compared to other ML algorithms, CNN is the most dominating. VGG16, VGG19, ResNet50, and UNet++ are among of the most prominent CNN architectures utilized widely in disease diagnosis.
In terms of databases, it was discovered that UCI repository data is the preferred option of academics and practitioners for constructing a Machine Learning-based Disease Diagnosis (MLBDD) model. However, while the current dataset frequently has shortcomings, several researchers have recently relied on additional data acquired from the hospital or clinic (i.e., imbalance data, missing data). To assist future researchers and practitioners interested in studying MLBDD, we have included a list of some of the most common datasets utilized in the reference literature in Table 11 , along with the link to the repository.
As previously indicated, there were several inconsistencies in terms of assessment measures published by the literature. For example, some research reported their results with accuracy [ 45 ]; others provided with accuracy, precision, recall, and F1-score [ 42 ]; while a few studies emphasized sensitivity, specificity, and true positive [ 67 ]. As a result, there were no criteria for the authors to follow in order to report their findings correctly and genuinely. Nonetheless, of all assessment criteria, accuracy is the most extensively utilized and recognized by academics.
With the emergence of COVID-19, MLBDD research switched mostly on Pneumonia and COVID-19 patient detection beginning in 2020, and COVID-19 remains a popular subject as the globe continues to battle this disease. As a result, it is projected that the application of ML and DL in the medical sphere for disease diagnosis would expand significantly in this domain in the future as well. Many questions have been raised due to the progress of ML and DL-based disease diagnosis. For example, if a doctor or other health practitioner incorrectly diagnoses a patient, he or she will be held accountable. However, if the machine does, who will be held accountable? Furthermore, fairness is an issue in ML because most ML models are skewed towards the majority class. As a result, future research should concentrate on ML ethics and fairness.
Model interpretation is absent in nearly all investigations, which is surprising. Interpreting machine learning models used to be difficult, but explainable and interpretable XAI have made it much easier. Despite the fact that the previous MLBDD lacked sufficient interpretations, it is projected that future researchers and practitioners would devote more attention to interpreting the machine learning model due to the growing demand for model interpretability.
The idea that ML alone will enough to construct an MLBDD model is a flawed one. To make the MLBDD model more dynamic, it may be anticipated that the model will need to be developed and stored on a cloud system, as the heath care industry generates a lot of data that is typically kept in cloud systems. As a result, the adversarial attack will focus on patients’ data, which is very sensitive. For future ML-based models, the data bridge and security challenges must be taken into consideration.
It is a major issue to analyze data if there is a large disparity in the data. As the ML-based diagnostic model deals with human life, every misdiagnosis is a possible danger to one’s health. However, despite the fact that many study used the imbalance dataset to perform their experiment, none of the cited literature highlights issues related to imbalance data. Thus, future work should demonstrate the validity of any ML models while developing with imbalanced data.
Within the many scopes this review paper also have some limitations which can be summarized as follows:
- 1. The study first searched the Scopus and WOS databases for relevant papers and then examined other papers that were pertinent to this investigation. If other databases like Google Scholar and Pubmed were used, the findings might be somewhat different. As a result, our study may provide some insight into MLBDD, but there is still a great deal of information that is outside of our control.
- 2. ML algorithms, DL algorithms, dataset, disease classifications, and evaluation metrics are highlighted in the review. Though the suggested ML process is thoroughly examined in reference literature, this paper does not go into that level of detail.
- 3. Only those publications that adhered to a systematic literature review technique were included in the study’s paper selection process. Using a more comprehensive range of keywords, on the other hand, might lead to higher search activity. However, our SLR approach will provide researchers and practitioners with a more thorough understanding of MLBDD.
8. Research Challenges and Future Agenda
While machine learning-based applications have been used extensively in disease diagnosis, researchers and practitioners still face several challenges when deploying them as a practical application in healthcare. In this section, the key challenges associated with ML in disease diagnosis have been summarized as follows:
8.1. Data Related Challenges
- 1. Data scarcity: Even though many patients’ data has been recorded by different hospitals and healthcare, due to the data privacy act, real-world data is not often available for global research purposes.
- 2. Noisy data: Frequently, the clinical data contains noise or missing values; therefore, such kind of data takes a reasonable amount of time to make it trainable.
- 3. Adversarial attack: Adversarial attack is one of the key issues in the disease dataset. Adversarial attack means the manipulation of training data, testing data, or machine learning model to result in wrong output from ML.
8.2. Disease Diagnosis-Related Challenges
- 1. Misclassification: While the machine learning model can be used to develop as a disease diagnosis model, any misclassification for a particular disease might bring severe damage. For instance, if a patient with stomach cancer is diagnosed as a non-cancer patient, it will have a huge impact.
- 2. Wrong image segmentation: One of the key challenges with the ML model is that the model often identifies the wrong region as an infected region. For instance, author Ahsan et al. (2020) shows that even though the accuracy is around 100% in detecting COVID-19 and non-COVID-19 patients, the pre-trained CNN models such as VGG16 and VGG19 often pay attention to the wrong region during the training process [ 2 ]. As a result, it also raises the question of the validity of the MLBDD.
- 3. Confusion: Some of the diseases such as COVID-19, pneumonia, edema in the chest often demonstrate similar symptoms; in these particular cases, many CNN models detect all of the data samples into one class, i.e., COVID-19.
8.3. Algorithm Related Challenges
- 1. Supervised vs. unsupervised: Most ML models (Linear regression, logistic regression) performed very well with the labeled data. However, similar algorithms’ performance was significantly reduced with the unlabeled data. On the other hand, popular algorithms that can perform well with unlabeled data such as K-means clustering, SVM, and KNNs performance also degraded with multidimensional data.
- 2. Blackbox-related challenges: One of the most widely used ML algorithms is convolutional neural networks. However, one of the key challenges associated with this algorithm is that it is often hard to interpret how the model adjusts internal parameters such as learning rate and weights. In healthcare, implementing such an algorithm-related model needs proper explanations.
8.4. Future Directions
The challenges addressed in the above section might give some future direction to future researchers and practitioners. Here we have introduced some of the possible algorithms and applications that might overcome existing MLBDD challenges.
- 1. GAN-based approach: Generative adversarial network is one of the most popular approaches in deep learning fields. Using this approach, it is possible to generate synthetic data which looks almost similar to the real data. Therefore, GAN might be a good option for handling data scarcity issues. Moreover, it will also reduce the dependency on real data and also will help to follow the data privacy act.
- 2. Explainable AI: Explainable AI is a popular domain that is now widely used to explain the algorithms’ behavior during training and prediction. Still, the explainable AI domains face many challenges; however, the implementation of interpretability and explainability clarifies the ML models’ deployment in the real world.
- 3. Ensemble-based approach: With the advancement of modern technology, we can now capture high resolutions and multidimensional data. While the traditional ML approach might not perform well with high-quality data, a combination of several machine learning models might be an excellent option to handle such high-dimensional data.
9. Conclusions and Potential Remarks
This study reviewed the papers published between 2012–2021 that focused on Machine Learning-based Disease Diagnosis (MLBDD). Researchers are particularly interested in some diseases, such as Heart disease, Breast cancer, Kidney disease, Diabetes, Alzheimer’s, and Parkinson’s diseases, which are discussed considering machine learning/deep learning-based techniques. Additionally, some other ML-based disease diagnosis approaches are discussed as well. Prior to that, A bibliometric analysis was performed, taking into account a variety of parameters such as subject area, publication year, journal, country, and identifying the most prominent contributors in the MLBDD field. According to our bibliometric research, machine learning applications in disease diagnosis have grown at an exponential rate since 2017. In terms of overall number of publications over the years, IEEE Access, Scientific Reports, and the International Journal of advanced computer science and applications are the three most productive journals. The three most-cited publications on MLBDD are those by Motwani et al. (2017), Gray et al. (2013), and Mohan et al. (2019). In terms of overall publications, China, the United States, and India are the three most productive countries. Kim J, the most influential author, published around 20 publications between 2012 and 2021, followed by Wang Y and Li J, who came in second and third place, respectively. Around 40% of the publication are from computer science domains and around 31% from engineering fields, demonstrating their domination in the MLBDD field.
Finally, we have systematically selected 102 papers for in-depth analysis. Our overall findings were highlighted in the discussion sections. Because of its remarkable performance in constructing a robust model, our primary conclusion implies that deep learning is the most popular method for researchers. Despite the fact that deep learning is widely applied in MLBDD fields, the majority of the research lacks sufficient explanations of the final predictions. As a result, future research in MLBDD needs focus on pre and post hoc analysis and model interpretation in order to use the ML model in healthcare.
Physical patient services are increasingly dangerous as a result of the emergence of COVID-19. At the same time, the health-care system must be maintained. While telemedicine and online consultation are becoming more popular, it is still important to consider an alternate strategy that may also highlight the importance of in-person health facilities. Many recent studies recommend home-robot service for patient care rather than hospitalization [ 138 ].
Many countries are increasingly worried about the privacy of patients’ data. Many nations have also raised legal concerns about the ethics of AI and ML when used with real-world patient data [ 139 ]. As a result, rather of depending on data gathering and processing, future study could try producing synthetic data. Some of the techniques that future researchers and practitioners may be interested in to produce synthetic data for the experiment include generative adversarial networks, ADASYN, SMOTE, and SVM-SMOTE.
Cloud systems are becoming potential threats as a result of data storage in it. As a result, any built ML models must safeguard patient access and transaction concerns. Many academics exploited blockchain technology to access and distribute data [ 140 , 141 ]. As a result, blockchain technology paired with deep learning and machine learning might be a promising study subject for constructing safe diagnostic systems.
We anticipate that our review will guide both novice and expert research and practitioners in MLBDD. It would be interesting to see some research work based on the limitations addressed in the discussion and conclusion section. Additionally, future works in MLBDD might focus on multiclass classification with highly imbalanced data along with highly missing data, explanation and Interpretation of multiclass data classification using XAI, and optimizing the big data containing numerical, categorical, and image data.
Author Contributions
Conceptualization—M.M.A.; methodology—M.M.A. and Z.S.; software—M.M.A.; validation—Z.S. and S.A.L.; formal analysis—S.A.L.; investigation—Z.S.; writing—original draft preparation—M.M.A.; writing—review and editing—M.M.A., S.A.L., Z.S.; supervision—Z.S. All authors have read and agreed to the published version of the manuscript.
This research received no external funding.
Conflicts of Interest
The authors declare no conflict of interest.
Publisher’s Note: MDPI stays neutral with regard to jurisdictional claims in published maps and institutional affiliations.
Explore advancements in Machine Learning
Javascript is disabled..
Please enable JavaScript for full page functionality.
All research
- Accessibility
- Computer Vision
- Data Science and Annotation
- Human-Computer Interaction
- Knowledge Bases and Search
- Methods and Algorithms
- Speech and Natural Language Processing
- Tools, Platforms, Frameworks
- Interspeech
- NeurIPS Workshop
- CVPR Workshop
- ICML Workshop
- EMNLP Workshop
- IEEE International Conference on Acoustics, Speech and Signal Processing
- ACM SIGSPATIAL
- ICCV Workshop
- KDD Workshop
- NAACL Workshop
- AAAI Workshop
- ACM Interaction Design and Children
- ACM Multimedia
- EACL Workshop
- IEEE BITS the Information Theory Magazine
- IEEE Signal Processing Letters
- IISE Transactions
- LREC-COLING
- Machine Learning: Science and Technology
- Nature Digital Medicine
- Nature - Scientific Reports
- Observational Studies
- Sane Workshop
- SIGGRAPH Asia
- SSW Workshop
- Transaction on Machine Learning Research
- When Creative AI Meets Conversational AI Workshop
MobileCLIP: Fast Image-Text Models through Multi-Modal Reinforced Training
Streaming anchor loss: augmenting supervision with temporal significance, towards a world-english language model, a multi-signal large language model for device-directed speech detection, tic-clip: continual training of clip models, mm1: methods, analysis & insights from multimodal llm pre-training, enhancing paragraph generation with a latent language diffusion model, construction of paired knowledge graph - text datasets informed by cyclic evaluation, personalizing health and fitness with hybrid modeling, corpus synthesis for zero-shot asr domain adaptation using large language models, motionprint: ready-to-use, device-agnostic, and location-invariant motion activity models, randomized algorithms for precise measurement of differentially-private, personalized recommendations, veclip: improving clip training via visual-enriched captions, interpreting and replaying accessibility tests from natural language for streamlined qa efforts, merge vision foundation models via multi-task distillation, moonwalk: advancing gait-based user recognition on wearable devices with metric learning, vision-based hand gesture customization from a single demonstration, humanizing word error rate for asr transcript readability and accessibility, human following in mobile platforms with person re-identification, privacy-preserving quantile treatment effect estimation for randomized controlled trials, synthdst: synthetic data is all you need for few-shot dialog state tracking, what can clip learn from task-specific experts, multichannel voice trigger detection based on transform-average-concatenate, keyframer: empowering animation design using large language models, resource-constrained stereo singing voice cancellation, efficient convbn blocks for transfer learning and beyond, the entity-deduction arena: a playground for probing the conversational reasoning and planning capabilities of llms, differentially private heavy hitter detection using federated analytics, scalable pre-training of large autoregressive image models, acoustic model fusion for end-to-end speech recognition, co-ml: collaborative machine learning model building for developing dataset design practices, flexible keyword spotting based on homogeneous audio-text embedding, investigating salient representations and label variance modeling in dimensional speech emotion analysis, large-scale training of foundation models for wearable biosignals, user-level differentially private stochastic convex optimization: efficient algorithms with optimal rates, bin prediction for better conformal prediction, fastsr-nerf: improving nerf efficiency on consumer devices with a simple super-resolution pipeline, hybrid model learning for cardiovascular biomarkers inference, improving vision-inspired keyword spotting using a streaming conformer encoder with input-dependent dynamic depth, simulation-based inference for cardiovascular models, unbalanced low-rank optimal transport solvers, personalization of ctc-based end-to-end speech recognition using pronunciation-driven subword tokenization, deploying attention-based vision transformers to apple neural engine, transformers learn through gradual rank increase, importance of smoothness induced by optimizers in fl4asr: towards understanding federated learning for end-to-end asr, advancing speech accessibility with personal voice, bootstrap your own variance, context tuning for retrieval augmented generation, datacomp: in search of the next generation of multimodal datasets, leveraging large language models for exploiting asr uncertainty, modality dropout for multimodal device directed speech detection using verbal and non-verbal features, training large-vocabulary neural language model by private federated learning for resource-constrained devices, lidar: sensing linear probing performance in joint embedding ssl architectures, deeppcr: parallelizing sequential operations in neural networks, hugs: human gaussian splats, multimodal data and resource efficient device-directed speech detection with large foundation models, controllable music production with diffusion models and guidance gradients, fast optimal locally private mean estimation via random projections, generating molecular conformers with manifold diffusion fields, how to scale your ema, manifold diffusion fields, pre-trained language models do not help auto-regressive text-to-image generation, 4m: massively multimodal masked modeling, adaptive weight decay, fleek: factual error detection and correction with evidence retrieved from external knowledge, one wide feedforward is all you need, relu strikes back: exploiting activation sparsity in large language models, agnostically learning single-index models using omnipredictors, characterizing omniprediction via multicalibration, federated learning for speech recognition: revisiting current trends towards large-scale asr, increasing coverage and precision of textual information in multilingual knowledge graphs, sam-clip: merging vision foundation models towards semantic and spatial understanding, what algorithms can transformers learn a study in length generalization, marrs: multimodal reference resolution system, automating behavioral testing in machine translation, diffusion models as masked audio-video learners, improved ddim sampling with moment matching gaussian mixtures, planner: generating diversified paragraph via latent language diffusion model, eelbert: tiny models through dynamic embeddings, semand: self-supervised anomaly detection in multimodal geospatial datasets, steer: semantic turn extension-expansion recognition for voice assistants, identifying controversial pairs in item-to-item recommendations, delphi: data for evaluating llms' performance in handling controversial issues, towards real-world streaming speech translation for code-switched speech, livepose: online 3d reconstruction from monocular video with dynamic camera poses, never-ending learning of user interfaces, slower respiration rate is associated with higher self-reported well-being after wellness training, when does optimizing a proper loss yield calibration, single-stage diffusion nerf: a unified approach to 3d generation and reconstruction, fastvit: a fast hybrid vision transformer using structural reparameterization, hyperdiffusion: generating implicit neural fields with weight-space diffusion, neilf++: inter-reflectable light fields for geometry and material estimation, gender bias in llms, reinforce data, multiply impact: improved model accuracy and robustness with dataset reinforcement, self-supervised object goal navigation with in-situ finetuning, all about sample-size calculations for a/b testing: novel extensions and practical guide, intelligent assistant language understanding on-device, rapid and scalable bayesian ab testing, consistent collaborative filtering via tensor decomposition, dataset and network introspection toolkit (dnikit), finerecon: depth-aware feed-forward network for detailed 3d reconstruction, improving the quality of neural tts using long-form content and multi-speaker multi-style modeling, voice trigger system for siri, conformalization of sparse generalized linear models, duet: 2d structured and equivariant representations, pdp: parameter-free differentiable pruning is all you need, population expansion for training language models with private federated learning, resolving the mixing time of the langevin algorithm to its stationary distribution for log-concave sampling, the role of entropy and reconstruction for multi-view self-supervised learning, upscale: unconstrained channel pruning, learning iconic scenes with differential privacy, nerfdiff: single-image view synthesis with nerf-guided distillation from 3d-aware diffusion, referring to screen texts with voice assistants, towards multimodal multitask scene understanding models for indoor mobile agents, 5ider: unified query rewriting for steering, intent carryover, disfluencies, entity carryover and repair, monge, bregman and occam: interpretable optimal transport in high-dimensions with feature-sparse maps, private online prediction from experts: separations and faster rates, spatial librispeech: an augmented dataset for spatial audio learning, stabilizing transformer training by preventing attention entropy collapse, the monge gap: a regularizer to learn all transport maps, cross-lingual knowledge transfer and iterative pseudo-labeling for low-resource speech recognition with transducers, symphony: composing interactive interfaces for machine learning, a unifying theory of distance from calibration, approximate nearest neighbour phrase mining for contextual speech recognition, latent phrase matching for dysarthric speech, matching latent encoding for audio-text based keyword spotting, near-optimal algorithms for private online optimization in the realizable regime, roomdreamer: text-driven 3d indoor scene synthesis with coherent geometry and texture, semi-supervised and long-tailed object detection with cascadematch, less is more: a unified architecture for device-directed speech detection with multiple invocation types, collaborative machine learning model building with families using co-ml, efficient multimodal neural networks for trigger-less voice assistants, fast class-agnostic salient object segmentation, application-agnostic language modeling for on-device asr, actionable data insights for machine learning, growing and serving large open-domain knowledge graphs, robustness in multimodal learning under train-test modality mismatch, learning language-specific layers for multilingual machine translation, modeling spoken information queries for virtual assistants: open problems, challenges and opportunities, learning to detect novel and fine-grained acoustic sequences using pretrained audio representations, pointconvformer: revenge of the point-based convolution, state spaces aren’t enough: machine translation needs attention, improved speech recognition for people who stutter, autofocusformer: image segmentation off the grid, joint speech transcription and translation: pseudo-labeling with out-of-distribution data, from robustness to privacy and back, generalization on the unseen, logic reasoning and degree curriculum, self-supervised temporal analysis of spatiotemporal data, considerations for distribution shift robustness in health, f-dm: a multi-stage diffusion model via progressive signal transformation, high-throughput vector similarity search in knowledge graphs, naturalistic head motion generation from speech, on the role of lip articulation in visual speech perception, facelit: neural 3d relightable faces, angler: helping machine translation practitioners prioritize model improvements, feedback effect in user interaction with intelligent assistants: delayed engagement, adaption and drop-out, text is all you need: personalizing asr models using controllable speech synthesis, pointersect: neural rendering with cloud-ray intersection, continuous pseudo-labeling from the start, mobileone: an improved one millisecond mobile backbone, neural transducer training: reduced memory consumption with sample-wise computation, tract: denoising diffusion models with transitive closure time-distillation, variable attention masking for configurable transformer transducer speech recognition, from user perceptions to technical improvement: enabling people who stutter to better use speech recognition, i see what you hear: a vision-inspired method to localize words, more speaking or more speakers, pre-trained model representations and their robustness against noise for speech emotion analysis, improvements to embedding-matching acoustic-to-word asr using multiple-hypothesis pronunciation-based embeddings, mast: masked augmentation subspace training for generalizable self-supervised priors, paedid: patch autoencoder-based deep image decomposition for unsupervised anomaly detection, rgi: robust gan-inversion for mask-free image inpainting and unsupervised pixel-wise anomaly detection, robust hybrid learning with expert augmentation, self supervision does not help natural language supervision at scale, audio-to-intent using acoustic-textual subword representations from end-to-end asr, fastfill: efficient compatible model update, loss minimization through the lens of outcome indistinguishability, mobilebrick: building lego for 3d reconstruction on mobile devices, diffusion probabilistic fields, heimdal: highly efficient method for detection and localization of wake-words, designing data: proactive data collection and iteration for machine learning, improving human annotation effectiveness for fact collection by identifying the most relevant answers, rangeaugment: efficient online augmentation with range learning, languages you know influence those you learn: impact of language characteristics on multi-lingual text-to-text transfer, active learning with expected error reduction, stable diffusion with core ml on apple silicon, supervised training of conditional monge maps, modeling heart rate response to exercise with wearable data, shift-curvature, sgd, and generalization, destseg: segmentation guided denoising student-teacher for anomaly detection, symbol guided hindsight priors for reward learning from human preferences, beyond cage: investigating generalization of learned autonomous network defense policies, rewards encoding environment dynamics improves preference-based reinforcement learning, homomorphic self-supervised learning, continuous soft pseudo-labeling in asr, elastic weight consolidation improves the robustness of self-supervised learning methods under transfer, mean estimation with user-level privacy under data heterogeneity, learning to break the loop: analyzing and mitigating repetitions for neural text generation, learning to reason with neural networks: generalization, unseen data and boolean measures, subspace recovery from heterogeneous data with non-isotropic noise, a large-scale observational study of the causal effects of a behavioral health nudge, improving generalization with physical equations, maeeg: masked auto-encoder for eeg representation learning, mbw: multi-view bootstrapping in the wild, statistical deconvolution for inference of infection time series, ape: aligning pretrained encoders to quickly learn aligned multimodal representations, emphasis control for parallel neural tts, the slingshot mechanism: an empirical study of adaptive optimizers and the grokking phenomenon, a treatise on fst lattice based mmi training, non-autoregressive neural machine translation: a call for clarity, prompting for a conversation: how to control a dialog model, fusion-id: a photoplethysmography and motion sensor fusion biometric authenticator with few-shot on-boarding, latent temporal flows for multivariate analysis of wearables data, the calibration generalization gap, spin: an empirical evaluation on sharing parameters of isotropic networks, learning bias-reduced word embeddings using dictionary definitions, low-rank optimal transport: approximation, statistics and debiasing, safe real-world reinforcement learning for mobile agent obstacle avoidance, speech emotion: investigating model representations, multi-task learning and knowledge distillation, texturify: generating textures on 3d shape surfaces, 3d parametric room representation with roomplan, generative multiplane images: making a 2d gan 3d-aware, flair: federated learning annotated image repository, privacy of noisy stochastic gradient descent: more iterations without more privacy loss, two-layer bandit optimization for recommendations, gaudi: a neural architect for immersive 3d scene generation, physiomtl: personalizing physiological patterns using optimal transport multi-task regression, toward supporting quality alt text in computing publications, providing insights for open-response surveys via end-to-end context-aware clustering, layer-wise data-free cnn compression, rgb-x classification for electronics sorting, aspanformer: detector-free image matching with adaptive span transformer, mel spectrogram inversion with stable pitch, multi-objective hyper-parameter optimization of behavioral song embeddings, improving voice trigger detection with metric learning, neilf: neural incident light field for material and lighting estimation, combining compressions for multiplicative size scaling on natural language tasks, integrating categorical features in end-to-end asr, cvnets: high performance library for computer vision, space-efficient representation of entity-centric query language models, a dense material segmentation dataset for indoor and outdoor scene parsing, benign, tempered, or catastrophic: a taxonomy of overfitting, forml: learning to reweight data for fairness, regularized training of nearest neighbor language models, minimax demographic group fairness in federated learning, neuman: neural human radiance field from a single video, device-directed speech detection: regularization via distillation for weakly-supervised models, vocal effort modeling in neural tts for improving the intelligibility of synthetic speech in noise, reachability embeddings: self-supervised representation learning from spatiotemporal motion trajectories for multimodal geospatial computer vision, dynamic memory for interpretable sequential optimization, artonomous: introducing middle school students to reinforcement learning through virtual robotics, efficient representation learning via adaptive context pooling, leveraging entity representations, dense-sparse hybrids, and fusion-in-decoder for cross-lingual question answering, optimal algorithms for mean estimation under local differential privacy, position prediction as an effective pre-training strategy, private frequency estimation via projective geometry, self-conditioning pre-trained language models, style equalization: unsupervised learning of controllable generative sequence models, critical regularizations for neural surface reconstruction in the wild, a multi-task neural architecture for on-device scene analysis, deploying transformers on the apple neural engine, neural face video compression using multiple views, efficient multi-view stereo via attention-driven 2d convolutions, robust joint shape and pose optimization for few-view object reconstruction, forward compatible training for large-scale embedding retrieval systems, bilingual end-to-end asr with byte-level subwords, end-to-end speech translation for code switched speech, streaming on-device detection of device directed speech from voice and touch-based invocation, training a tokenizer for free with private federated learning, utilizing imperfect synthetic data to improve speech recognition, data incubation - synthesizing missing data for handwriting recognition, a platform for continuous construction and serving of knowledge at scale, neo: generalizing confusion matrix visualization to hierarchical and multi-output labels, low-resource adaptation of open-domain generative chatbots, differentiable k-means clustering layer for neural network compression, enabling hand gesture customization on wrist-worn devices, learning compressed embeddings for on-device inference, neural fisher kernel: low-rank approximation and knowledge distillation, towards complete icon labeling in mobile applications, understanding screen relationships from screenshots of smartphone applications, mobilevit: light-weight, general-purpose, and mobile-friendly vision transformer, synthetic defect generation for display front-of-screen quality inspection: a survey, differential secrecy for distributed data and applications to robust differentially secure vector summation, information gain propagation: a new way to graph active learning with soft labels, can open domain question answering models answer visual knowledge questions, non-verbal sound detection for disordered speech, hierarchical prosody modeling and control in non-autoregressive parallel neural tts, element level differential privacy: the right granularity of privacy, learning spatiotemporal occupancy grid maps for lifelong navigation in dynamic scenes, collaborative filtering via tensor decomposition, modeling the impact of user mobility on covid-19 infection rates over time, fast and explicit neural view synthesis, federated evaluation and tuning for on-device personalization: system design & applications, lyric document embeddings for music tagging, acoustic neighbor embeddings, model stability with continuous data updates, reconstructing training data from diverse ml models by ensemble inversion, fair sa: sensitivity analysis for fairness in face recognition, learning invariant representations with missing data, interpretable adaptive optimization, robust robotic control from pixels using contrastive recurrent state-space models, challenges of adversarial image augmentations, self-supervised semi-supervised learning for data labeling and quality evaluation, batchquant: quantized-for-all architecture search with robust quantizer, high fidelity 3d reconstructions with limited physical views, arkitscenes - a diverse real-world dataset for 3d indoor scene understanding using mobile rgb-d data, do self-supervised and supervised methods learn similar visual representations, enforcing fairness in private federated learning via the modified method of differential multipliers, individual privacy accounting via a renyi filter, probabilistic attention for interactive segmentation, rim: reliable influence-based active learning on graphs, stochastic contrastive learning, it’s complicated: characterizing the time-varying relationship between cell phone mobility and covid-19 spread in the us, plan-then-generate: controlled data-to-text, randomized controlled trials without data retention, interdependent variables: remotely designing tactile graphics for an accessible workflow, breiman's two cultures: you don't have to choose sides, cross-domain data integration for entity disambiguation in biomedical text, evaluating the fairness of fine-tuning strategies in self-supervised learning, learning compressible subspaces for adaptive network compression at inference time, mmiu: dataset for visual intent understanding in multimodal assistants, on-device neural speech synthesis, on-device panoptic segmentation for camera using transformers, entity-based knowledge conflicts in question answering, finding experts in transformer models, deeppro: deep partial point cloud registration of objects, using pause information for more accurate entity recognition, conditional generation of synthetic geospatial images from pixel-level and feature-level inputs, multi-task learning with cross attention for keyword spotting, screen parsing: towards reverse engineering of ui models from screenshots, self-supervised learning of lidar segmentation for autonomous indoor navigation, a survey on privacy from statistical, information and estimation-theoretic views, improving neural network subspaces, combining speakers of multiple languages to improve quality of neural voices, audiovisual speech synthesis using tacotron2, user-initiated repetition-based recovery in multi-utterance dialogue systems, dexter: deep encoding of external knowledge for named entity recognition in virtual assistants, managing ml pipelines: feature stores and the coming wave of embedding ecosystems, smooth sequential optimization with delayed feedback, learning to generate radiance fields of indoor scenes, estimating respiratory rate from breath audio obtained through wearable microphones, online automatic speech recognition with listen, attend and spell model, subject-aware contrastive learning for biosignals, hypersim: a photorealistic synthetic dataset for holistic indoor scene understanding, model-based metrics: sample-efficient estimates of predictive model subpopulation performance, retrievalfuse: neural 3d scene reconstruction with a database, joint learning of portrait intrinsic decomposition and relighting, non-parametric differentially private confidence intervals for the median, recognizing people in photos through private on-device machine learning, unconstrained scene generation with locally conditioned radiance fields, trinity: a no-code ai platform for complex spatial datasets, a simple and nearly optimal analysis of privacy amplification by shuffling, when is memorization of irrelevant training data necessary for high-accuracy learning, implicit acceleration and feature learning in infinitely wide neural networks with bottlenecks, a discriminative entity aware language model for virtual assistants, analysis and tuning of a voice assistant system for dysfluent speech, implicit greedy rank learning in autoencoders via overparameterized linear networks, learning neural network subspaces, lossless compression of efficient private local randomizers, private adaptive gradient methods for convex optimization, private stochastic convex optimization: optimal rates in ℓ1 geometry, streaming transformer for hardware efficient voice trigger detection and false trigger mitigation, tensor programs iib: architectural universality of neural tangent kernel training dynamics, uncertainty weighted actor-critic for offline reinforcement learning, spatio-temporal context for action detection, bootleg: self-supervision for named entity disambiguation, instance-level task parameters: a robust multi-task weighting framework, morphgan: controllable one-shot face synthesis, evaluating entity disambiguation and the role of popularity in retrieval-based nlp, hdr environment map estimation for real-time augmented reality, extracurricular learning: knowledge transfer beyond empirical distribution, learning to optimize black-box evaluation metrics, on the role of visual cues in audiovisual speech enhancement, an attention free transformer, cread: combined resolution of ellipses and anaphora in dialogues, dynamic curriculum learning via data parameters for noise robust keyword spotting, error-driven pruning of language models for virtual assistants, knowledge transfer for efficient on-device false trigger mitigation, multimodal punctuation prediction with contextual dropout, noise-robust named entity understanding for virtual assistants, on the transferability of minimal prediction preserving inputs in question answering, open-domain question answering goes conversational via question rewriting, optimize what matters: training dnn-hmm keyword spotting model using end metric, progressive voice trigger detection: accuracy vs latency, sapaugment: learning a sample adaptive policy for data augmentation, making mobile applications accessible with machine learning, video frame interpolation via structure-motion based iterative feature fusion, when can accessibility help an exploration of accessibility feature recommendation on mobile devices, streaming models for joint speech recognition and translation, neural feature selection for learning to rank, generating natural questions from images for multimodal assistants, a comparison of question rewriting methods for conversational passage retrieval, screen recognition: creating accessibility metadata for mobile applications from pixels, set distribution networks: a generative model for sets of images, sep-28k: a dataset for stuttering event detection from podcasts with people who stutter, question rewriting for end to end conversational question answering, leveraging query resolution and reading comprehension for conversational passage retrieval, learning soft labels via meta learning, whispered and lombard neural speech synthesis, frame-level specaugment for deep convolutional neural networks in hybrid asr systems, cinematic l1 video stabilization with a log-homography model, on the generalization of learning-based 3d reconstruction, improving human-labeled data through dynamic automatic conflict resolution, what neural networks memorize and why: discovering the long tail via influence estimation, collegial ensembles, faster differentially private samplers via rényi divergence analysis of discretized langevin mcmc, on the error resistance of hinge-loss minimization, representing and denoising wearable ecg recordings, stability of stochastic gradient descent on nonsmooth convex losses, stochastic optimization with laggard data pipelines, a wrong answer or a wrong question an intricate relationship between question reformulation and answer selection in conversational question answering, conversational semantic parsing for dialog state tracking, efficient inference for neural machine translation, how effective is task-agnostic data augmentation for pre-trained transformers, generating synthetic images by combining pixel-level and feature-level geospatial conditional inputs, making smartphone augmented reality apps accessible, mage: fluid moves between code and graphical work in computational notebooks, rescribe: authoring and automatically editing audio descriptions, class lm and word mapping for contextual biasing in end-to-end asr, complementary language model and parallel bi-lrnn for false trigger mitigation, controllable neural text-to-speech synthesis using intuitive prosodic features, hybrid transformer and ctc networks for hardware efficient voice triggering, improving on-device speaker verification using federated learning with privacy, stacked 1d convolutional networks for end-to-end small footprint voice trigger detection, downbeat tracking with tempo-invariant convolutional neural networks, modality dropout for improved performance-driven talking faces, enhanced direct delta mush, learning insulin-glucose dynamics in the wild, double-talk robust multichannel acoustic echo cancellation using least squares mimo adaptive filtering: transversal, array, and lattice forms, mkqa: a linguistically diverse benchmark for multilingual open domain question answering, improving discrete latent representations with differentiable approximation bridges, adascale sgd: a user-friendly algorithm for distributed training, a generative model for joint natural language understanding and generation, equivariant neural rendering, learning to branch for multi-task learning, variational neural machine translation with normalizing flows, predicting entity popularity to improve spoken entity recognition by virtual assistants, robust multichannel linear prediction for online speech dereverberation using weighted householder least squares lattice adaptive filter, scalable multilingual frontend for tts, generalized reinforcement meta learning for few-shot optimization, learning to rank intents in voice assistants, detecting emotion primitives from speech and their use in discerning categorical emotions, lattice-based improvements for voice triggering using graph neural networks, automatic class discovery and one-shot interactions for acoustic activity recognition, tempura: query analysis with structural templates, understanding and visualizing data iteration in machine learning, multi-task learning for voice trigger detection, speech translation and the end-to-end promise: taking stock of where we are, embedded large-scale handwritten chinese character recognition, generating multilingual voices using speaker space translation based on bilingual speaker data, leveraging gans to improve continuous path keyboard input models, least squares binary quantization of neural networks, unsupervised style and content separation by minimizing mutual information for speech synthesis, sndcnn: self-normalizing deep cnns with scaled exponential linear units for speech recognition, on modeling asr word confidence, capsules with inverted dot-product attention routing, improving language identification for multilingual speakers, multi-task learning for speaker verification and voice trigger detection, stochastic weight averaging in parallel: large-batch training that generalizes well, adversarial fisher vectors for unsupervised representation learning, app usage predicts cognitive ability in older adults, filter distillation for network compression, multiple futures prediction, an exploration of data augmentation and sampling techniques for domain-agnostic question answering, data parameters: a new family of parameters for learning a differentiable curriculum, nonlinear conjugate gradients for scaling synchronous distributed dnn training, modeling patterns of smartphone usage and their relationship to cognitive health, worst cases policy gradients, empirical evaluation of active learning techniques for neural mt, skip-clip: self-supervised spatiotemporal representation learning by future clip order ranking, single training dimension selection for word embedding with pca, overton: a data system for monitoring and improving machine-learned products, leveraging user engagement signals for entity labeling in a virtual assistant, reverse transfer learning: can word embeddings trained for different nlp tasks improve neural language models, variational saccading: efficient inference for large resolution images, jointly learning to align and translate with transformer models, connecting and comparing language model interpolation techniques, active learning for domain classification in a commercial spoken personal assistant, coarse-to-fine optimization for speech enhancement, developing measures of cognitive impairment in the real world from consumer-grade multimodal sensor streams, raise to speak: an accurate, low-power detector for activating voice assistants on smartwatches, language identification from very short strings, learning conditional error model for simulated time-series data, bridging the domain gap for neural models, improving knowledge base construction from robust infobox extraction, protection against reconstruction and its applications in private federated learning, data platform for machine learning, speaker-independent speech-driven visual speech synthesis using domain-adapted acoustic models, addressing the loss-metric mismatch with adaptive loss alignment, lower bounds for locally private estimation via communication complexity, exploring retraining-free speech recognition for intra-sentential code-switching, parametric cepstral mean normalization for robust speech recognition, voice trigger detection from lvcsr hypothesis lattices using bidirectional lattice recurrent neural networks, neural network-based modeling of phonetic durations, mirroring to build trust in digital assistants, foundationdb record layer: a multi-tenant structured datastore, leveraging acoustic cues and paralinguistic embeddings to detect expression from voice, bandwidth embeddings for mixed-bandwidth speech recognition, sliced wasserstein discrepancy for unsupervised domain adaptation, towards learning multi-agent negotiations via self-play, optimizing siri on homepod in far‑field settings, can global semantic context improve neural language models, a new benchmark and progress toward improved weakly supervised learning, finding local destinations with siri’s regionally specific language models for speech recognition, personalized hey siri, structured control nets for deep reinforcement learning, learning with privacy at scale, an on-device deep neural network for face detection, hey siri: an on-device dnn-powered voice trigger for apple’s personal assistant, real-time recognition of handwritten chinese characters spanning a large inventory of 30,000 characters, deep learning for siri’s voice: on-device deep mixture density networks for hybrid unit selection synthesis, inverse text normalization as a labeling problem, improving neural network acoustic models by cross-bandwidth and cross-lingual initialization, learning from simulated and unsupervised images through adversarial training, improving the realism of synthetic images.
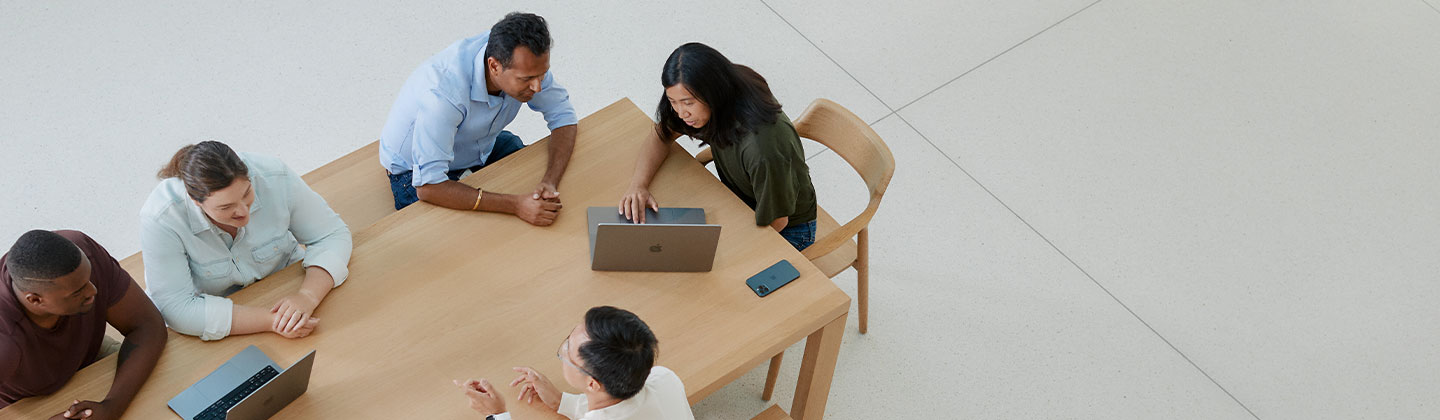
Discover opportunities in Machine Learning.
Our research in machine learning breaks new ground every day.
Work with us

A novel machine learning model for the characterization of material surfaces
Machine learning (ML) enables the accurate and efficient computation of fundamental electronic properties of binary and ternary oxide surfaces, as shown by scientists. Their ML-based model could be extended to other compounds and properties. The present research findings can aid in the screening of surface properties of materials as well as in the development of functional materials.
The design and development of novel materials with superior properties demands a comprehensive analysis of their atomic and electronic structures. Electron energy parameters such as ionization potential (IP), the energy needed to remove an electron from the valence band maximum, and electron affinity (EA), the amount of energy released upon the attachment of an electron to the conduction band minimum, reveal important information about the electronic band structure of surfaces of semiconductors, insulators, and dielectrics. The accurate estimation of IPs and EAs in such nonmetallic materials can indicate their applicability for use as functional surfaces and interfaces in photosensitive equipment and optoelectronic devices.
Additionally, IPs and EAs depend significantly on the surface structures, which adds another dimension to the complex procedure of their quantification. Traditional computation of IPs and EAs involves the use of accurate first-principles calculations, where the bulk and surface systems are separately quantified. This time-consuming process prevents quantifying IPs and EAs for many surfaces, which necessitates the use of computationally efficient approaches.
To address the wide-ranging issues affecting the quantification of IPs and EAs of nonmetallic solids, a team of scientists from Tokyo Institute of Technology (Tokyo Tech), led by Professor Fumiyasu Oba, have turned their focus towards machine learning (ML). Their research findings have been published in the Journal of the American Chemical Society .
Prof. Oba shares the motivation behind the present research, "In recent years, ML has gained a lot of attention in materials science research. The ability to virtually screen materials based on ML technology is a very efficient way to explore novel materials with superior properties. Also, the ability to train large datasets using accurate theoretical calculations allows for the successful prediction of important surface characteristics and their functional implications."
The researchers employed an artificial neural network to develop a regression model, incorporating the smooth overlap of atom positions (SOAPs) as numerical input data. Their model accurately and efficiently predicted the IPs and EAs of binary oxide surfaces by using the information on bulk crystal structures and surface termination planes.
Moreover, the ML-based prediction model could 'transfer learning,' a scenario where a model developed for a particular purpose can be made to incorporate newer datasets and reapplied for additional tasks. The scientists included the effects of multiple cations in their model by developing 'learnable' SOAPs and predicted the IPs and EAs of ternary oxides using transfer learning.
Prof. Oba concludes by saying, "Our model is not restricted to the prediction of surface properties of oxides but can be extended to study other compounds and their properties."
- Children's Health
- Controlled Substances
- Medical Topics
- Diseases and Conditions
- Pharmacology
- Tissue engineering
- Artificial neural network
- Computational neuroscience
- Nitrous oxide
Story Source:
Materials provided by Tokyo Institute of Technology . Note: Content may be edited for style and length.
Journal Reference :
- Shin Kiyohara, Yoyo Hinuma, Fumiyasu Oba. Band Alignment of Oxides by Learnable Structural-Descriptor-Aided Neural Network and Transfer Learning . Journal of the American Chemical Society , 2024; 146 (14): 9697 DOI: 10.1021/jacs.3c13574
Cite This Page :
Explore More
- Quantum Effects in Electron Waves
- Star Trek's Holodeck Recreated Using ChatGPT
- Cloud Engineering to Mitigate Global Warming
- Detecting Delayed Concussion Recovery
- Genes for Strong Muscles: Healthy Long Life
- Brightest Gamma-Ray Burst
- Stellar Winds of Three Sun-Like Stars Detected
- Fences Causing Genetic Problems for Mammals
- Ozone Removes Mating Barriers Between Fly ...
- Parkinson's: New Theory On Origins and Spread
Trending Topics
Strange & offbeat.
share this!
April 12, 2024
This article has been reviewed according to Science X's editorial process and policies . Editors have highlighted the following attributes while ensuring the content's credibility:
fact-checked
peer-reviewed publication
trusted source
A novel machine learning model for the characterization of material surfaces
by Tokyo Institute of Technology
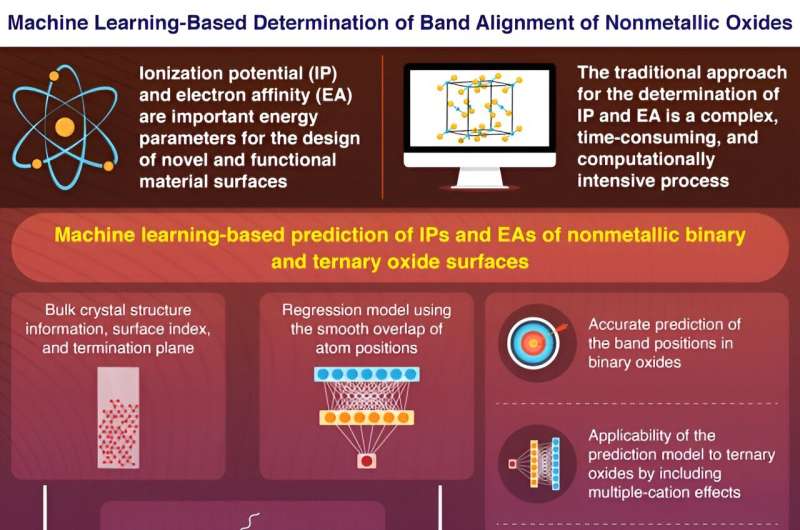
Machine learning (ML) enables the accurate and efficient computation of fundamental electronic properties of binary and ternary oxide surfaces, as shown by scientists from Tokyo Tech. Their ML-based model could be extended to other compounds and properties. The findings, published in the Journal of the American Chemical Society , could aid in the screening of surface properties of materials as well as in the development of functional materials.
The design and development of novel materials with superior properties demands a comprehensive analysis of their atomic and electronic structures.
Electron energy parameters such as ionization potential (IP), the energy needed to remove an electron from the valence band maximum, and electron affinity (EA), the amount of energy released upon the attachment of an electron to the conduction band minimum, reveal important information about the electronic band structure of surfaces of semiconductors, insulators, and dielectrics.
The accurate estimation of IPs and EAs in such nonmetallic materials can indicate their applicability for use as functional surfaces and interfaces in photosensitive equipment and optoelectronic devices.
Additionally, IPs and EAs depend significantly on the surface structures, which adds another dimension to the complex procedure of their quantification. Traditional computation of IPs and EAs involves the use of accurate first-principles calculations, where the bulk and surface systems are separately quantified. This time-consuming process prevents quantifying IPs and EAs for many surfaces, which necessitates the use of computationally efficient approaches.
To address the wide-ranging issues affecting the quantification of IPs and EAs of nonmetallic solids, a team of scientists from Tokyo Institute of Technology (Tokyo Tech), led by Professor Fumiyasu Oba, have turned their focus towards ML.
Prof. Oba says, "In recent years, ML has gained a lot of attention in materials science research. The ability to virtually screen materials based on ML technology is a very efficient way to explore novel materials with superior properties. Also, the ability to train large datasets using accurate theoretical calculations allows for the successful prediction of important surface characteristics and their functional implications."
The researchers employed an artificial neural network to develop a regression model , incorporating the smooth overlap of atom positions (SOAPs) as numerical input data. Their model accurately and efficiently predicted the IPs and EAs of binary oxide surfaces by using the information on bulk crystal structures and surface termination planes.
Moreover, the ML-based prediction model could "transfer learning," a scenario where a model developed for a particular purpose can be made to incorporate newer datasets and reapplied for additional tasks. The scientists included the effects of multiple cations in their model by developing "learnable" SOAPs and predicted the IPs and EAs of ternary oxides using transfer learning.
Prof. Oba concludes, "Our model is not restricted to the prediction of surface properties of oxides but can be extended to study other compounds and their properties."
Journal information: Journal of the American Chemical Society
Provided by Tokyo Institute of Technology
Explore further
Feedback to editors

Aboriginal people made pottery, sailed to distant islands thousands of years before Europeans arrived
6 hours ago

The experimental demonstration of a verifiable blind quantum computing protocol
Apr 13, 2024

A machine learning-based approach to discover nanocomposite films for biodegradable plastic alternatives

Saturday Citations: Listening to bird dreams, securing qubits, imagining impossible billiards

Physicists solve puzzle about ancient galaxy found by Webb telescope

Researchers study effects of solvation and ion valency on metallopolymers

Chemists devise easier new method for making a common type of building block for drugs

Research team discovers more than 50 potentially new deep-sea species in one of the most unexplored areas of the planet
Apr 12, 2024

New study details how starving cells hijack protein transport stations

New species of ant found pottering under the Pilbara named after Voldemort
Relevant physicsforums posts, can you eat the periodic table, zirconium versus zirconium carbide for use with galinstan.
Mar 29, 2024
Electrolysis: Dark blue oxide from steel?
Mar 28, 2024
Identification of HOMO/LUMO in radicals
Mar 27, 2024
What is the Role of Energy in Quantum Hybridized Orbitals?
New insight into the chemistry of solvents.
Mar 23, 2024
More from Chemistry
Related Stories

New method provides automated calculation of surface properties in crystals

Artificial intelligence helps to identify correct atomic structures
Nov 2, 2020

Using AI to develop hydrogen fuel cell catalysts more efficiently and economically
Oct 18, 2023

AI predicts extensive material properties to break down a previously insurmountable wall
Oct 18, 2021

Researchers uncover new avenues for finding unique class of insulators
Jul 25, 2017

Observing individual atoms in 3D nanomaterials and their surfaces
May 12, 2021
Recommended for you

Scientists find new paths to steer and optimize electrochemical processes
Apr 11, 2024

Scientists find new ways to convert inhibitors into degraders, paving the way for future drug discoveries

Liquid-metal transfer from anode to cathode without short circuiting

A new spin on organic shampoo makes it sudsier, longer lasting
Let us know if there is a problem with our content.
Use this form if you have come across a typo, inaccuracy or would like to send an edit request for the content on this page. For general inquiries, please use our contact form . For general feedback, use the public comments section below (please adhere to guidelines ).
Please select the most appropriate category to facilitate processing of your request
Thank you for taking time to provide your feedback to the editors.
Your feedback is important to us. However, we do not guarantee individual replies due to the high volume of messages.
E-mail the story
Your email address is used only to let the recipient know who sent the email. Neither your address nor the recipient's address will be used for any other purpose. The information you enter will appear in your e-mail message and is not retained by Phys.org in any form.
Newsletter sign up
Get weekly and/or daily updates delivered to your inbox. You can unsubscribe at any time and we'll never share your details to third parties.
More information Privacy policy
Donate and enjoy an ad-free experience
We keep our content available to everyone. Consider supporting Science X's mission by getting a premium account.
E-mail newsletter
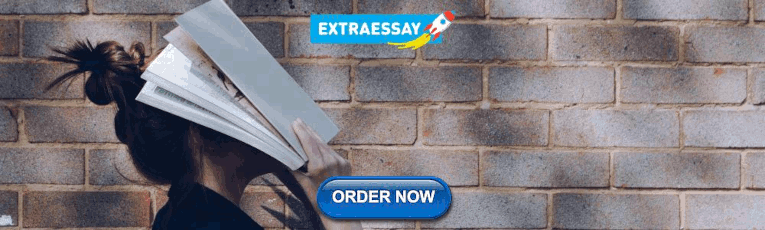
IMAGES
VIDEO
COMMENTS
mcgill-nlp/llm2vec • • 9 Apr 2024. We outperform encoder-only models by a large margin on word-level tasks and reach a new unsupervised state-of-the-art performance on the Massive Text Embeddings Benchmark (MTEB). Papers With Code highlights trending Machine Learning research and the code to implement it.
This paper is ranked #1 on Image Classification on ImageNet (using extra training data). For the research paper, read here. For code, see here. SwinIR: Image Restoration Using Swin Transformer. The authors of this article suggest the SwinIR image restoration model, which is based on the Swin Transformer. The model comprises three modules ...
Machine learning articles from across Nature Portfolio. Machine learning is the ability of a machine to improve its performance based on previous results. Machine learning methods enable computers ...
The Journal of Machine Learning Research (JMLR), , provides an international forum for the electronic and paper publication of high-quality scholarly articles in all areas of machine learning. All published papers are freely available online. JMLR has a commitment to rigorous yet rapid reviewing. Final versions are (ISSN 1533-7928) immediately ...
Realistic Continual Learning Approach using Pre-trained Models. Nadia Nasri, Carlos Gutiérrez-Álvarez, Sergio Lafuente-Arroyo, Saturnino Maldonado-Bascón, Roberto J. López-Sastre. Subjects: Machine Learning (cs.LG); Computer Vision and Pattern Recognition (cs.CV)
Improves how machine learning research is conducted. Prioritizes verifiable and replicable supporting evidence in all published papers. Editor-in-Chief. Hendrik Blockeel; Impact factor 7.5 (2022) 5 year impact factor 6.3 (2022) Submission to first decision (median) 28 days. Downloads 1,349,126 (2023)
Stay informed on the latest trending ML papers with code, research developments, libraries, methods, and datasets. Read previous issues. ... 2336 benchmarks • 663 tasks • 2017 datasets • 27970 papers with code Representation Learning Representation Learning. 16 benchmarks
Artificial intelligence (AI), particularly, machine learning (ML) have grown rapidly in recent years in the context of data analysis and computing that typically allows the applications to function in an intelligent manner [].ML usually provides systems with the ability to learn and enhance from experience automatically without being specifically programmed and is generally referred to as the ...
Subjects: Machine Learning (stat.ML); Artificial Intelligence (cs.AI); Machine Learning (cs.LG); Statistics Theory (math.ST) arXiv:2403.08941 [pdf, other] Title: Towards Model-Agnostic Posterior Approximation for Fast and Accurate Variational Autoencoders
The journal publishes research results in addition to new approaches to ML, with a focus on value and effectiveness. Application papers should demonstrate how ML can be used to solve important practical problems. Research methodology papers should demonstrate an improvement to the way in which existing ML research is conducted.
Machine learning (ML) is the ability of a system to automatically acquire, integrate, and then develop knowledge from large-scale data, and then expand the acquired knowledge autonomously by discovering new information, without being specifically programmed to do so. In short, the ML algorithms can find application
This 2022 ML paper presents an algorithm that teaches the meta-learner how to overcome the meta-optimization challenge and myopic meta goals. The algorithm's primary objective is meta-learning using gradients, which ensures improved performance. The research paper also examines the potential benefits due to bootstrapping.
Machine Intelligence. Google is at the forefront of innovation in Machine Intelligence, with active research exploring virtually all aspects of machine learning, including deep learning and more classical algorithms. Exploring theory as well as application, much of our work on language, speech, translation, visual processing, ranking and ...
However, we see strong diversity - only one author (Yoshua Bengio) has 2 papers, and the papers were published in many different venues: CoRR (3), ECCV (3), IEEE CVPR (3), NIPS (2), ACM Comp Surveys, ICML, IEEE PAMI, IEEE TKDE, Information Fusion, Int. J. on Computers & EE, JMLR, KDD, and Neural Networks. The top two papers have by far the ...
2. Literature review. The PubMed biomedical repository and the dblp computer science bibliography were selected to perform a literature overview on ML-based studies in cancer towards disease diagnosis, disease outcome prediction and patients' classification. We searched and selected original research journal papers excluding reviews and technical reports between 2016 (January) and 2020 ...
Additionally the disruptive character of AI and ML technology and research will required further research on business models and management of innovation capabilities. This special issue is based on submissions invited from the 17th Annual Learning and Technology Conference 2019 that was held at Effat University and open call jointly.
Current practices for selecting records for transfer to The National Archives (TNA) were developed to deal with paper records and are struggling to deal with this shift. This article examines the background to the problem and outlines a project that TNA undertook to research the feasibility of using commercially available artificial ...
Step 2: Finding research papers. One of the most excellent tools to use while looking at machine learning-related research papers, datasets, code, and other related materials is PapersWithCode. We use the search engine on the PapersWithCode website to get relevant research papers and content for our chosen topic, "Pose Estimation.".
The convergence of. AI and ML has propelled innovation to unprecedented heights, revolutionizing the way we perceive and. interact with the world around us. This paper embarks on a comprehensive ...
Step 2: Finding Research Papers. One of the most excellent tools to use while looking at machine learning-related research papers, datasets, code, and other related materials is PapersWithCode. We use the search engine on the PapersWithCode website to get relevant research papers and content for our chosen topic, "Pose Estimation."
Several papers offer various sorts of ML approaches [10,11]. The ML algorithms are generally classified into three categories such as supervised, unsupervised, ... As a result, future research should concentrate on ML ethics and fairness. Model interpretation is absent in nearly all investigations, which is surprising. Interpreting machine ...
Discover opportunities in Machine Learning. Our research in machine learning breaks new ground every day. Work with us. Explore advancements in state of the art machine learning research in speech and natural language, privacy, computer vision, health, and more.
Prof. Oba shares the motivation behind the present research, "In recent years, ML has gained a lot of attention in materials science research. The ability to virtually screen materials based on ML ...
Prof. Oba says, "In recent years, ML has gained a lot of attention in materials science research. The ability to virtually screen materials based on ML technology is a very efficient way to ...