Academia.edu no longer supports Internet Explorer.
To browse Academia.edu and the wider internet faster and more securely, please take a few seconds to upgrade your browser .
Enter the email address you signed up with and we'll email you a reset link.
- We're Hiring!
- Help Center
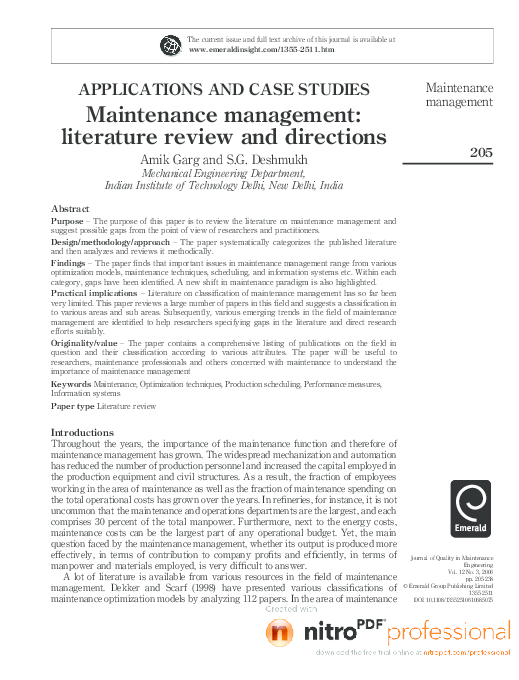
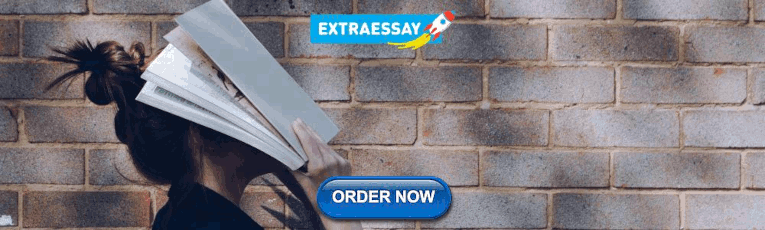
APPLICATIONS AND CASE STUDIES Maintenance management: literature review and directions

Purpose – The purpose of this paper is to review the literature on maintenance management and suggest possible gaps from the point of view of researchers and practitioners. Design/methodology/approach – The paper systematically categorizes the published literature and then analyzes and reviews it methodically. Findings – The paper finds that important issues in maintenance management range from various optimization models, maintenance techniques, scheduling, and information systems etc. Within each category, gaps have been identified. A new shift in maintenance paradigm is also highlighted. Practical implications – Literature on classification of maintenance management has so far been very limited. This paper reviews a large number of papers in this field and suggests a classification in to various areas and sub areas. Subsequently, various emerging trends in the field of maintenance management are identified to help researchers specifying gaps in the literature and direct research efforts suitably. Originality/value – The paper contains a comprehensive listing of publications on the field in question and their classification according to various attributes. The paper will be useful to researchers, maintenance professionals and others concerned with maintenance to understand the importance of maintenance management Introductions Throughout the years, the importance of the maintenance function and therefore of maintenance management has grown. The widespread mechanization and automation has reduced the number of production personnel and increased the capital employed in the production equipment and civil structures. As a result, the fraction of employees working in the area of maintenance as well as the fraction of maintenance spending on the total operational costs has grown over the years. In refineries, for instance, it is not uncommon that the maintenance and operations departments are the largest, and each comprises 30 percent of the total manpower. Furthermore, next to the energy costs, maintenance costs can be the largest part of any operational budget. Yet, the main question faced by the maintenance management, whether its output is produced more effectively, in terms of contribution to company profits and efficiently, in terms of manpower and materials employed, is very difficult to answer. A lot of literature is available from various resources in the field of maintenance management. Dekker and Scarf (1998) have presented various classifications of maintenance optimization models by analyzing 112 papers. In the area of maintenance
Related Papers
Journal of Quality in Maintenance Engineering
S.G. Deshmukh , Amik Garg
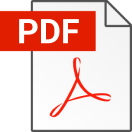
International Journal of Systems Assurance Engineering and Management
Peter Muchiri
Due to widespread automation and the high capital tied up in production equipment, the importance of maintenance is ever increasing. This makes maintenance an investment opportunity to be optimized, not a cost to be minimized. Academics have recognized this and many maintenance optimization models have been published over the years. Most of these models focus on one optimization criterion or objective, making multi-objective optimization models an underexplored area of maintenance optimization. Moreover, there is a big gap between academic models and application in practice. It is very difficult for industrial companies to adapt these models to their specific business context. This article reviews the literature on maintenance optimization models, with special focus on the optimization criteria and objectives used. To overcome flaws in present optimization models, a generic classification framework of maintenance optimization models is presented. All factors that have an influence on the optimization model will be made explicit and their links will be established. The framework is a starting point to develop business specific optimization models and enables decision making in e-maintenance. Moreover, it ensures a fit between the business model of a company and the maintenance optimization model right from the beginning. Future research will be on the development of a maintenance optimization model taking into account the most relevant optimization influence factors and criteria for a situation at hand.
European Journal of Operational Research
Liliane Pintelon , L. Gelders
Mahros Darsin
The purpose of this paper is to review from early days until recent time the development of maintenance management methods from maintenance textbooks to give a glance view for researcher and practitioner where maintenance start until recent development and practical application of maintenance management. The methodology approach is by reviewing an early age maintenance management, maintenance management on eighties, maintenance management on nineties and recent maintenance management. Comparing and categorizing maintenance management methods along with time will increase knowledge how to choose the right maintenance for practical application. From this review it found that the paper also revealed from time to time, maintenance management adapt and improve to gain more sustainable maintenance, including the new shift of paradigm of maintenance itself. The originality and contribution of this research is that the paper contains many maintenance management methods from maintenance text...
Parveen Persad
Journal of Manufacturing Systems
Usama Najeeb
Diana Barraza-Barraza , Mario G Beruvides
Fernando Espinosa
The importance of maintenance in the strategical structure of the companies for to increase the operational availability, is being stimulated for the productivity requirements, quality increase, competitiveness, markets opening, among others factors. It is asked, then: why the companies have had difficulties to improve its operational availability despite the investments in qualification and modernization? The raised hypotheses to answer this question indicate restrictions in methodologies in the field of the asset management, appropriate physical resources, computational resources, integrated qualification the culture of the "soil of plant" and adjusted scientific research. Based on these hypotheses, it is considered in this work to present a methodology that allows analyzing the different alternatives for maintenance managements with its more appropriate tools and techniques for the requirement of the company, in the industrial field that operates. The methodology presen...
IAEME Publication
This paper introduces methodology focused on improving of economic efficiency of the maintenance systems by choosing the perfect maintenance strategy for production departments. In first part of the research paper basic maintenance strategies are introduced and a global overview of the methodology is presented. The second part of the paper deals with the brief characteristic of the model MAM (Maintenance Analytical Module), which was developed on the MS Excel platform as a decision support tool for maintenance managers. Description of the model is primarily focused on characteristic of its structure and benefits of its application.
RELATED PAPERS
karina sugih arto
Krisdayanti Sianturi (C1C020158)
Jaap de Vries
Paola Termine
American Journal of Obstetrics and Gynecology
Leonardo Pereira
Journal of Power and Energy Systems
Jasmin Sudha
Analytical Letters
Cees Bruggink
International Journal of Data Analysis Techniques and Strategies
Nikos Tsotsolas
BioMed Research International
Muhammad Fathur Rizky
Journal of Biological Chemistry
Takeshi Uchida
Nicolò Bellanca
Brain, behavior, and immunity
Melissa Rose
Journal of Virology
Robert Ricciardi
Asian Pacific Journal of Tropical Biomedicine
Vinod Pawar
International Journal of Surgery Research & Practice
Hossam Soliman
Progress: Jurnal Pendidikan, Akuntansi dan Keuangan
Muhamad Riza
Journal of Contemporary Brachytherapy
Somsak Wanwilairat
Proceedings of the 2nd International Conference on Religion and Education, INCRE 2020, 11-12 November 2020, Jakarta, Indonesia
Muhamad Murtadlo
International Journal of Social Science And Human Research
Dudziro NHENGU
Iranian Journal of Insurance Research
Rainer Haldenwang
Materials Today: Proceedings
A Dhayal raj
Journal on Education
asrul bahar
Psychology of Retention
Mohammad Faraz Naim
Acta Paediatrica
Luis Carlos Machado
- We're Hiring!
- Help Center
- Find new research papers in:
- Health Sciences
- Earth Sciences
- Cognitive Science
- Mathematics
- Computer Science
- Academia ©2024

An official website of the United States government
The .gov means it’s official. Federal government websites often end in .gov or .mil. Before sharing sensitive information, make sure you’re on a federal government site.
The site is secure. The https:// ensures that you are connecting to the official website and that any information you provide is encrypted and transmitted securely.
- Publications
- Account settings
Preview improvements coming to the PMC website in October 2024. Learn More or Try it out now .
- Advanced Search
- Journal List
- Sensors (Basel)

Predictive Maintenance and Intelligent Sensors in Smart Factory: Review
Associated data.
Not applicable.
With the arrival of new technologies in modern smart factories, automated predictive maintenance is also related to production robotisation. Intelligent sensors make it possible to obtain an ever-increasing amount of data, which must be analysed efficiently and effectively to support increasingly complex systems’ decision-making and management. The paper aims to review the current literature concerning predictive maintenance and intelligent sensors in smart factories. We focused on contemporary trends to provide an overview of future research challenges and classification. The paper used burst analysis, systematic review methodology, co-occurrence analysis of keywords, and cluster analysis. The results show the increasing number of papers related to key researched concepts. The importance of predictive maintenance is growing over time in relation to Industry 4.0 technologies. We proposed Smart and Intelligent Predictive Maintenance (SIPM) based on the full-text analysis of relevant papers. The paper’s main contribution is the summary and overview of current trends in intelligent sensors used for predictive maintenance in smart factories.
1. Introduction
Industry sets the direction for the world economy, accounting for more than 70% of the world’s total material production [ 1 ], depending on the regional economy’s development. The continuous introduction of new technologies in developed countries has an impact on its relatively low level of employment of the population at the level of 20% [ 2 ]. Simultaneously, the share of products and semifinished products in international trade is continuously growing, despite the declining share of national Gross Domestic Product (GDP) in developed countries [ 3 ]. All these facts are caused by introducing new technologies, which in the current industrial era of Industry 4.0 are summarised by many authors under the name Smart Factory.
This review aims to give the reader a comprehensive view of maintenance and intelligent sensors in Smart Factory. As can be seen from the following, current literature reviews [ 4 , 5 , 6 , 7 , 8 , 9 , 10 ] have shown that the literature is focusing on specific topics only separately. The literature specializes in different types of sensors but does not consider them in relation to those technologies, and industry 4.0. Professional texts lack a summary of literature and texts that would bring the usability and potential of sensors closer to common practice, so that these findings can be clearly used for business management in the implementation of maintenance system planning. This would be beneficial for operational managers and engineers for the design of new maintenance systems. This article provides a comprehensive overview of current trends to help structure and guide future research. At the same time, it answers key questions related to contemporary trends in maintenance processes in smart factories. We define which Industry 4.0 technologies and intelligent sensors usually provide maintenance in smart factories. Moreover, it helps to find new trends in smart and intelligent predictive maintenance.
The article is organised into six sections ( Figure 1 ). After the Introduction ( Section 1 ), the Theoretical Background ( Section 2 ) discusses the relevant literature about intelligent sensors, smart factory, and predictive maintenance and defines key terms. In Materials and Methods ( Section 3 ), we explain the qualitative and quantitative methods used for the review. Section 4 is focused on the main results of the article, followed by a discussion ( Section 5 ). The last part presents the conclusion ( Section 6 ), contributions, limitations, and future research.

Organisation of the article.
2. Theoretical Background
A literature review discussing intelligent sensors for maintenance in smart factories has not been carried out. The literature currently offers reviews dealing with these areas separately. Song at al. [ 4 ] pays attention to smart sensors in monitoring the condition and integrity of rock bolts concerning economic and personnel losses. In the field of engineering, Jin [ 5 ] describes multifunctional sensors suitable for industrial production, Feng [ 11 ] describes sensors for intelligent gas sensing in the literature review, and Paidi [ 6 ] describes intelligent parking sensors replacing ultrasonic sensors in combination with machine learning. Sony and Talal [ 7 , 12 ] then characterise sensors for health monitoring. In literature reviews, we relatively often find a combination of smart sensors and smart factories, a key part of the 4.0 industry concept [ 13 ]. Lee [ 9 ] describes smart sensors’ use to evaluate and diagnose individual devices in a smart factory. Strozzi [ 10 ] expands the literature review emphasising the actual transition and implementation of large, intelligent factories. Pereira and Álvarez [ 14 , 15 ] also focus on implementing the principles of a smart factory and emphasise that effective value creation depends on the method of implementation. The implementation process about managing technological and organisational changes and desirable competencies is further addressed by Sousa [ 16 ], as well as Lee et al. [ 17 ]. They pay attention to the gap between recent researches on the actual level of deployment. In the field of maintenance of intelligent factories, we find several literature reviews with the resonant notion of predictive maintenance. In their overview, Carvalho [ 18 ] focuses on machine learning methods, which they consider a promising tool for predictive maintenance. Sakib [ 19 ] observes the shift from service activities to proactive, predictive maintenance and places [ 20 ] in the context of Industry 4.0. Olesen and Shaker [ 21 ] deals with practical use in thermal power plants, and Fei [ 22 ] in the field of aircraft systems.
2.1. Intelligent Sensors
We find a critical area in the concept of smart factories, and that is intelligent logistics—transport and warehousing. These operations include identification and detection of the location of resources across the company. We understand sensors as a technical reproduction of natural processes because human nerves transform external stimuli into electrical signals transmitted to the central nervous system—the brain. Smart sensors process aggregated data from production processes in real-time and enable self-determination of machines and other smart devices [ 23 , 24 ]. Data on production resources are used to monitor, collect, and evaluate the data obtained. The most common type of wireless sensors work on radio frequency identification (RFID), ZigBee, and Bluetooth technology [ 25 ]. Eifert [ 26 ] defines intelligent sensors as a multi-component measuring device that is self-calibrating, self-optimised, and easy to integrate into the environment for high connectivity. Besides, intelligent sensors also have process intelligence and can generate multidimensional data information. The literature of the most inflected RFID technology is also one of the key technologies for implementing the Internet of Things (IoT). In RFID technology, it is an active and passive tag, where the active sensor sends information for hundreds of meters, and the passive tag receives it. The integrated sensor in production enables a flexible and targeted strategy of predictive maintenance and control [ 27 ]. Sensors that are part of the IoT can proactively monitor the device and issue alerts when the device deviates from the specified parameters, we speak of so-called facility management [ 28 ]. Karabegovic [ 29 ] adds that the sensors convert physical parameters (temperature, speed, humidity) into signals that can be measured electronically. The use of smart sensors in Industry 4.0 is characterised by Schmitt [ 30 ]. The key element is knowledge of the current state of the system. Sensor data with other parameters of the given process in the sense of self-diagnostics and machine learning can be evaluated in real-time. The traceability of individual components is also essential—from screws to the uniformity of seals, this knowledge enables the measurement of tolerance and comprehensive monitoring of the condition of machines and other equipment.
2.2. Predictive Maintenance
Predictive maintenance (PdM) is the latest maintenance policy adopted by many industries. Above all, these are areas that require absolute reliability, such as power plants, public services, transport systems, and emergency services. Forecasted information is usually necessary for the long term and for planning various operational activities (maintenance, production, inventory, etc.). In addition, due to technological and logistical limitations, maintenance cannot always be performed everywhere [ 31 ]. Maintenance is a critical activity that takes place in production. Machine failures during production can lead to adverse effects on the production schedule, delivery delays, or employee overtime to compensate for the loss. PdM predicts system failures to optimize maintenance efforts [ 32 , 33 ]. According to Carvalho [ 18 , 34 ], PdM is a set of tools used to determine when specific maintenance is required. The tool is based on continuous monitoring of the machine or process, and this allows maintenance to be performed only when needed. A secondary, no less important function of PdM is the possibility of early detection of faults, thanks to tools based on historical data—machine learning—as well as visual aspects of faults—colour and wear. As a possible part of the Industry 4.0 concept, PdM aims to minimise maintenance costs, implement zero-waste production, and reduce the number of major failures [ 35 ]. Despite PdM’s benefits, Herrmann [ 36 ] highlights the potential risks of remote access to maintenance processes and cites Distributed Denial-of-Service (DDoS) attacks, for example. According to Zonta [ 20 ], we distinguish three approaches to PdM, namely: Based on a physical model, where the main feature is mathematical modelling requiring the timeliness of the state and statistical methods of evaluation. The second approach is the knowledge-based approach, which reduces the complexity of the physical model, and the last approach is the data-driven approach, which we find most often in the current development of PdM. This approach is based on artificial intelligence, i.e., machine learning and statistical modelling, and is a satisfactory approach in the conditions of Industry 4.0 [ 37 ]. Farooq et al. distinguish experience-driven and data-driven maintenance [ 38 ]. Experience-driven preventive maintenance is based on gathering knowledge about production equipment, which is then used to plan future maintenance. On the contrary, data-driven preventive maintenance is based on analysing a large volume of data ( Figure 2 ).

Experience- and data-driven predictive maintenance [ 38 ].
2.3. Smart Factory
One of the key components of Industry 4.0 is a smart factory, otherwise also a smart or digital factory. A smart factory represents the future state of fully interconnected production systems, without a significant amount of manpower [ 13 ]. According to Chen [ 25 ], a smart factory is a manufacturing solution that provides adaptive and flexible manufacturing processes that reflect the rapidly and dynamically changing conditions in the world. On the one hand, this solution can be understood as a combination of software and hardware, which should lead to the optimisation of production and the reduction of waste of scarce resources. On the other hand, the concept can be perceived as a space for perspective cooperation with business partners based on the formation of a dynamic organisation. The smart factory architecture itself includes a physical resource layer, a data and network layer, as well as an end layer. All elements of the intelligent factory are interconnected, exchanging information, and recognising and evaluating situations. Thus, physical and cyber technology is integrated, which results in improved controllability, control, transparency of production processes, maximises value for the customer, and in addition, there is communication between the factory and the market itself [ 39 , 40 ]. The core technologies of Industry 4.0 include IoT, cloud computing, and high-volume data analysis. IoT represents the integration of sensors and computer technology in the field of wireless communication, and cloud services allow access to the network respectively, as a shared pool of computing resources. The combination of these technologies allows the involvement of all devices in the concept of a smart factory, but the collection of huge amounts of data requires another technology, which is the analysis of high-volume data. With the help of analytical tools—data mining or machine learning—this technology is one of the most important elements of the entire concept of Industry 4.0 [ 41 ]. Other technologies related to the vision of a smart factory include autonomous robots, additive manufacturing, augmented reality, and cybersecurity. Drastic changes in technology are changing the concept of production. In the modern concept, traditional centrally controlled processes are replaced by decentralised control, which builds on the ability of individual elements of a smart factory to communicate with each other. In self-regulatory production, people, machines, equipment, and products communicate with each other [ 42 ].
3. Materials and Methods
The paper is based on a review of the literature focusing on intelligent sensors, maintenance, and smart factories. As part of this review, we analysed, evaluated, and discussed scientific publications from prestigious databases. This review focuses on combining three concepts and creating a comprehensive overview of studies to find possible research gaps. The literature review has shown that the current literature contains many reviews focusing on particular topics only separately. This paper provides an overview of the contemporary trends that will help structure and guide future research to fill this gap.
The scope of the paper is to review intelligent sensors and maintenance processes in smart factories. As part of the research, we formulated the following research questions:
- What are contemporary trends in maintenance processes in smart factories?
- How are Smart and Intelligent Predictive Maintenance characterised?
- Which Industry 4.0 technologies and intelligent sensors provide maintenance in smart factories?
3.1. Research Design
The research procedure and strategy phases are described in Figure 3 . First, we defined research questions that helped with keyword selection. Then, we analysed these keywords separately through burst detection analysis to reveal current research trends. Data was collected from database sources, filtered, and extracted based on predefined criteria. Further, we performed a content analysis and review of individual publications in our research team. Based on the analyses, we presented results of the synthesis.

Research design and procedure.
3.2. Keywords Selection
We used the modified Eligibility methodology [ 43 ] to prepare the research questions and achieve the review’s scientific quality. The four parts of this methodology (Problem/context, Intervention, Comparison, Outcomes) create the PICO logic grid [ 44 ]. We do not use the comparative part, and we have expanded the method into three main questions. The question “how” is related to the context smart factory. The question “which” refers to interventions via sensors that are drivers for bringing a change from the traditional concept of maintenance. Finally, the question “what” focus on outcomes for maintenance. We used software VOSviewer [ 45 ] for constructing keywords in the PICO logic grid ( Table 1 ).
PICO (Problem/context, Intervention, Comparison, Outcomes) logic grid.
3.3. Burst Detection Analysis
We examined research topics (smart factory, intelligent sensors, predictive maintenance) separately in this phase. We collected the required data from the Web of Science (WoS) and Scopus databases. Furthermore, we analysed the obtained publications concerning their trend over time, keywords, and burst detection. Only the most relevant research publications from the WoS database are analysed. The search strategy is shown in Table 2 .
Search strategy for burst detection analysis (1 December 2020).
Burst detection analysis is based on the analysis of keywords in a certain period of time [ 46 ]. Density of the frequency changes of keywords are determined for each monitored period. This analysis helps to identify the main research trends and helps to predict the future evolution of the literature. Burst analysis can be performed in the Sci2 Tool [ 47 ], which offers a display of the results of temporal bar graph promotions. The number of publications for burst analysis are presented in Table 3 .
Results of topics searched for burst detection analysis (1 December 2020).
3.4. Collection of Publications for Review
The systematic search strategy ( Table 4 ) combines various keywords and their synonyms to find out quality peer-reviewed journals. In this phase, we searched the publication’s databases Web of Science and Scopus to combine all three main terms. Our goal was to obtain publications that connect topics, smart factories, intelligent sensors, and maintenance.
Search strategy in the second phase (1 December 2020).
We present the total number of publications found in Table 5 . The result of the search was 890 publications, which we further filtered based on the selected criteria.
The number of papers from Web of Science (WoS) and Scopus bibliographic databases.
3.5. Data Extraction and Eligibility Criteria
Data extraction is based on the filtering criteria determining the selected publications in more in-depth research. We filtered search results based on the criteria:
- Not duplicated,
- Published from January 2010 to December 2020,
- Written in English,
- Type of publication: journal paper (not review, white paper, book, short survey, proceedings, conference paper, etc.) for higher quality of data,
- Publications with completed information (authors, year, journal name, etc.).
Furthermore, an objective screening was performed based on the title and keywords. To evaluate the eligibility, we analysed the title, keywords, and abstracts of publications. For this purpose, we have defined criteria for exclusion. We evaluated the publications at meetings of the research team. In case of discrepancies in the assessment of suitability in the title, keywords, or abstract, we compared the opinions of team members and, if necessary, performed a full-text analysis. To document the extraction process, we used the flow diagram in Figure 4 , which captures the entire review flow based on the Preferred Reporting Items for Systematic Reviews and Meta-Analyses (PRISMA) methodology [ 48 ] and Quality of Reporting of Meta-Analyses (QUORUM) methodology [ 49 ] from search to final selection. The PRISMA checklist is available in supplementary materials (Table S1) .

Flow diagram based on PRISMA [ 48 ] and QUORUM [ 49 ] flowchart.
The exclusion criteria are:
- The paper is a review (paper is focused only on challenges or future perspectives).
- The paper focused on maintenance, but not on “predictive” maintenance.
- The paper does not discuss Industry 4.0 technologies.
- The paper does not have a production/factory focus (for example, we eliminated papers related to smart cities and smart homes, agriculture, logistics, etc.).
- The paper has unavailable full text.
- The paper does not have a scientific structure (abstract, introduction, literature background, methodology, results, discussion, conclusion, references).
3.6. Content Analysis and Synthesis
The results of search query publications were further subject to analysis and synthesis. We performed the analysis in the Endnote software database and Microsoft Excel software, which were used to evaluate various aspects of the monitored topics. Our research team consisted of three members who participated in the evaluation and analysis of search results. This phase included evaluating the full texts of individual publications based on the set research goals.
We focused mainly on:
- The research method (case study, experiment, simulation)
- Objectives of the articles
- Used intelligent sensors and data
- Industry 4.0 technologies for smart factories
- Predictive maintenance processes characteristics
- Smart and intelligent keywords domain
We used the clustering method during the analysis, which is part of the VOSviewer software [ 45 ] and allows classification using keywords. Using VOSviewer, we performed a co-occurrence analysis of keywords, which is based on clustering methods. Cluster analysis enabled to find a number of paired keywords cited in the same publications. The results of the cluster analysis clearly capture the knowledge structure of the research frontiers. We evaluated the quality of publications included for the synthesis (final selection) thorough review of individual team members. In particular, the ranking of journals, citations of publications, and their possible biases were considered.
3.7. Excluded Studies
To avoid possible effects of bias, it is necessary to mention the most important publications, which were eliminated from the sample for qualitative synthesis reasons. Aheleroff et al. [ 50 ] examined the application of smart IoT sensors in the fridge (smart homes focus). Several publications are mainly focused on the logistic area [ 51 ], i.e., from the automotive industry [ 52 , 53 , 54 ] or the aviation industry [ 55 , 56 ]. We excluded from the analysis publications focused on agriculture, which do not deal with smart factories’ production processes. These are publications using intelligent sensors in milling [ 57 ] and aquaponics [ 58 ]. The review papers were eliminated from the final selection.
The results are divided into three parts according to the research question: an overview of the main topics, smart and intelligent predictive maintenance, and Industry 4.0 Technologies and Sensors for Smart Factory.
4.1. Main Topics and Trends Overview
First, we identified occurrences of the primary topics “smart factory/production,” “intelligent/smart sensors,” and “predictive/smart maintenance” in the Web of Science and Scopus databases. This part of the research related to the research question 1. All three concepts are connected in the research area by engineering, supplemented by telecommunications and predictive maintenance by medicine, or intelligent sensors in computer science. The total number of publications in the databases is shown in Figure 4 .
The results shown in Figure 5 show an increase in the number of publications over time. This increase has been apparent for smart factory publications since 2015 and intelligent sensors since 2012. Further, we performed burst detection analysis in the researched areas for Web of Science publications.

The development of the total number of Scopus and Web of Science publications. Note: square on the line (Web of Science), circle on the bold line (Scopus).
The burst detection analysis presents key terms for topics of intelligent sensors, smart factory, and predictive maintenance (see Figures S1–S3 in Supplementary Materials ). For a better overview, we compare the results in three time periods ( Figure 6 ), and then according to the individual importance of key concepts ( Figure 7 ). The importance of the terms was expressed using the obtained burst weights. Based on these findings and analysis, we tried to answer research question 1 responsibly.

The summary of burst detection analysis for main topics. The results are divided into three periods of time (1970–1990, 1990–2010, and 2010–2020). The terms in each period are sorted according to the burst weights.

The top terms in analysed areas based on the burst detection. Note: The top ten used terms are highlighted “bold” and top twenty terms are depicted “italic”.
Top cited papers from smart factory/production areas focus on using ion batteries for smart grids [ 59 , 60 ] and nanomaterials’ intelligent design [ 61 ]. The results show that the most used terms in the paper titles are intelligent, Industry 4.0, and agent. Based on Figure 5 , we conclude that the oldest wave in smart factories is associated with classical studies dealing with intelligent, flexible, and automation planning and scheduling of manufacturing systems. This wave is the period 1970–1990, characterised by the burst terms intelligent, system, knowledge, plan, and schedule. The second wave in 1990–2010 with the primary burst terms: agent, manufacturing, control, expert, and process, refers to papers using holon, RFID, or web technologies in factories. Publications on manufacturing control systems [ 62 , 63 ] were highly cited in this period. The current trend in smart factory is related to implementing intelligent manufacturing [ 64 ]. In this contemporary wave, burst terms Industry 4.0, digital twin, IoT, deep learning, digitalisation, smart grid, cyber, and sustain dominate. These terms are well-known Industry 4.0 technologies and processes. Top cited papers focused on operational planning of a smart grid [ 65 ], deep learning in agriculture [ 66 ], and big data for the self-organised multiagent system in the smart factory [ 67 ].
Top cited papers from smart/intelligent sensors were in areas related to the Internet of Things [ 68 ], wireless sensor networks [ 69 ], and nanotechnology applications [ 70 ]. We found that the important paper title terms are IoT, structure, and sensor in the burst analysis. Figure 5 shows that the early history of intelligent sensors, 1970–1990, emphasised the first application of sensors (burst terms sensor, intelligent, process). Later, in 1990–2010 came articles focused on the structure [ 71 ], optic, and control of sensors, and their usage for robots. Some essential publications in this period focused on structural health monitoring [ 72 ], piezoelectric laminate beam [ 73 ], and free vibration behaviour of the beam [ 74 ]. The most contemporary period from 2010 to 2020, similar to the smart factory/production, covered the area of Industry 4.0 new technologies. In addition to the mentioned Internet of Things [ 75 ], there is a significant representation of publications focused on wearable sensor-based systems [ 76 ], deep learning [ 77 ], edge technology [ 78 ], graphene-based smart materials, blockchain, smart city, and grid.
The last area focused on smart/predictive maintenance. After omitting medical and ecological articles, the most cited publications focused on proportional-integral-derivative (PID) control [ 79 ], monitoring, and fault diagnosis in production [ 80 ]. Based on the burst analysis results, we found that the most important terms are maintenance, learning, and predict. We identified three trend waves in area maintenance ( Figure 5 ). In the first wave from 1970 to 1990, the publications dealt with predictive maintenance. In engineering and production, maintenance is associated with predicting machines’ status [ 81 ] or deterioration of processes [ 82 ]. In the second wave in 1990 to 2010, we found that the publications dealt with burst terms program, diagnostics, intelligence, knowledge, and database. These publications focus, for example, on diagnostics, monitoring, or maintenance of intelligent computer numerical control (CNC) machine tools [ 83 ] or power transformers [ 84 ]. The current trend wave is characterised by Industry 4.0 technologies such as digital twins, deep machine learning, IoT, big data analytics, blockchain, and digitisation for maintenance. The most significant publications of this period focused on big data analytics in logistics and supply chain management [ 85 ], maintenance strategy selection [ 86 ], vibration analysis of rotating machinery, or cloud-enabled prognosis [ 87 ] for predictive maintenance in production.
Based on the burst analysis detection, we conclude that in all three areas in the last 10 years, the focus has been on the concept of Industry 4.0 and related technologies. We arranged the keywords with the highest burst weights into three research areas in Figure 7 . The results show that the terms Internet of Things and deep learning have the highest weight for all topics. The terms Big Data, grid, and intelligent are also common to the area. From this finding, we can conclude that the current trend in the monitored areas is related to the collecting of big data through intelligent sensors on IoT devices and their evaluation using learning algorithms.
The internet, smart grid, and blockchain technology are important for sensors used in maintenance. The use of sensors in smart factories lies mainly in the area of control, with a focus on processes. The sensors, together with actuators, are used to collect data to control and optimise conditions. Piezoelectric, optics, wearable, beam, graphene, and other sensors’ features are used. A special area of sensors lies in robotics, which has experienced rapid development in recent years. In the world’s most industrialised countries, such as South Korea, Japan, Germany, and Sweden, there is the largest share of robots per 10,000 employees in factories [ 88 ]. Automation in smart factories requires new types of sensors that have the ability to automatically calibrate and improve the functions of IoT devices. The IoT is not aiming only at connect two machines with pre-programmed functions. For IoT communication, it is important to connect embedded devices to the Internet and communicate with each other [ 89 ]. It is an intelligent connection of various products, devices, and facilities that provide a wide range of functions that evaluate certain conditions. The interaction between systems brings new possibilities. The key elements are miniature intelligent sensors [ 90 ]. Even though devices and systems were not originally designed to share data, the Internet of Things can. Connecting smart sensors and gateways to existing devices leads to data collection and analysis, understanding, and better decision making [ 91 ].
Publications about smart factory/production are related to cyber-physical systems, planning, scheduling, and sustaining them. Maintenance in smart factories relies on Industry 4.0 technologies, digitisation, data-driven manufacturing, agent-based systems, and digital twins. Predictive maintenance consists of programs for predicting, diagnosing, and analysing future maintenance needs. Based on the rules, features, and conditions, there are machines and devices controlled and repaired to maintain their life and future sustainability. Information and data are collected and shared through databases.
4.2. Smart and Intelligent Predictive Maintenance
We performed a co-occurrence analysis based on original papers’ keywords using VOSviewer. This part of the analysis related to research question 2. The results show that the keywords maintenance, optimisation, predictive maintenance, system, and big data were most often used in publications. Publications were grouped based on keywords into four clusters ( Figure 8 ).

Keywords of co-occurrence analysis.
- I4: Industry 4.0 for predictive maintenance in general (keywords: Industry 4.0, Big Data, prognostics, optimisation, performance, predictive maintenance, system).
- CbM: Smart manufacturing for condition-based maintenance (keywords: smart manufacturing, manufacture, condition-based maintenance).
- SFD: Condition, state, and fault diagnosis for maintenance (keywords: maintenance, condition monitoring, fault diagnosis).
- RUL: Prognostics and health management for RUL (keywords: prognostics and health management, signal processing, remaining useful lives).
The first cluster consists of publications that focus on intelligent sensors in smart maintenance factories without preferring specific methods. This cluster is represented, for example, by publications focused on data-driven simulation [ 92 ], big data in an Industry 4.0 environment [ 93 ], or performing predictive maintenance in a bottling plant [ 94 ]. The second cluster consists mainly of publications that emphasise the use of condition-based maintenance. The intelligent condition-based maintenance uses data fusion [ 95 ] and the Internet of Things in connection to learning techniques [ 96 ]. The third cluster related to publications mainly emphasised fault diagnosis’ importance for monitoring and maintenance. The fault diagnosis is used for prognosis in signal processing [ 97 ] and maintenance management systems [ 98 ]. The last cluster is characterised by a focus on determining the current health and the remaining life of devices and machines. This concept is described concerning edge-cloud platforms [ 99 ].
While the concept of condition monitoring has been around for some time, the market for more sophisticated predictive maintenance products is still very young. There are four types of maintenance classified in the literature: corrective, scheduled, condition-based, and statistical-based maintenance [ 100 , 101 ]. Predictive maintenance has evolved from corrective maintenance using new technologies and procedures for predicting and preventing failure. Corrective maintenance is based on the reactive strategy to the maintenance process—however, with a proactive strategy related to the preventive or opportunistic approach. Preventive maintenance is then seen as condition-based, dynamic predictive, or scheduled (periodic) maintenance. The corrective maintenance is based on the repair or replacement of assets ex-post. Condition-based maintenance means the decision-making process, usually in real-time, based on selected indicators computed from the gathered data.
Table 6 depicts maintenance process characteristics from analysed papers. The condition-based preventive maintenance is discussed in Farooq et al. [ 38 ], Kumar et al. [ 102 ], Li et al. [ 96 ], Lin et al. [ 103 ], Musselman and Djurdjanovic [ 104 ], Yan et al. [ 93 ], and Sadiki et al. [ 105 ]. Preventive maintenance is regular maintenance of machines, devices, and equipment to prevent their downtime concerning failure state. The preventive maintenance actions were classified by Doostparast et al. [ 106 ] as inspection, low-level repair, and replacement. These actions are based on fault prediction time statistically, upon failure accident, time-based (at the age for old machines), or cycle-based (periodically).
Maintenance process characteristics *.
* Acronyms: I4 (Industry 4.0 for predictive maintenance in general), CbM (Smart manufacturing for condition-based maintenance), SFD (Condition, state, and fault diagnosis for maintenance, RUL (Prognostics and health management for remaining useful life).
4.3. Industry 4.0 Technologies and Sensors for Smart Factory
Furthermore, full texts of articles concerning Industry 4.0 technologies were analysed. We performed a cluster analysis of the obtained keywords of Industry 4.0 technologies. The results of the analysis are shown in Figure 9 . The most common keywords in the articles were sensor, big data, Internet of Things, machine learning, and cloud. Through cluster analysis, we found three clusters:
- A: Intelligent sensors (keywords: sensor, actuator, intelligence, automation).
- B: Cloud-related technologies (keywords: cloud, cloud computing, Big Data, RFID, edge, PLC (programmable logic controller), 3D printer).
- C: Internet of Things technologies (keywords: Internet of Things, SCADA (Supervisory Control and Data Acquisition), CPS (cyber-physical system), machine learning, artificial intelligence, management, challenge).

Analysis of keywords related to Industry 4.0 technologies.
Table 7 presents the results of classification of researched papers according to belonging to the clusters. The intelligent cluster sensors mainly focused on sensors in general. Kumar et al. [ 102 ] analysed remaining useful life (RUL) of cutting machines by a polynomial regression method. Musselman and Djurdjanovic [ 104 ] analysed production belt for automation of material handling in the semiconductor industry. The second cluster is focused on Cloud-related technologies. It means that sensors based on RFID [ 92 ] and programmable logic controller [ 107 ] are used for cloud or edge computing [ 99 ] and analysis of big data [ 109 ]. The third cluster concerned the IoT technologies based on CPS systems [ 38 ], SCADA [ 94 ], and data for deep and machine learning.
Classification of Industry 4.0 technologies based on obtained keywords’ co-occurrences.
The different sensors’ data are used for prediction and diagnostics of devices, machines, facilities, and equipment. The results in Table 8 show that data are usually collected from SCADA systems, PLCs, CNC machine sensors, IoT devices, or other special sensors. Analysed papers mostly used case study and experimental research methods.
Methods and sensors’ data.
If we focus closely on individual types of sensors used for predictive maintenance, we will find a number of them, and we can categorize them according to the method of detection of the desired variable. The types and descriptions of sensors used in publications are shown in Table 9 . Furthermore, the sensors are elaborated in more details. Vibration and temperature sensors were most often used for predictive maintenance.
Sensors’ characteristics.
4.3.1. Motion, Position, Proximity, and Speed Sensors
The first type of sensors are motion-based probes. Position and movement sensors are mounted for monitoring the machine or product position on the production lines. Inductive, photoelectric, potentiometric (resistance-based), capacitive, optical, magnetic, and other sensing methods are used for detection of position. Sensors based on motion detection must meet the requirements in the areas of durability, weight, energy consumption, and at the same time, suitability for mass production regarding to the end user—customer [ 123 , 124 ]. Shoabid [ 125 ] describes motion-based sensors as a combination of an accelerometer, gyroscope, magnetometer, and linear acceleration. The application of these sensors can be found primarily in the field of healthcare systems, with various combinations of the above-mentioned sensors. A gyroscope is used mainly for gait analysis, fall detection, or gesture recognition, or in combination with an accelerometer. Proximity sensors detect the presence of an object without contact. These sensors are based on the optical, ultrasonic, inductive, and capacitive nature. Wearable sensors are able to monitor, for example, physiological parameters in real time.
Speed sensors have an opportunity for detecting object speed (usually for wheels, motor, or rotating particles). Enterprises use speedometers, accelerometers, light detection and ranging (LIDAR) sensors, tachometers, Doppler radars, etc. Farooq et al. [ 38 ] discussed genetic-algorithm-based prediction process for intelligent maintenance of textile spinning systems. They used vibration and speed data in a multiagent system for tracking discrepancies and error distribution of machine processing. Integrated Electronics Piezo-Electric accelerometers have been frequently used for machine vibration measurement. Peng et al. [ 113 ] use them for an automatic health condition diagnosis without field worker maintenance effort. The results show that dynamic response signals from the accelerometer increased the completeness and performance of the vibration diagnosis function. Further, Peng and Tsan [ 98 ] developed a sensor diagnosis and monitoring system to classify the health condition of the online integrated IEPE accelerometer. The solution was integrated into a production line.
Goodall et al. [ 92 ] developed, based on the RFID, a data-driven simulation for controlling work-in-process parts in a remanufacturing process and determining the time for operators to process them [ 104 ]. Park et al. [ 112 ] performed experiments on servo motor lifespan using an accelerated degradation testing method based on thermal stresses. The experimental data are used sensors for monitoring the torque, position, electrical resistance, and moment of inertia of rotor. Shan et al. [ 114 ] presented the system architecture and hardware for the welding line, which provides the real-time fine-grained visualisation of the welding robot operation status. The electrocardiogram of intelligent manufacturing equipment technology provides the maintenance of intelligent manufacturing equipment.
4.3.2. Vibration and Torque Sensors
Vibration sensors are used for monitoring the acceleration machine vibration, indicating a potential machine issue. Some sensors have modern fast Fourier transform signal processing to detect failures in machine components. Vibration sensors are the core of preventive maintenance and provide the condition of the device determination.
Barbieri et al. [ 99 ] proposed autonomous health management prognostics for smart manufacturing via on-board sensors. Kiangala and Wang [ 94 ] integrated a practical use of intelligent sensors in a small bottling plant. Predictive maintenance is used for detecting early faults and failures in conveyor motors. Uhlmann et al. [ 117 ] developed a smart sensor network for condition monitoring in factories. Collected data from MEMS sensors are processed in the cloud services and visualised on the mobile platform.
Torsion (torque, rotational) sensors convert a torque reaction and rotary into electrical signal. These sensors measure stationary or dynamic variables, usually in motors, turbines, or generators. Kozlowski et al. [ 110 ] used a torque sensor for designing a classifier for cutting tool condition assessment in RUL prediction. Kumar et al. [ 102 ] evaluated and estimated RUL for particular failures in distinct health states and faults. Vlasov et al. [ 119 ] used wireless vibration sensor networks that allow real-time analysis of the state of the electronic equipment (motor). The purpose of their approach is to minimize the cost of maintenance and develop a system of predictive maintenance for optimisation predictive repair. Zhang et al. [ 121 ] used vibration sensors for accurate prediction of the remaining useful life of the rotatory machines. Deep learning model combined a long short-term memory neural network with an attention mechanism for maintenance in mechanical manufacturing.
4.3.3. Acoustical, Sound, and Ultrasonic Sensors
Another group of sensors are sensors focused on sound detection, usually via microphone devices. When a signal is detected by a sound sensor, the level of voltage is translated to the appropriate sound level. Kaptan [ 126 ] describes the location of city buses using sound sensors and an accelerometer instead of the standard global positioning system (GPS) location. In such a scenario, the accelerometers detect the movement of the vehicle and the microphone sensing distinguishes the sound level inside and outside the vehicle. Compared to GPS location, energy savings of up to 46% occur. Another possible usage of acoustic sensors is described by Ryu [ 127 ] in the field of material detection. Using machine learning techniques, sound sensors are able to estimate relevant information such as the character of an object and its location. Ultrasonic sensors are non-contact devices using the flight of the sound wave greater than that of the human audible range. Similarly, as with sonar, the measurement is based on the Doppler Shift principle. Yan et al. [ 120 ] conducted fusion data mining to predict the remaining life of a key component of machining equipment by multisource sensors (acoustical, vibration, optical, or power).
4.3.4. Pressure, Force, Touch, and Tension Sensors
Pressure sensors identify the pressure deviations in the measurement objects or environment. The change detection is usually based on barometric, piezoelectric, capacitive, optical, or resonant sensing principles. Examples of these types of sensors are Bourdon tubes, diaphragms, pressure gauge, or manometers. Tension sensors help with the deformation and movement of the belt automated material handling system monitoring for intelligent condition-based maintenance [ 104 ].
Force sensors monitor tensile compressive force signal and translate it into an output electric signal. Their application includes lead cells, strain gauges, or sensing resistors. Very popular are piezoelectric and magnetostrictive technologies. Another way of sensing is based on induction, pneumatic, and hydraulic forces.
4.3.5. Optical, Light, and Machine Vision Sensors
Another type of sensors are sensors with a machine vision function. Machine vision technology has grown significantly in recent years and is becoming part of autonomous vehicles, intelligent systems, and robotics. Optical sensor input into the systems makes these systems intelligent. Visual data are captured in the form of a series of images and after the digitisation process are processed using a machine learning algorithm [ 128 , 129 ]. In the field of material wear detection, three-dimensional (3D) sensors are also used, which represent new technical means for obtaining information. Three-dimensional data provides more information and at the same time, reduces the deviation of the measured data [ 130 ]. A large group of sensors consists of chemical character sensors. Advances in chemical, sensing, and wireless technologies have accelerated the development of wireless chemical sensors. These devices allow the collection and distribution of biochemical information. The use of these types of sensors can be found in the areas of environmental or health monitoring [ 131 , 132 ].
Tarashioon et al. [ 115 ] described the design of solid-state lighting products based on the reliability system diagnostics (self-maintenance). The light sensor design using light-emitting diode technology is used to monitor system ambient light.
4.3.6. Temperature Sensors
Temperature sensors usually detect changes in machine condition or critical state in the factory (especially in hazardous environments.). The sensors obtain temperature information directly (resistive temperature detectors, thermistors, and thermocouples) or indirectly (infrared sensors). Some of these sensors have a temperature display. Infrared (IR) sensors work on the basis of the optical principle using light. We distinguish reflective and transmissive IR sensors. Reflective IR sensors’ transmitter and detector are positioned adjacent to each other facing the object. Transmissive sensors use LED and photo diodes to detect the object passing between them.
Another group of sensors consists of probes measuring temperature and humidity. Advances in biomaterials offer opportunities to design electronics with unique mechanical stability capabilities, i.e., sensors whose material composition offers the possibility of application in medical implants and disposable wearable devices. Suitable applications can be found in the accurate scanning of biological tissues, internal organs, but also in the textile and food industry [ 133 ].
Bekar et al. [ 108 ] analysed the real-world industrial data to implement the PdM strategy for the manufacturing enterprise. They evaluated the quality of the process, vibration and temperature data by understanding outliers, and developed maintenance solutions. Sadiki et al. [ 105 ] show the advantages of condition-based maintenance for real-time intelligent monitoring for the industrial machine. Tmote sky sensors network and Z1 mote operate through the edge router and enhance the maintenance simulation’s purpose. Villalobos et al. [ 118 ] introduced a flexible forecaster analyser system for anticipation of alarms’ activation based on the temperature sensor data. The deep learning techniques based on the short-term memory recurrent neural network contribute to the predictive maintenance approach.
4.3.7. Liquid, Flow, Gas, and Chemical Sensors
Flow sensors enable the possibility to analyse the cooling water and lubrication flow rate. These sensors use magnetic, ultrasonic, or thermal detectors to monitor the current intensity in the pipeline. Chien and Chen [ 109 ] used mass flow controllers for monitoring mode and position of silane reactant flow. Their research is related to a data-driven framework for monitoring equipment’s health condition (RUL).
Oil particle sensors enable the possibility of monitoring contamination levels in lubrication systems (for example, gear boxes). These sensors target to change the level of pollution based on the presence of the number of substances processed. They analysed the light intensity via a laser beam and photo detector.
Humidity (moisture) sensors focused diagnostics on water content in oils to prevent corrosion of machines. These sensors are usually installed in a lubrication or hydraulic tank. Humidity sensors play an essential role in the selected automated manufacturing processes. To achieve the desired atmosphere, it is necessary to detect, monitor, and regulate humidity in conditions of low and high temperatures. The use of sensors for moisture detection can be found, for example, in monitoring systems and networks, as a monitoring device in agriculture, and as a tool for determining soil moisture during irrigation. Furthermore, also in the field of corrosion diagnostics in the areas of infrastructure and construction. The key element in this type of sensor is the materials used and the associated availability of suitable production technologies [ 134 ].
4.3.8. Electronic, Current, Energy, and Magnetic Sensors
Energy and current measurement sensors ensure the density of electrical cable isolation. Their purpose is to measure the current draw of machines. These sensors have been used in many industrial areas but have had shortcomings regarding demands in the areas of miniaturisation, energy consumption, and insufficient stability. At present, optical fibres and magnetic fluids are widely used, due to their versatility of application [ 135 ]. In the case of electronic sensors, these can be, for example, gas sensors that are capable of performing sensitive analysis in real time, thanks to their flexibility and the possibility of integration with intelligent electronics and mechanical resistance in relation to energy consumption, and respectively, the performance of electronic components. However, balancing these variables offers applications not only in the above areas, but also in aviation, aerospace, and nuclear industry [ 136 ] display devices, and there is considerable potential in the area of environmental monitoring [ 137 ]. Zhang et al. [ 122 ] described a data-driven smart production line with installed energy consumption sensors for forecast and fault diagnosis of maintenance.
4.3.9. Virtual Sensors
Virtual sensors are advanced applications in the software layer of the machine that enhance the knowledge of the devices. These state-of-the-art sensors obtained data not only from physical machines (for example robots), but also the knowledge bases [ 138 ]. Al-Jlibawi et al. [ 107 ] call them adaptive soft sensors due to their low cost, parallel work, robust characteristics, easy implementation, and real-time estimation features. They used PLC and SCADA for collecting data in the refinery via a distribution control system.
4.3.10. Nuclear, Chemical, Microparticles, and Nanoparticles Sensors
The last category consists of sensors that are based on modern technologies using chemical processes. These are sensors based on nanoparticles and microparticles, which enable monitoring directly inside the monitored object. Lao et al. [ 111 ] present a robust moving horizon estimation of sensor maintenance based on the observation and monitoring of chemical processes. Comparison of four simulations demonstrates the economic performance advantages of sensor-predictive maintenance. Jia et al. [ 139 ] described the design of the nanosensors for detection of antibiotics to prevent the production of resistance to antibiotics. Nanomaterial chemistry is used for developing current arsenic detection nanostructures [ 140 ]. The main advantages of nanomaterials are the high flexibility, sensitivity, compatibility, and stretchability of sensors in electronics devices [ 141 ].
5. Discussion
The discussion is divided into two parts. First, we discuss the sensor-based smart factory to imagine the factories of the future. Then, in the second part, we focus on insights and future research issues related to intelligent sensors.
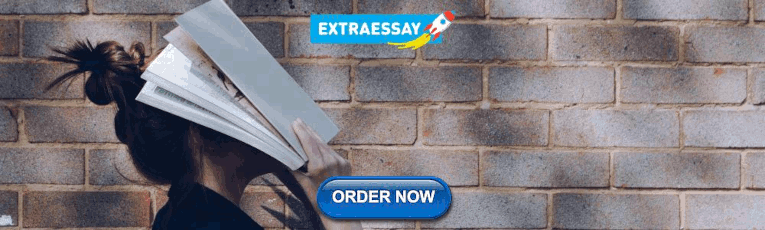
5.1. Sensor-Based Smart Factory Discussion
The essence of the use of intelligent sensors based on IoT lies in smart factories, which have modern sensor technology, intelligent analytical programs, and networking components of production (machines, supplies, components, final products, equipment, etc.). Smart factories are a new way of organizing production. Their goal is to better serve customers through greater production flexibility and resource optimisation. The factories of the future combine the efficiency of mass production with custom production and optimize the supply chain in real-time thanks to the Internet connection [ 142 ]. These factories handle fluctuations in demand, which are fully automated and fault-durable. The smart factory is connected to the Internet, however it has advanced security against cyberattacks that would jeopardize production.
We summarised the intelligent sensor advantages in Figure 10 based on Reference [ 143 ]. Sensitivity is defined as the relation unit change between output and input. Smart sensors such as IoT devices are wireless, using the internet and usually cloud. Intelligent sensors have low power consumption, automatic diagnostics, calibration, and the ability to process and share data in real-time. Robust means good durable material, solid welds, seals, potting, chemical compatibility, secured wires, and other situational protection. Automatic diagnostics are related to the possibility for making decisions or proceeding action-based actions for control. Some authors emphasise low cost as a feature for smart sensors, but we think that it depends on user experience and sensor value added.

Main characteristics of intelligent sensors.
The transformation of a traditional factory into a smart one brings with it a higher integration of physical production with digital technologies. Sensors and actuators bring factory communication capabilities and data collection and analysis capabilities [ 144 ]. The intelligent factory brings a change from traditional automation to a fully connected and flexible system that can use a continuous flow of data from connecting operations and production systems to learn and adapt to new requirements. The production system in smart factory is different—with more resources for small-lot products, dynamic routing of production line, comprehensive connections with high-speed network infrastructure, deep convergence of physical and digital world (digital twins), self-organisation control system, and big data analytics [ 64 ]. A flexible conveying system of the production lines is designed for the main production loops (cycles), with storage loops on the production line and branch loops for customizing products. The smart factory can integrate data from corporate assets to manage production, maintenance, inventory tracking, digitize operations through the digital twin, and other technologies. In the enterprise infrastructure, smart logistics, smart grids, smart buildings, and smart distribution are interconnected. Project management is important for the successful implementation and sustainability of these systems in smart factories [ 145 ].
Due to the frequent occurrence of extraordinary situations caused mainly by external elements, there is a need to deploy more demanding control systems. Management in smart factories is decentralised. Decentralisations can offer the ability to make decisions at process locations, independent of any central entity [ 146 ]. The complexity of these environments with many simultaneous processes, most of which follow each other, as well as the enormous amount of data that sensors generate in production, can no longer be served by existing control systems based on the simple technology of recording or processing transactions. Therefore, multi-agent systems come to the light, where intelligent information agents form a network of decentralised and distributed intelligence [ 147 , 148 ]. Beside the existing solutions, these systems are not based on centralised control but are capable of collective self-configuration. These systems interconnect individual autonomous agents (or their digital twins) to communicate, coordinate, and cooperate to achieve a set common goal. Individual communication elements collect data as needed, which they later use to improve and optimize production.
In smart factories, thanks to intelligent sensors, each product actively participates in the production process. The components to be processed contain digital information on how to process them. They, therefore, communicate directly with robots and production machines. With the help of a chip with radio frequency identification or other sensor technology, it can control its production flow. A smart product has access to knowledge related to its structure, composition, or purpose [ 149 ]. On the other hand, thanks to this connection, the customer uses the user interface and intervenes in production in real-time. The sensors allow the customer to obtain information for creating the product specification, and its adjustment according to needs and requirements [ 150 ]. Autonomous vehicles powered by electricity are also connected to the system, ensuring the transport of stock and final products around the factory. Vehicle control is provided by a sophisticated system of sensors. Parts, materials, and components needed for production are transported to the factory when they are really needed for production (Just-in-Time system). Sensors and possibly drones constantly check stock in a smart factory [ 151 ].
We performed profound words’ analysis of full-text papers to find phrases containing the terms “smart” or “intelligent”. Figure 11 shows that the obtained keywords form various clusters and subclusters related to the predictive maintenance process. We identified four main components of the Smart and Intelligent Predictive Maintenance (SIPM) system for smart factories based on cluster analysis. These are the production system, the monitoring system, the factory planning system, and the maintenance system. The production system of SIPM is based on energy saving control, transportation, and economics costs, with use of controllers for predictive maintenance based on data analysis, and equipment diagnostics. The monitoring system uses condition-based diagnostics, sensors network linking management, and production—the factory planning system concerning different components of objects and agents by using algorithms and meters. The maintenance system is related to using analysis and diagnostics sensor data for predictive maintenance.

Analysis of keywords “smart” and “intelligent”.
From the point of view of preventive maintenance, the machines and robots performing production communicate with each other continuously and inform each other about non-standard situations. The machines report themselves to the maintenance staff (in this sense it is a robot), besides, they precisely define the problem. The sensors in production are thus connected to other factory systems based on SCADA. All elements can minimize energy losses or use alternative energy sources for their activities [ 152 ]. Zero error rate is ensured in production using smart sensors. Smart sensors and testers monitor the quality of the final products.
We discussed the results and interpreted them in the perspective of previous studies and research. Predictive maintenances in Cavalieri-Salafia’s model [ 153 ] includes data acquisition from sensors, data manipulation (filtering, transforming, removing noise), aggregation, prediction, decision-making, scheduling, and further monitoring of status and configuration. Similarly, it describes the process of data acquisition, data processing, and machine decision-making [ 154 ]. Possible application of artificial intelligence (AI) for preventive maintenance is discussed by Carlson and Sakao [ 155 ]. Modern systems are based on the Internet of Things that enable real-time prediction and data sharing [ 96 ]. Uhlmann et al. [ 117 ] described the solution of sensor network enhanced by cloud. The edge technologies [ 156 ] allow integration between PLC and cloud for modern sensors. Miniaturisation of current sensors and nanotechnology [ 157 ] provides higher flexibility of maintenance systems. Fernandes et al. [ 158 ] emphasise the role of data visualisation, data mining, and data storage. These models have a standard data flow process which is a part of the possible prediction preparation. It is necessary to set a reliability model to analyse the dataset [ 159 ]. Ruhi and Karim [ 160 ] show that a suitable statistical model can be applied to estimate the optimum maintenance period at a minimum cost. Stodola and Stodola [ 161 ] pointed out that a useful model needs to consider human factors and related issues such as labour intensity, administrative, and human errors.
5.2. Discussion on Insights and Future Research Issues
We discussed practical considerations and potential avenues for future research. The following directions have been identified for potential further work.
5.2.1. Wireless Network of Sensors
One of the current trends is the use of a wireless network of sensors (WSN), which according to Vlasov et al. [ 119 ] consists of intelligent sensors for sensing physical parameters. The individual sensors act as network elements (nodes) that can read, process, and transmit wireless data in smart factories. They consist of processing, communication, and the sensor unit. WSN is a network of Micro-Electro-Mechanical systems (MEMS) of sensor devices [ 162 ]. MEMS offers a high potential for condition monitoring applications. These sensors are, in comparison to industrial sensors, cost-effective, wireless, and highly integrable and configurable [ 117 ]. Part of these networks could be process controllers (actuators) that communicate data and operate some units [ 163 ]. The cyber-physical systems integrate these sensors and actuator networks into a coherent form. Vlasov et al. [ 119 ] show that the use of wireless communication channels in the monitoring system is driven by the sensor network in the shortest possible time. Uhlmann et al. [ 117 ] pointed out their main advantage—low cost. Sadiki et al. [ 105 ] recommend to check the viability of these network applications before their implementation in factories to save operation costs and improve real-time monitoring performance. Main advantages of industrial WSN, summarising Kumar et al. [ 163 ], are: minimal cost and compactness, interoperability, resistance to noise (and co-existence), self-organizing, robustness (fault-tolerance), link-reliability, energy consumption, low-delay, service differentiation and quality, scalability, predictable behaviour, multiple sources, data aggregation, and specific protocols. However, the limitations of wireless sensors are the spatial arrangement in the environment, deployment time, maintenance cost of communication channels [ 119 ], scalability, and lack of protocols. Flammini et al. [ 164 ] emphasised that fault assumption and transmission errors are more frequent in wired communication than on wired links. Thus, data quality (in terms of validity, integrity, accuracy, and reliability) is an important factor of wired networks connected to the internet [ 165 ].
5.2.2. Dominance of Vibration and Temperature Sensors for Maintenance
The analysis of the types of sensors used for predictive maintenance in Section 4.3 showed that vibration and temperature sensors are most often used. According to Uhlmann [ 117 ], the number of powerful MEMS sensors such as vibration and temperature sensors has increased. Vibration can identify problems before other symptoms, such as temperature, sound, power consumption, and contaminated lubricants. When the first signs of a malfunction appear, there is usually only a few months left until a complete machine downtime. Vibration monitoring enables to determine which phase of the fault curve the machine is currently in. Excessive vibration is usually the first symptom of internal problems, such as defective bearings, imbalances, misalignments, and loose components, that shorten the life of the equipment. Only after detectable changes in vibration is it possible to detect possible errors using power, particle, or infrared sensors. Finally, errors also appear on the temperature sensors. According to Barbieri et al. [ 99 ], vibrational signals are a starting point of the component degradation model. Crucial decision lies in the selection of adequate signals for failure estimation and maintenance prediction. The further research challenge in infrared thermography is the signal and image processing to enhance the detection and simplify the interpretation of the results [ 166 ]. Akerberg et al. [ 167 ] pointed out that the requirements for vibration sensors, battery lifetime, update frequency, and security are lower than for flow, torque, or proximity sensors. The main benefits of vibration sensors are predictability of impending failures, machine safety, cost, extended maintenance intervals, machine reliability, and confidence in scheduled maintenance. In the future, these advantages resulting from the use of vibration sensors can be obtained by using new types of sensors, for example, virtual sensors or nanosensors, etc. For this reason, current research is focused on minimizing the costs and flexibility of vibration and temperature sensor solutions.
5.2.3. Challenges of the Deep Learning and Big Data Analytics
Components connected to the network generate a large amount of heterogeneous data that smart industry systems must process. Large industrial data is collected from multiple sources, such as devices, products, and customers, in heterogeneous forms [ 108 ]. Their analysis is crucial for companies in almost all market sectors. Advanced analysis of big data is a way to get to know customers and devices (machine state) in detail. Companies that use it will be able to provide customers with literally “tailor-made” services, lower moods, and increase efficiency in production, including maintenance. Thanks to the current capacity of computer technology, even large volumes of data can be processed faster, which allows smart industry systems to move information in real-time. Farooq et al. [ 38 ] consider industrial prognosis and applying predictive analytics using machine learning techniques as new challenges to identify failure modes and reduce downtime. The application of the simulation enhances more possibilities for after-market services, such as maintenance [ 92 ]. Learning and intelligent techniques such as deep learning to analyse industrial big data are proposed as future directions in maintenance [ 103 , 120 ]. We consider the main problem in the field of data analysis to be the inability to translate and create a business case for analysts with a comprehensive view. This means that there is no concept of how to evaluate the analytical results and translate them into actions (for example, maintenance schedules). Companies often make the mistake of supplementing random findings instead of a selective and targeted approach such as predictive maintenance. Results of the analysis must lead to conclusions, which requires a properly chosen tool for data visualisation. Other possible research directions are related to virtual metrology [ 109 ].
5.2.4. Challenge of Interoperability
Interconnectivity is a prerequisite for the existence of a smart industry. It is not just a peripheral connection of one device to another or a centralised connection of the machine to the control system, but a complex network. In a corporate environment, this means connecting machines, people, materials, products, information and communication technologies and systems, and, finally, data in the form of documents. Scalability enables possible network size enhancing or reduction based on the industrial requirements. This needs appropriate protocols, standards, and robust integration of hundreds of network nodes. Interoperability is linked to their compatibility, i.e., all elements of the industrial Internet of Things should be able to communicate with each other (exchange data) and interact. Through standardisation, disparate systems that work together can be soldered into a single process. Today’s factories already use sensor systems for various measurements and operations. The use of new solutions must work with these old systems. This problem is caused by a lack of robustness, time constraints, and service-differentiated protocols. One of the future solutions is, for example, the oneM2M [ 168 ] or OPC Unified Architecture [ 169 ] industry communication standards, which are platform-independent and support semantic interoperability [ 170 ]. On these platforms, data objects and each device have well-defined behaviour on the network, and the capability of horizontal communication between individual devices processing in real-time. There is a possibility to connect other components (such as plug and produce), which then have their image in the cloud.
5.2.5. Challenges of Control and Maintenance Systems Decentralisation
Many of the wireless sensor networks still have centralised control (so-called “network manager”). Centralised server-client systems had one central database, which leads to data consistency, easy administration, and a high level of security. However, centralisation can result in the failure of a single point. High traffic can overload the bottleneck. A centralised “network management” system can be slow and take a vest to lose data packets. It is essential to use decentralised solutions within complex digital ecosystems, such as production factories. The responsibility and need to make operational decisions is performed at lower levels. A distributed architecture based on decentralisation is suitable for their coordination and management [ 171 ]. Coordination between the individual nodes involved in cyber-physical systems requires communication between the elements. The benefit of the distributed architecture of components connected to the network and decentralised smart industry systems is the expandability (scalability) of the network, as well as the increased resistance to failures of the network itself, individual connected systems, as well as their components. For example, Kiangala and Wang [ 94 ] proposed a decentralised monitoring system with a cloud-based dashboard displaying real-time reports for every maintenance schedule generated. Zhang et al. [ 122 ] consider as a future trend services in cyberspace that control, plan, and schedule production line items in a timely way. Smart Industry systems already use intelligent algorithms to monitor, control, manage, and plan complex processes and operations throughout the production process and supply chain. Advanced cognitive technologies will be gradually implemented in production systems to increase the autonomy of individual components of the network. These systems use the principles of collective intelligence in industrial processes, especially solutions based on multiagent systems. The future of these systems lies in achieving a high level of artificial intelligence that will use the collective knowledge of all parts of the network.
5.2.6. High Potential of Virtual- and Nano-Sensors
Sensor technology, the elements informing about the state and activity of the object, is currently unprecedented and developed in various industries due to its reliability. Sensors as sources of primary information about the real environment are the input element of practically all control, measuring, and automation systems today, and together with microsystems, they accompany human activity (via biometric sensors) at almost every step. An interesting trend can be traced in the development of electronics and microelectronics in sensor systems, which include circuits for signal processing, analysis, and unification in a single compact design with a sensor element. In optimizing this process, a tighter system connection of electronics and microelectronics with related elements of optics or fine mechanics within mechatronics is increasingly being applied. The intention of such an interconnection is to achieve the development and production integration of entire systems on a single chip, from input sensors to various types of actuators. From another point of view, it is possible to see the influence of miniaturisation and new sensor types such as nanosensors. The higher resistance of the sensors to mechanical influences, acceleration, and vibrations also leads to their placement directly in the parts of the system, which in many cases gives the function of the sensor a wider range. Nanosensors have, due to miniaturisation, good preconditions for massive use in smart factories. These trends are currently aided by the search for ways to create wireless sensor networks with remote data transmission. Al-Jlibawi et al. [ 107 ] described the properties of soft (virtual) sensors and their function in parallel work with physical sensors and industrial processes. Virtual sensors are used for damage detection [ 172 ], industrial robot interaction [ 173 ], identification, and approximation tasks, or for digital twin applications. On-line evaluation of input effects directly in the actuator can then lead to a “sensor-less” system, whose other advantage, in addition to lower one-time investment costs, should be the greater reliability of the system with protection against possible outages. Li et al. [ 165 ] described automatic control systems without sensors based on wireless nodes, RIFID, and programmable logic controller (PLC). Automated devices perform their tasks based on industrial wireless nodes fully responsible for communication. Controllers have the automation control function based on the received commands from other devices in the network.
5.2.7. Challenges of Availability and Reconfigurability of Sensors
The development of industry systems is progressing towards their maximum modularity and capability of autonomous reconfiguration based on automatic situation recognition. The self-organisation of production processes therefore also includes reconfigurability, characterised as independent adaptability to internal and external conditions. Condition-based systems are being developed in the field of maintenance. The main problem in the future may be the use of old sensors (installed on older devices in production), which will lead to unacceptably high manufacturing and maintenance costs in the long term. Kozlowski et al. [ 110 ] mentioned the unavailability of devices (CNC machines) on the market to structurally optimally distributed sensors on machine parts. Related to this issue is the problem of today’s industrial practice—the lack of appropriate measurement signals. The result is the need for additional installation of sensors on the device. A problem with the weak signal from the sensors can lead to injury, product losses, and production outages. Even small and temporary communication errors can cause significant production interruptions [ 167 ]. This production issue can be caused by signal inference from devices that operate on the same frequency. It means that at the same time, waves and signals coexist in a given environment, which disrupt the function of the sensors. This problem can be described as a real-time availability issue. Lao et al. [ 111 ] show that preventive maintenance of sensors and actuators in real-time can significantly mitigate the damage from production losses, process upsets, and downtime based on specific routine regulations.
5.2.8. Security and Safety Challenge
The severity of cyber security issues is growing, along with our growing dependence on technology, both personally and socially. In production, important data is collected by increasingly connecting production systems or even the robots themselves to computer networks, sometimes with communication to the cloud, and new security risks arise. Basic cyber security principles can significantly improve the security of connected devices. The contemporary challenge of safety is the security of the stored data from sensors. In addition to the sensors that collect data, which is a physical prerequisite for the Industry 4.0 concept, the sovereignty of the data is important [ 174 ], especially from a psychological point of view. Only enterprises that trust the security of their networks are also willing to store and share their data. Sensor security does not require high demands on battery capacity. However, security is considered too complicated, especially for IoT, due to the use of various techniques such as keying to protect authentication, cryptographic code verification, security gateways [ 175 ], safety protocols, remote wireless security management [ 176 ], or security analysis to detect attacks. Almost all interconnected systems have their weaknesses that their creators are unaware of. For this reason, prevention is particularly important [ 167 ]. An important factor due to the number of interconnected devices is the cost of security. Gungor and Hancke [ 177 ] point out that security is a crucial feature in designing wireless sensor networks. Safe communication protects against external attacks and intrusion.
6. Conclusions
The fourth industrial revolution is permeating the industry, enabling an increasing number of enterprises to have an incomparably greater overview of their production and maintenance activities than ever before. The deployment of highly reliable and low-maintenance devices contributes to the precise planning of production capacity and equipment’s associated maintenance.
The first research question relates to the contemporary trends in the maintenance processes of smart factories. The number of papers discussing the key terms sensors, smart factories, and preventive maintenance increased over time, mostly in the last years. We found that the contemporary burst trend is related to Industry 4.0 technology. Predictive maintenance, smart factories, and intelligent sensors publications, together concerned topics mainly related to deep machine learning, Internet of Things, and big data analytics. The maintenance process in smart factories is based on digitisation, data-driven manufacturing, agent-based systems, and digital twins. Intelligent sensors in such factories use edge, fog, and deep learning methods for control of manufacturing processes. In the future, Internet and blockchain will be important for predictive maintenance.
Smart and intelligent predictive maintenance is characterised to answer a second research question. Here, the results show four different types of maintenance used in smart factories—Industry 4.0 for predictive maintenance, smart manufacturing for condition-based maintenance, fault diagnosis for maintenance and prognostics, and remaining useful life analysis. The importance of predictive maintenance is also growing due to the growing number of robots, digitisation, and artificial intelligence introduced into production lines to automate routine activities.
Following the third question’s answer, we can state that the three types of sensors are mainly used for predictive maintenance in smart factories. Firstly, intelligent sensors which have the potential to connect to higher-level systems. Furthermore, there is a possibility to connect these intelligent sensors to the internet—to build up the IoT devices. Finally, we can use the gathered data in cloud-related technologies. The most prevalent methods used for collecting and monitoring machines and devices are vibration analysis [ 120 ], SCADA systems, CNC machine sensors, and PLCs. Based on the deep analysis, we conclude that the current trend, insights, and future research issues are characterised by:
- Usage of multisource wireless networks of sensors in predictive maintenance.
- Dominance of vibration and temperature sensors for predictive maintenance.
- Challenges of the big data analytics and deep learning techniques.
- Challenges of interoperability of multiple sensors and maintenance systems.
- Decentralisation of maintenance control systems.
- High potential of virtual sensors and nanosensors for the future.
- Challenge of availability and reconfigurability of sensors.
- Security and safety of sensor data.
Based on the results synthesis, we proposed the Smart and Intelligent Predictive Maintenance (SIPM) system for smart factory concerning four major subsystems: production, monitoring, planning, and maintenance. These subsystems communicate and collaborate through modern IoT and cloud-based technologies. Their main advantage is real-time management and planning to reduce the economic costs caused by production downtime.
From a managerial point of view, the predictive maintenance system in smart factories is an early warning, especially in high-risk industries. The ability to detect weak signals of potentially significant strategic impact is a welcome positive in a turbulent business environment. The system of predictive maintenance does not reduce the responsibility or the possibility of personal development of employees, but it must be stimulated by responsible managers. It offers the possibility to reduce the number of hierarchical levels between managers and ordinary employees, so that you can bring about higher employee autonomy and help other innovation processes to be implemented effectively. The challenge for managers today is to select criteria based on which they will be able to select intelligent sensors for smart factories. There is a wide range of sensors on the market and the authors most often state the criteria of sensor sensitivity, sensor cost, flexibility, and size (miniaturity).
Further research may comprise the advanced machine learning methods as deep learning, data-driven algorithms. The new concept called “Machine as a Service” (MaaS) takes over the software as a service (SaaS) model and is another trend suitable for future research. An interesting direction of future research concerns building performance model evaluation related to the reasonable cost. The cost/benefit analysis of using preventive tools in contrast to sustainability requirements is challenging for research.
This work suffers from several limitations, notably related to publication collections, filtering, and analysis. The search strategy is biased by the problematic synonym of the term “factory”. Primarily, the term “plant” is not interchangeable in the same meaning. The study is limited because we omitted highly cited publications related to medicine in burst analysis. Our goal was to bring the reader closer manufacturing- and production-related publications. Investigated trends in burst detection analysis have weights based on occurrence in publication titles. Results do not show the quality of publication based on the times cited. Thus, we instead present examples of highly cited publications of most important burst terms. The lack of a comprehensive review due to a steadily increasing number of related works is another notable limitation of this study.
Acknowledgments
The authors thank the University of South Bohemia in Ceske Budejovice for technical support of the databases search.
Supplementary Materials
The PRISMA Checklist (Table S1) and burst analysis results (Figures S1–S3) are available online at https://www.mdpi.com/1424-8220/21/4/1470/s1 , Figure S1: The burst detection in topic Smart Factory/production based on WoS data, Figure S2: The burst detection in topic Smart Sensors based on WoS data, Figure S3: The burst detection in topic Smart/predictive maintenance based on WoS data, Table S1: The PRISMA Checklist.
Author Contributions
Conceptualisation, M.P. and J.V.; methodology, M.P.; software, M.P.; validation, M.P.; formal analysis, M.P.; investigation, M.P. and J.V.; resources, J.B.; data curation, M.P.; writing—original draft preparation, M.P., J.V. and J.B.; writing—review and editing, M.P. and J.V.; visualisation, M.P.; supervision, M.P.; project administration, J.V.; funding acquisition, J.B. and J.V. All authors have read and agreed to the published version of the manuscript.
This research was funded by “EF-150-GAJU 047/2019/S”.
Institutional Review Board Statement
Informed consent statement, data availability statement, conflicts of interest.
The authors declare no conflict of interest.
Publisher’s Note: MDPI stays neutral with regard to jurisdictional claims in published maps and institutional affiliations.

After being insulted, writing down your feelings on paper then getting rid of it reduces anger
A research group in Japan has discovered that writing down one's reaction to a negative incident on a piece of paper and then shredding it or throwing it away reduces feelings of anger.
"We expected that our method would suppress anger to some extent," lead researcher Nobuyuki Kawai said. "However, we were amazed that anger was eliminated almost entirely."
This research is important because controlling anger at home and in the workplace can reduce negative consequences in our jobs and personal lives. Unfortunately, many anger management techniques proposed by specialists lack empirical research support. They can also be difficult to recall when angry.
The results of this study, published in Scientific Reports , are the culmination of years of previous research on the association between the written word and anger reduction. It builds on work showing how interactions with physical objects can control a person's mood.
For their project, Kawai and his graduate student Yuta Kanaya, both at the Graduate School of Informatics, Nagoya University, asked participants to write brief opinions about important social problems, such as whether smoking in public should be outlawed. They then told them that a doctoral student at Nagoya University would evaluate their writing.
However, the doctoral students doing the evaluation were plants. Regardless of what the participants wrote, the evaluators scored them low on intelligence, interest, friendliness, logic, and rationality. To really drive home the point, the doctoral students also wrote the same insulting comment: "I cannot believe an educated person would think like this. I hope this person learns something while at the university."
After handing out these negative comments, the researchers asked the participants to write their thoughts on the feedback, focusing on what triggered their emotions. Finally, one group of participants was told to either dispose of the paper they wrote in a trash can or keep it in a file on their desk. A second group was told to destroy the document in a shredder or put it in a plastic box.
The students were then asked to rate their anger after the insult and after either disposing of or keeping the paper. As expected, all participants reported a higher level of anger after receiving insulting comments. However, the anger levels of the individuals who discarded their paper in the trash can or shredded it returned to their initial state after disposing of the paper. Meanwhile, the participants who held on to a hard copy of the insult experienced only a small decrease in their overall anger.
Kawai imagines using his research to help businesspeople who find themselves in stressful situations. "This technique could be applied in the moment by writing down the source of anger as if taking a memo and then throwing it away when one feels angry in a business situation," he explained.
Along with its practical benefits, this discovery may shed light on the origins of the Japanese cultural tradition known as hakidashisara ( hakidashi refers to the purging or spitting out of something, and sara refers to a dish or plate) at the Hiyoshi shrine in Kiyosu, Aichi Prefecture, just outside of Nagoya. Hakidashisara is an annual festival where people smash small discs representing things that make them angry. Their findings may explain the feeling of relief that participants report after leaving the festival.
- Anger Management
- Social Psychology
- Disorders and Syndromes
- Educational Psychology
- Consumer Behavior
- Anger management
- Social psychology
- Cognitive dissonance
- Self-awareness
- Obsessive-compulsive disorder
- Collaboration
Story Source:
Materials provided by Nagoya University . Note: Content may be edited for style and length.
Journal Reference :
- Yuta Kanaya, Nobuyuki Kawai. Anger is eliminated with the disposal of a paper written because of provocation . Scientific Reports , 2024; 14 (1) DOI: 10.1038/s41598-024-57916-z
Cite This Page :
Explore More
- Quantum Effects in Electron Waves
- Star Trek's Holodeck Recreated Using ChatGPT
- Cloud Engineering to Mitigate Global Warming
- Detecting Delayed Concussion Recovery
- Genes for Strong Muscles: Healthy Long Life
- Brightest Gamma-Ray Burst
- Stellar Winds of Three Sun-Like Stars Detected
- Fences Causing Genetic Problems for Mammals
- Ozone Removes Mating Barriers Between Fly ...
- Parkinson's: New Theory On Origins and Spread
Trending Topics
Strange & offbeat.
Overview and Discussion of Pavement Performance Prediction Techniques for Maintenance and Rehabilitation Decision-Making
- Original Research Paper
- Published: 11 April 2024
Cite this article
- Jeetendra Singh Khichad 1 &
- Rameshwar J. Vishwakarma ORCID: orcid.org/0000-0003-4362-6161 1
Explore all metrics
The pavement performance prediction techniques were studied for concrete and asphalt pavements. The future pavement conditions for optimized performance and maintenance can be predicted using various techniques. Different techniques including Mechanistic-Empirical Pavement Design Guide (MEPDG), Artificial Neural Network (ANN), Regression Analysis, Probabilistic Analysis, Expert Systems, Artificial Intelligence, Machine Learning, and Pavement Management Systems (PMS) were employed to systematically investigate and assess pavement performance. The performance of pavement is influenced by functional and structural factors of deterioration including materials, traffic, pavement type, and environmental conditions. Transport infrastructure development requires researcher collaboration for advancement. However, few studies used hybrid techniques and prediction models to accurately analyze pavement problems and make cost-effective, safe, and efficient repair plans within financial limits. Each performance technique has its advantages and limitations in systematic procedures to predict pavement performance. The focus of this study is on exploring approaches to predict pavement performance based on weather patterns and other variables, evaluating maintenance requirements based on actual pavement conditions, and optimizing maintenance costs. The research concludes that pavement engineers need advanced analysis models to precisely predict pavement performance for maintenance and repairs. To access the link between trends of pavement performance for maintenance, routine maintenance actions need to be properly documented. Based on varying pavement performance trends innovative materials, construction methods, and design methodologies may be incorporated for better performance are being used to improve structural integrity, durability, sustainability, and long-term performance. It would optimize the cost and frequency of the maintenance and repair requirements.
This is a preview of subscription content, log in via an institution to check access.
Access this article
Price includes VAT (Russian Federation)
Instant access to the full article PDF.
Rent this article via DeepDyve
Institutional subscriptions
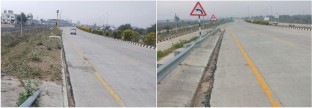
Data availability
All the data used during the present study appear in the published paper.
Abbreviations
Asphalt concrete
Asphalt concrete cement
Artificial intelligence
Artificial neural network
Coefficient of thermal expansion
Federal highway administration
Geographic information system
International roughness index
Life-cycle assessment
Lightweight cellular concrete
Life-cycle cost analysis
Long term pavement performance
Mechanistic-empirical pavement design guide
Machine learning
Multiple linear regression
Plain concrete
Portland cement concrete
Pavement management systems
Recycled concrete aggregate
Random forest
Root-mean-square error
Support vector machines
Sulphur waste
Weigh-in-motion
Waste poly ethylene
Waste poly propylene
Mills, L. (2010) Hierarchical Markov chain Monte Carlo and pavement roughness model, Doctoral dissertation, University of Delaware
Transportation Officials (2008) Mechanistic-empirical pavement design guide: a manual of practice, AASHTO
Ali, A., Heneash, U., Hussein, A., & Khan, S. (2023). Application of artificial neural network technique for prediction of pavement roughness as a performance indicator. Journal of King Saud University-Engineering Sciences . https://doi.org/10.1016/j.jksues.2023.01.001
Article Google Scholar
Schwartz, C. W., Forman, B. A., & Leininger, C. W. (2015). Alternative source of climate data for mechanistic–empirical pavement performance prediction. Transportation Research Record., 2524 (1), 83–91. https://doi.org/10.3141/2524-08
Hu, A., Bai, Q., Chen, L., Meng, S., Li, Q., & Xu, Z. (2022). A review on empirical methods of pavement performance modeling. Construction and Building Materials, 342 , 127968. https://doi.org/10.1016/j.conbuildmat.2022.127968
Ker, H. W., Lee, Y. H., & Lin, C. H. (2008). Development of faulting prediction models for rigid pavements using LTPP database. Statistics, 218 (0037.0), 0037–0130.
Google Scholar
Fuhaid, A. F. A., Arifuzzaman, M., & Gul, M. A. (2022). Application of mechanistic empirical pavement design guide software in Saudi Arabia. Applied Sciences., 12 (16), 8165. https://doi.org/10.3390/app12168165
Han, C., Tong, J., Ma, T., Tong, Z., & Wang, S. (2023). Rutting prediction model for semi-rigid base asphalt pavement based on a data-mechanistic dual driven method. International Journal of Pavement Engineering., 24 (1), 2173753. https://doi.org/10.1080/10298436.2023.2173753
Saha, S., Gu, F., Luo, X., & Lytton, R. L. (2023). Development of an artificial neural network-based k-value prediction model to improve the sensitivity of base layer on rigid pavement performance. Transportation Research Record . https://doi.org/10.1177/03611981221143114
Haider, S. W., Harichandran, R. S., & Dwaikat, M. B. (2010). Effect of axle load measurement errors on pavement performance and design reliability. Transportation Research Record., 2160 (1), 107–117. https://doi.org/10.3141/2160-12
Haider, S. W., Baladi, G. Y., Chatti, K., & Dean, C. M. (2010). Effect of frequency of pavement condition data collection on performance prediction. Transportation research record., 2153 (1), 67–80. https://doi.org/10.3141/2153-08
Yao, L., Leng, Z., Jiang, J., Fang, C., & Ni, F. (2023). Effects of traffic load amplitude sequence on the cracking performance of asphalt pavement with a semi-rigid base. International Journal of Pavement Engineering., 24 (1), 2152027. https://doi.org/10.1080/10298436.2022.2152027
El-Hakim, A., & El-Badawy, S. (2013). International roughness index prediction for rigid pavements: An artificial neural network application. In Advanced Materials Research, Trans Tech Publications Ltd, 723 , 854–860. https://doi.org/10.4028/www.scientific.net/AMR.723.854
Li, H., Ni, F., Dong, Q., & Zhu, Y. (2018). Application of analytic hierarchy process in network level pavement maintenance decision-making. International Journal of Pavement Research and Technology, 11 (4), 345–354. https://doi.org/10.1016/j.ijprt.2017.09.015
Jorge, D., & Ferreira, A. (2012). Road network pavement maintenance optimisation using the HDM-4 pavement performance prediction models. International Journal of Pavement Engineering, 13 (1), 39–51. https://doi.org/10.1080/10298436.2011.563851
Khichad, J. S., Vishwakarma, R. J., & Ingle, R. K. (2022). Load transfer mechanism for jointed plain concrete pavements: A review. The Indian Concrete Journal, 96 (7), 35–45.
Khichad, J. S., Vishwakarma, R. J., & Magade, S. B. (2023). Comparison of stresses in jointed plain concrete pavement without shoulder. Materials Today: Proceedings, 77 (03), 764–772. https://doi.org/10.1016/j.matpr.2022.11.445
Vishwakarma, R. J., & Ingle, R. K. (2020). Effect of non-uniform soil subgrade on critical stresses in concrete pavement. In T. V. Mathew, G. J. Joshi, N. R. Velaga, & S. Arkatkar (Eds.), Transportation Research: Proceedings of CTRG (pp. 805–817). Singapore: Springer Singapore.
Chapter Google Scholar
Vishwakarma, R. J., & Ingle, R. K. (2018). Effect of panel size and radius of relative stiffness on critical stresses in concrete pavement. Arabian Journal for Science and Engineering., 43 (10), 5677–5687. https://doi.org/10.1007/s13369-018-3308-x
Vishwakarma, R. J., Ingle, R. K. (2017) Evaluation of subgrade strength of soil below concrete pavement using non-destructive method, In Conference on numerical modeling in geo-mechanics, CoNMiG-2017, Roorkee. pp. 21–26.
Vishwakarma, R. J., & Ingle, R. K. (2019). Observations on evaluation of flexural stresses in rigid pavement. Indian Highways, 46 (4), 29–37.
Vishwakarma, R. J., & Ingle, R. K. (2017). Simplified approach for the evaluation of critical stresses in concrete pavement. Structural Engineering and Mechanics, An Int Journal, 61 (3), 389–396. https://doi.org/10.12989/sem.2017.61.3.389
Soltani, M., Moayedfar, R., & Vun, C. V. (2023). Using response surface methodology to assess the performance of the pervious concrete pavement. International Journal of Pavement Research and Technology, 16 (3), 576–591. https://doi.org/10.1007/s42947-021-00149-4
Al-Hadidy, A. I. (2023). Experimental investigation on performance of asphalt mixtures with waste materials. International Journal of Pavement Research and Technology . https://doi.org/10.1007/s42947-023-00288-w
Ali, B., Ahmed, H., Hafez, H., Brahmia, A., Ouni, M. H. E., & Raza, A. (2023). Life cycle impact assessment (cradle-to-gate) of fiber-reinforced concrete application for pavement use: A case study of Islamabad City. International Journal of Pavement Research and Technology., 16 (2), 247–263. https://doi.org/10.1007/s42947-021-00129-8
Al-Hadidy, A. I. (2023). Sustainable recycling of sulfur waste through utilization in asphalt paving applications. International Journal of Pavement Research and Technology., 16 (2), 474–486. https://doi.org/10.1007/s42947-021-00143-w
Sereewatthanawut, I., & Prasittisopin, L. (2020). Environmental evaluation of pavement system incorporating recycled concrete aggregate. International Journal of Pavement Research and Technology., 13 , 455–465. https://doi.org/10.1007/s42947-020-0002-7
Sabih, G., & Tarefder, R. A. (2016). Impact of variability of mechanical and thermal properties of concrete on predicted performance of jointed plain concrete pavements. International Journal of Pavement Research and Technology., 9 (6), 436–444. https://doi.org/10.1016/j.ijprt.2016.09.005
Marcelino, P., de Lurdes Antunes, M., Fortunato, E., & Gomes, M. C. (2020). Transfer learning for pavement performance prediction. International Journal of Pavement Research and Technology, 13 , 154–167. https://doi.org/10.1007/s42947-019-0096-z
Mallick, R. B., Radzicki, M. J., Daniel, J. S., & Jacobs, J. M. (2014). Use of system dynamics to understand long-term impact of climate change on pavement performance and maintenance cost. Transportation Research Record., 2455 (1), 1–9. https://doi.org/10.3141/2455-01
Yamany, M. S., & Abraham, D. M. (2021). Hybrid approach to incorporate preventive maintenance effectiveness into probabilistic pavement performance models, Journal of Transportation Engineering. Part B: Pavements., 147 (1), 04020077. https://doi.org/10.1061/JPEODX.0000227
Peterson, D. E. (1987) Pavement management practices. Transportation Research Board, National Research Council, Washington, D.C., (135)
Hicks, R. G., Moulthrop, J. S., & Daleiden, J. (1999). Selecting a preventive maintenance treatment for flexible pavements. Transportation research record., 1680 (1), 1–12. https://doi.org/10.3141/1680-01
Hall, K. T., Correa, C. E., & Simpson, A. L. (2003). Performance of flexible pavement maintenance treatments in the long-term pavement performance SPS-3 experiment. Transportation Research Record, 1823 (1), 47–54. https://doi.org/10.3141/1823-06
Fwa, T. F., & Sinha, K. C. (1986). Routine maintenance and pavement performance. Journal of Transportation Engineering., 112 (4), 329–344. https://doi.org/10.1061/(ASCE)0733-947X(1986)112:4(329)
Hein, D. K. (2010) Guidelines for the Implementation of a Pavement Preservation Program for Municipal Pavements in Canada, In First International Conference on Pavement Preservation, California Department of Transportation, Federal Highway Administration, Foundation for Pavement Preservation
Galehouse, L., Moulthrop, J. S., & Hicks, R. G. (2003) Principles of pavement preservation: Definitions, benefits, issues, and barriers, TR News. Transportation Research Board, National Research Council, Washington, D.C., (228), pp. 4–9
Chen, L. (2019). Q, Bai, Optimization in decision making in infrastructure asset management: A review. Applied Sciences, 9 (7), 1380. https://doi.org/10.3390/app9071380
Pourgholamali, M., Labi, S., & Sinha, K. C. (2023). Multi-objective optimization in highway pavement maintenance and rehabilitation project selection and scheduling: A state-of-the-art review. Journal of Road Engineering, 3 , 239–251. https://doi.org/10.1016/j.jreng.2023.05.003
Chen, W., & Zheng, M. (2021). Multi-objective optimization for pavement maintenance and rehabilitation decision-making: A critical review and future directions. Automation in construction, 130 , 103840. https://doi.org/10.1016/j.autcon.2021.103840
Chen, W., Zheng, M., Tian, N., Ding, X., Li, N., & Zhang, W. (2023). Project-based sustainable timing series decision-making for pavement maintenance using multi-objective optimization: An innovation in traditional solutions. Journal of Cleaner Production, 407 , 137172. https://doi.org/10.1016/j.jclepro.2023.137172
Chen, Z., Liang, Y., Wu, Y., & Sun, L. (2019). Research on comprehensive multi-infrastructure optimization in transportation asset management: The case of roads and bridges. Sustainability, 11 (16), 4430. https://doi.org/10.3390/su11164430
Saboori, A., Harvey, J., Lea, J., Wu, R., Mateos, A. (2021), Pavement ME sensitivity analysis (version 2.5. 3). https://doi.org/10.7922/G25D8Q4H
Yang, X., You, Z., Hiller, J. E., Mohd Hasan, M. R., Diab, A., & Luo, S. (2020). Sensitivity of rigid pavement performance predictions to individual climate variables using pavement ME design. Journal of Transportation Engineering, Part B: Pavements, 146 (3), 04020028. https://doi.org/10.1061/JPEODX.0000182
Li, Q., Xiao, D. X., Wang, K. C., Hall, K. D., & Qiu, Y. (2011). Mechanistic-empirical pavement design guide (MEPDG): A bird’s-eye view. Journal of Modern Transportation, 19 , 114–133. https://doi.org/10.1007/BF03325749
Wojtkiewicz, S. F., Khazanovich, L., Gaurav, G., & Velasquez, R. (2010). Probabilistic numerical simulation of pavement performance using MEPDG. Road Materials and Pavement Design, 11 (2), 291–306. https://doi.org/10.1080/14680629.2010.9690277
Aguiar-Moya, J. P., Prozzi, J. A., & de Fortier Smit, A. (2011). Mechanistic-empirical IRI model accounting for potential bias. Journal of Transportation Engineering, 137 (5), 297–304. https://doi.org/10.1061/(ASCE)TE.1943-5436.0000200
Hossain, Z., Zaman, M., Doiron, C., Cross, S. (2011) Development of flexible pavement database for local calibration of MEPDG, Final Report (Draft), SPR. 2209
Schwartz, C., Li, R., Ceylan, H., Kim, S., Gopalakrishnan, K. (2011) Sensitivity evaluation of MEPDG performance prediction
Sachs, S., Vandenbossche, J. M., & Snyder, M. B. (2015). Calibration of national rigid pavement performance models for the pavement mechanistic–empirical design guide. Transportation Research Record, 2524 (1), 59–67. https://doi.org/10.3141/2524-06
Guo, F., Zhao, X., Gregory, J., & Kirchain, R. (2022). A weighted multi-output neural network model for the prediction of rigid pavement deterioration. International Journal of Pavement Engineering, 23 (8), 2631–2643. https://doi.org/10.1080/10298436.2020.1867854
Pérez-Acebo, H., Gonzalo-Orden, H., Findley, D. J., & Rojí, E. (2021). Modeling the international roughness index performance on semi-rigid pavements in single carriageway roads. Construction and Building Materials, 272 , 121665. https://doi.org/10.1016/j.conbuildmat.2020.121665
McCarthy, L. M., Gudimettla, J. M., Crawford, G. L., Guercio, M. C., & Allen, D. (2015). Impacts of variability in coefficient of thermal expansion on predicted concrete pavement performance. Construction and Building Materials, 93 , 711–719. https://doi.org/10.1016/j.conbuildmat.2015.04.058
Haider, S. W., Brink, W. C., & Buch, N. (2017). Local calibration of rigid pavement performance models using resampling methods. International Journal of Pavement Engineering, 18 (7), 645–657. https://doi.org/10.1080/10298436.2015.1121777
Oyeyi, A. G., Achebe, J., Ni, F. M. W., & Tighe, S. (2023). Life cycle assessment of lightweight cellular concrete subbase pavements in Canada. International Journal of Pavement Engineering, 24 (1), 2168662. https://doi.org/10.1080/10298436.2023.2168662
Ioannides, A. M., & Tallapragada, P. K. (2013). An overview and a case study of pavement performance prediction. International Journal of Pavement Engineering, 14 (7), 629–644. https://doi.org/10.1080/10298436.2012.715644
Luo, X., Gu, F., Zhang, Y., Lytton, R. L., & Zollinger, D. (2017). Mechanistic-empirical models for better consideration of subgrade and unbound layers influence on pavement performance. Transportation Geotechnics, 13 , 52–68. https://doi.org/10.1016/j.trgeo.2017.06.002
Han, Z., Sha, A., Hu, L., & Jiang, W. (2023). Calibration of inverted asphalt pavement rut prediction model. Based on Full-Scale Accelerated Pavement Testing. Materials., 16 (2), 814. https://doi.org/10.3390/ma16020814
Kim, S. H., & Kim, N. (2006). Development of performance prediction models in flexible pavement using regression analysis method. KSCE Journal of Civil Engineering, 10 , 91–96.
Makendran, C., Murugasan, R., & Velmurugan, S. (2015). Performance prediction modelling for flexible pavement on low volume roads using multiple linear regression analysis. Journal of Applied Mathematics . https://doi.org/10.1155/2015/192485
Luo, Z. (2013). Pavement performance modelling with an auto-regression approach. International Journal of Pavement Engineering, 14 (1), 85–94. https://doi.org/10.1080/10298436.2011.617442
Article MathSciNet Google Scholar
Wang, X., Zhao, J., Li, Q., Fang, N., Wang, P., Ding, L., & Li, S. (2020). A hybrid model for prediction in asphalt pavement performance based on support vector machine and grey relation analysis. Journal of advanced transportation . https://doi.org/10.1155/2020/7534970
Abed, A., Thom, N., & Neves, L. (2019). Probabilistic prediction of asphalt pavement performance. Road Materials and Pavement Design, 20 (1), S247–S264. https://doi.org/10.1080/14680629.2019.1593229
Abaza, K. A. (2014). Back-calculation of transition probabilities for Markovian-based pavement performance prediction models. International Journal of Pavement Engineering, 17 (3), 253–264. https://doi.org/10.1080/10298436.2014.993185
Li, N., Haas, R., & Xie, W. C. (1997). Investigation of relationship between deterministic and probabilistic prediction models in pavement management. Transportation Research Record, 1592 (1), 70–79. https://doi.org/10.3141/1592-09
Fuentes, L., Camargo, R., Arellana, J., Velosa, C., & Martinez, G. (2021). Modelling pavement serviceability of urban roads using deterministic and probabilistic approaches. International Journal of Pavement Engineering, 22 (1), 77–86. https://doi.org/10.1080/10298436.2019.1577422
Soncim, S. P., de Oliveira, I. C. S., Santos, F. B., & Oliveira, C. A. D. S. (2018). Development of probabilistic models for predicting roughness in asphalt pavement. Road materials and pavement design, 19 (6), 1448–1457. https://doi.org/10.1080/14680629.2017.1304233
Al-Mansour, A., Lee, K. W. W., & Al-Qaili, A. H. (2022). Prediction of pavement maintenance performance using an expert system. Applied Sciences, 12 (10), 4802. https://doi.org/10.3390/app12104802
Ismail, N., Ismail, A., & Atiq, R. (2009). An overview of expert systems in pavement management. European Journal of Scientific Research, 30 (1), 99–111.
Mohammed, A. A., Ambak, K., Mosa, A. M., & Syamsunur, D. (2019). Expert system in engineering transportation: A review. Journal of Engineering Science and Technology., 14 (1), 229–252.
Hafez, M., Ksaibati, K., & Atadero, R. A. (2019). Optimizing expert-based decision-making of pavement maintenance using artificial neural networks with pattern-recognition algorithms. Transportation Research Record., 2673 (11), 90–100. https://doi.org/10.1177/0361198119851085
Kaya, O., Citir, N., Ceylan, H., Kim, S., & Waid, D. R. (2023). Development of pavement performance and remaining service life prediction tools for iowa jointed plain concrete pavement systems. Journal of Transportation Engineering, Part B: Pavements., 149 (1), 04022070. https://doi.org/10.1061/JPEODX.PVENG-1160
Kaloop, M. R., El-Badawy, S. M., Hu, J. W., & Abd El-Hakim, R. T. (2023). International Roughness Index prediction for flexible pavements using novel machine learning techniques. Engineering Applications of Artificial Intelligence., 122 , 106007. https://doi.org/10.1016/j.engappai.2023.106007
Huang, Y., Molavi Nojumi, M., Hashemian, L., & Bayat, A. (2023). Evaluation of a machine learning approach for temperature prediction in pavement base and subgrade layers in Alberta, Canada. Journal of Transportation Engineering, Part B: Pavements, 149 (1), 04022076. https://doi.org/10.1061/JPEODX.PVENG-1010
Mansour, E., Mousa, M. R., Dhasmana, H., & Hassan, M. (2023). Machine-learning-based framework for prediction of the long-term field performance of asphalt concrete overlays in a hot and humid climate. Transportation Research Record . https://doi.org/10.1177/03611981231161353
Tran, H., Robert, D., Gunarathna, P., Setunge, S. (2023) Multi-time step deterioration prediction of freeways using linear regression and Machine Learning approaches: a case study. International Journal of Pavement Research and Technology . 1–14
Alharbi, F. (2018) Predicting pavement performance utilizing artificial neural network (ANN) models
Bayat, R., Talatahari, S., Gandomi, A. H., Habibi, M., & Aminnejad, B. (2023). Artificial neural networks for flexible pavement. Information, 14 (2), 62. https://doi.org/10.3390/info14020062
Bayrak, M. B., & Ceylan, H. (2008). Neural network-based approach for analysis of rigid pavement systems using deflection data. Transportation Research Record., 2068 (1), 61–70. https://doi.org/10.3141/2068-07
Paterson, W. D. O. (1987). Road deterioration and maintenance effects: Models for planning and management, Baltimore . The Johns Hopkins University Press.
Bham, G. H., Nasir, G., & Darter, M. I. (2001). Hall, Illinois’s Experience with Pavement Analysis and Management Systems. In 80th Annual Conference of the Transportation Research Board , Washington, DC
Smith, R. E., Zulyaminayn, M., Pilson, C. C., Dossey, T., McCullough, B. F., & Mukherjee, B. (2001) Integration of Network and Project-Level Performance Models for TxDOT PMIS. No. FHWA/TX-01/1727–2. Texas Transportation Institute
Kulkarni, R. B., & Miller, R. W. (2003). Pavement management systems: Past, present, and future. Transportation Research Record, 1853 (1), 65–71. https://doi.org/10.3141/1853-08
Download references
Acknowledgements
Not applicable.
Author information
Authors and affiliations.
Department of Civil Engineering, Malaviya National Institute of Technology, Jaipur, Rajasthan, 302017, India
Jeetendra Singh Khichad & Rameshwar J. Vishwakarma
You can also search for this author in PubMed Google Scholar
Contributions
Jeetendra Singh Khichad: Conceptualization, Methodology, Formal analysis/review, Interpretation of data, Validation, Writing – original draft. Rameshwar J. Vishwakarma: Conceptualization, Writing – review & editing, Supervision.
Corresponding author
Correspondence to Rameshwar J. Vishwakarma .
Ethics declarations
Conflict of interest.
The authors declare that they have no conflict of interest.
Rights and permissions
Springer Nature or its licensor (e.g. a society or other partner) holds exclusive rights to this article under a publishing agreement with the author(s) or other rightsholder(s); author self-archiving of the accepted manuscript version of this article is solely governed by the terms of such publishing agreement and applicable law.
Reprints and permissions
About this article
Khichad, J.S., Vishwakarma, R.J. Overview and Discussion of Pavement Performance Prediction Techniques for Maintenance and Rehabilitation Decision-Making. Int. J. Pavement Res. Technol. (2024). https://doi.org/10.1007/s42947-024-00435-x
Download citation
Received : 22 December 2023
Revised : 09 March 2024
Accepted : 12 March 2024
Published : 11 April 2024
DOI : https://doi.org/10.1007/s42947-024-00435-x
Share this article
Anyone you share the following link with will be able to read this content:
Sorry, a shareable link is not currently available for this article.
Provided by the Springer Nature SharedIt content-sharing initiative
- Pavement performance prediction
- Pavement deterioration
- Cost-effective
- Decision-making
- Rehabilitation and maintenance
- Find a journal
- Publish with us
- Track your research
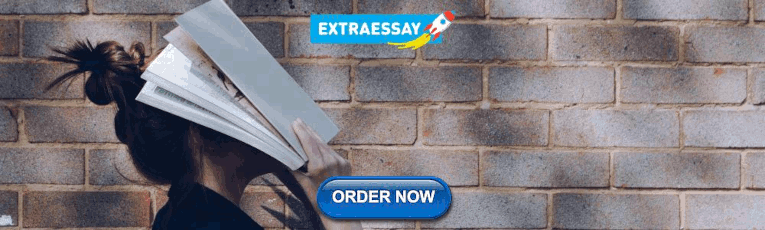
IMAGES
VIDEO
COMMENTS
Abstract. Purpose - The purpose of this paper is to review the literature on maintenance management and. suggest possible gaps from the point of view of researchers and practitioners. Design ...
Empirical research in industrial maintenance management. There is a general lack of empirical research within the field of maintenance. When Fraser et al. (2015) reviewed the literature, they found that out of several thousand articles on the most common maintenance concepts, only 82 displayed empirical real-world evidence. In contrast, the ...
The types of maintenance policies examined in research on Industry 4.0 maintenance were next examined. The result of the analysis of the relevant publications regarding maintenance policies is given in Fig. 3. From our analysis, we found that advanced maintenance practices such as preventive maintenance (around 28% of papers), predictive ...
The work presented in this paper is part of an ongoing project and aims to incorporate a new function in the existing computerized maintenance management (CMMS) of an automotive company to assist failure analysis and optimal periodicity definition of preventive interventions, considering costs [5].
The preventive maintenance (PdM) approach is a common strategy in maintenance management to avoid failures, as it provides maintenance actions based on a schedule. ... Table 1 shows the number of research papers used those models to study the benefits of predictive maintenance. The results of this literature paper show that between 2016 and ...
This paper introduces a research project which aims to identify and analyse various influences on maintenance management. The research (using contemporary social sciences investigation methods) is conducted by experienced engineers, and is part of a larger project on the nature of engineering work. The paper also provides a literature overview ...
Condition-based maintenance (CBM) detects early signs of failure and dictates when maintenance should be performed based on the actual condition of a system. In this paper, we first review some of the recent research on CBM under various physical structures and signal data. Then, we summarize several kinds of prognostic models that use monitoring information to estimate the reliability of ...
This paper presents an extensive literature review on the field of condition-based maintenance (CBM). The paper encompasses over 4000 contributions, analysed through bibliometric indicators and meta-analysis techniques. The review adopts Factor Analysis as a dimensionality reduction, concerning the metric of the co-citations of the papers. Four main research areas have been identified, able to ...
The standpoint of the paper by McComb, Kennedy, Green and Compton is that launching and supporting successful maintenance projects is an overlooked aspect of maintenance management research. So, the paper examines the effects of four important factors on project success, i.e. resource allocation, team leader authority, significant project ...
Furthermore, this research paper will be a great impetus for future academic and industrial researchers to implement the Smart Maintenance Management function in small and medium enterprises through their research and to implement smart factories. CRediT authorship contribution statement. K. Velmurugan: Writing - original draft. S.
From this review it found that the paper also revealed from time to time, maintenance management adapt and improve to gain more sustainable maintenance, including the new shift of paradigm of maintenance itself. The originality and contribution of this research is that the paper contains many maintenance management methods from maintenance text...
A total of two papers were found on Maintenance management 217 JQME 12,3 218 this type of maintenance. McKone and Weiss (2002) have presented detailed guidelines for implementing predictive maintenance in manufacturing industry. ... Prestige Journal of Management and Research, Vol. 5 No. 1, pp. 61-72. Gupta, S.M. and Turki, Y.A.Y. (1998 ...
The paper aims to review the current literature concerning predictive maintenance and intelligent sensors in smart factories. We focused on contemporary trends to provide an overview of future research challenges and classification. The paper used burst analysis, systematic review methodology, co-occurrence analysis of keywords, and cluster ...
Compared with data mining technology, OLAP is more intuitive and convenient, but it lacks the mining of hidden information and cannot achieve predictive operations. This paper focuses on the application of data mining technology in equipment operation and maintenance management. 3.2 Equipment Operation and Maintenance Data Mining Technology
Abstract: e purpose of this paper is to present the maintenance management status in Moroccan companies. e first part is based on the literature; it reviews the main maintenance models. e second part is resulted from a pilot survey about management maintenance in Moroccan companies. e study was performed by conducting a
The review included articles on issues affecting building maintenance practice and recommendations to minimize the issues. The maintenance practice issues were categorized into planning, management, staff, competency, technology, and technical capabilities. Besides, the study proposed recommendations from the literature for future studies ...
The purpose of this research paper is to study the impact of effective maintenance management on improvement of productivity, profitability of production system and effective workplace management in an ... Maintenance management tools such as KAIZEN, TPM, 5S, 6σ, etc. might be the only adequate model that identifies the relationship between ...
In this context, this paper proposed an MMI framework for asset management based on the ISO 55000 series. Thus, the proposed framework aims to improve maintenance management by treating the causes of the maintenance unwanted incidents (e.g., unexpected failure) and maintenance nonconformities (e.g., underperformed indicator) and addressing ...
A research group in Japan has discovered that writing down one's reaction to a negative incident on a piece of paper and then shredding it or throwing it away reduces feelings of anger.
For effective resource allocation in maintenance management, accurate pavement performance prognosis models are essential; however, ... From databases of digital scientific literature, more than 250 quality research papers/guidelines were extracted and reduced to 50 + quality papers related to pavement performance prediction. However, published ...