Have a language expert improve your writing
Run a free plagiarism check in 10 minutes, automatically generate references for free.
- Knowledge Base
- Methodology
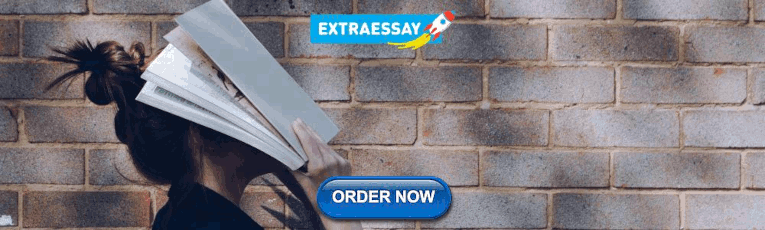
Research Design | Step-by-Step Guide with Examples
Published on 5 May 2022 by Shona McCombes . Revised on 20 March 2023.
A research design is a strategy for answering your research question using empirical data. Creating a research design means making decisions about:
- Your overall aims and approach
- The type of research design you’ll use
- Your sampling methods or criteria for selecting subjects
- Your data collection methods
- The procedures you’ll follow to collect data
- Your data analysis methods
A well-planned research design helps ensure that your methods match your research aims and that you use the right kind of analysis for your data.
Table of contents
Step 1: consider your aims and approach, step 2: choose a type of research design, step 3: identify your population and sampling method, step 4: choose your data collection methods, step 5: plan your data collection procedures, step 6: decide on your data analysis strategies, frequently asked questions.
- Introduction
Before you can start designing your research, you should already have a clear idea of the research question you want to investigate.
There are many different ways you could go about answering this question. Your research design choices should be driven by your aims and priorities – start by thinking carefully about what you want to achieve.
The first choice you need to make is whether you’ll take a qualitative or quantitative approach.
Qualitative research designs tend to be more flexible and inductive , allowing you to adjust your approach based on what you find throughout the research process.
Quantitative research designs tend to be more fixed and deductive , with variables and hypotheses clearly defined in advance of data collection.
It’s also possible to use a mixed methods design that integrates aspects of both approaches. By combining qualitative and quantitative insights, you can gain a more complete picture of the problem you’re studying and strengthen the credibility of your conclusions.
Practical and ethical considerations when designing research
As well as scientific considerations, you need to think practically when designing your research. If your research involves people or animals, you also need to consider research ethics .
- How much time do you have to collect data and write up the research?
- Will you be able to gain access to the data you need (e.g., by travelling to a specific location or contacting specific people)?
- Do you have the necessary research skills (e.g., statistical analysis or interview techniques)?
- Will you need ethical approval ?
At each stage of the research design process, make sure that your choices are practically feasible.
Prevent plagiarism, run a free check.
Within both qualitative and quantitative approaches, there are several types of research design to choose from. Each type provides a framework for the overall shape of your research.
Types of quantitative research designs
Quantitative designs can be split into four main types. Experimental and quasi-experimental designs allow you to test cause-and-effect relationships, while descriptive and correlational designs allow you to measure variables and describe relationships between them.
With descriptive and correlational designs, you can get a clear picture of characteristics, trends, and relationships as they exist in the real world. However, you can’t draw conclusions about cause and effect (because correlation doesn’t imply causation ).
Experiments are the strongest way to test cause-and-effect relationships without the risk of other variables influencing the results. However, their controlled conditions may not always reflect how things work in the real world. They’re often also more difficult and expensive to implement.
Types of qualitative research designs
Qualitative designs are less strictly defined. This approach is about gaining a rich, detailed understanding of a specific context or phenomenon, and you can often be more creative and flexible in designing your research.
The table below shows some common types of qualitative design. They often have similar approaches in terms of data collection, but focus on different aspects when analysing the data.
Your research design should clearly define who or what your research will focus on, and how you’ll go about choosing your participants or subjects.
In research, a population is the entire group that you want to draw conclusions about, while a sample is the smaller group of individuals you’ll actually collect data from.
Defining the population
A population can be made up of anything you want to study – plants, animals, organisations, texts, countries, etc. In the social sciences, it most often refers to a group of people.
For example, will you focus on people from a specific demographic, region, or background? Are you interested in people with a certain job or medical condition, or users of a particular product?
The more precisely you define your population, the easier it will be to gather a representative sample.
Sampling methods
Even with a narrowly defined population, it’s rarely possible to collect data from every individual. Instead, you’ll collect data from a sample.
To select a sample, there are two main approaches: probability sampling and non-probability sampling . The sampling method you use affects how confidently you can generalise your results to the population as a whole.
Probability sampling is the most statistically valid option, but it’s often difficult to achieve unless you’re dealing with a very small and accessible population.
For practical reasons, many studies use non-probability sampling, but it’s important to be aware of the limitations and carefully consider potential biases. You should always make an effort to gather a sample that’s as representative as possible of the population.
Case selection in qualitative research
In some types of qualitative designs, sampling may not be relevant.
For example, in an ethnography or a case study, your aim is to deeply understand a specific context, not to generalise to a population. Instead of sampling, you may simply aim to collect as much data as possible about the context you are studying.
In these types of design, you still have to carefully consider your choice of case or community. You should have a clear rationale for why this particular case is suitable for answering your research question.
For example, you might choose a case study that reveals an unusual or neglected aspect of your research problem, or you might choose several very similar or very different cases in order to compare them.
Data collection methods are ways of directly measuring variables and gathering information. They allow you to gain first-hand knowledge and original insights into your research problem.
You can choose just one data collection method, or use several methods in the same study.
Survey methods
Surveys allow you to collect data about opinions, behaviours, experiences, and characteristics by asking people directly. There are two main survey methods to choose from: questionnaires and interviews.
Observation methods
Observations allow you to collect data unobtrusively, observing characteristics, behaviours, or social interactions without relying on self-reporting.
Observations may be conducted in real time, taking notes as you observe, or you might make audiovisual recordings for later analysis. They can be qualitative or quantitative.
Other methods of data collection
There are many other ways you might collect data depending on your field and topic.
If you’re not sure which methods will work best for your research design, try reading some papers in your field to see what data collection methods they used.
Secondary data
If you don’t have the time or resources to collect data from the population you’re interested in, you can also choose to use secondary data that other researchers already collected – for example, datasets from government surveys or previous studies on your topic.
With this raw data, you can do your own analysis to answer new research questions that weren’t addressed by the original study.
Using secondary data can expand the scope of your research, as you may be able to access much larger and more varied samples than you could collect yourself.
However, it also means you don’t have any control over which variables to measure or how to measure them, so the conclusions you can draw may be limited.
As well as deciding on your methods, you need to plan exactly how you’ll use these methods to collect data that’s consistent, accurate, and unbiased.
Planning systematic procedures is especially important in quantitative research, where you need to precisely define your variables and ensure your measurements are reliable and valid.
Operationalisation
Some variables, like height or age, are easily measured. But often you’ll be dealing with more abstract concepts, like satisfaction, anxiety, or competence. Operationalisation means turning these fuzzy ideas into measurable indicators.
If you’re using observations , which events or actions will you count?
If you’re using surveys , which questions will you ask and what range of responses will be offered?
You may also choose to use or adapt existing materials designed to measure the concept you’re interested in – for example, questionnaires or inventories whose reliability and validity has already been established.
Reliability and validity
Reliability means your results can be consistently reproduced , while validity means that you’re actually measuring the concept you’re interested in.
For valid and reliable results, your measurement materials should be thoroughly researched and carefully designed. Plan your procedures to make sure you carry out the same steps in the same way for each participant.
If you’re developing a new questionnaire or other instrument to measure a specific concept, running a pilot study allows you to check its validity and reliability in advance.
Sampling procedures
As well as choosing an appropriate sampling method, you need a concrete plan for how you’ll actually contact and recruit your selected sample.
That means making decisions about things like:
- How many participants do you need for an adequate sample size?
- What inclusion and exclusion criteria will you use to identify eligible participants?
- How will you contact your sample – by mail, online, by phone, or in person?
If you’re using a probability sampling method, it’s important that everyone who is randomly selected actually participates in the study. How will you ensure a high response rate?
If you’re using a non-probability method, how will you avoid bias and ensure a representative sample?
Data management
It’s also important to create a data management plan for organising and storing your data.
Will you need to transcribe interviews or perform data entry for observations? You should anonymise and safeguard any sensitive data, and make sure it’s backed up regularly.
Keeping your data well organised will save time when it comes to analysing them. It can also help other researchers validate and add to your findings.
On their own, raw data can’t answer your research question. The last step of designing your research is planning how you’ll analyse the data.
Quantitative data analysis
In quantitative research, you’ll most likely use some form of statistical analysis . With statistics, you can summarise your sample data, make estimates, and test hypotheses.
Using descriptive statistics , you can summarise your sample data in terms of:
- The distribution of the data (e.g., the frequency of each score on a test)
- The central tendency of the data (e.g., the mean to describe the average score)
- The variability of the data (e.g., the standard deviation to describe how spread out the scores are)
The specific calculations you can do depend on the level of measurement of your variables.
Using inferential statistics , you can:
- Make estimates about the population based on your sample data.
- Test hypotheses about a relationship between variables.
Regression and correlation tests look for associations between two or more variables, while comparison tests (such as t tests and ANOVAs ) look for differences in the outcomes of different groups.
Your choice of statistical test depends on various aspects of your research design, including the types of variables you’re dealing with and the distribution of your data.
Qualitative data analysis
In qualitative research, your data will usually be very dense with information and ideas. Instead of summing it up in numbers, you’ll need to comb through the data in detail, interpret its meanings, identify patterns, and extract the parts that are most relevant to your research question.
Two of the most common approaches to doing this are thematic analysis and discourse analysis .
There are many other ways of analysing qualitative data depending on the aims of your research. To get a sense of potential approaches, try reading some qualitative research papers in your field.
A sample is a subset of individuals from a larger population. Sampling means selecting the group that you will actually collect data from in your research.
For example, if you are researching the opinions of students in your university, you could survey a sample of 100 students.
Statistical sampling allows you to test a hypothesis about the characteristics of a population. There are various sampling methods you can use to ensure that your sample is representative of the population as a whole.
Operationalisation means turning abstract conceptual ideas into measurable observations.
For example, the concept of social anxiety isn’t directly observable, but it can be operationally defined in terms of self-rating scores, behavioural avoidance of crowded places, or physical anxiety symptoms in social situations.
Before collecting data , it’s important to consider how you will operationalise the variables that you want to measure.
The research methods you use depend on the type of data you need to answer your research question .
- If you want to measure something or test a hypothesis , use quantitative methods . If you want to explore ideas, thoughts, and meanings, use qualitative methods .
- If you want to analyse a large amount of readily available data, use secondary data. If you want data specific to your purposes with control over how they are generated, collect primary data.
- If you want to establish cause-and-effect relationships between variables , use experimental methods. If you want to understand the characteristics of a research subject, use descriptive methods.
Cite this Scribbr article
If you want to cite this source, you can copy and paste the citation or click the ‘Cite this Scribbr article’ button to automatically add the citation to our free Reference Generator.
McCombes, S. (2023, March 20). Research Design | Step-by-Step Guide with Examples. Scribbr. Retrieved 29 April 2024, from https://www.scribbr.co.uk/research-methods/research-design/
Is this article helpful?
Shona McCombes

Research Design 101
Everything You Need To Get Started (With Examples)
By: Derek Jansen (MBA) | Reviewers: Eunice Rautenbach (DTech) & Kerryn Warren (PhD) | April 2023
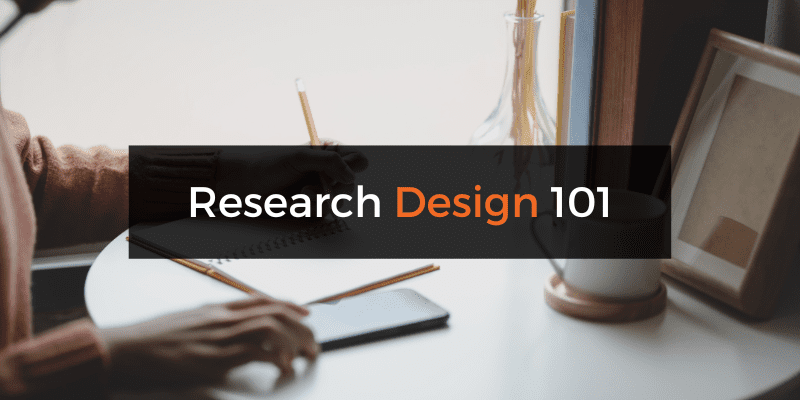
Navigating the world of research can be daunting, especially if you’re a first-time researcher. One concept you’re bound to run into fairly early in your research journey is that of “ research design ”. Here, we’ll guide you through the basics using practical examples , so that you can approach your research with confidence.
Overview: Research Design 101
What is research design.
- Research design types for quantitative studies
- Video explainer : quantitative research design
- Research design types for qualitative studies
- Video explainer : qualitative research design
- How to choose a research design
- Key takeaways
Research design refers to the overall plan, structure or strategy that guides a research project , from its conception to the final data analysis. A good research design serves as the blueprint for how you, as the researcher, will collect and analyse data while ensuring consistency, reliability and validity throughout your study.
Understanding different types of research designs is essential as helps ensure that your approach is suitable given your research aims, objectives and questions , as well as the resources you have available to you. Without a clear big-picture view of how you’ll design your research, you run the risk of potentially making misaligned choices in terms of your methodology – especially your sampling , data collection and data analysis decisions.
The problem with defining research design…
One of the reasons students struggle with a clear definition of research design is because the term is used very loosely across the internet, and even within academia.
Some sources claim that the three research design types are qualitative, quantitative and mixed methods , which isn’t quite accurate (these just refer to the type of data that you’ll collect and analyse). Other sources state that research design refers to the sum of all your design choices, suggesting it’s more like a research methodology . Others run off on other less common tangents. No wonder there’s confusion!
In this article, we’ll clear up the confusion. We’ll explain the most common research design types for both qualitative and quantitative research projects, whether that is for a full dissertation or thesis, or a smaller research paper or article.
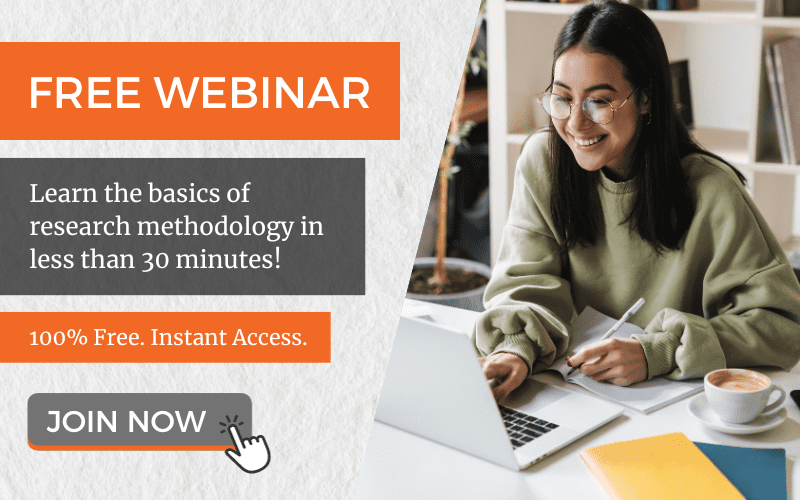
Research Design: Quantitative Studies
Quantitative research involves collecting and analysing data in a numerical form. Broadly speaking, there are four types of quantitative research designs: descriptive , correlational , experimental , and quasi-experimental .
Descriptive Research Design
As the name suggests, descriptive research design focuses on describing existing conditions, behaviours, or characteristics by systematically gathering information without manipulating any variables. In other words, there is no intervention on the researcher’s part – only data collection.
For example, if you’re studying smartphone addiction among adolescents in your community, you could deploy a survey to a sample of teens asking them to rate their agreement with certain statements that relate to smartphone addiction. The collected data would then provide insight regarding how widespread the issue may be – in other words, it would describe the situation.
The key defining attribute of this type of research design is that it purely describes the situation . In other words, descriptive research design does not explore potential relationships between different variables or the causes that may underlie those relationships. Therefore, descriptive research is useful for generating insight into a research problem by describing its characteristics . By doing so, it can provide valuable insights and is often used as a precursor to other research design types.
Correlational Research Design
Correlational design is a popular choice for researchers aiming to identify and measure the relationship between two or more variables without manipulating them . In other words, this type of research design is useful when you want to know whether a change in one thing tends to be accompanied by a change in another thing.
For example, if you wanted to explore the relationship between exercise frequency and overall health, you could use a correlational design to help you achieve this. In this case, you might gather data on participants’ exercise habits, as well as records of their health indicators like blood pressure, heart rate, or body mass index. Thereafter, you’d use a statistical test to assess whether there’s a relationship between the two variables (exercise frequency and health).
As you can see, correlational research design is useful when you want to explore potential relationships between variables that cannot be manipulated or controlled for ethical, practical, or logistical reasons. It is particularly helpful in terms of developing predictions , and given that it doesn’t involve the manipulation of variables, it can be implemented at a large scale more easily than experimental designs (which will look at next).
That said, it’s important to keep in mind that correlational research design has limitations – most notably that it cannot be used to establish causality . In other words, correlation does not equal causation . To establish causality, you’ll need to move into the realm of experimental design, coming up next…
Need a helping hand?
Experimental Research Design
Experimental research design is used to determine if there is a causal relationship between two or more variables . With this type of research design, you, as the researcher, manipulate one variable (the independent variable) while controlling others (dependent variables). Doing so allows you to observe the effect of the former on the latter and draw conclusions about potential causality.
For example, if you wanted to measure if/how different types of fertiliser affect plant growth, you could set up several groups of plants, with each group receiving a different type of fertiliser, as well as one with no fertiliser at all. You could then measure how much each plant group grew (on average) over time and compare the results from the different groups to see which fertiliser was most effective.
Overall, experimental research design provides researchers with a powerful way to identify and measure causal relationships (and the direction of causality) between variables. However, developing a rigorous experimental design can be challenging as it’s not always easy to control all the variables in a study. This often results in smaller sample sizes , which can reduce the statistical power and generalisability of the results.
Moreover, experimental research design requires random assignment . This means that the researcher needs to assign participants to different groups or conditions in a way that each participant has an equal chance of being assigned to any group (note that this is not the same as random sampling ). Doing so helps reduce the potential for bias and confounding variables . This need for random assignment can lead to ethics-related issues . For example, withholding a potentially beneficial medical treatment from a control group may be considered unethical in certain situations.
Quasi-Experimental Research Design
Quasi-experimental research design is used when the research aims involve identifying causal relations , but one cannot (or doesn’t want to) randomly assign participants to different groups (for practical or ethical reasons). Instead, with a quasi-experimental research design, the researcher relies on existing groups or pre-existing conditions to form groups for comparison.
For example, if you were studying the effects of a new teaching method on student achievement in a particular school district, you may be unable to randomly assign students to either group and instead have to choose classes or schools that already use different teaching methods. This way, you still achieve separate groups, without having to assign participants to specific groups yourself.
Naturally, quasi-experimental research designs have limitations when compared to experimental designs. Given that participant assignment is not random, it’s more difficult to confidently establish causality between variables, and, as a researcher, you have less control over other variables that may impact findings.
All that said, quasi-experimental designs can still be valuable in research contexts where random assignment is not possible and can often be undertaken on a much larger scale than experimental research, thus increasing the statistical power of the results. What’s important is that you, as the researcher, understand the limitations of the design and conduct your quasi-experiment as rigorously as possible, paying careful attention to any potential confounding variables .
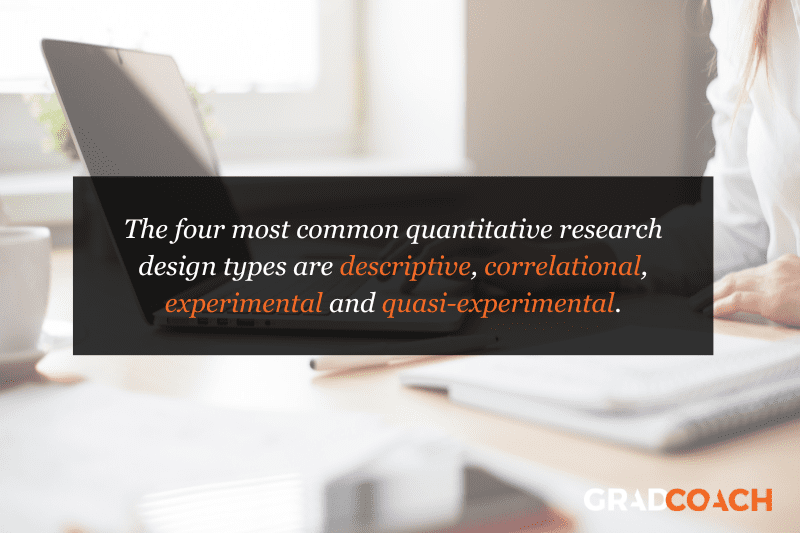
Research Design: Qualitative Studies
There are many different research design types when it comes to qualitative studies, but here we’ll narrow our focus to explore the “Big 4”. Specifically, we’ll look at phenomenological design, grounded theory design, ethnographic design, and case study design.
Phenomenological Research Design
Phenomenological design involves exploring the meaning of lived experiences and how they are perceived by individuals. This type of research design seeks to understand people’s perspectives , emotions, and behaviours in specific situations. Here, the aim for researchers is to uncover the essence of human experience without making any assumptions or imposing preconceived ideas on their subjects.
For example, you could adopt a phenomenological design to study why cancer survivors have such varied perceptions of their lives after overcoming their disease. This could be achieved by interviewing survivors and then analysing the data using a qualitative analysis method such as thematic analysis to identify commonalities and differences.
Phenomenological research design typically involves in-depth interviews or open-ended questionnaires to collect rich, detailed data about participants’ subjective experiences. This richness is one of the key strengths of phenomenological research design but, naturally, it also has limitations. These include potential biases in data collection and interpretation and the lack of generalisability of findings to broader populations.
Grounded Theory Research Design
Grounded theory (also referred to as “GT”) aims to develop theories by continuously and iteratively analysing and comparing data collected from a relatively large number of participants in a study. It takes an inductive (bottom-up) approach, with a focus on letting the data “speak for itself”, without being influenced by preexisting theories or the researcher’s preconceptions.
As an example, let’s assume your research aims involved understanding how people cope with chronic pain from a specific medical condition, with a view to developing a theory around this. In this case, grounded theory design would allow you to explore this concept thoroughly without preconceptions about what coping mechanisms might exist. You may find that some patients prefer cognitive-behavioural therapy (CBT) while others prefer to rely on herbal remedies. Based on multiple, iterative rounds of analysis, you could then develop a theory in this regard, derived directly from the data (as opposed to other preexisting theories and models).
Grounded theory typically involves collecting data through interviews or observations and then analysing it to identify patterns and themes that emerge from the data. These emerging ideas are then validated by collecting more data until a saturation point is reached (i.e., no new information can be squeezed from the data). From that base, a theory can then be developed .
As you can see, grounded theory is ideally suited to studies where the research aims involve theory generation , especially in under-researched areas. Keep in mind though that this type of research design can be quite time-intensive , given the need for multiple rounds of data collection and analysis.
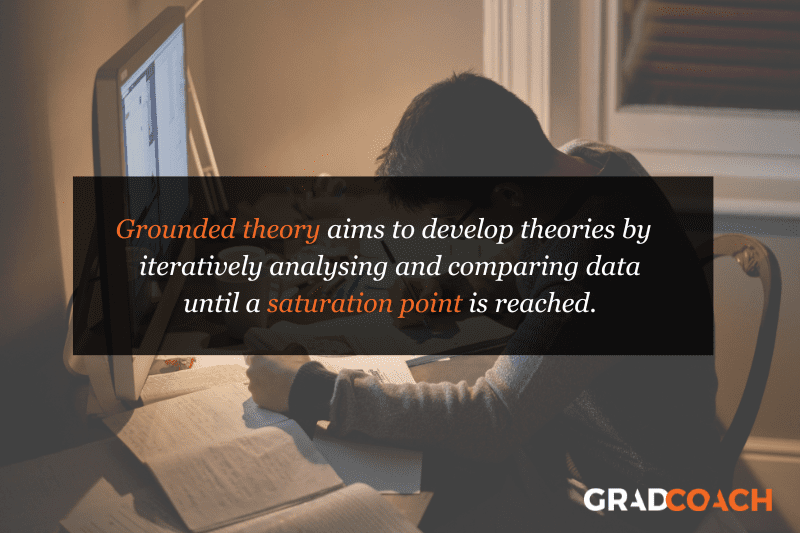
Ethnographic Research Design
Ethnographic design involves observing and studying a culture-sharing group of people in their natural setting to gain insight into their behaviours, beliefs, and values. The focus here is on observing participants in their natural environment (as opposed to a controlled environment). This typically involves the researcher spending an extended period of time with the participants in their environment, carefully observing and taking field notes .
All of this is not to say that ethnographic research design relies purely on observation. On the contrary, this design typically also involves in-depth interviews to explore participants’ views, beliefs, etc. However, unobtrusive observation is a core component of the ethnographic approach.
As an example, an ethnographer may study how different communities celebrate traditional festivals or how individuals from different generations interact with technology differently. This may involve a lengthy period of observation, combined with in-depth interviews to further explore specific areas of interest that emerge as a result of the observations that the researcher has made.
As you can probably imagine, ethnographic research design has the ability to provide rich, contextually embedded insights into the socio-cultural dynamics of human behaviour within a natural, uncontrived setting. Naturally, however, it does come with its own set of challenges, including researcher bias (since the researcher can become quite immersed in the group), participant confidentiality and, predictably, ethical complexities . All of these need to be carefully managed if you choose to adopt this type of research design.
Case Study Design
With case study research design, you, as the researcher, investigate a single individual (or a single group of individuals) to gain an in-depth understanding of their experiences, behaviours or outcomes. Unlike other research designs that are aimed at larger sample sizes, case studies offer a deep dive into the specific circumstances surrounding a person, group of people, event or phenomenon, generally within a bounded setting or context .
As an example, a case study design could be used to explore the factors influencing the success of a specific small business. This would involve diving deeply into the organisation to explore and understand what makes it tick – from marketing to HR to finance. In terms of data collection, this could include interviews with staff and management, review of policy documents and financial statements, surveying customers, etc.
While the above example is focused squarely on one organisation, it’s worth noting that case study research designs can have different variation s, including single-case, multiple-case and longitudinal designs. As you can see in the example, a single-case design involves intensely examining a single entity to understand its unique characteristics and complexities. Conversely, in a multiple-case design , multiple cases are compared and contrasted to identify patterns and commonalities. Lastly, in a longitudinal case design , a single case or multiple cases are studied over an extended period of time to understand how factors develop over time.
As you can see, a case study research design is particularly useful where a deep and contextualised understanding of a specific phenomenon or issue is desired. However, this strength is also its weakness. In other words, you can’t generalise the findings from a case study to the broader population. So, keep this in mind if you’re considering going the case study route.
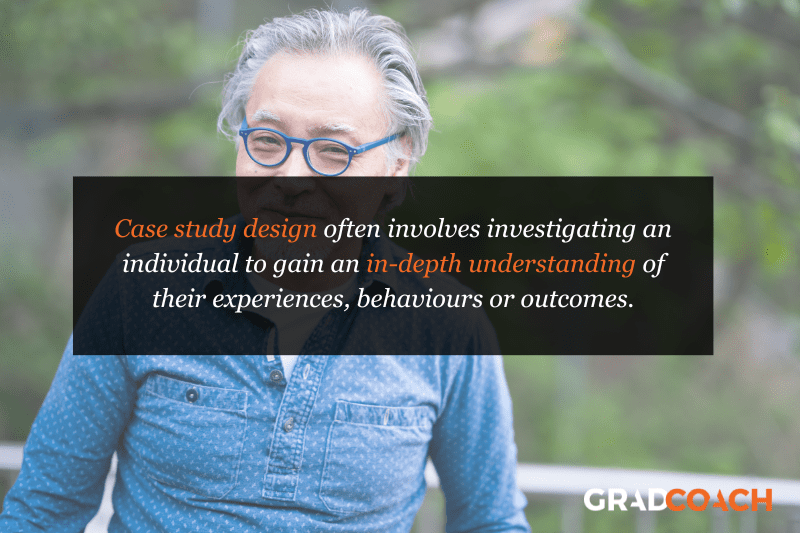
How To Choose A Research Design
Having worked through all of these potential research designs, you’d be forgiven for feeling a little overwhelmed and wondering, “ But how do I decide which research design to use? ”. While we could write an entire post covering that alone, here are a few factors to consider that will help you choose a suitable research design for your study.
Data type: The first determining factor is naturally the type of data you plan to be collecting – i.e., qualitative or quantitative. This may sound obvious, but we have to be clear about this – don’t try to use a quantitative research design on qualitative data (or vice versa)!
Research aim(s) and question(s): As with all methodological decisions, your research aim and research questions will heavily influence your research design. For example, if your research aims involve developing a theory from qualitative data, grounded theory would be a strong option. Similarly, if your research aims involve identifying and measuring relationships between variables, one of the experimental designs would likely be a better option.
Time: It’s essential that you consider any time constraints you have, as this will impact the type of research design you can choose. For example, if you’ve only got a month to complete your project, a lengthy design such as ethnography wouldn’t be a good fit.
Resources: Take into account the resources realistically available to you, as these need to factor into your research design choice. For example, if you require highly specialised lab equipment to execute an experimental design, you need to be sure that you’ll have access to that before you make a decision.
Keep in mind that when it comes to research, it’s important to manage your risks and play as conservatively as possible. If your entire project relies on you achieving a huge sample, having access to niche equipment or holding interviews with very difficult-to-reach participants, you’re creating risks that could kill your project. So, be sure to think through your choices carefully and make sure that you have backup plans for any existential risks. Remember that a relatively simple methodology executed well generally will typically earn better marks than a highly-complex methodology executed poorly.
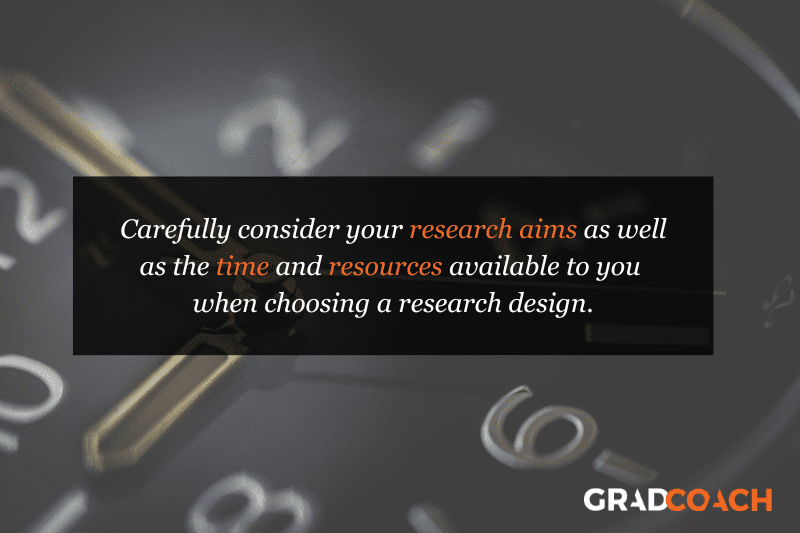
Recap: Key Takeaways
We’ve covered a lot of ground here. Let’s recap by looking at the key takeaways:
- Research design refers to the overall plan, structure or strategy that guides a research project, from its conception to the final analysis of data.
- Research designs for quantitative studies include descriptive , correlational , experimental and quasi-experimenta l designs.
- Research designs for qualitative studies include phenomenological , grounded theory , ethnographic and case study designs.
- When choosing a research design, you need to consider a variety of factors, including the type of data you’ll be working with, your research aims and questions, your time and the resources available to you.
If you need a helping hand with your research design (or any other aspect of your research), check out our private coaching services .
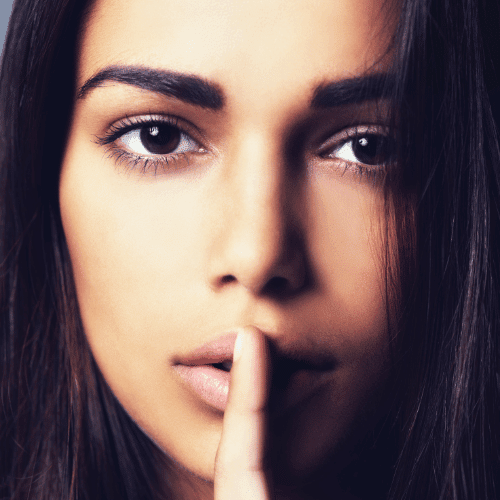
Psst... there’s more!
This post was based on one of our popular Research Bootcamps . If you're working on a research project, you'll definitely want to check this out ...
You Might Also Like:
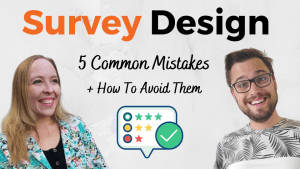
Is there any blog article explaining more on Case study research design? Is there a Case study write-up template? Thank you.
Thanks this was quite valuable to clarify such an important concept.
Thanks for this simplified explanations. it is quite very helpful.
This was really helpful. thanks
Thank you for your explanation. I think case study research design and the use of secondary data in researches needs to be talked about more in your videos and articles because there a lot of case studies research design tailored projects out there.
Please is there any template for a case study research design whose data type is a secondary data on your repository?
This post is very clear, comprehensive and has been very helpful to me. It has cleared the confusion I had in regard to research design and methodology.
This post is helpful, easy to understand, and deconstructs what a research design is. Thanks
how to cite this page
Thank you very much for the post. It is wonderful and has cleared many worries in my mind regarding research designs. I really appreciate .
Submit a Comment Cancel reply
Your email address will not be published. Required fields are marked *
Save my name, email, and website in this browser for the next time I comment.
- Print Friendly
- USC Libraries
- Research Guides
Organizing Your Social Sciences Research Paper
- Types of Research Designs
- Purpose of Guide
- Design Flaws to Avoid
- Independent and Dependent Variables
- Glossary of Research Terms
- Reading Research Effectively
- Narrowing a Topic Idea
- Broadening a Topic Idea
- Extending the Timeliness of a Topic Idea
- Academic Writing Style
- Applying Critical Thinking
- Choosing a Title
- Making an Outline
- Paragraph Development
- Research Process Video Series
- Executive Summary
- The C.A.R.S. Model
- Background Information
- The Research Problem/Question
- Theoretical Framework
- Citation Tracking
- Content Alert Services
- Evaluating Sources
- Primary Sources
- Secondary Sources
- Tiertiary Sources
- Scholarly vs. Popular Publications
- Qualitative Methods
- Quantitative Methods
- Insiderness
- Using Non-Textual Elements
- Limitations of the Study
- Common Grammar Mistakes
- Writing Concisely
- Avoiding Plagiarism
- Footnotes or Endnotes?
- Further Readings
- Generative AI and Writing
- USC Libraries Tutorials and Other Guides
- Bibliography
Introduction
Before beginning your paper, you need to decide how you plan to design the study .
The research design refers to the overall strategy and analytical approach that you have chosen in order to integrate, in a coherent and logical way, the different components of the study, thus ensuring that the research problem will be thoroughly investigated. It constitutes the blueprint for the collection, measurement, and interpretation of information and data. Note that the research problem determines the type of design you choose, not the other way around!
De Vaus, D. A. Research Design in Social Research . London: SAGE, 2001; Trochim, William M.K. Research Methods Knowledge Base. 2006.
General Structure and Writing Style
The function of a research design is to ensure that the evidence obtained enables you to effectively address the research problem logically and as unambiguously as possible . In social sciences research, obtaining information relevant to the research problem generally entails specifying the type of evidence needed to test the underlying assumptions of a theory, to evaluate a program, or to accurately describe and assess meaning related to an observable phenomenon.
With this in mind, a common mistake made by researchers is that they begin their investigations before they have thought critically about what information is required to address the research problem. Without attending to these design issues beforehand, the overall research problem will not be adequately addressed and any conclusions drawn will run the risk of being weak and unconvincing. As a consequence, the overall validity of the study will be undermined.
The length and complexity of describing the research design in your paper can vary considerably, but any well-developed description will achieve the following :
- Identify the research problem clearly and justify its selection, particularly in relation to any valid alternative designs that could have been used,
- Review and synthesize previously published literature associated with the research problem,
- Clearly and explicitly specify hypotheses [i.e., research questions] central to the problem,
- Effectively describe the information and/or data which will be necessary for an adequate testing of the hypotheses and explain how such information and/or data will be obtained, and
- Describe the methods of analysis to be applied to the data in determining whether or not the hypotheses are true or false.
The research design is usually incorporated into the introduction of your paper . You can obtain an overall sense of what to do by reviewing studies that have utilized the same research design [e.g., using a case study approach]. This can help you develop an outline to follow for your own paper.
NOTE : Use the SAGE Research Methods Online and Cases and the SAGE Research Methods Videos databases to search for scholarly resources on how to apply specific research designs and methods . The Research Methods Online database contains links to more than 175,000 pages of SAGE publisher's book, journal, and reference content on quantitative, qualitative, and mixed research methodologies. Also included is a collection of case studies of social research projects that can be used to help you better understand abstract or complex methodological concepts. The Research Methods Videos database contains hours of tutorials, interviews, video case studies, and mini-documentaries covering the entire research process.
Creswell, John W. and J. David Creswell. Research Design: Qualitative, Quantitative, and Mixed Methods Approaches . 5th edition. Thousand Oaks, CA: Sage, 2018; De Vaus, D. A. Research Design in Social Research . London: SAGE, 2001; Gorard, Stephen. Research Design: Creating Robust Approaches for the Social Sciences . Thousand Oaks, CA: Sage, 2013; Leedy, Paul D. and Jeanne Ellis Ormrod. Practical Research: Planning and Design . Tenth edition. Boston, MA: Pearson, 2013; Vogt, W. Paul, Dianna C. Gardner, and Lynne M. Haeffele. When to Use What Research Design . New York: Guilford, 2012.
Action Research Design
Definition and Purpose
The essentials of action research design follow a characteristic cycle whereby initially an exploratory stance is adopted, where an understanding of a problem is developed and plans are made for some form of interventionary strategy. Then the intervention is carried out [the "action" in action research] during which time, pertinent observations are collected in various forms. The new interventional strategies are carried out, and this cyclic process repeats, continuing until a sufficient understanding of [or a valid implementation solution for] the problem is achieved. The protocol is iterative or cyclical in nature and is intended to foster deeper understanding of a given situation, starting with conceptualizing and particularizing the problem and moving through several interventions and evaluations.
What do these studies tell you ?
- This is a collaborative and adaptive research design that lends itself to use in work or community situations.
- Design focuses on pragmatic and solution-driven research outcomes rather than testing theories.
- When practitioners use action research, it has the potential to increase the amount they learn consciously from their experience; the action research cycle can be regarded as a learning cycle.
- Action research studies often have direct and obvious relevance to improving practice and advocating for change.
- There are no hidden controls or preemption of direction by the researcher.
What these studies don't tell you ?
- It is harder to do than conducting conventional research because the researcher takes on responsibilities of advocating for change as well as for researching the topic.
- Action research is much harder to write up because it is less likely that you can use a standard format to report your findings effectively [i.e., data is often in the form of stories or observation].
- Personal over-involvement of the researcher may bias research results.
- The cyclic nature of action research to achieve its twin outcomes of action [e.g. change] and research [e.g. understanding] is time-consuming and complex to conduct.
- Advocating for change usually requires buy-in from study participants.
Coghlan, David and Mary Brydon-Miller. The Sage Encyclopedia of Action Research . Thousand Oaks, CA: Sage, 2014; Efron, Sara Efrat and Ruth Ravid. Action Research in Education: A Practical Guide . New York: Guilford, 2013; Gall, Meredith. Educational Research: An Introduction . Chapter 18, Action Research. 8th ed. Boston, MA: Pearson/Allyn and Bacon, 2007; Gorard, Stephen. Research Design: Creating Robust Approaches for the Social Sciences . Thousand Oaks, CA: Sage, 2013; Kemmis, Stephen and Robin McTaggart. “Participatory Action Research.” In Handbook of Qualitative Research . Norman Denzin and Yvonna S. Lincoln, eds. 2nd ed. (Thousand Oaks, CA: SAGE, 2000), pp. 567-605; McNiff, Jean. Writing and Doing Action Research . London: Sage, 2014; Reason, Peter and Hilary Bradbury. Handbook of Action Research: Participative Inquiry and Practice . Thousand Oaks, CA: SAGE, 2001.
Case Study Design
A case study is an in-depth study of a particular research problem rather than a sweeping statistical survey or comprehensive comparative inquiry. It is often used to narrow down a very broad field of research into one or a few easily researchable examples. The case study research design is also useful for testing whether a specific theory and model actually applies to phenomena in the real world. It is a useful design when not much is known about an issue or phenomenon.
- Approach excels at bringing us to an understanding of a complex issue through detailed contextual analysis of a limited number of events or conditions and their relationships.
- A researcher using a case study design can apply a variety of methodologies and rely on a variety of sources to investigate a research problem.
- Design can extend experience or add strength to what is already known through previous research.
- Social scientists, in particular, make wide use of this research design to examine contemporary real-life situations and provide the basis for the application of concepts and theories and the extension of methodologies.
- The design can provide detailed descriptions of specific and rare cases.
- A single or small number of cases offers little basis for establishing reliability or to generalize the findings to a wider population of people, places, or things.
- Intense exposure to the study of a case may bias a researcher's interpretation of the findings.
- Design does not facilitate assessment of cause and effect relationships.
- Vital information may be missing, making the case hard to interpret.
- The case may not be representative or typical of the larger problem being investigated.
- If the criteria for selecting a case is because it represents a very unusual or unique phenomenon or problem for study, then your interpretation of the findings can only apply to that particular case.
Case Studies. Writing@CSU. Colorado State University; Anastas, Jeane W. Research Design for Social Work and the Human Services . Chapter 4, Flexible Methods: Case Study Design. 2nd ed. New York: Columbia University Press, 1999; Gerring, John. “What Is a Case Study and What Is It Good for?” American Political Science Review 98 (May 2004): 341-354; Greenhalgh, Trisha, editor. Case Study Evaluation: Past, Present and Future Challenges . Bingley, UK: Emerald Group Publishing, 2015; Mills, Albert J. , Gabrielle Durepos, and Eiden Wiebe, editors. Encyclopedia of Case Study Research . Thousand Oaks, CA: SAGE Publications, 2010; Stake, Robert E. The Art of Case Study Research . Thousand Oaks, CA: SAGE, 1995; Yin, Robert K. Case Study Research: Design and Theory . Applied Social Research Methods Series, no. 5. 3rd ed. Thousand Oaks, CA: SAGE, 2003.
Causal Design
Causality studies may be thought of as understanding a phenomenon in terms of conditional statements in the form, “If X, then Y.” This type of research is used to measure what impact a specific change will have on existing norms and assumptions. Most social scientists seek causal explanations that reflect tests of hypotheses. Causal effect (nomothetic perspective) occurs when variation in one phenomenon, an independent variable, leads to or results, on average, in variation in another phenomenon, the dependent variable.
Conditions necessary for determining causality:
- Empirical association -- a valid conclusion is based on finding an association between the independent variable and the dependent variable.
- Appropriate time order -- to conclude that causation was involved, one must see that cases were exposed to variation in the independent variable before variation in the dependent variable.
- Nonspuriousness -- a relationship between two variables that is not due to variation in a third variable.
- Causality research designs assist researchers in understanding why the world works the way it does through the process of proving a causal link between variables and by the process of eliminating other possibilities.
- Replication is possible.
- There is greater confidence the study has internal validity due to the systematic subject selection and equity of groups being compared.
- Not all relationships are causal! The possibility always exists that, by sheer coincidence, two unrelated events appear to be related [e.g., Punxatawney Phil could accurately predict the duration of Winter for five consecutive years but, the fact remains, he's just a big, furry rodent].
- Conclusions about causal relationships are difficult to determine due to a variety of extraneous and confounding variables that exist in a social environment. This means causality can only be inferred, never proven.
- If two variables are correlated, the cause must come before the effect. However, even though two variables might be causally related, it can sometimes be difficult to determine which variable comes first and, therefore, to establish which variable is the actual cause and which is the actual effect.
Beach, Derek and Rasmus Brun Pedersen. Causal Case Study Methods: Foundations and Guidelines for Comparing, Matching, and Tracing . Ann Arbor, MI: University of Michigan Press, 2016; Bachman, Ronet. The Practice of Research in Criminology and Criminal Justice . Chapter 5, Causation and Research Designs. 3rd ed. Thousand Oaks, CA: Pine Forge Press, 2007; Brewer, Ernest W. and Jennifer Kubn. “Causal-Comparative Design.” In Encyclopedia of Research Design . Neil J. Salkind, editor. (Thousand Oaks, CA: Sage, 2010), pp. 125-132; Causal Research Design: Experimentation. Anonymous SlideShare Presentation; Gall, Meredith. Educational Research: An Introduction . Chapter 11, Nonexperimental Research: Correlational Designs. 8th ed. Boston, MA: Pearson/Allyn and Bacon, 2007; Trochim, William M.K. Research Methods Knowledge Base. 2006.
Cohort Design
Often used in the medical sciences, but also found in the applied social sciences, a cohort study generally refers to a study conducted over a period of time involving members of a population which the subject or representative member comes from, and who are united by some commonality or similarity. Using a quantitative framework, a cohort study makes note of statistical occurrence within a specialized subgroup, united by same or similar characteristics that are relevant to the research problem being investigated, rather than studying statistical occurrence within the general population. Using a qualitative framework, cohort studies generally gather data using methods of observation. Cohorts can be either "open" or "closed."
- Open Cohort Studies [dynamic populations, such as the population of Los Angeles] involve a population that is defined just by the state of being a part of the study in question (and being monitored for the outcome). Date of entry and exit from the study is individually defined, therefore, the size of the study population is not constant. In open cohort studies, researchers can only calculate rate based data, such as, incidence rates and variants thereof.
- Closed Cohort Studies [static populations, such as patients entered into a clinical trial] involve participants who enter into the study at one defining point in time and where it is presumed that no new participants can enter the cohort. Given this, the number of study participants remains constant (or can only decrease).
- The use of cohorts is often mandatory because a randomized control study may be unethical. For example, you cannot deliberately expose people to asbestos, you can only study its effects on those who have already been exposed. Research that measures risk factors often relies upon cohort designs.
- Because cohort studies measure potential causes before the outcome has occurred, they can demonstrate that these “causes” preceded the outcome, thereby avoiding the debate as to which is the cause and which is the effect.
- Cohort analysis is highly flexible and can provide insight into effects over time and related to a variety of different types of changes [e.g., social, cultural, political, economic, etc.].
- Either original data or secondary data can be used in this design.
- In cases where a comparative analysis of two cohorts is made [e.g., studying the effects of one group exposed to asbestos and one that has not], a researcher cannot control for all other factors that might differ between the two groups. These factors are known as confounding variables.
- Cohort studies can end up taking a long time to complete if the researcher must wait for the conditions of interest to develop within the group. This also increases the chance that key variables change during the course of the study, potentially impacting the validity of the findings.
- Due to the lack of randominization in the cohort design, its external validity is lower than that of study designs where the researcher randomly assigns participants.
Healy P, Devane D. “Methodological Considerations in Cohort Study Designs.” Nurse Researcher 18 (2011): 32-36; Glenn, Norval D, editor. Cohort Analysis . 2nd edition. Thousand Oaks, CA: Sage, 2005; Levin, Kate Ann. Study Design IV: Cohort Studies. Evidence-Based Dentistry 7 (2003): 51–52; Payne, Geoff. “Cohort Study.” In The SAGE Dictionary of Social Research Methods . Victor Jupp, editor. (Thousand Oaks, CA: Sage, 2006), pp. 31-33; Study Design 101. Himmelfarb Health Sciences Library. George Washington University, November 2011; Cohort Study. Wikipedia.
Cross-Sectional Design
Cross-sectional research designs have three distinctive features: no time dimension; a reliance on existing differences rather than change following intervention; and, groups are selected based on existing differences rather than random allocation. The cross-sectional design can only measure differences between or from among a variety of people, subjects, or phenomena rather than a process of change. As such, researchers using this design can only employ a relatively passive approach to making causal inferences based on findings.
- Cross-sectional studies provide a clear 'snapshot' of the outcome and the characteristics associated with it, at a specific point in time.
- Unlike an experimental design, where there is an active intervention by the researcher to produce and measure change or to create differences, cross-sectional designs focus on studying and drawing inferences from existing differences between people, subjects, or phenomena.
- Entails collecting data at and concerning one point in time. While longitudinal studies involve taking multiple measures over an extended period of time, cross-sectional research is focused on finding relationships between variables at one moment in time.
- Groups identified for study are purposely selected based upon existing differences in the sample rather than seeking random sampling.
- Cross-section studies are capable of using data from a large number of subjects and, unlike observational studies, is not geographically bound.
- Can estimate prevalence of an outcome of interest because the sample is usually taken from the whole population.
- Because cross-sectional designs generally use survey techniques to gather data, they are relatively inexpensive and take up little time to conduct.
- Finding people, subjects, or phenomena to study that are very similar except in one specific variable can be difficult.
- Results are static and time bound and, therefore, give no indication of a sequence of events or reveal historical or temporal contexts.
- Studies cannot be utilized to establish cause and effect relationships.
- This design only provides a snapshot of analysis so there is always the possibility that a study could have differing results if another time-frame had been chosen.
- There is no follow up to the findings.
Bethlehem, Jelke. "7: Cross-sectional Research." In Research Methodology in the Social, Behavioural and Life Sciences . Herman J Adèr and Gideon J Mellenbergh, editors. (London, England: Sage, 1999), pp. 110-43; Bourque, Linda B. “Cross-Sectional Design.” In The SAGE Encyclopedia of Social Science Research Methods . Michael S. Lewis-Beck, Alan Bryman, and Tim Futing Liao. (Thousand Oaks, CA: 2004), pp. 230-231; Hall, John. “Cross-Sectional Survey Design.” In Encyclopedia of Survey Research Methods . Paul J. Lavrakas, ed. (Thousand Oaks, CA: Sage, 2008), pp. 173-174; Helen Barratt, Maria Kirwan. Cross-Sectional Studies: Design Application, Strengths and Weaknesses of Cross-Sectional Studies. Healthknowledge, 2009. Cross-Sectional Study. Wikipedia.
Descriptive Design
Descriptive research designs help provide answers to the questions of who, what, when, where, and how associated with a particular research problem; a descriptive study cannot conclusively ascertain answers to why. Descriptive research is used to obtain information concerning the current status of the phenomena and to describe "what exists" with respect to variables or conditions in a situation.
- The subject is being observed in a completely natural and unchanged natural environment. True experiments, whilst giving analyzable data, often adversely influence the normal behavior of the subject [a.k.a., the Heisenberg effect whereby measurements of certain systems cannot be made without affecting the systems].
- Descriptive research is often used as a pre-cursor to more quantitative research designs with the general overview giving some valuable pointers as to what variables are worth testing quantitatively.
- If the limitations are understood, they can be a useful tool in developing a more focused study.
- Descriptive studies can yield rich data that lead to important recommendations in practice.
- Appoach collects a large amount of data for detailed analysis.
- The results from a descriptive research cannot be used to discover a definitive answer or to disprove a hypothesis.
- Because descriptive designs often utilize observational methods [as opposed to quantitative methods], the results cannot be replicated.
- The descriptive function of research is heavily dependent on instrumentation for measurement and observation.
Anastas, Jeane W. Research Design for Social Work and the Human Services . Chapter 5, Flexible Methods: Descriptive Research. 2nd ed. New York: Columbia University Press, 1999; Given, Lisa M. "Descriptive Research." In Encyclopedia of Measurement and Statistics . Neil J. Salkind and Kristin Rasmussen, editors. (Thousand Oaks, CA: Sage, 2007), pp. 251-254; McNabb, Connie. Descriptive Research Methodologies. Powerpoint Presentation; Shuttleworth, Martyn. Descriptive Research Design, September 26, 2008; Erickson, G. Scott. "Descriptive Research Design." In New Methods of Market Research and Analysis . (Northampton, MA: Edward Elgar Publishing, 2017), pp. 51-77; Sahin, Sagufta, and Jayanta Mete. "A Brief Study on Descriptive Research: Its Nature and Application in Social Science." International Journal of Research and Analysis in Humanities 1 (2021): 11; K. Swatzell and P. Jennings. “Descriptive Research: The Nuts and Bolts.” Journal of the American Academy of Physician Assistants 20 (2007), pp. 55-56; Kane, E. Doing Your Own Research: Basic Descriptive Research in the Social Sciences and Humanities . London: Marion Boyars, 1985.
Experimental Design
A blueprint of the procedure that enables the researcher to maintain control over all factors that may affect the result of an experiment. In doing this, the researcher attempts to determine or predict what may occur. Experimental research is often used where there is time priority in a causal relationship (cause precedes effect), there is consistency in a causal relationship (a cause will always lead to the same effect), and the magnitude of the correlation is great. The classic experimental design specifies an experimental group and a control group. The independent variable is administered to the experimental group and not to the control group, and both groups are measured on the same dependent variable. Subsequent experimental designs have used more groups and more measurements over longer periods. True experiments must have control, randomization, and manipulation.
- Experimental research allows the researcher to control the situation. In so doing, it allows researchers to answer the question, “What causes something to occur?”
- Permits the researcher to identify cause and effect relationships between variables and to distinguish placebo effects from treatment effects.
- Experimental research designs support the ability to limit alternative explanations and to infer direct causal relationships in the study.
- Approach provides the highest level of evidence for single studies.
- The design is artificial, and results may not generalize well to the real world.
- The artificial settings of experiments may alter the behaviors or responses of participants.
- Experimental designs can be costly if special equipment or facilities are needed.
- Some research problems cannot be studied using an experiment because of ethical or technical reasons.
- Difficult to apply ethnographic and other qualitative methods to experimentally designed studies.
Anastas, Jeane W. Research Design for Social Work and the Human Services . Chapter 7, Flexible Methods: Experimental Research. 2nd ed. New York: Columbia University Press, 1999; Chapter 2: Research Design, Experimental Designs. School of Psychology, University of New England, 2000; Chow, Siu L. "Experimental Design." In Encyclopedia of Research Design . Neil J. Salkind, editor. (Thousand Oaks, CA: Sage, 2010), pp. 448-453; "Experimental Design." In Social Research Methods . Nicholas Walliman, editor. (London, England: Sage, 2006), pp, 101-110; Experimental Research. Research Methods by Dummies. Department of Psychology. California State University, Fresno, 2006; Kirk, Roger E. Experimental Design: Procedures for the Behavioral Sciences . 4th edition. Thousand Oaks, CA: Sage, 2013; Trochim, William M.K. Experimental Design. Research Methods Knowledge Base. 2006; Rasool, Shafqat. Experimental Research. Slideshare presentation.
Exploratory Design
An exploratory design is conducted about a research problem when there are few or no earlier studies to refer to or rely upon to predict an outcome . The focus is on gaining insights and familiarity for later investigation or undertaken when research problems are in a preliminary stage of investigation. Exploratory designs are often used to establish an understanding of how best to proceed in studying an issue or what methodology would effectively apply to gathering information about the issue.
The goals of exploratory research are intended to produce the following possible insights:
- Familiarity with basic details, settings, and concerns.
- Well grounded picture of the situation being developed.
- Generation of new ideas and assumptions.
- Development of tentative theories or hypotheses.
- Determination about whether a study is feasible in the future.
- Issues get refined for more systematic investigation and formulation of new research questions.
- Direction for future research and techniques get developed.
- Design is a useful approach for gaining background information on a particular topic.
- Exploratory research is flexible and can address research questions of all types (what, why, how).
- Provides an opportunity to define new terms and clarify existing concepts.
- Exploratory research is often used to generate formal hypotheses and develop more precise research problems.
- In the policy arena or applied to practice, exploratory studies help establish research priorities and where resources should be allocated.
- Exploratory research generally utilizes small sample sizes and, thus, findings are typically not generalizable to the population at large.
- The exploratory nature of the research inhibits an ability to make definitive conclusions about the findings. They provide insight but not definitive conclusions.
- The research process underpinning exploratory studies is flexible but often unstructured, leading to only tentative results that have limited value to decision-makers.
- Design lacks rigorous standards applied to methods of data gathering and analysis because one of the areas for exploration could be to determine what method or methodologies could best fit the research problem.
Cuthill, Michael. “Exploratory Research: Citizen Participation, Local Government, and Sustainable Development in Australia.” Sustainable Development 10 (2002): 79-89; Streb, Christoph K. "Exploratory Case Study." In Encyclopedia of Case Study Research . Albert J. Mills, Gabrielle Durepos and Eiden Wiebe, editors. (Thousand Oaks, CA: Sage, 2010), pp. 372-374; Taylor, P. J., G. Catalano, and D.R.F. Walker. “Exploratory Analysis of the World City Network.” Urban Studies 39 (December 2002): 2377-2394; Exploratory Research. Wikipedia.
Field Research Design
Sometimes referred to as ethnography or participant observation, designs around field research encompass a variety of interpretative procedures [e.g., observation and interviews] rooted in qualitative approaches to studying people individually or in groups while inhabiting their natural environment as opposed to using survey instruments or other forms of impersonal methods of data gathering. Information acquired from observational research takes the form of “ field notes ” that involves documenting what the researcher actually sees and hears while in the field. Findings do not consist of conclusive statements derived from numbers and statistics because field research involves analysis of words and observations of behavior. Conclusions, therefore, are developed from an interpretation of findings that reveal overriding themes, concepts, and ideas. More information can be found HERE .
- Field research is often necessary to fill gaps in understanding the research problem applied to local conditions or to specific groups of people that cannot be ascertained from existing data.
- The research helps contextualize already known information about a research problem, thereby facilitating ways to assess the origins, scope, and scale of a problem and to gage the causes, consequences, and means to resolve an issue based on deliberate interaction with people in their natural inhabited spaces.
- Enables the researcher to corroborate or confirm data by gathering additional information that supports or refutes findings reported in prior studies of the topic.
- Because the researcher in embedded in the field, they are better able to make observations or ask questions that reflect the specific cultural context of the setting being investigated.
- Observing the local reality offers the opportunity to gain new perspectives or obtain unique data that challenges existing theoretical propositions or long-standing assumptions found in the literature.
What these studies don't tell you
- A field research study requires extensive time and resources to carry out the multiple steps involved with preparing for the gathering of information, including for example, examining background information about the study site, obtaining permission to access the study site, and building trust and rapport with subjects.
- Requires a commitment to staying engaged in the field to ensure that you can adequately document events and behaviors as they unfold.
- The unpredictable nature of fieldwork means that researchers can never fully control the process of data gathering. They must maintain a flexible approach to studying the setting because events and circumstances can change quickly or unexpectedly.
- Findings can be difficult to interpret and verify without access to documents and other source materials that help to enhance the credibility of information obtained from the field [i.e., the act of triangulating the data].
- Linking the research problem to the selection of study participants inhabiting their natural environment is critical. However, this specificity limits the ability to generalize findings to different situations or in other contexts or to infer courses of action applied to other settings or groups of people.
- The reporting of findings must take into account how the researcher themselves may have inadvertently affected respondents and their behaviors.
Historical Design
The purpose of a historical research design is to collect, verify, and synthesize evidence from the past to establish facts that defend or refute a hypothesis. It uses secondary sources and a variety of primary documentary evidence, such as, diaries, official records, reports, archives, and non-textual information [maps, pictures, audio and visual recordings]. The limitation is that the sources must be both authentic and valid.
- The historical research design is unobtrusive; the act of research does not affect the results of the study.
- The historical approach is well suited for trend analysis.
- Historical records can add important contextual background required to more fully understand and interpret a research problem.
- There is often no possibility of researcher-subject interaction that could affect the findings.
- Historical sources can be used over and over to study different research problems or to replicate a previous study.
- The ability to fulfill the aims of your research are directly related to the amount and quality of documentation available to understand the research problem.
- Since historical research relies on data from the past, there is no way to manipulate it to control for contemporary contexts.
- Interpreting historical sources can be very time consuming.
- The sources of historical materials must be archived consistently to ensure access. This may especially challenging for digital or online-only sources.
- Original authors bring their own perspectives and biases to the interpretation of past events and these biases are more difficult to ascertain in historical resources.
- Due to the lack of control over external variables, historical research is very weak with regard to the demands of internal validity.
- It is rare that the entirety of historical documentation needed to fully address a research problem is available for interpretation, therefore, gaps need to be acknowledged.
Howell, Martha C. and Walter Prevenier. From Reliable Sources: An Introduction to Historical Methods . Ithaca, NY: Cornell University Press, 2001; Lundy, Karen Saucier. "Historical Research." In The Sage Encyclopedia of Qualitative Research Methods . Lisa M. Given, editor. (Thousand Oaks, CA: Sage, 2008), pp. 396-400; Marius, Richard. and Melvin E. Page. A Short Guide to Writing about History . 9th edition. Boston, MA: Pearson, 2015; Savitt, Ronald. “Historical Research in Marketing.” Journal of Marketing 44 (Autumn, 1980): 52-58; Gall, Meredith. Educational Research: An Introduction . Chapter 16, Historical Research. 8th ed. Boston, MA: Pearson/Allyn and Bacon, 2007.
Longitudinal Design
A longitudinal study follows the same sample over time and makes repeated observations. For example, with longitudinal surveys, the same group of people is interviewed at regular intervals, enabling researchers to track changes over time and to relate them to variables that might explain why the changes occur. Longitudinal research designs describe patterns of change and help establish the direction and magnitude of causal relationships. Measurements are taken on each variable over two or more distinct time periods. This allows the researcher to measure change in variables over time. It is a type of observational study sometimes referred to as a panel study.
- Longitudinal data facilitate the analysis of the duration of a particular phenomenon.
- Enables survey researchers to get close to the kinds of causal explanations usually attainable only with experiments.
- The design permits the measurement of differences or change in a variable from one period to another [i.e., the description of patterns of change over time].
- Longitudinal studies facilitate the prediction of future outcomes based upon earlier factors.
- The data collection method may change over time.
- Maintaining the integrity of the original sample can be difficult over an extended period of time.
- It can be difficult to show more than one variable at a time.
- This design often needs qualitative research data to explain fluctuations in the results.
- A longitudinal research design assumes present trends will continue unchanged.
- It can take a long period of time to gather results.
- There is a need to have a large sample size and accurate sampling to reach representativness.
Anastas, Jeane W. Research Design for Social Work and the Human Services . Chapter 6, Flexible Methods: Relational and Longitudinal Research. 2nd ed. New York: Columbia University Press, 1999; Forgues, Bernard, and Isabelle Vandangeon-Derumez. "Longitudinal Analyses." In Doing Management Research . Raymond-Alain Thiétart and Samantha Wauchope, editors. (London, England: Sage, 2001), pp. 332-351; Kalaian, Sema A. and Rafa M. Kasim. "Longitudinal Studies." In Encyclopedia of Survey Research Methods . Paul J. Lavrakas, ed. (Thousand Oaks, CA: Sage, 2008), pp. 440-441; Menard, Scott, editor. Longitudinal Research . Thousand Oaks, CA: Sage, 2002; Ployhart, Robert E. and Robert J. Vandenberg. "Longitudinal Research: The Theory, Design, and Analysis of Change.” Journal of Management 36 (January 2010): 94-120; Longitudinal Study. Wikipedia.
Meta-Analysis Design
Meta-analysis is an analytical methodology designed to systematically evaluate and summarize the results from a number of individual studies, thereby, increasing the overall sample size and the ability of the researcher to study effects of interest. The purpose is to not simply summarize existing knowledge, but to develop a new understanding of a research problem using synoptic reasoning. The main objectives of meta-analysis include analyzing differences in the results among studies and increasing the precision by which effects are estimated. A well-designed meta-analysis depends upon strict adherence to the criteria used for selecting studies and the availability of information in each study to properly analyze their findings. Lack of information can severely limit the type of analyzes and conclusions that can be reached. In addition, the more dissimilarity there is in the results among individual studies [heterogeneity], the more difficult it is to justify interpretations that govern a valid synopsis of results. A meta-analysis needs to fulfill the following requirements to ensure the validity of your findings:
- Clearly defined description of objectives, including precise definitions of the variables and outcomes that are being evaluated;
- A well-reasoned and well-documented justification for identification and selection of the studies;
- Assessment and explicit acknowledgment of any researcher bias in the identification and selection of those studies;
- Description and evaluation of the degree of heterogeneity among the sample size of studies reviewed; and,
- Justification of the techniques used to evaluate the studies.
- Can be an effective strategy for determining gaps in the literature.
- Provides a means of reviewing research published about a particular topic over an extended period of time and from a variety of sources.
- Is useful in clarifying what policy or programmatic actions can be justified on the basis of analyzing research results from multiple studies.
- Provides a method for overcoming small sample sizes in individual studies that previously may have had little relationship to each other.
- Can be used to generate new hypotheses or highlight research problems for future studies.
- Small violations in defining the criteria used for content analysis can lead to difficult to interpret and/or meaningless findings.
- A large sample size can yield reliable, but not necessarily valid, results.
- A lack of uniformity regarding, for example, the type of literature reviewed, how methods are applied, and how findings are measured within the sample of studies you are analyzing, can make the process of synthesis difficult to perform.
- Depending on the sample size, the process of reviewing and synthesizing multiple studies can be very time consuming.
Beck, Lewis W. "The Synoptic Method." The Journal of Philosophy 36 (1939): 337-345; Cooper, Harris, Larry V. Hedges, and Jeffrey C. Valentine, eds. The Handbook of Research Synthesis and Meta-Analysis . 2nd edition. New York: Russell Sage Foundation, 2009; Guzzo, Richard A., Susan E. Jackson and Raymond A. Katzell. “Meta-Analysis Analysis.” In Research in Organizational Behavior , Volume 9. (Greenwich, CT: JAI Press, 1987), pp 407-442; Lipsey, Mark W. and David B. Wilson. Practical Meta-Analysis . Thousand Oaks, CA: Sage Publications, 2001; Study Design 101. Meta-Analysis. The Himmelfarb Health Sciences Library, George Washington University; Timulak, Ladislav. “Qualitative Meta-Analysis.” In The SAGE Handbook of Qualitative Data Analysis . Uwe Flick, editor. (Los Angeles, CA: Sage, 2013), pp. 481-495; Walker, Esteban, Adrian V. Hernandez, and Micheal W. Kattan. "Meta-Analysis: It's Strengths and Limitations." Cleveland Clinic Journal of Medicine 75 (June 2008): 431-439.
Mixed-Method Design
- Narrative and non-textual information can add meaning to numeric data, while numeric data can add precision to narrative and non-textual information.
- Can utilize existing data while at the same time generating and testing a grounded theory approach to describe and explain the phenomenon under study.
- A broader, more complex research problem can be investigated because the researcher is not constrained by using only one method.
- The strengths of one method can be used to overcome the inherent weaknesses of another method.
- Can provide stronger, more robust evidence to support a conclusion or set of recommendations.
- May generate new knowledge new insights or uncover hidden insights, patterns, or relationships that a single methodological approach might not reveal.
- Produces more complete knowledge and understanding of the research problem that can be used to increase the generalizability of findings applied to theory or practice.
- A researcher must be proficient in understanding how to apply multiple methods to investigating a research problem as well as be proficient in optimizing how to design a study that coherently melds them together.
- Can increase the likelihood of conflicting results or ambiguous findings that inhibit drawing a valid conclusion or setting forth a recommended course of action [e.g., sample interview responses do not support existing statistical data].
- Because the research design can be very complex, reporting the findings requires a well-organized narrative, clear writing style, and precise word choice.
- Design invites collaboration among experts. However, merging different investigative approaches and writing styles requires more attention to the overall research process than studies conducted using only one methodological paradigm.
- Concurrent merging of quantitative and qualitative research requires greater attention to having adequate sample sizes, using comparable samples, and applying a consistent unit of analysis. For sequential designs where one phase of qualitative research builds on the quantitative phase or vice versa, decisions about what results from the first phase to use in the next phase, the choice of samples and estimating reasonable sample sizes for both phases, and the interpretation of results from both phases can be difficult.
- Due to multiple forms of data being collected and analyzed, this design requires extensive time and resources to carry out the multiple steps involved in data gathering and interpretation.
Burch, Patricia and Carolyn J. Heinrich. Mixed Methods for Policy Research and Program Evaluation . Thousand Oaks, CA: Sage, 2016; Creswell, John w. et al. Best Practices for Mixed Methods Research in the Health Sciences . Bethesda, MD: Office of Behavioral and Social Sciences Research, National Institutes of Health, 2010Creswell, John W. Research Design: Qualitative, Quantitative, and Mixed Methods Approaches . 4th edition. Thousand Oaks, CA: Sage Publications, 2014; Domínguez, Silvia, editor. Mixed Methods Social Networks Research . Cambridge, UK: Cambridge University Press, 2014; Hesse-Biber, Sharlene Nagy. Mixed Methods Research: Merging Theory with Practice . New York: Guilford Press, 2010; Niglas, Katrin. “How the Novice Researcher Can Make Sense of Mixed Methods Designs.” International Journal of Multiple Research Approaches 3 (2009): 34-46; Onwuegbuzie, Anthony J. and Nancy L. Leech. “Linking Research Questions to Mixed Methods Data Analysis Procedures.” The Qualitative Report 11 (September 2006): 474-498; Tashakorri, Abbas and John W. Creswell. “The New Era of Mixed Methods.” Journal of Mixed Methods Research 1 (January 2007): 3-7; Zhanga, Wanqing. “Mixed Methods Application in Health Intervention Research: A Multiple Case Study.” International Journal of Multiple Research Approaches 8 (2014): 24-35 .
Observational Design
This type of research design draws a conclusion by comparing subjects against a control group, in cases where the researcher has no control over the experiment. There are two general types of observational designs. In direct observations, people know that you are watching them. Unobtrusive measures involve any method for studying behavior where individuals do not know they are being observed. An observational study allows a useful insight into a phenomenon and avoids the ethical and practical difficulties of setting up a large and cumbersome research project.
- Observational studies are usually flexible and do not necessarily need to be structured around a hypothesis about what you expect to observe [data is emergent rather than pre-existing].
- The researcher is able to collect in-depth information about a particular behavior.
- Can reveal interrelationships among multifaceted dimensions of group interactions.
- You can generalize your results to real life situations.
- Observational research is useful for discovering what variables may be important before applying other methods like experiments.
- Observation research designs account for the complexity of group behaviors.
- Reliability of data is low because seeing behaviors occur over and over again may be a time consuming task and are difficult to replicate.
- In observational research, findings may only reflect a unique sample population and, thus, cannot be generalized to other groups.
- There can be problems with bias as the researcher may only "see what they want to see."
- There is no possibility to determine "cause and effect" relationships since nothing is manipulated.
- Sources or subjects may not all be equally credible.
- Any group that is knowingly studied is altered to some degree by the presence of the researcher, therefore, potentially skewing any data collected.
Atkinson, Paul and Martyn Hammersley. “Ethnography and Participant Observation.” In Handbook of Qualitative Research . Norman K. Denzin and Yvonna S. Lincoln, eds. (Thousand Oaks, CA: Sage, 1994), pp. 248-261; Observational Research. Research Methods by Dummies. Department of Psychology. California State University, Fresno, 2006; Patton Michael Quinn. Qualitiative Research and Evaluation Methods . Chapter 6, Fieldwork Strategies and Observational Methods. 3rd ed. Thousand Oaks, CA: Sage, 2002; Payne, Geoff and Judy Payne. "Observation." In Key Concepts in Social Research . The SAGE Key Concepts series. (London, England: Sage, 2004), pp. 158-162; Rosenbaum, Paul R. Design of Observational Studies . New York: Springer, 2010;Williams, J. Patrick. "Nonparticipant Observation." In The Sage Encyclopedia of Qualitative Research Methods . Lisa M. Given, editor.(Thousand Oaks, CA: Sage, 2008), pp. 562-563.
Philosophical Design
Understood more as an broad approach to examining a research problem than a methodological design, philosophical analysis and argumentation is intended to challenge deeply embedded, often intractable, assumptions underpinning an area of study. This approach uses the tools of argumentation derived from philosophical traditions, concepts, models, and theories to critically explore and challenge, for example, the relevance of logic and evidence in academic debates, to analyze arguments about fundamental issues, or to discuss the root of existing discourse about a research problem. These overarching tools of analysis can be framed in three ways:
- Ontology -- the study that describes the nature of reality; for example, what is real and what is not, what is fundamental and what is derivative?
- Epistemology -- the study that explores the nature of knowledge; for example, by what means does knowledge and understanding depend upon and how can we be certain of what we know?
- Axiology -- the study of values; for example, what values does an individual or group hold and why? How are values related to interest, desire, will, experience, and means-to-end? And, what is the difference between a matter of fact and a matter of value?
- Can provide a basis for applying ethical decision-making to practice.
- Functions as a means of gaining greater self-understanding and self-knowledge about the purposes of research.
- Brings clarity to general guiding practices and principles of an individual or group.
- Philosophy informs methodology.
- Refine concepts and theories that are invoked in relatively unreflective modes of thought and discourse.
- Beyond methodology, philosophy also informs critical thinking about epistemology and the structure of reality (metaphysics).
- Offers clarity and definition to the practical and theoretical uses of terms, concepts, and ideas.
- Limited application to specific research problems [answering the "So What?" question in social science research].
- Analysis can be abstract, argumentative, and limited in its practical application to real-life issues.
- While a philosophical analysis may render problematic that which was once simple or taken-for-granted, the writing can be dense and subject to unnecessary jargon, overstatement, and/or excessive quotation and documentation.
- There are limitations in the use of metaphor as a vehicle of philosophical analysis.
- There can be analytical difficulties in moving from philosophy to advocacy and between abstract thought and application to the phenomenal world.
Burton, Dawn. "Part I, Philosophy of the Social Sciences." In Research Training for Social Scientists . (London, England: Sage, 2000), pp. 1-5; Chapter 4, Research Methodology and Design. Unisa Institutional Repository (UnisaIR), University of South Africa; Jarvie, Ian C., and Jesús Zamora-Bonilla, editors. The SAGE Handbook of the Philosophy of Social Sciences . London: Sage, 2011; Labaree, Robert V. and Ross Scimeca. “The Philosophical Problem of Truth in Librarianship.” The Library Quarterly 78 (January 2008): 43-70; Maykut, Pamela S. Beginning Qualitative Research: A Philosophic and Practical Guide . Washington, DC: Falmer Press, 1994; McLaughlin, Hugh. "The Philosophy of Social Research." In Understanding Social Work Research . 2nd edition. (London: SAGE Publications Ltd., 2012), pp. 24-47; Stanford Encyclopedia of Philosophy . Metaphysics Research Lab, CSLI, Stanford University, 2013.
Sequential Design
- The researcher has a limitless option when it comes to sample size and the sampling schedule.
- Due to the repetitive nature of this research design, minor changes and adjustments can be done during the initial parts of the study to correct and hone the research method.
- This is a useful design for exploratory studies.
- There is very little effort on the part of the researcher when performing this technique. It is generally not expensive, time consuming, or workforce intensive.
- Because the study is conducted serially, the results of one sample are known before the next sample is taken and analyzed. This provides opportunities for continuous improvement of sampling and methods of analysis.
- The sampling method is not representative of the entire population. The only possibility of approaching representativeness is when the researcher chooses to use a very large sample size significant enough to represent a significant portion of the entire population. In this case, moving on to study a second or more specific sample can be difficult.
- The design cannot be used to create conclusions and interpretations that pertain to an entire population because the sampling technique is not randomized. Generalizability from findings is, therefore, limited.
- Difficult to account for and interpret variation from one sample to another over time, particularly when using qualitative methods of data collection.
Betensky, Rebecca. Harvard University, Course Lecture Note slides; Bovaird, James A. and Kevin A. Kupzyk. "Sequential Design." In Encyclopedia of Research Design . Neil J. Salkind, editor. (Thousand Oaks, CA: Sage, 2010), pp. 1347-1352; Cresswell, John W. Et al. “Advanced Mixed-Methods Research Designs.” In Handbook of Mixed Methods in Social and Behavioral Research . Abbas Tashakkori and Charles Teddle, eds. (Thousand Oaks, CA: Sage, 2003), pp. 209-240; Henry, Gary T. "Sequential Sampling." In The SAGE Encyclopedia of Social Science Research Methods . Michael S. Lewis-Beck, Alan Bryman and Tim Futing Liao, editors. (Thousand Oaks, CA: Sage, 2004), pp. 1027-1028; Nataliya V. Ivankova. “Using Mixed-Methods Sequential Explanatory Design: From Theory to Practice.” Field Methods 18 (February 2006): 3-20; Bovaird, James A. and Kevin A. Kupzyk. “Sequential Design.” In Encyclopedia of Research Design . Neil J. Salkind, ed. Thousand Oaks, CA: Sage, 2010; Sequential Analysis. Wikipedia.
Systematic Review
- A systematic review synthesizes the findings of multiple studies related to each other by incorporating strategies of analysis and interpretation intended to reduce biases and random errors.
- The application of critical exploration, evaluation, and synthesis methods separates insignificant, unsound, or redundant research from the most salient and relevant studies worthy of reflection.
- They can be use to identify, justify, and refine hypotheses, recognize and avoid hidden problems in prior studies, and explain data inconsistencies and conflicts in data.
- Systematic reviews can be used to help policy makers formulate evidence-based guidelines and regulations.
- The use of strict, explicit, and pre-determined methods of synthesis, when applied appropriately, provide reliable estimates about the effects of interventions, evaluations, and effects related to the overarching research problem investigated by each study under review.
- Systematic reviews illuminate where knowledge or thorough understanding of a research problem is lacking and, therefore, can then be used to guide future research.
- The accepted inclusion of unpublished studies [i.e., grey literature] ensures the broadest possible way to analyze and interpret research on a topic.
- Results of the synthesis can be generalized and the findings extrapolated into the general population with more validity than most other types of studies .
- Systematic reviews do not create new knowledge per se; they are a method for synthesizing existing studies about a research problem in order to gain new insights and determine gaps in the literature.
- The way researchers have carried out their investigations [e.g., the period of time covered, number of participants, sources of data analyzed, etc.] can make it difficult to effectively synthesize studies.
- The inclusion of unpublished studies can introduce bias into the review because they may not have undergone a rigorous peer-review process prior to publication. Examples may include conference presentations or proceedings, publications from government agencies, white papers, working papers, and internal documents from organizations, and doctoral dissertations and Master's theses.
Denyer, David and David Tranfield. "Producing a Systematic Review." In The Sage Handbook of Organizational Research Methods . David A. Buchanan and Alan Bryman, editors. ( Thousand Oaks, CA: Sage Publications, 2009), pp. 671-689; Foster, Margaret J. and Sarah T. Jewell, editors. Assembling the Pieces of a Systematic Review: A Guide for Librarians . Lanham, MD: Rowman and Littlefield, 2017; Gough, David, Sandy Oliver, James Thomas, editors. Introduction to Systematic Reviews . 2nd edition. Los Angeles, CA: Sage Publications, 2017; Gopalakrishnan, S. and P. Ganeshkumar. “Systematic Reviews and Meta-analysis: Understanding the Best Evidence in Primary Healthcare.” Journal of Family Medicine and Primary Care 2 (2013): 9-14; Gough, David, James Thomas, and Sandy Oliver. "Clarifying Differences between Review Designs and Methods." Systematic Reviews 1 (2012): 1-9; Khan, Khalid S., Regina Kunz, Jos Kleijnen, and Gerd Antes. “Five Steps to Conducting a Systematic Review.” Journal of the Royal Society of Medicine 96 (2003): 118-121; Mulrow, C. D. “Systematic Reviews: Rationale for Systematic Reviews.” BMJ 309:597 (September 1994); O'Dwyer, Linda C., and Q. Eileen Wafford. "Addressing Challenges with Systematic Review Teams through Effective Communication: A Case Report." Journal of the Medical Library Association 109 (October 2021): 643-647; Okoli, Chitu, and Kira Schabram. "A Guide to Conducting a Systematic Literature Review of Information Systems Research." Sprouts: Working Papers on Information Systems 10 (2010); Siddaway, Andy P., Alex M. Wood, and Larry V. Hedges. "How to Do a Systematic Review: A Best Practice Guide for Conducting and Reporting Narrative Reviews, Meta-analyses, and Meta-syntheses." Annual Review of Psychology 70 (2019): 747-770; Torgerson, Carole J. “Publication Bias: The Achilles’ Heel of Systematic Reviews?” British Journal of Educational Studies 54 (March 2006): 89-102; Torgerson, Carole. Systematic Reviews . New York: Continuum, 2003.
- << Previous: Purpose of Guide
- Next: Design Flaws to Avoid >>
- Last Updated: Apr 29, 2024 1:49 PM
- URL: https://libguides.usc.edu/writingguide
- En español – ExME
- Em português – EME
An introduction to different types of study design
Posted on 6th April 2021 by Hadi Abbas
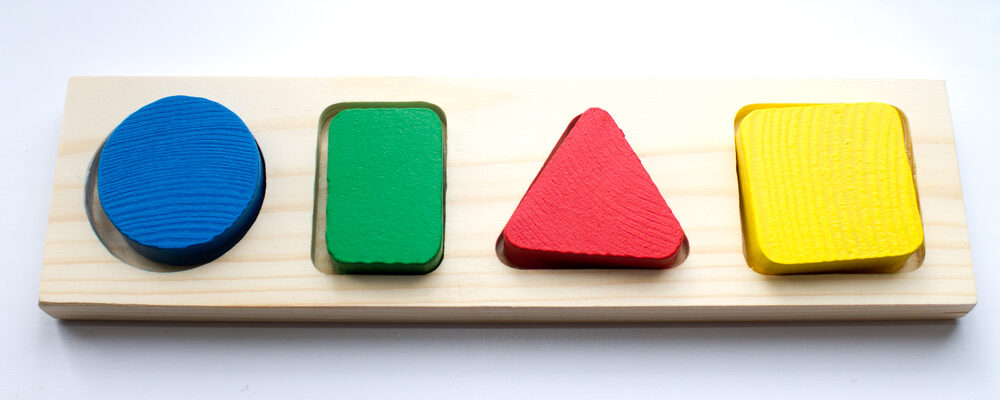
Study designs are the set of methods and procedures used to collect and analyze data in a study.
Broadly speaking, there are 2 types of study designs: descriptive studies and analytical studies.
Descriptive studies
- Describes specific characteristics in a population of interest
- The most common forms are case reports and case series
- In a case report, we discuss our experience with the patient’s symptoms, signs, diagnosis, and treatment
- In a case series, several patients with similar experiences are grouped.
Analytical Studies
Analytical studies are of 2 types: observational and experimental.
Observational studies are studies that we conduct without any intervention or experiment. In those studies, we purely observe the outcomes. On the other hand, in experimental studies, we conduct experiments and interventions.
Observational studies
Observational studies include many subtypes. Below, I will discuss the most common designs.
Cross-sectional study:
- This design is transverse where we take a specific sample at a specific time without any follow-up
- It allows us to calculate the frequency of disease ( p revalence ) or the frequency of a risk factor
- This design is easy to conduct
- For example – if we want to know the prevalence of migraine in a population, we can conduct a cross-sectional study whereby we take a sample from the population and calculate the number of patients with migraine headaches.
Cohort study:
- We conduct this study by comparing two samples from the population: one sample with a risk factor while the other lacks this risk factor
- It shows us the risk of developing the disease in individuals with the risk factor compared to those without the risk factor ( RR = relative risk )
- Prospective : we follow the individuals in the future to know who will develop the disease
- Retrospective : we look to the past to know who developed the disease (e.g. using medical records)
- This design is the strongest among the observational studies
- For example – to find out the relative risk of developing chronic obstructive pulmonary disease (COPD) among smokers, we take a sample including smokers and non-smokers. Then, we calculate the number of individuals with COPD among both.
Case-Control Study:
- We conduct this study by comparing 2 groups: one group with the disease (cases) and another group without the disease (controls)
- This design is always retrospective
- We aim to find out the odds of having a risk factor or an exposure if an individual has a specific disease (Odds ratio)
- Relatively easy to conduct
- For example – we want to study the odds of being a smoker among hypertensive patients compared to normotensive ones. To do so, we choose a group of patients diagnosed with hypertension and another group that serves as the control (normal blood pressure). Then we study their smoking history to find out if there is a correlation.
Experimental Studies
- Also known as interventional studies
- Can involve animals and humans
- Pre-clinical trials involve animals
- Clinical trials are experimental studies involving humans
- In clinical trials, we study the effect of an intervention compared to another intervention or placebo. As an example, I have listed the four phases of a drug trial:
I: We aim to assess the safety of the drug ( is it safe ? )
II: We aim to assess the efficacy of the drug ( does it work ? )
III: We want to know if this drug is better than the old treatment ( is it better ? )
IV: We follow-up to detect long-term side effects ( can it stay in the market ? )
- In randomized controlled trials, one group of participants receives the control, while the other receives the tested drug/intervention. Those studies are the best way to evaluate the efficacy of a treatment.
Finally, the figure below will help you with your understanding of different types of study designs.
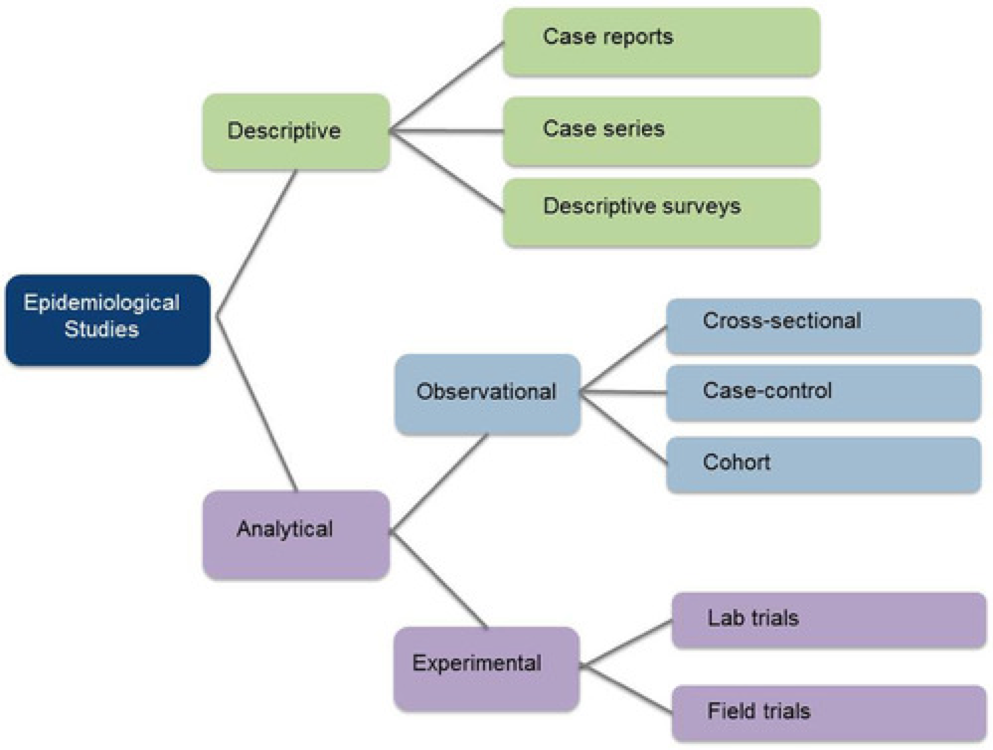
References (pdf)
You may also be interested in the following blogs for further reading:
An introduction to randomized controlled trials
Case-control and cohort studies: a brief overview
Cohort studies: prospective and retrospective designs
Prevalence vs Incidence: what is the difference?
Leave a Reply Cancel reply
Your email address will not be published. Required fields are marked *
Save my name, email, and website in this browser for the next time I comment.
No Comments on An introduction to different types of study design
you are amazing one!! if I get you I’m working with you! I’m student from Ethiopian higher education. health sciences student
Very informative and easy understandable
You are my kind of doctor. Do not lose sight of your objective.
Wow very erll explained and easy to understand
I’m Khamisu Habibu community health officer student from Abubakar Tafawa Balewa university teaching hospital Bauchi, Nigeria, I really appreciate your write up and you have make it clear for the learner. thank you
well understood,thank you so much
Well understood…thanks
Simply explained. Thank You.
Thanks a lot for this nice informative article which help me to understand different study designs that I felt difficult before
That’s lovely to hear, Mona, thank you for letting the author know how useful this was. If there are any other particular topics you think would be useful to you, and are not already on the website, please do let us know.
it is very informative and useful.
thank you statistician
Fabulous to hear, thank you John.
Thanks for this information
Thanks so much for this information….I have clearly known the types of study design Thanks
That’s so good to hear, Mirembe, thank you for letting the author know.
Very helpful article!! U have simplified everything for easy understanding
I’m a health science major currently taking statistics for health care workers…this is a challenging class…thanks for the simified feedback.
That’s good to hear this has helped you. Hopefully you will find some of the other blogs useful too. If you see any topics that are missing from the website, please do let us know!
Hello. I liked your presentation, the fact that you ranked them clearly is very helpful to understand for people like me who is a novelist researcher. However, I was expecting to read much more about the Experimental studies. So please direct me if you already have or will one day. Thank you
Dear Ay. My sincere apologies for not responding to your comment sooner. You may find it useful to filter the blogs by the topic of ‘Study design and research methods’ – here is a link to that filter: https://s4be.cochrane.org/blog/topic/study-design/ This will cover more detail about experimental studies. Or have a look on our library page for further resources there – you’ll find that on the ‘Resources’ drop down from the home page.
However, if there are specific things you feel you would like to learn about experimental studies, that are missing from the website, it would be great if you could let me know too. Thank you, and best of luck. Emma
Great job Mr Hadi. I advise you to prepare and study for the Australian Medical Board Exams as soon as you finish your undergrad study in Lebanon. Good luck and hope we can meet sometime in the future. Regards ;)
You have give a good explaination of what am looking for. However, references am not sure of where to get them from.
Subscribe to our newsletter
You will receive our monthly newsletter and free access to Trip Premium.
Related Articles
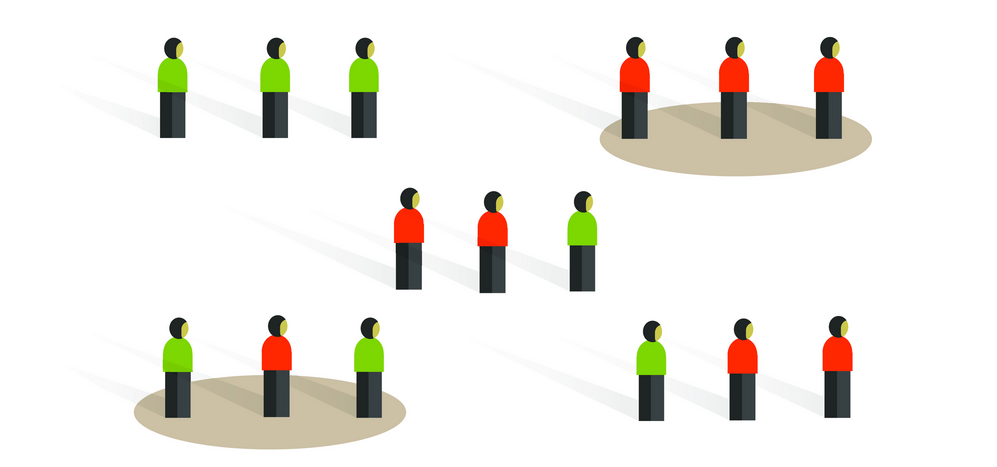
Cluster Randomized Trials: Concepts
This blog summarizes the concepts of cluster randomization, and the logistical and statistical considerations while designing a cluster randomized controlled trial.
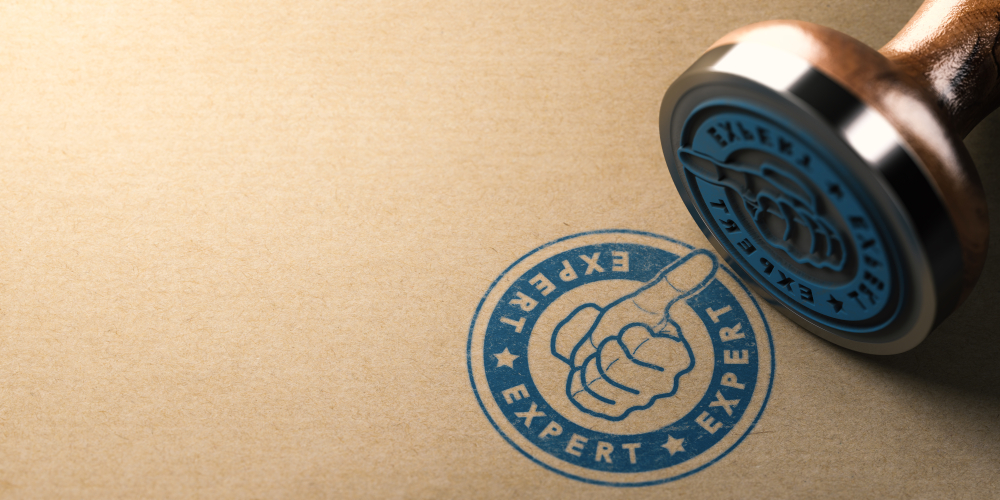
Expertise-based Randomized Controlled Trials
This blog summarizes the concepts of Expertise-based randomized controlled trials with a focus on the advantages and challenges associated with this type of study.
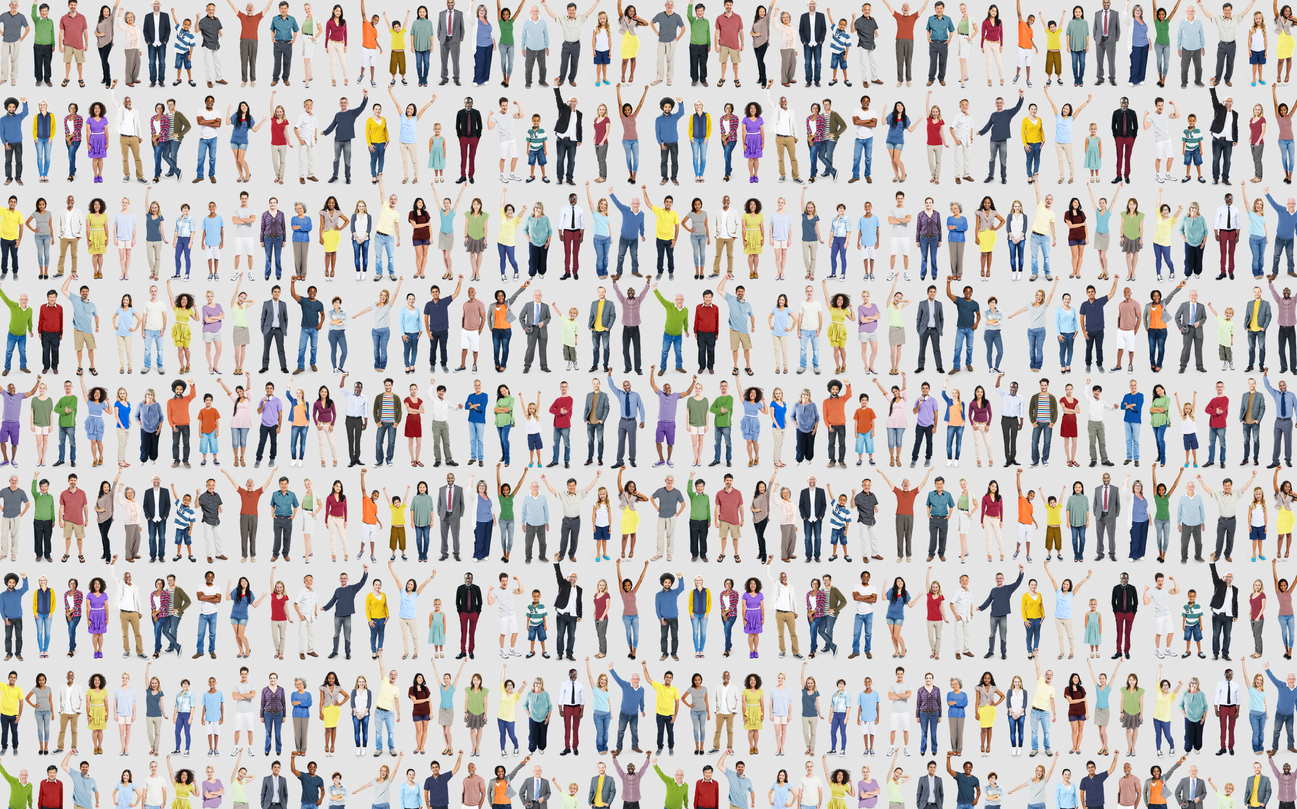
A well-designed cohort study can provide powerful results. This blog introduces prospective and retrospective cohort studies, discussing the advantages, disadvantages and use of these type of study designs.
- How it works
How to Write a Research Design – Guide with Examples
Published by Alaxendra Bets at August 14th, 2021 , Revised On October 3, 2023
A research design is a structure that combines different components of research. It involves the use of different data collection and data analysis techniques logically to answer the research questions .
It would be best to make some decisions about addressing the research questions adequately before starting the research process, which is achieved with the help of the research design.
Below are the key aspects of the decision-making process:
- Data type required for research
- Research resources
- Participants required for research
- Hypothesis based upon research question(s)
- Data analysis methodologies
- Variables (Independent, dependent, and confounding)
- The location and timescale for conducting the data
- The time period required for research
The research design provides the strategy of investigation for your project. Furthermore, it defines the parameters and criteria to compile the data to evaluate results and conclude.
Your project’s validity depends on the data collection and interpretation techniques. A strong research design reflects a strong dissertation , scientific paper, or research proposal .
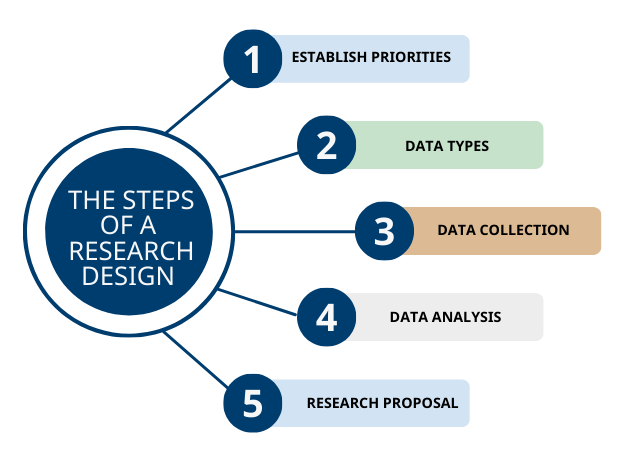
Step 1: Establish Priorities for Research Design
Before conducting any research study, you must address an important question: “how to create a research design.”
The research design depends on the researcher’s priorities and choices because every research has different priorities. For a complex research study involving multiple methods, you may choose to have more than one research design.
Multimethodology or multimethod research includes using more than one data collection method or research in a research study or set of related studies.
If one research design is weak in one area, then another research design can cover that weakness. For instance, a dissertation analyzing different situations or cases will have more than one research design.
For example:
- Experimental research involves experimental investigation and laboratory experience, but it does not accurately investigate the real world.
- Quantitative research is good for the statistical part of the project, but it may not provide an in-depth understanding of the topic .
- Also, correlational research will not provide experimental results because it is a technique that assesses the statistical relationship between two variables.
While scientific considerations are a fundamental aspect of the research design, It is equally important that the researcher think practically before deciding on its structure. Here are some questions that you should think of;
- Do you have enough time to gather data and complete the write-up?
- Will you be able to collect the necessary data by interviewing a specific person or visiting a specific location?
- Do you have in-depth knowledge about the different statistical analysis and data collection techniques to address the research questions or test the hypothesis ?
If you think that the chosen research design cannot answer the research questions properly, you can refine your research questions to gain better insight.
Step 2: Data Type you Need for Research
Decide on the type of data you need for your research. The type of data you need to collect depends on your research questions or research hypothesis. Two types of research data can be used to answer the research questions:
Primary Data Vs. Secondary Data
Qualitative vs. quantitative data.
Also, see; Research methods, design, and analysis .
Need help with a thesis chapter?
- Hire an expert from ResearchProspect today!
- Statistical analysis, research methodology, discussion of the results or conclusion – our experts can help you no matter how complex the requirements are.
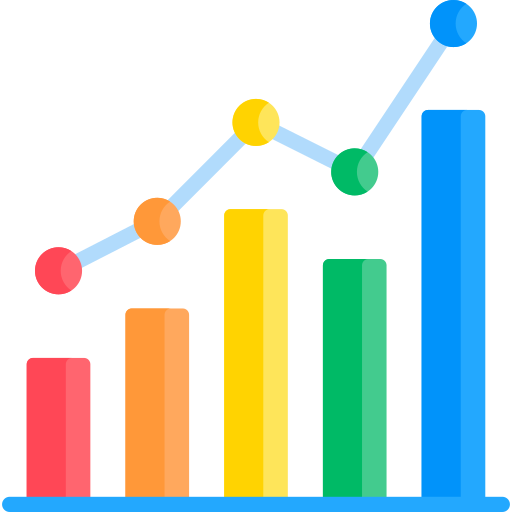
Step 3: Data Collection Techniques
Once you have selected the type of research to answer your research question, you need to decide where and how to collect the data.
It is time to determine your research method to address the research problem . Research methods involve procedures, techniques, materials, and tools used for the study.
For instance, a dissertation research design includes the different resources and data collection techniques and helps establish your dissertation’s structure .
The following table shows the characteristics of the most popularly employed research methods.
Research Methods
Step 4: Procedure of Data Analysis
Use of the correct data and statistical analysis technique is necessary for the validity of your research. Therefore, you need to be certain about the data type that would best address the research problem. Choosing an appropriate analysis method is the final step for the research design. It can be split into two main categories;
Quantitative Data Analysis
The quantitative data analysis technique involves analyzing the numerical data with the help of different applications such as; SPSS, STATA, Excel, origin lab, etc.
This data analysis strategy tests different variables such as spectrum, frequencies, averages, and more. The research question and the hypothesis must be established to identify the variables for testing.
Qualitative Data Analysis
Qualitative data analysis of figures, themes, and words allows for flexibility and the researcher’s subjective opinions. This means that the researcher’s primary focus will be interpreting patterns, tendencies, and accounts and understanding the implications and social framework.
You should be clear about your research objectives before starting to analyze the data. For example, you should ask yourself whether you need to explain respondents’ experiences and insights or do you also need to evaluate their responses with reference to a certain social framework.
Step 5: Write your Research Proposal
The research design is an important component of a research proposal because it plans the project’s execution. You can share it with the supervisor, who would evaluate the feasibility and capacity of the results and conclusion .
Read our guidelines to write a research proposal if you have already formulated your research design. The research proposal is written in the future tense because you are writing your proposal before conducting research.
The research methodology or research design, on the other hand, is generally written in the past tense.
How to Write a Research Design – Conclusion
A research design is the plan, structure, strategy of investigation conceived to answer the research question and test the hypothesis. The dissertation research design can be classified based on the type of data and the type of analysis.
Above mentioned five steps are the answer to how to write a research design. So, follow these steps to formulate the perfect research design for your dissertation .
ResearchProspect writers have years of experience creating research designs that align with the dissertation’s aim and objectives. If you are struggling with your dissertation methodology chapter, you might want to look at our dissertation part-writing service.
Our dissertation writers can also help you with the full dissertation paper . No matter how urgent or complex your need may be, ResearchProspect can help. We also offer PhD level research paper writing services.
Frequently Asked Questions
What is research design.
Research design is a systematic plan that guides the research process, outlining the methodology and procedures for collecting and analysing data. It determines the structure of the study, ensuring the research question is answered effectively, reliably, and validly. It serves as the blueprint for the entire research project.
How to write a research design?
To write a research design, define your research question, identify the research method (qualitative, quantitative, or mixed), choose data collection techniques (e.g., surveys, interviews), determine the sample size and sampling method, outline data analysis procedures, and highlight potential limitations and ethical considerations for the study.
How to write the design section of a research paper?
In the design section of a research paper, describe the research methodology chosen and justify its selection. Outline the data collection methods, participants or samples, instruments used, and procedures followed. Detail any experimental controls, if applicable. Ensure clarity and precision to enable replication of the study by other researchers.
How to write a research design in methodology?
To write a research design in methodology, clearly outline the research strategy (e.g., experimental, survey, case study). Describe the sampling technique, participants, and data collection methods. Detail the procedures for data collection and analysis. Justify choices by linking them to research objectives, addressing reliability and validity.
You May Also Like
This article is a step-by-step guide to how to write statement of a problem in research. The research problem will be half-solved by defining it correctly.
Find how to write research questions with the mentioned steps required for a perfect research question. Choose an interesting topic and begin your research.
Make sure that your selected topic is intriguing, manageable, and relevant. Here are some guidelines to help understand how to find a good dissertation topic.
USEFUL LINKS
LEARNING RESOURCES
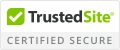
COMPANY DETAILS
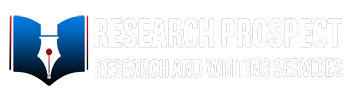
- How It Works
- Skip to main content
- Skip to primary sidebar
- Skip to footer
- QuestionPro

- Solutions Industries Gaming Automotive Sports and events Education Government Travel & Hospitality Financial Services Healthcare Cannabis Technology Use Case NPS+ Communities Audience Contactless surveys Mobile LivePolls Member Experience GDPR Positive People Science 360 Feedback Surveys
- Resources Blog eBooks Survey Templates Case Studies Training Help center

Home Market Research Research Tools and Apps
Research Design: What it is, Elements & Types
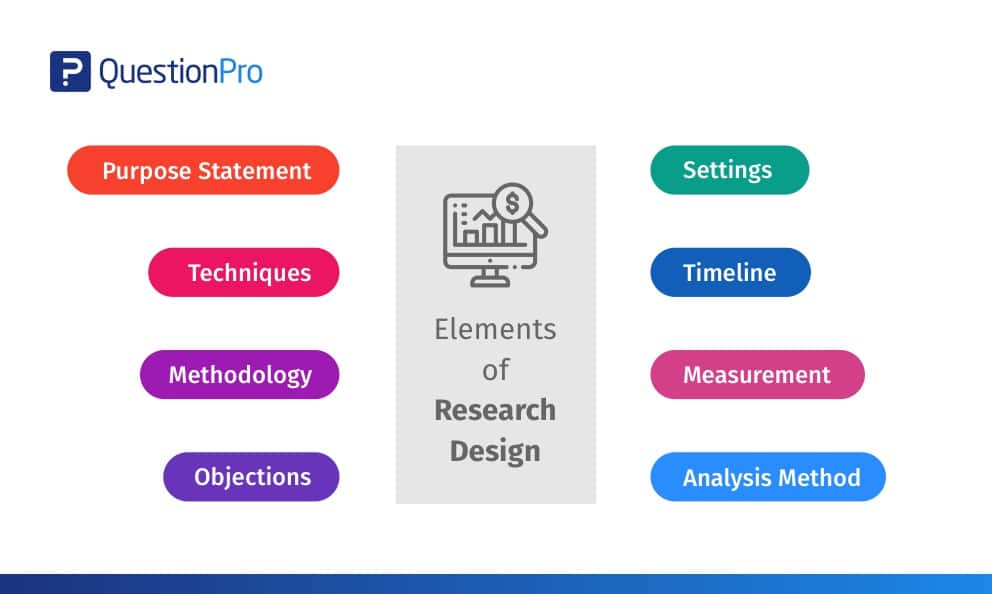
Can you imagine doing research without a plan? Probably not. When we discuss a strategy to collect, study, and evaluate data, we talk about research design. This design addresses problems and creates a consistent and logical model for data analysis. Let’s learn more about it.
What is Research Design?
Research design is the framework of research methods and techniques chosen by a researcher to conduct a study. The design allows researchers to sharpen the research methods suitable for the subject matter and set up their studies for success.
Creating a research topic explains the type of research (experimental, survey research , correlational , semi-experimental, review) and its sub-type (experimental design, research problem , descriptive case-study).
There are three main types of designs for research:
- Data collection
- Measurement
- Data Analysis
The research problem an organization faces will determine the design, not vice-versa. The design phase of a study determines which tools to use and how they are used.
The Process of Research Design
The research design process is a systematic and structured approach to conducting research. The process is essential to ensure that the study is valid, reliable, and produces meaningful results.
- Consider your aims and approaches: Determine the research questions and objectives, and identify the theoretical framework and methodology for the study.
- Choose a type of Research Design: Select the appropriate research design, such as experimental, correlational, survey, case study, or ethnographic, based on the research questions and objectives.
- Identify your population and sampling method: Determine the target population and sample size, and choose the sampling method, such as random , stratified random sampling , or convenience sampling.
- Choose your data collection methods: Decide on the data collection methods , such as surveys, interviews, observations, or experiments, and select the appropriate instruments or tools for collecting data.
- Plan your data collection procedures: Develop a plan for data collection, including the timeframe, location, and personnel involved, and ensure ethical considerations.
- Decide on your data analysis strategies: Select the appropriate data analysis techniques, such as statistical analysis , content analysis, or discourse analysis, and plan how to interpret the results.
The process of research design is a critical step in conducting research. By following the steps of research design, researchers can ensure that their study is well-planned, ethical, and rigorous.
Research Design Elements
Impactful research usually creates a minimum bias in data and increases trust in the accuracy of collected data. A design that produces the slightest margin of error in experimental research is generally considered the desired outcome. The essential elements are:
- Accurate purpose statement
- Techniques to be implemented for collecting and analyzing research
- The method applied for analyzing collected details
- Type of research methodology
- Probable objections to research
- Settings for the research study
- Measurement of analysis
Characteristics of Research Design
A proper design sets your study up for success. Successful research studies provide insights that are accurate and unbiased. You’ll need to create a survey that meets all of the main characteristics of a design. There are four key characteristics:
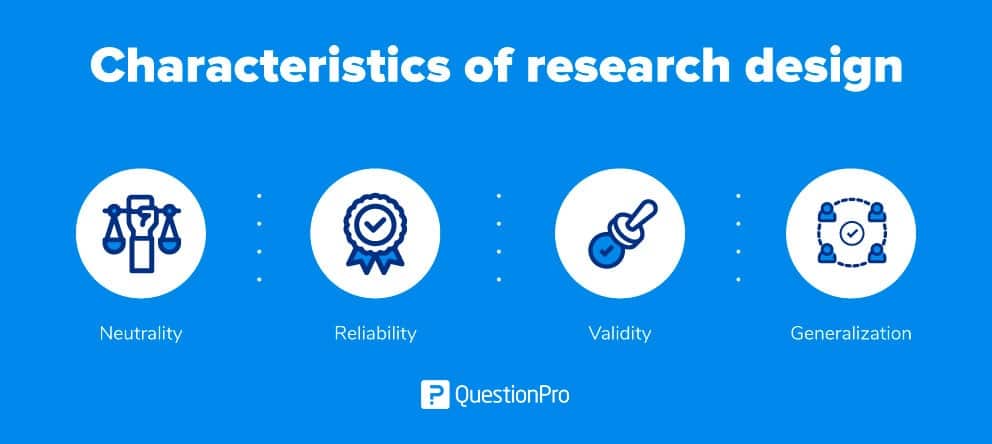
- Neutrality: When you set up your study, you may have to make assumptions about the data you expect to collect. The results projected in the research should be free from research bias and neutral. Understand opinions about the final evaluated scores and conclusions from multiple individuals and consider those who agree with the results.
- Reliability: With regularly conducted research, the researcher expects similar results every time. You’ll only be able to reach the desired results if your design is reliable. Your plan should indicate how to form research questions to ensure the standard of results.
- Validity: There are multiple measuring tools available. However, the only correct measuring tools are those which help a researcher in gauging results according to the objective of the research. The questionnaire developed from this design will then be valid.
- Generalization: The outcome of your design should apply to a population and not just a restricted sample . A generalized method implies that your survey can be conducted on any part of a population with similar accuracy.
The above factors affect how respondents answer the research questions, so they should balance all the above characteristics in a good design. If you want, you can also learn about Selection Bias through our blog.
Research Design Types
A researcher must clearly understand the various types to select which model to implement for a study. Like the research itself, the design of your analysis can be broadly classified into quantitative and qualitative.
Qualitative research
Qualitative research determines relationships between collected data and observations based on mathematical calculations. Statistical methods can prove or disprove theories related to a naturally existing phenomenon. Researchers rely on qualitative observation research methods that conclude “why” a particular theory exists and “what” respondents have to say about it.
Quantitative research
Quantitative research is for cases where statistical conclusions to collect actionable insights are essential. Numbers provide a better perspective for making critical business decisions. Quantitative research methods are necessary for the growth of any organization. Insights drawn from complex numerical data and analysis prove to be highly effective when making decisions about the business’s future.
Qualitative Research vs Quantitative Research
Here is a chart that highlights the major differences between qualitative and quantitative research:
In summary or analysis , the step of qualitative research is more exploratory and focuses on understanding the subjective experiences of individuals, while quantitative research is more focused on objective data and statistical analysis.
You can further break down the types of research design into five categories:
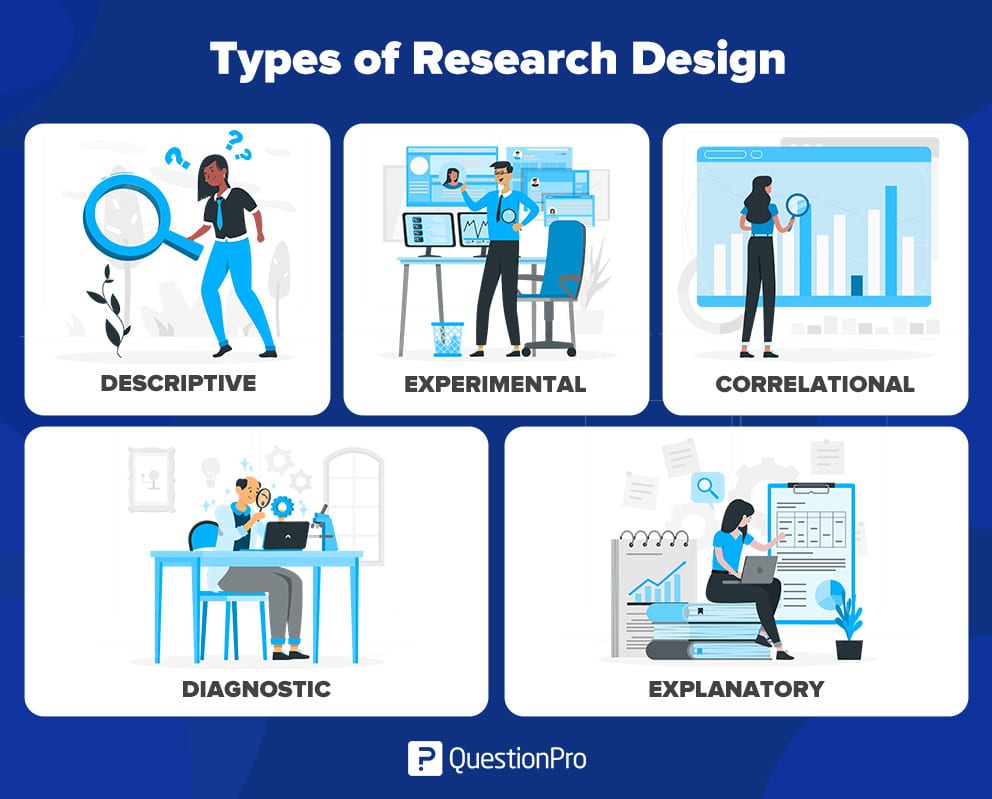
1. Descriptive: In a descriptive composition, a researcher is solely interested in describing the situation or case under their research study. It is a theory-based design method created by gathering, analyzing, and presenting collected data. This allows a researcher to provide insights into the why and how of research. Descriptive design helps others better understand the need for the research. If the problem statement is not clear, you can conduct exploratory research.
2. Experimental: Experimental research establishes a relationship between the cause and effect of a situation. It is a causal research design where one observes the impact caused by the independent variable on the dependent variable. For example, one monitors the influence of an independent variable such as a price on a dependent variable such as customer satisfaction or brand loyalty. It is an efficient research method as it contributes to solving a problem.
The independent variables are manipulated to monitor the change it has on the dependent variable. Social sciences often use it to observe human behavior by analyzing two groups. Researchers can have participants change their actions and study how the people around them react to understand social psychology better.
3. Correlational research: Correlational research is a non-experimental research technique. It helps researchers establish a relationship between two closely connected variables. There is no assumption while evaluating a relationship between two other variables, and statistical analysis techniques calculate the relationship between them. This type of research requires two different groups.
A correlation coefficient determines the correlation between two variables whose values range between -1 and +1. If the correlation coefficient is towards +1, it indicates a positive relationship between the variables, and -1 means a negative relationship between the two variables.
4. Diagnostic research: In diagnostic design, the researcher is looking to evaluate the underlying cause of a specific topic or phenomenon. This method helps one learn more about the factors that create troublesome situations.
This design has three parts of the research:
- Inception of the issue
- Diagnosis of the issue
- Solution for the issue
5. Explanatory research : Explanatory design uses a researcher’s ideas and thoughts on a subject to further explore their theories. The study explains unexplored aspects of a subject and details the research questions’ what, how, and why.
Benefits of Research Design
There are several benefits of having a well-designed research plan. Including:
- Clarity of research objectives: Research design provides a clear understanding of the research objectives and the desired outcomes.
- Increased validity and reliability: To ensure the validity and reliability of results, research design help to minimize the risk of bias and helps to control extraneous variables.
- Improved data collection: Research design helps to ensure that the proper data is collected and data is collected systematically and consistently.
- Better data analysis: Research design helps ensure that the collected data can be analyzed effectively, providing meaningful insights and conclusions.
- Improved communication: A well-designed research helps ensure the results are clean and influential within the research team and external stakeholders.
- Efficient use of resources: reducing the risk of waste and maximizing the impact of the research, research design helps to ensure that resources are used efficiently.
A well-designed research plan is essential for successful research, providing clear and meaningful insights and ensuring that resources are practical.
QuestionPro offers a comprehensive solution for researchers looking to conduct research. With its user-friendly interface, robust data collection and analysis tools, and the ability to integrate results from multiple sources, QuestionPro provides a versatile platform for designing and executing research projects.
Our robust suite of research tools provides you with all you need to derive research results. Our online survey platform includes custom point-and-click logic and advanced question types. Uncover the insights that matter the most.
FREE TRIAL LEARN MORE
MORE LIKE THIS
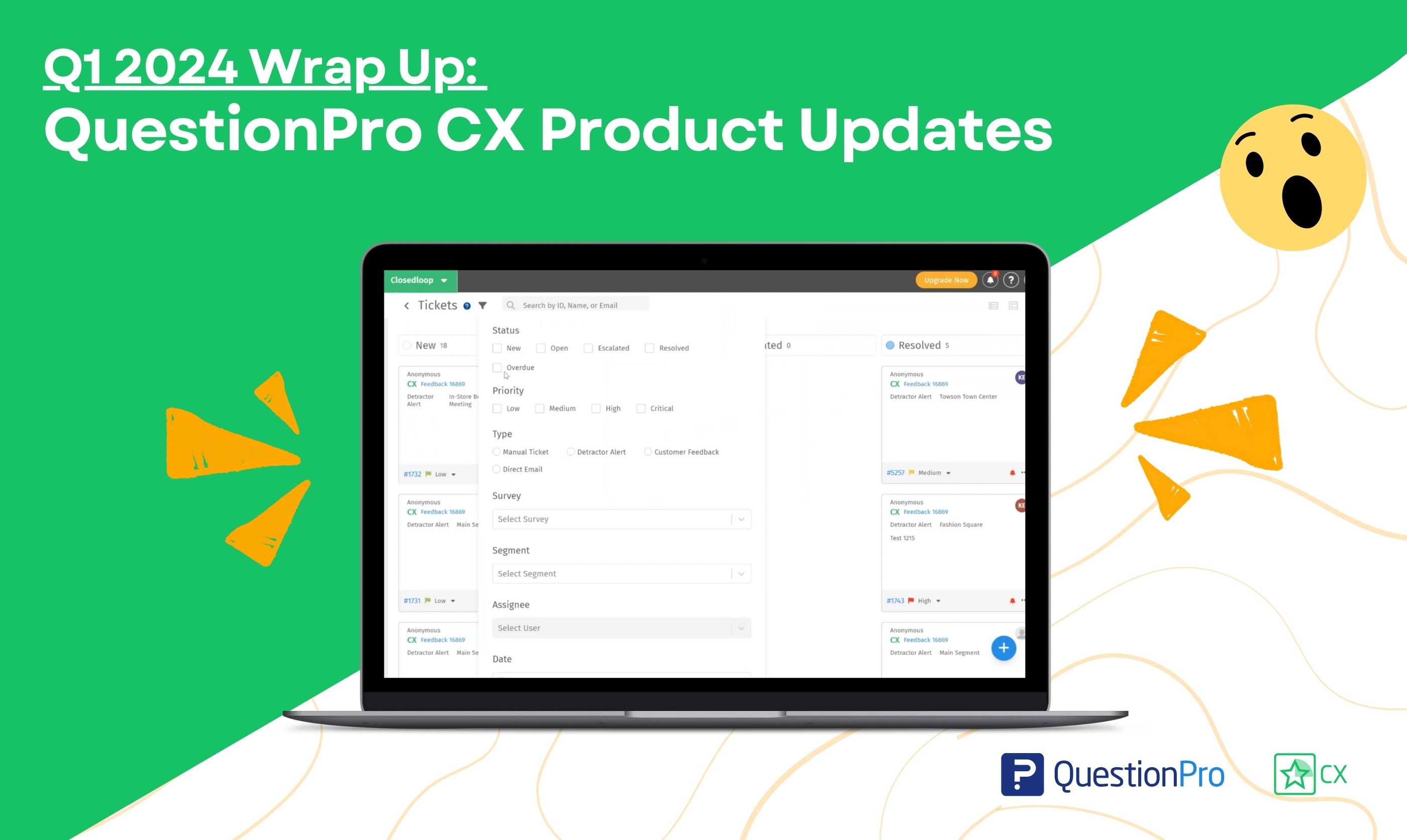
Like Never Seen Before: QuestionPro CX Product Updates – Quarter 1, 2024
Apr 29, 2024
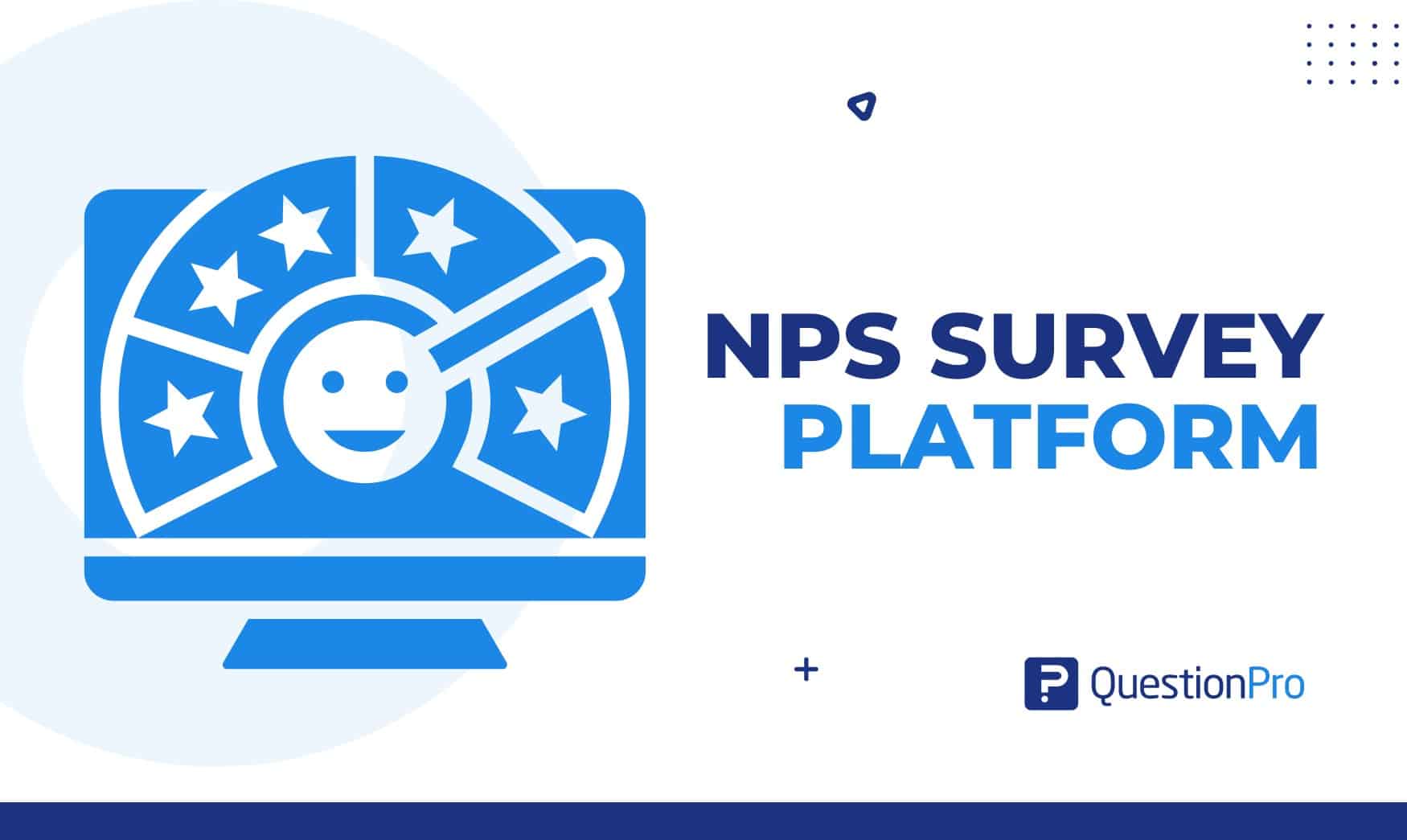
NPS Survey Platform: Types, Tips, 11 Best Platforms & Tools
Apr 26, 2024
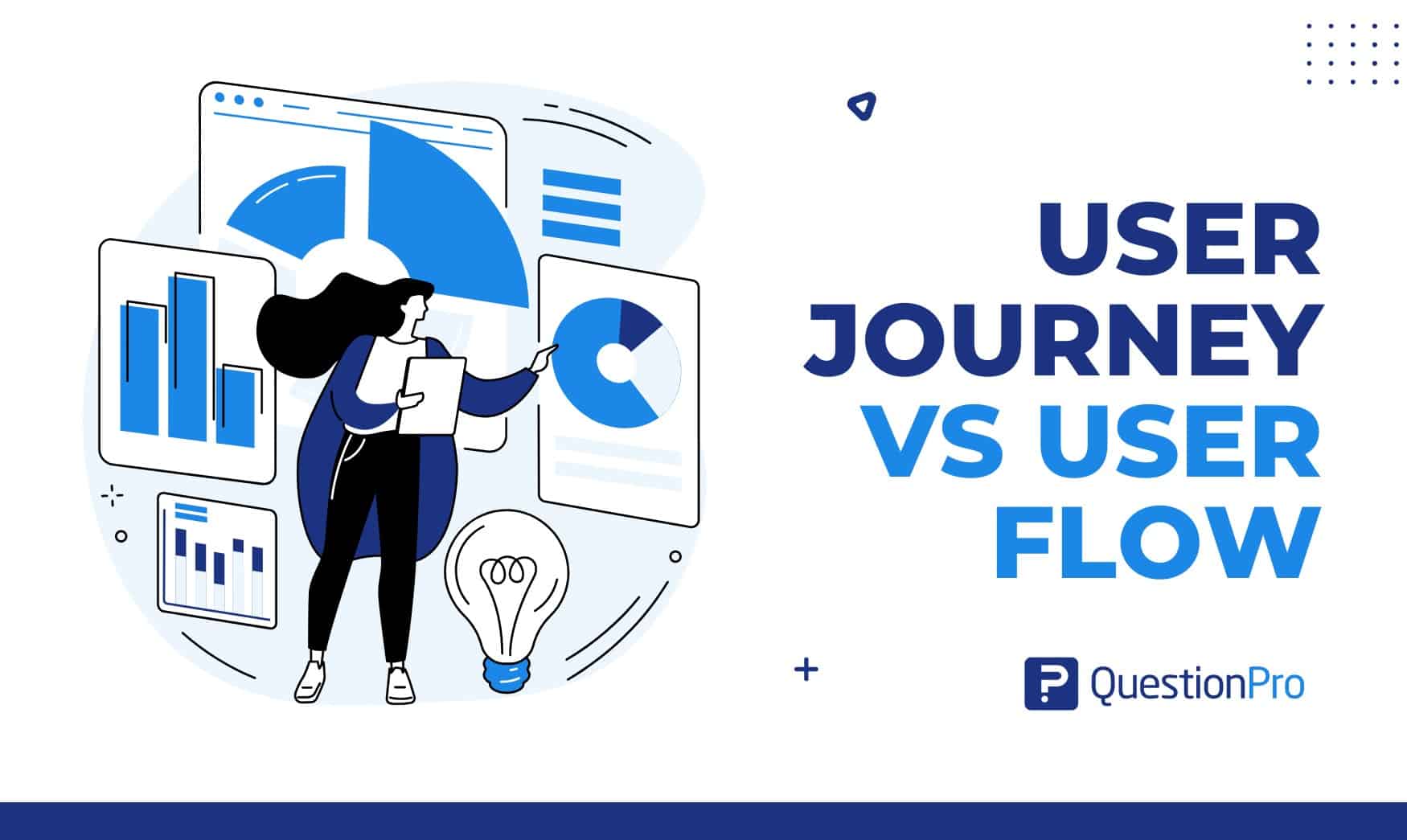
User Journey vs User Flow: Differences and Similarities
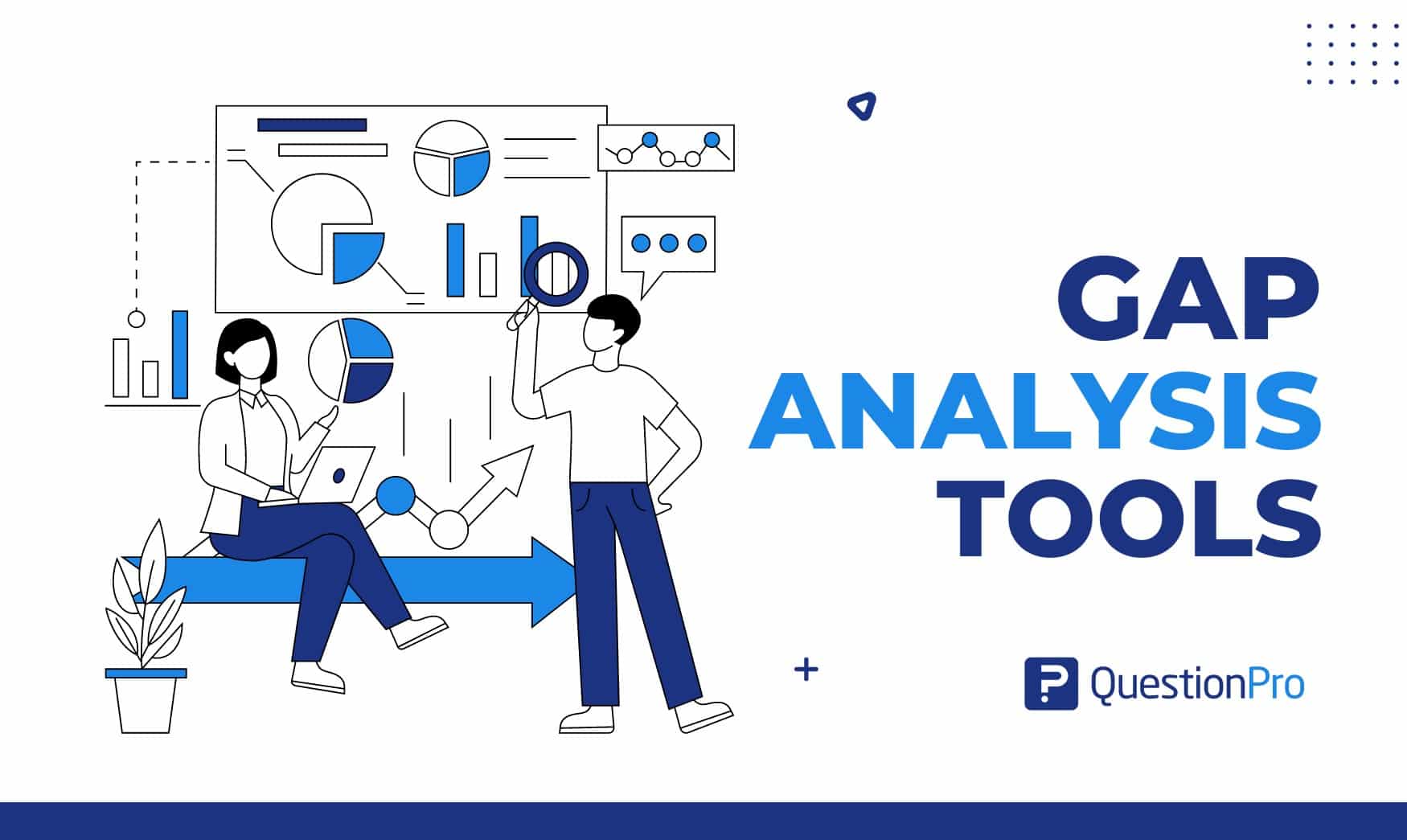
Best 7 Gap Analysis Tools to Empower Your Business
Apr 25, 2024
Other categories
- Academic Research
- Artificial Intelligence
- Assessments
- Brand Awareness
- Case Studies
- Communities
- Consumer Insights
- Customer effort score
- Customer Engagement
- Customer Experience
- Customer Loyalty
- Customer Research
- Customer Satisfaction
- Employee Benefits
- Employee Engagement
- Employee Retention
- Friday Five
- General Data Protection Regulation
- Insights Hub
- Life@QuestionPro
- Market Research
- Mobile diaries
- Mobile Surveys
- New Features
- Online Communities
- Question Types
- Questionnaire
- QuestionPro Products
- Release Notes
- Research Tools and Apps
- Revenue at Risk
- Survey Templates
- Training Tips
- Uncategorized
- Video Learning Series
- What’s Coming Up
- Workforce Intelligence
How to choose your study design
Affiliation.
- 1 Department of Medicine, Sydney Medical School, Faculty of Medicine and Health, University of Sydney, Sydney, New South Wales, Australia.
- PMID: 32479703
- DOI: 10.1111/jpc.14929
Research designs are broadly divided into observational studies (i.e. cross-sectional; case-control and cohort studies) and experimental studies (randomised control trials, RCTs). Each design has a specific role, and each has both advantages and disadvantages. Moreover, while the typical RCT is a parallel group design, there are now many variants to consider. It is important that both researchers and paediatricians are aware of the role of each study design, their respective pros and cons, and the inherent risk of bias with each design. While there are numerous quantitative study designs available to researchers, the final choice is dictated by two key factors. First, by the specific research question. That is, if the question is one of 'prevalence' (disease burden) then the ideal is a cross-sectional study; if it is a question of 'harm' - a case-control study; prognosis - a cohort and therapy - a RCT. Second, by what resources are available to you. This includes budget, time, feasibility re-patient numbers and research expertise. All these factors will severely limit the choice. While paediatricians would like to see more RCTs, these require a huge amount of resources, and in many situations will be unethical (e.g. potentially harmful intervention) or impractical (e.g. rare diseases). This paper gives a brief overview of the common study types, and for those embarking on such studies you will need far more comprehensive, detailed sources of information.
Keywords: experimental studies; observational studies; research method.
© 2020 Paediatrics and Child Health Division (The Royal Australasian College of Physicians).
- Case-Control Studies
- Cross-Sectional Studies
- Research Design*
- Privacy Policy

Home » Descriptive Research Design – Types, Methods and Examples
Descriptive Research Design – Types, Methods and Examples
Table of Contents

Descriptive Research Design
Definition:
Descriptive research design is a type of research methodology that aims to describe or document the characteristics, behaviors, attitudes, opinions, or perceptions of a group or population being studied.
Descriptive research design does not attempt to establish cause-and-effect relationships between variables or make predictions about future outcomes. Instead, it focuses on providing a detailed and accurate representation of the data collected, which can be useful for generating hypotheses, exploring trends, and identifying patterns in the data.
Types of Descriptive Research Design
Types of Descriptive Research Design are as follows:
Cross-sectional Study
This involves collecting data at a single point in time from a sample or population to describe their characteristics or behaviors. For example, a researcher may conduct a cross-sectional study to investigate the prevalence of certain health conditions among a population, or to describe the attitudes and beliefs of a particular group.
Longitudinal Study
This involves collecting data over an extended period of time, often through repeated observations or surveys of the same group or population. Longitudinal studies can be used to track changes in attitudes, behaviors, or outcomes over time, or to investigate the effects of interventions or treatments.
This involves an in-depth examination of a single individual, group, or situation to gain a detailed understanding of its characteristics or dynamics. Case studies are often used in psychology, sociology, and business to explore complex phenomena or to generate hypotheses for further research.
Survey Research
This involves collecting data from a sample or population through standardized questionnaires or interviews. Surveys can be used to describe attitudes, opinions, behaviors, or demographic characteristics of a group, and can be conducted in person, by phone, or online.
Observational Research
This involves observing and documenting the behavior or interactions of individuals or groups in a natural or controlled setting. Observational studies can be used to describe social, cultural, or environmental phenomena, or to investigate the effects of interventions or treatments.
Correlational Research
This involves examining the relationships between two or more variables to describe their patterns or associations. Correlational studies can be used to identify potential causal relationships or to explore the strength and direction of relationships between variables.
Data Analysis Methods
Descriptive research design data analysis methods depend on the type of data collected and the research question being addressed. Here are some common methods of data analysis for descriptive research:
Descriptive Statistics
This method involves analyzing data to summarize and describe the key features of a sample or population. Descriptive statistics can include measures of central tendency (e.g., mean, median, mode) and measures of variability (e.g., range, standard deviation).
Cross-tabulation
This method involves analyzing data by creating a table that shows the frequency of two or more variables together. Cross-tabulation can help identify patterns or relationships between variables.
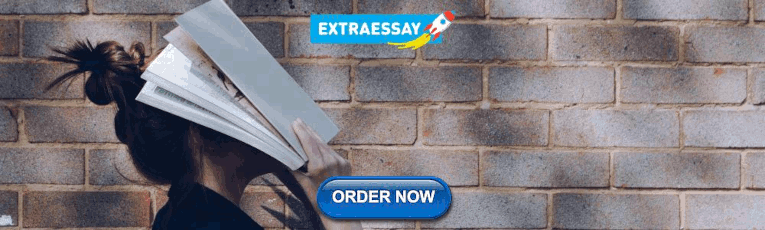
Content Analysis
This method involves analyzing qualitative data (e.g., text, images, audio) to identify themes, patterns, or trends. Content analysis can be used to describe the characteristics of a sample or population, or to identify factors that influence attitudes or behaviors.
Qualitative Coding
This method involves analyzing qualitative data by assigning codes to segments of data based on their meaning or content. Qualitative coding can be used to identify common themes, patterns, or categories within the data.
Visualization
This method involves creating graphs or charts to represent data visually. Visualization can help identify patterns or relationships between variables and make it easier to communicate findings to others.
Comparative Analysis
This method involves comparing data across different groups or time periods to identify similarities and differences. Comparative analysis can help describe changes in attitudes or behaviors over time or differences between subgroups within a population.
Applications of Descriptive Research Design
Descriptive research design has numerous applications in various fields. Some of the common applications of descriptive research design are:
- Market research: Descriptive research design is widely used in market research to understand consumer preferences, behavior, and attitudes. This helps companies to develop new products and services, improve marketing strategies, and increase customer satisfaction.
- Health research: Descriptive research design is used in health research to describe the prevalence and distribution of a disease or health condition in a population. This helps healthcare providers to develop prevention and treatment strategies.
- Educational research: Descriptive research design is used in educational research to describe the performance of students, schools, or educational programs. This helps educators to improve teaching methods and develop effective educational programs.
- Social science research: Descriptive research design is used in social science research to describe social phenomena such as cultural norms, values, and beliefs. This helps researchers to understand social behavior and develop effective policies.
- Public opinion research: Descriptive research design is used in public opinion research to understand the opinions and attitudes of the general public on various issues. This helps policymakers to develop effective policies that are aligned with public opinion.
- Environmental research: Descriptive research design is used in environmental research to describe the environmental conditions of a particular region or ecosystem. This helps policymakers and environmentalists to develop effective conservation and preservation strategies.
Descriptive Research Design Examples
Here are some real-time examples of descriptive research designs:
- A restaurant chain wants to understand the demographics and attitudes of its customers. They conduct a survey asking customers about their age, gender, income, frequency of visits, favorite menu items, and overall satisfaction. The survey data is analyzed using descriptive statistics and cross-tabulation to describe the characteristics of their customer base.
- A medical researcher wants to describe the prevalence and risk factors of a particular disease in a population. They conduct a cross-sectional study in which they collect data from a sample of individuals using a standardized questionnaire. The data is analyzed using descriptive statistics and cross-tabulation to identify patterns in the prevalence and risk factors of the disease.
- An education researcher wants to describe the learning outcomes of students in a particular school district. They collect test scores from a representative sample of students in the district and use descriptive statistics to calculate the mean, median, and standard deviation of the scores. They also create visualizations such as histograms and box plots to show the distribution of scores.
- A marketing team wants to understand the attitudes and behaviors of consumers towards a new product. They conduct a series of focus groups and use qualitative coding to identify common themes and patterns in the data. They also create visualizations such as word clouds to show the most frequently mentioned topics.
- An environmental scientist wants to describe the biodiversity of a particular ecosystem. They conduct an observational study in which they collect data on the species and abundance of plants and animals in the ecosystem. The data is analyzed using descriptive statistics to describe the diversity and richness of the ecosystem.
How to Conduct Descriptive Research Design
To conduct a descriptive research design, you can follow these general steps:
- Define your research question: Clearly define the research question or problem that you want to address. Your research question should be specific and focused to guide your data collection and analysis.
- Choose your research method: Select the most appropriate research method for your research question. As discussed earlier, common research methods for descriptive research include surveys, case studies, observational studies, cross-sectional studies, and longitudinal studies.
- Design your study: Plan the details of your study, including the sampling strategy, data collection methods, and data analysis plan. Determine the sample size and sampling method, decide on the data collection tools (such as questionnaires, interviews, or observations), and outline your data analysis plan.
- Collect data: Collect data from your sample or population using the data collection tools you have chosen. Ensure that you follow ethical guidelines for research and obtain informed consent from participants.
- Analyze data: Use appropriate statistical or qualitative analysis methods to analyze your data. As discussed earlier, common data analysis methods for descriptive research include descriptive statistics, cross-tabulation, content analysis, qualitative coding, visualization, and comparative analysis.
- I nterpret results: Interpret your findings in light of your research question and objectives. Identify patterns, trends, and relationships in the data, and describe the characteristics of your sample or population.
- Draw conclusions and report results: Draw conclusions based on your analysis and interpretation of the data. Report your results in a clear and concise manner, using appropriate tables, graphs, or figures to present your findings. Ensure that your report follows accepted research standards and guidelines.
When to Use Descriptive Research Design
Descriptive research design is used in situations where the researcher wants to describe a population or phenomenon in detail. It is used to gather information about the current status or condition of a group or phenomenon without making any causal inferences. Descriptive research design is useful in the following situations:
- Exploratory research: Descriptive research design is often used in exploratory research to gain an initial understanding of a phenomenon or population.
- Identifying trends: Descriptive research design can be used to identify trends or patterns in a population, such as changes in consumer behavior or attitudes over time.
- Market research: Descriptive research design is commonly used in market research to understand consumer preferences, behavior, and attitudes.
- Health research: Descriptive research design is useful in health research to describe the prevalence and distribution of a disease or health condition in a population.
- Social science research: Descriptive research design is used in social science research to describe social phenomena such as cultural norms, values, and beliefs.
- Educational research: Descriptive research design is used in educational research to describe the performance of students, schools, or educational programs.
Purpose of Descriptive Research Design
The main purpose of descriptive research design is to describe and measure the characteristics of a population or phenomenon in a systematic and objective manner. It involves collecting data that describe the current status or condition of the population or phenomenon of interest, without manipulating or altering any variables.
The purpose of descriptive research design can be summarized as follows:
- To provide an accurate description of a population or phenomenon: Descriptive research design aims to provide a comprehensive and accurate description of a population or phenomenon of interest. This can help researchers to develop a better understanding of the characteristics of the population or phenomenon.
- To identify trends and patterns: Descriptive research design can help researchers to identify trends and patterns in the data, such as changes in behavior or attitudes over time. This can be useful for making predictions and developing strategies.
- To generate hypotheses: Descriptive research design can be used to generate hypotheses or research questions that can be tested in future studies. For example, if a descriptive study finds a correlation between two variables, this could lead to the development of a hypothesis about the causal relationship between the variables.
- To establish a baseline: Descriptive research design can establish a baseline or starting point for future research. This can be useful for comparing data from different time periods or populations.
Characteristics of Descriptive Research Design
Descriptive research design has several key characteristics that distinguish it from other research designs. Some of the main characteristics of descriptive research design are:
- Objective : Descriptive research design is objective in nature, which means that it focuses on collecting factual and accurate data without any personal bias. The researcher aims to report the data objectively without any personal interpretation.
- Non-experimental: Descriptive research design is non-experimental, which means that the researcher does not manipulate any variables. The researcher simply observes and records the behavior or characteristics of the population or phenomenon of interest.
- Quantitative : Descriptive research design is quantitative in nature, which means that it involves collecting numerical data that can be analyzed using statistical techniques. This helps to provide a more precise and accurate description of the population or phenomenon.
- Cross-sectional: Descriptive research design is often cross-sectional, which means that the data is collected at a single point in time. This can be useful for understanding the current state of the population or phenomenon, but it may not provide information about changes over time.
- Large sample size: Descriptive research design typically involves a large sample size, which helps to ensure that the data is representative of the population of interest. A large sample size also helps to increase the reliability and validity of the data.
- Systematic and structured: Descriptive research design involves a systematic and structured approach to data collection, which helps to ensure that the data is accurate and reliable. This involves using standardized procedures for data collection, such as surveys, questionnaires, or observation checklists.
Advantages of Descriptive Research Design
Descriptive research design has several advantages that make it a popular choice for researchers. Some of the main advantages of descriptive research design are:
- Provides an accurate description: Descriptive research design is focused on accurately describing the characteristics of a population or phenomenon. This can help researchers to develop a better understanding of the subject of interest.
- Easy to conduct: Descriptive research design is relatively easy to conduct and requires minimal resources compared to other research designs. It can be conducted quickly and efficiently, and data can be collected through surveys, questionnaires, or observations.
- Useful for generating hypotheses: Descriptive research design can be used to generate hypotheses or research questions that can be tested in future studies. For example, if a descriptive study finds a correlation between two variables, this could lead to the development of a hypothesis about the causal relationship between the variables.
- Large sample size : Descriptive research design typically involves a large sample size, which helps to ensure that the data is representative of the population of interest. A large sample size also helps to increase the reliability and validity of the data.
- Can be used to monitor changes : Descriptive research design can be used to monitor changes over time in a population or phenomenon. This can be useful for identifying trends and patterns, and for making predictions about future behavior or attitudes.
- Can be used in a variety of fields : Descriptive research design can be used in a variety of fields, including social sciences, healthcare, business, and education.
Limitation of Descriptive Research Design
Descriptive research design also has some limitations that researchers should consider before using this design. Some of the main limitations of descriptive research design are:
- Cannot establish cause and effect: Descriptive research design cannot establish cause and effect relationships between variables. It only provides a description of the characteristics of the population or phenomenon of interest.
- Limited generalizability: The results of a descriptive study may not be generalizable to other populations or situations. This is because descriptive research design often involves a specific sample or situation, which may not be representative of the broader population.
- Potential for bias: Descriptive research design can be subject to bias, particularly if the researcher is not objective in their data collection or interpretation. This can lead to inaccurate or incomplete descriptions of the population or phenomenon of interest.
- Limited depth: Descriptive research design may provide a superficial description of the population or phenomenon of interest. It does not delve into the underlying causes or mechanisms behind the observed behavior or characteristics.
- Limited utility for theory development: Descriptive research design may not be useful for developing theories about the relationship between variables. It only provides a description of the variables themselves.
- Relies on self-report data: Descriptive research design often relies on self-report data, such as surveys or questionnaires. This type of data may be subject to biases, such as social desirability bias or recall bias.
About the author
Muhammad Hassan
Researcher, Academic Writer, Web developer
You may also like

Questionnaire – Definition, Types, and Examples

Case Study – Methods, Examples and Guide

Observational Research – Methods and Guide

Quantitative Research – Methods, Types and...

Qualitative Research Methods

Explanatory Research – Types, Methods, Guide
- Study Protocol
- Open access
- Published: 22 April 2024
Implementation and early effects of medicaid policy interventions to promote racial equity in pregnancy and early childhood outcomes in Pennsylvania: protocol for a mixed methods study
- Marian Jarlenski 1 ,
- Evan Cole 1 ,
- Christine McClure 1 ,
- Sarah Sanders 2 ,
- Marquita Smalls 2 &
- Dara D Méndez 2
BMC Health Services Research volume 24 , Article number: 498 ( 2024 ) Cite this article
127 Accesses
1 Altmetric
Metrics details
There are large racial inequities in pregnancy and early childhood health within state Medicaid programs in the United States. To date, few Medicaid policy interventions have explicitly focused on improving health in Black populations. Pennsylvania Medicaid has adopted two policy interventions to incentivize racial health equity in managed care (equity payment program) and obstetric service delivery (equity focused obstetric bundle). Our research team will conduct a mixed-methods study to investigate the implementation and early effects of these two policy interventions on pregnancy and infant health equity.
Qualitative interviews will be conducted with Medicaid managed care administrators and obstetric and pediatric providers, and focus groups will be conducted among Medicaid beneficiaries. Quantitative data on healthcare utilization, healthcare quality, and health outcomes among pregnant and parenting people will be extracted from administrative Medicaid healthcare data. Primary outcomes are stakeholder perspectives on policy intervention implementation (qualitative) and timely prenatal care, pregnancy and birth outcomes, and well-child visits (quantitative). Template analysis methods will be applied to qualitative data. Quantitative analyses will use an interrupted time series design to examine changes over time in outcomes among Black people, relative to people of other races, before and after adoption of the Pennsylvania Medicaid equity-focused policy interventions.
Findings from this study are expected to advance knowledge about how Medicaid programs can best implement policy interventions to promote racial equity in pregnancy and early childhood health.
Peer Review reports
Rates of maternal and infant morbidity and mortality in the United States far exceed those of comparable nations [ 1 ]. The burdens of racist policies have produced vastly worse outcomes for Black and Native, relative to White, populations [ 2 ]. For example, Black and Native birthing people are more than three times as likely to experience pregnancy-related mortality compared to white birthing people [ 3 ]. For every pregnancy-related death, there are thousands of birthing people who experience severe morbidity; including stark racial disparities where Black populations are more likely to experience stroke or serious cardiovascular events sending them on a trajectory of adverse health outcomes beyond pregnancy [ 4 , 5 ]. We also see similar racial inequities for infant mortality and morbidity. These racial inequities are not adequately explained by individual behaviors or other socio-economic factors, but are a complex intersection of factors shaped by structural and social determinants [ 2 , 6 ], policies and institutions carrying out such policies [ 7 ]. There is a long history of structural racism that has resulted in practices and policies that have had a detrimental impact on Black and Indigenous populations in the United States [ 8 ].
State Medicaid programs are the largest single payer for pregnancy and birth in the US, covering 68% of births to Black people [ 9 ]. As such, Medicaid programs have great potential to implement structural interventions to advance racial equity in healthcare and health outcomes during pregnancy and postpartum [ 10 ]. Historically, Medicaid policies have promoted equality, that is, providing equal benefits to all regardless of the distribution of need [ 11 ]. An equity-focused policy approach, however, will direct resources toward improving health and well-being among those with the greatest need [ 12 ]. Unfortunately, a vast body of research conducted among Medicaid-enrolled populations shows that healthcare systems do not provide the same quality of obstetric care to Black people and other people of color, relative to white people [ 13 , 14 , 15 , 16 , 17 , 18 ].
Pennsylvania’s Medicaid program is the fourth-largest in the United States, with 3.5 million people enrolled and expenditures of $35.1 billion [ 19 , 20 ]. Past research in the Pennsylvania Medicaid program has demonstrated Black people were less able to access prenatal and postpartum care relative to those in other race groups [ 15 ]. Reporting from the Pennsylvania Maternal Mortality Commission shows that in more than half of the cases of pregnancy-associated deaths, the decadents were enrolled in Medicaid [ 21 ]. Similar to national figures, pregnancy-associated death was far more common among Black people vs. those of other races ( [ 21 ].
To ameliorate these racial disparities, Pennsylvania Medicaid is currently implementing two novel policies with the goal to advance racial equity in pregnancy and child health. The first, the equity incentive payment program, was initiated in 2020. The equity incentive payment program makes available approximately $26 million in Medicaid managed care organization (MCO) payments each year to plans that improve access to timely prenatal care and well-child visits among Black beneficiaries. The second is the maternity care bundled payment model, initiated in 2021, designed to provide incentives to obstetric providers across a wide range of pregnancy health outcomes and specifically incentivizes improvements among Black beneficiaries.
Although these policy approaches are unique, it is possible that other state Medicaid programs or other health insurers could learn from the policies and adapt or expand these approaches. Our research team will conduct a mixed-methods study to investigate the implementation and early effects of the two aforementioned policy changes on pregnancy and infant health equity. Our research aims are to: (1) evaluate implementation and early effects of the equity incentive payment program prenatal and early childhood healthcare outcomes and experiences among Black Medicaid beneficiaries; and (2) determine the extent to which an equity-focused maternity care bundled payment model affects racial equity in obstetric care and pregnancy health outcomes. To achieve these aims, we will draw on established partnerships between university researchers, community organizations, and policymakers to collect and analyze data. First, we will collect qualitative data with diverse stakeholders including Medicaid beneficiaries, MCO plan representatives, and pediatric and obstetric care clinicians to study implementation of these equity-focused policy changes. Second, we will use a community-partnered approach to develop a quantitative analysis plan of Medicaid administrative data for an estimated 167,000 birthing person-child dyads to estimate early effects of these policies. Our cross-disciplinary, community-engaged partnerships will enable us to triangulate how the healthcare policy structures of state Medicaid programs can be changed to promote racial equity in health.
Theoretical framework
The proposed research seeks to advance knowledge about the causes of, and structural interventions to improve, health and well-being among Black pregnant and parenting persons and their children in Medicaid. The theoretical model underlying this work is informed by foundational literature from a range of disciplines. First, it incorporates Critical Race Theory and Public Health Critical Race Praxis, which posit structural determinants, such as racism and other forms of oppression (e.g., sexism, classism, poverty), as fundamental causes of adverse social environments that interact to make certain populations more susceptible to illness and resulting in suboptimal health [ 22 , 23 , 24 , 25 , 26 ]. Second, it incorporates political science theory that dominant social definitions of populations shape group empowerment and resulting health policies and material benefits [ 27 ]. Third, it draws on new scholarship suggesting the necessity of studying social welfare policies with a critical race lens centering the beneficiaries’ lived experiences [ 11 , 28 , 29 ].
As depicted in Fig. 1 , our research project identifies both the Medicaid policy environment as well as the beneficiary experiences of the policy environment as upstream factors that influence healthcare organization and beneficiaries’ interaction with healthcare systems. In particular, we aim to facilitate and further enhance the connection between beneficiaries’ lived experiences and policy decision-makers through our collaboration with community partners. This connection can influence the policymaking process that shapes how care is delivered both at the managed care and healthcare provider levels. Healthcare utilization and quality are conceptualized as intermediate outcomes which may influence pregnancy and birth outcomes.
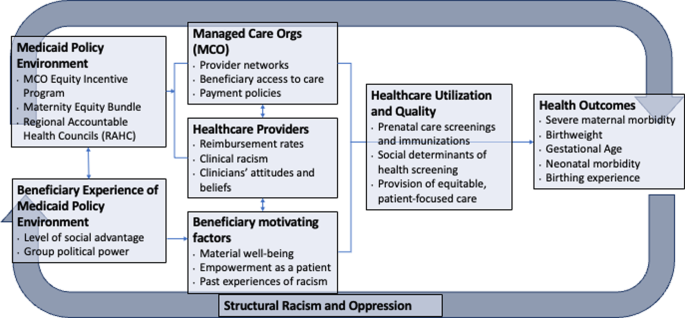
Conceptual model of the evaluation of structural interventions in Medicaid to promote racial equity in pregnancy and child health
Medicaid policy interventions
Nearly all Medicaid beneficiaries in Pennsylvania are enrolled in 1 of 8 Medicaid managed care plans, which manage the physical health care of enrollees and are subject to pay-for-performance requirements for healthcare quality measures. Currently, the Pennsylvania Medicaid program makes available 2% of total payments to MCO plans, contingent on MCO plan performance on 13 different healthcare quality metrics. An equity incentive payment program was added to this reimbursement scheme for two metrics in 2020: timely prenatal care and well-child visit utilization in the first 15 months of life (Fig. 2 ). Specifically, 2/13 (or 0.15%) of total payments are withheld for these two equity-focused metrics. MCO plans are assessed on overall performance and subsequently on the annual improvement on these measures among Black beneficiaries. MCO plans can be penalized (up to -0.12% of total payments) or rewarded (up to + 0.35% of total payments) for their performance on each of these two metrics.
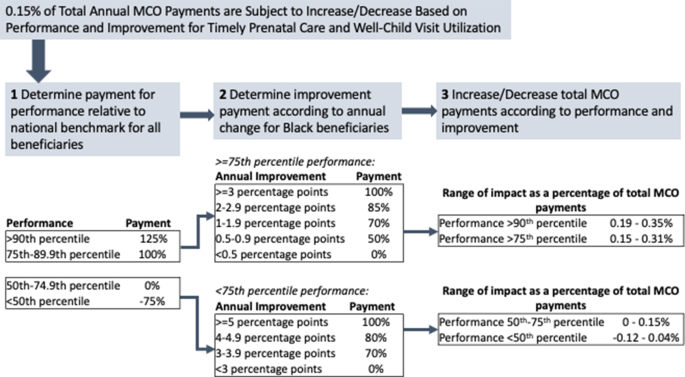
Pennsylvania Medicaid’s health equity incentive payment program will condition payments to managed care organizations based on overall performance as well as improvement among Black beneficiaries
Second, Pennsylvania Medicaid implemented a maternity care bundled payment model in 2021 that considers outcomes among Black beneficiaries (Fig. 3 ). Under maternity care bundled payment models, obstetric providers are incentivized to meet a total cost threshold and quality metrics for prenatal and delivery care [ 30 ]. Specifically, providers and payers agree on a target cost for low- or average-risk perinatal care, including pregnancy, delivery, and postpartum care. If total payments to providers are lower than the target cost while maintaining certain quality metrics, providers and payers share those savings. Under Pennsylvania’s new model, providers are able to achieve shared savings based on quality metric performance, as well as a health equity score reflecting performance on those metrics among Black beneficiaries.
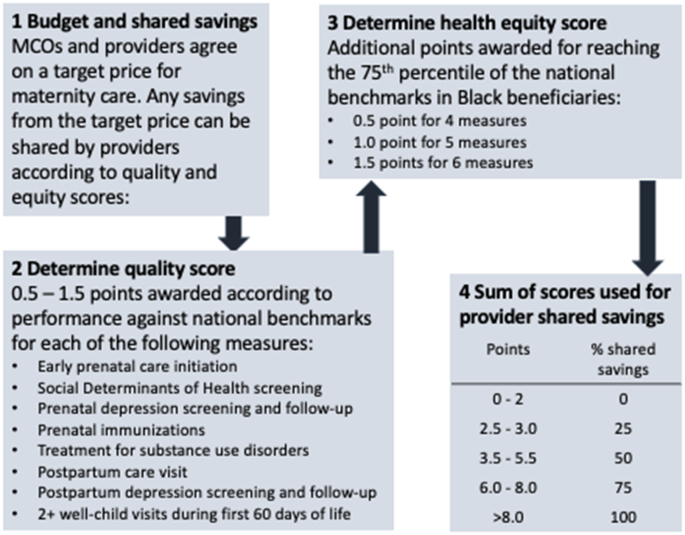
Pennsylvania Medicaid’s equity-focused maternity bundled payment model will allow for shared savings between obstetric care providers and managed care organizations, allowing for extra shared savings among providers whose Black patients experience better outcomes
Qualitative data Collection
To understand the interventions and responses to these policies, as well as related implementation barriers and facilitators, we will conduct interviews with each at least two representatives from each MCO ( n = 18). We will partner with colleagues from the Department of Human Services (DHS) to identify relevant MCO representatives. Interviews will elucidate MCOs’ perspectives, processes used by MCOs to design their interventions (e.g., review of existing evidence, input from community members or providers who serve them), anticipated effects, and sustainability of these payment policy changes. The goal is for some of the results of these interviews to inform our understanding of the implementation process which will be further explored in the interviews and focus groups with clinicians and Medicaid recipients.
In collaboration with the Community Health Advocates (CHA) program led by Healthy Start Pittsburgh, as well as other community and organizational partners across the state, we will recruit current and former Medicaid beneficiaries for focus group participation. We aim to recruit ∼ 50 community participants and will purposively oversample Black participants and will aim to recruit people of all ethnicities who identify as Black and multi-racial in order to achieve our aims of elucidating the experiences of Black parenting and pregnant people in Medicaid. Inclusion criteria are: current pregnancy or pregnant within the past 2 years; current or former enrollment in Pennsylvania Medicaid and/or Healthy Start; and ability to complete the interview in English.
Finally, we will partner with colleagues from DHS to identify pediatric and obstetric health professionals for interviews regarding the maternity bundled payment program and key outcomes related to the equity incentive payment. We will also use Medicaid administrative data to identify providers who serve Black beneficiaries and invite them to participate. We will aim to interview at least 20 obstetric and pediatric healthcare professionals to elucidate their perspectives on how structural racism in medicine affects patient outcomes, and the types of Medicaid policy changes that should be implemented.
We developed separate focus group/interview guides for community members, MCO leaders, and healthcare professionals. Each interview guide consists of open-ended questions to elucidate participants’ experiences with Medicaid; desired policy changes in Medicaid (among beneficiary participants); perceived steps that would be useful to combat anti-Black racism in healthcare and social services (especially among Black participants); and perspectives about the new Medicaid policies. Additionally, the interview guides ask demographic questions regarding gender identity, race, and ethnicity. We will first pilot-test the guide with our research partners and Healthy Start CHAs for clarity of question wording. All interviews will take place in-person in a private office space, or over the phone or videoconference, according to participants’ preferences and COVID-19 protocols. The interviewer will describe study objectives to each participant, obtain consent, and each interview will be audio-recorded and the interviewer will take notes throughout. Interview audio recordings will be transcribed verbatim, and transcripts spot-checked against the audio recordings for accuracy. The audio recording files will then be deleted to protect confidentiality of participants.
Qualitative data analysis
Study data will be analyzed and reported using the Consolidated Criteria for Reporting Qualitative Research (COREQ) Framework [ 31 ]. To analyze data, we will use template analysis, which combines features of deductive content analysis and inductive grounded theory, thereby allowing us to obtain specific information while also capturing any new or unanticipated themes [ 32 ]. Two coders will separately code the first 3 interview transcripts, meet to compare codes, discuss inconsistency in coding approaches, and then alter or add codes. This iterative process will be repeated until the coding scheme is fully developed. The coders will independently code all transcripts, and any coding discrepancies will be resolved via discussion. Once coding is complete, synthesis of content will begin by organizing codes under broader domains (meta-codes) as well as sub-codes. The primary analysis will be to convey qualitative data, including the use of illustrative quotes.
Quantitative healthcare data and analysis
Administrative healthcare data from the Pennsylvania Medicaid program, with linked birthing person-child dyads, will be used to create our quantitative analytic data. Medicaid data include a census of enrollment, hospital, outpatient/professional, pharmaceutical, and provider data for all beneficiaries in the Pennsylvania Medicaid program. Importantly, data contain self-reported race and ethnicity that is provided at the time of Medicaid enrollment (< 2% missing); as well as time-varying data on 9-digit ZIP code of residence. Data also include the amounts paid from Medicaid MCOs to healthcare providers for all medical services. Table 1 shows baseline data from Pennsylvania Medicaid-enrolled persons with a livebirth delivery in 2019, overall and stratified by race of the birthing person. We will also conduct sensitivity analyses to examine dyads that are multi-racial.
We will employ a comparative interrupted time series (ITS) analyses with a nonequivalent comparison group to estimate policy effects. Specifically, we will evaluate: (1) the extent to which the equity incentive payment program improved timely prenatal care and well-child visits among Black beneficiaries, relative to those of other races; and (2) the extent to which healthcare provider participation in the equity-focused maternity bundled payment model improved healthcare and health outcomes among Black beneficiaries, relative to those of other races.
For Aim 1, outcomes include binary measures of initiating prenatal care in the first trimester, and children receiving at least 6 well-child visits in the first six months of life. We will compare outcomes among Black beneficiaries relative to those of other racial groups, post- relative to pre- implementation of the equity incentive payment program. For Aim 2, outcomes include a composite of prenatal care quality measures (social determinants of health screening, prenatal and postpartum depression screening and follow-up, immunization, screening and treatment for substance use disorders, postpartum visit attendance), gestational age and birthweight, and severe maternal morbidity [ 33 ]. For both aims, multivariable regression models will control for maternal age, ethnicity, parity, ZIP code of residence, MCO plan enrollment, Medicaid eligibility category (expansion, pregnancy, disability, or others), and indices of obstetric and pediatric clinical comorbidities [ 34 , 35 ].
Sensitivity analyses
Analyses are designed to estimate early effects of the policies and should be interpreted alongside the qualitative results regarding policy implementation and beneficiary experiences. One assumption of ITS analyses is that our comparison groups approximate a counterfactual scenario for the intervention groups [ 36 , 37 , 38 ]. Although trends in Black-White inequities in pregnancy and child outcomes have, unfortunately, persisted over time [ 39 ], the COVID-19 pandemic has differentially burdened Black and Latina/x people relative to other race and ethnic groups [ 40 , 41 ]. Effects of the pandemic on pregnancy outcomes are only just beginning of what is to be explored [ 42 ]. It is therefore possible that we will not be able to disentangle policy effects from effects of COVID-19. To address this limitation, we will employ area-level rates of COVID-19 infection as control variables and for Aim 1 (equity incentive payment) we will conduct a sub-analysis investigating trends in 2021 vs. 2020. We chose to evaluate outcomes for Aim 2 (maternity care bundled payment) only in 2021, comparing the statistical intervention of race*provider. Finally, our qualitative analyses will provide context on differential impacts of COVID-19, which will inform interpretation of the quantitative results.
This study was approved by the University of Pittsburgh Institutional Review Board (Study # 23090108).
This mixed-methods research will investigate the extent to which changes in the Pennsylvania Medicaid program are associated with improvements in access to medical care and health outcomes among Black pregnant and birthing persons and their children. Our past research found that Black childbearing people in Pennsylvania Medicaid consistently experienced worse healthcare and health outcomes, compared to those of other racial and ethnic groups [ 43 , 44 ]. Racism in healthcare and other systems manifests in systematically worse access to and quality of care and other services for Black childbearing people [ 8 ]. In addition to suboptimal healthcare experiences, historical policies and practices such as residential redlining and segregation have resulted in lower wealth attainment in Black communities resulting in inequities in neighborhood factors and perinatal health [ 45 , 46 , 47 ].
The policies under study involve modifying common Medicaid reimbursement arrangements– namely, pay-for-performance programs and maternity care bundled payments. The policies are modified to embed financial incentives for Medicaid health plans and healthcare providers to improve the quality of care and health outcomes for Black pregnant and parenting persons and their children. These are the first such payment policies, to our knowledge, that explicitly aim to promote racial health equity with an explicit focus on addressing inequities that affect Black and Indigenous populations in Pennsylvania.
Interest from policymakers in payment reforms to improve health equity has increased recently; however, information on the implementation and effects of such models is sparse [ 48 , 49 ]. Although several state Medicaid programs have adopted maternity care bundled payment models, prior models have not considered racial inequities in pregnancy outcomes [ 30 , 50 ]. In 2012, Oregon adopted regional health equity coalitions as part of the state Medicaid program’s transition to Coordinated Care Organizations (CCOs). CCOs were required and given support to develop strategies that would address racial health disparities within the Medicaid population, and the regional health equity coalitions included underrepresented stakeholders to guide CCOs in the development of these interventions. While CCOs did reduce Black-white differences in primary care utilization and access to care within 3 years of policy implementation, it did not impact disparities in emergency department utilization [ 51 ]. The current research project will add to the extant evidence on how Medicaid programs can use policy to promote racial health equity.
Our study is limited in investigating the direct effects of the pandemic on racial inequities in perinatal and infant health and the intersections between the effects of the pandemic and the effects of the aforementioned Medicaid policies. However, we will have the ability to look at changes in outcomes over time. Additionally, these payment reform interventions focus largely on transforming the financing and delivery of healthcare, drawing attention to the structural and social determinants of health in the healthcare system. It is estimated that medical care contributes 10–20% to health outcomes; health and well-being are also shaped by factors such as environmental and socioeconomic conditions [ 52 ].
This study will contribute to the current body of knowledge about the recent interventions in Medicaid focused on racial equity. Specifically, findings will shed light on how the equity-focused obstetric care policies are being implemented and provide an evaluation of effects on health outcomes. These results can be used for future adaptions of the policy interventions or to inform the adoption of such equity-focused policies in different geographic regions of the United States.
Data availability
No datasets were generated or analysed during the current protocol study.
Abbreviations
Managed Care Organization
Community Health Advocate
Coordinated Care Organization
Alkema L, Chou D, Hogan D, Zhang S, Moller AB, Gemmill A, et al. Global, regional, and national levels and trends in maternal mortality between 1990 and 2015, with scenario-based projections to 2030: a systematic analysis by the UN Maternal Mortality Estimation Inter-agency Group. Lancet. 2016;387(10017):462–74.
Article PubMed Google Scholar
Wang E, Glazer KB, Howell EA, Janevic TM. Social determinants of pregnancy-related mortality and morbidity in the United States: a systematic review. Obstet Gynecol. 2020;135(4):896–915.
Article PubMed PubMed Central Google Scholar
Hoyert D. Maternal mortality rates in the United States, 2020. NCHS Health E-Stats; 2022.
Fingar K, Hambrick MM, Heslin KC, Moore JE. Trends and Disparities in Delivery Hospitalizations Involving Severe Maternal Morbidity, 2006–2015. Rockville, MD. 2018.
Thomas SB, Quinn SC, Butler J, Fryer CS, Garza MA. Toward a fourth generation of disparities research to achieve health equity. Annu Rev Public Health. 2011;32:399–416.
Crear-Perry J, Correa-de-Araujo R, Lewis Johnson T, McLemore MR, Neilson E, Wallace M. Social and Structural Determinants of Health Inequities in maternal health. J Womens Health (Larchmt). 2021;30(2):230–5.
Jones CP. Levels of racism: a theoretic framework and a gardener’s tale. Am J Public Health. 2000;90(8):1212–5.
Article CAS PubMed PubMed Central Google Scholar
Prather C, Fuller TR, Jeffries WLt, Marshall KJ, Howell AV, Belyue-Umole A, et al. Racism, African American Women, and their sexual and Reproductive Health: a review of historical and Contemporary Evidence and Implications for Health Equity. Health Equity. 2018;2(1):249–59.
Markus AR, Andres E, West KD, Garro N, Pellegrini C. Medicaid covered births, 2008 through 2010, in the context of the implementation of health reform. Womens Health Issues. 2013;23(5):e273–80.
Headen IE, Elovitz MA, Battarbee AN, Lo JO, Debbink MP. Racism and perinatal health inequities research: where we have been and where we should go. Am J Obstet Gynecol. 2022.
Michener JSM, Thurston C. From the Margins to the Center: A Bottom- Up Approach to Welfare State Scholarship. Perspectives on Politics. 2020:1–16.
Braveman PAE, Orleans T, Proctor D, Plough A. What is health equity? And what difference does a definition make? Princeton, NJ: Robert Wood Johnson Foundation; 2017.
Google Scholar
Thiel de Bocanegra H, Braughton M, Bradsberry M, Howell M, Logan J, Schwarz EB. Racial and ethnic disparities in postpartum care and contraception in California’s Medicaid program. Am J Obstet Gynecol. 2017;217(1):47. e1- e7.
Article Google Scholar
Gao YA, Drake C, Krans EE, Chen Q, Jarlenski MP. Explaining racial-ethnic disparities in the receipt of medication for opioid use disorder during pregnancy. J Addict Med. 2022.
Parekh N, Jarlenski M, Kelley D. Prenatal and Postpartum Care Disparities in a large Medicaid Program. Matern Child Health J. 2018;22(3):429–37.
Gavin NI, Adams EK, Hartmann KE, Benedict MB, Chireau M. Racial and ethnic disparities in the use of pregnancy-related health care among Medicaid pregnant women. Matern Child Health J. 2004;8(3):113–26.
Aseltine RH Jr., Yan J, Fleischman S, Katz M, DeFrancesco M. Racial and ethnic disparities in Hospital readmissions after Delivery. Obstet Gynecol. 2015;126(5):1040–7.
Wagner JL, White RS, Tangel V, Gupta S, Pick JS. Socioeconomic, racial, and ethnic disparities in Postpartum readmissions in patients with Preeclampsia: a multi-state analysis, 2007–2014. J Racial Ethn Health Disparities. 2019;6(4):806–20.
Kaiser Family Foundation. Total Monthly Medicaid/CHIP Enrollment and Pre-ACA Enrollment: Kaiser Family Foundation; 2022 [ https://www.kff.org/health-reform/state-indicator/total-monthly-medicaid-and-chip-enrollment/?currentTimeframe=0&sortModel=%7B%22colId%22:%22Location%22,%22sort%22:%22asc%22%7D .
Kaiser Family Foundation, Total Medicaid Spending. FY 2020 2022 [ https://www.kff.org/medicaid/state-indicator/total-medicaid-spending/?currentTimeframe=0&sortModel=%7B%22colId%22:%22Location%22,%22sort%22:%22asc%22%7D .
Pennsylvania Department of Health. Pregnancy-Associated Deaths in Pennsylvania, 2013–2018. Harrisburg, PA. 2020 December 2020.
Geronimus AT, Hicken MT, Pearson JA, Seashols SJ, Brown KL, Cruz TD. Do US Black women experience stress-related Accelerated Biological Aging? A Novel Theory and First Population-based test of black-white differences in telomere length. Hum Nat. 2010;21(1):19–38.
Marmot M. Health in an unequal world. Lancet. 2006;368(9552):2081–94.
Ekeke P, Mendez DD, Yanowitz TD, Catov JM. Racial differences in the biochemical effects of stress in pregnancy. Int J Environ Res Public Health. 2020;17:19.
Ford CL, Airhihenbuwa CO. Critical race theory, race equity, and public health: toward antiracism praxis. Am J Public Health. 2010;100(Suppl 1):S30–5.
Bailey ZD, Krieger N, Agenor M, Graves J, Linos N, Bassett MT. Structural racism and health inequities in the USA: evidence and interventions. Lancet. 2017;389(10077):1453–63.
Schneider AIH. Social Construction of Target populations: implications for politics and policy. Am Polit Sci Rev. 1993;87(2):334–47.
Michener J. Fragmented democracy: medicaid, federalism, and unequal politics. Cambridge, United Kingdom; New York, NY, USA: Cambridge University Press; 2018. xii, 226 pages p.
Ford CL, Airhihenbuwa CO. The public health critical race methodology: praxis for antiracism research. Soc Sci Med. 2010;71(8):1390–8.
Carroll C, Chernew M, Fendrick AM, Thompson J, Rose S. Effects of episode-based payment on health care spending and utilization: evidence from perinatal care in Arkansas. J Health Econ. 2018;61:47–62.
Tong A, Sainsbury P, Craig J. Consolidated criteria for reporting qualitative research (COREQ): a 32-item checklist for interviews and focus groups. Int J Qual Health Care. 2007;19(6):349–57.
Hsieh HF, Shannon SE. Three approaches to qualitative content analysis. Qual Health Res. 2005;15(9):1277–88.
Centers for Disease Control and Prevention. Severe Maternal Morbidity in the United States 2021 [ http://www.cdc.gov/reproductivehealth/maternalinfanthealth/severematernalmorbidity.html .
Bateman BT, Mhyre JM, Hernandez-Diaz S, Huybrechts KF, Fischer MA, Creanga AA, et al. Development of a comorbidity index for use in obstetric patients. Obstet Gynecol. 2013;122(5):957–65.
Feudtner C, Feinstein JA, Zhong W, Hall M, Dai D. Pediatric complex chronic conditions classification system version 2: updated for ICD-10 and complex medical technology dependence and transplantation. BMC Pediatr. 2014;14:199.
Ashenfelter OCD. Using the longitudinal structure of earnings to estimate the effect of training programs. Rev Econ Stat. 1985;67:648–60.
Donald SGLK. Inference with difference-in-differences and other panel data. Rev Econ Stat. 2007;89(2):221–33.
Imbens GWJ. Recent developments in the econometrics of program evaluation. J Econ Lit. 2009;47(1):5–86.
Goldfarb SS, Houser K, Wells BA, Brown Speights JS, Beitsch L, Rust G. Pockets of progress amidst persistent racial disparities in low birthweight rates. PLoS ONE. 2018;13(7):e0201658.
Prasannan L, Rochelson B, Shan W, Nicholson K, Solmonovich R, Kulkarni A et al. Social Determinants of Health and Coronavirus Disease 2019 in pregnancy: condensation: social determinants of health, including neighborhood characteristics such as household income and educational attainment, are associated with SARS-CoV-2 infection and severity of COVID-19 in pregnancy. Am J Obstet Gynecol MFM. 2021:100349.
Flores LE, Frontera WR, Andrasik MP, Del Rio C, Mondriguez-Gonzalez A, Price SA, et al. Assessment of the Inclusion of Racial/Ethnic Minority, female, and older individuals in Vaccine clinical trials. JAMA Netw Open. 2021;4(2):e2037640.
Janevic T, Glazer KB, Vieira L, Weber E, Stone J, Stern T, et al. Racial/Ethnic disparities in very Preterm Birth and Preterm Birth before and during the COVID-19 pandemic. JAMA Netw Open. 2021;4(3):e211816.
Krans EE, Kim JY, James AE 3rd, Kelley D, Jarlenski MP. Medication-assisted treatment use among pregnant women with opioid Use Disorder. Obstet Gynecol. 2019;133(5):943–51.
Parekh NJM, Kelley D. Disparities in access to care and emergency department utilization in a large Medicaid program. J Health Dispar Res Pract. 2018;11(4):1–13.
Assibey-Mensah V, Fabio A, Mendez DD, Lee PC, Roberts JM, Catov JM. Neighbourhood assets and early pregnancy cardiometabolic risk factors. Paediatr Perinat Epidemiol. 2019;33(1):79–87.
Mendez DD, Hogan VK, Culhane JF. Institutional racism, neighborhood factors, stress, and preterm birth. Ethn Health. 2014;19(5):479–99.
Mendez DD, Hogan VK, Culhane J. Institutional racism and pregnancy health: using Home Mortgage Disclosure act data to develop an index for mortgage discrimination at the community level. Public Health Rep. 2011;126(Suppl 3):102–14.
Huffstetler AN, Phillips RL. Jr. Payment structures that support Social Care Integration with Clinical Care: Social Deprivation indices and Novel Payment models. Am J Prev Med. 2019;57(6 Suppl 1):S82–8.
Anderson AC, O’Rourke E, Chin MH, Ponce NA, Bernheim SM, Burstin H. Promoting Health Equity and eliminating disparities through performance measurement and payment. Health Aff (Millwood). 2018;37(3):371–7.
Jarlenski M, Borrero S, La Charite T, Zite NB. Episode-based payment for Perinatal Care in Medicaid: implications for practice and policy. Obstet Gynecol. 2016;127(6):1080–4.
McConnell KJ, Charlesworth CJ, Meath THA, George RM, Kim H. Oregon’s emphasis on Equity Shows Signs of Early Success for Black and American Indian Medicaid Enrollees. Health Aff (Millwood). 2018;37(3):386–93.
Hood CM, Gennuso KP, Swain GR, Catlin BB. County Health rankings: relationships between determinant factors and Health outcomes. Am J Prev Med. 2016;50(2):129–35.
Download references
This study received funding from the National Institute of Nursing Research under award R01NR020670. The funder had no role in the study design, data collection or analysis, or decision to publish the study.
Author information
Authors and affiliations.
Department of Health Policy and Management, University of Pittsburgh School of Public Health, 130 DeSoto St, A619, 15261, Pittsburgh, PA, USA
Marian Jarlenski, Evan Cole & Christine McClure
Department of Epidemiology, University of Pittsburgh School of Public Health, Pittsburgh, PA, USA
Sarah Sanders, Marquita Smalls & Dara D Méndez
You can also search for this author in PubMed Google Scholar
Contributions
Jarlenski: Conceptualization; funding acquisition; investigation; methodology; supervision; writing-original draftCole: Conceptualization; data curation; investigation; resources; writing-reviewing and editingMcClure: Investigation; project administration; supervision; writing-reviewing and editingSanders: Investigation; methodology; visualization; writing-reviewing and editingSmalls: Investigation; project administration; visualization; writing-reviewing and editingMendez: Conceptualization; funding acquisition; investigation; validation; supervision; writing-original draft.
Corresponding author
Correspondence to Marian Jarlenski .
Ethics declarations
Ethical approval, competing interests.
The authors declare no competing interests.
Additional information
Publisher’s note.
Springer Nature remains neutral with regard to jurisdictional claims in published maps and institutional affiliations.
Rights and permissions
Open Access This article is licensed under a Creative Commons Attribution 4.0 International License, which permits use, sharing, adaptation, distribution and reproduction in any medium or format, as long as you give appropriate credit to the original author(s) and the source, provide a link to the Creative Commons licence, and indicate if changes were made. The images or other third party material in this article are included in the article’s Creative Commons licence, unless indicated otherwise in a credit line to the material. If material is not included in the article’s Creative Commons licence and your intended use is not permitted by statutory regulation or exceeds the permitted use, you will need to obtain permission directly from the copyright holder. To view a copy of this licence, visit http://creativecommons.org/licenses/by/4.0/ . The Creative Commons Public Domain Dedication waiver ( http://creativecommons.org/publicdomain/zero/1.0/ ) applies to the data made available in this article, unless otherwise stated in a credit line to the data.
Reprints and permissions
About this article
Cite this article.
Jarlenski, M., Cole, E., McClure, C. et al. Implementation and early effects of medicaid policy interventions to promote racial equity in pregnancy and early childhood outcomes in Pennsylvania: protocol for a mixed methods study. BMC Health Serv Res 24 , 498 (2024). https://doi.org/10.1186/s12913-024-10982-5
Download citation
Received : 12 December 2023
Accepted : 10 April 2024
Published : 22 April 2024
DOI : https://doi.org/10.1186/s12913-024-10982-5
Share this article
Anyone you share the following link with will be able to read this content:
Sorry, a shareable link is not currently available for this article.
Provided by the Springer Nature SharedIt content-sharing initiative
- Well-child visits
- Prenatal care
- Health policy
- Health equity
- Mixed methods
BMC Health Services Research
ISSN: 1472-6963
- General enquiries: [email protected]
Time-to-Event Genome-Wide Association Study for Incident Cardiovascular Disease in People With Type 2 Diabetes

- Split-Screen
- Article contents
- Figures & tables
- Supplementary Data
- Peer Review
- Open the PDF for in another window
- Cite Icon Cite
- Get Permissions
Soo Heon Kwak , Ryan B. Hernandez-Cancela , Daniel A. DiCorpo , David E. Condon , Jordi Merino , Peitao Wu , Jennifer A. Brody , Jie Yao , Xiuqing Guo , Fariba Ahmadizar , Mariah Meyer , Murat Sincan , Josep M. Mercader , Sujin Lee , Jeffrey Haessler , Ha My T. Vy , Zhaotong Lin , Nicole D. Armstrong , Shaopeng Gu , Noah L. Tsao , Leslie A. Lange , Ningyuan Wang , Kerri L. Wiggins , Stella Trompet , Simin Liu , Ruth J.F. Loos , Renae Judy , Philip H. Schroeder , Natalie R. Hasbani , Maxime M. Bos , Alanna C. Morrison , Rebecca D. Jackson , Alexander P. Reiner , JoAnn E. Manson , Ninad S. Chaudhary , Lynn K. Carmichael , Yii-Der Ida Chen , Kent D. Taylor , Mohsen Ghanbari , Joyce van Meurs , Achilleas N. Pitsillides , Bruce M. Psaty , Raymond Noordam , Ron Do , Kyong Soo Park , J. Wouter Jukema , Maryam Kavousi , Adolfo Correa , Stephen S. Rich , Scott M. Damrauer , Catherine Hajek , Nam H. Cho , Marguerite R. Irvin , James S. Pankow , Girish N. Nadkarni , Robert Sladek , Mark O. Goodarzi , Jose C. Florez , Daniel I. Chasman , Susan R. Heckbert , Charles Kooperberg , Josée Dupuis , Rajeev Malhotra , Paul S. de Vries , Ching-Ti Liu , Jerome I. Rotter , James B. Meigs , Cohorts for Heart and Aging Research in Genomic Epidemiology (CHARGE) Consortium; Time-to-Event Genome-Wide Association Study for Incident Cardiovascular Disease in People With Type 2 Diabetes. Diabetes Care 2024; dc232274. https://doi.org/10.2337/dc23-2274
Download citation file:
- Ris (Zotero)
- Reference Manager
To identify genetic risk factors for incident cardiovascular disease (CVD) among people with type 2 diabetes (T2D).
We conducted a multiancestry time-to-event genome-wide association study for incident CVD among people with T2D. We also tested 204 known coronary artery disease (CAD) variants for association with incident CVD.
Among 49,230 participants with T2D, 8,956 had incident CVD events (event rate 18.2%). We identified three novel genetic loci for incident CVD: rs147138607 (near CACNA1E/ZNF648 , hazard ratio [HR] 1.23, P = 3.6 × 10 −9 ), rs77142250 (near HS3ST1 , HR 1.89, P = 9.9 × 10 −9 ), and rs335407 (near TFB1M/NOX3 , HR 1.25, P = 1.5 × 10 −8 ). Among 204 known CAD loci, 5 were associated with incident CVD in T2D (multiple comparison–adjusted P < 0.00024, 0.05/204). A standardized polygenic score of these 204 variants was associated with incident CVD with HR 1.14 ( P = 1.0 × 10 −16 ).
The data point to novel and known genomic regions associated with incident CVD among individuals with T2D.
Graphical Abstract
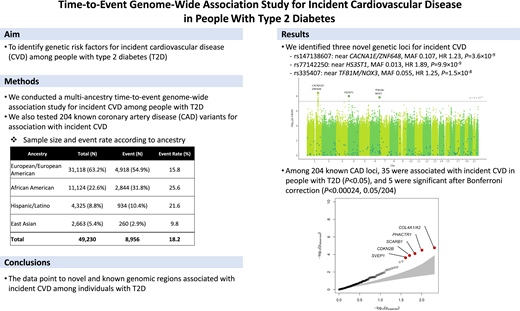
This article contains supplementary material online at https://doi.org/10.2337/figshare.25444327 .
Email alerts
- Online ISSN 1935-5548
- Print ISSN 0149-5992
- Diabetes Care
- Clinical Diabetes
- Diabetes Spectrum
- Standards of Medical Care in Diabetes
- Scientific Sessions Abstracts
- BMJ Open Diabetes Research & Care
- ShopDiabetes.org
- ADA Professional Books
Clinical Compendia
- Clinical Compendia Home
- Latest News
- DiabetesPro SmartBrief
- Special Collections
- DiabetesPro®
- Diabetes Food Hub™
- Insulin Affordability
- Know Diabetes By Heart™
- About the ADA
- Journal Policies
- For Reviewers
- Advertising in ADA Journals
- Reprints and Permission for Reuse
- Copyright Notice/Public Access Policy
- ADA Professional Membership
- ADA Member Directory
- Diabetes.org
- X (Twitter)
- Cookie Policy
- Accessibility
- Terms & Conditions
- Get Adobe Acrobat Reader
- © Copyright American Diabetes Association
This Feature Is Available To Subscribers Only
Sign In or Create an Account
Log in using your username and password
- Search More Search for this keyword Advanced search
- Latest content
- Current issue
- Hosted content
- BMJ Journals More You are viewing from: Google Indexer
You are here
- Volume 73, Issue 5
- Glucagon-like peptide-1 receptor agonists and risk of major adverse liver outcomes in patients with chronic liver disease and type 2 diabetes
- Article Text
- Article info
- Citation Tools
- Rapid Responses
- Article metrics

- http://orcid.org/0000-0003-3634-6591 Axel Wester 1 ,
- http://orcid.org/0000-0002-1496-1799 Ying Shang 1 ,
- Emilie Toresson Grip 1 , 2 ,
- Anthony A Matthews 3 ,
- http://orcid.org/0000-0002-8474-1759 Hannes Hagström 1 , 4
- 1 Department of Medicine Huddinge , Karolinska Institutet , Stockholm , Sweden
- 2 Quantify Research , Stockholm , Sweden
- 3 Unit of Epidemiology, Institute of Environmental Medicine , Karolinska Institutet , Stockholm , Sweden
- 4 Division of Hepatology, Department of Upper GI , Karolinska University Hospital , Stockholm , Sweden
- Correspondence to Dr Axel Wester, Department of Medicine Huddinge, Karolinska Institutet, Stockholm, Sweden; axel.wester{at}ki.se
Objective Phase II trials suggest glucagon-like peptide-1 receptor (GLP1) agonists resolve metabolic dysfunction-associated steatohepatitis but do not affect fibrosis regression. We aimed to determine the long-term causal effect of GLP1 agonists on the risk of major adverse liver outcomes (MALO) in patients with any chronic liver disease and type 2 diabetes.
Design We used observational data from Swedish healthcare registers 2010–2020 to emulate a target trial of GLP1 agonists in eligible patients with chronic liver disease and type 2 diabetes. We used an inverse-probability weighted marginal structural model to compare parametric estimates of 10-year MALO risk (decompensated cirrhosis, hepatocellular carcinoma, liver transplantation or MALO-related death) in initiators of GLP1 agonists with non-initiators. We randomly sampled 5% of the non-initiators to increase computational efficiency.
Results GLP1 agonist initiators had a 10-year risk of MALO at 13.3% (42/1026) vs 14.6% in non-initiators (1079/15 633) in intention-to-treat analysis (risk ratio (RR)=0.91, 95% CI=0.50 to 1.32). The corresponding 10-year per-protocol risk estimates were 7.4% (22/1026) and 14.4% (1079/15 633), respectively (RR=0.51, 95% CI=0.14 to 0.88). The per-protocol risk estimates at 6 years were 5.4% (21/1026) vs 9.0% (933/15 633) (RR=0.60, 95% CI=0.29 to 0.90) and at 8 years 7.2% (22/1026) vs 11.7% (1036/15 633) (RR=0.61, 95% CI=0.21 to 1.01).
Conclusion In patients with chronic liver disease and type 2 diabetes who adhered to therapy over time, GLP1 agonists may result in lower risk of MALO. This suggests that GLP1 agonists are promising agents to reduce risk of chronic liver disease progression in patients with concurrent type 2 diabetes, although this needs to be corroborated in randomised trials.
- PHARMACOTHERAPY
- FATTY LIVER
- EPIDEMIOLOGY
- DIABETES MELLITUS
Data availability statement
No data are available. No data are available due to Swedish regulations.
This is an open access article distributed in accordance with the Creative Commons Attribution Non Commercial (CC BY-NC 4.0) license, which permits others to distribute, remix, adapt, build upon this work non-commercially, and license their derivative works on different terms, provided the original work is properly cited, appropriate credit is given, any changes made indicated, and the use is non-commercial. See: http://creativecommons.org/licenses/by-nc/4.0/ .
https://doi.org/10.1136/gutjnl-2023-330962
Statistics from Altmetric.com
Request permissions.
If you wish to reuse any or all of this article please use the link below which will take you to the Copyright Clearance Center’s RightsLink service. You will be able to get a quick price and instant permission to reuse the content in many different ways.
Disclaimer: this video summarises a scientific article published by BMJ Publishing Group Limited (BMJ). The content of this video has not been peer-reviewed and does not constitute medical advice. Any opinions expressed are solely those of the contributors. Viewers should be aware that professionals in the field may have different opinions. BMJ does not endorse any opinions expressed or recommendations discussed. Viewers should not use the content of the video as the basis for any medical treatment. BMJ disclaims all liability and responsibility arising from any reliance placed on the content.
WHAT IS ALREADY KNOWN ON THIS TOPIC
Glucagon-like peptide-1 receptor (GLP1) agonists might resolve metabolic dysfunction-associated steatohepatitis, but their effect on hard clinical outcomes in patients with chronic liver diseases of any aetiology and concurrent type 2 diabetes is unknown.
WHAT THIS STUDY ADDS
Using Swedish register data, we emulated a target trial of GLP1 agonists in patients with chronic liver disease and type 2 diabetes and fitted an inverse-probability weighted marginal structural model to estimate 10-year risks of major adverse liver outcomes (MALO). The risk of MALO was 49% lower in initiators of GLP1 agonists in the per-protocol analysis, but our data were not compatible with a protective intention-to-treat effect.
HOW THIS STUDY MIGHT AFFECT RESEARCH, PRACTICE OR POLICY
GLP1 agonists might be a treatment option to reduce MALO risk in patients with type 2 diabetes and any chronic liver disease who adhere to the treatment over time, although this would need to be corroborated by randomised clinical trials.
Introduction
Chronic liver diseases are highly prevalent and can progress to decompensated cirrhosis, hepatocellular carcinoma (HCC) and liver-related death. 1–5 Type 2 diabetes strongly predicts presence and severity of metabolic dysfunction-associated steatotic liver disease (MASLD) and is also a major risk factor for disease progression in other liver diseases, likely due to interaction of hepatic steatosis and steatohepatitis with other liver diseases. 6–9
Currently, no approved pharmacotherapy exists for MASLD, but one promising drug class is glucagon-like peptide-1 receptor (GLP1) agonists, which are currently approved in patients with type 2 diabetes or obesity to achieve weight loss and control blood glucose. 10–12 Importantly, phase II trials indicate that GLP1 agonists resolve metabolic dysfunction-associated steatohepatitis (MASH) in patients with non-cirrhotic MASLD but do not cause fibrosis regression. 13 14 Large phase III trials that aim to estimate the effect of GLP1 agonists on resolving MASH or reducing hepatic fibrosis are, however, many years from completion. 15
Although achieving surrogate histopathological endpoints (eg, fibrosis regression) is considered by regulatory agencies to likely translate to improved prognosis, robust evidence is needed to understand if GLP1 agonists reduce the risk of long-term clinical outcomes, such as liver decompensation or HCC. 16 17 Given that the metabolic syndrome is a major driver of liver-related outcomes both in patients with MASLD and other chronic liver diseases such as alcohol-related liver disease or viral hepatitis C, there could be a similar effect of GLP1 agonists in patients with chronic liver diseases of any aetiology with concomitant metabolic traits, such as type 2 diabetes. 18–20 For instance, insulin resistance is the strongest predictor of liver fibrosis in patients with alcohol-related liver disease. 21 Therefore, we designed a target trial that would estimate the long-term causal effect of GLP1 agonists on major adverse liver outcomes (MALO) in patients with any chronic liver disease and type 2 diabetes, and then emulated it using observational data from Swedish healthcare registers.
Data sources
The Decoding the Epidemiology of LIVER disease in Sweden (DELIVER) cohort includes data from Swedish national healthcare registers on all patients with any chronic liver disease in Sweden 1964–2020. 22 The data include all International Classification of Diseases (ICD) codes from inpatient and specialised outpatient care, dates and causes of death, and automatically recorded information on filled prescriptions from any pharmacy in Sweden. 23–29 The positive predictive value is 96% for MASLD with comorbid type 2 diabetes, and >90% for most diagnoses related to cirrhosis. 30 31 A detailed overview of the registers is provided in online supplemental methods .
Supplemental material
Target trial specification and emulation.
A causal question is best answered by data from randomised trials, but when unavailable, researchers often resort to observational data from existing databases. To avoid common methodological pitfalls in observational studies, causal inference from such data can be viewed as an attempt to emulate a hypothetical pragmatic randomised trial—a target trial. 32 An overview of the target trial emulation concept is provided in reference. 33 After specifying the target trial protocol, it is emulated using the available observational data and appropriate methodology. For this observational study, we first specified the protocol of a target trial that would estimate the effect of GLP1 agonists on MALO risk in patients with chronic liver disease and type 2 diabetes. We then emulated the target trial using data from DELIVER. Table 1 summarises all protocol components from the target trial and its emulation, which we describe in detail below. All diagnoses and medications were defined by the ICD or Anatomical Therapeutic Chemical (ATC) codes in online supplemental tables 1–3 . Diagnoses were identified from January 1997 and forward (when ICD-10 was introduced in Sweden), and drugs from July 2005 and forward (when the Swedish Prescribed Drug Register was initiated).
- View inline
Protocol of a target trial and an emulated trial using observational data
Eligibility criteria
All Swedish residents ≥18 years of age between January 2010, when uptake of GLP1 agonists increased in Sweden, and November 2020 with any chronic liver disease and type 2 diabetes were identified. 22 To avoid structural positivity issues (ie, patients with zero probability of initiating a GLP1 agonist at baseline), patients were required to have filled at least one prescription of metformin within a year before baseline corresponding to a daily dose of ≥1 g (ie, patients potentially eligible for second-line treatment with a GLP1 agonist). Patients were excluded if they previously filled prescriptions of GLP1 agonists, had a history of a contraindication to GLP1 agonists (defined as pancreatitis, inflammatory bowel disease or severe chronic kidney disease) or prior MALO (defined below). As the non-initiator population was large, we randomly sampled 5% of this group to increase computational efficiency ( figure 1 ).
- Download figure
- Open in new tab
- Download powerpoint
Flow chart of the study population. Numbers represent study participants (initiators or non-initiators), while numbers in parentheses represent the corresponding number of unique patients. Note that the numbers of excluded study participants represent the total number of times that unique patients were non-eligible for any of the emulated target trials. If somebody was non-eligible for all the 131 emulated target trials, they would contribute with an addition of 131 to this total number.
Treatment strategies
We compared two treatment strategies: initiation of any GLP1 agonist (ATC code A10BJ) at baseline and continuation of treatment over follow-up unless a contraindication was diagnosed after baseline; and no initiation of a GLP1 agonist at baseline and continuation of no GLP1 agonist treatment during follow-up, unless indicated as deemed by the treating physician. Since we lacked data to specifically determine who were indicated for GLP1 agonists, we assumed that it was indicated in all non-initiators who started the drug during follow-up. We assessed drug continuation by summing the number of months of filled prescriptions. A gap between two successive prescriptions was allowed if it was less than twice the time intended for the most recently filled prescription. For example, an initiator who filled a prescription for 3 months treatment was considered to have stopped the treatment after 6 months, unless the prescription was refilled before that.
Treatment assignment
Patients were classified into two groups according to the strategy their data were compatible with at baseline, that is, GLP1 agonist initiators and non-initiators. We assumed groups were exchangeable at baseline conditional on baseline covariates (similar probability of initiating the drug in both arms, within levels of the covariates): age, sex, education (<10, 10–12 and >12 years), diabetes duration, liver disease aetiology, compensated cirrhosis, and a range of comorbidities and medications: obesity, cardiovascular disease, microvascular complications to diabetes, chronic obstructive pulmonary disease, alcohol use disorder, mental health disorder, the use of antidiabetic medications except metformin or GLP1 agonists and direct-acting antivirals in patients with viral hepatitis. As the relationship between age and the probability of initiating a GLP1 agonist might not be linear, we modelled age using linear, quadratic and cubic terms. If patients had coding for more than one liver disease aetiology, they were classified according to a predefined hierarchy ( online supplemental methods ).
The outcome of interest was the first MALO during follow-up, defined as decompensated cirrhosis (variceal bleeding, ascites, portal hypertension or hepatorenal syndrome), HCC, liver transplantation or MALO-related death. MALO was defined by ICD codes in the National Patient Register (main or secondary diagnosis), the Cancer Register or the Cause of Death Register (main or contributing cause) ( online supplemental table 3 ). These outcomes have been validated and found to have positive predictive values >90% ( online supplemental table 4 ). 31
Everyone was followed from baseline to the earliest of MALO, emigration from Sweden, 10 years of follow-up or December 2020. The follow-up was measured in calendar months.
Causal contrasts
We estimated observational analogues of the intention-to-treat and per-protocol effects.
Statistical analyses
We sequentially emulated the target trial as a series of separate target trials starting in each 131 calendar months between January 2010 and November 2020, meaning that patients could enter multiple target trials if eligible. To avoid immortal time bias, the baseline is best defined as the time when eligible patients initiate a treatment strategy. 32 The GLP agonist initiators naturally have one such time point. The definition of the baseline is, however, more challenging in the non-initiators since the same individual can be eligible at multiple times. One solution that avoids immortal time bias is to emulate a target trial that uses all those eligibility times as the baseline and consider each individual at each of those times as different individuals. 34 For example, a patient who fulfilled all eligibility criteria in our study in January 2010 and did not initiate a GLP1 agonist in that month would enter the target trial that started in January 2010 as a non-initiator. If still eligible in February 2010, this patient would also enter the target trial that started in this month as a non-initiator. If the same patient initiated a GLP1 agonist in March 2010 and still fulfilled all eligibility criteria, the patient would enter this target trial as an initiator and be non-eligible for all subsequent target trials as previous use of GLP1 agonists was an exclusion criterion. Target trials with only initiators or only non-initiators were excluded. Allowing repeated eligibility is statistically more efficient than choosing only 1 month as baseline and accounts for the fact that patients can be eligible in several different months during the study period. 35 36
We fitted a marginal structural model using parametric pooled logistic regression with an indicator for treatment group, a flexible time-varying intercept (linear and quadratic terms), product terms between treatment group and time, and a target trial indicator to pool data for all the emulated trials and estimate intention-to-treat and per-protocol effects. For estimation of the intention-to-treat effect, we weighted the model using inverse-probability of treatment weights (IPTW). The IPTW models included all baseline covariates, and the weights were stabilised. The balance between treatment groups was assessed using standardised mean differences (SMD) and inspection of kernel density plots. An SMD<0.1 is generally regarded to indicate good balance. 37 For estimation of the per-protocol effect, the same marginal structural model as above was used, but patients were additionally censored when deviating from their assigned treatment strategy and stabilised inverse-probability of censoring weights (IPCWs) were applied to adjust for baseline and time-varying covariates associated with adherence. 34 38 The marginal structural model was weighted using the product of the IPTWs and the IPCWs. The IPCW models included age, sex, education, diabetes duration and liver disease aetiology at baseline, and the following time-varying covariates: compensated cirrhosis and the same range of comorbidities and medications as described above. If patients stopped GLP1 agonist treatment because of a contraindication during follow-up (eg, an episode of pancreatitis), or started GLP1 agonist treatment, their censoring weights remained constant from that date forward. The weights are described in online supplemental table 5 .
The average 10-year absolute risks under each strategy were estimated using the predicted values from the marginal structural models, then resulting risk differences (RDs) and risk ratios (RRs) were calculated. Non-parametric bootstrapping with 500 replications was used to estimate 95% CIs.
We examined the intention-to-treat effect in subgroups according to liver disease aetiology (MASLD, other than MASLD), and liver disease severity at baseline (compensated cirrhosis, no cirrhosis). Several sensitivity analyses were done to assess the robustness of our results. First, we updated the eligibility criteria to include those with a lower daily dose of metformin (0.5 g instead of 1 g). Second, the time gap between two successive prescriptions was restricted to ≤30 days. Third, we censored non-initiators if they initiated a GLP1 agonist during follow-up, regardless of whether it was indicated, and IPCWs were applied as described above. Fourth, the inverse-probability weights were truncated at the 1st and 99th percentile before being applied to the marginal structural model, to avoid the impact of extreme values on the risk estimates. Fifth, we used standardisation to adjust for confounding at baseline, rather than IPTW. 39 In this analysis, the IPCWs were used in the per-protocol analysis as described above. Sixth, we estimated intention-to-treat and per-protocol point estimates including all non-initiators (without sampling 5%), to assess whether the sampling affected our risk estimates. Finally, to estimate how strongly an unmeasured confounder would need to be associated with both the exposure and outcome to fully explain any differences in risk between treatment groups, we calculated the E-value. 40
We additionally calculated the intention-to-treat and per-protocol effects at 2, 4, 6 and 8 years of follow-up. Because 2 years is a more plausible duration of a future randomised trial of GLP1 agonists in patients with chronic liver disease than 10 years, we computed the minimum sample size required in a clinical trial of GLP1 agonists to demonstrate an effect of equal strength as our estimated 2-year RR, using a 5% alpha and 80% power.
Analyses were done in Stata V.17.0 (StataCorp).
Patient and public involvement
Patients or the public were not involved in the design, conduct, reporting or dissemination plans of this research.
We included 1026 initiators of GLP1 agonists and 15 633 non-initiators, who participated in up to 123 target trials. The patient characteristics at baseline before and after weighting are summarised in table 2 . After IPTW, all baseline characteristics were well balanced (SMD<0.1). Kernel density plots also indicated good balance ( online supplemental figure 1 ). Of the initiators, 635 (61.9%) started treatment with liraglutide, 231 (22.5%) semaglutide, 120 (11.7%) dulaglutide, 25 (2.4%) exenatide and 15 (1.5%) lixisenatide at baseline.
Patient characteristics at baseline before and after inverse-probability of treatment weighting
In the intention-to-treat analysis, initiators and non-initiators were followed for a median (p25–p75) of 64 (36–96) and 76 months (50–100), respectively. 350 of 605 (57.9%) initiators still at risk at 2 years were continuous users at this time. The corresponding numbers at 4, 6, 8 and 10 years were 143 of 331 (43.2%), 64 of 188 (34.0%), 24 of 98 (24.5%) and 5 of 19 (26.3%), respectively. In the per-protocol analysis, where the patients were censored if they deviated from their assigned treatment strategy, the median follow-up was 43 (21–75) and 76 months (50–100), respectively. After a median of 14 months (8–26) follow-up, 517 (50.4%) initiators stopped the treatment; 21 (2.1%) initiators stopped the treatment after developing a contraindication, and 496 (48.3%) stopped the treatment without one of the prespecified contraindications. Of the 1026 initiators, 361 (35.2%) were censored in the per-protocol analysis because they stopped the treatment with a prespecified contraindication the first 2 years, another 92 (9.0%) between 2 and 4 years, 28 (2.7%) between 4 and 6 years, 11 (1.1%) between 6 and 8 years, and 4 (0.4%) between 8 and 10 years. Among the non-initiators, 2357 (15.1%) started treatment with a GLP1 agonist after a median follow-up of 31 months (14–52).
In the intention-to-treat analysis, MALO occurred in 42 initiators and 1079 non-initiators. The events in non-initiators corresponded to 486 distinct events (since participation in multiple target trials was allowed, some contributed with events to more than one target trial). The 10-year risk of MALO was 13.3% (95% CI=7.4% to 19.2%) in the initiators and 14.6% (95% CI=13.1% to 16.1%) in the non-initiators (RD=−1.3, 95% CI=−7.2 to 4.6, RR=0.91, 95% CI=0.50 to 1.32) ( table 3 , figure 2A ).
Inverse-probability weighted risk curves of major adverse liver outcomes comparing initiators of glucagon-like peptide-1 receptor (GLP1) agonists with non-initiators. (A) intention-to-treat effect, (B) per-protocol effect.
Risk of major adverse liver outcomes according to intention-to-treat and per-protocol analyses
In per-protocol analysis, we observed 22 events of MALO in the initiators and 1079 in the non-initiators. The 10-year MALO risk was 7.4% (95% CI=2.1% to 12.6%) in the initiators and 14.4% (95% CI=12.9% to 15.9%) in the non-initiators (RD=−7.1, 95% CI=−12.5 to –1.6; RR=0.51, 95% CI=0.14 to 0.88) ( table 3 , figure 2B ). This corresponds to a number needed to treat (initiate and continue with the GLP1 agonist treatment strategy) to avoid one event of MALO over the course of 10 years of 14 (95% CI=8 to 63).
Results from the intention-to-treat subgroup analyses are summarised in table 4 . In patients with MASLD, the 10-year risk of MALO was 15.8% in the initiators and 11.2% in the non-initiators (RR=1.41, 95% CI=0.53 to 2.30). The 10-year risk in patients with compensated cirrhosis was 36.5% and 34.6% in initiators and non-initiators, respectively (RR=1.05, 95% CI=0.20 to 1.91).
Risk of major adverse liver outcomes at 10 years according to intention-to-treat analyses in subgroups
The risk estimates from a sensitivity analysis that allowed patients to have a lower dose of metformin at baseline were similar to the main analyses (intention-to-treat RR=0.94, 95% CI=0.58 to 1.30; per-protocol RR=0.63, 95% CI=0.27 to 0.99) ( online supplemental table 6 ). The per-protocol estimates were also similar when restricting the gap between two successive prescriptions to ≤30 days (RR=0.48, 95% CI=0.11 to 0.85) ( online supplemental table 7 ). In addition, similar per-protocol estimates were found when the non-initiators were censored if they initiated a GLP1 agonist during follow-up (RR=0.55, 95% CI=0.18 to 0.92) ( online supplemental table 8 ). Both the intention-to-treat and per-protocol estimates were similar to the main analyses when the weights were truncated (intention-to-treat RR=0.84, 95% CI=0.48 to 1.20; per-protocol RR=0.55, 95% CI=0.16 to 0.93) ( online supplemental table 9 ). Moreover, results were similar to the main analysis when using standardisation to adjust for confounders at baseline (intention-to-treat RR=0.87, 95% CI=0.53 to 1.22; per-protocol RR=0.58, 95% CI=0.19 to 0.97) ( online supplemental table 10 ). Point estimates were similar to the main analysis when including all non-initiators (n=312 661) (intention-to-treat RR=1.01, per-protocol RR=0.53) ( online supplemental table 11 ). The E-value for the RRs in the main per-protocol analysis was 3.33 for the point estimate and 1.53 for the 95% CI, suggesting how strong an unmeasured confounder needs to be to fully explain the estimated per-protocol effect and shift the 95% CI to include the null.
Risk estimates for the intention-to-treat and per-protocol analyses at 2, 4, 6 and 8 years are presented in table 3 . At 2 years follow-up, a more plausible duration of a clinical trial than 10 years, the risk of MALO was 2.3% in the initiators and 3.2% in the non-initiators (overall event probability of 2.7%) when analysed by the intention-to-treat principle (RD=−0.9, 95% CI=−2.2 to 0.4; RR=0.72, 95% CI=0.31 to 1.13). A clinical trial of GLP1 agonists on the risk of MALO with 2 years of follow-up would need enrolment of at least 10 776 patients per arm to provide evidence for such effect.
We emulated a nationwide target trial to answer the question whether GLP1 agonists can prevent development of MALO in patients with chronic liver diseases and type 2 diabetes. The main finding was that the 10-year risk of MALO was 49% lower in patients who initiated and adhered to GLP1 agonists compared with non-initiators in the per-protocol analysis, but the estimates from the intention-to-treat analysis were imprecise with a 95% CI for the RR ranging from 0.50 to 1.32.
A placebo-controlled randomised phase II trial of 320 patients with MASH reported that the proportion achieving MASH resolution without worsening fibrosis after 72 weeks was more than tripled in the arm receiving 0.4 mg of the GLP1 agonist semaglutide compared with placebo (59% vs 17%). 14 We followed patients with any chronic liver disease and type 2 diabetes for up to 10 years and found a similarly strong effect of GLP1 agonists on the risk of MALO in per-protocol analysis, but an imprecise intention-to-treat effect. However, MALO risk was similar in the initiator arm when restricting the analysis to patients with MASLD, although this should be interpreted cautiously as we noted wide CIs for these estimates. The phase II trial only included patients with fibrosis stages F2–F3 in the primary outcome analysis, whereas we included patients of any fibrosis stage including compensated cirrhosis. 14 Our study did not provide support for a protective effect of GLP1 agonists in the subgroup with compensated cirrhosis, which is in line with a recent phase II trial in patients with MASH-related compensated cirrhosis. 41
Based on the observed probability of MALO, our data indicate that a clinical trial of GLP1 agonists in patients with any chronic liver disease and type 2 diabetes using 2-year MALO risk as the outcome would demand an immense number of patients, at least 10 776 patients per arm. This can be contrasted to the ongoing phase III trial of semaglutide looking at both histopathological endpoints (after 1.5 years) and MALO (after 4.5 years) that is planning to recruit 1200 patients with MASH (identifier NCT04822181 ). The emulation of a target trial is an appealing option to give timely answers to key research questions when data from large clinical trials are currently unavailable. 32 The difference between our intention-to-treat and per-protocol estimates is that many initiators stopped treatment without one of our prespecified contraindications. Patients who ended their treatment might have done so for good reasons, for example, severe gastrointestinal symptoms, but we were unable to capture this in our data. Moreover, since we lacked data to specifically determine whether GLP1 agonists were indicated for the non-initiators during follow-up, we assumed that it was indicated for all non-initiators that started treatment. Therefore, none of the non-initiators were censored for not adhering to protocol, whereas many initiators stopped treatment and were then censored in the per-protocol analysis. Large observational studies including detailed data with relevance for the choice of pharmacological treatment in type 2 diabetes, such as glycated haemoglobin, are warranted.
The main strength of our study was the use of an emulated target trial design to overcome common biases in observational analyses, including immortal time bias. 34 In fact, bias in observational studies often arise predominantly due to poor study design, rather than confounding due to lack of randomisation, and estimates from carefully emulated target trials closely resemble those from randomised trials. 36 There were two reasons why we designed a target trial that compared GLP1 agonists to non-initiators rather than an active comparator, another common approach. 42–44 First, it would have asked a different research question, that is, whether MALO risk differs from the active comparator. An ideal active comparator has an identical indication as the drug of interest and no effect on the outcome, but the effect of other antidiabetic medications on MALO risk is mostly unknown. Second, the average treatment effect in the whole study population is not identifiable when comparing to an active comparator. 45 We additionally fitted an inverse-probability weighted marginal structural model to account for possible time-varying confounders associated with adherence when estimating the per-protocol effect. Whereas the intention-to-treat effect is the most used causal contrast in clinical trials, the per-protocol analysis indicates what the effect of a treatment strategy would be if adhered to, which is of great importance in the real-world when physicians and patients decide on an appropriate treatment strategy. 38 When there is feedback between time-varying confounders and treatments, then standard regression models (eg, Cox) will produce biased estimates. 39 The family of g methods has been developed for this purpose (including inverse-probability weighted marginal structural models and standardisation). 39 In addition, we used the validated population-based Swedish national healthcare registers to minimise selection bias and estimate the long-term effect of GLP1 agonists. 23–25 29–31
Some limitations should be acknowledged. Despite a median follow-up of 5–6 years, few initiators experienced MALO, yielding estimates with low precision and preventing estimation of per-protocol effects in subgroups. The low number of events in initiators during late follow-up (only one event in the last 4 years and none in the last 2 years) could possibly explain part of the per-protocol effect, however, the RRs were stable across time from year 6 and forward. Additionally, we lacked data on some important covariates. First, we had no data on fibrosis stage, beyond classifying patients as cirrhotic (F4) or non-cirrhotic (F0–F3). Trials in MASLD are usually confined to patients with F2–F3, or cirrhosis, but we likely included some patients with F0–F1 where MALO is unlikely to occur. Second, we had no laboratory data, such as glycated haemoglobin, to assess diabetes severity and need for escalating to second-line treatment with GLP1 agonists. Diabetes duration, microvascular complications and other antidiabetic medications were, however, used as proxies for diabetes severity. The E-value suggested that an unmeasured confounder would need to increase the probability of both initiating a GLP1 agonist and experiencing MALO more than threefold to fully explain the estimated per-protocol effect. 40 This suggests that the observed RR in the per-protocol analysis might be explained by residual confounding (eg, fibrosis stage). For example, a study of patients with biopsy-proven MASLD found that patients with F3 had a fourfold higher hazard of MALO than patients with F0. 17 However, to fully explain the estimated effect, an unmeasured confounder would also need to be three times more likely to occur in either group. The presence of compensated cirrhosis was balanced between groups and other parameters associated with fibrosis such as age and cardiovascular disease were also well balanced and thus suggests that large differences in fibrosis are unlikely. Additionally, we sampled 5% of non-initiators to increase computational efficiency. Point estimates were, however, similar when including all non-initiators.
In conclusion, the risk of MALO in patients with chronic liver diseases and type 2 diabetes was lower if they initiated a GLP1 agonist and adhered to this treatment over time. The data were, however, not compatible with a protective intention-to-treat effect. Randomised trials using MALO as an outcome might be unfeasible, motivating further large observational studies using appropriate methodology to further delineate the effect of GLP1 agonists on the risk of MALO, complementing future phase III trials of GLP1 agonists.
Ethics statements
Patient consent for publication.
Not applicable.
Ethics approval
This study involves human participants and the Regional Ethics Board of Stockholm approved the study and waived informed consent (protocol number 2017/1019-31/1).
Acknowledgments
AW was supported by the Syskonen Svensson foundation for medical research (2021-00284), Mag-tarmfonden, the Bengt Ihre foundation (SLS-973809), Professor Nanna Svartz foundation (2022-00448) and the Stockholm County Council (FoUI-985859). AAM was supported by the Strategic Research Program in Epidemiology at Karolinska Institutet, Forte (2020-00029), and the Swedish Research Council (2021-02236). HH was supported by the Stockholm County Council (FoUI-960537), the Swedish Research Council (2021-01293) and the Swedish Cancer Society (22210 Pj01H).
- Collaborators GBDC
- Charette JH , et al
- Younossi ZM ,
- de Avila L , et al
- Koenig AB ,
- Abdelatif D , et al
- Anstee QM ,
- Arias-Loste MT , et al
- Tesfai K , et al
- Björkström K ,
- Franzén S ,
- Eliasson B , et al
- Elkrief L ,
- Rautou PE ,
- Sarin S , et al
- Rao Kondapally Seshasai S ,
- Kaptoge S ,
- Thompson A , et al
- Wilding JPH ,
- Batterham RL ,
- Calanna S , et al
- Andreadis P ,
- Karagiannis T ,
- Malandris K , et al
- Konerman MA ,
- Harrison SA
- Armstrong MJ ,
- Aithal GP , et al
- Newsome PN ,
- Buchholtz K ,
- Cusi K , et al
- Dufour J-F ,
- Bugianesi E , et al
- Patel J , et al
- Hagström H ,
- Ekstedt M , et al
- Pirola CJ , et al
- Israelsen M ,
- Detlefsen S , et al
- Wester A , et al
- Westergren K ,
- Holmberg L , et al
- Ludvigsson JF ,
- Almqvist C ,
- Bonamy A-KE , et al
- Andersson E ,
- Ekbom A , et al
- Otterblad-Olausson P ,
- Pettersson BU , et al
- Svedberg P ,
- Olén O , et al
- Wettermark B ,
- Fored CM , et al
- Brooke HL ,
- Talbäck M ,
- Hörnblad J , et al
- Bengtsson B ,
- Askling J ,
- Ludvigsson JF , et al
- Hernán MA ,
- Matthews AA ,
- Islam N , et al
- Hernández-Díaz S , et al
- García-Albéniz X ,
- Dickerman BA ,
- Logan RW , et al
- VanderWeele TJ ,
- Abdelmalek MF ,
- Armstrong MJ , et al
- Abrahami D ,
- McDonald EG ,
- Schnitzer ME , et al
- Huitfeldt A ,
- Hernan MA ,
- Kalager M , et al
Supplementary materials
Supplementary data.
This web only file has been produced by the BMJ Publishing Group from an electronic file supplied by the author(s) and has not been edited for content.
- Data supplement 1
AAM and HH are joint senior authors.
X @wester_axel, @YingShang1, @hanneshagstrom
Correction notice This article has been corrected since it published Online First. The senior author statement has been added.
Contributors Contributor and guarantor information:
Study conception and design: All
Acquisition of data: HH
Statistical analysis: AW and AAM.
Analysis and interpretation of data: All
Drafting of manuscript: AW.
Critical revision: All
Guarantors of article: AW and HH.
Funding AW was supported by the Syskonen Svensson foundation for medical research (2021-00284), Mag-tarmfonden, the Bengt Ihre foundation (SLS-973809), Professor Nanna Svartz foundation (2022-00448), and the Stockholm County Council (FoUI-985859). AAM was supported by the Strategic Research Program in Epidemiology at Karolinska Institutet, Forte (2020-00029), and the Swedish Research Council (2021-02236). HH was supported by the Stockholm County Council (FoUI-960537), the Swedish Research Council (2021-01293), and the Swedish Cancer Society (2 2210 Pj 01 H). The funders had no role in the conduct of this study.
Disclaimer The funders had no role in the conduct of this study.
Competing interests HH: institution has received research grants from Astra Zeneca, EchoSens, Gilead, Intercept, MSD, Novo Nordisk and Pfizer, all outside the current study. The other authors declare no conflicts.
Patient and public involvement Patients and/or the public were not involved in the design, or conduct, or reporting, or dissemination plans of this research.
Provenance and peer review Not commissioned; externally peer reviewed.
Supplemental material This content has been supplied by the author(s). It has not been vetted by BMJ Publishing Group Limited (BMJ) and may not have been peer-reviewed. Any opinions or recommendations discussed are solely those of the author(s) and are not endorsed by BMJ. BMJ disclaims all liability and responsibility arising from any reliance placed on the content. Where the content includes any translated material, BMJ does not warrant the accuracy and reliability of the translations (including but not limited to local regulations, clinical guidelines, terminology, drug names and drug dosages), and is not responsible for any error and/or omissions arising from translation and adaptation or otherwise.
Linked Articles
- Commentary Glucagon-like peptide-1 receptor agonists to treat chronic liver disease: real-world evidence or ambiguity? Samy Suissa Ruben Hernaez Gut 2024; 73 721-724 Published Online First: 18 Mar 2024. doi: 10.1136/gutjnl-2024-331976
Read the full text or download the PDF:

An official website of the United States government
The .gov means it’s official. Federal government websites often end in .gov or .mil. Before sharing sensitive information, make sure you’re on a federal government site.
The site is secure. The https:// ensures that you are connecting to the official website and that any information you provide is encrypted and transmitted securely.
- Publications
- Account settings
Preview improvements coming to the PMC website in October 2024. Learn More or Try it out now .
- Advanced Search
- Journal List
- J Korean Med Sci
- v.37(16); 2022 Apr 25

A Practical Guide to Writing Quantitative and Qualitative Research Questions and Hypotheses in Scholarly Articles
Edward barroga.
1 Department of General Education, Graduate School of Nursing Science, St. Luke’s International University, Tokyo, Japan.
Glafera Janet Matanguihan
2 Department of Biological Sciences, Messiah University, Mechanicsburg, PA, USA.
The development of research questions and the subsequent hypotheses are prerequisites to defining the main research purpose and specific objectives of a study. Consequently, these objectives determine the study design and research outcome. The development of research questions is a process based on knowledge of current trends, cutting-edge studies, and technological advances in the research field. Excellent research questions are focused and require a comprehensive literature search and in-depth understanding of the problem being investigated. Initially, research questions may be written as descriptive questions which could be developed into inferential questions. These questions must be specific and concise to provide a clear foundation for developing hypotheses. Hypotheses are more formal predictions about the research outcomes. These specify the possible results that may or may not be expected regarding the relationship between groups. Thus, research questions and hypotheses clarify the main purpose and specific objectives of the study, which in turn dictate the design of the study, its direction, and outcome. Studies developed from good research questions and hypotheses will have trustworthy outcomes with wide-ranging social and health implications.
INTRODUCTION
Scientific research is usually initiated by posing evidenced-based research questions which are then explicitly restated as hypotheses. 1 , 2 The hypotheses provide directions to guide the study, solutions, explanations, and expected results. 3 , 4 Both research questions and hypotheses are essentially formulated based on conventional theories and real-world processes, which allow the inception of novel studies and the ethical testing of ideas. 5 , 6
It is crucial to have knowledge of both quantitative and qualitative research 2 as both types of research involve writing research questions and hypotheses. 7 However, these crucial elements of research are sometimes overlooked; if not overlooked, then framed without the forethought and meticulous attention it needs. Planning and careful consideration are needed when developing quantitative or qualitative research, particularly when conceptualizing research questions and hypotheses. 4
There is a continuing need to support researchers in the creation of innovative research questions and hypotheses, as well as for journal articles that carefully review these elements. 1 When research questions and hypotheses are not carefully thought of, unethical studies and poor outcomes usually ensue. Carefully formulated research questions and hypotheses define well-founded objectives, which in turn determine the appropriate design, course, and outcome of the study. This article then aims to discuss in detail the various aspects of crafting research questions and hypotheses, with the goal of guiding researchers as they develop their own. Examples from the authors and peer-reviewed scientific articles in the healthcare field are provided to illustrate key points.
DEFINITIONS AND RELATIONSHIP OF RESEARCH QUESTIONS AND HYPOTHESES
A research question is what a study aims to answer after data analysis and interpretation. The answer is written in length in the discussion section of the paper. Thus, the research question gives a preview of the different parts and variables of the study meant to address the problem posed in the research question. 1 An excellent research question clarifies the research writing while facilitating understanding of the research topic, objective, scope, and limitations of the study. 5
On the other hand, a research hypothesis is an educated statement of an expected outcome. This statement is based on background research and current knowledge. 8 , 9 The research hypothesis makes a specific prediction about a new phenomenon 10 or a formal statement on the expected relationship between an independent variable and a dependent variable. 3 , 11 It provides a tentative answer to the research question to be tested or explored. 4
Hypotheses employ reasoning to predict a theory-based outcome. 10 These can also be developed from theories by focusing on components of theories that have not yet been observed. 10 The validity of hypotheses is often based on the testability of the prediction made in a reproducible experiment. 8
Conversely, hypotheses can also be rephrased as research questions. Several hypotheses based on existing theories and knowledge may be needed to answer a research question. Developing ethical research questions and hypotheses creates a research design that has logical relationships among variables. These relationships serve as a solid foundation for the conduct of the study. 4 , 11 Haphazardly constructed research questions can result in poorly formulated hypotheses and improper study designs, leading to unreliable results. Thus, the formulations of relevant research questions and verifiable hypotheses are crucial when beginning research. 12
CHARACTERISTICS OF GOOD RESEARCH QUESTIONS AND HYPOTHESES
Excellent research questions are specific and focused. These integrate collective data and observations to confirm or refute the subsequent hypotheses. Well-constructed hypotheses are based on previous reports and verify the research context. These are realistic, in-depth, sufficiently complex, and reproducible. More importantly, these hypotheses can be addressed and tested. 13
There are several characteristics of well-developed hypotheses. Good hypotheses are 1) empirically testable 7 , 10 , 11 , 13 ; 2) backed by preliminary evidence 9 ; 3) testable by ethical research 7 , 9 ; 4) based on original ideas 9 ; 5) have evidenced-based logical reasoning 10 ; and 6) can be predicted. 11 Good hypotheses can infer ethical and positive implications, indicating the presence of a relationship or effect relevant to the research theme. 7 , 11 These are initially developed from a general theory and branch into specific hypotheses by deductive reasoning. In the absence of a theory to base the hypotheses, inductive reasoning based on specific observations or findings form more general hypotheses. 10
TYPES OF RESEARCH QUESTIONS AND HYPOTHESES
Research questions and hypotheses are developed according to the type of research, which can be broadly classified into quantitative and qualitative research. We provide a summary of the types of research questions and hypotheses under quantitative and qualitative research categories in Table 1 .
Research questions in quantitative research
In quantitative research, research questions inquire about the relationships among variables being investigated and are usually framed at the start of the study. These are precise and typically linked to the subject population, dependent and independent variables, and research design. 1 Research questions may also attempt to describe the behavior of a population in relation to one or more variables, or describe the characteristics of variables to be measured ( descriptive research questions ). 1 , 5 , 14 These questions may also aim to discover differences between groups within the context of an outcome variable ( comparative research questions ), 1 , 5 , 14 or elucidate trends and interactions among variables ( relationship research questions ). 1 , 5 We provide examples of descriptive, comparative, and relationship research questions in quantitative research in Table 2 .
Hypotheses in quantitative research
In quantitative research, hypotheses predict the expected relationships among variables. 15 Relationships among variables that can be predicted include 1) between a single dependent variable and a single independent variable ( simple hypothesis ) or 2) between two or more independent and dependent variables ( complex hypothesis ). 4 , 11 Hypotheses may also specify the expected direction to be followed and imply an intellectual commitment to a particular outcome ( directional hypothesis ) 4 . On the other hand, hypotheses may not predict the exact direction and are used in the absence of a theory, or when findings contradict previous studies ( non-directional hypothesis ). 4 In addition, hypotheses can 1) define interdependency between variables ( associative hypothesis ), 4 2) propose an effect on the dependent variable from manipulation of the independent variable ( causal hypothesis ), 4 3) state a negative relationship between two variables ( null hypothesis ), 4 , 11 , 15 4) replace the working hypothesis if rejected ( alternative hypothesis ), 15 explain the relationship of phenomena to possibly generate a theory ( working hypothesis ), 11 5) involve quantifiable variables that can be tested statistically ( statistical hypothesis ), 11 6) or express a relationship whose interlinks can be verified logically ( logical hypothesis ). 11 We provide examples of simple, complex, directional, non-directional, associative, causal, null, alternative, working, statistical, and logical hypotheses in quantitative research, as well as the definition of quantitative hypothesis-testing research in Table 3 .
Research questions in qualitative research
Unlike research questions in quantitative research, research questions in qualitative research are usually continuously reviewed and reformulated. The central question and associated subquestions are stated more than the hypotheses. 15 The central question broadly explores a complex set of factors surrounding the central phenomenon, aiming to present the varied perspectives of participants. 15
There are varied goals for which qualitative research questions are developed. These questions can function in several ways, such as to 1) identify and describe existing conditions ( contextual research question s); 2) describe a phenomenon ( descriptive research questions ); 3) assess the effectiveness of existing methods, protocols, theories, or procedures ( evaluation research questions ); 4) examine a phenomenon or analyze the reasons or relationships between subjects or phenomena ( explanatory research questions ); or 5) focus on unknown aspects of a particular topic ( exploratory research questions ). 5 In addition, some qualitative research questions provide new ideas for the development of theories and actions ( generative research questions ) or advance specific ideologies of a position ( ideological research questions ). 1 Other qualitative research questions may build on a body of existing literature and become working guidelines ( ethnographic research questions ). Research questions may also be broadly stated without specific reference to the existing literature or a typology of questions ( phenomenological research questions ), may be directed towards generating a theory of some process ( grounded theory questions ), or may address a description of the case and the emerging themes ( qualitative case study questions ). 15 We provide examples of contextual, descriptive, evaluation, explanatory, exploratory, generative, ideological, ethnographic, phenomenological, grounded theory, and qualitative case study research questions in qualitative research in Table 4 , and the definition of qualitative hypothesis-generating research in Table 5 .
Qualitative studies usually pose at least one central research question and several subquestions starting with How or What . These research questions use exploratory verbs such as explore or describe . These also focus on one central phenomenon of interest, and may mention the participants and research site. 15
Hypotheses in qualitative research
Hypotheses in qualitative research are stated in the form of a clear statement concerning the problem to be investigated. Unlike in quantitative research where hypotheses are usually developed to be tested, qualitative research can lead to both hypothesis-testing and hypothesis-generating outcomes. 2 When studies require both quantitative and qualitative research questions, this suggests an integrative process between both research methods wherein a single mixed-methods research question can be developed. 1
FRAMEWORKS FOR DEVELOPING RESEARCH QUESTIONS AND HYPOTHESES
Research questions followed by hypotheses should be developed before the start of the study. 1 , 12 , 14 It is crucial to develop feasible research questions on a topic that is interesting to both the researcher and the scientific community. This can be achieved by a meticulous review of previous and current studies to establish a novel topic. Specific areas are subsequently focused on to generate ethical research questions. The relevance of the research questions is evaluated in terms of clarity of the resulting data, specificity of the methodology, objectivity of the outcome, depth of the research, and impact of the study. 1 , 5 These aspects constitute the FINER criteria (i.e., Feasible, Interesting, Novel, Ethical, and Relevant). 1 Clarity and effectiveness are achieved if research questions meet the FINER criteria. In addition to the FINER criteria, Ratan et al. described focus, complexity, novelty, feasibility, and measurability for evaluating the effectiveness of research questions. 14
The PICOT and PEO frameworks are also used when developing research questions. 1 The following elements are addressed in these frameworks, PICOT: P-population/patients/problem, I-intervention or indicator being studied, C-comparison group, O-outcome of interest, and T-timeframe of the study; PEO: P-population being studied, E-exposure to preexisting conditions, and O-outcome of interest. 1 Research questions are also considered good if these meet the “FINERMAPS” framework: Feasible, Interesting, Novel, Ethical, Relevant, Manageable, Appropriate, Potential value/publishable, and Systematic. 14
As we indicated earlier, research questions and hypotheses that are not carefully formulated result in unethical studies or poor outcomes. To illustrate this, we provide some examples of ambiguous research question and hypotheses that result in unclear and weak research objectives in quantitative research ( Table 6 ) 16 and qualitative research ( Table 7 ) 17 , and how to transform these ambiguous research question(s) and hypothesis(es) into clear and good statements.
a These statements were composed for comparison and illustrative purposes only.
b These statements are direct quotes from Higashihara and Horiuchi. 16
a This statement is a direct quote from Shimoda et al. 17
The other statements were composed for comparison and illustrative purposes only.
CONSTRUCTING RESEARCH QUESTIONS AND HYPOTHESES
To construct effective research questions and hypotheses, it is very important to 1) clarify the background and 2) identify the research problem at the outset of the research, within a specific timeframe. 9 Then, 3) review or conduct preliminary research to collect all available knowledge about the possible research questions by studying theories and previous studies. 18 Afterwards, 4) construct research questions to investigate the research problem. Identify variables to be accessed from the research questions 4 and make operational definitions of constructs from the research problem and questions. Thereafter, 5) construct specific deductive or inductive predictions in the form of hypotheses. 4 Finally, 6) state the study aims . This general flow for constructing effective research questions and hypotheses prior to conducting research is shown in Fig. 1 .

Research questions are used more frequently in qualitative research than objectives or hypotheses. 3 These questions seek to discover, understand, explore or describe experiences by asking “What” or “How.” The questions are open-ended to elicit a description rather than to relate variables or compare groups. The questions are continually reviewed, reformulated, and changed during the qualitative study. 3 Research questions are also used more frequently in survey projects than hypotheses in experiments in quantitative research to compare variables and their relationships.
Hypotheses are constructed based on the variables identified and as an if-then statement, following the template, ‘If a specific action is taken, then a certain outcome is expected.’ At this stage, some ideas regarding expectations from the research to be conducted must be drawn. 18 Then, the variables to be manipulated (independent) and influenced (dependent) are defined. 4 Thereafter, the hypothesis is stated and refined, and reproducible data tailored to the hypothesis are identified, collected, and analyzed. 4 The hypotheses must be testable and specific, 18 and should describe the variables and their relationships, the specific group being studied, and the predicted research outcome. 18 Hypotheses construction involves a testable proposition to be deduced from theory, and independent and dependent variables to be separated and measured separately. 3 Therefore, good hypotheses must be based on good research questions constructed at the start of a study or trial. 12
In summary, research questions are constructed after establishing the background of the study. Hypotheses are then developed based on the research questions. Thus, it is crucial to have excellent research questions to generate superior hypotheses. In turn, these would determine the research objectives and the design of the study, and ultimately, the outcome of the research. 12 Algorithms for building research questions and hypotheses are shown in Fig. 2 for quantitative research and in Fig. 3 for qualitative research.

EXAMPLES OF RESEARCH QUESTIONS FROM PUBLISHED ARTICLES
- EXAMPLE 1. Descriptive research question (quantitative research)
- - Presents research variables to be assessed (distinct phenotypes and subphenotypes)
- “BACKGROUND: Since COVID-19 was identified, its clinical and biological heterogeneity has been recognized. Identifying COVID-19 phenotypes might help guide basic, clinical, and translational research efforts.
- RESEARCH QUESTION: Does the clinical spectrum of patients with COVID-19 contain distinct phenotypes and subphenotypes? ” 19
- EXAMPLE 2. Relationship research question (quantitative research)
- - Shows interactions between dependent variable (static postural control) and independent variable (peripheral visual field loss)
- “Background: Integration of visual, vestibular, and proprioceptive sensations contributes to postural control. People with peripheral visual field loss have serious postural instability. However, the directional specificity of postural stability and sensory reweighting caused by gradual peripheral visual field loss remain unclear.
- Research question: What are the effects of peripheral visual field loss on static postural control ?” 20
- EXAMPLE 3. Comparative research question (quantitative research)
- - Clarifies the difference among groups with an outcome variable (patients enrolled in COMPERA with moderate PH or severe PH in COPD) and another group without the outcome variable (patients with idiopathic pulmonary arterial hypertension (IPAH))
- “BACKGROUND: Pulmonary hypertension (PH) in COPD is a poorly investigated clinical condition.
- RESEARCH QUESTION: Which factors determine the outcome of PH in COPD?
- STUDY DESIGN AND METHODS: We analyzed the characteristics and outcome of patients enrolled in the Comparative, Prospective Registry of Newly Initiated Therapies for Pulmonary Hypertension (COMPERA) with moderate or severe PH in COPD as defined during the 6th PH World Symposium who received medical therapy for PH and compared them with patients with idiopathic pulmonary arterial hypertension (IPAH) .” 21
- EXAMPLE 4. Exploratory research question (qualitative research)
- - Explores areas that have not been fully investigated (perspectives of families and children who receive care in clinic-based child obesity treatment) to have a deeper understanding of the research problem
- “Problem: Interventions for children with obesity lead to only modest improvements in BMI and long-term outcomes, and data are limited on the perspectives of families of children with obesity in clinic-based treatment. This scoping review seeks to answer the question: What is known about the perspectives of families and children who receive care in clinic-based child obesity treatment? This review aims to explore the scope of perspectives reported by families of children with obesity who have received individualized outpatient clinic-based obesity treatment.” 22
- EXAMPLE 5. Relationship research question (quantitative research)
- - Defines interactions between dependent variable (use of ankle strategies) and independent variable (changes in muscle tone)
- “Background: To maintain an upright standing posture against external disturbances, the human body mainly employs two types of postural control strategies: “ankle strategy” and “hip strategy.” While it has been reported that the magnitude of the disturbance alters the use of postural control strategies, it has not been elucidated how the level of muscle tone, one of the crucial parameters of bodily function, determines the use of each strategy. We have previously confirmed using forward dynamics simulations of human musculoskeletal models that an increased muscle tone promotes the use of ankle strategies. The objective of the present study was to experimentally evaluate a hypothesis: an increased muscle tone promotes the use of ankle strategies. Research question: Do changes in the muscle tone affect the use of ankle strategies ?” 23
EXAMPLES OF HYPOTHESES IN PUBLISHED ARTICLES
- EXAMPLE 1. Working hypothesis (quantitative research)
- - A hypothesis that is initially accepted for further research to produce a feasible theory
- “As fever may have benefit in shortening the duration of viral illness, it is plausible to hypothesize that the antipyretic efficacy of ibuprofen may be hindering the benefits of a fever response when taken during the early stages of COVID-19 illness .” 24
- “In conclusion, it is plausible to hypothesize that the antipyretic efficacy of ibuprofen may be hindering the benefits of a fever response . The difference in perceived safety of these agents in COVID-19 illness could be related to the more potent efficacy to reduce fever with ibuprofen compared to acetaminophen. Compelling data on the benefit of fever warrant further research and review to determine when to treat or withhold ibuprofen for early stage fever for COVID-19 and other related viral illnesses .” 24
- EXAMPLE 2. Exploratory hypothesis (qualitative research)
- - Explores particular areas deeper to clarify subjective experience and develop a formal hypothesis potentially testable in a future quantitative approach
- “We hypothesized that when thinking about a past experience of help-seeking, a self distancing prompt would cause increased help-seeking intentions and more favorable help-seeking outcome expectations .” 25
- “Conclusion
- Although a priori hypotheses were not supported, further research is warranted as results indicate the potential for using self-distancing approaches to increasing help-seeking among some people with depressive symptomatology.” 25
- EXAMPLE 3. Hypothesis-generating research to establish a framework for hypothesis testing (qualitative research)
- “We hypothesize that compassionate care is beneficial for patients (better outcomes), healthcare systems and payers (lower costs), and healthcare providers (lower burnout). ” 26
- Compassionomics is the branch of knowledge and scientific study of the effects of compassionate healthcare. Our main hypotheses are that compassionate healthcare is beneficial for (1) patients, by improving clinical outcomes, (2) healthcare systems and payers, by supporting financial sustainability, and (3) HCPs, by lowering burnout and promoting resilience and well-being. The purpose of this paper is to establish a scientific framework for testing the hypotheses above . If these hypotheses are confirmed through rigorous research, compassionomics will belong in the science of evidence-based medicine, with major implications for all healthcare domains.” 26
- EXAMPLE 4. Statistical hypothesis (quantitative research)
- - An assumption is made about the relationship among several population characteristics ( gender differences in sociodemographic and clinical characteristics of adults with ADHD ). Validity is tested by statistical experiment or analysis ( chi-square test, Students t-test, and logistic regression analysis)
- “Our research investigated gender differences in sociodemographic and clinical characteristics of adults with ADHD in a Japanese clinical sample. Due to unique Japanese cultural ideals and expectations of women's behavior that are in opposition to ADHD symptoms, we hypothesized that women with ADHD experience more difficulties and present more dysfunctions than men . We tested the following hypotheses: first, women with ADHD have more comorbidities than men with ADHD; second, women with ADHD experience more social hardships than men, such as having less full-time employment and being more likely to be divorced.” 27
- “Statistical Analysis
- ( text omitted ) Between-gender comparisons were made using the chi-squared test for categorical variables and Students t-test for continuous variables…( text omitted ). A logistic regression analysis was performed for employment status, marital status, and comorbidity to evaluate the independent effects of gender on these dependent variables.” 27
EXAMPLES OF HYPOTHESIS AS WRITTEN IN PUBLISHED ARTICLES IN RELATION TO OTHER PARTS
- EXAMPLE 1. Background, hypotheses, and aims are provided
- “Pregnant women need skilled care during pregnancy and childbirth, but that skilled care is often delayed in some countries …( text omitted ). The focused antenatal care (FANC) model of WHO recommends that nurses provide information or counseling to all pregnant women …( text omitted ). Job aids are visual support materials that provide the right kind of information using graphics and words in a simple and yet effective manner. When nurses are not highly trained or have many work details to attend to, these job aids can serve as a content reminder for the nurses and can be used for educating their patients (Jennings, Yebadokpo, Affo, & Agbogbe, 2010) ( text omitted ). Importantly, additional evidence is needed to confirm how job aids can further improve the quality of ANC counseling by health workers in maternal care …( text omitted )” 28
- “ This has led us to hypothesize that the quality of ANC counseling would be better if supported by job aids. Consequently, a better quality of ANC counseling is expected to produce higher levels of awareness concerning the danger signs of pregnancy and a more favorable impression of the caring behavior of nurses .” 28
- “This study aimed to examine the differences in the responses of pregnant women to a job aid-supported intervention during ANC visit in terms of 1) their understanding of the danger signs of pregnancy and 2) their impression of the caring behaviors of nurses to pregnant women in rural Tanzania.” 28
- EXAMPLE 2. Background, hypotheses, and aims are provided
- “We conducted a two-arm randomized controlled trial (RCT) to evaluate and compare changes in salivary cortisol and oxytocin levels of first-time pregnant women between experimental and control groups. The women in the experimental group touched and held an infant for 30 min (experimental intervention protocol), whereas those in the control group watched a DVD movie of an infant (control intervention protocol). The primary outcome was salivary cortisol level and the secondary outcome was salivary oxytocin level.” 29
- “ We hypothesize that at 30 min after touching and holding an infant, the salivary cortisol level will significantly decrease and the salivary oxytocin level will increase in the experimental group compared with the control group .” 29
- EXAMPLE 3. Background, aim, and hypothesis are provided
- “In countries where the maternal mortality ratio remains high, antenatal education to increase Birth Preparedness and Complication Readiness (BPCR) is considered one of the top priorities [1]. BPCR includes birth plans during the antenatal period, such as the birthplace, birth attendant, transportation, health facility for complications, expenses, and birth materials, as well as family coordination to achieve such birth plans. In Tanzania, although increasing, only about half of all pregnant women attend an antenatal clinic more than four times [4]. Moreover, the information provided during antenatal care (ANC) is insufficient. In the resource-poor settings, antenatal group education is a potential approach because of the limited time for individual counseling at antenatal clinics.” 30
- “This study aimed to evaluate an antenatal group education program among pregnant women and their families with respect to birth-preparedness and maternal and infant outcomes in rural villages of Tanzania.” 30
- “ The study hypothesis was if Tanzanian pregnant women and their families received a family-oriented antenatal group education, they would (1) have a higher level of BPCR, (2) attend antenatal clinic four or more times, (3) give birth in a health facility, (4) have less complications of women at birth, and (5) have less complications and deaths of infants than those who did not receive the education .” 30
Research questions and hypotheses are crucial components to any type of research, whether quantitative or qualitative. These questions should be developed at the very beginning of the study. Excellent research questions lead to superior hypotheses, which, like a compass, set the direction of research, and can often determine the successful conduct of the study. Many research studies have floundered because the development of research questions and subsequent hypotheses was not given the thought and meticulous attention needed. The development of research questions and hypotheses is an iterative process based on extensive knowledge of the literature and insightful grasp of the knowledge gap. Focused, concise, and specific research questions provide a strong foundation for constructing hypotheses which serve as formal predictions about the research outcomes. Research questions and hypotheses are crucial elements of research that should not be overlooked. They should be carefully thought of and constructed when planning research. This avoids unethical studies and poor outcomes by defining well-founded objectives that determine the design, course, and outcome of the study.
Disclosure: The authors have no potential conflicts of interest to disclose.
Author Contributions:
- Conceptualization: Barroga E, Matanguihan GJ.
- Methodology: Barroga E, Matanguihan GJ.
- Writing - original draft: Barroga E, Matanguihan GJ.
- Writing - review & editing: Barroga E, Matanguihan GJ.
- - Google Chrome
Intended for healthcare professionals
- Access provided by Google Indexer
- My email alerts
- BMA member login
- Username * Password * Forgot your log in details? Need to activate BMA Member Log In Log in via OpenAthens Log in via your institution

Search form
- Advanced search
- Search responses
- Search blogs
- Multiple adverse...
Multiple adverse outcomes associated with antipsychotic use in people with dementia: population based matched cohort study
Linked editorial.
Use of antipsychotics in adults with dementia
- Related content
- Peer review
- Pearl L H Mok , research fellow 1 2 ,
- Matthew J Carr , research fellow 1 2 3 ,
- Bruce Guthrie , professor 4 ,
- Daniel R Morales , Wellcome Trust clinical research fellow 5 ,
- Aziz Sheikh , professor 6 7 ,
- Rachel A Elliott , professor 3 8 ,
- Elizabeth M Camacho , senior research fellow 8 ,
- Tjeerd van Staa , professor 9 ,
- Anthony J Avery , professor 3 10 ,
- Darren M Ashcroft , professor 1 2 3
- 1 Centre for Pharmacoepidemiology and Drug Safety, Division of Pharmacy and Optometry, University of Manchester, Manchester, M13 9PT, UK
- 2 Manchester Academic Health Science Centre, Manchester, UK
- 3 NIHR Greater Manchester Patient Safety Research Collaboration, University of Manchester, Manchester, UK
- 4 Advanced Care Research Centre, Usher Institute, College of Medicine and Veterinary Medicine, University of Edinburgh, Edinburgh, UK
- 5 Population Health and Genomics, University of Dundee, Dundee, UK
- 6 Usher Institute, College of Medicine and Veterinary Medicine, University of Edinburgh, Edinburgh, UK
- 7 Nuffield Department of Primary Care Health Sciences, University of Oxford, Oxford, UK
- 8 Manchester Centre for Health Economics, Division of Population Health, Manchester, UK
- 9 Division of Informatics, Imaging and Data Sciences, University of Manchester, Manchester, UK
- 10 Centre for Primary Care, School of Medicine, University of Nottingham, Nottingham, UK
- Correspondence to: P L H Mok pearl.mok{at}manchester.ac.uk
- Accepted 29 February 2024
Objective To investigate risks of multiple adverse outcomes associated with use of antipsychotics in people with dementia.
Design Population based matched cohort study.
Setting Linked primary care, hospital and mortality data from Clinical Practice Research Datalink (CPRD), England.
Population Adults (≥50 years) with a diagnosis of dementia between 1 January 1998 and 31 May 2018 (n=173 910, 63.0% women). Each new antipsychotic user (n=35 339, 62.5% women) was matched with up to 15 non-users using incidence density sampling.
Main outcome measures The main outcomes were stroke, venous thromboembolism, myocardial infarction, heart failure, ventricular arrhythmia, fracture, pneumonia, and acute kidney injury, stratified by periods of antipsychotic use, with absolute risks calculated using cumulative incidence in antipsychotic users versus matched comparators. An unrelated (negative control) outcome of appendicitis and cholecystitis combined was also investigated to detect potential unmeasured confounding.
Results Compared with non-use, any antipsychotic use was associated with increased risks of all outcomes, except ventricular arrhythmia. Current use (90 days after a prescription) was associated with elevated risks of pneumonia (hazard ratio 2.19, 95% confidence interval (CI) 2.10 to 2.28), acute kidney injury (1.72, 1.61 to 1.84), venous thromboembolism (1.62, 1.46 to 1.80), stroke (1.61, 1.52 to 1.71), fracture (1.43, 1.35 to 1.52), myocardial infarction (1.28, 1.15 to 1.42), and heart failure (1.27, 1.18 to 1.37). No increased risks were observed for the negative control outcome (appendicitis and cholecystitis). In the 90 days after drug initiation, the cumulative incidence of pneumonia among antipsychotic users was 4.48% (4.26% to 4.71%) versus 1.49% (1.45% to 1.53%) in the matched cohort of non-users (difference 2.99%, 95% CI 2.77% to 3.22%).
Conclusions Antipsychotic use compared with non-use in adults with dementia was associated with increased risks of stroke, venous thromboembolism, myocardial infarction, heart failure, fracture, pneumonia, and acute kidney injury, but not ventricular arrhythmia. The range of adverse outcomes was wider than previously highlighted in regulatory alerts, with the highest risks soon after initiation of treatment.
Introduction
Dementia is a clinical syndrome characterised by progressive cognitive decline and functional disability, with estimates suggesting that by 2050 around 152.8 million people globally will be affected. 1 Behavioural and psychological symptoms of dementia are common aspects of the disease and include features such as apathy, depression, aggression, anxiety, irritability, delirium, and psychosis. Such symptoms can negatively impact the quality of life of patients and their carers and are associated with early admission to care. 2 3 Antipsychotics are commonly prescribed for the management of behavioural and psychological symptoms of dementia, despite longstanding concerns about their safety. 4 5 6 During the covid-19 pandemic, the proportion of people with dementia prescribed antipsychotics increased, possibly owing to worsened behavioural and psychological symptoms of dementia linked to lockdown measures or reduced availability of non-pharmaceutical treatment options. 7 According to guidelines from the UK’s National Institute for Health and Care Excellence, antipsychotics should only be prescribed for the treatment of behavioural and psychological symptoms of dementia if non-drug interventions have been ineffective, if patients are at risk of harming themselves or others or are experiencing agitation, hallucinations, or delusions causing them severe distress. 8 Antipsychotics should at most be prescribed at the lowest effective dose and for the shortest possible time. Only two antipsychotics, risperidone (an atypical, or second generation, antipsychotic) and haloperidol (a typical, or first generation, antipsychotic), are licensed in the UK for the treatment of behavioural and psychological symptoms of dementia, 9 although others have been commonly prescribed off-label. 5 10
Based on evidence from clinical trials of risperidone, the US Food and Drug Administration (FDA) first issued a warning in 2003 about the increased risks of cerebrovascular adverse events (eg, stroke, transient ischaemic attack) associated with use of atypical antipsychotics in older adults with dementia. 11 A meta-analysis of 17 trials among such patients subsequently found a 1.6-1.7-fold increased risk of mortality with atypical antipsychotics compared with placebo, which led the FDA to issue a “black box” warning in 2005 for all atypical antipsychotics. 11 This warning was extended to typical antipsychotics in 2008, after two observational studies reported that the risk of death associated with their use among older people might be even greater than for atypical antipsychotics. 12 13 14 The increased risks for stroke and mortality have been consistently reported by many observational studies and meta-analyses since, 11 15 16 17 18 19 20 21 and they have led to regulatory safety warnings and national interventions in the UK, US, and Europe, aiming to reduce inappropriate prescribing of these drugs for the treatment of behavioural and psychological symptoms of dementia. 8 11 22 23 24 25 26 Other adverse outcomes have also been investigated in observational studies, 27 28 29 although, with the exception of pneumonia, 14 30 31 32 the evidence is less conclusive or is more limited among people with dementia. For example, inconsistent or limited evidence has been found for risks of myocardial infarction, 33 34 ventricular arrhythmia, 35 36 venous thromboembolism, 37 38 39 40 fracture, 41 42 43 and acute kidney injury. 44 45 46 Most studies also reported only one outcome or type of outcomes. Examining multiple adverse events in a single cohort is needed to give a more comprehensive estimate of the total potential harm associated with use of antipsychotics in people with dementia.
Using linked primary and secondary care data in England, we investigated the risks of a range of adverse outcomes potentially associated with antipsychotic use in a large cohort of adults with dementia—namely, stroke, venous thromboembolism, myocardial infarction, heart failure, ventricular arrhythmia, fracture, pneumonia, and acute kidney injury. We report both relative and absolute risks.
Data sources
The study used anonymised electronic health records from Clinical Practice Research Datalink (CPRD). In the UK, residents are required to be registered with a primary care general practice to receive care from the NHS. The NHS is a publicly funded healthcare service, free at the point of use. More than 98% of the UK population are registered with a general practice, and their electronic health records are transferred when they change practice. 47 48 Community prescribing is most often done by the general practitioner, including antipsychotic treatment recommended by specialists. CPRD data are sourced from more than 2000 general practices covering around 20% of the UK population, and include information on diagnoses, primary healthcare contacts, prescribed drugs, laboratory test results, and referrals to secondary healthcare services. 47 48 CPRD contains two databases: Aurum and GOLD. CPRD Aurum includes data from contributing general practices in England that use the EMIS Web patient management software, and CPRD GOLD consists of patient data from practices across all four UK nations that use the Vision system. Both datasets are broadly representative of the UK population. 47 48 49 Primary care data from general practices in England can be linked to other datasets, including hospital admissions in Hospital Episode Statistics, and mortality and index of multiple deprivation data from the Office for National Statistics (ONS). Individual patients can opt-out of sharing their records with CPRD, and individual patient consent was not required as all data were deidentified.
Study population
We delineated two cohorts, one each from Aurum and GOLD. For the latter, we included patients from English practices only because linkage to hospital admission and mortality data were required in our analyses. To ensure that the study dataset would not contain any duplicate patient records, we used the bridging file provided by CPRD to identify English practices that have migrated from the GOLD to the Aurum dataset, and removed such practices from the GOLD dataset. For both cohorts, we included patients who had a first dementia diagnosis code between 1 January 1998 and 31 May 2018. Dementia was identified from Read, SNOMED, or EMIS codes used in the databases (see supplementary appendix). We defined the date of first dementia diagnosis as the date of first dementia code. Patients needed to be aged 50 years or over at the time of dementia diagnosis, have been registered with the CPRD practice for at least a year, not be prescribed an antipsychotic in the 365 days before their first dementia code, and have records that were eligible for linkage to Hospital Episodes Statistics, mortality, and index of multiple deprivation data. In addition, because anticholinesterases (such as donepezil, rivastigmine, and galantamine) may sometimes be prescribed to patients showing signs of dementia before their first dementia code, we excluded patients with an anticholinesterase prescription before their first dementia code. Supplementary figures S1 and S2 show how the two cohorts for Aurum and GOLD, respectively, were delineated.
Study design
Matched cohort design —We implemented a matched cohort design. Supplementary figure S3 shows the study design graphically. 50 For the Aurum and GOLD cohorts separately, patients who used antipsychotics were defined as patients in each cohort issued with an antipsychotic prescription after (or on the same day as) the date of their first dementia diagnosis, with the date of first antipsychotic prescription being the index date after which outcomes were measured. For each outcome, follow-up began from the date of the first antipsychotic prescription (the index date) and ended on the earliest of date of first diagnosis of outcome (ie, the earliest recording of the outcome whether it was from the patient’s primary or secondary care or mortality records), death, transfer out of the general practice, last data collection date of the general practice, two years from the date of antipsychotics initiation, or 31 May 2018. Because patients who have experienced an outcome were potentially at higher risk of subsequently experiencing the same event, which could confound any risks associated with antipsychotic use, we excluded those with a history of the specific outcome under investigation before the index date from the analysis of that outcome. For example, we excluded patients with a record of stroke before the index date from the analysis of stroke, but they would still be eligible for the study of other outcomes. For the analysis of acute kidney injury, patients with a diagnosis of end stage kidney disease before the index date were also excluded, and a diagnosis of end stage kidney disease after the index date was an additional condition for end of follow-up. 44
Matched comparators —Each patient who used antipsychotics on or after the date of their first dementia diagnosis was matched using incidence density sampling with up to 15 randomly selected patients who had the same date of first dementia diagnosis (or up to 56 days after) and who had not been prescribed an antipsychotic before diagnosis. Incidence density sampling involves matching on sampling time, with each antipsychotic user in our study being matched to one or more comparators who were eligible for an antipsychotic but had not become a user at the time of matching. 51 The selection of comparators was done with replacement—that is, an individual could be used as a comparator in multiple matched sets. In our study, this meant that patients were eligible to be a non-user matched comparator up to the date of their first antipsychotic prescription. We excluded matched comparators with a history of the specific outcome under investigation before the index date from the analysis of that event. For each outcome, follow-up of matched comparators began on the same day as the patient to whom they were matched (the index date) and ended on the earliest of date of their first antipsychotic prescription (if any), or date of one of the end of follow-up events described earlier for the antipsychotic users.
Use of antipsychotics
We included both typical and atypical antipsychotics, identified by product codes in Aurum and GOLD (see supplementary appendix for list of drugs included). Senior author DMA (pharmacist) reviewed the code lists. As previous studies have shown a temporal association between antipsychotic use and development of adverse outcomes, 30 31 52 we treated use of antipsychotics as a time varying variable, classified as current, recent, and past use. Current use was defined as the first 90 days from the date of an antipsychotic prescription, recent use as up to 180 days after current use ended, and past use as the time after the recent use period had ended. If a patient was issued another prescription during the 90 days after their last prescription, their current use period would be extended by 90 days from the date of their latest prescription. For example, if a patient had two prescriptions and the second was issued 60 days after the first, their current use period would be a total of 150 days: 60 days after the first prescription plus 90 days after the second. At the end of the 150 days current use period, the next 180 days would be the recent use period, and the time after this recent use period would be past use. As patients could have multiple prescriptions over time, they could move between the three antipsychotic use categories during follow-up, and they could therefore be defined as current, recent, or past users more than once. See the supplementary appendix for further information on how this definition is applied.
In post hoc analyses, we also investigated typical versus atypical antipsychotics, and specific drug substances: haloperidol, risperidone, quetiapine, and other antipsychotics (as a combined category).
Outcomes were stroke, venous thromboembolism (including deep vein thrombosis and pulmonary embolism), myocardial infarction, heart failure, ventricular arrhythmia, fracture, pneumonia, and acute kidney injury. With the exceptions of pneumonia and acute kidney injury, outcomes were identified by Read, SNOMED, or EMIS codes in the primary care records, and by ICD-10 (international classification of diseases, 10th revision) codes from linked secondary care data from Hospital Episodes Statistics, and cause of death data from the ONS mortality records. For pneumonia and acute kidney injury, we only included those that were diagnosed in hospitals or as a cause of death, ascertained from Hospital Episodes Statistics and ONS data.
We also investigated appendicitis and cholecystitis combined as an unrelated (negative control) outcome to detect potential unmeasured confounding. 53 These outcomes were chosen because evidence of an association with antipsychotic use is lacking from the literature. We identified appendicitis and cholecystitis from Read, SNOMED, EMIS, and ICD-10 codes. Clinicians (BG, AJA, DRM) checked all code lists (see supplementary appendix).
We used propensity score methods to control for imbalances in measurable patient characteristics between antipsychotic users and their matched non-users, with personal characteristics, lifestyle, comorbidities, and prescribed drugs included in the propensity score models. A counterfactual framework for causal inference was applied to estimate the average treatment effect adjusting for inverse probability of treatment weights generated from the propensity score models. 54 55 Selection of covariates was informed by the literature, based on their potential associations with antipsychotic initiation and study outcomes. 31 34 44 56 57 All variables were assessed before the index date (see supplementary figure S3). Variables for personal characteristics included sex, age at dementia diagnosis, age at start of follow-up, ethnicity, and index of multiple deprivation fifths based on the location of the general practice. Comorbidities were derived as dichotomous variables and included a history of hypertension, types 1 and 2 diabetes mellitus, chronic obstructive pulmonary disease, rheumatoid arthritis, moderate or severe renal disease, moderate or severe liver disease, atrial fibrillation, cancer, and serious mental illness (bipolar disorders, schizophrenia, schizoaffective disorders, and other psychotic disorders). Lifestyle factors included smoking status and alcohol use. Medication covariates were represented as dichotomous indicators, defined by at least two prescriptions for each of the following drugs in the 12 months before the index date: antiplatelets, oral anticoagulants, angiotensin converting enzyme inhibitors or angiotensin II receptor blockers, alpha blockers, beta blockers, calcium channel blockers, diuretics, lipid lowering drugs, insulin and antidiabetic drugs, non-steroidal anti-inflammatory drugs, antidepressants, benzodiazepines, and lithium. We also included the following potential confounders for the investigations of venous thromboembolism and fracture: prescriptions for hormone replacement therapy and selective oestrogen receptor modulators (for venous thromboembolism), 58 59 a history of inflammatory bowel disease (for pneumonia and fracture), 60 61 and prescriptions for immunosuppressants, oral corticosteroids, and inhaled corticosteroids (for pneumonia). 62 63
Statistical analysis
For each patient included in the study, we derived a propensity score representing the patient’s probability of receiving antipsychotic treatment. Propensity scores were estimated using multivariable logistic regression, with antipsychotic use as the dependent variable. Predictors included personal characteristics, lifestyle, comorbidities, and prescribed drugs. Patients with missing information on ethnicity, index of multiple deprivation, smoking, or alcohol use were grouped into an unknown category for each of these variables and included in the propensity score models. We used the Hosmer-Lemeshow test and likelihood ratio test to test the fit of the models, and interaction terms were included to improve the model fit. 64 The derived scores were used as inverse probability of treatment weights to reweigh the data, balancing the distribution of baseline covariates between antipsychotic users and non-users (matched comparators)—that is, standardised differences <0.1 after weighting. 65 Propensity score models were run for each outcome, and for the Aurum and GOLD cohorts separately. For further information, see the supplementary appendix section on propensity score methods to control for potential confounding.
Analyses for estimating harms were then conducted after combining (appending) the Aurum and GOLD datasets. We used Cox regression survival analyses to estimate the risks of each outcome associated with antipsychotic use relative to the comparator cohort, and we report the results as hazard ratios. Use of an antipsychotic was treated as a time varying variable. To account for the matched design, we fitted stratified models according to the matched sets and used robust variance estimation. In all models, we also included a covariate indicating whether the patient was from the Aurum or GOLD cohort and calculated hazard ratios with adjustments for inverse probability of treatment weights. Cox regression assumes proportional hazards—that is, the relative hazard of the outcome remains constant during the follow-up period. 66 We assessed this assumption using the Grambsch-Therneau test based on the Schoenfeld residuals. 67 Because this assumption did not hold for all outcomes examined, in addition to reporting the hazard ratios pertaining to the whole follow-up period, we estimated hazard ratios separately for the several time windows: the first seven days, 8-30 days, 31-180 days, 181-365 days, and 366 days to two years (see supplementary appendix for an illustration of stratification of follow-up time). For each outcome, we calculated the incidence rate and the number needed to harm (NNH) over the first 180 days as well as two years after start of follow-up. The NNH represents the number of patients needed to be treated with an antipsychotic for one additional patient to experience the outcome compared with no treatment. We also calculated cumulative incidence percentages (absolute risks) for each outcome accounting for competing mortality risks based on previous recommendations. 68 These were calculated at 90 days, 180 days, 365 days, and two years after start of follow-up for antipsychotic users and their matched comparators separately. We also reported the difference in cumulative incidence between antipsychotic users and their matched comparators at these time points. Analyses were conducted using Stata/MP v16.1.
Sensitivity analyses
We investigated two other definitions of antipsychotic use as sensitivity analyses: the first 60 days as current use followed by 120 days of recent use, and a current use period of 30 days followed by a recent use period of 60 days. We also conducted the following post hoc sensitivity analyses. Firstly, as levomepromazine is often prescribed in palliative care to treat distressing symptoms in the last days of life, 69 we censored individuals at the time of their first levomepromazine prescription. Secondly, we used Fine-Gray subdistribution hazard regression models to estimate the hazard of each adverse outcome, accounting for the competing risks of death. 70 These results were reported as subhazard ratios. Thirdly, we compared the incidence rates and hazards of adverse outcomes for male versus female individuals. For these sex specific analyses, we modified the existing matched cohort by excluding non-user comparators who were of a different sex from the antipsychotic user to whom they were matched. We then derived a new propensity score for each individual by excluding sex as a covariate in the propensity score models. Incidence rate ratios and corresponding 95% confidence intervals (CIs) for male versus female individuals were calculated using the ‘iri’ command in Stata. To investigate whether hazards of each adverse outcome associated with antipsychotic use differed by sex, we fitted Cox regression models with sex, antipsychotic use, and their interaction as covariates. Sex specific hazard ratios and ratios of male to female hazard ratios were reported.
Patient and public involvement
This study is part of a National Institute of Health and Care Research funded programme (RP-PG-1214-20012): Avoiding patient harm through the application of prescribing safety indicators in English general practices (PRoTeCT). Two patient and public involvement members in the project team contributed to the study design and protocol of this study. Our study was not, however, coproduced with people with dementia or their carers.
Characteristics of study population
A total of 173 910 adults (63.0% women) with dementia were eligible for inclusion in the study: 139 772 (62.9% women) in the Aurum dataset and 34 138 (63.4% women) in GOLD. The mean age at dementia diagnosis for individuals in both cohorts was 82.1 years (standard deviation (SD) 7.9 years), and the median age was 83 years (interquartile range (IQR) 78-88 years in Aurum and 78-87 years in GOLD). A total of 35 339 individuals (62.5% women; 28 187 in Aurum, 62.6% women; 7152 in GOLD, 62.5% women) were prescribed an antipsychotic during the study period, and a matched set was generated for each of these individuals. The mean number of days between first dementia diagnosis and date of a first antipsychotic prescription was 693.8 ((SD 771.1), median 443 days) in Aurum and 576.6 ((SD 670.0), median 342 days) in GOLD. A total of 544 203 antipsychotic prescriptions (433 694 in Aurum, 110 509 in GOLD) were issued, of which 25.3% were for a typical antipsychotic and 74.7% for an atypical antipsychotic. The most prescribed antipsychotics were risperidone (29.8% of all prescriptions), quetiapine (28.7%), haloperidol (10.5%), and olanzapine (8.8%), which together accounted for almost 80% of all prescriptions (see supplementary table S1).
Since we excluded people with a history of the event before the start of follow-up, the number of individuals and matched sets included in analysis varies by outcome. Table 1 shows the baseline characteristics of patients for the analysis of stroke, before and after inverse probability of treatment weighting. Antipsychotic users were more likely than their matched comparators to have a history of serious mental illness and to be prescribed antidepressants or benzodiazepines in the 12 months before start of follow-up. After inverse probability of treatment weighting, standardised differences were <0.1 for all covariates. Baseline characteristics of individuals included in the analyses of other outcomes were similar to those reported for stroke (see supplementary tables S2-S9).
Baseline characteristics of antipsychotic users and matched comparators included in the analysis of stroke (CPRD Aurum and GOLD combined data). Values are number (percentage) unless stated otherwise
- View inline
Incidence rates and relative hazards of adverse outcomes
All antipsychotics.
In the two years after initiation of antipsychotics, the highest incidence rates of adverse outcomes were for pneumonia, fracture, and stroke, and ventricular arrhythmias were rare ( table 2 ). Figure 1 shows the hazard ratios of adverse outcomes associated with current, recent, past, and any use of antipsychotics versus non-use (ie, matched comparators). Except for ventricular arrhythmia, any use of antipsychotics was associated with increased risks for all adverse outcomes, ranging from a hazard ratio of 2.03 (95% CI 1.96 to 2.10) for pneumonia to 1.16 (1.09 to 1.24) for heart failure. Current use (ie, prescribed in the previous 90 days) was associated with high risks for pneumonia (2.19, 2.10 to 2.28), acute kidney injury (1.72, 1.61 to 1.84), venous thromboembolism (1.62, 1.46 to 1.80), and stroke (1.61, 1.52 to 1.71). Recent antipsychotic use (ie, in the 180 days after current use ended) was also associated with increased risk for these outcomes, as well as for fracture, but past use of antipsychotics (ie, after recent use ended) was not associated with increased risks of the adverse outcomes examined, except for pneumonia. For the negative control outcome (appendicitis and cholecystitis), no significant associations were found with current, recent, or any antipsychotic use, but a statistically significant association was observed with past use (1.90, 1.01 to 3.56).
Incidence rate (per 10 000 person years) and number needed to harm of adverse outcomes associated with antipsychotic use during the first 180 days and two years of follow-up period

Hazard ratios (adjusted for inverse probability of treatment weights) of adverse outcomes associated with current, recent, and past antipsychotic use; with current use being defined as the first 90 days from the date of an antipsychotic prescription, recent use as up to 180 days after current use ended, and past use as after recent use. CI=confidence interval
- Download figure
- Open in new tab
- Download powerpoint
Table 2 shows that the NNH ranged from 9 (95% CI 9 to 10) for pneumonia to 167 (116 to 301) for myocardial infarction during the first 180 days after initiation of antipsychotics, and from 15 (14 to 16) for pneumonia to 254 (183 to 413) for myocardial infarction after two years. These figures suggest that over the 180 days after drug initiation, use of antipsychotics might be associated with one additional case of pneumonia for every nine patients treated, and one additional case of myocardial infarction for every 167 patients treated. At two years, there might be one additional case of pneumonia for every 15 patients treated, and one additional case of myocardial infarction for every 254 patients treated.
Table 3 shows hazard ratios stratified by follow-up time (except for ventricular arrhythmia and the negative control where the number of patients was very low). For almost all outcomes, relative hazards were highest in the first seven days after initiation of antipsychotic treatment. Risks for pneumonia were particularly increased in the first seven days (9.99, 8.78 to 11.40) and remained substantial afterwards (3.39, 3.04 to 3.77, 8-30 days). No increased risks for heart failure were found for current users after 180 days from start of treatment, nor for myocardial infarction one year after drug initiation. However, risks for stroke, venous thromboembolism, fracture, pneumonia, and acute kidney injury remained increased among continuous antipsychotic users up to two years after initiation of treatment.
Hazard ratios (adjusted for IPT weights) of adverse outcomes associated with current, recent, and past antipsychotic use stratified by follow-up period
Types of antipsychotics
During the current use period of 90 days after a prescription, both typical and atypical antipsychotics were associated with increased risks of all adverse outcomes compared with non-use, except for ventricular arrhythmia and the negative control (see supplementary table S10). Hazards were higher when current use of typical antipsychotics was directly compared with atypical antipsychotics for stroke (1.23, 1.09 to 1.40), heart failure (1.18, 1.01 to 1.39), fracture (1.22, 1.08 to 1.38), pneumonia (1.92, 1.77 to 2.08), and acute kidney injury (1.22, 1.05 to 1.42), but no significant differences between the two types of drug were found for the risks of venous thromboembolism or myocardial infarction.
Supplementary table S11 shows the risks of adverse outcomes associated with haloperidol (the most prescribed typical antipsychotic) and with risperidone and quetiapine (the two most prescribed atypical antipsychotics). Current use of risperidone and haloperidol compared with non-use was associated with increased risks of all adverse outcomes except for ventricular arrhythmia and the negative control. Current use of quetiapine compared with non-use was associated with increased risks for fracture, pneumonia, and acute kidney injury. Among current users of haloperidol or risperidone, risks for fracture, pneumonia, and acute kidney injury were higher for haloperidol versus risperidone, but risks for stroke, venous thromboembolism, myocardial infarction, and heart failure were similar for both drugs. With the exceptions of myocardial infarction, ventricular arrhythmia, and the negative control, risks of all adverse outcomes were higher for haloperidol than for quetiapine, especially for pneumonia (2.53, 2.21 to 2.89) and venous thromboembolism (1.99, 1.33 to 2.97). Among current users of quetiapine compared with risperidone, there were no significant differences in risks for myocardial infarction, heart failure, or fracture. However, risks for stroke (0.64, 0.53 to 0.78), venous thromboembolism (0.49, 0.36 to 0.68), pneumonia (0.72, 0.63 to 0.81), and acute kidney injury (0.81, 0.67 to 0.96) were lower for quetiapine than for risperidone.
Absolute risks of adverse outcomes
Cumulative incidence for all outcomes examined was higher for antipsychotic users versus matched comparators, except for ventricular arrhythmia and the negative control ( table 4 ). The absolute risk, as well as risk difference, was particularly large for pneumonia. In the 90 days after initiation of an antipsychotic, the cumulative incidence of pneumonia among antipsychotic users was 4.48% (95% CI 4.26% to 4.71%) v 1.49% (1.45% to 1.53%) in the matched cohort of non-users (difference 2.99%, 95% CI 2.77% to 3.22%). At one year, this increased to 10.41% (10.05% to 10.78%) for antipsychotic users compared with 5.63% (5.55% to 5.70%) for non-users (difference 4.78%, 4.41% to 5.16%).
Cumulative incidence of adverse outcomes associated with antipsychotic use at 90, 180, and 365 days and at two years after start of follow-up
Similar results were found in sensitivity analysis using two other definitions of antipsychotic use (see supplementary figures S4 and S5). Of the 544 203 antipsychotic prescriptions issued, 1.3% were for levomepromazine (see supplementary table S1). Results remained similar when patients were censored at the time of their first levomepromazine prescription (see supplementary figure S6). Results of the Fine-Gray models accounting for the competing risks of death also showed broadly similar patterns of hazards to those from the Cox models (see supplementary table S12 and figure S7). Sex specific analyses showed that male patients had higher incidence rates of all adverse outcomes than female patients, except for fracture and venous thromboembolism where incidence was higher for female patients than for male patients (see supplementary table S13). Compared with female antipsychotic users, male users had increased hazards for pneumonia and acute kidney injury (male to female hazard ratio 1.16, 95% CI 1.08 to 1.25 for pneumonia and 1.22, 1.08 to 1.37 for acute kidney injury), but lower hazards for stroke (0.81, 0.73 to 0.91). No significant differences were found by sex in the hazards for venous thromboembolism, myocardial infarction, heart failure, ventricular arrhythmia, or fracture (see supplementary table S14).
In this population based cohort study of adults (≥50 years) with dementia, use of antipsychotics compared with non-use was associated with increased risks for stroke, venous thromboembolism, myocardial infarction, heart failure, fracture, pneumonia, and acute kidney injury. Increased risks were observed among current and recent users and were highest in the first week after initiation of treatment. In the 90 days after a prescription, relative hazards were highest for pneumonia, acute kidney injury, stroke, and venous thromboembolism, with increased risks ranging from 1.5-fold (for venous thromboembolism) to twofold (for pneumonia) compared with non-use. No increased risk was found for ventricular arrhythmia or the negative control outcome (appendicitis and cholecystitis). Absolute risk differences between antipsychotic users and their matched comparators were substantial for most adverse events, and largest for pneumonia. In the 90 days after a prescription, risks of stroke, heart failure, fracture, pneumonia, and acute kidney injury were higher for typical antipsychotics versus atypical antipsychotics, whereas no significant differences between these two drug classes were found for risks of venous thromboembolism or myocardial infarction. Haloperidol was associated with higher risks for fracture, pneumonia, and acute kidney injury than risperidone, but no significant differences between the two drugs were found for the other outcomes. Risks of almost all adverse outcomes were higher for haloperidol than for quetiapine. No significant differences were found between risperidone and quetiapine for risks of myocardial infarction, heart failure, or fracture, but risks for stroke, venous thromboembolism, pneumonia, and acute kidney injury were lower for quetiapine versus risperidone.
Comparison with other studies
A population based study in Wales reported no increased risks for non-fatal acute cardiac events associated with antipsychotic use in patients with all cause dementia, although those with Alzheimer’s disease showed increased risks. 37 Systematic reviews and meta-analyses of studies not limited to patients with dementia have also reported inconsistent evidence for myocardial infarction, or lack of robustness of these data. 33 34 71 Our findings for myocardial infarction were similar to those in a study that first documented a modest and time limited increase in risk of this outcome associated with antipsychotic use among patients with dementia. 56 In a study of nursing home residents in the US, users of typical, but not atypical, antipsychotics were more likely than non-users to be admitted to hospital for ventricular arrhythmia or cardiac arrest, 35 and a study not limited to older people reported increased risks for ventricular arrhythmia or sudden cardiac death associated with both typical and atypical antipsychotics. 36 We did not find any association with ventricular arrhythmia, but the number of events was low and we did not examine cardiac arrest or sudden death.
Increased risks of venous thromboembolism associated with antipsychotic use have been reported in the general population, 38 but meta-analyses found increased risks of venous thromboembolism only among younger users. 39 40 Our findings are consistent with those of the Welsh study, which reported increased risks of venous thromboembolism in the 12 months after drug initiation (prior event rate ratio 1.95, 95% CI 1.83 to 2.0). 37 In absolute terms, however, these risks were relatively low compared with other outcomes examined in this study.
We found that both the relative and the absolute risks for pneumonia were highest among all outcomes examined. Current users of antipsychotics had a twofold increased risk compared with non-users ( fig 1 ), and although this magnitude of increased risk was comparable to previous reports, 14 31 32 we additionally observed that risks were greater in the first week after drug initiation. One study also reported a particularly high risk for patients with hospital diagnosed pneumonia in the first week, but the magnitude of increase (odds ratio 4.5, 95% CI 2.8 to 7.3) was much lower than our observation. 30 The mechanisms linking antipsychotic use and development of pneumonia is not well understood, and substantial heterogeneity exists among the drug substances, but antipsychotic induced extrapyramidal symptoms, sedation, xerostomia (dry mouth), and dyskinesia or impaired swallowing are commonly considered as potential risk factors. 72 In addition, because elderly people with pneumonia may be less likely than younger patients to present with respiratory symptoms but more likely to show signs of delirium, 73 it is possible that reverse causality might have contributed to the high risks observed in the early days after drug initiation, as delirium from the onset of pneumonia might have been treated with antipsychotics before pneumonia was diagnosed. 30 However, although causality cannot be inferred, the particularly high increased risks observed for a range of outcomes and not only for pneumonia in the early days after drug initiation are consistent with other studies. 28 This could be partly explained by further prescriptions being given only to patients who tolerated the first days of drug use.
The use of atypical antipsychotics in older adults (≥65 years) has been shown to be associated with increased risk of acute kidney injury. 44 45 46 Two studies reported significantly increased risks in users compared with non-users in the 90 days after initiation of atypical antipsychotics. 44 45 In contrast, another study observed no increased risks from use of the broad category of atypical antipsychotics, although a significantly increased risk was found with olanzapine. 46 In our study, we found increased risks of acute kidney injury with both typical and atypical antipsychotics, with risks being higher for haloperidol than for risperidone and quetiapine.
In a meta-analysis of observational studies, antipsychotic use was associated with increased risks of hip fracture among people with dementia. 41 A self-controlled case series study of older adult patients (≥65 years) also reported increased risks of falls and fracture after initiation of antipsychotics, but incidence was found to be even higher in the 14 days before treatment started. 43 Similar findings were also reported in another study, suggesting that the risks observed during the treatment periods might not be attributable to the antipsychotics alone. 42 Although we cannot eliminate confounding in our study, we minimised this risk by adjusting for a large number of both clinical and non-clinical characteristics that might have influenced treatment assignment. We also found no increased risks associated with current or recent antipsychotic use for the negative control outcome (appendicitis and cholecystitis).
Our study found that the risks of stroke and heart failure were higher for typical antipsychotics than for atypical antipsychotics, but risks of venous thromboembolism and myocardial infarction were similar between the two drug classes. We also found no significant differences between haloperidol and risperidone in risks of these four outcomes, but significantly increased risks for stroke, venous thromboembolism, and heart failure for haloperidol versus quetiapine. Previous studies of elderly patients have reported similar risks for cardiovascular or cerebrovascular events associated with use of typical and atypical antipsychotics, 17 74 75 76 but risks of these outcomes and of all cause mortality were increased with haloperidol versus risperidone. 21 76 For fracture and pneumonia, we found that risks were higher in association with typical antipsychotics than atypical antipsychotics and for haloperidol versus risperidone or quetiapine. The findings from previous studies comparing these risks by antipsychotic types have been inconsistent. 30 31 32 74 75
Strengths and limitations of this study
A key strength of this study was the investigation of a wide range of adverse events in a large population based cohort, and the reporting of both relative and absolute risk differences over multiple periods. Previous studies commonly focused on a single outcome or type of outcome, such as cerebrovascular events, and on the reporting of relative risks. By examining the same cohort at risk, we were able to directly compare the hazards of multiple outcomes without differential biases between the cohorts. In addition, we only included patients with a clinician recorded diagnosis of dementia, and we adjusted for many variables that might have influenced the probability of antipsychotic initiation, seeking to minimise confounding by indication. CPRD is one of the largest primary care databases in the world, and it is broadly representative of the UK population. 47 48 49 The database includes all prescriptions issued in participating primary care practices in the UK, and it is recognised as a high quality resource to support international pharmacovigilance. 77 The longitudinal nature of CPRD, with linked data from secondary care and mortality records, enabled us to capture the study outcomes from multiple sources, as well as information on prescribing and comorbidities. 78 79 Our findings were also robust to different classifications of usage periods and we found no associations between current and recent antipsychotic use with the development of the negative control outcome (appendicitis and cholecystitis). However, a significant association with past use was observed that we are unable to explain.
As with all observational studies, residual confounding cannot be excluded. For example, polypharmacy is common among elderly people, which could lead to drug-drug interactions and potentially confound our findings. 80 81 We also did not have information on indications for antipsychotics treatment. We minimised the risk of confounding using propensity score methods to control for imbalances in measurable patient characteristics between antipsychotic users and their matched comparators. However, unlike randomised control trials, which, if properly conducted, could account for both observed and unobserved differences between treated and untreated groups, the propensity score method can only adjust for the observed differences between two groups. Additionally, our choice of covariates was based on the literature and discussions with clinical experts and was not formally structured using, for example, a directed acyclic graph. Although the strong associations with pneumonia in the first seven days of antipsychotic initiation may partially be attributed to reverse causality, however, it is less likely to explain associations over longer periods. We also found no increased risk for appendicitis and cholecystitis during current and recent use—our negative control outcome that was included to detect potential unmeasured confounding. 53 Another limitation of our study is that although prescriptions issued in primary care are reliable in CPRD, information on dosage is not well recorded and information on drug adherence or prescriptions issued while patients are in hospital is not available. 48 Misclassification of drug use is therefore a potential problem. As with other electronic health data that are routinely collected for administrative rather than research purposes, potential issues exist with coding errors, missing or incomplete information, and variations in data quality between practices and healthcare settings. Although the data undergo quality checks before being released and our use of the linked data would have helped to deal with such problems, we were restricted to data coded in patients’ electronic health records. In addition, despite the representativeness of the CPRD data, care should be taken in making inferences beyond the population studied. Our sex specific investigations were also conducted as post hoc analyses. By using existing matched sets but restricting the comparators to those of the same sex as the antipsychotic user to whom they were matched, the number of comparators was greatly reduced. Although we found some evidence of differences in hazards for stroke, pneumonia, and acute kidney injury between male and female antipsychotic users, further research is needed to validate these findings.
Policy implications
The mechanisms underlying the links between antipsychotics and the outcomes in our study are not fully understood. In the UK, US, and Europe, current regulatory warnings for using antipsychotics to treat behavioural and psychological symptoms of dementia were mostly based on evidence of increased risks for stroke and mortality. 8 11 22 23 24 25 26 We found a considerably wider range of harms associated with antipsychotic use in people with dementia, and the risks of harm were highest soon after initiation. Our findings must be seen in the context of trial evidence of at best modest benefit on behavioural and psychological symptoms of dementia. The efficacy of antipsychotics in the management of behavioural and psychological symptoms of dementia remains inconclusive. 82 83 84 85 Atypical antipsychotics, including risperidone, which is one of two antipsychotics licensed in the UK for the treatment of behavioural and psychological symptoms of dementia, have the strongest evidence base, but the benefits are only modest. 82 85
Any potential benefits of antipsychotic treatment therefore need to be weighed against the risk of serious harm across multiple outcomes. Although there may be times when an antipsychotic prescription is the least bad option, clinicians should actively consider the risks, considering patients’ pre-existing comorbidities and living support. The NNH reported in this study can help to inform clinical judgements on the appropriateness of treatments, taking account of the modest potential benefits reported in clinical trials. When prescriptions of such drugs are needed, treatment plans should be reviewed regularly with patients and their carers to reassess the need for continuing treatment. 9 In addition, given the higher risks of adverse events in the early days after drug initiation, clinical examinations should be taken before, and clinical reviews conducted shortly after, the start of treatment. Our study reaffirms that these drugs should only be prescribed for the shortest period possible. 9 Although regulators have made efforts to limit the use of these drugs to people with the most severe behavioural and psychological symptoms of dementia, 8 82 86 antipsychotic prescribing in dementia remains common and has even increased in recent years. 4 5 87 88 If such trends continue, further communication on the associated risks could be considered by guideline developers or regulators after a review of the totality of evidence. Greater accountability and monitoring in the use of these drugs may be called for, and additional legal reforms may be required to regulate adherence. 89 In recent years, other psychotropic drugs such as antidepressants, benzodiazepines, mood stabilisers, and anticonvulsants have been prescribed instead of antipsychotics for the treatment of behavioural and psychological symptoms of dementia. 28 90 91 These drugs, however, also pose their own risks. Further research is needed into safer drug treatment of behavioural and psychological symptoms of dementia and more efficacious, easy to deliver, initial non-drug treatments.
Conclusions
Antipsychotic use is associated with a wide range of serious adverse outcomes in people with dementia, with relatively large absolute risks of harm for some outcomes. These risks should be considered in future regulatory decisions, alongside cerebrovascular events and mortality. Any potential benefits of antipsychotic treatment need to be weighed against risk of serious harm, and treatment plans should be reviewed regularly. The effect of antipsychotics on behavioural and psychological symptoms of dementia is modest at best, but the proportion of people with dementia prescribed antipsychotics has increased in recent years. Our finding that antipsychotics are associated with a wider range of risks than previously known is therefore of direct relevance to guideline developers, regulators, and clinicians considering the appropriateness of antipsychotic prescribing for behavioural and psychological symptoms of dementia.
What is already known on this topic
Despite safety concerns, antipsychotics continue to be frequently prescribed for the management of behavioural and psychological symptoms of dementia
Current regulatory warnings for the treatment of behavioural and psychological symptoms of dementia using antipsychotics are based on evidence of increased risks of stroke and death
Evidence for other adverse outcomes is less conclusive or is more limited among people with dementia, and comparisons of risks for multiple adverse events are also difficult owing to different study designs and populations
What this study adds
Antipsychotic use in people with dementia was associated with increased risks of stroke, venous thromboembolism, myocardial infarction, heart failure, fracture, pneumonia, and acute kidney injury, compared with non-use, but not ventricular arrhythmia
Relative hazards were highest for pneumonia, acute kidney injury, stroke, and venous thromboembolism, and absolute risk and risk difference between antipsychotic users and their matched comparators was largest for pneumonia
Risks of these wide ranging adverse outcomes need to be considered before prescribing antipsychotic drugs to people with dementia
Ethics statements
Ethical approval.
This study was approved by the Clinical Practice Research Datalink’s (CPRD) independent scientific advisory committee (protocol 18_168). CPRD also has ethical approval from the Health Research Authority to support research using anonymised patient data (research ethics committee reference 21/EM/0265). 92 Individual patient consent was not required as all data were deidentified.
Data availability statement
Electronic health records are, by definition, considered sensitive data in the UK by the Data Protection Act and cannot be shared via public deposition because of information governance restriction in place to protect patient confidentiality. Access to Clinical Practice Research Datalink (CPRD) data is subject to protocol approval via CPRD’s research data governance process. For more information see https://cprd.com/data-access . Linked secondary care data from Hospital Episodes Statistics, mortality data from the Office for National Statistics, and index of multiple deprivation data can also be requested from CPRD.
Acknowledgments
We thank Hayley Gorton and Thomas Allen for their contribution to the protocol development, Evan Kontopantelis for his statistical advice, and members of our patient and public involvement team, Antony Chuter and Jillian Beggs, for their contributions to this project. This study is based on data from the Clinical Practice Research Datalink obtained under licence from the UK Medicines and Healthcare Products Regulatory Agency (MHRA). The data are provided by patients and collected by the NHS as part of its care and support. Hospital Episode Statistics and Office for National Statistics mortality data are subject to Crown copyright (2022) protection, reused with the permission of The Health and Social Care Information Centre, all rights reserved. The interpretation and conclusions contained in this study are those of the authors alone, and not necessarily those of the MHRA, National Institute of Health and Care Research, NHS, or Department of Health and Social Care. The study protocol was approved by Clinical Practice Research Datalink’s independent scientific advisory committee (reference: 18_168). We would like to acknowledge all the data providers and general practices who make anonymised data available for research.
Contributors: All authors conceived and designed the study and acquired, analysed, or interpreted the data. BG, DRM, TvS, AJA, and DMA reviewed the clinical codes. PLHM conducted the statistical analyses and wrote the first draft of the manuscript. All authors critically revised the manuscript for important intellectual content and approved the final version. AJA, DMA, RAE, BG, DRM, AS, and TvS obtained the funding. PLHM is the guarantor. The corresponding author (PLHM) attests that all listed authors meet authorship criteria and that no others meeting the criteria have been omitted.
Funding: This study was funded by the National Institute for Health and Care Research (NIHR, RP-PG-1214-20012). MJC, AJA, and DMA were supported by the NIHR Greater Manchester Patient Safety Translational Research Centre (PSTRC-2016-003) at the time of this study and are now supported by the NIHR Greater Manchester Patient Safety Research Collaboration (NIHR204295). The funders had no role in the study design; in the collection, analysis, or interpretation of data; in the writing of the report; or in the decision to submit the article for publication. PLHM has full access to all data and all authors have full access to the statistical reports and tables in the study. PLHM takes responsibility for the integrity of the data and the accuracy of the data analysis.
Competing interests: All authors have completed the ICMJE uniform disclosure form at www.icmje.org/coi_disclosure.pdf and declare: BG reports research grants from the National Institute for Health and Care Research (NIHR). DRM was awarded a Wellcome Trust Clinical Research Development Fellowship (214588/Z/18/Z). AS reports a research grant from the NIHR. RAE reports research grants from the NIHR and NHS England, and travel costs to attend a roundtable dinner discussion on medication errors, House of Commons, Westminster, on 29 March 2022. TvS reports research grants from the NIHR. AJA is national clinical director for prescribing for NHS England and reports research grants from the NIHR. DMA reports research grants from the NIHR, AbbVie, Almirall, Celgene, Eli Lilly, Janssen, Novartis, UCB, and the Leo Foundation. All other authors declare no support from any organisation for the submitted work (except those listed in the funding section); no financial relationships with any organisations that might have an interest in the submitted work in the previous three years; no other relationships or activities that could appear to have influenced the submitted work.
Transparency: The lead author (PLHM: the manuscript’s guarantor) affirms that the manuscript is an honest, accurate, and transparent account of the study being reported; that no important aspects of the study have been omitted; and that any discrepancies from the study as planned (and, if relevant, registered) have been explained.
Dissemination to participants and related patient and public communities: This study used anonymised electronic health records from the CPRD and it is therefore not possible to disseminate the findings directly to individuals whose data we used. This study is part of a National Institute for Health and Care Research (NIHR) funded programme (RP-PG-1214-20012): Avoiding patient harm through the application of prescribing safety indicators in English general practices (PRoTeCT). We have experienced patient and public involvement members aligned to the programme who we will consult in the results dissemination. In addition, senior author DMA is director of NIHR Greater Manchester Patient Safety Research Collaboration (GMPSRC), and co-authors MJC and AJA are affiliated with it. The Patient Safety Research Collaboration has a community of public contributors including patients, carers, and people accessing health and social care services. The authors will work with this network to disseminate findings.
Provenance and peer review: Not commissioned; externally peer reviewed.
This is an Open Access article distributed in accordance with the terms of the Creative Commons Attribution (CC BY 4.0) license, which permits others to distribute, remix, adapt and build upon this work, for commercial use, provided the original work is properly cited. See: http://creativecommons.org/licenses/by/4.0/ .
- GBD 2019 Dementia Forecasting Collaborators
- McKeith I ,
- Stocks SJ ,
- Kontopantelis E ,
- Ashcroft DM
- Szczepura A ,
- Collings SL ,
- ↵ National Institute for Health and Care Excellence. Dementia: assessment, management and support for people living with dementia and their carers. NICE guideline NG97, 20 June 2018. www.nice.org.uk/guidance/ng97
- ↵ National Institute for Health and Care Excellence. Dementia: antipsychotics. Clinical knowledge summaries. December 2022. https://cks.nice.org.uk/topics/dementia/prescribing-information/antipsychotics/
- Marston L ,
- Nazareth I ,
- Petersen I ,
- Walters K ,
- Bronskill SE ,
- Normand SL ,
- Schneeweiss S ,
- Setoguchi S ,
- Brookhart A ,
- Dormuth C ,
- Dzahini O ,
- Schneider LS ,
- Dagerman KS ,
- Douglas IJ ,
- Sacchetti E ,
- Turrina C ,
- Valsecchi P
- Seyfried LS ,
- Kheirbek RE ,
- Little JT ,
- ↵ Medicines Healthcare Products Regulatory Agency. New advice issued on risperidone and olanzapine 2004. https://webarchive.nationalarchives.gov.uk/20141205150130/http://mhra.gov.uk/printpreview/defaultsp/con002047
- ↵ Medicines Healthcare Products Regulatory Agency. Antipsychotics: use in elderly patients with dementia. Drug safety update March 2009;2:8. https://webarchive.nationalarchives.gov.uk/20091114175357/http://mhra.gov.uk/Publications/Safetyguidance/DrugSafetyUpdate/CON041211
- ↵ Banerjee S. The use of antipsychotic medication for people with dementia: time for action. An independent report commissioned and funded by the Department of Health 2009.
- ↵ European Medicines Agency. Priorities for Drug Safety Research: Safety aspects of antipsychotics in demented patients. 2010. https://www.ema.europa.eu/en/documents/other/emea-2010-priorities-drug-safety-research-safety-aspects-antipsychotics-demented-patients_en.pdf
- Hessol NA ,
- Lexchin J ,
- Harrington C
- Lapeyre-Mestre M
- Rogowska M ,
- Thornton M ,
- van Marum RJ ,
- Jansen PA ,
- Souverein PC ,
- Schobben AF ,
- Trifirò G ,
- Gambassi G ,
- Tolppanen AM ,
- Koponen M ,
- Tanskanen A ,
- Zivkovic S ,
- Liperoti R ,
- Lapane KL ,
- Jönsson AK ,
- Spigset O ,
- Ostuzzi G ,
- Thabane L ,
- Brännström J ,
- Lövheim H ,
- Gustafson Y ,
- Nordström P
- Schuemie MJ ,
- Ramcharran D ,
- Brøgger Kristiansen S ,
- Baltzer Houlind M ,
- Herrett E ,
- Gallagher AM ,
- Bhaskaran K ,
- Campbell J ,
- Mahadevan P ,
- Fordyce S ,
- Rassen JA ,
- Rothman KJ ,
- Greenland S ,
- Kleijer BC ,
- Egberts AC ,
- Heerdink ER
- Lipsitch M ,
- Tchetgen Tchetgen E ,
- Austin PC ,
- Pariente A ,
- Fourrier-Réglat A ,
- Ducruet T ,
- Rigler SK ,
- Shireman TI ,
- Cook-Wiens GJ ,
- Balkwill A ,
- Million Women Study Collaborators
- Vinogradova Y ,
- Coupland C ,
- Hippisley-Cox J
- Sandler RS ,
- Kappelman MD
- Spoorenberg SMC ,
- Snijders D ,
- Ovidius Study Group ,
- Capisce Study Group ,
- STEP Study Group
- Koivula I ,
- ↵ Lunt M. Propensity analysis in Stata, revision: 1.1. 2014. University of Manchester. http://personalpages.manchester.ac.uk/staff/mark.lunt/propensity_guide.pdf
- Grambsch PM ,
- Therneau TM
- Gooley TA ,
- Leisenring W ,
- Crowley J ,
- Schmitz A ,
- Gastaldon C ,
- Sultana J ,
- Calabró M ,
- Garcia-Serna R ,
- Huybrechts KF ,
- Silliman RA ,
- Brookhart MA ,
- Schneeweiss S
- Gerhard T ,
- Sahlberg M ,
- Gislason GH ,
- Torp-Pedersen C ,
- Andersson C
- Crellin E ,
- Donegan K ,
- Padmanabhan S ,
- Cameron E ,
- Williams R ,
- Strongman H
- Nørgaard A ,
- Jensen-Dahm C ,
- Wimberley T ,
- Hansen ES ,
- Gitlin LN ,
- Lyketsos CG
- Goodarzi Z ,
- Veroniki AA ,
- Frederiksen KS ,
- Frisoni GB ,
- El Helou ML
- McDermid J ,
- Ballard C ,
- Schnier C ,
- McCarthy A ,
- Morales DR ,
- CVD-COVID-UK/COVID-IMPACT Consortium
- ↵ CPRD - Safeguarding patient data https://cprd.com/safeguarding-patient-data
Numbers, Facts and Trends Shaping Your World
Read our research on:
Full Topic List
Regions & Countries
- Publications
- Our Methods
- Short Reads
- Tools & Resources
Read Our Research On:
The American Trends Panel
What is the american trends panel (atp).
The ATP is Pew Research Center’s nationally representative online survey panel. The panel is composed of more than 10,000 adults selected at random from across the entire U.S.
Respondents have been recruited over the years, and they take our surveys frequently. The panel provides a relatively efficient method of data collection compared with fresh samples because the participants have already agreed to take part in more surveys. The major effort required with a fresh sample – making an initial contact, persuading respondents to take part and gathering the necessary demographic information for weighting – is not needed once a respondent has joined a panel. Another advantage of the ATP is that considerable information about panelists’ views and experiences can be accumulated over time. Because panelists may respond to multiple surveys on different topics, it is possible to build a much richer portrait of the public than is feasible in a single survey interview, which must be limited in length to prevent respondent fatigue.
But panels like the ATP have some limitations as well. They can be expensive to create and maintain, requiring more extensive technical skill and oversight than a one-off survey. A second concern is that panelists may drop out over time (known as attrition), making the panel less representative of the target population as time passes if the kinds of people who drop out are different from those who tend to remain. The ATP features an annual recruitment for new panelists from across the country to address this.
Another concern is that repeated questioning of the same individuals may yield different results than we would obtain with independent or “fresh” samples. If the same questions are asked repeatedly, respondents may remember their answers and feel some pressure to be consistent over time. The reverse is also a concern, as respondents might become “conditioned” to change their behavior because of questions asked previously. For example, questions about voting might spur them to register to vote. Respondents also become more skilled at answering particular kinds of questions. This may be beneficial in some instances, but to the extent it occurs, the panel results may be different from what would have been obtained from independent samples of people who have not had the practice in responding to surveys. Fortunately, research has detected no meaningful conditioning on the ATP .
Recruiting panelists to the ATP
The ATP was created in 2014, with the first cohort of panelists invited to join the panel at the end of a large, national, landline and cellphone random-digit-dial survey that was conducted in both English and Spanish. Two additional recruitments were conducted using the same method in 2015 and 2017, respectively.
In 2018, the ATP switched from telephone to address-based recruitment. Invitations are sent to a random, address-based sample (ABS) of households selected from the U.S. Postal Service’s Delivery Sequence File (DSF).
Randomization in sampling is carried through right down to the household level to maintain representativeness of sample. The adult with the next birthday in each selected household is asked to go online to complete a survey, at the end of which they are invited to join the panel.
For the online panel to be truly nationally representative, the share of those who do not use the internet must be represented on the panel somehow. In 2021, the share of non-internet users in the U.S was estimated to be 7%, and while this is a relatively small group, its members are quite different demographically from those who go online. In its early years, the ATP conducted interviews with non-internet users via paper questionnaires. However, in 2016, the Center switched to providing non-internet households with tablets which they could use to take the surveys online. The Center works with Ipsos , an international market and opinion research organization, to recruit panelists, manage the panel and conduct the surveys.
Drawing samples for ATP surveys
One of the benefits of a large panel like the ATP is that there are more panelists than a typical survey requires. Rather than selecting all 10,000-plus panelists each time, many ATP surveys interview only a subset (e.g., 2,500) of the panelists. This reduces the burden on individual panel members, sparing them from having to respond every time the Center fields a survey.
Drawing subsamples (rather than interviewing everyone on the panel) also allows Center researchers to make the samples more representative. Like most survey panels, the ATP has proportionately too many of some groups (e.g., college-educated individuals) and proportionately too few of others (e.g., young adults). ATP subsamples address these imbalances so that the responding sample looks quite like the U.S. public overall. This produces a sample that requires less weighting to align it with the population and, thus, a larger effective sample size.
Fielding ATP surveys
It takes about seven days for a questionnaire to be translated, programmed and made ready for its first phase of testing. The main objective during testing is to make sure that questions render on screen the way they ought to, and that question logic and skip patterns are all in place. For example, if the response to “How many people live in your household, including you?” is “1,” subsequent questions asking about other household members, such as “Are you the parent or guardian of any children under the age of 12 who live in your household?” should be skipped. Questions that require a number entry are also checked to make sure that incorrect formats (for example, decimals) are not an option when asking questions such as “What year were you born?” Lastly, this stage of testing is when most formatting changes and typos are caught and flagged for correction. Certain questions are programmed differently for mobile devices so that questions and text appear legibly without the need for horizontal, and sometimes (excessive) vertical scrolling.
A day or two are dedicated to fixing any programming errors that may have been detected during testing, as well as to updating the draft questionnaire and graduating it to a final stage. Programmers then send final test links (called “end to end” links) for final checking before survey launch. This round of testing verifies that the survey is working on all of the most common web browsers (e.g., Edge, Chrome, Mozilla, Safari) and devices (e.g., personal computers, Android phones, iPads, iPhones). Researchers also conduct breakoff tests to ensure that respondents can continue their survey at a later stage and on a different device.
Once testing is completed, the survey is ready for fielding. This process begins with a “soft launch,” wherein about 60 reliably fast ATP panelists are notified that the survey is ready. The soft launch typically takes place a day before full launch of the survey. Data quality checks are performed on this initial dataset to check the data format and to allow sufficient time to course correct any issues in the program that may be flagged before the survey gets sent out to all selected panelists for that survey. Survey invitations are sent out on the day the survey launches via email and, for panelists who consent to receiving SMS messages, via text messages as well. Several days after launch, panelists are sent up to two email or text reminders if they do not respond to the survey. From time to time, interactive voice recording reminder calls are also made to tablet households that previously provided consent to receive these reminders.
Data collection usually closes six to 14 days after full launch, depending on the research needs. Researchers then conduct a series of data quality checks to flag any issues with respondent satisficing or how answers were recorded in the dataset. All respondents are offered a post-paid incentive for their participation. Respondents choose to receive the post-paid incentive in the form of a check or a gift code to Amazon.com. Incentive amounts range depending on whether the respondent belongs to a part of the population that is harder or easier to reach. Differential incentive amounts are designed to increase panel survey participation among groups that traditionally have low survey response propensities.
Weighting ATP surveys
The ATP data is weighted in a multistep process that accounts for multiple stages of sampling and nonresponse that occur at different points in the survey process. First, each panelist begins with a base weight that reflects their probability of selection for their initial recruitment survey (and the probability of being invited to participate in the panel in cases where only a subsample of respondents were invited). The base weights for panelists recruited in different years are scaled to be proportionate to the effective sample size for all active panelists in their cohort. To correct for nonresponse to the initial recruitment surveys and gradual panel attrition, the base weights for all active panelists are calibrated to align with the population benchmarks identified in the accompanying table to create a full-panel weight.
For ATP waves in which only a subsample of panelists are invited to participate, a wave-specific base weight is created by adjusting the full-panel weights for subsampled panelists to account for any differential probabilities of selection for the particular panel wave. For waves in which all active panelists are invited to participate, the wave-specific base weight is identical to the full-panel weight.
In the final weighting step, the wave-specific base weights for panelists who completed the survey are again calibrated to match population benchmarks. The Center calibrates ATP surveys to both demographic benchmarks (e.g., age, education, sex, race, ethnicity, geography) and non-demographic benchmarks (e.g., political party affiliation, religious affiliation, registered voter status, volunteerism). These weights are then trimmed (typically at about the 1st and 99th percentiles) to reduce the loss in precision stemming from variance in the weights. Sampling errors and test of statistical significance take into account the effect of weighting.
Maintaining the ATP
Pew Research Center works with Ipsos to recruit panelists, manage the panel and conduct the surveys. Every year, we aim to “refresh” the panel by adding new panelists. Panelists also take an annual profile survey where they are given the opportunity to update certain aspects of their profile, such as their income, number of children, etc. If a panelist does not participate in the annual profile survey, they get retired from the panel. Occasionally, we also retire members that are demographically overrepresented on the panel, such as those who are more educated. We do this to ensure representation on the panel that resembles the general population, and to avoid having to weight certain panelists too heavily. We also retire panelists that have been inactive for a period and those who get flagged as repeat offenders on surveys for behaviors such as high refusal rates (skipping 80% or more questions) on two or more recent surveys.
We make a promise to our panelists to protect their identity. Several checks and balances are in place to make sure that Pew Research Center remains true to its word. Personal identifying information (PII) such as a panelist’s name or county of residence is maintained solely by the core panel administration team and is never made available to the general public. In some cases, additional steps such as data swapping – randomly swapping certain values among a small number of respondents with similar characteristics for sensitive questions – is also used to protect panelists’ information.
Learn more here about the history of the American Trends Panel , including its creation, development, and growth.
U.S. Surveys
Other research methods, sign up for our weekly newsletter.
Fresh data delivered Saturday mornings
1615 L St. NW, Suite 800 Washington, DC 20036 USA (+1) 202-419-4300 | Main (+1) 202-857-8562 | Fax (+1) 202-419-4372 | Media Inquiries
Research Topics
- Age & Generations
- Coronavirus (COVID-19)
- Economy & Work
- Family & Relationships
- Gender & LGBTQ
- Immigration & Migration
- International Affairs
- Internet & Technology
- Methodological Research
- News Habits & Media
- Non-U.S. Governments
- Other Topics
- Politics & Policy
- Race & Ethnicity
- Email Newsletters
ABOUT PEW RESEARCH CENTER Pew Research Center is a nonpartisan fact tank that informs the public about the issues, attitudes and trends shaping the world. It conducts public opinion polling, demographic research, media content analysis and other empirical social science research. Pew Research Center does not take policy positions. It is a subsidiary of The Pew Charitable Trusts .
Copyright 2024 Pew Research Center
Terms & Conditions
Privacy Policy
Cookie Settings
Reprints, Permissions & Use Policy
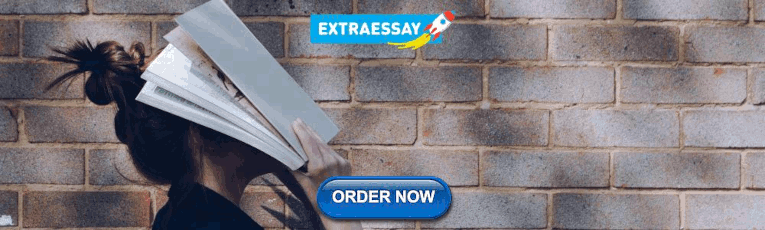
IMAGES
VIDEO
COMMENTS
A research design is a strategy for answering your research question using empirical data. Creating a research design means making decisions about: Your overall research objectives and approach. Whether you'll rely on primary research or secondary research. Your sampling methods or criteria for selecting subjects. Your data collection methods.
A research design is a strategy for answering your research question using empirical data. Creating a research design means making decisions about: ... For example, you might choose a case study that reveals an unusual or neglected aspect of your research problem, or you might choose several very similar or very different cases in order to ...
What is research design? Research design refers to the overall plan, structure or strategy that guides a research project, from its conception to the final data analysis.A good research design serves as the blueprint for how you, as the researcher, will collect and analyse data while ensuring consistency, reliability and validity throughout your study.
Research study design is a framework, or the set of methods and procedures used to collect and analyze data on variables specified in a particular research problem. Research study designs are of many types, each with its advantages and limitations. The type of study design used to answer a particular research question is determined by the ...
The purpose of research design is to plan and structure a research study in a way that enables the researcher to achieve the desired research goals with accuracy, validity, and reliability. Research design is the blueprint or the framework for conducting a study that outlines the methods, procedures, techniques, and tools for data collection ...
The research design refers to the overall strategy and analytical approach that you have chosen in order to integrate, in a coherent and logical way, the different components of the study, thus ensuring that the research problem will be thoroughly investigated. It constitutes the blueprint for the collection, measurement, and interpretation of ...
While many books and articles guide various qualitative research methods and analyses, there is currently no concise resource that explains and differentiates among the most common qualitative approaches. We believe novice qualitative researchers, students planning the design of a qualitative study or taking an introductory qualitative research course, and faculty teaching such courses can ...
Introduction. In clinical research, our aim is to design a study, which would be able to derive a valid and meaningful scientific conclusion using appropriate statistical methods that can be translated to the "real world" setting. 1 Before choosing a study design, one must establish aims and objectives of the study, and choose an appropriate target population that is most representative of ...
Types of Research Designs Compared | Guide & Examples. Published on June 20, 2019 by Shona McCombes.Revised on June 22, 2023. When you start planning a research project, developing research questions and creating a research design, you will have to make various decisions about the type of research you want to do.. There are many ways to categorize different types of research.
Research design refers to the overall strategy utilized to answer research questions. A research design typically outlines the theories and models underlying a project; the research question(s) of a project; a strategy for gathering data and information; and a strategy for producing answers from the data. A strong research design yields valid answers to research questions while weak designs ...
Ranganathan P. Understanding Research Study Designs. Indian J Crit Care Med 2019;23 (Suppl 4):S305-S307. Keywords: Clinical trials as topic, Observational studies as topic, Research designs. We use a variety of research study designs in biomedical research. In this article, the main features of each of these designs are summarized. Go to:
Research design methods refer to the systematic approaches and techniques used to plan, structure, and conduct a research study. The choice of research design method depends on the research questions, objectives, and the nature of the study. Here are some key research design methods commonly used in various fields: 1.
If your study system doesn't match these criteria, there are other types of research you can use to answer your research question. Step 3: Design your experimental treatments How you manipulate the independent variable can affect the experiment's external validity - that is, the extent to which the results can be generalized and applied ...
We may approach this study by 2 longitudinal designs: Prospective: we follow the individuals in the future to know who will develop the disease. Retrospective: we look to the past to know who developed the disease (e.g. using medical records) This design is the strongest among the observational studies. For example - to find out the relative ...
Step 1: Establish Priorities for Research Design. Before conducting any research study, you must address an important question: "how to create a research design.". The research design depends on the researcher's priorities and choices because every research has different priorities.
Research design is the framework of research methods and techniques chosen by a researcher to conduct a study. The design allows researchers to sharpen the research methods suitable for the subject matter and set up their studies for success. Creating a research topic explains the type of research (experimental,survey research,correlational ...
First, by the specific research question. That is, if the question is one of 'prevalence' (disease burden) then the ideal is a cross-sectional study; if it is a question of 'harm' - a case-control study; prognosis - a cohort and therapy - a RCT. Second, by what resources are available to you. This includes budget, time, feasibility re-patient ...
A sequential explanatory research design was chosen for this study, which combines qualitative and quantitative data collection and analysis strategies and integrates them into the interpretation ...
The design of a study defines the study type (descriptive, correlational, semi-experimental, experimental, review, meta-analytic) and sub-type (e.g., descriptive-longitudinal case study), research ...
As discussed earlier, common research methods for descriptive research include surveys, case studies, observational studies, cross-sectional studies, and longitudinal studies. Design your study: Plan the details of your study, including the sampling strategy, data collection methods, and data analysis plan.
Study data will be analyzed and reported using the Consolidated Criteria for Reporting Qualitative Research (COREQ) Framework . To analyze data, we will use template analysis, which combines features of deductive content analysis and inductive grounded theory, thereby allowing us to obtain specific information while also capturing any new or ...
research design and methods We conducted a multiancestry time-to-event genome-wide association study for incident CVD among people with T2D. We also tested 204 known coronary artery disease (CAD) variants for association with incident CVD.
Types of study design. Medical research is classified into primary and secondary research. Clinical/experimental studies are performed in primary research, whereas secondary research consolidates available studies as reviews, systematic reviews and meta-analyses. Three main areas in primary research are basic medical research, clinical research ...
Dr. Peter Chametzky, a professor of art history in the School of Visual Art and Design, has been honored with the prestigious Russell Research Award for Humanities and Social Sciences at the University of South Carolina.. The Russell Research Awards, established by Judge Donald S. Russell in 1957, are among the most esteemed accolades for research and scholarship at Carolina.
The main strength of our study was the use of an emulated target trial design to overcome common biases in observational analyses, including immortal time bias.34 In fact, bias in observational studies often arise predominantly due to poor study design, rather than confounding due to lack of randomisation, and estimates from carefully emulated ...
Problem statement, empathy map canvas, creativity techniques and a table full of prototyping material. - One of the most colorful and intensive workshops took place last week: In "Design Thinking Basics", our colleague Laura Martin equipped 10 highly motivated participants with the basics of design thinking and let them experience the mindset, process and tools on two use cases.
Existing studies have focused mainly on the environmental quality of scenic spots, such as sufficient oxygen content in the air and a high concentration of negative oxygen ions. The perceptions of soundscape in scenic areas are generally good, but there are few reports on the quantitative evaluation of soundscape quality in scenic areas. In this study, we analysed existing methods for ...
Consequently, these objectives determine the study design and research outcome. The development of research questions is a process based on knowledge of current trends, cutting-edge studies, and technological advances in the research field. Excellent research questions are focused and require a comprehensive literature search and in-depth ...
This study is part of a National Institute of Health and Care Research funded programme (RP-PG-1214-20012): Avoiding patient harm through the application of prescribing safety indicators in English general practices (PRoTeCT). Two patient and public involvement members in the project team contributed to the study design and protocol of this study.
The ATP is Pew Research Center's nationally representative online survey panel. The panel is composed of more than 10,000 adults selected at random from across the entire U.S. Respondents have been recruited over the years, and they take our surveys frequently. The panel provides a relatively efficient method of data collection compared with ...