Thank you for visiting nature.com. You are using a browser version with limited support for CSS. To obtain the best experience, we recommend you use a more up to date browser (or turn off compatibility mode in Internet Explorer). In the meantime, to ensure continued support, we are displaying the site without styles and JavaScript.
- View all journals
- Explore content
- About the journal
- Publish with us
- Sign up for alerts
- Published: 18 February 2021
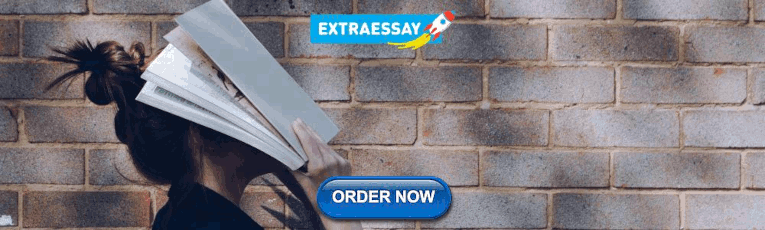
Essentials of data management: an overview
- Miren B. Dhudasia 1 , 2 ,
- Robert W. Grundmeier 2 , 3 , 4 &
- Sagori Mukhopadhyay 1 , 2 , 3
Pediatric Research volume 93 , pages 2–3 ( 2023 ) Cite this article
4184 Accesses
6 Citations
5 Altmetric
Metrics details
What is data management?
Data management is a multistep process that involves obtaining, cleaning, and storing data to allow accurate analysis and produce meaningful results. While data management has broad applications (and meaning) across many fields and industries, in clinical research the term data management is frequently used in the context of clinical trials. 1 This editorial is written to introduce early career researchers to practices of data management more generally, as applied to all types of clinical research studies.
Outlining a data management strategy prior to initiation of a research study plays an essential role in ensuring that both scientific integrity (i.e., data generated can accurately test the hypotheses proposed) and regulatory requirements are met. Data management can be divided into three steps—data collection, data cleaning and transformation, and data storage. These steps are not necessarily chronological and often occur simultaneously. Different aspects of the process may require the expertise of different people necessitating a team effort for the effective completion of all steps.
Data collection
Data source.
Data collection is a critical first step in the data management process and may be broadly classified as “primary data collection” (collection of data directly from the subjects specifically for the study) and “secondary use of data” (repurposing data that were collected for some other reason—either for clinical care in the subject’s medical record or for a different research study). While the terms retrospective and prospective data collection are occasionally used, 2 these terms are more applicable to how the data are utilized rather than how they are collected . Data used in a retrospective study are almost always secondary data; data collected as part of a prospective study typically involves primary data collection, but may also involve secondary use of data collected as part of ongoing routine clinical care for study subjects. Primary data collected for a specific study may be categorized as secondary data when used to investigate a new hypothesis, different from the question for which the data were originally collected. Primary data collection has the advantage of being specific to the study question, minimize missingness in key information, and provide an opportunity for data correction in real time. As a result, this type of data is considered more accurate but increases the time and cost of study procedures. Secondary use of data includes data abstracted from medical records, administrative data such as from the hospital’s data warehouse or insurance claims, and secondary use of primary data collected for a different research study. Secondary use of data offers access to large amounts of data that are already collected but often requires further cleaning and codification to align the data with the study question.
A case report form (CRF) is a powerful tool for effective data collection. A CRF is a paper or electronic questionnaire designed to record pertinent information from study subjects as outlined in the study protocol. 3 CRFs are always required in primary data collection but can also be useful in secondary use of data to preemptively identify, define, and, if necessary, derive critical variables for the study question. For instance, medical records provide a wide array of information that may not be required or be useful for the study question. A CRF with well-defined variables and parameters helps the chart reviewer focus only on the relevant data, and makes data collection more objective and unbiased, and, in addition, optimize patient confidentiality by minimizing the amount of patient information abstracted. Tools like REDCap (Research Electronic Data Capture) provide electronic CRFs and offer some advanced features like setting validation rules to minimize errors during data collection. 4 Designing an effective CRF upfront during the study planning phase helps to streamline the data collection process, and make it more efficient. 3
Data cleaning and transformation
Quality checks.
Data collected may have errors that arise from multiple sources—data manually entered in a CRF may have typographical errors, whereas data obtained from data warehouses or administrative databases may have missing data, implausible values, and nonrandom misclassification errors. Having a systematic approach to identify and rectify these errors, while maintaining a log of the steps performed in the process, can prevent many roadblocks during analysis.
First, it is important to check for missing data. Missing data are defined as values that are not available and that would be meaningful for analysis if they were observed. 5 Missing data can bias the results of the study depending on how much data is missing and what is the pattern of distribution of missing data in the study cohort. Many methods for handling missing data have been published. Kang 6 provide a practical review of methods for handling missing data. If missing data cannot be retrieved and is limited to only a small number of subjects, one approach is to exclude these subjects from the study. Missing data in different variables across many subjects often require more sophisticated approaches to account for the “missingness.” These may include creating a category of “missing” (for categorical variables), simple imputation (e.g., substituting missing values in a variable with an average of non-missing values in the variable), or multiple imputations (substituting missing values with the most probable value derived from other variables in the dataset). 7
Second, errors in the data can be identified by running a series of data validation checks. Some examples of data validation rules for identifying implausible values are shown in Table 1 . Automated algorithms for detection and correction of implausible values may be available for cleaning specific variables in large datasets (e.g., growth measurements). 8 After identification, data errors can either be corrected, if possible, or can be marked for deletion. Other approaches, similar to those for dealing with missing data, can also be used for managing data errors.
Data transformation
The data collected may not be in the form required for analysis. The process of data transformation includes recategorization and recodification of the data, which has been collected along with derivation of new variables, to align with the study analytic plan. Examples include categorizing body mass index collected as a continuous variable into under- and overweight categories, recoding free-text values such as “growth of an organism” or “no growth,” and into a binary “positive” or “negative,” or deriving new variables such as average weight per year from multiple weight values over time available in the dataset. Maintaining a code-book of definitions for all variables, predefined and derived, can help a data analyst better understand the data.
Data storage
Securely storing data is especially important in clinical research as the data may contain protected health information of the study subjects. 9 Most institutes that support clinical research have guidelines for safeguards to prevent accidental data breaches.
Data are collected in paper or electronic formats. Paper data should be stored in secure file cabinets inside a locked office at the site approved by the institutional review board. Electronic data should be stored on a secure approved institutional server, and should never be transported using unencrypted portable media devices (e.g., “thumb drives”). If all study team members do not require access to study data, then selective access should be granted to the study team members based on their roles.
Another important aspect of data storage is data de-identification. Data de-identification is a process by which identifying characteristics of the study participants are removed from the data, in order to mitigate privacy risks to individuals. 10 Identifying characteristics of a study subject includes name, medical record number, date of birth/death, and so on. To de-identify data, these characteristics should either be removed from the data or modified (e.g., changing the medical record number to study IDs, changing dates to age/duration, etc.). If feasible, study data should be de-identified when storing. If you anticipate that reidentification of the study participants may be required in future, then the data can be separated into two files, one containing only the de-identified data of the study participants, and one containing all the identifying information, with both files containing a common linking variable (e.g., study ID), which is unique for every subject or record in the two files. The linking variable can be used to merge the two files when reidentification is required to carry out additional analyses or to get further data. The link key should be maintained in a secure institutional server accessible only to authorized individuals who need access to the identifiers.
To conclude, effective data management is important to the successful completion of research studies and to ensure the validity of the results. Outlining the steps of the data management process upfront will help streamline the process and reduce the time and effort subsequently required. Assigning team members responsible for specific steps and maintaining a log, with date/time stamp to document each action as it happens, whether you are collecting, cleaning, or storing data, can ensure all required steps are done correctly and identify any errors easily. Effective documentation is a regulatory requirement for many clinical trials and is helpful for ensuring all team members are on the same page. When interpreting results, it will serve as an important tool to assess if the interpretations are valid and unbiased. Last, it will ensure the reproducibility of the study findings.
Krishnankutty, B., Bellary, S., Kumar, N. B. & Moodahadu, L. S. Data management in clinical research: an overview. Indian J. Pharm. 44 , 168–172 (2012).
Article Google Scholar
Weinger, M. B. et al. Retrospective data collection and analytical techniques for patient safety studies. J. Biomed. Inf. 36 , 106–119 (2003).
Avey, M. in Clinical Data Management 2nd edn. (eds Rondel, R. K., Varley, S. A. & Webb, C. F.) 47–73 (Wiley, 1999).
Harris, P. A. et al. Research electronic data capture (REDCap)—a metadata-driven methodology and workflow process for providing translational research informatics support. J. Biomed. Inf. 42 , 377–381 (2009).
Little, R. J. et al. The prevention and treatment of missing data in clinical trials. N. Engl. J. Med. 367 , 1355–1360 (2012).
Article CAS Google Scholar
Kang, H. The prevention and handling of the missing data. Korean J. Anesthesiol. 64 , 402 (2013).
Rubin, D. B. Inference and missing data. Biometrika 63 , 581–592 (1976).
Daymont, C. et al. Automated identification of implausible values in growth data from pediatric electronic health records. J. Am. Med. Inform. Assoc. 24 , 1080–1087 (2017).
Office for Civil Rights, Department of Health and Human Services. Health insurance portability and accountability act (HIPAA) privacy rule and the national instant criminal background check system (NICS). Final rule. Fed. Regist. 81 , 382–396 (2016).
Google Scholar
Office for Civil Rights (OCR). Methods for de-identification of PHI. https://www.hhs.gov/hipaa/for-professionals/privacy/special-topics/de-identification/index.html (2012).
Download references
Acknowledgements
This work was partially supported in part by the Eunice Kennedy Shriver National Institute of Child Health & Human Development of the National Institutes of Health grant (K23HD088753).
Author information
Authors and affiliations.
Division of Neonatology, Children’s Hospital of Philadelphia, Philadelphia, PA, USA
Miren B. Dhudasia & Sagori Mukhopadhyay
Center for Pediatric Clinical Effectiveness, Children’s Hospital of Philadelphia, Philadelphia, PA, USA
Miren B. Dhudasia, Robert W. Grundmeier & Sagori Mukhopadhyay
Department of Pediatrics, University of Pennsylvania Perelman School of Medicine, Philadelphia, PA, USA
Robert W. Grundmeier & Sagori Mukhopadhyay
Department of Biomedical and Health Informatics, Children’s Hospital of Philadelphia, Philadelphia, PA, USA
Robert W. Grundmeier
You can also search for this author in PubMed Google Scholar
Corresponding author
Correspondence to Sagori Mukhopadhyay .
Ethics declarations
Competing interests.
The authors declare no competing interests.
Additional information
Publisher’s note Springer Nature remains neutral with regard to jurisdictional claims in published maps and institutional affiliations.
Rights and permissions
Reprints and permissions
About this article
Cite this article.
Dhudasia, M.B., Grundmeier, R.W. & Mukhopadhyay, S. Essentials of data management: an overview. Pediatr Res 93 , 2–3 (2023). https://doi.org/10.1038/s41390-021-01389-7
Download citation
Received : 11 December 2020
Revised : 27 December 2020
Accepted : 06 January 2021
Published : 18 February 2021
Issue Date : January 2023
DOI : https://doi.org/10.1038/s41390-021-01389-7
Share this article
Anyone you share the following link with will be able to read this content:
Sorry, a shareable link is not currently available for this article.
Provided by the Springer Nature SharedIt content-sharing initiative
This article is cited by
Advancing clinical and translational research in germ cell tumours (gct): recommendations from the malignant germ cell international consortium.
- Adriana Fonseca
- Matthew J. Murray
British Journal of Cancer (2022)
Quick links
- Explore articles by subject
- Guide to authors
- Editorial policies

Qualitative Methods of Validation
- Reference work entry
- First Online: 16 May 2017
- Cite this reference work entry
- Anne Lazaraton 5
Part of the book series: Encyclopedia of Language and Education ((ELE))
5669 Accesses
3 Citations
It is not surprising that there are continuing tensions between the disciplinary research paradigms in which language testers situate themselves: psychometrics, which, by definition, involves the objective measurement of psychological traits, processes, and abilities and is based on the analysis of sophisticated, quantitative data, and applied linguistics, where the study of language in use, and especially the construction of discourse, often demands a more interpretive, qualitative approach to the research process. This chapter looks at qualitative research techniques that are increasingly popular choices for designing, revising, and validating performance tests – those in which test takers write or speak, the latter of which is the primary focus of this chapter. It traces the history of qualitative research in language testing from 1990 to the present and describes some of the main findings about face-to-face speaking tests that have emerged from this scholarship. Several recent qualitative research papers on speaking tests are summarized, followed by an examination of three mixed methods studies, where both qualitative and quantitative techniques are carefully and consciously mixed in order to further elucidate findings that could not be derived from either method alone. I conclude by considering challenges facing qualitative language testing researchers, especially in terms of explicating research designs and determining appropriate evaluative criteria, and speculating on areas for future research, including studies that tap other methodological approaches such as critical language testing and ethnography and that shed light on World Englishes (WEs) and the Common European Framework of Reference (CEFR).
This is a preview of subscription content, log in via an institution to check access.
Access this chapter
- Available as PDF
- Read on any device
- Instant download
- Own it forever
- Available as EPUB and PDF
- Durable hardcover edition
- Dispatched in 3 to 5 business days
- Free shipping worldwide - see info
Tax calculation will be finalised at checkout
Purchases are for personal use only
Institutional subscriptions
Brown, J. D. (2014a). Mixed methods research for TESOL . Edinburgh: Edinburgh University Press.
Google Scholar
Brown, J. D. (2014b). The future of World Englishes in language testing. Language Assessment Quarterly, 11 (1), 5–26. doi:10.1080/15434303.2013.869817.
Article Google Scholar
Creswell, J. W. (2014). A concise introduction to mixed methods research . Los Angeles: Sage.
Gan, Z. (2010). Interaction in group assessment: A case study of higher- and lower-scoring students. Language Testing, 27 (4), 585–602. doi:10.1177/0265532210364049.
Green, A. (1998). Verbal protocol analysis in language testing research . Cambridge: Cambridge University Press.
Harding, L. (2014). Communicative language testing: Current issues and future research. Language Assessment Quarterly, 11 (2), 186–197. doi:10.1080/15434303.2014.895829.
Hill, K., & McNamara, T. (2012). Developing a comprehensive, empirically based research framework for classroom-based assessment. Language Testing, 29 (3), 395–420. doi:10.1177/0265532211428317.
Jacoby, S., & Ochs, E. (1995). Co-construction: An introduction. Research on Language and Social Interaction, 28 (3), 171–183. doi:10.1207/s15327973rlsi2803_1.
Kane, M. (2012). Validating score interpretations and uses: Messick Lecture. Language Testing, 29 (1), 3–17. doi:10.1177/0265532211417210.
Kim, Y.-H. (2009). An investigation into native and non-native teachers’ judgments of oral English performance: A mixed methods approach. Language Testing, 26 (2), 187–217. doi:10.1177/0265532208101010.
Lazaraton, A. (2002). A qualitative approach to the validation of oral language tests . Cambridge: Cambridge University Press.
Lazaraton, A. (2008). Utilizing qualitative methods for assessment. In E. Shohamy & N. H. Hornberger (Eds.), Encyclopedia of language and education (Language testing and assessment 2nd ed., Vol. 7, pp. 197–209). New York: Springer.
Li, H., & He, L. (2015). A comparison of EFL raters’ essay-rating processes across two types of rating scales. Language Assessment Quarterly, 12 , 178–212. doi:10.1080/15434303.2015.1011738.
Luk, J. (2010). Talking to score: Impression management in L2 oral assessment and the co-construction of a test discourse genre. Language Assessment Quarterly, 7 (1), 25–53. doi:10.1080/15434300903473997.
May, L. (2011). Interactional competence in a paired speaking test: Features salient to raters. Language Assessment Quarterly, 8 (2), 127–145. doi:10.1080/15434303.2011.565845.
McNamara, T. (2011). Applied linguistics and measurement: A dialogue. Language Testing, 28 (4), 435–440. doi:10.1177/0265532211413446.
McNamara, T. (2014). 30 years on – Evolution or revolution? Language Assessment Quarterly, 11 (2), 226–232. doi:10.1080/15434303.2014.895830.
Norton, J. (2013). Performing identities in speaking tests: Co-construction revisited. Language Assessment Quarterly, 10 (3), 309–330. doi:10.1080/15434303.2013.769549.
O’Loughlin, K. (2011). The interpretation and use of proficiency test scores in university selection: How valid and ethical are they? Language Assessment Quarterly, 8 (2), 146–160. doi:10.1080/15434303.2011.564698.
Sasaki, M. (2014). Introspective methods. In A. Kunnan (Ed.), Companion to language assessment. Wiley. doi:10.1002/9781118411360.wbcla076.
Shohamy, E. (2001). The power of tests: A critical perspective on the use of language tests . New York: Pearson.
Shohamy, E., & McNamara, T. (2009). Language assessment for immigration, citizenship, and asylum. Language Assessment Quarterly,6 (4). doi:10.1080/15434300802606440.
Sidnell, J., & Stivers, T. (Eds.). (2013). The handbook of conversation analysis . West Sussex: Wiley-Blackwell.
Taylor, L., & Galaczi, E. (2011). Scoring validity. In L. Taylor (Ed.), Examining speaking: Research and practice in assessing second language speaking (pp. 171–233). Cambridge: Cambridge University Press.
Tsagari, D. (2012). FCE exam preparation discourses: Insights from an ethnographic study. UCLES Research Notes, 47 , 36–48.
Turner, C. (2014). Mixed methods research. In A. Kunnan (Ed.), Companion to language assessment . Wiley. doi:10.1002/9781118411360.wbcla142.
Van Lier, L. (1989). Reeling, writhing, drawling, stretching, and fainting in coils: Oral proficiency interviews as conversation. TESOL Quarterly, 23 (3), 489–508. doi:10.2307/3586922.
Winke, P., & Gass, S. (2013). The influence of second language experience and accent familiarity on oral proficiency rating: A qualitative investigation. TESOL Quarterly, 47 (4), 762–789. doi:10.1002/tesq.73.
Youn, S. J. (2015). Validity argument for assessing L2 pragmatics in interaction using mixed methods. Language Testing, 32 (2), 199–225. doi:10.1177/0265532214557113.
Zhao, C. G. (2013). Measuring authorial voice strength in L2 argumentative writing: The development and validation of an analytic rubric. Language Testing, 30 , 201–230. doi:10.1177/0265532212456965.
Download references
Author information
Authors and affiliations.
Department of Writing Studies, University of Minnesota, 200 Nolte Center, Minneapolis, MN, 55455, USA
Anne Lazaraton
You can also search for this author in PubMed Google Scholar
Corresponding author
Correspondence to Anne Lazaraton .
Editor information
Editors and affiliations.
School of Education, Tel Aviv University, Tel Aviv, Israel
Elana Shohamy
Faculty of Education and Social Work, The University of Auckland, Auckland, New Zealand
Stephen May
Rights and permissions
Reprints and permissions
Copyright information
© 2017 Springer International Publishing AG
About this entry
Cite this entry.
Lazaraton, A. (2017). Qualitative Methods of Validation. In: Shohamy, E., Or, I., May, S. (eds) Language Testing and Assessment. Encyclopedia of Language and Education. Springer, Cham. https://doi.org/10.1007/978-3-319-02261-1_15
Download citation
DOI : https://doi.org/10.1007/978-3-319-02261-1_15
Published : 16 May 2017
Publisher Name : Springer, Cham
Print ISBN : 978-3-319-02260-4
Online ISBN : 978-3-319-02261-1
eBook Packages : Education Reference Module Humanities and Social Sciences Reference Module Education
Share this entry
Anyone you share the following link with will be able to read this content:
Sorry, a shareable link is not currently available for this article.
Provided by the Springer Nature SharedIt content-sharing initiative
- Publish with us
Policies and ethics
- Find a journal
- Track your research

An official website of the United States government
The .gov means it’s official. Federal government websites often end in .gov or .mil. Before sharing sensitive information, make sure you’re on a federal government site.
The site is secure. The https:// ensures that you are connecting to the official website and that any information you provide is encrypted and transmitted securely.
- Publications
- Account settings
Preview improvements coming to the PMC website in October 2024. Learn More or Try it out now .
- Advanced Search
- Journal List
- J Family Med Prim Care
- v.4(3); Jul-Sep 2015
Validity, reliability, and generalizability in qualitative research
Lawrence leung.
1 Department of Family Medicine, Queen's University, Kingston, Ontario, Canada
2 Centre of Studies in Primary Care, Queen's University, Kingston, Ontario, Canada
In general practice, qualitative research contributes as significantly as quantitative research, in particular regarding psycho-social aspects of patient-care, health services provision, policy setting, and health administrations. In contrast to quantitative research, qualitative research as a whole has been constantly critiqued, if not disparaged, by the lack of consensus for assessing its quality and robustness. This article illustrates with five published studies how qualitative research can impact and reshape the discipline of primary care, spiraling out from clinic-based health screening to community-based disease monitoring, evaluation of out-of-hours triage services to provincial psychiatric care pathways model and finally, national legislation of core measures for children's healthcare insurance. Fundamental concepts of validity, reliability, and generalizability as applicable to qualitative research are then addressed with an update on the current views and controversies.
Nature of Qualitative Research versus Quantitative Research
The essence of qualitative research is to make sense of and recognize patterns among words in order to build up a meaningful picture without compromising its richness and dimensionality. Like quantitative research, the qualitative research aims to seek answers for questions of “how, where, when who and why” with a perspective to build a theory or refute an existing theory. Unlike quantitative research which deals primarily with numerical data and their statistical interpretations under a reductionist, logical and strictly objective paradigm, qualitative research handles nonnumerical information and their phenomenological interpretation, which inextricably tie in with human senses and subjectivity. While human emotions and perspectives from both subjects and researchers are considered undesirable biases confounding results in quantitative research, the same elements are considered essential and inevitable, if not treasurable, in qualitative research as they invariable add extra dimensions and colors to enrich the corpus of findings. However, the issue of subjectivity and contextual ramifications has fueled incessant controversies regarding yardsticks for quality and trustworthiness of qualitative research results for healthcare.
Impact of Qualitative Research upon Primary Care
In many ways, qualitative research contributes significantly, if not more so than quantitative research, to the field of primary care at various levels. Five qualitative studies are chosen to illustrate how various methodologies of qualitative research helped in advancing primary healthcare, from novel monitoring of chronic obstructive pulmonary disease (COPD) via mobile-health technology,[ 1 ] informed decision for colorectal cancer screening,[ 2 ] triaging out-of-hours GP services,[ 3 ] evaluating care pathways for community psychiatry[ 4 ] and finally prioritization of healthcare initiatives for legislation purposes at national levels.[ 5 ] With the recent advances of information technology and mobile connecting device, self-monitoring and management of chronic diseases via tele-health technology may seem beneficial to both the patient and healthcare provider. Recruiting COPD patients who were given tele-health devices that monitored lung functions, Williams et al. [ 1 ] conducted phone interviews and analyzed their transcripts via a grounded theory approach, identified themes which enabled them to conclude that such mobile-health setup and application helped to engage patients with better adherence to treatment and overall improvement in mood. Such positive findings were in contrast to previous studies, which opined that elderly patients were often challenged by operating computer tablets,[ 6 ] or, conversing with the tele-health software.[ 7 ] To explore the content of recommendations for colorectal cancer screening given out by family physicians, Wackerbarth, et al. [ 2 ] conducted semi-structure interviews with subsequent content analysis and found that most physicians delivered information to enrich patient knowledge with little regard to patients’ true understanding, ideas, and preferences in the matter. These findings suggested room for improvement for family physicians to better engage their patients in recommending preventative care. Faced with various models of out-of-hours triage services for GP consultations, Egbunike et al. [ 3 ] conducted thematic analysis on semi-structured telephone interviews with patients and doctors in various urban, rural and mixed settings. They found that the efficiency of triage services remained a prime concern from both users and providers, among issues of access to doctors and unfulfilled/mismatched expectations from users, which could arouse dissatisfaction and legal implications. In UK, a care pathways model for community psychiatry had been introduced but its benefits were unclear. Khandaker et al. [ 4 ] hence conducted a qualitative study using semi-structure interviews with medical staff and other stakeholders; adopting a grounded-theory approach, major themes emerged which included improved equality of access, more focused logistics, increased work throughput and better accountability for community psychiatry provided under the care pathway model. Finally, at the US national level, Mangione-Smith et al. [ 5 ] employed a modified Delphi method to gather consensus from a panel of nominators which were recognized experts and stakeholders in their disciplines, and identified a core set of quality measures for children's healthcare under the Medicaid and Children's Health Insurance Program. These core measures were made transparent for public opinion and later passed on for full legislation, hence illustrating the impact of qualitative research upon social welfare and policy improvement.
Overall Criteria for Quality in Qualitative Research
Given the diverse genera and forms of qualitative research, there is no consensus for assessing any piece of qualitative research work. Various approaches have been suggested, the two leading schools of thoughts being the school of Dixon-Woods et al. [ 8 ] which emphasizes on methodology, and that of Lincoln et al. [ 9 ] which stresses the rigor of interpretation of results. By identifying commonalities of qualitative research, Dixon-Woods produced a checklist of questions for assessing clarity and appropriateness of the research question; the description and appropriateness for sampling, data collection and data analysis; levels of support and evidence for claims; coherence between data, interpretation and conclusions, and finally level of contribution of the paper. These criteria foster the 10 questions for the Critical Appraisal Skills Program checklist for qualitative studies.[ 10 ] However, these methodology-weighted criteria may not do justice to qualitative studies that differ in epistemological and philosophical paradigms,[ 11 , 12 ] one classic example will be positivistic versus interpretivistic.[ 13 ] Equally, without a robust methodological layout, rigorous interpretation of results advocated by Lincoln et al. [ 9 ] will not be good either. Meyrick[ 14 ] argued from a different angle and proposed fulfillment of the dual core criteria of “transparency” and “systematicity” for good quality qualitative research. In brief, every step of the research logistics (from theory formation, design of study, sampling, data acquisition and analysis to results and conclusions) has to be validated if it is transparent or systematic enough. In this manner, both the research process and results can be assured of high rigor and robustness.[ 14 ] Finally, Kitto et al. [ 15 ] epitomized six criteria for assessing overall quality of qualitative research: (i) Clarification and justification, (ii) procedural rigor, (iii) sample representativeness, (iv) interpretative rigor, (v) reflexive and evaluative rigor and (vi) transferability/generalizability, which also double as evaluative landmarks for manuscript review to the Medical Journal of Australia. Same for quantitative research, quality for qualitative research can be assessed in terms of validity, reliability, and generalizability.
Validity in qualitative research means “appropriateness” of the tools, processes, and data. Whether the research question is valid for the desired outcome, the choice of methodology is appropriate for answering the research question, the design is valid for the methodology, the sampling and data analysis is appropriate, and finally the results and conclusions are valid for the sample and context. In assessing validity of qualitative research, the challenge can start from the ontology and epistemology of the issue being studied, e.g. the concept of “individual” is seen differently between humanistic and positive psychologists due to differing philosophical perspectives:[ 16 ] Where humanistic psychologists believe “individual” is a product of existential awareness and social interaction, positive psychologists think the “individual” exists side-by-side with formation of any human being. Set off in different pathways, qualitative research regarding the individual's wellbeing will be concluded with varying validity. Choice of methodology must enable detection of findings/phenomena in the appropriate context for it to be valid, with due regard to culturally and contextually variable. For sampling, procedures and methods must be appropriate for the research paradigm and be distinctive between systematic,[ 17 ] purposeful[ 18 ] or theoretical (adaptive) sampling[ 19 , 20 ] where the systematic sampling has no a priori theory, purposeful sampling often has a certain aim or framework and theoretical sampling is molded by the ongoing process of data collection and theory in evolution. For data extraction and analysis, several methods were adopted to enhance validity, including 1 st tier triangulation (of researchers) and 2 nd tier triangulation (of resources and theories),[ 17 , 21 ] well-documented audit trail of materials and processes,[ 22 , 23 , 24 ] multidimensional analysis as concept- or case-orientated[ 25 , 26 ] and respondent verification.[ 21 , 27 ]
Reliability
In quantitative research, reliability refers to exact replicability of the processes and the results. In qualitative research with diverse paradigms, such definition of reliability is challenging and epistemologically counter-intuitive. Hence, the essence of reliability for qualitative research lies with consistency.[ 24 , 28 ] A margin of variability for results is tolerated in qualitative research provided the methodology and epistemological logistics consistently yield data that are ontologically similar but may differ in richness and ambience within similar dimensions. Silverman[ 29 ] proposed five approaches in enhancing the reliability of process and results: Refutational analysis, constant data comparison, comprehensive data use, inclusive of the deviant case and use of tables. As data were extracted from the original sources, researchers must verify their accuracy in terms of form and context with constant comparison,[ 27 ] either alone or with peers (a form of triangulation).[ 30 ] The scope and analysis of data included should be as comprehensive and inclusive with reference to quantitative aspects if possible.[ 30 ] Adopting the Popperian dictum of falsifiability as essence of truth and science, attempted to refute the qualitative data and analytes should be performed to assess reliability.[ 31 ]
Generalizability
Most qualitative research studies, if not all, are meant to study a specific issue or phenomenon in a certain population or ethnic group, of a focused locality in a particular context, hence generalizability of qualitative research findings is usually not an expected attribute. However, with rising trend of knowledge synthesis from qualitative research via meta-synthesis, meta-narrative or meta-ethnography, evaluation of generalizability becomes pertinent. A pragmatic approach to assessing generalizability for qualitative studies is to adopt same criteria for validity: That is, use of systematic sampling, triangulation and constant comparison, proper audit and documentation, and multi-dimensional theory.[ 17 ] However, some researchers espouse the approach of analytical generalization[ 32 ] where one judges the extent to which the findings in one study can be generalized to another under similar theoretical, and the proximal similarity model, where generalizability of one study to another is judged by similarities between the time, place, people and other social contexts.[ 33 ] Thus said, Zimmer[ 34 ] questioned the suitability of meta-synthesis in view of the basic tenets of grounded theory,[ 35 ] phenomenology[ 36 ] and ethnography.[ 37 ] He concluded that any valid meta-synthesis must retain the other two goals of theory development and higher-level abstraction while in search of generalizability, and must be executed as a third level interpretation using Gadamer's concepts of the hermeneutic circle,[ 38 , 39 ] dialogic process[ 38 ] and fusion of horizons.[ 39 ] Finally, Toye et al. [ 40 ] reported the practicality of using “conceptual clarity” and “interpretative rigor” as intuitive criteria for assessing quality in meta-ethnography, which somehow echoed Rolfe's controversial aesthetic theory of research reports.[ 41 ]
Food for Thought
Despite various measures to enhance or ensure quality of qualitative studies, some researchers opined from a purist ontological and epistemological angle that qualitative research is not a unified, but ipso facto diverse field,[ 8 ] hence any attempt to synthesize or appraise different studies under one system is impossible and conceptually wrong. Barbour argued from a philosophical angle that these special measures or “technical fixes” (like purposive sampling, multiple-coding, triangulation, and respondent validation) can never confer the rigor as conceived.[ 11 ] In extremis, Rolfe et al. opined from the field of nursing research, that any set of formal criteria used to judge the quality of qualitative research are futile and without validity, and suggested that any qualitative report should be judged by the form it is written (aesthetic) and not by the contents (epistemic).[ 41 ] Rolfe's novel view is rebutted by Porter,[ 42 ] who argued via logical premises that two of Rolfe's fundamental statements were flawed: (i) “The content of research report is determined by their forms” may not be a fact, and (ii) that research appraisal being “subject to individual judgment based on insight and experience” will mean those without sufficient experience of performing research will be unable to judge adequately – hence an elitist's principle. From a realism standpoint, Porter then proposes multiple and open approaches for validity in qualitative research that incorporate parallel perspectives[ 43 , 44 ] and diversification of meanings.[ 44 ] Any work of qualitative research, when read by the readers, is always a two-way interactive process, such that validity and quality has to be judged by the receiving end too and not by the researcher end alone.
In summary, the three gold criteria of validity, reliability and generalizability apply in principle to assess quality for both quantitative and qualitative research, what differs will be the nature and type of processes that ontologically and epistemologically distinguish between the two.
Source of Support: Nil.
Conflict of Interest: None declared.
Data Validation for Machine Learning
Research areas.
Machine Intelligence
Data Management
Learn more about how we conduct our research
We maintain a portfolio of research projects, providing individuals and teams the freedom to emphasize specific types of work.
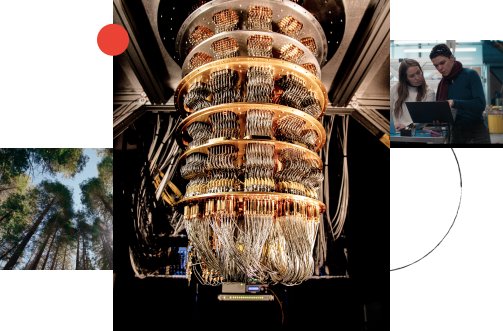
- Open access
- Published: 10 May 2024
Cross-site validation of lung cancer diagnosis by electronic nose with deep learning: a multicenter prospective study
- Meng-Rui Lee 1 , 2 ,
- Mu-Hsiang Kao 3 ,
- Ya-Chu Hsieh 3 ,
- Min Sun 3 ,
- Kea-Tiong Tang 3 ,
- Jann-Yuan Wang 1 ,
- Chao-Chi Ho 1 ,
- Jin-Yuan Shih 1 &
- Chong-Jen Yu 1 , 2
Respiratory Research volume 25 , Article number: 203 ( 2024 ) Cite this article
262 Accesses
1 Altmetric
Metrics details
Although electronic nose (eNose) has been intensively investigated for diagnosing lung cancer, cross-site validation remains a major obstacle to be overcome and no studies have yet been performed.
Patients with lung cancer, as well as healthy control and diseased control groups, were prospectively recruited from two referral centers between 2019 and 2022. Deep learning models for detecting lung cancer with eNose breathprint were developed using training cohort from one site and then tested on cohort from the other site. Semi-Supervised Domain-Generalized (Semi-DG) Augmentation (SDA) and Noise-Shift Augmentation (NSA) methods with or without fine-tuning was applied to improve performance.
In this study, 231 participants were enrolled, comprising a training/validation cohort of 168 individuals (90 with lung cancer, 16 healthy controls, and 62 diseased controls) and a test cohort of 63 individuals (28 with lung cancer, 10 healthy controls, and 25 diseased controls). The model has satisfactory results in the validation cohort from the same hospital while directly applying the trained model to the test cohort yielded suboptimal results (AUC, 0.61, 95% CI: 0.47─0.76). The performance improved after applying data augmentation methods in the training cohort (SDA, AUC: 0.89 [0.81─0.97]; NSA, AUC:0.90 [0.89─1.00]). Additionally, after applying fine-tuning methods, the performance further improved (SDA plus fine-tuning, AUC:0.95 [0.89─1.00]; NSA plus fine-tuning, AUC:0.95 [0.90─1.00]).
Our study revealed that deep learning models developed for eNose breathprint can achieve cross-site validation with data augmentation and fine-tuning. Accordingly, eNose breathprints emerge as a convenient, non-invasive, and potentially generalizable solution for lung cancer detection.
Clinical trial registration
This study is not a clinical trial and was therefore not registered.
Introduction
Lung cancer remains a predominant cause of cancer-related mortality worldwide, accounting for an estimated 2.2 million new cases and 1.8 million deaths in 2020 [ 1 ]. In its early stages, lung cancer often presents no symptoms, making it challenging to detect during routine health examinations. Although low-dose computed tomography (CT) of chest has been employed for lung cancer screening to facilitate earlier diagnosis and reduce mortality, a significant number of lung cancer patients remain undiagnosed until the disease has advance [ 2 ]. Furthermore, low-dose CT of chest has its limitations, including high cost, radiation exposure, and limited availability in many clinics. Consequently, there is a pressing need for a non-invasive, cost-effective, and readily accessible screening tool for early detection of lung cancer.
Electronic nose (eNose) is a novel device using sensors to generate breathprints that reflect patterns of volatile organic compounds [ 3 ]. eNose has the advantage of being non-invasive, easy to operate, short turnaround time and point-of-care. eNose has been applied in diagnosis of various diseases, encompassing communicable diseases such as COVID-19, tuberculosis and non-communicable diseases including diabetes and cancer. eNose has also been investigated in lung cancer diagnosis and treatment monitoring in previous studies.
Earlier studies evaluating eNose in lung cancer detection were mainly single center and compare between lung cancer and healthy control [ 4 ]. Previous studies also have shortcomings of lack of validation, especially cross-site validation [ 5 ]. While breathomics are prone to change in environment, external validation remains a major obstacle to clinical application. While more recent studies usually include a multicenter design of recruiting participants, cross site and independent validation were still not readily available [ 6 , 7 ].
On the other hand, algorithms for eNose breathprint analysis is also in evolution [ 8 ]. Deep learning involving convoluted neural network (CNN) is novel and emerging technique for breathprint analysis [ 8 , 9 ]. Some analytic approaches such as transfer learning and data augmentation have been applied in other aspects of biomedical imaging researches [ 10 ]. These methods could potentially propagate sample size, enhance performance and ameliorate the drop of performance in domain shift [ 11 , 12 ]. Most eNose studies have not yet incorporated this into analytic methods of eNose breathprint for lung cancer identification.
This study, therefore, aimed to validate eNose breathprint for lung cancer diagnosis in a cross-site setting with deep learning techniques including data augmentation and fine-tuning incorporated into the analytic methods. We aimed to expand generalizability of eNose breathprint in lung cancer diagnosis and advance eNose further in clinical practice.
Patient selection and study setting
This study was conducted prospectively at two facilities: the National Taiwan University Hospital (NTUH; test cohort, S2, site 2) and its Hsin-Chu branch (NTUH-HC; training/validation cohort, S1, site 1), both of which are referral centers for individuals with lung cancer and lung cancer suspects in Taiwan. The NTUH, a 2300-bed medical center in northern Taiwan, and the NTUH-HC, a regional hospital located 60 km away with a 700-bed capacity, have actively participated in eNose breathprint studies. The personnel at these institutions are well-acquainted with the eNose collection process and equipment operation. The institutional review boards (IRB) of participating hospitals approved this study (IRB no. 202112057RINB, 108-011-E). Inform consent was obtained from all participants who agreed to participate in this study.
For this study, we enlisted participants from three groups: individuals diagnosed with lung cancer, healthy controls, and diseased controls with either structural lung diseases confirmed on chest CTs or spirometry-confirmed chronic obstructive pulmonary disease. We confirmed the absence of lung cancer in the diseased control group through chest CT imaging and follow-up evaluations. During a two-year follow-up period, all control participants, encompassing both healthy and diseased controls, remained free from lung cancer.
Definition of diseases and data collection
For lung cancer patients, pathological confirmation was required for establishing the diagnosis. The stage was classified according to the 8th edition of the American Joint Committee on Cancer staging system for lung cancer [ 13 ]. We collected the data from a prospectively maintained database and medical records. Comorbidities included chronic obstructive pulmonary disease (COPD), asthma, diabetes mellitus (DM), and end-stage renal disease (ESRD). For healthy participants, a screening interview was performed to exclude underlying lung diseases and smoking habits. Chest x-rays of healthy participants, if available, were also reviewed to exclude structural lung disease. For diseased controls, participants must have either structural lung diseases confirmed on chest computed tomography or spirometry-confirmed chronic obstructive pulmonary disease.
Breath sample collection
The breath sample collection process has been described in our previous study [ 9 ]. Briefly, the breath sampling system included a one-way VBMax™ filter and two one-litre multi-layer foil gas sampling bags. Participants fasted for 4 h and avoided smoking and alcohol before testing. Each individual took a deep breath, then used the blow-to-breath sampling system connected to two Robert Clamps: the first collecting dead space air (not analyzed) and the second collecting end-tidal breath for analysis.
Breath analysis using eNose
The eNose system, developed by SEXTANT (Enosim Bio-Tech Co., Ltd., Hsinchu City, Taiwan), builds upon previous work and incorporates a total of 14 metal-oxide gas sensors. This system, which also includes flow meters and temperature and humidity sensors, is designed to work seamlessly with the necessary interface circuits. Leveraging Metal-Oxide-Semiconductor (MOS) gas sensors sourced from Figaro USA, Inc. and Nissha FIS, Inc., the SEXTANT system operates based on oxidation-reduction sensing mechanisms. These sensors have been enhanced with different materials to optimize both selectivity and sensitivity in detecting various gases [ 9 ]. A video describing the process of breath analysis using eNose is also available as Additional File 1 : Supplementary Video.
CNN model construction
For eNose breathprint, we first pre-processed the raw data of eNose into 14-channel \(16\times 16\) images and use a parallelizable calculation model, the convolution neural network, as the training model. We chose the rectified linear units (ReLUs) as the activation function to improve the training speed, and applied three layers of CNN to extract binary output from input images. Positive and negative outputs refer to whether this patient has lung cancer or not, respectively. The structure of CNN is shown in Additional File 2 : Figure S1
Data augmentation and fine-tuning
In this study, we applied two methods of data augmentation including Semi-supervised Domain Generalized (Semi-DG) Augmentation (SDA) and Noise-Shift Augmentation (NSA) methods. In SDA, Fourier transformation was applied and while in the NSA, we added Gaussian noise to the breathprint and performed a backward shift operation [ 14 , 15 , 16 , 17 ]. The detailed techniques of data augmentation were described in Additional File 3 : Supplementary File, Additional File 4 : Figure S2 and Additional File 5 : Figure S3 . We augmented eNose breathprint at an 1:1 ratio.
For fine-tuning, we first trained the model on the training cohort to obtain the initial weight of the model. Then, we used 10 test cohort to fine-tune the model to obtain new model weights. We chose to fine-tune our dataset using 10 samples based on our previous study, where we aimed to use a small proportion of our dataset, approximately 10–20% of the samples, for tuning [ 18 ]. We also conducted another analysis using 20 samples but observed only marginal improvement in the results. Additionally, data used for fine-tuning were separated from the test data and not used for testing.
Dataset definition and analytic flow
The training cohort was divided at 7:3 ratio, with 70% used for model training and the remaining 30% for model validation, according to time frame of recruitment. For the analysis, data augmentation (at a 1:1 ratio) was applied to the training portion. After training and validation, the model was tested with or without fine-tuning on the test dataset. The rest of the test dataset served to evaluate the model’s performance. The detailed process was described in Fig. 1 .
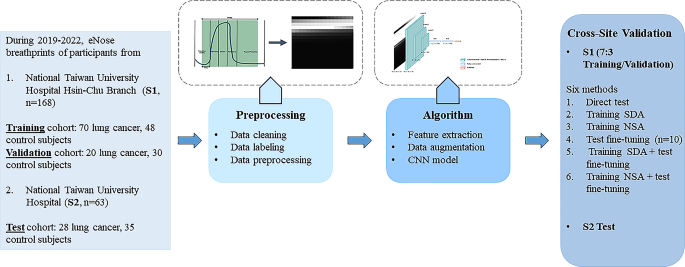
Flowchart and analytic flow. CNN, convoluted neural network; NSA, noise-shift augmentation; SDA, semi-supervised domain generalized augmentation
Statistical analysis
All variables were presented as either numbers (percentages) or as the mean ± standard deviation, depending on their nature. For categorical variables, the chi-square test was employed. For continuous variables, either the student’s t-test or the one-way analysis of variance (ANOVA) was used for comparison. To evaluate the model’s performance, we assessed accuracy, sensitivity, and specificity. Additionally, the area under the receiver operating characteristic (AU-ROC) curves were constructed to showcase the model’s performance. Confidence intervals (CI) were provided for analysis using the bootstrapping procedure. For the machine learning method, we used the scikit-learn package (version 0.23.2) in Python (version 3.8.5). All p-values were two-sided, with statistical significance set at p < 0.05.
Demographics of participants
A total of 231 participants were enrolled (168 in the training/validation cohort (Site 1, National Taiwan University Hospital Hsin-Chu branch cohort) and 63 in the test cohort (Site 2, National Taiwan University Hospital cohort)). Table 1 . describes the demographic data of all participants in the training, validation and test cohort. In the training cohort (S1), there were 70 (59.3%) lung cancer patients and 48 (33.9%) non-lung cancer control subjects (including 10 healthy control and 38 diseased control). In the validation cohort (S1), there were 20 (40%) lung cancer and 30 (60%) control subjects (including 6 healthy control and 24 diseased control). On the other hand, there were 28 (44.4%) lung cancer and 35 (55.6%) non lung cancer patients (including 10 healthy control and 25 diseased control) in the test cohort (S2).
In the training cohort, the smoking status were different between the lung cancer and control subjects. In the validation test, the demographic data were similar between the lung cancer and control subjects. In the test cohort, there is a slight female preponderance not reaching statistical significance in the lung cancer subjects compared with the control subjects (Table 1 ).
For the lung cancer patients in training/validation cohort, 70 (77.8%) were adenocarcinoma, 12 (13.3%) were squamous cell carcinoma, 4 (4.4%) were small cell lung cancer while 4 (4.4%) were other histology type. In the test cohort, 15 (53.6%) were adenocarcinoma, 4 (14.3%) were squamous cell carcinoma, 4 (14.3%) were small cell lung cancer while 5 (17.9%) were other histology type. The distribution of histology type was different in the training/validation cohort and test cohort ( p = 0.0165). For cancer stage, the two cohorts were not different ( p = 0.5444) while the majority was stage IV cancer patients (Additional File 3 : Table S1 ).
eNose breathprints PCA
Figure 2 illustrates the PCA plots of breathprints in this study. Breathprints from the two individual sites were distinct. Within each site, the breathprints of both the lung cancer and non-lung cancer groups were interspersed and scattered.
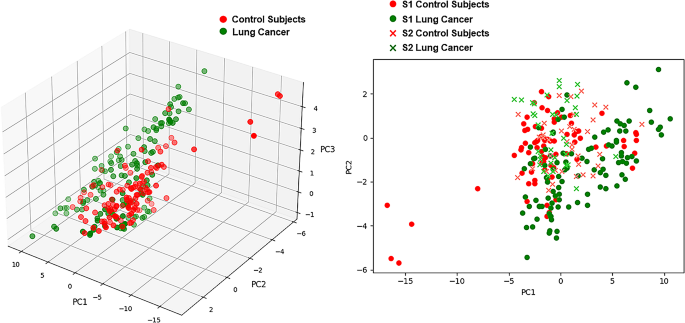
Principal component analysis plots of eNose breathprints
Performance of eNose
In the validation cohort (S1), the performance of eNose achieved an AUC of 0.89 (95% CI:0.84─0.93) with sensitivity of 0.90 (95% CI:0.85─0.95) and specificity of 0.83 (95% CI:0.73─0.87). While applying to the test cohort (S2), the performance was suboptimal with an AUC of 0.61 (95% CI:0.47─0.76), sensitivity of 0.43 (95% CI:0.36─0.50), specificity 0.43 (95% CI:0.37─0.54). With SDA, the AUC improved to 0.89 (95% CI: 0.81─0.97) with sensitivity of 0.82 (95% CI: 0.75─0.86) and specificity of 0.69 (95% CI: 0.60─0.80). With NSA, the AUC improved to 0.90 (95% CI: 0.83─0.98) with sensitivity of 0.82 (95% CI:0.75─0.86) and specificity of 0.69 (95% CI: 0.60─0.80). Applying fine-tuning, the AUC improved to 0.83 (95% CI: 0.72─0.94) and sensitivity of 0.78 (95% CI:0.70─0.83) and specificity of 0.6 (95% CI: 0.53─0.73). With SDA and fine-tuning, the performance further improved to AUC of 0.95 (95% CI: 0.89─1.00), sensitivity of 0.91 (95% CI: 0.83─0.96) and specificity of 0.77 (95% CI: 0.67─0.90). With NSA and fine-tuning, the performance also improved to AUC of 0.95 (95% CI: 0.90─1.00), sensitivity of 0.91 (95% CI: 0.83─0.96) and specificity of 0.77 (95% CI: 0.67─0.90) (Table 2 ). The AU-ROC of the test cohort (S2) is illustrated in Fig. 3 .
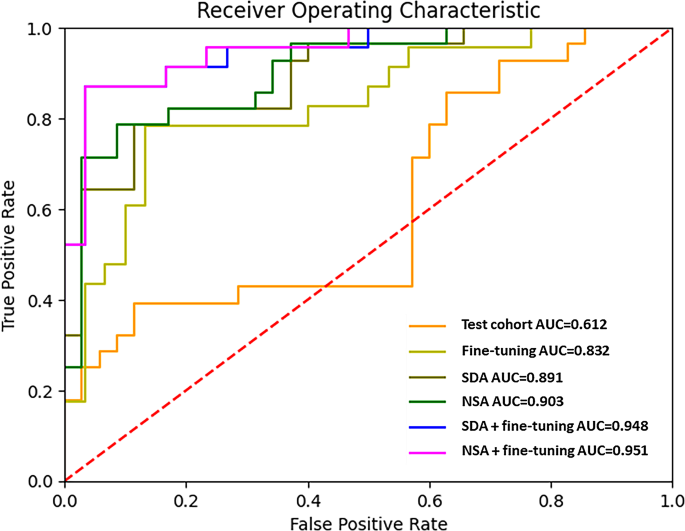
Area under the receiver operating characteristic curve of the test cohort (S2). AUC, area under the receiver operating characteristic curve; NSA, noise-shift augmentation; SDA, semi-supervised domain generalized augmentation
Reversing the training/validation and test cohort (the training/validation cohort (S1) then became the test cohort, while the test cohort (S2) became the training validation cohort), we found that the performance of eNose achieved an AUC of 0.91 (95% CI: 0.81─1.00) with sensitivity of 0.89 (95% CI: 0.80─1.00) and specificity of 0.80 (95% CI: 0.60─1.00) in the new validation cohort. Again, the performance was unsatisfactory in the test cohort with an AUC of 0.56 (95% CI: 0.44─0.73), sensitivity of 0.63 (95% CI: 0.52─0.76), specificity 0.54 (95% CI: 0.48─0.60). SDA or NSA plus fine-tuning both achieved an AUC of 0.84 (95% CI: 0.78─0.90), sensitivity of 0.82 (95% CI: 0.73─0.90) and specificity of 0.79 (95% CI: 0.70─0.89) (Additional File 3 : Table S2 ). The AU-ROC of the test cohort (S1) is illustrated in Additional File 6 : Supplementary Fig. 4 .
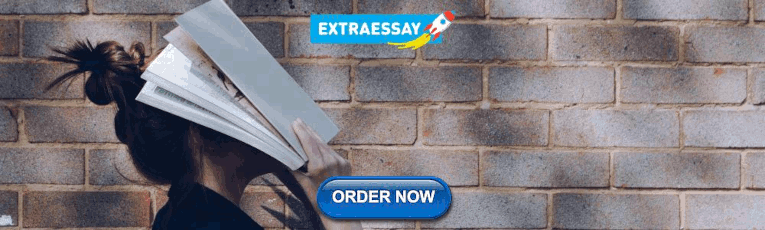
Subgroup analysis
In subgroup analysis (Fig. 4 ), we found that patients aged above 65-year-old had worse eNose performance compared with age less than 65-year-old (Accuracy: 0.76, 0.64─0.92 vs. 0.89, 0.79─1.00). While female and male patients had similar performance, the eNose had performed less satisfactory among those who ever or actively smoked than never smokers (Accuracy: 0.77, 95% CI: 0.59─0.91 vs. 0.87, 95% CI: 0.74─0.97). The performance was also best in the healthy control (accuracy: 1.00, 95% CI:0.89─1.00), followed by lung cancer patients (accuracy: 0.91, 95% CI:0.64─1.00) and diseased control patients (accuracy: 0.67, 95% CI:0.57─0.80). Among different histology types of lung cancer, the eNOSE correctly identifies all adenocarcinoma, SCLC, SqCC but incorrectly identifies two of the four lung cancer patients with other histologic classification.
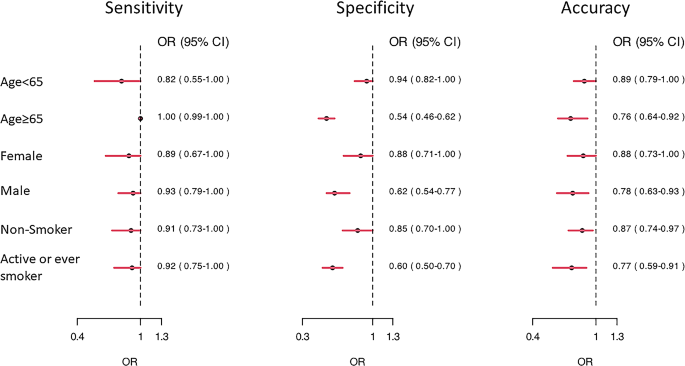
Forest plot of subgroup analysis. OR, odds ratio
Two patients were in their early stage (stage I and II) in the test cohort and they were all correctly classified as lung cancer (100%, 2/2). Also, the accuracy rate was 83.3% (5/6) for stage III lung cancer and 93.3% (14/15) for stage IV lung cancer in the test cohort.
Also, our model correctly identified 16 out of 17 (94.1%) lung cancer patients under treatment and 5 out of 6 (83.3%) fresh lung cancer patients not yet receiving anti-cancer treatment.
Detailed subgroup analysis of age, smoking status and comorbidities were further described in Additional File 3 : Table S3 .
In our study, we found that combining deep learning with transfer learning and data augmentation enables eNose to effectively tackle cross-site validation challenges. Using an eNose model trained at one site directly on another led to suboptimal results. Yet, by utilizing data augmentation and transfer learning, the eNose’s performance notably improved, achieving an AUC exceeding 0.9. As a result, electronic noses can accurately differentiate between lung cancer patients and those without the condition.
Breathomics has undergone extensive research for the purpose of detecting lung cancer. This approach is grounded in the theory that lung cancer patients may exhibit distinct metabolites and exhaled volatile organic compounds (VOCs) compared to persons without lung cancer [ 19 ]. In one prior investigation also conducted in the same participating hospital, the authors employed selected ion flow tube mass spectrometry (SIFT-MS) to identify and quantify 116 VOCs. Subsequently, the authors developed a predictive model for determining the likelihood of lung cancer based on quantitative VOC measurements. This approach yielded a commendable AUC and accuracy, with further enhancements achieved through the adjustment of confounding VOC effects [ 20 ]. It is worth noting, however, that this earlier study remained limited to a single-center setting and lacked external validation.
Cross-site validation of electronic nose has always been an important issue to be overcome. In earlier studies, the differentiation between lung cancer and non-lung cancer patients was performed without validation [ 4 ]. Some studies split one single cohort into training and validation part [ 5 , 21 , 22 ]. In one study, for instance, 199 participants were randomly split into an 80% training cohort and 20% validation cohort. A classification accuracy of 79% was subsequently attained by using XGBoost method [ 22 ]. In another study, by including 60 patients with lung cancer and 107 controls and assigning participants either to training or blinded validation cohort, the blinded validation cohort yielded diagnostic accuracy of 86%, sensitivity of 88% and specificity of 86%. For this approach, one may refer to the results of 86% accuracy obtained in our validation cohort.
Other studies used pooled data from multi-cohorts and then randomly split into training and validation cohort. In one study including multi-center cohorts with total of 575 patients, 376 patients were assigned to the training cohort and 199 patients assigned to the validation cohort. The training model then achieved an AUC-ROC of 0.79 (0.72–0.85) with a sensitivity of 88.2% and specificity of 48.3% in the validation. The study further achieved a better performance after integrating clinical data [ 6 ]. These approaches, however, do not really tackle with the issue of cross-site validation.
Cross-site validation is crucial due to several challenges associated with the generation of eNose breathprints. One significant challenge is the pervasive influence of environmental VOCs, which are constantly inhaled and participate in metabolic processes. This can modify the VOCs exhaled in human breath, subsequently affecting the generation of breathprints [ 20 , 23 ]. Another challenge stems from the device itself, encompassing issues such as sensor drift and the complexities of achieving absolute calibration [ 24 ]. Although the PCA plot revealed a distinct breathprint distribution, it also highlighted the challenges of achieving cross-site validation. Our study indicated that using data-augmentation techniques could significantly reduce the load of data collection and improved model performance. With combination of fine-tuning using data from individual sites, the performance of eNose further improved. Importantly, in our research, we only utilized a small portion of the test dataset for fine-tuning, making a clinical approach feasible.
The appropriate selection of a control group is paramount in ensuring the validity of research findings. Differentiating between healthy individuals and those diagnosed with lung cancer may seem straightforward. However, such differentiation may not encapsulate the complexities of real-world scenarios. To enhance the representativeness of our study, we incorporated individuals with other pulmonary conditions into our control cohort. While smoking is predominantly identified as a primary risk factor for lung cancer among Caucasians, another distinct demographic—non-smoking Asian females with lung adenocarcinoma—emerges as notably susceptible [ 25 ]. In an effort to account for this, our control group integrated patients with structural lung disease primary consisting of bronchiectasis. Additionally, patients with COPD were incorporated into our cohort. By combining different groups with healthy people, we believe our control group more closely matches the variety of individuals with lung screenings in real life.
Subgroup analysis revealed that the eNose exhibited less satisfactory performance in elderly participants and smokers. This finding holds particular significance, as elderly participants often present with a higher prevalence of comorbidities compared to their younger counterparts. These comorbidities may have introduced complexity into the eNose breathprint profiles [ 26 ]. It is noteworthy that elderly patients constitute an emerging demographic among lung cancer patients, and early lung cancer detection could enhance the feasibility of surgical interventions and further improvement of performance of eNose may be warranted [ 27 ]. Additionally, it’s worth highlighting that eNose demonstrated less satisfactory performance in the smoker subgroup. This finding was consistent with our previous which also found inferior performance in the smoker group [ 9 ]. Considering smoking remains a major risk factor for lung cancer [ 28 ], Detecting lung cancer in individuals who smoke or have chronic obstructive pulmonary disease is crucial for early intervention and treatment of lung cancer [ 29 ]. Therefore, our findings highlight areas of weakness that need to be strengthened in our eNose device. eNose technology simulates the human olfactory system.
In real environments, gas mixtures can be influenced by numerous factors, such as environmental volatile organic compounds and humidity. Therefore, data enhancement methods are valuable as they can simulate these variations, making the model more adaptable and reducing the need for extensive data collection. Common data enhancement techniques for eNose encompass noise addition, data rotation and translation, and synthetic data generation. For instance, a study with focus on eNose’s classification of alternative herbal medicines employed several data enhancement strategies to minimize the heavy dependency on training materials [ 17 ]. One method involved augmenting the training dataset by adding Gaussian noise and data shifting [ 17 ]. In another study exploring the use of eNose to identify ripe tomatoes, the collected gas’s concentration value was converted into a grayscale value, synthesized into a grayscale image, and then augmented using methods such as cropping and zooming [ 30 ]. These data augmentation techniques successfully improved the performance of eNose.
There were studies utilizing data augmentation methods in human disease research to enhance domain generalization, bolster model robustness, and minimize overfitting risks. For instance, one study employed a continuous frequency domain spatial interpolation approach for data augmentation, achieving state-of-the-art results in retinal fundus and prostate magnetic resonance imaging segmentations [ 31 ]. More recently, another study explored six data augmentation techniques for electromyography signals: trial averaging, time slice recombination, frequency slice recombination, noise addition, cropping, and the use of a variational autoencoder. This research aimed to enrich data diversity, enabling the model to better adapt to real-world variations, thereby boosting its robustness and domain generalization. Subsequently, the model’s accuracy improved by 3% and 12% on two motor imagery datasets [ 32 ].
Fine-tuning was used in our study to improve the versatility of our model. Fine-tuning is one of the domain adaptation techniques which can help the model better adapt to the features and distribution of new data and improve the performance of the model in new environments [ 33 ]. In one landscape study, a deep learning model was pre-trained on the ImageNet dataset, being fine-tuned and applied to different medical imaging data. The pre-trained model was successfully applied to retinal optical coherence tomography and pneumonia diagnosis [ 34 ]. In our previous studies, we also successfully demonstrated the capability of fine-tuning in improving model performance on external cohort [ 10 , 18 ].
We did not have information on potential confounding various such as BMI, alcohol intake, and dietary habits for our study participants. Although BMI is less frequently reported to affect the results of eNose breathprints, it can be associated with other diseases, such as diabetes, that may lead to distinct breathprints [ 35 ]. Dietary habits have previously been reported to influence VOC metabolites [ 36 ]. Lifestyle has also been noted to affect fecal VOCs [ 37 ]. On the other hand, one study investigating the impact of food intake on eNose breathprints suggested that the impact would be significant if the food intake occurred very recently, and two hours might be sufficient to avoid food-induced alterations in eNose breathprints [ 38 ]. In our study, we requested that participants fast for four hours prior to testing. However, the impact of the aforementioned factors may still warrant special attention and could be evaluated in future studies.
Our study has limitations. Firstly, the majority of lung cancer patients we studied in the study were in advanced stages, limiting the validation of eNose performance in early-stage lung cancer. Though the case number is limited, we have corrected identified two early stage lung cancer in our test cohort. Another limitation concerns transfer learning, which still necessitates some samples from the test cohort, potentially leading to inconvenience. While using data augmentation without fine-tuning yielded satisfactory results, fine-tuning can be viewed as a means to further optimize these results. Also, the study was confined to a Taiwanese population and the generalizability of the findings to other ethnicities remains uncertain. Finally, the reduced performance of eNose among elderly individuals and smokers also necessitates further investigation and strategies for improvement.
In conclusion, our study has shown that cross-site validation of the electronic nose for diagnosing lung cancer is attainable. Data augmentation and fine-tuning have demonstrated to be crucial methods for improving the performance when applying the eNose across different sites. Consequently, the electronic nose holds promise as a valuable tool for accurately identifying lung cancer patients in clinical practice. Future researches were warranted to further assess the generalization of eNose, minimize influence of confounding factors and validate eNose in early-stage lung cancer, diverse populations as well as high-risk groups.
Data availability
All data will be available upon reasonable request. Part of this study has been presented in IEEE BioSensors 2023 conference.
Abbreviations
Electronic Nose
Semi-supervised Domain-generalized Augmentation
Noise-Shift Augmentation
Area Under the Curve
Area Under the Receiver Operating Characteristic
Computed Tomography
Convoluted Neural Network
National Taiwan University Hospital
National Taiwan University Hospital Hsin-Chu branch
Chronic Obstructive Pulmonary Disease
Diabetes Mellitus
End-Stage Renal Disease
Metal-Oxide-Semiconductor
Rectified Linear Units
Confidence Intervals
Principal Component Analysis
Small Cell Lung Cancer
Squamous Cell Carcinoma
Volatile Organic Compounds
Selected Ion Flow Tube Mass Spectrometry
Sharma R. Mapping of global, regional and national incidence, mortality and mortality-to-incidence ratio of lung cancer in 2020 and 2050. Int J Clin Oncol. 2022;27:665–75.
Article PubMed PubMed Central Google Scholar
Jonas DE, Reuland DS, Reddy SM, Nagle M, Clark SD, Weber RP, et al. Screening for Lung Cancer with Low-Dose Computed Tomography: updated evidence report and systematic review for the US Preventive Services Task Force. JAMA. 2021;325:971–87.
Article PubMed Google Scholar
van der Sar IG, Wijbenga N, Nakshbandi G, Aerts J, Manintveld OC, Wijsenbeek MS, et al. The smell of lung disease: a review of the current status of electronic nose technology. Respir Res. 2021;22:246.
Di Natale C, Macagnano A, Martinelli E, Paolesse R, D’Arcangelo G, Roscioni C, et al. Lung cancer identification by the analysis of breath by means of an array of non-selective gas sensors. Biosens Bioelectron. 2003;18:1209–18.
Machado RF, Laskowski D, Deffenderfer O, Burch T, Zheng S, Mazzone PJ, et al. Detection of lung cancer by sensor array analyses of exhaled breath. Am J Respir Crit Care Med. 2005;171:1286–91.
Kort S, Brusse-Keizer M, Schouwink H, Citgez E, de Jongh FH, van Putten JWG, et al. Diagnosing Non-small Cell Lung Cancer by Exhaled Breath profiling using an electronic nose: a Multicenter Validation Study. Chest. 2023;163:697–706.
de Vries R, Farzan N, Fabius T, De Jongh FHC, Jak PMC, Haarman EG, et al. Prospective detection of early lung Cancer in patients with COPD in regular care by electronic nose analysis of exhaled breath. Chest. 2023;164:1315–24.
Chen H, Huo D, Zhang J. Gas recognition in E-Nose system: a review. IEEE Trans Biomed Circuits Syst. 2022;16:169–84.
Lee MR, Huang HL, Huang WC, Wu SY, Liu PC, Wu JC, et al. Electronic nose in differentiating and ascertaining clinical status among patients with pulmonary nontuberculous mycobacteria: a prospective multicenter study. J Infect. 2023;87:255–8.
Liu CJ, Tsai CC, Kuo LC, Kuo PC, Lee MR, Wang JY, et al. A deep learning model using chest X-ray for identifying TB and NTM-LD patients: a cross-sectional study. Insights Imaging. 2023;14:67.
Garcea F, Serra A, Lamberti F, Morra L. Data augmentation for medical imaging: a systematic literature review. Comput Biol Med. 2023;152:106391.
Kim HE, Cosa-Linan A, Santhanam N, Jannesari M, Maros ME, Ganslandt T. Transfer learning for medical image classification: a literature review. BMC Med Imaging. 2022;22:69.
Detterbeck FC, Boffa DJ, Kim AW, Tanoue LT. The eighth edition lung cancer stage classification. Chest. 2017;151:193–203.
Yao HHX, Li X. Enhancing pseudo label quality for semi-supervised domain-generalized medical image segmentation. In Proceedings of the AAAI Conference on Artificial Intelligence. 2022;36:3099–3107.
Stéphane M. A Wavelet Tour of Signal Processing the sparse way. 3rd ed. Elsevier; 2009.
N BR. The Fourier transform and its applications. New York: McGraw-Hill; 1978.
Google Scholar
Li Liu XZ, Wu R, Guan X, Wang Z, Zhang W, Pilanci M, et al. a Case in Alternative Herbal Medicine Discrimination With Electronic Nose. IEEE Sens J. 2021;21:22995–3005. Boost AI Power: Data Augmentation Strategies With Unlabeled Data and Conformal Prediction,.
Yu KL, Tseng YS, Yang HC, Liu CJ, Kuo PC, Lee MR, et al. Deep learning with test-time augmentation for radial endobronchial ultrasound image differentiation: a multicentre verification study. BMJ Open Respir Res. 2023;10:e001602.
Jia Z, Zhang H, Ong CN, Patra A, Lu Y, Lim CT, et al. Detection of Lung Cancer: concomitant volatile Organic compounds and Metabolomic Profiling of Six Cancer Cell lines of different histological origins. ACS Omega. 2018;3:5131–40.
Article CAS PubMed PubMed Central Google Scholar
Tsou PH, Lin ZL, Pan YC, Yang HC, Chang CJ, Liang SK, et al. Exploring volatile Organic compounds in Breath for High-Accuracy Prediction of Lung Cancer. Cancers (Basel). 2021;13:1431.
van de Goor R, van Hooren M, Dingemans AM, Kremer B, Kross K. Training and validating a Portable Electronic nose for Lung Cancer Screening. J Thorac Oncol. 2018;13:676–81.
V AB, Subramoniam M, Mathew L. Detection of COPD and Lung Cancer with electronic nose using ensemble learning methods. Clin Chim Acta. 2021;523:231–8.
Article Google Scholar
Beauchamp J. Inhaled today, not gone tomorrow: pharmacokinetics and environmental exposure of volatiles in exhaled breath. J Breath Res. 2011;5:037103.
Article CAS PubMed Google Scholar
Harper WJ. The strengths and weaknesses of the electronic nose. Adv Exp Med Biol. 2001;488:59–71.
Saito S, Espinoza-Mercado F, Liu H, Sata N, Cui X, Soukiasian HJ. Current status of research and treatment for non-small cell lung cancer in never-smoking females. Cancer Biol Ther. 2017;18:359–68.
Temerdashev AZ, Gashimova EM, Porkhanov VA, Polyakov IS, Perunov DV, Dmitrieva EV. Non-invasive lung Cancer Diagnostics through metabolites in Exhaled Breath: influence of the Disease variability and comorbidities. Metabolites. 2023;13:203.
Blanco R, Maestu I, de la Torre MG, Cassinello A, Nunez I. A review of the management of elderly patients with non-small-cell lung cancer. Ann Oncol. 2015;26:451–63.
Walser T, Cui X, Yanagawa J, Lee JM, Heinrich E, Lee G, et al. Smoking and lung cancer: the role of inflammation. Proc Am Thorac Soc. 2008;5:811–5.
Choi E, Ding VY, Luo SJ, Ten Haaf K, Wu JT, Aredo JV, et al. Risk model-based lung Cancer screening and racial and ethnic disparities in the US. JAMA Oncol. 2023;9:1640–8.
Anticuando MK, D DCKR, Padilla D. Electronic Nose and Deep Learning Approach in Identifying Ripe Lycopersicum esculentum L. TomatoFruit. In 13th International Conference on Computing Communication and Networking Technologies (ICCCNT). pp. 1–6; 2022:1–6.
Liu QCC, Qin J, Dou Q, Heng PA, Feddg. Federated domain generalization on medical image segmentation via episodic learning in continuous frequency space. n Proceedings of the IEEE/CVF Conference on Computer Vision and Pattern Recognition 2021:1013–1023.
George O, Smith R, Madiraju P, Yahyasoltani N, Ahamed SI. Data augmentation strategies for EEG-based motor imagery decoding. Heliyon. 2022;8:e10240.
Sundaresan V, Zamboni G, Dinsdale NK, Rothwell PM, Griffanti L, Jenkinson M. Comparison of domain adaptation techniques for white matter hyperintensity segmentation in brain MR images. Med Image Anal. 2021;74:102215.
Kermany DS, Goldbaum M, Cai W, Valentim CCS, Liang H, Baxter SL et al. Identifying Medical Diagnoses and Treatable Diseases by Image-Based Deep Learning. Cell. 2018;172:1122–1131.
Gudino-Ochoa A, Garcia-Rodriguez JA, Ochoa-Ornelas R, Cuevas-Chavez JI, Sanchez-Arias DA. Noninvasive diabetes detection through human breath using TinyML-Powered E-Nose. Sens (Basel). 2024;24:1294.
Article CAS Google Scholar
Ajibola OA, Smith D, Spanel P, Ferns GA. Effects of dietary nutrients on volatile breath metabolites. J Nutr Sci. 2013;2:e34.
Bosch S, Lemmen JP, Menezes R, van der Hulst R, Kuijvenhoven J, Stokkers PC, et al. The influence of lifestyle factors on fecal volatile organic compound composition as measured by an electronic nose. J Breath Res. 2019;13:046001.
Dragonieri S, Quaranta VN, Portacci A, Ahroud M, Di Marco M, Ranieri T, et al. Effect of Food Intake on Exhaled Volatile Organic compounds Profile analyzed by an electronic nose. Molecules. 2023;28:5755.
Download references
Acknowledgements
We would like to thank all the participants who agreed to take part in this study. The authors would like to thank the Data Science Statistical Cooperation Center of Academia Sinica (AS-CFII-111-215) for statistical support.
This study was funded by National Taiwan University Hospital Hsin-Chu Branch (109-HCH034) and National Tsing-Hua University (110F7MAHE1).
Author information
Authors and affiliations.
Department of Internal Medicine, National Taiwan University Hospital, Taipei, Taiwan
Meng-Rui Lee, Jann-Yuan Wang, Chao-Chi Ho, Jin-Yuan Shih & Chong-Jen Yu
Department of Internal Medicine, National Taiwan University Hospital Hsin-Chu Branch, Hsin-Chu, Taiwan
Meng-Rui Lee & Chong-Jen Yu
Department. of Electrical Engineering, National Tsing Hua University, No. 101, Sec. 2, Kuang-Fu Road, Hsinchu, 30013, Taiwan
Mu-Hsiang Kao, Ya-Chu Hsieh, Min Sun & Kea-Tiong Tang
You can also search for this author in PubMed Google Scholar
Contributions
M.R.L., K.T.T. and M.S. designed all the experiments. M.R.L., M.H.K. and Y.C.H. conducted the experiments and analyzed and interpreted the results. K.T.T, M.S., J.Y.W., C.C.H., J.Y.S. and C.J.Y. supervised the project. M.R.L., M.H.K. and Y.C.H. prepared the manuscript. M.R.L., K.T.T, M.S., J.Y.W., C.C.H.,J.Y.S and C.J.Y. reviewed and edited the manuscript. All the authors have read and agreed to the published version of the manuscript.
Corresponding authors
Correspondence to Min Sun or Kea-Tiong Tang .
Ethics declarations
Ethics approval and consent to participate.
The institutional review boards (IRB) of participating hospitals approved this study (IRB no. 202112057RINB, 108-011-E). Inform consent was obtained from all participants who agreed to participate in this study.
Competing for publication
Not applicable.
Competing interests
The original eNose technology of Enosim Bio-tech Co., Ltd. was licensed by National Tsing Hua University and this technology was owned by K.T.T. who serves as a faculty member at National Tsing Hua University’s department of electrical engineering. K.T.T. also received advisory fee from Enosim biotech.
Additional information
Publisher’s note.
Springer Nature remains neutral with regard to jurisdictional claims in published maps and institutional affiliations.
Electronic supplementary material
Below is the link to the electronic supplementary material.
Supplementary Material 1
Supplementary material 2, supplementary material 3, supplementary material 4, supplementary material 5, supplementary material 6, rights and permissions.
Open Access This article is licensed under a Creative Commons Attribution 4.0 International License, which permits use, sharing, adaptation, distribution and reproduction in any medium or format, as long as you give appropriate credit to the original author(s) and the source, provide a link to the Creative Commons licence, and indicate if changes were made. The images or other third party material in this article are included in the article’s Creative Commons licence, unless indicated otherwise in a credit line to the material. If material is not included in the article’s Creative Commons licence and your intended use is not permitted by statutory regulation or exceeds the permitted use, you will need to obtain permission directly from the copyright holder. To view a copy of this licence, visit http://creativecommons.org/licenses/by/4.0/ . The Creative Commons Public Domain Dedication waiver ( http://creativecommons.org/publicdomain/zero/1.0/ ) applies to the data made available in this article, unless otherwise stated in a credit line to the data.
Reprints and permissions
About this article
Cite this article.
Lee, MR., Kao, MH., Hsieh, YC. et al. Cross-site validation of lung cancer diagnosis by electronic nose with deep learning: a multicenter prospective study. Respir Res 25 , 203 (2024). https://doi.org/10.1186/s12931-024-02840-z
Download citation
Received : 29 January 2024
Accepted : 06 May 2024
Published : 10 May 2024
DOI : https://doi.org/10.1186/s12931-024-02840-z
Share this article
Anyone you share the following link with will be able to read this content:
Sorry, a shareable link is not currently available for this article.
Provided by the Springer Nature SharedIt content-sharing initiative
- Electronic nose
- Cross-site validation
- Lung cancer
- Breathprint
- Deep learning
- Data augmentation
Respiratory Research
ISSN: 1465-993X
- General enquiries: [email protected]
- Open access
- Published: 09 May 2024
Development and validation of a predictive model for the risk of sarcopenia in the older adults in China
- Qiugui Li 1 na1 ,
- Hongtao Cheng 1 na1 ,
- Wenjiao Cen 1 ,
- Tao Yang 2 &
- Shengru Tao 3
European Journal of Medical Research volume 29 , Article number: 278 ( 2024 ) Cite this article
192 Accesses
Metrics details
Sarcopenia is a progressive age-related disease that can cause a range of adverse health outcomes in older adults, and older adults with severe sarcopenia are also at increased short-term mortality risk. The aim of this study was to construct and validate a risk prediction model for sarcopenia in Chinese older adults.
This study used data from the 2015 China Health and Retirement Longitudinal Study (CHARLS), a high-quality micro-level data representative of households and individuals aged 45 years and older adults in China. The study analyzed 65 indicators, including sociodemographic indicators, health-related indicators, and biochemical indicators.
3454 older adults enrolled in the CHARLS database in 2015 were included in the final analysis. A total of 997 (28.8%) had phenotypes of sarcopenia. Multivariate logistic regression analysis showed that sex, Body Mass Index (BMI), Mean Systolic Blood Pressure (MSBP), Mean Diastolic Blood Pressure (MDBP) and pain were predictive factors for sarcopenia in older adults. These factors were used to construct a nomogram model, which showed good consistency and accuracy. The AUC value of the prediction model in the training set was 0.77 (95% CI = 0.75–0.79); the AUC value in the validation set was 0.76 (95% CI = 0.73–0.79). Hosmer–Lemeshow test values were P = 0.5041 and P = 0.2668 (both P > 0.05). Calibration curves showed significant agreement between the nomogram model and actual observations. ROC and DCA showed that the nomograms had good predictive properties.
Conclusions
The constructed sarcopenia risk prediction model, incorporating factors such as sex, BMI, MSBP, MDBP, and pain, demonstrates promising predictive capabilities. This model offers valuable insights for clinical practitioners, aiding in early screening and targeted interventions for sarcopenia in Chinese older adults.
With the rapid development of China’s economy, the country has gradually transitioned into an aging society. According to the findings of the seventh census conducted in 2020, China’s population aged 60 and above is projected to exceed 260 million, accounting for 18.7% of the total population. Within this demographic, those aged 65 and above are expected to surpass 190 million, making up 13.5% of the total population [ 1 ]. The increasing number of older adults will significantly escalate expenditures within the social security system, imposing a considerable financial burden on the government. Among the various health-related factors contributing to disability in older adults, sarcopenia and cognitive impairment have attracted significant academic and clinical attention [ 2 ]. Currently, China’s focus on sarcopenia has started relatively late, with general hospitals showing evident specialization and insufficient understanding of sarcopenia, which has yet to be categorized into a specific field.
Sarcopenia was traditionally defined by a reduction in muscle mass, but current research highlights the significance of muscle strength and its impact on physical function [ 3 ]. Since 2016, the World Health Organization has officially recognized sarcopenia as a disease and a pressing public health concern in aging populations [ 4 , 5 , 6 ]. The loss of skeletal muscle mass is central to sarcopenia and can lead to physical dysfunction [ 7 ]. Studies indicate that sarcopenia affects over a quarter of older adults in Chinese communities [ 8 ], with a global incidence among individuals over 60 ranging from 10 to 27% [ 9 ]. Various factors contribute to the onset of sarcopenia, including age, nutrition intake, physical inactivity, diseases, and iatrogenic factors [ 10 ]. Risk factors such as aging, malnutrition, smoking, and low BMI have been identified [ 11 , 12 ], with higher prevalence observed in patients with chronic obstructive pulmonary disease [ 13 ], chronic heart failure [ 14 ] and chronic liver disease [ 15 ]. Furthermore, sarcopenia is associated with adverse outcomes like falls, functional decline, frailty, and mortality [ 16 , 17 ].
Although there are a number of sarcopenia risk prediction models, they all have some limitations. For example, some models have small sample sizes, which may limit their generalizability and applicability to different older adults [ 18 ]. Additionally, some models rely on predictor variables that are difficult and time-consuming to collect, limiting their usefulness in real-world clinical applications [ 19 ]. Furthermore, there are models that do not capture all risk factors for sarcopenia, which may affect their predictive accuracy [ 20 ]. These limitations underscore the need for further research to develop more comprehensive and practical prediction models for sarcopenia.
In contrast to foreign studies primarily focused on disease-specific correlations with sarcopenia, research in our country is predominantly centered on current situations and influencing factors. Key factors such as age, exercise habits, number of diseases, malnutrition, risk of falls, and fatigue are identified as easily inducible factors for sarcopenia. This study aims to identify and incorporate these factors into the construction of a sarcopenia risk prediction model, providing valuable insights for early screening and intervention by clinical medical staff.
Data source
We utilized data from the China Health and Retirement Longitudinal Study (CHARLS), publicly accessible at http://charls.pku.edu.cn . CHARLS is an ongoing longitudinal survey encompassing families and individuals aged 45 and older across 150 counties and 450 communities (villages) within 28 provinces, autonomous regions, and municipalities nationwide. Its comprehensive content spans demographic, economic, health, pension, and other pertinent information. Approval for this project was granted by the Biomedical Ethics Committee of Peking University (Beijing, China) (IRB00001052-11015), with our study adhering strictly to the principles outlined in the Declaration of Helsinki, and obtaining informed consent from all participants. Our analysis specifically focused on CHARLS2015 data, wherein after excluding subjects with missing data, a total of 3454 participants were ultimately included in our study cohort. Notably, our research targeted individuals aged 60 and above. The flowchart of the study is outlined in Fig. 1 .
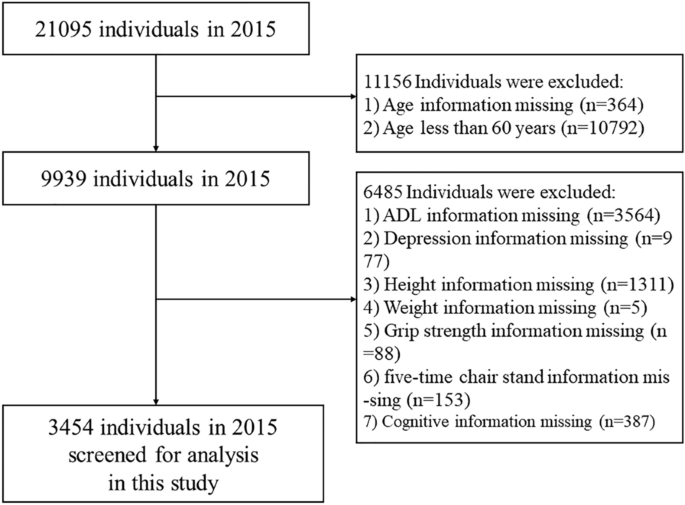
Flowchart of the study
Data extraction
Assessment of symptoms of sarcopenia.
Sarcopenia was evaluated according to the criteria recommended by the AWGS2019 [ 7 ], which encompass muscle strength, physical performance and appendicular skeletal muscle mass (ASM). Handgrip strength (unit: kg) was assessed in both the dominant and non-dominant hand using the YuejianTM WL-1000 dynamometer. Participants were instructed to squeeze the handle as firmly as possible for 3 s. Measurements were taken twice for each hand, with a minimum interval of 15 s between trials. The recorded value represents the average of the maximum grip strength from both hands. The thresholds for low grip strength established by AWGS are < 28 kg for men and < 18 kg for women [ 21 ]. Physical performance decline, as per AWGS criteria, is defined as 5 times sitting time > 12 s or 6-m walking speed < 1 m/s [ 22 ]. ASM measurements were derived using validated anthropometric equations specifically developed for the Chinese population [ 23 , 24 ]. The study demonstrated strong concordance between the ASM equation and Dual-Energy X-ray Absorptiometry (DXA) [ 23 , 24 ]. In our study cohort, the cutoff for low muscle mass was determined based on sex-specific criteria, equating to a minimum of 20% of height-adjusted muscle mass (ASM/Ht 2 ) [ 23 , 24 , 25 , 26 ]. Height and weight were recorded in centimeters and kilograms, respectively. Regarding sex, a value of 1 represents male and a value of 2 represents female. Consequently, individuals with ASM/Ht 2 < 5.69 kg/m 2 for females and ASM/Ht 2 < 6.88 kg/m 2 for males were classified as having low muscle mass. The ASM equation utilized is:
Sarcopenia manifests through a blend of diminished muscle strength, impaired physical performance, or decreased appendicular skeletal muscle mass. Diagnosis typically hinges on identifying low muscle strength, either alone or accompanied by reduced appendicular skeletal muscle mass. Individuals displaying low muscle strength, compromised physical performance, and diminished appendicular skeletal muscle mass were classified as having severe sarcopenia. For the purposes of this study, participants were segregated into two main groups: those with sarcopenia and those without.
Assessment of depressive symptoms
Depressive symptoms were assessed with a 10-item stream using the Center for Epidemiologic Studies Depression Scale (CES-D) to assess depressive mood and behavior. CESD pay attention to the individual's situation in the past week and rate it as “Rarely or none of the time (< 1 day)”, “Some or a little of the time (1-2 days)”, “Occasionally or a moderate amount of the time (3-4 days)”, and “Most or all of the time (5-7 days)” according to the frequency of symptoms, and assign 0, 1, 2, and 3 points, respectively, with higher scores representing individuals with more severe depressive symptoms. In this study, according to the research results of ROBERTS and his colleagues [ 27 ], CESD ≥ 16 is considered to have depressive symptoms, and < 16 is considered to have no depressive symptoms.
Assessment of cognitive function
CHARLS measures cognitive function in three parts: Telephone Interview for Cognitive Status (TICS), Word Recall, and Picture Drawing. The higher the score, the better the cognitive function. The TICS requires the subject to correctly name the year, month, day, day of the week, and season, and each correct answer is worth 1 point; the subject is required to correctly calculate 100-7, and each correct answer is worth 1 point, and the scores of the two parts are added together, the total score is 0–10 points, which mainly evaluates the subject’s orientation, calculation ability and attention. Word recall: the researchers read 10 words and asked the subjects to recall the 10 words in a short time and after answering several other questions, each correct recall of a word was recorded as 1 point, and the average score of the two words recalled was taken. A total score of 0–10 points was used to assess episodic memory ability. Picture drawing: the researcher provides a picture of two overlapping five-pointed stars and asks the subjects to draw the figure on a white piece of paper. If they can draw a similar figure, they get 1 point, and if they cannot, they get 0 points. It is used to evaluate the subject's visuospatial ability.
Assessment of activities of daily living
Activities of daily living include physical self-maintenance scale (PSMS) and instrumental activities of daily living (IADL). PSMS evaluates essential tasks like dressing, bathing, eating, getting out of bed, going to the toilet, controlling bowel and urine. Meanwhile, IADL assesses more complex activities such as shopping, cooking, doing housework, taking medicine, managing money and making phone calls. According to the degree, it is divided into “No, I don’t have any difficulty”, “I have difficulty but can still do it”, “Yes, I have difficulty and need help” and “I cannot do it”. These options correspond to scores of 1, 2, 3, and 4, respectively, indicating higher scores reflect greater impairment in the skill.
Socio-demographic information
Socio-demographics include sex, age, marital status, education level, address and residence. Sex is defined as male and female. Education level was divided into no schooling, primary school, junior high school and above. Marital status was defined as married if the subject was currently married and living with a spouse; unmarried if the subject was currently separated, divorced, widowed, or never married. Address is divided into “Family house”, “Nursing home” and “Other”. Residence is divided into “The center of city/town”, “Combination zone between urban and rural areas”, “Village” and “Special area”.
Health-related information
Within the health-related data examined as potential risk factors, a broad spectrum of conditions and indicators were included. These encompassed physical disabilities, neurological impairments such as brain damage, sensory deficits like blindness, deafness and muteness, as well as prevalent medical conditions including hypertension, dyslipidemia, diabetes, cancer and various chronic diseases affecting organs such as the lungs, liver, heart and kidneys. Mental health aspects such as emotional disturbances, memory-related ailments, and joint diseases or rheumatism were also considered. Other factors such as asthma, pain (specifically chronic pain), history of surgeries like cataract or hip fracture, usage of assistive devices like hearing aids, dental health indicators like tooth loss and lifestyle habits like smoking status, alcohol consumption, and social activity levels were evaluated. Additionally, variables related to accidents, falls, vision and hearing impairments and subjective health assessments were included. Specifically, aspects like distant vision, near vision, hearing ability and self-assessment of health status were categorized as “good”, “fair” or “poor”, while the remaining variables were dichotomized as “yes” or “no”. These variables can be directly obtained from the CHARLS questionnaire.
Statistical methods
In this study, data from the CHARLS database in 2015 were selected for analysis. Continuous variables were expressed as medians and interquartile ranges, and rank sum tests were used to compare between groups; categorical variables were expressed as percentages, and χ 2 tests or Fisher's exact tests were used to compare between groups. First, the data set is randomly divided into training set ( n = 2417) and verification set ( n = 1037) according to the ratio of 7:3. During this process, we set a random seed to ensure the randomization and repeatability of the sampling [ 28 ].
Utilizing a nomogram to depict the risk of sarcopenia among the older adults in China, we employed Least Absolute Selection and Shrinkage Operator (LASSO) regression analysis to construct and validate the model. We chose LASSO regression due to its capability to manage high-dimensional datasets with multicollinearity, effectively selecting variables and improving model interpretability. In contrast to Rigid and Elastic Net models, LASSO provides greater flexibility in variable selection and sparsity, making it the preferred choice for our specific research objectives and dataset characteristics. This choice ultimately leads to a more accurate and concise model. The primary R packages utilized in this study include: “mice”, “tableone”, “glmnet”, “rms”, “pROC” and “rmda”. First, LASSO regression analysis was performed on the training set data to select predictors of sarcopenia in Chinese older adults [ 29 , 30 ]. Then, the tuning parameter (λ) suitable for LASSO regression analysis was determined by tenfold cross-validation, and the most significant features were screened using the LASSO algorithm. Finally, the selected predictors were included in the multivariate logistic regression analysis and the predictors with P -value < 0.05 were included in the nomogram model. The maximum missing value of all extracted variables does not exceed 20%, and multiple imputation is used to handle missing data [ 31 ].
In this study, the area under the receiver operating characteristic curve (AUROC) was used to determine the discriminative ability of the model. Calibration curves are used to determine the degree of agreement between predicted probabilities and observed results. Clinical validity was assessed by decision curve analysis (DCA). All data in this study were analyzed using R software (version 4.1.0). All tests were two-tailed and P < 0.05 was considered statistically significant.
General information and clinical characteristics of the older adults
A total of 3454 older adult subjects (aged 60 years and older) were enrolled in this study, and the screening process for specific subjects is shown in Fig. 1 . The general information and clinical characteristics of the subjects are listed in Table 1 . There were 1708 men (49.4%) and 1746 women (50.6%). More detailed information is provided in a separate document (see supplement information).
Prevalence and associated variables of sarcopenia
The prevalence of sarcopenia was 28.8% (997/3454). There were significant differences in sex, BMI, and MDBP between the two groups of older adults ( P < 0.05). According to clinical experience [ 32 , 33 ], pain and MSBP were included in the model, and significant differences were found between the two groups of older adults. In the older adults, 2417 (70%) and 1037 (30%) were randomly assigned to the training and validation sets, respectively. The comparison of training and validation sets in the supplement information shows no significant difference between the two groups ( P > 0.05).
LASSO logistic regression
In this investigation, non-zero coefficients were identified as potential predictors of frailty through the LASSO regression model (Fig. 2 A and Fig. 2 B). Subsequently, these underlying factors linked with sarcopenia were incorporated into multiple logistic regression models utilizing the ‘rms’ package within the ‘R’ software environment. Ultimately, it was found that sex ( P < 0.001), BMI ( P < 0.001), MSBP ( P < 0.001), MDBP ( P < 0.001) and pain ( P = 0.015) were correlated with sarcopenia in the older adults (Table 2 ).
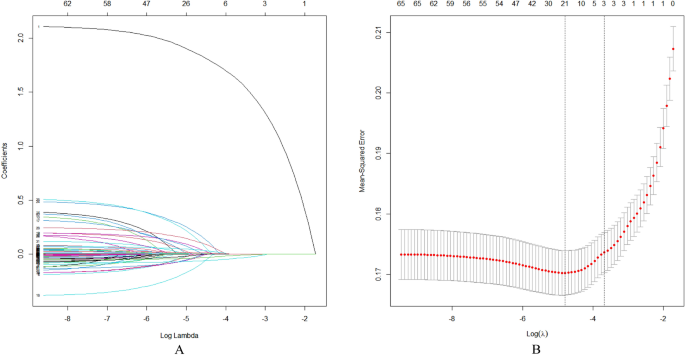
Demographic and clinical feature selection using the LASSO regression model. A According to the logarithmic (lambda) sequence, a coefficient profile was generated, and non-zero coefficients were produced by the optimal lambda. B The optimal parameter (lambda) in the LASSO model was selected via tenfold cross-validation using minimum criteria. The partial likelihood deviation (binomial deviation) curve relative to log (lambda) was plotted. A virtual vertical line at the optimal value was drawn using one SE of minimum criterion (the 1-SE criterion)
Developing predictive models
Based on tenfold cross-validation, LASSO regression analysis was used to screen the best predictors of the model. Multiple logistic regression was used to build the prediction model. The variance inflation factor (VIF) test was performed, and the VIF values of all variables were < 4. Without covariance, the model fits well. The predictive model consists of variables with a P -value of less than 0.05 in a multivariate logistic regression. These variables included sex, BMI, MSBP, MDBP, pain as predictors. The prediction model adopts nomogram, which can be used to quantitatively predict the risk of sarcopenia in the older adults (Fig. 3 ).
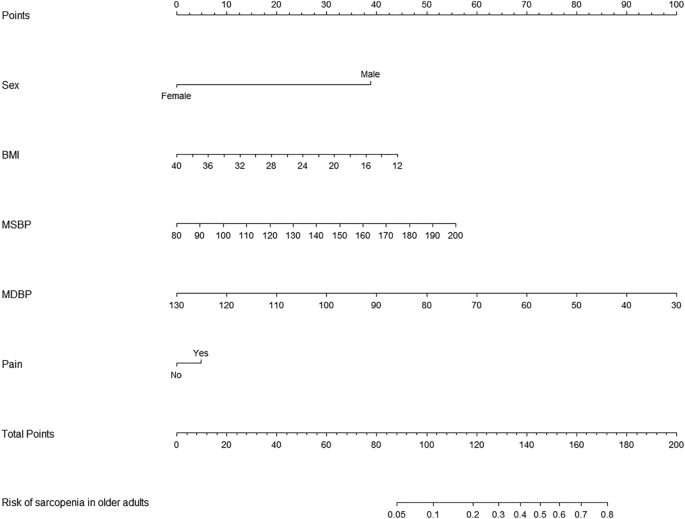
A nomogram for predicting sarcopenia in the older adults in China
Validating predictive models
AUC (area under curve) is a statistical metric that measures the performance of a classifier, specifically indicating the probability that a randomly chosen positive sample will rank higher than a randomly chosen negative sample. It is commonly utilized to assess the effectiveness of machine learning models. AUC values were computed to evaluate the discriminative power of the prediction model, by examining the incidence of sarcopenia among older adults in both the training and validation datasets. As shown in Fig. 4 A, B, the AUC value of the predictive model in the training set was 0.77 (95% CI = 0.75–0.7901); the AUC value in the validation set was 0.76 (95% CI = 0.7287–0.7904). These data suggest that the nomogram has good discriminative power and predictive value, correctly identifying sarcopenic and non-sarcopenic patients.
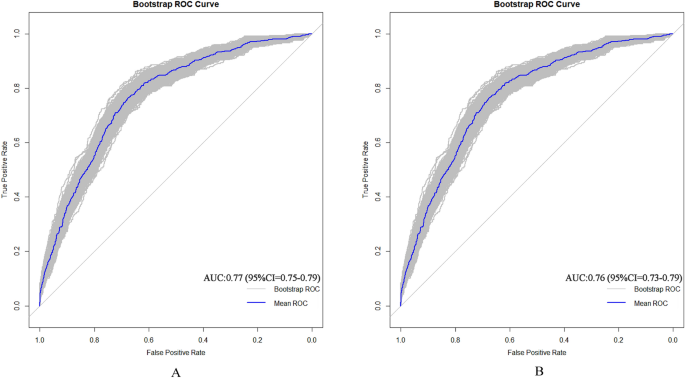
A Nomogram ROC curves generated from the training dataset. B Nomogram ROC curves generated using the validation dataset
Correcting the predictive model
Calibration plots and the Hosmer–Lemeshow goodness-of-fit test were used to evaluate the model plots ( P > 0.05 indicated that the model fit was very good). The test results show that the model fits both the training set ( χ 2 = 7.305, df = 8, p = 0.5041) and the validation set ( χ 2 = 9.9748, df = 8, p = 0.2668) well. The calibration plots of the training and validation sets based on the multivariate logistic regression model are shown in Fig. 5 A, B. The calibration curves of the modality maps showed that the predicted probability of sarcopenia for the training set (Fig. 5 A) and the validation set (Fig. 5 B) were highly consistent with the actual probability of sarcopenia.
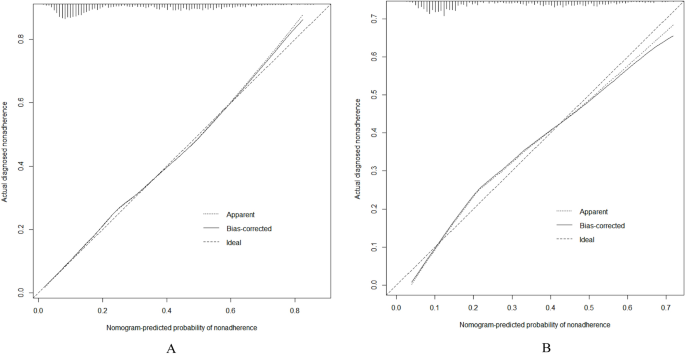
A Calibration plots for training dataset. B Calibration plots for validation dataset
Clinical validity assessment
The DCA method was used to evaluate the clinical validity of the model, and the results are shown in Figs. 6 A, B. From the decision curve, the net benefit of the prediction model on the internal validation set is significantly higher than that of the two extreme cases, indicating that the nomogram model has better net benefit and prediction accuracy.
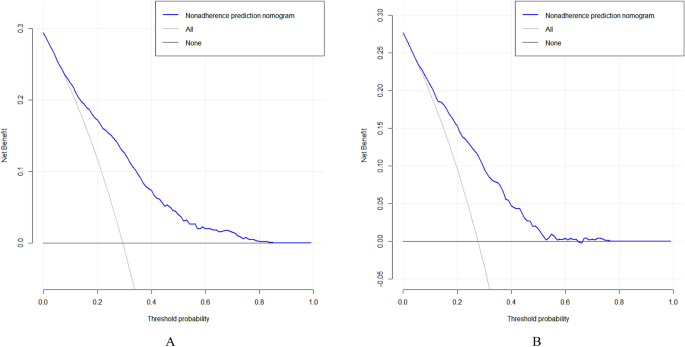
A DCA curves for training data set. B DCA curves for validation data set
This study reveals that the prevalence of sarcopenia among the older adults in China stands at 28.8%, aligning with the findings reported by Cruz-Jentoft and his colleagues [ 34 ], which ranged from 1 to 29%. Sarcopenia can lead to reduced mobility, increased disability, falls, and risk of death [ 35 , 36 ]. Therefore, identification of high-risk individuals is critical to preventing sarcopenia and its associated adverse outcomes.
This study shows that sex is a predictor of sarcopenia, and the results show that sarcopenia is related to sex, and the male population suffers from sarcopenia more often, which is consistent with previous research findings [ 37 , 38 ]. The reason for the analysis may be that sarcopenia is caused by genetic inheritance or gene mutation. Since the disease-causing gene is located on the sex chromosome, and men have only one chromosome, one gene mutation is enough to cause the disease; women have two chromosomes, so two copies of muscular dystrophy can only be caused by a mutation in the gene. It is rare for a woman to inherit two disease-causing genes on her chromosomes, so men are more likely to have muscular dystrophy than women [ 39 ].Whether it is genetic or a mutation, there is currently no way to change the gene. The only way to control it is to delay development, improve symptoms, increase muscle strength, and prolong life through medication.
Our study revealed that BMI serves as a predictor of sarcopenia, with a lower BMI indicating a higher risk of sarcopenia, consistent with findings reported by Wu LC and colleagues [ 40 ]. This suggests a potential association between higher BMI and improved prognosis among older adults. However, it is important to note that while higher BMI may confer certain benefits, such as reduced risk of sarcopenia, it can also contribute to metabolic syndrome, posing physiological challenges for older individuals. Moreover, metabolic disorders associated with obesity may exacerbate malnutrition, perpetuating a detrimental cycle. A meta-analysis encompassing 26 studies [ 41 ] underscored the significant impact of different training modalities on muscle strength and physical performance in older adults with sarcopenia. Similarly, a systematic review [ 42 ] highlighted the positive effects of appropriate physical activity in enhancing muscle strength and flexibility, averting muscle atrophy and degeneration, and promoting blood circulation and metabolism, thereby fostering overall health in older adults aged 60 years and above [ 43 ]. Furthermore, the role of supplements in enhancing muscle mass and preventing metabolic syndrome onset is noteworthy. Selenium and magnesium, investigated in randomized controlled trials and dietary observational studies [ 44 , 45 ], have shown potential associations with improved physical activity and muscle performance in older adults. Additionally, randomized controlled trials [ 46 ] have consistently demonstrated the efficacy of omega-3 fatty acids in preserving muscle mass and mitigating age-related muscle loss. In addressing the role of supplements, it is pertinent to mention Beta-hydroxy-beta-methylbutyrate (HMB), a metabolite of the essential amino acid leucine, which has garnered attention for its potential benefits in muscle health. Several studies have explored the effects of HMB supplementation on muscle mass preservation and physical function in older adults [ 47 , 48 ]. These findings suggest that HMB may serve as a valuable adjunct to nutritional interventions and muscle training in mitigating the risk of sarcopenia among older adults. Therefore, early nutritional intervention and muscle training should be offered to older adults at risk for sarcopenia to reduce the risk of sarcopenia.
At the same time, this study also found that blood pressure is closely related to the occurrence of sarcopenia. High systolic blood pressure may reflect the stiffness of the blood vessels, which may reduce the ability of blood to flow to the muscles, resulting in an inadequate supply of nutrients to the muscles, thus increasing muscle loss. Low diastolic blood pressure may indicate that the heart is not pumping enough blood to the body during diastole, which may also affect the supply of nutrients to the muscles [ 49 ]. Both high systolic blood pressure and low diastolic blood pressure can be signs of physical decline in the older adults, and physical decline is closely related to sarcopenia. Therefore, in the future, in addition to paying attention to heart, brain and kidney complications, hypertensive patients should also pay attention to muscle loss.
In addition, this study shows that chronic pain, particularly chronic low back pain, is also associated with sarcopenia, which has a critical impact on spinal health because maintaining spinal function requires the involvement of strong lower back muscles. On the one hand, the decrease in muscle quantity and quality reduces muscle tolerance to exercise and makes muscles more susceptible to fatigue, which reduces their ability to maintain overall spinal stability. Spinal instability greatly increases the incidence of chronic low back pain. On the other hand, the decline in the function of the trunk muscles, especially the dorsi extensors, leads to a weakening of the muscles' suspension force on the spine, making it difficult for the body to maintain a normal upright posture, resulting in a severe forward tilt of the body. Leaning forward increases the work of the posterior muscles, fatigues the muscle tissue, and makes it impossible to keep the body upright, creating a vicious cycle that affects the patient's quality of life [ 50 ]. Therefore, the prevention and treatment of sarcopenia is a very important and urgent issue for spinal health in older adults.
The nomogram, constructed through multifactorial regression analysis, amalgamates various predictive indicators to represent the relationships between variables in the predictive model using scaled line segments on a common plane according to a predetermined ratio. It serves as a tool to forecast the probability of a clinical outcome event by summing the scores assigned to each predictor to derive a total score. Widely employed across diverse clinical domains, the nomogram stands as a common predictive model utilized in research endeavors. To further bolster the credibility of our findings, we recognize the importance of engaging with previous studies that have developed and validated nomograms, as this interaction could enhance the robustness of our research outcomes. In this study, we identified sex, BMI, MSBP, MDBP and pain as the main factors predicting sarcopenia in Chinese older adults. Our prediction model, constructed based on these five factors influencing sarcopenia development, exhibited good discrimination, calibration, and clinical validity. This suggests that the prediction model holds value for effectively identifying high-risk older adults with sarcopenia. The nomogram specifically quantifies the hazard ratio in terms of scores, allowing for the calculation of the probability of a certain outcome through simple calculations. It provides individualized risk assessment for each person, enhancing relevance and accuracy.
Therefore, the establishment of a prediction model for sarcopenia in older adults constitutes a novel achievement of this study. Nomograms, as efficient and accurate assessment tools, can assist clinical medical staff in objectively screening older adults at risk of sarcopenia, thereby providing a theoretical basis and starting point for formulating early prevention and intervention measures. Their clinical applicability is robust, aiding in the identification of patients at high risk for sarcopenia, enabling the implementation of early intervention plans, and facilitating individual health management in older adults.
This study has several limitations. Firstly, the absence of age-specific analysis based on different age groups is a notable gap. Sarcopenia, which involves a decrease in muscle mass due to age-related hormonal changes, would have benefited from a more granular examination across age brackets. Secondly, the CHARLS database lacked some potential predictors, such as dietary habits and nutritional status, limiting the scope of our analysis. Thirdly, the nomogram developed in this study is specific to data from China, and its generalizability to other regions and countries remains to be determined through external validation. Additionally, while pain was identified as an important factor in sarcopenia, the study did not delve into the specifics of pain, such as its location, intensity, and duration, which could have provided deeper insights. Furthermore, patients with impaired cognitive function were not excluded, and in some cases, family members assisted with self-reporting, potentially introducing biases into the results. Given these limitations, future research should aim to conduct prospective studies, incorporate more comprehensive predictor variables, and externally validate the model to enhance its generalizability and accuracy.
Our sarcopenia risk prediction model based on CHARLS data provides a reliable and accurate tool for Chinese older adults. This model can help clinicians to identify high-risk patients earlier and take timely preventive and interventional measures to improve the quality of life and health outcomes of the older adults.
Availability of data and materials
The datasets generated during and/or analyzed during the current study are available in the CHARLS repository, http://charls.pku.edu.cn .
Ren R, Qi J, Lin S, et al. The China alzheimer report 2022. General Psychiatry. 2022;35(1): e100751.
Article PubMed PubMed Central Google Scholar
Liu YZZ, Rao K, Wang S. Blue book of elderly health: annual report on elderly health in China (2018). China: Social Science Academic Press; 2019.
Google Scholar
Damluji AA, Alfaraidhy M, Alhajri N, et al. Sarcopenia and cardiovascular diseases. Circulation. 2023;147(20):1534–53.
Cruz-Jentoft AJ, Sayer AA. Sarcopenia. Lancet (London, England). 2019;393(10191):2636–46.
Article PubMed Google Scholar
Bauer J, Morley JE, Schols A, et al. Sarcopenia: a time for action. An SCWD position paper. J Cachexia Sarcopenia Muscle. 2019;10(5):956–61.
Anker SD, Morley JE, von Haehling S. Welcome to the ICD-10 code for sarcopenia. J Cachexia Sarcopenia Muscle. 2016;7(5):512–4.
Chen LK, Woo J, Assantachai P, et al. Asian working group for sarcopenia: 2019 consensus update on sarcopenia diagnosis and treatment. J Am Med Directors Assoc. 2020;21(3):300-7.e2.
Article Google Scholar
Xu W, Chen T, Cai Y, et al. Sarcopenia in community-dwelling oldest old is associated with disability and poor physical function. J Nutr Health Aging. 2020;24(23):339–45.
Article CAS PubMed Google Scholar
Petermann-Rocha F, Balntzi V, Gray SR, et al. Global prevalence of sarcopenia and severe sarcopenia: a systematic review and meta-analysis. J Cachexia Sarcopenia Muscle. 2022;13(1):86–99.
Cesari M, Kuchel GA. Role of sarcopenia definition and diagnosis in clinical care: moving from risk assessment to mechanism-guided interventions. J Am Geriatr Soc. 2020;68(7):1406–9.
Shimokata H, Ando F. Sarcopenia and its risk factors in epidemiological study. Nihon Ronen Igakkai zasshi Japanese journal of geriatrics. 2012;49(6):721–5.
PubMed Google Scholar
Dodds RM, Granic A, Davies K, et al. Prevalence and incidence of sarcopenia in the very old: findings from the Newcastle 85+ Study. J Cachexia Sarcopenia Muscle. 2017;8(2):229–37.
Bone AE, Hepgul N, Kon S, et al. Sarcopenia and frailty in chronic respiratory disease. Chron Respir Dis. 2017;14(1):85–99.
Springer J, Springer JI, Anker SD. Muscle wasting and sarcopenia in heart failure and beyond: update 2017. ESC Heart Failure. 2017;4(4):492–8.
Bhanji RA, Narayanan P, Allen AM, et al. Sarcopenia in hiding: the risk and consequence of underestimating muscle dysfunction in nonalcoholic steatohepatitis. Hepatology (Baltimore, MD). 2017;66(6):2055–65.
Cruz-Jentoft AJ, Bahat G, Bauer J, et al. Sarcopenia: revised European consensus on definition and diagnosis. Age Ageing. 2019;48(1):16–31.
Gao K, Cao LF, Ma WZ, et al. Association between sarcopenia and cardiovascular disease among middle-aged and older adults: findings from the China health and retirement longitudinal study. EClinicalMedicine. 2022;44: 101264.
Cai G, Ying J, Pan M, et al. Development of a risk prediction nomogram for sarcopenia in hemodialysis patients. BMC Nephrol. 2022;23(1):319.
Article CAS PubMed PubMed Central Google Scholar
Yin G, Qin J, Wang Z, et al. A nomogram to predict the risk of sarcopenia in older people. Medicine. 2023;102(16): e33581.
Mo YH, Su YD, Dong X, et al. Development and validation of a nomogram for predicting sarcopenia in community-dwelling older adults. J Am Med Dir Assoc. 2022;23(5):715-21.e5.
Zhao Y, Hu Y, Smith JP, et al. Cohort profile: the China Health and Retirement Longitudinal Study (CHARLS). Int J Epidemiol. 2014;43(1):61–8.
Chen LK, Woo J, Assantachai P, et al. Asian working group for sarcopenia: 2019 consensus update on sarcopenia diagnosis and treatment. J Am Med Dir Assoc. 2020;21(3):300-7.e2.
Wen X, Wang M, Jiang CM, et al. Anthropometric equation for estimation of appendicular skeletal muscle mass in Chinese adults. Asia Pac J Clin Nutr. 2011;20(4):551–6.
Yang M, Hu X, Wang H, et al. Sarcopenia predicts readmission and mortality in elderly patients in acute care wards: a prospective study. J Cachexia Sarcopenia Muscle. 2017;8(2):251–8.
Alexandre Tda S, Duarte YA, Santos JL, et al. Sarcopenia according to the European Working Group on Sarcopenia in Older People (EWGSOP) versus dynapenia as a risk factor for mortality in the elderly. J Nutr Health Aging. 2014;18(8):751–6.
Wu X, Li X, Xu M, et al. Sarcopenia prevalence and associated factors among older Chinese population: Findings from the China Health and Retirement Longitudinal Study. PLoS ONE. 2021;16(3): e0247617.
Roberts RE, Rhoades HM, Vernon SW. Using the CES-D scale to screen for depression and anxiety: effects of language and ethnic status. Psychiatry Res. 1990;31(1):69–83.
Wu WT, Li YJ, Feng AZ, et al. Data mining in clinical big data: the frequently used databases, steps, and methodological models. Mil Med Res. 2021;8(1):44.
PubMed PubMed Central Google Scholar
Lyu J, Li Z, Wei H, et al. A potent risk model for predicting new-onset acute coronary syndrome in patients with type 2 diabetes mellitus in Northwest China. Acta Diabetol. 2020;57(6):705–13.
Morris TP, White IR, Royston P. Tuning multiple imputation by predictive mean matching and local residual draws. BMC Med Res Methodol. 2014;14:75.
Xue QL. The frailty syndrome: definition and natural history. Clin Geriatr Med. 2011;27(1):1–15.
Chen J, Wang X, Xu Z. Sarcopenia and chronic pain in the elderly: a systematic review and meta-analysis. J Pain Res. 2023;16:3569–81.
Du Y, Oh C, No J. Associations between sarcopenia and metabolic risk factors: a systematic review and meta-analysis. J Obes Metab Syndr. 2018;27(3):175–85.
Cruz-Jentoft AJ, Landi F, Schneider SM, et al. Prevalence of and interventions for sarcopenia in ageing adults: a systematic review. Report of the International Sarcopenia Initiative (EWGSOP and IWGS). Age Ageing. 2014;43(6):748–59.
Papadopoulou SK. Sarcopenia: a contemporary health problem among older adult populations. Nutrients. 2020;12(5):1293.
Senior HE, Henwood TR, Beller EM, et al. Prevalence and risk factors of sarcopenia among adults living in nursing homes. Maturitas. 2015;82(4):418–23.
Gao Q, Hu K, Yan C, et al. Associated factors of sarcopenia in community-dwelling older adults: a systematic review and meta-analysis. Nutrients. 2021;13(12):4291.
Bouchard DR, Dionne IJ, Brochu M. Sarcopenic/obesity and physical capacity in older men and women: data from the Nutrition as a Determinant of Successful Aging (NuAge)-the Quebec longitudinal Study. Obesity (Silver Spring, Md). 2009;17(11):2082–8.
Laurent MR, Dedeyne L, Dupont J, et al. Age-related bone loss and sarcopenia in men. Maturitas. 2019;122:51–6.
Wu LC, Kao HH, Chen HJ, et al. Preliminary screening for sarcopenia and related risk factors among the elderly. Medicine. 2021;100(19): e25946.
Lu L, Mao L, Feng Y, et al. Effects of different exercise training modes on muscle strength and physical performance in older people with sarcopenia: a systematic review and meta-analysis. BMC Geriatr. 2021;21(1):708.
Montero-Fernández N, Serra-Rexach JA. Role of exercise on sarcopenia in the elderly. Eur J Phys Rehabil Med. 2013;49(1):131–43.
Beaudart C, Dawson A, Shaw SC, et al. Nutrition and physical activity in the prevention and treatment of sarcopenia: systematic review. Osteoporosis Int. 2017;28(6):1817–33.
Article CAS Google Scholar
Rederstorff M, Krol A, Lescure A. Understanding the importance of selenium and selenoproteins in muscle function. CMLS. 2006;63(1):52–9.
Brown MR, Cohen HJ, Lyons JM, et al. Proximal muscle weakness and selenium deficiency associated with long term parenteral nutrition. Am J Clin Nutr. 1986;43(4):549–54.
Lalia AZ, Dasari S, Robinson MM, et al. Influence of omega-3 fatty acids on skeletal muscle protein metabolism and mitochondrial bioenergetics in older adults. Aging. 2017;9(4):1096–129.
Yang C, Song Y, Li T, et al. Effects of beta-hydroxy-beta-methylbutyrate supplementation on older adults with sarcopenia: a randomized, double-blind, placebo-controlled study. J Nutr Health Aging. 2023;27(5):329–39.
Landi F, Calvani R, Picca A, et al. Beta-hydroxy-beta-methylbutyrate and sarcopenia: from biological plausibility to clinical evidence. Curr Opin Clin Nutr Metab Care. 2019;22(1):37–43.
Zhang XZ, Xie WQ, Chen L, et al. Blood flow restriction training for the intervention of sarcopenia: current stage and future perspective. Front Med. 2022;9: 894996.
Lin T, Dai M, Xu P, et al. Prevalence of sarcopenia in pain patients and correlation between the two conditions: a systematic review and meta-analysis. J Am Med Directors Assoc. 2022;23(5):902.e1-e20.
Download references
Acknowledgements
This research received no specific grant from any funding agency in the public, commercial, or not-for-profit sectors.
No funding.
Author information
Qiugui Li and Hongtao Cheng equally contributed to this work.
Authors and Affiliations
School of Nursing, Jinan University, Guangzhou, Guangdong, China
Qiugui Li, Hongtao Cheng & Wenjiao Cen
Department of Neurosurgery, The First Affiliated Hospital of Jinan University, Guangzhou, Guangdong, China
Department of Healthcare-Associated Infection Management, The First Affiliated Hospital of Jinan University, Guangzhou, Guangdong, China
Shengru Tao
You can also search for this author in PubMed Google Scholar
Contributions
Qiugui Li, Hongtao Cheng: raise questions, screen variables, analyze data, write papers; Wenjiao Cen: screen variables; Tao Yang, Shengru Tao: Revise the thesis.
Corresponding author
Correspondence to Shengru Tao .
Ethics declarations
Ethics approval and consent to participate.
This is a retrospective study based on CHARLS database. The patient's information has been hidden before the study. The original CHARLS was approved by the Ethical Review Committee of Peking University (IRB00001052–11015), and all participants signed the informed consent at the time of participation. This research followed the guidance of the Declaration of Helsinki.
Consent for publication
Not applicable.
Competing interests
The authors declare that they have no known competing financial interests or personal relationships that could have appeared to influence the work reported in this paper.
Additional information
Publisher's note.
Springer Nature remains neutral with regard to jurisdictional claims in published maps and institutional affiliations.
Supplementary Information
Supplementary material 1. comparison between variables in the training and validation datasets, rights and permissions.
Open Access This article is licensed under a Creative Commons Attribution 4.0 International License, which permits use, sharing, adaptation, distribution and reproduction in any medium or format, as long as you give appropriate credit to the original author(s) and the source, provide a link to the Creative Commons licence, and indicate if changes were made. The images or other third party material in this article are included in the article's Creative Commons licence, unless indicated otherwise in a credit line to the material. If material is not included in the article's Creative Commons licence and your intended use is not permitted by statutory regulation or exceeds the permitted use, you will need to obtain permission directly from the copyright holder. To view a copy of this licence, visit http://creativecommons.org/licenses/by/4.0/ . The Creative Commons Public Domain Dedication waiver ( http://creativecommons.org/publicdomain/zero/1.0/ ) applies to the data made available in this article, unless otherwise stated in a credit line to the data.
Reprints and permissions
About this article
Cite this article.
Li, Q., Cheng, H., Cen, W. et al. Development and validation of a predictive model for the risk of sarcopenia in the older adults in China. Eur J Med Res 29 , 278 (2024). https://doi.org/10.1186/s40001-024-01873-w
Download citation
Received : 30 January 2024
Accepted : 26 April 2024
Published : 09 May 2024
DOI : https://doi.org/10.1186/s40001-024-01873-w
Share this article
Anyone you share the following link with will be able to read this content:
Sorry, a shareable link is not currently available for this article.
Provided by the Springer Nature SharedIt content-sharing initiative
- Predictive model
European Journal of Medical Research
ISSN: 2047-783X
- Submission enquiries: [email protected]
- General enquiries: [email protected]
This paper is in the following e-collection/theme issue:
Published on 17.5.2024 in Vol 26 (2024)
Machine Learning–Based Prediction of Suicidal Thinking in Adolescents by Derivation and Validation in 3 Independent Worldwide Cohorts: Algorithm Development and Validation Study
Authors of this article:

There are no citations yet available for this article according to Crossref .
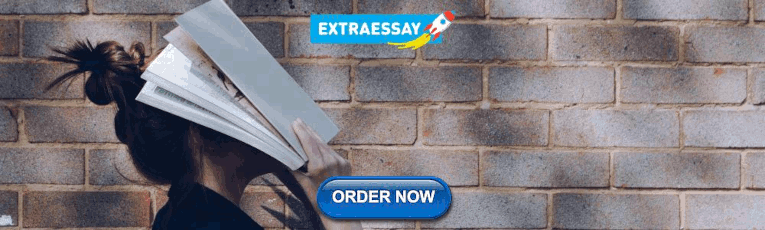
IMAGES
VIDEO
COMMENTS
Abstract. Data validation is the activity where one decides whether or not a particular data set is fit for a given purpose. Formalizing the requirements that drive this decision process allows ...
The precision in which the findings accurately reflect the data. Recognises that multiple realities exist; the researchers' outline personal experiences and viewpoints that may have resulted in methodological bias; clearly and accurately presents participants perspectives. '. Reliability.
of validity in the research process to obtain scientific rigor, such as a strongly formulated research question, a well-described theory, a research design that potentiates the scientific aspects of the study, and a well-described process of collecting and analyzing data. This paper is divided into four sections.
Abstract. This article provides a discussion on the question of validity in qualitative evaluation. Although validity in qualitative inquiry has been widely reflected upon in the methodological literature (and is still often subject of debate), the link with evaluation research is underexplored. Elaborating on epistemological and theoretical ...
Good qualitative research contributes to science via a logical chain of reasoning, multiple sources of converging evidence to support an explanation, and ruling out rival hypotheses with convincing arguments and solid data. Sampling of research par-ticipants in qualitative research is described as purposive, meaning there is far less emphasis on
sophisticated, quantitative data collection, analysis, and interpretations. In contrast, the study of language in use, and especially the construction of discourse, often demands a more interpretive, qualitative approach to the research process. While language assessment research remains primarily a quantitative endeavor focused
PRO Development Process. Ensuring content validity to develop a new PRO is a multistep process including qualitative data collection, item generation based on the patient perspective, and cognitive debriefing. Psychometric validation should not be conducted until the concept elicitation phase is complete.
Pediatric Research - Essentials of data management: an overview. ... Some examples of data validation rules for identifying implausible values are shown in Table ... Download PDF. Associated content.
It is not surprising that there are continuing tensions between the disciplinary research paradigms in which language testers situate themselves: psychometrics, which, by definition, involves the objective measurement of psychological traits, processes, and abilities and is based on the analysis of sophisticated, quantitative data, and applied linguistics, where the study of language in use ...
Abstract. The criteria for the validation of qualitative research are still open to discussion. This article has two aims: first, to present a summary of concepts, emerging from the field of qualitative research that present answers regarding issues of validation, reliability, and generalization; and second, to propose six concepts that allow the monitoring of the validation of ...
Refocusing the qualitative research process to verification strategies is not without profound implications. It will, for example, enhance researcher's responsiveness to data and constantly remind researchers to be proactive, and take responsibility for rigor. 6 Student projects, although necessarily smaller in scope, must also be responsive to ...
Definition 1. The state of completeness, validity, consistency, timeliness and accuracy that makes data appropriate for a. specific use. (Government of British Columbia) Definition 2. The quality of a particular dataset or record is to describe the. fitness. of that dataset or record for a particular use that one may have in mind for the data.
Validity in Qualitative 2. Feedback: "Soliciting feedback from others is an extremely useful strategy for identifying validity threats, your own biases and assumptions, and flaws in your logic or methods" (Maxwell, 1996, p. 94). Member Checks: "systematically soliciting feedback about one's data and conclusions from the people you are ...
Abstract. Data entry can result in errors that cause analytic problems and delays in disseminating research. Invalid responses can lead to incorrect statistics and statistical conclusions. The purpose of this article is to provide researchers some basic strategies for avoiding out-of-range data entry errors and streamlining data collection.
Validity in qualitative research means "appropriateness" of the tools, processes, and data. Whether the research question is valid for the desired outcome, the choice of methodology is appropriate for answering the research question, the design is valid for the methodology, the sampling and data analysis is appropriate, and finally the ...
A Step-By-Step Guide to Questionnaire Validation Research 2022. A S t e p -By- S t e p G u i d e t o Q u e s t i o n n a ire Va l i d a t i o n Re s e a rc h. Authors Mohamad Adam Bujang Hon Yoon Khee Lee Keng Yee. Editor Prof. Dr. Shamsul Azhar Shah.
This argument points to a data-centric approach to machine learning that treats training and serving data as an important production asset, on par with the algorithm and infrastructure used for learning. In this paper, we tackle this problem and present a data validation system that is designed to detect anomalies specifically in data fed into ...
models using bad data, research and industrial practice suggest incorporating a data validation process and tool in ML system development process. Aim: The study investigates the adoption of a data validation process and tool in industrial ML projects. The data validation process demands significant engineering resources for tool devel-
Background Although electronic nose (eNose) has been intensively investigated for diagnosing lung cancer, cross-site validation remains a major obstacle to be overcome and no studies have yet been performed. Methods Patients with lung cancer, as well as healthy control and diseased control groups, were prospectively recruited from two referral centers between 2019 and 2022. Deep learning ...
Sarcopenia is a progressive age-related disease that can cause a range of adverse health outcomes in older adults, and older adults with severe sarcopenia are also at increased short-term mortality risk. The aim of this study was to construct and validate a risk prediction model for sarcopenia in Chinese older adults. This study used data from the 2015 China Health and Retirement Longitudinal ...
In this validation study, we examined the factor structure of the mediated learning observation (MLO) used during the teaching phase of dynamic assessment. As an indicator of validity, we evaluated whether the MLO factor structure was consistent across children with and without developmental language disorder (DLD).
For validation, performance-intensity functions of each word list were fitted with responses from 60 NH subjects under six presentation levels (−1, 3, 5, 7, 11, and 20 dB HL). The final material set was determined by the intelligibility scores at each decibel level and the mean slopes.
www.vichealth.vic.gov.au
Calibration and validation of a hybrid traffic flow model based on vehicle trajectory data from a field car-following experiment. ... MISSIONE 4 COMPONENTE 2, INVESTIMENTO 1.4 - D.D. 1033 17/06/2022, CN00000023). This research was partially funded by the University of Salerno, under local grants no. ORSA214124-2021, no. ORSA223793- 2022 and ...
Background: Suicide is the second-leading cause of death among adolescents and is associated with clusters of suicides. Despite numerous studies on this preventable cause of death, the focus has primarily been on single nations and traditional statistical methods. Objective: This study aims to develop a predictive model for adolescent suicidal thinking using multinational data sets and machine ...
workforce. This brief provides detailed data on the occupations within three major health care disciplines in the U.S. health care workforce: medicine, nursing, and oral health. For these critical occupations, this brief presents the most recent data on adequacy, distribution, and the educational pipeline of these future health care providers.