- Open access
- Published: 04 February 2022
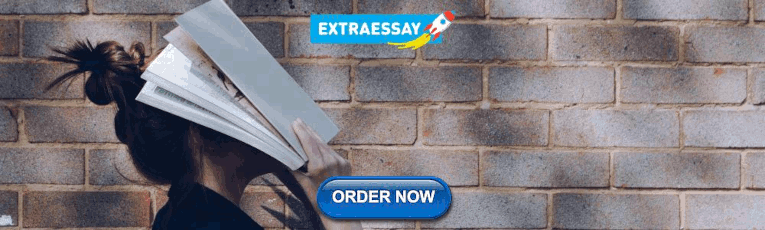
Analysis of the COVID-19 pandemic: lessons towards a more effective response to public health emergencies
- Yibeltal Assefa ORCID: orcid.org/0000-0003-2393-1492 1 ,
- Charles F. Gilks 1 ,
- Simon Reid 1 ,
- Remco van de Pas 2 ,
- Dereje Gedle Gete 1 &
- Wim Van Damme 2
Globalization and Health volume 18 , Article number: 10 ( 2022 ) Cite this article
38k Accesses
34 Citations
10 Altmetric
Metrics details
The pandemic of Coronavirus Disease 2019 (COVID-19) is a timely reminder of the nature and impact of Public Health Emergencies of International Concern. As of 12 January 2022, there were over 314 million cases and over 5.5 million deaths notified since the start of the pandemic. The COVID-19 pandemic takes variable shapes and forms, in terms of cases and deaths, in different regions and countries of the world. The objective of this study is to analyse the variable expression of COVID-19 pandemic so that lessons can be learned towards an effective public health emergency response.
We conducted a mixed-methods study to understand the heterogeneity of cases and deaths due to the COVID-19 pandemic. Correlation analysis and scatter plot were employed for the quantitative data. We used Spearman’s correlation analysis to determine relationship strength between cases and deaths and socio-economic and health systems. We organized qualitative information from the literature and conducted a thematic analysis to recognize patterns of cases and deaths and explain the findings from the quantitative data.
We have found that regions and countries with high human development index have higher cases and deaths per million population due to COVID-19. This is due to international connectedness and mobility of their population related to trade and tourism, and their vulnerability related to older populations and higher rates of non-communicable diseases. We have also identified that the burden of the pandemic is also variable among high- and middle-income countries due to differences in the governance of the pandemic, fragmentation of health systems, and socio-economic inequities.
The COVID-19 pandemic demonstrates that every country remains vulnerable to public health emergencies. The aspiration towards a healthier and safer society requires that countries develop and implement a coherent and context-specific national strategy, improve governance of public health emergencies, build the capacity of their (public) health systems, minimize fragmentation, and tackle upstream structural issues, including socio-economic inequities. This is possible through a primary health care approach, which ensures provision of universal and equitable promotive, preventive and curative services, through whole-of-government and whole-of-society approaches.
The pandemic of Coronavirus Disease 2019 (COVID-19) is a timely reminder of the nature and impact of emerging infectious diseases that become Public Health Emergency of International Concern (PHEIC) [ 1 ]. The COVID-19 pandemic takes variable shapes and forms in how it affects communities in different regions and countries [ 2 , 3 ]. As of 12 January, 2022, there were over 314 million cases and over 5.5 million deaths notified around the globe since the start of the pandemic. The number of cases per million population ranged from 7410 in Africa to 131,730 in Europe while the number of deaths per million population ranged from 110 in Oceania to 2740 in South America. Case-fatality rates (CFRs) ranged from 0.3% in Oceania to 2.9% in South America [ 4 , 5 ]. Regions and countries with high human development index (HDI), which is a composite index of life expectancy, education, and per capita income indicators [ 6 ], are affected by COVID-19 more than regions with low HDI. North America and Europe together account for 55 and 51% of cases and deaths, respectively. Regions with high HDI are affected by COVID-19 despite their high universal health coverage index (UHCI) and Global Health Security index (GHSI) [ 7 ].
This seems to be a paradox (against the established knowledge that countries with weak (public) health systems capacity will have worse health outcomes) in that the countries with higher UHCI and GHSI have experienced higher burdens of COVID-19 [ 7 ]. The paradox can partially be explained by variations in testing algorithms, capacity for testing, and reporting across different countries. Countries with high HDI have health systems with a high testing capacity; the average testing rate per million population is less than 32, 000 in Africa and 160,000 in Asia while it is more than 800, 000 in HICs (Europe and North America). This enables HICs to identify more confirmed cases that will ostensibly increase the number of reported cases [ 3 ]. Nevertheless, these are insufficient to explain the stark differences between countries with high HDI and those with low HDI. Many countries with high HDI have a high testing rate and a higher proportion of symptomatic and severe cases, which are also associated with higher deaths and CFRs [ 7 ]. On the other hand, there are countries with high HDI that sustain a lower level of the epidemic than others with a similar high HDI. It is, therefore, vital to analyse the heterogeneity of the COVID-19 pandemic and explain why some countries with high HDI, UHCI and GHSI have the highest burden of COVID-19 while others are able to suppress their epidemics and mitigate its impacts.
The objective of this study was to analyse the COVID-19 pandemic and understand its variable expression with the intention to learn lessons for an effective and sustainable response to public health emergencies. We hypothesised that high levels of HDI, UHCI and GHSI are essential but not sufficient to prevent and control COVID-19.
We conducted an explanatory mixed-methods study to understand and explain the heterogeneity of the pandemic around the world. The study integrated quantitative and qualitative secondary data. The following steps were included in the research process: (i) collecting and analysing quantitative epidemiological data, (ii) conducting literature review of qualitative secondary data and (iii) evaluating countries’ pandemic responses to explain the variability in the COVID-19 epidemiological outcomes. The study then illuminated specific factors that were vital towards an effective and sustainable epidemic response.
We used the publicly available secondary data sources from Johns Hopkins University ( https://coronavirus.jhu.edu/data/new-cases ) for COVID-19 and UNDP 2020 HDI report ( http://hdr.undp.org/en/2019-report ) for HDI, demographic and epidemiologic variables. These are open data sources which are regularly updated and utilized by researchers, policy makers and funders. We performed a correlation analysis of the COVID-19 pandemic. We determined the association between COVID-19 cases, severity, deaths and CFRs at the 0.01 and 0.05 levels (2-tailed). We used Spearman’s correlation analysis, as there is no normal distribution of the variables [ 8 ].
The UHCI is calculated as the geometric mean of the coverage of essential services based on 17 tracer indicators from: (1) reproductive, maternal, newborn and child health; (2) infectious diseases; (3) non-communicable diseases; and, (4) service capacity and access and health security [ 9 ]. The GHSI is a composite measure to assess a country’s capability to prevent, detect, and respond to epidemics and pandemics [ 10 ].
We then conducted a document review to explain the epidemic patterns in different countries. Secondary data was obtained from peer-reviewed journals, reputable online news outlets, government reports and publications by public health-related associations, such as the WHO. To explain the variability of COVID-19 across countries, a list of 14 indicators was established to systematically assess country’s preparedness, actual pandemic response, and overall socioeconomic and demographic profile in the context of COVID-19. The indicators used in this study include: 1) Universal Health Coverage Index, 2) public health capacity, 3) Global Health Security Index, 4) International Health Regulation, 5) leadership, governance and coordination of response, 6) community mobilization and engagement, 7) communication, 8) testing, quarantines and social distancing, 9) medical services at primary health care facilities and hospitals, 10) multisectoral actions, 11) social protection services, 12) absolute and relative poverty status, 13) demography, and 14) burden of communicable and non-communicable diseases. These indicators are based on our previous studies and recommendation from the World Health Organization [ 3 , 4 ]. We conducted thematic analysis and synthesis to identify the factors that may explain the heterogeneity of the pandemic.
Heterogeneity of COVID-19 cases and deaths around the world: what can explain it?
Table 1 indicates that the pandemic of COVID-19 is heterogeneous around regions of the world. Figure 1 also shows that there is a strong and significant correlation between HDI and globalisation (with an increase in trade and tourism as proxy indicators) and a corresponding strong and significant correlation with COVID-19 burden.
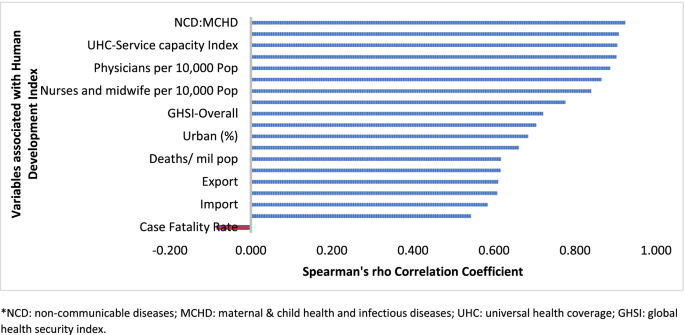
Human development index and its correlates associated with COVID-19 in 189 countries*
Globalisation and pandemics interact in various ways, including through international trade and mobility, which can lead to multiple waves of infections [ 11 ]. In at least the first waves of the pandemic, countries with high import and export of consumer goods, food products and tourism have high number of cases, severe cases, deaths and CFRs. Countries with high HDI are at a higher risk of importing (and exporting) COVID-19 due to high mobility linked to trade and tourism, which are drivers of the economy. These may have led to multiple introductions of COVID-19 into these countries before border closures.
The COVID-19 pandemic was first identified in China, which is central to the global network of trade, from where it spread to all parts of the world, especially those countries with strong links with China [ 12 ]. The epidemic then spread to Europe. There is very strong regional dimension to manufacturing and trading, which could be facilitate the spread of the virus. China is the heart of ‘Factory Asia’; Italy is in the heart of ‘Factory Europe’; the United States is the heart of ‘Factory North America’; and Brazil is the heart of ‘Factory Latin America’ [ 13 ]. These are the countries most affected by COVID-19 during the first wave of the pandemic [ 2 , 3 , 14 ].
It is also important to note that two-third of the countries currently reporting more than a million cases are middle-income countries (MICs), which are not only major emerging market economies but also regional political powers, including the BRICS countries (Brazil, Russia, India and South Africa) [ 3 , 15 ]. These countries participate in the global economy, with business travellers and tourists. They also have good domestic transportation networks that facilitate the internal spread of the virus. The strategies that helped these countries to become emerging markets also put them at greater risk for importing and spreading COVID-19 due to their connectivity to the rest of the world.
In addition, countries with high HDI may be more significantly impacted by COVID-19 due to the higher proportion of the elderly and higher rates of non-communicable diseases. Figure 1 shows that there is a strong and significant correlation between HDI and demographic transition (high proportion of old-age population) and epidemiologic transition (high proportion of the population with non-communicable diseases). Countries with a higher proportion of people older than 65 years and NCDs (compared to communicable diseases) have higher burden of COVID-19 [ 16 , 17 , 18 , 19 , 20 ]. Evidence has consistently shown a higher risk of severe COVID-19 in older individuals and those with underlying health conditions [ 21 , 22 , 23 , 24 , 25 ]. CFR is age-dependent; it is highest in persons aged ≥85 years (10 to 27%), followed by those among persons aged 65–84 years (3 to 11%), and those among persons aged 55-64 years (1 to 3%) [ 26 ].
On the other hand, regions and countries with low HDI have, to date, experienced less severe epidemics. For instance, as of January 12, 2022, the African region has recorded about 10.3 million cases and 233,000 deaths– far lower than other regions of the world (Table 1 ) [ 27 ]. These might be due to lower testing rates in Africa, where only 6.5% of the population has been tested for the virus [ 14 , 28 ], and a greater proportion of infections may remain asymptomatic [ 29 ]. Indeed, the results from sero-surveys in Africa show that more than 80% of people infected with the virus were asymptomatic compared to an estimated 40-50% asymptomatic infections in HICs [ 30 , 31 ]. Moreover, there is a weak vital registration system in the region indicating that reports might be underestimating and underreporting the disease burden [ 32 ]. However, does this fully explain the differences observed between Africa and Europe or the Americas?
Other possible factors that may explain the lower rates of cases and deaths in Africa include: (1) Africa is less internationally connected than other regions; (2) the imposition of early strict lockdowns in many African countries, at a time when case numbers were relatively small, limited the number of imported cases further [ 2 , 33 , 34 ]; (3) relatively poor road network has also limited the transmission of the virus to and in rural areas [ 35 ]; (4) a significant proportion of the population resides in rural areas while those in urban areas spend a lot of their time mostly outdoors; (5) only about 3% of Africans are over the age of 65 (so only a small proportion are at risk of severe COVID-19) [ 36 ]; (6) lower prevalence of NCDs, as disease burden in Africa comes from infectious causes, including coronaviruses, which may also have cross-immunity that may reduce the risk of developing symptomatic cases [ 37 ]; and (7) relative high temperature (a major source of vitamin D which influences COVID-19 infection and mortality) in the region may limit the spread of the virus [ 38 , 39 ]. We argue that a combination of all these factors might explain the lower COVID-19 burden in Africa.
The early and timely efforts by African leaders should not be underestimated. The African Union, African CDC, and WHO convened an emergency meeting of all African ministers of health to establish an African taskforce to develop and implement a coordinated continent-wide strategy focusing on: laboratory; surveillance; infection prevention and control; clinical treatment of people with severe COVID-19; risk communication; and supply chain management [ 40 ]. In April 2021, African Union and Africa CDC launched the Partnerships for African Vaccine Manufacturing (PAVM), framework to expanding Africa’s vaccine manufacturing capacity for health security [ 41 ].
Heterogeneity of the pandemic among countries with high HDI: what can explain it?
Figures 2 and 3 illustrate the variability of cases and deaths due to the COVID-19 pandemic across high-income countries (HICs). Contrary to the overall positive correlation between high HDI and cases, deaths and fatality rates due to COVID-19, there are outlier HICs, which have been able to control the epidemic. Several HICs, such as New Zealand, Australia, South Korea, Japan, Denmark, Iceland, and Norway, managed to contain their epidemics (Figs. 2 and 3 ) [ 15 , 42 , 43 ]. It is important to note that most of these countries (especially the island states) have far less cross-border mobility than other HICs.
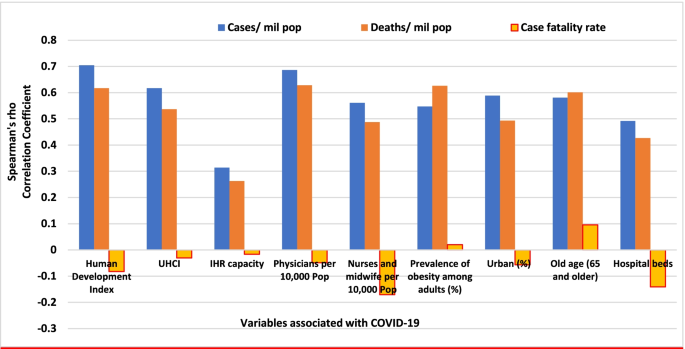
Scatter plot of COVID-19 cases per million population in countries with high human development index (> 0.70)
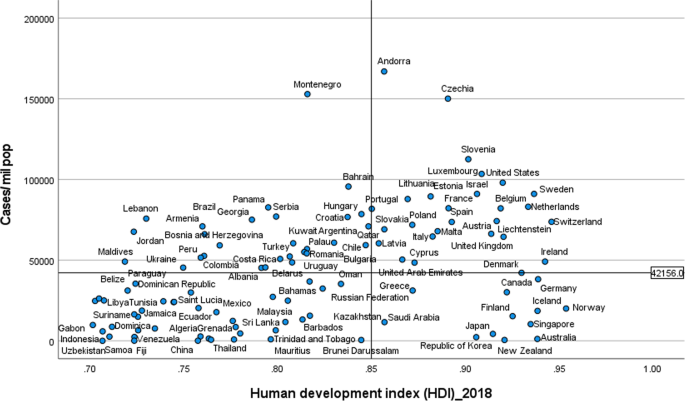
Scatter plot of COVID-19 deaths per million population in countries with high human development index (> 0.70)
HICs that have been successful at controlling their epidemics have similar characteristics, which are related to governance of the response [ 44 ], synergy between UHC and GHS, and existing relative socio-economic equity in the country. Governance and leadership is a crucial factor to explain the heterogeneity of the epidemic among countries with high HDI [ 45 ]. There has been substantial variation in the nature and timing of the public health responses implemented [ 46 ]. Adaptable and agile governments seem better able to respond to their epidemics [ 47 , 48 ]. Countries that have fared the best are the ones with good governance and public support [ 49 ]. Countries with an absence of coherent leadership and social trust have worse outcomes than countries with collective action, whether in a democracy or autocracy, and rapid mobilisation of resources [ 50 ]. The erosion of trust in the United States government has hurt the country’s ability to respond to the COVID-19 crisis [ 51 , 52 ]. The editors of the New England Journal of Medicine argued that the COVID-19 crisis has produced a test of leadership; but, the leaders in the United States had failed that test [ 47 ].
COVID-19 has exposed the fragility of health systems, not only in the public health and primary care, but also in acute and long-term care systems [ 49 ]. Fragmentation of health systems, defined here to mean inadequate synergy and/ or integration between GHS and UHC, is typical of countries most affected by the COVID-19 pandemic. Even though GHS and UHC agendas are convergent and interdependent, they tend to have different policies and practices [ 53 ]. The United States has the highest index for GHS preparedness; however, it has reported the world’s highest number of COVID-19 cases and deaths due to its greatly fragmented health system [ 54 , 55 ]. Countries with health systems and policies that are able to integrate International Health Regulations (IHR) core capacities with primary health care (PHC) services have been effective at mitigating the effects of COVID-19 [ 50 , 53 ]. Australia has been able to control its COVID-19 epidemic through a comprehensive primary care response, including protection of vulnerable people, provision of treatment and support services to affected people, continuity of regular healthcare services, protection and support of PHC workers and primary care services, and provision of mental health services to the community and the primary healthcare workforce [ 56 ]. Strict implementation of public health and social intervention together with UHC systems have ensured swift control of the epidemics in Singapore, South Korea, and Thailand [ 57 ].
The heterogeneity of cases and deaths, due to COVID-19, is also explained by differences in levels of socio-economic inequalities, which increase susceptibility to acquiring the infection and disease progression as well as worsening of health outcomes [ 58 ]. COVID-19 has been a stress test for public services and social protection systems. There is a higher burden of COVID-19 in Black, Asian and Minority Ethnic individuals due to socio-economic inequities in HICs [ 59 , 60 ]. Poor people are more likely to live in overcrowded accommodation, are more likely to have unstable work conditions and incomes, have comorbidities associated with poverty and precarious living conditions, and reduced access to health care [ 59 ].
The epidemiology of COVID-19 is also variable across MICs, with HDI between 0.70 and 0.85, around the world. Overall, the epidemic in MICs is exacerbated by the rapid demographic and epidemiologic transitions as well as high prevalence of obesity. While India and Brazil witnessed rapidly increasing rates of cases and deaths, China, Thailand, Vietnam have experienced a relatively lower disease burden [ 15 ]. This heterogeneity may be attributed to a number of factors, including governance, communication and service delivery. Thailand, China and Vietnam have implemented a national harmonized strategic response with decentralized implementation through provincial and district authorities [ 61 ]. Thailand increased its testing capacity from two to over 200 certified facilities that could process between 10,000 to 100,000 tests per day; moreover, over a million village health volunteers in Thailand supported primary health services [ 62 , 63 ]. China’s swift and decisive actions enabled the country to contain its epidemic though there was an initial delay in detecting the disease. China has been able to contain its epidemic through community-based measures, very high public cooperation and social mobilization, strategic lockdown and isolation, multi-sector action [ 64 ]. Overall, multi-level governance (effective and decisive leadership and accountability) of the response, together with coordination of public health and socio-economic services, and high levels of citizen adherence to personal protection, have enabled these countries to successfully contain their epidemics [ 61 , 65 , 66 ].
On the other hand, the Brazilian leadership was denounced for its failure to establish a national surveillance network early in the pandemic. In March 2020, the health minister was reported to have stated that mass testing was a waste of public funding, and to have advised against it [ 67 ]. This was considered as a sign of a collapse of public health leadership, characterized by ignorance, neoliberal authoritarianism [ 68 ]. There were also gaps in the public health capacity in different municipalities, which varied greatly, with a considerable number of Brazilian regions receiving less funding from the federal government due to political tension [ 69 ]. The epidemic has a disproportionate adverse burden on states and municipalities with high socio-economic vulnerability, exacerbated by the deep social and economic inequalities in Brazil [ 70 ].
India is another middle-income country with a high burden of COVID-19. It was one of the countries to institute strict measures in the early phase of the pandemic [ 71 , 72 ]. However, the government eased restrictions after the claim that India had beaten the pandemic, which lead to a rapid increase in disease incidence. Indeed, on 12 January 2022, India reported 36 million cumulative cases and almost 485,000 total deaths [ 15 ]. The second wave of the epidemic in India exposed weaknesses in governance and inadequacies in the country’s health and other social systems [ 73 ]. The nature of the Indian federation, which is highly centripetal, has prevented state and local governments from tailoring a policy response to suit local needs. A centralized one-size-fits-all strategy has been imposed despite high variations in resources, health systems capacity, and COVID-19 epidemics across states [ 74 ]. There were also loose social distancing and mask wearing, mass political rallies and religious events [ 75 ]. Rapid community transmission driven by high population density and multigenerational households has been a feature of the current wave in India [ 76 ]. In addition, several new variants of the virus, including the UK (B.1.1.7), the South Africa (20H/501Y or B.1.351), and Brazil (P.1), alongside a newly identified Indian variant (B.1.617), are circulating in India and have been implicated as factors in the second wave of the pandemic [ 75 , 76 ].
Heterogeneity of case-fatality rates around the world: what can explain it?
The pandemic is characterized by variable CFRs across regions and countries that are negatively associated with HDI (Fig. 1 ). The results presented in Fig. 4 show that the proportion of elderly population and rate of obesity are important factors which are positively associated with CFR. On the other hand, UHC, IHR capacity and other indicators of health systems capacity (health workforce density and hospital beds) are negatively associated with the CFR (Figs. 1 and 4 ).
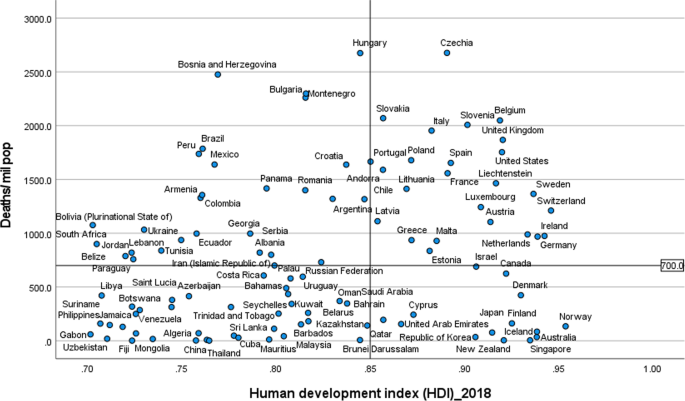
Correlates of COVID-19 cases, deaths and case-fatality rates in 189 countries
The evidence from several research indicates that heterogeneity can be explained by several factors, including differences in age-pyramid, socio-economic status, access to health services, or rates of undiagnosed infections. Differences in age-pyramid may explain some of the observed variation in epidemic severity and CFR between countries [ 77 ]. CFRs across countries look similar when taking age into account [ 78 ]. The elderly and other vulnerable populations in Africa and Asia are at a similar risk as populations in Europe and Americas [ 79 ]. Data from European countries suggest that as high as 57% of all deaths have happened in care homes and many deaths in the US have also occurred in nursing homes. On the other hand, in countries such as Mexico and India, individuals < 65 years contributed the majority of deaths [ 80 ].
Nevertheless, CFR also depends on the quality of hospital care, which can be used to judge the health system capacity, including the availability of healthcare workers, resources, and facilities, which affects outcomes [ 81 ]. The CFR can increase if there is a surge of infected patients, which adds to the strain on the health system [ 82 ]. COVID-19 fatality rates are affected by numerous health systems factors, including bed capacity, existence and capacity of intensive care unit (ICU), and critical care resources (such as oxygen and dexamethasone) in a hospital. Regions and countries with high HDI have a greater number of acute care facilities, ICU, and hospital bed capacities compared to lower HDI regions and countries [ 83 ]. Differences in health systems capacity could explain why North America and Europe, which have experienced much greater number of cases and deaths per million population, reported lower CFRs than the Southern American and the African regions, partly also due to limited testing capacity in these regions (Table 1 ) [ 84 , 85 , 86 ]. The higher CFR in Southern America can be explained by the relatively lower health systems surge capacity that could not adequately respond to the huge demand for health services [ 69 , 86 ]. The COVID-19 pandemic has highlighted existing health systems’ weaknesses, which are not able to effectively prepare for and respond to PHEs [ 87 ]. The high CFRs in the region are also exacerbated by the high social inequalities [ 69 ].
On the other hand, countries in Asia recorded lower CFRs (~ 1.4%) despite sharing many common risk factors (including overcrowding and poverty, weak health system capacity etc) with Africa. The Asian region shares many similar protective factors to the African region. They have been able to minimize their CFR by suppressing the transmission of the virus and flattening the epidemic curve of COVID-19 cases and deaths. Nevertheless, the epidemic in India is likely to be different because it has exceeded the health system capacity to respond and provide basic medical care and medical supplies such as oxygen [ 88 ]. Overall, many Asian countries were able to withstand the transmission of the virus and its effect due to swift action by governments in the early days of the pandemic despite the frequency of travel between China and neighbouring countries such as Hong Kong, Taiwan and Singapore [ 89 ]. This has helped them to contain the pandemic to ensure case numbers remain within their health systems capacity. These countries have benefited from their experience in the past in the prevention and control of epidemics [ 90 ].
There are a number of issues with the use of the CFR to compare the management of the pandemic between countries and regions [ 91 ], as it does not depict the true picture of the mortality burden of the pandemic. A major challenge with accurate calculation of the CFR is the denominator on number of identified cases, as asymptomatic infections and patients with mild symptoms are frequently left untested, and therefore omitted from CFR calculations. Testing might not be widely available, and proactive contact tracing and containment might not be employed, resulting in a smaller denominator, and skewing to a higher CFR [ 82 ]. It is, therefore, far more relevant to estimate infection fatality rate (IFR), the proportion of all infected individuals who have died due to the infection [ 91 ], which is central to understanding the public health impact of the pandemic and the required policies for its prevention and control [ 92 ].
Estimates of prevalence based on sero-surveys, which includes asymptomatic and mildly symptomatic infections, can be used to estimate IFR [ 93 ]. In a systematic review of 17 studies, seroprevalence rates ranged from 0.22% in Brazil to 53% in Argentina [ 94 ]. The review also identified that the seroprevalence estimate was higher than the cumulative reported case incidence, by a factor between 1.5 times in Germany to 717 times in Iran, in all but two studies (0.56 times in Brazil and 0.88 times in Denmark) [ 94 , 95 ]. The difference between seroprevalence and cumulative reported cases might be due to asymptomatic cases, atypical or pauci-symptomatic cases, or the lack of access to and uptake of testing [ 94 ]. There is only a modest gap between the estimated number of infections from seroprevalence surveys and the cumulative reported cases in regions with relatively thorough symptom-based testing. Much of the gap between reported cases and seroprevalence is likely to be due to undiagnosed symptomatic or asymptomatic infections [ 94 ].
Collateral effects of the COVID-19 pandemic
It is important to note that the pandemic has significant collateral effects on the provision of essential health services, in addition to the direct health effects [ 96 ]. Disruptions in the provision of essential health services, due to COVID-19, were reported by nearly all countries, though it is more so in lower-income than higher-income countries [ 97 , 98 ]. The biggest impact reported is on provision of day-to-day primary care to prevent and manage some of the most common health problems [ 99 ].
The causes of disruptions in service delivery were a mix of demand and supply factors [ 100 ]. Countries reported that just over one-third of services were disrupted due to health workforce-related reasons (the most common causes of service disruptions), supply chains, community mistrust and fears of becoming infected, and financial challenge s[ 101 ]. Cognizant of the disruptive effects of the pandemic, countries have reorganized their health system.
Countries with better response to COVID-19 have mobilized, trained and reallocated their health workforce in addition to hiring new staff, using volunteers and medical trainees and mobilizing retirees [ 102 ]. Several strategies have also been implemented to mitigate disruptions in service delivery and utilization, including: triaging to identify the most urgent patient needs, and postponing elective medical procedures; switching to alternative models of care, such as providing more home-based care and telemedicine [ 101 ].
This study identifies that the COVID-19 pandemic, in terms f cases and deaths, is heterogeneous around the world. This variability is explained by differences in vulnerability, preparedness, and response. It confirms that a high level of HDI, UHCI and GHSI are essential but not sufficient to control epidemics [ 103 ]. An effective response to public health emergencies requires a joint and reinforcing implementation of UHC, health emergency and disease control priorities [ 104 , 105 ], as well as good governance and social protection systems [ 106 ]. Important lessons have been learned to cope better with the COVID-19 pandemic and future emerging or re-emerging pandemics. Countries should strengthen health systems, minimize fragmentation of public health, primary care and secondary care, and improve coordination with other sectors. The pandemic has exposed the health effects of longstanding social inequities, which should be addressed through policies and actions to tackle vulnerability in living and working conditions [ 106 ].
The shift in the pandemic epicentre from high-income to MICs was observed in the second global wave of the pandemic. This is due to in part to the large-scale provision of vaccines in HICs [ 15 ] as well as the limitations in the response in LMICs, including inadequate testing, quarantine and isolation, contact tracing, and social distancing. The second wave of the pandemic in low- and middle-income countries spread more rapidly than the first wave and affected younger and healthier populations due to factors, including poor government decision making, citizen behaviour, and the emergence of highly transmissible SARS-CoV-2 variants [ 107 ]. It has become catastrophic in some MICs to prematurely relax key public health measures, such as mask wearing, physical distancing, and hand hygiene [ 108 ].
There is consensus that global vaccination is essential to ending the pandemic. Universal and equitable vaccine delivery, implemented with high volume, speed and quality, is vital for an effective and sustainable response to the current pandemic and future public health emergencies. There is, however, ongoing concern regarding access to COVID-19 vaccines in low-income countries [ 109 ]. Moreover, there is shortage of essential supplies, including oxygen, which has had a major impact on the prevention and control of the pandemic. It is, therefore, vital to transform (through good governance and financing mechanisms) the ACT-A platform to deliver vaccines, therapeutics, diagnostics, and other essential supplies [ 109 , 110 ]. The global health community has the responsibility to address these inequalities so that we can collectively end the pandemic [ 107 ].
The Omicron variant has a huge role in the current wave around the world despite high vaccine coverage [ 111 ]. Omicron appears to spread rapidly around the world ever since it was identified in November 2021 [ 112 ]. It becomes obvious that vaccination alone is inadequate for controlling the infection. This has changed our understanding of the COVID-19 pandemic endgame. The emergence of new variants of concern and their spread around the world has highlighted the importance of combination prevention, including high vaccination coverage in combination with other public health prevention measures [ 112 ].
Overall, the COVID-19 pandemic and the response to it emphasise valuable lessons towards an effective and sustainable response to public health emergencies. We argue that the PHC approach captures the different preparedness and response strategies required towards ensuring health security and UHC [ 113 ]. The PHC approach enables countries to progressively realize universal access to good-quality health services (including essential public health functions) and equity, empower people and communities, strengthen multi-sectoral policy and action for health, and enhance good governance [ 114 ]. These are essential in the prevention and control of public health emergencies, to suppress transmission, and reduce morbidity and mortality [ 115 ]. Access to high-quality primary care is at the foundation of any strong health system [ 116 ], which will, in turn, have effect on containing the epidemic, and reducing mortality and CFR [ 117 ]. Australia is a good example in this regard because it has implemented a comprehensive PHC approach in combination with border restrictions to ensure health system capacity is not exceeded [ 56 ]. The PHC approach will enable countries to develop and implement a context-specific health strategy, enhance governance, strengthen their (public) health systems, minimize segmentation and fragmentation, and tackle upstream structural issues, including discrimination and socio-economic inequities [ 118 ]. This is the type of public health approach (comprehensive, equity-focused and participatory) that will be effective and sustainable to tackle public health emergencies in the twenty-first century [ 119 , 120 ]. In addition, it is vital to transform the global and regional health systems, with a strong IHR and an empowered WHO at the apex [ 121 ]. We contend that this is the way towards a healthier and safer country, region and world.
The COVID-19 pandemic demonstrates that the world remains vulnerable to public health emergencies with significant health and other socio-economic impacts. The pandemic takes variable shapes and forms across regions and countries around the world. The pandemic has impacted countries with inadequate governance of the epidemic, fragmentation of their health systems and higher socio-economic inequities more than others. We argue that adequate response to public health emergencies requires that countries develop and implement a context-specific national strategy, enhance governance of public health emergency, build the capacity of their health systems, minimize fragmentation, and tackle socio-economic inequities. This is possible through a PHC approach that provides universal access to good-quality health services through empowered communities and multi-sectoral policy and action for health development. The pandemic has affected every corner of the world; it has demonstrated that “no country is safe unless other countries are safe”. This should be a call for a strong global health system based on the values of justice and capabilities for health.
Availability of data and materials
Data are available in a public, open access repository: Johns Hopkins University: https://coronavirus.jhu.edu/data/new-cases , and UNDP: http://hdr.undp.org/en/2019-report ; WHO: https://www.who.int/publications/m/item/weekly-epidemiological-update%2D%2D-22-december-2020
Abbreviations
Coronavirus Disease 2019
Case-fatality rates
Human development index
Universal health coverage index
Global Health Security index
High-income countries
Middle-income countries
El Zowalaty ME, Järhult JD. From SARS to COVID-19: A previously unknown SARS-CoV-2 virus of pandemic potential infecting humans–Call for a One Health approach. One Health. 2020;9:100124. https://doi.org/10.1016/j.onehlt.2020.100124 .
Van Damme W, Dahake R, Delamou A, Ingelbeen B, Wouters E, Vanham G, et al. The COVID-19 pandemic: diverse contexts; different epidemics—how and why? BMJ Glob Health. 2020;5(7):e003098.
Article PubMed Google Scholar
World Health Organization (WHO): Coronavirus disease ( COVID-19): situation report, 150. 2020. https://www.who.int/docs/default-source/coronaviruse/situation-reports/20200618-covid-19-sitrep-150.pdf .
Weekly epidemiological update - 22 December 2020 [ https://www.who.int/publications/m/item/weekly-epidemiological-update%2D%2D-22-december-2020 ].
Worldometer: COVID-19 coronavirus pandemic. 2020. https://www.worldometers.info/coronavirus/ .
Anand S, Sen A. Human Development Index: Methodology and Measurement. 1994. http://hdr.undp.org/en/content/human-development-index-methodology-and-measurement .
De Larochelambert Q, Marc A, Antero J, Le Bourg E, Toussaint J-F. Covid-19 mortality: a matter of vulnerability among nations facing limited margins of adaptation. Public Health. 2020;8. https://doi.org/10.3389/fpubh.2020.604339 .
de Winter JC, Gosling SD, Potter J. Comparing the Pearson and Spearman correlation coefficients across distributions and sample sizes: a tutorial using simulations and empirical data. Psychol Methods. 2016;21(3):273.
World Health Organization. Universal health coverage [ http://www.who.int/universal_health_coverage/en/ ].
Johns Hopkins Center for Health Security. Global Health Security Index [ https://www.ghsindex.org/ ].
Pol Antràs SJR. Esteban Rossi-Hansberg: how do globalisation and pandemics interact? Surprising insights from a new model. In: #LSEThinks | CEP | global development vol. 2020. London: London School of Economics; 2020.
Google Scholar
Cai P. Understanding China’s belt and road initiative; 2017.
Baldwin R, Tomiura E. Thinking ahead about the trade impact of COVID-19. Economics in the Time of COVID-19. 2020;59. https://repository.graduateinstitute.ch/record/298220?ln=en .
Organization WH: Coronavirus disease ( COVID-19): situation report, 182. 2020.
Johns Hopkins University: COVID-19 Map - Johns Hopkins Coronavirus Resource Center. In. Edited by Security JHUCfH; 2021. https://coronavirus.jhu.edu/map.html .
Wang T, Du Z, Zhu F, Cao Z, An Y, Gao Y, et al. Comorbidities and multi-organ injuries in the treatment of COVID-19. Lancet. 2020;395(10228):e52.
Article CAS PubMed PubMed Central Google Scholar
Wang D, Hu B, Hu C, Zhu F, Liu X, Zhang J, et al. Clinical characteristics of 138 hospitalized patients with 2019 novel coronavirus–infected pneumonia in Wuhan, China. Jama. 2020;323(11):1061–9.
Huang C, Wang Y, Li X, Ren L, Zhao J, Hu Y, et al. Clinical features of patients infected with 2019 novel coronavirus in Wuhan, China. Lancet. 2020;395(10223):497–506.
Chen N, Zhou M, Dong X, Qu J, Gong F, Han Y, et al. Epidemiological and clinical characteristics of 99 cases of 2019 novel coronavirus pneumonia in Wuhan, China: a descriptive study. Lancet. 2020;395(10223):507–13.
Hussain A, Bhowmik B, Do Vale Moreira NC. COVID-19 and diabetes: knowledge in progress. Diabetes Res Clin Pract. 2020;162:108142. https://doi.org/10.1016/j.diabres.2020.108142 . Epub 2020 Apr 9.
Covid C, COVID C, COVID C, Chow N, Fleming-Dutra K, Gierke R, Hall A, et al. Preliminary estimates of the prevalence of selected underlying health conditions among patients with coronavirus disease 2019—United States, February 12–march 28, 2020. Morb Mortal Wkly Rep. 2020;69(13):382.
Group C-S. Characteristics of COVID-19 patients dying in Italy: report based on available data on March 20th, 2020. Rome: Instituto Superiore Di Sanita; 2020. https://www.epicentro.iss.it/coronavirus/bollettino/Report-COVID-2019_20_marzo_eng.pdf .
Guan W-j, Ni Z-y, Hu Y, Liang W-H, Ou C-Q, He J-X, et al. Clinical characteristics of coronavirus disease 2019 in China. N Engl J Med. 2020;382(18):1708–20.
Article CAS PubMed Google Scholar
Arentz M, Yim E, Klaff L, Lokhandwala S, Riedo FX, Chong M, et al. Characteristics and outcomes of 21 critically ill patients with COVID-19 in Washington state. Jama. 2020;323(16):1612–4.
Richardson S, Hirsch JS, Narasimhan M, Crawford JM, McGinn T, Davidson KW, et al. Presenting characteristics, comorbidities, and outcomes among 5700 patients hospitalized with COVID-19 in the new York City area. JAMA. 2020;323(20):2052–9. https://doi.org/10.1001/jama.2020.6775 .
Covid C, Team R. Severe outcomes among patients with coronavirus disease 2019 (COVID-19)—United States, February 12–march 16, 2020. MMWR Morb Mortal Wkly Rep. 2020;69(12):343–6.
Article Google Scholar
Mbow M, Lell B, Jochems SP, Cisse B, Mboup S, Dewals BG, et al. COVID-19 in Africa: dampening the storm? Science. 2020;369(6504):624–6.
Kavanagh MM, Erondu NA, Tomori O, Dzau VJ, Okiro EA, Maleche A, et al. Access to lifesaving medical resources for African countries: COVID-19 testing and response, ethics, and politics. Lancet. 2020;395(10238):1735–8.
Nordling L. Africa's pandemic puzzle: why so few cases and deaths? In: American Association for the Advancement of Science; 2020.
Oran DP, Topol EJ. Prevalence of asymptomatic SARS-CoV-2 infection: a narrative review. Ann Intern Med. 2020;173(5):362–7. https://doi.org/10.7326/M20-3012 . Epub 2020 Jun 3.
Nikolai LA, Meyer CG, Kremsner PG, Velavan TP. Asymptomatic SARS coronavirus 2 infection: invisible yet invincible. Int J Infect Dis. 2020;100:112–6. https://doi.org/10.1016/j.ijid.2020.08.076 . Epub 2020 Sep 3.
Rao C, Bradshaw D, Mathers CD. Improving death registration and statistics in developing countries: lessons from sub-Saharan Africa. Southern Afr J Demography. 2004:81–99.
Mehtar S, Preiser W, Lakhe NA, Bousso A, TamFum J-JM, Kallay O, et al. Limiting the spread of COVID-19 in Africa: one size mitigation strategies do not fit all countries. Lancet Glob Health. 2020;8(7):e881–3. Published online 2020 Apr 28. https://doi.org/10.1016/S2214-109X(20)30212-6 .
Nachega J, Seydi M, Zumla A. The late arrival of coronavirus disease 2019 (COVID-19) in Africa: mitigating pan-continental spread. Clin Infect Dis. 2020;71(15):875–8.
Gwilliam K, Foster V, Archondo-Callao R, Briceno-Garmendia C, Nogales A, Sethi K. Africa infrastructure country diagnostic: roads in sub-Saharan Africa: The World Bank; 2008.
Guengant J-P. Africa’s population: history, current status, and projections. In: Africa's Population: In Search of a Demographic Dividend: Springer; 2017. p. 11–31.
Collaborators GOD, Bernabe E, Marcenes W, Hernandez C, Bailey J, Abreu L, et al. Global, regional, and national levels and trends in burden of oral conditions from 1990 to 2017: a systematic analysis for the global burden of disease 2017 study. J Dent Res. 2020;99(4):362–73.
Cambaza EM, Viegas GC, Cambaza C. Manuel a: potential impact of temperature and atmospheric pressure on the number of cases of COVID-19 in Mozambique, southern Africa. J Public Health Epidemiol. 2020;12(3):246–60.
Lawal Y. Africa’s low COVID-19 mortality rate: a paradox? Int J Infect Dis. 2021;102:118–22.
Nkengasong JN, Mankoula W. Looming threat of COVID-19 infection in Africa: act collectively, and fast. Lancet. 2020;395(10227):841–2.
African Union and Africa CDC. African union and Africa CDC launches partnerships for African vaccine manufacturing (PAVM), framework to achieve it and signs 2 MoUs: African Union and Africa CDC; 2021.
Forbes. What Do Countries With The Best Coronavirus Responses Have In Common? Women Leaders [ https://www.forbes.com/sites/avivahwittenbergcox/2020/04/13/what-do-countries-with-the-best-coronavirus-reponses-have-in-common-women-leaders/#603bd9433dec ].
University of Notre Dame. What can we learn from Austria’s response to COVID-19? [ https://keough.nd.edu/what-can-we-learn-from-austrias-response-to-covid-19/ ].
Stoller JK. Reflections on leadership in the time of COVID-19. BMJ Leader. 2020. https://doi.org/10.1136/leader-2020-000244 .
Houston Public Media. What 6 Of The 7 Countries With The Most COVID-19 Cases Have In Common [ https://www.npr.org/sections/goatsandsoda/2020/07/31/896879448/the-nations-with-the-most-to-lose-from-covid-19 ].
Hale T, Petherick A, Phillips T, Webster S. Variation in government responses to COVID-19. Blavatnik school of government working paper. 2020;31. https://en.unesco.org/inclusivepolicylab/sites/default/files/learning/document/2020/4/BSG-WP-2020-031-v3.0.pdf .
The Editors. Dying in a leadership vacuum. N Engl J Med. 2020;383(15):1479–80.
The Cable News Network. US and UK are bottom of the pile in rankings of governments' handling of coronavirus pandemic [ https://edition.cnn.com/2020/08/27/world/global-coronavirus-attitudes-pew-intl/index.html ].
Huston P, Campbell J, Russell G, Goodyear-Smith F, Phillips RL, van Weel C, et al. COVID-19 and primary care in six countries. BJGP Open. 2020;4(4):bjgpopen20X101128. https://doi.org/10.3399/bjgpopen20X101128 .
Goodyear-Smith F, Kinder K, Eden AR, Strydom S, Bazemore A, Phillips R, et al. Primary care perspectives on pandemic politics. Glob Public Health. 2021;16(8-9):1304–19. https://doi.org/10.1080/17441692.2021.1876751 . Epub 2021 Jan 24.
Rutledge PE. Trump, COVID-19, and the war on expertise. Am Rev Public Adm. 2020;50(6-7):505–11.
Ugarte DA, Cumberland WG, Flores L, Young SD. Public attitudes about COVID-19 in response to president trump's social media posts. JAMA Netw Open. 2021;4(2):e210101.
Article PubMed PubMed Central Google Scholar
Lal A, Erondu NA, Heymann DL, Gitahi G, Yates R. Fragmented health systems in COVID-19: rectifying the misalignment between global health security and universal health coverage. Lancet. 2021;397(10268):61–7. https://doi.org/10.1016/S0140-6736(20)32228-5 . Epub 2020 Dec 1.
Tromberg BJ, Schwetz TA, Pérez-Stable EJ, Hodes RJ, Woychik RP, Bright RA, et al. Rapid scaling up of Covid-19 diagnostic testing in the United States—the NIH RADx initiative. N Engl J Med. 2020;383(11):1071–7.
Marwaha J, Halamka J, Brat G. Lifesaving ventilators will sit unused without a national data-sharing effort; 2020.
Kidd MR. Five principles for pandemic preparedness: lessons from the Australian COVID-19 primary care response. Br J Gen Pract. 2020;70(696):316–7. https://doi.org/10.3399/bjgp20X710765 . Print 2020 Jul.
Hsu LY, Tan M-H. What Singapore can teach the US about responding to COVID-19. STAT News. 2020. https://www.statnews.com/2020/03/23/singapore-teach-united-states-about-covid-19-response/ .
Horton R. Offline: COVID-19 is not a pandemic. Lancet (London, England). 2020;396(10255):874.
Article CAS Google Scholar
Raisi-Estabragh Z, McCracken C, Bethell MS, Cooper J, Cooper C, Caulfield MJ, et al. Greater risk of severe COVID-19 in black, Asian and minority ethnic populations is not explained by cardiometabolic, socioeconomic or behavioural factors, or by 25 (OH)-vitamin D status: study of 1326 cases from the UK biobank. J Public Health. 2020;42(3):451–60.
Hamidianjahromi A. Why African Americans are a potential target for COVID-19 infection in the United States. J Med Internet Res. 2020;22(6):e19934.
Tangcharoensathien V, Bassett MT, Meng Q, Mills A. Are overwhelmed health systems an inevitable consequence of covid-19? Experiences from China, Thailand, and New York State. BMJ. 2021;372. https://doi.org/10.1136/bmj.n83 .
Organization WH: COVID-19 health system response monitor, Thailand. 2020.
Narkvichien M. Thailand’s 1 million village health volunteers-“unsung heroes”-are helping guard communities nationwide from COVID-19. Nonthaburi: World Health Organization; 2020.
Kupferschmidt K, Cohen J. Can China's COVID-19 strategy work elsewhere? In: American Association for the Advancement of Science; 2020.
Al Saidi AMO, Nur FA, Al-Mandhari AS, El Rabbat M, Hafeez A, Abubakar A. Decisive leadership is a necessity in the COVID-19 response. Lancet. 2020;396(10247):295–8.
Forman R, Atun R, McKee M, Mossialos E. 12 lessons learned from the management of the coronavirus pandemic. Health Policy. 2020;124(6):577–80. https://doi.org/10.1016/j.healthpol.2020.05.008 .
Barberia LG, Gómez EJ. Political and institutional perils of Brazil's COVID-19 crisis. Lancet. 2020;396(10248):367–8.
Ortega F, Orsini M. Governing COVID-19 without government in Brazil: ignorance, neoliberal authoritarianism, and the collapse of public health leadership. Global Public Health. 2020;15(9):1257–77.
Ezequiel GE, Jafet A, Hugo A, Pedro D, Ana Maria M, Carola OV, et al. The COVID-19 pandemic: a call to action for health systems in Latin America to strengthen quality of care. Int J Qual Health Care. 2021;33(1):mzaa062.
Rocha R, Atun R, Massuda A, Rache B, Spinola P, Nunes L, et al. Effect of socioeconomic inequalities and vulnerabilities on health-system preparedness and response to COVID-19 in Brazil: a comprehensive analysis. Lancet Glob Health. 2021;9(6):e782–92. https://doi.org/10.1016/S2214-109X(21)00081-4 . Epub 2021 Apr 12.
Lancet T. India under COVID-19 lockdown. Lancet (London, England). 2020;395(10233):1315.
Siddiqui AF, Wiederkehr M, Rozanova L, Flahault A. Situation of India in the COVID-19 pandemic: India’s initial pandemic experience. Int J Environ Res Public Health. 2020;17(23):8994.
Article CAS PubMed Central Google Scholar
Taneja P, Bali AS. India’s domestic and foreign policy responses to COVID-19. Round Table. 2021;110(1):46–61.
Choutagunta A, Manish G, Rajagopalan S. Battling COVID-19 with dysfunctional federalism: lessons from India. South Econ J. 2021;87(4):1267–99.
Mallapaty S. India's massive COVID surge puzzles scientists. Nature. 2021;592(7856):667–8.
Thiagarajan K. Why is India having a covid-19 surge? In: British Medical Journal Publishing Group; 2021.
Fisman DN, Greer AL, Tuite AR. Age is just a number: a critically important number for COVID-19 case fatality. Ann Intern Med. 2020;173(9):762–3.
Sudharsanan N, Didzun O, Bärnighausen T, Geldsetzer P. The contribution of the age distribution of cases to COVID-19 case fatality across countries: a 9-country demographic study. Ann Intern Med. 2020;173(9):714–20. https://doi.org/10.7326/M20-2973 . Epub 2020 Jul 22.
Think Global Health. The Myth of South Asian Exceptionalism. In: He Myth of South Asian Exceptionalism: South Asia's young population conceals the effects that COVID-19 has on its older and more vulnerable people. vol. 2020; 2020.
Ioannidis JP, Axfors C, Contopoulos-Ioannidis DG. Population-level COVID-19 mortality risk for non-elderly individuals overall and for non-elderly individuals without underlying diseases in pandemic epicenters. Environ Res. 2020;188:109890.
Kim D-H, Choe YJ, Jeong J-Y. Understanding and interpretation of case fatality rate of coronavirus disease 2019. J Korean Med Sci. 2020;35(12):e137. https://doi.org/10.3346/jkms.2020.35.e137 .
Rajgor DD, Lee MH, Archuleta S, Bagdasarian N, Quek SC. The many estimates of the COVID-19 case fatality rate. Lancet Infect Dis. 2020;20(7):776–7.
Sorci G, Faivre B, Morand S. Explaining among-country variation in COVID-19 case fatality rate. Sci Rep. 2020;10(1):1–11.
Sen-Crowe B, Sutherland M, McKenney M, Elkbuli A. A closer look into global hospital beds capacity and resource shortages during the COVID-19 pandemic. J Surg Res. 2021;260:56–63.
Li M, Zhang Z, Cao W, Liu Y, Du B, Chen C, et al. Identifying novel factors associated with COVID-19 transmission and fatality using the machine learning approach. Sci Total Environ. 2021;764:142810.
Undurraga EA, Chowell G, Mizumoto K. COVID-19 case fatality risk by age and gender in a high testing setting in Latin America: Chile, march–august 2020. Infect Dis Poverty. 2021;10(1):1–11.
Taylor L. How Latin America is fighting covid-19, for better and worse. BMJ. 2020;370:m3319. https://doi.org/10.1136/bmj.m3319 .
Bhuyan A. Covid-19: India looks to import oxygen as cases surge, overwhelming hospitals. In: British Medical Journal Publishing Group; 2021.
An BY, Tang S-Y. Lessons from COVID-19 responses in East Asia: institutional infrastructure and enduring policy instruments. Am Rev Public Adm. 2020;50(6-7):790–800.
Chen H, Shi L, Zhang Y, Wang X, Sun G. A cross-country core strategy comparison in China, Japan, Singapore and South Korea during the early COVID-19 pandemic. Glob Health. 2021;17(1):1–10.
Kahathuduwa CN, Dhanasekara CS, Chin S-H. Case fatality rate in COVID-19: a systematic review and meta-analysis. J Prev Med Hyg. 2021;62(2):E311–20. https://doi.org/10.15167/2421-4248/jpmh2021.62.2.1627 . eCollection 2021 Jun.
Meyerowitz-Katz G, Merone L. A systematic review and meta-analysis of published research data on COVID-19 infection-fatality rates. Int J Infect Dis. 2020.
Seoane B. A scaling approach to estimate the COVID-19 infection fatality ratio from incomplete data. PLoS One. 2021;16(2):e0246831. https://doi.org/10.1371/journal.pone.0246831 . eCollection 2021.
Byambasuren O, Dobler CC, Bell K, Rojas DP, Clark J, McLaws M-L, et al. Comparison of seroprevalence of SARS-CoV-2 infections with cumulative and imputed COVID-19 cases: systematic review. PLoS One. 2021;16(4):e0248946.
Shakiba M, Nazemipour M, Salari A, Mehrabian F, Nazari SSH, Rezvani SM, et al. Seroprevalence of SARS-CoV-2 in Guilan Province, Iran, April 2020. Emerg Infect Dis. 2021;27(2):636.
Blanchet K, Alwan A, Antoine C, Cros MJ, Feroz F, Guracha TA, et al. Protecting essential health services in low-income and middle-income countries and humanitarian settings while responding to the COVID-19 pandemic. BMJ Glob Health. 2020;5(10):e003675.
Thome J, Coogan AN, Fischer M, Tucha O, Faltraco F. Challenges for mental health services during the 2020 COVID-19 outbreak in Germany. Psychiatry Clin Neurosci. 2020;74(7):407. https://doi.org/10.1111/pcn.13019 . Epub 2020 May 26.
Riley T, Sully E, Ahmed Z, Biddlecom A. Estimates of the potential impact of the COVID-19 pandemic on sexual and reproductive health in low-and middle-income countries. Int Perspect Sex Reprod Health. 2020;46:73–6.
World Health Organization (WHO): Maintaining essential health services: operational guidance for the COVID-19 context: interim guidance, 1 June 2020. In.: World Health Organization; 2020. https://www.who.int/publications/i/item/WHO-2019-nCoV-essential_health_services-2020.2 .
World Health Organization (WHO): Pulse survey on continuity of essential health services during the COVID-19 pandemic: interim report, 27 August 2020. In.: World Health Organization; 2020. https://www.who.int/publications-detail-redirect/WHO-2019-nCoV-EHS_continuity-survey-2020.1 .
World Health Organization (WHO): Second round of the national pulse survey on continuity of essential health services during the COVID-19 pandemic: interim report, 22 April 2021. In.: World Health Organization; 2021. https://www.who.int/publications/i/item/WHO-2019-nCoV-EHS-continuity-survey-2021.1 .
Organization WH. COVID-19: operational guidance for maintaining essential health services during an outbreak: interim guidance, 25 march 2020: World Health Organization; 2020.
Chen Y-Y, Assefa Y. The heterogeneity of the COVID-19 pandemic and national responses: an explanatory mixed-methods study. BMC Public Health. 2021;21(1):1–15.
World Health Organization (WHO). Thirteenth general programme of work 2019–2023. The seventy-first world health assembly. Geneva (Switzerland): World Health Organization; 2018.
Mahjour J, Mirza Z, Rashidian A, Atta H, Hajjeh R, Thieren M, et al. " promote health, keep the world safe, serve the vulnerable" in the eastern Mediterranean region. East Mediterr Health J. 2018;24(4):323–4.
Rollston R, Galea S. COVID-19 and the social determinants of health. Am J Health Promot. 2020;34(6):687–9. https://doi.org/10.1177/0890117120930536b .
Nachega JB, Sam-Agudu NA, Masekela R, van der Zalm MM, Nsanzimana S, Condo J, et al. Addressing challenges to rolling out COVID-19 vaccines in African countries. Lancet Glob Health. 2021;9(6):e746–8. https://doi.org/10.1016/S2214-109X(21)00097-8 . Epub 2021 Mar 10.
Skegg D, Gluckman P, Boulton G, Hackmann H, Karim SSA, Piot P, et al. Future scenarios for the COVID-19 pandemic. Lancet. 2021;397(10276):777–8.
Nhamo G, Chikodzi D, Kunene HP, Mashula N. COVID-19 vaccines and treatments nationalism: challenges for low-income countries and the attainment of the SDGs. Global Public Health. 2021;16(3):319–39.
Figueroa JP, Bottazzi ME, Hotez P, Batista C, Ergonul O, Gilbert S, et al. Urgent needs of low-income and middle-income countries for COVID-19 vaccines and therapeutics. Lancet. 2021;397(10274):562–4.
He X, Hong W, Pan X, Lu G, Wei X. SARS-CoV-2 omicron variant: characteristics and prevention. Med Comm (2020). 2021;2(4):838–45. https://doi.org/10.1002/mco2.110 .
Karim SSA, Karim QA. Omicron SARS-CoV-2 variant: a new chapter in the COVID-19 pandemic. Lancet. 2021;398(10317):2126–8.
Sanders D, Nandi S, Labonté R, Vance C, Van Damme W. From primary health care to universal health coverage—one step forward and two steps back. Lancet. 2019;394(10199):619–21.
Hone T, Macinko J, Millett C. Revisiting Alma-Ata: what is the role of primary health care in achieving the sustainable development goals? Lancet. 2018;392(10156):1461–72.
Redwood-Campbell L, Abrahams J. Primary health care and disasters—the current state of the literature: what we know, gaps and next steps. Prehospital Disaster Med. 2011;26(3):184–91.
Bitton A, Ratcliffe HL, Veillard JH, Kress DH, Barkley S, Kimball M, et al. Primary health care as a foundation for strengthening health systems in low-and middle-income countries. J Gen Intern Med. 2017;32(5):566–71.
Dunlop C, Howe A, Li D, Allen LN. The coronavirus outbreak: the central role of primary care in emergency preparedness and response. BJGP Open. 2020;4(1):bjgpopen20X101041. https://doi.org/10.3399/bjgpopen20X101041 .
Assefa Y, Gilks CF, van de Pas R, Reid S, Gete DG, Van Damme W. Reimagining global health systems for the 21st century: lessons from the COVID-19 pandemic. BMJ Glob Health. 2021;6(4):e004882.
Loewenson R, Accoe K, Bajpai N, Buse K, Abi Deivanayagam T, London L, et al. Reclaiming comprehensive public health. BMJ Glob Health. 2020;5(9):e003886.
Rawaf S, Allen LN, Stigler FL, Kringos D, Quezada Yamamoto H, van Weel C, et al. Lessons on the COVID-19 pandemic, for and by primary care professionals worldwide. Eur J Gen Pract. 2020;26(1):129–33.
Gostin LO, Friedman EA. A retrospective and prospective analysis of the west African Ebola virus disease epidemic: robust national health systems at the foundation and an empowered WHO at the apex. Lancet. 2015;385(9980):1902–9.
Download references
Acknowledgements
Not applicable.
No funding was obtained for this study.
Author information
Authors and affiliations.
School of Public Health, the University of Queensland, Brisbane, Australia
Yibeltal Assefa, Charles F. Gilks, Simon Reid & Dereje Gedle Gete
Institute of Tropical Medicine, Antwerp, Belgium
Remco van de Pas & Wim Van Damme
You can also search for this author in PubMed Google Scholar
Contributions
YA conceived the idea of the research, collected and analysed the data, and prepared the first and subsequent drafts. DG participated in data collection and analysis. CFG, SR, RVDP, DG and WVD provided comments during subsequent drafts. All authors read and approved the final manuscript.
Corresponding author
Correspondence to Yibeltal Assefa .
Ethics declarations
Ethics approval and consent to participate, consent for publication, competing interests.
The authors declare that they have no competing interests.
Additional information
Publisher’s note.
Springer Nature remains neutral with regard to jurisdictional claims in published maps and institutional affiliations.
Rights and permissions
Open Access This article is licensed under a Creative Commons Attribution 4.0 International License, which permits use, sharing, adaptation, distribution and reproduction in any medium or format, as long as you give appropriate credit to the original author(s) and the source, provide a link to the Creative Commons licence, and indicate if changes were made. The images or other third party material in this article are included in the article's Creative Commons licence, unless indicated otherwise in a credit line to the material. If material is not included in the article's Creative Commons licence and your intended use is not permitted by statutory regulation or exceeds the permitted use, you will need to obtain permission directly from the copyright holder. To view a copy of this licence, visit http://creativecommons.org/licenses/by/4.0/ . The Creative Commons Public Domain Dedication waiver ( http://creativecommons.org/publicdomain/zero/1.0/ ) applies to the data made available in this article, unless otherwise stated in a credit line to the data.
Reprints and permissions
About this article
Cite this article.
Assefa, Y., Gilks, C.F., Reid, S. et al. Analysis of the COVID-19 pandemic: lessons towards a more effective response to public health emergencies. Global Health 18 , 10 (2022). https://doi.org/10.1186/s12992-022-00805-9
Download citation
Received : 04 August 2021
Accepted : 14 January 2022
Published : 04 February 2022
DOI : https://doi.org/10.1186/s12992-022-00805-9
Share this article
Anyone you share the following link with will be able to read this content:
Sorry, a shareable link is not currently available for this article.
Provided by the Springer Nature SharedIt content-sharing initiative
- Heterogeneity
Globalization and Health
ISSN: 1744-8603
- Submission enquiries: [email protected]
- General enquiries: [email protected]
- Research article
- Open access
- Published: 04 June 2021
Coronavirus disease (COVID-19) pandemic: an overview of systematic reviews
- Israel Júnior Borges do Nascimento 1 , 2 ,
- Dónal P. O’Mathúna 3 , 4 ,
- Thilo Caspar von Groote 5 ,
- Hebatullah Mohamed Abdulazeem 6 ,
- Ishanka Weerasekara 7 , 8 ,
- Ana Marusic 9 ,
- Livia Puljak ORCID: orcid.org/0000-0002-8467-6061 10 ,
- Vinicius Tassoni Civile 11 ,
- Irena Zakarija-Grkovic 9 ,
- Tina Poklepovic Pericic 9 ,
- Alvaro Nagib Atallah 11 ,
- Santino Filoso 12 ,
- Nicola Luigi Bragazzi 13 &
- Milena Soriano Marcolino 1
On behalf of the International Network of Coronavirus Disease 2019 (InterNetCOVID-19)
BMC Infectious Diseases volume 21 , Article number: 525 ( 2021 ) Cite this article
16k Accesses
28 Citations
13 Altmetric
Metrics details
Navigating the rapidly growing body of scientific literature on the SARS-CoV-2 pandemic is challenging, and ongoing critical appraisal of this output is essential. We aimed to summarize and critically appraise systematic reviews of coronavirus disease (COVID-19) in humans that were available at the beginning of the pandemic.
Nine databases (Medline, EMBASE, Cochrane Library, CINAHL, Web of Sciences, PDQ-Evidence, WHO’s Global Research, LILACS, and Epistemonikos) were searched from December 1, 2019, to March 24, 2020. Systematic reviews analyzing primary studies of COVID-19 were included. Two authors independently undertook screening, selection, extraction (data on clinical symptoms, prevalence, pharmacological and non-pharmacological interventions, diagnostic test assessment, laboratory, and radiological findings), and quality assessment (AMSTAR 2). A meta-analysis was performed of the prevalence of clinical outcomes.
Eighteen systematic reviews were included; one was empty (did not identify any relevant study). Using AMSTAR 2, confidence in the results of all 18 reviews was rated as “critically low”. Identified symptoms of COVID-19 were (range values of point estimates): fever (82–95%), cough with or without sputum (58–72%), dyspnea (26–59%), myalgia or muscle fatigue (29–51%), sore throat (10–13%), headache (8–12%) and gastrointestinal complaints (5–9%). Severe symptoms were more common in men. Elevated C-reactive protein and lactate dehydrogenase, and slightly elevated aspartate and alanine aminotransferase, were commonly described. Thrombocytopenia and elevated levels of procalcitonin and cardiac troponin I were associated with severe disease. A frequent finding on chest imaging was uni- or bilateral multilobar ground-glass opacity. A single review investigated the impact of medication (chloroquine) but found no verifiable clinical data. All-cause mortality ranged from 0.3 to 13.9%.
Conclusions
In this overview of systematic reviews, we analyzed evidence from the first 18 systematic reviews that were published after the emergence of COVID-19. However, confidence in the results of all reviews was “critically low”. Thus, systematic reviews that were published early on in the pandemic were of questionable usefulness. Even during public health emergencies, studies and systematic reviews should adhere to established methodological standards.
Peer Review reports
The spread of the “Severe Acute Respiratory Coronavirus 2” (SARS-CoV-2), the causal agent of COVID-19, was characterized as a pandemic by the World Health Organization (WHO) in March 2020 and has triggered an international public health emergency [ 1 ]. The numbers of confirmed cases and deaths due to COVID-19 are rapidly escalating, counting in millions [ 2 ], causing massive economic strain, and escalating healthcare and public health expenses [ 3 , 4 ].
The research community has responded by publishing an impressive number of scientific reports related to COVID-19. The world was alerted to the new disease at the beginning of 2020 [ 1 ], and by mid-March 2020, more than 2000 articles had been published on COVID-19 in scholarly journals, with 25% of them containing original data [ 5 ]. The living map of COVID-19 evidence, curated by the Evidence for Policy and Practice Information and Co-ordinating Centre (EPPI-Centre), contained more than 40,000 records by February 2021 [ 6 ]. More than 100,000 records on PubMed were labeled as “SARS-CoV-2 literature, sequence, and clinical content” by February 2021 [ 7 ].
Due to publication speed, the research community has voiced concerns regarding the quality and reproducibility of evidence produced during the COVID-19 pandemic, warning of the potential damaging approach of “publish first, retract later” [ 8 ]. It appears that these concerns are not unfounded, as it has been reported that COVID-19 articles were overrepresented in the pool of retracted articles in 2020 [ 9 ]. These concerns about inadequate evidence are of major importance because they can lead to poor clinical practice and inappropriate policies [ 10 ].
Systematic reviews are a cornerstone of today’s evidence-informed decision-making. By synthesizing all relevant evidence regarding a particular topic, systematic reviews reflect the current scientific knowledge. Systematic reviews are considered to be at the highest level in the hierarchy of evidence and should be used to make informed decisions. However, with high numbers of systematic reviews of different scope and methodological quality being published, overviews of multiple systematic reviews that assess their methodological quality are essential [ 11 , 12 , 13 ]. An overview of systematic reviews helps identify and organize the literature and highlights areas of priority in decision-making.
In this overview of systematic reviews, we aimed to summarize and critically appraise systematic reviews of coronavirus disease (COVID-19) in humans that were available at the beginning of the pandemic.
Methodology
Research question.
This overview’s primary objective was to summarize and critically appraise systematic reviews that assessed any type of primary clinical data from patients infected with SARS-CoV-2. Our research question was purposefully broad because we wanted to analyze as many systematic reviews as possible that were available early following the COVID-19 outbreak.
Study design
We conducted an overview of systematic reviews. The idea for this overview originated in a protocol for a systematic review submitted to PROSPERO (CRD42020170623), which indicated a plan to conduct an overview.
Overviews of systematic reviews use explicit and systematic methods for searching and identifying multiple systematic reviews addressing related research questions in the same field to extract and analyze evidence across important outcomes. Overviews of systematic reviews are in principle similar to systematic reviews of interventions, but the unit of analysis is a systematic review [ 14 , 15 , 16 ].
We used the overview methodology instead of other evidence synthesis methods to allow us to collate and appraise multiple systematic reviews on this topic, and to extract and analyze their results across relevant topics [ 17 ]. The overview and meta-analysis of systematic reviews allowed us to investigate the methodological quality of included studies, summarize results, and identify specific areas of available or limited evidence, thereby strengthening the current understanding of this novel disease and guiding future research [ 13 ].
A reporting guideline for overviews of reviews is currently under development, i.e., Preferred Reporting Items for Overviews of Reviews (PRIOR) [ 18 ]. As the PRIOR checklist is still not published, this study was reported following the Preferred Reporting Items for Systematic Reviews and Meta-Analyses (PRISMA) 2009 statement [ 19 ]. The methodology used in this review was adapted from the Cochrane Handbook for Systematic Reviews of Interventions and also followed established methodological considerations for analyzing existing systematic reviews [ 14 ].
Approval of a research ethics committee was not necessary as the study analyzed only publicly available articles.
Eligibility criteria
Systematic reviews were included if they analyzed primary data from patients infected with SARS-CoV-2 as confirmed by RT-PCR or another pre-specified diagnostic technique. Eligible reviews covered all topics related to COVID-19 including, but not limited to, those that reported clinical symptoms, diagnostic methods, therapeutic interventions, laboratory findings, or radiological results. Both full manuscripts and abbreviated versions, such as letters, were eligible.
No restrictions were imposed on the design of the primary studies included within the systematic reviews, the last search date, whether the review included meta-analyses or language. Reviews related to SARS-CoV-2 and other coronaviruses were eligible, but from those reviews, we analyzed only data related to SARS-CoV-2.
No consensus definition exists for a systematic review [ 20 ], and debates continue about the defining characteristics of a systematic review [ 21 ]. Cochrane’s guidance for overviews of reviews recommends setting pre-established criteria for making decisions around inclusion [ 14 ]. That is supported by a recent scoping review about guidance for overviews of systematic reviews [ 22 ].
Thus, for this study, we defined a systematic review as a research report which searched for primary research studies on a specific topic using an explicit search strategy, had a detailed description of the methods with explicit inclusion criteria provided, and provided a summary of the included studies either in narrative or quantitative format (such as a meta-analysis). Cochrane and non-Cochrane systematic reviews were considered eligible for inclusion, with or without meta-analysis, and regardless of the study design, language restriction and methodology of the included primary studies. To be eligible for inclusion, reviews had to be clearly analyzing data related to SARS-CoV-2 (associated or not with other viruses). We excluded narrative reviews without those characteristics as these are less likely to be replicable and are more prone to bias.
Scoping reviews and rapid reviews were eligible for inclusion in this overview if they met our pre-defined inclusion criteria noted above. We included reviews that addressed SARS-CoV-2 and other coronaviruses if they reported separate data regarding SARS-CoV-2.
Information sources
Nine databases were searched for eligible records published between December 1, 2019, and March 24, 2020: Cochrane Database of Systematic Reviews via Cochrane Library, PubMed, EMBASE, CINAHL (Cumulative Index to Nursing and Allied Health Literature), Web of Sciences, LILACS (Latin American and Caribbean Health Sciences Literature), PDQ-Evidence, WHO’s Global Research on Coronavirus Disease (COVID-19), and Epistemonikos.
The comprehensive search strategy for each database is provided in Additional file 1 and was designed and conducted in collaboration with an information specialist. All retrieved records were primarily processed in EndNote, where duplicates were removed, and records were then imported into the Covidence platform [ 23 ]. In addition to database searches, we screened reference lists of reviews included after screening records retrieved via databases.
Study selection
All searches, screening of titles and abstracts, and record selection, were performed independently by two investigators using the Covidence platform [ 23 ]. Articles deemed potentially eligible were retrieved for full-text screening carried out independently by two investigators. Discrepancies at all stages were resolved by consensus. During the screening, records published in languages other than English were translated by a native/fluent speaker.
Data collection process
We custom designed a data extraction table for this study, which was piloted by two authors independently. Data extraction was performed independently by two authors. Conflicts were resolved by consensus or by consulting a third researcher.
We extracted the following data: article identification data (authors’ name and journal of publication), search period, number of databases searched, population or settings considered, main results and outcomes observed, and number of participants. From Web of Science (Clarivate Analytics, Philadelphia, PA, USA), we extracted journal rank (quartile) and Journal Impact Factor (JIF).
We categorized the following as primary outcomes: all-cause mortality, need for and length of mechanical ventilation, length of hospitalization (in days), admission to intensive care unit (yes/no), and length of stay in the intensive care unit.
The following outcomes were categorized as exploratory: diagnostic methods used for detection of the virus, male to female ratio, clinical symptoms, pharmacological and non-pharmacological interventions, laboratory findings (full blood count, liver enzymes, C-reactive protein, d-dimer, albumin, lipid profile, serum electrolytes, blood vitamin levels, glucose levels, and any other important biomarkers), and radiological findings (using radiography, computed tomography, magnetic resonance imaging or ultrasound).
We also collected data on reporting guidelines and requirements for the publication of systematic reviews and meta-analyses from journal websites where included reviews were published.
Quality assessment in individual reviews
Two researchers independently assessed the reviews’ quality using the “A MeaSurement Tool to Assess Systematic Reviews 2 (AMSTAR 2)”. We acknowledge that the AMSTAR 2 was created as “a critical appraisal tool for systematic reviews that include randomized or non-randomized studies of healthcare interventions, or both” [ 24 ]. However, since AMSTAR 2 was designed for systematic reviews of intervention trials, and we included additional types of systematic reviews, we adjusted some AMSTAR 2 ratings and reported these in Additional file 2 .
Adherence to each item was rated as follows: yes, partial yes, no, or not applicable (such as when a meta-analysis was not conducted). The overall confidence in the results of the review is rated as “critically low”, “low”, “moderate” or “high”, according to the AMSTAR 2 guidance based on seven critical domains, which are items 2, 4, 7, 9, 11, 13, 15 as defined by AMSTAR 2 authors [ 24 ]. We reported our adherence ratings for transparency of our decision with accompanying explanations, for each item, in each included review.
One of the included systematic reviews was conducted by some members of this author team [ 25 ]. This review was initially assessed independently by two authors who were not co-authors of that review to prevent the risk of bias in assessing this study.
Synthesis of results
For data synthesis, we prepared a table summarizing each systematic review. Graphs illustrating the mortality rate and clinical symptoms were created. We then prepared a narrative summary of the methods, findings, study strengths, and limitations.
For analysis of the prevalence of clinical outcomes, we extracted data on the number of events and the total number of patients to perform proportional meta-analysis using RStudio© software, with the “meta” package (version 4.9–6), using the “metaprop” function for reviews that did not perform a meta-analysis, excluding case studies because of the absence of variance. For reviews that did not perform a meta-analysis, we presented pooled results of proportions with their respective confidence intervals (95%) by the inverse variance method with a random-effects model, using the DerSimonian-Laird estimator for τ 2 . We adjusted data using Freeman-Tukey double arcosen transformation. Confidence intervals were calculated using the Clopper-Pearson method for individual studies. We created forest plots using the RStudio© software, with the “metafor” package (version 2.1–0) and “forest” function.
Managing overlapping systematic reviews
Some of the included systematic reviews that address the same or similar research questions may include the same primary studies in overviews. Including such overlapping reviews may introduce bias when outcome data from the same primary study are included in the analyses of an overview multiple times. Thus, in summaries of evidence, multiple-counting of the same outcome data will give data from some primary studies too much influence [ 14 ]. In this overview, we did not exclude overlapping systematic reviews because, according to Cochrane’s guidance, it may be appropriate to include all relevant reviews’ results if the purpose of the overview is to present and describe the current body of evidence on a topic [ 14 ]. To avoid any bias in summary estimates associated with overlapping reviews, we generated forest plots showing data from individual systematic reviews, but the results were not pooled because some primary studies were included in multiple reviews.
Our search retrieved 1063 publications, of which 175 were duplicates. Most publications were excluded after the title and abstract analysis ( n = 860). Among the 28 studies selected for full-text screening, 10 were excluded for the reasons described in Additional file 3 , and 18 were included in the final analysis (Fig. 1 ) [ 25 , 26 , 27 , 28 , 29 , 30 , 31 , 32 , 33 , 34 , 35 , 36 , 37 , 38 , 39 , 40 , 41 , 42 ]. Reference list screening did not retrieve any additional systematic reviews.
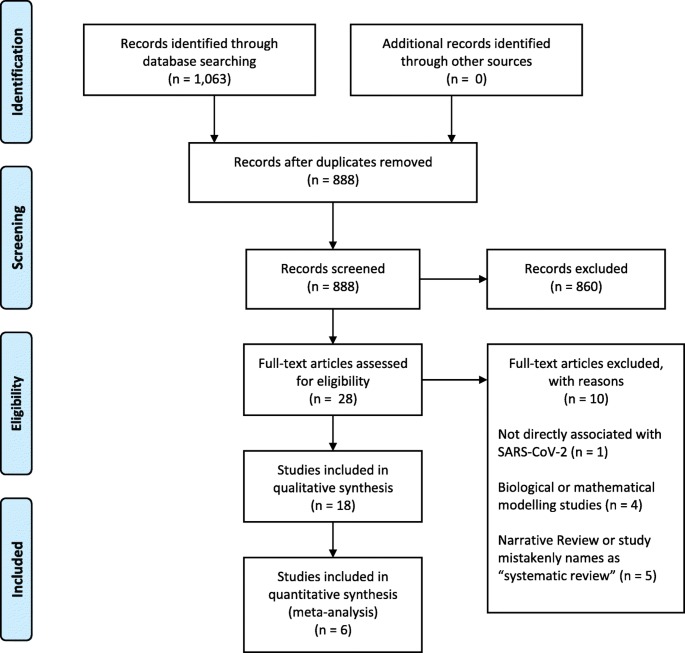
PRISMA flow diagram
Characteristics of included reviews
Summary features of 18 systematic reviews are presented in Table 1 . They were published in 14 different journals. Only four of these journals had specific requirements for systematic reviews (with or without meta-analysis): European Journal of Internal Medicine, Journal of Clinical Medicine, Ultrasound in Obstetrics and Gynecology, and Clinical Research in Cardiology . Two journals reported that they published only invited reviews ( Journal of Medical Virology and Clinica Chimica Acta ). Three systematic reviews in our study were published as letters; one was labeled as a scoping review and another as a rapid review (Table 2 ).
All reviews were published in English, in first quartile (Q1) journals, with JIF ranging from 1.692 to 6.062. One review was empty, meaning that its search did not identify any relevant studies; i.e., no primary studies were included [ 36 ]. The remaining 17 reviews included 269 unique studies; the majority ( N = 211; 78%) were included in only a single review included in our study (range: 1 to 12). Primary studies included in the reviews were published between December 2019 and March 18, 2020, and comprised case reports, case series, cohorts, and other observational studies. We found only one review that included randomized clinical trials [ 38 ]. In the included reviews, systematic literature searches were performed from 2019 (entire year) up to March 9, 2020. Ten systematic reviews included meta-analyses. The list of primary studies found in the included systematic reviews is shown in Additional file 4 , as well as the number of reviews in which each primary study was included.
Population and study designs
Most of the reviews analyzed data from patients with COVID-19 who developed pneumonia, acute respiratory distress syndrome (ARDS), or any other correlated complication. One review aimed to evaluate the effectiveness of using surgical masks on preventing transmission of the virus [ 36 ], one review was focused on pediatric patients [ 34 ], and one review investigated COVID-19 in pregnant women [ 37 ]. Most reviews assessed clinical symptoms, laboratory findings, or radiological results.
Systematic review findings
The summary of findings from individual reviews is shown in Table 2 . Overall, all-cause mortality ranged from 0.3 to 13.9% (Fig. 2 ).
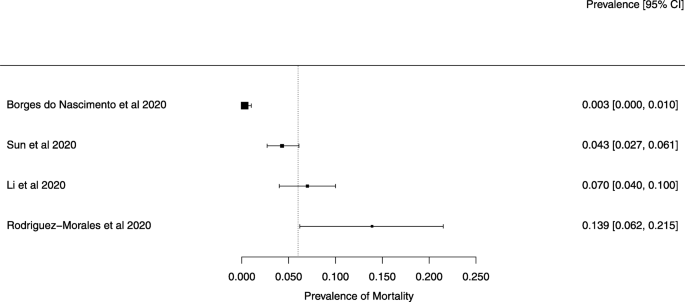
A meta-analysis of the prevalence of mortality
Clinical symptoms
Seven reviews described the main clinical manifestations of COVID-19 [ 26 , 28 , 29 , 34 , 35 , 39 , 41 ]. Three of them provided only a narrative discussion of symptoms [ 26 , 34 , 35 ]. In the reviews that performed a statistical analysis of the incidence of different clinical symptoms, symptoms in patients with COVID-19 were (range values of point estimates): fever (82–95%), cough with or without sputum (58–72%), dyspnea (26–59%), myalgia or muscle fatigue (29–51%), sore throat (10–13%), headache (8–12%), gastrointestinal disorders, such as diarrhea, nausea or vomiting (5.0–9.0%), and others (including, in one study only: dizziness 12.1%) (Figs. 3 , 4 , 5 , 6 , 7 , 8 and 9 ). Three reviews assessed cough with and without sputum together; only one review assessed sputum production itself (28.5%).
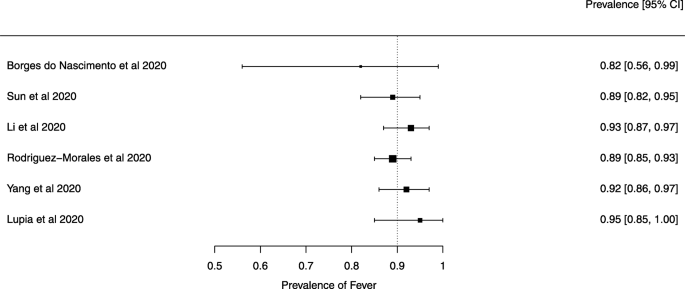
A meta-analysis of the prevalence of fever
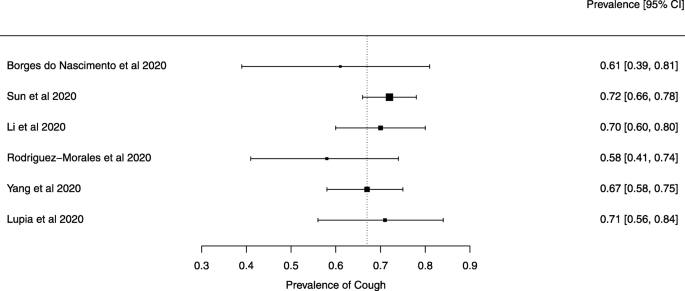
A meta-analysis of the prevalence of cough
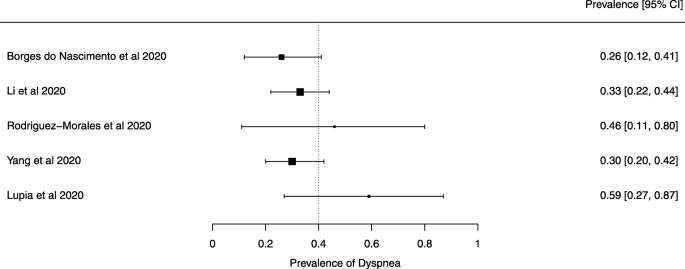
A meta-analysis of the prevalence of dyspnea
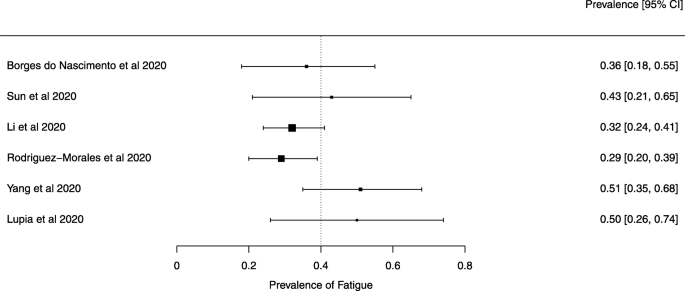
A meta-analysis of the prevalence of fatigue or myalgia
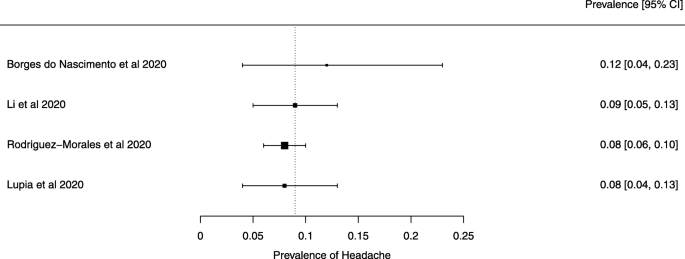
A meta-analysis of the prevalence of headache
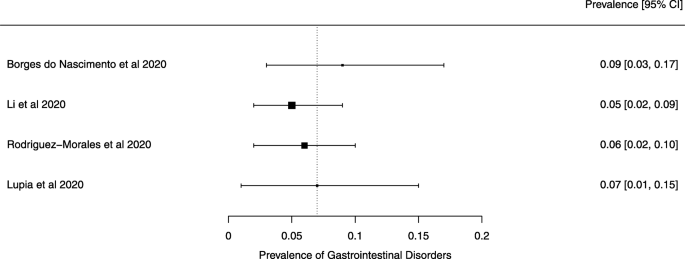
A meta-analysis of the prevalence of gastrointestinal disorders
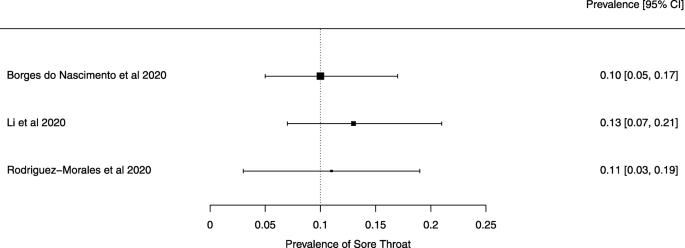
A meta-analysis of the prevalence of sore throat
Diagnostic aspects
Three reviews described methodologies, protocols, and tools used for establishing the diagnosis of COVID-19 [ 26 , 34 , 38 ]. The use of respiratory swabs (nasal or pharyngeal) or blood specimens to assess the presence of SARS-CoV-2 nucleic acid using RT-PCR assays was the most commonly used diagnostic method mentioned in the included studies. These diagnostic tests have been widely used, but their precise sensitivity and specificity remain unknown. One review included a Chinese study with clinical diagnosis with no confirmation of SARS-CoV-2 infection (patients were diagnosed with COVID-19 if they presented with at least two symptoms suggestive of COVID-19, together with laboratory and chest radiography abnormalities) [ 34 ].
Therapeutic possibilities
Pharmacological and non-pharmacological interventions (supportive therapies) used in treating patients with COVID-19 were reported in five reviews [ 25 , 27 , 34 , 35 , 38 ]. Antivirals used empirically for COVID-19 treatment were reported in seven reviews [ 25 , 27 , 34 , 35 , 37 , 38 , 41 ]; most commonly used were protease inhibitors (lopinavir, ritonavir, darunavir), nucleoside reverse transcriptase inhibitor (tenofovir), nucleotide analogs (remdesivir, galidesivir, ganciclovir), and neuraminidase inhibitors (oseltamivir). Umifenovir, a membrane fusion inhibitor, was investigated in two studies [ 25 , 35 ]. Possible supportive interventions analyzed were different types of oxygen supplementation and breathing support (invasive or non-invasive ventilation) [ 25 ]. The use of antibiotics, both empirically and to treat secondary pneumonia, was reported in six studies [ 25 , 26 , 27 , 34 , 35 , 38 ]. One review specifically assessed evidence on the efficacy and safety of the anti-malaria drug chloroquine [ 27 ]. It identified 23 ongoing trials investigating the potential of chloroquine as a therapeutic option for COVID-19, but no verifiable clinical outcomes data. The use of mesenchymal stem cells, antifungals, and glucocorticoids were described in four reviews [ 25 , 34 , 35 , 38 ].
Laboratory and radiological findings
Of the 18 reviews included in this overview, eight analyzed laboratory parameters in patients with COVID-19 [ 25 , 29 , 30 , 32 , 33 , 34 , 35 , 39 ]; elevated C-reactive protein levels, associated with lymphocytopenia, elevated lactate dehydrogenase, as well as slightly elevated aspartate and alanine aminotransferase (AST, ALT) were commonly described in those eight reviews. Lippi et al. assessed cardiac troponin I (cTnI) [ 25 ], procalcitonin [ 32 ], and platelet count [ 33 ] in COVID-19 patients. Elevated levels of procalcitonin [ 32 ] and cTnI [ 30 ] were more likely to be associated with a severe disease course (requiring intensive care unit admission and intubation). Furthermore, thrombocytopenia was frequently observed in patients with complicated COVID-19 infections [ 33 ].
Chest imaging (chest radiography and/or computed tomography) features were assessed in six reviews, all of which described a frequent pattern of local or bilateral multilobar ground-glass opacity [ 25 , 34 , 35 , 39 , 40 , 41 ]. Those six reviews showed that septal thickening, bronchiectasis, pleural and cardiac effusions, halo signs, and pneumothorax were observed in patients suffering from COVID-19.
Quality of evidence in individual systematic reviews
Table 3 shows the detailed results of the quality assessment of 18 systematic reviews, including the assessment of individual items and summary assessment. A detailed explanation for each decision in each review is available in Additional file 5 .
Using AMSTAR 2 criteria, confidence in the results of all 18 reviews was rated as “critically low” (Table 3 ). Common methodological drawbacks were: omission of prospective protocol submission or publication; use of inappropriate search strategy: lack of independent and dual literature screening and data-extraction (or methodology unclear); absence of an explanation for heterogeneity among the studies included; lack of reasons for study exclusion (or rationale unclear).
Risk of bias assessment, based on a reported methodological tool, and quality of evidence appraisal, in line with the Grading of Recommendations Assessment, Development, and Evaluation (GRADE) method, were reported only in one review [ 25 ]. Five reviews presented a table summarizing bias, using various risk of bias tools [ 25 , 29 , 39 , 40 , 41 ]. One review analyzed “study quality” [ 37 ]. One review mentioned the risk of bias assessment in the methodology but did not provide any related analysis [ 28 ].
This overview of systematic reviews analyzed the first 18 systematic reviews published after the onset of the COVID-19 pandemic, up to March 24, 2020, with primary studies involving more than 60,000 patients. Using AMSTAR-2, we judged that our confidence in all those reviews was “critically low”. Ten reviews included meta-analyses. The reviews presented data on clinical manifestations, laboratory and radiological findings, and interventions. We found no systematic reviews on the utility of diagnostic tests.
Symptoms were reported in seven reviews; most of the patients had a fever, cough, dyspnea, myalgia or muscle fatigue, and gastrointestinal disorders such as diarrhea, nausea, or vomiting. Olfactory dysfunction (anosmia or dysosmia) has been described in patients infected with COVID-19 [ 43 ]; however, this was not reported in any of the reviews included in this overview. During the SARS outbreak in 2002, there were reports of impairment of the sense of smell associated with the disease [ 44 , 45 ].
The reported mortality rates ranged from 0.3 to 14% in the included reviews. Mortality estimates are influenced by the transmissibility rate (basic reproduction number), availability of diagnostic tools, notification policies, asymptomatic presentations of the disease, resources for disease prevention and control, and treatment facilities; variability in the mortality rate fits the pattern of emerging infectious diseases [ 46 ]. Furthermore, the reported cases did not consider asymptomatic cases, mild cases where individuals have not sought medical treatment, and the fact that many countries had limited access to diagnostic tests or have implemented testing policies later than the others. Considering the lack of reviews assessing diagnostic testing (sensitivity, specificity, and predictive values of RT-PCT or immunoglobulin tests), and the preponderance of studies that assessed only symptomatic individuals, considerable imprecision around the calculated mortality rates existed in the early stage of the COVID-19 pandemic.
Few reviews included treatment data. Those reviews described studies considered to be at a very low level of evidence: usually small, retrospective studies with very heterogeneous populations. Seven reviews analyzed laboratory parameters; those reviews could have been useful for clinicians who attend patients suspected of COVID-19 in emergency services worldwide, such as assessing which patients need to be reassessed more frequently.
All systematic reviews scored poorly on the AMSTAR 2 critical appraisal tool for systematic reviews. Most of the original studies included in the reviews were case series and case reports, impacting the quality of evidence. Such evidence has major implications for clinical practice and the use of these reviews in evidence-based practice and policy. Clinicians, patients, and policymakers can only have the highest confidence in systematic review findings if high-quality systematic review methodologies are employed. The urgent need for information during a pandemic does not justify poor quality reporting.
We acknowledge that there are numerous challenges associated with analyzing COVID-19 data during a pandemic [ 47 ]. High-quality evidence syntheses are needed for decision-making, but each type of evidence syntheses is associated with its inherent challenges.
The creation of classic systematic reviews requires considerable time and effort; with massive research output, they quickly become outdated, and preparing updated versions also requires considerable time. A recent study showed that updates of non-Cochrane systematic reviews are published a median of 5 years after the publication of the previous version [ 48 ].
Authors may register a review and then abandon it [ 49 ], but the existence of a public record that is not updated may lead other authors to believe that the review is still ongoing. A quarter of Cochrane review protocols remains unpublished as completed systematic reviews 8 years after protocol publication [ 50 ].
Rapid reviews can be used to summarize the evidence, but they involve methodological sacrifices and simplifications to produce information promptly, with inconsistent methodological approaches [ 51 ]. However, rapid reviews are justified in times of public health emergencies, and even Cochrane has resorted to publishing rapid reviews in response to the COVID-19 crisis [ 52 ]. Rapid reviews were eligible for inclusion in this overview, but only one of the 18 reviews included in this study was labeled as a rapid review.
Ideally, COVID-19 evidence would be continually summarized in a series of high-quality living systematic reviews, types of evidence synthesis defined as “ a systematic review which is continually updated, incorporating relevant new evidence as it becomes available ” [ 53 ]. However, conducting living systematic reviews requires considerable resources, calling into question the sustainability of such evidence synthesis over long periods [ 54 ].
Research reports about COVID-19 will contribute to research waste if they are poorly designed, poorly reported, or simply not necessary. In principle, systematic reviews should help reduce research waste as they usually provide recommendations for further research that is needed or may advise that sufficient evidence exists on a particular topic [ 55 ]. However, systematic reviews can also contribute to growing research waste when they are not needed, or poorly conducted and reported. Our present study clearly shows that most of the systematic reviews that were published early on in the COVID-19 pandemic could be categorized as research waste, as our confidence in their results is critically low.
Our study has some limitations. One is that for AMSTAR 2 assessment we relied on information available in publications; we did not attempt to contact study authors for clarifications or additional data. In three reviews, the methodological quality appraisal was challenging because they were published as letters, or labeled as rapid communications. As a result, various details about their review process were not included, leading to AMSTAR 2 questions being answered as “not reported”, resulting in low confidence scores. Full manuscripts might have provided additional information that could have led to higher confidence in the results. In other words, low scores could reflect incomplete reporting, not necessarily low-quality review methods. To make their review available more rapidly and more concisely, the authors may have omitted methodological details. A general issue during a crisis is that speed and completeness must be balanced. However, maintaining high standards requires proper resourcing and commitment to ensure that the users of systematic reviews can have high confidence in the results.
Furthermore, we used adjusted AMSTAR 2 scoring, as the tool was designed for critical appraisal of reviews of interventions. Some reviews may have received lower scores than actually warranted in spite of these adjustments.
Another limitation of our study may be the inclusion of multiple overlapping reviews, as some included reviews included the same primary studies. According to the Cochrane Handbook, including overlapping reviews may be appropriate when the review’s aim is “ to present and describe the current body of systematic review evidence on a topic ” [ 12 ], which was our aim. To avoid bias with summarizing evidence from overlapping reviews, we presented the forest plots without summary estimates. The forest plots serve to inform readers about the effect sizes for outcomes that were reported in each review.
Several authors from this study have contributed to one of the reviews identified [ 25 ]. To reduce the risk of any bias, two authors who did not co-author the review in question initially assessed its quality and limitations.
Finally, we note that the systematic reviews included in our overview may have had issues that our analysis did not identify because we did not analyze their primary studies to verify the accuracy of the data and information they presented. We give two examples to substantiate this possibility. Lovato et al. wrote a commentary on the review of Sun et al. [ 41 ], in which they criticized the authors’ conclusion that sore throat is rare in COVID-19 patients [ 56 ]. Lovato et al. highlighted that multiple studies included in Sun et al. did not accurately describe participants’ clinical presentations, warning that only three studies clearly reported data on sore throat [ 56 ].
In another example, Leung [ 57 ] warned about the review of Li, L.Q. et al. [ 29 ]: “ it is possible that this statistic was computed using overlapped samples, therefore some patients were double counted ”. Li et al. responded to Leung that it is uncertain whether the data overlapped, as they used data from published articles and did not have access to the original data; they also reported that they requested original data and that they plan to re-do their analyses once they receive them; they also urged readers to treat the data with caution [ 58 ]. This points to the evolving nature of evidence during a crisis.
Our study’s strength is that this overview adds to the current knowledge by providing a comprehensive summary of all the evidence synthesis about COVID-19 available early after the onset of the pandemic. This overview followed strict methodological criteria, including a comprehensive and sensitive search strategy and a standard tool for methodological appraisal of systematic reviews.
In conclusion, in this overview of systematic reviews, we analyzed evidence from the first 18 systematic reviews that were published after the emergence of COVID-19. However, confidence in the results of all the reviews was “critically low”. Thus, systematic reviews that were published early on in the pandemic could be categorized as research waste. Even during public health emergencies, studies and systematic reviews should adhere to established methodological standards to provide patients, clinicians, and decision-makers trustworthy evidence.
Availability of data and materials
All data collected and analyzed within this study are available from the corresponding author on reasonable request.
World Health Organization. Timeline - COVID-19: Available at: https://www.who.int/news/item/29-06-2020-covidtimeline . Accessed 1 June 2021.
COVID-19 Dashboard by the Center for Systems Science and Engineering (CSSE) at Johns Hopkins University (JHU). Available at: https://coronavirus.jhu.edu/map.html . Accessed 1 June 2021.
Anzai A, Kobayashi T, Linton NM, Kinoshita R, Hayashi K, Suzuki A, et al. Assessing the Impact of Reduced Travel on Exportation Dynamics of Novel Coronavirus Infection (COVID-19). J Clin Med. 2020;9(2):601.
Chinazzi M, Davis JT, Ajelli M, Gioannini C, Litvinova M, Merler S, et al. The effect of travel restrictions on the spread of the 2019 novel coronavirus (COVID-19) outbreak. Science. 2020;368(6489):395–400. https://doi.org/10.1126/science.aba9757 .
Article CAS PubMed PubMed Central Google Scholar
Fidahic M, Nujic D, Runjic R, Civljak M, Markotic F, Lovric Makaric Z, et al. Research methodology and characteristics of journal articles with original data, preprint articles and registered clinical trial protocols about COVID-19. BMC Med Res Methodol. 2020;20(1):161. https://doi.org/10.1186/s12874-020-01047-2 .
EPPI Centre . COVID-19: a living systematic map of the evidence. Available at: http://eppi.ioe.ac.uk/cms/Projects/DepartmentofHealthandSocialCare/Publishedreviews/COVID-19Livingsystematicmapoftheevidence/tabid/3765/Default.aspx . Accessed 1 June 2021.
NCBI SARS-CoV-2 Resources. Available at: https://www.ncbi.nlm.nih.gov/sars-cov-2/ . Accessed 1 June 2021.
Gustot T. Quality and reproducibility during the COVID-19 pandemic. JHEP Rep. 2020;2(4):100141. https://doi.org/10.1016/j.jhepr.2020.100141 .
Article PubMed PubMed Central Google Scholar
Kodvanj, I., et al., Publishing of COVID-19 Preprints in Peer-reviewed Journals, Preprinting Trends, Public Discussion and Quality Issues. Preprint article. bioRxiv 2020.11.23.394577; doi: https://doi.org/10.1101/2020.11.23.394577 .
Dobler CC. Poor quality research and clinical practice during COVID-19. Breathe (Sheff). 2020;16(2):200112. https://doi.org/10.1183/20734735.0112-2020 .
Article Google Scholar
Bastian H, Glasziou P, Chalmers I. Seventy-five trials and eleven systematic reviews a day: how will we ever keep up? PLoS Med. 2010;7(9):e1000326. https://doi.org/10.1371/journal.pmed.1000326 .
Lunny C, Brennan SE, McDonald S, McKenzie JE. Toward a comprehensive evidence map of overview of systematic review methods: paper 1-purpose, eligibility, search and data extraction. Syst Rev. 2017;6(1):231. https://doi.org/10.1186/s13643-017-0617-1 .
Pollock M, Fernandes RM, Becker LA, Pieper D, Hartling L. Chapter V: Overviews of Reviews. In: Higgins JPT, Thomas J, Chandler J, Cumpston M, Li T, Page MJ, Welch VA (editors). Cochrane Handbook for Systematic Reviews of Interventions version 6.1 (updated September 2020). Cochrane. 2020. Available from www.training.cochrane.org/handbook .
Higgins JPT, Thomas J, Chandler J, Cumpston M, Li T, Page MJ, et al. Cochrane handbook for systematic reviews of interventions version 6.1 (updated September 2020). Cochrane. 2020; Available from www.training.cochrane.org/handbook .
Pollock M, Fernandes RM, Newton AS, Scott SD, Hartling L. The impact of different inclusion decisions on the comprehensiveness and complexity of overviews of reviews of healthcare interventions. Syst Rev. 2019;8(1):18. https://doi.org/10.1186/s13643-018-0914-3 .
Pollock M, Fernandes RM, Newton AS, Scott SD, Hartling L. A decision tool to help researchers make decisions about including systematic reviews in overviews of reviews of healthcare interventions. Syst Rev. 2019;8(1):29. https://doi.org/10.1186/s13643-018-0768-8 .
Hunt H, Pollock A, Campbell P, Estcourt L, Brunton G. An introduction to overviews of reviews: planning a relevant research question and objective for an overview. Syst Rev. 2018;7(1):39. https://doi.org/10.1186/s13643-018-0695-8 .
Pollock M, Fernandes RM, Pieper D, Tricco AC, Gates M, Gates A, et al. Preferred reporting items for overviews of reviews (PRIOR): a protocol for development of a reporting guideline for overviews of reviews of healthcare interventions. Syst Rev. 2019;8(1):335. https://doi.org/10.1186/s13643-019-1252-9 .
Moher D, Liberati A, Tetzlaff J, Altman DG, PRISMA Group. Preferred reporting items for systematic reviews and meta-analyses: the PRISMA statement. Open Med. 2009;3(3):e123–30.
Krnic Martinic M, Pieper D, Glatt A, Puljak L. Definition of a systematic review used in overviews of systematic reviews, meta-epidemiological studies and textbooks. BMC Med Res Methodol. 2019;19(1):203. https://doi.org/10.1186/s12874-019-0855-0 .
Puljak L. If there is only one author or only one database was searched, a study should not be called a systematic review. J Clin Epidemiol. 2017;91:4–5. https://doi.org/10.1016/j.jclinepi.2017.08.002 .
Article PubMed Google Scholar
Gates M, Gates A, Guitard S, Pollock M, Hartling L. Guidance for overviews of reviews continues to accumulate, but important challenges remain: a scoping review. Syst Rev. 2020;9(1):254. https://doi.org/10.1186/s13643-020-01509-0 .
Covidence - systematic review software. Available at: https://www.covidence.org/ . Accessed 1 June 2021.
Shea BJ, Reeves BC, Wells G, Thuku M, Hamel C, Moran J, et al. AMSTAR 2: a critical appraisal tool for systematic reviews that include randomised or non-randomised studies of healthcare interventions, or both. BMJ. 2017;358:j4008.
Borges do Nascimento IJ, et al. Novel Coronavirus Infection (COVID-19) in Humans: A Scoping Review and Meta-Analysis. J Clin Med. 2020;9(4):941.
Article PubMed Central Google Scholar
Adhikari SP, Meng S, Wu YJ, Mao YP, Ye RX, Wang QZ, et al. Epidemiology, causes, clinical manifestation and diagnosis, prevention and control of coronavirus disease (COVID-19) during the early outbreak period: a scoping review. Infect Dis Poverty. 2020;9(1):29. https://doi.org/10.1186/s40249-020-00646-x .
Cortegiani A, Ingoglia G, Ippolito M, Giarratano A, Einav S. A systematic review on the efficacy and safety of chloroquine for the treatment of COVID-19. J Crit Care. 2020;57:279–83. https://doi.org/10.1016/j.jcrc.2020.03.005 .
Li B, Yang J, Zhao F, Zhi L, Wang X, Liu L, et al. Prevalence and impact of cardiovascular metabolic diseases on COVID-19 in China. Clin Res Cardiol. 2020;109(5):531–8. https://doi.org/10.1007/s00392-020-01626-9 .
Article CAS PubMed Google Scholar
Li LQ, Huang T, Wang YQ, Wang ZP, Liang Y, Huang TB, et al. COVID-19 patients’ clinical characteristics, discharge rate, and fatality rate of meta-analysis. J Med Virol. 2020;92(6):577–83. https://doi.org/10.1002/jmv.25757 .
Lippi G, Lavie CJ, Sanchis-Gomar F. Cardiac troponin I in patients with coronavirus disease 2019 (COVID-19): evidence from a meta-analysis. Prog Cardiovasc Dis. 2020;63(3):390–1. https://doi.org/10.1016/j.pcad.2020.03.001 .
Lippi G, Henry BM. Active smoking is not associated with severity of coronavirus disease 2019 (COVID-19). Eur J Intern Med. 2020;75:107–8. https://doi.org/10.1016/j.ejim.2020.03.014 .
Lippi G, Plebani M. Procalcitonin in patients with severe coronavirus disease 2019 (COVID-19): a meta-analysis. Clin Chim Acta. 2020;505:190–1. https://doi.org/10.1016/j.cca.2020.03.004 .
Lippi G, Plebani M, Henry BM. Thrombocytopenia is associated with severe coronavirus disease 2019 (COVID-19) infections: a meta-analysis. Clin Chim Acta. 2020;506:145–8. https://doi.org/10.1016/j.cca.2020.03.022 .
Ludvigsson JF. Systematic review of COVID-19 in children shows milder cases and a better prognosis than adults. Acta Paediatr. 2020;109(6):1088–95. https://doi.org/10.1111/apa.15270 .
Lupia T, Scabini S, Mornese Pinna S, di Perri G, de Rosa FG, Corcione S. 2019 novel coronavirus (2019-nCoV) outbreak: a new challenge. J Glob Antimicrob Resist. 2020;21:22–7. https://doi.org/10.1016/j.jgar.2020.02.021 .
Marasinghe, K.M., A systematic review investigating the effectiveness of face mask use in limiting the spread of COVID-19 among medically not diagnosed individuals: shedding light on current recommendations provided to individuals not medically diagnosed with COVID-19. Research Square. Preprint article. doi : https://doi.org/10.21203/rs.3.rs-16701/v1 . 2020 .
Mullins E, Evans D, Viner RM, O’Brien P, Morris E. Coronavirus in pregnancy and delivery: rapid review. Ultrasound Obstet Gynecol. 2020;55(5):586–92. https://doi.org/10.1002/uog.22014 .
Pang J, Wang MX, Ang IYH, Tan SHX, Lewis RF, Chen JIP, et al. Potential Rapid Diagnostics, Vaccine and Therapeutics for 2019 Novel coronavirus (2019-nCoV): a systematic review. J Clin Med. 2020;9(3):623.
Rodriguez-Morales AJ, Cardona-Ospina JA, Gutiérrez-Ocampo E, Villamizar-Peña R, Holguin-Rivera Y, Escalera-Antezana JP, et al. Clinical, laboratory and imaging features of COVID-19: a systematic review and meta-analysis. Travel Med Infect Dis. 2020;34:101623. https://doi.org/10.1016/j.tmaid.2020.101623 .
Salehi S, Abedi A, Balakrishnan S, Gholamrezanezhad A. Coronavirus disease 2019 (COVID-19): a systematic review of imaging findings in 919 patients. AJR Am J Roentgenol. 2020;215(1):87–93. https://doi.org/10.2214/AJR.20.23034 .
Sun P, Qie S, Liu Z, Ren J, Li K, Xi J. Clinical characteristics of hospitalized patients with SARS-CoV-2 infection: a single arm meta-analysis. J Med Virol. 2020;92(6):612–7. https://doi.org/10.1002/jmv.25735 .
Yang J, Zheng Y, Gou X, Pu K, Chen Z, Guo Q, et al. Prevalence of comorbidities and its effects in patients infected with SARS-CoV-2: a systematic review and meta-analysis. Int J Infect Dis. 2020;94:91–5. https://doi.org/10.1016/j.ijid.2020.03.017 .
Bassetti M, Vena A, Giacobbe DR. The novel Chinese coronavirus (2019-nCoV) infections: challenges for fighting the storm. Eur J Clin Investig. 2020;50(3):e13209. https://doi.org/10.1111/eci.13209 .
Article CAS Google Scholar
Hwang CS. Olfactory neuropathy in severe acute respiratory syndrome: report of a case. Acta Neurol Taiwanica. 2006;15(1):26–8.
Google Scholar
Suzuki M, Saito K, Min WP, Vladau C, Toida K, Itoh H, et al. Identification of viruses in patients with postviral olfactory dysfunction. Laryngoscope. 2007;117(2):272–7. https://doi.org/10.1097/01.mlg.0000249922.37381.1e .
Rajgor DD, Lee MH, Archuleta S, Bagdasarian N, Quek SC. The many estimates of the COVID-19 case fatality rate. Lancet Infect Dis. 2020;20(7):776–7. https://doi.org/10.1016/S1473-3099(20)30244-9 .
Wolkewitz M, Puljak L. Methodological challenges of analysing COVID-19 data during the pandemic. BMC Med Res Methodol. 2020;20(1):81. https://doi.org/10.1186/s12874-020-00972-6 .
Rombey T, Lochner V, Puljak L, Könsgen N, Mathes T, Pieper D. Epidemiology and reporting characteristics of non-Cochrane updates of systematic reviews: a cross-sectional study. Res Synth Methods. 2020;11(3):471–83. https://doi.org/10.1002/jrsm.1409 .
Runjic E, Rombey T, Pieper D, Puljak L. Half of systematic reviews about pain registered in PROSPERO were not published and the majority had inaccurate status. J Clin Epidemiol. 2019;116:114–21. https://doi.org/10.1016/j.jclinepi.2019.08.010 .
Runjic E, Behmen D, Pieper D, Mathes T, Tricco AC, Moher D, et al. Following Cochrane review protocols to completion 10 years later: a retrospective cohort study and author survey. J Clin Epidemiol. 2019;111:41–8. https://doi.org/10.1016/j.jclinepi.2019.03.006 .
Tricco AC, Antony J, Zarin W, Strifler L, Ghassemi M, Ivory J, et al. A scoping review of rapid review methods. BMC Med. 2015;13(1):224. https://doi.org/10.1186/s12916-015-0465-6 .
COVID-19 Rapid Reviews: Cochrane’s response so far. Available at: https://training.cochrane.org/resource/covid-19-rapid-reviews-cochrane-response-so-far . Accessed 1 June 2021.
Cochrane. Living systematic reviews. Available at: https://community.cochrane.org/review-production/production-resources/living-systematic-reviews . Accessed 1 June 2021.
Millard T, Synnot A, Elliott J, Green S, McDonald S, Turner T. Feasibility and acceptability of living systematic reviews: results from a mixed-methods evaluation. Syst Rev. 2019;8(1):325. https://doi.org/10.1186/s13643-019-1248-5 .
Babic A, Poklepovic Pericic T, Pieper D, Puljak L. How to decide whether a systematic review is stable and not in need of updating: analysis of Cochrane reviews. Res Synth Methods. 2020;11(6):884–90. https://doi.org/10.1002/jrsm.1451 .
Lovato A, Rossettini G, de Filippis C. Sore throat in COVID-19: comment on “clinical characteristics of hospitalized patients with SARS-CoV-2 infection: a single arm meta-analysis”. J Med Virol. 2020;92(7):714–5. https://doi.org/10.1002/jmv.25815 .
Leung C. Comment on Li et al: COVID-19 patients’ clinical characteristics, discharge rate, and fatality rate of meta-analysis. J Med Virol. 2020;92(9):1431–2. https://doi.org/10.1002/jmv.25912 .
Li LQ, Huang T, Wang YQ, Wang ZP, Liang Y, Huang TB, et al. Response to Char’s comment: comment on Li et al: COVID-19 patients’ clinical characteristics, discharge rate, and fatality rate of meta-analysis. J Med Virol. 2020;92(9):1433. https://doi.org/10.1002/jmv.25924 .
Download references
Acknowledgments
We thank Catherine Henderson DPhil from Swanscoe Communications for pro bono medical writing and editing support. We acknowledge support from the Covidence Team, specifically Anneliese Arno. We thank the whole International Network of Coronavirus Disease 2019 (InterNetCOVID-19) for their commitment and involvement. Members of the InterNetCOVID-19 are listed in Additional file 6 . We thank Pavel Cerny and Roger Crosthwaite for guiding the team supervisor (IJBN) on human resources management.
This research received no external funding.
Author information
Authors and affiliations.
University Hospital and School of Medicine, Universidade Federal de Minas Gerais, Belo Horizonte, Minas Gerais, Brazil
Israel Júnior Borges do Nascimento & Milena Soriano Marcolino
Medical College of Wisconsin, Milwaukee, WI, USA
Israel Júnior Borges do Nascimento
Helene Fuld Health Trust National Institute for Evidence-based Practice in Nursing and Healthcare, College of Nursing, The Ohio State University, Columbus, OH, USA
Dónal P. O’Mathúna
School of Nursing, Psychotherapy and Community Health, Dublin City University, Dublin, Ireland
Department of Anesthesiology, Intensive Care and Pain Medicine, University of Münster, Münster, Germany
Thilo Caspar von Groote
Department of Sport and Health Science, Technische Universität München, Munich, Germany
Hebatullah Mohamed Abdulazeem
School of Health Sciences, Faculty of Health and Medicine, The University of Newcastle, Callaghan, Australia
Ishanka Weerasekara
Department of Physiotherapy, Faculty of Allied Health Sciences, University of Peradeniya, Peradeniya, Sri Lanka
Cochrane Croatia, University of Split, School of Medicine, Split, Croatia
Ana Marusic, Irena Zakarija-Grkovic & Tina Poklepovic Pericic
Center for Evidence-Based Medicine and Health Care, Catholic University of Croatia, Ilica 242, 10000, Zagreb, Croatia
Livia Puljak
Cochrane Brazil, Evidence-Based Health Program, Universidade Federal de São Paulo, São Paulo, Brazil
Vinicius Tassoni Civile & Alvaro Nagib Atallah
Yorkville University, Fredericton, New Brunswick, Canada
Santino Filoso
Laboratory for Industrial and Applied Mathematics (LIAM), Department of Mathematics and Statistics, York University, Toronto, Ontario, Canada
Nicola Luigi Bragazzi
You can also search for this author in PubMed Google Scholar
Contributions
IJBN conceived the research idea and worked as a project coordinator. DPOM, TCVG, HMA, IW, AM, LP, VTC, IZG, TPP, ANA, SF, NLB and MSM were involved in data curation, formal analysis, investigation, methodology, and initial draft writing. All authors revised the manuscript critically for the content. The author(s) read and approved the final manuscript.
Corresponding author
Correspondence to Livia Puljak .
Ethics declarations
Ethics approval and consent to participate.
Not required as data was based on published studies.
Consent for publication
Not applicable.
Competing interests
The authors declare no conflict of interest.
Additional information
Publisher’s note.
Springer Nature remains neutral with regard to jurisdictional claims in published maps and institutional affiliations.
Supplementary Information
Additional file 1: appendix 1..
Search strategies used in the study.
Additional file 2: Appendix 2.
Adjusted scoring of AMSTAR 2 used in this study for systematic reviews of studies that did not analyze interventions.
Additional file 3: Appendix 3.
List of excluded studies, with reasons.
Additional file 4: Appendix 4.
Table of overlapping studies, containing the list of primary studies included, their visual overlap in individual systematic reviews, and the number in how many reviews each primary study was included.
Additional file 5: Appendix 5.
A detailed explanation of AMSTAR scoring for each item in each review.
Additional file 6: Appendix 6.
List of members and affiliates of International Network of Coronavirus Disease 2019 (InterNetCOVID-19).
Rights and permissions
Open Access This article is licensed under a Creative Commons Attribution 4.0 International License, which permits use, sharing, adaptation, distribution and reproduction in any medium or format, as long as you give appropriate credit to the original author(s) and the source, provide a link to the Creative Commons licence, and indicate if changes were made. The images or other third party material in this article are included in the article's Creative Commons licence, unless indicated otherwise in a credit line to the material. If material is not included in the article's Creative Commons licence and your intended use is not permitted by statutory regulation or exceeds the permitted use, you will need to obtain permission directly from the copyright holder. To view a copy of this licence, visit http://creativecommons.org/licenses/by/4.0/ . The Creative Commons Public Domain Dedication waiver ( http://creativecommons.org/publicdomain/zero/1.0/ ) applies to the data made available in this article, unless otherwise stated in a credit line to the data.
Reprints and permissions
About this article
Cite this article.
Borges do Nascimento, I.J., O’Mathúna, D.P., von Groote, T.C. et al. Coronavirus disease (COVID-19) pandemic: an overview of systematic reviews. BMC Infect Dis 21 , 525 (2021). https://doi.org/10.1186/s12879-021-06214-4
Download citation
Received : 12 April 2020
Accepted : 19 May 2021
Published : 04 June 2021
DOI : https://doi.org/10.1186/s12879-021-06214-4
Share this article
Anyone you share the following link with will be able to read this content:
Sorry, a shareable link is not currently available for this article.
Provided by the Springer Nature SharedIt content-sharing initiative
- Coronavirus
- Evidence-based medicine
- Infectious diseases
BMC Infectious Diseases
ISSN: 1471-2334
- Submission enquiries: [email protected]
- General enquiries: [email protected]
Log in using your username and password
- Search More Search for this keyword Advanced search
- Latest content
- Current issue
- BMJ Journals More You are viewing from: Google Indexer
You are here
- Volume 76, Issue 2
- COVID-19 pandemic and its impact on social relationships and health
- Article Text
- Article info
- Citation Tools
- Rapid Responses
- Article metrics

- http://orcid.org/0000-0003-1512-4471 Emily Long 1 ,
- Susan Patterson 1 ,
- Karen Maxwell 1 ,
- Carolyn Blake 1 ,
- http://orcid.org/0000-0001-7342-4566 Raquel Bosó Pérez 1 ,
- Ruth Lewis 1 ,
- Mark McCann 1 ,
- Julie Riddell 1 ,
- Kathryn Skivington 1 ,
- Rachel Wilson-Lowe 1 ,
- http://orcid.org/0000-0002-4409-6601 Kirstin R Mitchell 2
- 1 MRC/CSO Social and Public Health Sciences Unit , University of Glasgow , Glasgow , UK
- 2 MRC/CSO Social and Public Health Sciences Unit, Institute of Health & Wellbeing , University of Glasgow , Glasgow , UK
- Correspondence to Dr Emily Long, MRC/CSO Social and Public Health Sciences Unit, University of Glasgow, Glasgow G3 7HR, UK; emily.long{at}glasgow.ac.uk
This essay examines key aspects of social relationships that were disrupted by the COVID-19 pandemic. It focuses explicitly on relational mechanisms of health and brings together theory and emerging evidence on the effects of the COVID-19 pandemic to make recommendations for future public health policy and recovery. We first provide an overview of the pandemic in the UK context, outlining the nature of the public health response. We then introduce four distinct domains of social relationships: social networks, social support, social interaction and intimacy, highlighting the mechanisms through which the pandemic and associated public health response drastically altered social interactions in each domain. Throughout the essay, the lens of health inequalities, and perspective of relationships as interconnecting elements in a broader system, is used to explore the varying impact of these disruptions. The essay concludes by providing recommendations for longer term recovery ensuring that the social relational cost of COVID-19 is adequately considered in efforts to rebuild.
- inequalities
Data availability statement
Data sharing not applicable as no data sets generated and/or analysed for this study. Data sharing not applicable as no data sets generated or analysed for this essay.
This is an open access article distributed in accordance with the Creative Commons Attribution 4.0 Unported (CC BY 4.0) license, which permits others to copy, redistribute, remix, transform and build upon this work for any purpose, provided the original work is properly cited, a link to the licence is given, and indication of whether changes were made. See: https://creativecommons.org/licenses/by/4.0/ .
https://doi.org/10.1136/jech-2021-216690
Statistics from Altmetric.com
Request permissions.
If you wish to reuse any or all of this article please use the link below which will take you to the Copyright Clearance Center’s RightsLink service. You will be able to get a quick price and instant permission to reuse the content in many different ways.
Introduction
Infectious disease pandemics, including SARS and COVID-19, demand intrapersonal behaviour change and present highly complex challenges for public health. 1 A pandemic of an airborne infection, spread easily through social contact, assails human relationships by drastically altering the ways through which humans interact. In this essay, we draw on theories of social relationships to examine specific ways in which relational mechanisms key to health and well-being were disrupted by the COVID-19 pandemic. Relational mechanisms refer to the processes between people that lead to change in health outcomes.
At the time of writing, the future surrounding COVID-19 was uncertain. Vaccine programmes were being rolled out in countries that could afford them, but new and more contagious variants of the virus were also being discovered. The recovery journey looked long, with continued disruption to social relationships. The social cost of COVID-19 was only just beginning to emerge, but the mental health impact was already considerable, 2 3 and the inequality of the health burden stark. 4 Knowledge of the epidemiology of COVID-19 accrued rapidly, but evidence of the most effective policy responses remained uncertain.
The initial response to COVID-19 in the UK was reactive and aimed at reducing mortality, with little time to consider the social implications, including for interpersonal and community relationships. The terminology of ‘social distancing’ quickly became entrenched both in public and policy discourse. This equation of physical distance with social distance was regrettable, since only physical proximity causes viral transmission, whereas many forms of social proximity (eg, conversations while walking outdoors) are minimal risk, and are crucial to maintaining relationships supportive of health and well-being.
The aim of this essay is to explore four key relational mechanisms that were impacted by the pandemic and associated restrictions: social networks, social support, social interaction and intimacy. We use relational theories and emerging research on the effects of the COVID-19 pandemic response to make three key recommendations: one regarding public health responses; and two regarding social recovery. Our understanding of these mechanisms stems from a ‘systems’ perspective which casts social relationships as interdependent elements within a connected whole. 5
Social networks
Social networks characterise the individuals and social connections that compose a system (such as a workplace, community or society). Social relationships range from spouses and partners, to coworkers, friends and acquaintances. They vary across many dimensions, including, for example, frequency of contact and emotional closeness. Social networks can be understood both in terms of the individuals and relationships that compose the network, as well as the overall network structure (eg, how many of your friends know each other).
Social networks show a tendency towards homophily, or a phenomenon of associating with individuals who are similar to self. 6 This is particularly true for ‘core’ network ties (eg, close friends), while more distant, sometimes called ‘weak’ ties tend to show more diversity. During the height of COVID-19 restrictions, face-to-face interactions were often reduced to core network members, such as partners, family members or, potentially, live-in roommates; some ‘weak’ ties were lost, and interactions became more limited to those closest. Given that peripheral, weaker social ties provide a diversity of resources, opinions and support, 7 COVID-19 likely resulted in networks that were smaller and more homogenous.
Such changes were not inevitable nor necessarily enduring, since social networks are also adaptive and responsive to change, in that a disruption to usual ways of interacting can be replaced by new ways of engaging (eg, Zoom). Yet, important inequalities exist, wherein networks and individual relationships within networks are not equally able to adapt to such changes. For example, individuals with a large number of newly established relationships (eg, university students) may have struggled to transfer these relationships online, resulting in lost contacts and a heightened risk of social isolation. This is consistent with research suggesting that young adults were the most likely to report a worsening of relationships during COVID-19, whereas older adults were the least likely to report a change. 8
Lastly, social connections give rise to emergent properties of social systems, 9 where a community-level phenomenon develops that cannot be attributed to any one member or portion of the network. For example, local area-based networks emerged due to geographic restrictions (eg, stay-at-home orders), resulting in increases in neighbourly support and local volunteering. 10 In fact, research suggests that relationships with neighbours displayed the largest net gain in ratings of relationship quality compared with a range of relationship types (eg, partner, colleague, friend). 8 Much of this was built from spontaneous individual interactions within local communities, which together contributed to the ‘community spirit’ that many experienced. 11 COVID-19 restrictions thus impacted the personal social networks and the structure of the larger networks within the society.
Social support
Social support, referring to the psychological and material resources provided through social interaction, is a critical mechanism through which social relationships benefit health. In fact, social support has been shown to be one of the most important resilience factors in the aftermath of stressful events. 12 In the context of COVID-19, the usual ways in which individuals interact and obtain social support have been severely disrupted.
One such disruption has been to opportunities for spontaneous social interactions. For example, conversations with colleagues in a break room offer an opportunity for socialising beyond one’s core social network, and these peripheral conversations can provide a form of social support. 13 14 A chance conversation may lead to advice helpful to coping with situations or seeking formal help. Thus, the absence of these spontaneous interactions may mean the reduction of indirect support-seeking opportunities. While direct support-seeking behaviour is more effective at eliciting support, it also requires significantly more effort and may be perceived as forceful and burdensome. 15 The shift to homeworking and closure of community venues reduced the number of opportunities for these spontaneous interactions to occur, and has, second, focused them locally. Consequently, individuals whose core networks are located elsewhere, or who live in communities where spontaneous interaction is less likely, have less opportunity to benefit from spontaneous in-person supportive interactions.
However, alongside this disruption, new opportunities to interact and obtain social support have arisen. The surge in community social support during the initial lockdown mirrored that often seen in response to adverse events (eg, natural disasters 16 ). COVID-19 restrictions that confined individuals to their local area also compelled them to focus their in-person efforts locally. Commentators on the initial lockdown in the UK remarked on extraordinary acts of generosity between individuals who belonged to the same community but were unknown to each other. However, research on adverse events also tells us that such community support is not necessarily maintained in the longer term. 16
Meanwhile, online forms of social support are not bound by geography, thus enabling interactions and social support to be received from a wider network of people. Formal online social support spaces (eg, support groups) existed well before COVID-19, but have vastly increased since. While online interactions can increase perceived social support, it is unclear whether remote communication technologies provide an effective substitute from in-person interaction during periods of social distancing. 17 18 It makes intuitive sense that the usefulness of online social support will vary by the type of support offered, degree of social interaction and ‘online communication skills’ of those taking part. Youth workers, for instance, have struggled to keep vulnerable youth engaged in online youth clubs, 19 despite others finding a positive association between amount of digital technology used by individuals during lockdown and perceived social support. 20 Other research has found that more frequent face-to-face contact and phone/video contact both related to lower levels of depression during the time period of March to August 2020, but the negative effect of a lack of contact was greater for those with higher levels of usual sociability. 21 Relatedly, important inequalities in social support exist, such that individuals who occupy more socially disadvantaged positions in society (eg, low socioeconomic status, older people) tend to have less access to social support, 22 potentially exacerbated by COVID-19.
Social and interactional norms
Interactional norms are key relational mechanisms which build trust, belonging and identity within and across groups in a system. Individuals in groups and societies apply meaning by ‘approving, arranging and redefining’ symbols of interaction. 23 A handshake, for instance, is a powerful symbol of trust and equality. Depending on context, not shaking hands may symbolise a failure to extend friendship, or a failure to reach agreement. The norms governing these symbols represent shared values and identity; and mutual understanding of these symbols enables individuals to achieve orderly interactions, establish supportive relationship accountability and connect socially. 24 25
Physical distancing measures to contain the spread of COVID-19 radically altered these norms of interaction, particularly those used to convey trust, affinity, empathy and respect (eg, hugging, physical comforting). 26 As epidemic waves rose and fell, the work to negotiate these norms required intense cognitive effort; previously taken-for-granted interactions were re-examined, factoring in current restriction levels, own and (assumed) others’ vulnerability and tolerance of risk. This created awkwardness, and uncertainty, for example, around how to bring closure to an in-person interaction or convey warmth. The instability in scripted ways of interacting created particular strain for individuals who already struggled to encode and decode interactions with others (eg, those who are deaf or have autism spectrum disorder); difficulties often intensified by mask wearing. 27
Large social gatherings—for example, weddings, school assemblies, sporting events—also present key opportunities for affirming and assimilating interactional norms, building cohesion and shared identity and facilitating cooperation across social groups. 28 Online ‘equivalents’ do not easily support ‘social-bonding’ activities such as singing and dancing, and rarely enable chance/spontaneous one-on-one conversations with peripheral/weaker network ties (see the Social networks section) which can help strengthen bonds across a larger network. The loss of large gatherings to celebrate rites of passage (eg, bar mitzvah, weddings) has additional relational costs since these events are performed by and for communities to reinforce belonging, and to assist in transitioning to new phases of life. 29 The loss of interaction with diverse others via community and large group gatherings also reduces intergroup contact, which may then tend towards more prejudiced outgroup attitudes. While online interaction can go some way to mimicking these interaction norms, there are key differences. A sense of anonymity, and lack of in-person emotional cues, tends to support norms of polarisation and aggression in expressing differences of opinion online. And while online platforms have potential to provide intergroup contact, the tendency of much social media to form homogeneous ‘echo chambers’ can serve to further reduce intergroup contact. 30 31
Intimacy relates to the feeling of emotional connection and closeness with other human beings. Emotional connection, through romantic, friendship or familial relationships, fulfils a basic human need 32 and strongly benefits health, including reduced stress levels, improved mental health, lowered blood pressure and reduced risk of heart disease. 32 33 Intimacy can be fostered through familiarity, feeling understood and feeling accepted by close others. 34
Intimacy via companionship and closeness is fundamental to mental well-being. Positively, the COVID-19 pandemic has offered opportunities for individuals to (re)connect and (re)strengthen close relationships within their household via quality time together, following closure of many usual external social activities. Research suggests that the first full UK lockdown period led to a net gain in the quality of steady relationships at a population level, 35 but amplified existing inequalities in relationship quality. 35 36 For some in single-person households, the absence of a companion became more conspicuous, leading to feelings of loneliness and lower mental well-being. 37 38 Additional pandemic-related relational strain 39 40 resulted, for some, in the initiation or intensification of domestic abuse. 41 42
Physical touch is another key aspect of intimacy, a fundamental human need crucial in maintaining and developing intimacy within close relationships. 34 Restrictions on social interactions severely restricted the number and range of people with whom physical affection was possible. The reduction in opportunity to give and receive affectionate physical touch was not experienced equally. Many of those living alone found themselves completely without physical contact for extended periods. The deprivation of physical touch is evidenced to take a heavy emotional toll. 43 Even in future, once physical expressions of affection can resume, new levels of anxiety over germs may introduce hesitancy into previously fluent blending of physical and verbal intimate social connections. 44
The pandemic also led to shifts in practices and norms around sexual relationship building and maintenance, as individuals adapted and sought alternative ways of enacting sexual intimacy. This too is important, given that intimate sexual activity has known benefits for health. 45 46 Given that social restrictions hinged on reducing household mixing, possibilities for partnered sexual activity were primarily guided by living arrangements. While those in cohabiting relationships could potentially continue as before, those who were single or in non-cohabiting relationships generally had restricted opportunities to maintain their sexual relationships. Pornography consumption and digital partners were reported to increase since lockdown. 47 However, online interactions are qualitatively different from in-person interactions and do not provide the same opportunities for physical intimacy.
Recommendations and conclusions
In the sections above we have outlined the ways in which COVID-19 has impacted social relationships, showing how relational mechanisms key to health have been undermined. While some of the damage might well self-repair after the pandemic, there are opportunities inherent in deliberative efforts to build back in ways that facilitate greater resilience in social and community relationships. We conclude by making three recommendations: one regarding public health responses to the pandemic; and two regarding social recovery.
Recommendation 1: explicitly count the relational cost of public health policies to control the pandemic
Effective handling of a pandemic recognises that social, economic and health concerns are intricately interwoven. It is clear that future research and policy attention must focus on the social consequences. As described above, policies which restrict physical mixing across households carry heavy and unequal relational costs. These include for individuals (eg, loss of intimate touch), dyads (eg, loss of warmth, comfort), networks (eg, restricted access to support) and communities (eg, loss of cohesion and identity). Such costs—and their unequal impact—should not be ignored in short-term efforts to control an epidemic. Some public health responses—restrictions on international holiday travel and highly efficient test and trace systems—have relatively small relational costs and should be prioritised. At a national level, an earlier move to proportionate restrictions, and investment in effective test and trace systems, may help prevent escalation of spread to the point where a national lockdown or tight restrictions became an inevitability. Where policies with relational costs are unavoidable, close attention should be paid to the unequal relational impact for those whose personal circumstances differ from normative assumptions of two adult families. This includes consideration of whether expectations are fair (eg, for those who live alone), whether restrictions on social events are equitable across age group, religious/ethnic groupings and social class, and also to ensure that the language promoted by such policies (eg, households; families) is not exclusionary. 48 49 Forethought to unequal impacts on social relationships should thus be integral to the work of epidemic preparedness teams.
Recommendation 2: intelligently balance online and offline ways of relating
A key ingredient for well-being is ‘getting together’ in a physical sense. This is fundamental to a human need for intimate touch, physical comfort, reinforcing interactional norms and providing practical support. Emerging evidence suggests that online ways of relating cannot simply replace physical interactions. But online interaction has many benefits and for some it offers connections that did not exist previously. In particular, online platforms provide new forms of support for those unable to access offline services because of mobility issues (eg, older people) or because they are geographically isolated from their support community (eg, lesbian, gay, bisexual, transgender and queer (LGBTQ) youth). Ultimately, multiple forms of online and offline social interactions are required to meet the needs of varying groups of people (eg, LGBTQ, older people). Future research and practice should aim to establish ways of using offline and online support in complementary and even synergistic ways, rather than veering between them as social restrictions expand and contract. Intelligent balancing of online and offline ways of relating also pertains to future policies on home and flexible working. A decision to switch to wholesale or obligatory homeworking should consider the risk to relational ‘group properties’ of the workplace community and their impact on employees’ well-being, focusing in particular on unequal impacts (eg, new vs established employees). Intelligent blending of online and in-person working is required to achieve flexibility while also nurturing supportive networks at work. Intelligent balance also implies strategies to build digital literacy and minimise digital exclusion, as well as coproducing solutions with intended beneficiaries.
Recommendation 3: build stronger and sustainable localised communities
In balancing offline and online ways of interacting, there is opportunity to capitalise on the potential for more localised, coherent communities due to scaled-down travel, homeworking and local focus that will ideally continue after restrictions end. There are potential economic benefits after the pandemic, such as increased trade as home workers use local resources (eg, coffee shops), but also relational benefits from stronger relationships around the orbit of the home and neighbourhood. Experience from previous crises shows that community volunteer efforts generated early on will wane over time in the absence of deliberate work to maintain them. Adequately funded partnerships between local government, third sector and community groups are required to sustain community assets that began as a direct response to the pandemic. Such partnerships could work to secure green spaces and indoor (non-commercial) meeting spaces that promote community interaction. Green spaces in particular provide a triple benefit in encouraging physical activity and mental health, as well as facilitating social bonding. 50 In building local communities, small community networks—that allow for diversity and break down ingroup/outgroup views—may be more helpful than the concept of ‘support bubbles’, which are exclusionary and less sustainable in the longer term. Rigorously designed intervention and evaluation—taking a systems approach—will be crucial in ensuring scale-up and sustainability.
The dramatic change to social interaction necessitated by efforts to control the spread of COVID-19 created stark challenges but also opportunities. Our essay highlights opportunities for learning, both to ensure the equity and humanity of physical restrictions, and to sustain the salutogenic effects of social relationships going forward. The starting point for capitalising on this learning is recognition of the disruption to relational mechanisms as a key part of the socioeconomic and health impact of the pandemic. In recovery planning, a general rule is that what is good for decreasing health inequalities (such as expanding social protection and public services and pursuing green inclusive growth strategies) 4 will also benefit relationships and safeguard relational mechanisms for future generations. Putting this into action will require political will.
Ethics statements
Patient consent for publication.
Not required.
- Office for National Statistics (ONS)
- Ford T , et al
- Riordan R ,
- Ford J , et al
- Glonti K , et al
- McPherson JM ,
- Smith-Lovin L
- Granovetter MS
- Fancourt D et al
- Stadtfeld C
- Office for Civil Society
- Cook J et al
- Rodriguez-Llanes JM ,
- Guha-Sapir D
- Patulny R et al
- Granovetter M
- Winkeler M ,
- Filipp S-H ,
- Kaniasty K ,
- de Terte I ,
- Guilaran J , et al
- Wright KB ,
- Martin J et al
- Gabbiadini A ,
- Baldissarri C ,
- Durante F , et al
- Sommerlad A ,
- Marston L ,
- Huntley J , et al
- Turner RJ ,
- Bicchieri C
- Brennan G et al
- Watson-Jones RE ,
- Amichai-Hamburger Y ,
- McKenna KYA
- Page-Gould E ,
- Aron A , et al
- Pietromonaco PR ,
- Timmerman GM
- Bradbury-Jones C ,
- Mikocka-Walus A ,
- Klas A , et al
- Marshall L ,
- Steptoe A ,
- Stanley SM ,
- Campbell AM
- ↵ (ONS), O.f.N.S., Domestic abuse during the coronavirus (COVID-19) pandemic, England and Wales . Available: https://www.ons.gov.uk/peoplepopulationandcommunity/crimeandjustice/articles/domesticabuseduringthecoronaviruscovid19pandemicenglandandwales/november2020
- Rosenberg M ,
- Hensel D , et al
- Banerjee D ,
- Bruner DW , et al
- Bavel JJV ,
- Baicker K ,
- Boggio PS , et al
- van Barneveld K ,
- Quinlan M ,
- Kriesler P , et al
- Mitchell R ,
- de Vries S , et al
Twitter @karenmaxSPHSU, @Mark_McCann, @Rwilsonlowe, @KMitchinGlasgow
Contributors EL and KM led on the manuscript conceptualisation, review and editing. SP, KM, CB, RBP, RL, MM, JR, KS and RW-L contributed to drafting and revising the article. All authors assisted in revising the final draft.
Funding The research reported in this publication was supported by the Medical Research Council (MC_UU_00022/1, MC_UU_00022/3) and the Chief Scientist Office (SPHSU11, SPHSU14). EL is also supported by MRC Skills Development Fellowship Award (MR/S015078/1). KS and MM are also supported by a Medical Research Council Strategic Award (MC_PC_13027).
Competing interests None declared.
Provenance and peer review Not commissioned; externally peer reviewed.
Read the full text or download the PDF:
- - Google Chrome
Intended for healthcare professionals
- Access provided by Google Indexer
- My email alerts
- BMA member login
- Username * Password * Forgot your log in details? Need to activate BMA Member Log In Log in via OpenAthens Log in via your institution

Search form
- Advanced search
- Search responses
- Search blogs
- Effectiveness of...
Effectiveness of public health measures in reducing the incidence of covid-19, SARS-CoV-2 transmission, and covid-19 mortality: systematic review and meta-analysis
Linked editorial.
Public health measures for covid-19
- Related content
- Peer review
- Stella Talic , lecturer in clinical epidemiology and public health 1 2 ,
- Shivangi Shah , honours student 1 ,
- Holly Wild , lecturer and honours student 1 3 ,
- Danijela Gasevic , senior lecturer in epidemiology and chronic disease prevention 1 4 ,
- Ashika Maharaj , lecturer quality and safety and cancer epidemiology 1 ,
- Zanfina Ademi , associate professor of medical outcomes and health economics 1 2 ,
- Xue Li , assistant professor 4 6 ,
- Wei Xu , research student 4 ,
- Ines Mesa-Eguiagaray , statistical geneticist 4 ,
- Jasmin Rostron , research student 4 ,
- Evropi Theodoratou , professor of cancer epidemiology and global health 4 5 ,
- Xiaomeng Zhang , research student 4 ,
- Ashmika Motee , research student 4 ,
- Danny Liew , professor of medical outcomes and health economics 1 2 ,
- Dragan Ilic , professor of medical education and public health 1
- 1 School of Public Health and Preventive Medicine, Monash University, Melbourne, 3004 VIC, Australia
- 2 Monash Outcomes Research and health Economics (MORE) Unit, Monash University, VIC, Australia
- 3 Torrens University, VIC, Australia
- 4 Centre for Global Health, The Usher Institute, University of Edinburgh, Edinburgh, UK
- 5 Cancer Research UK Edinburgh Centre, MRC Institute of Genetics and Molecular Medicine, University of Edinburgh, Edinburgh, UK
- 6 School of Public Health and The Second Affiliated Hospital, Zhejiang University School of Medicine, Hangzhou, China
- Correspondence to: S Talic stella.talic{at}monash.edu
- Accepted 21 October 2021
Objective To review the evidence on the effectiveness of public health measures in reducing the incidence of covid-19, SARS-CoV-2 transmission, and covid-19 mortality.
Design Systematic review and meta-analysis.
Data sources Medline, Embase, CINAHL, Biosis, Joanna Briggs, Global Health, and World Health Organization COVID-19 database (preprints).
Eligibility criteria for study selection Observational and interventional studies that assessed the effectiveness of public health measures in reducing the incidence of covid-19, SARS-CoV-2 transmission, and covid-19 mortality.
Main outcome measures The main outcome measure was incidence of covid-19. Secondary outcomes included SARS-CoV-2 transmission and covid-19 mortality.
Data synthesis DerSimonian Laird random effects meta-analysis was performed to investigate the effect of mask wearing, handwashing, and physical distancing measures on incidence of covid-19. Pooled effect estimates with corresponding 95% confidence intervals were computed, and heterogeneity among studies was assessed using Cochran’s Q test and the I 2 metrics, with two tailed P values.
Results 72 studies met the inclusion criteria, of which 35 evaluated individual public health measures and 37 assessed multiple public health measures as a “package of interventions.” Eight of 35 studies were included in the meta-analysis, which indicated a reduction in incidence of covid-19 associated with handwashing (relative risk 0.47, 95% confidence interval 0.19 to 1.12, I 2 =12%), mask wearing (0.47, 0.29 to 0.75, I 2 =84%), and physical distancing (0.75, 0.59 to 0.95, I 2 =87%). Owing to heterogeneity of the studies, meta-analysis was not possible for the outcomes of quarantine and isolation, universal lockdowns, and closures of borders, schools, and workplaces. The effects of these interventions were synthesised descriptively.
Conclusions This systematic review and meta-analysis suggests that several personal protective and social measures, including handwashing, mask wearing, and physical distancing are associated with reductions in the incidence covid-19. Public health efforts to implement public health measures should consider community health and sociocultural needs, and future research is needed to better understand the effectiveness of public health measures in the context of covid-19 vaccination.
Systematic review registration PROSPERO CRD42020178692.

- Download figure
- Open in new tab
- Download powerpoint
Introduction
The impact of SARS-CoV-2 on global public health and economies has been profound. 1 As of 14 October 2021, there were 239 007 759 million cases of confirmed covid-19 and 4 871 841 million deaths with covid-19 worldwide. 2
A variety of containment and mitigation strategies have been adopted to adequately respond to covid-19, with the intention of deferring major surges of patients in hospitals and protecting the most vulnerable people from infection, including elderly people and those with comorbidities. 3 Strategies to achieve these goals are diverse, commonly based on national risk assessments that include estimation of numbers of patients requiring hospital admission and availability of hospital beds and ventilation support.
Globally, vaccination programmes have proved to be safe and effective and save lives. 4 5 Yet most vaccines do not confer 100% protection, and it is not known how vaccines will prevent future transmission of SARS-CoV-2, 6 given emerging variants. 7 8 9 The proportion of the population that must be vaccinated against covid-19 to reach herd immunity depends greatly on current and future variants. 10 This vaccination threshold varies according to the country and population’s response, types of vaccines, groups prioritised for vaccination, and viral mutations, among other factors. 6 Until herd immunity to covid-19 is reached, regardless of the already proven high vaccination rates, 11 public health preventive strategies are likely to remain as first choice measures in disease prevention, 12 particularly in places with a low uptake of covid-19 vaccination. Measures such as lockdown (local and national variant), physical distancing, mandatory use of face masks, and hand hygiene have been implemented as primary preventive strategies to curb the covid-19 pandemic. 13
Public health (or non-pharmaceutical) interventions have been shown to be beneficial in fighting respiratory infections transmitted through contact, droplets, and aerosols. 14 15 Given that SARS-CoV-2 is highly transmissible, it is a challenge to determine which measures might be more effective and sustainable for further prevention.
Substantial benefits in reducing mortality were observed in countries with universal lockdowns in place, such as Australia, New Zealand, Singapore, and China. Universal lockdowns are not, however, sustainable, and more tailored interventions need to be considered; the ones that maintain social lives and keep economies functional while protecting high risk individuals. 16 17 Substantial variation exists in how different countries and governments have applied public health measures, 18 and it has proved a challenge for assessing the effectiveness of individual public health measures, particularly in policy decision making. 19
Previous systematic reviews on the effectiveness of public health measures to treat covid-19 lacked the inclusion of analytical studies, 20 a comprehensive approach to data synthesis (focusing only on one measure), 21 a rigorous assessment of effectiveness of public health measures, 22 an assessment of the certainty of the evidence, 23 and robust methods for comparative analysis. 24 To tackle these gaps, we performed a systematic review of the evidence on the effectiveness of both individual and multiple public health measures in reducing the incidence of covid-19, SARS-CoV-2 transmission, and covid-19 mortality. When feasible we also did a critical appraisal of the evidence and meta-analysis.
This systematic review and meta-analysis were conducted in accordance with PRISMA 25 (supplementary material 1, table 1) and with PROSPERO (supplementary material 1, table 2).
Eligibility criteria
Articles that met the population, intervention, comparison, outcome, and study design criteria were eligible for inclusion in this systematic review (supplementary material 1, table 3). Specifically, preventive public health measures that were tested independently were included in the main analysis. Multiple measures, which generally contain a “package of interventions”, were included as supplementary material owing to the inability to report on the individual effectiveness of measures and comparisons on which package led to enhanced outcomes. The public health measures were identified from published World Health Organization sources that reported on the effectiveness of such measures on a range of communicable diseases, mostly respiratory infections, such as influenza.
Given that the scientific community is concerned about the ability of the numerous mathematical models, which are based on assumptions, to predict the course of virus transmission or effectiveness of interventions, 26 this review focused only on empirical studies. We excluded case reports and case studies, modelling and simulation studies, studies that provided a graphical summary of measures without clear statistical assessments or outputs, ecological studies that provided a descriptive summary of the measures without assessing linearity or having comparators, non‐empirical studies (eg, commentaries, editorials, government reports), other reviews, articles involving only individuals exposed to other pathogens that can cause respiratory infections, such as severe acute respiratory syndrome or Middle East respiratory syndrome, and articles in a language other than English.
Information sources
We carried out electronic searches of Medline, Embase, CINAHL (Cumulative Index to Nursing and Allied Health Literature, Ebsco), Global Health, Biosis, Joanna Briggs, and the WHO COVID-19 database (for preprints). A clinical epidemiologist (ST) developed the initial search strategy, which was validated by two senior medical librarians (LR and MD) (supplementary material 1, table 4). The updated search strategy was last performed on 7 June 2021. All citations identified from the database searches were uploaded to Covidence, an online software designed for managing systematic reviews, 27 for study selection.
Study selection
Authors ST, DG, SS, AM, ET, JR, XL, WX, IME, and XZ independently screened the titles and abstracts and excluded studies that did not match the inclusion criteria. Discrepancies were resolved in discussion with the main author (ST). The same authors retrieved full text articles and determined whether to include or exclude studies on the basis of predetermined selection criteria. Using a pilot tested data extraction form, authors ST, SS, AM, JR, XL, WX, AM, IME, and XZ independently extracted data on study design, intervention, effect measures, outcomes, results, and limitations. ST, SS, AM, and HW verified the extracted data. Table 5 in supplementary material 1 provides the specific criteria used to assess study designs. Given the heterogeneity and diversity in how studies defined public health measures, we took a common approach to summarise evidence of these interventions (supplementary material 1, table 6).
Risk of bias within individual studies
SS, JR, XL, WX, IME, and XZ independently assessed risk of bias for each study, which was cross checked by ST and HW. For non-interventional observational studies, a ROBINS-I (risk of bias in non-randomised studies of interventions) risk of bias tool was used. 28 For interventional studies, a revised tool for assessing risk of bias in randomised trials (RoB 2) tool was used. 29 Reviewers rated each domain for overall risk of bias as low, moderate, high, or serious/critical.
Data synthesis
The DerSimonian and Laird method was used for random effects meta-analysis, in which the standard error of the study specific estimates was adjusted to incorporate a measure of the extent of variation, or heterogeneity, among the effects observed for public health measures across different studies. It was assumed that the differences between studies are a result of different, yet related, intervention effects being estimated. If fewer than five studies were included in meta-analysis, we applied a recommended modified Hartung-Knapp-Sidik-Jonkman method. 30
Statistical analysis
Because of the differences in the effect metrics reported by the included studies, we could only perform quantitative data synthesis for three interventions: handwashing, face mask wearing, and physical distancing. Odds ratios or relative risks with corresponding 95% confidence intervals were reported for the associations between the public health measures and incidence of covid-19. When necessary, we transformed effect metrics derived from different studies to allow pooled analysis. We used the Dersimonian Laird random effects model to estimate pooled effect estimates along with corresponding 95% confidence intervals for each measure. Heterogeneity among individual studies was assessed using the Cochran Q test and the I 2 test. 31 All statistical analyses were conducted in R (version 4.0.3) and all P values were two tailed, with P=0.05 considered to be significant. For the remaining studies, when meta-analysis was not feasible, we reported the results in a narrative synthesis.
Public and patient involvement
No patients or members of the public were directly involved in this study as no primary data were collected. A member of the public was, however, asked to read the manuscript after submission.
A total of 36 729 studies were initially screened, of which 36 079 were considered irrelevent. After exclusions, 650 studies were eligible for full text review and 72 met the inclusion criteria. Of these studies, 35 assessed individual interventions and were included in the final synthesis of results ( fig 1 ) and 37 assessed multiple interventions as a package and are included in supplementary material 3, tables 2 and 3. The included studies comprised 34 observational studies and one interventional study, eight of which were included in the meta-analysis.

Flow of articles through the review. WHO=World Health Organization
Risk of bias
According to the ROBINS-I tool, 28 the risk of bias was rated as low in three studies, 32 33 34 moderate in 24 studies, 35 36 37 38 39 40 41 42 43 44 45 46 47 48 49 50 51 52 53 54 55 56 57 58 and high to serious in seven studies. 59 60 61 62 63 64 65 One important source of serious or critical risk of bias in most of the included studies was major confounding, which was difficult to control for because of the novel nature of the pandemic (ie, natural settings in which multiple interventions might have been enforced at once, different levels of enforcement across regions, and uncaptured individual level interventions such as increased personal hygiene). Variations in testing capacity and coverage, changes to diagnostic criteria, and access to accurate and reliable outcome data on covid-19 incidence and covid-19 mortality, was a source of measurement bias for numerous studies ( fig 2 ). These limitations were particularly prominent early in the pandemic, and in low income environments. 47 52 62 63 65 The randomised controlled trial 66 was rated as moderate risk of bias according to the ROB-2 tool. Missing data, losses to follow-up, lack of blinding, and low adherence to intervention all contributed to the reported moderate risk. Tables 1 and 2 in supplementary material 2 summarise the risk of bias assessment for each study assessing individual measures.

Summary of risk of bias across studies assessing individual measures using risk of bias in non-randomised studies of interventions (ROBINS-I) tool
Study characteristics
Studies assessing individual measures.
Thirty five studies provided estimates on the effectiveness of an individual public health measures. The studies were conducted in Asia (n=11), the United States (n=9), Europe (n=7), the Middle East (n=3), Africa (n=3), South America (n=1), and Australia (n=1). Thirty four of the studies were observational and one was a randomised controlled trial. The study designs of the observational studies comprised natural experiments (n=11), quasi-experiments (n=3), a prospective cohort (n=1), retrospective cohorts (n=8), case-control (n=2), and cross sectional (n=9). Twenty six studies assessed social measures, 32 34 35 37 38 39 40 41 42 44 46 47 48 52 53 55 56 57 58 59 60 61 63 64 65 67 12 studies assessed personal protective measures, 36 43 45 49 50 57 58 60 63 66 68 three studies assessed travel related measures, 54 58 62 and one study assessed environmental measures 57 (some interventions overlapped across studies). The most commonly measured outcome was incidence of covid-19 (n=18), followed by SARS-CoV-2 transmission, measured as reproductive number, growth number, or epidemic doubling time (n=13), and covid-19 mortality (n=8). Table 1 in supplementary material 3 provides detailed information on each study.
Effects of interventions
Personal protective measures.
Handwashing and covid-19 incidence —Three studies with a total of 292 people infected with SARS-CoV-2 and 10 345 participants were included in the analysis of the effect of handwashing on incidence of covid-19. 36 60 63 Overall pooled analysis suggested an estimated 53% non-statistically significant reduction in covid-19 incidence (relative risk 0.47, 95% confidence interval 0.19 to 1.12, I 2 =12%) ( fig 3 ). A sensitivity analysis without adjustment showed a significant reduction in covid-19 incidence (0.49, 0.33 to 0.72, I 2 =12%) ( fig 4 ). Risk of bias across the three studies ranged from moderate 36 60 to serious or critical 63 ( fig 2 ).

Meta-analysis of evidence on association between handwashing and incidence of covid-19 using modified Hartung-Knapp-Sidik-Jonkman adjusted random effect model

Meta-analysis of evidence on association between handwashing and incidence of covid-19 using unadjusted random effect model
Mask wearing and covid-19 incidence —Six studies with a total of 2627 people with covid-19 and 389 228 participants were included in the analysis examining the effect of mask wearing on incidence of covid-19 ( table 1 ). 36 43 57 60 63 66 Overall pooled analysis showed a 53% reduction in covid-19 incidence (0.47, 0.29 to 0.75), although heterogeneity between studies was substantial (I 2 =84%) ( fig 5 ). Risk of bias across the six studies ranged from moderate 36 57 60 66 to serious or critical 43 63 ( fig 2 ).
Study characteristics and main results from studies that assessed individual personal protective and environmental measures
- View inline

Meta-analysis of evidence on association between mask wearing and incidence of covid-19 using unadjusted random effect model
Mask wearing and transmission of SARS-CoV-2, covid-19 incidence, and covid-19 mortality —The results of additional studies that assessed mask wearing (not included in the meta-analysis because of substantial differences in the assessed outcomes) indicate a reduction in covid-19 incidence, SARS-CoV-2 transmission, and covid-19 mortality. Specifically, a natural experiment across 200 countries showed 45.7% fewer covid-19 related mortality in countries where mask wearing was mandatory ( table 1 ). 49 Another natural experiment study in the US reported a 29% reduction in SARS-CoV-2 transmission (measured as the time varying reproductive number Rt) (risk ratio 0.71, 95% confidence interval 0.58 to 0.75) in states where mask wearing was mandatory. 58
A comparative study in the Hong Kong Special Administrative Region reported a statistically significant lower cumulative incidence of covid-19 associated with mask wearing than in selected countries where mask wearing was not mandatory ( table 1 ). 68 Similarly, another natural experiment involving 15 US states reported a 2% statistically significant daily decrease in covid-19 transmission (measured as case growth rate) at ≥21 days after mask wearing became mandatory, 50 whereas a cross sectional study reported that a 10% increase in self-reported mask wearing was associated with greater odds for control of SARS-CoV-2 transmission (adjusted odds ratio 3.53, 95% confidence interval 2.03 to 6.43). 45 The five studies were rated at moderate risk of bias ( fig 2 ).
Environmental measures
Disinfection in household and covid-19 incidence.
Only one study, from China, reported the association between disinfection of surfaces and risk of secondary transmission of SARS-CoV-2 within households ( table 1 ). 57 The study assessed disinfection retrospectively by asking participants about their “daily use of chlorine or ethanol-based disinfectant in households,” and observed that use of disinfectant was 77% effective at reducing SARS-CoV-2 transmission (odds ratio 0.23, 95% confidence interval 0.07 to 0.84). The study did not collect data on the concentration of the disinfectant used by participants and was rated at moderate risk of bias ( fig 2 ).
Social measures
Physical distancing and covid-19 incidence.
Five studies with a total of 2727 people with SARS-CoV-2 and 108 933 participants were included in the analysis that examined the effect of physical distancing on the incidence of covid-19. 37 53 57 60 63 Overall pooled analysis indicated a 25% reduction in incidence of covid-19 (relative risk 0.75, 95% confidence interval 0.59 to 0.95, I 2 =87%) ( fig 6 ). Heterogeneity among studies was substantial, and risk of bias ranged from moderate 37 53 57 60 to serious or critical 63 ( fig 2 ).

Meta-analysis of evidence on association between physical distancing and incidence of covid-19 using unadjusted random effect model
Physical distancing and transmission of SARS-CoV-2 and covid-19 mortality
Studies that assessed physical distancing but were not included in the meta-analysis because of substantial differences in outcomes assessed, generally reported a positive effect of physical distancing ( table 2 ). A natural experiment from the US reported a 12% decrease in SARS-CoV-2 transmission (relative risk 0.88, 95% confidence interval 0.86 to 0.89), 40 and a quasi-experimental study from Iran reported a reduction in covid-19 related mortality (β −0.07, 95% confidence interval −0.05 to −0.10; P<0.001). 47 Another comparative study in Kenya also reported a reduction in transmission of SARS-CoV-2 after physical distancing was implemented, reporting 62% reduction in overall physical contacts (reproductive number pre-intervention was 2.64 and post-intervention was 0.60 (interquartile range 0.50 to 0.68)). 61 These three studies were rated at moderate risk of bias 40 61 to serious or critical risk of bias 47 ( fig 2 ).
Study characteristics and main results from studies assessing individual social measures
Stay at home or isolation and transmission of SARS-CoV-2
All the studies that assessed stay at home or isolation measures reported reductions in transmission of SARS-CoV-2 ( table 2 ). A retrospective cohort study from the US reported a significant reduction in the odds of having a positive reproductive number (R0) result (odds ratio 0.07, 95% confidence interval 0.01 to 0.37), 41 and a natural experiment reported a 51% reduction in time varying reproductive number (Rt) (risk ratio 0.49, 95% confidence interval 0.43 to 0.54). 58
A study from the UK reported a 74% reduction in the average daily number of contacts observed for each participant and estimated a decrease in reproductive number: the reproductive number pre-intervention was 3.6 and post-intervention was 0.60 (95% confidence interval 0.37 to 0.89). 65 Similarly, an Iranian study projected the reproductive number using serial interval distribution and the number of incidence cases and found a significant decrease: the reproductive number pre-intervention was 2.70 and post-intervention was 1.13 (95% confidence interval 1.03 to 1.25). 55 Three of the studies were rated at moderate to serious or critical risk of bias, 55 58 65 and one study was rated at low risk of bias 41 ( fig 2 ).
Quarantine and incidence and transmission of SARS-CoV-2
Quarantine was assessed in two studies ( table 2 ). 34 59 A prospective cohort study from Saudi Arabia reported a 4.9% decrease in the incidence of covid-19 at eight weeks after the implementation of quarantine. 34 This study was rated at low risk of bias ( fig 2 ). A retrospective cohort study from India reported a 14 times higher risk of SARS-CoV-2 transmission associated with no quarantine compared with strict quarantine (odds ratio 14.44, 95% confidence interval 2.42 to 86.17). 59 This study was rated at moderate risk of bias ( fig 2 ).
School closures and covid-19 incidence and covid-19 mortality
Two studies assessed the effectiveness of school closures on transmission of SARS-CoV-2, incidence of covid-19, or covid-19 mortality ( table 2 ). 44 48 A US population based longitudinal study reported on the effectiveness of state-wide closure of primary and secondary schools and observed a 62% decrease (95% confidence interval −49% to −71%) in incidence of covid-19 and a 58% decrease (−46% to−68%) in covid-19 mortality. 48 Conversely, a natural experiment from Japan reported no effect of school closures on incidence of covid-19 (α coefficient 0.08, 95% confidence interval −0.36 to 0.65). 44 Both studies were rated at moderate risk of bias ( fig 2 ).
School closures and transmission of SARS-CoV-2
Two natural experiments from the US reported a reduction in transmission (ie, reproductive number); with one study reporting a reduction of 13% (relative risk 0.87, 95% confidence interval 0.86 to 0.89) 40 and another reporting a 10% (0.90, 0.86 to 0.93) reduction ( table 2 ). 58 A Swedish study reported an association between school closures and a small increase in confirmed SARS-CoV-2 infections in parents (odds ratio 1.17, 95% confidence interval 1.03 to 1.32), but observed that teachers in lower secondary schools were twice as likely to become infected than teachers in upper secondary schools (2.01, 1.52 to 2.67). 32 All three studies were rated at moderate risk of bias ( fig 2 ).
Business closures and transmission of SARS-CoV-2
Two natural experiment studies assessed business closures across 50 US states and reported reductions in transmission of SARS-CoV-2 ( table 2 ). 40 58 One of the studies observed a significant reduction in transmission of 12% (relative risk 0.88, 95% confidence interval 0.86 to 0.89) 40 and the other reported a significant 16% (risk ratio 0.84, 0.79 to 0.90) reduction. 58 Both studies were rated at moderate risk of bias ( fig 2 ).
Lockdown and incidence of covid-19
A natural experiment involving 202 countries suggested that countries that implemented universal lockdown had fewer new cases of covid-19 than countries that did not (β coefficient −235.8 (standard error −11.04), P<0.01) ( table 2 ). 52 An Indian quasi-experimental study reported a 10.8% reduction in incidence of covid-19 post-lockdown, 56 whereas a South African retrospective cohort study observed a 14.1% reduction in risk after implementation of universal lockdown ( table 2 ). 46 These studies were rated at high risk of bias 52 and moderate risk of bias 46 56 ( fig 2 ).
Lockdown and covid-19 mortality
The three studies that assessed universal lockdown and covid-19 mortality generally reported a decrease in mortality ( table 2 ). 35 38 42 A natural experiment study involving 45 US states reported a decrease in covid-19 related mortality of 2.0% (95% confidence interval −3.0% to 0.9%) daily after lockdown had been made mandatory. 35 A Brazilian quasi-experimental study reported a 27.4% average difference in covid-19 related mortality rates in the first 25 days of lockdown. 42 In addition, a natural experiment study reported about 30% and 60% reductions in covid-19 related mortality post-lockdown in Italy and Spain over four weeks post-intervention, respectively. 38 All three studies were rated at moderate risk of bias ( fig 2 ).
Lockdown and transmission of SARS-CoV-2
Four studies assessed universal lockdown and transmission of SARS-CoV-2 during the first few months of the pandemic ( table 2 ). The decrease in reproductive number (R0) ranged from 1.27 in Italy (pre-intervention 2.03, post-intervention 0.76) 39 to 2.09 in India (pre-intervention 3.36, post-intervention 1.27), 64 and 3.97 in China (pre-intervention 4.95, post-intervention 0.98). 33 A natural experiment from the US reported that lockdown was associated with an 11% reduction in transmission of SARS-CoV-2 (relative risk 0.89, 95% confidence interval 0.88 to 0.91). 40 All the studies were rated at low risk of bias 33 39 to moderate risk 40 64 ( fig 2 ).
Travel related measures
Restricted travel and border closures.
Border closure was assessed in one natural experiment study involving nine African countries ( table 3 ). 62 Overall, the countries recorded an increase in the incidence of covid-19 after border closure. These studies concluded that the implementation of border closures within African countries had minimal effect on the incidence of covid-19. The study had important limitations and was rated at serious or critical risk of bias. In the US, a natural experiment study reported that restrictions on travel between states contributed about 11% to a reduction in SARS-CoV-2 transmission ( table 3 ). 36 The study was rated at moderate risk of bias ( fig 2 ).
Study characteristics and main results from studies that assessed individual travel measures
Entry and exit screening (virus or symptom screening)
One retrospective cohort study assessed screening of symptoms, which involved testing 65 000 people for fever ( table 3 ). 54 The study found that screening for fever lacked sensitivity (ranging from 18% to 24%) in detecting people with SARS-CoV-2 infection. This translated to 86% of the population with SARS-CoV-2 remaining undetected when screening for fever. The study was rated at moderate risk of bias ( fig 2 ).
Multiple public health measures
Overall, 37 studies provided estimates on the effectiveness of multiple public health measures, assessed as a collective group. Studies were mostly conducted in Asia (n=15), the US (n=11), Europe (n=6), Africa (n=4), and South America (n=1). All the studies were observational. The most commonly measured outcome was transmission of disease (ie, measured as reproductive number, growth number, or epidemic doubling time) (n=23), followed by covid-19 incidence (n=19) and covid-19 mortality (n=8). This review attempted to assess the overall effectiveness of the public health intervention packages by reporting the percentage difference in outcome before and after implementation of measures or between regions or countries studied. Eleven of the 37 included studies noted a difference of between 26% and 50% in transmission of SARS-CoV-2 and incidence of covid-19, 70 71 72 73 74 75 76 77 78 79 80 nine noted a difference of between 51% and 75% in SARS-CoV-2 transmission, covid-19 incidence, and covid-19 mortality, 81 82 83 84 85 86 87 88 89 and 14 noted a difference of more than 75% in transmission of SARS-CoV-2, covid-19 incidence and covid-19 mortality. 79 80 89 90 91 92 93 94 95 96 97 98 99 100 For the remaining studies, the overall effectiveness was not assessed owing to a lack of comparators (see supplementary material 3, table 3). Two studies that assessed universal lockdown and physical distancing reported a decrease of between 0% and 25% in SARS-CoV-2 transmission and covid-19 incidence. 79 101 Studies that included school and workplace closures, 91 95 96 isolation or stay at home measures, 80 94 or a combination of both 79 89 93 97 98 99 reported decreases of more than 75% in SARS-CoV-2 transmission. Supplementary material 3, table 2 provides detailed information on each study.
Worldwide, government and public health organisations are mitigating the spread of SARS-CoV-2 by implementing various public health measures. This systematic review identified a statistically significant reduction in the incidence of covid-19 through the implementation of mask wearing and physical distancing. Handwashing interventions also indicated a substantial reduction in covid-19 incidence, albeit not statistically significant in the adjusted model. As the random effects model tends to underestimate confidence intervals when a meta-analysis includes a small number of individual studies (<5), the adjusted model for handwashing showed a statistically non-significant association in reducing the incidence of covid-19 compared with the unadjusted model.
Overall effectiveness of these interventions was affected by clinical heterogeneity and methodological limitations, such as confounding and measurement bias. It was not possible to evaluate the impact of type of face maks (eg, surgical, fabric, N95 respirators) and compliance and frequency of wearing masks owing to a lack of data. Similarly, it was not feasible to assess the differences in effect that different recommendations for physical distancing (ie, 1.5 m, 2m, or 3 m) have as preventive strategies.
The effectiveness of measures such as universal lockdowns and closures of businesses and schools for the containment of covid-19 have largely been effective, but depended on early implementation when incidence rates of covid-19 were still low. 42 52 58 Only Japan reported no decrease in covid-19 incidence after school closures, 44 and other studies found that different public health measures were sometimes implemented simultaneously or soon after one another, thus the results should be interpreted with caution. 32 46 56
Isolation or stay at home was an effective measure in reducing the transmission of SARS-CoV-2, but the included studies used results for mobility to assess stay at home or isolation and therefore could have been limited by potential flaws in publicly available phone data, 41 58 102 and variations in the enforcement of public health measures in different states or regions were not assessed. 55 58 102 Quarantine was found to be as effective in reducing the incidence of covid-19 and transmission of SARS-CoV-2, yet variation in testing and case detection in low income environments was substantial. 59 96 98 Another study reported that quarantine was effective in reducing the transmission of SARS-CoV-2 in a cohort with a low prevalence of the virus, yet it is unknown if the same effect would be observed with higher prevalence. 34
It was not possible to draw conclusions about the effectiveness of restricted travel and full border closures because the number of empirical studies was insufficient. Single studies identified that border closure in Africa had a minimal effect in reducing SARS-CoV-2 transmission, but the study was assessed as being at high risk of bias. 62 Screening for fever was also identified to be ineffective, with only 24% of positive cases being captured by screening. 54
Comparison with other studies
Previous literature reviews have identified mask wearing as an effective measure for the containment of SARS-CoV-2 103 ; the caveat being that more high level evidence is required to provide unequivocal support for the effectiveness of the universal use of face masks. 104 105 Additional empirical evidence from a recent randomised controlled trial (originally published as a preprint) indicates that mask wearing achieved a 9.3% reduction in seroprevalence of symptomatic SARS-CoV-2 infection and an 11.9% reduction in the prevalence of covid-19-like symptoms. 106 Another systematic review showed stronger effectiveness with the use of N95, or similar, respirators than disposable surgical masks, 107 and a study evaluating the protection offered by 18 different types of fabric masks found substantial heterogeneity in protection, with the most effective mask being multilayered and tight fitting. 108 However, transmission of SARS-CoV-2 largely arises in hospital settings in which full personal protective measures are in place, which suggests that when viral load is at its highest, even the best performing face masks might not provide adequate protection. 51 Additionally, most studies that assessed mask wearing were prone to important confounding bias, which might have altered the conclusions drawn from this review (ie, effect estimates might have been underestimated or overestimated or can be related to other measures that were in place at the time the studies were conducted). Thus, the extent of such limitations on the conclusions drawn remain unknown.
A 2020 rapid review concluded that quarantine is largely effective in reducing the incidence of covid-19 and covid-19 mortality. However, uncertainty over the magnitude of such an effect still remains, 109 with enhanced management of quality quarantine facilities for improved effective control of the epidemics urgently needed. 110 In addition, findings on the application of school and workplace closures are still inconclusive. Policy makers should be aware of the ambiguous evidence when considering school closures, as other potentially less disruptive physical distancing interventions might be more appropriate. 21 Numerous findings from studies on the efficacy of school closures showed that the risk of transmission within the educational environment often strongly depends on the incidence of covid-19 in the community, and that school closures are most successfully associated with control of SARS-CoV-2 transmission when other mitigation strategies are in place in the community. 111 112 113 114 115 116 117 School closures have been reported to be disruptive to students globally and are likely to impair children’s social, psychological, and educational development 118 119 and to result in loss of income and productivity in adults who cannot work because of childcare responsibilities. 120
Speculation remains as how best to implement physical distancing measures. 121 Studies that assess physical distancing measures might interchangeably study physical distancing with lockdown 35 52 56 64 and other measures and thus direct associations are difficult to assess.
Empirical evidence from restricted travel and full border closures is also limited, as it is almost impossible to study these strategies as single measures. Current evidence from a recent narrative literature review suggested that control of movement, along with mandated quarantine, travel restrictions, and restricting nationals from entering areas of high infection, are effective measures, but only with good compliance. 122 A narrative literature review of travel bans, partial lockdowns, and quarantine also suggested effectiveness of these measures, 123 and another rapid review further supported travel restrictions and cross border restrictions to stop the spread of SARS-CoV-2. 124 It was impossible to make such observations in the current review because of limited evidence. A German review, however, suggested that entry, exit, and symptom screening measures to prevent transmission of SARS-CoV-2 are not effective at detecting a meaningful proportion of cases, 125 and another review using real world data from multiple countries found that border closures had minimal impact on the control of covid-19. 126
Although universal lockdowns have shown a protective effect in lowering the incidence of covid-19, SARS-CoV-2 transmission, and covid-19 mortality, these measures are also disruptive to the psychosocial and mental health of children and adolescents, 127 global economies, 128 and societies. 129 Partial lockdowns could be an alternative, as the associated effectiveness can be high, 125 especially when implemented early in an outbreak, 85 and such measures would be less disruptive to the general population.
It is important to also consider numerous sociopolitical and socioeconomic factors that have been shown to increase SARS-CoV-2 infection 130 131 and covid-19 mortality. 132 Immigration status, 82 economic status, 81 101 and poverty and rurality 98 can influence individual and community compliance with public health measures. Poverty can impact the ability of communities to physically distance, 133 especially in crowded living environments, 134 135 as well as reduce access to personal protective measures. 134 135 A recent study highlights that “a one size fits all” approach to public health measures might not be effective at reducing the spread of SARS-CoV-2 in vulnerable communities 136 and could exacerbate social and economic inequalities. 135 137 As such, a more nuanced and community specific approach might be required. Even though screening is highly recommended by WHO 138 because a proportion of patients with covid-19 can be asymptomatic, 138 screening for symptoms might miss a larger proportion of the population with covid-19. Hence, temperature screening technologies might need to be reconsidered and evaluated for cost effectiveness, given such measures are largely depended on symptomatic fever cases.
Strengths and limitations of this review
The main strength of this systematic review was the use of a comprehensive search strategy to identify and select studies for review and thereby minimise selection bias. A clinical epidemiologist developed the search strategy, which was validated by two senior medical librarians. This review followed a comprehensive appraisal process that is recommended by the Cochrane Collaboration 31 to assess the effectiveness of public health measures, with specifically validated tools used to independently and individually assess the risk of bias in each study by study design.
This review has some limitations. Firstly, high quality evidence on SARS CoV-2 and the effectiveness of public health measures is still limited, with most studies having different underlying target variables. Secondly, information provided in this review is based on current evidence, so will be modified as additional data become available, especially from more prospective and randomised studies. Also, we excluded studies that did not provide certainty over the effect measure, which might have introduced selection bias and limited the interpretation of effectiveness. Thirdly, numerous studies measured interventions only once and others multiple times over short time frames (days v month, or no timeframe). Additionally, the meta-analytical portion of this study was limited by significant heterogeneity observed across studies, which could neither be explored nor explained by subgroup analyses or meta-regression. Finally, we quantitatively assessed only publications that reported individual measures; studies that assessed multiple measures simultaneously were narratively analysed with a broader level of effectiveness (see supplementary material 3, table 3). Also, we excluded studies in languages other than English.
Methodological limitations of studies included in the review
Several studies failed to define and assess for potential confounders, which made it difficult for our review to draw a one directional or causal conclusion. This problem was mainly because we were unable to study only one intervention, given that many countries implemented several public health measures simultaneously; thus it is a challenge to disentangle the impact of individual interventions (ie, physical distancing when other interventions could be contributing to the effect). Additionally, studies measured different primary outcomes and in varied ways, which limited the ability to statistically analyse other measures and compare effectiveness.
Further pragmatic randomised controlled trials and natural experiment studies are needed to better inform the evidence and guide the future implementation of public health measures. Given that most measures depend on a population’s adherence and compliance, it is important to understand and consider how these might be affected by factors. A lack of data in the assessed studies meant it was not possible to understand or determine the level of compliance and adherence to any of the measures.
Conclusions and policy implications
Current evidence from quantitative analyses indicates a benefit associated with handwashing, mask wearing, and physical distancing in reducing the incidence of covid-19. The narrative results of this review indicate an effectiveness of both individual or packages of public health measures on the transmission of SARS-CoV-2 and incidence of covid-19. Some of the public health measures seem to be more stringent than others and have a greater impact on economies and the health of populations. When implementing public health measures, it is important to consider specific health and sociocultural needs of the communities and to weigh the potential negative effects of the public health measures against the positive effects for general populations. Further research is needed to assess the effectiveness of public health measures after adequate vaccination coverage has been achieved. It is likely that further control of the covid-19 pandemic depends not only on high vaccination coverage and its effectiveness but also on ongoing adherence to effective and sustainable public health measures.
What is already known on this topic
Public health measures have been identified as a preventive strategy for influenza pandemics
The effectiveness of such interventions in reducing the transmission of SARS-CoV-2 is unknown
What this study adds
The findings of this review suggest that personal and social measures, including handwashing, mask wearing, and physical distancing are effective at reducing the incidence of covid-19
More stringent measures, such as lockdowns and closures of borders, schools, and workplaces need to be carefully assessed by weighing the potential negative effects of these measures on general populations
Further research is needed to assess the effectiveness of public health measures after adequate vaccination coverage
Ethics statements
Ethical approval.
Not required.
Data availability statement
No additional data available.
Acknowledgments
We thank medical subject librarians Lorena Romero (LR) and Marshall Dozier (MD) for their expert advice and assistance with the study search strategy.
Contributors: ST, DG, DI, DL, and ZA conceived and designed the study. ST, DG, SS, AM, HW, WX, JR, ET, AM, XL, XZ, and IME collected and screened the data. ST, DG, and DI acquired, analysed, or interpreted the data. ST, HW, and SS drafted the manuscript. All authors critically revised the manuscript for important intellectual content.. XL and ST did the statistical analysis. NA obtained funding. LR and MD provided administrative, technical, or material support. ST and DI supervised the study. ST and DI had full access to all the data in the study and take responsibility for the integrity of the data and the accuracy of the data analysis. ST is the guarantor. The corresponding author attests that all listed authors meet authorship criteria and that no others meeting the criteria have been omitted.
Funding: No funding was available for this research. ET is supported by a Cancer Research UK Career Development Fellowship (grant No C31250/A22804). XZ is supported by The Darwin Trust of Edinburgh.
Competing interests: All authors have completed the ICMJE uniform disclosure form at www.icmje.org/disclosure-of-interest/ and declare: ET is supported by a Cancer Research UK Career Development Fellowship and XZ is supported by The Darwin Trust of Edinburgh; no financial relationships with any organisations that might have an interest in the submitted work in the previous three years; and no other relationships or activities that could appear to have influenced the submitted work.
The lead author (ST) affirms that the manuscript is an honest, accurate, and transparent account of the study reported; no important aspects of the study have been omitted. Dissemination to participants and related patient and public communities: It is anticipated to disseminate the results of this research to wider community via press release and social media platforms.
Provenance and peer review: Not commissioned; externally peer reviewed.
This is an Open Access article distributed in accordance with the Creative Commons Attribution Non Commercial (CC BY-NC 4.0) license, which permits others to distribute, remix, adapt, build upon this work non-commercially, and license their derivative works on different terms, provided the original work is properly cited and the use is non-commercial. See: http://creativecommons.org/licenses/by-nc/4.0/ .
- ↵ World Health Organization. WHO Coronavirus (COVID-19) Dashboard. 2021. https://covid19.who.int/
- Parodi SM ,
- Bernal JL ,
- Andrews N ,
- Chodick G ,
- Patalon T ,
- Anderson RM ,
- Vegvari C ,
- Truscott J ,
- Khateeb J ,
- McArthur AG ,
- Banerjee A ,
- Sanyaolu A ,
- Marinkovic A ,
- ↵ World Health Organization. Coronavirus disease (COVID-19): Herd immunity, lockdowns and COVID-19. 2020. www.who.int/news-room/q-a-detail/herd-immunity-lockdowns-and-covid-19
- Stehlik P ,
- Glasziou PP
- ↵ World Health Organization. COVID-19 strategy update. 2020. www.who.int/docs/default-source/coronaviruse/covid-strategy-update-14april2020.pdf?sfvrsn=29da3ba0_19
- Hollingsworth TD ,
- Klinkenberg D ,
- Heesterbeek H ,
- Anderson RM
- Aledort JE ,
- Wasserman J ,
- Bozzette SA
- ↵ World Health Organization. Non-pharmaceutical public health measures for mitigating the risk and impact of epidemic and pandemic influenza 2019. 2019. https://apps.who.int/iris/bitstream/handle/10665/329438/9789241516839-eng.pdf?ua=1 .
- Yang Chan EY ,
- Shahzada TS ,
- Hellewell J ,
- Centre for the Mathematical Modelling of Infectious Diseases COVID-19 Working Group
- Mendez-Brito A ,
- El Bcheraoui C ,
- Pozo-Martin F
- Russell SJ ,
- Craig KJT ,
- Maatoug J ,
- Liberati A ,
- Tetzlaff J ,
- Altman DG ,
- PRISMA Group
- Holmdahl I ,
- ↵ Covidence Systematic Review Software. Veritas Health Innovation, Melbourne Australia. www.covidence.org
- Sterne JA ,
- Hernán MA ,
- Reeves BC ,
- Sterne JAC ,
- Savović J ,
- ↵ Higgins JPTTJ, Chandler J, Cumpston M, Li T, Page MJ. Welch VA, ed. Cochrane Handbook for Systematic Reviews of Interventions. : Chichester, UK: Wiley; 2019. 2nd edn. https://training.cochrane.org/handbook .
- Vlachos J ,
- Hertegård E ,
- B Svaleryd H
- Al-Tawfiq JA ,
- Al-Khadra H ,
- Siedner MJ ,
- Harling G ,
- Reynolds Z ,
- Cheong HH ,
- Van den Berg P ,
- Schechter-Perkins EM ,
- Guzzetta G ,
- Riccardo F ,
- Marziano V ,
- COVID-19 Working Group,2
- McAuley FM ,
- Figueiredo Filho D ,
- Fernandes A
- Krishnamachari B ,
- Zastrow D ,
- Santella AJ
- Miyakoshi C
- Motloba P ,
- Motaung KSC ,
- Alimohamadi Y ,
- Holakouie-Naieni K ,
- Sepandi M ,
- Richardson T ,
- Leffler CT ,
- Lykins JD ,
- McKeown CA ,
- Grzybowski A
- Luckhoff C ,
- Mitchell RD ,
- O’Reilly GM ,
- Khosravi A ,
- Rohani-Rasaf M ,
- Mehravaran S ,
- Thayer WM ,
- Sankhla P ,
- Valamparampil MJ ,
- Varghese B ,
- van Zandvoort K ,
- CMMID COVID-19 Working Group
- Ilesanmi OS
- Doung-Ngern P ,
- Suphanchaimat R ,
- Panjangampatthana A ,
- Salvatore M ,
- Jarvis CI ,
- Van Zandvoort K ,
- CMMID COVID-19 working group
- Bundgaard H ,
- Bundgaard JS ,
- Raaschou-Pedersen DET ,
- Cheng VC-C ,
- Chuang VW-M ,
- Malheiro R ,
- Figueiredo AL ,
- Magalhães JP ,
- Dasgupta S ,
- Kassem AM ,
- Sunshine G ,
- Bendavid E ,
- Bhattacharya J ,
- Ioannidis JPA
- Rothenbühler M ,
- Fisher BT ,
- Khosrawipour V ,
- Kocbach P ,
- Athotra A ,
- Vaisakh TP ,
- NCDC COVID Incident Management Team
- Courtemanche C ,
- Garuccio J ,
- Pinkston J ,
- Al Wahaibi A ,
- Al Manji A ,
- Al Maani A ,
- Timelli L ,
- Undurraga EA ,
- Laborde CC ,
- Bhattacharyya R ,
- Castillo RC ,
- Staguhn ED ,
- Weston-Farber E
- Cruz-Cano R ,
- Venkataramani A ,
- Gilbert RF ,
- Tchole AIM ,
- Cheeloo EcoHealth Consortium (CLEC)
- McCreesh N ,
- Dlamini V ,
- Edwards A ,
- Haapanen M ,
- McGrail DJ ,
- McAndrews KM ,
- Clipman SJ ,
- Wesolowski AP ,
- Gibson DG ,
- Brainard J ,
- Camargo MC ,
- Martinez-Silveira MS ,
- ↵ Abaluck J, Kwong LH, Styczynskyi A, et al. The Impact of Community Masking on COVID-19: A Cluster-Randomized Trial in Bangladesh. 2021. www.poverty-action.org/sites/default/files/publications/Mask_RCT____Symptomatic_Seropositivity_083121.pdf
- MacDougall CC ,
- Johnstone J ,
- Schwartz B ,
- O’Kelly E ,
- Nussbaumer-Streit B ,
- Dobrescu AI ,
- ↵ Nafees M, Khan F. Pakistan’s response to COVID-19 pandemic and efficacy of quarantine and partial lockdown: A review. Electronic Journal of General Medicine 2020;17:em240.
- Macartney K ,
- Pillsbury AJ ,
- NSW COVID-19 Schools Study Team
- Link-Gelles R ,
- DellaGrotta AL ,
- Fontanet A ,
- Tondeur L ,
- ↵ National Centre for Immunisation Research and Surveliance. COVID-19 in schools and early childhood education and care services – the Term 3 experience in NSW. 2020, NSW government. www.ncirsorgau/sites/default/files/2020-10/COVID-19%20Transmission%20in%20educational%20settings%20in%20NSW%20Term%203%20report_0pdf
- ↵ National Centre for Immunisation Research and Surveliance. COVID-19 in schools and early childhood education and care services – the Term 1 experience in NSW. 2020, NSW government. www.ncirsorgau/sites/default/files/2020-08/COVID-19%20Transmission%20in%20educational%20settings%20in%20NSW%20Term%201%20report_0pdf
- ↵ National Centre for Immunisation Research and Surveliance. COVID-19 in schools and early childhood education and care services – the Term 2 experience in NSW. 2020, NSW government. www.ncirsorgau/sites/default/files/2020-08/COVID-19%20Transmission%20in%20educational%20settings%20in%20NSW%20Term%202%20report_0pdf
- Chatterjee S ,
- Fenichel EP
- Bhattacharya S ,
- Chakraborty A
- Stephen S ,
- Movsisyan A ,
- Stratil JM ,
- Geyrhofer L ,
- Patiño-Lugo DF ,
- Velásquez Salazar P ,
- Parveen S ,
- ↵ Felsenthal M. COVID-19 to plunge global economy into worst recession since World War II 2020. www.worldbank.org/en/news/press-release/2020/06/08/covid-19-to-plunge-global-economy-into-worst-recession-since-world-war-ii .
- Brodeur A ,
- Powdthavee N
- Niedzwiedz CL ,
- O’Donnell CA ,
- Chadeau-Hyam M ,
- Bodinier B ,
- Elliott J ,
- Williamson E ,
- Walker AJ ,
- Bhaskaran K ,
- Garnier R ,
- Benetka JR ,
- Kraemer J ,
- Brasher C ,
- Chikumba E ,
- McDougall R ,
- Mellin-Olsen J ,
- Corburn J ,
- Cooney RE ,
- ↵ World Health Organization. Transmission of COVID-19 by asymptomatic cases. 2020. www.emro.who.int/health-topics/corona-virus/transmission-of-covid-19-by-asymptomatic-cases.html
Click through the PLOS taxonomy to find articles in your field.
For more information about PLOS Subject Areas, click here .
Loading metrics
Open Access
Peer-reviewed
Research Article
A statistical analysis of the novel coronavirus (COVID-19) in Italy and Spain
Roles Conceptualization, Data curation, Formal analysis, Investigation, Methodology, Project administration, Resources, Software, Validation, Visualization, Writing – original draft, Writing – review & editing
* E-mail: [email protected]
Affiliation School of Statistics, Renmin University of China, Beijing, China

- Jeffrey Chu
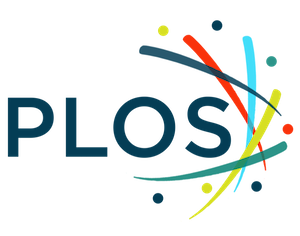
- Published: March 25, 2021
- https://doi.org/10.1371/journal.pone.0249037
- Reader Comments
The novel coronavirus (COVID-19) that was first reported at the end of 2019 has impacted almost every aspect of life as we know it. This paper focuses on the incidence of the disease in Italy and Spain—two of the first and most affected European countries. Using two simple mathematical epidemiological models—the Susceptible-Infectious-Recovered model and the log-linear regression model, we model the daily and cumulative incidence of COVID-19 in the two countries during the early stage of the outbreak, and compute estimates for basic measures of the infectiousness of the disease including the basic reproduction number, growth rate, and doubling time. Estimates of the basic reproduction number were found to be larger than 1 in both countries, with values being between 2 and 3 for Italy, and 2.5 and 4 for Spain. Estimates were also computed for the more dynamic effective reproduction number, which showed that since the first cases were confirmed in the respective countries the severity has generally been decreasing. The predictive ability of the log-linear regression model was found to give a better fit and simple estimates of the daily incidence for both countries were computed.
Citation: Chu J (2021) A statistical analysis of the novel coronavirus (COVID-19) in Italy and Spain. PLoS ONE 16(3): e0249037. https://doi.org/10.1371/journal.pone.0249037
Editor: Abdallah M. Samy, Faculty of Science, Ain Shams University (ASU), EGYPT
Received: July 14, 2020; Accepted: March 9, 2021; Published: March 25, 2021
Copyright: © 2021 Jeffrey Chu. This is an open access article distributed under the terms of the Creative Commons Attribution License , which permits unrestricted use, distribution, and reproduction in any medium, provided the original author and source are credited.
Data Availability: The raw data files for the incidence of COVID-19 in Italy and Spain are available from the following links: https://github.com/pcm-dpc/COVID-19 https://github.com/datadista/datasets/tree/master/COVID%2019 .
Funding: The author(s) received no specific funding for this work.
Competing interests: The authors have declared that no competing interests exist.
Introduction
The novel coronavirus (COVID-19) was widely reported to have first been detected in Wuhan (Hebei province, China) in December 2019. After the initial outbreak, COVID-19 continued to spread to all provinces in China and very quickly spread to other countries within and outside of Asia. At present, over 45 million cases of infected individuals have been confirmed in over 180 countries with in excess of 1 million deaths [ 1 ]. Although the foundations of this disease are very similar to the severe acute respiratory syndrome (SARS) virus that took hold of Asia in 2003, it is shown to spread much more easily and there currently exists no vaccine.
Since the first confirmed cases were reported in China, much of the literature has focused on the outbreak in China including the transmission of the disease, the risk factors of infection, and the biological properties of the virus—see for example key literature such as [ 2 – 6 ]. However, more recent literature has started to cover an increasing number of regions outside of China.
For example, studies covering the wider Asia region include: investigations into the outbreak on board the Diamond Princess cruise ship in Japan, using a Bayesian framework with a Hamiltonian Monte Carlo algorithm [ 7 ]; estimation of the ascertainment rate in Japan using a Poisson process [ 8 ]; modelling the evolution of the basic and effective reproduction numbers in South Korea using Susceptible-Infected-Susceptible models [ 9 ] and generalised growth models with varying growth rates [ 10 ]; modelling the basic reproduction number in India with a classical Susceptible-Exposed-Infectious-Recovered-type compartmental model [ 11 ]; forecasting numbers of cases in Indian states using deep learning-based models [ 12 ].
Analyses on North and South America have also used similar classical methods, for example [ 13 ] model the progression of the outbreak in the United States until the end of 2021 with the simple Susceptible-Infected-Recovered model, and [ 14 ] predict epidemic trends in Brazil and Peru using a logistic growth model and machine learning techniques. However, other studies include: analysis of the spatial variability of the incidence in the United States using spatial lag and error models, and geographically weighted regression [ 15 ]; estimation of the number of deaths in the United States using a modified logistic fault-dependent detection model [ 16 ]; estimating prevalence and infection rates across different states in the United States using a sample selection model [ 17 ]; investigating the relationship between social media communication and the incidence in Colombia using non-linear regression models.
Focusing on Africa, [ 18 ] simulate and predict the spread of the disease in South Africa, Egypt, Algeria, Nigeria, Senegal, and Kenya, using a modified Susceptible-Exposed-Infectious-Recovered model; [ 19 ] apply a six-compartmental model to model the transmission in South Africa; [ 20 ] predict the spread of the disease in West Africa using a deterministic Susceptible-Exposed-Infectious-Recovered model; [ 21 ] implement Autoregressive Integrated Moving Average models to forecast the prevalence of COVID-19 in East Africa; [ 22 ] predict the spread of the disease using travel history and personal contact in Nigeria through ordinary least squares regression; [ 23 ] use logistic growth and Susceptible-Infected-Recovered models to generate real-time forecasts of daily confirmed cases in Saudi Arabia.
Aside from many of the classical models mentioned above, recent developments in the econometrics and statistics literature have led to a number of new models that could potentially be applied in the modelling of infectious diseases. These include (but are not limited to) mixed frequency analysis, model selection and combination, and dynamic time warping. Mixed frequency analysis is an iterative approach proposed for dealing with the joint dynamics of time series data which are sampled at different frequencies [ 24 ]. In the economic literature, the common example is quarterly gross domestic product (GDP) and monthly inflation. [ 25 ] notes that studying the co-movements between mixed frequency data usually involves analysing the joint process sampled at a common low frequency, however, this can mis-specify the relationship. [ 24 , 25 ] propose vector autoregressive models for mixed frequency analysis that operate at the highest sampling frequency of all the time series in the model. These models allow for the modelling of the joint dynamics of the dependent and independent variables using time disaggregation, where the low frequency variables are interpolated and time-aggregated into a higher frequency. In the context of infectious diseases, such models could be beneficial for modelling the relationship between higher frequency data such as the number of daily cases or deaths and lower frequency data relating to, say, weekly cases or deaths, news and information about health prevention measures, etc. [ 26 , 27 ] propose the use of Bayesian Predictive Synthesis (BPS) for model selection and combination. They note that there are many scenarios that generate multiple, interrelated time series, where the dependence has a significant impact on decisions, policies, and their outcomes. In addition, methods need to learn and integrate information about forecasters and models, bias, etc. and how they change over time, to improve their accuracy [ 26 ]. Decision and policy makers often use multiple sources, models, and forecasters to generate forecasts, in particular, probabilistic density forecasts. However, although complex estimation methods may have useful properties for policy makers, large standard deviations may be a result of the complexity of the data, model, etc., and it may be difficult to know the source. The aim is to use the dependencies between time series to improve forecasts over multiple horizons for policy decisions [ 27 ]. For example, in the economic literature, setting interest rates based on utility or loss that account for inflation, real economy measures, employment, etc. BPS relates to a decision maker that accounts for multiple models as providers of “forecast data” to be used for prior-posterior updating. The decision maker learns over time about relationships between agents, forecasts, and dependencies, which are incorporated into the model, and dynamically calibrate, learn, and update weights for ranges of forecasts from dynamic models, with multiple lags and predictors [ 26 ]. In epidemiology, BPS could potentially be used in a similar context to analyse the dependency between various interrelated time series such as daily cases and deaths, hospital capacity, number vaccinations, etc. Different models and sources of data could then be combined and characterised in one single model improving the accuracy of forecasts. Dynamic time warping as noted by [ 28 , 29 ] is a technique that has not been widely used outside of speech and gesture recognition. It can be used to identify the relation structure between two time series by describing their non-linear alignment with warping paths [ 28 ]. The procedure involves a local cost measure characterising the sum of the differences between pairs of realisations of data at each time point, where an optimal warping path gives the lowest total cost. The optimal path is found under a variable lead-lag structure, where the most suitable lag can then be found [ 28 ]. This then reveals and identifies the lead-lag effects between the time series data. Indeed, dynamic time warping has recently been used in the modelling of COVID-19 by [ 30 ]. [ 30 ] use the method to determine the lead-lag relation between the cumulative number of daily cases of COVID-19 in various countries, in addition to forecasting the future incidence in selected countries. This allows for the classification of countries as being in the early, middle, and late stages of an outbreak.
Controlling an infectious disease such as COVID-19 is an important, time-critical but difficult issue. The health of the global population is, perhaps, the most important factor as research is directed towards vaccines and governments scramble to implement public health measures to reduce the spread of the disease. In most countries around the world, these measures have come in the form of local or national lockdowns where individuals are advised or required to remain at home unless they have good reason not to—e.g. for educational or medical purposes, or if they are unable to work from home. However, the implications of trying to control COVID-19 are being felt not only by the health sector, but also in areas such as the economy, environment, and society.
As the number of cases of infected individuals has risen rapidly, there has been an increase in pressure on medical services as healthcare providers seek to test and diagnose infected individuals, in addition to the normal load of medical services that are offered in general. In many cases, trying to control COVID-19 has led to a backlog for and deprivation of other medical procedures [ 31 ], with healthcare providers needing to find a balance between the two. [ 32 ] note that this conflict may change the nature of healthcare with public and private health sectors working together more often. The implementation of restrictions on the movement of individuals has also led to many suggesting that anxiety and distress may lead to increased psychiatric disorders. These may be related to suicidal behaviour and morbidity and may have a long-term negative impact on the mental health of individuals [ 33 , 34 ].
In addition to restrictions on the movement of individuals, governments have required most non-essential businesses to close. This has negatively impacted national economies with many businesses permanently closing leading to a significant increase in unemployment. Limits on travel have severely affected the tourism and travel industries, and countries and economies that are dependent on these for income. Whilst many of the implications of controlling COVID-19 on the economy are negative, there have been some positive changes as businesses adapt to the ‘new normal’. For example, the banking industry is dealing with increased credit risks, while the insurance industry is developing more digital products and pandemic-focused solutions [ 32 ]. The automotive industry is expected to see profits reduced by approximately $100 billion, which may be offset by the development of software subscription services of modern vehicles [ 32 ]. Some traditional office-based businesses have been able to reduce costs by shifting to remote working, while the restaurant industry has shifted towards takeaway and delivery services [ 32 ].
In terms of the environment, the limitations on businesses that have been able to continue operating throughout the epidemic has led to possible improvements in the environment—mainly from the reduction in pollution [ 35 ]. However, societal issues have been exacerbated. [ 32 ] note that the reduction in the labour force that has resulted from controlling for COVID-19 has affected ethnic minorities and women most significantly. Furthermore, in many countries health services employ more women than men creating a dilemma for working mothers—either leave the labour force and provide childcare for their families or remain in employment and pay extra costs for childcare.
In Europe, Italy and Spain were two of the first European countries to be significantly affected by COVID-19. However, the majority of the literature covering the two countries focuses on the clinical aspects of the disease, [ 36 – 40 ], with only a limited number exploring the prevalence of the disease, [ 41 – 43 ].
As as a result of this on going pandemic, new results and reports are being produced and published daily. Thus, our motivation stems from wanting to contribute to the statistical analysis of the incidence of COVID-19 in Italy and Spain, where the literature is limited. The main contributions of this paper are: i) to model the incidence of COVID-19 in Italy and Spain using simple mathematical models in epidemiology; ii) to provide estimates of basic measures of the infectiousness and severity of COVID-19 in Italy and Spain; iii) to investigate the predictive ability of simple mathematical models and provide simple forecasts for the future incidence of COVID-19 in Italy and Spain.
The contents of this paper are organised as follows. In the data section, we describe the incidence data used in the main analysis and provide a brief summary analysis. The method section outlines the Susceptible-Infectious-Recovered model and the log-linear model used to model the incidence of COVID-19, and introduces the basic reproduction number and effective reproduction number as measures of the infectiousness of diseases. In the results section, we present the main results for the fitted models and estimates of the measures of infectiousness, in addition to simple predictions for the future incidence of COVID-19. Some concluding remarks are given in the conclusion.
The data used in this analysis consists of the daily and cumulative incidence (confirmed cases) of COVID-19 for Italy and Spain (nationally), and their respective regions or autonomous provinces. For Italy, this data covers 21 regions for 37 days from 21st February 2020 to 28th March 2020, inclusive; for Spain, this data covers 19 regions for 34 days from 27th February to 31st March 2020, inclusive. The data for Italy was obtained from [ 44 ] where the raw data was sourced from the Italian Department of Civil Protection; the data for Spain was obtained from [ 45 ] where the raw data was sourced from the Spanish Ministry of Health. The starting dates for both sets of data indicate the dates on which the first cases were confirmed in each country, however, it should be noted that in some regions cases were not confirmed until after these dates. These particular time periods were chosen as they cover over one month since the initial outbreaks in both countries and were the most up to date data available at the time of writing. In the remainder of this section, we provide a simple exploratory analysis of the incidence data.
Fig 1 plots the daily cumulative incidence for Italy and its 21 regions over the whole sample period. All cumulative incidence appears to show an exponential trend, increasing slowly for the first 14 days after the first cases are confirmed before growing rapidly. Checking the same plot on a log-linear scale, shown in Fig 2 , we find that the logarithm of cumulative incidence in some regions exhibits an approximate linear trend suggesting that cumulative incidence is growing exponentially. However, in the majority of regions (and nationally) this trend is not exactly linear, suggesting a slightly sub-exponential growth in cumulative incidence.
- PPT PowerPoint slide
- PNG larger image
- TIFF original image
https://doi.org/10.1371/journal.pone.0249037.g001
https://doi.org/10.1371/journal.pone.0249037.g002
Of all the regions in Italy, the northern region of Lombardy is one of the worst affected and Fig 3 plots the daily incremental incidence for both Lombardy and Italy, respectively. In terms of the number of new cases confirmed each day, the trends are very similar and, again, possibly exponential until peaking around 21st March 2020 before levelling off. Comparing the trends for the other regions in Fig 4 , it can be seen that other significantly affected northern regions such as Piedmont and Emilia-Romagna exhibit similarities to Lombardy—growing, peaking, and levelling around the same times. However, many other regions show some slight differences such as peaking at earlier or later dates, and even exhibiting an erratic trend.
https://doi.org/10.1371/journal.pone.0249037.g003
https://doi.org/10.1371/journal.pone.0249037.g004
In Fig 5 , things are put in perspective when the cumulative incidence of all Italian regions are plotted on the same scale. It is clear that Lombardy is the most affected region contributing to the largest share of national cumulative incidence, and indeed it is the epicentre of the outbreak in Italy.
https://doi.org/10.1371/journal.pone.0249037.g005
In the case of Spain, Fig 6 plots the daily cumulative incidence nationally and for all 19 Spanish regions over the whole sample period. The trend appears to be exponential and is similar between regions, but is also similar to that of the daily cumulative incidence in Italy. On a log-linear scale, in Fig 7 , the growth of the daily cumulative incidence appears to be closer to an exponential trend compared with Italy, due to the plots arguably exhibiting a more linear trend. It can be seen that there is a slight difference with Italy in that it appears as though most Spanish regions were affected at approximately the same time—when the country’s first cases were confirmed. This is reflected by the majority of plots starting from the very left of the x-axis, with the exception of the plots for a few regions such as Ceuta and Melilla. In Italy only a small number of regions were affected when the country’s first cases were confirmed, with the growth in cumulative incidence for the majority of the other regions coming later on.
https://doi.org/10.1371/journal.pone.0249037.g006
https://doi.org/10.1371/journal.pone.0249037.g007
The worst affected regions in Spain are Madrid and Catalonia, and Fig 8 plots the daily incremental incidence for both regions and the national trend. The growth in daily incidence, in all three cases, could be classed as being approximately exponential, however, daily incidence appears to peak on 26th March 2020 before falling and peaking again on 31st March 2020. It is confirmed that the true peak daily incidence does indeed occur on 31st March 2020 and we return to this point later on in the analysis. In comparison to other Spanish regions, it seems that Madrid and Catalonia are the exceptions as the majority of regions exhibit an exponential rise in daily incidence and peak around 26th and 27th March 2020 before falling.
https://doi.org/10.1371/journal.pone.0249037.g008
Plotting the daily incidence of all regions on the same scale in Fig 9 , it is clear that Madrid and Catalonia are the most affected regions contributing the largest share of the national cumulative incidence. Whilst Madrid and Catalonia are the main epicentres of the outbreak in Spain, many coastal regions also show significant numbers of confirmed cases, although not quite on the same scale.
https://doi.org/10.1371/journal.pone.0249037.g009
The SIR (Susceptible-Infectious-Recovered) model
In the mathematical modelling of infectious diseases, there exist many compartmental models that can be used to describe the spread of a disease within a population. One of the simplest models is the SIR (Susceptible-Infectious-Recovered) model proposed by [ 46 ], in which the population is split into three groups or compartments: those who are susceptible ( S ) but not yet infected with the disease; those who are infectious ( I ); those who have recovered ( R ) and are immune to the disease or who have deceased.
The SIR model has been extensively researched and applied in practice, thus it would not be practical to mention and cover all of the literature. However, some of the most prominent literature covers areas such as the stability and optimality of the simple SIR model ([ 47 – 51 ]); pulse vaccination strategy in the SIR model ([ 52 – 55 ]); applications of the SIR in the modelling of infectious diseases ([ 56 – 64 ]).
With regards to COVID-19, many have applied the basic SIR model (or slightly modified versions) to model the outbreak. Some particular examples include (but are not limited to): [ 2 ] who estimate the overall symptomatic case fatality risk of COVID-19 in Wuhan and use the SIR model to generate simulations of the COVID-19 outbreak in Wuhan; [ 65 ] who apply a modified SIR model to identify contagion, recovery, and death rates of COVID-19 in Italy; [ 66 ] who combine the SIR model with probabilistic and statistical methods to estimate the true number of infected individuals in France; [ 67 ] who use a number of methods including the SIR model to estimate the basic and controlled reproduction numbers for the COVID-19 outbreak in Wuhan, China; [ 68 ] who show that the basic SIR model performs better than extended versions in modelling confirmed cases of COVID-19 and present predictions for cases after the lockdown of Wuhan, China; [ 69 ] who model the temporal dynamics of COVID-19 in China, Italy, and France, and find that although the rate of recovery appears to be similar in the three countries, infection and death rates are more variable; [ 70 ] who simulate the outbreak in Wuhan, China, using an extended SIR model and investigate the age distribution of cases; [ 71 ] who study the number of infections and deaths from COVID-19 in Sweden using the SIR model; [ 72 ] who use the SIR model, with an additional parameter for social distancing, to model and forecast the early stages of the COVID-19 outbreak in Brazil.
In reference to the SIR model, [ 74 ] note that it “examines only the temporal dynamics of the infection cycle and should thus be appropriate for the description of a well-localised epidemic outburst”, therefore, it would appear to be reasonable for use in analysis at city, province, or country level. In the form above, the dynamics of the model are controlled by the parameters β and γ , representing the rates of transition from S to I (susceptibility to infection), and I to R (infection to recovery or death), respectively.
To fit the model and find the optimal parameter values of β and γ , we use the optim function in R [ 75 ] to solve the minimisation problem. The system of differential equations, Eqs ( 1 ) to ( 3 ), are set up as a single function. The model is then initialised with starting values for S , I , and R , with parameters β and γ unknown. We obtain the daily cumulative incidence for the sample period, total population ( N ), and the susceptible population ( S ) as the total population minus the number of currently infected individuals. This is defined as the cumulative number of infected individuals minus the number of recovered or dead, however, these exact values are difficult to obtain. Thus, the cumulative number of infected individuals at the start date of the sample period is used as a proxy—since at the start date of the disease, this is likely to be close to the true value, as the number of recovered or dead should be very small (if not zero).
The residual sum of squares is then defined and set up as a function of β and γ . The optim package is used for general purpose optimisation problems, and in this case it is used to minimise the function RSS with respect to the sample of cumulative incidence. More specifically, we use the limited memory Broyden-Fletcher-Goldfarb-Shanno (L-BFGS-B) algorithm for the minimisation, which allows us to specify box constraints (lower and upper bounds) for the unknown parameters β and γ . The lower and upper bounds of zero and one, respectively, were selected for both parameters. The optim function then searches for the β and γ that minimise the RSS function, given starting values of 0.5 for both parameters. The optimal solution is found via the gradient method by repeatedly improving the estimates of RSS to try and find a solution with a lower value. The function makes small changes to the parameters in the direction of where RSS changes the fastest, where in this direction the lowest value of RSS is. This is repeated until no further improvement can be made or the improvement is below a threshold.
We consider convergence as the main criteria for finding an optimal solution in the minimisation of RSS —when the lowest RSS has been found, and no further improvement can be found or the improvement is below a threshold. In the case where convergence is not achieved, or there is some related error, then we use the parscale function in the optimisation. As the true values of β and γ are unknown, in the default case, the parameters are adjusted by a fixed step starting from their initial values. Most common issues were addressed using the parscale function to rescale—alter the sensitivity/magnitude of the parameters on the objective function. In other words, it allows the algorithm to compute the gradient at a finer scale (similar to the ndeps parameter—used to adjust step sizes for the finite-difference approximation to the gradient). In most cases, issues were solved by using a step size of 10 −4 . Of course, smaller step sizes could be used, but there is a risk that selecting too small a step size will lead to the optimal values of β and γ being found at their starting values. However, the results should be interpreted with caution. It is possible that estimates will vary with different population sizes N and the starting values specified for β and γ , which may also cause the optimisation process to be unstable.
It should be noted that the application of the basic SIR model to COVID-19 simplifies the analysis and makes the strong assumption that individuals who become infected but recover are immune to COVID-19. This is assumed purely for the simplification of modelling and we do not claim this to be true in reality. At present, it remains unclear whether those who recover from infection are immune [ 76 ]. Indeed, there have been studies and unconfirmed reports of individuals who have possibly recovered but then subsequently tested positive for the virus again, see for example [ 77 – 79 ].
The basic reproduction number R 0
Whilst the fitted model and optimal parameters allow us to make a simple prediction about how the trajectory of the number of susceptible, infectious, and recovered individuals evolves over time, a more useful statistic or parameter that can be computed from the fitted model is the basic reproduction number R 0 . Originally developed for the study of demographics in the early 20th century, it was adapted for use in the study of infectious diseases in the 1950’s [ 80 ]. It is defined as the “expected number of secondary infections arising from a single individual during his or her entire infectious period, in a population of susceptibles” [ 80 ], and is widely considered to be a fundamental concept in the study of epidemiology. In other words, it is the estimated number of people that an individual will go on to infect after becoming infected.
The R 0 value can provide an indication of the severity of the outbreak of an infectious disease: if R 0 < 1, each infected individual will go on to infect less than one individual (on average) and the disease will die out; if R 0 = 1, each infected individual will go on to infect one individual (on average) and the disease will continue to spread but will be stable; if R 0 > 1, each infected individual will go on to infect more than one individual (on average) and the disease will continue to spread and grow, with the possibility of becoming a pandemic ([ 80 , 81 ]).
Log-linear model
https://doi.org/10.1371/journal.pone.0249037.g010
To fit the log-linear model, we use the incidence package [ 82 ] in R [ 75 ] to obtain the optimal values of the parameters. Using the estimated parameters, the fitted model can be used to predict the trajectory of the incidence up until the peak incidence in the growth phase. However, although the log-linear model allows for the modelling and prediction of the incidence, compared with the SIR model it does not provide any indication about the number of susceptible or recovered individuals.
We are able to use the epitrix R package [ 84 ] to implement the method by [ 83 ] for empirical distributions to estimate R 0 from the growth rate r . However, [ 83 ] note that an “epidemic model implicitly specifies a generation interval distribution” (also known as the serial interval distribution), which is defined as “the time between the onset of symptoms in a primary case and the onset of symptoms in secondary cases” [ 85 ]. As we do not have access to more detailed COVID-19 patient data, we are not able to compute the parameters of the serial interval distribution directly. However, a number of existing analyses of COVID-19 patient data report some preliminary estimates of the best fitting serial interval distributions and their corresponding model parameters. These are: i) gamma distribution with mean μ = 7.5 and standard deviation σ = 3.4 [ 81 ]; ii) gamma distribution with mean μ = 7 and standard deviation σ = 4.5 [ 2 ]; iii) gamma distribution with mean μ = 6.3 and standard deviation σ = 4.2 [ 86 ]. By using these three serial intervals in conjunction with the above method, we are able to obtain estimates of R 0 from estimates of the growth rate r . It should be noted that serial interval distributions are not only restricted to the gamma distribution—other common distributions used include the Weibull and log-normal distributions, and that the parameters are dependent on a number of factors including the time to isolation [ 86 ].
The effective reproduction number R e
As mentioned above, the estimation of the R 0 value is not always ideal, due to it being a single fixed value reflecting a specific period of growth (in the log-linear model) or requiring assumptions that only hold true in specific time periods (in the basic SIR model). In other words, it is “time and situation specific” [ 85 ]. In reality, the reproduction number will vary over time but it will also be influenced by governments and health authorities implementing measures in order to reduce the impact of the disease. Therefore, a more useful approach for measuring the severity of an infectious disease is to track the reproduction number over time. The effective reproduction number R e is one way to achieve this, and thus allows us to see how the reproduction number changes over time in response to the development of the disease itself but also effectiveness of interventions. Although there are numerous methods that can be used to analyse the severity of a disease over time, the majority are not straightforward to implement (especially in software) [ 85 ].
One popular method for estimating R e is that proposed by [ 85 ]. The basic premise of this method is that “once infected, individuals have an infectivity profile given by a probability distribution w s , dependent on time since infection of the case, s , but independent of calendar time, t . For example, an individual will be most infectious at time s when w s is the largest. The distribution w s typically depends on individual biological factors such as pathogen shedding or symptom severity” [ 85 ].
The function models the transmissibility of a disease with a Poisson process, such that an individual infected at time t − s will generate new infections at time t at a rate of R t w s , where R t is the instantaneous (effective) reproduction number at time t . Thus, the incidence at time t is defined to be Poisson distributed with mean equal to the average daily incidence (number of new cases) at time t . This value is just for a single time period t , however, estimates for a single time period can be highly variable meaning that it is not easy to interpret, especially for making policy decisions. Therefore, we consider longer time periods of one week (seven days)—assuming that within a rolling window the instantaneous reproduction number remains constant. Note that there is a potential trade off, as using longer rolling windows gives more precise estimates of R t but this means fewer estimates can be computed (requires more incidence values to start with) and a more delayed trend reducing the ability to detect changes in transmissibility. Whereas shorter rolling windows lead to more rapid detection in changes but with more noise. Using this method, it is recommended that a minimum cumulative daily incidence of 12 cases have been observed before attempting to estimate R e . For the data sets used, this does not pose a problem as a cumulative total of 16 and 17 cases, respectively, exist on the first day of the sample at the country level, and by the seventh day the totals are around 200 and 650 for Spain and Italy, respectively.
From the posterior distribution, the posterior mean R t , τ can be computed at time t for the rolling window of [ t − τ , t ] by the ratio of the gamma distribution parameters. We refer the readers to the supplementary information of [ 85 ] for further details regarding the Bayesian framework. As noted by [ 85 ], this method works best when times of infection are known and the infectivity profile or distribution can be estimated from patient level data. However, as mentioned above, we do not have access to this level of data, and instead utilise three different serial intervals from the literature that have been estimated from real data.
In practice, the transmission of a disease will vary over time especially when health prevention measures are implemented. However, this method is the only reproduction number that can be easily computed in real-time, and in comparison to similar methods, it captures the effect of control measures since it will cause sudden decreases in estimates compared with other methods.
In this analysis, we use the most basic version of this method and estimate the effective reproduction number over a rolling window of seven days. This appears to be sufficient and in line with our results, as we do not suffer from the problem of small sample sizes as the samples are sufficiently large and we start computing the effective reproduction number after one mean serial interval. It should be noted that estimates of this reproduction number are dependent on the distribution of the infectiousness profile w s . In addition, it is known that this distribution may not always be well documented, especially in the early parts of an epidemic. However, here we assume that the serial interval is defined for our sample period and the use of the three serial intervals from the literature appears to give satisfactory results.
If problems did arise, or to account for uncertainty in the serial interval distribution, an alternative method is to implement a modified procedure by [ 85 ], which allows for uncertainty in the serial interval distribution. This modified method assumes that the serial interval is gamma distributed but the mean and standard deviation are allowed to vary according to a standard normal distribution. Some N * pairs of means and standard deviations are simulated—mean first and standard deviation second, with the constraint that the mean is less than the standard deviation to ensure that for each pair the probability density function of the serial interval distribution is null at time t = 0. Then, for each rolling window 1000 realisations are sampled of the instantaneous reproduction number using the posterior distribution conditional on the pair of parameters.
The SIR model and R 0
For both Italy and Spain, we set up and solve the minimisation problem for the SIR model described in Section for region-level and national-level COVID-19 incidence for the first 14 days after the first cases were confirmed in each respective country and region. The first 14 days after the first cases are detected can be considered to be the early stage of an outbreak, and it is reasonable to assume that there are few, if no, infected or immune individuals prior to this. However, it is a rather strong assumption as it is possible that individuals may be infected but do not display any symptoms. Tables 1 and 2 show the output corresponding to each region/country including the date that the first cases were confirmed, the population size (obtained from [ 88 ]), the cumulative number of cases at the 14th day after the first cases were confirmed, the fitted estimates for the parameters β and γ , and estimates for R 0 .
https://doi.org/10.1371/journal.pone.0249037.t001
https://doi.org/10.1371/journal.pone.0249037.t002
From Tables 1 and 2 , we observe that many of the first regions to be affected in both countries are those with the largest population sizes, however, the cumulative number of cases (after the first 14 days) in these regions are not always the highest among all regions. The estimates of the parameters β and γ also do not show any particular trends and this is reflected in the estimated R 0 values. It can be seen that for all regions in both Italy and Spain, the estimated R 0 values fall between one and three. This suggests that, according to the thresholds described above, the disease is spreading and growing in all Italian and Spanish regions during the 14 days after the first localised cases were confirmed. At a national level, the estimated values of R 0 are greater than two for both countries, again, suggesting a spreading and growing disease. This is perhaps not surprising since this time period reflects the early stages of the spread of the disease, thus we would expect it to be growing and spreading quickly before any preventative action is taken.
We note that in Tables 1 and 2 , there are some cases where the estimated value of β is very close to or at the upper limit of 1.000—e.g. Lombardy (Italy) and Madrid (Spain). This leads to the consequence that the parameter estimates appear to be bound by the upper limit. However, all parameter estimates are dependent on the starting values defined for β and γ , and the upper and lower bounds specified. For all cases of estimating the parameters in Tables 1 and 2 , we used the same optimisation procedure and criteria for determining a satisfactory estimate that is the convergence in the minimisation of the RSS ( Eq (4) ). In all cases, convergence was achieved but this is still slightly problematic. For cases where the estimated value of β is 1.000, although convergence was achieved, this indicates only that it generates the lowest RSS within the upper and lower limits defined. Therefore, there may or may not exist values of the parameter outside of this range that may be more optimal. Indeed, the results may vary depending on the upper and lower bounds, and the starting values that are selected. Thus, there is also the question of how to change the starting values and bounds appropriately (instead of, say, simply increasing them). Furthermore, as the R 0 value in the SIR model is computed as β / γ , another consequence of the estimated value of β being 1.000 is that the true value of β may actually be larger than this, and so the true value of R 0 may be larger than the estimated value.
Using the estimated parameters for the best fitted models, the predicted trajectories of the numbers in each of the compartments of the model can be generated. For brevity, in the remainder of the analysis, we show only the results for Italy, Spain, and their worst affected regions. Fig 11 plots the observed and predicted cumulative incidence for the 14 days immediately following the first confirmed cases in Lombardy and Italy, respectively. It can be seen that the model appears to under predict the true total number of cases in both cases during the early part of the outbreak before over estimating towards the end of the 14 days. In Fig 12 the SIR model trajectories are plotted along with the observed cumulative incidence on a logarithmic scale for Lombardy and Italy. The under prediction of the cumulative incidence in the first 14 days (to the left of the vertical dashed black line) is indicated by the solid red line (predicted cumulative incidence) lying below the black points (observed cumulative incidence) however, after the initial 14 days and after the implementation of a nationwide lock down (vertical dashed red line), the observed cumulative incidence grows at a slower rate than predicted by the fitted model. Indeed, this reflects the fact that the model is based only on the initial 14 days and does not account for any interventions.
https://doi.org/10.1371/journal.pone.0249037.g011
https://doi.org/10.1371/journal.pone.0249037.g012
In Fig 13 , the observed and predicted cumulative incidence for the 14 days immediately following the first confirmed cases in Catalonia, Madrid, and Italy, respectively, are shown. In contrast to the results for Italy, the fitted model for all three appears to predict the true total number of cases across the whole of the first 14 days reasonably well. Fig 14 plots the SIR model trajectories and the observed cumulative incidence on a logarithmic scale for Catalonia, Madrid, and Spain. Here, the more accurate predictions of the cumulative incidence are reflected in the area to the left of the vertical dashed black line. However, it can be seen that at the time when the nationwide lock down came into force (vertical dashed red line) the growth of the true total number of cases slowed down. It is likely that this is coincidental, since it is known that the effect on the incidence of infectious diseases from health interventions is not immediate, but instead lags behind.
https://doi.org/10.1371/journal.pone.0249037.g013
https://doi.org/10.1371/journal.pone.0249037.g014
Log-linear model and R 0
Following the SIR model, we implemented the log-linear model as described above for region-level and national-level COVID-19 daily incidence for the entire growth phase (from the time of the first confirmed cases until the time at which daily incidence peaks). The estimated parameters of the fitted log-linear models for the daily incidence of Lombardy and Italy, respectively, are shown in Table 3 . It can be seen that the peak daily incidence in both Lombardy and at country level occurred on the same day (21st March 2020), however, the growth rate (doubling time) is found to be slightly greater (shorter) at country level (0.18 and 3.88) compared with the Lombardy region (0.16 and 4.34). In comparison to the SIR model and modelling the cumulative incidence, the log-linear model modelling the daily incidence in the growth phase (as shown in Fig 15 ) appears to be slightly more accurate.
Upper and lower limits of the 95% confidence intervals are indicated by the dashed red lines.
https://doi.org/10.1371/journal.pone.0249037.g015
https://doi.org/10.1371/journal.pone.0249037.t003
In Table 4 , the estimated parameters of the fitted log-linear models for the daily incidence of Madrid, Catalonia, and Spain, respectively, are given. Similarly, the peak daily incidence occurs on the same day (31st March 2020) for Madrid, Catalonia, and Spain, although this is later than that for Italy. Interestingly, the growth rate (doubling time) is greatest (shortest) for Catalonia (0.24 and 3.85), whilst Madrid and Spain share similar growth rates and doubling times (0.21/0.22 and 3.24/3.21). It should be noted that there appears to be a slight difference in the observed daily incidence compared with the case of Italy and its regions. In Fig 16 , it can be seen that the observed daily incidence appears to initially peak in the last few days of March in all cases before falling, but then increases to a higher peak at the end of the growth phase. This seems to throw off the fitted log-linear model, as after the initial (approximate) 14 days the fitted model under predicts and then over predicts the daily incidence.
https://doi.org/10.1371/journal.pone.0249037.g016
https://doi.org/10.1371/journal.pone.0249037.t004
As with the SIR model, we are also able to use the fitted log-linear models in conjunction with the three serial intervals mentioned above to compute estimates of the R 0 value. Table 5 shows the mean estimates of the R 0 value for Italy, Spain, and their most affected regions, computed from the fitted log-linear models and the three serial intervals. In each case, the mean estimates are computed from 10,000 samples of R 0 values generated from the log-linear regression of the incidence data in the growth phase, and the distributions of these samples are plotted in S1 Fig . Compared with the estimates from the SIR model, we find that in all but the case of Italy, the estimates of R 0 from the log-linear model are greater than that from the SIR model—in these cases, the lowest estimates of R 0 from the log-linear models are larger by between 0.5 to 1. In the case of Italy, we find that the estimate of R 0 computed from the SIR model is approximately the same as that computed from the log-linear model using a serial interval using a gamma distribution with mean μ = 7 and standard deviation σ = 4.5 [ 2 ]. Using the log-linear models, the largest R 0 values computed are for Catalonia, whereas the smallest values are for Lombardy. It can also be seen that serial distributions with a lower mean appear to correspond with lower R 0 values. A possible explanation for the difference between the estimated R 0 values computed from the SIR models and the log-linear models is that the only incidence data from the first 14 days was used in the former, whereas incidence data from the whole growth phase was used in the latter—almost double the data. Therefore, it is arguable that the R 0 estimates from the log-linear models could be considered to be more accurate.
https://doi.org/10.1371/journal.pone.0249037.t005
Effective reproductive number R e .
Turning towards the more dynamic measure of the infectiousness of diseases, Figs 17 and 18 plot the estimated reproductive numbers computed for Lombardy, Italy, Madrid, Catalonia, and Spain, over the entire sample period. Using the method proposed by [ 85 ], in each case estimates were computed using rolling windows of the daily incidence over the previous 7 days and the same three serial distributions as for the log-linear models. As a result, no estimates are computed for the first 7 days of each respective sample period. In all cases, we analyse and compute the R e values over the whole sample period available allowing us to see how the infectiousness of COVID-19 varies during the initial outbreak stages and the effect of any interventions implemented by the respective governments. In Fig 17 , we observe that for both Lombardy and Italy, R e is generally decreasing over the time (under any of the three serial distributions), and although it is initially larger for Italy, after approximately the first 7 days the R e values are similar. However, the trend of R e both to the left and right (before and after) of the nationwide lockdown (indicated by the dotted line) shows some differences. Prior to the nationwide lockdown, R e decreases rapidly towards a value of between three and four, which could be attributed to the fact that northern Italy (including Lombardy) was the most affected area in the early stages of the outbreak and lockdowns local to the area were already being enforced from 21st February 2020. Thus, this is likely to have contributed (in part) to the initial reduction in the R e value. After the nationwide lockdown came into force on 9th March 2020, R e continues to decrease but at a slower pace and appears to level off approximately 14 days later—this coincides with the peak in daily incidence on 21st March 2020. After this point, it is likely that the effects of the nationwide lockdown are starting to appear with R e appearing to decrease again more rapidly towards the critical value of one (solid horizontal line)—suggesting that the disease is still spreading but stabilising.
Upper and lower limits of the 95% confidence intervals for the mean are indicated by the red dashed lines, and the grey dotted line indicates the date at which the national lock down becomes effective.
https://doi.org/10.1371/journal.pone.0249037.g017
https://doi.org/10.1371/journal.pone.0249037.g018
In Fig 18 , we observe a different trend in the R e value for Madrid, Catalonia, and Spain, compared with Lombardy and Italy. Whilst R e exhibits a decrease over the sample time period (under any of the three serial distributions), the initial values are actually larger for Madrid and Catalonia, however, the values for all three are similar after the initial 7 days. The trend in the estimated R e values before and after the nationwide lockdown again show some differences, but also differ to those for the cases of Lombardy and Italy. Prior to the nationwide lockdown (indicated by the dotted line), the trend of the estimated R e values is very erratic: decreasing, increasing, and then decreasing again. This could be due to the daily incidence for Madrid, Catalonia, and Spain, showing greater variation compared with that for Italy before the respective lockdowns. It is found that in the period before the lockdowns, Spanish daily incidence appears to show more alternation between increases and decreases compared with the previous day’s incidence, whilst Italian daily incidence shows much less. After the nationwide lockdown on 14th March 2020, for all three cases the estimated R e decreases significantly towards a value of two. More specifically, in mid-March 2020 daily incidence for Madrid, Catalonia, and Spain, levels off corresponding to the reduction in R e , but in the run up to 23rd March 2020 daily incidence again becomes more variable and alternates between significantly larger and smaller daily incidence, with R e levelling off. After 23rd March 2020, this levelling off is more sustained for Madrid and Spain compared with Catalonia. This may be attributed to the daily incidence initially peaking and then decreasing much more significantly for Catalonia, leading to a more significant decrease in R e at the latter end of the sample period. In general, the estimated R e values are larger for Spain than Italy, since Spain is lagging behind in terms of the start of the outbreak, however, it is found that the estimated R e is larger for Italy than Spain, but larger for Madrid and Catalonia than Lombardy.
Predictive ability of models.
Whilst the results regarding the estimated reproduction values ( R 0 and R e ) provide useful indicators about the infectiousness of COVID-19 and the variability over time, the predictive ability of models is also key—especially in the decay phase of an outbreak after the daily incidence has peaked and is in decline. Predictions about the daily incidence in the decay phase can contribute to determining whether health interventions are working, but can additionally provide time frames for when daily incidence may reach certain thresholds—e.g. below which the disease may be considered under control. To compare the predictive ability of the SIR and log-linear models, we use the projections package [ 89 ] in R [ 75 ]. As this section acts to provide only a brief analysis of the predictive ability of the models, we refer the readers to [ 89 ] for in-depth documentation regarding the finer details of the computations. The initial step is to consider which of the two models provides the best predictive ability in the growth phase of the COVID-19 outbreak and for simplicity, we analyse only Italy and Spain at country level. Using the estimated R 0 values for Italy and Spain from the SIR and log-linear models above, we combine these with the three serial distributions mentioned earlier. We then use the projections package [ 89 ] to forecast and predict the daily incidence for Italy and Spain from the 14th day (since the first cases in each location) until the day of peak incidence.
Plots of the true daily incidence in Italy and Spain during their respective growth phases and the predicted values using the SIR and log-linear models are shown in Figs 19 and 20 . In each figure, the first row plots the predictions using the SIR model; the second row plots the predictions using the log-linear model. For the case of Italy, the plots in Fig 19 appear to show that the predictions using the R 0 value estimated from the SIR model and the serial interval of a gamma distribution with mean μ = 7.5 and standard deviation σ = 3.4 [ 81 ] provide the most accurate general predictions. However, although using the R 0 value estimated from the log-linear model generates predictions which are accurate up until the last 7 days of the growth phase (where all three cases show over prediction), these results are more consistent compared with those using the SIR model. For the case of Spain, the plots in Fig 20 show that the predictions using the R 0 value estimated from the SIR model are consistent but significantly under predicting the observed daily incidence. In contrast, predictions using the R 0 value estimated from the log-linear model are consistent and accurate up until the initial peak in daily incidence a few days before the true peak at the end of the growth phase. Based on these results for the growth phase of the outbreak, we propose to use the log-linear model to compute basic predictions for the decay phase.
95% confidence intervals for the predicted incidence are indicated by the shaded light purple regions.
https://doi.org/10.1371/journal.pone.0249037.g019
https://doi.org/10.1371/journal.pone.0249037.g020
At the time of conducting this part of the analysis, approximately one month of daily incidence data was available for the decay phase (following peak daily incidence) of both Italy and Spain. Similarly, we follow the methodology for fitting the log-linear model but now apply it to the decay phase daily incidence. The model is fitted to the decay phase daily incidence in the same way, and model parameters can be computed. Note that for the decay phase, the values and interpretation of the estimated parameters change—the growth rate takes a negative value and the doubling time becomes the halving time (both reflecting the decay and decrease in daily incidence). The fitted log-linear regressions for Italy and Spain are shown in the left hand plots of Figs 21 and 22 , respectively. The fitted models appear to provide reasonable fits to the observed decay phase daily incidence much like the case for the growth phase.
Plots of the observed (dot-dashed black line) and projected daily incidence for the next 180 days using the log-linear model and serial interval distributions SI 1 (green line), SI 2 (blue line), and SI 3 (red line) (right).
https://doi.org/10.1371/journal.pone.0249037.g021
https://doi.org/10.1371/journal.pone.0249037.g022
Also, as in the growth phase, the R 0 value can still be computed for the log-linear model during the decay phase, and for consistency we obtain mean estimates of R 0 from 10,000 samples of R 0 generated from the log-linear regressions of the daily incidence during the decay phase in conjunction with the three serial distributions. Distributions of these estimates are plotted in S2 Fig and it can be seen that (in contrast to the growth phase) the mean estimates of R 0 for Italy and Spain, individually, are very similar (under the three serial distributions)—between 0.85 and 0.87 for Italy, and 0.77 and 0.83 for Spain. Using the mean estimated R 0 values and the three serial distributions, we computed projections of the daily incidence for the 180 days immediately following the end of the decay phase sample period on 22nd April 2020. The paths of these projections for Italy and Spain are shown in the right hand plots of Figs 21 and 22 , respectively.
A simple comparison of the projected daily incidence for both countries is given in Table 6 , at one and two months following the end of the decay phase sample period. Observed daily incidence for the remainder of the decay phase was obtained from [ 44 , 90 , 91 ]. In general, it appears that the predictions for future daily incidence (under all three serial distributions) in both Italy and Spain are significantly greater than the observed daily incidence. At the one month time point (21st May 2020) projections of daily incidence for Italy are approximately twice as large as the true incidence; projections of daily incidence for Spain are approximately two to three times as large as the true incidence. Moving forward to the two month time point (21st June 2020) projections of the daily incidence for Italy are approximately two to three times as large as the true incidence; projections of the daily incidence for Spain are up to twice as large as the true incidence. However, the projection of Spanish daily incidence using the serial interval of a gamma distribution with mean μ = 6.3 and standard deviation σ = 4.2 [ 86 ] is almost identical to the true incidence.
https://doi.org/10.1371/journal.pone.0249037.t006
Whilst the results of the projections generally show significant over estimation of future daily incidence in both Italy and Spain, they do provide some additional information to the reproduction values regarding the trends of daily incidence. However, such forecasts should be not be taken directly at face value as there are a number of pitfalls that will influence the predictions. Limited decay phase incidence data was available at the time of the original analysis, which is likely to have led to less accurate estimates of R 0 and thus predictions. On a related note, the predictions are conditional on the data up until the end of the sample decay phase data and thus do not account for any health policies or interventions implemented after this, likely leading to the over estimation.
In this paper, we have provided a simple statistical analysis of the novel Coronavirus (COVID-19) outbreak in Italy and Spain—two of the worst affected countries in Europe. Using data of the daily and cumulative incidence in both countries over approximately the first month after the first cases were confirmed in each respective country, we have analysed the trends and modelled the incidence and estimated the basic reproduction value using two common approaches in epidemiology—the SIR model and a log-linear model.
Results from the SIR model showed an adequate fit to the cumulative incidence of Spain and its most affected regions in the early stages of the outbreak, however, it showed significant under estimation in the case of Italy and its most affected regions. Estimates of the basic reproduction number in the early stage of the outbreak from the model were found to be greater than one in all cases, suggesting a growing infectiousness of COVID-19—in line with expectations. Applying the log-linear regression model to the daily incidence, results for the growth phase of the outbreak in Italy and Spain revealed a greater growth rate for Spain compared with Italy (and their most affected regions)—approximately between 0.21 to 0.24 for the former and 0.15 to 0.18 for the latter. The time for the daily incidence to double for Spain was also found to be shorter than Italy (approximately three days compared to four days).
With the lack of detailed clinical COVID-19 data for the two countries, we utilised existing results regarding the serial interval distribution of COVID-19 from the literature to estimate the basic reproduction number via the log-linear model. Estimates of this value were found to be between 2.1 and 3 for Italy and its most affected region Lombardy, and between 2.5 and approximately 4 for Spain and its most affected regions of Madrid and Catalonia. Further analysis of the effective reproduction number (based on the incidence over the previous seven days) indicated that in both countries the infectious of COVID-19 was decreasing and reflecting the positive impact of health interventions such as nationwide lock downs.
Basic predictions of future daily incidence in Italy and Spain were estimated using the log-linear regression model for the decay phase of the outbreak. Estimates of the projected daily incidence at various time points in the future were generally found to be between two to three times larger than the true levels of daily incidence. These results highlight the fact that the estimates may only give reasonable indications in the short term, since they are based on past data which may or may not account for factors which change in the short term—e.g. new health interventions, public policy, etc.
Despite the simplicity of our results, we believe that they provide an interesting insight into the statistics of the COVID-19 outbreak in two of the worst affected countries in Europe. Our results appear to indicate that the log-linear model may be more suitable in modelling the incidence of COVID-19 and other infectious diseases in both the growth and decay phases, and for short term predictions of the growth (or decay) of the number of new cases when no intervention measures have recently been implemented. In addition, the results could be useful in contributing to health policy decisions or government interventions—especially in the case of a significant second wave of COVID-19. However, these results should be used in conjunction with the results from other more complex mathematical and epidemiological models.
Supporting information
S1 fig. plots of the distributions of samples of r 0 values computed from the fitted log-linear regressions of growth phase incidence..
i) Lombardy (top left); ii) Italy (top right); iii) Madrid (middle left); iv) Catalonia (middle right); v) Spain (bottom). a) SI 1 (blue); b) SI 2 (red) c) SI 3 (green).
https://doi.org/10.1371/journal.pone.0249037.s001
S2 Fig. Plots of the distributions of samples of R 0 values computed from the fitted log-linear regressions of decay phase incidence.
i) Italy (left); ii) Spain (right). a) SI 1 (green); b) SI 2 (red) c) SI 3 (blue).
https://doi.org/10.1371/journal.pone.0249037.s002
- 1. Center for Systems Science and Engineering (CSSE) at Johns Hopkins University (JHU), 2020. Coronavirus COVID-19 (2019-nCoV). Available at: https://gisanddata.maps.arcgis.com/apps/opsdashboard/index.html#/bda7594740fd40299423467b48e9ecf6 .
- View Article
- Google Scholar
- PubMed/NCBI
- 13. Atkeson, A., 2020. What Will Be the Economic Impact of COVID-19 in the US? Rough Estimates of Disease Scenarios. National Bureau of Economic Research, Working Paper 26867.
- 17. Benatia, D., Godefroy, R. and Lewis, J., 2020. Estimating COVID-19 Prevalence in the United States: A Sample Selection Model Approach. Available at: https://ssrn.com/abstract=3578760 .
- 32. McKinsey & Company, 2020. COVID-19: Implications for business. Available at: https://www.mckinsey.com/business-functions/risk/our-insights/covid-19-implications-for-business .
- 44. GitHub, 2020a. pcm-dpc/COVID-19: COVID-19 Italia—Monitoraggio situazione. Available at: https://github.com/pcm-dpc/COVID-19 .
- 45. GitHub, 2020b. datasets/COVID 19 at master ⋅ datadista/datasets. Available at: https://github.com/datadista/datasets/tree/master/COVID%2019 .
- 64. Correia A.M., Mena F.C., Soares A.J., 2011. An Application of the SIR Model to the Evolution of Epidemics in Portugal. In: M. Peixoto, A. Pinto and D. Rand eds. Dynamics, Games and Science II. Springer Proceedings in Mathematics, vol 2. Berlin: Springer. pp. 247-250.
- 65. Calafiore, G.C., Novara, C. and Possieri, C., 2020. A Modified SIR Model for the COVID-19 Contagion in Italy. arXiv:2003.14391v1.
- 66. Roques, L., Klein, E., Papax, J., Sar, A. and Soubeyrand, S., 2020. Using early data to estimate the actual infection fatality ratio from COVID-19 in France (Running title: Infection fatality ratio from COVID-19). arXiv:2003.10720v3.
- 67. You, C., Deng, Y., Hu, Y., Sun, J., Lin, Q., Zhou, F., et al. Estimation of the Time-Varying Reproduction Number of COVID-19 Outbreak in China. Available at SSRN: https://ssrn.com/abstract=3539694 .
- 71. Qi, C., Karlsson, D., Sallmen, K. and Wyss, R., 2020. Model studies on the COVID-19 pandemic in Sweden. arXiv:2004.01575v1.
- 72. Bastos, S.B. and Cajuero, D.O., 2020. Modeling and forecasting the early evolution of the Covid-19 pandemic in Brazil. arXiv:2003.14288v2.
- 75. R Development Core Team, 2020. R: A Language and Environment for Statistical Computing. R Foundation for Statistical Computing, Vienna, Austria (2020).
- 76. World Health Organization, 2020. “‘Immunity passports” in the context of COVID-19’. Available at: https://www.who.int/news-room/commentaries/detail/immunity-passports-in-the-context-of-covid-19 .
- 79. Reuters, 2020. “Explainer: Coronavirus reappears in discharged patients, raising questions in containment fight”. Available at: https://uk.reuters.com/article/us-china-health-reinfection-explainer/explainer-coronavirus-reappears-in-discharged-patients-raising-questions-in-containment-fight-idUKKCN20M124 .
- 82. Jombart, T., Kamvar, Z.N., FitzJohn, R., Cai, J., Bhatia, S., Schumacher, J, et al. 2020. incidence: Compute, Handle, Plot and Model Incidence of Dated Events. R package version 1.7.1. https://CRAN.R-project.org/package=incidence .
- 84. Jombart, T., Cori, A., Kamvar, Z.N. and Schumacher, D., 2019. epitrix: Small Helpers and Tricks for Epidemics Analysis. R package version 0.2.2. https://CRAN.R-project.org/package=epitrix .
- 87. Cori, A., Cauchemez, S., Ferguson, N.M., Fraser, C., Dahlqwist, E., Demarsh, P.A., et al. 2019. EpiEstim: Estimate Time Varying Reproduction Numbers from Epidemic Curves. R package version 2.2-1 https://cran.r-project.org/package=EpiEstim .
- 88. Eurostat, 2019. Population: demography, population projections, census, asylum & migration—Overview. Available at: https://ec.europa.eu/eurostat/web/population/overview .
- 89. Jombart, T., Nouvellat, P., Bhatia, S. and Kamvar, Z.N., 2018. projections: Project Future Case Incidence. R package version 0.3.1. https://CRAN.R-project.org/package=projections .
- 90. Worldometer, 2020. Worldometer—real time world statistics. Available at: https://www.worldometers.info/ .
- 91. Ministerio de Sanidad, Consumo y Bienestar Social. Enfermedad por nuevo coronavirus, COVID-19. Available at: https://www.mscbs.gob.es/profesionales/saludPublica/ccayes/alertasActual/nCov-China/ .
Analytical insights of COVID-19 pandemic
Affiliation.
- 1 Department of Bionanotechnology, Gachon University, Gyeonggi-do, 13120, Republic of Korea.
- PMID: 33100439
- PMCID: PMC7567672
- DOI: 10.1016/j.trac.2020.116072
Coronavirus disease-19 (COVID-19) is caused by the severe acute respiratory syndrome coronavirus 2 (2019-nCoV or SARS-CoV-2). Genomic analysis has revealed that bat and pangolin coronaviruses are phylogenetically related to SARS-CoV-2. The actual origin and passage history of the virus are unknown, but human-human transmission of the virus has been confirmed. Several diagnostic techniques have been developed to detect COVID-19 in this prevailing pandemic period. In this review, we provide an overview of SARS-CoV-2 and other coronaviruses. The origin, structure, current diagnostic techniques, such as molecular assays based on oligonucleotides, immunoassay-based detection, nanomaterial-based biosensing, and distinctive sample based detection are also discussed. Furthermore, our review highlights the admissible treatment strategies for COVID-19 and future perspectives on the development of biosensing techniques and vaccines for the diagnosis and prevention of the disease, respectively.
Keywords: COVID-19; Diagnostic techniques; Genome; Pandemic outbreak; Treatment; Vaccines.
© 2020 Elsevier B.V. All rights reserved.
Publication types
8.4 Annotated Student Sample: "U.S. Response to COVID-19" by Trevor Garcia
Learning outcomes.
By the end of this section, you will be able to:
- Identify the genre conventions of an informal analytical report.
- Analyze the organizational structure of a report and how writers develop ideas.
- Recognize how writers use evidence and objectivity to build credibility.
- Identify sources of evidence within a text and in source citations.
Introduction
The analytical report that follows was written by a student, Trevor Garcia, for a first-year composition course. Trevor’s assignment was to research and analyze a contemporary issue in terms of its causes or effects. He chose to analyze the causes behind the large numbers of COVID-19 infections and deaths in the United States in 2020. The report is structured as an essay, and its format is informal.
Living by Their Own Words
Successes and failures.
student sample text With more than 83 million cases and 1.8 million deaths at the end of 2020, COVID-19 has turned the world upside down. By the end of 2020, the United States led the world in the number of cases, at more than 20 million infections and nearly 350,000 deaths. In comparison, the second-highest number of cases was in India, which at the end of 2020 had less than half the number of COVID-19 cases despite having a population four times greater than the U.S. (“COVID-19 Coronavirus Pandemic,” 2021). How did the United States come to have the world’s worst record in this pandemic? An examination of the U.S. response shows that a reduction of experts in key positions and programs, inaction that led to equipment shortages, and inconsistent policies were three major causes of the spread of the virus and the resulting deaths. end student sample text
annotated text Introduction. Informal reports follow essay structure and open with an overview. end annotated text
annotated text Statistics as Evidence. The writer gives statistics about infection rates and numbers of deaths; a comparison provides context. end annotated text
annotated text Source Citation in APA Style: No Author. A web page without a named author is cited by the title and the year. end annotated text
annotated text Thesis Statement. The rhetorical question leads to the thesis statement in the last sentence of the introduction. The thesis statement previews the organization and indicates the purpose—to analyze the causes of the U.S. response to the virus. end annotated text
Reductions in Expert Personnel and Preparedness Programs
annotated text Headings. This heading and those that follow mark sections of the report. end annotated text
annotated text Body. The three paragraphs under this heading support the first main point in the thesis statement. end annotated text
student sample text Epidemiologists and public health officials in the United States had long known that a global pandemic was possible. end student sample text
annotated text Topic Sentence. The paragraph opens with a sentence stating the topic. The rest of this paragraph and the two that follow develop the topic chronologically. end annotated text
student sample text In 2016, the National Security Council (NSC) published Playbook for Early Response to High-Consequence Emerging Infectious Disease Threats and Biological Incidents , a 69-page document on responding to diseases spreading within and outside of the United States. On January 13, 2017, the joint transition teams of outgoing president Barack Obama and then president-elect Donald Trump performed a pandemic preparedness exercise based on the playbook; however, it was never adopted by the incoming administration (Goodman & Schulkin, 2020). A year later, in February 2018, the Trump administration began to cut funding for the Prevention and Public Health Fund at the Centers for Disease Control and Prevention, leaving key positions unfilled. Other individuals who were fired or resigned in 2018 were the homeland security adviser, whose portfolio included global pandemics; the director for medical and biodefense preparedness; and the top official in charge of a pandemic response. None of them were replaced, thus leaving the White House with no senior person who had experience in public health (Goodman & Schulkin, 2020). Experts voiced concerns, among them Luciana Borio, director of medical and biodefense preparedness at the NSC, who spoke at a symposium marking the centennial of the 1918 influenza pandemic in May 2018: “The threat of pandemic flu is the number one health security concern,” she said. “Are we ready to respond? I fear the answer is no” (Sun, 2018, final para.). end student sample text
annotated text Audience. The writer assumes that his readers have a strong grasp of government and agencies within the government. end annotated text
annotated text Synthesis. The paragraph synthesizes factual evidence from two sources and cites them in APA style. end annotated text
annotated text Expert Quotation as Supporting Evidence. The expert’s credentials are given, her exact words are placed in quotation marks, and the source is cited in parentheses. end annotated text
annotated text Source Citation in APA Style: No Page Numbers. Because the source of the quotation has no page numbers, the specific paragraph within the source (“final para.”; alternatively, “para. 18”) is provided in the parenthetical citation. end annotated text
student sample text Cuts continued in 2019, among them a maintenance contract for ventilators in the federal emergency supply and PREDICT, a U.S. agency for international development designed to identify and prevent pandemics (Goodman & Schulkin, 2020). In July 2019, the White House eliminated the position of an American public health official in Beijing, China, who was working with China’s disease control agency to help detect and contain infectious diseases. The first case of COVID-19 emerged in China four months later, on November 17, 2019. end student sample text
annotated text Development of First Main Point. This paragraph continues the chronological development of the first point, using a transitional sentence and evidence to discuss the year 2019. end annotated text
student sample text After the first U.S. coronavirus case was confirmed in 2020, the secretary of the Department of Health and Human Services (HHS) was named to lead a task force on a response, but after several months, he was replaced when then vice president Mike Pence was officially charged with leading the White House Coronavirus Task Force (Ballhaus & Armour, 2020). Experts who remained, including Dr. Deborah Birx and Dr. Anthony Fauci of the National Institutes of Health, were sidelined. Turnover of personnel in related government departments and agencies continued throughout 2020, leaving the country without experts in key positions to lead the pandemic response. end student sample text
annotated text Development of First Main Point. This paragraph continues the chronological development of the first point, using a transitional sentence and evidence to discuss the start of the pandemic in 2020. end annotated text
Inaction and Equipment Shortages
annotated text Body. The three paragraphs under this heading support the second main point in the thesis statement. end annotated text
student sample text In January and February of 2020, the president’s daily brief included more than a dozen detailed warnings, based on wire intercepts, computer intercepts, and satellite images by the U.S. intelligence community (Miller & Nakashima, 2020). Although senior officials began to assemble a task force, no direct action was taken until mid-March. end student sample text
annotated text Topic Sentences. The paragraph opens with two sentences stating the topic that is developed in the following paragraphs. end annotated text
student sample text The stockpile of medical equipment and personal protective equipment was dangerously low before the pandemic began. Although the federal government had paid $9.8 million to manufacturers in 2018 and 2019 to develop and produce protective masks, by April 2020 the government had not yet received a single mask (Swaine, 2020). Despite the low stockpile, a request by the head of the Food and Drug Administration (FDA) in early 2020 to begin contacting companies about possible shortages of necessary medical equipment, including personal protective equipment, was denied. This decision was made to avoid alarming the industry and the public and to avoid giving the impression that the administration was not prepared for the pandemic (Ballhaus & Armour, 2020). end student sample text
annotated text Topic Sentence. The paragraph opens with a sentence stating the topic that is developed in the paragraph. end annotated text
annotated text Objective Stance. The writer presents evidence (facts, statistics, and examples) in mostly neutral, unemotional language, which builds trustworthiness, or ethos , with readers. end annotated text
annotated text Synthesis. The paragraph synthesizes factual evidence from two sources. end annotated text
student sample text When former President Trump declared a national emergency on March 13, federal agencies began placing bulk orders for masks and other medical equipment. These orders led to critical shortages throughout the nation. In addition, states were instructed to acquire their own equipment and found themselves bidding against each other for the limited supplies available, leading one head of a coronavirus team composed of consulting and private equity firms to remark that “the federal stockpile was . . . supposed to be our stockpile. It’s not supposed to be states’ stockpiles that they then use” (Goodman & Schulkin, 2020, April 2, 2020). end student sample text
Policy Decisions
annotated text Body. The paragraph under this heading addresses the third main point in the thesis statement. end annotated text
student sample text Policy decisions, too, hampered the U.S. response to the pandemic. end student sample text
student sample text Although the HHS and NSC recommended stay-at-home directives on February 14, directives and guidelines for social distancing were not announced until March 16, and guidelines for mask wearing were inconsistent and contradictory (Goodman & Schulkin, 2020). Implementing the recommendations was left to the discretion of state governors, resulting in uneven stay-at-home orders, business closures, school closures, and mask mandates from state to state. The lack of a consistent message from the federal government not only delegated responsibility to state and local governments but also encouraged individuals to make their own choices, further hampering containment efforts. Seeing government officials and politicians without masks, for example, led many people to conclude that masks were unnecessary. Seeing large groups of people standing together at political rallies led people to ignore social distancing in their own lives. end student sample text
annotated text Synthesis. The paragraph synthesizes factual evidence from a source and examples drawn from the writer’s observation. end annotated text
student sample text Although the first cases of COVID-19 were detected in the United States in January, genetic researchers later determined that the viral strain responsible for sustained transmission of the disease did not enter the country until around February 13 (Branswell, 2020), providing further evidence that the failed U.S. response to the pandemic could have been prevented. Cuts to public health staff reduced the number of experts in leadership positions. Inaction in the early months of the pandemic led to critical shortages of medical equipment and supplies. Mixed messages and inconsistent policies undermined efforts to control and contain the disease. Unfortunately, the response to the disease in 2020 cannot be changed, but 2021 looks brighter. Most people who want the vaccine—nonexistent at the beginning of the pandemic and unavailable until recently—will have received it by the end of 2021. Americans will have experienced two years of living with the coronavirus, and everyone will have been affected in some way. end student sample text
annotated text Conclusion. The report concludes with a restatement of the main points given in the thesis and points to the future. end annotated text
Ballhaus, R., & Armour, S. (2020, April 22). Health chief’s early missteps set back coronavirus response. Wall Street Journal . https://www.wsj.com/articles/health-chiefs-early-missteps-set-back-coronavirus-response-11587570514
Branswell, H. (2020, May 26). New research rewrites history of when COVID-19 took off in the U.S.—and points to missed chances to stop it . STAT. https://www.statnews.com/2020/05/26/new-research-rewrites-history-of-when-covid-19-arrived-in-u-s-and-points-to-missed-chances-to-stop-it/
COVID-19 coronavirus pandemic . (2021, January 13). Worldometer. https://www.worldometers.info/coronavirus/#countries
Goodman, R., & Schulkin, D. (2020, November 3). Timeline of the coronavirus pandemic and U.S. response . Just Security. https://www.justsecurity.org/69650/timeline-of-the-coronavirus-pandemic-and-u-s-response/
Miller, G., & Nakashima, E. (2020, April 27). President’s intelligence briefing book repeatedly cited virus threat. Washington Post . https://www.washingtonpost.com/national-security/presidents-intelligence-briefing-book-repeatedly-cited-virus-threat/2020/04/27/ca66949a-8885-11ea-ac8a-fe9b8088e101_story.html
Sun, L. H. (2018, May 10). Top White House official in charge of pandemic response exits abruptly. Washington Post . https://www.washingtonpost.com/news/to-your-health/wp/2018/05/10/top-white-house-official-in-charge-of-pandemic-response-exits-abruptly/
Swaine, J. (2020, April 3). Federal government spent millions to ramp up mask readiness, but that isn’t helping now. Washington Post . https://www.washingtonpost.com/investigations/federal-government-spent-millions-to-ramp-up-mask-readiness-but-that-isnt-helping-now/2020/04/03/d62dda5c-74fa-11ea-a9bd-9f8b593300d0_story.html
annotated text References Page in APA Style. All sources cited in the text of the report, and only those sources, are listed in alphabetical order with full publication information. See the Handbook for more on APA documentation style. end annotated text
Discussion Questions
As an Amazon Associate we earn from qualifying purchases.
This book may not be used in the training of large language models or otherwise be ingested into large language models or generative AI offerings without OpenStax's permission.
Want to cite, share, or modify this book? This book uses the Creative Commons Attribution License and you must attribute OpenStax.
Access for free at https://openstax.org/books/writing-guide/pages/1-unit-introduction
- Authors: Michelle Bachelor Robinson, Maria Jerskey, featuring Toby Fulwiler
- Publisher/website: OpenStax
- Book title: Writing Guide with Handbook
- Publication date: Dec 21, 2021
- Location: Houston, Texas
- Book URL: https://openstax.org/books/writing-guide/pages/1-unit-introduction
- Section URL: https://openstax.org/books/writing-guide/pages/8-4-annotated-student-sample-u-s-response-to-covid-19-by-trevor-garcia
© Dec 19, 2023 OpenStax. Textbook content produced by OpenStax is licensed under a Creative Commons Attribution License . The OpenStax name, OpenStax logo, OpenStax book covers, OpenStax CNX name, and OpenStax CNX logo are not subject to the Creative Commons license and may not be reproduced without the prior and express written consent of Rice University.
COVID-19’s Economic Impact around the World
Key takeaways.
- Although the COVID-19 pandemic affected all parts of the world in 2020, low-, middle- and high-income nations were hit in different ways.
- In low-income countries, average excess mortality reached 34%, followed by 14% in middle-income countries and 10% in high-income ones.
- However, middle-income nations experienced the largest hit to their gross domestic product (GDP) growth, followed by high-income nations.
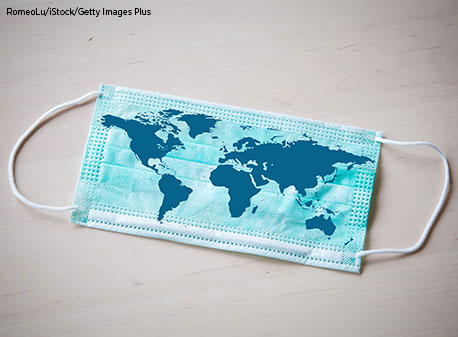
Since the COVID-19 pandemic began in March 2020, the world economy has been affected in many ways. Poorer countries have suffered the most, but, despite their greater resources, wealthier countries have faced their own challenges. This article looks at the impact of COVID-19 in different areas of the world.
First, I put 171 nations into three groups according to per capita income: low, middle and high income. Second, I examined health statistics to show how hard-hit by the virus these nations were. Then, by comparing economic forecasts the International Monetary Fund (IMF) made in October 2019 (pre-pandemic) for 2020 with their actual values, I obtained estimates for the pandemic’s impact on growth and key economic policy variables.
Low- and high-income groups each compose 25% of the world’s countries, and the middle-income group makes up 50%. Average income per capita in 2019 was more than five times larger in the middle-income group than in the low-income group. In the high-income countries, it was almost 20 times larger.
Health Outcomes and Policies
The first table shows that COVID-19 had a significant impact on all three groups. Average excess mortality, which indicates how much larger the number of deaths was relative to previous years, was more than 34% in low-income countries, almost 14% in middle-income countries and about 10% in high-income countries. And even though poorer countries were more affected by deaths, their COVID-19 testing was much more limited given their smaller resources.
Since the beginning of the pandemic, high-income countries did more than one test per person, while low-income countries did only one test per 27 people (or 0.037 per person). Given the significant differences in testing, it is not surprising that reported cases were much higher in wealthier countries. Finally, note that there were significant differences in the progress of vaccination. As of June 2021, nearly 20% of the population in the wealthiest countries was fully vaccinated compared to about 2% in the poorest countries.
Impact on GDP Growth
COVID-19-related lockdowns were very common during 2020-21, directly impacting economic activity. The figure below shows the impact on GDP. To isolate the impact of COVID-19 from previous trends, I plotted the difference between the actual GDP growth in 2020 and the IMF forecast made in October 2019.
The immediate consequence of closing many sectors of the economy was a significant decline in GDP growth, which was as large as 8.7 percentage points for the median middle-income countries. Wealthier countries suffered a bit less, with a median of 6.4 percentage points, mainly because they began to recover before the end of 2020. The impact of COVID-19 was smaller in poorer countries because many did not have the resources to implement strict lockdowns. However, even in this group of countries, median GDP growth was 5.2 percentage points lower than expected.
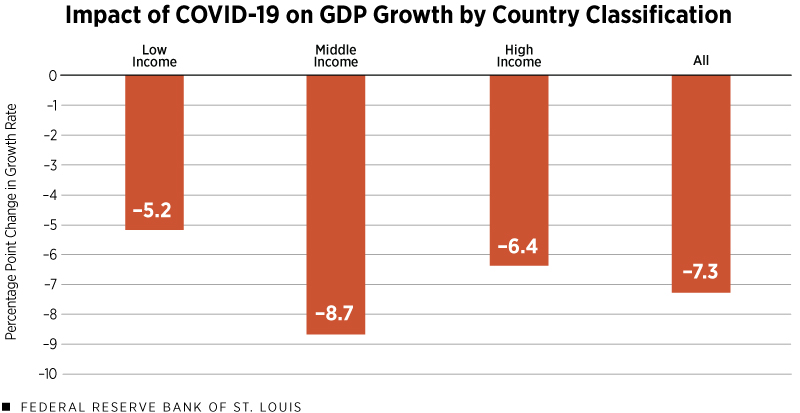
SOURCES: IMF World Economic Outlook Reports (April 2021 and October 2019), Penn World Table (version 10.0) and author’s calculations.
NOTE: The COVID-19 impact is the difference between the actual gross domestic product growth rate in 2020 and the IMF forecast for it made in October 2019.
Economic Policies
Differences in GDP performance are not only related to lockdowns but also to economic policy responses. The second table contains information about six policy variables.
In particular, the first three rows present the fiscal response to the pandemic computed as the difference between the actual value in 2020 and the IMF forecast made before the pandemic in October 2019 relative to GDP. Revenue relative to GDP declined slightly in all regions, but mostly in middle-income countries, reaching more than 1 percentage point of GDP.
Expenditures relative to GDP, however, increased in middle- and high-income countries while remaining stable in low-income countries. These expenditures increased by nearly 7 percentage points of GDP in high-income countries. The more significant fiscal deficit relative to GDP implied a larger increase in net government borrowing, which reached 7 percentage points of GDP in the median high-income countries.
Finally, COVID-19 also had a clear impact on the evolution of monetary aggregates such as cash and deposits. In the table, to isolate the impact of COVID-19 from previous trends, I present the growth rate of M1 and M2 M1 generally includes physical currency, demand deposits, traveler’s checks and other checkable deposits. M2 generally includes M1 plus savings deposits, money market securities, mutual funds and other time deposits. Note that the above definitions can differ slightly by country. net of the yearly growth rates of these variables between 2017 and 2019. The pandemic implied an increase in the growth rate of monetary aggregates across countries in all income groups, but more significantly in wealthier countries.
For instance, the growth rate in M1 was over 10 percentage points larger than in the previous two years in the median high-income countries. Without a change in money demand, such an acceleration in the quantity of money would have implied increasing inflation.
However, the last row of the table shows that inflation remained stable in 2020. In fact, for middle- and high-income countries, inflation in 2020 was lower than the IMF forecast made in October 2019.
Conclusions
COVID-19 impacted health outcomes in all regions of the world. Wealthier countries responded with more testing and quicker vaccination rates. Comparing actual outcomes with pre-pandemic forecasts, I found a significant impact of the pandemic on GDP growth, which is more prominent in middle-income countries.
I conjecture that the impact on GDP growth was less significant in the poorest countries because of less restrictive lockdowns and in the wealthiest countries because of more aggressive economic policies.
- M1 generally includes physical currency, demand deposits, traveler’s checks and other checkable deposits. M2 generally includes M1 plus savings deposits, money market securities, mutual funds and other time deposits. Note that the above definitions can differ slightly by country.
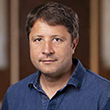
Juan M. Sánchez is an economist and senior economic policy advisor at the Federal Reserve Bank of St. Louis. He has conducted research on several topics in macroeconomics involving financial decisions by firms, households and countries. He has been at the St. Louis Fed since 2010. View more about the author and his research.
Related Topics
Views expressed in Regional Economist are not necessarily those of the St. Louis Fed or Federal Reserve System.
For the latest insights from our economists and other St. Louis Fed experts, visit On the Economy and subscribe .
Media questions
How to Write About Coronavirus in a College Essay
Students can share how they navigated life during the coronavirus pandemic in a full-length essay or an optional supplement.
Writing About COVID-19 in College Essays

Getty Images
Experts say students should be honest and not limit themselves to merely their experiences with the pandemic.
The global impact of COVID-19, the disease caused by the novel coronavirus, means colleges and prospective students alike are in for an admissions cycle like no other. Both face unprecedented challenges and questions as they grapple with their respective futures amid the ongoing fallout of the pandemic.
Colleges must examine applicants without the aid of standardized test scores for many – a factor that prompted many schools to go test-optional for now . Even grades, a significant component of a college application, may be hard to interpret with some high schools adopting pass-fail classes last spring due to the pandemic. Major college admissions factors are suddenly skewed.
"I can't help but think other (admissions) factors are going to matter more," says Ethan Sawyer, founder of the College Essay Guy, a website that offers free and paid essay-writing resources.
College essays and letters of recommendation , Sawyer says, are likely to carry more weight than ever in this admissions cycle. And many essays will likely focus on how the pandemic shaped students' lives throughout an often tumultuous 2020.
But before writing a college essay focused on the coronavirus, students should explore whether it's the best topic for them.
Writing About COVID-19 for a College Application
Much of daily life has been colored by the coronavirus. Virtual learning is the norm at many colleges and high schools, many extracurriculars have vanished and social lives have stalled for students complying with measures to stop the spread of COVID-19.
"For some young people, the pandemic took away what they envisioned as their senior year," says Robert Alexander, dean of admissions, financial aid and enrollment management at the University of Rochester in New York. "Maybe that's a spot on a varsity athletic team or the lead role in the fall play. And it's OK for them to mourn what should have been and what they feel like they lost, but more important is how are they making the most of the opportunities they do have?"
That question, Alexander says, is what colleges want answered if students choose to address COVID-19 in their college essay.
But the question of whether a student should write about the coronavirus is tricky. The answer depends largely on the student.
"In general, I don't think students should write about COVID-19 in their main personal statement for their application," Robin Miller, master college admissions counselor at IvyWise, a college counseling company, wrote in an email.
"Certainly, there may be exceptions to this based on a student's individual experience, but since the personal essay is the main place in the application where the student can really allow their voice to be heard and share insight into who they are as an individual, there are likely many other topics they can choose to write about that are more distinctive and unique than COVID-19," Miller says.
Opinions among admissions experts vary on whether to write about the likely popular topic of the pandemic.
"If your essay communicates something positive, unique, and compelling about you in an interesting and eloquent way, go for it," Carolyn Pippen, principal college admissions counselor at IvyWise, wrote in an email. She adds that students shouldn't be dissuaded from writing about a topic merely because it's common, noting that "topics are bound to repeat, no matter how hard we try to avoid it."
Above all, she urges honesty.
"If your experience within the context of the pandemic has been truly unique, then write about that experience, and the standing out will take care of itself," Pippen says. "If your experience has been generally the same as most other students in your context, then trying to find a unique angle can easily cross the line into exploiting a tragedy, or at least appearing as though you have."
But focusing entirely on the pandemic can limit a student to a single story and narrow who they are in an application, Sawyer says. "There are so many wonderful possibilities for what you can say about yourself outside of your experience within the pandemic."
He notes that passions, strengths, career interests and personal identity are among the multitude of essay topic options available to applicants and encourages them to probe their values to help determine the topic that matters most to them – and write about it.
That doesn't mean the pandemic experience has to be ignored if applicants feel the need to write about it.
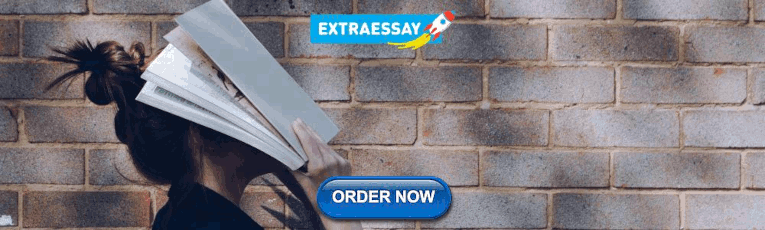
Writing About Coronavirus in Main and Supplemental Essays
Students can choose to write a full-length college essay on the coronavirus or summarize their experience in a shorter form.
To help students explain how the pandemic affected them, The Common App has added an optional section to address this topic. Applicants have 250 words to describe their pandemic experience and the personal and academic impact of COVID-19.
"That's not a trick question, and there's no right or wrong answer," Alexander says. Colleges want to know, he adds, how students navigated the pandemic, how they prioritized their time, what responsibilities they took on and what they learned along the way.
If students can distill all of the above information into 250 words, there's likely no need to write about it in a full-length college essay, experts say. And applicants whose lives were not heavily altered by the pandemic may even choose to skip the optional COVID-19 question.
"This space is best used to discuss hardship and/or significant challenges that the student and/or the student's family experienced as a result of COVID-19 and how they have responded to those difficulties," Miller notes. Using the section to acknowledge a lack of impact, she adds, "could be perceived as trite and lacking insight, despite the good intentions of the applicant."
To guard against this lack of awareness, Sawyer encourages students to tap someone they trust to review their writing , whether it's the 250-word Common App response or the full-length essay.
Experts tend to agree that the short-form approach to this as an essay topic works better, but there are exceptions. And if a student does have a coronavirus story that he or she feels must be told, Alexander encourages the writer to be authentic in the essay.
"My advice for an essay about COVID-19 is the same as my advice about an essay for any topic – and that is, don't write what you think we want to read or hear," Alexander says. "Write what really changed you and that story that now is yours and yours alone to tell."
Sawyer urges students to ask themselves, "What's the sentence that only I can write?" He also encourages students to remember that the pandemic is only a chapter of their lives and not the whole book.
Miller, who cautions against writing a full-length essay on the coronavirus, says that if students choose to do so they should have a conversation with their high school counselor about whether that's the right move. And if students choose to proceed with COVID-19 as a topic, she says they need to be clear, detailed and insightful about what they learned and how they adapted along the way.
"Approaching the essay in this manner will provide important balance while demonstrating personal growth and vulnerability," Miller says.
Pippen encourages students to remember that they are in an unprecedented time for college admissions.
"It is important to keep in mind with all of these (admission) factors that no colleges have ever had to consider them this way in the selection process, if at all," Pippen says. "They have had very little time to calibrate their evaluations of different application components within their offices, let alone across institutions. This means that colleges will all be handling the admissions process a little bit differently, and their approaches may even evolve over the course of the admissions cycle."
Searching for a college? Get our complete rankings of Best Colleges.
10 Ways to Discover College Essay Ideas

Tags: students , colleges , college admissions , college applications , college search , Coronavirus
2024 Best Colleges

Search for your perfect fit with the U.S. News rankings of colleges and universities.
College Admissions: Get a Step Ahead!
Sign up to receive the latest updates from U.S. News & World Report and our trusted partners and sponsors. By clicking submit, you are agreeing to our Terms and Conditions & Privacy Policy .
Ask an Alum: Making the Most Out of College
You May Also Like
Find a job in the age of ai.
Angie Kamath April 25, 2024

Protests Boil Over on College Campuses
Lauren Camera April 22, 2024

Supporting Low-Income College Applicants
Shavar Jeffries April 16, 2024

Supporting Black Women in Higher Ed
Zainab Okolo April 15, 2024

Law Schools With the Highest LSATs
Ilana Kowarski and Cole Claybourn April 11, 2024

Today NAIA, Tomorrow Title IX?
Lauren Camera April 9, 2024

Grad School Housing Options
Anayat Durrani April 9, 2024

How to Decide if an MBA Is Worth it
Sarah Wood March 27, 2024

What to Wear to a Graduation
LaMont Jones, Jr. March 27, 2024

FAFSA Delays Alarm Families, Colleges
Sarah Wood March 25, 2024


- SUGGESTED TOPICS
- The Magazine
- Newsletters
- Managing Yourself
- Managing Teams
- Work-life Balance
- The Big Idea
- Data & Visuals
- Reading Lists
- Case Selections
- HBR Learning
- Topic Feeds
- Account Settings
- Email Preferences
Bringing an Analytics Mindset to the Pandemic
- Nico Neumann

How to collect, weigh, and report complex data.
The Covid-19 crisis reminds us that data analytics problems are never simple. Analysts usually need to make difficult and to some extent subjective judgments about how how much data to collect (and from how wide a universe), how much relative weight to give different factors when evaluating the causal chain, and how to report the results of the analysis.
In these difficult times, we’ve made a number of our coronavirus articles free for all readers. To get all of HBR’s content delivered to your inbox, sign up for the Daily Alert newsletter.
Spend just 10 minutes on Twitter to catch up with Covid-19 news, and you’ll run into updated numbers and loud (sometimes angry) arguments about what all the data we’re collecting means. It’s proving difficult to pin down how infectious the virus is, what its mortality rate is, how effective different mitigation efforts are, and why different regions are seeing such different patterns of infection, mortality, and recurrence.

- NN Nico Neumann is a fellow at the Centre for Business Analytics and an assistant professor at the Melbourne Business School, where he teaches business analytics and marketing communications.
Partner Center
- CBSE Class 10th
- CBSE Class 12th
- UP Board 10th
- UP Board 12th
- Bihar Board 10th
- Bihar Board 12th
- Top Schools in India
- Top Schools in Delhi
- Top Schools in Mumbai
- Top Schools in Chennai
- Top Schools in Hyderabad
- Top Schools in Kolkata
- Top Schools in Pune
- Top Schools in Bangalore
Products & Resources
- JEE Main Knockout April
- Free Sample Papers
- Free Ebooks
- NCERT Notes
- NCERT Syllabus
- NCERT Books
- RD Sharma Solutions
- Navodaya Vidyalaya Admission 2024-25
- NCERT Solutions
- NCERT Solutions for Class 12
- NCERT Solutions for Class 11
- NCERT solutions for Class 10
- NCERT solutions for Class 9
- NCERT solutions for Class 8
- NCERT Solutions for Class 7
- JEE Main 2024
- MHT CET 2024
- JEE Advanced 2024
- BITSAT 2024
- View All Engineering Exams
- Colleges Accepting B.Tech Applications
- Top Engineering Colleges in India
- Engineering Colleges in India
- Engineering Colleges in Tamil Nadu
- Engineering Colleges Accepting JEE Main
- Top IITs in India
- Top NITs in India
- Top IIITs in India
- JEE Main College Predictor
- JEE Main Rank Predictor
- MHT CET College Predictor
- AP EAMCET College Predictor
- GATE College Predictor
- KCET College Predictor
- JEE Advanced College Predictor
- View All College Predictors
- JEE Main Question Paper
- JEE Main Cutoff
- JEE Main Answer Key
- JEE Main Result
- Download E-Books and Sample Papers
- Compare Colleges
- B.Tech College Applications
- JEE Advanced Registration
- MAH MBA CET Exam
- View All Management Exams
Colleges & Courses
- MBA College Admissions
- MBA Colleges in India
- Top IIMs Colleges in India
- Top Online MBA Colleges in India
- MBA Colleges Accepting XAT Score
- BBA Colleges in India
- XAT College Predictor 2024
- SNAP College Predictor
- NMAT College Predictor
- MAT College Predictor 2024
- CMAT College Predictor 2024
- CAT Percentile Predictor 2023
- CAT 2023 College Predictor
- CMAT 2024 Registration
- TS ICET 2024 Registration
- CMAT Exam Date 2024
- MAH MBA CET Cutoff 2024
- Download Helpful Ebooks
- List of Popular Branches
- QnA - Get answers to your doubts
- IIM Fees Structure
- AIIMS Nursing
- Top Medical Colleges in India
- Top Medical Colleges in India accepting NEET Score
- Medical Colleges accepting NEET
- List of Medical Colleges in India
- List of AIIMS Colleges In India
- Medical Colleges in Maharashtra
- Medical Colleges in India Accepting NEET PG
- NEET College Predictor
- NEET PG College Predictor
- NEET MDS College Predictor
- DNB CET College Predictor
- DNB PDCET College Predictor
- NEET Application Form 2024
- NEET PG Application Form 2024
- NEET Cut off
- NEET Online Preparation
- Download Helpful E-books
- LSAT India 2024
- Colleges Accepting Admissions
- Top Law Colleges in India
- Law College Accepting CLAT Score
- List of Law Colleges in India
- Top Law Colleges in Delhi
- Top Law Collages in Indore
- Top Law Colleges in Chandigarh
- Top Law Collages in Lucknow
Predictors & E-Books
- CLAT College Predictor
- MHCET Law ( 5 Year L.L.B) College Predictor
- AILET College Predictor
- Sample Papers
- Compare Law Collages
- Careers360 Youtube Channel
- CLAT Syllabus 2025
- CLAT Previous Year Question Paper
- AIBE 18 Result 2023
- NID DAT Exam
- Pearl Academy Exam
Animation Courses
- Animation Courses in India
- Animation Courses in Bangalore
- Animation Courses in Mumbai
- Animation Courses in Pune
- Animation Courses in Chennai
- Animation Courses in Hyderabad
- Design Colleges in India
- Fashion Design Colleges in Bangalore
- Fashion Design Colleges in Mumbai
- Fashion Design Colleges in Pune
- Fashion Design Colleges in Delhi
- Fashion Design Colleges in Hyderabad
- Fashion Design Colleges in India
- Top Design Colleges in India
- Free Design E-books
- List of Branches
- Careers360 Youtube channel
- NIFT College Predictor
- UCEED College Predictor
- NID DAT College Predictor
- IPU CET BJMC
- JMI Mass Communication Entrance Exam
- IIMC Entrance Exam
- Media & Journalism colleges in Delhi
- Media & Journalism colleges in Bangalore
- Media & Journalism colleges in Mumbai
- List of Media & Journalism Colleges in India
- CA Intermediate
- CA Foundation
- CS Executive
- CS Professional
- Difference between CA and CS
- Difference between CA and CMA
- CA Full form
- CMA Full form
- CS Full form
- CA Salary In India
Top Courses & Careers
- Bachelor of Commerce (B.Com)
- Master of Commerce (M.Com)
- Company Secretary
- Cost Accountant
- Charted Accountant
- Credit Manager
- Financial Advisor
- Top Commerce Colleges in India
- Top Government Commerce Colleges in India
- Top Private Commerce Colleges in India
- Top M.Com Colleges in Mumbai
- Top B.Com Colleges in India
- IT Colleges in Tamil Nadu
- IT Colleges in Uttar Pradesh
- MCA Colleges in India
- BCA Colleges in India
Quick Links
- Information Technology Courses
- Programming Courses
- Web Development Courses
- Data Analytics Courses
- Big Data Analytics Courses
- RUHS Pharmacy Admission Test
- Top Pharmacy Colleges in India
- Pharmacy Colleges in Pune
- Pharmacy Colleges in Mumbai
- Colleges Accepting GPAT Score
- Pharmacy Colleges in Lucknow
- List of Pharmacy Colleges in Nagpur
- GPAT Result
- GPAT 2024 Admit Card
- GPAT Question Papers
- NCHMCT JEE 2024
- Mah BHMCT CET
- Top Hotel Management Colleges in Delhi
- Top Hotel Management Colleges in Hyderabad
- Top Hotel Management Colleges in Mumbai
- Top Hotel Management Colleges in Tamil Nadu
- Top Hotel Management Colleges in Maharashtra
- B.Sc Hotel Management
- Hotel Management
- Diploma in Hotel Management and Catering Technology
Diploma Colleges
- Top Diploma Colleges in Maharashtra
- UPSC IAS 2024
- SSC CGL 2024
- IBPS RRB 2024
- Previous Year Sample Papers
- Free Competition E-books
- Sarkari Result
- QnA- Get your doubts answered
- UPSC Previous Year Sample Papers
- CTET Previous Year Sample Papers
- SBI Clerk Previous Year Sample Papers
- NDA Previous Year Sample Papers
Upcoming Events
- NDA Application Form 2024
- UPSC IAS Application Form 2024
- CDS Application Form 2024
- CTET Admit card 2024
- HP TET Result 2023
- SSC GD Constable Admit Card 2024
- UPTET Notification 2024
- SBI Clerk Result 2024
Other Exams
- SSC CHSL 2024
- UP PCS 2024
- UGC NET 2024
- RRB NTPC 2024
- IBPS PO 2024
- IBPS Clerk 2024
- IBPS SO 2024
- Top University in USA
- Top University in Canada
- Top University in Ireland
- Top Universities in UK
- Top Universities in Australia
- Best MBA Colleges in Abroad
- Business Management Studies Colleges
Top Countries
- Study in USA
- Study in UK
- Study in Canada
- Study in Australia
- Study in Ireland
- Study in Germany
- Study in China
- Study in Europe
Student Visas
- Student Visa Canada
- Student Visa UK
- Student Visa USA
- Student Visa Australia
- Student Visa Germany
- Student Visa New Zealand
- Student Visa Ireland
- CUET PG 2024
- IGNOU B.Ed Admission 2024
- DU Admission 2024
- UP B.Ed JEE 2024
- LPU NEST 2024
- IIT JAM 2024
- IGNOU Online Admission 2024
- Universities in India
- Top Universities in India 2024
- Top Colleges in India
- Top Universities in Uttar Pradesh 2024
- Top Universities in Bihar
- Top Universities in Madhya Pradesh 2024
- Top Universities in Tamil Nadu 2024
- Central Universities in India
- CUET Exam City Intimation Slip 2024
- IGNOU Date Sheet
- CUET Mock Test 2024
- CUET Admit card 2024
- CUET PG Syllabus 2024
- CUET Participating Universities 2024
- CUET Previous Year Question Paper
- CUET Syllabus 2024 for Science Students
- E-Books and Sample Papers
- CUET Exam Pattern 2024
- CUET Exam Date 2024
- CUET Syllabus 2024
- IGNOU Exam Form 2024
- IGNOU Result
- CUET Courses List 2024
Engineering Preparation
- Knockout JEE Main 2024
- Test Series JEE Main 2024
- JEE Main 2024 Rank Booster
Medical Preparation
- Knockout NEET 2024
- Test Series NEET 2024
- Rank Booster NEET 2024
Online Courses
- JEE Main One Month Course
- NEET One Month Course
- IBSAT Free Mock Tests
- IIT JEE Foundation Course
- Knockout BITSAT 2024
- Career Guidance Tool
Top Streams
- IT & Software Certification Courses
- Engineering and Architecture Certification Courses
- Programming And Development Certification Courses
- Business and Management Certification Courses
- Marketing Certification Courses
- Health and Fitness Certification Courses
- Design Certification Courses
Specializations
- Digital Marketing Certification Courses
- Cyber Security Certification Courses
- Artificial Intelligence Certification Courses
- Business Analytics Certification Courses
- Data Science Certification Courses
- Cloud Computing Certification Courses
- Machine Learning Certification Courses
- View All Certification Courses
- UG Degree Courses
- PG Degree Courses
- Short Term Courses
- Free Courses
- Online Degrees and Diplomas
- Compare Courses
Top Providers
- Coursera Courses
- Udemy Courses
- Edx Courses
- Swayam Courses
- upGrad Courses
- Simplilearn Courses
- Great Learning Courses
Access premium articles, webinars, resources to make the best decisions for career, course, exams, scholarships, study abroad and much more with
Plan, Prepare & Make the Best Career Choices
Covid 19 Essay in English
Essay on Covid -19: In a very short amount of time, coronavirus has spread globally. It has had an enormous impact on people's lives, economy, and societies all around the world, affecting every country. Governments have had to take severe measures to try and contain the pandemic. The virus has altered our way of life in many ways, including its effects on our health and our economy. Here are a few sample essays on ‘CoronaVirus’.
100 Words Essay on Covid 19
200 words essay on covid 19, 500 words essay on covid 19.
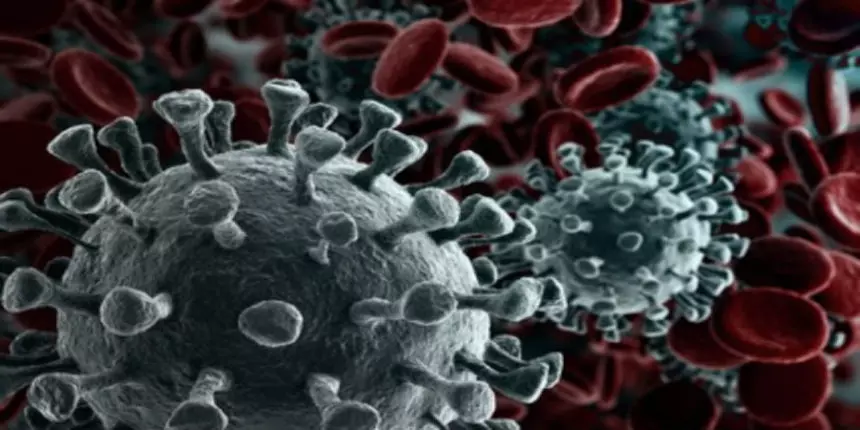
COVID-19 or Corona Virus is a novel coronavirus that was first identified in 2019. It is similar to other coronaviruses, such as SARS-CoV and MERS-CoV, but it is more contagious and has caused more severe respiratory illness in people who have been infected. The novel coronavirus became a global pandemic in a very short period of time. It has affected lives, economies and societies across the world, leaving no country untouched. The virus has caused governments to take drastic measures to try and contain it. From health implications to economic and social ramifications, COVID-19 impacted every part of our lives. It has been more than 2 years since the pandemic hit and the world is still recovering from its effects.
Since the outbreak of COVID-19, the world has been impacted in a number of ways. For one, the global economy has taken a hit as businesses have been forced to close their doors. This has led to widespread job losses and an increase in poverty levels around the world. Additionally, countries have had to impose strict travel restrictions in an attempt to contain the virus, which has resulted in a decrease in tourism and international trade. Furthermore, the pandemic has put immense pressure on healthcare systems globally, as hospitals have been overwhelmed with patients suffering from the virus. Lastly, the outbreak has led to a general feeling of anxiety and uncertainty, as people are fearful of contracting the disease.
My Experience of COVID-19
I still remember how abruptly colleges and schools shut down in March 2020. I was a college student at that time and I was under the impression that everything would go back to normal in a few weeks. I could not have been more wrong. The situation only got worse every week and the government had to impose a lockdown. There were so many restrictions in place. For example, we had to wear face masks whenever we left the house, and we could only go out for essential errands. Restaurants and shops were only allowed to operate at take-out capacity, and many businesses were shut down.
In the current scenario, coronavirus is dominating all aspects of our lives. The coronavirus pandemic has wreaked havoc upon people’s lives, altering the way we live and work in a very short amount of time. It has revolutionised how we think about health care, education, and even social interaction. This virus has had long-term implications on our society, including its impact on mental health, economic stability, and global politics. But we as individuals can help to mitigate these effects by taking personal responsibility to protect themselves and those around them from infection.
Effects of CoronaVirus on Education
The outbreak of coronavirus has had a significant impact on education systems around the world. In China, where the virus originated, all schools and universities were closed for several weeks in an effort to contain the spread of the disease. Many other countries have followed suit, either closing schools altogether or suspending classes for a period of time.
This has resulted in a major disruption to the education of millions of students. Some have been able to continue their studies online, but many have not had access to the internet or have not been able to afford the costs associated with it. This has led to a widening of the digital divide between those who can afford to continue their education online and those who cannot.
The closure of schools has also had a negative impact on the mental health of many students. With no face-to-face contact with friends and teachers, some students have felt isolated and anxious. This has been compounded by the worry and uncertainty surrounding the virus itself.
The situation with coronavirus has improved and schools have been reopened but students are still catching up with the gap of 2 years that the pandemic created. In the meantime, governments and educational institutions are working together to find ways to support students and ensure that they are able to continue their education despite these difficult circumstances.
Effects of CoronaVirus on Economy
The outbreak of the coronavirus has had a significant impact on the global economy. The virus, which originated in China, has spread to over two hundred countries, resulting in widespread panic and a decrease in global trade. As a result of the outbreak, many businesses have been forced to close their doors, leading to a rise in unemployment. In addition, the stock market has taken a severe hit.
Effects of CoronaVirus on Health
The effects that coronavirus has on one's health are still being studied and researched as the virus continues to spread throughout the world. However, some of the potential effects on health that have been observed thus far include respiratory problems, fever, and coughing. In severe cases, pneumonia, kidney failure, and death can occur. It is important for people who think they may have been exposed to the virus to seek medical attention immediately so that they can be treated properly and avoid any serious complications. There is no specific cure or treatment for coronavirus at this time, but there are ways to help ease symptoms and prevent the virus from spreading.
Explore Career Options (By Industry)
- Construction
- Entertainment
- Manufacturing
- Information Technology
Data Administrator
Database professionals use software to store and organise data such as financial information, and customer shipping records. Individuals who opt for a career as data administrators ensure that data is available for users and secured from unauthorised sales. DB administrators may work in various types of industries. It may involve computer systems design, service firms, insurance companies, banks and hospitals.
Bio Medical Engineer
The field of biomedical engineering opens up a universe of expert chances. An Individual in the biomedical engineering career path work in the field of engineering as well as medicine, in order to find out solutions to common problems of the two fields. The biomedical engineering job opportunities are to collaborate with doctors and researchers to develop medical systems, equipment, or devices that can solve clinical problems. Here we will be discussing jobs after biomedical engineering, how to get a job in biomedical engineering, biomedical engineering scope, and salary.
Ethical Hacker
A career as ethical hacker involves various challenges and provides lucrative opportunities in the digital era where every giant business and startup owns its cyberspace on the world wide web. Individuals in the ethical hacker career path try to find the vulnerabilities in the cyber system to get its authority. If he or she succeeds in it then he or she gets its illegal authority. Individuals in the ethical hacker career path then steal information or delete the file that could affect the business, functioning, or services of the organization.
GIS officer work on various GIS software to conduct a study and gather spatial and non-spatial information. GIS experts update the GIS data and maintain it. The databases include aerial or satellite imagery, latitudinal and longitudinal coordinates, and manually digitized images of maps. In a career as GIS expert, one is responsible for creating online and mobile maps.
Data Analyst
The invention of the database has given fresh breath to the people involved in the data analytics career path. Analysis refers to splitting up a whole into its individual components for individual analysis. Data analysis is a method through which raw data are processed and transformed into information that would be beneficial for user strategic thinking.
Data are collected and examined to respond to questions, evaluate hypotheses or contradict theories. It is a tool for analyzing, transforming, modeling, and arranging data with useful knowledge, to assist in decision-making and methods, encompassing various strategies, and is used in different fields of business, research, and social science.
Geothermal Engineer
Individuals who opt for a career as geothermal engineers are the professionals involved in the processing of geothermal energy. The responsibilities of geothermal engineers may vary depending on the workplace location. Those who work in fields design facilities to process and distribute geothermal energy. They oversee the functioning of machinery used in the field.
Database Architect
If you are intrigued by the programming world and are interested in developing communications networks then a career as database architect may be a good option for you. Data architect roles and responsibilities include building design models for data communication networks. Wide Area Networks (WANs), local area networks (LANs), and intranets are included in the database networks. It is expected that database architects will have in-depth knowledge of a company's business to develop a network to fulfil the requirements of the organisation. Stay tuned as we look at the larger picture and give you more information on what is db architecture, why you should pursue database architecture, what to expect from such a degree and what your job opportunities will be after graduation. Here, we will be discussing how to become a data architect. Students can visit NIT Trichy , IIT Kharagpur , JMI New Delhi .
Remote Sensing Technician
Individuals who opt for a career as a remote sensing technician possess unique personalities. Remote sensing analysts seem to be rational human beings, they are strong, independent, persistent, sincere, realistic and resourceful. Some of them are analytical as well, which means they are intelligent, introspective and inquisitive.
Remote sensing scientists use remote sensing technology to support scientists in fields such as community planning, flight planning or the management of natural resources. Analysing data collected from aircraft, satellites or ground-based platforms using statistical analysis software, image analysis software or Geographic Information Systems (GIS) is a significant part of their work. Do you want to learn how to become remote sensing technician? There's no need to be concerned; we've devised a simple remote sensing technician career path for you. Scroll through the pages and read.
Budget Analyst
Budget analysis, in a nutshell, entails thoroughly analyzing the details of a financial budget. The budget analysis aims to better understand and manage revenue. Budget analysts assist in the achievement of financial targets, the preservation of profitability, and the pursuit of long-term growth for a business. Budget analysts generally have a bachelor's degree in accounting, finance, economics, or a closely related field. Knowledge of Financial Management is of prime importance in this career.
Underwriter
An underwriter is a person who assesses and evaluates the risk of insurance in his or her field like mortgage, loan, health policy, investment, and so on and so forth. The underwriter career path does involve risks as analysing the risks means finding out if there is a way for the insurance underwriter jobs to recover the money from its clients. If the risk turns out to be too much for the company then in the future it is an underwriter who will be held accountable for it. Therefore, one must carry out his or her job with a lot of attention and diligence.
Finance Executive
Product manager.
A Product Manager is a professional responsible for product planning and marketing. He or she manages the product throughout the Product Life Cycle, gathering and prioritising the product. A product manager job description includes defining the product vision and working closely with team members of other departments to deliver winning products.
Operations Manager
Individuals in the operations manager jobs are responsible for ensuring the efficiency of each department to acquire its optimal goal. They plan the use of resources and distribution of materials. The operations manager's job description includes managing budgets, negotiating contracts, and performing administrative tasks.
Stock Analyst
Individuals who opt for a career as a stock analyst examine the company's investments makes decisions and keep track of financial securities. The nature of such investments will differ from one business to the next. Individuals in the stock analyst career use data mining to forecast a company's profits and revenues, advise clients on whether to buy or sell, participate in seminars, and discussing financial matters with executives and evaluate annual reports.
A Researcher is a professional who is responsible for collecting data and information by reviewing the literature and conducting experiments and surveys. He or she uses various methodological processes to provide accurate data and information that is utilised by academicians and other industry professionals. Here, we will discuss what is a researcher, the researcher's salary, types of researchers.
Welding Engineer
Welding Engineer Job Description: A Welding Engineer work involves managing welding projects and supervising welding teams. He or she is responsible for reviewing welding procedures, processes and documentation. A career as Welding Engineer involves conducting failure analyses and causes on welding issues.
Transportation Planner
A career as Transportation Planner requires technical application of science and technology in engineering, particularly the concepts, equipment and technologies involved in the production of products and services. In fields like land use, infrastructure review, ecological standards and street design, he or she considers issues of health, environment and performance. A Transportation Planner assigns resources for implementing and designing programmes. He or she is responsible for assessing needs, preparing plans and forecasts and compliance with regulations.
Environmental Engineer
Individuals who opt for a career as an environmental engineer are construction professionals who utilise the skills and knowledge of biology, soil science, chemistry and the concept of engineering to design and develop projects that serve as solutions to various environmental problems.
Safety Manager
A Safety Manager is a professional responsible for employee’s safety at work. He or she plans, implements and oversees the company’s employee safety. A Safety Manager ensures compliance and adherence to Occupational Health and Safety (OHS) guidelines.
Conservation Architect
A Conservation Architect is a professional responsible for conserving and restoring buildings or monuments having a historic value. He or she applies techniques to document and stabilise the object’s state without any further damage. A Conservation Architect restores the monuments and heritage buildings to bring them back to their original state.
Structural Engineer
A Structural Engineer designs buildings, bridges, and other related structures. He or she analyzes the structures and makes sure the structures are strong enough to be used by the people. A career as a Structural Engineer requires working in the construction process. It comes under the civil engineering discipline. A Structure Engineer creates structural models with the help of computer-aided design software.
Highway Engineer
Highway Engineer Job Description: A Highway Engineer is a civil engineer who specialises in planning and building thousands of miles of roads that support connectivity and allow transportation across the country. He or she ensures that traffic management schemes are effectively planned concerning economic sustainability and successful implementation.
Field Surveyor
Are you searching for a Field Surveyor Job Description? A Field Surveyor is a professional responsible for conducting field surveys for various places or geographical conditions. He or she collects the required data and information as per the instructions given by senior officials.
Orthotist and Prosthetist
Orthotists and Prosthetists are professionals who provide aid to patients with disabilities. They fix them to artificial limbs (prosthetics) and help them to regain stability. There are times when people lose their limbs in an accident. In some other occasions, they are born without a limb or orthopaedic impairment. Orthotists and prosthetists play a crucial role in their lives with fixing them to assistive devices and provide mobility.
Pathologist
A career in pathology in India is filled with several responsibilities as it is a medical branch and affects human lives. The demand for pathologists has been increasing over the past few years as people are getting more aware of different diseases. Not only that, but an increase in population and lifestyle changes have also contributed to the increase in a pathologist’s demand. The pathology careers provide an extremely huge number of opportunities and if you want to be a part of the medical field you can consider being a pathologist. If you want to know more about a career in pathology in India then continue reading this article.
Veterinary Doctor
Speech therapist, gynaecologist.
Gynaecology can be defined as the study of the female body. The job outlook for gynaecology is excellent since there is evergreen demand for one because of their responsibility of dealing with not only women’s health but also fertility and pregnancy issues. Although most women prefer to have a women obstetrician gynaecologist as their doctor, men also explore a career as a gynaecologist and there are ample amounts of male doctors in the field who are gynaecologists and aid women during delivery and childbirth.
Audiologist
The audiologist career involves audiology professionals who are responsible to treat hearing loss and proactively preventing the relevant damage. Individuals who opt for a career as an audiologist use various testing strategies with the aim to determine if someone has a normal sensitivity to sounds or not. After the identification of hearing loss, a hearing doctor is required to determine which sections of the hearing are affected, to what extent they are affected, and where the wound causing the hearing loss is found. As soon as the hearing loss is identified, the patients are provided with recommendations for interventions and rehabilitation such as hearing aids, cochlear implants, and appropriate medical referrals. While audiology is a branch of science that studies and researches hearing, balance, and related disorders.
An oncologist is a specialised doctor responsible for providing medical care to patients diagnosed with cancer. He or she uses several therapies to control the cancer and its effect on the human body such as chemotherapy, immunotherapy, radiation therapy and biopsy. An oncologist designs a treatment plan based on a pathology report after diagnosing the type of cancer and where it is spreading inside the body.
Are you searching for an ‘Anatomist job description’? An Anatomist is a research professional who applies the laws of biological science to determine the ability of bodies of various living organisms including animals and humans to regenerate the damaged or destroyed organs. If you want to know what does an anatomist do, then read the entire article, where we will answer all your questions.
For an individual who opts for a career as an actor, the primary responsibility is to completely speak to the character he or she is playing and to persuade the crowd that the character is genuine by connecting with them and bringing them into the story. This applies to significant roles and littler parts, as all roles join to make an effective creation. Here in this article, we will discuss how to become an actor in India, actor exams, actor salary in India, and actor jobs.
Individuals who opt for a career as acrobats create and direct original routines for themselves, in addition to developing interpretations of existing routines. The work of circus acrobats can be seen in a variety of performance settings, including circus, reality shows, sports events like the Olympics, movies and commercials. Individuals who opt for a career as acrobats must be prepared to face rejections and intermittent periods of work. The creativity of acrobats may extend to other aspects of the performance. For example, acrobats in the circus may work with gym trainers, celebrities or collaborate with other professionals to enhance such performance elements as costume and or maybe at the teaching end of the career.
Video Game Designer
Career as a video game designer is filled with excitement as well as responsibilities. A video game designer is someone who is involved in the process of creating a game from day one. He or she is responsible for fulfilling duties like designing the character of the game, the several levels involved, plot, art and similar other elements. Individuals who opt for a career as a video game designer may also write the codes for the game using different programming languages.
Depending on the video game designer job description and experience they may also have to lead a team and do the early testing of the game in order to suggest changes and find loopholes.
Radio Jockey
Radio Jockey is an exciting, promising career and a great challenge for music lovers. If you are really interested in a career as radio jockey, then it is very important for an RJ to have an automatic, fun, and friendly personality. If you want to get a job done in this field, a strong command of the language and a good voice are always good things. Apart from this, in order to be a good radio jockey, you will also listen to good radio jockeys so that you can understand their style and later make your own by practicing.
A career as radio jockey has a lot to offer to deserving candidates. If you want to know more about a career as radio jockey, and how to become a radio jockey then continue reading the article.
Choreographer
The word “choreography" actually comes from Greek words that mean “dance writing." Individuals who opt for a career as a choreographer create and direct original dances, in addition to developing interpretations of existing dances. A Choreographer dances and utilises his or her creativity in other aspects of dance performance. For example, he or she may work with the music director to select music or collaborate with other famous choreographers to enhance such performance elements as lighting, costume and set design.
Social Media Manager
A career as social media manager involves implementing the company’s or brand’s marketing plan across all social media channels. Social media managers help in building or improving a brand’s or a company’s website traffic, build brand awareness, create and implement marketing and brand strategy. Social media managers are key to important social communication as well.
Photographer
Photography is considered both a science and an art, an artistic means of expression in which the camera replaces the pen. In a career as a photographer, an individual is hired to capture the moments of public and private events, such as press conferences or weddings, or may also work inside a studio, where people go to get their picture clicked. Photography is divided into many streams each generating numerous career opportunities in photography. With the boom in advertising, media, and the fashion industry, photography has emerged as a lucrative and thrilling career option for many Indian youths.
An individual who is pursuing a career as a producer is responsible for managing the business aspects of production. They are involved in each aspect of production from its inception to deception. Famous movie producers review the script, recommend changes and visualise the story.
They are responsible for overseeing the finance involved in the project and distributing the film for broadcasting on various platforms. A career as a producer is quite fulfilling as well as exhaustive in terms of playing different roles in order for a production to be successful. Famous movie producers are responsible for hiring creative and technical personnel on contract basis.
Copy Writer
In a career as a copywriter, one has to consult with the client and understand the brief well. A career as a copywriter has a lot to offer to deserving candidates. Several new mediums of advertising are opening therefore making it a lucrative career choice. Students can pursue various copywriter courses such as Journalism , Advertising , Marketing Management . Here, we have discussed how to become a freelance copywriter, copywriter career path, how to become a copywriter in India, and copywriting career outlook.
In a career as a vlogger, one generally works for himself or herself. However, once an individual has gained viewership there are several brands and companies that approach them for paid collaboration. It is one of those fields where an individual can earn well while following his or her passion.
Ever since internet costs got reduced the viewership for these types of content has increased on a large scale. Therefore, a career as a vlogger has a lot to offer. If you want to know more about the Vlogger eligibility, roles and responsibilities then continue reading the article.
For publishing books, newspapers, magazines and digital material, editorial and commercial strategies are set by publishers. Individuals in publishing career paths make choices about the markets their businesses will reach and the type of content that their audience will be served. Individuals in book publisher careers collaborate with editorial staff, designers, authors, and freelance contributors who develop and manage the creation of content.
Careers in journalism are filled with excitement as well as responsibilities. One cannot afford to miss out on the details. As it is the small details that provide insights into a story. Depending on those insights a journalist goes about writing a news article. A journalism career can be stressful at times but if you are someone who is passionate about it then it is the right choice for you. If you want to know more about the media field and journalist career then continue reading this article.
Individuals in the editor career path is an unsung hero of the news industry who polishes the language of the news stories provided by stringers, reporters, copywriters and content writers and also news agencies. Individuals who opt for a career as an editor make it more persuasive, concise and clear for readers. In this article, we will discuss the details of the editor's career path such as how to become an editor in India, editor salary in India and editor skills and qualities.
Individuals who opt for a career as a reporter may often be at work on national holidays and festivities. He or she pitches various story ideas and covers news stories in risky situations. Students can pursue a BMC (Bachelor of Mass Communication) , B.M.M. (Bachelor of Mass Media) , or MAJMC (MA in Journalism and Mass Communication) to become a reporter. While we sit at home reporters travel to locations to collect information that carries a news value.
Corporate Executive
Are you searching for a Corporate Executive job description? A Corporate Executive role comes with administrative duties. He or she provides support to the leadership of the organisation. A Corporate Executive fulfils the business purpose and ensures its financial stability. In this article, we are going to discuss how to become corporate executive.
Multimedia Specialist
A multimedia specialist is a media professional who creates, audio, videos, graphic image files, computer animations for multimedia applications. He or she is responsible for planning, producing, and maintaining websites and applications.
Quality Controller
A quality controller plays a crucial role in an organisation. He or she is responsible for performing quality checks on manufactured products. He or she identifies the defects in a product and rejects the product.
A quality controller records detailed information about products with defects and sends it to the supervisor or plant manager to take necessary actions to improve the production process.
Production Manager
A QA Lead is in charge of the QA Team. The role of QA Lead comes with the responsibility of assessing services and products in order to determine that he or she meets the quality standards. He or she develops, implements and manages test plans.
Process Development Engineer
The Process Development Engineers design, implement, manufacture, mine, and other production systems using technical knowledge and expertise in the industry. They use computer modeling software to test technologies and machinery. An individual who is opting career as Process Development Engineer is responsible for developing cost-effective and efficient processes. They also monitor the production process and ensure it functions smoothly and efficiently.
AWS Solution Architect
An AWS Solution Architect is someone who specializes in developing and implementing cloud computing systems. He or she has a good understanding of the various aspects of cloud computing and can confidently deploy and manage their systems. He or she troubleshoots the issues and evaluates the risk from the third party.
Azure Administrator
An Azure Administrator is a professional responsible for implementing, monitoring, and maintaining Azure Solutions. He or she manages cloud infrastructure service instances and various cloud servers as well as sets up public and private cloud systems.
Computer Programmer
Careers in computer programming primarily refer to the systematic act of writing code and moreover include wider computer science areas. The word 'programmer' or 'coder' has entered into practice with the growing number of newly self-taught tech enthusiasts. Computer programming careers involve the use of designs created by software developers and engineers and transforming them into commands that can be implemented by computers. These commands result in regular usage of social media sites, word-processing applications and browsers.
Information Security Manager
Individuals in the information security manager career path involves in overseeing and controlling all aspects of computer security. The IT security manager job description includes planning and carrying out security measures to protect the business data and information from corruption, theft, unauthorised access, and deliberate attack
ITSM Manager
Automation test engineer.
An Automation Test Engineer job involves executing automated test scripts. He or she identifies the project’s problems and troubleshoots them. The role involves documenting the defect using management tools. He or she works with the application team in order to resolve any issues arising during the testing process.
Applications for Admissions are open.

Aakash iACST Scholarship Test 2024
Get up to 90% scholarship on NEET, JEE & Foundation courses

JEE Main Important Chemistry formulas
As per latest 2024 syllabus. Chemistry formulas, equations, & laws of class 11 & 12th chapters

PACE IIT & Medical, Financial District, Hyd
Enrol in PACE IIT & Medical, Financial District, Hyd for JEE/NEET preparation

ALLEN JEE Exam Prep
Start your JEE preparation with ALLEN

ALLEN NEET Coaching
Ace your NEET preparation with ALLEN Online Programs

SAT® | CollegeBoard
Registeration closing on 19th Apr for SAT® | One Test-Many Universities | 90% discount on registrations fee | Free Practice | Multiple Attempts | no penalty for guessing
Everything about Education
Latest updates, Exclusive Content, Webinars and more.
Download Careers360 App's
Regular exam updates, QnA, Predictors, College Applications & E-books now on your Mobile

Cetifications
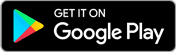
We Appeared in

Thank you for visiting nature.com. You are using a browser version with limited support for CSS. To obtain the best experience, we recommend you use a more up to date browser (or turn off compatibility mode in Internet Explorer). In the meantime, to ensure continued support, we are displaying the site without styles and JavaScript.
- View all journals
- Explore content
- About the journal
- Publish with us
- Sign up for alerts
Latest science news, discoveries and analysis
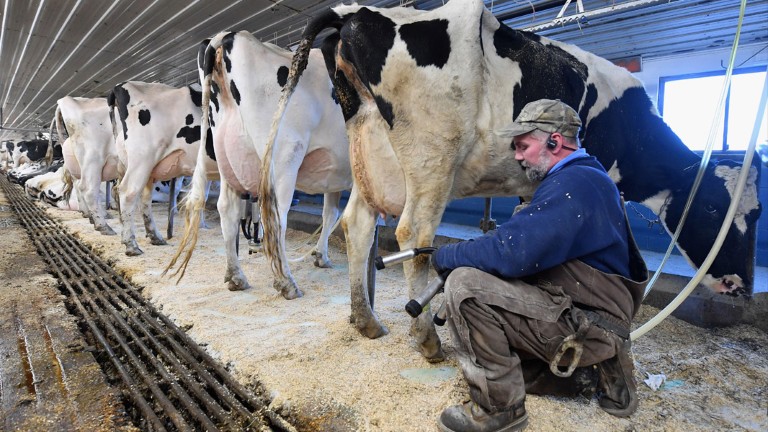
Bird flu in US cows: is the milk supply safe?
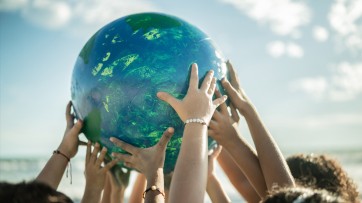
Future of Humanity Institute shuts: what's next for ‘deep future’ research?
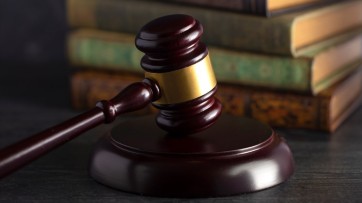
Judge dismisses superconductivity physicist’s lawsuit against university
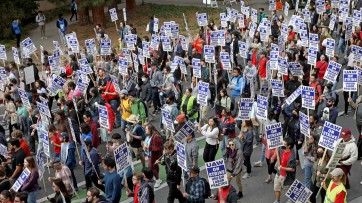
NIH pay raise for postdocs and PhD students could have US ripple effect
Hello puffins, goodbye belugas: changing arctic fjord hints at our climate future, china's moon atlas is the most detailed ever made, ‘shut up and calculate’: how einstein lost the battle to explain quantum reality, rat neurons repair mouse brains — and restore sense of smell, ecologists: don’t lose touch with the joy of fieldwork chris mantegna.
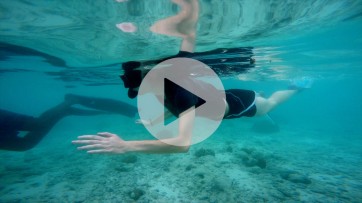
Should the Maldives be creating new land?
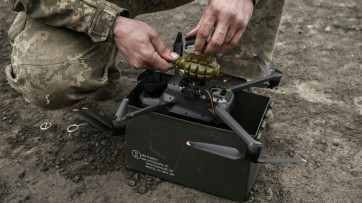
Lethal AI weapons are here: how can we control them?
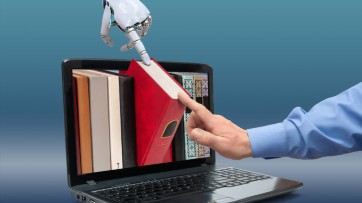
Algorithm ranks peer reviewers by reputation — but critics warn of bias
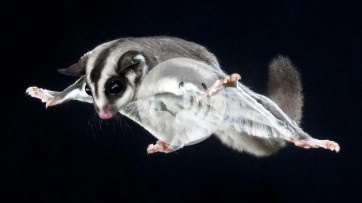
How gliding marsupials got their ‘wings’
Audio long read: why loneliness is bad for your health, nato is boosting ai and climate research as scientific diplomacy remains on ice, plastic pollution: three numbers that support a crackdown, the maldives is racing to create new land. why are so many people concerned.
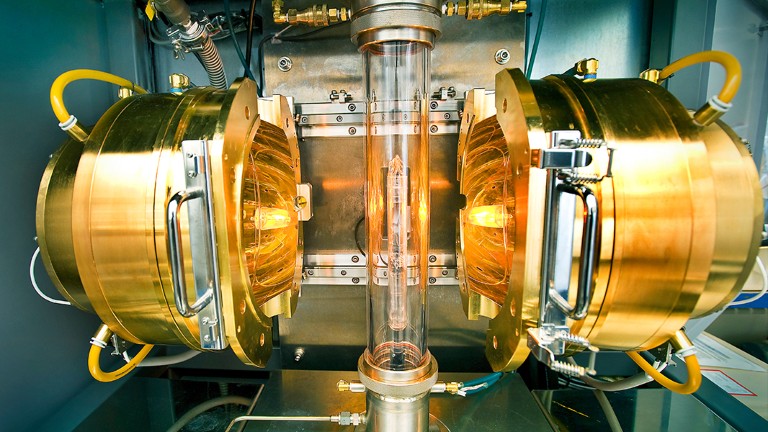
Retractions are part of science, but misconduct isn’t — lessons from a superconductivity lab
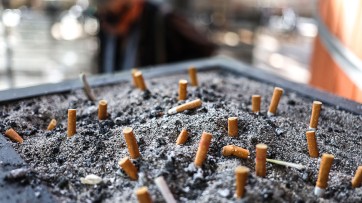
Any plan to make smoking obsolete is the right step
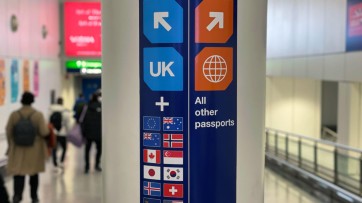
Citizenship privilege harms science
European ruling linking climate change to human rights could be a game changer — here’s how charlotte e. blattner, will ai accelerate or delay the race to net-zero emissions, current issue.
Surprise hybrid origins of a butterfly species
Stripped-envelope supernova light curves argue for central engine activity, optical clocks at sea, research analysis.
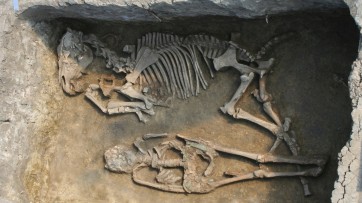
Ancient DNA traces family lines and political shifts in the Avar empire
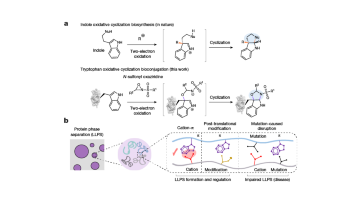
A chemical method for selective labelling of the key amino acid tryptophan
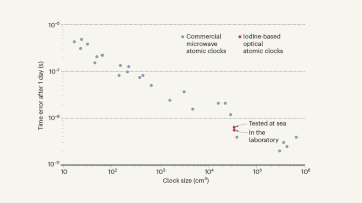
Robust optical clocks promise stable timing in a portable package
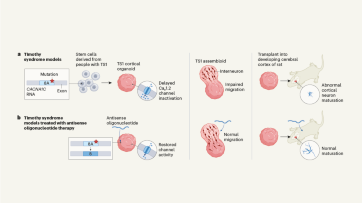
Targeting RNA opens therapeutic avenues for Timothy syndrome
Bioengineered ‘mini-colons’ shed light on cancer progression, galaxy found napping in the primordial universe, tumours form without genetic mutations, marsupial genomes reveal how a skin membrane for gliding evolved.
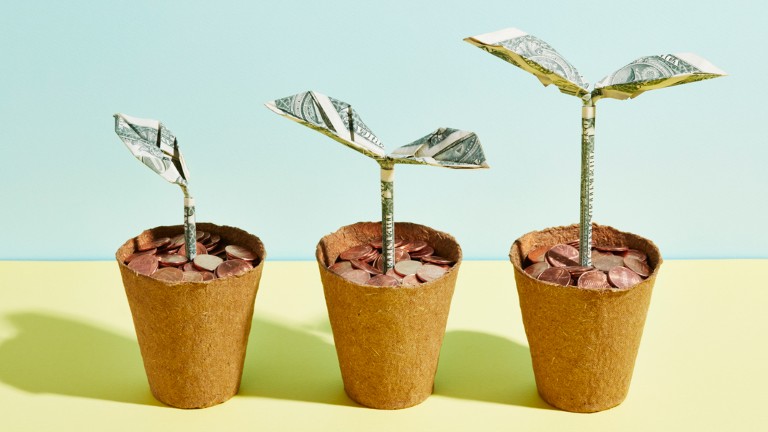
Scientists urged to collect royalties from the ‘magic money tree’
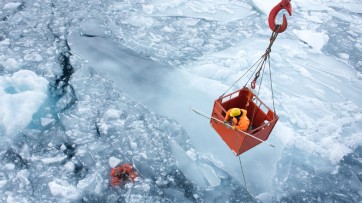
Breaking ice, and helicopter drops: winning photos of working scientists
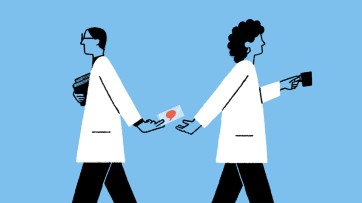
Shrouded in secrecy: how science is harmed by the bullying and harassment rumour mill
How ground glass might save crops from drought on a caribbean island, londoners see what a scientist looks like up close in 50 photographs, books & culture.
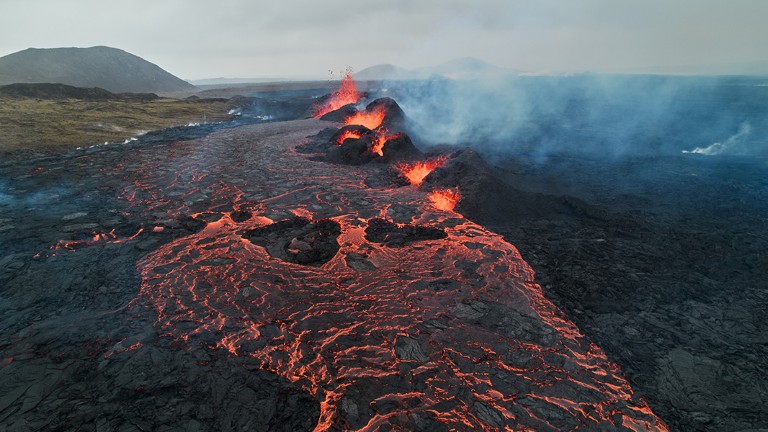
How volcanoes shaped our planet — and why we need to be ready for the next big eruption
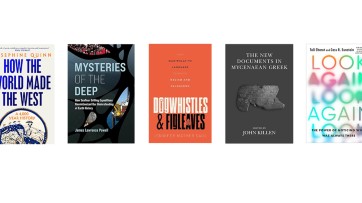
Dogwhistles, drilling and the roots of Western civilization: Books in brief
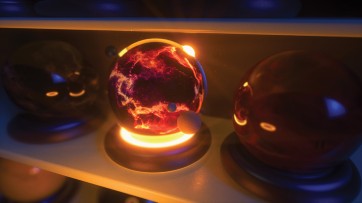
Cosmic rentals
Las borinqueñas remembers the forgotten puerto rican women who tested the first pill, dad always mows on summer saturday mornings, nature podcast.

Latest videos
Nature briefing.
An essential round-up of science news, opinion and analysis, delivered to your inbox every weekday.

Quick links
- Explore articles by subject
- Guide to authors
- Editorial policies

An official website of the United States government
The .gov means it’s official. Federal government websites often end in .gov or .mil. Before sharing sensitive information, make sure you’re on a federal government site.
The site is secure. The https:// ensures that you are connecting to the official website and that any information you provide is encrypted and transmitted securely.
- Publications
- Account settings
Preview improvements coming to the PMC website in October 2024. Learn More or Try it out now .
- Advanced Search
- Journal List
- JMIR Public Health Surveill
- v.6(3); Jul-Sep 2020

COVID-19 in India: Statewise Analysis and Prediction
Palash ghosh.
1 Department of Mathematics, Indian Institute of Technology, Guwahati, India
2 Centre for Quantitative Medicine, Duke-National University of Singapore Medical School, Singapore, Singapore
Bibhas Chakraborty
3 Centre for Quantitative Medicine & Programme in Health Services and Systems Research, Duke-National University of Singapore Medical School, Singapore, Singapore
4 Department of Statistics and Applied Probability, National University of Singapore, Singapore, Singapore
5 Department of Biostatistics and Bioinformatics, Duke University, Durham, NC, United States
Associated Data
Supplementary material.
The highly infectious coronavirus disease (COVID-19) was first detected in Wuhan, China in December 2019 and subsequently spread to 212 countries and territories around the world, infecting millions of people. In India, a large country of about 1.3 billion people, the disease was first detected on January 30, 2020, in a student returning from Wuhan. The total number of confirmed infections in India as of May 3, 2020, is more than 37,000 and is currently growing fast.
Most of the prior research and media coverage focused on the number of infections in the entire country. However, given the size and diversity of India, it is important to look at the spread of the disease in each state separately, wherein the situations are quite different. In this paper, we aim to analyze data on the number of infected people in each Indian state (restricted to only those states with enough data for prediction) and predict the number of infections for that state in the next 30 days. We hope that such statewise predictions would help the state governments better channelize their limited health care resources.
Since predictions from any one model can potentially be misleading, we considered three growth models, namely, the logistic, the exponential, and the susceptible-infectious-susceptible models, and finally developed a data-driven ensemble of predictions from the logistic and the exponential models using functions of the model-free maximum daily infection rate (DIR) over the last 2 weeks (a measure of recent trend) as weights. The DIR is used to measure the success of the nationwide lockdown. We jointly interpreted the results from all models along with the recent DIR values for each state and categorized the states as severe, moderate, or controlled.
We found that 7 states, namely, Maharashtra, Delhi, Gujarat, Madhya Pradesh, Andhra Pradesh, Uttar Pradesh, and West Bengal are in the severe category. Among the remaining states, Tamil Nadu, Rajasthan, Punjab, and Bihar are in the moderate category, whereas Kerala, Haryana, Jammu and Kashmir, Karnataka, and Telangana are in the controlled category. We also tabulated actual predicted numbers from various models for each state. All the R 2 values corresponding to the logistic and the exponential models are above 0.90, indicating a reasonable goodness of fit. We also provide a web application to see the forecast based on recent data that is updated regularly.
Conclusions
States with nondecreasing DIR values need to immediately ramp up the preventive measures to combat the COVID-19 pandemic. On the other hand, the states with decreasing DIR can maintain the same status to see the DIR slowly become zero or negative for a consecutive 14 days to be able to declare the end of the pandemic.
Introduction
The world is now facing an unprecedented crisis due to the novel coronavirus, first detected in Wuhan, China in December 2019 [ 1 ]. The World Health Organization (WHO) defined coronavirus as a family of viruses that range from the common cold to the Middle East respiratory syndrome coronavirus and the severe acute respiratory syndrome coronavirus [ 2 ]. Coronaviruses circulate in some wild animals and have the capability to transmit from animals to humans. These viruses can cause respiratory symptoms in humans, along with other symptoms of the common cold and fever [ 3 ]. There are no specific treatments for coronaviruses to date. However, one can avoid infection by maintaining basic personal hygiene and social distancing from infected persons.
The WHO declared the coronavirus disease (COVID-19) as a global pandemic on March 11, 2020 [ 4 ]. The disease has spread across 212 countries and territories around the world, with a total of more than 3 million confirmed cases [ 5 , 6 ]. In India, the disease was first detected on January 30, 2020, in Kerala in a student who returned from Wuhan [ 7 , 8 ]. The total (cumulative) number of confirmed infected people is more than 37,000 to date (May 3, 2020) across India. The bar chart in Figure 1 shows the daily growth of the COVID-19 cases in India. After the first 3 cases from January 30 to February 3, 2020, there were no confirmed COVID-19 cases for about a month. The COVID-19 cases appeared again from March 2, 2020, onwards. These cases are related to people who have been evacuated or have arrived from COVID-19–affected countries. From March 20, 2020, onwards, there is an exponential growth in the daily number of COVID-19 cases at the pan-India level.

Bar chart of daily infected cases (blue) in India. Red bar denotes death. The black curve is a fitted smooth curve on the daily cases.
There are four stages of COVID-19 depending on the types of virus transmission [ 9 , 10 ]. During the first stage, a country or region experiences imported infected cases with travel history from virus-hit countries. During the second stage, a country or region gets new infections from persons who did not have a travel history but came in contact with persons defined in stage 1. Stage 3 is community transmission; in this period, new infection occurs in a person who has not been in contact with an infected person or anyone with a travel history of virus-hit countries. At stage 4, the virus spread is practically uncontrollable, and the country can have many major clusters of infection.
Many news agencies are repeatedly saying or questioning whether India is now at stage 3 [ 9 , 11 , 12 ]. In reality, different Indian states are or will be at various stages of infection at different points in time. Labeling a COVID-19 stage at the pan-India level is problematic. It will spread misinformation to common people. Those states that are at stage 3 require more rapid action compared to others. On the other hand, states that are in stages 1 and 2 need to focus on stopping the community spread of COVID-19.
In this paper, we first discuss the importance of statewise consideration, contemplating all the states together. Second, we will focus on the infected people in each state (considering only those states with enough data for prediction) and build growth models to predict infected people for that state in the next 30 days.
Why Statewise Consideration?
India is a vast country with a geographic area of 3,287,240 square kilometers and a total population of about 1.3 billion [ 13 ]. Most of the Indian states are quite large in geographic area and population. Analyzing coronavirus infection data, considering the entirety of India to be on the same page may not provide us the right picture. This is because the first infection, new infection rate, progression over time, and preventive measures taken by state governments and the common public for each state are different. We need to address each state separately. It will enable the government to use the limited available resources optimally. For example, currently, Maharashtra already has more than 10,000 confirmed infected cases, whereas West Bengal has less than 800 confirmed cases (May 1, 2020). The approaches to addressing the two states must be different due to limited resources. One way to separate the statewise trajectories is to look at when each state was first infected.
In Figure 2 , we present the first infection date along with the infected person’s travel history in each of the Indian states. All the states and the union territories, except Assam, Tripura, Nagaland, Meghalaya, and Arunachal Pradesh, observed their first confirmed infected case from a person who had travel history from one or more already COVID-19–infected countries. The Indian government imposed a complete ban on international flights to India on March 22, 2020 [ 14 ]. Figure 2 justifies government action to international flight suspension. Had it been taken earlier, we could have restricted the disease to only a few states compared to the current scenario.

When the first case in each state happened with their travel histories. UAE: United Arab Emirates.
Figure 3 shows the curve of the cumulative number of infected people in those Indian states having at least 10 total infected people. Currently, Maharashtra, Delhi, Gujarat, Tamil Nadu, Madhya Pradesh, Rajasthan, and Uttar Pradesh are the states where the cumulative number of infected people have crossed the 2000 mark, with Maharashtra having more than 10,000 cases. Kerala, the first state to have a COVID-19 confirmed case, seems to have restricted the growth rate. There are few states with cumulative infected people in the range of 500-1500. Depending on how those states strictly follow the preventive measures, we may see a rise in the confirmed cases.

Cumulative number of infected people over time in states with at least 10 infected cases.
Preventive Measures
In Textbox 1 , we list the major preventive measures taken by the Indian Government [ 15 ].
List of major preventive measures taken by the Indian Government.
January 25-March 13, 2020
Health screenings at airports and border crossings
February 26-March 20, 2020
Introduction of quarantine policies: gradually for passengers coming from different countries
February 26-March 13, 2020
Visa restrictions: gradually for different countries
March 5, 2020
Limit public gatherings (closure of some selected public institutions like museums, religious places, and postponing of several local elections to stop public gatherings)
March 11, 2020
Border checks
March 13-15, 2020
Border closure
March 16, 2020
Limit public gatherings (ban on all sorts of public gatherings and meetings, and stopping people from making any congregation)
March 18, 2020
Travel restrictions
March 20, 2020
Testing for the coronavirus disease (before this point, only people who had traveled from abroad were tested; this point onwards, testing was also introduced for symptomatic contacts of laboratory-confirmed cases, symptomatic health care workers, and all hospitalized patients with severe acute respiratory illness)
March 22, 2020
Flight suspensions
Cancellation of passenger train services until March 31, 2020
March 24, 2020
Suspension of domestic airplane operations
March 25, 2020
21-day lockdown of entire country
Cancellation of passenger train services extended to April 14, 2020
March 30, 2020
Increase of quarantine/isolation facilities
April 14, 2020
Extension of lockdown until May 3, 2020
May 1, 2020
Extension of lockdown until May 17, 2020
Data Source
We have used Indian COVID-19 data available publicly. The three primary sources of the data are the Ministry of Health and Family Welfare, India [ 16 ]; COVID-19 India [ 17 ]; and Wikipedia [ 18 ].
Statistical Models
In this paper, we consider the exponential model, the logistic model, and the susceptible-infectious-susceptible (SIS) model for COVID-19 pandemic prediction at the state level. These models have already been used to predict epidemics like COVID-19 around the world, including in China, and for the Ebola outbreak in Bomi, Liberia in 2014 [ 19 - 21 ]. See Multimedia Appendix 1 [ 20 - 22 ] for details about the models.
Using the Models in State-Level Data
The previously mentioned three models will provide a different prediction perspective for each state. The exponential model–based prediction will give a picture of what could be the cumulative number of infected people in the next 30 days if we do not take any preventive measures. We can consider the forecast from the exponential model as an estimate of the upper bound of the total number of infected people in the next 30 days. The logistic model–based prediction will capture the effect of preventive measures that have already been taken by the respective state governments as well as the central government. The logistic model assumes that the infection rate will slow down in the future with an overall “S” type growth curve. In other words, the logistic model tries to explore a situation where there is a full lockdown in the country, leading to an extreme restraint on the people’s movement, hence reducing the rate of infection considerably. Under the effective implementation of the lockdown, it is appropriate to use a logistic model. In this scenario, many people have already been infected; the virus may find it hard to spot more susceptible people. Thus, the virus slows down its spread, causing the flattening in the S-curve at a later stage. Several research papers have used the logistic model in the context of COVID-19 [ 23 - 26 ].

Study the Effect of Lockdown Using the Daily Infection Rate and SIS Model
Kumar et al [ 31 ] reported the estimated number of people that a person may come in contact with within a day (24 hours) in a rural community in Haryana, India to be 17. They defined contact as having a face-to-face conversation within 3 feet, which may or may not have included physical contact. The estimate of the contact-rate parameter from their paper is 0.70. In practice, only some of all the people who come in contact with a person infected with COVID-19 may be actually infected by the virus. Note that India has already taken many preventive measures to ensure social distancing. In the current scenario, the infection rate based on Kumar et al’s [ 31 ] study could be an overestimate of its present value. However, despite nationwide lockdown, banks, hospitals, and grocery stores are still open to cater to the essential needs of people. We consider here two approaches to study the effect of lockdown and other preventive measures jointly in each state. First , we plot the daily infection rates (DIRs) for each state. The DIR for a given day is defined as:

India implemented a nationwide lockdown on March 25, 2020. We first considered the incubation period of the novel coronavirus to study the effect of the lockdown. The incubation period of an infectious disease is defined as the time between infection and the first appearance of signs and symptoms [ 32 ]. Using the incubation period, health researchers can decide on the quarantine periods and halt a potential pandemic without the aid of a vaccine or treatment [ 33 ]. The estimated median incubation period for COVID-19 is 5.1 (95% CI 4.5-5.8) days, and 97.5% of those who develop symptoms will do so within 11.5 (95% CI 8.2-15.6) days of infection [ 34 ]. The WHO recommends that a person with laboratory-confirmed COVID-19 be quarantined for 14 days from the last time they were exposed to the patient [ 35 ]. Therefore, if a person was infected before the lockdown (March 25, 2020), they should not infect others except their family members if that person is entirely inside their house for more than 14 days. The WHO also recommends common people to maintain a distance of at least 1 meter from each other in a public place to avoid COVID-19 infection. The effective implementation of social distancing can stop the spread of the virus from an infected person, even when they are outside for some essential business. However, given a highly dense population in most of India, particularly in cities, it may not always be possible to maintain adequate social distance.
Statewise Analysis and Prediction Report
In this section, we depend on inputs from the exponential, logistic, and SIS models along with DIRs for each state. Remembering the words of the famous statistician George Box “All models are wrong, but some are useful,” we interpreted the results from different models jointly. We consider different states with at least 300 cumulative infected cases. For each state, we present four graphs. We have used the state-level data until May 1, 2020. The first and second graphs are based on the logistic and the exponential models, respectively, with the next 30-day predictions. The third graph is the plot of DIRs for a state. Finally, the fourth graph is showing the growth of the active infected patients using SIS model prediction ( “pred” ) along with the observed active infected patients. Table 1 represents the 30-day prediction of the cumulative infected number of people for each state using the logistic model, the exponential model, and a data-driven combination of the two. The corresponding measures of goodness of fit ( R 2 and deviance) are presented in the table in Multimedia Appendix 1 .
Data-driven assessment and 30-day prediction using the logistic and exponential models, and their linear combination.
a DIR: daily infection rate.
b R 0 : basic reproduction number.
c SIS: susceptible-infectious-susceptible.
d COVID-19: coronavirus disease.
e LC pred : linear combination prediction.
Maharashtra
The situation in Maharashtra is currently very severe with respect to the active number of cases (see Figure 4 ). As of May 1, 2020, the total number of infected cases is 10,498. The logistic model indicates that, in another 30 days from now, the state could observe around 17,100 cumulative infected cases. The DIRs for this state were between 0.03 and 0.15 in the last 2 weeks, and it was more than 0.4 for 2 days at the beginning of April. Note that, for Maharashtra, the lower DIR values of 0.03 may not indicate a good sign since the total number of active infected cases is above 8000. Thus, a DIR value of 0.03 for a day implies 8000 x 0.03 = 240 new infected cases. The curves from the SIS model are alarming as the observed active infected patients (red line, fourth panel) line is far above the predicted line with estimated infection rate at the 80th percentile of observed DIRs (β=0.22). It is apparent from the graphs that even after 30 days of lockdown, Maharashtra has not seen any decline in the number of active cases. The estimated R 0 for Maharashtra obtained from the fitted SIS model is 3.5, which is the highest among all the states. This may also indicate that there could be a large number of people who are in the community without knowing that they are carrying the virus. The state can be considered to be in stage 3.

Graphs for the state of Maharashtra. SIS: susceptible-infectious-susceptible.
Delhi, being a state of high population density, has already observed 3515 confirmed COVID-19 cases (see Figure 5 ). Based on the logistic model, the predicted number of cumulative infected cases could reach around 4200 in the next 30 days. The DIR has not seen a downward trend in the past few days. The curve (red line, fourth panel) of observed active infected patients was showing a downward trend from April 20 to April 23, 2020. However, the same graph has picked up exponential growth in the last few days. This is an important observation that illustrates why we need a continuous downward trend of active cases for at least 14 days and that a slight relaxation may put a state in the same severe condition where it was earlier. The estimated R 0 for the state obtained from the fitted SIS model being 2.94 is quite alarming. The observed DIR has been currently fluctuating between –0.06 and 0.17 in the last 2 weeks. The occasional high DIR may suggest that there could be many people who are in the community without knowing that they are already infected with COVID-19. The state could be heading to community spread of COVID-19 (stage 3).

Graphs for the state of Delhi. SIS: susceptible-infectious-susceptible.
The cumulative infected cases in Tamil Nadu is 2323 (see Figure 6 ). The state has observed a high DIR of more than 0.7 for some days in March. Tamil Nadu is one of the states where the effect of lockdown is visible from the declining DIRs from the beginning to the end of April. However, there was again an increasing trend in DIR over the last 3 days. The DIRs were between –0.13 and 0.12 over the previous 2 weeks. The latter part of the curve (red line, fourth panel) of observed active infected patients is showing a decreasing trend first but then an increasing trend again. The estimated R 0 for this southern state obtained from the fitted SIS model is 3.22, which is quite high. The preventive measures need to be maintained to bring down the active cases as well as to stop new infections in this state.

Graphs for the state of Tamil Nadu. SIS: susceptible-infectious-susceptible.
Madhya Pradesh
This state currently has 2719 cumulative COVID-19 cases (see Figure 7 ). In the later part of the lockdown, after April 10, 2020, the state observed a few days with a DIR more than 0.4. Until now, there is no sight of a declining trend in the DIRs. The same type of conclusion can be drawn from the curves of the SIS model. The curve (red line, fourth panel) of observed active infected patients is in between the curves of the SIS model corresponding to the 50th-75th percentiles’ curves. The same curve is maintaining an exponential growth after April 10. Note that, for Madhya Pradesh, the 50th percentile of observed DIRs was 0.14, which is higher than the 50th percentile of some other states. The estimated R 0 for this state obtained from the fitted SIS model was 3.36, which is pretty high. The high growth of active cases in the latter part of the lockdown is a major concern for this state. It could be a signal of a community spread of COVID-19.

Graphs for the state of Madhya Pradesh. SIS: susceptible-infectious-susceptible.
The western state of India, Rajasthan, reported 2584 cumulative infected COVID-19 cases (see Figure 8 ). The logistic model indicates that in another 30 days from now, the state could observe around 2800 cumulative infected cases. The state has seen a declining trend in the DIRs during the last part of April. The curve (red line, fourth panel) of observed active infected patients is increasing and is in between the curves of the SIS model corresponding to the 50th-75th percentiles of observed DIRs (0.14-0.27) using the SIS model. In the last 2 weeks, the DIRs for Rajasthan have been fluctuating between –0.05 and 0.12. The active cases in this state have not increased too much in the latter part of April. An increase in recovery cases is one of the reasons. The estimated R 0 for Rajasthan obtained from the fitted SIS model was 2.94. Therefore, the current COVID-19 situation in the state is not controlled yet.

Graphs for the state of Rajasthan. SIS: susceptible-infectious-susceptible.
The state is currently experiencing exponential growth with 4395 as the cumulative number of COVID-19 cases (see Figure 9 ). Using the logistic model, the cumulative infected cases could reach around 5206 in the next 30 days. There is apparently a stable rather than a declining trend in the DIRs in the last few days. The DIRs were in the range of 0.03-0.27 in the last 2 weeks, which are on the higher side. The curve (redline, fourth panel) of observed active infected patients is close to the curve of the SIS model corresponding to the estimated 75th percentile of observed DIR (β=.26). Surprisingly, in the latter part of the lockdown, the red line is experiencing exponential growth. The estimated R 0 for Gujarat obtained from the fitted SIS model was 3.5, which is one of the highest. This state needs immediate intervention to implement all the preventive measures already taken by the Government strictly.

Graphs for the state of Gujarat. SIS: susceptible-infectious-susceptible.
Uttar Pradesh
This northern state of India has experienced 2281 cumulative COVID-19 cases (see Figure 10 ). Using the logistic model, the predicted number of cumulative confirmed cases could be around 3000 in the next 30 days. The curve (red line, fourth panel) of observed active infected patients was in between the curves of the SIS model corresponding to the 50th and 75th percentiles of observed DIRs (β=0.12 and 0.23, respectively). The DIR was in the range of –0.02 to 0.13 without a moderately decreasing trend in the last 2 weeks. The overall growth of active cases was still exponential, which is a major concern for the state. The estimated R 0 for the state obtained from the fitted SIS model was 2.52. There could be many unreported cases in the state. In the absence of preventive measures, unreported cases can contribute to spreading the virus in the community.

Graphs for the state of Uttar Pradesh. SIS: susceptible-infectious-susceptible.
The southern Indian state of Telangana has reported 1039 cumulative infected cases until now (see Figure 11 ). The logistic model predicts that the number of cases for the state will be around 1063 in the next 30 days. In the fourth graph, the curve (red line, fourth panel) shows that the active number of cases has continuously remained below the curve of the SIS model corresponding to the 75th percentile of the observed DIRs (β=0.25). The estimated R 0 for Telangana obtained from the fitted SIS model was 2.66. From April 23, 2020, onwards, there is a visible downward trend in the same line graph. This evidence is also supported by a clear decreasing trend in the DIR for more than 2 weeks. The state is going in the right direction to control the COVID-19 pandemic. However, preventive measures need to be in place to see long-term success against the virus.

Graphs for the state of Telangana. SIS: susceptible-infectious-susceptible.
Andhra Pradesh
This state has observed 1463 confirmed cumulative infected cases so far (see Figure 12 ). The curve (red line, fourth panel) shows that the number of active cases is now below and close to the curve of the SIS model corresponding to the 75th percentile of the observed DIR (β=0.23). The logistic model predicted that the maximum number of cumulative infected people will be around 2313 in the next 30 days. Despite showing good progress in mid-April, the state is again showing an exponential type growth rate. This state has seen DIRs between –0.04 and 0.17 during the last 2 weeks. The estimated R 0 for this state obtained from the fitted SIS model was 3.22, which is quite high. The state has shown a few short declining trends, without any long-term declining trend in the DIR values. It could be due to many unreported infected cases in the community that is spreading the virus.

Graphs for the state of Andhra Pradesh. SIS: susceptible-infectious-susceptible.
The southern state of Kerala is one of the few states of India where the effect of the lockdown is observed strongly. The state reported the first COVID-19 case in India. However, Kerala has been able to control the spread of the virus to a large extent to date. The cumulative number of cases reported until now is 497 (see Figure 13 ). It is a state where the curve (red line, fourth panel) of observed active infected patients is going down, which shows that the lockdown and other preventive measures have been effective for this state. The DIR has declined steadily from positive to negative values. However, some spikes in the DIR values can be noticed in the last few days. The estimated R 0 for Kerala obtained from the fitted SIS model was 1.96, which is quite low compared to other states. It can be expected that with the present scenario of the extended lockdown the number of active cases will be few at the end of May.

Graphs for the state of Kerala. SIS: susceptible-infectious-susceptible.
The state has managed to restrict the cumulative infected cases to 576 until now (see Figure 14 ). The curve (red line, fourth panel) of observed active infected patients is now below the curve of the SIS model corresponding to the 75th percentile of the observed DIRs (β=0.18). Compared to other states, the 75th percentile DIR is on the lower side. The estimated R 0 for the state obtained from the fitted SIS model was 2.38. We can observe the ups and downs of the DIR with an upper bound of 0.2 from early April. This state has seen DIRs between –0.04 and 0.06 during the last 2 weeks. However, the preventive measures need to be maintained to control the spread of the virus.

Graphs for the state of Karnataka. SIS: susceptible-infectious-susceptible.
Jammu and Kashmir
The northernmost state of Jammu and Kashmir has seen 614 cumulative infected cases so far (see Figure 15 ). The curve (red line, fourth panel) of observed active infected patients has been far below the curve of the SIS model corresponding to the 75th percentile of the observed DIR (β=0.35). The estimated R 0 for the state obtained from the fitted SIS model was 2.66. From April 9, 2020, onwards, the DIR was apparently decreasing. There are some spikes in DIR values occasionally. It could be due to many unreported cases, which are allowing the infection to spread even during the lockdown period. The DIR was in the range of –0.02 to 0.09 in the last 2 weeks.

Graphs for the state of Jammu and Kashmir. SIS: susceptible-infectious-susceptible.
West Bengal
The state of West Bengal is standing at 795 cumulative infected cases as of now (see Figure 16 ). The DIR values do not show any trend of slowing down in recent times. Based on the logistic model, the predicted cumulative infected cases could be around 1261 in the next 30 days. The curve (red line, fourth panel) of observed active infected patients was above the curve of the SIS model corresponding to the 75th percentile of the DIR (β=0.21). The DIRs were between 0.03 and 0.17 in the last 2 weeks. The cumulative infected cases graphs based on logistic and exponential models (first and second panels), as well as the active cases–based curve (red line, fourth panel) were all showing exponential type growth rates. The estimated R 0 for West Bengal obtained from the fitted SIS model was 3.22, which is quite high. Strict implementation of preventive measures is needed to control the spread of COVID-19 in the state.

Graphs for the state of West Bengal. SIS: susceptible-infectious-susceptible.
The state of Haryana has observed 313 cumulative infected COVID-19 cases so far (see Figure 17 ). It has reported a very low rate of infection in the latter part of the lockdown except for the last reported day. In the fourth panel, the curve (red line) of observed active infected patients is now far below the curve of the SIS model corresponding to the 50th percentile of observed DIRs (β=0.15) and is showing a decreasing trend in the latter part. The estimated R 0 for the state obtained from the fitted SIS model was 1.82, which is on the lower side. The DIRs were between –0.28 and 0.18 in the last 2 weeks. Under the assumption that there are not too many unreported cases, the situation in Haryana seems to be under control.

Graphs for the state of Haryana. SIS: susceptible-infectious-susceptible.
The state of Punjab has reported 357 cumulative infected cases until now (see Figure 18 ). Based on the logistic model, the predicted cumulative confirmed cases could be around 419 in the next 30 days. The curve (red line) of observed active infected patients was in between the SIS model curves corresponding to the estimated 75th and 80th percentiles of observed DIRs (β=0.15 and 0.28, respectively). The estimated R 0 for Punjab obtained from the fitted SIS model was 2.52. The DIRs were between –0.05 and 0.14 in the last 2 weeks, which is good given the low number of active infected cases in the state.

Graphs for the state of Punjab. SIS: susceptible-infectious-susceptible.
The state has reported 426 cumulative infected cases until now (see Figure 19 ). Based on the logistic model, Bihar could see 16,452 total infected cases in the next 30 days. The estimated R 0 for the state obtained from the fitted SIS model was 3.08. It may be an overestimate. However, the DIRs showed no sign to decline in the last 2 weeks, with the highest reported value of 0.39. It may indicate many unreported cases in the state. However, the cumulative infected cases are still low for this state. Effective implementation of preventive measures is needed for the state.

Graphs for the state of Bihar. SIS: susceptible-infectious-susceptible.
Joint Interpretation of Results From all Models
We consider a data-driven assessment of the COVID-19 situation based on the growth of active cases in recent times (red line, fourth panel in each state plot) along with the DIR values for each state (see Table 1 ). We labeled the condition of a state as severe if we observed a nondecreasing trend in DIR values over the last 2 weeks and a near exponential growth in active infected cases, as moderate if we observed an almost decreasing trend in DIR values over the last 2 weeks and neither increasing nor decreasing growth in active infected cases, and as controlled if we observed a decreasing trend in the last 2 weeks’ DIR values and a decreasing growth in active infected cases. It can be noticed that the logistic model is underpredicting the next 30-day prediction, whereas the exponential model is overpredicting the same. As we have argued earlier, despite nationwide lockdown, people are out of their homes for essential businesses, which can contribute to the spreading of the virus. The maximum value of DIR in the last 2 weeks can capture how severely COVID-19 is spreading in recent times. Note that, for example, a DIR value of 0.10 cannot be interpreted in the same way for two different states with, for example, 500 and 5000 active cases. For the first state, we see 500 x 0.10 = 50 new cases, and for the second state, we observe 5000 x 0.10 = 500 new cases. In an attempt to capture these various subtleties in a realistic prediction, we propose a linear combination prediction (LC pred ) of the logistic and the exponential predictions using the maximum value of DIR over the last 2 weeks (DIR max ) as a weighting coefficient (tuning parameter) as follows:
Such a choice of the tuning parameter λ makes the LC pred equal to the logistic prediction when DIR max is negative with λ=0. On the other hand, the LC pred is equal to the exponential prediction when DIR max is more than 1 with λ=1. When DIR max is in between 0 and 1, the LC pred is a combination of the predictions from the logistic and the exponential models. Given the situation in the entirety India, we recommend LC pred along with the exponential predictions (particularly for states in severe condition) to be used for assessment purposes in each state.
Extensive testing may not be logistically feasible given India’s large population and limited health care budget. The undertesting can significantly impact the logistic prediction and less so the exponential prediction since the first one is underforecasting and the second one is overforecasting. The DIR indirectly captures the undertesting phenomenon. Thus, the LC pred with (a truncated version of) DIR as the weight (λ) can be thought of as a treatment for undertesting, albeit in a limited fashion.
From Table 1 , we can see that out of 16 states for which we have predictions, 10 states lay between the linear combination (LC pred ) and the exponential predictions, 4 states are below the LC preds , and 2 states are above the exponential predictions.
India, a country of approximately 1.3 billion people, has reported 17,615 confirmed COVID-19 cases after 80 days (from January 30, 2020) from the first reported case in Kerala [ 36 ]. In a similar duration from the first case, the United States reported more than 400,000 cases, and both Spain and Italy reported more than 150,000 confirmed COVID-19 cases. To gain some more perspective, note that, the United States has around one-fourth of the Indian population size. Therefore, according to the reported data so far, India seems to have managed the COVID-19 pandemic better compared to many other countries. One can argue that India has conducted too few tests compared to its population size [ 37 ]. However, a smaller number of testing may not be the only reason behind the low number of COVID-19–confirmed cases in India so far. India has taken many preventive measures to combat COVID-19 in much earlier stages compared to other countries, including a nationwide lockdown from March 25, 2020. Apart from the lockdown, people have certain conjectures about possible reasons behind India’s relative success (eg, measures like the travel ban relatively early, use of Bacille Calmette-Guerin vaccination to combat tuberculosis in the population that may have secondary effects against COVID-19 [ 38 , 39 ], exposure to malaria and antimalarial drugs [ 40 ], and hot and humid weather slowing the transmission [ 41 , 42 ]). However, as of now, there is no concrete evidence to support these conjectures, although some clinical trials are currently underway to investigate some of these [ 43 ].
Note that India may have seen fewer COVID-19 cases until now, but the war is not over yet. There are many states like Maharashtra, Delhi, Madhya Pradesh, Rajasthan, Gujarat, Uttar Pradesh, and West Bengal who are still at high risk. These states may see a significant increase in confirmed COVID-19 cases in the coming days if preventive measures are not implemented properly. On the positive side, Kerala has shown how to effectively “flatten” or even “crush the curve” of COVID-19 cases. We hope India can limit the spread and impact of COVID-19 with a strong determination in policies as already shown by the central and state governments.
There are a few other works that are based explicitly on Indian COVID-19 data. Das [ 30 ] has used the epidemiological model to estimate the R 0 at national and some state levels. Ray et al [ 44 ] used a predictive model for case counts in India. They also discussed hypothetical interventions with various intensities and provided projections over a time horizon. Both the papers have used the susceptible-infected-recovered model (or some extension) for their analysis and prediction. As we discussed earlier, considering the great diversity in every aspect of India, along with its vast population, it would be a better idea to look at each of the states individually. The study of each of the states individually would help decide further actions to contain the spread of the disease, which can be crucial for the specific states only. In this paper, we have mainly focused on the SIS model along with the logistic and the exponential models at each state (restricting to only those states with enough data for prediction). The SIS model takes into account the possibility that an infected individual can return to the susceptible class on recovery because the disease confers no long-standing immunity against reinfection. In South Korea, the health authorities discovered 163 patients who tested positive again after a full recovery [ 45 , 46 ]. The WHO is aware of these reports of patients who were first tested negative for COVID-19 using polymerase chain reaction testing and then after some days, tested positive again [ 47 ]. In a scientific brief, dated April 24, 2020, the WHO said, “there is currently no evidence that people who have recovered from COVID-19 and have antibodies are protected from a second infection” [ 48 ]. Several research papers have reported that, even though being infected by the virus may build immunity against the disease in the short-term, it is not a guaranteed fact, and it may not be long-lasting protection [ 49 - 51 ].
A report based on one particular model can mislead us. Here, we have considered the exponential, the logistic, and the SIS models along with the DIR. We have interpreted the results jointly from all models rather than individually. We expect the DIR to be zero or negative to conclude that COVID-19 is not spreading in a certain state. Even a small positive DIR such as 0.01 indicates that the virus is still spreading in the community and can potentially increase the DIR anytime. The states without a decreasing trend in DIR and near exponential growth in active infected cases are Maharashtra, Delhi, Gujarat, Madhya Pradesh, Andhra Pradesh, Uttar Pradesh, and West Bengal. The states with an almost decreasing trend in DIR and nonincreasing growth in active infected cases are Tamil Nadu, Rajasthan, Punjab, and Bihar. The states with a decreasing trend in DIR and decreasing growth in active infected cases in the last few days are Kerala, Haryana, Jammu and Kashmir, Karnataka, and Telangana. States with nondecreasing DIR need to do much more in terms of the preventive measures immediately to combat the COVID-19 pandemic. On the other hand, the states with decreasing DIR can maintain the same status to see the DIR become zero or negative for a consecutive 14 days to be able to declare the end of the pandemic.
Based on the modeling approaches presented in this paper, we have developed a web application [ 52 ] to see the Indian statewise forecast based on recent data that is updated regularly. The web application also offers a 30-day prediction of cumulative cases at the pan-India level by summing up the predicted cumulative cases of considered states.
Abbreviations
Multimedia appendix 1.
Conflicts of Interest: None declared.
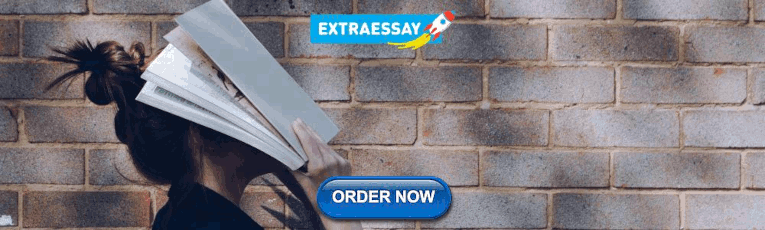
IMAGES
VIDEO
COMMENTS
1. Introduction. The world woke up to a perilous reality on the 11th of March, 2020 when the World Health Organization (WHO) declared novel coronavirus (COVID-19) a pandemic (Sohrabi et al., 2020; WHO, 2020a).Originating from Wuhan, China, cases rapidly spread to Japan, South Korea, Europe and the United States as it reached global proportions.
The pandemic of Coronavirus Disease 2019 (COVID-19) is a timely reminder of the nature and impact of Public Health Emergencies of International Concern. As of 12 January 2022, there were over 314 million cases and over 5.5 million deaths notified since the start of the pandemic. The COVID-19 pandemic takes variable shapes and forms, in terms of cases and deaths, in different regions and ...
The spread of the "Severe Acute Respiratory Coronavirus 2" (SARS-CoV-2), the causal agent of COVID-19, was characterized as a pandemic by the World Health Organization (WHO) in March 2020 and has triggered an international public health emergency [].The numbers of confirmed cases and deaths due to COVID-19 are rapidly escalating, counting in millions [], causing massive economic strain ...
Coronavirus disease-19 (COVID-19) is caused by the severe acute respiratory syndrome coronavirus 2 (2019-nCoV or SARS-CoV-2). Genomic analysis has revealed that bat and pangolin coronaviruses are phylogenetically related to SARS-CoV-2. The actual origin and passage history of the virus are unknown, but human-human transmission of the virus has ...
This essay examines key aspects of social relationships that were disrupted by the COVID-19 pandemic. It focuses explicitly on relational mechanisms of health and brings together theory and emerging evidence on the effects of the COVID-19 pandemic to make recommendations for future public health policy and recovery. We first provide an overview of the pandemic in the UK context, outlining the ...
1. Introduction. The coronavirus disease 2019 (COVID-19) pandemic has led to unprecedented changes in people's daily lives, with implications for mental health and well-being [1-4], both at the level of a given country's population, and when considering specific vulnerable groups [5-7].In order to mitigate the untoward impact of the pandemic (including lockdown) and support mental health ...
Transmission. The role of the Huanan Seafood Wholesale Market in propagating disease is unclear. Many initial COVID-19 cases were linked to this market suggesting that SARS-CoV-2 was transmitted from animals to humans .However, a genomic study has provided evidence that the virus was introduced from another, yet unknown location, into the market where it spread more rapidly, although human-to ...
Objective To review the evidence on the effectiveness of public health measures in reducing the incidence of covid-19, SARS-CoV-2 transmission, and covid-19 mortality. Design Systematic review and meta-analysis. Data sources Medline, Embase, CINAHL, Biosis, Joanna Briggs, Global Health, and World Health Organization COVID-19 database (preprints). Eligibility criteria for study selection ...
Download MP3. In the final Coronapod of 2020, we dive into the scientific literature to reflect on the COVID-19 pandemic. Researchers have discovered so much about SARS-CoV-2 - information that ...
Article selection. A total of 14787 COVID-19 papers were identified as of May 14, 2020 and 4892 duplicate articles were removed. In total, 9895 titles and abstracts were screened, and 9101 ...
The novel coronavirus (COVID-19) that was first reported at the end of 2019 has impacted almost every aspect of life as we know it. This paper focuses on the incidence of the disease in Italy and Spain—two of the first and most affected European countries. Using two simple mathematical epidemiological models—the Susceptible-Infectious-Recovered model and the log-linear regression model, we ...
Abstract. Coronavirus disease-19 (COVID-19) is caused by the severe acute respiratory syndrome coronavirus 2 (2019-nCoV or SARS-CoV-2). Genomic analysis has revealed that bat and pangolin coronaviruses are phylogenetically related to SARS-CoV-2. The actual origin and passage history of the virus are unknown, but human-human transmission of the ...
During the COVID-19 pandemic, medicine and public health have grappled with challenges related to individual behaviour and decision making, both in terms of infection and more recently concerning the uptake of COVID-19 vaccines. Social theory has long pointed to the role of narrative when trying to grasp the complexity of understanding and decision making in times of crisis.
Nearly every 100 years, humans collectively face a pandemic crisis. After the Spanish flu, now the world is in the grip of coronavirus disease 2019 (COVID-19). First detected in 2019 in the Chinese city of Wuhan, COVID-19 causes severe acute respiratory distress syndrome. Despite the initial evidence indicating a zoonotic origin, the contagion ...
The analytical report that follows was written by a student, Trevor Garcia, for a first-year composition course. ... Informal reports follow essay structure and open with an overview. end annotated text. ... New research rewrites history of when COVID-19 took off in the U.S.—and points to missed chances to stop it. STAT. https://www.statnews ...
Impact of Covid-19: Analytical Essay. This essay sample was donated by a student to help the academic community. Papers provided by EduBirdie writers usually outdo students' samples. Covid-19 is a new disease caused by a new strain of coronavirus that has proven to be a life-threatening worldwide pandemic causing millions of infections and ...
Although the COVID-19 pandemic affected all parts of the world in 2020, low-, middle- and high-income nations were hit in different ways. In low-income countries, average excess mortality reached 34%, followed by 14% in middle-income countries and 10% in high-income ones. However, middle-income nations experienced the largest hit to their gross ...
Students can choose to write a full-length college essay on the coronavirus or summarize their experience in a shorter form. To help students explain how the pandemic affected them, The Common App ...
Editor in Chief's Introduction to Essays on the Impact of COVID-19 on Work and Workers. On March 11, 2020, the World Health Organization declared that COVID-19 was a global pandemic, indicating significant global spread of an infectious disease ( World Health Organization, 2020 ). At that point, there were 118,000 confirmed cases of the ...
The Covid-19 crisis reminds us that data analytics problems are never simple. Analysts usually need to make difficult and to some extent subjective judgments about how how much data to collect ...
100 Words Essay on Covid 19. COVID-19 or Corona Virus is a novel coronavirus that was first identified in 2019. It is similar to other coronaviruses, such as SARS-CoV and MERS-CoV, but it is more contagious and has caused more severe respiratory illness in people who have been infected. The novel coronavirus became a global pandemic in a very ...
The novel COVID-19 pandemic's impact on college students is unprecedented. College students are a priority population for health promotion and disease prevention [], and universities are unique settings that can affect the health of a larger segment of the population.College campuses are densely populated, with students living in close proximity to others; this means that college students can ...
Find breaking science news and analysis from the world's leading research journal.
The highly infectious coronavirus disease (COVID-19) was first detected in Wuhan, China in December 2019 and subsequently spread to 212 countries and territories around the world, infecting millions of people. In India, a large country of about 1.3 billion people, the disease was first detected on January 30, 2020, in a student returning from ...