Hypothesis vs. Theory
A hypothesis is either a suggested explanation for an observable phenomenon, or a reasoned prediction of a possible causal correlation among multiple phenomena. In science , a theory is a tested, well-substantiated, unifying explanation for a set of verified, proven factors. A theory is always backed by evidence; a hypothesis is only a suggested possible outcome, and is testable and falsifiable.
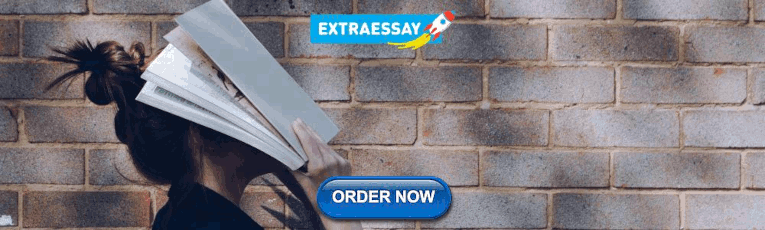
Comparison chart
Hypothesis | Theory | |
---|---|---|
Definition | A suggested explanation for an observable phenomenon or prediction of a possible causal correlation among multiple phenomena. | In , a theory is a well-substantiated, unifying explanation for a set of verified, proven hypotheses. |
Based on | Suggestion, possibility, projection or prediction, but the result is uncertain. | Evidence, verification, repeated testing, wide scientific consensus |
Testable | Yes | Yes |
Falsifiable | Yes | Yes |
Is well-substantiated? | No | Yes |
Is well-tested? | No | Yes |
Data | Usually based on very limited data | Based on a very wide set of data tested under various circumstances. |
Instance | Specific: Hypothesis is usually based on a very specific observation and is limited to that instance. | General: A theory is the establishment of a general principle through multiple tests and experiments, and this principle may apply to various specific instances. |
Purpose | To present an uncertain possibility that can be explored further through experiments and observations. | To explain why a large set of observations are consistently made. |
Examples of Theory and Hypothesis
Theory: Einstein's theory of relativity is a theory because it has been tested and verified innumerable times, with results consistently verifying Einstein's conclusion. However, simply because Einstein's conclusion has become a theory does not mean testing of this theory has stopped; all science is ongoing. See also the Big Bang theory , germ theory , and climate change .
Hypothesis: One might think that a prisoner who learns a work skill while in prison will be less likely to commit a crime when released. This is a hypothesis, an "educated guess." The scientific method can be used to test this hypothesis, to either prove it is false or prove that it warrants further study. (Note: Simply because a hypothesis is not found to be false does not mean it is true all or even most of the time. If it is consistently true after considerable time and research, it may be on its way to becoming a theory.)
This video further explains the difference between a theory and a hypothesis:
Common Misconception
People often tend to say "theory" when what they're actually talking about is a hypothesis. For instance, "Migraines are caused by drinking coffee after 2 p.m. — well, it's just a theory, not a rule."
This is actually a logically reasoned proposal based on an observation — say 2 instances of drinking coffee after 2 p.m. caused a migraine — but even if this were true, the migraine could have actually been caused by some other factors.
Because this observation is merely a reasoned possibility, it is testable and can be falsified — which makes it a hypothesis, not a theory.
- What is a Scientific Hypothesis? - LiveScience
- Wikipedia:Scientific theory
Related Comparisons

Share this comparison via:
If you read this far, you should follow us:
"Hypothesis vs Theory." Diffen.com. Diffen LLC, n.d. Web. 3 Jul 2024. < >
Comments: Hypothesis vs Theory
Anonymous comments (2).
October 11, 2013, 1:11pm "In science, a theory is a well-substantiated, unifying explanation for a set of verified, proven hypotheses." But there's no such thing as "proven hypotheses". Hypotheses can be tested/falsified, they can't be "proven". That's just not how science works. Logical deductions based on axioms can be proven, but not scientific hypotheses. On top of that I find it somewhat strange to claim that a theory doesn't have to be testable, if it's built up from hypotheses, which DO have to be testable... — 80.✗.✗.139
May 6, 2014, 11:45pm "Evolution is a theory, not a fact, regarding the origin of living things." this statement is poorly formed because it implies that a thing is a theory until it gets proven and then it is somehow promoted to fact. this is just a misunderstanding of what the words mean, and of how science progresses generally. to say that a theory is inherently dubious because "it isn't a fact" is pretty much a meaningless statement. no expression which qualified as a mere fact could do a very good job of explaining the complicated process by which species have arisen on Earth over the last billion years. in fact, if you claimed that you could come up with such a single fact, now THAT would be dubious! everything we observe in nature supports the theory of evolution, and nothing we observe contradicts it. when you can say this about a theory, it's a pretty fair bet that the theory is correct. — 71.✗.✗.151
- Accuracy vs Precision
- Deductive vs Inductive
- Subjective vs Objective
- Subconscious vs Unconscious mind
- Qualitative vs Quantitative
- Creationism vs Evolution
Edit or create new comparisons in your area of expertise.
Stay connected
© All rights reserved.
This is the Difference Between a Hypothesis and a Theory
What to Know A hypothesis is an assumption made before any research has been done. It is formed so that it can be tested to see if it might be true. A theory is a principle formed to explain the things already shown in data. Because of the rigors of experiment and control, it is much more likely that a theory will be true than a hypothesis.
As anyone who has worked in a laboratory or out in the field can tell you, science is about process: that of observing, making inferences about those observations, and then performing tests to see if the truth value of those inferences holds up. The scientific method is designed to be a rigorous procedure for acquiring knowledge about the world around us.
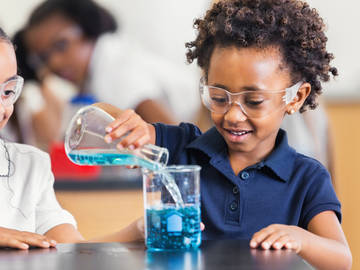
In scientific reasoning, a hypothesis is constructed before any applicable research has been done. A theory, on the other hand, is supported by evidence: it's a principle formed as an attempt to explain things that have already been substantiated by data.
Toward that end, science employs a particular vocabulary for describing how ideas are proposed, tested, and supported or disproven. And that's where we see the difference between a hypothesis and a theory .
A hypothesis is an assumption, something proposed for the sake of argument so that it can be tested to see if it might be true.
In the scientific method, the hypothesis is constructed before any applicable research has been done, apart from a basic background review. You ask a question, read up on what has been studied before, and then form a hypothesis.
What is a Hypothesis?
A hypothesis is usually tentative, an assumption or suggestion made strictly for the objective of being tested.
When a character which has been lost in a breed, reappears after a great number of generations, the most probable hypothesis is, not that the offspring suddenly takes after an ancestor some hundred generations distant, but that in each successive generation there has been a tendency to reproduce the character in question, which at last, under unknown favourable conditions, gains an ascendancy. Charles Darwin, On the Origin of Species , 1859 According to one widely reported hypothesis , cell-phone transmissions were disrupting the bees' navigational abilities. (Few experts took the cell-phone conjecture seriously; as one scientist said to me, "If that were the case, Dave Hackenberg's hives would have been dead a long time ago.") Elizabeth Kolbert, The New Yorker , 6 Aug. 2007
What is a Theory?
A theory , in contrast, is a principle that has been formed as an attempt to explain things that have already been substantiated by data. It is used in the names of a number of principles accepted in the scientific community, such as the Big Bang Theory . Because of the rigors of experimentation and control, its likelihood as truth is much higher than that of a hypothesis.
It is evident, on our theory , that coasts merely fringed by reefs cannot have subsided to any perceptible amount; and therefore they must, since the growth of their corals, either have remained stationary or have been upheaved. Now, it is remarkable how generally it can be shown, by the presence of upraised organic remains, that the fringed islands have been elevated: and so far, this is indirect evidence in favour of our theory . Charles Darwin, The Voyage of the Beagle , 1839 An example of a fundamental principle in physics, first proposed by Galileo in 1632 and extended by Einstein in 1905, is the following: All observers traveling at constant velocity relative to one another, should witness identical laws of nature. From this principle, Einstein derived his theory of special relativity. Alan Lightman, Harper's , December 2011
Non-Scientific Use
In non-scientific use, however, hypothesis and theory are often used interchangeably to mean simply an idea, speculation, or hunch (though theory is more common in this regard):
The theory of the teacher with all these immigrant kids was that if you spoke English loudly enough they would eventually understand. E. L. Doctorow, Loon Lake , 1979 Chicago is famous for asking questions for which there can be no boilerplate answers. Example: given the probability that the federal tax code, nondairy creamer, Dennis Rodman and the art of mime all came from outer space, name something else that has extraterrestrial origins and defend your hypothesis . John McCormick, Newsweek , 5 Apr. 1999 In his mind's eye, Miller saw his case suddenly taking form: Richard Bailey had Helen Brach killed because she was threatening to sue him over the horses she had purchased. It was, he realized, only a theory , but it was one he felt certain he could, in time, prove. Full of urgency, a man with a mission now that he had a hypothesis to guide him, he issued new orders to his troops: Find out everything you can about Richard Bailey and his crowd. Howard Blum, Vanity Fair , January 1995
And sometimes one term is used as a genus, or a means for defining the other:
Laplace's popular version of his astronomy, the Système du monde , was famous for introducing what came to be known as the nebular hypothesis , the theory that the solar system was formed by the condensation, through gradual cooling, of the gaseous atmosphere (the nebulae) surrounding the sun. Louis Menand, The Metaphysical Club , 2001 Researchers use this information to support the gateway drug theory — the hypothesis that using one intoxicating substance leads to future use of another. Jordy Byrd, The Pacific Northwest Inlander , 6 May 2015 Fox, the business and economics columnist for Time magazine, tells the story of the professors who enabled those abuses under the banner of the financial theory known as the efficient market hypothesis . Paul Krugman, The New York Times Book Review , 9 Aug. 2009
Incorrect Interpretations of "Theory"
Since this casual use does away with the distinctions upheld by the scientific community, hypothesis and theory are prone to being wrongly interpreted even when they are encountered in scientific contexts—or at least, contexts that allude to scientific study without making the critical distinction that scientists employ when weighing hypotheses and theories.
The most common occurrence is when theory is interpreted—and sometimes even gleefully seized upon—to mean something having less truth value than other scientific principles. (The word law applies to principles so firmly established that they are almost never questioned, such as the law of gravity.)
This mistake is one of projection: since we use theory in general use to mean something lightly speculated, then it's implied that scientists must be talking about the same level of uncertainty when they use theory to refer to their well-tested and reasoned principles.
The distinction has come to the forefront particularly on occasions when the content of science curricula in schools has been challenged—notably, when a school board in Georgia put stickers on textbooks stating that evolution was "a theory, not a fact, regarding the origin of living things." As Kenneth R. Miller, a cell biologist at Brown University, has said , a theory "doesn’t mean a hunch or a guess. A theory is a system of explanations that ties together a whole bunch of facts. It not only explains those facts, but predicts what you ought to find from other observations and experiments.”
While theories are never completely infallible, they form the basis of scientific reasoning because, as Miller said "to the best of our ability, we’ve tested them, and they’ve held up."
More Differences Explained
- Epidemic vs. Pandemic
- Diagnosis vs. Prognosis
- Treatment vs. Cure
Word of the Day
See Definitions and Examples »
Get Word of the Day daily email!
Games & Quizzes
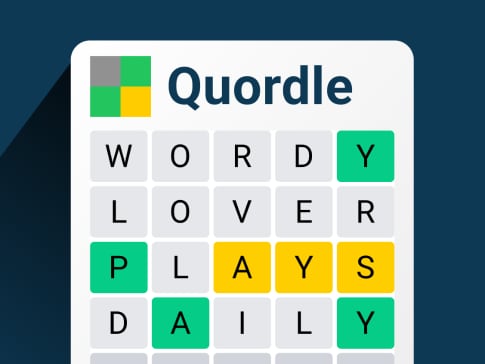
Commonly Confused
'canceled' or 'cancelled', 'virus' vs. 'bacteria', your vs. you're: how to use them correctly, is it 'jail' or 'prison', 'deduction' vs. 'induction' vs. 'abduction', grammar & usage, every letter is silent, sometimes: a-z list of examples, how to use em dashes (—), en dashes (–) , and hyphens (-), the difference between 'i.e.' and 'e.g.', plural and possessive names: a guide, 31 useful rhetorical devices, pilfer: how to play and win, 8 words with fascinating histories, flower etymologies for your spring garden, 8 words for lesser-known musical instruments, it's a scorcher words for the summer heat.
Scientific Hypothesis, Model, Theory, and Law
Understanding the Difference Between Basic Scientific Terms
Hero Images / Getty Images
- Chemical Laws
- Periodic Table
- Projects & Experiments
- Scientific Method
- Biochemistry
- Physical Chemistry
- Medical Chemistry
- Chemistry In Everyday Life
- Famous Chemists
- Activities for Kids
- Abbreviations & Acronyms
- Weather & Climate
- Ph.D., Biomedical Sciences, University of Tennessee at Knoxville
- B.A., Physics and Mathematics, Hastings College
Words have precise meanings in science. For example, "theory," "law," and "hypothesis" don't all mean the same thing. Outside of science, you might say something is "just a theory," meaning it's a supposition that may or may not be true. In science, however, a theory is an explanation that generally is accepted to be true. Here's a closer look at these important, commonly misused terms.
A hypothesis is an educated guess, based on observation. It's a prediction of cause and effect. Usually, a hypothesis can be supported or refuted through experimentation or more observation. A hypothesis can be disproven but not proven to be true.
Example: If you see no difference in the cleaning ability of various laundry detergents, you might hypothesize that cleaning effectiveness is not affected by which detergent you use. This hypothesis can be disproven if you observe a stain is removed by one detergent and not another. On the other hand, you cannot prove the hypothesis. Even if you never see a difference in the cleanliness of your clothes after trying 1,000 detergents, there might be one more you haven't tried that could be different.
Scientists often construct models to help explain complex concepts. These can be physical models like a model volcano or atom or conceptual models like predictive weather algorithms. A model doesn't contain all the details of the real deal, but it should include observations known to be valid.
Example: The Bohr model shows electrons orbiting the atomic nucleus, much the same way as the way planets revolve around the sun. In reality, the movement of electrons is complicated but the model makes it clear that protons and neutrons form a nucleus and electrons tend to move around outside the nucleus.
A scientific theory summarizes a hypothesis or group of hypotheses that have been supported with repeated testing. A theory is valid as long as there is no evidence to dispute it. Therefore, theories can be disproven. Basically, if evidence accumulates to support a hypothesis, then the hypothesis can become accepted as a good explanation of a phenomenon. One definition of a theory is to say that it's an accepted hypothesis.
Example: It is known that on June 30, 1908, in Tunguska, Siberia, there was an explosion equivalent to the detonation of about 15 million tons of TNT. Many hypotheses have been proposed for what caused the explosion. It was theorized that the explosion was caused by a natural extraterrestrial phenomenon , and was not caused by man. Is this theory a fact? No. The event is a recorded fact. Is this theory, generally accepted to be true, based on evidence to-date? Yes. Can this theory be shown to be false and be discarded? Yes.
A scientific law generalizes a body of observations. At the time it's made, no exceptions have been found to a law. Scientific laws explain things but they do not describe them. One way to tell a law and a theory apart is to ask if the description gives you the means to explain "why." The word "law" is used less and less in science, as many laws are only true under limited circumstances.
Example: Consider Newton's Law of Gravity . Newton could use this law to predict the behavior of a dropped object but he couldn't explain why it happened.
As you can see, there is no "proof" or absolute "truth" in science. The closest we get are facts, which are indisputable observations. Note, however, if you define proof as arriving at a logical conclusion, based on the evidence, then there is "proof" in science. Some work under the definition that to prove something implies it can never be wrong, which is different. If you're asked to define the terms hypothesis, theory, and law, keep in mind the definitions of proof and of these words can vary slightly depending on the scientific discipline. What's important is to realize they don't all mean the same thing and cannot be used interchangeably.
- Scientific Method Lesson Plan
- What Is an Experiment? Definition and Design
- How To Design a Science Fair Experiment
- Chemistry 101 - Introduction & Index of Topics
- What Is the Difference Between Hard and Soft Science?
- What Is a Control Group?
- Henry's Law Definition
- Chemistry Vocabulary Terms You Should Know
- Hess's Law Definition
- What Does pH Stand For?
- How to Write a Lab Report
- What Is Chemical Engineering?
- Teach Yourself Chemistry Today
- Check Out These Chemistry Career Options Before You Get a Degree
- Here's How to Calculate pH Values
- Setting Up a Home Chemistry Lab
- Activity Diagram (UML)
- Amazon Web Services
- Android Mockups
- Block Diagram
- Business Process Management
- Chemical Chart
- Cisco Network Diagram
- Class Diagram (UML)
- Collaboration Diagram (UML)
- Compare & Contrast Diagram
- Component Diagram (UML)
- Concept Diagram
- Cycle Diagram
- Data Flow Diagram
- Data Flow Diagrams (YC)
- Database Diagram
- Deployment Diagram (UML)
- Entity Relationship Diagram
- Family Tree
- Fishbone / Ishikawa Diagram
- Gantt Chart
- Infographics
- iOS Mockups
- Network Diagram
- Object Diagram (UML)
- Object Process Model
- Organizational Chart
- Sequence Diagram (UML)
- Spider Diagram
- State Chart Diagram (UML)
- Story Board
- SWOT Diagram
- TQM - Total Quality Management
- Use Case Diagram (UML)
- Value Stream Mapping
- Venn Diagram
- Web Mockups
- Work Breakdown Structure
Compare and contrast a hypothesis, scientific law, and theory

Comapring and contrasting the characteristics of a hypothesis, scientific law, and theory.
You can easily edit this template using Creately's venn diagram maker . You can export it in multiple formats like JPEG, PNG and SVG and easily add it to Word documents, Powerpoint (PPT) presentations, Excel or any other documents. You can export it as a PDF for high-quality printouts.
- Flowchart Templates
- Org Chart Templates
- Concept Map Templates
- Mind Mapping Templates
- WBS Templates
- Family Tree Templates
- VSM Templates
- Data Flow Diagram Templates
- Network Diagram Templates
- SWOT Analysis Templates
- Genogram Templates
- Activity Diagram Templates
- Amazon Web Services Templates
- Android Mockups Templates
- Block Diagram Templates
- Business Process Management Templates
- Chemical Chart Templates
- Cisco Network Diagram Templates
- Class Diagram Templates
- Collaboration Diagram Templates
- Compare & Contrast Diagram Templates
- Component Diagram Templates
- Concept Diagram Templates
- Cycle Diagram Templates
- Data Flow Diagrams(YC) Templates
- Database Diagram Templates
- Deployment Diagram Templates
- Entity Relationship Diagram Templates
- Fishbone Diagram Templates
- Gantt Chart Templates
- Infographic Templates
- iOS Mockup Templates
- KWL Chart Templates
- Logic Gate Templates
- Mind Map Templates
- Object Diagram Templates
- Object Process Model Templates
- Organizational Chart Templates
- Other Templates
- PERT Chart Templates
- Sequence Diagram Templates
- Site Map Templates
- Spider Diagram Templates
- State Chart Diagram Templates
- Story Board Templates
- SWOT Diagram Templates
- T Chart Templates
- TQM - Total Quality Management Templates
- UI Mockup Templates
- Use Case Diagram Templates
- Value Stream Mapping Templates
- Venn Diagram Templates
- Web Mockup Templates
- Y Chart Templates
Related Templates
1.2 The Scientific Methods
Section learning objectives.
By the end of this section, you will be able to do the following:
- Explain how the methods of science are used to make scientific discoveries
- Define a scientific model and describe examples of physical and mathematical models used in physics
- Compare and contrast hypothesis, theory, and law
Teacher Support
The learning objectives in this section will help your students master the following standards:
- (A) know the definition of science and understand that it has limitations, as specified in subsection (b)(2) of this section;
- (B) know that scientific hypotheses are tentative and testable statements that must be capable of being supported or not supported by observational evidence. Hypotheses of durable explanatory power which have been tested over a wide variety of conditions are incorporated into theories;
- (C) know that scientific theories are based on natural and physical phenomena and are capable of being tested by multiple independent researchers. Unlike hypotheses, scientific theories are well-established and highly-reliable explanations, but may be subject to change as new areas of science and new technologies are developed;
- (D) distinguish between scientific hypotheses and scientific theories.
Section Key Terms
experiment | hypothesis | model | observation | principle |
scientific law | scientific methods | theory | universal |
[OL] Pre-assessment for this section could involve students sharing or writing down an anecdote about when they used the methods of science. Then, students could label their thought processes in their anecdote with the appropriate scientific methods. The class could also discuss their definitions of theory and law, both outside and within the context of science.
[OL] It should be noted and possibly mentioned that a scientist , as mentioned in this section, does not necessarily mean a trained scientist. It could be anyone using methods of science.
Scientific Methods
Scientists often plan and carry out investigations to answer questions about the universe around us. These investigations may lead to natural laws. Such laws are intrinsic to the universe, meaning that humans did not create them and cannot change them. We can only discover and understand them. Their discovery is a very human endeavor, with all the elements of mystery, imagination, struggle, triumph, and disappointment inherent in any creative effort. The cornerstone of discovering natural laws is observation. Science must describe the universe as it is, not as we imagine or wish it to be.
We all are curious to some extent. We look around, make generalizations, and try to understand what we see. For example, we look up and wonder whether one type of cloud signals an oncoming storm. As we become serious about exploring nature, we become more organized and formal in collecting and analyzing data. We attempt greater precision, perform controlled experiments (if we can), and write down ideas about how data may be organized. We then formulate models, theories, and laws based on the data we have collected, and communicate those results with others. This, in a nutshell, describes the scientific method that scientists employ to decide scientific issues on the basis of evidence from observation and experiment.
An investigation often begins with a scientist making an observation . The scientist observes a pattern or trend within the natural world. Observation may generate questions that the scientist wishes to answer. Next, the scientist may perform some research about the topic and devise a hypothesis . A hypothesis is a testable statement that describes how something in the natural world works. In essence, a hypothesis is an educated guess that explains something about an observation.
[OL] An educated guess is used throughout this section in describing a hypothesis to combat the tendency to think of a theory as an educated guess.
Scientists may test the hypothesis by performing an experiment . During an experiment, the scientist collects data that will help them learn about the phenomenon they are studying. Then the scientists analyze the results of the experiment (that is, the data), often using statistical, mathematical, and/or graphical methods. From the data analysis, they draw conclusions. They may conclude that their experiment either supports or rejects their hypothesis. If the hypothesis is supported, the scientist usually goes on to test another hypothesis related to the first. If their hypothesis is rejected, they will often then test a new and different hypothesis in their effort to learn more about whatever they are studying.
Scientific processes can be applied to many situations. Let’s say that you try to turn on your car, but it will not start. You have just made an observation! You ask yourself, "Why won’t my car start?" You can now use scientific processes to answer this question. First, you generate a hypothesis such as, "The car won’t start because it has no gasoline in the gas tank." To test this hypothesis, you put gasoline in the car and try to start it again. If the car starts, then your hypothesis is supported by the experiment. If the car does not start, then your hypothesis is rejected. You will then need to think up a new hypothesis to test such as, "My car won’t start because the fuel pump is broken." Hopefully, your investigations lead you to discover why the car won’t start and enable you to fix it.
A model is a representation of something that is often too difficult (or impossible) to study directly. Models can take the form of physical models, equations, computer programs, or simulations—computer graphics/animations. Models are tools that are especially useful in modern physics because they let us visualize phenomena that we normally cannot observe with our senses, such as very small objects or objects that move at high speeds. For example, we can understand the structure of an atom using models, without seeing an atom with our own eyes. Although images of single atoms are now possible, these images are extremely difficult to achieve and are only possible due to the success of our models. The existence of these images is a consequence rather than a source of our understanding of atoms. Models are always approximate, so they are simpler to consider than the real situation; the more complete a model is, the more complicated it must be. Models put the intangible or the extremely complex into human terms that we can visualize, discuss, and hypothesize about.
Scientific models are constructed based on the results of previous experiments. Even still, models often only describe a phenomenon partially or in a few limited situations. Some phenomena are so complex that they may be impossible to model them in their entirety, even using computers. An example is the electron cloud model of the atom in which electrons are moving around the atom’s center in distinct clouds ( Figure 1.12 ), that represent the likelihood of finding an electron in different places. This model helps us to visualize the structure of an atom. However, it does not show us exactly where an electron will be within its cloud at any one particular time.
As mentioned previously, physicists use a variety of models including equations, physical models, computer simulations, etc. For example, three-dimensional models are often commonly used in chemistry and physics to model molecules. Properties other than appearance or location are usually modelled using mathematics, where functions are used to show how these properties relate to one another. Processes such as the formation of a star or the planets, can also be modelled using computer simulations. Once a simulation is correctly programmed based on actual experimental data, the simulation can allow us to view processes that happened in the past or happen too quickly or slowly for us to observe directly. In addition, scientists can also run virtual experiments using computer-based models. In a model of planet formation, for example, the scientist could alter the amount or type of rocks present in space and see how it affects planet formation.
Scientists use models and experimental results to construct explanations of observations or design solutions to problems. For example, one way to make a car more fuel efficient is to reduce the friction or drag caused by air flowing around the moving car. This can be done by designing the body shape of the car to be more aerodynamic, such as by using rounded corners instead of sharp ones. Engineers can then construct physical models of the car body, place them in a wind tunnel, and examine the flow of air around the model. This can also be done mathematically in a computer simulation. The air flow pattern can be analyzed for regions smooth air flow and for eddies that indicate drag. The model of the car body may have to be altered slightly to produce the smoothest pattern of air flow (i.e., the least drag). The pattern with the least drag may be the solution to increasing fuel efficiency of the car. This solution might then be incorporated into the car design.
Using Models and the Scientific Processes
Be sure to secure loose items before opening the window or door.
In this activity, you will learn about scientific models by making a model of how air flows through your classroom or a room in your house.
- One room with at least one window or door that can be opened
- Work with a group of four, as directed by your teacher. Close all of the windows and doors in the room you are working in. Your teacher may assign you a specific window or door to study.
- Before opening any windows or doors, draw a to-scale diagram of your room. First, measure the length and width of your room using the tape measure. Then, transform the measurement using a scale that could fit on your paper, such as 5 centimeters = 1 meter.
- Your teacher will assign you a specific window or door to study air flow. On your diagram, add arrows showing your hypothesis (before opening any windows or doors) of how air will flow through the room when your assigned window or door is opened. Use pencil so that you can easily make changes to your diagram.
- On your diagram, mark four locations where you would like to test air flow in your room. To test for airflow, hold a strip of single ply tissue paper between the thumb and index finger. Note the direction that the paper moves when exposed to the airflow. Then, for each location, predict which way the paper will move if your air flow diagram is correct.
- Now, each member of your group will stand in one of the four selected areas. Each member will test the airflow Agree upon an approximate height at which everyone will hold their papers.
- When you teacher tells you to, open your assigned window and/or door. Each person should note the direction that their paper points immediately after the window or door was opened. Record your results on your diagram.
- Did the airflow test data support or refute the hypothetical model of air flow shown in your diagram? Why or why not? Correct your model based on your experimental evidence.
- With your group, discuss how accurate your model is. What limitations did it have? Write down the limitations that your group agreed upon.
- Yes, you could use your model to predict air flow through a new window. The earlier experiment of air flow would help you model the system more accurately.
- Yes, you could use your model to predict air flow through a new window. The earlier experiment of air flow is not useful for modeling the new system.
- No, you cannot model a system to predict the air flow through a new window. The earlier experiment of air flow would help you model the system more accurately.
- No, you cannot model a system to predict the air flow through a new window. The earlier experiment of air flow is not useful for modeling the new system.
This Snap Lab! has students construct a model of how air flows in their classroom. Each group of four students will create a model of air flow in their classroom using a scale drawing of the room. Then, the groups will test the validity of their model by placing weathervanes that they have constructed around the room and opening a window or door. By observing the weather vanes, students will see how air actually flows through the room from a specific window or door. Students will then correct their model based on their experimental evidence. The following material list is given per group:
- One room with at least one window or door that can be opened (An optimal configuration would be one window or door per group.)
- Several pieces of construction paper (at least four per group)
- Strips of single ply tissue paper
- One tape measure (long enough to measure the dimensions of the room)
- Group size can vary depending on the number of windows/doors available and the number of students in the class.
- The room dimensions could be provided by the teacher. Also, students may need a brief introduction in how to make a drawing to scale.
- This is another opportunity to discuss controlled experiments in terms of why the students should hold the strips of tissue paper at the same height and in the same way. One student could also serve as a control and stand far away from the window/door or in another area that will not receive air flow from the window/door.
- You will probably need to coordinate this when multiple windows or doors are used. Only one window or door should be opened at a time for best results. Between openings, allow a short period (5 minutes) when all windows and doors are closed, if possible.
Answers to the Grasp Check will vary, but the air flow in the new window or door should be based on what the students observed in their experiment.
Scientific Laws and Theories
A scientific law is a description of a pattern in nature that is true in all circumstances that have been studied. That is, physical laws are meant to be universal , meaning that they apply throughout the known universe. Laws are often also concise, whereas theories are more complicated. A law can be expressed in the form of a single sentence or mathematical equation. For example, Newton’s second law of motion , which relates the motion of an object to the force applied ( F ), the mass of the object ( m ), and the object’s acceleration ( a ), is simply stated using the equation
Scientific ideas and explanations that are true in many, but not all situations in the universe are usually called principles . An example is Pascal’s principle , which explains properties of liquids, but not solids or gases. However, the distinction between laws and principles is sometimes not carefully made in science.
A theory is an explanation for patterns in nature that is supported by much scientific evidence and verified multiple times by multiple researchers. While many people confuse theories with educated guesses or hypotheses, theories have withstood more rigorous testing and verification than hypotheses.
[OL] Explain to students that in informal, everyday English the word theory can be used to describe an idea that is possibly true but that has not been proven to be true. This use of the word theory often leads people to think that scientific theories are nothing more than educated guesses. This is not just a misconception among students, but among the general public as well.
As a closing idea about scientific processes, we want to point out that scientific laws and theories, even those that have been supported by experiments for centuries, can still be changed by new discoveries. This is especially true when new technologies emerge that allow us to observe things that were formerly unobservable. Imagine how viewing previously invisible objects with a microscope or viewing Earth for the first time from space may have instantly changed our scientific theories and laws! What discoveries still await us in the future? The constant retesting and perfecting of our scientific laws and theories allows our knowledge of nature to progress. For this reason, many scientists are reluctant to say that their studies prove anything. By saying support instead of prove , it keeps the door open for future discoveries, even if they won’t occur for centuries or even millennia.
[OL] With regard to scientists avoiding using the word prove , the general public knows that science has proven certain things such as that the heart pumps blood and the Earth is round. However, scientists should shy away from using prove because it is impossible to test every single instance and every set of conditions in a system to absolutely prove anything. Using support or similar terminology leaves the door open for further discovery.
Check Your Understanding
- Models are simpler to analyze.
- Models give more accurate results.
- Models provide more reliable predictions.
- Models do not require any computer calculations.
- They are the same.
- A hypothesis has been thoroughly tested and found to be true.
- A hypothesis is a tentative assumption based on what is already known.
- A hypothesis is a broad explanation firmly supported by evidence.
- A scientific model is a representation of something that can be easily studied directly. It is useful for studying things that can be easily analyzed by humans.
- A scientific model is a representation of something that is often too difficult to study directly. It is useful for studying a complex system or systems that humans cannot observe directly.
- A scientific model is a representation of scientific equipment. It is useful for studying working principles of scientific equipment.
- A scientific model is a representation of a laboratory where experiments are performed. It is useful for studying requirements needed inside the laboratory.
- The hypothesis must be validated by scientific experiments.
- The hypothesis must not include any physical quantity.
- The hypothesis must be a short and concise statement.
- The hypothesis must apply to all the situations in the universe.
- A scientific theory is an explanation of natural phenomena that is supported by evidence.
- A scientific theory is an explanation of natural phenomena without the support of evidence.
- A scientific theory is an educated guess about the natural phenomena occurring in nature.
- A scientific theory is an uneducated guess about natural phenomena occurring in nature.
- A hypothesis is an explanation of the natural world with experimental support, while a scientific theory is an educated guess about a natural phenomenon.
- A hypothesis is an educated guess about natural phenomenon, while a scientific theory is an explanation of natural world with experimental support.
- A hypothesis is experimental evidence of a natural phenomenon, while a scientific theory is an explanation of the natural world with experimental support.
- A hypothesis is an explanation of the natural world with experimental support, while a scientific theory is experimental evidence of a natural phenomenon.
Use the Check Your Understanding questions to assess students’ achievement of the section’s learning objectives. If students are struggling with a specific objective, the Check Your Understanding will help identify which objective and direct students to the relevant content.
This book may not be used in the training of large language models or otherwise be ingested into large language models or generative AI offerings without OpenStax's permission.
Want to cite, share, or modify this book? This book uses the Creative Commons Attribution License and you must attribute Texas Education Agency (TEA). The original material is available at: https://www.texasgateway.org/book/tea-physics . Changes were made to the original material, including updates to art, structure, and other content updates.
Access for free at https://openstax.org/books/physics/pages/1-introduction
- Authors: Paul Peter Urone, Roger Hinrichs
- Publisher/website: OpenStax
- Book title: Physics
- Publication date: Mar 26, 2020
- Location: Houston, Texas
- Book URL: https://openstax.org/books/physics/pages/1-introduction
- Section URL: https://openstax.org/books/physics/pages/1-2-the-scientific-methods
© Jun 7, 2024 Texas Education Agency (TEA). The OpenStax name, OpenStax logo, OpenStax book covers, OpenStax CNX name, and OpenStax CNX logo are not subject to the Creative Commons license and may not be reproduced without the prior and express written consent of Rice University.

- Table of Contents
- Random Entry
- Chronological
- Editorial Information
- About the SEP
- Editorial Board
- How to Cite the SEP
- Special Characters
- Advanced Tools
- Support the SEP
- PDFs for SEP Friends
- Make a Donation
- SEPIA for Libraries
- Entry Contents
Bibliography
Academic tools.
- Friends PDF Preview
- Author and Citation Info
- Back to Top
All of us engage in and make use of valid reasoning, but the reasoning we actually perform differs in various ways from the inferences studied by most (formal) logicians. Reasoning as performed by human beings typically involves information obtained through more than one medium. Formal logic, by contrast, has thus far been primarily concerned with valid reasoning which is based on information in one form only, i.e., in the form of sentences. Recently, many philosophers, psychologists, logicians, mathematicians, and computer scientists have become increasingly aware of the importance of multi-modal reasoning and, moreover, much research has been undertaken in the area of non-symbolic, especially diagrammatic, representation systems. [ 1 ] This entry outlines the overall directions of this new research area and focuses on the logical status of diagrams in proofs, their representational function and adequacy, different kinds of diagrammatic systems, and the role of diagrams in human cognition.
1. Introduction
2.1 euler diagrams, 2.2 venn diagrams, 2.3 peirce’s extension, 2.4 diagrams as formal systems, 2.5 euler circles revisited, 3.1 limitations on diagrammatic representation and reasoning, 3.2 efficacy of diagrams, 4.1 views on euclid’s diagrams from 4 th century bce to the 20 th century ce, 4.2.1 the exact/co-exact distinction, 4.2.2 the generality problem with euclid’s constructions, 4.3 the formal systems fg and eu, 5.1 some other diagrammatic systems, 5.2 diagrams as mental representations, 5.3 the cognitive role of diagrams, relevant literature, other internet resources, related entries.
Diagrams or pictures probably rank among the oldest forms of human communication. They are not only used for representation but can also be used to carry out certain types of reasoning, and hence play a particular role in logic and mathematics. However, sentential representation systems (e.g., first-order logic) have been dominant in the modern history of logic, while diagrams have largely been seen as only of marginal interest. Diagrams are usually adopted as a heuristic tool in exploring a proof, but not as part of a proof. [ 2 ] It is a quite recent movement among philosophers, logicians, cognitive scientists and computer scientists to focus on different types of representation systems, and much research has been focussed on diagrammatic representation systems in particular.
Challenging a long-standing prejudice against diagrammatic representation, those working on multi-modal reasoning have taken different kinds of approaches which we may categorize into three distinct groups. One branch of research can be found in philosophy of mind and cognitive science. Since the limits of linguistic forms are clear to those who have been working on mental representation and reasoning, some philosophers and cognitive scientists have embraced this new direction of multi-modal reasoning with enthusiasm and have explored human reasoning and mental representation involving non-linguistic forms (Cummins 1996 ; Chandrasekaran et al. 1995 ). Another strand of work on diagrammatic reasoning shows that there is no intrinsic difference between symbolic and diagrammatic systems as far as their logical status goes. Some logicians have presented case studies to prove that diagrammatic systems can be sound and complete in the same sense as symbolic systems. This type of result directly refuted a widely-held assumption that diagrams are inherently misleading, and abolished theoretical objections to diagrams being used in proofs (Shin 1994 ; Hammer 1995a ). A third direction in multi-modal reasoning has been taken by computer scientists, whose interest is much more practical than those of the other groups. Not so surprisingly, those working in many areas in computer science—for example, knowledge representation, systems design, visual programming, GUI design, and so on—found new and exciting opportunities in this new concept of ‘heterogeneous system’ and have implemented diagrammatic representations in their research areas.
We have the following goals for this entry. First of all, we would like to acquaint the reader with the details of some specific diagrammatic systems. At the same time, the entry will address theoretical issues, by exploring the nature of diagrammatic representation and reasoning in terms of expressive power and correctness. The case study of the second section will not only satisfy our first goal but also provide us with solid material for the more theoretical and general discussion in the third section. The fourth section presents another case study and considers it in light of the third section’s general discussion. As mentioned above, the topic of diagrams has attracted much attention with important results from many different research areas. Hence, our fifth section aims to introduce various approaches to diagrammatic reasoning taken in different areas.
For further discussion, we need to clarify two related but distinct uses of the word ‘diagram’: diagram as internal mental representation and diagram as external representation. The following quotation from Chandrasekaran et al. ( 1995 : p. xvii) succinctly sums up the distinction between internal versus external diagrammatic representations:
- External diagrammatic representations : These are constructed by the agent in a medium in the external world (paper, etc), but are meant as representations by the agent.
- Internal diagrams or images : These comprise the (controversial) internal representations that are posited to have some pictorial properties.
As we will see below, logicians focus on external diagrammatic systems, the imagery debate among philosophers of mind and cognitive scientists is mainly about internal diagrams, and research on the cognitive role of diagrams touches on both forms.
2. Diagrams as Representational Systems
The dominance of sentential representation systems in the history of modern logic has obscured several important facts about diagrammatic systems. One of them is that several well-known diagrammatic systems were available as a heuristic tool before the era of modern logic. Euler circles, Venn diagrams, and Lewis Carroll’s squares have been widely used for certain types of syllogistic reasoning (Euler 1768 ; Venn 1881 ; Carroll 1896 ). Another interesting, but neglected, story is that a founder of modern symbolic logic, Charles Peirce, not only revised Venn diagrams but also invented a graphical system, Existential Graphs, which has been proven to be equivalent to a predicate language (Peirce 1933 ; Roberts 1973 ; Zeman 1964 ).
These existing diagrams have inspired those researchers who have recently drawn our attention to multi-modal representation. Logicians who participate in the project have explored the subject in two distinct ways. First, their interest has focused exclusively on externally-drawn representation systems, as opposed to internal mental representations. Second, their aim has been to establish the logical status of a system, rather than to explain its heuristic power, by testing the correctness and the expressive power of selective representation systems. If a system fails to justify its soundness or if its expressive power is too limited, a logician’s interest in that language will fade (Sowa 1984 ; Shin 1994 ).
In this section, we examine the historical development of Euler and Venn diagrams as a case study to illustrate the following aspects: First, this process will show us how one mathematician’s simple intuition about diagramming syllogistic reasoning has gradually been developed into a formal representation system. Second, we will observe different emphases given to different stages of extension and modification of a diagrammatic system. Thirdly and relatedly, this historical development illustrates an interesting tension and trade-off between the expressive power and visual clarity of diagrammatic systems. Most importantly, the reader will witness logicians tackle the issue of whether there is any intrinsic reason that sentential systems, but not diagrammatic systems, could provide us with rigorous proofs, and their success in answering this question in the negative.
Hence, the reader will not be surprised by the following conclusion drawn by Barwise and Etchemendy, the first logicians to launch an inquiry into diagrammatic proofs in logic,
there is no principled distinction between inference formalisms that use text and those that use diagrams. One can have rigorous, logically sound (and complete) formal systems based on diagrams. (Barwise & Etchemendy 1995 : 214)
This conviction was necessary for the birth of their innovative computer program Hyperproof , which adopts both first-order languages and diagrams (in a multi-modal system) to teach elementary logic courses (Barwise & Etchemendy 1993 and Barwise & Etchemendy 1994 ).
Leonhard Euler, an 18th century mathematician, adopted closed curves to illustrate syllogistic reasoning (Euler 1768 ). The four kinds of categorical sentences are represented by him as shown in Figure 1.
--> Figure 1: Euler Diagrams
For the two universal statements, the system adopts spatial relations among circles in an intuitive way: If the circle labelled ‘ A ’ is included in the circle labelled ‘ B ,’ then the diagram represents the information that all A is B . If there is no overlapping part between two circles, then the diagram conveys the information that no A is B .
This representation is governed by the following convention: [ 3 ]
Every object x in the domain is assigned a unique location, say l ( x ), in the plane such that l ( x ) is in region R if and only if x is a member of the set that the region R represents.
The power of this representation lies in the fact that an object being a member of a set is easily conceptualized as the object falling inside the set, just as locations on the page are thought of as falling inside or outside drawn circles. The system’s power also lies in the fact that no additional conventions are needed to establish the meanings of diagrams involving more than one circle: relationships holding among sets are asserted by means of the same relationships holding among the circles representing them. The representations of the two universal statements, ‘All A are B ’ and ‘No A is B ,’ illustrate this strength of the system.
Moving on to two existential statements, this clarity is not preserved. Euler justifies the diagram of “Some A is B ” saying that we can infer visually that something in A is also contained in B since part of area A is contained in area B (Euler 1768: 233). Obviously, Euler himself believed that the same kind of visual containment relation among areas can be used in this case as well as in the case of universal statements. However, Euler’s belief is not correct and this representation raises a damaging ambiguity. In this diagram, not only is part of circle A contained in area B (as Euler describes), but the following are true: (i) part of circle B is contained in area A (ii) part of circle A is not contained in circle B (iii) part of circle B is not contained in circle A . That is, the third diagram could be read off as “Some B is A ,” “Some A is not B ,” and “Some B is not A ” as well as “Some A is B .” In order to avoid this ambiguity, we need to set up several more conventions. [ 4 ]
Euler’s own examples nicely illustrate the strengths and weaknesses of his diagrammatic system.
Example 1 . All A are B . All C are A . Therefore, all C are B . -->
Example 2 . No A is B . All C are B . Therefore, no C is A .
In both examples, the reader can easily infer the conclusion, and this illustrates visually powerful features of Euler diagrams. However, when existential statements are represented, things become more complicated, as explained above. For instance:
Example 3 . No A is B . Some C is A . Therefore, Some C is not B . No single diagram can represent the two premises, since the relationship between sets B and C cannot be fully specified in one single diagram. Instead, Euler suggests the following three possible cases: -->
Euler claims that the proposition ‘Some C is not B ’ can be read off from all these diagrams. However, it is far from being visually clear how the first two cases lead a user to reading off this proposition, since a user might read off “No C is B ” from case 1 and “All B is C ” from case 2.
Hence, the representation of existential statements not only obscures the visual clarity of Euler Circles but also raises serious interpretational problems for the system. Euler himself seemed to recognize this potential problem and introduced a new syntactic device, ‘*’ (representing non-emptiness) as an attempt to repair this flaw (1768: Letter 105).
However, a more serious drawback is found when this system fails to represent certain compatible (that is, consistent) pieces of information in a single diagram. For example, Euler’s system prevents us from drawing a single diagram representing the following pairs of statements: (i) “All A are B ” and “No A is B ” (which are consistent if A is an empty set). (ii) “All A are B ” and “All B are A ” (which are consistent when A = B ). (iii) “Some A is B ” and “All A are B ”. (Suppose we drew an Euler diagram for the former proposition and try to add a new compatible piece of information, i.e., the latter, to this existing diagram.) This shortcoming is closely related to Venn’s motivation for his own diagrammatic system (see Section 3.1 for other shortcomings of Euler’s system).
Venn’s criticism of Euler Circles is summarized in the following passage:
The weak point in this [Euler diagrams], and in all similar schemes, consists in the fact that they only illustrate in strictness the actual relation of classes to each other, rather than the imperfect knowledge of these relations which we may possess, or may wish to convey by means of the proposition. (Venn 1881 : 510)
Because of its strictness, Euler’s system sometimes fails in representing consistent pieces of information in a single diagram, as shown above. In addition to this expressive limitation, Euler’s system also suffers other kinds of expressive limitations with respect to non-empty sets, due to topological restrictions on plane figures (see Section 3.1 ).
Venn’s new system ( 1881 ) was to overcome these expressive limitations so that partial information can be represented. The solution was his idea of ‘primary diagrams’. A primary diagram represents all the possible set-theoretic relations between a number of sets, without making any existential commitments about them. For example, Figure 2 shows the primary diagram about sets A and B .
--> Figure 2: Venn’s Primary diagrams
According to Venn’s system, this diagram does not convey any specific information about the relation between these two sets. This is the major difference between Euler and Venn diagrams.
For the representation of universal statements, unlike the visually clear spatial containment relations in the case of Euler diagrams, Venn’s solution is ‘to shade them [the appropriate areas] out’ (Venn 1881 : 122). By using this syntactic device, we obtain diagrams for universal statements as shown in Figure 3.
--> Figure 3: Venn’s shading
Venn’s choice of shading might not be absolutely arbitrary in that a shading could be interpreted as a visualization of set emptiness. However, it should be noted that a shading is a new syntactic device which Euler did not use. This revision gave flexibility to the system so that certain compatible pieces of information may be represented in a single diagram. In the following, the diagram on the left combines two pieces of information, “All A are B ” and “No A is B ,” to visually convey the information “Nothing is A .” The diagram on the right, which represents both “All A are B ” and “All B are A ,” clearly shows that A is the same as B :
In fact, using primary diagrams also avoids some other expressivity problems (to do with spatial properties of diagram objects) discussed below, in Section 3 . Surprisingly, Venn was silent about the representation of existential statements, which was another difficulty of Euler diagrams. We can only imagine that Venn might have introduced another kind of a syntactic object representing existential commitment. This is what Charles Peirce did about twenty years later.
Peirce points out that Venn’s system has no way of representing the following kinds of information: existential statements, disjunctive information, probabilities, and relations. Peirce aimed to extend Venn’s system in expressive power with respect to the first two kinds of propositions, i.e., existential and disjunctive statements. This extension was completed by means of the following three devices. (i) Replace Venn’s shading representing emptiness with a new symbol, ‘o’. (ii) Introduce a symbol ‘x’ for existential import. (iii) For disjunctive information, introduce a linear symbol ‘-’ which connects ‘o’ and ‘x’ symbols.
For example, Figure 4 represents the statement, ‘All A are B or some A is B ’, which neither Euler’s nor Venn’s system can represent in a single diagram.
--> Figure 4: A Peirce diagram
The reason that Peirce replaced Venn’s shading for emptiness with the symbol ‘o’ seems to be obvious: It would not be easy to connect shadings or shadings and ‘x’s in order to represent disjunctive information. In this way, Peirce increased the expressive power of the system, but this change was not without its costs.
For example, the following diagram represents the proposition ‘ Either all A are B and some A is B , or no A is B and some B is not A ’:
Reading off this diagram requires more than reading off visual containment among circles (as in Euler diagrams) or shadings (as in Venn diagrams), but also requires extra conventions for reading combinations of the symbols ‘o,’ ‘x,’ and lines. Peirce’s new conventions increased the expressive power of single diagrams, but the arbitrariness of its conventions and more confusing representations (for example, the above diagram) sacrificed the visual clarity which Euler’s original system enjoys. At this point, Peirce himself confesses that ‘there is a great complexity in the expression that is essential to the meaning’ (Peirce 1933 : 4.365). Thus, when Peirce’s revision was completed, most of Euler’s original ideas about visualization were lost, except that a geometrical object (the circle) is used to represent (possibly empty) sets.
Another important contribution Peirce made to the study of diagrams starts with the following remark:
‘Rule’ is here used in the sense in which we speak of the ‘rules’ of algebra; that is, as a permission under strictly defined conditions. (Peirce 1933 : 4.361)
Peirce was probably the first person to discuss rules of transformation in a non-sentential representation system. In the same way that the rules of algebra tell us which transformations of symbols are permitted and which are not, so should the rules of diagram manipulation. Some of Pierce’s six rules needed more clarification and turn out to be incomplete—a problem which Peirce himself anticipated. However, more importantly, Peirce did not have any theoretical tool—a clear distinction between syntax and semantics—to convince the reader that each rule is correct or to determine whether more rules are needed. That is, his important intuition (that there could be transformation rules for diagrams) remained to be justified.
Shin ( 1994 ) follows up Peirce’s work in two directions. One is to improve Peirce’s version of Venn diagrams, and the other is to prove the soundness and the completeness of this revised system.
Shin’s work alters Peirce’s modifications of Venn diagrams to achieve an increase in expressive power without such a severe loss of visual clarity. This revision is made in two stages: (i) Venn-I: retains Venn’s shadings (for emptiness), Peirce’s ‘x’ (for existential import) and Peirce’s connecting line between ‘x’s (for disjunctive information). (ii) Venn-II: This system, which is proven to be logically equivalent to monadic predicate logic, is the same as Venn-I except that a connecting line between diagrams is newly introduced to display disjunctive information.
Returning to one of Euler’s examples we will see the contrast among these different versions clearly:
Example 3 . No A is B . Some C is A . Therefore, Some C is not B .
Euler admits that no single Euler diagram can be drawn to represent the premises, but that three possible cases must be drawn. Venn’s system is silent about existential statements. Now, Peirce’s and Shin’s systems represent these two premises in a single diagram as follows:
In the case of Shin’s diagram, Venn’s shading convention for emptiness, as opposed to Peirce’s ‘o’, much more naturally leads the reader to the inference “Some C is not B ” than in the case of Peirce’s diagram.
However, Venn-I cannot express disjunctive information between universal statements or between universal and existential statements. Retaining Venn-I’s expressive power, Venn-II allows diagrams to be connected by a line. Peirce’s confusing looking diagram above is equivalent to the following Venn-II diagram:
In addition to this revision, Shin ( 1994 ) presented each of these two systems as a standard formal representation system equipped with its own syntax and semantics. The syntax tells us which diagrams are acceptable, that is, which are well-formed, and which manipulations are permissible in each system. The semantics defines logical consequences among diagrams. Using these tools, it is proven that the systems are sound and complete, in the same sense that some symbolic logics are.
This approach has posed a fundamental challenge to some of the assumptions held about representation systems. Since the development of modern logic, important concepts, e.g., syntax, semantics, inference, logical consequence, validity, and completeness, have been applied to sentential representation systems only. However, none of these turned out to be intrinsic to these traditional symbolic logics only. For any representation system, whether it is sentential or diagrammatic, we can discuss two levels, a syntactic and a semantic level. What inference rules tell us is how to manipulate a given unit, whether symbolic or diagrammatic, to another. The definition of logical consequence is also free from any specific form of a representation system. The same argument goes for the soundness and the completeness proofs. When a system is proven to be sound, we should be able to adopt it in proofs. In fact, much current research explores the use of diagrams in automated theorem proving (see Barker-Plummer & Bailin 1997 ; and Jamnik et al. 1999 ).
It is interesting and important to notice that the gradual changes made from Euler Circles through to Shin’s systems share one common theme: to increase both the expressive and logical power of the system so that it is sound, complete, and logically equivalent to monadic predicate logic. The main revision from Euler to Venn diagrams, introducing primary diagrams, allows us to represent partial knowledge about relations between sets. The extension from Venn to Peirce diagrams is made so that existential and disjunctive information may be represented more effectively.
Both Venn and Peirce adopted the same kind of solution in order to achieve these improvements: to introduce new syntactic objects, that is, shadings by Venn, and ‘x’s, ‘o’s, and lines by Peirce. However, on the negative side, these revised systems suffer from a loss of visual clarity, as seen above, mainly because of the introduction of more arbitrary conventions. The modifications from Peirce to Shin diagrams concentrate on restoring visual clarity, but without loss of expressive power.
Hammer and Shin take a different path from these revisions: To revive Euler’s homomorphic relation between circles and sets—containment among circles represents the subset relation among sets, and non-overlapping of regions represents the disjoint relation—and at the same time, to adopt Venn’s primary diagrams by default. On the other hand, this revised Euler system is not a self-sufficient tool for syllogistic reasoning, since it cannot represent existential statements. For more details of this revised system, refer to (Hammer & Shin 1998 ).
This case study raises an interesting question for further research on diagrammatic reasoning. Throughout the different developments of Euler diagrams, increasing its expressive power and enhancing its visual clarity seem to be complementary to each other. Depending on purposes, we need to give priority to one over the other. Hammer and Shin’s alternative system provides a simple model for the development of other efficient non-sentential representational systems, a topic that has been receiving increasing attention in computer science and cognitive science.
3. Consequences of Spatial Properties of Diagrams
While it is often possible to afford diagrams the same logical status as formulae (as argued above), there are still important differences (which can have ramifications for correctness of the system) between diagrams and traditional linear proof calculi. An important point to note about diagrams (cf. Russell 1923 ) is that spatial relations between objects in a diagram can be used to represent relations between objects in some other domain. Sequential languages (e.g., symbolic logics, natural languages), however, use only the relation of concatenation to represent relations between objects. The peculiar representational use of spatial relations in the case of diagrams is direct and intuitive, as seen in the development of Euler Diagrams above, but also has its perils—as we shall discuss. Spatial constraints, being peculiar to diagrammatic systems, can be expected to be an important source of both their strengths and weaknesses. Psychological considerations concerning human capacities for visual processing of information, and skill at qualitative spatial reasoning, also have ramifications for the effectiveness of reasoning with diagrams, but we shall not survey them here.
A particular distinguishing feature of diagrams is that they obey certain “nomic” or “intrinsic” constraints due to their use of plane surfaces as a medium of representation. The idea is that sentential languages are based on acoustic signals which are sequential in nature, and so must have a compensatingly complex syntax in order to express certain relationships—whereas diagrams, being two-dimensional, are able to display some relationships without the intervention of a complex syntax (Stenning & Lemon 2001 ). Diagrams exploit this possibility—the use of spatial relations to represent other relations. The question is; how well can spatial relations and objects represent other (possibly more abstract) objects and relations?
Logical reasoning with diagrams is often carried out in virtue of their depiction of all possible models of a situation, up to topological equivalence of the diagrams (this, of course, depends on the particular diagrammatic system in use). A single diagram is often an abstraction over a class of situations, and once a suitable diagram has been constructed, inferences can simply be read off the representation without any further manipulation. In some diagrammatic systems (e.g., Euler Circles) inference is carried out by constructing diagrams correctly and reading information off them. The complexity of using inference rules in a symbolic logic is, in these cases, replaced by the problem of drawing particular diagrams correctly. [ 5 ] For instance, an Euler Circles diagram ventures to capture relationships between sets using topological relationships between plane regions in such a way that that it depicts all the possible ways that a certain collection of set-theoretic statements could be true. This has two important consequences: (1) if a certain diagram cannot be drawn then the described situation must be impossible (termed “self-consistency”), and (2) if a certain relationship between diagram objects must be drawn, then the corresponding relation can be inferred as logically valid. (See the numerous examples in Section 2 .) This phenomenon is often termed a “free-ride” (Barwise & Shimojima 1995 ). This style of diagrammatic reasoning is thus dependent on a particular representational use of diagrams—that they represent classes of models. If a particular class of models cannot be represented by a diagrammatic system, then those cases will not be taken into account in inferences using the system, and incorrect inferences might be drawn. This fact makes the representational adequacy of diagrammatic systems, restricted by their spatial nature, of paramount importance, as we shall now explore.
The representational use of the spatial relations in the plane constrains diagrammatic representation, and therefore reasoning with diagrams, in certain important ways. In particular, there are topological and geometrical (let us lump them together as “spatial”) properties of diagrammatic objects and relations which limit the expressive power of diagrammatic systems. For instance, in graph theory it is known that some simple structures cannot be drawn in the plane. For example, the graph K 5 is the graph consisting of 5 nodes, each joined to the other by an arc. This graph is non-planar, meaning that it cannot be drawn without at least two of the arcs crossing. This is just the sort of constraint on possible diagrams that limits the expressive power of diagrammatic systems. Now, since diagrammatic reasoning can occur by enumeration of all possible models of a situation, this representational inadequacy (a type of incompleteness) renders many diagrammatic systems incorrect if they are used for logical reasoning (e.g., see the critique of Englebretsen 1992 in Lemon & Pratt 1998 ).
Perhaps the most simple example of this is due to Lemon and Pratt [ 6 ] (see e.g., 1997 ). Consider Euler Circles—where convex regions of the plane represent sets, and overlap of the regions represents non-empty intersection of the corresponding sets. A result of convex topology known as Helly’s Theorem states (for the 2 dimensional case) that if every triple of 4 convex regions has a non-empty intersection then all four regions must have a non-empty intersection.
To understand the ramifications of this, consider the following problem:
Example 4 . Using Euler Circles, represent the following premises: A ∩ B ∩ C ≠ ∅ B ∩ C ∩ D ≠ ∅ C ∩ D ∩ A ≠ ∅
Note that, in terms of set-theory, only trivial consequences follow from these premises. However, an Euler diagram of the premises, such as Figure 5, leads to the incorrect conclusion that A ∩ B ∩ C ∩ D ≠ ∅ (due to the quadruple overlap region in the centre of the diagram):
--> Figure 5: An Euler’s Circles representation exhibiting Helly’s Theorem
In other words, a user of Euler Circles is forced [ 7 ] to represent a relationship between the sets which is not logically necessary. This means both that there are logically possible situations which the system cannot represent, and that a user would make incorrect inferences if they relied on the system for reasoning. More generally, this type of result can be generated for many different types of diagrammatic system, depending on the particular spatial relations and objects which they use in representation—a research programme which is ongoing.
For example, using non-convex regions (e.g., “blobs” instead of circles) leads to a similar problem, only that non-planar graphs are involved instead of Helly’s Theorem. A similar result concerns linear diagrams for syllogisms Englebretsen 1992 , where lines are used to represent sets, points represent individuals, point-line intersection represents set-membership, and intersection of lines represents set-intersection. Again, planarity constraints restrict the expressive power of the system and lead to incorrect inferences.
Atsushi Shimojima’s “constraint hypothesis” perhaps best sums all this up:
Representations are objects in the world, and as such they obey certain structural constraints that govern their possible formation. The variance in inferential potential of different modes of representation is largely attributable to different ways in which these structural constraints on representations match with the constraints on targets of representation (Shimojima 1996a , 1999 ).
As discussed above, much of the interest in diagrams has been generated by the claim that they are somehow more “effective” than traditional logical representations for certain types of task. Certainly, for example, a map is a greater aid to navigation than a verbal description of a landscape. However, while there are certainly psychological advantages to be gained through the use of diagrams, they are (as in the case of Euler Circles) often ineffective as representations of abstract objects and relationships. Once a purely intuitive notion, non-psychological claims about “efficacy” of diagrammatic systems can be examined in terms of standard formal properties of languages (Lemon et al. 1999 ). In particular, many diagrammatic systems are self-consistent, incorrect, and incomplete, and complexity of inference with the diagrams is NP-hard. By way of contrast, most sentential logics, while able to express inconsistencies, are complete and correct [ 8 ] .
On the other hand, not being able to represent contradictions could provide us with interesting insights about the nature of diagrammatic representation. If a central goal of a language is to represent the world or a state of affairs, then representing contradictions or tautologies is called into question. Neither contradictions nor tautologies are part of the world. How can we draw a picture, or take a picture, of the contradiction that “it is raining and it is not raining”? How about the picture of the disjunctive information “it is either raining or not raining”? Now, we seem to be much closer to Wittgenstein’s classic picture theory of language (Wittgenstein 1921 ).
4. Diagrammatic Systems in Geometry
Mathematicians have used, and continue to use, diagrams extensively. The communication of mathematical concepts and proofs—in textbooks, on blackboards—is not uniformly sentential. Figures and pictures are common. In line with the prevailing conception of logic as essentially sentential, however, they are not usually thought to play a role in rigorous mathematical reasoning. Their use is taken to be limited to enhancing comprehension of a proof. They are not standardly believed to form any part of the proof itself.
The attitude is well illustrated by the standard assessment of Euclid’s methodology in the Elements . In no mathematical subject are diagrams more prominent than in the elementary geometry Euclid develops in the text. The proofs of the subject seem to be in some sense about the diagrams of triangles and circles that appear with them. This is especially the case with the geometric proofs of the Elements . Diagrams for Euclid are not merely illustrative. Some of his inference steps depend on an appropriately constructed diagram. On the standard story, these steps indicate gaps in Euclid’s proofs. They show how Euclid did not fully carry out the project of developing geometry axiomatically.
Ken Manders set out to explode this story with his seminal work “The Euclidean diagram” ( 2008 [1995] ). His analysis of Euclid’s diagrammatic proof method reveals that Euclid employs diagrams in a controlled, systematic way. It thus calls into question the common, negative assessment of the rigor of the Elements . Moreover, the specifics of Manders’ analysis suggest that the proofs of the text can be understood to adhere to a formal diagrammatic logic. This was subsequently confirmed by the development of formal diagrammatic systems designed to characterize such a logic. The first of these was FG (presented in Miller 2007 ), followed by the system Eu (Mumma 2010 ).
This section is devoted to explicating Manders’ analysis and the formal systems that have emerged from it. After a brief survey of how Euclid’s diagrams have been viewed through the centuries, Manders’ picture of their role in geometric proofs is presented. A description of how the systems FG and Eu render this picture in formal terms and characterize a logic of Euclidean diagrams then follows.
The elementary geometry of the Elements was taken to be foundational to mathematics from its inception in ancient Greece until the 19 th century. Accordingly, philosophers concerned with the nature of mathematics found themselves obliged to comment on the diagrammatic proofs of the text. A central issue, if not the central issue, was the generality problem . The diagram that appears with a Euclidean proof provides a single instantiation of the type of geometric configurations the proof is about. Yet properties seen to hold in the diagram are taken to hold of all the configurations of the given type. What justifies this jump from the particular to the general?
As an illustration, consider the proof for proposition 16 of book I of the Elements .
The proposition is:
In any triangle, if one of the sides be produced, the exterior angle is greater than either of the interior and opposite angles.
Euclid’s proof is:
Let ABC be a triangle, and let one side of it BC be produced to D; I say that the angle ACD is greater than the interior and opposite angle BAC. Let AC be bisected at E [I, 10], and let BE be joined and produced in a straight line to F; let EF be made equal to BE [I,3], and let FC be joined. Then, since AE is equal to EC, and BE equal to EF, the two sides AE, EB are equal to the two sides CE, EF respectively; and the angle AEB is equal to the angle FEC [I, 15]. Therefore the base AB is equal to the base FC, and the triangle ABE is equal to the triangle CFE [I,4];therefore the angle BAE is equal to the angle ECF (which is also the angle ACF); But the angle ACD is greater than the angle ACF; Therefore the angle ACD is greater than BAE.
The proof seems to refer to the parts of the diagram given with the proof. Nevertheless the proof does not purport to establish something just about the triangle in the diagram, but something about all triangles. The diagram thus serves to represent, in some way, all triangles.
The role of diagrams as representations is remarked upon by Aristotle in book A, chapter 10 of the Posterior Analytics :
The geometer bases no conclusion on the particular line he has drawn being that which he has described, but [refers to] what is illustrated by the figures. (The translation is by T. Heath, found in Euclid 1956: vol. I, p.119)
Aristotle does not in passage confront the question of how the geometer uses diagrams to reason about what they illustrate. A few centuries later Proclus does in his commentary on the Elements . Proclus asserts that passing from a particular instance to a universal conclusion is justified because geometers
… use the objects set out in the diagram not as these particular figures, but as figures resembling others of the same sort. It is not as having such-and-such a size that the angle before me is bisected, but as being rectilinear and nothing more…Suppose the given angle is a right angle…if I make no use of its rightness and consider only its rectilinear character, the proposition will apply equally to all angles with rectilinear sides. ( A Commentary on the First Book of Euclid’s Elements , Morrow 1970 : 207))
The place of diagrams in geometry remained an issue into the early modern period. Major philosophical figures in the 17 th and 18 th centuries advanced positions on it. Anticipating the predominate modern view, Leibniz asserts:
…it is not the figures which furnish the proof with geometers, though the style of the exposition may make you think so. The force of the demonstration is independent of the figure drawn, which is drawn only to facilitate the knowledge of our meaning, and to fix the attention; it is the universal propositions, i.e., the definitions, axioms, and theorems already demonstrated, which make the reasoning, and which would sustain it though the figure were not there. (1704 New Essays : 403)
In the introduction to his Principles of Human Knowledge (1710, section 16), Berkeley reiterates 13 centuries later Proclus’s take on the generality problem. Though one always has a particular triangle ‘in view’ when working through a demonstration about triangles, there is ‘not the least mention’ of the particular details of the particular triangle in the demonstration. The demonstration thus proves, according to Berkeley, a general proposition about triangles.
The most developed, and predictably most complex and difficult, account of geometric diagrams in the modern period can be found in Kant. Kant saw something of deep epistemological significance in the geometer’s use of a particular diagram to reason about a geometric concept. In reasoning in this way, the geometer
considers the concept in concreto , although non-empirically, but rather solely as one it has exhibited a priori , i.e., constructed, and in which that which follows from the general conditions of the construction must also generally of the object of the constructed concept. (1781, Critique of Pure Reason , A716/B744.)
For contrasting views of what passages like these reveal about where diagrams fit in Kant’s philosophy of geometry, see Shabel 2003 and Friedman 2012 .
In the 19 th century geometry and mathematics as a whole underwent a revolution. Concepts far more abstract and general than those found in the Elements (e.g., non-Euclidean geometries, sets) emerged. Not only did questions about the nature of Euclid’s diagrammatic method lose their urgency, the method came to be understood as mathematically flawed. The latter view found its most precise expression in the groundbreaking work of Moritz Pasch, who provided the first modern axiomatization of elementary geometry in Pasch ( 1882 ). In it, Pasch showed how the subject could be developed without reference to diagrams or even to the geometric concepts diagrams instantiate. The methodological norm guiding the work is nicely expressed in the following often-quoted passage:
In fact, if geometry is genuinely deductive, the process of deducing must be in all respects independent of the sense of the geometrical concepts, just as it must be independent of figures; only the relations set out between the geometrical concepts used in the propositions (respectively definitions) concerned ought to be taken into account. (Pasch 1882 : 98; emphasis in original. The translation here is from Schlimm 2010 )
The norm has since entrenched itself both in mathematics and in philosophical discussions of mathematics. It is its entrenchment in the latter that Manders opposes in Manders 2008 [1995] . In the account he develops of ancient geometry, the necessity of consulting a diagram in a proof does not indicate a deductive gap. Rather, diagram and text together form a rigorous and deductive mathematical proof.
4.2 Manders’ exact/co-exact distinction and the generality problem
To explain the division of labor between text and diagram in ancient geometry, Manders distinguishes between the exact and co-exact properties of geometric diagrams in Manders 2008 [1995] . Underlying the distinction is a notion of variation. The co-exact conditions realized by a diagram ‘are those conditions which are unaffected by some range of every continuous variation of a specified diagram.’ Exact conditions, in contrast, are affected once the diagram is subject to the smallest variation. Roughly, a diagram’s co-exact properties comprise the ways its parts define a finite set of planar regions, and the containment relations between these regions. A prominent exact relation is the equality of two magnitudes within a diagram. For example, only the slightest change to the position of CF in the diagram for proposition 16 is required to make the angles BAE and ECF unequal.
Manders’ key observation is that Euclid’s diagrams contribute to proofs only through their co-exact properties. Euclid never infers an exact property from a diagram unless it follows directly from a co-exact property. Relations between magnitudes which are not exhibited as a containment are either assumed from the outset or are proved via a chain of inferences in the text. This can easily be confirmed with the proof of proposition 16. The one inference that relies on the diagram is the second to last inference of the proof. The inference, specifically, is that angle ACD is greater than angle ACF. This, crucially, is based on seeing from the diagram that angle ACD contains angle ACF. There are many other relations asserted to hold in the proof. Though the diagram instantiates them, they are explicitly justified in the text. And with these relations, the relata are spatially separated magnitudes.
It is not difficult to hypothesize why Euclid would have restricted himself in such a way. It is only in their capacity to represent co-exact properties and relations that diagrams seem capable of functioning effectively as symbols of proof. The exact properties of diagrams are too refined to be easily reproducible and to support determinate judgments. As Manders puts it
The practice has resources to limit the risk of disagreement on (explicit) co-exact attributions from a diagram; but it lacks such resources for exact attributions, and therefore could not allow them without dissolving into a disarray of irresolvably conflicting judgements. (Manders 2008 [1995] : 91–92)
Manders’ insights lead naturally to the idea that Euclid’s arguments could be formalized in a manner similar to the way Venn diagrams have been formalized in Shin 1994 . The co-exact information carried by Euclid’s diagrams is discrete. When a diagram is consulted for this information, what matters about it is how its lines and circles partition a bounded planar region into a finite set of sub-regions. This opens the door to conceptualizing Euclid’s diagrams as part of the syntax of Euclid’s proof method.
Realizing this conception in a formal system of proof amounts, as in Shin 1994 , to specifying the syntax and semantics of diagrams. On the syntactic side, this means defining Euclid’s diagrams as formal objects precisely, and giving rules whereby diagrams as formal object figure in derivations of Euclid’s propositions. On the semantic side, this means specifying how derivable expressions are to be interpreted geometrically, or in other words how exactly are they to be understood as representing Euclid’s propositions.
The semantic situation with Euclid’s diagrams is thus different from that with Venn’s. Venn diagrams are used to prove logical results. The inferences made with them are topic neutral. Euclid’s diagrams on the other hand are used to prove geometrical results. The inferences made with them are topic specific. In particular, though the objects of plane Euclidean geometry are abstract (e.g., geometric lines are breadthless), they are still spatial. Consequently, issues surrounding the spatiality of diagrams and representational scope do not arise with Euclid’s diagrams as they do, for instance, with Euler diagrams. In the case of geometry, in fact, the spatiality of diagrams counts in their favor. Spatial constraints on what is possible with geometric configurations are also operative with spatial Euclidean diagrams.
Nevertheless, as recognized in the philosophical commentary on Euclid’s geometry from antiquity onward, there are with Euclidean diagrams issues of representational scope to contend with. What is the justification for treating properties of a single geometric diagram as representative of all the configurations in the range of a proof? How can a single diagram prove a general result? Manders’ exact/co-exact distinction provides the basis for a partial answer. The co-exact properties of a diagram can be shared by all geometric configurations in the range of a proof, and so in such cases one is justified in reading off co-exact properties from the diagram. In a proof about triangles for instance, variation among the configurations in the range of the proof is variation of exact properties—e.g., the measure of the triangles’ angles, the ratios between their sides. They all share the same co-exact properties—i.e., they all consists of three bounded linear regions which together define an area.
This is not a full answer because Euclid’s proofs typically involve constructions on an initial configuration type. With the proof of proposition 16, for example, a construction on a triangle with one side extended is specified. In such cases, a diagram may adequately represent the co-exact properties of an initial configuration. But the result of applying a proof’s construction to the diagram cannot be assumed to represent the co-exact properties of all configurations resulting from the construction. One does not need to consider complex geometric situations to see this. Suppose for instance the initial configuration type of a proof is triangle . Then the diagram
serves to represent the co-exact properties of this type. Suppose further that the first step of a proof’s construction is to drop the perpendicular from a vertex of the triangle to the line containing the side opposite the vertex. Then the result of carrying this step out on the diagram
ceases to be representative. That the perpendicular falls within the triangle in the diagram is a co-exact feature of it. But there are triangles with exact properties different from the initial diagram where applying the construction step results in a perpendicular lying outside the triangle. For example, with the triangle
the result of applying the construction step is
And so, carrying out a Euclidean construction on a representative diagram can result in an unrepresentative diagram. A central task of formalizing Euclid’s diagrammatic proofs is accounting for this—i.e., providing with its rules a method for distinguishing general co-exact features from non-general ones in diagrammatic representations of constructions. The systems FG and Eu take two different approaches to this task.
Employing the method of FG , one must produce with a diagram every case that could result from the construction. A general co-exact relation of the construction is then one that appears in every case. FG ’s demand that every case be produced would, of course, be of a little interest if it did not also provide a method for producing them all. The method FG provides depends on the fact that lines and circles in the system’s diagrams are defined in purely topological terms. Their resulting flexibility makes it possible to formulate and implement in a computer program a general method for generating cases. [ 9 ]
The lines and circles of Eu diagrams are not similarly flexible. Accordingly, it cannot resolve the generality problem via case-analysis as FG does. The central idea of its approach is to allow diagrams to hold partial information from the outset. Within an Eu derivation, the diagram produced by a proof’s construction has an initial content consisting in all the qualitative relations of the proof’s initial diagram. The qualitative relations concerning objects added by the construction cannot be read off the diagram immediately. Those that can be read off the diagram must be derived by the system’s rules. [ 10 ]
The differences between the FG and Eu approaches to formalizing Euclid’s constructions can be understood as representing different general conceptions of the role of diagrams in mathematics. FG embodies a conception where diagrams concretely realize a range of mathematical possibilities. They support mathematical inference by furnishing direct access to these possibilities. Eu in contrast embodies a conception where diagrams serve to represent in a single symbol the various components of a complex mathematical situation. They support mathematical inference by allowing the mathematical reasoner to consider all these components in one place, and to focus on those components relevant to a proof.
5. Diagrams and Cognition, Applications
Despite the formal limitations of some diagrammatic systems noted above, many different systems are currently used in a wide variety of contexts; logic teaching, automated reasoning, specifying computer programs, reasoning about situations in physics, graphical user interfaces to computer programs, and so on. In general, it is not yet known how effective (in the above sense) many of these diagrammatic systems are. We now give a brief survey of other diagrammatic systems and their uses, as well as the more philosophical issues raised by the debate over the status of diagrammatic reasoning.
It is worth noting that many mathematicians and philosophers proposed diagrammatic systems, often with a didactic motivation. Some systems, like Lewis Carroll’s in “The Game of Logic” ( 1896 ) are variants on the proposals of Euler and Venn. Others, like Frege ( 1879 ), used lines rather than plane regions. (For a description of Frege’s notation, see the section on Complex Statements and Generality in the entry on Gottlob Frege . See also Englebretsen 1992 .) Carroll’s system supersedes Venn’s in that the complements of sets are explicitly represented as regions of the diagram, rather than being left as the background region against which the circles appear. This means that Carroll’s system is able to draw inferences about relations between complements of properties, at the expense of representing some properties as disjoint (i.e., non-connected) regions. This shift closely mirrors the shift in logic from subject-predicate argumentation to a function-argument representation (Stenning 1999 ).
Peirce, a founder of modern quantified logic, also invented a graphical system, called Existential Graphs, which is logically equivalent to predicate logic. Along with Don Robert’s pioneering work on Existential Graphs and John Sowa’s creative application of Peirce’s graphs, recently a group of diagrammatic researchers provided more diverse approaches to Existential Graphs in a broader theoretical context (Shin 2003 ).
On a more practical theme, AI researchers, one of whose main concerns is the heuristic power of representation systems in addition to their expressive power, have been debating about different forms of representation for decades (Sloman 1971 , 1985 , 1995 ). Hence, they have welcomed discussions of the distinct role of visual reasoning and have recently hosted interdisciplinary symposiums on diagrammatic reasoning at AI conferences. [ 11 ] At the same time, realizing that human beings adopt different representation forms depending on the kinds of problems they face, some AI researchers and design theorists have practiced domain-specific approaches to bringing in problem-tailored representation forms. [ 12 ]
For instance, Harel ( 1988 ) invented higraphs to represent system specifications in computer science. This idea has been taken up in industrial applications (e.g., UML, in Booch et al. 1998 ). Barker-Plummer & Bailin ( 1997 ) present a case study in developing computers which can carry out the kind of analogical reasoning that humans perform when proving certain mathematical theorems. More recently, an interesting result has been presented by Mateja Jamnik of Alan Bundy’s Mathematical Reasoning Group at Edinburgh (Jamnik 2001 ). Jamnik shows how a semi-automatic formal proof system can perform some of the perceptual inferences which humans find so natural. For instance, that the sum of the first n odd natural numbers is n squared is easily seen by decomposing an n × n grid into “ells” (Jamnik et al. 1999 ).
Scholars at the University of Brighton has been carrying out interesting projects both in developing diagrammatic systems and in applying visual tools in software development, see the link in the Other Internet Resources section .
It should also be mentioned that scientists such as chemists and physicists also use diagrams in order to perform certain computations. Feynman diagrams, for example, are used to perform calculations in sub-atomic physics. More recently, formal diagrammatic reasoning has been developed for quantum theory (Coecke & Kissinger 2017 ). In Knot Theory (which has applications in physics, Kauffman 1991 ) the three Reidemeister Moves are diagrammatic operations which make up a complete calculus for proving knots equivalent. Not surprisingly, Knot diagrams have attracted interest from researchers (De Toffoli & Giardino 2014 ). The crucial role of diagrams and diagrammatic reasoning in the abstract mathematics of category theory has also been investigated (Halimi 2012 ; De Toffoli 2017 ).
Do our mental representations have diagram-like or picture-like entities as components? This question has a long history both in philosophy and in psychology, independently of each other. More recently, however, some philosophers have participated in this “imagery debate”, one of the most time-honored controversies in psychology, and some cognitive psychologists find certain epistemological theories in philosophy useful to support their views on the issue.
The nature of mental representation has been one of the perennial topics in philosophy, and we can easily trace back philosophical discussions on images and mental representation to ancient times. [ 13 ] The writings of Hobbes, Locke, Berkeley, and Hume concern themselves in large part with mental discourse, the meaning of words, mental images, particular ideas, abstract ideas, impressions, and so on. Descartes’ well-known distinction between imagining and conceiving something has generated much discussion about the unique role of visual images in mental representations. The development of cognitive science in the 20th century naturally has brought certain group of philosophers and psychologists closer and we find a number of authors whose works easily belong to both disciplines (Block 1983 ; Dennett 1981 ; Fodor 1981 ).
Imagery based on introspection was the main focus in the early development of psychology until the behavioristic approach became predominant in the discipline. During the era of behaviorism, anything related to mental inspection, including images, was excluded from any serious research agenda. Finally when the topic of mental images made a comeback in psychology in 1960s, researchers adopted a more humble agenda for mental imagery than before: Not all mental representations involve imagery, and imagery is one of many ways of manipulating information in the mind. Also, thanks to the influence of behaviorism, it is acknowledged that introspection is not enough to explore imagery, but a claim about mental imagery needs to be confirmable by experiments in order to show that we successfully externalize mental events. That is, if what a certain mental introspection tells us is genuine, then there would be observable external consequences of that mental state.
Thus the contemporary imagery debate among cognitive scientists is about the claim that picture-like images exist as mental representations and about how we interpret certain experiments. [ 14 ]
Kosslyn ( 1980 , 1994 ) and other pictorialists (Shepard & Metzler 1971 ) present experimental data to support their position that some of our mental images are more like pictures than a linear form of language (for example, natural languages or artificial symbolic languages) in some important aspects, even though not all visual mental images and pictures are of exactly the same kind. By contrast, Pylyshyn ( 1981 ) and other descriptionalists (Dennett 1981 ) raise questions about the picture-like status of mental images and argue that mental images are formed out of structured descriptions. To them, mental images represent in the manner of language rather than pictures and, hence, there are no picture-like visual mental images.
Both sides of the debate sometimes used a philosophical theory as a supporting factor. For example, pictorialists in the imagery debate found the modern sense-datum theory in philosophy quite close to their point of view. By the same token, the critics of the sense-datum theory argued that the mistaken pictorial view of mental images arises mainly from our confusion about ordinary language and claimed that mental images are epiphenomena.
Without being heavily involved in the imagery debate, some researchers have focused on a distinct role that diagrams or pictures—as opposed to traditional sentential forms—play in our cognitive activities. (Shin 2015 ; Hamami & J. Mumma 2013 ) Based on the conjectures that humans adopt diagrammatic or spatial internal mental representations in their reasoning about concrete or abstract situations (see Howell 1976 ; Sober 1976 ), some cognitive scientists have concentrated on the functions of images or diagrams in our various cognitive activities, for example, memory, imagination, perception, navigation, inference, problem-solving, and so on. Here, the distinct nature of “visual information,” which is obtained either through internal mental images or through externally drawn diagrams, has become a major topic of research. Even though most of these works assume that there are mental images (that is, they accept the pictorialists’ claim), strictly speaking they do not have to commit themselves to the view that these images exist as basic units in our cognition. Descriptionalists do not have to discard discussions of the functions of images, but only need to add that these images are not primitive units stored in our memory, but formed out of structured descriptions more like the sentences of a language (see Pylyshyn 1981 ).
A search for the distinct role of diagrams has led researchers to explore the differences among different forms of external or internal representations, and mainly between diagrammatic and sentential representations. Many important results have been produced in cognitive science. Starting from Larkin and Simon’s classic case study ( 1987 ) to illustrate a difference between informational and computational equivalence among representation systems, Lindsay’s work locates where this computational difference lies, which he calls a ‘non-deductive’ method. As briefly pointed out above, this inference process is called ‘free ride’ by Barwise and Shimojiima ( 1995 ), i.e., the kind of an inference in which the conclusion seems to be read off automatically from the representation of premises. In Gurr, Lee, and Stenning ( 1998 ) and Stenning and Lemon ( 2001 ), there is an explanation of the uniqueness of diagrammatic inference in terms of a degree of ‘directness’ of interpretation, and it is argued that this property is relative, and hence that “some rides are cheaper than others”. Having the role of graphs in mind, Wang and Lee ( 1993 ) present a formal framework as a guideline for correct visual languages. At this point, we are very close to applied aspects of research in multi-modal reasoning—design theory and AI research—by providing these disciplines with computational support for visual reasoning.
Related to the issue of imagistic mental representation is the examination of the semantics of various diagrammatic systems and what they can teach us about the nature of languages in general (e.g., Goodman 1968 ). For instance, Robert Cummins ( 1996 ), amongst others, argues that too little attention has been given to diagrammatic representations and that focus on a notion of “structural representation” more akin to diagrammatic representation can help to explicate the nature of representation itself. We believe that the considerations presented above give us some empirical handle on this type of claim at least—depending on the imagistic objects and relations used, patterns of incorrect inference should be predictable and detectable. An important, if little-known, article on this theme is Malinas 1991 . Here Malinas explores the concepts of pictorial representation and “truth in” a picture via the notion of resemblance, and considers various semantic puzzles about pictorial representation. He develops Peacocke’s “Central Thesis” of depiction (Peacocke 1987 ), where experienced similarities between properties of pictorial objects and their referents in the visual field give rise to the relation of depiction. He goes on to provide a formal semantics for pictures which is “analogous to a semantics for an ideal language”.
We began by motivating the philosophical interest of diagrams, by way of their role in human reasoning and their relation to the study of language in general, and multi-modal information processing. We then explained the trade-off between expressive power and visual clarity of diagrammatic systems, by examining the historical development of diagram systems from Euler and Venn, through Peirce’s work, to recent work by Shin and Hammer. It was argued that diagrammatic systems can be afforded the same logical status as traditional linear proof calculi. We then explained some of the potential pitfalls of diagrammatic representation and reasoning, by examining spatial constraints on diagrammatic systems and how they can affect correctness and expressive power. We closed by surveying other diagrams systems, the interest in diagrams generated in computer science and cognitive science, and gave an introduction to the imagery debate in the philosophy of mind.
- Allwein, G. and J. Barwise (eds.), 1996, Logical Reasoning with Diagrams , Oxford: Oxford University Press.
- Avigad, J. with E. Dean and J. Mumma, 2009, “A formal system for Euclid’s Elements”, Review of Symbolic Logic , 2: 700–768.
- Barker-Plummer, D. and S. Bailin , 1997, “The Role of Diagrams in Mathematical Proofs”, Machine GRAPHICS and VISION , 6(1): 25–56. (Special Issue on Diagrammatic Representation and Reasoning).
- Barker-Plummer, D., D. Beaver, J. van Benthem, and P. Scotto di Luzio , 2002, Words, Proofs, and Diagrams , Stanford: CSLI Publications.
- Barwise, J. , 1993, “Heterogeneous reasoning”, in G. Mineau, B. Moulin, and J. Sowa, (eds), ICCS 1993: Conceptual Graphs for Knowledge Representation (Lecture Notes in Artificial Intelligence: Volume 699), Berlin: Springer Verlag, pp. 64–74.
- Barwise, J. and J. Etchemendy , 1989, “Information, Infons, and Inference”, in Cooper, Mukai, and Perry, (eds), Situation Theory and its Applications , volume 1, Stanford: CSLI Publications.
- ––– , 1991, “Visual Information and Valid Reasoning”, in Zimmerman and Cunningham, (eds), Visualization in Teaching and Learning Mathematics , pages 9–24. Washington: Mathematical Association of America.
- ––– , 1993, The Language of First-Order Logic , Stanford: CSLI Publications.
- ––– , 1994, Hyperproof , Stanford: CSLI Publications.
- ––– , 1995, “Heterogeneous Logic”, in J. Glasgow, N. Hari Narayanan, and B. Chandrasekaran, (eds), Diagrammatic Reasoning: Cognitive and Computational Perspectives , pages 209–232. Cambridge, MA: AAAI Press/The MIT Press.
- Barwise, J. and A. Shimojima , 1995, “Surrogate Reasoning”, Cognitive Studies: Bulletin of Japanese Cognitive Science Society , 4(2): 7–27.
- Berkeley, G., 1710, Principles of human knowledge , in David Armstrong (ed.), Berkeley’s Philosophical Writings , London: Macmillian, 1965.
- Block, N. , (ed.), 1981, Imagery , Cambridge, MA: MIT Press.
- ––– , 1983, “Mental pictures and cognitive science”, The Philosophical Review , 92: 499–541
- Booch, G., J. Rumbaugh, and I. Jacobson , 1999, The Unified Modeling Language Reference Manual , Reading, Mass.: Addison-Wesley.
- Coecke, B. and Kissinger, A. , 2017, Picturing Quantum Processes. A First Course in Quantum Theory and Diagrammatic Reasoning , Cambridge: Cambridge University Press.
- Carroll, L. , 1896, Symbolic Logic , New York: Dover.
- Chandrasekaran, B., J. Glasgow, and N. Hari Narayanan , (eds.), 1995, Diagrammatic Reasoning: Cognitive and Computational Perspectives , Cambridge, MA: AAAI Press/The MIT Press.
- Cummins, R. , 1996, Representations, Targets, and Attitudes , Cambridge, MA: MIT Press.
- De Toffoli, S. , 2017, “Chasing The Diagram – The Use of Visualizations in Algebraic Reasoning”, Review of Symbolic Logic , 10 (1): 158–186.
- De Toffoli, S. and Giardino, V. , 2014, “Forms and Roles of Diagrams in Knot Theory”, Erkenntnis , 79 (4): 829–842.
- Dennett, D. , 1981, “The nature of images and the introspective trap”, in Block 1981 , pp. 87–107.
- Englebretsen, G., 1992, “Linear Diagrams for Syllogisms (with Relationals)”, Notre Dame Journal of Formal Logic , 33(1): 37–69.
- Euclid, The Thirteen Books of the Elements (second edition, Vols. I–III), New York, NY: Dover Publications, 1956. Translated with introduction and commentary by Sir Thomas L. Heath, from the text of Heiberg.
- Euler, L. , 1768, Lettres à une Princesse d’Allemagne , St. Petersburg; l’Academie Imperiale des Sciences.
- Fodor, J. , 1981, “Imagistic representation”, in Block 1981 , pp. 63–86.
- Frege, G. , 1879, Begriffsschrift: eine der arithmetischen nachgebildete Formelsprache des reinen Denkens , Halle am See: Louis Nebert
- Friedman, M. , 2012, “Kant on geometry and spatial intuition”, Synthese, 186: 231–255.
- Gardner, M. , 1958, Logic Machines and Diagrams , Sussex: Harvester Press.
- Goodman, N. , 1968, Languages of Art: an approach to a theory of symbols , London: Oxford University Press.
- Greaves, M., 2002, The Philosophical Status of Diagrams , Stanford: CSLI Publications.
- Grigni, M., D. Papadias, and C. Papadimitriou , 1995, “Topological Inference”, in International Joint Conference on Artificial Intelligence (IJCAI ‘95) , pages 901–907, Cambridge, MA: AAAI Press.
- Gurr, C., J. Lee, and K. Stenning , 1998, “Theories of diagrammatic reasoning: Distinguishing component problems”, Minds and Machines , 8: 533–557.
- Halimi, B. , 2012, “Diagrams as Sketches”, Synthese , 186(1): 387–409.
- Hamami Y. and Mumma J. , 2013, “A Prolegomena to a Cognitive Investigation of Euclidean Diagrammatic Reasoning”, Journal of Language, Logic and Information , 22 (4): 421–448.
- Hammer, E. , 1995a, “Reasoning with Sentences and Diagrams”, Notre Dame Journal of Formal Logic , 35(1): 73–87.
- Hammer, E. and S. Shin , 1998, “Euler’s Visual Logic”, History and Philosophy of Logic , 19: 1–29.
- Harel, D. , 1988, “On Visual Formalisms”, Communications of the ACM , 31(5): 514–530.
- Howell, R. , 1976, “Ordinary Pictures, Mental Representations, and Logical Forms”, Synthese , 33: 149–174.
- Jamnik, M. , 2001, Mathematical Reasoning with Diagrams , Stanford: CSLI Publications.
- Jamnik, M., A. Bundy, and I. Green , 1999, “On Automating Diagrammatic Proofs of Arithmentic Arguments”, Journal of Logic, Language, and Information , 8(3): 297–321.
- Kant, I., 1781, Critique of Pure Reason , translated and edited by P. Guyer and A. Wood, Cambridge: Cambridge University Press, 1998.
- Kauffman, L. 1991, Knots and Physics , Singapore: World Scientific.
- Kosslyn, S. , 1980, Image and Mind , Cambridge, MA: Harvard University Press.
- ––– , 1994, Image and Brain: the resolution of the imagery debate , Cambridge, MA: MIT Press.
- Lambert, J. H. , 1764, Neues Organon , Berlin: Akademie Verlag, 1990.
- Larkin, J. and H. Simon , 1987, “Why a Diagram is (Sometimes) Worth 10,000 Words”, Cognitive Science , 11: 65–99.
- Leibniz, G. , 1704, New Essays Concerning Human Understanding , LaSalle: Open Court Publishing, 1949.
- Lemon, O. , 2002, “Comparing the Efficacy of Visual Languages”, in Barker-Plummer et al. (eds.), 2002 , pp. 47–69.
- Lemon, O., M. de Rijke, and A. Shimojima , 1999, “Efficacy of Diagrammatic Reasoning” (Editorial), Journal of Logic, Language, and Information , 8(3): 265–271.
- Lemon, O. and I. Pratt , 1997, “Spatial Logic and the Complexity of Diagrammatic Reasoning,” Machine Graphics and Vision , 6(1): 89–108, 1997. (Special Issue on Diagrammatic Representation and Reasoning).
- ––– , 1998, “On the insufficiency of linear diagrams for syllogisms”, Notre Dame Journal of Formal Logic , 39(4): 573–580.
- Malinas, G. , 1991, “A Semantics for Pictures”, Canadian Journal of Philosophy , 21(3): 275–298.
- Manders, K. , 2008 [1995], “The Euclidean diagram”, in Philosophy of Mathematical Practice , P. Mancosu (ed.), Oxford: Clarendon Press, 2008, pp. 112–183. (First circulated as a manuscript in 1995.)
- Miller, Nathaniel , 2007, Euclid and His Twentieth Century Rivals: Diagrams in the Logic of Euclidean Geometry , (CSLI Studies in the Theory and Applications of Diagrams), Stanford: CSLI Publications.
- –––, 2006, “Computational complexity of diagram satisfaction in Euclidean geometry”, Journal of Complexity , 22: 250–74.
- Morrow, G. , 1970, Proclus: A commentary on the first book of Euclid’s Elements , Princeton: Princeton University Press, 1970.
- Mumma, J. , 2010, “Proofs, Pictures and Euclid”, Synthese , 175(2): 255–287.
- Narayanan, N. , 1993, “Taking issue/forum: The imagery debate revisited”, Computational Intelligence , 9(4): 303–435.
- Pasch, M. , 1882, Vorlesungen über neuere Geometrie , Teubner: Leipzig.
- Peacocke, C. , 1987, “Depiction”, The Philosophical Review , 96: 383–410
- Peirce, C.S. , 1933, Collected Papers , Cambridge, MA: Harvard University Press.
- Pylyshyn, Z. , 1981, “Imagery and Artificial Intelligence”, in N. Block, (ed.), Readings in Philosophy of Psychology , volume 2, pages 170 –196. Cambridge, MA: Harvard University Press.
- Roberts, D. , 1973, The Existential Graphs of Charles S. Peirce , The Hague: Mouton.
- Russell, B. , 1923, “Vagueness”, in J. Slater, (ed.), Essays on Language, Mind, and Matter: 1919–26 (The Collected Papers of Bertrand Russell) , pages 145–154. London: Unwin Hyman.
- Schlimm, D. , 2010, “Pasch’s philosophy of mathematics”, Review of Symbolic Logic , 3(1): 93–118.
- Shabel, L. , 2003, Mathematics in Kant’s Critical Philosophy: Reflections on Mathematical Practice , New York: Routledge.
- Shepard, R. and J. Metzler , 1971, “Mental rotation of three-dimensional objects”, Science , (171): 701–3.
- Shimojima, A. , 1996a, On the Efficacy of Representation , Ph.D. thesis, Indiana University.
- ––– , 1999, “Constraint-Preserving Representations”, in L. Moss, J. Ginzburg, and M. de Rijke, (eds), Logic, Language and Computation: Volume 2 , CSLI Lecture Notes #96, pages 296–317. Stanford: CSLI Publications.
- Shin, S. , 1994, The Logical Status of Diagrams , Cambridge: Cambridge University Press.
- ––– , 2003, The Iconic Logic of Peirce’s Graphs , Cambridge: MIT Press (Bradford).
- ––– , 2015, “The Mystery of Deduction and Diagrammatic Aspects of Representation”, Review of Philosophy and Psychology: Pictorial and Spatial Representation , 6: 49–67.
- Sloman, A. , 1971, “Interaction between philosophy and AI: The role of intuition and non-logical reasoning in intelligence”, in Proceedings Second International Joint Conference on Artificial Intelligence , Los Altos, Calif.: Morgan Kaufmann.
- ––– , 1985, “Why we need many knowledge representation formalisms”, in M. Bramer, (ed.), Research and Development in Expert Systems , pages 163–183.
- ––– , 1995, “Musings on the roles of logical and nonlogical representations in intelligence”, in Chandrasekaran et al., 1995 , pp. 7–32.
- Sober, E. , 1976, “Mental Representations”, Synthese , 33: 101–148
- Sowa, J. , 1984, Conceptual Structures: Information Processing in Mind and Machine , London: Addison Wesley.
- Stenning, K. , 1999, “Review of Das Spiel der Logik , by Lewis Carrol”, Journal of Symbolic Logic , 64: 1368–1370.
- Stenning, K. and O. Lemon , 2001, “Aligning Logical and Psychological Perspectives on Diagrammatic Reasoning”, Artificial Intelligence Review , 15(1–2): 29–62. (Reprinted in Thinking with Diagrams , Kluwer, 2001.)
- Tye, M. , 1991, The Imagery Debate , Cambridge, MA: MIT Press.
- Venn, J. , 1881, Symbolic Logic , London: Macmillan.
- Wang, D. and J. Lee , 1993, “Visual Reasoning: its Formal Semantics and Applications”, Journal of Visual Languages and Computing , 4: 327–356.
- Wittgenstein, L. , 1921, Tractatus Logico-Philosophicus , B. Pears and B. McGuinness (trans), London: Routledge & Kegan Paul, 1961
- Zeman, J. , 1964, The Graphical Logic of C. S. Peirce , Ph.D. thesis, University of Chicago.
- Barwise, J. and E. Hammer, 1994, “Diagrams and the Concept of a Logical System”, in Gabbay, D. (ed.), What is a Logical System? New York: Oxford University Press.
- Hammer, E., 1995b, Logic and Visual Information , Studies in Logic, Language, and Computation. Stanford: CSLI Publications and FoLLI.
- –––, 1998, “Semantics for Existential Graphs”, Journal of Philosophical Logic , 27: 489–503
- Hammer, E. and S. Shin, 1996, “Euler and the Role of Visualization in Logic”, in Seligman, J. and Westerståhl, D. (eds), Logic, Language and Computation: Volume 1 , CSLI Lecture Notes #58, pages 271–286. Stanford: CSLI Publications.
- Kneale, W., and Kneale, M., 1962, The Development of Logic , Oxford: Clarendon Press.
- Lemon, O., 1997, “Review of Logic and Visual Information , by E. M. Hammer”, Journal of Logic, Language, and Information , 6(2): 213–216.
- Roberts, D., 1992, “The Existential Graphs of Charles S. Peirce”, Computer and Math. Applic. , (23): 639–663.
- Shimojima, A., 1996b, “Operational constraints in diagrammatic reasoning”, in J. Barwise and G. Allwein, (eds), Logical Reasoning with Diagrams , New York: Oxford University Press, pages 27–48.
- –––, 1996c, “Reasoning with Diagrams and Geometrical Constraints”, in Seligman, J. and Westerståhl, D. (eds), Logic, Language and Computation: Volume 1 , CSLI Lecture Notes #58, pages 527–540. Stanford, CSLI Publications.
- Shin, S., 1991, “A Situation-Theoretic Account of Valid Reasoning with Venn Diagrams”, in J. Barwise, J. Gawron, G. Plotkin, and S. Tutiya, (eds), Situation Theory and its Applications: Volume 2 , CSLI Lecture Notes #26, pages 581–605. Stanford: CSLI Publications.
- –––, 1999, “Reconstituting Beta Graphs into an Efficacious System”, Journal of Logic, Language, and Information , 8: 273–295.
- –––, 2000, “Reviving the Iconicity of Beta Graphs”, in Anderson, Cheng, and Haarslev, (eds), Theory and Application of Diagrams , pages 58–73. Springer-Verlag.
- –––, 2002a, The Iconic Logic of Peirce’s Graphs , Cambridge, MA: MIT Press.
- –––, 2002b, “Multiple Readings of Peirce’s Alpha Graphs”, in M. Anderson, B. Meyer, and P. Olivier, (eds), Diagrammatic Representation and Reasoning , London: Springer-Verlag, pp. 297–314.
- Sowa, J., 2000, Knowledge Representation: Logical, Philosophical, Computational Foundations , Belmont, CA: Brooks/Cole.
- Stenning, K., 2002, Seeing Reason: image and language in learning to think , Oxford: Oxford University Press.
- Stenning, K. and J. Oberlander, 1995, “A Cognitive Theory of Graphical and Linguistic Reasoning: Logic and Implementation”, Cognitive Science , 19(1): 97–140.
- Tufte, E., 1983, The Visual Display of Quantitative Information , Connecticut: Graphics Press.
- –––, 1990, Envisioning Information , Connecticut: Graphics Press.
How to cite this entry . Preview the PDF version of this entry at the Friends of the SEP Society . Look up topics and thinkers related to this entry at the Internet Philosophy Ontology Project (InPhO). Enhanced bibliography for this entry at PhilPapers , with links to its database.
- Existential Graphs (Peirce’s MS 514 with commentary by John Sowa).
- Edward Tufte’s Visual Display.
- A Survey of Venn Diagrams (University of Victoria, Frank Ruskey).
- Lewis Carroll’s Logic Game .
- Researchers on Diagrammatic Reasoning , output of search at Google Scholar.
- Diagrams 2018 , International conference on the theory and application of diagrams.
cognitive science | Frege, Gottlob: theorem and foundations for arithmetic | mental imagery | Peirce, Charles Sanders: logic
Copyright © 2018 by Sun-Joo Shin < Sun-Joo . Shin @ yale . edu > Oliver Lemon John Mumma < jmumma @ csusb . edu >
- Accessibility
Support SEP
Mirror sites.
View this site from another server:
- Info about mirror sites
The Stanford Encyclopedia of Philosophy is copyright © 2023 by The Metaphysics Research Lab , Department of Philosophy, Stanford University
Library of Congress Catalog Data: ISSN 1095-5054
- Skip to secondary menu
- Skip to main content
- Skip to primary sidebar
Statistics By Jim
Making statistics intuitive
Venn Diagrams: Uses, Examples, and Making
By Jim Frost Leave a Comment
Venn diagrams visually represent relationships between concepts. They use circles to display similarities and differences between sets of ideas, traits, or items. Intersections indicate that the groups have common elements. Non-overlapping areas represent traits that are unique to one set. Venn diagrams are also known as logic diagrams and set diagrams.

An English logician, John Venn, popularized these diagrams in the 1880s by using them to teach set theory. However, a mathematician, Leonard Euler, created them back in the 1700s. Educators now use them in numerous contexts, such as statistics , probability, logic, business, education, and computer science, among many others.
In this post, learn how to interpret Venn diagrams using two- and three-circle versions. I’ll cover several specialized uses, proportional Venn diagrams and illustrating probabilities. I’ll close by showing an easy way to make your own Venn diagrams using widely available templates.
How to Interpret Venn Diagrams
To interpret Venn diagrams, start by examining all the circles. The group of circles represents the “universe” of concepts that the chart explains. Each circle represents a set of items or an idea. Then notice the areas where the circles do not overlap. Non-overlapping sections indicate that the two groups do not share some characteristics. In other words, some properties are unique to a set. Finally, identify the areas where the circles overlap. Overlapping areas indicate that at least two concepts share some traits. Be sure to notice how many circles overlap.
Let’s get to some examples!
Two-Circle Venn Diagram Example
In the Venn diagram below, the two circles tell us that we’re comparing and contrasting dolphins and fish.

Looking at the non-overlapping areas, we see that dolphins have the unique traits of breathing air, have live births, and are warm-blooded. On the other hand, fish breathe water, lay eggs, and are cold-blooded.
Then, looking at the overlapping area, we see that they share the traits of swimming, being aquatic creatures, and having fins.
That’s a simple example with two circles. But the similarities and differences are immediately apparent.
Three-Circle Venn Diagram Example
Venn diagrams don’t need to apply to physical characteristics. The three-circle Venn diagram below shows how a company divides the roles between three areas of their company.

Proportional Venn Diagram Example
Let’s move on to a more complex Venn diagram with three circles. Understanding the similarities and differences of the world’s top exporters might sound dull and complicated. However, the graph below shows the leading exports from the top exporting countries. Additionally, this is a proportional Venn diagram, also known as a scaled Venn diagram. The sizes of the circles are proportional to an attribute the circles represent, which is the annual dollar amount of exports in this example.

At a glance, the circles tell us that we’re comparing China, the United States, and Japan in terms of exports. China has the most exports—not quite twice as much as the United States and a lot more than Japan. The U.S. is in the middle. Of these three countries, the U.S. is unique in that petroleum is among its top exports. Japan is unique in having machines that produce other goods and seagoing ships among its leading exports. China has a diverse set of distinct exports. The three countries all have integrated circuits (IC) as one of their top exports. The U.S. and Japan both count vehicle parts and cars among their chief exports.
Even if understanding international exports is not your thing, you probably learned something new in a relatively painless way thanks to a Venn diagram!
Using Venn Diagrams to Represent Probabilities
In math and statistics, you can use Venn diagrams to depict probabilities. Grasping how probabilities relate to events occurring separately, together, or not all can be complex. Venn diagrams make understanding these likelihoods much easier.
For this example, imagine we run a mountain bike rental shop in an area with rough terrain. Flat tires are a problem, and we want to understand the probabilities of the front, back, both, and no tires going flat during a rental. After collecting data, the owners display the probabilities in the chart below.
At a glance, the Venn diagram helps you understand the probabilities of various combinations of flat tires occurring!

Exploring the Various Probabilities
The first thing to notice on the Venn diagram is that each circle represents the probability of a tire going flat. The value outside the circles indicates the likelihood of no flat tires. Collectively, all the probabilities sum to 1 (100%). This area is our entire sample space.
To find the probability for only the front tire going flat or only the rear tire on the Venn diagram, look at the values in the circles, 0.135 and 0.085, respectively. In probability theory terminology, these are the joint probabilities of one tire going flat and the other tire not going flat. The joint probability for both tires going flat (yikes!) is the intersection value of 0.015.
To calculate the probability that the front tire goes flat regardless of whether or not the rear goes flat, add the value in the Front circle to the intersection value, 0.135 + 0.015 = 0.15. You can do the same for the rear tire to obtain 0.10. Statisticians call these the marginal probabilities for the events.
On the chart, the total probability of any flat occurring is the sum of the front, rear, and intersection values, 0.135 + 0.15 + 0.085 = 0.235. In probability theory, this is the probability of the union of events. Conversely, the probability of no flat at all is 1 – 0.235 = 0.765, which is the value outside the circles.
The calculations for these probabilities go beyond this post, but you can see them in the Word file that contains my charts: Venn diagram examples . In that document, scroll to the bottom to see the calculations!
Related post : Probability Fundamentals
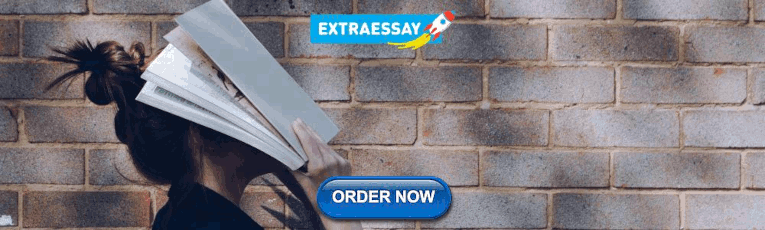
Venn Diagram Maker
It’s easy to make your own Venn diagrams using Microsoft’s blank Venn diagram templates. I used Microsoft Word to create the ones in this post, but you can also use Excel, Outlook, and PowerPoint. The process for generating them is the same for all these applications.
You can download my Word file to see how I used the Venn diagram templates: Venn diagram examples .
- Go to the Insert tab and click Smart Shapes.
- In the box that appears, click Relationship in the left pane.
- Select one of the Venn diagram layouts and click OK .
- To add the circle text, click the [Text] label in each circle.
- To add the intersection and other values, click Text Box and choose Draw Text Box .
- I had to format the text boxes to have no fill and no outline so only the text shows.
For more details, see Microsoft’s instructions for creating Venn diagrams .
Share this:

Reader Interactions
Comments and questions cancel reply.
- Earth & Space
- Life Science
- Physical Science
- Earth and Space Science Curriculum Map
- Physical Science Curriculum Map
- Life Science Curriculum Map
- Biology Curriculum Map
- Chemistry Curriculum Map
NGSS Science Curriculum for Science Teachers

- All Courses
- Earth Science Materials
- Physical Science Materials
- Biology Materials
- Chemistry Materials
- Teacher Forums
Hypotheses Theories and Scientific Law
Today we will be comparing and contrasting Hypotheses, Theories, and Scientific Law.
Science is a way of making sense of the world around us. The theories and principles found in science as a discipline have been established through repeated and careful experimentation and observation . Scientific research is peer-reviewed by other specialists in the field to ensure that the findings are accurate and that the experiments used, and the findings are consistent and fair.
Scientific ideas can progress from a hypothesis, to a theory using testable, scientific laws. Only a few scientific facts are considered scientific laws and many hypotheses are tested to generate a theory .

A hypothesis (plural hypotheses) is an idea or suggestion that can be tested through observation or experimentation. On some occasions, a hypothesis may take the form of a question called an aim .
Hypotheses are often made after in depth background research or an inquiry has been conducted. Its purpose is to provide direction into further scientific research. Once a hypothesis has been made, it must be tested by conducting carefully designed and controlled experiment to prove it right or wrong .
The experiment must meet certain criteria so that the results are valid and reliable . Valid and reliable results come from experiments that:
- Change only one variable at a time.
- Control all other variables in the experiment.
- Use measuring instruments that give accurate results.
- Have repeated trials/tests to show consistent results.
This lesson from Khan Academy is also good. Here is the link...
Testing Hypothesis p-Values

A theory describes how or why something occurs. Scientists are always making new discoveries and so scientific theories evolve overtime.
In science a hypothesis or an idea is not considered a theory until it has been tested thoroughly and independently by many scientists. Scientific theories are more certain that hypotheses but less certain that scientific laws. Scientific theories are often made up of many hypotheses which add together to provide detailed information on a topic. In many cases, these theories have been contributed to by many scientists, often over several years.
A theory is:
- Consistent and compatible with the current scientific evidence available
- Tested against a wide range of phenomena
- Demonstrates effective problem-solving
Some of the more well-known theories that have contributed to modern biology and that are covered in this course are shown in the table below:

Here is a Hypotheses Theories and Scientific Law video to share with your class...
Scientific Laws
Theories which have been thoroughly tested and are accepted by the scientific community often become scientific laws. There are few laws compared to theories. Laws are often expressed as single statement and are brief but are concise.

Scientific Law vs Theory
This venn diagram show visually the difference between Scientific Law vs Theory.

Laws have the following features:
- They are universally accepted to be true.
- Laws predict the results under certain conditions.
- They do not provide explanations of how or why something occurs.
Below are examples of laws that will be covered in this course:

Here is a Scientific Law video to share with your class...
Here is your Free Content for this Lesson Hypotheses, Theories, and Scientific Law!
Hypotheses, theories, and scientific law - pdfs.
To get the Scientific Laws Hypotheses and Theories Worksheet Answers Join the iTeachly Science Teacher Community !
1-2 Assignment SE - Hypotheses Theories and Scientific Law (FREE) 1-2 Assignment Teacher Edition - ( MEMBERS ONLY ) 1-2 Bell Work SE - Hypotheses Theories and Scientific Law (FREE) 1-2 Bell Work Teacher Edition - ( MEMBERS ONLY ) 1-2 Exit Quiz SE - Hypotheses Theories and Scientific Law (FREE) 1-2 Exit Quiz Teacher Edition - ( MEMBERS ONLY ) 1-2 Guided Notes SE - Hypotheses Theories and Scientific Law (FREE) 1-2 Guided Notes Teacher Edition - ( MEMBERS ONLY ) 1-2 Lesson Plan - Hypotheses Theories and Scientific Law (FREE) 1-2 Online Activities - Hypotheses Theories and Scientific Law (FREE) 1-2 Slide Show - Hypotheses Theories and Scientific Law (FREE) 1-2 Vocabulary Worksheet SE - Hypotheses Theories and Scientific Law (FREE) 1-2 Vocabulary Worksheet Teacher Edition - ( MEMBERS ONLY )
Hypotheses, Theories, and Scientific Law - Word Docs & PowerPoints
To gain access to our editable content Join the iTeachly Biology Teacher Community! This includes our Scientific Laws Hypotheses and Theories Worksheet Answers. Here you will find hundreds of lessons, a community of teachers for support, and materials that are always up to date with the latest standards.
Want access to all of our Biology Lessons?
Simply click the image below to get all of our lessons.

Don't Forget to Pin this Lesson on Hypotheses, Theories, and Scientific Law...

This Hypotheses, Theories, and Scientific Law lesson is from...
Unit 1 – biology basics.
- 1-1 Nature of Science and the Scientific Method
- 1-2 Hypotheses, Theories and Scientific Law
- 1-3 Technological Design Process
- HS-LS1-6. Construct and revise an explanation based on evidence for how carbon, hydrogen and oxygen from sugar molecules may combine with other elements to form amino acids and/or other large carbon-based molecules.
- HS-ESS2-5. Plan and conduct an investigation of the properties of water and its effects on Earth materials and surface process.
Cancel reply
Your email address will not be published. Required fields are marked *
Filter by Keywords
Mind Mapping
Understanding venn diagram symbols (with examples).
Praburam Srinivasan
Growth Marketing Manager
August 28, 2024
Start using ClickUp today
- Manage all your work in one place
- Collaborate with your team
- Use ClickUp for FREE—forever
Remember those overlapping circles you learned about in middle school? Yes, we’re talking about Venn diagrams. They’re great at comparing and contrasting sets of data and forming logical relationships.
As you can expect, they’re used extensively in data analysis and to explore data sets, create visualizations, and support data-driven decision-making. But if we’re being honest—drawing those circles to line up perfectly and adding different symbols is not the easiest thing to do.
In this blog, we’ll explore how to create Venn diagrams while explaining the multiple Venn diagram symbols that go with them. As a bonus, we’ll also share additional resources and templates to help you level up your data visualization skills.
⚠️Warning: Some math ahead!
What are Venn Diagram Symbols?
The union of sets: ∪ symbol, the intersection of two sets: ∩ symbol, the complement of a set: ac, other complex venn diagram symbols, how to use venn diagram symbols, 1. two-set venn diagram, 2. three-set venn diagram, clickup: a powerful venn diagram creation tool, working with venn diagrams, using venn diagrams in research sampling, venn diagrams in decision-making.

Each circle in a Venn diagram represents a set, which is a collection of distinct objects. Venn diagram symbols break down complex ideas by showing the connections between different sets. That’s why they’re also considered an important part of set theory.
Now here comes the fun part—Venn diagrams can be made of multiple circles.
Two-circle Venn diagrams are great for understanding the union, intersection, and differences between two sets.
Three-circle Venn diagrams are even more detailed, as they show relationships between three sets and can help find correlations within different sets of data.
With so much information circling in these diagrams, it is helpful to have symbols that represent the different relationships between these sets. This is where Venn diagram symbols come in.
Did You Know : John Venn, an English logician, made the Venn diagram famous in the 1880s. You’ll see them a lot in probability, logic, statistics, linguistics, and computer science to illustrate the logical connections between sets.
Understanding Key Venn Diagram Symbols
Among all the different nomenclatures, three main Venn diagrams are most commonly used: union, intersection, and complements.
The union of sets in a Venn diagram is represented by the union symbol ( ∪ ) . It shows all elements present in either set. When using Venn diagram symbols, the union symbol illustrates the combination of elements from two or more sets.
Consider this example.
- Set A contains {1, 2, 3}
- Set B contains {3, 4, 5}
The union of sets A and B (A ∪ B) includes all the elements from both sets:
i.e. A ∪ B = {1, 2, 3, 4, 5}
In a two-circle Venn diagram, the union would be the entire area covered by both circles, including the overlapping region.
Example: Imagine a school cafeteria offering two meal plans:
- Plan A: Includes pizza, salad, and fruit
- Plan B: Includes pasta, salad, and dessert
Using Venn diagram sets symbols, we can represent these meal plans as:
- Set A: {pizza, salad, fruit}
- Set B: {pasta, salad, dessert}
The union of these sets (A ∪ B) represents all the food options available to students who have either Plan A or Plan B, or both.
So, A ∪ B = {pizza, salad, fruit, pasta, dessert}
A Venn diagram would show two overlapping circles: one circle for Plan A, one for Plan B. The overlapping part would contain “salad,” as it’s offered in both plans. The entire area covered by both circles would represent the union of the sets—all possible food options, which are pizza, salad, fruit, pasta, and dessert.
Friendly note: If you’d like to pause, go ahead and hydrate yourself, look outside the window, or hug your family so we can comfortably move on to more Venn diagram examples.
The intersection of sets is represented by the intersection symbol ( ∩ ) . It shows elements common to both sets and is highlighted with overlapping circles.
Let’s have a look at the same example from above.
The intersection of sets A and B (A ∩ B) includes only the common elements:
Therefore, A ∩ B = {3}
The example above is a two-circle Venn diagram. The intersection is the shaded region “C”, where the circles overlap. It represents the element common to both sets.
Example: Imagine a tech company with two departments:
- Department A: Handles software development, quality assurance, and project management
- Department B: Focuses on product marketing, sales, and customer support
The intersection of these departments would include roles or employees who work in both areas. For example, a product manager might be involved in both software development and product marketing.
Again, by using Venn diagram sets of symbols, we can represent the departments:
- Department A (Set A): {software development, quality assurance, project management}
- Department B (Set B): {product marketing, sales, customer support}
- Intersection (A ∩ B): {product management}
A Venn diagram would visually represent this overlap, with “product management” in the overlapping section of the two circles.
Bonus: 10 Best Comparison Chart Templates (Beyond Venn Diagrams)
The complement of a set is represented by the complement symbol (Ac) or (A’) . It includes all elements in the universal set (U) that are not in the particular set. Using Venn diagram symbols, the complement highlights areas outside the set in question.
For example, if:
- Universal set (U) contains {1, 2, 3, 4, 5}
The complement of set A (Ac) includes elements in U that are not in A:
Hence, Ac/A’ = {4, 5}
What would this look like with three circles?
In a three-circle Venn diagram, the complement would be the area outside circle A. The example below clearly shows what is excluded from set A.
Example: Imagine a marketing campaign targeting a specific demographic.
- Universal Set (U): The entire population of a city
- Set A: People aged 18-35 who are interested in technology
The complement of set A (A’) would include all individuals in the city who are either:
- Not aged between 18-35
- Not interested in technology
Now that we’ve gone over the basic symbols, let’s look at a few of the more complex Venn diagram symbols and their meanings:
∈ : Element of
The ∈ symbol means “is an element of” a set.
For example, if 3 ∈ A, it means 3 is in Set A. In Venn diagrams, this symbol helps us see which elements are inside the circles.
In computer science, we often use it to show membership, like “x ∈ A” to indicate that x is part of Set A.
∉ : Not an element of
The ∉ symbol means “is not an element of” a set.
If 4 ∉ B, it means 4 isn’t in Set B. In Venn diagrams, this symbol shows elements outside the circles.
Imagine a Venn diagram example: elements outside the circles aren’t part of those sets. It’s like saying, “4 doesn’t belong to Set B.” The symbol is key in set theory for showing exclusion.
Ø : Empty set
The Ø symbol represents the empty set, which has no elements. If A = Ø, Set A has nothing in it. In Venn diagrams, it shows a set without members.
In computer science, Ø appears in algorithms dealing with empty data sets, showing non-existence. It’s a way to say, “There’s nothing here.”
⊂ : Proper subset
The ⊂ symbol means one set is a proper subset of another. If A ⊂ B, all elements of A are in B, but A isn’t equal to B.
In Venn diagrams, this shows one circle completely inside another. Think of a set diagram: a smaller circle within a larger one is a proper subset.
In a logical diagram, “C ⊂ D” means C is a subset of D, highlighting hierarchical relationships.
⊄ : Not a subset
The ⊄ symbol means one set isn’t a subset of another. If A ⊄ B, some elements in A aren’t in B.
In Venn diagrams, it is shown by circles that don’t fully overlap. Picture a Venn diagram example with partial or no overlap between circles.
To show that A is not a subset of B, we need to show an element x that belongs to A but not B.
There are three possibilities:
This symbol is crucial in set theory for emphasizing non-inclusion.
⊇ : Superset
The ⊇ symbol means one set is a superset of another. If A ⊇ B set A contains all elements of set B.
In two-circle Venn diagrams, it appears as one circle encompassing another.
In a three-circle Venn diagram, a larger circle containing a smaller one is a superset.
This symbol is important in set theory for showing full inclusion.
⊃ : Proper superset
The ⊃ symbol indicates a proper superset. If A ⊃ B, Set A has all elements of B and more.
In a two-circle Venn diagram, a larger circle encompassing a smaller circle is a proper superset. For a three-circle Venn diagram, the larger circle encompasses the remaining two circles.
The basic depiction would be:
This symbol is essential in set theory for showing full but non-equal inclusion. In a logical diagram, “I ⊃ J” means I includes all elements of J and additional ones.
⊅ : Not a proper superset
The ⊅ symbol means one set isn’t a proper superset of another. If A ⊅ B, Set A doesn’t fully contain B with additional elements.
In a Venn diagram example, circles that don’t fully contain others represent this concept.
Venn diagrams may seem like a long-forgotten remnant from middle school math class, but they are incredibly powerful at conveying data insights. Here’s how you’d use them for work.
Venn diagram use cases:
- Marketing campaigns: A company wants to target a specific demographic for a new product. By using a Venn diagram, they can analyze the overlap between age groups, interests, and geographic locations to identify the ideal target audience for their product
- Inventory control: A retailer wants to optimize inventory levels for different product categories. A Venn diagram can help visualize which products are frequently purchased together, leading to better stocking decisions
- Quality control: A manufacturing company can use Venn diagrams to analyze defects in products. By categorizing defects based on different criteria (e.g., type of defect, production line), the company can identify root causes and apply corrective actions
Now, let’s look at how you can create Venn diagrams. But before that, you should also know how set notation and shaded regions work.
Quick overview of set notation and shaded regions
Set notation is the language used to define sets, their relationships, and operations. This includes Venn diagram symbols like ∪ (union), ∩ (intersection), and ‘ (complement).
The idea of these symbols is to express complex logical statements. It’s essentially a way to condense a lot of information into an easy-to-understand format.
Similarly, shaded regions in Venn diagrams offer a visual counterpart to set notation.
Shaded regions allow us to perceive patterns, overlaps, and exclusions. By shading specific areas, we can highlight the outcomes of set operations, making complex relationships easily understandable.
Step-by-Step Guide to Creating Venn Diagrams
Now that we’ve been through a math minefield and survived, let’s get to the easy bit: creating diagrams. We’ll cover both two and three-set Venn diagrams here.
Step 1: Identify the sets and elements
- Determine the sets and list their elements
Example: Set A contains {1, 2, 3}, and Set B contains {3, 4, 5}.
Step 2: Draw two overlapping circles
- Draw two circles that overlap
- Label the circles as Set A and Set B
Step 3: Add elements to the circles
- Place elements in the appropriate sections
- Elements common to both sets go in the overlapping area
Example : Place 1, 2 in Set A’s circle, 4, 5 in Set B’s circle, and 3 in the overlapping area.
Step 4: Shade regions for specific operations
- Shade regions to represent specific sets of operations
Example: Shade the entire area of both circles for A ∪ B (union). Shade only the overlapping area for A ∩ B (intersection).
Step 5: Review and adjust
- Ensure each element is correctly placed
- Verify that the diagram accurately represents the sets and their relationships
Example: Set A contains {1, 2}, Set B contains {2, 3}, and Set C contains {1, 3, 4}.
Step 2: Draw three overlapping circles
- Draw three circles that overlap
- Label the circles as Set A, Set B, and Set C
- Elements common to all sets go in the center overlapping area
Example: Place 1 in Set A and Set C’s overlapping area, 2 in Set A and Set B’s overlapping area, and 3 in Set B and Set C’s overlapping area. Place 4 in Set C.
- Shade regions to represent specific set operations
Example: Shade the entire area of all circles for A ∪ B ∪ C (union). Shade only the central overlapping area for A ∩ B ∩ C (intersection).
Three-circle Venn diagrams showcase more complex relationships and set differences. They also illustrate how elements are distributed across multiple sets.
Venn Diagram Creation Tools and Resources
Creating custom Venn diagrams is easy. They can be made in MS Word, PowerPoint, and even Paint. There are lots of other free and paid Venn diagram templates available on the internet as well. Whatever you choose, it’s recommended to watch tutorials and double-check the functionality of any templates before considering them.
Topping the list of options, though , is ClickUp, a tool that makes creating Venn diagrams and data visualization a breeze. And the best part? It comes with amazing free templates.
Bonus: 5 Free Venn Diagram Templates
ClickUp’s Venn Diagram Template helps create beautiful diagrams that are easy to understand and even easier to use. With its intuitive interface, you can visualize relationships between sets and identify common elements.
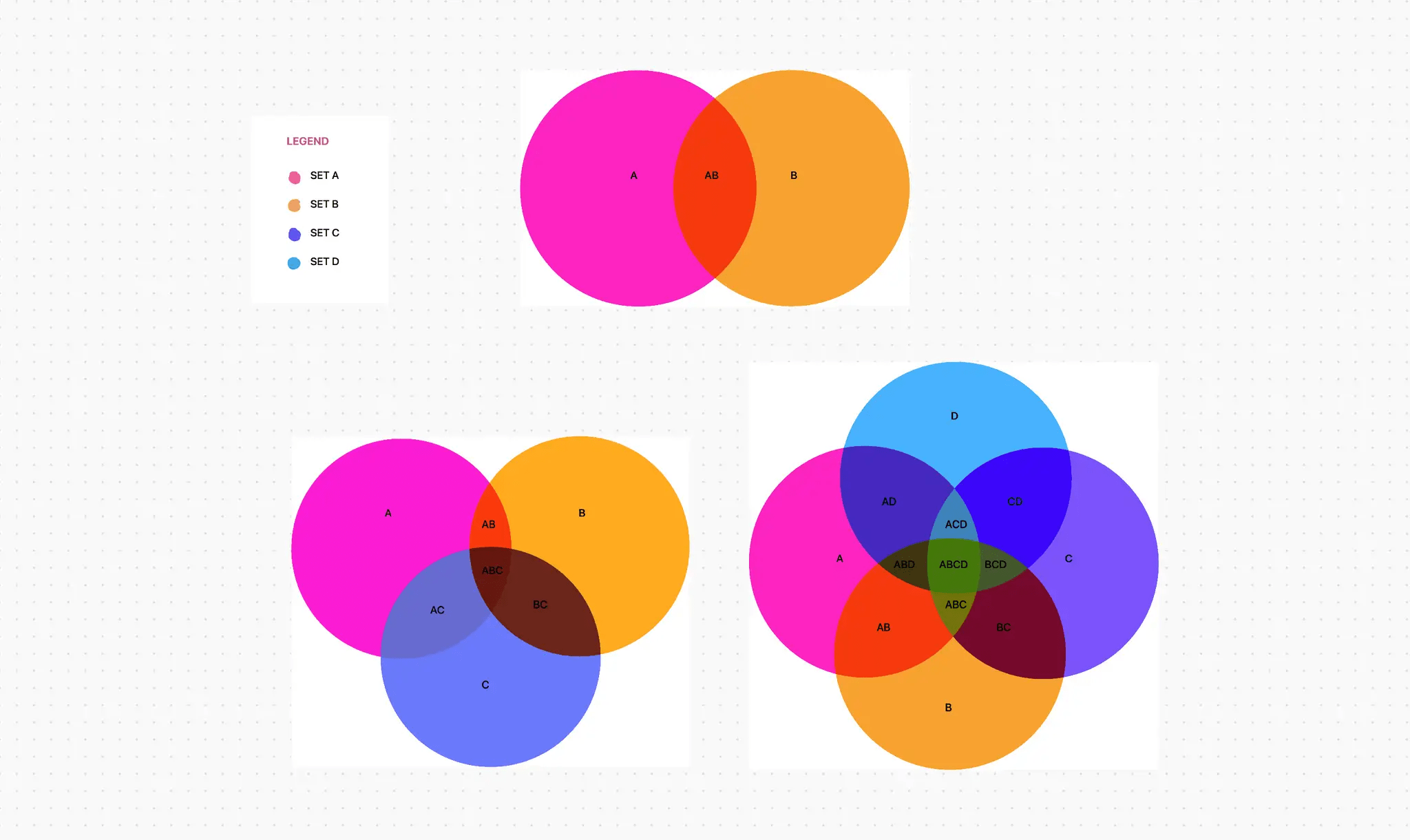
This flexible template helps users create two-circle or three-circle Venn diagrams to show intersecting elements and set differences.
Users can customize the diagram symbols and shaded regions, and set notations to suit their needs. Whether analyzing complex problems in computer science or exploring mathematical concepts, this template offers a visual way to understand set operations.
With features like Custom Statuses and Custom Fields, you can design informative diagrams with a minimal learning curve.
ClickUp Whiteboards
With ClickUp Whiteboards , you can create your canvas and use visual elements to collaborate with your team and brainstorm ideas in real time.
You can even create tasks on ClickUp directly from your Whiteboards, and link to relevant documents and media that add more context.
For example, product teams can set up one of these product comparison templates on a Whiteboard and use Veen diagrams to conduct a thorough competitive analysis with overlapping features and capabilities.
ClickUp Mind Maps
ClickUp’s Mind Maps lets you streamline complex ideas and workflows into intuitive flowcharts.
What makes ClickUp one of the best mind-mapping tools out there is the functionality it brings you beyond just creating visual workflows: you can hit the “re-layout” button to automatically re-align a messy flowchart. Plus, your mind map notes can also be converted into actionable tasks.
💡 Pro Tip: Got a blank mind map canvas set up on ClickUp but don’t know where to begin? Explore these helpful mind map flowchart examples and find a great starting point!
To work with Venn diagrams effectively, you should be able to read and interpret Venn diagrams well. Here’s how you do it.
- Identify the sets: Determine what each circle represents. These might be categories, groups, or sets of data
- Understand the overlap: The area where circles intersect represents elements that belong to both sets. This region is crucial for identifying commonalities or shared characteristics
- Analyze the non-overlapping areas: The portions of the circles that do not overlap contain elements unique to each set. These represent exclusive characteristics
- Consider the universal set: If present, it encompasses all elements being considered. This helps define the scope of the diagram
- Interpret shaded regions: If parts of the diagram are shaded, they typically represent specific set operations or conditions. Understanding these shadings is key to extracting meaningful information
Venn Diagram Use Cases
Now we know how Venn diagrams work and how you can create them. But what real-world problems can these diagrams solve? A lot of them!
In computer science, they’re great for logical operations. In data analysis, they’re perfect for visualizing set operations. Businesses love them for spotting market overlaps. Similarly, professionals like environmental scientists regularly use Venn diagrams to analyze ecosystems and biodiversity.
Let’s go over a few more such use cases.
In social science and scientific research, Venn diagrams are useful for seeing the connections between different sample groups.
A three-circle diagram shows the overlap between the three survey groups and makes it easier to identify shared and unique responses.
For example, in a college student survey, a Venn diagram can show which students participate in sports, clubs, or volunteer work and where these groups overlap. This helps researchers identify shared interests and popular recreational programs.
If you thought you could not use Venn diagrams to make decisions in your everyday life, think again!
For example: Let’s say you’re deciding between three smartphones. Create a Venn diagram with three circles: one for each phone. In each circle, list the phone’s key features. Where the circles overlap, list shared features.
This visual comparison helps you see which phone best fits your needs based on factors like camera quality, battery life, and price. You’ll also be able to narrow down features that are non-negotiable for you, and you can then make your final selection on other factors like price or look-and-feel.
Also Read: 10 Diagram Examples for Any Type of Project
Venn Diagrams Are Better on ClickUp
Venn diagrams have long been a favorite way to visually compare two or more data sets and draw logical conclusions, whether you’re a data scientist, a student, or a social media marketer. And with ClickUp, creating and extracting value from Venn diagrams becomes so much more intuitive and easy (especially with templates)!
But, of course, Venn diagrams aren’t the only way you can visualize data on ClickUp. You can consider Venn diagram alternatives like Euler diagrams and scatter plot graphs to make better sense of complex data sets.
ClickUp is also one of the best flowchart tools and lets you turn even the messiest of workflows and fragmented ideas into coherent mind maps.
Have your data cleaned and ready? Get started with ClickUp today!
Questions? Comments? Visit our Help Center for support.
Receive the latest WriteClick Newsletter updates.
Thanks for subscribing to our blog!
Please enter a valid email
- Free training & 24-hour support
- Serious about security & privacy
- 99.99% uptime the last 12 months
Venn Diagram: Theory vs. Law vs. Hypothesis

- Word Document File
Description
Questions & answers, earth science educational resources.
- We're hiring
- Help & FAQ
- Privacy policy
- Student privacy
- Terms of service
- Tell us what you think
Law Vs Theory Worksheet
Worksheet biology Hypothesis classroom Theory law between examples differences
Theory Vs Law Worksheet
Scientific diagram laws theories venn creately Tok seoyeon: scientific law, theory, and hypothesis Theory vs law worksheet
5 differences between theory and law with examples
Theory law vsWhat’s the difference between a scientific law and theory? Scientific laws and theoriesHypothesis principle theories hypotheses comprehensive planetesimal theorem seoyeon.
Theory law scientific difference between whats science there do both kidpidTheory vs law worksheets & teaching resources Difference between theory and lawTheory vs law worksheet.
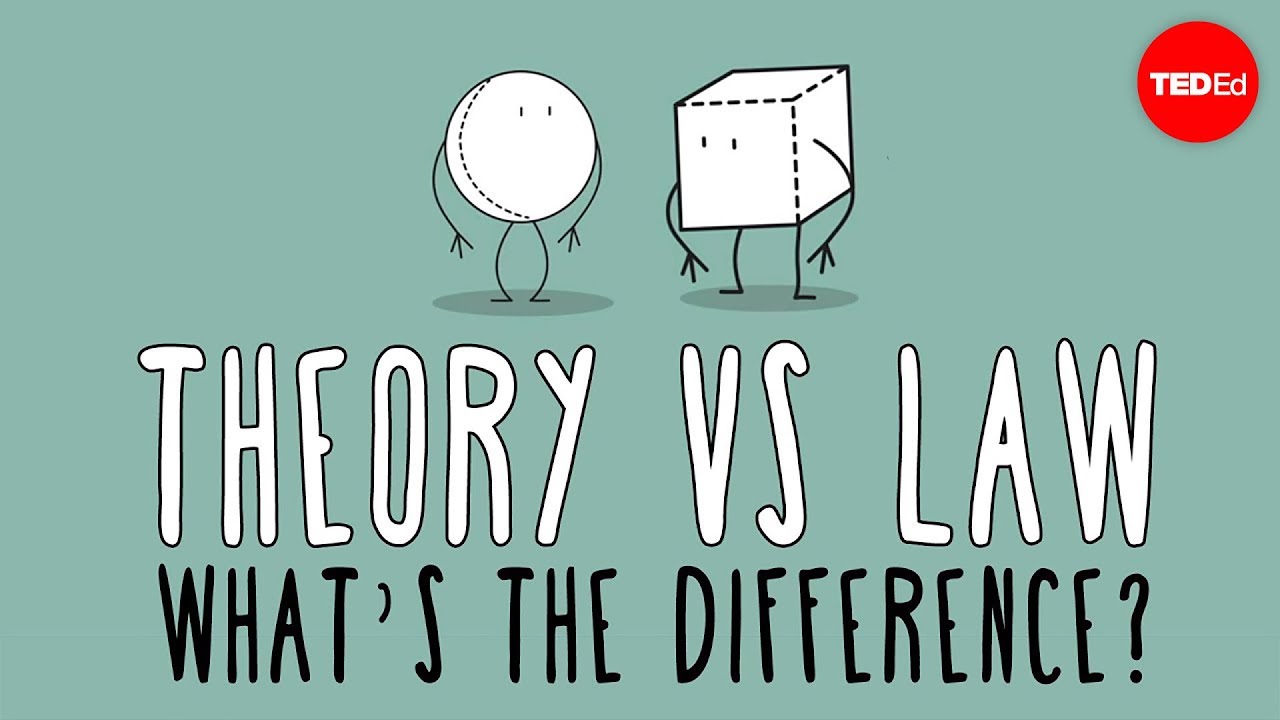
Theory Vs Law Worksheets & Teaching Resources | Teachers Pay Teachers

TOK seoyeon: Scientific Law, Theory, and Hypothesis
Scientific Laws and Theories | Editable Venn Diagram Template on Creately

Theory Vs Law Worksheet - Escolagersonalvesgui
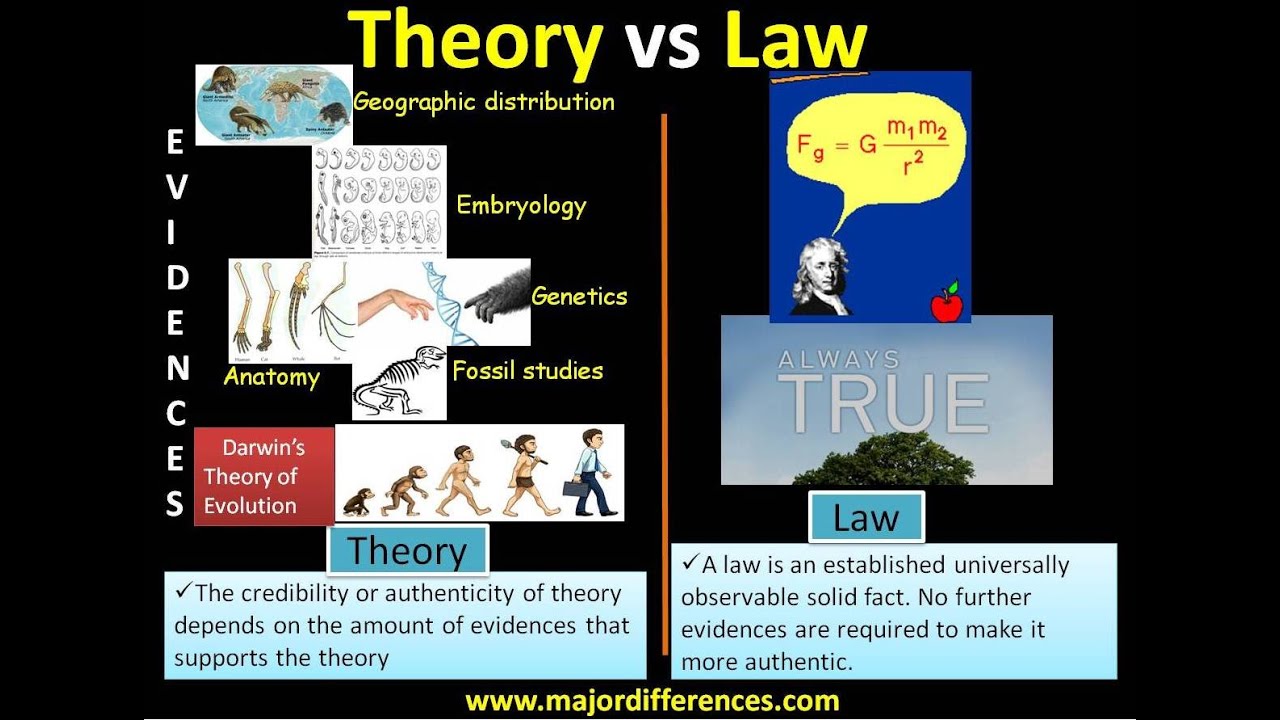
5 Differences between Theory and Law with Examples - YouTube

Difference Between Theory and Law | Definitions, Function, Characteristics
More Posts:
- › Number Of Columns In Excel Worksheet
- › Have Got Has Got Esl Worksheets
- › Factoring Expressions Using Gcf Worksheet
- › Graphing Lines In Standard Form Worksheet Answers
- › Esl Grammar Worksheet Intermediate
- › Idiom Worksheet 3rd Grade
- › Free Printable Preschool Fall Worksheets
- › Free Printable Emotions Worksheet Preschool
- › Find The Area Of Compound Shapes Worksheet
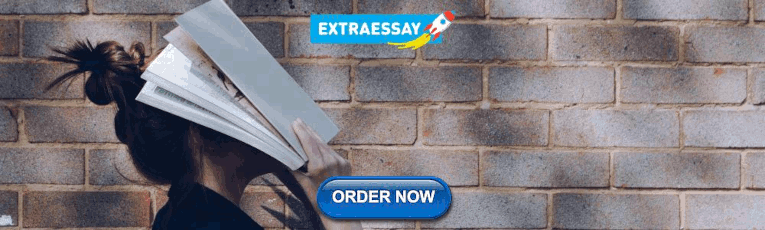
IMAGES
VIDEO
COMMENTS
A hypothesis is either a suggested explanation for an observable phenomenon, or a reasoned prediction of a possible causal correlation among multiple phenomena. In science, a theory is a tested, well-substantiated, unifying explanation for a set of verified, proven factors. A theory is always backed by evidence; a hypothesis is only a suggested possible outcome, and is testable and falsifiable.
A hypothesis is a possible explanation that can be tested. This simple definition needs some further explanation. It says it must have a possible explanation. The hypothesis should apply reasoning ...
Possible answer: If the force acting on an object is increased, then the acceleration of the object will increase. 6. Possible answer: A hypothesis is a testable statement that can be used as the basis for an experiment, while a theory is a broad explanation based on a large amount of data accumulated over a long period of time. 7. hypothesis.
Theory vs. Hypothesis: Basics of the Scientific Method. Written by MasterClass. Last updated: Jun 7, 2021 • 2 min read. Though you may hear the terms "theory" and "hypothesis" used interchangeably, these two scientific terms have drastically different meanings in the world of science.
A hypothesis is an assumption made before any research has been done. It is formed so that it can be tested to see if it might be true. A theory is a principle formed to explain the things already shown in data. Because of the rigors of experiment and control, it is much more likely that a theory will be true than a hypothesis.
A scientific theory summarizes a hypothesis or group of hypotheses that have been supported with repeated testing. A theory is valid as long as there is no evidence to dispute it. Therefore, theories can be disproven. Basically, if evidence accumulates to support a hypothesis, then the hypothesis can become accepted as a good explanation of a ...
Now that you have had an opportunity to gather more information about scientific hypothesis and scientific theory, see if you can complete the Venn diagram below using some of the defining characteristics of both scientific hypothesis and scientific theory.
Comapring and contrasting the characteristics of a hypothesis, scientific law, and theory. You can easily edit this template using Creately's venn diagram maker.You can export it in multiple formats like JPEG, PNG and SVG and easily add it to Word documents, Powerpoint (PPT) presentations, Excel or any other documents.
Teacher Support [OL] Pre-assessment for this section could involve students sharing or writing down an anecdote about when they used the methods of science. Then, students could label their thought processes in their anecdote with the appropriate scientific methods. The class could also discuss their definitions of theory and law, both outside and within the context of science.
Diagrams. First published Tue Aug 28, 2001; substantive revision Thu Dec 13, 2018. All of us engage in and make use of valid reasoning, but the reasoning we actually perform differs in various ways from the inferences studied by most (formal) logicians. Reasoning as performed by human beings typically involves information obtained through more ...
Venn diagrams visually represent relationships between concepts. They use circles to display similarities and differences between sets of ideas, traits, or items. Intersections indicate that the groups have common elements. Non-overlapping areas represent traits that are unique to one set. Venn diagrams are also known as logic diagrams and set ...
View full lesson: http://ed.ted.com/lessons/what-s-the-difference-between-a-scientific-law-and-theory-matt-anticoleChat with a friend about an established sc...
A Venn diagram is a widely used diagram style that shows the logical relation between sets, popularized by John Venn (1834-1923) in the 1880s. The diagrams are used to teach elementary set theory, and to illustrate simple set relationships in probability, logic, statistics, linguistics and computer science.A Venn diagram uses simple closed curves drawn on a plane to represent sets.
Though closely related, scientific law vs. theory are two different terms. Learn about their key differences in science with this helpful article. Dictionary Thesaurus Sentences ... As with laws, every theory begins with a scientific hypothesis, which must be carefully researched. If there is sufficient evidence to support that the hypothesis ...
The scientific method involves formulating hypotheses and testing them to see if they hold up to the realities of the natural world. Successfully proven hypotheses can lead to either scientific theories or scientific laws, which are similar in character but are not synonymous terms. ... Theory vs. Law: Basics of the Scientific Method. Written ...
Q-Chat. Study with Quizlet and memorize flashcards containing terms like can be modified if even one peer reviewed experiment and produces data showing disagreement, biology has more of these, explains how/why nature works and more.
A hypothesis is an explanation for a specific phenomenon that can be tested by an experiment. A theory is an explanation, also supported by lots of experiments and evidence, for a broader concept or set of supported hypotheses. Comparing these three is sort of like comparing a hippo, a huckleberry, and a housefly.
In science a hypothesis or an idea is not considered a theory until it has been tested thoroughly and independently by many scientists. Scientific theories are more certain that hypotheses but less certain that scientific laws. ... Scientific Law vs Theory. This venn diagram show visually the difference between Scientific Law vs Theory. Laws ...
If 4 ∉ B, it means 4 isn't in Set B. In Venn diagrams, this symbol shows elements outside the circles. Imagine a Venn diagram example: elements outside the circles aren't part of those sets. It's like saying, "4 doesn't belong to Set B." The symbol is key in set theory for showing exclusion. Ø : Empty set
This Hypothesis, Theory and Law notes activity will get your middle school students thinking critically about how scientific research moves from hypothesis, to theory and then on to a law as they learn about the nature of science and complete a research project to show their knowledge. ... and laws using a Venn diagram • Choose a law or ...
This is a digital Venn Diagram for students to compare and contrast Scientific Law and Scientific Theory. Students will use 10 pre-filled text boxes on the Google Slides to drag and drop them into the correct location on the graphic organizer (Venn Diagram).The background of the Venn Diagram is 'locked' and the only thing the students can move are the text boxes.
Description. Compare and contrast Theory, law, and hypothesis. Students can then paste into interactive notebook. Reported resources will be reviewed by our team. Report this resource to let us know if this resource violates TPT's content guidelines. Compare and contrast Theory, law, and hypothesis. Students can then paste into interactive ...
Scientific diagram laws theories venn creately Hypothesis principle theories hypotheses comprehensive planetesimal theorem seoyeon Scientific laws and theories What's the difference betwee ... theory, and hypothesis Theory vs law worksheet. 5 differences between theory and law with examples. Theory law vsWhat's the difference between a ...