Our websites may use cookies to personalize and enhance your experience. By continuing without changing your cookie settings, you agree to this collection. For more information, please see our University Websites Privacy Notice .
Neag School of Education
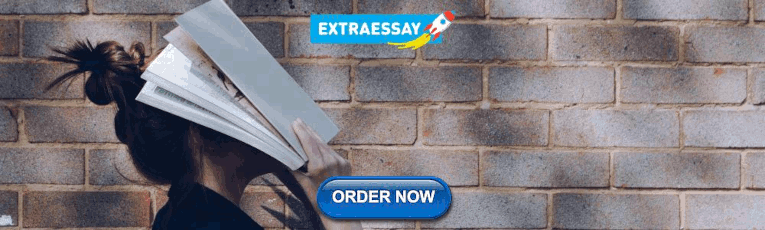
Educational Research Basics by Del Siegle
Instrument reliability.
Reliability (visit the concept map that shows the various types of reliability)
A test is reliable to the extent that whatever it measures, it measures it consistently. If I were to stand on a scale and the scale read 15 pounds, I might wonder. Suppose I were to step off the scale and stand on it again, and again it read 15 pounds. The scale is producing consistent results. From a research point of view, the scale seems to be reliable because whatever it is measuring, it is measuring it consistently. Whether those consistent results are valid is another question. However, an instrument cannot be valid if it is not reliable.
There are three major categories of reliability for most instruments: test-retest, equivalent form, and internal consistency. Each measures consistency a bit differently and a given instrument need not meet the requirements of each. Test-retest measures consistency from one time to the next. Equivalent-form measures consistency between two versions of an instrument. Internal-consistency measures consistency within the instrument (consistency among the questions). A fourth category (scorer agreement) is often used with performance and product assessments. Scorer agreement is consistency of rating a performance or product among different judges who are rating the performance or product. Generally speaking, the longer a test is, the more reliable it tends to be (up to a point). For research purposes, a minimum reliability of .70 is required for attitude instruments. Some researchers feel that it should be higher. A reliability of .70 indicates 70% consistency in the scores that are produced by the instrument. Many tests, such as achievement tests, strive for .90 or higher reliabilities.
Relationship of Test Forms and Testing Sessions Required for Reliability Procedures
Test-Retest Method (stability: measures error because of changes over time) The same instrument is given twice to the same group of people. The reliability is the correlation between the scores on the two instruments. If the results are consistent over time, the scores should be similar. The trick with test-retest reliability is determining how long to wait between the two administrations. One should wait long enough so the subjects don’t remember how they responded the first time they completed the instrument, but not so long that their knowledge of the material being measured has changed. This may be a couple weeks to a couple months.
If one were investigating the reliability of a test measuring mathematics skills, it would not be wise to wait two months. The subjects probably would have gained additional mathematics skills during the two months and thus would have scored differently the second time they completed the test. We would not want their knowledge to have changed between the first and second testing.
Equivalent-Form (Parallel or Alternate-Form) Method (measures error because of differences in test forms) Two different versions of the instrument are created. We assume both measure the same thing. The same subjects complete both instruments during the same time period. The scores on the two instruments are correlated to calculate the consistency between the two forms of the instrument.
Internal-Consistency Method (measures error because of idiosyncrasies of the test items) Several internal-consistency methods exist. They have one thing in common. The subjects complete one instrument one time. For this reason, this is the easiest form of reliability to investigate. This method measures consistency within the instrument three different ways.
– Split-Half A total score for the odd number questions is correlated with a total score for the even number questions (although it might be the first half with the second half). This is often used with dichotomous variables that are scored 0 for incorrect and 1 for correct.The Spearman-Brown prophecy formula is applied to the correlation to determine the reliability.
– Kuder-Richardson Formula 20 (K-R 20) and Kuder-Richardson Formula 21 (K-R 21) These are alternative formulas for calculating how consistent subject responses are among the questions on an instrument. Items on the instrument must be dichotomously scored (0 for incorrect and 1 for correct). All items are compared with each other, rather than half of the items with the other half of the items. It can be shown mathematically that the Kuder-Richardson reliability coefficient is actually the mean of all split-half coefficients (provided the Rulon formula is used) resulting from different splittings of a test. K-R 21 assumes that all of the questions are equally difficult. K-R 20 does not assume that. The formula for K-R 21 can be found on page 179.
– Cronbach’s Alpha When the items on an instrument are not scored right versus wrong, Cronbach’s alpha is often used to measure the internal consistency. This is often the case with attitude instruments that use the Likert scale. A computer program such as SPSS is often used to calculate Cronbach’s alpha. Although Cronbach’s alpha is usually used for scores which fall along a continuum, it will produce the same results as KR-20 with dichotomous data (0 or 1).
I have created an Excel spreadsheet that will calculate Spearman-Brown, KR-20, KR-21, and Cronbach’s alpha. The spreadsheet will handle data for a maximum 1000 subjects with a maximum of 100 responses for each.
Scoring Agreement (measures error because of the scorer) Performance and product assessments are often based on scores by individuals who are trained to evaluate the performance or product. The consistency between rating can be calculated in a variety of ways.
– Interrater Reliability Two judges can evaluate a group of student products and the correlation between their ratings can be calculated (r=.90 is a common cutoff).
– Percentage Agreement Two judges can evaluate a group of products and a percentage for the number of times they agree is calculated (80% is a common cutoff).
———
All scores contain error. The error is what lowers an instrument’s reliability. Obtained Score = True Score + Error Score
———-
There could be a number of reasons why the reliability estimate for a measure is low. Four common sources of inconsistencies of test scores are listed below:
Test Taker — perhaps the subject is having a bad day Test Itself — the questions on the instrument may be unclear Testing Conditions — there may be distractions during the testing that detract the subject Test Scoring — scores may be applying different standards when evaluating the subjects’ responses
Del Siegle, Ph.D. Neag School of Education – University of Connecticut [email protected] www.delsiegle.info
Created 9/24/2002 Edited 10/17/2013

- My Bookings
- How to Determine the Validity and Reliability of an Instrument
How to Determine the Validity and Reliability of an Instrument By: Yue Li
Validity and reliability are two important factors to consider when developing and testing any instrument (e.g., content assessment test, questionnaire) for use in a study. Attention to these considerations helps to insure the quality of your measurement and of the data collected for your study.
Understanding and Testing Validity
Validity refers to the degree to which an instrument accurately measures what it intends to measure. Three common types of validity for researchers and evaluators to consider are content, construct, and criterion validities.
- Content validity indicates the extent to which items adequately measure or represent the content of the property or trait that the researcher wishes to measure. Subject matter expert review is often a good first step in instrument development to assess content validity, in relation to the area or field you are studying.
- Construct validity indicates the extent to which a measurement method accurately represents a construct (e.g., a latent variable or phenomena that can’t be measured directly, such as a person’s attitude or belief) and produces an observation, distinct from that which is produced by a measure of another construct. Common methods to assess construct validity include, but are not limited to, factor analysis, correlation tests, and item response theory models (including Rasch model).
- Criterion-related validity indicates the extent to which the instrument’s scores correlate with an external criterion (i.e., usually another measurement from a different instrument) either at present ( concurrent validity ) or in the future ( predictive validity ). A common measurement of this type of validity is the correlation coefficient between two measures.
Often times, when developing, modifying, and interpreting the validity of a given instrument, rather than view or test each type of validity individually, researchers and evaluators test for evidence of several different forms of validity, collectively (e.g., see Samuel Messick’s work regarding validity).
Understanding and Testing Reliability
Reliability refers to the degree to which an instrument yields consistent results. Common measures of reliability include internal consistency, test-retest, and inter-rater reliabilities.
- Internal consistency reliability looks at the consistency of the score of individual items on an instrument, with the scores of a set of items, or subscale, which typically consists of several items to measure a single construct. Cronbach’s alpha is one of the most common methods for checking internal consistency reliability. Group variability, score reliability, number of items, sample sizes, and difficulty level of the instrument also can impact the Cronbach’s alpha value.
- Test-retest measures the correlation between scores from one administration of an instrument to another, usually within an interval of 2 to 3 weeks. Unlike pre-post tests, no treatment occurs between the first and second administrations of the instrument, in order to test-retest reliability. A similar type of reliability called alternate forms , involves using slightly different forms or versions of an instrument to see if different versions yield consistent results.
- Inter-rater reliability checks the degree of agreement among raters (i.e., those completing items on an instrument). Common situations where more than one rater is involved may occur when more than one person conducts classroom observations, uses an observation protocol or scores an open-ended test, using a rubric or other standard protocol. Kappa statistics, correlation coefficients, and intra-class correlation (ICC) coefficient are some of the commonly reported measures of inter-rater reliability.
Developing a valid and reliable instrument usually requires multiple iterations of piloting and testing which can be resource intensive. Therefore, when available, I suggest using already established valid and reliable instruments, such as those published in peer-reviewed journal articles. However, even when using these instruments, you should re-check validity and reliability, using the methods of your study and your own participants’ data before running additional statistical analyses. This process will confirm that the instrument performs, as intended, in your study with the population you are studying, even though they are identical to the purpose and population for which the instrument was initially developed. Below are a few additional, useful readings to further inform your understanding of validity and reliability.
Resources for Understanding and Testing Reliability
- American Educational Research Association, American Psychological Association, & National Council on Measurement in Education. (1985). Standards for educational and psychological testing . Washington, DC: Authors.
- Bond, T. G., & Fox, C. M. (2001). Applying the Rasch model: Fundamental measurement in the human sciences . Mahwah, NJ: Lawrence Erlbaum.
- Cronbach, L. (1990). Essentials of psychological testing . New York, NY: Harper & Row.
- Carmines, E., & Zeller, R. (1979). Reliability and Validity Assessment . Beverly Hills, CA: Sage Publications.
- Messick, S. (1987). Validity . ETS Research Report Series, 1987: i–208. doi: 10.1002/j.2330-8516.1987.tb00244.x
- Liu, X. (2010). Using and developing measurement instruments in science education: A Rasch modeling approach . Charlotte, NC: Information Age.
- Search for:
Recent Posts
- Avoiding Data Analysis Pitfalls
- Advice in Building and Boasting a Successful Grant Funding Track Record
- Personal History of Miami University’s Discovery and E & A Centers
- Center Director’s Message
Recent Comments
- November 2016
- September 2016
- February 2016
- November 2015
- October 2015
- Uncategorized
- Entries feed
- Comments feed
- WordPress.org

Validity & Reliability In Research
A Plain-Language Explanation (With Examples)
By: Derek Jansen (MBA) | Expert Reviewer: Kerryn Warren (PhD) | September 2023
Validity and reliability are two related but distinctly different concepts within research. Understanding what they are and how to achieve them is critically important to any research project. In this post, we’ll unpack these two concepts as simply as possible.
This post is based on our popular online course, Research Methodology Bootcamp . In the course, we unpack the basics of methodology using straightfoward language and loads of examples. If you’re new to academic research, you definitely want to use this link to get 50% off the course (limited-time offer).
Overview: Validity & Reliability
- The big picture
- Validity 101
- Reliability 101
- Key takeaways
First, The Basics…
First, let’s start with a big-picture view and then we can zoom in to the finer details.
Validity and reliability are two incredibly important concepts in research, especially within the social sciences. Both validity and reliability have to do with the measurement of variables and/or constructs – for example, job satisfaction, intelligence, productivity, etc. When undertaking research, you’ll often want to measure these types of constructs and variables and, at the simplest level, validity and reliability are about ensuring the quality and accuracy of those measurements .
As you can probably imagine, if your measurements aren’t accurate or there are quality issues at play when you’re collecting your data, your entire study will be at risk. Therefore, validity and reliability are very important concepts to understand (and to get right). So, let’s unpack each of them.
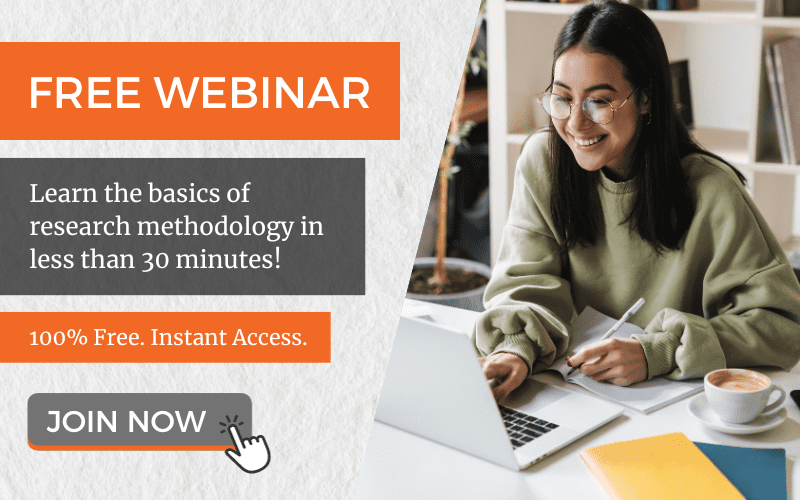
What Is Validity?
In simple terms, validity (also called “construct validity”) is all about whether a research instrument accurately measures what it’s supposed to measure .
For example, let’s say you have a set of Likert scales that are supposed to quantify someone’s level of overall job satisfaction. If this set of scales focused purely on only one dimension of job satisfaction, say pay satisfaction, this would not be a valid measurement, as it only captures one aspect of the multidimensional construct. In other words, pay satisfaction alone is only one contributing factor toward overall job satisfaction, and therefore it’s not a valid way to measure someone’s job satisfaction.
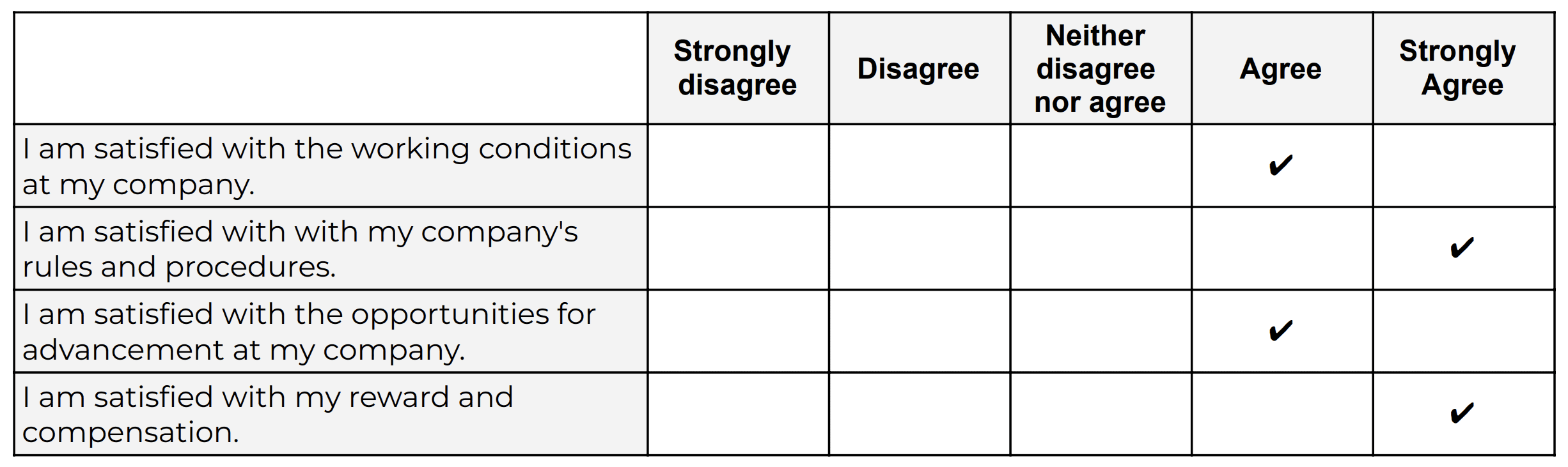
Oftentimes in quantitative studies, the way in which the researcher or survey designer interprets a question or statement can differ from how the study participants interpret it . Given that respondents don’t have the opportunity to ask clarifying questions when taking a survey, it’s easy for these sorts of misunderstandings to crop up. Naturally, if the respondents are interpreting the question in the wrong way, the data they provide will be pretty useless . Therefore, ensuring that a study’s measurement instruments are valid – in other words, that they are measuring what they intend to measure – is incredibly important.
There are various types of validity and we’re not going to go down that rabbit hole in this post, but it’s worth quickly highlighting the importance of making sure that your research instrument is tightly aligned with the theoretical construct you’re trying to measure . In other words, you need to pay careful attention to how the key theories within your study define the thing you’re trying to measure – and then make sure that your survey presents it in the same way.
For example, sticking with the “job satisfaction” construct we looked at earlier, you’d need to clearly define what you mean by job satisfaction within your study (and this definition would of course need to be underpinned by the relevant theory). You’d then need to make sure that your chosen definition is reflected in the types of questions or scales you’re using in your survey . Simply put, you need to make sure that your survey respondents are perceiving your key constructs in the same way you are. Or, even if they’re not, that your measurement instrument is capturing the necessary information that reflects your definition of the construct at hand.
If all of this talk about constructs sounds a bit fluffy, be sure to check out Research Methodology Bootcamp , which will provide you with a rock-solid foundational understanding of all things methodology-related. Remember, you can take advantage of our 60% discount offer using this link.
Need a helping hand?
What Is Reliability?
As with validity, reliability is an attribute of a measurement instrument – for example, a survey, a weight scale or even a blood pressure monitor. But while validity is concerned with whether the instrument is measuring the “thing” it’s supposed to be measuring, reliability is concerned with consistency and stability . In other words, reliability reflects the degree to which a measurement instrument produces consistent results when applied repeatedly to the same phenomenon , under the same conditions .
As you can probably imagine, a measurement instrument that achieves a high level of consistency is naturally more dependable (or reliable) than one that doesn’t – in other words, it can be trusted to provide consistent measurements . And that, of course, is what you want when undertaking empirical research. If you think about it within a more domestic context, just imagine if you found that your bathroom scale gave you a different number every time you hopped on and off of it – you wouldn’t feel too confident in its ability to measure the variable that is your body weight 🙂
It’s worth mentioning that reliability also extends to the person using the measurement instrument . For example, if two researchers use the same instrument (let’s say a measuring tape) and they get different measurements, there’s likely an issue in terms of how one (or both) of them are using the measuring tape. So, when you think about reliability, consider both the instrument and the researcher as part of the equation.
As with validity, there are various types of reliability and various tests that can be used to assess the reliability of an instrument. A popular one that you’ll likely come across for survey instruments is Cronbach’s alpha , which is a statistical measure that quantifies the degree to which items within an instrument (for example, a set of Likert scales) measure the same underlying construct . In other words, Cronbach’s alpha indicates how closely related the items are and whether they consistently capture the same concept .
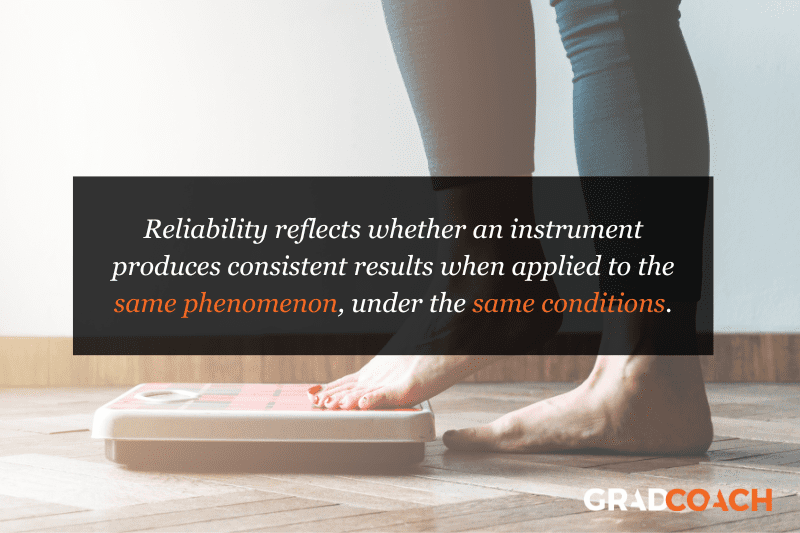
Recap: Key Takeaways
Alright, let’s quickly recap to cement your understanding of validity and reliability:
- Validity is concerned with whether an instrument (e.g., a set of Likert scales) is measuring what it’s supposed to measure
- Reliability is concerned with whether that measurement is consistent and stable when measuring the same phenomenon under the same conditions.
In short, validity and reliability are both essential to ensuring that your data collection efforts deliver high-quality, accurate data that help you answer your research questions . So, be sure to always pay careful attention to the validity and reliability of your measurement instruments when collecting and analysing data. As the adage goes, “rubbish in, rubbish out” – make sure that your data inputs are rock-solid.
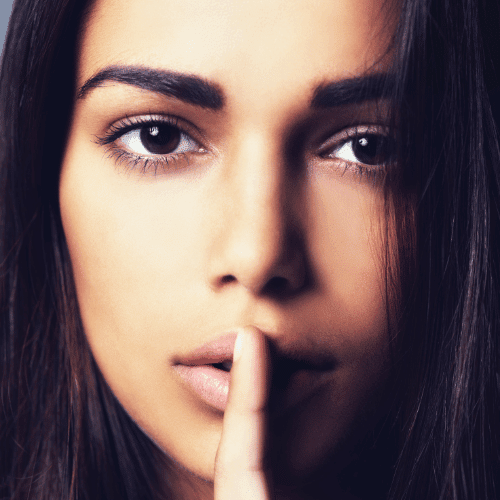
Psst… there’s more!
This post is an extract from our bestselling short course, Methodology Bootcamp . If you want to work smart, you don't want to miss this .
You Might Also Like:
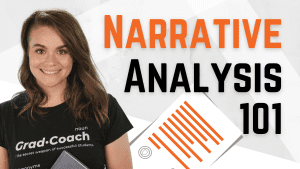
THE MATERIAL IS WONDERFUL AND BENEFICIAL TO ALL STUDENTS.
THE MATERIAL IS WONDERFUL AND BENEFICIAL TO ALL STUDENTS AND I HAVE GREATLY BENEFITED FROM THE CONTENT.
Submit a Comment Cancel reply
Your email address will not be published. Required fields are marked *
Save my name, email, and website in this browser for the next time I comment.
- Print Friendly
- How it works
Reliability and Validity – Definitions, Types & Examples
Published by Alvin Nicolas at August 16th, 2021 , Revised On October 26, 2023
A researcher must test the collected data before making any conclusion. Every research design needs to be concerned with reliability and validity to measure the quality of the research.
What is Reliability?
Reliability refers to the consistency of the measurement. Reliability shows how trustworthy is the score of the test. If the collected data shows the same results after being tested using various methods and sample groups, the information is reliable. If your method has reliability, the results will be valid.
Example: If you weigh yourself on a weighing scale throughout the day, you’ll get the same results. These are considered reliable results obtained through repeated measures.
Example: If a teacher conducts the same math test of students and repeats it next week with the same questions. If she gets the same score, then the reliability of the test is high.
What is the Validity?
Validity refers to the accuracy of the measurement. Validity shows how a specific test is suitable for a particular situation. If the results are accurate according to the researcher’s situation, explanation, and prediction, then the research is valid.
If the method of measuring is accurate, then it’ll produce accurate results. If a method is reliable, then it’s valid. In contrast, if a method is not reliable, it’s not valid.
Example: Your weighing scale shows different results each time you weigh yourself within a day even after handling it carefully, and weighing before and after meals. Your weighing machine might be malfunctioning. It means your method had low reliability. Hence you are getting inaccurate or inconsistent results that are not valid.
Example: Suppose a questionnaire is distributed among a group of people to check the quality of a skincare product and repeated the same questionnaire with many groups. If you get the same response from various participants, it means the validity of the questionnaire and product is high as it has high reliability.
Most of the time, validity is difficult to measure even though the process of measurement is reliable. It isn’t easy to interpret the real situation.
Example: If the weighing scale shows the same result, let’s say 70 kg each time, even if your actual weight is 55 kg, then it means the weighing scale is malfunctioning. However, it was showing consistent results, but it cannot be considered as reliable. It means the method has low reliability.
Internal Vs. External Validity
One of the key features of randomised designs is that they have significantly high internal and external validity.
Internal validity is the ability to draw a causal link between your treatment and the dependent variable of interest. It means the observed changes should be due to the experiment conducted, and any external factor should not influence the variables .
Example: age, level, height, and grade.
External validity is the ability to identify and generalise your study outcomes to the population at large. The relationship between the study’s situation and the situations outside the study is considered external validity.
Also, read about Inductive vs Deductive reasoning in this article.
Looking for reliable dissertation support?
We hear you.
- Whether you want a full dissertation written or need help forming a dissertation proposal, we can help you with both.
- Get different dissertation services at ResearchProspect and score amazing grades!
Threats to Interval Validity
Threats of external validity, how to assess reliability and validity.
Reliability can be measured by comparing the consistency of the procedure and its results. There are various methods to measure validity and reliability. Reliability can be measured through various statistical methods depending on the types of validity, as explained below:
Types of Reliability
Types of validity.
As we discussed above, the reliability of the measurement alone cannot determine its validity. Validity is difficult to be measured even if the method is reliable. The following type of tests is conducted for measuring validity.
Does your Research Methodology Have the Following?
- Great Research/Sources
- Perfect Language
- Accurate Sources
If not, we can help. Our panel of experts makes sure to keep the 3 pillars of Research Methodology strong.
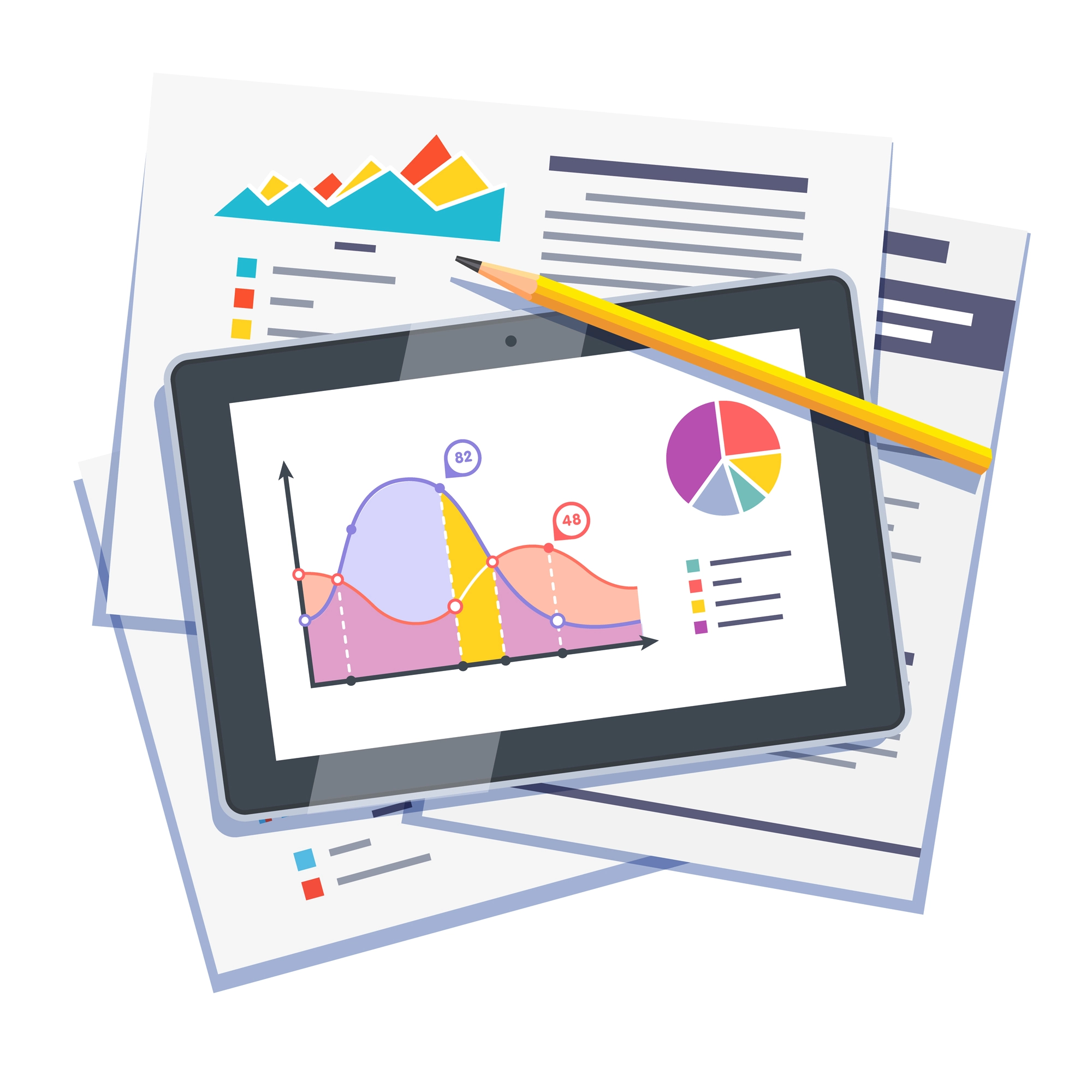
How to Increase Reliability?
- Use an appropriate questionnaire to measure the competency level.
- Ensure a consistent environment for participants
- Make the participants familiar with the criteria of assessment.
- Train the participants appropriately.
- Analyse the research items regularly to avoid poor performance.
How to Increase Validity?
Ensuring Validity is also not an easy job. A proper functioning method to ensure validity is given below:
- The reactivity should be minimised at the first concern.
- The Hawthorne effect should be reduced.
- The respondents should be motivated.
- The intervals between the pre-test and post-test should not be lengthy.
- Dropout rates should be avoided.
- The inter-rater reliability should be ensured.
- Control and experimental groups should be matched with each other.
How to Implement Reliability and Validity in your Thesis?
According to the experts, it is helpful if to implement the concept of reliability and Validity. Especially, in the thesis and the dissertation, these concepts are adopted much. The method for implementation given below:
Frequently Asked Questions
What is reliability and validity in research.
Reliability in research refers to the consistency and stability of measurements or findings. Validity relates to the accuracy and truthfulness of results, measuring what the study intends to. Both are crucial for trustworthy and credible research outcomes.
What is validity?
Validity in research refers to the extent to which a study accurately measures what it intends to measure. It ensures that the results are truly representative of the phenomena under investigation. Without validity, research findings may be irrelevant, misleading, or incorrect, limiting their applicability and credibility.
What is reliability?
Reliability in research refers to the consistency and stability of measurements over time. If a study is reliable, repeating the experiment or test under the same conditions should produce similar results. Without reliability, findings become unpredictable and lack dependability, potentially undermining the study’s credibility and generalisability.
What is reliability in psychology?
In psychology, reliability refers to the consistency of a measurement tool or test. A reliable psychological assessment produces stable and consistent results across different times, situations, or raters. It ensures that an instrument’s scores are not due to random error, making the findings dependable and reproducible in similar conditions.
What is test retest reliability?
Test-retest reliability assesses the consistency of measurements taken by a test over time. It involves administering the same test to the same participants at two different points in time and comparing the results. A high correlation between the scores indicates that the test produces stable and consistent results over time.
How to improve reliability of an experiment?
- Standardise procedures and instructions.
- Use consistent and precise measurement tools.
- Train observers or raters to reduce subjective judgments.
- Increase sample size to reduce random errors.
- Conduct pilot studies to refine methods.
- Repeat measurements or use multiple methods.
- Address potential sources of variability.
What is the difference between reliability and validity?
Reliability refers to the consistency and repeatability of measurements, ensuring results are stable over time. Validity indicates how well an instrument measures what it’s intended to measure, ensuring accuracy and relevance. While a test can be reliable without being valid, a valid test must inherently be reliable. Both are essential for credible research.
Are interviews reliable and valid?
Interviews can be both reliable and valid, but they are susceptible to biases. The reliability and validity depend on the design, structure, and execution of the interview. Structured interviews with standardised questions improve reliability. Validity is enhanced when questions accurately capture the intended construct and when interviewer biases are minimised.
Are IQ tests valid and reliable?
IQ tests are generally considered reliable, producing consistent scores over time. Their validity, however, is a subject of debate. While they effectively measure certain cognitive skills, whether they capture the entirety of “intelligence” or predict success in all life areas is contested. Cultural bias and over-reliance on tests are also concerns.
Are questionnaires reliable and valid?
Questionnaires can be both reliable and valid if well-designed. Reliability is achieved when they produce consistent results over time or across similar populations. Validity is ensured when questions accurately measure the intended construct. However, factors like poorly phrased questions, respondent bias, and lack of standardisation can compromise their reliability and validity.
You May Also Like
Experimental research refers to the experiments conducted in the laboratory or under observation in controlled conditions. Here is all you need to know about experimental research.
Disadvantages of primary research – It can be expensive, time-consuming and take a long time to complete if it involves face-to-face contact with customers.
You can transcribe an interview by converting a conversation into a written format including question-answer recording sessions between two or more people.
USEFUL LINKS
LEARNING RESOURCES
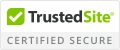
COMPANY DETAILS
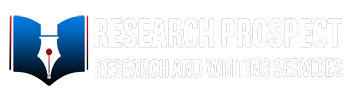
- How It Works
Reliability and validity: Importance in Medical Research
Affiliations.
- 1 Al-Nafees Medical College,Isra University, Islamabad, Pakistan.
- 2 Fauji Foundation Hospital, Foundation University Medical College, Islamabad, Pakistan.
- PMID: 34974579
- DOI: 10.47391/JPMA.06-861
Reliability and validity are among the most important and fundamental domains in the assessment of any measuring methodology for data-collection in a good research. Validity is about what an instrument measures and how well it does so, whereas reliability concerns the truthfulness in the data obtained and the degree to which any measuring tool controls random error. The current narrative review was planned to discuss the importance of reliability and validity of data-collection or measurement techniques used in research. It describes and explores comprehensively the reliability and validity of research instruments and also discusses different forms of reliability and validity with concise examples. An attempt has been taken to give a brief literature review regarding the significance of reliability and validity in medical sciences.
Keywords: Validity, Reliability, Medical research, Methodology, Assessment, Research tools..
Publication types
- Biomedical Research*
- Reproducibility of Results
- Privacy Policy
Buy Me a Coffee

Home » Reliability – Types, Examples and Guide
Reliability – Types, Examples and Guide
Table of Contents

Reliability
Definition:
Reliability refers to the consistency, dependability, and trustworthiness of a system, process, or measurement to perform its intended function or produce consistent results over time. It is a desirable characteristic in various domains, including engineering, manufacturing, software development, and data analysis.
Reliability In Engineering
In engineering and manufacturing, reliability refers to the ability of a product, equipment, or system to function without failure or breakdown under normal operating conditions for a specified period. A reliable system consistently performs its intended functions, meets performance requirements, and withstands various environmental factors, stress, or wear and tear.
Reliability In Software Development
In software development, reliability relates to the stability and consistency of software applications or systems. A reliable software program operates consistently without crashing, produces accurate results, and handles errors or exceptions gracefully. Reliability is often measured by metrics such as mean time between failures (MTBF) and mean time to repair (MTTR).
Reliability In Data Analysis and Statistics
In data analysis and statistics, reliability refers to the consistency and repeatability of measurements or assessments. For example, if a measurement instrument consistently produces similar results when measuring the same quantity or if multiple raters consistently agree on the same assessment, it is considered reliable. Reliability is often assessed using statistical measures such as test-retest reliability, inter-rater reliability, or internal consistency.
Research Reliability
Research reliability refers to the consistency, stability, and repeatability of research findings . It indicates the extent to which a research study produces consistent and dependable results when conducted under similar conditions. In other words, research reliability assesses whether the same results would be obtained if the study were replicated with the same methodology, sample, and context.
What Affects Reliability in Research
Several factors can affect the reliability of research measurements and assessments. Here are some common factors that can impact reliability:
Measurement Error
Measurement error refers to the variability or inconsistency in the measurements that is not due to the construct being measured. It can arise from various sources, such as the limitations of the measurement instrument, environmental factors, or the characteristics of the participants. Measurement error reduces the reliability of the measure by introducing random variability into the data.
Rater/Observer Bias
In studies involving subjective assessments or ratings, the biases or subjective judgments of the raters or observers can affect reliability. If different raters interpret and evaluate the same phenomenon differently, it can lead to inconsistencies in the ratings, resulting in lower inter-rater reliability.
Participant Factors
Characteristics or factors related to the participants themselves can influence reliability. For example, factors such as fatigue, motivation, attention, or mood can introduce variability in responses, affecting the reliability of self-report measures or performance assessments.
Instrumentation
The quality and characteristics of the measurement instrument can impact reliability. If the instrument lacks clarity, has ambiguous items or instructions, or is prone to measurement errors, it can decrease the reliability of the measure. Poorly designed or unreliable instruments can introduce measurement error and decrease the consistency of the measurements.
Sample Size
Sample size can affect reliability, especially in studies where the reliability coefficient is based on correlations or variability within the sample. A larger sample size generally provides more stable estimates of reliability, while smaller samples can yield less precise estimates.
Time Interval
The time interval between test administrations can impact test-retest reliability. If the time interval is too short, participants may recall their previous responses and answer in a similar manner, artificially inflating the reliability coefficient. On the other hand, if the time interval is too long, true changes in the construct being measured may occur, leading to lower test-retest reliability.
Content Sampling
The specific items or questions included in a measure can affect reliability. If the measure does not adequately sample the full range of the construct being measured or if the items are too similar or redundant, it can result in lower internal consistency reliability.
Scoring and Data Handling
Errors in scoring, data entry, or data handling can introduce variability and impact reliability. Inaccurate or inconsistent scoring procedures, data entry mistakes, or mishandling of missing data can affect the reliability of the measurements.
Context and Environment
The context and environment in which measurements are obtained can influence reliability. Factors such as noise, distractions, lighting conditions, or the presence of others can introduce variability and affect the consistency of the measurements.
Types of Reliability
There are several types of reliability that are commonly discussed in research and measurement contexts. Here are some of the main types of reliability:
Test-Retest Reliability
This type of reliability assesses the consistency of a measure over time. It involves administering the same test or measure to the same group of individuals on two separate occasions and then comparing the results. If the scores are similar or highly correlated across the two testing points, it indicates good test-retest reliability.
Inter-Rater Reliability
Inter-rater reliability examines the degree of agreement or consistency between different raters or observers who are assessing the same phenomenon. It is commonly used in subjective evaluations or assessments where judgments are made by multiple individuals. High inter-rater reliability suggests that different observers are likely to reach the same conclusions or make consistent assessments.
Internal Consistency Reliability
Internal consistency reliability assesses the extent to which the items or questions within a measure are consistent with each other. It is commonly measured using techniques such as Cronbach’s alpha. High internal consistency reliability indicates that the items within a measure are measuring the same construct or concept consistently.
Parallel Forms Reliability
Parallel forms reliability assesses the consistency of different versions or forms of a test that are intended to measure the same construct. Two equivalent versions of a test are administered to the same group of individuals, and the scores are compared to determine the level of agreement between the forms.
Split-Half Reliability
Split-half reliability involves splitting a measure into two halves and examining the consistency between the two halves. It can be done by dividing the items into odd-even pairs or by randomly splitting the items. The scores from the two halves are then compared to assess the degree of consistency.
Alternate Forms Reliability
Alternate forms reliability is similar to parallel forms reliability, but it involves administering two different versions of a test to the same group of individuals. The two forms should be equivalent and measure the same construct. The scores from the two forms are then compared to assess the level of agreement.
Applications of Reliability
Reliability has several important applications across various fields and disciplines. Here are some common applications of reliability:
Psychological and Educational Testing
Reliability is crucial in psychological and educational testing to ensure that the scores obtained from assessments are consistent and dependable. It helps to determine the accuracy and stability of measures such as intelligence tests, personality assessments, academic exams, and aptitude tests.
Market Research
In market research, reliability is important for ensuring consistent and dependable data collection. Surveys, questionnaires, and other data collection instruments need to have high reliability to obtain accurate and consistent responses from participants. Reliability analysis helps researchers identify and address any issues that may affect the consistency of the data.
Health and Medical Research
Reliability is essential in health and medical research to ensure that measurements and assessments used in studies are consistent and trustworthy. This includes the reliability of diagnostic tests, patient-reported outcome measures, observational measures, and psychometric scales. High reliability is crucial for making valid inferences and drawing reliable conclusions from research findings.
Quality Control and Manufacturing
Reliability analysis is widely used in industries such as manufacturing and quality control to assess the reliability of products and processes. It helps to identify and address sources of variation and inconsistency, ensuring that products meet the required standards and specifications consistently.
Social Science Research
Reliability plays a vital role in social science research, including fields such as sociology, anthropology, and political science. It is used to assess the consistency of measurement tools, such as surveys or observational protocols, to ensure that the data collected is reliable and can be trusted for analysis and interpretation.
Performance Evaluation
Reliability is important in performance evaluation systems used in organizations and workplaces. Whether it’s assessing employee performance, evaluating the reliability of scoring rubrics, or measuring the consistency of ratings by supervisors, reliability analysis helps ensure fairness and consistency in the evaluation process.
Psychometrics and Scale Development
Reliability analysis is a fundamental step in psychometrics, which involves developing and validating measurement scales. Researchers assess the reliability of items and subscales to ensure that the scale measures the intended construct consistently and accurately.
Examples of Reliability
Here are some examples of reliability in different contexts:
Test-Retest Reliability Example: A researcher administers a personality questionnaire to a group of participants and then administers the same questionnaire to the same participants after a certain period, such as two weeks. The scores obtained from the two administrations are highly correlated, indicating good test-retest reliability.
Inter-Rater Reliability Example: Multiple teachers assess the essays of a group of students using a standardized grading rubric. The ratings assigned by the teachers show a high level of agreement or correlation, indicating good inter-rater reliability.
Internal Consistency Reliability Example: A researcher develops a questionnaire to measure job satisfaction. The researcher administers the questionnaire to a group of employees and calculates Cronbach’s alpha to assess internal consistency. The calculated value of Cronbach’s alpha is high (e.g., above 0.8), indicating good internal consistency reliability.
Parallel Forms Reliability Example: Two versions of a mathematics exam are created, which are designed to measure the same mathematical skills. Both versions of the exam are administered to the same group of students, and the scores from the two versions are highly correlated, indicating good parallel forms reliability.
Split-Half Reliability Example: A researcher develops a survey to measure self-esteem. The survey consists of 20 items, and the researcher randomly divides the items into two halves. The scores obtained from each half of the survey show a high level of agreement or correlation, indicating good split-half reliability.
Alternate Forms Reliability Example: A researcher develops two versions of a language proficiency test, which are designed to measure the same language skills. Both versions of the test are administered to the same group of participants, and the scores from the two versions are highly correlated, indicating good alternate forms reliability.
Where to Write About Reliability in A Thesis
When writing about reliability in a thesis, there are several sections where you can address this topic. Here are some common sections in a thesis where you can discuss reliability:
Introduction :
In the introduction section of your thesis, you can provide an overview of the study and briefly introduce the concept of reliability. Explain why reliability is important in your research field and how it relates to your study objectives.
Theoretical Framework:
If your thesis includes a theoretical framework or a literature review, this is a suitable section to discuss reliability. Provide an overview of the relevant theories, models, or concepts related to reliability in your field. Discuss how other researchers have measured and assessed reliability in similar studies.
Methodology:
The methodology section is crucial for addressing reliability. Describe the research design, data collection methods, and measurement instruments used in your study. Explain how you ensured the reliability of your measurements or data collection procedures. This may involve discussing pilot studies, inter-rater reliability, test-retest reliability, or other techniques used to assess and improve reliability.
Data Analysis:
In the data analysis section, you can discuss the statistical techniques employed to assess the reliability of your data. This might include measures such as Cronbach’s alpha, Cohen’s kappa, or intraclass correlation coefficients (ICC), depending on the nature of your data and research design. Present the results of reliability analyses and interpret their implications for your study.
Discussion:
In the discussion section, analyze and interpret the reliability results in relation to your research findings and objectives. Discuss any limitations or challenges encountered in establishing or maintaining reliability in your study. Consider the implications of reliability for the validity and generalizability of your results.
Conclusion:
In the conclusion section, summarize the main points discussed in your thesis regarding reliability. Emphasize the importance of reliability in research and highlight any recommendations or suggestions for future studies to enhance reliability.
Importance of Reliability
Reliability is of utmost importance in research, measurement, and various practical applications. Here are some key reasons why reliability is important:
- Consistency : Reliability ensures consistency in measurements and assessments. Consistent results indicate that the measure or instrument is stable and produces similar outcomes when applied repeatedly. This consistency allows researchers and practitioners to have confidence in the reliability of the data collected and the conclusions drawn from it.
- Accuracy : Reliability is closely linked to accuracy. A reliable measure produces results that are close to the true value or state of the phenomenon being measured. When a measure is unreliable, it introduces error and uncertainty into the data, which can lead to incorrect interpretations and flawed decision-making.
- Trustworthiness : Reliability enhances the trustworthiness of measurements and assessments. When a measure is reliable, it indicates that it is dependable and can be trusted to provide consistent and accurate results. This is particularly important in fields where decisions and actions are based on the data collected, such as education, healthcare, and market research.
- Comparability : Reliability enables meaningful comparisons between different groups, individuals, or time points. When measures are reliable, differences or changes observed can be attributed to true differences in the underlying construct, rather than measurement error. This allows for valid comparisons and evaluations, both within a study and across different studies.
- Validity : Reliability is a prerequisite for validity. Validity refers to the extent to which a measure or assessment accurately captures the construct it is intended to measure. If a measure is unreliable, it cannot be valid, as it does not consistently reflect the construct of interest. Establishing reliability is an important step in establishing the validity of a measure.
- Decision-making : Reliability is crucial for making informed decisions based on data. Whether it’s evaluating employee performance, diagnosing medical conditions, or conducting research studies, reliable measurements and assessments provide a solid foundation for decision-making processes. They help to reduce uncertainty and increase confidence in the conclusions drawn from the data.
- Quality Assurance : Reliability is essential for maintaining quality assurance in various fields. It allows organizations to assess and monitor the consistency and dependability of their processes, products, and services. By ensuring reliability, organizations can identify areas of improvement, address sources of variation, and deliver consistent and high-quality outcomes.
Limitations of Reliability
Here are some limitations of reliability:
- Limited to consistency: Reliability primarily focuses on the consistency of measurements and findings. However, it does not guarantee the accuracy or validity of the measurements. A measurement can be consistent but still systematically biased or flawed, leading to inaccurate results. Reliability alone cannot address validity concerns.
- Context-dependent: Reliability can be influenced by the specific context, conditions, or population under study. A measurement or instrument that demonstrates high reliability in one context may not necessarily exhibit the same level of reliability in a different context. Researchers need to consider the specific characteristics and limitations of their study context when interpreting reliability.
- Inadequate for complex constructs: Reliability is often based on the assumption of unidimensionality, which means that a measurement instrument is designed to capture a single construct. However, many real-world phenomena are complex and multidimensional, making it challenging to assess reliability accurately. Reliability measures may not adequately capture the full complexity of such constructs.
- Susceptible to systematic errors: Reliability focuses on minimizing random errors, but it may not detect or address systematic errors or biases in measurements. Systematic errors can arise from flaws in the measurement instrument, data collection procedures, or sample selection. Reliability assessments may not fully capture or address these systematic errors, leading to biased or inaccurate results.
- Relies on assumptions: Reliability assessments often rely on certain assumptions, such as the assumption of measurement invariance or the assumption of stable conditions over time. These assumptions may not always hold true in real-world research settings, particularly when studying dynamic or evolving phenomena. Failure to meet these assumptions can compromise the reliability of the research.
- Limited to quantitative measures: Reliability is typically applied to quantitative measures and instruments, which can be problematic when studying qualitative or subjective phenomena. Reliability measures may not fully capture the richness and complexity of qualitative data, limiting their applicability in certain research domains.
Also see Reliability Vs Validity
About the author
Muhammad Hassan
Researcher, Academic Writer, Web developer
You may also like

Validity – Types, Examples and Guide

Alternate Forms Reliability – Methods, Examples...

Construct Validity – Types, Threats and Examples

Internal Validity – Threats, Examples and Guide

Reliability Vs Validity

Internal Consistency Reliability – Methods...
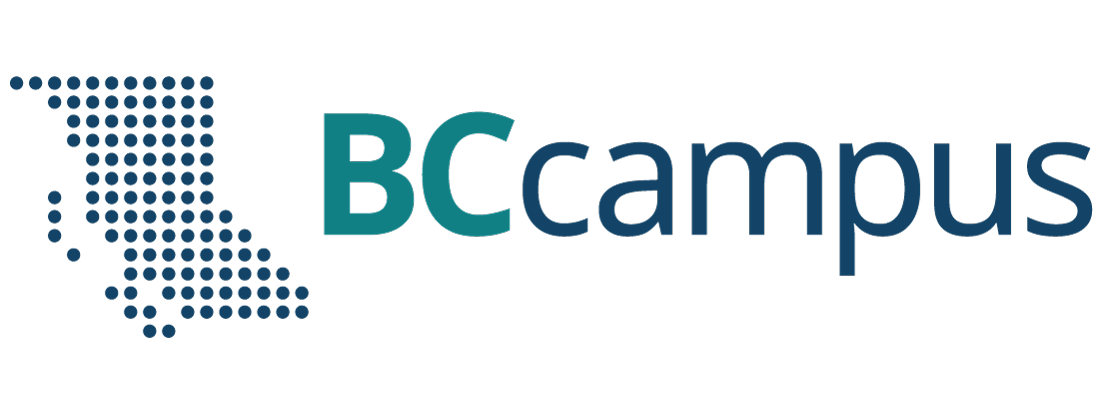
Want to create or adapt books like this? Learn more about how Pressbooks supports open publishing practices.
Chapter 5: Psychological Measurement
Reliability and Validity of Measurement
Learning Objectives
- Define reliability, including the different types and how they are assessed.
- Define validity, including the different types and how they are assessed.
- Describe the kinds of evidence that would be relevant to assessing the reliability and validity of a particular measure.
Again, measurement involves assigning scores to individuals so that they represent some characteristic of the individuals. But how do researchers know that the scores actually represent the characteristic, especially when it is a construct like intelligence, self-esteem, depression, or working memory capacity? The answer is that they conduct research using the measure to confirm that the scores make sense based on their understanding of the construct being measured. This is an extremely important point. Psychologists do not simply assume that their measures work. Instead, they collect data to demonstrate that they work. If their research does not demonstrate that a measure works, they stop using it.
As an informal example, imagine that you have been dieting for a month. Your clothes seem to be fitting more loosely, and several friends have asked if you have lost weight. If at this point your bathroom scale indicated that you had lost 10 pounds, this would make sense and you would continue to use the scale. But if it indicated that you had gained 10 pounds, you would rightly conclude that it was broken and either fix it or get rid of it. In evaluating a measurement method, psychologists consider two general dimensions: reliability and validity.
Reliability
Reliability refers to the consistency of a measure. Psychologists consider three types of consistency: over time (test-retest reliability), across items (internal consistency), and across different researchers (inter-rater reliability).
Test-Retest Reliability
When researchers measure a construct that they assume to be consistent across time, then the scores they obtain should also be consistent across time. Test-retest reliability is the extent to which this is actually the case. For example, intelligence is generally thought to be consistent across time. A person who is highly intelligent today will be highly intelligent next week. This means that any good measure of intelligence should produce roughly the same scores for this individual next week as it does today. Clearly, a measure that produces highly inconsistent scores over time cannot be a very good measure of a construct that is supposed to be consistent.
Assessing test-retest reliability requires using the measure on a group of people at one time, using it again on the same group of people at a later time, and then looking at test-retest correlation between the two sets of scores. This is typically done by graphing the data in a scatterplot and computing Pearson’s r . Figure 5.2 shows the correlation between two sets of scores of several university students on the Rosenberg Self-Esteem Scale, administered two times, a week apart. Pearson’s r for these data is +.95. In general, a test-retest correlation of +.80 or greater is considered to indicate good reliability.
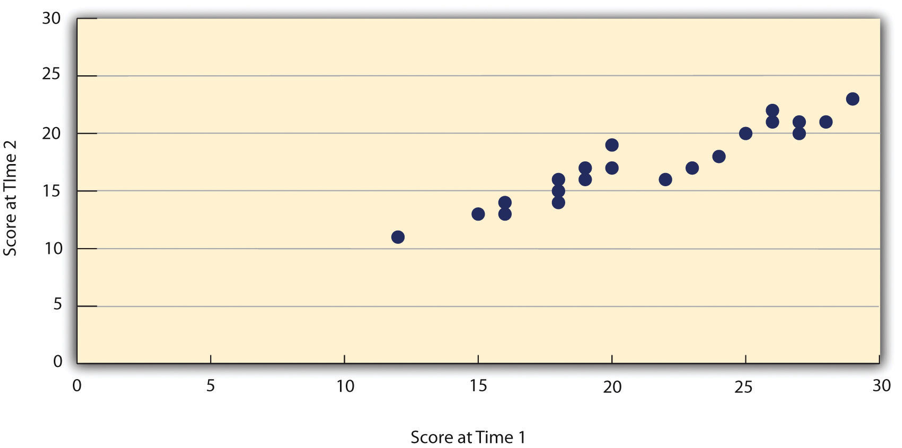
Again, high test-retest correlations make sense when the construct being measured is assumed to be consistent over time, which is the case for intelligence, self-esteem, and the Big Five personality dimensions. But other constructs are not assumed to be stable over time. The very nature of mood, for example, is that it changes. So a measure of mood that produced a low test-retest correlation over a period of a month would not be a cause for concern.
Internal Consistency
A second kind of reliability is internal consistency , which is the consistency of people’s responses across the items on a multiple-item measure. In general, all the items on such measures are supposed to reflect the same underlying construct, so people’s scores on those items should be correlated with each other. On the Rosenberg Self-Esteem Scale, people who agree that they are a person of worth should tend to agree that that they have a number of good qualities. If people’s responses to the different items are not correlated with each other, then it would no longer make sense to claim that they are all measuring the same underlying construct. This is as true for behavioural and physiological measures as for self-report measures. For example, people might make a series of bets in a simulated game of roulette as a measure of their level of risk seeking. This measure would be internally consistent to the extent that individual participants’ bets were consistently high or low across trials.
Like test-retest reliability, internal consistency can only be assessed by collecting and analyzing data. One approach is to look at a split-half correlation . This involves splitting the items into two sets, such as the first and second halves of the items or the even- and odd-numbered items. Then a score is computed for each set of items, and the relationship between the two sets of scores is examined. For example, Figure 5.3 shows the split-half correlation between several university students’ scores on the even-numbered items and their scores on the odd-numbered items of the Rosenberg Self-Esteem Scale. Pearson’s r for these data is +.88. A split-half correlation of +.80 or greater is generally considered good internal consistency.
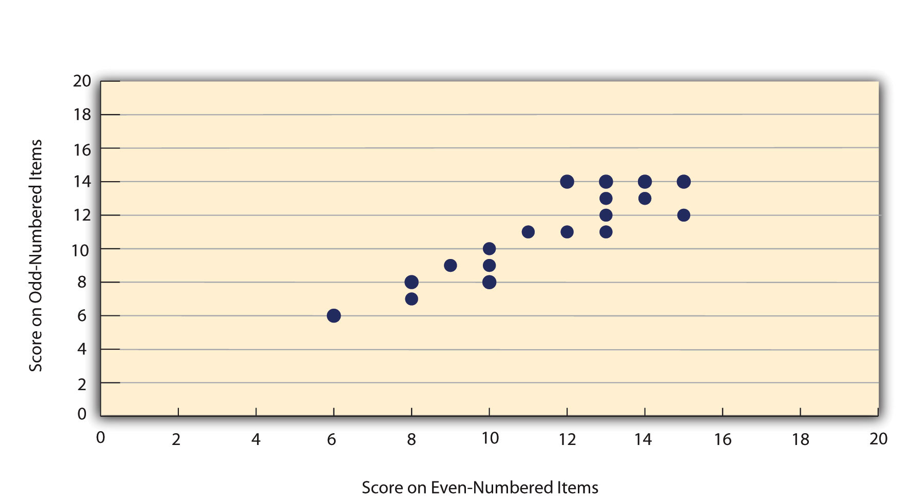
Perhaps the most common measure of internal consistency used by researchers in psychology is a statistic called Cronbach’s α (the Greek letter alpha). Conceptually, α is the mean of all possible split-half correlations for a set of items. For example, there are 252 ways to split a set of 10 items into two sets of five. Cronbach’s α would be the mean of the 252 split-half correlations. Note that this is not how α is actually computed, but it is a correct way of interpreting the meaning of this statistic. Again, a value of +.80 or greater is generally taken to indicate good internal consistency.
Interrater Reliability
Many behavioural measures involve significant judgment on the part of an observer or a rater. Inter-rater reliability is the extent to which different observers are consistent in their judgments. For example, if you were interested in measuring university students’ social skills, you could make video recordings of them as they interacted with another student whom they are meeting for the first time. Then you could have two or more observers watch the videos and rate each student’s level of social skills. To the extent that each participant does in fact have some level of social skills that can be detected by an attentive observer, different observers’ ratings should be highly correlated with each other. Inter-rater reliability would also have been measured in Bandura’s Bobo doll study. In this case, the observers’ ratings of how many acts of aggression a particular child committed while playing with the Bobo doll should have been highly positively correlated. Interrater reliability is often assessed using Cronbach’s α when the judgments are quantitative or an analogous statistic called Cohen’s κ (the Greek letter kappa) when they are categorical.
Validity is the extent to which the scores from a measure represent the variable they are intended to. But how do researchers make this judgment? We have already considered one factor that they take into account—reliability. When a measure has good test-retest reliability and internal consistency, researchers should be more confident that the scores represent what they are supposed to. There has to be more to it, however, because a measure can be extremely reliable but have no validity whatsoever. As an absurd example, imagine someone who believes that people’s index finger length reflects their self-esteem and therefore tries to measure self-esteem by holding a ruler up to people’s index fingers. Although this measure would have extremely good test-retest reliability, it would have absolutely no validity. The fact that one person’s index finger is a centimetre longer than another’s would indicate nothing about which one had higher self-esteem.
Discussions of validity usually divide it into several distinct “types.” But a good way to interpret these types is that they are other kinds of evidence—in addition to reliability—that should be taken into account when judging the validity of a measure. Here we consider three basic kinds: face validity, content validity, and criterion validity.
Face Validity
Face validity is the extent to which a measurement method appears “on its face” to measure the construct of interest. Most people would expect a self-esteem questionnaire to include items about whether they see themselves as a person of worth and whether they think they have good qualities. So a questionnaire that included these kinds of items would have good face validity. The finger-length method of measuring self-esteem, on the other hand, seems to have nothing to do with self-esteem and therefore has poor face validity. Although face validity can be assessed quantitatively—for example, by having a large sample of people rate a measure in terms of whether it appears to measure what it is intended to—it is usually assessed informally.
Face validity is at best a very weak kind of evidence that a measurement method is measuring what it is supposed to. One reason is that it is based on people’s intuitions about human behaviour, which are frequently wrong. It is also the case that many established measures in psychology work quite well despite lacking face validity. The Minnesota Multiphasic Personality Inventory-2 (MMPI-2) measures many personality characteristics and disorders by having people decide whether each of over 567 different statements applies to them—where many of the statements do not have any obvious relationship to the construct that they measure. For example, the items “I enjoy detective or mystery stories” and “The sight of blood doesn’t frighten me or make me sick” both measure the suppression of aggression. In this case, it is not the participants’ literal answers to these questions that are of interest, but rather whether the pattern of the participants’ responses to a series of questions matches those of individuals who tend to suppress their aggression.
Content Validity
Content validity is the extent to which a measure “covers” the construct of interest. For example, if a researcher conceptually defines test anxiety as involving both sympathetic nervous system activation (leading to nervous feelings) and negative thoughts, then his measure of test anxiety should include items about both nervous feelings and negative thoughts. Or consider that attitudes are usually defined as involving thoughts, feelings, and actions toward something. By this conceptual definition, a person has a positive attitude toward exercise to the extent that he or she thinks positive thoughts about exercising, feels good about exercising, and actually exercises. So to have good content validity, a measure of people’s attitudes toward exercise would have to reflect all three of these aspects. Like face validity, content validity is not usually assessed quantitatively. Instead, it is assessed by carefully checking the measurement method against the conceptual definition of the construct.
Criterion Validity
Criterion validity is the extent to which people’s scores on a measure are correlated with other variables (known as criteria ) that one would expect them to be correlated with. For example, people’s scores on a new measure of test anxiety should be negatively correlated with their performance on an important school exam. If it were found that people’s scores were in fact negatively correlated with their exam performance, then this would be a piece of evidence that these scores really represent people’s test anxiety. But if it were found that people scored equally well on the exam regardless of their test anxiety scores, then this would cast doubt on the validity of the measure.
A criterion can be any variable that one has reason to think should be correlated with the construct being measured, and there will usually be many of them. For example, one would expect test anxiety scores to be negatively correlated with exam performance and course grades and positively correlated with general anxiety and with blood pressure during an exam. Or imagine that a researcher develops a new measure of physical risk taking. People’s scores on this measure should be correlated with their participation in “extreme” activities such as snowboarding and rock climbing, the number of speeding tickets they have received, and even the number of broken bones they have had over the years. When the criterion is measured at the same time as the construct, criterion validity is referred to as concurrent validity ; however, when the criterion is measured at some point in the future (after the construct has been measured), it is referred to as predictive validity (because scores on the measure have “predicted” a future outcome).
Criteria can also include other measures of the same construct. For example, one would expect new measures of test anxiety or physical risk taking to be positively correlated with existing measures of the same constructs. This is known as convergent validity .
Assessing convergent validity requires collecting data using the measure. Researchers John Cacioppo and Richard Petty did this when they created their self-report Need for Cognition Scale to measure how much people value and engage in thinking (Cacioppo & Petty, 1982) [1] . In a series of studies, they showed that people’s scores were positively correlated with their scores on a standardized academic achievement test, and that their scores were negatively correlated with their scores on a measure of dogmatism (which represents a tendency toward obedience). In the years since it was created, the Need for Cognition Scale has been used in literally hundreds of studies and has been shown to be correlated with a wide variety of other variables, including the effectiveness of an advertisement, interest in politics, and juror decisions (Petty, Briñol, Loersch, & McCaslin, 2009) [2] .
Discriminant Validity
Discriminant validity , on the other hand, is the extent to which scores on a measure are not correlated with measures of variables that are conceptually distinct. For example, self-esteem is a general attitude toward the self that is fairly stable over time. It is not the same as mood, which is how good or bad one happens to be feeling right now. So people’s scores on a new measure of self-esteem should not be very highly correlated with their moods. If the new measure of self-esteem were highly correlated with a measure of mood, it could be argued that the new measure is not really measuring self-esteem; it is measuring mood instead.
When they created the Need for Cognition Scale, Cacioppo and Petty also provided evidence of discriminant validity by showing that people’s scores were not correlated with certain other variables. For example, they found only a weak correlation between people’s need for cognition and a measure of their cognitive style—the extent to which they tend to think analytically by breaking ideas into smaller parts or holistically in terms of “the big picture.” They also found no correlation between people’s need for cognition and measures of their test anxiety and their tendency to respond in socially desirable ways. All these low correlations provide evidence that the measure is reflecting a conceptually distinct construct.
Key Takeaways
- Psychological researchers do not simply assume that their measures work. Instead, they conduct research to show that they work. If they cannot show that they work, they stop using them.
- There are two distinct criteria by which researchers evaluate their measures: reliability and validity. Reliability is consistency across time (test-retest reliability), across items (internal consistency), and across researchers (interrater reliability). Validity is the extent to which the scores actually represent the variable they are intended to.
- Validity is a judgment based on various types of evidence. The relevant evidence includes the measure’s reliability, whether it covers the construct of interest, and whether the scores it produces are correlated with other variables they are expected to be correlated with and not correlated with variables that are conceptually distinct.
- The reliability and validity of a measure is not established by any single study but by the pattern of results across multiple studies. The assessment of reliability and validity is an ongoing process.
- Practice: Ask several friends to complete the Rosenberg Self-Esteem Scale. Then assess its internal consistency by making a scatterplot to show the split-half correlation (even- vs. odd-numbered items). Compute Pearson’s r too if you know how.
- Discussion: Think back to the last college exam you took and think of the exam as a psychological measure. What construct do you think it was intended to measure? Comment on its face and content validity. What data could you collect to assess its reliability and criterion validity?
- Cacioppo, J. T., & Petty, R. E. (1982). The need for cognition. Journal of Personality and Social Psychology, 42 , 116–131. ↵
- Petty, R. E, Briñol, P., Loersch, C., & McCaslin, M. J. (2009). The need for cognition. In M. R. Leary & R. H. Hoyle (Eds.), Handbook of individual differences in social behaviour (pp. 318–329). New York, NY: Guilford Press. ↵
The consistency of a measure.
The consistency of a measure over time.
The consistency of a measure on the same group of people at different times.
Consistency of people’s responses across the items on a multiple-item measure.
Method of assessing internal consistency through splitting the items into two sets and examining the relationship between them.
A statistic in which α is the mean of all possible split-half correlations for a set of items.
The extent to which different observers are consistent in their judgments.
The extent to which the scores from a measure represent the variable they are intended to.
The extent to which a measurement method appears to measure the construct of interest.
The extent to which a measure “covers” the construct of interest.
The extent to which people’s scores on a measure are correlated with other variables that one would expect them to be correlated with.
In reference to criterion validity, variables that one would expect to be correlated with the measure.
When the criterion is measured at the same time as the construct.
when the criterion is measured at some point in the future (after the construct has been measured).
When new measures positively correlate with existing measures of the same constructs.
The extent to which scores on a measure are not correlated with measures of variables that are conceptually distinct.
Research Methods in Psychology - 2nd Canadian Edition Copyright © 2015 by Paul C. Price, Rajiv Jhangiani, & I-Chant A. Chiang is licensed under a Creative Commons Attribution-NonCommercial-ShareAlike 4.0 International License , except where otherwise noted.
Share This Book
Log in using your username and password
- Search More Search for this keyword Advanced search
- Latest content
- Current issue
- Write for Us
- BMJ Journals More You are viewing from: Google Indexer
You are here
- Volume 18, Issue 3
- Validity and reliability in quantitative studies
- Article Text
- Article info
- Citation Tools
- Rapid Responses
- Article metrics

- Roberta Heale 1 ,
- Alison Twycross 2
- 1 School of Nursing, Laurentian University , Sudbury, Ontario , Canada
- 2 Faculty of Health and Social Care , London South Bank University , London , UK
- Correspondence to : Dr Roberta Heale, School of Nursing, Laurentian University, Ramsey Lake Road, Sudbury, Ontario, Canada P3E2C6; rheale{at}laurentian.ca
https://doi.org/10.1136/eb-2015-102129
Statistics from Altmetric.com
Request permissions.
If you wish to reuse any or all of this article please use the link below which will take you to the Copyright Clearance Center’s RightsLink service. You will be able to get a quick price and instant permission to reuse the content in many different ways.
Evidence-based practice includes, in part, implementation of the findings of well-conducted quality research studies. So being able to critique quantitative research is an important skill for nurses. Consideration must be given not only to the results of the study but also the rigour of the research. Rigour refers to the extent to which the researchers worked to enhance the quality of the studies. In quantitative research, this is achieved through measurement of the validity and reliability. 1
- View inline
Types of validity
The first category is content validity . This category looks at whether the instrument adequately covers all the content that it should with respect to the variable. In other words, does the instrument cover the entire domain related to the variable, or construct it was designed to measure? In an undergraduate nursing course with instruction about public health, an examination with content validity would cover all the content in the course with greater emphasis on the topics that had received greater coverage or more depth. A subset of content validity is face validity , where experts are asked their opinion about whether an instrument measures the concept intended.
Construct validity refers to whether you can draw inferences about test scores related to the concept being studied. For example, if a person has a high score on a survey that measures anxiety, does this person truly have a high degree of anxiety? In another example, a test of knowledge of medications that requires dosage calculations may instead be testing maths knowledge.
There are three types of evidence that can be used to demonstrate a research instrument has construct validity:
Homogeneity—meaning that the instrument measures one construct.
Convergence—this occurs when the instrument measures concepts similar to that of other instruments. Although if there are no similar instruments available this will not be possible to do.
Theory evidence—this is evident when behaviour is similar to theoretical propositions of the construct measured in the instrument. For example, when an instrument measures anxiety, one would expect to see that participants who score high on the instrument for anxiety also demonstrate symptoms of anxiety in their day-to-day lives. 2
The final measure of validity is criterion validity . A criterion is any other instrument that measures the same variable. Correlations can be conducted to determine the extent to which the different instruments measure the same variable. Criterion validity is measured in three ways:
Convergent validity—shows that an instrument is highly correlated with instruments measuring similar variables.
Divergent validity—shows that an instrument is poorly correlated to instruments that measure different variables. In this case, for example, there should be a low correlation between an instrument that measures motivation and one that measures self-efficacy.
Predictive validity—means that the instrument should have high correlations with future criterions. 2 For example, a score of high self-efficacy related to performing a task should predict the likelihood a participant completing the task.
Reliability
Reliability relates to the consistency of a measure. A participant completing an instrument meant to measure motivation should have approximately the same responses each time the test is completed. Although it is not possible to give an exact calculation of reliability, an estimate of reliability can be achieved through different measures. The three attributes of reliability are outlined in table 2 . How each attribute is tested for is described below.
Attributes of reliability
Homogeneity (internal consistency) is assessed using item-to-total correlation, split-half reliability, Kuder-Richardson coefficient and Cronbach's α. In split-half reliability, the results of a test, or instrument, are divided in half. Correlations are calculated comparing both halves. Strong correlations indicate high reliability, while weak correlations indicate the instrument may not be reliable. The Kuder-Richardson test is a more complicated version of the split-half test. In this process the average of all possible split half combinations is determined and a correlation between 0–1 is generated. This test is more accurate than the split-half test, but can only be completed on questions with two answers (eg, yes or no, 0 or 1). 3
Cronbach's α is the most commonly used test to determine the internal consistency of an instrument. In this test, the average of all correlations in every combination of split-halves is determined. Instruments with questions that have more than two responses can be used in this test. The Cronbach's α result is a number between 0 and 1. An acceptable reliability score is one that is 0.7 and higher. 1 , 3
Stability is tested using test–retest and parallel or alternate-form reliability testing. Test–retest reliability is assessed when an instrument is given to the same participants more than once under similar circumstances. A statistical comparison is made between participant's test scores for each of the times they have completed it. This provides an indication of the reliability of the instrument. Parallel-form reliability (or alternate-form reliability) is similar to test–retest reliability except that a different form of the original instrument is given to participants in subsequent tests. The domain, or concepts being tested are the same in both versions of the instrument but the wording of items is different. 2 For an instrument to demonstrate stability there should be a high correlation between the scores each time a participant completes the test. Generally speaking, a correlation coefficient of less than 0.3 signifies a weak correlation, 0.3–0.5 is moderate and greater than 0.5 is strong. 4
Equivalence is assessed through inter-rater reliability. This test includes a process for qualitatively determining the level of agreement between two or more observers. A good example of the process used in assessing inter-rater reliability is the scores of judges for a skating competition. The level of consistency across all judges in the scores given to skating participants is the measure of inter-rater reliability. An example in research is when researchers are asked to give a score for the relevancy of each item on an instrument. Consistency in their scores relates to the level of inter-rater reliability of the instrument.
Determining how rigorously the issues of reliability and validity have been addressed in a study is an essential component in the critique of research as well as influencing the decision about whether to implement of the study findings into nursing practice. In quantitative studies, rigour is determined through an evaluation of the validity and reliability of the tools or instruments utilised in the study. A good quality research study will provide evidence of how all these factors have been addressed. This will help you to assess the validity and reliability of the research and help you decide whether or not you should apply the findings in your area of clinical practice.
- Lobiondo-Wood G ,
- Shuttleworth M
- ↵ Laerd Statistics . Determining the correlation coefficient . 2013 . https://statistics.laerd.com/premium/pc/pearson-correlation-in-spss-8.php
Twitter Follow Roberta Heale at @robertaheale and Alison Twycross at @alitwy
Competing interests None declared.
Read the full text or download the PDF:

An official website of the United States government
The .gov means it’s official. Federal government websites often end in .gov or .mil. Before sharing sensitive information, make sure you’re on a federal government site.
The site is secure. The https:// ensures that you are connecting to the official website and that any information you provide is encrypted and transmitted securely.
- Publications
- Account settings
Preview improvements coming to the PMC website in October 2024. Learn More or Try it out now .
- Advanced Search
- Journal List
- J Grad Med Educ
- v.3(2); 2011 Jun
A Primer on the Validity of Assessment Instruments
1. what is reliability 1.
Reliability refers to whether an assessment instrument gives the same results each time it is used in the same setting with the same type of subjects. Reliability essentially means consistent or dependable results. Reliability is a part of the assessment of validity.
2. What is validity? 1
Validity in research refers to how accurately a study answers the study question or the strength of the study conclusions. For outcome measures such as surveys or tests, validity refers to the accuracy of measurement. Here validity refers to how well the assessment tool actually measures the underlying outcome of interest. Validity is not a property of the tool itself, but rather of the interpretation or specific purpose of the assessment tool with particular settings and learners.
Assessment instruments must be both reliable and valid for study results to be credible. Thus, reliability and validity must be examined and reported, or references cited, for each assessment instrument used to measure study outcomes. Examples of assessments include resident feedback survey, course evaluation, written test, clinical simulation observer ratings, needs assessment survey, and teacher evaluation. Using an instrument with high reliability is not sufficient; other measures of validity are needed to establish the credibility of your study.
3. How is reliability measured? 2 – 4
Reliability can be estimated in several ways; the method will depend upon the type of assessment instrument. Sometimes reliability is referred to as internal validity or internal structure of the assessment tool.
For internal consistency 2 to 3 questions or items are created that measure the same concept, and the difference among the answers is calculated. That is, the correlation among the answers is measured.
Cronbach alpha is a test of internal consistency and frequently used to calculate the correlation values among the answers on your assessment tool. 5 Cronbach alpha calculates correlation among all the variables, in every combination; a high reliability estimate should be as close to 1 as possible.
For test/retest the test should give the same results each time, assuming there are no interval changes in what you are measuring, and they are often measured as correlation, with Pearson r.
Test/retest is a more conservative estimate of reliability than Cronbach alpha, but it takes at least 2 administrations of the tool, whereas Cronbach alpha can be calculated after a single administration. To perform a test/retest, you must be able to minimize or eliminate any change (ie, learning) in the condition you are measuring, between the 2 measurement times. Administer the assessment instrument at 2 separate times for each subject and calculate the correlation between the 2 different measurements.
Interrater reliability is used to study the effect of different raters or observers using the same tool and is generally estimated by percent agreement, kappa (for binary outcomes), or Kendall tau.
Another method uses analysis of variance (ANOVA) to generate a generalizability coefficient, to quantify how much measurement error can be attributed to each potential factor, such as different test items, subjects, raters, dates of administration, and so forth. This model looks at the overall reliability of the results. 6
5. How is the validity of an assessment instrument determined? 4 – 7 , 8
Validity of assessment instruments requires several sources of evidence to build the case that the instrument measures what it is supposed to measure. , 9,10 Determining validity can be viewed as constructing an evidence-based argument regarding how well a tool measures what it is supposed to do. Evidence can be assembled to support, or not support, a specific use of the assessment tool. Evidence can be found in content, response process, relationships to other variables, and consequences.
Content includes a description of the steps used to develop the instrument. Provide information such as who created the instrument (national experts would confer greater validity than local experts, who in turn would have more validity than nonexperts) and other steps that support the instrument has the appropriate content.
Response process includes information about whether the actions or thoughts of the subjects actually match the test and also information regarding training for the raters/observers, instructions for the test-takers, instructions for scoring, and clarity of these materials.
Relationship to other variables includes correlation of the new assessment instrument results with other performance outcomes that would likely be the same. If there is a previously accepted “gold standard” of measurement, correlate the instrument results to the subject's performance on the “gold standard.” In many cases, no “gold standard” exists and comparison is made to other assessments that appear reasonable (eg, in-training examinations, objective structured clinical examinations, rotation “grades,” similar surveys).
Consequences means that if there are pass/fail or cut-off performance scores, those grouped in each category tend to perform the same in other settings. Also, if lower performers receive additional training and their scores improve, this would add to the validity of the instrument.
Different types of instruments need an emphasis on different sources of validity evidence. 7 For example, for observer ratings of resident performance, interrater agreement may be key, whereas for a survey measuring resident stress, relationship to other variables may be more important. For a multiple choice examination, content and consequences may be essential sources of validity evidence. For high-stakes assessments (eg, board examinations), substantial evidence to support the case for validity will be required. 9
There are also other types of validity evidence, which are not discussed here.
6. How can researchers enhance the validity of their assessment instruments?
First, do a literature search and use previously developed outcome measures. If the instrument must be modified for use with your subjects or setting, modify and describe how, in a transparent way. Include sufficient detail to allow readers to understand the potential limitations of this approach.
If no assessment instruments are available, use content experts to create your own and pilot the instrument prior to using it in your study. Test reliability and include as many sources of validity evidence as are possible in your paper. Discuss the limitations of this approach openly.
7. What are the expectations of JGME editors regarding assessment instruments used in graduate medical education research?
JGME editors expect that discussions of the validity of your assessment tools will be explicitly mentioned in your manuscript, in the methods section. If you are using a previously studied tool in the same setting, with the same subjects, and for the same purpose, citing the reference(s) is sufficient. Additional discussion about your adaptation is needed if you (1) have modified previously studied instruments; (2) are using the instrument for different settings, subjects, or purposes; or (3) are using different interpretation or cut-off points. Discuss whether the changes are likely to affect the reliability or validity of the instrument.
Researchers who create novel assessment instruments need to state the development process, reliability measures, pilot results, and any other information that may lend credibility to the use of homegrown instruments. Transparency enhances credibility.
In general, little information can be gleaned from single-site studies using untested assessment instruments; these studies are unlikely to be accepted for publication.
8. What are useful resources for reliability and validity of assessment instruments?
The references for this editorial are a good starting point.
Gail M. Sullivan, MD, MPH, is Editor-in-Chief, Journal of Graduate Medical Education .
Uncomplicated Reviews of Educational Research Methods
- Instrument, Validity, Reliability
.pdf version of this page
Part I: The Instrument
Instrument is the general term that researchers use for a measurement device (survey, test, questionnaire, etc.). To help distinguish between instrument and instrumentation, consider that the instrument is the device and instrumentation is the course of action (the process of developing, testing, and using the device).
Instruments fall into two broad categories, researcher-completed and subject-completed, distinguished by those instruments that researchers administer versus those that are completed by participants. Researchers chose which type of instrument, or instruments, to use based on the research question. Examples are listed below:
Usability refers to the ease with which an instrument can be administered, interpreted by the participant, and scored/interpreted by the researcher. Example usability problems include:
- Students are asked to rate a lesson immediately after class, but there are only a few minutes before the next class begins (problem with administration).
- Students are asked to keep self-checklists of their after school activities, but the directions are complicated and the item descriptions confusing (problem with interpretation).
- Teachers are asked about their attitudes regarding school policy, but some questions are worded poorly which results in low completion rates (problem with scoring/interpretation).
Validity and reliability concerns (discussed below) will help alleviate usability issues. For now, we can identify five usability considerations:
- How long will it take to administer?
- Are the directions clear?
- How easy is it to score?
- Do equivalent forms exist?
- Have any problems been reported by others who used it?
It is best to use an existing instrument, one that has been developed and tested numerous times, such as can be found in the Mental Measurements Yearbook . We will turn to why next.
Part II: Validity
Validity is the extent to which an instrument measures what it is supposed to measure and performs as it is designed to perform. It is rare, if nearly impossible, that an instrument be 100% valid, so validity is generally measured in degrees. As a process, validation involves collecting and analyzing data to assess the accuracy of an instrument. There are numerous statistical tests and measures to assess the validity of quantitative instruments, which generally involves pilot testing. The remainder of this discussion focuses on external validity and content validity.
External validity is the extent to which the results of a study can be generalized from a sample to a population. Establishing eternal validity for an instrument, then, follows directly from sampling. Recall that a sample should be an accurate representation of a population, because the total population may not be available. An instrument that is externally valid helps obtain population generalizability, or the degree to which a sample represents the population.
Content validity refers to the appropriateness of the content of an instrument. In other words, do the measures (questions, observation logs, etc.) accurately assess what you want to know? This is particularly important with achievement tests. Consider that a test developer wants to maximize the validity of a unit test for 7th grade mathematics. This would involve taking representative questions from each of the sections of the unit and evaluating them against the desired outcomes.
Part III: Reliability
Reliability can be thought of as consistency. Does the instrument consistently measure what it is intended to measure? It is not possible to calculate reliability; however, there are four general estimators that you may encounter in reading research:
- Inter-Rater/Observer Reliability : The degree to which different raters/observers give consistent answers or estimates.
- Test-Retest Reliability : The consistency of a measure evaluated over time.
- Parallel-Forms Reliability: The reliability of two tests constructed the same way, from the same content.
- Internal Consistency Reliability: The consistency of results across items, often measured with Cronbach’s Alpha.
Relating Reliability and Validity
Reliability is directly related to the validity of the measure. There are several important principles. First, a test can be considered reliable, but not valid. Consider the SAT, used as a predictor of success in college. It is a reliable test (high scores relate to high GPA), though only a moderately valid indicator of success (due to the lack of structured environment – class attendance, parent-regulated study, and sleeping habits – each holistically related to success).
Second, validity is more important than reliability. Using the above example, college admissions may consider the SAT a reliable test, but not necessarily a valid measure of other quantities colleges seek, such as leadership capability, altruism, and civic involvement. The combination of these aspects, alongside the SAT, is a more valid measure of the applicant’s potential for graduation, later social involvement, and generosity (alumni giving) toward the alma mater.
Finally, the most useful instrument is both valid and reliable. Proponents of the SAT argue that it is both. It is a moderately reliable predictor of future success and a moderately valid measure of a student’s knowledge in Mathematics, Critical Reading, and Writing.
Part IV: Validity and Reliability in Qualitative Research
Thus far, we have discussed Instrumentation as related to mostly quantitative measurement. Establishing validity and reliability in qualitative research can be less precise, though participant/member checks, peer evaluation (another researcher checks the researcher’s inferences based on the instrument ( Denzin & Lincoln, 2005 ), and multiple methods (keyword: triangulation ), are convincingly used. Some qualitative researchers reject the concept of validity due to the constructivist viewpoint that reality is unique to the individual, and cannot be generalized. These researchers argue for a different standard for judging research quality. For a more complete discussion of trustworthiness, see Lincoln and Guba’s (1985) chapter .
Share this:
- How To Assess Research Validity | Windranger5
- How unreliable are the judges on Strictly Come Dancing? | Delight Through Logical Misery
Comments are closed.
About Research Rundowns
Research Rundowns was made possible by support from the Dewar College of Education at Valdosta State University .
- Experimental Design
- What is Educational Research?
- Writing Research Questions
- Mixed Methods Research Designs
- Qualitative Coding & Analysis
- Qualitative Research Design
- Correlation
- Effect Size
- Mean & Standard Deviation
- Significance Testing (t-tests)
- Steps 1-4: Finding Research
- Steps 5-6: Analyzing & Organizing
- Steps 7-9: Citing & Writing
- Writing a Research Report
Create a free website or blog at WordPress.com.
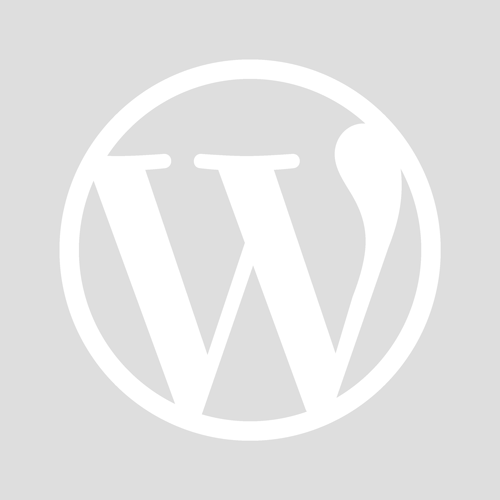
- Already have a WordPress.com account? Log in now.
- Subscribe Subscribed
- Copy shortlink
- Report this content
- View post in Reader
- Manage subscriptions
- Collapse this bar
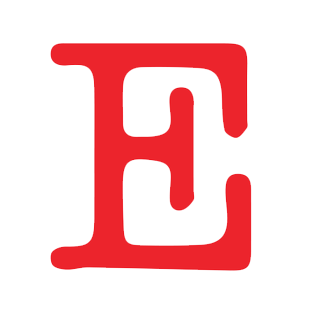
- Scholarly Community Encyclopedia
- Log in/Sign up

Video Upload Options
- MDPI and ACS Style
- Chicago Style
Questionnaire is one of the most widely used tools to collect data in especially social science research. The main objective of questionnaire in research is to obtain relevant information in most reliable and valid manner. Thus the accuracy and consistency of survey/questionnaire forms a significant aspect of research methodology which are known as validity and reliability. Often new researchers are confused with selection and conducting of proper validity type to test their research instrument (questionnaire/survey).
1. Introduction
Validity explains how well the collected data covers the actual area of investigation [ 1 ] . Validity basically means “measure what is intended to be measured” [ 2 ] .
2. Face Validity
Face validity is a subjective judgment on the operationalization of a construct. Face validity is the degree to which a measure appears to be related to a specific construct, in the judgment of non-experts such as test takers and representatives of the legal system. That is, a test has face validity if its content simply looks relevant to the person taking the test. It evaluates the appearance of the questionnaire in terms of feasibility, readability, consistency of style and formatting, and the clarity of the language used.
In other words, face validity refers to researchers’ subjective assessments of the presentation and relevance of the measuring instrument as to whether the items in the instrument appear to be relevant, reasonable, unambiguous and clear [ 3 ] .
In order to examine the face validity, the dichotomous scale can be used with categorical option of “Yes” and “No” which indicate a favourable and unfavourable item respectively. Where favourable item means that the item is objectively structured and can be positively classified under the thematic category. Then the collected data is analysed using Cohen’s Kappa Index (CKI) in determining the face validity of the instrument. DM. et al. [ 4 ] recommended a minimally acceptable Kappa of 0.60 for inter-rater agreement. Unfortunately, face validity is arguably the weakest form of validity and many would suggest that it is not a form of validity in the strictest sense of the word.
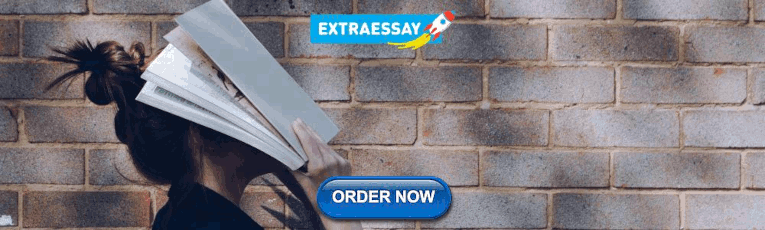
3. Content Validity
Content validity is defined as “the degree to which items in an instrument reflect the content universe to which the instrument will be generalized” (Straub, Boudreau et al. [ 5 ] ). In the field of IS, it is highly recommended to apply content validity while the new instrument is developed. In general, content validity involves evaluation of a new survey instrument in order to ensure that it includes all the items that are essential and eliminates undesirable items to a particular construct domain [ 6 ] ). The judgemental approach to establish content validity involves literature reviews and then follow-ups with the evaluation by expert judges or panels. The procedure of judgemental approach of content validity requires researchers to be present with experts in order to facilitate validation. However it is not always possible to have many experts of a particular research topic at one location. It poses a limitation to conduct validity on a survey instrument when experts are located in different geographical areas (Choudrie and Dwivedi [ 7 ] ). Contrastingly, a quantitative approach may allow researchers to send content validity questionnaires to experts working at different locations, whereby distance is not a limitation. In order to apply content validity following steps are followed:
1.An exhaustive literature reviews to extract the related items.
2.A content validity survey is generated (each item is assessed using three point scale (not necessary, useful but not essential and essential).
3.The survey should sent to the experts in the same field of the research.
4.The content validity ratio (CVR) is then calculated for each item by employing Lawshe [ 8 ] (1975) ‘s method.
5.Items that are not significant at the critical level are eliminated. In following the critical level of Lawshe method is explained.
4. Construct Validity
If a relationship is causal, what are the particular cause and effect behaviours or constructs involved in the relationship? Construct validity refers to how well you translated or transformed a concept, idea, or behaviour that is a construct into a functioning and operating reality, the operationalization. Construct validity has two components: convergent and discriminant validity.
4.1 Discriminant Validity
Discriminant validity is the extent to which latent variable A discriminates from other latent variables (e.g., B, C, D). Discriminant validity means that a latent variable is able to account for more variance in the observed variables associated with it than a) measurement error or similar external, unmeasured influences; or b) other constructs within the conceptual framework. If this is not the case, then the validity of the individual indicators and of the construct is questionable (Fornell and Larcker [ 9 ] ). In brief, Discriminant validity (or divergent validity) tests that constructs that should have no relationship do, in fact, not have any relationship.
4.2 Convergent Validity
Convergent validity, a parameter often used in sociology, psychology, and other behavioural sciences, refers to the degree to which two measures of constructs that theoretically should be related, are in fact related. In brief, Convergent validity tests that constructs that are expected to be related are, in fact, related.
With the purpose of verifying the construct validity (discriminant and convergent validity), a factor analysis can be conducted utilizing principal component analysis (PCA) with varimax rotation method (Koh and Nam [ 9 ] , Wee and Quazi, [ 10 ] ). Items loaded above 0.40, which is the minimum recommended value in research are considered for further analysis. Also, items cross loading above 0.40 should be deleted. Therefore, the factor analysis results will satisfy the criteria of construct validity including both the discriminant validity (loading of at least 0.40, no cross-loading of items above 0.40) and convergent validity (eigenvalues of 1, loading of at least 0.40, items that load on posited constructs) (Straub et al., [ 11 ] ). There are also other methods to test the convergent and discriminant validity.
5. Criterion Validity
Criterion or concrete validity is the extent to which a measure is related to an outcome. It measures how well one measure predicts an outcome for another measure. A test has this type of validity if it is useful for predicting performance or behavior in another situation (past, present, or future).
Criterion validity is an alternative perspective that de-emphasizes the conceptual meaning or interpretation of test scores. Test users might simply wish to use a test to differentiate between groups of people or to make predictions about future outcomes. For example, a human resources director might need to use a test to help predict which applicants are most likely to perform well as employees. From a very practical standpoint, she focuses on the test’s ability to differentiate good employees from poor employees. If the test does this well, then the test is “valid” enough for her purposes. From the traditional three-faceted view of validity, criterion validity refers to the degree to which test scores can predict specific criterion variables. The key to validity is the empirical association between test scores and scores on the relevant criterion variable, such as “job performance.”
Messick [ 12 ] suggests that “even for purposes of applied decision making, reliance on criterion validity or content coverage is not enough. The meaning of the measure, and hence its construct validity, must always be pursued – not only to support test interpretation but also to justify test use”. There are two types of criterion validity namely; concurrent validity, predictive and postdictive validity.
6. Reliability
Reliability concerns the extent to which a measurement of a phenomenon provides stable and consist result (Carmines and Zeller [ 13 ] ). Reliability is also concerned with repeatability. For example, a scale or test is said to be reliable if repeat measurement made by it under constant conditions will give the same result (Moser and Kalton [ 14 ] ).
Testing for reliability is important as it refers to the consistency across the parts of a measuring instrument (Huck [ 15 ] ). A scale is said to have high internal consistency reliability if the items of a scale “hang together” and measure the same construct (Huck [ 16 ] Robinson [ 17 ] ). The most commonly used internal consistency measure is the Cronbach Alpha coefficient. It is viewed as the most appropriate measure of reliability when making use of Likert scales (Whitley [ 18 ] , Robinson [ 19 ] ). No absolute rules exist for internal consistencies, however most agree on a minimum internal consistency coefficient of .70 (Whitley [ 20 ] , Robinson [ 21 ] ).
For an exploratory or pilot study, it is suggested that reliability should be equal to or above 0.60 (Straub et al. [ 22 ] ). Hinton et al. [ 23 ] have suggested four cut-off points for reliability, which includes excellent reliability (0.90 and above), high reliability (0.70-0.90), moderate reliability (0.50-0.70) and low reliability (0.50 and below)(Hinton et al., [ 24 ] ). Although reliability is important for study, it is not sufficient unless combined with validity. In other words, for a test to be reliable, it also needs to be valid [ 25 ] .
- ACKOFF, R. L. 1953. The Design of Social Research, Chicago, University of Chicago Press.
- BARTLETT, J. E., KOTRLIK, J. W. & HIGGINS, C. C. 2001. Organizational research: determining appropriate sample size in survey research. Learning and Performance Journal, 19, 43-50.
- BOUDREAU, M., GEFEN, D. & STRAUB, D. 2001. Validation in IS research: A state-of-the-art assessment. MIS Quarterly, 25, 1-24.
- BREWETON, P. & MILLWARD, L. 2001. Organizational Research Methods, London, SAGE.
- BROWN, G. H. 1947. A comparison of sampling methods. Journal of Marketing, 6, 331-337.
- BRYMAN, A. & BELL, E. 2003. Business research methods, Oxford, Oxford University Press.
- CARMINES, E. G. & ZELLER, R. A. 1979. Reliability and Validity Assessment, Newbury Park, CA, SAGE.
- CHOUDRIE, J. & DWIVEDI, Y. K. Investigating Broadband Diffusion in the Household: Towards Content Validity and Pre-Test of the Survey Instrument. Proceedings of the 13th European Conference on Information Systems (ECIS 2005), May 26-28, 2005 2005 Regensburg, Germany.
- DAVIS, D. 2005. Business Research for Decision Making, Australia, Thomson South-Western.
- DM., G., DP., H., CC., C., CL., S. & ., P. B. 1975. The effects of instructional prompts and praise on children's donation rates. Child Development 46, 980-983.
- ENGELLANT, K., HOLLAND, D. & PIPER, R. 2016. Assessing Convergent and Discriminant Validity of the Motivation Construct for the Technology Integration Education (TIE) Model. Journal of Higher Education Theory and Practice 16, 37-50.
- FIELD, A. P. 2005. Discovering Statistics Using SPSS, Sage Publications Inc.
- FORNELL, C. & LARCKER, D. F. 1981. Evaluating structural equation models with unobservable variables and measurement error. Journal of Marketing Research, 18, 39-50.
- FOWLER, F. J. 2002. Survey research methods, Newbury Park, CA, SAGE.
- GHAURI, P. & GRONHAUG, K. 2005. Research Methods in Business Studies, Harlow, FT/Prentice Hall.
- GILL, J., JOHNSON, P. & CLARK, M. 2010. Research Methods for Managers, SAGE Publications.
- HINTON, P. R., BROWNLOW, C., MCMURRAY, I. & COZENS, B. 2004. SPSS explained, East Sussex, England, Routledge Inc.
- HUCK, S. W. 2007. Reading Statistics and Research, United States of America, Allyn & Bacon.
- KOH, C. E. & NAM, K. T. 2005. Business use of the internet: a longitudinal study from a value chain perspective. Industrial Management & Data Systems, 105 85-95.
- LAWSHE, C. H. 1975. A quantitative approach to content validity. Personnel Psychology, 28, 563-575.
- LEWIS, B. R., SNYDER, C. A. & RAINER, K. R. 1995. An empirical assessment of the Information Resources Management construct. Journal of Management Information Systems, 12, 199-223.
- MALHOTRA, N. K. & BIRKS, D. F. 2006. Marketing Research: An Applied Approach, Harlow, FT/Prentice Hall.
- MAXWELL, J. A. 1996. Qualitative Research Design: An Intractive Approach London, Applied Social Research Methods Series.
- MESSICK, S. 1989. Validity. In: LINN, R. L. (ed.) Educational measurement. New York: Macmillan.
- MOSER, C. A. & KALTON, G. 1989. Survey methods in social investigation, Aldershot, Gower.
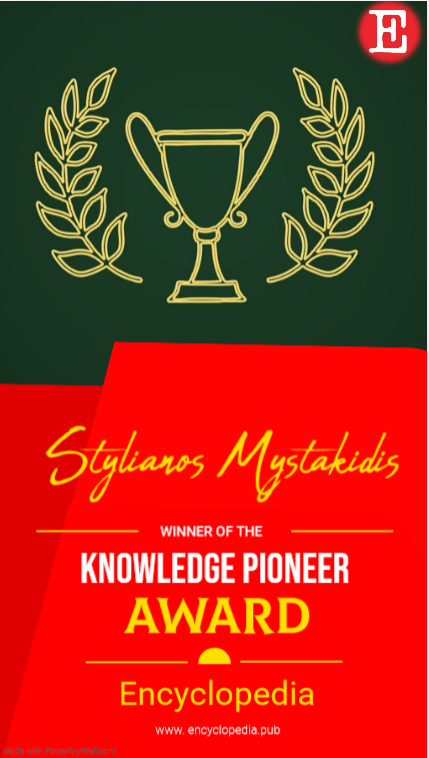
- Terms and Conditions
- Privacy Policy
- Advisory Board
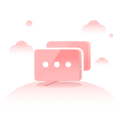
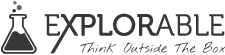
- Foundations
- Write Paper
Search form
- Experiments
- Anthropology
- Self-Esteem
- Social Anxiety

Instrument Reliability
Instrument reliability is a way of ensuring that any instrument used for measuring experimental variables gives the same results every time.
This article is a part of the guide:
- Validity and Reliability
- Types of Validity
- Definition of Reliability
- Content Validity
- Construct Validity
Browse Full Outline
- 1 Validity and Reliability
- 2 Types of Validity
- 3.1 Population Validity
- 3.2 Ecological Validity
- 4 Internal Validity
- 5.1.1 Concurrent Validity
- 5.1.2 Predictive Validity
- 6 Content Validity
- 7.1 Convergent and Discriminant Validity
- 8 Face Validity
- 9 Definition of Reliability
- 10.1 Reproducibility
- 10.2 Replication Study
- 11 Interrater Reliability
- 12 Internal Consistency Reliability
- 13 Instrument Reliability
In the physical sciences, the term is self-explanatory, and it is a matter of making sure that every piece of hardware, from a mass spectrometer to a set of weighing scales, is properly calibrated.
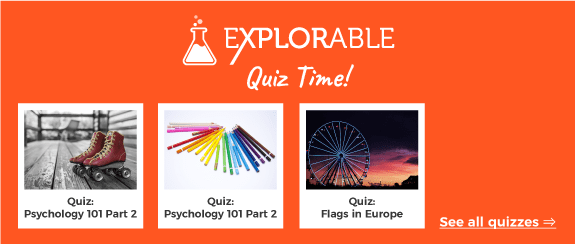
Instruments in Research
As an example, a researcher will always test the instrument reliability of weighing scales with a set of calibration weights, ensuring that the results given are within an acceptable margin of error .
Some of the highly accurate balances can give false results if they are not placed upon a completely level surface, so this calibration process is the best way to avoid this.
In the non-physical sciences, the definition of an instrument is much broader, encompassing everything from a set of survey questions to an intelligence test. A survey to measure reading ability in children must produce reliable and consistent results if it is to be taken seriously.
Political opinion polls, on the other hand, are notorious for producing inaccurate results and delivering a near unworkable margin of error.
In the physical sciences, it is possible to isolate a measuring instrument from external factors , such as environmental conditions and temporal factors. In the social sciences, this is much more difficult, so any instrument must be tested with a reasonable range of reliability.
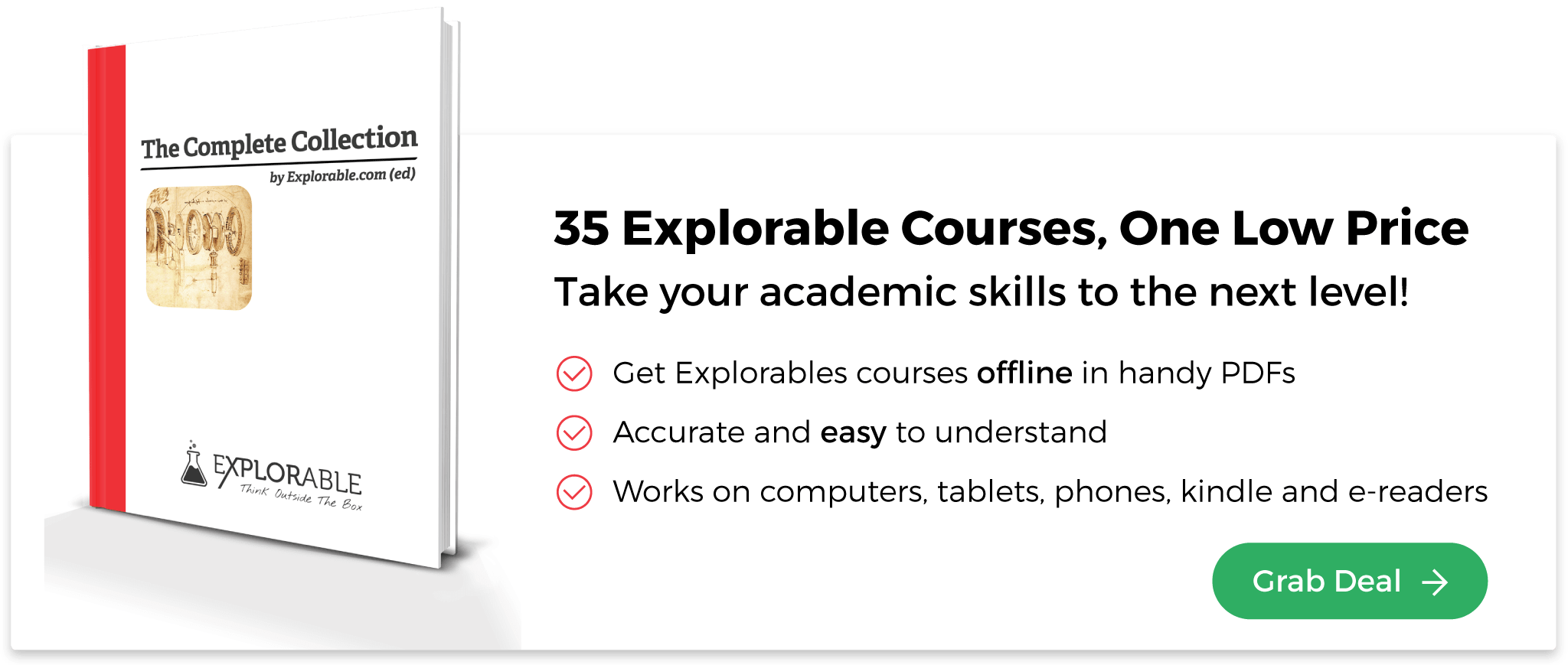
Test of Stability
Any test of instrument reliability must test how stable the test is over time, ensuring that the same test performed upon the same individual gives exactly the same results.
The test-retest method is one way of ensuring that any instrument is stable over time.
Of course, there is no such thing as perfection and there will be always be some disparity and potential for regression , so statistical methods are used to determine whether the stability of the instrument is within acceptable limits.
Test of Equivalence
Testing equivalence involves ensuring that a test administered to two people, or similar tests administered at the same time give similar results.
Split-testing is one way of ensuring this, especially in tests or observations where the results are expected to change over time. In a school exam, for example, the same test upon the same subjects will generally result in better results the second time around, so testing stability is not practical.
Checking that two researchers observe similar results also falls within the remit of the test of equivalence.
Test of Internal Consistency
The test of internal consistency involves ensuring that each part of the test generates similar results, and that each part of a test measures the correct construct.
For example, a test of IQ should measure IQ only, and every single question must also contribute. One way of doing this is with the variations upon the split-half tests, where the test is divided into two sections, which are checked against each other. The odd-even reliability is a similar method used to check internal consistency .
Physical sciences often use tests of internal consistency, and this is why sports drugs testers take two samples, each measured independently by different laboratories, to ensure that experimental or human error did not skew or influence the results .
- Psychology 101
- Flags and Countries
- Capitals and Countries
Martyn Shuttleworth (Apr 16, 2009). Instrument Reliability. Retrieved Apr 21, 2024 from Explorable.com: https://explorable.com/instrument-reliability
You Are Allowed To Copy The Text
The text in this article is licensed under the Creative Commons-License Attribution 4.0 International (CC BY 4.0) .
This means you're free to copy, share and adapt any parts (or all) of the text in the article, as long as you give appropriate credit and provide a link/reference to this page.
That is it. You don't need our permission to copy the article; just include a link/reference back to this page. You can use it freely (with some kind of link), and we're also okay with people reprinting in publications like books, blogs, newsletters, course-material, papers, wikipedia and presentations (with clear attribution).
Related articles
Internal Consistency
Interrater Reliability
Reproducibility
Test-Retest Reliability
Want to stay up to date? Follow us!
Save this course for later.
Don't have time for it all now? No problem, save it as a course and come back to it later.
Footer bottom
- Privacy Policy
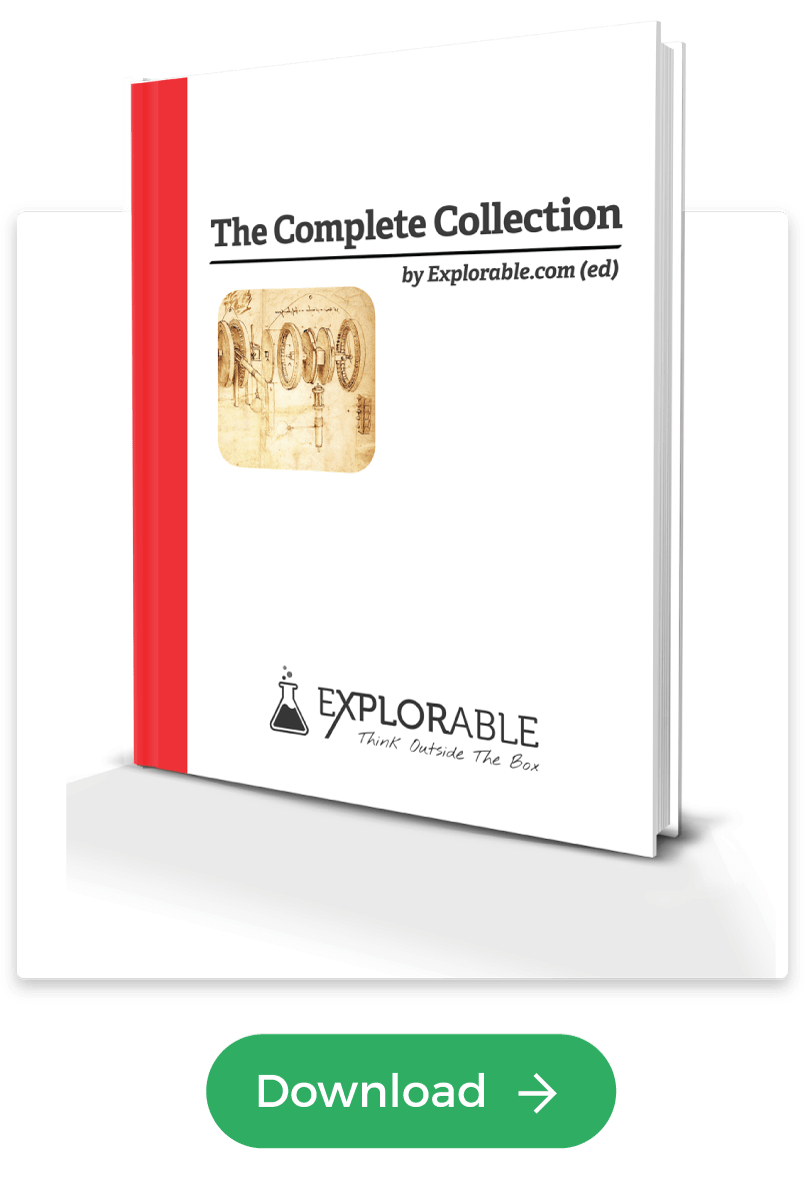
- Subscribe to our RSS Feed
- Like us on Facebook
- Follow us on Twitter
- Open access
- Published: 20 April 2024
Development and validation of the hospice professional coping scale among Chinese nurses
- Yanting Zhang 1 na1 ,
- Li Zheng 2 na1 ,
- Yanling He 3 ,
- Min Han 3 ,
- Yu Wang 3 ,
- Jinyu Xv 3 ,
- Hui Qiu 3 na2 &
- Liu Yang 3 na2
BMC Health Services Research volume 24 , Article number: 491 ( 2024 ) Cite this article
Metrics details
Hospice care professionals often experience trauma patient deaths and multiple patient deaths in a short period of time (more so than other nurses). This repeated exposure to the death process and the death of patients leads to greater psychological pressure on hospice care professionals. But at present, people pay more attention to the feelings and care burden of the family members of dying patients but pay less attention to medical staff. Thus, this study aimed to develop a scale on the burden of care for hospice care providers and assess the coping capacity of hospice professionals. Raising awareness of the psychological burden of hospice professionals.
Through a literature review, research group discussion, Delphi method and a pre-survey of professional coping skills among nurses, 200 hospice professionals who had received training in hospice care from pilot institutions engaged in or providing hospice care were selected for investigation. Cronbach’s α coefficient and split-half reliability were used to test the internal consistency of the scale, and content validity and explore factor analysis (EFA) were used to test the construct validity of the scale.
Two rounds of Delphi methods were carried out, and the effective recovery rate was 100%. The expert authority coefficients of the two rounds were 0.838 and 0.833, respectively. The Kendall’s W coefficient of experts in the first round was 0.121 ~ 0.200 ( P < 0.05), and the Kendall’s W coefficient of the second round was 0.115–0.136 ( P < 0.05), indicating a good level of expert coordination. The final survey scale for the care burden of hospice professionals included four dimensions—working environment (9 items), professional roles (8 items), clinical nursing (9 items) and psychological burden (7 items)—with a total of 33 items. The total Cronbach’s α coefficient of the scale was 0.963, and the Cronbach’s α coefficients of the working environment, professional roles, clinical nursing and psychological burden dimensions were 0.920, 0.889, 0.936 and 0.910, respectively. The total split-half reliability of the scale was 0.927, and the split-half reliability of each dimension was 0.846, 0.817, 0.891, and 0.832. The content validity of the scale items ranged from 0.90 to 1.00. Exploratory factor analysis revealed 5 common factors, with a total cumulative contribution rate of 68.878%. The common degree of each item in the scale was > 0.4, and the factor loading of each item was also > 0.4.
The scale is an open-access, short, easy-to-administer scale. And which for assessing hospice care burden among hospice professionals developed in this study demonstrated strong reliability and validity. This tool can serve as a dependable instrument for evaluating the burden of hospice care for terminally ill patients by professionals in the hospice setting.
Peer Review reports
Hospice care refers to providing patients with terminal diseases with physical, psychological, and spiritual care, as well as humanistic care, by controlling the symptoms of pain and discomfort to improve their quality of life and help them die comfortably, calmly, and with dignity [ 1 ]. In June 2020, hospice care was incorporated into Chinese law for the first time. Article 36 of the Law on the Promotion of Basic Medicine and Health clearly stipulates that medical institutions provide hospice care and other medical and health services to citizens [ 2 ]. As early as 2016, the National Health and Family Planning Commission issued the National Nursing Development Plan (2016–2020) [ 3 ], noting the need to strengthen capacity-building for hospice care and improve relevant mechanisms. While the state vigorously promoted the development of hospice care, it also exposed many problems. These problems include the relatively traditional concept of death for our citizens, uneven development in the field of hospice care, and a lack of human resources and teams. The legal provisions on hospice care are relatively broad, and a lack of understanding of hospice care services can easily lead to medical disputes [ 4 , 5 ]. This not only poses numerous obstacles to the practical development of hospice care but also exposes hospice nursing staff to complex clinical situations [ 6 ].
According to previous studies, hospice care professionals often experience traumatic patient deaths and multiple patient deaths in a short period of time (more so than other nurses) [ 7 , 8 ]. This repeated exposure to the death process and the death of patients leads to greater psychological pressure on hospice care professionals [ 9 , 10 ]. In different groups, social support alleviates many adverse outcomes of hospice care professionals, such as high psychological stress and high emotional burnout [ 11 , 12 ]. In addition, nurses in oncology departments and palliative care departments need to continue to provide empathy and care for patients, not only to bear psychological pressure but also to undertake the emotional work of patients’ families, which easily results in empathy fatigue [ 13 , 14 ]. The psychological stress caused by empathy fatigue seriously affects the mental health and nurse‒patient relationships of nurses and may even lead to their resignation [ 15 ]. The assessment of the care burden of hospice care professionals can provide a reference for the formulation of relevant policies, provide guidance for terminally ill patients and their families to implement better hospice care services, provide comfort and respect for people in the final stages of life, and promote the development of hospice care [ 16 ].
At present, people pay more attention to the feelings and care burden of the family members of dying patients but pay less attention to medical staff. In addition, the related assessment tools in China are mainly aimed at assessing nurses’ knowledge, attitudes and behaviors related to hospice care. For example, the assessment tool used by Zheng is the self-developed hospice care attitude scale [ 17 , 18 ], and few studies have assessed the psychological stress of medical staff. However, due to cultural differences, assessment tools such as the Zarit Nursing Burden Scale (ZBI) [ 19 ] are not applicable in other countries. In recent years, some scholars have developed and verified self-care ability assessment tools for hospice care practitioners, but there is still a lack of assessments of care burden [ 20 , 21 ]. Therefore, this study provides a tool for assessing the care burden level of hospice care professionals by developing a scale for hospice care professionals and testing its reliability and validity. In addition, this study provides a clearer understanding of the current situation and influencing factors of hospice care burden in China and evaluates the effectiveness of interventions to reduce hospice care burden.
Development and procedure
Constructing a scale item pool.
Under the guidance of the Zarit Nursing Burden Scale (ZBI) [ 19 ], which uses hospice care/hospice care/health care personnel/nurses care/stress/empathy/psychological burden/fatigue as the key words, a large number of related studies were consulted through Pubmed/Web of Science/CINAL/China Knowledge Network/Wan Fang and other databases. To form a pool of items in the nursing burden scale for hospice care staff. The scale pool consists of 32 items, including working environment, professional role, clinical nursing and psychological burden. All the items were scored on a 5-point Likert scale, and they were all positive.
Delphi method
Expert inclusion criteria.
Bachelor’s degree or above; intermediate or above professional title; engaged in clinical work ≥ 5 years; were familiar with hospice care treatment and highly enthusiastic about this study; and voluntarily participated in and completed multiple rounds of inquiries.
Delphi method expert consultation form
The expert consultation form consisted of four parts: an introduction, basic information from the experts, a nursing burden scale for hospice care professionals, and an expert authority scale. The preface introduces the purpose, significance, and instructions of this survey. The basic expert information table includes age, sex, educational background, professional title, clinical working years, research field, and whether he or she is a graduate tutor. The nursing burden scale of hospice care professionals includes four dimensions: working environment, professional role, clinical nursing, and psychological burden. The importance and relevance of the scale items were evaluated by experts. For items that need to be modified, deleted, or added, experts can write down their comments in the corresponding “modified comments” column. Importance is divided into 5 levels: level 5 is highly important, level 1 is highly unimportant, relevance is divided into 4 levels, level 4 is highly relevant, and level 1 is highly irrelevant.
The expert authority scale includes the degree of experts’ familiarity with hospice care (very familiar = 1, relatively familiar = 0.8, generally familiar = 0.6, unfamiliar = 0.4, very unfamiliar = 0.2) and the influence of judgment basis (work experience judgment/theoretical knowledge analysis/domestic and foreign relevant data) on expert judgment.
Distribution and recycling of scales
During the first round of Delphi, the items of the scale were made into an expert consultation form and sent to all the experts by email. The experts were invited to provide responses within a week and to integrate, analyse and discuss their views. After an interval of 2 weeks, the second round of the credit scale is sent to all the experts via the same process as the first round. The selection criteria for the items were as follows: mean importance ≥ 4, coefficient of variation (CV) ≤ 0.25, and full score ratio > 0.20. Items that met all three criteria were retained. If only 1–2 criteria are met, further confirmation or panel discussion with the expert is required to decide whether to retain the criterion, and if none of the three criteria are met, the criterion is deleted [ 22 ].
Item modification content
After the first round of Delphi method, the items were added or modified according to the experts’ scores on the importance and relevance of the items as well as the expert’s advice. Three items with a coefficient of variation > 0.25 and a full score ratio < 0.2 were excluded (see supplement 1: Tables 1 and 2 for specific results). In the clinical nursing dimension, there is an item that does not meet the above criteria: “Do you think the terminally ill patients or their families you care for will require too much care for you?” After discussion with the working group, this item was retained because of its importance. The languages of 10 items had to be revised. One new item was added to each of the three dimensions of working environment, professional role and clinical nursing, and the new item was “Do you think that hospice care currently lacks the support of social recognition and other social forces?”, “Do you think it is more difficult for hospice workers to gain a sense of professional achievement?”, “Do you think that family members’ recognition and compatibility with hospice care is an important factor in carrying out work?”
After the second round of Delphi method, only one of the items in the clinical nursing dimension was modified: “strong death identity” was replaced by “patients who are pessimistic about death”. Finally, the nursing burden survey scale of hospice care professionals was developed, which included working environment (9 items), professional role (8 items), clinical nursing (9 items) and psychological burden (7 items), for a total of 33 items.
Pre-investigation
Using a convenience sampling method, 50 hospice care professionals who were engaged in or who received hospice care training in pilot hospice care institutions were selected as the research subjects in October 2022. In the course of the survey, the participants were closely observed for difficulty in understanding the scale and their opinions. After the last 2 rounds of Delphi method, all the entries were retained for formal investigation.
Sample size
According to the rough estimation method of sample size proposed by clinical epidemiology, the sample size is 5 ∼ 10 times the number of items in the scale [ 23 ], and the final number of items in this scale is 33, so the sample size is 165 ∼ 330.
Characteristics of participants
Using a convenience sampling method, 200 hospice care professionals who were engaged in or who received hospice care training in several hospitals or hospice pilot institutions were selected in December 2022, of which 150 were used for supplementary investigation. It should be noted that the supplementary survey objects here are the sample sizes collected after the presurvey. The inclusion criteria for participants were medical staff who participated in hospice care and who had received training, were aged ≥ 18 years, were clearly conscious, had good expression, provided informed consent, and had more than 2 years of work experience. The exclusion criteria were working for ≤ 2 years; not providing informed consent; only professionals who understood but did not participate in the hospice care system; and who had received training in the hospice care system.
Survey tools
① The general and basic conditions of hospice care and nursing staff. ② The scale of care burden of hospice nurses included four dimensions: working environment (9 items), professional role (8 items), clinical nursing (9 items) and psychological burden (7 items). On a 5-point Likert scale, 1 indicates complete lack (very disagree), and 5 indicates proficiency (very much agree).
Investigation procedure
The scale survey method was as follows: To ensure the smooth progress of the study, informed consent was obtained from the respondents before the scale survey, and the purpose and significance of this study were explained to the respondents to obtain cooperation. All the scales distributed in this study were distributed and completed through the scale stars. It can only be submitted after answering the set questions. It can only answer each time to ensure the rigor, authenticity and completeness of the scale. The scale collected must be reviewed by the research team, and if all the answers are the same, it will be determined to be invalid. A total of 250 copies were distributed in this study, and 200 copies were recovered.
Statistical methods
The data were inputted by two people using EpiData 3.0 software, and SPSS 23.0 statistical software was used for descriptive analysis, project analysis, exploratory factor analysis [ 24 ], correlation analysis, reliability and validity testing. The specific contents of the analysis were as follows: the items of the scale were screened by the differentiation method, and the items were sorted according to their scores. The first 27% of the scores are high, and the remaining 27% are low. Then, the average score of each item was calculated for the high score and low score groups. Using the independent sample t test, if the average score of an item has no significant difference between the high score and the low score (0.05), the importance and differentiation of the item are not significant, and the entry is excluded [ 22 ]. Cronbach’s α coefficient and the Spearman Brown method were used to test the reliability. Content validity and construct validity were used to test the validity of the scale, item-level content validity (I-CVI) and average scale-level content validity (S-CVI/Ave) were used as content validity indicators, and exploratory factor analysis was used to determine the number of common factors, cumulative contribution rate and eigenvalues of the scale. The screening criteria for each item were cumulative contribution rate > 60%, eigenvalue > 1, common variance > 0.4, and factor load > 0.4 for each entry.
Ethical considerations
All participants provided signed informed consent when reliability and validity tests were conducted. This study was approved by the Ethics Committee of Zhongnan Hospital of Wuhan University [2,022,119 K].
Basic characteristics of the experts
A total of 20 experts were selected for this study, and the details are shown in Table 1 .
Basic characteristics of the study subjects
Table 2 shows the general characteristics of the hospice care professionals.
Delphi results
A total of 2 rounds of Delphi method were conducted, 20 scales were distributed in each round, and the effective recovery rate was 100%. In the first round, 10 experts put forward their opinions, and in the second round, two experts put forward their opinions, and the experts were highly motivated. The authority coefficients of the two rounds of experts are 0.838 and 0.833 respectively. The expert authority coefficient of Delphi method is 0.75 ∼ 1. It is generally believed that an expert authority coefficient greater than 0.7 indicates the degree of expert authority [ 22 ], so the degree of expert authority in this study is greater. The Kendall consistency of the experts in the first round was 0.121 ∼ 0.200, and the reliability of the experts in the second round ranged from 0.115 to 0.136 ( P < 0.05).
Analysis of scale entries
The t values of each item in the high-score group and the low-score group ranged from 5.442 to 10.170 ( P < 0.05), and there was no item that could be deleted.
Scale reliability
The reliability of the scale is based on Cronbach’s α coefficient and the half-and-half reliability coefficient, which are commonly used to determine the reliability of the index. It is generally believed that Cronbach’s α coefficient and half-and-half reliability coefficient are greater than 0.7, indicating that the scale has good reliability. (Table 3 ).
The Cronbach’s α coefficients of each dimension of the scale were 0.920, 0.889, 0.938 and 0.910 respectively, and the half-and-half reliability coefficients were 0.846, 0.817, 0.891 and 0.832, respectively, while the Cronbach’s α coefficient and half-and-half reliability coefficient of the total scale were 0.963 and 0.927, respectively, all ≥ 0.7, indicating that the scale had good reliability, internal consistency and stability.
Content validity (correlation score 1–4)
The validity of the scale was expressed by the content validity index (CVI), including the content validity index of the item level (I-CVI) and the average content validity index of the scale level (S-CVI) [ 25 ]. When the I-CVI > 0.78, the content validity at the item level is better [ 26 ]. S-CVI/Ave is the average I-CVI for all projects. When the S-CVI/Ave > 0.9, the scale has good content validity at the average level [ 27 ].
The I-CVI was 0.90-1 > 0.78, and the content validity at the item level was good. The S-CVI/Ave was 0.967, and the S-CVI/Ave of each dimension was > 0.90, ranging from 0.964 to 0.980. The content validity of the average scale was good.
Structural validity - exploratory factor analysis
Kmo and bartlett tests (table 4 ).
Table 4 shows that the KMO values are all greater than 0.7, the validity is good, and P < 0.001. There is a correlation between variables, so exploratory factor analysis can be carried out.
Using principal component maximum variance rotation factor analysis
According to the analysis of the overall structural validity of the scale, the scale has five common factors, and the total cumulative contribution rate is 68.878%. After principal component analysis and maximum orthogonal rotation of variance, the common variance (commensurate) of the scale was more than 0.4, and the factor load of each item was also more than 0.4. Factor 1 is the clinical nursing dimension, factor 2 is the psychological burden dimension, factor 3 and factor 5 are the working environment dimension, and factor 4 is the professional role dimension. It should be noted that the B1 entry in factor 3 is slightly different from the structure of the original scale. However, considering that B1 reflects the content related to professional roles, after expert discussion, the entry remains in the professional role dimension. The specific analysis is shown in Table 5 below. (A is the working environment dimension, B is the professional role dimension, C is the clinical nursing dimension, and D is the psychological burden dimension).
Quality control of scale preparation
In the process of developing the scale, we first consulted a large number of related studies at home and abroad under the guidance of the Zarit Nursing Burden Scale (ZBI) to ensure the standardization, rigor and rationality of the scale. After 2 rounds of Delphi method, the relevant items of the scale were further revised. We selected experts in the fields of clinical nursing, geriatric nursing, nursing management, nursing education, nursing research, oncology clinics, etc., and proposed constructive suggestions for the revision of the contents of the scale to ensure its quality. In the process of sending the scale to the expert, we carefully checked whether there were missing items in each scale to ensure the effectiveness of the scale collection. After 2 rounds of Delphi method, the effective recovery rate of the scale was 100%. In the first round, 10 experts put forward their opinions, and in the second round, 2 experts put forward their opinions. The authority coefficients of the two rounds of experts are 0.838 and 0.833, respectively, indicating a high degree of authority. Kendall’s W coefficient of the first-round expert opinion test was 0.121-0.200 ( P < 0.05), and Kendall’s W coefficient of the second-round expert opinion test was 0.115–0.136 ( P < 0.05).
Reliability evaluation of the scale
In terms of reliability, it is generally believed that the reliability of a scale is good when the Cronbach’s α coefficient and Spearman-Brown coefficient are above 0.7. The Cronbach’s α coefficients of each dimension of the scale are 0.920, 0.889, 0.938, 0.910, 0.86, 0.817, 0.891, 0.832 and 0.927, indicating that the reliability, internal consistency and stability of the scale are good.
Validity evaluation of the scale
Content validity.
Content validity, also known as apparent validity or logical validity, refers to whether each item of the scale measures what it wants to measure, that is, whether the object’s understanding and answer to the question is consistent with what the item designer wants to ask [ 28 ]. In this study, the Delphi method was used to invite experts to score the relevance of the scale and evaluate its content validity. When I-CVI/Ave > 0.78 and S-CVI/Ave > 0.9, the content validity of the scale is good. According to the results of expert evaluation, the item-level content validity (I-CVI) is 0.90-1.00, and the average scale-level content validity (S-CVI) of the total scale is 0.967, indicating that the scale has good content validity.
Structural validity
Construct validity, also known as construct validity or feature validity, refers to whether the structure of the scale is consistent with the theoretical hypothesis of tabulation and whether the internal components of the measurement results are consistent with the field that the designer intends to measure; the commonly used statistical method is factor analysis, which reflects the contribution of a project to the field. The greater the factor load value is, the closer the relationship is to the domain [ 29 ]. Five common factors were extracted based on a characteristic root > 1, which explained 68.878% of the total variation. The commonness of 33 items in the scale is ≥ 0.4, and the factor load of each item is also ≥ 0.4, indicating that the construct validity of the scale is good.
The practicality and significance of the scale
On the basis of an extensive literature review and Delphi method, the nursing burden scale of hospice care professionals in China was developed. To clarify the current situation and influencing factors of the care burden of hospice care professionals in China, and to evaluate the effect of intervention measures on the care burden of hospice care professionals. At present, hospice care has received increasing attention, and a series of problems have emerged. One of the problems related to health care staff is the nursing burden. The scale developed in this study is practical and helpful for nursing managers to formulate intervention measures to reduce their nursing burden and improve the efficiency of hospice care.
Limitations and further research
As with any study, this study had several important limitations. In this study, exploratory factor analysis was used to develop and verify the scale, which ensured the scientific nature of the study in terms of methodology. However, in the actual investigation process, because there are many nurses involved in hospice care in the oncology department, most of the population was selected from the oncology department, which may have biased the results. There are 33 items in total. In the future, a short version of the scale will be further developed and verified in multiple centers to ensure the popularization of the scale.
Conclusions
The reliability and validity test showed that the care burden scale of hospice care professionals developed in this study has good reliability and validity and can be used to evaluate the level of care burden of hospice care professionals in China. However, confirmatory factor analysis was not performed for the scale, and the selected samples were mainly medical staff engaged in or carrying out hospice care pilot institutions in Hubei Province. The representativeness of the sample size needs to be studied, and the sample size will be further expanded in multiple centers to improve the content of the scale.
Data availability
The datasets used and/or analysed during the current study are available from the corresponding author upon reasonable request.
World Health Organization. WHO definition of palliative care [EB/OL]. [2019-07-10] http://www.who.int/cancer/palliative/definition/e .
Xinhua News Agency Basic Medical and Health Care. and Health Promotion Law of the People’s Republic of China [EB/OL].[2020-06-01]. http://www.xinhuanet.com/legal/2019-12/28/c_1125399629.htm .
National Health and Family Planning Commission. Notice on Issuing the National Nursing Development Plan (2016–2020) [EB/OL].
Li Fangfang. Qualitative research on hospice care experience of medical staff. J Nurs Adm. 2018;18(08):549–53.
Google Scholar
Liang LJ, Zhang G. Research on the Status of Hospice Care in Chinese Mainland. Practical Geriatr. 2018;32(01):20–2.
Wang, Mengying. Wang Xian.Development status and suggestions of domestic hospice care. J Nurs Adm. 2018;18(12):878–82.
Harris DG, Flowers S, Noble SI. Nurses’ views of the coping and support mechanisms experienced in managing terminal haemorrhage. Int J Palliat Nurs. 2011;17(1):7–13. https://doi.org/10.12968/ijpn.2011.17.1.7 .
Article PubMed Google Scholar
Abendroth M, Flannery J. Predicting the risk of compassion fatigue: a study of hospice professional. J Hospice Palliat Nurs. 2006;8(6):346–56.
Article Google Scholar
Funk LM, Peters S, Roger KS. The emotional labor of personal grief in palliative care: balancing caring and professional identities. Qual Health Res. 2017;27(14):2211–21.
Melvin CS. Professional compassion fatigue: what is the true cost of nurses caring for the dying? Int J Palliat Nurs. 2012;18(12):606–11.
Ferguson M, Carlson D, Zivnuska S, et al. Support at work and home: the path to satisfaction through balance. J Vocat Behav. 2012;80(2):299–307.
Huynh JY, Winefield AH, Xanthopoulou D, et al. Burnout and connectedness in the job demands–resources model: studying palliative care volunteers and their families. Am J Hospice Palliat Medicine®. 2012;29(6):462–75.
Jung M Y,Matthews A. K.Understanding nurses’experi-ences and perceptions of end-of-life care for cancer patients inKorea:a scoping review. J Palliat Care,2 0 2 1,3 6 (4):2 5 5 – 2 6.
Hotchkiss JT. Mindful self-care and secondary traumatic stress mediate a relationship between Compassion satisfaction and burnout risk among Hospice Care professionals. Am J Hospice Palliat Medicine®. 2018;35(8):1099–108. https://doi.org/10.1177/10499091187566 .
Yu H, Qiao A. Gui L. Predictors of compassion fatigue, burn-out, and compassion satisfaction among emergency nurses: a cross-sectional survey. Int Emerg Nurs. 2021, 55:100961. https://doi.org/10.1016/j.ienj.2020.100961 .
National Library of Medicine.Hospice Care[EB. /OL].[2018-08-24] http://medlinePlus.gov/hospicecare . html#summary.
Zheng Yue-ping, Ying-lan LI, Yao-hui WANG, et al. Attitudes of medical staff towards death and hospice care and its influencing factors [J]. Chin J Gerontol. 2011;31(24):4879–81. https://doi.org/10.3969/j.issn.1005-9202.2011.24.061 . (in Chinese).
Zheng Yue-ping, Ying-lan LI, Yang ZHOU. Chin Nurs Manage. 2010;10(4):53–5. https://doi.org/10.3969/j.issn.1672-1756.2010.04.019 .
Baugartez M, Hanley JA, Infante- Rivard I. Health of family members caring for elderly persons with dementia: a longitudinal study[J]. Ann Intern Med. 1994;120:126–32.
Hotchkiss JT. Mindful self-care and secondary traumatic stress mediate a relationship between Compassion satisfaction and burnout risk among Hospice Care professionals. Am J Hosp Palliat Care. 2018;35(8):1099–108. https://doi.org/10.1177/1049909118756657 .
Duan Qingnan Wang, Ziyu Xue, Yunzhen et al. Research progress on the correlation between mindfulness self-care and professional quality of life in hospice care practitioners. Nurs Res 2023,37(14):2557–63. https://doi.org/10.12102/j.issn.1009-6493.2023.14.013 .
Wu ML. Scale statistical analysis Practice[M]. Chongqing: Chongqing University; 2010. p. 1.
Wu ML. Statistical application practice of SPSS: Scale analysis and applied statistics. Beijing: Science; 2003. pp. 23–5.
Watkins MW. Exploratory factor analysis: a guide to best practice. J Black Psychol. 2018;44(3):219–46. https://doi.org/10.1177/0095798418771807 .
Li Zheng. Nursing research methods. Beijing: People’s Medical; 2018. p. 1.
Liu Ke. How to test the content validity. J Nurses’ Adv Study. 2010;25(1):37–9.
Kamer RS, Dieck EM, McClung J, et al. Effect of New York State’s donotresus citatelegislation in-hospital cardiopulmonary resuscitation practice[J]. Am J Med. 1990;88(2):108–11.
Article CAS PubMed Google Scholar
Polit D F, Beck C. T. The content validity index: are you sure you know what’s being reported? Critique and recommendations. Res Nurs Health. 2006;29(5):489–97.
Jiang Xiaohua S, Zhuozhi Z, Nannan L, Hongxiu. Xu Haiyan. Reliability and Validity Analysis of the Scale. Mod Prev Med. 2010;37(03):429–31.
Download references
Acknowledgements
In this study, the participants were medical staff, whose cooperation throughout the study was appreciated.
The study did not receive any funding.
Author information
Yanting Zhang and Li Zheng contributed equally to this work.
Hui Qiu and Liu Yang contributed equally to this work.
Authors and Affiliations
Department of Critical Care Medicine, Hubei Clinical Research Center for Critical Care Medicine, Zhongnan Hospital of Wuhan University, Wuhan, Hubei, 430071, China
Yanting Zhang
Department of Lung Cancer Radiotherapy and Chemotherapy, Zhongnan Hospital of Wuhan University, Wuhan, Hubei, 430071, China
Department of Gynecological Tumor Radiotherapy and Chemotherapy, Zhongnan Hospital of Wuhan University, Wuhan, Hubei, 430071, China
Yanling He, Min Han, Yu Wang, Jinyu Xv, Hui Qiu & Liu Yang
You can also search for this author in PubMed Google Scholar
Contributions
All listed authors have contributed substantially to the manuscript in the following ways: Z.Y.T (Conception, Design, Data Collection, Writer, Analysis and Interpretation); Z.L (Design, Data Processing, Writer, Analysis and Interpretation); H.Y.L, H.M, W.Y,X.J.Y, (Analysis and Interpretation, Literature Review); Q.H.(Literature Review, Writer, Critical Review); Y.L (Literature Review, Writer, Critical Review).
Corresponding authors
Correspondence to Hui Qiu or Liu Yang .
Ethics declarations
Ethics approval and consent to participate.
The purpose of this study was to develop and validate scales. All participants signed informed consent during the reliability and validity tests. This study was approved by the Ethics Committee of Zhongnan Hospital of Wuhan University [2022119 K], and the implementation of all methods in this study complied with the Declaration of Helsinki.
Consent to publish
Not applicable.
Competing interests
The authors declare no competing interests.
Additional information
Publisher’s note.
Springer Nature remains neutral with regard to jurisdictional claims in published maps and institutional affiliations.
Electronic supplementary material
Below is the link to the electronic supplementary material.
Supplementary Material 1
Rights and permissions.
Open Access This article is licensed under a Creative Commons Attribution 4.0 International License, which permits use, sharing, adaptation, distribution and reproduction in any medium or format, as long as you give appropriate credit to the original author(s) and the source, provide a link to the Creative Commons licence, and indicate if changes were made. The images or other third party material in this article are included in the article’s Creative Commons licence, unless indicated otherwise in a credit line to the material. If material is not included in the article’s Creative Commons licence and your intended use is not permitted by statutory regulation or exceeds the permitted use, you will need to obtain permission directly from the copyright holder. To view a copy of this licence, visit http://creativecommons.org/licenses/by/4.0/ . The Creative Commons Public Domain Dedication waiver ( http://creativecommons.org/publicdomain/zero/1.0/ ) applies to the data made available in this article, unless otherwise stated in a credit line to the data.
Reprints and permissions
About this article
Cite this article.
Zhang, Y., Zheng, L., He, Y. et al. Development and validation of the hospice professional coping scale among Chinese nurses. BMC Health Serv Res 24 , 491 (2024). https://doi.org/10.1186/s12913-024-10970-9
Download citation
Received : 25 July 2023
Accepted : 09 April 2024
Published : 20 April 2024
DOI : https://doi.org/10.1186/s12913-024-10970-9
Share this article
Anyone you share the following link with will be able to read this content:
Sorry, a shareable link is not currently available for this article.
Provided by the Springer Nature SharedIt content-sharing initiative
- Hospice care
- End-of-life patients
- Care burden
- Reliability
- Scale research
BMC Health Services Research
ISSN: 1472-6963
- General enquiries: [email protected]
- Open access
- Published: 19 April 2024
A first look at the reliability, validity and responsiveness of L-PF-35 dyspnea domain scores in fibrotic hypersensitivity pneumonitis
- Jeffrey J. Swigris ORCID: orcid.org/0000-0002-2643-8110 1 ,
- Kerri Aronson 2 &
- Evans R. Fernández Pérez 1
BMC Pulmonary Medicine volume 24 , Article number: 188 ( 2024 ) Cite this article
15 Accesses
Metrics details
Dyspnea impairs quality of life (QOL) in patients with fibrotic hypersensitivity pneumonitis (FHP). The Living with Pulmonary Fibrosis questionnaire (L-PF) assesses symptoms, their impacts and PF-related QOL in patients with any form of PF. Its scores have not undergone validation analyses in an FHP cohort.
We used data from the Pirfenidone in FHP trial to examine reliability, validity and responsiveness of the L-PF-35 Dyspnea domain score (Dyspnea) and to estimate its meaningful within-patient change (MWPC) threshold for worsening. Lack of suitable anchors precluded conducting analyses for other L-PF-35 scores.
At baseline, Dyspnea’s internal consistency (Cronbach’s coefficient alpha) was 0.85; there were significant correlations with all four anchors (University of California San Diego Shortness of Breath Questionnaire scores r = 0.81, St. George’s Activity domain score r = 0.82, percent predicted forced vital capacity r = 0.37, and percent predicted diffusing capacity of the lung for carbon monoxide r = 0.37). Dyspnea was significantly different between anchor subgroups (e.g., lowest percent predicted forced vital capacity (FVC%) vs. highest, 33.5 ± 18.5 vs. 11.1 ± 9.8, p = 0.01). There were significant correlations between changes in Dyspnea and changes in anchor scores at all trial time points. Longitudinal models further confirmed responsiveness. The MWPC threshold estimate for worsening was 6.6 points (range 5–8).
The L-PF-35 Dyspnea domain appears to possess acceptable psychometric properties for assessing dyspnea in patients with FHP. Because instrument validation is never accomplished with one study, additional research is needed to build on the foundation these analyses provide.
Trial registration
The data for the analyses presented in this manuscript were generated in a trial registered on ClinicalTrials.gov; the identifier was NCT02958917.
Peer Review reports
Introduction
Fibrotic hypersensitivity pneumonitis (FHP) is a form of fibrosing interstitial lung disease (fILD) that, like other fILDs is incurable, induces burdensome symptoms, confers the risk of shortened survival [ 1 , 2 ], and robs patients of their quality of life (QOL) [ 3 , 4 ]. Although in FHP there has not been as much research into the patient experience as with idiopathic pulmonary fibrosis (IPF), available data reveal that FHP-induced dyspnea, fatigue and cough affect how patients feel and function in their daily lives [ 3 , 4 ].
Given the potential for FHP to progress and respond poorly to immunosuppression and antigen avoidance (if one can be identified), Fernández Pérez and colleagues conducted a placebo-controlled trial of the antifibrotic, pirfenidone, in patients with FHP [ 5 ]. In that trial (Pirfenidone in FHP), among other patient-reported outcome measures (PROMs), the Living with Pulmonary Fibrosis (L-PF) questionnaire was used to examine the effects of pirfenidone on FHP-related QOL, symptoms and their impacts.
Here, we present findings from a hypothesis-based analysis of the reliability, validity and responsiveness of the Dyspnea domain from the 35-item L-PF (or L-PF-35; these 35 items are the same 35 that compose the Living with Idiopathic Pulmonary Fibrosis questionnaire (L-IPF) [ 6 ]).
The design and primary results for the single-center, double-blinded Pirfenidone in FHP trial (ClinicalTrials.gov identifier NCT02958917) from which the data for our analyses were generated have been published [ 5 ]. Briefly, 40 subjects with FHP were randomized 2:1 to receive pirfenidone or a matching placebo for 52 weeks. Study visits occurred at baseline, 13, 26, 39 and 52 weeks. At each visit, subjects completed three patient-reported outcome measures (PROMs) and performed spirometry to capture forced vital capacity (FVC). Diffusing capacity (DLCO) was assessed at baseline, 26 and 52 weeks only. This analysis was performed under an approved research protocol by the National Jewish Health central Institutional Review Board (HS# 3034).
PROMs used in the Pirfenidone in FHP trial
The l-pf-35 (living with pulmonary fibrosis 35-item questionnaire).
The L-PF-35 is designed to assess PF-related QOL, symptoms and their impacts. L-PF-35 is equivalent to the Living with Idiopathic Pulmonary Fibrosis Questionnaire (L-IPF) but with the word “idiopathic” removed from the title and a single item from the Impacts Module. L-IPF began as a 44-item questionnaire, but in a previously published validation study that included 125 patients with IPF, psychometric analyses supported reducing numbers from 44 to 35 items [ 6 ]. The intent of the developer of the L-PF is to have a single, 35-item questionnaire for all forms of PF (IPF and non-IPF, including FHP). Thus, although the 44-item version (again, with the word “idiopathic” removed) was administered in the Pirfenidone in FHP trial, our analyses here were conducted on the Dyspnea domain from the 35-item version resulting from the IPF analysis. From here on, we refer to this instrument as the L-PF-35.
Percentage-of-total-possible points is used to generate the Dyspnea domain, Cough domain, Energy/Fatigue domain, and Impacts module from the L-PF-35. The Symptoms module score is derived as the average of the Dyspnea, Cough and Energy/Fatigue domain scores. The total score is the average of the Symptoms and Impacts module scores. The Symptoms module contains 15 items (Dyspnea domain 7 items, Cough domain 5 items, Energy/Fatigue domain 3 items), each with a 24-hour recall period. The Impacts module contains 20 items, each with a 7-day recall period. The range for each of the six scores is 0-100, and higher scores connote greater impairment.
The SGRQ (St. George’s Respiratory Questionnaire)
The SGRQ is a 50-item questionnaire that yields four scores (total, Symptoms, Activity, Impacts). For the version used in the trial, the recall period for some items is three months and for others, it is “these days”. The range for each score is 0-100, and higher scores indicate worse respiratory health status [ 7 , 8 ].
The UCSD (University of California San Diego Shortness of Breath Questionnaire)
The UCSD is a 24-item questionnaire that assesses dyspnea severity while performing each of 21 activities, and it includes another 3 items that ask about limitations induced by shortness of breath [ 9 ]. Each item is scored on a 0–5 scale. There is no stated recall period. Scores range from 0 to 120, and higher scores indicate greater dyspnea severity.
Statistical analyses
Baseline data were tabulated and summarized using counts, percentages and measures of central tendency. We formulated hypotheses (included in the Supplementary material) for the L-PF-35 Dyspnea domain and conducted analyses in accordance with COSMIN recommendations for studies on the measurement properties of PROMs [ 10 , 11 ]. We used SGRQ Activity domain change scores, UCSD change scores, percent predicted FVC (FVC%) change, and percent predicted DLCO (DLCO%) change as anchors. Analyses included the following: (1) internal consistency and test-retest reliability, (2) convergent and known-groups analyses to assess content validity, (3) responsiveness, and (4) an estimation of the meaningful within-patient change (MWPC) threshold for worsening.
For applicable analyses, we defined worsening for the anchors in the following way: 1) ≥ 5 point increase for SGRQ Activity domain [ 12 , 13 ]; 2) ≥ 5 point increase in UCSD score [ 14 ]; 3) > 2% drop in FVC% (e.g., 70% to less than 68%) [ 15 ]; and 4) ≥ 5% drop in DLCO% (e.g., 70–65% or lower). Analyses were conducted in SAS, version 9.4 (SAS Institute Inc.; Cary, NC).
Internal consistency
We used Cronbach’s raw coefficient alpha as the measure of internal consistency (IC). Values > 0.7 are considered acceptable.
Test-retest reliability
We used a two-way mixed effects model for absolute agreement to generate the intraclass correlation coefficient (ICC (2,1)) as a measure of test-retest reliability of L-PF-35 Dyspnea domain scores (from baseline to week 26) among subjects considered stable according to change (also from baseline to week 26) scores for the various anchors. Values > 0.7 are considered acceptable.
Convergent and known-groups validity
Convergent validity was examined using pairwise Spearman correlations between L-PF-35 Dyspnea domain scores and anchors at baseline. We used analysis of variance with secondary, p-value corrected (Tukey) pairwise comparisons to look for statistically significant differences in L-PF-35 Dyspnea domain scores between most and least severe anchor subgroup strata (with anchors di- or trichotomized based on clinically relevant cut-points; e.g., FVC: ≤55, 55 < FVC < 70, or ≥ 70).
Responsiveness
We used pairwise correlation, longitudinal models and empirical cumulative distribution function (eCDF) plots to assess the responsiveness of L-PF-35 Dyspnea domain scores among subjects whose dyspnea changed as defined by the applicable anchor. In the correlational analyses, for 13-, 26-, 39- and 52-week timepoints, we examined pairwise Spearman correlations between L-PF-35 Dyspnea domain change scores and anchor change. In the modeling analyses, for each anchor, we built a repeated-measures, longitudinal model with L-PF-35 Dyspnea domain change score (from baseline to each subsequent time point) as the outcome variable and anchor change (from baseline to each subsequent time point) as the lone predictor variable. Visit (week 13, 26, 39, 52) was included in each model as a class variable, and an unstructured covariance structure was used (i.e., type = un in SAS). For the eCDF, we graphed the cumulative distribution of L-PF-35 Dyspnea domain change scores from baseline to week 26 for each of two dichotomized anchor change strata (worse vs. not at week 26 as defined above).
Meaningful within patient change (MWPC) threshold
We used predictive modeling (anchor as the outcome and L-PF-35 Dyspnea domain as the lone predictor) and adjustment for the correlation between L-PF-35 Dyspnea domain score change and anchor score change [ 16 ] to generate MWPC threshold estimates for worsening at 26 weeks. We used the method of Trigg and Griffiths [ 17 ] to generate a correlation-weighted point estimate.
Baseline characteristics and PROM scores from the trial population are presented in Table 1 . Most subjects were of non-Hispanic white ethnicity/race and supplemental oxygen users, with moderate pulmonary physiological impairment.
Internal consistency and test-retest reliability
IC for the L-PF-35 Dyspnea domain was at least 0.85 at all time points. Test-retest reliability (TRR) coefficients for L-PF-35 Dyspnea were 0.81 or greater for each anchor. Table S1 contains IC and TRR values.
Pairwise correlations at baseline are presented in Table 2 . Correlations between L-PF-35 Dyspnea domain scores and UCSD or SGRQ Activity scores are very strong, statistically significant and in the expected directions. Correlations between L-PF-35 Dyspnea and FVC% or DLCO% are low-moderately strong, statistically significant and in the expected directions.
Table 3 shows results for known-groups validity analyses. For each of the four anchors, compared to the least impaired anchor subgroup, L-PF-35 Dyspnea scores were significantly worse (i.e., higher and of large effect; e.g., worse by > 1 standard deviation) for the more impaired anchor subgroup.
Across study timepoints, 12 of 14 correlations between L-PF-35 Dyspnea domain score change and anchor change values were statistically significant and at least moderately strong (Table S2).
Longitudinal modeling showed significant ( p < 0.0001 for all) associations between L-PF-35 Dyspnea domain score change and anchor change values over the course of the trial (Fig. 1 ). Table S3 shows results for all longitudinal models.
eCDF plots of L-PF-35 Dyspnea domain 26-week change scores are displayed in Fig. 2 . They show separation between subgroups that worsened vs. not at 26 weeks according to each of the four anchors. Table 4 provides values of L-PF-35 Dyspnea domain 26-week change scores for the cohort using percentile cut-points.
MWPC threshold
Predictive modeling yielded estimates for MWPC for worsening in L-PF-35 Dyspnea domain scores of 6.3, 4.8, 8.0 and 6.9 for the four anchors: UCSD, SGRQ Activity, FVC%, and DLCO% respectively. The corresponding point-biserial correlations between L-PF-35 Dyspnea domain score change and the dichotomized UCSD, SGRQ Activity, FVC%, and DLCO% anchors (worse vs. not) were the following: 0.30, 0.49, 0.47, and 0.65. Thus, the weighted MWPC threshold estimate for worsening of L-PF-35 Dyspnea domain scores was 6.6 points (range 5–8).
In this study, we conducted analyses whose results offer a first glance at the psychometric properties of the L-PF-35 Dyspnea domain and support its reliability, validity and the responsiveness of its score as a measure of dyspnea in patients with FHP. Measurement experts and regulatory bodies have compiled criteria that, when met, deem clinical outcome assessments (COAs)– like PROMs– fit for the purpose of measuring outcomes in a target population [ 10 , 18 ]. The internal structure of the PROM must be sound, with sufficiently strong correlations among grouped items (internal consistency); PROM scores from respondents who are stable on the construct being measured should be similarly stable (test-retest reliability); PROM scores should differ between subgroups of respondents known– or hypothesized– to differ on the construct being measured (known-groups validity); and PROM scores should change for respondents who change on the underlying construct (responsiveness).
Because there are no gold standards for any of the constructs assessed by L-PF-35 scores (including dyspnea), anchors are employed as surrogates for gold standards, and hypotheses are formulated around the surrogates while incorporating the fit-for-purpose criteria outlined above. Anchors, themselves, must be suitable and ideally have undergone validity assessments of their own. Reassuringly, in their studies of patients with PF, other investigators have employed the anchors we used in our analyses [ 19 ]. Additionally, self-report anchors (like the UCSD and SGRQ Activity domain) generally surpass expert-endorsed suitability criteria [ 20 ], and the FVC and DLCO are universally accepted metrics of PF severity.
As hypothesized, the L-PF-35 Dyspnea domain surpassed the acceptability criteria (0.7) for internal consistency and test-retest reliability. Likewise, L-PF-35 Dyspnea domain scores distinguished respondents hypothesized to have the greatest dyspnea severity (e.g., those with the highest (worst) UCSD scores, highest (worst) SGRQ Activity scores, lowest FVC% or lowest DLCO%) from those with the least dyspnea severity. L-PF-35 Dyspnea domain change scores correlated with anchor change scores, and longitudinal modeling and eCDF plots further supported the L-PF-35 Dyspnea domain score as responsive to changes in dyspnea severity over time.
When the recall period for a PROM is 24 h, variability can be accommodated by averaging scores over a given time frame (e.g., a week). That was not done in the Pirfenidone in FHP trial. However, reassuringly, despite the difference in recall periods (L-PF-35 Dyspnea domain 24 h, UCSD no timeframe, SGRQ Activity domain three months), correlations between anchor change scores were generally moderately strong, statistically significant and always in the hypothesized directions. These results, and previously published data showing a < 1 point day-to-day variability in scores from the L-IPF Dyspnea domain scores over a 14 day period in 125 patients with IPF [ 6 ], provide indirect evidence that a single administration of L-PF-35 at each data collection timepoint/visit will likely suffice. And administration on consecutive days with averaging of scores is unlikely to yield significant differences from single administration.
In a previously published study, using different methodology than us, the MWPC threshold for deterioration in the L-PF-44 Dyspnea domain was estimated at 6–7 points in the INBUILD trial population (which included patients with all forms of PF, including FHP, who had progressed within 24 months of enrollment) [ 21 ]. The population in the Pirfenidone in FHP trial was similar to the INBUILD population; in both trials, subjects had to have fibrosis and meet the same progression criteria. In our MWPC analysis, we employed predictive modeling, which is argued to yield the most precise MWPC estimates [ 16 ]. We did not include distribution-based estimates, because they fail to capture patients’ perspectives, ignore the concept of “minimal”, and arguably, should not be included at all in MWPC estimates [ 22 , 23 ]. We used a weighting approach that appropriately incorporated the correlation between the L-PF-35 Dyspnea domain score change and anchor change. Doing so yields a less biased estimate than taking the mean or median of all estimates [ 17 ]. Regardless, it is reassuring that our point estimate perfectly aligns with the estimate generated from the INBUILD data.
Limitations
A lack of suitable anchors were available to conduct analyses for the other L-PF-35 scores, so those must be left for future studies (e.g., there were no cough or fatigue questionnaires included in the trial; SGRQ “total” and L-PF-35 “total” are similar in name but not necessarily in the constructs they capture. The same is true for the L-PF-35 Symptoms module and the SGRQ Symptoms domain). Moving forward, investigators would greatly help advance the science of measurement in the ILD field by including patient global impression (PGI) items for all the constructs being evaluated (e.g., here, these could have included PGI Dyspnea Severity, PGI Cough Severity/Frequency, PGI Fatigue Severity, PGI pulmonary fibrosis-related QOL or PGI general QOL). Additional limitations in our study include the low number of subjects (of predominantly the same ethnic/racial background) and the single-center design of the trial that generated the data, both of which potentially limit generalizing results to the broader FHP population. Because “validation” is not a threshold phenomenon and can not be achieved in a single study, our results should be viewed as only a first– but important– step in the process of confirming L-PF-35 Dyspnea domain scores as fit-for-purpose in this population. Additional research, including validation work, concept elicitation, and cognitive debriefing studies in patients with FHP and other non-IPF populations, is encouraged.
Conclusions
L-PF-35 Dyspnea domain scores appear to possess acceptable reliability, validity and responsiveness for assessing dyspnea severity in patients with FHP. Additional studies are needed to further support its validity and to assess the psychometric properties of the other five L-PF-35 scores for assessing their respective constructs. For now, it is reasonable to use 5–8 points as the estimated range for the MWPC threshold for worsening for the L-PF-35 Dyspnea domain in patients with FHP.
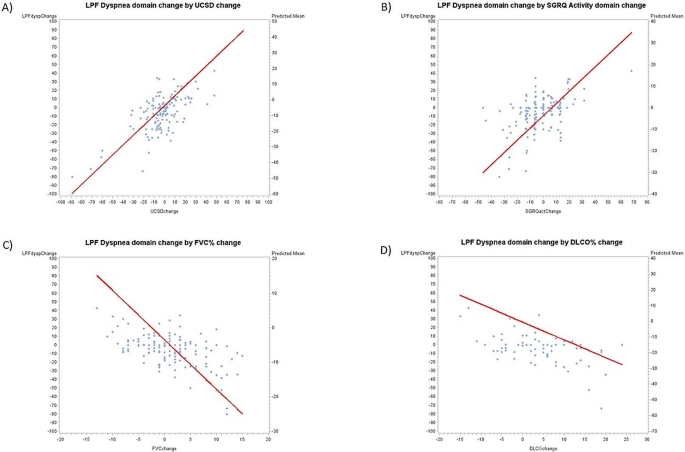
Results for mixed-effects longitudinal models showing the relationship between baseline-to-weeks 13/26/39/52 changes in L-PF-35 Dyspnea domain scores and baseline-to-weeks 13/26/39/52 changes in anchor values (Panel A: UCSD anchor, Panel B: SGRQ Activity Domain anchor, Panel C: FVC% anchor, Panel D: DLCO% anchor). Footnote: UCSD = University of California San Diego Shortness of Breath Questionnaire; SGRQ = St. George’s Respiratory Questionnaire; FVC% = percentage of the predicted forced vital capacity; DLCO% = percentage of the predicted diffusing capacity of the lung for carbon monoxide; L-PF = 35-item Living with Pulmonary Fibrosis Questionnaire
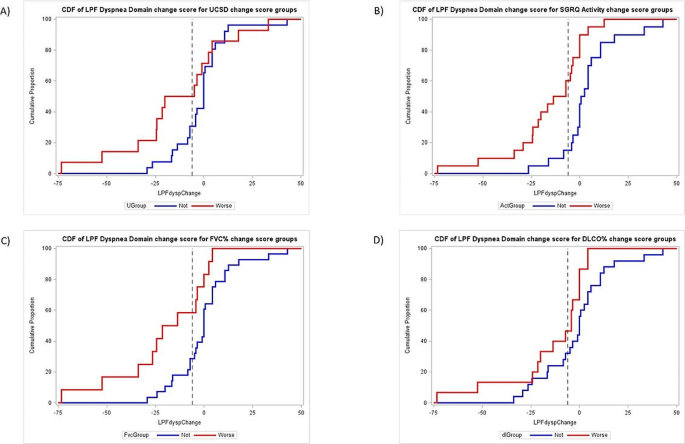
CDF (Cumulative Distribution Function) plots showing baseline-to-week 26 changes in L-PF-35 Dyspnea domain scores for subgroups defined by anchor change, worse or not from baseline to week 26 (Panel A: UCSD anchor, Panel B: SGRQ Activity Domain anchor, Panel C: FVC% anchor, Panel D: DLCO% anchor) values. Footnote: Red = worsened according to anchor; Blue = not worsened (stable/improved) according to anchor; UCSD = University of California San Diego Shortness of Breath Questionnaire; SGRQ = St. George’s Respiratory Questionnaire; FVC% = percentage of the predicted forced vital capacity; DLCO% = percentage of the predicted diffusing capacity of the lung for carbon monoxide; L-PF = 35-item Living with Pulmonary Fibrosis Questionnaire. Definitions for anchors worsened: 1) ≥ 5 point increase for SGRQ Activity domain; 2) ≥ 5 point increase in UCSD score; 3) > 2% drop in FVC% (e.g., 70% to less than 68%); and 4) ≥ 5% drop in DLCO% (e.g., 70–65% or lower)
Data availability
Data are not publicly available. Parties interested in accessing the data used in this study are encouraged to contact Dr. Fernandez Perez ([email protected]).
Fernandez Perez ER, Swigris JJ, Forssen AV, Tourin O, Solomon JJ, Huie TJ, Olson AL, Brown KK. Identifying an inciting antigen is associated with improved survival in patients with chronic hypersensitivity pneumonitis. Chest. 2013;144:1644–51.
Article PubMed PubMed Central Google Scholar
Hanak V, Golbin JM, Ryu JH. Causes and presenting features in 85 consecutive patients with hypersensitivity pneumonitis. Mayo Clin Proc. 2007;82:812–6.
Article PubMed Google Scholar
Aronson KI, Hayward BJ, Robbins L, Kaner RJ, Martinez FJ, Safford MM. It’s difficult, it’s life changing what happens to you’ patient perspective on life with chronic hypersensitivity pneumonitis: a qualitative study. BMJ Open Resp Res. 2019;6:e000522.
Article PubMed Central Google Scholar
Lubin M, Chen H, Elicker B, Jones KD, Collard HR, Lee JS. A Comparison of Health-Related Quality of Life in Idiopathic Pulmonary Fibrosis and Chronic Hypersensitivity Pneumonitis. Chest. 2014.
Fernandez Perez ER, Crooks JL, Lynch DA, Humphries SM, Koelsch TL, Swigris JJ, Solomon JJ, Mohning MP, Groshong SD, Fier K. Pirfenidone in fibrotic hypersensitivity pneumonitis: a double-blind, randomised clinical trial of efficacy and safety. Thorax. 2023.
Swigris JJ, Andrae DA, Churney T, Johnson N, Scholand MB, White ES, Matsui A, Raimundo K, Evans CJ. Development and initial validation analyses of the living with idiopathic pulmonary fibrosis questionnaire. Am J Respir Crit Care Med. 2020;202:1689–97.
Jones PW, Quirk FH, Baveystock CM. The St George’s Respiratory Questionnaire. Respiratory medicine 1991; 85 Suppl B: 25–31; discussion 33– 27.
Jones PW, Quirk FH, Baveystock CM, Littlejohns P. A self-complete measure of health status for chronic airflow limitation. The St. George’s respiratory questionnaire. Am Rev Respir Dis. 1992;145:1321–7.
Article CAS PubMed Google Scholar
Eakin EG, Resnikoff PM, Prewitt LM, Ries AL, Kaplan RM. Validation of a new dyspnea measure: the UCSD Shortness of Breath Questionnaire. University of California, San Diego. Chest. 1998; 113: 619–624.
Mokkink LB, Terwee CB, Patrick DL, Alonso J, Stratford PW, Knol DL, Bouter LM, de Vet HC. The COSMIN checklist for assessing the methodological quality of studies on measurement properties of health status measurement instruments: an international Delphi study. Qual life Research: Int J Qual life Aspects Treat care Rehabilitation. 2010;19:539–49.
Article Google Scholar
Terwee CB, Prinsen CAC, Chiarotto A, Westerman MJ, Patrick DL, Alonso J, Bouter LM, de Vet HCW, Mokkink LB. COSMIN methodology for evaluating the content validity of patient-reported outcome measures: a Delphi study. Qual life Research: Int J Qual life Aspects Treat care Rehabilitation. 2018;27:1159–70.
Article CAS Google Scholar
Swigris JJ, Brown KK, Behr J, du Bois RM, King TE, Raghu G, Wamboldt FS. The SF-36 and SGRQ: validity and first look at minimum important differences in IPF. Respir Med. 2010;104:296–304.
Swigris JJ, Wilson H, Esser D, Conoscenti CS, Stansen W, Kline Leidy N, Brown KK. Psychometric properties of the St George’s respiratory questionnaire in patients with idiopathic pulmonary fibrosis: insights from the INPULSIS trials. BMJ open Respiratory Res. 2018;5:e000278.
Chen T, Tsai APY, Hur SA, Wong AW, Sadatsafavi M, Fisher JH, Johannson KA, Assayag D, Morisset J, Shapera S, Khalil N, Fell CD, Manganas H, Cox G, To T, Gershon AS, Hambly N, Halayko AJ, Wilcox PG, Kolb M, Ryerson CJ. Validation and minimum important difference of the UCSD Shortness of Breath Questionnaire in fibrotic interstitial lung disease. Respir Res. 2021;22:202.
Article CAS PubMed PubMed Central Google Scholar
du Bois RM, Weycker D, Albera C, Bradford WZ, Costabel U, Kartashov A, King TE, Lancaster L, Noble PW, Sahn SA, Thomeer M, Valeyre D, Wells AU. Forced Vital Capacity in Patients with Idiopathic Pulmonary Fibrosis: Test Properties and Minimal Clinically Important Difference. American journal of respiratory and critical care medicine. 2011.
Terluin B, Eekhout I, Terwee C, De Vet H. Minimal important change (MIC) based on a predictive modeling approach was more precise than MIC based on ROC analysis. J Clin Epidemiol 2015; 68.
Trigg A, Griffiths P. Triangulation of multiple meaningful change thresholds for patient-reported outcome scores. Qual life Research: Int J Qual life Aspects Treat care Rehabilitation. 2021;30:2755–64.
US Department of Health and Human Services and the Food and Drug Administration (CDER). Guidance for Industry, Food and Drug Administration Staff, and other stakeholders: patient-focused Drug Development. Incorporating Clinical Outcome Assessments Into Endpoints for Regulatory Decision-Making Silver Spring, MD; 2023.
Swigris JJ, Esser D, Conoscenti CS, Brown KK. The psychometric properties of the St George’s respiratory questionnaire (SGRQ) in patients with idiopathic pulmonary fibrosis: a literature review. Health Qual Life Outcomes. 2014;12:124.
Devji T, Carrasco-Labra A, Qasim A, Phillips M, Johnston BC, Devasenapathy N, Zeraatkar D, Bhatt M, Jin X, Brignardello-Petersen R, Urquhart O, Foroutan F, Schandelmaier S, Pardo-Hernandez H, Vernooij RW, Huang H, Rizwan Y, Siemieniuk R, Lytvyn L, Patrick DL, Ebrahim S, Furukawa T, Nesrallah G, Schunemann HJ, Bhandari M, Thabane L, Guyatt GH. Evaluating the credibility of anchor based estimates of minimal important differences for patient reported outcomes: instrument development and reliability study. BMJ. 2020;369:m1714.
Swigris JJ, Bushnell DM, Rohr K, Mueller H, Baldwin M, Inoue Y. Responsiveness and meaningful change thresholds of the living with pulmonary fibrosis (L-PF) questionnaire Dyspnoea and Cough scores in patients with progressive fibrosing interstitial lung diseases. BMJ open Respiratory Res 2022; 9.
Swigris J, Foster B, Johnson N. Determining and reporting minimal important change for patient-reported outcome instruments in pulmonary medicine. Eur Respir J 2022; 60.
Terwee CB, Peipert JD, Chapman R, Lai JS, Terluin B, Cella D, Griffith P, Mokkink LB. Minimal important change (MIC): a conceptual clarification and systematic review of MIC estimates of PROMIS measures. Qual life Research: Int J Qual life Aspects Treat care Rehabilitation. 2021;30:2729–54.
Download references
Acknowledgements
Authors’ information (This is optional): N/A.
There was no funding for this study. Genentech/Roche was the sponsor of the Pirfenidone in Chronic HP trial.
Author information
Authors and affiliations.
Center for Interstitial Lung Disease, National Jewish Health, 1400 Jackson Street, G07, 80206, Denver, CO, USA
Jeffrey J. Swigris & Evans R. Fernández Pérez
Division of Pulmonary and Critical Care Medicine, Weill Cornell College of Medicine, New York, NY, USA
Kerri Aronson
You can also search for this author in PubMed Google Scholar
Contributions
Study conceptualization: JJS, KA, ERFP. Data acquisition: ERFP. Data analysis: JJS. Interpretation of results: JJS, KA, ERFP. Manuscript preparation and approval of submitted version: JJS, KA, ERFP.
Corresponding author
Correspondence to Jeffrey J. Swigris .
Ethics declarations
Ethics approval and consent to participate.
This analysis was performed under an approved research protocol by the National Jewish Health central Institutional Review Board (HS# 3034). All methods were carried out in accordance with relevant guidelines and regulations. Informed consent was obtained from all subjects enrolled in the trial.
Consent for publication
Not applicable.
Competing interests
JJS is the developer of L-PF-44, L-PF-35 and other questionnaires designed to assess outcomes in patients with various forms of interstitial lung disease. KA and ERFP report no conflict related to this study.
Additional information
Publisher’s note.
Springer Nature remains neutral with regard to jurisdictional claims in published maps and institutional affiliations.
Take-home message: Our analyses begin to build the foundation supporting scores from the 35-item Living with Pulmonary Fibrosis Dyspnea domain as possessing psychometric characteristics that make it a suitable measure of dyspnea severity in patients with fibrotic hypersensitivity pneumonitis. The estimate for the meaningful within patient threshold for deterioration in this patient population is 6.6 points with a range of 5–8.
Electronic supplementary material
Below is the link to the electronic supplementary material.
Supplementary Material 1
Rights and permissions.
Open Access This article is licensed under a Creative Commons Attribution 4.0 International License, which permits use, sharing, adaptation, distribution and reproduction in any medium or format, as long as you give appropriate credit to the original author(s) and the source, provide a link to the Creative Commons licence, and indicate if changes were made. The images or other third party material in this article are included in the article’s Creative Commons licence, unless indicated otherwise in a credit line to the material. If material is not included in the article’s Creative Commons licence and your intended use is not permitted by statutory regulation or exceeds the permitted use, you will need to obtain permission directly from the copyright holder. To view a copy of this licence, visit http://creativecommons.org/licenses/by/4.0/ . The Creative Commons Public Domain Dedication waiver ( http://creativecommons.org/publicdomain/zero/1.0/ ) applies to the data made available in this article, unless otherwise stated in a credit line to the data.
Reprints and permissions
About this article
Cite this article.
Swigris, J.J., Aronson, K. & R. Fernández Pérez, E. A first look at the reliability, validity and responsiveness of L-PF-35 dyspnea domain scores in fibrotic hypersensitivity pneumonitis. BMC Pulm Med 24 , 188 (2024). https://doi.org/10.1186/s12890-024-02991-1
Download citation
Received : 25 July 2023
Accepted : 02 April 2024
Published : 19 April 2024
DOI : https://doi.org/10.1186/s12890-024-02991-1
Share this article
Anyone you share the following link with will be able to read this content:
Sorry, a shareable link is not currently available for this article.
Provided by the Springer Nature SharedIt content-sharing initiative
- Hypersensitivity pneumonitis
- Patient-reported outcome
BMC Pulmonary Medicine
ISSN: 1471-2466
- Submission enquiries: [email protected]
- General enquiries: [email protected]
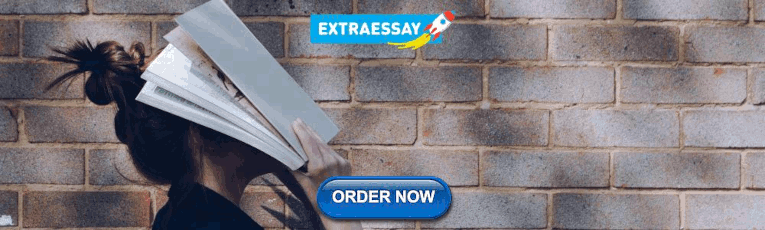
IMAGES
VIDEO
COMMENTS
Reliability is about the consistency of a measure, and validity is about the accuracy of a measure.opt. It's important to consider reliability and validity when you are creating your research design, planning your methods, and writing up your results, especially in quantitative research. Failing to do so can lead to several types of research ...
For research purposes, a minimum reliability of .70 is required for attitude instruments. Some researchers feel that it should be higher. A reliability of .70 indicates 70% consistency in the scores that are produced by the instrument. Many tests, such as achievement tests, strive for .90 or higher reliabilities.
Reliability is a key concept in research that measures how consistent and trustworthy the results are. In this article, you will learn about the four types of reliability in research: test-retest, inter-rater, parallel forms, and internal consistency. You will also find definitions and examples of each type, as well as tips on how to improve reliability in your own research.
A similar type of reliability called alternate forms, involves using slightly different forms or versions of an instrument to see if different versions yield consistent results. Inter-rater reliability checks the degree of agreement among raters (i.e., those completing items on an instrument). Common situations where more than one rater is ...
What Is Reliability? As with validity, reliability is an attribute of a measurement instrument - for example, a survey, a weight scale or even a blood pressure monitor. But while validity is concerned with whether the instrument is measuring the "thing" it's supposed to be measuring, reliability is concerned with consistency and stability.In other words, reliability reflects the degree ...
Reliability refers to the consistency of the measurement. Reliability shows how trustworthy is the score of the test. If the collected data shows the same results after being tested using various methods and sample groups, the information is reliable. If your method has reliability, the results will be valid. Example: If you weigh yourself on a ...
Reliability and validity are among the most important and fundamental domains in the assessment of any measuring methodology for data-collection in a good research. Validity is about what an instrument measures and how well it does so, whereas reliability concerns the truthfulness in the data obtained and the degree to which any measuring tool ...
Research reliability refers to the consistency, stability, and repeatability of research findings. It indicates the extent to which a research study produces consistent and dependable results when conducted under similar conditions. ... The quality and characteristics of the measurement instrument can impact reliability. If the instrument lacks ...
Reliability is consistency across time (test-retest reliability), across items (internal consistency), and across researchers (interrater reliability). Validity is the extent to which the scores actually represent the variable they are intended to. Validity is a judgment based on various types of evidence.
Learn how to design and measure quantitative research with excellence and validity from this comprehensive article.
Hence, the essence of reliability for qualitative research lies with consistency.[24,28] A margin of variability for results is tolerated in qualitative research provided the methodology and epistemological logistics consistently yield data that are ontologically similar but may differ in richness and ambience within similar dimensions.
Validity. Validity is defined as the extent to which a concept is accurately measured in a quantitative study. For example, a survey designed to explore depression but which actually measures anxiety would not be considered valid. The second measure of quality in a quantitative study is reliability, or the accuracy of an instrument.In other words, the extent to which a research instrument ...
2. Research Objectives The objectives of the study are as follows: i) To analyse the reliability of the ILS, SPCD, and CMAT instruments; ii) To analyse the value of separation index in the ILS, SPCD, and CMAT instruments; iii) To distinguish the sufficiency of PTMEA and item fit in defining the terms in research instruments; and iv) To analyse ...
In (1), J denotes the output of the integration process, s i is the scale value assigned to consideration i, and n is the number of considerations taken into account. The scale values represent the implications of the consideration—that is, the answer it points to—for the particular question. Equation (1) applies equally well when an existing evaluation is the only consideration taken into ...
Validity in research refers to how accurately a study answers the study question or the strength of the study conclusions. ... Discuss whether the changes are likely to affect the reliability or validity of the instrument. Researchers who create novel assessment instruments need to state the development process, reliability measures, pilot ...
The research instrument is comprised of six structural parts to examine every kind of skills noticed above. The time to fulfill the tasks is regulated. The sufficient validity and reliability of ...
Validity is the extent to which an instrument measures what it is supposed to measure and performs as it is designed to perform. It is rare, if nearly impossible, that an instrument be 100% valid, so validity is generally measured in degrees. As a process, validation involves collecting and analyzing data to assess the accuracy of an instrument.
Validity and Reliability of the Research Instrument. This entry is adapted from the peer-reviewed paper 10.2139/ssrn.3205040. Questionnaire is one of the most widely used tools to collect data in especially social science research. The main objective of questionnaire in research is to obtain relevant information in most reliable and valid manner.
the validity and reliability of a questionnaire/survey and also discusses various forms of validity and reliability tests. Key Words Research Instrument, Questionnaire, Survey, Survey Validity, Questionnaire Reliability, Content Validity, Face Validity, Construct Validity, and Criterion Validity. I. INTRODUCTION
Abstract and Figures. The validity and reliability of the scales used in research are important factors that enable the research to yield healthy results. For this reason, it is useful to ...
The aim of this paper is to investigate the problem of reliability for non-material instruments, the instruments being applied in the social sciences. Any possible lack of reliability of the ...
Instrument Reliability. Instrument reliability is a way of ensuring that any instrument used for measuring experimental variables gives the same results every time. In the physical sciences, the term is self-explanatory, and it is a matter of making sure that every piece of hardware, from a mass spectrometer to a set of weighing scales, is ...
The Cronbach's α coefficients of each dimension of the scale were 0.920, 0.889, 0.938 and 0.910 respectively, and the half-and-half reliability coefficients were 0.846, 0.817, 0.891 and 0.832, respectively, while the Cronbach's α coefficient and half-and-half reliability coefficient of the total scale were 0.963 and 0.927, respectively, all ≥ 0.7, indicating that the scale had good ...
This paper primarily focuses explicitly on two terms namely; reliability and validity as used in. the field of educational research. When conducting any educa tional study it is worth noting that ...
The MWPC threshold estimate for worsening was 6.6 points (range 5-8). The L-PF-35 Dyspnea domain appears to possess acceptable psychometric properties for assessing dyspnea in patients with FHP. Because instrument validation is never accomplished with one study, additional research is needed to build on the foundation these analyses provide.
This study aimed to describe people's panic in a closed area building during a disaster. The research conducted is using descriptive methods with the instrument Panic Spreading Questionnaire. The panic-spreading questionnaire has 14 items already valid and has a very high level of reliability based on the field test. The result is that people will panic when a disaster happens in a closed-area ...