Prescriptive analytics: Literature review and research challenges
- Related Documents
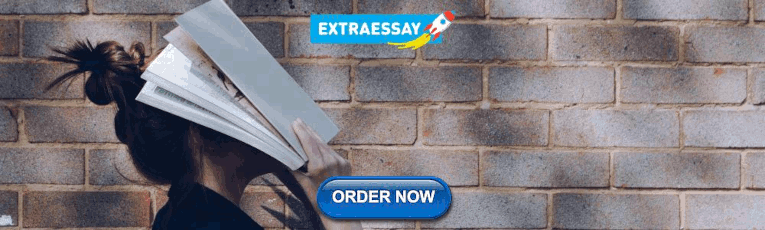
A Systematic Literature Review of Open Government Data Research: Challenges, Opportunities and Gaps
Optimization of fast fashion retail supply chain processes: overall literature review and future research challenges.
Fast fashion trends are changing in nonstop. Brands are consequently faced to big challenges. Processes must be fluent, while costs must be optimized within all the constraints. This article is a literature review, about the fast fashion process engineering challenges, from production to retail. We explore a review analysis of the main problematic nodes, of the whole chain, that retailers focus on to improve their productivity and profit. We examine the literature review of solutions proposed to these problems. Finally we propose the untapped fields, and the potential research scopes to work on in future.
Extended Reality in Spatial Sciences: A Review of Research Challenges and Future Directions
This manuscript identifies and documents unsolved problems and research challenges in the extended reality (XR) domain (i.e., virtual (VR), augmented (AR), and mixed reality (MR)). The manuscript is structured to include technology, design, and human factor perspectives. The text is visualization/display-focused, that is, other modalities such as audio, haptic, smell, and touch, while important for XR, are beyond the scope of this paper. We further narrow our focus to mainly geospatial research, with necessary deviations to other domains where these technologies are widely researched. The main objective of the study is to provide an overview of broader research challenges and directions in XR, especially in spatial sciences. Aside from the research challenges identified based on a comprehensive literature review, we provide case studies with original results from our own studies in each section as examples to demonstrate the relevance of the challenges in the current research. We believe that this paper will be of relevance to anyone who has scientific interest in extended reality, and/or uses these systems in their research.
Research Challenges in Adaptive Case Management: A Literature Review
Estimating peak water demand: literature review of current standing and research challenges, who gives “voice” or “empowers migrants” in participatory action research challenges and solutions.
Given the power relationships between researchers and participants in Participatory Action Research (PAR), this chapter challenges the assumption that migration researchers “give voice” or “empower” participants, and advances the idea that such researchers need to uncover their own voice in the research process through dialogue, interaction and reflection with their partners. In the literature review on PAR, the concept of “giving voice” is quite prevalent yet based on the author’s own qualitative/migration research, she would argue that the actual voice of participants themselves is seldom emphasized or revealed in qualitative/migration research. Paulo Freire’s concepts of dialogue, conscientization, and action for change underscored by his interpretation of voice, which recognizes that marginalized people’s voices emerge out of the conditioned silence created by differential power dynamics, is critically needed as grounding for PAR researchers. In critiquing the use of voice, the conclusion makes a plea for PAR researchers to engage in finding their own voice by embracing the notion of cultural humility.
The Tornado Warning Process: A Review of Current Research, Challenges, and Opportunities
With the unusually violent tornado season of 2011, there has been a renewed national interest, through such programs as NOAA's Weather Ready Nation initiative, to reevaluate and improve our tornado warning process. This literature review provides an interdisciplinary, end-to-end examination of the tornado warning process. Following the steps outlined by the Integrated Warning System, current research in tornado prediction and detection, the warning decision process, warning dissemination, and public response are reviewed, and some of the major challenges for improving each stage are highlighted. The progress and challenges in multi-day to short-term tornado prediction are discussed, followed by an examination of tornado detection, focused primarily upon the contributions made by weather radar and storm spotters. Next is a review of the warning decision process and the challenges associated with dissemination of the warning, followed by a discussion of the complexities associated with understanding public response. Finally, several research opportunities are considered, with emphases on understanding acceptable risk, greater community and personal preparation, and personalization of the hazard risk.
Ground-coupled heat pumps: Part 1 – Literature review and research challenges in modeling and optimal control
Internet of things for smart cities: current issues and research challenges.
Every day, we are stepping towards to lead a smart life within a smart world, thanks of IoT smart applications. The continually need for new urban systems including smart infrastructures, smart energy grids and smart mobility systems makes appear of a new concept, named: “Smart City”. This concept represents one of the most promising challenges of IoT applications since it involves the enhancement of our lifestyle. Among its promising advantage we can cites: the reducing resource consumption, the real-time guidance for citizens, the transportation facilities, etc. In this paper, we propose, first, a literature review on researches addressing many aspects of Smart City. Second, we provide a comparative study between these researches on the basic of multiple criteria like interoperability, scalability, security, etc.
Social innovation for biodiversity: A literature review and research challenges
Export citation format, share document.
Academia.edu no longer supports Internet Explorer.
To browse Academia.edu and the wider internet faster and more securely, please take a few seconds to upgrade your browser .
Enter the email address you signed up with and we'll email you a reset link.
- We're Hiring!
- Help Center
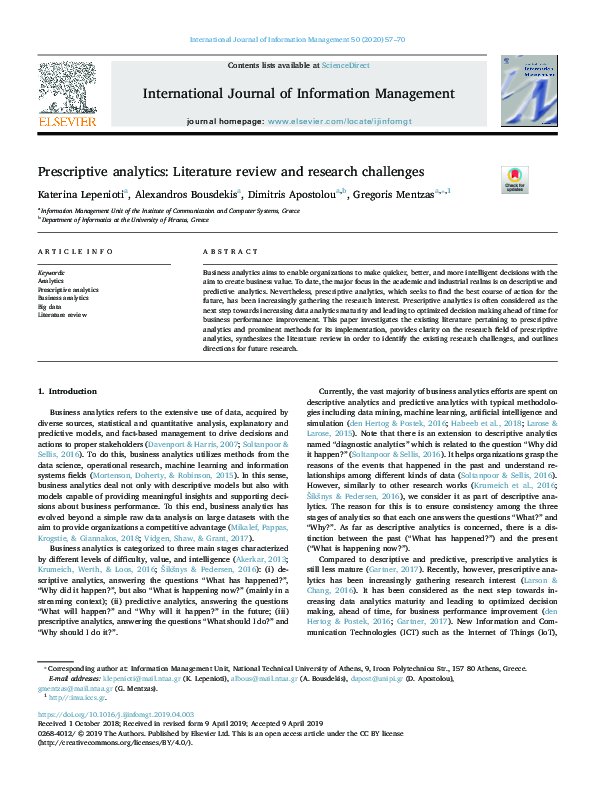
Prescriptive analytics: Literature review and research challenges

2020, International Journal of Information Management
Related Papers
The VLDB Journal
Laurynas Siksnys
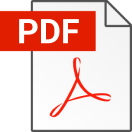
Journal of Computer Science IJCSIS
Data is vantage and valuable. Across world, " big data " and analytics are helping businesses to become smarter, more productive, and better at making predictions. The internet has engendered an explosion in data growth in the form of data sets, called Big Data, that are so large they are difficult to store, manage and analyze using traditional RDBMS which are tuned for Online Transaction Processing (OLTP) only. Big Data is changing the way analytics were commonly viewed, from data mining to Advanced Analytics. Big Data is composed of text, image, video, audio, and mobile or other forms of data collected from multiple datasets, and is rapidly growing in size and complexity. It has created a huge volume of multidimensional data within a very short time period. A real-world application could be modelled as a multi objective, dynamic, large scale optimization problem. It is recognized that the prescriptive analytics based techniques are good ways to handle this kind of problems. Based on the utilization of business intelligence systems, the real-world system will be more efficient and effective. We crystallize the availability of new in-memory technology high-performance analytics and prescriptive analytics that works on business intelligence systems in providing a better way to analyze data more quickly than ever.
Dr-Kartick Mondal
A B S T R A C T The effective development of advanced data analytics solutions requires tackling challenges such as eliciting analytical requirements, designing the machine learning solution, and ensuring the alignment between analytics initiatives and business strategies, among others. The use of conceptual modeling methods and techniques is seen to be of considerable value in overcoming such challenges. This paper proposes a modeling framework (including a set of metamodels and a set of design catalogues) for requirements analysis and design of data analytics systems. It consists of three complementary modeling views: business view, analytics design view, and data preparation view. These views are linked together to connect enterprise strategies to analytics algorithms and to data preparation activities. The framework includes a set of design catalogues that codify and represent an organized body of business analytics design knowledge. As the first attempt to validate the framework, three real-world data analytics case studies are used to illustrate the expressiveness and usability of the framework. Findings suggest that the framework provides an adequate set of concepts to support the design and implementation of analytics solutions. 1. Introduction The effective design and implementation of advanced data analytics solutions has proven to be difficult. Despite the hype around data analytics, many organizations struggle to find how to use analytics to derive value and gain competitive advantage [1]. Requirements elicitation for data analytics systems is a complex task [2,3]. Analytics requirements are often vague and incomplete at the early phases of projects. While business stakeholders often have a clear understanding of their strategic goals (e.g., improve marketing campaigns, reduce inventory levels), they are not clear on how analytics can help them achieve those goals. This is, to a great extent, due to the huge conceptual distance between business strategies, decision processes and organizational performance on one hand, and the implementation of analytics systems in terms of databases, preprocessing activities, and machine learning algorithms on the other hand. Previous researches report that the leading barrier to using analytics techniques is the lack of understanding of how to use analytics and unlock its value to improve the business [4,5]. Moreover, designing analytics solutions involves making critical design decisions, taking into account quality requirements (i.e., softgoals) and tradeoffs [6]. Analytics requirements, once discovered and elaborated, must lead to solution design and implementations that includes choice of algorithms, machine learning experimentations, and data preparation activities. A large number of machine learning and data mining algorithms exist and new ones are being developed continuously. During analytics projects, one needs to make design choices such as: what are potential algorithms that can address the problem at hand? What criteria should be considered to evaluate those algorithms? What/how data should be prepared to be used by algorithms? These decisions have important implications in several aspects of the eventual analytics solution, such as scalability, understandability, tolerance to noisy data and missing values.
Massimo Ruffolo , Saverino Verteramo
Abstract In many industries and business areas the demand of Business Analytics (BA) tools is growing as executives and managers from strategy to operations demand better means to critically validate their intuition with data so to increase confidence in decision-making. Nevertheless currently existing BA tools are not broadly adopted across the organizations.
International Journal for Research in Applied Science & Engineering Technology (IJRASET)
IJRASET Publication
Business analytics is primarily about getting the most out of data. Data has lately been dubbed "the new oil" rather than the "sludge of the information era." While data can be used to develop new products and services, identify market niches, and spot new opportunities, it is also notoriously amorphous and difficult to extract value from. It involves different steps to get the insights from the data present majorly involving approaches like Aligning strategy, desired behaviors, and business performance management with analytical activities and capabilities is necessary to derive value from data. This article uses both conventional and qualitative research methods to examine the expanding body of work on business analytics (BA).In this paper, an attempt is being made to review several viewpoints on how business analytics is defined and how it relates to business intelligence. Additionally, we highlight business education and demonstrate how business analytics are applied in both company and industrial sectors. I. INTRODUCTION Big data is a fast-growing discipline being used to define and analyze huge quantities of data present in various forms organized, semistructured, and non-structured data from multiple enormous and complex sources. Data is rapidly growing in every sector, making it a quickly growing to enforce used to define and analyze massive volumes of data present in various forms of organized, semistructured, and non-structured data from various immense and complicated sources. The method also necessitates the use of sophisticated data-processing technologies and advanced analytic programs. Big data has changed the way businesses and organizations operate. Companies of all sizes and industries may benefit from big data applications. According to corporate groups, such benefits might boost productivity, revenue, and growth.1 Many organizations are implementing big data tools and complicated statistical applications to improve quality in areas such as operations, customer happiness or satisfaction from the deployed process, and loyalty, as well as to strengthen overall standards of corporate governance and combat malicious activities such as fraud, cyber attacks, embezzlement, and other financial crimes, which have recently increased. Apart from that, big data has a variety of business applications. High-quality essays on both theoretical and practical knowledge of big data in business will be included in this special issue.2 Statistical procedures (analysis of variance (ANOVA, tables and charts, and so on), data software applications (data mining, sorting routines), and market methodological approaches are all used to explore, visualize, identify, and further communicate the patterns or trends existing in data (linear programming). Broadly said, analytics is the conversion of data into useful knowledge. Analytics is an older term that today refers to a wide number of disciplines, not only business. A notable example of how analytics can be employed is the collecting and translation of meteorological data into statistics, which are then used to anticipate weather patterns.3 Business analytics is described as the art of discovering insight via the use of complex mathematical, analytical, machine learning, and network science methodologies, as well as a variety of data and expert knowledge. It aids in speedy decision-making. Business analytics may be considered a tool for resolving problems and making decisions. Indeed, business analytics is a subgroup of analytics that employs the utilization of tools, techniques, and other statistical ideas to solve more complicated business problems. Analytics is often used by businesses to explain, predict, and improve their performance. As data grows, it contains insights that, when used properly, may result in productive outputs and provide value for the firm. Because of its growing popularity as a term, analytics is being used to replace a variety of previously popular ideas such as intelligence, mining, and discovery. For example, business intelligence is now known as business analytics, whereas customer intelligence is now known as customer analytics, Web mining is now known as Web analytics, and knowledge discovery is now known as data analytics. Because of the number, diversity, and speed with which data is generated-i.e., big data-modern analytics may necessitate a significant amount of computation, as well as the tools, methodologies, and algorithms used in analytics projects. The special issue on big data in company or organization accepted five accomplishments in the areas of business innovativeness in the big data era, non-structured big data analytical methods and techniques in firms, advanced analytical approach for business analytics in big data, geospatial deep insight for retail proposition using similarity metric, and big data as well as modifications through interactive data visualisation.4
Main focus of any organization functioning in today's competitive marketplace is to gain and sustain competitive advantage. With the huge volumes of data stored in databases, data marts and data warehouses coupled with advanced data analysis tools, managers are now in a better position to make smart and effective decisions which result in competitive advantage for their organizations. Business Analytics (BA) is a new and upcoming area of advanced data analysis that has emerged as a significant area of study for both researchers as well as practitioners over the last two decades. BA is the process of transforming huge volumes of data into new knowledge through analysis and using that knowledge for effective decision making and problem solving which ultimately results in value-creating competitive actions. Keeping in view the importance of Business Analytics, this paper discusses the concept of Business Analytics, its framework and application.
Decision Support Systems
Technical Report, University of Tallinn, 2013.
Amir Mosavi
By utilizing the statistical analysis, analytics, information processing and business intelligence the business processes are understood and decisions are made aiming to improve profitability. Yet due to the involvement of big data, highly non-linear and multicriteria nature of decision making scenarios in today’s governance programs the complex analytics models create significant business, operational and technology risks as well as modeling errors presenting the lack of effective modeling system to governance programs. Consequently the traditional approaches have been reported less useful in proper guiding decision-making communication and in drawing insights from big data. Alternatively here the proposed methodology of integration of data mining, modeling and interactive decision-making is studied as an effective approach where what-if scenarios are evaluated and optimization-based decisions are made.
Ethnographic Praxis in Industry Conference Proceedings
Samy Megahed
RELATED TOPICS
- We're Hiring!
- Help Center
- Find new research papers in:
- Health Sciences
- Earth Sciences
- Cognitive Science
- Mathematics
- Computer Science
- Academia ©2024
See discussions, stats, and author profiles for this publication at: https://www.researchgate.net/publication/338970341
Prescriptive analytics : Literature review and research challenges
Article in International Journal of Information Management · February 2020 DOI: 10.1016/j.ijinfomgt.2019.04.003
CITATIONS READS 8 336
4 authors, including:
Alexandros Bousdekis National Technical University of Athens
24 PUBLICATIONS 175 CITATIONS
SEE PROFILE
Some of the authors of this publication are also working on these related projects:
UPTIME - Unified Predictive Maintenance System View project
All content following this page was uploaded by Alexandros Bousdekis on 05 February 2020.
The user has requested enhancement of the downloaded file. International Journal of Information Management 50 (2020) 57–70
Contents lists available at ScienceDirect
International Journal of Information Management
journal homepage: www.elsevier.com/locate/ijinfomgt
Prescriptive analytics: Literature review and research challenges T ⁎ Katerina Lepeniotia, Alexandros Bousdekisa, Dimitris Apostoloua,b, Gregoris Mentzasa, ,1 a Information Management Unit of the Institute of Communication and Computer Systems, Greece b Department of Informatics at the University of Piraeus, Greece
ARTICLE INFO ABSTRACT
Keywords: Business analytics aims to enable organizations to make quicker, better, and more intelligent decisions with the Analytics aim to create business value. To date, the major focus in the academic and industrial realms is on descriptive and Prescriptive analytics predictive analytics . Nevertheless, prescriptive analytics, which seeks to find the best course of action forthe Business analytics future, has been increasingly gathering the research interest. Prescriptive analytics is often considered as the Big data next step towards increasing data analytics maturity and leading to optimized decision making ahead of time for Literature review business performance improvement. This paper investigates the existing literature pertaining to prescriptive analytics and prominent methods for its implementation, provides clarity on the research field of prescriptive analytics, synthesizes the literature review in order to identify the existing research challenges, and outlines directions for future research.
1. Introduction Currently, the vast majority of business analytics efforts are spent on descriptive analytics and predictive analytics with typical methodolo- Business analytics refers to the extensive use of data, acquired by gies including data mining , machine learning, artificial intelligence and diverse sources, statistical and quantitative analysis, explanatory and simulation (den Hertog & Postek, 2016; Habeeb et al., 2018; Larose & predictive models, and fact-based management to drive decisions and Larose, 2015). Note that there is an extension to descriptive analytics actions to proper stakeholders (Davenport & Harris, 2007; Soltanpoor & named “diagnostic analytics” which is related to the question “Why did Sellis, 2016). To do this, business analytics utilizes methods from the it happen?” (Soltanpoor & Sellis, 2016). It helps organizations grasp the data science, operational research, machine learning and information reasons of the events that happened in the past and understand re- systems fields (Mortenson, Doherty, & Robinson, 2015). In this sense, lationships among different kinds of data (Soltanpoor & Sellis, 2016). business analytics deal not only with descriptive models but also with However, similarly to other research works (Krumeich et al., 2016; models capable of providing meaningful insights and supporting deci- Šikšnys & Pedersen, 2016), we consider it as part of descriptive ana- sions about business performance. To this end, business analytics has lytics. The reason for this is to ensure consistency among the three evolved beyond a simple raw data analysis on large datasets with the stages of analytics so that each one answers the questions “What?” and aim to provide organizations a competitive advantage (Mikalef, Pappas, “Why?”. As far as descriptive analytics is concerned, there is a dis- Krogstie, & Giannakos, 2018; Vidgen, Shaw, & Grant, 2017). tinction between the past (“What has happened?”) and the present Business analytics is categorized to three main stages characterized (“What is happening now?”). by different levels of difficulty, value, and intelligence (Akerkar, 2013; Compared to descriptive and predictive, prescriptive analytics is Krumeich, Werth, & Loos, 2016; Šikšnys & Pedersen, 2016): (i) de- still less mature (Gartner, 2017). Recently, however, prescriptive ana- scriptive analytics, answering the questions “What has happened?”, lytics has been increasingly gathering research interest (Larson & “Why did it happen?”, but also “What is happening now?” (mainly in a Chang, 2016). It has been considered as the next step towards in- streaming context); (ii) predictive analytics, answering the questions creasing data analytics maturity and leading to optimized decision “What will happen?” and “Why will it happen?” in the future; (iii) making, ahead of time, for business performance improvement (den prescriptive analytics, answering the questions “What should I do?” and Hertog & Postek, 2016; Gartner, 2017). New Information and Com- “Why should I do it?”. munication Technologies (ICT) such as the Internet of Things (IoT),
⁎ Corresponding author at: Information Management Unit, National Technical University of Athens, 9, Iroon Polytechniou Str., 157 80 Athens, Greece. E-mail addresses: [email protected] (K. Lepenioti), [email protected] (A. Bousdekis), [email protected] (D. Apostolou), [email protected] (G. Mentzas). 1 http//:imu.iccs.gr. https://doi.org/10.1016/j.ijinfomgt.2019.04.003 Received 1 October 2018; Received in revised form 9 April 2019; Accepted 9 April 2019 Available online 22 May 2019 0268-4012/ © 2019 The Authors. Published by Elsevier Ltd. This is an open access article under the CC BY license (http://creativecommons.org/licenses/BY/4.0/). K. Lepenioti, et al. International Journal of Information Management 50 (2020) 57–70 real-time streaming and sensor-driven business operations have bol- whether an event will happen, when it is about to happen as well as the stered prescriptive analytics and have provided businesses with accu- reason why it will happen. As shown in Fig. 1, predictive analytics has rate prescriptions facilitating reliable and effective decision making. proved to contribute significantly to the business value. However, thisis ICT advancements are also challenging the operational research com- closely related to the decisions that are taken and the actions that are munity toward the development of methods and algorithms that can implemented. In case of human decisions, it is very much depending on take advancement of available technologies and work in synergy with their knowledge and experience. prescriptive analytics, helping business know which solution is best Full exploitation of predictive analytics can be achieved in con- suited for a given business problem. junction with prescriptive analytics for optimized decision making Although, there are several literature review papers about de- ahead of time. However, even in this case, it is not possible to achieve scriptive (Batrinca & Treleaven, 2015; Duan & Xiong, 2015; Sun, Wu, the maximum potential of predictive analytics since there is a time Liang, & Liu, 2013; Tsai, Lai, Chao, & Vasilakos, 2015) and predictive interval until the decision. Therefore, there is an inevitably lost business analytics (Lu et al., 2017; Mishra & Silakari, 2012), to the best of our value between the event prediction and the proactive decision. The knowledge this paper is the first that targets a systematic literature time scale of this interval may vary depending on the computational review on prescriptive analytics. The objectives of our work are: (i) to environment, e.g., real-time vs. offline, and the application domain. In investigate the existing literature regarding prescriptive analytics and any case, the minimization of this interval is of outmost importance. prominent methods for its implementation; (ii) to provide clarity on the As shown in Fig. 1, prescriptive analytics generate proactive deci- research field of prescriptive analytics; (iii) to synthesize the literature sions on the basis of the predictive analytics outcomes. Between the review in order to identify the existing research challenges; (iv) to decision and the implementation of the action, there is a time interval outline directions for future research. for the preparation of the action. Moreover, it should be noted that an The rest of the paper is organized as follows: Section 2 provides a action may be better to be implemented at a specific time before the synthesis of background related to business analytics with a focus on predicted event occurrence when the expected utility/ loss is optimized. prescriptive analytics. Section 3 presents the methodology that we When the event actually occurs, descriptive analytics is applied in order followed for the literature review, while Section 4 describes the analysis to derive insights about what happened and why it happened. In this of the reviewed papers. Section 5 outlines directions for future research case, descriptive analytics may be applied in a different timescale. In work, while Section 6 concludes the paper. this sense, it may deal with near real-time reactive actions or with more long-term actions. Therefore, a timely detection of the current state of a business and a timely prediction of emerging events are crucial in terms 2. Towards prescriptive analytics of potential loss of business value.
Prescriptive analytics, which is the focus of the current literature 3. Literature review methodology review, is the most sophisticated type of business analytics and can bring the greatest intelligence and value to businesses (Šikšnys & In this Section, we outline the methodology of the literature review Pedersen, 2016). It aims at suggesting (prescribing) the best decision which is based on Tranfield, Denyer, and Smart (2003). This metho- options in order to take advantage of the predicted future utilizing large dology has been widely used in literature reviews for big data analytics, amounts of data (Šikšnys & Pedersen, 2016). To do this, it incorporates operational research and management science (Barbosa, Vicente, the predictive analytics output and utilizes artificial intelligence, opti- Ladeira, & Oliveira, 2018; Howick & Ackermann, 2011; Nguyen, Li, mization algorithms and expert systems in a probabilistic context in Spiegler, Ieromonachou, & Lin, 2018; Seifert, Seifert, & Protopappa- order to provide adaptive, automated, constrained, time-dependent and Sieke, 2013), while similar methodologies have been followed in other optimal decisions (Basu, 2013; Engel, Etzion, & Feldman, 2012; domains (Duan, Edwards, & Dwivedi, 2019; Ismagilova, Hughes, Gartner, 2017). Dwivedi, & Raman, 2019; Koivisto & Hamari, 2019; Senyo, Liu, & Effah, Prescriptive analytics has two levels of human intervention: deci- 2019; Tamilmani, Rana, Prakasam, & Dwivedi, 2019). sion support, e.g. providing recommendations; decision automation, We searched the literature using the query term “prescriptive ana- e.g. implementing the prescribed action (Gartner, 2017). The effec- lytics”. Although the query was quite generic, the limited amount of tiveness of the prescriptions depends on how well these models in- research works on this field made it sufficient for our literature review. corporate a combination of structured and unstructured data , represent We searched in the following scientific databases: ACM, ArXiv, the domain under study and capture impacts of decisions being ana- Emerald, IEEE, ScienceDirect and SpringerLink. We limited our search lyzed (Basu, 2013; Šikšnys & Pedersen, 2016). space to include only journals, books and conferences publications. We The business value of the three stages of business analytics with exclude any grey literature like white papers and blog posts because respect to time is depicted in Fig. 1. Beginning from the left side, de- their quality may vary and can affect the validity of our results. scriptive analytics aims to determine what is happening now by gath- More specifically, we follow the procedure described below: ering and analyzing parameters related to the root causes of the event to be eliminated or mitigated. Descriptive analytics is able to detect • We select the papers to be reviewed according to the criteria of the patterns that indicate a potential problem or a future opportunity for literature review methodology (Section 3). the business. On this basis, predictive analytics is able to predict
Fig. 1. The business value of analytics with respect to time (Source: Adapted from (Krumeich et al., 2016)).
58 K. Lepenioti, et al. International Journal of Information Management 50 (2020) 57–70
• Since prescriptive analytics takes as input the outcome of predictive Table 2 analytics algorithms, the vast majority of the reviewed research The distribution of the third phase’s papers throughout the years. works include methods for predictive analytics as well. Therefore, Year 2010 2011 2012 2013 2014 2015 2016 2017 2018 2019 we separate the methods used for predictive analytics and the methods Papers 1 0 0 2 8 7 9 12 15 2 used for prescriptive analytics for each reviewed paper (Section 4.1). • We classify the identified methods for predictive analytics and the ones for prescriptive analytics in 7 categories: Probabilistic Models, January 2010 and January 2019; (iii) the publication type is scientific Machine Learning/Data Mining, Statistical Analysis, Mathematical journal, conference or book chapter. The third phase resulted in 56 Programming, Evolutionary Computation, Simulation, Logic-based papers, consisting of 19 journal articles, 36 conference papers and 1 Models (Section 4.1). book chapter. • Since most of the reviewed research works utilize combinations of The distribution of the number of papers with respect to years is methods (instead of a single method), we identify the categories of shown in Table 2. This trend shows that there is also an increasing trend methods and their combinations in the literature for both predictive in publications contributing to the research field of prescriptive ana- and prescriptive analytics (Section 4.1). Then, we focused on the lytics. It should be noted that no journal or conference gathers speci- methods that are used for performing prescriptive analytics. fically research works on prescriptive analytics. The publications ex- • We also classify the reviewed papers per application domain in order to amined exist in several different journals and conference proceedings show the application domains that have gathered the most and the from various research fields. least interest, as well as the categories of methods that have been used (Section 4.1). 4. Analysis of reviewed papers • For each category of methods and for each identified combination, we extensively discuss how they have been used in the context of prescriptive As we mentioned in Sections 1 and 2, prescriptive analytics takes as analytics in the related papers, the problems that they solved, while input the outcome of predictive analytics algorithms. For this reason, we identified the key contributions. We also distinguished pro- the vast majority of the reviewed research works include methods for mising methods and approaches (Section 4.2). predictive analytics as well, with the aim to provide the required input • Based on the literature review, we conclude in a synthesis, in which we to prescriptive analytics. In this Section, we present the methods that also discuss the existing research challenges and potential directions for we have identified in the reviewed papers for both predictive and future research (Section 5). prescriptive analytics.
The identification and selection of documents is conducted inthree 4.1. Categories of methods for predictive analytics and prescriptive analytics phases in order to filter out the relevant documents. The number of papers identified in each phase is shown in Table 1. For the first phase, Fig. 2 depicts the classification of the methods for predictive ana- we queried the scientific databases to find papers that contain the query lytics that we have identified in the reviewed papers. We classified in their full record, including the full text of the publication, without these methods in three categories: Probabilistic Models, Machine any other constraints. The first phase of our search resulted in917 Learning/Data Mining and Statistical Analysis. Fig. 3 depicts the clas- papers. We found that there is almost an exponential growth of the use sification of the methods for prescriptive analytics that we haveiden- of the term “prescriptive analytics” in publications throughout the last tified in the reviewed papers. We classified these methods in sixcate- years. This trend outlines an increasing research interest and con- gories: Probabilistic Models, Machine Learning/Data Mining, tributes to the argumentation of the need for a literature review on Mathematical Programming, Evolutionary Computation, Simulation prescriptive analytics. and Logic-based Models. Since the first phase of the search includes works that do notne- Since the boundaries among these categories are not always clear, cessarily contribute to the field of prescriptive analytics, we conducted we provide the following definitions according to which we classified a second phase in order to search for research works with the query the methods for predictive and prescriptive analytics that we found in term in their metadata, i.e. title, abstract, keywords or other metadata the literature. of their record. The second phase resulted in 99 papers, as shown in Table 1. However, several papers refer the term “prescriptive analytics” • Probabilistic models without contributing to the field. For example, in many cases, it exists in introductory paragraphs (as background information) about business A probabilistic model quantifies the uncertainty by integrating first- analytics. principle knowledge with data in order to capture the dynamics in a Therefore, the third phase of our search aimed at examining more distribution over model predictions for state transitions between sam- in-depth the content of these papers. To this end, it is conducted ac- ples in a batch run (Martinez, Cristaldi, & Grau, 2009; Martínez, cording to the following inclusion criteria: (i) The papers contribute to Cristaldi, & Grau, 2013). In this sense, this category includes models the field of prescriptive analytics; (ii) the publication date is between that represent uncertain causal relationships (i.e. between causes and effects). In predictive and prescriptive analytics, probabilistic models are used to calculate the likelihood of certain events occurring Instead Table 1 of monitoring actual data in search of events and data points that The three phases of search. conform to a set of rules defined by historical analysis. Number of papers Machine learning/data mining Source First phase Second phase Third phase •
ACM 15 11 6 Machine learning refers to algorithms that rely on models and in- ArXiv 5 5 4 ference based upon data processing without using explicit instructions Emerald 42 1 0 (Nasrabadi, 2007). Machine learning algorithms build a mathematical IEEE 260 40 17 model of sample data, known as "training data", in order to make pre- ScienceDirect 186 23 8 dictions or decisions without being explicitly programmed to perform SpringerLink 409 20 21 TOTAL 917 99 56 the task. It has been considered as a subset of artificial intelligence. Data mining is the process of discovering patterns in large data sets with
59 K. Lepenioti, et al. International Journal of Information Management 50 (2020) 57–70
Fig. 2. Classification of the methods for predictive analytics. the aim to extract information and transform it into a comprehensible Mathematical programming deals with the optimal allocation of structure for further use (Chakrabarti et al., 2006). It involves analysis, limited resources among competing activities, under a set of constraints database and data management aspects, data pre-processing, model and imposed by the nature of the problem being studied. In broad terms, inference considerations, interestingness metrics, complexity con- mathematical programming can be defined as a mathematical re- siderations, post-processing of discovered structures, visualization, and presentation aimed at programming or planning the best possible al- online updating (Chakrabarti et al., 2006). Since machine learning and location of scarce resources. It is considered to be a branch of mathe- data mining are terms that are closely interrelated (Chakrabarti et al., matics, management science and operational research that aims at 2006), we treat them as one category of methods. Essentially, using enabling better decisions by arriving at optimal or near-optimal solu- machine learning and data mining techniques, we can build algorithms tions to complex decision-making problems (Chong & Zak, 2008). to extract data and see important hidden information from them. In predictive analytics, we seek information that can predict future out- • Evolutionary computation come from data based on previous patterns while in prescriptive ana- lytics we seek information that can help in finding the best course of In computer science, evolutionary computation is a family of algo- action for a given situation. rithms for global optimization inspired by biological evolution, and the subfield of artificial intelligence and soft computing studying theseal- • Statistical analysis gorithms (Bäck, Fogel, & Michalewicz, 1997). In technical terms, they are a family of population-based trial and error problem solvers with a Statistical analysis is a branch of mathematics dealing with data metaheuristic or stochastic optimization character. An initial set of collection, organization, analysis, interpretation and presentation candidate solutions is generated and iteratively updated. Each new (Dodge, 2006; Romijn, 2014). Statistical analysis deals with all aspects generation is produced by stochastically removing less desired solu- of data, including the planning of data collection in terms of the design tions, and introducing small random changes. In the context of pre- of surveys and experiments studies, and solves problems related to a scriptive analytics, evolutionary computation is used for solving com- statistical population or a statistical model process (Romijn, 2014). In plex problems in data-rich environments in which exact solutions predictive analytics, statistical analysis is used to extract information cannot be derived. from data and using it to predict trends and behavior patterns. • Simulation • Mathematical programming Simulation is referred to the process of modelling a real-life or
60 K. Lepenioti, et al. International Journal of Information Management 50 (2020) 57–70
Fig. 3. Classification of the methods for prescriptive analytics. hypothetical situation on a computer so that it can be studied to see • Logic-based models how the system works (Jerry, 2005). By changing variables in mathe- matical models along with expert knowledge, predictions about the Logic-based models are hypothesized descriptions of the chain of behaviour of the system can be generated and decisions can be fa- causes and effects leading to an outcome of interest. In the context of cilitated. Simulation is used in prescriptive analytics in order to im- the information systems, they may include rule-based systems, re- prove the effectiveness of decisions made by humans or by decision presentation of expert knowledge and elicitation of domain knowledge logic embedded in applications. In this respect, simulation is useful for supporting proactive decision making in prescriptive analytics ap- because it can be used to test new ideas about business decisions and plications. actions to mitigate risk pertaining to a process or system, or how Most of the reviewed research works utilize combinations of modifications will affect an existing process or system. Simulation is methods in order to provide a solution for the problem under ex- often used in prescriptive analytics applications related to safety of amination, while the same methods may be used for addressing dif- infrastructure as well as safety, quality, and design of products. ferent research challenges. To this end, Fig. 4 illustrates a Venn diagram
61 K. Lepenioti, et al. International Journal of Information Management 50 (2020) 57–70
Fig. 4. Venn diagram for the representation of the methods used in literature.
Table 3 Classification of papers according to predictive analytics categories of methods.
A. Predictive Analytics
Categories of methods References Count
A1 Probabilistic Models Ayhan et al., 2018 1 A2 Machine Learning/Data Mining Anderson, 2017; Aref et al., 2015; Bertsimas & Kallus, 2014; Bertsimas & Van Parys, 2017; Christ et al., 22 2016; de Aguiar, Greve, & Costa, 2017; Dey et al., 2019; Giurgiu et al., 2017; Gröger et al., 2014; Harikumar et al., 2018b; Huang et al., 2018; Ito & Fujimaki, 2017; Jank et al., 2018; Kawas et al., 2013; Laude, 2018; Lo & Pachamanova, 2015; Mendes et al., 2014; Schwartz, York, Nowakowski-Sims, & Ramos-Hernandez, 2017; Srinivas & Ravindran, 2018; Stein, Meller, & Flath, 2018; Thammaboosadee & Wongpitak, 2018; von Bischhoffshausen et al., 2015 A3 Statistical Analysis Baur et al., 2014; Ceravolo & Zavatarelli, 2015; Du et al., 2016; Matyas et al., 2017 4 A2∩A3 Machine Learning/Data Mining AND Statistical Goyal et al., 2016; Hupfeld, Maccioni, Sesemann, & Ravazzolo, 2016 2 Analysis A1∩A3 Probabilistic Models AND Statistical Analysis Shroff et al., 2014 1 A1∩A2∩A3 Probabilistic Models AND Machine Learning/Data Wang et al., 2018 1 Mining AND Statistical Analysis which depicts the categories of methods and their combinations that the research interest in the context of prescriptive analytics in order to have been identified in the literature for both predictive andpre- derive optimal solutions based on several objectives. The objective most scriptive analytics. Each circle refers to a category of methods, while often considered is the operational cost to be minimized (Achenbach & the size of each circle represents the amount of research works be- Spinler, 2018). longing to this specific category. The intersections of the circles illus- Arguably, linear programming and its extensions are the most used trate the combinations of the categories. Fig. 4 is separated into two optimization methods in prescriptive analytics. Linear programming is parts: Predictive Analytics and Prescriptive Analytics. Tables 3 and 4 a technique for the optimization of a linear objective function, subject show the associated references for each circle and for each intersection to linear equality and linear inequality constraints (Sierksma & Zwols, of circles for predictive and prescriptive analytics respectively. More- 2015). Specifically, the linear integer programming model has been over, Table 5 presents the categories of methods used per application used in the context of prescriptive analytics for finding a feasible domain. combination of environmental alternatives that minimizes the emis- sions of transport fleets (Wu & Yang, 2017) and for planning sales force assignments on the basis of predictions about the sales impact (von 4.2. Analysis of research works and discussion Bischhoffshausen, Paatsch, Reuter, Satzger, & Fromm, 2015). Linear integer programming has also been used in the context of information In this section, we further discuss the methods that have been used systems for redesigning the enterprise software stack centered around a in the literature, with an emphasis on the methods used for prescriptive unified declarative programming model in order to support mathema- analytics, which is the focus of our work. tical optimization (Aref et al., 2015). Linear integer programming has been also used for capacity planning in stadiums for sports events 4.2.1. Mathematical programming (Ghoniem, Ali, Al-Salem, & Khallouli, 2017) as well as for optimally As it can be seen in Fig. 4 and in Table 4, Mathematical Program- determining hotel room prices to be published in the tour operator’s ming has been widely used in the context of prescriptive analytics. brochure (Baur, Klein, & Steinhardt, 2014). Particularly, the vast majority of the reviewed papers deal with opti- Mixed integer linear programming differs from linear integer mization methods and algorithms. Optimization methods have gathered
62 K. Lepenioti, et al. International Journal of Information Management 50 (2020) 57–70
Table 4 Classification of papers according to prescriptive analytics categories of methods.
B. Prescriptive Analytics
A1 Probabilistic Models – 0 A2 Machine Learning/Data Mining Revathy & Mukesh, 2018 1 B1 Mathematical Programming Aref et al., 2015; Baur et al., 2014; Berk et al., 2019; Bertsimas & Kallus, 2014; Bertsimas & Van 17 Parys, 2017; Chalamalla, Ilyas, Ouzzani, & Papotti, 2014; Dey et al., 2019; Ghoniem et al., 2017; Goyal et al., 2016; Harikumar et al., 2018a, 2018b; Huang et al., 2018; Ito & Fujimaki, 2017; Kawas et al., 2013; Lo & Pachamanova, 2015; von Bischhoffshausen et al., 2015; Wu & Yang, 2017 B2 Evolutionary Computation Dey et al., 2019 1 B3 Simulation Giurgiu et al., 2017; Jank et al., 2018; Wang et al., 2018 3 B4 Logic-based Models Ceravolo & Zavatarelli, 2015; Cho et al., 2015; Du et al., 2016; Du et al., 2018; Gröger et al., 2014; 11 Lee et al., 2014; Matyas et al., 2017; Ramannavar & Sidnal, 2018; Song et al., 2013, 2014; Srinivas & Ravindran, 2018 A1∩B1 Probabilistic Models AND Mathematical Programming Ayhan et al., 2018; Jiang et al., 2010 2 A2∩B4 Machine Learning/Data Mining AND Logic-based Gröger et al., 2014; Mendes et al., 2014 2 Models A2∩B2 Machine Learning/Data Mining AND Evolutionary Thammaboosadee & Wongpitak, 2018 1 Computation B1∩B3 Mathematical Programming AND Simulation Hupfeld et al., 2016; Stein et al., 2018 2 B3∩B4 Simulation AND Logic-based Models Srinivas & Ravindran, 2018 1 A2∩B2∩B4 Machine Learning/Data Mining AND Evolutionary Laude, 2018 1 Computation AND Logic-based Models A2∩B1∩B4 Mathematical Programming AND Machine Learning/ Ghosh et al., 2016 1 Data Mining AND Logic-based Models A2∩B1∩B3 Machine Learning/Data Mining AND Mathematical Shroff et al., 2014 1 Programming AND Simulation
Table 5 Classification of papers based on application domains for prescriptive analytics methods.
Application domain Count Categories References
Manufacturing 12 B1 Anderson, 2017; Goyal et al., 2016 B2 Dey et al., 2019 B4 Ceravolo & Zavatarelli, 2015; Gröger et al., 2014; Matyas et al., 2017 A2∩B2 Thammaboosadee & Wongpitak, 2018 B1∩B3 Brodsky et al., 2017; Krumeich et al., 2016; Stein et al., 2018 B1∩B4 Ringsquandl et al., 2016 A2∩B1∩B3 Shroff et al., 2014 Sales/ Marketing 14 B1 Aref et al., 2015; Baur et al., 2014; Bertsimas & Kallus, 2014; Bertsimas & Van Parys, 2017; Hong et al., 2015; Huang et al., 2018; Ito & Fujimaki, 2017; Kawas et al., 2013; Lo & Pachamanova, 2015; von Bischhoffshausen et al., 2015 B3 Jank et al., 2018 B4 Du et al., 2018 B1∩B3 Hupfeld et al., 2016 A2∩B1∩B3 Shroff et al., 2014 Education/Research 7 B1 Harikumar et al., 2018a B4 Cho et al., 2015; Du et al., 2016; Lee et al., 2014; Song et al., 2013, 2014 Health/Social Policy 6 A2 Schwartz et al., 2017 B1 Harikumar et al., 2018a, 2018b B4 Srinivas & Ravindran, 2018 A2∩B4 de Aguiar et al., 2017; Osmani, Forti, Mayora, & Conforti, 2017 Human Resources 3 B1 Berk et al., 2019 B4 Ramannavar & Sidnal, 2018 A1∩B1 Jiang et al., 2010 Transport 3 B1 Achenbach & Spinler, 2018; Wu & Yang, 2017 A1∩B1 Ayhan et al., 2018 Business Strategy 3 B3 Wang et al., 2018 B4 Ghosh et al., 2016 Data Engineering/ Information 3 A2 Revathy & Mukesh, 2018 Systems B1 Chalamalla et al., 2014 B3 Giurgiu et al., 2017 Capacity Planning 1 B1 Ghoniem et al., 2017 Social Media 1 B4 Mendes et al., 2014 Agriculture 1 A2∩B2∩ B4 Laude, 2018 programming in that only some of the variables are constrained to be derived from mining historical selling data in order to capture the be- integers, while other variables are allowed to be non-integers and hence havioral relationship between the size and composition of the sales is suitable in prescriptive analytics applications such as allocating sales team and the revenue earned for different types of clients and oppor- teams to sales opportunities with the aim to maximize business revenue tunities subjected to the business constraints. and profit (Kawas, Squillante, Subramanian, & Varshney, 2013). In this Binary linear programming has been applied in cases when all of the case, the prescriptive analytics algorithm incorporates predictions variables are binary such when recommending products or services to
63 K. Lepenioti, et al. International Journal of Information Management 50 (2020) 57–70 customers (Lo & Pachamanova, 2015). In this research work, the pro- combination with constraint programming, i.e. Constrained Bayesian blem was formulated as a multiple treatment optimization model with Optimization, for solving a nested global optimization problem aiming binary decision variables. at guarantying the privacy of the data. Further, Harikumar et al. Non-linear programming, i.e. when some of the constraints or the (2018b) proposed a promising method for prescriptive analytics aiming objective function is non-linear, was used by Goyal et al. (2016) in the at finding the minimum change that should be made in order tomove context of asset health management. More specifically, the authors from an undesirable state to a desirable state. In this sense, the pro- proposed a method based on mixed integer non-linear programming for posed prescriptive analytics method is generic and thus, capable of scheduling and planning preventive, proactive and corrective main- being applied in different application domains and problems. The au- tenance in an electrical network considering budgetary constraints. On thors compared their results with the Improved Stochastic Ranking the other hand, Ito and Fujimaki (2017) proposed a prescriptive ana- Evolution Strategy Genetic Algorithm and concluded that the latter lytics model based on binary quadratic non-linear programming for failed to converge in the case of expensive function evaluation but deriving the optimal price strategy, i.e. the one which maximizes the converges faster in the absence of it. future profit on the basis of a customer-centric pricing approach. The problem was solved by a combination of semi-definite programming 4.2.2. Evolutionary computation relaxation and rounding techniques (fast approximation algorithm). For problems that are too complicated to get the optimal solution Finally, Huang, Bergman, and Gopal (2018) addressed the problem of with mathematical programming, evolutionary computation methods selecting expansion locations for retailers selling add-on products can be used for providing approximate solutions efficiently (Duan & whose demand is dependent on the demand of another base product. Xiong, 2015). Evolutionary computation methods have been estab- They concluded in a non-linear binary quadratic optimization problem lished as optimization tools since they have been proved to be powerful affected by the non-linear predictive model. global optimizers. Evolutionary approaches such as genetic algorithms A traditional technique for decision making under uncertainty is have been studied as a prescriptive analytics tool by Dey, Gupta, stochastic optimization (Birge & Louveaux, 2011). Stochastic optimi- Pathak, Kela, and Datta (2019). Their work proposes a genetic algo- zation refers to a collection of methods for minimizing or maximizing rithm for performing the search of the composition of the steel to an objective function when randomness is present. In this sense, it fo- achieve a target property, based on the prediction of steel features si- cuses on decision making under uncertainty (Bertsimas & Kallus, 2014; milar to strength, ductility and hardness. Kawas et al., 2013). Bertsimas and Kallus (2014) defined prescriptive analytics from an operational research point of view as a conditional 4.2.3. Simulation stochastic optimization problem given imperfect observations, where Another important category of methods for prescriptive analytics is the joint probability distributions that specify the problem are un- Simulation. Giurgiu et al. (2017) applied simulation over the random known. However, stochastic optimization has been difficult to imple- forest model for prescribing the required improvement actions to ser- ment in practice (Berk, Bertsimas, Weinstein, & Yan, 2019). Multi- vers by using the information available from the incident tickets. In period problems suffer from the curse of dimensionality; although op- addition, stochastic simulation was exploited by Jank, Dölle, and Schuh timal solutions can be computed using the Bellman equations, these (2018) in order to maximize the benefits of a company’s product equations grow exponentially in size with the dimension of the state portfolio in accordance with the corporate objectives. On the other space (Berk et al., 2019). Moreover, the required probability distribu- hand, Wang, Cheng, and Deng (2018) proposed a method based on tions are difficult to estimate without copious amounts of data, which stochastic simulation over a Bayesian Belief Network in order to con- could be a barrier for companies just beginning forays into predictive duct fact-based decision making based on KPIs in a data-driven way. In and prescriptive analytics. this way, they moved beyond expert-oriented or multi-criteria decision Adaptive robust optimization has started to be used in the literature making. for solving prescriptive analytics problems. Adaptive robust optimiza- tion is an improved version of the static robust optimization in which 4.2.4. Machine learning/data mining instead of assigning probability distribution to handle uncertainty, it Machine Learning/Data Mining algorithms have been widely used treats uncertainty as a function of ellipsoid, polyhedron, or any other in predictive analytics during the last years. However, their exploitation ways that might best serve a specific case of interest. Adaptive robust in the context of prescriptive analytics is rather scarce. We found that optimization is inspired by the statistical bootstrap and deals with op- only one paper relies solely on machine learning and data mining al- timization problems in which a certain measure of robustness is sought gorithms (Revathy & Mukesh, 2018). The authors present an approach against uncertainty that can be represented as deterministic variability based on k-means clustering in order to address the problem of adaptive in the value of the parameters of the problem itself and/or its solution. data placement across distributed nodes in a secure way by considering Furthermore, the characterization of the uncertainty sets can be data- the sensitivity and security of the underlying data. Moreover, Shroff driven and is often interpretable (Bertsimas, Brown, & Caramanis, et al. (2014) proposed a framework based upon reinforcement learning, 2011). Therefore, Bertsimas and Van Parys (2017) proposed a generic i.e. learning the behavior of an agent through trial-and-error interac- robust and adaptive optimization solution. Further, Berk et al. (2019) tions within a dynamic environment, integrated with simulation and got involved with the optimization component of the human resources optimization in an online learning scenario. planning problem in order to model the forecasting uncertainty within the optimization problem. Their aim was to dynamically make hiring 4.2.5. Logic-based models decisions that maximize profit while remaining as flexible as possible Logic-based Models, especially rule-based systems, have been used Finally, an increasingly emerging optimization method is Bayesian in the prescriptive analytics literature for incorporating the expert optimization. Bayesian optimization is a technique for efficiently opti- knowledge into the prescriptive analytics models. Ceravolo and mizing multiple continuous parameters with computationally expensive Zavatarelli (2015) developed a knowledge acquisition process as an function evaluations. Typically, this process begins by evaluating a investigation over the process executions. The prescriptive knowledge small number of randomly selected function values, and fitting a base evaluates the achievement of the business rules or the objectives Gaussian process regression model to the results (Letham, Karrer, associated to a process and identifies unexpected patterns. Matyas, Ottoni, & Bakshy, 2019). It is able to solve a variety of optimization Nemeth, Kovacs, and Glawar (2017) proposed a procedural approach problems where traditional numerical methods and global optimizers for realizing prescriptive maintenance planning. The approach is based are insufficient (Gardner, Kusner, Xu, Weinberger, & Cunningham, on rules represented by mathematical functions for each machine 2014). Harikumar et al. (2018a) exploited this technique in component, taking into account the prognoses of the wear reserve for
64 K. Lepenioti, et al. International Journal of Information Management 50 (2020) 57–70 machine components, condition based monitoring and variations in process execution. product quality. On the other hand, Mendes et al. (2014) investigated how the word Ramannavar and Sidnal (2018) proposed a distributed architecture choice in Twitter messages contributes to their propagation and pro- which integrates advanced analytics to map a job to resumes utilizing posed an approach for prescribing changes to the tweet wording in semantic technologies. For a given job description, every resume is order to get more retweets. To do this, they used generalized linear ranked according to two measures, coverage and comprehensibility, models, i.e. a flexible generalization of ordinary linear regression that denoting the number of concepts from a predefined class and the allows for response variables that have error distribution models other number of sections and subsections covered in a resume, respectively. than a normal distribution, embedded in a rule-based system. An ad- Du, Plaisant, Spring, and Shneiderman (2016) focused on the pre- vantage of generalized linear models is that they are transparent and sentation and explanation of recommendations of temporal event se- friendly for user interaction since the weights can be displayed as knobs quences using a prescriptive analytics interface. They developed the or sliders on an interface, giving the users the freedom to disagree with EventAction system, which identifies similar records, estimates the our model’s suggestions. current record’s potential outcomes based on the outcome distribution Ghosh, Gupta, Chattopadhyay, Banerjee, and Dasgupta (2016) of the similar archived records and recommends actions by summar- combined methods from the Mathematical Programming, Machine izing the activities of those who achieved the desired outcome. This Learning/ Data Mining and Logic-based Models in order to define, approach was applied in an education scenario as well as in a digital monitor, rank and classify a set of KPIs measuring the operational ef- marketing scenario (Du, Malik, Koh, & Theocharous, 2018). In the ficiency of business process outsourcing service providers. To dothis, latter, the authors evaluated the effectiveness of their tool in helping they used the Kemeny rank aggregation method in order to generate an marketers prescribing personalized marketing interventions. Further, aggregate ranked list that minimizes the number of pair-wise dis- prescriptive analytics have been utilized the 5W1H (What to do, with agreements between client pairs between the individual rank lists. They whom, where, how/when) methodology embedded in an information combined it with integer linear optimization and rule-based systems. system for the purpose of providing recommendations and advice on Jiang, Jensen, Cao, and Kumar (2010) combined Mathematical research directions to researchers (Cho, Song, Weber, Jung, & Lee, Programming and Probabilistic Models in order to build business in- 2015; Lee, Cho, Gim, Jeong, & Jung, 2014; Song et al., 2013, 2014). telligence applications having predictive and prescriptive analytics capabilities. Ayhan, Costas, and Samet (2018) developed a prescriptive 4.2.6. Combinations of categories analytics method for the long-range aircraft conflict detection and re- As seen in Fig. 4 and Table 4, there are various combinations of the solution problem. Their proposed approach learns from descriptive aforementioned categories of methods in order to perform prescriptive patterns of historical trajectories and pertinent weather observations analytics. Shroff et al. (2014) proposed a framework that combines and builds a Hidden Markov Model using a variant of the Viterbi al- Mathematical Programming, Simulation and Machine Learning/ Data gorithm. The system avoids the airspace volume in which the conflict is Mining. This framework is based upon reinforcement learning, i.e. detected and generates a new optimal trajectory that is conflict-free. learning the behavior of an agent through trial-and-error interactions within a dynamic environment, integrated with simulation and opti- 5. Synthesis, challenges and future directions mization in an online learning scenario. Srinivas and Ravindran (2018) proposed a method which combines the categories of Mathematical Based on our literature review, there is a clear trend towards pre- Programming and Logic-based Models. More specifically, they proposed scriptive analytics models capable of being executed in a real-time, a prescriptive analytics model which involves scheduling rules eval- streaming computational environment in the context of IoT. In addi- uated using simulation. Simulation models are used to generate the tion, the wide availability of big data from heterogeneous data sources schedule and determine the weighted score of the generated schedule. paves the way for minimizing human judgement, that suffers from In this way, they achieve to improve the performance of an appoint- subjectivity and for exploiting the information that can be derived from ment system with respect to patient satisfaction and resource utiliza- data processing. In this way, the prescriptive analytics models will be tion. able to be built and updated dynamically as soon as new data are ac- Thammaboosadee and Wongpitak (2018) combined Evolutionary quired. Therefore, there is the need for generic prescriptive analytics Computation and Machine Learning/ Data Mining, and particularly k- models and systems capable of fulfilling the aforementioned require- means clustering, in order to allocate electrical equipment’s parts ments. taking into consideration the customer behavior and the company Further, there is a trend of applying artificial intelligence methods policy. Moreover, Laude (2018) focused on the application of pre- and tools to prescriptive analytics (Lee, Davari, Singh, & Pandhare, scriptive analytics for automated agriculture systems. For this, they 2018). Up to now, the full potential of these capabilities has been in- defined a prescriptive analytics engine as the aggregation of several vestigated only conceptually (Anderson, 2017; Bertsimas & Van Parys, actionable insights to generate a set of trigger events, consisting of any 2017; Giurgiu et al., 2017; Nechifor, Puiu, Tarnauca, & Moldoveanu, combination of ensemble methods, particle swarm optimization and 2015; Shroff et al., 2014; Soltanpoor & Sellis, 2016). Although de- fuzzy linear programming or fuzzy rule-based systems. scriptive and predictive analytics have evolved during the last years Gröger, Schwarz, and Mitschang (2014) combined methods of Ma- towards these directions in order to be applicable to data-rich en- chine Learning/ Data Mining and Logic-based Models, and specifically vironments, prescriptive analytics is still at its dawn. For example, our data mining techniques and rules derived from decision trees, in order survey revealed only sparse advanced techniques for uncertainty to perform recommendation-based business process optimization on top handling of streaming data that are useful for prescriptive analytics. In of a holistic process warehouse. They also presented a case study in the order to contribute to further advancements in prescriptive analytics, in manufacturing domain. It was shown that the proposed recommenda- the following we synthesize the challenges derived from the literature tion-based business process optimization approach moves beyond pat- review and we outline potential directions for future research. tern-based optimization, recommendation-based process mining, and risk-based decision support. This research work provides a significant 5.1. Offline vs. real-time processing approaches contribution since it exploits prescriptive analytics methods in order to transform analysis results into concrete improvement actions without According to our literature review, real-time processing has not leaving this step completely up to the subjective judgment of the user. been well exploited in prescriptive analytics research, since 64% of the Another contribution is that it integrates process data and operational reviewed papers deal with offline prescriptive analytics models. data, while it is able to perform a proactive improvement during However, the emergence of IoT in modern enterprises has led to an
65 K. Lepenioti, et al. International Journal of Information Management 50 (2020) 57–70 increasing demand for real-time systems. Apart from the technical judgement. challenges that exist in developing scalable and efficient sensor-driven Due to the uncertain nature of prescriptive analytics, most of the information systems (Biegler, Yang, & Fischer, 2015; Yaqoob et al., problems can be represented in a probabilistic structure. Probabilistic 2016), there is also the need for recursive algorithms for prescriptive graphical models, and especially Bayesian Networks, have been con- analytics, which are able to process data with time-varying character- sidered a powerful tool for representing the graphical structure among istics and thus, to solve large-scale problems. Recursion deals with the random variables in terms of the conditional dependencies solving a problem that depends on solving smaller instances of the same (Gudivada, Irfan, Fathi, & Rao, 2016) and thus, they can enhance problem. prescriptive analytics models. Proposition 1. The development of real-time, sensor-driven information On the other hand, Markov Decision Processes (MDP), which have systems and recursive algorithms can promote the application of prescriptive been used for proactive decision making by representing the decision analytics in large-scale problems. making process instead of the physical process itself (Bousdekis, Papageorgiou, Magoutas, Apostolou, & Mentzas, 2018), can be utilized The computational challenges are even higher when predictive and in prescriptive analytics for handling uncertainty. MDP is a discrete prescriptive algorithms need to be developed for distributed platforms time stochastic control process which provides a mathematical frame- (Biegler et al., 2015), i.e. platforms with components that are located in work for modeling decision making in situations where outcomes are different networked computers, communicating and coordinating their partly random and partly under the control of a decision maker (Meyn, actions by passing messages to each other (Tanenbaum & Van Steen, 2008). MDPs are useful for studying optimization problems solved via 2007). According to our literature review, there is only one paper dynamic programming and reinforcement learning. In general, in (Hong et al., 2015) that proposes an implementation of a prescriptive comparison to physical models (e.g. simulation of the physical process analytics approach in a distributed parallel computing platform. Pre- of a manufacturing equipment), probabilistic generative models enable scriptive analytics can significantly benefit from distributed computing also inference using statistical predictive models to ease the search for for processing large amounts of unstructured, semi-structured and optimal parameter settings (Shroff et al., 2014). structured big data. Proposition 5. Due to the uncertain nature of prescriptive analytics, most Proposition 2. Prescriptive analytics can benefit from distributed of the problems can be represented in a probabilistic structure. computing for processing large amounts of data. Moreover, during the last years, there have been some attempts for Various research communities have independently arrived at stream coupling probabilistic logic and uncertainty into CEP engines in order processing as a programming model for efficient and parallel com- to react to predictions about future events before they occur (Cugola, puting (Hirzel, Soulé, Schneider, Gedik, & Grimm, 2014). In this con- Margara, Matteucci, & Tamburrelli, 2015; Engel et al., 2012; Lee & text, Complex Event Processing (CEP) technologies can play a sig- Jung, 2017; Mousheimish, Taher, & Zeitouni, 2016; Wang, Cao, & nificant role. CEP tracks and processes streams of information (data) Zhang, 2013). Still, there is very limited work on how to integrate the about things that happen (events) by combining multiple sources with probabilistic results pertaining to event predictions within CEP systems the aim to infer events or patterns that reveal meaningful events, such (Christ, Krumeich, & Kempa-Liehr, 2016). Therefore, similarly to the as opportunities or threats, and to react to them (Bates, 2012; Etzion, approaches using CEP for detecting important patterns and situations Niblett, & Luckham, 2011; Luckham, 2011; Schmerken, 2008). and reacting to them, prescriptive analytics can significantly benefit Proposition 3. Stream processing and Complex Event Processing can from these advancements in order to utilize CEP for prescribing ap- enable the development of prescriptive analytics in a real-time environment. propriate actions in a proactive way, on the basis of predictions. In this direction, machine learning can be coupled to CEP in order to enrich the knowledge base beyond the knowledge of the domain experts. 5.2. Deterministic vs. probabilistic approaches Proposition 6. Prescriptive analytics can benefit from coupling probabilistic logic with CEP engines for prescribing actions in a proactive The uncertainty that is handled by prescriptive analytics ap- way. proaches, methods and algorithms has three main sources: (i) the un- certainty of the prediction, which is estimated by a prediction evalua- tion method in predictive analytics algorithms; (ii) the uncertainty of 5.3. Domain knowledge vs. data-driven models the quality of data; and, (iii) the subjectivity in human judgement when building the prescriptive analytics model. Although uncertainty is While the use of big data adds significant value to business considered a crucial aspect of prescriptive analytics by 76% of the re- throughout the entire value chain, the integration of big data analytics viewed papers, it is usually limited to data cleaning techniques for to the decision-making process remains a challenge (Akter et al., 2019). tackling issues of incomplete data. In this sense, the prescriptive ana- In this sense, prescriptive analytics has the potential to play a crucial lytics model that represents the decision making process is considered role towards this direction. According to our literature review, 82% of deterministic. existing research on prescriptive analytics relies on models that have Although prediction evaluation algorithms have gathered recent been developed solely or mainly on the basis of expert knowledge. research attention, it is not clear how to go from a good prediction to a However, such models depend on human subjectivity and are cum- good decision (Bertsimas & Kallus, 2014), since prescriptive analytics bersome to develop because there are several challenges associated algorithms incorporate additional uncertainty sources in information with expert knowledge elicitation. processing. Currently, dealing with incomplete and noisy data, elicita- Although there is an increasing trend towards the use of the avail- tion and modelling of expert knowledge and uncertainty in decision ability of data for model development, this is currently an under- making process are three fields that are developed in parallel topre- explored area. The emergence of big data applications paves the way scriptive analytics research without clear connections among them. for the development of prescriptive analytics models less dependent on This fact becomes even more important when analyzing real-time data domain expert knowledge and based more upon data analytics. and there is the need for timely decisions in day-to-day business op- Although there have been a lot of descriptive and predictive algorithms erations. utilizing data mining, machine learning and artificial intelligence Proposition 4. It is necessary to address the uncertainty introduced by the (Larson & Chang, 2016; Tsai et al., 2015), prescriptive analytics has not predictions, the incomplete and noisy data and the subjectivity in human gathered the same attention yet. In most reviewed papers, prescriptive
66 K. Lepenioti, et al. International Journal of Information Management 50 (2020) 57–70 analytics relies on an optimization problem with an objective function applications, dynamic, flexible and adaptive response to changes plays created by the expert. a key role in organizations’ success. Although adaptation mechanisms providing feedback to descriptive and predictive algorithms are a well- Proposition 7. Prescriptive analytics models have the potential to become studied area, their application to prescriptive analytics is rarely in- less dependent on domain expert knowledge and more dependent upon big vestigated. However, adaptation of prescriptive analytics is of outmost data analytics. importance, especially in today’s complex and dynamic business en- Further research is also needed towards the direction of combining vironment with lots and heterogeneous data and information sources. A the “learned knowledge” of machine learning and data mining methods dynamic prescriptive model refers to the adaptation of the model in with the “engineered knowledge” elicited from domain experts. To this order to be valid when new requirements arise or when new knowledge end, the combined use of machine learning and knowledge engineering is gained, e.g., new types of constraints have to be added or there is a can complement each other’s strengths and mitigate their weaknesses, change in problem environment. since explicitly represented application knowledge could assist data- Adaptation of prescriptive analytics can be defined in terms of driven machine-learning approaches to converge faster on sparse data amended decisions, changed recommendations or new courses of ac- and to be more robust against noise. tions (Soltanpoor & Sellis, 2016). Such feedback mechanisms need to track the suggested recommendations as well as unprecedented events Proposition 8. Further research is needed towards the direction of occurrence in system’s lifetime (Soltanpoor & Sellis, 2016). Although combining the “learned knowledge” of machine learning and data mining adaptation of predictive analytics has been identified as an important methods with the “engineered knowledge” elicited from domain experts. factor for their reliability, implementations in algorithms are presented As already mentioned, apart from the aforementioned methods and only in 29% of the reviewed papers, while implementations in in- algorithms, we have also identified in the literature some conceptual formation systems are non-existent. Even in the aforementioned papers, approaches which pave the way for the development of more advanced the capabilities of the adaptation mechanisms are limited. prescriptive analytics models. In all conceptual approaches, the full Notable approaches aiming at exploiting the full potential of data- potential of big data to prescriptive analytics is outlined with the aim to rich environments are those by Nechifor et al. (2015) and Soltanpoor reduce the input by the domain expert in a way that the human has a and Sellis (2016), albeit still at a conceptual level. The remaining re- more supervisory role in the data analytics lifecycle. To this end, sto- levant publications investigate extensions to domain-specific, user-de- chastic simulation and business rules (Soltanpoor & Sellis, 2016), CEP fined prescriptive models in order to derive some values thatwere (Krumeich et al., 2016), autonomic networking (Nechifor et al., 2015) previously estimated by the user. The significance of a closed-loop and graph-based analytics (Ringsquandl, Lamparter, & Lepratti, 2016) feeding the recommendations back into a process control system was in a stream processing computational environment have been con- identified by Gröger et al. (2014), while an attempt to tackle it is the sidered as key enablers for the further development of prescriptive Prescriptive Information Fusion framework which incorporates adap- analytics in various application domains. In addition, there are several tive closed-loop prescriptive analytics approaches (Shroff et al., 2014). attempts for designing conceptual architectures addressing real-time Proposition 11. Adaptation mechanisms for prescriptive analytics are of data analytics with a particular focus on prescriptive analytics (Brodsky outmost importance in dynamic environments. et al., 2017; Deshpande, 2019; Pospieszny, 2017; Tan, Ang, Lu, Gan, & Corral, 2016). Adaptation mechanisms aiming to improve performance and relia- With the advancements in big data technologies, artificial in- bility of prescriptive analytics become even more important in a real- telligence has become an important element of digital systems, because, time/ streaming computational environment and especially when the among others, they make a profound impact on human decision making decision is implemented automatically. However, the direction of de- (Duan et al., 2019). As a result, there is an increasing demand for in- cision automation has not been explored yet. We found only one paper formation embedding artificial intelligence and machine learning al- (Nechifor et al., 2015) with a conceptual approach that deals with gorithms for decision making (Duan et al., 2019). In this way, there will decision automation. A major challenge in this area is the fact that the be the possibility to develop generic, domain-agnostic, data-driven uncertainty and the probabilistic nature of prescriptive analytics may methodologies and algorithms for performing prescriptive analytics. result in implementing inappropriate actions. Moreover, very complex application domains are not mature to address decision automation. For Proposition 9. There is the need for generic prescriptive analytics methods example, the automatic reproduction of an inappropriate video has and algorithms utilizing artificial intelligence and machine learning. incomparably lower risk than the automatic inappropriate re- Some prominent machine learning methods that have been utilized configuration of an industrial machine. In the first case, the negative effectively in decision making problems in different contexts are: impact will probably be the instant disturbance of the user, while, in the Artificial Neural Networks (Domingos, Burguillo, & Lenaerts, 2017), second case, it will probably be a failure which can lead to high costs, Bayesian Networks (Said, Shahzad, Zamai, Hubac, & Tollenaere, 2016), but also to accidents and environmental pollution. topic models (Roberts et al., 2014), fuzzy sets (Pal & Ceglarek, 2013), Proposition 12. Prescriptive analytics can enable decision automation, Markov models (Bousdekis et al., 2018). In addition, constraint pro- provided that the challenges of uncertainty, dynamicity and complexity are gramming is a promising approach to this direction. Constraint pro- faced effectively. gramming is a programming paradigm wherein relations between variables are stated in the form of constraints (Ceberio & Kreinovich, 2016). Constraints differ from the common primitives of imperative 6. Conclusions programming languages in that they do not specify a step or sequence of steps to execute, but rather the properties of a solution to be found Business analytics is an evolving area which gathers the interest of (Ceberio & Kreinovich, 2016). both researchers and practitioners. With the increasing availability of large amounts of data within organizations, many research works aim Proposition 10. Constraint programming is a promising approach for to contribute to the business analytics field in order to enable organi- building prescriptive models. zations gain meaningful insights about their performance. In this paper, we investigated the literature on prescriptive analytics, analyzed the 5.4. Static vs. dynamic prescriptive models results, identified the existing research gaps and outlined directions for future research, depicted in Table 6 in the form of research proposi- Since change is an indispensable aspect of modern real-world tions. Our review has shown that prescriptive analytics is a critical
67 K. Lepenioti, et al. International Journal of Information Management 50 (2020) 57–70
Table 6 A summary of research propositions.
Challenges Propositions
Offline vs. real-time processing Proposition 1: The development of real-time, sensor-driven information systems and recursive algorithms can promote the application of prescriptive analytics in large-scale problems. Proposition 2: Prescriptive analytics can benefit from distributed computing for processing large amounts ofdata. Proposition 3: Stream processing and Complex Event Processing can enable the development of prescriptive analytics in a real-time environment. Deterministic vs. probabilistic Proposition 4: It is necessary to address the uncertainty introduced by the predictions, the incomplete and noisy data and the subjectivity in human judgement. Proposition 5: Due to the uncertain nature of prescriptive analytics, most of the problems can be represented in a probabilistic structure. Proposition 6: Prescriptive analytics can benefit from coupling probabilistic logic with CEP engines for prescribing actions in aproactive way. Domain knowledge vs. data-driven models Proposition 7: Prescriptive analytics models have the potential to become less dependent on domain expert knowledge and more dependent upon big data analytics. Proposition 8: Further research is needed towards the direction of combining the “learned knowledge” of machine learning and data mining methods with the “engineered knowledge” elicited from domain experts. Proposition 9: There is the need for generic prescriptive analytics methods and algorithms utilizing artificial intelligence and machine learning. Proposition 10: Constraint programming is a promising approach for building prescriptive models. Static vs. dynamic prescriptive models Proposition 11: Adaptation mechanisms for prescriptive analytics are of outmost importance in dynamic environments. Proposition 12: Prescriptive analytics can enable decision automation, provided that the challenges of uncertainty, dynamicity and complexity are faced effectively.
advancement in analytics. It can improve decision making and process Perspectives, 5, 265–279. effectiveness by helping analysts get closer to tying outcomes to specific Advanced data analytics for business. In B. Akerkar (Ed.). Big data computing (pp. 373– 397). Boca Raton: CRC Press. situations. Currently, prescriptive analytics appears to have attracted Akter, S., Bandara, R., Hani, U., Wamba, S. F., Foropon, C., & Papadopoulos, T. (2019). attention in a rather restricted range of application domains. Analytics-based decision-making for service systems: A qualitative study and agenda The review presented herein is limited to works explicitly scoped as for future research. International Journal of Information Management, 48, 85–95. Anderson, R. N. (2017). ’Petroleum analytics learning machine’ for optimizing the in- prescriptive analytics. It does not deal with works from different re- ternet of things of today’s digital oil field-to-refinery petroleum system. Big data (Big search fields that can potentially contribute to the field of prescriptive Data), 2017 IEEE international conference on, 4542–4545 December. analytics. There is a plethora of advancements in areas such as opti- Aref, M., ten Cate, B., Green, T. J., Kimelfeld, B., Olteanu, D., Pasalic, E., ... Washburn, G. mization, simulation, machine learning, recommender systems and (2015). Design and implementation of the LogicBlox system. Proceedings of the 2015 ACM sigmod international conference on management of data, 1371–1382. uncertain decision making, which should be examined from the view of Ayhan, S., Costas, P., & Samet, H. (2018). Prescriptive analytics system for long-range prescriptive analytics and assessed in the context of the challenges that aircraft conflict detection and resolution. Proceedings of the 26th ACM SIGSPATIAL prescriptive analytics problems present, as well as the opportunities international conference on advances in geographic information systems, 239–248. Bäck, T., Fogel, D. B., & Michalewicz, Z. (1997). Handbook of evolutionary computation. provided by recent advancements in information technology. CRC Press. Prescriptive analytics is expected to become much more common Barbosa, M. W., Vicente, A. D. L. C., Ladeira, M. B., & Oliveira, M. P. V. D. (2018). and understood by a wide variety of managers. Capturing business Managing supply chain resources with big data analytics: A systematic review. International Journal of Logistics Research and Applications, 21(3), 177–200. value from data requires action quickly on real-time events, or the value Basu, A. T. A. N. U. (2013). Five pillars of prescriptive analytics success. Analytics ma- disappears. But taking intelligent actions quickly requires more than a gazine, 8, 8–12. prediction; it requires knowing exactly what to do and when to do it. Bates, J. (2012). John Bates of Progress explains how complex event processing works and how it can simplify the use of algorithms for finding and capturing trading opportunities. Fix Our review has shown that prescriptive analytics fills this critical gap Global Trading Retrieved 14 May. for business analytics. With recent advancements in event processing Batrinca, B., & Treleaven, P. C. (2015). Social media analytics: A survey of techniques, technology as well as distributed, pervasive computing infrastructures, tools and platforms. AI & Society, 30(1), 89–116. Baur, A., Klein, R., & Steinhardt, C. (2014). Model-based decision support for optimal we expect that next generation prescriptive analytics systems will ex- brochure pricing: Applying advanced analytics in the tour operating industry. OR ploit sophisticated features such as distributed processing and data Spektrum, 36(3), 557–584. management and will embed scalable operational research and ma- Berk, L., Bertsimas, D., Weinstein, A. M., & Yan, J. (2019). Prescriptive analytics for chine learning algorithms to facilitate actionability. Through dis- human resource planning in the professional services industry. European Journal of Operational Research, 272(2), 636–641. tributed processing and data management and in conjunction with Bertsimas, D., & Kallus, N. (2014). From predictive to prescriptive analytics. arXiv preprint advanced algorithms, next generation analytics systems will be able not arXiv:1402.5481. only to identify risks and potential problems in business situations, but Bertsimas, D., & Van Parys, B. (2017). Bootstrap Robust Prescriptive Analytics. arXiv preprint arXiv:1711.09974. also recommend mitigating actions, effectively delivering clear, defi- Bertsimas, D., Brown, D. B., & Caramanis, C. (2011). Theory and applications of robust nitive, real-time decision support to business users. optimization. SIAM Review, 53(3), 464–501. Biegler, L. T., Yang, X., & Fischer, G. A. G. (2015). Advances in sensitivity-based nonlinear model predictive control and dynamic real-time optimization. Journal of Process Declarations of interest Control, 30, 104–116. Birge, J. R., & Louveaux, F. (2011). Introduction to stochastic programming. Springer None. Science & Business Media. Bousdekis, A., Papageorgiou, N., Magoutas, B., Apostolou, D., & Mentzas, G. (2018). Enabling condition-based maintenance decisions with proactive event-driven com- Acknowledgement puting. Computers in Industry, 100, 173–183. Brodsky, A., Shao, G., Krishnamoorthy, M., Narayanan, A., Menascé, D., & Ak, R. (2017). Analysis and optimization based on reusable knowledge base of process performance This work is partly funded by the European Commission project models. The International Journal of Advanced Manufacturing Technology, 88(1–4), H2020 UPTIME “Unified Predictive Maintenance System” (768634). 337–357. Ceberio, M., & Kreinovich, V. (2016). Constraint programming and decision making. References Springer. Ceravolo, P., & Zavatarelli, F. (2015). Knowledge acquisition in process intelligence. Information and communication technology research (ICTRC), 2015 international con- Achenbach, A., & Spinler, S. (2018). Prescriptive analytics in airline operations: Arrival ference on, 218–221. time prediction and cost index optimization for short-haul flights. Operations Research Chakrabarti, S., Ester, M., Fayyad, U., Gehrke, J., Han, J., Morishita, S., ... Wang, W.
68 K. Lepenioti, et al. International Journal of Information Management 50 (2020) 57–70
(2006). Data mining curriculum: A proposal (Version 1.0). Intensive Working Group of future directions. European Journal of Operational Research, 215(3), 503–511. ACM SIGKDD Curriculum Committee140. Huang, T., Bergman, D., & Gopal, R. (2018). Predictive and prescriptive analytics for location Chalamalla, A., Ilyas, I. F., Ouzzani, M., & Papotti, P. (2014). Descriptive and prescriptive selection of add-on retail products. arXiv preprint arXiv:1804.01182. data cleaning. Proceedings of the 2014 ACM SIGMOD international conference on Hupfeld, D., Maccioni, R., Sesemann, R., & Ravazzolo, D. (2016). Fleet asset capacity management of data, 445–456. analysis and revenue management optimization using advanced prescriptive analy- Cho, M., Song, S. K., Weber, J., Jung, H., & Lee, M. (2015). Prescriptive analytics for tics. Journal of Revenue and Pricing Management, 15(6), 516–522. planning research-performance strategy. Computer science and its applications. Berlin, Ismagilova, E., Hughes, L., Dwivedi, Y. K., & Raman, K. R. (2019). Smart cities: Advances Heidelberg: Springer1123–1129. in research—An information systems perspective. International Journal of Information Chong, E. K. P., & Zak, S. H. (2008). An introduction to optimization (Wiley-Interscience Management, 47, 88–100. series in discrete mathematics and optimization). Ito, S., & Fujimaki, R. (2017). Optimization beyond prediction: Prescriptive price opti- Christ, M., Krumeich, J., & Kempa-Liehr, A. W. (2016). Integrating predictive analytics mization. Proceedings of the 23rd ACM SIGKDD international conference on knowledge into complex event processing by using conditional density estimations. Enterprise discovery and data mining, 1833–1841. distributed object - computing workshop (EDOCW), 2016 IEEE 20th international, 1–8. Jank, M. H., Dölle, C., & Schuh, G. (2018). Product portfolio design using prescriptive Cugola, G., Margara, A., Matteucci, M., & Tamburrelli, G. (2015). Introducing uncertainty analytics. Congress of the German academic association for production technology, in complex event processing: Model, implementation, and validation. Computing, 584–593. 97(2), 103–144. Jerry, B. (2005). Discrete event system simulation. India: Pearson Education. Davenport, T. H., & Harris, J. G. (2007). Competing on analytics: The new science of winning. Jiang, C., Jensen, D. L., Cao, H., & Kumar, T. (2010). Building business intelligence ap- Harvard Business Press. plications having prescriptive and predictive capabilities. International conference on de Aguiar, M., Greve, F., & Costa, G. (2017). PrescStream: A framework for streaming soft web-age information management, 376–385. Real-time predictive and prescriptive analytics. International conference on computa- Kawas, B., Squillante, M. S., Subramanian, D., & Varshney, K. R. (2013). Prescriptive tional science and its applications, 325–341. analytics for allocating sales teams to opportunities. Data mining workshops (ICDMW), den Hertog, D., & Postek, K. (2016). Bridging the gap between predictive and prescriptive 2013 IEEE 13th international conference on, 211–218. analytics-new optimization methodology neededAvailable at:. Netherlands: Technical Koivisto, J., & Hamari, J. (2019). The rise of motivational information systems: A review report, Tilburg University. http://www.optimization-online.org/DB_HTML/2016/ of gamification research. International Journal of Information Management, 45, 12/5779.html. 191–210. Deshpande, P. (2019). Predictive and prescriptive analytics in big data era. Computing, Krumeich, J., Werth, D., & Loos, P. (2016). Prescriptive control of business processes. communication and signal processing. Singapore: Springer123–132. Business & Information Systems Engineering, 58(4), 261–280. Dey, S., Gupta, N., Pathak, S., Kela, D. H., & Datta, S. (2019). Data-driven design optimi- Larose, D. T., & Larose, C. D. (2015). Data mining and predictive analytics. John Wiley & zation for industrial products. Optimization in industry. Cham: Springer253–267. Sons. Dodge, Y. (Ed.). (2006). The Oxford dictionary of statistical terms. Oxford University Press. Larson, D., & Chang, V. (2016). A review and future direction of agile, business in- Domingos, E. F., Burguillo, J. C., & Lenaerts, T. (2017). Reactive versus anticipative decision telligence, analytics and data science. International Journal of Information making in a novel gift-giving game. AAAI4399–4405. Management, 36(5), 700–710. Du, F., Malik, S., Koh, E., & Theocharous, G. (2018). Interactive campaign planning for Laude, H. (2018). France’s governmental big data analytics: From predictive to prescriptive marketing analysts. Extended abstracts of the 2018 CHI conference on human factors in using R. Federal data science. Academic Press81–94. computing systems, LBW006. Lee, O. J., & Jung, J. E. (2017). Sequence clustering-based automated rule generation for Du, F., Plaisant, C., Spring, N., & Shneiderman, B. (2016). EventAction: Visual analytics adaptive complex event processing. Future Generation Computer Systems, 66, 100–109. for temporal event sequence recommendation. Visual analytics science and technology Lee, M., Cho, M., Gim, J., Jeong, D. H., & Jung, H. (2014). Prescriptive analytics system (VAST), 2016 IEEE conference on, 61–70. for scholar research performance enhancement. International conference on human- Duan, L., & Xiong, Y. (2015). Big data analytics and business analytics. Journal of computer interaction, 186–190. Management Analytics, 2(1), 1–21. Lee, J., Davari, H., Singh, J., & Pandhare, V. (2018). Industrial artificial intelligence for Duan, Y., Edwards, J. S., & Dwivedi, Y. K. (2019). Artificial intelligence for decision industry 4.0-based manufacturing systems. Manufacturing Letters, 18, 20–23. making in the era of Big Data–evolution, challenges and research agenda. Letham, B., Karrer, B., Ottoni, G., & Bakshy, E. (2019). Constrained Bayesian optimization International Journal of Information Management, 48, 63–71. with noisy experiments. Bayesian Analysis, 14(2), 495–519. Engel, Y., Etzion, O., & Feldman, Z. (2012). A basic model for proactive event-driven com- Lo, V. S., & Pachamanova, D. A. (2015). From predictive uplift modeling to prescriptive puting. Proceedings of the 6th ACM international conference on distributed event-based uplift analytics: A practical approach to treatment optimization while accounting for systems - DEBS’ 12. estimation risk. Journal of Marketing Analytics, 3(2), 79–95. Etzion, O., Niblett, P., & Luckham, D. C. (2011). Event processing in action. Greenwich: Lu, J., Chen, W., Ma, Y., Ke, J., Li, Z., Zhang, F., & Maciejewski, R. (2017). Recent pro- Manning. gress and trends in predictive visual analytics. Frontiers of Computer Science, 11(2), Gardner, J. R., Kusner, M. J., Xu, Z. E., Weinberger, K. Q., & Cunningham, J. P. (2014). 192–207. Bayesian optimization with inequality constraints. ICML, 937–945. Luckham, D. C. (2011). Event processing for business: Organizing the real-time enterprise. Gartner (2017). Planning guide for data and analytics. Last Accessed: 03 April 2018 https:// John Wiley & Sons. www.gartner.com/binaries/content/assets/events/keywords/catalyst/catus8/2017_ Martinez, E. C., Cristaldi, M. D., & Grau, R. J. (2009). Design of dynamic experiments in planning_guide_for_data_analytics.pdf. modeling for optimization of batch processes. Industrial & Engineering Chemistry Ghoniem, A., Ali, A. I., Al-Salem, M., & Khallouli, W. (2017). Prescriptive analytics for Research, 48(7), 3453–3465. FIFA World Cup lodging capacity planning. Journal of the Operational Research Society, Martínez, E. C., Cristaldi, M. D., & Grau, R. J. (2013). Dynamic optimization of bior- 68(10), 1183–1194. eactors using probabilistic tendency models and Bayesian active learning. Computers Ghosh, R., Gupta, A., Chattopadhyay, S., Banerjee, A., & Dasgupta, K. (2016). CoCOA: A & Chemical Engineering, 49, 37–49. framework for comparing aggregate client operations in BPO services. Services com- Matyas, K., Nemeth, T., Kovacs, K., & Glawar, R. (2017). A procedural approach for puting (SCC), 2016 IEEE international conference on, 539–546. realizing prescriptive maintenance planning in manufacturing industries. CIRP Giurgiu, I., Wiesmann, D., Bogojeska, J., Lanyi, D., Stark, G., Wallace, R. B., ... Hidalgo, A. Annals, 66(1), 461–464. A. (2017). On the adoption and impact of predictive analytics for server incident Mendes, P. N., Gruhl, D., Drews, C., Kau, C., Lewis, N., Nagarajan, M., ... Welch, S. (2014). reduction. IBM Journal of Research and Development, 61(1), 9–98. Sonora: A prescriptive model for message authoring on Twitter. International conference on Goyal, A., Aprilia, E., Janssen, G., Kim, Y., Kumar, T., Mueller, R., ... Zhang, R. (2016). web information systems engineering. Cham: Springer17–31 October. Asset health management using predictive and prescriptive analytics for the electric Meyn, S. (2008). Control techniques for complex networks. Cambridge University Press. power grid. IBM Journal of Research and Development, 60(1), 4–11. Mikalef, P., Pappas, I., Krogstie, J., & Giannakos, M. (2018). Big data analytics cap- Gröger, C., Schwarz, H., & Mitschang, B. (2014). Prescriptive analytics for re- abilities: A systematic literature review and research agenda. Information Systems and commendation-based business process optimization. International Conference on e-Business Management. 16(3), 547–578. Business Information Systems, 25–37. Mishra, N., & Silakari, S. (2012). Predictive analytics: A survey, trends, applications, Gudivada, V. N., Irfan, M. T., Fathi, E., & Rao, D. L. (2016). Cognitive analytics: Going opportunities & challenges. International Journal of Computer Science and Information beyond big data analytics and machine learning. Handbook of statistics , Vol. 35, Technologies, 3(3), 4434–4438. Elsevier169–205. Mortenson, M. J., Doherty, N. F., & Robinson, S. (2015). Operational research from Habeeb, R. A. A., Nasaruddin, F., Gani, A., Hashem, I. A. T., Ahmed, E., & Imran, M. Taylorism to Terabytes: A research agenda for the analytics age. European Journal of (2018). Real-time big data processing for anomaly detection: A survey. International Operational Research, 241(3), 583–595. Journal of Information Management, 45, 289–307. Mousheimish, R., Taher, Y., & Zeitouni, K. (2016). Autocep: Automatic learning of pre- Harikumar, H., Rana, S., Gupta, S., Nguyen, T., Kaimal, R., & Venkatesh, S. (2018a). dictive rules for complex event processing. International conference on service-oriented Differentially private prescriptive analytics. 2018 IEEE international conference on data computing, 586–593. mining (ICDM), 995–1000. Nasrabadi, N. M. (2007). Pattern recognition and machine learning. Journal of Electronic Harikumar, H., Rana, S., Gupta, S., Nguyen, T., Kaimal, R., & Venkatesh, S. (2018b). Imaging, 16(4), 049901. Prescriptive analytics through constrained Bayesian optimization. Pacific-Asia con- Nechifor, S., Puiu, D., Tarnauca, B., & Moldoveanu, F. (2015). Prescriptive analytics based ference on knowledge discovery and data mining, 335–347. autonomic networking for urban streams services provisioning. Vehicular Technology Hirzel, M., Soulé, R., Schneider, S., Gedik, B., & Grimm, R. (2014). A catalog of stream Conference (VTC Spring), 2015 IEEE 81st, 1–5. processing optimizations. ACM Computing Surveys (CSUR), 46(4), 46. Nguyen, T., Li, Z. H. O. U., Spiegler, V., Ieromonachou, P., & Lin, Y. (2018). Big data Hong, S., Shin, S., Kim, Y. M., Seon, C. N., ho Um, J., & Song, S. K. (2015). Design of analytics in supply chain management: A state-of-the-art literature review. Computers marketing scenario planning based on business big data analysis. International con- & Operations Research, 98, 254–264. ference on HCI in business, 585–592. Osmani, V., Forti, S., Mayora, O., & Conforti, D. (2017). Enabling prescription-based health Howick, S., & Ackermann, F. (2011). Mixing OR methods in practice: Past, present and apps. arXiv preprint arXiv:1706.09407.
69 K. Lepenioti, et al. International Journal of Information Management 50 (2020) 57–70
Pal, A., & Ceglarek, D. (2013). Modeling of decision making process for product service decision-support for manual processes. Journal of Business Economics, 1–24. failure diagnosis. Procedia CIRP, 11, 32–37. Sun, G. D., Wu, Y. C., Liang, R. H., & Liu, S. X. (2013). A survey of visual analytics Pospieszny, P. (2017). Software estimation: Towards prescriptive analytics. Proceedings of techniques and applications: State-of-the-art research and future challenges. Journal the 27th international workshop on software measurement and 12th international con- of Computer Science and Technology, 28(5), 852–867. ference on software process and product measurement, 221–226. Tamilmani, K., Rana, N. P., Prakasam, N., & Dwivedi, Y. K. (2019). The battle of Brain vs. Ramannavar, M., & Sidnal, N. S. (2018). A proposed contextual model for Big data analysis Heart: A literature review and meta-analysis of “hedonic motivation” use in UTAUT2. using advanced analytics. Big data analytics. Singapore: Springer329–339. International Journal of Information Management, 46, 222–235. Revathy, P., & Mukesh, R. (2018). HadoopSec: Sensitivity-aware secure data placement Tan, J. S., Ang, A. K., Lu, L., Gan, S. W., & Corral, M. G. (2016). Quality analytics in a big strategy for big Data/Hadoop platform using prescriptive analytics. GSTF Journal on data supply chain: Commodity data analytics for quality engineering. Region 10 Computing (JoC), 6(2), 5. Conference (TENCON), 2016 IEEE, 3455–3463. Ringsquandl, M., Lamparter, S., & Lepratti, R. (2016). Graph-based predictions and re- Tanenbaum, A. S., & Van Steen, M. (2007). Distributed systems: Principles and paradigms. commendations in flexible manufacturing systems. Industrial electronics society, Prentice-Hall. IECON 2016-42nd annual conference of the IEEE, 6937–6942. Thammaboosadee, S., & Wongpitak, P. (2018). An integration of requirement forecasting Roberts, M. E., Stewart, B. M., Tingley, D., Lucas, C., Leder-Luis, J., Gadarian, S. K., ... and customer segmentation models towards prescriptive analytics for electrical de- Rand, D. G. (2014). Structural topic models for open-ended survey responses. vices production. 2018 international conference on information technology (InCIT), 1–5. American Journal of Political Science, 58(4), 1064–1082. Tranfield, D., Denyer, D., & Smart, P. (2003). Towards a methodology for developing Romijn, J. W. (2014). Philosophy of statistics. Stanford Encyclopedia of Philosophy. evidence-informed management knowledge by means of systematic review. British Said, A. B., Shahzad, M. K., Zamai, E., Hubac, S., & Tollenaere, M. (2016). Towards Journal of Management, 14(3), 207–222. proactive maintenance actions scheduling in the Semiconductor Industry (SI) using Tsai, C. W., Lai, C. F., Chao, H. C., & Vasilakos, A. V. (2015). Big data analytics: A survey. Bayesian approach. IFAC-PapersOnLine, 49(12), 544–549. Journal of Big Data, 2(1), 21. Schmerken, I. (2008). Deciphering the myths around complex event processing. Wall Street & Vidgen, R., Shaw, S., & Grant, D. B. (2017). Management challenges in creating value Technology. from business analytics. European Journal of Operational Research, 261(2), 626–639. Schwartz, I. M., York, P., Nowakowski-Sims, E., & Ramos-Hernandez, A. (2017). von Bischhoffshausen, J. K., Paatsch, M., Reuter, M., Satzger, G., & Fromm, H. (2015).An Predictive and prescriptive analytics, machine learning and child welfare risk as- information system for sales team assignments utilizing predictive and prescriptive sessment: The Broward County experience. Children and Youth Services Review, 81, analytics. Business informatics (CBI), 2015 IEEE 17th conference on, Vol. 1, 68–76. 309–320. Wang, C. H., Cheng, H. Y., & Deng, Y. T. (2018). Using Bayesian belief network and time- Seifert, D., Seifert, R. W., & Protopappa-Sieke, M. (2013). A review of trade credit lit- series model to conduct prescriptive and predictive analytics for computer industries. erature: Opportunities for research in operations. European Journal of Operational Computers & Industrial Engineering, 115, 486–494. Research, 231(2), 245–256. Wang, Y. H., Cao, K., & Zhang, X. M. (2013). Complex event processing over distributed Senyo, P. K., Liu, K., & Effah, J. (2019). Digital business ecosystem: Literature review and probabilistic event streams. Computers & Mathematics with Applications, 66(10), a framework for future research. International Journal of Information Management, 47, 1808–1821. 52–64. Wu, P. J., & Yang, C. K. (2017). The green fleet optimization model for a low-carbon Shroff, G., Agarwal, P., Singh, K., Kazmi, A. H., Shah, S., & Sardeshmukh, A.(2014). economy: A prescriptive analytics. Applied system innovation (ICASI), 2017 interna- Prescriptive information fusion. Information fusion (Fusion), 2014 17th international tional conference on, 107–110. conference on, 1–8. Yaqoob, I., Hashem, I. A. T., Gani, A., Mokhtar, S., Ahmed, E., Anuar, N. B., & Vasilakos, Sierksma, G., & Zwols, Y. (2015). Linear and integer optimization: Theory and practice. A. V. (2016). Big data: From beginning to future. International Journal of Information Chapman and Hall/CRC. Management, 36(6), 1231–1247. Šikšnys, L., & Pedersen, T. B. (2016). Prescriptive analytics. In L. Liu, & M. Özsu (Eds.). Encyclopedia of database systems. New York, NY: Springer. Katerina Lepenioti is a researcher and a Ph.D. candidate at the Information Management Soltanpoor, R., & Sellis, T. (2016). Prescriptive analytics for big data. In M. A. Cheema, W. Unit of the Institute of Communication and Computer Systems in Greece. Zhang, & L. Chang (Vol. Eds.), 27th Australasian database conference: ADC 2016. Databases theory and applications, LNCS: Vol. 9877, (pp. 245–325). Sydney, NSW: Springer International Publishing. Alexandros Bousdekis is a researcher at the Information Management Unit of the Institute of Communication and Computer Systems in Greece. Song, S. K., Jeong, D. H., Kim, J., Hwang, M., Gim, J., & Jung, H. (2014). Research advising system based on prescriptive analytics. Future information technology. Berlin, Heidelberg: Springer569–574. Dimitris Apostolou is associate professor in the Department of Informatics at the Song, S. K., Kim, D. J., Hwang, M., Kim, J., Jeong, D. H., Lee, S., ... Sung, W. (2013). University of Piraeus and a Senior Researcher at the Institute of Communication and Prescriptive analytics system for improving research power. Computational science and Computer Systems in Greece. engineering (CSE), 2013 IEEE 16th international conference on, 1144–1145. Srinivas, S., & Ravindran, A. R. (2018). Optimizing outpatient appointment system using Gregoris Mentzas is full Professor of Management Information Systems, School of machine learning algorithms and scheduling rules: A prescriptive analytics frame- Electrical and Computer Engineering, National Technical University of Athens and work. Expert Systems with Applications, 102, 245–261. Director of the Information Management Unit (IMU), a multidisciplinary research unit. Stein, N., Meller, J., & Flath, C. M. (2018). Big data on the shop-floor: Sensor-based
View publication stats
Advertisement
Prescriptive analytics applications in sustainable operations research: conceptual framework and future research challenges
- Original - Survey or Exposition
- Published: 01 March 2023
Cite this article
- Deepa Bhatt Mishra 1 ,
- Sameen Naqvi 2 ,
- Angappa Gunasekaran ORCID: orcid.org/0000-0002-6335-1962 3 &
- Vartika Dutta 4
4171 Accesses
2 Citations
Explore all metrics
In the broad sphere of Analytics, prescriptive analytics is one of the emerging areas of interest for both academicians and practitioners. As prescriptive analytics has transitioned from its inception to an emerging topic, there is a need to review existing literature in order to ascertain development in this area. There are a very few reviews in the related field but not specifically on the applications of prescriptive analytics in sustainable operations research using content analysis. To address this gap, we performed a review of 147 articles published in peer-reviewed academic journals from 2010 to August 2021. Using content analysis, we have identified the five emerging research themes. Through this study, we aim to contribute to the literature on prescriptive analytics by identifying and proposing emerging research themes and future research directions. Based on our literature review, we propose a conceptual framework for studying the impacts of the adoption of prescriptive analytics and its impact on sustainable supply chain resilience, sustainable supply chain performance and competitive advantage. Finally, the paper acknowledges the managerial implications, theoretical contribution and the limitations of this study.
Similar content being viewed by others
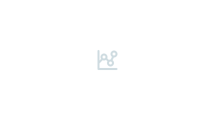
Reporting reliability, convergent and discriminant validity with structural equation modeling: A review and best-practice recommendations
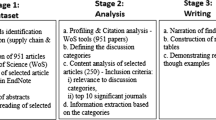
Supply chain disruptions and resilience: a major review and future research agenda
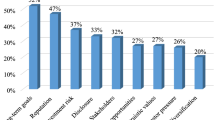
Environmental-, social-, and governance-related factors for business investment and sustainability: a scientometric review of global trends
Avoid common mistakes on your manuscript.
1 Introduction
Analytics is not a new term—it has been around since many decades (Davenport & Soulard, 2014 ; Zamani et al., 2022 ). Over this period, it has transitioned from limited number of data sources to the unlimited data being produced with every click on the website, from saving data in the repositories to using data lakes for saving Big Data. As Big Data is not only about the size, organizations began focusing on how quickly they can acquire and extract meaningful information from this data which is continuously evolving in terms of volume, velocity and veracity (Hazen et al., 2018 ; Hindle et al., 2020 ). This has led to the growth of analytics from descriptive to predictive and prescriptive (Ashrafi & Zareravasan, 2022 ; Larson & Chang, 2016 ).
Descriptive analytics is the traditional technique of data collection and classification, and also helps analysts to identify and visualize patterns in the data (Frazzetto et al., 2019 ). However, it lacks the ability to predict the future events. To overcome this drawback, Predictive Analytics was proposed which not only models the past events but also gives indication on what will happen in the future. Now, once the data has been collected (via descriptive analytics) and the future event has been predicted (via Predictive analytics), it become crucial to understand the possible actions that need to be taken in order to make it happen (Frazzetto et al., 2019 ). This last stage is achieved via Prescriptive analytics which allows decision makers to select and adapt the best possible business opportunity. Thus, it not only minimizes the associated risk by suggesting the optimal sequence of actions, but also assists the organizations in maximizing their business value (Soltanpoor & Sellis, 2016 ).
Although descriptive and predictive analytics have been around for years utilizing machine learning, data mining, and simulation techniques, prescriptive analytics is still in nascent stage (Gartner, 2017 ; Ivanov et al., 2022 ). It combines and integrates optimization, simulation and mathematical tools in a synergetic way so that complex real-world problems can be tackled, and better decisions can be made by relying on the accuracy of the predictions (Frazzetto et al., 2019 ; Šikšnys & Pedersen, 2016 ). This is the reason why tech giants like Microsoft, Oracle, and Google want to leverage the benefits of prescriptive analytics and achieve competitive advantage (Jiang et al., 2010 ; Larson & Chang, 2016 ; Lepenioti et al., 2020 ). Consequently, there has been increased interest among the research community to tackle problems related to supply chain management (Akter et al., 2016 ), disaster operations management (Nagarajan et al., 2012 ), revenue management (Besbes & Zeevi, 2009 ), healthcare (Nahra et al., 2009 ), and customer relationship management (Moon & Kamakura, 2017 ). More importantly, with the pressing need to create a sustainable environment, organizations are now forced to adopt procedures and practices that would not have adverse impact on the surroundings. Decision makers have recognized that sustainable operations management, encompassing green supply chain, green procurement, and reverse logistics, is the key to gain competitive advantage while safeguarding the natural resources for future generations, and thus, achieve long term success (Kamble et al., 2020 ; Seuring and Müller, 2008 ; Srivastava, 2008 ; Gunasekaran & Irani, 2014 ). In this situation, prescriptive analytics is extremely beneficial to decision makers as it would help them in selecting the best possible option while planning for waste management, evaluating strategies for water resource management, finding the optimal location for setting up the waste treatment plant, ranking the alternatives in a vendor selection process, and so on (Achillas et al., 2010a , b ; Montazer et al., 2009 ; Mysiak, 2006 ). Thus, prescriptive analytics is an important asset and poses a number of novel questions to decision makers and researchers that are relevant amid the growing demand to create a sustainable environment.
The motivation for our study stems from the current need to perform sustainable operations, along with the significant role of prescriptive analytics in attaining it. This necessitates a quick response to questions surrounding sustainable operations research and comprehend how prescriptive analytics can assist decision makers in creating a sustainable environment. Over the last decade, an enormous array of reviews, offering important insights, has been conducted on descriptive (Batrinca & Treleaven, 2015 ; Duan & Xiong, 2015 ; Sun et al., 2013 ; Tsai et al., 2015 ), predictive (Lu et al., 2017 ; Mishra & Silakari, 2012 ) and prescriptive analytics (Lepenioti et al., 2020 ). Moreover, separate literature reviews have been conducted in the area of sustainable operations research (Jia et al., 2021 ; Srivastava, 2007 ; Taghikhah et al., 2017 ; Thies et al., 2019 ; White & Lee, 2009 ). However, there is no published survey comprehensively encompassing prescriptive analytics and sustainable operations research. At present, this topic is at a nascent stage, even though it is a promising and crucial research area. Therefore, this work aims to help bridge the gap by investigating the available prescriptive analytics literature and its role in attaining a sustainable environment.
Our study is devoted to exploring the applications of prescriptive analytics through the lens of sustainable operations research and identify the avenues for future research. It thus aims to contribute in the following four ways: First, we collate state-of the-art research on prescriptive analytics and sustainable operations research and investigate the role of prescriptive analytics in carrying out sustainable operations. Second, we streamline the literature by classifying the studies in various themes and sub-themes as the existing knowledge on prescriptive analytics and sustainable operations research is multi-faceted and deserves to be thoroughly reviewed and analyzed. Third, we intend to offer a robust research agenda by investigating the past, present and future, of prescriptive analytics and sustainable operations-related field. This will assist in advancing the extant literature and, at the same time, provide decision makers significant insights while focusing on the sustainability aspect. Fourth, based on this literature analysis, we propose a conceptual framework that can be adopted by supply chain managers and decision makers to gain sustainable supply chain performance, sustainable supply chain resilience and competitive advantage by incorporating sustainable supply chain practices and prescriptive analytical capabilities.
We carefully examine 147 articles published in peer-reviewed academic journals during 2010 to August 2021. Streamlining the literature allowed us to reveal several new research themes and subthemes building connections between prescriptive analytics and sustainable operations research. To this end, our study aims to address four main research questions (RQ):
RQ1: How far prescriptive analytics has diffused in Sustainable operations research? This research question can be helpful in understanding the significance of prescriptive analytics in carrying out sustainable operations. RQ2: What are the main themes linking prescriptive analytics and Sustainable operations? Insights from this question will make it possible to see the dominant topics and their interconnections. RQ3: What is the past, current and future research trends for prescriptive analytics and sustainable operations? This point allows for an understanding of the research agenda, which will be useful for developing the topic further. RQ4: Based on the current knowledge of the literature, what would be a suitable conceptual framework to study the role of prescriptive analytical capabilities in understanding sustainable supply chain performance, sustainable supply chain resilience and competitive advantage by utilizing all three pillars of sustainable supply chain practices: environmental practices, social practices, economic practices?
In particular, we see that prescriptive analytics continues to diffuse in the areas of healthcare, inventory management, revenue management, supply chain management, waste management, water management, energy conservation, disaster management and so on. We also find that the relevant literature can be classified into five main themes: applications of optimization techniques, sustainable operations management, data mining and statistical techniques, sustainable supply chain management, and disaster management. Corresponding to each theme, various sub-themes with the current and potential future research gaps and objectives are proposed and discussed. Our findings and proposed framework can be used by decision makers and researchers to propose avenues for improvements and bridge the gap between the theory and practice.
The paper is organized as follows. The next section presents the prior reviews conducted in the area; Sect. 3 shows the methodological approach to the review; Sect. 4 synthesizes the principal themes drawn from the body of research reviewed; and Sect. 5 discusses the main issues to future research on prescriptive analytics and in its application in sustainable operations research.
2 Prior reviews
To synthesize the literature and gain insights, it is important to conduct literature review-based studies (Kazemi et al., 2019 ). It accelerates the development of the research area by providing quick access to related issues and exploring the major contributions (Creswell, 2013 ; Machi & McEvoy, 2012 ). Owing to the significant interest in the areas of prescriptive analytics and sustainable operations research, various literature reviews have been conducted which target one of these two areas. In the direction of analytics (descriptive, predictive, and prescriptive analytics), social media tools and techniques were comprehensively reviewed by Batrinca and Treleaven ( 2015 ), big data and business analytics captured the attention of Duan and Xiong ( 2015 ), Tsai et al. ( 2015 ), Mikalef et al. ( 2018 ), and Ajah and Nweke ( 2019 ), visual analytics techniques and applications were surveyed by Sun et al. ( 2013 ), predictive visual analytics recent trends and developments were explored by Mishra and Silakari ( 2012 ) and Lu et al. ( 2017 ), and more recently, the literature on prescriptive analytics was reviewed by Lepenioti et al. ( 2020 ) to identify the research and implementation challenges. In the direction of SOR, Srivastava ( 2007 ) reviewed the literature on green supply chain management, White and Lee ( 2009 ) discussed the potential of operations research in the area of sustainable development, Gunasekaran and Irani ( 2014 ) gave a call for papers on sustainable operations management in order to understand the strategies, tactics and implementation processes, Thies et al. ( 2019 ) reviewed 142 articles that use Operations Research methods for assessing the environmental and social impacts of the products, and Jia et al. ( 2021 ) systematically reviewed 83 articles on sustainability-oriented supplier development and adopted contingency theory to understand how these initiatives can be incorporated in the supply chains. However, to the best of our knowledge, no review study has appeared which conjoins prescriptive analytics and sustainable operations research areas together. Thus, we aim to fill this gap in the literature by providing a comprehensive review of the applications of prescriptive analytics in the area of sustainable operations research.
3 Research design
In this section, we describe the research methodology adopted in this study. We begin with the systematic literature review methodology framework, then define the search terms and database, followed by the search results, while describing the inclusion and exclusion criteria of this refinement and finally, discuss about the literature review analysis, conceptual framework and future research directions.
3.1 Step 1: Selecting systematic literature review methodology
The aim of a Systematic Literature Review (SLR) is to examine the body of literature, highlight the major gaps and underline the boundaries of knowledge (Tranfield et al., 2003 ; Ambilkar et al., 2022 ; Dohale et al., 2022 ; Paul & Criado, 2020 ). SLR has been considered as one of the most rigorous methods for conducting the literature reviews as its applications can be seen in various fields, including the recent works in the area of supply chain (Sawyerr & Harrison, 2022 ; Suryawanshi & Dutta, 2022 ).
In this study, we adopted a research protocol highlighted in Table 1 from Queiroz et al. ( 2020 ). Research background is first established for prescriptive analytics applications in sustainable operations research and then the search terms are defined for the data collection. For data extraction, we used bibliometric software Bib Excel and, using Endnote, the data is further cleaned by removing any duplicated and invalid documents. Next, the content analysis is presented to discuss the major themes, followed by the avenues for future research.
3.2 Step 2: Defining the appropriate search terms and database
This research is conducted to explore the applications of prescriptive analytics in sustainable operations research. The topic consists of two terms: prescriptive analytics and sustainable operations research. To ensure that both aspects are fully captured by the keywords, we included two search strings, which are shown in Table 2 . The first string is related to prescriptive analytics, including machine learning and data analytics. The second search string contains "sustainable operations research" and "operations research". The selection of keywords was based on previous literature reviews on similar topics and the authors' own research experience.
The data used in this research was collected from the well-established academic database, Scopus, to ensure no major relevant works are missed out as it has been considered one of the largest bibliographic databases. It provides seamless access to articles published since 1996 and covers approximately 58 + million records. Even though there are other databases like Web of Science, EBSCO, we considered only Scopus database because it includes all sort of journals with or without an impact factor, thus fulfilling our objective to identify maximum number of articles for the literature review. However, the debate on which database is better is outside the scope of this study. Also, the user-friendly search engine of Scopus facilitated the article refinement process because it enabled us to incorporate specific search conditions.
3.3 Step 3: Data search results
The initial search attempts identified 3089 titles (see Fig. 1 ). Next, the data is cleaned by focusing on articles and reviews published between 2010 and August 2021 and removing all the articles not written in English. The reason for selecting the above timeline is that post-2010 only, the area of prescriptive analytics actually gained the popularity and most of the seminal articles appeared after that. This screening resulted in 250 papers. Next, the articles which did not mainly focus on the role of prescriptive analytics in sustainable operations research were excluded. To facilitate this, a team of co-authors prepared a table with all 250 papers and a column to decide 'include' or 'exclude' the article. This search resulted in 147 papers which were stored in CSV format to include all the essential paper information such as paper title, authors' names and affiliations, abstract, keywords and references. The inclusion and exclusion criteria can be clearly seen in Table 2 .
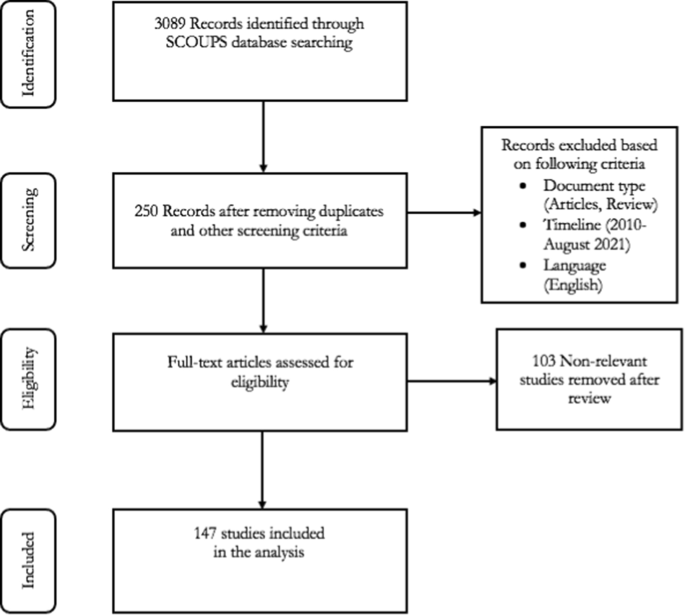
Methodology framework
3.4 Step 4: Literature analysis
All the shortlisted articles are used for data analysis. We performed content analysis and identified five broad themes. We also conducted a descriptive analysis to highlight the journal publication trend, top countries and subject areas.
3.5 Step 5: Example conceptual framework
Based on the literature review analysis, we propose a research framework in Sect. 4 for studying the role of adoption of prescriptive analytics in creating a sustainable environment in terms of sustainable supply chain resilience, sustainable supply chain performance and competitive advantage.
3.6 Step 6: Future research directions
The most important aspect of any literature review is to provide future research opportunities for other scholars who are working in the same research area. For this purpose, we have provided opportunities for future research in Sect. 5 .
3.6.1 Descriptive statistics
This part of the study focuses on the descriptive statistics of the reviewed literature. Figure 2 shows the distribution of the publications over the years starting from 2010 to August 2021. It is quite evident that the number of publications has increased drastically in the last 3 years thereby reflecting the actual growth in this area. It is also expected that this publication trend will continue to increase in the coming years as post COVID-19 pandemic, companies and researchers are focussing more in this area of research.
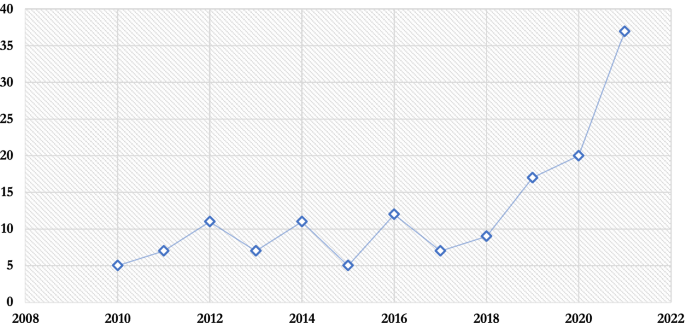
Publication by year
A further bibliometric analysis was performed on the subject area. It is evident from Fig. 3 that the maximum number of papers are in decision sciences, followed by computer science, mathematics, engineering and business management area. Other subject areas, such as, social sciences, material science and finance are still in the nascent stage. Similarly, we used Bib Excel software to extract the country wise information for further analysis. Figure 4 highlights the top countries that have contributed maximum in this area of research. The top four countries are USA, UK, China and Canada. Nonetheless, scholars from across the world are attracted towards the area of prescriptive analytics and sustainable operations research.
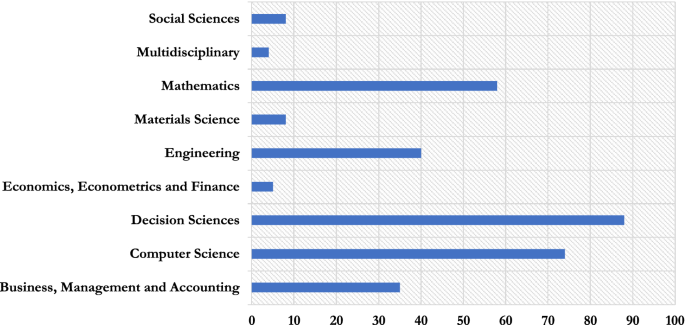
Publication by subject
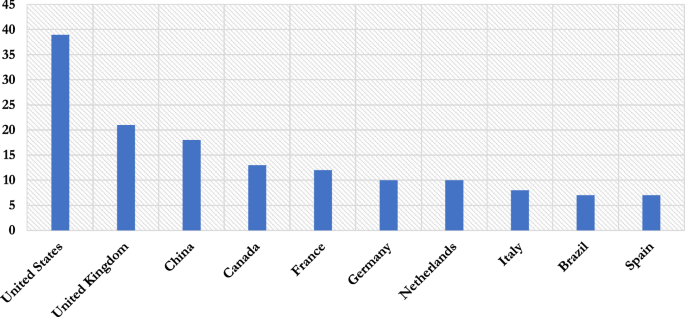
Country-wise publications
4 Findings from content analysis
Content analysis is one of the most important methods for conducting literature reviews and, specifically, for generating different research themes. In content analysis, different set of information is collected in order to provide deeper insights (Krippendorff, 2004 ). To synthesize the content, periods, keywords, abstract, applications and methodologies for more reliable results, it is recommended that more than one researcher should be involved in this process (Govindan & Hasanagic, 2018 ; Seuring & Gold, 2012 ). For the purpose of this study, the selected articles were segregated based on their research issues, methodologies and applications and five broad themes were developed, and within each of these themes, we identified sub-themes. The details of our research themes can be seen in the below mentioned subsections:
4.1 Theme 1: Applications of optimization techniques
There are 29 papers in this theme and these papers prominently discuss about the applications of optimization techniques in various sectors. Due to the homogeneous connections of the papers in this theme, we conduct content analysis by dividing and reviewing papers according to five subsections: (1) Optimization in Inventory Management, (2) Optimization in Revenue management, (3) Optimization in Healthcare (4) Operations Management and Operations Research.
4.1.1 Optimization in inventory management
Overall, there are 7 papers (24.1%) in this subtheme. It is clear that the papers in the previous decade focused on application of optimization techniques in inventory management (Kök and Fisher, 2007 ; Dehoratius et al., 2008 ; Balakrishnan et al., 2008 ; Olsen & Parker, 2008 ; Pastor and Olivella, 2008 ; Beutel & Minner, 2012 ; Naseraldin & Herer, 2008 ).
Retailers often come across problems such as inventory record inaccuracy, assortment planning, demand uncertainty, inventory stockout. To help retailers with assortment planning, Kök and Fisher ( 2007 ) developed an algorithm to compute the best assortment of the products. Under the assumption that substitution of a product with a similar product of the same category is feasible, optimal results were obtained through the proposed iterative optimization heuristic. To tackle inventory record inaccuracy, Dehoratius et al. ( 2008 ) adopted a Bayesian approach and proposed an intelligent inventory management tool. By performing simulation, the authors showed that the proposed replenishment policy recovers the cost incurred due to record inaccuracy, and that their inventory audit policy performs better than the famous “zero balance walk” audit policy. Balakrishnan et al. ( 2008 ) highlighted the significance of maintaining higher inventories in stimulating demand. They considered profit-maximization policy for a stochastic inventory model and showed that demand stimulation can increase the service level and thereby generate higher profits. To capture the economic effect of a customer leaving the market at the time of inventory stockout, Olsen and Parker ( 2008 ) proposed models to study the stochastic demand distribution in a time-dynamic context. As manual allocation and adaptation of weekly work schedules is difficult, Pastor and Olivella ( 2008 ) proposed a mixed linear program along with a local optimization process. To check the effectiveness of their proposed method, the authors considered the two franchises of a retail clothing chain and the results were found to be satisfactory.
The traditional inventory management approaches assumed a demand distribution and a time series-based framework was used to parameterize the demand model. However, the demand may depend upon various external factors, specifically, in case of retail inventory management system. Thus, to incorporate these factors, Beutel and Minner ( 2012 ) compared regression model approach to forecast demand and linear programming approach to optimize the target inventory function. It was shown that if sample size is small, then the former method outperforms the latter, whereas, if some of the assumptions of the former method are violated, the latter approach gives more robust inventory levels. To further assist retailers with replenishment decisions, Naseraldin and Herer ( 2008 ) integrate the number and location of each retail outlet and analyse the system using expected cost. They showed that an improved solution can be obtained by combining strategic and operational decisions and expressing in terms of the number of retail outlets.
4.1.2 Optimization in revenue management
There are four papers (13.8%) in this subtheme and mainly focus on revenue management through the use of optimization techniques (Besbes & Zeevi, 2009 ; Liu & van Ryzin, 2008 ; Mukhopadhyay et al., 2007 ; Netessine et al., 2006 ).
In e-commerce industry, dynamic cross-selling of products and services has become increasingly common. Selection of the complimentary product and it’s pricing are the two major issues faced by a company. To formulate this problem of revenue management, Netessine et al. ( 2006 ) propose a stochastic dynamic program with combinatorial optimization and subsequently, suggest various pricing and packaging heuristics. Another aspect of maximizing revenue can be seen in Mukhopadhyay et al. ( 2007 ) where the focus is on airline revenue management. As revenue can be improved by making accurate passenger demand forecasts, the authors propose a method to adjust system forecast by taking into account the unseen demand and evaluate the performance using forecast monitoring system. While studying network revenue management, Liu and van Ryzin ( 2008 ) included the concept of efficiency for determining the optimal policy in the choice-based revenue management problem. To further improve the performance of the static linear programming solution, the authors proposed a practical decomposition heuristic for converting the static choice-based deterministic linear programming model solution into a dynamic control policy. In order to maximize the expected revenue, Besbes and Zeevi ( 2009 ) studied single-product revenue management problem where the demand function is unknown. On measuring the efficiency of the proposed algorithms in terms of regret, it was found to be very close to the lower bound for any admissible pricing policy.
4.1.3 Optimization in healthcare
Also, in this theme, we review 13 papers (44.8%) addressing the healthcare sector where a few have focused on sustainable ways to improve healthcare system (Jagesar et al., 2021 ; Kohno et al., 2019 ; Zolbanin et al., 2020 ).
Appointment scheduling has come at the forefront of the healthcare sector as it not only provides access to service providers but also to clinics for scheduling the surgeries (Gupta & Denton, 2008 ). For instance, a clinic scheduler might use it to schedule the appointments for outpatients. This can be formulated using a stochastic overbooking model with the idea that patient no-show will be compensated by overbooking (Muthuraman & Lawley, 2008 ). Another study on outpatients which employs optimization techniques is Nahra et al. ( 2009 ). The authors highlight the drawback of the traditional methodology and propose a super efficiency Data envelopment analysis to treat outpatients dealing with substance abuse. Zolbanin et al. ( 2020 ) suggested that one way in which hospitals can utilize the resources sustainably is by predicting patient’s duration of stay at the hospital using deep learning neural network. To optimize the outcome of patients suffering from brain trauma, Kunene and Weistroffer ( 2008 ) modelled various factors affecting the outcome using multicriteria decision making. The results suggested that better prediction can be made if a priori information is available when using data mining tools. To identify the application of Analytic hierarchy process (AHP) in healthcare, Liberatore and Nydick ( 2008 ) conducted a literature review and identified that the number of articles has increased significantly in the recent years. They also pointed that AHP has been used in doctor-patient decision making, selection of correct medical treatment, and evaluation of the related technologies and policies. To reduce the problem of nurse rerostering in hospitals, Moz and Pato ( 2004 ) proposed two integer multicommodity flow models. These models were tested on real data from a public hospital in Portugal and it was found that the second outperformed the first in terms of solution quality and computational time. Jagesar et al. ( 2021 ) presented requirements and guidelines to achieve a secure and sustainable digital phenotyping. To further benefit the healthcare system, Kohno et al. ( 2019 ) integrated big data analytics and information and communication technology, and proposed a medical healthcare platform that regulates sustainable operations.
Time is a critical factor in any healthcare setting, and hence, transportation needs of patients should be given utmost priority. To address this issue, Melachrinoudis et al. ( 2007 ) propose a double request dial-a-ride model where coordination and central dispatching system eases the process and also minimizes the transportation cost and inconvenience time. Further, a decision support tool was designed by Ahmed and Alkhamis ( 2009 ) to enhance the service efficiency of an emergency unit in a hospital. For this purpose, they integrated simulation and optimization and proposed an optimal allocation which would maximize patient throughput and also reduce their waiting time. As the location of nomads keeps changing seasonally, it is a challenge for health care units to provide them with the proper medical facilities. To determine the optimal number and location of health care units in such a situation, Ndiaye and Alfares ( 2008 ) formulated a binary integer programming model. The model gave efficient solution when applied to nomadic groups in Middle East. Nicholson et al. ( 2004 ) highlighted the benefit of inventory management in healthcare systems. They compared inventory cost and service level of an in-house three-echelon distribution network with that of an outsourced one and showed that outsourcing non-critical inventory items is a better choice.
4.1.4 Operations management (OM) and operations research (OR)
There are five papers (17.3%) in this subtheme. Although OM and OR overlap in terms of the employed techniques, they differ at the conceptual level. OM is more oriented towards the management of resources which are critical to firm’s growth and performance, while OR deals with modelling, problem solving and decision making (Anderson et al., 2002 ). In this regard, Meredith ( 1998 ) emphasized that OM should not be only studied by traditional techniques of optimization and simulation but also through case and field-based studies as they are inter-related and can offer important insights. Tranfield et al. ( 2003 ) provided step by step methodology to perform systematic review and is now being adopted by OM researchers. With the increasing usage of analytics in organizations, academicians and practitioners have shifted their attention to study the impacts and opportunities of OR. Fortun and Schweber ( 1993 ) analysed OR through the lens of World war II by studying its relation with system engineering and comparing the developments in the US and Great Britain post the world war. Kirby ( 2000 ) noted that understanding the history of OR is equally important to further expand the knowledge in this research area. With this in mind, the author connected the history of OR in Britain and United States over the span of 50 years, i.e., 1940s to 1990s. Continuing this further, Liberatore and Luo ( 2010 ) discussed the drivers of this analytic shift and also studied the opportunities and implications it offers for promoting and expanding operations research.
4.2 Theme 2: Sustainable operations management
Forty papers make theme 2 the largest theme. At the first glance, researchers mainly seek to solve sustainability related issues using MCDA, a branch of operations research, which is an efficient decision-making method to evaluate multiple criteria and find the best feasible solution, because majority of the papers (21) appear in this direction. Another direction of research which is evident from this theme is the use of Machine Learning techniques for sustainable operations management. We structure this theme as (1) MCDA and Sustainability, and (2) Machine Learning and Sustainability.
4.3 MCDA and sustainability
There are 25 papers (62.5%) in this subtheme which includes papers on MCDA, as well as ELECTRE, which is a family of MCDA techniques, and on MCDA review papers from the perspective of sustainability.
From the perspective of creating a sustainable environment, MCDA has been used in assessing eutrophication (Moriki & Karydis, 1994 ), evaluating water resource management strategies and selecting the best one (Nijkamp & Vos, 1977 ), identifying risk prone areas in mining locations (Merad et al., 2004 ), recognizing the optimal location for setting up electrical and electronic waste treatment plants (Achillas et al., 2010a , 2010b ) and for waste management (Morrissey & Browne, 2004 ), developing risk assessment approach for sustainable constructions engineering projects (Marhavilas et al., 2020 ), for searching the optimal setpoints and the ideal gas fuel for sustainable operation of wastewater treatment plants (Heo et al., 2021 ; Akbaş and Bilgen, 2017 ). To better predict water demand, Hu et al. ( 2021 ) proposed a preprocessing framework using neural network and Balkhair et al. ( 2016 ) developed a cost-effective sustainable operation policy of the plant during the failure of any production pump. All these works emphasized on the need of cooperation between the authorities and scientists in order to create a sustainable environment. Other applications of MCDA include selection of a final solution from a set of solutions during software release planning process (Ngo-The & Ruhe, 2008 ), evaluation of the credibility of the credit card applicant (Matsatsinis, 2002 ), and in manufacturing of products as for the development of a new product, manufacturers often use old parts with similar specifications. In order to efficiently search for such parts from a database, Mun and Ramani ( 2011 ) employed MCDA and ontology, and also validated their approach by considering a case study of ejector pins.
ELECTRE belongs to the family of multi-criteria decision analysis. In recent years, evolutions of ELECTRE, such as ELECTRE III and ELECTRE Tri, have received considerable attention from the researchers and practitioners. For instance, Montazer et al. ( 2009 ) used the fuzzy version of ELECTRE III for ranking the alternatives in a vendor selection process, Mróz and Thiel ( 2005 ) employed ELECTRE III for evaluating the heating system of educational buildings. In a similar way, ELECTRE TRI was studied by Mousseau and Slowinski ( 1998 ) to assign a given alternative to some pre-defined categories. The authors proposed an interactive approach where the desired parameters (importance coefficients, weights, thresholds, category limits, etc.) are determined via assignment examples, and then this problem is solved using non-linear optimization technique. Through this approach, the decision makers have the feasibility to modify the assignment problem and draw more information for optimization. ELECTRE TRI was further modified by Mousseau et al. ( 2000 ) who proposed ELECTRE TRI Assistant method to ease the process of preference selection by the decision maker. Their method mainly targeted determination of importance coefficients by keeping other parameters fixed and then solved the problem as linear program. Later, Moussea et al. ( 2001 ) and Ngo-The and Moussea ( 2002 ) considered subproblems to determine the weights and category limit, respectively, and formulated it as a linear programming problem. The problem of inconsistency among the constraints on these parameters was addressed by Mousseau et al. ( 2003 ). The above two methods, ELECTRE III and ELECTRE Tri, were integrated in Mousseau and Dias ( 2004 ) where a modified version of valued outranking relation was considered. To obtain the values of the parameters, authors adopt disaggregation approach which has been rarely used in case of outranking methods. These modifications were then showed to make the computation of inference problems easier.
Authors have also reviewed MCDA approaches to create a sustainable environment by saving the environment, forest, and natural resources (Mendoza & Martins, 2006 ; Mysiak, 2006 ). For instance, Mysiak ( 2006 ) focused on application of MCDA in environmental and natural resource management. The author reviewed various experiments that were conducted to make comparisons between the results of different MCDA methods. It was highlighted that multimethod MCDA should be given preference in comparison to traditional sensitivity analysis while dealing with unstructured decision problems. A similar review on MCDA methods in natural resource management was conducted by Mendoza and Martins ( 2006 ) where the methods were classified based on its characteristics and properties. The point of differentiation from the review of Mysiak ( 2006 ) is that Mendoza and Martins ( 2006 ) described new MCDA approaches in forest management and pointed out the need to shift from the concept of problem solving to that on problem structuring. Other set of reviews include those by Malczewski ( 2006 ) and Farahani et al. ( 2010 ), where the former integrated MCDA with GIS and reviewed the relevant papers to identify the trends and developments in this domain, and the latter reviewed articles on the multi-criteria location problems.
4.3.1 Machine learning and sustainability
There are 15 papers (37.5%) in this subset. With the shift towards the digital era, the role of Machine Learning in attaining sustainability is deemed to receive much attention (Breiman, 2001 ; Ceballos-Fuentealba et al., 2019 ; Chahidi et al., 2021 ; Chu et al., 2019 ; Diaz et al., 2021 ; Duan et al., 2020 ; Fallah et al., 2018 ; Fujimoto et al., 2019 ; Hegedűs et al., 2021 ; Lim et al., 2017 ; Rafique & Jianhua, 2018 ; Schreiber et al., 2021 ; Shaharum et al., 2020 ; Trivedi et al., 2021 ; Tsai et al., 2011 ).
To promote sustainable development of non-residential buildings, Ceballos-Fuentealba et al. ( 2019 ) proposed a simulation and optimization methodology to predict the energy consumed in any building and the impact various energy conservation measures have on that building. Anther work to monitor energy consumption was done by Tsai et al. ( 2011 ) where life cycle assessment is used to analyse the cost of carbon dioxide emission and mathematical programming technique is used to study the distribution of limited resources. Rafique and Jianhua ( 2018 ) reviewed the literature on energy management, generation and consumption for microgrids. As greenhouse cultivation has greatly increased, Chahidi et al. ( 2021 ) compared different ML models such as, Artificial Neural Networks, Gaussian Process Regression, Support Vector Machine and Boosting trees, to predict the greenhouse energy demand and production. Moreover, Schreiber et al. ( 2021 ) utilized ML methods to generate automated data-driven model that can optimize the cooling system of the entire campus. For distribution of oil-palm over a large area, Shaharum et al. ( 2020 ) adopted non-linear ML techniques and showed that Support Vector Machine and Random Forest are equally effective, Trivedi et al. ( 2021 ) utilized random forest ensemble learning model based on mobile sensing and crowdsensing to predict the ambient temperature in buildings, Diaz et al. ( 2021 ) proposed a random forest model predictive control for paste thickening, a new method in mineral processing, to ensure an environmentally sustainable operation, and Breiman ( 2001 ) studied Random Forests to solve regression and classification problems.
ML based approach has also been used to assess the crop quality and consumption of electricity (Fujimoto et al., 2019 ), to forecast energy load in smart energy management grids (Fallah et al., 2018 ), to address the sustainable operations of power control by incorporating energy harvesting and internet of things (Chu et al., 2019 ), to reduce waste and conserve industrial resources and implement process graph framework to deal with resource conservation network issues (Lim et al., 2017 ), to evaluate the relationship between sustainable operation of rail transit and land use by employing data envelopment analysis (Duan et al., 2020 ) and to achieve sustainable transportation, explore vehicle automation through closed loop matching where the dataset is generated, trained and evaluated using neural network (Hegedűs et al., 2021 ).
4.4 Theme 3: Data mining and statistical techniques
With 30 papers, this is the second largest theme. In today’s digital world, huge amounts of data is being produced every nano second and to develop patterns and relationships from this data, data mining techniques have come to the forefront. Another set of technique which is being employed to analyze data is statistical techniques. Thus, content analysis is done by dividing and studying this theme according to two subsections: (1) Data mining techniques and Customer Relationship Management (CRM), and (2) Multivariate Statistical Techniques.
4.4.1 Data mining techniques and CRM
There are seven papers (20%) which solely studied the role of data mining techniques in customer relationship management. To be more specific, Moon and Russell ( 2008 ) proposed a product recommendation model using joint space mapping and spatial choice modeling to predict product purchase behavior. The model when applied on a customer database of an insurance firm provided good forecasts results. With the idea that number of previous buyers effect the purchase timing of new product, Bass ( 1969 ) developed a growth model for consumer durables and forecasted the sales of television sets. Another step in the direction of customer relationship was taken by Neslin et al. ( 2006 ) and Holtrop et al. ( 2017 ) who studied the effectiveness of customer churn predicted models. Here, Neslin et al. ( 2006 ) noted significant differences among various modelling approaches and Holtrop et al. ( 2017 ) developed a method to predict churn without sacrificing consumer privacy. Moreover, Moon and Kamakura ( 2017 ) emphasized that online product reviews reflect information about the reviewer. The authors translated wine and hotel reviews to a product positioning map by combining ontology learning-based text mining and psychometric techniques. This framework is beneficial to product managers as it offers insights into consumer segmentation strategy. For sustainable operation of social networking sites, Chin et al. ( 2018 ) conducted a survey on 1108 users to determine the intention behind self-disclosure as privacy of these websites has recently come under scrutiny. More insight into the application of data mining techniques in CRM was provided by Ngai et al. ( 2009 ) who reviewed the literature for a period of 2000–2006. The papers were categorized on four dimensions of CRM (customer identification, attraction, retention and development) and the review revealed that consumer retention has been widely studied by researchers and, data mining functions of classification and association are the two most commonly used techniques in CRM.
4.4.2 Statistical techniques
In this subset, a total of 23 papers (77%) are explored. Multivariate statistical techniques, such as Cluster analysis, discriminant analysis, correspondence analysis, have attracted the attention of researchers over decades. For instance, in order to provide an effective measuring scale for input variables, Morrison ( 1967 ) modified the cluster analysis procedure so that statistically meaningful results can be obtained. Later, Morey et al. ( 1983 ) compared different methods of cluster analysis with respect to derivation, replication, external validation and cross-validation, Tibshirani et al. ( 2001 ) proposed a method, The Gap Statistic, for estimating the number of clusters in a dataset, and McNicholas et al. ( 2010 ) proposed an algorithm, by modifying the expectation–maximization (EM) algorithm, to implement model-based clustering via Gaussian mixture models and discuss the issue of model selection and convergence criteria. Further, Morrison ( 1969 ) provided guidance to interpret the results of linear discriminant analysis, Nenadic and Greenacre ( 2007 ) introduced a package for simple, multiple, and joint correspondence analysis in R, Murtagh ( 2005 ) studied the mathematics and algorithms behind correspondence analysis, highlighted its significance in artificial intelligence and discussed data coding in Java and R, Mclachlan and Krishnan ( 2007 ) focused on the theory and applications of the EM algorithm and its extensions, and Roig-Tierno et al. ( 2013 ) used Analytical Hierarchy Process with GIS to determine the retail site location. In many real situations, the random variables representing lifetime of a component, unit or a system are dependent, and hence a separate treatment of this subject is required. In this direction, Nelsen ( 2003 ) studied the concept of copulas, which is an efficient way to express multivariate distribution in terms of their marginals. This concept is mainly used to describe the dependence and association between random variables. Further, to model dependency between random variables, Meeuwissen and Bedford ( 1997 ) considered bivariate distributions with uniform marginals and a specified rank correlation, and Morales et al. ( 2008 ) suggested the use of conditional and unconditional rank correlations. Another work in the multivariate set-up was done by Morales-Nápoles and Worm ( 2013 ) who conducted hypothesis testing of multivariate probability distributions.
For forecasting a time series, Newbold and Granger ( 1974 ) compared the performance of Box-Jenkins, Holt-Winters and Stepwise Autoregression, and suggested that a combination of these individual forecasts is often profitable. Murphy and Winkler ( 1970 ) studied the role of Scoring Rules in probability assessment and evaluation, as well as, in probability forecasts and assessors, Mcnees ( 1987 ) studied consensus forecast, Martino ( 1992 ) showed how technological forecasting is essential in all domains, engineering, management, and strategic planning, and Myung et al. ( 1996 ) employed Shannon’s information measure to aggregate opinions of experts on the prediction of outcome of an uncertain event. To assist decision makers, Morris ( 1974 ) introduced the concept of involving expert’s opinion with their own. This can be done by applying the tools of Bayesian inference. Later, Morris ( 1977 ) adopted Bayesian approach to incorporate different probability assessments by experts. In case of single expert, decision maker can multiply expert’s probability assessment with his own prior probability assessment and normalize while in case of multi-experts, multiplicative rule is applied. Morris ( 1983 ) proposed axioms to combine expert probability assessments and developed a method to merge these probabilities into a single estimate. Nadkarni and Shenoy ( 2004 ) constructed Bayesian networks by utilizing the knowledge of experts via casual mapping approach. The authors highlighted how knowledge can be represented as causal maps and their graphical structure can be used to construct Bayes nets. As maintaining the cooling system of data centres is key to sustainable operation, Patnaik et al. ( 2011 ) proposed a data mining solution incorporating motif mining, association analysis and dynamic Bayesian network analysis.
4.5 Theme 4: Sustainable supply chain management
Twenty-six papers make theme 4 the third largest theme. As the theme name itself speaks, researchers mainly seek to develop ways in which supply chains can be made sustainable. We structure this theme as (1) Supply chain management and Sustainability, and (2) Applications of Artificial Intelligence (AI) in Sustainable Supply Chains.
4.5.1 Supply chain management and sustainability
There are 16 papers (61.5%) in this subtheme with a focus on sustainable supply chain management, supplier selection, order allocation and firm performance. With the aim to present guidelines for logistics and supply chain managers to adapt to the circular economy business models, Lopes de Sousa et al. ( 2019 ) conducted a systematic literature review and highlighted the changes that are required in the decision making process of operations management so as to support the circular economy business models, Jabbour et al. ( 2019 ) integrated big data and circular economy, and Kumar et al. ( 2021 ) focused on post-COVID-19 era and analysed barriers in adopting big data analytics for sustainable manufacturing operations. Shaharudin et al. ( 2019 ) focussed on the aspect of sustainability by conducting a literature review and social network analysis on low carbon supply chain management. The authors identified six main domains in the area, specifically, sustainability, climate change, green supply chain management, supply chain management, innovation, sustainable development, and environmental management. They also highlighted the benefit of including sustainable management practices and carbon performance indicators in enhancing the integrated performance.
Another important aspect of supply chain is supplier selection and order allocation. In this regard, Scott et al. ( 2015 ) proposed a method for supplier selection that integrates stochastic, multi criteria decision analysis and multi stakeholder approaches. It is based on AHP-Quality Process–Quality Function Deployment and optimization algorithm which selects suitable suppliers and then allocates them order optimally. For solving the multi-objective inventory control problem, Moslemi and Zandieh ( 2011 ) compared strategies on multi-objective particle swarm optimization and showed that the proposed method significantly reduces computational time and gives optimal solutions. Beyond what has been discussed, researchers also study some mathematical ways to guarantee sustainable operations. For instance, mathematical programming model for addressing the problem faced by service providers in designing an urban electric vehicle sharing system (He et al., 2017 ), hybrid modelling framework for routing one-commodity pick-up and delivery by bike sharing systems (Lei & Ouyang, 2018 ), mathematical programming approach to asses process improvement capability index of products (Chen et al., 2015 ), and mixed integer programming approach to obtain the optimum product mix in a process industry (Galal & Moneim, 2015 ). Other works on optimization problems include algorithms for simulation based objective functions with large number of parameters (Nedelkova et al., 2018 ), and three-stage fixed charge multi-objective problem for sustainable transportation (Mehlawat et al., 2019 ).
Enhancing firm performance is of prime concern to firm managers. In this direction, Akter et al. ( 2016 ) and Wamba et al. ( 2017 ) proposed models that examine how companies can develop Big data analytics capability to enhance firm performance. Here, Akter et al. ( 2016 ) based the model on Resource Based Theory and the entanglement view of sociomaterialism and highlighted the moderating role of business strategy in this relationship, while Wamba et al. ( 2017 ) examined direct effect, as well as, mediating effect of process oriented dynamic capabilities, and provided insights to enhance firm performance. Moreover, it should be noted that “big data” is not only about the size, rather it is a combination of 5Vs, i.e., volume, variety, velocity, veracity, valence, value. Chen et al. ( 2012 ). A broader definition of big data was provided by Gandomi and Haider ( 2015 ) and methods were developed for analysing unstructured data which accounts for 95% of big data, and at the same time, emphasis was given on the necessity to develop new tools for structured big data.
4.5.2 Applications of AI in sustainable supply chains
There are 10 papers (38.5%) in this subtheme. AI is greatly known to improve the decision-making process of human beings in the areas of supply chain management, supply chain risk management, banks and medicine. Similarly, its sub-field, Deep Learning plays a significant role in speech recognition, object recognition and detection (Lecun et al., 2015 ). Lee ( 2021 ) reviewed the literature and linked sustainability and OM by highlighting the benefits of adopting AI in product design, and that social sustainability is the basis for economic and environmental sustainability. To understand its application in supply chain management, Min ( 2010 ) reviewed previous papers on the successful application on AI and identified the core areas of SCM where it can be applied. In the context of textile and apparel supply chains, Ngai et al. ( 2014 ) reviewed the literature on applications of decision support and AI systems in these industries. The articles were categorized based on different sectors of production, manufacture and distribution, and research gaps were identified. To further explore the benefits of AI in supply chain risk management (SCRM), Baryannis et al. ( 2019 ) reviewed the literature, categorized the papers based on the methodology (i.e., mathematical programming, Machine Learning, Big Data Analytics) and different SCRM tasks they handle, and proposed future research directions.
Fethi and Pasiouras ( 2010 ) studied the role of AI and OR techniques for assessing the efficiency and performance of banks. For this purpose, the authors reviewed the relevant literature and pointed the applications of techniques such as data envelopment analysis, neural networks, support vector machines, and MCDA. Srebro et al. ( 2021 ) applied different Z-score models to calculate the risk of bankruptcy in agricultural companies. To ensure sustainable operations, it is important these companies report correct financial figures and also provide detailed information on environmental protection. Nazemi et al. ( 2017 ) compared the predictions of support vector regression, regression trees and OLS regression, and showed that fuzzy logic approach enhance prediction accuracy of loss-given-default of corporate bonds. To see the role of AI technologies in medical decision making, proposed case-based reasoning as an approach to design a modular architecture. Further, applications of AI in medicine were reviewed by Hamet and Tremblay ( 2017 ), and these applications range from robotics to medical diagnosis and statistics to biology branches of omics. A knowledge warehouse architecture was proposed by Nemati et al. ( 2002 ) to not only capture, store and code knowledge but also to retrieve and share it across the organization. This model is an architectural integration of knowledge management, decision support, AI and data warehousing.
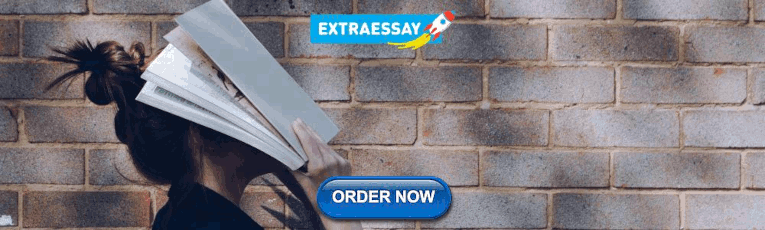
4.6 Theme 5: Disaster management
With 22 papers, this theme focusses on disaster management, which is a broad area of research in operations management. Due to the frequent occurrences of natural and man-made disasters, it is important to minimize their impact and deliver relief material at the right time to the right people (Mishra et al., 2019 ). This whole process is divided into four phases: mitigation, preparedness, response and recovery (Araya-Córdova & Vásquez, 2018 ). Likewise, we categorize the papers based on these four phases as follows: (1) mitigation and preparedness, and (2) response and recovery.
4.6.1 Mitigation and preparedness
There are four papers (18.2%) in this subtheme, two each in mitigation and preparedness phases. Mitigation refers to the measures which are adopted to either delay the onset of disaster or reduce its impact once it occurs whereas, preparedness basically aims to prepare the community to respond if any such event occurs (Altay & Green, 2006 ).
With respect to the mitigation phase, Das et al. ( 2008 ) presented a large-scale simulation model to develop mitigation strategies for communicable disease like influenza. The results of the experiment can be utilized during decision making by healthcare professionals. Moreover, Nigmatulina and Larson ( 2009 ) used mathematical models to study the impact of travel restrictions, social distancing and improved hygiene during influenza outbreak. It was suggested that if these precautions are strictly performed by the society then the spread of the disease can be significantly mitigated. To prepare for natural disasters, Rawls and Turnquist ( 2010 ) proposed a two-stage stochastic mixed integer program so that the location and quantity of relief supplies can be determined. Matopoulos et al. ( 2014 ) emphasized that during humanitarian operations, it is important to identify what resources are needed and how to procure them. In this regard, authors conducted an empirical study on housing reconstruction projects and showed that procurement of local resources has positive influence on supply chain performance.
4.6.2 Response and recovery
With 18 papers (81.8%), this is the largest subtheme in this theme. In the response phase, majority of the papers focus on either the ways to evacuate the threatened populations (6 papers) or investigate the channels through which relief material can be delivered in a sustainable way (9 papers). However, only 1 paper has appeared in the recovery phase with regard to the fund allocation post disaster. In addition, two review papers have also appeared.
In order to respond to the disaster, Chiu et al. ( 2007 ) formulated a no-notice mass evacuation model using a dynamic traffic flow optimization technique. As the model has a single-destination linear structure, it is computationally efficient than the other models available in the literature. Yi and Ozdamar ( 2007 ) undertook a different approach for evacuation at the time of disaster. They emphasized that logistics coordination is an important issue as medicines and medical personnel have to be allocated in an optimal way in disasters. A mixed integer multi-commodity network flow model is proposed to coordinate evacuation and support and operations. Other works on evacuation planning include discrete event simulation for emergency evacuation in case of offshore oil industry (Mould, 2001 ), multiobjective evolutionary algorithms and GIS to propose a three-step approach for evacuation planning (Saadatseresht et al., 2009 ), a framework for reliable evacuation planning by taking into account the fluctuation in number of evacuees and the road capacities (Ng & Waller, 2010 ), and an agent based simulation model to see the behavior of neighborhoods when the warning message to evacuate is circulated (Nagarajan et al., 2012 ).
To design a relief delivery system, Tzeng et al. ( 2007 ) adopted multi-objective programming approach to ensure efficient distribution of relief materials to all the demand centers. In addition, Lee et al. ( 2006 ) developed a simulation and decision support system to distribute medicines at the time infectious disease outbreaks and biological threats, Naji-Azimi et al. ( 2012 ) suggested a covering tour approach to locate the satellite distribution centers so that medical aid can be provided in a split delivery fashion. As transportation routes and distribution of relief material play a critical role during relief operations, Huang et al. ( 2012 ) measured their performance in terms of efficiency, efficacy and equity, Najafi et al. ( 2013 ) proposed a multi-objective model and Najafi et al. ( 2014 ) proposed a dynamic model to plan logistic activities at the time of earthquake. Another framework for transportation planning was proposed by Barbarosoğlu and Arda ( 2004 ) wherein a two-stage programming model was introduced to disperse medical aid at the time of disaster. To improve decision making during large scale disasters, Moskowitz et al. ( 2011 ) employed machine learning tool of multistage linear support vector machine to quickly assess the response strategy, and Mendonça ( 2007 ) developed a decision support system which can be improvised based on the response to natural disasters.
With regards to the Recovery phase, Natarajan and Swaminathan ( 2014 ) viewed humanitarian operations through the lens of funding as these operations are heavily affected by the amount, timings and regularity of the funding provided by various organizations. The authors present an optimal procurement policy with the emphasis that funding delays should be avoided, and donors should not be forced to pay the entire amount upfront.
In addition, two review papers have also appeared. Altay and Green ( 2006 ) reviewed the literature and explored the possible future avenues of research in OR/MS. Later, Galindo and Batta ( 2013 ) also reviewed the literature on OR/MS in disaster operations management and revealed that no drastic development has been made in this area since the previous review of Altay and Green ( 2006 ). However, they evaluated various assumptions of the literature and provided future research directions.
Now, we try to highlight the major relationships among the above five themes (see Fig. 5 ). To provide more clarity, we have used different colors and numberings for each of these themes; red for theme 1, green for theme 2, purple for theme 3, orange for theme 4 and blue for theme 5. It is evident that different types of connection exist between each of these themes, and it is represented by the thickness of the arrows reflecting the amount of work published between the themes. For instance, scholars have published a good number of optimization studies for disaster management, and it is highlighted by the thick purple arrow. However, lesser work has been shown using thinner blue arrows such as, the area of data mining and its role in disaster management, sustainable supply chain management and sustainable operations management have been comparatively explored less.
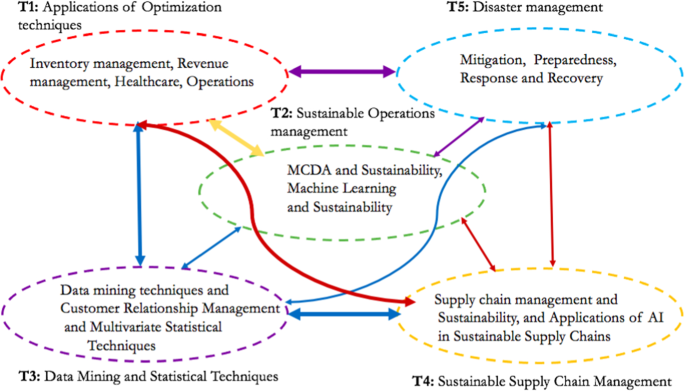
Relationships between five themes
It is important to emphasize that these research gaps are highlighted in Table 3 along with the detailed research objectives for scholars to explore in future.
5 Conceptual framework and propositions
From Fig. 5 , it can be seen that there is a scope of future research linking Theme 2 and 4 (reflected by a thin arrow between these themes). We make an attempt to address one such research direction by proposing a conceptual framework and propositions highlighting the connection between sustainable practices, prescriptive analytics and sustainable supply chain resilience and performance. The idea to propose a conceptual framework with clearly defined constructs is also backed by researchers working in the area of operations and supply chain management as it helps in bridging the gap between the theory and practice (Babbar & Prasad, 1998 ; Chen & Paulraj, 2004 ; Chen & Small, 1996 ; Meredith, 1993 ; New et al., 2000 ).
The proposed framework will help practitioners in deciding upon the type of resources that the company should focus in terms of adopting prescriptive analytical capability and achieve competitive advantage, Sustainable Supply Chain Resilience and Sustainable Supply Chain Performance. The conceptual framework has nine constructs: Environmental Practices (EP), Social Practices (SP), Circular Economic Practices (CEP), Prescriptive Analytical Capabilities (PAC), Sustainable Supply Chain Resilience (SSCR), Competitive Advantage (CA), Sustainable Supply Chain Performance (SSCP), Flexible Orientation (FO) and Control Orientation (CO). Here, FO and CO act as mediator variables. By considering all these constructs together, we intend to offer a thorough insight on the impact of sustainable practices on PAC and then the final effect on SSCR, CA and SSCP. We also try to understand the role of organizational orientation (FO, CO) in terms of implementation or adoption of Prescriptive analytical techniques. Subsequently, we unify the defined propositions in a conceptual framework as depicted in Fig. 6 . The following subsections discuss the theoretical background of the framework and the proposed research propositions.
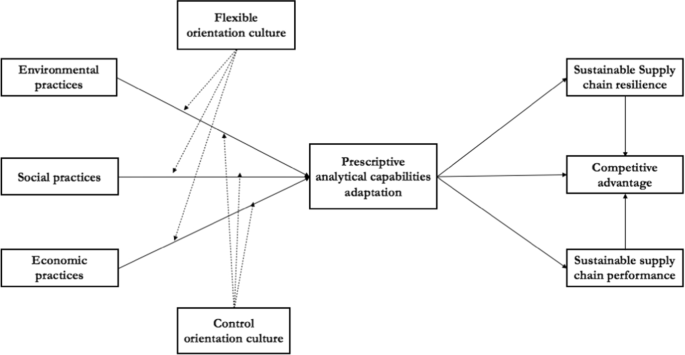
Conceptual framework
5.1 Underpinning theory
The proposed framework can be understood from the perspective of resource based view (RBV). It focuses on firms’ external and internal resources and capabilities for gaining competitive advantage (Hitt et al., 2016 ; Taylor & Taylor, 2009 ). These resources can be defined as the assets, capabilities, processes and information controlled by a firm in order to enhance its efficiency and effectiveness (Sarkis et al., 2011 ). In the last few years, RBV has gained significant attention among the scholars working in the area of operations and supply chain management (Bowen et al., 2001 ; Rungtusanatham et al., 2003 ; Taylor & Taylor, 2009 ; Hunt & Davis, 2012 ; Gligor & Holcomb, 2014 ; Brandon-Jones et al., 2014 ). Through RBV, firms can better explain the importance of focusing on the internal strength and weaknesses (Foss & Eriksen, 1995 ; Grant, 1996 ) which can be easily controlled rather than focusing on the external opportunities and threats (Grant, 1996 ). There is no harm in saying that RBV provides the best way to achieve competitive advantage by focusing on the resources. The main reason behind is that the competitive advantage can be sustained by utilizing the resources that are unique, important and irreplaceable by any competitors (Barney, 1991 ). Based on RBV, we suggest that firms can achieve the prescriptive analytic capability by utilizing their resources which are; environmental, economic and social practices and consequently, gain competitive advantage. The development of resources and capabilities may be exemplified through improvements in various organizational performance metrics. As an example, for attaining sustainable supply chain, firms need to focus on the three dimensions; environmental, economic and social practices, and these three resources are valuable, unique and irreplaceable for any firms that supports the aspect of RBV (Sarkis et al., 2011 ). Studies have found and argued that the above mentioned resources can help a firm in implementing technological capabilities, such as, big data analytics, blockchain technology, green information systems and later, prescriptive analytics capability can help a firm in achieving competitive advantage as per RBV concept.
5.2 Research propositions
In line with Edwin Cheng et al. ( 2021 ), we consider three types of sustainability practices in the supply chain—environment, social and economic. Environment friendly practices are embodied in water management, waste management, reduction in energy usage, eco design and pollution prevention. Adopting socially sustainable practices ensure that the human rights of workers are intact, their health and safety are promoted, and ethical norms are practiced providing them a safe working environment (Sodhi, 2015 ). Lastly, economic practices focus on creating a balance between firm's assets and liabilities and thus improving sales and market share and efficiently maintaining the resources (Ageron et al., 2012 ). As Elkington and Fennell ( 1998 ) and Seuring and Müller ( 2008 ) articulated, all three practices have to be considered together in order to have a sustainable supply chain. These practices reflect essential aspects of business (Raut et al., 2019 ) as responding to social, environmental and financial demands has become a primary challenge for firms (Dubey et al., 2019 ; Gunasekaran & Irani, 2014 ; Park-Poaps & Rees, 2010 ). Khan et al. ( 2021 ) argued that sustainable supply chain practices have a significant positive association with blockchain technology and green information systems, and hence, have a significant impact on organizational performance. In addition, literature suggests that sustainability can be improved with the application of big data predictive analytics (Liu & Zhang, 2017 ; Song et al., 2017a , 2017b ; Zhang et al., 2017 ). Benzidia et al. ( 2021 ) also insisted that making big data analytics and AI enabled decisions would enhance the environmental performance, and hence, firms should adopt practices that respect the environment (Singh & El-Kassar, 2019 ). Nonetheless, there is a noticeable paucity of empirical research investigating the association between big data predictive analytical capability and social and environmental sustainability practices (Song et al., 2017a , b ). When firms value these practices as an instrument to gain competitive advantage, they can move towards sustainable growth and development (Carvalho et al., 2018 ) and those who are yet to adopt, witness difficulties (Mittal et al., 2018 ). Since our actions and decisions generate data which in turn can drive business practices (Pappas et al., 2018 ), it is important for firms to follow sustainable practices as it would influence the adoption of prescriptive analytics capabilities. As Petrini and Pozzebon ( 2009 ) articulated, sustainability practices and business intelligence and analytics have to be integrated to attain firm’s strategic goals. Thus, sustainable practices and prescriptive analytics capabilities can prove rewarding for the firms. In light of the above arguments, the first set of propositions are:
P1 : Environmental practices positively influence the adaptation of prescriptive analytical capabilities. P2: Social practices positively influence the adaptation of prescriptive analytical capabilities. P3 : Economic practices positively influence the adaptation of prescriptive analytical capabilities.
Next, organizational culture allows the firms to ensure their rules, regulation, perspectives and procedures are properly implemented (Dowty & Wallace, 2010 ; Prasanna & Haavisto, 2018 ) and, is unique due to firms individual unique history, mission, structure and leadership (Wentz, 2006 ). It can also be viewed as a combination of assumption, values and beliefs which is clearly depicted in the practices and goals of a firm (Schein, 2010 ; Shao, 2019 ; White et al., 2003 ). Various frameworks have been proposed by scholars to empirically measure organizational culture and among them, Competing Values Model, proposed by Quinn and Rohrbaugh ( 1983 ), emerges as one of the most widely used, validated and accepted frameworks (Dubey et al., 2019 ; Shao, 2019 ). The most important feature of their framework is it focusses on the two dimensions of organizational culture rather than having a single point (Khazanchi et al., 2007 ; Stock et al., 2007 ) as; flexible focus and internal activities or external environments focus (Quinn & Spreitzer, 1991 ). These focuses are termed as flexible oriented (FO) culture and control oriented (CO) culture. Firms having FO culture are more risk takers and oriented toward creativity (Khazanchi et al., 2007 ) whereas firms with CO culture give more preference to hierarchy, productivity and efficiency (Khazanchi et al., 2007 ; Liu et al., 2010 ).
In a similar vein, Prasanna and Haavisto ( 2018 ) highlighted the difference between FO and CO culture based on firm's interpretations of external events, and the way they respond to environmental needs (Deshpandé et al., 1993 ; Khazanchi et al., 2007 ). For instance, military organizations are more particular on following traditions and hierarchy whereas the relief providing organizations have a flat structure and follow an informal approach to traditions with lesser authoritarian attitude (Tatham & Rietjens, 2016 ; Wentz, 2006 ). The literature has proved that firms should align organizational culture and supply chain practices in order to gain supply chain performance (Braunscheidel et al., 2010 ; Schwartz & Davis, 1981 ). As the FO firms focuses more on creativity and innovation, it is suitable for visionary leaders having strategic focus and high expectations to the members of the organization. On the other side, CO firms are more suitable for leaders who are more process oriented and have problem solving skills as it is necessary for these firms to increase efficiency and productivity (Shao, 2019 ). CO firms are not very keen in terms of implementing any new or risky technique (cloud computing, machine learning) as it can impact their productivity and processes as well while, firms having FO culture are more inclined towards these tools for developing new products and services (Albanese & Manning, 2015 ).
Moreover, firms with FO culture give more importance to information systems strategy as compared to CO culture firms (Shao, 2019 ). In this direction, Leidner and Kayworth ( 2006 ) highlighted the role of organizational culture in adopting Information technology and diffusion. For instance, firms with FO culture are more interested in adopting any new advanced manufacturing technology as compared to firms with CO culture (Kitchell, 1995 ). Thus, the adoption of internet is more successful in firms with innovation and flexible culture (Ruppel & Harrington, 2001 ). Based on the arguments mentioned above, we support the idea that FO culture and sustainable practices have a positive effect on prescriptive analytical capabilities adoption, while CO culture has a negative effect. On this line of reasoning, we propose our next set of propositions as:
P4: Flexible orientated culture positively moderates the relationship between environmental, social and economic practices and prescriptive analytical capabilities adoption. P5: Control orientated culture negatively moderates the relationship between environmental, social and economic practices and prescriptive analytical capabilities adoption.
From the perspective of Resource Based Theory, capabilities are developed in order to gain competitive advantage (Barney, 2001 ; Benzidia et al., 2021 ; Wernerfelt, 1984 ). Large and Thomsen ( 2011 ) also insisted on the need to develop capabilities in order to have a sustainable supply chain.
As articulated by De Oliveira et al. ( 2012 ) and Lepenioti et al. ( 2020 ), business analytics, in general, is known to create capabilities and empower supply chains by leveraging its sustainable performance. Further, it has been shown that big data analytics capability have a significant positive relation with supply chain agility, supply chain risk resilience, competitive advantage and firm performance (Dubey et al., 2019 ; Gu et al., 2021 ; Singh & Singh, 2019 ). Following the same rationale, with the adaption of PAC, it becomes easier for firms to achieve their goals of cost reduction, customer satisfaction, maximization of profit and enhanced efficiency (Kuttapa, 2020 ). Since prescriptive analytics identifies the optimal course of action, it takes the business decision making to another level, and thus, leads to superior performance (den Gartner, 2017 ; Hertog & Postek, 2016 ). Moreover, it is known that superior performance is one of the indicators to adjudge competitive advantage (Schilke, 2014 ). As the label itself suggests, competitive advantage is the manner in which a firm implements business strategies in order to gain leverage over the competitors (Porter, 1985 ). It can be derived by identifying appropriate resources and/or capabilities, which can be then exploited to achieve superior performance and hence, competitive advantage (Barney, 1991 ; Reed & DeFillippi, 1990 ; Schilke, 2014 ).
To excel in this competitive environment, firms need to distinguish themselves from others by making their supply chains sustainable. Sustainable supply chains can effectively reduce their operational cost, build image and improve efficiency and effectiveness by making data driven decisions (Jose et al., 2020 ; Tsai et al., 2021 ). With the application of prescriptive analytics, supply chain activities can be monitored, and corrective actions can be taken to manage issues of resilience and risk management, thus attain the sustainability agenda (Mageto, 2021 ). Supply chain resilience, which is known as the ability of a supply chain to regain its original state and meet the needs of customers (Ambulkar et al., 2015 ; Christopher & Peck, 2004 ; Holling, 1973 ), has recently gained attention because in the current uncertain environment, supply chains have become vulnerable to disruptions, thereby creating financial loses (Adobor & McMullen, 2018 ; Ivanov & Dolgui, 2021 ). Therefore, it is important for a supply chain to quickly respond and survive in a turbulent environment. It is not only a tool to minimize risk and vulnerability but also a source of competitive advantage (Klibi et al., 2010 ). As supply chain resilience can be built by making sustainable use of resources (Edgeman & Wu, 2016 ), firm’s performance can be enhanced by incorporating sustainability in supply chains (Negri et al., 2021 ; Pinto, 2020 ). Sustainable supply chain provides an opportunity to achieve competitive advantage by enhancing performance and earning the loyalty of its customers (D’Agostini et al., 2017 ; Markley & Davis, 2007 ). Hence, it is vital to measure and understand sustainable supply chain performance (Kumar & Goswami, 2019 ). Following Kumar and Goswami ( 2019 ) and Schilke ( 2014 ), we argue that sustainable supply chain resilience and sustainable supply chain performance are two important building blocks of competitive advantage. Based on this discussion, we have the following set of propositions:
P6: Adoption of prescriptive analytical capabilities positively influence sustainable supply chain resilience, performance and competitive advantage. P7: Sustainable supply chain resilience and sustainable supply chain performance positively influence competitive advantage.
6 Future research directions
This section highlights some interesting research opportunities for scholars working in the area of prescriptive analytics and sustainable operations research. In order to answer RQ3, the suggestions for future directions are developed as follows:
From the content analysis, it is clear that research in applications of optimization technique in sustainable operations management is an emerging topic. In order to accelerate this adoption and maximise the full potential, it is critical to conduct theory-based research as it will provide a firm foundation to explore future avenues of research. This will also assist in developing theoretical frameworks for understanding the impact of employing deep learning tools in sustainability and operations research. Another aspect which is evident from the content analysis of theme 1 is the lack of studies on applications of blockchain in improving the healthcare by maintain the privacy of the patients and also in order to build smart healthcare.
The content analysis of theme 2 exposes potential gaps in the area of sustainable operations management. Develop case studies with more emphasis on the application of prescriptive analytics tools like AI, machine learning and digital twin, in sustainable operation research area. Although there have been few studies on natural resource management, research should investigate specific problems of applications of prescriptive analytics on carbon emission, closed-loop supply chains and green supply chain management. Quantitative and case studies in this direction can be a valuable and fruitful area for future scholars. In addition, a thorough comparative study on different ranking tools would create transparency and add more values to decision makers. Another interesting research gap is the selection and usage of appropriate technique to identify the right analytical tool for firms with different strategical orientation.
Although data mining techniques have been there for quite some time, safety and privacy issues associated with data are still largely understudied. This aspect when explored together with data quality issues would lead to an efficient decision-making process. Researchers should also identify the role of Deep Learning in understanding customer relationships management. In addition, application of latest statistical tools for data analysis would add more value to the services and products and enhance customer experience.
In-depth content analysis has exposed research gap in sustainable supply chain management. It is witnessed that although research in this area has been evolving in these years, more empirical research is needed to see the role of AI in achieving sustainability in supply chain. In addition, research should further investigate and develop models for emission evaluation and social welfare associated with sustainability. With the concept of integrating analytical tools and supply chain, it would be interesting to further look into other supply chain aspects such as reverse logistics and closed-loop supply chain.
From the content analysis, it is clear that research in disaster management has potential gaps. It is evident that usage of data analytics tools would be highly beneficial in all four phases of disaster management. For instance, more studies should be done to investigate the role of data in predicting disasters in advance for better preparedness and in locating injured people post-disaster. More specifically, virtual reality is tested so that better preparation can be fixed before any actual disasters. These studies can be also extended to different types of disasters like hurricanes, floods, and terrorist attacks. It is also evident that analytics and data mining techniques for risk mitigation and risk recovery is scant. Better modelling techniques for the recovery phase of the disaster, specifically post disaster housing, and comparison of different models and techniques would assist decision makers in better managing the situation.
7 Conclusions
We began this article with a brief overview of prescriptive analytics and its applications in sustainable operations research and highlighted the uniqueness of the current work. A content analysis was performed on 147 articles and from the five major themes, we identified the current research area and the scope for future research directions. Our study makes the following contributions that can help sustainable operations researchers position their research against this mapping.
From the five main themes and the sub-themes developed in Sect. 3 , it is evident that prescriptive analytics has substantially diffused in sustainable operations research (RQ1). The applications of prescriptive analytics can be seen in the various areas of healthcare, inventory management, revenue management, supply chain management, wate management, water management, energy conservation, disaster management and many more.
Five main themes (RQ2) and their sub-themes connecting prescriptive analytics and sustainable operations have been identified using content analysis. These are applications of optimization techniques, sustainable operations management, data mining and statistical techniques, sustainable supply chain management, and disaster management. By utilizing these themes, researchers can avoid saturated research ideas while working on their own research work.
Having studied the past works on prescriptive analytics and sustainable operations in RQ2, the present and future (RQ3) of this research area can be found in Sect. 4 . Five actionable future research directions are developed based on the content analysis for the scholars working in this area of research.
A conceptual framework (RQ4) and a couple of propositions have been established in order to enhance our understanding about prescriptive analytics and its role in sustainable operations management. The framework is grounded in DCV and utilizes the three pillars of sustainable supply chain practices: environmental, social, and economic practices. We believe that this framework would bridge the gap between the theory and practice.
As rightly pointed out by Terwiesch ( 2019 ), Fisher et al. ( 2020 ) and Dubey et al. ( 2020 ), the primary objective of any theory-based study is to identify its relevance in the practical-world and explore the differences in theory and practice. Thus, we make an attempt to offer some practical implications of our study. First, by collating the recent published articles on prescriptive analytics and its applications in sustainable operations research, we intend to provide an overview to professionals and scholars trying to implement digital transformation strategy in their respective firms with the intent to achieve sustainable supply chain practices. Second, from the proposed conceptual framework, managers can understand the role of investing in supply chain practices: "environmental", "economic" and "social"; as these practices can accelerate the adoption of prescriptive analytical capabilities in a firm. Third, managers would realise how a firm's orientation can help them in implementing prescriptive analytical capabilities and gaining competitive advantage. It would be helpful for top managers to take decision of investing in these three supply chain practices.
Further, from the bibliometric analysis, it is quite evident that the pattern of publications is increasing over time which indicates that the applications of prescriptive analytics in sustainable operations research are growing significantly and prescriptive analytics has become an important tool for achieving sustainable supply chain performance, sustainable supply chain resilience and competitive advantage. Also, it is noticeable that the maximum number of articles that are published is from computer science followed by mathematics, engineering and business management area. Last, every country is working towards the area of prescriptive analytics, majorly countries like USA, UK, China and Canada are the most contributing ones. The study not only guides senior managers to focus on sustainable supply chain practices; environmental, economic and social, for the successful adaptation of prescriptive analytical capabilities, but also, helps in understanding the importance of firms orientation which acts as a mediating factor for implementing prescriptive analytics. From our descriptive statistics, practitioners can see which countries have made significant achievements in the area of prescriptive analytics and its application in sustainable operations. Also, the top subject areas will provide better understanding of the applications of prescriptive analytics in various fields and that would provide various future opportunities.
We believe that our study gives an accurate information of the recent development in the area of prescriptive analytics literature. We sincerely hope that our results and suggestions will contribute towards the improvement of the area and also inspire other scholars to develop work in order to fill the gaps identified. This study provides interesting research avenues for scholars or practitioners who want to explore the area of prescriptive analytics and sustainable operations research.
This study has its own limitations, some of which can stimulate future research. First, the data is collected using Scopus, so there could be a possibility of not including relevant research articles not registered in this database. Second, this study only focuses on peer-reviewed articles published in English which might not be enough for managers to understand this area and the challenges completely. In future, scholars could extend this work by including non-academic literature i.e., government and public sectors reports, white papers, newspaper articles, conference papers, editorials and books, and also the ones published in other languages for understanding problems from global perspective.
Achillas, C., Vlachokostas, C., Aidonis, D., Moussiopoulos, Ν, Iakovou, E., & Banias, G. (2010a). Optimising reverse logistics network to support policy-making in the case of electrical and electronic equipment. Waste Management, 30 (12), 2592–2600.
Article Google Scholar
Achillas, C., Vlachokostas, C., Moussiopoulos, N., & Banias, G. (2010b). Decision support system for the optimal location of electrical and electronic waste treatment plants: a case study In Greece. Waste Management, 30 (5), 870–879.
Adobor, H., & McMullen, R. S. (2018). Supply chain resilience: A dynamic and multidimensional approach. The International Journal of Logistics Management, 29 (4), 1451–1471.
Ageron, B., Gunasekaran, A., & Spalanzani, A. (2012). Sustainable supply management: An empirical study. International Journal of Production Economics, 140 (1), 168–182.
Ahmed, M. A., & Alkhamis, T. M. (2009). Simulation optimization for an emergency Department Healthcare Unit In Kuwait. European Journal of Operational Research, 198 (3), 936–942.
Ajah, I. A., & Nweke, H. F. (2019). Big data and business analytics: Trends, platforms, success factors and applications. Big Data and Cognitive Computing, 3 (2), 32.
Akbaş, H., & Bilgen, B. (2017). An integrated fuzzy QFD and TOPSIS methodology for choosing the ideal gas fuel at WWTPs. Energy, 125 , 484–497.
Akter, S., Wamba, S. F., Gunasekaran, A., Dubey, R., & Childe, S. J. (2016). How to improve firm performance using big data analytics capability and business strategy alignment? International Journal of Production Economics, 182 , 113–131.
Albanese, J. & Manning, B. (2015). Revive: how to transform traditional businesses into digital leaders . FT Press.
Altay, N., & Green III, W. G. (2006). Or/Ms research in disaster operations management. European Journal of Operational Research, 175 (1), 475–493.
Ambilkar, P., Dohale, V., Gunasekaran, A., & Bilolikar, V. (2022). Product returns management: A comprehensive review and future research agenda. International Journal of Production Research, 60 (12), 3920–3944.
Ambulkar, S., Blackhurst, J., & Grawe, S. (2015). Firm’s resilience to supply chain disruptions: Scale development and empirical examination. Journal of Operations Management, 33 , 111–122.
Anderson, D., Sweeney, D., & Williams, T. (2002). An Introduction to Management Science: Quantitative Approaches to Decision Making (10th ed.). South-Western Publishing Company.
Google Scholar
Araya-Córdova, P. J., & Vásquez, Ó. C. (2018). The disaster emergency unit scheduling problem to control wildfires. International Journal of Production Economics, 200 , 311–317.
Ashrafi, A., & Zareravasan, A. (2022). An ambidextrous approach on the business analytics-competitive advantage relationship: Exploring the moderating role of business analytics strategy. Technological Forecasting and Social Change, 179 , 121665.
Babbar, S., & Prasad, S. (1998). International purchasing, inventory management and logistics research: An assessment and agenda. International Journal of Operations & Production Management, 18 (1), 6–36.
Balakrishnan, A., Pangburn, M. S., & Stavrulaki, E. (2008). Integrating the promotional and service roles of retail inventories. Manufacturing and Service Operations Management, 10 (2), 218–235.
Balkhair, K. S., AlMaghrabi, H., & Kamis, A. S. (2016). Cost-effective sustainable operation policy of Jeddah RO desalination plant under production pumps failure using mathematical programming. Desalination and Water Treatment, 57 (1), 28–36.
Barbarosoǧlu, G., & Arda, Y. (2004). A two-stage stochastic programming framework for transportation planning in disaster response. Journal of Operations Research SocIety, 55 (1), 43–53.
Barney, J. (1991). Firm resources and sustained competitive advantage. Journal of Management, 17 (1), 99–120.
Barney, J. B. (2001). Resource-based theories of competitive advantage: A ten-year retrospective on the resource-based view. Journal of Management, 27 (6), 643–650.
Baryannis, G., Validi, S., Dani, S., & Antoniou, G. (2019). Supply chain risk management and artificial intelligence: state of the art and future research directions. International Journal of Production Research, 57 (7), 2179–2202.
Bass, F. M. (1969). A new product growth for model consumer durables. Management Science, 15 (5), 215–227.
Batrinca, B., & Treleaven, P. C. (2015). Social media analytics: A survey of techniques, tools and platforms. Ai & Society, 30 (1), 89–116.
Benzidia, S., Makaoui, N., & Bentahar, O. (2021). The impact of big data analytics and artificial intelligence on green supply chain process integration and hospital environmental performance. Technological Forecasting and Social Change, 165 , 120557.
Besbes, O., & Zeevi, A. (2009). Dynamic pricing without knowing the demand function: Risk bounds and near-optimal algorithms. Operations Research, 57 (6), 1407–1420.
Beutel, A.-L., & Minner, S. (2012). Safety stock planning under causal demand forecasting. International Journal of Production Economics, 140 (2), 637–645.
Bowen, F. E., Cousins, P. D., Lamming, R. C., & Farukt, A. C. (2001). The role of supply management capabilities in green supply. Production and Operations Management, 10 (2), 174–189.
Brandon-Jones, E., Squire, B., Autry, C. W., & Petersen, K. J. (2014). A contingent resource-based perspective of supply chain resilience and robustness. Journal of Supply Chain Management, 50 (3), 55–73.
Braunscheidel, M. J., Suresh, N. C., & Boisnier, A. D. (2010). Investigating the impact of organizational culture on supply chain integration. Human Resource Management, 49 (5), 883–911.
Breiman, L. (2001). Random Forests. Machine Learning, 45 (1), 5–32.
Carvalho, N., Chaim, O., Cazarini, E., & Gerolamo, M. (2018). Manufacturing in the fourth industrial revolution: A positive prospect in sustainable manufacturing. Procedia Manufacturing, 21 , 671–678.
Ceballos-Fuentealba, I., Álvarez-Miranda, E., Torres-Fuchslocher, C., del Campo-Hitschfeld, M. L., & Díaz-Guerrero, J. (2019). Simulation and optimisation methodology for choosing energy efficiency measures in non-residential buildings. Applied Energy, 256 , 113953.
Chahidi, L. O., Fossa, M., Priarone, A., & Mechaqrane, A. (2021). Evaluation of supervised learning models in predicting greenhouse energy demand and production for intelligent and sustainable operations. Energies, 14 (19), 6297.
Chen, I. J., & Paulraj, A. (2004). Towards a theory of supply chain management: The constructs and measurements. Journal of Operations Management, 22 (2), 119–150.
Chen, I. J., & Small, M. H. (1996). Planning for advanced manufacturing technology: A research framework. International Journal of Operations & Production Management, 16 (5), 4–24.
Chen, H., Chiang, R. H. L., & Storey, V. C. (2012). Business intelligence and analytics: From big data to big impact. Mis Quarterly, 36 (4), 1165–1188.
Chen, K. S., Yang, S. L., & Chen, H. T. (2015). Process improvement capability index with cost—A modeling method of mathematical programming. Applied Mathematical Modelling, 39 (5–6), 1577–1586.
Chin, Y. C., Su, W. Z., Chen, S. C., Hou, J., & Huang, Y. C. (2018). Exploring users’ self-disclosure intention on social networking applying novel soft computing theories. Sustainability, 10 (11), 3928.
Chiu, Y. C., Zheng, H., Villalobos, J., & Gautam, B. (2007). Modeling no-notice mass evacuation using a dynamic traffic flow optimization model. Iie Transactions, 39 (1), 83–94.
Christopher, M., & Peck, H. (2004). Building the resilient supply chain. International Journal of Logistics Management, 15 (2), 1–13.
Chu, M., Liao, X., Li, H., & Cui, S. (2019). Power control in energy harvesting multiple access system with reinforcement learning. IEEE Internet of Things Journal, 6 (5), 9175–9186.
Creswell, J. W. (2013). Research Design: Qualitative, Quantitative, and Mixed Methods Approaches . SAGE Publications Inc.
D’Agostini, M., Tondolo, V. A. G., Camargo, M. E., dos Santos Dullius, A. I., Tondolo, R. D. R. P., & Russo, S. L. (2017). Relationship between sustainable operations practices and performance: A meta-analysis. International Journal of Productivity and Performance Management, 66 (8), 1020–1042.
Das, T. K., Savachkin, A. A., & Zhu, Y. (2008). A large-scale simulation model of pandemic influenza outbreaks for development of dynamic mitigation strategies. Iie Transactions, 40 (9), 893–905.
Davenport, T. H., & Soulard, H. (2014). Big Data Strategy . Pearson.
De Oliveira, M. P. V., McCormack, K., & Trkman, P. (2012). Business analytics in supply chains—The contingent effect of business process maturity. Expert Systems with Applications, 39 (5), 5488–5498.
de Sousa, L., Jabbour, A. B., Rojas Luiz, J. V., Rojas, L. O., Jabbour, C. J. C., Ndubisi, N. O., Caldeira de Oliveira, J. H., & Junior, F. H. (2019). Circular economy business models and operations management. Journal of Cleaner Production, 235 , 1525–1539.
Dehoratius, N., Mersereau, A. J., & Schrage, L. (2008). Retail inventory management when records are inaccurate. Manufacturing & Service Operations Management, 10 (2), 257–277.
den Hertog, D., & Postek, K. (2016). Bridging the gap between predictive and prescriptive analytics-new optimization methodology needed . Tilburg Univ.
Deshpandé, R., Farley, J. U., & Webster, F. E., Jr. (1993). Corporate culture, customer orientation, and innovativeness in Japanese firms: A quadrad analysis. Journal of Marketing, 57 (1), 23–37.
Diaz, P., Salas, J. C., Cipriano, A., & Núñez, F. (2021). Random forest model predictive control for paste thickening. Minerals Engineering, 163 , 106760.
Dohale, V., Gunasekaran, A., Akarte, M. M., & Verma, P. (2022). 52 Years of manufacturing strategy: An evolutionary review of literature (1969–2021). International Journal of Production Research, 60 (2), 569–594.
Dowty, R. A., & Wallace, W. A. (2010). Implications of organizational culture for supply chain disruption and restoration. International Journal of Production Economics, 126 (1), 57–65.
Duan, L., & Xiong, Y. (2015). Big data analytics and business analytics. Journal of Management Analytics, 2 (1), 1–21.
Duan, Y. Q., Fan, X. Y., Liu, J. C., & Hou, Q. H. (2020). Operating efficiency-based data mining on intensive land use in smart city. IEEE Access, 8 , 17253–17262.
Dubey, R., Gunasekaran, A., Childe, S. J., Roubaud, D., Wamba, S. F., Giannakis, M., & Foropon, C. (2019). Big data analytics and organizational culture as complements to swift trust and collaborative performance in the humanitarian supply chain. International Journal of Production Economics, 210 , 120–136.
Dubey, R., Gunasekaran, A., Bryde, D. J., Dwivedi, Y. K., & Papadopoulos, T. (2020). Blockchain technology for enhancing swift-trust, collaboration and resilience within a humanitarian supply chain setting. International Journal of Production Research, 58 (11), 3381–3398.
Edgeman, R., & Wu, Z. (2016). Supply chain criticality in sustainable and resilient enterprises. Journal of Modelling in Management, 11 (4), 869–888.
Edwin Cheng, T.C., Kamble, S.S., Belhadi, A., Ndubisi, N.O., Lai, K.H. & Kharat, M.G. (2021). Linkages between big data analytics, circular economy, sustainable supply chain flexibility, and sustainable performance in manufacturing firms . International Journal of Production Research , pp.1–15.
Elkington, J., & Fennell, S. (1998). Partners for sustainability. Greener Management International , pp.48–48.
Fallah, S. N., Deo, R. C., Shojafar, M., Conti, M., & Shamshirband, S. (2018). Computational intelligence approaches for energy load forecasting in smart energy management grids: State of the art, future challenges, and research directions. Energies, 11 (3), 596.
Farahani, R. Z., Steadieseifi, M., & Asgari, N. (2010). Multiple criteria facility location problems: A survey. Applied Mathematical Modelling, 34 (7), 1689–1709.
Fethi, M. D., & Pasiouras, F. (2010). Assessing bank efficiency and performance with operational research and artificial intelligence techniques: a survey. European Journal of Operational Research, 204 (2), 189–198.
Fisher, M., Olivares, M., & Staats, B. R. (2020). Why empirical research is good for operations management, and what is good empirical operations management? Manufacturing & Service Operations Management, 22 (1), 170–178.
Fortun, M., & Schweber, S. S. (1993). Scientists and the Legacy Of World War Ii: The Case Of Operations Research (Or). Social Studies of Science, 23 (4), 595–642.
Foss, N.J. & Eriksen, B. (1995). Competitive advantage and industry capabilities. In Resource-based and evolutionary theories of the firm: Towards a synthesis (pp. 43–69). Springer, Boston, MA.
Frazzetto, D., Nielsen, T. D., Pedersen, T. B., & Šikšnys, L. (2019). Prescriptive analytics: A survey of emerging trends and technologies. The VLDB Journal, 28 (4), 575–595.
Fujimoto, Y., Murakami, S., Kaneko, N., Fuchikami, H., Hattori, T., & Hayashi, Y. (2019). Machine learning approach for graphical model-based analysis of energy-aware growth control in plant factories. IEEE Access, 7 , 32183–32196.
Galal, N. M., & Moneim, A. F. A. (2015). A mathematical programming approach to the optimal sustainable product mix for the process industry. Sustainability, 7 (10), 13085–13103.
Galindo, G., & Batta, R. (2013). Review of recent developments in Or/Ms research in disaster operations management. European Journal of Operations Research, 230 (2), 201–211.
Gandomi, A., & Haider, M. (2015). Beyond the Hype: Big data concepts, methods, and analytics. International Journal of Information Management, 35 (2), 137–144.
Gartner (2017). Planning guide for data and analytics. Last Accessed: 03 April 2018. www.gartner.com/binaries/content/assets/events/keywords/catalyst/catus8/2017_planning_guide_for_data_analytics.pdf .
Gligor, D. M., & Holcomb, M. (2014). The road to supply chain agility: An RBV perspective on the role of logistics capabilities. The International Journal of Logistics Management, 25 (1), 160–179.
Govindan, K., & Hasanagic, M. (2018). A systematic review on drivers, barriers, and practices towards circular economy: A supply chain perspective. International Journal of Production Research, 56 (1–2), 278–311.
Grant, R. M. (1996). Toward a knowledge-based theory of the firm. Strategic Management Journal, 17 (S2), 109–122.
Gu, V. C., Zhou, B., Cao, Q., & Adams, J. (2021). Exploring the relationship between supplier development, big data analytics capability, and firm performance. Annals of Operations Research, 302 (1), 151–172.
Gunasekaran, A., & Irani, Z. (2014). Sustainable operations management: Design, modelling and analysis. Journal of the Operational Research Society, 65 (6), 801–805.
Gupta, D., & Denton, B. (2008). Appointment scheduling in health care: Challenges and opportunities. Iie Transactions, 40 (9), 800–819.
Hamet, P., & Tremblay, J. (2017). Artificial intelligence in medicine. Metabolism, 69 , S36–S40.
Hazen, B. T., Skipper, J. B., Boone, C. A., & Hill, R. R. (2018). Back in business: Operations research in support of big data analytics for operations and supply chain management. Annals of Operations Research, 270 (1), 201–211.
He, L., Mak, H.-Y., Rong, Y., & Shen, Z.-J.M. (2017). Service region design for urban electric vehicle sharing systems. Manufacturing and Service Operations Management, 19 (2), 309–327.
Hegedűs, T., Fényes, D., Németh, B., & Gáspár, P. (2021). Improving sustainable safe transport via automated vehicle control with closed-loop matching. Sustainability, 13 (20), 11264.
Heo, S., Nam, K., Tariq, S., Lim, J. Y., Park, J., & Yoo, C. (2021). A hybrid machine learning–based multi-objective supervisory control strategy of a full-scale wastewater treatment for cost-effective and sustainable operation under varying influent conditions. Journal of Cleaner Production, 291 , 125853.
Hindle, G., Kunc, M., Mortensen, M., Oztekin, A., & Vidgen, R. (2020). Business analytics: Defining the field and identifying a research agenda. European Journal of Operational Research, 281 (3), 483–490.
Hitt, M. A., Carnes, C. M., & Xu, K. (2016). A current view of resource based theory in operations management: A response to Bromiley and Rau. Journal of Operations Management, 41 (10), 107–109.
Holling, C. S. (1973). Resilience and stability of ecological systems. Annual Review of Ecology and Systematics, 4 (1), 1–23.
Holtrop, N., Wieringa, J. E., Gijsenberg, M. J., & Verhoef, P. C. (2017). No future without the past? Predicting Churn in the face of customer privacy. International Journal of Research in Marketing, 34 (1), 154–172.
Hu, S., Gao, J., Zhong, D., Deng, L., Ou, C. & Xin P., 2021. An innovative hourly water demand forecasting preprocessing framework with local outlier correction and adaptive decomposition techniques. Water (Switzerland),13(5), pp. 582.
Huang, M., Smilowitz, K., & Balcik, B. (2012). Models for relief routing: Equity, efficiency and efficacy. Transportation Research Part E, 48 (1), 2–18.
Hunt, S. D., & Davis, D. F. (2012). Grounding supply chain management in resource-advantage theory: In defense of a resource‐based view of the firm. Journal of Supply Chain Management, 48 (2), 14–20.
Ivanov, D., & Dolgui, A. (2021). OR-methods for coping with the ripple effect in supply chains during COVID-19 pandemic: Managerial insights and research implications. International Journal of Production Economics, 232 , 107921.
Ivanov, D., Dolgui, A., & Sokolov, B. (2022). Cloud supply chain: Integrating industry 4.0 and digital platforms in the “Supply Chain-as-a-Service”. Transportation Research Part E: Logistics and Transportation Review, 160 , 102676.
Jabbour, C. J. C., Jabbour, A. B. L. D. S., Sarkis, J., & Filho, M. G. (2019). Unlocking the circular economy through new business models based on large-scale data: An integrative framework and research agenda. Technological Forecasting and Social Change, 144 , 546–552.
Jagesar, R. R., Vorstman, J. A., & Kas, M. J. (2021). Requirements and operational guidelines for secure and sustainable digital phenotyping: Design and development study. Journal of Medical Internet Research, 23 (4), e20996.
Jia, M., Stevenson, M., & Hendry, L. (2021). A systematic literature review on sustainability-oriented supplier development. Production Planning and Control , pp.1–21.
Jiang, C., Jensen, D. L., Cao, H., & Kumar, T. (2010). Building business intelligence applications having prescriptive and predictive capabilities. International conference on web-age information management, Springer, pp. 376–385.
Jose, R., Panigrahi, S. K., Patil, R. A., Fernando, Y., & Ramakrishna, S. (2020). Artificial intelligence-driven circular economy as a key enabler for sustainable energy management. Materials Circular Economy, 2 (1), 1–7.
Kamble, S. S., Gunasekaran, A., & Gawankar, S. A. (2020). Achieving sustainable performance in a data-driven agriculture supply chain: A review for research and applications. International Journal of Production Economics, 219 , 179–194.
Kazemi, N., Modak, N. M., & Govindan, K. (2019). A review of reverse logistics and closed loop supply chain management studies published in IJPR: A bibliometric and content analysis. International Journal of Production Research, 57 (15–16), 4937–4960.
Khan, S.A.R., Godil, D.I., Jabbour, C.J.C., Shujaat, S., Razzaq, A. & Yu, Z. (2021). Green data analytics, blockchain technology for sustainable development, and sustainable supply chain practices: evidence from small and medium enterprises. Annals of Operations Research , pp.1–25.
Khazanchi, S., Lewis, M. W., & Boyer, K. K. (2007). Innovation-supportive culture: The impact of organizational values on process innovation. Journal of Operations Management, 25 (4), 871–884.
Kirby, M. W. (2000). Operations research trajectories: The Anglo-American experience from the 1940S to the 1990S. Operations Research, 48 (5), 661–670.
Kitchell, S. (1995). Corporate culture, environmental adaptation, and innovation adoption: A qualitative/quantitative approach. Journal of the Academy of Marketing Science, 23 (3), 195–205.
Klibi, W., Martel, A., & Guitouni, A. (2010). The design of robust value-creating supply chain networks: A critical review. European Journal of Operational Research, 203 (2), 283–293.
Kohno, R., Kobayashi, T., Sugimoto, C., Kinjo, Y., Hämäläinen, M., & Iinatti, J. (2019). Medical healthcare network platform and big data analysis based on integrated ICT and data science with regulatory science. IEICE Transactions on Communications, 102 (6), 1078–1087.
Kök, A. G., & Fisher, M. L. (2007). Demand estimation and assortment optimization under substitution: Methodology and application. Operations Research, 55 (6), 1001–1021.
Krippendorff, K. (2004). Reliability in content analysis: Some common misconceptions and recommendations. Human Communication Research, 30 (3), 411–433.
Kumar, G., & Goswami, M. (2019). Sustainable supply chain performance, its practice and impact on barriers to collaboration. International Journal of Productivity and Performance Management, 68 (8), 1434–1456.
Kumar, N., Kumar, G., & Singh, R. K. (2021). Analysis of barriers intensity for investment in big data analytics for sustainable manufacturing operations in post-COVID-19 pandemic era. Journal of Enterprise Information Management, 35 (1), 179–213.
Kunene, K. N., & Weistroffer, H. R. (2008). An approach for predicting and describing patient outcome using multicriteria decision analysis and decision rules. European Journal of Operational Research, 185 (3), 984–997.
Kuttapa, S. (2020). Why-prescriptive-analytics-and-decision-optimization-are-crucial? https://www.ibm.com/blogs/journey-to-ai/2020/04/why-prescriptive-analytics-and-decision-optimization-are-crucial/
Large, R. O., & Thomsen, C. G. (2011). Drivers of green supply management performance: Evidence from Germany. Journal of Purchasing and Supply Management, 17 (3), 176–184.
Larson, D., & Chang, V. (2016). A review and future direction of agile, business intelligence, analytics and data science. International Journal of Information Management, 36 (5), 700–710.
Lecun, Y., Bengio, Y., & Hinton, G. (2015). Deep learning. Nature, 521 (7553), 436.
Lee, K. (2021). A systematic review on social sustainability of artificial intelligence in product design. Sustainability, 13 (5), 2668.
Lee, E. K., Maheshwary, S., Mason, J., & Glisson, W. (2006). Decision support system for mass dispensing of medications for infectious disease outbreaks and bioterrorist attacks. Annals of Operations Research, 148 (1), 25–53.
Lei, C., & Ouyang, Y. (2018). Continuous approximation for demand balancing in solving large-scale one-commodity pickup and delivery problems. Transportation Research Part b: Methodological, 109 , 90–109.
Leidner, D.E. & Kayworth, T. (2006). A review of culture in information systems research: Toward a theory of information technology culture conflict. MIS quarterly, pp. 357–399.
Lepenioti, K., Bousdekis, A., Apostolou, D., & Mentzas, G. (2020). Prescriptive analytics: Literature review and research challenges. International Journal of Information Management, 50 , 57–70.
Liberatore, M. J., & Luo, W. (2010). The analytics movement: Implications for operations research. Interfaces, 40 (4), 313–324.
Liberatore, M. J., & Nydick, R. L. (2008). The analytic hierarchy process in medical and health care decision making: A literature review. European Journal of Operational Research, 189 (1), 194–207.
Lim, C. H., Pereira, P. S., Shum, C. K., Ong, W. J., Tan, R. R., Lam, H. L., & Foo, D. C. (2017). Synthesis of resource conservation networks with P-graph approach—direct reuse/recycle. Process Integration and Optimization for Sustainability, 1 (1), 69–86.
Liu, Q., & Van Ryzin, G. (2008). On the choice-based linear programming model for network revenue management. Manufacturing & Service Operations Management, 10 (2), 288–310.
Liu, H., Ke, W., Wei, K. K., Gu, J., & Chen, H. (2010). The role of institutional pressures and organizational culture in the firm’s intention to adopt internet-enabled supply chain management systems. Journal of Operations Management, 28 (5), 372–384.
Liu, X., & Zhang, C. (2017). Corporate governance, social responsibility information disclosure, and enterprise value in China. Journal of Cleaner Production, 142 , 1075–1084.
Lu, J., Chen, W., Ma, Y., Ke, J., Li, Z., Zhang, F., & Maciejewski, R. (2017). Recent progress and trends in predictive visual analytics. Frontiers of Computer Science, 11 (2), 192–207.
Machi, L. A., & McEvoy, B. T. (2012). The Literature Review: Six Steps to Success (2nd ed.). Corwin Press.
Mageto, J. (2021). Big data analytics in sustainable supply chain management: A focus on manufacturing supply chains. Sustainability, 13 (13), 7101.
Malczewski, J. (2006). Gis-based multicriteria decision analysis: A survey of the literature. International Journal of Geographical Information Science, 20 (7), 703–726.
Marhavilas, P. K., Tegas, M. G., Koulinas, G. K., & Koulouriotis, D. E. (2020). A joint stochastic/deterministic process with multi-objective decision making risk-assessment framework for sustainable constructions engineering projects—A case study. Sustainability, 12 (10), 4280.
Markley, M. J., & Davis, L. (2007). Exploring future competitive advantage through sustainable supply chains. International Journal of Physical Distribution & Logistics Management, 37 (9), 763–774.
Martino, J.P. (1992). Technological Forecasting For Decision Making . Mcgraw-Hill.
Matopoulos, A., KovÔøΩCs, G., & Hayes, O. (2014). Local resources and procurement practices in humanitarian supply chains: an empirical examination of large-scale house reconstruction projects. Decision Science, 45 (4), 621–646.
Matsatsinis, N. F. (2002). An intelligent decision support system for credit card assessment based on a machine learning technique. Operational Research An International Journal, 2 (2), 243–260.
McLachlan, G.J. & Krishnan, T. (2007). The EM algorithm and extensions (Vol. 382). Wiley.
Mcnees, S.K. (1987). Consensus Forecasts: Tyranny Of The Majority? New England Economic Review, Nov/Dec, pp. 15–21.
Mcnicholas, P. D., Murphy, T. B., Mcdaid, A. F., & Frost, D. (2010). Serial and parallel implementations of model-based clustering via Parsimonious Gaussian mixture models. Computational Statistics & Data Analysis, 54 (3), 711–723.
Meeuwissen, A. M., & Bedford, T. (1997). Minimally informative distributions with given rank correlation for use in uncertainty analysis. Journal of Statistical Computation and Simulation, 57 (1–4), 143–174.
Mehlawat, M.K., Kannan, D., Gupta, P. & Aggarwal, U. (2019). Sustainable transportation planning for a three-stage fixed charge multi-objective transportation problem. Annals of Operations Research , pp. 1–37.
Melachrinoudis, E., Ilhan, A. B., & Min, H. (2007). A dial-a-ride problem for client transportation in a Health-Care Organization. Computers and Operations Research, 34 (3), 742–759.
Mendonça, D. (2007). Decision support for improvisation in response to extreme events: Learning from The Response To The 2001 World Trade Center Attack. Decision Support System, 43 (3), 952–967.
Mendoza, G. A., & Martins, H. (2006). Multi-criteria decision analysis in natural resource management: A critical review of methods and new modelling paradigms. Forest Ecology and Management, 230 (1–3), 1–22.
Merad, M. M., Verdel, T., Roy, B., & Kouniali, S. (2004). Use of multi-criteria decision-aids for risk zoning and management of large area subjected to mining-induced hazards. Tunnelling and Underground Space Technology, 19 (2), 125–138.
Meredith, W. (1993). Measurement invariance, factor analysis and factorial invariance. Psychometrika, 58 (4), 525–543.
Meredith, J. (1998). Building operations management theory through case and field research. Journal of Operations Management, 16 (4), 441–454.
Mikalef, P., Pappas, I. O., Krogstie, J., & Giannakos, M. (2018). Big data analytics capabilities: A systematic literature review and research agenda. Information Systems and e-Business Management, Springer, 16 (3), 547–578.
Min, H. (2010). Artificial intelligence in Supply Chain Management: Theory and applications. International Journal of Logistics Research and Applications, 13 (1), 13–39.
Mishra, N., & Silakari, S. (2012). Predictive analytics: A survey, trends, applications, oppurtunities & challenges. International Journal of Computer Science and Information Technologies, 3 (3), 4434–4438.
Mishra, D., Kumar, S., & Hassini, E. (2019). Current trends in disaster management simulation modelling research. Annals of Operations Research, 283 (1), 1387–1411.
Mittal, S., Khan, M. A., Romero, D., & Wuest, T. (2018). A critical review of smart manufacturing & Industry 4.0 maturity models: Implications for small and medium-sized enterprises (SMEs). Journal of Manufacturing Systems, 49 , 194–214.
Montazer, G. A., Saremi, H. Q., & Ramezani, M. (2009). Design a new mixed expert decision aiding system using fuzzy ELECTRE III method for vendor selection. Expert Systems with Applications, 36 (8), 10837–10847.
Moon, S., & Kamakura, W. A. (2017). A picture is worth a thousand words: translating product reviews into a product positioning map. International Journal of Research in Marketing, 34 (1), 265–285.
Moon, S., & Russell, G. J. (2008). Predicting product purchase from inferred customer similarity: An autologistic model approach. Management Science, 54 (1), 71–82.
Morales, N. O., Kurowicka, D., & Roelen, A. (2008). Eliciting conditional and unconditional rank correlations from conditional probabilities. Reliability Engineering and System Safety, 93 , 699–710.
Morales-Nápoles, O. & Worm, D. (2013). Hypothesis testing of multidimensional probability distributions. WP4 GAMES2R TNO Report , (0100003764).
Morey, L. C., Blashfield, R. K., & Skinner, H. A. (1983). A comparison of cluster analysis techniques withing a sequential validation framework. Multivariate Behavioral Research, 18 (3), 309–329.
Moriki, A., & Karydis, M. (1994). Application of multicriteria choice-methods in assessing eutrophication. Environmental Monitoring and Assessment, 33 (1), 1–18.
Morris, P. A. (1974). Decision analysis expert use. Management Science., 20 , 1233–1241.
Morris, P. A. (1977). Combining expert judgements: A Bayesian approach. Management Science, 23 , 679–693.
Morris, P. A. (1983). An Axiomatic Approach to Expert Resolution. Management Science, 29 , 24–32.
Morrison, D. G. (1967). Measurement problems in cluster analysis. Management Science, 13 (12), B775–B780.
Morrison, D. G. (1969). On the interpretation of discriminant analysis. Journal of Marketing Research, 6 (2), 156–163.
Morrissey, A. J., & Browne, J. (2004). Waste management models and their application to sustainable waste management. Waste Management, 24 (3), 297–308.
Moskowitz, H., Drnevich, P., Ersoy, O., Altinkemer, K., & Chaturvedi, A. (2011). Using real-time decision tools to improve distributed decision-making capabilities in high-magnitude crisis situations. Decision Sciences, 42 (2), 477–493.
Moslemi, H., & Zandieh, M. (2011). Comparisons of some improving strategies on Mopso for multi-objective (R, Q) inventory system. Expert Systems with Applications, 38 (10), 12051–12057.
Mould, G. I. (2001). Assessing systems for offshore emergency evacuation. Journal of the Operational Research Society, 52 (4), 401–408.
Mousseau, V., & Dias, L. (2004). Valued outranking relations in electre providing manageable disaggregation procedures. European Journal of Operational Research, 156 (2), 467–482.
Mousseau, V., & Slowinski, R. (1998). Inferring an Electre tri model from assignment examples. Journal of Global Optimization, 12 (2), 157–174.
Mousseau, V., Slowinski, R., & Zielniewicz, P. (2000). A user-oriented implementation of the Electre-tri method integrating preference elicitation support. Computers & Operations Research, 27 (7–8), 757–777.
Mousseau, V., Figueira, J., & Naux, J. P. (2001). Using assignment examples to infer weights for Electre tri method: some experimental results. European Journal of Operational Research, 130 (2), 263–275.
Mousseau, V., Figueira, J., Dias, L., Gomes Da Silva, C., & ClÔøΩMaco, J. (2003). Resolving inconsistencies among constraints on the parameters of an Mcda model. European Journal of Operational Research, 147 (1), 72–93.
Moz, M., & Pato, M. V. (2004). Solving the problem of rerostering nurse schedules with hard constraints: new multicommodity flow models. Annals of Operations Research, 128 (1), 179–197.
Mróz, T., & Thiel, T. (2005). Evaluation of a heating system for buildings using multiple criteria decision analysis. Archives of Civil Engineering, 51 (2), 281–298.
Mukhopadhyay, S., Samaddar, S., & Colville, G. (2007). Improving revenue management decision making for airlines by evaluating analyst-adjusted passenger demand forecasts. Decision Sciences, 38 (2), 309–327.
Mun, D., & Ramani, K. (2011). Knowledge-based part similarity measurement utilizing ontology and multi-criteria decision making technique. Advanced Engineering Informatics, 25 (2), 119–130.
Murphy, A. H., & Winkler, R. L. (1970). Scoring rules in probability assessment and evaluation. Acta Psychologica, 34 , 273–286.
Murtagh, F. (2005). Correspondence Analysis And Data Coding With Java And R . 1St Chapman & Hall Boca Raton, USA.
Muthuraman, K., & Lawley, M. (2008). A stochastic overbooking model for outpatient clinical scheduling with no-shows. IIE Transactions, 40 (9), 820–837.
Mysiak, J. (2006). Consistency of the results of different Mca methods: A critical review. Environment and Planning c: Government and Policy, 24 (2), 257–277.
Myung, I. J., Ramamoorti, S., & Bailey, A. D., Jr. (1996). Maximum entropy aggregation of expert predictions. Management Science, 42 (10), 1420–1436.
Nadkarni, S., & Shenoy, P. P. (2004). A causal mapping approach to constructing Bayesian networks. Decision Support System, 38 , 259–281.
Nagarajan, M., Shaw, D., & Albores, P. (2012). Disseminating a warning message to evacuate: A simulation study of the behaviour of neighbours. European Journal of Operational Research, 220 (3), 810–819.
Nahra, T. A., Mendez, D., Alexander, J. A., & Jeffrey, A. (2009). Employing super-efficiency analysis as an alternative to Dea: An application in outpatient substance abuse treatment. European Journal of Operational Research, 196 (3), 1097–1106.
Najafi, M., Eshghi, K., & Dullaert, W. (2013). A multi-objective robust optimization model for logistics planning in the earthquake response phase. Transportation Research Part E, 49 (1), 217–249.
Najafi, M., Eshghi, K., & De Leeuw, S. (2014). A dynamic dispatching and routing model to plan/re-plan logistics activities in response to an earthquake. Or Spectrum, 36 (2), 323–356.
Naji-Azimi, Z., Renaud, J., Ruiz, A., & Salari, M. (2012). A covering tour approach to the location of satellite distribution centers to supply humanitarian aid. European Journal of Operational Research, 222 (3), 596–605.
Naseraldin, H., & Herer, Y. T. (2008). Integrating the number and location of retail outlets on a line with replenishment decisions. Management Science, 54 (9), 1666–1683.
Natarajan, K. V., & Swaminathan, J. M. (2014). Inventory management in humanitarian operations: Impact of amount, schedule, and uncertainty in funding. Manufacturing & Service Operations Management, 16 (4), 595–603.
Nazemi, A., Fatemi, P. F., Heidenreich, K., & Fabozzi, F. J. (2017). Fuzzy decision fusion approach for loss-given-default modeling. European Journal of Operational Research, 262 (2), 780–791.
Ndiaye, M., & Alfares, H. (2008). Modeling Health Care facility location for moving population groups. Computers and Operations Research, 35 (7), 2154–2161.
Nedelkova, Z., Lindroth, P., Patriksson, M., & Stromberg, A. B. (2018). Efficient solution of many instances of a simulation-based optimization problem utilizing a partition of the decision space. Annals of Operations Research, 265 (1), 93–118.
Negri, M., Cagno, E., Colicchia, C., & Sarkis, J. (2021). Integrating sustainability and resilience in the supply chain: A systematic literature review and a research agenda. Business Strategy and the Environment, 30 (7), 2858–2886.
Nelsen, R. B. (2013). An Introduction to Copulas . Springer.
Nemati, H. R., Steiger, D. M., Iyer, L. S., & Herschel, R. T. (2002). Knowledge warehouse: An architectural integration of knowledge management, decision support, artificial intelligence and data warehousing. Decision Support Systems, 33 (2), 143–161.
Nenadic, O., & Greenacre, M. (2007). Correspondence analysis in R, with two- and three-dimensional graphics: The Ca package. Journal of Statistical Software, 20 (3), 1–13.
Neslin, S. A., Gupta, S., Kamakura, W. A., Lu, J., & Mason, C. H. (2006). Defection detection: measuring and understanding the predictive accuracy of customer churn models. Journal of Marketing Research, 43 (2), 204–211.
Netessine, S., Savin, S., & Xiao, W. (2006). Revenue management through dynamic cross selling in E-Commerce retailing. Operations Research, 54 (5), 893–913.
New, M., Hulme, M. & Jones, P. (2000). Representing twentieth-century space–time climate variability. Part II: Development of 1901–96 monthly grids of terrestrial surface climate. Journal of climate, 13 (13), 2217–2238.
Newbold, P., & Granger, C. (1974). Experience with forecasting univariate time series and the combination of forecasts. Journal of the Royal Statistical Society Series A (general), 137 , 131–149.
Ng, M., & Waller, S. T. (2010). Reliable evacuation planning via demand inflation and supply deflation. Transportation Research Part E, 46 (6), 1086–1094.
Ngai, E. W. T., Peng, S., Alexander, P., & Moon, K. K. (2014). Decision support and intelligent systems in the textile and apparel supply chain: An academic review of research articles. Expert Systems with Applications, 41 (1), 81–91.
Ngai, E.W.T., Xiu, L. & Chau, D.C.K. (2009). Application of data mining techniques in customer relationship management: a literature review and classification. Expert Systems with Applications, 36 (2, Part 2), 2592–2602.
Ngo-The, A., & Mousseau, V. (2002). Using assignment examples to infer category limits for the Electre tri method. Journal of Multi-Criteria Decision Analysis, 11 (1), 29–43.
Ngo-The, A., & Ruhe, G. (2008). A systematic approach for solving the wicked problem of software release planning. Soft Computing, 12 (1), 95–108.
Nicholson, L., Vakharia, A. J., & Erengus, S. S. (2004). Outsourcing inventory management decisions in healthcare: Models and application. European Journal of Operational Research, 154 (1), 271–290.
Nigmatulina, K. R., & Larson, R. C. (2009). Living with influenza: impacts of government imposed and voluntarily selected interventions. European Journal of Operational Research, 195 (2), 613–627.
Nijkamp, P., & Vos, J. B. (1977). A multicriteria analysis for water resource and land use development. Water Resources Research, 13 (3), 513–518.
Olsen, T. L., & Parker, R. P. (2008). Inventory management under market size dynamics. Management Science, 54 (10), 1805–1821.
Pappas, I. O., Mikalef, P., Giannakos, M. N., Krogstie, J., & Lekakos, G. (2018). Big data and business analytics ecosystems: Paving the way towards digital transformation and sustainable societies. Information Systems and e-Business Management, 16 (3), 479–491.
Park-Poaps, H., & Rees, K. (2010). Stakeholder forces of socially responsible supply chain management orientation. Journal of Business Ethics, 92 (2), 305–322.
Pastor, R., & Olivella, J. (2008). Selecting and adapting weekly work schedules with working time accounts: A case of a retail clothing chain. European Journal of Operational Research, 184 (1), 1–12.
Patnaik, D., Marwah, M., Sharma, R. K., & Ramakrishnan, N. (2011). Temporal data mining approaches for sustainable chiller management in data centers. ACM Transactions on Intelligent Systems and Technology, 2 (4), 34.
Paul, J., & Criado, A. R. (2020). The art of writing literature review: What do we know and what do we need to know? International Business Review, 29 (4), 101717.
Petrini, M., & Pozzebon, M. (2009). Managing sustainability with the support of business intelligence: Integrating socio-environmental indicators and organisational context. The Journal of Strategic Information Systems, 18 (4), 178–191.
Pinto, L. (2020). Green supply chain practices and company performance in Portuguese manufacturing sector. Business Strategy and the Environment, 29 (5), 1832–1849.
Porter, M. E. (1985). Technology and competitive advantage. Journal of Business Strategy, 5 (3), 60–78.
Prasanna, S. R., & Haavisto, I. (2018). Collaboration in humanitarian supply chains: An organisational culture framework. International Journal of Production Research, 56 (17), 5611–5625.
Queiroz, M.M., Ivanov, D., Dolgui, A. & Fosso Wamba, S. (2020). Impacts of epidemic outbreaks on supply chains: mapping a research agenda amid the COVID-19 pandemic through a structured literature review. Annals of Operations Research , pp.1–38.
Quinn, R.E. & Spreitzer, G.M. (1991). The psychometrics of the competing values culture instrument and an analysis of the impact of organizational culture on quality of life . Emerald.
Quinn, R. E., & Rohrbaugh, J. (1983). A spatial model of effectiveness criteria: Towards a competing values approach to organizational analysis. Management Science, 29 (3), 363–377.
Rafique, S. F., & Jianhua, Z. (2018). Energy management system, generation and demand predictors: A review. IET Generation, Transmission and Distribution, 12 (3), 519–530.
Raut, R. D., Mangla, S. K., Narwane, V. S., Gardas, B. B., Priyadarshinee, P., & Narkhede, B. E. (2019). Linking big data analytics and operational sustainability practices for sustainable business management. Journal of Cleaner Production, 224 , 10–24.
Rawls, C. G., & Turnquist, M. A. (2010). Pre-positioning of emergency supplies for disaster response. Transportation Research Part B, 44 (4), 521–534.
Reed, R., & DeFillippi, R. J. (1990). Causal ambiguity, barriers to imitation, and sustainable competitive advantage. Academy of Management Review, 15 (1), 88–102.
Roig-Tierno, N., Baviera-Puig, A., Buitrago-Vera, J., & Mas-Verdu, F. (2013). The retail site location decision process using GIS and the analytical hierarchy process. Applied Geography, 40 , 191–198.
Rungtusanatham, M., Salvador, F., Forza, C. and Choi, T.Y., 2003. Supply‐chain linkages and operational performance: A resource‐based‐view perspective. International Journal of Operations & Production Management.
Ruppel, C. P., & Harrington, S. J. (2001). Sharing knowledge through intranets: A study of organizational culture and intranet implementation. IEEE Transactions on Professional Communication, 44 (1), 37–52.
Saadatseresht, M., Mansourian, A., & Taleai, M. (2009). Evacuation planning using multiobjective evolutionary optimization approach. European Journal of Operational Research, 198 (1), 305–314.
Sarkis, J., Zhu, Q., & Lai, K. H. (2011). An organizational theoretic review of green supply chain management literature. International Journal of Production Economics, 130 (1), 1–15.
Sawyerr, E. & Harrison, C. (2022). Resilience in healthcare supply chains: a review of the UK’s response to the COVID19 pandemic. International Journal of Physical Distribution & Logistics Management , (ahead-of-print).
Schein, E.H. (2010). Organizational culture and leadership (Vol. 2). John Wiley & Sons.
Schilke, O. (2014). On the contingent value of dynamic capabilities for competitive advantage: The nonlinear moderating effect of environmental dynamism. Strategic Management Journal, 35 (2), 179–203.
Schreiber, T., Netsch, C., Eschweiler, S., Wang, T., Storek, T., Baranski, M., & Müller, D. (2021). Application of data-driven methods for energy system modelling demonstrated on an adaptive cooling supply system. Energy, 230 , 120894.
Schwartz, H., & Davis, S. M. (1981). Matching corporate culture and business strategy. Organizational Dynamics, 10 (1), 30–48.
Scott, J., Ho, W., Dey, P. K., & Talluri, S. (2015). A decision support system for supplier selection and order allocation in stochastic, multi-stakeholder and multi-criteria environments. International Journal of Production Economics, 166 , 226–237.
Seuring, S., & Gold, S. (2012). Conducting content-analysis based literature reviews in supply chain management. Supply Chain Management: An International Journal, 17 (5), 544–555.
Seuring, S., & Müller, M. (2008). From a literature review to a conceptual framework for sustainable supply chain management. Journal of Cleaner Production, 16 (15), 1699–1710.
Shaharudin, M. S., Fernando, Y., Chiappetta Jabbour, C. J., Sroufe, R., & Jasmi, M. F. A. (2019). Past, present, and future low carbon supply chain management: A content review using social network analysis. Journal of Cleaner Production, 218 , 629–643.
Shaharum, N. S. N., Shafri, H. Z. M., Ghani, W. A. W. A. K., Samsatli, S., Al-Habshi, M. M. A., & Yusuf, B. (2020). Oil palm mapping over Peninsular Malaysia using Google Earth Engine and machine learning algorithms. Remote Sensing Applications: Society and Environment, 17 , 100287.
Shao, Z. (2019). Interaction effect of strategic leadership behaviors and organizational culture on IS-Business strategic alignment and Enterprise Systems assimilation. International Journal of Information Management, 44 , 96–108.
Šikšnys, L., & Pedersen, T. B. (2016). Prescriptive analytics. In L. Liu, & M. Özsu (Eds.). Encyclopedia of database systems . Springer.
Singh, N. P. & Singh, S. (2019). Building supply chain risk resilience: Role of big data analytics in supply chain disruption mitigation. Benchmarking: An International Journal, 26 (7), 2318–2342.
Singh, S. K., & El-Kassar, A. N. (2019). Role of big data analytics in developing sustainable capabilities. Journal of Cleaner Production, 213 , 1264–1273.
Sodhi, M. S. (2015). Conceptualizing social responsibility in operations via stakeholder resource-based view. Production and Operations Management, 24 (9), 1375–1389.
Soltanpoor, R. & Sellis, T. (2016). Prescriptive analytics for big data. In Australasian database conference (pp. 245–256). Springer.
Song, M., Du, Q., & Zhu, Q. (2017a). A theoretical method of environmental performance evaluation in the context of big data. Production Planning & Control, 28 (11–12), 976–984.
Song, M., Cen, L., Zheng, Z., Fisher, R., Liang, X., Wang, Y., and Huisingh, D., 2017b. How would big data support societal development and environmental sustainability? Insights and practices. Journal of Cleaner Production , 142, pp. 489–500.
Srebro, B., Mavrenski, B., Bogojević Arsić, V., Knežević, S., Milašinović, M., & Travica, J. (2021). Bankruptcy risk prediction in ensuring the sustainable operation of agriculture companies. Sustainability, 13 (14), 7712.
Srivastava, S. K. (2007). Green supply-chain management: A state-of the-art literature review. International Journal of Management Reviews, 9 (1), 53–80.
Srivastava, S. K. (2008). Network design for reverse logistics. Omega, 36 (4), 535–548.
Stock, G. N., McFadden, K. L., & Gowen, C. R., III. (2007). Organizational culture, critical success factors, and the reduction of hospital errors. International Journal of Production Economics, 106 (2), 368–392.
Sun, G. D., Wu, Y. C., Liang, R. H., & Liu, S. X. (2013). A survey of visual analytics techniques and applications: State-of-the-art research and future challenges. Journal of Computer Science and Technology, 28 (5), 852–867.
Suryawanshi, P., & Dutta, P. (2022). Optimization models for supply chains under risk, uncertainty, and resilience: A state-of-the-art review and future research directions. Transportation Research Part e: Logistics and Transportation Review, 157 , 102553.
Taghikhah, F., Daniel, J., & Mooney, G. (2017). Sustainable supply chain analytics: Grand challenges and future opportunities. In Pacific Asia Conference on Information Systems (PACIS) 2017 Proceedings , 44.
Tatham, P., & Rietjens, S. (2016). Integrated disaster relief logistics: A stepping stone towards viable civil–military networks? Disasters, 40 (1), 7–25.
Taylor, A. & Taylor, M. (2009). Operations management research: contemporary themes, trends and potential future directions. International Journal of Operations & Production Management.
Terwiesch, C. (2019). OM forum—Empirical research in operations management: From field studies to analyzing digital exhaust. Manufacturing & Service Operations Management, 21 (4), 713–722.
Thies, C., Kieckhäfer, K., Spengler, T. S., & Sodhi, M. S. (2019). Operations research for sustainability assessment of products: A review. European Journal of Operational Research, 274 (1), 1–21.
Tibshirani, R., Walther, G., & Hastie, T. (2001). Estimating the number of clusters in a data set via the gap statistic. Journal of the Royal Statistical Society: Series B (statistical Methodology), 63 (2), 411–423.
Tranfield, D., Denyer, D., & Smart, P. (2003). Towards a methodology for developing evidence-informed management knowledge by means of systematic review. British Journal of Management, 14 (3), 207–222.
Trivedi, A., Bovornkeeratiroj, P., Breda, J., Shenoy, P., Taneja, J., & Irwin, D. (2021). Phone-based ambient temperature sensing using opportunistic crowdsensing and machine learning. Sustainable Computing: Informatics and Systems, 29 , 100479.
Tsai, W.-H., Lin, S.-J., Liu, J.-Y., Lin, W.-R., & Lee, K.-C. (2011). Incorporating life cycle assessments into building project decision-making: An energy consumption and CO2 emission perspective. Energy, 36 (5), 3022–3029.
Tsai, C. W., Lai, C. F., Chao, H. C., & Vasilakos, A. V. (2015). Big data analytics: A survey. Journal of Big Data, 2 (1), 1–32.
Tsai, F. M., Bui, T. D., Tseng, M. L., Ali, M. H., Lim, M. K., & Chiu, A. S. (2021). Sustainable supply chain management trends in world regions: A data-driven analysis. Resources, Conservation and Recycling, 167 , 105421.
Tzeng, G. H., Cheng, H. J., & Huang, T. D. (2007). Multi-objective optimal planning for designing relief delivery systems. Transportation Research Part E, 43 (6), 673–686.
Wamba, S. F., Gunasekaran, A., Akter, S., Ren, S. J. F., Dubey, R., & Childe, S. J. (2017). Big data analytics and firm performance: Effects of dynamic capabilities. Journal of Business Research, 70 , 356–365.
Wentz, L., 2006. An ICT primer: Information and communication technologies for civil-military coordination in disaster relief and stabilization and reconstruction. National Defense Univ Washington Dc Center For Technology And National Security Policy.
Wernerfelt, B. (1984). A resource-based view of the firm. Strategic Management Journal, 5 (2), 171–180.
White, L., & Lee, G. J. (2009). Operational research and sustainable development: Tackling the social dimension. European Journal of Operational Research, 193 (3), 683–682.
White, J. C., Varadarajan, P. R., & Dacin, P. A. (2003). Market situation interpretation and response: The role of cognitive style, organizational culture, and information use. Journal of Marketing, 67 (3), 63–79.
Yi, W., & Ozdamar, L. (2007). A dynamic logistics coordination model for evacuation and support in disaster response activities. European Journal Operational Research, 179 (3), 1177–1193.
Zamani, E.D., Smyth, C., Gupta, S., & Dennehy, D. (2022). Artificial intelligence and big data analytics for supply chain resilience: a systematic literature review. Annals of Operations Research , pp.1–28.
Zhang, Y., Ren, S., Liu, Y., Sakao, T., & Huisingh, D. (2017). A framework for Big Data driven product lifecycle management. Journal of Cleaner Production, 159 , 229–240.
Zolbanin, H.M., Davazdahemami, B., Delen, D. & Zadeh, A.H. (2020). Data analytics for the sustainable use of resources in hospitals: Predicting the length of stay for patients with chronic diseases. Information and Management , pp. 103282.
Download references
Author information
Authors and affiliations.
Montpellier Business School, 2300 Avenue Des Moulins, 34185, Montpellier, France
Deepa Bhatt Mishra
Indian Institute of Technology Hyderabad, Sangareddy, India
Sameen Naqvi
School of Business Administration, Penn State Harrisburg, 777 West Harrisburg Pike, Middletown, PA, 17057-4846, USA
Angappa Gunasekaran
Indian Institute of Management Amritsar, Amritsar, India
Vartika Dutta
You can also search for this author in PubMed Google Scholar
Corresponding author
Correspondence to Angappa Gunasekaran .
Additional information
Publisher's note.
Springer Nature remains neutral with regard to jurisdictional claims in published maps and institutional affiliations.
Rights and permissions
Springer Nature or its licensor (e.g. a society or other partner) holds exclusive rights to this article under a publishing agreement with the author(s) or other rightsholder(s); author self-archiving of the accepted manuscript version of this article is solely governed by the terms of such publishing agreement and applicable law.
Reprints and permissions
About this article
Bhatt Mishra, D., Naqvi, S., Gunasekaran, A. et al. Prescriptive analytics applications in sustainable operations research: conceptual framework and future research challenges. Ann Oper Res (2023). https://doi.org/10.1007/s10479-023-05251-3
Download citation
Accepted : 20 February 2023
Published : 01 March 2023
DOI : https://doi.org/10.1007/s10479-023-05251-3
Share this article
Anyone you share the following link with will be able to read this content:
Sorry, a shareable link is not currently available for this article.
Provided by the Springer Nature SharedIt content-sharing initiative
- Prescriptive analytics
- Systematic literature review
- Content analysis
- Sustainable supply chain practices
- Sustainable operations research
- Find a journal
- Publish with us
- Track your research

An official website of the United States government
The .gov means it’s official. Federal government websites often end in .gov or .mil. Before sharing sensitive information, make sure you’re on a federal government site.
The site is secure. The https:// ensures that you are connecting to the official website and that any information you provide is encrypted and transmitted securely.
- Publications
- Account settings
Preview improvements coming to the PMC website in October 2024. Learn More or Try it out now .
- Advanced Search
- Journal List
- Springer Nature - PMC COVID-19 Collection

Machine Learning for Predictive and Prescriptive Analytics of Operational Data in Smart Manufacturing
Katerina lepenioti.
8 Information Management Unit (IMU), Institute of Communication and Computer Systems (ICCS), National Technical University of Athens (NTUA), Athens, Greece
Minas Pertselakis
9 Suite5 Data Intelligence Solutions Limited, Limassol, Cyprus
Alexandros Bousdekis
Andreas louca, fenareti lampathaki, dimitris apostolou.
10 Department of Informatics, University of Piraeus, Piraeus, Greece
Gregoris Mentzas
Stathis anastasiou.
11 M. J. Maillis S.A., Inofyta, Greece
Perceiving information and extracting insights from data is one of the major challenges in smart manufacturing. Real-time data analytics face several challenges in real-life scenarios, while there is a huge treasure of legacy, enterprise and operational data remaining untouched. The current paper exploits the recent advancements of (deep) machine learning for performing predictive and prescriptive analytics on the basis of enterprise and operational data aiming at supporting the operator on the shopfloor. To do this, it implements algorithms, such as Recurrent Neural Networks for predictive analytics, and Multi-Objective Reinforcement Learning for prescriptive analytics. The proposed approach is demonstrated in a predictive maintenance scenario in steel industry.
Introduction
Perceiving information and extracting business insights and knowledge from data is one of the major challenges in smart manufacturing [ 1 ]. In this sense, advanced data analytics is a crucial enabler of Industry 4.0 [ 2 ]. More specifically, among the major challenges for smart manufacturing are: (deep) machine learning, prescriptive analytics in industrial plants, and analytics-based decision support in manufacturing operations [ 3 ]. The wide adoption of IoT devices, sensors and actuators in manufacturing environments has fostered an increasing research interest on real-time data analytics. However, these approaches face several challenges in real-life scenarios: (i) They require a large amount of sensor data that already have experienced events (e.g. failures of -ideally- all possible causes); (ii) They require an enormous computational capacity that cannot be supported by existing computational infrastructure of factories; (iii) In most cases, the sensor data involve only a few components of a production line, or a small number of parameters related to each component (e.g. temperature, pressure, vibration), making impossible to capture the whole picture of the factory shop floor and the possible correlations among all the machines; (iv) The cold-start problem is rarely investigated. On the other hand, there is a huge treasure of legacy, enterprise and operational systems data remaining untouched. Manufacturers are sitting on a goldmine of unexplored historical, legacy and operational data from their Manufacturing Execution Systems (MES), Enterprise Resource Planning systems (ERP), etc. and they cannot afford to miss out on its unexplored potential. However, only 20–30% of the value from such available data-at-rest is currently accrued [ 4 ].
Legacy data contain information regarding the whole factory cycle and store events from all machines, whether they have sensors installed or not (e.g. products per day, interruption times of production line, maintenance logs, causalities, etc.) [ 5 ]. Therefore, legacy data analytics have the credentials to move beyond KPIs calculations of business reports (e.g. OEE, uptime, etc.), towards providing an all-around view of manufacturing operations on the shopfloor in a proactive manner. In this direction, the recent advancements of machine learning can have a substantial contribution in performing predictive and prescriptive analytics on the basis of enterprise and operational data aiming at supporting the operator on the shopfloor and at extracting meaningful insights. Combining predictive and prescriptive analytics is essential for smarter decisions in manufacturing [ 2 ]. In addition mobile computing (with the use of mobile devices, such as smartphones and tablets) can significantly enable timely, comfortable, non-intrusive and reliable interaction with the operator on the shopfloor [ 6 ], e.g. for generating alerts, guiding their work, etc. through dedicated mobile apps.
The current paper proposes an approach for predictive and prescriptive analytics on the basis of enterprise and operational data for smart manufacturing. To do this, it develops algorithms based on Recurrent Neural Networks (RNN) for predictive analytics, and Multi-Objective Reinforcement Learning (MORL) for prescriptive analytics. The rest of the paper is organized as follows: Sect. 2 presents the background, the challenges and prominent methods for predictive and prescriptive analytics of enterprise and operational data for smart manufacturing. Section 3 describes the proposed approach, while Sect. 4 shows a walkthrough scenario of the proposed approach in the steel industry. Section 5 presents the experimental results, while Sect. 6 concludes the paper and outlines the plans for future research.
Background, Existing Challenges and Novel Methods
Predictive analytics for smart manufacturing, background..
Intelligent and automated data analysis which aims to discover useful insights from data has become a best practice for modern factories. It is supported today by many software tools and data warehouses, and it is known by the name “descriptive analytics”. A step further, however, is to use the same data to feed models that can make predictions with similar or better accuracy than a human expert. In the framework of smart manufacturing, prognostics related to machines’ health status is a critical research domain that often leverages machine learning methods and data mining tools. In most of the cases, this is related to the analysis of streaming sensor data mainly for health monitoring [ 7 – 9 ], but also for failure prediction [ 10 – 12 ] as part of a predictive maintenance strategy. However, in all of these approaches, the prediction is produced only minutes or even seconds before the actual failure, which, is not often a realistic and practical solution for a real industrial case. The factory managers need to have this information hours or days before the event, so that there is enough time for them to act proactively and prevent it. One way to achieve this is to perform data mining on maintenance and operational data that capture the daily life-cycle of the shop floor in order to make more high-level predictions [ 13 – 15 ].
Existing Challenges.
The most notable challenges related to predictive analytics for smart manufacturing include: (a) Predictions always involve a degree of uncertainty, especially when the data available are not sufficient quantity-wise or quality-wise; (b) Inconsistent, incomplete or missing data with low dimensionality often result into overfitting or underfitting that can lead to misleading conclusions; (c) Properly preparing and manipulating the data in order to conclude to the most appropriate set of features to be used as input to the model is the most time-consuming, yet critical to the accuracy of the algorithms, activity; (d) Lack of a common “language” between data scientists and domain experts hinders the extraction of appropriate hypothesis from the beginning and the correct interpretation and explainability of results.
Novel Methods.
Time series forecasting involves prediction models that analyze time series data and usually infer future data trends. A time series is a sequence of data points indexed in time order. Unlike regression predictive modeling, time series also adds the complexity of a sequence dependence among the input variables. Recurrent Neural Networks (RNN) are considered to be powerful neural networks designed to handle sequence dependence. Long Short-Term Memory Network (LSTM) is a type of RNN that is typically used in deep learning for its ability to learn long-term dependencies and handle multiple input and output variables.
Prescriptive Analytics for Smart Manufacturing
Prescriptive analytics aims at answering the questions “What should I do?” and “Why should I do it?”. It is able to bring business value through adaptive, time-dependent and optimal decisions on the basis of predictions about future events [ 16 ]. During the last years, there is an increasing interest on prescriptive analytics for smart manufacturing [ 17 ], and is considered to be the next evolutionary step towards increasing data analytics maturity for optimized decision making, ahead of time.
The most important challenges of prescriptive analytics include [ 2 , 17 , 18 ]: (i) Addressing the uncertainty introduced by the predictions, the incomplete and noisy data and the subjectivity in human judgement; (ii) Combining the “learned knowledge” of machine learning and data mining methods with the “engineered knowledge” elicited from domain experts; (iii) Developing generic prescriptive analytics methods and algorithms utilizing artificial intelligence and machine learning instead of problem-specific optimization models; (iv) Incorporating adaptation mechanisms capable of processing data and human feedback to continuously improve decision making process over time and to generate non-intrusive prescriptions; (v) Recommending optimal plans out of a list of alternative (sets of) actions.
Reinforcement Learning (RL) is considered to be a third machine learning paradigm, alongside supervised learning and unsupervised learning [ 19 ]. RL shows an increasing trend in research literature as a tool for optimal policies in manufacturing problems (e.g. [ 20 , 21 ]). In RL, the problem is represented by an environment consisting of states and actions and learning agents with a defined goal state. The agents aim to reach the goal state while maximizing the rewards by selecting actions and moving to different states. In Interactive RL , there is the additional capability of incorporating evaluative feedback by a human observer so that the RL agent learns from both human feedback and environmental reward [ 22 ]. Another extension is Multi-Objective RL (MORL) , which is a sequential decision making problem with multiple objectives. MORL requires a learning agent to obtain action policies that can optimize multiple objectives at the same time [ 23 ].
The Proposed Approach
The proposed approach consists of a predictive analytics component (Sect. 3.1 ) and a prescriptive analytics component (Sect. 3.2 ) that process enterprise and operational data from manufacturing legacy systems, as depicted in Fig. 1 . The communication is conducted through an event broker for the event predictions and the actions prescriptions, while other parameters (i.e. objective values and alternative actions) become available through RESTful APIs. The results are communicated to business users and shopfloor operators through intuitive interfaces addressed to both computers and mobile devices.

The architecture of the proposed approach.
Recurrent Neural Network for Predictive Analytics
The proposed predictive analytics approach aims to: (i) exploit hidden correlations inside the data that derive from the day-to-day shop floor operations, (ii) create and adjust a predictive model able to identify future machinery failures, and (iii) make estimations regarding the timing of the failure, i.e. when a failure of the machinery may occur, given the history of operations on the factory. This type of data usually contains daily characteristics that derive from the production line operations and are typically collected as part of a world-wide best practice for monitoring, evaluation and improvement of the effectiveness of the production process. The basic measurement of this process is an industry standard known as Overall Equipment Effectiveness (OEE) and is computed as: OEE(%) = Availability(%) × Performance(%) × Quality(%). Availability is the ratio of actual operational time versus the planned operational time, Performance is the ratio of actual throughput of products versus the maximum potential throughput, and the Quality is the ratio of the not-rejected items produced vs the total production. The OEE factor can be computed for the whole production line as an indication of the factory’s effectiveness or per machine or a group of machines. The proposed methodology takes advantage of these commonly extracted indicators and processes them in two steps: in predictive model building (learning) and predictive model deployment.
Predictive Model Building.
The predictive analytics model incorporates LSTM and exploits its unique ability to “remember” a sequence of patterns and its relative insensitivity to possible time gaps in the time series. As in most neural network algorithms, LSTM networks are able to seamlessly model non-linear problems with multiple input variables through the iterative training of their parameters (weights). Since the predictive analytics model deals with time-series, the LSTM model is trained using supervised learning on a set of training sequences assigned to a known output value. Therefore, an analyst feeds the model with a set of daily features for a given machine (e.g. the factors that produce the OEE) and use as outcome the number of days until the next failure. This number is known since historical data can hold this information. Nevertheless, when the model is finally built and put in operation, it will use new input data and will have to estimate the new outcome.
Predictive Model Deployment.
When the LSTM model is fed with new data it can produce an estimation of when the next failure will occur (i.e. number of days or hours) and what is the expected interruption duration in the following days. Although this estimation may not be 100% accurate, it could help factory managers to program maintenance actions proactively in a flexible and dynamic manner, compared to an often rigid and outdated schedule that is currently the common practice. This estimation feeds into prescriptive analytics aiming at automating the whole decision-making process and provide optimal plans.
Multi-Objective Reinforcement Learning for Prescriptive Analytics
The proposed prescriptive analytics approach is able to: (i) recommend (prescribe) both perfect and imperfect actions (e.g. maintenance actions with various degrees of restoration); (ii) model the decision making process under uncertainty instead of the physical manufacturing process, thus making it applicable to various industries and production processes; and, (iii) incorporate the preference of the domain expert into the decision making process (e.g. according to their skills, experience, etc.), in the form of feedback over the generated prescriptions. To do these, it incorporates Multi-Objective Reinforcement Learning (MORL). Unlike most of the Multi-objective Optimization approaches which result in the Pareto front set of optimal solutions [ 24 ], the proposed approach provides a single optimal solution (prescription), thus generating more concrete insights to the user. The proposed prescriptive analytics algorithm consists of three steps: prescriptive model building, prescriptive model solving, and prescriptive model adapting, which are described in detail below.
Prescriptive Model Building.
An example of the prescriptive analytics model with 3 alternative (sets of) actions.
Prescriptive Model Deployment.
Prescriptive Model Adaptation.
A Predictive Maintenance Scenario in the Steel Industry
The case examined is the cold rolling production line of M. J. Maillis S.A. Cold rolling is a process of reduction of the cross-sectional area through the deformation caused by a pair of rotating in opposite directions metal rolls in order to produce rolling products with the closest possible thickness tolerances and an excellent surface finish. In the milling station, there is one pair of back up rolls and one pair of work rolls. The deformation takes place through force of the rolls supported by adjustable strip tension in both coilers and de-coilers. Over the life of the roll some wear will occur due to normal processing, and some wear will occur due to extraneous conditions. During replacement, the rolls are removed for grinding, during which some roll diameter is lost, and then are stored in the warehouse for future use. After several regrinding, the diameter of the roll becomes so small that is no longer operational.
The LSTM model of predictive analytics was created using the Keras library with Tensorflow as backend and the MORL using Brown-UMBC Reinforcement Learning and Planning (BURLAP) library, while the event communication between them is performed with a Kafka broker. In the M. J. Maillis S.A case, the system predicts the time of the next breakdown and the RUL of the available rolls. For the latter, the operator can select one of the repaired rollers, having been subject to grinding, or a new one. Therefore, the alternative actions are created dynamically according to the available repaired rollers existing in the warehouse. Each one has a different RUL, according to its previous operation, and a different cost (retrieved from enterprise systems) due to its depreciation. Each roller has an ID and is assigned to its characteristics/objectives of MORL (i.e. cost to be minimized and RUL to be maximized) in order to facilitate its traceability. The available rolls along with the aforementioned objectives values are retrieved on the basis of a predicted breakdown event trigger.
The alternative actions for the current scenario along with their costs and RULs are shown in Table 1 . The action “Replace with new roller” represents a perfect maintenance action, while the rest ones represent imperfect maintenance actions. Figure 3 depicts an example of the process in which the prescription “Replace with repaired roller ID3” is generated on the basis of a breakdown prediction and previously received feedback and instantly communicated to the operators through a dedicated mobile app. The operators are also expected to provide feedback so that their knowledge and preferences are incorporated in the system and the models are adapted accordingly.
Table 1.
The alternative actions, their costs and their RULs.

Illustration of the scenario under examination.
Experimental Results
Evaluation of the predictions about the timing of interruptions.
The legacy datasets used are related to the OEE of M. J. Maillis S.A. factory for the years 2017, 2018 and 2019. The datasets included a total of 21 features and some of the most useful were the real operational time, the time of interruptions and the duration of the breakdown events. A multivariate time series model was designed, as several input features were used in order to predict one output feature. To this direction, the first time series analysis performed in order to predict when the next interruption will occur (‘ When do we expect the next interruption ?’). A new feature named ‘Days from next breakdown event’ was created, and the model was trained. The input features selected for this prediction were: Availability, Performance, Minutes of Breakdown, Minutes of Interruptions, Real Gross Production and the date. After preprocessing the data, the appropriate sequences were extracted that would allow for several timesteps to be selected and tested for the analysis. In our case, timestep represented the last “n” days that the model will use. The LSTM model was then created by testing several layers, optimizers, neurons, batch sizes and epochs until the best performing model was designed. The final result ended being a sequential model with a first LSTM layer of 32 neurons, a second LSTM layer of 16 neurons, a dropout layer with rate 0.1, and finally a dense layer. The model was then trained using data from 2017, 2018 and six months of 2019; with an rmsprop optimizer, a batch size of 1, a timestep of 1, an epochs value of 300, and an early stopping that reached the best performance around 100 epochs. Predictions deal with the last six months of 2019, and the result can be seen in Fig. 4 . The blue line represents the actual values and the orange line represents the predicted values. The RMSE came to be 1.26, meaning that there is an average of 1.26 days uncertainty in every result predicted.

LSTM result - prediction of when the next interruption will occur. (Color figure online)
Evaluation of the Predictions About the Duration of Interruptions
The second analysis aimed to predict the expected interruption duration for the following day (‘ Which is the expected interruption duration for the following day? ’). The input features used in this LSTM model were: Availability, Performance, Minutes of breakdown, Real Gross Production, Number of breakdowns, and month (date). Again, several LSTM parameters and layers were tested and the final model resulted to be a sequential model with a first LSTM layer of 24 neurons and an activation function ‘relu’, a second layer of 12 neurons with a ‘relu’ activation function, a dropout layer of 0.1 rate, and finally a dense layer. The model was trained using data from 2017 and 2018; using a batch size of 20, 100 epochs, a timestep of 3 and an rmsprop optimizer. Predictions were performed in 2019 data and results are depicted in Fig. 5 . The blue line represents the actual value whereas the orange line represents the predicted value. The overall RMSE is 107.57, meaning that there is an average of 107.57 min uncertainty in each prediction.

LSTM result - prediction of the expected interruption duration for the following day. (Color figure online)
Evaluation of Feedback Impact on Prescriptions
For this experiment, the actor-critic algorithm, which calculates the associated optimal policy sequentially within 10000 episodes, consists of a Boltzmann actor and a TD-Lambda critic with learning rate = 0.3, lambda = 0.4 and gamma = 0.99. The generated policies are then integrated into a single policy taking into account the consistency (C = 0.7) and likelihood (L = 0.8) values. Table 2 presents five “snapshots” of positive and negative feedback along with the resulting shaped prescriptions and their respective policies. Each “snapshot” is compared to the previous one.
Table 2.
Evaluation of feedback impact on prescriptive analytics.
Conclusion and Future Work
In this paper, we proposed an approach for predictive and prescriptive analytics aiming at exploiting the huge treasure of legacy enterprise and operational data and to overcome some challenges of real-time data analytics. The potential of the proposed approach is high, especially in traditional industries that have not benefit from the advancements of Industry 4.0 and that have just started investigating the potential of data analytics and machine learning for the optimization of their production processes. The traditional manufacturing sectors (e.g. textile, furniture, packaging, steel processing) have usually older factories with limited capacity on investing in modern production technologies. Since the neural networks are inherently adaptive, the proposed approach could be applied to similar production lines (e.g. at a newly established factory of the same type) overcoming the cold-start problem, due to which other techniques usually fail. It also exploits both the “voice of data” and the “voice of experts”. Regarding future work, we plan to evaluate our proposed approach in additional use cases, with different requirements, as well as to investigate approaches and algorithms for fusion of the outcomes derived from real-time data analytics and operational data analytics that represent different levels of information.
Acknowledgments
This work is funded by the European Commission project H2020 UPTIME “Unified Predictive Maintenance System” (768634).
Contributor Information
Sophie Dupuy-Chessa, Email: [email protected] .
Henderik A. Proper, Email: [email protected] .
Katerina Lepenioti, Email: rg.autn.liam@itoinepelk .
Minas Pertselakis, Email: ue.5etius@sanim .
Alexandros Bousdekis, Email: rg.autn.liam@suobla .
Andreas Louca, Email: ue.5etius@saerdna .
Fenareti Lampathaki, Email: ue.5etius@iteranef .
Dimitris Apostolou, Email: rg.ipinu@tsopad .
Gregoris Mentzas, Email: rg.autn.liam@saztnemg .
Stathis Anastasiou, Email: [email protected] .
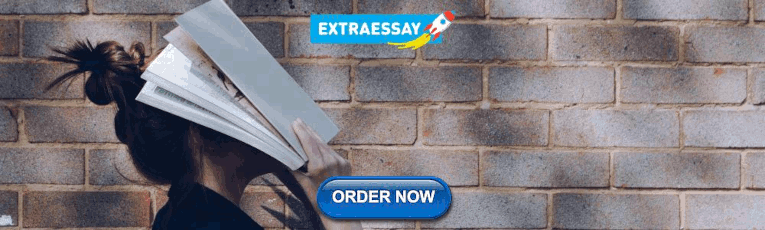
IMAGES
VIDEO
COMMENTS
This paper investigates the existing literature pertaining to prescriptive analytics and prominent methods for its implementation, provides clarity on the research field of prescriptive analytics, synthesizes the literature review in order to identify the existing research challenges, and outlines directions for future research. Previous.
This paper investigates the existing literature pertaining to prescriptive. analytics and prominent methods for its implementation, provides clarity on the research field of prescriptive ...
2023. TLDR. A four-step prescriptive analytics conceptual framework is introduced, which facilitates the development and deployment of prescriptive models in smart factories and emphasizes the existing gap between prescriptive analytics and decision theory in the current state of the art. Expand. Highly Influenced.
In this paper, we present a literature review on prescriptive analytics, we frame the prescriptive analytics lifecycle and we identify the existing research challenges on this topic. To the best of our knowledge, this is the first literature review on prescriptive analytics. Until now, prescriptive analytics applications are usually developed ...
Research Challenges . Comprehensive Literature Review. This manuscript identifies and documents unsolved problems and research challenges in the extended reality (XR) domain (i.e., virtual (VR), augmented (AR), and mixed reality (MR)). The manuscript is structured to include technology, design, and human factor perspectives.
The objectives of our work are: (i) to investigate the existing literature regarding prescriptive analytics and prominent methods for its implementation; (ii) to provide clarity on the research field of prescriptive analytics; (iii) to synthesize the literature review in order to identify the existing research challenges; (iv) to outline ...
areas, prescriptive analytics has just started to emerge in an increasing rate. In this paper, we present a literature review on prescriptive analytics, we frame the prescriptive analytics lifecycle and we identify the existing research challenges on this topic. To the best of our knowledge, this is the first literature review on prescriptive ...
Prescriptive analytics: Literature review and research challenges. Abstract: Business analytics aims to enable organizations to make quicker, better, and more intelligent decisions with the aim to create business value. To date, the major focus in the academic and industrial realms is on descriptive and predictive analytics.
(DOI: 10.1016/J.IJINFOMGT.2019.04.003) This article is published in International Journal of Information Management.The article was published on 2020-02-01 and is currently open access. It has received 232 citations till now. The article focuses on the topics: Prescriptive analytics & Business analytics.
We combine ideas from machine learning (ML) and operations research and management science (OR/MS) ... Prescriptive analytics: Literature review and research challenges. Highlights . This article focuses on prescriptive analytics, which is the less mature area of business analytics in comparison with descriptive and predictive analytics. ...
These challenges are particularly salient in the context of data-driven prescriptive analytics. Prescriptive analytics leverages data and mathematical algorithms for the prescription of specific actions (Delen & Demirkan, 2013). Thereby, the focus is shifted from understanding (diagnostic analytics) and forecasting (predictive analytics) to the ...
This paper investigates the existing literature pertaining to prescriptive analytics and prominent methods for its implementation, provides clarity on the research field of prescriptive analytics, synthesizes the literature review in order to identify the existing research challenges, and outlines directions for future research.
Prescriptive analytics: Literature review and research challenges. Highlights . This article focuses on prescriptive analytics, which is the less mature area of business analytics in comparison with descriptive and predictive analytics. Abstract ...
prescriptive analytics lifecycle and we identify the existing research challenges on this topic. To the best of our knowledge, this is the fi rst literature review on
See discussions, stats, and author profiles for this publication at: Prescriptive analytics: Literature. See discussions, stats, and author profiles for this publication at: Prescriptive analytics: Literature ... Literature review and research challenges. Article in International Journal of Information Management · February 2020 DOI: 10.1016/j ...
One challenge of prescriptive analytics is to rigorously show that the optimal decision prescribed is indeed better than that derived using the currently used approach (i.e., to make a fair comparison). ... Prescriptive analytics: literature review and research challenges. Int. J. Inf. Manag. (2020) M.W. Ng
Prescriptive analytics: Literature review and research challenges Highlights This article focuses on prescriptive analytics, which is the less mature area of business analytics in comparison with descriptive and predictive analytics.
In the broad sphere of Analytics, prescriptive analytics is one of the emerging areas of interest for both academicians and practitioners. As prescriptive analytics has transitioned from its inception to an emerging topic, there is a need to review existing literature in order to ascertain development in this area. There are a very few reviews in the related field but not specifically on the ...
K. Lepenioti, A. Bousdekis, D. Apostolou, G. Mentzas, Prescriptive analytics: Literature review and research challenges, Int. J. Inf. Nasim Sadat Mosavi et al. / Procedia Computer Science 177 (2020) 528â€"533 533 Author name / Procedia Computer Science 00 (2018) 000â€"000 5 change the parameters for meeting the objectives [7]. 4.
Prescriptive analytics: Literature review and research challenges Highlights This article focuses on prescriptive analytics, which is the less mature area of business analytics in comparison with descriptive and predictive analytics.
Prior reviews. To synthesize the literature and gain insights, it is important to conduct literature review-based studies (Kazemi et al., 2019).It accelerates the development of the research area by providing quick access to related issues and exploring the major contributions (Creswell, 2013; Machi & McEvoy, 2012).Owing to the significant interest in the areas of prescriptive analytics and ...
Introduction. Perceiving information and extracting business insights and knowledge from data is one of the major challenges in smart manufacturing [].In this sense, advanced data analytics is a crucial enabler of Industry 4.0 [].More specifically, among the major challenges for smart manufacturing are: (deep) machine learning, prescriptive analytics in industrial plants, and analytics-based ...
This paper investigates the existing literature pertaining to prescriptive analytics and prominent methods for its implementation, provides clarity on the research field of prescriptive analytics, synthesizes the literature review in order to identify the existing research challenges, and outlines directions for future research. 1.