General insurance marketing: A review and future research agenda
- Original Article
- Published: 23 October 2015
- Volume 20 , pages 282–291, ( 2015 )
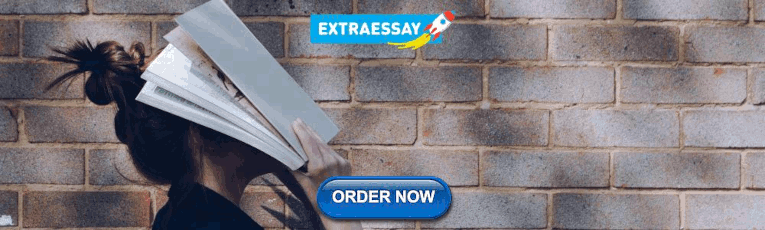
Cite this article
- Julie Robson 1
605 Accesses
10 Citations
Explore all metrics
The financial services sector is a huge and diverse industry comprising many different forms of organisations and product offerings. Yet, a review of past papers in the Journal of Financial Services Marketing ( JFSM ) reveals a heavy bias towards articles on banking, to the neglect of other equally important financial services categories. The purpose of this article is to address this imbalance and to call for more research to be conducted in a wider range of financial services categories. In particular, general insurance is singled out as a category worthy of further research. Looking to the past, this article reviews research published to-date on general insurance in the JFSM to establish a benchmark and explore theoretical contributions. Attention is then turned to the future to identify a research agenda for the general insurance sector going forward. Five important themes are identified: trust, transparency and simplification, technology, HNW, and Takaful.
This is a preview of subscription content, log in via an institution to check access.
Access this article
Price includes VAT (Russian Federation)
Instant access to the full article PDF.
Rent this article via DeepDyve
Institutional subscriptions
Similar content being viewed by others
Marketing Financial Services: Preliminary Findings from Northern Ireland.
Financial Services Marketing in a Changing Deregulated Environment
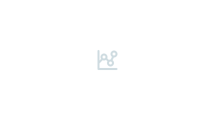
Diversification Versus Strategic Focus: Evidence from Insurance Intermediaries in Taiwan
Jin Lung Peng, Lih Ru Chen, … Larry Y. Tzeng
ABI (2015) Is your home underinsured? http://www.abi.org.uk , accessed 17 July 2015.
Ali, N. (2012) An exploratory study into the implementation of internal marketing in small insurance brokers in the United Kingdom. Journal of Financial Services Marketing 17 (3): 242–254.
Article Google Scholar
AMBest (2015) Takaful Operators Struggle with Growth and Profitability. Middle East – Non-Life and Life Special Report. Market Review, 13 April, New Jersey: A.M. Best Company.
Beaverstock, J.V. (2004) ‘Managing across borders’: Knowledge management and expatriation in professional service legal firms. Journal of Economic Geography 4 (2): 157–179.
Beaverstock, J.V. and Faulconbridge, J. (2014) Wealth segmentation and the mobilities of the super-rich: A conceptual framework. Elite mobilities. London: Routledge, pp. 40–61.
Google Scholar
Beaverstock, J.V., Faulconbridge, J.R. and Hall, S.J. (2014) The Globalization of Executive Search: Professional Services Strategy and Dynamics in the Contemporary World. Vol. 59. New York: Routledge.
Beloucif, A., Donaldson, B. and Kazanci, U. (2004) Insurance broker–client relationships: An assessment of quality and duration. Journal of Financial Services Marketing 8 (4): 327–342.
Blanc, A. (2012) Responding to Challenges in General Insurance in Upon the Door of Every Cottage … Protecting the Public through General Insurance. London: Chartered Insurance Institute.
Bond, A. and Stone, M. (2004) How the automotive insurance claims experience affects customer retention. Journal of Financial Services Marketing 9 (2): 160–171.
Brophy, R. (2015) A collection of insurance brands: The story of RSA in Ireland. Cogent Business & Management 2 (1): 1067567.
CII (2012) Upon the Door of Every Cottage … Protecting the Public through General Insurance. London: Chartered Insurance Institute.
Dunkley, E. (2015) A million UK customers switched banks in 2014. 22 January, http://www.Ft.com , accessed 14 July 2015.
Dusuki, A.W. and Abdullah, N.I. (2007) Why do Malaysian customers patronise Islamic banks? International Journal of Bank Marketing 25 (3): 142–160.
Edwards, K., Chisholm, L. and Smith, T. (2003) Craving for coaching: A case study from Kwik-Fit insurance services. Journal of Financial Services Marketing 8 (2): 167–173.
Ehrlich, I. and Becker, G.S. (1972) Market insurance, self-insurance, and self-protection. Journal of Political Economy 80 (4): 623–648.
Ennew, C., Kharouf, H. and Sekhon, H. (2011) Trust in UK financial services: A longitudinal analysis. Journal of Financial Services Marketing 16 (2): 65–75.
Ennew, C. and Sekhon, H. (2007) Measuring trust in financial services: The trust index. Consumer Policy Review 17 (2): 62.
Ennew, C. and Waite, N. (2007) Financial Services Marketing: An International Guide to Principles and Practice. Oxford: Butterworth-Heinemann.
EY (2015) 2015 Global Insurance Outlook. Ernest and Young, http://www.ey.com , accessed 17 July 2015.
Farquhar, J. and Robson, J. (2014) A brave new world: Branding in financial services. In: T. Harrison and H. Estelami (eds.) Routledge Companion to Financial Services Marketing. Abington, UK: Routledge.
Featherstone, M. (2014) The rich and the super-rich: Mobility, consumption and luxury lifestyles. In: N. Mathur (ed.) Consumer Culture, Modernity and Identity. India: Sage.
IIB Insurance (2015) The dangers of being underinsured. Independent insurance bureau ltd, http://www.iibinsurance.co.uk , accessed 28 July 2015.
Insurance Times (2015) Underinsurance is a business problem but a broker opportunity, http://www.insurancetimes.co.uk .
Jabnoun, N. and Khalifa, A. (2005) A customized measure of service quality in the UAE. Managing Service Quality 15 (4): 374–388.
Krikler, S., Dolberger, D. and Eckel, J. (2004) Method and tools for insurance price and revenue optimisation. Journal of Financial Services Marketing 9 (1): 68–79.
Kunreuther, H.C., Pauly, M.V. and McMorrow, S. (2013) Insurance and Behavioral Economics: Improving Decisions in the Most Misunderstood Industry. Cambridge University Press.
Lymberopoulos, K., Chaniotakis, I.E. and Soureli, M. (2004) Opportunities for banks to cross-sell insurance products in Greece. Journal of Financial Services Marketing 9 (1): 34–48.
Mankaby, M. (2015) London – A global Takaful centre. Global reinsurance. 20 August, www.globalreinsurance.com .
Market Line (2015) Global non-life insurance. MarketLine Industry Profile, February, Datamonitor.
Marroun, S., Wilkinson, I. and Young, L. (2014) Researching consumer behaviour at the top of the pyramid: Cultures of consumption of the super rich. Australian New Zealand Marketing Academy Annual Conference , Griffith, Australia.
Middelstaedt, J.D. (2002) A framework for understanding the relationships between religion and markets. Journal of Macromarketing 22 (1): 6–8.
Mirshekary, S., Yaftian, A.M. and Cross, D. (2005) Australian corporate collapse: The case of HIH Insurance. Journal of Financial Services Marketing 9 (3): 249–258.
Pew Forum (2015) The future of world religions: Population growth projections, 2010–2050, http://www.pewforum.org , accessed 28 July 2015.
Post Magazine (2015) Trust insurance campaign: A thirst for knowledge. Available from Insurance hound at http://www.insurancehound.co.uk .
Rajatanavin, R. and Speece, M. (2004) The sales force as an information transfer mechanism for new service development in the Thai insurance industry. Journal of Financial Services Marketing 8 (3): 244–258.
Robson, J. and Sekhon, Y. (2011) Addressing the research needs of the insurance sector. International Journal of Bank Marketing Special Ediition on Insurance 29 (7): 512–516.
Schwarcz, D. (2013) Transparency opaque: Understanding the lack of transparency in insurance consumer protection. UCLA Law Review 61: 394–396.
Sekhon, H. and Kennington, C. (2001) Buyer seller relationships in the UK insurance market: Is this the key competitive advantage? Journal of Financial Services Marketing 5 (3): 207–214.
Spencer, R. (2013) General Insurance in the twenty-first century: Meeting the challenges. The Chartered Insurance Institute Thinkpiece No. 94, May.
Taylor, S.A. (2013) Affect and marketing stimuli in consumer loyalty decisions to automobile insurers. Journal of Financial Services Marketing 18 (1): 4–16.
Thambiah, S., Malarvizhi, C. and Ismail, H. (2011) Islamic retail banking adoption: A conceptual framework. Australian Journal of Basic And Applied Sciences 5 (12): 649–657.
Trowbridge, C.L. (1975) Insurance as a transfer mechanism. The Journal of Risk and Insurance 42 (1): (March) 1–15.
Waite, N. (2001) New entrants in the financial services sector: The case of Kwik-Fit insurance services. International Journal of Bank Marketing 19 (5): 213–216.
Worthington, S. (2014) ‘Challenger banks’: Are they for real? In: T. Harrison and H. Estelami (eds.) Routledge Companion to Financial Services Marketing. Abington: Routledge.
Download references
Author information
Authors and affiliations.
Executive Business Centre, Bournemouth University, 89 Holdenhurst Road, Bournemouth, BH8 8EB, UK
Julie Robson
You can also search for this author in PubMed Google Scholar
Corresponding author
Correspondence to Julie Robson .
Additional information
1 specialises in financial services marketing, particularly general insurance. She has secured grants from the ESRC, HEIF and AMRC to support this work. She is on the editorial board of the International Journal of Bank Marketing and the Journal of Financial Service Marketing . She is also Senior Associate of the Centre for Risk, Banking and Financial Services, University of Nottingham. She is based at Bournemouth University where she has been Associate Dean, Director of Enterprise and Head of Department, Strategy and Marketing within the Business School. She is currently Chair of the Qualifications Examination and Assessments Committee of the Chartered Insurance Institute (CII), a member of the Chartered Institute of Loss Adjusters Examination Board and a past President of the Bournemouth Insurance Institute.
Rights and permissions
Reprints and permissions
About this article
Robson, J. General insurance marketing: A review and future research agenda. J Financ Serv Mark 20 , 282–291 (2015). https://doi.org/10.1057/fsm.2015.20
Download citation
Received : 21 August 2015
Revised : 21 August 2015
Published : 23 October 2015
Issue Date : 01 December 2015
DOI : https://doi.org/10.1057/fsm.2015.20
Share this article
Anyone you share the following link with will be able to read this content:
Sorry, a shareable link is not currently available for this article.
Provided by the Springer Nature SharedIt content-sharing initiative
- general insurance
- transparency and simplification
- Find a journal
- Publish with us
- Track your research
general insurance Recently Published Documents
Total documents.
- Latest Documents
- Most Cited Documents
- Contributed Authors
- Related Sources
- Related Keywords
IPO of life insurance company and privatisation of a general insurance company
Analysis of the efficiency of insurance companies in indonesia.
Financial system stability is not only supported by the banking sector, but also the role of insurance companies that operate efficiently. The study aims to analyze the efficiency performance of general insurance companies using two stages of data envelopment analysis during the 2017 – 2018 period. The first stage of efficiency measurement using a non-parametric data envelopment analysis (DEA) approach shows the efficiency level of general insurance companies experiencing a positive trend. The performance of general insurance companies in 2018 was more efficient than in 2017 based on the value of technical efficiency (CRS) and the value of pure technical efficiency (VRS). This means that in general there has been an increase in the efficiency of general insurance companies in Indonesia from 2017 to 2018. Testing the efficiency determinants in the second stage using the Tobit regression model found that the cost ratio is the only factor that significantly influences the efficiency level of general insurance companies in Indonesia. Meanwhile, company ownership and investment adequacy ratio have no significant effect on the efficiency level of general insurance companies in Indonesia. The results of the study provide recommendations to the management of general insurance companies that efficiency performance has not reached the maximum, and to improve it, it is necessary to control costs without disturbing routine operations and development activities.
FORMULATION OF STRATEGY FOR COMPETITIVENESS IMPROVEMENT OF GENERAL INSURANCE COMPANY IN INDONESIA
The gross direct premium income under general and health insurance: a segment – wise analysis of indian public sector insurers.
Insurance business is broadly classified under the two heads – Life Insurance and General Insurance. Life Insurance Corporation of India (LICI) and General Insurance Corporation of India (GICI) are the key players in the public sector. The Indian insurance market consists of many private players as well. The gross direct premium has been the income sources for the insurance business. This study shall highlight the gross direct premium income specifically under the General and Health insurance business of the Indian public sector insurers.
Risk Underwriting, Crisis Management, Regulatory Framework and Performance of Insurance Companies in Kenya: A Case of Sanlam General Insurance Company
Unparalleled volatility in sector-wise return in the chittagong stock exchange: a covid-19 perspective.
This study examined the effect of the Covid-19 health crisis on the volatility of sector-wise securities return listed in the Chittagong Stock Exchange (CSE) and compared this volatility with the pre-pandemic context. This study focused on the Chittagong Stock Exchange because this bourse offers a platform for negotiability and transferability of securities to investors in Chittagong and also plays a significant role in capital mobilization and the industrial development of Bangladesh. A sample of 90 securities under 19 sectors listed in the CSE were examined. The trend analysis indicated that Bank, Food, Footwear, Leasing, Life Insurance, Electrical and Engineering and Mutual Funds had same level of volatility between the Pre-Covid and Post-Covid time periods. Only four sectors, including Energy, Telecommunication, General Insurance and Miscellaneous sectors displayed a higher Post-Covid volatility relative to the Pre-Covid context. The result indicated that volatility of return was not the same for 19 sectors in the CSE over the selected time period. The researcher discovered that high and low periods of deaths had a significant impact on weekly volatility of return on 19 sectors of the CSE. However, the difference in volatility of return across all sectors between the Pre-Covid and Post-Covid time periods were not statistically significant from each other. Keywords: volatility of return, stock market, covid-19
PENERAPAN METODE BAYES DALAM MENGESTIMASI PREMI KREDIBILITAS PADA ASURANSI UMUM
Determination of insurance premiums is very important the calculation must be done carefully so that there is experience losses. The purpose of this research is to find out the application of empirical Bayes credibility theory Model 1 and estimate of the credibility premium on general insurance. A method that can help in overcoming these problems, that is empirical Bayes credibility theory Model 1, results of the estimated credibility premium credibility (in Euros) for insurance companies Alianz, Csob, Generali, Koop, Unisqa, and Wusten respectively as follows: 46.774811, 7.801307, 10.368991, 58.812250, 6.703035, and 5.091605. These results, the average claim is greater than the credibility premium, so that insurance companies can reserve premiums for the future.
Life insurance: features, present state and problems
The essence of life insurance, its features and advantages in comparison with other kinds of insurance is considered. Influence of changes of insurance payments on change of level of payments is analyzed and the interrelation between insurance premiums and insurance payments is found out. The forecast for 2021–2023 on insurance premiums and payments accordingly is constructed. In connection with this, the study of the life insurance market in Ukraine and the identification of the main problems of its development is currently quite relevant. Life insurance plays an important role in ensuring security and social stability in the country. Considering the economic essence of life insurance, it should be noted its feature, which is a combination of properties of insurance protection and savings. In this regard, in the general insurance market of the country, its structural segment has specific features in defining the basic concepts of insurance risk and insured event. Yes, insurance risk is based on the unpredictability of the event. The brake on the development of the life insurance market in Ukraine is that the capitalization and financial condition of the vast majority of insurance companies is unsatisfactory and does not ensure their competitiveness even in the domestic market. The limited amount and imperfect structure of financial savings of most insurers prevent them from becoming an effective institution of social protection and investment in the Ukrainian economy. Among the inhibiting factors should also be noted the limited investment activities of insurers. It is defined the basic problems that hinder the development of life insurance in Ukraine.
Two-Stage Data Envelopment Analysis Efficiency of Indian General Insurance Companies
The present study compares efficiency-related performance of 15 Indian general insurance companies using a two-stage efficiency evaluation model. Efficiency evaluation has been made for the span 2009–2010 to 2017–2018 using network DEA (data envelopment analysis). The results indicate that the in-sample private sector general insurance companies outcompeted the public sector insurers with regard to first-stage activity (premium mobilization), while the reverse was observed in terms of the second-stage activity (asset management and provision of claim benefits). The study also carried out regression of efficiency scores on several contextual variables. The results indicate that ownership is an influential contextual variable in both stages of productivity while solvency significantly impacts efficiency in the second stage.
Efficiency Analysis of Malaysian General Insurance Companies Using Data Envelopment and Super-efficiency Approach
Export citation format, share document.
Academia.edu no longer supports Internet Explorer.
To browse Academia.edu and the wider internet faster and more securely, please take a few seconds to upgrade your browser .
- We're Hiring!
- Help Center
General Insurance
- Most Cited Papers
- Most Downloaded Papers
- Newest Papers
- Save to Library
- Insurance Business Follow Following
- Risk Management and Insurance Follow Following
- Bootstrapping method Follow Following
- Financial Institutions Follow Following
- Bangladesh Follow Following
- Insurance Follow Following
- Insurance and reinsurance Follow Following
- Life Insurance Follow Following
- Wealth Management Follow Following
- Asuransi Follow Following
Enter the email address you signed up with and we'll email you a reset link.
- Academia.edu Publishing
- We're Hiring!
- Help Center
- Find new research papers in:
- Health Sciences
- Earth Sciences
- Cognitive Science
- Mathematics
- Computer Science
- Academia ©2024
Health insurance sector in India: an analysis of its performance
Vilakshan - XIMB Journal of Management
ISSN : 0973-1954
Article publication date: 30 November 2020
Issue publication date: 16 December 2020
Health insurance is one of the major contributors of growth of general insurance industry in India. It alone accounts for around 29% of total general insurance premium income earned in India. The growth of this sector is important from the perspective of overall growth of general insurance Industry. At the same time, problems in this sector are also many which are affecting its performance.
Design/methodology/approach
The paper provides an understanding on performance of health insurance sector in India. This study attempts to find out how much claims and commission and management expenses it has to incur to earn certain amount of premium. Methodology used for the study is regression analysis to establish relationship between dependent variable (Profit/Loss) and independent variable (Health Insurance Premium earned).
Findings of the study indicate that there is significant relationship between earned premium and underwriting loss. There has been increase of premium earnings which instead of increasing profit for the sector in fact has increased underwriting loss over the years. The earnings of the sector is growing at compounded annual growth rate of 27% still it is unable to earn underwriting profit.
Originality/value
This study is self-driven based on secondary data obtained from insurance regulatory and development authority site.
- Health insurance premium
- Management expenses
- Insurance regulatory and development authority
- Underwriting loss
- Compound annual growth rate
Dutta, M.M. (2020), "Health insurance sector in India: an analysis of its performance", Vilakshan - XIMB Journal of Management , Vol. 17 No. 1/2, pp. 97-109. https://doi.org/10.1108/XJM-07-2020-0021
Emerald Publishing Limited
Copyright © 2020, Madan Mohan Dutta.
Published in Vilakshan - XIMB Journal of Management . Published by Emerald Publishing Limited. This article is published under the Creative Commons Attribution (CC BY 4.0) licence. Anyone may reproduce, distribute, translate and create derivative works of this article (for both commercial and non-commercial purposes), subject to full attribution to the original publication and authors. The full terms of this licence maybe seen at http://creativecommons.org/licences/by/4.0/legalcode
1. Introduction
1.1 meaning of insurance.
Insurance is a contract between two parties where by one party agrees to undertake the risk of the other in exchange for consideration known as premium and promises to indemnify the party on happening of an uncertain event. The great advantage of insurance is that it spreads the risk of a few people over a large group of people exposed to risk of similar type.
Insurance has been identified as a sunrise sector by the financial planners of India. The insurance industry has lot of potential to grow, penetrate and service the masses of India. Insurance is all about protection. An insured needs two types of protection life and non-life. General insurance industry deals with non-life protection of the insured of which health insurance is a part.
1.2 Meaning of health insurance
Health insurance is a part of general insurance which contributes about 29% of premium amongst all other sectors of general insurance. But problems in this sector are many which is the driving force behind this study. This study will help the insurance companies to understand their performance and the quantum of losses that this sector is making over the years.
A plan that covers or shares the expenses associated with health care can be described as health insurance. These plans fall into commercial health insurance, which is provided by government, private and stand-alone health insurance companies.
Health insurance in India typically pays for only inpatient hospitalization and for treatment at hospitals in India. Outpatient services are not payable under health policies in India. The first health policy in India was Mediclaim Policy. In 2000, the Government of India liberalized insurance and allowed private players into the insurance sector. The advent of private insurers in India saw the introduction of many innovative products like family floater plans, critical illness plans, hospital cash and top-up policies.
Health insurance in India is an emerging insurance sector after life and automobile insurance sector. Rise in middle class, higher hospitalization cost, expensive health care, digitization and increase in awareness level are some important drivers for the growth of health insurance market in India.
Lifestyle diseases are on the rise. A sedentary lifestyle has pervaded our being. There is lower physical labour today than earlier and there is no reason why this would not be the trend going forward. The implication is the advent of lifestyle chronic diseases such as cardiac problems and diabetes.
In the context of the Indian health insurance industry, one could look at it both ways. Mired by low penetration and negative consumer perception about its utility are affecting the prospect of this industry. The flipside though is that we have hardly scratched the surface of the opportunity that lies in the future. It is as if the glass is half full. Much remains to be conquered and even more remains to be accomplished.
Health insurance companies needs to be optimistic and have courage to bring in innovation in the areas of product, services and distribution system. Bring it to the fold as the safety net that smartly covers and craft a health insurance plan befitting the need of the customers.
1.3 Background of health insurance sector in India
India’s tryst with health insurance programme goes back to the late 1940s and early 1950s when the civil servants (Central Government Health Scheme) and formal sector workers (Employees’ State Insurance Scheme) were enrolled into a contributory but heavily subsidized health insurance programmes. As a consequence of liberalization of the economy since the early 1990s, the government opened up private sector (including health insurance) in 1999. This development threw open the possibility for higher income groups to access quality care from private tertiary care facilities. However, India in the past five years (since 2007) has witnessed a plethora of new initiatives, both by the central government and a host of state governments also entering the bandwagon of health insurance. One of the reasons for initiating such programs may be traced to the commitment of the governments in India to scale up public spending in health care.
1.4 The need for health insurance in India
1.4.1 lifestyles have changed..
Indians today suffer from high levels of stress. Long hours at work, little exercise, disregard for a healthy balanced diet and a consequent dependence on junk food have weakened our immune systems and put us at an increased risk of contracting illnesses.
1.4.2 Rare non-communicable diseases are now common.
Obesity, high blood pressure, strokes and heart attacks, which were earlier considered rare, now affect an increasing number of urban Indians.
1.4.3 Medical care is unbelievably expensive.
Medical breakthroughs have resulted in cures for dreaded diseases. These cures however are available only to a select few. This is because of high operating and treatment expenses.
1.4.4 Indirect costs add to the financial burden.
Indirect sources of expense like travel, boarding and lodging, and even temporary loss of income account for as much as 35% of the overall cost of treatment. These facts are overlooked when planning for medical expenses.
1.4.5 Incomplete financial planning.
Most of us have insured our home, vehicle, child’s education and even our retirement years. Ironically however we have not insured our health. We ignore the fact that illnesses strike without warning and seriously impact our finances and eat into our savings in the absence of a good health insurance or medical insurance plan.
1.5 Classification of health insurance plans in India
Health insurance plans in India today can be broadly classified into the following categories:
1.5.1 Hospitalization.
Hospitalization plans are indemnity plans that pay cost of hospitalization and medical costs of the insured subject to the sum insured. There is another type of hospitalization policy called a top-up policy . Top-up policies have a high deductible typically set a level of existing cover.
1.5.2 Family floater health insurance.
Family health insurance plan covers entire family in one health insurance plan. It works under assumption that not all member of a family will suffer from illness in one time.
1.5.3 Pre-existing disease cover plans.
It offers covers against disease that policyholder had before buying health policy. Pre-existing disease cover plans offers cover against pre-existing disease, e.g. diabetes, kidney failure and many more. After waiting for two to four years, it gives covers to the insured.
1.5.4 Senior citizen health insurance.
This type of health insurance plan is for older people in the family. It provides covers and protection from health issues during old age.
1.5.5 Maternity Health insurance.
Maternity health insurance ensures coverage for maternity and other additional expenses.
1.5.6 Hospital daily cash benefit plans.
Daily cash benefits are a defined benefit policy that pays a defined sum of money for every day of hospitalization.
1.5.7 Critical illness plans.
These are benefit-based policies which pay a lump sum amount on certain critical illnesses, e.g. heart attack, cancer and stroke.
1.5.8 Disease-specific special plans.
Some companies offer specially designed disease-specific plans such as Dengue Care and Corona Kavach policy.
1.6 Strength, weakness, opportunity and threat analysis of health insurance sector (SWOT analysis)
The strengths, weaknesses, opportunities and threats (SWOT) is a study undertaken to identify internal strengths and weaknesses as well as external opportunities and threats of the health insurance sector.
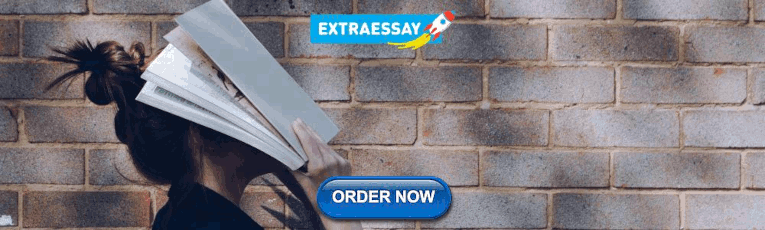
1.6.1 Strengths.
The growth trend of the health insurance sector is likely to be high due to rise in per capita income and emerging middle-income group in India. New products are being launched in this sector by different insurance companies which will help to satisfy customers need. Customers will be hugely benefited when cash less facility will be provided to all across the country by all the insurance companies.
1.6.2 Weaknesses.
The financial condition of this sector is weak due to low investment in this sector. The public sector insurance companies are still dominating this industry due to their greater infrastructure facilities. This sector is prone to high claim ratio and many false claims are also made.
1.6.3 Opportunities.
The possibility of future growth of this sector is high, as penetration in the rural sector is low. The improvement of technology and the use of internet facility are helping this sector to grow in magnitude and move towards environment-friendly paperless regime.
1.6.4 Threats.
The biggest threat of this sector lies in the change in the government regulations. The profitability of this sector is affected due to increasing expenses and claims. The economic slowdown and recession in the economy can affect growth of this sector adversely. The increasing losses and need for insurance might reach a point of no return where insurance companies may be compelled to decline an insurance policy.
1.7 Political economic socio cultural and technological analysis of health insurance sector (PEST analysis)
This analysis describes a framework of macro-environmental factors used as strategic tool for understanding business position, growth potential and direction for operations.
1.7.1 Political factors.
Service tax on premium on insurance policies is being increased by the government for past few years during budget. Government monopoly in this sector came to an end after insurance companies were opened up for private participation in the year 2000. Foreign players were allowed to enter into joint venture with their Indian counterpart with 26% holding and which was further increased to 49% in the year 2015.
1.7.2 Economic factors.
The gross savings of people in India have increased significantly thereby encouraging people to buy insurance policy to cover their risks. Insurance companies are fast becoming prominent players in the security market. As these companies have huge disposable income which they are investing in the security market.
1.7.3 Socio-cultural factors.
Increase in insurance knowledge is helping people to increase their awareness about the risk to be covered through insurance. Change in lifestyle is leading to increase in risk thereby giving an opportunity to insurance companies to innovate newer products. Societal benefit is derived by transfer of risk through insurance due to improved socio-cultural environment.
1.7.4 Technological factors.
Insurance companies deals in large database and maintaining it by the application of latest technology is huge gain for this sector. Technological advancement has helped insurance companies to sale their products through their electronic portals. This has made their task of providing service to the customers easier and faster.
2. Review of literature
After opening up of the insurance industry health insurance sector has become significant both from economic and social point of view and researchers have explored and probed these aspects.
Ellis et al. (2000) reviewed a variety of health insurance systems in India. It was revealed that there is a need for a competitive environment which can only happen with the opening up of the insurance sector. Aubu (2014) conducted a comparative study on public and private companies towards marketing of health insurance policies. Study revealed that private sector services evoked better response than that of public sector because of new strategies and technologies adopted by them. Nair (2019) has made a comparative study of the satisfaction level of health insurance claimants of public and private sector general insurance companies. It was revealed that majority of the respondents had claim of reimbursement nature through third party administrator. Satisfaction with respect to settlement of claim was found relatively higher for public sector than private sector. Devadasan et al. (2004) studied community health insurance to be an important intermediate step in the evolution of an equitable health financing mechanism in Europe and Japan. It was concluded that community health insurance programmes in India offer valuable lessons for its policy makers. Kumar (2009) examined the role of insurance in financing health care in India. It was found that insurance can be an important means of mobilizing resources, providing risk protection and health insurance facilities. But for this to happen, it will require systemic reforms of this sector from the end of the Government of India. Dror et al. (2006) studied about willingness among rural and poor persons in India to pay for their health insurance. Study revealed that insured persons were more willing to pay for their insurance than the uninsured persons. Jayaprakash (2007) examined to understand the hurdles preventing the people to purchase health insurance policies in the country and methods to reduce claims ratio in this sector. Yadav and Sudhakar (2017) studied personal factors influencing purchase decision of health insurance policies in India. It was found that factors such as awareness, tax benefit, financial security and risk coverage has significant influence on purchase decision of health insurance policy holders. Thomas (2017) examined health insurance in India from the perspective of consumer insights. It was found that consumers consider various aspects before choosing a health insurer like presence of a good hospital network, policy coverage and firm with wide product choice and responsive employees. Savita (2014) studied the reason for the decline of membership of micro health insurance in Karnataka. Major reason for this decline was lack of money, lack of clarity on the scheme and intra house-hold factors. However designing the scheme according to the need of the customer is the main challenge of the micro insurance sector. Shah (2017) analysed health insurance sector post liberalization in India. It was found that significant relationship exists between premiums collected and claims paid and demographic variables impacted policy holding status of the respondents. Binny and Gupta (2017) examined opportunities and challenges of health insurance in India. These opportunities are facilitating market players to expand their business and competitiveness in the market. But there are some structural problems faced by the companies such as high claim ratio and changing need of the customers which entails companies to innovate products for the satisfaction of the customers. Chatterjee et al. (2018) have studied health insurance sector in India. The premise of this paper was to study the current situation of the health-care insurance industry in India. It was observed that India is focusing more on short-term care of its citizens and must move from short-term to long-term care. Gambhir et al. (2019) studied out-patient coverage of private sector insurance in India. It was revealed that the share of the private health insurance companies has increased considerably, despite of the fact that health insurance is not a good deal. Chauhan (2019) examined medical underwriting and rating modalities in health insurance sector. It was revealed that while underwriting a health policy one has to keep in mind the various aspects of insured including lifestyle, occupation, health condition and habits. There have been substantial studies on health insurance done in India and abroad. But there has not been any work on performance of health insurance sector based on underwriting profit or loss.
3. Research gap
After extensive review of literature it is understood that there has not been substantial study on the performance of health insurance sector taking underwriting profit or loss into consideration. In spite of high rate of growth of earned premium, this sector is unable to make underwriting profit. This is mainly because growth of premium is more than compensated by claims incurred and commission and other expenses paid. Thereby leading to growth of underwriting loss over the years across the different insurance companies covered under both public and private sector. This unique feature of negative performance of this sector has not been studied so far in India.
4. Objectives
review health insurance scenario in India; and
study the performance of health insurance sector in India with respect to underwriting profit or loss by the application of regression analysis.
5. Research methodology
The study is based on secondary data sourced from the annual reports of Insurance Regulatory Development Authority (IRDA), various journals, research articles and websites. An attempt has been made to evaluate the performance of the health insurance sector in India. Appropriate research tools have been used as per the need and type of the study. The information so collected has been classified, tabulated and analysed as per the objectives of the study.
The data is based on a time period of 12 years ranging from 2006–2007 to 2018–2019.
Secondary data analysis has been done using regression of the form: Y = a + b X
The research has used SPSS statistics software package for carrying out regression and for the various graphs Microsoft Excel software has been used.
5.1 The problem statement
It is taken to be a general assumption that whenever the premium increases the profit also increases. This determines that profits are actually dependent on the premium income. Hence, whenever the premium tends to increase, the profit made also supposed to increase.
The aim of the study is to find out whether the underwriting profit of the health insurance sector is increasing or there is an underwriting loss.
The problem statement is resolved by applying regression analysis between the premium earned and underwriting profit or loss incurred. It is assumed that if the underwriting profit increases along with the premium received, then the pattern forms a normal distribution and alternate hypothesis can be accepted and if this pattern of dependability is not found then the null hypothesis will be accepted stating that there is no relation between the premium and the underwriting loss or the underwriting profit by the sector. But what is happening in this sector is the increase in premium is leading to increase in underwriting loss. So premium is negatively impacting underwriting profit which is astonishing thing to happen and is the crux of the problem of this sector.
5.1.1 Underwriting profit/loss = net premium earned – (claim settled + commission and management expenses incurred).
Underwriting profit is a term used in the insurance industry to indicate earned premium remaining after claims have been settled and commission and administrative expenses have been paid. It excludes income from investment earned on premium held by the company. It is the profit generated by the insurance company in the normal course of its business.
5.2 Data analysis
Table 1 shows that health insurance premium increased from Rs.1910 crores in 2006–2007 to Rs. 33011 crores in 2018–2019. But claims incurred together with commission and management expenses have grown from Rs. 3349 crores to Rs. 40076 crores during the same period. So the claims and management expenses incurred together is more than the health insurance premium earned in all the years of our study thereby leading to underwriting loss.
Claim incurred shown above is the outcome of the risk covered against which premium is received and commission and management expenses are incurred to obtain contract of insurance. Both these expenses are important for insurance companies to generate new business as stiff competition exists in this sector since it was opened up in the year 2000.
Figure 1 depicts the relationship between health insurance premium earned and claims and management expenses incurred by the insurance companies of the health insurance sector for the period 2006–2007 to 2018–2019.
Bar chart between premiums earned and claims and management expenses incurred show that claims and management expenses together is higher than premium earned in all the years of the study thereby leading to losses. Claims, commission and management expenses are important factors leading to the sale of insurance policies thereby earning revenue for the insurance companies in the form of premium. But proper management of claims and commission and management expenses will help this sector to improve its performance.
Table 2 provides insight into the performance of health insurance sector in India. The growth of health insurance in India has been from Rs.1909 crores for the financial year 2006–2007 to Rs. 33011crores for the financial year 2018–2019. The growth percentage is 1629% i.e. growing at an average rate of 135% per annum. Compounded Annual Growth Rate (CAGR) is working out to be 27%.
From the same table, it can be inferred that health insurance sector is making underwriting loss in all the financial years. There is no specific trend can be seen, it has increased in some years and decreased in some other years. Here underwriting loss is calculated by deducting claims and commission and management expenses incurred from health insurance premium earned during these periods.
With every unit of increase in premium income the claims incurred together with commission and management expenses paid increased more than a unit. Thereby up setting the bottom line. So instead of earning profit due to better business through higher premium income, it has incurred losses.
Underwriting principles needs to be streamlined so that proper scrutiny of each policy is carried out so that performance of this sector improves.
It is seen from Figure 2 that there is stiff rise in premium earned over the years but claims and commission and management expenses incurred have also grown equally and together surpassed earned premium. So the net impact resulted in loss to this sector which can also be seen in the figure. It is also seen that loss is increasing over the years. So, increase in earnings of revenue in the form of premium is leading to increase in losses in this sector which is normally not seen in any other sectors.
But a time will come when commission and management expenses will stabilize through market forces to minimize underwriting losses. On the other hand, it will also require proper management of claims so that health insurance sector can come of this unprofitable period.
5.3 Interpretation of regression analysis
5.3.1 regression model..
Where Y = Dependent variable
X = Independent variablea = Intercept of the lineb = Slope of the line
5.3.2 Regression fit.
Here, Y is dependent variable (Underwriting Profit or Loss) which is to be predicted, X is the known independent variable (Health Insurance Premium earned) on which predictions are to be based and a and b are parameters, the value of which are to be determined ( Table 3 ). Y = − 1028.737 − 0.226 X
5.3.3 Predictive ability of the model.
The value of R 2 = 0.866 which explains 86.6% relationship between health insurance premium earned and loss made by this sector ( Table 4 ). In other words, 13.4% of the total variation of the relationship has remained unexplained.
4.1 Regression coefficients ( Table 5 ).
H1.1 : β = 0 (No influence of Health Insurance Premium earned on Underwriting Profit or Loss made)
5.4.1.2 Alternative hypothesis.
H1.2 : β ≠ 0 (Health Insurance Premium earned influences underwriting Profit or Loss made by this sector)
The computed p -value at 95% confidence level is 0.000 which is less than 0.05. This is the confidence with which the alternative hypothesis is accepted and the null hypothesis is rejected. Thus regression equation shows that there is influence of health insurance premium earned on loss incurred by this sector.
The outcome obtained in this analysis is not what happens normally in the industry. With the increase of revenue income in the form of premium, it may lead to either profit or loss. But what is happening surprisingly here is that increase of revenue income is leading to increase of losses. So growth of premium income instead of influencing profit is actually influencing growth of losses.
6.1 Findings
The finding from the analysis is listed below:
The average growth of net premium for the health insurance has been around 135% per annum even then this sector is unable to earn underwriting profit.
The CAGR works out to around 27%. CAGR of 27% for insurance sector is considered to be very good rate of growth by any standard.
Along with high growth of premium, claims and commission and management expenses incurred in this sector have also grown substantially and together it surpassed in all the years of the study.
Thus, growth of claims and commission and management expenses incurred has more than compensated high rate of growth of health insurance premium earned. This resulted into underwriting loss that this sector is consistently making.
Astonishing findings has been higher rate of increase of premium earnings leading to higher rate of underwriting loss incurred over the years. Even though the sector is showing promise in terms of its revenue collection, but it is not enough to earn underwriting profit.
6.2 Recommendations
COVID 19 outbreak in India has led to a spike in health-care costs in the country. So, upward revision of premium charges must be considered to see bottom line improvement in this sector.
Immediate investigation of the claim is required. This will enable the insurers to curb unfair practice and dishonest means of making a claim which is rampant in this sector.
Health insurance market is not able to attract younger generation of the society. So entry age-based pricing might attract this group of customers. An individual insured at the age 30 and after 10 years of continuous coverage the premium will be less than the other individual buying a policy at the age of 40 for the first time.
6.3 Limitations and scope of future studies
The analysis of performance of health insurance sector in India taking underwriting profit into consideration is the only study of its kind in this sector. As a result, adequate literature on the subject was not available.
Health insurance and health care are part of medical care industry and are inter dependent with each other. So performance of health insurance sector can be better understood by taking health-care industry into consideration which is beyond the scope of the study.
This sector is consistently incurring losses. So, new ideas need to be incorporated to reduce losses if not making profits.
Opportunity of the insurance companies in this sector lies in establishing innovative product, services and distribution channels. So, continuous modification by the application of research is required to be undertaken.
Health insurance sector will take a massive hit, as tax benefit is going to be optional from this financial year. This can be a subject of study for the future.
6.4 Conclusion
This sector is prone to claims and its bottom line is always under tremendous pressure. In recent times, IRDA has taken bold step by increasing the premium rate of health insurance products. This will help in the growth of this sector.
With better technological expertise coming in from the foreign partners and involvement by the IRDA the health insurance sector in India must turn around and start to earn profit.
The COVID-19 pandemic is a challenge for the health insurance industry on various fronts at the same time it provides an opportunity to the insurers to fetch in new customers.
The main reason for high commission and management expense being cut-throat competition brought in after opening up of the insurance sector in the year 2000. So, new companies are offering higher incentives to the agents and brokers to penetrate into the market. This trend needs to be arrested as indirectly it is affecting profitability of this sector.
The study will richly contribute to the existing literature and help insurance companies to know about their performance and take necessary measures to rectify the situation.
Chart on health insurance premium earned and claims and management expenses paid
Chart on performance of health insurance sector in India
Data showing health insurance premium earned and claims and management expenses paid
. Dependent variable: Underwriting profit or loss;
. Predictors: (Constant), Health insurance premium earned
Aubu , R. ( 2014 ), “ Marketing of health insurance policies: a comparative study on public and private insurance companies in Chennai city ”, UGC Thesis, Shodgganga.inflibnet.ac.in .
Chatterjee , S. , Giri , A. and Bandyopadhyay , S.N. ( 2018 ), “ Health insurance sector in India: a study ”, Tech Vistas , Vol. 1 , pp. 105 - 115 .
Chauhan , V. ( 2019 ), “ Medical underwriting and rating modalities in health insurance ”, The Journal of Inssurance Institute of India , Vol. VI , pp. 14 - 18 .
Devadasan , N. , Ranson , K. , Damme , W.V. and Criel , B. ( 2004 ), “ Community health insurance in India: an overview ”, Health Policy , Vol. 29 No. 2 , pp. 133 - 172 .
Dror , D.M. , Radermacher , R. and Koren , R. ( 2006 ), “ Willingness to pay for health insurance among rural and poor persons: Field evidence form seven micro health insurance units in India ”, Health Policy , pp. 1 - 16 .
Ellis , R.P. , Alam , M. and Gupta , I. ( 2000 ), “ Health insurance in India: Prognosis and prospectus ”, Economic and Political Weekly , Vol. 35 No. 4 , pp. 207 - 217 .
Gambhir , R.S. , Malhi , R. , Khosla , S. , Singh , R. , Bhardwaj , A. and Kumar , M. ( 2019 ), “ Out-patient coverage: Private sector insurance in India ”, Journal of Family Medicine and Primary Care , Vol. 8 No. 3 , pp. 788 - 792 .
Gupta , D. and Gupta , M.B. ( 2017 ), “ Health insurance in India-Opportunities and challenges ”, International Journal of Latest Technology in Engineering, Management and Applied Science , Vol. 6 , pp. 36 - 43 .
Hand book on India Insurance Statistics revisited ( 2020 ), “ Insurance regulatory and development authority website ”, available at: www.irda.gov.in ( accessed 2 July 2020 ).
Jayaprakash , S. ( 2007 ), “ An explorative study on health insurance industry in India ”, UGC Thesis, Shodgganga.inflibnet.ac.in .
Kumar , A. ( 2009 ), “ Health insurance in India: is it the way forward? ”, World Health Statistics (WHO) , pp. 1 - 25 .
Nair , S. ( 2019 ), “ A comparative study of the satisfaction level of health insurance claimants of public and private sector general insurance companies ”, The Journal of Insurance Institute of India) , Vol. VI , pp. 33 - 42 .
Savita ( 2014 ), “ A qualitative analysis of declining membership in micro health insurance in Karmataka ”, SIES Journal of Management , Vol. 10 , pp. 12 - 21 .
Shah , A.Y.C. ( 2017 ), “ Analysis of health insurance sector post liberalisation in India ”, UGC Thesis, Shodgganga.inflibnet.ac.in .
Thomas , K.T. ( 2017 ), “ Health insurance in India: a study on consumer insight ”, IRDAI Journal , Vol. XV , pp. 25 - 31 .
Yadav , S.C. and Sudhakar , A. ( 2017 ), “ Personal factors influencing purchase decision making: a study of health insurance sector in India ”, BIMAQUEST , Vol. 17 , pp. 48 - 59 .
Further reading
Beri , G.C. ( 2010 ), Marketing Research , TATA McGraw Hill Education Private , New Delhi, ND .
Dutta , M.M. and Mitra , G. ( 2017 ), “ Performance of Indian automobile insurance sector ”, KINDLER , Vol. 17 , pp. 160 - 168 .
Majumdar , P.I. and Diwan , M.G. ( 2001 ), Principals of Insurance , Insurance Institute of India , Mumbai, MM .
Pai , V.A. and Diwan , M.G. ( 2001 ), “ Practice of general insurance ”, Insurance Institute of India , Mumbai, MM .
Shahi , A.K. and Gill , H.S. ( 2013 ), “ Origin, growth, pattern and trends: a study of Indian health insurance sector ”, IOSR Journal of Humanities and Social Science , Vol. 12 , pp. 1 - 9 .
Corresponding author
Related articles, we’re listening — tell us what you think, something didn’t work….
Report bugs here
All feedback is valuable
Please share your general feedback
Join us on our journey
Platform update page.
Visit emeraldpublishing.com/platformupdate to discover the latest news and updates
Questions & More Information
Answers to the most commonly asked questions here

An official website of the United States government
The .gov means it’s official. Federal government websites often end in .gov or .mil. Before sharing sensitive information, make sure you’re on a federal government site.
The site is secure. The https:// ensures that you are connecting to the official website and that any information you provide is encrypted and transmitted securely.
- Publications
- Account settings
Preview improvements coming to the PMC website in October 2024. Learn More or Try it out now .
- Advanced Search
- Journal List
- Wiley - PMC COVID-19 Collection

Did COVID‐19 change life insurance offerings?
Timothy f. harris.
1 Department of Economics, Illinois State University, Normal Illinois, USA
Aaron Yelowitz
2 Department of Economics, Gatton College of Business and Economics, University of Kentucky, Lexington Kentucky, USA
Charles Courtemanche
Associated data.
The profitability of life insurance offerings is contingent on accurate projections and pricing of mortality risk. The COVID‐19 pandemic created significant uncertainty, with dire mortality predictions from early forecasts resulting in widespread government intervention and greater individual precaution that reduced the projected death toll. We analyze how life insurance companies changed pricing and offerings in response to COVID‐19 using monthly data on term life insurance policies from Compulife. We estimate event‐study models that exploit well‐established variation in the COVID‐19 mortality rate based on age and underlying health status. Despite the increase in mortality risk and significant uncertainty, the results generally indicate that life insurance companies did not increase premiums or decrease policy offerings due to COVID‐19. Nonetheless, we find some evidence that premiums differentially increased for individuals with very high risk and that some policies were removed for the oldest of the old.
1. INTRODUCTION
Since the 2019 novel coronavirus (SARS‐CoV‐2) first emerged, there has been substantial uncertainty regarding the magnitude of the increase in mortality risk. In March 2020, a highly cited study from Imperial College (Ferguson et al., 2020 ) reported that uncontrolled spread of coronavirus in the United States could lead to 2.2 million fatalities, based on key assumptions such as 80% of the population ultimately getting COVID‐19 and an infection fatality rate (IFR) of 0.9%. The modeling led to widespread action by policymakers in the United States and other countries to reduce transmission; within 3 days of the publication, California implemented the first‐in‐the‐nation shelter‐in‐place order (Friedson et al., 2020 ), and most other states followed quickly thereafter.
As of March 2021, the COVID‐19 death toll in the United States has been substantially below this projection. The difference between the most pessimistic forecasts and actual fatalities is likely due to changes in behavior—such as better handwashing, staying home more, and wearing facemasks or social distancing when outside the home—that are partly voluntary and partly induced by government suppression and mitigation policies (Courtemanche et al., 2020 ; Hsiang et al., 2020 ; Lyu & Wehby, 2020 ). While the average IFR has been the subject of debate in the literature due to different methods of accounting for undetected mild or asymptomatic infections, most studies put it in the range of 0.5% to 1%—similar to the rate used by the Imperial College report, and an order of magnitude deadlier than the flu (Abbott & Douglas, 2020 ; Meyerowitz‐Katz & Merone, 2020 ).
The duration and magnitude of increased mortality risk from COVID‐19 are contingent on many uncertain events, such as the availability and efficacy of vaccines (Corum et al., 2020 ), the ability to implement technological innovations like pooled testing (Augenblick et al., 2020 ; Mandavilli, 2020 ), at‐home testing, and contact tracing, and innovations in treating those who contract COVID‐19 with therapeutics like Remdesivir (Beigel et al., 2020 ). In addition to these factors, health messaging has been conflated with political considerations, contributing to more uncertainty.
Underlying uncertainty about the direct and indirect effects of the virus, policy missteps, incorrect forecasts, and uncertainty about longer‐run consequences all provide challenges for the life insurance industry, which relies on accurate estimates of mortality risk. In this study, we use monthly data on approximately 800,000 policies from 96 distinct companies listed on Compulife, a key distributor of life insurance quotes, to analyze the influence of COVID‐19 on both term life insurance pricing and policy offerings. One key prediction is that insurance premiums should respond to exogenous changes in overall risk, which is precisely what happened due to COVID‐19. Such short‐run changes are well documented for automobile insurance, where reductions in driving and accident claims led to premium refunds early during the pandemic (Scism, 2020 ).
To analyze the influence of increased mortality risk on life insurance premiums and offerings, we exploit well‐known and widely accepted variation in mortality risks from COVID‐19 originating from age and comorbidities. 1 Those with chronic conditions or advanced age are far more likely than others to be hospitalized or die from the virus (CDC, US Centers for Disease Control, and Prevention, 2020 ). Early evidence from mainland China estimated IFR of 7.8% for those aged over 80 and over, 4.28% for those aged 70–79, and 1.93% for those aged 60–69, compared to 0.03% for young adults aged 20–29 (Verity et al., 2020 ). As a consequence, the direct health consequences of the virus (such as through mortality) and indirect effects (such as through foregone preventative care, mental health consequences, or rising obesity) are far more pronounced for older, less‐healthy individuals than for younger, healthier individuals, especially for mortality in the short‐run. 2
This variation in mortality risk allows for the construction of treatment and control groups that we analyze using event‐study models. Specifically, we estimate hedonic insurance pricing models by comparing the changes in premiums of policies offered to older individuals to those of policies offered to younger individuals. In addition, we compare the response of policies offered to relatively healthy younger individuals compared to relatively unhealthy older individuals (e.g., individuals in the lowest health category and those that smoke). This estimation approach allows us to difference out variation in pricing that might have occurred due to pandemic‐induced changes in the bond and stock markets (which influence the profitability of life insurance products). In addition to analyzing changes in premiums, we also estimate the impact of the pandemic on policy offerings.
Overall, the analysis shows that life insurance companies generally did not respond to the changes in mortality risk by increasing premiums or reducing policy offerings for those that experienced the greatest change in mortality risk. However, we do find evidence that policies with the lowest prices and those offered to smokers in the worst health category did differentially increase premiums for policies offered to older individuals. This implies that the lack of an overall response is caused in part by market competition. In addition, we find that policies offered to the oldest of the old (age 75+) were differentially removed from the market consistent with Hendren ( 2013 ).
Although one might initially have expected large effects on life insurance premiums for the high‐risk groups, several explanations—in addition to market competition—may account for the lack of meaningful overall responses. First, life insurance premiums account for the rise in mortality, unconditional on the infection. Although the case fatality rate (CFR) or IFR is much higher than other illnesses like the flu, there have been unprecedented steps such as lockdowns and behavior changes that should reduce the probability of infection. Put differently, with extensive underwriting, there should be very little adverse selection from individuals who currently have COVID‐19 being able to successfully obtain policies (Cawley & Philipson, 1999 ; Harris & Yelowitz, 2014 ) and conditional on not yet receiving the diagnosis, mortality risk has not significantly increased. 3 Second, it is possible that even a “high‐risk” life insurance customer who purchases a policy listed in our data is quite different from those who are currently dying from COVID‐19 in terms of mortality risk. To date, more than one‐third of deaths nationwide are from those residing in nursing homes (NYT, 2020 ), individuals who almost certainly would be rejected if applying for a new term life insurance policy. Recent commentary suggests that even accounting for excess deaths in New York City, the increased odds of death in 2020 for a 70‐year‐old are approximately 1 in 30 (NYC DOHMH, 2020 ; Rosenkranz, 2020 ). Third, even sizable transitory increases in risk would reflect small increases in annual premium as the price increase is spread over the term of the policy (e.g., 15 years).
Another potential possibility for the lack of strong effects in our analysis is that the pandemic risk was largely in line with expectations and already taken into account in the premium structure. Several industry studies in the decade before the coronavirus pandemic recognize the need for pricing pandemic risk into life insurance premiums (e.g., SOA, 2007 ; Swiss, 2007 ), where once in 100‐year benchmarks like the 1918 flu pandemic are considered in formulating appropriate reserve requirements. Pandemics, far more than other risks like natural disasters, industrial accidents, or terrorist attacks, pose solvency issues for insurers due to correlated risks (Huynh et al., 2013 ). By pricing in pandemic risk, overall insolvency issues from the coronavirus pandemic are unlikely to arise from life insurance claims; indeed, actual life insurance claims have been in line with the pre‐COVID‐19 scenarios (Richter & Wilson, 2020 ). However, given the rarity of pandemics (approximately 3%–4% per year per Huynh et al., 2013 ), the realized distribution of age‐specific excess mortality can vary from one pandemic to the next; for example, the 1918 flu pandemic disproportionately affected young adults. Thus, the mortality pattern that emerged in early 2020 opens the possibility for life insurance companies to adjust their premiums based on observable characteristics like age and health because “the contributions to a mutual pooling arrangement or the premiums paid to a private insurer would need to vary based on the expected losses of each entity.” (Hartwig et al., 2020 ).
The paper is organized as follows. Section 2 gives an overview of the life insurance market and a theoretical model of pricing. Section 3 describes the Compulife data. Section 4 presents the empirical specifications and results, and Section 5 concludes.
2. BACKGROUND AND THEORY
The fundamental purpose of life insurance is to protect family members—often surviving spouses—against earning losses due to a breadwinner's mortality (Harris & Yelowitz, 2018 ), although there are hybrid life insurance products that also serve other purposes such as tax planning and investing. Life insurance coverage in the United States is widespread, with approximately 70% of households having some form of life insurance coverage in either the individual or group markets (Harris & Yelowitz, 2017 ). The two markets differ in that individual market premiums are experience‐rated with extensive underwriting, while group markets typically have some form of community rating and guaranteed issue. There is considerable discussion of adverse selection and asymmetric information in the individual term life insurance market (see Cawley & Philipson, 1999 ; Harris & Yelowitz, 2014 ; He, 2009 , 2011 ; Hedengren & Stratmann, 2016 ), and there are explicit mechanisms in contracts to discourage moral hazard (e.g., riders on suicide for the first several years).
Life insurance markets have evolved over the years, and many companies offer premium quotes online, which in turn allows for lower search costs, greater comparison shopping, and more vigorous price competition (Brown & Goolsbee, 2002 ). Industry studies find that one‐half of all adults sought life insurance information online in recent years, and nearly one‐third attempted to purchase coverage. 4 Although some features of term life insurance—such as a company's reputation and financial health—may enter into purchasing decisions, the key factors that contribute to annual premiums are the face value of the policy, term length, and probability of death proxied by measurable risk factors such as age, sex, underlying health, and risk behavior. Term life insurance policies would then appear to be much like a commodity, where policies from different companies are very close substitutes for each other. In an online setting, with comparison shopping, small premium adjustments may lead to leapfrogging, which in turn could dramatically affect demand. Of course, life insurance companies screen most customers extensively offline in the individual market (e.g., medical exams), potentially leading to reclassification risk (Handel et al., 2015 ), which is not observable in the data on policy offerings. In such a market, where there is clearly an exogenous increase in mortality for defined groups—such as those who are older and less healthy in 2020 (relative to earlier years and other groups)—we would expect larger premium increases. 5 It is also possible for insurers to withdraw policies—essentially universal rejection for various groups—if the regulatory environment, competitive market pressure, or private information (Hendren, 2013 ) made premium increases infeasible. Of course, given that different companies make different projections about the overall aggregate risk from COVID‐19, the magnitude of adjustment might be quite different. Nonetheless, it is hard to envision another market where the consequences of making a forecasting mistake are higher or where those making premium adjustments are more expert on mortality consequences.
To understand the influence of an increase in mortality risk on premiums, we use a basic model of term life insurance pricing in a competitive market where companies set premiums such that the expected net present value (ENPV) of premiums equals the ENPV of payouts from the company's perspective. 6 The following equation presents the ENPV of total premiums, D , for level annual premiums, d .
T is the term length in years, β is the discount factor, ρ t is the annual probability of death (with ρ 0 = 0 ). The equation merely discounts future premium payments and takes into account that annual premiums are only collected conditional on survival.
The next equation represents the expected net present value of costs, C , for a term life insurance policy with face value v .
Setting Equation ( 1 ) equal to Equation ( 2 ) and solving for the annual premium gives the following expression for term life insurance premiums
We use this simplified framework and resulting solution to get a sense of how large the premium response theoretically should be based on the expectation of a transitory shock to mortality risk. As inputs for 1‐year probability of death, we use general actuarial tables from the Social Security Administration. 7 Based on the simplified model, a 10 year $100,000 term policy for a 60‐year old male, with a 3% discount rate, would have an annual premium of $1550. 8 If life insurance companies anticipated a 10% increase in mortality risk for the first year of a policy, then the model implies a 0.9% increase in premiums (to $1564). Consequently, the 1‐year mortality shock elasticity of premiums is 0.09 for a 10‐year term policy for a 60‐year‐old male. As the term of the policy increases, the responsiveness to an increase in the 1‐year mortality risk lessens. For example, holding all the other above conditions constant, the elasticity is 1.00, 0.19, 0.06, and 0.04 for 1, 5, 15, and 20‐year policies, respectively. The elasticity is nearly identical for policies sold to different aged individuals.
If life insurance companies anticipated that the increased mortality risk would extend past the first year, then the responsiveness of term premiums significantly increases (e.g., 10‐year term policy with 2 years of increased mortality has an elasticity of premiums of 0.20). Furthermore, the elasticity of premiums increases as the discount rate increases, but are unchanged with different face values.
Overall, the premium response of life insurance companies is contingent on the projected increase in mortality rates, the anticipated persistence of the increased risk, the term length, and discounting.
Using statistics on actual deaths involving COVID‐19 and deaths from all causes as reported by the CDC, the mortality rate for individuals aged 15–34 increased by 2.8% while the mortality rate for individuals aged 55–74 increased by 10.6%. 9 Taken together with the elasticity, for a 10‐year term policy, the model predicts that premiums would increase by 0.95% for a 60‐year‐old and 0.25% for a 20‐year‐old. A back‐of‐the‐envelope calculation implies that an analysis comparing the old to the young would result in premiums differentially increasing by 0.70% for the old relative to the young.
We use data from Compulife to analyze the influence of the pandemic on life insurance premiums and offerings. 10 Compulife is a quotation software used by life insurance agents to compare premiums and generate quotes for potential customers. We use monthly data from January 2014 to February 2021 from 96 companies and 814,730 unique policies. 11 The Compulife database has information on at least one subsidiary for 19 out of the top 25 groups/companies in terms of market share. 12
In addition to company and policy names, these data provide information on the general characteristics of the policy, including term length, face value, smoking status, health category (Regular, Regular Plus, Preferred, and Preferred Plus), gender, and age at purchase. New purchasers of life insurance taper off drastically for individuals older than 60, with very few new policies initiated for individuals older than 70 (Harris & Yelowitz, 2014 ). Given the distribution of new purchasers, in the main analysis we specifically use data extracted on premiums and offerings for individuals from age 20 to 70 in 5‐year increments (i.e., 20, 25, …, 70). 13
Premiums vary both based on the company issuing the policy and policy characteristics. Nonetheless, the vast majority of premium variation is based on general characteristics. A model that regresses the general characteristics (indicators for age, health category, term, face value, etc.) on log premiums has an R 2 of 0.98 using our estimation sample. 14
In the main analysis, we restrict the data to common term lengths, including 10, 15, and 20‐year term policies, which reduces the total policy count to 610,880 policies. Of those unique policies, 402,943 policies started being offered and were removed in the sample window, with only 48,580 policies active for the entire 86 months. For the 216,364 policies that were offered in January 2014, 88% were active 1‐year later, with 70% still active after 2 years. By 2020 only 27% of those initially in the sample were offered on Compulife. Overall, the median duration of a policy being offered is 18 months.
Firms that actively change their policy offerings might be more likely to be responsive to changes in expected mortality resulting from the pandemic. To gauge the premium change activity level in a company, we analyze the frequency of premium adjustments from 2014 to 2019, before the pandemic. We analyze policies that are offered for more than 13 months to capture annual price adjustments. As our metric for premium change activity, we use the proportion of policies that experienced a premium change over a year. The median company altered 13.5% of their policies' premiums with an average of 27.8%. We define a company as active if they are in the upper quartile of premium change activity ( > 44.4%).
In addition to actively changing premiums, a company might respond to variations in the market or risk by no longer offering a particular policy and potentially replacing it with a new one. Hendren ( 2013 ) notes that in some contexts, including life insurance, companies choose not to sell insurance to potential customers who have certain observable, often high‐risk characteristics. Such rejections, potentially withdrawing policies in our context, can be explained by private information, where premium adjustments are not sustainable. To measure activity on this extensive margin, we aggregate the number of times a company changes a policy offering (i.e., add or drop a policy) annually. We then take the ratio of the median annual changes to the median number of policies offered. 15 Over a year, the median company altered the equivalent of 14.6% of their median policies offerings. We define a company as being active in offerings if the company is in the top quartile of alterations (greater than 49.5%). 16
4. EMPIRICAL ANALYSES
4.1. premium adjustments.
Before estimating a formal regression, we first plot changes in premiums over time. To do this, we first create an index to normalize premiums such that modifications can be viewed as percent changes. The premium index for policy i in time t is given by:
where t 0 is the premium in the first month of the estimation sample. We then get a measure of premiums at the company level ( P r e m i u m _ I n d e x j t ) by averaging the premium index ( P r e m i u m _ I n d e x i t ) across all policies within a company. The main reason to use the average index rather than the average of premiums is that policies with larger premiums (e.g., higher face values) would implicitly receive more weight in an average of the premiums. A change in the index represents the average percent change in premiums rather than the change in dollar amounts.
Figure 1a plots the average of the company premium indexes for a balanced panel of policies offered from January 2019 until February 2021. 17 The figure shows minimal changes on average premiums from January 2019 to February 2021. There is a small overall increase in the average premium index in 2020 and the beginning of 2021, which would be consistent with a premium response to COVID‐19. Nonetheless, one of the most substantial increases in the index occurs from November to December 2019, which predates meaningful news of the pandemic. 18

Average company premium changes. The figure presents the average company premium index from January 2019 to July 2020 originating from a balanced panel of 10, 15, and 20‐year term policies that were continuously offered from July 2019 until February 2021. Low Risk is defined as individuals in the worst health category (Regular) that smoke and High Risk is defined as individuals in the best health categories (Preferred and Preferred Plus) that do not smoke [Color figure can be viewed at wileyonlinelibrary.com ]
To better understand any change in premium in 2020 and 2021, we investigate further and narrow the sample window to December 2019 to February 2021. Of the 74 companies that continuously offered policies for the shorter window, 49 did not change premiums and only 15 had higher premium indexes in February 2021 relative to December 2019. Table 1 reports the changes for companies that altered premiums from December 2019 to February 2021. As shown, the increase in premiums is attributed mainly to the premium changes of 5 out of 74 companies analyzed for the table.
Premium changes by company (Index = 100 in December 2019)
Note : The sample used to generate the table includes policies that were continuously active from December 2019 to February 2021 with premiums normalized to 100 in December 2019. We report the average of these indexed premiums across companies. We only present the 24 companies that changed their premiums out of 74 companies analyzed. The table is sorted descending based on the February 2021 premium index.
Theory would suggest that life insurance companies would be most likely to adjust premiums for the demographic that experienced the greatest change in mortality risk and that companies would be less likely to increase premiums for demographics that do not experience significant changes in mortality risk. With this in mind, Figure 1b plots the change in premiums for the oldest individuals in the main sample compared to younger individuals whose mortality is less affected by the virus. Mortality rate changes from COVID‐19 gradually increase (at an increasing rate) with age. Consequently, we exclude policies offered to individuals aged 40 to 55 in these figures as well as the regression analysis to compare individuals that experienced modest changes in mortality risk to individuals that experienced much greater increases from COVID‐19. 19 As shown in the figure, the increase in premiums in 2020 occurred more for younger individuals than the older individuals, inconsistent with a COVID‐19 explanation.
In addition to age, COVID‐19 disproportionately increases the risk for individuals who have pre‐existing conditions or smoke. Figure 1c compares the change in premiums for older individuals in the worst health category (Regular) that smoke to younger individuals in the best health categories (Preferred and Preferred Plus) that do not smoke. As shown, the premiums for high‐risk older consumers do not experience any meaningful change from January to September 2020, whereas there is a slight increase in the early months of 2020 for low‐risk younger policies. The early months of 2021 show an increase in premiums for high‐risk policies with a slight decrease in premiums for low‐risk policies. Nonetheless, these figures do not provide any compelling evidence of changes in premiums caused by COVID‐19.
Our formal empirical strategy relies on the assumption that COVID‐19‐induced changes to the stock and bond markets influenced the profitability of policies sold to both younger and older individuals. Under this assumption, our empirical specification differences out the shock to financial assets by using policies offered to younger individuals as controls for those offered to older consumers. We hypothesize that any differential changes in premiums would be the result of differences in age‐specific mortality risk. The following equation illustrates the event study specification we use to estimate the impact of COVID‐19 on life insurance premiums.
where log ( P r e m i u m i t ) is the log of annual premiums for policy i in month t and O l d e r i is an indicator for policies offered to individuals aged 60, 65, or 70. 1 [ s = t ] is an variable equal one if s = t and zero otherwise for s ∈ [ Jan 2019 , Feb 2021 ] . α i and γ t , respectively, represent policy and month/year fixed effects. The coefficients of interest are β s , which represent the monthly treatment effects relative to the base month of December 2019 (directly before the earliest information about COVID‐19). If the monthly treatment effects are positive for months in 2020 or 2021, then there is evidence that life insurance companies differentially increased premiums for the old relative to the young. Any statistical significance for months in 2019 would indicate potential violations of the parallel trends assumption. Standard errors are clustered at the company level to account for coordinated pricing strategies inside a company.
Figure 2 illustrates the finding from the main event study. As shown, there is no evidence of differential increases in premiums for the old relative to the young. Also, the point estimates before COVID are statistically insignificant, which provides evidence supporting the parallel trends assumption.

Event study, influence of COVID on log premiums. The unbalanced panel includes 10, 15, and 20‐year term policies listed on Compulife from January 2019 to February 2021 that were offered to individuals aged 20, 25, 30, 35, 60, 65, and 70. There were 57,161 unique policies from 32 companies and 755,904 total observations. Controls included policy and month fixed effects and standard errors were clustered at the company level [Color figure can be viewed at wileyonlinelibrary.com ]
Table 2 reports the findings of several subsample analyses, with the main dependent variables being interactions of months in 2020 and 2021 with an indicator for the policy being sold to older individuals (age 60–70). 20 Specifically, we analyze companies that have the largest market shares, companies that are the most active in terms of modifying premiums and offerings, and those with different AM Best ratings. Across each of these subgroups, there is not a statistically significant increase in premiums for the old relative to the young. (Companies that actively changed premiums actually marginally decreased prices for the old relative to the young in March.) The latter two columns of Table 2 , use additional variation in health status that theoretically could differentially increase premiums. For both of these specifications, we use policies sold to younger non‐smokers in the best health categories as the control group. For the treatment group, we use older individuals in the worst health category and older individuals in the worst health category that also smoke for the treatment groups, respectively. The main statistically significant premium effect is observed from October 2020 to February 2021, ranging from 0.9% to 1.4% for the specification that uses policies sold to relatively unhealthy older smokers as the treatment group. These results indicate that life insurance companies differentially increased premiums for individuals with very high risk (worst health older smokers), whereas premiums in general remained unaffected.
Premium response to COVID‐19: dependent variable log premiums
Note : The unbalanced panel includes 10, 15, and 20‐year term policies listed on Compulife from January 2019 to February 2021 that were offered to individuals aged 20, 25, 30, 35, 60, 65, and 70. Top Share refers to companies listed in the top 25 for market share (or whose parent company is listed) by National Association of Insurance Commissioners. The top modifier of premiums and offerings indicate companies in the top quartile of activity respectively based on changes from 2014 to 2018. AM Best Ratings are indicators of a companies ability to meet financial obligations. The second to last column includes policies sold to younger individuals (age 20–35) who do not smoke in the best health categories and older individuals in the worst health category. The last column further restrict the policies sold to older individuals to those that smoke. Policy and month fixed effects were included but not reported here. Standard errors are clustered at the company level and are shown in parentheses.
The theoretical model predicts that shorter‐term policies should, all else equal, have larger responses to temporary mortality shocks. Also, it is reasonable to assume that companies might be more sensitive regarding policies with higher face values as they represent larger potential losses per policy. Consequently, we analyze and present policies stratified by term length and face value in Table 3 . Across each of the specifications, there is no evidence that COVID‐19 caused differential increases in premiums for the old relative to the young. Of the 140 event study coefficients presented in this table, virtually all are “wrong‐signed” (negative), with point estimates very close to zero (often changes of 0.4% or less), with standard errors that preclude the possibility of large premium increases.
Log premium response by term length and face value
Note : The unbalanced panel includes 10, 15, and 20‐year term policies listed on Compulife from January 2019 to February 2021 that were offered to individuals aged 20, 25, 30, 35, 60, 65, and 70. Policy and month fixed effects were included but not reported here. Standard errors are clustered at the company level and are shown in parentheses.
One possible explanation for the lack of response in premiums from the pandemic is that market competition prevents companies from increasing premiums. Conditional on policy characteristics (face value, term length, etc.) and applicant risk (age, sex, health, etc.), life insurance very much resembles a commodity, where consumers are likely to be extremely sensitive to price. To explore this possibility, we analyze if there are differential responses by low‐price leaders and other market participants. We restrict the sample to companies with either A++ or A+ AM Best Ratings to decrease the chances that differences in premiums result from different likelihoods of default. Within a particular product type (e.g., 15‐year term, age 30, male, nonsmoker, preferred health), the median difference between the lowest price and the second‐lowest price is 1.2% (mean of 5.2%), with 22.6% of unique product types having at least two firms that offer the lowest price. If the low‐price leader increased premiums such that their product was no longer the cheapest, they could lose a considerable share of price‐sensitive customers. Alternatively, it could be argued that firms with the low price advantage have a small amount of latitude to increase their premiums without as much concern for losing customers.
Products that do not have the low price advantage and compete across different dimensions (e.g., customer service, additional riders, etc.) might have more flexibility to increase their prices in response to the pandemic. Alternatively, those without a price advantage might be unwilling to increase their price as there already exists cheaper options for their potential customers.
To analyze if life insurance policies with the low price behave differently, we estimate regression models that limit the sample based on the product's price ordering in December 2019, immediately before the first news of COVID‐19. Table 4 presents results first for policies that did not offer the lowest premium within a given product type and shows no statistically significant response. The second column reports results for analysis using only policies that offer the lowest within product premium. For this subset of policies, there is a statistically significant increase in premiums for policies offered to an older group relative to policies offered to younger individuals, with point estimates implying that annual premiums raised by 0.2 to 1.1% between March 2020 and February 2021. The last column further restricts the sample to policies that had the low price advantage, with the next lowest premium being at least 1% more expensive. Consistent with the gap allowing for more adjustments, the premium for policies offered to older individuals increased more relative to changes in premiums offered to younger individuals with the highest monthly effect of a 1.6% relative increase in premiums. In both of the lowest price specifications, there were not statistically significant responses in the early months of 2020 consistent with the timing of information on COVID‐19 and its likely effects on US residents.
Price competition: Dependent variable log premiums
Note : The unbalanced panel includes 10, 15, and 20‐year term policies listed on Compulife from January 2019 to February 2021 that were offered to individuals aged 20, 25, 30, 35, 60, 65, and 70. The sample is restricted to companies with A++ or A+ AM Best Ratings. Premium comparisons in December 2019 were used to determine if the policy was the low price leader or not. Policy and month fixed effects were included but not reported here. Standard errors are clustered at the company level and are shown in parentheses.
Overall, the results imply that the premium response was minimal due to COVID‐19, with some evidence that price competition inhibited life insurance companies from adjusting their premiums in response to increased mortality risk.
4.2. Policy offerings
Rather than changing premiums for a policy that became riskier due to COVID‐19, companies could have responded by not offering the policy to new customers. There do not appear to be any regulatory hurdles to discontinue offering a policy to new customers. Given the looming uncertainty surrounding COVID‐19 that makes products difficult to price, this option might be attractive for policies targeting demographics at a higher risk of death from COVID‐19.
In an innovative study, Hendren ( 2013 ) explores circumstances under which insurance companies reject applicants rather than adjusting premiums. In our context, with the changing mortality risk with COVID‐19 in 2020 and 2021 relative to earlier years, withdrawing life insurance offerings for applicants with observable characteristics such as older age or poorer health is creating a new “rejection” group. Hendren argues that private information known by potential applicants—beyond what can be captured by their observable characteristics—has a key role in such rejections. Much like Hendren's motivation with long‐term care insurance, there are specific factors and preferences related to the pandemic—such as the ability or willingness to be vigilant about COVID‐19 safety—that are difficult for an insurance company to obtain and verify.
Indeed, underwriting guidelines did change in recognition of the pandemic (BUA, 2020 ). Companies generally postponed offerings (e.g., created new rejection groups) based on a combination of age, health status, and face value. For example, AIG postponed the issuance of policies for those over age 75 and for those aged 66‐75 inclusive of “medical flat extras” (essentially risks that the standard rating tables do not cover).
We once again analyze 10, 15, and 20‐year term policies from January 2019 to February 2021. If a company either starts or stops listing policies on Compulife, we do not have data on any of their policy offerings. We hesitate to assume that all of the policies were discontinued if not listed on Compulife. Consequently, we restrict the analysis to the 78 companies that continuously listed policies on Compulife during the sample period. 21
Figure 3 plots the proportion of all policies that were active for each month based on age groups. As shown, there is a slight decrease in offerings for younger individuals over time, whereas there is a larger decrease in offerings to older individuals. Nonetheless, the decrease in net policies offered to older individuals begins prior to any news of COVID‐19.

Proportion of policies offered by month and age group. The figure shows the proportion of the 199,685 unique policies that were actively offered on Compulife for the 78 companies that continuously listed policies on the platform from January 2019 to February 2021 by age group [Color figure can be viewed at wileyonlinelibrary.com ]
To estimate the impact of COVID‐19 on offerings formally, we use an event study specification analogous to the model used to estimate the pandemic's influence on premiums.
Where A c t i v e i t is an indicator for a company offering policy i on Compulife at time t .
Figure 4a presents the results of the base specification. While the point estimates are statistically significant (at the 10% level) and negative in 2020, consistent with a COVID‐19 effect, there is also evidence of a pretrend, which violates a main assumption of the analysis. To control for this pretrend, we first estimate a model with A c t i v e as the dependent variable and age‐group fixed effects (i.e., older and younger) and age‐group by month trends using data from the preperiod, January 2019 to December 2019 (Kleven et al., 2014 ). We generate residuals for the full time period using the estimated coefficients and then use these residuals as the dependent variable for the event study presented in Figure 4b . 22 As shown, after controlling for linear pretrends, there is no statistically significant evidence of differential policy removal for the old relative to the young due to the pandemic.

Event study, influence of COVID on offerings. The sample includes 10, 15, and 20‐year term policies for individuals aged 20, 25, 30, 35, 60, 65, 70 that appeared at least once from January 2019 to February 2021. Only policies from companies that continuously listed policies on Compulife during the sample period were included. There were 199,685 unique policies from 78 companies for a total of 5,191,810 observations. Controls included policy and month fixed effects and standard errors were clustered at the company level. In panel (a), the dependent variable is an indicator for the policy actively being offered in a given month. In panel (b), the dependent variable is the residuals that result from an estimation of an indicator of policy offering on age‐group (i.e., younger and older) fixed effects and age‐group by date linear trends on the pretreatment period (2019) [Color figure can be viewed at wileyonlinelibrary.com ]
Table 5 presents the results for several different subsets, with each specification accounting for a linear pretrend as described above. In the table, there is no evidence of differential decreases in offerings for the elderly. The only statistically significant results indicate that, if anything, offerings to the oldest individuals actually increased for top share companies and those that adjusted offerings frequently.
Subsample analysis: The influence of COVID‐19 on policy offerings
Note : As the dependent variable, the specifications use the residuals that result from an estimation of an indicator of policy offering on age‐group (i.e., younger and older) fixed effects and age‐group by date linear trends on the pretreatment period. The sample includes 10, 15, and 20‐year term policies for individuals aged 20, 25, 30, 35, 60, 65, 70 that appeared at least once from January 2019 to February 2021. Only policies from companies that continuously listed policies on Compulife during the sample period were included. Policy and month fixed effects were included but not reported here. Standard errors are clustered at the company level and are shown in parentheses.
Table 6 further analyzes changes in offerings based on term length and face value. Stratifying by term length does not change the main findings. However, there is some evidence that companies differentially decreased policy offerings for the largest face value policies ( ≥ $1 million). For $1 million term policies, offerings decreased for the elderly by 1.6, 1.7, and 3.0 percentage points, respectively for May, June, and July 2020. This decrease in the largest face value policies could represent increased scrutiny of policies that could represent the biggest losses for a company. Alternatively, the statistically significant result could merely be the product of random chance given the large number of reported estimates.
Policy offering analysis by term and face value
Overall, the offerings analysis indicates that the pandemic did not cause widespread adjustments to offerings.
4.3. The New York market
In the main analysis, we use quotes from the entire United States. In general, companies offer the same life insurance policy to residents of all or almost all states, limiting analysis that uses geographic variation in the pandemic's severity. New York, however, presents an interesting case study as it was harder‐hit early on by the pandemic and also because the life insurance regulatory structure leads to subsidiaries being created specifically to offer policies to residents of New York (Pottier & Sommer, 1998 ).
We analyze policies offered in New York but not in New Mexico to isolate policies that have a greater potential to respond to the early effects of COVID‐19 specific to New York. 23 Table 7 presents the results for both changes in premiums and offerings. 24 As illustrated, life insurance companies did not increase premiums or differentially discontinue policies offered to older individuals, consistent with the main analysis. These results indicate that companies did not significantly alter premiums or offering even in localities that were impacted the most early on in the pandemic.
Influence of COVID‐19 on New York policies
Note : The sample is restricted to policies that were offered in New York but not in New Mexico. The panel includes 10, 15, and 20‐year term policies listed on Compulife from April 2019 to February 2021 that were offered to individuals aged 20, 25, 30, 35, 60, 65, and 70. Only policies from companies that continuously listed policies on Compulife during the sample period were included in the policy offered specification. As the dependent variable for the offering specification, we use the residuals that result from an estimation of an indicator of policy offering on age‐group (i.e., younger and older) fixed effects and age‐group by date linear trends on the pretreatment period. Standard errors are clustered at the company level and are shown in parentheses.
4.4. Policies listed for the oldest of the old
The main sample includes policies sold to individuals between the ages of 20 and 70, which captures the vast majority of commonly purchased policies (Harris & Yelowitz, 2014 ). In this section, we analyze the response for policies sold to individuals aged 75, 80, and 85, who experience the greatest increase in mortality risk due to COVID‐19. Given the large mortality risk, both the number of policies and companies offering policies are comparatively small for these advanced ages. 25
For analysis of premium changes for policies sold to individuals aged 75 through 85 compared to policies sold to younger individuals (aged 20–35), we find no significant response to COVID. However, when we analyze policy offerings, we find that offerings significantly decreased for the oldest age group starting in May 2020, as shown in Figure 5 . In contrast to the null result for the main specification, this oldest group experienced significant decreases with point estimates, implying that the oldest policies offerings differentially decreased in July by 15.5 percentage points for the sample using 10, 15, and 20‐year term policy and by 13.4 percentage points for 1, 5, and 10‐year policies (more typical term lengths for the oldest age groups). These large results indicate that companies decreased offerings to the most vulnerable, but it likely influenced a small minority of atypical purchasers.

Event study, influence of COVID on offerings for age 75+. The sample includes term policies for individuals aged 20, 25, 30, 35, 75, 80, and 85 that appeared at least once from January 2019 to February 2021. Only policies from companies that continuously listed policies on Compulife during the sample period were included. There were 139,200 unique policies from 80 companies for a total of 3,619,200 observations for the specification presented in panel (a). There were 60,077 unique policies from 78 companies for a total of 1,562,002 observations for the specification presented in panel (b). Controls included policy and month fixed effects and standard errors were clustered at the company level. The dependent variable is an indicator for the policy actively being offered in a given month [Color figure can be viewed at wileyonlinelibrary.com ]
5. CONCLUSION
The coronavirus pandemic has created unparalleled short‐run disruption across virtually every segment of society, both in the United States and elsewhere. If the earliest predictions of the increase in mortality rate from COVID‐19 would have come to fruition, then theory suggests that life insurance companies would have been forced to significantly adjust life insurance premiums or offerings to account for the increased risk. Our findings—from an analysis starting with nearly 100 companies and over 800,000 policies—suggest minimal observable adjustments through February 2021. Nonetheless, we do find evidence that low price leaders raised premiums in response to increased mortality risk, premiums were raised for unhealthy older smokers, and policies offered to individuals age 75 and above were differentially removed from the market. Overall, these results are consistent with a combination of market competition in life insurance and anticipation of meaningful precautionary behavior to contain the spread among the vulnerable, leading to only modest short‐run increases in mortality risk.
Our expectation is that market‐driven adjustments in the life insurance industry represent some of the most informed expectations on the path of the pandemic. Nonetheless, our findings of relatively small adjustments in the term life insurance market—perhaps unexpected—should not be interpreted as dismissing the individual risk from COVID‐19, especially for more vulnerable members of society. Although there are a host of reasons that could explain our results, the most likely one is that as of early March 2021, there have been approximately 28.8 million confirmed cases and 523,850 fatalities in the United States, in a country with 330 million individuals and 2.8 million annual deaths. 26 The most dire mortality forecasts—using either the CFR or IFR—rely on much larger percentages of the population being infected.
Supporting information
Supplementary Information
ACKNOWLEDGEMENTS
We thank the senior editor and two anonymous referees for helpful comments and Compulife for generously providing life insurance pricing data.
Harris, T. F. , Yelowitz, A. , & Courtemanche, C. (2021). Did COVID‐19 change life insurance offerings? J Risk Insur . 88 , 831–861. 10.1111/jori.12344 [ PMC free article ] [ PubMed ] [ CrossRef ] [ Google Scholar ]
1 There also appear to be stark COVID‐19 disparities in the United States by race and ethnicity (Benitez et al., 2020 ; Selden & Berdahl, 2020 ), where the causes are only partially explained by current economic, health, and transmission factors.
2 That said, some other possible health consequences arising from the spread of the virus include reduced economic activity, less pollution, fewer car accidents, more drug use, more domestic violence, and less capacity in the health care system for non‐COVID‐19 issues, some of which certainly affects younger and healthier groups. Almond ( 2006 ) shows long‐lasting consequences from the 1918 influenza pandemic. In addition, some of the precautions that individuals take against COVID‐19 might also reduce the harm from other illnesses like influenza (Luhnow & Uribe, 2020 ; Olsen et al., 2020 ).
3 Early in the pandemic, life insurance companies rapidly changed their underwriting guidelines, postponing issuance of life insurance for applicants with a COVID‐19 diagnosis or exposure. For example, as of April 2020, AIG would only consider applicants 30 days after a full recovery from COVID‐19 and a return to previous health status (BUA, 2020 ). See https://www.buaweb.com/news/AIG-underwriting-updates.html .
4 https://www.iii.org/fact-statistic/facts-statistics-life-insurance
5 “Premiums for life insurance and annuity products generally are not subject to regulatory approval, although regulators may seek to ensure that policy benefits are commensurate with the premiums charged.” See https://www.naic.org/documents/consumer_state_reg_brief.pdf .
6 See Mao et al. ( 2004 ), for an example of a more sophisticated life insurance pricing model.
7 See https://www.ssa.gov/oact/STATS/table4c6.html .
8 An annual premium of $1550 is higher than premiums offered for 60‐year old males listed on Compulife, except for individuals in “regular” health who are smokers. This difference in premiums is likely a result of life insurance companies not offering policies to individuals in “poor” and “very poor” health who greatly influence mortality rates for the general population captured in the Social Security actuarial tables.
9 See https://www.cdc.gov/nchs/nvss/vsrr/covid_weekly/index.htm#AgeAndSex . Accessed September 4, 2020.
10 See Cawley and Philipson ( 1999 ), Hendel and Lizzeri ( 2003 ), Cox et al. ( 2013 ), and Koijen and Yogo ( 2015 ) for additional studies that conduct analysis using Compulife data.
11 To enhance the data quality, we make some minor corrections and remove outliers as outlined in Appendix A.
12 The National Association of Insurance Commissioners reports on the top groups/companies in terms of market share for life insurance in 2019. See https://www.naic.org/documents/web_market_share_life_fraternal.pdf?81 . Compulife data do not contain information on Dai‐Ichi Life Holdings Inc Group, Globe Life Inc Group, Metropolitan Group, Penn Mutual Group, State Farm Group, or Voya Financial Group during the main analysis period. The report on market share does not differentiate based on product type, which explains the omission of, for example, Voya Financial group as it sells employer‐sponsored life insurance but does not sell individual term life insurance.
13 Policies offered for common term lengths (e.g., 10, 15, and 20 years) dwindle significantly for policies offered to individuals older than 70, with only 1‐year term policies available for 85‐year‐old individuals.
14 A model that excludes the indicator for smoking has an R‐squared of 0.93, and a model that excludes health status has an R‐squared of 0.97. The R 2 is insensitive to the exclusion of observations from 2020.
15 We do not count initial inclusion or exit from Compulife as changes as we do not know if the policies were already in effect or if they continue after the company stopped listing with Compulife. If a company is only listed for a portion of a year, we scale the changes assuming the observed months are typical of the year.
16 There is a weakly positive correlation between the two activity metrics (0.15) indicating some companies that both actively adjust premiums and actively alter offerings, with the majority of active companies primarily focusing on one or the other.
17 For this balanced panel, there are 62 companies and 103,161 policies.
18 Premiums for a given month are released the first of the month. Consequently, changes in premiums in December 2019 would predate the earliest news of COVID‐19.
19 The main empirical findings are not sensitive to this exclusion with only marginal changes to the point estimates. See Appendix Table A1.
20 Similar results are found if we estimate a full event study with interactions for the months in 2019 as well.
21 We also require that a policy be offered at least once in the sample period.
22 We use this method rather than directly including a group by month trend in the specification, as the group by year trend could absorb any potential treatment effect, thus attenuating any result.
23 We selected New Mexico because it is the default state in the Compulife software. Nonetheless, there is significant overlap in policies offered across the United States due in part to the Interstate Insurance Compact. Only a handful of states—including New York—do not participate in the compact. See https://www.insurancecompact.org/about.htm for more information.
24 The table reports point estimates for analysis starting in March 2019 rather than January 2019 as in the main analysis due to an insufficient number of companies in the offering specification to cluster at the company level and estimate all of the coefficients.
25 Appendix Figure A2 illustrates the distribution of policies and the number of companies that offer policies by age.
26 See https://covid.cdc.gov/covid-data-tracker/#datatracker-home .
- Abbott, B. , & Douglas, J. (2020). How deadly is COVID‐19? Researchers are getting closer to an answer . The Wall Street Journal . https://www.wsj.com/articles/how-deadly-is-covid-19-researchers-are-getting-closer-to-an-answer-11595323801?mod=hp_lead_pos5 [ Google Scholar ]
- Almond, D. (2006). Is the 1918 Influenza pandemic over? Long‐term effects of in utero influenza exposure in the post‐1940 Us population . Journal of Political Economy , 114 ( 4 ), 672–712. [ Google Scholar ]
- Augenblick, N. , Kolstad, J. T. , Obermeyer, Z. , & Wang, A. (2020). Group testing in a pandemic: The role of frequent testing, correlated risk, and machine learning . National Bureau of Economic Research. [ Google Scholar ]
- Beigel, J. H. , Tomashek, K. M. , Dodd, L. E. , Mehta, A. K. , Zingman, B. S. , Kalil, A. C. , Hohmann, E. , Chu, H. Y. , Luetkemeyer, A. , Kline, S. , de Castilla, D. L. , Finberg, R. W. , Dierberg, K. , Tapson, V. , Hsieh, L. , Patterson, T. F. , Paredes, R. , Sweeney, D. A. , Short, W. R. … Lane, H. C. (2020). Remdesivir for the treatment of Covid‐19 preliminary report . New England Journal of Medicine , 383 , 1813–1826. [ PMC free article ] [ PubMed ] [ Google Scholar ]
- Benitez, J. , Courtemanche, C. , & Yelowitz, A. (2020). Racial and ethnic disparities in COVID‐19: Evidence from six large cities . Journal of Economics, Race, and Policy , 3 ( 4 ), 243–261. [ PMC free article ] [ PubMed ] [ Google Scholar ]
- Brown, J. R. , & Goolsbee, A. (2002). Does the internet make markets more competitive? Evidence from the life insurance industry . Journal of Political Economy , 110 ( 3 ), 481–507. [ Google Scholar ]
- BUA . (2020). COVID‐19 life insurance carrier updates. https://www.buaweb.com/pages/COVID-19-Life-Updates.html
- Cawley, J. , & Philipson, T. (1999). An empirical examination of information barriers to trade in insurance . American Economic Review , 89 ( 4 ), 827–846. [ Google Scholar ]
- CDC, U.S. Centers for Disease Control, and Prevention . (2020). People who are at higher risk for severe illness ∣ CDC. @CDCgov. https://www.cdc.gov/coronavirus/2019-ncov/need-extra-precautions/people-at-higher-risk.html
- Corum, J. , Grady, D. , Wee, S.‐L. , & Zimmer, C. (2020). Coronavirus vaccine tracker . The New York Times . [ Google Scholar ]
- Courtemanche, C. , Garuccio, J. , Le, A. , Pinkston, J. , & Yelowitz, A. (2020). Strong social distancing measures in the United States reduced the Covid‐19 growth rate: Study evaluates the impact of social distancing measures on the growth rate of confirmed Covid‐19 cases across the United States . Health Affairs , 39 ( 7 ), 1237–1246. [ PubMed ] [ Google Scholar ]
- Cox, S. H. , Lin, Y. , Tian, R. , & Zuluaga, L. F. (2013). Mortality portfolio risk management . Journal of Risk and Insurance , 80 ( 4 ), 853–890. [ Google Scholar ]
- Ferguson, N. , Laydon, D. , Gilani, G. N. , Imai, N. , Ainslie, K. , Baguelin, M. , Bhatia, S. , Boonyasiri, A. , Cucunubá, Z. , Cuomo‐Dannenburg, G. , Dighe, A. , Dorigatti, I. , Fu, H. , Gaythorpe, K. , Green, W. , Hamlet, A. , Hinsley, W. , Okell, L. C. , van Elsland, S. , … Ghani, C. (2020). Report 9: Impact of non‐pharmaceutical interventions (NPIs) to reduce covid19 mortality and healthcare demand.
- Friedson, A. I. , McNichols, D. , Sabia, J. J. , & Dave, D. (2020). Did Californias shelter in place order work? Early evidence on coronavirus‐related health benefits . National Bureau of Economic Research . 10.3386/w26992. http://www.nber.org/papers/w26992 [ CrossRef ] [ Google Scholar ]
- Handel, B. , Hendel, I. , & Whinston, M. D. (2015). Equilibria in health exchanges: Adverse selection versus reclassification risk . Econometrica , 83 ( 4 ), 1261–1313. [ Google Scholar ]
- Harris, T. F. , & Yelowitz, A. (2014). Is there adverse selection in the life insurance market? Evidence from a representative sample of purchasers . Economics Letters , 124 ( 3 ), 520–522. [ Google Scholar ]
- Harris, T. F. , & Yelowitz, A. (2017). Nudging life insurance holdings in the workplace . Economic Inquiry , 55 ( 2 ), 951–981. [ Google Scholar ]
- Harris, T. F , & Yelowitz, A. (2018). Life insurance holdings and well‐being of surviving spouses . Contemporary Economic Policy , 36 ( 3 ), 526–538. [ Google Scholar ]
- Hartwig, R. , Niehaus, G. , & Qiu, J. (2020). Insurance for economic losses caused by pandemics . Geneva Risk and Insurance Review , 45 ( 2 ), 134–170. [ PMC free article ] [ PubMed ] [ Google Scholar ]
- He, D. (2009). The life insurance market: Asymmetric information revisited . Journal of Public Economics , 93 ( 9‐10 ), 1090–1097. [ Google Scholar ]
- He, D. (2011). Is there dynamic adverse selection in the life insurance market? Economics Letters , 112 ( 1 ), 113–115. [ Google Scholar ]
- Hedengren, D. , & Stratmann, T. (2016). Is there adverse selection in life insurance markets? Economic Inquiry , 54 ( 1 ), 450–463. [ Google Scholar ]
- Hendel, I. , & Lizzeri, A. (2003). The role of commitment in dynamic contracts: Evidence from life insurance . Quarterly Journal of Economics , 118 ( 1 ), 299–328. [ Google Scholar ]
- Hendren, N. (2013). Private information and insurance rejections . Econometrica , 81 ( 5 ), 1713–1762. [ PMC free article ] [ PubMed ] [ Google Scholar ]
- Hsiang, S. , Allen, D. , Annan‐Phan, S. , Bell, K. , Bolliger, I. , Chong, T. , Druckenmiller, H. , Huang, L. Y. , Hultgren, A. , Krasovich, E. , Lau, P. , Lee, J. , Rolf, E. , Tseng, J , & Wu, T. (2020). The effect of large‐scale anti‐contagion policies on the Covid‐19 pandemic . Nature , 584 , 262–267. [ PubMed ] [ Google Scholar ]
- Huynh, A. , Bruhn, A. , & Browne, B. (2013). A review of catastrophic risks for life insurers . Risk Management and Insurance Review , 16 ( 2 ), 233–266. [ Google Scholar ]
- Kleven, H. J. , Landais, C. , Saez, E. , & Schultz, E. (2014). Migration and wage effects of taxing top earners: evidence from the foreigners tax scheme in denmark . Quarterly Journal of Economics , 129 ( 1 ), 333–378. [ Google Scholar ]
- Koijen, R. S. J. , & Yogo, M. (2015). The cost of financial frictions for life insurers . American Economic Review , 105 ( 1 ), 445–75. [ Google Scholar ]
- Luhnow, D. , & Uribe, A. (2020). Covid‐19 measures have all but wiped out the flu in the southern hemisphere . The Wall Street Journal . https://www.wsj.com/articles/covid-19-measures-have-all-but-wiped-out-the-flu-in-the-southern-hemisphere-11595440682 [ Google Scholar ]
- Lyu, W. , & Wehby, G. L. (2020). Shelter‐in‐place orders reduced Covid‐19 mortality and reduced the rate of growth in hospitalizations: Study examine effects of shelter‐in‐places orders on daily growth rates of Covid‐19 deaths and hospitalizations using event study models . Health Affairs , 39 , 1615–1625. [ PubMed ] [ Google Scholar ]
- Mandavilli, A. (2020). Federal officials turn to a new testing strategy as infections surge. The New York Times . https://www.nytimes.com/2020/07/01/health/coronavirus-pooled-testing.html
- Mao, H. , Carson, J. M. , Ostaszewski, K. M. , & Shoucheng, L. (2004). Pricing life insurance: Combining economic, financial, and actuarial approaches . Journal of Insurance Issues , 27 , 134–159. [ Google Scholar ]
- Meyerowitz‐Katz, G. , & Merone, L. (2020). A systematic review and meta‐analysis of published research data on COVID‐19 infection‐fatality rates . International Journal of Infectious Diseases , 101 , 138–148. [ PMC free article ] [ PubMed ] [ Google Scholar ]
- NYC DOHMH . (2020). Preliminary estimate of excess mortality during the COVID‐19 outbreak–New York City, March 11‐May 2, 2020 . MMWR Morb Mortal Wkly Rep , 69 , 603–605. [ PubMed ] [ Google Scholar ]
- NYT . (2020). More than one‐third of U.S. coronavirus deaths are linked to nursing homes . The New York Times . https://www.nytimes.com/interactive/2020/us/coronavirus-nursing-homes.html [ Google Scholar ]
- Olsen, S. J. , Azziz‐Baumgartner, E. , Budd, A. P. , Brammer, L. , Sullivan, S. , Pineda, R. F. , Cohen, C. , & Fry, A. M. (2020). Decreased influenza activity during the COVID‐19 pandemic–United States, Australia, Chile, and South Africa, 2020 . American Journal of Transplantation , 20 ( 12 ), 3681–3685. [ PMC free article ] [ PubMed ] [ Google Scholar ]
- Pottier, S. W. , & Sommer, D. W. (1998). Regulatory stringency and New York licensed life insurers . Journal of Risk and Insurance , 65 , 485–502. [ Google Scholar ]
- Richter, A. , & Wilson, T. C. (2020). Covid‐19: Implications for insurer risk management and the insurability of pandemic risk . Geneva risk and insurance review , 45 ( 2 ), 171–199. [ PMC free article ] [ PubMed ] [ Google Scholar ]
- Rosenkranz, R. (2020). The measure of New York's coronavirus devastation. Wall Street Journal . https://www.wsj.com/articles/the-measure-of-new-yorks-coronavirus-devastation-11591140254 [ Google Scholar ]
- Scism, L. (2020). Less driving, fewer accidents: Car insurers give millions in coronavirus refunds . The Wall Street Journal . https://www.wsj.com/articles/car-insurer-american-family-gives-200-million-in-coronavirus-refunds-as-accidents-decline-11586175602 [ Google Scholar ]
- Selden, T. M. , & Berdahl, T. A. (2020). COVID‐19 and racial/ethnic disparities in health risk, employment, and household composition: Study examines potential explanations for racial‐ethnic disparities in Covid‐19 hospitalizations and mortality . Health Affairs , 39 , 1624–1632. [ PubMed ] [ Google Scholar ]
- SOA . (2007). Potential impact of pandemic influenza on the U.S. life insurance industry. Society of Actuaries. https://www.soa.org/globalassets/assets/files/research/projects/resrch-li-rep-pan-life.pdf
- Swiss RE . (2007). Pandemic influenza: A 21st century model for mortality shocks.
- Verity, R. , Okell, L. C. , Dorigatti, I. , Winskill, P. , Whittaker, C. , Imai, N. , Cuomo‐Dannenburg, G. , Thompson, H. , Walker, P. G. T. , Fu, H. , Dighe, A. , Griffin, J. T. , Baguelin, M. , Bhatia, S. , Boonyasiri, A. , Cori, A. , Cucunubá, Z. , FitzJohn, R. , Gaythorpe, K. … Ferguson, N. M. (2020). Estimates of the severity of coronavirus disease 2019: A model‐based analysis . The Lancet Infectious Diseases , 20 , 669–677. [ PMC free article ] [ PubMed ] [ Google Scholar ]
Journal of Advanced Research in Law and Economics
- Announcements
- AUTHOR'S GUIDELINES
Balance of Interests as a Principle of Civil Law: Some Aspects of Legal Consciousness
- Yury Alexandrovich SVIRIN Department Civil Procedural Law and Bailiff Organization Department, All-Russian State University of Justice, Moscow, Russian Federation
- Vladimir Viktorovich KULAKOV Department of Civil Law, Russian State University of Justice, Moscow, Russia Federation
- Alexandr Anatolievich MOKHOV Kutafin Moscow State Law University, Moscov, Russian Federation
- Sergej Nikolaevich SHESTOV Institute of Economics and Law, Academy of Labor and Social Relations, Sevastopol, Russian Federation
- Vladislav Petrovich SOROKIN Department of Civil Law and Process Ows, Academy of Labour and Social Relations, Moscow, Russian Federation
The research considers the category of reasonable balance of interests in the context of civil relations. The authors of the article highlight the need to restrict permissibility as a method of civil regulation aimed at protecting the rights and interests of the weaker party in some legal relations. A reasonable balance of interests is ensured by laws and agreements, whose conditions become the subject of a judicial dispute in the absence of a mandatory rule. The authors have analyzed judicial acts conditioned by the need to maintain a reasonable balance of interests. As a result, they have determined that the first condition for applying the fair balance principle is the equivalence of counter-performance in the absence of both excessive benefits and excessive losses for the parties. The second condition is the party-related division in some civil relations. The authors have concluded that the risks of negative consequences should not be borne only by the weaker party if the latter could not reasonably foresee the consequences upon concluding the relevant agreement. Methods. The study is based on the comparative analysis of the Russian scientific doctrine and judicial practice. The main approach to the analysis of the legal tools in question is the method of system analysis. In addition, the authors used the structural-functional method and general scientific methods of cognition. The study aims at determining the principle of a reasonable balance of interests in civil law, its essence, necessity and expediency in the system of law enforcement. The authors aim to define conditions for applying legal norms to achieve a reasonable balance of interests among all parties in disputed legal relations. Results. The study results let the authors claim that the risks of negative consequences should not be borne only by the weaker party if it could not reasonably foresee such consequences upon concluding the agreement and the imbalance of interests among parties in civil-legal relations is caused by the violation by one or another party of the principles of fair practice and reasonableness . Although a reasonable balance of interests is a counterweight to the principles of contractual freedom and free will, courts should apply it to ensure the right of justice.
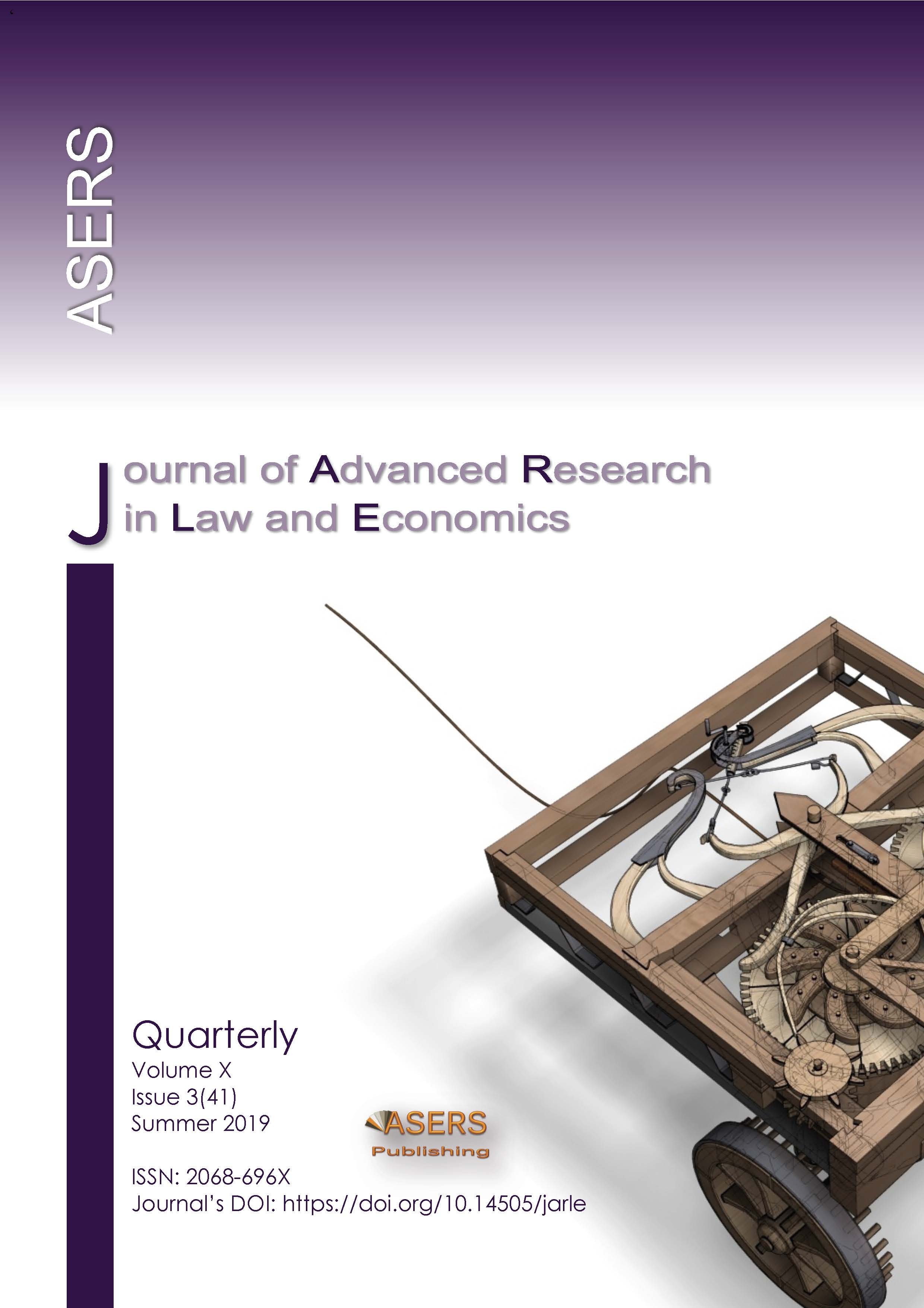
- EndNote - EndNote format (Macintosh & Windows)
- ProCite - RIS format (Macintosh & Windows)
- Reference Manager - RIS format (Windows only)
The Copyright Transfer Form to ASERS Publishing (The Publisher) This form refers to the manuscript, which an author(s) was accepted for publication and was signed by all the authors. The undersigned Author(s) of the above-mentioned Paper here transfer any and all copyright-rights in and to The Paper to The Publisher. The Author(s) warrants that The Paper is based on their original work and that the undersigned has the power and authority to make and execute this assignment. It is the author's responsibility to obtain written permission to quote material that has been previously published in any form. The Publisher recognizes the retained rights noted below and grants to the above authors and employers for whom the work performed royalty-free permission to reuse their materials below. Authors may reuse all or portions of the above Paper in other works, excepting the publication of the paper in the same form. Authors may reproduce or authorize others to reproduce the above Paper for the Author's personal use or for internal company use, provided that the source and The Publisher copyright notice are mentioned, that the copies are not used in any way that implies The Publisher endorsement of a product or service of an employer, and that the copies are not offered for sale as such. Authors are permitted to grant third party requests for reprinting, republishing or other types of reuse. The Authors may make limited distribution of all or portions of the above Paper prior to publication if they inform The Publisher of the nature and extent of such limited distribution prior there to. Authors retain all proprietary rights in any process, procedure, or article of manufacture described in The Paper. This agreement becomes null and void if and only if the above paper is not accepted and published by The Publisher, or is with drawn by the author(s) before acceptance by the Publisher.
- Come and join our team! become an author
- Soon, we launch the books app stay tune!
- Online support 24/7 +4077 033 6758
- Tell Friends and get $5 a small gift for you
- Privacy Policy
- Customer Service
- Refunds Politics
Mail to: [email protected]
Phone: +40754 027 417
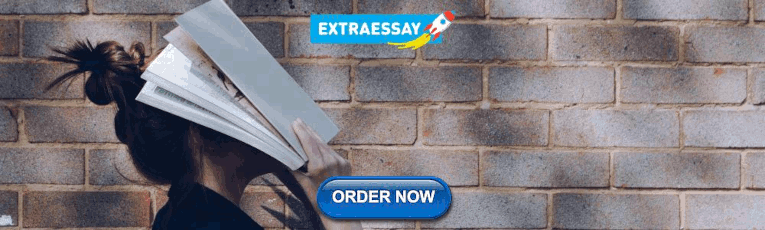
IMAGES
VIDEO
COMMENTS
Looking to the past, this paper reviews research published to-date on general insurance in the JFSM to establish a benchmark and explore theoretical contributions. Attention is then turned to the ...
The Journal of Risk and Insurance (JRI) is the premier outlet for theoretical and empirical research on the topics of insurance economics and risk management.Research in the JRI informs practice, policy-making, and regulation in insurance markets as well as corporate and household risk management. JRI is the flagship journal for the American Risk and Insurance Association, and is currently ...
Future Actuaries. Education & Exams. Professional Development. Research Institute. Professional Sections. Tools & Resources. About SOA. Risk management reports that are relevant to general insurance matters are available for download below.
Ms. Tnr. Kavitha, Dr. A. Latha and Ms. S.Jamuna in their research paper, "Customers' Attitude towards General Insurance - A Factor Analysis Approach" analysed the attitude of customers towards the insurance offered by general insurance companies where 750 policy holders opinion was collected on the basis of 5 point scaling.
The JFSM is a natural home for empirical papers on general insurance marketing. An online search of all available papers using common general insurance terms (that is, insurance, insurer, broker, claims and underwriting, and excluding papers on life insurance) identified just nine papers on general insurance in the JFSM.This equates to less than 3 per cent of all papers published.
2023. TLDR. This paper demonstrates the data-driven construction of an insurance pricing model when hierarchically structured risk factors, contract-specific as well as externally collected risk factors are available and improves the predictive performance and the risk differentiation in the workers' compensation insurance portfolio. Expand. 4.
Second Stage . General Insurance. The present study compares efficiency-related performance of 15 Indian general insurance companies using a two-stage efficiency evaluation model. Efficiency evaluation has been made for the span 2009-2010 to 2017-2018 using network DEA (data envelopment analysis).
The paper derives a formula to estimate the optimal trading strategy of a portfolio with a general insurance product and a life insurance product. Application of Predictive Modeling for the Local Government Property Insurance Fund—This report was sponsored by the General Insurance Research Committee and authored by Gee Lee and Edward Frees ...
Claims reserving by joint modelling. Actuarial Research Paper No. 72, Department of Actuarial Science and Statistics, City University, London. Google Scholar. Renshaw, A. E. ( 1994 b). On the second moment properties and the implementation of certain GLIM based stochastic claims reserving models.
The general insurance industry in India has a rich heritage. Modern form of general insurance business was established in 1850. India had more than hundred general insurance companies when the industry was nationalised in 1973, which led to the formation of 4 state-sponsored general insurance companies through the amalgamation of 107 private general insurance companies.
General insurance penetration has not shown much change over the years. For developed countries the average General insurance penetration for 2008 was 3.40 while that of developing nations was just 2.90. Also, there have been no major changes in these values since 2001. Among developing countries, South Africa and Taiwan are fast gaining ...
There are 15 general insurance companies after the segregation regulation while three companies still operating as composite insurers,accounting 55.3 billion rupees of growth written premium and industry has grown by 3.66% in general insurance in 2014.Insurance Board of Sri Lanka has planned to strengthen the non viable insurance companies by ...
This paper is largely devoted to aspects of the pricing of property reinsurance, namely experience rating, exposure rating and the specific topics of reinstatements and annual aggregate limits, aggregate annual deductibles and no-claim bonuses. 11.4. NAIC Review Team. Lloyd's: a review by US state insurance regulators.
1.The Highest Average Operating Expenses Ratio of Hdfc Ergo General Insurance Company Shown 38.73%. and the lowest Average Operating Expenses Ratio of Bajaj Allianz General Insurance company shown 27.71%. 2.Combined Ratio of Cholamandalam General Insurance company show72.97 %. 3.The Highest Average Return on Capital Employed of Bajaj Allianz ...
insurance and other financial liabilities. 5 Actuarial Models and Applications ... Applying this talent to the general insurance business will lead to a stronger industry. Title: IAA Overview for SOA Board Author: Stuart Wason Created Date: 12/21/2009 1:08:47 PM ...
Table 2 provides insight into the performance of health insurance sector in India. The growth of health insurance in India has been from Rs.1909 crores for the financial year 2006-2007 to Rs. 33011crores for the financial year 2018-2019. The growth percentage is 1629% i.e. growing at an average rate of 135% per annum.
As inputs for 1‐year probability of death, we use general actuarial tables from the Social Security Administration. 7 Based on the simplified model, a 10 year $100,000 term policy for a 60‐year old male, with a 3% discount rate, would have an annual premium of $1550. 8 If life insurance companies anticipated a 10% increase in mortality risk ...
political authority of the backing monetary sovereign through deposit insurance, discount window access, lender of last resort support and a general commitment to maintain at-par convertibility between fiat currency and bank deposits. Thus, as Hockett and Omarova have ... (2016), Bank of England Staff Working Paper No. 605, 15, https://papers ...
The research considers the category of reasonable balance of interests in the context of civil relations. The authors of the article highlight the need to restrict permissibility as a method of civil regulation aimed at protecting the rights and interests of the weaker party in some legal relations. A reasonable balance of interests is ensured by laws and agreements, whose conditions become ...
General Directorate 27 Feb, 2013, 11:24 ET. Share this article. Share to X. Share this article. Share to X. MOSCOW, February 27, 2013 /PRNewswire/ --