From David E. Gray \(2014\). Doing Research in the Real World \(3rd ed.\) London, UK: Sage.
- Experimental Research Designs: Types, Examples & Methods

Experimental research is the most familiar type of research design for individuals in the physical sciences and a host of other fields. This is mainly because experimental research is a classical scientific experiment, similar to those performed in high school science classes.
Imagine taking 2 samples of the same plant and exposing one of them to sunlight, while the other is kept away from sunlight. Let the plant exposed to sunlight be called sample A, while the latter is called sample B.
If after the duration of the research, we find out that sample A grows and sample B dies, even though they are both regularly wetted and given the same treatment. Therefore, we can conclude that sunlight will aid growth in all similar plants.
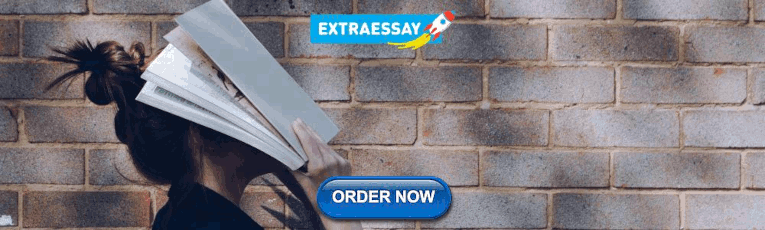
What is Experimental Research?
Experimental research is a scientific approach to research, where one or more independent variables are manipulated and applied to one or more dependent variables to measure their effect on the latter. The effect of the independent variables on the dependent variables is usually observed and recorded over some time, to aid researchers in drawing a reasonable conclusion regarding the relationship between these 2 variable types.
The experimental research method is widely used in physical and social sciences, psychology, and education. It is based on the comparison between two or more groups with a straightforward logic, which may, however, be difficult to execute.
Mostly related to a laboratory test procedure, experimental research designs involve collecting quantitative data and performing statistical analysis on them during research. Therefore, making it an example of quantitative research method .
What are The Types of Experimental Research Design?
The types of experimental research design are determined by the way the researcher assigns subjects to different conditions and groups. They are of 3 types, namely; pre-experimental, quasi-experimental, and true experimental research.
Pre-experimental Research Design
In pre-experimental research design, either a group or various dependent groups are observed for the effect of the application of an independent variable which is presumed to cause change. It is the simplest form of experimental research design and is treated with no control group.
Although very practical, experimental research is lacking in several areas of the true-experimental criteria. The pre-experimental research design is further divided into three types
- One-shot Case Study Research Design
In this type of experimental study, only one dependent group or variable is considered. The study is carried out after some treatment which was presumed to cause change, making it a posttest study.
- One-group Pretest-posttest Research Design:
This research design combines both posttest and pretest study by carrying out a test on a single group before the treatment is administered and after the treatment is administered. With the former being administered at the beginning of treatment and later at the end.
- Static-group Comparison:
In a static-group comparison study, 2 or more groups are placed under observation, where only one of the groups is subjected to some treatment while the other groups are held static. All the groups are post-tested, and the observed differences between the groups are assumed to be a result of the treatment.
Quasi-experimental Research Design
The word “quasi” means partial, half, or pseudo. Therefore, the quasi-experimental research bearing a resemblance to the true experimental research, but not the same. In quasi-experiments, the participants are not randomly assigned, and as such, they are used in settings where randomization is difficult or impossible.
This is very common in educational research, where administrators are unwilling to allow the random selection of students for experimental samples.
Some examples of quasi-experimental research design include; the time series, no equivalent control group design, and the counterbalanced design.
True Experimental Research Design
The true experimental research design relies on statistical analysis to approve or disprove a hypothesis. It is the most accurate type of experimental design and may be carried out with or without a pretest on at least 2 randomly assigned dependent subjects.
The true experimental research design must contain a control group, a variable that can be manipulated by the researcher, and the distribution must be random. The classification of true experimental design include:
- The posttest-only Control Group Design: In this design, subjects are randomly selected and assigned to the 2 groups (control and experimental), and only the experimental group is treated. After close observation, both groups are post-tested, and a conclusion is drawn from the difference between these groups.
- The pretest-posttest Control Group Design: For this control group design, subjects are randomly assigned to the 2 groups, both are presented, but only the experimental group is treated. After close observation, both groups are post-tested to measure the degree of change in each group.
- Solomon four-group Design: This is the combination of the pretest-only and the pretest-posttest control groups. In this case, the randomly selected subjects are placed into 4 groups.
The first two of these groups are tested using the posttest-only method, while the other two are tested using the pretest-posttest method.
Examples of Experimental Research
Experimental research examples are different, depending on the type of experimental research design that is being considered. The most basic example of experimental research is laboratory experiments, which may differ in nature depending on the subject of research.
Administering Exams After The End of Semester
During the semester, students in a class are lectured on particular courses and an exam is administered at the end of the semester. In this case, the students are the subjects or dependent variables while the lectures are the independent variables treated on the subjects.
Only one group of carefully selected subjects are considered in this research, making it a pre-experimental research design example. We will also notice that tests are only carried out at the end of the semester, and not at the beginning.
Further making it easy for us to conclude that it is a one-shot case study research.
Employee Skill Evaluation
Before employing a job seeker, organizations conduct tests that are used to screen out less qualified candidates from the pool of qualified applicants. This way, organizations can determine an employee’s skill set at the point of employment.
In the course of employment, organizations also carry out employee training to improve employee productivity and generally grow the organization. Further evaluation is carried out at the end of each training to test the impact of the training on employee skills, and test for improvement.
Here, the subject is the employee, while the treatment is the training conducted. This is a pretest-posttest control group experimental research example.
Evaluation of Teaching Method
Let us consider an academic institution that wants to evaluate the teaching method of 2 teachers to determine which is best. Imagine a case whereby the students assigned to each teacher is carefully selected probably due to personal request by parents or due to stubbornness and smartness.
This is a no equivalent group design example because the samples are not equal. By evaluating the effectiveness of each teacher’s teaching method this way, we may conclude after a post-test has been carried out.
However, this may be influenced by factors like the natural sweetness of a student. For example, a very smart student will grab more easily than his or her peers irrespective of the method of teaching.
What are the Characteristics of Experimental Research?
Experimental research contains dependent, independent and extraneous variables. The dependent variables are the variables being treated or manipulated and are sometimes called the subject of the research.
The independent variables are the experimental treatment being exerted on the dependent variables. Extraneous variables, on the other hand, are other factors affecting the experiment that may also contribute to the change.
The setting is where the experiment is carried out. Many experiments are carried out in the laboratory, where control can be exerted on the extraneous variables, thereby eliminating them.
Other experiments are carried out in a less controllable setting. The choice of setting used in research depends on the nature of the experiment being carried out.
- Multivariable
Experimental research may include multiple independent variables, e.g. time, skills, test scores, etc.
Why Use Experimental Research Design?
Experimental research design can be majorly used in physical sciences, social sciences, education, and psychology. It is used to make predictions and draw conclusions on a subject matter.
Some uses of experimental research design are highlighted below.
- Medicine: Experimental research is used to provide the proper treatment for diseases. In most cases, rather than directly using patients as the research subject, researchers take a sample of the bacteria from the patient’s body and are treated with the developed antibacterial
The changes observed during this period are recorded and evaluated to determine its effectiveness. This process can be carried out using different experimental research methods.
- Education: Asides from science subjects like Chemistry and Physics which involves teaching students how to perform experimental research, it can also be used in improving the standard of an academic institution. This includes testing students’ knowledge on different topics, coming up with better teaching methods, and the implementation of other programs that will aid student learning.
- Human Behavior: Social scientists are the ones who mostly use experimental research to test human behaviour. For example, consider 2 people randomly chosen to be the subject of the social interaction research where one person is placed in a room without human interaction for 1 year.
The other person is placed in a room with a few other people, enjoying human interaction. There will be a difference in their behaviour at the end of the experiment.
- UI/UX: During the product development phase, one of the major aims of the product team is to create a great user experience with the product. Therefore, before launching the final product design, potential are brought in to interact with the product.
For example, when finding it difficult to choose how to position a button or feature on the app interface, a random sample of product testers are allowed to test the 2 samples and how the button positioning influences the user interaction is recorded.
What are the Disadvantages of Experimental Research?
- It is highly prone to human error due to its dependency on variable control which may not be properly implemented. These errors could eliminate the validity of the experiment and the research being conducted.
- Exerting control of extraneous variables may create unrealistic situations. Eliminating real-life variables will result in inaccurate conclusions. This may also result in researchers controlling the variables to suit his or her personal preferences.
- It is a time-consuming process. So much time is spent on testing dependent variables and waiting for the effect of the manipulation of dependent variables to manifest.
- It is expensive.
- It is very risky and may have ethical complications that cannot be ignored. This is common in medical research, where failed trials may lead to a patient’s death or a deteriorating health condition.
- Experimental research results are not descriptive.
- Response bias can also be supplied by the subject of the conversation.
- Human responses in experimental research can be difficult to measure.
What are the Data Collection Methods in Experimental Research?
Data collection methods in experimental research are the different ways in which data can be collected for experimental research. They are used in different cases, depending on the type of research being carried out.
1. Observational Study
This type of study is carried out over a long period. It measures and observes the variables of interest without changing existing conditions.
When researching the effect of social interaction on human behavior, the subjects who are placed in 2 different environments are observed throughout the research. No matter the kind of absurd behavior that is exhibited by the subject during this period, its condition will not be changed.
This may be a very risky thing to do in medical cases because it may lead to death or worse medical conditions.
2. Simulations
This procedure uses mathematical, physical, or computer models to replicate a real-life process or situation. It is frequently used when the actual situation is too expensive, dangerous, or impractical to replicate in real life.
This method is commonly used in engineering and operational research for learning purposes and sometimes as a tool to estimate possible outcomes of real research. Some common situation software are Simulink, MATLAB, and Simul8.
Not all kinds of experimental research can be carried out using simulation as a data collection tool . It is very impractical for a lot of laboratory-based research that involves chemical processes.
A survey is a tool used to gather relevant data about the characteristics of a population and is one of the most common data collection tools. A survey consists of a group of questions prepared by the researcher, to be answered by the research subject.
Surveys can be shared with the respondents both physically and electronically. When collecting data through surveys, the kind of data collected depends on the respondent, and researchers have limited control over it.
Formplus is the best tool for collecting experimental data using survey s. It has relevant features that will aid the data collection process and can also be used in other aspects of experimental research.
Differences between Experimental and Non-Experimental Research
1. In experimental research, the researcher can control and manipulate the environment of the research, including the predictor variable which can be changed. On the other hand, non-experimental research cannot be controlled or manipulated by the researcher at will.
This is because it takes place in a real-life setting, where extraneous variables cannot be eliminated. Therefore, it is more difficult to conclude non-experimental studies, even though they are much more flexible and allow for a greater range of study fields.
2. The relationship between cause and effect cannot be established in non-experimental research, while it can be established in experimental research. This may be because many extraneous variables also influence the changes in the research subject, making it difficult to point at a particular variable as the cause of a particular change
3. Independent variables are not introduced, withdrawn, or manipulated in non-experimental designs, but the same may not be said about experimental research.
Experimental Research vs. Alternatives and When to Use Them
1. experimental research vs causal comparative.
Experimental research enables you to control variables and identify how the independent variable affects the dependent variable. Causal-comparative find out the cause-and-effect relationship between the variables by comparing already existing groups that are affected differently by the independent variable.
For example, in an experiment to see how K-12 education affects children and teenager development. An experimental research would split the children into groups, some would get formal K-12 education, while others won’t. This is not ethically right because every child has the right to education. So, what we do instead would be to compare already existing groups of children who are getting formal education with those who due to some circumstances can not.
Pros and Cons of Experimental vs Causal-Comparative Research
- Causal-Comparative: Strengths: More realistic than experiments, can be conducted in real-world settings. Weaknesses: Establishing causality can be weaker due to the lack of manipulation.
2. Experimental Research vs Correlational Research
When experimenting, you are trying to establish a cause-and-effect relationship between different variables. For example, you are trying to establish the effect of heat on water, the temperature keeps changing (independent variable) and you see how it affects the water (dependent variable).
For correlational research, you are not necessarily interested in the why or the cause-and-effect relationship between the variables, you are focusing on the relationship. Using the same water and temperature example, you are only interested in the fact that they change, you are not investigating which of the variables or other variables causes them to change.
Pros and Cons of Experimental vs Correlational Research
3. experimental research vs descriptive research.
With experimental research, you alter the independent variable to see how it affects the dependent variable, but with descriptive research you are simply studying the characteristics of the variable you are studying.
So, in an experiment to see how blown glass reacts to temperature, experimental research would keep altering the temperature to varying levels of high and low to see how it affects the dependent variable (glass). But descriptive research would investigate the glass properties.
Pros and Cons of Experimental vs Descriptive Research
4. experimental research vs action research.
Experimental research tests for causal relationships by focusing on one independent variable vs the dependent variable and keeps other variables constant. So, you are testing hypotheses and using the information from the research to contribute to knowledge.
However, with action research, you are using a real-world setting which means you are not controlling variables. You are also performing the research to solve actual problems and improve already established practices.
For example, if you are testing for how long commutes affect workers’ productivity. With experimental research, you would vary the length of commute to see how the time affects work. But with action research, you would account for other factors such as weather, commute route, nutrition, etc. Also, experimental research helps know the relationship between commute time and productivity, while action research helps you look for ways to improve productivity
Pros and Cons of Experimental vs Action Research
Conclusion .
Experimental research designs are often considered to be the standard in research designs. This is partly due to the common misconception that research is equivalent to scientific experiments—a component of experimental research design.
In this research design, one or more subjects or dependent variables are randomly assigned to different treatments (i.e. independent variables manipulated by the researcher) and the results are observed to conclude. One of the uniqueness of experimental research is in its ability to control the effect of extraneous variables.
Experimental research is suitable for research whose goal is to examine cause-effect relationships, e.g. explanatory research. It can be conducted in the laboratory or field settings, depending on the aim of the research that is being carried out.
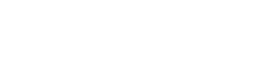
Connect to Formplus, Get Started Now - It's Free!
- examples of experimental research
- experimental research methods
- types of experimental research
- busayo.longe
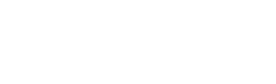
You may also like:
What is Experimenter Bias? Definition, Types & Mitigation
In this article, we will look into the concept of experimental bias and how it can be identified in your research

Response vs Explanatory Variables: Definition & Examples
In this article, we’ll be comparing the two types of variables, what they both mean and see some of their real-life applications in research
Simpson’s Paradox & How to Avoid it in Experimental Research
In this article, we are going to look at Simpson’s Paradox from its historical point and later, we’ll consider its effect in...
Experimental Vs Non-Experimental Research: 15 Key Differences
Differences between experimental and non experimental research on definitions, types, examples, data collection tools, uses, advantages etc.
Formplus - For Seamless Data Collection
Collect data the right way with a versatile data collection tool. try formplus and transform your work productivity today..
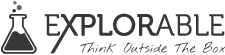
- Foundations
- Write Paper
Search form
- Experiments
- Anthropology
- Self-Esteem
- Social Anxiety

Case Study Research Design
The case study research design have evolved over the past few years as a useful tool for investigating trends and specific situations in many scientific disciplines.
This article is a part of the guide:
- Research Designs
- Quantitative and Qualitative Research
- Literature Review
- Quantitative Research Design
- Descriptive Research
Browse Full Outline
- 1 Research Designs
- 2.1 Pilot Study
- 2.2 Quantitative Research Design
- 2.3 Qualitative Research Design
- 2.4 Quantitative and Qualitative Research
- 3.1 Case Study
- 3.2 Naturalistic Observation
- 3.3 Survey Research Design
- 3.4 Observational Study
- 4.1 Case-Control Study
- 4.2 Cohort Study
- 4.3 Longitudinal Study
- 4.4 Cross Sectional Study
- 4.5 Correlational Study
- 5.1 Field Experiments
- 5.2 Quasi-Experimental Design
- 5.3 Identical Twins Study
- 6.1 Experimental Design
- 6.2 True Experimental Design
- 6.3 Double Blind Experiment
- 6.4 Factorial Design
- 7.1 Literature Review
- 7.2 Systematic Reviews
- 7.3 Meta Analysis
The case study has been especially used in social science, psychology, anthropology and ecology.
This method of study is especially useful for trying to test theoretical models by using them in real world situations. For example, if an anthropologist were to live amongst a remote tribe, whilst their observations might produce no quantitative data, they are still useful to science.
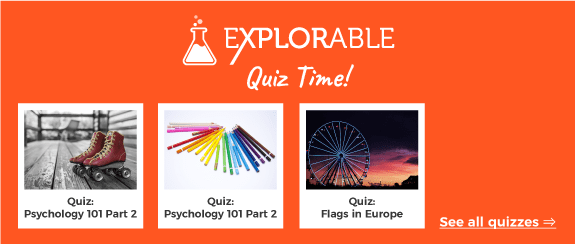
What is a Case Study?
Basically, a case study is an in depth study of a particular situation rather than a sweeping statistical survey . It is a method used to narrow down a very broad field of research into one easily researchable topic.
Whilst it will not answer a question completely, it will give some indications and allow further elaboration and hypothesis creation on a subject.
The case study research design is also useful for testing whether scientific theories and models actually work in the real world. You may come out with a great computer model for describing how the ecosystem of a rock pool works but it is only by trying it out on a real life pool that you can see if it is a realistic simulation.
For psychologists, anthropologists and social scientists they have been regarded as a valid method of research for many years. Scientists are sometimes guilty of becoming bogged down in the general picture and it is sometimes important to understand specific cases and ensure a more holistic approach to research .
H.M.: An example of a study using the case study research design.
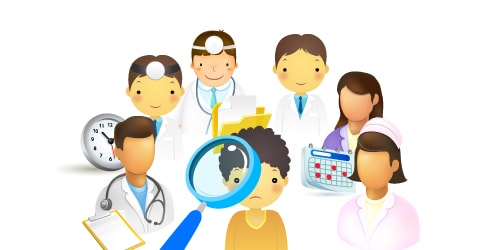
The Argument for and Against the Case Study Research Design
Some argue that because a case study is such a narrow field that its results cannot be extrapolated to fit an entire question and that they show only one narrow example. On the other hand, it is argued that a case study provides more realistic responses than a purely statistical survey.
The truth probably lies between the two and it is probably best to try and synergize the two approaches. It is valid to conduct case studies but they should be tied in with more general statistical processes.
For example, a statistical survey might show how much time people spend talking on mobile phones, but it is case studies of a narrow group that will determine why this is so.
The other main thing to remember during case studies is their flexibility. Whilst a pure scientist is trying to prove or disprove a hypothesis , a case study might introduce new and unexpected results during its course, and lead to research taking new directions.
The argument between case study and statistical method also appears to be one of scale. Whilst many 'physical' scientists avoid case studies, for psychology, anthropology and ecology they are an essential tool. It is important to ensure that you realize that a case study cannot be generalized to fit a whole population or ecosystem.
Finally, one peripheral point is that, when informing others of your results, case studies make more interesting topics than purely statistical surveys, something that has been realized by teachers and magazine editors for many years. The general public has little interest in pages of statistical calculations but some well placed case studies can have a strong impact.
How to Design and Conduct a Case Study
The advantage of the case study research design is that you can focus on specific and interesting cases. This may be an attempt to test a theory with a typical case or it can be a specific topic that is of interest. Research should be thorough and note taking should be meticulous and systematic.
The first foundation of the case study is the subject and relevance. In a case study, you are deliberately trying to isolate a small study group, one individual case or one particular population.
For example, statistical analysis may have shown that birthrates in African countries are increasing. A case study on one or two specific countries becomes a powerful and focused tool for determining the social and economic pressures driving this.
In the design of a case study, it is important to plan and design how you are going to address the study and make sure that all collected data is relevant. Unlike a scientific report, there is no strict set of rules so the most important part is making sure that the study is focused and concise; otherwise you will end up having to wade through a lot of irrelevant information.
It is best if you make yourself a short list of 4 or 5 bullet points that you are going to try and address during the study. If you make sure that all research refers back to these then you will not be far wrong.
With a case study, even more than a questionnaire or survey , it is important to be passive in your research. You are much more of an observer than an experimenter and you must remember that, even in a multi-subject case, each case must be treated individually and then cross case conclusions can be drawn .
How to Analyze the Results
Analyzing results for a case study tends to be more opinion based than statistical methods. The usual idea is to try and collate your data into a manageable form and construct a narrative around it.
Use examples in your narrative whilst keeping things concise and interesting. It is useful to show some numerical data but remember that you are only trying to judge trends and not analyze every last piece of data. Constantly refer back to your bullet points so that you do not lose focus.
It is always a good idea to assume that a person reading your research may not possess a lot of knowledge of the subject so try to write accordingly.
In addition, unlike a scientific study which deals with facts, a case study is based on opinion and is very much designed to provoke reasoned debate. There really is no right or wrong answer in a case study.
- Psychology 101
- Flags and Countries
- Capitals and Countries
Martyn Shuttleworth (Apr 1, 2008). Case Study Research Design. Retrieved Aug 18, 2024 from Explorable.com: https://explorable.com/case-study-research-design
You Are Allowed To Copy The Text
The text in this article is licensed under the Creative Commons-License Attribution 4.0 International (CC BY 4.0) .
This means you're free to copy, share and adapt any parts (or all) of the text in the article, as long as you give appropriate credit and provide a link/reference to this page.
That is it. You don't need our permission to copy the article; just include a link/reference back to this page. You can use it freely (with some kind of link), and we're also okay with people reprinting in publications like books, blogs, newsletters, course-material, papers, wikipedia and presentations (with clear attribution).
Want to stay up to date? Follow us!
Get all these articles in 1 guide.
Want the full version to study at home, take to school or just scribble on?
Whether you are an academic novice, or you simply want to brush up your skills, this book will take your academic writing skills to the next level.
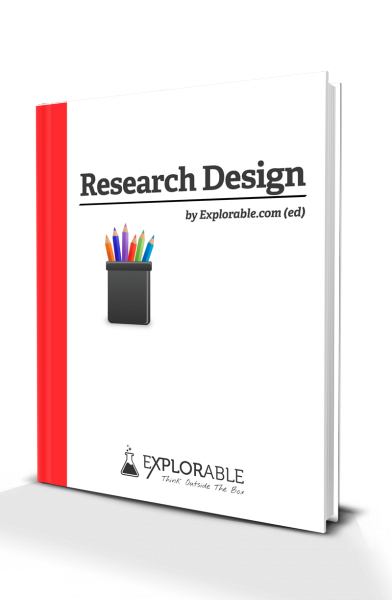
Download electronic versions: - Epub for mobiles and tablets - PDF version here
Save this course for later
Don't have time for it all now? No problem, save it as a course and come back to it later.
Footer bottom
- Privacy Policy
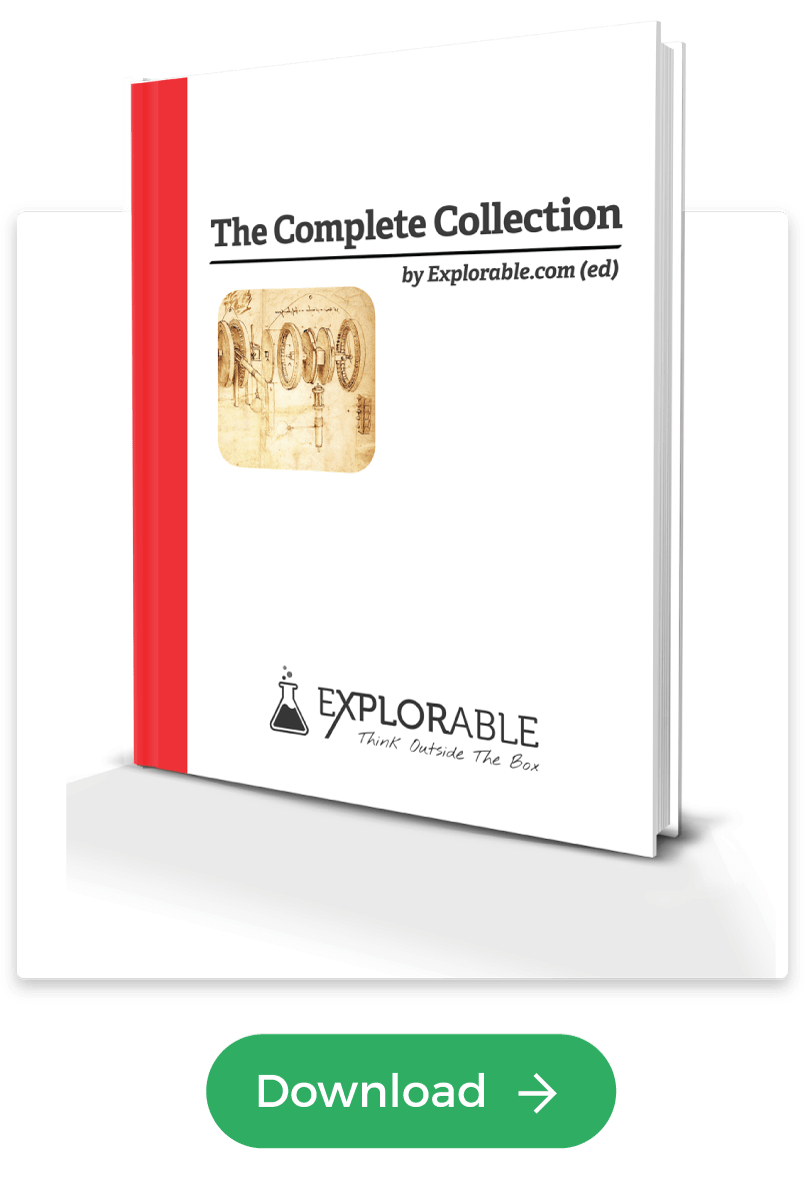
- Subscribe to our RSS Feed
- Like us on Facebook
- Follow us on Twitter

Want to create or adapt books like this? Learn more about how Pressbooks supports open publishing practices.
Part 3: Using quantitative methods
13. Experimental design
Chapter outline.
- What is an experiment and when should you use one? (8 minute read)
- True experimental designs (7 minute read)
- Quasi-experimental designs (8 minute read)
- Non-experimental designs (5 minute read)
- Critical, ethical, and critical considerations (5 minute read)
Content warning : examples in this chapter contain references to non-consensual research in Western history, including experiments conducted during the Holocaust and on African Americans (section 13.6).
13.1 What is an experiment and when should you use one?
Learning objectives.
Learners will be able to…
- Identify the characteristics of a basic experiment
- Describe causality in experimental design
- Discuss the relationship between dependent and independent variables in experiments
- Explain the links between experiments and generalizability of results
- Describe advantages and disadvantages of experimental designs
The basics of experiments
The first experiment I can remember using was for my fourth grade science fair. I wondered if latex- or oil-based paint would hold up to sunlight better. So, I went to the hardware store and got a few small cans of paint and two sets of wooden paint sticks. I painted one with oil-based paint and the other with latex-based paint of different colors and put them in a sunny spot in the back yard. My hypothesis was that the oil-based paint would fade the most and that more fading would happen the longer I left the paint sticks out. (I know, it’s obvious, but I was only 10.)
I checked in on the paint sticks every few days for a month and wrote down my observations. The first part of my hypothesis ended up being wrong—it was actually the latex-based paint that faded the most. But the second part was right, and the paint faded more and more over time. This is a simple example, of course—experiments get a heck of a lot more complex than this when we’re talking about real research.
Merriam-Webster defines an experiment as “an operation or procedure carried out under controlled conditions in order to discover an unknown effect or law, to test or establish a hypothesis, or to illustrate a known law.” Each of these three components of the definition will come in handy as we go through the different types of experimental design in this chapter. Most of us probably think of the physical sciences when we think of experiments, and for good reason—these experiments can be pretty flashy! But social science and psychological research follow the same scientific methods, as we’ve discussed in this book.
As the video discusses, experiments can be used in social sciences just like they can in physical sciences. It makes sense to use an experiment when you want to determine the cause of a phenomenon with as much accuracy as possible. Some types of experimental designs do this more precisely than others, as we’ll see throughout the chapter. If you’ll remember back to Chapter 11 and the discussion of validity, experiments are the best way to ensure internal validity, or the extent to which a change in your independent variable causes a change in your dependent variable.
Experimental designs for research projects are most appropriate when trying to uncover or test a hypothesis about the cause of a phenomenon, so they are best for explanatory research questions. As we’ll learn throughout this chapter, different circumstances are appropriate for different types of experimental designs. Each type of experimental design has advantages and disadvantages, and some are better at controlling the effect of extraneous variables —those variables and characteristics that have an effect on your dependent variable, but aren’t the primary variable whose influence you’re interested in testing. For example, in a study that tries to determine whether aspirin lowers a person’s risk of a fatal heart attack, a person’s race would likely be an extraneous variable because you primarily want to know the effect of aspirin.
In practice, many types of experimental designs can be logistically challenging and resource-intensive. As practitioners, the likelihood that we will be involved in some of the types of experimental designs discussed in this chapter is fairly low. However, it’s important to learn about these methods, even if we might not ever use them, so that we can be thoughtful consumers of research that uses experimental designs.
While we might not use all of these types of experimental designs, many of us will engage in evidence-based practice during our time as social workers. A lot of research developing evidence-based practice, which has a strong emphasis on generalizability, will use experimental designs. You’ve undoubtedly seen one or two in your literature search so far.
The logic of experimental design
How do we know that one phenomenon causes another? The complexity of the social world in which we practice and conduct research means that causes of social problems are rarely cut and dry. Uncovering explanations for social problems is key to helping clients address them, and experimental research designs are one road to finding answers.
As you read about in Chapter 8 (and as we’ll discuss again in Chapter 15 ), just because two phenomena are related in some way doesn’t mean that one causes the other. Ice cream sales increase in the summer, and so does the rate of violent crime; does that mean that eating ice cream is going to make me murder someone? Obviously not, because ice cream is great. The reality of that relationship is far more complex—it could be that hot weather makes people more irritable and, at times, violent, while also making people want ice cream. More likely, though, there are other social factors not accounted for in the way we just described this relationship.
Experimental designs can help clear up at least some of this fog by allowing researchers to isolate the effect of interventions on dependent variables by controlling extraneous variables . In true experimental design (discussed in the next section) and some quasi-experimental designs, researchers accomplish this w ith the control group and the experimental group . (The experimental group is sometimes called the “treatment group,” but we will call it the experimental group in this chapter.) The control group does not receive the intervention you are testing (they may receive no intervention or what is known as “treatment as usual”), while the experimental group does. (You will hopefully remember our earlier discussion of control variables in Chapter 8 —conceptually, the use of the word “control” here is the same.)
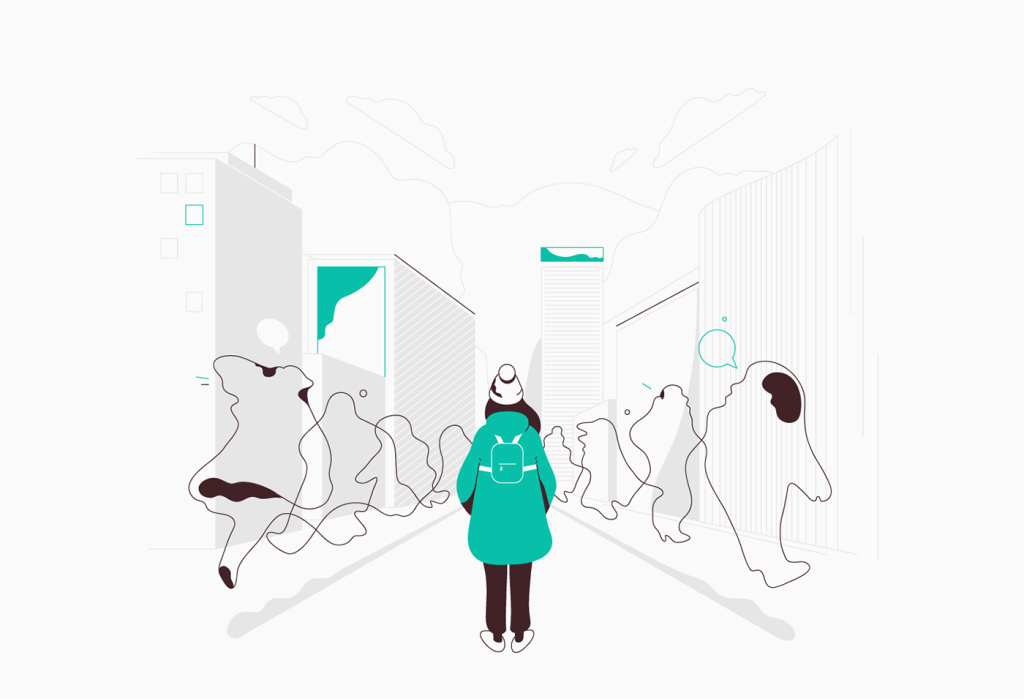
In a well-designed experiment, your control group should look almost identical to your experimental group in terms of demographics and other relevant factors. What if we want to know the effect of CBT on social anxiety, but we have learned in prior research that men tend to have a more difficult time overcoming social anxiety? We would want our control and experimental groups to have a similar gender mix because it would limit the effect of gender on our results, since ostensibly, both groups’ results would be affected by gender in the same way. If your control group has 5 women, 6 men, and 4 non-binary people, then your experimental group should be made up of roughly the same gender balance to help control for the influence of gender on the outcome of your intervention. (In reality, the groups should be similar along other dimensions, as well, and your group will likely be much larger.) The researcher will use the same outcome measures for both groups and compare them, and assuming the experiment was designed correctly, get a pretty good answer about whether the intervention had an effect on social anxiety.
You will also hear people talk about comparison groups , which are similar to control groups. The primary difference between the two is that a control group is populated using random assignment, but a comparison group is not. Random assignment entails using a random process to decide which participants are put into the control or experimental group (which participants receive an intervention and which do not). By randomly assigning participants to a group, you can reduce the effect of extraneous variables on your research because there won’t be a systematic difference between the groups.
Do not confuse random assignment with random sampling. Random sampling is a method for selecting a sample from a population, and is rarely used in psychological research. Random assignment is a method for assigning participants in a sample to the different conditions, and it is an important element of all experimental research in psychology and other related fields. Random sampling also helps a great deal with generalizability , whereas random assignment increases internal validity .
We have already learned about internal validity in Chapter 11 . The use of an experimental design will bolster internal validity since it works to isolate causal relationships. As we will see in the coming sections, some types of experimental design do this more effectively than others. It’s also worth considering that true experiments, which most effectively show causality , are often difficult and expensive to implement. Although other experimental designs aren’t perfect, they still produce useful, valid evidence and may be more feasible to carry out.
Key Takeaways
- Experimental designs are useful for establishing causality, but some types of experimental design do this better than others.
- Experiments help researchers isolate the effect of the independent variable on the dependent variable by controlling for the effect of extraneous variables .
- Experiments use a control/comparison group and an experimental group to test the effects of interventions. These groups should be as similar to each other as possible in terms of demographics and other relevant factors.
- True experiments have control groups with randomly assigned participants, while other types of experiments have comparison groups to which participants are not randomly assigned.
- Think about the research project you’ve been designing so far. How might you use a basic experiment to answer your question? If your question isn’t explanatory, try to formulate a new explanatory question and consider the usefulness of an experiment.
- Why is establishing a simple relationship between two variables not indicative of one causing the other?
13.2 True experimental design
- Describe a true experimental design in social work research
- Understand the different types of true experimental designs
- Determine what kinds of research questions true experimental designs are suited for
- Discuss advantages and disadvantages of true experimental designs
True experimental design , often considered to be the “gold standard” in research designs, is thought of as one of the most rigorous of all research designs. In this design, one or more independent variables are manipulated by the researcher (as treatments), subjects are randomly assigned to different treatment levels (random assignment), and the results of the treatments on outcomes (dependent variables) are observed. The unique strength of experimental research is its internal validity and its ability to establish ( causality ) through treatment manipulation, while controlling for the effects of extraneous variable. Sometimes the treatment level is no treatment, while other times it is simply a different treatment than that which we are trying to evaluate. For example, we might have a control group that is made up of people who will not receive any treatment for a particular condition. Or, a control group could consist of people who consent to treatment with DBT when we are testing the effectiveness of CBT.
As we discussed in the previous section, a true experiment has a control group with participants randomly assigned , and an experimental group . This is the most basic element of a true experiment. The next decision a researcher must make is when they need to gather data during their experiment. Do they take a baseline measurement and then a measurement after treatment, or just a measurement after treatment, or do they handle measurement another way? Below, we’ll discuss the three main types of true experimental designs. There are sub-types of each of these designs, but here, we just want to get you started with some of the basics.
Using a true experiment in social work research is often pretty difficult, since as I mentioned earlier, true experiments can be quite resource intensive. True experiments work best with relatively large sample sizes, and random assignment, a key criterion for a true experimental design, is hard (and unethical) to execute in practice when you have people in dire need of an intervention. Nonetheless, some of the strongest evidence bases are built on true experiments.
For the purposes of this section, let’s bring back the example of CBT for the treatment of social anxiety. We have a group of 500 individuals who have agreed to participate in our study, and we have randomly assigned them to the control and experimental groups. The folks in the experimental group will receive CBT, while the folks in the control group will receive more unstructured, basic talk therapy. These designs, as we talked about above, are best suited for explanatory research questions.
Before we get started, take a look at the table below. When explaining experimental research designs, we often use diagrams with abbreviations to visually represent the experiment. Table 13.1 starts us off by laying out what each of the abbreviations mean.
R | Randomly assigned group (control/comparison or experimental) |
O | Observation/measurement taken of dependent variable |
X | Intervention or treatment |
X | Experimental or new intervention |
X | Typical intervention/treatment as usual |
A, B, C, etc. | Denotes different groups (control/comparison and experimental) |
Pretest and post-test control group design
In pretest and post-test control group design , participants are given a pretest of some kind to measure their baseline state before their participation in an intervention. In our social anxiety experiment, we would have participants in both the experimental and control groups complete some measure of social anxiety—most likely an established scale and/or a structured interview—before they start their treatment. As part of the experiment, we would have a defined time period during which the treatment would take place (let’s say 12 weeks, just for illustration). At the end of 12 weeks, we would give both groups the same measure as a post-test .
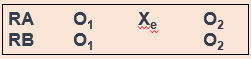
In the diagram, RA (random assignment group A) is the experimental group and RB is the control group. O 1 denotes the pre-test, X e denotes the experimental intervention, and O 2 denotes the post-test. Let’s look at this diagram another way, using the example of CBT for social anxiety that we’ve been talking about.

In a situation where the control group received treatment as usual instead of no intervention, the diagram would look this way, with X i denoting treatment as usual (Figure 13.3).
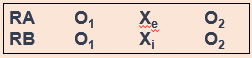
Hopefully, these diagrams provide you a visualization of how this type of experiment establishes time order , a key component of a causal relationship. Did the change occur after the intervention? Assuming there is a change in the scores between the pretest and post-test, we would be able to say that yes, the change did occur after the intervention. Causality can’t exist if the change happened before the intervention—this would mean that something else led to the change, not our intervention.
Post-test only control group design
Post-test only control group design involves only giving participants a post-test, just like it sounds (Figure 13.4).
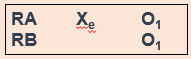
But why would you use this design instead of using a pretest/post-test design? One reason could be the testing effect that can happen when research participants take a pretest. In research, the testing effect refers to “measurement error related to how a test is given; the conditions of the testing, including environmental conditions; and acclimation to the test itself” (Engel & Schutt, 2017, p. 444) [1] (When we say “measurement error,” all we mean is the accuracy of the way we measure the dependent variable.) Figure 13.4 is a visualization of this type of experiment. The testing effect isn’t always bad in practice—our initial assessments might help clients identify or put into words feelings or experiences they are having when they haven’t been able to do that before. In research, however, we might want to control its effects to isolate a cleaner causal relationship between intervention and outcome.
Going back to our CBT for social anxiety example, we might be concerned that participants would learn about social anxiety symptoms by virtue of taking a pretest. They might then identify that they have those symptoms on the post-test, even though they are not new symptoms for them. That could make our intervention look less effective than it actually is.
However, without a baseline measurement establishing causality can be more difficult. If we don’t know someone’s state of mind before our intervention, how do we know our intervention did anything at all? Establishing time order is thus a little more difficult. You must balance this consideration with the benefits of this type of design.
Solomon four group design
One way we can possibly measure how much the testing effect might change the results of the experiment is with the Solomon four group design. Basically, as part of this experiment, you have two control groups and two experimental groups. The first pair of groups receives both a pretest and a post-test. The other pair of groups receives only a post-test (Figure 13.5). This design helps address the problem of establishing time order in post-test only control group designs.
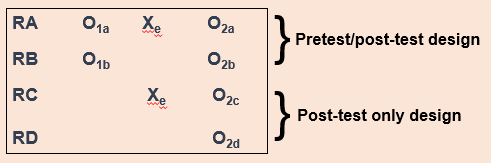
For our CBT project, we would randomly assign people to four different groups instead of just two. Groups A and B would take our pretest measures and our post-test measures, and groups C and D would take only our post-test measures. We could then compare the results among these groups and see if they’re significantly different between the folks in A and B, and C and D. If they are, we may have identified some kind of testing effect, which enables us to put our results into full context. We don’t want to draw a strong causal conclusion about our intervention when we have major concerns about testing effects without trying to determine the extent of those effects.
Solomon four group designs are less common in social work research, primarily because of the logistics and resource needs involved. Nonetheless, this is an important experimental design to consider when we want to address major concerns about testing effects.
- True experimental design is best suited for explanatory research questions.
- True experiments require random assignment of participants to control and experimental groups.
- Pretest/post-test research design involves two points of measurement—one pre-intervention and one post-intervention.
- Post-test only research design involves only one point of measurement—post-intervention. It is a useful design to minimize the effect of testing effects on our results.
- Solomon four group research design involves both of the above types of designs, using 2 pairs of control and experimental groups. One group receives both a pretest and a post-test, while the other receives only a post-test. This can help uncover the influence of testing effects.
- Think about a true experiment you might conduct for your research project. Which design would be best for your research, and why?
- What challenges or limitations might make it unrealistic (or at least very complicated!) for you to carry your true experimental design in the real-world as a student researcher?
- What hypothesis(es) would you test using this true experiment?
13.4 Quasi-experimental designs
- Describe a quasi-experimental design in social work research
- Understand the different types of quasi-experimental designs
- Determine what kinds of research questions quasi-experimental designs are suited for
- Discuss advantages and disadvantages of quasi-experimental designs
Quasi-experimental designs are a lot more common in social work research than true experimental designs. Although quasi-experiments don’t do as good a job of giving us robust proof of causality , they still allow us to establish time order , which is a key element of causality. The prefix quasi means “resembling,” so quasi-experimental research is research that resembles experimental research, but is not true experimental research. Nonetheless, given proper research design, quasi-experiments can still provide extremely rigorous and useful results.
There are a few key differences between true experimental and quasi-experimental research. The primary difference between quasi-experimental research and true experimental research is that quasi-experimental research does not involve random assignment to control and experimental groups. Instead, we talk about comparison groups in quasi-experimental research instead. As a result, these types of experiments don’t control the effect of extraneous variables as well as a true experiment.
Quasi-experiments are most likely to be conducted in field settings in which random assignment is difficult or impossible. They are often conducted to evaluate the effectiveness of a treatment—perhaps a type of psychotherapy or an educational intervention. We’re able to eliminate some threats to internal validity, but we can’t do this as effectively as we can with a true experiment. Realistically, our CBT-social anxiety project is likely to be a quasi experiment, based on the resources and participant pool we’re likely to have available.
It’s important to note that not all quasi-experimental designs have a comparison group. There are many different kinds of quasi-experiments, but we will discuss the three main types below: nonequivalent comparison group designs, time series designs, and ex post facto comparison group designs.
Nonequivalent comparison group design
You will notice that this type of design looks extremely similar to the pretest/post-test design that we discussed in section 13.3. But instead of random assignment to control and experimental groups, researchers use other methods to construct their comparison and experimental groups. A diagram of this design will also look very similar to pretest/post-test design, but you’ll notice we’ve removed the “R” from our groups, since they are not randomly assigned (Figure 13.6).
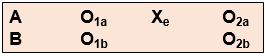
Researchers using this design select a comparison group that’s as close as possible based on relevant factors to their experimental group. Engel and Schutt (2017) [2] identify two different selection methods:
- Individual matching : Researchers take the time to match individual cases in the experimental group to similar cases in the comparison group. It can be difficult, however, to match participants on all the variables you want to control for.
- Aggregate matching : Instead of trying to match individual participants to each other, researchers try to match the population profile of the comparison and experimental groups. For example, researchers would try to match the groups on average age, gender balance, or median income. This is a less resource-intensive matching method, but researchers have to ensure that participants aren’t choosing which group (comparison or experimental) they are a part of.
As we’ve already talked about, this kind of design provides weaker evidence that the intervention itself leads to a change in outcome. Nonetheless, we are still able to establish time order using this method, and can thereby show an association between the intervention and the outcome. Like true experimental designs, this type of quasi-experimental design is useful for explanatory research questions.
What might this look like in a practice setting? Let’s say you’re working at an agency that provides CBT and other types of interventions, and you have identified a group of clients who are seeking help for social anxiety, as in our earlier example. Once you’ve obtained consent from your clients, you can create a comparison group using one of the matching methods we just discussed. If the group is small, you might match using individual matching, but if it’s larger, you’ll probably sort people by demographics to try to get similar population profiles. (You can do aggregate matching more easily when your agency has some kind of electronic records or database, but it’s still possible to do manually.)
Time series design
Another type of quasi-experimental design is a time series design. Unlike other types of experimental design, time series designs do not have a comparison group. A time series is a set of measurements taken at intervals over a period of time (Figure 13.7). Proper time series design should include at least three pre- and post-intervention measurement points. While there are a few types of time series designs, we’re going to focus on the most common: interrupted time series design.

But why use this method? Here’s an example. Let’s think about elementary student behavior throughout the school year. As anyone with children or who is a teacher knows, kids get very excited and animated around holidays, days off, or even just on a Friday afternoon. This fact might mean that around those times of year, there are more reports of disruptive behavior in classrooms. What if we took our one and only measurement in mid-December? It’s possible we’d see a higher-than-average rate of disruptive behavior reports, which could bias our results if our next measurement is around a time of year students are in a different, less excitable frame of mind. When we take multiple measurements throughout the first half of the school year, we can establish a more accurate baseline for the rate of these reports by looking at the trend over time.
We may want to test the effect of extended recess times in elementary school on reports of disruptive behavior in classrooms. When students come back after the winter break, the school extends recess by 10 minutes each day (the intervention), and the researchers start tracking the monthly reports of disruptive behavior again. These reports could be subject to the same fluctuations as the pre-intervention reports, and so we once again take multiple measurements over time to try to control for those fluctuations.
This method improves the extent to which we can establish causality because we are accounting for a major extraneous variable in the equation—the passage of time. On its own, it does not allow us to account for other extraneous variables, but it does establish time order and association between the intervention and the trend in reports of disruptive behavior. Finding a stable condition before the treatment that changes after the treatment is evidence for causality between treatment and outcome.
Ex post facto comparison group design
Ex post facto (Latin for “after the fact”) designs are extremely similar to nonequivalent comparison group designs. There are still comparison and experimental groups, pretest and post-test measurements, and an intervention. But in ex post facto designs, participants are assigned to the comparison and experimental groups once the intervention has already happened. This type of design often occurs when interventions are already up and running at an agency and the agency wants to assess effectiveness based on people who have already completed treatment.
In most clinical agency environments, social workers conduct both initial and exit assessments, so there are usually some kind of pretest and post-test measures available. We also typically collect demographic information about our clients, which could allow us to try to use some kind of matching to construct comparison and experimental groups.
In terms of internal validity and establishing causality, ex post facto designs are a bit of a mixed bag. The ability to establish causality depends partially on the ability to construct comparison and experimental groups that are demographically similar so we can control for these extraneous variables .
Quasi-experimental designs are common in social work intervention research because, when designed correctly, they balance the intense resource needs of true experiments with the realities of research in practice. They still offer researchers tools to gather robust evidence about whether interventions are having positive effects for clients.
- Quasi-experimental designs are similar to true experiments, but do not require random assignment to experimental and control groups.
- In quasi-experimental projects, the group not receiving the treatment is called the comparison group, not the control group.
- Nonequivalent comparison group design is nearly identical to pretest/post-test experimental design, but participants are not randomly assigned to the experimental and control groups. As a result, this design provides slightly less robust evidence for causality.
- Nonequivalent groups can be constructed by individual matching or aggregate matching .
- Time series design does not have a control or experimental group, and instead compares the condition of participants before and after the intervention by measuring relevant factors at multiple points in time. This allows researchers to mitigate the error introduced by the passage of time.
- Ex post facto comparison group designs are also similar to true experiments, but experimental and comparison groups are constructed after the intervention is over. This makes it more difficult to control for the effect of extraneous variables, but still provides useful evidence for causality because it maintains the time order of the experiment.
- Think back to the experiment you considered for your research project in Section 13.3. Now that you know more about quasi-experimental designs, do you still think it’s a true experiment? Why or why not?
- What should you consider when deciding whether an experimental or quasi-experimental design would be more feasible or fit your research question better?
13.5 Non-experimental designs
- Describe non-experimental designs in social work research
- Discuss how non-experimental research differs from true and quasi-experimental research
- Demonstrate an understanding the different types of non-experimental designs
- Determine what kinds of research questions non-experimental designs are suited for
- Discuss advantages and disadvantages of non-experimental designs
The previous sections have laid out the basics of some rigorous approaches to establish that an intervention is responsible for changes we observe in research participants. This type of evidence is extremely important to build an evidence base for social work interventions, but it’s not the only type of evidence to consider. We will discuss qualitative methods, which provide us with rich, contextual information, in Part 4 of this text. The designs we’ll talk about in this section are sometimes used in qualitative research but in keeping with our discussion of experimental design so far, we’re going to stay in the quantitative research realm for now. Non-experimental is also often a stepping stone for more rigorous experimental design in the future, as it can help test the feasibility of your research.
In general, non-experimental designs do not strongly support causality and don’t address threats to internal validity. However, that’s not really what they’re intended for. Non-experimental designs are useful for a few different types of research, including explanatory questions in program evaluation. Certain types of non-experimental design are also helpful for researchers when they are trying to develop a new assessment or scale. Other times, researchers or agency staff did not get a chance to gather any assessment information before an intervention began, so a pretest/post-test design is not possible.
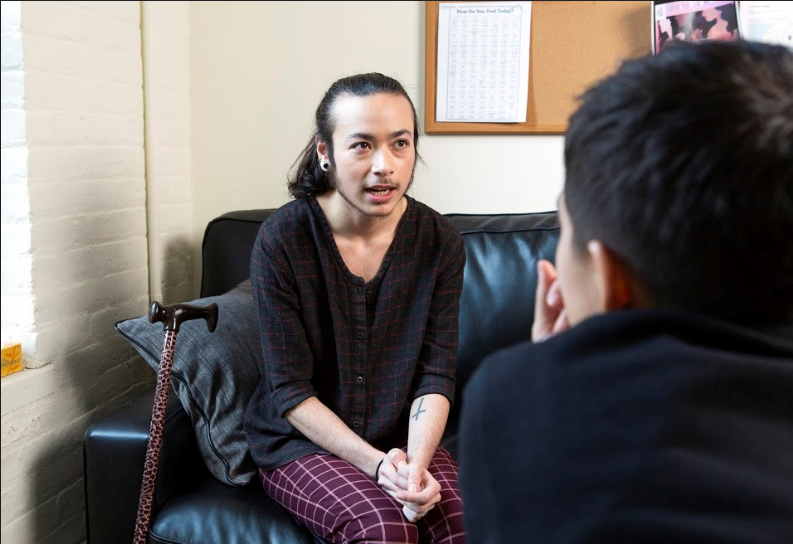
A significant benefit of these types of designs is that they’re pretty easy to execute in a practice or agency setting. They don’t require a comparison or control group, and as Engel and Schutt (2017) [3] point out, they “flow from a typical practice model of assessment, intervention, and evaluating the impact of the intervention” (p. 177). Thus, these designs are fairly intuitive for social workers, even when they aren’t expert researchers. Below, we will go into some detail about the different types of non-experimental design.
One group pretest/post-test design
Also known as a before-after one-group design, this type of research design does not have a comparison group and everyone who participates in the research receives the intervention (Figure 13.8). This is a common type of design in program evaluation in the practice world. Controlling for extraneous variables is difficult or impossible in this design, but given that it is still possible to establish some measure of time order, it does provide weak support for causality.

Imagine, for example, a researcher who is interested in the effectiveness of an anti-drug education program on elementary school students’ attitudes toward illegal drugs. The researcher could assess students’ attitudes about illegal drugs (O 1 ), implement the anti-drug program (X), and then immediately after the program ends, the researcher could once again measure students’ attitudes toward illegal drugs (O 2 ). You can see how this would be relatively simple to do in practice, and have probably been involved in this type of research design yourself, even if informally. But hopefully, you can also see that this design would not provide us with much evidence for causality because we have no way of controlling for the effect of extraneous variables. A lot of things could have affected any change in students’ attitudes—maybe girls already had different attitudes about illegal drugs than children of other genders, and when we look at the class’s results as a whole, we couldn’t account for that influence using this design.
All of that doesn’t mean these results aren’t useful, however. If we find that children’s attitudes didn’t change at all after the drug education program, then we need to think seriously about how to make it more effective or whether we should be using it at all. (This immediate, practical application of our results highlights a key difference between program evaluation and research, which we will discuss in Chapter 23 .)
After-only design
As the name suggests, this type of non-experimental design involves measurement only after an intervention. There is no comparison or control group, and everyone receives the intervention. I have seen this design repeatedly in my time as a program evaluation consultant for nonprofit organizations, because often these organizations realize too late that they would like to or need to have some sort of measure of what effect their programs are having.
Because there is no pretest and no comparison group, this design is not useful for supporting causality since we can’t establish the time order and we can’t control for extraneous variables. However, that doesn’t mean it’s not useful at all! Sometimes, agencies need to gather information about how their programs are functioning. A classic example of this design is satisfaction surveys—realistically, these can only be administered after a program or intervention. Questions regarding satisfaction, ease of use or engagement, or other questions that don’t involve comparisons are best suited for this type of design.
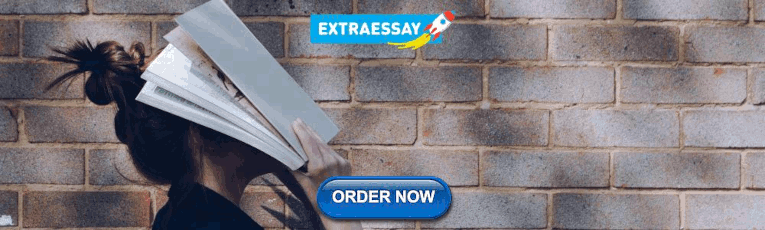
Static-group design
A final type of non-experimental research is the static-group design. In this type of research, there are both comparison and experimental groups, which are not randomly assigned. There is no pretest, only a post-test, and the comparison group has to be constructed by the researcher. Sometimes, researchers will use matching techniques to construct the groups, but often, the groups are constructed by convenience of who is being served at the agency.
Non-experimental research designs are easy to execute in practice, but we must be cautious about drawing causal conclusions from the results. A positive result may still suggest that we should continue using a particular intervention (and no result or a negative result should make us reconsider whether we should use that intervention at all). You have likely seen non-experimental research in your daily life or at your agency, and knowing the basics of how to structure such a project will help you ensure you are providing clients with the best care possible.
- Non-experimental designs are useful for describing phenomena, but cannot demonstrate causality.
- After-only designs are often used in agency and practice settings because practitioners are often not able to set up pre-test/post-test designs.
- Non-experimental designs are useful for explanatory questions in program evaluation and are helpful for researchers when they are trying to develop a new assessment or scale.
- Non-experimental designs are well-suited to qualitative methods.
- If you were to use a non-experimental design for your research project, which would you choose? Why?
- Have you conducted non-experimental research in your practice or professional life? Which type of non-experimental design was it?
13.6 Critical, ethical, and cultural considerations
- Describe critiques of experimental design
- Identify ethical issues in the design and execution of experiments
- Identify cultural considerations in experimental design
As I said at the outset, experiments, and especially true experiments, have long been seen as the gold standard to gather scientific evidence. When it comes to research in the biomedical field and other physical sciences, true experiments are subject to far less nuance than experiments in the social world. This doesn’t mean they are easier—just subject to different forces. However, as a society, we have placed the most value on quantitative evidence obtained through empirical observation and especially experimentation.
Major critiques of experimental designs tend to focus on true experiments, especially randomized controlled trials (RCTs), but many of these critiques can be applied to quasi-experimental designs, too. Some researchers, even in the biomedical sciences, question the view that RCTs are inherently superior to other types of quantitative research designs. RCTs are far less flexible and have much more stringent requirements than other types of research. One seemingly small issue, like incorrect information about a research participant, can derail an entire RCT. RCTs also cost a great deal of money to implement and don’t reflect “real world” conditions. The cost of true experimental research or RCTs also means that some communities are unlikely to ever have access to these research methods. It is then easy for people to dismiss their research findings because their methods are seen as “not rigorous.”
Obviously, controlling outside influences is important for researchers to draw strong conclusions, but what if those outside influences are actually important for how an intervention works? Are we missing really important information by focusing solely on control in our research? Is a treatment going to work the same for white women as it does for indigenous women? With the myriad effects of our societal structures, you should be very careful ever assuming this will be the case. This doesn’t mean that cultural differences will negate the effect of an intervention; instead, it means that you should remember to practice cultural humility implementing all interventions, even when we “know” they work.
How we build evidence through experimental research reveals a lot about our values and biases, and historically, much experimental research has been conducted on white people, and especially white men. [4] This makes sense when we consider the extent to which the sciences and academia have historically been dominated by white patriarchy. This is especially important for marginalized groups that have long been ignored in research literature, meaning they have also been ignored in the development of interventions and treatments that are accepted as “effective.” There are examples of marginalized groups being experimented on without their consent, like the Tuskegee Experiment or Nazi experiments on Jewish people during World War II. We cannot ignore the collective consciousness situations like this can create about experimental research for marginalized groups.
None of this is to say that experimental research is inherently bad or that you shouldn’t use it. Quite the opposite—use it when you can, because there are a lot of benefits, as we learned throughout this chapter. As a social work researcher, you are uniquely positioned to conduct experimental research while applying social work values and ethics to the process and be a leader for others to conduct research in the same framework. It can conflict with our professional ethics, especially respect for persons and beneficence, if we do not engage in experimental research with our eyes wide open. We also have the benefit of a great deal of practice knowledge that researchers in other fields have not had the opportunity to get. As with all your research, always be sure you are fully exploring the limitations of the research.
- While true experimental research gathers strong evidence, it can also be inflexible, expensive, and overly simplistic in terms of important social forces that affect the resources.
- Marginalized communities’ past experiences with experimental research can affect how they respond to research participation.
- Social work researchers should use both their values and ethics, and their practice experiences, to inform research and push other researchers to do the same.
- Think back to the true experiment you sketched out in the exercises for Section 13.3. Are there cultural or historical considerations you hadn’t thought of with your participant group? What are they? Does this change the type of experiment you would want to do?
- How can you as a social work researcher encourage researchers in other fields to consider social work ethics and values in their experimental research?
Media Attributions
- Being kinder to yourself © Evgenia Makarova is licensed under a CC BY-NC-ND (Attribution NonCommercial NoDerivatives) license
- Original by author is licensed under a CC BY-NC-SA (Attribution NonCommercial ShareAlike) license
- Original by author. is licensed under a CC BY-NC-SA (Attribution NonCommercial ShareAlike) license
- Orginal by author. is licensed under a CC BY-NC-SA (Attribution NonCommercial ShareAlike) license
- therapist © Zackary Drucker is licensed under a CC BY-NC-ND (Attribution NonCommercial NoDerivatives) license
- nonexper-pretest-posttest is licensed under a CC BY-NC-SA (Attribution NonCommercial ShareAlike) license
- Engel, R. & Schutt, R. (2016). The practice of research in social work. Thousand Oaks, CA: SAGE Publications, Inc. ↵
- Sullivan, G. M. (2011). Getting off the “gold standard”: Randomized controlled trials and education research. Journal of Graduate Medical Education , 3 (3), 285-289. ↵
an operation or procedure carried out under controlled conditions in order to discover an unknown effect or law, to test or establish a hypothesis, or to illustrate a known law.
explains why particular phenomena work in the way that they do; answers “why” questions
variables and characteristics that have an effect on your outcome, but aren't the primary variable whose influence you're interested in testing.
the group of participants in our study who do not receive the intervention we are researching in experiments with random assignment
in experimental design, the group of participants in our study who do receive the intervention we are researching
the group of participants in our study who do not receive the intervention we are researching in experiments without random assignment
using a random process to decide which participants are tested in which conditions
The ability to apply research findings beyond the study sample to some broader population,
Ability to say that one variable "causes" something to happen to another variable. Very important to assess when thinking about studies that examine causation such as experimental or quasi-experimental designs.
the idea that one event, behavior, or belief will result in the occurrence of another, subsequent event, behavior, or belief
An experimental design in which one or more independent variables are manipulated by the researcher (as treatments), subjects are randomly assigned to different treatment levels (random assignment), and the results of the treatments on outcomes (dependent variables) are observed
a type of experimental design in which participants are randomly assigned to control and experimental groups, one group receives an intervention, and both groups receive pre- and post-test assessments
A measure of a participant's condition before they receive an intervention or treatment.
A measure of a participant's condition after an intervention or, if they are part of the control/comparison group, at the end of an experiment.
A demonstration that a change occurred after an intervention. An important criterion for establishing causality.
an experimental design in which participants are randomly assigned to control and treatment groups, one group receives an intervention, and both groups receive only a post-test assessment
The measurement error related to how a test is given; the conditions of the testing, including environmental conditions; and acclimation to the test itself
a subtype of experimental design that is similar to a true experiment, but does not have randomly assigned control and treatment groups
In nonequivalent comparison group designs, the process by which researchers match individual cases in the experimental group to similar cases in the comparison group.
In nonequivalent comparison group designs, the process in which researchers match the population profile of the comparison and experimental groups.
a set of measurements taken at intervals over a period of time
Research that involves the use of data that represents human expression through words, pictures, movies, performance and other artifacts.
Graduate research methods in social work Copyright © 2021 by Matthew DeCarlo, Cory Cummings, Kate Agnelli is licensed under a Creative Commons Attribution-NonCommercial-ShareAlike 4.0 International License , except where otherwise noted.
Share This Book
Experimental Design: Types, Examples & Methods
Saul McLeod, PhD
Editor-in-Chief for Simply Psychology
BSc (Hons) Psychology, MRes, PhD, University of Manchester
Saul McLeod, PhD., is a qualified psychology teacher with over 18 years of experience in further and higher education. He has been published in peer-reviewed journals, including the Journal of Clinical Psychology.
Learn about our Editorial Process
Olivia Guy-Evans, MSc
Associate Editor for Simply Psychology
BSc (Hons) Psychology, MSc Psychology of Education
Olivia Guy-Evans is a writer and associate editor for Simply Psychology. She has previously worked in healthcare and educational sectors.
On This Page:
Experimental design refers to how participants are allocated to different groups in an experiment. Types of design include repeated measures, independent groups, and matched pairs designs.
Probably the most common way to design an experiment in psychology is to divide the participants into two groups, the experimental group and the control group, and then introduce a change to the experimental group, not the control group.
The researcher must decide how he/she will allocate their sample to the different experimental groups. For example, if there are 10 participants, will all 10 participants participate in both groups (e.g., repeated measures), or will the participants be split in half and take part in only one group each?
Three types of experimental designs are commonly used:
1. Independent Measures
Independent measures design, also known as between-groups , is an experimental design where different participants are used in each condition of the independent variable. This means that each condition of the experiment includes a different group of participants.
This should be done by random allocation, ensuring that each participant has an equal chance of being assigned to one group.
Independent measures involve using two separate groups of participants, one in each condition. For example:
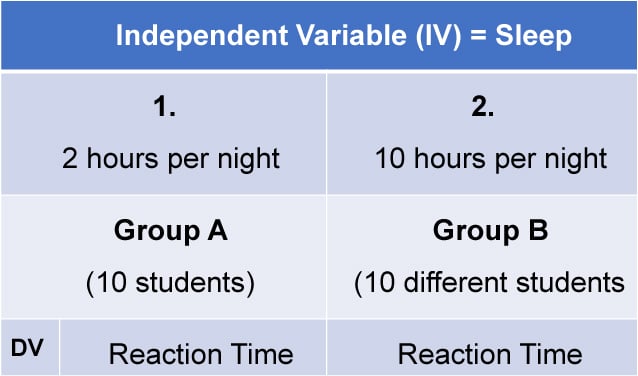
- Con : More people are needed than with the repeated measures design (i.e., more time-consuming).
- Pro : Avoids order effects (such as practice or fatigue) as people participate in one condition only. If a person is involved in several conditions, they may become bored, tired, and fed up by the time they come to the second condition or become wise to the requirements of the experiment!
- Con : Differences between participants in the groups may affect results, for example, variations in age, gender, or social background. These differences are known as participant variables (i.e., a type of extraneous variable ).
- Control : After the participants have been recruited, they should be randomly assigned to their groups. This should ensure the groups are similar, on average (reducing participant variables).
2. Repeated Measures Design
Repeated Measures design is an experimental design where the same participants participate in each independent variable condition. This means that each experiment condition includes the same group of participants.
Repeated Measures design is also known as within-groups or within-subjects design .
- Pro : As the same participants are used in each condition, participant variables (i.e., individual differences) are reduced.
- Con : There may be order effects. Order effects refer to the order of the conditions affecting the participants’ behavior. Performance in the second condition may be better because the participants know what to do (i.e., practice effect). Or their performance might be worse in the second condition because they are tired (i.e., fatigue effect). This limitation can be controlled using counterbalancing.
- Pro : Fewer people are needed as they participate in all conditions (i.e., saves time).
- Control : To combat order effects, the researcher counter-balances the order of the conditions for the participants. Alternating the order in which participants perform in different conditions of an experiment.
Counterbalancing
Suppose we used a repeated measures design in which all of the participants first learned words in “loud noise” and then learned them in “no noise.”
We expect the participants to learn better in “no noise” because of order effects, such as practice. However, a researcher can control for order effects using counterbalancing.
The sample would be split into two groups: experimental (A) and control (B). For example, group 1 does ‘A’ then ‘B,’ and group 2 does ‘B’ then ‘A.’ This is to eliminate order effects.
Although order effects occur for each participant, they balance each other out in the results because they occur equally in both groups.
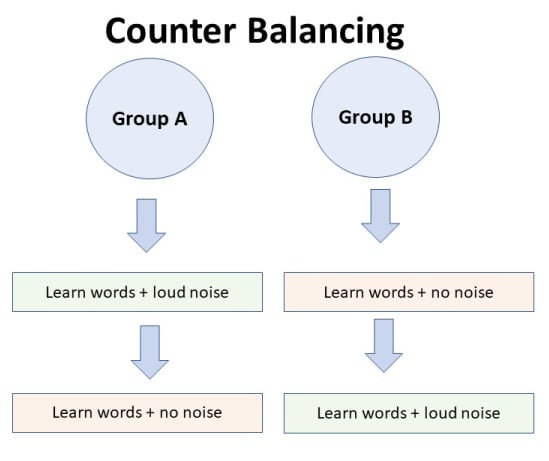
3. Matched Pairs Design
A matched pairs design is an experimental design where pairs of participants are matched in terms of key variables, such as age or socioeconomic status. One member of each pair is then placed into the experimental group and the other member into the control group .
One member of each matched pair must be randomly assigned to the experimental group and the other to the control group.
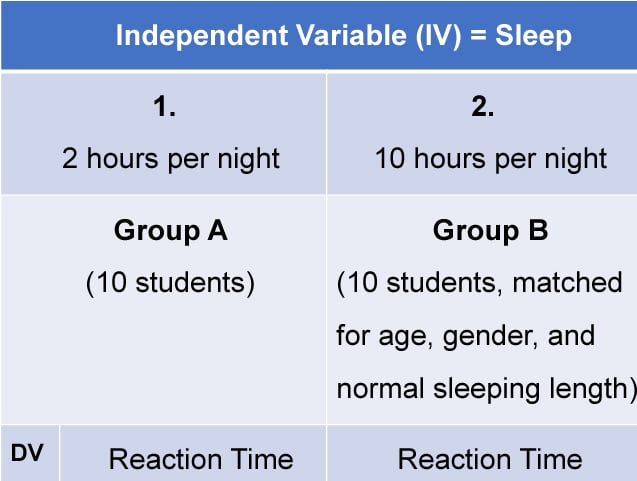
- Con : If one participant drops out, you lose 2 PPs’ data.
- Pro : Reduces participant variables because the researcher has tried to pair up the participants so that each condition has people with similar abilities and characteristics.
- Con : Very time-consuming trying to find closely matched pairs.
- Pro : It avoids order effects, so counterbalancing is not necessary.
- Con : Impossible to match people exactly unless they are identical twins!
- Control : Members of each pair should be randomly assigned to conditions. However, this does not solve all these problems.
Experimental design refers to how participants are allocated to an experiment’s different conditions (or IV levels). There are three types:
1. Independent measures / between-groups : Different participants are used in each condition of the independent variable.
2. Repeated measures /within groups : The same participants take part in each condition of the independent variable.
3. Matched pairs : Each condition uses different participants, but they are matched in terms of important characteristics, e.g., gender, age, intelligence, etc.
Learning Check
Read about each of the experiments below. For each experiment, identify (1) which experimental design was used; and (2) why the researcher might have used that design.
1 . To compare the effectiveness of two different types of therapy for depression, depressed patients were assigned to receive either cognitive therapy or behavior therapy for a 12-week period.
The researchers attempted to ensure that the patients in the two groups had similar severity of depressed symptoms by administering a standardized test of depression to each participant, then pairing them according to the severity of their symptoms.
2 . To assess the difference in reading comprehension between 7 and 9-year-olds, a researcher recruited each group from a local primary school. They were given the same passage of text to read and then asked a series of questions to assess their understanding.
3 . To assess the effectiveness of two different ways of teaching reading, a group of 5-year-olds was recruited from a primary school. Their level of reading ability was assessed, and then they were taught using scheme one for 20 weeks.
At the end of this period, their reading was reassessed, and a reading improvement score was calculated. They were then taught using scheme two for a further 20 weeks, and another reading improvement score for this period was calculated. The reading improvement scores for each child were then compared.
4 . To assess the effect of the organization on recall, a researcher randomly assigned student volunteers to two conditions.
Condition one attempted to recall a list of words that were organized into meaningful categories; condition two attempted to recall the same words, randomly grouped on the page.
Experiment Terminology
Ecological validity.
The degree to which an investigation represents real-life experiences.
Experimenter effects
These are the ways that the experimenter can accidentally influence the participant through their appearance or behavior.
Demand characteristics
The clues in an experiment lead the participants to think they know what the researcher is looking for (e.g., the experimenter’s body language).
Independent variable (IV)
The variable the experimenter manipulates (i.e., changes) is assumed to have a direct effect on the dependent variable.
Dependent variable (DV)
Variable the experimenter measures. This is the outcome (i.e., the result) of a study.
Extraneous variables (EV)
All variables which are not independent variables but could affect the results (DV) of the experiment. Extraneous variables should be controlled where possible.
Confounding variables
Variable(s) that have affected the results (DV), apart from the IV. A confounding variable could be an extraneous variable that has not been controlled.
Random Allocation
Randomly allocating participants to independent variable conditions means that all participants should have an equal chance of taking part in each condition.
The principle of random allocation is to avoid bias in how the experiment is carried out and limit the effects of participant variables.
Order effects
Changes in participants’ performance due to their repeating the same or similar test more than once. Examples of order effects include:
(i) practice effect: an improvement in performance on a task due to repetition, for example, because of familiarity with the task;
(ii) fatigue effect: a decrease in performance of a task due to repetition, for example, because of boredom or tiredness.


An official website of the United States government
The .gov means it’s official. Federal government websites often end in .gov or .mil. Before sharing sensitive information, make sure you’re on a federal government site.
The site is secure. The https:// ensures that you are connecting to the official website and that any information you provide is encrypted and transmitted securely.
- Publications
- Account settings
Preview improvements coming to the PMC website in October 2024. Learn More or Try it out now .
- Advanced Search
- Journal List
- HHS Author Manuscripts
- PMC10016625

The Family of Single-Case Experimental Designs
Leonard h. epstein.
1 Jacobs School of Medicine and Biomedical Sciences, Division of Behavioral Medicine, Department of Pediatrics, University at Buffalo, Buffalo, New York, United States of America,
Jesse Dallery
2 Department of Psychology, University of Florida, Gainesville, Florida, United States of America
Single-case experimental designs (SCEDs) represent a family of research designs that use experimental methods to study the effects of treatments on outcomes. The fundamental unit of analysis is the single case—which can be an individual, clinic, or community—ideally with replications of effects within and/or between cases. These designs are flexible and cost-effective and can be used for treatment development, translational research, personalized interventions, and the study of rare diseases and disorders. This article provides a broad overview of the family of single-case experimental designs with corresponding examples, including reversal designs, multiple baseline designs, combined multiple baseline/reversal designs, and integration of single-case designs to identify optimal treatments for individuals into larger randomized controlled trials (RCTs). Personalized N-of-1 trials can be considered a subcategory of SCEDs that overlaps with reversal designs. Relevant issues for each type of design—including comparisons of treatments, design issues such as randomization and blinding, standards for designs, and statistical approaches to complement visual inspection of single-case experimental designs—are also discussed.
1. Introduction
Single-case experimental designs (SCEDs) represent a family of experimental designs to examine the relationship between one or more treatments or levels of treatment and changes in biological or behavioral outcomes. These designs originated in early experimental psychology research ( Boring, 1929 ; Ebbinghaus, 1913 ; Pavlov, 1927 ), and were later expanded and formalized in the fields of basic and applied behavior analysis ( Morgan & Morgan, 2001 ; Sidman, 1960 ). SCEDs have been extended to a number of fields, including medicine ( Lillie et al., 2011 ; Schork, 2015 ), public health ( Biglan et al., 2000 ; Duan et al., 2013 ), education ( Horner et al., 2005 ), counseling psychology ( Lundervold & Belwood, 2000 ), clinical psychology ( Vlaeyen et al., 2020 ), health behavior ( McDonald et al., 2017 ), and neuroscience ( Soto, 2020 ).
SCEDs provide a framework to determine whether changes in a target behavior(s) or symptom are in fact a function of the intervention. The fundamentals of an SCED involve repeated measurement, replication of conditions (e.g., baseline and intervention conditions), and the analysis of effects with respect to each individual serving as his or her own control. This process can be useful for identifying the optimal treatment for an individual ( Dallery & Raiff, 2014 ; Davidson et al., 2021 ), treating rare diseases ( Abrahamyan et al., 2016 ), and implementing early phase translational research ( Czajkowski et al., 2015 ). SCEDs can be referred to as ‘personalized (N-of-1) trials’ when used this way, but they also have broad applicability to a range of scientific questions. Results from SCEDs can be aggregated using meta-analytic techniques to establish generalizable methods and treatment guidelines ( Shadish, 2014 ; Vannest et al., 2018 ). Figure 1 presents the main family of SCEDs, and shows how personalized (N-of-1) trials fit into these designs ( Vohra et al., 2016 ). The figure also distinguishes between experimental and nonexperimental single-case designs. In the current article, we provide an overview of SCEDs and thus a context for the articles in this special issue focused on personalized (N-of-1) trials. Our focus is to provide the fundamentals of these designs, and more detailed treatments of data analysis ( Moeyaert & Fingerhut, 2022 ; Schork, 2022 ) conduct and reporting standards ( Kravitz & Duan, 2022 ; Porcino & Vohra, 2022 ), and other methodological considerations are provided in this special issue. Our hope is that this article will inspire a diverse array of students, engineers, scientists, and practitioners to further explore the utility, rigor, and flexibility of these designs.

A = Baseline, B and C refer to different treatments.
The most common approach to evaluating the effectiveness of interventions on outcomes is using randomized controlled trials (RCTs). RCTs provide an idea of the average effect of an intervention on outcomes. People do not all change at the same rate or in the same way, however; variability in both how people change and the effect of the intervention is inevitable ( Fisher et al., 2018 ; Normand, 2016 ; Roustit et al., 2018 ). These sources of variability are conflated in a typical RCT, leading to heterogeneity of treatment effects (HTE). Research on HTE has shown variability in outcomes in RCTs, and in some studies very few people actually exhibit the benefits of that treatment ( Williams, 2010 ). One approach in RCTs is to assess moderators of treatment response to identify individual differences that may predict response to a treatment. This approach may not limit variability in response, and substantial reduction in variability of treatment for subgroups in comparison to the group as a whole is far from assured. Even if variability is reduced, the average effect for that subgroup may not be representative of individual members of the subgroup.
SCEDs can identify the optimal treatment for an individual person rather than the average person in a group ( Dallery & Raiff, 2014 ; Davidson et al., 2021 ; Hekler et al., 2020 ). SCEDs are multiphase experimental designs in which a great deal of data is collected on a single person, said person serves as his or her own control ( Kazdin, 2011 , 2021 ), and the order of presentation of conditions can be randomized to enhance experimental control. That is, a person’s outcomes in one phase are compared to outcomes in another phase. In a typical study, replications are achieved within and/or across several individuals; this allows for strong inferences about causation between behavior and the treatment (or levels thereof). Achieving replications is synonymous with achieving experimental control.
We provide an overview of three experimental designs that can be adapted for personalized medicine: reversal, multiple baseline, and combined reversal and multiple baseline designs, and we discuss how SCEDs can be integrated into RCTs. These designs focus on demonstrating experimental control of the relationship between treatment and outcome. Several general principles common to all of the designs are noteworthy ( Lobo et al., 2017 ). First, in many studies, treatment effects are compared with control conditions with a no- intervention baseline as the initial condition. To reduce threats to internal validity of the study, the order of assignment of interventions can be randomized ( Kratochwill & Levin, 2010 ) and, when possible, the intervention and data collection can be blinded. The demonstration of experimental control across conditions or people needs to be replicated several times (three replications is the minimum) to ensure confidence of the relationship between treatment and outcome ( Kratochwill et al., 2010 ; Kratochwill & Levin, 2015 ). Demonstrating stability of data within a phase or, otherwise, no trend in the direction of treatment effects prior to starting treatment is particularly important. Stability refers to the degree of variability in the data path over time (e.g., data points must fall within a 15% range of the median for a condition). Thus, phase length needs to be flexible for the sake of determining stability and trend within a phase, but a minimum of 5 data points per phase has been recommended ( Kratochwill et al., 2013 ). The focus of the intervention’s effects is on clinically rather than statistically significant effects with the target effect prespecified and considered in interpretation of the relevance of the effect for clinical practice ( Epstein et al., 2021 ). In addition, multiple dependent outcomes can be simultaneously measured ( Epstein et al., 2021 ). SCEDs can be used to test whether a variable mediates the effect of a treatment on symptoms or behavior ( Miočević et al., 2020 ; Riley & Gaynor, 2014 ). Visual inspection of graphical data is typically used to determine treatment effects, and statistical methods are commonly used to assist in interpretation of graphical data ( Epstein et al., 2021 ). Furthermore, a growing number of statistical approaches can summarize treatment effects and provide effect sizes ( Kazdin, 2021 ; Moeyaert & Fingerhut, this issue; Pustejovsky, 2019 ; Shadish et al., 2014 ). Data across many SCED trials can be aggregated to assess the generality of the treatment effects to help address for whom and under what conditions an intervention is effective ( Branch & Pennypacker, 2013 ; Shadish, 2014 ; Van den Noortgate & Onghena, 2003 ).
2. Reversal Designs
A reversal design collects behavioral or biological outcome data in at least two phases: a baseline or no treatment phase (labeled as ‘A’) and the experimental or treatment phase (labeled as ‘B’). The design is called a reversal design because there must be reversals or replications of phases for each individual; for example, in an ABA design, the baseline phase is replicated ( Kazdin, 2011 ). Ideally, three replications of treatment effects are used to demonstrate experimental control ( Kratochwill et al., 2010 ; Kratochwill & Levin, 1992 ). Figure 2 shows hypothetical results from an A1B1A2B2 design. The graph shows three replications of treatment effects (A1 versus B1, B1 versus A2, A2 versus B2) across four participants. Each phase was carried out until stability was evident from visual inspection of the data as well as absence of trends in the direction of the desired effect. The replication across participants increases the confidence in the effectiveness of the intervention. Extension of this design is possible by comparing multiple interventions, as well. The order of the treatments should be randomized, especially when the goal is to combine SCEDs across participants.

A1 = First Baseline, B1 First Treatment, A2 = Return to Baseline, B2 = Return to Treatment. P1–P4 represent different hypothetical participants.
Reversal designs can be more dynamic and compare several treatments. A common approach in personalized medicine would be to compare two or more doses of or different components of the same treatment ( Ward-Horner & Sturmey, 2010 ). For example, two drug doses could be compared using an A1B1C1B2C2 design, where A represents placebo and B and C represent the different drug doses ( Guyatt et al., 1990 ). In the case of drug studies, the drug/placebo administration can be double blinded. A more complex design could be A1B1A2C1A3C2A4B2, which would yield multiple replications of the comparison between drug and placebo. Based on the kinetics of the drug and the need for a washout period, the design could also be A1B1C1B2C2. This would provide three demonstration of treatment effects: B1 to C1, C1 to B2, and B2 to C2. Other permutations could be planned strategically to identify the optimal dose for each individual.
Advantages of SCED reversal designs are their ability to experimentally show that a particular treatment was functionally related to a particular change in an outcome variable for that person . This is the core principle of personalized medicine: an optimal treatment for an individual can be identified ( Dallery & Raiff, 2014 ; Davidson et al., 2021 ; Guyatt et al., 1990 ; Hekler et al., 2020 ; Lillie et al., 2011 ). These designs can work well for studying the effect of interventions on rare diseases in which collecting enough participants with similar characteristics for an RCT would be unlikely. An additional strength is the opportunity for the clinical researcher who also delivers clinical care to translate basic science findings or new findings from RCTs to their patients, who can potentially benefit ( Dallery & Raiff, 2014 ; Hayes, 1981 ). Research suggests that the trickledown of new developments and hypotheses to their support in RCTs can take more than 15 years; many important advancements in the medical and behavior sciences are likely not to be implemented rapidly enough ( Riley et al., 2013 ). The ability to test new intervention developments using scientific principles could speed up their translation into practice.
Limitations to SCED designs, however, are worth noting. Firstly, in line with the expectation that the outcome returns to baseline levels, reversals may require removal of the treatment. If the effect is not quickly reversible, then the designs are not relevant. A washout period may be placed in-between phases if the effect is not immediately reversible; for example, a drug washout period could be planned based on the half-life of drug. Secondly, the intervention should have a relatively immediate effect on the outcome. If many weeks to months are needed for some interventions to show effects, a reversal design may not be optimal unless the investigator is willing to plan a lengthy study. Thirdly, the design depends on comparing stable data over conditions. If achieving stability due to uncontrolled sources of biological or environmental variation is not possible, a reversal design may not be appropriate to evaluate a treatment, though it may be useful to identify the sources of variability ( Sidman, 1960 ). Finally, for a reversal to a baseline, a no-treatment phase may be inappropriate in investigating treatment effects for a very ill patient.
3. Multiple Baseline Designs
An alternative to a reversal design is the multiple baseline design, which does not require reversal of conditions to establish experimental control. There are three types of multiple baseline designs: multiple baseline across people, behaviors, and settings. The most popular is the multiple baseline across people, in which baselines are established for three or more people for the same outcome ( Cushing et al., 2011 ; Meredith et al., 2011 ). Treatment is implemented after different durations of baseline across individuals. The order of treatment implementation across people can be randomized ( Wen et al., 2019 ). Figure 3 shows an example across three individuals. In this hypothetical example, baseline data for each person are relatively stable and not decreasing, and reductions in the dependent variable are only observed after introduction of the intervention. Inclusion of one control person, who remains in baseline throughout the study and provides a control for extended monitoring, is also possible. Another variation is to collect baseline data intermittently in a ‘probe’ design, which can minimize burden associated with simultaneous and repeated measurement of outcomes ( Byiers et al., 2012 ; Horner & Baer, 1978 ). If the outcomes do not change during baseline conditions and the changes only occur across participants after the treatment has been implemented—and this sequence is replicated across several people—change in the outcome may be safely attributed to the treatment. The length of the baselines still must be long enough to show stability and no trend toward improvement until the treatment is implemented.

P1–P3 represent different hypothetical participants.
The two other multiple baseline designs focus on individual people: the multiple baseline across settings and the multiple baseline across behaviors ( Boles et al., 2008 ; Lane-Brown & Tate, 2010 ). An example of a multiple baseline across settings would be a dietary intervention implemented across meals. An intervention that targets a reduction in consumption of high–glycemic index foods, or foods with added sugar across meals, could be developed with the order of meals randomized. For example, someone may be randomized to reduce sugar-added or high–glycemic index foods for breakfast without any implementation at lunch or dinner. Implementation of the diet at lunch and then dinner would occur after different durations of baselines in these settings. An example of multiple baseline across behaviors might be to use feedback to develop a comprehensive exercise program that involves stretching, aerobic exercise, and resistance training. Feedback could target improvement in one of these randomly selected behaviors, implemented in a staggered manner.
The main limitation to a multiple baseline design is that some people (or behaviors) may be kept in baseline or control conditions for extended periods before treatment is implemented. Of course, failure to receive an effective treatment is common in RCTs for people who are randomized to control conditions, but unlike control groups in RCTs, all participants eventually receive treatment.
Finally, while the emphasis in personalized medicine is the identification of an optimal treatment plan for an individual person, situations in which multiple baselines across people prove relevant for precision medicine may arise. For example, identification of a small group of people with common characteristics—perhaps with a rare disease and for which a multiple-baseline-across-people design could be used to test an intervention more effectively than a series of personalized designs—is possible. In a similar vein, differential response to a common treatment in a multiple-baseline-across-people design can help to identify individual differences that can compromise the response to a treatment.
4. Integrating Multiple Baseline and Reversal Designs
While reversal designs can be used to compare effects of interventions, multiple baseline designs provide experimental control for testing one intervention but do not compare different interventions. One way to take advantage of the strengths of both designs is to combine them. For example, the effects of a first treatment could be studied using a multiple-baseline format and, after experimental control has been established, return to baseline prior to the commencement of a different treatment, which may be introduced in a different order. These comparisons can be made for several different interventions with the combination of both designs to demonstrate experimental control and compare effects of the interventions.
Figure 4 shows a hypothetical example of a combined approach to identify the best drug to decrease blood pressure. Baseline blood pressures are established for three people under placebo conditions before new drug X is introduced across participants in a staggered fashion to establish relative changes in blood pressure. All return to placebo after blood pressures reach stability, drug Y is introduced in a staggered sequence, participants are returned to placebo, and the most effective intervention for each individual (drug X or Y) is reintroduced to replicate the most important result: the most effective medication. This across-subjects design establishes experimental control for two different new drug interventions across three people while also establishing experimental control for five comparisons within subjects (placebo—drug X, drug Y—placebo, placebo—drug Y, drug Y—placebo, placebo—more effective drug). Though this combined design strengthens confidence beyond either reversal or multiple baseline designs, in many situations, experimental control demonstrated using a reversal design is sufficient.

BL = Baseline. Drug X and Drug Y represent hypothetical drugs to lower blood pressure, and Best Drug represents a reversal to the most effective drug as identified for each hypothetical participant, labeled P1–P3.
5. Other Varieties of Single-Case Experimental Designs
Other less commonly used designs within the family of SCEDs may be useful for personalized medicine. One of the most relevant may be the alternating treatment design ( Barlow & Hayes, 1979 ; Manolov et al., 2021 ), in which people are exposed to baseline and one or more treatments for very brief periods without the concern about stability before changing conditions. While the treatment period may be short, many more replications of treatments—and ineffective treatments—can be identified quickly. This type of design may be relevant for drugs that have rapid effects with a short half-life and behavioral interventions that have rapid effects ( Coyle & Robertson, 1998 )—for example, the effects of biofeedback on heart rate ( Weems, 1998 ). Another design is the changing criterion design, in which experimental control is demonstrated when the outcome meets certain preselected criteria that can be systematically increased or decreased over time ( Hartmann & Hall, 1976 ). The design is especially useful when learning a new skill or when outcomes change slowly over time ( Singh & Leung, 1988 )—for example, gradually increasing the range of foods chosen in a previously highly selective eater ( Russo et al., 2019 ).
6. Integrating Single-Case Experimental Designs Into Randomized Controlled Trials
SCEDs can be integrated into RCTs to compare the efficacy of treatments chosen for someone based on SCEDs versus a standardized or usual care treatment ( Epstein et al., 2021 ; Schork & Goetz, 2017 ). Such innovative designs may capture the best in SCEDs and randomized controlled designs. Kravitz et al. (2018) used an RCT in which one group ( n = 108) experienced a series of reversal AB conditions, or a personalized (N-of-1) trial. The specific conditions were chosen for each patient from among eight categories of treatments to reduce chronic musculoskeletal pain (e.g., acetaminophen, any nonsteroidal anti-inflammatory drug, acetaminophen/oxycodone, tramadol). The other group ( n = 107) received usual care. The study also incorporated mobile technology to record pain-related data daily (see Dallery et al., 2013 , for a discussion of technology and SCEDs). The results suggested that the N-of-1 approach was feasible and acceptable, but it did not yield statistically significant superior results in pain measures compared to the usual care group. However, as noted by Vohra and Punja (2019) , the results do not indicate a flaw in the methodological approach: finding that two treatments do not differ in superiority is a finding worth knowing.
Another example of a situation where an integrated approach may be useful is selecting a diet for weight control. Many diets for weight control that vary in their macronutrient intake—such as low carb, higher fat versus low fat, and higher carb—have their proponents and favorable biological mechanisms. However, direct comparisons of these diets basically show that they achieve similar weight control with large variability in outcome. Thus, while the average person on a low-fat diet does about the same as the average person on a lowcarb diet, some people on the low-carb diet do very well, while some fail. Some of the people who fail on the low-fat diet would undoubtedly do well on the low-carb diet, and some who fail on the low-fat diet would do well on the low-carb diet. Further, some would fail on both diets due to general problems in adherence.
Personalized medicine suggests that diets should be individualized to achieve the best results. SCEDs would be one way to show ‘proof of concept’ that a particular diet is better than a standard healthy diet. First, people would be randomized to experimental (including SCEDs) or control (not basing diet on SCEDs). Subject selection criteria would proceed as in any RCT. For the first 3 months, people in the experimental group would engage in individual reversal designs in which 2-week intervals of low-carb and low-fat diets would be interspersed with their usual eating, and weight loss, diet adherence, food preferences, and the reinforcing value of foods in the diet would be measured to assess biological, behavioral, and subjective changes.
Participants in the control group would experience a similar exposure to the different types of diets, but the diet to which they are assigned would be randomly chosen rather than chosen using SCED methods. In this way, they would have similar exposure to diets during the first 3 months of the study, but this experience would not impact group assignment. As with any RCT, the study would proceed with regular measures (e.g., 6, 12, 24 months) and the hypothesis that those assigned to a diet that results in better initial weight loss, and that they like and are motivated to continue, would do better than those receiving a randomly selected diet. The study could also be designed with three groups: a single-case design experimental group similar to the approach in the hypothetical study above and two control groups, one low-fat and one low-carb.
An alternative design would be to have everyone experience SCEDs for the first 3 months and then be randomized to either the optimal treatment identified during the first 3 months or an intervention randomly chosen among the interventions to be studied. This design has the advantage of randomization being after 3 months of study so that dropouts and non-adherers within the first 3 months would not be randomized in an intent-to-treat format.
The goal of either hypothesized study, or any study that attempts to incorporate SCEDs into RCTs, is that matching participants to treatments will provide superior results in comparison to providing the same treatment to everyone in a group. Two hypotheses can be generated in these types of designs: first, that the mean changes will differ between groups, and second, that the variability will differ between groups with less variability in outcome for people who have treatment selected after a single-case trial than people who have a treatment randomly selected. A reduction in variability plus mean differences in outcome should increase the effect size for people treated using individualized designs, increase power, and allow for a smaller sample size to ensure confidence about the differences observed between groups.
7. Limitations of Single-Case Experimental Designs
Single-case experimental designs have their common limitations. If a measure changes with repeated testing without intervention, it may not be useful for an SCED unless steps can be taken to mitigate such reactivity, such as more unobtrusive monitoring ( Kazdin, 2021 ). Given that the effects of interventions are evaluated over time, systematic environmental changes or maturation could influence the relationship between a treatment and outcome and thereby obscure the effect of a treatment. However, the design logic of reversal and multiple baseline designs largely control for such influences. Since SCEDs rely on repeated measures and a detailed study of the relationship between treatment and outcome, studies that use dependent measures that cannot be sampled frequently are not candidates for SCEDs. Likewise, the failure to identify a temporal relationship between the introduction of treatment and initiation of change in the outcome can make attribution of changes to the intervention challenging. A confounding variable’s association with introduction or removal of the intervention, which may cause inappropriate decisions about the effects of the intervention, is always possible. Dropout or uncontrolled events that occur to individuals can introduce confounding variables to the SCED. These problems are not unique to SCEDs and also occur with RCTs.
8. Single-Case Experimental Designs in Early Stage Translational Research
The emphasis of a research program may be on translating basic science findings to clinical interventions. The goal may be to collect early phase translational research as a step toward a fully powered RCT—( Epstein et al., 2021 ). The fact that a large amount of basic science does not get translated into clinical interventions is well known ( Butler, 2008 ; Seyhan, 2019 ); this served in part as the stimulus for the National Institutes of Health (NIH) to develop a network of clinical and translational science institutes in medical schools and universities throughout the United States. A common approach to early phase translational research is to implement a small, underpowered RCT to secure a ‘signal’ of a treatment effect and an effect size. This is a problematic approach to pilot research, and it is not advocated by the NIH as an approach to early phase translational research ( National Center for Complementary and Integrative Health, 2020 ). The number of participants needed for a fully powered RCT may be substantially different from the number projected from a small-sample RCT. These small, underpowered, early phase translational studies may provide too large an estimate of an effect size, leading to an underpowered RCT. Likewise, a small-sample RCT can lead to a small effect size that can, in turn, lead to a failure to implement a potentially effective intervention ( Kraemer et al., 2006 ). Therefore, SCEDs—especially reversal and multiple baseline designs—are evidently ideally suited to early phase translational research. This use complements the utility of SCEDs for identifying the optimal treatment for an individual or small group of individuals.
9. Conclusion
Single-case experimental designs provide flexible, rigorous, and cost-effective approaches that can be used in personalized medicine to identify the optimal treatment for an individual patient. SCEDs represent a broad array of designs, and personalized (N-of-1) designs are a prominent example, particularly in medicine. These designs can be incorporated into RCTs, and they can be integrated using meta-analysis techniques. SCEDs should become a standard part of the toolbox for clinical researchers to improve clinical care for their patients, and they can lead to the next generation of interventions that show maximal effects for individual cases as well as for early phase translational research to clinical practice.
Acknowledgments
We thank Lesleigh Stinson and Andrea Villegas for preparing the figures.
Disclosure Statement
Preparation of this special issue was supported by grants R01LM012836 from the National Library of Medicine of the National Institutes of Health and P30AG063786 from the National Institute on Aging of the National Institutes of Health. Funding to authors of this article was supported by grants U01 HL131552 from the National Heart, Lung, and Blood Institute, UH3 DK109543 from the National Institute of Diabetes, Digestive and Kidney Diseases, and RO1HD080292 and RO1HD088131 from the Eunice Kennedy Shriver National Institute of Child Health and Human Development. The funders had no role in the design and conduct of the study; collection, management, analysis, and interpretation of the data; preparation, review, or approval of the manuscript; or decision to submit the manuscript for publication. The views expressed in this paper are those of the authors and do not represent the views of the National Institutes of Health, the U.S. Department of Health and Human Services, or any other government entity.
- Abrahamyan L, Feldman BM, Tomlinson G, Faughnan ME, Johnson SR, Diamond IR, & Gupta S (2016). Alternative designs for clinical trials in rare diseases . American Journal of Medical Genetics, Part C: Seminars in Medical Genetics , 172 ( 4 ), 313–331. 10.1002/ajmg.c.31533 [ PubMed ] [ CrossRef ] [ Google Scholar ]
- Barlow DH, & Hayes SC (1979). Alternating treatments design: One strategy for comparing the effects of two treatments in a single subject . Journal of Applied Behavior Analysis , 12 ( 2 ), 199–210. 10.1901/jaba.1979.12-199 [ PMC free article ] [ PubMed ] [ CrossRef ] [ Google Scholar ]
- Biglan A, Ary D, & Wagenaar AC (2000). The value of interrupted time-series experiments for community intervention research . Prevention Science , 1 ( 1 ), 31–49. 10.1023/a:1010024016308 [ PMC free article ] [ PubMed ] [ CrossRef ] [ Google Scholar ]
- Boles RE, Roberts MC, & Vernberg EM (2008). Treating non-retentive encopresis with rewarded scheduled toilet visits . Behavior Analysis in Practice , 1 ( 2 ), 68–72. 10.1007/bf03391730 [ PMC free article ] [ PubMed ] [ CrossRef ] [ Google Scholar ]
- Boring EG (1929). A history of experimental psychology . Appleton-Century-Crofts. [ Google Scholar ]
- Branch MN, & Pennypacker HS (2013). Generality and generalization of research findings. In Madden GJ, Dube WV, Hackenberg TD, Hanley GP, & Lattal KA (Eds.), APA handbook of behavior analysis, Vol. 1. Methods and principles (pp. 151–175). American Psychological Association. 10.1037/13937-007 [ CrossRef ] [ Google Scholar ]
- Butler D (2008). Translational research: Crossing the valley of death . Nature , 453 ( 7197 ), 840–842. 10.1038/453840a [ PubMed ] [ CrossRef ] [ Google Scholar ]
- Byiers BJ, Reichle J, & Symons FJ (2012). Single-subject experimental design for evidence-based practice . American Journal of Speech-Language Pathology , 21 ( 4 ), 397–414. 10.1044/1058-0360(2012/11-0036) [ PMC free article ] [ PubMed ] [ CrossRef ] [ Google Scholar ]
- Coyle JA, & Robertson VJ (1998). Comparison of two passive mobilizing techniques following Colles’ fracture: A multi-element design . Manual Therapy , 3 ( 1 ), 34–41. 10.1054/math.1998.0314 [ PubMed ] [ CrossRef ] [ Google Scholar ]
- Cushing CC, Jensen CD, & Steele RG (2011). An evaluation of a personal electronic device to enhance self-monitoring adherence in a pediatric weight management program using a multiple baseline design . Journal of Pediatric Psychology , 36 ( 3 ), 301–307. 10.1093/jpepsy/jsq074 [ PubMed ] [ CrossRef ] [ Google Scholar ]
- Czajkowski SM, Powell LH, Adler N, Naar-king S, Reynolds KD, Hunter CM, Laraia B, Olster DH, Perna FM, Peterson JC, Epel E, Boyington JE, Charlson ME, Related O, Czajkowski SM, Powell LH, Adler N, Reynolds KD, Hunter CM, … Boyington JE (2015). From ideas to efficacy: The ORBIT model for developing behavioral treatments for chronic diseases . Health Psychology , 34 ( 10 ), 971–982. 10.1037/hea0000161 [ PMC free article ] [ PubMed ] [ CrossRef ] [ Google Scholar ]
- Dallery J, Cassidy RN, & Raiff BR (2013). Single-case experimental designs to evaluate novel technology-based health interventions . Journal of Medical Internet Research , 15 ( 2 ), Article e22. 10.2196/jmir.2227 [ PMC free article ] [ PubMed ] [ CrossRef ] [ Google Scholar ]
- Dallery J, & Raiff BR (2014). Optimizing behavioral health interventions with single-case designs: From development to dissemination . Translational Behavioral Medicine , 4 ( 3 ), 290–303. 10.1007/s13142-014-0258-z [ PMC free article ] [ PubMed ] [ CrossRef ] [ Google Scholar ]
- Davidson KW, Silverstein M, Cheung K, Paluch RA, & Epstein LH (2021). Experimental designs to optimize treatments for individuals . JAMA Pediatrics , 175 ( 4 ), 404–409. 10.1001/jamapediatrics.2020.5801 [ PMC free article ] [ PubMed ] [ CrossRef ] [ Google Scholar ]
- Duan N, Kravitz RL, & Schmid CH (2013). Single-patient (n-of-1) trials: A pragmatic clinical decision methodology for patient-centered comparative effectiveness research . Journal of Clinical Epidemiology , 66 ( 8 Suppl ), S21–S28. 10.1016/j.jclinepi.2013.04.006 [ PMC free article ] [ PubMed ] [ CrossRef ] [ Google Scholar ]
- Ebbinghaus H (1913). Memory; A contribution to experimental psychology . Teachers College, Columbia University. [ Google Scholar ]
- Epstein LH, Bickel WK, Czajkowski SM, Paluch RA, Moeyaert M, & Davidson KW (2021). Single case designs for early phase behavioral translational research in health psychology . Health Psychology , 40 ( 12 ), 858–874. 10.1037/hea0001055 [ PMC free article ] [ PubMed ] [ CrossRef ] [ Google Scholar ]
- Fisher AJ, Medaglia JD, & Jeronimus BF (2018). Lack of group-to-individual generalizability is a threat to human subjects research . Proceedings of the National Academy of Sciences of the United States of America , 115 ( 27 ), E6106–E6115. 10.1073/pnas.1711978115 [ PMC free article ] [ PubMed ] [ CrossRef ] [ Google Scholar ]
- Guyatt GH, Heyting A, Jaeschke R, Keller J, Adachi JD, & Roberts RS (1990). N of 1 randomized trials for investigating new drugs . Controlled Clinical Trials , 11 ( 2 ), 88–100. 10.1016/0197-2456(90)90003-k [ PubMed ] [ CrossRef ] [ Google Scholar ]
- Hartmann D, & Hall RV (1976). The changing criterion design . Journal of Applied Behavior Analysis , 9 ( 4 ), 527–532. 10.1901/jaba.1976.9-527 [ PMC free article ] [ PubMed ] [ CrossRef ] [ Google Scholar ]
- Hayes SC (1981). Single case experimental design and empirical clinical practice . Journal of Consulting and Clinical Psychology , 49 ( 2 ), 193–211. 10.1037/0022-006X.49.2.193 [ PubMed ] [ CrossRef ] [ Google Scholar ]
- Hekler E, Tiro JA, Hunter CM, & Nebeker C (2020). Precision health: The role of the social and behavioral sciences in advancing the vision . Annals of Behavioral Medicine , 54 ( 11 ), 805–826. 10.1093/abm/kaaa018 [ PMC free article ] [ PubMed ] [ CrossRef ] [ Google Scholar ]
- Horner RD, & Baer DM (1978). Multiple-probe technique: A variation on the multiple baseline1 . Journal of Applied Behavior Analysis , 11 ( 1 ), 189–196. 10.1901/jaba.1978.11-189 [ PMC free article ] [ PubMed ] [ CrossRef ] [ Google Scholar ]
- Horner RH, Carr EG, Halle J, McGee G, Odom S, & Wolery M (2005). The use of single-subject research to identify evidence-based practice in special education . Exceptional Children , 71 ( 2 ), 165–179. https://search.ebscohost.com/login.aspx?direct=true&db=psyh&AN=2004-22378-004&site=ehost-live [ Google Scholar ]
- Kazdin AE (2011). Single-case research designs: Methods for clinical and applied settings. In Single-case research designs: Methods for clinical and applied settings (2nd ed.). Oxford University Press. https://search.ebscohost.com/login.aspx?direct=true&db=psyh&AN=2010-18971-000&site=ehost-live [ Google Scholar ]
- Kazdin AE (2021). Single-case experimental designs: Characteristics, changes, and challenges . Journal of the Experimental Analysis of Behavior , 115 ( 1 ), 56–85. 10.1002/jeab.638 [ PubMed ] [ CrossRef ] [ Google Scholar ]
- Kraemer HC, Mintz J, Noda A, Tinklenberg J, & Yesavage JA (2006). Caution regarding the use of pilot studies to guide power calculations for study proposals . In Archives of General Psychiatry , 63 ( 5 ), 484–489. 10.1001/archpsyc.63.5.484 [ PubMed ] [ CrossRef ] [ Google Scholar ]
- Kratochwill TR, Hitchcock J, Horner RH, Levin JR, Odom SL, Rindskopf DM, & Shadish WR (2010). Single-case designs technical documentation . What Works Clearinghouse. [ Google Scholar ]
- Kratochwill TR & Levin JR (1992). Single-case research design and analysis: New directions for psychology and education . Lawrence Erlbaum. [ Google Scholar ]
- Kratochwill TR, & Levin JR (2010). Enhancing the scientific credibility of single-case intervention research: Randomization to the rescue . Psychological Methods , 15 ( 2 ), 124–144. 10.1037/a0017736 [ PubMed ] [ CrossRef ] [ Google Scholar ]
- Kratochwill TR, & Levin JR (2015). Single-case research design and analysis: New directions for psychology and education . Routledge. 10.4324/9781315725994 [ CrossRef ] [ Google Scholar ]
- Kratochwill TR, Hitchcock JH, Horner RH, Levin JR, Odom SL, Rindskopf DM, & Shadish WR (2013). Single-case intervention research design standards . Remedial and Special Education , 34 ( 1 ), 26–38. 10.1177/0741932512452794 [ CrossRef ] [ Google Scholar ]
- Kravitz R, & Duan N (2022). Conduct and implementation of personalized trials in research and practice . Harvard Data Science Review , ( Special Issue 3 ). 10.1162/99608f92.901255e7 [ CrossRef ] [ Google Scholar ]
- Kravitz RL, Schmid CH, Marois M, Wilsey B, Ward D, Hays RD, Duan N, Wang Y, MacDonald S, Jerant A, Servadio JL, Haddad D, & Sim I (2018). Effect of mobile device-supported single-patient multi-crossover trials on treatment of chronic musculoskeletal pain: A randomized clinical trial . JAMA Internal Medicine , 178 ( 10 ), 1368–1377. 10.1001/jamainternmed.2018.3981 [ PMC free article ] [ PubMed ] [ CrossRef ] [ Google Scholar ]
- Lane-Brown A, & Tate R (2010). Evaluation of an intervention for apathy after traumatic brain injury: A multiple-baseline, single-case experimental design . Journal of Head Trauma Rehabilitation , 25 ( 6 ), 459–469. 10.1097/HTR.0b013e3181d98e1d [ PubMed ] [ CrossRef ] [ Google Scholar ]
- Lillie EO, Patay B, Diamant J, Issell B, Topol EJ, & Schork NJ (2011). The n-of-1 clinical trial: the ultimate strategy for individualizing medicine? Personalized Medicine , 8 ( 2 ), 161–173. 10.2217/pme.11.7 [ PMC free article ] [ PubMed ] [ CrossRef ] [ Google Scholar ]
- Lobo MA, Moeyaert M, Cunha AB, & Babik I (2017). Single-case design, analysis, and quality assessment for intervention research . Journal of Neurologic Physical Therapy , 41 ( 3 ), 187–197. 10.1097/NPT.0000000000000187 [ PMC free article ] [ PubMed ] [ CrossRef ] [ Google Scholar ]
- Lundervold DA, & Belwood MF (2000). The best kept secret in counseling: Single-case (N = 1) experimental designs . Journal of Counseling and Development , 78 ( 1 ), 92–102. 10.1002/j.1556-6676.2000.tb02565.x [ CrossRef ] [ Google Scholar ]
- Manolov R, Tanious R, & Onghena P (2021). Quantitative techniques and graphical representations for interpreting results from alternating treatment design . Perspectives on Behavior Science . Advance online publication. 10.1007/s40614-021-00289-9 [ PMC free article ] [ PubMed ] [ CrossRef ] [ Google Scholar ]
- McDonald S, Quinn F, Vieira R, O’Brien N, White M, Johnston DW, & Sniehotta FF (2017). The state of the art and future opportunities for using longitudinal n-of-1 methods in health behaviour research: A systematic literature overview . Health Psychology Review , 11 ( 4 ), 307–323. 10.1080/17437199.2017.1316672 [ PubMed ] [ CrossRef ] [ Google Scholar ]
- Meredith SE, Grabinski MJ, & Dallery J (2011). Internet-based group contingency management to promote abstinence from cigarette smoking: A feasibility study . Drug and Alcohol Dependence , 118 ( 1 ), 23–30. 10.1016/j.drugalcdep.2011.02.012 [ PMC free article ] [ PubMed ] [ CrossRef ] [ Google Scholar ]
- Miočević M, Klaassen F, Geuke G, Moeyaert M, & Maric M (2020). Using Bayesian methods to test mediators of intervention outcomes in single-case experimental designs . Evidence-Based Communication Assessment and Intervention , 14 ( 1–2 ), 52–68. 10.1080/17489539.2020.1732029 [ CrossRef ] [ Google Scholar ]
- Moeyaert M, & Fingerhut J (2022). Quantitative synthesis of personalized trials studies: Meta-analysis of aggregated data versus individual patient data . Harvard Data Science Review , ( Special Issue 3 ). 10.1162/99608f92.3574f1dc [ CrossRef ] [ Google Scholar ]
- Morgan DL, & Morgan RK (2001). Single-participant research design: Bringing science to managed care . American Psychologist , 56 ( 2 ), 119–127. 10.1037/0003-066X.56.2.119 [ PubMed ] [ CrossRef ] [ Google Scholar ]
- National Center for Complementary and Integrative Health. (2020, May 18). Pilot studies: Common uses and misuses . National Institutes of Health. https://www.nccih.nih.gov/grants/pilot-studies-common-uses-andmisuses [ Google Scholar ]
- Normand MP (2016). Less is more: Psychologists can learn more by studying fewer people . In Frontiers in Psychology , 7 ( 94 ). 10.3389/fpsyg.2016.00934 [ PMC free article ] [ PubMed ] [ CrossRef ] [ Google Scholar ]
- Pavlov IP (1927). Conditioned reflexes . Clarendon Press. [ Google Scholar ]
- Porcino A, & Vohra S (2022). N-of-1 trials, their reporting guidelines, and the advancement of open science principles . Harvard Data Science Review , ( Special Issue 3 ). 10.1162/99608f92.a65a257a [ CrossRef ] [ Google Scholar ]
- Pustejovsky JE (2019). Procedural sensitivities of effect sizes for single-case designs with directly observed behavioral outcome measures . Psychological Methods , 24 ( 2 ), 217–235. 10.1037/met0000179 [ PubMed ] [ CrossRef ] [ Google Scholar ]
- Riley AR, & Gaynor ST (2014). Identifying mechanisms of change: Utilizing single-participant methodology to better understand behavior therapy for child depression . Behavior Modification , 38 ( 5 ), 636–664. 10.1177/0145445514530756 [ PubMed ] [ CrossRef ] [ Google Scholar ]
- Riley WT, Glasgow RE, Etheredge L, & Abernethy AP (2013). Rapid, responsive, relevant (R3) research: A call for a rapid learning health research enterprise . Clinical and Translational Medicine , 2 ( 1 ), Article e10. 10.1186/2001-1326-2-10 [ PMC free article ] [ PubMed ] [ CrossRef ] [ Google Scholar ]
- Roustit M, Giai J, Gaget O, Khouri C, Mouhib M, Lotito A, Blaise S, Seinturier C, Subtil F, Paris A, Cracowski C, Imbert B, Carpentier P, Vohra S, & Cracowski JL (2018). On-demand sildenafil as a treatment for raynaud phenomenon: A series of n -of-1 trials . Annals of Internal Medicine , 169 ( 10 ), 694–703. 10.7326/M18-0517 [ PubMed ] [ CrossRef ] [ Google Scholar ]
- Russo SR, Croner J, Smith S, Chirinos M, & Weiss MJ (2019). A further refinement of procedures addressing food selectivity . Behavioral Interventions , 34 ( 4 ), 495–503. 10.1002/bin.1686 [ CrossRef ] [ Google Scholar ]
- Schork NJ (2015). Personalized medicine: Time for one-person trials . Nature , 520 ( 7549 ), 609–611. 10.1038/520609a [ PubMed ] [ CrossRef ] [ Google Scholar ]
- Schork N (2022). Accommodating serial correlation and sequential design elements in personalized studies and aggregated personalized studies . Harvard Data Science Review , ( Special Issue 3 ). 10.1162/99608f92.f1eef6f4 [ CrossRef ] [ Google Scholar ]
- Schork NJ, & Goetz LH (2017). Single-subject studies in translational nutrition research . Annual Review of Nutrition , 37 , 395–422. 10.1146/annurev-nutr-071816-064717 [ PMC free article ] [ PubMed ] [ CrossRef ] [ Google Scholar ]
- Seyhan AA (2019). Lost in translation: The valley of death across preclinical and clinical divide – Identification of problems and overcoming obstacles . Translational Medicine Communications , 4 ( 1 ), Article 18. 10.1186/s41231-019-0050-7 [ CrossRef ] [ Google Scholar ]
- Shadish WR (2014). Analysis and meta-analysis of single-case designs: An introduction . Journal of School Psychology , 52 ( 2 ), 109–122. 10.1016/j.jsp.2013.11.009 [ PubMed ] [ CrossRef ] [ Google Scholar ]
- Shadish WR, Hedges LV, & Pustejovsky JE (2014). Analysis and meta-analysis of single-case designs with a standardized mean difference statistic: A primer and applications . Journal of School Psychology , 52 ( 2 ), 123–147. 10.1016/j.jsp.2013.11.005 [ PubMed ] [ CrossRef ] [ Google Scholar ]
- Sidman M (1960). Tactics of scientific research . Basic Books. [ Google Scholar ]
- Singh NN, & Leung JP (1988). Smoking cessation through cigarette-fading, self-recording, and contracting: Treatment, maintenance and long-term followup . Addictive Behaviors , 13 ( 1 ), 101–105. 10.1016/0306-4603(88)90033-0 [ PubMed ] [ CrossRef ] [ Google Scholar ]
- Soto PL (2020). Single-case experimental designs for behavioral neuroscience . Journal of the Experimental Analysis of Behavior , 114 ( 3 ), 447–467. 10.1002/jeab.633 [ PubMed ] [ CrossRef ] [ Google Scholar ]
- Van den Noortgate W, & Onghena P (2003). Hierarchical linear models for the quantitative integration of effect sizes in single-case research . Behavior Research Methods, Instruments, & Computers , 35 ( 1 ), 1–10. 10.3758/bf03195492 [ PubMed ] [ CrossRef ] [ Google Scholar ]
- Vannest KJ, Peltier C, & Haas A (2018). Results reporting in single case experiments and single case meta-analysis . Research in Developmental Disabilities , 79 , 10–18. 10.1016/j.ridd.2018.04.029 [ PubMed ] [ CrossRef ] [ Google Scholar ]
- Vlaeyen JWS, Wicksell RK, Simons LE, Gentili C, De TK, Tate RL, Vohra S, Punja S, Linton SJ, Sniehotta FF, & Onghena P (2020). From boulder to stockholm in 70 years: Single case experimental designs in clinical research . Psychological Record , 70 ( 4 ), 659–670. 10.1007/s40732-020-00402-5 [ CrossRef ] [ Google Scholar ]
- Vohra S, Punja S (2019). A case for n-of-1 trials . JAMA Internal Medicine , 179 ( 3 ), 452. 10.1001/jamainternmed.2018.7166 [ PubMed ] [ CrossRef ] [ Google Scholar ]
- Vohra S, Shamseer L, Sampson M, Bukutu C, Schmid CH, Tate R, Nikles J, Zucker DR, Kravitz R, Guyatt G, Altman DG, Moher D, & CENT Group (2016). CONSORT extension for reporting N-of-1 trials (CENT) 2015 Statement . Journal of Clinical Epidemiology , 76 , 9–17. 10.1016/j.jclinepi.2015.05.004 [ PubMed ] [ CrossRef ] [ Google Scholar ]
- Ward-Horner J, & Sturmey P (2010). Component analyses using single-subject experimental designs: A review . Journal of Applied Behavior Analysis , 43 ( 4 ), 685–704. 10.1901/jaba.2010.43-685 [ PMC free article ] [ PubMed ] [ CrossRef ] [ Google Scholar ]
- Weems CF (1998). The evaluation of heart rate biofeedback using a multi-element design . Journal of Behavior Therapy and Experimental Psychiatry , 29 ( 2 ), 157–162. 10.1016/S0005-7916(98)00005-6 [ PubMed ] [ CrossRef ] [ Google Scholar ]
- Wen X, Eiden RD, Justicia-Linde FE, Wang Y, Higgins ST, Thor N, Haghdel A, Peters AR, & Epstein LH (2019). A multicomponent behavioral intervention for smoking cessation during pregnancy: A nonconcurrent multiple-baseline design . Translational Behavioral Medicine , 9 ( 2 ), 308–318. 10.1093/tbm/iby027 [ PMC free article ] [ PubMed ] [ CrossRef ] [ Google Scholar ]
- Williams BA (2010). Perils of evidence-based medicine . Perspectives in Biology and Medicine , 53 ( 1 ), 106–120. 10.1353/pbm.0.0132 [ PubMed ] [ CrossRef ] [ Google Scholar ]
More From Forbes
Governance by design: three case studies on privacy, security and grc.

- Share to Facebook
- Share to Twitter
- Share to Linkedin
Jimmie founded JLEE with the mission to "Enhance life for all through innovative, disruptive technologies." Learn more at jlee.com .
The software, IT, network and cloud industries have undergone evolutionary change for years. We have outpaced any expectation of usage and application of the original foundation of the internet and networks. Thus, we have been playing catch-up to build tools, policies, awareness and services to provide privacy, security and GRC (governance, risk and compliance) to aging technologies awaiting replacement, transformation and modernization.
Here are three case studies on strategies to apply governance by design, starting with privacy, security and GRC for every product.
Scenario 1: Brand-New Startup Beginning From Scratch
The core decision point is to understand which industry and what data access and computing are required.
Starting with a no-code or low-code front end makes the most sense. A CRM application may be best if there is a high touch with customers or clients, as startups often have more extended trial periods.
If possible, focus on one country to start and build in privacy and GRC up front. Review and outline compliance and regulation requirements and confirm architecture can be adjusted to meet them without expending energy to comply.
Security is simple if most of your product can be contained within the chosen no-code/low-code platform or CRM. Only use approved integrations by OEMs if possible. Minimize the sensitive data from customers initially and avoid any PII, PCI or HIPAA data by using integrations with other platforms that already maintain compliance, especially payment processors.
Scenario 2: New Product Innovation To Drive Revenue For A Growth Stage Or Emerging Company
Larger Fortune 500 companies will likely already have most of the infrastructure required. The challenge may be that the NPI requires mostly modern technologies, and the infrastructure in place may not be readily compatible. Here, the core is to build the proof of concept and alpha product with current technologies within the lab or development environment.
Partnerships, relationships, leadership and trust are essential in this scenario when working cross-functionally. Empathy, support and offering a temporary headcount may be tools to accelerate the other departments' projects, which most often have out-prioritized the updates and transformation required for the new product.
Here, network, security and privacy segmentation will be the foundation of the GRC plan for this area. Compliance with current strategy and architectures is required.
Your North Stars are highlighting and identifying when this new NPI and core infrastructure merge. Mapping expected cost reductions and revenue generation to the infrastructure merge plan presents the baseline for conversations, cross-functional strategy planning and, ultimately, budget approval.
Scenario 3: Finding Alignment In A Fortune 500 Or Fortune 1000 With Tech Debt And Competing Priorities
Similar in many ways to the NPI scenario, this one carries much more risk and requires more empathy, relationship building, trust and leadership up front.
The highest priority as a director or executive coming into a new role in a Fortune 500 is to quickly establish yourself as a strong listener, partner and trust builder. The more understanding you have of the company’s mission, vision, strategy and roadmap, the better.
Often, pushing disruptive innovation and NPI is a core competency aligned and assigned to specific departments with their strategy, roadmap and vision. At this stage, the initiatives in sight have often been investigated, researched, estimated, reviewed and prioritized out of current scope. Going in, you are likely pushing a large, heavy object uphill.
This is where understanding the priorities of your peer organizations and what it means for company growth, market share and overall evolution is vital.
Map the company’s priorities against your peer organization’s priorities, then the intersections of the new product. Estimate risk reduction, cost reduction, revenue growth, security, privacy and GRC enhancements for the new product. Then, scale the benefits of the security, privacy and GRC company-wide. Be sure to calculate risk based on current events and case studies for financial impact.
The financial impact is best framed with a few variables: current laws and regulations (especially those the company is not compliant with); the amount of fines, the diversion of company resources from strategic goals due to those fines and the consequential damage to the brand, with case studies on lost revenue for multiple years; loss of customer trust; and loss of market share with current examples on if any regained their previous hold on the market.
With this information, build a document or slides featuring high-level data. Make primary use of white space for a clean and visually appealing layout. Provide detailed information as a supplementary backup to support the main content.
Final Thoughts
Advocating for GRC can be a costly endeavor. Most often, it is a cost reduction once implemented properly with automation. With the proper use of AI and assisted platforms, the continual improvement and enhancement of privacy, security and GRC is more achievable now than ever. It is vital for growth-stage, emerging and Fortune 500 companies to build trust, relationships and partnerships cross-functionally early. For those in roles for many years, it may be too late. So, a strategic hire with personality, empathy, patience and relationship building can be your front-person agent for change.
In all cases, governance by design takes humility, patience and iterations. The greater the prep, the greater the implementation and adherence to policies and regulations, and the tighter security and privacy for all.
Forbes Technology Council is an invitation-only community for world-class CIOs, CTOs and technology executives. Do I qualify?
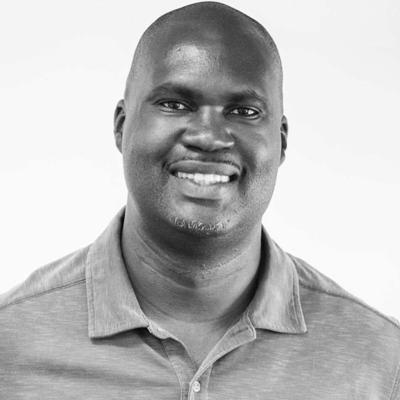
- Editorial Standards
- Reprints & Permissions
Have a language expert improve your writing
Run a free plagiarism check in 10 minutes, generate accurate citations for free.
- Knowledge Base
Methodology
- Quasi-Experimental Design | Definition, Types & Examples
Quasi-Experimental Design | Definition, Types & Examples
Published on July 31, 2020 by Lauren Thomas . Revised on January 22, 2024.
Like a true experiment , a quasi-experimental design aims to establish a cause-and-effect relationship between an independent and dependent variable .
However, unlike a true experiment, a quasi-experiment does not rely on random assignment . Instead, subjects are assigned to groups based on non-random criteria.
Quasi-experimental design is a useful tool in situations where true experiments cannot be used for ethical or practical reasons.
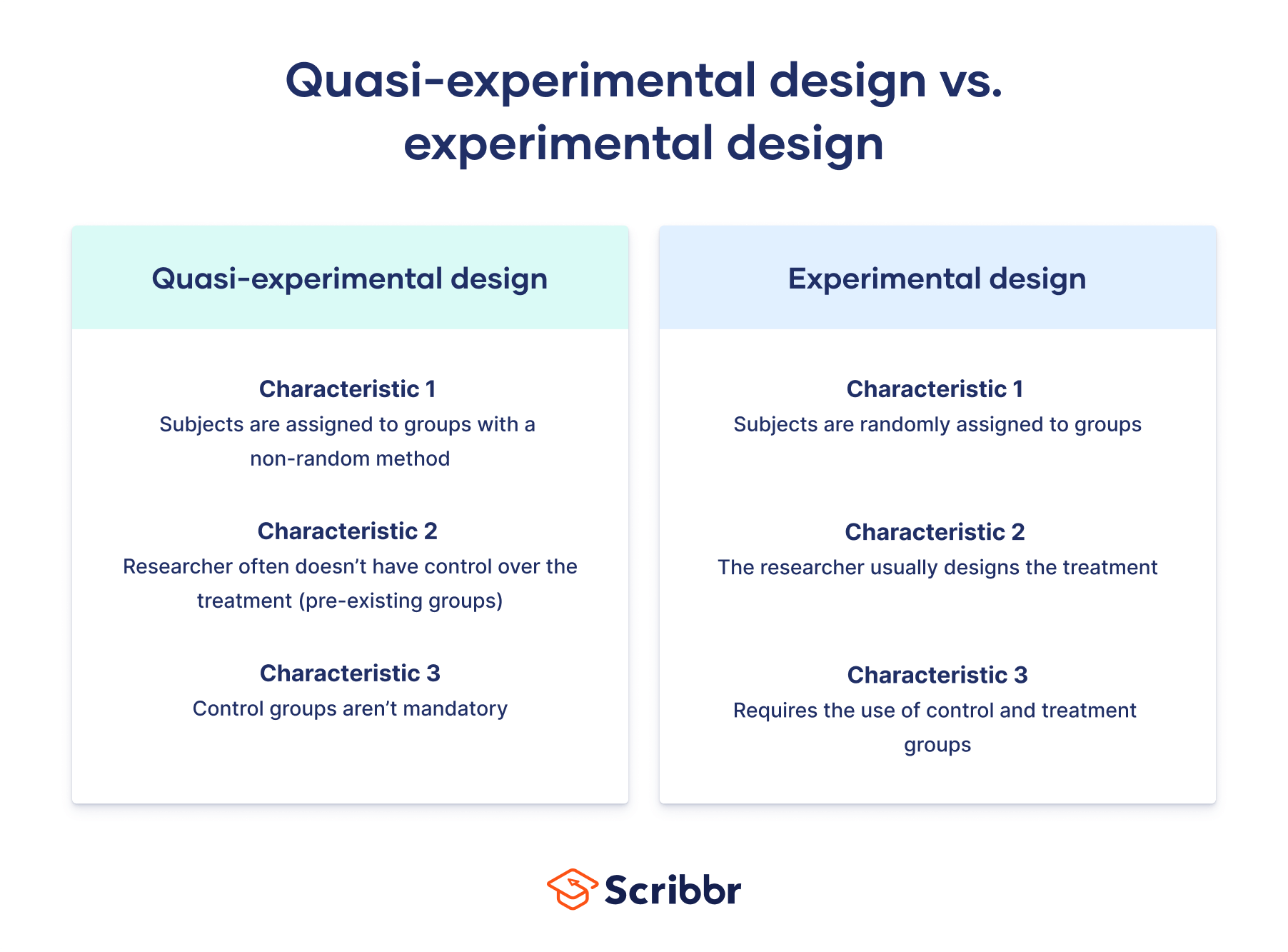
Table of contents
Differences between quasi-experiments and true experiments, types of quasi-experimental designs, when to use quasi-experimental design, advantages and disadvantages, other interesting articles, frequently asked questions about quasi-experimental designs.
There are several common differences between true and quasi-experimental designs.
True experimental design | Quasi-experimental design | |
---|---|---|
Assignment to treatment | The researcher subjects to control and treatment groups. | Some other, method is used to assign subjects to groups. |
Control over treatment | The researcher usually . | The researcher often , but instead studies pre-existing groups that received different treatments after the fact. |
Use of | Requires the use of . | Control groups are not required (although they are commonly used). |
Example of a true experiment vs a quasi-experiment
However, for ethical reasons, the directors of the mental health clinic may not give you permission to randomly assign their patients to treatments. In this case, you cannot run a true experiment.
Instead, you can use a quasi-experimental design.
You can use these pre-existing groups to study the symptom progression of the patients treated with the new therapy versus those receiving the standard course of treatment.
Receive feedback on language, structure, and formatting
Professional editors proofread and edit your paper by focusing on:
- Academic style
- Vague sentences
- Style consistency
See an example
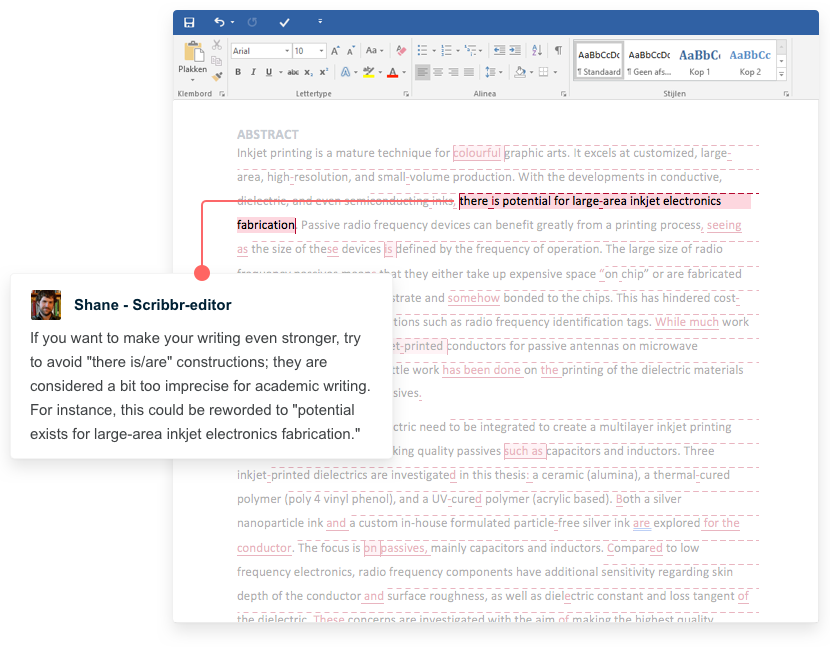
Many types of quasi-experimental designs exist. Here we explain three of the most common types: nonequivalent groups design, regression discontinuity, and natural experiments.
Nonequivalent groups design
In nonequivalent group design, the researcher chooses existing groups that appear similar, but where only one of the groups experiences the treatment.
In a true experiment with random assignment , the control and treatment groups are considered equivalent in every way other than the treatment. But in a quasi-experiment where the groups are not random, they may differ in other ways—they are nonequivalent groups .
When using this kind of design, researchers try to account for any confounding variables by controlling for them in their analysis or by choosing groups that are as similar as possible.
This is the most common type of quasi-experimental design.
Regression discontinuity
Many potential treatments that researchers wish to study are designed around an essentially arbitrary cutoff, where those above the threshold receive the treatment and those below it do not.
Near this threshold, the differences between the two groups are often so minimal as to be nearly nonexistent. Therefore, researchers can use individuals just below the threshold as a control group and those just above as a treatment group.
However, since the exact cutoff score is arbitrary, the students near the threshold—those who just barely pass the exam and those who fail by a very small margin—tend to be very similar, with the small differences in their scores mostly due to random chance. You can therefore conclude that any outcome differences must come from the school they attended.
Natural experiments
In both laboratory and field experiments, researchers normally control which group the subjects are assigned to. In a natural experiment, an external event or situation (“nature”) results in the random or random-like assignment of subjects to the treatment group.
Even though some use random assignments, natural experiments are not considered to be true experiments because they are observational in nature.
Although the researchers have no control over the independent variable , they can exploit this event after the fact to study the effect of the treatment.
However, as they could not afford to cover everyone who they deemed eligible for the program, they instead allocated spots in the program based on a random lottery.
Although true experiments have higher internal validity , you might choose to use a quasi-experimental design for ethical or practical reasons.
Sometimes it would be unethical to provide or withhold a treatment on a random basis, so a true experiment is not feasible. In this case, a quasi-experiment can allow you to study the same causal relationship without the ethical issues.
The Oregon Health Study is a good example. It would be unethical to randomly provide some people with health insurance but purposely prevent others from receiving it solely for the purposes of research.
However, since the Oregon government faced financial constraints and decided to provide health insurance via lottery, studying this event after the fact is a much more ethical approach to studying the same problem.
True experimental design may be infeasible to implement or simply too expensive, particularly for researchers without access to large funding streams.
At other times, too much work is involved in recruiting and properly designing an experimental intervention for an adequate number of subjects to justify a true experiment.
In either case, quasi-experimental designs allow you to study the question by taking advantage of data that has previously been paid for or collected by others (often the government).
Quasi-experimental designs have various pros and cons compared to other types of studies.
- Higher external validity than most true experiments, because they often involve real-world interventions instead of artificial laboratory settings.
- Higher internal validity than other non-experimental types of research, because they allow you to better control for confounding variables than other types of studies do.
- Lower internal validity than true experiments—without randomization, it can be difficult to verify that all confounding variables have been accounted for.
- The use of retrospective data that has already been collected for other purposes can be inaccurate, incomplete or difficult to access.
If you want to know more about statistics , methodology , or research bias , make sure to check out some of our other articles with explanations and examples.
- Normal distribution
- Degrees of freedom
- Null hypothesis
- Discourse analysis
- Control groups
- Mixed methods research
- Non-probability sampling
- Quantitative research
- Ecological validity
Research bias
- Rosenthal effect
- Implicit bias
- Cognitive bias
- Selection bias
- Negativity bias
- Status quo bias
A quasi-experiment is a type of research design that attempts to establish a cause-and-effect relationship. The main difference with a true experiment is that the groups are not randomly assigned.
In experimental research, random assignment is a way of placing participants from your sample into different groups using randomization. With this method, every member of the sample has a known or equal chance of being placed in a control group or an experimental group.
Quasi-experimental design is most useful in situations where it would be unethical or impractical to run a true experiment .
Quasi-experiments have lower internal validity than true experiments, but they often have higher external validity as they can use real-world interventions instead of artificial laboratory settings.
Cite this Scribbr article
If you want to cite this source, you can copy and paste the citation or click the “Cite this Scribbr article” button to automatically add the citation to our free Citation Generator.
Thomas, L. (2024, January 22). Quasi-Experimental Design | Definition, Types & Examples. Scribbr. Retrieved August 14, 2024, from https://www.scribbr.com/methodology/quasi-experimental-design/
Is this article helpful?
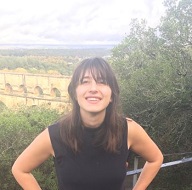
Lauren Thomas
Other students also liked, guide to experimental design | overview, steps, & examples, random assignment in experiments | introduction & examples, control variables | what are they & why do they matter, "i thought ai proofreading was useless but..".
I've been using Scribbr for years now and I know it's a service that won't disappoint. It does a good job spotting mistakes”
Information
- Author Services
Initiatives
You are accessing a machine-readable page. In order to be human-readable, please install an RSS reader.
All articles published by MDPI are made immediately available worldwide under an open access license. No special permission is required to reuse all or part of the article published by MDPI, including figures and tables. For articles published under an open access Creative Common CC BY license, any part of the article may be reused without permission provided that the original article is clearly cited. For more information, please refer to https://www.mdpi.com/openaccess .
Feature papers represent the most advanced research with significant potential for high impact in the field. A Feature Paper should be a substantial original Article that involves several techniques or approaches, provides an outlook for future research directions and describes possible research applications.
Feature papers are submitted upon individual invitation or recommendation by the scientific editors and must receive positive feedback from the reviewers.
Editor’s Choice articles are based on recommendations by the scientific editors of MDPI journals from around the world. Editors select a small number of articles recently published in the journal that they believe will be particularly interesting to readers, or important in the respective research area. The aim is to provide a snapshot of some of the most exciting work published in the various research areas of the journal.
Original Submission Date Received: .
- Active Journals
- Find a Journal
- Proceedings Series
- For Authors
- For Reviewers
- For Editors
- For Librarians
- For Publishers
- For Societies
- For Conference Organizers
- Open Access Policy
- Institutional Open Access Program
- Special Issues Guidelines
- Editorial Process
- Research and Publication Ethics
- Article Processing Charges
- Testimonials
- Preprints.org
- SciProfiles
- Encyclopedia
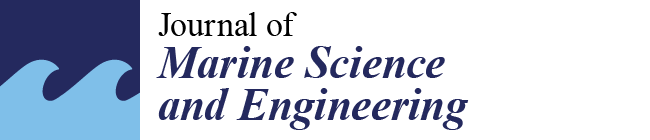
Article Menu

- Subscribe SciFeed
- Recommended Articles
- Google Scholar
- on Google Scholar
- Table of Contents
Find support for a specific problem in the support section of our website.
Please let us know what you think of our products and services.
Visit our dedicated information section to learn more about MDPI.
JSmol Viewer
Experimental research on the low-cycle fatigue crack growth rate for a stiffened plate of eh36 steel for use in ship structures.
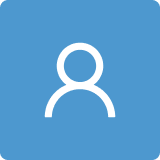
1. Introduction
2. low cycle fatigue crack growth experiment for stiffened plate, 3. result and discussion, 3.1. experimental results of stiffened plates with single-edge crack, 3.2. experimental results of stiffened plates with central crack, 4. conclusions, author contributions, institutional review board statement, informed consent statement, data availability statement, conflicts of interest.
- Dong, Q.; Xu, G.; Zhao, J.; Hu, Y. Experimental and numerical study on crack propagation of cracked plates under low cycle fatigue loads. J. Mar. Sci. Eng. 2023 , 11 , 1436. [ Google Scholar ] [ CrossRef ]
- Dong, Q.; Lu, X.; Xu, G. Experimental study on low-cycle fatigue characteristics of marine structural steel. J. Mar. Sci. Eng. 2024 , 12 , 651. [ Google Scholar ] [ CrossRef ]
- Gan, J.; Sun, D.; Deng, H.; Wang, Z.; Wang, X.; Yao, L.; Wu, W. Fatigue characteristics of designed T-type specimen under two-step repeating variable amplitude load with low-amplitude load below the fatigue limit. J. Mar. Sci. Eng. 2021 , 9 , 107. [ Google Scholar ] [ CrossRef ]
- Wang, Q.; Huber, N.; Liu, X.; Kashaev, N. On the analysis of plasticity induced crack closure in welded specimens: A mechanism controlled by the stress intensity factor resulting from residual stresses. Int. J. Fatigue 2022 , 162 , 106940. [ Google Scholar ] [ CrossRef ]
- Sistaninia, M.; Kolednik, O. A novel approach for determining the stress intensity factor for cracks in multilayered cantilevers. Eng. Fract. Mech. 2022 , 266 , 108386. [ Google Scholar ] [ CrossRef ]
- Nassiraei, H.; Rezadoost, P. Stress concentration factors in tubular T-joints reinforced with external ring under in-plane bending moment. Ocean. Eng. 2022 , 266 , 112551. [ Google Scholar ] [ CrossRef ]
- Nassiraei, H.; Rezadoost, P. Probabilistic analysis of the SCFs in tubular T/Y-joints reinforced with FRP under axial, in-plane bending, and out-of-plane bending loads. Structures 2022 , 35 , 1078–1097. [ Google Scholar ] [ CrossRef ]
- Nassiraei, H.; Rezadoost, P. Stress concentration factors in tubular T/Y-connections reinforced with FRP under in-plane bending load. Mar. Struct. 2021 , 76 , 102871. [ Google Scholar ] [ CrossRef ]
- Nassiraei, H.; Rezadoost, P. Static capacity of tubular X-joints reinforced with fiber reinforced polymer subjected to compressive load. Eng. Struct. 2021 , 236 , 112041. [ Google Scholar ] [ CrossRef ]
- Nassiraei, H.; Rezadoost, P. Stress concentration factors in tubular T/Y-joints strengthened with FRP subjected to compressive load in offshore structures. Int. J. Fatigue 2020 , 140 , 105719. [ Google Scholar ] [ CrossRef ]
- Dong, Q.; Yang, P.; Xu, G. Low cycle fatigue crack growth analysis by CTOD under variable amplitude loading for AH32 steel. Mar. Struct. 2019 , 63 , 257–268. [ Google Scholar ] [ CrossRef ]
- Dowling, N.E. Geometry effects and the J-integral approach to elastic-plastic fatigue crack growth. In Cracks and Fracture ; Swedlow, J., Williams, M., Eds.; ASTM STP 601; American Society for Testing and Materials: Philadelphia, PA, USA, 1976; pp. 19–32. [ Google Scholar ]
- Gonzales, G.L.G.; González, J.A.O.; Antunes, F.V.; Neto, D.M.; Díaz, F.A. Experimental determination of the reversed plastic zone around fatigue crack using digital image correlation. Theor. Appl. Fract. Mech. 2023 , 125 , 103901. [ Google Scholar ] [ CrossRef ]
- Dunham, F.W. Fatigue Testing of Large-Scale Models of Submarine Structural Details. Mar. Technol. SNAME News 1965 , 2 , 299–307. [ Google Scholar ] [ CrossRef ]
- Chen, L.; Chen, X. The low cycle fatigue tests on submarine structures. Ship Sci. Technol. 1991 , 2 , 19–20. [ Google Scholar ]
- Li, C. Research on low cycle fatigue properties of several types of steel for submarine pressure shell. Dev. Appl. Mater. 1986 , 12 , 28–37. [ Google Scholar ]
- Liu, Y.; Zhu, X.; Huang, X. Experimental research on low frequency fatigue crack propagation rate of 921A hull steel structure. J. Nav. Univ. Eng. 2008 , 20 , 69–74. [ Google Scholar ]
- Jandejsek, I.; Gajdoš, L.; Šperl, M.; Vavřík, D. Analysis of standard fracture toughness test based on digital image correlation data. Eng. Fract. Mech. 2017 , 182 , 607–620. [ Google Scholar ] [ CrossRef ]
- Zhang, W.; Liu, Y. Plastic zone size estimation under cyclic loadings using in situ optical microscopy fatigue testing. Fatigue Fract. Eng. Mater. Struct. 2011 , 34 , 717–727. [ Google Scholar ] [ CrossRef ]
- Vasco-Olmo, J.M.; Díaz, F.A.; Antunes, F.V.; James, M.N. Characterization of fatigue crack growth using digital image correlation measurements of plastic CTOD. Theor. Appl. Fract. Mech. 2019 , 101 , 332–341. [ Google Scholar ] [ CrossRef ]
- Belytschko, T.; Black, T. Elastic crack growth in finite elements with minimal remeshing. Int. J. Numer. Methods Eng. 1999 , 45 , 601–620. [ Google Scholar ] [ CrossRef ]
- Melenk, J.M.; Babuška, I. The partition of unity finite element method: Basic theory and applications. Comput. Methods Appl. Mech. 1996 , 139 , 289–314. [ Google Scholar ] [ CrossRef ]
- He, L.; Liu, Z.; Gu, J.; Wang, J.; Men, K. Fatigue crack propagation path and life prediction based on XFEM. J. Northwestern Polytech. Univ. 2019 , 37 , 737–743. [ Google Scholar ] [ CrossRef ]
- Tu, W.; Yue, J.; Xie, H.; Tang, W. Fatigue crack propagation behavior of high-strength steel under variable amplitude loading. Eng. Fract. Mech. 2021 , 247 , 107642. [ Google Scholar ] [ CrossRef ]
- Huang, X.; Zhang, X.; Bai, G.; Xu, W.; Wang, H. Residual strength analysis of thin-walled structures with multiple site damage based on crack tip opening angle method. J. Shanghai Jiao Tong Univ. 2013 , 47 , 519–524+531. [ Google Scholar ]
- Hwang, J.H.; Kim, H.T.; Kim, Y.J.; Nam, H.S.; Kim, J.W. Crack tip fields at crack initiation and growth under monotonic and large amplitude cyclic loading: Experimental and FE analyses. Int. J. Fatigue 2020 , 141 , 105889. [ Google Scholar ] [ CrossRef ]
- Xiong, K.; Deng, J.; Pei, Z.; Yang, P.; Dong, Q. Analysis of accumulative plasticity and fracture behavior of hull cracked plates subjected to biaxial low-cycle fatigue loading. J. Ship Mech. 2022 , 26 , 113–124. [ Google Scholar ]
- Wei, X. Fatigue Reliability Analysis of Ship Stiffened Panel Structure Subjected to Multiple Cracks. Master’s Thesis, Harbin Engineering University, Harbin, China, 2017. [ Google Scholar ]
- Soares, C.G.; Garbatov, Y.; Safety, S. Fatigue reliability of the ship hull girder accounting for inspection and repair. Reliab. Eng. 1996 , 51 , 341–351. [ Google Scholar ] [ CrossRef ]
- Duncheva, G.; Maximov, J.; Ganev, N.; Ivanova, M. Fatigue life enhancement of welded stiffened S355 steel plates with noncircular openings. J. Constr. Steel Res. 2015 , 112 , 93–107. [ Google Scholar ] [ CrossRef ]
- Lei, J.; Yue, J.; Xu, Z.; Fang, X.; Liu, H. Theoretical and Experimental Analysis on Low-Cycle Fatigue Crack Initiation for High Strength Steel Stiffened Plates. In Proceedings of the ASME 2022 41st International Conference on Ocean, Offshore and Arctic Engineering, Hamburg, Germany, 5–10 June 2022. [ Google Scholar ]
- Dexter, R.J.; Pilarski, P.J. Crack propagation in welded stiffened panels. J. Constr. Steel Res. 2002 , 58 , 1081–1102. [ Google Scholar ] [ CrossRef ]
- Jiang, W.; Yang, P. Experimental studies on crack propagation and accumulative mean strain of cracked stiffened plates under low-cycle fatigue loads. Ocean. Eng. 2020 , 214 , 107744. [ Google Scholar ] [ CrossRef ]
- Song, Y.; Yang, P.; Hu, K.; Jiang, W.; Zhang, G. Study of low-cycle fatigue crack growth behavior of central-cracked stiffened plates. Ocean. Eng. 2021 , 241 , 110083. [ Google Scholar ] [ CrossRef ]
- Dong, Q.; Yang, P.; Deng, J.L.; Wang, D. The theoretical and numerical research on CTOD for ship plate under cyclic loading considering accumulative plastic strain. J. Ship Mech. 2015 , 19 , 1507–1516. [ Google Scholar ]
- Deng, J.; Yang, P.; Dong, Q.; Wang, D. Research on CTOD for low cycle fatigue analysis of central through cracked plates considering accumulative plastic strain. Eng. Fract. Mech. 2016 , 154 , 128–139. [ Google Scholar ] [ CrossRef ]
- Dong, Q.; Yang, P.; Xu, G.; Deng, J.L. Mechanisms and modeling of low cycle fatigue crack propagation in a pressure vessel steel Q345. Int. J. Fatigue 2016 , 89 , 2–10. [ Google Scholar ] [ CrossRef ]
Click here to enlarge figure
Elastic Modulus/GPa | Poisson’s Ratio | Yield Stress/MPa | Ultimate Tensile Strength/MPa |
---|---|---|---|
206 | 0.3 | 434.94 | 548.91 |
Specimen Number | P /kN | R = P /P | Nominal Stress/MPa | Crack Location | Stiffener Height |
---|---|---|---|---|---|
P1 | 84.24 | −1 | 120 | single-edge crack | 30 mm |
P2 | 90.72 | −1 | 130 | single-edge crack | 30 mm |
P3 | 97.20 | −1 | 140 | single-edge crack | 30 mm |
P4 | 384.00 | 0.031 | 280 | central crack | 30 mm |
P5 | 420.00 | 0.2 | 300 | central crack | 30 mm |
P6 | 420.00 | 0.2 | 300 | central crack | 0 mm |
The statements, opinions and data contained in all publications are solely those of the individual author(s) and contributor(s) and not of MDPI and/or the editor(s). MDPI and/or the editor(s) disclaim responsibility for any injury to people or property resulting from any ideas, methods, instructions or products referred to in the content. |
Share and Cite
Dong, Q.; Xu, G.; Chen, W. Experimental Research on the Low-Cycle Fatigue Crack Growth Rate for a Stiffened Plate of EH36 Steel for Use in Ship Structures. J. Mar. Sci. Eng. 2024 , 12 , 1365. https://doi.org/10.3390/jmse12081365
Dong Q, Xu G, Chen W. Experimental Research on the Low-Cycle Fatigue Crack Growth Rate for a Stiffened Plate of EH36 Steel for Use in Ship Structures. Journal of Marine Science and Engineering . 2024; 12(8):1365. https://doi.org/10.3390/jmse12081365
Dong, Qin, Geng Xu, and Wei Chen. 2024. "Experimental Research on the Low-Cycle Fatigue Crack Growth Rate for a Stiffened Plate of EH36 Steel for Use in Ship Structures" Journal of Marine Science and Engineering 12, no. 8: 1365. https://doi.org/10.3390/jmse12081365
Article Metrics
Article access statistics, further information, mdpi initiatives, follow mdpi.
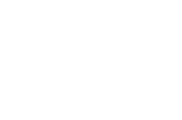
Subscribe to receive issue release notifications and newsletters from MDPI journals
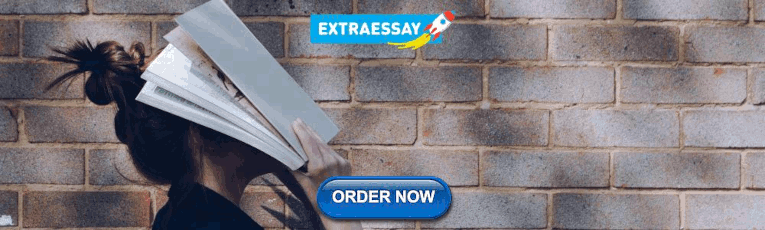
COMMENTS
Abstract. Single-case experimental designs (SCEDs) represent a family of research designs that use experimental methods to study the effects of treatments on outcomes. The fundamental unit of analysis is the single case—which can be an individual, clinic, or community—ideally with replications of effects within and/or between cases.
Single-case studies can provide a viable alternative to large group studies such as randomized clinical trials. Single case studies involve repeated measures, and manipulation of and independent variable. They can be designed to have strong internal validity for assessing causal relationships between interventions and outcomes, and external ...
Experimental study design. The basic concept of experimental study design is to study the effect of an intervention. In this study design, the risk factor/exposure of interest/treatment is controlled by the investigator. Therefore, these are hypothesis testing studies and can provide the most convincing demonstration of evidence for causality.
This article systematically reviews the research design and methodological characteristics of single-case experimental design (SCED) research published in peer-reviewed journals between 2000 and 2010. SCEDs provide researchers with a flexible and viable alternative to group designs with large sample sizes. However, methodological challenges ...
Guide to Experimental Design | Overview, 5 steps & Examples. Published on December 3, 2019 by Rebecca Bevans.Revised on June 21, 2023. Experiments are used to study causal relationships.You manipulate one or more independent variables and measure their effect on one or more dependent variables.. Experimental design create a set of procedures to systematically test a hypothesis.
Tactics of Scientific Research (Sidman, 1960) provides a visionary treatise on single-case designs, their scientific underpinnings, and their critical role in understanding behavior. Since the foundational base was provided, single-case designs have proliferated especially in areas of application where they have been used to evaluate interventions with an extraordinary range of clients ...
When rigorously designed, single-case studies can be particularly useful experimental designs in a variety of situations, such as when research resources are limited, studied conditions have low incidences, or when examining effects of novel or expensive interventions. Readers will be directed to ex …
MH20012/MH/NIMH NIH HHS/United States. This article systematically reviews the research design and methodological characteristics of single-case experimental design (SCED) research published in peer-reviewed journals between 2000 and 2010. SCEDs provide researchers with a flexible and viable alternative to group designs with large sample ….
A case study is one of the most commonly used methodologies of social research. This article attempts to look into the various dimensions of a case study research strategy, the different epistemological strands which determine the particular case study type and approach adopted in the field, discusses the factors which can enhance the effectiveness of a case study research, and the debate ...
Revised on June 22, 2023. A case-control study is an experimental design that compares a group of participants possessing a condition of interest to a very similar group lacking that condition. Here, the participants possessing the attribute of study, such as a disease, are called the "case," and those without it are the "control.".
Revised on November 20, 2023. A case study is a detailed study of a specific subject, such as a person, group, place, event, organization, or phenomenon. Case studies are commonly used in social, educational, clinical, and business research. A case study research design usually involves qualitative methods, but quantitative methods are ...
McMaster University, West Hamilton, Ontario, Canada. Qualitative case study methodology prov ides tools for researchers to study. complex phenomena within their contexts. When the approach is ...
which case study research designs attempt to mimic the virtues of experimental design and the degree to which they succeed. The classic experiment, with manipulated treatment and randomized control, thus provides a useful template for discussion. about methodological issues in experimental and observational contexts.
Chapter objectives. After reading this chapter you will be able to: Describe the purpose of case studies. Plan a systematic approach to case study design. Recognize the strengths and limitations of case studies as a research method. Compose a case study report that is appropriately structured and presented.
Case Studies We will introduce aspects of experimental design on the basis of these case studies: An education example; An Arabidopsis fruit length example; A starling song length example; A dairy cow nutrition study; A weight loss study. Designing Experiments Case Studies 3 / 31 Biology Education Case Study
The pre-experimental research design is further divided into three types. One-shot Case Study Research Design. In this type of experimental study, only one dependent group or variable is considered. The study is carried out after some treatment which was presumed to cause change, making it a posttest study.
How to Design and Conduct a Case Study. The advantage of the case study research design is that you can focus on specific and interesting cases. This may be an attempt to test a theory with a typical case or it can be a specific topic that is of interest. Research should be thorough and note taking should be meticulous and systematic.
A case study is a research approach that is used to generate an in-depth, multi-faceted understanding of a complex issue in its real-life context. It is an established research design that is used extensively in a wide variety of disciplines, particularly in the social sciences. A case study can be defined in a variety of ways (Table 5 ), the ...
Key Takeaways. Experimental designs are useful for establishing causality, but some types of experimental design do this better than others. Experiments help researchers isolate the effect of the independent variable on the dependent variable by controlling for the effect of extraneous variables.; Experiments use a control/comparison group and an experimental group to test the effects of ...
Experimental Design. Experimental design is a process of planning and conducting scientific experiments to investigate a hypothesis or research question. It involves carefully designing an experiment that can test the hypothesis, and controlling for other variables that may influence the results. Experimental design typically includes ...
Three types of experimental designs are commonly used: 1. Independent Measures. Independent measures design, also known as between-groups, is an experimental design where different participants are used in each condition of the independent variable. This means that each condition of the experiment includes a different group of participants.
Research projects can be designed and conducted using different techniques and methodologies. Explore quasi-experimental, case studies, and correlational research designs, and recognize how they ...
Case Studies; Quasi-Experimental Designs; Researchers who are simply interested in describing characteristics of people, describing relationships between variables, and using those relationships to make predictions can use a non-experimental research design. Using the non-experimental approach, the researcher simply measures variables as they ...
BACKGROUND AND AIM[|]The statistical method development for estimating the complex association between mixed environmental exposures and health outcomes is an area of ongoing research that has garnered significant attention in recent years. However, a statistical framework that can estimate the acute effects of mixed exposures at different lag times in the case-crossover design remains lacking ...
The study could also be designed with three groups: a single-case design experimental group similar to the approach in the hypothetical study above and two control groups, one low-fat and one low-carb. ... Single-case research design and analysis: New directions for psychology and education. Routledge. 10.4324/9781315725994 [Google ...
Here are three case studies on strategies to apply governance by design, starting with privacy, security and GRC for every product. Scenario 1: Brand-New Startup Beginning From Scratch
Revised on January 22, 2024. Like a true experiment, a quasi-experimental design aims to establish a cause-and-effect relationship between an independent and dependent variable. However, unlike a true experiment, a quasi-experiment does not rely on random assignment. Instead, subjects are assigned to groups based on non-random criteria.
Temperature uniformity inside the autoclave and in the manufactured thermoset composite part is the key to enhancing curing performance. The present study incorporated experimental setup, multiphysics and computational fluid dynamics (CFD) models to provide insight into the gas flow pattern and temperature distribution inside the autoclave besides temperature and curing evolution in composite ...
The experimental results offer new insights into methane-coal dust explosions and help explain explosion accidents in turbulent and complex scenarios during investigations. The research also demonstrates the potential of flow visualization and emission spectroscopy techniques for studying gas-solid two-phase explosions. 2. Experimental 2.1.
This paper presents a straightforward approach for determining the low-cycle fatigue (LCF) crack propagation rate in stiffened plate structures containing cracks. The method relies on both the crack tip opening displacement (CTOD) and the accumulative plastic strain, offering valuable insights for ship structure design and assessing LCF strength. Meanwhile, the LCF crack growth tests for the ...