8.2 Non-Equivalent Groups Designs
Learning objectives.
- Describe the different types of nonequivalent groups quasi-experimental designs.
- Identify some of the threats to internal validity associated with each of these designs.
Recall that when participants in a between-subjects experiment are randomly assigned to conditions, the resulting groups are likely to be quite similar. In fact, researchers consider them to be equivalent. When participants are not randomly assigned to conditions, however, the resulting groups are likely to be dissimilar in some ways. For this reason, researchers consider them to be nonequivalent. A nonequivalent groups design , then, is a between-subjects design in which participants have not been randomly assigned to conditions. There are several types of nonequivalent groups designs we will consider.
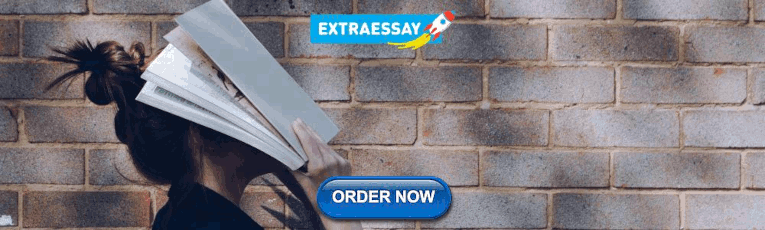
Posttest Only Nonequivalent Groups Design
The first nonequivalent groups design we will consider is the posttest only nonequivalent groups design. In this design, participants in one group are exposed to a treatment, a nonequivalent group is not exposed to the treatment, and then the two groups are compared. Imagine, for example, a researcher who wants to evaluate a new method of teaching fractions to third graders. One way would be to conduct a study with a treatment group consisting of one class of third-grade students and a control group consisting of another class of third-grade students. This design would be a nonequivalent groups design because the students are not randomly assigned to classes by the researcher, which means there could be important differences between them. For example, the parents of higher achieving or more motivated students might have been more likely to request that their children be assigned to Ms. Williams’s class. Or the principal might have assigned the “troublemakers” to Mr. Jones’s class because he is a stronger disciplinarian. Of course, the teachers’ styles, and even the classroom environments might be very different and might cause different levels of achievement or motivation among the students. If at the end of the study there was a difference in the two classes’ knowledge of fractions, it might have been caused by the difference between the teaching methods—but it might have been caused by any of these confounding variables.
Of course, researchers using a posttest only nonequivalent groups design can take steps to ensure that their groups are as similar as possible. In the present example, the researcher could try to select two classes at the same school, where the students in the two classes have similar scores on a standardized math test and the teachers are the same sex, are close in age, and have similar teaching styles. Taking such steps would increase the internal validity of the study because it would eliminate some of the most important confounding variables. But without true random assignment of the students to conditions, there remains the possibility of other important confounding variables that the researcher was not able to control.
Pretest-Posttest Nonequivalent Groups Design
Another way to improve upon the posttest only nonequivalent groups design is to add a pretest. In the pretest-posttest nonequivalent groups design t here is a treatment group that is given a pretest, receives a treatment, and then is given a posttest. But at the same time there is a nonequivalent control group that is given a pretest, does not receive the treatment, and then is given a posttest. The question, then, is not simply whether participants who receive the treatment improve, but whether they improve more than participants who do not receive the treatment.
Imagine, for example, that students in one school are given a pretest on their attitudes toward drugs, then are exposed to an anti-drug program, and finally, are given a posttest. Students in a similar school are given the pretest, not exposed to an anti-drug program, and finally, are given a posttest. Again, if students in the treatment condition become more negative toward drugs, this change in attitude could be an effect of the treatment, but it could also be a matter of history or maturation. If it really is an effect of the treatment, then students in the treatment condition should become more negative than students in the control condition. But if it is a matter of history (e.g., news of a celebrity drug overdose) or maturation (e.g., improved reasoning), then students in the two conditions would be likely to show similar amounts of change. This type of design does not completely eliminate the possibility of confounding variables, however. Something could occur at one of the schools but not the other (e.g., a student drug overdose), so students at the first school would be affected by it while students at the other school would not.
Returning to the example of evaluating a new measure of teaching third graders, this study could be improved by adding a pretest of students’ knowledge of fractions. The changes in scores from pretest to posttest would then be evaluated and compared across conditions to determine whether one group demonstrated a bigger improvement in knowledge of fractions than another. Of course, the teachers’ styles, and even the classroom environments might still be very different and might cause different levels of achievement or motivation among the students that are independent of the teaching intervention. Once again, differential history also represents a potential threat to internal validity. If asbestos is found in one of the schools causing it to be shut down for a month then this interruption in teaching could produce a difference across groups on posttest scores.
If participants in this kind of design are randomly assigned to conditions, it becomes a true between-groups experiment rather than a quasi-experiment. In fact, it is the kind of experiment that Eysenck called for—and that has now been conducted many times—to demonstrate the effectiveness of psychotherapy.
Interrupted Time-Series Design with Nonequivalent Groups
One way to improve upon the interrupted time-series design is to add a control group. The interrupted time-series design with nonequivalent groups involves taking a set of measurements at intervals over a period of time both before and after an intervention of interest in two or more nonequivalent groups. Once again consider the manufacturing company that measures its workers’ productivity each week for a year before and after reducing work shifts from 10 hours to 8 hours. This design could be improved by locating another manufacturing company who does not plan to change their shift length and using them as a nonequivalent control group. If productivity increased rather quickly after the shortening of the work shifts in the treatment group but productivity remained consistent in the control group, then this provides better evidence for the effectiveness of the treatment.
Similarly, in the example of examining the effects of taking attendance on student absences in a research methods course, the design could be improved by using students in another section of the research methods course as a control group. If a consistently higher number of absences was found in the treatment group before the intervention, followed by a sustained drop in absences after the treatment, while the nonequivalent control group showed consistently high absences across the semester then this would provide superior evidence for the effectiveness of the treatment in reducing absences.
Pretest-Posttest Design With Switching Replication
Some of these nonequivalent control group designs can be further improved by adding a switching replication. Using a pretest-posttest design with switching replication design, nonequivalent groups are administered a pretest of the dependent variable, then one group receives a treatment while a nonequivalent control group does not receive a treatment, the dependent variable is assessed again, and then the treatment is added to the control group, and finally the dependent variable is assessed one last time.
As a concrete example, let’s say we wanted to introduce an exercise intervention for the treatment of depression. We recruit one group of patients experiencing depression and a nonequivalent control group of students experiencing depression. We first measure depression levels in both groups, and then we introduce the exercise intervention to the patients experiencing depression, but we hold off on introducing the treatment to the students. We then measure depression levels in both groups. If the treatment is effective we should see a reduction in the depression levels of the patients (who received the treatment) but not in the students (who have not yet received the treatment). Finally, while the group of patients continues to engage in the treatment, we would introduce the treatment to the students with depression. Now and only now should we see the students’ levels of depression decrease.
One of the strengths of this design is that it includes a built in replication. In the example given, we would get evidence for the efficacy of the treatment in two different samples (patients and students). Another strength of this design is that it provides more control over history effects. It becomes rather unlikely that some outside event would perfectly coincide with the introduction of the treatment in the first group and with the delayed introduction of the treatment in the second group. For instance, if a change in the weather occurred when we first introduced the treatment to the patients, and this explained their reductions in depression the second time that depression was measured, then we would see depression levels decrease in both the groups. Similarly, the switching replication helps to control for maturation and instrumentation. Both groups would be expected to show the same rates of spontaneous remission of depression and if the instrument for assessing depression happened to change at some point in the study the change would be consistent across both of the groups. Of course, demand characteristics, placebo effects, and experimenter expectancy effects can still be problems. But they can be controlled for using some of the methods described in Chapter 5.
Switching Replication with Treatment Removal Design
In a basic pretest-posttest design with switching replication, the first group receives a treatment and the second group receives the same treatment a little bit later on (while the initial group continues to receive the treatment). In contrast, in a switching replication with treatment removal design , the treatment is removed from the first group when it is added to the second group. Once again, let’s assume we first measure the depression levels of patients with depression and students with depression. Then we introduce the exercise intervention to only the patients. After they have been exposed to the exercise intervention for a week we assess depression levels again in both groups. If the intervention is effective then we should see depression levels decrease in the patient group but not the student group (because the students haven’t received the treatment yet). Next, we would remove the treatment from the group of patients with depression. So we would tell them to stop exercising. At the same time, we would tell the student group to start exercising. After a week of the students exercising and the patients not exercising, we would reassess depression levels. Now if the intervention is effective we should see that the depression levels have decreased in the student group but that they have increased in the patient group (because they are no longer exercising).
Demonstrating a treatment effect in two groups staggered over time and demonstrating the reversal of the treatment effect after the treatment has been removed can provide strong evidence for the efficacy of the treatment. In addition to providing evidence for the replicability of the findings, this design can also provide evidence for whether the treatment continues to show effects after it has been withdrawn.
Key Takeaways
- Quasi-experimental research involves the manipulation of an independent variable without the random assignment of participants to conditions or counterbalancing of orders of conditions.
- There are three types of quasi-experimental designs that are within-subjects in nature. These are the one-group posttest only design, the one-group pretest-posttest design, and the interrupted time-series design.
- There are five types of quasi-experimental designs that are between-subjects in nature. These are the posttest only design with nonequivalent groups, the pretest-posttest design with nonequivalent groups, the interrupted time-series design with nonequivalent groups, the pretest-posttest design with switching replication, and the switching replication with treatment removal design.
- Quasi-experimental research eliminates the directionality problem because it involves the manipulation of the independent variable. However, it does not eliminate the problem of confounding variables, because it does not involve random assignment to conditions or counterbalancing. For these reasons, quasi-experimental research is generally higher in internal validity than non-experimental studies but lower than true experiments.
- Of all of the quasi-experimental designs, those that include a switching replication are highest in internal validity.
- Practice: Imagine that two professors decide to test the effect of giving daily quizzes on student performance in a statistics course. They decide that Professor A will give quizzes but Professor B will not. They will then compare the performance of students in their two sections on a common final exam. List five other variables that might differ between the two sections that could affect the results.
- regression to the mean
- spontaneous remission

Share This Book
- Increase Font Size
Have a language expert improve your writing
Run a free plagiarism check in 10 minutes, generate accurate citations for free.
- Knowledge Base
Methodology
- Random Assignment in Experiments | Introduction & Examples
Random Assignment in Experiments | Introduction & Examples
Published on March 8, 2021 by Pritha Bhandari . Revised on June 22, 2023.
In experimental research, random assignment is a way of placing participants from your sample into different treatment groups using randomization.
With simple random assignment, every member of the sample has a known or equal chance of being placed in a control group or an experimental group. Studies that use simple random assignment are also called completely randomized designs .
Random assignment is a key part of experimental design . It helps you ensure that all groups are comparable at the start of a study: any differences between them are due to random factors, not research biases like sampling bias or selection bias .
Table of contents
Why does random assignment matter, random sampling vs random assignment, how do you use random assignment, when is random assignment not used, other interesting articles, frequently asked questions about random assignment.
Random assignment is an important part of control in experimental research, because it helps strengthen the internal validity of an experiment and avoid biases.
In experiments, researchers manipulate an independent variable to assess its effect on a dependent variable, while controlling for other variables. To do so, they often use different levels of an independent variable for different groups of participants.
This is called a between-groups or independent measures design.
You use three groups of participants that are each given a different level of the independent variable:
- a control group that’s given a placebo (no dosage, to control for a placebo effect ),
- an experimental group that’s given a low dosage,
- a second experimental group that’s given a high dosage.
Random assignment to helps you make sure that the treatment groups don’t differ in systematic ways at the start of the experiment, as this can seriously affect (and even invalidate) your work.
If you don’t use random assignment, you may not be able to rule out alternative explanations for your results.
- participants recruited from cafes are placed in the control group ,
- participants recruited from local community centers are placed in the low dosage experimental group,
- participants recruited from gyms are placed in the high dosage group.
With this type of assignment, it’s hard to tell whether the participant characteristics are the same across all groups at the start of the study. Gym-users may tend to engage in more healthy behaviors than people who frequent cafes or community centers, and this would introduce a healthy user bias in your study.
Although random assignment helps even out baseline differences between groups, it doesn’t always make them completely equivalent. There may still be extraneous variables that differ between groups, and there will always be some group differences that arise from chance.
Most of the time, the random variation between groups is low, and, therefore, it’s acceptable for further analysis. This is especially true when you have a large sample. In general, you should always use random assignment in experiments when it is ethically possible and makes sense for your study topic.
Receive feedback on language, structure, and formatting
Professional editors proofread and edit your paper by focusing on:
- Academic style
- Vague sentences
- Style consistency
See an example
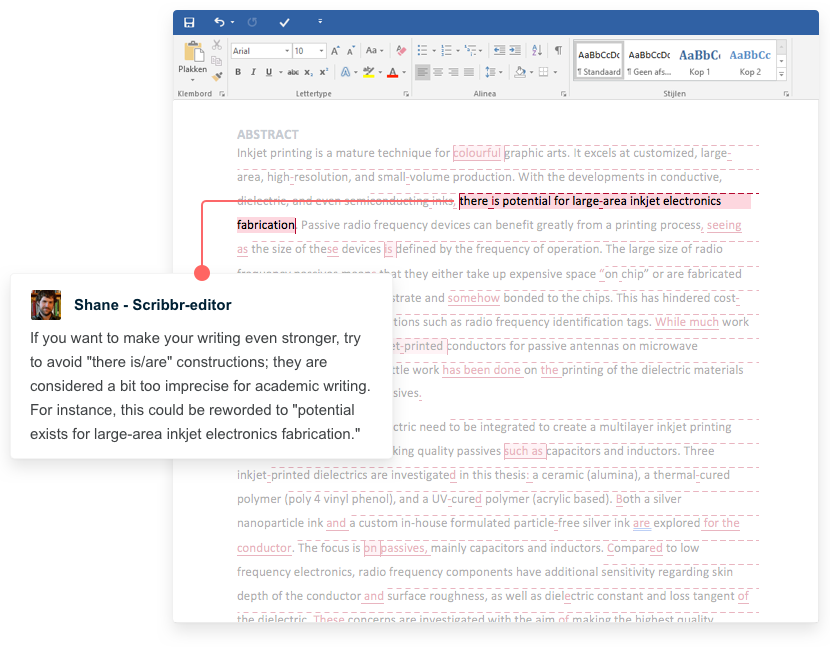
Random sampling and random assignment are both important concepts in research, but it’s important to understand the difference between them.
Random sampling (also called probability sampling or random selection) is a way of selecting members of a population to be included in your study. In contrast, random assignment is a way of sorting the sample participants into control and experimental groups.
While random sampling is used in many types of studies, random assignment is only used in between-subjects experimental designs.
Some studies use both random sampling and random assignment, while others use only one or the other.
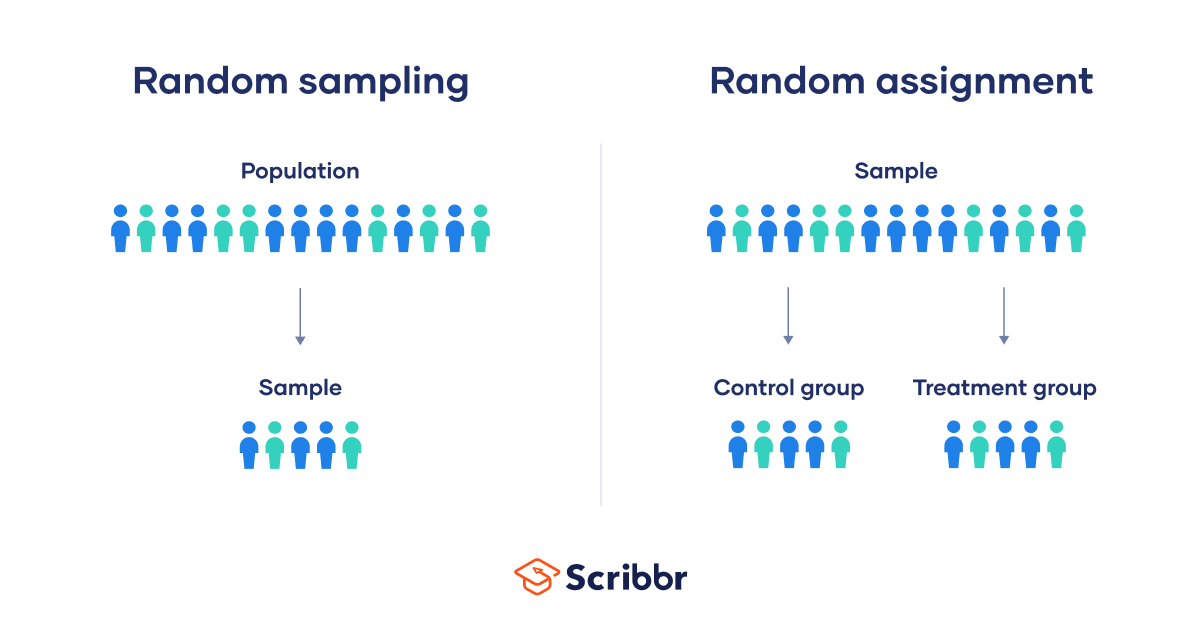
Random sampling enhances the external validity or generalizability of your results, because it helps ensure that your sample is unbiased and representative of the whole population. This allows you to make stronger statistical inferences .
You use a simple random sample to collect data. Because you have access to the whole population (all employees), you can assign all 8000 employees a number and use a random number generator to select 300 employees. These 300 employees are your full sample.
Random assignment enhances the internal validity of the study, because it ensures that there are no systematic differences between the participants in each group. This helps you conclude that the outcomes can be attributed to the independent variable .
- a control group that receives no intervention.
- an experimental group that has a remote team-building intervention every week for a month.
You use random assignment to place participants into the control or experimental group. To do so, you take your list of participants and assign each participant a number. Again, you use a random number generator to place each participant in one of the two groups.
To use simple random assignment, you start by giving every member of the sample a unique number. Then, you can use computer programs or manual methods to randomly assign each participant to a group.
- Random number generator: Use a computer program to generate random numbers from the list for each group.
- Lottery method: Place all numbers individually in a hat or a bucket, and draw numbers at random for each group.
- Flip a coin: When you only have two groups, for each number on the list, flip a coin to decide if they’ll be in the control or the experimental group.
- Use a dice: When you have three groups, for each number on the list, roll a dice to decide which of the groups they will be in. For example, assume that rolling 1 or 2 lands them in a control group; 3 or 4 in an experimental group; and 5 or 6 in a second control or experimental group.
This type of random assignment is the most powerful method of placing participants in conditions, because each individual has an equal chance of being placed in any one of your treatment groups.
Random assignment in block designs
In more complicated experimental designs, random assignment is only used after participants are grouped into blocks based on some characteristic (e.g., test score or demographic variable). These groupings mean that you need a larger sample to achieve high statistical power .
For example, a randomized block design involves placing participants into blocks based on a shared characteristic (e.g., college students versus graduates), and then using random assignment within each block to assign participants to every treatment condition. This helps you assess whether the characteristic affects the outcomes of your treatment.
In an experimental matched design , you use blocking and then match up individual participants from each block based on specific characteristics. Within each matched pair or group, you randomly assign each participant to one of the conditions in the experiment and compare their outcomes.
Sometimes, it’s not relevant or ethical to use simple random assignment, so groups are assigned in a different way.
When comparing different groups
Sometimes, differences between participants are the main focus of a study, for example, when comparing men and women or people with and without health conditions. Participants are not randomly assigned to different groups, but instead assigned based on their characteristics.
In this type of study, the characteristic of interest (e.g., gender) is an independent variable, and the groups differ based on the different levels (e.g., men, women, etc.). All participants are tested the same way, and then their group-level outcomes are compared.
When it’s not ethically permissible
When studying unhealthy or dangerous behaviors, it’s not possible to use random assignment. For example, if you’re studying heavy drinkers and social drinkers, it’s unethical to randomly assign participants to one of the two groups and ask them to drink large amounts of alcohol for your experiment.
When you can’t assign participants to groups, you can also conduct a quasi-experimental study . In a quasi-experiment, you study the outcomes of pre-existing groups who receive treatments that you may not have any control over (e.g., heavy drinkers and social drinkers). These groups aren’t randomly assigned, but may be considered comparable when some other variables (e.g., age or socioeconomic status) are controlled for.
Prevent plagiarism. Run a free check.
If you want to know more about statistics , methodology , or research bias , make sure to check out some of our other articles with explanations and examples.
- Student’s t -distribution
- Normal distribution
- Null and Alternative Hypotheses
- Chi square tests
- Confidence interval
- Quartiles & Quantiles
- Cluster sampling
- Stratified sampling
- Data cleansing
- Reproducibility vs Replicability
- Peer review
- Prospective cohort study
Research bias
- Implicit bias
- Cognitive bias
- Placebo effect
- Hawthorne effect
- Hindsight bias
- Affect heuristic
- Social desirability bias
In experimental research, random assignment is a way of placing participants from your sample into different groups using randomization. With this method, every member of the sample has a known or equal chance of being placed in a control group or an experimental group.
Random selection, or random sampling , is a way of selecting members of a population for your study’s sample.
In contrast, random assignment is a way of sorting the sample into control and experimental groups.
Random sampling enhances the external validity or generalizability of your results, while random assignment improves the internal validity of your study.
Random assignment is used in experiments with a between-groups or independent measures design. In this research design, there’s usually a control group and one or more experimental groups. Random assignment helps ensure that the groups are comparable.
In general, you should always use random assignment in this type of experimental design when it is ethically possible and makes sense for your study topic.
To implement random assignment , assign a unique number to every member of your study’s sample .
Then, you can use a random number generator or a lottery method to randomly assign each number to a control or experimental group. You can also do so manually, by flipping a coin or rolling a dice to randomly assign participants to groups.
Cite this Scribbr article
If you want to cite this source, you can copy and paste the citation or click the “Cite this Scribbr article” button to automatically add the citation to our free Citation Generator.
Bhandari, P. (2023, June 22). Random Assignment in Experiments | Introduction & Examples. Scribbr. Retrieved March 20, 2024, from https://www.scribbr.com/methodology/random-assignment/
Is this article helpful?
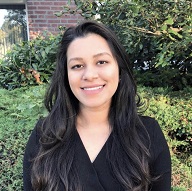
Pritha Bhandari
Other students also liked, guide to experimental design | overview, steps, & examples, confounding variables | definition, examples & controls, control groups and treatment groups | uses & examples, "i thought ai proofreading was useless but..".
I've been using Scribbr for years now and I know it's a service that won't disappoint. It does a good job spotting mistakes”
Random versus nonrandom assignment in controlled experiments: do you get the same answer?
Affiliation.
- 1 Department of Psychology, University of Memphis, Tennessee 38152, USA.
- PMID: 8991316
Psychotherapy meta-analyses commonly combine results from controlled experiments that use random and nonrandom assignment without examining whether the 2 methods give the same answer. Results from this article call this practice into question. With the use of outcome studies of marital and family therapy, 64 experiments using random assignment yielded consistently higher mean post-test effects and less variable posttest effects than 36 studies using nonrandom assignment. This difference was reduced by about half by taking into account various covariates, especially pretest effect size levels and various characteristics of control groups. The importance of this finding depends on (a) whether one is discussing meta-analysis or primary experiments, (b) how precise an answer is desired, and (c) whether some adjustment to the data from studies using nonrandom assignment is possible. It is concluded that studies using nonrandom assignment may produce acceptable approximations to results from randomized experiments under some circumstances but that reliance on results from randomized experiments as the gold standard is still well founded.
Publication types
- Comparative Study
- Family Therapy
- Marital Therapy
- Meta-Analysis as Topic*
- Random Allocation*
IResearchNet
Nonexperimental Designs
The most frequently used experimental design type for research in industrial and organizational psychology and a number of allied fields is the nonexperiment. This design type differs from that of both the randomized experiment and the quasi-experiment in several important respects. Prior to describing the nonexperimental design type, we note that the article on experimental designs in this section considers basic issues associated with (a) the validity of inferences stemming from empirical research and (b) the settings within which research takes place. Thus, the same set of issues is not addressed in this entry.
Attributes of Nonexperimental Designs
Nonexperimental designs differ from both quasi-experimental designs and randomized experimental designs in several important respects. Overall, these differences lead research using nonexperimental designs to be far weaker than that using alternative designs, in terms of internal validity and several other criteria.
Academic Writing, Editing, Proofreading, And Problem Solving Services
Get 10% off with 24start discount code, measurement of assumed causes.
In nonexperimental research, variables that are assumed causes are measured, as opposed to being manipulated. For example, a researcher interested in testing the relation between organizational commitment (an assumed cause) and worker productivity (an assumed effect) would have to measure the levels of these variables. Because of the fact that commitment levels were measured, the study would have little if any internal validity. Note, moreover, that the internal validity of such research would not be at all improved by a host of data analytic strategies (e.g., path analysis, structural equation modeling) that purport to allow for inferences about causal connections between and among variables (Stone-Romero, 2002; Stone-Romero & Rosopa, 2004).
Nonrandom Assignment of Participants and Absence of Conditions
In nonexperiments, there are typically no explicitly defined research conditions. For example, a researcher interested in assessing the relation between job satisfaction (an assumed cause) and organizational commitment (an assumed effect) would simply measure the level of both such variables. Because participants were not randomly assigned to conditions in which the level of job satisfaction was manipulated, the researcher would be left in the uncomfortable position of not having information about the many variables that were confounded with job satisfaction. Thus, the internal validity of the study would be a major concern. Moreover, even if the study involved the comparison of scores on one or more dependent variables across existing conditions over which the researcher had no control, the researcher would have no control over the assignment of participants to the conditions. For example, a researcher investigating the assumed effects of incentive systems on firm productivity in several manufacturing firms would have no control over the attributes of such systems. Again, this would serve to greatly diminish the internal validity of the study.
Measurement of Assumed Dependent Variables
In nonexperimental research, assumed dependent variables are measured. Note that the same is true of both randomized experiments and quasi-experiments. However, there are very important differences among the three experimental design types that warrant attention. More specifically, in the case of well-conducted randomized experiments, the researcher can be highly confident that the scores on the dependent variable(s) were a function of the study’s manipulations. Moreover, in quasi-experiments with appropriate design features, the investigator can be fairly confident that the study’s manipulations were responsible for observed differences on the dependent variable(s). However, in nonexperimental studies, the researcher is placed in the uncomfortable position of having to assume that what he or she views as dependent variables are indeed effects. Regrettably, in virtually all nonexperimental research, this assumption rests on a very shaky foundation. Thus, for example, in a study of the assumed effect of job satisfaction on intentions to quit a job, what the researcher assumes to be the effect may in fact be the cause. That is, individuals who have decided to quit for reasons that were not based on job satisfaction could, in the interest of cognitive consistency, view their jobs as not being satisfying.
Control Over Extraneous or Confounding Variables
Because of the fact that nonexperimental research does not benefit from the controls (e.g., random assignment to conditions) that are common to studies using randomized experimental designs, there is relatively little potential to control extraneous variables. As a result, the results of nonexperimental research tend to have little, if any, internal validity. For instance, assume that a researcher did a nonexperimental study of the assumed causal relation between negative affectivity and job-related strain and found these variables to be positively related. It would be inappropriate to conclude that these variables were causally related. At least one important reason for this is that the measures of these constructs have common items. Thus, any detected relation between them could well be spurious, as noted by Eugene F. Stone-Romero in 2005.
In hopes of bolstering causal inference, researchers who do nonexperimental studies often measure variables that are assumed to be confounds and then use such procedures as hierarchical multiple regression, path analysis, and structural equation modeling to control them. Regrettably, such procedures have little potential to control confounds. There are at least four reasons for this. First, researchers are seldom aware of all of the relevant confounds. Second, even if all of them were known, it is seldom possible to measure more than a few of them in any given study and use them as controls. Third, to the degree that the measures of confounds are unreliable, procedures such as multiple regression will fail to fully control for the effects of measured confounds. Fourth, and finally, because a large number of causal models may be consistent with a given set of covariances among a set of variables, statistical procedures are incapable of providing compelling evidence about the superiority of any given model over alternative models.
References:
- Cook, T. D., & Campbell, D. T. (1979). Quasi-experimentation: Design and analysis issues for field settings. Boston: Houghton Mifflin.
- Shadish, W. R., Cook, T. D., & Campbell, D. T. (2002). Experimental and quasi-experimental designs for generalized causal inference. Boston: Houghton Mifflin.
- Stone-Romero, E. F. (2002). The relative validity and usefulness of various empirical research designs. In
- G. Rogelberg (Ed.), Handbook of research methods in industrial and organizational psychology (pp. 77-98). Malden, MA: Blackwell.
- Stone-Romero, E. F. (2005). Personality-based stigmas and unfair discrimination in work organizations. In R. L. Dipboye & A. Colella (Eds.), Discrimination at work: The psychological and organizational bases (pp. 255-280). Mahwah, NJ: Lawrence Erlbaum.
- Stone-Romero, E. F., & Rosopa, P. (2004). Inference problems with hierarchical multiple regression-based tests of mediating effects. Research in Personnel and Human Resources Management, 23, 249-290.
Have a language expert improve your writing
Run a free plagiarism check in 10 minutes, automatically generate references for free.
- Knowledge Base
- Methodology
- Random Assignment in Experiments | Introduction & Examples
Random Assignment in Experiments | Introduction & Examples
Published on 6 May 2022 by Pritha Bhandari . Revised on 13 February 2023.
In experimental research, random assignment is a way of placing participants from your sample into different treatment groups using randomisation.
With simple random assignment, every member of the sample has a known or equal chance of being placed in a control group or an experimental group. Studies that use simple random assignment are also called completely randomised designs .
Random assignment is a key part of experimental design . It helps you ensure that all groups are comparable at the start of a study: any differences between them are due to random factors.
Table of contents
Why does random assignment matter, random sampling vs random assignment, how do you use random assignment, when is random assignment not used, frequently asked questions about random assignment.
Random assignment is an important part of control in experimental research, because it helps strengthen the internal validity of an experiment.
In experiments, researchers manipulate an independent variable to assess its effect on a dependent variable, while controlling for other variables. To do so, they often use different levels of an independent variable for different groups of participants.
This is called a between-groups or independent measures design.
You use three groups of participants that are each given a different level of the independent variable:
- A control group that’s given a placebo (no dosage)
- An experimental group that’s given a low dosage
- A second experimental group that’s given a high dosage
Random assignment to helps you make sure that the treatment groups don’t differ in systematic or biased ways at the start of the experiment.
If you don’t use random assignment, you may not be able to rule out alternative explanations for your results.
- Participants recruited from pubs are placed in the control group
- Participants recruited from local community centres are placed in the low-dosage experimental group
- Participants recruited from gyms are placed in the high-dosage group
With this type of assignment, it’s hard to tell whether the participant characteristics are the same across all groups at the start of the study. Gym users may tend to engage in more healthy behaviours than people who frequent pubs or community centres, and this would introduce a healthy user bias in your study.
Although random assignment helps even out baseline differences between groups, it doesn’t always make them completely equivalent. There may still be extraneous variables that differ between groups, and there will always be some group differences that arise from chance.
Most of the time, the random variation between groups is low, and, therefore, it’s acceptable for further analysis. This is especially true when you have a large sample. In general, you should always use random assignment in experiments when it is ethically possible and makes sense for your study topic.
Prevent plagiarism, run a free check.
Random sampling and random assignment are both important concepts in research, but it’s important to understand the difference between them.
Random sampling (also called probability sampling or random selection) is a way of selecting members of a population to be included in your study. In contrast, random assignment is a way of sorting the sample participants into control and experimental groups.
While random sampling is used in many types of studies, random assignment is only used in between-subjects experimental designs.
Some studies use both random sampling and random assignment, while others use only one or the other.
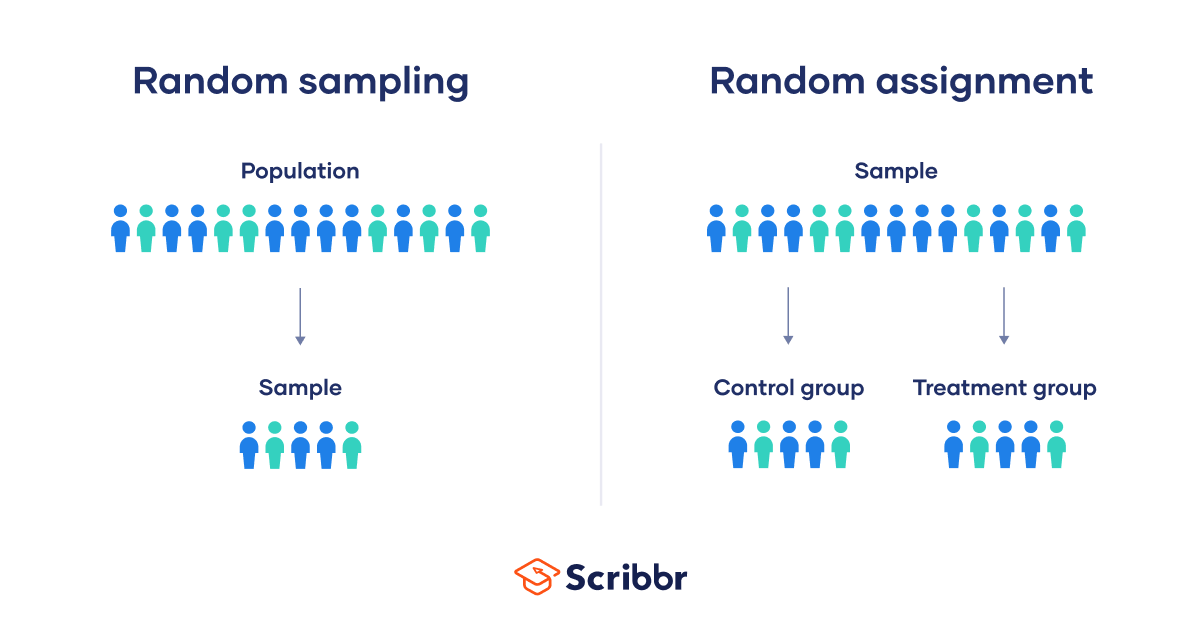
Random sampling enhances the external validity or generalisability of your results, because it helps to ensure that your sample is unbiased and representative of the whole population. This allows you to make stronger statistical inferences .
You use a simple random sample to collect data. Because you have access to the whole population (all employees), you can assign all 8,000 employees a number and use a random number generator to select 300 employees. These 300 employees are your full sample.
Random assignment enhances the internal validity of the study, because it ensures that there are no systematic differences between the participants in each group. This helps you conclude that the outcomes can be attributed to the independent variable .
- A control group that receives no intervention
- An experimental group that has a remote team-building intervention every week for a month
You use random assignment to place participants into the control or experimental group. To do so, you take your list of participants and assign each participant a number. Again, you use a random number generator to place each participant in one of the two groups.
To use simple random assignment, you start by giving every member of the sample a unique number. Then, you can use computer programs or manual methods to randomly assign each participant to a group.
- Random number generator: Use a computer program to generate random numbers from the list for each group.
- Lottery method: Place all numbers individually into a hat or a bucket, and draw numbers at random for each group.
- Flip a coin: When you only have two groups, for each number on the list, flip a coin to decide if they’ll be in the control or the experimental group.
- Use a dice: When you have three groups, for each number on the list, roll a die to decide which of the groups they will be in. For example, assume that rolling 1 or 2 lands them in a control group; 3 or 4 in an experimental group; and 5 or 6 in a second control or experimental group.
This type of random assignment is the most powerful method of placing participants in conditions, because each individual has an equal chance of being placed in any one of your treatment groups.
Random assignment in block designs
In more complicated experimental designs, random assignment is only used after participants are grouped into blocks based on some characteristic (e.g., test score or demographic variable). These groupings mean that you need a larger sample to achieve high statistical power .
For example, a randomised block design involves placing participants into blocks based on a shared characteristic (e.g., college students vs graduates), and then using random assignment within each block to assign participants to every treatment condition. This helps you assess whether the characteristic affects the outcomes of your treatment.
In an experimental matched design , you use blocking and then match up individual participants from each block based on specific characteristics. Within each matched pair or group, you randomly assign each participant to one of the conditions in the experiment and compare their outcomes.
Sometimes, it’s not relevant or ethical to use simple random assignment, so groups are assigned in a different way.
When comparing different groups
Sometimes, differences between participants are the main focus of a study, for example, when comparing children and adults or people with and without health conditions. Participants are not randomly assigned to different groups, but instead assigned based on their characteristics.
In this type of study, the characteristic of interest (e.g., gender) is an independent variable, and the groups differ based on the different levels (e.g., men, women). All participants are tested the same way, and then their group-level outcomes are compared.
When it’s not ethically permissible
When studying unhealthy or dangerous behaviours, it’s not possible to use random assignment. For example, if you’re studying heavy drinkers and social drinkers, it’s unethical to randomly assign participants to one of the two groups and ask them to drink large amounts of alcohol for your experiment.
When you can’t assign participants to groups, you can also conduct a quasi-experimental study . In a quasi-experiment, you study the outcomes of pre-existing groups who receive treatments that you may not have any control over (e.g., heavy drinkers and social drinkers).
These groups aren’t randomly assigned, but may be considered comparable when some other variables (e.g., age or socioeconomic status) are controlled for.
In experimental research, random assignment is a way of placing participants from your sample into different groups using randomisation. With this method, every member of the sample has a known or equal chance of being placed in a control group or an experimental group.
Random selection, or random sampling , is a way of selecting members of a population for your study’s sample.
In contrast, random assignment is a way of sorting the sample into control and experimental groups.
Random sampling enhances the external validity or generalisability of your results, while random assignment improves the internal validity of your study.
Random assignment is used in experiments with a between-groups or independent measures design. In this research design, there’s usually a control group and one or more experimental groups. Random assignment helps ensure that the groups are comparable.
In general, you should always use random assignment in this type of experimental design when it is ethically possible and makes sense for your study topic.
To implement random assignment , assign a unique number to every member of your study’s sample .
Then, you can use a random number generator or a lottery method to randomly assign each number to a control or experimental group. You can also do so manually, by flipping a coin or rolling a die to randomly assign participants to groups.
Cite this Scribbr article
If you want to cite this source, you can copy and paste the citation or click the ‘Cite this Scribbr article’ button to automatically add the citation to our free Reference Generator.
Bhandari, P. (2023, February 13). Random Assignment in Experiments | Introduction & Examples. Scribbr. Retrieved 21 March 2024, from https://www.scribbr.co.uk/research-methods/random-assignment-experiments/
Is this article helpful?
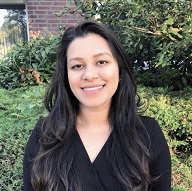
Pritha Bhandari
Other students also liked, a quick guide to experimental design | 5 steps & examples, controlled experiments | methods & examples of control, control groups and treatment groups | uses & examples.
Thank you for visiting nature.com. You are using a browser version with limited support for CSS. To obtain the best experience, we recommend you use a more up to date browser (or turn off compatibility mode in Internet Explorer). In the meantime, to ensure continued support, we are displaying the site without styles and JavaScript.
- View all journals
- My Account Login
- Explore content
- About the journal
- Publish with us
- Sign up for alerts
- Review Article
- Open access
- Published: 29 July 2021
Clinical Research
Errors in the implementation, analysis, and reporting of randomization within obesity and nutrition research: a guide to their avoidance
- Colby J. Vorland ORCID: orcid.org/0000-0003-4225-372X 1 ,
- Andrew W. Brown ORCID: orcid.org/0000-0002-1758-8205 1 ,
- John A. Dawson 2 ,
- Stephanie L. Dickinson ORCID: orcid.org/0000-0002-2998-4467 3 ,
- Lilian Golzarri-Arroyo ORCID: orcid.org/0000-0002-1221-6701 3 ,
- Bridget A. Hannon 4 ,
- Moonseong Heo ORCID: orcid.org/0000-0001-7711-1209 5 ,
- Steven B. Heymsfield ORCID: orcid.org/0000-0003-1127-9425 6 ,
- Wasantha P. Jayawardene ORCID: orcid.org/0000-0002-8798-0894 1 ,
- Chanaka N. Kahathuduwa 7 ,
- Scott W. Keith 8 ,
- J. Michael Oakes 9 ,
- Carmen D. Tekwe 3 ,
- Lehana Thabane 10 &
- David B. Allison ORCID: orcid.org/0000-0003-3566-9399 3
International Journal of Obesity volume 45 , pages 2335–2346 ( 2021 ) Cite this article
13k Accesses
17 Citations
74 Altmetric
Metrics details
- Nutrition disorders
Randomization is an important tool used to establish causal inferences in studies designed to further our understanding of questions related to obesity and nutrition. To take advantage of the inferences afforded by randomization, scientific standards must be upheld during the planning, execution, analysis, and reporting of such studies. We discuss ten errors in randomized experiments from real-world examples from the literature and outline best practices for their avoidance. These ten errors include: representing nonrandom allocation as random, failing to adequately conceal allocation, not accounting for changing allocation ratios, replacing subjects in nonrandom ways, failing to account for non-independence, drawing inferences by comparing statistical significance from within-group comparisons instead of between-groups, pooling data and breaking the randomized design, failing to account for missing data, failing to report sufficient information to understand study methods, and failing to frame the causal question as testing the randomized assignment per se. We hope that these examples will aid researchers, reviewers, journal editors, and other readers to endeavor to a high standard of scientific rigor in randomized experiments within obesity and nutrition research.
Similar content being viewed by others
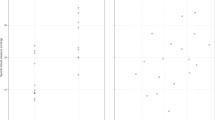
Statistical analysis of continuous outcomes from parallel-arm randomized controlled trials in nutrition—a tutorial
Christian Ritz
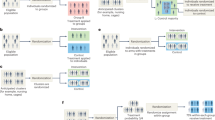
Randomization, design and analysis for interdependency in aging research: no person or mouse is an island
Daniella E. Chusyd, Steven N. Austad, … David B. Allison
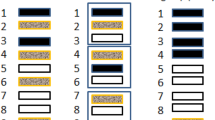
The “completely randomised” and the “randomised block” are the only experimental designs suitable for widespread use in pre-clinical research
Michael F. W. Festing
Introduction
Randomization in scientific experiments bolsters causal inference. Determining a true causal effect would require observing the difference between two outcomes within a single unit (e.g., person, animal) in one case after exogenous manipulation (e.g., “treatment”) and in another case without the manipulation, with all else, including the time of observation, held constant [ 1 ]. However, this true causal effect would require parallel universes in which the same unit at the same time undergoes manipulation in one universe but does not in the other. In the absence of parallel universes, we can estimate average causal effects by balancing all differences between multiple units, such that one group looks as similar as possible to the other group. In practice, however, balancing all variables is likely impossible. For practical application, randomization is an alternative because the selection process is independent of the individual’s pre-randomization (observed and unobserved) characteristics that could confound the outcome, and also balances in the long run the distributions of variables that would otherwise be potential confounders, thereby providing unbiased estimation of treatment effects [ 2 ]. Randomization and exogenous treatment allow inferential statistics to create unbiased effect estimates [ 3 ]. Departures from randomization may increase uncertainty and yield bias.
Randomization is a seemingly simple concept: just assign people (or more generically, “units” [e.g., mice, rats, flies, classrooms, clinics, families]) randomly to one treatment or intervention versus another. The importance of randomization may have been first recognized at the end of the nineteenth century, and formalized in the 1920s [ 4 ]. Yet since its inception there have been errors in the implementation or interpretation of randomized experiments. In 1930, the Lanarkshire Milk investigation tested whether raw or pasteurized milk altered weight and height vs. a control condition in 20,000 schoolchildren [ 5 ]. After publication of the experiment, William Gosset (writing as “Student” of “Student’s t -test” fame) critiqued the study [ 6 ], noting that while there was some random selection of students, a subset of the children were selected on the basis of being either “well fed or ill nourished,” which favored more of the smaller and lighter children being selected, rather than randomized, to the milk groups. Thus, the greater growth in individuals assigned to the milk groups could have been from receiving the milk intervention, or the result of selection bias, an invalidating design flaw. This violates the assumption that the intervention is independent of pre-randomization characteristics of the person being assigned.
Methodologists continue to improve our understanding of the implications of effective randomization, including random sequence generation, implementation (like allocation concealment and blinding), special randomization situations (e.g., randomizing groups of individuals), analysis (e.g., how to analyze an experiment with missing data), and reporting (e.g., how to describe the randomization procedures). Herein, we identify recent publications within obesity and nutrition literature that contain errors in these aspects (see Supplementary Table 1 for a structured list). These examples largely focus on errors arising in the context of null hypothesis significance testing; while there are misconceptions associated with the understanding of p values per se [ 7 , 8 ], it is the framework by which authors typically draw conclusions. The examples span randomized experiments and trials, without or with control groups (i.e., randomized controlled trials [RCTs]). We use these examples to discuss how errors can bias study findings and fail to meet best practices for performing and reporting randomized studies. We clarify that the examples represent a convenience sample, and we make no claims about the frequency of these errors other than that they are frequent enough to have caught our attention. Our categories of errors are neither exhaustive nor in any rank order of severity. Furthermore, we make no assumptions about the circumstances that led to the errors. Rather, we share these examples in the spirit of Gosset who wrote in 1931 on the Lanarkshire Milk experiment, “…but what follows is written not so much in criticism of what was done…as in the hope that in any further work full advantage may be taken of the light which may be thrown on the best methods of arrangement by the defects as well as by the merits” [ 6 ].
Errors in implementing group allocation
1. error: representing nonrandom allocation methods as random, description.
Participants are allocated into treatment groups by use of methods that are not random, but the study is labeled as randomized.
Explanation
Allocation refers to the assignment of subjects into experimental groups. The use of random methods gives each study participant a known probability of being assigned to any experimental group. When any nonrandom allocation is used, studies should not be labeled as randomized.
Authors of studies published in a sample of Chinese journals that were labeled as randomized were interviewed about their methods, and in only ~7% was randomization determined to be properly implemented [ 9 ]. Improperly labeling studies as randomized is not uncommon in both human and animal research on topics of nutrition and obesity, and can occur in different ways.
In one instance, a vitamin D supplementation trial used a nonrandomized convenience sample from a different hospital as a control group, yet labeled the trial as randomized [ 10 ]. In a reply [ 11 ], the authors suggested that no selection bias occurred during the allocation because they detected no significant differences between groups on measured covariates. However, this assumption is unjustified because (a) unobserved or mismeasured covariates can potentially introduce bias, or measurement of a covariate may be imperfect, (b) the inferential validity of randomization rests on the assumption that the distributions of all pre-randomization variables are the same in the long run across levels of the treatment groups, not that the distributions are the same across groups in any one sample, and (c) concluding that groups are identical at baseline because no significant differences were detected entails fallaciously “accepting the null.” Regardless of the lack of observed statistical differences between groups, treatment allocation was not randomized and should not be labeled as such.
In another example, researchers first allocated all participants to the intervention to ensure a sufficient sample size and then randomized future participants [ 12 ]. This violates the assumption that every subject has some probability of being assigned to every group [ 13 ]; the participants first allocated had no probability of being in the control group. In addition, those in the initial allocation wave may have had different characteristics from those with later enrollment.
If units are not all concurrently randomized (e.g., one group is enrolled at a different time), there is also a time-associated confound [ 14 ]. This is exemplified by a study of the effects of a nutraceutical formulation on hair growth that was labeled as randomized [ 15 ]. Participants were randomized to one of two treatment groups, and then each group underwent placebo and treatment sequentially (essentially a pretest-posttest design). The sequential order suggested a hair growth-by-time confound, with hair growth differing by season [ 16 ].
Nonrandom allocation can leave a signature in baseline between-group differences. With randomization, on average, the p values of baseline group comparisons will be uniform for independent measurements. While there are limitations to applying this principle broadly to assessing literature [ 17 , 18 , 19 ], in some cases it has proved useful as a prompt for more information about how and whether randomization was actually employed. An analysis by Carlisle of baseline p value distributions in over 5000 trials flagged apparent deviations from this expectation [ 20 ], suggesting that many studies labeled as randomized may not be. One trial flagged [ 21 ] was the Primary Prevention of Cardiovascular Disease with a Mediterranean Diet (PREDIMED) trial, which highlighted the significant impact of advice to consume a Mediterranean-style diet coupled with additional intake of extra-virgin olive oil or mixed nuts on risk for cardiovascular disease, compared with advice to consume a low-fat diet [ 22 ]. An audit by the PREDIMED authors discovered that members of some of the households were nonrandomly assigned to the same group as the randomized member. Furthermore, one intervention site switched from individuals to clinics as the randomization unit [ 23 ] (see section 5, “Error: failing to account for non-independence” for discussion of non-independence). Thus, the original analysis at the individual level was inappropriate for these participants because some did not have a known probability of being assigned to one of the treatment groups or the control. A retraction and reanalysis did not change the main results or conclusions [ 23 ], although causal language in the article was tempered. Conclusions from secondary analyses were affected, however, such as the 5-year change in body weight and waist circumference, which changed statistical significance for the olive oil group [ 24 ]. Use of statistical principles to examine the likelihood that randomization was properly implemented has flagged other studies related to nutrition and obesity, too [ 25 , 26 , 27 , 28 ]. In at least four cases, publications were retracted [ 22 , 26 , 29 , 30 ].
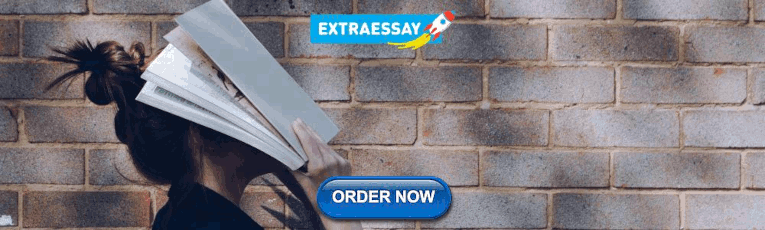
Best practices
Where randomization is impossible, methods should be clearly stated so that there is no conflation of nonrandomized with randomized experiments. Investigators should establish procedures a priori to monitor how randomization is implemented. Furthermore, although a given randomized sample may not appear balanced on all measurable baseline variables, by definition those imbalances have occurred by chance. Altering the allocation process to enforce balance with the use of nonrandom methods may introduce bias. Importantly, use of nonrandom methods may warrant changing how study results are communicated. At a practical level, most methodologists and statisticians would agree that if an RCT is properly randomized, it is reasonable to make causal claims about intervention assignment and outcomes. Whereas the purpose of most research is to seek causal effects [ 31 ], errors discussed herein break randomization, and thereby introduce additional concerns that must be satisfied to increase the confidence in unbiased estimates. While a nuanced discussion of the use of causal language is outside the scope of this review, from a purist perspective, the description of relationships as causal from nonrandom methods is inappropriate [ 32 ].
Where important pre-randomization factors are identified that could influence results if they are imbalanced (such as animal body weight), forms of restricted randomization exist to maintain the benefits of randomization with control over such factors, instead of using haphazard methods that may introduce bias. These include blocking and stratification [ 33 , 34 ], which necessitate additional consideration at the analysis stage beyond a simple randomization scheme (see section 5, “Error: failing to account for non-independence”).
2. Error: failing to adequately conceal allocation from investigators
Investigators who assign treatments, and the participants receiving them, are inadequately concealed from knowing what condition was assigned.
Allocation concealment, when implemented properly, prevents researchers from foreknowing the allocation of the next participant. Furthermore, it prevents participants from foreknowing their assignment, who may choose to dropout if they do not receive a preferred treatment. Thus, concealment prevents selection bias and confounding [ 35 , 36 , 37 ]. Whereas randomization is a method to create unbiased estimates of effect, allocation concealment is necessary to remove the human element of decisions (whether conscious or unconscious) when participants are assigned to groups, and both are important for a rigorous trial. When concealment is broken, sample estimates can become biased in different ways.
Even with the use of random allocation methods, the failure to conceal allocation means that the researchers, and sometimes participants, will know upcoming assignments. The audit of PREDIMED, as discussed in section 1, “Error: representing nonrandom allocation methods as random,” also clarified that allocation was not concealed [ 23 ], despite using computer-generated randomization tables. In the case of the Lanarkshire study as described above [ 5 , 6 ], the failure to conceal allocation led to conscious bias in how schoolchildren were assigned to the interventions. In other cases, researchers may unconsciously bias allocations if they have any involvement in the allocation. For example, if the researcher who is doing the allocation is using a physical method of randomization such as rolling a die or flipping a coin in the presence of the subject, their perception of how the die or coin is rolled or flipped, or how it falls, leaves room to redo it in ways that may select for certain subjects being allocated to particular assignments.
Nonrandom allocation also may make concealment impossible; examples and explanations are presented in Table 1 .
Appropriate concealment strategies may vary by study, but it is ideal that concealment be implemented. The random generation and storage of allocation codes is essential to allocation concealment, using generic numerals or letters unknown to the investigator. Electronic generation and storage of allocations in a protected centralized database is sometimes preferred [ 33 , 38 ] to opaque sealed envelopes [ 39 , 40 ], which is not completely immune to breach and can bias the results if poorly carried out or intentionally compromised [ 41 , 42 , 43 ]. Furthermore, if feasible, real-time generation may be favored over pre-generated allocations [ 44 ]. Regardless of physical or electronic concealment, the allocation codes and other important information about the assignment scheme, such as block size in permuted block randomization [ 45 ], should remain concealed from all research staff and participants. Initial allocation concealment can still be implemented and would improve the rigor of trials even if blinding (i.e., preventing post-randomization knowledge of group assignments) throughout the trial cannot be maintained.
3. Error: not accounting for changes in allocation ratios
The allocation ratio or number of treatment groups is changed partway through a study, but the change is not accounted for in the statistical analysis.
Over the course of a study, researchers may intentionally change treatment group allocations, such as adding, dropping, or combining treatment arms, for various reasons. When researchers change allocation ratios mid-study, this must be taken into account during statistical analysis [ 46 ]. Allocation ratios also change in “adaptive trials,” which have specific methods and concerns beyond what we can cover here (see [ 47 ] for more information).
A study evaluating effects of weight loss on telomere length performed one phase by randomizing participants to three treatment groups (in-person counseling, telephone counseling, and usual care) with 1:1:1 allocation. After no significant difference was found between in-person and telephone counseling, participants in the next phase of the study were randomized with 1:1 allocation into a combined intervention of in-person and telephone counseling or usual care [ 48 ]. In addition to the authors’ choice of analyzing interim results before starting another phase (which risks increasing false-positive findings and should be accounted for in statistical analysis [ 49 ]), the analysis combined these two phases, effectively analyzing 2:1 and 1:1 allocations together [ 50 ]. Another study of low-calorie sweeteners and sucrose and weight-related outcomes [ 51 ] started by randomly allocating participants evenly to five treatment groups with 1:1:1:1:1 allocation, but changed to 2:1:1:1:1 midway through after one group had a higher attrition rate. Neither of these two studies reported accounting for these different phases of study in the statistical analysis. Using different allocation ratios for different groups can bias study results [ 46 , 50 ]. This is because differences may exist between the different periods of recruitment in participant characteristics, such as baseline BMI [ 46 , 50 ]. Thus, baseline differences in the wave of participants allocated at the 2:1 ratio, when pooled with the ratio of those allocated at the 1:1 ratio, would exaggerate the differences when analyzed as though all participants were allocated at the same time.
When allocation ratios change within studies or between randomized experiments that are pooled, caution should be used in combining data. Changes in allocation ratios must be properly taken into account in statistical analysis (see section 7, “Error: improper pooling of data”).
4. Error: replacements are not randomly selected
Participants who dropout are replaced in ways that are nonrandom, for instance, by allocating individuals to a single treatment that experienced a high percentage of participant dropout.
Nonrandom replacement of dropouts is another example of changing allocation ratios. Dropout is common in real-world studies and often leads to missing data, bias, and potentially the loss of power. A meta-analysis of pharmaceutical trials for obesity estimated an average 1-year dropout rate of 37% [ 52 ]. Similarly, a secondary analysis of a diet intervention estimated that the probability of completing the trial was only 60% after just 12 weeks [ 53 ]. Analytical approaches like intention-to-treat [ITT] analysis and imputation of data (described in the Errors in analysis section below) may obviate the need to consider replacing subjects after the initial randomization [ 52 , 54 ]. Yet replacement is sometimes observed in the literature and failing to use random methods to do so introduces another source of potential bias.
In a properly implemented simple RCT, every subject will have the same a priori probability of belonging to any group as any other subject. When a subject who has dropped out is replaced with the next person enrolled instead of by using randomization for assignment, the new participant did not have the same chances as the other subjects in the study of being allocated to that group. This corrupts the process of randomization, potentially introducing bias, and compromises causal inference. Furthermore, allocating participants this way makes allocation concealment impossible.
It is vital to account for dropout in the calculation of sample size and allocation ratios when designing the study. Nevertheless, if dropout was not accounted for a priori, one option is that for the number of dropouts encountered, new participants are enrolled, but each new participant is randomly assigned to groups with the same allocation ratios as the originals [ 55 ]. Note that if dropouts are higher from a particular group and if completers only are analyzed, this may result in an imbalance in the final sample group allocation, but this is not an issue if the ITT principle is adhered to (see section 8, “Error: failing to account for missing data”).
Often, studies do not specify the methods used to replace subjects and use nondescript sentences similar to “subjects who dropped out were replaced” [ 56 , 57 , 58 , 59 ]. As discussed in regard to a trial on green tea ointment and pain and wound healing [ 60 ], such vagueness might suggest introduction of bias and lead to questionable conclusions.
Although replacing subjects may indeed help with the problem of power, the consequences can be detrimental if not properly implemented. Therefore, the decision to replace participants should be thoroughly considered, preplanned if at all possible, and performed by using correct methods, if found to be necessary.
Errors in the analysis of randomized experiments
5. error: failing to account for non-independence.
Groups of subjects (e.g., classrooms, schools, cages of animals) are randomly assigned to experimental conditions together but the data are analyzed as if they were randomized individually, or repeated within-subject measures are treated as independent. Or, measures are treated as independent when subjects individually randomized have repeated within-subject measures or are treated in groups.
The use of cluster randomized trial (cRCT) designs is increasing in nutrition and obesity studies, particularly for the study of school-based interventions, and in contexts where participants are exposed to the other group(s) and as such there is a lack of independence. Similarly, animals are commonly housed together (e.g., in cages, tanks) or grouped by litter. If investigators randomize all animals to treatments by groups instead of individually, this correlation must be addressed in the analysis, but is often unrecognized or ignored. These concerns also exist in cell culture experiments, for example, if treatments are randomized to an entire plate instead of individual wells. In cluster designs, the unit of randomization is the cluster, and not the individual. A frequent error in such interventions is to power and analyze the study at the individual (e.g., person, animal) level instead of the cluster level. Failing to account for within-cluster correlation (often measured by the intraclass correlation coefficient) and cluster-level impacts during study planning leads to an overestimation of statistical power [ 61 ] and typically leads to p values and associated confidence intervals that are artificially small [ 62 , 63 ].
If cRCTs are implemented incorrectly to start, valid inferential analysis for treatment effects is not possible without untestable assumptions [ 61 ]. For instance, randomly assigning one school to an intervention and one to a control yields no degrees of freedom, akin to randomizing one individual to treatment and one to control and treating multiple measurements on each of the two individuals as though those measurements were independent [ 61 ].
Studies that randomize at the individual level may also have correlated observations that should be considered in the analysis, and so it is important to identify potential sources of clustering. For example, outcome measures may be correlated when animals are individually randomized but then group housed for treatment. Likewise, participants individually randomized may be treated in group sessions (such as classes related to the intervention), or may be grouped together within surgeons that do not equally operate in all study arms. These types of scenarios require consideration in statistical analysis [ 64 ]. When repeated measurements are taken on subjects, they similarly must account for within-subject correlation. Taking multiple measurements within individuals (e.g., measuring eyesight in the left and right eye or longitudinal data within person over time) and treating them as independent will lead to invalid inferences [ 64 ].
A distinct issue exists when using forms of restricted randomization (e.g., stratification, blocking, minimization) that are employed to have tighter control over particular factors of interest. In such situations, it is important to include the factors on which randomization restrictions occur as covariates in the statistical model to account for the added correlation between groups [ 65 , 66 ]. Not doing so can result in p values and associated confidence intervals that are artificially large and reduced statistical power. On the other hand, given that one is likely employing restricted randomization because of a small number of units of randomization, losing even a few “denominator” degrees of freedom due to the inclusion of additional covariates in the model may also adversely affect power [ 67 , 68 ].
Failing to account for clustering is one of the most pervasive errors in nutrition and obesity studies that we observe [ 6 , 61 , 69 , 70 , 71 , 72 , 73 , 74 , 75 , 76 , 77 , 78 , 79 ]. A review of school-based randomized trials with weight-related outcomes found that only 21.5% of studies used intracluster correlation coefficients in their power analysis, and only 68.6% applied multilevel models to account for clustering [ 80 ]. In the most severe cases that we observe, a failure to appropriately focus on the cluster as the unit of randomization invalidates any hope of deriving causal inferences [ 70 , 75 , 81 ]. For additional discussion of errors in implementation and reporting in cRCTs, see ref. [ 61 ].
In an example of clustering within participants, a study of vitamin E on diabetic neuropathy randomized participants to the intervention or placebo, but for outcomes related to nerve conduction, the authors conducted measurements in limbs, stating that “left and right sides were treated independently” [ 82 ]. Because these measures were taken within the same participants, within-subject correlations must be taken into account in statistical analyses. Treating non-independent measurements as independent in statistical analysis is sometimes called “pseudoreplication” and is also a common error in animal and cell culture experiments [ 83 ].
When planning cRCTs, it is critical to perform a power calculation that incorporates the number of clusters in the design [ 61 ]. Moreover, analyses of such designs, as well as individually randomized designs, need to include the correlations from clustering for proper treatment inferences, just as repeated measurements of outcomes within subjects must be treated as non-independent.
6. Error: basing conclusions on within-group statistical tests instead of between-groups tests
Experimental groups are analyzed separately for significant differences in the change from baseline and a difference is concluded if one is significant and the other(s) not, instead of comparing directly between groups.
The probative comparison for RCTs is between groups. Sometimes, however, researchers use pre-post within-group tests and draw conclusions based on whether the within-group significance is different, for example, significant in one group but not the other (the so-called “Difference in Nominal Significance” or DINS error [ 84 ]). Using these within-group tests to imply differences between groups increases the false-positive rate of 5% for equal group sizes to up to 50% (and higher for unequal groups) [ 85 ] and is therefore invalid.
The DINS error was identified in an RCT testing isomaltulose vs. sucrose in the context of effects of an energy-reduced diet on weight and fat mass, where some conclusions, such as the outcome of fat mass, were drawn from within-group comparisons but the between-group comparison was not statistically different [ 86 ]. We observe this error frequently in nutrition and obesity research [ 87 , 88 , 89 , 90 , 91 , 92 , 93 , 94 , 95 , 96 , 97 , 98 , 99 , 100 , 101 , 102 , 103 ]. Sometimes using this logic still reaches the correct conclusions (i.e., the between-group and within-group comparisons are both statistically significant or not), but often it does not, and therefore it is an unreliable approach for inferences.
For proper analysis of RCTs, within-group testing should not be represented as the comparison of interest [ 71 , 84 , 85 , 87 , 102 ]. Journal editors, reviewers, and readers should request that conclusions be drawn from between-group comparisons.
7. Error: improper pooling of data
Data for a single RCT are pooled without maintaining the randomized design, or data from multiple RCTs are pooled (i.e., meta-analysis) without accounting for study in statistical analysis.
Data for statistical analysis can be pooled either within one or multiple RCTs, but errors can arise when the random elements of assignment are disregarded. Pooling within one study refers to the process of combining data across different groups, subgroups, or sites to include in a single analysis. When a single RCT is performed across multiple sites or subgroups and the same allocation ratio is not used across all sites or subgroups, or the randomization allocation to study arms changes during the course of an RCT, these different sites, subgroups, or phases of the study need to be taken into account during data analysis. This is because assignment probability is confounded with subset. If data are pooled simply with no account for subsets, any differences between subsets can bias effect estimation [ 50 ].
When combining multiple RCTs, individual participant data (IPD) can be used (i.e., IPD meta-analysis). However, if they are treated as though they came from a single RCT without accounting for site, at best it will increase the residual variance and make the analysis inefficient, and at worst will confound the results and make the effect estimates biased [ 104 ]. Another error in IPD meta-analyses is the use of data pooled across trials to compare intervention effects in one subgroup of participants with another (e.g., to test the interaction between intervention and pre-randomization subgroups) without accounting for trial in the analysis. This increases the risk of bias, owing to lack of knowledge of individual within- and across-trial interaction effects and inability to separate them, as well as inappropriate standard errors for the interaction effect [ 105 ]. This differs from “typical” meta-analyses because the effect estimates already account for the fact that both treatment groups existed in the same study.
In the trial of how weight loss affects telomere length in women with breast cancer (see subsection “Examples” under section 3, “Error: not accounting for changes in allocation ratios”), data were pooled from two different phases of an RCT that had different allocation ratios, which was not taken into account in the analysis [ 50 ]. Another example is a pooling study that combined IPD from multiple RCTs to examine the effects of a school-based weight management program on summer weight gain among students but ignored “study” as a factor in the analysis [ 106 ].
When pooling data under the umbrella of one study (e.g., allocation ratio change during the study), statistical analysis should include variables for subgroups to prevent confounding [ 46 ]. When pooling IPD from multiple RCTs, care must be taken to include a term for “study” when group conditions or group allocation ratios are not identical across all included RCTs [ 106 ]. For additional information on methods for IPD meta-analysis, see ref. [ 105 ].
8. Error: failing to account for missing data
Missing data (due to dropouts, errors in measurement, or other reasons) are not accounted for in an RCT.
The integrity of the randomization of subjects must be maintained throughout a study. Any post-randomization exclusion of subjects or observations, or any instances of missingness in post-randomization measurements, violates both randomization and the ITT principle (analyzing all subjects according to their original treatment assignments) and thus potentially compromises the validity of any statistical analyses and the conclusions drawn from them. There are two main reasons for this. Whereas randomization minimizes potential confounding by providing similar distributions in baseline participant characteristics, missing data that are not completely at random breaks the randomization, introduces potential bias in various ways, and degrades the confidence that the effect (or lack thereof) is the result only of the experimental condition [ 107 , 108 ]. Consider as an example reported income. If individuals with very low or very high incomes are less likely to report their incomes, then non-missing income values and their corresponding covariate values cannot provide valid inference for individuals who did not report income, because the populations are simply not the same. Missing data are extremely common in RCTs, as discussed in section 4, “Error: replacements are not randomly selected.” Regardless of the intervention, investigators need to be prepared to handle missing data based on assumptions about how data are missing.
One review found that only 50% of trials use adequate methods to account for missing data [ 109 ], and studies of obesity and nutrition are no exception. For example, in a trial of intermittent vs. continuous energy restriction on body composition and resting metabolic rate with a 50% dropout rate, reanalysis of all participants halved the magnitude of effect estimates compared with analyses of completers only [ 99 ]. As in this case, investigators will often report analyses performed only on participants who have completed the study, without also reporting an ITT analysis that includes all subjects who were randomized. Investigators may dismiss ITT analyses because they perceive them as “diluting” the effect of the treatment [ 110 ]. However, this presumes that there is an effect of treatment at all. Dropouts may result in an apparent effect that is actually an artifact. If dropouts are nonrandom, then groups may simply appear different because people remaining in the treatment group are different people from those who dropped out. Attempts to estimate whether those who dropped out differ from those who stayed in are often underpowered.
Furthermore, some investigators may not understand ITT and mislabel their analysis. For instance, in an RCT of a ketogenic diet in patients with breast cancer, the authors reported that “[s]tatistical analysis was carried out according to the intention-to-treat protocol” of the 80 randomized participants, yet the flow diagram and results suggest that the analyses were restricted to completers only [ 111 ]. Surveys of ITT practices suggest that there is a general lack of adequate reporting of information pertaining to how missing data is handled [ 112 ].
Many analyses can be conducted on randomized data including “per protocol” (removing data from noncompliant subjects) and ITT. However, simply comparing per protocol to ITT analyses as a sensitivity analysis is suboptimal; they estimate different things [ 113 ]. As such, the Food and Drug Administration has recently focused on the concept of estimands to clearly establish the question being tested [ 114 ]. ITT can estimate the effect of assignment, not treatment per se, in an unbiased manner, whereas the per protocol analysis can only estimate in a way that allows the possibility for bias.
In an oft-paraphrased maxim of Lachin [ 108 ], “the best way to deal with the problem [of missing data] is to have as little missing data as possible.” This goal may be furthered through diligent administrative follow-up and constant contact with subjects; further considerations on minimization of loss-to-follow-up and other missingness may be found elsewhere [ 115 , 116 ]. However, having no missing data whatsoever is often not achievable in practice, especially for large, randomized studies. Thus, something must be done when missing data exist. In general, the simplest and best way to mitigate the problem of missing data is through the ITT principle when conducting the statistical analysis.
Statistical approaches for handling missing data require untestable assumptions, assumptions that lack face validity and hence are unfounded, or both [ 108 ]. Complete case analyses, where subjects with missing data are ignored, require assumptions that the data are missing completely at random that are not recommended [ 108 ]. Multiple imputation fills in missing data repeatedly, with relationship and predictions guided by other covariates, and is recommended under the assumption that data are missing at random (MAR); that is, the missingness or not of an observation is not directly impacted by its true value. Methods commonly used in obesity trials such as last observation carried forward (LOCF) [ 117 ] or baseline observation carried forward (BOCF) are not recommended because of the strict or unreasonable assumptions required to yield valid conclusions [ 108 , 117 , 118 ]. In such cases where values are missing not at random (MNAR; this set of assumptions may also be referred to as “not missing at random”, NMAR), explicit modeling for the missingness process is required [ 119 ], requiring stronger assumptions that may not be valid.
Finally, when it is apparent that data are MNAR, when the integrity of randomization is no longer intact, or both, estimates are no longer represented as a causal effect afforded by randomization and care should be taken that causal language is tempered. Even in cases where the assumptions are violated, however, ignoring the missingness (e.g., completers only analyses) is generally not recommended.
In summary, minimizing missing data should be a key goal in any randomized study. But when data are missing, thoughtful approaches are necessary to respect the ITT principle and produce unbiased effect estimates. Additional discussion about best practices to handle missing data in the nutrition context is available at ref. [ 107 ].
Errors in the reporting of randomization
9. error: failing to fully describe randomization.
Published reports fail to provide sufficient information so that readers can assess the methods used for randomization.
Studies cannot be adequately evaluated unless methods used for randomization are reported in sufficient detail. Indeed, many examples described herein were obscured by poor reporting until we or others were able to gain clarification from the study authors through personal communication or post-publication discourse. Accepted guidelines that define the standards of reporting the results of clinical trials (i.e., Consolidated Standards of Reporting Trials for human trials (CONSORT) [ 120 ]), animal research (i.e., Animal Research: Reporting of In Vivo Experiments (ARRIVE) [ 121 ]), and others [ 122 ] have emphasized the importance of adequate reporting of randomization methods. Researchers should, to the fullest extent possible, report according to accepted guidelines as part of responsible research conduct [ 123 ].
Most authors (including historically us), however, do not report adequately, and this includes randomization sequence generation and allocation concealment in human and animal research [ 124 , 125 ]. We have noted specific examples of a failure to include sufficient details about the method of randomization and allocation ratio in a study of dairy- and berry-based snacks on nutritional status and grip strength [ 126 ], which were clarified in a reply [ 127 ]. In a personal communication regarding another trial of a nutritional intervention on outcomes in individuals with autism spectrum disorder, we learned that the authors had used additional blocking factors, and randomized some siblings as pairs, neither of which were reported in the paper nor accounted for in the statistical analysis [ 128 ]. In another study that pooled RCTs of school-based weight management programs, the reported number of participants of the included studies was inconsistent with the original publications [ 106 ]. In other cases, the methods used to account for clustering may not be appropriately described for readers to assess them [ 129 , 130 ]. In one case, the authors reported randomizing in pairs, yet the number randomized was an odd number and differed between groups ( n = 21 and n = 24) [ 131 ], to which the authors reported a coding error [ 132 ]. Other vague language descriptions include statements such as “the samples were randomly divided into two groups” [ 27 ].
The use of non-specific language to describe allocation methods may also lead to confusion as to whether randomized methods were actually used. For example, we observed the term “semi-random” used to reflect stratified randomization [ 133 ] or minimization [ 134 ], whereas elsewhere it may describe methods that are nonrandom or not clearly stated [ 135 ].
Neglecting to report essential components of how randomization was implemented hinders the ability of a reader from fully evaluating the trial and hence from interpreting the validity of the reported findings. We emphasize that reporting guidelines such as CONSORT [ 120 ] should be consulted during the study planning and publication preparation stages to ensure that essential components related to randomization are reported, such as methods used to generate the allocation sequence, implement randomization, and conceal allocation; any matching or blocking procedures used; accuracy and consistency of the numbers in flow diagrams; and reporting baseline demographic and clinical variables. With regard to the last point, a common error is to report p values of baseline statistical comparisons and conclude covariate imbalance between groups if they are <0.05. An example of this type of thinking is as follows: “[a]s randomization was not fully successful concerning age, it was included as covariate in the main analyses.” [ 136 ], or conversely, “The similarity between the exercise plus supplement and exercise plus placebo groups for both demographic composition and pre-intervention fitness and cognitive scores provides strong evidence that participants were randomly assigned into groups” [ 137 ]. However, as discussed in section 1, “Error: representing nonrandom allocation methods as random,” the distribution of p values from baseline group comparisons is uniform in the long run with randomization and therefore we would expect on average that 1/20 p values will be <0.05 by chance, with some caveats [ 17 , 18 , 19 ]. In other words, per CONSORT, “[s]uch significance tests assess the probability that observed baseline differences could have occurred by chance; however, we already know that any differences are caused by chance” [ 120 ], and should not be reported. Baseline p values do not reflect whether imbalances might affect the results; imbalanced variables that are prognostic on the outcome that are not p < 0.05 can still have a strong effect on the result [ 138 , 139 ]. Thus, statistical tests should not be used to determine prognostic covariates; such covariates should preferably be identified and included in an analysis plan prior to executing the study [ 139 ].
10. Error: failing to properly communicate inferences from randomized studies
The causal question is not framed as testing the randomized assignment per se.
The appropriate execution and analysis of a randomized experiment tests the effect of treatment assignment on the outcome of interest. The causal effect being tested is what participants are assigned to, not what they actually did. That is, if some participants drop out, do not comply with the intervention, are accidentally given the wrong treatment, or in other ways do not complete the intended treatment, the proper analysis maintains the randomized assignment of the subjects and tests the effect of assigning subjects to the treatment, which includes factors beyond the treatment itself. Indeed, it may be that dropout or non-compliance is caused by the assignment itself. This distinction is particularly important in nutrition trials, which often suffer from poor compliance, and is discussed in part in subsection “Explanation” under section 8, “Error: failing to account for missing data” with respect to the ITT principle. For instance, researchers may be interested in discussing the effect of eating their diet, when in fact what was tested was being assigned to eat the diet.
As discussed in section 8, “Error: failing to account for missing data,” there is often a perception among authors that including subjects that are, e.g., noncompliant or incorrectly assigned will preclude an understanding of the true effect of the intervention on the outcome(s) of interest. But the realization of unbiased effect estimates that the principles of randomization afford us is only achieved when subjects are analyzed as they are randomized. For example, the random assignment to 25% energy restriction of participants in a 2-year trial resulted in an average reduction of about 12% (~300 kcal) [ 140 ]. The public discussion of this trial advertised that “Cutting 300 Calories a Day Shows Health Benefits” [ 141 ]. Yet it is possible that assigning participants to cut only 300 kcal would not have produced the same benefits if they once again achieved only half of that assigned. In another example, the random assignment of high phytate bread did not lead to a statistically significant difference in whole body iron status as compared to dephytinized bread when missing data was imputed, but it was significantly higher when dropouts were excluded [ 98 , 142 , 143 ]. A difference cannot be concluded from these data based on the causal question of the assignment of high phytate bread, particularly because dropout was significantly higher in one group, which may create an artificial effect.
The appropriate framing of the treatment assignment (i.e., following the ITT principle) as the causal effect of interest is important when communicating and interpreting results of RCTs. From this perspective, maximizing the validity of randomized studies from planning, execution, and analysis is a matter of maintaining the randomized assignments to the greatest extent possible. To this end, randomized studies should be communicated carefully that the causal question is assignment to treatment.
Randomization is a powerful tool to examine causal relationships in nutrition and obesity research. Empirical evidence supports the use of both randomization and allocation concealment for unbiased effect estimates. Trials with inadequate concealment are associated with larger effect estimates than are those with adequate concealment [ 144 , 145 , 146 , 147 ], likely reflecting bias. Despite such undesirable potential consequences, many randomized studies of humans and animals do not adequately conceal allocation [ 43 , 124 , 148 ]. Although more difficult to compare in human studies, the results of nonrandomized studies sometimes differ from those of randomized trials [ 149 ], while nonrandomized animal studies are associated with increased effect sizes [ 148 ]. These empirical observations are suggestive of biased estimates, and when coupled with the theoretical arguments, indicate that randomization should be implemented whenever possible. For these reasons, where randomization is implemented per the best practices described herein, the use of causal language to communicate results is appropriate. But where it is not correctly implemented or maintained, the greater potential for bias in the effect estimates and additional assumptions that need to be met to increase confidence in causal relationships invariably changes how such effects should be communicated.
Even when randomization is implemented, errors related to randomization are common, suggesting that researchers in nutrition and obesity may benefit from statistical support during the design, execution, analysis, and reporting of randomized experiments for more rigorous, reproducible, and replicable research [ 150 ]. When errors are discovered, authors and editors have a responsibility to correct the scientific record, and journals should have procedures in place to do so expeditiously [ 151 ]. The severity of the error, ranging from invalidating the conclusions [ 152 ] to simply requiring clarification, means that different considerations exist for each type of error. For example, some invalidating errors are consequent to the design and cannot be fixed, and retractions have been issued [ 29 , 153 , 154 ]. For other examples such as PREDIMED, for which errors in randomization required a reanalysis as a quasi-experimental design, the reanalysis, retraction, and republication serve as an important example of scientific questioning and transparency of research methods [ 155 ]. Other cases require reanalysis or reporting of the appropriate statistical analyses but are otherwise not invalidated by design flaws [ 88 , 156 ]. Yet others need clarity on the methods, for instance when a study did not really use random allocation but reported as such [ 157 ].
The involvement of professional biostatisticians and others with methodological expertise from the planning stages of a study will prevent many of these errors. The use of trial and analysis plan preregistration can aid in thinking through decisions a priori while simultaneously increasing transparency and guarding against unpublished results and inflated false positives from analytic flexibility by pre-specifying outcomes and analyses [ 71 ]. Being cognizant of these errors and becoming familiar with CONSORT and other reporting guidelines enhance the value of the time, effort, and financial investment we devote to obesity and nutrition research.
Imbens GW, Rubin DB. Rubin causal model. In: Durlauf SN, Blume LE, (eds.) Microeconometrics. London: Springer; 2010. p. 229–41 https://doi.org/10.1057/9780230280816 .
Senn S. Seven myths of randomisation in clinical trials. Stat Med. 2013;32:1439–50.
Article PubMed Google Scholar
Greenland S. Randomization, statistics, and causal inference. Epidemiology. 1990;1:421–9.
CG P, Gluud C. The controlled clinical trial turns 100 years: Fibiger’s trial of serum treatment of diphtheria. BMJ. 1998;317:1243–5.
Article Google Scholar
Leighton G, McKinlay PL. Milk consumption and the growth of school children. Report on an investigation in Lanarkshire schools. Scotland, Edinburgh: H.M.S.O.; 1930. p. 20.
Student. The Lanarkshire Milk experiment. Biometrika. 1931;23:398–406.
Wasserstein RL, Lazar NA. The ASA statement on p-values: context, process, and purpose. Am Stat. 2016;70:129–33.
Greenland S, Senn SJ, Rothman KJ, Carlin JB, Poole C, Goodman SN, et al. Statistical tests, P values, confidence intervals, and power: a guide to misinterpretations. Eur J Epidemiol. 2016;31:337–50.
Article PubMed PubMed Central Google Scholar
Wu T, Li Y, Bian Z, Liu G, Moher D. Randomized trials published in some Chinese journals: how many are randomized? Trials. 2009;10:1–8.
Shub A, McCarthy EA. Letter to the Editor: “Effectiveness of prenatal vitamin D deficiency screening and treatment program: a stratified randomized field trial”. J Clin Endocrinol Metab. 2018;104:337–8.
Ramezani Tehrani F, Minooee S, Rostami M, Bidhendi Yarandi R, Hosseinpanah F. Response to Letter to the Editor: “Effectiveness of prenatal vitamin D deficiency screening and treatment program: a stratified randomized field trial”. J Clin Endocrinol Metab. 2018;104:339–40.
Williams LK, Abbott G, Thornton LE, Worsley A, Ball K, Crawford D. Improving perceptions of healthy food affordability: results from a pilot intervention. Int J Behav Nutr Phys Act. 2014;11:33.
Westreich D, Cole SR. Invited commentary: positivity in practice. Am J Epidemiol. 2010;171:674–7.
Campbell DT, Stanley JC. Experimental and quasi-experimental designs for research. Wilmington, MA: Houghton Mifflin Company; 1963.
Tenore GC, Caruso D, Buonomo G, D’Avino M, Santamaria R, Irace C, et al. Annurca apple nutraceutical formulation enhances keratin expression in a human model of skin and promotes hair growth and tropism in a randomized clinical trial. J Med Food. 2018;21:90–103.
Article CAS PubMed PubMed Central Google Scholar
Keith SW, Brown AW, Heo M, Heymsfield SB, Allison DB. Re: “Annurca apple nutraceutical formulation enhances keratin expression in a human model of skin and promotes hair growth and tropism in a randomized clinical trial” by Tenore et al. (J Med Food 2018;21:90–103). J Med Food. 2019;22:1301–2.
Bolland MJ, Gamble GD, Avenell A, Grey A. Rounding, but not randomization method, non-normality, or correlation, affected baseline P-value distributions in randomized trials. J Clin Epidemiol. 2019;110:50–62.
Bolland MJ, Gamble GD, Avenell A, Grey A, Lumley T. Baseline P value distributions in randomized trials were uniform for continuous but not categorical variables. J Clin Epidemiol. 2019;112:67–76.
Mascha EJ, Vetter TR, Pittet J-F. An appraisal of the Carlisle-Stouffer-Fisher method for assessing study data integrity and fraud. Anesth Analg. 2017;125:1381–5.
Carlisle JB. Data fabrication and other reasons for non-random sampling in 5087 randomised, controlled trials in anaesthetic and general medical journals. Anaesthesia. 2017;72:944–52.
Article CAS PubMed Google Scholar
The Editors of the Lancet Diabetes & Endocrinology. Retraction and republication—effect of a high-fat Mediterranean diet on bodyweight and waist circumference: a prespecified secondary outcomes analysis of the PREDIMED randomised controlled trial. Lancet Diabetes Endocrinol. 2019;7:334
Article CAS Google Scholar
Estruch R, Ros E, Salas-Salvadó J, Covas M-I, Corella D, Arós F, et al. Primary prevention of cardiovascular disease with a Mediterranean diet. N Engl J Med. 2013;368:1279–90.
Estruch R, Ros E, Salas-Salvado J, Covas MI, Corella D, Aros F, et al. Primary prevention of cardiovascular disease with a Mediterranean diet supplemented with extra-virgin olive oil or nuts. N Engl J Med. 2018;378:e34.
Estruch R, Martínez-González MA, Corella D, Salas-Salvadó J, Fitó M, Chiva-Blanch G, et al. Effect of a high-fat Mediterranean diet on bodyweight and waist circumference: a prespecified secondary outcomes analysis of the PREDIMED randomised controlled trial. Lancet Diabetes Endocrinol. 2019;7:e6–17.
Mestre LM, Dickinson SL, Golzarri-Arroyo L, Brown AW, Allison DB. Data anomalies and apparent reporting errors in ‘Randomized controlled trial testing weight loss and abdominal obesity outcomes of moxibustion’. Biomed Eng Online. 2020;19:1–3.
Abou-Raya A, Abou-Raya S, Helmii M. The effect of vitamin D supplementation on inflammatory and hemostatic markers and disease activity in patients with systemic lupus erythematosus: a randomized placebo-controlled trial. J Rheumatol. 2013;40:265–72.
George BJ, Brown AW, Allison DB. Errors in statistical analysis and questionable randomization lead to unreliable conclusions. J Paramed Sci. 2015;6:153–4.
PubMed PubMed Central Google Scholar
Bolland M, Gamble GD, Grey A, Avenell A. Empirically generated reference proportions for baseline p values from rounded summary statistics. Anaesthesia. 2020;75:1685–7.
Hsieh C-H, Tseng C-C, Shen J-Y, Chuang P-Y. Retraction Note to: randomized controlled trial testing weight loss and abdominal obesity outcomes of moxibustion. Biomed Eng Online. 2020;19:1.
Hosseini R, Mirghotbi M, Pourvali K, Kimiagar SM, Rashidkhani B, Mirghotbi T. The effect of food service system modifications on staff body mass index in an industrial organization. J Paramed Sci. 2015;6:2008–4978.
Google Scholar
Hernán MA. The C-word: scientific euphemisms do not improve causal inference from observational data. Am J Public Health. 2018;108:616–9.
Lazarus C, Haneef R, Ravaud P, Boutron I. Classification and prevalence of spin in abstracts of non-randomized studies evaluating an intervention. BMC Med Res Methodol. 2015;15:85.
Moher D, Hopewell S, Schulz KF, Montori V, Gotzsche PC, Devereaux PJ, et al. CONSORT 2010 explanation and elaboration: updated guidelines for reporting parallel group randomised trials. BMJ. 2010;340:c869.
Altman DG, Bland JM. How to randomise. BMJ. 1999;319:703–4.
Kahan BC, Rehal S, Cro S. Risk of selection bias in randomised trials. Trials. 2015;16:405.
McKenzie JE. Randomisation is more than a coin toss: the role of allocation concealment. BJOG. 2019;126:1288.
Chalmers I. Why transition from alternation to randomisation in clinical trials was made. BMJ. 1999;319:1372.
Torgerson DJ, Roberts C. Understanding controlled trials. Randomisation methods: concealment. BMJ. 1999;319:375–6.
Doig GS, Simpson F. Randomization and allocation concealment: a practical guide for researchers. J Crit Care. 2005;20:187–91. discussion 91–3.
Swingler GH, Zwarenstein M. An effectiveness trial of a diagnostic test in a busy outpatients department in a developing country: issues around allocation concealment and envelope randomization. J Clin Epidemiol. 2000;53:702–6.
Altman DG, Schulz KF. Statistics notes: concealing treatment allocation in randomised trials. BMJ. 2001;323:446–7.
Kennedy ADM, Torgerson DJ, Campbell MK, Grant AM. Subversion of allocation concealment in a randomised controlled trial: a historical case study. Trials. 2017;18:204.
Clark L, Fairhurst C, Torgerson DJ. Allocation concealment in randomised controlled trials: are we getting better? BMJ. 2016;355:i5663.
Zhao W. Selection bias, allocation concealment and randomization design in clinical trials. Contemp Clin Trials. 2013;36:263–5.
Broglio K. Randomization in clinical trials: permuted blocks and stratification. JAMA. 2018;319:2223–4.
Altman DG. Avoiding bias in trials in which allocation ratio is varied. J R Soc Med. 2018;111:143–4.
Pallmann P, Bedding AW, Choodari-Oskooei B, Dimairo M, Flight L, Hampson LV, et al. Adaptive designs in clinical trials: why use them, and how to run and report them. BMC Med. 2018;16:1–15.
Sanft T, Usiskin I, Harrigan M, Cartmel B, Lu L, Li F-Y, et al. Randomized controlled trial of weight loss versus usual care on telomere length in women with breast cancer: the lifestyle, exercise, and nutrition (LEAN) study. Breast Cancer Res Treat. 2018;172:105–12.
Demets DL, Lan KG. Interim analysis: the alpha spending function approach. Stat Med. 1994;13:1341–52.
Dickinson SL, Golzarri-Arroyo L, Brown AW, McComb B, Kahathuduwa CN, Allison DB. Change in study randomization allocation needs to be included in statistical analysis: comment on ‘Randomized controlled trial of weight loss versus usual care on telomere length in women with breast cancer: the lifestyle, exercise, and nutrition (LEAN) study’. Breast Cancer Res Treat. 2019;175:263–4.
Higgins KA, Mattes RD. A randomized controlled trial contrasting the effects of 4 low-calorie sweeteners and sucrose on body weight in adults with overweight or obesity. Am J Clin Nutr. 2019;109:1288–301.
Elobeid MA, Padilla MA, McVie T, Thomas O, Brock DW, Musser B, et al. Missing data in randomized clinical trials for weight loss: scope of the problem, state of the field, and performance of statistical methods. PLoS One. 2009;4:e6624.
Article PubMed PubMed Central CAS Google Scholar
Landers PS, Landers TL. Survival analysis of dropout patterns in dieting clinical trials. J Am Diet Assoc. 2004;104:1586–8.
Wood AM, White IR, Thompson SG. Are missing outcome data adequately handled? A review of published randomized controlled trials in major medical journals. Clin Trials. 2004;1:368–76.
Biswal S, Jose VM. An overview of clinical trial operation: fundamentals of clinical trial planning and management in drug development. 2nd ed. 2018.
Lichtenstein AH, Jalbert SM, Adlercreutz H, Goldin BR, Rasmussen H, Schaefer EJ, et al. Lipoprotein response to diets high in soy or animal protein with and without isoflavones in moderately hypercholesterolemic subjects. Arterioscler Thromb Vasc Biol. 2002;22:1852–8.
Shahrahmani H, Kariman N, Jannesari S, Rafieian‐Kopaei M, Mirzaei M, Ghalandari S, et al. The effect of green tea ointment on episiotomy pain and wound healing in primiparous women: a randomized, double‐blind, placebo‐controlled clinical trial. Phytother Res. 2018;32:522–30.
Draijer R, de Graaf Y, Slettenaar M, de Groot E, Wright C. Consumption of a polyphenol-rich grape-wine extract lowers ambulatory blood pressure in mildly hypertensive subjects. Nutrients. 2015;7:3138–53.
de Clercq NC, van den Ende T, Prodan A, Hemke R, Davids M, Pedersen HK, et al. Fecal microbiota transplantation from overweight or obese donors in cachectic patients with advanced gastroesophageal cancer: a randomized, double-blind, placebo-controlled, phase II atudy. Clin Cancer Res. 2021;27:3784–92.
Golzarri-Arroyo L, Dickinson SL, Allison DB. Replacement of dropouts may bias results: Comment on “The effect of green tea ointment on episiotomy pain and wound healing in primiparous women: A randomized, double-blind, placebo-controlled clinical trial”. Phytother Res. 2019;33:1955–6.
Brown AW, Li P, Bohan Brown MM, Kaiser KA, Keith SW, Oakes JM, et al. Best (but oft-forgotten) practices: designing, analyzing, and reporting cluster randomized controlled trials. Am J Clin Nutr. 2015;102:241–8.
Donner A, Klar N. Pitfalls of and controversies in cluster randomization trials. Am J Public Health. 2004;94:416–22.
Campbell M, Donner A, Klar N. Developments in cluster randomized trials and Statistics in Medicine. Stat Med. 2007;26:2–19.
Kahan BC, Morris TP. Assessing potential sources of clustering in individually randomised trials. BMC Med Res Methodol. 2013;13:1–9.
Kahan BC, Morris TP. Reporting and analysis of trials using stratified randomisation in leading medical journals: review and reanalysis. BMJ. 2012;345:e5840.
Kahan BC, Morris TP. Improper analysis of trials randomised using stratified blocks or minimisation. Stat Med. 2012;31:328–40.
Allison DR. When is it worth measuring a covariate in a randomized clinical trial? J Consult Clin Psychol. 1995;63:339.
Kahan BC, Jairath V, Doré CJ, Morris TP. The risks and rewards of covariate adjustment in randomized trials: an assessment of 12 outcomes from 8 studies. Trials. 2014;15:1–7.
Vorland CJ, Brown AW, Dickinson SL, Gelman A, Allison DB. Comment on: Comprehensive nutritional and dietary intervention for autism spectrum disorder—a randomized, controlled 12-month trial, Nutrients 2018, 10, 369. Nutrients. 2019;11:1126.
Article PubMed Central Google Scholar
Koretz RL. JPEN Journal Club 45. Cluster randomization. JPEN J Parenter Enter Nutr. 2019;43:941–3.
Brown AW, Altman DG, Baranowski T, Bland JM, Dawson JA, Dhurandhar NV, et al. Childhood obesity intervention studies: a narrative review and guide for investigators, authors, editors, reviewers, journalists, and readers to guard against exaggerated effectiveness claims. Obes Rev. 2019;20:1523–41.
Golzarri-Arroyo L, Oakes JM, Brown AW, Allison DB. Incorrect analyses of cluster-randomized trials that do not take clustering and nesting into account likely lead to p-values that are too small. Child Obes. 2020;16:65–6.
Li P, Brown AW, Oakes JM, Allison DB. Comment on “Intervention effects of a school-based health promotion programme on obesity related behavioural outcomes”. J Obes. 2015;2015:708181.
Vorland CJ, Brown AW, Kahathuduwa CN, Dawson JA, Gletsu-Miller N, Kyle TK, et al. Questions on ‘Intervention effects of a kindergarten-based health promotion programme on obesity related behavioural outcomes and BMI percentiles’. Prev Med Rep. 2019;17:101022.
Golzarri-Arroyo L, Vorland CJ, Thabane L, Oakes JM, Hunt ET, Brown AW, et al. Incorrect design and analysis render conclusion unsubstantiated: comment on “A digital movement in the world of inactive children: favourable outcomes of playing active video games in a pilot randomized trial”. Eur J Pediatr. 2020;179:1487–8.
Golzarri-Arroyo L, Chen X, Dickinson SL, Short KR, Thompson DM, Allison DB. Corrected analysis of ‘Using financial incentives to promote physical activity in American Indian adolescents: a randomized controlled trial’confirms conclusions. PLos One. 2020;15:e0233273.
Li P, Brown AW, Oakes JM, Allison DB. Comment on “School-based obesity prevention intervention in chilean children: effective in controlling, but not reducing obesity”. J Obes. 2015;2015:183528.
Wood AC, Brown AW, Li P, Oakes JM, Pavela G, Thomas DM, et al. A Comment on Scherr et al “A multicomponent, school-based intervention, the shaping healthy choices program, improves nutrition-related outcomes”. J Nutr Educ Behav. 2018;50:324–5.
Mietus-Snyder M, Narayanan N, Krauss RM, Laine-Graves K, McCann JC, Shigenaga MK, et al. Randomized nutrient bar supplementation improves exercise-associated changes in plasma metabolome in adolescents and adult family members at cardiometabolic risk. PLoS One. 2020;15:e0240437.
Heo M, Nair SR, Wylie-Rosett J, Faith MS, Pietrobelli A, Glassman NR, et al. Trial characteristics and appropriateness of statistical methods applied for design and analysis of randomized school-based studies addressing weight-related issues: a literature review. J Obes. 2018;2018:8767315.
Meurer ST, Lopes ACS, Almeida FA, Mendonça RdD, Benedetti TRB. Effectiveness of the VAMOS strategy for increasing physical activity and healthy dietary habits: a randomized controlled community trial. Health Educ Behav. 2019;46:406–16.
Ng YT, Phang SCW, Tan GCJ, Ng EY, Botross Henien NP, Palanisamy UDM. et al. The effects of tocotrienol-rich vitamin E (Tocovid) on diabetic neuropathy: a phase II randomized controlled trial. Nutrients.2020;12:1522
Article CAS PubMed Central Google Scholar
Lazic SE, Clarke-Williams CJ, Munafò MR. What exactly is ‘N’ in cell culture and animal experiments? PLoS Biol. 2018;16:e2005282.
George BJ, Beasley TM, Brown AW, Dawson J, Dimova R, Divers J, et al. Common scientific and statistical errors in obesity research. Obesity (Silver Spring). 2016;24:781–90.
Bland JM, Altman DG. Comparisons against baseline within randomised groups are often used and can be highly misleading. Trials. 2011;12:264.
Vorland CJ, Kyle TK, Brown AW. Comparisons of within-group instead of between-group affect the conclusions. Comment on: “Changes in weight and substrate oxidation in overweight adults following isomaltulose intake during a 12-week weight loss intervention: a randomized, double-blind, controlled trial”. Nutrients 2019, 11 (10), 2367. Nutrients. 2020;12:2335.
Bland JM, Altman DG. Best (but oft forgotten) practices: testing for treatment effects in randomized trials by separate analyses of changes from baseline in each group is a misleading approach. Am J Clin Nutr. 2015;102:991–4.
Kroeger CM, Brown AW, Allison DB. Differences in Nominal Significance (DINS) Error leads to invalid conclusions: Letter regarding, “Diet enriched with fresh coconut decreases blood glucose levels and body weight in normal adults”. J Complement Integr Med. 2019;16:2.
Koretz RL. JPEN Journal Club 40. Differences in nominal significance. JPEN J Parenter Enter Nutr. 2019;43:311.
Dickinson SL, Brown AW, Mehta T, Heymsfield SB, Ebbeling CB, Ludwig DS, et al. Incorrect analyses were used in “Different enteral nutrition formulas have no effect on glucose homeostasis but on diet-induced thermogenesis in critically ill medical patients: a randomized controlled trial” and corrected analyses are requested. Eur J Clin Nutr. 2019;73:152–3.
Brown AW, Allison DB. Letter to the Editor and Response Letter to the Editor and Author Response of assessment of a health promotion model on obese turkish children. The Journal of Nursing Research, 25, 436-446. J Nurs Res. 2018;26:373–4.
Kaiser KA, George BJ, Allison DB. Re: Errors in Zhao et al (2015), Impact of enteral nutrition on energy metabolism in patients with Crohn’s disease. World J Gastroenterol. 2016;22:2867.
Allison DB, Brown AW, George BJ, Kaiser KA. Reproducibility: a tragedy of errors. Nature. 2016;530:27–9.
Dawson JA, Brown AW, Allison DB. The stated conclusions are contradicted by the data, based on inappropriate statistics, and should be corrected: Comment on “Intervention for childhood obesity based on parents only or parents and child compared with follow-up alone”. Pediatr Obes. 2018;13:656.
Allison D. The conclusions are unsupported by the data, are based on invalid analyses, are incorrect, and should be corrected: Letter regarding “Sleep quality and body composition variations in obese male adults after 14 weeks of yoga intervention: a randomized controlled trial”. Int J Yoga. 2018;11:83–4.
Dimova RB, Allison DB. Inappropriate statistical method in a parallel-group randomized controlled trial results in unsubstantiated conclusions. Nutr J. 2015;15:58.
Dickinson SL, Foote G, Allison DB. Commentary: studying a possible placebo effect of an imaginary low-calorie diet. Front Psychiatry. 2020;11:329.
Vorland CJ, Mestre LM, Mendis SS, Brown AW. Within-group comparisons led to unsubstantiated conclusions in “Low-phytate wholegrain bread instead of high-phytate wholegrain bread in a total diet context did not improve iron status of healthy Swedish females: a 12-week, randomized, parallel-design intervention study”. Eur J Nutr. 2020;59:2813–4.
Peos J, Brown AW, Vorland CJ, Allison DB, Sainsbury A. Contrary to the conclusions stated in the paper, only dry fat-free mass was different between groups upon reanalysis. Comment on: “Intermittent energy restriction attenuates the loss of fat-free mass in resistance trained individuals. a randomized controlled trial”. J Funct Morphol Kinesiol. 2020;5:85.
Eckert I. Letter to the editor: Inadequate statistical inferences in the randomized clinical trial by Canheta et al. Clin Nutr. 2021;40:338.
Vorland CJ, Foote G, Dickinson SL, Mayo-Wilson E, Allison DB, Brown AW. Letter to the Editor Medicine Correspondence. Blog2020. https://journals.lww.com/md-journal/Blog/MedicineCorrespondenceBlog/pages/post.aspx?PostID=126 .
Sainani K. Misleading comparisons: the fallacy of comparing statistical significance. PM R. 2010;2:559–62.
Allison DB, Antoine LH, George BJ. Incorrect statistical method in parallel-groups RCT led to unsubstantiated conclusions. Lipids Health Dis. 2016;15:1–5.
Tierney JF, Vale C, Riley R, Smith CT, Stewart L, Clarke M, et al. Individual participant data (IPD) meta-analyses of randomised controlled trials: guidance on their use. PLos Med. 2015;12:e1001855.
Fisher D, Copas A, Tierney J, Parmar M. A critical review of methods for the assessment of patient-level interactions in individual participant data meta-analysis of randomized trials, and guidance for practitioners. J Clin Epidemiol. 2011;64:949–67.
Jayawardene WP, Brown AW, Dawson JA, Kahathuduwa CN, McComb B, Allison DB. Conditioning on “study” is essential for valid inference when combining individual data from multiple randomized controlled trials: a comment on Reesor et al’s School-based weight management program curbs summer weight gain among low-income Hispanic middle school students. J Sch Health. 2019;89(1):59–67. J Sch Health. 2019;89:515–8.
Li P, Stuart EA. Best (but oft-forgotten) practices: missing data methods in randomized controlled nutrition trials. Am J Clin Nutr. 2019;109:504–8.
Lachin JM. Statistical considerations in the intent-to-treat principle. Control Clin Trials. 2000;21:167–89.
Powney M, Williamson P, Kirkham J, Kolamunnage-Dona R. A review of the handling of missing longitudinal outcome data in clinical trials. Trials. 2014;15:237.
Hoppe M, Ross AB, Svelander C, Sandberg AS, Hulthen L. Reply to the comments by Vorland et al. on our paper: “low-phytate wholegrain bread instead of high-phytate wholegrain bread in a total diet context did not improve iron status of healthy Swedish females: a 12-week, randomized, parallel-design intervention study”. Eur J Nutr. 2020;59:2815–7.
Khodabakhshi A, Akbari ME, Mirzaei HR, Seyfried TN, Kalamian M, Davoodi SH. Effects of Ketogenic metabolic therapy on patients with breast cancer: a randomized controlled clinical trial. Clin Nutr. 2021;40:751–8.
Hollis S, Campbell F. What is meant by intention to treat analysis? Survey of published randomised controlled trials. BMJ. 1999;319:670–4.
Morris TP, Kahan BC, White IR. Choosing sensitivity analyses for randomised trials: principles. BMC Med Res Methodol. 2014;14:1–5.
ICH Expert Working Group. Addendum on estimands and sensitivity analysis in clinical trials to the guideline on statistical principles for clinical trials; E9(R1) 2019. https://database.ich.org/sites/default/files/E9-R1_Step4_Guideline_2019_1203.pdf .
Gupta SK. Intention-to-treat concept: a review. Perspect Clin Res. 2011;2:109.
Lichtenstein AH, Petersen K, Barger K, Hansen KE, Anderson CA, Baer DJ, et al. Perspective: design and conduct of human nutrition randomized controlled trials. Adv Nutr. 2021;12:4–20.
Gadbury G, Coffey C, Allison D. Modern statistical methods for handling missing repeated measurements in obesity trial data: beyond LOCF. Obes Rev. 2003;4:175–84.
Veberke G, Molenberghs G, Bijnens L, Shaw D. Linear mixed models in practice. New York: Springer; 1997.
Linero AR, Daniels MJ. Bayesian approaches for missing not at random outcome data: the role of identifying restrictions. Statist Sci. 2018;33:198.
Schulz KF, Altman DG, Moher D. CONSORT 2010 statement: updated guidelines for reporting parallel group randomised trials. BMC Med. 2010;8:18.
Percie du Sert N, Hurst V, Ahluwalia A, Alam S, Avey MT, Baker M, et al. The ARRIVE guidelines 2.0: updated guidelines for reporting animal research. J Cereb Blood Flow Metab. 2020;40:1769–77.
Enhancing the QUAlity and Transparency Of health Research. https://www.equator-network.org/ .
Altman DG, Simera I. Responsible reporting of health research studies: transparent, complete, accurate and timely. J Antimicrob Chemother. 2010;65:1–3.
Dechartres A, Trinquart L, Atal I, Moher D, Dickersin K, Boutron I, et al. Evolution of poor reporting and inadequate methods over time in 20 920 randomised controlled trials included in Cochrane reviews: research on research study. BMJ. 2017;357:j2490.
Kilkenny C, Parsons N, Kadyszewski E, Festing MF, Cuthill IC, Fry D, et al. Survey of the quality of experimental design, statistical analysis and reporting of research using animals. PLoS One. 2009;4:e7824.
Kahathuduwa CN, Allison DB. Letter to the editor: Insufficient reporting of randomization procedures and unexplained unequal allocation: a commentary on “Dairy-based and energy-enriched berry-based snacks improve or maintain nutritional and functional status in older people in home care. J Nutr Health Aging. 2019;23:396.
Nykänen I. Insufficient reporting of randomization procedures and unexplained unequal allocation: a commentary on “Dairy-based and energy-enriched berry-based snacks improve or maintain nutritional and functional status in older people in home care”. J Nutr Health Aging. 2019;23:397.
Vorland CJ, Brown AW, Dickinson SL, Gelman A, Allison DB. The implementation of randomization requires corrected analyses. Comment on “Comprehensive nutritional and dietary intervention for autism spectrum disorder—a randomized, controlled 12-month trial, Nutrients 2018, 10, 369”. Nutrients. 2019;11:1126.
Tekwe CD, Allison DB. Randomization by cluster, but analysis by individual without accommodating clustering in the analysis is incorrect: comment. Ann Behav Med. 2020;54:139.
Morgan PJ, Young MD, Barnes AT, Eather N, Pollock ER, Lubans DR. Correction that the analyses were adjusted for clustering: a response to Tekwe et al. Ann Behav Med. 2020;54:140.
Barnard ND, Levin SM, Gloede L, Flores R. Turning the waiting room into a classroom: weekly classes using a vegan or a portion-controlled eating plan improve diabetes control in a randomized translational study. J Acad Nutr Diet. 2018;118:1072–9.
Erratum. J Acad Nutr Diet. 2019;119:1391–3.
Douglas SM, Byers AW, Leidy HJ. Habitual breakfast patterns do not influence appetite and satiety responses in normal vs. high-protein breakfasts in overweight adolescent girls. Nutrients. 2019;11:1223.
Dalenberg JR, Patel BP, Denis R, Veldhuizen MG, Nakamura Y, Vinke PC, et al. Short-term consumption of sucralose with, but not without, carbohydrate impairs neural and metabolic sensitivity to sugar in humans. Cell Metab. 2020;31:493–502 e7.
Quin C, Erland BM, Loeppky JL, Gibson DL. Omega-3 polyunsaturated fatty acid supplementation during the pre and post-natal period: a meta-analysis and systematic review of randomized and semi-randomized controlled trials. J Nutr Intermed Metab. 2016;5:34–54.
Folkvord F, Anschütz D, Geurts M. Watching TV cooking programs: effects on actual food intake among children. J Nutr Educ Behav. 2020;52:3–9.
Zwilling CE, Strang A, Anderson E, Jurcsisn J, Johnson E, Das T, et al. Enhanced physical and cognitive performance in active duty Airmen: evidence from a randomized multimodal physical fitness and nutritional intervention. Sci Rep. 2020;10:1–13.
Altman DG. Comparability of randomised groups. J R Stat Soc Series D. 1985;34:125–36.
Senn S. Testing for baseline balance in clinical trials. Stat Med. 1994;13:1715–26.
Kraus WE, Bhapkar M, Huffman KM, Pieper CF, Das SK, Redman LM, et al. 2 years of calorie restriction and cardiometabolic risk (CALERIE): exploratory outcomes of a multicentre, phase 2, randomised controlled trial. Lancet Diabetes Endocrinol. 2019;7:673–83.
O’Connor A. Cutting 300 calories a day shows health benefits. 2019. https://www.nytimes.com/2019/07/16/well/eat/cutting-300-calories-a-day-shows-health-benefits.html .
Hoppe M, Ross AB, Svelander C, Sandberg AS, Hulthen L. Correction to: Low-phytate wholegrain bread instead of high-phytate wholegrain bread in a total diet context did not improve iron status of healthy Swedish females: a 12 week, randomized, parallel-design intervention study. Eur J Nutr. 2020;59:2819–20.
Hoppe M, Ross AB, Svelander C, Sandberg A-S, Hulthén L. Low-phytate wholegrain bread instead of high-phytate wholegrain bread in a total diet context did not improve iron status of healthy Swedish females: a 12-week, randomized, parallel-design intervention study. Eur J Nutr. 2019;58:853–64.
Schulz KF, Chalmers I, Hayes RJ, Altman DG. Empirical evidence of bias. Dimensions of methodological quality associated with estimates of treatment effects in controlled trials. JAMA. 1995;273:408–12.
Hewitt C, Hahn S, Torgerson DJ, Watson J, Bland JM. Adequacy and reporting of allocation concealment: review of recent trials published in four general medical journals. BMJ. 2005;330:1057–8.
Savović J, Jones HE, Altman DG, Harris RJ, Jüni P, Pildal J, et al. Influence of reported study design characteristics on intervention effect estimates from randomized, controlled trials. Ann Intern Med. 2012;157:429–38.
Page MJ, Higgins JP, Clayton G, Sterne JA, Hróbjartsson A, Savović J. Empirical evidence of study design biases in randomized trials: systematic review of meta-epidemiological studies. PLoS One. 2016;11:e0159267.
Hirst JA, Howick J, Aronson JK, Roberts N, Perera R, Koshiaris C, et al. The need for randomization in animal trials: an overview of systematic reviews. PLoS One. 2014;9:e98856.
Peinemann F, Tushabe DA, Kleijnen J. Using multiple types of studies in systematic reviews of health care interventions—a systematic review. PLoS One. 2013;8:e85035.
National Academies of Sciences, Engineering, and Medicine. Reproducibility and replicability in science. Washington, DC: The National Academies Press; 2019. p. 218.
Vorland CJ, Brown AW, Ejima K, Mayo-Wilson E, Valdez D, Allison DB. Toward fulfilling the aspirational goal of science as self-correcting: a call for editorial courage and diligence for error correction. Eur J Clin Invest. 2020;50:e13190.
Brown AW, Kaiser KA, Allison DB. Issues with data and analyses: errors, underlying themes, and potential solutions. Proc Natl Acad Sci USA. 2018;115:2563–70.
Retraction Statement. LA sprouts randomized controlled nutrition, cooking and gardening program reduces obesity and metabolic risk in Latino youth. Obesity (Silver Spring). 2015;23:2522.
The effect of vitamin D supplementation on inflammatory and hemostatic markers and disease activity in patients with systemic lupus erythematosus: a randomized placebo-controlled trial. J Rheumatol. 2018;45:1713.
Estruch R, Ros E, Salas-Salvadó J, Covas M-I, Corella D, Arós F, et al. Retraction and republication: primary prevention of cardiovascular disease with a Mediterranean diet. N Engl J Med. 2013;368:1279–90.
Kroeger CM, Brown AW, Allison DB. Unsubstantiated conclusions in randomized controlled trial of bingeeating program due to Differences in Nominal Significance (DINS) Error. https://pubpeer.com/publications/3596ABE0460E074A8FA5063606FFAB .
Zhang J, Wei Y, Allison DB. Comment on: "Chronic exposure to air pollution particles increases the risk ofobesity and metabolic syndrome: findings from a natural experiment in Beijing". https://hypothes.is/a/AQKsEg1lEeiymitN4n0bQQ .
Hannon BA, Oakes JM, Allison DB. Alternating assignment was incorrectly labeled as randomization. J Alzheimers Dis. 2019;62:1767–75.
Ito N, Saito H, Seki S, Ueda F, Asada T. Effects of composite supplement containing astaxanthin and sesamin on cognitive functions in people with mild cognitive impairment: a randomized, double-blind, placebo-controlled trial. J Alzheimers Dis. 2018;62:1767–75.
Rae P, Robb P. Megaloblastic anaemia of pregnancy: a clinical and laboratory study with particular reference to the total and labile serum folate levels. J Clin Pathol. 1970;23:379–91.
Griffen WO Jr, Young VL, Stevenson CC. A prospective comparison of gastric and jejunoileal bypass procedures for morbid obesity. Surg Obes Relat Dis. 2005;1:163–72.
Lang TA, Secic M. How to report statistics in medicine: annotated guidelines for authors, editors, and reviewers. Philadelphia, PA: ACP Press; 2006.
Altman DG. Randomisation. BMJ. 1991;302:1481.
Download references
Acknowledgements
We thank Zad Rafi for insightful feedback on an earlier draft and Jennifer Holmes for editing our manuscript.
CJV is supported in part by the Gordon and Betty Moore Foundation. DBA and AWB are supported in part by NIH grants R25HL124208 and R25DK099080. SBH is supported in part by National Institutes of Health NORC Center Grants P30DK072476, Pennington/Louisiana and P30DK040561, Harvard. CDT research is supported by National Cancer Institute Supplemental Award Number U01-CA057030-29S2. Other authors received no specific funding for this work. The opinions expressed are those of the authors and do not necessarily represent those of the NIH or any other organization.
Author information
Authors and affiliations.
Department of Applied Health Science, Indiana University School of Public Health-Bloomington, Bloomington, IN, USA
Colby J. Vorland, Andrew W. Brown & Wasantha P. Jayawardene
Department of Nutritional Sciences, Texas Tech University, Lubbock, TX, USA
John A. Dawson
Department of Epidemiology and Biostatistics, Indiana University School of Public Health-Bloomington, Bloomington, IN, USA
Stephanie L. Dickinson, Lilian Golzarri-Arroyo, Carmen D. Tekwe & David B. Allison
Division of Nutritional Sciences, University of Illinois at Urbana-Champaign, Urbana, IL, USA
Bridget A. Hannon
Department of Public Health Sciences, Clemson University, Clemson, SC, USA
Moonseong Heo
Pennington Biomedical Research Center, Louisiana State University, Baton Rouge, LA, USA
Steven B. Heymsfield
Department of Psychiatry, School of Medicine, Texas Tech University Health Sciences Center, Lubbock, TX, USA
Chanaka N. Kahathuduwa
Department of Pharmacology and Experimental Therapeutics, Division of Biostatistics, Thomas Jefferson University, Philadelphia, PA, USA
Scott W. Keith
Department of Epidemiology, School of Public Health, University of Minnesota, Minneapolis, MN, USA
J. Michael Oakes
Department of Health Research Methods, Evidence and Impact, McMaster University, Hamilton, ON, Canada
Lehana Thabane
You can also search for this author in PubMed Google Scholar
Contributions
Conceptualization, D.B.A.; writing - original draft preparation & review and editing, all authors. All authors haveread and agreed to the published version of the manuscript.
Corresponding authors
Correspondence to Colby J. Vorland or David B. Allison .
Ethics declarations
Competing interests.
In the 36 months prior to the initial submission, DBA has received personal payments or promises for the same from: American Society for Nutrition; Alkermes, Inc.; American Statistical Association; Biofortis; California Walnut Commission; Clark Hill PLC; Columbia University; Fish & Richardson, P.C.; Frontiers Publishing; Gelesis; Henry Stewart Talks; IKEA; Indiana University; Arnold Ventures (formerly the Laura and John Arnold Foundation); Johns Hopkins University; Law Offices of Ronald Marron; MD Anderson Cancer Center; Medical College of Wisconsin; National Institutes of Health (NIH); Medpace; National Academies of Science; Sage Publishing; The Obesity Society; Sports Research Corp.; The Elements Agency, LLC; Tomasik, Kotin & Kasserman LLC; University of Alabama at Birmingham; University of Miami; Nestle; WW (formerly Weight Watchers International, LLC). Donations to a foundation have been made on his behalf by the Northarvest Bean Growers Association. DBA was previously a member (unpaid) of the International Life Sciences Institute North America Board of Trustees. In the last 36 months prior to the initial submission, AWB has received travel expenses from University of Louisville; speaking fees from Kentuckiana Health Collaborative, Purdue University, and Rippe Lifestyle Institute, Inc.; consulting fees from Epigeum (Oxford University Press), LA NORC, and Pennington Biomedical Research Center. The institution of DBA, AWB, CJV, SLD, and LG-A, Indiana University, has received funds to support their research or educational activities from: NIH; USDA; Soleno Therapeutics; National Cattlemen’s Beef Association; Eli Lilly and Co.; Reckitt Benckiser Group PLC; Alliance for Potato Research and Education; American Federation for Aging Research; Dairy Management Inc; Arnold Ventures; the Gordon and Betty Moore Foundation; the Alfred P. Sloan Foundation; Indiana CTSI, and numerous other for-profit and non-profit organizations to support the work of the School of Public Health and the university more broadly. BAH is an employee of Abbott Nutrition in Columbus, OH. SBH is on the Medical Advisory Board of Medifast Corporation. Other authors declare no competing interests.
Additional information
Publisher’s note Springer Nature remains neutral with regard to jurisdictional claims in published maps and institutional affiliations.
Supplementary information
Supplementary table – highlighted changes, rights and permissions.
Open Access This article is licensed under a Creative Commons Attribution 4.0 International License, which permits use, sharing, adaptation, distribution and reproduction in any medium or format, as long as you give appropriate credit to the original author(s) and the source, provide a link to the Creative Commons license, and indicate if changes were made. The images or other third party material in this article are included in the article’s Creative Commons license, unless indicated otherwise in a credit line to the material. If material is not included in the article’s Creative Commons license and your intended use is not permitted by statutory regulation or exceeds the permitted use, you will need to obtain permission directly from the copyright holder. To view a copy of this license, visit http://creativecommons.org/licenses/by/4.0/ .
Reprints and permissions
About this article
Cite this article.
Vorland, C.J., Brown, A.W., Dawson, J.A. et al. Errors in the implementation, analysis, and reporting of randomization within obesity and nutrition research: a guide to their avoidance. Int J Obes 45 , 2335–2346 (2021). https://doi.org/10.1038/s41366-021-00909-z
Download citation
Received : 10 November 2020
Revised : 26 June 2021
Accepted : 06 July 2021
Published : 29 July 2021
Issue Date : November 2021
DOI : https://doi.org/10.1038/s41366-021-00909-z
Share this article
Anyone you share the following link with will be able to read this content:
Sorry, a shareable link is not currently available for this article.
Provided by the Springer Nature SharedIt content-sharing initiative
This article is cited by
Optimizing clinical nutrition research: the role of adaptive and pragmatic trials.
- Camila E. Orsso
- Katherine L. Ford
- Carla M. Prado
European Journal of Clinical Nutrition (2023)
Quality Assessment of Randomized Controlled Trials Published In Journal of Maxillofacial and Oral Surgery (MAOS) From 2009–2021 Using RoB-2.0 Tool
- Amanjot Kaur
- Rishi Kumar Bali
- Kirti Chaudhry
Journal of Maxillofacial and Oral Surgery (2022)
Quick links
- Explore articles by subject
- Guide to authors
- Editorial policies


An official website of the United States government
The .gov means it’s official. Federal government websites often end in .gov or .mil. Before sharing sensitive information, make sure you’re on a federal government site.
The site is secure. The https:// ensures that you are connecting to the official website and that any information you provide is encrypted and transmitted securely.
- Publications
- Account settings
Preview improvements coming to the PMC website in October 2024. Learn More or Try it out now .
- Advanced Search
- Journal List
- HHS Author Manuscripts

Preference in Random Assignment: Implications for the Interpretation of Randomized Trials
Cathaleene macias.
Community Intervention Research, McLean Hospital, Belmont, MA 02478, USA, ude.dravrah.naelcm@saicamc
Paul B. Gold
Department of Counseling and Personnel Services, University of Maryland, College Park, MD 20742, USA, ude.dmu@dlogp
William A. Hargreaves
Department of Psychiatry, University of California, San Francisco, CA, USA, ten.tsacmoc@grahllib
Elliot Aronson
Department of Psychology, University of California, Santa Cruz, CA, USA, ude.cscu.STAC@toille
Leonard Bickman
Center for Evaluation and Program Improvement, Vanderbilt University, Nashville, TN, USA, [email protected]
Paul J. Barreira
Harvard University Health Services, Harvard University, Boston, MA, USA, ude.dravrah.shu@arierrabp
Danson R. Jones
Institutional Research, Wharton County Junior College, Wharton, TX 77488, USA, ude.cjcw@dsenoj
Charles F. Rodican
Community Intervention Research, McLean Hospital, Belmont, MA 02478, USA, ude.dravrah.naelcm@nacidorc
William H. Fisher
Department of Psychiatry, University of Massachusetts Medical School, Worcester, MA, USA, [email protected]
Random assignment to a preferred experimental condition can increase service engagement and enhance outcomes, while assignment to a less-preferred condition can discourage service receipt and limit outcome attainment. We examined randomized trials for one prominent psychiatric rehabilitation intervention, supported employment, to gauge how often assignment preference might have complicated the interpretation of findings. Condition descriptions, and greater early attrition from services-as-usual comparison conditions, suggest that many study enrollees favored assignment to new rapid-job-placement supported employment, but no study took this possibility into account. Reviews of trials in other service fields are needed to determine whether this design problem is widespread.
The validity of research in any field depends on the extent to which studies rule out alternative explanations for findings and provide meaningful explanations of how and why predicted outcomes were attained (e.g., Bickman 1987 ; Lewin 1943 ; Shadish et al. 2002 ; Trist and Sofer 1959 ). In mental health services research, participants’ expectations about the pros and cons of being randomly assigned to each experimental intervention can offer post hoc explanations for study findings that rival the explanations derived from study hypotheses. Unlike most drug studies that can ‘blind’ participants to their condition assignment, studies that evaluate behavioral or psychosocial interventions typically tell each participant his or her experimental assignment soon after randomization, and being assigned to a non-preferred intervention could be disappointing, or even demoralizing ( Shapiro et al. 2002 ), and thereby reduce participants’ interest in services or motivation to pursue service goals ( Cook and Campbell 1979 ; Shadish 2002 ). On the other hand, if most participants randomly assigned to one experimental condition believe they are fortunate, this condition may have an unfair advantage in outcome comparisons.
Reasons for preferring assignment to a particular experimental condition can be idiosyncratic and diverse, but as long as each condition is assigned the same percentage of participants who are pleased or displeased with their condition assignment, then there will be no overall pattern of condition preferences that could explain differences in outcomes. The greater threat to a valid interpretation of findings occurs when most study enrollees share a general preference for random assignment to one particular condition. Greater preference for one experimental condition over another could stem from general impressions of relative service model effectiveness, or from information that is tangential, e.g., program location on a main bus route or in a safer area of town. Even if random assignment distributes service preferences in equal proportions across conditions, the less attractive experimental condition will receive a higher percentage of participants who are mismatched to their preference, and the more attractive condition will receive a higher percentage of participants matched to their preference. For example, if 60% of all study enrollees prefer condition A and 40% prefer condition B, then, with true equivalence across conditions, service A would have 60% pleased and 40% disappointed assignees, while service B would have 40% pleased and 60% disappointed assignees.
There is potential to engender a general preference for assignment to a particular experimental intervention whenever a study’s recruitment posters, information sheets, or consent documents depict one intervention as newer or seemingly better, even if no evidence yet supports a difference in intervention effectiveness. For instance, in a supported housing study, if a comparison condition is described as offering familiar ‘services-as-usual’ help with moving into supervised housing, participants might reasonably prefer assignment to a more innovative experimental intervention designed to help individuals find their own independent apartments.
Methodologists have proposed protocol adaptations to the typical randomized trial to measure and monitor the impact of participants’ intervention preferences on study enrollment and engagement in assigned experimental conditions ( Braver and Smith 1996 ; Corrigan and Salzer 2003 ; Lambert and Wood 2000 ; Marcus 1997 ; Staines et al. 1999 ; TenHave et al. 2003 ). Nevertheless, few mental health service studies have adopted these design modifications, and even fewer have followed recommendations to measure, report, and, if necessary, statistically control for enrollees’ expressed preferences for assignment to a particular condition ( Halpern 2002 ; King et al. 2005 ; Shapiro et al. 2002 ; Torgerson et al. 1996 ).
In this article, we begin by describing several ways that participants’ preferences for random assignment to a specific service intervention can complicate the interpretation of findings. We then review one field of services research to estimate the prevalence of some of these problems. Obstacles to a valid interpretation of findings include the likelihood of (a) lower service engagement and/or greater early attrition from less-preferred conditions, and (b) similarities among people who refuse or leave a non-preferred program and, hence, condition differences in types of retained participants. Even if all randomized participants do receive assigned services, those who preferred assignment to a certain condition may be unique in ways (e.g., functioning, motivation) that predict outcomes over and above the impact of services, and (c) certain program designs may ameliorate or intensify the effects of disappointment in service assignment. Finally, (d) preference for assignment to one condition over another may reflect a clash between program characteristics (e.g., attendance requirements) and participants’ situational considerations (e.g., time constraints, residential location) so that participants assigned to a non-preferred condition may tend to encounter similar difficulties in attaining outcomes and may choose the same alternative activities. We now discuss each of these issues.
How Participants’ Service Preferences Can Influence Outcomes
Impact of assignment preference on service engagement and retention.
Research participants who are disappointed in their random assignment to a non-preferred experimental condition may refuse to participate, or else withdraw from assigned services or treatment early in the study ( Hofmann et al. 1998 ; Kearney and Silverman 1998 ; Laengle et al. 2000 ; Macias et al. 2005 ; Shadish et al. 2000 ; Wahlbeck et al. 2001 ). If this occurs more often for one experimental condition than another, such differential early attrition can quickly transform a randomized controlled trial into a quasi-experiment ( Corrigan and Salzer 2003 ; Essock et al. 2003 ; West and Sagarin 2000 ). Unless participants’ preferences for assignment to experimental interventions are measured prior to randomization, it will be impossible to distinguish the emotional impact on participants of being matched or mismatched to intervention preference from each intervention’s true ability to engage and retain its assigned participants. If participants who reject their service assignments tend to refuse research interviews, the least-preferred intervention may also have a disproportionately higher incidence of ‘false negatives’ (undetected positive outcomes), and this can further bias the interpretation of findings.
Researchers can statistically control for intervention preferences if these attitudes are measured prior to randomization and one intervention is not greatly preferred over another. Even if a study is unable to measure and statistically control participants’ pre-existing preferences for assignment to experimental conditions, statistically adjusting for differential attrition from assigned services can help to rule out disappointment or satisfaction with random assignment as an alternative explanation for findings. However, rather than statistically controlling (erasing) the impact of intervention preferences on service retention and outcomes, it may be far more informative to investigate whether preference in random assignment might have modified a program’s potential to engage and motivate participants ( Sosin 2002 ). For instance, a statistically significant ‘program assignment-by-program preference’ interaction term in a regression analysis ( Aguinis 2004 ; Aiken and West 1991 ) might reveal a demoralization effect (e.g., a combination of less effort, lower service satisfaction, poorer outcomes) for participants randomly assigned to a comparison condition that was not their preference. A more complex program-by-preference interaction analysis might reveal that an assertive program is better at engaging and retaining consumers who are disappointed in their service assignment, while a less assertive program, when it is able to hang onto its disappointed assignees, is better at helping them attain service goals ( Delucchi and Bostrom 2004 ; Lachenbruch 2002 ). Ability to engage and retain participants is a prerequisite for effectiveness, but, in the same way that medication compliance is distinguished from medication efficacy in pharmaceutical trials, service retention should not be confused with the impact of services received ( Little and Rubin 2000 ).
Similarities Between People with the Same Preferences
Even if rates of early attrition are comparable across study conditions, experimental groups may differ in the types of people who decide to accept assigned services ( Magidson 2000 ). If participants who reject a service intervention resemble one another in some way, then the intervention samples of service-active participants will likely differ on these same dimensions.
As yet, we know very little about the effectiveness of different types of community interventions for engaging various types of consumers ( Cook 1999a , b ; Mark et al. 1992 ), but mobile services and programs that provide assertive community outreach appear to have stronger engagement and retention, presumably because staff schedule and initiate most service contacts on a routine basis ( McGrew et al. 2003 ). If these program characteristics match participants’ reasons for preferring one experimental condition over another, then a bias can exist whether or not intervention preference is balanced across conditions. For instance, consumers who are physically disabled, old, or agoraphobic may prefer home-based service delivery and are likely to be disappointed if assigned to a program that requires regular attendance. Greater retention of these more disabled individuals could put a mobile intervention at a disadvantage in a direct evaluation of service outcomes, like employment, that favor able-bodied, younger, or less anxious individuals. On the other hand, in rehabilitation fields like supported housing, education, or employment that depend strongly on consumer initiative and self-determination, higher functioning or better educated consumers may drop out of control conditions because they are not offered needed opportunities soon enough ( Shadish 2002 ). This was evident in a recent study of supported housing ( McHugo et al. 2004 ), which reported a higher proportion of ‘shelter or street’ homeless participants in the control condition relative to a focal supported housing condition, presumably because participants who were more familiar with local services (e.g., those temporarily homeless following eviction or hospital discharge) considered the control condition services inadequate and sought housing on their own outside the research project.
Service model descriptions and intervention theories suggest many interactions between program characteristics and participant preferences that could be tested as research hypotheses if proposed prior to data analysis. Unfortunately, such hypotheses are rarely formulated and tested.
It is also rare for a randomized trial to compare experimental interventions on sample characteristics at a point in time later than baseline, after every participant has had an opportunity to accept or reject his or her experimental assignment, so that sample differences that emerge early in the project can be statistically controlled in outcome analyses.
Interaction Between Responses to Service Assignment and Service Characteristics
A more subtle threat to research validity exists whenever participants disappointed in their intervention assignment do not drop out of services, but instead remain half-heartedly engaged ( Corrigan and Salzer 2003 ). Participants randomized to a preferred intervention are likely to be pleased and enthusiastic, ready to engage with service providers, while those randomized to a non-preferred intervention are more likely to be disappointed and less motivated to succeed. However, the strength of participant satisfaction or disappointment in service assignment can vary greatly depending on service program characteristics ( Brown et al. 2002 ; Calsyn et al. 2000 ; Grilo et al. 1998 ; Macias et al. 2005 ; Meyer et al. 2002 ). For instance, in a randomized comparison of assertive community treatment (PACT) to a certified clubhouse ( Macias et al. 2009 ), we found that being randomly assigned to the less preferred program decreased service engagement more often in the clubhouse condition than in PACT. However, clubhouse members who had not wanted this service assignment, but nevertheless used clubhouse services to find a job, ended up employed longer and were more satisfied with services than other study enrollees. Study hypotheses based on program differences in staff assertiveness (PACT) and consumer self-determination (clubhouse) predicted this rare three-way interaction prior to data collection, and offer a theory-based (dissonance theory; Aronson 1999 ; Festinger 1957 ) explanation of the complex finding. Presumably, clubhouse members not wanting assignment to this service needed to rationalize their voluntary participation in a non-preferred program by viewing the clubhouse as a means-to-an-end. They tried harder than usual to get a job and stay employed, and gave the clubhouse some credit for their personal success. By contrast, PACT participants who had not wanted this service assignment could credit assertive program staff for keeping them involved, so they experienced less cognitive dissonance and had less need to justify their continued receipt of a non-preferred service. Whether being assigned to a non-preferred program turns out to have negative or positive consequences can depend on a complex interplay between participant motivation and program characteristics. The generation of useful hypotheses for any mental health service trial depends on thoughtful reflection on experimental program differences, as well as familiarity with research in disciplines that study human motivation, such as psychiatry, social psychology, and advertising ( Krause and Howard 2003 ).
Alternative Outcomes Related to Service Preferences
If participants who prefer a certain service condition share similar characteristics, they may also share similar life circumstances and make similar life choices. Individuals who have the same personal responsibilities or physical limitations may prefer not to be assigned to a particular intervention because they cannot fully comply with the requirements for participation, even if they try to do so. For instance, some research participants may have difficulty with regular program attendance because they have competing time commitments, such as caring for an infant or seriously ill relative, or attending school to pursue work credentials ( Collins et al. 2000 ; Mowbray et al. 1999 ; Wolf et al. 2001 ). These productive alternative activities could also compete with the research study’s targeted outcomes, and be misinterpreted as outcome ‘failures.’ For instance, in supported employment trials, unemployment should not be considered a negative outcome if the individual is attending college or pursuing job-related training, or if she has chosen to opt out of the job market for a while to take care of small children or an ill or handicapped relative. These alternative pursuits will be coded simply as ‘unemployed,’ and interpreted as program failure, unless they are tracked and reported as explanations for why work was not obtained. For this reason, it is important to examine relationships between participant circumstances and service preferences at the outset of a study to identify what additional life events and occupations might need to be documented to fully explain intervention outcome differences.
Scope of the Assignment Preference Problem
Regardless of the reason for research participant preference in random assignment, condition differences in service attractiveness can be statistically controlled if (a) preference is measured prior to randomization and (b) if there is sufficient variability in preferences so that the vast majority of study enrollees do not prefer the same intervention. Unfortunately, most randomized service trials have neither measured pre-randomization service preference nor taken it into account when comparing intervention outcomes. Therefore, it is important to assess whether undetected participant preference in random assignment might have existed in published randomized trials, and, if so, whether it might have compromised the interpretation of findings.
As a case example, we review the empirical support for one evidence-based practice, supported employment for adults with severe mental illness, to obtain a qualitative estimate of the extent to which unmeasured service preference for a focal intervention might offer an alternative explanation for published findings. Supported employment offers an ideal starting point for our inquiry given its extensive body of research, which includes a $20 million multi-site randomized study (EIDP, Cook et al. 2002 ), and consensus among psychiatric rehabilitation stakeholders that supported employment is an evidence-based practice ready for dissemination and implementation (Bond et al. 2001). Consumer receptivity and participation in supported employment has been studied in depth through ethnographies ( Alverson et al. 1998 ; Alverson et al. 1995 ; Quimby et al. 2001 ), structured interviews ( McQuilken et al. 2003 ; Secker et al. 2002 ), and personal essays ( Honey 2000 ), and these publications suggest that most consumers know what they need and should expect from a quality vocational program. For this reason, consumer service preferences should be a salient consideration in the design of supported employment research.
Sample of Randomized Trials of Supported Employment
The evidence base for supported employment effectiveness consists of a series of randomized controlled studies of the Individual Placement and Support (IPS) service model (Bond et al. 1997, 2001). One reason research on supported employment has been restricted to a single service delivery model is the ready availability of standardized IPS training and fidelity measures ( Bond et al. 2002 ; McGrew and Griss 2005 ). As a result of a substantial body of research evidence that IPS produces good employment outcomes, this service model has become synonymous with ‘supported employment’ in much of the psychiatric rehabilitation literature (Bond et al. 1997; Crowther et al. 2001 ; Drake et al. 2003 ), and many state departments of mental health in the United States now endorse a definition of supported employment as Individual Placement and Support (IPS).
Table 1 presents a recently published list of all randomized controlled trials of supported employment programs recognized as having high fidelity to Individual Placement and Support (IPS) by the designers of this service delivery model (Bond et al. 2008). Every study has the IPS model as its focal intervention, and IPS experts provided staff training and verified the fidelity of each focal intervention using a supported employment (IPS) fidelity scale (Bond et al. 2008). Research study eligibility was generally limited to unemployed individuals with severe mental illness who had an expressed interest in finding a mainstream job. Most study samples had a mean age of about 40, except that the Twamley et al. (2008) sample was older ( M = 50 years) and the Killackey et al. (2008) sample was younger ( M = 21 years). Except for the study by Lehman et al. (2002) , all studies discouraged enrollment of participants who had major physical limitations or substance use problems.
Randomized trials of high fidelity IPS supported employment: indicators of possible participant preference in condition assignment
As reported in the IPS review article by Bond et al. (2008), or in these original study publications
Possible Indicators of Differential Service Preference
Table 1 lists verbatim service descriptions of the comparison condition in each of the eleven original study publications, along with condition labels derived from the Bond et al. (2008) review of these same randomized trials. Although we do not know the language used to describe the service interventions in recruitment flyers or induction meetings, we assumed there was a strong possibility that most study enrollees would prefer assignment to a new IPS program whenever the comparison condition was an existing sheltered workshop, traditional psychosocial rehabilitation program, or conventional vocational rehabilitation that had been routinely offered by the state or a local authority over several previous years. Since all study enrollees had an expressed interest in obtaining competitive work, we also assumed the possibility of greater preference for IPS if the comparison condition were designed primarily to provide non-competitive jobs, or if program activities delayed entry into competitive employment. Most studies (8 of 11) reported mandatory attendance of all study applicants at one or more research project induction groups in which the experimental conditions were described and questions answered ( Drake et al. 1994 ).
Next, we documented whether each study reported greater early service attrition, or a lower service engagement, for its comparison condition. We report the percentage of study enrollees who were ever active in assigned services at the earliest post-randomization point reported in the original publication or in the summary review article by Bond et al.(2008). We chose the earliest report period so that it would be reasonable to attribute low service contact to disappointment in service assignment. Early service attrition can also be attributed to service ineffectiveness (e.g., poor outreach, slow development of staff-client relationships, or lack of immediate efforts to help participants get a job), so we assume that lower engagement in comparison services is a probable, but not conclusive indication that a comparison condition was generally less appealing than IPS. Our assumption that disappointment in service assignment is a reasonable explanation for early service attrition is based on a demonstrated temporal relationship between random assignment to a non-preferred intervention and subsequently low rates of service engagement within two very different supported employment interventions that had comparable employment rates ( Macias et al. 2005 ).
We also provide research study retention rates for each condition at the latest measurement point as a check on the possibility that loss of participants from services was attributable to the same causes that prevented participation in research interviews and/or the tracking of study outcomes. If research study retention rates at a later point in time are as good or better than service intervention retention rates at an earlier time point, we will assume that factors that typically restrict or enhance research study participation (e.g., program differences in outcome tracking, deaths, hospitalizations, residential mobility) do not account for early differential attrition from experimental and control conditions.
We will consider a study to be at high risk for misinterpretation of findings if the condition labels or descriptions were less favorable for the comparison condition(s), and if there is greater early attrition from comparison services in spite of high research retention.
Review Findings
Descriptions of comparison conditions.
The comparison condition for every study listed in Table 1 was a pre-existing conventional or traditional vocational rehabilitation service that would have been familiar to many participants and did not prioritize rapid placement into mainstream employment. By contrast, each IPS program was a new intervention introduced to the local service system through the research project that was designed to offer fast entry into mainstream work. Although no study recorded participants’ service assignment preference prior to research enrollment or randomization, we might reasonably assume that, in some studies, satisfaction with service assignment to IPS, or disappointment in assignment to the non-supported employment comparison condition, contributed to differences in mainstream employment rates between experimental conditions.
Differential Early Attrition/Retention
Six of the eleven studies reported a 20% or greater advantage in service retention for the focal IPS intervention within the first 8 weeks following randomization. Two other studies that assessed service retention at the 6-months point in the research project reported 17 and 19% differences in favor of IPS. Only the South Carolina and Hong Kong studies ( Gold et al. 2006 ; Wong et al. 2008 ) reported comparably high rates of service retention across experimental interventions, possibly because both studies required all participants to be active in a local mental health program at the time of research study enrollment.
Overall, the majority of participants remained active in each research study for the duration of the trial, with comparable research retention across study conditions. This comparability suggests that factors known to increase research attrition (e.g., residential mobility, chronic illness) cannot explain early differential attrition from services.
IPS interventions may have had better service retention rates in eight of these eleven randomized trials because IPS had more assertive outreach, provided more useful services, or IPS staff collaborated more closely with clinicians than staff in the comparison conditions (Bond et al. 2008; Gold et al. 2006 ; McGurk et al. 2007 ). However, greater intensity or quality of IPS services cannot reasonably account for the very low service retention rates for most comparison conditions relative to research project retention, so disappointment in assignment remains a credible additional explanation for greater early attrition from comparison services.
Only the South Carolina study statistically controlled for variation in participant exposure to vocational services, which might be considered a proxy for the effects of differential attrition attributable to service preference. No study reported whether early attrition resulted in the loss of different types of people from each study condition, and every study compared study conditions on participant characteristics only at baseline.
Our review of research in one dominant field of adult psychiatric rehabilitation reveals that every randomized controlled trial of high-fidelity supported employment had a ‘services-as-usual’ comparison condition that might have predisposed work-interested participants to prefer random assignment to the new ‘rapid-job-placement’ IPS intervention. We cannot be certain that IPS was preferred by most participants over comparison conditions in any of these studies because no study measured participants’ pre-randomization service preferences or satisfaction with condition assignment. However, neither does any original publication offer evidence that would refute our assumption of greater preference for IPS. Eight of these 11 studies reported 15% or greater service attrition from the comparison condition early in the project that could reflect disappointment in service assignment, but no study reporting early differential attrition statistically controlled for exposure to services, examined how attrition might have changed sample characteristics, or distinguished between service retention and outcome attainment in data analyses.
We cannot conclude that the outcomes for any of these eleven studies would differ from the reported findings if service preference, service receipt, or the effects of early attrition on sample characteristics had been measured and, assuming sufficient variability in these measures, intervention differences had been statistically controlled. Moreover, design factors other than program descriptions provided in study advertisements, research induction sessions, or consent documents might have engendered a general preference for assignment to IPS. For instance, in the Bond et al. (2007) study, IPS services were located at the same health center that provided most participants’ clinical care, while comparison services were off-site, and so condition differences in service convenience could also explain better retention rates and outcomes for IPS. Regardless, the published labels and descriptions of comparison interventions presented in Table 1 , and early condition differences in service retention rates, suggest the possibility that outcome differences between study conditions that consistently favor IPS might be partially explained by corresponding differences in participant expectations about services, and, ultimately, satisfaction or disappointment in service assignment. If these same research designs problems are prevalent in other fields of mental health services research, we need to consider what widespread impact these alternative explanations may have had on the interpretation of research findings.
Variability in Impact of Participant Preferences on Outcomes
Unmeasured participant preference in random assignment may not pose the same threat in other service trials, even if informed consent procedures are similar to those used in these supported employment trials, and even if service descriptions inadvertently induce a general preference for one intervention over another. The direct impact of service preference on outcomes may depend a great deal on whether the primary study outcome is measured subjectively or objectively, and on the type of intervention under evaluation, including its frequency, intensity, or duration ( Torgerson and Moffett 2005 ). Moreover, if study outcomes do not depend on participant attitudes or motivation, then disappointment in service assignment may have no impact on outcomes at all.
A mismatch to service preference is likely to have the strongest impact on study outcomes whenever participants are expected to improve their own lives in observable ways that demand strong commitment and self-determination, as is the case for supported employment. By contrast, the impact of a mismatch to service preference on outcomes is probably least discernable when participation is passive or condition assignment remains unknown, as is the case in most drug and medical treatment trials ( King et al. 2005 ; Leykin et al. 2007 ). Whether disappointment in service assignment reduces or enhances outcomes may also depend on prevailing attitudes toward cooperation with service professionals ( Nichols and Maner 2008 ) or perceived pressure to participate from program staff ( Macias et al. 2009 ). However, the impact of service preference on outcomes should almost always be strong when the reason for preferring an intervention is based on expectations of relative efficacy, since even medication trials have shown better outcomes when participants believe a drug will be efficacious ( Krell et al. 2004 ), as well as worse outcomes when they suspect a drug is a placebo ( Sneed et al. 2008 ).
Research reviews are needed to estimate the potential impact of unmeasured service preference in other service fields, and to identify moderating variables that deserve further study. Until the relative threat of participant service preference can be determined for a specific field, pre-randomization service preference should be measured routinely in every randomized controlled trial and, if there is sufficient variability in preference measurement, condition differences in preference should be statistically controlled, and tests of interaction effects conducted to identify moderating variables. Examples of statistical control for service preference in logistic regression and event history analysis can be found in reports on a supported employment randomized trial that compared two SE interventions ( Macias et al. 2005 ; Macias et al. 2006 ). A third publication from this same trial illustrates a theory-driven test of moderation effects ( Macias et al. 2009 ). However, whenever one experimental condition is greatly preferred over another, there is no statistical remedy that will allow an unbiased comparison of outcomes.
New Directions for Employment Research
The body of research on supported employment (SE) offers compelling evidence that most adults with severe mental illness do not find prevocational training or standard vocational rehabilitation attractive routes to mainstream employment ( Cook 1999a , b ; Noble et al. 1997 ). It may be time to relinquish ‘SE vs. no SE’ research designs that evoke preference for assignment to SE and move on to compare different ways of delivering the same high quality SE job-hunting services and on-the-job supports ( Bickman 2002 ; Lavori 2000 ). Comparisons of alternative modalities of the same service typically provide less striking, statistically weaker contrasts in outcomes, but they preserve the ethical principle of equipoise and help to ensure that all participants receive adequate care and comparable opportunities for life improvement ( Lavori et al. 2001 ; Lilford and Jackson 1995 ; Schwartz and Sprangers 1999 ).
We would learn more about why supported employment is effective, and what aspects of SE are most attractive to prospective research participants, if studies would provide more detailed descriptions of service implementation so that the same key concepts (e.g., rapid job placement, service integration, frequent contact) could be compared across separate studies and in meta-analyses ( Campbell and Fiske 1959 ; Sechrest et al. 2000 ; TenHave et al. 2003 ). Such studies would also help to justify specificity in fidelity measurement during dissemination and implementation of evidence-based practices ( Rosenheck 2001 ; West et al. 2002 ). It would be especially advantageous to compare ways to increase access to mainstream work in specific service environments, since the heterogeneity in IPS employment rates, internationally and across the USA, suggests that social, political, economic, and organizational factors are far greater predictors of the work attainment of disabled individuals than receipt of employment services, or even disability itself.
Conclusions
The randomized controlled trial is still the gold standard of research designs ( Cook 1999a , b ), and randomization greatly strengthens causal inference ( Abelson 1995 ; Beveridge 1950 ). However, cause-effect inference depends on the measurement of all plausibly potent causal factors, including study participants’ attitudes toward their assigned interventions. Ironically, while consumer advocates champion the individual’s right to choose services, researchers rarely examine the contribution of consumer self-direction to outcomes considered indicative of service effectiveness. It may well be a legitimate responsibility of institutional review boards to assess the potential impact of study designs and research enrollment documents on participants’ preferences in random assignment and, hence, their eventual well-being as research participants and role in determining study outcomes ( Adair et al. 1983 ).
Our review of one prominent field of mental health services research suggests a general need to reexamine published randomized controlled trials to gauge the extent to which research protocols or descriptions of experimental conditions might have predisposed participants to prefer assignment to one particular condition over another, and whether participant responses to these research design elements might have moderated, or even mediated, service effectiveness.
Acknowledgments
Work on this article was funded by National Institute of Mental Health grants to the first and second authors (MH62628; MH01903). We are indebted to Ann Hohmann, Ph.D. for her supportive monitoring of the NIMH research grant that fostered this interdisciplinary collaboration, and to anonymous reviewers who offered invaluable insights during manuscript preparation.
Contributor Information
Cathaleene Macias, Community Intervention Research, McLean Hospital, Belmont, MA 02478, USA, ude.dravrah.naelcm@saicamc .
Paul B. Gold, Department of Counseling and Personnel Services, University of Maryland, College Park, MD 20742, USA, ude.dmu@dlogp .
William A. Hargreaves, Department of Psychiatry, University of California, San Francisco, CA, USA, ten.tsacmoc@grahllib .
Elliot Aronson, Department of Psychology, University of California, Santa Cruz, CA, USA, ude.cscu.STAC@toille .
Leonard Bickman, Center for Evaluation and Program Improvement, Vanderbilt University, Nashville, TN, USA, [email protected] .
Paul J. Barreira, Harvard University Health Services, Harvard University, Boston, MA, USA, ude.dravrah.shu@arierrabp .
Danson R. Jones, Institutional Research, Wharton County Junior College, Wharton, TX 77488, USA, ude.cjcw@dsenoj .
Charles F. Rodican, Community Intervention Research, McLean Hospital, Belmont, MA 02478, USA, ude.dravrah.naelcm@nacidorc .
William H. Fisher, Department of Psychiatry, University of Massachusetts Medical School, Worcester, MA, USA, [email protected] .
- Abelson RP. Statistics as principled argument. Hillsdale, NJ: Lawrence Erlbaum; 1995. [ Google Scholar ]
- Adair JG, Lindsay RCL, Carlopio J. Social artifact research and ethical regulation: Their impact on the teaching of experimental methods. Teaching of Psychology. 1983; 10 :159–162. doi: 10.1207/s15328023top1003_10. [ PubMed ] [ CrossRef ] [ Google Scholar ]
- Aguinis H. Regression analysis for categorical moderators. New York: Guilford Press; 2004. [ Google Scholar ]
- Aiken LS, West SG. Multiple regression: Testing and interpreting interactions. Newbury Park, CA: Sage; 1991. [ Google Scholar ]
- Alverson H, Alverson M, Drake RE, Becker DR. Social correlates of competitive employment among people with severe mental illness. Psychosocial Rehabilitation Journal. 1998; 22 (1):34–40. [ Google Scholar ]
- Alverson M, Becker DR, Drake RE. An ethnographic study of coping strategies used by people with severe mental illness participating in supported employment. Psychosocial Rehabilitation Journal. 1995; 18 (4):115–127. [ Google Scholar ]
- Aronson E. The power of self-persuasion. The American Psychologist. 1999; 54 (11):873–875. doi: 10.1037/h0088188. [ CrossRef ] [ Google Scholar ]
- Beveridge WIB. The art of scientific investigation. New York: Vintage Books; 1950. [ Google Scholar ]
- Bickman L. The functions of program theory. In: Bickman L, editor. Using program theory in evaluation. San Francisco: Jossey-Bass; 1987. [ Google Scholar ]
- Bickman L. The death of treatment as usual: An excellent first step on a long road. Clinical Psychology: Science and Practice. 2002; 9 (2):195–199. doi: 10.1093/clipsy/9.2.195. [ CrossRef ] [ Google Scholar ]
- Bond GR, Becker DR, Drake RE, Rapp C, Meisler N, Lehman AF. Implementing supported employment as an evidence-based practice. Psychiatric Services. 2001a; 52 (3):313–322. doi: 10.1176/appi.ps.52.3.313. [ PubMed ] [ CrossRef ] [ Google Scholar ]
- Bond GR, Becker DR, Drake RE, Vogler K. A fidelity scale for the individual placement and support model of supported employment. Rehabilitation Counseling Bulletin. 1997a; 40 (4):265–284. [ Google Scholar ]
- Bond GR, Campbell K, Evans LJ, Gervey R, Pascaris A, Tice S, et al. A scale to measure quality of supported employment for persons with severe mental illness. Journal of Vocational Rehabilitation. 2002; 17 (4):239–250. [ Google Scholar ]
- Bond GR, Drake R, Becker D. An update on randomized controlled trials of evidence-based supported employment. Psychiatric Rehabilitation Journal. 2008a; 31 (4):280–290. doi: 10.2975/31.4.2008.280.290. [ PubMed ] [ CrossRef ] [ Google Scholar ]
- Bond GR, Drake RE, Mueser KT, Becker DR. An update on supported employment for people with severe mental illness. Psychiatric Services. 1997b; 48 (3):335–346. [ PubMed ] [ Google Scholar ]
- Bond GR, McHugo GH, Becker D, Rapp CA, Whitley R. Fidelity of supported employment: Lessons learned from the national evidence-based practice project. Psychiatric Rehabilitation Journal. 2008b; 31 (4):300–305. doi: 10.2975/31.4.2008.300.305. [ PubMed ] [ CrossRef ] [ Google Scholar ]
- Bond GR, Salyers MP, Roudebush RL, Dincin J, Drake RE, Becker DR, et al. A randomized controlled trial comparing two vocational models for persons with severe mental illness. Journal of Consulting and Clinical Psychology. 2007; 75 (6):968–982. doi: 10.1037/0022-006X.75.6.968. [ PubMed ] [ CrossRef ] [ Google Scholar ]
- Bond GR, Vogler K, Resnick SG, Evans L, Drake R, Becker D. Dimensions of supported employment: Factor structure of the IPS fidelity scale. Journal of Mental Health. 2001b; 10 (4):383–393. doi: 10.1080/09638230120041146. [ CrossRef ] [ Google Scholar ]
- Braver SL, Smith MC. Maximizing both external and internal validity in longitudinal true experiments with voluntary treatments: The ‘combined modified’ design. Evaluation and Program Planning. 1996; 19 :287–300. doi: 10.1016/S0149-7189(96)00029-8. [ CrossRef ] [ Google Scholar ]
- Brown TG, Seraganian P, Tremblay J, Annis H. Matching substance abuse aftercare treatments to client characteristics. Addictive Behaviors. 2002; 27 :585–604. doi: 10.1016/S0306-4603(01)00195-2. [ PubMed ] [ CrossRef ] [ Google Scholar ]
- Burns T, Catty J, Becker T, Drake RE, Fioritti A, Knapp M, et al. The effectiveness of supported employment for people with severe mental illness: A randomised controlled trial. Lancet. 2007; 370 :1146–1152. doi: 10.1016/S0140-6736(07)61516-5. [ PubMed ] [ CrossRef ] [ Google Scholar ]
- Calsyn R, Winter J, Morse G. Do consumers who have a choice of treatment have better outcomes? Community Mental Health Journal. 2000; 36 (2):149–160. doi: 10.1023/A:1001890210218. [ PubMed ] [ CrossRef ] [ Google Scholar ]
- Campbell DT, Fiske DW. Convergent and discriminant validation by the multitrait–multimethod matrix. Psychological Bulletin. 1959; 56 :81–105. doi: 10.1037/h0046016. [ PubMed ] [ CrossRef ] [ Google Scholar ]
- Collins ME, Mowbray C, Bybee D. Characteristics predicting successful outcomes of participants with severe mental illness in supported education. Psychiatric Services. 2000; 51 (6):774–780. doi: 10.1176/appi.ps.51.6.774. [ PubMed ] [ CrossRef ] [ Google Scholar ]
- Cook JA. Understanding the failure of vocational rehabilitation: What do we need to know and how can we learn it? Journal of Disability Policy Studies. 1999a; 10 (1):127–132. [ Google Scholar ]
- Cook TD. Considering the major arguments against random assignment: An analysis of the intellectual culture surrounding evaluation in American schools of education. Paper presented at the Harvard Faculty Seminar on Experiments in Education; Cambridge, MA. 1999b. [ Google Scholar ]
- Cook TD, Campbell DT. Quasi-experimentation: Design & analysis issues for field settings. Boston: Houghton Mifflin; 1979. [ Google Scholar ]
- Cook JA, Carey MA, Razzano L, Burke J, Blyler CR. The pioneer: The employment intervention demonstration program. New Directions for Evaluation. 2002; 94 :31–44. doi: 10.1002/ev.49. [ CrossRef ] [ Google Scholar ]
- Corrigan PW, Salzer MS. The conflict between random assignment and treatment preference: Implications for internal validity. Evaluation and Program Planning. 2003; 26 :109–121. doi: 10.1016/S0149-7189(03)00014-4. [ PubMed ] [ CrossRef ] [ Google Scholar ]
- Crowther RE, Marshall M, Bond GR, Huxley P. Helping people with severe mental illness to obtain work: Systematic review. BMJ: British Medical Journal. 2001; 322 (7280):204–208. doi: 10.1136/bmj.322.7280.204. [ PMC free article ] [ PubMed ] [ CrossRef ] [ Google Scholar ]
- Delucchi KL, Bostrom A. Methods for analysis of skewed data distributions in psychiatric clinical studies: Working with many zero values. The American Journal of Psychiatry. 2004; 161 (7):1159–1168. doi: 10.1176/appi.ajp.161.7.1159. [ PubMed ] [ CrossRef ] [ Google Scholar ]
- Drake RE, Becker DR, Anthony WA. A research induction group for clients entering a mental health services research project. Hospital & Community Psychiatry. 1994; 45 (5):487–489. [ PubMed ] [ Google Scholar ]
- Drake RE, Becker D, Bond GR. Recent research on vocational rehabilitation for persons with severe mental illness. Current Opinion in Psychiatry. 2003; 16 :451–455. doi: 10.1097/00001504-200307000-00012. [ CrossRef ] [ Google Scholar ]
- Drake RE, McHugo GJ, Bebout RR, Becher DR, Harris M, Bond GR, et al. A randomized clinical trial of supported employment for inner-city patients with severe mental disorders. Archives of General Psychiatry. 1999; 56 :627–633. doi: 10.1001/archpsyc.56.7.627. [ PubMed ] [ CrossRef ] [ Google Scholar ]
- Drake RE, McHugo GJ, Becker D, Anthony WA, Clark RE. The new Hampshire study of supported employment for people with severe mental illness. Journal of Consulting and Clinical Psychology. 1996; 64 (2):391–399. doi: 10.1037/0022-006X.64.2.391. [ PubMed ] [ CrossRef ] [ Google Scholar ]
- Essock SM, Drake R, Frank RG, McGuire TG. Randomized controlled trials in evidence-based mental health care: Getting the right answer to the right question. Schizophrenia Bulletin. 2003; 29 (1):115–123. [ PubMed ] [ Google Scholar ]
- Festinger L. A theory of cognitive dissonance. Stanford, CA: Stanford University Press; 1957. [ Google Scholar ]
- Gold PB, Meisler N, Santos AB, Carnemolla MA, Williams OH, Keleher J. Randomized trial of supported employment integrated with assertive community treatment for rural adults with severe mental illness. Schizophrenia Bulletin. 2006; 32 (2):378–395. doi: 10.1093/schbul/sbi056. [ PMC free article ] [ PubMed ] [ CrossRef ] [ Google Scholar ]
- Grilo CM, Money R, Barlow DH, Goddard AW, Gorman JM, Hofmann SG, et al. Pretreatment patient factors predicting attrition from a multicenter randomized controlled treatment study for panic disorder. Comprehensive Psychiatry. 1998; 39 (6):323–332. doi: 10.1016/S0010-440X(98)90043-8. [ PubMed ] [ CrossRef ] [ Google Scholar ]
- Halpern SD. Prospective preference assessment: a method to enhance the ethics and efficiency of randomized controlled trials. Controlled Clinical Trials. 2002; 23 :274–288. doi: 10.1016/S0197-2456(02)00191-5. [ PubMed ] [ CrossRef ] [ Google Scholar ]
- Hofmann SG, Barlow DH, Papp LA, Detweiler MF, Ray SE, Shear MK, et al. Pretreatment attrition in a comparative treatment outcome study on panic disorder. The American Journal of Psychiatry. 1998; 155 (1):43–47. [ PubMed ] [ Google Scholar ]
- Honey A. Psychiatric vocational rehabilitation: Where are the customers’ views. Psychiatric Rehabilitation Journal. 2000; 23 (3):270–279. [ PubMed ] [ Google Scholar ]
- Kearney C, Silverman W. A critical review of pharmacotherapy for youth with anxiety disorders: Things are not as they seem. Journal of Anxiety Disorders. 1998; 12 (2):83–102. doi: 10.1016/S0887-6185(98)00005-X. [ PubMed ] [ CrossRef ] [ Google Scholar ]
- Killackey E, Jackson HJ, McGorry PD. Vocational intervention in first-episode psychosis: Individual placement and support versus treatment as usual. The British Journal of Psychiatry. 2008; 193 :114–120. doi: 10.1192/bjp.bp.107.043109. [ PubMed ] [ CrossRef ] [ Google Scholar ]
- King M, Nazareth I, Lampe F, Bower P, Chandler M, Morou M, et al. Impact of participant and physician intervention preferences on randomized trials: A systematic review. Journal of the American Medical Association. 2005; 293 (9):1089–1099. doi: 10.1001/jama.293.9.1089. [ PubMed ] [ CrossRef ] [ Google Scholar ]
- Krause MS, Howard KI. What random assignment does and does not do. Journal of Clinical Psychology. 2003; 59 :751–766. doi: 10.1002/jclp.10170. [ PubMed ] [ CrossRef ] [ Google Scholar ]
- Krell HV, Leuchter AF, Morgan M, Cook IA, Abrams M. Subject expectations of treatment effectiveness and outcome of treatment with an experimental antidepressant. The Journal of Clinical Psychiatry. 2004; 65 (9):1174–1179. [ PubMed ] [ Google Scholar ]
- Lachenbruch PA. Analysis of data with excess zeros. Statistical Methods in Medical Research. 2002; 11 :297–302. doi: 10.1191/0962280202sm289ra. [ PubMed ] [ CrossRef ] [ Google Scholar ]
- Laengle G, Welte W, Roesger U, Guenthner A, U’Ren R. Chronic psychiatric patients without psychiatric care: A pilot study. Social Psychiatry and Psychiatric Epidemiology. 2000; 35 (10):457–462. doi: 10.1007/s001270050264. [ PubMed ] [ CrossRef ] [ Google Scholar ]
- Lambert MF, Wood J. Incorporating patient preferences into randomized trials. Journal of Clinical Epidemiology. 2000; 53 :163–166. doi: 10.1016/S0895-4356(99)00146-8. [ PubMed ] [ CrossRef ] [ Google Scholar ]
- Latimer EA, LeCompte MD, Becker DR, Drake RE, Duclos I, Piat M, et al. Generalizability of the individual placement and support model of supported employment: Results of a canadian randomised controlled trial. The British Journal of Psychiatry. 2006; 189 :65–73. doi: 10.1192/bjp.bp.105.012641. [ PubMed ] [ CrossRef ] [ Google Scholar ]
- Lavori PW. Placebo control groups in randomized treatment trials: A statistician’s perspective. Biological Psychiatry. 2000; 47 :717–723. doi: 10.1016/S0006-3223(00)00838-6. [ PubMed ] [ CrossRef ] [ Google Scholar ]
- Lavori PW, Rush AJ, Wisniewski SR, Alpert J, Fava M, Kupfer DJ, et al. Strengthening clinical effectiveness trials: Equipoise-stratified randomization. Biological Psychiatry. 2001; 50 (10):792–801. doi: 10.1016/S0006-3223(01)01223-9. [ PubMed ] [ CrossRef ] [ Google Scholar ]
- Lehman AF, Goldberg RW, Dixon LB, NcNary S, Postrado L, Hackman A, et al. Improving employment outcomes for persons with severe mental illnesses. Archives of General Psychiatry. 2002; 59 (2):165–172. doi: 10.1001/archpsyc.59.2.165. [ PubMed ] [ CrossRef ] [ Google Scholar ]
- Lewin K. Forces behind food habits and methods of change. Bulletin of the National Research Council. 1943; 108 :35–65. [ Google Scholar ]
- Leykin Y, DeRubeis RJ, Gallop R, Amsterdam JD, Shelton RC, Hollon SD. The relation of patients’ treatment preferences to outcome in a randomized clinical trial. Behavior Therapy. 2007; 38 :209–217. doi: 10.1016/j.beth.2006.08.002. [ PubMed ] [ CrossRef ] [ Google Scholar ]
- Lilford R, Jackson J. Equipoise and the ethics of randomisation. Journal of the Royal Society of Medicine. 1995; 88 :552–559. [ PMC free article ] [ PubMed ] [ Google Scholar ]
- Little RJ, Rubin DB. Causal effects in clinical and epidemiological studies via potential outcomes: Concepts and analytical approaches. Annual Review of Public Health. 2000; 21 :121–145. doi: 10.1146/annurev.publhealth.21.1.121. [ PubMed ] [ CrossRef ] [ Google Scholar ]
- Macias C, Aronson E, Hargreaves W, Weary G, Barreira P, Harvey JH, et al. Transforming dissatisfaction with services into self-determination: A social psychological perspective on community program effectiveness. Journal of Applied Social Psychology. 2009; 39 (7) [ PMC free article ] [ PubMed ] [ Google Scholar ]
- Macias C, Barreira P, Hargreaves W, Bickman L, Fisher WH, Aronson E. Impact of referral source and study applicants’ preference for randomly assigned service on research enrollment, service engagement, and evaluative outcomes. The American Journal of Psychiatry. 2005; 162 (4):781–787. doi: 10.1176/appi.ajp.162.4.781. [ PMC free article ] [ PubMed ] [ CrossRef ] [ Google Scholar ]
- Macias C, Rodican CF, Hargreaves WA, Jones DR, Barreira PJ, Wang Q. Supported employment outcomes of a randomized controlled trial of assertive community treatment and clubhouse models. Psychiatric Services. 2006; 57 (10):1406–1415. doi: 10.1176/appi.ps.57.10.1406. [ PMC free article ] [ PubMed ] [ CrossRef ] [ Google Scholar ]
- Magidson J. On models used to adjust for preexisting differences. In: Bickman L, editor. Research design. Vol. 2. Thousand Oaks, CA: Sage; 2000. [ Google Scholar ]
- Marcus SM. Assessing non-consent bias with parallel randomized and nonrandomized clinical trials. Journal of Clinical Epidemiology. 1997; 50 (7):823–828. doi: 10.1016/S0895-4356(97)00068-1. [ PubMed ] [ CrossRef ] [ Google Scholar ]
- Mark MM, Hofmann DA, Reichardt CS. Testing theories in theory-driven evaluations: Tests of moderation in all things. In: Chen H, Rossi PH, editors. Using theory to improve program and policy evaluations. New York: Greenwood Press; 1992. [ Google Scholar ]
- McGrew JH, Griss ME. Concurrent and predictive validity of two scales to assess the fidelity of implementation of supported employment. Psychiatric Rehabilitation Journal. 2005; 29 (1):41–47. doi: 10.2975/29.2005.41.47. [ PubMed ] [ CrossRef ] [ Google Scholar ]
- McGrew JH, Pescosolido BA, Wright E. Case managers’ perspectives on critical ingredients of Assertive Community Treatment and on its implementation. Psychiatric Services. 2003; 54 (3):370–376. doi: 10.1176/appi.ps.54.3.370. [ PubMed ] [ CrossRef ] [ Google Scholar ]
- McGurk S, Mueser K, Feldman K, Wolfe R, Pascaris A. Cognitive training for supported employment: 2–3 year outcomes of a randomized controlled trial. The American Journal of Psychiatry. 2007; 164 :437–441. doi: 10.1176/appi.ajp.164.3.437. [ PubMed ] [ CrossRef ] [ Google Scholar ]
- McHugo GJ, Bebout RR, Harris M, Cleghorn S, Herring G, Xie H, et al. A randomized controlled trial of integrated versus parallel housing services for homeless adults with severe mental illness. Schizophrenia Bulletin. 2004; 30 (4):969–982. [ PubMed ] [ Google Scholar ]
- McQuilken M, Zahniser JH, Novak J, Starks RD, Olmos A, Bond GR. The work project survey: Consumer perspectives on work. Journal of Vocational Rehabilitation. 2003; 18 :59–68. [ Google Scholar ]
- Meyer B, Pilkonis PA, Krupnick JL, Egan MK, Simmens SJ, Sotsky SM. Treatment expectancies, patient alliance and outcome: Further analyses from the national institute of mental health treatment of depression collaborative research program. Journal of Consulting and Clinical Psychology. 2002; 70 (4):1051–1055. doi: 10.1037/0022-006X.70.4.1051. [ PubMed ] [ CrossRef ] [ Google Scholar ]
- Mowbray CT, Collins M, Deborah B. Supported education for individuals with psychiatric disabilities: Long-term outcomes from an experimental study. Social Work Research. 1999; 23 (2):89–100. [ Google Scholar ]
- Mueser KT, Clark RE, Haines M, Drake RE, McHugo GJ, GR B, et al. The Hartford study of supported employment for persons with severe mental illness. Journal of Consulting and Clinical Psychology. 2004; 72 (3):479–490. doi: 10.1037/0022-006X.72.3.479. [ PubMed ] [ CrossRef ] [ Google Scholar ]
- Nichols AL, Maner JK. The good-subject effect: Investigating participant demand characteristics. The Journal of General Psychology. 2008; 135 (2):151–165. doi: 10.3200/GENP.135.2.151-166. [ PubMed ] [ CrossRef ] [ Google Scholar ]
- Noble JH, Honberg RS, Hall LL, Flynn LM. A legacy of failure: The inability of the federal-state vocational rehabilitation system to serve people with severe mental illness. Arlington: VA: National Alliance for the Mentally Ill; 1997. [ Google Scholar ]
- Quimby E, Drake R, Becker D. Ethnographic findings from the Washington, DC vocational services study. Psychiatric Rehabilitation Journal. 2001; 24 (4):368–374. [ PubMed ] [ Google Scholar ]
- Rosenheck RA. Organizational process: A missing link between research and practice. Psychiatric Services. 2001; 52 (12):1607–1612. doi: 10.1176/appi.ps.52.12.1607. [ PubMed ] [ CrossRef ] [ Google Scholar ]
- Schwartz CE, Sprangers M. Methodological approaches for assessing response shift in longitudinal quality of life research. Social Science & Medicine. 1999; 48 :1531–1548. doi: 10.1016/S0277-9536(99)00047-7. [ PubMed ] [ CrossRef ] [ Google Scholar ]
- Sechrest L, Davis M, Stickle T, McKnight P. Understanding ‘method’ variance. In: Bickman L, editor. Research design. Thousand Oaks, CA: Sage; 2000. [ Google Scholar ]
- Secker J, Membrey H, Grove B, Seebohm P. Recovering from illness or recovering your life? Implications of clinical versus social models of recovery from mental health problems for employment support services. Disability & Society. 2002; 17 (4):403–418. doi: 10.1080/09687590220140340. [ CrossRef ] [ Google Scholar ]
- Shadish WR. Revisiting field experimentation: Field notes for the future. Psychological Methods. 2002; 7 (1):3–18. doi: 10.1037/1082-989X.7.1.3. [ PubMed ] [ CrossRef ] [ Google Scholar ]
- Shadish WR, Cook TD, Campbell DT. Experimental and quasi-experimental designs for generalized causal inference. New York: Houghton Mifflin; 2002. [ Google Scholar ]
- Shadish WR, Matt GE, Navarro AM, Phillips G. The effects of psychological therapies under clinically representative conditions: A meta-analysis. Psychological Bulletin. 2000; 126 (4):512–529. doi: 10.1037/0033-2909.126.4.512. [ PubMed ] [ CrossRef ] [ Google Scholar ]
- Shapiro SL, Figueredo AJ, Caspi O, Schwartz GE, Bootzin RR, Lopez AM, et al. Going quasi: The premature disclosure effect in a randomized clinical trial. Journal of Behavioral Medicine. 2002; 25 (6):605–621. doi: 10.1023/A:1020693417427. [ PubMed ] [ CrossRef ] [ Google Scholar ]
- Sneed JR, Rutherford BR, Rindskopf D, Lane DT, Sackeim HA, Roose SP. Design makes a difference: A meta-analysis of antidepressant response rates in placebo-controlled versus comparator trials in late-life depression. The American Journal of Geriatric Psychiatry. 2008; 16 :65–73. doi: 10.1097/JGP.0b013e3181256b1d. [ PubMed ] [ CrossRef ] [ Google Scholar ]
- Sosin MR. Outcomes and sample selection: The case of a homelessness and substance abuse intervention. The British Journal of Mathematical and Statistical Psychology. 2002; 55 (1):63–92. doi: 10.1348/000711002159707. [ PubMed ] [ CrossRef ] [ Google Scholar ]
- Staines G, McKendrick K, Perlis T, Sacks S, DeLeon G. Sequential assignment and treatment as usual: Alternatives to standard experimental designs in field studies of treatment efficacy. Evaluation Review. 1999; 23 (1):47–76. doi: 10.1177/0193841X9902300103. [ PubMed ] [ CrossRef ] [ Google Scholar ]
- TenHave T, Coyne J, Salzer M, Katz I. Research to improve the quality of care for depression: Alternatives to the simple randomized clinical trial. General Hospital Psychiatry. 2003; 25 :115–123. doi: 10.1016/S0163-8343(02)00275-X. [ PubMed ] [ CrossRef ] [ Google Scholar ]
- Torgerson D, Klaber Moffett JA, Russell IT. Including patient preferences in randomized clinical trials. Journal of Health Services Research & Policy. 1996; 1 :194–197. [ PubMed ] [ Google Scholar ]
- Torgerson D, Moffett JK. Patient Preference and Validity of Randomized Controlled Trials: Letter to the Editor. Journal of the American Medical Association. 2005; 294 (1):41. doi: 10.1001/jama.294.1.41-b. [ PubMed ] [ CrossRef ] [ Google Scholar ]
- Trist E, Sofer C. Exploration in group relations. Leicester: Leicester University Press; 1959. [ Google Scholar ]
- Twamley EW, Narvaez JM, Becker DR, Bartels SJ, Jeste DV. Supported employment for middle-aged and older people with schizophrenia. American Journal of Psychiatric Rehabilitation. 2008; 11 (1):76–89. doi: 10.1080/15487760701853326. [ PMC free article ] [ PubMed ] [ CrossRef ] [ Google Scholar ]
- Wahlbeck K, Tuunainen A, Ahokas A, Leucht S. Dropout rates in randomised antipsychotic drug trials. Psychopharmacology. 2001; 155 (3):230–233. doi: 10.1007/s002130100711. [ PubMed ] [ CrossRef ] [ Google Scholar ]
- West SG, Aiken LS, Todd M. Probing the effects of individual components in multiple component prevention programs. In: Revenson & T, D’Agostino RB, editors. Ecological research to promote social change: Methodological advances from community psychology. New York, NY: Kluwer; 2002. [ PubMed ] [ Google Scholar ]
- West SG, Sagarin BJ. Participant selection and loss in randomized experiments. In: Bickman L, editor. Research design: Donald Campbell’s legacy. II. Thousand Oaks, CA: Sage; 2000. pp. 117–154. [ Google Scholar ]
- Wolf J, Coba C, Cirella M. Education as psychosocial rehabilitation: Supported education program partnerships with mental health and behavioral healthcare certificate programs. Psychiatric Rehabilitation Skills. 2001; 5 (3):455–476. [ Google Scholar ]
- Wong KK, Chiu R, Tang B, Mak D, Liu J, Chiu SN. A randomized controlled trial of a supported employment program for persons with long-term mental illness in Hong Kong. Psychiatric Services (Washington, DC) 2008; 59 (1):84–90. doi: 10.1176/appi.ps.59.1.84. [ PubMed ] [ CrossRef ] [ Google Scholar ]
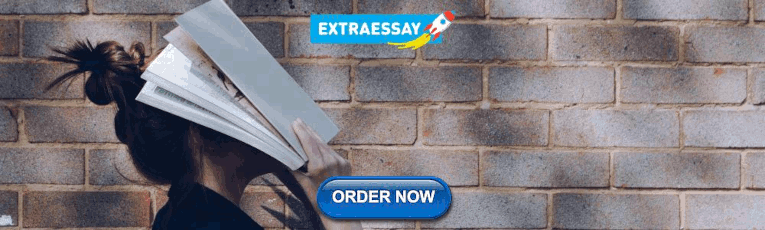
IMAGES
VIDEO
COMMENTS
The researcher knows the assignments of the participants enrolled without randomization: Alternating, such as allocating every other individual (e.g., [158, 159]) Participants may enroll in groups in nonrandom ways, and with small numbers of groups this can create imbalances: The researcher knows the next group assignment
Abstract. A nonrandomized control study is a clinical trial where participants are not assigned by randomization. Each group in the study is assigned by the researcher or chosen by the participant to receive a certain treatment, procedure, or intervention. Often, these studies analyze if a new treatment is more effective than standard treatment ...
Hence, nonrandomized controlled trials (NCTs)—a quasi-experimental study design that does not utilize random assignment—can be good alternatives when RCTs are not feasible. It should be noted that NCTs can also fall under prospective studies in addition to experimental studies. This chapter will focus on NCTs including basic principles ...
The finding that randomized research participants and non-participants tend to achieve similar clinical outcomes also contradicts prior studies suggesting that trial ... McLellan AT, Snider EC, O'Brien CP. Effect of random versus nonrandom assignment in a comparison of inpatient and day hospital rehabilitation for male alcoholics. J ...
Sometimes, the nonrandomized trial study design is used, whereby participants are allocated to treatment groups using nonrandom methods. The design has the potential for confounding with the consequence that internal validity may be compromised. Nonetheless, some nonrandom methods have benefits.
The first nonequivalent groups design we will consider is the posttest only nonequivalent groups design. In this design, participants in one group are exposed to a treatment, a nonequivalent group is not exposed to the treatment, and then the two groups are compared. Imagine, for example, a researcher who wants to evaluate a new method of ...
Some research areas refer to the use of random assignment of participants, whereas others use the term random allocation. Another example involves the terms multilevel model, hierarchical linear model, and mixed effects model, all of which are used to identify a similar approach to data analysis.
the best approximation to this true counterfactual may be a group of participants whose assignment method (random or nonrandom) was itself randomly assigned to them, where all other features of the experiment are held equal. This was not done in Dehejia and Wahba (1999), Heckman et al. (1997) and Hill et al. (2004), or any other such studies.
to conduct a randomized study if the investigator must accept the assignment of people to treatment or comparison groups as determined by nature or by some institutional process (some examples will be given in Sections 4.4 and 4.5). ... sometimes want to consider nonrandom allocations. For example, it is possible
Random Assignment in Experiments | Introduction & Examples. Published on March 8, 2021 by Pritha Bhandari.Revised on June 22, 2023. In experimental research, random assignment is a way of placing participants from your sample into different treatment groups using randomization. With simple random assignment, every member of the sample has a known or equal chance of being placed in a control ...
Abstract. Psychotherapy meta-analyses commonly combine results from controlled experiments that use random and nonrandom assignment without examining whether the 2 methods give the same answer. Results from this article call this practice into question. With the use of outcome studies of marital and family therapy, 64 experiments using random ...
Nonrandom Assignment of Participants and Absence of Conditions In nonexperiments, there are typically no explicitly defined research conditions. For example, a researcher interested in assessing the relation between job satisfaction (an assumed cause) and organizational commitment (an assumed effect) would simply measure the level of both such ...
Abstract. Nonrandom sampling, also called "nonprobabilistic" or "nonprobability sampling," is any sampling method in which the process that determines whether a member of the population is selected for inclusion in the sample is guided by a nonchance or nonrandom process. Such nonrandom processes can include the investigator choosing ...
Random sampling is a process for obtaining a sample that accurately represents a population. Random assignment uses a chance process to assign subjects to experimental groups. Using random assignment requires that the experimenters can control the group assignment for all study subjects. For our study, we must be able to assign our participants ...
Sixty research investigations published in the biomedical literature were analyzed to examine the effect of design attributes on outcome. ... Thirty of the trials used random assignment of participants to treat- ment or control conditions and 30 trials employed some nonrandom method of subject assignment Trial results were aggregated and ...
participants who were randomly assigned to the nonrandom- ized treatment condition were asked to privately select which training session they would prefer to attend and list the rea-
Random sampling (also called probability sampling or random selection) is a way of selecting members of a population to be included in your study. In contrast, random assignment is a way of sorting the sample participants into control and experimental groups. While random sampling is used in many types of studies, random assignment is only used ...
Consideration of challenges encountered in implementing random assignment suggests that 1) researcher communication with program staff improves compliance, but may not overcome the need for learning through experience; 2) in keeping with arguments in favor of random assignment-based research, random assignment may control for diverse selection ...
Randomization is an important tool used to establish causal inferences in studies designed to further our understanding of questions related to obesity and nutrition. To take advantage of the ...
A specific form of nonrandom assignment to treatment groups, the "alternate ranks" design, was investigated. This design eliminates the possibility of a correlation between the covariate and ...
This hypothesis has not been consistently supported by empirical studies; however, previous methods used to study this hypothesis have confounded assignment method with other study features. To avoid these confounding factors, this study randomly assigned participants to be in a randomized experiment or a nonrandomized experiment.
Impact of Assignment Preference on Service Engagement and Retention. Research participants who are disappointed in their random assignment to a non-preferred experimental condition may refuse to participate, or else withdraw from assigned services or treatment early in the study (Hofmann et al. 1998; Kearney and Silverman 1998; Laengle et al. 2000; Macias et al. 2005; Shadish et al. 2000 ...
A key justification for using nonrandomized experiments is that, with proper adjustment, their results can well approximate results from randomized experiments. This hypothesis has not been consistently supported by empirical studies; however, previous methods used to study this hypothesis have confounded assignment method with other study features. To avoid these confounding factors, this ...