- Search Menu
- Sign in through your institution
- Advance articles
- Featured articles
- Virtual Issues
- Browse content in B - History of Economic Thought, Methodology, and Heterodox Approaches
- Browse content in B4 - Economic Methodology
- B49 - Other
- Browse content in C - Mathematical and Quantitative Methods
- Browse content in C0 - General
- C01 - Econometrics
- Browse content in C1 - Econometric and Statistical Methods and Methodology: General
- C10 - General
- C11 - Bayesian Analysis: General
- C12 - Hypothesis Testing: General
- C13 - Estimation: General
- C14 - Semiparametric and Nonparametric Methods: General
- C15 - Statistical Simulation Methods: General
- Browse content in C2 - Single Equation Models; Single Variables
- C21 - Cross-Sectional Models; Spatial Models; Treatment Effect Models; Quantile Regressions
- C22 - Time-Series Models; Dynamic Quantile Regressions; Dynamic Treatment Effect Models; Diffusion Processes
- C23 - Panel Data Models; Spatio-temporal Models
- C26 - Instrumental Variables (IV) Estimation
- Browse content in C3 - Multiple or Simultaneous Equation Models; Multiple Variables
- C31 - Cross-Sectional Models; Spatial Models; Treatment Effect Models; Quantile Regressions; Social Interaction Models
- C33 - Panel Data Models; Spatio-temporal Models
- C34 - Truncated and Censored Models; Switching Regression Models
- C35 - Discrete Regression and Qualitative Choice Models; Discrete Regressors; Proportions
- C36 - Instrumental Variables (IV) Estimation
- Browse content in C4 - Econometric and Statistical Methods: Special Topics
- C41 - Duration Analysis; Optimal Timing Strategies
- C44 - Operations Research; Statistical Decision Theory
- Browse content in C5 - Econometric Modeling
- C50 - General
- C51 - Model Construction and Estimation
- C52 - Model Evaluation, Validation, and Selection
- C53 - Forecasting and Prediction Methods; Simulation Methods
- C54 - Quantitative Policy Modeling
- C55 - Large Data Sets: Modeling and Analysis
- C57 - Econometrics of Games and Auctions
- Browse content in C6 - Mathematical Methods; Programming Models; Mathematical and Simulation Modeling
- C61 - Optimization Techniques; Programming Models; Dynamic Analysis
- C62 - Existence and Stability Conditions of Equilibrium
- C63 - Computational Techniques; Simulation Modeling
- C67 - Input-Output Models
- C68 - Computable General Equilibrium Models
- Browse content in C7 - Game Theory and Bargaining Theory
- C70 - General
- C72 - Noncooperative Games
- C73 - Stochastic and Dynamic Games; Evolutionary Games; Repeated Games
- C78 - Bargaining Theory; Matching Theory
- Browse content in C8 - Data Collection and Data Estimation Methodology; Computer Programs
- C83 - Survey Methods; Sampling Methods
- Browse content in C9 - Design of Experiments
- C90 - General
- C91 - Laboratory, Individual Behavior
- C92 - Laboratory, Group Behavior
- C93 - Field Experiments
- Browse content in D - Microeconomics
- Browse content in D0 - General
- D01 - Microeconomic Behavior: Underlying Principles
- D02 - Institutions: Design, Formation, Operations, and Impact
- D03 - Behavioral Microeconomics: Underlying Principles
- Browse content in D1 - Household Behavior and Family Economics
- D11 - Consumer Economics: Theory
- D12 - Consumer Economics: Empirical Analysis
- D13 - Household Production and Intrahousehold Allocation
- D14 - Household Saving; Personal Finance
- D15 - Intertemporal Household Choice: Life Cycle Models and Saving
- D18 - Consumer Protection
- Browse content in D2 - Production and Organizations
- D21 - Firm Behavior: Theory
- D22 - Firm Behavior: Empirical Analysis
- D23 - Organizational Behavior; Transaction Costs; Property Rights
- D24 - Production; Cost; Capital; Capital, Total Factor, and Multifactor Productivity; Capacity
- D25 - Intertemporal Firm Choice: Investment, Capacity, and Financing
- D29 - Other
- Browse content in D3 - Distribution
- D30 - General
- D31 - Personal Income, Wealth, and Their Distributions
- Browse content in D4 - Market Structure, Pricing, and Design
- D40 - General
- D41 - Perfect Competition
- D42 - Monopoly
- D43 - Oligopoly and Other Forms of Market Imperfection
- D44 - Auctions
- D47 - Market Design
- Browse content in D5 - General Equilibrium and Disequilibrium
- D50 - General
- D51 - Exchange and Production Economies
- D52 - Incomplete Markets
- D53 - Financial Markets
- D57 - Input-Output Tables and Analysis
- D58 - Computable and Other Applied General Equilibrium Models
- Browse content in D6 - Welfare Economics
- D60 - General
- D61 - Allocative Efficiency; Cost-Benefit Analysis
- D62 - Externalities
- D63 - Equity, Justice, Inequality, and Other Normative Criteria and Measurement
- D64 - Altruism; Philanthropy
- Browse content in D7 - Analysis of Collective Decision-Making
- D70 - General
- D71 - Social Choice; Clubs; Committees; Associations
- D72 - Political Processes: Rent-seeking, Lobbying, Elections, Legislatures, and Voting Behavior
- D73 - Bureaucracy; Administrative Processes in Public Organizations; Corruption
- D74 - Conflict; Conflict Resolution; Alliances; Revolutions
- D78 - Positive Analysis of Policy Formulation and Implementation
- Browse content in D8 - Information, Knowledge, and Uncertainty
- D80 - General
- D81 - Criteria for Decision-Making under Risk and Uncertainty
- D82 - Asymmetric and Private Information; Mechanism Design
- D83 - Search; Learning; Information and Knowledge; Communication; Belief; Unawareness
- D84 - Expectations; Speculations
- D85 - Network Formation and Analysis: Theory
- D86 - Economics of Contract: Theory
- Browse content in D9 - Micro-Based Behavioral Economics
- D90 - General
- D91 - Role and Effects of Psychological, Emotional, Social, and Cognitive Factors on Decision Making
- D92 - Intertemporal Firm Choice, Investment, Capacity, and Financing
- Browse content in E - Macroeconomics and Monetary Economics
- Browse content in E0 - General
- E03 - Behavioral Macroeconomics
- Browse content in E1 - General Aggregative Models
- E10 - General
- E12 - Keynes; Keynesian; Post-Keynesian
- E13 - Neoclassical
- E17 - Forecasting and Simulation: Models and Applications
- Browse content in E2 - Consumption, Saving, Production, Investment, Labor Markets, and Informal Economy
- E20 - General
- E21 - Consumption; Saving; Wealth
- E22 - Investment; Capital; Intangible Capital; Capacity
- E23 - Production
- E24 - Employment; Unemployment; Wages; Intergenerational Income Distribution; Aggregate Human Capital; Aggregate Labor Productivity
- E25 - Aggregate Factor Income Distribution
- E26 - Informal Economy; Underground Economy
- E27 - Forecasting and Simulation: Models and Applications
- Browse content in E3 - Prices, Business Fluctuations, and Cycles
- E30 - General
- E31 - Price Level; Inflation; Deflation
- E32 - Business Fluctuations; Cycles
- E37 - Forecasting and Simulation: Models and Applications
- Browse content in E4 - Money and Interest Rates
- E40 - General
- E41 - Demand for Money
- E42 - Monetary Systems; Standards; Regimes; Government and the Monetary System; Payment Systems
- E43 - Interest Rates: Determination, Term Structure, and Effects
- E44 - Financial Markets and the Macroeconomy
- E49 - Other
- Browse content in E5 - Monetary Policy, Central Banking, and the Supply of Money and Credit
- E50 - General
- E51 - Money Supply; Credit; Money Multipliers
- E52 - Monetary Policy
- E58 - Central Banks and Their Policies
- Browse content in E6 - Macroeconomic Policy, Macroeconomic Aspects of Public Finance, and General Outlook
- E60 - General
- E61 - Policy Objectives; Policy Designs and Consistency; Policy Coordination
- E62 - Fiscal Policy
- E65 - Studies of Particular Policy Episodes
- Browse content in E7 - Macro-Based Behavioral Economics
- E70 - General
- E71 - Role and Effects of Psychological, Emotional, Social, and Cognitive Factors on the Macro Economy
- Browse content in F - International Economics
- Browse content in F1 - Trade
- F10 - General
- F11 - Neoclassical Models of Trade
- F12 - Models of Trade with Imperfect Competition and Scale Economies; Fragmentation
- F13 - Trade Policy; International Trade Organizations
- F14 - Empirical Studies of Trade
- F15 - Economic Integration
- F16 - Trade and Labor Market Interactions
- F17 - Trade Forecasting and Simulation
- F18 - Trade and Environment
- F19 - Other
- Browse content in F2 - International Factor Movements and International Business
- F20 - General
- F21 - International Investment; Long-Term Capital Movements
- F22 - International Migration
- F23 - Multinational Firms; International Business
- Browse content in F3 - International Finance
- F30 - General
- F31 - Foreign Exchange
- F32 - Current Account Adjustment; Short-Term Capital Movements
- F33 - International Monetary Arrangements and Institutions
- F34 - International Lending and Debt Problems
- F36 - Financial Aspects of Economic Integration
- Browse content in F4 - Macroeconomic Aspects of International Trade and Finance
- F40 - General
- F41 - Open Economy Macroeconomics
- F42 - International Policy Coordination and Transmission
- F43 - Economic Growth of Open Economies
- F44 - International Business Cycles
- Browse content in F5 - International Relations, National Security, and International Political Economy
- F50 - General
- F53 - International Agreements and Observance; International Organizations
- Browse content in F6 - Economic Impacts of Globalization
- F60 - General
- F62 - Macroeconomic Impacts
- F63 - Economic Development
- F64 - Environment
- F65 - Finance
- Browse content in G - Financial Economics
- Browse content in G0 - General
- G01 - Financial Crises
- G02 - Behavioral Finance: Underlying Principles
- Browse content in G1 - General Financial Markets
- G10 - General
- G11 - Portfolio Choice; Investment Decisions
- G12 - Asset Pricing; Trading volume; Bond Interest Rates
- G13 - Contingent Pricing; Futures Pricing
- G14 - Information and Market Efficiency; Event Studies; Insider Trading
- G15 - International Financial Markets
- G18 - Government Policy and Regulation
- Browse content in G2 - Financial Institutions and Services
- G20 - General
- G21 - Banks; Depository Institutions; Micro Finance Institutions; Mortgages
- G22 - Insurance; Insurance Companies; Actuarial Studies
- G23 - Non-bank Financial Institutions; Financial Instruments; Institutional Investors
- G24 - Investment Banking; Venture Capital; Brokerage; Ratings and Ratings Agencies
- G28 - Government Policy and Regulation
- Browse content in G3 - Corporate Finance and Governance
- G30 - General
- G31 - Capital Budgeting; Fixed Investment and Inventory Studies; Capacity
- G32 - Financing Policy; Financial Risk and Risk Management; Capital and Ownership Structure; Value of Firms; Goodwill
- G33 - Bankruptcy; Liquidation
- G34 - Mergers; Acquisitions; Restructuring; Corporate Governance
- G38 - Government Policy and Regulation
- Browse content in G4 - Behavioral Finance
- G40 - General
- G41 - Role and Effects of Psychological, Emotional, Social, and Cognitive Factors on Decision Making in Financial Markets
- Browse content in H - Public Economics
- Browse content in H0 - General
- H00 - General
- Browse content in H1 - Structure and Scope of Government
- H11 - Structure, Scope, and Performance of Government
- Browse content in H2 - Taxation, Subsidies, and Revenue
- H20 - General
- H21 - Efficiency; Optimal Taxation
- H23 - Externalities; Redistributive Effects; Environmental Taxes and Subsidies
- H24 - Personal Income and Other Nonbusiness Taxes and Subsidies; includes inheritance and gift taxes
- H25 - Business Taxes and Subsidies
- H26 - Tax Evasion and Avoidance
- Browse content in H3 - Fiscal Policies and Behavior of Economic Agents
- H30 - General
- H31 - Household
- Browse content in H4 - Publicly Provided Goods
- H41 - Public Goods
- Browse content in H5 - National Government Expenditures and Related Policies
- H50 - General
- H51 - Government Expenditures and Health
- H52 - Government Expenditures and Education
- H53 - Government Expenditures and Welfare Programs
- H55 - Social Security and Public Pensions
- H56 - National Security and War
- Browse content in H6 - National Budget, Deficit, and Debt
- H60 - General
- H63 - Debt; Debt Management; Sovereign Debt
- Browse content in H7 - State and Local Government; Intergovernmental Relations
- H71 - State and Local Taxation, Subsidies, and Revenue
- H75 - State and Local Government: Health; Education; Welfare; Public Pensions
- Browse content in H8 - Miscellaneous Issues
- H81 - Governmental Loans; Loan Guarantees; Credits; Grants; Bailouts
- Browse content in I - Health, Education, and Welfare
- Browse content in I0 - General
- I00 - General
- Browse content in I1 - Health
- I10 - General
- I11 - Analysis of Health Care Markets
- I12 - Health Behavior
- I13 - Health Insurance, Public and Private
- I14 - Health and Inequality
- I15 - Health and Economic Development
- I18 - Government Policy; Regulation; Public Health
- Browse content in I2 - Education and Research Institutions
- I20 - General
- I21 - Analysis of Education
- I22 - Educational Finance; Financial Aid
- I23 - Higher Education; Research Institutions
- I24 - Education and Inequality
- I25 - Education and Economic Development
- I26 - Returns to Education
- I28 - Government Policy
- Browse content in I3 - Welfare, Well-Being, and Poverty
- I30 - General
- I31 - General Welfare
- I32 - Measurement and Analysis of Poverty
- I38 - Government Policy; Provision and Effects of Welfare Programs
- I39 - Other
- Browse content in J - Labor and Demographic Economics
- Browse content in J0 - General
- J00 - General
- J01 - Labor Economics: General
- J08 - Labor Economics Policies
- Browse content in J1 - Demographic Economics
- J10 - General
- J11 - Demographic Trends, Macroeconomic Effects, and Forecasts
- J12 - Marriage; Marital Dissolution; Family Structure; Domestic Abuse
- J13 - Fertility; Family Planning; Child Care; Children; Youth
- J14 - Economics of the Elderly; Economics of the Handicapped; Non-Labor Market Discrimination
- J15 - Economics of Minorities, Races, Indigenous Peoples, and Immigrants; Non-labor Discrimination
- J16 - Economics of Gender; Non-labor Discrimination
- J17 - Value of Life; Forgone Income
- Browse content in J2 - Demand and Supply of Labor
- J20 - General
- J21 - Labor Force and Employment, Size, and Structure
- J22 - Time Allocation and Labor Supply
- J23 - Labor Demand
- J24 - Human Capital; Skills; Occupational Choice; Labor Productivity
- Browse content in J3 - Wages, Compensation, and Labor Costs
- J30 - General
- J31 - Wage Level and Structure; Wage Differentials
- J32 - Nonwage Labor Costs and Benefits; Retirement Plans; Private Pensions
- J33 - Compensation Packages; Payment Methods
- J38 - Public Policy
- Browse content in J4 - Particular Labor Markets
- J41 - Labor Contracts
- J42 - Monopsony; Segmented Labor Markets
- J44 - Professional Labor Markets; Occupational Licensing
- J47 - Coercive Labor Markets
- Browse content in J5 - Labor-Management Relations, Trade Unions, and Collective Bargaining
- J50 - General
- J52 - Dispute Resolution: Strikes, Arbitration, and Mediation; Collective Bargaining
- Browse content in J6 - Mobility, Unemployment, Vacancies, and Immigrant Workers
- J60 - General
- J61 - Geographic Labor Mobility; Immigrant Workers
- J62 - Job, Occupational, and Intergenerational Mobility
- J63 - Turnover; Vacancies; Layoffs
- J64 - Unemployment: Models, Duration, Incidence, and Job Search
- J65 - Unemployment Insurance; Severance Pay; Plant Closings
- J68 - Public Policy
- Browse content in J7 - Labor Discrimination
- J71 - Discrimination
- Browse content in J8 - Labor Standards: National and International
- J81 - Working Conditions
- J82 - Labor Force Composition
- J83 - Workers' Rights
- Browse content in K - Law and Economics
- Browse content in K0 - General
- K00 - General
- Browse content in K1 - Basic Areas of Law
- K14 - Criminal Law
- Browse content in K3 - Other Substantive Areas of Law
- K31 - Labor Law
- K33 - International Law
- K35 - Personal Bankruptcy Law
- Browse content in K4 - Legal Procedure, the Legal System, and Illegal Behavior
- K40 - General
- K41 - Litigation Process
- K42 - Illegal Behavior and the Enforcement of Law
- Browse content in L - Industrial Organization
- Browse content in L0 - General
- L00 - General
- Browse content in L1 - Market Structure, Firm Strategy, and Market Performance
- L10 - General
- L11 - Production, Pricing, and Market Structure; Size Distribution of Firms
- L12 - Monopoly; Monopolization Strategies
- L13 - Oligopoly and Other Imperfect Markets
- L14 - Transactional Relationships; Contracts and Reputation; Networks
- L15 - Information and Product Quality; Standardization and Compatibility
- Browse content in L2 - Firm Objectives, Organization, and Behavior
- L20 - General
- L22 - Firm Organization and Market Structure
- L23 - Organization of Production
- L25 - Firm Performance: Size, Diversification, and Scope
- Browse content in L3 - Nonprofit Organizations and Public Enterprise
- L31 - Nonprofit Institutions; NGOs; Social Entrepreneurship
- Browse content in L4 - Antitrust Issues and Policies
- L41 - Monopolization; Horizontal Anticompetitive Practices
- L42 - Vertical Restraints; Resale Price Maintenance; Quantity Discounts
- L43 - Legal Monopolies and Regulation or Deregulation
- Browse content in L5 - Regulation and Industrial Policy
- L50 - General
- L51 - Economics of Regulation
- Browse content in L6 - Industry Studies: Manufacturing
- L60 - General
- L62 - Automobiles; Other Transportation Equipment; Related Parts and Equipment
- L63 - Microelectronics; Computers; Communications Equipment
- Browse content in L7 - Industry Studies: Primary Products and Construction
- L71 - Mining, Extraction, and Refining: Hydrocarbon Fuels
- Browse content in L8 - Industry Studies: Services
- L81 - Retail and Wholesale Trade; e-Commerce
- L82 - Entertainment; Media
- L86 - Information and Internet Services; Computer Software
- Browse content in L9 - Industry Studies: Transportation and Utilities
- L93 - Air Transportation
- L94 - Electric Utilities
- L96 - Telecommunications
- Browse content in M - Business Administration and Business Economics; Marketing; Accounting; Personnel Economics
- Browse content in M0 - General
- M00 - General
- Browse content in M1 - Business Administration
- M11 - Production Management
- M14 - Corporate Culture; Social Responsibility
- Browse content in M2 - Business Economics
- M21 - Business Economics
- Browse content in M3 - Marketing and Advertising
- M31 - Marketing
- M37 - Advertising
- Browse content in M5 - Personnel Economics
- M50 - General
- M51 - Firm Employment Decisions; Promotions
- M52 - Compensation and Compensation Methods and Their Effects
- M54 - Labor Management
- M55 - Labor Contracting Devices
- Browse content in N - Economic History
- Browse content in N1 - Macroeconomics and Monetary Economics; Industrial Structure; Growth; Fluctuations
- N10 - General, International, or Comparative
- N13 - Europe: Pre-1913
- Browse content in N2 - Financial Markets and Institutions
- N20 - General, International, or Comparative
- Browse content in N3 - Labor and Consumers, Demography, Education, Health, Welfare, Income, Wealth, Religion, and Philanthropy
- N31 - U.S.; Canada: Pre-1913
- N32 - U.S.; Canada: 1913-
- N33 - Europe: Pre-1913
- N34 - Europe: 1913-
- Browse content in N4 - Government, War, Law, International Relations, and Regulation
- N42 - U.S.; Canada: 1913-
- N43 - Europe: Pre-1913
- N44 - Europe: 1913-
- N45 - Asia including Middle East
- Browse content in N9 - Regional and Urban History
- N90 - General, International, or Comparative
- N92 - U.S.; Canada: 1913-
- N94 - Europe: 1913-
- Browse content in O - Economic Development, Innovation, Technological Change, and Growth
- Browse content in O1 - Economic Development
- O10 - General
- O11 - Macroeconomic Analyses of Economic Development
- O12 - Microeconomic Analyses of Economic Development
- O13 - Agriculture; Natural Resources; Energy; Environment; Other Primary Products
- O14 - Industrialization; Manufacturing and Service Industries; Choice of Technology
- O15 - Human Resources; Human Development; Income Distribution; Migration
- O16 - Financial Markets; Saving and Capital Investment; Corporate Finance and Governance
- O17 - Formal and Informal Sectors; Shadow Economy; Institutional Arrangements
- O18 - Urban, Rural, Regional, and Transportation Analysis; Housing; Infrastructure
- Browse content in O2 - Development Planning and Policy
- O23 - Fiscal and Monetary Policy in Development
- Browse content in O3 - Innovation; Research and Development; Technological Change; Intellectual Property Rights
- O30 - General
- O31 - Innovation and Invention: Processes and Incentives
- O32 - Management of Technological Innovation and R&D
- O33 - Technological Change: Choices and Consequences; Diffusion Processes
- O34 - Intellectual Property and Intellectual Capital
- O38 - Government Policy
- Browse content in O4 - Economic Growth and Aggregate Productivity
- O40 - General
- O41 - One, Two, and Multisector Growth Models
- O42 - Monetary Growth Models
- O43 - Institutions and Growth
- O44 - Environment and Growth
- O47 - Empirical Studies of Economic Growth; Aggregate Productivity; Cross-Country Output Convergence
- Browse content in O5 - Economywide Country Studies
- O51 - U.S.; Canada
- O55 - Africa
- Browse content in P - Economic Systems
- Browse content in P0 - General
- P00 - General
- Browse content in P1 - Capitalist Systems
- P16 - Political Economy
- Browse content in P2 - Socialist Systems and Transitional Economies
- P26 - Political Economy; Property Rights
- Browse content in Q - Agricultural and Natural Resource Economics; Environmental and Ecological Economics
- Browse content in Q1 - Agriculture
- Q15 - Land Ownership and Tenure; Land Reform; Land Use; Irrigation; Agriculture and Environment
- Q16 - R&D; Agricultural Technology; Biofuels; Agricultural Extension Services
- Browse content in Q3 - Nonrenewable Resources and Conservation
- Q33 - Resource Booms
- Browse content in Q4 - Energy
- Q41 - Demand and Supply; Prices
- Q43 - Energy and the Macroeconomy
- Browse content in Q5 - Environmental Economics
- Q51 - Valuation of Environmental Effects
- Q52 - Pollution Control Adoption Costs; Distributional Effects; Employment Effects
- Q53 - Air Pollution; Water Pollution; Noise; Hazardous Waste; Solid Waste; Recycling
- Q54 - Climate; Natural Disasters; Global Warming
- Q55 - Technological Innovation
- Q56 - Environment and Development; Environment and Trade; Sustainability; Environmental Accounts and Accounting; Environmental Equity; Population Growth
- Q58 - Government Policy
- Browse content in R - Urban, Rural, Regional, Real Estate, and Transportation Economics
- Browse content in R1 - General Regional Economics
- R10 - General
- R11 - Regional Economic Activity: Growth, Development, Environmental Issues, and Changes
- R12 - Size and Spatial Distributions of Regional Economic Activity
- R13 - General Equilibrium and Welfare Economic Analysis of Regional Economies
- R15 - Econometric and Input-Output Models; Other Models
- Browse content in R2 - Household Analysis
- R21 - Housing Demand
- R23 - Regional Migration; Regional Labor Markets; Population; Neighborhood Characteristics
- Browse content in R3 - Real Estate Markets, Spatial Production Analysis, and Firm Location
- R31 - Housing Supply and Markets
- R33 - Nonagricultural and Nonresidential Real Estate Markets
- Browse content in R4 - Transportation Economics
- R41 - Transportation: Demand, Supply, and Congestion; Travel Time; Safety and Accidents; Transportation Noise
- R48 - Government Pricing and Policy
- Browse content in R5 - Regional Government Analysis
- R51 - Finance in Urban and Rural Economies
- Browse content in Z - Other Special Topics
- Browse content in Z1 - Cultural Economics; Economic Sociology; Economic Anthropology
- Z10 - General
- Z12 - Religion
- Z13 - Economic Sociology; Economic Anthropology; Social and Economic Stratification
- Author Guidelines
- Submission Site
- Open Access
- About The Review of Economic Studies
- Editorial Board
- Advertising and Corporate Services
- Self-Archiving Policy
- Dispatch Dates
- Journals on Oxford Academic
- Books on Oxford Academic

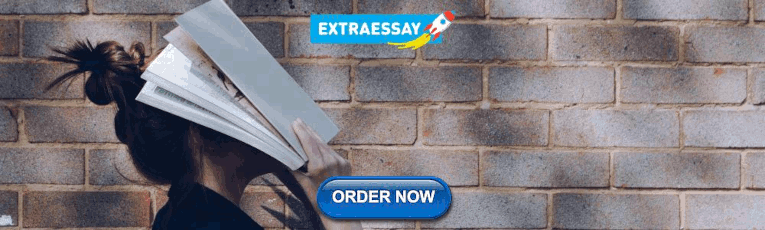
Article Contents
1. introduction, 2. teacher assignment to schools in france, 3. model and basic definitions, 4. theoretical results, 5. empirical analysis, 6. relationship to the literature, 7. concluding remarks, data availability statement, supplementary data, acknowledgments.
- < Previous
The Design of Teacher Assignment: Theory and Evidence
- Article contents
- Figures & tables
Julien Combe, Olivier Tercieux, Camille Terrier, The Design of Teacher Assignment: Theory and Evidence, The Review of Economic Studies , Volume 89, Issue 6, November 2022, Pages 3154–3222, https://doi.org/10.1093/restud/rdac002
- Permissions Icon Permissions
To assign teachers to schools, a modified version of the well-known deferred acceptance mechanism has been proposed in the literature and is used in practice. We show that this mechanism fails to be fair and efficient for both teachers and schools. We identify a class of strategy-proof mechanisms that cannot be improved upon in terms of both efficiency and fairness. Using a rich dataset on teachers’ applications in France, we estimate teachers preferences and perform a counterfactual analysis. The results show that these mechanisms perform much better than the modified version of deferred acceptance. For instance, the number of teachers moving from their positions more than triples under our mechanism.
Teachers are a key determinant of student achievement, and their distribution across schools can have a major impact on achievement gaps between students from different ethnic and/or social backgrounds. Growing concerns that disadvantaged students may have less access to effective teachers have given rise to policies intended to better distribute effective teachers across schools. 1 However, such policies must be implemented with caution, as they might have unexpected effects on teachers satisfaction and, ultimately, on the overall appeal of the teaching profession. 2 This raises a central question: How can be designed a teacher assignment procedure that takes into account both teachers preferences and administration concerns about the distribution of (effective) teachers? This article introduces a new assignment procedure and assesses its performance.
In many countries, the labour market for teachers is highly regulated by a central administration that is in charge of assigning teachers to schools. 3 Within such systems, teachers submit ranked lists of school preferences, and each school ranks teachers. When policymakers design assignment processes, they must consider several objectives, some of which might be difficult to reconcile. A first intuitive objective is to maximize teachers satisfaction by implementing all beneficial exchanges of positions across teachers. Yet, policymakers must ensure that such position exchanges do not unintentially harm some disadvantaged schools. In many countries, teachers priorities at schools are primarily determined by their experience, which reflects the administration’s effort to not assign novice teachers to disadvantaged students. By implementing all beneficial exchanges, one may increase the number of inexperienced teachers in disadvantaged schools, which is a key concern for policymakers. Hence, in this context, one natural objective is to allow teachers to exchange positions only if it does not increase the number of inexperienced teachers in disadvantaged schools.
More generally, the criteria used to define teachers priorities reflect clear social objectives. 4 A relevant objective is therefore to allow teachers to exchange positions only if schools are not assigned teachers with lower priorities. We name this requirement two-sided efficiency . While this is formally equivalent to an efficiency notion that considers both teachers and schools as welfare-relevant entities, in our context this requirement is mainly instrumental. It allows us to produce teacher assignments that respect the administration objectives, as reflected by the priority system. In the empirical section (Section 5 ) of this article, we examine the French teacher assignment system and show that its underlying social objectives are better fulfilled when using assignment schemes that satisfy two-sided efficiency.
The central administration in charge of designing the assignment mechanism faces an additional fundamental constraint. Many teachers already have positions and want to be reassigned. In practice, tenured teachers have the right to keep their initial positions if they do not obtain any of the schools they asked for. Thus, the administration has to offer a teacher a position at a school that he or she likes at least as much as the one to which he or she is currently assigned. In other words, the assignment of teachers must be individually rational . To fulfil this constraint, a standard approach is to use a variation on the well-known deferred acceptance mechanism ( Gale and Shapley, 1962 )—DA, for short—to make the assignment individually rational ( Compte and Jehiel, 2008 ; Pereyra, 2013 ). This variation consists of, first, artificially modifying the school’s ordering of teachers such that all teachers initially assigned to a school are moved to the top of that school’s ranking. In a second step, we run the DA mechanism using the modified priorities. This mechanism is used to assign teachers to schools in France, as well as for the assignment of on-campus housing in several places around the world ( Guillen and Kesten, 2012 ), and, in many countries, to assign students to schools when students have the right to stay in their district school.
While, by construction, the modified version of DA is individually rational, a first objective of this article is to show that the modification loses an important property of DA by failing to be two-sided efficient in that one can find alternative assignments whereby all teachers would be better-off and schools would get matched to teachers to which they assign higher priority. 5 Importantly, this “Pareto improvement” can be achieved while simultaneously improving fairness; i.e., we can reduce the number of teachers who are refused by a school while other teachers with lower rankings at that school are accepted. (We use standard terminology and say that such teachers and schools form a blocking pair .) Hence, the modified DA can be improved both in terms of efficiency—beneficial exchanges of positions across teachers—and fairness. This lack of trade-off between these two core notions is in stark contrast with what we know from the college admission and school choice environments and makes clear that the teacher assignment problem is quite different from previously studied settings.
The main goal of this article is therefore to design mechanisms that do not suffer from the same limitations as the modified version of DA, while keeping the good incentive properties of this mechanism, that is, strategy-proofness (meaning that teachers have straightforward incentives to report their preferences truthfully). 6 As we will make clear, merely tweaking the DA mechanism is not enough to fulfil this objective. We say that a matching is two-sided maximal if (1) compared to the initial assignment, all teachers are better-off and all schools get teachers with higher priorities, and (2) the matching cannot be improved in terms of (2i) (two-sided) efficiency and (2ii) fairness. This requirement is actually quite weak, and two-sided maximal matchings are easily shown to correspond to assignments that are both two-sided efficient and individually rational on both sides of the market.
To characterize two-sided maximal matchings, we provide an algorithm called the block exchange (BE) algorithm. The idea is simple: starting from the initial assignment, if two teachers block one another’s schools, we allow these teachers to exchange their schools. Obviously, larger exchanges involving many teachers are possible. As a teacher may be involved in several cycles, the outcome of the BE algorithm depends on the order in which we select the cycles. A first natural result shows that any possible outcome of the BE algorithm is a two-sided maximal matching, and, conversely, any two-sided maximal matching can be achieved with an appropriate selection of cycles. 7 While we obtain a plethora of different possible matchings depending on how we select exchange cycles in the BE algorithm, our main result shows that there are ways to select cycles that make this algorithm strategy-proof for teachers. Such mechanisms are called teacher-optimal BE algorithms (TO-BE). The name emphasizes the fact that TO-BE is a teacher-optimal selection of BE. Our results also give a sense in which adding strategy-proofness drastically reduces the set of possible mechanisms: in the one-to-one environment, there is a unique two-sided maximal mechanism that is strategy-proof.
We provide additional theoretical results in two respects. First, we consider a case in which only teachers are welfare-relevant entities, and we want to improve their welfare as well as the fairness of the allocation. In this context, we provide a similar characterization to that obtained with the BE algorithm. Depending on how exchange cycles are selected, we once again identify a large class of mechanisms. Although this approach obviously favours teachers, we show that no mechanism in this class is strategy proof. 8 Second, we consider a large market approach in which preferences and schools rankings are drawn randomly from a rich class of distributions. 9 We show that when the market size increases, our mechanisms perform quantitatively better than the modified DA in terms of utilitarian efficiency and number of blocking pairs. We also identify the potential cost of adopting a strategy-proof mechanism in terms of utilitarian outcomes and number of blocking pairs compared to a first-best approach whereby one could select any two-sided maximal mechanism. 10
We then use a nationwide labour market to empirically estimate the magnitude of gains and trade-offs in a real teacher assignment problem. In France, like in several other countries, the central administration manages teachers assignment to schools. We start by estimating teachers preferences over regions using an estimation method recently developed by Fack et al. (2019) . We compare estimations under (i) the standard truth telling assumption , which assumes that teachers truthfully rank regions, and (ii) the weaker stability assumption , which assumes that teachers may skip regions that are unachievable for them, an omission that is outcome-irrelevant. We provide supporting evidence in favour of the second assumption by using formal statistical tests and by showing that stability-based estimations are much better at predicting teachers submitted rank order lists (ROL). We estimate the preferences of 6,302 teachers in the nine largest subjects (like French, mathematics, or English). Then, we use our estimates of teachers preferences to run counterfactuals and quantify our mechanisms performance. The results confirm that the modified version of DA (DA |$^{\ast }$| for short) is never two-sided maximal, meaning that assignments can be simultaneously improved in terms of teachers welfare, “schools welfare,” and fairness. In addition, we show that DA |$^{\ast }$| is far from being two-sided maximal: almost four times more teachers (953.3 vs. 237.9) can be matched when running a two-sided maximal mechanism Pareto-dominating DA |$^{\ast }$| . The results also show that TO-BE performs significantly better than DA |$^{\ast }$| . The number of teachers moving from their initial assignment increases by 274.9 |$\%$| under TO-BE, compared to DA |$^{\ast }$| , and the distribution of teachers ranks (over regions they obtain) stochastically dominates that of DA |$^{\ast }$| up to rank 23 (out of 25). Regarding fairness, the number of teachers who are not blocking with any region increases by 34.1 |$\%$| . Finally, contrary to DA |$^{\ast }$| , under TO-BE, no region has a position for which the teacher assigned to it has a lower priority than the teacher initially assigned to that position. More importantly, we show that the administration’s objectives are better fulfilled when using mechanisms that are two-sided maximal. Indeed, the percentage of unexperienced teachers in disadvantaged regions diminishes under all the mechanisms we suggest, and the number of teachers who are assigned a position closer to their partner increases. This provides some justification for our two-sided maximality notion which, as underlined before, is an instrument to produce assignments respecting the administration’s objectives, as reflected by the priority system. Finally, we underline that all the gains we obtain are statistically significant.
Our work stands at the crossroads of different strands of the literature. Our theoretical setup covers two standard models in matching theory. The first is the college admission problem , in which schools have preferences that are taken into account for both efficiency considerations and fairness issues ( Gale and Shapley, 1962 ). Second, our model also embeds the house allocation problem (e.g. Shapley and Scarf, 1974 ; Abdulkadiroğlu and Sonmez, 1999 ; Sönmez and Ünver, 2010 ). In this framework, individuals own a house and are willing to exchange their initial assignments. Our article builds on these two lines of research by incorporating both the initial assignment and the two-sided efficiency criteria. This mixed model covers important applications. While our article focuses on re-assignment of teachers to positions, the theory we develop applies more broadly to any transfer of agents between jobs, especially when transfers are based on priorities. Both public and private organizations increasingly rely on centralized transfer processes on the ground that they provide more transparent and fair rules. To give an example in each sector, Ireland is currently introducing the “Civil Service Mobility” scheme that allows Clerical Officers and Executive Officers to apply for mobility, while EasyJet uses a “Base Transfer Process” to let its pilots transfer base location. 11
Despite covering important applications, this mixed model remains understudied, though Guillen and Kesten (2012) note that the modified version of the DA mechanism is used to allocate on-campus housing at MIT. Compte and Jehiel (2008) and Pereyra (2013) provide results on the properties of this mechanism. After explaining that fairness and individual rationality are not compatible, they propose weakening the notion of blocking pairs and show that the modified version of DA maximizes fairness under this weakening. By contrast, our work retains the standard definition of blocking pairs and addresses notions of maximal fairness using the usual definition. More importantly, our theoretical and empirical results highlight that maximizing their notion of fairness can have high costs in terms of efficiency and the traditional notion of fairness (see Section 6 for further details). Dur and Ünver (2019) introduce a matching model to study two-sided exchange markets, such as tuition or temporary worker exchanges. We discuss the similarities and differences between our papers in more details in the Section 6 .
Our work also relates to the literature on the design of school choice allocation mechanisms. In this literature, efficiency (where students are the only welfare relevant entities) and fairness have received considerable attention. The two goals are incompatible (see Roth, 1982 , Abdulkadiroğlu and Sonmez, 2003 and Abdulkadiroğlu et al. , 2009 ). Efficient matching mechanisms, such as the school choice version of top trading cycle (defined by Abdulkadiroğlu and Sonmez, 2003 ), attain efficiency but fail to be fair. Fair mechanisms, such as the DA algorithm do not guarantee efficiency. This trade-off between efficiency and fairness is well studied, with particular focus on how to attain one objective with the minimum possible sacrifice of the other. 12 The designer’s task often boils down to a choice between two mechanisms: the student-proposing DA mechanism or the top trading cycle mechanism. 13 Yet our work shows that, in the teacher assignment problem, the individually rational version of DA can be improved in terms of both efficiency and fairness. 14 In addition, we identify a class of mechanisms, closely related to the top trading cycle mechanism (TO-BE), as natural alternatives for a designer concerned with these two core notions. Our conclusion is in striking contrast with the previous literature and confirms that the teacher assignment problem is novel and presents important conceptual differences from previously studied contexts.
Finally, our article builds on a recent literature that has developed preference estimation methods, notably for settings in which the reported preferences might fail to be truthfull ( Abdulkadiroğlu et al. , 2017 ; Agarwal and Somaini, 2018 ; Fack et al. , 2019 ; Calsamiglia et al. , 2020 ).
France, like several other countries, has a highly centralized labour market for teachers. The 400,000 public school teachers are civil servants. The French Ministry of Education is responsible for their recruitment, assignment to schools, and salary scale. 15 This gives us the opportunity to use a nationwide labour market to compare the performance of different assignment algorithms. Prior to assignment, the central administration defines teachers priorities using a point system that takes into account three legal priorities (spousal reunification, disability, and having a position in a disadvantaged or violent school), as well as teacher characteristics, including total seniority in teaching, seniority in the current school, time away from the spouse and/or children. This score determines schools rankings or preferences. We will use the terms “priorities” and “preferences” interchangeably (for the rationale behind this terminology, see Section 1). The point system, defined by the central administration, is well known by all teachers wishing to change schools. 16
The Ministry divides French territory into 31 regions (see the map in the Supplementary material S.1 ). Since 1999, the matching process has comprised two successive phases. First, during a region assignment phase, newly tenured teachers and teachers who wish to move to another region submit an ordered list of regions. A matching mechanism (described in the next section) is used to match teachers to regions, using priorities defined by the point system. Then, during the second phase, each region proceeds to school assignment . In each region, teachers matched to the region after the first phase and teachers who already have a position but wish to change schools within the same region report their preferences for schools in the region. Matching is completed using the same mechanism as in Phase 1 and a similar priority-defining point system as in Phase 1. The main difference is that teachers are limited in the number of schools that they can rank during this phase.
In 2013, just over 25,000 teachers applied in Phase 1, and 75,000 in Phase 2. In practice, the assignment process is decomposed into as many markets as there are subjects (107), and market size can differ quite substantially (from Sports, French, and Math that have between 2,000 and 2,500 teachers each to smaller subjects like Esthetics or Thermal Engineering that have between 15 and 60 teachers). As a teacher teaches only one subject and positions are specific to a subject, the markets are independent from one another. 17
A lack of mobility has emerged as a concern for the Ministry. In 2013, of the 17,000 tenured teachers requesting a new assignment, only 40.9 |$\%$| had their requests satisfied and 29 |$\%$| of the teachers asking to move closer to their families did not obtain a new assignment, many of them for several consecutive years. Due to this lack of mobility, the Mediator of the French Ministry of Education (2015) , responsible for resolving conflicts between the Ministry and teachers, receives approximately 700 complaints from primary and secondary school teachers every year related to assignment issues. In his annual report, he states that “the assignment algorithm opens doors to difficult personal situations that can eventually tarnish the quality and the investment of human resources.” The lack of mobility discourages students from becoming teachers ( Périer and Gurgand, 2016 ). This leaves some students without teachers and regularly requires regions to hire last-minute replacement staff who are not trained to teach. In the least attractive schools, labelled “priority education,” 30 |$\%$| of teachers do not have teaching certification, vs. 7.6 |$\%$| in other schools. One of the objectives of this article is to show that using an alternative mechanism can significantly reduce the current lack of mobility.
Consider a problem in which a finite set of teachers |$T$| has to be assigned to a finite set |$S$| of schools. Each school |$s$| has |$q_{s}$| available seats. Each teacher |$t$| has a strict preference relation |$\succ _{t}$| over the set of schools and being unmatched (being unmatched is denoted by |$\emptyset$| ). For any teacher |$t$| , we write |$s\succeq _{t}s^{\prime }$| if and only if |$ s\succ _{t}s^{\prime }$| or |$s=s^{\prime }$| . Similarly, each school |$s$| has a strict preference relation |$\succ _{s}$| over teachers and being unmatched. 18 For simplicity, we assume that all teachers and schools prefer to be matched rather than being unmatched. A matching |$\mu$| is a mapping from |$ T\cup S$| into |$T\cup S\cup \{\emptyset \}$| such that (i) for each |$t\in T$| , |$ \mu (t)\in S\cup \{\emptyset \}$| and for each |$s\in S$| , |$\mu (s)\subset T$| , |$ |\mu _{0}(s)|\leq q_{s}$| and (ii) |$\mu (t)=s$| iff |$t\in \mu (s)$| . That is, a matching simply specifies the school to which each teacher is assigned or that a teacher is unmatched. It also specifies the teachers assigned to each school, if any. We also sometimes use the term “assignment” instead of “matching.” Thus far, our environment does not differ from the college admission problem ( Gale and Shapley, 1962 ).
However, in a teacher assignment problem, there is an additional component: teachers have an initial assignment. Let us denote the corresponding matching by |$\mu _{0}$| . We assume that |$\mu _{0}(t)\neq \emptyset$| for each teacher |$t$| and |$|\mu _{0}(s)|=q_{s}$| for each school |$s$| . Thus, we focus on a pure reassignment process among teachers. We further discuss this assumption in Section 5.5 . All teachers are initially assigned a school (there is no incoming flow of teachers into the market), and there is no available seat at schools (there is no outgoing flow of teachers out of the market). We define a teacher allocation problem as a quadruplet |$[T,S,\mu_0,\succ,\mathbf{q}]$| where |$ \succ :=(\succ _{a})_{a\in S\cup T}$| and |$\mathbf{q}:=(q_s)_{s \in S}$| .
Since we are in a many-to-one setting, one has to define schools preferences over groups of teachers. We adopt a (standard) conservative approach. Consider a school |$s$| with |$q$| positions to fill and two vectors of size |$q$| , say |$\mathbf{x}:=(t_{1},\dots,t_{q})$| and |$\mathbf{y} :=(t_{1}^{\prime },\dots,t_{q}^{\prime })$| . Let us assume that each of these vectors is ordered in such a way that for each |$k=1,\dots q-1$| , the |$k$| th element of vector |$\mathbf{x}$| is preferred by |$s$| to its |$k+1$| th element; we make analogous assumptions for vector |$\mathbf{y}$| . We say that |$ \mathbf{x}$| is (weakly) preferred by the school to |$\mathbf{y}$| if, for each |$k=1,\dots q$| , the |$k$| th element of vector |$\mathbf{x}$| is (weakly) preferred by |$s$| to the |$k$| th element of vector |$\mathbf{y}$| , i.e., |$ t_{k}\succeq _{s}t_{k}^{\prime }$| . The preference is strict if |$t_{k}\succ _{s}t_{k}^{\prime }$| for at least one coordinate |$k$| . In the following, when comparing two matchings |$\mu$| and |$\mu ^{\prime }$| for a school |$s$| , we will abuse notations and note |$\mu ^{\prime }(s)\succeq _{s}\mu (s)$| if |$\mu ^{\prime }(s)$| is (weakly) preferred by |$s$| to |$\mu (s)$| . We will say that school |$s$| weakly prefers or is weakly better under the matching |$\mu ^{\prime }$| . 19 Again, we use the terms “preferences” and “priorities” interchangeably even though, in our context, schools’ ordering over teachers are priorities given by law. Many of our welfare notions (e.g. two-sided efficiency defined below) will do “as if” these priorities were the schools’ true preferences with the motivation that priorities reflect the administration’s normative criteria and so are welfare relevant (see Section 1). Again, Section 5 will show that the administration’s objectives are better fulfilled when using this approach.
We are interested in different efficiency and fairness criteria, depending on whether we regard both teachers and schools or only teachers as welfare-relevant entities. First, we say that a matching |$\mu$| is two-sided individually rational (2-IR) if, for each teacher |$t$| , |$\mu (t)$| is acceptable to |$t$| , i.e., |$\mu (t)\succeq _{t}\mu _{0}(t)$| and, in addition, for each school |$s$| , |$\mu (s)$| is acceptable to |$s$| , i.e., |$\mu (s)\succeq _{s}\mu _{0}(s)$| . 20 Similarly, a matching is one-sided individually rational (1-IR) if each teacher finds his assignment acceptable. We say that a matching |$\mu$| 2-Pareto dominates (respectively 1-Pareto dominates) another matching |$\mu ^{\prime }$| if all teachers and schools (respectively teachers) are weakly better of—and some strictly better of—under |$ \mu$| than under |$\mu ^{\prime }$| . A matching is two-sided Pareto-efficient (2-PE) if there is no other matching that 2-Pareto dominates it. Similarly, we define one-sided Pareto-efficient (1-PE) matchings as assignments for which no alternative matching exists that 1-Pareto dominates it. We say that under matching |$\mu$| , a teacher |$t$| has justified envy toward teacher |$t^{\prime }$| if |$t$| prefers the assignment of |$t^{\prime }$| , i.e., |$\mu (t^{\prime })=:s$| , to his own assignment |$\mu (t)$| and |$s$| prefers |$t$| to |$t^{\prime }$| . Using the standard terminology from the literature, we say that |$(t,s)$| blocks matching |$\mu$| . A matching |$\mu$| is stable if there is no pair |$ (t,s)$| blocking |$\mu$| . 21 We will sometimes say that a matching |$\mu$| dominates another matching |$\mu ^{\prime }$| in terms of stability if the set of blocking pairs of |$\mu$| is included in that of |$\mu ^{\prime }$| .
A matching mechanism is a function |$\varphi $| that maps problems into matchings. We write |$\varphi (\succ )$| for the matching obtained in problem |$[T,S,\mu_0,\succ,\mathbf{q}]$| . We also write |$\varphi _{t}(\succ )$| for the school that teacher |$t$| obtains under matching |$\varphi (\succ )$| . It is 2-IR/1-IR/1-PE/2-PE/stable if, for each problem, it selects a matching that is 2-IR/1-IR/1-PE/2-PE/stable. Finally, a matching mechanism |$ \varphi$| is strategy-proof if, for each profile of preferences |$ \succ$| and teacher |$t$| , |$\varphi _{t}(\succ )\succeq _{t}\varphi _{t}(\succ _{t}^{\prime },\succ _{-t})$| for any possible report |$\succ _{t}^{\prime }$| of teacher |$t$| . 22
In the next sections, we explain why DA is not individually rational and describe its modification which makes it individually rational. We explain the drawbacks of this modified DA and define new algorithms which avoid these drawbacks.
4.1. The modified DA and its drawbacks
One of the standard matching mechanisms is DA, as proposed by Gale and Shapley (1962) . Because we discuss a closely related mechanism, we first recall its definition.
Step 1. Each teacher |$t$| applies to his most preferred school. Each school tentatively accepts, up to its capacity, its most preferred teachers among the offers it receives and rejects all other offers.
In general,
Step |$\mathbf{k\geq 1}$| . Each teacher |$t$| who was rejected at step |$k-1$| applies to his most preferred school among those to which he has not yet applied. Each school tentatively accepts, up to its capacity, its most favourite teachers among the new offers in the current step and the applicants tentatively selected from the previous step (if any), and rejects all other offers.
While DA is known to be stable and 2-PE, it fails to be 1-IR (and thus 2-IR). This is unavoidable: in general, there is a conflict between individual rationality and stability. The basic intuition is as follows: imposing 1-IR on a mechanism yields situations in which a teacher |$t$| may be able to keep his initial assignment |$\mu _{0}(t)=:s$| , while school |$s$| may perfectly prefer other teachers to |$t$| . These other teachers may rank |$s$| at the top of their preference relation and hence block with school |$s$| . We summarize this discussion in the following observation. 23
( Gale and Shapley, 1962 ) DA is a stable and 2-PE mechanism. There is no mechanism that is both 1-IR and stable. Hence, DA is not 1-IR.
Because there is a fundamental trade-off between 1-IR and stability, one may wish to find a mechanism that restores individual rationality while retaining DA’s other desirable properties, such as its stability and 2-Pareto efficiency, to the greatest extent possible. An approach followed in the literature (see, for instance, Pereyra, 2013 or Compte and Jehiel, 2008 ) and used in practice tries to achieve this balance by artificially modifying schools preferences such that each teacher |$t$| is ranked, in the (modified) ranking of his initial school |$s:=\mu _{0}(t)$| , above any teacher |$t^{\prime }\notin \mu _{0}(s)$| . Other than this modification, the schools’ preference relations remain unchanged. 24 With this modification in place, DA proceeds, as defined above, using schools’ modified preferences. We denote this mechanism as DA |$^{\ast }$| . By construction, this is a 1-IR mechanism. It is used in several real-world situations, including assigning on-campus housing at MIT ( Guillen and Kesten, 2012 ) and, more pertinent to our interests here, assigning teachers to public schools in France.
As noted above, this mechanism is 1-IR by construction, and therefore, by Proposition 1, we know it is not stable. Yet, is there a sense in which the violation of stability is minimal? What about efficiency: Is DA |$^{\ast }$| 2-PE? Furthermore, if the answers to these questions are negative, can we find ways to improve upon DA |$^{\ast }$| ? The following example illustrates an important drawback of DA |$^{\ast }$| to which we will return in both our theoretical analysis and in our empirical assessment.
We consider a simple environment with |$n$| teachers and |$n$| schools with initial assignment |$\mu _{0}$| . Let us assume that a teacher |$t^{\ast }$| is initially assigned to school |$s^{\ast }$| (i.e. |$\mu _{0}(t^{\ast })=s^{\ast }$| ) and is ranked first by all schools. In addition, school |$s^{\ast }$| is ranked at the bottom of each teacher preference relation, including |$t^{\ast }$| ; hence, |$t^{\ast }$| is willing to move. Under these assumptions, no teacher will move from his initial assignment if we use DA |$^{\ast }$| to assign teachers. To see this, note first that |$t^{\ast }$| does not move from his initial assignment. Indeed, because DA |$^{\ast }$| is 1-IR, if |$t^{\ast }$| were to move, then some teacher |$t$| would have to take the seat at school |$s^{\ast }$| (or be unmatched), but since |$s^{\ast }$| is the worst school for every teacher (and teachers prefer to be matched rather than unmatched), this assignment would violate the individual rationality condition for teacher |$t$| , a contradiction. Note that this implies that, under the DA |$ ^{\ast }$| algorithm, |$t^{\ast }$| applies to every school |$s$| (but is eventually rejected). Now, to see that no teacher other than |$t^{\ast }$| moves, assume on the contrary that |$t\neq t^{\ast }$| is assigned a school |$ s\neq \mu _{0}(t)$| . As mentioned above, at some step of the DA |$^{\ast }$| algorithm, |$t^{\ast }$| applies to |$s$| . Since |$t^{\ast }$| is ranked above |$t$| in the preference relation of school |$s$| (recall that |$s\neq \mu _{0}(t)$| ), |$ t$| cannot eventually be matched to school |$s$| , a contradiction.
To recap, under our assumptions, no teacher moves from his initial assignment. Since the initial assignment can perform very poorly in terms of basic criteria such as stability or 2-Pareto efficiency, we can easily imagine the existence of alternative matchings that would make both teachers and schools better off and, thereby, shrink the set of blocking pairs. 25
The driving force in this example is the existence of a teacher who is ranked at the top of each school’s ranking and is initially assigned to the worst school. 26 This is, of course, a stylized example, and one can easily imagine less extreme examples in which a similar phenomenon would occur. The basic idea is that, for DA |$^{\ast }$| to perform poorly, it is enough to have one teacher (a single one is enough) who has a fairly high ranking for a relatively large fraction of the schools, being assigned an unpopular school. Our theoretical analysis and our empirical assessment will show that the described phenomenon is not pathological.
The above example identifies a weakness of DA |$^{\ast }$| : it can be improved upon in terms of both efficiency (on both sides) and fairness (i.e. we can shrink its set of blocking pairs). This is an important difference with the standard college admission DA, which is known to be 2-PE. In |$\mbox{DA} ^{\ast }$| , the change in schools preferences made before running the algorithm to ensure the 1-IR property leads to the failure of the 2-PE property. Thus, we are interested in mechanisms that do not have this type of disadvantage. We also wish to retain the elementary property that our mechanism improves on the initial assignment. This suggests the following definition.
A matching |$\mu$| is two-sided maximal if |$\mu$| is 2-IR, 27 and there is no other matching |$\mu ^{\prime }$| such that (1) all teachers and schools are weakly better off and some strictly better off, and (2) the set of blocking pairs under |$\mu ^{\prime }$| is a subset of that under |$\mu$| .
Consistent with our previous notions, we say that a mechanism is two-sided maximal if it selects a two-sided maximal matching. Let us note that, if there is a matching |$\mu ^{\prime }$| under which all teachers and schools are weakly better off and some strictly better off than under a matching |$\mu$| , then the set of blocking pairs under |$\mu ^{\prime }$| is a subset of that under |$\mu$| . Thus, in the definition of two-sided maximality, requirement (2) can be dropped. Hence, a matching |$\mu$| is two-sided maximal if and only if |$\mu$| is 2-IR and 2-PE.
4.2. Alternative mechanisms and main result
We define a class of mechanisms that characterizes the set of two-sided maximal mechanisms. The mechanism will sequentially clear cycles of an appropriately constructed directed graph in the spirit of Gale’s top trading cycle (TTC hereafter), originally introduced in Shapley and Scarf (1974) .
4.2.1. The BE algorithm
The basic idea behind the mechanisms we define is the following: starting from the initial assignment, if a teacher |$t$| has a justified envy toward |$ t^{\prime }$| and |$t^{\prime }$| also has a justified envy toward |$t$| , then we allow |$t$| and |$t^{\prime }$| to trade their initial assignments. This is a pairwise exchange between |$t$| and |$t^{\prime }$| , but three-way or even larger exchanges could also occur. Once such an exchange has been made, we obtain a new matching and can again search for possible trades. More precisely, our class of mechanisms is induced by the following algorithm, named the BE:
Step |$0:$| set |$\mu (0):=\mu _{0}$| .
Step |$k\geq 1:$| Given |$\mu (k-1)$| , let the teachers and their assignments stand for the vertices of a directed graph where, for each pair of nodes |$(t,s)$| and |$(t^{\prime },s^{\prime })$| , there is an edge |$(t,s)\longrightarrow (t^{\prime },s^{\prime })$| if and only if teacher |$t$| has a justified envy toward teacher |$t^{\prime}$| at |$s^{\prime}$| , i.e., |$t$| prefers |$s^{\prime}$| to |$s$| and |$s^{\prime}$| prefers |$t$| to |$t^{\prime}$| . If there is no cycle, then set |$\mu (k-1)$| as the outcome of the algorithm. Otherwise, select a cycle in this directed graph. For each edge |$(t,s)\longrightarrow (t^{\prime },s^{\prime })$| in the cycle, assign teacher |$t$| to school |$s^{\prime }$| . Let |$\mu (k)$| be the matching so obtained. Go to step |$k+1$| .
It is easy to verify that this algorithm converges in (finite and) polynomial time. 28 In the above description, we do not specify how the algorithm should select the cycle of the directed graph. Therefore, the above description can be thought to define a class of mechanisms, wherein a mechanism is determined only after we fully specify how to act when confronted with multiple cycles. These selections may be random or dependent on earlier selections. In general, for each preference profile for teachers and schools |$\succ$| , a possible outcome of BE is a matching that can be obtained by an appropriate selection of cycles in the above procedure. Thus, we consider the following correspondence BE: |$\succ \rightrightarrows \mu$| where |${\rm BE}(\succ )$| stands for the set of all possible outcomes of BE. A selection of the BE algorithm is a mapping |$\varphi :$| |$\succ \mapsto \mu$| s.t. |$\varphi (\succ )\in BE(\succ )$| . Obviously, each selection |$\varphi$| of BE defines a mechanism.
As mentioned above, our class of mechanisms shares some similarities with Gale’s TTC, but there are two important differences. 29 The first and the most minor difference is that a teacher in a node can point to several nodes and thus, implicitly, to several schools. This is why, contrary to TTC, we have an issue regarding cycle selection and our algorithm does not define a unique mechanism. However, as we will see in the next result, this is necessary for our characterization. Second, and certainly more importantly, our algorithm takes into account welfare on both sides of the market. Indeed, a teacher in a node |$(t,s)$| can point to a school in |$(t^{\prime },s^{\prime })$| only if |$s^{\prime }$| prefers |$t$| to its assignment |$ t^{\prime }$| . This is what ensures, contrary to TTC, that each time we carry out a cycle, both teachers and schools become better off. This has the desirable implication that each time a cycle is cleared, the set of blocking pairs shrinks.
As we will see, the selections of the BE algorithm are two-sided maximal. In particular, those selections will avoid the drawback of DA |$^{\ast }$| illustrated in Example 1.
(Example 1 continued.) Let us further specify Example 1 and assume that |$n$| (the number of teachers and schools) is equal to |$3$| . Recall that teacher |$ t^{\ast }$| is initially assigned to school |$s^{\ast }$| (i.e. |$\mu _{0}(t^{\ast })=s^{\ast }$| ) and is ranked first by all schools. In addition, school |$s^{\ast }$| is ranked at the bottom of each teacher’s preference relation, including |$t^{\ast }$| . We further assume that the two other teachers, |$t_{1}$| and |$t_{2}$| , are initially assigned school |$s_{1}$| and |$ s_{2}$| respectively and |$t_{1}$| justifiably envies |$t_{2}$| at |$s_{2}$| while |$ t_{2}$| justifiably envies |$t_{1}$| at |$s_{1}$| . Since both teachers |$t_{1}$| and |$t_{2}$| as well as schools |$s_{1}$| and |$s_{2}$| would prefer the matching where |$t_{1}$| and |$t_{2}$| swap their assigned schools to the initial assignment, |$\mu _{0}$| is not 2-PE. Since, as we already claimed, no teacher moves from his initial assignment when using DA |$^{\ast }$| , DA |$^{\ast }$| is not two-sided maximal. The directed graph constructed in Step |$1$| of the |$BE$| algorithm is as follows.
Since, there is a unique cycle, at the end of Step |$1$| , |$\mu (1)$| assigns teacher |$t_{1}$| to school |$s_{2}$| and teacher |$t_{2}$| to school |$s_{1}$| . It is easy to check that the allocation achieved is 2-PE. Hence, there is no longer any cycle in the directed graph constructed at Step |$2$| of the BE algorithm. The outcome of |$BE$| is thus two-sided maximal and, as we will see, this is a general feature.

This leaves us with a fairly large set of two-sided maximal matching mechanisms. However, one issue with many selections of the BE algorithm is that they are not strategy proof. This is illustrated in the following example.
Consider an environment with three teachers |$\{t_{1},t_{2},t_{3}\}$| and three schools |$\{s_{1},s_{2},s_{3}\}$| . For each |$i=1,2,3$| , we assume that teacher |$t_{i}$| is initially assigned to school |$s_{i}$| . Teacher |$t_{1}$| ’s most preferred school is |$s_{2}$| , and he ranks his initial school |$s_{1}$| second. Teacher |$t_{2}$| ranks |$s_{1}$| first, followed by |$s_{3}$| . Teacher |$ t_{3}$| ranks |$s_{2}$| first and his initial assignment |$s_{3}$| second. Finally, we assume that each teacher is ranked in last position by the school to which he is initially assigned. We obtain the following graph for the BE algorithm.
There are two possible cycles that intersect at |$(t_{2},s_{2})$| . Consider a selection of the BE algorithm that picks cycle |$(t_{2},s_{2}) \leftrightarrows (t_{3},s_{3})$| . In that case, the algorithm ends at the end of Step |$1$| , and teacher |$t_{2}$| is eventually matched to school |$s_{3}$| , his second most preferred school. However, if teacher |$t_{2}$| lies and claims that he ranks |$s_{3}$| below his initial assignment, the directed graph associated with the BE algorithm has a single cycle |$ (t_{1},s_{1})\leftrightarrows (t_{2},s_{2})$| . In that case, the unique selection of the BE algorithm assigns |$t_{2}$| to his most preferred school |$ s_{1}$| . Hence, |$t_{2}$| has a profitable deviation under the selection of the BE algorithm considered here.

4.2.2. Strategy-proof selection of the BE algorithm
We define a class of mechanisms that are selections of the BE algorithm and are strategy proof. Before defining the mechanism, we need an additional piece of notation. Given a matching |$\mu$| , a set of teachers |$T^{\prime }$| , a set of school |$S^{\prime }\subseteq S$| and a teacher |$t$| , we let Opp |$ (t,\mu,T^{\prime },S'):=\{s\in S^{\prime }\left\vert t\succeq _{s}t^{\prime } \text{ for some }t^{\prime }\in \mu (s)\cap T^{\prime }\right. \}$| be the opportunity set of teacher |$t$| within schools in |$S^{\prime }$| when the set of teachers considered is |$T^{\prime }$| . Note that for each teacher |$t$| , if |$ \mu _{0}(t)\in S^{\prime }$| and |$t\in T^{\prime }$| , then Opp |$(t,\mu _{0},T^{\prime },S')\neq \emptyset$| since |$\mu _{0}(t)\in$| Opp |$(t,\mu _{0}$| , |$T^{\prime },S')$| .
For each school |$s\in S$| , fix an ordering over teachers |$ f_{s}:\{1,\dots,|T|\}\rightarrow T$| . We denote |$f=(f_{s})_{s\in S}$| the collection of the orderings, sometimes referred to simply as a collection, one for each school. |$f$| is the index for our class of mechanisms. 30
We define the Teacher Optimal Block Exchange (TO-BE) algorithm as follows:
Step |$CDATA[0:$| Set |$CDATA[\mu (0)=\mu _{0}$| , |$CDATA[T(0):=T$| and |$CDATA[S(0):=S$| .
Step |$CDATA[k\geq 1:$| Given |$CDATA[T(k-1)$| and |$CDATA[S(k-1)$| , let the teachers in |$CDATA[T(k-1)$| and their assignments stand for the vertices of a directed graph where, for each pair of nodes |$CDATA[(t,s)$| and |$CDATA[(t^{\prime },s^{\prime })$| , there is an edge |$CDATA[(t,s)\longrightarrow (t^{\prime },s^{\prime })$| if and only if:
teacher |$t$| ranks school |$s^{\prime }$| first in his opportunity set Opp |$(t,\mu (k-1),T(k-1),S(k-1))$|
teacher |$t^{\prime}$| has a lower priority than teacher |$t$| at school |$s^{\prime }$|
teacher |$t^{\prime}$| has the lowest ordering according to |$f_{s^{\prime }}$| among all teachers in school |$s^{\prime }$| who have a lower priority than |$t$| at |$s^{\prime }$| (i.e. |$f_{s^{\prime }}(t^{\prime }) \leq f_{s^{\prime }}(t^{\prime \prime })$| for all |$t^{\prime \prime }$| such that |$\mu(k-1)(t^{\prime \prime })=s^{\prime }$| and |$t \succeq _{s^{\prime }} t^{\prime \prime }$| )
The obtained directed graph has out-degree one and, as such, at least one cycle; cycles are pairwise disjoint. For each edge |$(t,s)\longrightarrow (t^{\prime },s^{\prime })$| in a cycle, assign teacher |$t$| to school |$s^{\prime }$| . Let |$\mu (k)$| be the assignment obtained and |$T(k)$| be the set of teachers who are not part of any cycle at the current step. The number of seats of each school is reduced consistently, and we let |$S(k)$| be the set of schools with positive remaining capacities. If |$T(k)$| is empty, then set |$\mu (k)$| as the outcome of the algorithm. Otherwise, go to step |$k+1$| .
The following example illustrates how the TO-BE algorithm works and how its outcome may depend on the collection |$f$| .
With this example, the graph of BE starting at the initial matching is:
|$f_{s_{1}}(t_{1}) < f_{s_{1}}(t_{1}^{\prime })$| and |$f_{s_{2}}(t_{2}) < f_{s_{2}}(t_{2}^{\prime })$| : then, at the first step of |$\mbox{TO-BE}_{f}$| , the implemented cycle is |$(t_{1},s_{1}) \leftrightarrows (t_{2},s_{2})$| and, at the second step, it is |$(t_{1}^{\prime },s_{1}) \leftrightarrows (t_{2}^{\prime },s_{2})$| . In the final matching, every teacher is assigned his top-ranked school.
|$f_{s_{1}}(t_{1}^{\prime }) < f_{s_{1}}(t_{1})$| and |$f_{s_{2}}(t_{2}) < f_{s_{2}}(t_{2}^{\prime })$| : then, at the first step of |$\mbox{TO-BE}_{f}$| , the implemented cycle is |$(t^{\prime }_{1},s_{1}) \leftrightarrows(t_{2},s_{2})$| . In the second step, since |$t_{1} \succ_{s_{1}}t_{2}^{\prime }$| , |$t_{2}^{\prime }$| is assigned to |$s_2$| . The algorithm stops afterwards with |$t_{1}$| and |$t_{2}^{\prime }$| assigned at their initial school.

The TO-BE algorithm has some similarities with the TTC mechanism as defined in Shapley and Scarf (1974) . As TTC, it sequentially clears cycles. In the case of TTC, the assignment obtained after cycles are cleared Pareto-improves the teacher side. However, schools can be worse-off. In case of TO-BE, both sides of the market (teachers and schools) get better-off when we clear cycles. 31 The fact that TTC can hurt schools can have far reaching consequences in practice. Indeed, in our empirical analysis (Section 5 ), we show this is the case empirically and explain how it relates to the failure of TTC to achieve important social objectives (such as having teachers getting closer to their families or having more experienced teachers in deprived areas). We also explore the formal connection between TO-BE and TTC in Appendix B .
Each mechanism in the above-defined class is indexed by its collection of orderings, |$f$| , and is denoted |$\mbox{TO-BE}_{f}$| . We sometimes omit the collection and simply note |$\mbox{TO-BE}$| when there is no risk of confusion. We further describe the role played by orderings |$f$| for the final outcome in Section 4.3.1 .
4.2.3. Main theoretical result
We are now in a position to state our main theoretical result giving a sense in which the TO-BE mechanism avoids the drawback of DA |$^{\ast }$| (described in Section 4.1 ) while keeping its incentive properties.
DA |$^{\ast }$| is strategy-proof but not two-sided maximal;
The set of two-sided maximal mechanisms coincides with the set of selections of BE;
For any collection |$f$| , |$\mbox{TO-BE}_{f}$| is strategy-proof and is a selection of the BE algorithm.
In the next few lines, we explain the arguments behind each point of the above theorem. First, point |$i.$| simply comes from the observation made in Example 1 where under DA |$^{\ast }$| no teacher is able to move from his initial assignment and the initial assignment is not 2-PE. 32 Point |$ii.$| uses the following natural result stating that the BE algorithm does characterize the set of two-sided maximal matchings.
Fix a preference profile. The set of possible outcomes of the BE algorithm coincides with the set of two-sided maximal matchings.
The proof uses the following technical lemma:
Assume that |$\mu ^{\prime }$| 2-Pareto dominates |$\mu$| . Starting from |$\mu (0)=\mu$| , there is a collection of disjoint cycles in the directed graph associated with the BE algorithm that, once carried out, yields matching |$\mu ^{\prime }$| .
The proof can be found in Appendix A . ■
We can complete the proof of Proposition 2 which yields point |$ii.$| of Theorem 1.
If |$\mu$| is an outcome of BE, then it must be two-sided maximal. Indeed, if this were not the case, then by the above lemma, there would exist a cycle, in the directed graph associated with the BE algorithm starting from |$\mu$| , which contradicts our assumption that |$\mu$| is an outcome of the BE algorithm. Now, if |$\mu$| is two-sided maximal, it 2-Pareto dominates the initial assignment |$\mu _{0}$| . Hence, appealing again to the above lemma, there is a collection of disjoint cycles in the directed graph associated with the BE algorithm starting from |$\mu _{0}$| that, once carried out, yields the assignment |$\mu$| . Clearly, once |$\mu$| is achieved by the BE algorithm, there are no more cycles in the associated graph. ■
Finally, point |$iii.$| of Theorem 1 is intuitive given the proximity between TO-BE and TTC, the latter being strategy-proof. Indeed, all teachers matched in the first step of TO-BE get their most preferred teacher–school pair (clearly, they are indifferent between two pairs involving the same school) within all pairs |$ (t,\mu _{0}(t))$| for which these teachers have a higher priority than |$t$| at |$\mu _{0}(t)$| . Hence, the only way for teachers matched in the first step to get better-off through a deviation is to get matched to a pair |$(t,\mu _{0}(t))$| for which these teachers would have a lower priority than |$t$| at |$ \mu _{0}(t)$| . However, this is clearly impossible by definition of TO-BE. Hence, these agents matched in the first step have no incentives to misreport their preferences. Under our assumption that the ordering |$f$| does not depend on teachers preferences, by deviating, agents matched in the second step of TO-BE cannot get a seat of a teacher matched in the first step. Then, the argument is similar for these agents matched in the second step and we can proceed inductively. While the argument is fairly standard, the proof can be found in Appendix C .
4.3. Further results
We now present additional theoretical results. First, we explain how the choice of the collection of orderings |$f$| for TO-BE can make the mechanism teacher-optimal in a well-defined sense. Second, we provide economically relevant environments under which TO-BE characterizes the whole class of strategy-proof and two-sided maximal mechanisms. We also introduce a notion of maximality which relaxes the constraint that schools must also get better-off compared to the initial assignment. We characterize this weakening via a class of mechanisms which we show does not contain any strategy-proof selection. Finally, we analyse our algorithms in a large market environment and provide further comparisons between DA |$^{\ast }$| , BE, and TO-BE in this setting.
4.3.1. Teacher-optimality
In this section, we show that if the collection of orderings |$f$| is chosen appropriately, TO-BE is optimal for teachers in a well-defined sense. This justifies our terminology: Teacher-Optimal Block Exchange. We say that a selection |$\varphi$| of the BE algorithm is teacher-optimal if there is no 2-IR matching mechanism that 1-Pareto dominates |$\varphi$| . Let |$f^{\ast }:=(f_{s}^{\ast })_{s\in S}$| be the collection of orderings under which for each school |$s\in S$| and each pair of teachers |$t,t^{\prime }\in T$| , |$ f_{s}^{\ast }(t^{\prime })<f_{s}^{\ast }(t)\Leftrightarrow t^{\prime }\succ _{s}t$| , i.e., the orderings of the schools follow their priorities. The ordering |$f$| can match schools priorities, but it does not have to. |$f$| is used to break ties between teachers of a given school and therefore determines which teacher might be pointed at first in an exchange cycle. As such, |$f$| might be used as an instrument to achieve certain policy goals, for example, retaining some teachers in specific schools. For instance, in the first case of Example 4, the schools orderings match the schools preferences, and TO-BE produces the teacher-optimal matching. In the second case, however, the schools’ orderings differ from the schools’ preferences, and TO-BE does not produce the teacher-optimal matching. In that case, however, both schools prefer the resulting assignment compared to the teacher-optimal matching.
Consistently, we show that, when schools’ orderings follow schools’ priorities, i.e., the collection is |$f^{\ast }$| , |$\mbox{TO-BE}_{f^{\ast }}$| is a teacher-optimal mechanism. We further show that TO-BE is not teacher-optimal if a different collection of orderings is used.
Let |$\varphi$| be a 2-IR mechanism. |$\mbox{TO-BE}_{f^{*}}$| is not 1-Pareto dominated by |$ \varphi$| . Moreover, for any other collection |$f \neq f^{*}$| , |$\mbox{TO-BE} _{f}$| is 1-Pareto dominated by some alternative 2-IR mechanism.
The proof can be found in Appendix D . ■
|$\mbox{TO-BE}_{f^{*}}$| is a teacher-optimal selection of BE. |$\mbox{TO-BE}_{f}$| with |$f \neq f^{*}$| is not a teacher-optimal selection of BE. In a one-to-one environment, |$ \mbox{TO-BE}_{f}$| is a teacher-optimal selection of BE for any |$f$| . 33
4.3.2. Characterizations
We show that the class of TO-BE mechanisms we introduce fully characterizes the strategy-proof and two-sided maximal mechanisms in two cases. First, in a many-to-one environment, we obtain a characterization when each teacher finds a single school acceptable beyond his initial assignment. While this is a very special preference structure, it can be satisfied in several natural environments. In particular, as we will further explain, our application is close to satisfying this assumption. 34 Second, in a one-to-one setting, we prove that the class of TO-BE mechanisms reduces to a singleton and coincides with BE’s unique strategy-proof selection. 35
Let us denote by |$\mathcal{P}$| the restricted domain of preferences under which each teacher finds acceptable at most one school beyond his initial assignment. A mechanism in this context is a mapping from |$\mathcal{P}$| to matchings. In the sequel, we consider an algorithm that gives the outcome set that our collections of TO-BE can achieve. The algorithm follows the same steps as TO-BE but does not refer to any collection |$f$| . Formally, along the steps, |$(t,s)$| is allowed to point to |$(t^{\prime },s^{\prime })$| if and only if |$t$| ranks |$s^{\prime }$| first in his opportunity set and |$ t^{\prime }$| has a lower priority than |$t$| at school |$s^{\prime }$| . At any step, cycles may intersect, so that the outcome of this algorithm is not uniquely defined. Here again, we do not specify how the algorithm selects cycles in the graph. Hence, we consider a correspondence TO-BE |$:\succ \rightrightarrows \mu$| where TO-BE |$(\succ )$| stands for the set of all possible outcomes of this algorithm. A selection of the TO-BE algorithm is then a mapping |$\varphi :$| |$\succ \mapsto \mu$| s.t. |$\varphi (\succ )\in$| TO-BE |$(\succ )$| .
In the restricted domain |$\mathcal{P}$| , the set of two-sided maximal and strategy-proof mechanisms coincides with all selections of TO-BE. 36
The proof can be found in Appendix E . ■
The intuition behind this result is simple. In essence, BE lets teachers point to schools with which they can form a blocking pair. TO-BE, however, lets teachers point to their favourite school among those with which they can block. Hence, when each teacher ranks a single school as acceptable, the two mechanisms are very similar. The domain restriction in the above statement is obviously strong, but in the teacher labour market presented in Section 5 , teachers are assigned to regions, and most teachers rank only one region acceptable beyond the one to which they are currently assigned. This result is therefore particularly relevant to the type of environment we consider.
We now present a characterization result in a one-to-one environment wherein each school is initially assigned one teacher. Note that, in the previous many-to-one setting, the orderings were used to choose which pair to point to among pairs of the form |$(t^{\prime },s^{\prime })$| and |$(t^{\prime \prime },s^{\prime })$| , i.e., pairs with different teachers assigned to the same school. In a one-to-one framework, such cases do not arise since there is only one seat per school and so the collection |$f$| is not needed. Thus, the set of TO-BE algorithms is a singleton. We refer to this unique algorithm as simply TO-BE. Theorem 4 below shows that in this setting, TO-BE is the unique selection of BE that is strategy-proof.
In a one-to-one environment, TO-BE is the unique selection of the BE algorithm that is strategy-proof.
The proof can be found in Appendix F . ■
This result shows that the teacher assignment problem is structurally similar to the college admission problem. Indeed, in the college admission problem, imposing two-sided efficiency and stability yields a large set of stable mechanisms. Some of these mechanisms favour students whilst others favour colleges. Our characterization of two-sided maximal matchings is similar (i.e. Theorem 1). We end up with a plethora of possible mechanisms, some favouring teachers and others favouring schools. In the college admission problem, imposing (one-sided) strategy-proofness produces a unique mechanism: the stable mechanism that favours students (i.e. DA). Similarly, Theorem 4 shows that, in the one-to-one teacher assignment problem, imposing the same incentive constraints generates a unique mechanism: TO-BE, which favours teachers. While the structure is very similar, the two mechanisms (DA and TO-BE) are very different, as are the underlying arguments.
4.3.3. One-sided maximality
Accounting for both teachers and schools welfare is quite conservative. In particular, some teachers may be unable to leave their positions because no other teacher with a higher priority will be willing to replace them. Accordingly, we will examine the cost that our two-sided efficiency requirement may impose on teachers welfare. When we ignore the school side, we obtain the following natural counterpart of our two-sided maximality notion.
A matching is one-sided maximal if |$\mu$| is 1-IR, the set of blocking pairs under |$\mu$| is a subset of that under |$\mu _{0}$| and there is no other matching |$\mu ^{\prime }$| such that (1) all teachers are weakly better off and some strictly better off, and (2) the set of blocking pairs under |$\mu ^{\prime }$| is a subset of that under |$\mu$| .
We now turn to the characterization of one-sided maximality. As we did for two-sided maximality, we introduce a class of mechanisms with possible outcomes spanning the whole set of one-sided maximal matchings. With two-sided maximality, the underlying criteria targeted by the designer are teacher welfare, school welfare, and the set of blocking pairs. In contrast, with one-sided maximality, the designer only targets teacher welfare and the set of blocking pairs. The basic idea behind the mechanism described in this section is as follows: under the BE algorithm, two teachers can exchange their assignments if and only if they justifiably envy each other. However, one can imagine a pair of teachers |$t$| and |$t^{\prime }$| who each desire the other’s school – say |$s$| and |$s^{\prime }$| , respectively—and, while school |$s$| does not necessarily rank |$t^{\prime }$| above |$t$| , it does rank first |$t^{\prime }$| among the individuals who desire |$s$| . 37 Similarly, if |$s^{\prime }$| ranks |$t$| first among the individuals who desire |$s^{\prime }$| , then an exchange between |$t$| and |$ t^{\prime }$| increases the teachers welfare and shrinks the set of blocking pairs. Hence, based on a similar idea, we will weaken the definition of the pointing behaviour in the directed graph defined in BE in such a way that—although schools may become worse off—both teachers welfare increases and the set of blocking pairs shrinks each time we carry out a cycle. The following algorithm, named one-sided BE (1S-BE for short), accomplishes this weakening, and Proposition 3 below shows how this is the best weakening one can hope to achieve.
Step |$k\geq 1:$| Given |$\mu (k-1)$| , let the teachers and their assignments stand for the vertices of a directed graph in which, for each pair of nodes |$(t,s)$| and |$(t^{\prime },s^{\prime })$| , there is an edge |$(t,s)\longrightarrow (t^{\prime },s^{\prime })$| if and only if either (1) teacher |$t$| has justified envy toward |$t^{\prime}$| at |$s^{\prime}$| or (2) |$t$| desires |$s^{\prime }$| and |$t$| is ranked higher by |$s^{\prime }$| than each teacher who also desires |$s^{\prime }$| and does not block with |$s^{\prime }.$| 38 |$^{,}$| 39 If there is no cycle, then set |$\mu (k-1)$| as the outcome of the algorithm. Otherwise, select a cycle in this directed graph. For each edge |$(t,s)\longrightarrow (t^{\prime },s^{\prime })$| in the cycle, assign teacher |$t$| to school |$s^{\prime }$| . Let |$\mu (k)$| be the matching so obtained. Go to step |$k+1$| .
Here, again, it is easy to verify that this algorithm converges in (finite and) polynomial time. Similar to our process for the BE algorithm, we do not specify how the algorithm should select the cycle of the directed graph, and thus this algorithm defines a class of mechanisms. Each mechanism in this class is a selection from the correspondence between preference profiles and matchings that corresponds to the whole set of possible outcomes that can be achieved by the 1S-BE algorithm.
By construction, starting from |$\mu (k-1)$| , the directed graph defined above is a supergraph of the directed graph that would have been built under the BE algorithm. Hence, there will be more cycles in our graph and more possibilities for improving teachers welfare and shrinking the set of blocking pairs. This reflects the fact that we dropped the constraint that schools welfare must increase along the algorithm, so that more can be achieved in terms of teachers welfare and reduction of justified envy. This is illustrated in the following example.
This example has a similar feature as Example 1: |$ t_{4}$| is the best teacher and is matched to the worst school. Thus, we know that, in that case, |$\mbox{DA}^{\ast }$| coincides with the initial assignment. We have six blocking pairs: |$(t_{1},s_{2})$| , |$(t_{2},s_{1})$| , |$ (t_{3},s_{2})$| , and |$(t_{4},s_{k})$| for |$k=1,2,3$| which determine the graph of BE. There is one cycle in this graph |$(t_{1},s_{1})\leftrightarrows (t_{2},s_{2})$| . The graph of 1S-BE contains the edges of the graph of BE but now has two new additional edges. Indeed, |$t_{1}$| and |$t_{2}$| both desire |$s_{3}$| but do not block with it under |$\mu _{0}$| , and |$t_{2}$| is preferred to |$ t_{1}$| at |$s_{3}$| ; thus, the node |$(t_{2},s_{2})$| can point to |$ (t_{3},s_{3})$| . Since |$t_{3}$| is the only one who desires |$s_{1}$| and does not block with it, |$(t_{3},s_{3})$| can point to |$(t_{1},s_{1})$| . Therefore, the graph of 1S-BE is as follows:
There are now only three blocking pairs: |$(t_{4},s_{k})$| for |$k=1,2,3$| .

We now turn to our characterization result. We note that, while the argument in the proof of Proposition 2 is simple, the proof of the characterization result below is non-trivial.
Fix a preference profile. The set of possible outcomes of the 1S-BE algorithm coincides with the set of one-sided maximal matchings.
The proof is relegated to Appendix G . ■
This result also provides a computationally simple procedure to find one-sided maximal matchings. As for the BE algorithm, we can easily construct selections of the 1S-BE algorithm that are not strategy-proof. However, while there are strategy-proof selections of BE, we show that there is no strategy-proof selection of the 1S-BE algorithm.
There is no strategy-proof selection of the 1S-BE algorithm.
The proof can be found in Appendix H . ■
This result highlights an important difference between the classes of two-sided and one-sided maximal mechanisms. In contrast to the graph of BE, the graph of 1S-BE can have an edge |$(t,s)\longrightarrow (t^{\prime },s^{\prime })$| if |$t$| desires |$s^{\prime }$| and |$t$| is ranked first by |$s^{\prime }$| among teachers who both desire |$s^{\prime }$| and do not block with |$s^{\prime }$| . Because of this condition, a teacher can modify the pointing behaviour of others: indeed, if |$t$| is ranked first by |$s^{\prime }$| among teachers who both desire |$s^{\prime }$| and do not block with |$s^{\prime }$| , then teacher |$ t$| can change other teachers’ set of outgoing edges depending on whether he claims to desire |$s^{\prime }$| . The argument for Theorem 5 relies on this additional feature. Consider that, for each possible cycle selection under the 1S-BE algorithm, one teacher can profitably misreport his preferences. Two manipulations are used in that case: one is basic and consists of ranking as acceptable an unacceptable school in order to be able, once matched with it, to exchange it for a better one. However, for some cycle selection, another manipulation is needed whereby a teacher ranks as unacceptable an acceptable school in order to expand other teachers’ sets of outgoing edges. Again, this new type of manipulation is central to the argument in Theorem 5 and is not available under the BE algorithm.
4.3.4. Large markets
Theorem 1 states that DA |$^{\ast }$| is not two-sided maximal while BE and TO-BE are. As mentioned above, these results essentially show that DA |$^{\ast }$| is not on the Pareto frontier (when both teachers and schools are welfare-relevant entities), while selections of BE and TO-BE are. This theoretical finding raises a new set of questions concerning both the magnitude of DA |$^{\ast }$| ’s underperformance and the performance of the selections of the BE algorithm, in particular TO-BE. Our main goal is to answer these questions by conducting an empirical analysis (Section 5 ); in the current section, we also provide some theoretical answers by adopting a large-market approach.
For simplicity, we assume we are in a one-to-one setting. However, all our results hold in a many-to-one setting as long as the number of school seats has an upper bound that does not grow with the number |$n$| of students or at least does not grow too quickly.
Note that schools preferences are based only on idiosyncratic shocks and not on common values. We discuss the possibility to relax this assumption in Remark 2 below. Finally, the initial assignment |$\mu _{0}$| is selected at random among all possible |$n!$| matchings, where |$n:=\left\vert T\right\vert =\left\vert S\right\vert$| . A random environment is thus characterized by the number of tiers, their size, common values, and utility functions for teachers and schools: |$[K,\left\{ x_{k}\right\} _{k=1}^{K},\left\{ u_{k}\right\} _{k=1}^{K},U,V]$| . The maximum normalized sum of teachers payoffs that can be achieved in this society is |$\bar{U}_{T}:=\displaystyle \sum_{k=1}^{K}x_{k}U\left( u_{k},1\right)$| , which is attained if all teachers are matched to schools with which they enjoy the highest possible idiosyncratic payoff. The maximum normalized sum of schools payoffs that can be achieved in this society is |$\bar{V}_{S}:=V(1)$| , which is attained if all schools are matched to teachers with whom they enjoy the highest possible idiosyncratic payoff. Clearly, in our environment, in which preferences are drawn randomly, a mechanism can be seen as a random variable. In the sequel, we let |$\varphi (t)$| be the random assignment that teacher |$t$| obtains under mechanism |$\varphi$| .
The following results show that the comparison between DA |$^{\ast }$| and TO-BE in Section 4.2 can be strengthened in this large market environment. The proofs of these results are relegated to Appendix I .
DA |$^{\ast }$| does not maximize movement, and thus is not asymptotically teacher-efficient, asymptotically school-efficient, or asymptotically stable;
Each selection of BE asymptotically maximizes movement. There is a selection of BE that is asymptotically teacher-efficient, asymptotically school-efficient, and asymptotically stable;
TO-BE is asymptotically teacher-efficient. TO-BE is neither asymptotically school-efficient nor asymptotically stable.
The proof of point i. exploits the idea of Example 1: with non-vanishing probability, if a teacher |$t$| initially assigned a top tier school applies to a school in that tier other than his initial assignment, some teacher in the lower tiers will be preferred by that school (like teacher |$t^{\ast }$| in Example 1). Hence, under DA |$^{\ast }$| , teacher |$t$| will not be able to access that school. This simple argument implies that, among teachers initially assigned to schools in tier |$1$| , the expected fraction of teachers staying at their initial assignments is bounded away from |$0$| . Point ii. confirms that this issue does not occur with any selection of BE. The novelty of Theorem 6 though is that TO-BE can fail to be asymptotically stable even though some selections of BE are. Since, as we have already proved, in our one-to-one environment, TO-BE is the unique selection of BE which is strategy-proof, we interpret this as a cost of strategy-proofness. 43 Where does this cost come from? As we have already noted, while the BE algorithm treats teachers and schools symmetrically, TO-BE favours teachers at the expense of schools. Indeed, TO-BE only ensures that schools are assigned a teacher they weakly prefer over their initial assignment. Hence, each school’s assignment under TO-BE is a random draw within the set of teachers it finds acceptable. Given the school’s idiosyncratic payoff for its initial assignment |$\eta _{\mu _{0}(s)s}$| , the expected idiosyncratic payoff for a school |$s$| under TO-BE |$ (s)$| is |$\mathbb{E}\left[ \eta _{st}\left\vert \eta _{st}\geq \eta _{\mu _{0}(s)s}\right. \right] =\frac{1}{2}\left( 1+\eta _{\mu _{0}(s)s}\right)$| . Thus, the (unconditional) expected idiosyncratic payoff of school |$s$| under TO-BE |$(s)$| is |$\mathbb{E}\left[ \frac{1}{2}\left( 1+\eta _{\mu _{0}(s)s}\right) \right] =\frac{3}{4}$| , therefore TO-BE cannot be asymptotically school-efficient or asymptotically stable.
Let us recall that TTC, as defined in Shapley and Scarf (1974) , does not take into account the school side at all, that is, the outcome achieved by TTC does not depend on the realization of the collection |$\{\eta _{ts}\}_{t,s}$| . This simple observation implies that each school’s assignment under TTC is a random draw within the whole set of teachers. Thus, the expected idiosyncratic payoff of a school |$s$| under TTC is 1/2 which is 2/3 of what is achieved under TO-BE. Because of this, one can prove that the expected fraction of blocking pairs under TTC is always larger than under TO-BE. 44
Schools preferences could be drawn in a similar way as students preferences (allowing tiers). The only issue when introducing richer schools preferences is that asymptotic stability and individual rationality become incompatible. To understand this, consider two tiers |$S_{1}$| and |$S_{2}$| for schools, each of cardinality |$n/2$| , and two tiers for teachers |$T_{1}$| and |$T_{2}$| , each of size |$n/2$| . Further assume that common values are chosen in such a way that all schools (respectively teachers) in the first tier are preferred by all teachers (respectively schools) to schools (respectively teachers) in the second tier irrespective of the realizations of the idiosyncratic shocks. Given that the initial assignment is drawn at random, with a probability which is bounded away from |$0$| , the fraction of teachers in the second tier initially matched to a school in the first tier is bounded away from |$0$| . This implies that at any individually rational matching, with probability bounded away from |$0$| , there is a non-vanishing fraction of teachers from the first tier matched to schools in the second tier and a non-vanishing fraction of teachers in the second tier matched to schools in the first tier. Thus, with probability bounded away from |$0$| , we will have a non-vanishing fraction of blocking pairs. Therefore, the second part of point ii. of Theorem 6 would not extend to this richer environment. We conjecture, however, that there is a selection of BE which is asymptotically teacher-efficient and has an expected fraction of blocking pairs strictly smaller than that under TO-BE.
This empirical section aims at estimating how much the mechanisms we suggest would change assignments in a real teacher assignment problem. We start by motivating our focus on region assignment and providing a brief presentation of the dataset. We then estimate teachers preferences over regions and run counterfactual scenarios for our mechanisms to measure the extent of the improvements they may yield.
5.1. Focus on region assignment
Teachers are assigned to schools in two successive phases. In the first phase, they can apply to a region if they want to transfer from their current region to another one. In case they participated in the first phase, teachers have to apply in the second phase to a school within the region they obtained in the first phase. If they did not participate in the first phase, teachers can also apply to a school within the region they are currently assigned to. We estimate teachers preferences over regions to predict the matching outcome of the first phase when we change the algorithm used in that phase. Of course, one may wonder if preferences over regions are well-defined objects since what matters for teachers is their assigned school within a region. Note that there is no limit on the size of the list teachers can submit in the first phase. One simplistic assumption is that teachers have preferences over schools that are lexicographic: what matters foremost for them is the region where the school is located and then the school within that region. Some evidence supports this assumption. First, regions are quite large (there are only 31 of them in France) and tenured teachers are very picky on their region choice: 78 |$\%$| of them rank a single region, and many of them simply ask for a transfer to the region where they grew up (46 |$\%$| ) or to the region where their family is located (30 |$\%$| ). This may suggest that getting closer to a teacher’s hometown or family members is of first-order importance in many teachers preferences over regions while the exact school obtained within the region may only be of second order.
Of course, we do not have definitive evidence that preferences are lexicographic. However, if teachers’ preferences are not lexicographic, their preferences over regions may still be well-defined by backward induction, meaning that teachers assess their school assignment probabilities in the second phase and then use these probabilities to form their preferences over regions. 45 In that case, it is important that teachers’ assessments of their school assignment probabilities do not vary too much when we run counterfactuals so that teachers’ preferences over regions remain the same. This assumption is relatively plausible for several reasons. First, our counterfactual exercises only change the assignment mechanism in the first phase (not in the second). In addition, changes of algorithms in the first phase only marginally impact the pool of participants in the second phase. This is because (1) there are significantly more participants in the second phase than in the first phase (about three times more) and (2) in the first phase, the distribution of teachers’ priorities assigned to each region only marginally changes when we change mechanism. 46 This suggests that a change of mechanism from DA* to TO-BE in the first phase would only marginally change competition across teachers in the second phase.
We use data on teacher assignment to regions in 2013 including (1) teachers reported preferences, (2) regions priorities, (3) each teacher’s initial assignment (if any), and (4) regions’ vacant positions. We also use data on teachers and regions characteristics to estimate teachers preferences ( DEPP, 2013 , 2014 ). We keep teachers in the nine largest subjects, such as sports, literature, or maths. 47 Smaller subjects often yield estimates that are too unprecisely estimated and we were often unable to estimate region fixed effects because no teacher is initially assigned one of the 31 regions. In addition, we suppress all teachers who do not have an initial assignment (newly tenured) and all empty seats in regions. 48 Hence, in our market, each teacher is initially assigned to a region, and each seat is initially assigned to a teacher. We also remove couples from our sample. Spouses in two different subjects can submit joint mobility applications (by submitting two identical lists), which creates dependencies between the markets. As is well-known the presence of couples can create additional complications, and we ignore this issue. After ignoring 1,042 spouses, our final sample contains 6,302 teachers.
Table 1 reports descriptive statistics on teachers, their initial assignment, and the region they rank first. Appendix J.1 provides a description of each variable. The share of female teachers varies substantially across subjects (from 15.1 |$\%$| in technology to 84 |$\%$| in English). Compared to the region they are initially assigned to, teachers’ first-choice regions tend to have students from more privileged backgrounds and students of higher ability (as measured by the share of students who pass the national exam at the end of middle school, called DNB). Teachers’ first-choice regions also tend to be much more likely to be the region in which they were born, a region situated in the South of France, a region where the share of private schools is higher, and where the share of inexperienced teachers is lower. Conversely, compared to the region they are initially assigned, teachers’ first-choice regions are significantly less likely to have a large share of students enrolled in a priority-education school which is the label given to the most disadvantaged schools. Finally, while a large share of the teachers who submit a mobility request come from the two most unattractive regions of Créteil or Versailles (70.6 |$\%$| in Sports and 37.7 |$\%$| in French for instance), a very small share of the teachers rank these two regions first (1 |$\%$| in Sports and 2.8 |$\%$| in French).
Descriptive statistics for teachers and regions
Notes: This table reports descriptive statistics for teachers and regions in each of the nine subjects we use for the demand estimations, i.e., from column (1) to column (9): sports (SPO), literature (LIT), English (ENG), mathematics (MAT), Spanish (SPA), history and geography (HG), biology (BIO), physics (PHY), and technology (TEC). Statistics are reported for the sample of teachers we use for the demand estimations. These are teachers with an initial assignment. We omit teachers who submit a joint list with their partner, teachers who are from one of the five regions that are overseas, and teachers for whom one of the individual characteristics is missing. The last row reports the number of teachers in each subject. Panels A, B, and C, respectively present descriptive statistics on teachers, on the region in which they are initially assigned, and on the region they rank first.
5.3. Estimation of teachers’ preferences
5.3.1. identifying assumptions..
We considered two identifying approaches to estimate our parameters. First, given that preferences over regions are well-defined and the mechanism used to assign teachers to regions (DA |$^{\ast }$| ) is strategy-proof, we assume that teachers report their preferences sincerely. 49 Because teachers’ choices are ranked ordered lists and thanks to the functional form assumptions, one can get closed form solutions for teachers’ choice probabilities (e.g. Hausman and Ruud, 1987 ) and estimate the parameters by maximum likelihood. This has been a standard approach in the literature so far (e.g. Abdulkadiroğlu et al. , 2017 ).
The second approach relaxes the truthtelling assumption. In our context where French teachers have reasonably accurate information on their acceptance probabilities in each region, one may be concerned that teachers simply do not rank regions where their chances to be accepted are too low. 50 This behaviour would bias the estimated parameters when assuming that teachers are truthful. To address this potential bias, we use a second estimation method recently developed by Fack et al. (2019) . 51 To describe the approach, let us define the feasible set of each teacher as the set of regions that have a cutoff—that is, the lowest priority of the teachers assigned to a region—smaller than his own score. These are regions a teacher could be assigned to if he was ranking the region first in his rank order list. The new identifying assumption is that, for each teacher, the region obtained is his most preferred region among all regions that are in his feasible set. This assumption is theoretically founded: Artemov, Che and He (2019) show that, in a large market environment, any (regular) equilibrium outcome of DA |$^{\ast }$| must have this property. Hence, we have a discrete choice model with personalized choice sets. Here again, choice probabilities have closed form solutions and we estimate parameters using maximum likelihood.
We performed two tests and the results lead us to use the second approach for our analysis. First, we run the statistical test based on Hausman (1978) and proposed by Fack et al. (2019) to test truthtelling (i.e. the first identifying assumption). The test strongly rejects truthtelling in each of the nine subjects we consider. This test is a joint test of the truthtelling assumption and the functional form assumptions, so we provide additional evidence by testing the goodness of fit of the estimated model. To do so, we look at the top two schools that a teacher has included in his submitted rank order list (ROL), and we compute the probability of observing this particular preference ordering in the ROL predicted with our estimations, under both the truthtelling and stability assumptions. In the latter, in the nine subjects in which we run the estimations, the ordering of teachers’ top two choices has a mean predicted probability of 66 |$\%$| (ranging from 63 |$\%$| in technology to 72 |$\%$| in Spanish). On average, that measure of goodness of fit is 25 percentage points higher when using the stability-based estimates (second assumption) than when using the truthtelling assumption (first assumption). Based on these results, we only use the second identifying assumption for the estimations and counterfactuals. We also present in the Supplementary Appendix S.3 additional fit measures for subgroups of teachers as well as out-of-sample fit measures that compare the characteristics of the regions that teachers rank.
We report preference estimates for French, math, and English teachers in Table 2 and additional sets of estimates for teachers in other subjects in Tables A.1 and A.2 . Supplementary Appendix S.2 provides a detailed description of the variables and final specification we use for preference estimations. The first nine rows of Table 2 report coefficients for a selected set of region fixed effects. The bottom part of the table reports estimates for region–teacher interaction terms (the first term of the interaction always refers to regions characteristics and the second term to teachers characteristics). Although our goal is mainly to use these estimates for counterfactuals rather than to interpret the sign and magnitude of each variable, there are several interesting patterns in Table 2 . First, we find very negative fixed effects for the three regions of Créteil, Versailles, and Amiens (relative to Paris) that are well known for being disadvantaged and unattractive. Second, teachers have a strong preference for the region in which they were born, which is consistent with the fact that 46 |$\%$| of them rank their birth region first. Third, teachers dislike regions that are further away from their initial region. The results finally suggest that birth regions are significantly less attractive when they gather a large fraction of young teachers.
Teachers preference estimates
Notes: This table reports selected coefficients from estimations of teachers preferences for regions characteristics based on Equation 5.1 . We use the estimation method recently developed by Fack et al. (2019) . We use a discrete choice model with personalized feasible choice sets. For each teacher, his feasible choice set is the set of regions that have a cutoff smaller than his own score. We estimate parameters in columns 1, 3, and 5 using maximum likelihood. We set the fixed effect of the Paris region to |$0$| . The last row reports our goodness of fit measure, that we compute by looking at the top two regions that a teacher has included in his submitted rank order list (ROL). We measure, for each teacher, the probability of observing this particular preference ordering in the ROL predicted with our estimations. We then average these probabilities across teachers. Stars correspond to the following p -values: * |$p<.05$| ; ** |$p<.01$| ; *** |$p<.001$| .
5.4. Counterfactual results
5.4.1. methodology and preliminary results.
We use our estimates of teachers preferences to draw their rank ordered list 5,000 times using equation ( 5.1 ). After having drawn preferences, we keep the entire set of regions without imposing any truncation. 52 In each of the nine subjects and for each preference draw, we use these simulated preferences and the priorities from our data to run |$\mbox{DA}^{*}$| , BE, TO-BE, and 1S-BE. 53 |$^{,}$| 54 All the results we report in this section correspond to averages over the 5,000 preference draws and nine subjects. 55 This empirical section aims at testing our theoretical results. We therefore focus on three main outcomes: teachers welfare, regions welfare, and number of blocking pairs. We start by reporting a few preliminary results.
(i) Under the regular |$\mbox{DA}$| mechanism, individual rationality is violated for 1,413 teachers, that is, they are assigned to a region that they consider worse than their initial region. 1,433 teachers obtain a region that they prefer to their initial one.
(ii) The individually rational mechanism that maximizes movement allows on average 1,817 teachers to move from their initial assignment, that is, 29 |$\%$| of the teachers. 56
This fact confirms that the regular DA mechanism is not individually rational, but most importantly it shows that the violation of individual rationality is quite strong. The second point shows that there is congestion on the markets: if we focus only on individually rational matchings and attempt to ensure as much movement as possible, only 29 |$\%$| of teachers will be able to move. We should bear in mind this upper bound when considering the performance of our algorithms and the scope of their improvement. The relatively small fraction of teachers able to move is explained primarily by the high proportion of teachers reporting few acceptable regions (i.e. regions ranked ahead of the region they are currently assigned to) combined with correlation in their preferences. Under the estimated preferences, half of the teachers rank only 6 regions (out of 31) above their initial region. 57
5.4.2. The inefficiency of |$\mbox{DA}^{\ast }$| and its cause
Our theoretical results show that |$\mbox{DA}^{\ast }$| is not two-sided maximal. To assess how far |$\mbox{DA}^{\ast }$| is from the two-sided efficiency frontier, we run the TO-BE algorithm starting from the matching obtained by |$\mbox{DA}^{\ast}$| . We call the resulting mechanism TO-BE |$\circ \mbox{DA}^{\ast }$| . Running TO-BE on top of |$\mbox{DA}^{\ast}$| guarantees that we obtain a matching that dominates that of |$\mbox{DA}^{\ast}$| in terms of both teacher and region welfare. Every time the assignment under TO-BE |$\circ \mbox{DA}^{\ast }$| differs from that under |$\mbox{DA}^{\ast }$| , |$\mbox{DA}^{\ast }$| is not two-sided maximal. A first striking result is that |$\mbox{DA}^{\ast }$| is noticeably inefficient.
|$\mbox{DA}^{\ast }$| is never two-sided maximal. 58 TO-BE |$\circ \mbox{DA}^{\ast }$| matches four times more teachers (953.3) than |$\mbox{DA}^{\ast }$| (237.9).
This large difference is statistically significant at the 1 |$\%$| level. Next, we investigate the origin of |$\mbox{DA}^{\ast }$| inefficiency. Our Example 1 suggests a possible explanation: some teachers initially assigned to unpopular regions do not get into some of the regions they ranked (for instance region A and B), and therefore stay in their initial region. Because some of these teachers have a relatively high priority, they block the exchanges of seats for other teachers (between region A and B), and therefore limit the overall mobility. We check the role played by this mechanism in our data by keeping track of the cycles implemented when running the TO-BE mechanism on top of the assignment we get under |$\mbox{DA}^{\ast }$| . We identify all cycles implemented at some step of the TO-BE |$\circ \mbox{DA}^{\ast }$| mechanism and check how many times each cycle is blocked by a teacher who is prevented from moving away from Créteil or Versailles under |$\mbox{DA}^{\ast}$| (the two most unattractive regions in France). 59
This exercise confirms that |$\mbox{DA}^{\ast}$| ’s inefficiency is largely driven by our stylized example. Non-movers from unattractive regions can have a harmful effect on mobility by blocking a large number of cycles exactly like in Example 1. On average, 99.5 |$\%$| of the cycles of |$\mbox{TO-BE} \circ \mbox{DA}^{\ast }$| are blocked by teachers initially assigned in Créteil or Versailles. Put differently, from the assignment given by |$\mbox{DA}^{\ast}$| , almost all exchanges which would make both sides of the markets better-off are not realized because some high priority teachers stuck in a disadvantaged region block the exchange. 60 These figures explain the under-performance of |$\mbox{DA}^{\ast}$| but also the magnitudes of improvement we report for our alternative mechanisms in the next sections.
5.4.3. Performance of the TO-BE mechanism
We now turn to discussing the performance of the TO-BE mechanism. Before commenting on the results, it is worth briefly discussing why comparing TO-BE to |$\mbox{DA}^{\ast }$| is relevant. We should bear in mind that, for an arbitrary outcome of the BE mechanism (in particular the outcome of TO-BE), its set of blocking pairs may differ from that of |$\mbox{DA}^{\ast }$| , and similarly, the outcome may not 2-Pareto dominate |$\mbox{DA}^{\ast }$| . Nevertheless, the comparison remains interesting for two reasons. First, we know from the above results that |$\mbox{DA}^{\ast }$| is far from being two-sided maximal, so that BE and TO-BE, which are two-sided maximal, can be expected to perform much better in terms of welfare and fairness. Second, our large market theoretical results (Theorem 6) suggest that BE and TO-BE perform better than |$\mbox{DA}^{\ast }$| in these two dimensions. In particular, it suggests that the welfare of teachers should be much higher under TO-BE than under |$\mbox{DA}^{\ast }$| in terms of the average ranks of the region teachers obtain. This is indeed consistent with the following empirical finding.
Teachers welfare. We use two measures of teacher welfare: the number of teachers who move under each mechanism and the rank of the region a teacher obtains. TO-BE performs significantly better than |$\mbox{DA}^{\ast}$| in both dimensions, as reported in Table 3 . While this is not implied by our theoretical results, we observe the following fact: 61
Teacher welfare
Notes: This table presents the cumulative distribution of teachers who obtain region rank |$k$| under their initial assignment in column 1, under |$\mbox{DA}^{\ast}$| in column 2, TO-BE in column 3, BE in column 4, and 1S-BE in column 5. The data come from our simulations of the French teacher assignments to regions in 2013. The standard errors reported in parentheses account for simulation errors but not for estimation errors in the coefficients.
The distribution of ranks that teachers obtain under TO-BE first-order stochastically dominates the distribution under |$\mbox{DA}^{\ast}$| , up to rank 23 (out of 25). 62 652.5 additional teachers move under TO-BE compared to |$\mbox{DA}^{\ast}$| , which corresponds to an increase of 274.92 |$\%$| . The number of teachers who obtain their most preferred region is also 53.51 |$\%$| larger under TO-BE than under |$\mbox{DA}^{\ast}$| .
We perform a test to check the statistical significance of the stochastic dominance up to a given rank |$K$| . Note that the number of teachers who obtain rank |$k$| is not independent of the number of teachers who obtain rank |$k+1$| , so we cannot directly use the standard errors reported in Table 3 to perform a test. Hence, we proceed as follows. For each of the 5,000 preference draws, we compute the rank distribution for each mechanism. Then, for each pair of mechanisms, we compare the two cumulative rank distributions by computing, for each rank |$k$| , the difference in the number of teachers assigned rank |$k$| or lower. We keep the minimal distance over ranks |$k=1,...,K$| . Repeating this 5,000 times gives us 5,000 draws of an i.i.d. variable. Using its standard error, we perform a t-test of whether the variable is positive, that is, whether one distribution stochastically dominates the other up to rank |$K$| .
Up to rank 23, the rank distribution under TO-BE significantly dominates that under |$\mbox{DA}^{\ast}$| at the 1 |$\%$| significance level. Finally, note that there is no 2-Pareto domination between TO-BE and |$\mbox{DA}^{\ast}$| . In particular, some teachers may prefer the latter assignment. 63
We investigate what drives the magnitudes of reassignments under TO-BE. First, quite intuitively, the larger the number of applicants to a region, the larger the number of teachers who can leave that region as the latter can be replaced. Figure A.5 shows a strong correlation between the ratio of entering over exiting requests in a region and the share of teachers initially assigned that region who obtain a new assignment. The priority of the teachers requesting to move is also likely to determine the performance of the TO-BE mechanism. Figure A.5 confirms that several regions with similar entering over exiting ratios have large differences in mobility. Grenoble and Lyon, for instance, have respective mobility rates of 47 |$\%$| and 53 |$\%$| despite having the same requests ratio. That difference in performance seems partly driven by a large difference in the age profile, and therefore in the priority, of the teachers initially assigned to these two regions. Teachers from Grenoble have an average priority of 97 vs. 131 in Lyon, a difference equivalent to 1.3 years of experience. The good performance of TO-BE (keeping entering over exiting requests constant) usually correlates with high priority of exiting teachers. This is true when comparing Lyon and Grenoble, Dijon and Rouen, or the trio Montpellier–Toulouse–Limoges, hence suggesting that higher priorities help teachers leave their region. 64

Mobility vs. entering/exiting requests ratio.
Fairness. Recall that Theorem 6 suggests that TO-BE performs better than |$\mbox{DA}^{\ast }$| in terms of fairness. In particular, it suggests that the number of teachers with justified envy should be smaller under TO-BE than under |$\mbox{DA}^{\ast }$| . This is indeed consistent with the following empirical finding. Table 4 reports the cumulative distribution of the number of regions with which teachers block.
Notes: The upper part of this table presents the cumulative distribution of the number of regions with which teachers block w.r.t. true preferences. The data are from our simulations of the French teacher assignments to regions in 2013. Column 1 reports the cumulative distribution of the number of regions with which teachers block under their initial assignment. The following columns report the cumulative distribution of the number of regions with which teachers block under |$\mbox{DA}^{\ast}$| , TO-BE, BE, and 1S-BE. The standard errors reported in parentheses account for simulation errors but not for estimation errors in the coefficients.
The distributions of the number of regions teachers can block with under TO-BE stochastically dominates that under |$\mbox{DA}^{\ast }$| up to rank 23 (out of 25). The number of teachers who are not part of a blocking pair increases from 1,075 under |$\mbox{DA}^{\ast }$| to 1,442 under TO-BE which represents an increase of 34.1 |$\%$| .
Here again, the dominance is statistically significant at the 1 |$\%$| level up to rank 23. As for teachers welfare, some teachers may block with a region under BE or TO-BE but not under |$\mbox{DA}^{\ast }$| (and, of course, the other way around).
Regions welfare. Comparing regions welfare across mechanisms is of particular interest, as we know that |$\mbox{DA}^{\ast }$| can harm some regions compared to their initial allocation, in contrast to the BE and TO-BE mechanisms. We start by building a measure of regions welfare by looking at the number of positions that receive a higher ranked teacher. More specifically, trying to match the theoretical definition of 2-Pareto domination, for each region, we first take the initial assignment and sort it by decreasing order of priority. We obtain a vector in which the first element/position is the teacher with the highest priority in that region at the initial assignment, the second element/position is the teacher with the second highest, and so forth. Call this vector |$\mathbf{x}$| . We perform the same operation for this region’s assignment with the mechanism under study. Let us call this vector |$\mathbf{y}$| . Finally, we say that a position |$k$| is assigned a teacher with higher (respectively lower) priority if the |$k$| th element of vector |$\mathbf{y}$| has a higher (respectively lower) priority than the |$k$| th element of vector |$\mathbf{y}$| . Based on this, we compute the percentage of net improvement in positions, that is, the percentage of positions receiving a teacher with higher priority minus the percentage of positions being assigned a teacher with lower priority. Table 5 reports the cumulative distribution of regions with a net percentage of positions of less than |$x$| |$\%$| (where |$x$| can be negative if a region has more positions receiving lower ranked teachers). By construction, TO-BE never hurts any region. In line with our Theorem 6, we also find that TO-BE performs better than |$\mbox{DA}^{\ast }$| in terms of regions welfare and find, here again, a stochastic dominance relation.
Regions’ welfare
Notes: This table presents the cumulative number of regions with a net welfare improvement (relative to their initial assignment). For each region, we compute the number of positions assigned to teachers with higher priority, from which we subtract the number of positions assigned to teachers with lower priority. Then, the net total is divided by the total number of positions to obtain the percentage of positions with net improvement. We average results over iterations. For instance, on average, under |$\mbox{DA}^{\ast}$| , 1.7 regions have more than 10 |$\%$| of their seats assigned teachers with lower priority (in net terms). The standard errors reported in parentheses account for simulation errors but not for estimation errors in the coefficients.
The distribution of regions’ welfare under TO-BE stochastically dominates the distribution under |$\mbox{DA}^{\ast}$| . 65 Under |$\mbox{DA}^{\ast}$| , on average 1.7 regions have more than 10 |$\%$| of their seats assigned to teachers with a net lower priority, while no region has seats assigned lower-priority teachers under TO-BE.
The stochastic dominance of the distribution of TO-BE over the one of |$\mbox{DA}^{\ast}$| is significant at the 1 |$\%$| level. We highlighted in the theoretical section that TO-BE bears some similarities to the TTC mechanism. 66 One main difference, however, is that TO-BE does not allow exchanges that harm regions, whereas TTC does. We verify this empirically by running TTC and find that the distribution of regions welfare under TTC is indeed stochastically dominated by the distribution of TO-BE. For instance, on average, 9.3 regions under TTC have more positions assigned to teachers with lower priority than positions assigned to higher priority teachers. This never happens under TO-BE.
Administration objectives. We motivated the two-sided efficiency notion by its better ability to fulfil the administration’s objectives, as reflected by the three main criteria defining the priority system: (i) experience in teaching, (ii) spousal reunification, and (iii) years of teaching in a disadvantaged school. For instance, under the two-sided efficiency notion, a reassignment of teachers that, ceteris paribus, decreases the number of experienced teachers in disadvantaged regions would not meet the administration’s objective to better distribute experienced teachers across regions. We look more closely at these three criteria in Table 6 .
Fulfilment of the administration’s objectives
Notes: The upper part of this table presents the share of teachers who have only one or two years of experience under |$\mbox{DA}^{\ast}$| , TO-BE, BE, and 1S-BE. We successively report statistics for all teachers post re-assignment (i.e. those who arrive and those who stay in a region), for incoming teachers only, and for exiting teachers. The lowest two rows of the table present statistics on the percentage of teachers who move closer to their spouses and who leave a disadvantaged school. The standard errors reported in parentheses account for simulation errors but not for estimation errors in the coefficients.
The first criterion gives more points to more experienced teachers. Obviously, it is not possible to increase teachers experience in all regions. However, as some regions are more disadvantaged than others, one objective is to control the share of inexperienced teachers in these regions. As discussed earlier, Créteil and Versailles are the two most unattractive regions. 67 The upper part of Table 6 reports, for both disadvantaged and non-disadvantaged regions, the percentage of teachers with only 1 or 2 years of experience under the current algorithm and the alternative mechanisms we suggest. 68 Under |$\mbox{DA}^{\ast}$| , 27.6 |$\%$| of the teachers have only one or two years of experience in non-disadvantaged regions. This rate goes up to 50.7 |$\%$| in disadvantaged regions. A first interesting result is that, under the TO-BE mechanism, the share of inexperienced teachers slightly goes down in disadvantaged regions but goes up in non-disadvantaged regions. This fulfils the policy-maker objective not to increase the share of inexperienced teachers in deprived regions. 69 Interestingly, 45 |$\%$| of the teachers who leave Créteil or Versailles under TO-BE have less than 2 years of experience, against 14.4 |$\%$| under |$\mbox{DA}^{\ast}$| . This is explained by the requirement that an exiting teacher must be replaced by a higher-priority incoming teacher under TO-BE but not under |$\mbox{DA}^{\ast}$| .
Finally, we look at performance for two additional criteria: spousal reunification and having spent more than 5 or 7 years in a disadvantaged school. The objective behind these criteria is to help teachers get closer to their spouse or reward them for having dedicated several years to disadvantaged students. The bottom part of Table 6 shows that TO-BE significantly increases the percentage of teachers who move closer to their spouses compared to |$\mbox{DA}^{\ast}$| (from 2 |$\%$| to 5.9 |$\%$| ). On the other hand, the percentage of experienced teachers who move away from disadvantaged schools goes down under TO-BE. However, it is important to keep in mind that less than 10 |$\%$| of the teachers stayed long enough in a disadvantaged school to benefit from this criterion.
5.4.4. Performance of BE and 1S-BE
We briefly comment on the results for the BE and 1S-BE algorithms. As a note of caution, selections of these algorithms are not strategy-proof while our results assume that agents truthfully report their preferences. Hence, the results reported below are useful to test some of the insights of the theory but should be taken with care given that agents may not be truthful in reality. Also, because each BE and 1S-BE defines a class of mechanisms, we need to randomly pick selections in these classes. In order to do so, for each of these algorithms BE and 1S-BE, we randomly select a sequence of cycles that leads to a matching on the two-sided and one-sided maximality frontier. Therefore, for each of these algorithms, for each preference draw, we randomly draw 100 selections. All results reported in this section correspond to averages over the 5,000 preference draws, 100 selections draws, and 9 subjects.
First, we observe that, compared with the BE algorithm, TO-BE assigns more teachers to their most-preferred region and has a teachers’ rank distribution that stochastically dominates the distribution under BE. This is natural given that TO-BE is teacher-optimal while our selections of BE may not be. Theorem 6 suggests a cost of using a strategy-proof selection of the BE algorithm such as TO-BE. In particular, based on this result, one may expect the BE algorithm to outperform TO-BE in terms of regions welfare. Our results confirm this. Table 5 shows that regions’ welfare is higher under BE than under TO-BE. 70
We now discuss the performance of 1S-BE, keeping in mind that 1S-BE ignores regions welfare constraints. Consistent with expectations, the results show an improved performance in terms of teachers’s welfare, but at the cost of a significantly worse performance in terms of regions’ welfare and administration objectives. The number of teachers who move is 40.2 |$\%$| larger under 1S-BE than under TO-BE, and 5 times larger than under |$\mbox{DA}^{\ast}$| . 71 The rank distribution under 1S-BE also stochastically dominates that under both TO-BE and |$\mbox{DA}^{\ast}$| . The number of teachers who are part of a blocking pair drops from 4,859.6 under TO-BE to 4,788.8 under 1S-BE. However, 1S-BE hurts regions. As shown in Table 5 , 5.3 regions (out of 25) have more seats receiving a lower ranked teacher than seats receiving a higher ranked one. 1S-BE also makes fewer teachers move closer to their spouses than TO-BE.
5.5. Incorporating vacant positions and newly recruited teachers
Our empirical results so far focus on markets in which all teachers have an initial position and there are no vacant seats. There are two reasons for this. First, our main theoretical results show that DA |$ ^{\ast }$| fails to implement exchanges of positions among initially assigned teachers that would benefit both sides of the market, whereas TO-BE does not have this drawback. Since this point specifically focuses on exchanges among tenured teachers, it makes more sense for the empirical application to match this environment by focusing on a market with no newcomers and no vacant seats. Second, in many markets, newly recruited teachers have a lower priority than tenured teachers. 72 In such a case, two-sided maximality implies that the assignment of newcomers and that of tenured teachers are essentially made on separate markets: we first assign tenured teachers without vacant positions and then newcomers are assigned to vacant seats. In this context, it is straightforward to define our algorithms. 73 In this section, we test the robustness of our empirical results to the introduction of vacant seats and newcomers. We explain in Appendix K how we extend TO-BE to the environment with newcomers and vacant positions, and we present the results below. Adopting the methodology of Section 5 , we estimate teachers preferences and run counterfactuals. We explain our methodology in detail in Supplementary Section S.7.4 . 74 The gains that TO-BE brings upon DA |$^{\ast }$| are mostly preserved when we keep newcomers and vacant positions. First, in every subject, DA |$^{\ast }$| is not two-sided maximal. Second, TO-BE continues to generate more movement than DA |$^{\ast }$| as shown in Table 7 .
Results with vacant positions and newly recruited teachers
Because DA |$^{\ast }$| is not two-sided maximal (and so not 2-IR), many tenured teachers leave their initial position to fill a vacant position in another region. These teachers are often replaced by newcomers with lower priority. While this type of reassignment clearly hurts regions (since newcomers have lower priorities than tenured teachers), this is also an important source of movement of teachers under DA |$^{\ast }$| , in particular, for teachers initially assigned disadvantaged regions for which demand from tenured teachers is low. 75 Because TO-BE is two-sided maximal, replacement of tenured teachers by newcomers (with lower priority) is not possible and this, to the contrary, limits movement for teachers initially assigned disadvantaged regions. Hence, to get a deeper understanding of the mobility gains, we disaggregate the results by regions types. On average, across all subjects, mobility decreases in disadvantaged regions when moving from DA |$^{\ast }$| to TO-BE (it is reduced by 36 |$\%$| in Créteil but increases by 33 |$\%$| in Versailles). For regions that are not disadvantaged, the increase in movement from DA |$^{\ast }$| to TO-BE is significant (+56 |$\%$| ). In other words, except for the disadvantaged region of Créteil, the mobility gains of switching from DA |$^{\ast }$| to TO-BE are still very significant.
While our two-sided maximality requirement clearly limits mobility in some disadvantaged regions, it achieves its goal: the fraction of inexperienced teachers in disadvantaged regions is 10 percentage points higher under DA |$^{\ast }$| than under TO-BE. This may be seen as an important advantage of TO-BE over DA |$^{\ast }$| . However, as we already pointed out, there is a trade-off between teachers mobility in disadvantaged regions and the requirement to Pareto-improve the region side upon the initial assignment. If, for a policy maker, the trade-off goes in favour of teachers mobility, that is, one is willing to allow tenured teachers to leave disadvantaged regions and be replaced by teachers with lower priority, TO-BE can easily be modified to accommodate this more permissive requirement. 76 The results of this relaxed TO-BE are reported in Table 7 . We get a significant increase in movement in non-disadvantaged but also in disadvantaged regions while the fraction of inexperienced teachers in disadvantaged regions remains essentially the same as under DA |$^{\ast }$| . 77
To conclude, our empirical results continue to hold and the above exercise reveals that the gains of TO-BE over DA |$^{\ast }$| are mainly driven by non-disadvantaged regions. If improving the region welfare is a hard constraint, DA |$^{\ast }$| violates this constraint while TO-BE does not which may lead to lower mobility in disadvantaged regions. If the constraint is soft, a simple modification of TO-BE allows movement to increase upon DA |$^{\ast }$| in both disadvantaged and non-disadvantaged regions.
Before concluding, we discuss the relationships with the existing literature.
6.1. Stability and individual rationality.
Our definition of stability follows the standard definition in the college admission literature. In that case, we know that no matching is both stable and individually rational. Given this conflict, one approach is to weaken stability to make it compatible with individual rationality. This is the path followed by Guillen and Kesten (2012) , Pereyra (2013) , and Compte and Jehiel (2008) . To understand the approach, note that two types of blocking pairs |$(t,s)$| may exist. (i) One where teacher |$t$| desires school |$s$| and has a higher priority in that school than a teacher who was initially assigned to school |$s$| and stays in that school. (ii) One where |$t$| also desires |$s$| but now has a higher priority than a teacher assigned to |$s$| but who was not initially assigned to that school. Pereyra (2013) refers to the second type of blocking pairs as inappropriate blocking pairs . In that case, |$\mbox{DA}^{\ast}$| is a mechanism with no inappropriate blocking pairs. Put differently, |$\mbox{DA}^{\ast}$| satisfies a weakening of stability. The basic idea behind this notion is that teacher |$t$| will feel no or less justified envy when the envied teacher is initially matched to school |$s$| . If one believes this is the case in reality, one may naturally be interested in such a weakening of stability. Guillen and Kesten (2012) showed that |$\mbox{DA}^{\ast}$| Pareto-dominates any other mechanism that has no inappropriate blocking pairs. So, if one only wants to forbid inappropriate blocking pairs, |$\mbox{DA}^{\ast}$| achieves the highest mobility and cannot be improved upon. However, our results highlight two important points. First, sometimes the movement of |$\mbox{DA}^{\ast}$| is only obtained by hurting the school side, that is, |$\mbox{DA}^{\ast}$| does not Pareto-dominate the initial assignment for schools, which, as we already argued, in our teacher assignment framework, is an important policy objective. Second, there is a high welfare cost to require no inappropriate blocking pairs since, under the assignment given by |$\mbox{DA}^{\ast}$| , both teachers and schools can be made better-off. This is confirmed by our theoretical and empirical findings. Hence, our work also quantifies the trade-off between efficiency and the elimination of inappropriate blocking pairs. In our counterfactual results, while the number of teachers who are part of a blocking pair in the standard sense goes from 5,295.8 under |$\mbox{DA}^{\ast}$| to 4,958.4 under TO-BE, 3,995.26 teachers are part of an inappropriate blocking pair under TO-BE and this number is, by construction, zero under |$\mbox{DA}^{\ast}$| . However, TO-BE generates 4 times more mobility than |$\mbox{DA}^{\ast}$| and dominates the latter in terms of teachers welfare, regions welfare, fairness, and administrative objectives.
6.2. Two-sided improvement cycles.
Proposition 2 provides a simple and computationally easy procedure to find two-sided maximal matchings. The idea of implementing cycles to achieve two-sided maximality is natural. Erdil and Ergin (2017) already identified a similar algorithm to characterize 2-Pareto-efficient stable matchings in two-sided matching environments where agents may not have strict preferences. 78 Our motivations, however, are very different. They aim at Pareto-improving stable assignments while we Pareto-improve on an initial assignment. As we saw in Section 4.2.2 , this difference has far reaching consequences once we deal with incentive issues. In particular, in their environment, no 2-Pareto-efficient stable matching mechanism is strategy-proof even if incentives are restricted to one side of the market as we assume (see, Erdil, 2014 ). Yet, in our environment, it is possible to find two-sided maximal matchings that are strategy-proof for teachers.
6.3. Characterization of TO-BE.
Theorem 4 is closely related to Ma (1994) and Dur and Ünver (2019) . Ma shows that in the Shapley–Scarf economy, TTC is the unique mechanism that is 1-IR, 1-PE, and strategy-proof. Intuitively, in a one-to-one setting, Theorem 4 applies to richer environments in which schools have non-trivial preferences that are taken into account when determining welfare. This suggests that Theorem 4 is a generalization of Ma’s. Indeed, to see this, note that in the specific situation in which each school ranks its initial assignment at the bottom of its ranking, TO-BE and TTC coincide. In this context, 1-IR and 2-IR are obviously equivalent. In addition, since 1-PE implies 2-PE, the class of mechanisms considered by Ma is a subset of BE algorithm’s selections. Applying Theorem 4 to these selections yields Ma’s result. While our argument builds upon that of Ma’s, there are a number of crucial differences. As mentioned above, even in the very specific environment in which each school ranks its initial assignment at the bottom of its preferences, the BE algorithm contains many other mechanisms that include, in particular, all those that are 2-PE but not 1-PE and all 1-PE mechanisms that are sensitive to schools preferences. 79 In addition, our result applies to settings in which schools preferences are arbitrary and, thus, to many other types of mechanisms that are not well defined in Ma’s environment.
6.4. Balanced exchanges.
Our article is also closely related to Dur and Ünver (2019) , who study two-sided matchings via balanced exchanges, and use tuition and worker exchanges as applications. They propose an algorithm, called the Two-Sided Top Trading Cycle (2S-TTC), that ensures that imports and exports are balanced. Further, they prove that 2S-TTC is the only mechanism that is balanced-efficient, worker strategy-proof, acceptable, individually rational, and that respects internal priorities. Although our article pertains to the same two-sided environment with initial assignment, two key features differentiate our analysis. First, the main focus of our article is the conflict between efficiency and fairness. We show that DA |$^{\ast }$| can be improved upon in these two dimensions, while our alternative mechanism cannot. This focus of our article is thus independent from Dur and Ünver (2019) ’s main purposes. Second, we differentiate ourselves from Dur and Ünver (2019) ’s setting by taking into account a richer set of schools priorities. To capture the specificities of worker-exchange programs, Dur and Ünver (2019) make assumptions about the preferences of workers and firms. They notably assume that firms do not have strong preferences over acceptable workers, who are all equally desirable for firms. This assumption of coarse preferences over incoming agents is certainly plausible in the environment of temporary worker exchange, as the cost of not being matched with the best candidate is relatively limited. However, when assignments are permanent, firms (or schools) are likely to have finer preferences over applicants. Schools know that teachers’ characteristics can vary widely, notably in terms of years of experience, experience teaching in disadvantaged schools, family situation, and so on. These characteristics are all used to define a teacher’s priority in a school. Unlike Dur and Ünver (2019) , we account for schools’ finer preferences over incoming teachers. In our context, this is particularly important when the distribution of experienced teachers differs across schools. Schools may want to maintain a balance in their teachers characteristics and experience. If an experienced teacher wishes to leave, the school may want to replace him with an equally experienced teacher.
We refer to Dur and Ünver (2019) ’s coarse preferences over acceptable and non-acceptable workers as group preferences . 80 In practice, non-grouped preferences arise quite frequently. In our dataset, for the ten largest disciplines, 53.2 |$\%$| of the applicants have an “intermediate priority,” meaning their priority is strictly higher than internal teachers’ lowest priority and strictly lower than internal teachers’ highest priority. On average, 91.3 |$\%$| of the regions receive at least one application with intermediate priority. 81
Incorporating schools fine preferences has two important consequences. First, with finer preferences, 2S-TTC is not two-sided maximal, and this mechanism could create new blocking pairs due to the possibility to recruit teachers who have lower priority than those leaving. Second, the characterization result in Dur and Ünver (2019) relies on the axiom of respect of internal priorities (see Dur and Ünver (2019) for a formal definition). In our environment, as we show in Supplementary Appendix S.5 , no mechanism is two-sided maximal, strategy-proof, and respects internal priorities; accordingly, our two approaches radically differ in a many-to-one setting.
6.5. One-sided maximality.
The 1S-BE algorithm shares some similarities with—and can indeed be seen as a generalization of—the stable improvement cycle (SIC) algorithm defined by Erdil and Ergin (2008) . The SIC algorithm is designed to improve stable outcomes whenever an outcome is not teacher-optimal, as is the case, for instance, with the outcome of the teacher-proposing DA when schools have weak preferences. Starting from a stable outcome, SIC and 1S-BE are the same. However, our mechanism extends the SIC algorithm’s properties to cases in which the starting assignment is arbitrary. To illustrate why this is true and why the SIC algorithm does not suit our purposes, consider one of our initial motivations, which is to improve the outcome of DA |$^{\ast }$| . Although both BE |$\circ$| DA |$^{\ast }$| and 1S-BE |$\circ$| DA |$^{\ast }$| successfully improve that outcome, the SIC algorithm (starting from the outcome of DA |$^{\ast }$| ) does not. Given the individual rationality of DA |$^{\ast }$| , no teacher desires his initial assignment under the matching achieved by DA |$^{\ast }$| . Therefore, the pointing behaviour associated with SIC (starting from DA |$^{\ast }$| ) remains unchanged if we use the modified schools preferences used to run DA |$ ^{\ast }$| as opposed to the true schools preferences. Under the modified preferences, by definition, DA |$^{\ast }$| yields the teacher-optimal stable matching. Hence, there cannot be any cycle in the graph associated with SIC (again, starting from DA |$^{\ast }$| ).
Last, Dur et al. (2019) also proposed a similar extension of the SIC algorithm. For each school |$s,$| they fix a set of teachers |$C(s)$| under which, if |$t \in C(s)$| then one can violate the priority of teacher |$t$| at school |$s$| . They define an algorithm which, for teachers, Pareto-improves on DA while respecting priorities of teachers outside |$C(s)$| for each school |$s$| . Their algorithm is close to the 1S-BE algorithm we propose but there is one crucial difference: in our algorithm, at each step, we want to ensure that the set of blocking pairs shrinks. In their constrained efficiency notion, they only require that the set of blocking pairs is a subset of those defined by the above sets |$(C(s))_{s}$| . More importantly, our class of mechanisms starts from an arbitrary initial assignment, while given their motivation, they are interested only in the class improving upon DA, and thus, they begin from the DA allocation. Our main message concerns the non-existence of a strategy-proof selection in our class of mechanisms. When starting from an arbitrary exogenous initial assignment (which excludes starting from the DA allocation), this result is non-trivial.
In many countries, a central administration is in charge of assigning teachers to schools. In an attempt to ensure that every teacher is assigned to a school that he weakly prefers to his current one, several countries have adopted a modified version of the well-known deferred acceptance mechanism (DA) for teacher assignment. In this article, we show that this mechanism fails to be fair and efficient for both teachers and schools. Ensuring that schools are not “harmed” by teacher reassignments is important as schools’ priorities partly reflect a social objective, notably in terms of the experience of teachers assigned to deprived schools. To address the weakness of the modified version of DA, we characterize the class of mechanisms that cannot be improved upon in terms of both efficiency and fairness, and we identify the subclass of strategy-proof mechanisms. We further test and confirm the performance of these alternative mechanisms by showing that, when the market size grows, they perform much better in terms of utilitarian efficiency and fairness. Finally, we use a rich dataset on teachers applications for transfers in France to measure the relevant gains. As our counterfactual analysis shows, the alternative mechanisms, compared to the modified version of DA, generate significant gains in efficiency, and fairness. In particular, the number of teachers moving from their initial assignments more than triples under our mechanism.
7.1. Dynamic environment.
One may argue that the teacher assignment problem is a fundamentally dynamic situation, and this is important for two reasons. First, as teachers change positions several times throughout their careers this is important if one believes that incentives to truthfully report preferences can be affected by this dynamic aspect. 82 Pereyra (2013) defined a dynamic overlapping generation model wherein newly recruited teachers arrive, are assigned to schools, and can later ask for re-assignments during a certain number of periods before exiting the market. 83 In this environment, assuming that all newly tenured teachers entering at a given date are less preferred by all schools than the tenured teachers who already have an assignment, he showed that |$\mbox{DA}^{\ast}$| is dynamically strategy-proof; that is, no teacher can ever misreport his preferences and obtain a better-school at the current or some later date. If the TO-BE algorithm is properly extended to account for newly recruited teachers and vacant positions (as done in Section 5.5 ), then, in the same setting as in Pereyra (2013) , one can show that TO-BE is also dynamically strategy-proof.
The second reason why dynamics is important is because, each year, the initial assignment is defined by the previous year assignment. In our context, this means that the initial assignment in our data is very inefficient because under |$\mbox{DA}^{\ast}$| many teachers are unable to move and, as we showed, important two-sided Pareto improvements are possible. However, once TO-BE is used, this source of inefficiency will be removed in the subsequent years. While there will certainly be gains from using TO-BE rather than |$\mbox{DA}^{\ast}$| , the gains will naturally be smaller than what we obtained in Section 5 since the initial assignment will be less inefficient.
7.2. Teacher participation decisions.
In reality, tenured teachers have the choice of participating in the assignment scheme. While one may think that participating is a dominant strategy for teachers, in practice, this is probably more complicated. For instance, if a teacher believes her odds of moving are too low, it is likely that the teacher will decide to settle in the region where she is currently assigned to and pay some fixed cost associated with settling down (e.g. buy a house, have his family move close to him, have his partner search for a permanent job, etc...). Put differently, teachers with low chances of seeing their transfer requests satisfied will simply not participate and, probably, for several consecutive years. 84 In this context, a change of mechanism which increases mobility rates will certainly impact participation of teachers: those with a higher chance of moving may participate more while those with lower chances may participate less. Hence, a change from |$\mbox{DA}^{\ast}$| to TO-BE is likely to change the participation of teachers. While precisely estimating the changes in participation decisions is beyond the scope of the article and would further require additional data, our analysis and simulations can provide some hints on the resulting effects.
The first important element we want to point out is that (1) the number of teachers who lose from a switch of algorithm (from |$\mbox{DA}^{\ast}$| to TO-BE) is very small. We only have 116.4 teachers who lose on average, which represents 1.8 |$\%$| of the teachers. The second point we want to stress is that (2) among these teachers who lose from a switch, 88 |$\%$| simply remain in their initial region under TO-BE. This being said, considering a pessimistic scenario where we assume that all teachers losing from the switch leave the market, by point (1) above, on average, only 1.8 |$\%$| of teachers leave. One may be concerned by “propagation effects,” that is, once teachers losing leave the market some remaining teachers may now wish to leave the market. Put differently, the behaviour of those leaving the market may propagate and the market may eventually become much thinner which may eventually lead to less efficient outcomes. However, the impact of teachers leaving the market on the outcome is likely to be small since, by point (2) above, 88 |$\%$| of those leaving would have no impact on the outcome of TO-BE. This indeed holds true because removing teachers who stay at their initial assignment (together with their initial position) has no impact on the outcome of TO-BE. Hence, the likelihood that their behaviour propagates to other teachers may be small as well. On this last point, using our estimations, we randomly drew preference profiles and removed iteratively all teachers who were worse-off after the switch of algorithm. 85 This led to an average of 867.3 teachers moving under TO-BE (vs. 890.4 in Table 3 ), still three times more than under |$\mbox{DA}^{\ast}$| . 86
While we see these results as encouraging and suggesting that our results may be strengthened once endogenous participation is accounted for, we acknowledge that these are still speculative and a full analysis (and additional data) is required to fully understand how participation impacts our results. 87
7.3. Centralized and decentralized systems.
In many countries across the world, the assignment of teachers to public schools is regulated by a central administration (e.g. France, Germany, Czech Republic, Italy, Turkey, Mexico, Peru, Uruguay, and Portugal. See references given in Footnote 3.). The main concerns of the administrations in charge of designing the assignment schemes are similar across the world: typically, satisfying teachers mobility requests while taking into account the distribution of (effective) teachers ( Hanushek et al. , 2004 ; Jackson, 2009 ). Even though France may have additional specific policy objectives, our setup and algorithms are clearly flexible enough to allow for many alternative objectives. 88 Of course, more empirical work based on data from other countries may be needed, but we believe that our study has important policy implications for all these countries using a centralized assignment system by making clear what a teacher-optimal mechanism may be in this context. Finally, our work also helps envision the potential impact of transitioning from a decentralized to a centralized assignment system in other countries. More specifically, we show that adopting the modified version of DA, rather than one of the alternative mechanisms we suggest, would largely underestimate the performance of a centralized system (for instance, in terms of teacher mobility).
The editor in charge of this paper was Christian Hellwig.
The replication package for this research is available on Zenodo at https://dx.doi.org/10.5281/zenodo.5658604 .
Supplementary data are available at Review of Economic Studies online. And the replication packages are available at https://dx.doi.org/10.5281/zenodo.5658604 .
We are grateful to Christian Hellwig, the Editor, as well as to three anonymous referees for careful readings and helpful recommendations. We also thank Francis Bloch, Yeon-Koo Che, Yinghua He, Jinwoo Kim, Jacob Leshno, Parag Pathak, Juan Pereyra, and seminar participants at Columbia University, Princeton University, MIT, the London School of Economics, the Paris School of Economics, University College London and Seoul National University for their helpful comments. We owe a special gratitude to Garbielle Fack, Julien Grenet, and Yinghua He for sharing programming codes for demand estimation. This work is supported by a public grant overseen by the French National Research Agency (ANR) as part of the Investissements d’avenir program (reference : ANR-10-EQPX-17 “Centre d’accès sécurisé aux données” (CASD). Combe is grateful to Vasiliki Skreta for the support from the ERC Grant No. 682417, Frontiers in Design - Frontiers in Mechanism Design: Methodology and Applications. Terrier acknowledges support from the Walton Family Foundation under grant 2015-1641. Tercieux is grateful for the support from ANR grant SCHOOL_CHOICE (ANR-12-JSH1-0004-01).
A. Proof of Lemma 1
Consider the directed graph |$G := (N,E)$| of BE starting at the matching |$ \mu(0) = \mu$| . For each school |$s$| s.t. |$\mu^{\prime }(s) \neq \mu(s)$| , consider the sets of teachers |$T_{s} := \{ t \in T : t \in \mu(s) \backslash \mu^{\prime }(s) \}$| and |$T^{\prime }_{s} := \{ t \in T : t \in \mu^{\prime }(s)\backslash \mu(s) \}$| . Since we are in a re-assignment setting, we have that |$|T_{s}| = |T^{\prime }_{s}| := m_{s}$| for some |$m_{s} \leq q_{s}$| . Let |$\mathbf{x} := (t_{1},\dots, t_{m})$| and |$\mathbf{x^{\prime }} := (t_{1}^{\prime },\dots,t_{m}^{\prime })$| be the two ordered vectors corresponding to |$T_{s}$| and |$T^{\prime }_{s}$| , where each vector orders the teachers in each set in a decreasing order according to |$\succ_{s}$| . Since |$ \mu^{\prime }$| 2-Pareto dominates |$\mu$| , we have that |$\forall k = 1, \dots, m$| , |$t^{\prime }_{k} \succ_{s} t_{k}$| . 89 So under |$G$| , the graph of BE, we have that |$ (t_{k}^{\prime },\mu(t_{k}^{\prime }))$| points to |$(t_{k},s)$| , i.e., |$ [(t_{k}^{\prime },\mu(t_{k}^{\prime })),(t_{k},s)] \in E$| . Let |$e_{k}^{s} := [(t_{k}^{\prime },\mu(t_{k}^{\prime })),(t_{k},s)]$| . Consider the subgraph |$ G^{\prime }:= (N^{\prime },E^{\prime })$| where |$N^{\prime }:= \{ (t,\mu(t)) : \mu(t) \neq \mu^{\prime }(t) \}$| and |$E^{\prime }:= \{ e_{k}^{s} : s \in S, k = 1,\dots,m_{s} \}$| . Note that, by construction of |$G^{\prime }$| , each node |$(t,s) \in N^{\prime }$| has a unique incoming edge from another node |$ (t^{\prime },s^{\prime }) \in E^{\prime }$| and that |$s = \mu^{\prime }(t^{\prime })$| . If all graph nodes have an in-degree of exactly one, then there exists a collection of disjoint cycles that includes all graph nodes. Indeed, start from any node in |$N^{\prime }$| : call it |$n_{1}$| . Then, since |$ n_{1}$| has in-degree one, there is a unique node |$n_{2}$| s.t. |$(n_{2},n_{1}) \in E^{\prime }$| . Applying the same argument for |$n_{2}$| , there is a unique node |$n_{3}$| s.t. |$(n_{3},n_{2}) \in E^{\prime }$| . Iterating the argument, we can identify a cycle in |$G^{\prime }$| involving |$L \geq 2$| nodes of |$ N^{\prime }$| . Let |$A := \{ n_{1}, \dots, n_{L} \} \subset N^{\prime }$| be such nodes. Note that since the nodes of |$G^{\prime }$| have an in-degree of exactly one, there is no node |$n \in N^{\prime }\backslash A$| that points to a node in |$A$| since otherwise, it would imply that a node in |$A$| has an in-degree of at least two. So if one deletes nodes in |$A$| together with their edges, the resulting subgraph of |$G^{\prime }$| will still have edges with an in-degree of exactly one, and we can iterate the argument to find a new cycle in this subgraph, and so on. This process will lead to a collection of disjoint cycles in |$G^{\prime }$| that involve all the nodes in |$N^{\prime }$| . To conclude the proof, note that all these disjoint cycles are actual cycles of |$G$| , the original graph of the BE algorithm starting at |$\mu$| , and that each teacher matched using these cycles is assigned his school under |$\mu^{\prime }$| . By implementing these cycles under the BE algorithm, then, one indeed goes from |$\mu$| to |$\mu^{\prime }$| .
B. Relationship between TO-BE and TTC
The TO-BE algorithm shares some similarities with the (Shapley–Scarf) TTC mechanism. TTC is well-defined only in a one-to-one environment (i.e. when |$ q_s=1$| for each school |$s$| ). But even in this environment, TTC and TO-BE are different. Indeed, TTC operates in the same manner as the TO-BE algorithm but does not refer to schools preferences/priorities: an edge |$ (t,s)\longrightarrow (t^{\prime },s^{\prime })$| is added if and only if teacher |$t$| ranks school |$s^{\prime }$| first within the set of all remaining schools (i.e. at step |$k$| , those are the schools in |$S(k-1)$| ). The other additional feature is that TO-BE is defined in a many-to-one setting while TTC is defined in a one-to-one environment.
Yet, TTC and TO-BE can be formally related. Starting from our many-to-one environment, one can construct a one-to-one environment in which the outcome of TTC will give us the outcome of TO-BE. To see this, fix a preference profile and a collection |$f$| and let us build this one-to-one environment. We assume that each teacher |$t$| is “endowed” with |$(t,\mu_0(t))$| , which we refer to as an object. Each teacher |$t$| has preferences over possible objects in |$\{(t^{\prime },\mu_0(t^{\prime }))\}_{t^{\prime }\in T}$| that are given as follows. Teacher |$t$| finds unacceptable any object |$(t^{\prime },s^{\prime })$| for which |$t^{\prime }\succ_{s^{\prime }} t$| while any other object is acceptable. For any pair of objects |$(t^{\prime },s^{\prime })$| and |$(t^{\prime \prime },s^{\prime \prime })$| that are acceptable to |$t$| , we consider two cases. First, if |$s^{\prime }\neq s^{\prime \prime }$| then |$ (t^{\prime },s^{\prime })$| is preferred to |$(t^{\prime \prime },s^{\prime \prime })$| if and only if |$s^{\prime }\succ_{t} s^{\prime \prime }$| . Second, if |$s^{\prime }= s^{\prime \prime }$| then |$(t^{\prime },s^{\prime })$| is preferred to |$(t^{\prime \prime },s^{\prime \prime })$| if and only if |$ f_{s^{\prime }}(t^{\prime }) < f_{s^{\prime }}(t^{\prime \prime })$| . In the sequel, if |$\succ_t$| denotes the original preferences of teacher |$t$| , we let |$\succ^{\prime }_t$| be his preferences in this modified environment. In this one-to-one environment with strict preferences, standard TTC is well-defined, and it is easily checked that teacher |$t$| is matched to an object |$(t^{\prime },s^{\prime })$| under TTC for some teacher |$t^{\prime }\in \mu_0(s^{\prime })$| if and only if teacher |$t$| is matched to |$s^{\prime }$| under |$\mbox{TO-BE}_{f}$| in our original environment.
We summarize this discussion in the following Lemma:
Fix a preference profile |$\succ$| and a collection |$f$| . |$\mbox{TO-BE}_{f}(\succ )(t)=s^{\prime }$| if and only if TTC( |$\succ ^{\prime })(t)=(t^{\prime },s^{\prime })$| for some |$t^{\prime }\in \mu_0(s^{\prime })$| where the preference relation |$\succ ^{\prime }$| is constructed according to the procedure described above.
C. Proof of Point iii. of Theorem 1
Given the connection with TTC, a mechanism known to be strategy-proof, pointed out in Lemma 2, it follows that |$ \mbox{TO-BE}_{f}$| is likewise strategy-proof. Indeed, |$\mbox{TO-BE} _{f}(\succ)[t]$| , the school obtained by teacher |$t$| under |$\mbox{TO-BE}_{f}$| , corresponds to the element in the second dimension of |$\mbox{TTC} (\succ^{\prime })[t]=(t^{\prime },s)$| . Similarly, when teacher |$t$| misreports his preferences to |$\tilde{\succ}_t$| , he obtains school |$ \mbox{TO-BE}_{f}(\tilde{\succ}_t,\succ_{-t})[t]$| under |$\mbox{TO-BE}_{f}$| corresponding to the element in the second dimension of |$\mbox{TTC}(\tilde{ \succ}^{\prime }_t,\succ_{-t})[t]=(t^{\prime \prime },s^{\prime })$| . By strategy-proofness of TTC, we obtain that |$\mbox{TTC}(\succ^{\prime })[t]=(t^{\prime },s) \succeq^{\prime }_t \mbox{TTC}(\tilde{\succ}^{\prime }_t,\succ_{-t})[t]=(t^{\prime \prime },s^{\prime })$| . By definition of |$ \succ^{\prime }$| , this implies that |$\mbox{TO-BE}_{f}(\succ)[t]=s \succeq_t s^{\prime }=\mbox{TO-BE}_{f}(\tilde{\succ}_t,\succ_{-t})[t]$| .
Now, we show that |$\mbox{TO-BE}_{f}$| is a selection of BE. Assume by contradiction that TO-BE |$_{f}$| is not a selection of BE. Appealing to Proposition 2, this implies that TO-BE |$ _{f}$| is not two-sided maximal. By construction, TO-BE |$_{f}$| is 2-IR so we obtain that TO-BE |$_{f}$| is 2-Pareto dominated at some preference profile |$ \succ$| by an alternative assignment, say |$\mu$| . Now, let us consider the one-to-one environment described in Section B under which the outcome of TTC corresponds to the outcome of TO-BE |$_{f}$| in our original many-to-one environment. Recall that we denote the modified preference profile in the one-to-one environment by |$\succ ^{\prime }$| . We claim that, in this modified environment, TTC is 1-Pareto-dominated by some matching |$\mu ^{\prime }$| which contradicts the well-known 1-Pareto efficiency of TTC. In the sequel, we build |$\mu ^{\prime }$| .
For each school |$s$| , we define |$T^{\rm out}(s)$| as the set of teachers who exit school |$s$| when we move from TO-BE |$_{f}(\succ )$| to the assignment given by |$ \mu$| , i.e., |$T^{\rm out}(s):=$| TO-BE |$_{f}(\succ )[s]\backslash \mu (s)$| . We similarly denote |$T^{\rm in}(s)$| for the set of teachers entering into school |$s$| , i.e., |$T^{\rm in}(s):=\mu (s)\backslash$| TO-BE |$_{f}(\succ )[s]$| . Clearly, for each school |$s$| , we must have that |$\left \vert T^{\rm out}(s)\right \vert =\left \vert T^{\rm in}(s)\right \vert$| . Without loss of generality, we denote |$ T^{\rm out}(s)=\{t_{1},...,t_{k}\},$| where teacher |$t_{\ell }$| has the |$\ell$| th highest preference ranking / priority in |$T^{\rm out}(s)$| . We similarly denote |$ T^{\rm in}(s)=\{t_{1}^{\prime },...,t_{k}^{\prime }\}$| , where teacher |$t_{\ell }^{\prime }$| has the |$\ell$| th highest preference ranking / priority in |$ T^{\rm in}(s)$| . We then build assignment |$\mu ^{\prime }$| as follows. Pick any teacher |$t$| . If |$\mu (t)=$| TO-BE |$_{f}(\succ )[t]$| , then let |$\mu ^{\prime }(t)=$| TTC |$(\succ ^{\prime })[t]$| . Trivially, |$\mu ^{\prime }(t)\succeq _{t}^{\prime }$| TTC |$(\succ ^{\prime })[t]$| . Now, if |$\mu (t)\neq$| TO-BE |$ _{f}(\succ )[t]$| , then |$t$| must belong to |$T^{\rm in}(s),$| where |$s:=\mu (t)$| . Assume that |$t$| has the |$\ell$| th highest priority in |$T^{\rm in}(s)$| . We set |$ \mu ^{\prime }(t)$| to TTC |$(\succ ^{\prime })[t^{\prime }],$| where |$t^{\prime }$| has the |$\ell$| th highest priority in |$T^{\rm out}(s)$| . 90 Now, let us show that |$\mu ^{\prime }(t)\succ _{t}^{\prime }$| TTC |$(\succ ^{\prime })[t]$| . Because we let TTC |$(\succ ^{\prime })[t^{\prime }]=:(t^{\prime \prime },s)$| , in the sequel, we would like to show that |$(t^{\prime \prime },s)\succ _{t}^{\prime }$| TTC |$(\succ ^{\prime })[t]$| . Since |$s\succ _{t}$| TO-BE |$_{f}(\succ )[t]$| , we only have to show that |$(t^{\prime \prime },s)$| is acceptable to |$t$| under |$\succ_{t}^{\prime }$| . Since |$\mu$| 2-Pareto dominates TO-BE |$_{f}(\succ )$| , |$t\succ_{s}t^{\prime }$| . In addition, by definition, TTC |$(\succ ^{\prime})[t^{\prime }]$| is acceptable to |$t^{\prime }$| under |$\succ _{t^{\prime }}^{\prime }$| and so, by construction of |$ \succ_{t^{\prime }}^{\prime }$| , we must have that |$t^{\prime }\succeq_{s}t^{\prime \prime }$| . We conclude that |$t\succ_{s}t^{\prime \prime }$| and so |$(t^{\prime \prime },s)$| is acceptable to |$t$| under preference profile |$\succ ^{\prime }$| . This proves that |$\mu ^{\prime }$| 1-Pareto dominates TTC |$(\succ ^{\prime })$| , thereby yielding our contradiction.
D. Proof of Theorem 2
For the first part of the proof of Theorem 2, we proceed by contradiction and fix a preference profile |$\succ$| under which |$\mbox{TO-BE}_{f^{*}}(\succ)$| is 1-Pareto dominated by another 2-IR matching, say |$\mu$| . Consider the one-to-one environment described in Section B , under which the outcome of TTC corresponds to that of |$\mbox{TO-BE}_{f^{*}}$| in the original many-to-one environment (see Lemma 2). The modified teacher preference profile in this environment is |$\succ^{\prime }$| . We will show that, in this modified environment, TTC is 1-Pareto dominated by some matching |$\mu^{\prime }$| , which contradicts the well-known 1-Pareto efficiency of TTC. Let us build |$\mu^{\prime }$| .
For each school |$s$| , we let |$T_{0}^{\rm out}(s) := \mu_{0}(s) \backslash \mu(s)$| and |$T_{0}^{\rm in}(s) := \mu(s) \backslash \mu_{0}(s)$| . 91 Remember that for each school |$s$| we must have |$|T_{0}^{\rm in}(s)| = |T_{0}^{\rm out}(s)| :=m$| . Without loss of generality, let |$T^{\rm out}(s) = \{ t_{1}, \dots, t_{m} \}$| where teacher |$t_{\ell}$| has the |$\ell$| -th highest priority in |$T^{\rm out}_{0}(s)$| . Similarly, let |$T^{\rm in}_{0}(s) = \{ t_{1}^{\prime }, \dots, t_{m}^{\prime }\}$| . Note that, since |$\mu$| is 2-IR and so 2-Pareto dominates |$\mu_{0}$| , we have that |$t^{\prime }_{\ell} \succ_{s} t_{\ell}$| for all |$\ell = 1, \dots, m$| . Finally, let |$T_{0}(s) := \mu_{0}(s) \cap \mu(s)$| . We define a first matching |$\mu_{1}^{\prime }$| as follows.
Fix a teacher |$t$| . If |$t := t_{\ell}^{\prime} \in T_{0}^{\rm in}(s)$| for some school |$s$| , then let |$\mu_{1}^{\prime }(t) := (t_{\ell},s)$| . If |$t$| does not appear in any set |$T^{\rm in}_{0}(s)$| for all schools |$s \in S$| , then it implies that the teacher belongs to |$T_{0}(s)$| for some school |$s$| . In that case, let |$\mu_{1}^{\prime }(t) = (t,s)$| . Note that, under the latter case, we have that |$\mu_{0}(t) = \mu(t) \succeq_{t} \mbox{TO-BE}_{f^{*}}(\succ)[t] \succeq_{t} \mu_{0}(t)$| so that |$\mu_{0}(t) = \mbox{TO-BE}_{f^{*}}(\succ)[t] = \mu(t)$| and, by definition of TTC |$(\succ^{\prime })$| , we have that TTC |$ (\succ^{\prime })[t] = (t,s)$| .
For a teacher |$t$| , if |$s:= \mu(t) \succ_{t} \mbox{TO-BE} _{f^{*}}(\succ)[t] := \tilde{s}$| , then |$t_{\ell}^{\prime} \in T^{\rm in}_{0}(s)$| and |$\mu_{1}^{\prime }(t) = (t_{\ell},s)$| . Let |$(\tilde{t},\tilde{s}) := \mbox{TTC}(\succ^{\prime })[t]$| . Since |$s \succ_{t} \tilde{s}$| and |$t = t_{\ell}^{\prime }\succ_{s} t_{\ell}$| , we indeed have that |$(t_{\ell},s)$| is acceptable for |$t$| under |$\succ_{t}^{\prime }$| and |$(t_{\ell},s) \succ_{t} ( \tilde{t},\tilde{s})$| .
If |$t \in T_{0}(s)$| for some |$s$| , then, as described above, we trivially have |$\mu_{1}^{\prime }(t) = \mbox{TTC}(\succ^{\prime })[t] = (t,s)$| .
Now, assume that teacher |$t$| is s.t. |$\mu(t) = \mbox{TO-BE}_{f^{*}}(\succ)[t] =: s \succ_{t} \mu_{0}(t)$| so that |$t := t_{\ell}^{\prime} \in T^{\rm in}_{0}(s)$| . So |$\mu_{1}^{\prime }(t_{\ell}^{\prime }) = (t_{\ell},s)$| and |$\mbox{TTC}(\succ^{\prime })[t] := (t_{k},s)$| for some |$k$| . By construction of |$\mbox{TTC}(\succ^{\prime })$| , we have that |$t \succ_{s} t_{k}$| . We also know that |$t = t_{\ell}^{\prime }\succ_{s} t_{\ell}$| so that |$(t_{\ell},s)$| and |$(t_{k},s)$| are both acceptable under |$ \succ_{t}^{\prime }$| . If |$(t_{\ell},s) \succeq_{t}^{\prime }(t_{k},s)$| then |$ \mu_{1}^{\prime }(t) \succeq_{t}^{\prime }\mbox{TTC}(\succ^{\prime })[t]$| . 92
Assume that |$(t_{k},s) \succ_{t}^{\prime }(t_{\ell},s)$| . We will build a matching |$\mu^{\prime }_{2}$| s.t. all teachers who preferred their assignments under |$\mu_{1}^{\prime }$| to those under |$\mbox{TTC} (\succ^{\prime })$| continue to do so and teacher |$t$| will weakly prefer his assignment under |$\mu_{2}^{\prime }$| to the one under |$\mbox{TTC} (\succ^{\prime })$| . Let |$k_{1} := k$| , by construction of the profile |$ \succ^{\prime }$| , since |$(t_{k},s) \succ_{t}^{\prime }(t_{\ell},s)$| then |$ f^{*}_{s}(t_{k_{1}}) < f^{*}_{s}(t_{\ell})$| . By definition of |$f^{*}_{s}$| , it means that |$t_{k_{1}} \succ_{s} t_{\ell}$| , so that |$k_{1} < \ell$| . Let |$ O_{< \ell} := \{ (t_{1},s), \dots, (t_{\ell - 1},s) \}$| , |$O_{\geq \ell} := \{ (t_{\ell},s), \dots, (t_{m},s) \}$| , and |$\tilde{O} := O_{\geq \ell} \cup O_{< \ell}$| . We build a bipartite digraph |$(N,E)$| where |$N := T^{\rm in}_{0}(s) \cup \tilde{O}$| . Let |$t_{\ell}^{\prime }:= n_{1} \in T^{\rm in}_{0}(s)$| and let him point to |$n_{1}^{\prime }:= (t_{k_{1}},s) \in \tilde{O}$| . Now, let |$ n_{1}^{\prime }$| point to |$n_{2} := t_{k_{1}}^{\prime }$| where |$ t_{k_{1}}^{\prime }$| the teacher assigned to |$(t_{k_{1}},s)$| under |$ \mu^{\prime }_{1}$| . Now, there are three cases:
If |$s = \mu(t_{k_{1}}^{\prime }) \neq \mbox{TO-BE}_{f^{*}}(\succ)[t_{k_{1}}^{\prime }]$| , then let |$n_{2} = t_{k_{1}}^{\prime }$| point to |$n_{2}^{\prime }:= (t_{\ell},s)$| . Since |$k_{1} < \ell$| , we have that |$t_{k_{1}}^{\prime }\succ_{s} t_{\ell}^{\prime }\succ_{s} t_{\ell}$| , so that |$(t_{\ell},s)$| is acceptable under |$\succ_{t_{k_{1}}^{\prime }}^{\prime }$| and so |$(t_{\ell},s) \succ_{t_{k_{1}}^{\prime }}^{\prime }\mbox{TTC} (\succ^{\prime })[t_{k_{1}}^{\prime }]$| .
If |$s =\mu(t_{k_{1}}^{\prime }) = \mbox{TO-BE}_{f^{*}}(\succ)[t_{k_{1}}^{\prime }]$| and |$(t_{\ell},s) \succ_{t_{k_{1}}^{\prime}}^{\prime }\mbox{TTC}(\succ^{\prime })[t_{k_{1}}^{\prime }] =: (t_{k_{2}},s)$| , then let |$n_{2} = t_{k_{1}}^{\prime }$| point to |$n_{2}^{\prime }:= (t_{\ell},s)$| .
If |$s =\mu(t_{k_{1}}^{\prime }) = \mbox{TO-BE}_{f^{*}}(\succ)[t_{k_{1}}^{\prime }]$| and |$\mbox{TTC}(\succ^{\prime})[t_{k_{1}}^{\prime }] =: (t_{k_{2}},s) \succ_{t_{k_{1}}^{\prime }}^{\prime}(t_{\ell},s)$| , then let |$n_{2} = t_{k_{1}}^{\prime }$| point to |$(t_{k_{2}},s)$| . Since |$(t_{\ell},s)$| is acceptable at |$\succ_{t_{k_{1}}^{\prime }}^{\prime }$| , we must have that |$k_{2} < \ell$| so that |$(t_{k_{2}},s) \in O_{<\ell}$| . Let |$n_{2}^{\prime }:= (t_{k_{2}},s)$| and |$n_{3} := t_{k_{2}}^{\prime }$| , where |$t_{k_{2}}^{\prime }$| is the teacher assigned to |$(t_{k_{2}},s)$| under |$\mu_{1}^{\prime }$| , and let the former point to the latter.
If Case 3 holds, if one starts with |$t_{k_{2}}^{\prime }$| rather than |$ t_{k_{1}}^{\prime }$| and since |$k_{2} < \ell$| , then it is possible to use the same argument as above. If Case 3 still happens, then one can iterate until the pointing reaches |$(t_{\ell},s)$| . Note that it does reach |$ (t_{\ell},s)$| only once it reaches Case 1 or 2 and that, by finiteness of the set |$O_{< \ell}$| , Case 3 cannot holds indefinitely. 93 Let |$p-1$| be the number of iterations needed before reaching Case 1 or 2. Once it does, we have |$p$| nodes |$ n_{1},n_{1}^{\prime }, \dots, n_{p},n_{p}^{\prime }$| where, for |$i = 1, \dots, p$| , |$n_{i} = t_{k_{i-1}}^{\prime }$| points to |$n_{i}^{\prime }= (t_{k_{i}},s)$| (where |$t_{k_{0}}^{\prime }$| stands for |$t = t_{\ell}^{\prime }$| ) and the latter points to |$n_{i+1} = t_{k_{i}}^{\prime }$| . Note that |$ n_{p}^{\prime }= (t_{\ell},s)$| . Let |$\mu_{2}^{\prime }$| be the same matching as |$\mu_{1}^{\prime }$| except that, for |$i= 1,\dots, p$| , the teacher |$ t_{k_{i}}^{\prime }$| in node |$n_{i}$| is assigned the object |$(t_{k_{i}},s)$| . 94 Note that, by construction, for each teacher |$t_{k_{i-1}}^{\prime }$| with |$i = 1, \dots, p-1$| , we have that |$\mu_{2}^{\prime }(t_{k_{i-1}}^{\prime }) = \mbox{TTC} (\succ^{\prime })[t_{k_{i-1}}^{\prime }]$| and that, for teacher |$ t_{k_{p-1}}^{\prime }$| , we have |$\mu_{2}^{\prime }(t_{k_{p-1}}^{\prime }) = (t_{\ell},s) \succ_{t_{k_{p-1}}^{\prime }}^{\prime }\mbox{TTC}(\succ^{\prime })[t_{k_{p-1}}^{\prime }]$| .
Now, we can iterate the entire above argument with |$\mu^{\prime }_{2}$| if there is still a teacher |$t$| and a school |$s$| s.t. |$t := t_{\ell}^{\prime} \in T_{0}(s)$| , |$\mu(t) = s = \mbox{TO-BE}_{f^{*}}(\succ)[t]$| but |$\mbox{TTC} (\succ^{\prime })[t] \succ_{t}^{\prime }\mu_{2}^{\prime }(t)$| . Note that such teacher |$t$| cannot be one of those treated above. So at some point, this process will stop and identify a matching |$\mu_{K}^{\prime }$| where for all |$t \in T$| , |$\mu_{K}^{\prime }(t) \succeq_{t}^{\prime }\mbox{TTC} (\succ^{\prime })[t]$| . Since by assumption there is at least one teacher |$t$| s.t. |$\mu(t) \succ_{t} \mbox{TO-BE}_{f^{*}}(\succ)[t]$| , then our above construction implies that |$\mu_{K}^{\prime }(t) \succ_{t} \mbox{TTC} (\succ^{\prime })[t]$| . Setting |$\mu^{\prime }= \mu_{K}^{\prime }$| , we obtain the desired contradiction.
For the second part of the theorem, assume that |$f \neq f^{*}$| , then one can construct an instance of preferences wherein the outcome |$ \mbox{TO-BE}_{f}$| is 1-Pareto dominated by a 2-IR matching |$\mu$| . This is enough for our purpose since we can build a 2-IR mechanism that selects |$\mu$| at this specific instance and coincides with |$\mbox{TO-BE}_{f}$| at any other instance. Since |$f \neq f^{*}$| , then |$\exists s^{*} \in S$| and two teachers |$ t_{1}^{*},t_{2}^{*}$| s.t.
|$\mu_{0}(t_{1}^{*}) = \mu_{0}(t_{2}^{*}) = s^{*}$|
|$t_{1}^{*} \succ_{s^{*}} t_{2}^{*}$|
|$f_{s^{*}}(t_{1}^{*}) > f_{s^{*}}(t_{2}^{*})$|
At the first step of |$\mbox{TO-BE}_{f^{*}}(\succ)$| , the only cycle is |$(t,s) \rightarrow (t_{2}^{*},s^{*}) \rightarrow (t,s)$| . Once implemented, |$ (t^{\prime },s^{\prime })$| cannot point to |$(t_{1}^{*},s^{*})$| since |$ t_{1}^{*} \succ_{s^{*}} t^{\prime }$| so the final matching matches |$t$| and |$ t_{1}^{*}$| to |$s^{*}$| , |$t_{2}^{*}$| to |$s$| and |$t^{\prime }$| to |$s^{\prime }$| . But in matching |$t^{\prime }$| to |$s^{*}$| and |$t_{1}^{*}$| to |$s^{\prime }$| , we would obtain a 2-IR matching |$\mu$| that 1-Pareto dominates |$\mbox{TO-BE} _{f^{*}}(\succ)$| . Note, however, that, in doing so, school |$s^{*}$| would be worse off compared to under |$\mbox{TO-BE}_{f^{*}}$| . Indeed, its assignment under |$\mbox{TO-BE}_{f^{*}}(\succ)$| is |$\{t,t_{1}^{*}\}$| , while the one under |$\mu$| is |$\{t,t^{\prime }\}$| and |$t_{1}^{*} \succ_{s^{*}} t^{\prime }$| .
E. Proof of Theorem 3
The proof of Theorem 3 directly follows from the proposition below.
Consider any two-sided maximal (and strategy-proof) mechanism |$\varphi$| . Fix any profile |$\succ$| which lies in |$\mathcal{P}$| . We must have |$\varphi (\succ )\in$| TO-BE |$(\succ )$| .
Consider the graph in the first step of TO-BE |$(\succ )$| . We claim that there is a cycle of TO-BE |$(\succ )$| such that, under |$\varphi (\succ )$| , any teacher who is part of the cycle gets assigned the object he points to. First, note that if there is a self-cycle in this step, i.e., a node |$(t,s)$| pointing to itself, then, by 2-IR of |$\varphi$| , |$\varphi (\succ )$| must assign |$t$| to |$s$| . So let us assume that there is no self-cycle in the graph. Pick an arbitrary cycle denoted |$ (t_{1},s_{1}),(t_{2},s_{2}),...,(t_{K},s_{K})$| .
We claim that in this cycle, there must be a node |$(t_{k},s_{k})$| such that |$ \varphi (\succ )[t_{k}]\neq s_{k}$| . Indeed, if this was not the case, we would have that for all |$k=1,...,K$| , |$\varphi (\succ )[t_{k}]=s_{k}$| . But then |$\varphi (\succ )$| is not two-sided maximal since we can assign each |$ t_{k}$| to |$s_{k+1}$| . Noticing that this gives a 2-IR assignment, the assignment we obtain 2-Pareto dominates |$\varphi (\succ )$| , a contradiction with the assumption that |$\varphi$| is a selection of BE and, hence, two-sided maximal.
So there must be a node |$(t_{k},s_{k})$| such that |$\varphi (\succ )[t_{k}]\neq s_{k}$| , wlog, let us assume that |$k=1$| . The following simple lemma shows that there must be a cycle of TO-BE |$(\succ )$| such that, under |$ \varphi (\succ )$| , any teacher who is part of the cycle gets assigned the object he points to.
If there exists |$t_{1}$| such that |$\varphi (\succ )[t_{1}]\neq \mu _{0}(t_{1})$| then there exists a cycle |$(t_{1},s_{1}),(t_{2},s_{2}),...,(t_{K},s_{K})$| in the graph such that |$ \varphi (\succ )[t_{k}]=s_{k+1}$| for any |$k=1,...,K$| .
Assume that there exists |$t_{1}$| such that |$\varphi (\succ )[t_{1}]\neq \mu _{0}(t_{1})$| . Because |$\succ$| lies in |$\mathcal{P}$| , |$\varphi (\succ )[t_{1}]=:s_{2}$| where |$s_{2}$| is |$t_{1}$| ’s top choice. In addition, since, under |$\varphi (\succ )$| , one seat of school |$s_{2}$| is taken by |$t_{1}$| , there must be a teacher |$t_{2}$| such that |$\mu _{0}(t_{2})=s_{2}$| and |$ \varphi (\succ )[t_{2}]\neq s_{2}$| . In addition, because |$\varphi (\succ )$| is two-sided maximal, this teacher can be chosen so that |$t_{1}\succ _{s_{2}}t_{2}$| . More specifically, we pick |$t_{2}$| the teacher with the highest priority at |$s$| among all those who have a lower priority than |$ t_{1}$| at school |$s$| . By definition of the graph, |$(t_{1},s_{1})$| points to |$(t_{2},s_{2})$| . Now, since |$\varphi (\succ )[t_{2}]\neq s_{2}=\mu _{0}(t_{2})$| , we can iterate the reasoning to induce a path |$ (t_{1},s_{1}),(t_{2},s_{2}),...$| in the graph such that |$\varphi (\succ )[t_{k}]=s_{k+1}$| for any |$k\geq 1$| . Since the graph is finite, this path will cycle at some point. ■
Now, consider the new graph obtained after we removed the teachers who are part of this cycle and the seats they point to in the cycle. The exact same reasoning holds here. Hence, we can iterate the reasoning until we exhaust the market. We obtain a sequence of cycles selected in the graphs associated with TO-BE which, once implemented, yields the assignment given by |$\varphi (\succ )$| . This shows that |$\varphi (\succ )\in$| TO-BE |$(\succ )$| . ■
F. Proof of Theorem 4
We want to prove the following proposition. Remember that, for this result, we assume that we are in a one-to-one setting so that the set of TO-BE mechanisms is a singleton. We refer to the unique mechanism of this class as simply TO-BE.
Before moving to the proof, we further connect TTC and TO-BE in the one-to-one environment. As is well-known, in a Shapley–Scarf economy (in which schools are replaced by objects with no preferences but are initially owned by the other side of the market), TTC is the unique element of the core ( Shapley and Scarf, 1974 ; Roth and Postlewaite, 1977 ). Because TO-BE is related to TTC, it can be related to some notion of the core. This notion will be used in the course of the argument for the proof of Proposition 5 below. Define the two-sided notion of the core as the set of matchings |$\mu$| s.t. there is no (two-sided blocking) coalition |$B\subseteq T$| for which there is a matching |$\nu $| s.t. for each |$t\in B$| , |$\nu (t)$| is a school to which a teacher in |$B$| is initially matched and for all |$t\in B:\nu (t)\succeq _{t}\mu (t)$| and, for |$s:=\nu (t)$| , |$t\succeq _{s}\mu _{0}(s)$| with a strict equality for some teacher (or school). Given a profile of preferences, it is easily verified that a matching is in the two-sided core if and only if it is in the (standard) core when preferences are modified in such a way that each teacher |$t$| ranks schools outside his opportunity set Opp |$(t,\mu _{0},T,S)$| below his initial assignment. Thus, appealing to the results mentioned above (i.e. Shapley and Scarf, 1974 ; Roth and Postlewaite, 1977 ), we conclude that the two-sided core is a singleton and coincides with TO-BE.
Let |$\varphi$| be any selection of BE. If |$ \varphi \neq$| TO-BE then |$\varphi$| is not strategy-proof.
Let |$\varphi$| be any selection of BE. Fix any profile of preferences |$\succ $| and assume that |$\varphi (\succ )\neq$| TO-BE |$(\succ )$| . Let |$x$| be the outcome of TO-BE |$(\succ )$| and let |$y$| be that of |$\varphi (\succ )$| . There exists |$t$| s.t. |$x(t)\succ _{t}y(t)\succ _{t}\mu _{0}(t)$| .
Let |$T(x,y)$| be the set of teachers for which |$x(t)\succ _{t}y(t)\succeq _{t}\mu _{0}(t)$| . We know that |$x$| is not 1-Pareto dominated by |$y$| (by Proposition 2), and since |$y$| is individually rational and |$ x\neq y$| , we must have |$T(x,y)\neq \emptyset$| . Proceed by contradiction and assume that, for all |$t\in T(x,y)$| , we have |$y(t)=\mu _{0}(t)$| . Let |$ B:=T\backslash T(x,y)$| . Note that for any |$t\in B$| , |$y(t)$| is a school initially assigned to some teacher in |$B$| . In addition, by definition, for all |$t\in B$| , |$y(t)\succeq _{t}x(t)$| . If there was no teacher |$t\in B$| for which |$y(t)\succ _{t}x(t)$| , then we would have the following situation: |$y$| would select the initial allocation for all |$t\in T(x,y)$| and would be identical to |$x$| for all |$t\notin T(x,y)$| . Given that |$x\neq y$| , we must have |$\ x(t)\neq y(t)=\mu _{0}(t)$| for some |$t\in T(x,y)$| . Since |$x$| is individually rational, we have |$x(t)\succ _{t}y(t)=\mu _{0}(t)$| for those |$ t\in T(x,y)$| . Hence, |$x$| 1-Pareto-dominates |$y$| . However, all schools are also better off under |$x$| than under |$y$| . Indeed, for each school |$s$| s.t. |$ y(s)\notin T(x,y)$| , |$y(s)=x(s)$| and for each school |$s$| s.t. |$y(s)\in T(x,y) $| , because |$x$| is individually rational on both sides, |$x(s)\succeq _{s}y(s)=\mu _{0}(s)$| with a strict inequality for |$s$| satisfying |$x(s)\neq y(s)$| (and this |$s$| must exist since |$x\neq y$| ). Thus, |$x$| is individually rational on both sides and 2-Pareto-dominates |$y$| , which is not possible, given that |$y$| is an outcome of BE.
To recap, we have that, for any |$t\in B$| , |$y(t)$| is a school initially assigned to some teacher in |$B$| and for all |$t\in B$| , |$y(t)\succeq _{t}x(t)$| with a strict inequality for some |$t\in B$| . In addition, since |$y$| is the outcome of |$\varphi (\succ )$| and |$\varphi$| 2-Pareto-dominates the initial allocation |$\mu _{0}$| , we must have that, for all schools |$s$| , |$y(s)\succeq _{s}\mu _{0}(s)$| . Hence, |$B$| is a two-sided blocking coalition for |$x$| , which is a contradiction since |$x$| must be a point in the two-sided core. ■
Proof of Proposition 5. We start from a profile of preferences |$\succ$| under which |$\varphi (\succ )\neq$| TO-BE |$ (\succ )$| which must exist because of our assumption that |$\varphi \neq$| TO-BE. Given our profile of preferences |$\succ$| , we let the profile of preferences |$\succ ^{\prime }$| be defined as follows. For any |$t$| , any school |$s$| other than TO-BE |$(\succ )[t]$| are ranked as unacceptable for |$t$| under |$\succ ^{\prime }$| . We must have TO-BE |$(\succ )=$| TO-BE |$(\succ ^{\prime })$| . Now, we are in a position to prove the following lemma.
TO-BE |$(\succ ^{\prime })=\varphi (\succ ^{\prime })$| .
Suppose |$x:=$| TO-BE |$(\succ ^{\prime })\neq \varphi (\succ ^{\prime })=:y$| . By the above lemma, there exists |$t$| s.t. |$x(t)\succ _{t}^{\prime }y(t)\succ _{t}^{\prime }\mu _{0}(t)$| , which yields a contradiction, by construction of |$\succ _{t}^{\prime }$| . ■
Note that TO-BE also satisfies the following property: for any profile of preferences |$\succ$| , for any teacher |$t$| , TO-BE |$(\succ )(t)=$| TO-BE |$(\succ _{-t},\succ _{t}^{\prime })(t)$| . This will be used in the following lemma.
If |$\varphi$| is strategy-proof, then TO-BE |$(\succ _{Z},\succ _{-Z}^{\prime })=\varphi (\succ _{Z},\succ _{-Z}^{\prime })$| for any |$Z\subseteq T$| .
Assume |$\varphi$| is strategy-proof. The proof is, by induction, on the size of |$Z$| . For |$\left\vert Z\right\vert =0$| , the result is given by the previous lemma. Now, the induction hypothesis is that TO-BE |$(\succ _{Z},\succ _{-Z}^{\prime })=\varphi (\succ _{Z},\succ _{-Z}^{\prime })$| for any subset |$Z$| with |$\left\vert Z\right\vert =k$| . Proceed by contradiction and suppose that there is |$Z$| s.t. |$\left\vert Z\right\vert =k+1$| for which |$ x:=$| TO-BE |$(\succ _{Z},\succ _{-Z}^{\prime })\neq \varphi (\succ _{Z},\succ _{-Z}^{\prime })=:y$| . By the first lemma above, there exists |$t$| s.t. TO-BE |$ (\succ _{Z},\succ _{-Z}^{\prime })(t)\vartriangleright _{t}\varphi (\succ _{Z},\succ _{-Z}^{\prime })(t)\vartriangleright _{t}\mu _{0}(t)$| where |$ \vartriangleright _{t}=\succ _{t}^{\prime }$| if |$t\notin Z$| while |$ \vartriangleright _{t}=\succ _{t}$| otherwise. If |$t\notin Z$| , then there is a straightforward contradiction since, under |$\succ _{t}^{\prime }$| , there is a single school ranked above |$\mu _{0}(t)$| for teacher |$t$| . Now, assume that |$t\in Z$| . By the property noted just before the lemma statement, we must have TO-BE |$(\succ _{Z\backslash \{t\}},\succ _{-Z}^{\prime },\succ _{t}^{\prime })(t)=$| TO-BE |$(\succ _{Z},\succ _{-Z}^{\prime })(t)$| and, by our induction hypothesis, |$\varphi (\succ _{Z\backslash \{t\}},\succ _{-Z}^{\prime },\succ _{t}^{\prime })(t)=$| TO-BE |$(\succ _{Z\backslash \{t\}},\succ _{-Z}^{\prime },\succ _{t}^{\prime })(t)$| . Thus, we obtain |$ \varphi (\succ _{Z\backslash \{t\}},\succ _{-Z}^{\prime },\succ _{t}^{\prime })(t)=$| TO-BE |$(\succ _{Z\backslash \{t\}},\succ _{-Z}^{\prime },\succ _{t}^{\prime })(t)=$| TO-BE |$(\succ _{Z},\succ _{-Z}^{\prime })(t)\succ _{t}\varphi (\succ _{Z},\succ _{-Z}^{\prime })(t)$| , which is a contradiction with the assumption that |$\varphi$| is strategy-proof (indeed, at ( |$\succ _{Z},\succ _{-Z}^{\prime }$| ), teacher |$t\in Z$| has an incentive to report |$ \succ _{t}^{\prime }$| instead of |$\succ _{t}$| ). ■
Taking |$Z=T$| in the above lemma, given that |$\varphi (\succ )\neq$| TO-BE |$ (\succ )$| , we obtain the following corollary, which completes the proof of our proposition.
|$\varphi$| is not strategy-proof.
G. Proof of Proposition 3
In the sequel, we prove our characterization result of one-sided maximal matchings given in Proposition 3. Our proof is divided into two parts. We start by showing that any outcome of the 1S-BE algorithm is a one-sided maximal matching (Section G.1 ):
If |$\mu$| is an outcome of the 1S-BE algorithm then |$\mu$| is one-sided maximal.
Then, we move to the proof that any one-sided maximal matching corresponds to a possible outcome of the 1S-BE algorithm (Section G.2 ):
If |$\mu$| is one-sided maximal then |$\mu$| is an outcome of the 1S-BE algorithm.
G.1. Proof of Proposition 6
Before moving to the proof we introduce a new notation. Given matching |$\mu$| , we denote |$\mathcal{B}_{\mu}$| for the set of blocking pairs of |$\mu$| .
In the sequel, we fix two matchings |$\mu$| and |$\mu ^{\prime }$| such that |$ \mu ^{\prime }$| Pareto-dominates |$\mu$| for teachers and |$\mathcal{B}_{\mu ^{\prime }}\subseteq \mathcal{B}_{\mu }$| . We show below that starting from |$ \mu$| , the graph associated with the 1S-BE algorithm must have a cycle. Hence, any outcome of 1S-BE must be one-sided maximal, as claimed in Proposition 6.
As in Lemma 1, we can exhibit “cycles of exchanges” that can be used to go from |$\mu$| to |$\mu^{\prime }$| in the proposition. It is worth noting that in the many-to-one environment, these cycles of exchanges are not uniquely defined. Indeed, if for a given selection of cycles of exchanges, there are two nodes that involve the same school, then this cycle can be decomposed into two cycles of exchanges. Figure A.1 illustrates this simple fact: in the left part of the figure, starting from |$\mu$| , there is a a cycle of exchanges that, once implemented leads to |$\mu^{\prime }$| . It is easy to see that we can decompose this cycle into two smaller cycles of exchanges, shown in the right part of the figure, that also lead to |$\mu^{\prime }$| once implemented.

Two equivalent cycles of exchanges in many-to-one.
So for the rest of the proof, we fix (a collection of) exchange cycles that takes us from |$\mu$| to |$\mu ^{\prime }$| once implemented. To fix ideas, in the example, we consider the one on the left part of Figure A.1 . In Lemma 1, these cycles of exchanges were actual cycles in the graph associated with BE. However, when considering the graph associated with 1S-BE, this is no longer the case: the cycles of exchanges are not necessarily cycles of the graph associated with 1S-BE. Before moving to the first lemma, we note that all nodes that are not part of cycles of exchanges are those where the teacher of that node has the same allocation between |$\mu$| and |$\mu ^{\prime }$| . In the following, the “nodes of the cycles of exchanges” will be all the nodes |$(t,s)$| s.t |$\mu (t)\neq \mu ^{\prime }(t)$| . We will say that a node |$(t,s)$| 1S-BE-points to another node |$(t^{\prime },s^{\prime })$| if |$(t,s)$| points toward |$(t^{\prime },s^{\prime })$| in the graph associated with the 1S-BE algorithm (starting from |$\mu$| ).
either its predecessor according to the the cycles of exchanges 1S-BE-points toward |$(t,s)$| ;
or there is a node |$(t^{\prime },s^{\prime })$| in the cycles of exchanges that such that |$t^{\prime }$| does not block with |$s$| under |$\mu$| , |$s\succ _{t^{\prime }}s^{\prime }$| and |$t^{\prime }$| has the highest priority among those who desire |$s$| but do not block with it under |$\mu$| . And so |$(t^{\prime },s^{\prime })$| 1S-BE-points toward |$(t,s)$| .
Before moving to the proof, let us illustrate this lemma in the example. Assume that all nodes except |$(t_{3},s_{3})$| are 1S-BE-pointed by their predecessors in the cycle of exchanges. According to Lemma 7, there must be a node |$(t^{\prime },s^{\prime })$| in the cycle of exchanges that 1S-BE-points toward |$(t_{3},s_{3})$| . In the graph of Figure A.2 , this node is assumed to be |$(t_{4},s_{4})$| . The dashed edge from |$(t_{2},s_{2})$| to |$ (t_{3},s_{3})$| is here to show that this is not an edge of the 1S-BE graph but rather is an edge corresponding to the exchange cycle.

Cycles of exchanges and |$(N^{\prime },E_{1})$| .
Call |$(t^{\prime \prime },s^{\prime \prime })$| the predecessor of node |$ (t,s)$| in the cycles of exchanges so that |$s^{\prime \prime }:=\mu (t^{\prime \prime })$| and |$s:=\mu ^{\prime }(t^{\prime \prime })$| . Because |$ \mu ^{\prime }$| Pareto-dominates for teachers |$\mu$| , we know that |$s\succ _{t^{\prime \prime }}s^{\prime \prime }$| so that |$t^{\prime \prime }$| desires |$s$| under |$\mu$| . Assume that |$(t^{\prime \prime },s^{\prime \prime })$| does not 1S-BE-point to |$(t,s)$| . This means that |$t^{\prime \prime }$| does not block with |$s$| under |$\mu$| and that there is another teacher |$ t^{\prime }$| who does not block with |$s$| and has the highest priority among those who desire |$s$| and do not block with it. Thus, |$(t^{\prime },s^{\prime })$| (where |$s^{\prime }:=\mu (t^{\prime })$| ) 1S-BE points toward |$(t,s)$| . It remains to show that |$(t^{\prime },s^{\prime })$| is part of the cycles of exchanges. If this was not the case, it would mean that |$\mu (t^{\prime })=\mu ^{\prime }(t^{\prime })=s^{\prime }$| . Let us recap. We have that |$ t^{\prime }$| does not block with |$s$| under |$\mu$| . In addition, by definition of |$t^{\prime }$| , we must have that |$t^{\prime }\succ _{s}t^{\prime \prime }$| (since |$t^{\prime \prime }$| does not block with |$s$| under |$\mu$| and desires |$s$| ). In addition, |$t^{\prime }$| desires |$s$| under |$ \mu$| , and so |$\mu (t^{\prime })=\mu ^{\prime }(t^{\prime })$| implies that |$ t^{\prime }$| also desires |$s$| under |$\mu^{\prime }$| . Hence, because |$ t^{\prime \prime }\in \mu ^{\prime }(s)$| , we obtain that |$t^{\prime }$| blocks with |$s$| under |$\mu ^{\prime }$| . This contradicts our assumption that |$\mathcal{B}_{\mu ^{\prime }}\subseteq \mathcal{B}_{\mu }$| . ■
Lemma 7 allows us to identify a subgraph |$(N^{\prime },E_{1})$| of the 1S-BE graph starting from |$\mu$| such that |$N^{\prime }$| are the nodes of the cycles of exchanges and the set of edges |$E_{1}$| is built as follows. We start from |$E_{1}=\emptyset$| and add the following edges: for each node |$(t,s)$| in the cycles of exchange, if its predecessor |$(\tilde{t},\tilde{s})$| under the cycles of exchanges 1S-BE-points to |$(t,s)$| then |$((\tilde{t},\tilde{s}),(t,s))$| is added to |$ E_{1}$| . If, on the contrary, |$(\tilde{t},\tilde{s})$| does no not 1S-BE-point to |$(t,s)$| , then we pick the node |$(t^{\prime },s^{\prime })$| in the cycles of exchanges, identified in the second condition of Lemma 7, that 1S-BE-points toward |$(t,s)$| and we add |$((\tilde{t },\tilde{s}),(t,s))$| to |$E_{1}$| . Note that, by construction, each node in |$ N^{\prime }$| has a unique in-going edge in |$(N^{\prime },E_{1})$| . In the example, this subgraph |$(N^{\prime },E_{1})$| is given by the right graph of Figure A.2 (the solid arrows). Note that this graph admits a cycle: |$(t_{3},s_{3})\rightarrow (t_{1}^{\prime },s_{1})\rightarrow (t_{4},s_{4})\rightarrow (t_{3},s_{3})$| . This is a simple property of digraphs with in-degree one:
Fix a finite digraph |$(N,E)$| such that each node has in-degree one. There is a cycle in this graph.
Fix a node |$n_{1}$| in the graph |$(N,E)$| . Because it has in-degree one, we can let |$n_{2}$| be the unique node pointing to |$n_{1}$| . Again, from |$n_{2}$| we can let |$n_{3}$| be the unique node pointing to |$n_{2}$| . Because there are a finite number of nodes in the graph, this process must cycle at some point. ■
As the example illustrates, applying this lemma to |$ (N^{\prime },E_{1})$| leads to the following corollary:
There is a cycle in the graph associated with 1S-BE starting from |$\mu$| .
We are now in a position to prove Proposition 6.
Completion of the Proof of Proposition 6 Let |$\mu$| be an outcome of the 1S-BE algorithm. Proceed by contradiction and assume that |$\mu$| is not one-sided maximal. Thus, there must be a matching |$ \mu^{\prime }$| such that |$\mu^{\prime }$| Pareto-dominates |$\mu$| for teachers and |$\mathcal{B}_{\mu^{\prime }} \subseteq \mathcal{B}_{\mu}$| . Corollary 3 implies that there must be a cycle in the graph associated with 1S-BE starting from |$\mu$| , contradicting the fact that |$\mu$| is an outcome of 1S-BE. ■
G.2. Proof of Proposition 7
In the sequel, we fix a one-sided maximal matching |$\mu ^{\prime }$| . We let |$ \mu$| be a matching such that |$\mu ^{\prime }$| Pareto-dominates for teachers |$\mu$| and satisfies |$\mathcal{B}_{\mu ^{\prime }}\subseteq \mathcal{B}_{\mu }$| . We claim there is a cycle in the graph associated with 1S-BE starting from |$\mu$| which, once implemented, leads to a matching |$\tilde{\mu}$| such that |$\mu ^{\prime }$| Pareto-dominates |$\tilde{\mu}$| for teachers and satisfies |$\mathcal{B}_{\mu ^{\prime }}\subseteq \mathcal{B}_{\tilde{\mu}}$| . Note that this implies Proposition 7. Indeed, because, by definition, |$\mu ^{\prime }$| Pareto-dominates |$\mu _{0}$| and |$ \mathcal{B}_{\mu ^{\prime }}\subseteq \mathcal{B}_{\mu _{0}}$| , we must have a cycle in the graph associated with 1S-BE starting from |$\mu _{0}$| , which, once implemented, yields to a matching say |$\tilde{\mu}_{1}$| such that |$\mu ^{\prime }$| Pareto-dominates |$\tilde{\mu}_{1}$| for teachers and satisfies |$ \mathcal{B}_{\mu ^{\prime }}\subseteq \mathcal{B}_{\tilde{\mu}_{1}}$| . Now, we can iterate the reasoning, and we again see that there is a cycle in the graph associated with 1S-BE starting from |$\tilde{\mu}_{1}$| , which, once implemented, yields to a matching say |$\tilde{\mu}_{2}$| such that |$\mu ^{\prime }$| Pareto-dominates |$\tilde{\mu}_{2}$| for teachers and satisfies |$ \mathcal{B}_{\mu ^{\prime }}\subseteq \mathcal{B}_{\tilde{\mu}_{2}}$| . We can pursue this reasoning: at some point, because the environment is finite, we must reach matching |$\mu ^{\prime }$| , as we intended to show.
We start by proving a lemma that will be useful in the subsequent arguments. Starting from any matching |$\mu$| , in the graph associated to the 1S-BE algorithm, if a node points to another node involving school |$s$| then it also points to all other nodes involving school |$s$| .
Let |$(t,s)$| be a node in the graph associated with the 1S-BE algorithm, starting from a matching |$\mu$| . If |$(t,s)$| points to |$(t^{\prime },s^{\prime })$| then |$(t,s)$| points to |$ (t^{\prime \prime },s^{\prime })$| for all |$t^{\prime \prime }\in \mu(s^{\prime })$| .
Let us assume that |$(t,s)$| points to |$(t^{\prime },s^{\prime })$| and consider any node |$(t^{\prime \prime },s^{\prime })$| . Let us first consider the case where |$t\succ _{s^{\prime }}t^{\prime \prime }$| . Given that |$(t,s)$| points to |$(t^{\prime },s^{\prime })$| , we must have that |$t$| desires |$ s^{\prime }$| . Hence, |$t$| has justified envy toward |$t^{\prime \prime }$| and so, by definition of 1S-BE, |$(t,s)$| must point to |$(t^{\prime \prime },s^{\prime })$| . Now, consider the other case in which |$t^{\prime \prime }\succ _{s^{\prime }}t$| . We have to check that |$t$| is preferred by |$ s^{\prime }$| to each teacher who desires |$s^{\prime }$| and does not block with it. If |$t\succ _{s^{\prime }}t^{\prime }$| , then for any teacher |$\tilde{ t}$| who desires |$s^{\prime }$| and does not block with |$s^{\prime }$| , we have that |$t^{\prime }\succ _{s^{\prime }}\tilde{t}$| which implies |$t\succ _{s^{\prime }}\tilde{t}$| so that |$t$| is preferred by |$s^{\prime }$| to those who desire |$s^{\prime }$| and do not block with it. Hence, |$(t,s)$| must point to |$(t^{\prime \prime },s^{\prime })$| . Now, if |$t^{\prime }\succ _{s^{\prime }}t$| , because we know that |$(t,s)$| points to |$(t^{\prime },s^{\prime })$| , |$t$| must be preferred by |$s^{\prime }$| to those who desire |$s^{\prime }$| and do not block with it so that |$(t,s)$| must also point to |$(t^{\prime \prime },s^{\prime })$| . ■
We first show the following lemma.
|$\mu ^{\prime }$| Pareto-dominates |$ \mu _{1}$| for teachers.
Case 1: |$s=\mu _{1}(t)\neq s^{\prime }$| . Because |$\mu^{\prime }$| Pareto-dominates |$\mu$| for teachers, we have that |$\mu ^{\prime}(t)=s^{\prime }\succeq _{t}\mu _{1}(t)=\mu (t)=s$| .
Case 2: |$s\neq \mu _{1}(t)=s^{\prime }$| . In such a case, we trivially have |$\mu ^{\prime }(t)\succeq _{t}\mu _{1}(t)$| .
Case 3: |$s\neq \mu _{1}(t):=s_{1}\neq s^{\prime }$| . By construction of the graph |$(N^{\prime },E_{1})$| when we implement cycle |$C_{1}$| , we know that there is a unique edge |$\big((t,s),(t_{1},s_{1})\big)$| in |$C_{1}$| and that |$(t,s)$| is not the predecessor of |$(t_{1},s_{1})$| under the cycles of exchanges, since otherwise, |$t$| would be matched to |$s^{\prime }$| under |$\mu _{1}$| , which is not the case by assumption. Hence, by construction of |$(N^{\prime },E_{1})$| , the predecessor of |$(t_{1},s_{1})$| under the cycles of exchanges, say |$(t^{\prime \prime },s^{\prime \prime })$| , does not 1S-BE point to |$(t_{1},s_{1})$| and, in addition, |$t$| does not block with |$s_{1}$| under |$\mu$| , |$s_{1}\succ _{t}s$| , and |$t$| has the highest priority among those who desire |$s_{1}$| but do not block with it under |$\mu$| and 1S-BE-points to |$(t_{1},s_{1})$| . Because |$(t^{\prime \prime },s^{\prime \prime })$| does not 1S-BE point to |$(t_{1},s_{1})$| , we know that |$t^{\prime \prime }$| does not block with |$s_{1}$| . While because |$(t^{\prime \prime },s^{\prime \prime })$| points to |$(t_{1},s_{1})$| under the cycles of exchange, we must have that |$t^{\prime \prime }$| desires |$s_{1}$| . Thus, we conclude that |$t\succ _{s_{1}}t^{\prime \prime }$| .
Now, proceed by contradiction and assume that |$(\mu _{1}(t)=)s_{1}\succ _{t}s^{\prime }(=\mu ^{\prime }(t))$| . Because |$t^{\prime \prime }\in \mu ^{\prime }(s_{1})$| (recall that |$(t^{\prime \prime },s^{\prime \prime })$| is the predecessor of |$(t_{1},s_{1})$| under the cycles of exchange) and |$t\succ _{s_{1}}t^{\prime \prime }$| , we have that |$t$| blocks with |$s_{1}$| under |$\mu ^{\prime }$| i.e. |$(t,s_{1})\in \mathcal{B}_{\mu ^{\prime }}$| . But, as already claimed, |$(t,s_{1})\notin \mathcal{B}_{\mu }$| . This contradicts that |$\mathcal{B}_{\mu ^{\prime }}\subseteq \mathcal{B}_{\mu }$| . Thus, we must have |$\mu ^{\prime }(t)\succeq _{t}\mu _{1}(t)$| . 96
So we have shown that |$\forall t$| , |$\mu ^{\prime }(t)\succeq _{t}\mu _{1}(t)$| . ■
If we were sure that it is always the case that |$\mathcal{ B}_{\mu ^{\prime }}\subseteq \mathcal{B}_{\mu _{1}}$| , the proof would be completed. Unfortunately, even if this is true in the one-to-one environment, it may not be true in the many-to-one case. To give an intuition, assume that in the example we have |$t_{1}\succ _{s_{1}}t_{3}\succ _{s_{1}}t_{2}\succ _{s_{1}}t_{2}^{\prime }\succ _{s_{1}}t_{1}^{\prime }$| and |$s_{1}\succ _{t_{2}}s_{3}\succ _{t_{2}}s_{2}$| . So |$t_{2}$| blocks with |$s_{1}$| under both |$\mu$| and |$\mu ^{\prime }$| . But after implementing cycle |$C_{1}$| , we see that |$t_{2}$| does not block with |$s_{1}$| anymore. Indeed, the only teacher for whom |$t_{2}$| feels justified envy under |$\mu$| is |$t_{1}^{\prime }$| . But |$t_{1}^{\prime }$| is replaced by |$t_{3}$| once |$C_{1}$| is implemented, and |$t_{1}$| has a higher priority than |$t_{2}$| at |$s_{1}$| (while |$t_{1}$| stays matched to |$s_{1}$| ). We will show that if this arises, we can find another subgraph of the 1S-BE graph starting from |$\mu$| , call it |$(N^{\prime },E_{2})$| , still with in-degree one for each node so that there is a cycle |$C_{2}$| in this subgraph and the matching |$\mu _{2}$| obtained with this cycle keeps the blocking pair |$(t_{2},s_{1})$| .
Assume there is |$ (t_{1},s_{1})\in \mathcal{B}_{\mu ^{\prime }}$| but not in |$\mathcal{B}_{\mu _{1}}$| . Then there is a teacher |$t_{1}^{\ast }$| with |$s_{1}^{\ast }:=\mu (t_{1}^{\ast })$| s.t |$t_{1}\succ _{s_{1}}t_{1}^{\ast }$| , and |$(t_{1}^{\ast },s_{1}^{\ast })$| is part of the cycles of exchanges and points to all nodes of the form |$(t,s_{1})$| in the graph associated with 1S-BE starting from |$ \mu$| .
Before moving to the proof, let us illustrate the lemma in the example. As explained above, the “problem” in the graph |$(N^{\prime },E_{1})$| (which yields to |$(t_{2},s_{1})\notin \mathcal{B}_{\mu _{1}}$| ) is that the node pointing to |$(t_{1}^{\prime },s_{1})$| is |$(t_{3},s_{3})$| and that |$t_{3}\succ _{s_{1}}t_{2}$| . But we have teacher |$t_{2}^{\prime }$| who is less preferred by |$s_{1}$| than |$t_{2}$| , is not matched to |$s_{1}$| under |$\mu$| , but is under |$\mu ^{\prime }$| . In addition, since we assumed that node |$ (t_{2}^{\prime },s_{2})$| is 1S-BE-pointing to |$(t_{1},s_{1})$| under |$\mu$| , we can use Lemma 9 to be sure it is also pointing to |$(t_{1}^{\prime },s_{1})$| so that |$t_{1}^{\ast }$| in the above lemma would be |$t_{2}^{\prime }$| in the example. The argument in the proof below shows that this construction can be made in general.
Note first that because |$t_{1}$| desires |$s_{1}$| under |$\mu$| and |$\mu ^{\prime }$| , |$t_{1}$| must also desire |$s_{1}$| under |$\mu _{1}$| because, by Lemma 10, |$\mu ^{\prime }(t_{1})\succeq _{t_{1}}\mu _{1}(t_{1})$| . Now, because |$t_{1}$| blocks with |$s_{1}$| under |$ \mu$| , it means that there is |$t\in \mu (s_{1})$| s.t |$t_{1}\succ _{s_{1}}t$| . Fix one such teacher |$t$| . Since, by assumption, |$(t_{1},s_{1})\notin \mathcal{B}_{\mu _{1}}$| , it means that |$t$| is not matched to |$s_{1}$| under |$ \mu _{1}$| and so, when implementing |$C_{1}$| , |$t$| has been replaced by a teacher |$t^{\prime }$| such that |$t^{\prime }\succ _{s_{1}}t_{1}$| since |$ t_{1}$| does not block with |$s_{1}$| under |$\mu _{1}$| but desires |$s_{1}$| under |$\mu _{1}$| . Since |$t_{1}$| blocks with |$s_{1}$| under |$\mu ^{\prime }$| it means that there is a teacher |$t_{1}^{\prime }\in \mu ^{\prime }(s_{1})$| s.t |$t_{1}\succ _{s_{1}}t_{1}^{\prime }$| , let |$s_{1}^{\prime }:=\mu (t_{1}^{\prime })$| . Note first that |$(t_{1}^{\prime },s_{1}^{\prime })$| is part of the cycles of exchanges. To see this, observe that if it was not the case then we would have that |$t_{1}^{\prime }\in \mu (s_{1})$| , but because |$ (t_{1},s_{1})$| does not block |$\mu _{1}$| , |$t_{1}^{\prime }\notin \mu _{1}(s_{1})$| . Because |$\mu _{1}(t_{1})\succ _{t_{1}}\mu (t_{1})=s_{1}$| and, by Lemma 10, |$\mu ^{\prime }(t_{1})\succeq _{t_{1}}\mu _{1}(t_{1})$| , we conclude that |$t_{1}$| cannot be matched to |$s_{1}$| under |$\mu ^{\prime }$| , a contradiction. Hence, if the node |$(t_{1}^{\prime },s_{1}^{\prime })$| 1S-BE-points to |$(t,s_{1})$| then we can set |$t_{1}^{\ast }:=t_{1}^{\prime }$| and |$s_{1}^{\ast }:=s_{1}^{\prime }$| , and the argument is complete using Lemma 9. Now consider the case in which node |$(t_{1}^{\prime },s_{1}^{\prime })$| does not 1S-BE-point to |$(t,s_{1})$| . We already know that |$(t_{1}^{\prime },s_{1}^{\prime })$| is part of the cycles of exchanges, so let |$(\tilde{t},s_{1})$| be its successor under these cycles of exchanges ( |$s_{1}$| has to be part of this node since |$t_{1}^{\prime }\in \mu ^{\prime }(s_{1})$| ). If |$(t_{1}^{\prime },s_{1}^{\prime })$| was 1S-BE-pointing to |$( \tilde{t},s_{1})$| , then by Lemma 9 it would also point to |$(t,s_{1})$| , a contradiction. So node |$(t_{1}^{\prime },s_{1}^{\prime })$| does not 1S-BE-point to its successor under the cycles of exchanges, i.e., |$(\tilde{t},s_{1})$| . Thus, we have that |$t_{1}^{\prime }$| does not block with |$s_{1}$| under |$\mu$| (if he were to block with |$s_{1}$| , |$ (t_{1}^{\prime },s_{1}^{\prime })$| would be 1S-BE-pointing to some node which includes school |$s_{1}$| and so toward |$(\tilde{t},s_{1})$| , a contradiction) and, by condition 2 of Lemma 7, there is a teacher |$t_{1}^{\prime \prime }$| , whose node is part of the cycles of exchanges, who does not block with |$s_{1}$| under |$\mu$| , desires |$s_{1}$| , and has the highest priority among those who do not block with |$s_{1}$| under |$\mu$| and desire it. In particular, the node |$ (t_{1}^{\prime \prime },\mu (t_{1}^{\prime \prime }))$| 1S-BE-points to |$( \tilde{t},s_{1})$| , and so by Lemma 9 points also to |$(t,s_{1})$| . Since |$t_{1}^{\prime \prime }$| does not block with |$s_{1}$| under |$\mu$| but |$t_{1}$| does, it means that |$t_{1}\succ _{s_{1}}t_{1}^{\prime \prime }$| , so we can set |$t_{1}^{\ast }:=t_{1}^{\prime \prime }$| and |$s_{1}^{\ast }:=\mu (t_{1}^{\prime \prime })$| . Here again, we can use Lemma 9 to make sure that |$ (t_{1}^{\ast },s_{1}^{\ast })$| indeed points to all the nodes |$(t,s_{1})$| under the graph of 1S-BE. ■
Coming back to our example, we can modify the graph |$ (N^{\prime },E_{1})$| by deleting the edge |$\big((t_{3},s_{3}),(t_{1}^{\prime },s_{1})\big)$| and replacing it with |$\big((t_{2}^{\prime },s_{2}),(t_{1}^{\prime },s_{1})\big)$| . In doing so, we obtain a new subgraph of 1S-BE wherein each node still has in-degree one and so still has a cycle. But, by constructing this new graph, the matching once the new cycle is implemented keeps |$(t_{2},s_{1})$| as a blocking pair. This is illustrated in the left graph in Figure A.3 , and the new cycle is now |$(t_{2}^{\prime },s_{2})\rightarrow (t_{1}^{\prime },s_{1})\rightarrow (t_{4},s_{4})\rightarrow (t_{2}^{\prime },s_{2})$| . The general procedure is given below.

Graphs of |$(N^{\prime },E_{2})$| and |$(N^{\prime },E_{3})$| .
Let us assume there is a node |$(t_{1},s_{1})$| such that it is in |$\mathcal{B} _{\mu ^{\prime }}$| but not in |$\mathcal{B}_{\mu _{1}}$| . Fix a teacher |$t\in \mu (s_{1})$| such that |$t_{1}\succ _{s_{1}}t$| . We know that |$t$| must leave |$ s_{1}$| under |$\mu _{1}$| (because |$(t_{1},s_{1})$| does not block |$\mu _{1}$| ) and is replaced by a teacher |$t^{\prime }$| such that |$t^{\prime }\succ _{s_{1}}t_{1}$| . Since the teacher |$t_{1}^{\ast }$| identified in Lemma 11 satisfies |$t_{1}\succ _{s_{1}}t_{1}^{\ast }$| , we have that |$t^{\prime }\neq t_{1}^{\ast }$| . So under the graph |$(N^{\prime },E_{1})$| , because |$t^{\prime }$| replaces |$t$| when at |$s_{1}$| when we implement |$C_{1}$| , we must have that |$\big( (t^{\prime },\mu (t^{\prime })),(t,s_{1})\big)\in E_{1}$| , and because each node has in-degree one, |$\big((t_{1}^{\ast },s_{1}^{\ast }),(t,s_{1})\big) \notin E_{1}$| . But that node is an edge in the graph of 1S-BE by construction. We therefore define a new graph |$(N^{\prime },E_{2})$| where |$ E_{2}$| corresponds to |$E_{1}$| , all edges of the form |$\big((t^{\prime },\mu (t^{\prime })),(t,s_{1})\big)$| with |$t_{1}\succ _{s_{1}}t$| have been replaced by |$\big((t_{1}^{\ast },s_{1}^{\ast }),(t,s_{1})\big)$| , and |$ (t_{1}^{\ast },s_{1}^{\ast })$| is as in Lemma 11. So |$(N^{\prime },E_{2})$| is still a subgraph of the 1S-BE graph starting from |$\mu$| , and all the nodes in |$N^{\prime }$| still have in-degree one so that, using Lemma 8, we have a cycle |$C_{2}$| . We let |$\mu _{2}$| be the matching obtained once |$ C_{2}$| is implemented.
|$\mu ^{\prime }$| Pareto-dominates |$\mu _{2}$| for teachers.
|$(t_{1},s_{1}) \in \mathcal{B}_{\mu_{2}}$| .
Case 1: |$t_{1}^{\ast }=t_{1}^{\prime }$| . In that case, we know that |$t_{1}^{\prime }\in \mu ^{\prime }(s_{1})$| and so, trivially, that |$s_{1}=\mu ^{\prime }(t_{1}^{\ast })\succeq _{t_{1}^{\ast }}\mu_{2}(t_{1}^{\ast })=s_{1}$| .
Case 2: |$t_{1}^{\ast }=t_{1}^{\prime \prime }$| . If |$\mu ^{\prime }(t_{1}^{\prime \prime })=s_{1}$| then trivially, |$\mu ^{\prime }(t_{1}^{\prime \prime })\succeq _{t_{1}^{\prime \prime }}\mu _{2}(t_{1}^{\prime \prime })=s_{1}$| . Assume that |$\mu ^{\prime }(t_{1}^{\prime \prime })\neq \mu _{2}(t_{1}^{\prime \prime })$| and toward a contradiction that, |$\mu _{2}(t_{1}^{\prime \prime })=s_{1}\succ _{t_{1}^{\prime \prime }}\mu ^{\prime }(t_{1}^{\prime \prime })$| . By the proof of Lemma 11, we know that |$t_{1}^{\prime \prime }$| does not block with |$s_{1}$| under |$\mu$| , and since |$\mathcal{B}_{\mu ^{\prime }}\subseteq \mathcal{B}_{\mu }$| , he does not block with |$s_{1}$| under |$\mu ^{\prime }$| either. Again, using the proof of Lemma 11, we know that because, by assumption, |$(t_{1}^{\prime },s_{1}^{\prime })$| does not 1S-BE-point to |$(t,s_{1})$| , |$t_{1}^{\prime }$| therefore does not block with |$s_{1}$| under |$\mu$| . In addition, since |$t_{1}^{\prime }\in \mu ^{\prime }(s_{1})$| , we must have |$t_{1}^{\prime }$| desires |$s_{1}$| Thus, because, by construction of |$t_{1}^{\prime \prime }$| , teacher |$t_{1}^{\prime \prime }$| has the highest priority among those who do not block with |$s_{1}$| under |$\mu$| and desire |$s_{1}$| , we must have |$t_{1}^{\prime \prime }\succ _{s_{1}}t_{1}^{\prime }$| . Because |$t_{1}^{\prime }\in \mu ^{\prime }(s_{1})$| and by assumption |$t_{1}^{\prime \prime }$| desires |$s_{1}$| under |$\mu ^{\prime }$| , we obtain that |$(t_{1}^{\prime \prime },s_{1})\in \mathcal{B} _{\mu ^{\prime }}$| , which yields a contradiction since, again by construction of |$t_{1}^{\prime \prime }$| , we must have |$(t_{1}^{\prime \prime },s_{1})\notin \mathcal{B}_{\mu }$| .
For part 2 assume that |$(t_{1},s_{1})\notin \mathcal{B} _{\mu _{2}}$| . Since |$(t_{1},s_{1})\in \mathcal{B}_{\mu ^{\prime }}$| , we have that |$s_{1}\succ _{t_{1}}\mu ^{\prime }(t_{1})$| . In addition, by Lemma 10, |$\mu ^{\prime }(t_{1})\succeq _{t_{1}}\mu _{2}(t_{1})\succeq _{t_{1}}\mu (t_{1})$| and so |$s_{1}\succ _{t_{1}}\mu _{2}(t_{1})$| . Then because |$(t_{1},s_{1})\notin \mathcal{B}_{\mu _{2}}$| , we must have that all teachers |$t$| s.t |$t\in \mu (s_{1})$| and |$ t_{1}\succ _{s_{1}}t$| are not matched to |$s_{1}$| anymore under |$\mu _{2}$| , i.e., once cycle |$C_{2}$| is implemented. But under |$(N^{\prime },E_{2})$| the only incoming edge for a node |$(t,s_{1})$| with |$t_{1}\succ _{s_{1}}t$| is |$\big((t_{1}^{\ast },s_{1}^{\ast }),(t,s_{1})\big)$| , and, since |$t_{1}\succ _{s_{1}}t_{1}^{\ast }$| , it contradicts that |$(t_{1},s_{1})\notin \mathcal{B} _{\mu _{2}}$| since |$t_{1}$| feels justified envy toward |$t_{1}^{\ast }$| under |$\mu _{2}$| . ■
As for |$\mu _{1}$| , if we were sure that |$\mathcal{B}_{\mu ^{\prime }}\subseteq \mathcal{B}_{\mu _{2}}$| , the proof would be completed. However, as for |$\mu _{1}$| , this may not be the case. For instance, in the example, if we assume that |$t_{2}\succ _{s_{2}}t_{4}\succ _{s_{2}}t_{3}\succ _{s_{2}}t_{1}\succ _{s_{2}}t_{2}^{\prime }$| and |$s_{2}\succ _{t_{3}}s_{1}\succ _{t_{3}}s_{3}$| , we have that |$(t_{3},s_{2})\in \mathcal{B} _{\mu ^{\prime }}\subset \mathcal{B}_{\mu }$| . Then, when we implement the cycle |$C_{2}$| given in the left graph of Figure A.3 , we can see that we delete the blocking pair |$(t_{3},s_{2})$| and so |$(t_{3},s_{2})\notin \mathcal{B}_{\mu _{2}}$| . With this observation in mind, the idea now is to define a new graph, as we did when we constructed |$(N^{\prime },E_{2})$| from |$(N^{\prime },E_{1})$| , in order to be sure that this is a subgraph of 1S-BE and that it contains a cycle |$C_{3}$| , which, once implemented, yields a matching that keeps the desired blocking pairs.
For the general case, assume there is a pair |$ (t_{2},s_{2})$| s.t |$(t_{2},s_{2})\in \mathcal{B}_{\mu ^{\prime }}\subseteq \mathcal{B}_{\mu }$| but |$(t_{2},s_{2})\notin \mathcal{B}_{\mu _{2}}$| . In that case, we can apply exactly the same argument as in Lemma 11 and exhibit a teacher |$t_{2}^{\ast }$| such that |$t_{2}\succ _{s_{2}}t_{2}^{\ast }$| and |$(t_{2}^{\ast },s_{2}^{\ast })$| 1S-BE-points to all the nodes of the form |$(t,s_{2})$| under the graph of 1S-BE starting at |$\mu$| . However, when |$s_{2}=s_{1}$| if |$t_{2}^{\ast }\succ _{s_{1}}t_{1}^{\ast }$| then we reset |$t_{2}^{\ast }$| to be |$t_{1}^{\ast }$| . Then, we define a new graph |$(N^{\prime },E_{3})$| with |$E_{3}$| , where |$E_{3}$| corresponds to |$E_{2}$| where all edges of the form |$\big((t^{\prime },\mu (t^{\prime })),(t,s^{\prime })\big)$| with |$t_{2}\succ _{s_{2}}t$| are replaced by |$\big((t_{2}^{\ast },s_{2}^{\ast }),(t,s_{1})\big)$| , where |$ (t_{2}^{\ast },s_{2}^{\ast })$| as in the above paragraph. Here again, |$ (N^{\prime },E_{3})$| is indeed a subgraph of the graph associated with 1S-BE starting from |$\mu$| , and each node still has in-degree one. Applying Lemma 8, we get the existence of a cycle |$ C_{3}$| which, once implemented, yields to a matching |$\mu _{3}$| . In the example, |$t_{2}^{\ast }$| would be |$t_{1}$| and |$(N^{\prime },E_{3})$| is shown in the right graph of Figure A.3 .
It is easy to see that we can mimic the proof of Lemma 12 in order to obtain the following lemma.
|$\mu ^{\prime }$| Pareto-dominates |$\mu _{3}$| for teachers.
|$\{(t_{1},s_{1}),(t_{2},s_{2})\}\subset \mathcal{B}_{\mu _{3}}$| .
In the example, the unique |$t_{2}^{\ast }$| is |$t_{1}$| and the graph of |$(N^{\prime },E_{3})$| is given in the right graph of Figure A.3 . In that case, the cycle |$C_{3}$| is |$(t_{1},s_{1})\leftrightarrows (t_{2}^{\prime },s_{2})$| . Note that, once |$ C_{3}$| is implemented, we indeed have |$\{(t_{2},s_{1}),(t_{3},s_{2})\} \subset \mathcal{B}_{\mu _{3}}$| and |$\mathcal{B}_{\mu ^{\prime }}\subseteq \mathcal{B}_{\mu _{3}}$| so that we have found the desired matching.
Of course, in full generality, it is possible to have a pair |$(t_{3},s_{3})$| satisfying |$(t_{3},s_{3})\in \mathcal{B}_{\mu ^{\prime }}\subseteq \mathcal{B}_{\mu }$| while |$(t_{3},s_{3})\notin \mathcal{B}_{\mu _{3}}$| . In order to prove the desired result—namely that there is a cycle in the graph associated with 1S-BE starting from |$\mu$| which, once implemented, leads to a matching |$\tilde{\mu}$| such that |$\mu ^{\prime }$| Pareto-dominates |$\tilde{\mu}$| for teachers and satisfies |$\mathcal{B}_{\mu ^{\prime }}\subseteq \mathcal{B}_{\tilde{\mu}}$| —we would continue to apply the same logic. Because we have a finite environment, at some point we must find a matching |$\tilde{\mu}$| with the desired property.
H. Proof of Theorem 5
Starting from the initial assignment, the solid arrows in the graph below correspond to the graph associated with 1S-BE.

We add dashed arrows from one node to another if the teacher in the origin of the arrow prefers the school in the pointed node. These arrows are not actual arrows of the graph associated with 1S-BE and therefore cannot be used to select a cycle. These arrows only facilitate understanding of the argument.
When |$\succ$| is submitted, there are two possible choices of cycles in the graph:
A large cycle given by |$(t_{2},s_{2})\rightarrow(t_{3},s_{3})\rightarrow (t_{4},s_{4})\rightarrow (t_{5},s_{5})\rightarrow(t_{2},s_{2})$| . Denote this cycle by |$\bar{C}$| .
A small cycle given by |$(t_{2},s_{2})\rightarrow(t_{3},s_{3})\rightarrow (t_{5},s_{5})\rightarrow (t_{2},s_{2})$| . Denote this cycle by |$\underline{C}$| .
We decompose the analysis for these two cases.
Case A: Under |$\succ$| , |$\bar{C}$| is selected:
Now, assume that teacher |$t_{2}$| reports the following preference relation |$ \succ _{t_{2}}^{\prime }:s_{1},s_{5},s_{2}$| , while others report according to |$\succ$| . Under this profile, starting from the initial assignment, the graph associated with 1S-BE is

Now, there are two possible cycle choices.
Case A.1: The cycle chosen is |$ (t_{2},s_{2})\leftrightarrows (t_{5},s_{5})$| . Once carried out, the graph associated with 1S-BE starting from the new matching is

Clearly, there is a unique cycle |$(t_{4},s_{4})\leftrightarrows (t_{3},s_{3})$| . Consider the new matching once this cycle is implemented. Teacher |$t_{3}$| obtains his most favourite school. Hence, in the graph associated with 1S-BE starting from the new matching, node |$(t_1,s_1)$| will now point to node |$(t_{2},s_{5})$| . In this graph, the only cycle is |$ (t_{2},s_{5})\leftrightarrows (t_{1},s_{1})$| ; therefore, |$t_{2}$| is eventually matched to school |$s_{1}$| . Hence, |$t_{2}$| obtains his most preferred school under |$\succ_{t_2}$| , and we exhibit a profitable misreport.
Case A.2: The cycle chosen is |$ (t_{4},s_{4})\leftrightarrows (t_{3},s_{3})$| . Once carried out, the graph associated with 1S-BE starting from the new matching is

In this graph, there are three possible cycle choices:
|$(t_{2},s_{2})\rightarrow (t_{1},s_{1})\rightarrow (t_{5},s_{5})\rightarrow (t_{2},s_{2})$| : in that case, |$t_{2}$| is matched to |$s_{1}$| and so, again, we identified a profitable misreport.
|$(t_{2},s_{2})\leftrightarrows (t_{5},s_{5})$| : Once cleared, the only cycle that is left is |$(t_{1},s_{1})\leftrightarrows (t_{2},s_{5})$| ; therefore, |$t_{2}$| will be matched to |$s_{1}$| , leading to a successful manipulation.
|$(t_{1},s_{1})\leftrightarrows (t_{5},s_{5})$| : Once cleared, since |$t_{5}$| prefers |$s_{2}$| to |$s_{1}$| , there is a unique cycle left: |$(t_{5},s_{1})\leftrightarrows (t_{2},s_{2})$| . Once again, the manipulation of |$t_{2}$| is successful.
Thus, we have shown that, when cycle |$\overline{C} $| is selected under the profile |$\succ$| , teacher |$t_{2}$| has a profitable misreport irrespective of the possible selections of cycles performed after |$ t_2$| ’s deviation. Let us now move to the other case.
Case B: Under |$\succ$| , |$\underline{C}$| is selected:
Once this cycle is carried out, the graph associated with 1S-BE starting from the new matching is

There are two possible cycle choices.
Case B.1: Choose |$(t_{3},s_{5})\leftrightarrows (t_{4},s_{4})$| . Then, the matching obtained is the same as the one obtained when we selected cycle |$\bar{C}$| . Therefore, we can come back to Case A , and we know that |$t_{2}$| has a successful misreport.
Case B.2: Choose |$(t_{1},s_{1})\rightarrow (t_{3},s_{5})\rightarrow (t_{4},s_{4})\rightarrow (t_{2},s_{3})\rightarrow (t_{1},s_{1})$| . In this case, each teacher but teacher |$t_{4}$| gets his most preferred school. Hence, there are no more cycles in the new graph associated with 1S-BE. In particular, teacher |$t_{4}$| is matched to school |$ s_3$| . Now, assume that |$t_{4}$| submits the following preferences: |$\succ _{t_{4}}^{\prime }:s_{5},s_{4}$| . The graph associated with 1S-BE starting from the initial assignment is the same as the one under truthful reports (note that, although these are not the arrows of the graph of 1S-BE, the dashed arrow from |$(t_{4},s_{4})$| disappears). Therefore, again, we are left with a choice between cycle |$\bar{C}$| and |$\underline{C}$| .
If we carry out |$\underline{C}$| , the graph starting from the new matching will be given by the graph just above, except that |$(t_{4},s_{4})$| no longer points to |$(t_{2},s_{3})$| . Hence, we can pick only cycle |$(t_{3},s_{5})\leftrightarrows (t_{4},s_{4})$| ; therefore, |$t_{4}$| obtains his best school, and we identify a profitable misreport for teacher |$t_4$| .
If we select |$\bar{C}$| , we already know that we end up with matching |$\bar{\mu}$| , as defined above. Therefore, here again, |$t_{4}$| obtains his best school |$s_{5}$| and the manipulation is also a success.
To sum up, we have shown that, for each possible cycle selection under 1S-BE, there is a teacher who has a profitable misreport. Thus, no selection of the 1S-BE algorithm is strategy-proof, as we intended to show.
I. Proof of Theorem 6
I.1. preliminaries in random graph.
In the sequel, we will exploit two standard results in random graph theory that are stated in this section. It is thus worth introducing the relevant model of random graph. A graph |$G(n)$| consists of |$n$| vertices, |$V$| , and edges |$E\subseteq V\times V$| across |$V$| . A bipartite graph |$ G_{b}(n)$| consists of |$2n$| vertices |$V_{1}\cup V_{2}$| (each of equal size) and edges |$E\subset V_{1}\times V_{2}$| across |$V_{1}$| and |$V_{2}$| (with no possible edges within vertices in each side). Random (bipartite) graphs can be seen as random variables over the space of (bipartite) graphs. We will see two asymptotic properties of random graphs: one based on the notion of perfect matchings, the other on that of independent sets.
A perfect matching of |$G_{b}(n)$| is a subset |$E^{\prime }$| of |$E$| such that each node in |$V_{1}\cup V_{2}$| is contained in a single edge of |$ E^{\prime }$| .
(Erdös–Rényi) Fix |$p\in (0,1)$| . Consider a random graph that selects a graph |$G_{b}(n)$| with the following procedure. Each pair |$ (v_{1},v_{2})\in V_{1}\times V_{2}$| is linked by an edge with probability |$p$| independently (of edges created for all other pairs). The probability that there is a perfect matching in a realization of this random graph tends to |$ 1$| as |$n\rightarrow \infty$| .
The second important technical result is about so-called independent sets. An independent set of |$G(n)$| is |$\bar{V}\subseteq V$| such that for any |$(v_{1},v_{2})\in \bar{V}\times \bar{V}$| , |$(v_{1},v_{2})$| is not in |$E$| .
I.2. Proof of i. of Theorem 6
Thus, the |$\lim \inf$| of the expected fraction of teachers not moving is bounded away from |$0$| .
Note that the lower bound computed here can be improved. Indeed, for |$t$| not to move, one only needs that, for each school |$s\in S_{1}$| that |$t$| finds acceptable , there is a teacher |$r\in T_{2}$| s.t. |$r$| is ranked above |$t$| (according to |$s$| ’s true preferences). In general, simulations suggest that a much larger fraction of teachers are not moving. These simulations also show that the assumption we made above that |$ u_{1}>u_{2}+1$| is not necessary and that the result seems to hold in much broader contexts. 97
I.3. Proof of ii. of Theorem 6
In the sequel, we fix |$\mu _{0}$| and let |$T_{k}$| be |$\mu _{0}(S_{k})$| , where |$\mu _{0}$| is the initial allocation. We will prove the following result, which implies the first part of point ii. of Theorem 6.
Put another way, both |$(t,\mu _{0}(t^{\prime }))$| and |$(t^{\prime },\mu _{0}(t))$| block |$\varphi$| . Since, by definition, under |$\varphi$| , |$t$| is assigned |$\mu _{0}(t)$| and |$t^{\prime }$| is assigned |$\mu _{0}(t^{\prime })$| , there are still cycles in the graph associated with BE when starting from the assignment given by |$\varphi$| , which contradicts the fact that |$ \varphi$| is a selection of BE.
We know that, for the left hand-side above, |$\frac{|\hat{T}_{k}|}{|T_{k}|} \overset{p}{\longrightarrow }0$| as |$n\rightarrow \infty$| . By the law of large numbers, |$\frac{|\tilde{T}_{k}^{c}|}{|T_{k}|}\overset{p}{ \longrightarrow }1-\left( 1-\varepsilon \right) ^{2}$| , which can be made arbitrarily close to |$0$| , given that |$\varepsilon >0$| is arbitrary. Hence, we obtain that |$\frac{|\bar{T}_{k}^{c}|}{|T_{k}|}\overset{p}{\longrightarrow }0$| as |$n\rightarrow \infty$| , as we intended to prove.
Let us now move to the other part of point ii. of Theorem 6. We aim to show that there is a selection of BE that is asymptotically teacher-efficient, asymptotically school-efficient, and asymptotically stable. Note that, in our environment, asymptotic school-efficiency implies asymptotic stability. Hence, the following proposition is sufficient for this purpose.
In the sequel, we condition w.r.t. a realization of the random set |$ T_{k}^{0}\times S_{k}^{0}$| assuming that both |$\frac{|T_{k}^{0}|}{|T_{k}|}$| and |$\frac{|S_{k}^{0}|}{|S_{k}|}$| are greater than |$1-\delta$| . Now, fix |$ \varepsilon _{0}^{\prime }>0$| and note that, conditional on this, each teacher |$t\in T_{k}^{0}$| draws randomly 98 in |$S_{k}^{0}$| his |$\varepsilon _{0}^{\prime }|S_{k}^{0}|$| most favourite schools in |$S_{k}^{0}$| . Similarly, each school |$s\in S_{k}^{0}$| draws randomly in |$T_{k}^{0}$| its |$\varepsilon _{0}^{\prime }|T_{k}^{0}|$| most favourite teachers in |$T_{k}^{0}$| . We build a random bipartite graph on |$T_{k}^{0}\cup S_{k}^{0}$| where the edge |$(t,s)\in T_{k}^{0}\times S_{k}^{0}$| is added if and only if |$t$| ranks |$ s$| within his |$\varepsilon _{0}^{\prime }|S_{k}^{0}|$| most favourite schools in |$S_{k}^{0}$| and, similarly, |$s$| ranks |$t$| within its |$\varepsilon _{0}^{\prime }\left\vert T_{k}^{0}\right\vert$| most favourite teachers in |$ T_{k}^{0}$| . This random bipartite graph can be seen as a mapping from the set of ordinal preferences into the set of bipartite graph |$G_{b}(\left\vert T_{k}^{0}\right\vert )$| . We denote this random graph by |$\tilde{G}_{b}$| . While Lemma 14 does not apply directly to this type of random graph, we will claim below that this random graph has a perfect matching with probability approaching one as the market grows. Before stating and proving this result, we must define the following lemma.
With probability approaching one, for any teacher |$t\in T_{k}^{0}$| , any school |$s\in S_{k}^{0}$| with which |$\xi _{ts}\geq 1-\frac{\varepsilon _{0}^{\prime }}{2}$| must be within his |$ \varepsilon _{0}^{\prime }|S_{k}^{0}|$| most favourite schools in |$S_{k}^{0}$| . Similarly, with probability approaching one, for any school |$s\in S_{k}^{0}$| , any teacher |$t\in T_{k}^{0}$| , with whom |$\eta _{ts}\geq 1-\frac{ \varepsilon _{0}^{\prime }}{2}$| must be within its |$\varepsilon _{0}^{\prime }|S_{k}^{0}|$| most favourite teachers in |$T_{k}^{0}$| .
We now move to our statement on the existence of perfect matching in |$\tilde{ G}_{b}$| .
With probability going to |$1$| as |$n\rightarrow \infty$| , the realization of |$ \tilde{G}_{b}$| has a perfect matching.
Now, we build the mechanism |$\psi$| as follows. For each realization of ordinal preferences (for each |$k=1,...,K$| ), we build a graph on |$ T_{k}^{0}\cup S_{k}^{0}$| as defined above, i.e., where the edge |$(t,s)\in T_{k}^{0}\times S_{k}^{0}$| is added if and only if |$t$| ranks |$s$| within his |$ \varepsilon _{0}^{\prime }\left\vert S_{k}^{0}\right\vert$| most favourite schools in |$S_{k}^{0}$| and, similarly, |$s$| ranks |$t$| within its |$\varepsilon _{0}^{\prime }\left\vert T_{k}^{0}\right\vert$| most favourite teachers in |$ T_{k}^{0}$| . If there is perfect matching, then under |$\psi$| , teachers in |$ T_{k}^{0}$| are matched according to this perfect matching, while teachers in |$T_{k}\backslash T_{k}^{0}$| remain at their initial assignments. If there is no perfect matching, then under |$\psi$| , all teachers in |$T_{k}$| remain at their initial assignments. Assuming that |$\varepsilon _{0}^{\prime }+\delta <\varepsilon _{0}$| , we obtain that the mechanism built in that way is 2-IR. 99 To see this, consider a teacher |$t$| who is not matched to his initial school. This means that |$t$| is matched to a school |$s$| given by a perfect matching of the random bipartite graph. By construction, this means that |$t$| ranks |$s$| within his |$ \varepsilon _{0}^{\prime }\left\vert S_{k}^{0}\right\vert$| most favourite schools in |$S_{k}^{0}$| . Hence, |$s$| is within his |$\varepsilon _{0}^{\prime }\left\vert S_{k}^{0}\right\vert +\delta \left\vert S_{k}\right\vert$| most favourite schools in |$S_{k}$| . Since |$\varepsilon _{0}^{\prime }\left\vert S_{k}^{0}\right\vert +\delta \left\vert S_{k}\right\vert \leq (\varepsilon _{0}^{\prime }+\delta )\left\vert S_{k}\right\vert <\varepsilon _{0}\left\vert S_{k}\right\vert$| and because |$t\in T_{k}^{0}$| implies that |$ \mu _{0}(t)$| is not within |$t$| ’s |$\varepsilon _{0}|S_{k}|$| most favourite schools in |$S_{k}$| , we obtain that |$s$| is preferred by |$t$| to his initial assignment. Since a similar reasoning holds for schools, we obtain that |$ \psi$| is 2-IR.
Given that the conditioning event has a probability approaching |$1$| as |$ n\rightarrow \infty$| , this is even true without conditioning.
Now, without loss of generality, let us assume that |$\delta$| is small enough so that |$\varepsilon _{0}^{\prime }+\delta <\varepsilon$| . It remains to show that these |$\left( \varepsilon _{0}^{\prime }+\delta \right) \left\vert S_{k}\right\vert$| favourite partners in |$S_{k}$| (resp. |$T_{k}$| ) yield an idiosyncratic payoff greater than |$1-\varepsilon$| . The following lemma completes the argument.
With probability approaching |$1$| as |$n\rightarrow \infty$| , the |$\left( \varepsilon _{0}^{\prime }+\delta \right) \left\vert S_{k}\right\vert$| most favourite schools of each teacher in |$T_{k}$| yield an idiosyncratic payoff higher than |$1-\varepsilon$| and the |$\left( \varepsilon _{0}^{\prime }+\delta \right) \left\vert T_{k}\right\vert$| most favourite teachers of each school in |$S_{k}$| yield an idiosyncratic payoff higher than |$ 1-\varepsilon$| .
I.4. Proof of iii. of Theorem 6
Recall that |$T_{k}$| stands for |$\mu _{0}(S_{k})$| , where |$\mu _{0}$| is the initial allocation. Fix |$\zeta >0$| . We want to show that for any |$k=1,...,K$| , |$|\{t\in T_{k}\left\vert U_{t}(\text{TO-BE}(t))\geq U(u_{k},1)-\zeta \right. \}|/|T_{k}|$| converges to |$1$| in probability. In the sequel, we let |$ \varepsilon >0$| be small enough so that |$U(u_{k},1-\varepsilon )>U(u_{k},1)-\zeta$| for each |$k=1,...,K$| which is well-defined since functions |$U$| is continuous. Now, the following proposition is enough for our purpose.
Thus, |$\Pr (E)\rightarrow 1$| . Let |$T_{k}^{0}:=\{t\in T_{k}\left\vert \eta _{t\mu _{0}(t)}\leq 1-\delta \right. \}$| .
Hence, given that |$\frac{|\bar{T}_{k}^{0}|}{|T_{k}^{0}|}\overset{p}{ \longrightarrow }0$| , we must have that, with probability approaching |$1$| as |$ n$| approaches infinity, |$\frac{|\bar{T}_{k}^{c}|}{|T_{k}|}\leq 3\delta$| and so |$\frac{|\bar{T}_{k}|}{|T_{k}|}\geq 1-3\delta$| .
To recap, given event |$E$| and any realization of |$\left\{ \eta _{\mu _{0}(s)s}\right\} _{s\in S}$| , we have |$\frac{|\bar{T}_{k}|}{|T_{k}|}\geq 1-3\delta$| with probability approaching |$1$| as |$n\rightarrow \infty$| . Since the realization of |$\left\{ \eta _{\mu _{0}(s)s}\right\} _{s\in S}$| is arbitrary, we obtain that, given event |$E$| , |$\frac{|\bar{T}_{k}|}{|T_{k}|} \geq 1-3\delta$| with probability approaching |$1$| as |$n\rightarrow \infty$| . Since |$\Pr (E)\rightarrow 1\mbox{ as }n\rightarrow \infty$| , we obtain that |$ \frac{|\bar{T}_{k}|}{|T_{k}|}\geq 1-3\delta$| with probability approaching |$ 1$| as |$n\rightarrow \infty$| . Since |$\delta >0$| is arbitrarily small, we obtain |$\frac{|\bar{T}_{k}|}{|T_{k}|}\overset{p}{\longrightarrow }1$| as |$ n\rightarrow \infty$| , as claimed.
The statement is related to that of Che and Tercieux (2018) . However, since |$ \text{TO-BE}$| is not Pareto-efficient, their proof/argument does not apply.
The argument relies on the fact that |$\text{TO-BE}$| is not blocked by any coalition of size |$2$| . Hence, the result applies beyond the |$\text{TO-BE}$| mechanism and applies to any mechanism that cannot be blocked by any coalition of size |$2$| .

Correlation of region-entry cutoffs between 2012 and 2013
Notes: Figures A.4 and A.5 display correlation between the cutoff in 2012 and the cutoff in 2013 in math and French. In each region, the cutoff corresponds to the minimum value of the entering teachers priorities. In each subject, there is one cutoff per region and per year. The coefficient of correlation between the cutoffs in 2012 and 2013 is 0.98 in both subjects.
J. Teacher Preference Estimations
J.1. variables used for teacher preference estimations.
This Appendix describes the variables we use for teacher preference estimations. See Supplementary Appendix S.2 for further details.
We use the following regions’ characteristics:
Share of students classified as disadvantaged (labelled as “ |$\%$| disadv stud”).
Share of students living in an urban area (labelled as “ |$\%$| stud urban”).
Share of students who attend a school classified as “priority education” (labelled as “ |$\%$| stud in priority educ”). Priority education is a label given to the most disadvantaged schools in France.
Share of students who attend a private school (labelled as “ |$\%$| stud in private school”).
Share of teachers who are younger than 30 (labelled as “ |$\%$| teachers younger than 30”)
Region is in South of France (labelled as “Region in South of France”). The following 5 regions are classified as being in the South of France: Aix-Marseille, Bordeaux, Montpellier, Toulouse, and Nice.
We use the following teachers characteristics:
Current region of the teacher (labelled as “Current region”). This is the region a teacher is initially assigned to.
Region where a teacher was born (labelled as “Birth region”).
Distance between the region ranked and the current region of a teacher (labelled as “Distance current region”).
Number of years of teaching experience (labelled as “Teach exp”).
Squared number of years of teaching experience (labelled as “Teach exp sq”).
Teacher’s current region is Créteil or Versailles, which are the two least attractive regions (labelled as “Teach from CV”). The attractiveness of a region is measured by the ratio of the number of teachers who rank the region divided by the number of teachers who ask to leave the region.
Teacher is married (labelled as “Married”).
Teacher has spent at least 5 years in a school labelled as priority education (labelled as “Teach in disadv sch”).
Teacher has an advanced teaching qualification (labelled as “Advanced qualif”).
J.2. Estimation and counterfactual results
We report in this section preference estimates for teachers in sports, Spanish, history–geography, biology, physics–chemistry, and technology.
Notes: This table reports selected coefficients from estimations of teachers preferences for regions characteristics based on Equation 5.1 . We use the estimation method recently developed by Fack et al. (2019) . We use a discrete choice model with personalized feasible choice sets. For each teacher, his feasible choice set is the set of regions that have a cutoff smaller than his own score. We estimate parameters in columns 1, 3, and 5 using maximum likelihood. We set the fixed effect of the Paris region to |$0$| . The last row reports our goodness of fit measure, that we compute by looking at the top two schools that a teacher has included in his submitted rank order list (ROL). We then measure the probability of observing this particular preference ordering in the ROL predicted with our estimations. Stars correspond to the following p -values: * |$p<.05$| ; ** |$p<.01$| ; |$^{***}$| |$p<.001$| .
K. Extension of TO-BE with Newcomers and Vacant Seats
Definition of TO-BE with newcomers and vacant seats. There are several ways to extend TO-BE to an environment with newcomers and vacant seats. Recall that TO-BE is a version of TTC where, in essence, teachers preferences are modified in such a way that any region which finds him unacceptable is dropped from his preference list (see Appendix B for additional details). TTC has been extended to the environment with vacant seats and newcomers by Abdulkadiroğlu and Sonmez (1999) . A natural extension of TO-BE would therefore be a version of the algorithm they define in which regions finding a teacher unacceptable would be dropped from this teacher rank order list. This would be a straightforward approach to extend TO-BE while keeping its properties. However, we propose another extension. The motivation for this alternative is given by the following two requirements which are in line both with our theoretical approach so far as well as with our understanding of basic policy objectives in the context of teacher assignment. (However, we also ran the extension based on Abdulkadiroğlu and Sonmez (1999) on our data and the results are similar to what we obtain with our proposal.)
Requirement (1). One of the main requirements of our analysis is to Pareto-improve both sides of the market upon the initial assignment, which should be thought of as the previous year assignment. In our context, because most open seats correspond to teachers retiring (i.e. highly experienced teachers leaving their position), Pareto-improving the region side upon the initial assignment may simply not be feasible: there may be no teacher with (weakly) higher experience willing to replace the retired teachers. 100 However, even if this is not feasible, our first natural requirement is that the vacant positions of the regions should not be filled by newcomers, whenever possible. 101
Requirement (2). A key motivation in this paper is to design a mechanism which satisfies as much as possible the criteria of the college admission problem (i.e. strategy-proofness, 2-PE, and stability). With tenured teachers, this is non-trivial since, as already explained, these criteria conflict with individual rationality. However, in an environment with only newcomers and vacant seats, we know that the unique mechanism satisfying these criteria is the regular DA. Hence, we would like the extended version of TO-BE to coincide with DA in the environment with no tenured teachers.
Description of the mechanism. Given these two requirements, our proposed mechanism is a simple variation on the TO-BE mechanism defined in Section 4 and we thus only informally describe it below (details can be found in Supplementary Section S.7 ). We enrich the graph of TO-BE with nodes corresponding to each newcomer and vacant seat (we add node of the form |$(\emptyset,s)$| for each region |$s$| having at least one vacant seat). As in TO-BE, a newcomer points to a node |$(t,s)$| if he has a higher priority than teacher |$t$| at region |$s$| and |$s$| is his favourite region in his opportunity set. 102 We do not allow newcomers to point to a node corresponding to a vacant seat. However, any node |$(t,s)$| can point to a node |$(\emptyset,s^{\prime })$| if |$s^{\prime }$| is |$t$| ’s most favourite region in his opportunity set. In a first phase, the mechanism searches for cycles of exchange among tenured teachers—like TO-BE defined in Section 4 —and also searches for chains . Chains are sequences of nodes |$ \{t_{N},(t_{1},s_{1}),(t_{2},s_{2}),...,(t_{K},s_{K}),(\emptyset,s)\}$| starting with a newcomer |$t_{N}$| and ending with an empty seat where each node points to his successor, if any. The mechanism implements the cycles and chains iteratively. The fact that a newcomer cannot point to a node |$(\emptyset,s)$| ensures that vacant seats are only filled by tenured teachers in this first phase. Obviously, the assignment at the end of this phase may be wasteful, that is, there may be unmatched newcomers together with unfilled seats that are acceptable to these newcomers. Hence, in a second phase we simply run regular DA for unmatched newcomers and remaining unfilled positions.
The existence of the second phase not only solves the wastefulness issue, it also ensures that our mechanism trivially coincides with regular DA in the environment with no tenured teachers. This fulfils Requirement (2) above. Further, by construction, the mechanism tries to match unfilled positions first to tenured teachers. It eventually matches unfilled positions to newcomers only to avoid wastefulness. This is in line with our Requirement (1). Under the natural assumption that newcomers have a lower priority than tenured teachers, we show in the supplementary material that this mechanism is two-sided maximal and strategy-proof. Indeed, in our counter-factual analysis, this TO-BE mechanism is statistically indistinguishable from a two-sided maximal matching. 103 We also prove in this supplementary material that this extension of TO-BE is strategy-proof under the assumption that newcomers must rank all regions, a condition fulfilled in France. 104 Hence, this mechanism is a natural extension of the TO-BE mechanism defined in Section 4 which, in the French context, preserves its main properties.
Recent initiatives in the US have intended to measure teacher effectiveness and ensure that disadvantaged students have equal access to effective teachers. See for instance, Teach for America, Teach First in the UK, and, more generally, Race to the Top, the Teacher Incentive Fund, and the flexibility policy of the Elementary and Secondary Education Act.
Two important issues facing the teaching profession are the increasing shortage of qualified teachers ( Corcoran et al. , 1994 ) and the difficulty of retaining new teachers in the profession ( Boyd et al. , 2005 ).
This is the case, for example, in France ( Terrier, 2014 ), Germany, Czech Republic ( Cechlárová et al. , 2015 ), Italy ( Barbieri et al. , 2011 ), Turkey ( Dur and Kesten, 2014 ), Mexico ( Pereyra, 2013 ), Peru, Uruguay ( Vegas et al. , 2006 ), and Portugal.
In practice, several other criteria used to determine teacher priorities might also reflect broader social objectives. For instance, in France, spousal reunification and children reunification give a priority bonus to teachers at schools close to where their spouse or children live. Again, one can easily see the social objective motivating these priorities, namely, to allow for position exchanges that are not at the expense of teachers experience in (possibly disadvantaged) schools, except when an exchange can allow a teacher to join his/her family. This is what our approach will ensure.
Under the (standard) DA, it is well known that one can reassign teachers and make all of them better off, some strictly. However, this will be done at the expense of schools, given that the (standard) DA is in the Core and, hence, two-sided efficient. Here, in stark contrast with the standard DA, we show that, under the modified DA, two-sided efficiency is violated; i.e., both teachers and schools can be made better-off.
Teachers are the only strategic agents in this teacher assignment context.
This result is related to Erdil and Ergin (2017) , who characterize two-sided Pareto-efficient stable matchings in a two-sided matching framework with indifferences. We discuss the connection in more depth later in Section 6 . In particular, while there is no two-sided Pareto-efficient stable matching mechanism that is strategy-proof for agents on one side of the market (see, Erdil, 2014 ), we show that, in our environment, some two-sided maximal matching mechanisms are strategy-proof for teachers.
In a school choice setting, Dur et al. (2019) characterize a class of constrained efficient and partially stable matchings which shares some similarities with ours. We discuss the differences in details in Section 6 .
These markets can involve a large number of agents. For instance, in France, approximately 65,000 teachers ask for an assignment every year.
Our arguments build on techniques from random graph theory, as in Lee (2016) , Che and Tercieux (2018) , and Che and Tercieux (2019) .
See this link for a presentation of the “Base Transfer Process” and this link for a presentation of the Irish civil servant mobility scheme.
For instance, DA selects a fair matching that Pareto dominates all other fair mechanisms for the proposing side ( Gale and Shapley, 1962 ), and the top trading cycle mechanism ( Abdulkadiroğlu and Sonmez, 2003 ), which allows agents to sequentially trade their priorities, can be considered efficient with minimal unfairness ( Abdulkadiroğlu et al. , 2019 ).
See also Che and Tercieux (2019) for additional perspectives on this topic.
Noticeably, both sides of the market are Pareto improved.
Public sector teachers are civil servants. Their salary is completely regulated by a detailed pay scale. Neither schools nor teachers can influence salary or promotions. All teachers with the same number of years of experience and who have passed the same exam earn the same salary. Further details on the recruitment and assignment process are available on the Matching in Practice website.
An official list of criteria used to compute the point system is available on the government website .
In practice, couples from different subjects can submit joint applications. Since members of a couple may potentially teach in different fields, this creates some dependencies across subjects. We ignore the issues related to couples in this paper. In particular, we eliminated all couples from our sample. Details are provided in Supplementary Appendix S.6 .
Our results easily extend to the case of weak preferences for schools.
This is a strong notion. However, even with this conservative notion, we can significantly improve on the standard mechanisms. Using such a strong notion only strengthen this result.
Requiring individual rationality on both the teacher and school sides is non-standard but is equivalent to the requirement that the assignment Pareto dominates the initial assignment for both teachers and schools. This is consistent with our motivation for considering both sides of the market as welfare-relevant entities (see Section 1). Our empirical analysis indeed points out that this notion allows to better fulfil the administration objectives.
Usually, one also adds a non-wastefulness condition that requires that there must be no teacher |$t$| and school |$s$| such that |$s\succ _{t}\mu (t)$| and |$|\mu (s)|<q_{s}$| . Since we assumed that for any school |$s$| , |$|\mu _{0}(s)|=q_{s}$| and all teachers and schools are willing to be matched, under any 1-IR or 2-IR matching |$\mu$| , we have |$|\mu (s)|=q_{s}$| . Because our analysis focuses on such matchings, we can omit the non-wastefulness condition.
Using standard notation, |$\succ _{-t}$| denotes the vector of preference relations |$(\succ _{t^{\prime }})_{t^{\prime }\neq t}$| .
This is highlighted in Compte and Jehiel (2008) and Pereyra (2013) .
Formally, for each school |$s$| , a new preference relation |$\succ _{s}^{\prime }$| is defined such that |$t\succ _{s}^{\prime }t^{\prime }$| for each |$t\in \mu _{0}(s)$| and |$t^{\prime }\notin \mu _{0}(s)$| , and for each |$t,t^{\prime }$| not in the school’s initial assignment |$\mu _{0}(s)$| , we have |$t\succ _{s}^{\prime }t^{\prime }$| if and only if |$t\succ _{s}t^{\prime }$| . If |$t,t^{\prime }\in \mu _{0}(s)$| , we assume similarly that these teachers are ranked according to |$\succ _{s}$| . This is not necessary for the results.
One may argue that the definition of blocking pairs in our setting has to be changed to account for the individual rationality criterion. This is indeed the route taken by Compte and Jehiel (2008) and Pereyra (2013) . We discuss in details the differences with their approach in Section 6 .
Calsamiglia and Miralles (2016) study a school choice model and show that neighbourhood priorities when there is an unpopular school can decrease students’ welfare by leading most of them to stay at their neighbourhood school. The mechanism driving this welfare loss is similar to that of our example. The main difference is that, in our setting, we show that one can improve the welfare of both teachers and schools, which is not possible in a school choice framework.
Recall that the motivation for imposing 2-IR is to ensure that our assignments 2-Pareto-dominate the initial assignment and that, compared to the initial assignment, the set of blocking pairs shrinks.
To see that this algorithm converges in a finite number of steps, observe that, whenever we carry out a cycle, at least one teacher is strictly better off. Hence, in the worst case, one needs |$(n-1)n$| steps for this algorithm to end. Because finding a cycle in a directed graph can be solved in polynomial time, the algorithm converges in polynomial time.
The TTC mechanism is defined in the one-to-one environment and corresponds to TO-BE where the opportunity set of all teachers corresponds to the whole set of regions.
Our results would also go through with a collection |$(f_{(t,s)})_{(t,s)\in T\times S}$| of orderings over the teachers which are teacher–school specific. The class of strategy-proof selections of BE would be larger. However, as Theorem 2 will show below, only one collection, that is school-specific, is teacher-optimal as detailed in Section 4.3.1 .
However, TO-BE takes into account the school side in a weak sense: it only ensures that schools get assigned a teacher that is weakly preferred to the teacher they were initially assigned to. As Theorems 3 and 4 below show, in many contexts, this property is an implication of strategy-proofness and two-sided maximality.
As we already mentioned, the matching |$\mu ^{\prime }$| which Pareto-dominates |$\mu$| must also have a set of blocking pairs included in that of |$\mu$| .
In the one-to-one environment, since there is only one teacher per school, the ordering |$f$| is irrelevant.
In practice, 78 |$\%$| of teachers rank only one additional region above their initial one.
In a general many-to-one setting, one can exhibit a selection of BE that is strategy-proof but that is not in the class of TO-BE mechanisms.
Note that two-sided maximality implies 1-IR which, under domain restriction |$\mathcal{P}$| , implies strategy-proofness. Hence, all selections of BE are strategy-proof under domain restriction |$\mathcal{P}$| .
Henceforth, given a matching |$\mu$| , we say that |$t$| desires |$s$| if |$s\succ _{t}\mu (t)$| .
Note that, here, teacher |$t$| may block with |$s^{\prime}$| under condition (2). Thus, it is easy to see that, if (1) is satisfied, then (2) is also satisfied. Hence, one could simplify the definition and suppress condition (1). We keep this definition just to have a parallel with the definition of BE.
This algorithm shares some similarities with the Stable Improvement Cycle algorithm of Erdil and Ergin (2008) . We discuss how they relate in Section 6 .
Note that even if one wished to select one of the two other cycles, another cycle would lead to the same matching.
To see this, note that if |$F$| is the cumulative distribution of |$\xi _{ts}$| , then by the probability integral transform Theorem, |$F(\xi _{ts})$| is uniform over |$[0,1]$| . In addition, since |$F$| is strictly increasing and continuous, |$F^{-1}$| is well-defined and continuous. Hence, $$ \begin{eqnarray*} U_{t}(s) &=&U(u_{k},\xi _{ts}) \\ &=&U(u_{k},F^{-1}\circ F(\xi _{ts})) \\ &=&\hat{U}(u_{k},\hat{\xi}_{ts}) \end{eqnarray*}$$ where |$\hat{U}:=U(\cdot,F^{-1}(\cdot ))$| and |$\hat{\xi}_{ts}:=F(\xi _{ts})$| . Now, |$\hat{U}$| is strictly increasing and continuous and |$\hat{\xi}_{ts}$| follows a uniform distribution over |$[0,1]$| . Our assumption that the distribution of |$\xi_{ts}$| has bounded support is not used here. It ensures, however, that |$\hat{U}(u_{k},1)<\infty$| which will be useful for us to define asymptotic versions of efficiency or stability.
In France, in our dataset, we also observe that some regions are systematically preferred to others, as measured by the share of teachers ranking these regions first. This shows a pattern of tiers: whereas less than 0.5 |$\%$| of the teachers rank the unattractive regions of Amiens and Créteil first, more than 9 |$\%$| of the teachers rank each of the attractive regions of Paris, Bordeaux, or Rennes as their first choices. The differences observed are likely related to cross-regional differences in the proportion of students from disadvantaged social backgrounds and/or minority students.
While the implementation of the asymptotically stable selection of BE may not be practical, we consider this a benchmark and want to compare it to what can be achieved by a mechanism such as TO-BE.
Specifically, fix any |$\varepsilon >0$| , the expectation (when |$n$| goes to infinity) of |$\left\vert \{(t,s)\in T\times S\mid U_{t}(s)>U(\varphi (t))+\varepsilon \text{ and }V_{s}(t)>V(\varphi (t))+\varepsilon \}\right\vert$| divided by |$\left\vert \{T\times S\}\right\vert$| is greater when |$\varphi =$| TTC than when |$\varphi =$| TO-BE.
For each region R, a teacher has beliefs over the schools he may obtain provided that he gets assigned region R in Phase 1. These assessments can be based on annual publications by the ministry and the teacher unions of the cutoff score needed to enter each school (i.e. the lowest priority of the teachers who entered the region). Teachers know their own score in each school, so they can easily compare it to the cutoffs, which are fairly stable over time, as shown in Figures A.4 and A.5. The coefficient of correlation between the cutoffs in 2012 and 2013 is 0.93 in math and 0.98 in French. Coefficients are similar for other fields and other years.
Regarding (1), in 2013, about 25,000 teachers participated in the first phase and 65,000 in the second phase. 5,400 tenured teachers were assigned to a new region in the first phase, which represents only 8 |$\%$| of the teachers participating in the second phase. We also provide suggestive evidence regarding (2). In math and English, we find that for 71 |$\%$| and 83 |$\%$| of the regions, the average change in teacher priority that would be experienced by moving from DA |$^{\ast }$| to TO-BE is smaller than the average cutoff difference between districts of a given region (each region is partitioned into districts). This means that, for a teacher, his set of feasible districts is likely to remain similar when moving from DA |$^{\ast }$| to TO-BE.
Among all subjects, the number of teachers ranges from 2 to 1,753 teachers. The nine subjects we keep are sports, literature, English, mathematics, Spanish, history and geography, biology, physics, and technology.
This keeps the exercise in line with the model used in Section 3 . Further, our main theoretical insights in Section 4.1 show that DA |$^{\ast }$| (the algorithm currently used to assign teachers in France) fails to implement exchanges of positions across tenured teachers which can make both sides of the market better-off. These exchanges are made across tenured teachers and the analysis is thus made clearer by first ignoring newcomers and vacant positions. We discuss the robustness of our empirical results to the introduction of 4,627 newcomers in Section 5.5 .
To be accurate, the mechanism used in France is equivalent to DA |$^{\ast }$| . The ministry uses the following steps: (1) It considers teachers’ rankings and their modified priorities . (2) It runs the school-proposing deferred acceptance mechanism using the modified priorities and the reported preferences. (3) From this outcome, it runs stable improvement cycles (as defined in Erdil and Ergin, 2008 ) using the modified priorities. Using Theorem 1 in Erdil and Ergin (2008) , we know this process yields the outcome of the teacher-proposing deferred acceptance mechanism with the modified priorities.
Cutoff values for entry in each region are published every year. Figures A.4 and A.5 show that these cutoffs are relatively persistent over time, which provides reasonably accurate information to teachers on their chances to enter each region. The coefficient of correlation between the cutoffs in 2012 and 2013 is 0.93 in math and 0.98 in French. They are similar for other subjects and years.
For more references on estimations that do not require truthtelling, see Akyol and Krishna (2017) , Artemov et al. (2019), Agarwal and Somaini (2018) , and Calsamiglia et al. (2020) .
This means that teachers can rank unacceptable regions. In practice, teachers would rank regions up to their initially assigned region; this is the case, for instance, for the reported preferences in our dataset. Obviously, for individually rational mechanisms, the outcomes are the same.
To determine a teacher’s priority in his initial region, we only use the seniority criteria (total seniority in teaching and seniority in the current school). The other criteria currently used by the ministry (like spousal reunification or having a position in a disadvantaged school) are supposed to help a teacher leave his current region. It would not make sense to use these criteria for the region a teacher is initially assigned to.
Recall that we view teachers’ priorities as capturing social objectives of the designer. If one wants to take regions rankings as real regions preferences, our definition of priorities assumes that a region may not like incumbent teachers more than external teachers. In other words, we assume that the modified priorities used to run |$\mbox{DA}^{*}$| (in which incumbent teachers get the highest priority) may not reflect regions’ true preferences. With this interpretation, we acknowledge that there are arguments in favour and against this assumption: on one hand, an existing teacher might possess region-specific knowledge that might be valuable for a region. On the other hand, retaining teachers who deeply wish to leave a region can give rise to discontent and disengagement from teachers. So overall, we do take the interpretation that teachers⣙ priorities reflect the designer’s social objective. Our view is that the high priority that incumbent teachers are given under |$\mbox{DA}^{*}$| is not the actual priority, but just an artificial priority to guarantee the individual rationality of |$\mbox{DA}^{*}$| .
Note that our preference estimates (presented in Section 5.3 ) assume that each teacher obtains his most preferred region in his feasible set. Under this assumption, teachers may not sincerely report their preferences, which might seem at odds with our counterfactual analysis that assumes that teachers sincerely report their preferences. Yet, it is important to keep in mind that, under our identifying assumption, agents misreport only in an outcome irrelevant way (i.e. the matching obtained when misreporting is the same as the matching obtained when reporting sincerely). In our counterfactual analysis, we implicitly assume that this is the way in which teachers behave which is consistent with the assumption we make for preference estimations ( Artemov et al. , 2019 ).
To find such an assignment, we simply solve a linear assignment problem, see for instance Martello and Toth (1987) .
The first column of Table 3 shows that, in our simulated preferences, on average, 263.7 teachers rank their initial region first. This is because our estimation approach does not account for the fact that, in the reported preferences, all teachers rank at least one region above their initial one. In practice, because 78 |$\%$| of teachers rank only one additional region above their initial one, our estimation leads to a very high utility for the initial region. Combined with the unbounded gumbel shocks, this can lead some teachers to rank their initial region first.
This result holds for each subject and preference draw. Put in another way, with empirical frequency equal to 1, |$\mbox{DA}^{\ast }$| is not two-sided maximal. This, of course, implies that the result also holds when we average results across all subjects and preference draws.
More formally, let |$\mu := \mbox{DA}^{\ast }$| and |$\mu' := \mbox{TO-BE} \circ \mbox{DA}^{\ast }$| . Fix a cycle |$C$| implemented at some step of the TO-BE |$\circ \mbox{DA}^{\ast }$| mechanism. We say that a teacher |$t$| blocks cycle |$C$| if (i) |$\mu(t) = \mu_{0}(t)$| and there exists a teacher |$t'$| who is part of cycle |$C$| such that (ii) |$\mu'(t') \succ_{t} \mu(t)$| and |$t \succ_{\mu'(t')} t'$| . In other words, a cycle is blocked by a teacher if this teacher stays at his initial assignment under |$\mbox{DA}^{\ast}$| , envies region |$\mu'(t')$| and would justifiably envy teachers |$t'$| if the latter was matched to this region.
The teachers who are part of these blocked cycles represent 99.7 |$\%$| of the additional movement generated by |$\mbox{TO-BE} \circ \mbox{DA}^{\ast }$| .
The TO-BE mechanisms are parameterized by a collection of orderings over teachers, one for each region. We use that in which teachers are ordered according to their priority points within each region so that, following Theorem 2, the outcome is teacher optimal.
Note that the numbers we report are averaged across all subjects and preference draws. The rank distribution under TO-BE dominates that under |$\mbox{DA}^{\ast }$| in all preference draws and subjects up to rank 22.
Only 116.3 teachers (1.8 |$\%$| of the market) prefer their assignment under |$\mbox{DA}^{\ast}$| . 834.5 teachers prefer their TO-BE assignment to their |$\mbox{DA}^{\ast}$| assignment. We also checked which groups of teachers lose or gain. We find that all groups of teachers have a higher number of teachers who prefer their TO-BE assignment, except for teachers initially assigned to the disadvantaged regions of Créteil and Versailles. Out of the 3561 teachers initially assigned these regions, 33.3 are better-off under TO-BE and 65.8 are worse-off. This is because TO-BE is 2-IR whereas |$\mbox{DA}^{\ast}$| is not, which can restrict the mobility of teachers from disadvantaged regions. This effect is extensively discussed in Section 5.5 in which we incorporate vacant positions and teachers without an initial assignment. It can easily be solved by introducing a version of TO-BE that partly relaxes the 2-IR constraint as discussed in this section.
Priority differences between two regions can affect the mobility through two channels under TO-BE. First, high-priority teachers are able to point towards a larger number of the regions they list–remember that a teacher can only point if he has a higher priority than a teacher currently assigned that region. On the other hand, for a teacher to be able to exit his region, it must be that another teacher with a higher priority replaces him. Hence, having a high priority can sometimes lower the chances that a teacher will be able to move. This is the case when comparing the two regions of Nantes and Caen for instance. The same number of teachers move away from Caen (46.9 |$\%$| ) and from Nantes (46.1.6 |$\%$| ) despite the higher priority of teachers in Caen (125.3) than in Nantes (114.4).
Again, this is an average over our 5,000 draws. The region welfare distribution under TO-BE statistically dominates that under |$\mbox{DA}^{\ast }$| in 95 |$\%$| of the preference draws.
We think of TTC here as corresponding to TO-BE where the opportunity set of all teachers corresponds to the whole set of regions.
Their unattractiveness partly stems from the highly disadvantaged student populations they serve. Créteil and Versailles have the largest shares of students enrolled in “priority education” (25 |$\%$| and 35 |$\%$| ), a label given to the most disadvantaged schools.
We distinguish between teachers having only one or two years of experience and more experienced teachers based on evidence that teachers in their first year tend not to perform as well as more experienced teachers ( Chetty et al. , 2014 ; Rockoff, 2004 ). To report the results, we merge the two disadvantaged regions together on one side and the other regions on the other side and report the overall percentage of inexperienced teachers matched to each of these groups. Movements within a group of regions therefore do not count.
Note that the share of inexperienced teachers in disadvantaged regions is larger under TTC (50.5 |$\%$| ) than under TO-BE (50.2 |$\%$| ). More importantly, in non-disadvantaged regions, that share is significantly lower under TTC (26.8 |$\%$| ) than under TO-BE (29.5 |$\%$| ). This is consistent with the fact that TTC allows exchanges that harm regions, while TO-BE does not.
In terms of blocking pairs, BE leads to slightly fewer teachers blocking with at least one region compared with TO-BE which, here again, is consistent with Theorem 6.
|$\mbox{DA}^{\ast}$| is never two-sided maximal in our simulations, which implies that it is also never one-sided maximal.
Indeed, it is a standard assumption made in the theoretical literature (see for instance Pereyra, 2013 ). In our data, when considering the 8 subjects (we drop the subject Technology in our empirical analysis when dealing with newly recruited teachers and vacant positions because the number of newcomers is too small for estimation purposes; see the Supplementary Appendix S.7.4 ) and 25 regions, on average, newcomers have a lower priority than tenured teachers. When considering teacher rank in region priorities (on a scale of 0 to 1), we also find that in more than 75 |$\%$| of the regions, the rank difference between tenured and newcomers is larger than 0.3, which confirms that newly recruited teachers tend to have a much lower priority than tenured teachers.
Recall that DA |$^{\ast }$| is not two-sided maximal. However, under the same assumption that newcomers have a lower priority than tenured teachers, it is easy to show that |$\mbox{DA}^{\ast}$| will also assign tenured teachers first and newcomers to the remaining vacant seats.
The fit quality we obtain for the preference estimations remains very close to those we have when running the estimations on the market of tenured teachers. On average, for the eight subjects, our fit measure only goes down by 0.04 (which represents a reduction of 5.8 |$\%$| ).
By design, newcomers rank all regions (in particular disadvantaged regions) as acceptable. The ministry does so to ensure that a newcomer will be matched at the end of the procedure.
We can modify the algorithm described in Appendix K by simply allowing chains to start from tenured teachers who are initially matched to disadvantaged regions. When starting with a tenured teacher, this teacher is allowed to point directly to a school with a vacant seat.
All facts in Section 5 remain true except for Fact 3. The distribution of the number of regions with which teachers block under TO-BE does not stochastically dominate the one under DA.
Technically, Erdil and Ergin (2017) start from a stable matching and, then, run the BE algorithm on top of this matching. As long as agents preferences are not strict, the BE algorithm may exhibit cycles.
That is, 1-PE mechanisms that select two different matchings for two different preference profiles in which teachers preferences remain unchanged.
Formally, for a school |$s$| , its preference |$\succ_{s}$| is a group preference if for any teacher |$t^{\prime }\notin \mu_{0}(s)$| , either: i) |$\forall t \in \mu_{0}(s)$| , |$t^{\prime }\succ_{s} t$| or ii) |$\forall t \in \mu_{0}(s)$| , |$t \succ_{s} t^{\prime }$| .
To compute this statistic, for every discipline-by-region combination, we have defined the minimum and the maximum of the internal teachers priorities. Then, for every applicant teacher, we define his priority as “intermediate” if it is strictly higher than the minimum and strictly lower than the maximum.
However, in our application, teachers are unlikely to apply many times during their careers. Indeed, most teachers target either the region where their family lives or the region of their home town. Hence, once a desired region is obtained, they are unlikely to reapply in the short or medium term.
In his setting, teachers preferences are fixed over time while schools preferences/priorities can evolve.
In the French system, if a teacher gets assigned the school/region she asked for, she must transfer to that school/region.
More specifically, we start removing all teachers who get worst-off under TO-BE. Once removed we run TO-BE and again remove all teachers who get worse-off compared to what they were initially getting under DA*. We continue this procedure until no teacher is removed. We then averaged the number of teachers leaving over our draws. We provide further details on these simulations in Supplementary Section S.8 .
Our discussion suggests that there will be few teachers dropping out once we switch algorithms and these teachers may have only a negligible effect on the outcome of TO-BE. This is under a worst-case scenario where all teachers who are worse-off leave the market and no other teacher enters. We ran additional simulations where we increase participation by bootstrapping a fixed additional number of teachers in those teachers getting strictly better-off after the switch. As expected, the gap in terms of teachers’ mobility between TO-BE and DA* widens.
In order to argue that teachers’ preferences over regions are well-defined (assuming that these are not lexicographic), in Section 5.1 , we provided evidence showing how a change of mechanism in the first phase only marginally impacts the pool of participants in the second phase. We acknowledge that an implicit assumption here is that teachers participation is invariant to the counterfactual policy. This is one more reason why a full empirical analysis of teachers’ participation is important to understand the robustness of our empirical results.
Rich policy objectives trading-off mobility and equal geographic distributions in various two sided matching markets are further explored in our ongoing work ( Combe et al. , 2020 ).
While the statement is fairly intuitive, we provide the formal argument in Supplementary Appendix S.4 .
One can easily check that |$\mu ^{\prime }$| is a well-defined matching.
Note that the construction is different from the proof of point iii. of Theorem 1 since here, we consider the move from |$\mu_{0}$| to |$\mu$| and not the one from |$\mbox{TO-BE}_{f^{*}}(\succ)$| to |$\mu$| .
Remember that we are trying to build a matching |$\mu^{\prime }$| of objects that Pareto dominates at |$\succ^{\prime }$| the matching given by TTC |$(\succ^{\prime })$| .
Note that it is not possible for the pointing defined in Case 3 to cycle. Indeed, take the first iteration with |$\mu_{1}^{\prime }$| , in which each object points to the assigned teacher assigned under |$\mu_{1}^{\prime }$| , and each teacher in the Case 3 points to his assigned object under |$\mu_{1}^{\prime }$| . If the pointing does cycle, it means that a teacher, say for instance |$t_{k_{4}}$| , points to an object that a previous iteration of the Case 3 used, say for instance |$(t_{k_{2}},s)$| . In that case, it would mean that the object |$(t_{k_{2}},s)$| was assigned to both teacher |$t_{k_{2}}^{\prime }$| and teacher |$t_{k_{4}}^{\prime }$| under |$\mu_{1}^{\prime }$| , a contradiction.
This indeed defines a matching since |$\mu^{\prime }_{2}$| just reassign the objects corresponding to seats inside the same school |$s$| .
One needs at least two additional teachers into two different schools. If there are more teachers and more schools, one can trivially set their preferences s.t. they rank their initial school first so that the exchanges below are the only possible ones.
Case 3 in Lemma 10 can be illustrated in the example. The node |$(t,s)$| would be |$(t_{4},s_{4})$| in the right graph of Figure A.2 . |$t_{4}$| is matched to |$s_{3}$| under |$\mu _{1}$| but is matched to |$s_{2}$| under |$\mu ^{\prime }$| . Under |$C_{1}$| (i.e. |$(t_{3},s_{3})\rightarrow (t_{1}^{\prime },s_{1})\rightarrow (t_{4},s_{4})\rightarrow (t_{3},s_{3})$| ), node |$(t_{4},s_{4})$| points to |$(t_{3},s_{3})$| while |$(t_{2},s_{2})$| does not 1S-BE-point to |$(t_{3},s_{3})$| . Because |$(t_{2},s_{2})$| points to |$(t_{3},s_{3})$| in the cycle of exchanges, it means that |$t_{2}\in \mu ^{\prime }(s_{3})$| so that if |$t_{4}$| preferred |$s_{3}$| to his match under |$\mu ^{\prime }$| , |$s_{2}$| , it would imply that |$t_{4}$| blocks with |$s_{3}$| under |$\mu ^{\prime }$| while he does not under |$\mu$| , and so this would yield the contradiction.
Available upon request.
In the following, by randomly, we mean uniformly i.i.d.
This is without loss of generality because, if |$\Pr \left\{ \frac{\left\vert \left\{ t\in T_{k}\left\vert \xi _{t\psi (t)}\geq 1-\varepsilon \right. \right\} \right\vert }{\left\vert T_{k}\right\vert }>1-\delta \right\} \rightarrow 1$| then, |$\Pr \left\{ \frac{\left\vert \left\{ t\in T_{k}\left\vert \xi _{t\psi (t)}\geq 1-\varepsilon \right. \right\} \right\vert }{\left\vert T_{k}\right\vert }>1-\delta ^{\prime }\right\} \rightarrow 1$| for any |$\delta ^{\prime }>\delta$| .
In 2013, statistics from the French ministry of education (for the entire market) show that 9,468 teachers retired and there were 8,974 vacant positions.
We do not have characteristics of retired teachers associated to open seats. However, as we already pointed out, we do know that teachers in their early career tend not to perform as well as more experienced teachers ( Chetty et al. , 2014 ; Rockoff, 2004 ). This is the rationale for avoiding to replace retired teachers with newcomers.
All ties are broken using a collection of ordering |$f$| like in the TO-BE defined in Section 4 .
More specifically, out of our 5,000 preference draws, it is two-sided maximal in 69 |$\%$| of them. Moreover, on average, we can make 0.8 teachers better-off via a two-sided maximal matching Pareto-dominating TO-BE. With a variance of 0.54, it is not statistically different from zero. Finally, over all draws, a maximum of 3 teachers can be made better-off. Recall that we have 10,460 teachers in our data. For further details, see Supplementary Section S.7 .
In France, when teachers do not rank all regions, the ministry fills the list until it reaches 31 regions. This is to ensure that no new teacher remains unassigned because he ranked too few regions The methodology used by the ministry to fill the list is described at this link .
ABDULKADIROĞLU, A. and SONMEZ, T. ( 1999 ), “ House Allocation with Existing Tenants ”, Journal of Economic Theory , 1999, 88 , 233 – 260 .
Google Scholar
ABDULKADIROĞLU, A. and SONMEZ, T. , ( 2003 ), “ School Choice: A Mechanism Design Approach ”, American Economic Review , 93 , 729 – 747 .
AGARWAL, N. , and PATHAK, P. A. ( 2017 ), “ The Welfare Effects of Coordinated Assignment: Evidence from the New York City High School Match ”, American Economic Review , 107 , 3635 – 3689 .
Pathak, P. A. and Roth, A. E. ( 2009 ), “ Strategy-proofness versus Efficiency in Matching with Indifferences: Redesigning the New York City High School Match ”, American Economic Review , 99 , 1954 – 1978 .
CHE, Y.-K. , PATHAK, P. , ROTH, A. and TERCIEUX, O. ( 2019 ), “Efficiency, Justified Envy, and Incentives in Priority-based Matching ”, American Economic Review: Insights , 2 , 425 – 442 .
AGARWAL, N. and SOMAINI, P. ( 2018 ), “ Demand Analysis Using Strategic Reports: An Application to a School Choice Mechanism ”, Econometrica , 86 , 391 – 444 .
AKYOL, P. and KRISHNA, K. ( 2017 ), “ Preferences, Selection, and Value Added: A Structural Approach ”, European Economic Review , 91 , 89 – 117 .
ARTEMOV, G. , CHE, Y.-K. and HE, Y. ( 2019 ), “ Strategic ‘Mistakes’:: Implications for Market Design Research ” (Technical Report, mimeo).
BARBIERI, G. , ROSSETTI, C. , and SESTITO, P. ( 2011 ), “ The Determinants of Teacher Mobility: Evidence using Italian Teachers’ Transfer Applications ”, Economics of Education Review , 30 , 1430 – 1444 .
BOYD, D. , LANKFORD, H. , LOEB, S. and WYCKOFF, J. ( 2013 ), “ Analyzing the Determinants of the Matching of Public School Teachers to Jobs: Disentangling the Preferences of Teachers and Employers ”, Journal of Labor Economics , 2013, 31 , 83 – 117 .
HAMILTON, L. , LOEB, S. and WYCKOFF, J. ( 2005 ), “ Explaining the Short Careers of High-Achieving Teachers in Schools with Low-Performing Students ”, American Economic Review , 95 , 166 – 171 .
CALSAMIGLIA, C. and MIRALLES, A. ( 2016 ), “ Catchment Areas and Access to Better Schools ” ( Technical Report, mimeo ).
FU, C. and GELL, M. ( 2020 ), “ Structural Estimation of a Model of School Choices: The Boston Mechanism versus Its Alternatives ”, Journal of Political Economy , 128 , 642 – 680 .
CECHLÁROVÁ, K. , FLEINER, T. , MANLOVE, D. F. , MCBRIDE, I. and POTPINKOVÁ, E. ( 2015 ), “ Modelling Practical Placement of Trainee Teachers to Schools ”, Central European Journal of Operations Research , 23 , 547 – 562 .
CHE, Y.-K. and TERCIEUX, O. ( 2018 ), “ Payoff Equivalence of Efficient Mechanisms in Large Matching Markets ”, Theoretical Economics , 13 , 239 – 271 .
CHE, Y.-K. and TERCIEUX, O. , ( 2019 ), “ Efficiency and Stability in Large Matching Markets ”, Journal of Political Economy , 127 , 2301 – 2342 .
CHETTY, R. , FRIEDMAN, J. N. and ROCKOFF, J. E. ( 2014 ), “ Measuring the Impacts of Teachers I: Evaluating Bias in Teacher Value-Added Estimates ”, American Economic Review , 104 , 2593 – 2632 .
COMBE, J. , DUR, U. , TERCIEUX, O. , TERRIER, C. and UTKU ÜNVER, M. ( 2020 ), “ A Market Design Solution to Unfair Distribution of Teachers in Schools ” ( Technical Report, mimeo ).
COMPTE, O. and JEHIEL, P. ( 2008 ), “ Voluntary Participation and Re-assignment in Two-sided Matching ” ( Paris School of Economics , Unpublished mimeo ).
CORCORAN, S. P. , EVANS, W. N. and SCHWAB, R. M. ( 1994 ), “ Changing Labor-market Opportunities for Women and the Quality of Teachers, 1957–2000 ”, American Economic Review , 94 , 230 – 235 .
DEPP ( 2013 ), “ Bases sur la mouvement national inter-academique des enseignants ”, Direction de l Evaluation de la Prospective et de la Performance (DEPP) France .
DEPP ( 2014 ), “ Geographie de l’Ecole ”, Direction de l Evaluation de la Prospective et de la Performance (DEPP) France . https://www.data.gouv.fr/fr/datasets/geographie-de-lecole/ .
DUR, U. , ARDA GITMEZ, A. and YıLMAZ, Ö. ( 2019 ), “ School Choice under Partial Fairness ”, Theoretical Economics , 14 , 1309 – 1346 .
DUR, U. , ARDA GITMEZ, A. and KESTEN, O. ( 2014 ), “ Pareto Optimal, Strategy-proof, and Non-bossy Matching Mechanisms ” ( Technical Report, mimeo ).
DUR, U. M. and UTKU ÜNVER, M. ( 2019 ), “ Two-sided Matching via Balanced Exchange ”, Journal of Political Economy , 127 , 1156 – 1177 .
ERDIL, A. ( 2014 ), “ Strategy-proof Stochastic Assignment ”, Journal of Economic Theory , 151 , 146 – 162 .
ERDIL, A. and ERGIN, H. ( 2008 ), “ What’s the Matter with Tie-breaking? Improving Efficiency in School Choice ”, American Economic Review , 98 , 669 – 689 .
ERDIL, A. and ERGIN, H. , ( 2017 ), “ Two-sided Matching with Indifferences ”, Journal of Economic Theory , 171 ( Supplement C ), 268 – 292 .
FACK, G. , GRENET, J. and HE, Y. ( 2019 ), “ Beyond Truth-Telling: Preference Estimation with Centralized School Choice and College Admissions ”, American Economic Review , 109 , 1486 – 1529 .
GALE, D. and SHAPLEY, L. S. ( 1962 ), “ College Admissions and the Stability of Marriage ”, American Mathematical Monthly , 69 , 9 – 15 .
GRIMMETT, G. R. and McDIARMID, C. J. H. ( 1975 ), “ On Colouring Random Graphs ”, Mathematical Proceedings of the Cambridge Philosophical Society , 77 , 313 – 324 .
GUILLEN, P. and KESTEN, O. ( 2012 ), “ Matching Markets with Mixed Ownership: The Case for a Real-life Assignment Mechanism ”, International Economic Review , 53 , 1027 – 1046 .
HANUSHEK, E. A. , KAIN, J. F. and RIVKIN, S. G. ( 2004 ), “ Why Public Schools Lose Teachers ”, The Journal of Human Resources , 39 , 326 – 354 .
HAUSMAN, J. A. , ( 1978 ), “ Specification Tests in Econometrics ”, Econometrica , 46 , 1251 – 1271 .
HAUSMAN, J. A. and RUUD, P. A. ( 1987 ), “ Specifying and Testing Econometric Models for Rank-ordered Data ”, Journal of Econometrics , 34 , 83 – 104 .
JACKSON, K. C. ( 2009 ), “ Student Demographics, Teacher Sorting, and Teacher Quality: Evidence from the End of School Desegregation ”, Journal of Labor Economics , 27 , 213 – 256 .
LEE, S. ( 2016 ), “ Incentive Compatibility of Large Centralized Matching Markets ”, The Review of Economic Studies , 84 , 444 – 463 .
MA, J. ( 1994 ), “ Strategy-Proofness and the Strict Core in a Market with Indivisibilities ”, International Journal of Game Theory , 23 , 75 – 83 .
MARTELLO, S. and TOTH, P. ( 1987 ), “ Linear Assignment Problems ”, Surveys in Combinatorial Optimization , 132 , 259 – 282 .
Mediator of the French Ministry of Education ( 2015 ) “ Rapport du médiateur de l’éducation nationale et de l’enseignement supérieur ” ( Technical Report ).
PEREYRA, J. S. ( 2013 ), “ A Dynamic School Choice Model ”, Games and Economic Behavior , 80 , 100 – 114 .
Périer, P. and GURGAND, M. ( 2016 ), “ Attractivité du métier d’enseignant. Etat des lieux et perspectives ”, Conseil National d’Evaluation du Système Scolaire .
ROCKOFF, J. E. ( 2004 ), “ The Impact of Individual Teachers on Student Achievement: Evidence from Panel Data ”, American Economic Review , 94 , 247 – 252 .
ROTH, A. E. ( 1982 ), “ The Economics of Matching: Stability and Incentives ”, Mathematics of Operations Research , 7 , 617 – 628 .
ROTH, A. E. and POSTLEWAITE, A. ( 1977 ) “ Weak versus Strong Domination in a Market with Indivisible Goods ”, Journal of Mathematical Economics , 4 , 131 – 137 .
SHAPLEY, L. and SCARF, H. ( 1974 ), “ On Cores and Indivisibility ”, Journal of Mathematical Economics , 1 , 22 – 37 .
SÖNMEZ, T. and UTKU ÜNVER, M. ( 2010 ), “ House Allocation with Existing Tenants: A Characterization ”, Games and Economic Behavior , 69 , 425 – 445 .
TERRIER, C. ( 2014 ), “ Matching Practices for Secondary Public School Teachers “France ”, Matching in Practice . https://www.matching-in-practice.eu/matching-practices-of-teachers-to-schools-france/ .
VEGAS, E. , URQUIOLA, M. and CERDÃ N-INFANTES, P. ( 2006 ), “ Teacher Assignment, Mobility and their Impact on Equity and Quality of Education in Uruguay ” (Unpublished mimeo).
Supplementary data
Email alerts, citing articles via.
- Recommend to your Library
- Journals Career Network
Affiliations
- Online ISSN 1467-937X
- Print ISSN 0034-6527
- Copyright © 2024 Review of Economic Studies Ltd
- About Oxford Academic
- Publish journals with us
- University press partners
- What we publish
- New features
- Open access
- Institutional account management
- Rights and permissions
- Get help with access
- Accessibility
- Advertising
- Media enquiries
- Oxford University Press
- Oxford Languages
- University of Oxford
Oxford University Press is a department of the University of Oxford. It furthers the University's objective of excellence in research, scholarship, and education by publishing worldwide
- Copyright © 2024 Oxford University Press
- Cookie settings
- Cookie policy
- Privacy policy
- Legal notice
This Feature Is Available To Subscribers Only
Sign In or Create an Account
This PDF is available to Subscribers Only
For full access to this pdf, sign in to an existing account, or purchase an annual subscription.
- New Findings
- Health Care
- 2023 Annual Report
- 2022 Annual Report
- 2021 Annual Report
- Charter School Research Collaborative
- School Access and Quality Fellowship
The Design of Teacher Assignment: Theory and Evidence
Peer-reviewed publication.
Julien Combe, Olivier Tercieux, and Camille Terrier
To assign teachers to schools, a modified version of the well-known deferred acceptance mechanism has been proposed in the literature and is used in practice. The research shows that this mechanism fails to be fair and efficient for both teachers and schools. The researchers identify a class of strategy-proof mechanisms that cannot be improved upon in terms of both efficiency and fairness. Using a rich dataset on teachers’ applications in France, the researchers estimate teacher preferences and perform a counterfactual analysis. The results show that these mechanisms perform much better than the modified version of deferred acceptance. For instance, the number of teachers moving from their positions more than triples under our mechanism.
Subscribe for Updates
Browse Econ Literature
- Working papers
- Software components
- Book chapters
- JEL classification
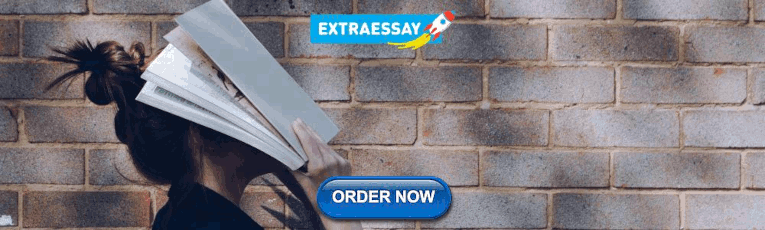
More features
- Subscribe to new research
RePEc Biblio
Author registration.
- Economics Virtual Seminar Calendar NEW!
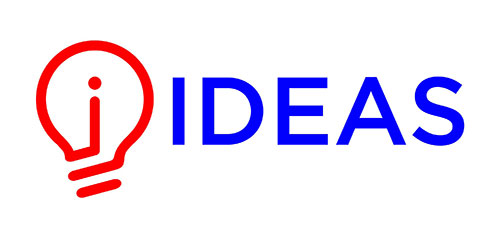
The Design of Teacher Assignment: Theory and Evidence [House Allocation with Existing Tenants]
- Author & abstract
- Download & other version
- 17 References
- 5 Citations
- Most related
- Related works & more
Corrections
- Julien Combe
- Olivier Tercieux
- Camille Terrier
Suggested Citation
Download full text from publisher, other versions of this item:, references listed on ideas.
Follow serials, authors, keywords & more
Public profiles for Economics researchers
Various research rankings in Economics
RePEc Genealogy
Who was a student of whom, using RePEc
Curated articles & papers on economics topics
Upload your paper to be listed on RePEc and IDEAS
New papers by email
Subscribe to new additions to RePEc
EconAcademics
Blog aggregator for economics research
Cases of plagiarism in Economics
About RePEc
Initiative for open bibliographies in Economics
News about RePEc
Questions about IDEAS and RePEc
RePEc volunteers
Participating archives
Publishers indexing in RePEc
Privacy statement
Found an error or omission?
Opportunities to help RePEc
Get papers listed
Have your research listed on RePEc
Open a RePEc archive
Have your institution's/publisher's output listed on RePEc
Get RePEc data
Use data assembled by RePEc
- By discipline
- By publishing or writing date
- By publication type
- Browse HAL SHS known laboratories
- HAL documentation
The Design of Teacher Assignment: Theory and Evidence
- Fonction : Auteur
- PersonId : 1183575
- IdHAL : combejumd
- PersonId : 1183578
Caroline Bauer : Connectez-vous pour contacter le contributeur
https://shs.hal.science/halshs-03917996
Soumis le : lundi 2 janvier 2023 à 12:06:01
Dernière modification le : mardi 21 mai 2024 à 03:18:00
Dates et versions
Identifiants.
- HAL Id : halshs-03917996 , version 1
- DOI : 10.1093/restud/rdac002
Collections
The Design of Teacher Assignment: Theory and Evidence.
Recommended format for most reference management software
Recommended format for BibTeX-specific software
- Combe, Julien (Author)
- Tercieux, Olivier (Author)
- Terrier, Camille (Author)
- Education Workforce
- Geodata in Education
- Teacher Education
- United States
- Czech Republic
- Haßler, B. (2022, April 27). Commonwealth Collaboration to Build Back Better . 21CCEM, Nairobi, Kenya. https://doi.org/10.53832/opendeved.0275
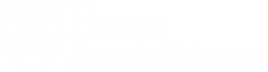
- University of Pennsylvania
- School of Arts and Sciences
Search Results
The design of teacher assignment: theory and evidence.
Tuesday, January 29, 2019 - 4:00pm - Tuesday, January 29, 2019 - 5:30pm
PCPSE Room 100 United States
More on Julien Combe
Sign Up Sheet
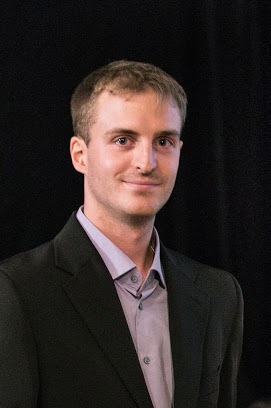
Julien Combe
University college london.
Download Paper
Latest News
- Unveiling Gender Inequalities March 8, 2024
- Mesurer le pluralisme à la télé avec l’IA : facile, à condition de savoir ce que l’on cherche March 7, 2024
- Récit écologique et conditions de vie March 6, 2024
- CRESTive Minds – Épisode 4 – Samuel Coavoux March 1, 2024
- Adaptation climatique : pourquoi a t-on besoin d’investissements privés ? March 1, 2024
- A panel organised and chaired by sociologist Paola Tubaro at the European Parliament in Brussels February 28, 2024
- Le Cercle des Économistes : Une interview de Pauline Rossi pour le podcast Génération Économie. February 28, 2024
- COP28 Sécurité de l’eau : question locale à impact international February 21, 2024
Announcements
We provide orientation programs to help students become a part of the campus community. Click here for more orientation details .
Financial Aid is available for all students enrolled in classes. Click here for financial aid information .
The Design of Teacher Assignment: Theory and Evidence
Journal: Review of Economics Studies
Author(s): Julien Combe, Olivier Tercieux, Camille Terrier
URL: https://academic.oup.com/restud/article/89/6/3154/6520472
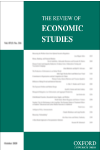
- Maps & Directions
- Faculty Emeritus
- Affiliated Members
- Support Staff
- PhD Students
- Undergraduate
- Job Market Candidates
- Job Openings
- CNRS Positions
- Job Placement
Skip to main content
Centre for Economic Performance
- Research programmes
- Community wellbeing
- Education and skills
- Labour markets
- Green Growth
- Related centres and projects
- Centre for Vocational Education Research (CVER)
- Economy 2030 Inquiry
- Programme on Innovation and Diffusion (POID)
- What Works Centre for Local Economic Growth
- Publications
- Academic research papers
- Discussion papers
- Occasional papers
- Journal articles
- Wider audience publications
- CentrePiece magazine
- Blogs and podcasts
- Policy analyses and reports
- Submissions to parliamentary inquiries
- External publications
- Audio and video
- Events calendar
- Public events, lectures and conferences
- Applications seminars
- Capabilities, competition and innovation seminars
- International economics seminars
- International economics workshops
- Labour markets workshops
- Policing and crime workshops
- Urban and regional economics seminars
- Education and skills seminars
- Vocational education research seminars
- Wellbeing seminars
- Press releases
- Media coverage
- Announcements
- Centre and programme directors
- All staff A-Z
- Support Staff
- Staff search
- CEP history
- Work for CEP
Sign up for updates
- +44 (0)20 7955 7673
- [email protected]
Search the Centre for Economic Performance Website
Labour Markets Workshops
The design of teacher assignment : theory and evidence
Camille terrier (university of lausanne and cep), tuesday 24 november 2015 12:45 - 14:00, sal 2.04, 2nd floor conference room, sir arthur lewis building, lse, 32 lincoln's inn fields, london wc2a 3ph.
Participants are expected to adhere to the CEP Events Code of Conduct.
This event will take place in SAL 2.04, 2nd Floor Conference Room, Sir Arthur Lewis Building, LSE, 32 Lincoln's Inn Fields, London WC2A 3PH.

Labour Markets Workshops are part of the CEP's Labour Markets programme.
All Labour Markets Workshops
Search all events, cep events code of conduct.
Aligning Learner-Centered Design Philosophy, Theory, Research, and Practice
- Reference work entry
- First Online: 15 October 2023
- Cite this reference work entry
- Cliff Zintgraff 4 &
- Atsusi Hirumi 5
143 Accesses
Learner-centered approaches to teaching and learning are favored by many educators and instructional designers. However, even those who favor learner-centered design (LCD) recognize that there are multiple interpretations and applications, and that some designs work better than others. Why is there such variance in LCD? One reason is the lack of alignment in LCD philosophy, theory, research, and practice. Lack of alignment begins with confusion over the plethora of constructs associated with LCD. Then, as the LCD process unfolds, alignment can suffer in two ways, top-down and bottom-up. Alignment should flow down from philosophies, to theory and research, and into the practice of instructional design, curriculum development, and delivery. Alignment should also flow bottom-up, from the practical constraints of the classroom, to those of curriculum development and instructional design, and then further to inform research, theory, and educational philosophies. Within a course, the learning objectives, strategies, and assessments must also be aligned to ensure the kind of internal consistency that is fundamental to the design of effective, efficient, and engaging learning experiences. Finally, when different researchers examine evidence, multiple overlapping interpretations contribute to differing conclusions about the effectiveness of LCD and resulting implications. To facilitate the alignment of LCD philosophy, theory, research, and practice, the authors pursue a coherent framework that captures the key elements of LCD and their relationships. Building on the framework, a simple model is proposed for assessing the artifacts of LCD, including documentation of LCD techniques, methods, curricula, and implementations in online and classroom settings. The framework and related assessments can guide future LCD research and practice.
This is a preview of subscription content, log in via an institution to check access.
Access this chapter
- Available as PDF
- Read on any device
- Instant download
- Own it forever
- Available as EPUB and PDF
- Durable hardcover edition
- Dispatched in 3 to 5 business days
- Free shipping worldwide - see info
Tax calculation will be finalised at checkout
Purchases are for personal use only
Institutional subscriptions
Abras, C., Maloney-Krichmar, D., & Preece, J. (2004). User-centered design. In W. Bainbridge (Ed.), Encyclopedia of human-computer interaction . Thousand Oaks, CA: Sage.
Google Scholar
Albion, P., Jamieson-Proctor, R., & Finger, G. (2010, March). Auditing the TPACK competence and confidence of Australian teachers: The teaching with ICT audit survey (TWICTAS). In Society for Information Technology and Teacher Education Conference (SITE), San Diego, California .
Alexander, H. (2006). A view from somewhere: Explaining the paradigms of educational research. Journal of Philosophy of Education, 40 (2), 205–221.
Article Google Scholar
American Psychological Association. (1993). Learner-centered psychological principles (ERIC Document Reproduction Service No. ED371994). ERIC Clearinghouse).
Atkinson, R. C., & Shiffrin, R. M. (1968). Human memory: A proposed system and its control processes. Psychology of Learning and Motivation, 2 , 89–195.
Bandura, A. (1994). Self-efficacy. In V. S. Ramachaudran (Ed.), Encyclopedia of Human Behavior, 4 , 71–81. New York: Academic Press.
Bannon, L., Bardzell, J., & Bødker, S. (2019). Reimagining participatory design. ACM Interactions, 26 (1), 27–32. ACM.
Barab, S., & Squire, K. (2004). Design-based research: Putting a stake in the ground. The Journal of the Learning Sciences, 13 (1), 1–14.
Barker, L., & Borko, H. (2011). Conclusion: Presence and the art of improvisational teaching. In R. K. Sawyer (Ed.), Structure and improvisation in creative teaching (pp. 279–298). New York, NY: Cambridge University Press.
Chapter Google Scholar
Barrows, H. S. (1985). How to design a problem based curriculum for the preclinical years . New York, NY: Springer Publishing.
Bednar, A., Cunningham, D. J., Duffy, T., & Perry, D. (1995). Theory in practice: How do we link? In G. Anglin (Ed.), Instructional technology: Past, present, and future (2nd ed., pp. 100–112). Englewood, CO: Libraries Unlimited.
Beghetto, R. A., & Kaufman, J. C. (2011). Teaching for creativity with disciplined improvisation. In R. K. Sawyer (Ed.), Structure and improvisation in creative teaching (pp. 94–109). New York, NY: Cambridge University Press.
Bernstein, R. J. (1995). The new constellation: The ethical-political horizons of modernity/postmodernity . Cambridge, MA: MIT Press.
Box, G. E. P. (1976). Science and statistics. Journal of the American Statistical Association, 71 , 791–799.
Brown, A. L. (1992). Design experiments: Theoretical and methodological challenges in creating complex interventions. Journal of the Learning Sciences, 2 , 141–178.
Brown, J. S., Collins, A., & Duguid, P. (1989). Situated cognition and the culture of learning. Educational Researcher, 18 (1), 32–42.
Bruner, J. S. (1961). The act of discovery. Harvard Educational Review, 31 , 21–32.
Burnard, P. (2011). Creativity, pedagogic partnerships, and the improvisatory space of teaching. In R. K. Sawyer (Ed.), Structure and improvisation in creative teaching (pp. 51–72). New York, NY: Cambridge University Press.
Bybee, R. W. (2002). Scientific inquiry, student learning, and the science curriculum. In R. W. Bybee (Ed.), Learning science and the science of learning (pp. 25–36). Arlington, VA: NSTA Press.
Chaiklin, S. (2003). The zone of proximal development in Vygotsky’s analysis of learning and instruction. In Vygotsky’s educational theory in cultural context (Vol. 1, pp. 39–64). New York, NY: Cambridge University Press.
Christou, C., Eliophotou-Menon, M., & Philippou, G. (2004). Teachers’ concerns regarding the adoption of a new mathematics curriculum: An application of CBAM. Educational Studies in Mathematics, 57 (2), 157–176.
Cobb, P., Confrey, J., diSessa, A., Lehrer, R., & Schauble, L. (2003). Design experiments in educational research. Educational Researcher, 32 (1), 9–13.
Cochrane, T., & Narayan, V. (2017). Design considerations for mobile learning. In C. M. Reigeluth, B. J. Beatty, & R. D. Myers (Eds.), Instructional-design theories and models, Volume IV: The learner-centered paradigm of education . New York, NY: Routledge.
Collins, A., Brown, J. S., & Newman, S. E. (1988). Cognitive apprenticeship: Teaching the craft of reading, writing and mathematics. Thinking: The Journal of Philosophy for Children, 8 (1), 2–10.
Computer Professionals for Social Responsibility. (n.d.). Participatory design. Retrieved November 1, 2020, from http://cpsr.org/issues/pd/
Cross, K. P. (1991, June 12). Every teacher a researcher, every classroom a laboratory. The Chronicle of Higher Education , p. B2.
CTGV (Cognition & Technology Group at Vanderbilt). (1992). The Jasper experiment: An exploration of issues in learning and instructional design. Educational Technology Research and Development, 40 (1), 65–80.
Cuban, L. (1986). Teachers and machines: The classroom use of technology since 1920 . New York, NY: Teachers College Press.
Cuban, L. (2001). Oversold and underused: Reforming schools through technology, 1980–2000 . Cambridge, MA: Harvard University.
Book Google Scholar
Dewey, J. (1900). Psychology and social practice. The Psychological Review, VII (2), 105–124.
Dewey, J. (1997). My pedagogic creed. In The curriculum studies reader (pp. 17–23). New York, NY: Routledge.
DeZutter, S. (2011). Professional improvisation and teacher education: Opening the conversation. In R. K. Sawyer (Ed.), Structure and improvisation in creative teaching (pp. 27–50). New York, NY: Cambridge University Press.
Dick, W., Carey, L., & Carey, J. O. (2015). The systematic design of instruction (8th ed.). Upper Saddle River, NJ: Pearson.
Dodge, B. (1998). The WebQuest page . Retrieved April 3, 2000, from http://edweb.sdsu.edu/webquest/webquest.html
Driscoll, M. P. (1994). Psychology of learning for instruction . New York, NY: Wiley.
Duffy, T. M. (2009). Building lines of communication and a research agenda. In S. Tobias & T. M. Duffy (Eds.), Constructivist instruction: Success or failure? New York, NY: Routledge.
Erickson, F. (1984). School literacy, reasoning, and civility: An anthropologist’s perspective. Review of Educational Research, 54 (4), 525–546.
Ertmer, P. A. (2005). Teacher pedagogical beliefs: The final frontier in our quest for technology integration? Educational Technology Research and Development, 53 (4), 25–39.
Ertmer, P. A., & Newby, T. (1993). Behaviorism, cognitivism, constructivism: Comparing critical features from an instructional design perspective. Performance Improvement Quarterly, 6 (4), 50–72.
Ertmer, P. A., & Newby, T. (1996). Students’ responses and approaches to case-based instruction: The role of reflective self-regulation. American Educational Research Journal, 33 (3), 719–752.
Ertmer, P. A., & Ottenbreit-Leftwich, A. T. (2010). Teacher technology change: How knowledge, confidence, beliefs, and culture intersect. Journal of Research on Technology and Education, 42 (3), 255–284.
FIRST. (2016). Vision and mission. Retrieved April 24, 2016, from http://www.firstinspires.org/about/vision-and-mission
Francom, G. M. (2017). Principles for task-centered instruction. In C. M. Reigeluth, B. J. Beatty, & R. D. Myers (Eds.), Instructional-design theories and models, Volume IV: The learner-centered paradigm of education . New York, NY: Routledge.
Gibbons, A. S., McConkie, M., Seo, K. K., & Wiley, D. A. (2009). Simulation approach to instruction. In C. M. Reigeluth & A. A. Carr-Chellman (Eds.), Instructional-design theories and models, Volume III: Building a common knowledge base . New York, NY: Routledge.
Gibson, J. T. (2009). Discussion approach to instruction. In C. M. Reigeluth & A. A. Carr-Chellman (Eds.), Instructional-design theories and models, Volume III: Building a common knowledge base (Vol. 3) [Kindle DX Version]. New York, NY: Routledge.
Grayling, A. C. (1998). Philosophy 1: A guide through the subject . Oxford, UK: Oxford University Press.
Guba, E. G., & Lincoln, Y. S. (1994). Competing paradigms in qualitative research. In Handbook of qualitative research (Vol. 2, No. 163–194, p. 105). Thousand Oaks, CA: Sage.
Gustafson, K. L., & Branch, R. M. (2002). What is instructional design? In R. A. Reiser & J. V. Dempsey (Eds.), Trends and issues in instructional design and technology (Vol. 1, pp. 16–25). Upper Saddle River, NJ: Prentice-Hall.
Hall, G. E., & Hord, S. M. (Eds.). (1987). Change in schools: Facilitating the process . New York, NY: SUNY Press.
Hall, G. E., & Hord, S. M. (2006). Implementing change: Patterns, principles, and potholes . Boston, MA: Pearson.
Hannafin, M. J., Hannafin, K. M., Land, S., & Oliver, K. (1997). Grounded practice in the design of learning systems. Educational Technology Research and Development, 45 (3), 101–117.
Harris, K. S. (2005). Teachers’ perceptions of modular technology education laboratories. Journal of Industrial Teacher Education, 42 (4), 52–71.
Harris, J., Mishra, P., & Koehler, M. (2009). Teachers’ technological pedagogical content knowledge and learning activity types: Curriculum-based technology integration reframed. Journal of Research on Technology in Education, 41 (4), 393–416.
Hirumi, A. (2002). Student-centered, technology-rich, learning environments (SCenTRLE): Operationalizing constructivist approaches to teaching and learning. Journal for Technology and Teacher Education, 10 (4), 497–537.
Hirumi, A. (Ed.). (2014a). Grounded designs for online and hybrid learning: Practical guidelines for educators and instructional designers. Book I – Design fundamentals . Eugene, WA: International Society for Technology in Education.
Hirumi, A. (Ed.). (2014b). Grounded designs for online and hybrid learning: Practical guidelines for educators and instructional designers. Book II – Designs in action . Eugene, WA: International Society for Technology in Education.
Hirumi, A. (Ed.). (2014c). Grounded designs for online and hybrid learning: Practical guidelines for educators and instructional designers. Book III – Trends and technology . Eugene, WA: International Society for Technology in Education.
Hirumi, A., Johnson, K., Kleinsmith, A., Reyes, R., Rivera-Gutierrez, D., Kubovec, S., … Cendan, J. (2017). Advancing virtual patient simulations and experiential learning with InterPLAY: Examining how theory informs design and design informs theory. Journal of Applied Instructional Design, 6 (1), 49–65. https://doi.org/10.28990/jaid2017.061005 .
Hmelo-Silver, C. E., Duncan, R. G., & Chinn, C. A. (2007). Scaffolding and achievement in problem-based and inquiry learning: A response to Kirschner, Sweller, and Clark (2006). Educational Psychologist, 42 , 99–107.
Holmes Group. (1990). Tomorrow’s schools: Principles for the design of professional development schools . East Lansing, MI: The H.G. Inc.
Hoy, W. K., & Adams, C. M. (2015). Quantitative research in education: A primer . Los Angeles, CA: Sage.
Interaction Design Foundation. (n.d.). User-centered design. Retrieved November 1, 2020, from https://www.interaction-design.org/literature/topics/user-centered-design#:~:text=User%2Dcentered%20design%20(UCD),and%20accessible%20products%20for%20them
Jenlink, P. M. (2013). Situated cognition theory. In B. Irby, G. H. Brown, R. Lara-Alecio, & S. A. Jackson (Eds.), The handbook of educational theories (pp. 185–198). Charlotte, NC: Information Age Publishing.
Jonassen, D. (1999). Designing constructivist learning environments. In Instructional design theories and models: A new paradigm of instructional theory (Vol. 2, pp. 215–239). Erlbaum.
Kirschner, P. A., Sweller, J., & Clark, R. E. (2006). Why minimal guidance during instruction does not work: An analysis of the failure of constructivist, discovery, problem-based, experiential, and inquiry-based teaching. Educational Psychologist, 41 (2), 75–86.
Kolb, D. A. (1984). Experiential learning: Experience as a source of learning and development . Englewood Cliffs, NJ: Prentice-Hall.
Kozulin, A., Gindis, B., Ageyev, V. S., & Miller, S. M. (2003). Vygotsky’s educational theory in cultural context . New York, NY: Cambridge University Press.
Kuniavsky, M. (2010). Smart things: Ubiquitous computing user experience design . Amsterdam, Netherlands: Elsevier Science.
Lave, J., & Wenger, E. (1991). Situated learning: Legitimate peripheral participation . Cambridge, UK: Cambridge University Press.
Learning-theories.com (2020a). Educational robotics and constructionism (Papert). Retrieved January 9, 2021 from https://www.learning-theories.com/educational-robotics-and-constructionism.html .
Learning-theories.com (2020b). Situated cognition (Brown, Collins, & Duguid). Retrieved January 9, 2021 from https://www.learning-theories.com/situated-cognition-brown-collins-duguid.html .
Lefrancois, G. R. (1997). Psychology for teachers (9th ed.). Belmont, CA: Wadsworth.
Lexico. (2019). Practice. Retrieved July 30, 2019, from https://www.lexico.com/en/definition/practice
Lindsey, L., & Berger, N. (2009). Simulation approach to instruction. In C. M. Reigeluth & A. A. Carr-Chellman (Eds.), Instructional-design theories and models, Volume III: Building a common knowledge base . New York, NY: Routledge.
Markham, T., Larmer, J., & Ravitz, J. (2003). Project based learning handbook: A guide to standards-focused project based learning for middle and high school teachers . Novato, CA: Buck Institute for Education.
Martin, L. C., & Towers, J. (2011). Improvisational understanding in the mathematics classroom. In R. K. Sawyer (Ed.), Structure and improvisation in creative teaching (pp. 252–278). New York, NY: Cambridge University Press.
McKay, C. S., & Glazewski, K. D. (2017). Designing maker-based instruction. In C. M. Reigeluth, B. J. Beatty, & R. D. Myers (Eds.), Instructional-design theories and models, Volume IV: The learner-centered paradigm of education . New York, NY: Routledge.
McKenney, S. E., & Reeves, T. (2018). Conducting educational design research: What, why and how . London, England: Taylor & Francis.
Melchior, A., Burack, C., Hoover, M., & Marcus, J. (2016). FIRST longitudinal study: Findings at follow-up (year 3 report) . Waltham, MA: The Center for Youth and Communities, Heller School for Social Policy and Management, Brandeis University.
Meyers, C., & Jones, T. B. (1993). Promoting active learning: Strategies for the college classroom . San Francisco, CA: Jossey-Bass.
Mishra, P., & Koehler, M. (2006). Technological pedagogical content knowledge: A framework for teacher knowledge. The Teachers College Record, 108 (6), 1017–1054.
Moos, D. C. (2011). Self-regulated learning and externally generated feedback with hypermedia. Journal of Educational Computing Research, 44 (3), 265–297.
Morrison, G. R., Ross, S. M., Kemp, J. E., & Kalman, H. (2010). Designing effective instruction . Hoboken, NJ: Wiley.
Myers, R. D., & Reigeluth, C. M. (2017). Designing games for learning. In C. M. Reigeluth, B. J. Beatty, & R. D. Myers (Eds.), Instructional-design theories and models, Volume IV: The learner-centered paradigm of education . New York, NY: Routledge.
Nix, R. K. (2012). Cultivating constructivist classrooms through evaluation of an integrated science learning environment. In B. J. Fraser, K. G. Tobin, & C. J. McRobbie (Eds.), Second international handbook of science education (pp. 1291–1303). Dordrecht, The Netherlands: Springer.
Norman, D. (2013). The design of everyday things: Revised and expanded edition . New York, NY: Basic Books.
Novak, G. M., & Beatty, B. J. (2017). Designing just-in-time instruction. In C. M. Reigeluth, B. J. Beatty, & R. D. Myers (Eds.), Instructional-design theories and models, Volume IV: The learner-centered paradigm of education . New York, NY: Routledge.
Palincsar, A. S. (1986). The role of dialogue in providing scaffolded instruction. Educational Psychologist, 21 , 261–277.
Palincsar, A. S. (1998). Social constructivist perspectives on teaching and learning. Annual Review of Psychology, 49 (1), 345–375.
Palincsar, A. S., & Brown, A. L. (1984). Reciprocal teaching of comprehension-fostering and monitoring activities. Cognition and Instruction, 1 , 117–175.
Papert, S., & Harel, I. (1991). Situating constructionism. Constructionism, 36 , 1–11.
Peirce, C. S. (1878). How to make our ideas clear. Popular Science Monthly, 12 , 286–302.
Prince, M. J., & Felder, R. M. (2006). Inductive teaching and learning methods: Definitions, comparisons, and research bases. Journal of Engineering Education, 95 (2), 123–138.
Project Lead the Way. (2014). PLTW . Retrieved November 25, 2014, from http://www.pltw.org
Reigeluth, C. M., Beatty, B. J., & Myers, R. D. (Eds.). (2017). Instructional-design theories and models, Volume IV: The learner-centered paradigm of education [Kindle DX Version]. New York, NY: Routledge.
Reigeluth, C. M., & Carr-Chellman, A. A. (Eds.). (2009a). Instructional-design theories and models, Volume III: Building a common knowledge base . New York, NY: Routledge.
Reigeluth, C. M., & Carr-Chellman, A. A. (2009b). Understanding instructional theory. In C. M. Reigeluth & A. A. Carr-Chellman (Eds.), Instructional-design theories and models, Volume III: Building a common knowledge base (Vol. 3, pp. 3–26) [Kindle DX Version]. New York, NY: Routledge.
Reigeluth, C. M., Myers, R., & Lee, D. (2017). Chapter 1, The learner-centered paradigm of education. In C. M. Reigeluth, B. J. Beatty, & R. D. Myers. (Eds.), Instructional-design theories and models, Volume IV: The learner-centered paradigm of education (Vol. 4) [Kindle DX Version]. New York, NY: Routledge.
Savery, J. R. (2015). Overview of problem-based learning: Definitions and distinctions. In Essential readings in problem-based learning: Exploring and extending the legacy of Howard S. Barrows (pp. 5–15). Purdue University Press.
Sawyer, R. K. (Ed.). (2011). Structure and improvisation in creative teaching . New York, NY: Cambridge University Press.
Schmidt, H. G., Loyens, S. M. M., van Gog, T., & Paas, F. (2007). Problem-based learning is compatible with human cognitive architecture: Commentary on Kirschner, Sweller, and Clark (2006). Educational Psychologist, 42 , 91–97.
Schwartz, D. L., Lindgren, R., & Lewis, S. (2009). Constructivism in an age of non-constructivist assessments. In Constructivist instruction (pp. 46–73). New York, NY: Routledge.
Scott, C. (2012). An investigation of science, technology, engineering and mathematics (STEM) focused high schools in the US. Journal of STEM Education: Innovations and Research, 13 (5), 30–39.
Siemens, G. (2014). Connectivism: A learning theory for the digital age. International Journal of Instructional Technology and Distance Learning, 2 (1). Retrieved May 22, 2017, from http://www.itdl.org/journal/jan_05/article01.htm
Smith, P. L., & Ragan, T. J. (1999). Instructional design (2nd ed.). New York, NY: Wiley.
Spector, J. M. (2014). Program and project evaluation. In J. M. Spector (Ed.), Handbook of research on educational communications and technology (pp. 195–201). New York, NY: Springer.
Spinuzzi, C. (2005). The methodology of participatory design. Technical Communications, 52 (2), 163–174.
Strayer, J. F. (2017). Chapter 12, Designing instruction for flipped classrooms. In C. M. Reigeluth, B. J. Beatty, & R. D. Myers (Eds.), Instructional-design theories and models, Volume IV: The learner-centered paradigm of education (Vol. 4). New York, NY: Routledge.
Sweller, J. (1988). Cognitive load during problem solving: Effects on learning. Cognitive Science, 12 (2), 257–285.
Sweller, J., Kirschner, P. A., & Clark, R. E. (2007). Why minimally guided teaching techniques do not work: A reply to commentaries. Educational Psychologist, 42 (2), 115–121.
Taylor, P. C., Fraser, B. J., & White, L. R. (1994). CLES: An instrument for monitoring the development of constructivist learning environments. In Annual meeting of the American Educational Research Association , New Orleans, LA.
Teichmann, J., & Evans, K. C. (1999). Philosophy: A beginner’s guide . Oxford, UK: Blackwell Publishing.
Texas Education Agency. (2014, August). TEA news releases online . Retrieved December 15, 2014, from http://tea.texas.gov/news_release.aspx?id=25769815392
Tobias, S., & Duffy, T. M. (Eds.). (2009). Constructivist instruction: Success or failure? New York, NY: Routledge.
Van de Pol, J., Volman, M., & Beishuizen, J. (2010). Scaffolding in teacher–student interaction: A decade of research. Educational Psychology Review, 22 (3), 271–296.
Veenman, M. V., Van Hout-Wolters, B. H., & Afflerbach, P. (2006). Metacognition and learning: Conceptual and methodological considerations. Metacognition and Learning, 1 (1), 3–14.
Verenikina, I. (2003). Understanding scaffolding and the ZPD in educational research. In Proceedings of the international education research conference (AARE – NZARE), 30 November–3 December 2003, Auckland, New Zealand.
Voorhees, R. A., & Voorhees, A. B. (2017). Principles for competency-based education. In C. M. Reigeluth, B. J. Beatty, & R. D. Myers (Eds.), Instructional-design theories and models, Volume IV: The learner-centered paradigm of education . New York, NY: Routledge.
Vygotsky, L. S. (1978). Mind in society . Cambridge, MA: Harvard University Press.
Waring, S. M. (2011). Conducting authentic historical investigations in the digital age. In A. Hirumi (Ed.), Grounded designs for online and hybrid learning: Practical guidelines for educators and instructional designers . Eugene, WA: International Society for Technology in Education.
Wiley, D. A. (2000). Connecting learning objects to instructional design theory: A definition, a metaphor, and a taxonomy. In D. A. Wiley (Ed.), The instructional use of learning objects . Bloomington, IN: Agency for Instructional Technology and Association for Educational Communications & Technology.
Wise, A. F., & O’Neill, K. (2009). Beyond more versus less: A reframing of the debate on instructional guidance. In Constructivist instruction (pp. 94–117). New York, NY: Routledge.
Zintgraff, A. C., Jr. (2016). STEM professional volunteers in K-12 competition programs: Educator practices and impact on pedagogy (Doctoral dissertation).
Download references
Acknowledgments
The authors acknowledge the role of Dr. Michael Spector and the National Science Foundation-funded Cyberlearning Early Career Workshop-University of North Texas for organizing the activities that led to this publication.
Author information
Authors and affiliations.
IC2 Institute, The University of Texas at Austin, Austin, TX, USA
Cliff Zintgraff
University of Central Florida, Orlando, FL, USA
Atsusi Hirumi
You can also search for this author in PubMed Google Scholar
Corresponding author
Correspondence to Cliff Zintgraff .
Editor information
Editors and affiliations.
Department of Learning Technologies, University of North Texas, Denton, TX, USA
J. Michael Spector
Instructional Design and Technology, Virginia Tech School of Education, Blacksburg, VA, USA
Barbara B. Lockee
Marcus D. Childress
Section Editor information
Department of Educational Technology, Boise State University, Boise, Idaho, USA
Ross A. Perkins
Rights and permissions
Reprints and permissions
Copyright information
© 2023 Springer Nature Switzerland AG
About this entry
Cite this entry.
Zintgraff, C., Hirumi, A. (2023). Aligning Learner-Centered Design Philosophy, Theory, Research, and Practice. In: Spector, J.M., Lockee, B.B., Childress, M.D. (eds) Learning, Design, and Technology. Springer, Cham. https://doi.org/10.1007/978-3-319-17461-7_119
Download citation
DOI : https://doi.org/10.1007/978-3-319-17461-7_119
Published : 15 October 2023
Publisher Name : Springer, Cham
Print ISBN : 978-3-319-17460-0
Online ISBN : 978-3-319-17461-7
eBook Packages : Education Reference Module Humanities and Social Sciences Reference Module Education
Share this entry
Anyone you share the following link with will be able to read this content:
Sorry, a shareable link is not currently available for this article.
Provided by the Springer Nature SharedIt content-sharing initiative
- Publish with us
Policies and ethics
- Find a journal
- Track your research
Fachportal Pädagogik

- in Literatur suchen
- in Forschungsdaten suchen
- in Forschungsinformation suchen

Groß-/Kleinschreibung : wird nicht unterschieden.
Verknüpfung von Suchbegriffen: Und-Verknüpfung ist voreingestellt
Platzhalter:
- * für mehrere Zeichen
- _ für ein einzelnes Zeichen innerhalb eines Wortes
Suche auf Angebotsteil einschränken Oberhalb der Eingabezeile finden Sie 3 Checkboxen, mit deren Hilfe Sie die Suche auf einen oder mehrere Angebotsteile einschränken oder erweitern können. Die Voreinstellung variiert je nach Standort. Die gewünschte Treffermenge können Sie nach der Suche per Tab auswählen.
Suche mit Autocomplete (Suchwortvervollständigung) Nach Eingabe der ersten Buchstaben wird eine Liste mit den am häufigsten vorkommenden Indextermen (Schlagwörter, Personen) der FIS Bildung Literaturdatenbank angezeigt. Es können mehrere Werte in ein Feld übernommen werden. Die Eingabe eines eigenen Suchbegriffs ist möglich.
Suchphrase : muss in " " eingeschlossen werden.
Manual zur Literaturrecherche auf YouTube Dank an die Universitätsbibliothek der Justus-Liebig-Universität Gießen
Informationen über den Suchraum
Ariadne Pfad:
Literaturnachweis - detailanzeige.
Feld Schlagwörter - Suchen Sie Ihr Suchwort? (fallweise eingeblendet)
Hinter dem Link verbirgt sich jeweils eine Menge an Stichworten, die aus den oberhalb angezeigten englischsprachigen Schlagworten abgeleitet wurden. Falls der Abgleich Ihrer Suchworte mit dem Nachweis keine Gemeinsamkeiten zeigte, können Sie hier nachschauen (+ klicken) und fündig werden.
Es handelt sich zum Einen um Übersetzungen ins Deutsche, die dem FIS Bildung-Schlagwortbestand entnommen wurden. Zum Anderen wurden zusammengesetzte englische Schlagworte in Terme zerlegt, die in der Regel nur einen inhaltlichen Aspekt repräsentieren. Ergänzend wurden Synonyme und vereinzelt zusätzliche Pluralformen hinzugefügt. Diese Anreicherung geht auf die Nutzung intellektueller Vorarbeiten zurück.
Sinn und Zweck dieser Maßnahme ist es, die bislang in der Regel deutschsprachigen Suchworte einerseits mit der englischsprachigen Sacherschließung der ergänzenden Datenquellen des Fachinformationsdienstes Erziehungswissenschaft und Bildungsforschung andererseits zu verbinden.
- Suchverlauf
Literaturbeschaffung und Bestandsnachweise in Bibliotheken prüfen
Standortgebundene Dienste
Suchen im Datenbestand Ihrer Institution
Falls Ihr Rechner sich im Netzwerk einer bei uns registrierten Einrichtung befindet, wird Ihnen automatisch ein Link angeboten, über den Sie die Literatur in den Beständen Ihrer Einrichtung suchen bzw. finden können. Dazu vergleichen wir die IP-Adresse Ihres Rechners mit den Einträgen unserer Registrierung. Eine Speicherung Ihrer IP-Adresse findet nicht statt. Von außerhalb der registrierten Institutionennetzwerke können Sie sich mit Hilfe der Liste "Institution wählen" manuell zuordnen um o.g. Link zu erzeugen.
Elektronische Zeitschriftendatenbank (EZB) UB Regensburg
Falls Ihr Rechner sich im Netzwerk einer bei uns registrierten Einrichtung befindet und der Zeitschriftentitel des gewählten Artikel-Nachweises durch die EZB erfasst ist, bekommen Sie einen Link angeboten, der Sie zum entsprechenden Eintrag leitet. Dort bekommen Sie weitere Hinweise zur Verfügbarkeit.
Standortunabhängige Dienste
Die Anzeige der Links ist abhängig vom Dokumenttyp: Zeitschriftenartikel sind, sofern verfügbar, mit einem Link auf den passenden Eintrag des Zeitschriftentitels in der Zeitschriftendatenbank (ZDB) der Staatsbibliothek Berlin versehen. Dort kann man sich über die Einrichtungen informieren, die die jeweilige Zeitschrift lizensiert haben. Der Link auf das Bestellformular von Subito überträgt die Daten direkt in das Bestellformular. Die Bestellung einer Artikelkopie setzt ein Konto dort voraus. Die Bestellung ist kostenpflichtig. Publikationen in Buchform erzeugen einen Link auf die ISBN-Suchseite der Wikipedia. Von dort aus haben Sie die Möglichkeit die Verfügbarkeit in einer Vielzahl von Katalogen zu prüfen.
Dateien exportieren oder versenden Informationen zu dauerhaft gespeicherten Einstellungen Markieren Sie in der Trefferlisteliste die Einträge, deren Nachweise Sie exportieren wollen. Im Bereich "Exportieren" wählen Sie in der Auswahlliste "System/Format wählen" eines der Exportformate bzw. -systeme. Wollen Sie die gewählten Nachweise direkt in Ihre Literaturverwaltung importieren, so wählen Sie das entsprechende System aus und drücken den Schalter "exportieren" . Die weiteren Schritte sind abhängig von der installierten Software. Eine detaillierte Beschreibung finden Sie, zusammen mit den einzelnen Formatbeschreibungen auf unserer Hilfeseite . Die Formate können Sie auf Ihrem Rechner als Textdatei abspeichern. Alle Formate können zusätzlich auch als Datei an eine beliebige E-Mailadresse versendet werden. Gehen Sie vor wie beim normalen Export. Tragen Sie zusätzlich die E-Mailadresse ein und aktivieren Sie den Schalter "versenden" . Der Versand der Datei erfolgt mit der passenden Dateiextension im Dateinamen. Achten Sie auf die korrekte Eingabe Ihrer Mailadresse , um den Verlust Ihrer Rechercheergebnisse zu vermeiden. Das Fachportal Pädagogik unterstützt die Übernahme bibliografischer Daten in andere Systeme durch Einbettung so genannter COinS in den HTML-Quellcode. Sie erkennen die Möglichkeit der Übernahme durch eine Meldung Ihres Browsers.
Permalink als qr-code.
Inhalt auf sozialen Plattformen teilen (nur vorhanden, wenn Javascript eingeschaltet ist)

Jump to navigation
Formulaire de recherche
The design of teacher assignment: theory and evidence.
Julien COMBE (UCL)
21 janvier 2019, 14h00–15h30
Salle MS001
Job Market Seminar
The reassignment of teachers to schools is a central issue in education policies. In several countries, this assignment is managed by a central administration that faces a key constraint: ensuring that teachers obtain an assignment that they weakly prefer to their current position. To satisfy this constraint a variation on the Deferred Acceptance (DA) mechanism of Gale and Shapley (1962) has been proposed in the literature and used in practice|for example, in the assignment of French teachers to schools. We show that this mechanism fails to be efficient in a strong sense: we can reassign teachers in a way that i) makes them better-off and ii) better fulfills the administration's objectives represented by the priority rankings of the schools. To address this weakness, we characterize the class of mechanisms that do not suffer from this efficiency loss and elicit a set of strategy-proof mechanisms within this class. To empirically assess the extent of potential gains associated with the adoption of our mechanisms, we use a rich dataset on teachers' applications for transfers in France. These empirical results confirm both the poor performance of the modified DA mechanism and the significant improvements that our alternative mechanisms deliver in terms of teachers' mobility and administration's objectives.
- Smartphone Calendrier
You are using an outdated browser. Please upgrade your browser to improve your experience.
There is a newer version of the record available.
Replication package for: The Design of Teacher Assignment: Theory and Evidence
- Camille Terrier 2
- Olivier Tercieux 3
- 1. CREST & Ecole polytechnique
- 2. University of Lausanne
- 3. CNRS & Paris School of Economics
Description
Replication files for the paper Combe, J., Tercieux, O., & Terrier, C. (2021). The design of teacher assignment: Theory and evidence. Review of Economic Studies
The file contains 5 folders and 1 Readme file, please read the readme file:
- The folder 0 contains all the tables and graphs of the paper
- The folder 1 contains a readme and all the Matlab codes and inputs used to run the estimation of preferences
- The folder 2 contains a readme and all the Matlab codes and inputs used to run the counterfactual simulations (to be done after the previous step)
- Folders 3 & 4 contain Stata do files used to produce minor descriptive tables/graphs of the paper (Table 1 and Graph A.1)
NOTE: many input data cannot be shared due to legal restrictions. The readme files contain the ministry in charge of the production of the data.
Replication_package_CTT.zip
Files (646.8 kb).
This site uses cookies. Find out more on how we use cookies
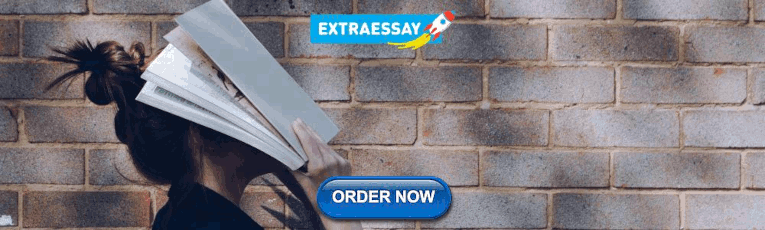
IMAGES
VIDEO
COMMENTS
Julien Combe, Olivier Tercieux, Camille Terrier, The Design of Teacher Assignment: Theory and Evidence, The Review of Economic Studies, Volume 89, Issue 6, November 2022, ... The Design of Teacher Assignment: Theory and Evidence - 24 Hours access EUR €51.00 GBP £44.00 ...
THE DESIGN OF TEACHER ASSIGNMENT: THEORY AND EVIDENCE Julien Combe, Olivier Tercieux and Camille Terrier APPENDIX. A Proof of Lemma1. Consider the directed graph G := (N;E) of BE starting at the matching (0) = . For each school ss.t. 0(s) 6= (s), consider the sets of teachers T.
Peer-reviewed Publication. To assign teachers to schools, a modified version of the well-known deferred acceptance mechanism has been proposed in the literature and is used in practice. The research shows that this mechanism fails to be fair and efficient for both teachers and schools. The researchers identify a class of strategy-proof ...
teacher assignments that respect the administration objectives, as reflected by the priority system. In the empirical section (Section 5) of this article, we examine the French teacher assignment system and show that its underlying social objectives are better fulfilled when using assignment schemes that satisfy two-sided efficiency.
Abstract. To assign teachers to schools, a modified version of the well-known deferred acceptance mechanism has been proposed in the literature and is used in practice. We show that this mechanism ...
Julien Combe & Olivier Tercieux & Camille Terrier, 2022. " The Design of Teacher Assignment: Theory and Evidence [House Allocation with Existing Tenants] ," The Review of Economic Studies, Review of Economic Studies Ltd, vol. 89 (6), pages 3154-3222. Handle: RePEc:oup:restud:v:89:y:2022:i:6:p:3154-3222. Downloadable (with restrictions)! To ...
The Design of Teacher Assignment: Theory and Evidence Julien Combey Olivier Tercieuxz Camille Terrierx December 21, 2016 Abstract To assign teachers to schools, a modi ed version of the well-known deferred accep-tance mechanism has been proposed in the literature and is used in practice. We show
The Design of Teacher Assignment: Theory and Evidence. In several countries, teachers' assignment to schools is managed by a central administration. One of the objectives of this reassignment process is to make sure that teachers obtain an assignment which they weakly prefer to their current position. The Deferred-Acceptance mechanism (DA ...
The Design of Teacher Assignment: Theory and Evidence House Allocation with Existing Tenants. Julien Combe, Olivier Tercieux and Camille Terrier. The Review of Economic Studies, 2022, vol. 89, issue 6, 3154-3222 . Abstract: To assign teachers to schools, a modified version of the well-known deferred acceptance mechanism has been proposed in the literature and is used in practice.
The Design of Teacher Assignment: Theory and Evidence Résumé: en. To assign teachers to schools, a modified version of the well-known deferred acceptance mechanism has been proposed in the literature and is used in practice. ... The Design of Teacher Assignment: Theory and Evidence. Review of Economic Studies, 2022, 89 (6), pp.3154-3222. 10. ...
Corpus ID: 53579719; The Design of Teacher Assignment: Theory and Evidence @inproceedings{Combe2015TheDO, title={The Design of Teacher Assignment: Theory and Evidence}, author={Julien Combe and Olivier Tercieux and Camille Terrier}, year={2015} }
The Design of Teacher Assignment: Theory and Evidence. Abstract To assign teachers to schools, a modified version of the well-known deferred acceptance mechanism has been proposed in the literature and is used in practice.
In several countries, teachers' assignment to schools is managed by a central administration. One of the objectives of the reassignment process is to make sure that teachers obtain an assignment which they weakly prefer to their current position. The Deferred-Acceptance mechanism (DA) proposed Gale and Shapley (1962) fails to satisfy this ...
The Design of Teacher Assignment: Theory and Evidence. Julien Combe, Olivier Tercieux and Camille Terrier Additional contact information Olivier Tercieux: PSE - Paris School of Economics - UP1 - Université Paris 1 Panthéon-Sorbonne - ENS-PSL - École normale supérieure - Paris - PSL - Université Paris sciences et lettres - EHESS - École des hautes études en sciences sociales - ENPC ...
The reassignment of teachers to schools is a central issue in education policies. In several countries, this assignment is managed by a central administration that faces a key constraint: ensuring that teachers obtain an assignment that they weakly prefer to their current position.
The Design of Teacher Assignment: Theory and Evidence. Tuesday, January 29, 2019 - 4:00pm - Tuesday, January 29, 2019 - 5:30pm. Junior Recruiting Seminar Micro Theory Seminar. PCPSE Room 100. United States.
Department of economics IP Paris. 5 Avenue Henry Le Chatelier TSA 96642 91764 Palaiseau Cedex FRANCE
The design of teacher assignment : theory and evidence | Camille Terrier | Tuesday 24 November 2015 12:45 - 14:00 | CEP Event
The Topic of the Overall Framework: LCD. Smith and Ragan defined instructional design as the "systematic and reflective process of translating principles of learning and instruction into plans for instructional materials, activities, information resources, and evaluation" (p. 2).For LCD, the principles referenced by Smith and Ragan are those specific to learner-centered instructional methods.
The Design of Teacher Assignment: Theory and Evidence Julien Combey Olivier Tercieuxz Camille Terrierx June 10, 2016 Abstract The (re-)assignment of teachers to schools is a centr
The design of teacher assignment. Theory and evidence. Quelle: Cambridge, MA: MIT Department of Economics (2022), ca. 90 S. PDF als Volltext kostenfreie Datei (1); PDF als Volltext kostenfreie Datei (2) Link als defekt melden Verfügbarkeit : Reihe: Discussion paper / Blueprint Labs. 2022, 11: Beigaben: Illustrationen: Sprache: englisch ...
Julien COMBE (UCL), « The Design of Teacher Assignment: Theory and Evidence », Job Market Seminar, Toulouse : TSE, 21 janvier 2019, 14h00-15h30, salle MS001.
Replication files for the paper Combe, J., Tercieux, O., & Terrier, C. (2021). The design of teacher assignment: Theory and evidence. Review of Economic Studies The file contains 5 folders and 1 Readme file, please read the readme file: - The folder 0 contains all the tables and graphs of the paper - The folder 1 contains a readme and all the Matlab codes and inputs used to run the estimation ...