
An official website of the United States government
The .gov means it’s official. Federal government websites often end in .gov or .mil. Before sharing sensitive information, make sure you’re on a federal government site.
The site is secure. The https:// ensures that you are connecting to the official website and that any information you provide is encrypted and transmitted securely.
- Publications
- Account settings
Preview improvements coming to the PMC website in October 2024. Learn More or Try it out now .
- Advanced Search
- Journal List
- J Korean Med Sci
- v.37(16); 2022 Apr 25

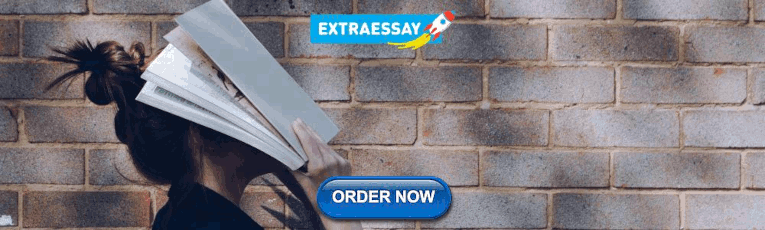
A Practical Guide to Writing Quantitative and Qualitative Research Questions and Hypotheses in Scholarly Articles
Edward barroga.
1 Department of General Education, Graduate School of Nursing Science, St. Luke’s International University, Tokyo, Japan.
Glafera Janet Matanguihan
2 Department of Biological Sciences, Messiah University, Mechanicsburg, PA, USA.
The development of research questions and the subsequent hypotheses are prerequisites to defining the main research purpose and specific objectives of a study. Consequently, these objectives determine the study design and research outcome. The development of research questions is a process based on knowledge of current trends, cutting-edge studies, and technological advances in the research field. Excellent research questions are focused and require a comprehensive literature search and in-depth understanding of the problem being investigated. Initially, research questions may be written as descriptive questions which could be developed into inferential questions. These questions must be specific and concise to provide a clear foundation for developing hypotheses. Hypotheses are more formal predictions about the research outcomes. These specify the possible results that may or may not be expected regarding the relationship between groups. Thus, research questions and hypotheses clarify the main purpose and specific objectives of the study, which in turn dictate the design of the study, its direction, and outcome. Studies developed from good research questions and hypotheses will have trustworthy outcomes with wide-ranging social and health implications.
INTRODUCTION
Scientific research is usually initiated by posing evidenced-based research questions which are then explicitly restated as hypotheses. 1 , 2 The hypotheses provide directions to guide the study, solutions, explanations, and expected results. 3 , 4 Both research questions and hypotheses are essentially formulated based on conventional theories and real-world processes, which allow the inception of novel studies and the ethical testing of ideas. 5 , 6
It is crucial to have knowledge of both quantitative and qualitative research 2 as both types of research involve writing research questions and hypotheses. 7 However, these crucial elements of research are sometimes overlooked; if not overlooked, then framed without the forethought and meticulous attention it needs. Planning and careful consideration are needed when developing quantitative or qualitative research, particularly when conceptualizing research questions and hypotheses. 4
There is a continuing need to support researchers in the creation of innovative research questions and hypotheses, as well as for journal articles that carefully review these elements. 1 When research questions and hypotheses are not carefully thought of, unethical studies and poor outcomes usually ensue. Carefully formulated research questions and hypotheses define well-founded objectives, which in turn determine the appropriate design, course, and outcome of the study. This article then aims to discuss in detail the various aspects of crafting research questions and hypotheses, with the goal of guiding researchers as they develop their own. Examples from the authors and peer-reviewed scientific articles in the healthcare field are provided to illustrate key points.
DEFINITIONS AND RELATIONSHIP OF RESEARCH QUESTIONS AND HYPOTHESES
A research question is what a study aims to answer after data analysis and interpretation. The answer is written in length in the discussion section of the paper. Thus, the research question gives a preview of the different parts and variables of the study meant to address the problem posed in the research question. 1 An excellent research question clarifies the research writing while facilitating understanding of the research topic, objective, scope, and limitations of the study. 5
On the other hand, a research hypothesis is an educated statement of an expected outcome. This statement is based on background research and current knowledge. 8 , 9 The research hypothesis makes a specific prediction about a new phenomenon 10 or a formal statement on the expected relationship between an independent variable and a dependent variable. 3 , 11 It provides a tentative answer to the research question to be tested or explored. 4
Hypotheses employ reasoning to predict a theory-based outcome. 10 These can also be developed from theories by focusing on components of theories that have not yet been observed. 10 The validity of hypotheses is often based on the testability of the prediction made in a reproducible experiment. 8
Conversely, hypotheses can also be rephrased as research questions. Several hypotheses based on existing theories and knowledge may be needed to answer a research question. Developing ethical research questions and hypotheses creates a research design that has logical relationships among variables. These relationships serve as a solid foundation for the conduct of the study. 4 , 11 Haphazardly constructed research questions can result in poorly formulated hypotheses and improper study designs, leading to unreliable results. Thus, the formulations of relevant research questions and verifiable hypotheses are crucial when beginning research. 12
CHARACTERISTICS OF GOOD RESEARCH QUESTIONS AND HYPOTHESES
Excellent research questions are specific and focused. These integrate collective data and observations to confirm or refute the subsequent hypotheses. Well-constructed hypotheses are based on previous reports and verify the research context. These are realistic, in-depth, sufficiently complex, and reproducible. More importantly, these hypotheses can be addressed and tested. 13
There are several characteristics of well-developed hypotheses. Good hypotheses are 1) empirically testable 7 , 10 , 11 , 13 ; 2) backed by preliminary evidence 9 ; 3) testable by ethical research 7 , 9 ; 4) based on original ideas 9 ; 5) have evidenced-based logical reasoning 10 ; and 6) can be predicted. 11 Good hypotheses can infer ethical and positive implications, indicating the presence of a relationship or effect relevant to the research theme. 7 , 11 These are initially developed from a general theory and branch into specific hypotheses by deductive reasoning. In the absence of a theory to base the hypotheses, inductive reasoning based on specific observations or findings form more general hypotheses. 10
TYPES OF RESEARCH QUESTIONS AND HYPOTHESES
Research questions and hypotheses are developed according to the type of research, which can be broadly classified into quantitative and qualitative research. We provide a summary of the types of research questions and hypotheses under quantitative and qualitative research categories in Table 1 .
Research questions in quantitative research
In quantitative research, research questions inquire about the relationships among variables being investigated and are usually framed at the start of the study. These are precise and typically linked to the subject population, dependent and independent variables, and research design. 1 Research questions may also attempt to describe the behavior of a population in relation to one or more variables, or describe the characteristics of variables to be measured ( descriptive research questions ). 1 , 5 , 14 These questions may also aim to discover differences between groups within the context of an outcome variable ( comparative research questions ), 1 , 5 , 14 or elucidate trends and interactions among variables ( relationship research questions ). 1 , 5 We provide examples of descriptive, comparative, and relationship research questions in quantitative research in Table 2 .
Hypotheses in quantitative research
In quantitative research, hypotheses predict the expected relationships among variables. 15 Relationships among variables that can be predicted include 1) between a single dependent variable and a single independent variable ( simple hypothesis ) or 2) between two or more independent and dependent variables ( complex hypothesis ). 4 , 11 Hypotheses may also specify the expected direction to be followed and imply an intellectual commitment to a particular outcome ( directional hypothesis ) 4 . On the other hand, hypotheses may not predict the exact direction and are used in the absence of a theory, or when findings contradict previous studies ( non-directional hypothesis ). 4 In addition, hypotheses can 1) define interdependency between variables ( associative hypothesis ), 4 2) propose an effect on the dependent variable from manipulation of the independent variable ( causal hypothesis ), 4 3) state a negative relationship between two variables ( null hypothesis ), 4 , 11 , 15 4) replace the working hypothesis if rejected ( alternative hypothesis ), 15 explain the relationship of phenomena to possibly generate a theory ( working hypothesis ), 11 5) involve quantifiable variables that can be tested statistically ( statistical hypothesis ), 11 6) or express a relationship whose interlinks can be verified logically ( logical hypothesis ). 11 We provide examples of simple, complex, directional, non-directional, associative, causal, null, alternative, working, statistical, and logical hypotheses in quantitative research, as well as the definition of quantitative hypothesis-testing research in Table 3 .
Research questions in qualitative research
Unlike research questions in quantitative research, research questions in qualitative research are usually continuously reviewed and reformulated. The central question and associated subquestions are stated more than the hypotheses. 15 The central question broadly explores a complex set of factors surrounding the central phenomenon, aiming to present the varied perspectives of participants. 15
There are varied goals for which qualitative research questions are developed. These questions can function in several ways, such as to 1) identify and describe existing conditions ( contextual research question s); 2) describe a phenomenon ( descriptive research questions ); 3) assess the effectiveness of existing methods, protocols, theories, or procedures ( evaluation research questions ); 4) examine a phenomenon or analyze the reasons or relationships between subjects or phenomena ( explanatory research questions ); or 5) focus on unknown aspects of a particular topic ( exploratory research questions ). 5 In addition, some qualitative research questions provide new ideas for the development of theories and actions ( generative research questions ) or advance specific ideologies of a position ( ideological research questions ). 1 Other qualitative research questions may build on a body of existing literature and become working guidelines ( ethnographic research questions ). Research questions may also be broadly stated without specific reference to the existing literature or a typology of questions ( phenomenological research questions ), may be directed towards generating a theory of some process ( grounded theory questions ), or may address a description of the case and the emerging themes ( qualitative case study questions ). 15 We provide examples of contextual, descriptive, evaluation, explanatory, exploratory, generative, ideological, ethnographic, phenomenological, grounded theory, and qualitative case study research questions in qualitative research in Table 4 , and the definition of qualitative hypothesis-generating research in Table 5 .
Qualitative studies usually pose at least one central research question and several subquestions starting with How or What . These research questions use exploratory verbs such as explore or describe . These also focus on one central phenomenon of interest, and may mention the participants and research site. 15
Hypotheses in qualitative research
Hypotheses in qualitative research are stated in the form of a clear statement concerning the problem to be investigated. Unlike in quantitative research where hypotheses are usually developed to be tested, qualitative research can lead to both hypothesis-testing and hypothesis-generating outcomes. 2 When studies require both quantitative and qualitative research questions, this suggests an integrative process between both research methods wherein a single mixed-methods research question can be developed. 1
FRAMEWORKS FOR DEVELOPING RESEARCH QUESTIONS AND HYPOTHESES
Research questions followed by hypotheses should be developed before the start of the study. 1 , 12 , 14 It is crucial to develop feasible research questions on a topic that is interesting to both the researcher and the scientific community. This can be achieved by a meticulous review of previous and current studies to establish a novel topic. Specific areas are subsequently focused on to generate ethical research questions. The relevance of the research questions is evaluated in terms of clarity of the resulting data, specificity of the methodology, objectivity of the outcome, depth of the research, and impact of the study. 1 , 5 These aspects constitute the FINER criteria (i.e., Feasible, Interesting, Novel, Ethical, and Relevant). 1 Clarity and effectiveness are achieved if research questions meet the FINER criteria. In addition to the FINER criteria, Ratan et al. described focus, complexity, novelty, feasibility, and measurability for evaluating the effectiveness of research questions. 14
The PICOT and PEO frameworks are also used when developing research questions. 1 The following elements are addressed in these frameworks, PICOT: P-population/patients/problem, I-intervention or indicator being studied, C-comparison group, O-outcome of interest, and T-timeframe of the study; PEO: P-population being studied, E-exposure to preexisting conditions, and O-outcome of interest. 1 Research questions are also considered good if these meet the “FINERMAPS” framework: Feasible, Interesting, Novel, Ethical, Relevant, Manageable, Appropriate, Potential value/publishable, and Systematic. 14
As we indicated earlier, research questions and hypotheses that are not carefully formulated result in unethical studies or poor outcomes. To illustrate this, we provide some examples of ambiguous research question and hypotheses that result in unclear and weak research objectives in quantitative research ( Table 6 ) 16 and qualitative research ( Table 7 ) 17 , and how to transform these ambiguous research question(s) and hypothesis(es) into clear and good statements.
a These statements were composed for comparison and illustrative purposes only.
b These statements are direct quotes from Higashihara and Horiuchi. 16
a This statement is a direct quote from Shimoda et al. 17
The other statements were composed for comparison and illustrative purposes only.
CONSTRUCTING RESEARCH QUESTIONS AND HYPOTHESES
To construct effective research questions and hypotheses, it is very important to 1) clarify the background and 2) identify the research problem at the outset of the research, within a specific timeframe. 9 Then, 3) review or conduct preliminary research to collect all available knowledge about the possible research questions by studying theories and previous studies. 18 Afterwards, 4) construct research questions to investigate the research problem. Identify variables to be accessed from the research questions 4 and make operational definitions of constructs from the research problem and questions. Thereafter, 5) construct specific deductive or inductive predictions in the form of hypotheses. 4 Finally, 6) state the study aims . This general flow for constructing effective research questions and hypotheses prior to conducting research is shown in Fig. 1 .

Research questions are used more frequently in qualitative research than objectives or hypotheses. 3 These questions seek to discover, understand, explore or describe experiences by asking “What” or “How.” The questions are open-ended to elicit a description rather than to relate variables or compare groups. The questions are continually reviewed, reformulated, and changed during the qualitative study. 3 Research questions are also used more frequently in survey projects than hypotheses in experiments in quantitative research to compare variables and their relationships.
Hypotheses are constructed based on the variables identified and as an if-then statement, following the template, ‘If a specific action is taken, then a certain outcome is expected.’ At this stage, some ideas regarding expectations from the research to be conducted must be drawn. 18 Then, the variables to be manipulated (independent) and influenced (dependent) are defined. 4 Thereafter, the hypothesis is stated and refined, and reproducible data tailored to the hypothesis are identified, collected, and analyzed. 4 The hypotheses must be testable and specific, 18 and should describe the variables and their relationships, the specific group being studied, and the predicted research outcome. 18 Hypotheses construction involves a testable proposition to be deduced from theory, and independent and dependent variables to be separated and measured separately. 3 Therefore, good hypotheses must be based on good research questions constructed at the start of a study or trial. 12
In summary, research questions are constructed after establishing the background of the study. Hypotheses are then developed based on the research questions. Thus, it is crucial to have excellent research questions to generate superior hypotheses. In turn, these would determine the research objectives and the design of the study, and ultimately, the outcome of the research. 12 Algorithms for building research questions and hypotheses are shown in Fig. 2 for quantitative research and in Fig. 3 for qualitative research.

EXAMPLES OF RESEARCH QUESTIONS FROM PUBLISHED ARTICLES
- EXAMPLE 1. Descriptive research question (quantitative research)
- - Presents research variables to be assessed (distinct phenotypes and subphenotypes)
- “BACKGROUND: Since COVID-19 was identified, its clinical and biological heterogeneity has been recognized. Identifying COVID-19 phenotypes might help guide basic, clinical, and translational research efforts.
- RESEARCH QUESTION: Does the clinical spectrum of patients with COVID-19 contain distinct phenotypes and subphenotypes? ” 19
- EXAMPLE 2. Relationship research question (quantitative research)
- - Shows interactions between dependent variable (static postural control) and independent variable (peripheral visual field loss)
- “Background: Integration of visual, vestibular, and proprioceptive sensations contributes to postural control. People with peripheral visual field loss have serious postural instability. However, the directional specificity of postural stability and sensory reweighting caused by gradual peripheral visual field loss remain unclear.
- Research question: What are the effects of peripheral visual field loss on static postural control ?” 20
- EXAMPLE 3. Comparative research question (quantitative research)
- - Clarifies the difference among groups with an outcome variable (patients enrolled in COMPERA with moderate PH or severe PH in COPD) and another group without the outcome variable (patients with idiopathic pulmonary arterial hypertension (IPAH))
- “BACKGROUND: Pulmonary hypertension (PH) in COPD is a poorly investigated clinical condition.
- RESEARCH QUESTION: Which factors determine the outcome of PH in COPD?
- STUDY DESIGN AND METHODS: We analyzed the characteristics and outcome of patients enrolled in the Comparative, Prospective Registry of Newly Initiated Therapies for Pulmonary Hypertension (COMPERA) with moderate or severe PH in COPD as defined during the 6th PH World Symposium who received medical therapy for PH and compared them with patients with idiopathic pulmonary arterial hypertension (IPAH) .” 21
- EXAMPLE 4. Exploratory research question (qualitative research)
- - Explores areas that have not been fully investigated (perspectives of families and children who receive care in clinic-based child obesity treatment) to have a deeper understanding of the research problem
- “Problem: Interventions for children with obesity lead to only modest improvements in BMI and long-term outcomes, and data are limited on the perspectives of families of children with obesity in clinic-based treatment. This scoping review seeks to answer the question: What is known about the perspectives of families and children who receive care in clinic-based child obesity treatment? This review aims to explore the scope of perspectives reported by families of children with obesity who have received individualized outpatient clinic-based obesity treatment.” 22
- EXAMPLE 5. Relationship research question (quantitative research)
- - Defines interactions between dependent variable (use of ankle strategies) and independent variable (changes in muscle tone)
- “Background: To maintain an upright standing posture against external disturbances, the human body mainly employs two types of postural control strategies: “ankle strategy” and “hip strategy.” While it has been reported that the magnitude of the disturbance alters the use of postural control strategies, it has not been elucidated how the level of muscle tone, one of the crucial parameters of bodily function, determines the use of each strategy. We have previously confirmed using forward dynamics simulations of human musculoskeletal models that an increased muscle tone promotes the use of ankle strategies. The objective of the present study was to experimentally evaluate a hypothesis: an increased muscle tone promotes the use of ankle strategies. Research question: Do changes in the muscle tone affect the use of ankle strategies ?” 23
EXAMPLES OF HYPOTHESES IN PUBLISHED ARTICLES
- EXAMPLE 1. Working hypothesis (quantitative research)
- - A hypothesis that is initially accepted for further research to produce a feasible theory
- “As fever may have benefit in shortening the duration of viral illness, it is plausible to hypothesize that the antipyretic efficacy of ibuprofen may be hindering the benefits of a fever response when taken during the early stages of COVID-19 illness .” 24
- “In conclusion, it is plausible to hypothesize that the antipyretic efficacy of ibuprofen may be hindering the benefits of a fever response . The difference in perceived safety of these agents in COVID-19 illness could be related to the more potent efficacy to reduce fever with ibuprofen compared to acetaminophen. Compelling data on the benefit of fever warrant further research and review to determine when to treat or withhold ibuprofen for early stage fever for COVID-19 and other related viral illnesses .” 24
- EXAMPLE 2. Exploratory hypothesis (qualitative research)
- - Explores particular areas deeper to clarify subjective experience and develop a formal hypothesis potentially testable in a future quantitative approach
- “We hypothesized that when thinking about a past experience of help-seeking, a self distancing prompt would cause increased help-seeking intentions and more favorable help-seeking outcome expectations .” 25
- “Conclusion
- Although a priori hypotheses were not supported, further research is warranted as results indicate the potential for using self-distancing approaches to increasing help-seeking among some people with depressive symptomatology.” 25
- EXAMPLE 3. Hypothesis-generating research to establish a framework for hypothesis testing (qualitative research)
- “We hypothesize that compassionate care is beneficial for patients (better outcomes), healthcare systems and payers (lower costs), and healthcare providers (lower burnout). ” 26
- Compassionomics is the branch of knowledge and scientific study of the effects of compassionate healthcare. Our main hypotheses are that compassionate healthcare is beneficial for (1) patients, by improving clinical outcomes, (2) healthcare systems and payers, by supporting financial sustainability, and (3) HCPs, by lowering burnout and promoting resilience and well-being. The purpose of this paper is to establish a scientific framework for testing the hypotheses above . If these hypotheses are confirmed through rigorous research, compassionomics will belong in the science of evidence-based medicine, with major implications for all healthcare domains.” 26
- EXAMPLE 4. Statistical hypothesis (quantitative research)
- - An assumption is made about the relationship among several population characteristics ( gender differences in sociodemographic and clinical characteristics of adults with ADHD ). Validity is tested by statistical experiment or analysis ( chi-square test, Students t-test, and logistic regression analysis)
- “Our research investigated gender differences in sociodemographic and clinical characteristics of adults with ADHD in a Japanese clinical sample. Due to unique Japanese cultural ideals and expectations of women's behavior that are in opposition to ADHD symptoms, we hypothesized that women with ADHD experience more difficulties and present more dysfunctions than men . We tested the following hypotheses: first, women with ADHD have more comorbidities than men with ADHD; second, women with ADHD experience more social hardships than men, such as having less full-time employment and being more likely to be divorced.” 27
- “Statistical Analysis
- ( text omitted ) Between-gender comparisons were made using the chi-squared test for categorical variables and Students t-test for continuous variables…( text omitted ). A logistic regression analysis was performed for employment status, marital status, and comorbidity to evaluate the independent effects of gender on these dependent variables.” 27
EXAMPLES OF HYPOTHESIS AS WRITTEN IN PUBLISHED ARTICLES IN RELATION TO OTHER PARTS
- EXAMPLE 1. Background, hypotheses, and aims are provided
- “Pregnant women need skilled care during pregnancy and childbirth, but that skilled care is often delayed in some countries …( text omitted ). The focused antenatal care (FANC) model of WHO recommends that nurses provide information or counseling to all pregnant women …( text omitted ). Job aids are visual support materials that provide the right kind of information using graphics and words in a simple and yet effective manner. When nurses are not highly trained or have many work details to attend to, these job aids can serve as a content reminder for the nurses and can be used for educating their patients (Jennings, Yebadokpo, Affo, & Agbogbe, 2010) ( text omitted ). Importantly, additional evidence is needed to confirm how job aids can further improve the quality of ANC counseling by health workers in maternal care …( text omitted )” 28
- “ This has led us to hypothesize that the quality of ANC counseling would be better if supported by job aids. Consequently, a better quality of ANC counseling is expected to produce higher levels of awareness concerning the danger signs of pregnancy and a more favorable impression of the caring behavior of nurses .” 28
- “This study aimed to examine the differences in the responses of pregnant women to a job aid-supported intervention during ANC visit in terms of 1) their understanding of the danger signs of pregnancy and 2) their impression of the caring behaviors of nurses to pregnant women in rural Tanzania.” 28
- EXAMPLE 2. Background, hypotheses, and aims are provided
- “We conducted a two-arm randomized controlled trial (RCT) to evaluate and compare changes in salivary cortisol and oxytocin levels of first-time pregnant women between experimental and control groups. The women in the experimental group touched and held an infant for 30 min (experimental intervention protocol), whereas those in the control group watched a DVD movie of an infant (control intervention protocol). The primary outcome was salivary cortisol level and the secondary outcome was salivary oxytocin level.” 29
- “ We hypothesize that at 30 min after touching and holding an infant, the salivary cortisol level will significantly decrease and the salivary oxytocin level will increase in the experimental group compared with the control group .” 29
- EXAMPLE 3. Background, aim, and hypothesis are provided
- “In countries where the maternal mortality ratio remains high, antenatal education to increase Birth Preparedness and Complication Readiness (BPCR) is considered one of the top priorities [1]. BPCR includes birth plans during the antenatal period, such as the birthplace, birth attendant, transportation, health facility for complications, expenses, and birth materials, as well as family coordination to achieve such birth plans. In Tanzania, although increasing, only about half of all pregnant women attend an antenatal clinic more than four times [4]. Moreover, the information provided during antenatal care (ANC) is insufficient. In the resource-poor settings, antenatal group education is a potential approach because of the limited time for individual counseling at antenatal clinics.” 30
- “This study aimed to evaluate an antenatal group education program among pregnant women and their families with respect to birth-preparedness and maternal and infant outcomes in rural villages of Tanzania.” 30
- “ The study hypothesis was if Tanzanian pregnant women and their families received a family-oriented antenatal group education, they would (1) have a higher level of BPCR, (2) attend antenatal clinic four or more times, (3) give birth in a health facility, (4) have less complications of women at birth, and (5) have less complications and deaths of infants than those who did not receive the education .” 30
Research questions and hypotheses are crucial components to any type of research, whether quantitative or qualitative. These questions should be developed at the very beginning of the study. Excellent research questions lead to superior hypotheses, which, like a compass, set the direction of research, and can often determine the successful conduct of the study. Many research studies have floundered because the development of research questions and subsequent hypotheses was not given the thought and meticulous attention needed. The development of research questions and hypotheses is an iterative process based on extensive knowledge of the literature and insightful grasp of the knowledge gap. Focused, concise, and specific research questions provide a strong foundation for constructing hypotheses which serve as formal predictions about the research outcomes. Research questions and hypotheses are crucial elements of research that should not be overlooked. They should be carefully thought of and constructed when planning research. This avoids unethical studies and poor outcomes by defining well-founded objectives that determine the design, course, and outcome of the study.
Disclosure: The authors have no potential conflicts of interest to disclose.
Author Contributions:
- Conceptualization: Barroga E, Matanguihan GJ.
- Methodology: Barroga E, Matanguihan GJ.
- Writing - original draft: Barroga E, Matanguihan GJ.
- Writing - review & editing: Barroga E, Matanguihan GJ.
- Cookies & Privacy
- GETTING STARTED
- Introduction
- FUNDAMENTALS
- Acknowledgements
- Research questions & hypotheses
- Concepts, constructs & variables
- Research limitations
- Getting started
- Sampling Strategy
- Research Quality
- Research Ethics
- Data Analysis
How to structure quantitative research questions
There is no "one best way" to structure a quantitative research question. However, to create a well-structured quantitative research question, we recommend an approach that is based on four steps : (1) Choosing the type of quantitative research question you are trying to create (i.e., descriptive, comparative or relationship-based); (2) Identifying the different types of variables you are trying to measure, manipulate and/or control, as well as any groups you may be interested in; (3) Selecting the appropriate structure for the chosen type of quantitative research question, based on the variables and/or groups involved; and (4) Writing out the problem or issues you are trying to address in the form of a complete research question. In this article, we discuss each of these four steps , as well as providing examples for the three types of quantitative research question you may want to create: descriptive , comparative and relationship-based research questions .
- STEP ONE: Choose the type of quantitative research question (i.e., descriptive, comparative or relationship) you are trying to create
- STEP TWO: Identify the different types of variable you are trying to measure, manipulate and/or control, as well as any groups you may be interested in
- STEP THREE: Select the appropriate structure for the chosen type of quantitative research question, based on the variables and/or groups involved
- STEP FOUR: Write out the problem or issues you are trying to address in the form of a complete research question
STEP ONE Choose the type of quantitative research question (i.e., descriptive, comparative or relationship) you are trying to create
The type of quantitative research question that you use in your dissertation (i.e., descriptive , comparative and/or relationship-based ) needs to be reflected in the way that you write out the research question; that is, the word choice and phrasing that you use when constructing a research question tells the reader whether it is a descriptive, comparative or relationship-based research question. Therefore, in order to know how to structure your quantitative research question, you need to start by selecting the type of quantitative research question you are trying to create: descriptive, comparative and/or relationship-based.
STEP TWO Identify the different types of variable you are trying to measure, manipulate and/or control, as well as any groups you may be interested in
Whether you are trying to create a descriptive, comparative or relationship-based research question, you will need to identify the different types of variable that you are trying to measure , manipulate and/or control . If you are unfamiliar with the different types of variable that may be part of your study, the article, Types of variable , should get you up to speed. It explains the two main types of variables: categorical variables (i.e., nominal , dichotomous and ordinal variables) and continuous variables (i.e., interval and ratio variables). It also explains the difference between independent and dependent variables , which you need to understand to create quantitative research questions.
To provide a brief explanation; a variable is not only something that you measure , but also something that you can manipulate and control for. In most undergraduate and master's level dissertations, you are only likely to measure and manipulate variables. You are unlikely to carry out research that requires you to control for variables, although some supervisors will expect this additional level of complexity. If you plan to only create descriptive research questions , you may simply have a number of dependent variables that you need to measure. However, where you plan to create comparative and/or relationship-based research questions , you will deal with both dependent and independent variables . An independent variable (sometimes called an experimental or predictor variable ) is a variable that is being manipulated in an experiment in order to observe the effect this has on a dependent variable (sometimes called an outcome variable ). For example, if we were interested in investigating the relationship between gender and attitudes towards music piracy amongst adolescents , the independent variable would be gender and the dependent variable attitudes towards music piracy . This example also highlights the need to identify the group(s) you are interested in. In this example, the group of interest are adolescents .
Once you identifying the different types of variable you are trying to measure, manipulate and/or control, as well as any groups you may be interested in, it is possible to start thinking about the way that the three types of quantitative research question can be structured . This is discussed next.
STEP THREE Select the appropriate structure for the chosen type of quantitative research question, based on the variables and/or groups involved
The structure of the three types of quantitative research question differs, reflecting the goals of the question, the types of variables, and the number of variables and groups involved. By structure , we mean the components of a research question (i.e., the types of variables, groups of interest), the number of these different components (i.e., how many variables and groups are being investigated), and the order that these should be presented (e.g., independent variables before dependent variables). The appropriate structure for each of these quantitative research questions is set out below:
Structure of descriptive research questions
- Structure of comparative research questions
- Structure of relationship-based research questions
There are six steps required to construct a descriptive research question: (1) choose your starting phrase; (2) identify and name the dependent variable; (3) identify the group(s) you are interested in; (4) decide whether dependent variable or group(s) should be included first, last or in two parts; (5) include any words that provide greater context to your question; and (6) write out the descriptive research question. Each of these steps is discussed in turn:
Choose your starting phrase
Identify and name the dependent variable
Identify the group(s) you are interested in
Decide whether the dependent variable or group(s) should be included first, last or in two parts
Include any words that provide greater context to your question
Write out the descriptive research question
FIRST Choose your starting phrase
You can start descriptive research questions with any of the following phrases:
How many? How often? How frequently? How much? What percentage? What proportion? To what extent? What is? What are?
Some of these starting phrases are highlighted in blue text in the examples below:
How many calories do American men and women consume per day?
How often do British university students use Facebook each week?
What are the most important factors that influence the career choices of Australian university students?
What proportion of British male and female university students use the top 5 social networks?
What percentage of American men and women exceed their daily calorific allowance?
SECOND Identify and name the dependent variable
All descriptive research questions have a dependent variable. You need to identify what this is. However, how the dependent variable is written out in a research question and what you call it are often two different things. In the examples below, we have illustrated the name of the dependent variable and highlighted how it would be written out in the blue text .
The first two examples highlight that while the name of the dependent variable is the same, namely daily calorific intake , the way that this dependent variable is written out differs in each case.
THIRD Identify the group(s) you are interested in
All descriptive research questions have at least one group , but can have multiple groups . You need to identify this group(s). In the examples below, we have identified the group(s) in the green text .
What are the most important factors that influence the career choices of Australian university students ?
The examples illustrate the difference between the use of a single group (e.g., British university students ) and multiple groups (e.g., American men and women ).
FOURTH Decide whether the dependent variable or group(s) should be included first, last or in two parts
Sometimes it makes more sense for the dependent variable to appear before the group(s) you are interested in, but sometimes it is the opposite way around. The following examples illustrate this, with the group(s) in green text and the dependent variable in blue text :
Group 1st; dependent variable 2nd:
How often do British university students use Facebook each week ?
Dependent variable 1st; group 2nd:
Sometimes, the dependent variable needs to be broken into two parts around the group(s) you are interested in so that the research question flows. Again, the group(s) are in green text and the dependent variable is in blue text :
How many calories do American men and women consume per day ?
Of course, you could choose to restructure the question above so that you do not have to split the dependent variable into two parts. For example:
How many calories are consumed per day by American men and women ?
When deciding whether the dependent variable or group(s) should be included first or last, and whether the dependent variable should be broken into two parts, the main thing you need to think about is flow : Does the question flow? Is it easy to read?
FIFTH Include any words that provide greater context to your question
Sometimes the name of the dependent variable provides all the explanation we need to know what we are trying to measure. Take the following examples:
In the first example, the dependent variable is daily calorific intake (i.e., calories consumed per day). Clearly, this descriptive research question is asking us to measure the number of calories American men and women consume per day. In the second example, the dependent variable is Facebook usage per week. Again, the name of this dependent variable makes it easy for us to understand that we are trying to measure the often (i.e., how frequently; e.g., 16 times per week) British university students use Facebook.
However, sometimes a descriptive research question is not simply interested in measuring the dependent variable in its entirety, but a particular component of the dependent variable. Take the following examples in red text :
In the first example, the research question is not simply interested in the daily calorific intake of American men and women, but what percentage of these American men and women exceeded their daily calorific allowance. So the dependent variable is still daily calorific intake, but the research question aims to understand a particular component of that dependent variable (i.e., the percentage of American men and women exceeding the recommend daily calorific allowance). In the second example, the research question is not only interested in what the factors influencing career choices are, but which of these factors are the most important.
Therefore, when you think about constructing your descriptive research question, make sure you have included any words that provide greater context to your question.
SIXTH Write out the descriptive research question
Once you have these details ? (1) the starting phrase, (2) the name of the dependent variable, (3) the name of the group(s) you are interested in, and (4) any potential joining words ? you can write out the descriptive research question in full. The example descriptive research questions discussed above are written out in full below:
In the section that follows, the structure of comparative research questions is discussed.
Quantitative Research
- Reference work entry
- First Online: 13 January 2019
- Cite this reference work entry
- Leigh A. Wilson 2 , 3
4 Citations
Quantitative research methods are concerned with the planning, design, and implementation of strategies to collect and analyze data. Descartes, the seventeenth-century philosopher, suggested that how the results are achieved is often more important than the results themselves, as the journey taken along the research path is a journey of discovery. High-quality quantitative research is characterized by the attention given to the methods and the reliability of the tools used to collect the data. The ability to critique research in a systematic way is an essential component of a health professional’s role in order to deliver high quality, evidence-based healthcare. This chapter is intended to provide a simple overview of the way new researchers and health practitioners can understand and employ quantitative methods. The chapter offers practical, realistic guidance in a learner-friendly way and uses a logical sequence to understand the process of hypothesis development, study design, data collection and handling, and finally data analysis and interpretation.
This is a preview of subscription content, log in via an institution to check access.
Access this chapter
- Available as PDF
- Read on any device
- Instant download
- Own it forever
- Available as EPUB and PDF
- Durable hardcover edition
- Dispatched in 3 to 5 business days
- Free shipping worldwide - see info
Tax calculation will be finalised at checkout
Purchases are for personal use only
Institutional subscriptions
Babbie ER. The practice of social research. 14th ed. Belmont: Wadsworth Cengage; 2016.
Google Scholar
Descartes. Cited in Halverston, W. (1976). In: A concise introduction to philosophy, 3rd ed. New York: Random House; 1637.
Doll R, Hill AB. The mortality of doctors in relation to their smoking habits. BMJ. 1954;328(7455):1529–33. https://doi.org/10.1136/bmj.328.7455.1529 .
Article Google Scholar
Liamputtong P. Research methods in health: foundations for evidence-based practice. 3rd ed. Melbourne: Oxford University Press; 2017.
McNabb DE. Research methods in public administration and nonprofit management: quantitative and qualitative approaches. 2nd ed. New York: Armonk; 2007.
Merriam-Webster. Dictionary. http://www.merriam-webster.com . Accessed 20th December 2017.
Olesen Larsen P, von Ins M. The rate of growth in scientific publication and the decline in coverage provided by Science Citation Index. Scientometrics. 2010;84(3):575–603.
Pannucci CJ, Wilkins EG. Identifying and avoiding bias in research. Plast Reconstr Surg. 2010;126(2):619–25. https://doi.org/10.1097/PRS.0b013e3181de24bc .
Petrie A, Sabin C. Medical statistics at a glance. 2nd ed. London: Blackwell Publishing; 2005.
Portney LG, Watkins MP. Foundations of clinical research: applications to practice. 3rd ed. New Jersey: Pearson Publishing; 2009.
Sheehan J. Aspects of research methodology. Nurse Educ Today. 1986;6:193–203.
Wilson LA, Black DA. Health, science research and research methods. Sydney: McGraw Hill; 2013.
Download references
Author information
Authors and affiliations.
School of Science and Health, Western Sydney University, Penrith, NSW, Australia
Leigh A. Wilson
Faculty of Health Science, Discipline of Behavioural and Social Sciences in Health, University of Sydney, Lidcombe, NSW, Australia
You can also search for this author in PubMed Google Scholar
Corresponding author
Correspondence to Leigh A. Wilson .
Editor information
Editors and affiliations.
Pranee Liamputtong
Rights and permissions
Reprints and permissions
Copyright information
© 2019 Springer Nature Singapore Pte Ltd.
About this entry
Cite this entry.
Wilson, L.A. (2019). Quantitative Research. In: Liamputtong, P. (eds) Handbook of Research Methods in Health Social Sciences. Springer, Singapore. https://doi.org/10.1007/978-981-10-5251-4_54
Download citation
DOI : https://doi.org/10.1007/978-981-10-5251-4_54
Published : 13 January 2019
Publisher Name : Springer, Singapore
Print ISBN : 978-981-10-5250-7
Online ISBN : 978-981-10-5251-4
eBook Packages : Social Sciences Reference Module Humanities and Social Sciences Reference Module Business, Economics and Social Sciences
Share this entry
Anyone you share the following link with will be able to read this content:
Sorry, a shareable link is not currently available for this article.
Provided by the Springer Nature SharedIt content-sharing initiative
- Publish with us
Policies and ethics
- Find a journal
- Track your research

How To Write The Results/Findings Chapter
For quantitative studies (dissertations & theses).
By: Derek Jansen (MBA) | Expert Reviewed By: Kerryn Warren (PhD) | July 2021
So, you’ve completed your quantitative data analysis and it’s time to report on your findings. But where do you start? In this post, we’ll walk you through the results chapter (also called the findings or analysis chapter), step by step, so that you can craft this section of your dissertation or thesis with confidence. If you’re looking for information regarding the results chapter for qualitative studies, you can find that here .
Overview: Quantitative Results Chapter
- What exactly the results chapter is
- What you need to include in your chapter
- How to structure the chapter
- Tips and tricks for writing a top-notch chapter
- Free results chapter template
What exactly is the results chapter?
The results chapter (also referred to as the findings or analysis chapter) is one of the most important chapters of your dissertation or thesis because it shows the reader what you’ve found in terms of the quantitative data you’ve collected. It presents the data using a clear text narrative, supported by tables, graphs and charts. In doing so, it also highlights any potential issues (such as outliers or unusual findings) you’ve come across.
But how’s that different from the discussion chapter?
Well, in the results chapter, you only present your statistical findings. Only the numbers, so to speak – no more, no less. Contrasted to this, in the discussion chapter , you interpret your findings and link them to prior research (i.e. your literature review), as well as your research objectives and research questions . In other words, the results chapter presents and describes the data, while the discussion chapter interprets the data.
Let’s look at an example.
In your results chapter, you may have a plot that shows how respondents to a survey responded: the numbers of respondents per category, for instance. You may also state whether this supports a hypothesis by using a p-value from a statistical test. But it is only in the discussion chapter where you will say why this is relevant or how it compares with the literature or the broader picture. So, in your results chapter, make sure that you don’t present anything other than the hard facts – this is not the place for subjectivity.
It’s worth mentioning that some universities prefer you to combine the results and discussion chapters. Even so, it is good practice to separate the results and discussion elements within the chapter, as this ensures your findings are fully described. Typically, though, the results and discussion chapters are split up in quantitative studies. If you’re unsure, chat with your research supervisor or chair to find out what their preference is.
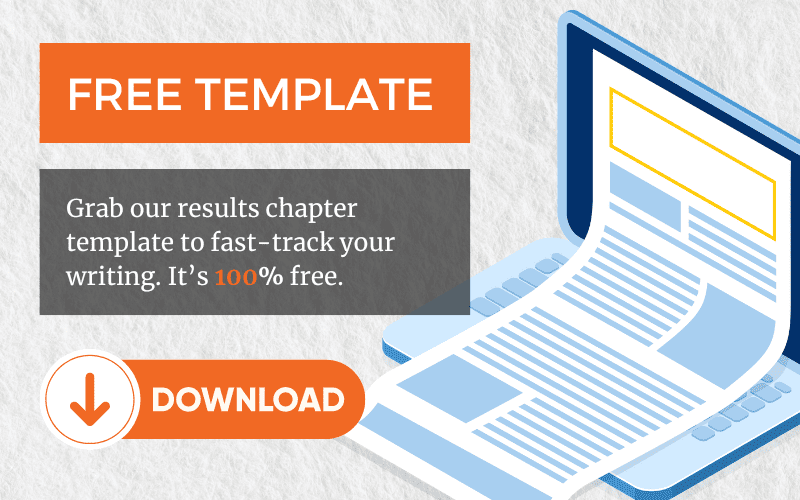
What should you include in the results chapter?
Following your analysis, it’s likely you’ll have far more data than are necessary to include in your chapter. In all likelihood, you’ll have a mountain of SPSS or R output data, and it’s your job to decide what’s most relevant. You’ll need to cut through the noise and focus on the data that matters.
This doesn’t mean that those analyses were a waste of time – on the contrary, those analyses ensure that you have a good understanding of your dataset and how to interpret it. However, that doesn’t mean your reader or examiner needs to see the 165 histograms you created! Relevance is key.
How do I decide what’s relevant?
At this point, it can be difficult to strike a balance between what is and isn’t important. But the most important thing is to ensure your results reflect and align with the purpose of your study . So, you need to revisit your research aims, objectives and research questions and use these as a litmus test for relevance. Make sure that you refer back to these constantly when writing up your chapter so that you stay on track.
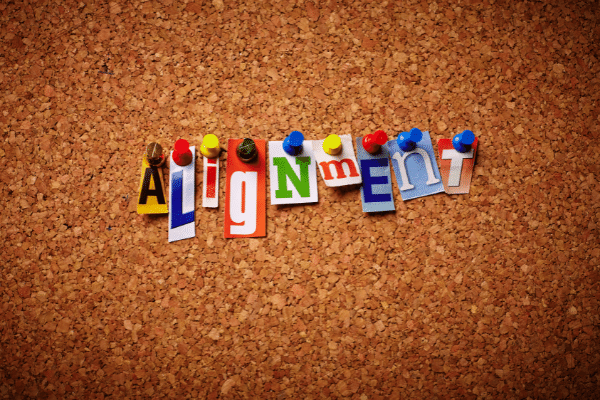
As a general guide, your results chapter will typically include the following:
- Some demographic data about your sample
- Reliability tests (if you used measurement scales)
- Descriptive statistics
- Inferential statistics (if your research objectives and questions require these)
- Hypothesis tests (again, if your research objectives and questions require these)
We’ll discuss each of these points in more detail in the next section.
Importantly, your results chapter needs to lay the foundation for your discussion chapter . This means that, in your results chapter, you need to include all the data that you will use as the basis for your interpretation in the discussion chapter.
For example, if you plan to highlight the strong relationship between Variable X and Variable Y in your discussion chapter, you need to present the respective analysis in your results chapter – perhaps a correlation or regression analysis.
Need a helping hand?
How do I write the results chapter?
There are multiple steps involved in writing up the results chapter for your quantitative research. The exact number of steps applicable to you will vary from study to study and will depend on the nature of the research aims, objectives and research questions . However, we’ll outline the generic steps below.
Step 1 – Revisit your research questions
The first step in writing your results chapter is to revisit your research objectives and research questions . These will be (or at least, should be!) the driving force behind your results and discussion chapters, so you need to review them and then ask yourself which statistical analyses and tests (from your mountain of data) would specifically help you address these . For each research objective and research question, list the specific piece (or pieces) of analysis that address it.
At this stage, it’s also useful to think about the key points that you want to raise in your discussion chapter and note these down so that you have a clear reminder of which data points and analyses you want to highlight in the results chapter. Again, list your points and then list the specific piece of analysis that addresses each point.
Next, you should draw up a rough outline of how you plan to structure your chapter . Which analyses and statistical tests will you present and in what order? We’ll discuss the “standard structure” in more detail later, but it’s worth mentioning now that it’s always useful to draw up a rough outline before you start writing (this advice applies to any chapter).
Step 2 – Craft an overview introduction
As with all chapters in your dissertation or thesis, you should start your quantitative results chapter by providing a brief overview of what you’ll do in the chapter and why . For example, you’d explain that you will start by presenting demographic data to understand the representativeness of the sample, before moving onto X, Y and Z.
This section shouldn’t be lengthy – a paragraph or two maximum. Also, it’s a good idea to weave the research questions into this section so that there’s a golden thread that runs through the document.
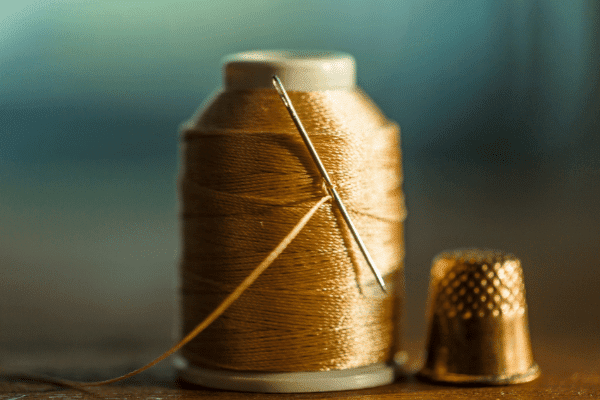
Step 3 – Present the sample demographic data
The first set of data that you’ll present is an overview of the sample demographics – in other words, the demographics of your respondents.
For example:
- What age range are they?
- How is gender distributed?
- How is ethnicity distributed?
- What areas do the participants live in?
The purpose of this is to assess how representative the sample is of the broader population. This is important for the sake of the generalisability of the results. If your sample is not representative of the population, you will not be able to generalise your findings. This is not necessarily the end of the world, but it is a limitation you’ll need to acknowledge.
Of course, to make this representativeness assessment, you’ll need to have a clear view of the demographics of the population. So, make sure that you design your survey to capture the correct demographic information that you will compare your sample to.
But what if I’m not interested in generalisability?
Well, even if your purpose is not necessarily to extrapolate your findings to the broader population, understanding your sample will allow you to interpret your findings appropriately, considering who responded. In other words, it will help you contextualise your findings . For example, if 80% of your sample was aged over 65, this may be a significant contextual factor to consider when interpreting the data. Therefore, it’s important to understand and present the demographic data.
Step 4 – Review composite measures and the data “shape”.
Before you undertake any statistical analysis, you’ll need to do some checks to ensure that your data are suitable for the analysis methods and techniques you plan to use. If you try to analyse data that doesn’t meet the assumptions of a specific statistical technique, your results will be largely meaningless. Therefore, you may need to show that the methods and techniques you’ll use are “allowed”.
Most commonly, there are two areas you need to pay attention to:
#1: Composite measures
The first is when you have multiple scale-based measures that combine to capture one construct – this is called a composite measure . For example, you may have four Likert scale-based measures that (should) all measure the same thing, but in different ways. In other words, in a survey, these four scales should all receive similar ratings. This is called “ internal consistency ”.
Internal consistency is not guaranteed though (especially if you developed the measures yourself), so you need to assess the reliability of each composite measure using a test. Typically, Cronbach’s Alpha is a common test used to assess internal consistency – i.e., to show that the items you’re combining are more or less saying the same thing. A high alpha score means that your measure is internally consistent. A low alpha score means you may need to consider scrapping one or more of the measures.
#2: Data shape
The second matter that you should address early on in your results chapter is data shape. In other words, you need to assess whether the data in your set are symmetrical (i.e. normally distributed) or not, as this will directly impact what type of analyses you can use. For many common inferential tests such as T-tests or ANOVAs (we’ll discuss these a bit later), your data needs to be normally distributed. If it’s not, you’ll need to adjust your strategy and use alternative tests.
To assess the shape of the data, you’ll usually assess a variety of descriptive statistics (such as the mean, median and skewness), which is what we’ll look at next.
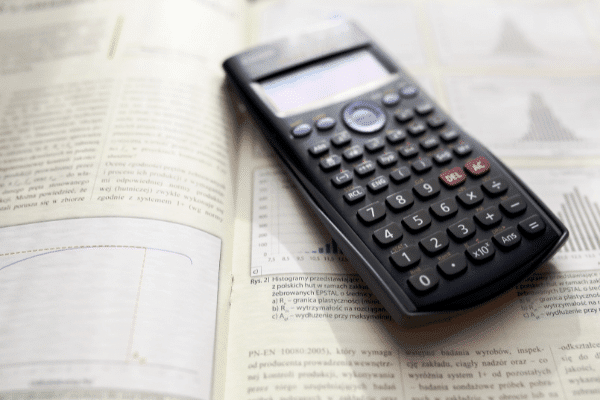
Step 5 – Present the descriptive statistics
Now that you’ve laid the foundation by discussing the representativeness of your sample, as well as the reliability of your measures and the shape of your data, you can get started with the actual statistical analysis. The first step is to present the descriptive statistics for your variables.
For scaled data, this usually includes statistics such as:
- The mean – this is simply the mathematical average of a range of numbers.
- The median – this is the midpoint in a range of numbers when the numbers are arranged in order.
- The mode – this is the most commonly repeated number in the data set.
- Standard deviation – this metric indicates how dispersed a range of numbers is. In other words, how close all the numbers are to the mean (the average).
- Skewness – this indicates how symmetrical a range of numbers is. In other words, do they tend to cluster into a smooth bell curve shape in the middle of the graph (this is called a normal or parametric distribution), or do they lean to the left or right (this is called a non-normal or non-parametric distribution).
- Kurtosis – this metric indicates whether the data are heavily or lightly-tailed, relative to the normal distribution. In other words, how peaked or flat the distribution is.
A large table that indicates all the above for multiple variables can be a very effective way to present your data economically. You can also use colour coding to help make the data more easily digestible.
For categorical data, where you show the percentage of people who chose or fit into a category, for instance, you can either just plain describe the percentages or numbers of people who responded to something or use graphs and charts (such as bar graphs and pie charts) to present your data in this section of the chapter.
When using figures, make sure that you label them simply and clearly , so that your reader can easily understand them. There’s nothing more frustrating than a graph that’s missing axis labels! Keep in mind that although you’ll be presenting charts and graphs, your text content needs to present a clear narrative that can stand on its own. In other words, don’t rely purely on your figures and tables to convey your key points: highlight the crucial trends and values in the text. Figures and tables should complement the writing, not carry it .
Depending on your research aims, objectives and research questions, you may stop your analysis at this point (i.e. descriptive statistics). However, if your study requires inferential statistics, then it’s time to deep dive into those .
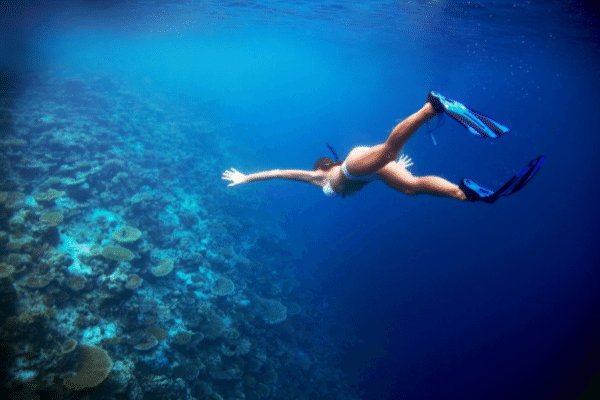
Step 6 – Present the inferential statistics
Inferential statistics are used to make generalisations about a population , whereas descriptive statistics focus purely on the sample . Inferential statistical techniques, broadly speaking, can be broken down into two groups .
First, there are those that compare measurements between groups , such as t-tests (which measure differences between two groups) and ANOVAs (which measure differences between multiple groups). Second, there are techniques that assess the relationships between variables , such as correlation analysis and regression analysis. Within each of these, some tests can be used for normally distributed (parametric) data and some tests are designed specifically for use on non-parametric data.
There are a seemingly endless number of tests that you can use to crunch your data, so it’s easy to run down a rabbit hole and end up with piles of test data. Ultimately, the most important thing is to make sure that you adopt the tests and techniques that allow you to achieve your research objectives and answer your research questions .
In this section of the results chapter, you should try to make use of figures and visual components as effectively as possible. For example, if you present a correlation table, use colour coding to highlight the significance of the correlation values, or scatterplots to visually demonstrate what the trend is. The easier you make it for your reader to digest your findings, the more effectively you’ll be able to make your arguments in the next chapter.
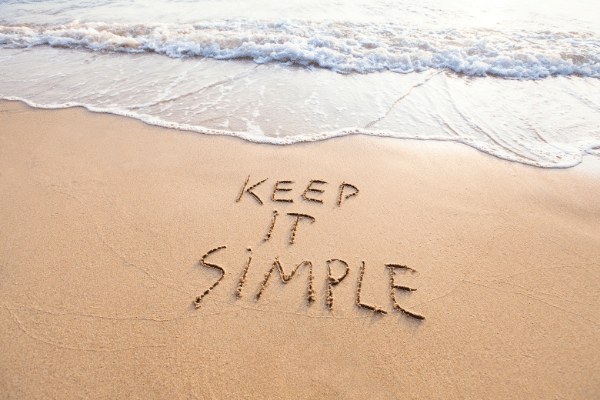
Step 7 – Test your hypotheses
If your study requires it, the next stage is hypothesis testing. A hypothesis is a statement , often indicating a difference between groups or relationship between variables, that can be supported or rejected by a statistical test. However, not all studies will involve hypotheses (again, it depends on the research objectives), so don’t feel like you “must” present and test hypotheses just because you’re undertaking quantitative research.
The basic process for hypothesis testing is as follows:
- Specify your null hypothesis (for example, “The chemical psilocybin has no effect on time perception).
- Specify your alternative hypothesis (e.g., “The chemical psilocybin has an effect on time perception)
- Set your significance level (this is usually 0.05)
- Calculate your statistics and find your p-value (e.g., p=0.01)
- Draw your conclusions (e.g., “The chemical psilocybin does have an effect on time perception”)
Finally, if the aim of your study is to develop and test a conceptual framework , this is the time to present it, following the testing of your hypotheses. While you don’t need to develop or discuss these findings further in the results chapter, indicating whether the tests (and their p-values) support or reject the hypotheses is crucial.
Step 8 – Provide a chapter summary
To wrap up your results chapter and transition to the discussion chapter, you should provide a brief summary of the key findings . “Brief” is the keyword here – much like the chapter introduction, this shouldn’t be lengthy – a paragraph or two maximum. Highlight the findings most relevant to your research objectives and research questions, and wrap it up.
Some final thoughts, tips and tricks
Now that you’ve got the essentials down, here are a few tips and tricks to make your quantitative results chapter shine:
- When writing your results chapter, report your findings in the past tense . You’re talking about what you’ve found in your data, not what you are currently looking for or trying to find.
- Structure your results chapter systematically and sequentially . If you had two experiments where findings from the one generated inputs into the other, report on them in order.
- Make your own tables and graphs rather than copying and pasting them from statistical analysis programmes like SPSS. Check out the DataIsBeautiful reddit for some inspiration.
- Once you’re done writing, review your work to make sure that you have provided enough information to answer your research questions , but also that you didn’t include superfluous information.
If you’ve got any questions about writing up the quantitative results chapter, please leave a comment below. If you’d like 1-on-1 assistance with your quantitative analysis and discussion, check out our hands-on coaching service , or book a free consultation with a friendly coach.
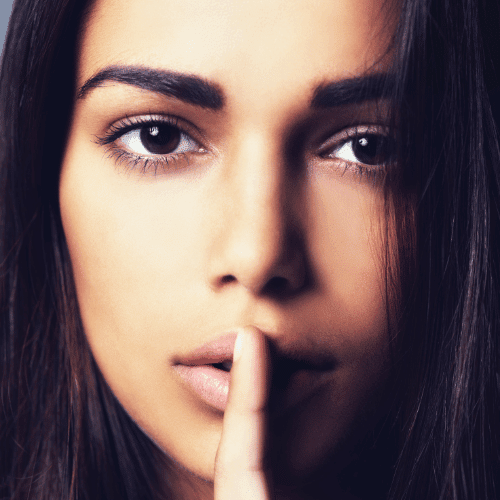
Psst... there’s more!
This post was based on one of our popular Research Bootcamps . If you're working on a research project, you'll definitely want to check this out ...
You Might Also Like:
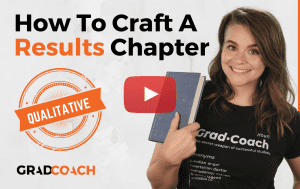
Thank you. I will try my best to write my results.
Awesome content 👏🏾
this was great explaination
Submit a Comment Cancel reply
Your email address will not be published. Required fields are marked *
Save my name, email, and website in this browser for the next time I comment.
- Print Friendly

Want to create or adapt books like this? Learn more about how Pressbooks supports open publishing practices.
Quantitative Data Analysis
9 Presenting the Results of Quantitative Analysis
Mikaila Mariel Lemonik Arthur
This chapter provides an overview of how to present the results of quantitative analysis, in particular how to create effective tables for displaying quantitative results and how to write quantitative research papers that effectively communicate the methods used and findings of quantitative analysis.
Writing the Quantitative Paper
Standard quantitative social science papers follow a specific format. They begin with a title page that includes a descriptive title, the author(s)’ name(s), and a 100 to 200 word abstract that summarizes the paper. Next is an introduction that makes clear the paper’s research question, details why this question is important, and previews what the paper will do. After that comes a literature review, which ends with a summary of the research question(s) and/or hypotheses. A methods section, which explains the source of data, sample, and variables and quantitative techniques used, follows. Many analysts will include a short discussion of their descriptive statistics in the methods section. A findings section details the findings of the analysis, supported by a variety of tables, and in some cases graphs, all of which are explained in the text. Some quantitative papers, especially those using more complex techniques, will include equations. Many papers follow the findings section with a discussion section, which provides an interpretation of the results in light of both the prior literature and theory presented in the literature review and the research questions/hypotheses. A conclusion ends the body of the paper. This conclusion should summarize the findings, answering the research questions and stating whether any hypotheses were supported, partially supported, or not supported. Limitations of the research are detailed. Papers typically include suggestions for future research, and where relevant, some papers include policy implications. After the body of the paper comes the works cited; some papers also have an Appendix that includes additional tables and figures that did not fit into the body of the paper or additional methodological details. While this basic format is similar for papers regardless of the type of data they utilize, there are specific concerns relating to quantitative research in terms of the methods and findings that will be discussed here.
In the methods section, researchers clearly describe the methods they used to obtain and analyze the data for their research. When relying on data collected specifically for a given paper, researchers will need to discuss the sample and data collection; in most cases, though, quantitative research relies on pre-existing datasets. In these cases, researchers need to provide information about the dataset, including the source of the data, the time it was collected, the population, and the sample size. Regardless of the source of the data, researchers need to be clear about which variables they are using in their research and any transformations or manipulations of those variables. They also need to explain the specific quantitative techniques that they are using in their analysis; if different techniques are used to test different hypotheses, this should be made clear. In some cases, publications will require that papers be submitted along with any code that was used to produce the analysis (in SPSS terms, the syntax files), which more advanced researchers will usually have on hand. In many cases, basic descriptive statistics are presented in tabular form and explained within the methods section.
The findings sections of quantitative papers are organized around explaining the results as shown in tables and figures. Not all results are depicted in tables and figures—some minor or null findings will simply be referenced—but tables and figures should be produced for all findings to be discussed at any length. If there are too many tables and figures, some can be moved to an appendix after the body of the text and referred to in the text (e.g. “See Table 12 in Appendix A”).
Discussions of the findings should not simply restate the contents of the table. Rather, they should explain and interpret it for readers, and they should do so in light of the hypothesis or hypotheses that are being tested. Conclusions—discussions of whether the hypothesis or hypotheses are supported or not supported—should wait for the conclusion of the paper.
Creating Effective Tables
When creating tables to display the results of quantitative analysis, the most important goals are to create tables that are clear and concise but that also meet standard conventions in the field. This means, first of all, paring down the volume of information produced in the statistical output to just include the information most necessary for interpreting the results, but doing so in keeping with standard table conventions. It also means making tables that are well-formatted and designed, so that readers can understand what the tables are saying without struggling to find information. For example, tables (as well as figures such as graphs) need clear captions; they are typically numbered and referred to by number in the text. Columns and rows should have clear headings. Depending on the content of the table, formatting tools may need to be used to set off header rows/columns and/or total rows/columns; cell-merging tools may be necessary; and shading may be important in tables with many rows or columns.
Here, you will find some instructions for creating tables of results from descriptive, crosstabulation, correlation, and regression analysis that are clear, concise, and meet normal standards for data display in social science. In addition, after the instructions for creating tables, you will find an example of how a paper incorporating each table might describe that table in the text.
Descriptive Statistics
When presenting the results of descriptive statistics, we create one table with columns for each type of descriptive statistic and rows for each variable. Note, of course, that depending on level of measurement only certain descriptive statistics are appropriate for a given variable, so there may be many cells in the table marked with an — to show that this statistic is not calculated for this variable. So, consider the set of descriptive statistics below, for occupational prestige, age, highest degree earned, and whether the respondent was born in this country.
To display these descriptive statistics in a paper, one might create a table like Table 2. Note that for discrete variables, we use the value label in the table, not the value.
If we were then to discuss our descriptive statistics in a quantitative paper, we might write something like this (note that we do not need to repeat every single detail from the table, as readers can peruse the table themselves):
This analysis relies on four variables from the 2021 General Social Survey: occupational prestige score, age, highest degree earned, and whether the respondent was born in the United States. Descriptive statistics for all four variables are shown in Table 2. The median occupational prestige score is 47, with a range from 16 to 80. 50% of respondents had occupational prestige scores scores between 35 and 59. The median age of respondents is 53, with a range from 18 to 89. 50% of respondents are between ages 37 and 66. Both variables have little skew. Highest degree earned ranges from less than high school to a graduate degree; the median respondent has earned an associate’s degree, while the modal response (given by 39.8% of the respondents) is a high school degree. 88.8% of respondents were born in the United States.
Crosstabulation
When presenting the results of a crosstabulation, we simplify the table so that it highlights the most important information—the column percentages—and include the significance and association below the table. Consider the SPSS output below.
Table 4 shows how a table suitable for include in a paper might look if created from the SPSS output in Table 3. Note that we use asterisks to indicate the significance level of the results: * means p < 0.05; ** means p < 0.01; *** means p < 0.001; and no stars mean p > 0.05 (and thus that the result is not significant). Also note than N is the abbreviation for the number of respondents.
If we were going to discuss the results of this crosstabulation in a quantitative research paper, the discussion might look like this:
A crosstabulation of respondent’s class identification and their highest degree earned, with class identification as the independent variable, is significant, with a Spearman correlation of 0.419, as shown in Table 4. Among lower class and working class respondents, more than 50% had earned a high school degree. Less than 20% of poor respondents and less than 40% of working-class respondents had earned more than a high school degree. In contrast, the majority of middle class and upper class respondents had earned at least a bachelor’s degree. In fact, 50% of upper class respondents had earned a graduate degree.
Correlation
When presenting a correlating matrix, one of the most important things to note is that we only present half the table so as not to include duplicated results. Think of the line through the table where empty cells exist to represent the correlation between a variable and itself, and include only the triangle of data either above or below that line of cells. Consider the output in Table 5.
Table 6 shows what the contents of Table 5 might look like when a table is constructed in a fashion suitable for publication.
If we were to discuss the results of this bivariate correlation analysis in a quantitative paper, the discussion might look like this:
Bivariate correlations were run among variables measuring age, occupational prestige, the highest year of school respondents completed, and family income in constant 1986 dollars, as shown in Table 6. Correlations between age and highest year of school completed and between age and family income are not significant. All other correlations are positive and significant at the p<0.001 level. The correlation between age and occupational prestige is weak; the correlations between income and occupational prestige and between income and educational attainment are moderate, and the correlation between education and occupational prestige is strong.
To present the results of a regression, we create one table that includes all of the key information from the multiple tables of SPSS output. This includes the R 2 and significance of the regression, either the B or the beta values (different analysts have different preferences here) for each variable, and the standard error and significance of each variable. Consider the SPSS output in Table 7.
The regression output in shown in Table 7 contains a lot of information. We do not include all of this information when making tables suitable for publication. As can be seen in Table 8, we include the Beta (or the B), the standard error, and the significance asterisk for each variable; the R 2 and significance for the overall regression; the degrees of freedom (which tells readers the sample size or N); and the constant; along with the key to p/significance values.
If we were to discuss the results of this regression in a quantitative paper, the results might look like this:
Table 8 shows the results of a regression in which age, occupational prestige, and highest year of school completed are the independent variables and family income is the dependent variable. The regression results are significant, and all of the independent variables taken together explain 15.6% of the variance in family income. Age is not a significant predictor of income, while occupational prestige and educational attainment are. Educational attainment has a larger effect on family income than does occupational prestige. For every year of additional education attained, family income goes up on average by $3,988.545; for every one-unit increase in occupational prestige score, family income goes up on average by $522.887. [1]
- Choose two discrete variables and three continuous variables from a dataset of your choice. Produce appropriate descriptive statistics on all five of the variables and create a table of the results suitable for inclusion in a paper.
- Using the two discrete variables you have chosen, produce an appropriate crosstabulation, with significance and measure of association. Create a table of the results suitable for inclusion in a paper.
- Using the three continuous variables you have chosen, produce a correlation matrix. Create a table of the results suitable for inclusion in a paper.
- Using the three continuous variables you have chosen, produce a multivariate linear regression. Create a table of the results suitable for inclusion in a paper.
- Write a methods section describing the dataset, analytical methods, and variables you utilized in questions 1, 2, 3, and 4 and explaining the results of your descriptive analysis.
- Write a findings section explaining the results of the analyses you performed in questions 2, 3, and 4.
- Note that the actual numberical increase comes from the B values, which are shown in the SPSS output in Table 7 but not in the reformatted Table 8. ↵
Social Data Analysis Copyright © 2021 by Mikaila Mariel Lemonik Arthur is licensed under a Creative Commons Attribution-NonCommercial-ShareAlike 4.0 International License , except where otherwise noted.
- (855) 776-7763
Training Maker
All Products
Qualaroo Insights
ProProfs.com
- Sign Up Free
Do you want a free Survey Software?
We have the #1 Online Survey Maker Software to get actionable user insights.
How to Write Quantitative Research Questions: Types With Examples
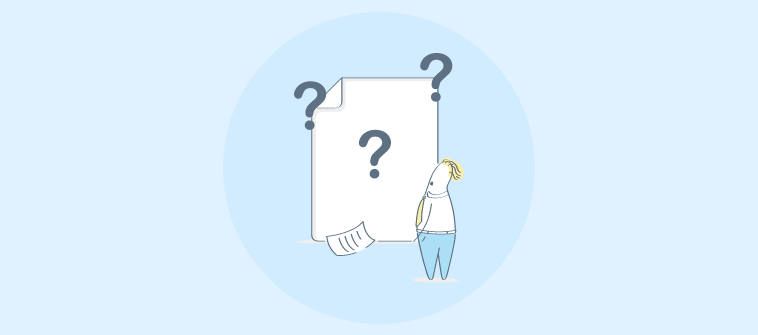
For research to be effective, it becomes crucial to properly formulate the quantitative research questions in a correct way. Otherwise, you will not get the answers you were looking for.
Has it ever happened that you conducted a quantitative research study and found out the results you were expecting are quite different from the actual results?
This could happen due to many factors like the unpredictable nature of respondents, errors in calculation, research bias, etc. However, your quantitative research usually does not provide reliable results when questions are not written correctly.
We get it! Structuring the quantitative research questions can be a difficult task.
Hence, in this blog, we will share a few bits of advice on how to write good quantitative research questions. We will also look at different types of quantitative research questions along with their examples.
Let’s start:
How to Write Quantitative Research Questions?
When you want to obtain actionable insight into the trends and patterns of the research topic to make sense of it, quantitative research questions are your best bet.
Being objective in nature, these questions provide you with detailed information about the research topic and help in collecting quantifiable data that can be easily analyzed. This data can be generalized to the entire population and help make data-driven and sound decisions.
Respondents find it easier to answer quantitative survey questions than qualitative questions. At the same time, researchers can also analyze them quickly using various statistical models.
However, when it comes to writing the quantitative research questions, one can get a little overwhelmed as the entire study depends on the types of questions used.
There is no “one good way” to prepare these questions. However, to design well-structured quantitative research questions, you can follow the 4-steps approach given below:
1. Select the Type of Quantitative Question
The first step is to determine which type of quantitative question you want to add to your study. There are three types of quantitative questions:
- Descriptive
- Comparative
- Relationship-based
This will help you choose the correct words and phrases while constructing the question. At the same time, it will also assist readers in understanding the question correctly.
2. Identify the Type of Variable
The second step involves identifying the type of variable you are trying to measure, manipulate, or control. Basically, there are two types of variables:
- Independent variable (a variable that is being manipulated)
- Dependent variable (outcome variable)
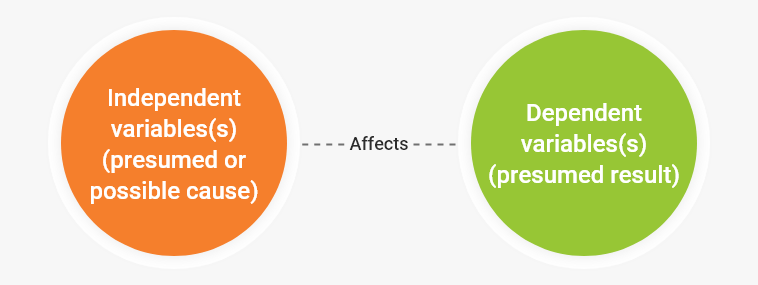
If you plan to use descriptive research questions, you have to deal with a number of dependent variables. However, where you plan to create comparative or relationship research questions, you will deal with both dependent and independent variables.
3. Select the Suitable Structure
The next step is determining the structure of the research question. It involves:
- Identifying the components of the question. It involves the type of dependent or independent variable and a group of interest (the group from which the researcher tries to conclude the population).
- The number of different components used. Like, as to how many variables and groups are being examined.
- Order in which these are presented. For example, the independent variable before the dependent variable or vice versa.
4. Draft the Complete Research Question
The last step involves identifying the problem or issue that you are trying to address in the form of complete quantitative survey questions . Also, make sure to build an exhaustive list of response options to make sure your respondents select the correct response. If you miss adding important answer options, then the ones chosen by respondents may not be entirely true.
Types of Quantitative Research Questions With Examples
Quantitative research questions are generally used to answer the “who” and “what” of the research topic. For quantitative research to be effective, it is crucial that the respondents are able to answer your questions concisely and precisely. With that in mind, let’s look in greater detail at the three types of formats you can use when preparing quantitative market research questions.
1. Descriptive
Descriptive research questions are used to collect participants’ opinions about the variable that you want to quantify. It is the most effortless way to measure the particular variable (single or multiple variables) you are interested in on a large scale. Usually, descriptive research questions begin with “ how much,” “how often,” “what percentage,” “what proportion,” etc.
Examples of descriptive research questions include:
2. Comparative
Comparative research questions help you identify the difference between two or more groups based on one or more variables. In general, a comparative research question is used to quantify one variable; however, you can use two or more variables depending on your market research objectives.
Comparative research questions examples include:
3. Relationship-based
Relationship research questions are used to identify trends, causal relationships, or associations between two or more variables. It is not vital to distinguish between causal relationships, trends, or associations while using these types of questions. These questions begin with “What is the relationship” between independent and dependent variables, amongst or between two or more groups.
Relationship-based quantitative questions examples include:
Ready to Write Your Quantitative Research Questions?
So, there you have it. It was all about quantitative research question types and their examples. By now, you must have figured out a way to write quantitative research questions for your survey to collect actionable customer feedback.
Now, the only thing you need is a good survey maker tool , like ProProfs Survey Maker , that will glide your process of designing and conducting your surveys . You also get access to various survey question types, both qualitative and quantitative, that you can add to any kind of survey along with professionally-designed survey templates .
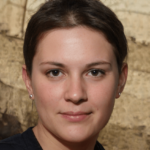
About the author
Emma David is a seasoned market research professional with 8+ years of experience. Having kick-started her journey in research, she has developed rich expertise in employee engagement, survey creation and administration, and data management. Emma believes in the power of data to shape business performance positively. She continues to help brands and businesses make strategic decisions and improve their market standing through her understanding of research methodologies.
Popular Posts in This Category
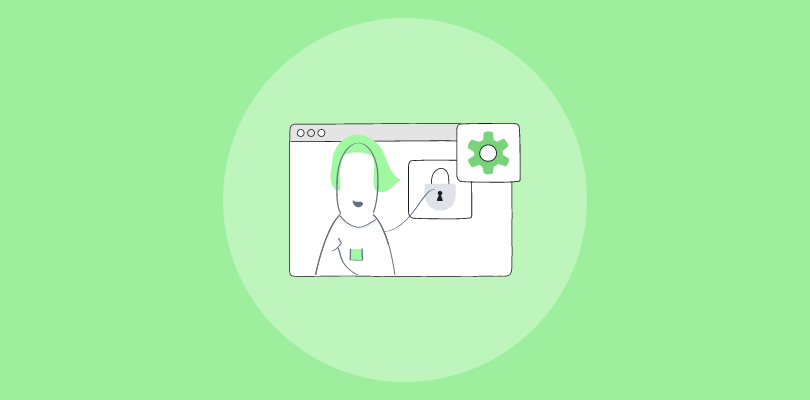
Top 10 Delighted Alternatives & Competitors in 2024
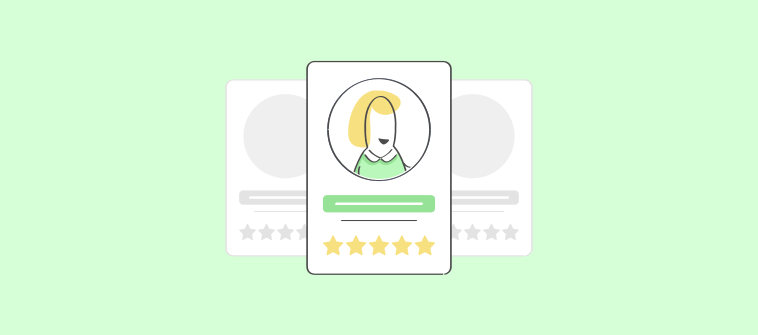
How to Measure Customer Satisfaction In 5 Simple Steps
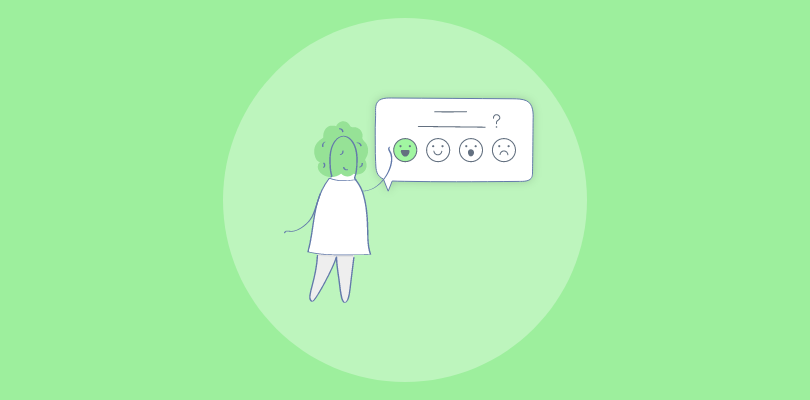
Guide to Create an Effective Employee Surveys [Questions + Templates]
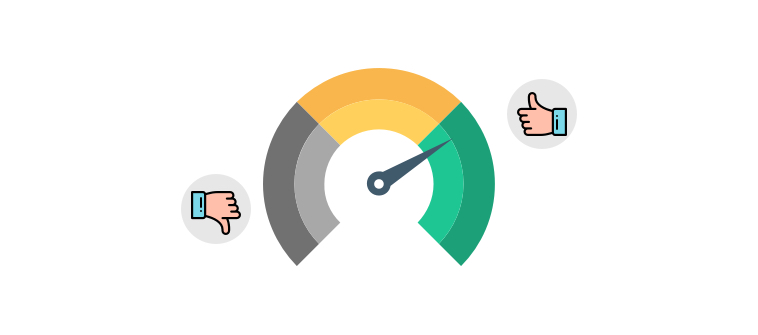
What Is the Difference Between CSAT, CES, and NPS? Pros & Cons Explained
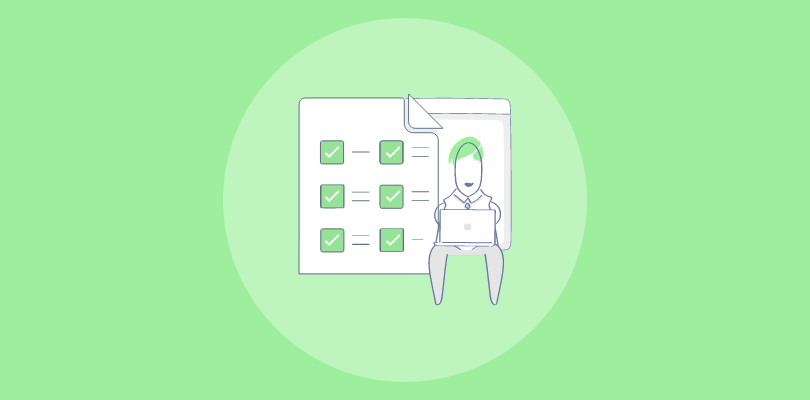
10 Best Form Builder Software to Simplify Data Collection
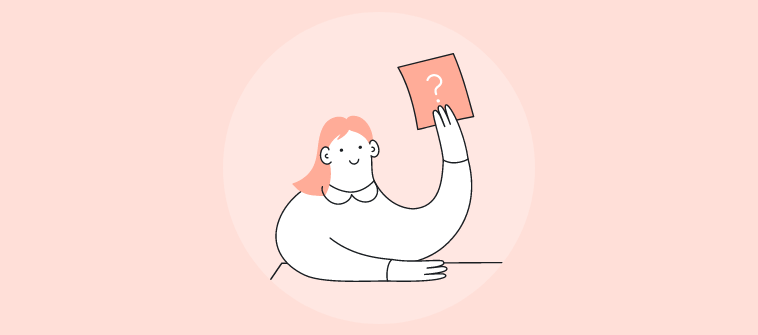
How to Ask Sensitive Questions in Surveys
Have a language expert improve your writing
Run a free plagiarism check in 10 minutes, automatically generate references for free.
- Knowledge Base
- Methodology
Research Design | Step-by-Step Guide with Examples
Published on 5 May 2022 by Shona McCombes . Revised on 20 March 2023.
A research design is a strategy for answering your research question using empirical data. Creating a research design means making decisions about:
- Your overall aims and approach
- The type of research design you’ll use
- Your sampling methods or criteria for selecting subjects
- Your data collection methods
- The procedures you’ll follow to collect data
- Your data analysis methods
A well-planned research design helps ensure that your methods match your research aims and that you use the right kind of analysis for your data.
Table of contents
Step 1: consider your aims and approach, step 2: choose a type of research design, step 3: identify your population and sampling method, step 4: choose your data collection methods, step 5: plan your data collection procedures, step 6: decide on your data analysis strategies, frequently asked questions.
- Introduction
Before you can start designing your research, you should already have a clear idea of the research question you want to investigate.
There are many different ways you could go about answering this question. Your research design choices should be driven by your aims and priorities – start by thinking carefully about what you want to achieve.
The first choice you need to make is whether you’ll take a qualitative or quantitative approach.
Qualitative research designs tend to be more flexible and inductive , allowing you to adjust your approach based on what you find throughout the research process.
Quantitative research designs tend to be more fixed and deductive , with variables and hypotheses clearly defined in advance of data collection.
It’s also possible to use a mixed methods design that integrates aspects of both approaches. By combining qualitative and quantitative insights, you can gain a more complete picture of the problem you’re studying and strengthen the credibility of your conclusions.
Practical and ethical considerations when designing research
As well as scientific considerations, you need to think practically when designing your research. If your research involves people or animals, you also need to consider research ethics .
- How much time do you have to collect data and write up the research?
- Will you be able to gain access to the data you need (e.g., by travelling to a specific location or contacting specific people)?
- Do you have the necessary research skills (e.g., statistical analysis or interview techniques)?
- Will you need ethical approval ?
At each stage of the research design process, make sure that your choices are practically feasible.
Prevent plagiarism, run a free check.
Within both qualitative and quantitative approaches, there are several types of research design to choose from. Each type provides a framework for the overall shape of your research.
Types of quantitative research designs
Quantitative designs can be split into four main types. Experimental and quasi-experimental designs allow you to test cause-and-effect relationships, while descriptive and correlational designs allow you to measure variables and describe relationships between them.
With descriptive and correlational designs, you can get a clear picture of characteristics, trends, and relationships as they exist in the real world. However, you can’t draw conclusions about cause and effect (because correlation doesn’t imply causation ).
Experiments are the strongest way to test cause-and-effect relationships without the risk of other variables influencing the results. However, their controlled conditions may not always reflect how things work in the real world. They’re often also more difficult and expensive to implement.
Types of qualitative research designs
Qualitative designs are less strictly defined. This approach is about gaining a rich, detailed understanding of a specific context or phenomenon, and you can often be more creative and flexible in designing your research.
The table below shows some common types of qualitative design. They often have similar approaches in terms of data collection, but focus on different aspects when analysing the data.
Your research design should clearly define who or what your research will focus on, and how you’ll go about choosing your participants or subjects.
In research, a population is the entire group that you want to draw conclusions about, while a sample is the smaller group of individuals you’ll actually collect data from.
Defining the population
A population can be made up of anything you want to study – plants, animals, organisations, texts, countries, etc. In the social sciences, it most often refers to a group of people.
For example, will you focus on people from a specific demographic, region, or background? Are you interested in people with a certain job or medical condition, or users of a particular product?
The more precisely you define your population, the easier it will be to gather a representative sample.
Sampling methods
Even with a narrowly defined population, it’s rarely possible to collect data from every individual. Instead, you’ll collect data from a sample.
To select a sample, there are two main approaches: probability sampling and non-probability sampling . The sampling method you use affects how confidently you can generalise your results to the population as a whole.
Probability sampling is the most statistically valid option, but it’s often difficult to achieve unless you’re dealing with a very small and accessible population.
For practical reasons, many studies use non-probability sampling, but it’s important to be aware of the limitations and carefully consider potential biases. You should always make an effort to gather a sample that’s as representative as possible of the population.
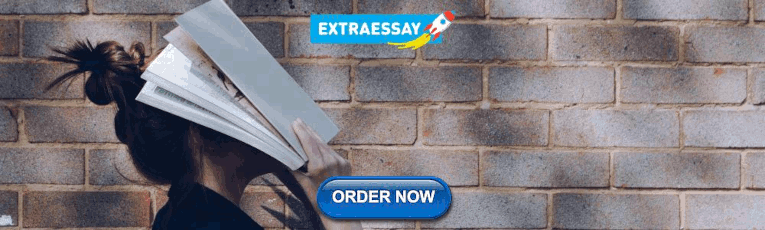
Case selection in qualitative research
In some types of qualitative designs, sampling may not be relevant.
For example, in an ethnography or a case study, your aim is to deeply understand a specific context, not to generalise to a population. Instead of sampling, you may simply aim to collect as much data as possible about the context you are studying.
In these types of design, you still have to carefully consider your choice of case or community. You should have a clear rationale for why this particular case is suitable for answering your research question.
For example, you might choose a case study that reveals an unusual or neglected aspect of your research problem, or you might choose several very similar or very different cases in order to compare them.
Data collection methods are ways of directly measuring variables and gathering information. They allow you to gain first-hand knowledge and original insights into your research problem.
You can choose just one data collection method, or use several methods in the same study.
Survey methods
Surveys allow you to collect data about opinions, behaviours, experiences, and characteristics by asking people directly. There are two main survey methods to choose from: questionnaires and interviews.
Observation methods
Observations allow you to collect data unobtrusively, observing characteristics, behaviours, or social interactions without relying on self-reporting.
Observations may be conducted in real time, taking notes as you observe, or you might make audiovisual recordings for later analysis. They can be qualitative or quantitative.
Other methods of data collection
There are many other ways you might collect data depending on your field and topic.
If you’re not sure which methods will work best for your research design, try reading some papers in your field to see what data collection methods they used.
Secondary data
If you don’t have the time or resources to collect data from the population you’re interested in, you can also choose to use secondary data that other researchers already collected – for example, datasets from government surveys or previous studies on your topic.
With this raw data, you can do your own analysis to answer new research questions that weren’t addressed by the original study.
Using secondary data can expand the scope of your research, as you may be able to access much larger and more varied samples than you could collect yourself.
However, it also means you don’t have any control over which variables to measure or how to measure them, so the conclusions you can draw may be limited.
As well as deciding on your methods, you need to plan exactly how you’ll use these methods to collect data that’s consistent, accurate, and unbiased.
Planning systematic procedures is especially important in quantitative research, where you need to precisely define your variables and ensure your measurements are reliable and valid.
Operationalisation
Some variables, like height or age, are easily measured. But often you’ll be dealing with more abstract concepts, like satisfaction, anxiety, or competence. Operationalisation means turning these fuzzy ideas into measurable indicators.
If you’re using observations , which events or actions will you count?
If you’re using surveys , which questions will you ask and what range of responses will be offered?
You may also choose to use or adapt existing materials designed to measure the concept you’re interested in – for example, questionnaires or inventories whose reliability and validity has already been established.
Reliability and validity
Reliability means your results can be consistently reproduced , while validity means that you’re actually measuring the concept you’re interested in.
For valid and reliable results, your measurement materials should be thoroughly researched and carefully designed. Plan your procedures to make sure you carry out the same steps in the same way for each participant.
If you’re developing a new questionnaire or other instrument to measure a specific concept, running a pilot study allows you to check its validity and reliability in advance.
Sampling procedures
As well as choosing an appropriate sampling method, you need a concrete plan for how you’ll actually contact and recruit your selected sample.
That means making decisions about things like:
- How many participants do you need for an adequate sample size?
- What inclusion and exclusion criteria will you use to identify eligible participants?
- How will you contact your sample – by mail, online, by phone, or in person?
If you’re using a probability sampling method, it’s important that everyone who is randomly selected actually participates in the study. How will you ensure a high response rate?
If you’re using a non-probability method, how will you avoid bias and ensure a representative sample?
Data management
It’s also important to create a data management plan for organising and storing your data.
Will you need to transcribe interviews or perform data entry for observations? You should anonymise and safeguard any sensitive data, and make sure it’s backed up regularly.
Keeping your data well organised will save time when it comes to analysing them. It can also help other researchers validate and add to your findings.
On their own, raw data can’t answer your research question. The last step of designing your research is planning how you’ll analyse the data.
Quantitative data analysis
In quantitative research, you’ll most likely use some form of statistical analysis . With statistics, you can summarise your sample data, make estimates, and test hypotheses.
Using descriptive statistics , you can summarise your sample data in terms of:
- The distribution of the data (e.g., the frequency of each score on a test)
- The central tendency of the data (e.g., the mean to describe the average score)
- The variability of the data (e.g., the standard deviation to describe how spread out the scores are)
The specific calculations you can do depend on the level of measurement of your variables.
Using inferential statistics , you can:
- Make estimates about the population based on your sample data.
- Test hypotheses about a relationship between variables.
Regression and correlation tests look for associations between two or more variables, while comparison tests (such as t tests and ANOVAs ) look for differences in the outcomes of different groups.
Your choice of statistical test depends on various aspects of your research design, including the types of variables you’re dealing with and the distribution of your data.
Qualitative data analysis
In qualitative research, your data will usually be very dense with information and ideas. Instead of summing it up in numbers, you’ll need to comb through the data in detail, interpret its meanings, identify patterns, and extract the parts that are most relevant to your research question.
Two of the most common approaches to doing this are thematic analysis and discourse analysis .
There are many other ways of analysing qualitative data depending on the aims of your research. To get a sense of potential approaches, try reading some qualitative research papers in your field.
A sample is a subset of individuals from a larger population. Sampling means selecting the group that you will actually collect data from in your research.
For example, if you are researching the opinions of students in your university, you could survey a sample of 100 students.
Statistical sampling allows you to test a hypothesis about the characteristics of a population. There are various sampling methods you can use to ensure that your sample is representative of the population as a whole.
Operationalisation means turning abstract conceptual ideas into measurable observations.
For example, the concept of social anxiety isn’t directly observable, but it can be operationally defined in terms of self-rating scores, behavioural avoidance of crowded places, or physical anxiety symptoms in social situations.
Before collecting data , it’s important to consider how you will operationalise the variables that you want to measure.
The research methods you use depend on the type of data you need to answer your research question .
- If you want to measure something or test a hypothesis , use quantitative methods . If you want to explore ideas, thoughts, and meanings, use qualitative methods .
- If you want to analyse a large amount of readily available data, use secondary data. If you want data specific to your purposes with control over how they are generated, collect primary data.
- If you want to establish cause-and-effect relationships between variables , use experimental methods. If you want to understand the characteristics of a research subject, use descriptive methods.
Cite this Scribbr article
If you want to cite this source, you can copy and paste the citation or click the ‘Cite this Scribbr article’ button to automatically add the citation to our free Reference Generator.
McCombes, S. (2023, March 20). Research Design | Step-by-Step Guide with Examples. Scribbr. Retrieved 27 May 2024, from https://www.scribbr.co.uk/research-methods/research-design/
Is this article helpful?
Shona McCombes
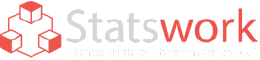
How To Write Methodology For A Quantitative Study?
Methodology Chapter Provides Information On All The Steps Taken For The Study Of A Problem And The Justifications Given For Specific Steps Taken To Gather, Process, And Analyze Data Related To Understanding The Problem. The Chapter Allows The Reader To Understand And Evaluate The Research Design And Thus Validate It.
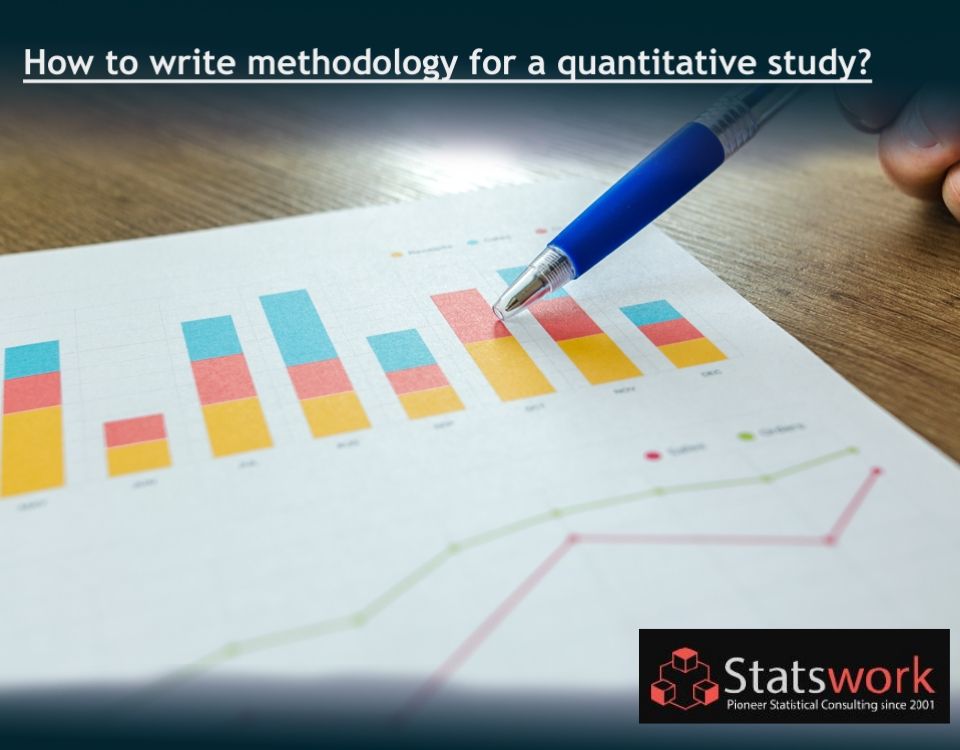
Quantitative Study: Quantitative Research Is The Quantification Of A Problem Via Generating Numerical Data That Are Most Often Converted Into Statistics. The Statistical Analysis In Research Methodology Helps In Proving Or Disproving A Thesis. The Research Is Usually Conducted Among A Broad Populace In The Form Of Questionnaire, Polls And Other Surveys Which Involves The Participants To Reply In Numbers. This Means Quantitative Research Falls Under The Headings Of Empirical Or Statistical Studies. Its Main Characteristics Are :
- Use Of Standard Instruments To Gather Data.
- Sizes Of The Samples Are Proportional To That Of The Population In Question.
- Research Is Conducted On A Well-Defined Question.
- Studies Are Well Designed Before The Process.
- Numerical Data That Quantify A Problem Or An Area Of Research Using Various Means Like Charts, Tables, Graphs, Etc. Data Collection Carried Out Through Surveys, Questionnaires, Etc.
A Methodology Chapter For A Quantitative Study Explains The Following In A Direct And Precise Manner:
How Was The Data Generated? How Was It Processed? Why Was The Design, That Was Used, Used?
Any Methodology Chapter Must Be Written In The Past Tense And Follows The Following Guidelines: Chapter Provides Information On The Methods Of Data Collection To Enable The Readers To Understand The Process.
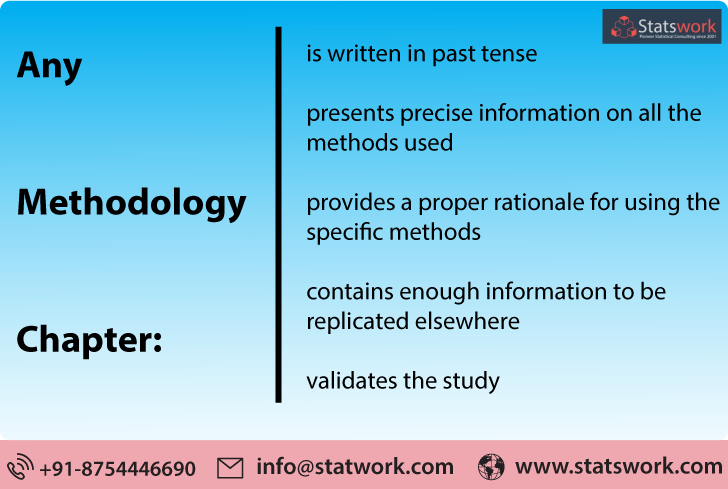
- Significance Of The Methodology Is That A Proper Methodology Validates The Study As A Whole.
- Methodology Section Should Provide A Rationale For Choosing A Particular Design.
- Methods Used Must Be Valid And Conforming To The Conventional Norms Of Research. As Such, The Methodology Chapter Must Provide Enough Evidence To Support The Credibility Of Each Method Used.
- The Appropriateness Of The Methods Chosen Must Be Stated. Only The Methods That Are Appropriate To The Scale Of The Subject In Investigation Must Be Chosen.
- The Chapter Must Contain Information On The Constraints And Challenges That Were Anticipated Before The Beginning Of The Study, The Steps Taken To Prevent The Problems From Occurring, And The Ways The Constraints That Did Occur Were Tackled With Minimal Impact And How The Said Impact Doesn’t Affect The Outcome Of The Study.
- Necessary Information To Replicate Any Particular Method Must Be Provided Concisely In The Chapter.
A methodology for a quantitative study begins with a reiteration of the research question and its context.
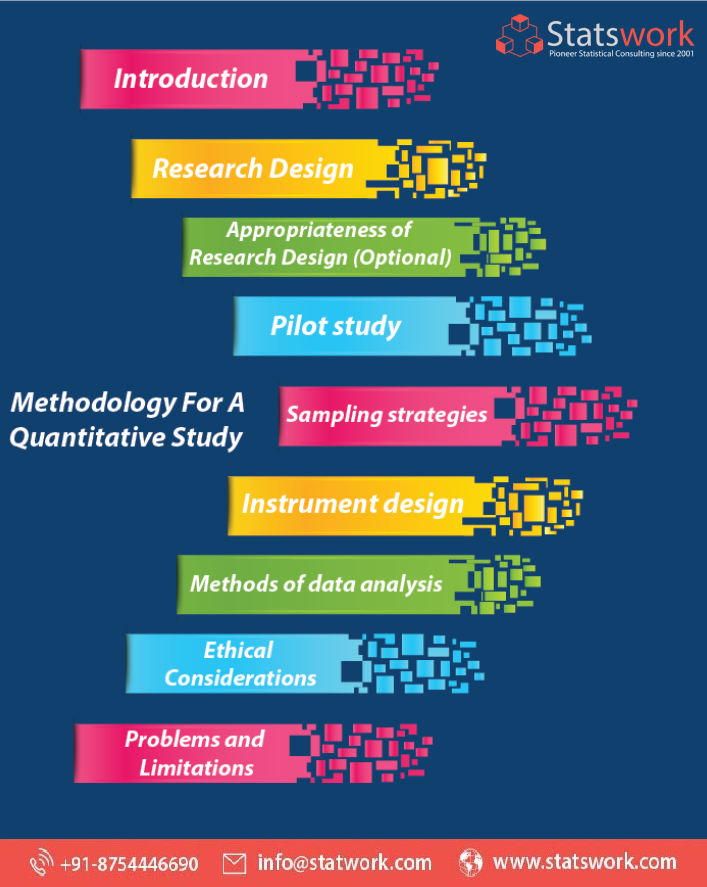
This is followed by the research design , where the methods used to gather, process and analyze the data are given. This is usually preceded or followed by a justification of the appropriateness of the research design . Then, the information on the pilot study is given. It is followed by the precise information on sampling strategies, instrument design, and methods of data analysis . ‘ Ethical considerations ’ comes next . Here the researcher informs the reader of the measures taken to ensure participants privacy and consent, etc. Penultimate to concluding the methodology chapter is problems and limitations, where the reader is informed of all the constraints the researcher anticipated and all the problems that occurred and the limitations methods may pose to the overall study.
Links, Related Posts
– indispensable qualitative research methods.
- Qualitative Research Design Methodology
- Quantitative Research Design Methodology
- Research Methodology Help
- Research Methodology Services
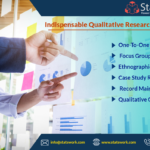
- A global market analysis (1)
- Academic (22)
- Algorithms (1)
- Big Data Analytics (4)
- Bio Statistics (3)
- Clinical Prediction Model (1)
- Corporate (9)
- Corporate statistics service (1)
- Data Analyses (23)
- Data collection (11)
- Genomics & Bioinformatics (1)
- Guidelines (2)
- Machine Learning – Blogs (1)
- Meta-analysis service (2)
- Network Analysis (1)
- Predictive analyses (2)
- Qualitative (1)
- Quantitaive (2)
- Quantitative Data analysis service (1)
- Research (59)
- Shipping & Logistics (1)
- Statistical analysis service (7)
- Statistical models (1)
- Statistical Report Writing (1)
- Statistical Software (10)
- Statistics (64)
- Survey & Interview from Statswork (1)
- Uncategorized (1)
Recent Posts
- Top 10 Machine Learning Algorithms Expected to Shape the Future of AI
- Data-Driven Governance: Revolutionizing State Youth Policies through Web Scraping
- The Future is Now: The Potential of Predictive Analytics Models and Algorithms
- 2024 Vision: Exploring the Impact and Evolution of Advanced Analytics Tools
- Application of machine learning in marketing
Statswork is a pioneer statistical consulting company providing full assistance to researchers and scholars. Statswork offers expert consulting assistance and enhancing researchers by our distinct statistical process and communication throughout the research process with us.
Functional Area
– Research Planning – Tool Development – Data Mining – Data Collection – Statistics Coursework – Research Methodology – Meta Analysis – Data Analysis
- – Corporate
- – Statistical Software
- – Statistics
Corporate Office
#10, Kutty Street, Nungambakkam, Chennai, Tamil Nadu – 600034, India No : +91 4433182000, UK No : +44-1223926607 , US No : +1-9725029262 Email: [email protected]
Website: www.statswork.com
© 2024 Statswork. All Rights Reserved
- UR Research
- Citation Management
- NIH Public Access
More information on Research
- Apps and Mobile Resources
- Med Students Phase 1
- School of Nursing
- Faculty Resources
More information on Guides and Tutorials
- iPad Information and Support
More information on Computing and iPads
- Library Hours
- Make A Gift
- A-Z Site Index
More information on us
- Miner Library Classrooms
- Multimedia Tools and Support
- Meet with a Librarian
More information on Teaching and Learning
- History of Medicine
More information on Historical Services
- Order Articles and Books
- Find Articles
- Access Resources from Off Campus
- Get Info for my Patient
- Contact my Librarian
More information on our services
- Research and Publishing
- Guides and Tutorials
- Computing and iPads
- Teaching and Learning
- How Do I..?
Medical Education Research and Scholarship: Quantitative Methods
- Literature Reviews
- Program Evaluation
- Quantitative Methods
- Qualitative Methods
- Dissemination
Overview of Quantitative Research Methods in Medical Education
- Conducting Quantitative Medical Education Research: From Design to Dissemination
- 23 Questions to Guide the Writing of a Quantitative Medical Education Research Proposal
- Quantitative Research Methods in Medical Education
Survey Design
- How to conduct surveys: A step-by-step guide
- Educator's blueprint: A how-to guide for survey design
- Step-by-step survey design for medical education research
- Pitfalls to avoid in survey design
- << Previous: Program Evaluation
- Next: Qualitative Methods >>
- Last Updated: May 24, 2024 2:42 PM
- URL: https://libguides.urmc.rochester.edu/meded-research-scholarship
- USC Libraries
- Research Guides
Organizing Your Social Sciences Research Paper
Glossary of research terms.
- Purpose of Guide
- Design Flaws to Avoid
- Independent and Dependent Variables
- Reading Research Effectively
- Narrowing a Topic Idea
- Broadening a Topic Idea
- Extending the Timeliness of a Topic Idea
- Academic Writing Style
- Applying Critical Thinking
- Choosing a Title
- Making an Outline
- Paragraph Development
- Research Process Video Series
- Executive Summary
- The C.A.R.S. Model
- Background Information
- The Research Problem/Question
- Theoretical Framework
- Citation Tracking
- Content Alert Services
- Evaluating Sources
- Primary Sources
- Secondary Sources
- Tiertiary Sources
- Scholarly vs. Popular Publications
- Qualitative Methods
- Quantitative Methods
- Insiderness
- Using Non-Textual Elements
- Limitations of the Study
- Common Grammar Mistakes
- Writing Concisely
- Avoiding Plagiarism
- Footnotes or Endnotes?
- Further Readings
- Generative AI and Writing
- USC Libraries Tutorials and Other Guides
- Bibliography
This glossary is intended to assist you in understanding commonly used terms and concepts when reading, interpreting, and evaluating scholarly research. Also included are common words and phrases defined within the context of how they apply to research in the social and behavioral sciences.
- Acculturation -- refers to the process of adapting to another culture, particularly in reference to blending in with the majority population [e.g., an immigrant adopting American customs]. However, acculturation also implies that both cultures add something to one another, but still remain distinct groups unto themselves.
- Accuracy -- a term used in survey research to refer to the match between the target population and the sample.
- Affective Measures -- procedures or devices used to obtain quantified descriptions of an individual's feelings, emotional states, or dispositions.
- Aggregate -- a total created from smaller units. For instance, the population of a county is an aggregate of the populations of the cities, rural areas, etc. that comprise the county. As a verb, it refers to total data from smaller units into a large unit.
- Anonymity -- a research condition in which no one, including the researcher, knows the identities of research participants.
- Baseline -- a control measurement carried out before an experimental treatment.
- Behaviorism -- school of psychological thought concerned with the observable, tangible, objective facts of behavior, rather than with subjective phenomena such as thoughts, emotions, or impulses. Contemporary behaviorism also emphasizes the study of mental states such as feelings and fantasies to the extent that they can be directly observed and measured.
- Beliefs -- ideas, doctrines, tenets, etc. that are accepted as true on grounds which are not immediately susceptible to rigorous proof.
- Benchmarking -- systematically measuring and comparing the operations and outcomes of organizations, systems, processes, etc., against agreed upon "best-in-class" frames of reference.
- Bias -- a loss of balance and accuracy in the use of research methods. It can appear in research via the sampling frame, random sampling, or non-response. It can also occur at other stages in research, such as while interviewing, in the design of questions, or in the way data are analyzed and presented. Bias means that the research findings will not be representative of, or generalizable to, a wider population.
- Case Study -- the collection and presentation of detailed information about a particular participant or small group, frequently including data derived from the subjects themselves.
- Causal Hypothesis -- a statement hypothesizing that the independent variable affects the dependent variable in some way.
- Causal Relationship -- the relationship established that shows that an independent variable, and nothing else, causes a change in a dependent variable. It also establishes how much of a change is shown in the dependent variable.
- Causality -- the relation between cause and effect.
- Central Tendency -- any way of describing or characterizing typical, average, or common values in some distribution.
- Chi-square Analysis -- a common non-parametric statistical test which compares an expected proportion or ratio to an actual proportion or ratio.
- Claim -- a statement, similar to a hypothesis, which is made in response to the research question and that is affirmed with evidence based on research.
- Classification -- ordering of related phenomena into categories, groups, or systems according to characteristics or attributes.
- Cluster Analysis -- a method of statistical analysis where data that share a common trait are grouped together. The data is collected in a way that allows the data collector to group data according to certain characteristics.
- Cohort Analysis -- group by group analytic treatment of individuals having a statistical factor in common to each group. Group members share a particular characteristic [e.g., born in a given year] or a common experience [e.g., entering a college at a given time].
- Confidentiality -- a research condition in which no one except the researcher(s) knows the identities of the participants in a study. It refers to the treatment of information that a participant has disclosed to the researcher in a relationship of trust and with the expectation that it will not be revealed to others in ways that violate the original consent agreement, unless permission is granted by the participant.
- Confirmability Objectivity -- the findings of the study could be confirmed by another person conducting the same study.
- Construct -- refers to any of the following: something that exists theoretically but is not directly observable; a concept developed [constructed] for describing relations among phenomena or for other research purposes; or, a theoretical definition in which concepts are defined in terms of other concepts. For example, intelligence cannot be directly observed or measured; it is a construct.
- Construct Validity -- seeks an agreement between a theoretical concept and a specific measuring device, such as observation.
- Constructivism -- the idea that reality is socially constructed. It is the view that reality cannot be understood outside of the way humans interact and that the idea that knowledge is constructed, not discovered. Constructivists believe that learning is more active and self-directed than either behaviorism or cognitive theory would postulate.
- Content Analysis -- the systematic, objective, and quantitative description of the manifest or latent content of print or nonprint communications.
- Context Sensitivity -- awareness by a qualitative researcher of factors such as values and beliefs that influence cultural behaviors.
- Control Group -- the group in an experimental design that receives either no treatment or a different treatment from the experimental group. This group can thus be compared to the experimental group.
- Controlled Experiment -- an experimental design with two or more randomly selected groups [an experimental group and control group] in which the researcher controls or introduces the independent variable and measures the dependent variable at least two times [pre- and post-test measurements].
- Correlation -- a common statistical analysis, usually abbreviated as r, that measures the degree of relationship between pairs of interval variables in a sample. The range of correlation is from -1.00 to zero to +1.00. Also, a non-cause and effect relationship between two variables.
- Covariate -- a product of the correlation of two related variables times their standard deviations. Used in true experiments to measure the difference of treatment between them.
- Credibility -- a researcher's ability to demonstrate that the object of a study is accurately identified and described based on the way in which the study was conducted.
- Critical Theory -- an evaluative approach to social science research, associated with Germany's neo-Marxist “Frankfurt School,” that aims to criticize as well as analyze society, opposing the political orthodoxy of modern communism. Its goal is to promote human emancipatory forces and to expose ideas and systems that impede them.
- Data -- factual information [as measurements or statistics] used as a basis for reasoning, discussion, or calculation.
- Data Mining -- the process of analyzing data from different perspectives and summarizing it into useful information, often to discover patterns and/or systematic relationships among variables.
- Data Quality -- this is the degree to which the collected data [results of measurement or observation] meet the standards of quality to be considered valid [trustworthy] and reliable [dependable].
- Deductive -- a form of reasoning in which conclusions are formulated about particulars from general or universal premises.
- Dependability -- being able to account for changes in the design of the study and the changing conditions surrounding what was studied.
- Dependent Variable -- a variable that varies due, at least in part, to the impact of the independent variable. In other words, its value “depends” on the value of the independent variable. For example, in the variables “gender” and “academic major,” academic major is the dependent variable, meaning that your major cannot determine whether you are male or female, but your gender might indirectly lead you to favor one major over another.
- Deviation -- the distance between the mean and a particular data point in a given distribution.
- Discourse Community -- a community of scholars and researchers in a given field who respond to and communicate to each other through published articles in the community's journals and presentations at conventions. All members of the discourse community adhere to certain conventions for the presentation of their theories and research.
- Discrete Variable -- a variable that is measured solely in whole units, such as, gender and number of siblings.
- Distribution -- the range of values of a particular variable.
- Effect Size -- the amount of change in a dependent variable that can be attributed to manipulations of the independent variable. A large effect size exists when the value of the dependent variable is strongly influenced by the independent variable. It is the mean difference on a variable between experimental and control groups divided by the standard deviation on that variable of the pooled groups or of the control group alone.
- Emancipatory Research -- research is conducted on and with people from marginalized groups or communities. It is led by a researcher or research team who is either an indigenous or external insider; is interpreted within intellectual frameworks of that group; and, is conducted largely for the purpose of empowering members of that community and improving services for them. It also engages members of the community as co-constructors or validators of knowledge.
- Empirical Research -- the process of developing systematized knowledge gained from observations that are formulated to support insights and generalizations about the phenomena being researched.
- Epistemology -- concerns knowledge construction; asks what constitutes knowledge and how knowledge is validated.
- Ethnography -- method to study groups and/or cultures over a period of time. The goal of this type of research is to comprehend the particular group/culture through immersion into the culture or group. Research is completed through various methods but, since the researcher is immersed within the group for an extended period of time, more detailed information is usually collected during the research.
- Expectancy Effect -- any unconscious or conscious cues that convey to the participant in a study how the researcher wants them to respond. Expecting someone to behave in a particular way has been shown to promote the expected behavior. Expectancy effects can be minimized by using standardized interactions with subjects, automated data-gathering methods, and double blind protocols.
- External Validity -- the extent to which the results of a study are generalizable or transferable.
- Factor Analysis -- a statistical test that explores relationships among data. The test explores which variables in a data set are most related to each other. In a carefully constructed survey, for example, factor analysis can yield information on patterns of responses, not simply data on a single response. Larger tendencies may then be interpreted, indicating behavior trends rather than simply responses to specific questions.
- Field Studies -- academic or other investigative studies undertaken in a natural setting, rather than in laboratories, classrooms, or other structured environments.
- Focus Groups -- small, roundtable discussion groups charged with examining specific topics or problems, including possible options or solutions. Focus groups usually consist of 4-12 participants, guided by moderators to keep the discussion flowing and to collect and report the results.
- Framework -- the structure and support that may be used as both the launching point and the on-going guidelines for investigating a research problem.
- Generalizability -- the extent to which research findings and conclusions conducted on a specific study to groups or situations can be applied to the population at large.
- Grey Literature -- research produced by organizations outside of commercial and academic publishing that publish materials, such as, working papers, research reports, and briefing papers.
- Grounded Theory -- practice of developing other theories that emerge from observing a group. Theories are grounded in the group's observable experiences, but researchers add their own insight into why those experiences exist.
- Group Behavior -- behaviors of a group as a whole, as well as the behavior of an individual as influenced by his or her membership in a group.
- Hypothesis -- a tentative explanation based on theory to predict a causal relationship between variables.
- Independent Variable -- the conditions of an experiment that are systematically manipulated by the researcher. A variable that is not impacted by the dependent variable, and that itself impacts the dependent variable. In the earlier example of "gender" and "academic major," (see Dependent Variable) gender is the independent variable.
- Individualism -- a theory or policy having primary regard for the liberty, rights, or independent actions of individuals.
- Inductive -- a form of reasoning in which a generalized conclusion is formulated from particular instances.
- Inductive Analysis -- a form of analysis based on inductive reasoning; a researcher using inductive analysis starts with answers, but formulates questions throughout the research process.
- Insiderness -- a concept in qualitative research that refers to the degree to which a researcher has access to and an understanding of persons, places, or things within a group or community based on being a member of that group or community.
- Internal Consistency -- the extent to which all questions or items assess the same characteristic, skill, or quality.
- Internal Validity -- the rigor with which the study was conducted [e.g., the study's design, the care taken to conduct measurements, and decisions concerning what was and was not measured]. It is also the extent to which the designers of a study have taken into account alternative explanations for any causal relationships they explore. In studies that do not explore causal relationships, only the first of these definitions should be considered when assessing internal validity.
- Life History -- a record of an event/events in a respondent's life told [written down, but increasingly audio or video recorded] by the respondent from his/her own perspective in his/her own words. A life history is different from a "research story" in that it covers a longer time span, perhaps a complete life, or a significant period in a life.
- Margin of Error -- the permittable or acceptable deviation from the target or a specific value. The allowance for slight error or miscalculation or changing circumstances in a study.
- Measurement -- process of obtaining a numerical description of the extent to which persons, organizations, or things possess specified characteristics.
- Meta-Analysis -- an analysis combining the results of several studies that address a set of related hypotheses.
- Methodology -- a theory or analysis of how research does and should proceed.
- Methods -- systematic approaches to the conduct of an operation or process. It includes steps of procedure, application of techniques, systems of reasoning or analysis, and the modes of inquiry employed by a discipline.
- Mixed-Methods -- a research approach that uses two or more methods from both the quantitative and qualitative research categories. It is also referred to as blended methods, combined methods, or methodological triangulation.
- Modeling -- the creation of a physical or computer analogy to understand a particular phenomenon. Modeling helps in estimating the relative magnitude of various factors involved in a phenomenon. A successful model can be shown to account for unexpected behavior that has been observed, to predict certain behaviors, which can then be tested experimentally, and to demonstrate that a given theory cannot account for certain phenomenon.
- Models -- representations of objects, principles, processes, or ideas often used for imitation or emulation.
- Naturalistic Observation -- observation of behaviors and events in natural settings without experimental manipulation or other forms of interference.
- Norm -- the norm in statistics is the average or usual performance. For example, students usually complete their high school graduation requirements when they are 18 years old. Even though some students graduate when they are younger or older, the norm is that any given student will graduate when he or she is 18 years old.
- Null Hypothesis -- the proposition, to be tested statistically, that the experimental intervention has "no effect," meaning that the treatment and control groups will not differ as a result of the intervention. Investigators usually hope that the data will demonstrate some effect from the intervention, thus allowing the investigator to reject the null hypothesis.
- Ontology -- a discipline of philosophy that explores the science of what is, the kinds and structures of objects, properties, events, processes, and relations in every area of reality.
- Panel Study -- a longitudinal study in which a group of individuals is interviewed at intervals over a period of time.
- Participant -- individuals whose physiological and/or behavioral characteristics and responses are the object of study in a research project.
- Peer-Review -- the process in which the author of a book, article, or other type of publication submits his or her work to experts in the field for critical evaluation, usually prior to publication. This is standard procedure in publishing scholarly research.
- Phenomenology -- a qualitative research approach concerned with understanding certain group behaviors from that group's point of view.
- Philosophy -- critical examination of the grounds for fundamental beliefs and analysis of the basic concepts, doctrines, or practices that express such beliefs.
- Phonology -- the study of the ways in which speech sounds form systems and patterns in language.
- Policy -- governing principles that serve as guidelines or rules for decision making and action in a given area.
- Policy Analysis -- systematic study of the nature, rationale, cost, impact, effectiveness, implications, etc., of existing or alternative policies, using the theories and methodologies of relevant social science disciplines.
- Population -- the target group under investigation. The population is the entire set under consideration. Samples are drawn from populations.
- Position Papers -- statements of official or organizational viewpoints, often recommending a particular course of action or response to a situation.
- Positivism -- a doctrine in the philosophy of science, positivism argues that science can only deal with observable entities known directly to experience. The positivist aims to construct general laws, or theories, which express relationships between phenomena. Observation and experiment is used to show whether the phenomena fit the theory.
- Predictive Measurement -- use of tests, inventories, or other measures to determine or estimate future events, conditions, outcomes, or trends.
- Principal Investigator -- the scientist or scholar with primary responsibility for the design and conduct of a research project.
- Probability -- the chance that a phenomenon will occur randomly. As a statistical measure, it is shown as p [the "p" factor].
- Questionnaire -- structured sets of questions on specified subjects that are used to gather information, attitudes, or opinions.
- Random Sampling -- a process used in research to draw a sample of a population strictly by chance, yielding no discernible pattern beyond chance. Random sampling can be accomplished by first numbering the population, then selecting the sample according to a table of random numbers or using a random-number computer generator. The sample is said to be random because there is no regular or discernible pattern or order. Random sample selection is used under the assumption that sufficiently large samples assigned randomly will exhibit a distribution comparable to that of the population from which the sample is drawn. The random assignment of participants increases the probability that differences observed between participant groups are the result of the experimental intervention.
- Reliability -- the degree to which a measure yields consistent results. If the measuring instrument [e.g., survey] is reliable, then administering it to similar groups would yield similar results. Reliability is a prerequisite for validity. An unreliable indicator cannot produce trustworthy results.
- Representative Sample -- sample in which the participants closely match the characteristics of the population, and thus, all segments of the population are represented in the sample. A representative sample allows results to be generalized from the sample to the population.
- Rigor -- degree to which research methods are scrupulously and meticulously carried out in order to recognize important influences occurring in an experimental study.
- Sample -- the population researched in a particular study. Usually, attempts are made to select a "sample population" that is considered representative of groups of people to whom results will be generalized or transferred. In studies that use inferential statistics to analyze results or which are designed to be generalizable, sample size is critical, generally the larger the number in the sample, the higher the likelihood of a representative distribution of the population.
- Sampling Error -- the degree to which the results from the sample deviate from those that would be obtained from the entire population, because of random error in the selection of respondent and the corresponding reduction in reliability.
- Saturation -- a situation in which data analysis begins to reveal repetition and redundancy and when new data tend to confirm existing findings rather than expand upon them.
- Semantics -- the relationship between symbols and meaning in a linguistic system. Also, the cuing system that connects what is written in the text to what is stored in the reader's prior knowledge.
- Social Theories -- theories about the structure, organization, and functioning of human societies.
- Sociolinguistics -- the study of language in society and, more specifically, the study of language varieties, their functions, and their speakers.
- Standard Deviation -- a measure of variation that indicates the typical distance between the scores of a distribution and the mean; it is determined by taking the square root of the average of the squared deviations in a given distribution. It can be used to indicate the proportion of data within certain ranges of scale values when the distribution conforms closely to the normal curve.
- Statistical Analysis -- application of statistical processes and theory to the compilation, presentation, discussion, and interpretation of numerical data.
- Statistical Bias -- characteristics of an experimental or sampling design, or the mathematical treatment of data, that systematically affects the results of a study so as to produce incorrect, unjustified, or inappropriate inferences or conclusions.
- Statistical Significance -- the probability that the difference between the outcomes of the control and experimental group are great enough that it is unlikely due solely to chance. The probability that the null hypothesis can be rejected at a predetermined significance level [0.05 or 0.01].
- Statistical Tests -- researchers use statistical tests to make quantitative decisions about whether a study's data indicate a significant effect from the intervention and allow the researcher to reject the null hypothesis. That is, statistical tests show whether the differences between the outcomes of the control and experimental groups are great enough to be statistically significant. If differences are found to be statistically significant, it means that the probability [likelihood] that these differences occurred solely due to chance is relatively low. Most researchers agree that a significance value of .05 or less [i.e., there is a 95% probability that the differences are real] sufficiently determines significance.
- Subcultures -- ethnic, regional, economic, or social groups exhibiting characteristic patterns of behavior sufficient to distinguish them from the larger society to which they belong.
- Testing -- the act of gathering and processing information about individuals' ability, skill, understanding, or knowledge under controlled conditions.
- Theory -- a general explanation about a specific behavior or set of events that is based on known principles and serves to organize related events in a meaningful way. A theory is not as specific as a hypothesis.
- Treatment -- the stimulus given to a dependent variable.
- Trend Samples -- method of sampling different groups of people at different points in time from the same population.
- Triangulation -- a multi-method or pluralistic approach, using different methods in order to focus on the research topic from different viewpoints and to produce a multi-faceted set of data. Also used to check the validity of findings from any one method.
- Unit of Analysis -- the basic observable entity or phenomenon being analyzed by a study and for which data are collected in the form of variables.
- Validity -- the degree to which a study accurately reflects or assesses the specific concept that the researcher is attempting to measure. A method can be reliable, consistently measuring the same thing, but not valid.
- Variable -- any characteristic or trait that can vary from one person to another [race, gender, academic major] or for one person over time [age, political beliefs].
- Weighted Scores -- scores in which the components are modified by different multipliers to reflect their relative importance.
- White Paper -- an authoritative report that often states the position or philosophy about a social, political, or other subject, or a general explanation of an architecture, framework, or product technology written by a group of researchers. A white paper seeks to contain unbiased information and analysis regarding a business or policy problem that the researchers may be facing.
Elliot, Mark, Fairweather, Ian, Olsen, Wendy Kay, and Pampaka, Maria. A Dictionary of Social Research Methods. Oxford, UK: Oxford University Press, 2016; Free Social Science Dictionary. Socialsciencedictionary.com [2008]. Glossary. Institutional Review Board. Colorado College; Glossary of Key Terms. Writing@CSU. Colorado State University; Glossary A-Z. Education.com; Glossary of Research Terms. Research Mindedness Virtual Learning Resource. Centre for Human Servive Technology. University of Southampton; Miller, Robert L. and Brewer, John D. The A-Z of Social Research: A Dictionary of Key Social Science Research Concepts London: SAGE, 2003; Jupp, Victor. The SAGE Dictionary of Social and Cultural Research Methods . London: Sage, 2006.
- << Previous: Independent and Dependent Variables
- Next: 1. Choosing a Research Problem >>
- Last Updated: May 25, 2024 4:09 PM
- URL: https://libguides.usc.edu/writingguide
EducationalWave
20 pros and cons of quantitative research.
The pros of quantitative research include swift data collection for rapid decision-making, reduced bias through randomized sampling, reliable forecasting from consistent findings, and broad market extrapolation from statistical analysis. These methods provide objective data that inform clear strategies and offer comprehensive market insights.
The cons of quantitative research involve limited qualitative feedback, potentially missing nuanced consumer insights, and a tendency to overlook long-term trends. Data-driven strategies may underrepresent participant experiences, and complex business motivations can be oversimplified. Additionally, the significant investment required for market research can be a drawback.
Quantitative Research Fact Sheet
- Nature of Quantitative Research : Expressed in numbers and graphs, allowing for visualization of data.
- Purpose : Utilized to test or confirm theories and assumptions, providing a basis for generalizations.
- Usage : Establishes generalizable facts about various subjects.
- Common Methods : Includes experiments, numerical observations, and surveys with closed-ended questions.
- Data Collection and Analysis : Involves collecting numerical data and employing statistical methods for analysis.
- Objective : Aims to produce objective, empirical data that is quantifiable.
- Sample Size : Data is often collected from large samples to enhance reliability and generalizability.
- Applications : Used to test hypotheses, identify patterns, and make predictions.
- Widely applied in psychology, economics, demography, sociology, marketing, community health, health & human development, gender studies, and political science.
- Can be repeated and reproduced due to standardized protocols and definitions, allowing for cross-cultural, temporal, or demographic comparisons.
Pros of Quantitative Research
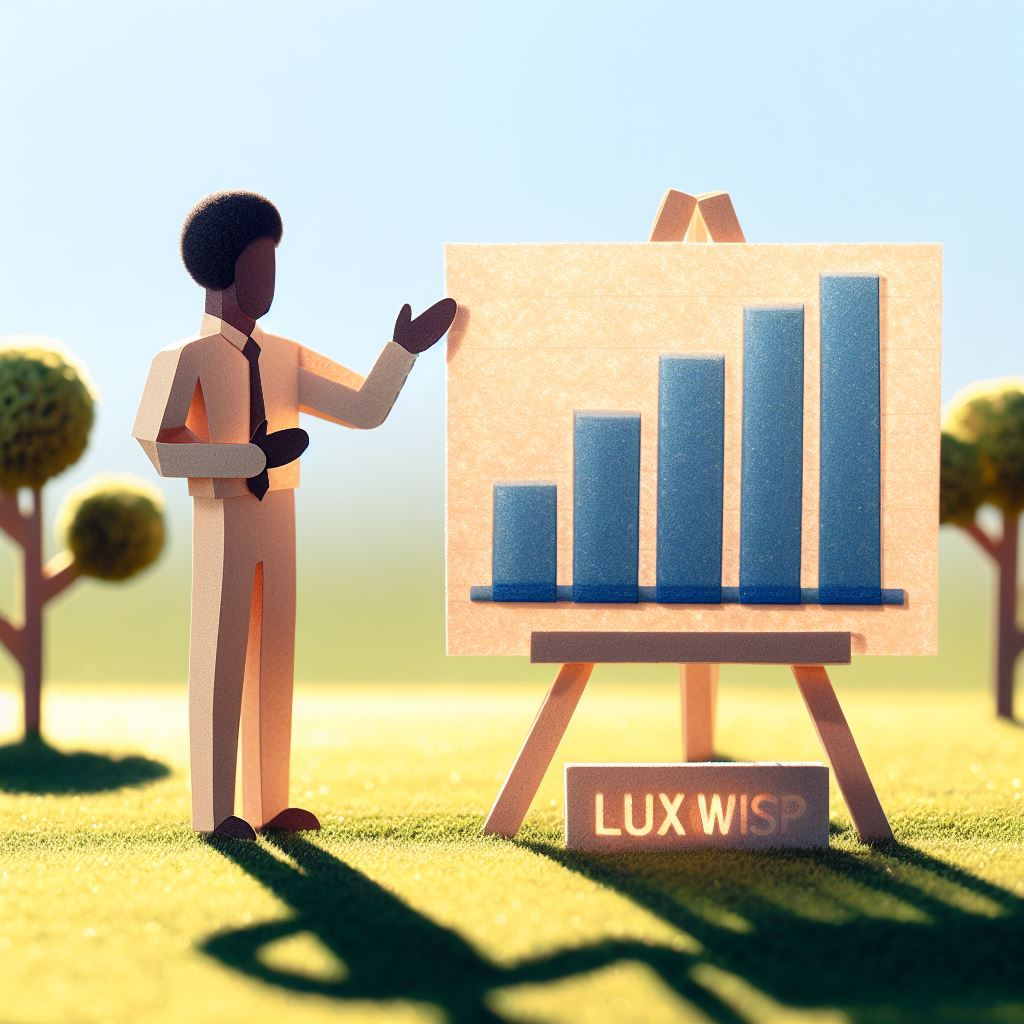
- Swift and Extensive Data Collection: Quantitative research methodologies, such as surveys and structured observations, facilitate the swift collection of vast amounts of data. For instance, utilizing online survey platforms enables researchers to reach thousands of participants across the globe in a matter of hours, expediting the research timeline significantly. This method proves invaluable in fields such as market research, where understanding consumer trends quickly can lead to significant competitive advantage.
- Minimization of Subjectivity and Bias: Quantitative research’s structured design, often involving random sampling and assignment, inherently guards against bias, thereby enhancing the credibility of the research findings. For example, in clinical trials, the use of a double-blind placebo-controlled design ensures that neither the subjects nor the researchers know who is receiving the treatment, which eliminates the risk of placebo effects or researcher-induced bias.
- Consistency and Replicability: The standardized approach of quantitative research, which includes replicable measures and observational techniques, ensures that studies can be repeated and verified. In the scientific community, this is paramount; for example, the consistent results across repeated trials of a new drug are what allow for its approval and widespread clinical use. Replicability also reinforces the strength of evidence when policies or decisions are based on research outcomes.
- Statistical Significance and Wider Applicability: Quantitative research results can be extrapolated to the broader population with statistical techniques, assuming the sample is representative. This is seen in national census data, where a stratified sample can provide insights into demographic changes and trends that inform policy decisions at the highest levels of government.
- Objective Data Analysis: The objective nature of quantitative research, with its emphasis on numbers and statistical analysis, limits the scope for researcher’s subjectivity. For example, in customer satisfaction research, using a Likert scale to quantify satisfaction levels allows for objective analysis of customer feedback, rather than relying on more subjective narrative responses.
- Comprehensive Population Insights: Quantitative studies can encompass broad aspects of population behaviors and characteristics, thereby providing a macro-level view of trends and patterns. For example, epidemiological studies examining the incidence of a disease across different regions and time periods rely on quantitative methods to track and predict health trends on a global scale.
- Generalizability of Findings: The structured nature of quantitative research often allows for findings to be generalized to larger groups beyond the sample when conditions are controlled. In educational research, standardized tests can provide data that, when analyzed, contribute to understanding educational outcomes across different populations and geographies.
- Clear, Focused Research Aims: The specificity with which quantitative research is designed ensures a clear and focused exploration of hypotheses. For instance, in economic research, specific variables such as interest rates or employment levels can be isolated and their impact on economic growth can be quantified and analyzed with precision.
- Enhanced Reliability Through Quantification: The use of statistical models and computational analysis enhances the reliability of quantitative research. For example, in finance, quantitative models are used to predict stock market trends, and the reliability of these models is continually tested against historical data and current market conditions.
- Identification and Analysis of Cause-and-Effect Relationships: Quantitative research is adept at identifying and rigorously testing cause-and-effect relationships. For instance, in social sciences, researchers can use statistical methods to determine the likelihood that a particular educational intervention causes improvements in student outcomes, controlling for other variables.
Cons of Quantitative Research
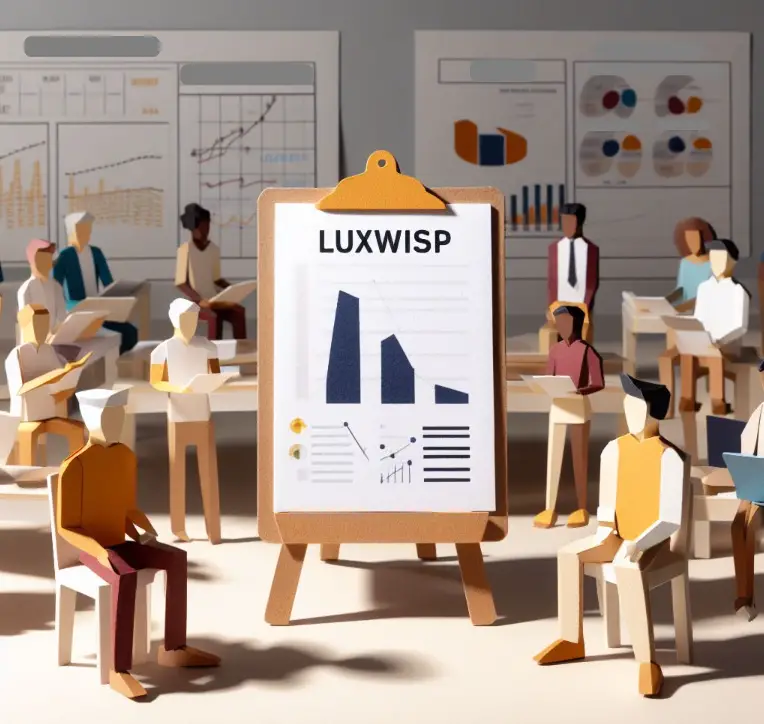
- Restricted Depth in Responses: Quantitative research typically doesn’t allow for probing or follow-up questions, which limits the depth of responses. For example, in a survey on job satisfaction, a participant may not have the opportunity to explain the unique factors influencing their satisfaction, potentially overlooking nuances that could be vital for organizational change.
- Potentially Artificial Settings: Conducting studies in controlled or unnatural settings can affect the behavior of participants, leading to outcomes that do not accurately reflect real-world scenarios. In market research, a focus group might react differently to a product in a testing facility than they would in their homes, which could skew the data about product usability.
- Risk of Oversimplification: The numerical focus of quantitative research may not capture the full scope of human experience, leading to an oversimplification of complex issues. For example, quantifying grief or happiness on a numeric scale can overlook the subjective intensity or personal significance of these emotions.
- Vulnerability to External Influences: Quantitative studies can be affected by external variables not accounted for in the research design, such as measurement errors or sampling biases. An educational assessment, for instance, could yield skewed results if the test environment is too noisy, potentially misrepresenting the students’ actual abilities.
- Intensive Resource Requirements: Gathering and analyzing quantitative data can be resource-heavy, demanding significant time, financial investment, and specialized expertise. In environmental studies, large-scale data collection efforts to monitor pollution levels can require costly equipment and numerous personnel to manage the data.
- Overemphasis on Numerical Data: There’s a risk in quantitative research of over-reliance on numbers, which may lead to neglecting important non-quantifiable factors. In employee performance metrics, focusing solely on sales figures might overlook critical elements such as customer service quality or team collaboration.
- Elevated Costs: The expenses associated with conducting comprehensive quantitative research, including materials and logistics, can be prohibitive. Large-scale health research often requires funding for medical equipment, staff, and the processing of numerous tests, which can amount to substantial sums.
- Artificial Research Conditions: The structured nature of quantitative research may create an artificial environment that does not authentically represent natural conditions. In behavioral studies, for example, participants might alter their natural responses due to the awareness of being observed or tested.
- Contextual Limitations: Quantitative data may fail to capture the full context of the research subject, possibly overlooking factors that influence the results. In social research, quantitative methods might not fully reflect the impact of cultural nuances on social behavior patterns.
- Inflexibility in Data Collection: The rigid structure of quantitative research instruments means there’s little room for adaptation once data collection has begun. If initial surveys in a consumer study don’t capture emerging trends, researchers can’t adjust questions mid-study to explore these new developments, potentially missing critical insights.
Type of Quantitative Data
Benefits of rapid data collection.
The efficiency of rapid data collection in quantitative research offers significant advantages for businesses, enabling them to respond quickly to market changes and make informed decisions. This speed and precision provide opportunities for immediate action, a clear advantage in today’s fast-paced commercial landscape.
One of the significant benefits of rapid data collection is the ability to seize opportunities as they arise. By quickly analyzing data, companies can spot market trends and patterns before their competitors do. This advantage gives them a head start in adjusting their strategies, launching new products, or targeting new market segments.
Quantitative research is also effective in spotting potential problems. If a product isn’t selling as expected or a marketing campaign isn’t getting the desired response, quick data collection allows businesses to identify the issue and implement corrections promptly.
Moreover, rapid data collection can improve customer service. By promptly gathering and analyzing customer feedback, companies can swiftly address any concerns or complaints, enhancing customer satisfaction and loyalty.
Importance of Randomized Sampling
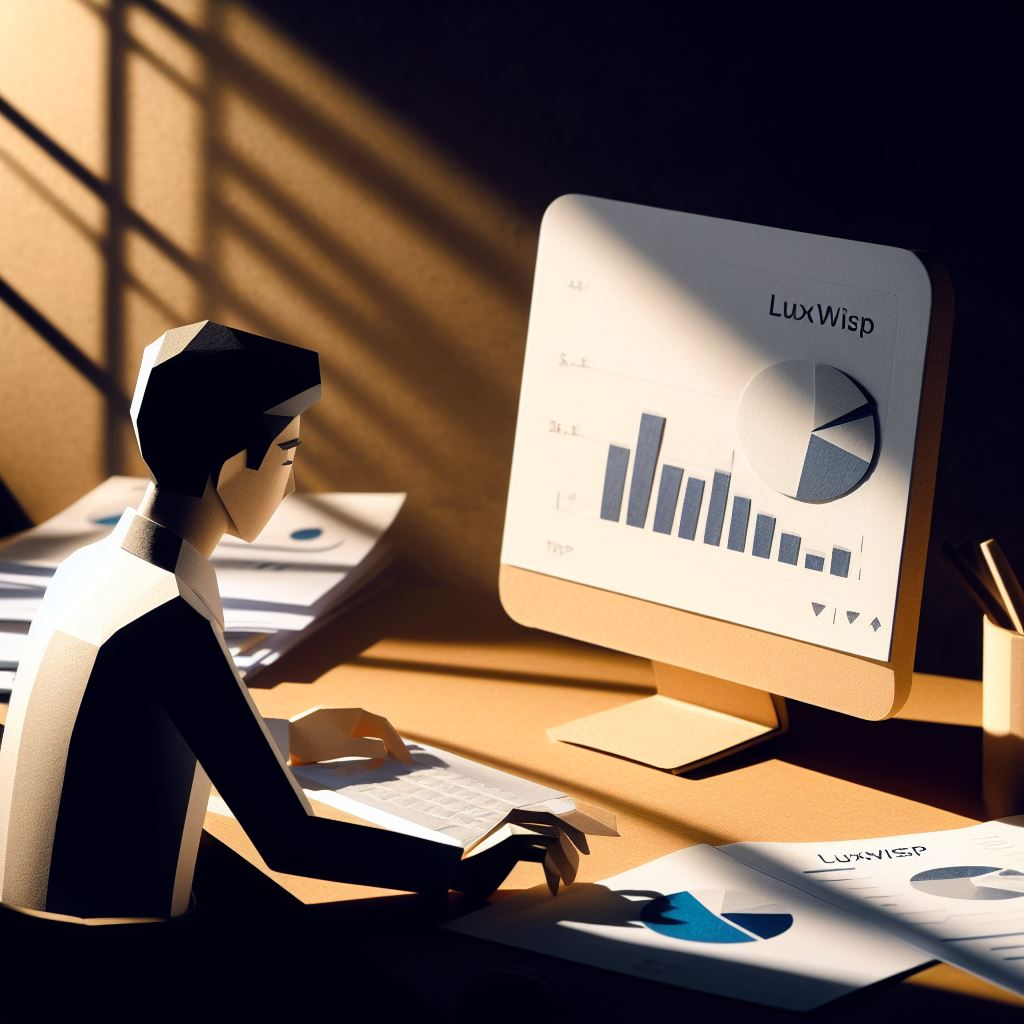
Building on the benefits of rapid data collection, it’s crucial to consider how randomized sampling in quantitative research substantially enhances the quality and reliability of gathered data. The importance of randomized sampling can’t be overlooked when discussing the pros and cons of quantitative research.
- Reduction of bias : Researchers often favor randomized sampling as it minimizes selection bias, ensuring a fair representation of the population in the study.
- Enhanced reliability : Randomized sampling strengthens the reliability of obtained data, as it reduces the chance of systematic errors.
- Validity : By giving every member of a population an equal chance of being selected, randomized sampling enhances the validity of the research findings.
- Generalizability : This approach allows researchers to apply their findings to the broader population from which the sample was drawn, increasing the study’s generalizability.
The Role of Consistent Findings
In numerous business scenarios, consistent findings in quantitative research play a crucial role in underpinning reliable forecasting and strategic planning. These findings, derived from a multitude of data points, offer a solid foundation for making informed business decisions.
The role of consistent findings in quantitative research can’t be understated. Consistency provides a level of certainty, allowing businesses to predict trends, understand customer behavior, and develop effective strategies. However, it’s essential to consider the pros and cons of quantitative research. As beneficial as consistent findings are, they’re dependent on the accuracy and reliability of the data collected.
If the results are skewed or biased, the findings won’t provide an accurate reflection of the reality, which can lead to misguided strategies. This highlights another crucial role of consistent findings – they act as a check and balance system. They can identify anomalies or inconsistencies in the data that may indicate issues with collection or interpretation.
While the benefits of consistent findings in quantitative research are clear, they’re not without their drawbacks. Therefore, it’s important for businesses to understand and account for these potential pitfalls when leveraging quantitative research for strategic planning.
Drawbacks of Limited Qualitative Feedback
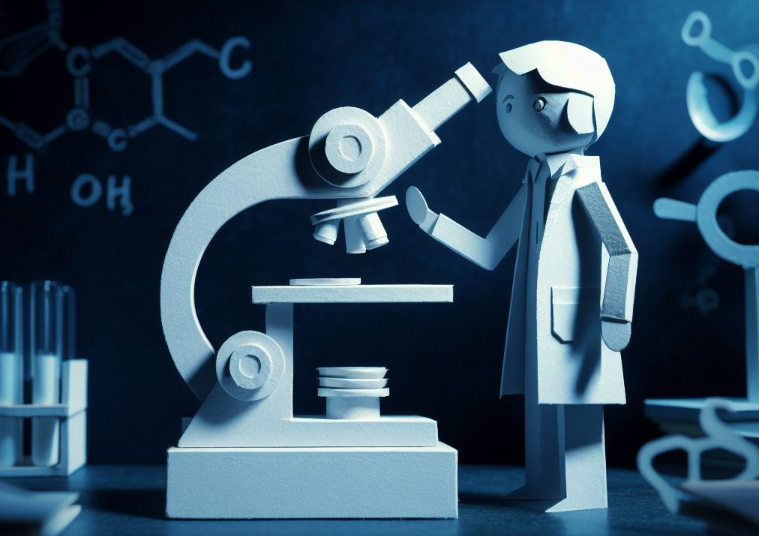
Despite the strengths of consistent findings, one potential pitfall of quantitative research lies in its limited capacity for qualitative feedback. This limitation can have a profound impact on research outcomes, rendering them less insightful than they might be with the inclusion of qualitative data.
- Missed nuances: The first disadvantage of limited qualitative feedback is the potential to miss nuanced elements of the data. Quantitative research thrives on numerical data, often overlooking the subtleties that qualitative research can highlight.
- Limited understanding of motivations: Without qualitative insights, quantitative research may not fully understand the motivations behind certain behaviors, leading to incomplete or even erroneous conclusions.
- Lack of context: Thirdly, the absence of qualitative feedback can leave data devoid of context, making it challenging to interpret results accurately.
- Reduced participant representation: Lastly, quantitative research can fail to represent individual participant experiences and perspectives, thereby limiting the depth of the research findings.
Issues With Snapshot Approach
Often, quantitative research’s snapshot approach doesn’t accurately reflect long-term market trends, causing potentially significant misinterpretations in business decision-making. This method, a common feature of quantitative research, collects data at a single point in time, providing a ‘snapshot’ of the market. While this approach is valuable for capturing immediate information, it often overlooks evolving trends and fluctuations, leading to a potential skew in market research.
One of the significant drawbacks of using quantitative research is this snapshot approach. It assumes that the market, consumer behaviors, and business operations remain static over time. This assumption is often incorrect as markets continuously evolve and change. Thus, the snapshot taken today may not be representative of the market’s state tomorrow, next week, or next month.
The snapshot approach’s limitations highlight the need for a balanced use of research methods. While quantitative research offers swift, generalizable results, it should be complemented with methods that capture ongoing changes. Using quantitative research over a more extended period, or combining it with qualitative methods, can yield a more comprehensive, accurate picture of the market, thereby informing more effective business strategies.
Impact of External Market Variables
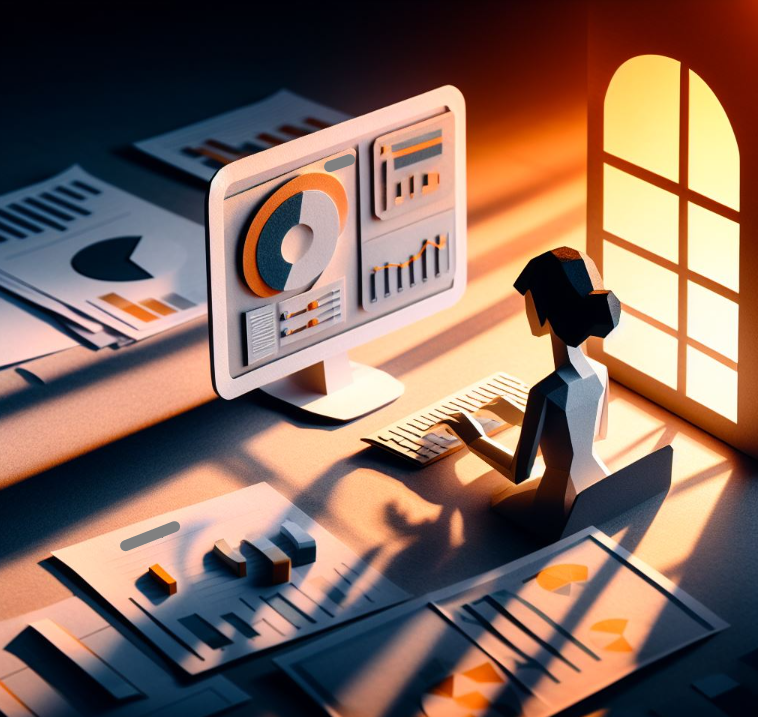
Quantitative research, although robust, isn’t immune to external market variables that can skew data. Factors such as market fluctuations can impact the accuracy of findings, presenting challenges in data interpretation.
It’s essential, then, to investigate strategies for mitigating the effects of these variables to ensure sound research conclusions.
Data Skewness Risks
Market fluctuations present a significant risk to the accuracy of quantitative research data, potentially skewing results and leading to misleading conclusions. The impact of these external market variables reveals the limitations of quantitative research over qualitative. Notably, four significant risks arise:
- Inflation or Deflation : Rapid changes in the economy can distort projections, diluting the advantages of quantitative research.
- Market Volatility : Unpredictable shifts can invalidate data collected, diminishing reliability.
- Sudden Market Events : Unexpected occurrences like product recalls, scandals or natural disasters can radically alter consumer behavior, skewing data.
- Regulatory Changes : Governmental policy alterations can impact market trends, affecting data relevance.
These risks highlight the importance of considering market dynamics and external variables when conducting quantitative research.
Mitigating Variable Impact
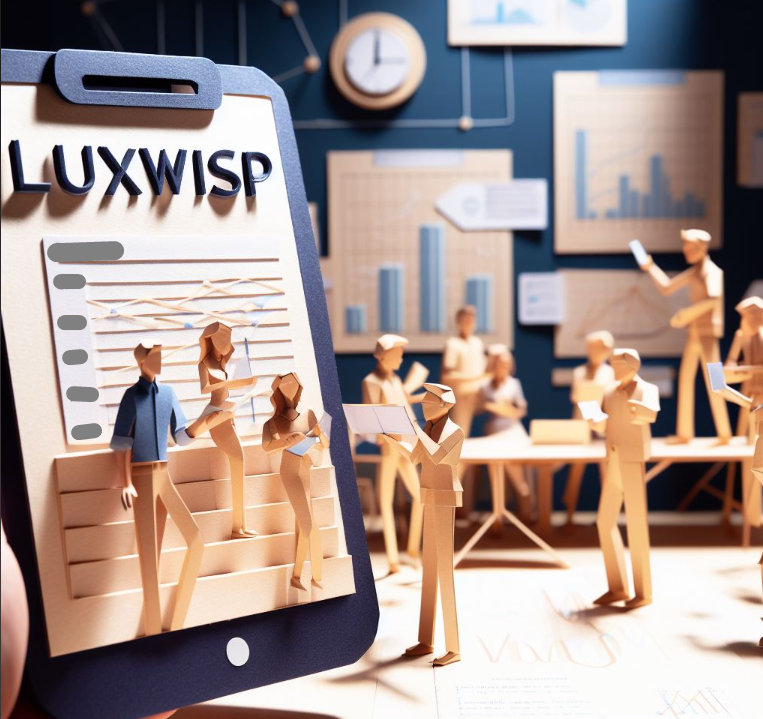
Mitigating the impact of external market variables requires a strategic approach to quantitative research, ensuring that data remains relevant and accurate despite unpredictable market changes. Researchers must be vigilant, anticipating potential fluctuations and adjusting methodologies accordingly.
This can involve developing dynamic models that factor in variables like economic conditions, competitor activities, or technological advancements. By doing so, they can isolate and assess the impact of these factors on their data. This proactive approach reduces the risk of skewed results, enhancing the reliability of the research.
However, it’s not foolproof. The ever-changing nature of markets means some variables may still slip through, impacting data accuracy. Therefore, constant monitoring and adjustment are crucial to mitigating variable impact in quantitative research.
Frequently Asked Questions
What are some practical examples of quantitative research in business”.
Quantitative research’s practicality shines in business. It helps firms understand customer preferences through surveys, informs stock decisions with sales data analysis, and guides marketing strategies through social media metrics. It’s not perfect, but it’s invaluable.
How Does Quantitative Research Compare to Qualitative Research in Terms of Cost and Time Efficiency?”
Quantitative research typically costs more and takes longer than qualitative due to its scale. However, it’s often more time-efficient in the long run as it provides broad, generalizable results for strategic decision-making.
What Are Some Common Methodologies Used in Quantitative Research in Business?”
In business, common quantitative research methodologies include surveys, experiments, and observational studies. They’re chosen based on their ability to provide precise, objective data for decision-making and forecasting.
Can Quantitative Research Be Combined With Qualitative Methods for a More Holistic Market Analysis?”
Yes, they can combine quantitative research with qualitative methods for a more holistic market analysis. It’ll give them a more in-depth understanding of their market, enhancing the accuracy of their business decisions.
What Are Some Possible Solutions to the Disadvantages of Quantitative Research in Business?”
To counteract disadvantages of quantitative research in business, one could integrate qualitative methods, consider long-term trends, deepen consumer understanding, account for complex motivations, and adjust for market changes. This requires both resources and a nuanced approach.
Related Posts:
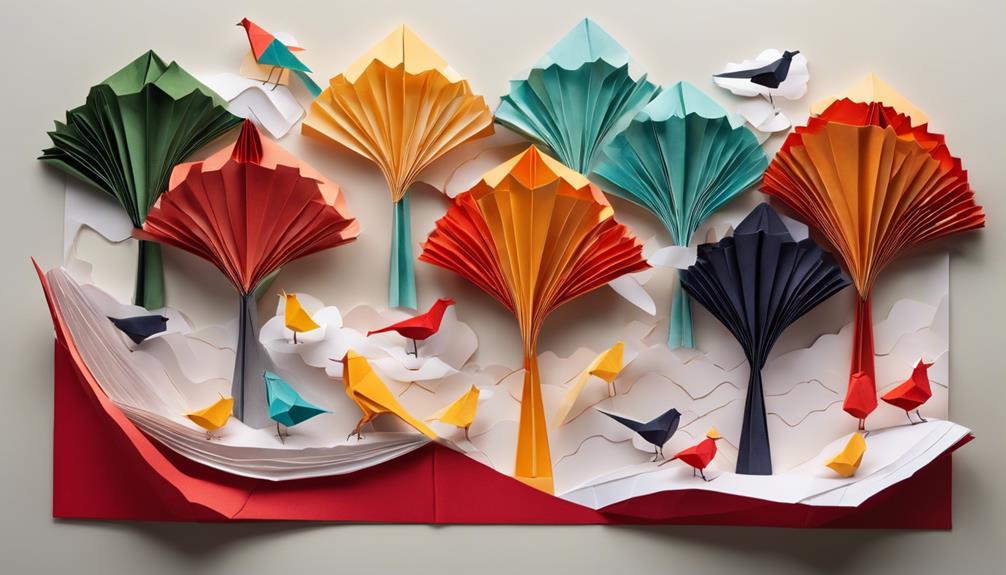
educationalwave.com
Frequent Searches
Academic Programs
Academic Calendar
Search Directory
Find contact details for people, departments and facilities information.
$50.00 USD application fee (grad only)
Official high school or college transcript from all previous schools
Test Scores (may be optional) AP or IB results
3 letters of recommendation (grad only)
Apply by 3/1 for Fall start
Apply by 12/1 for Spring start
10 Tips on Creating a Research Program and Proposal Writing
Office of sponsored programs and research.
Start small and early. As a new faculty member-reach out to mentors (on campus and off) and inquire what might be a great first proposal to write. Given that grant funding today is more difficult to obtain than ever before, starting early in your career and capitalizing on the advantages of your "early-career" status is key. Reach out to the Office of Sponsored Programs and Research for a listening session and find grant programs specifically aimed at new faculty members. These grant programs typically do not require significant preliminary data. Instead, funding decisions rely most heavily on your promise and potential as a candidate — your training to date, your mentors, and your topic’s importance.
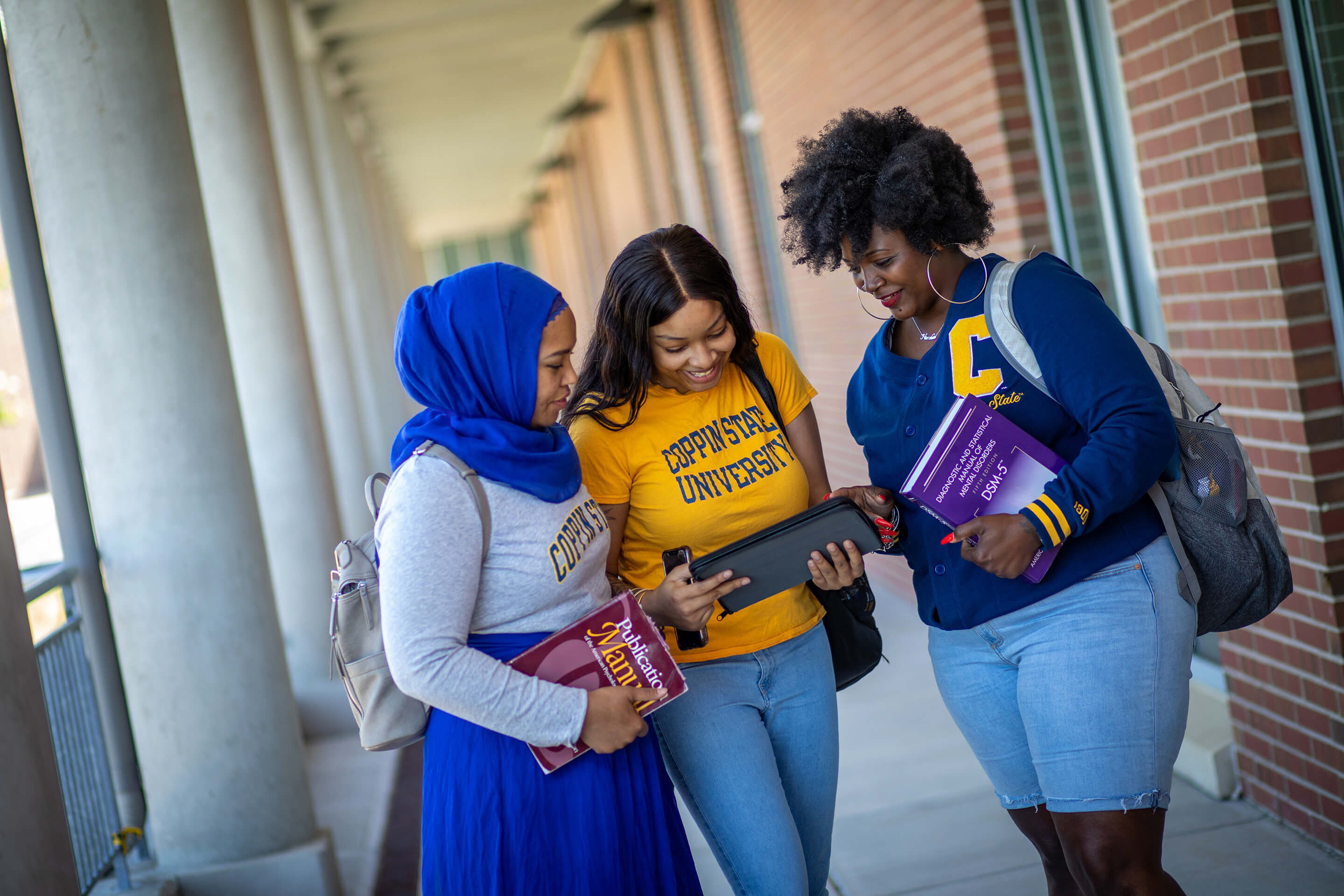
Early on in your career, it’s critical to envision your ultimate large grant. Drawing a Venn diagram of your research program could help communicate your research expertise to collaborators. Typically a major grant would include five aims. Once you’ve envisioned your big grant and its five aims, your next steps become clear: create component projects of that larger project by writing small grants designed to support one or more of your five specific aims. Small grants will show that each of your aims is feasible. This approach is critical as grant-review panels often see a large grant as the culmination of a growing body of work progressing from modest seed grants to larger and larger awards.
A key factor in developing a vision of your ultimate large grant will be the advice of your mentor(s). If you do not have a mentor in your department ask the chair to assign you one. It is also usually considered acceptable to seek out your own mentor. Indeed, many early-career academics assemble a mentorship team, in which each member provides guidance on different career facets (i.e., a teaching mentor, a research mentor, a work-life mentor). Consider approaching people on other campuses as well as at Coppin.
Look at who and what got funded before. Grant agencies typically list previous award recipients online. This list is critical as it shows the agency’s potential interest in supporting your area of research. Some agencies post abstracts online of both active and prior awards. They can give you a critical sense of what has been successful. Looking at the number of specific aims and the range of acceptable sample sizes will provide you with key insights as to what has appealed to your target agency in the past.
Funding agencies may post a list of prior and current grant reviewers and their affiliations online. Review the list and ask yourself if their expertise overlaps with the aims and methodology of your study. Reach out to program directors with a potential idea many months in advance of the deadline—ask for their feedback.
Spend half of your time on the abstract and aims. Writers of successful grant applications typically report that they spent 50 percent of their time on writing and revising their abstract and aims. When you finally start drafting your proposal, the specific aims should be the first thing you write — well before the background or methods sections.
Send a one-page sketch of your project abstract and aims to your mentor and co-investigators early in the grant-writing process with the goal of kicking off an iterative process of review and revision.
Why is this page so critical? Because of the nature of the peer-review process. Typically, only three or four academics are assigned as primary and secondary reviewers of your grant. The majority of review-panel members will only have read your proposal’s abstract. Therefore, it must not only provide a clear snapshot of the entire study, but also convey what is novel about your application.
Demonstrate your strengths and capabilities to do the research. These skills are a critical factor for reviewers. How do you demonstrate you can feasibly conduct the work?
- First, if possible, collaborate on the grant with senior investigators who have conducted similar projects. A senior scholar’s involvement will be a key factor supporting your potential for success, particularly if you are early in your career.
- Co-investigators should not appear in name only. Show established working relationships with them either via co-authored publications, co-presentations, and/or via an established mentoring relationship (e.g., as part of a training grant). Of course, much of this information will appear in the bio sketches in your proposal, but you cannot rely upon reviewers to connect the dots. Make it easy for reviewers by clearly noting these prior collaborations in your "preliminary studies" section.
- Finally, present evidence that you have conducted smaller-scale feasibility studies. That reassures reviewers that you, as a principal investigator, will be able to conduct your proposed aims and, ideally, translate that work into publications.
Take a focused methodological plan directly tied to your specific aims will be the most impressive to reviewers. For example, include methods in the proposal that relate directly to each of your study’s aims and don’t include additional methods that do not correspond to any aims.
You can never have too many figures or tables. They make it easy for a reviewer to quickly grasp your proposal, as compared with dense text. In addition, the act of creating them will help you to cross check your specific aims and study methods. Figures and tables can save space — reducing the amount of text necessary — which is critical to meeting the page limitations of most grant submissions. This tip is relevant for every section of your grant application: Figures can be used to show how your specific aims interrelate, to depict study designs, and to demonstrate your anticipated results.
Seek external reviews prior to submission. The same person cannot write a grant and review it for clarity. You will miss errors, simply by virtue of your familiarity with the material. So ask colleagues to read the application. Even a generalist can read your grant proposal with the following questions in mind: Are the goals clearly stated? Does the grant extend prior work in the field? What is the impact of your potential findings?
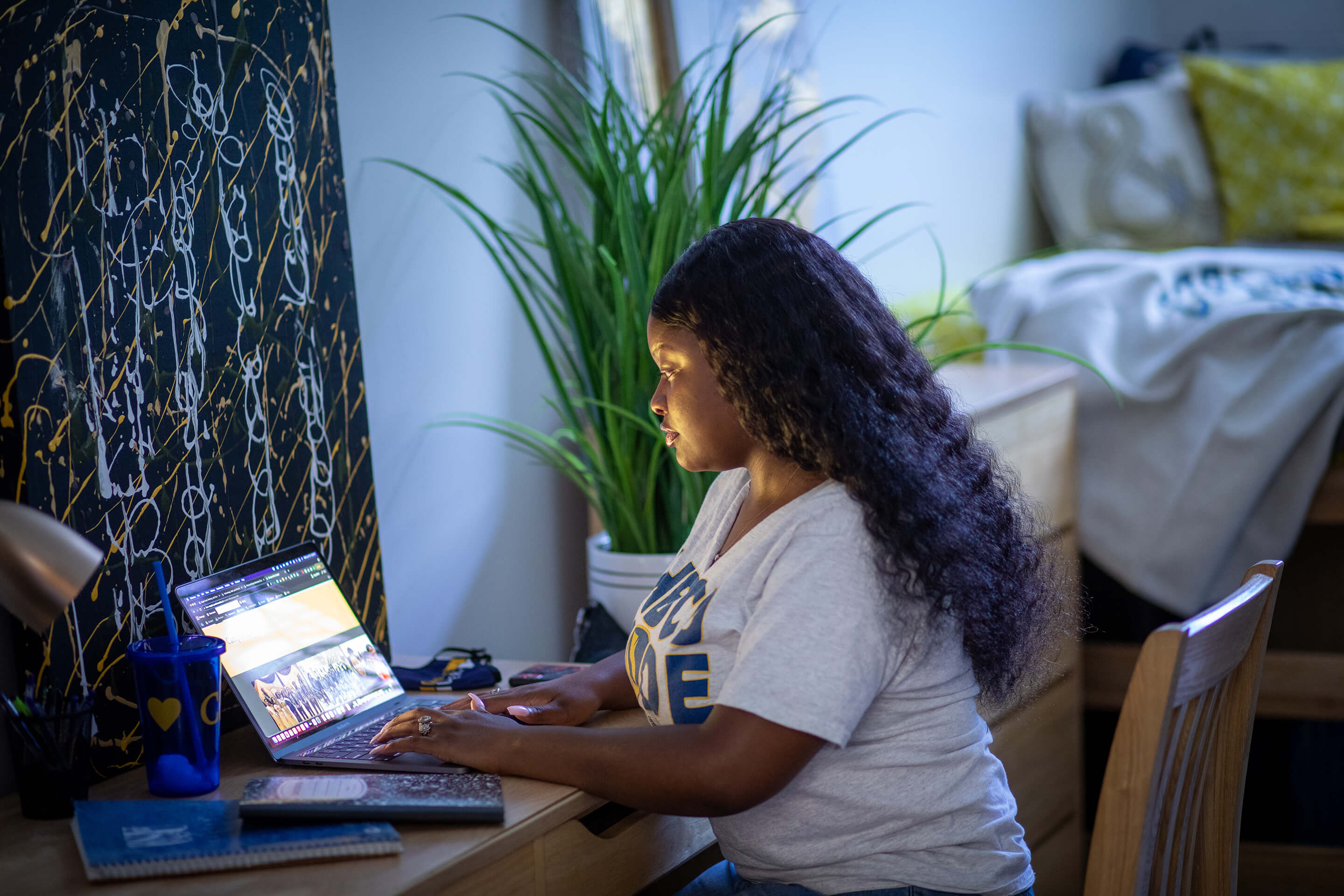
In fact, it may be preferable for some of your proofreaders not to have expertise in your area at all — given that members of the grant-review panel will not have expertise in every aspect of your proposal.
Use the grant-review criteria as subheadings in your proposal, making it easier for the panelists to fill out their review forms. For example, reviewers typically have to complete a section on "Innovation." A clearly labeled subsection on "Innovation" not only saves the reviewer time, but gives you the opportunity to share your vision with the reviewer on innovative aspects they may not have recognized on their own.
Choose a topic that you find interesting—your expertise and passion will come through in your grant proposal. Having several grants in the pipeline and under review at the same time can help stack the deck in your favor.
Related links

CRO Platform
Test your insights. Run experiments. Win. Or learn. And then win.

eCommerce Customer Analytics Platform

Acquisition matters. But retention matters more. Understand, monitor & nurture the best customers.
- Case Studies
- Ebooks, Tools, Templates
- Digital Marketing Glossary
- eCommerce Growth Stories
- eCommerce Growth Show
- Help & Technical Documentation
CRO Guide > Chapter 3.1
Qualitative Research: Definition, Methodology, Limitation & Examples
Qualitative research is a method focused on understanding human behavior and experiences through non-numerical data. Examples of qualitative research include:
- One-on-one interviews,
- Focus groups, Ethnographic research,
- Case studies,
- Record keeping,
- Qualitative observations
In this article, we’ll provide tips and tricks on how to use qualitative research to better understand your audience through real world examples and improve your ROI. We’ll also learn the difference between qualitative and quantitative data.

Table of Contents
Marketers often seek to understand their customers deeply. Qualitative research methods such as face-to-face interviews, focus groups, and qualitative observations can provide valuable insights into your products, your market, and your customers’ opinions and motivations. Understanding these nuances can significantly enhance marketing strategies and overall customer satisfaction.
What is Qualitative Research
Qualitative research is a market research method that focuses on obtaining data through open-ended and conversational communication. This method focuses on the “why” rather than the “what” people think about you. Thus, qualitative research seeks to uncover the underlying motivations, attitudes, and beliefs that drive people’s actions.
Let’s say you have an online shop catering to a general audience. You do a demographic analysis and you find out that most of your customers are male. Naturally, you will want to find out why women are not buying from you. And that’s what qualitative research will help you find out.
In the case of your online shop, qualitative research would involve reaching out to female non-customers through methods such as in-depth interviews or focus groups. These interactions provide a platform for women to express their thoughts, feelings, and concerns regarding your products or brand. Through qualitative analysis, you can uncover valuable insights into factors such as product preferences, user experience, brand perception, and barriers to purchase.
Types of Qualitative Research Methods
Qualitative research methods are designed in a manner that helps reveal the behavior and perception of a target audience regarding a particular topic.
The most frequently used qualitative analysis methods are one-on-one interviews, focus groups, ethnographic research, case study research, record keeping, and qualitative observation.
1. One-on-one interviews
Conducting one-on-one interviews is one of the most common qualitative research methods. One of the advantages of this method is that it provides a great opportunity to gather precise data about what people think and their motivations.
Spending time talking to customers not only helps marketers understand who their clients are, but also helps with customer care: clients love hearing from brands. This strengthens the relationship between a brand and its clients and paves the way for customer testimonials.
- A company might conduct interviews to understand why a product failed to meet sales expectations.
- A researcher might use interviews to gather personal stories about experiences with healthcare.
These interviews can be performed face-to-face or on the phone and usually last between half an hour to over two hours.
When a one-on-one interview is conducted face-to-face, it also gives the marketer the opportunity to read the body language of the respondent and match the responses.
2. Focus groups
Focus groups gather a small number of people to discuss and provide feedback on a particular subject. The ideal size of a focus group is usually between five and eight participants. The size of focus groups should reflect the participants’ familiarity with the topic. For less important topics or when participants have little experience, a group of 10 can be effective. For more critical topics or when participants are more knowledgeable, a smaller group of five to six is preferable for deeper discussions.
The main goal of a focus group is to find answers to the “why”, “what”, and “how” questions. This method is highly effective in exploring people’s feelings and ideas in a social setting, where group dynamics can bring out insights that might not emerge in one-on-one situations.
- A focus group could be used to test reactions to a new product concept.
- Marketers might use focus groups to see how different demographic groups react to an advertising campaign.
One advantage that focus groups have is that the marketer doesn’t necessarily have to interact with the group in person. Nowadays focus groups can be sent as online qualitative surveys on various devices.
Focus groups are an expensive option compared to the other qualitative research methods, which is why they are typically used to explain complex processes.
3. Ethnographic research
Ethnographic research is the most in-depth observational method that studies individuals in their naturally occurring environment.
This method aims at understanding the cultures, challenges, motivations, and settings that occur.
- A study of workplace culture within a tech startup.
- Observational research in a remote village to understand local traditions.
Ethnographic research requires the marketer to adapt to the target audiences’ environments (a different organization, a different city, or even a remote location), which is why geographical constraints can be an issue while collecting data.
This type of research can last from a few days to a few years. It’s challenging and time-consuming and solely depends on the expertise of the marketer to be able to analyze, observe, and infer the data.
4. Case study research
The case study method has grown into a valuable qualitative research method. This type of research method is usually used in education or social sciences. It involves a comprehensive examination of a single instance or event, providing detailed insights into complex issues in real-life contexts.
- Analyzing a single school’s innovative teaching method.
- A detailed study of a patient’s medical treatment over several years.
Case study research may seem difficult to operate, but it’s actually one of the simplest ways of conducting research as it involves a deep dive and thorough understanding of the data collection methods and inferring the data.
5. Record keeping
Record keeping is similar to going to the library: you go over books or any other reference material to collect relevant data. This method uses already existing reliable documents and similar sources of information as a data source.
- Historical research using old newspapers and letters.
- A study on policy changes over the years by examining government records.
This method is useful for constructing a historical context around a research topic or verifying other findings with documented evidence.
6. Qualitative observation
Qualitative observation is a method that uses subjective methodologies to gather systematic information or data. This method deals with the five major sensory organs and their functioning, sight, smell, touch, taste, and hearing.
- Sight : Observing the way customers visually interact with product displays in a store to understand their browsing behaviors and preferences.
- Smell : Noting reactions of consumers to different scents in a fragrance shop to study the impact of olfactory elements on product preference.
- Touch : Watching how individuals interact with different materials in a clothing store to assess the importance of texture in fabric selection.
- Taste : Evaluating reactions of participants in a taste test to identify flavor profiles that appeal to different demographic groups.
- Hearing : Documenting responses to changes in background music within a retail environment to determine its effect on shopping behavior and mood.
Below we are also providing real-life examples of qualitative research that demonstrate practical applications across various contexts:
Qualitative Research Real World Examples
Let’s explore some examples of how qualitative research can be applied in different contexts.
1. Online grocery shop with a predominantly male audience
Method used: one-on-one interviews.
Let’s go back to one of the previous examples. You have an online grocery shop. By nature, it addresses a general audience, but after you do a demographic analysis you find out that most of your customers are male.
One good method to determine why women are not buying from you is to hold one-on-one interviews with potential customers in the category.
Interviewing a sample of potential female customers should reveal why they don’t find your store appealing. The reasons could range from not stocking enough products for women to perhaps the store’s emphasis on heavy-duty tools and automotive products, for example. These insights can guide adjustments in inventory and marketing strategies.
2. Software company launching a new product
Method used: focus groups.
Focus groups are great for establishing product-market fit.
Let’s assume you are a software company that wants to launch a new product and you hold a focus group with 12 people. Although getting their feedback regarding users’ experience with the product is a good thing, this sample is too small to define how the entire market will react to your product.
So what you can do instead is holding multiple focus groups in 20 different geographic regions. Each region should be hosting a group of 12 for each market segment; you can even segment your audience based on age. This would be a better way to establish credibility in the feedback you receive.
3. Alan Pushkin’s “God’s Choice: The Total World of a Fundamentalist Christian School”
Method used: ethnographic research.
Moving from a fictional example to a real-life one, let’s analyze Alan Peshkin’s 1986 book “God’s Choice: The Total World of a Fundamentalist Christian School”.
Peshkin studied the culture of Bethany Baptist Academy by interviewing the students, parents, teachers, and members of the community alike, and spending eighteen months observing them to provide a comprehensive and in-depth analysis of Christian schooling as an alternative to public education.
The study highlights the school’s unified purpose, rigorous academic environment, and strong community support while also pointing out its lack of cultural diversity and openness to differing viewpoints. These insights are crucial for understanding how such educational settings operate and what they offer to students.
Even after discovering all this, Peshkin still presented the school in a positive light and stated that public schools have much to learn from such schools.
Peshkin’s in-depth research represents a qualitative study that uses observations and unstructured interviews, without any assumptions or hypotheses. He utilizes descriptive or non-quantifiable data on Bethany Baptist Academy specifically, without attempting to generalize the findings to other Christian schools.
4. Understanding buyers’ trends
Method used: record keeping.
Another way marketers can use quality research is to understand buyers’ trends. To do this, marketers need to look at historical data for both their company and their industry and identify where buyers are purchasing items in higher volumes.
For example, electronics distributors know that the holiday season is a peak market for sales while life insurance agents find that spring and summer wedding months are good seasons for targeting new clients.
5. Determining products/services missing from the market
Conducting your own research isn’t always necessary. If there are significant breakthroughs in your industry, you can use industry data and adapt it to your marketing needs.
The influx of hacking and hijacking of cloud-based information has made Internet security a topic of many industry reports lately. A software company could use these reports to better understand the problems its clients are facing.
As a result, the company can provide solutions prospects already know they need.
Real-time Customer Lifetime Value (CLV) Benchmark Report
See where your business stands compared to 1,000+ e-stores in different industries.
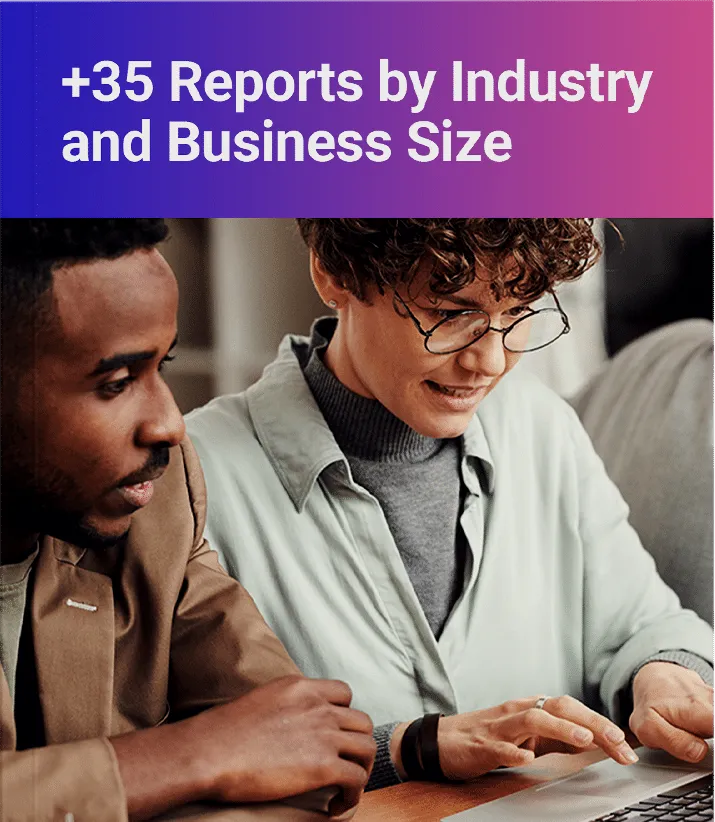
Qualitative Research Approaches
Once the marketer has decided that their research questions will provide data that is qualitative in nature, the next step is to choose the appropriate qualitative approach.
The approach chosen will take into account the purpose of the research, the role of the researcher, the data collected, the method of data analysis , and how the results will be presented. The most common approaches include:
- Narrative : This method focuses on individual life stories to understand personal experiences and journeys. It examines how people structure their stories and the themes within them to explore human existence. For example, a narrative study might look at cancer survivors to understand their resilience and coping strategies.
- Phenomenology : attempts to understand or explain life experiences or phenomena; It aims to reveal the depth of human consciousness and perception, such as by studying the daily lives of those with chronic illnesses.
- Grounded theory : investigates the process, action, or interaction with the goal of developing a theory “grounded” in observations and empirical data.
- Ethnography : describes and interprets an ethnic, cultural, or social group;
- Case study : examines episodic events in a definable framework, develops in-depth analyses of single or multiple cases, and generally explains “how”. An example might be studying a community health program to evaluate its success and impact.
How to Analyze Qualitative Data
Analyzing qualitative data involves interpreting non-numerical data to uncover patterns, themes, and deeper insights. This process is typically more subjective and requires a systematic approach to ensure reliability and validity.
1. Data Collection
Ensure that your data collection methods (e.g., interviews, focus groups, observations) are well-documented and comprehensive. This step is crucial because the quality and depth of the data collected will significantly influence the analysis.
2. Data Preparation
Once collected, the data needs to be organized. Transcribe audio and video recordings, and gather all notes and documents. Ensure that all data is anonymized to protect participant confidentiality where necessary.
3. Familiarization
Immerse yourself in the data by reading through the materials multiple times. This helps you get a general sense of the information and begin identifying patterns or recurring themes.
Develop a coding system to tag data with labels that summarize and account for each piece of information. Codes can be words, phrases, or acronyms that represent how these segments relate to your research questions.
- Descriptive Coding : Summarize the primary topic of the data.
- In Vivo Coding : Use language and terms used by the participants themselves.
- Process Coding : Use gerunds (“-ing” words) to label the processes at play.
- Emotion Coding : Identify and record the emotions conveyed or experienced.
5. Thematic Development
Group codes into themes that represent larger patterns in the data. These themes should relate directly to the research questions and form a coherent narrative about the findings.
6. Interpreting the Data
Interpret the data by constructing a logical narrative. This involves piecing together the themes to explain larger insights about the data. Link the results back to your research objectives and existing literature to bolster your interpretations.
7. Validation
Check the reliability and validity of your findings by reviewing if the interpretations are supported by the data. This may involve revisiting the data multiple times or discussing the findings with colleagues or participants for validation.
8. Reporting
Finally, present the findings in a clear and organized manner. Use direct quotes and detailed descriptions to illustrate the themes and insights. The report should communicate the narrative you’ve built from your data, clearly linking your findings to your research questions.
Limitations of qualitative research
The disadvantages of qualitative research are quite unique. The techniques of the data collector and their own unique observations can alter the information in subtle ways. That being said, these are the qualitative research’s limitations:
1. It’s a time-consuming process
The main drawback of qualitative study is that the process is time-consuming. Another problem is that the interpretations are limited. Personal experience and knowledge influence observations and conclusions.
Thus, qualitative research might take several weeks or months. Also, since this process delves into personal interaction for data collection, discussions often tend to deviate from the main issue to be studied.
2. You can’t verify the results of qualitative research
Because qualitative research is open-ended, participants have more control over the content of the data collected. So the marketer is not able to verify the results objectively against the scenarios stated by the respondents. For example, in a focus group discussing a new product, participants might express their feelings about the design and functionality. However, these opinions are influenced by individual tastes and experiences, making it difficult to ascertain a universally applicable conclusion from these discussions.
3. It’s a labor-intensive approach
Qualitative research requires a labor-intensive analysis process such as categorization, recording, etc. Similarly, qualitative research requires well-experienced marketers to obtain the needed data from a group of respondents.
4. It’s difficult to investigate causality
Qualitative research requires thoughtful planning to ensure the obtained results are accurate. There is no way to analyze qualitative data mathematically. This type of research is based more on opinion and judgment rather than results. Because all qualitative studies are unique they are difficult to replicate.
5. Qualitative research is not statistically representative
Because qualitative research is a perspective-based method of research, the responses given are not measured.
Comparisons can be made and this can lead toward duplication, but for the most part, quantitative data is required for circumstances that need statistical representation and that is not part of the qualitative research process.
While doing a qualitative study, it’s important to cross-reference the data obtained with the quantitative data. By continuously surveying prospects and customers marketers can build a stronger database of useful information.
Quantitative vs. Qualitative Research

Image source
Quantitative and qualitative research are two distinct methodologies used in the field of market research, each offering unique insights and approaches to understanding consumer behavior and preferences.
As we already defined, qualitative analysis seeks to explore the deeper meanings, perceptions, and motivations behind human behavior through non-numerical data. On the other hand, quantitative research focuses on collecting and analyzing numerical data to identify patterns, trends, and statistical relationships.
Let’s explore their key differences:
Nature of Data:
- Quantitative research : Involves numerical data that can be measured and analyzed statistically.
- Qualitative research : Focuses on non-numerical data, such as words, images, and observations, to capture subjective experiences and meanings.
Research Questions:
- Quantitative research : Typically addresses questions related to “how many,” “how much,” or “to what extent,” aiming to quantify relationships and patterns.
- Qualitative research: Explores questions related to “why” and “how,” aiming to understand the underlying motivations, beliefs, and perceptions of individuals.
Data Collection Methods:
- Quantitative research : Relies on structured surveys, experiments, or observations with predefined variables and measures.
- Qualitative research : Utilizes open-ended interviews, focus groups, participant observations, and textual analysis to gather rich, contextually nuanced data.
Analysis Techniques:
- Quantitative research: Involves statistical analysis to identify correlations, associations, or differences between variables.
- Qualitative research: Employs thematic analysis, coding, and interpretation to uncover patterns, themes, and insights within qualitative data.

Do Conversion Rate Optimization the Right way.
Explore helps you make the most out of your CRO efforts through advanced A/B testing, surveys, advanced segmentation and optimised customer journeys.

If you haven’t subscribed yet to our newsletter, now is your chance!

Like what you’re reading?
Join the informed ecommerce crowd.
We will never bug you with irrelevant info.
By clicking the Button, you confirm that you agree with our Terms and Conditions .
Continue your Conversion Rate Optimization Journey
- Last modified: January 3, 2023
- Conversion Rate Optimization , User Research
Valentin Radu

We’re a team of people that want to empower marketers around the world to create marketing campaigns that matter to consumers in a smart way. Meet us at the intersection of creativity, integrity, and development, and let us show you how to optimize your marketing.
Our Software
- > Book a Demo
- > Partner Program
- > Affiliate Program
- Blog Sitemap
- Terms and Conditions
- Privacy & Security
- Cookies Policy
- REVEAL Terms and Conditions
Quantitative Researcher Resume Samples
Quantitative Researcher is a professional specializing in designing and conducting quantitative research studies to analyze and interpret numerical data. The duties expected from these professionals are listed on the Quantitative Researcher Resume as – formulating research questions, developing methodologies, and utilizing statistical techniques to collect, process, and analyze data . The Quantitative Researcher often works in various fields such as finance, economics, social sciences, or market research, and provides valuable insights through the use of statistical models and data-driven approaches.
The nature of the job demands the following skills and abilities – proficiency in statistical software, the ability to derive meaningful conclusions from data; experience in statistical modeling, data analysis, and research methodologies; strong programming skills, the ability to use languages like Python, or R; the ability to use statistical tools like SAS, or SPSS; effective communication skills, and staying updated on advancements in statistical methods and data analysis techniques. Education-wise, a Master’s degree or Ph.D. in a relevant field such as Statistics, Mathematics, or Economics is required.
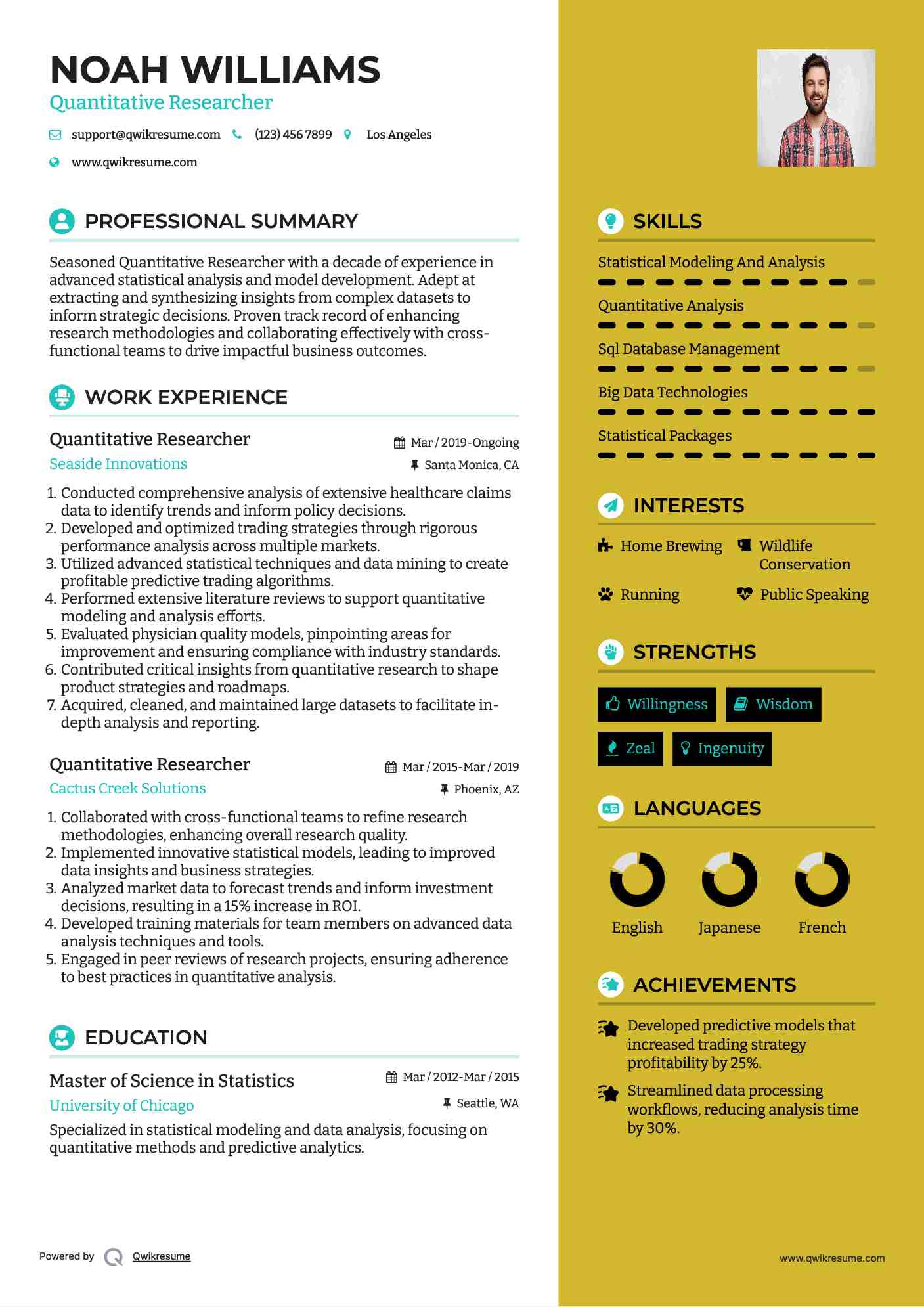
- Resume Samples
- Quantitative Researcher
Quantitative Researcher Resume
Summary : As a Quantitative Researcher, supports the development and implementation of a comprehensive and ambitious research plan, methodologies, and data collection techniques to investigate various aspects of unsheltered homelessness.
Skills : Math skills, Financial skills.
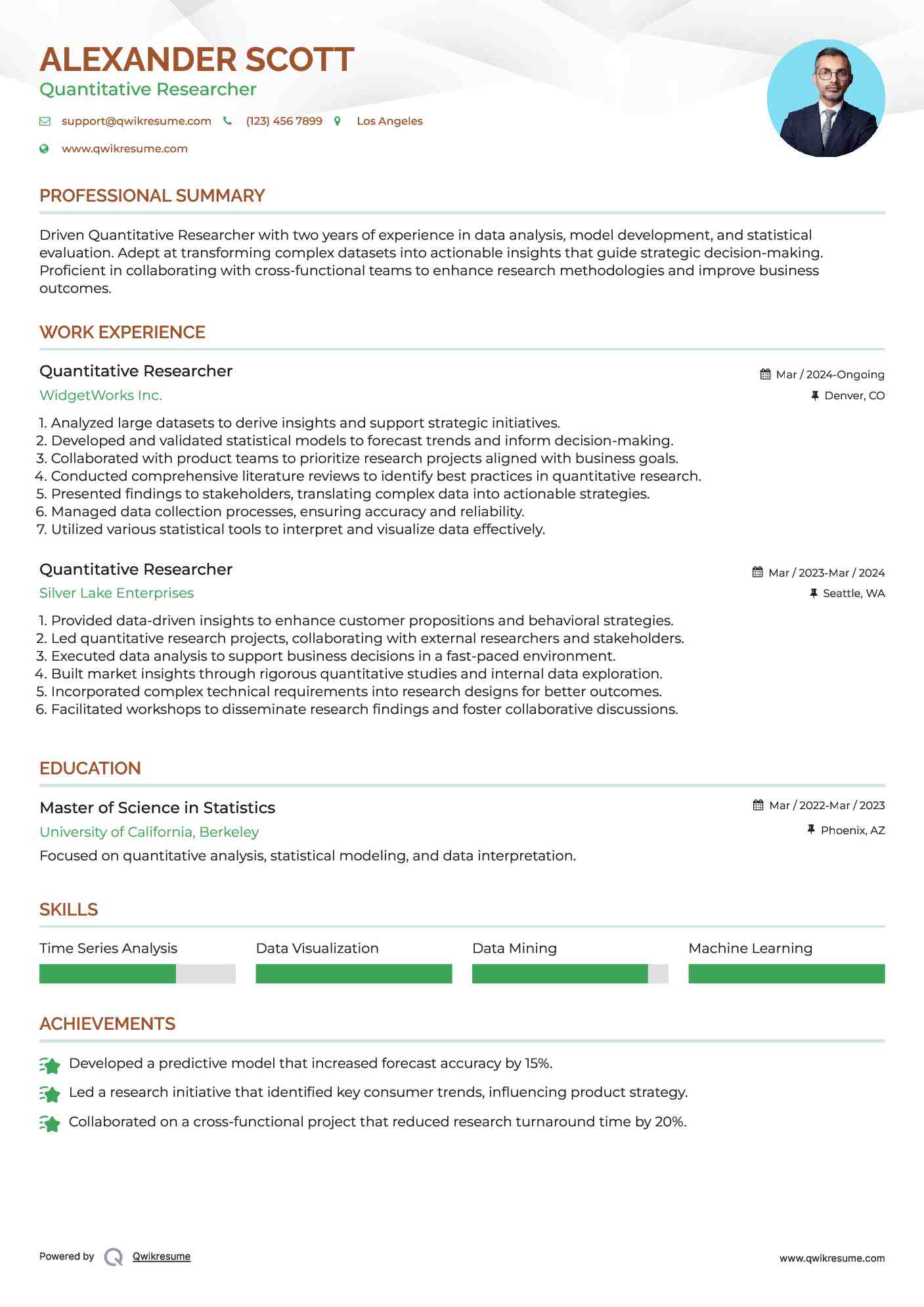
Description :
- Managed research and evaluation activities including planning and executing research, data analysis, reporting, and improving and managing data quality.
- Established research programs and practices to increase the efficiency of data production and use.
- Professionalized research and evaluation activities using data science principles.
- Supported the development of new operational mechanisms for efficient and effective data gathering and data management processes.
- Developed and applied appropriate conceptual and analytical frameworks in research analysis and program development processes.
- Provided advice and support based on both internal and external data sources and research.
- Generated a range of options based on research results, assessed the implications and feasibility of these options, and recommended viable solutions and implementation strategies for new research.
Objective : As a Quantitative Researcher, responsible for analyzing and interpreting data and prepare high-quality research reports, and policy briefs that present research findings, recommendations, and actionable insights to relevant stakeholders, policymakers, and the public.
Skills : Problem-solving skills, Communication skills.
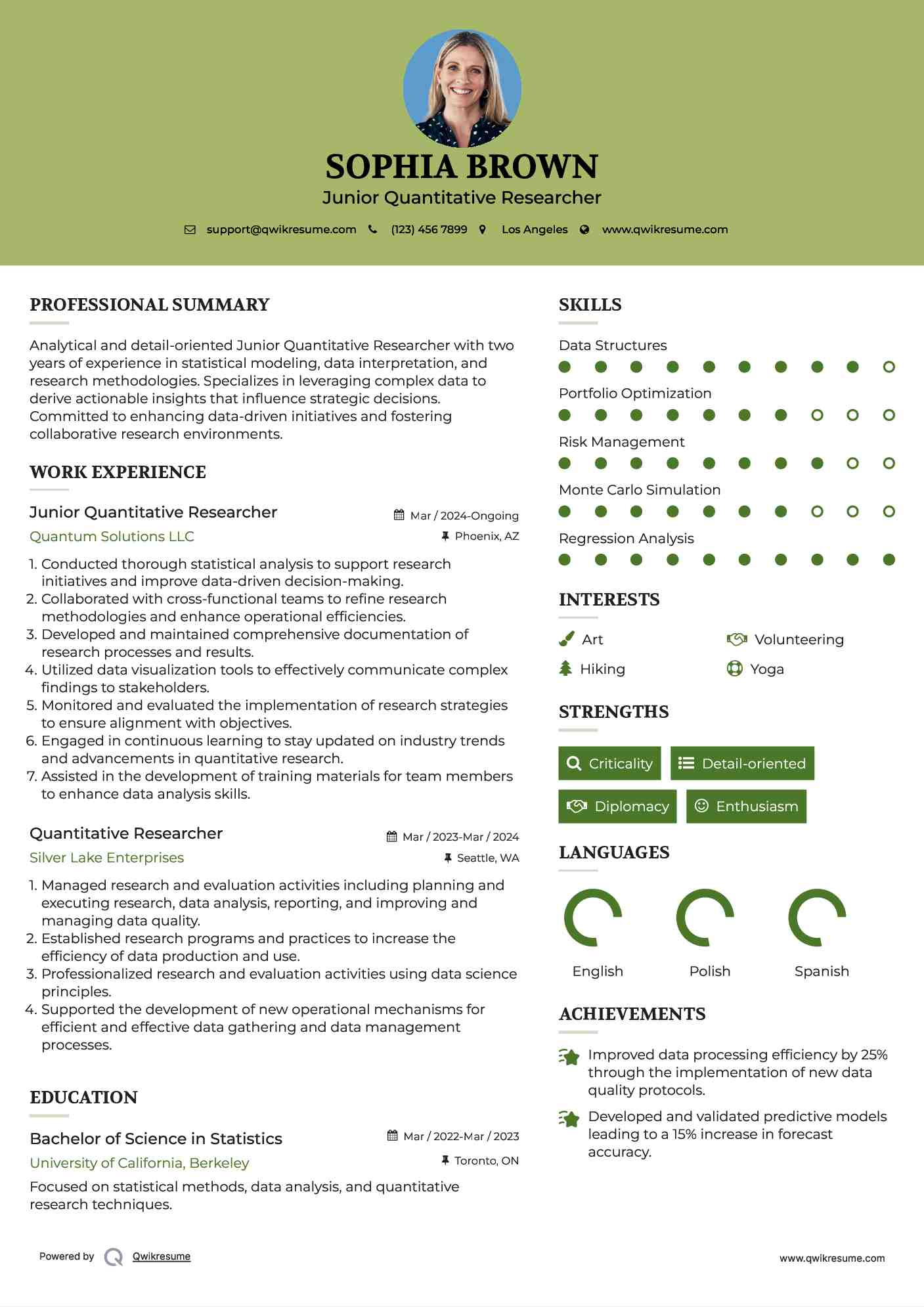
- Provided data and information as required to colleagues.
- Collaborated with external researchers, evaluators, and contractors.
- Ran quantitative research programs that informed the customer propositions and behavioral bets that the business made.
- Worked with Design and Product to prioritize research opportunities in a fast-paced, rapidly changing environment.
- Led and advised on research plans, overall user research strategy, and methods.
- Built thorough market and consumer insight through a series of quantitative research initiatives, published research, and internal data sources.
- Understood and incorporated complex technical and business requirements into research.
Summary : As a Quantitative Researcher, supported all strategies from alpha generation through to trade execution and implementation and work closely with other team members to create new and support existing strategies by identifying new investment ideas or innovative data sources.
Skills : Independence, Programming.
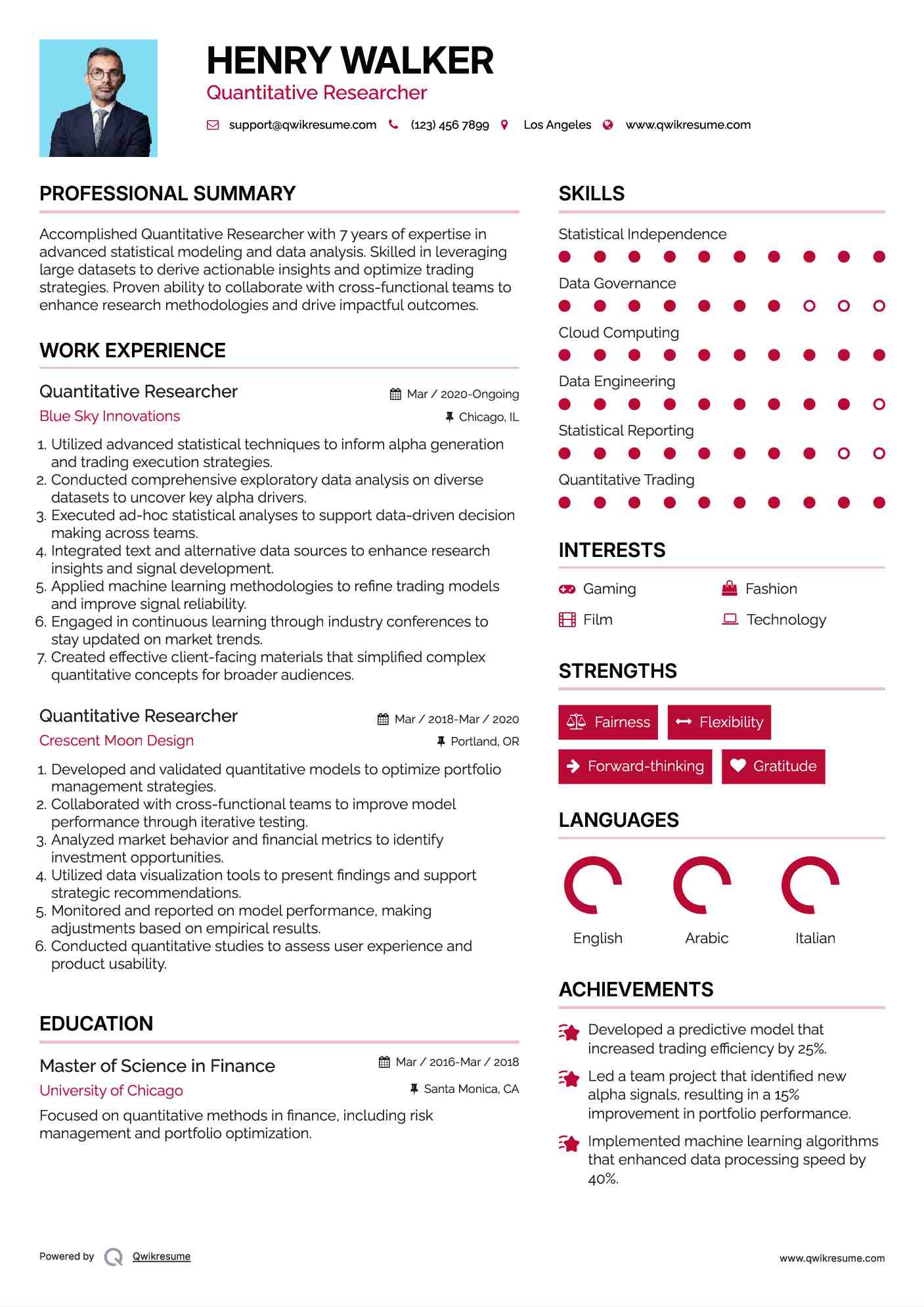
- Advocated and provided guidance on how to use customer insights and data to inform decisions.
- Presented and communicated consumer and market insight and recommendations to the larger organization.
- Supported and developed best practices, research methodologies, and frameworks to drive efficiency across the organization.
- Conducted independent, innovative quantitative research across asset classes, focusing on options markets and capturing alpha opportunities.
- Developed and implemented systematic trading strategies based on research insights, primarily focusing on options markets.
- Supported, monitored, and explained the performance of a suite of factor strategies across diverse asset classes to internal and external clients.
- Evaluated and onboarded new datasets (including alternative data and economic indicators) to improve strategy signals across asset classes.
Objective : As a Quantitative Researcher, responsible for gathering and refining complex data for modelling, coding and performing statistical analysis to build and refine models, and interpreting, presenting, and implementing the results.
Skills : Data analysis, Communication.
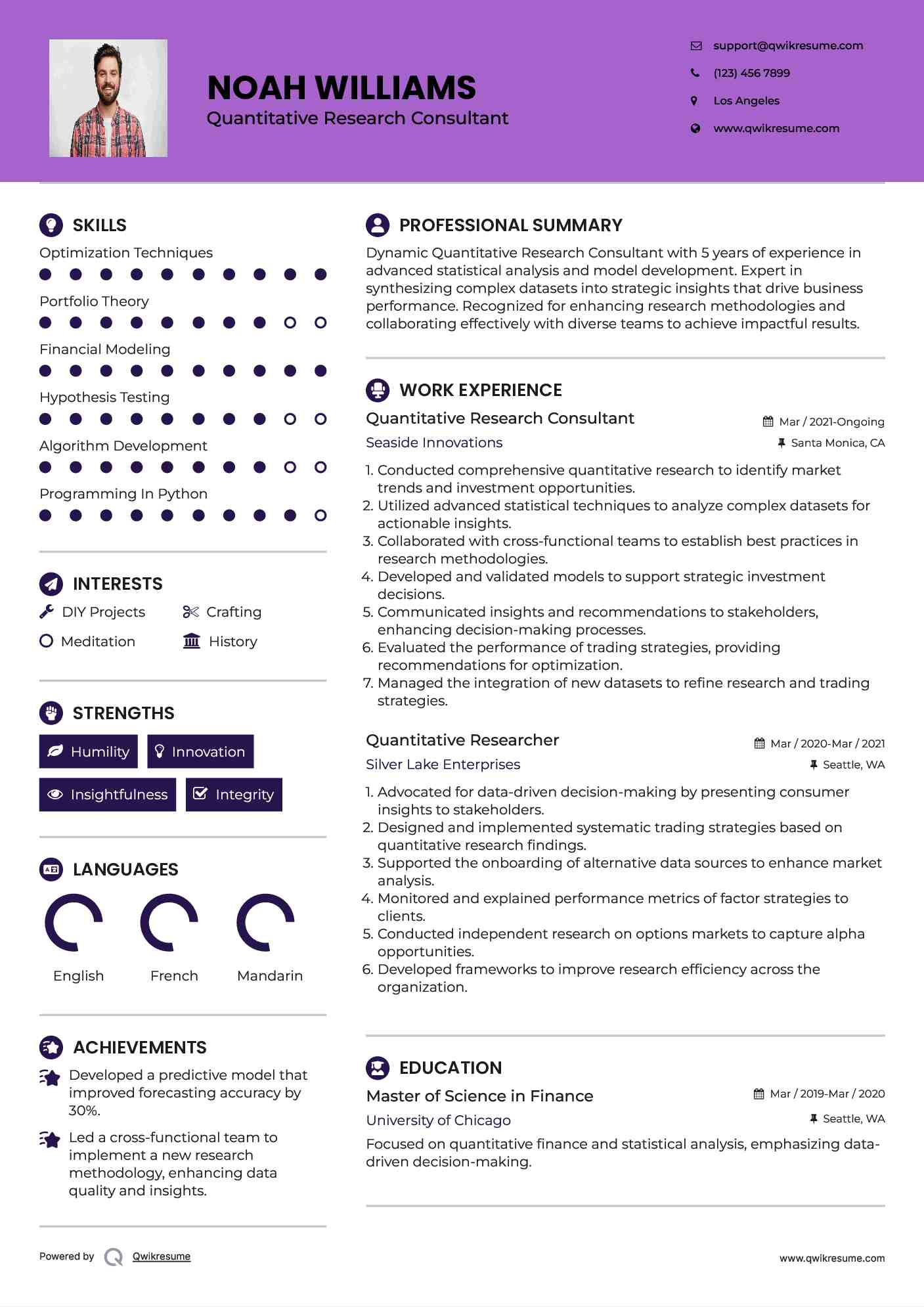
- Crafted innovative strategies using advanced mathematical techniques, primarily in derivatives markets (futures, forwards, CDX, swaps).
- Performed exploratory data analysis on structured and unstructured data to identify alpha drivers and build robust signals.
- Performed ad-hoc exploratory statistical analysis across multiple large complex data sets.
- Explored and integrated text data into research to drive alpha generation.
- Applied machine learning techniques (deep, reinforcement, causal) to build and refine monetization systems for trading signals.
- Attended industry events and conferences to stay ahead of the curve and gain local market insights.
- Developed and maintained client marketing materials, effectively communicating complex concepts to diverse audiences.
Objective : As a Quantitative Researcher, researchs, creates, and evaluates mathematical optimization models for tax-smart portfolio management products. Implements and provides expertise on model implementation, testing, performance tuning and enhancements.
Skills : Statistical analysis, Math.
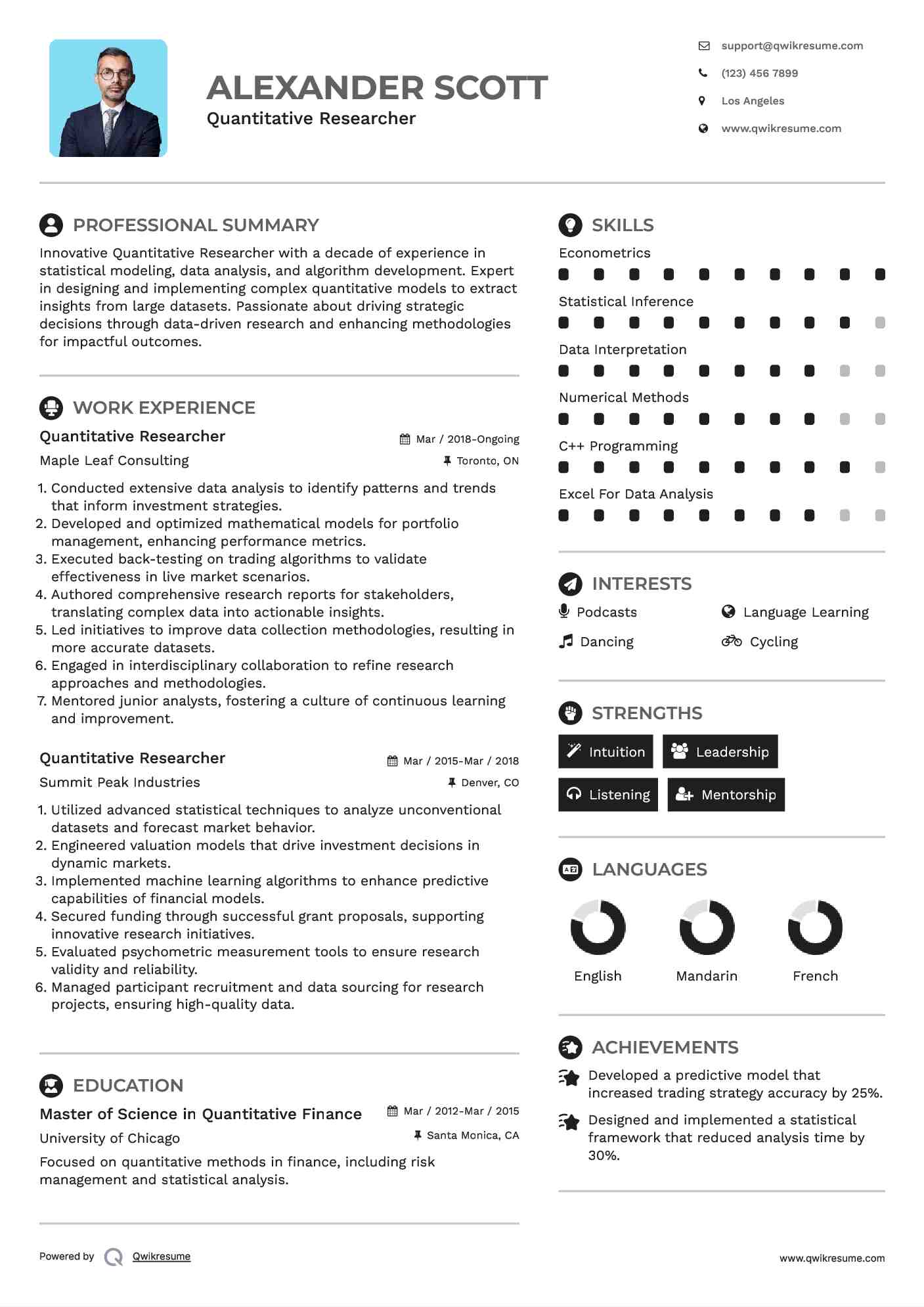
- Presented quantitative results to research and non-technical audiences.
- Wrote model specifications and documentation.
- Provided clear and concise oral and written communication across various functions and levels.
- Assisted in initiatives related to the improvement of the model development processes and infrastructure in a production environment.
- Assisted in initiatives related to investment product design, feature enhancements, and testing.
- Supported live account trading, responded to trading and debugging requests.
- Conducted research and statistical analyses in the evaluation of securities.
Summary : As a Quantitative Researcher, responsible for analyzing data from a variety of sources from behavior to user demographics to weather to the global economy and help companies understand which metrics to pay attention to when making business decisions.
Skills : Data modeling, Problem solving.
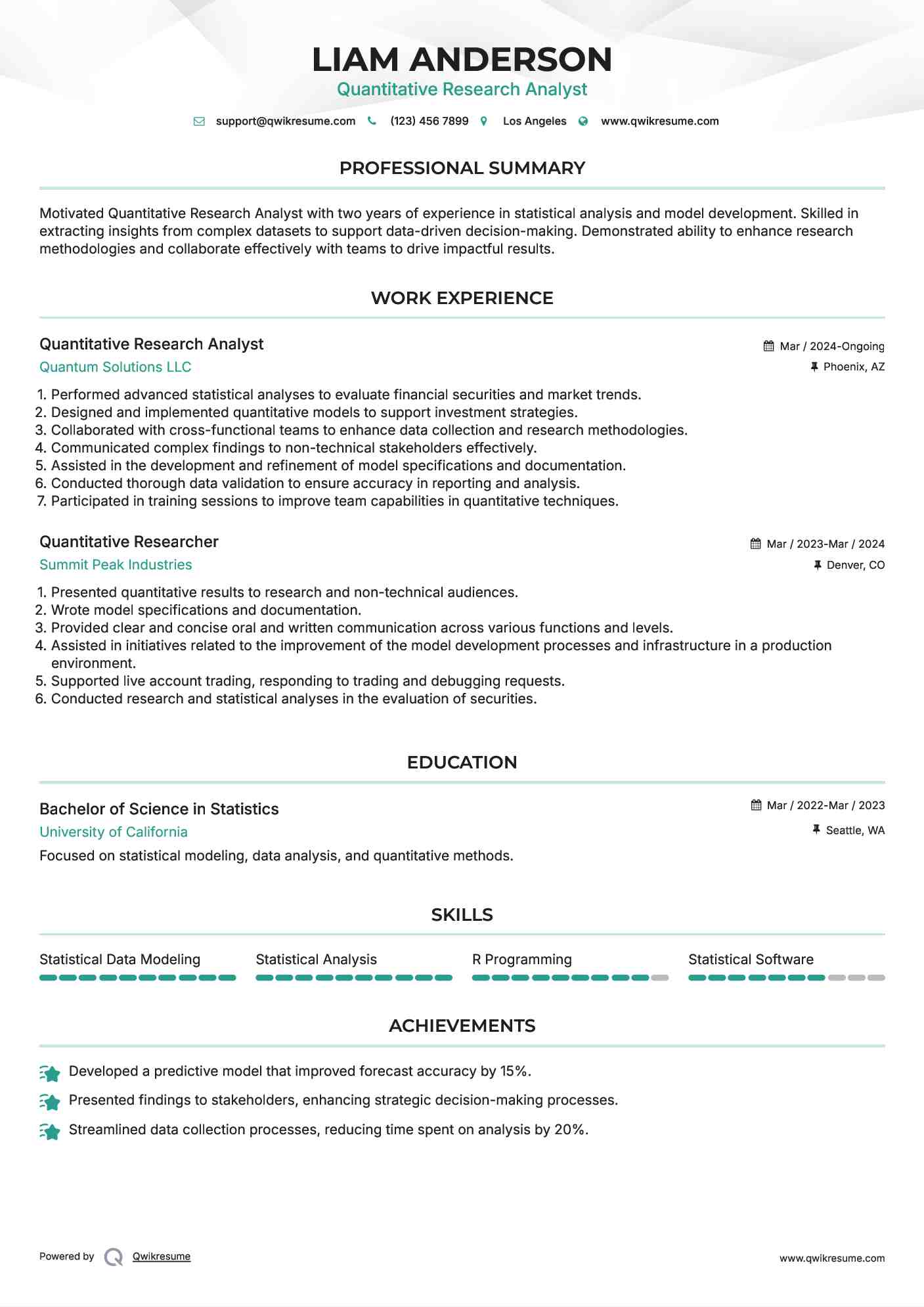
- Worked with large data sets, including unconventional data sources, to predict and test statistical market patterns.
- Conceptualized valuation strategies, developed and continuously improved mathematical models, and translated algorithms into code.
- Back-tested and implemented trading models and signals in a live trading environment.
- Wrote research proposals that catered to experts and non-specialists.
- Procured funding from within the department and from outside sources.
- Selected psychometrically robust and contextually sensitive measurement instruments.
- Sourced, vetted, and oriented research participants.
Summary : As a Quantitative Researcher, responsible for doing market research and finding solutions to difficult financial issues using descriptive and inferential statistics. They use environmental studies and financial models to examine and evaluate the performance of enterprises.
Skills : Research, Organization.
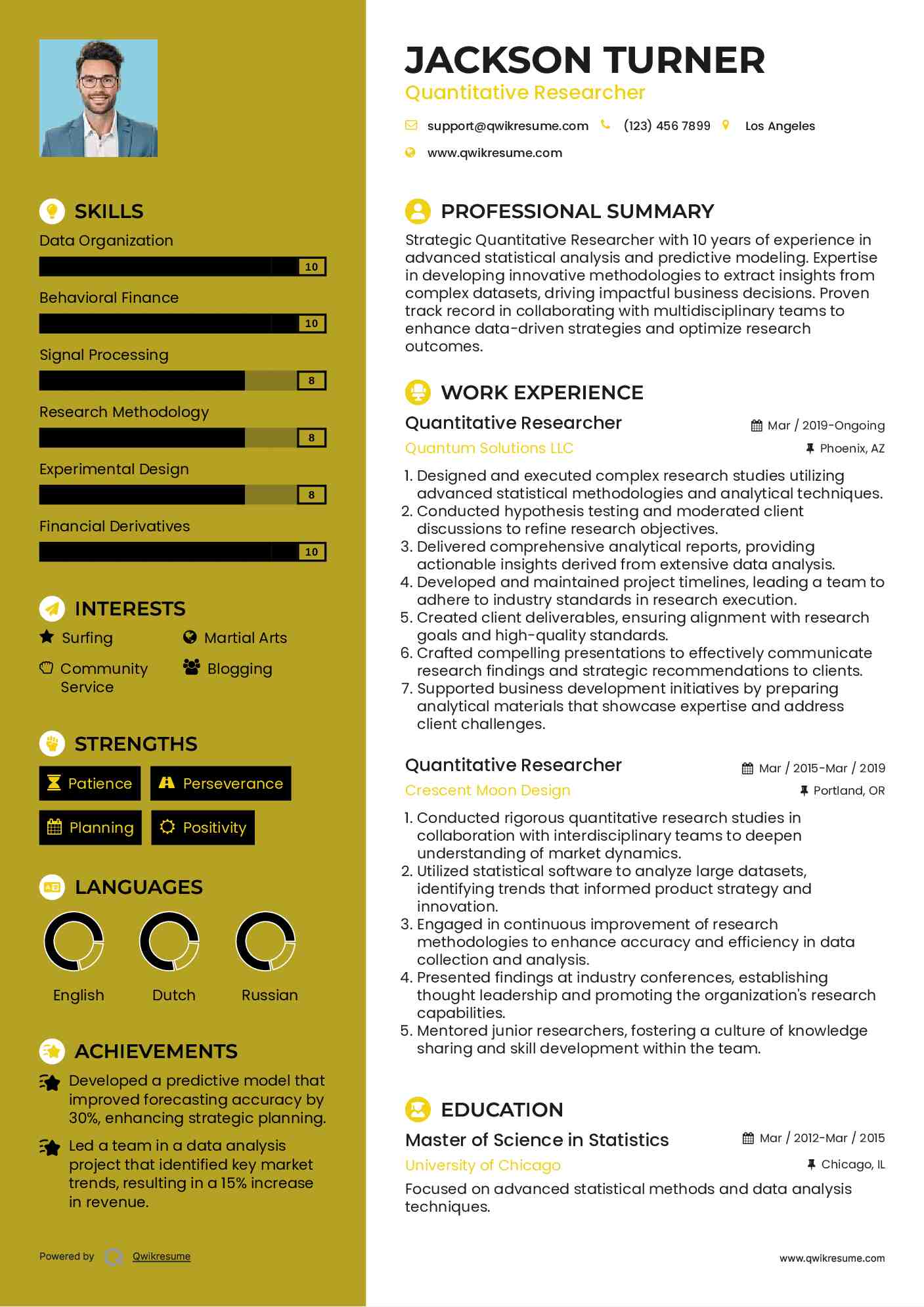
- Developed new or improved existing trading models using in-house platforms.
- Used advanced mathematical techniques to model and predict market movements.
- Analyzed large financial datasets to identify trading opportunities.
- Provided real-time analytical support to experienced traders.
- Evaluated trading data, including pre-processing, feature engineering, variable selection, dimensionality reduction.
- Leveraged machine learning to extract insights from data and worked with investment managers to put those into action.
- Designed and developed models/ML solutions for trading and liquidity.
Summary : As a Quantitative Researcher, provides in-depth reports that detail their findings and offer interpretations of the analyzed financial data to help with decision-making. They also verify the accuracy of data processing techniques.
Skills : Teamwork and Time management.
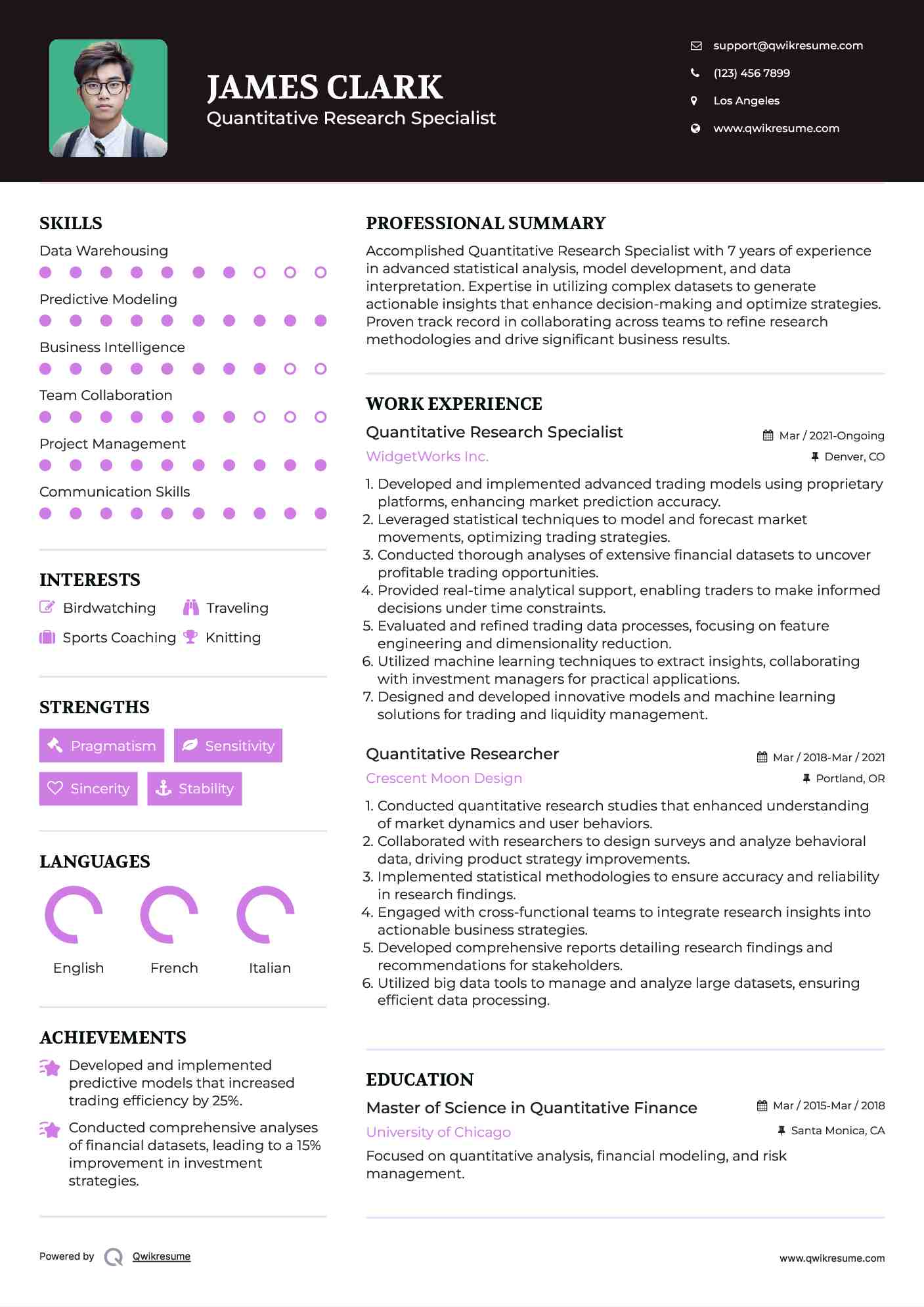
- Designed research studies using a variety of primary research methodologies and analytical techniques.
- Led/participated in telephonic or in-person hypothesis gathering sessions, and moderated client discussions.
- Delivered projects and executed tasks such as analytical planning, survey analysis, expert interview moderation, and generation of insights from research data.
- Prepared realistic work plans and led a team to conduct research as per standard industry protocols.
- Prepared client deliverables (PowerPoint reports, research instruments) for quality and consistency with the original scope/research objectives.
- Developed PowerPoint presentations to assure effective storytelling, and presented insights to clients.
- Supported new business development efforts with timely materials to showcase experience and thinking around client problems.
Objective : As a Quantitative Researcher, prioritizes research needs and approaches across multiple work streams to work on the right project, scale, and scope at the right time and ensure impact by delivering actionable insights.
Skills : Data collection, Financial knowledge.
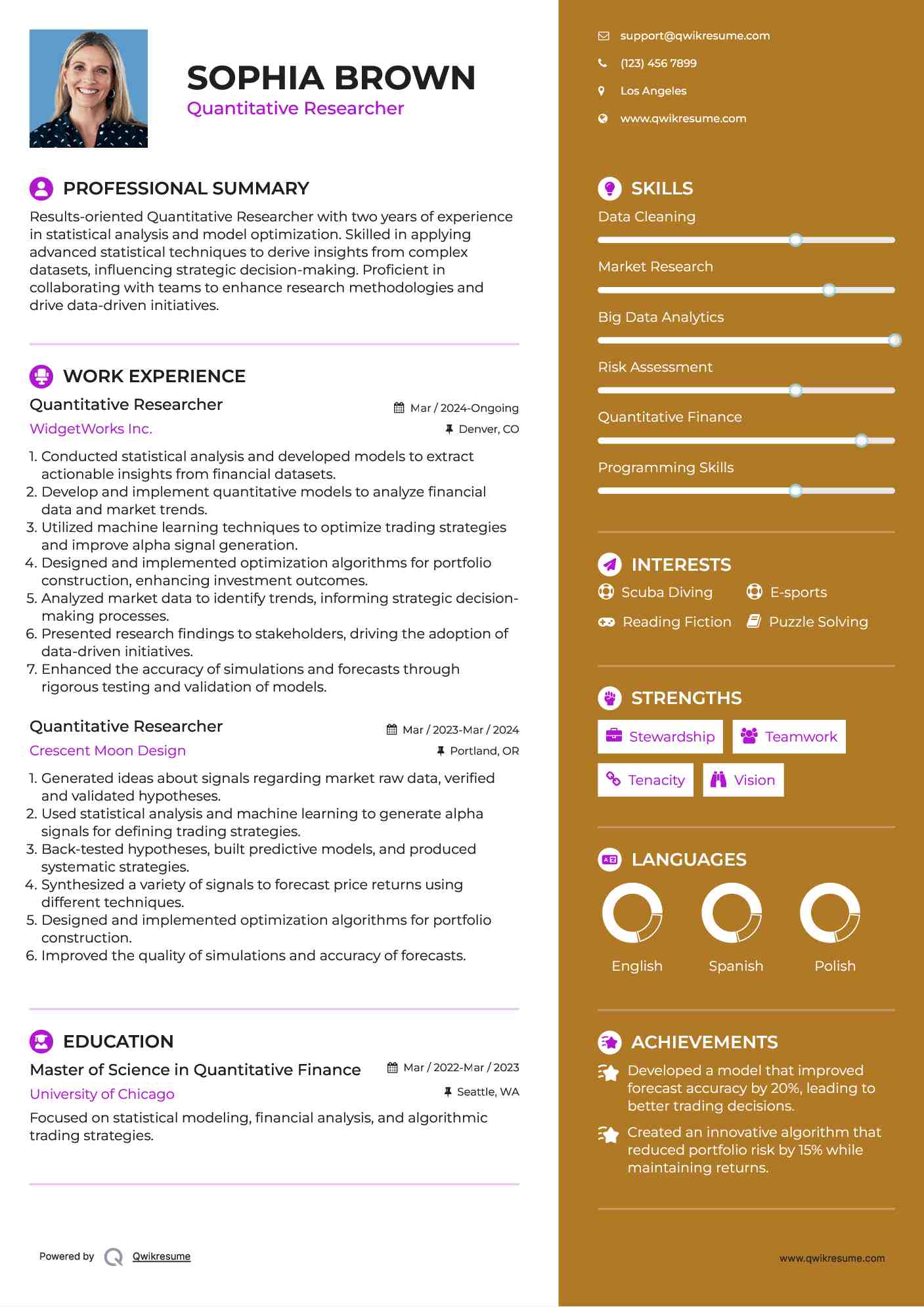
- Worked with extensive administrative healthcare claims data.
- Analyzed the performance of trading strategies over different markets and optimized strategies based on the analysis.
- Utilized statistical analysis and data mining skills to research, make forecasts, and develop profitable predictive trading algorithms.
- Performed literature research to support modeling or analysis efforts.
- Evaluated physician quality models and identified areas for improvement.
- Impacted the product roadmap with key insights distilled from quantitative research.
- Acquired, cleaned, maintained, and analyzed data sets to identify trends and patterns.
Summary : As a Quantitative Researcher, conducts quantitative research studies in partnership with other researchers using methods such as survey research and behavioral/log data analysis that deepen our understanding of the user experience and drive product strategy.
Skills : Mathematical and Statistical skills.
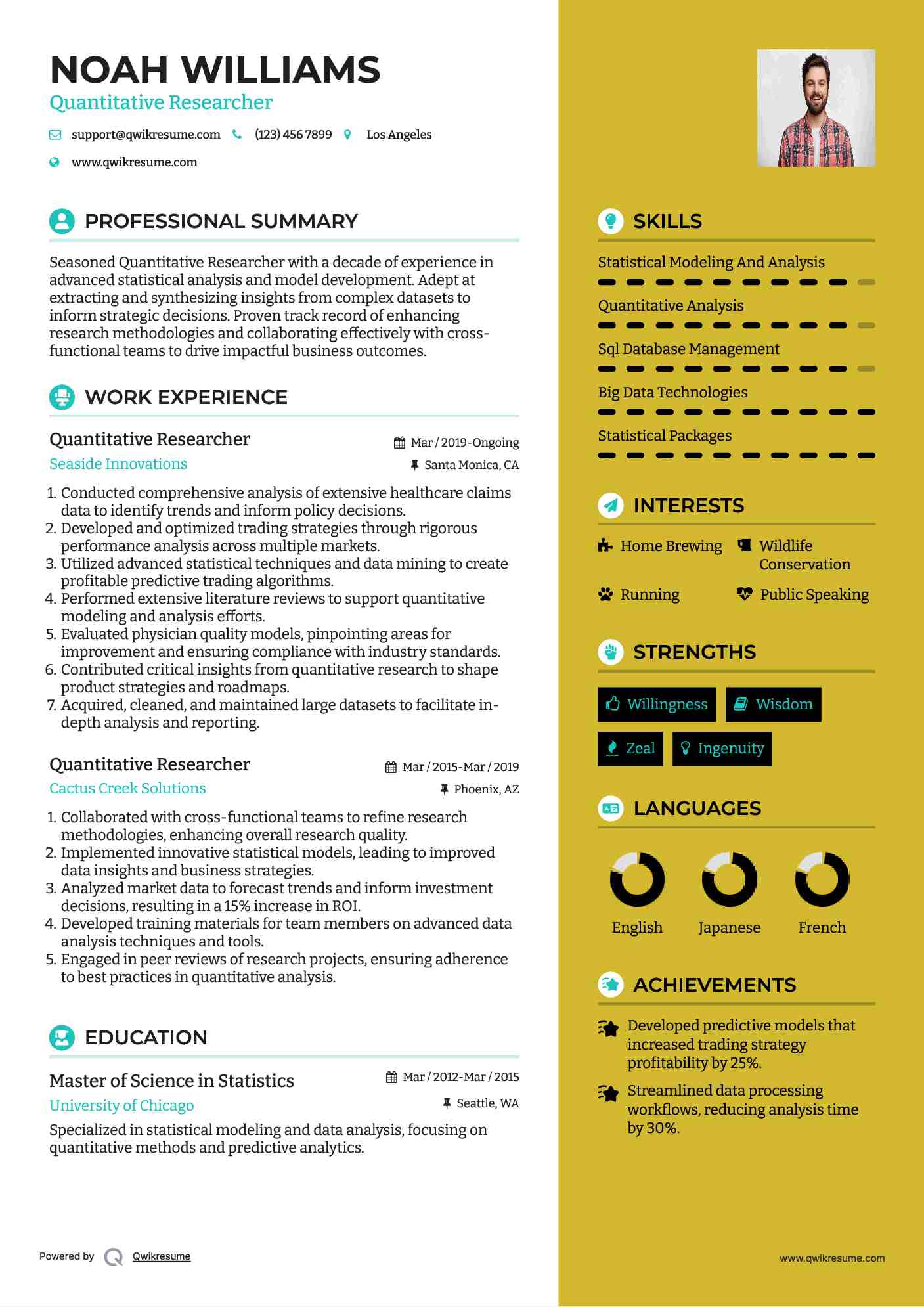
- Generated ideas about signals regarding market raw data, verified and validated hypotheses.
- Used statistical analysis and machine learning to generate alpha signals for defining trading strategies.
- Back-tested hypotheses, built predictive models, and produced systematic strategies.
- Synthesized a variety of signals to forecast price returns using different techniques.
- Designed and implemented optimization algorithms for portfolio construction.
- Improved the quality of simulations and accuracy of forecasts.
- Shaped the trading footprint by identifying new markets to trade on.
Table of Contents
Recent posts, download this pdf template., creating an account is free and takes five seconds. you'll get access to the pdf version of this resume template., choose an option., unlock the power of over 10,000 resume samples., take your job search to the next level with our extensive collection of 10,000+ resume samples. find inspiration for your own resume and gain a competitive edge in your job search., get hired faster with resume assistant., make your resume shine with our resume assistant. you'll receive a real-time score as you edit, helping you to optimize your skills, experience, and achievements for the role you want., get noticed with resume templates that beat the ats., get past the resume screeners with ease using our optimized templates. our professional designs are tailored to beat the ats and help you land your dream job..
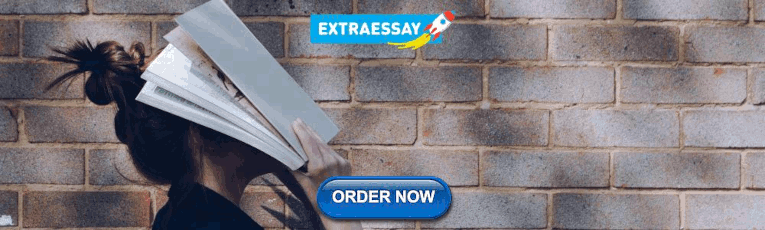
IMAGES
VIDEO
COMMENTS
INTRODUCTION. Scientific research is usually initiated by posing evidenced-based research questions which are then explicitly restated as hypotheses.1,2 The hypotheses provide directions to guide the study, solutions, explanations, and expected results.3,4 Both research questions and hypotheses are essentially formulated based on conventional theories and real-world processes, which allow the ...
Quantitative research methods. You can use quantitative research methods for descriptive, correlational or experimental research. In descriptive research, you simply seek an overall summary of your study variables.; In correlational research, you investigate relationships between your study variables.; In experimental research, you systematically examine whether there is a cause-and-effect ...
Quantitative research methods provide an relatively conclusive answer to the research questions. When the data is collected and analyzed in accordance with standardized, reputable methodology, the results are usually trustworthy. With statistically significant sample sizes, the results can be generalized to an entire target group.
Quantitative methods emphasize objective measurements and the statistical, mathematical, or numerical analysis of data collected through polls, questionnaires, and surveys, or by manipulating pre-existing statistical data using computational techniques.Quantitative research focuses on gathering numerical data and generalizing it across groups of people or to explain a particular phenomenon.
Quantitative research is the process of collecting and analyzing numerical data to describe, predict, or control variables of interest. This type of research helps in testing the causal relationships between variables, making predictions, and generalizing results to wider populations. The purpose of quantitative research is to test a predefined ...
STEP ONE: Choose the type of quantitative research question (i.e., descriptive, comparative or relationship) you are trying to create. STEP TWO: Identify the different types of variable you are trying to measure, manipulate and/or control, as well as any groups you may be interested in. STEP THREE: Select the appropriate structure for the ...
Summarizing quantitative data and its effective presentation and discussion can be challenging for students and researchers. This chapter provides a framework for adequately reporting findings from quantitative analysis in a research study for those contemplating to write a research paper. The rationale underpinning the reporting methods to ...
Revised on 10 October 2022. Quantitative research is the process of collecting and analysing numerical data. It can be used to find patterns and averages, make predictions, test causal relationships, and generalise results to wider populations. Quantitative research is the opposite of qualitative research, which involves collecting and ...
Controlled collection and analysis of information in order to understand a phenomenon. Originates with a question, a problem, a puzzling fact. Requires both theory and data. Previous theory helps us form an understanding of the data we see (no blank slate). Data lets us tests our hypotheses.
Quantitative research methods are concerned with the planning, design, and implementation of strategies to collect and analyze data. Descartes, the seventeenth-century philosopher, suggested that how the results are achieved is often more important than the results themselves, as the journey taken along the research path is a journey of discovery. . High-quality quantitative research is ...
How do I write the results chapter? There are multiple steps involved in writing up the results chapter for your quantitative research. The exact number of steps applicable to you will vary from study to study and will depend on the nature of the research aims, objectives and research questions.However, we'll outline the generic steps below.
9 Presenting the Results of Quantitative Analysis . Mikaila Mariel Lemonik Arthur. This chapter provides an overview of how to present the results of quantitative analysis, in particular how to create effective tables for displaying quantitative results and how to write quantitative research papers that effectively communicate the methods used and findings of quantitative analysis.
A good research question is essential to guide your research paper, dissertation, or thesis. All research questions should be: Focused on a single problem or issue. Researchable using primary and/or secondary sources. Feasible to answer within the timeframe and practical constraints. Specific enough to answer thoroughly.
Read More - 90+ Market Research Questions to Ask Your Customers. 1. Select the Type of Quantitative Question. The first step is to determine which type of quantitative question you want to add to your study. There are three types of quantitative questions: Descriptive. Comparative. Relationship-based.
Quantitative Research Design (JARS-Quant) The current JARS-Quant standards, released in 2018, expand and revise the types of research methodologies covered in the original JARS, which were published in 2008. JARS-Quant include guidance for manuscripts that report. In addition, JARS-Quant now divides hypotheses, analyses, and conclusions ...
Mixed-methods research is a flexible approach, where the research design is determined by what we want to find out rather than by any predetermined epistemological position. In mixed-methods research, qualitative or quantitative components can predominate, or both can have equal status. 1.4. Units and variables.
nominal. It is important to know w hat kind of data you are planning to collect or analyse as this w ill. affect your analysis method. A 12 step approach to quantitative data analysis. Step 1 ...
Step 2: Choose a type of research design. Within both qualitative and quantitative approaches, there are several types of research design to choose from. Each type provides a framework for the overall shape of your research. Types of quantitative research designs. Quantitative designs can be split into four main types.
Step 1: Consider your aims and approach. Step 2: Choose a type of research design. Step 3: Identify your population and sampling method. Step 4: Choose your data collection methods. Step 5: Plan your data collection procedures. Step 6: Decide on your data analysis strategies. Other interesting articles.
Instruments. This section should include the instruments you plan on using to measure the variables in the research questions. (a) the source or developers of the instrument. (b) validity and reliability information. •. (c) information on how it was normed. •. (d) other salient information (e.g., number of. items in each scale, subscales ...
A methodology for a quantitative study begins with a reiteration of the research question and its context. Methodology for a Quantitative study. This is followed by the research design, where the methods used to gather, process and analyze the data are given. This is usually preceded or followed by a justification of the appropriateness of the ...
Conducting Quantitative Medical Education Research: From Design to Dissemination; 23 Questions to Guide the Writing of a Quantitative Medical Education Research Proposal; Quantitative Research Methods in Medical Education; Survey Design. How to conduct surveys: A step-by-step guide;
It can appear in research via the sampling frame, random sampling, or non-response. It can also occur at other stages in research, such as while interviewing, in the design of questions, or in the way data are analyzed and presented. Bias means that the research findings will not be representative of, or generalizable to, a wider population.
Pros of Quantitative Research. Swift and Extensive Data Collection: Quantitative research methodologies, such as surveys and structured observations, facilitate the swift collection of vast amounts of data. For instance, utilizing online survey platforms enables researchers to reach thousands of participants across the globe in a matter of hours, expediting the research timeline significantly.
Tip #2. Early on in your career, it's critical to envision your ultimate large grant. Drawing a Venn diagram of your research program could help communicate your research expertise to collaborators. Typically a major grant would include five aims. Once you've envisioned your big grant and its five aims, your next steps become clear: create ...
When collecting and analyzing data, quantitative research deals with numbers and statistics, while qualitative research deals with words and meanings. Both are important for gaining different kinds of knowledge. Quantitative research. Quantitative research is expressed in numbers and graphs. It is used to test or confirm theories and assumptions.
Qualitative research is a method focused on understanding human behavior and experiences through non-numerical data. Examples of qualitative research include: One-on-one interviews, Focus groups, Ethnographic research, Case studies, Record keeping, Qualitative observations. In this article, we'll provide tips and tricks on how to use ...
Quantitative Researcher is a professional specializing in designing and conducting quantitative research studies to analyze and interpret numerical data. The duties expected from these professionals are listed on the Quantitative Researcher Resume as - formulating research questions, developing methodologies, and utilizing statistical ...
Qualitative research is a type of research that aims to gather and analyse non-numerical (descriptive) data in order to gain an understanding of individuals' social reality, including understanding their attitudes, beliefs, and motivation. This type of research typically involves in-depth interviews, focus groups, or observations in order to collect data that is rich in detail and context.
Table of contents. Step 1: Introduce your topic. Step 2: Describe the background. Step 3: Establish your research problem. Step 4: Specify your objective (s) Step 5: Map out your paper. Research paper introduction examples. Frequently asked questions about the research paper introduction.