- Business Essentials
- Leadership & Management
- Credential of Leadership, Impact, and Management in Business (CLIMB)
- Entrepreneurship & Innovation
- Digital Transformation
- Finance & Accounting
- Business in Society
- For Organizations
- Support Portal
- Media Coverage
- Founding Donors
- Leadership Team
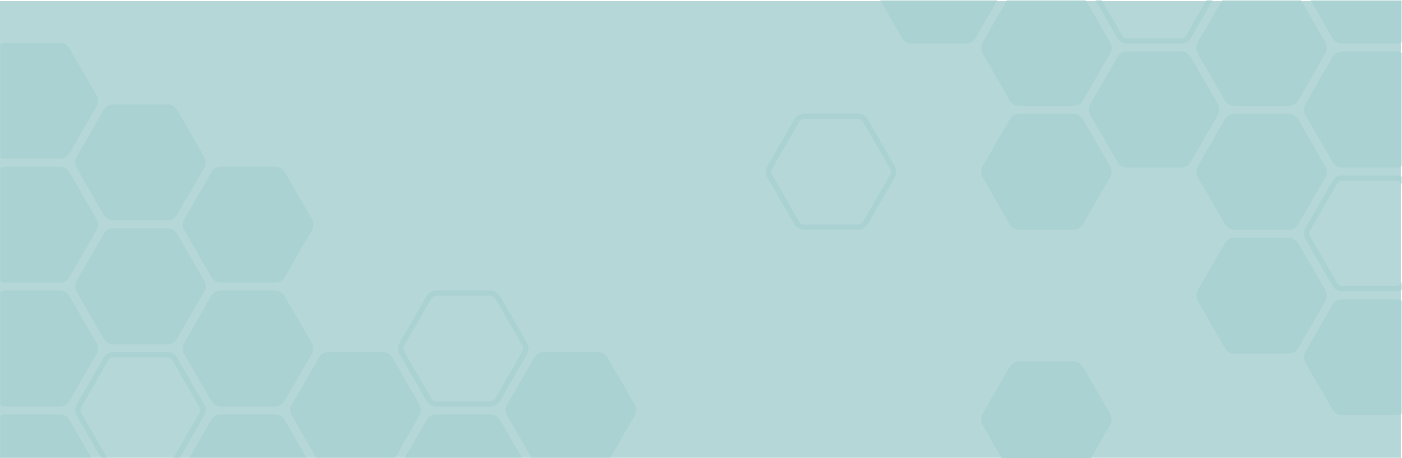
- Harvard Business School →
- HBS Online →
- Business Insights →
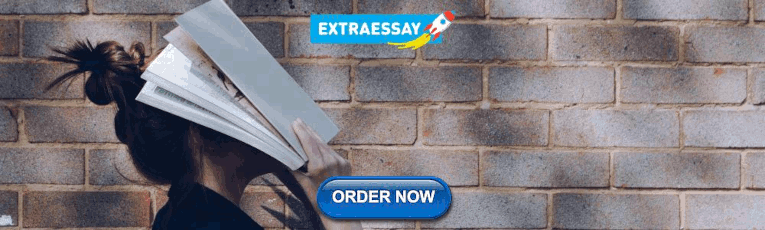
Business Insights
Harvard Business School Online's Business Insights Blog provides the career insights you need to achieve your goals and gain confidence in your business skills.
- Career Development
- Communication
- Decision-Making
- Earning Your MBA
- Negotiation
- News & Events
- Productivity
- Staff Spotlight
- Student Profiles
- Work-Life Balance
- AI Essentials for Business
- Alternative Investments
- Business Analytics
- Business Strategy
- Business and Climate Change
- Design Thinking and Innovation
- Digital Marketing Strategy
- Disruptive Strategy
- Economics for Managers
- Entrepreneurship Essentials
- Financial Accounting
- Global Business
- Launching Tech Ventures
- Leadership Principles
- Leadership, Ethics, and Corporate Accountability
- Leading Change and Organizational Renewal
- Leading with Finance
- Management Essentials
- Negotiation Mastery
- Organizational Leadership
- Power and Influence for Positive Impact
- Strategy Execution
- Sustainable Business Strategy
- Sustainable Investing
- Winning with Digital Platforms
What Is Predictive Analytics? 5 Examples
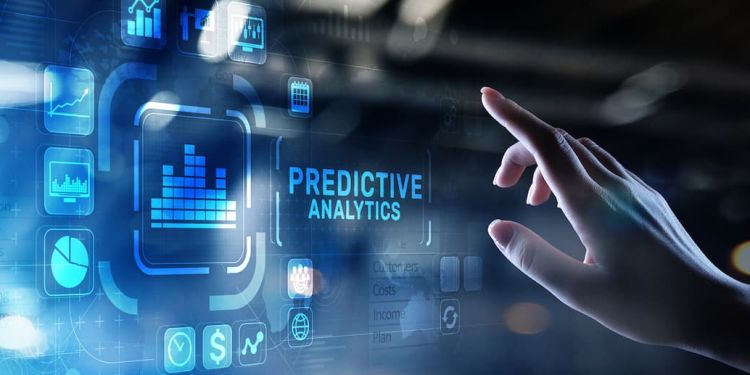
- 26 Oct 2021
Data analytics —the practice of examining data to answer questions, identify trends, and extract insights—can provide you with the information necessary to strategize and make impactful business decisions.
There are four key types of data analytics :
- Descriptive , which answers the question, “What happened?”
- Diagnostic , which answers the question, “Why did this happen?”
- Prescriptive , which answers the question, “What should we do next?”
- Predictive , which answers the question, “What might happen in the future?”
The ability to predict future events and trends is crucial across industries. Predictive analytics appears more often than you might assume—from your weekly weather forecast to algorithm-enabled medical advancements. Here’s an overview of predictive analytics to get you started on the path to data-informed strategy formulation and decision-making.
Access your free e-book today.
What Is Predictive Analytics?
Predictive analytics is the use of data to predict future trends and events. It uses historical data to forecast potential scenarios that can help drive strategic decisions.
The predictions could be for the near future—for instance, predicting the malfunction of a piece of machinery later that day—or the more distant future, such as predicting your company’s cash flows for the upcoming year.
Predictive analysis can be conducted manually or using machine-learning algorithms. Either way, historical data is used to make assumptions about the future.
One predictive analytics tool is regression analysis , which can determine the relationship between two variables ( single linear regression ) or three or more variables ( multiple regression ). The relationships between variables are written as a mathematical equation that can help predict the outcome should one variable change.
“Regression allows us to gain insights into the structure of that relationship and provides measures of how well the data fit that relationship,” says Harvard Business School Professor Jan Hammond, who teaches the online course Business Analytics , one of the three courses that make up the Credential of Readiness (CORe) program . “Such insights can prove extremely valuable for analyzing historical trends and developing forecasts.”
Forecasting can enable you to make better decisions and formulate data-informed strategies. Here are several examples of predictive analytics in action to inspire you to use it at your organization.

5 Examples of Predictive Analytics in Action
1. finance: forecasting future cash flow.
Every business needs to keep periodic financial records, and predictive analytics can play a big role in forecasting your organization’s future health. Using historical data from previous financial statements, as well as data from the broader industry, you can project sales, revenue, and expenses to craft a picture of the future and make decisions.
HBS Professor V.G. Narayanan mentions the importance of forecasting in the course Financial Accounting , which is also part of CORe .
“Managers need to be looking ahead in order to plan for the future health of their business,” Narayanan says. “No matter the field in which you work, there is always a great amount of uncertainty involved in this process.”
2. Entertainment & Hospitality: Determining Staffing Needs
One example explored in Business Analytics is casino and hotel operator Caesars Entertainment’s use of predictive analytics to determine venue staffing needs at specific times.
In entertainment and hospitality, customer influx and outflux depend on various factors, all of which play into how many staff members a venue or hotel needs at a given time. Overstaffing costs money, and understaffing could result in a bad customer experience, overworked employees, and costly mistakes.
To predict the number of hotel check-ins on a given day, a team developed a multiple regression model that considered several factors. This model enabled Caesars to staff its hotels and casinos and avoid overstaffing to the best of its ability.
3. Marketing: Behavioral Targeting
In marketing, consumer data is abundant and leveraged to create content, advertisements, and strategies to better reach potential customers where they are. By examining historical behavioral data and using it to predict what will happen in the future, you engage in predictive analytics.
Predictive analytics can be applied in marketing to forecast sales trends at various times of the year and plan campaigns accordingly.
Additionally, historical behavioral data can help you predict a lead’s likelihood of moving down the funnel from awareness to purchase. For instance, you could use a single linear regression model to determine that the number of content offerings a lead engages with predicts—with a statistically significant level of certainty—their likelihood of converting to a customer down the line. With this knowledge, you can plan targeted ads at various points in the customer’s lifecycle.
Related: What Is Marketing Analytics?
4. Manufacturing: Preventing Malfunction
While the examples above use predictive analytics to take action based on likely scenarios, you can also use predictive analytics to prevent unwanted or harmful situations from occurring. For instance, in the manufacturing field, algorithms can be trained using historical data to accurately predict when a piece of machinery will likely malfunction.
When the criteria for an upcoming malfunction are met, the algorithm is triggered to alert an employee who can stop the machine and potentially save the company thousands, if not millions, of dollars in damaged product and repair costs. This analysis predicts malfunction scenarios in the moment rather than months or years in advance.
Some algorithms even recommend fixes and optimizations to avoid future malfunctions and improve efficiency, saving time, money, and effort. This is an example of prescriptive analytics; more often than not, one or more types of analytics are used in tandem to solve a problem.
5. Health Care: Early Detection of Allergic Reactions
Another example of using algorithms for rapid, predictive analytics for prevention comes from the health care industry . The Wyss Institute at Harvard University partnered with the KeepSmilin4Abbie Foundation to develop a wearable piece of technology that predicts an anaphylactic allergic reaction and automatically administers life-saving epinephrine.
The sensor, called AbbieSense, detects early physiological signs of anaphylaxis as predictors of an ensuing reaction—and it does so far quicker than a human can. When a reaction is predicted to occur, an algorithmic response is triggered. The algorithm can predict the reaction’s severity, alert the individual and caregivers, and automatically inject epinephrine when necessary. The technology’s ability to predict the reaction at a faster speed than manual detection could save lives.

Using Data to Strategize for the Future
No matter your industry, predictive analytics can provide the insights needed to make your next move. Whether you’re driving financial decisions, formulating marketing strategies, changing your course of action, or working to save lives, building a foundation in analytical skills can serve you well.
For hands-on practice and a deeper understanding of how you can put analytics to work for your organization, consider taking Business Analytics , one of three online courses that make up HBS Online’s CORe program .
Do you want to become a data-driven professional? Explore our eight-week Business Analytics course and our three-course Credential of Readiness (CORe) program to deepen your analytical skills and apply them to real-world business problems.

About the Author
Introduction to Predictive Analytics
- First Online: 01 January 2022
Cite this chapter
- Richard V. McCarthy 4 ,
- Mary M. McCarthy 5 &
- Wendy Ceccucci 4
2277 Accesses
There are few technologies that have the ability to revolutionize how business operates. Predictive analytics is one of those technologies. Predictive analytics consists primarily of the “Big 3” techniques: regression analysis, decision trees, and neural networks. Although several other techniques, such as random forests and ensemble models, have become increasingly popular in their use, predictive analytics focuses on building and evaluating predictive models resulting in key output fit statistics that are used to solve business problems. This chapter defines essential analytics terminology, walks through the nine-step process for building predictive analytics models, introduces the “Big 3” techniques, and discusses careers within business analytics.
This is a preview of subscription content, log in via an institution to check access.
Access this chapter
- Available as EPUB and PDF
- Read on any device
- Instant download
- Own it forever
- Compact, lightweight edition
- Dispatched in 3 to 5 business days
- Free shipping worldwide - see info
- Durable hardcover edition
Tax calculation will be finalised at checkout
Purchases are for personal use only
Institutional subscriptions
Bacchner J (2013) Predictive policing preventing crimes with data and analytics. White paper from the IBM Center for the business of government. Available at http://businessofgovernment.org/sites/default/files/Predictive%20Policing.pdf . Accessed 15 June 2021
Baesens B, Van Vlasselaer V, Verbeke W (2015) Fraud analytics: using descriptive, predictive, and social network techniques: a guide to data science for fraud detection. Wiley & Sons, Hoboken
Book Google Scholar
Carr D (2013) Giving viewers what they want. http://www.nytimes.com/2013/02/25/business/media/for-house-of-cards-using-big-data-to-guarantee-its-popularity.html . Accessed 15 June 2021
Earley C (2015) Data analytics in auditing: opportunities and challenges. Bus Horiz 58:493–500
Article Google Scholar
Gartner (n.d.-a). https://www.gartner.com/it-glossary/business-analytics/ . Accessed 15 June 2021
Gartner (n.d.-b). https://www.gartner.com/it-glossary/big-data . Accessed 15 June 2021
Goldsmith S (2016) The power of data and predictive analytics. Government Technology. http://www.govtech.com/data/The-Power-of-Data-and-Predictive-Analytics.html . Accessed 15 June 2021
Hays C (2004) What Wal-Mart knows about customers’ habits. New York Times. Nov 14, 2004. https://www.nytimes.com/2004/11/14/business/yourmoney/what-walmart-knows-about-customers-habits.html . Accessed 15 June 2021
Houser K, Sanders D (2017) Vanderbilt. Journal of Entertainment and Technology Law, 19(4). Available via http://www.jetlaw.org/wp-content/uploads/2017/04/Houser-Sanders_Final.pdf . Accessed 15 June 2021
IBM (n.d.) What is big data analytics? https://www.ibm.com/analytics/hadoop/big-data-analytics . Accessed 15 June 2021
Insurance Information Institute (2018) Background On: Insurance Fraud. https://www.iii.org/article/background-on-insurance-fraud . Accessed Jan 1, 2018
Kent J (2020) 3 ways healthcare is using predictive analytics to combat COVID-19, Health IT Analytics. https://healthitanalytics.com/news/3-ways-healthcare-is-using-predictive-analytics-to-combat-covid-19 . Accessed 15 June 2021
Komorowski M (2014) A history of history of storage cost (update). http://www.mkomo.com/cost-per-gigabyte-update . Accessed 9 Sept 2018
Kopalle P (2014) Why Amazon’s anticipatory shipping is pure genius. Forbes online January 28, 2014. https://www.forbes.com/sites/onmarketing/2014/01/28/why-amazons-anticipatory-shipping-is-pure-genius/#3b3f13704605 . Accessed 9 Sept 2018
Marr B (2015) How big data is changing the insurance industry forever. Forbes online December 16, 2015. Retrieved at https://www.forbes.com/sites/bernardmarr/2015/12/16/how-big-data-is-changing-the-insurance-industry-forever/#28c1225d289b
Marr B (2017) How Big Data helps to tackle the number 1 cause of accidental death in the U.S. Forbes online January 17, 2017 https://www.forbes.com/sites/bernardmarr/2017/01/16/how-big-data-helps-to-tackle-the-no-1-cause-of-accidental-death-in-the-u-s/#76e20ad639ca . Accessed 9 Sept 2018
McAfee A, Brynjolfsson (2012) Big data: the management revolution. Harvard Business Review. October 2012
Google Scholar
McKinsey Global Institute (2011) Big data: the next frontier for innovation, competition, and productivity. https://www.mckinsey.com/business-functions/digital-mckinsey/our-insights/big-data-the-next-frontier-for-innovation . Accessed 9 Sept 2018
O*Net (2018). https://www.onetonline.org/link/summary/15-1199.08?redir=15-1099 . Accessed 9 Sept 2018
Power B (2017) How Harley-Davidson used artificial intelligence to increase New York sales leads by 2,930%. Harvard Business Review. May 30, 2017. https://hbr.org/2017/05/how-harley-davidson-used-predictive-analytics-to-increase-new-york-sales-leads-by-2930 . Accessed 9 Sept 2018
Price-Waterhouse Coopers & Business-Higher Education Forum (2017) Investing in Americas data science and analytics talent. Retrieved at pwc.com/us/dsa-skills . Accessed 15 June 2021
Reuters (2018) Six-year-old Youtube star brings his own toyline to Walmart. https://www.reuters.com/article/us-usa-toys/six-year-old-youtube-star-brings-his-own-toy-line-to-walmart-idUSKBN1KK1IQ . Accessed 9 Sept 2018
Richardson J, Schlegel K, Sallam R, Kronz A, Sun J (2021) Magic quadrant for analytics and business intelligence platforms, Gartner Group. Retrieved from: https://www.gartner.com/doc/reprints?id=1-1YOXON7Q&ct=200330&st=sb
Samuels M (2017) Big data case study: how UPS is using analytics to improve performance. ZDNet.com https://www.zdnet.com/article/big-data-case-study-how-ups-is-using-analytics-to-improve-performance/ . Accessed 9 Sept 2018
Saporito P (2015) Applied insurance analytics. Pearson Education, Upper Saddle River
Syvaniemi A (2015) Predictive analytics change internal audit practices. Blog newsletter. Retrieved at http://blog.houston-analytics.com/blog/predictive-analytics-change-internal-audit-practices
Troester M (n.d.) Big Data meets data analytics. SAS White Paper https://slidelegend.com/big-data-meets-big-data-analytics-sas_5a09b8481723ddee444f4116.html . Accessed 9 Sept 2018
van Rijmenjam M (2018) Why UPS spends $1 billion on data annually. https://datafloq.com/read/ups-spends-1.billion-big-dataannually/273 . Accessed 9 Sept 2018
Walmart Staff (2017) 5 ways Walmart uses Big Data to help customers. https://blog.walmart.com/innovation/20170807/5-ways-walmart-uses-big-data-to-help-customers . Accessed 9 Sept 2018
Download references
Author information
Authors and affiliations.
Quinnipiac University, Hamden, CT, USA
Richard V. McCarthy & Wendy Ceccucci
Central Connecticut State University, New Britain, CT, USA
Mary M. McCarthy
You can also search for this author in PubMed Google Scholar
Rights and permissions
Reprints and permissions
Copyright information
© 2022 The Author(s), under exclusive license to Springer Nature Switzerland AG
About this chapter
McCarthy, R.V., McCarthy, M.M., Ceccucci, W. (2022). Introduction to Predictive Analytics. In: Applying Predictive Analytics. Springer, Cham. https://doi.org/10.1007/978-3-030-83070-0_1
Download citation
DOI : https://doi.org/10.1007/978-3-030-83070-0_1
Published : 01 January 2022
Publisher Name : Springer, Cham
Print ISBN : 978-3-030-83069-4
Online ISBN : 978-3-030-83070-0
eBook Packages : Engineering Engineering (R0)
Share this chapter
Anyone you share the following link with will be able to read this content:
Sorry, a shareable link is not currently available for this article.
Provided by the Springer Nature SharedIt content-sharing initiative
- Publish with us
Policies and ethics
- Find a journal
- Track your research

An official website of the United States government
The .gov means it’s official. Federal government websites often end in .gov or .mil. Before sharing sensitive information, make sure you’re on a federal government site.
The site is secure. The https:// ensures that you are connecting to the official website and that any information you provide is encrypted and transmitted securely.
- Publications
- Account settings
Preview improvements coming to the PMC website in October 2024. Learn More or Try it out now .
- Advanced Search
- Journal List
- J Am Med Inform Assoc
- v.26(12); 2019 Dec
Predictive analytics in health care: how can we know it works?
Ben van calster.
1 Department of Development and Regeneration, KU Leuven, Leuven, Belgium
2 Department of Biomedical Data Sciences, Leiden University Medical Center (LUMC), Leiden, The Netherlands
Laure Wynants
Dirk timmerman.
3 Department of Obstetrics and Gynaecology, University Hospitals Leuven, Leuven, Belgium
Ewout W Steyerberg
Gary s collins.
4 Centre for Statistics in Medicine, Nuffield, Department of Orthopaedics, Rheumatology and Musculoskeletal Sciences, University of Oxford, UK
5 Oxford University Hospitals NHS Foundation Trust, Oxford, UK
Associated Data
There is increasing awareness that the methodology and findings of research should be transparent. This includes studies using artificial intelligence to develop predictive algorithms that make individualized diagnostic or prognostic risk predictions. We argue that it is paramount to make the algorithm behind any prediction publicly available. This allows independent external validation, assessment of performance heterogeneity across settings and over time, and algorithm refinement or updating. Online calculators and apps may aid uptake if accompanied with sufficient information. For algorithms based on “black box” machine learning methods, software for algorithm implementation is a must. Hiding algorithms for commercial exploitation is unethical, because there is no possibility to assess whether algorithms work as advertised or to monitor when and how algorithms are updated. Journals and funders should demand maximal transparency for publications on predictive algorithms, and clinical guidelines should only recommend publicly available algorithms.
The current interest in predictive analytics for improving health care is reflected by a surge in long-term investment in developing new technologies using artificial intelligence and machine learning to forecast future events (possibly in real time) to improve the health of individuals. Predictive algorithms or clinical prediction models, as they have historically been called, help identify individuals at increased likelihood of disease for diagnosis and prognosis (see Supplementary Material Table S1 for a glossary of terms used in this manuscript). 1 In an era of personalized medicine, predictive algorithms are used to make clinical management decisions based on individual patient characteristics (rather than on population averages) and to counsel patients. The rate at which new algorithms are published shows no sign of abating, particularly with the increasing availability of Big Data, medical imaging, routinely collected electronic health records, and national registry data. 2–4 The scientific community is making efforts to improve data sharing, increase study registration beyond clinical trials, and make reporting transparent and comprehensive with full disclosure of study results. 5 , 6 We discuss the importance of transparency in the context of medical predictive analytics.
ALGORITHM PERFORMANCE IS NOT GUARANTEED: FULLY INDEPENDENT EXTERNAL VALIDATION IS KEY
Before recommending a predictive algorithm for clinical practice, it is important to know whether and for whom it works well. First, predictions should discriminate between individuals with and without the disease (ie, higher predictions in those with the disease compared to those without the disease). Risk predictions should be also accurate (often referred to as calibrated). 7 Algorithm development may suffer from overfitting, which usually results in poorer discrimination and calibration when evaluated on new data. 8 Although the clinical literature tends to focus on discrimination, calibration is clearly crucial. Inaccurate risk predictions can lead to inappropriate decisions or expectations, even when discrimination is good. 7 Calibration has therefore been labeled the Achilles heel of prediction. 2
In addition, there is often substantial heterogeneity between populations, as well as changes in populations over time. 9 , 10 For example, there may be differences between patients in academic hospitals compared with patients at regional hospitals, ethnicities, or past versus contemporary patients due to advances in patient care. 11–13 Recent work indicated that the half-life of clinical data relevance can be remarkably short. 14 , 15 Hence, algorithms are likely to perform differently across centers, settings, and time. On top of overfitting and heterogeneity between populations, operational heterogeneity can affect algorithm performance. Different hospitals may, for example, use different EHR software, imaging machines, or marker kits. 2 , 10 , 16 As a result, the clinical utility of predictive algorithms for decision-making may vary greatly. It is well established that “internal validation” of performance using, for example, a train–test split of available data is insufficient. Rather, algorithms should undergo “external validation” on a different data set. 17 , 18 Notably, algorithms developed using traditional study designs may not validate well when applied on electronic health record data. 4 , 19 It is important to stress 3 issues. First, external validation should be extensive: it should take place at various sites in contemporary cohorts of patients from the targeted population. Second, performance should be monitored over time. 11 Third, external validation by independent investigators is imperative. 20 It is a good evolution to include an external validation as part of the algorithm development study, 18 but one can imagine that algorithms with poor performance on a different data set may be less likely to get published in the first place. If performance in a specific setting is poor, an algorithm can be updated—specifically, its calibration. 1 , 7 To counter temporal changes in populations, continual updating strategies may help. 1 For example, QRISK2 models ( www.qrisk.org ) are updated regularly as new data are continually being collected.
POTENTIAL HURDLES FOR MAKING PREDICTIVE ALGORITHMS PUBLICLY AVAILABLE
To allow others to independently evaluate the predictive accuracy, it is important to describe in full detail how the algorithm was developed. 21 Algorithms should be available in a format that can readily be implemented by others. Not adhering to these principles severely limits the usefulness of the findings—surely a research waste. 22 An analogous situation would be an article describing the findings from a randomized clinical trial without actually reporting the intervention effect or how to implement the intervention.
Transparent and full reporting
The Transparent Reporting of a multivariable prediction model for Individual Prognosis Or Diagnosis (TRIPOD) statement, a reporting guideline for studies on predictive algorithms, recommends that the equation behind an algorithm is presented in the publication describing its development. 21 More explicitly, the mathematical formula of an algorithm should be available in full. This includes details such as which predictors are included, how they are coded (including ranges of any continuous predictors, units of measurement), and the values of the regression coefficients. Publications presenting new algorithms often fail to include key information such as specification of the baseline risk (namely, the intercept in logistic regression models for binary outcomes; the baseline hazard at 1 or more clinically relevant time points for time-to-event regression models). 23 Without this information, making predictions is not possible. Below, we expand on modern artificial intelligence methods that do not produce straightforward mathematical equations.
Online calculators and mobile apps
It has become customary to implement algorithms as online calculators or mobile apps. Then, we depend on the researchers’ openness to provide clear and honest information about algorithm development and results of validation studies, with references to relevant publications. For example, FRAX predicts the 10-year probability of hip fracture and major osteoporotic fracture ( www.sheffield.ac.uk/FRAX/ ). FRAX is a collection of algorithms (eg, 68 country-specific equations), which are both freely available via a website interface or commercially available via a desktop application. However, none of these algorithms has been published in full. The release notes indicate that the algorithms are continually revised, but do not offer detailed information. This lack of full disclosure prohibits independent evaluation. 24 In theory, we can try “reverse engineering” by reconstructing the equation based on risk estimates for a sample of patients (see Supplementary Material ). However, such reverse engineering is not a realistic solution. The solution is to avoid hidden algorithms.
Online or mobile calculators allow the inclusion of algorithms into daily clinical routine, which is a positive evolution. However, it is impractical for large-scale independent validation studies, because information for every single patient has to be entered manually.
Machine learning algorithms
Machine learning methods, such as random forests or deep learning, are becoming increasingly popular to develop predictive algorithms. 3 , 25 The architecture of these algorithms is often too complex to fully disentangle and report the relation between a set of predictors and the outcome (“black box”). This is the commonly addressed problem when discussing transparency of predictive analytics based on machine learning. 26 We argue that algorithm availability is at least as important. A similar problem can affect regression-based algorithms that use complex spline functions to model continuous predictors. Software implementations are therefore imperative for validation purposes, in particular, because these algorithms have a higher risk of overfitting and instable performance. 8 , 17 Machine learning algorithms can be stored in computer files that may be transferred to other computers to allow validation studies. Recently, initiatives in this direction are being set up. 27 , 28
Proprietary algorithms
Developers may choose not to disclose an algorithm, and to offer the algorithm on a fee-for-service basis. 16 For example, a biomarker-based algorithm to diagnose ovarian cancer has a cost of $897 per patient ( http://vermillion.com/2436-2/ ). Assume we want to validate this algorithm in a center that has 20% malignancies in the target population. If we want to recruit at least 100 patients in each outcome group, following current recommendations for validation studies, the study needs at least 500 patients. 7 This implies a minimum cost of $448 500 in order to obtain useful information about whether this algorithm works in this particular center. It is important to emphasize this is just the cost required to judge whether the algorithm has any validity in this setting; there is no guarantee that it will be clinically useful.
Many predictive algorithms have been developed using financial support from public institutions. Then we believe that the results belong to the community and should be fully and publicly available. If this is the case, asking a small installation fee for an attractive and user-friendly calculator is defendable to cover software development and generate resources for maintenance and improvements. Such implementations facilitate uptake and inclusion into daily workflow.
Private companies may invest in the development of an algorithm that uses predictors for which the company offers measurement tools (eg, kits, biomarkers). In these instances, the return on investment should focus on the measurement tools, not on selling the algorithm. We argue that it is ethically unacceptable to have a business model that focuses on selling an algorithm. 29 However, such business models may facilitate Food and Drug Administration (FDA) approval or Conformité Européenne (CE) marking of predictive algorithms (eg, https://www.hcanews.com/news/predictive-patient-surveillance-system-receives-fda-clearance ). It is important to realize that regulatory approval does not imply clinical validity or usefulness of a predictive algorithm in a specific clinical setting. 30
THE IMPORTANCE OF ALGORITHM METADATA IN ORDER TO MAKE ALGORITHMS WORK
Although making algorithms fully and publicly available is imperative, the context of the algorithm is equally important. This extends the abovementioned issue of full and transparent reporting according to the TRIPOD guidelines. Reporting should provide full details of algorithm development practices. This includes—but is not limited to—the source of study data (e.g., retrospective EHR, randomized controlled trial data, or prospectively collected cohort data), the number and type of participating centers, the patient recruitment period, inclusion and exclusion criteria, clear definitions of predictors and the outcome, details on how variables were measured, detailed information on missing values and how these were handled, and a full account of the modeling strategy (eg, predictor selection, handling of continuous variables, hyperparameter tuning). Unfortunately, studies reveal time and again that such metadata are poorly reported. 21 , 31 Even when authors develop an algorithm using sensible procedures (eg ,with low risk of overfitting), poor reporting will lead to poor understanding of the context, which may contribute to decreased performance on external validation. Initiatives such as the Observational Health Data Sciences and Informatics (OHDSI; http://ohdsi.org ) focus on such contextual differences and aim to standardize procedures (eg, in terms of terminology, data formats, and definitions of variables) in order to lead to better and more applicable predictive algorithms. 27 , 32 In addition, when an algorithm is made available electronically, we recommend it include an indication of the extent to which the algorithm has been validated.
Predictive algorithms should be fully and publicly available to facilitate independent external validation across various settings ( Table 1 ). For complex algorithms, alternative and innovative solutions are needed; a calculator is a minimal requirement, but downloadable software to batch process multiple records is more efficient. We believe that selling predictions from an undisclosed algorithm is unethical. This article does not touch on legal consequences of using predictive algorithms, where issues such as algorithm availability or black-box predictions cannot be easily ignored. 33 When journals consider manuscripts introducing a predictive algorithm, its availability should be a minimum requirement before acceptance. Clinical guideline documents should focus on publicly available algorithms that have been independently validated.
Summary of arguments in favor of making predictive algorithms fully available, hurdles for doing so, and reasons why developers choose to hide and sell algorithms
SUPPLEMENTARY MATERIAL
Supplementary material is available at Journal of the American Medical Informatics Association online.
This work was funded by Research Foundation – Flanders (grant G0B4716N), Internal Funds KU Leuven (grant C24/15/037). The funders had no role in study design, data collection and analysis, decision to publish, or preparation of the manuscript.
CONTRIBUTIONS
Conception: BVC, LW, DT, EWS, GSC. Writing—original draft preparation: BVC. Writing—review and editing: BVC, LW, DT, EWS, GSC. All authors approved the submitted version and agreed to be accountable.
Conflict of interest statement
LW is a postdoctoral fellow of the Research Foundation – Flanders. GSC was supported by the NIHR Biomedical Research Centre, Oxford.
Supplementary Material
Ocz130_supplementary_data.
- Search Search Please fill out this field.
- Behavioral Economics
Predictive Analytics: Definition, Model Types, and Uses
:max_bytes(150000):strip_icc():format(webp)/Clay_Halton_BW_web_ready-13-69177d5045e84abd939938c80735cd05.jpg)
Erika Rasure is globally-recognized as a leading consumer economics subject matter expert, researcher, and educator. She is a financial therapist and transformational coach, with a special interest in helping women learn how to invest.
:max_bytes(150000):strip_icc():format(webp)/CSP_ER9-ErikaR.-dce5c7e19ef04426804e6b611fb1b1b4.jpg)
Investopedia / Julie Bang
What Is Predictive Analytics?
Predictive analytics is the use of statistics and modeling techniques to forecast future outcomes. Current and historical data patterns are examined and plotted to determine the likelihood that those patterns will repeat.
Businesses use predictive analytics to fine-tune their operations and decide whether new products are worth the investment. Investors use predictive analytics to decide where to put their money. Internet retailers use predictive analytics to fine-tune purchase recommendations to their users and increase sales.
Key Takeaways
- Industries from insurance to marketing use predictive techniques to make important decisions.
- Predictive models help make weather forecasts, develop video games, translate voice-to-text messages, make customer service decisions, and develop investment portfolios.
- Predictive analytics determines a likely outcome based on an examination of current and historical data.
- Decision trees, regression, and neural networks all are types of predictive models.
- People often confuse predictive analytics with machine learning even though the two are different disciplines.
Understanding Predictive Analytics
Predictive analytics looks for past patterns to measure the likelihood that those patterns will reoccur. It draws on a series of techniques to make these determinations, including artificial intelligence (AI), data mining , machine learning, modeling, and statistics. For instance, data mining involves the analysis of large sets of data to detect patterns from it. Text analysis does the same using large blocks of text.
Predictive models are used for many applications, including weather forecasts, creating video games, translating voice to text, customer service, and investment portfolio strategies. All of these applications use descriptive statistical models of existing data to make predictions about future data.
Predictive analytics helps businesses manage inventory, develop marketing strategies , and forecast sales . It also helps businesses survive, especially in highly competitive industries such as health care and retail. Investors and financial professionals draw on this technology to help craft investment portfolios and reduce their overall risk potential.
These models determine relationships, patterns, and structures in data that are used to draw conclusions as to how changes in the underlying processes that generate the data will change the results. Predictive models build on these descriptive models and look at past data to determine the likelihood of certain future outcomes, given current conditions or a set of expected future conditions.
Uses of Predictive Analytics
Predictive analytics is a decision-making tool in many industries. Following are some examples.
Manufacturing
Forecasting is essential in manufacturing to optimize the use of resources in a supply chain . Critical spokes of the supply chain wheel, whether it is inventory management or the shop floor, require accurate forecasts for functioning.
Predictive modeling is often used to clean and optimize the quality of data used for such forecasts. Modeling ensures that more data can be ingested by the system, including from customer-facing operations, to ensure a more accurate forecast.
Credit scoring makes extensive use of predictive analytics. When a consumer or business applies for credit, data on the applicant's credit history and the credit record of borrowers with similar characteristics are used to predict the risk that the applicant might fail to repay any new credit that is approved.
Underwriting
Data and predictive analytics play an important role in underwriting. Insurance companies examine applications for new policies to determine the likelihood of having to pay out for a future claim . The analysis is based on the current risk pool of similar policyholders as well as past events that have resulted in payouts.
Predictive models that consider characteristics in comparison to data about past policyholders and claims are routinely used by actuaries .
Marketing professionals planning a new campagn look at how consumers have reacted to the overall economy. They can use these shifts in demographics to determine if the current mix of products will entice consumers to make a purchase.
Stock Traders
Active traders look at a variety of historical metrics when deciding whether to buy a particular stock or other asset.
Moving averages, bands, and breakpoints all are based on historical data and are used to forecast future price movements.
Fraud Detection
Financial services use predictive analytics to examine transactions for irregular trends and patterns. The irregularities pinpointed can then be examined as potential signs of fraudulent activity.
This may be done by analyzing activity between bank accounts or analyzing when certain transactions occur.
Supply Chain
Supply chain analytics is used to manage inventory levels and set pricing strategies. Supply chain predictive analytics use historical data and statistical models to forecast future supply chain performance, demand, and potential disruptions.
This helps businesses proactively identify and address risks, optimize resources and processes, and improve decision-making. Companies can forecast what materials should be on hand at any given moment and whether there will be any shortages.
Human Resources
Human resources uses predictive analytics to improve various processes such as identifying future workforce skill requirements or identifying factors that contribute to high staff turnover.
Predictive analytics can also analyze an employee's performance, skills, and preferences to predict their career progression and help with career development.
Predictive Analytics vs. Machine Learning
A common misconception is that predictive analytics and machine learning are the same. Predictive analytics help us understand possible future occurrences by analyzing the past. At its core, predictive analytics includes a series of statistical techniques (including machine learning, predictive modeling, and data mining) and uses statistics (both historical and current) to estimate, or predict, future outcomes.
Thus, machine learning is a tool used in predictive analysis.
Machine learning is a subfield of computer science that means "the programming of a digital computer to behave in a way which, if done by human beings or animals, would be described as involving the process of learning." That's a 1959 definition by Arthur Samuel, a pioneer in computer gaming and artificial intelligence.
The most common predictive models include decision trees, regressions (linear and logistic), and neural networks, which is the emerging field of deep learning methods and technologies.
Types of Predictive Analytical Models
There are three common techniques used in predictive analytics: Decision trees, neural networks, and regression.
Decision Trees
If you want to understand what leads to someone's decisions, you may find it useful to build a decision tree .
This type of model places data into different sections based on certain variables, such as price or market capitalization . Just as the name implies, it looks like a tree with individual branches and leaves. Branches indicate the choices available while individual leaves represent a particular decision.
Decision trees are easy to understand and dissect. They're useful when you need to make a decision quickly.
This is the model that is used the most in statistical analysis. Use it when you want to decipher patterns in large sets of data and when there's a linear relationship between the inputs.
This method works by figuring out a formula, which represents the relationship between all the inputs found in the dataset.
For example, you can use regression to figure out how price and other key factors can shape the performance of a stock .
Neural Networks
Neural networks were developed as a form of predictive analytics by imitating the way the human brain works. This model can deal with complex data relationships using artificial intelligence and pattern recognition.
Use this method if you have any of several hurdles that you need to overcome. For example, you may have too much data on hand, or don't have the formula you need to find a relationship between the inputs and outputs in your dataset, or need to make predictions rather than come up with explanations.
If you've already used decision trees and regression as models, you can confirm your findings with neural networks.
Cluster Models
Clustering is a method of aggregating data that share similar attributes. For example, Amazon.com can cluster sales based on the quantity purchased, or on the average account age of its consumers.
separating data into similar groups based on shared features, analysts may be able to identify other characteristics that define future activity.
Time Series Modeling
In some cases, data relates to time, and specific predictive analytics rely on the relationship between what happens when. These types of models assess inputs at specific frequencies such as daily, weekly, or monthly iterations.
Then, analytical models can seek seasonality, trends, or behavioral patterns based on timing.
This type of predictive model is useful to predict when peak customer service periods are needed or when specific sales can be expected to jump.
How Businesses Can Use Predictive Analytics
As noted above, predictive analysis can be used in a number of different applications. Businesses can capitalize on models to help advance their interests and improve their operations. Predictive models are frequently used by businesses to help improve customer service and outreach.
Executives and business owners can take advantage of this kind of statistical analysis to determine customer behavior. For instance, the owner of a business can use predictive techniques to identify and target regular customers who might otherwise defect to a competitor.
Predictive analytics plays a key role in advertising and marketing . Companies can use models to determine which customers are likely to respond positively to marketing and sales campaigns. Business owners can save money by targeting customers who will respond positively rather than doing blanket campaigns.
Benefits of Predictive Analytics
As mentioned above, predictive analytics can help anticipate outcomes when there are no obvious answers available.
Investors, financial professionals, and business leaders use models to help reduce risk. For instance, an investor or an advisor can use models to help craft an investment portfolio with an appropriate level of risk, considering factors such as age, family responsibilities, and goals.
Businesses use them to keep their costs down. They can determine the likelihood of success or failure of a product before it is developed. Or they can set aside capital for production improvements before the manufacturing process begins.
Criticism of Predictive Analytics
The use of predictive analytics has been criticized and, in some cases, legally restricted due to perceived inequities in its outcomes. Most commonly, this involves predictive models that result in statistical discrimination against racial or ethnic groups in areas such as credit scoring, home lending, employment, or risk of criminal behavior.
A famous example of this is the now illegal practice of redlining in home lending by banks. Regardless of the accuracy of the predictions, their use is discouraged as they perpetuate discriminatory lending practices and contribute to the decline of redlined neighborhoods.
How Does Netflix Use Predictive Analytics?
Data collection is important to a company like Netflix. It collects data from its customers based on their behavior and past viewing patterns. It uses that information to make recommendations based on their preferences.
This is the basis of the "Because you watched..." lists you'll find on the site. Other sites, notably Amazon, use their data for "Others who bought this also bought..." lists.
What Are the 3 Pillars of Data Analytics?
The three pillars of data analytics are the needs of the entity that is using the model, the data and technology used to study it, and the actions and insights that result from the analysis.
What Is Predictive Analytics Good For?
Predictive analytics is good for forecasting, risk management, customer behavior analytics, fraud detection, and operational optimization. Predictive analytics can help organizations improve decision-making, optimize processes, and increase efficiency and profitability. This branch of analytics is used to leverage data to forecast what may happen in the future.
What Is the Best Model for Predictive Analytics?
The best model for predictive analytics depends on several factors, such as the type of data, the objective of the analysis, the complexity of the problem, and the desired accuracy of the results. The best model to choose from may range from linear regression, neural networks, clustering, or decision trees.
The Bottom Line
The goal of predictive analytics is to make predictions about future events, then use those predictions to improve decision-making. Predictive analytics is used in a variety of industries including finance, healthcare, marketing, and retail. Different methods are used in predictive analytics such as regression analysis, decision trees, or neural networks.
Predictive Analytics Today. " WHAT IS PREDICTIVE ANALYSIS? "
IBM. " Predictive analytics ."
Global Newswire. " Trends in Predictive Analytics Market Size & Share will Reach $10.95 Billion by 2022 ."
PWC. " Big data: innovation in investing ."
Samule, Arthur. " Some Studies in Machine Learning Using the Game of Checkers. " IBM Journal of Research and Development, vol. 3, no. 3, July 1959, pp. 210-229.
SAS. " Predictive Analysis ."
Logi Analytics. " What Is Predictive Analysis? "
Utreee. What is Predictive Analytics, its Benefits and Challenges? "
:max_bytes(150000):strip_icc():format(webp)/autoregressive-integrated-moving-average-arima.asp-Final-cda3fcc744084e08808bc96d87b1f496.png)
- Terms of Service
- Editorial Policy
- Privacy Policy
- Your Privacy Choices
- Español – América Latina
- Português – Brasil
What is predictive analytics?
Predictive analytics is an advanced form of data analytics that attempts to answer the question, “What might happen next?” As a branch of data science for business, the growth of predictive and augmented analytics coincides with that of big data systems, where larger, broader pools of data enable increased data mining activities to provide predictive insights. Advancements in big data machine learning have also helped expand predictive analytics capabilities.
The growth of predictive and augmented analytics coincides with that of big data systems, where broader pools of data enable increased data mining activities to provide predictive insights. Advancements in big data machine learning have also helped expand predictive analytics capabilities.
Learn how Google Cloud data analytics , machine learning, and artificial intelligence solutions can help your business run smoother and faster with predictive analytics.
Predictive analytics defined
Predictive analytics is the process of using data to forecast future outcomes. The process uses data analysis, machine learning, artificial intelligence, and statistical models to find patterns that might predict future behavior. Organizations can use historic and current data to forecast trends and behaviors seconds, days, or years into the future with a great deal of precision.
How does predictive analytics work?
Data scientists use predictive models to identify correlations between different elements in selected datasets. once data collection is complete, a statistical model is formulated, trained, and modified to generate predictions..
The workflow for building predictive analytics frameworks follows five basic steps:
- Define the problem : A prediction starts with a good thesis and set of requirements. For instance, can a predictive analytics model detect fraud? Determine optimal inventory levels for the holiday shopping season? Identify potential flood levels from severe weather? A distinct problem to solve will help determine what method of predictive analytics should be used.
- Acquire and organize data : An organization may have decades of data to draw upon, or a continual flood of data from customer interactions. Before predictive analytics models can be developed, data flows must be identified, and then datasets can be organized in a repository such as a data warehouse like BigQuery .
- Pre-process data : Raw data is only nominally useful by itself. To prepare the data for the predictive analytics models, it should be cleaned to remove anomalies, missing data points, or extreme outliers, any of which might be the result of input or measurement errors.
- Develop predictive models : Data scientists have a variety of tools and techniques to develop predictive models depending on the problem to be solved and nature of the dataset. Machine learning, regression models, and decision trees are some of the most common types of predictive models.
- Validate and deploy results : Check on the accuracy of the model and adjust accordingly. Once acceptable results have been achieved, make them available to stakeholders via an app, website, or data dashboard.
What are predictive analytics techniques?
In general, there are two types of predictive analytics models: classification and regression models. Classification models attempt to put data objects (such as customers or potential outcomes) into one category or another. For instance, if a retailer has a lot of data on different types of customers, they may try to predict what types of customers will be receptive to market emails. Regression models try to predict continuous data, such as how much revenue that customer will generate during their relationship with the company.
Predictive analytics tends to be performed with three main types of techniques:
Regression analysis
Regression is a statistical analysis technique that estimates relationships between variables. Regression is useful to determine patterns in large datasets to determine the correlation between inputs. It is best employed on continuous data that follows a known distribution. Regression is often used to determine how one or more independent variables affects another, such as how a price increase will affect the sale of a product.
Decision trees
Decision trees are classification models that place data into different categories based on distinct variables. The method is best used when trying to understand an individual's decisions. The model looks like a tree, with each branch representing a potential choice, with the leaf of the branch representing the result of the decision. Decision trees are typically easy to understand and work well when a dataset has several missing variables.
Neural networks
Neural networks are machine learning methods that are useful in predictive analytics when modeling very complex relationships. Essentially, they are powerhouse pattern recognition engines. Neural networks are best used to determine nonlinear relationships in datasets, especially when no known mathematical formula exists to analyze the data. Neural networks can be used to validate the results of decision trees and regression models.
Solve your business challenges with Google Cloud
Uses and examples of predictive analytics, fraud detection.
Predictive analytics examines all actions on a company’s network in real time to pinpoint abnormalities that indicate fraud and other vulnerabilities.
Conversion and purchase prediction
Companies can take actions, like retargeting online ads to visitors, with data that predicts a greater likelihood of conversion and purchase intent.
Risk reduction
Credit scores, insurance claims, and debt collections all use predictive analytics to assess and determine the likelihood of future defaults.
Operational improvement
Companies use predictive analytics models to forecast inventory, manage resources, and operate more efficiently.
Customer segmentation
By dividing a customer base into specific groups, marketers can use predictive analytics to make forward-looking decisions to tailor content to unique audiences.
Maintenance forecasting
Organizations use data to predict when routine equipment maintenance will be required and can then schedule it before a problem or malfunction arises.
Related products and services
Take the next step
Start building on Google Cloud with $300 in free credits and 20+ always free products.
Start your next project, explore interactive tutorials, and manage your account.
- Need help getting started? Contact sales
- Work with a trusted partner Find a partner
- Continue browsing See all products
- Get tips & best practices See tutorials
- Privacy Policy

Home » Predictive Analytics – Techniques, Tools and Examples
Predictive Analytics – Techniques, Tools and Examples
Table of Contents

Predictive Analytics
Definition:
Predictive analytics is the practice of extracting information from existing data sets in order to predict future probabilities and trends. The goal is to go beyond what has happened and provide a best assessment on what will happen in the future. This is accomplished through various statistical and machine learning techniques.
Predictive Analytics Methodology
Predictive analytics methodology involves a series of steps from defining the problem to model deployment and evaluation. The specific steps can vary somewhat depending on the context, but a standard methodology might look something like this:
- Problem Definition: Before you can start analyzing data, you need to clearly define the problem you are trying to solve. This could be predicting customer churn, forecasting sales, detecting fraud, or any number of other predictive tasks.
- Data Gathering: The next step is to gather the necessary data. This could involve aggregating data from different sources, such as databases, files, and external data sources. The data should be relevant to the problem you are trying to solve.
- Data Preparation: Once you have the data, you need to clean it and prepare it for analysis. This could involve dealing with missing data, removing outliers, transforming variables, and other data preparation tasks.
- Exploratory Data Analysis (EDA): This is the process of exploring the data, checking assumptions, and getting a feel for the data. This might involve statistical summaries, data visualization, or other techniques for understanding the data.
- Feature Engineering and Selection: Features are the variables or attributes that the model will use to make predictions. Feature engineering involves creating new features from existing ones, while feature selection involves choosing the most relevant features for your model.
- Modeling: This involves selecting a suitable predictive model, training the model on your data, and tuning the model’s parameters to improve its predictive performance. This could involve a variety of machine learning techniques such as regression, decision trees, random forests, neural networks, etc.
- Model Validation: Once the model has been trained, it needs to be validated. This typically involves dividing your data into a training set and a test set. The model is trained on the training set, and then its predictions are compared to the actual values in the test set to measure its performance.
- Model Deployment: Once the model has been validated and you are satisfied with its performance, it can be deployed. This involves integrating the model into your existing systems so that it can start making predictions on new data.
- Model Monitoring and Updating: Even after a model has been deployed, it should be monitored to ensure that it continues to perform well as new data comes in. If the model’s performance starts to decline, it may need to be updated or replaced.
Predictive Analytics Techniques
Predictive analytics techniques can be divided into several categories, including statistical techniques, machine learning techniques, and artificial intelligence techniques.
Here are some of the most commonly used techniques:
Statistical Techniques
These techniques involve the application of statistical methods to interpret and analyze data. Some examples are:
- Regression Analysis : This technique predicts a dependent variable based on independent variables. For example, predicting sales based on advertising spend.
- Time Series Analysis: This method analyzes data that’s collected or recorded over a specified period to detect trends, patterns, or seasonal variances. For example, forecasting stock prices over time.
- Bayesian Statistics: This technique is based on the Bayes theorem and provides a probabilistic framework to update prior beliefs given new data. It’s often used when we have prior knowledge or certain degrees of belief about some scenario.
Machine Learning Techniques
Machine learning algorithms make predictions or decisions based on data. Some common techniques include:
- Decision Trees: A decision tree is a flowchart-like model where each internal node represents a feature (or attribute), each branch represents a decision rule, and each leaf node represents an outcome. This is a simple but powerful technique for classification and regression tasks.
- Random Forests: A random forest is a meta-estimator that fits a number of decision tree classifiers on various sub-samples of the dataset and uses averaging to improve the predictive accuracy and control over-fitting.
- Support Vector Machines (SVM): SVMs are powerful models used for classification and regression tasks. They work well in high-dimensional spaces and can be used for complex decision boundaries.
- K-Nearest Neighbors (KNN): KNN is a type of instance-based learning that classifies new instances based on a similarity measure with known instances.
- Gradient Boosting Algorithms: These are ensemble techniques that combine the predictions of multiple models to create a more accurate and robust prediction. They work by sequentially adding predictors to an ensemble, each one correcting its predecessor.
Artificial Intelligence Techniques
These are typically more complex and powerful techniques, including:
- Neural Networks: These are inspired by the human brain and are used for tasks such as image and speech recognition. They work well with large amounts of data and can handle complex patterns.
- Deep Learning: This is a subset of neural networks with more layers, which enables it to learn and represent more complex patterns. It’s often used in fields such as image recognition, natural language processing, and voice recognition.
Predictive Analytics Tools
There are numerous tools available for predictive analytics, which cater to different skill levels, use cases, and budgets. Here are a few of the most popular ones:
Python: Python is a programming language that has become one of the standard languages for predictive analytics and data science. It has strong capabilities for data manipulation, data visualization, and machine learning through libraries such as pandas, matplotlib, seaborn, scikit-learn, and TensorFlow.
R: R is another programming language that is widely used in the data science community. It has numerous packages for data manipulation, statistical modeling, and machine learning, and is known for its strong data visualization capabilities.
SAS: SAS is a software suite developed by SAS Institute for advanced analytics, multivariate analyses, business intelligence, data management, and predictive analytics. SAS offers a wide range of data analysis and manipulation tools, though it can be more expensive and have a steeper learning curve compared to Python and R.
SPSS: IBM’s SPSS is a predictive analytics software that offers advanced analytics like hypothesis testing, ad-hoc analysis, predictive models, and more. SPSS Modeler is IBM’s visual interface for building predictive models.
Apache Hadoop: This is an open-source software framework that enables processing of large data sets across clusters of computers. It is useful for handling big data, but it requires substantial setup and expertise.
Tableau: Tableau is a business intelligence and data visualization tool that also offers some predictive analytics features. It is used to create interactive dashboards and reports, and it can connect to a wide range of data sources.
Microsoft Excel: Excel is a common tool that offers basic data analysis and visualization capabilities, as well as more advanced predictive capabilities through its data analysis toolpak. While not as powerful or flexible as some of the other tools mentioned, it is widely available and accessible to non-programmers.
RapidMiner: RapidMiner is a data science platform that provides an integrated environment for data preparation, machine learning, deep learning, text mining, and predictive analytics. It’s a very user-friendly tool, allowing users to design a data mining process without programming.
Alteryx: Alteryx is an advanced data analysis program that integrates with your existing data for comprehensive future insight. It combines predictive analytics with reporting, data manipulation, and data visualization.
KNIME: KNIME is a free and open-source data analytics, reporting, and integration platform. KNIME integrates various components for machine learning and data mining through its modular data pipelining concept.
Predictive Analytics Examples
Predictive Analytics Examples are as follows:
Predictive Maintenance in Manufacturing: In the manufacturing industry, companies use predictive analytics to estimate when equipment failures might occur. By monitoring sensor data from machines, predictive models can identify patterns that precede failure. This approach, known as predictive maintenance, can help prevent unexpected equipment downtime, reduce maintenance costs, and improve operational efficiency. For instance, an automobile manufacturing plant might use predictive analytics to forecast when certain parts are likely to fail based on variables like usage, age, and environmental conditions.
Customer Churn Prediction in Telecommunications: Telecom companies use predictive analytics to identify customers who are likely to cancel their service, an event known as churn. By analyzing customer behavior and usage patterns, predictive models can identify the signs that a customer may be about to churn. The company can then take proactive measures, such as reaching out to the customer with special offers or addressing their issues. This can lead to improved customer retention and reduced acquisition costs.
Credit Scoring in Finance: Banks and financial institutions use predictive analytics to assess the risk associated with lending to a particular customer. This is done by creating a credit score, which predicts the likelihood of a customer defaulting on their loan based on features like their credit history, income, employment status, and other variables. This use of predictive analytics helps financial institutions make more informed lending decisions, reduce the risk of bad loans, and ensure that customers are given credit terms they can manage.
Predictive Analytics Case Study Example
let’s look at a case study from the retail sector: Walmart’s use of predictive analytics.
Walmart, being the world’s largest retailer with over 11,000 stores worldwide, deals with millions of transactions every day. The company wanted to better understand customer buying patterns and behaviors to improve inventory management and sales.
To accomplish this, Walmart turned to predictive analytics. They collected data from various sources including in-store transactions, online sales, and social media. They also considered external factors such as weather patterns, which can significantly affect retail sales.
Walmart used this data to build predictive models to forecast demand for different products at different times and in different locations. For example, they could predict increased demand for umbrellas if the weather forecast called for rain, or for specific products around certain holidays.
They also used predictive analytics to understand more nuanced customer behaviors. For example, they found that before a hurricane, not only do sales of emergency items like flashlights increase, but there’s also a spike in demand for strawberry Pop-Tarts.
Walmart’s application of predictive analytics led to more efficient inventory management, reducing the costs associated with overstocking or understocking items. It also enabled better targeted marketing by providing insights into what, when, and why customers buy.
Applications of Predictive Analytics
Predictive analytics can be applied in numerous ways across a variety of sectors and industries. Here are some applications:
Healthcare: Predictive analytics can be used in disease prediction and prevention, patient readmission, drug discovery, and hospital resource allocation. For example, predictive models can help identify patients at risk of chronic diseases like diabetes or heart disease based on factors such as age, weight, blood pressure, and lifestyle habits.
Retail: Retailers use predictive analytics for inventory management, demand forecasting, price optimization, customer segmentation, and personalized marketing. They can predict which products are likely to be popular in the future, optimize prices based on predicted demand, and target customers with personalized offers based on their purchasing history and preferences.
Finance: Banks and other financial institutions use predictive analytics for credit scoring, risk assessment, fraud detection, and algorithmic trading. For example, they can predict the likelihood of a customer defaulting on a loan based on their credit history and other personal information.
Transportation and Logistics: Airlines, shipping companies, and delivery services use predictive analytics for route optimization, demand forecasting, price optimization, and predictive maintenance of vehicles. They can predict the most efficient routes for deliveries or flights, optimize prices based on predicted demand, and predict when vehicles are likely to need maintenance or repair.
Energy: Energy companies use predictive analytics for demand forecasting, price optimization, grid optimization, and predictive maintenance. They can predict energy demand based on factors such as time of day, weather, and economic activity, optimize prices based on predicted demand, and predict when equipment is likely to need maintenance or repair.
Telecommunications: Telecom companies use predictive analytics to predict customer churn, optimize network performance, and target customers with personalized offers. They can predict which customers are likely to switch to a different provider, optimize network performance based on predicted demand, and target customers with personalized offers based on their usage patterns and preferences.
Manufacturing: Predictive analytics is used in quality control, demand forecasting, supply chain management, and predictive maintenance. Manufacturers can predict when equipment is likely to fail, forecast demand for their products, and optimize their supply chain based on these predictions.
Cybersecurity: Predictive analytics in cybersecurity is used for threat detection and prevention, risk assessment, and incident prediction. It helps in predicting, identifying, and mitigating potential threats before they can cause significant damage.
- Threat Detection and Prevention: Predictive models can be used to identify patterns of malicious activity, such as repeated login attempts, abnormal network traffic, or patterns consistent with known malware. This enables companies to respond to and neutralize threats before they can do serious harm.
- Risk Assessment: By evaluating historical incident data along with real-time activity, predictive analytics can identify areas of the network that are most vulnerable to attack. This helps prioritize security efforts to areas where they are most needed.
- Incident Prediction: Predictive models can forecast future security incidents based on patterns observed in historical data. For example, if data shows that a certain type of attack often follows a specific sequence of events, companies can take proactive steps when they see that sequence beginning to unfold.
- User Behavior Analytics (UBA): By analyzing normal user behavior, predictive analytics can identify anomalous activity that may indicate a security threat. For example, if a user who normally accesses the network during regular business hours suddenly logs in at midnight, this could be a sign of a compromised account.
When to use Predictive Analytics
Here are some situations where the use of predictive analytics can be particularly beneficial:
Future Forecasting: When you want to understand future trends or behaviors based on historical data, predictive analytics is the go-to solution. For example, a retailer could use predictive analytics to forecast future sales based on historical sales data, trends, and seasonality.
Risk Assessment: Predictive analytics can help assess the risk associated with different decisions or scenarios. Banks often use predictive analytics to predict the likelihood of a borrower defaulting on a loan.
Optimizing Marketing Campaigns: If you want to target customers more effectively, predictive analytics can help. By understanding customer behavior and preferences, you can create more personalized and effective marketing campaigns.
Improving Operations: Predictive analytics can also be used to improve operational efficiency. For example, a manufacturer could use predictive analytics to anticipate maintenance needs, reducing downtime and improving productivity.
Enhancing Customer Retention: Predictive analytics can identify the signs that a customer may be about to churn, allowing a company to take proactive measures to retain that customer.
Detecting Fraud: Predictive analytics can identify patterns and anomalies that may indicate fraudulent activity. This is particularly useful in areas like credit card transactions or insurance claims.
Decision-Making Support: In any situation where decisions need to be made and there’s a wealth of data that can inform those decisions, predictive analytics can help. Whether it’s deciding where to open a new store, how to allocate resources, or which product features to prioritize, predictive analytics can provide data-driven insights to guide these decisions.
Advantages of Predictive Analytics
Predictive analytics brings numerous advantages to organizations and businesses. Here are some of the key benefits:
- Informed Decision Making: Predictive analytics uses data, statistical algorithms, and machine learning techniques to identify future risks and opportunities, enabling organizations to make data-driven decisions.
- Efficient Resource Utilization: With predictive analytics, businesses can optimize their resources based on forecasted demand, improving efficiency and reducing costs. This could be applied in areas like inventory management, staff scheduling, and capacity planning.
- Risk Reduction: Predictive analytics can identify potential risks and threats, allowing businesses to take proactive measures to mitigate them. For example, credit risk models can predict the likelihood of a customer defaulting on a loan, enabling the lender to adjust the loan terms or deny the loan if necessary.
- Improved Customer Relationship: By understanding customer behavior and preferences, businesses can create personalized marketing campaigns and offers, improving customer engagement and retention.
- Increased Revenue: By predicting future trends, optimizing pricing, and better targeting marketing efforts, businesses can increase their revenue and profitability.
- Enhanced Operational Efficiency: Predictive maintenance models can predict when equipment is likely to fail, enabling proactive maintenance and reducing downtime.
- Fraud Detection: Predictive analytics can help detect and prevent fraudulent activities by identifying patterns and anomalies that may suggest fraud.
- Competitive Advantage: Businesses that use predictive analytics can gain a competitive edge by leveraging data to discover insights and make more informed decisions.
- Proactive Approach: Predictive analytics enables a more proactive approach to decision-making. Instead of reacting to events after they happen, businesses can anticipate outcomes and trends and act accordingly.
Disadvantages of Predictive Analytics
While predictive analytics offers numerous benefits, it’s not without its challenges and limitations. Here are some potential disadvantages to consider:
- Data Quality and Relevance: The accuracy of predictive analytics heavily relies on the quality and relevance of the data used. If the data is inaccurate, incomplete, or biased, it can lead to misleading predictions.
- Complexity: Predictive analytics often involves complex mathematical and statistical methods, which require a deep understanding to implement effectively. It also requires significant computational resources, especially when dealing with big data.
- Resource Intensive: Setting up predictive analytics can be resource-intensive, requiring significant investments in technology and skilled personnel. Small businesses, in particular, may find it challenging to afford these resources.
- Privacy Concerns: Predictive analytics often involves processing large amounts of personal data, raising concerns about privacy and data protection. It’s important for organizations to comply with all relevant privacy laws and regulations and to use the data ethically.
- Over-reliance on Technology: There’s a risk that decision-makers may over-rely on predictive analytics and ignore their intuition or other important non-quantitative factors. Predictive analytics should be used as a tool to support decision-making, not as a substitute for human judgment.
- False Positives or Negatives: Predictive models may sometimes generate false positives (predicting an event that doesn’t happen) or false negatives (not predicting an event that does happen). This could lead to unnecessary actions or missed opportunities.
- Uncertainty: Predictive analytics involves a degree of uncertainty, as predictions are based on probabilities. It’s essential to understand this uncertainty and to communicate it effectively when using predictive analytics to inform decision-making.
About the author
Muhammad Hassan
Researcher, Academic Writer, Web developer
You may also like

Digital Ethnography – Types, Methods and Examples

Big Data Analytics -Types, Tools and Methods

Diagnostic Analytics – Methods, Tools and...

Blockchain Research – Methods, Types and Examples

Social Network Analysis – Types, Tools and...

What is Data Science? Components, Process and Tools

Research Topics & Ideas: Data Science
50 Topic Ideas To Kickstart Your Research Project
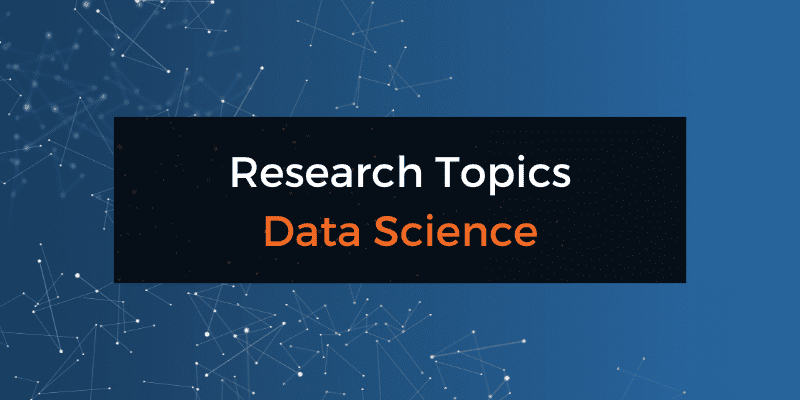
If you’re just starting out exploring data science-related topics for your dissertation, thesis or research project, you’ve come to the right place. In this post, we’ll help kickstart your research by providing a hearty list of data science and analytics-related research ideas , including examples from recent studies.
PS – This is just the start…
We know it’s exciting to run through a list of research topics, but please keep in mind that this list is just a starting point . These topic ideas provided here are intentionally broad and generic , so keep in mind that you will need to develop them further. Nevertheless, they should inspire some ideas for your project.
To develop a suitable research topic, you’ll need to identify a clear and convincing research gap , and a viable plan to fill that gap. If this sounds foreign to you, check out our free research topic webinar that explores how to find and refine a high-quality research topic, from scratch. Alternatively, consider our 1-on-1 coaching service .
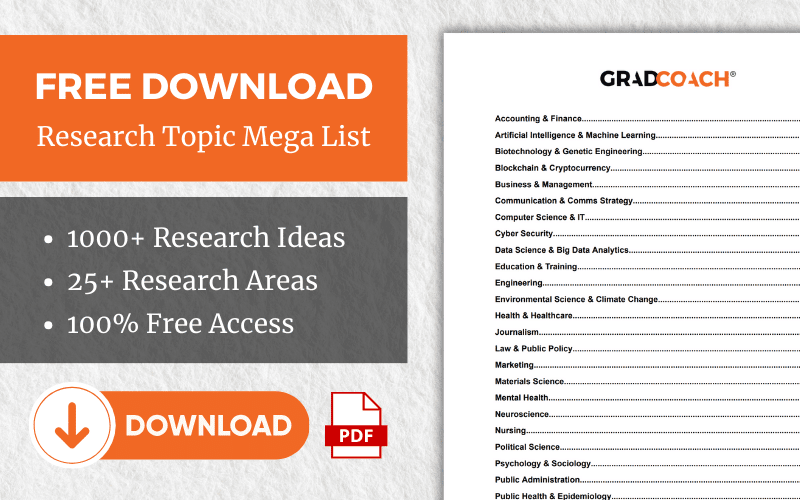
Data Science-Related Research Topics
- Developing machine learning models for real-time fraud detection in online transactions.
- The use of big data analytics in predicting and managing urban traffic flow.
- Investigating the effectiveness of data mining techniques in identifying early signs of mental health issues from social media usage.
- The application of predictive analytics in personalizing cancer treatment plans.
- Analyzing consumer behavior through big data to enhance retail marketing strategies.
- The role of data science in optimizing renewable energy generation from wind farms.
- Developing natural language processing algorithms for real-time news aggregation and summarization.
- The application of big data in monitoring and predicting epidemic outbreaks.
- Investigating the use of machine learning in automating credit scoring for microfinance.
- The role of data analytics in improving patient care in telemedicine.
- Developing AI-driven models for predictive maintenance in the manufacturing industry.
- The use of big data analytics in enhancing cybersecurity threat intelligence.
- Investigating the impact of sentiment analysis on brand reputation management.
- The application of data science in optimizing logistics and supply chain operations.
- Developing deep learning techniques for image recognition in medical diagnostics.
- The role of big data in analyzing climate change impacts on agricultural productivity.
- Investigating the use of data analytics in optimizing energy consumption in smart buildings.
- The application of machine learning in detecting plagiarism in academic works.
- Analyzing social media data for trends in political opinion and electoral predictions.
- The role of big data in enhancing sports performance analytics.
- Developing data-driven strategies for effective water resource management.
- The use of big data in improving customer experience in the banking sector.
- Investigating the application of data science in fraud detection in insurance claims.
- The role of predictive analytics in financial market risk assessment.
- Developing AI models for early detection of network vulnerabilities.
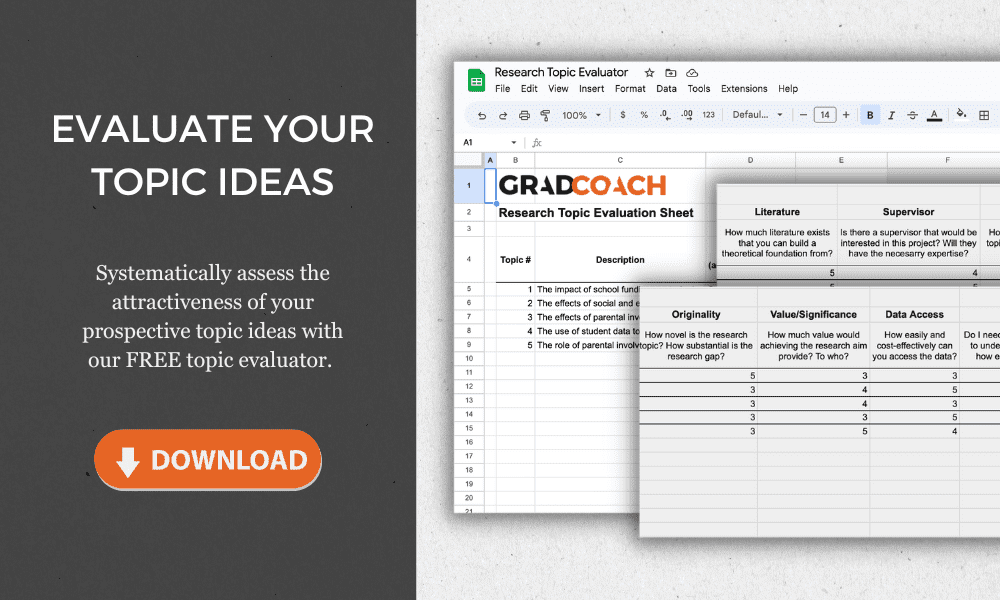
Data Science Research Ideas (Continued)
- The application of big data in public transportation systems for route optimization.
- Investigating the impact of big data analytics on e-commerce recommendation systems.
- The use of data mining techniques in understanding consumer preferences in the entertainment industry.
- Developing predictive models for real estate pricing and market trends.
- The role of big data in tracking and managing environmental pollution.
- Investigating the use of data analytics in improving airline operational efficiency.
- The application of machine learning in optimizing pharmaceutical drug discovery.
- Analyzing online customer reviews to inform product development in the tech industry.
- The role of data science in crime prediction and prevention strategies.
- Developing models for analyzing financial time series data for investment strategies.
- The use of big data in assessing the impact of educational policies on student performance.
- Investigating the effectiveness of data visualization techniques in business reporting.
- The application of data analytics in human resource management and talent acquisition.
- Developing algorithms for anomaly detection in network traffic data.
- The role of machine learning in enhancing personalized online learning experiences.
- Investigating the use of big data in urban planning and smart city development.
- The application of predictive analytics in weather forecasting and disaster management.
- Analyzing consumer data to drive innovations in the automotive industry.
- The role of data science in optimizing content delivery networks for streaming services.
- Developing machine learning models for automated text classification in legal documents.
- The use of big data in tracking global supply chain disruptions.
- Investigating the application of data analytics in personalized nutrition and fitness.
- The role of big data in enhancing the accuracy of geological surveying for natural resource exploration.
- Developing predictive models for customer churn in the telecommunications industry.
- The application of data science in optimizing advertisement placement and reach.
Recent Data Science-Related Studies
While the ideas we’ve presented above are a decent starting point for finding a research topic, they are fairly generic and non-specific. So, it helps to look at actual studies in the data science and analytics space to see how this all comes together in practice.
Below, we’ve included a selection of recent studies to help refine your thinking. These are actual studies, so they can provide some useful insight as to what a research topic looks like in practice.
- Data Science in Healthcare: COVID-19 and Beyond (Hulsen, 2022)
- Auto-ML Web-application for Automated Machine Learning Algorithm Training and evaluation (Mukherjee & Rao, 2022)
- Survey on Statistics and ML in Data Science and Effect in Businesses (Reddy et al., 2022)
- Visualization in Data Science VDS @ KDD 2022 (Plant et al., 2022)
- An Essay on How Data Science Can Strengthen Business (Santos, 2023)
- A Deep study of Data science related problems, application and machine learning algorithms utilized in Data science (Ranjani et al., 2022)
- You Teach WHAT in Your Data Science Course?!? (Posner & Kerby-Helm, 2022)
- Statistical Analysis for the Traffic Police Activity: Nashville, Tennessee, USA (Tufail & Gul, 2022)
- Data Management and Visual Information Processing in Financial Organization using Machine Learning (Balamurugan et al., 2022)
- A Proposal of an Interactive Web Application Tool QuickViz: To Automate Exploratory Data Analysis (Pitroda, 2022)
- Applications of Data Science in Respective Engineering Domains (Rasool & Chaudhary, 2022)
- Jupyter Notebooks for Introducing Data Science to Novice Users (Fruchart et al., 2022)
- Towards a Systematic Review of Data Science Programs: Themes, Courses, and Ethics (Nellore & Zimmer, 2022)
- Application of data science and bioinformatics in healthcare technologies (Veeranki & Varshney, 2022)
- TAPS Responsibility Matrix: A tool for responsible data science by design (Urovi et al., 2023)
- Data Detectives: A Data Science Program for Middle Grade Learners (Thompson & Irgens, 2022)
- MACHINE LEARNING FOR NON-MAJORS: A WHITE BOX APPROACH (Mike & Hazzan, 2022)
- COMPONENTS OF DATA SCIENCE AND ITS APPLICATIONS (Paul et al., 2022)
- Analysis on the Application of Data Science in Business Analytics (Wang, 2022)
As you can see, these research topics are a lot more focused than the generic topic ideas we presented earlier. So, for you to develop a high-quality research topic, you’ll need to get specific and laser-focused on a specific context with specific variables of interest. In the video below, we explore some other important things you’ll need to consider when crafting your research topic.
Get 1-On-1 Help
If you’re still unsure about how to find a quality research topic, check out our Research Topic Kickstarter service, which is the perfect starting point for developing a unique, well-justified research topic.
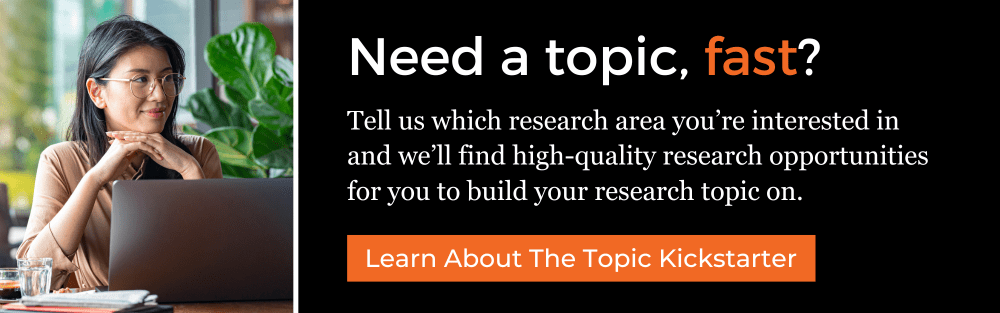
You Might Also Like:
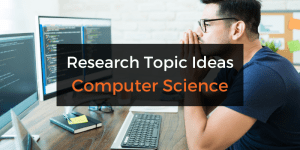
Submit a Comment Cancel reply
Your email address will not be published. Required fields are marked *
Save my name, email, and website in this browser for the next time I comment.
- Print Friendly
predictive analytics Recently Published Documents
Total documents.
- Latest Documents
- Most Cited Documents
- Contributed Authors
- Related Sources
- Related Keywords
Predictive Analytics of Energy Usage by IoT-Based Smart Home Appliances for Green Urban Development
Green IoT primarily focuses on increasing IoT sustainability by reducing the large amount of energy required by IoT devices. Whether increasing the efficiency of these devices or conserving energy, predictive analytics is the cornerstone for creating value and insight from large IoT data. This work aims at providing predictive models driven by data collected from various sensors to model the energy usage of appliances in an IoT-based smart home environment. Specifically, we address the prediction problem from two perspectives. Firstly, an overall energy consumption model is developed using both linear and non-linear regression techniques to identify the most relevant features in predicting the energy consumption of appliances. The performances of the proposed models are assessed using a publicly available dataset comprising historical measurements from various humidity and temperature sensors, along with total energy consumption data from appliances in an IoT-based smart home setup. The prediction results comparison show that LSTM regression outperforms other linear and ensemble regression models by showing high variability ( R 2 ) with the training (96.2%) and test (96.1%) data for selected features. Secondly, we develop a multi-step time-series model using the auto regressive integrated moving average (ARIMA) technique to effectively forecast future energy consumption based on past energy usage history. Overall, the proposed predictive models will enable consumers to minimize the energy usage of home appliances and the energy providers to better plan and forecast future energy demand to facilitate green urban development.
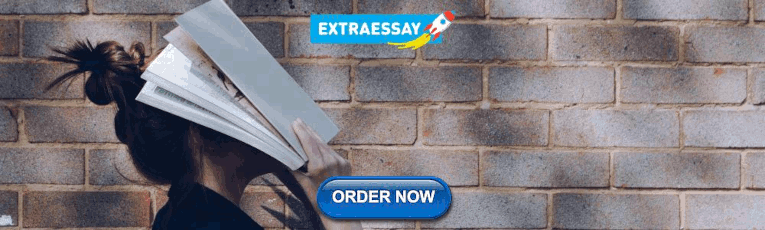
Influence of AI and Machine Learning in Insurance Sector
The Aim of this research is to identify influence, usage, and the benefits of AI (Artificial Intelligence) and ML (Machine learning) using big data analytics in Insurance sector. Insurance sector is the most volatile industry since multiple natural influences like Brexit, pandemic, covid 19, Climate changes, Volcano interruptions. This research paper will be used to explore potential scope and use cases for AI, ML and Big data processing in Insurance sector for Automate claim processing, fraud prevention, predictive analytics, and trend analysis towards possible cause for business losses or benefits. Empirical quantitative research method is used to verify the model with the sample of UK insurance sector analysis. This research will conclude some practical insights for Insurance companies using AI, ML, Big data processing and Cloud computing for the better client satisfaction, predictive analysis, and trending.
Can HRM predict mental health crises? Using HR analytics to unpack the link between employment and suicidal thoughts and behaviors
PurposeThe aim of this research is to determine the extent to which the human resource (HR) function can screen and potentially predict suicidal employees and offer preventative mental health assistance.Design/methodology/approachDrawing from the 2019 National Survey of Drug Use and Health (N = 56,136), this paper employs multivariate binary logistic regression to model the work-related predictors of suicidal ideation, planning and attempts.FindingsThe results indicate that known periods of joblessness, the total number of sick days and absenteeism over the last 12 months are significantly associated with various suicidal outcomes while controlling for key psychosocial correlates. The results also indicate that employee assistance programs are associated with a significantly reduced likelihood of suicidal ideation. These findings are consistent with conservation of resources theory.Research limitations/implicationsThis research demonstrates preliminarily that the HR function can unobtrusively detect employee mental health crises by collecting data on key predictors.Originality/valueIn the era of COVID-19, employers have a duty of care to safeguard employee mental health. To this end, the authors offer an innovative way through which the HR function can employ predictive analytics to address mental health crises before they result in tragedy.
An AI-Enabled Predictive Analytics Dashboard for Acute Neurosurgical Referrals
Abstract Healthcare dashboards make key information about service and clinical outcomes available to staff in an easy-to-understand format. Most dashboards are limited to providing insights based on group-level inference, rather than individual prediction. Here, we evaluate a dashboard which could analyze and forecast acute neurosurgical referrals based on 10,033 referrals made to a large volume tertiary neurosciences center in central London, U.K., from the start of the Covid-19 pandemic lockdown period until October 2021. As anticipated, referral volumes significantly increased in this period, largely due to an increase in spinal referrals. Applying a range of validated time-series forecasting methods, we found that referrals were projected to increase beyond this time-point. Using a mixed-methods approach, we determined that the dashboard was usable, feasible, and acceptable to key stakeholders. Dashboards provide an effective way of visualizing acute surgical referral data and for predicting future volume without the need for data-science expertise.
Price Bubbles in the Real Estate Markets - Analysis and Prediction
The article concerns the issue of price bubbles on the markets, with particular emphasis on the specificity of the real estate market. Up till now, more than a decade after the subprime crisis, there is no accurate enough method to predict price movements, their culmination and, eventually, the burst of price and speculative bubbles on the markets. Hence, the main goal of the article is to present the possibility of early detection of price bubbles and their consequences from the point of view of the surveyed managers. The following research hypothesis was verified: price bubbles on the real estate market cannot be excluded, therefore constant monitoring and predictive analytics of this market are needed. In addition to standard research methods (desk research or statistical analysis), the authors conducted their own survey on a group of randomly selected managers from Portugal and Poland in the context of their attitude to crises and price bubbles. The obtained results allowed us to conclude that managers in both analysed countries are different relating the effects of price bubbles to the activities of their own companies but are similar (about 40% of respondents) expecting quick detection and deactivation of emerging bubbles by the government or by central bank. Nearly 40% of Polish and Portuguese managers claimed that the consequences of crises must include an increased responsibility of managers for their decisions, especially those leading to failures.
Covid-19 Impact and Implications on Traffic: Smart Predictive Analytics for Mobility Navigation
Empirical study on classifiers for earlier prediction of covid-19 infection cure and death rate in the indian states.
Machine Learning methods can play a key role in predicting the spread of respiratory infection with the help of predictive analytics. Machine Learning techniques help mine data to better estimate and predict the COVID-19 infection status. A Fine-tuned Ensemble Classification approach for predicting the death and cure rates of patients from infection using Machine Learning techniques has been proposed for different states of India. The proposed classification model is applied to the recent COVID-19 dataset for India, and a performance evaluation of various state-of-the-art classifiers to the proposed model is performed. The classifiers forecasted the patients’ infection status in different regions to better plan resources and response care systems. The appropriate classification of the output class based on the extracted input features is essential to achieve accurate results of classifiers. The experimental outcome exhibits that the proposed Hybrid Model reached a maximum F1-score of 94% compared to Ensembles and other classifiers like Support Vector Machine, Decision Trees, and Gaussian Naïve Bayes on a dataset of 5004 instances through 10-fold cross-validation for predicting the right class. The feasibility of automated prediction for COVID-19 infection cure and death rates in the Indian states was demonstrated.
People Analytics: Augmenting Horizon from Predictive Analytics to Prescriptive Analytics
Analytics techniques: descriptive analytics, predictive analytics, and prescriptive analytics, unlocking drivers for employee engagement through human resource analytics.
The authors have discussed in detail the meaning of employee engagement and its relevance for the organizations in the present scenario. The authors also highlighted the various factors that predict the employee engagement of the employees in the varied organizations. The authors have emphasized on the role that HR analytics can play to identify the reasons for low level of engagement among employees and suggesting ways to improve the same using predictive analytics. The authors have also advocated the benefits that organizations can reap by making use of HR analytics in measuring the engagement levels of the employees and improving the engagement levels of diverse workforce in the existing organizations. The authors have also proposed the future perspectives of the proposed study that help the organizations and officials from the top management to tap the benefits of analytics in the function of human resource management and to address the upcoming issues related to employee behavior.
Export Citation Format
Share document.
- Survey Paper
- Open access
- Published: 25 July 2020
Predictive big data analytics for supply chain demand forecasting: methods, applications, and research opportunities
- Mahya Seyedan 1 &
- Fereshteh Mafakheri ORCID: orcid.org/0000-0002-7991-4635 1
Journal of Big Data volume 7 , Article number: 53 ( 2020 ) Cite this article
113k Accesses
126 Citations
23 Altmetric
Metrics details
Big data analytics (BDA) in supply chain management (SCM) is receiving a growing attention. This is due to the fact that BDA has a wide range of applications in SCM, including customer behavior analysis, trend analysis, and demand prediction. In this survey, we investigate the predictive BDA applications in supply chain demand forecasting to propose a classification of these applications, identify the gaps, and provide insights for future research. We classify these algorithms and their applications in supply chain management into time-series forecasting, clustering, K-nearest-neighbors, neural networks, regression analysis, support vector machines, and support vector regression. This survey also points to the fact that the literature is particularly lacking on the applications of BDA for demand forecasting in the case of closed-loop supply chains (CLSCs) and accordingly highlights avenues for future research.
Introduction
Nowadays, businesses adopt ever-increasing precision marketing efforts to remain competitive and to maintain or grow their margin of profit. As such, forecasting models have been widely applied in precision marketing to understand and fulfill customer needs and expectations [ 1 ]. In doing so, there is a growing attention to analysis of consumption behavior and preferences using forecasts obtained from customer data and transaction records in order to manage products supply chains (SC) accordingly [ 2 , 3 ].
Supply chain management (SCM) focuses on flow of goods, services, and information from points of origin to customers through a chain of entities and activities that are connected to one another [ 4 ]. In typical SCM problems, it is assumed that capacity, demand, and cost are known parameters [ 5 ]. However, this is not the case in reality, as there are uncertainties arising from variations in customers’ demand, supplies transportation, organizational risks and lead times. Demand uncertainties, in particular, has the greatest influence on SC performance with widespread effects on production scheduling, inventory planning, and transportation [ 6 ]. In this sense, demand forecasting is a key approach in addressing uncertainties in supply chains [ 7 , 8 , 9 ].
A variety of statistical analysis techniques have been used for demand forecasting in SCM including time-series analysis and regression analysis [ 10 ]. With the advancements in information technologies and improved computational efficiencies, big data analytics (BDA) has emerged as a means of arriving at more precise predictions that better reflect customer needs, facilitate assessment of SC performance, improve the efficiency of SC, reduce reaction time, and support SC risk assessment [ 11 ].
The focus of this meta-research (literature review) paper is on “demand forecasting” in supply chains. The characteristics of demand data in today’s ever expanding and sporadic global supply chains makes the adoption of big data analytics (and machine learning) approaches a necessity for demand forecasting. The digitization of supply chains [ 12 ] and incoporporation Blockchain technologies [ 13 ] for better tracking of supply chains further highlights the role of big data analytics. Supply chain data is high dimensional generated across many points in the chain for varied purposes (products, supplier capacities, orders, shipments, customers, retailers, etc.) in high volumes due to plurality of suppliers, products, and customers and in high velocity reflected by many transactions continuously processed across supply chain networks. In the sense of such complexities, there has been a departure from conventional (statistical) demand forecasting approaches that work based on identifying statistically meannignful trends (characterized by mean and variance attributes) across historical data [ 14 ], towards intelligent forecasts that can learn from the historical data and intelligently evolve to adjust to predict the ever changing demand in supply chains [ 15 ]. This capability is established using big data analytics techniques that extract forecasting rules through discovering the underlying relationships among demand data across supply chain networks [ 16 ]. These techniques are computationally intensive to process and require complex machine-programmed algorithms [ 17 ].
With SCM efforts aiming at satisfying customer demand while minimizing the total cost of supply, applying machine-learning/data analytics algorithms could facilitate precise (data-driven) demand forecasts and align supply chain activities with these predictions to improve efficiency and satisfaction. Reflecting on these opportunities, in this paper, first a taxonmy of data sources in SCM is proposed. Then, the importance of demand management in SCs is investigated. A meta-research (literature review) on BDA applications in SC demand forecasting is explored according to categories of the algorithms utilized. This review paves the path to a critical discussion of BDA applications in SCM highlighting a number of key findings and summarizing the existing challenges and gaps in BDA applications for demand forecasting in SCs. On that basis, the paper concludes by presenting a number of avenues for future research.
Data in supply chains
Data in the context of supply chains can be categorized into customer, shipping, delivery, order, sale, store, and product data [ 18 ]. Figure 1 provides the taxonomy of supply chain data. As such, SC data originates from different (and segmented) sources such as sales, inventory, manufacturing, warehousing, and transportation. In this sense, competition, price volatilities, technological development, and varying customer commitments could lead to underestimation or overestimation of demand in established forecasts [ 19 ]. Therefore, to increase the precision of demand forecast, supply chain data shall be carefully analyzed to enhance knowledge about market trends, customer behavior, suppliers and technologies. Extracting trends and patterns from such data and using them to improve accuracy of future predictions can help minimize supply chain costs [ 20 , 21 ].
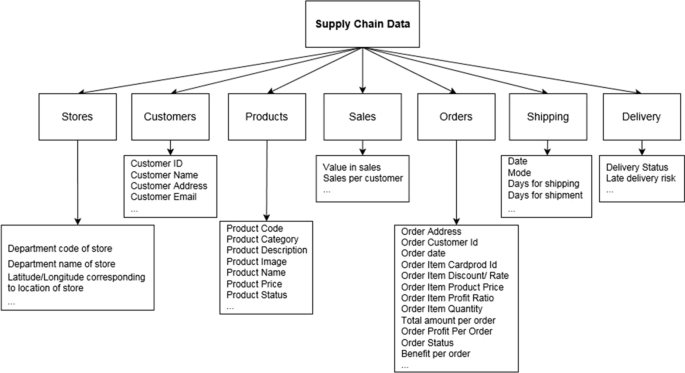
Taxonomy of supply chain data
Analysis of supply chain data has become a complex task due to (1) increasing multiplicity of SC entities, (2) growing diversity of SC configurations depending on the homogeneity or heterogeneity of products, (3) interdependencies among these entities (4) uncertainties in dynamical behavior of these components, (5) lack of information as relate to SC entities; [ 11 ], (6) networked manufacturing/production entities due to their increasing coordination and cooperation to achieve a high level customization and adaptaion to varying customers’ needs [ 22 ], and finally (7) the increasing adoption of supply chain digitization practices (and use of Blockchain technologies) to track the acitivities across supply chains [ 12 , 13 ].
Big data analytics (BDA) has been increasingly applied in management of SCs [ 23 ], for procurement management (e.g., supplier selection [ 24 ], sourcing cost improvement [ 25 ], sourcing risk management [ 26 ], product research and development [ 27 ], production planning and control [ 28 ], quality management [ 29 ], maintenance, and diagnosis [ 30 ], warehousing [ 31 ], order picking [ 32 ], inventory control [ 33 ], logistics/transportation (e.g., intelligent transportation systems [ 34 ], logistics planning [ 35 ], in-transit inventory management [ 36 ], demand management (e.g., demand forecasting [ 37 ], demand sensing [ 38 ], and demand shaping [ 39 ]. A key application of BDA in SCM is to provide accurate forecasting, especially demand forecasting, with the aim of reducing the bullwhip effect [ 14 , 40 , 41 , 42 ].
Big data is defined as high-volume, high-velocity, high-variety, high value, and high veracity data requiring innovative forms of information processing that enable enhanced insights, decision making, and process automation [ 43 ]. Volume refers to the extensive size of data collected from multiple sources (spatial dimension) and over an extended period of time (temporal dimension) in SCs. For example, in case of freight data, we have ERP/WMS order and item-level data, tracking, and freight invoice data. These data are generated from sensors, bar codes, Enterprise resource planning (ERP), and database technologies. Velocity can be defined as the rate of generation and delivery of specific data; in other words, it refers to the speed of data collection, reliability of data transferring, efficiency of data storage, and excavation speed of discovering useful knowledge as relate to decision-making models and algorithms. Variety refers to generating varied types of data from diverse sources such as the Internet of Things (IoT), mobile devices, online social networks, and so on. For instance, the vast data from SCM are usually variable due to the diverse sources and heterogeneous formats, particularly resulted from using various sensors in manufacturing sites, highways, retailer shops, and facilitated warehouses. Value refers to the nature of the data that must be discovered to support decision-making. It is the most important yet the most elusive, of the 5 Vs. Veracity refers to the quality of data, which must be accurate and trustworthy, with the knowledge that uncertainty and unreliability may exist in many data sources. Veracity deals with conformity and accuracy of data. Data should be integrated from disparate sources and formats, filtered and validated [ 23 , 44 , 45 ]. In summary, big data analytics techniques can deal with a collection of large and complex datasets that are difficult to process and analyze using traditional techniques [ 46 ].
The literature points to multiple sources of big data across the supply chains with varied trade-offs among volume, velocity, variety, value, and veracity attributes [ 47 ]. We have summarized these sources and trade-offs in Table 1 . Although, the demand forecasts in supply chains belong to the lower bounds of volume, velocity, and variety, however, these forecasts can use data from all sources across the supply chains from low volume/variety/velocity on-the-shelf inventory reports to high volume/variety/velocity supply chain tracking information provided through IoT. This combination of data sources used in SC demand forecasts, with their diverse temporal and spatial attributes, places a greater emphasis on use of big data analytics in supply chains, in general, and demand forecasting efforts, in particular.
The big data analytics applications in supply chain demand forecasting have been reported in both categories of supervised and unsupervised learning. In supervised learning, data will be associated with labels, meaning that the inputs and outputs are known. The supervised learning algorithms identify the underlying relationships between the inputs and outputs in an effort to map the inputs to corresponding outputs given a new unlabeled dataset [ 48 ]. For example, in case of a supervised learning model for demand forecasting, future demand can be predicted based on the historical data on product demand [ 41 ]. In unsupervised learning, data are unlabeled (i.e. unknown output), and the BDA algorithms try to find the underlying patterns among unlabeled data [ 48 ] by analyzing the inputs and their interrelationships. Customer segmentation is an example of unsupervised learning in supply chains that clusters different groups of customers based on their similarity [ 49 ]. Many machine-learning/data analytics algorithms can facilitate both supervised learning (extracting the input–output relationships) and unsupervised learning (extracting inputs, outputs and their relationships) [ 41 ].
Demand management in supply chains
The term “demand management” emerged in practice in the late 1980s and early 1990s. Traditionally, there are two approaches for demand management. A forward approach which looks at potential demand over the next several years and a backward approach that relies on past or ongoing capabilities in responding to demand [ 50 ].
In forward demand management, the focus will be on demand forecasting and planning, data management, and marketing strategies. Demand forecasting and planning refer to predicting the quantities and timings of customers’ requests. Such predictions aim at achieving customers’ satisfaction by meeting their needs in a timely manner [ 51 ]. Accurate demand forecasting could improve the efficiency and robustness of production processes (and the associated supply chains) as the resources will be aligned with requirements leading to reduction of inventories and wastes [ 52 , 53 ].
In the light of the above facts, there are many approaches proposed in the literature and practice for demand forecasting and planning. Spreadsheet models, statistical methods (like moving averages), and benchmark-based judgments are among these approaches. Today, the most widely used demand forecasting and planning tool is Excel. The most widespread problem with spreadsheet models used for demand forecasting is that they are not scalable for large-scale data. In addition, the complexities and uncertainties in SCM (with multiplicity and variability of demand and supply) cannot be extracted, analyzed, and addressed through simple statistical methods such as moving averages or exponential smoothing [ 50 ]. During the past decade, traditional solutions for SC demand forecasting and planning have faced many difficulties in driving the costs down and reducing inventories [ 50 ]. Although, in some cases, the suggested solutions have improved the day’s payable, they have pushed up the SC costs as a burden to suppliers.
The era of big data and high computing analytics has enabled data processing at a large scale that is efficient, fast, easy, and with reduced concerns about data storage and collection due to cloud services. The emergence of new technologies in data storage and analytics and the abundance of quality data have created new opportunities for data-driven demand forecasting and planning. Demand forecast accuracy can be significantly improved with data-mining algorithms and tools that can sift through data, analyze the results, and learn about the relationships involved. This could lead to highly accurate demand forecasting models that learn from data and are scalable for application in SCM. In the following section, a review of BDA applications in SCM is presented. These applications are categorized based on the employed techniques in establishing the data-drive demand forecasts.
BDA for demand forecasting in SCM
This survey aims at reviewing the articles published in the area of demand and sales forecasting in SC in the presence of big data to provide a classification of the literature based on algorithms utilized as well as a survey of applications. To the best of our knowledge, no comprehensive review of the literature specifically on SC demand forecasting has been conducted with a focus on classification of techniques of data analytics and machine learning. In doing so, we performed a thorough search of the existing literature, through Scopus, Google Scholar, and Elsevier, with publication dates ranging from 2005 to 2019. The keywords used for the search were supply chain, demand forecasting, sales forecasting, big data analytics, and machine learning.
Figure 2 shows the trend analysis of publications in demand forecasting for SC appeared from 2005 to 2019. There is a steadily increasing trend in the number of publications from 2005 to 2019. It is expected that such growth continues in 2020. Reviewing the past 15 years of research on big data analysis/machine learning applications in SC demand forecasting, we identified 64 research papers (excluding books, book chapters, and review papers) and categorized them with respect to the methodologies adopted for demand forecasting. The five most frequently used techniques are listed in Table 2 that includes “Neural Network,” “Regression”, “Time-series forecasting (ARIMA)”, “Support Vector Machine”, and “Decision Tree” methods. This table implies the growing use of big data analysis techniques in SC demand forecasting. It shall be mentioned that there were a few articles using multiple of these techniques.
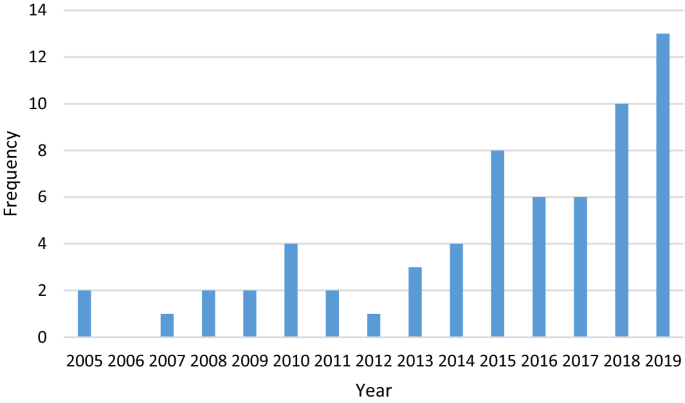
Distribution of literature in supply chain demand forecasting from 2005 to 2019
It shall be mentioned that there are literature review papers exploring the use of big data analytics in SCM [ 10 , 16 , 23 , 54 , 55 , 56 , 57 , 58 , 59 , 60 , 61 , 62 , 63 , 64 , 65 , 66 , 67 ]. However, this study focuses on the specific topic of “demand forecasting” in SCM to explore BDA applications in line with this particular subtopic in SCM.
As Hofmann and Rutschmann [ 58 ] indicated in their literature review, the key questions to answer are why, what and how big data analytics/machine-learning algorithms could enhance forecasts’ accuracy in comparison to conventional statistical forecasting approaches.
Conventional methods have faced a number of limitations for demand forecasting in the context of SCs. There are a lot of parameters influencing the demand in supply chains, however, many of them were not captured in studies using conventional methods for the sake of simplicity. In this regard, the forecasts could only provide a partial understanding of demand variations in supply chains. In addition, the unexplained demand variations could be simply considered as statistical noise. Conventional approaches could provide shorter processing times in exchange for a compromise on robustness and accuracy of predictions. Conventional SC demand forecasting approaches are mostly done manually with high reliance on the planner’s skills and domain knowledge. It would be worthwhile to fully automate the forecasting process to reduce such a dependency [ 58 ]. Finally, data-driven techniques could learn to incorporate non-linear behaviors and could thus provide better approximations in demand forecasting compared to conventional methods that are mostly derived based on linear models. There is a significant level of non-linearity in demand behavior in SC particularly due to competition among suppliers, the bullwhip effect, and mismatch between supply and demand [ 40 ].
To extract valuable knowledge from a vast amount of data, BDA is used as an advanced analytics technique to obtain the data needed for decision-making. Reduced operational costs, improved SC agility, and increased customer satisfaction are mentioned among the benefits of applying BDA in SCM [ 68 ]. Researchers used various BDA techniques and algorithms in SCM context, such as classification, scenario analysis, and optimization [ 23 ]. Machine-learning techniques have been used to forecast demand in SCs, subject to uncertainties in prices, markets, competitors, and customer behaviors, in order to manage SCs in a more efficient and profitable manner [ 40 ].
BDA has been applied in all stages of supply chains, including procurement, warehousing, logistics/transportation, manufacturing, and sales management. BDA consists of descriptive analytics, predictive analytics, and prescriptive analytics. Descriptive analysis is defined as describing and categorizing what happened in the past. Predictive analytics are used to predict future events and discover predictive patterns within data by using mathematical algorithms such as data mining, web mining, and text mining. Prescriptive analytics apply data and mathematical algorithms for decision-making. Multi-criteria decision-making, optimization, and simulation are among the prescriptive analytics tools that help to improve the accuracy of forecasting [ 10 ].
Predictive analytics are the ones mostly utilized in SC demand and procurement forecasting [ 23 ]. In this sense, in the following subsections, we will review various predictive big data analytics approaches, presented in the literature for demand forecasting in SCM, categorized based on the employed data analytics/machine learning technique/algorithm, with elaborations of their purpose and applications (summarized in Table 3 ).
Time-series forecasting
Time series are methodologies for mining complex and sequential data types. In time-series data, sequence data, consisting of long sequences of numeric data, recorded at equal time intervals (e.g., per minute, per hour, or per day). Many natural and human-made processes, such as stock markets, medical diagnosis, or natural phenomenon, can generate time-series data. [ 48 ].
In case of demand forecasting using time-series, demand is recorded over time at equal size intervals [ 69 , 70 ]. Combinations of time-series methods with product or market features have attracted much attention in demand forecasting with BDA. Ma et al. [ 71 ] proposed and developed a demand trend-mining algorithm for predictive life cycle design. In their method, they combined three models (a) a decision tree model for large-scale historical data classification, (b) a discrete choice analysis for present and past demand modeling, and (c) an automated time-series forecasting model for future trend analysis. They tested and applied their 3-level approach in smartphone design, manufacturing and remanufacturing.
Time-series approach was used for forecasting of search traffic (service demand) subject to changes in consumer attitudes [ 37 ]. Demand forecasting has been achieved through time-series models using exponential smoothing with covariates (ESCov) to provide predictions for short-term, mid-term, and long-term demand trends in the chemical industry SCs [ 7 ]. In addition, Hamiche et al. [ 72 ] used a customer-responsive time-series approach for SC demand forecasting.
In case of perishable products, with short life cycles, having appropriate (short-term) forecasting is extremely critical. Da Veiga et al. [ 73 ] forecasted the demand for a group of perishable dairy products using Autoregressive Integrated Moving Average (ARIMA) and Holt-Winters (HW) models. The results were compared based on mean absolute percentage error (MAPE) and Theil inequality index (U-Theil). The HW model showed a better goodness-of-fit based on both performance metrics.
In case of ARIMA, the accuracy of predictions could diminish where there exists a high level of uncertainty in future patterns of parameters [ 42 , 74 , 75 , 76 ]. HW model forecasting can yield better accuracy in comparison to ARIMA [ 73 ]. HW is simple and easy to use. However, data horizon could not be larger than a seasonal cycle; otherwise, the accuracy of forecasts will decrease sharply. This is due to the fact that inputs of an HW model are themselves predicted values subject to longer-term potential inaccuracies and uncertainties [ 45 , 73 ].
Clustering analysis
Clustering analysis is a data analysis approach that partitions a group of data objects into subgroups based on their similarities. Several applications of clustering analysis has been reported in business analytics, pattern recognition, and web development [ 48 ]. Han et al. [ 48 ] have emphasized the fact that using clustering customers can be organized into groups (clusters), such that customers within a group present similar characteristic.
A key target of demand forecasting is to identify demand behavior of customers. Extraction of similar behavior from historical data leads to recognition of customer clusters or segments. Clustering algorithms such as K-means, self-organizing maps (SOMs), and fuzzy clustering have been used to segment similar customers with respect to their behavior. The clustering enhances the accuracy of SC demand forecasting as the predictions are established for each segment comprised of similar customers. As a limitation, the clustering methods have the tendency to identify the customers, that do not follow a pattern, as outliers [ 74 , 77 ].
Hierarchical forecasts of sales data are performed by clustering and categorization of sales patterns. Multivariate ARIMA models have been used in demand forecasting based on point-of-sales data in industrial bakery chains [ 19 ]. These bakery goods are ordered and clustered daily with a continuous need to demand forecasts in order to avoid both shortage or waste [ 19 ]. Fuel demand forecasting in thermal power plants is another domain with applications of clustering methods. Electricity consumption patterns are derived using a clustering of consumers, and on that basis, demand for the required fuel is established [ 77 ].
K-nearest-neighbor (KNN)
KNN is a method of classification that has been widely used for pattern recognition. KNN algorithm identifies the similarity of a given object to the surrounding objects (called tuples) by generating a similarity index. These tuples are described by n attributes. Thus, each tuple corresponds to a point in an n-dimensional space. The KNN algorithm searches for k tuples that are closest to a given tuple [ 48 ]. These similarity-based classifications will lead to formation of clusters containing similar objects. KNN can also be integrated into regression analysis problems [ 78 ] for dimensionality reduction of the data [ 79 ]. In the realm of demand forecasting in SC, Nikolopoulos et al. [ 80 ] applied KNN for forecasting sporadic demand in an automotive spare parts supply chain. In another study, KNN is used to forecast future trends of demand for Walmart’s supply chain planning [ 81 ].
Artificial neural networks
In artificial neural networks, a set of neurons (input/output units) are connected to one another in different layers in order to establish mapping of the inputs to outputs by finding the underlying correlations between them. The configuration of such networks could become a complex problem, due to a high number of layers and neurons, as well as variability of their types (linear or nonlinear), which needs to follow a data-driven learning process to be established. In doing so, each unit (neuron) will correspond to a weight, that is tuned through a training step [ 48 ]. At the end, a weighted network with minimum number of neurons, that could map the inputs to outputs with a minimum fitting error (deviation), is identified.
As the literature reveals, artificial neural networks (ANN) are widely applied for demand forecasting [ 82 , 83 , 84 , 85 ]. To improve the accuracy of ANN-based demand predictions, Liu et al. [ 86 ] proposed a combination of a grey model and a stacked auto encoder applied to a case study of predicting demand in a Brazilian logistics company subject to transportation disruption [ 87 ]. Amirkolaii et al. [ 88 ] applied neural networks in forecasting spare parts demand to minimize supply chain shortages. In this case of spare parts supply chain, although there were multiple suppliers to satisfy demand for a variety of spare parts, the demand was subject to high variability due to a varying number of customers and their varying needs. Their proposed ANN-based forecasting approach included (1) 1 input demand feature with 1 Stock-Keeping Unit (SKU), (2) 1 input demand feature with all SKUs, (3) 16 input demand features with 1 SKU, and (4) 16 input demand features with all SKUs. They applied neural networks with back propagation and compared the results with a number of benchmarks reporting a Mean Square Error (MSE) for each configuration scenario.
Huang et al. [ 89 ] compared a backpropagation (BP) neural network and a linear regression analysis for forecasting of e-logistics demand in urban and rural areas in China using data from 1997 to 2015. By comparing mean absolute error (MAE) and the average relative errors of backpropagation neural network and linear regression, they showed that backpropagation neural networks could reach higher accuracy (reflecting lower differences between predicted and actual data). This is due to the fact that a Sigmoid function was used as the transfer function in the hidden layer of BP, which is differentiable for nonlinear problems such as the one presented in their case study, whereas the linear regression works well with linear problems.
ANNs have also been applied in demand forecasting for server models with one-week demand prediction ahead of order arrivals. In this regard, Saha et al. [ 90 ] proposed an ANN-based forecasting model using a 52-week time-series data fitted through both BP and Radial Basis Function (RBF) networks. A RBF network is similar to a BP network except for the activation/transfer function in RBF that follows a feed-forward process using a radial basis function. RBF results in faster training and convergence to ANN weights in comparison with BP networks without compromising the forecasting precision.
Researchers have combined ANN-based machine-learning algorithms with optimization models to draw optimal courses of actions, strategies, or decisions for future. Chang et al. [ 91 ] employed a genetic algorithm in the training phase of a neural network using sales/supply chain data in the printed circuit board industry in Taiwan and presented an evolving neural network-forecasting model. They proposed use of a Genetic Algorithms (GA)-based cost function optimization to arrive at the best configuration of the corresponding neural network for sales forecast with respect to prediction precision. The proposed model was then compared to back-propagation and linear regression approaches using three performance indices of MAPE, Mean Absolute Deviation (MAD), and Total Cost Deviation (TCD), presenting its superior prediction precision.
Regression analysis
Regression models are used to generate continuous-valued functions utilized for prediction. These methods are used to predict the value of a response (dependent) variable with respect to one or more predictor (independent) variables. There are various forms of regression analysis, such as linear, multiple, weighted, symbolic (random), polynomial, nonparametric, and robust. The latter approach is useful when errors fail to satisfy normalcy conditions or when we deal with big data that could contain significant number of outliers [ 48 ].
Merkuryeva et al. [ 92 ] analyzed three prediction approaches for demand forecasting in the pharmaceutical industry: a simple moving average model, multiple linear regressions, and a symbolic regression with searches conducted through an evolutionary genetic programming. In this experiment, symbolic regression exhibited the best fit with the lowest error.
As perishable products must be sold due to a very short preservation time, demand forecasting for this type of products has drawn increasing attention. Yang and Sutrisno [ 93 ] applied and compared regression analysis and neural network techniques to derive demand forecasts for perishable goods. They concluded that accurate daily forecasts are achievable with knowledge of sales numbers in the first few hours of the day using either of the above methods.
Support vector machine (SVM)
SVM is an algorithm that uses a nonlinear mapping to transform a set of training data into a higher dimension (data classes). SVM searches for an optimal separating hyper-plane that can separate the resulting class from another) [ 48 ]. Villegas et al. [ 94 ] tested the applicability of SVMs for demand forecasting in household and personal care SCs with a dataset comprised of 229 weekly demand series in the UK. Wu [ 95 ] applied an SVM, using a particle swarm optimization (PSO) to search for the best separating hyper-plane, classifying the data related to car sales and forecasting the demand in each cluster.
Support vector regression (SVR)
Continuous variable classification problems can be solved by support vector regression (SVR), which is a regression implementation of SVM. The main idea behind SVR regression is the computation of a linear regression function within a high-dimensional feature space. SVR has been applied in financial/cost prediction problems, handwritten digit recognition, and speaker identification, object recognition, etc. [ 48 ].
Guanghui [ 96 ] used the SVR method for SC needs prediction. The use of SVR in demand forecasting can yield a lower mean square error than RBF neural networks due to the fact that the optimization (cost) function in SVR does not consider the points beyond a margin of distance from the training set. Therefore, this method leads to higher forecast accuracy, although, similar to SVM, it is only applicable to a two-class problem (such as normal versus anomaly detection/estimation problems). Sarhani and El Afia [ 97 ] sought to forecast SC demand using SVR and applied Particle swarm optimization (PSO) and GA to optimize SVR parameters. SVR-PSO and SVR-GA approaches were compared with respect to accuracy of predictions using MAPE. The results showed a superior performance by PSO in terms time intensity and MAPE when configuring the SVR parameters.
Mixed approaches
Some works in the literature have used a combination of the aforementioned techniques. In these studies, the data flow into a sequence of algorithms and the outputs of one stage become inputs of the next step. The outputs are explanatory in the form of qualitative and quantitative information with a sequence of useful information extracted out of each algorithm. Examples of such studies include [ 15 , 98 , 99 , 100 , 101 , 102 , 103 , 104 , 105 ].
In more complex supply chains with several points of supply, different warehouses, varied customers, and several products, the demand forecasting becomes a high dimensional problem. To address this issue, Islek and Oguducu [ 100 ] applied a clustering technique, called bipartite graph clustering, to analyze the patterns of sales for different products. Then, they combined a moving average model and a Bayesian belief network approaches to improve the accuracy of demand forecasting for each cluster. Kilimci et al. [ 101 ] developed an intelligent demand forecasting system by applying time-series and regression methods, a support vector regression algorithm, and a deep learning model in a sequence. They dealt with a case involving big amount of data accounting for 155 features over 875 million records. First, they used a principal component analysis for dimension reduction. Then, data clustering was performed. This is followed by demand forecasting for each cluster using a novel decision integration strategy called boosting ensemble. They concluded that the combination of a deep neural network with a boosting strategy yielded the best accuracy, minimizing the prediction error for demand forecasting.
Chen and Lu [ 98 ] combined clustering algorithms of SOM, a growing hierarchical self-organizing mapping (GHSOM), and K-means, with two machine-learning techniques of SVR and extreme learning machine (ELM) in sales forecasting of computers. The authors found that the combination of GHSOM and ELM yielded better accuracy and performance in demand forecasts for their computer retailing case study. Difficulties in forecasting also occur in cases with high product variety. For these types of products in an SC, patterns of sales can be extracted for clustered products. Then, for each cluster, a machine-learning technique, such as SVR, can be employed to further improve the prediction accuracy [ 104 ].
Brentan et al. [ 106 ] used and analyzed various BDA techniques for demand prediction; including support vector machines (SVM), and adaptive neural fuzzy inference systems (ANFIS). They combined the predicted values derived from each machine learning techniques, using a linear regression process to arrive at an average prediction value adopted as the benchmark forecast. The performance (accuracy) of each technique is then analyzed with respect to their mean square root error (RMSE) and MAE values obtained through comparing the target values and the predicted ones.
In summary, Table 3 provides an overview of the recent literature on the application of Predictive BDA in demand forecasting.
Discussions
The data produced in SCs contain a great deal of useful knowledge. Analysis of such massive data can help us to forecast trends of customer behavior, markets, prices, and so on. This can help organizations better adapt to competitive environments. To forecast demand in an SC, with the presences of big data, different predictive BDA algorithms have been used. These algorithms could provide predictive analytics using time-series approaches, auto-regressive methods, and associative forecasting methods [ 10 ]. The demand forecasts from these BDA methods could be integrated with product design attributes as well as with online search traffic mapping to incorporate customer and price information [ 37 , 71 ].
Predictive BDA algorithms
Most of the studies examined, developed and used a certain data-mining algorithm for their case studies. However, there are very few comparative studies available in the literature to provide a benchmark for understanding of the advantages and disadvantages of these methodologies. Additionally, as depicted by Table 3 , there is no clear trend between the choice of the BDA algorithm/method and the application domain or category.
Predictive BDA applicability
Most data-driven models used in the literature consider historical data. Such a backward-looking forecasting ignores the new trends and highs and lows in different economic environments. Also, organizational factors, such as reputation and marketing strategies, as well as internal risks (related to availability of SCM resources), could greatly influence the demand [ 107 ] and thus contribute to inaccuracy of BDA-based demand predictions using historical data. Incorporating existing driving factors outside the historical data, such as economic instability, inflation, and purchasing power, could help adjust the predictions with respect to unseen future scenarios of demand. Combining predictive algorithms with optimization or simulation can equip the models with prescriptive capabilities in response to future scenarios and expectations.
Predictive BDA in closed-loop supply chains (CLSC)
The combination of forward and reverse flow of material in a SC is referred to as a closed-loop supply chain (CLSC). A CLSC is a more complex system than a traditional SC because it consists of the forward and reverse SC simultaneously [ 108 ]. Economic impact, environmental impact, and social responsibility are three significant factors in designing a CLSC network with inclusion of product recycling, remanufacturing, and refurbishment functions. The complexity of a CLSC, compared to a common SC, results from the coordination between backward and forward flows. For example, transportation cost, holding cost, and forecasting demand are challenging issues because of uncertainties in the information flows from the forward chain to the reverse one. In addition, the uncertainties about the rate of returned products and efficiencies of recycling, remanufacturing, and refurbishment functions are some of the main barriers in establishing predictions for the reverse flow [ 5 , 6 , 109 ]. As such, one key finding from this literature survey is that CLSCs particularly deal with the lack of quality data for remanufacturing. Remanufacturing refers to the disassembly of products, cleaning, inspection, storage, reconditioning, replacement, and reassembling. As a result of deficiencies in data, optimal scheduling of remanufacturing functions is cumbersome due to uncertainties in the quality and quantity of used products as well as timing of returns and delivery delays.
IoT-based approaches can overcome the difficulties of collecting data in a CLSC. In an IoT environment, objects are monitored and controlled remotely across existing network infrastructures. This enables more direct integration between the physical world and computer-based systems. The results include improved efficiency, accuracy, and economic benefit across SCs [ 50 , 54 , 110 ].
Radio frequency identification (RFID) is another technology that has become very popular in SCs. RFID can be used for automation of processes in an SC, and it is useful for coordination of forecasts in CLSCs with dispersed points of return and varied quantities and qualities of returned used products [ 10 , 111 , 112 , 113 , 114 ].
Conclusions
The growing need to customer behavior analysis and demand forecasting is deriven by globalization and increasing market competitions as well as the surge in supply chain digitization practices. In this study, we performed a thorough review for applications of predictive big data analytics (BDA) in SC demand forecasting. The survey overviewed the BDA methods applied to supply chain demand forecasting and provided a comparative categorization of them. We collected and analyzed these studies with respect to methods and techniques used in demand prediction. Seven mainstream techniques were identified and studied with their pros and cons. The neural networks and regression analysis are observed as the two mostly employed techniques, among others. The review also pointed to the fact that optimization models or simulation can be used to improve the accuracy of forecasting through formulating and optimizing a cost function for the fitting of the predictions to data.
One key finding from reviewing the existing literature was that there is a very limited research conducted on the applications of BDA in CLSC and reverse logistics. There are key benefits in adopting a data-driven approach for design and management of CLSCs. Due to increasing environmental awareness and incentives from the government, nowadays a vast quantity of returned (used) products are collected, which are of various types and conditions, received and sorted in many collection points. These uncertainties have a direct impact on the cost-efficiency of remanufacturing processes, the final price of the refurbished products and the demand for these products [ 115 ]. As such, design and operation of CLSCs present a case for big data analytics from both supply and demand forecasting perspectives.
Availability of data and materials
The paper presents a review of the literature extracted from main scientific databases without presenting data.
Abbreviations
Adaptive neural fuzzy inference systems
Auto regressive integrated moving average
Artificial neural network
- Big data analytics
Backpropagation
Closed-loop supply chain
Extreme learning machine
Enterprise resource planning
Genetic algorithms
Growing hierarchical self-organizing map
Holt-winters
Internet of things
K-nearest-neighbor
Mean absolute deviation
Mean absolute error
Mean absolute percentage error
Mean square error
Mean square root error
Radial basis function
Particle swarm optimization
Self-organizing maps
Stock-keeping unit
Supply chain analytics
Supply chain
- Supply chain management
Support vector machine
Support vector regression
Total cost deviation
Theil inequality index
You Z, Si Y-W, Zhang D, Zeng X, Leung SCH, Li T. A decision-making framework for precision marketing. Expert Syst Appl. 2015;42(7):3357–67. https://doi.org/10.1016/J.ESWA.2014.12.022 .
Article Google Scholar
Guo ZX, Wong WK, Li M. A multivariate intelligent decision-making model for retail sales forecasting. Decis Support Syst. 2013;55(1):247–55. https://doi.org/10.1016/J.DSS.2013.01.026 .
Wei J-T, Lee M-C, Chen H-K, Wu H-H. Customer relationship management in the hairdressing industry: an application of data mining techniques. Expert Syst Appl. 2013;40(18):7513–8. https://doi.org/10.1016/J.ESWA.2013.07.053 .
Lu LX, Swaminathan JM. Supply chain management. Int Encycl Soc Behav Sci. 2015. https://doi.org/10.1016/B978-0-08-097086-8.73032-7 .
Gholizadeh H, Tajdin A, Javadian N. A closed-loop supply chain robust optimization for disposable appliances. Neural Comput Appl. 2018. https://doi.org/10.1007/s00521-018-3847-9 .
Tosarkani BM, Amin SH. A possibilistic solution to configure a battery closed-loop supply chain: multi-objective approach. Expert Syst Appl. 2018;92:12–26. https://doi.org/10.1016/J.ESWA.2017.09.039 .
Blackburn R, Lurz K, Priese B, Göb R, Darkow IL. A predictive analytics approach for demand forecasting in the process industry. Int Trans Oper Res. 2015;22(3):407–28. https://doi.org/10.1111/itor.12122 .
Article MathSciNet MATH Google Scholar
Boulaksil Y. Safety stock placement in supply chains with demand forecast updates. Oper Res Perspect. 2016;3:27–31. https://doi.org/10.1016/J.ORP.2016.07.001 .
Article MathSciNet Google Scholar
Tang CS. Perspectives in supply chain risk management. Int J Prod Econ. 2006;103(2):451–88. https://doi.org/10.1016/J.IJPE.2005.12.006 .
Wang G, Gunasekaran A, Ngai EWT, Papadopoulos T. Big data analytics in logistics and supply chain management: certain investigations for research and applications. Int J Prod Econ. 2016;176:98–110. https://doi.org/10.1016/J.IJPE.2016.03.014 .
Awwad M, Kulkarni P, Bapna R, Marathe A. Big data analytics in supply chain: a literature review. In: Proceedings of the international conference on industrial engineering and operations management, 2018(SEP); 2018, p. 418–25.
Büyüközkan G, Göçer F. Digital Supply Chain: literature review and a proposed framework for future research. Comput Ind. 2018;97:157–77.
Kshetri N. 1 Blockchain’s roles in meeting key supply chain management objectives. Int J Inf Manage. 2018;39:80–9.
Michna Z, Disney SM, Nielsen P. The impact of stochastic lead times on the bullwhip effect under correlated demand and moving average forecasts. Omega. 2019. https://doi.org/10.1016/J.OMEGA.2019.02.002 .
Zhu Y, Zhao Y, Zhang J, Geng N, Huang D. Spring onion seed demand forecasting using a hybrid Holt-Winters and support vector machine model. PLoS ONE. 2019;14(7):e0219889. https://doi.org/10.1371/journal.pone.0219889 .
Govindan K, Cheng TCE, Mishra N, Shukla N. Big data analytics and application for logistics and supply chain management. Transport Res Part E Logist Transport Rev. 2018;114:343–9. https://doi.org/10.1016/J.TRE.2018.03.011 .
Bohanec M, Kljajić Borštnar M, Robnik-Šikonja M. Explaining machine learning models in sales predictions. Expert Syst Appl. 2017;71:416–28. https://doi.org/10.1016/J.ESWA.2016.11.010 .
Constante F, Silva F, Pereira A. DataCo smart supply chain for big data analysis. Mendeley Data. 2019. https://doi.org/10.17632/8gx2fvg2k6.5 .
Huber J, Gossmann A, Stuckenschmidt H. Cluster-based hierarchical demand forecasting for perishable goods. Expert Syst Appl. 2017;76:140–51. https://doi.org/10.1016/J.ESWA.2017.01.022 .
Ali MM, Babai MZ, Boylan JE, Syntetos AA. Supply chain forecasting when information is not shared. Eur J Oper Res. 2017;260(3):984–94. https://doi.org/10.1016/J.EJOR.2016.11.046 .
Bian W, Shang J, Zhang J. Two-way information sharing under supply chain competition. Int J Prod Econ. 2016;178:82–94. https://doi.org/10.1016/J.IJPE.2016.04.025 .
Mourtzis D. Challenges and future perspectives for the life cycle of manufacturing networks in the mass customisation era. Logist Res. 2016;9(1):2.
Nguyen T, Zhou L, Spiegler V, Ieromonachou P, Lin Y. Big data analytics in supply chain management: a state-of-the-art literature review. Comput Oper Res. 2018;98:254–64. https://doi.org/10.1016/J.COR.2017.07.004 .
Choi Y, Lee H, Irani Z. Big data-driven fuzzy cognitive map for prioritising IT service procurement in the public sector. Ann Oper Res. 2018;270(1–2):75–104. https://doi.org/10.1007/s10479-016-2281-6 .
Huang YY, Handfield RB. Measuring the benefits of erp on supply management maturity model: a “big data” method. Int J Oper Prod Manage. 2015;35(1):2–25. https://doi.org/10.1108/IJOPM-07-2013-0341 .
Miroslav M, Miloš M, Velimir Š, Božo D, Đorđe L. Semantic technologies on the mission: preventing corruption in public procurement. Comput Ind. 2014;65(5):878–90. https://doi.org/10.1016/J.COMPIND.2014.02.003 .
Zhang Y, Ren S, Liu Y, Si S. A big data analytics architecture for cleaner manufacturing and maintenance processes of complex products. J Clean Prod. 2017;142:626–41. https://doi.org/10.1016/J.JCLEPRO.2016.07.123 .
Shu Y, Ming L, Cheng F, Zhang Z, Zhao J. Abnormal situation management: challenges and opportunities in the big data era. Comput Chem Eng. 2016;91:104–13. https://doi.org/10.1016/J.COMPCHEMENG.2016.04.011 .
Krumeich J, Werth D, Loos P. Prescriptive control of business processes: new potentials through predictive analytics of big data in the process manufacturing industry. Bus Inform Syst Eng. 2016;58(4):261–80. https://doi.org/10.1007/s12599-015-0412-2 .
Guo SY, Ding LY, Luo HB, Jiang XY. A Big-Data-based platform of workers’ behavior: observations from the field. Accid Anal Prev. 2016;93:299–309. https://doi.org/10.1016/J.AAP.2015.09.024 .
Chuang Y-F, Chia S-H, Wong J-Y. Enhancing order-picking efficiency through data mining and assignment approaches. WSEAS Transactions on Business and Economics. 2014;11(1):52–64.
Google Scholar
Ballestín F, Pérez Á, Lino P, Quintanilla S, Valls V. Static and dynamic policies with RFID for the scheduling of retrieval and storage warehouse operations. Comput Ind Eng. 2013;66(4):696–709. https://doi.org/10.1016/J.CIE.2013.09.020 .
Alyahya S, Wang Q, Bennett N. Application and integration of an RFID-enabled warehousing management system—a feasibility study. J Ind Inform Integr. 2016;4:15–25. https://doi.org/10.1016/J.JII.2016.08.001 .
Cui J, Liu F, Hu J, Janssens D, Wets G, Cools M. Identifying mismatch between urban travel demand and transport network services using GPS data: a case study in the fast growing Chinese city of Harbin. Neurocomputing. 2016;181:4–18. https://doi.org/10.1016/J.NEUCOM.2015.08.100 .
Shan Z, Zhu Q. Camera location for real-time traffic state estimation in urban road network using big GPS data. Neurocomputing. 2015;169:134–43. https://doi.org/10.1016/J.NEUCOM.2014.11.093 .
Ting SL, Tse YK, Ho GTS, Chung SH, Pang G. Mining logistics data to assure the quality in a sustainable food supply chain: a case in the red wine industry. Int J Prod Econ. 2014;152:200–9. https://doi.org/10.1016/J.IJPE.2013.12.010 .
Jun S-P, Park D-H, Yeom J. The possibility of using search traffic information to explore consumer product attitudes and forecast consumer preference. Technol Forecast Soc Chang. 2014;86:237–53. https://doi.org/10.1016/J.TECHFORE.2013.10.021 .
He W, Wu H, Yan G, Akula V, Shen J. A novel social media competitive analytics framework with sentiment benchmarks. Inform Manage. 2015;52(7):801–12. https://doi.org/10.1016/J.IM.2015.04.006 .
Marine-Roig E, Anton Clavé S. Tourism analytics with massive user-generated content: a case study of Barcelona. J Destination Market Manage. 2015;4(3):162–72. https://doi.org/10.1016/J.JDMM.2015.06.004 .
Carbonneau R, Laframboise K, Vahidov R. Application of machine learning techniques for supply chain demand forecasting. Eur J Oper Res. 2008;184(3):1140–54. https://doi.org/10.1016/J.EJOR.2006.12.004 .
Article MATH Google Scholar
Munir K. Cloud computing and big data: technologies, applications and security, vol. 49. Berlin: Springer; 2019.
Rostami-Tabar B, Babai MZ, Ali M, Boylan JE. The impact of temporal aggregation on supply chains with ARMA(1,1) demand processes. Eur J Oper Res. 2019;273(3):920–32. https://doi.org/10.1016/J.EJOR.2018.09.010 .
Beyer MA, Laney D. The importance of ‘big data’: a definition. Stamford: Gartner; 2012. p. 2014–8.
Benabdellah AC, Benghabrit A, Bouhaddou I, Zemmouri EM. Big data for supply chain management: opportunities and challenges. In: Proceedings of IEEE/ACS international conference on computer systems and applications, AICCSA, no. 11, p. 20–26; 2016. https://doi.org/10.1109/AICCSA.2016.7945828 .
Kumar M. Applied big data analytics in operations management. Appl Big Data Anal Oper Manage. 2016. https://doi.org/10.4018/978-1-5225-0886-1 .
Zhong RY, Huang GQ, Lan S, Dai QY, Chen X, Zhang T. A big data approach for logistics trajectory discovery from RFID-enabled production data. Int J Prod Econ. 2015;165:260–72. https://doi.org/10.1016/J.IJPE.2015.02.014 .
Varela IR, Tjahjono B. Big data analytics in supply chain management: trends and related research. In: 6th international conference on operations and supply chain management, vol. 1, no. 1, p. 2013–4; 2014. https://doi.org/10.13140/RG.2.1.4935.2563 .
Han J, Kamber M, Pei J. Data mining: concepts and techniques. Burlington: Morgan Kaufmann Publishers; 2013. https://doi.org/10.1016/B978-0-12-381479-1.00001-0 .
Book MATH Google Scholar
Arunachalam D, Kumar N. Benefit-based consumer segmentation and performance evaluation of clustering approaches: an evidence of data-driven decision-making. Expert Syst Appl. 2018;111:11–34. https://doi.org/10.1016/J.ESWA.2018.03.007 .
Chase CW. Next generation demand management: people, process, analytics, and technology. Hoboken: Wiley; 2016.
Book Google Scholar
SAS Institute. Demand-driven forecasting and planning: take responsiveness to the next level. 13; 2014. https://www.sas.com/content/dam/SAS/en_us/doc/whitepaper2/demand-driven-forecasting-planning-107477.pdf .
Acar Y, Gardner ES. Forecasting method selection in a global supply chain. Int J Forecast. 2012;28(4):842–8. https://doi.org/10.1016/J.IJFORECAST.2011.11.003 .
Ma S, Fildes R, Huang T. Demand forecasting with high dimensional data: the case of SKU retail sales forecasting with intra- and inter-category promotional information. Eur J Oper Res. 2016;249(1):245–57. https://doi.org/10.1016/J.EJOR.2015.08.029 .
Addo-Tenkorang R, Helo PT. Big data applications in operations/supply-chain management: a literature review. Comput Ind Eng. 2016;101:528–43. https://doi.org/10.1016/J.CIE.2016.09.023 .
Agrawal S, Singh RK, Murtaza Q. A literature review and perspectives in reverse logistics. Resour Conserv Recycl. 2015;97:76–92. https://doi.org/10.1016/J.RESCONREC.2015.02.009 .
Gunasekaran A, Kumar Tiwari M, Dubey R, Fosso Wamba S. Big data and predictive analytics applications in supply chain management. Comput Ind Eng. 2016;101:525–7. https://doi.org/10.1016/J.CIE.2016.10.020 .
Hazen BT, Skipper JB, Ezell JD, Boone CA. Big data and predictive analytics for supply chain sustainability: a theory-driven research agenda. Comput Ind Eng. 2016;101:592–8. https://doi.org/10.1016/J.CIE.2016.06.030 .
Hofmann E, Rutschmann E. Big data analytics and demand forecasting in supply chains: a conceptual analysis. Int J Logist Manage. 2018;29(2):739–66. https://doi.org/10.1108/IJLM-04-2017-0088 .
Jain A, Sanders NR. Forecasting sales in the supply chain: consumer analytics in the big data era. Int J Forecast. 2019;35(1):170–80. https://doi.org/10.1016/J.IJFORECAST.2018.09.003 .
Jin J, Liu Y, Ji P, Kwong CK. Review on recent advances in information mining from big consumer opinion data for product design. J Comput Inf Sci Eng. 2018;19(1):010801. https://doi.org/10.1115/1.4041087 .
Kumar R, Mahto D. Industrial forecasting support systems and technologies in practice: a review. Glob J Res Eng. 2013;13(4):17–33.
MathSciNet Google Scholar
Mishra D, Gunasekaran A, Papadopoulos T, Childe SJ. Big Data and supply chain management: a review and bibliometric analysis. Ann Oper Res. 2016;270(1):313–36. https://doi.org/10.1007/s10479-016-2236-y .
Ren S, Zhang Y, Liu Y, Sakao T, Huisingh D, Almeida CMVB. A comprehensive review of big data analytics throughout product lifecycle to support sustainable smart manufacturing: a framework, challenges and future research directions. J Clean Prod. 2019;210:1343–65. https://doi.org/10.1016/J.JCLEPRO.2018.11.025 .
Singh Jain AD, Mehta I, Mitra J, Agrawal S. Application of big data in supply chain management. Mater Today Proc. 2017;4(2):1106–15. https://doi.org/10.1016/J.MATPR.2017.01.126 .
Souza GC. Supply chain analytics. Bus Horiz. 2014;57(5):595–605. https://doi.org/10.1016/J.BUSHOR.2014.06.004 .
Tiwari S, Wee HM, Daryanto Y. Big data analytics in supply chain management between 2010 and 2016: insights to industries. Comput Ind Eng. 2018;115:319–30. https://doi.org/10.1016/J.CIE.2017.11.017 .
Zhong RY, Newman ST, Huang GQ, Lan S. Big Data for supply chain management in the service and manufacturing sectors: challenges, opportunities, and future perspectives. Comput Ind Eng. 2016;101:572–91. https://doi.org/10.1016/J.CIE.2016.07.013 .
Ramanathan U, Subramanian N, Parrott G. Role of social media in retail network operations and marketing to enhance customer satisfaction. Int J Oper Prod Manage. 2017;37(1):105–23. https://doi.org/10.1108/IJOPM-03-2015-0153 .
Coursera. Supply chain planning. Coursera E-Learning; 2019. https://www.coursera.org/learn/planning .
Villegas MA, Pedregal DJ. Supply chain decision support systems based on a novel hierarchical forecasting approach. Decis Support Syst. 2018;114:29–36. https://doi.org/10.1016/J.DSS.2018.08.003 .
Ma J, Kwak M, Kim HM. Demand trend mining for predictive life cycle design. J Clean Prod. 2014;68:189–99. https://doi.org/10.1016/J.JCLEPRO.2014.01.026 .
Hamiche K, Abouaïssa H, Goncalves G, Hsu T. A robust and easy approach for demand forecasting in supply chains. IFAC-PapersOnLine. 2018;51(11):1732–7. https://doi.org/10.1016/J.IFACOL.2018.08.206 .
Da Veiga CP, Da Veiga CRP, Catapan A, Tortato U, Da Silva WV. Demand forecasting in food retail: a comparison between the Holt-Winters and ARIMA models. WSEAS Trans Bus Econ. 2014;11(1):608–14.
Murray PW, Agard B, Barajas MA. Forecasting supply chain demand by clustering customers. IFAC-PapersOnLine. 2015;48(3):1834–9. https://doi.org/10.1016/J.IFACOL.2015.06.353 .
Ramos P, Santos N, Rebelo R. Performance of state space and ARIMA models for consumer retail sales forecasting. Robot Comput Integr Manuf. 2015;34:151–63. https://doi.org/10.1016/J.RCIM.2014.12.015 .
Schaer O, Kourentzes N. Demand forecasting with user-generated online information. Int J Forecast. 2019;35(1):197–212. https://doi.org/10.1016/J.IJFORECAST.2018.03.005 .
Pang Y, Yao B, Zhou X, Zhang Y, Xu Y, Tan Z. Hierarchical electricity time series forecasting for integrating consumption patterns analysis and aggregation consistency; 2018. In: IJCAI international joint conference on artificial intelligence; 2018, p. 3506–12.
Goyal R, Chandra P, Singh Y. Suitability of KNN regression in the development of interaction based software fault prediction models. IERI Procedia. 2014;6:15–21. https://doi.org/10.1016/J.IERI.2014.03.004 .
Runkler TA. Data analytics (models and algorithms for intelligent data analysis). In: Revista Espanola de las Enfermedades del Aparato Digestivo (Vol. 26, Issue 4). Springer Fachmedien Wiesbaden; 2016. https://doi.org/10.1007/978-3-658-14075-5 .
Nikolopoulos KI, Babai MZ, Bozos K. Forecasting supply chain sporadic demand with nearest neighbor approaches. Int J Prod Econ. 2016;177:139–48. https://doi.org/10.1016/j.ijpe.2016.04.013 .
Gaur M, Goel S, Jain E. Comparison between nearest Neighbours and Bayesian network for demand forecasting in supply chain management. In: 2015 international conference on computing for sustainable global development, INDIACom 2015, May; 2015, p. 1433–6.
Burney SMA, Ali SM, Burney S. A survey of soft computing applications for decision making in supply chain management. In: 2017 IEEE 3rd international conference on engineering technologies and social sciences, ICETSS 2017, 2018, p. 1–6. https://doi.org/10.1109/ICETSS.2017.8324158 .
González Perea R, Camacho Poyato E, Montesinos P, Rodríguez Díaz JA. Optimisation of water demand forecasting by artificial intelligence with short data sets. Biosyst Eng. 2019;177:59–66. https://doi.org/10.1016/J.BIOSYSTEMSENG.2018.03.011 .
Vhatkar S, Dias J. Oral-care goods sales forecasting using artificial neural network model. Procedia Comput Sci. 2016;79:238–43. https://doi.org/10.1016/J.PROCS.2016.03.031 .
Wong WK, Guo ZX. A hybrid intelligent model for medium-term sales forecasting in fashion retail supply chains using extreme learning machine and harmony search algorithm. Int J Prod Econ. 2010;128(2):614–24. https://doi.org/10.1016/J.IJPE.2010.07.008 .
Liu C, Shu T, Chen S, Wang S, Lai KK, Gan L. An improved grey neural network model for predicting transportation disruptions. Expert Syst Appl. 2016;45:331–40. https://doi.org/10.1016/J.ESWA.2015.09.052 .
Yuan WJ, Chen JH, Cao JJ, Jin ZY. Forecast of logistics demand based on grey deep neural network model. Proc Int Conf Mach Learn Cybern. 2018;1:251–6. https://doi.org/10.1109/ICMLC.2018.8527006 .
Amirkolaii KN, Baboli A, Shahzad MK, Tonadre R. Demand forecasting for irregular demands in business aircraft spare parts supply chains by using artificial intelligence (AI). IFAC-PapersOnLine. 2017;50(1):15221–6. https://doi.org/10.1016/J.IFACOL.2017.08.2371 .
Huang L, Xie G, Li D, Zou C. Predicting and analyzing e-logistics demand in urban and rural areas: an empirical approach on historical data of China. Int J Performabil Eng. 2018;14(7):1550–9. https://doi.org/10.23940/ijpe.18.07.p19.15501559 .
Saha C, Lam SS, Boldrin W. Demand forecasting for server manufacturing using neural networks. In: Proceedings of the 2014 industrial and systems engineering research conference, June 2014; 2015.
Chang P-C, Wang Y-W, Tsai C-Y. Evolving neural network for printed circuit board sales forecasting. Expert Syst Appl. 2005;29(1):83–92. https://doi.org/10.1016/J.ESWA.2005.01.012 .
Merkuryeva G, Valberga A, Smirnov A. Demand forecasting in pharmaceutical supply chains: a case study. Procedia Comput Sci. 2019;149:3–10. https://doi.org/10.1016/J.PROCS.2019.01.100 .
Yang CL, Sutrisno H. Short-term sales forecast of perishable goods for franchise business. In: 2018 10th international conference on knowledge and smart technology: cybernetics in the next decades, KST 2018, p. 101–5; 2018. https://doi.org/10.1109/KST.2018.8426091 .
Villegas MA, Pedregal DJ, Trapero JR. A support vector machine for model selection in demand forecasting applications. Comput Ind Eng. 2018;121:1–7. https://doi.org/10.1016/J.CIE.2018.04.042 .
Wu Q. The hybrid forecasting model based on chaotic mapping, genetic algorithm and support vector machine. Expert Syst Appl. 2010;37(2):1776–83. https://doi.org/10.1016/J.ESWA.2009.07.054 .
Guanghui W. Demand forecasting of supply chain based on support vector regression method. Procedia Eng. 2012;29:280–4. https://doi.org/10.1016/J.PROENG.2011.12.707 .
Sarhani M, El Afia A. Intelligent system based support vector regression for supply chain demand forecasting. In: 2014 2nd world conference on complex systems, WCCS 2014; 2015, p. 79–83. https://doi.org/10.1109/ICoCS.2014.7060941 .
Chen IF, Lu CJ. Sales forecasting by combining clustering and machine-learning techniques for computer retailing. Neural Comput Appl. 2017;28(9):2633–47. https://doi.org/10.1007/s00521-016-2215-x .
Fasli M, Kovalchuk Y. Learning approaches for developing successful seller strategies in dynamic supply chain management. Inf Sci. 2011;181(16):3411–26. https://doi.org/10.1016/J.INS.2011.04.014 .
Islek I, Oguducu SG. A retail demand forecasting model based on data mining techniques. In: IEEE international symposium on industrial electronics; 2015, p. 55–60. https://doi.org/10.1109/ISIE.2015.7281443 .
Kilimci ZH, Akyuz AO, Uysal M, Akyokus S, Uysal MO, Atak Bulbul B, Ekmis MA. An improved demand forecasting model using deep learning approach and proposed decision integration strategy for supply chain. Complexity. 2019;2019:1–15. https://doi.org/10.1155/2019/9067367 .
Loureiro ALD, Miguéis VL, da Silva LFM. Exploring the use of deep neural networks for sales forecasting in fashion retail. Decis Support Syst. 2018;114:81–93. https://doi.org/10.1016/J.DSS.2018.08.010 .
Punam K, Pamula R, Jain PK. A two-level statistical model for big mart sales prediction. In: 2018 international conference on computing, power and communication technologies, GUCON 2018; 2019. https://doi.org/10.1109/GUCON.2018.8675060 .
Puspita PE, İnkaya T, Akansel M. Clustering-based Sales Forecasting in a Forklift Distributor. In: Uluslararası Muhendislik Arastirma ve Gelistirme Dergisi, 1–17; 2019. https://doi.org/10.29137/umagd.473977 .
Thomassey S. Sales forecasts in clothing industry: the key success factor of the supply chain management. Int J Prod Econ. 2010;128(2):470–83. https://doi.org/10.1016/J.IJPE.2010.07.018 .
Brentan BM, Ribeiro L, Izquierdo J, Ambrosio JK, Luvizotto E, Herrera M. Committee machines for hourly water demand forecasting in water supply systems. Math Probl Eng. 2019;2019:1–11. https://doi.org/10.1155/2019/9765468 .
Mafakheri F, Breton M, Chauhan S. Project-to-organization matching: an integrated risk assessment approach. Int J IT Project Manage. 2012;3(3):45–59. https://doi.org/10.4018/jitpm.2012070104 .
Mafakheri F, Nasiri F. Revenue sharing coordination in reverse logistics. J Clean Prod. 2013;59:185–96. https://doi.org/10.1016/J.JCLEPRO.2013.06.031 .
Bogataj M. Closed Loop Supply Chain (CLSC): economics, modelling, management and control. Int J Prod Econ. 2017;183:319–21. https://doi.org/10.1016/J.IJPE.2016.11.020 .
Hopkins J, Hawking P. Big Data Analytics and IoT in logistics: a case study. Int J Logist Manage. 2018;29(2):575–91. https://doi.org/10.1108/IJLM-05-2017-0109 .
de Oliveira CM, Soares PJSR, Morales G, Arica J, Matias IO. RFID and its applications on supply chain in Brazil: a structured literature review (2006–2016). Espacios. 2017;38(31). https://www.scopus.com/inward/record.uri?eid=2-s2.0-85021922345&partnerID=40&md5=f062191611541391ded4cdb73eea55cb .
Griva A, Bardaki C, Pramatari K, Papakiriakopoulos D. Retail business analytics: customer visit segmentation using market basket data. Expert Syst Appl. 2018;100:1–16. https://doi.org/10.1016/J.ESWA.2018.01.029 .
Lee CKM, Ho W, Ho GTS, Lau HCW. Design and development of logistics workflow systems for demand management with RFID. Expert Syst Appl. 2011;38(5):5428–37. https://doi.org/10.1016/J.ESWA.2010.10.012 .
Mohebi E, Marquez L. Application of machine learning and RFID in the stability optimization of perishable foods; 2008.
Jiao Z, Ran L, Zhang Y, Li Z, Zhang W. Data-driven approaches to integrated closed-loop sustainable supply chain design under multi-uncertainties. J Clean Prod. 2018;185:105–27.
Levis AA, Papageorgiou LG. Customer demand forecasting via support vector regression analysis. Chem Eng Res Des. 2005;83(8):1009–18. https://doi.org/10.1205/CHERD.04246 .
Chi H-M, Ersoy OK, Moskowitz H, Ward J. Modeling and optimizing a vendor managed replenishment system using machine learning and genetic algorithms. Eur J Oper Res. 2007;180(1):174–93. https://doi.org/10.1016/J.EJOR.2006.03.040 .
Sun Z-L, Choi T-M, Au K-F, Yu Y. Sales forecasting using extreme learning machine with applications in fashion retailing. Decis Support Syst. 2008;46(1):411–9. https://doi.org/10.1016/J.DSS.2008.07.009 .
Efendigil T, Önüt S, Kahraman C. A decision support system for demand forecasting with artificial neural networks and neuro-fuzzy models: a comparative analysis. Expert Syst Appl. 2009;36(3):6697–707. https://doi.org/10.1016/J.ESWA.2008.08.058 .
Lee CC, Ou-Yang C. A neural networks approach for forecasting the supplier’s bid prices in supplier selection negotiation process. Expert Syst Appl. 2009;36(2):2961–70. https://doi.org/10.1016/J.ESWA.2008.01.063 .
Chen F-L, Chen Y-C, Kuo J-Y. Applying Moving back-propagation neural network and Moving fuzzy-neuron network to predict the requirement of critical spare parts. Expert Syst Appl. 2010;37(9):6695–704. https://doi.org/10.1016/J.ESWA.2010.04.037 .
Wu Q. Product demand forecasts using wavelet kernel support vector machine and particle swarm optimization in manufacture system. J Comput Appl Math. 2010;233(10):2481–91. https://doi.org/10.1016/J.CAM.2009.10.030 .
Babai MZ, Ali MM, Boylan JE, Syntetos AA. Forecasting and inventory performance in a two-stage supply chain with ARIMA(0,1,1) demand: theory and empirical analysis. Int J Prod Econ. 2013;143(2):463–71. https://doi.org/10.1016/J.IJPE.2011.09.004 .
Kourentzes N. Intermittent demand forecasts with neural networks. Int J Prod Econ. 2013;143(1):198–206. https://doi.org/10.1016/J.IJPE.2013.01.009 .
Lau HCW, Ho GTS, Zhao Y. A demand forecast model using a combination of surrogate data analysis and optimal neural network approach. Decis Support Syst. 2013;54(3):1404–16. https://doi.org/10.1016/J.DSS.2012.12.008 .
Arunraj NS, Ahrens D. A hybrid seasonal autoregressive integrated moving average and quantile regression for daily food sales forecasting. Int J Prod Econ. 2015;170:321–35. https://doi.org/10.1016/J.IJPE.2015.09.039 .
Di Pillo G, Latorre V, Lucidi S, Procacci E. An application of support vector machines to sales forecasting under promotions. 4OR. 2016. https://doi.org/10.1007/s10288-016-0316-0 .
da Veiga CP, da Veiga CRP, Puchalski W, dos Coelho LS, Tortato U. Demand forecasting based on natural computing approaches applied to the foodstuff retail segment. J Retail Consumer Serv. 2016;31:174–81. https://doi.org/10.1016/J.JRETCONSER.2016.03.008 .
Chawla A, Singh A, Lamba A, Gangwani N, Soni U. Demand forecasting using artificial neural networks—a case study of American retail corporation. In: Applications of artificial intelligence techniques in wind power generation. Integrated Computer-Aided Engineering; 2018, p. 79–90. https://doi.org/10.3233/ica-2001-8305 .
Pereira MM, Machado RL, Ignacio Pires SR, Pereira Dantas MJ, Zaluski PR, Frazzon EM. Forecasting scrap tires returns in closed-loop supply chains in Brazil. J Clean Prod. 2018;188:741–50. https://doi.org/10.1016/J.JCLEPRO.2018.04.026 .
Fanoodi B, Malmir B, Jahantigh FF. Reducing demand uncertainty in the platelet supply chain through artificial neural networks and ARIMA models. Comput Biol Med. 2019;113:103415. https://doi.org/10.1016/J.COMPBIOMED.2019.103415 .
Sharma R, Singhal P. Demand forecasting of engine oil for automotive and industrial lubricant manufacturing company using neural network. Mater Today Proc. 2019;18:2308–14. https://doi.org/10.1016/J.MATPR.2019.07.013 .
Tanizaki T, Hoshino T, Shimmura T, Takenaka T. Demand forecasting in restaurants using machine learning and statistical analysis. Procedia CIRP. 2019;79:679–83. https://doi.org/10.1016/J.PROCIR.2019.02.042 .
Wang C-H, Chen J-Y. Demand forecasting and financial estimation considering the interactive dynamics of semiconductor supply-chain companies. Comput Ind Eng. 2019;138:106104. https://doi.org/10.1016/J.CIE.2019.106104 .
Download references
Acknowledgements
The authors are very much thankful to anonymous reviewers whose comments and suggestion were very helpful in improving the quality of the manuscript.
Author information
Authors and affiliations.
Concordia Institute for Information Systems Engineering (CIISE), Concordia University, Montreal, H3G 1M8, Canada
Mahya Seyedan & Fereshteh Mafakheri
You can also search for this author in PubMed Google Scholar
Contributions
The authors contributed equally to the writing of the paper. First author conducted the literature search. Both authors read and approved the final manuscript.
Corresponding author
Correspondence to Fereshteh Mafakheri .
Ethics declarations
Ethics approval.
Not applicable.
Competing interests
The authors declare no competing or conflicting interests.
Additional information
Publisher's note.
Springer Nature remains neutral with regard to jurisdictional claims in published maps and institutional affiliations.
Rights and permissions
Open Access This article is licensed under a Creative Commons Attribution 4.0 International License, which permits use, sharing, adaptation, distribution and reproduction in any medium or format, as long as you give appropriate credit to the original author(s) and the source, provide a link to the Creative Commons licence, and indicate if changes were made. The images or other third party material in this article are included in the article's Creative Commons licence, unless indicated otherwise in a credit line to the material. If material is not included in the article's Creative Commons licence and your intended use is not permitted by statutory regulation or exceeds the permitted use, you will need to obtain permission directly from the copyright holder. To view a copy of this licence, visit http://creativecommons.org/licenses/by/4.0/ .
Reprints and permissions
About this article
Cite this article.
Seyedan, M., Mafakheri, F. Predictive big data analytics for supply chain demand forecasting: methods, applications, and research opportunities. J Big Data 7 , 53 (2020). https://doi.org/10.1186/s40537-020-00329-2
Download citation
Received : 05 April 2020
Accepted : 17 July 2020
Published : 25 July 2020
DOI : https://doi.org/10.1186/s40537-020-00329-2
Share this article
Anyone you share the following link with will be able to read this content:
Sorry, a shareable link is not currently available for this article.
Provided by the Springer Nature SharedIt content-sharing initiative
- Demand forecasting
- Closed-loop supply chains
- Machine-learning
Root out friction in every digital experience, super-charge conversion rates, and optimize digital self-service
Uncover insights from any interaction, deliver AI-powered agent coaching, and reduce cost to serve
Increase revenue and loyalty with real-time insights and recommendations delivered to teams on the ground
Know how your people feel and empower managers to improve employee engagement, productivity, and retention
Take action in the moments that matter most along the employee journey and drive bottom line growth
Whatever they’re are saying, wherever they’re saying it, know exactly what’s going on with your people
Get faster, richer insights with qual and quant tools that make powerful market research available to everyone
Run concept tests, pricing studies, prototyping + more with fast, powerful studies designed by UX research experts
Track your brand performance 24/7 and act quickly to respond to opportunities and challenges in your market
Explore the platform powering Experience Management
- Free Account
- For Digital
- For Customer Care
- For Human Resources
- For Researchers
- Financial Services
- All Industries
Popular Use Cases
- Customer Experience
- Employee Experience
- Net Promoter Score
- Voice of Customer
- Customer Success Hub
- Product Documentation
- Training & Certification
- XM Institute
- Popular Resources
- Customer Stories
- Artificial Intelligence
- Market Research
- Partnerships
- Marketplace
The annual gathering of the experience leaders at the world’s iconic brands building breakthrough business results, live in Salt Lake City.
- English/AU & NZ
- Español/Europa
- Español/América Latina
- Português Brasileiro
- REQUEST DEMO
- Experience Management
- Survey Data Analysis & Reporting
- Predictive Analytics
Predictive analytics definition
Predictive analytics vs other types of business analytics, predictive analytics – a human trait, what are predictive models, the importance of current data, how does predictive analytics work, predictive analytics benefits in commercial business, predictive analytics: operational benefits, predictive analytics: getting started, how expert partners can help, predictive analytics with qualtrics, try qualtrics for free, what is predictive analytics.
19 min read Predictive analytics combines powerful statistical techniques and artificial intelligence to help you anticipate future outcomes. Here’s how predictive analytics works, what it can do for your business, and how you can use it to stay one step ahead of trouble.
Predictive analytics is the art of using historical and current data to make projections about what might happen in the future. By looking at what’s happening in the present and what has happened historically, and then applying statistical analysis techniques to the data, researchers can make predictions about what the future might hold.
Predictive analytics is used in a wide variety of business contexts, including experience management programs, to model the impact of possible future actions on a business. Using predictive analytics can transform the way organizations make decisions, since they’re able to ‘foresee’ the results of possible courses of action before choosing which path to take.
Of course, predictive analytics isn’t foolproof. Sometimes the predictions will be wrong, although it still presents a powerful alternative to blind guesses.
Automatically identify at-risk customers and take action with Predict iQ
Predictive analysis sits alongside a few other types of data analysis which are increasingly becoming mainstream in the world of business. It can be easy to get them confused, especially when the names are used interchangeably. Here’s a quick glossary of the main types.
- Descriptive analytics tells you what has been happening leading up to the present
- Real-time analytics gives you moment-by-moment data on what is happening
- Diagnostic analytics helps you assess the causal factors relating to an event or situation
- Predictive analytics uses past and current events to forecast what might happen next
- Prescriptive analytics makes recommendations on the best course of action in the future. Prescriptive analytics can be considered one of the most advanced forms of predictive analytics.
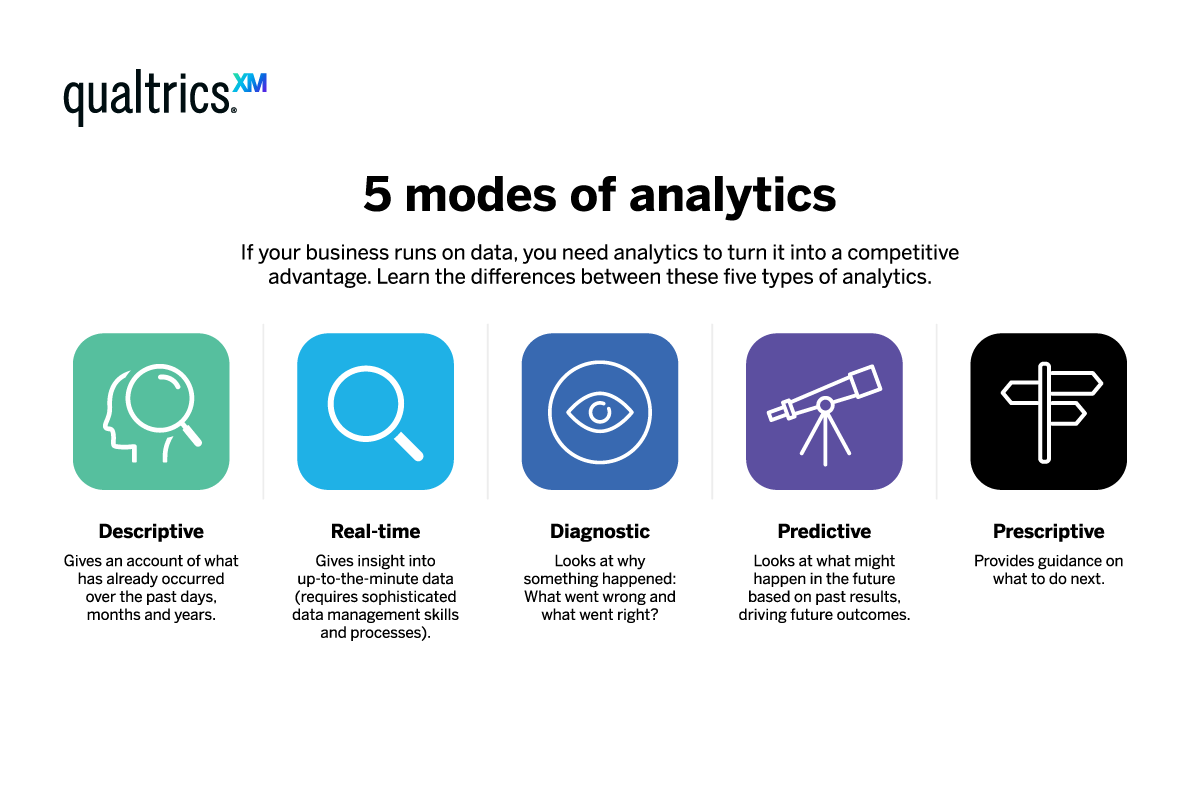
As human beings, we’re hard-wired to find ways to predict future events. We take our past experiences, quickly assess how they’re similar to the current situation, and use that information to make an educated guess about what’s likely to happen next. In that sense, the human brain is already primed for predictive analytics, although it’s only relatively recently that predictive models and the data analysis tools to create them for business purposes have been developed.
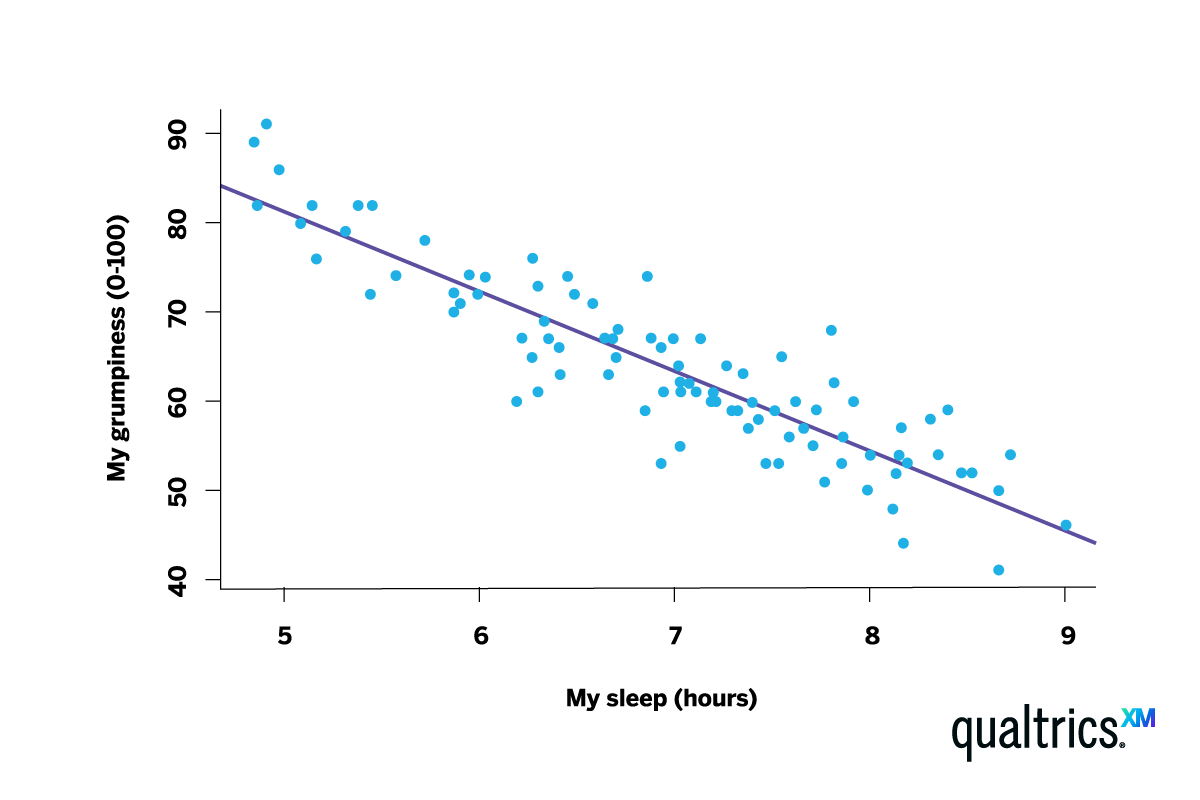
See what’s in store, and take action
Until recently, that kind of data required for high quality predictive analytics was in limited supply. However, with the emergence of data mining, data analytics, and intelligent software suites, predictive analytics has become not only more accessible but more powerful than ever before.
We can now collect huge volumes of data – a phenomenon often called ‘big data’ – and we have the processing power to analyze it rapidly and easily. We also have an array of technologies, including machine learning and multiple kinds of predictive model.
Making predictions from data involves constructing a mathematical model (AKA predictive model). This is a tool for finding out what you want to know based on historical data, the target outcome, and the known facts about the scenario.
You can think of a predictive model as a mathematical representation of reality. Like a scale model or architectural model, it replicates a real-world scenario or idea and scales it down so that only the parts you’re interested in are included.
Predictive models are objective, repeatable, based on real information, and use statistics to identify and organize what matters most, to make the prediction accurate. Predictive models are what we use in predictive analytics because they’re much better than human “gut” predictions, which are subject to personal bias and human error.
Predictive models need to be kept up to date in order to stay effective. Predictive analytics software requires a steady stream of up-to-date information in order to be able to make predictions, since it relies on past data and present data to make accurate forecasts.
That’s part of the reason ‘big data’ capabilities are so important. The more data collected, the more accurate your predictive analytics process will be. Naturally, then, organizations are increasingly looking to collect more data on their employees , customers , products, and brands so they can continue to make predictions about future events.
One of the most straightforward examples of predictive analytics – and one that is highly popular and effective – is regression analysis.
Regression analysis, which is divided into linear and nonlinear regression dependeing on the method used, looks at causal relationships between variables. It charts how an independent variable affects dependent variables over time. If there is a consistent pattern, the regression analysis will identify that pattern. It can then make predictions that the same kind of effect will occur in the future, according to the pattern observed in the past.
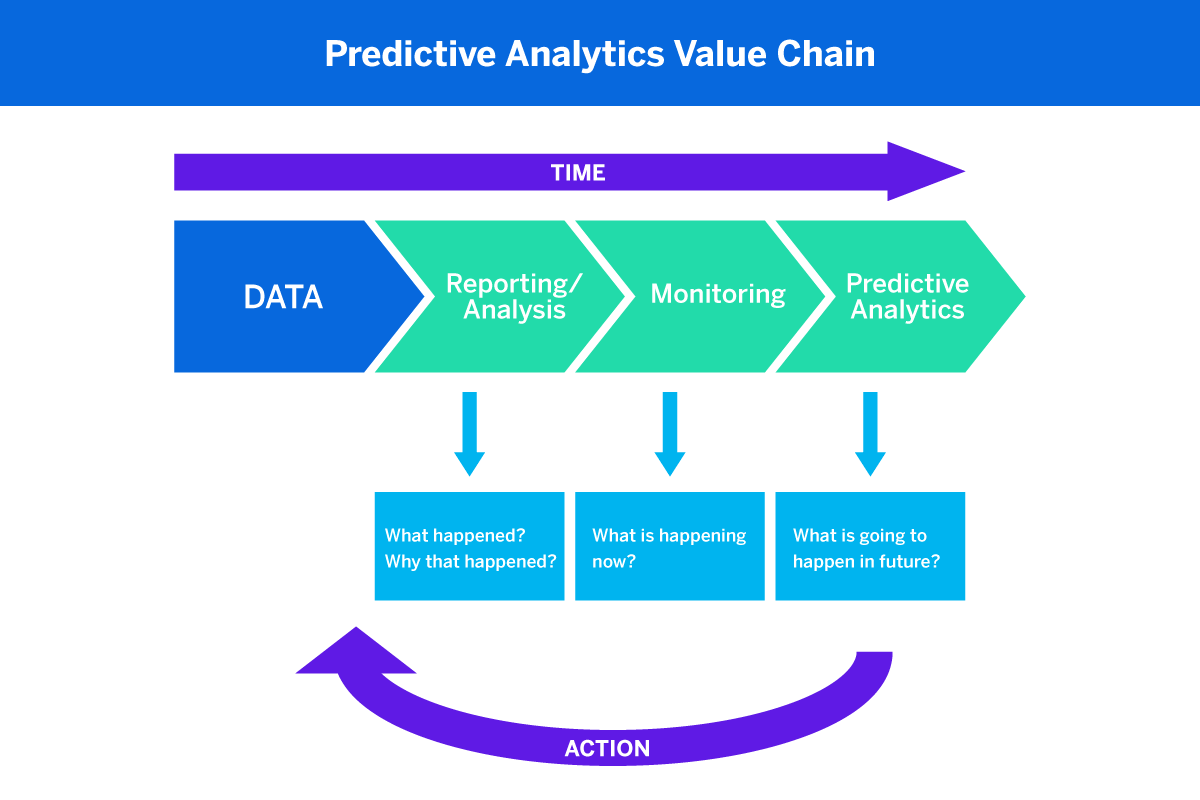
In short, there are a few key steps to any predictive modeling process:
- Decide what you’d like to predict
Whether it’s customer churn or future trends, you need to pick an outcome that you’d like your predictive analytics software to monitor.
- Collect data
As with any kind of data analysis, the more data mining you can do, the more accurate your predictive modeling will be, as per the principle of ‘big data’. We recommend using experience management software to automate this process as much as possible.
- Train and test
Put your model to the test, and use the results to train it to be more accurate in the future – on an ongoing basis. Predictive analytics applications that use artificial intelligence and machine learning should be able to self-improve over time.
Building predictive models
Begin the predictive analytics process by gathering all the data you have on the variables that you think might predict some outcome of interest.
If you are training your predictive modeling using machine learning, you’ll need to have some source of ‘ground truth’ to train it against. This is basically a dataset for a desired outcome, so that the model can learn from what happened in the past.
‘Ground truth’ definition:
Ground truth is any information that is known to be true, provided by direct measurement (i.e. empirical evidence) – that’s as opposed to information provided anecdotally.
For example, let’s say you’re developing predictive modeling techniques for finding out whether it will be sunny on a certain day. To train it, you’d provide data that covered things like:
- How often it was sunny on the same date in past years
- What the weather was like leading up to sunny days in the past
- Any known weather systems such as storms or areas of low pressure
Testing and training the model
When you then introduce new data or the ‘test data set,’ you’ll provide the model with your desired outcome and see how well it predicts it based on the ‘ground truth.’
It’s important to split the dataset into ‘training’ and ‘test’ sets, so that when you give it your training data set – all the predictors without the knowledge of whether it was sunny that day or not – you can assess how well it predicted a sunny day.
The model would also need clear parameters to define the outcome you’re interested in. For example, you might specify the hours of sunshine and the temperature range that would qualify a day as sunny.
At the end of the training period, your model would hopefully be able to predict that, for example, sunny days are most likely after a thunderstorm, and happen more often now than they did 50 years ago.
Using predictive ‘drivers’
A key benefit of predictive modeling is scale. A human can look at a small dataset and identify key indicators that something will happen, but it’s impossible for us to extract predictors out of millions of data points – we just don’t have the processing capacity.
That’s why, as well as using your model to predict if an outcome occurs, you can also use it to extract the most predictive elements in the model and surface them as ‘drivers.’
XM Discover Drivers, for instance, can return the key predictors of your chosen outcome. In that sense, you can think of predictive drivers as a ‘hypothesis generation tool’. In a business environment, it means that instead of spending days slicing and dicing data looking for correlations with a key outcome (like churn, for example), you can use drivers to sniff out leads proactively.
With so many possible applications of predictive technology, the benefits are theoretically endless. Here’s how predictive analytics can help in commercial settings…
Predicting customer churn
Predictive models can use historical and transactional data to learn the behavioral patterns that precede customer churn , and flag up when they’re happening. By acting promptly, a company may then be able to retain the customer by taking action.
Boosting the customer experience
Predictive technology can help businesses provide a personalized experience to customers by learning what they like and anticipating what they may want next. It can also boost the customer experience more generally by building an understanding of typical consumer behaviors and preferences that businesses can use to help them plan and design experiences.
As well as this, predictive analysis can also help with acting on customer support. Free text (e.g. responses typed into an open field on a survey or as part of a customer review) is information-rich but harder to process than numbers and rating scales because it varies in form and structure.
Predictive technology is now capable of processing both structured and unstructured data. It can process text data at scale and identify clusters of words and phrases that represent certain sentiments or ideas. It can then generalize them to create a big-picture analysis that can be understood at a glance.
Detecting and preventing fraud
The strength of predictive analytics is its ability to recognize patterns, which means it can also spot when something is out of place. Predictive technology can help businesses detect unusual patterns of behavior that might indicate fraud.
If a banking customer based in the US suddenly seems to be making purchases in many other continents in a short space of time, the company can intervene to ensure the account is secure.
Assessing risk
Whether you want to predict credit risk before offering a loan to a customer, or you are a health care clinician who needs to decide the right treatment pathway for a patient, applying predictive analytics can give you the ability to predict future outcomes in an unbiased and consistent way.
Because these kinds of assessments can materially affect people’s lives, it’s paramount that the data sets provided to clinical decision support systems and business analytics for lending and borrowing money are of the highest quality. The training data sets the behavior of the model, so it must be kept up-to-date and actively reviewed by qualified data scientists to make sure that inherent bias in the model doesn’t lead to poor choices.
Implementing predictive data analytics into your day-to-day operations – and using it to anticipate future events – can have real, tangible benefits to organizations of all shapes and sizes.
Planning ahead
Maybe the most obvious reason to use predictive analytics in business is its ability to help you see into the future and plan accordingly across a wide range of verticals like stock, staffing, and customer behavior . Predictive technologies can tell you what’s likely to be over the horizon so that you can prepare in advance and adjust how you allocate your resources.
Predictive analytics example
Let’s say you’re a fashion retailer, and an advanced analytics model tells you that natural materials are about to rise in popularity. You can start working with designers and manufacturers who make these kinds of clothes and cut back on your synthetic lines.
Time-saving and efficiency
Businesses can turn a lot of the work involved in low-risk, routine decision-making over to predictive technologies, freeing up humans for more valuable or high-risk strategic tasks.
Predictive analytics can do much of the work of generating a credit score or deciding whether a straightforward insurance claim can be paid out. In healthcare, predictive analytics can be used to automatically map the likely success rates for new treatments, identify patients that would benefit, or help predict the outcomes of trials based on what’s gone before.
Predicting and preventing risks
By looking at trends and patterns from your operational past, predictive analytics models can spot potential threats, what causes them, and how likely they are to arise. You can then use that information to build out risk or crisis-management processes ahead of time.
You’re a food retailer who relies on a steady supply of inventory to meet customer needs. Predictive insights making use of Big Data can track factors that affect shipping and distribution – like weather or sea conditions. This can help you adjust your stock orders dynamically, as well as to prepare what you’ll do if shortages arise.
Right now, we’re living in a sweet spot for predictive analytics. The technology is affordable, the know-how is accessible and there’s enough accessible historical data to make truly valuable predictions for business, governance, and the organization of everything from ecological conservation work to education and healthcare.
But although these capabilities are more accessible than they used to be, they are not yet standard. Now is a time when the mastery of predictive analytics still offers companies a competitive advantage, particularly if they make wise choices when choosing their predictive analytics applications.
Most businesses are aware that predictive technology is of value to themselves and their customers, but not everyone is using it – yet .
So how can you start to use predictive analytics in your business?
Luckily, the solution is simple: bring predictive analytics applications into your tech stack. You’ll usually find predictive analytics capabilities built into experience management software – either as a managed, user-directed tool, or an automatic one that uses machine learning algorithms to do a lot of the heavy lifting for you.
Using machine learning to model future events, these software suites will be able to do the hard work for you, and then – ideally – suggest actions as a result, all while using continuous data collection to self-improve. You can use these action points to get ahead of trouble before it arises.
Leading providers have developed predictive analytics tools that put the power of advanced predictive analysis in the hands of just about anyone. Machine learning and ‘big data’ mining techniques to run predictive analysis on a constant, ever-evolving basis in order to make predictions about future events, identify risks and even offer guidance on the right choices via prescriptive analytics.
It’s important to choose a predictive analytics partner with a human-first ethos, which means you can take the often intimidating world of data science and turn it into a powerful, everyday tool.
Here are a few ways our tools can help with your predictive analytics process:
Text iQ can parse text from surveys, reviews, social media, and just about any other natural language source, before unearthing big-picture trends and patterns. That can help you understand how customers are feeling about a product, brand, or experience, or pinpoint where to focus when you’re improving customer journeys.
Driver iQ uses powerful statistical analysis to uncover which aspects of your business matter most – so you can devote more of your energies to the drivers of key metrics like customer satisfaction, repeat purchase, brand advocacy, and more.
Voice iQ uses data mining, voice recognition, and a sophisticated index of known customer effort markers to take unstructured voice data and turn it into insights.
Stats iQ automatically scours through advanced analytics options and selects the appropriate statistical tests you need to run.
Predict iQ , meanwhile, uses advanced machine learning to build a detailed picture of customer behavior, so you can anticipate customers leaving your company even before they do. And when you know a customer is at risk of leaving, you can reach out and repair the relationship before it reaches breaking point.
Concerned about data privacy and compliance? Fret not: all of XM Discover’s machine learning features are optional, so you can decide for yourself what acceptable, compliant dataset inputs look like for your business.
The result? Continuous predictive analytics with clear, concise, and actionable insights. Click below to learn more.
Related resources
Analysis & Reporting
Margin of error 11 min read
Data saturation in qualitative research 8 min read, thematic analysis 11 min read, behavioral analytics 12 min read, statistical significance calculator: tool & complete guide 18 min read, regression analysis 19 min read, data analysis 31 min read, request demo.
Ready to learn more about Qualtrics?
- Artificial Intelligence
- Generative AI
- Business Operations
- Cloud Computing
- Data Center
- Data Management
- Emerging Technology
- Enterprise Applications
- IT Leadership
- Digital Transformation
- IT Strategy
- IT Management
- Diversity and Inclusion
- IT Operations
- Project Management
- Software Development
- Vendors and Providers
- Enterprise Buyer’s Guides
- United States
- Middle East
- España (Spain)
- Italia (Italy)
- Netherlands
- United Kingdom
- New Zealand
- Data Analytics & AI
- Newsletters
- Foundry Careers
- Terms of Service
- Privacy Policy
- Cookie Policy
- Copyright Notice
- Member Preferences
- About AdChoices
- Your California Privacy Rights
Our Network
- Computerworld
- Network World
7 projects primed for predictive analytics
The advanced techniques of predictive analytics are becoming widely available, bringing forecasting power within reach of almost any business. here are key areas where predictive analytics can have an impact..

Predictive analytics isn’t just for oil and gas exploration anymore. The power of predictive analytics is being injected into a wide range of revenue-focused initiatives across all industries.
In 2018, a third of businesses in the EIU’s Intelligent Economies study said predictive analytics was already the most frequently used AI technology in their organization. Almost two thirds of the CIOs in Capgemini’s most recent World Quality Report said they’d be focusing on predictive analytics in 2019, but what differentiates predictive analytics and where can you get value from it in your organization?
[ Find out how to get started with predictive analytics and the 7 secrets of predictive analytics success . | Get the latest on data analytics by signing up for CIO newsletters . ]
Predictive analytics differs from business intelligence primarily in perspective: whether you’re looking forward or backwards with data. With BI, the emphasis is on reporting and visualization — slicing historical data to understand what has happened. But with predictive analytics, “you’re no longer talking about descriptive analytics and you’re mostly focused on building a model for predictions,” says Kjell Carlsson, a senior analyst at Forrester.
Many of those algorithms are also used for machine learning, and Carlsson views predictive analytics and machine learning technologies as complementary. But predictive analytics doesn’t have to be complex. Salesforce Einstein Discovery and the insights feature in Microsoft’s Power BI both use regression analysis, but because they can work on massive data sets, they can find insights that would be too tedious for business users to discover on their own.
“If I’ve got a solution that guides salespeople to focus on the accounts that have the highest likelihood to convert and gives them reasons why this is a good account to reach out to right now, like they just downloaded a white paper, then that becomes extremely valuable from a business point of view,” Carlsson points out.
Predictive analytics is likely already in use at your organization, driven by lines of business rather than IT, he warns. “There is an incredible amount of shadow IT here,” Carlsson says. That can be a problem if poor data governance leads to a data breach, but there can also be issues when successful prototypes need wider deployment and longer-term maintenance.
At that point, CIOs and chief enterprise architects are being asked to take over. To keep ahead of the game, here are seven key projects primed for use of predictive analytics today.
1. Predictive equipment maintenance
Knowing when industrial or manufacturing equipment is likely to break down can help save money and improve customer satisfaction. Elevator manufacturers, air conditioning systems, national railways and oil well operators use IoT sensors and digital twins to provide predictive, proactive maintenance.
Here, predictive analytics doesn’t just help you avoid outages and repair bills. Knowing which spare parts, equipment and trained staff will be needed means work can be planned more efficiently, with fewer trips to the site and no delays waiting for the right part. Plus, it’s faster to repair a part before it fails because there can be damage caused by the failure. Avoiding that also extends the life of the machinery.
The information you collect can also feed forward into product design for the next version or help you develop better operating procedures.
2. Predictive IT
Predictive maintenance is also a boon for IT. Data center management tools, such as Nlyte or Virtual Power Systems, can warn you to replace UPS batteries or perform maintenance on a cooling unit.
“If you buy storage from Dell, the ProSupport Plus service uses predive analytics to predict when drives are going to fail and they pre-emptively send you replacement drives before they fail rather than afterwards,” Carlsson says. Similarly, Veritas offers Predictive Insights for it storage appliances that creates system reliability scores. When those drop, the IT team might get a notification to install a patch — or Veritas might send out a technician to replace a part before it fails. HPE’s 3PAR InfoSight management and DataDirect’s Tintri Analytics use predictive analytics to improve storage performance and handle routine storage management.
This is one area where a third-party service is likely better than building your own because if you may not have enough data to predict problems, Carlsson points out. “External vendors have the advantage of collecting data from different customers. If there’s an update for your particular hardware that’s causing problems for other companies with the same configuration as you and you haven’t applied that patch yet, you will never know from your internal data that there’s anomalous behavior.”
Predictive IT doesn’t have to be hardware either. Windows Server 2019 has predictive analytics built into the Windows Admin Center to help you perform capacity planning for compute, networking and storage, including clusters. System Insights uses local data such as performance counters and system events, and you can write your own predictive maintenance capabilities for performance, say, and then use Azure Monitor or System Center Operations Manager to view predictions across groups of servers.
3. Forecasting HVAC needs
Combine the weather forecast with what your building automation system tells you about how your facilities are used by staff and the data you can get from your HVAC system and you can reduce costs for heating, ventilation and air conditioning.
It takes time to get a building to the temperature you want when people are at work (especially if you’re saving energy by not heating or cooling them out of hours), and that varies for each building and depends on the weather. Plus, not every building is fully occupied all year round. Instead of starting the systems at the same time every day for every building, you can save money and keep employees more comfortable at work by predicting the right time to ramp up the HVAC system. When Microsoft’s real estate team applied this to just three buildings, they saw savings of $15,000 annually; that will turn into more than $500,000 once the system is in 43 buildings — and 60 fewer hours when employees are sweating or shivering.
4. Customer service and support
Predictive analytics is common in sales tools like Salesforce, but you can also use it to handle the customers you already have, whether that’s field service or call centers. Adobe Analytics uses predictive analytics to forecast future customer behavior down to when you’ll run into special shipping requirements.
MTD makes outdoor equipment like lawn mowers and snow ploughs and credits the predictive analytics and real-time information it’s added to call center systems with reducing call abandonment by 65 percent and cutting the average time to handle a call by 40 percent, thanks to better agent scheduling — because managers know in advance when they’ll need more agents at work.
Ecommerce sites have long had the advantage of being able to track customer behavior to help predict sales figures. Jet.com even models how likely it is that a supplier will have the right amount of inventory in stock before listing products in its marketplace. Now retail stores are turning to IoT sensors and predictive analytics to forecast what, when and where customers will buy, to help with inventory management. Polo and Urban Outfitters are using shelf-counted cameras and Trax’s predictive analytics system (running on Google Cloud) to do real-time stock tracking and management.
Dr Martens is using a mix of IoT, predictive analytics, machine learning and Dynamics 365 to understand more about the demographics and buying patterns of the customers who are browsing their stores. Sales staff can then use this information to make suggestions or even rearrange where products are displayed using custom schematics for the store.
6. Quality Assurance
Predictive analytics is ideal for QA, because whether it’s testing physical products or part of DevOps, QA is about avoiding defects, problems and mistakes by assuring risk. You can determine patterns and predict potential risks based on trends and use predictive analytics to reduce cycle times and cost by targeting testing where defects are most likely to occur, says Darren Coupland, Deputy CEO and COO at Sogeti UK (part of Capgemini).
“CIOs should be using predictive analytics, along with AI and cognitive solutions, to truly understand the quality of their overall operation and make informed decisions based upon insights. In order to take this one step further, CIOs should consider combining additional data sources, such as PPM [project portfolio management] tools, SCM [source code management] tools and operational tools, in order to predict the successful delivery of projects and provide important information into the overall business risk associated with a change,” Coupland says.
7. Alongside business intelligence
If you want to give business teams the freedom to work with predictive analytics alongside the more familiar visualization and analytics tools, and still have central oversight, the new no-code AI tools that will be in public preview for Microsoft Power BI soon may be what you’re looking for.
Power BI has been able to do simple predictive analytics like forecasting future patterns for time series data, with sliders for the confidence level and how strong you expect seasonal trends to be. You currently need to build more sophisticated models in a tool such as Azure Machine Learning Studio and use R scripts to extract data from SQL Azure and send it to the machine learning model and then extract the scores into Power BI. With the new no-code connection, business analysts will be able to choose and train a model in Azure Machine Learning Studio and apply it to Power BI data without leaving the Power BI interface. Your data science team can also create and train models with the Azure machine learning tools for them to use that will show up in in Power BI automatically if a business user has access to them.
More on predictive analytics:
- What is predictive analytics? Transforming data into future insights
- 7 secrets of predictive analytics success
- How to get started with predictive analytics
- Top 8 predictive analytics tools compared
- 7 ways predictive analytics can improve customer experience
- 7 tips for overcoming predictive analytics challenges
- How predictive analytics can help prevent network failures
- 12 myths of data analytics debunked
- 7 sure-fire ways to fail at data analytics
Related content
State of it jobs: mixed signals, changes ahead, project manager salary: 5 key tips to earn more, cyber resilience: a business imperative cios must get right, shine a spotlight on your team’s it excellence with cio awards canada, from our editors straight to your inbox, show me more, camunda simplifies process automation with new ai-powered natural language features.

What’s holding CTOs back?

Baldor’s first-ever CIO sets the transformation agenda

CIO Leadership Live with Satya Jayadev, Vice President & CIO, Skyworks Solutions

Principal Financial CIO Kathy Kay on balancing traditional AI and genAI

CIO Leadership Live Middle East with Ramadan Mohamad, Digital infrastructure specialist at Public Transport Corp.

Alteryx adds genAI to enable analytics creation via no-code platform

Sponsored Links
- Everybody's ready for AI except your data. Unlock the power of AI with Informatica

Published: 5 April 2024 Contributors: Tim Mucci, Cole Stryker
Big data analytics refers to the systematic processing and analysis of large amounts of data and complex data sets, known as big data, to extract valuable insights. Big data analytics allows for the uncovering of trends, patterns and correlations in large amounts of raw data to help analysts make data-informed decisions. This process allows organizations to leverage the exponentially growing data generated from diverse sources, including internet-of-things (IoT) sensors, social media, financial transactions and smart devices to derive actionable intelligence through advanced analytic techniques.
In the early 2000s, advances in software and hardware capabilities made it possible for organizations to collect and handle large amounts of unstructured data. With this explosion of useful data, open-source communities developed big data frameworks to store and process this data. These frameworks are used for distributed storage and processing of large data sets across a network of computers. Along with additional tools and libraries, big data frameworks can be used for:
- Predictive modeling by incorporating artificial intelligence (AI) and statistical algorithms
- Statistical analysis for in-depth data exploration and to uncover hidden patterns
- What-if analysis to simulate different scenarios and explore potential outcomes
- Processing diverse data sets, including structured, semi-structured and unstructured data from various sources.
Four main data analysis methods – descriptive, diagnostic, predictive and prescriptive – are used to uncover insights and patterns within an organization's data. These methods facilitate a deeper understanding of market trends, customer preferences and other important business metrics.
IBM named a Leader in the 2024 Gartner® Magic Quadrant™ for Augmented Data Quality Solutions.
Structured vs unstructured data
What is data management?
The main difference between big data analytics and traditional data analytics is the type of data handled and the tools used to analyze it. Traditional analytics deals with structured data, typically stored in relational databases . This type of database helps ensure that data is well-organized and easy for a computer to understand. Traditional data analytics relies on statistical methods and tools like structured query language (SQL) for querying databases.
Big data analytics involves massive amounts of data in various formats, including structured, semi-structured and unstructured data. The complexity of this data requires more sophisticated analysis techniques. Big data analytics employs advanced techniques like machine learning and data mining to extract information from complex data sets. It often requires distributed processing systems like Hadoop to manage the sheer volume of data.
These are the four methods of data analysis at work within big data:
The "what happened" stage of data analysis. Here, the focus is on summarizing and describing past data to understand its basic characteristics.
The “why it happened” stage. By delving deep into the data, diagnostic analysis identifies the root patterns and trends observed in descriptive analytics.
The “what will happen” stage. It uses historical data, statistical modeling and machine learning to forecast trends.
Describes the “what to do” stage, which goes beyond prediction to provide recommendations for optimizing future actions based on insights derived from all previous.
The following dimensions highlight the core challenges and opportunities inherent in big data analytics.
The sheer volume of data generated today, from social media feeds, IoT devices, transaction records and more, presents a significant challenge. Traditional data storage and processing solutions are often inadequate to handle this scale efficiently. Big data technologies and cloud-based storage solutions enable organizations to store and manage these vast data sets cost-effectively, protecting valuable data from being discarded due to storage limitations.
Data is being produced at unprecedented speeds, from real-time social media updates to high-frequency stock trading records. The velocity at which data flows into organizations requires robust processing capabilities to capture, process and deliver accurate analysis in near real-time. Stream processing frameworks and in-memory data processing are designed to handle these rapid data streams and balance supply with demand.
Today's data comes in many formats, from structured to numeric data in traditional databases to unstructured text, video and images from diverse sources like social media and video surveillance. This variety demans flexible data management systems to handle and integrate disparate data types for comprehensive analysis. NoSQL databases , data lakes and schema -on-read technologies provide the necessary flexibility to accommodate the diverse nature of big data.
Data reliability and accuracy are critical, as decisions based on inaccurate or incomplete data can lead to negative outcomes. Veracity refers to the data's trustworthiness, encompassing data quality, noise and anomaly detection issues. Techniques and tools for data cleaning, validation and verification are integral to ensuring the integrity of big data, enabling organizations to make better decisions based on reliable information.
Big data analytics aims to extract actionable insights that offer tangible value. This involves turning vast data sets into meaningful information that can inform strategic decisions, uncover new opportunities and drive innovation. Advanced analytics, machine learning and AI are key to unlocking the value contained within big data, transforming raw data into strategic assets.
Data professionals, analysts, scientists and statisticians prepare and process data in a data lakehouse, which combines the performance of a data lakehouse with the flexibility of a data lake to clean data and ensure its quality. The process of turning raw data into valuable insights encompasses several key stages:
- Collect data: The first step involves gathering data, which can be a mix of structured and unstructured forms from myriad sources like cloud, mobile applications and IoT sensors. This step is where organizations adapt their data collection strategies and integrate data from varied sources into central repositories like a data lake, which can automatically assign metadata for better manageability and accessibility.
- Process data: After being collected, data must be systematically organized, extracted, transformed and then loaded into a storage system to ensure accurate analytical outcomes. Processing involves converting raw data into a format that is usable for analysis, which might involve aggregating data from different sources, converting data types or organizing data into structure formats. Given the exponential growth of available data, this stage can be challenging. Processing strategies may vary between batch processing, which handles large data volumes over extended periods and stream processing, which deals with smaller real-time data batches.
- Clean data: Regardless of size, data must be cleaned to ensure quality and relevance. Cleaning data involves formatting it correctly, removing duplicates and eliminating irrelevant entries. Clean data prevents the corruption of output and safeguard’s reliability and accuracy.
- Analyze data: Advanced analytics, such as data mining, predictive analytics, machine learning and deep learning, are employed to sift through the processed and cleaned data. These methods allow users to discover patterns, relationships and trends within the data, providing a solid foundation for informed decision-making.
Under the Analyze umbrella, there are potentially many technologies at work, including data mining, which is used to identify patterns and relationships within large data sets; predictive analytics, which forecasts future trends and opportunities; and deep learning , which mimics human learning patterns to uncover more abstract ideas.
Deep learning uses an artificial neural network with multiple layers to model complex patterns in data. Unlike traditional machine learning algorithms, deep learning learns from images, sound and text without manual help. For big data analytics, this powerful capability means the volume and complexity of data is not an issue.
Natural language processing (NLP) models allow machines to understand, interpret and generate human language. Within big data analytics, NLP extracts insights from massive unstructured text data generated across an organization and beyond.
Structured Data
Structured data refers to highly organized information that is easily searchable and typically stored in relational databases or spreadsheets. It adheres to a rigid schema, meaning each data element is clearly defined and accessible in a fixed field within a record or file. Examples of structured data include:
- Customer names and addresses in a customer relationship management (CRM) system
- Transactional data in financial records, such as sales figures and account balances
- Employee data in human resources databases, including job titles and salaries
Structured data's main advantage is its simplicity for entry, search and analysis, often using straightforward database queries like SQL. However, the rapidly expanding universe of big data means that structured data represents a relatively small portion of the total data available to organizations.
Unstructured Data
Unstructured data lacks a pre-defined data model, making it more difficult to collect, process and analyze. It comprises the majority of data generated today, and includes formats such as:
- Textual content from documents, emails and social media posts
- Multimedia content, including images, audio files and videos
- Data from IoT devices, which can include a mix of sensor data, log files and time-series data
The primary challenge with unstructured data is its complexity and lack of uniformity, requiring more sophisticated methods for indexing, searching and analyzing. NLP, machine learning and advanced analytics platforms are often employed to extract meaningful insights from unstructured data.
Semi-structured data
Semi-structured data occupies the middle ground between structured and unstructured data. While it does not reside in a relational database, it contains tags or other markers to separate semantic elements and enforce hierarchies of records and fields within the data. Examples include:
- JSON (JavaScript Object Notation) and XML (eXtensible Markup Language) files, which are commonly used for web data interchange
- Email, where the data has a standardized format (e.g., headers, subject, body) but the content within each section is unstructured
- NoSQL databases, can store and manage semi-structured data more efficiently than traditional relational databases
Semi-structured data is more flexible than structured data but easier to analyze than unstructured data, providing a balance that is particularly useful in web applications and data integration tasks.
Ensuring data quality and integrity, integrating disparate data sources, protecting data privacy and security and finding the right talent to analyze and interpret data can present challenges to organizations looking to leverage their extensive data volumes. What follows are the benefits organizations can realize once they see success with big data analytics:
Real-time intelligence
One of the standout advantages of big data analytics is the capacity to provide real-time intelligence. Organizations can analyze vast amounts of data as it is generated from myriad sources and in various formats. Real-time insight allows businesses to make quick decisions, respond to market changes instantaneously and identify and act on opportunities as they arise.
Better-informed decisions
With big data analytics, organizations can uncover previously hidden trends, patterns and correlations. A deeper understanding equips leaders and decision-makers with the information needed to strategize effectively, enhancing business decision-making in supply chain management, e-commerce, operations and overall strategic direction.
Cost savings
Big data analytics drives cost savings by identifying business process efficiencies and optimizations. Organizations can pinpoint wasteful expenditures by analyzing large datasets, streamlining operations and enhancing productivity. Moreover, predictive analytics can forecast future trends, allowing companies to allocate resources more efficiently and avoid costly missteps.
Better customer engagement
Understanding customer needs, behaviors and sentiments is crucial for successful engagement and big data analytics provides the tools to achieve this understanding. Companies gain insights into consumer preferences and tailor their marketing strategies by analyzing customer data.
Optimized risk management strategies
Big data analytics enhances an organization's ability to manage risk by providing the tools to identify, assess and address threats in real time. Predictive analytics can foresee potential dangers before they materialize, allowing companies to devise preemptive strategies.
As organizations across industries seek to leverage data to drive decision-making, improve operational efficiencies and enhance customer experiences, the demand for skilled professionals in big data analytics has surged. Here are some prominent career paths that utilize big data analytics:
Data scientist
Data scientists analyze complex digital data to assist businesses in making decisions. Using their data science training and advanced analytics technologies, including machine learning and predictive modeling, they uncover hidden insights in data.
Data analyst
Data analysts turn data into information and information into insights. They use statistical techniques to analyze and extract meaningful trends from data sets, often to inform business strategy and decisions.
Data engineer
Data engineers prepare, process and manage big data infrastructure and tools. They also develop, maintain, test and evaluate data solutions within organizations, often working with massive datasets to assist in analytics projects.
Machine learning engineer
Machine learning engineers focus on designing and implementing machine learning applications. They develop sophisticated algorithms that learn from and make predictions on data.
Business intelligence analyst
Business intelligence (BI) analysts help businesses make data-driven decisions by analyzing data to produce actionable insights. They often use BI tools to convert data into easy-to-understand reports and visualizations for business stakeholders.
Data visualization specialist
These specialists focus on the visual representation of data. They create data visualizations that help end users understand the significance of data by placing it in a visual context.
Data architect
Data architects design, create, deploy and manage an organization's data architecture. They define how data is stored, consumed, integrated and managed by different data entities and IT systems.
IBM and Cloudera have partnered to create an industry-leading, enterprise-grade big data framework distribution plus a variety of cloud services and products — all designed to achieve faster analytics at scale.
IBM Db2 Database on IBM Cloud Pak for Data combines a proven, AI-infused, enterprise-ready data management system with an integrated data and AI platform built on the security-rich, scalable Red Hat OpenShift foundation.
IBM Big Replicate is an enterprise-class data replication software platform that keeps data consistent in a distributed environment, on-premises and in the hybrid cloud, including SQL and NoSQL databases.
A data warehouse is a system that aggregates data from different sources into a single, central, consistent data store to support data analysis, data mining, artificial intelligence and machine learning.
Business intelligence gives organizations the ability to get answers they can understand. Instead of using best guesses, they can base decisions on what their business data is telling them — whether it relates to production, supply chain, customers or market trends.
Cloud computing is the on-demand access of physical or virtual servers, data storage, networking capabilities, application development tools, software, AI analytic tools and more—over the internet with pay-per-use pricing. The cloud computing model offers customers flexibility and scalability compared to traditional infrastructure.
Purpose-built data-driven architecture helps support business intelligence across the organization. IBM analytics solutions allow organizations to simplify raw data access, provide end-to-end data management and empower business users with AI-driven self-service analytics to predict outcomes.
Artificial intelligence in strategy
Can machines automate strategy development? The short answer is no. However, there are numerous aspects of strategists’ work where AI and advanced analytics tools can already bring enormous value. Yuval Atsmon is a senior partner who leads the new McKinsey Center for Strategy Innovation, which studies ways new technologies can augment the timeless principles of strategy. In this episode of the Inside the Strategy Room podcast, he explains how artificial intelligence is already transforming strategy and what’s on the horizon. This is an edited transcript of the discussion. For more conversations on the strategy issues that matter, follow the series on your preferred podcast platform .
Joanna Pachner: What does artificial intelligence mean in the context of strategy?
Yuval Atsmon: When people talk about artificial intelligence, they include everything to do with analytics, automation, and data analysis. Marvin Minsky, the pioneer of artificial intelligence research in the 1960s, talked about AI as a “suitcase word”—a term into which you can stuff whatever you want—and that still seems to be the case. We are comfortable with that because we think companies should use all the capabilities of more traditional analysis while increasing automation in strategy that can free up management or analyst time and, gradually, introducing tools that can augment human thinking.
Joanna Pachner: AI has been embraced by many business functions, but strategy seems to be largely immune to its charms. Why do you think that is?
Subscribe to the Inside the Strategy Room podcast
Yuval Atsmon: You’re right about the limited adoption. Only 7 percent of respondents to our survey about the use of AI say they use it in strategy or even financial planning, whereas in areas like marketing, supply chain, and service operations, it’s 25 or 30 percent. One reason adoption is lagging is that strategy is one of the most integrative conceptual practices. When executives think about strategy automation, many are looking too far ahead—at AI capabilities that would decide, in place of the business leader, what the right strategy is. They are missing opportunities to use AI in the building blocks of strategy that could significantly improve outcomes.
I like to use the analogy to virtual assistants. Many of us use Alexa or Siri but very few people use these tools to do more than dictate a text message or shut off the lights. We don’t feel comfortable with the technology’s ability to understand the context in more sophisticated applications. AI in strategy is similar: it’s hard for AI to know everything an executive knows, but it can help executives with certain tasks.
When executives think about strategy automation, many are looking too far ahead—at AI deciding the right strategy. They are missing opportunities to use AI in the building blocks of strategy.
Joanna Pachner: What kind of tasks can AI help strategists execute today?
Yuval Atsmon: We talk about six stages of AI development. The earliest is simple analytics, which we refer to as descriptive intelligence. Companies use dashboards for competitive analysis or to study performance in different parts of the business that are automatically updated. Some have interactive capabilities for refinement and testing.
The second level is diagnostic intelligence, which is the ability to look backward at the business and understand root causes and drivers of performance. The level after that is predictive intelligence: being able to anticipate certain scenarios or options and the value of things in the future based on momentum from the past as well as signals picked in the market. Both diagnostics and prediction are areas that AI can greatly improve today. The tools can augment executives’ analysis and become areas where you develop capabilities. For example, on diagnostic intelligence, you can organize your portfolio into segments to understand granularly where performance is coming from and do it in a much more continuous way than analysts could. You can try 20 different ways in an hour versus deploying one hundred analysts to tackle the problem.
Predictive AI is both more difficult and more risky. Executives shouldn’t fully rely on predictive AI, but it provides another systematic viewpoint in the room. Because strategic decisions have significant consequences, a key consideration is to use AI transparently in the sense of understanding why it is making a certain prediction and what extrapolations it is making from which information. You can then assess if you trust the prediction or not. You can even use AI to track the evolution of the assumptions for that prediction.
Those are the levels available today. The next three levels will take time to develop. There are some early examples of AI advising actions for executives’ consideration that would be value-creating based on the analysis. From there, you go to delegating certain decision authority to AI, with constraints and supervision. Eventually, there is the point where fully autonomous AI analyzes and decides with no human interaction.
Because strategic decisions have significant consequences, you need to understand why AI is making a certain prediction and what extrapolations it’s making from which information.
Joanna Pachner: What kind of businesses or industries could gain the greatest benefits from embracing AI at its current level of sophistication?
Yuval Atsmon: Every business probably has some opportunity to use AI more than it does today. The first thing to look at is the availability of data. Do you have performance data that can be organized in a systematic way? Companies that have deep data on their portfolios down to business line, SKU, inventory, and raw ingredients have the biggest opportunities to use machines to gain granular insights that humans could not.
Companies whose strategies rely on a few big decisions with limited data would get less from AI. Likewise, those facing a lot of volatility and vulnerability to external events would benefit less than companies with controlled and systematic portfolios, although they could deploy AI to better predict those external events and identify what they can and cannot control.
Third, the velocity of decisions matters. Most companies develop strategies every three to five years, which then become annual budgets. If you think about strategy in that way, the role of AI is relatively limited other than potentially accelerating analyses that are inputs into the strategy. However, some companies regularly revisit big decisions they made based on assumptions about the world that may have since changed, affecting the projected ROI of initiatives. Such shifts would affect how you deploy talent and executive time, how you spend money and focus sales efforts, and AI can be valuable in guiding that. The value of AI is even bigger when you can make decisions close to the time of deploying resources, because AI can signal that your previous assumptions have changed from when you made your plan.
Joanna Pachner: Can you provide any examples of companies employing AI to address specific strategic challenges?
Yuval Atsmon: Some of the most innovative users of AI, not coincidentally, are AI- and digital-native companies. Some of these companies have seen massive benefits from AI and have increased its usage in other areas of the business. One mobility player adjusts its financial planning based on pricing patterns it observes in the market. Its business has relatively high flexibility to demand but less so to supply, so the company uses AI to continuously signal back when pricing dynamics are trending in a way that would affect profitability or where demand is rising. This allows the company to quickly react to create more capacity because its profitability is highly sensitive to keeping demand and supply in equilibrium.
Joanna Pachner: Given how quickly things change today, doesn’t AI seem to be more a tactical than a strategic tool, providing time-sensitive input on isolated elements of strategy?
Yuval Atsmon: It’s interesting that you make the distinction between strategic and tactical. Of course, every decision can be broken down into smaller ones, and where AI can be affordably used in strategy today is for building blocks of the strategy. It might feel tactical, but it can make a massive difference. One of the world’s leading investment firms, for example, has started to use AI to scan for certain patterns rather than scanning individual companies directly. AI looks for consumer mobile usage that suggests a company’s technology is catching on quickly, giving the firm an opportunity to invest in that company before others do. That created a significant strategic edge for them, even though the tool itself may be relatively tactical.
Joanna Pachner: McKinsey has written a lot about cognitive biases and social dynamics that can skew decision making. Can AI help with these challenges?
Yuval Atsmon: When we talk to executives about using AI in strategy development, the first reaction we get is, “Those are really big decisions; what if AI gets them wrong?” The first answer is that humans also get them wrong—a lot. [Amos] Tversky, [Daniel] Kahneman, and others have proven that some of those errors are systemic, observable, and predictable. The first thing AI can do is spot situations likely to give rise to biases. For example, imagine that AI is listening in on a strategy session where the CEO proposes something and everyone says “Aye” without debate and discussion. AI could inform the room, “We might have a sunflower bias here,” which could trigger more conversation and remind the CEO that it’s in their own interest to encourage some devil’s advocacy.
We also often see confirmation bias, where people focus their analysis on proving the wisdom of what they already want to do, as opposed to looking for a fact-based reality. Just having AI perform a default analysis that doesn’t aim to satisfy the boss is useful, and the team can then try to understand why that is different than the management hypothesis, triggering a much richer debate.
In terms of social dynamics, agency problems can create conflicts of interest. Every business unit [BU] leader thinks that their BU should get the most resources and will deliver the most value, or at least they feel they should advocate for their business. AI provides a neutral way based on systematic data to manage those debates. It’s also useful for executives with decision authority, since we all know that short-term pressures and the need to make the quarterly and annual numbers lead people to make different decisions on the 31st of December than they do on January 1st or October 1st. Like the story of Ulysses and the sirens, you can use AI to remind you that you wanted something different three months earlier. The CEO still decides; AI can just provide that extra nudge.
Joanna Pachner: It’s like you have Spock next to you, who is dispassionate and purely analytical.
Yuval Atsmon: That is not a bad analogy—for Star Trek fans anyway.
Joanna Pachner: Do you have a favorite application of AI in strategy?
Yuval Atsmon: I have worked a lot on resource allocation, and one of the challenges, which we call the hockey stick phenomenon, is that executives are always overly optimistic about what will happen. They know that resource allocation will inevitably be defined by what you believe about the future, not necessarily by past performance. AI can provide an objective prediction of performance starting from a default momentum case: based on everything that happened in the past and some indicators about the future, what is the forecast of performance if we do nothing? This is before we say, “But I will hire these people and develop this new product and improve my marketing”— things that every executive thinks will help them overdeliver relative to the past. The neutral momentum case, which AI can calculate in a cold, Spock-like manner, can change the dynamics of the resource allocation discussion. It’s a form of predictive intelligence accessible today and while it’s not meant to be definitive, it provides a basis for better decisions.
Joanna Pachner: Do you see access to technology talent as one of the obstacles to the adoption of AI in strategy, especially at large companies?
Yuval Atsmon: I would make a distinction. If you mean machine-learning and data science talent or software engineers who build the digital tools, they are definitely not easy to get. However, companies can increasingly use platforms that provide access to AI tools and require less from individual companies. Also, this domain of strategy is exciting—it’s cutting-edge, so it’s probably easier to get technology talent for that than it might be for manufacturing work.
The bigger challenge, ironically, is finding strategists or people with business expertise to contribute to the effort. You will not solve strategy problems with AI without the involvement of people who understand the customer experience and what you are trying to achieve. Those who know best, like senior executives, don’t have time to be product managers for the AI team. An even bigger constraint is that, in some cases, you are asking people to get involved in an initiative that may make their jobs less important. There could be plenty of opportunities for incorporating AI into existing jobs, but it’s something companies need to reflect on. The best approach may be to create a digital factory where a different team tests and builds AI applications, with oversight from senior stakeholders.
The big challenge is finding strategists to contribute to the AI effort. You are asking people to get involved in an initiative that may make their jobs less important.
Joanna Pachner: Do you think this worry about job security and the potential that AI will automate strategy is realistic?
Yuval Atsmon: The question of whether AI will replace human judgment and put humanity out of its job is a big one that I would leave for other experts.
The pertinent question is shorter-term automation. Because of its complexity, strategy would be one of the later domains to be affected by automation, but we are seeing it in many other domains. However, the trend for more than two hundred years has been that automation creates new jobs, although ones requiring different skills. That doesn’t take away the fear some people have of a machine exposing their mistakes or doing their job better than they do it.
Joanna Pachner: We recently published an article about strategic courage in an age of volatility that talked about three types of edge business leaders need to develop. One of them is an edge in insights. Do you think AI has a role to play in furnishing a proprietary insight edge?
Yuval Atsmon: One of the challenges most strategists face is the overwhelming complexity of the world we operate in—the number of unknowns, the information overload. At one level, it may seem that AI will provide another layer of complexity. In reality, it can be a sharp knife that cuts through some of the clutter. The question to ask is, Can AI simplify my life by giving me sharper, more timely insights more easily?
Joanna Pachner: You have been working in strategy for a long time. What sparked your interest in exploring this intersection of strategy and new technology?
Yuval Atsmon: I have always been intrigued by things at the boundaries of what seems possible. Science fiction writer Arthur C. Clarke’s second law is that to discover the limits of the possible, you have to venture a little past them into the impossible, and I find that particularly alluring in this arena.
AI in strategy is in very nascent stages but could be very consequential for companies and for the profession. For a top executive, strategic decisions are the biggest way to influence the business, other than maybe building the top team, and it is amazing how little technology is leveraged in that process today. It’s conceivable that competitive advantage will increasingly rest in having executives who know how to apply AI well. In some domains, like investment, that is already happening, and the difference in returns can be staggering. I find helping companies be part of that evolution very exciting.
Explore a career with us
Related articles.

Strategic courage in an age of volatility
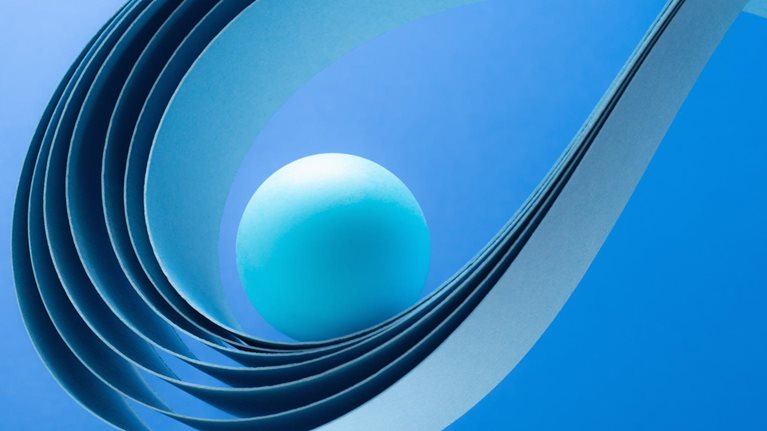
Bias Busters Collection
- Frontiers in Molecular Biosciences
- Molecular Diagnostics and Therapeutics
- Research Topics
Recent Advances in Breath Analysis: Exploring Exhaled Breath Biomarkers for Disease Diagnostics
Total Downloads
Total Views and Downloads
About this Research Topic
Breathomics is a branch of metabolomics that analyzes various volatile organic compounds (VOCs) from exhaled breath samples. It has been rapidly growing as a non-invasive diagnostic tool to probe or infer the pathogenic or physiological status of the human body, often yielding crucial information for disease diagnostics. Unlike traditional diagnostic methods that often require invasive procedures or complex laboratory analyses, breath analysis offers a simple, cost-effective, and patient-friendly approach that can be easily integrated into routine clinical practice. The non-invasive nature of breath sample collection makes breathomics particularly attractive for disease screening, monitoring, and personalized medicine. Understanding the link between breath molecules and diseases has gained significant advances due to recent developments in more reliable detection techniques and standardized breath sample collection methods. Additionally, the recent rapid progress in algorithm development, including machine learning and artificial intelligence, has unveiled intriguing associations between breath VOCs and diseases that were previously convoluted by multiple factors. With successful clinical applications of gastrointestinal and respiratory diagnostics, breath analysis has expanded to broader fields such as thoracic diseases, neurological disorders, pharmacokinetics, and more. The surge in registered clinical trials employing breath analysis and breathomics underscores the growing significance and potential of this innovative approach in modern healthcare. The objective of this Research Topic is to provide a comprehensive overview of the recent advancements in breathomics, with a particular focus on exploring the association between exhaled breath VOCs and diseases. The aim is to delve deeper into the scientific underpinnings of this emerging field and to assess its potential in modern healthcare. Focusing on the following four areas: • Investigating the mechanism of breath biomarkers and diseases, including assessing unique VOC signatures associated with different diseases, potential VOC profiles for early disease screening, and influences of various factors such as diet, lifestyle, and environmental exposure on breath VOC composition. • Advancements in breath analysis technologies, including novel analytical techniques for the detection and quantification of VOCs in exhaled breath and applications of standardized protocols for breath sample collection, storage, and analysis. • Machine learning and AI-driven data processing in breathomics, including the development of predictive models for disease classification and risk prediction, integration of multi-omics data with breathomics data for comprehensive disease profiling, and validation strategies for assessing the robustness and generalizability of AI-driven models. • Clinical applications of breathomics, including evaluation of breathomics-based diagnostic tools in clinical settings, monitoring disease progression and treatment response through longitudinal breath analysis or pharmacokinetics studies, and exploring the potential of breathomics in personalized medicine and disease screening. Topic editor Meixiu Sun is employed by WIM Spirare Health Technology Limited. The other Topic Editors declare no potential conflicts of interest with regards to the Research.
Keywords : breath analysis, breathomics, volatile organic compounds (VOC), disease diagnostics, machine learning, artificial intelligence
Important Note : All contributions to this Research Topic must be within the scope of the section and journal to which they are submitted, as defined in their mission statements. Frontiers reserves the right to guide an out-of-scope manuscript to a more suitable section or journal at any stage of peer review.
Topic Editors
Topic coordinators, submission deadlines, participating journals.
Manuscripts can be submitted to this Research Topic via the following journals:
total views
- Demographics
No records found
total views article views downloads topic views
Top countries
Top referring sites, about frontiers research topics.
With their unique mixes of varied contributions from Original Research to Review Articles, Research Topics unify the most influential researchers, the latest key findings and historical advances in a hot research area! Find out more on how to host your own Frontiers Research Topic or contribute to one as an author.
- Latest News
- Artificial Intelligence
- Big Data and Analytics
- Cybersecurity
- Applications
- IT Management
- Small Business
- Development
- PC Hardware
- Search Engines
- Virtualization
Predictive Sales Analytics: Using AI to Drive Sales
Unlock sales potential with predictive analytics. Discover strategies and AI applications for predictive sales success.
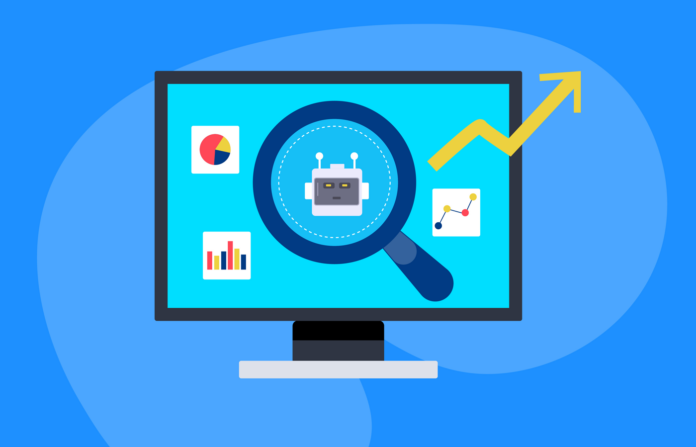
eWEEK content and product recommendations are editorially independent. We may make money when you click on links to our partners. Learn More .
Predictive sales analysis refers to the AI software and processes that businesses use to make accurate predictions about the future by analyzing data corresponding to various factors, including historical sales data, economic conditions, customer trends, and more.
These AI-based predictions help sales leaders and managers make accurate sales forecasts they can trust, which enables sales teams to effectively plan, budget, and anticipate pipeline risks and opportunities.
Predictive sales analytics projects are delicate and multi-step, so it’s common for businesses to use sales analytics software to streamline the process. These AI-powered platforms typically integrate with your CRM and other data sources and analyze that data according to the insights you want to generate. Some CRMs also come with predictive analytics features.
Let’s look at the many facets of predictive AI sales analysis, including how it works, benefits, challenges, key tools, and the future of this emerging use of AI in sales.
TABLE OF CONTENTS
6 Benefits of AI-Based Predictive Sales Analysis
Predictive sales analysis using artificial intelligence has many benefits for all types of businesses, from increasing the accuracy of your sales forecasts to helping you spot and plan for potential risks like cash flow slumps or economic downturns.
Below are five of its biggest benefits:
Increase the Precision of Your Sales Forecast
Predictive sales analytics tools improve the accuracy of your sales forecasts in various ways:
- Automatically gather relevant past and current sales, marketing, and financial data from your data sources and AI CRM .
- Clean the data, enrich datasets, remove redundancies, and fill in missing fields.
- Apply machine learning and statistical techniques to effectively turn the data into insights and sales predictions.
- Predict industry and competitor trends that will help you make better forecasts.
Using predictive analysis software also ensures that your predictions aren’t corrupted by human error.
And when you’re confident in your forecasts, you’ll be more likely to use them to inform your business and sales plans. Doubt is the enemy of effective execution.
Understand Your Customers on a Deeper Level
By analyzing customer data such as past purchases, survey feedback, and social media activity, AI-based sales analytics helps you form a clearer picture of your customer segments and how they’ll change going forward.
With a better idea of their future needs and behavior, you can build sales processes and offers that resonate with those buyers.
For example, you might predict that in 2025 your target customers will be seeking out more self-service options for exploring your solutions.
In that case, you could integrate a better AI chatbot into your website that can automatically send them content and recorded demos related to their interests. This helps you win customers from competitors who aren’t taking this into account.
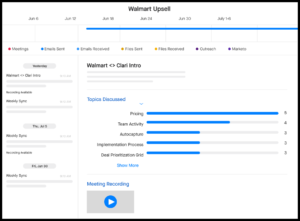
This predictive sales analytics tool by Clari summarizes topics and offers additional guidance for sales reps. Source: Clari.
Enhance Your Marketing Campaigns
Insights into the future desires and situations of your audience will help you create data-driven marketing campaigns that reach and engage your buyers.
By reviewing past campaigns and other data, predictive analysis can help you find the marketing channel with the best ROI, identify the attributes of your highest-quality leads, and write copy that moves buyers to take action.
To learn about AI for customer relationship management, read our guide: Top 8 AI CRM Software
Effectively Allocate Your Assets
AI-based analysis can help you predict future events like slumps in sales numbers in a certain territory, which will enable you to improve inventory management and staff assignment.
With predictions like that at your disposal, you’ll also be able to put your effort and money into high ROI-activities and market segments while avoiding the ones that look unpromising.
Improve Sales Performance
Predictive analytics tools can tie into your CRM and the other platforms your sales teams use.
This enables you to track key sales metrics and figure out which processes and tactics will work in the future and which ones need adjustment.
These tools can also assist in predicting shifts in the marketplace, which will affect which sales skills you focus on developing.
If, for example, it looks like your leads are going to increasingly come from social media, it’s important to train your sales team in social selling.
More Proactive Risk Management
Imagine a meteor was heading for Earth.
With predictive analytics, the scientists could identify the timeframe and likelihood the meteor strikes, but that’s not all — they’d also be able to examine the effectiveness of the various meteor-prevention strategies.
For instance, the military’s option might be to “blow the darn thing up.” Using predictive analysis, the scientists could then assess the effectiveness by predicting the weather patterns at that time, where the pieces would fall, and how likely their missiles were to hit the target.
This is the power of predictive analytics for businesses.
Sales operations leaders can spot potential causes of future drops in sales and make predictions about how well each avoidance strategy would work. This gives them the greatest chance of eliminating or at least mitigating the risk to the pipeline.
To gain a deeper understanding of today’s AI software for sales, read our guide to AI Sales Tools and Software
How does Predictive Sales Analytics Work?
Predictive sales analytics uses AI, machine learning, and statistical models to find patterns in data and forecast future sales trends.
The data it analyzes depends on your forecasting goals but often includes things like:
- Customer information
- Market trends
- Economic conditions
- Past sales performance
- Marketing data
- Financial numbers
Usually, businesses use a predictive analytics software, or sales forecasting tool, to automatically gather and update relevant data, clean it, analyze it, and auto-generate reports based on questions they need answered.
Some businesses may still use spreadsheets. However, this method is comparatively inefficient and prone to human error compared with using an AI-based analytics approach.
Example of a Predictive Sales Analysis Project
Imagine you run a retail chain. In order to forecast demand for seasonal products, you use predictive sales analytics to analyze historical sales data, customer behavior, and external factors that affect foot traffic, like weather patterns.
The results empower you to optimize inventory levels, plan promotions, and intelligently allocate resources, resulting in increased sales and fewer inventory shortages. The value here is leaving behind the classic retail “gut instinct” approach and focus on a strictly data driven approach, bolstered by AI.
6 Effective Ways To Use Predictive Sales Analytics For Sales Forecasting
There are many ways predictive analytics and AI sales tools can help you create more accurate sales forecasts in less time:
- Create Ongoing Forecast Refinement: Predictive analytics tools can tap into real-time data and adjust your forecasts accordingly.
- Leverage AI Chatbots: Use AI chatbots to gather more customer data and feedback that will fuel your predictive analyses and sales forecasts.
- Uncover Relevant Economic Trends: Track economic indicators to predict certain fluctuations in market demand, and use this to inform your sales forecasts.
- Use Detailed Deal Tracking: Sales analytics platforms can estimate the likelihood that a deal in your pipeline will close and bring these estimates into the sales forecast.
- Collect More Customer Data: Set up your predictive analytics tool to auto-collect customer purchase and behavioral data so you have more data for your forecasts.
- Create Better Lead Scoring: Improve your lead scoring model with AI-based lead generation tools that measure a wide range of variables; this will help you more accurately predict which leads will turn into sales, once again improving your sales forecasting.
Whether it’s forecasting next year’s customer behavior trends or estimating the number of sales in a given future quarter, predictive analytics software can dramatically improve your process by making it easier to collect and make sense of data from various disparate sources.
3 Predictive Analytics Tools For Your Team
Below are three powerful predictive analytics tools that will help your sales team make accurate sales forecasts in an efficient manner.
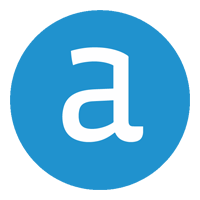
Alteryx is an AI platform for enterprise analytics. Its automated data preparation, AI-powered predictive analytics, and easy-to-use interface allows analysts to create predictive models and transform data into sales insights.
If you’re a big organization looking to create a more data-driven culture that uses AI-based predictive analytics across departments, this is a great option for you.
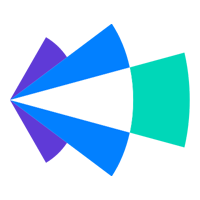
Clari Forecast is a predictive revenue and sales forecasting software that uses AI-based sales analytics and deal inspection to make accurate sales forecasts.
It takes into account rep inputs, sales performance across segments, deal context, and other predictions into one powerful forecasting model. The tool will also recommend tactics for hitting your revenue targets.
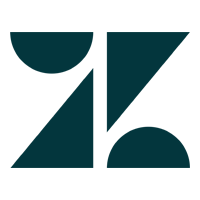
Zendesk Sell
Zendesk Sell is an affordable sales CRM with various sales forecasting features, including win probability forecasting, sales forecasting, monthly forecast analytics, and pre-built analytics dashboards.
It’s designed to give salespeople their sales performance via accurate data-driven insights and predictions based on various CRM metrics, like salesperson performance and pipeline value.
3 Challenges of Predictive Analytics In Sales Forecasting
The major challenges of predictive analytics in sales forecasting include poor data quality, complexity of the projects, and the unpredictability of significant market-altering events (also known as “black swans”).
Lacking Accurate or Sufficient Data
Poor data quality and insufficient data are common hurdles for businesses attempting to do predictive analytics. After all, these AI tools require sound inputs to make their predictions.
If you haven’t been tracking relevant metrics, say the open rate of past email campaigns, then the analysis won’t be able to use that data in its predictions.
To compensate for a lack of in-house data, sometimes companies use data scraping tools, which allow them to extract data like product prices or lead data from public sources.
They may also use tools like B2B database platforms like ZoomInfo to gain more information about their customers or to enrich their current database with more information.
Also important: remember to make sure that your predictive analytics tool integrates with your various data sources. If not, your project will quickly run into limitations.
Predictive Analytics Projects Are Delicate, Multi-Step Processes
Due to the complexity of predictive analytics, expertise and care are required to properly integrate it into your sales forecasting methodology.
Sales teams will likely need training and support to get to a skill level where they feel comfortable using predictive analytics and the required tools.
Of course, there are user-friendly predictive analytics platforms and CRM features that make it easier for non-techies to conduct predictive sales forecasting.
Global Events, Economic Conditions Are Highly Unpredictable
Regardless of the soundness of your methodology and the quality of your data, the sales forecasts you make with predictive analytics are still not guarantees; they’re estimates.
Many unforeseen factors, from political shifts to technological advancements, can pop up and render your forecast inaccurate.
That said, quality data is becoming increasingly available, and so are the AI tools that allow you to use it to understand the future.
Therefore, you may be able to integrate economic and other types of data into your sales forecasts to make them extremely robust.
Future Trends of Predictive Sales Analytics
Predictive sales analytics will continue to become more available and advanced as AI companies continue to develop and offer more robust predictive capabilities.
Further, in the next few years, expect to see more CRMs coming out with AI-based sales forecasting to meet the rising demand among sales professionals and executives for artificial intelligence.
Also, large language models (LLMs) and AI chatbots like Chat-GPT will make it easier for users of all technical skill sets to make sales predictions.
Assuming the generative AI tool is trained on company data, a VP of Sales can simply ask the generative AI certain questions, such as, “what will be our sales in Chicago in May 2026?”
Overall, predictive sales analytics will become more democratized as software companies aim to make their platforms easier for professionals to use.
Bottom Line: AI Drives Improved Sales Analytics
Predictive sales analytics and AI sales tools are making it easier than ever for businesses to capture data from various sources and make predictions, including sales forecasts, about the future.
This helps businesses prepare for potential risks, budget accordingly, and make sales plans that better fit future market trends and customer expectations.
To see a list of the leading generative AI apps, read our guide: Top 20 Generative AI Tools and Apps 2024
Get the Free Newsletter!
Subscribe to Daily Tech Insider for top news, trends & analysis
MOST POPULAR ARTICLES
10 best artificial intelligence (ai) 3d generators, ringcentral expands its collaboration platform, 8 best ai data analytics software &..., zeus kerravala on networking: multicloud, 5g, and..., datadog president amit agarwal on trends in....

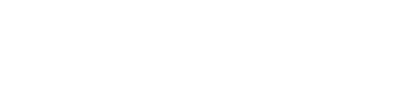
Along with Stanford news and stories, show me:
- Student information
- Faculty/Staff information
We want to provide announcements, events, leadership messages and resources that are relevant to you. Your selection is stored in a browser cookie which you can remove at any time using “Clear all personalization” below.
Image credit: Claire Scully
New advances in technology are upending education, from the recent debut of new artificial intelligence (AI) chatbots like ChatGPT to the growing accessibility of virtual-reality tools that expand the boundaries of the classroom. For educators, at the heart of it all is the hope that every learner gets an equal chance to develop the skills they need to succeed. But that promise is not without its pitfalls.
“Technology is a game-changer for education – it offers the prospect of universal access to high-quality learning experiences, and it creates fundamentally new ways of teaching,” said Dan Schwartz, dean of Stanford Graduate School of Education (GSE), who is also a professor of educational technology at the GSE and faculty director of the Stanford Accelerator for Learning . “But there are a lot of ways we teach that aren’t great, and a big fear with AI in particular is that we just get more efficient at teaching badly. This is a moment to pay attention, to do things differently.”
For K-12 schools, this year also marks the end of the Elementary and Secondary School Emergency Relief (ESSER) funding program, which has provided pandemic recovery funds that many districts used to invest in educational software and systems. With these funds running out in September 2024, schools are trying to determine their best use of technology as they face the prospect of diminishing resources.
Here, Schwartz and other Stanford education scholars weigh in on some of the technology trends taking center stage in the classroom this year.
AI in the classroom
In 2023, the big story in technology and education was generative AI, following the introduction of ChatGPT and other chatbots that produce text seemingly written by a human in response to a question or prompt. Educators immediately worried that students would use the chatbot to cheat by trying to pass its writing off as their own. As schools move to adopt policies around students’ use of the tool, many are also beginning to explore potential opportunities – for example, to generate reading assignments or coach students during the writing process.
AI can also help automate tasks like grading and lesson planning, freeing teachers to do the human work that drew them into the profession in the first place, said Victor Lee, an associate professor at the GSE and faculty lead for the AI + Education initiative at the Stanford Accelerator for Learning. “I’m heartened to see some movement toward creating AI tools that make teachers’ lives better – not to replace them, but to give them the time to do the work that only teachers are able to do,” he said. “I hope to see more on that front.”
He also emphasized the need to teach students now to begin questioning and critiquing the development and use of AI. “AI is not going away,” said Lee, who is also director of CRAFT (Classroom-Ready Resources about AI for Teaching), which provides free resources to help teach AI literacy to high school students across subject areas. “We need to teach students how to understand and think critically about this technology.”
Immersive environments
The use of immersive technologies like augmented reality, virtual reality, and mixed reality is also expected to surge in the classroom, especially as new high-profile devices integrating these realities hit the marketplace in 2024.
The educational possibilities now go beyond putting on a headset and experiencing life in a distant location. With new technologies, students can create their own local interactive 360-degree scenarios, using just a cell phone or inexpensive camera and simple online tools.
“This is an area that’s really going to explode over the next couple of years,” said Kristen Pilner Blair, director of research for the Digital Learning initiative at the Stanford Accelerator for Learning, which runs a program exploring the use of virtual field trips to promote learning. “Students can learn about the effects of climate change, say, by virtually experiencing the impact on a particular environment. But they can also become creators, documenting and sharing immersive media that shows the effects where they live.”
Integrating AI into virtual simulations could also soon take the experience to another level, Schwartz said. “If your VR experience brings me to a redwood tree, you could have a window pop up that allows me to ask questions about the tree, and AI can deliver the answers.”
Gamification
Another trend expected to intensify this year is the gamification of learning activities, often featuring dynamic videos with interactive elements to engage and hold students’ attention.
“Gamification is a good motivator, because one key aspect is reward, which is very powerful,” said Schwartz. The downside? Rewards are specific to the activity at hand, which may not extend to learning more generally. “If I get rewarded for doing math in a space-age video game, it doesn’t mean I’m going to be motivated to do math anywhere else.”
Gamification sometimes tries to make “chocolate-covered broccoli,” Schwartz said, by adding art and rewards to make speeded response tasks involving single-answer, factual questions more fun. He hopes to see more creative play patterns that give students points for rethinking an approach or adapting their strategy, rather than only rewarding them for quickly producing a correct response.
Data-gathering and analysis
The growing use of technology in schools is producing massive amounts of data on students’ activities in the classroom and online. “We’re now able to capture moment-to-moment data, every keystroke a kid makes,” said Schwartz – data that can reveal areas of struggle and different learning opportunities, from solving a math problem to approaching a writing assignment.
But outside of research settings, he said, that type of granular data – now owned by tech companies – is more likely used to refine the design of the software than to provide teachers with actionable information.
The promise of personalized learning is being able to generate content aligned with students’ interests and skill levels, and making lessons more accessible for multilingual learners and students with disabilities. Realizing that promise requires that educators can make sense of the data that’s being collected, said Schwartz – and while advances in AI are making it easier to identify patterns and findings, the data also needs to be in a system and form educators can access and analyze for decision-making. Developing a usable infrastructure for that data, Schwartz said, is an important next step.
With the accumulation of student data comes privacy concerns: How is the data being collected? Are there regulations or guidelines around its use in decision-making? What steps are being taken to prevent unauthorized access? In 2023 K-12 schools experienced a rise in cyberattacks, underscoring the need to implement strong systems to safeguard student data.
Technology is “requiring people to check their assumptions about education,” said Schwartz, noting that AI in particular is very efficient at replicating biases and automating the way things have been done in the past, including poor models of instruction. “But it’s also opening up new possibilities for students producing material, and for being able to identify children who are not average so we can customize toward them. It’s an opportunity to think of entirely new ways of teaching – this is the path I hope to see.”
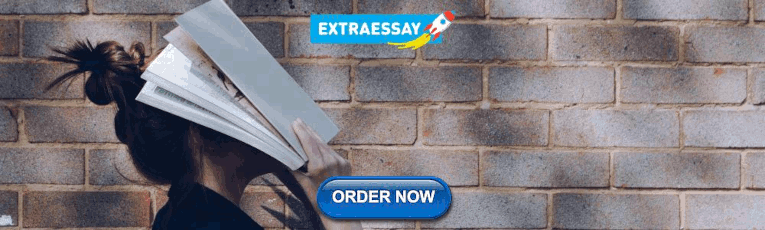
IMAGES
VIDEO
COMMENTS
Predictive analytics, a branch in the domain of advanced. analytics, is used in predicting the fut ure events. It analyzes. the current and historical data in order to make predictions. about the ...
Abstract —Artificial Intelligence (AI) has been growing con-. siderably over the last ten years. Machine Learning (ML) is. probably the most popular branch of AI to date. Most systems. that use ...
Predictive analytics is an umbrella term that describes various statistical and data analytics techniques - including data mining, predictive modeling, and machine learning. The primary purpose of predictive analytics is to make predictions about outcomes, trends, or events based on patterns and insights from historical data. Predictive ...
6 Roundtable Report - Analytics of the Future: Predictive Analytics November 2020 A Leading Organization's Approach A large technology hardware, software, and service company shared its extensive efforts in using data science and predictive analytics, which were part of its company-wide four-year digital transformation journey.
Three steps are typically involved in the big data analytics ( Table 1 ). The first step is the formulation of clinical questions ( 4 ), which can be categorized into three types: (I) epidemiological question on prevalence and incidence and risk factors; (II) effectiveness and/or safety of an intervention; and (III) predictive analytics.
What is predictive analytics? Predictive analytics is a branch of advanced analytics that makes predictions about future outcomes using historical data combined with statistical modeling, data mining techniques and machine learning. Companies employ predictive analytics to find patterns in this data to identify risks and opportunities ...
5 Examples of Predictive Analytics in Action. 1. Finance: Forecasting Future Cash Flow. Every business needs to keep periodic financial records, and predictive analytics can play a big role in forecasting your organization's future health. Using historical data from previous financial statements, as well as data from the broader industry, you ...
The last few years have witnessed an upsurge in the number of studies using Machine and Deep learning models to predict vital academic outcomes based on different kinds and sources of student-related data, with the goal of improving the learning process from all perspectives. This has led to the emergence of predictive modelling as a core practice in Learning Analytics and Educational Data ...
Full size image. Step 1 in the model is to determine the business problem. The business problem in this example is to develop a predictive model to detect and mitigate potentially fraudulent automobile insurance claims. Step 2 in the model is to narrow down the business problem and develop the hypotheses.
Predictive analytics is one of the four key types of data analytics, and typically forecasts what will happen in the future, such as how sales will shift during different seasons or how consumers will respond to a change in price. Businesses often use predictive analytics to make data-driven decisions and optimize outcomes.
This includes studies using artificial intelligence to develop predictive algorithms that make individualized diagnostic or prognostic risk predictions. We argue that it is paramount to make the algorithm behind any prediction publicly available. This allows independent external validation, assessment of performance heterogeneity across ...
Predictive analytics is a branch of data science that applies various techniques including statistical inference, machine learning, data mining, and information visualization toward the ultimate goal of forecasting, modeling, and understanding the future behavior of a system based on historical and/or real-time data.
Predictive Analytics: The use of statistics and modeling to determine future performance based on current and historical data. Predictive analytics look at patterns in data to determine if those ...
Predictive analytics is the process of using data to forecast future outcomes. The process uses data analysis, machine learning, artificial intelligence, and statistical models to find patterns that might predict future behavior. Organizations can use historic and current data to forecast trends and behaviors seconds, days, or years into the ...
The Actionable Mining and Predictive Analysis model just presented differs from the first two models in its specificity to the public safety and security domains, as well as in the inclusion of operationally relevant preprocessing and output. Specifically, this model includes operationally relevant recoding and variable selection, public safety and security-specific model evaluation, and an ...
Definition: Predictive analytics is the practice of extracting information from existing data sets in order to predict future probabilities and trends. The goal is to go beyond what has happened and provide a best assessment on what will happen in the future. This is accomplished through various statistical and machine learning techniques.
Data Science-Related Research Topics. Developing machine learning models for real-time fraud detection in online transactions. The use of big data analytics in predicting and managing urban traffic flow. Investigating the effectiveness of data mining techniques in identifying early signs of mental health issues from social media usage.
They also argue the textual analysis of market research reports can provide predictive information. There is a growing body of literature that makes use of ML techniques in such an approach. 53 Most scholars classify the optimization challenge as a supervised learning problem. 54 , 103 For example, Ban et al 55 propose a Performance Based ...
Empirical quantitative research method is used to verify the model with the sample of UK insurance sector analysis. This research will conclude some practical insights for Insurance companies using AI, ML, Big data processing and Cloud computing for the better client satisfaction, predictive analysis, and trending. Download Full-text.
Big data analytics (BDA) in supply chain management (SCM) is receiving a growing attention. This is due to the fact that BDA has a wide range of applications in SCM, including customer behavior analysis, trend analysis, and demand prediction. In this survey, we investigate the predictive BDA applications in supply chain demand forecasting to propose a classification of these applications ...
Predictive analytics definition. Predictive analytics is the art of using historical and current data to make projections about what might happen in the future. By looking at what's happening in the present and what has happened historically, and then applying statistical analysis techniques to the data, researchers can make predictions about ...
To keep ahead of the game, here are seven key projects primed for use of predictive analytics today. 1. Predictive equipment maintenance. Knowing when industrial or manufacturing equipment is ...
Future Actuaries. Education & Exams. Professional Development. Research Institute. Professional Sections. Tools & Resources. About SOA. Examples of SOA experience studies and research reports that have made use of predictive analytic techniques.
Moreover, predictive analytics can forecast future trends, allowing companies to allocate resources more efficiently and avoid costly missteps. Better customer engagement. Understanding customer needs, behaviors and sentiments is crucial for successful engagement and big data analytics provides the tools to achieve this understanding. Companies ...
In the ongoing discussion about how learning analytics can effectively support self-regulated student learning and which types of data are most suitable for this purpose, this empirical study aligns with the framework proposed by Buckingham Shum and Deakin Crick (2012) who advocated the inclusion of both behavioural trace data and survey data in learning analytics studies. By incorporating ...
Yuval Atsmon: When people talk about artificial intelligence, they include everything to do with analytics, automation, and data analysis. Marvin Minsky, the pioneer of artificial intelligence research in the 1960s, talked about AI as a "suitcase word"—a term into which you can stuff whatever you want—and that still seems to be the case.
Keywords: breath analysis, breathomics, volatile organic compounds (VOC), disease diagnostics, machine learning, artificial intelligence . Important Note: All contributions to this Research Topic must be within the scope of the section and journal to which they are submitted, as defined in their mission statements.
Predictive sales analysis refers to the AI software and processes that businesses use to make accurate predictions about the future by analyzing data corresponding to various factors, including ...
In 2023 K-12 schools experienced a rise in cyberattacks, underscoring the need to implement strong systems to safeguard student data. Technology is "requiring people to check their assumptions ...
This paper pioneers a novel approach in electromagnetic (EM) system analysis by synergistically combining Bayesian Neural Networks (BNNs) informed by Latin Hypercube Sampling (LHS) with advanced thermal-mechanical surrogate modeling within COMSOL simulations for high-frequency low-pass filter modeling. Our methodology transcends traditional EM characterization by integrating physical ...