Big Ideas 2021: 10 Broad Trends in K-12 Education in 10 Charts
Each year, for the past four years, Education Week has produced a special report on big ideas in K-12 education. The reports focus on important and timely issues that schools have grappled with in the past 12 months. Not surprisingly, the coronavirus pandemic was a major emphasis in the 2021 edition, Big Ideas for Education’s Urgent Challenges . Initially published in September 2021, this report examined such pandemic-related topics as remote learning, educator stress, and student home environments.
Drawing upon the results of a nationally representative survey of nearly 900 teachers, principals, and district leaders that was incorporated into Education Week’s fourth annual report on big ideas in K-12 education, this Spotlight sums up 10 broad trends in 10 accompanying charts. These ideas include the transformation of educational technology, educators’ knowledge of students’ lives outside of school, and educator stress. Given the time period covered by the survey, all of these broad trends were deeply impacted by the coronavirus pandemic, a theme that resonates throughout this report.
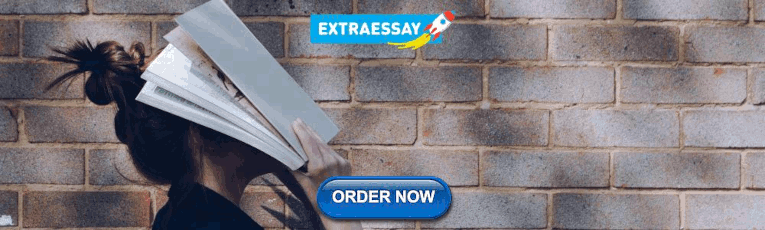
Sign Up for The Savvy Principal
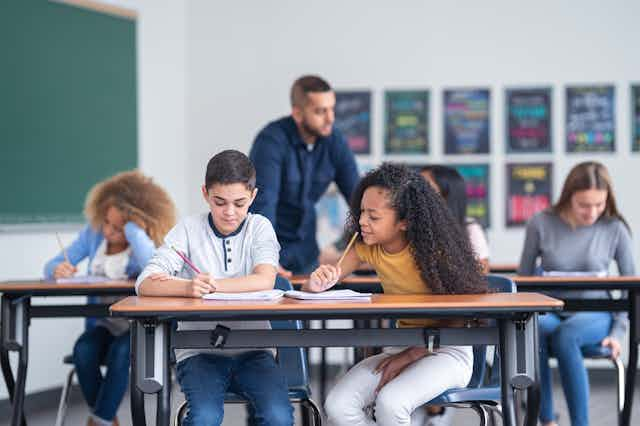
How educational research could play a greater role in K-12 school improvement
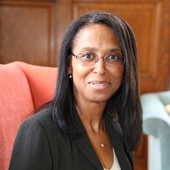
Clinical Professor of Applied Human Development, Boston University
Disclosure statement
Detris Honora Adelabu does not work for, consult, own shares in or receive funding from any company or organisation that would benefit from this article, and has disclosed no relevant affiliations beyond their academic appointment.
Boston University provides funding as a founding partner of The Conversation US.
View all partners
For the past 20 years, I have taught research methods in education to students here in the U.S. and in other countries. While the purpose of the course is to show students how to do effective research, the ultimate goal of the research is to get better academic results for the nation’s K-12 students and schools.
Vast resources are already being spent on this goal. Between 2019 and 2022, the Institute of Educational Sciences , the research and evaluation arm of the U.S. Education Department, distributed US$473 million in 255 grants to improve educational outcomes.
In 2021, colleges and universities spent approximately $1.6 billion on educational research .
The research is not hard to find. The Educational Research Information Center, a federally run repository, houses 1.6 million educational research sources in over 1,000 scholarly journals.
And there are plenty of opportunities for educational researchers to network and collaborate. Each year, for instance, more than 15,000 educators and researchers gather to present or discuss educational research findings at the annual meeting of the American Educational Research Association .
Yet, for all the time, money and effort that have been spent on producing research in the field of education, the nation seems to have little to show for it in terms of improvements in academic achievement.
Growing gaps
Even prior to the COVID-19 pandemic, test scores were beginning to decline. Results from the 2019 National Assessment of Educational Progress, , or NAEP – the most representative assessment of what elementary and middle school students know across specific subjects – show a widening gap between the highest and lowest achievement levels on the NAEP for fourth grade mathematics and eighth grade reading between 2017-19. During the same period, NAEP outcomes show stagnated growth in reading achievement among fourth graders. By eighth grade, there is a greater gap in reading achievement between the highest- and lowest-achieving students.
Some education experts have even suggested that the chances for progress get dimmer for students as they get older. For instance, in a 2019-2020 report to Congress , Mark Schneider, the Institute of Educational Sciences director, wrote: “for science and math, the longer students stay in school, the more likely they are to fail to meet even NAEP’s basic performance level.”
Scores on the International Assessment of Adult Competencies , a measure of literacy, numeracy and problem-solving skills, suggest a similar pattern of achievement. Achievement levels on the assessment show a slight decline in literacy and numeracy between 2012-14 and 2017. Fewer Americans are scoring at the highest levels of proficiency in literacy and numeracy.
As an educational researcher who focuses on academic outcomes for low-income students and students of color , I believe these troubling results raise serious questions about whether educational research is being put to use.
Are school leaders and policymakers actually reading any of the vast amount of educational research that exists? Or does it go largely unnoticed in voluminous virtual vaults? What, if anything, can be done to make sure that educational research findings and recommendations are actually being tried?
Here are four things I believe can be done in order to make sure that educational research is actually being applied.
1. Build better relationships with school leaders
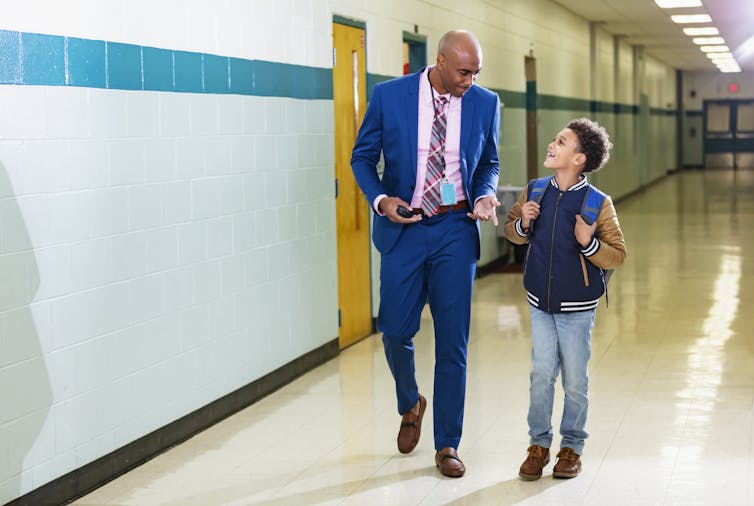
Educational researchers can reach out to school leaders before doing their research in order to design research based on the needs of schools and schoolchildren. If school leaders can see how educational research can specifically benefit their school community, they may be more likely to implement findings and recommendations from the research.
2. Make policy and practice part of the research process
By implementing new policies and practices based on research findings, researchers can work with school leaders to do further research to see if the new policies and practices actually work.
For example, The Investing in Innovation (i3) Fund was established by the American Recovery and Reinvestment Act of 2009 to fund the implementation and evaluation of education interventions with a record of improving student achievement. Through the fund, $679 million was distributed through 67 grants – and 12 of those 67 funded projects improved student outcomes. The key to success? Having a “tight implementation” plan, which was shown to produce at least one positive student outcome.
3. Rethink how research impact is measured
As part of the national rankings for colleges of education – that is, the schools that prepare schoolteachers for their careers – engagement with public schools could be made a factor in the rankings. The rankings could also include measurable educational impact.
4. Rethink and redefine how research is distributed
Evidence-based instruction can improve student outcomes . However, public school teachers often can’t afford to access the evidence or the time to make sense of it. Research findings written in everyday language could be distributed at conferences frequented by public school teachers and in the periodicals that they read.
If research findings are to make a difference, I believe there has to be a stronger focus on using research to bring about real-world change in public schools.
- Academic research
- Education research
- Academic results
- Proficiency Level
- K-12 education
- Student test scores
- Higher ed attainment
- Federal role in K-12 education
- K-12 schools

Case Management Specialist

Lecturer / Senior Lecturer - Marketing

Assistant Editor - 1 year cadetship

Executive Dean, Faculty of Health

Lecturer/Senior Lecturer, Earth System Science (School of Science)
- Our Program Divisions
- Our Three Academies
- Government Affairs
- Statement on Diversity and Inclusion
- Our Study Process
- Conflict of Interest Policies and Procedures
- Project Comments and Information
- Read Our Expert Reports and Published Proceedings
- Explore PNAS, the Flagship Scientific Journal of NAS
- Access Transportation Research Board Publications
- Coronavirus Disease 2019 (COVID-19)
- Diversity, Equity, and Inclusion
- Economic Recovery
- Fellowships and Grants
- Publications by Division
- Division of Behavioral and Social Sciences and Education
- Division on Earth and Life Studies
- Division on Engineering and Physical Sciences
- Gulf Research Program
- Health and Medicine Division
- Policy and Global Affairs Division
- Transportation Research Board
- National Academy of Sciences
- National Academy of Engineering
- National Academy of Medicine
- Publications by Topic
- Agriculture
- Behavioral and Social Sciences
- Biography and Autobiography
- Biology and Life Sciences
- Computers and Information Technology
- Conflict and Security Issues
- Earth Sciences
- Energy and Energy Conservation
- Engineering and Technology
- Environment and Environmental Studies
- Food and Nutrition
- Health and Medicine
- Industry and Labor
- Math, Chemistry, and Physics
- Policy for Science and Technology
- Space and Aeronautics
- Surveys and Statistics
- Transportation and Infrastructure
- Searchable Collections
- New Releases
VIEW LARGER COVER
STEM Integration in K-12 Education
Status, prospects, and an agenda for research.
STEM Integration in K-12 Education examines current efforts to connect the STEM disciplines in K-12 education. This report identifies and characterizes existing approaches to integrated STEM education, both in formal and after- and out-of-school settings. The report reviews the evidence for the impact of integrated approaches on various student outcomes, and it proposes a set of priority research questions to advance the understanding of integrated STEM education. STEM Integration in K-12 Education proposes a framework to provide a common perspective and vocabulary for researchers, practitioners, and others to identify, discuss, and investigate specific integrated STEM initiatives within the K-12 education system of the United States.
STEM Integration in K-12 Education makes recommendations for designers of integrated STEM experiences, assessment developers, and researchers to design and document effective integrated STEM education. This report will help to further their work and improve the chances that some forms of integrated STEM education will make a positive difference in student learning and interest and other valued outcomes.
Winner of ITEEA 's Council on Technology and Engineering Teacher Education 2015 Outstanding Research Award
RESOURCES AT A GLANCE
- Press Release
- Report Brief
- Education — Math and Science Education
- Education — K-12 Education
- Engineering and Technology — Engineering Education
Suggested Citation
National Academy of Engineering and National Research Council. 2014. STEM Integration in K-12 Education: Status, Prospects, and an Agenda for Research . Washington, DC: The National Academies Press. https://doi.org/10.17226/18612. Import this citation to: Bibtex EndNote Reference Manager
Publication Info
- Paperback: 978-0-309-29796-7
- Ebook: 978-0-309-29799-8
What is skim?
The Chapter Skim search tool presents what we've algorithmically identified as the most significant single chunk of text within every page in the chapter. You may select key terms to highlight them within pages of each chapter.
What is STEM? It's the acronym for science, technology, engineering and mathematics. But while kindergarten through 12th grade education usually focuses on science or mathematics in isolation, all four of these disciplines are closely intertwined in the real world. Imagine if K-12 students were taught in ways that highlighted these connections, making their education more relevant to their lives and opening doors to new and exciting careers. Watch this video and tell us what you think!
Copyright Information
The National Academies Press (NAP) has partnered with Copyright Clearance Center's Marketplace service to offer you a variety of options for reusing NAP content. Through Marketplace, you may request permission to reprint NAP content in another publication, course pack, secure website, or other media. Marketplace allows you to instantly obtain permission, pay related fees, and print a license directly from the NAP website. The complete terms and conditions of your reuse license can be found in the license agreement that will be made available to you during the online order process. To request permission through Marketplace you are required to create an account by filling out a simple online form. The following list describes license reuses offered by the NAP through Marketplace:
- Republish text, tables, figures, or images in print
- Post on a secure Intranet/Extranet website
- Use in a PowerPoint Presentation
- Distribute via CD-ROM
Click here to obtain permission for the above reuses. If you have questions or comments concerning the Marketplace service, please contact:
Marketplace Support International +1.978.646.2600 US Toll Free +1.855.239.3415 E-mail: [email protected] marketplace.copyright.com
To request permission to distribute a PDF, please contact our Customer Service Department at [email protected] .
What is a prepublication?
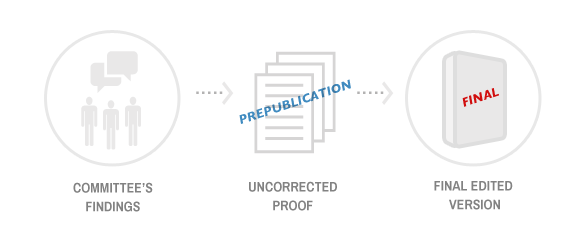
An uncorrected copy, or prepublication, is an uncorrected proof of the book. We publish prepublications to facilitate timely access to the committee's findings.
What happens when I pre-order?
The final version of this book has not been published yet. You can pre-order a copy of the book and we will send it to you when it becomes available. We will not charge you for the book until it ships. Pricing for a pre-ordered book is estimated and subject to change. All backorders will be released at the final established price. As a courtesy, if the price increases by more than $3.00 we will notify you. If the price decreases, we will simply charge the lower price. Applicable discounts will be extended.
Downloading and Using eBooks from NAP
What is an ebook.
An ebook is one of two file formats that are intended to be used with e-reader devices and apps such as Amazon Kindle or Apple iBooks.
Why is an eBook better than a PDF?
A PDF is a digital representation of the print book, so while it can be loaded into most e-reader programs, it doesn't allow for resizable text or advanced, interactive functionality. The eBook is optimized for e-reader devices and apps, which means that it offers a much better digital reading experience than a PDF, including resizable text and interactive features (when available).
Where do I get eBook files?
eBook files are now available for a large number of reports on the NAP.edu website. If an eBook is available, you'll see the option to purchase it on the book page.
View more FAQ's about Ebooks
Types of Publications
Consensus Study Report: Consensus Study Reports published by the National Academies of Sciences, Engineering, and Medicine document the evidence-based consensus on the study’s statement of task by an authoring committee of experts. Reports typically include findings, conclusions, and recommendations based on information gathered by the committee and the committee’s deliberations. Each report has been subjected to a rigorous and independent peer-review process and it represents the position of the National Academies on the statement of task.
Thank you for visiting nature.com. You are using a browser version with limited support for CSS. To obtain the best experience, we recommend you use a more up to date browser (or turn off compatibility mode in Internet Explorer). In the meantime, to ensure continued support, we are displaying the site without styles and JavaScript.
- View all journals
- My Account Login
- Explore content
- About the journal
- Publish with us
- Sign up for alerts
- Review Article
- Open access
- Published: 03 November 2023
Subject integration and theme evolution of STEM education in K-12 and higher education research
- Zehui Zhan ORCID: orcid.org/0000-0002-6936-1977 1 , 2 &
- Shijing Niu 1
Humanities and Social Sciences Communications volume 10 , Article number: 781 ( 2023 ) Cite this article
3100 Accesses
5 Citations
1 Altmetric
Metrics details
- Science, technology and society
Over the past two decades, the field of STEM education has produced a wealth of research findings. This study systematically reviewed the published literature from the perspective of subject integration and theme evolution, considering both K-12 and higher education. It was found that STEM education originated from higher education, but the main emphasis is gradually shifting to the K-12 stage. There were mainly sixteen subjects involved in STEM education, showing the gradual in-depth integration of science, engineering, technology, math, humanities, and social sciences, in which humanism is increasingly emphasized. Culture is a new perspective for understanding the diversity of participants, which also gives STEM education a distinctive regional character. In addition, in the K-12 stage, research related to computer science and art stands out alongside the four main subjects, demonstrating relatively even distribution across research themes. Conversely, in higher education, engineering, and chemistry garner considerable attention, with research themes predominantly concentrated on learning outcomes and social relevance. On a holistic scale, researchers exhibit a pronounced interest in learning outcomes, yet relatively less emphasis is placed on pedagogical aspects. Regarding prospective trends, there should be a heightened focus on the cultivation of students’ thinking competencies, students’ career development, and pedagogy.
Similar content being viewed by others
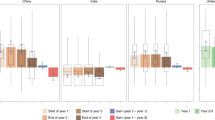
Skill levels and gains in university STEM education in China, India, Russia and the United States
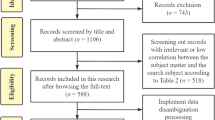
Education reform and change driven by digital technology: a bibliometric study from a global perspective
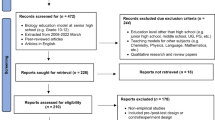
A meta-analysis to gauge the impact of pedagogies employed in mixed-ability high school biology classrooms
Introduction.
In response to the global challenges, the promotion of economic development, and the need to meet modern society’s demands for knowledge and skills within the realms of STEM, the emergence of STEM education aimed to develop employment opportunities in STEM fields while bolstering national competitiveness. The acronym STEM education originated from the four subjects (i.e., science, mathematics, engineering, and technology) that were proposed in the report “ Undergraduate Science, Mathematics, and Engineering Education ” (National Science Board 1986 ). Essentially, STEM education stands as an innovation-oriented education that prevailed in Western countries, spearheaded by the United States.
Subsequently, Yakman ( 2008 ) introduced the addition of the “A” element, representing arts, to STEM education, thereby incorporating humanities subjects such as history, philosophy, and religion. The fundamental objective of STEM education is to amalgamate multiple subjects into a cohesive framework (Morrison 2006 ). According to the National Science Foundation ( 2014 ), STEM entails a comprehensive integration of various disciplines, encompassing not only the subjects of natural sciences (e.g., computers and information, engineering, and mathematics), but also the subjects of social sciences (e.g., psychology, economics, sociology, and political science). With an increasing number of disciplines becoming intertwined in STEM education, its interdisciplinary essence has become progressively prominent. As a result, STEM education is increasingly acknowledged as interdisciplinary education with a focus on engineering, where subject integration plays a central role.
In the past two decades, STEM education has witnessed a large number of research achievements, and many scholars have conducted comprehensive reviews on the topic. These studies have focused either on curriculum reform (Uskoković 2023 ), teaching methods (Li and Wong 2023 ), or technology applications (Salas-Pilco et al. 2022 ; Conde et al. 2021 ). At the research level, especially in teaching and learning, many researchers have recognized the interdisciplinary nature of STEM education, but almost no research has focused on the development of STEM education from the perspective of subject integration (Perignat and Katz-Buonincontro 2019 ). The evolution of STEM disciplines and the development of their themes are closely interrelated, but the underlying coupling relationships and reasons for their formation remain unexplored.
Moreover, there exist significant differences in the disciplinary systems of K-12 education and higher education, including teaching objectives, methods, breadth, and depth. As a result, STEM education at different educational levels exhibits distinct characteristics, making it necessary to conduct a segmented analysis. Although some researchers have analyzed the development trends in STEM education from a macro perspective and recognized differences between educational stages, this has not been the primary focus of their work, and there has also been a lack of emphasis on specific disciplines (Zhan et al. 2022a ).
Based on these considerations, this study attempts to examine and explore the developmental trajectories and trends of STEM education at various educational stages from the perspective of disciplinary evolution. Specifically, the following questions will be addressed:
RQ1: How were subjects integrated into STEM education in K-12 and higher education?
RQ2: What is the distribution of the subject themes involved in STEM education at the K-12 and higher education levels?
Keyword search
Papers related to STEM education were searched on 10 July 2023 from the Science Web Core Collection. The query started with the search statement TI = (STEM education) OR TI = (STEAM education) OR AK = (STEM education) OR AK = (STEAM education) OR AK = (STEAM education) OR KP= (STEM education) OR KP = (STEAM education), which yielded a total of 3668 publications. The search results were further refined according to the research area, while duplicates, poorly indexed documents, and documents inconsistent with STEM Education/STEAM Education research were removed, leaving a final total of 2188 publications.
Research process
WOS (Web of Science) was selected as the data source for this study. This database covers a wide range of journals, has a high impact, and can provide a complete sample for this study (Martín-Páez et al. 2019 ). Then, the following steps were used to analyze the data.
Step 1: Data classification (education stage classification). It has been shown that K-12 and higher education systems have different focuses on STEM education (Zhan et al. 2022a ). To clarify the characteristics of the different stages, the data was divided into K-12 and higher education levels based on field information such as title, keywords (including author keywords and keywords plus), journal, and abstract. After discarding the data that could not be categorized, 903 valid data were obtained for the K-12 stage, with the time range from 2009 to 2023, and 873 valid data for the higher education stage, with the time range from 2004 to 2023.
Step 2: Keywords cleaning. In the collected data, some keywords have the same meaning but may be analyzed as different words, such as math, mathematics; model, models, etc., and some words have similar semantics, which may also lead to inaccurate analysis results when analyzed separately, so it was necessary to build a synonym database for synonym replacement so that they could be more accurately counted and visualized.
Step 3: Data classification (time and theme classification). The data at different stages were sub-categorized by time and theme respectively. Time division according to a time slice for a year. The keywords with the top 10 frequency in each subject were screened as alternative theme terms, and the alternative subject terms of each subject were integrated, then the remaining keywords were used as subject terms to participate in the final statistics.
Step 4: Data statistics and visualization. The categorized subject time and subject themes of different sections were counted separately, and the statistical results were visualized and described using heat maps. The heat map used in this study is a kind of statistical chart that shows the frequency of a certain word by the relative shades of color blocks, with dark colors representing the high frequency of occurrence and light colors representing the low frequency of occurrence. Finally, four maps were created to depict the time distribution and theme distribution at the K-12 and higher education levels. The research process is shown in Fig. 1 .
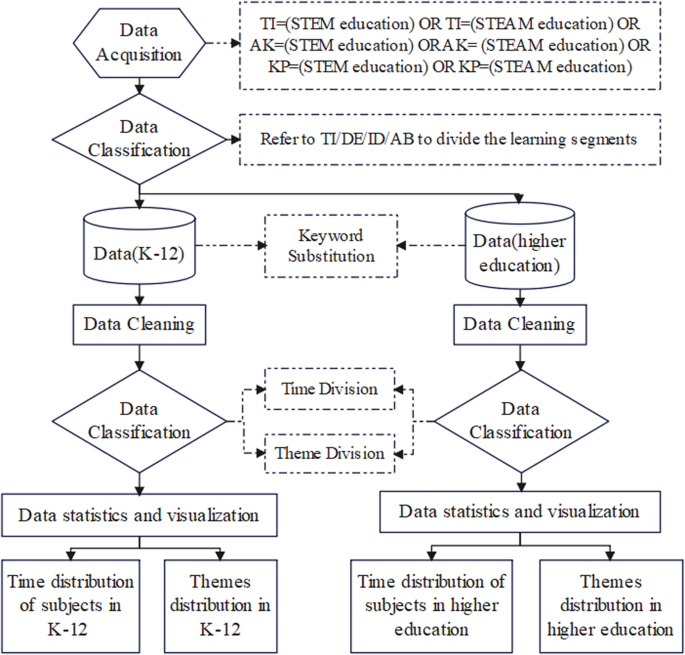
The entire research process went through five stages: data acquisition, data classification (education stage classification), keyword cleaning, data classification (time and theme classification), and data statistics and visualization.
Research findings
Analysis of the temporal evolution of the subject.
STEM education originated from higher education, but in recent years, there has been rapid development in the K-12 stage. Both levels show a similar trend of overall integration, starting with a focus on science, technology, engineering, and mathematics, and later, an increasing involvement of humanities and social sciences. Interdisciplinary integration has become prominent, particularly in higher education. As shown in Fig. 2 .
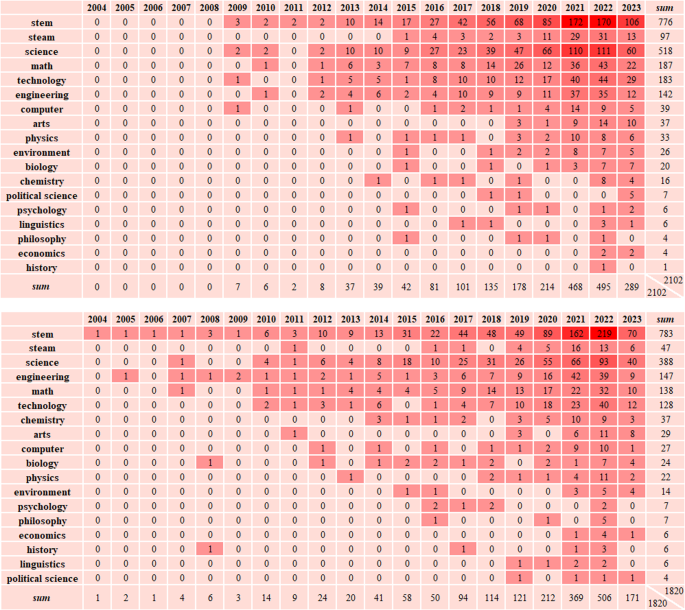
The first column displays the subjects involved in STEM education, and the first row is the timeline. This figure illustrates the time and subject distribution of STEM-related literature. Darker colors indicate a greater number of documents related to the corresponding time node and subject.
Subject integration of STEM in K-12 Education
Subject integration refers to the methods and processes of cross-fertilization of different subjects, which is specifically expressed as the mutual integration of a subject with one or more subjects through knowledge, concepts, skills, methods, etc. at a certain time node, so time node is one important element of subject integration path analysis. Figure 2(1) illustrates the integration of different subjects at different time points at the K12 level. In the early stages (2009 to 2014), the subjects of science, technology, engineering, and mathematics played a dominant role, and these subjects were considered to be the core of STEM education. Over time, science subjects such as computer science, arts, physics, and environmental science were gradually incorporated into the STEM education integration pathway. In the post-2019 period, more and more research has emerged in the humanities and social sciences.
Different subjects played different roles in STEM education at the K-12 level. Science and technology provided a rich foundation of knowledge and practice for students involved in STEM education. Engineering developed students’ design thinking and problem-solving skills, while mathematics provided the foundation for quantitative and logical thinking. Early STEM education has not yet shown a clear trend of cross-fertilization of disciplines. Science courses, such as physics, chemistry, and biology, were considered the main foci of STEM education, with students exploring basic science concepts through participation in experiments and educational games.
As time went on, computer science and environmental science became important subjects for STEM education, and they facilitated the development of computational thinking and environmental awareness in students at the basic education level (Zhan et al. 2022b ). In 2013, Grover and Pea ( 2013 ) published a study entitled “Computational Thinking in K-12: A Review of the State of the Field”, which explored the importance of including computational thinking as a content and goal of STEM education and had a profound impact on subsequent research regarding the integration of computing into STEM education. In 2022, the U.S. Department of Education proposed “ Science, Technology, Engineering, and Math, including Computer Science ”, also hinting at the importance of computer science in STEM (Department of Education, 2022 ).
Environmental issues have always been important social topics and are closely related to the development of engineering and technology. The integration of environmental science emphasized the importance of environmental awareness and sustainable development, making students conscious of environmental problems and proposing solutions through scientific and technological means. At the K-12 level, researchers have focused on green skills elements in STEM curricula and the integration of STEM educational approaches in environmental curricula (Sümen and Çalisici 2016 ).
After 2019, the integration of humanities and social sciences brought more dimensions and diversity to STEM education. At this stage, STEM education showed a clear interdisciplinary character. Compared to science courses that are involved in STEM education in the form of teaching content, humanities, and social sciences are integrated in a way that is more on the level of research methods and educational philosophy.
Psychological research explored the impact of spatial thinking, spatial skills, and spatial abilities on STEM learning, recognizing the importance of students’ mental states and cognitive abilities for learning (Buckley et al. 2018 ; Gilligan et al. 2017 ; Taylor and Hutton 2013 ). The inclusion of arts enhanced students’ understanding of creativity and encouraged them to use their imagination and creative abilities in the practice of science and engineering (Yakman 2010 ). The inclusion of political science primarily conducted a comparative study of STEM education across different regions from the perspective of policies (Sharma and Yarlagadda 2018 ).
Philosophy created a framework for analyzing and synthesizing STEM education goals and discourses, encouraging students to think deeply about the value and impact of science and technology (Ortiz-Revilla et al. 2020 ). The incorporation of history offered students diverse learning objectives that enabled them to understand the context and social impact of the development of science and technology (Park and Cho 2022 ). The inclusion of linguistics promoted the engagement of culturally and linguistically diverse students in STEM education, encouraging cross-cultural communication and collaboration across linguistic and cultural boundaries (Mallinson and Hudley 2018 ).
In the K-12 stage, there is a significant concentration of disciplines in STEM education, with computer science and arts receiving the most attention alongside the four main subjects. Additionally, interdisciplinary teaching in this stage is guided by conceptual instruction. In 2013, the United States released the milestone document “ K-12 Science Education Framework ”, initiating a major reform in science education. This document became the blueprint for the formal launch of the new era of science education reform known as the “ Next Generation Science Standards ” (NGSS). NGSS proposed a paradigm for science education in the U.S., integrating three dimensions: practices, cross-cutting concepts, and disciplinary core ideas. Seven powerful cross-cutting concepts were selected from these dimensions to bridge the boundaries between different subjects. These concepts include patterns, cause and effect relationships, systems and system models, matter and energy, structure and function, stability and change, and scale, proportion, and quantity (National Research Council 2013 ). The document brought new guidance and direction to STEM education in the United States, emphasizing comprehensive and interdisciplinary educational principles.
Subject integration of STEM in higher education
Figure 2(2) illustrates the time distribution of subjects at the higher education level. Since 2004, a total of 16 subjects have been involved in STEM studies at the higher education level. Similar to the K-12 level, the integration in higher education also shows an intersection of science, technology, engineering, humanities, and social sciences.
STEM subjects (science, technology, engineering, and mathematics) continued to play an important role at the higher education level, covering a wide range of fields of study. Unlike at the K-12 level, STEM education in higher education has exhibited a blend of disciplines at the beginning because of the strong interdisciplinary nature of the courses offered at universities themselves (for example, biochemistry). Students were exposed to more specialized and in-depth knowledge of science, technology, engineering, and mathematics disciplines in their areas of specialization. The focus of disciplinary integration was on combining theories and methods from different disciplines for cross-disciplinary research and innovation. For example, researchers in the multidisciplinary education (ME) course selected undergraduate students in engineering, pre-nursing, and pre-occupational health to collaborate in a maker space to solve health problems and create practical solutions to health-related problems facing the community through their backgrounds and competencies (Ludwig et al. 2017 ).
The development and disciplinary integration of STEM education was influenced by educational reform and societal needs. With the continuous advancement of technology and globalization, there was an increasing demand for comprehensive ability and interdisciplinary thinking. Traditional science and engineering education could no longer meet the current social and professional needs. Therefore, the integration of humanities and social science disciplines has become an important trend in the development of STEM education. For example, art subjects have promoted the integration of innovation and esthetics by providing creative expression and the development of design thinking. The prominence of gender, race, and economic issues, cultural background conflict in higher education has called for the inclusion of social science disciplines such as psychology, economics, and philosophy, linguistics, political science.
In higher education, the distribution of subjects was relatively diverse, with engineering receiving significant attention among the four main subjects. Additionally, chemistry has also been highly regarded, while comparatively, computer science’s involvement is not as prominent.
Comparing the temporal evolution of subjects at different educational levels
In summary, the concept of STEM education was gradually evolving from an initial bias toward engineering education to a more integrated and diverse educational paradigm. Since 2004, there have been 16 subjects involved in STEM (i.e., science, technology, engineering, mathematics, art, physics, chemistry, biology, psychology, computer science, environmental science, linguistics, economics, political science, philosophy, and history). In the analysis of subject integration, the overall integration trend was similar between the K-12 stage and the higher education stage. However, there were still some differences between K-12 education and higher education.
First, STEM education arose in higher education, but there seems to be a trend of research focus shifting from higher education to K-12 education. From 2004 to 2009, STEM research was focused on higher education, and after 2016, the number of papers in K-12 surpassed higher education. The reason for this phenomenon may be that the rise of STEM education sprung from the lack of talent in STEM careers, and higher education was directly oriented to society, so it was reasonable for research and reform to start from higher education, while government policies lead and funding investment largely promoted the rapid development of STEM education in K-12 education stage. Higher education points to the current talent needs of society, while K-12 education points to the future talent needs of society. The inclusion of STEM education in the education strategy of several countries also indicates that STEM talents are an important component of future national competitiveness, so it is very necessary to emphasize the K-12 stage.
Second, at the level of pedagogy and practice, disciplinary integration in STEM education at the K-12 level was often achieved through interdisciplinary projects and activities, such as engineering design challenges, science experiments, and mathematical modeling. These activities were usually classroom-cantered, with teachers guiding students through practice and inquiry. In contrast, in STEM education at the higher education level, disciplinary integration was focused more on the integration of research and practice. Students explored and applied integrated disciplinary knowledge in depth through participation in research projects, hands-on internships, and interdisciplinary courses.
In addition, the concept of STEAM education was more popular at the K-12 stage. “STEAM” was more frequently used in the K-12 stage, which could be said to a certain extent that the STEAM education concept was more popular in the K-12 stage, but may not necessarily indicate a deeper level of interdisciplinary integration in this stage.
Analysis of the evolution of subject themes
Research hotspots are reflected, to some extent, by the frequency of scientific theme terms. In this study, 32 keywords were selected as subject themes at the K-12 level and 33 keywords were selected at the higher education level. To facilitate the analysis, these keywords were grouped into “learning outcomes”, “teachers’ professional development”, “technology empowerment”, “social relevance”, and “pedagogy”. As shown in Fig. 3 .
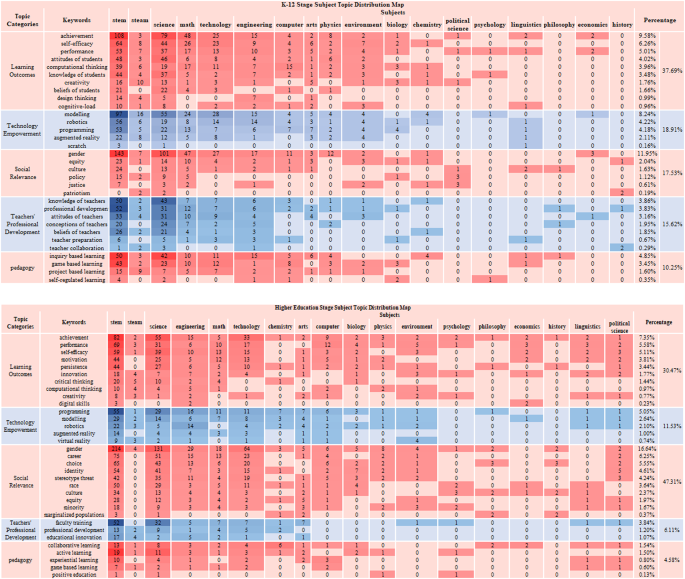
The first column represents topic categories, the second column contains relevant keywords, and the third row displays the subjects involved in STEM education. This figure illustrates the theme and subject distribution of STEM-related literature. Darker colors indicate a greater number of documents related to the corresponding subject and theme.
Subject theme evolution in K-12 education
Overall, STEM research topics at the K-12 level predominantly emphasize “learning outcomes”, while maintaining a relatively balanced distribution across “teachers’ professional development”, “technology empowerment”, “social relevance”, and “pedagogy”. The dimension of “learning outcomes” primarily encompassed keywords such as students’ academic performance, thinking skills, and associated influencing factors. “Teachers’ professional development” involved aspects related to teachers’ preparedness for STEM education and collaborative efforts among educators. “Technology empowerment” focused on the impact of various technologies such as modeling, robotics, programming, and augmented reality on both the teaching environment and instructional content. “Pedagogy” primarily revolved around inquiry based and game based learning. Furthermore, research related to social themes primarily aimed to foster educational equity from multiple dimensions, including aspects like gender, culture, and policy.
At the K-12 level, the theme of “learning outcomes” account for the largest proportion with 37.69%, under which the theme words included “achievement”, “self-efficacy”, “performance”, “attitudes”, “computational thinking”, “knowledge”, “creativity”, “beliefs”, “design thinking” and “cognitive-load”. In 2009, Obama proposed the “ Competing for Excellence ” initiative, which aimed to improve students’ achievement in STEM. This initiative has led to more researchers exploring different teaching models, activities, and tools to improve student achievement and performance. Also, students’ attitudes, knowledge, beliefs, self-efficacy, and cognitive-load were important factors influencing STEM performance and interest and have received close attention from researchers. Self-efficacy refers to one’s perceived ability to perform specific behaviors that may contain difficulties and stress (Bandura et al. 1999 ). Cognitive load is a multidimensional structure that represents the burden placed on a learner’s cognitive system when processing specific tasks, often appearing alongside keywords like motivation, performance, etc., in educational research with technical support (Kao and Ruan 2022 ).
Computational thinking, creativity (Zhan et al. 2023 ), and design thinking were goals of STEM education and were closely related to the disciplines. Computational thinking (CT) could be seen as a thinking pattern for solving problems with computational tools, and it is a fundamental skill required in everyday life (Wing 2006 ). It has the most direct relationship with computers, and the “ Next Generation Science Standards ” emphasized its significance by considering computational thinking as a core scientific practice. In China, computational thinking is recognized as a core competency in the curriculum standards for information technology. In addition, there is also increasing research focusing on the connection between CT and mathematics (Lv et al. 2023 ). Weintrop et al. defined computational thinking in mathematical and scientific practices using a taxonomy that includes four main categories: data practices, modeling and simulation practices, computational problem-solving practices, and systems thinking practices, which had a broad impact on K-12 education (Weintrop et al. 2016 ).
Furthermore, there was a clear association between creativity and the arts, as well as between design thinking and engineering disciplines. Some scholars argued that creativity plays one of three roles that arts assume in STEM education, with the other two being arts/esthetic learning and contextual understanding (Liu et al. 2021 ). Design is a prerequisite for making and the first step in the formation of STEM work, often found in studies of engineering subjects (Hernandez et al. 2014 ), and design thinking also plays an important role in engineering education, especially in high school (Li and Zhan 2022 ).
“Technology empowerment” (18.91%) was the second most popular theme, with the following themes: “modeling”, “robotics”, “programming”, “augmented reality”, and “scratch”. “Technology empowerment” emphasized the development of student literacy such as information awareness and computational thinking on the one hand, and laid the foundation for students’ STEM education practices on the other. Researchers have explored that robotics education has the potential to cultivate transferable skills in the STEM field (Nelson 2014 ) and narrow the gender gap in STEM, particularly by promoting girls’ learning (Zhong et al. 2023 ). The use of modeling tools can help students visualize abstract scientific and mathematical concepts or objects, which has a positive impact on learners’ academic and personal growth.
In addition, programming is a fundamental requirement for learning computer subjects, and the development of skills related to computer programming and robotics, as well as the introduction of computational thinking principles in STEM education, were considered by researchers as trends in today’s world (Bermúdez et al. 2019 ). AR (Augmented Reality) is the technology that allows virtual objects to be overlaid on real images, enriching students’ learning experiences. AR-STEM research was primarily conducted among K-12 students and typically relies on marker-based AR. However, location-based AR has significant advantages in supporting student learning beyond the classroom and facilitating scientific inquiry-based learning (Sırakaya and Alsancak Sırakaya 2022 ). Scratch is a graphical programming tool. In the K-12 stage, the abstract nature of programming concepts and languages makes it challenging for students to grasp them directly. Graphical programming significantly reduces the complexity of programming, making Scratch widely adopted (Kao and Ruan 2022 ).
The theme of “social relevance” ranked third with 17.53%, with the main themes related to “gender”, “equity”, “culture”, “policy”, “justice” and “patriotism”. Equality has always been an important topic in education, ensuring that individuals of different genders and races can participate in STEM education without discrimination. The Obama administration launched “ the Teach for Innovation program ” in 2009, which aimed to increase access to STEM education and employment opportunities for disadvantaged groups, and has contributed in part to researchers’ attention to gender. The topic of justice was multifaceted, with environmental justice being particularly prominent. Its purpose was to encourage readers to reframe societal and environmental issues as an ethical responsibility, fostering the construction of this responsibility through care, recognition, openness, and responsiveness to both human and non-human vitality (Kayumova et al. 2019 ).
Furthermore, since STEM education was a national priority, many researchers have analyzed the development of STEM education through policy analysis (Zhong et al. 2022 ), particularly focusing on different countries and regions such as South Korea (Park et al. 2016 ), the United States, Europe (Subotnik et al. 2017 ), India, Australia (Sharma and Yarlagadda 2018 ), etc. In South Korea, researchers have combined history education with traditional STEM education to inspire students’ patriotism (Park and Cho, 2022 ).
STEM education originated in the United States, and its evolution is determined by a variety of factors, including national economy, politics, and culture (Zhong et al. 2022 ). As STEM education was increasingly promoted worldwide, it faced challenges of cultural conflicts and international exchanges. “Culture” was a broadly encompassing term, and research about culture could be divided into two categories. First, it served as a research methodology, such as sociocultural theory, exploring social issues like gender and race and aiming at promoting educational equity for students of diverse cultures and languages (Eisenhart and Allen 2020 ).
Second, culture served as the background and content carrier for STEM activities. In China, researchers have developed C-STEAM, or culturally oriented disciplinary integration education, based on STEM education and considering the reality and needs of China’s development. This concept emphasized exploring and creating cultural concepts using related disciplines in the context of traditional Chinese culture, cultivating students’ humanistic spirit, and enhancing their cultural identity and understanding. At the same time, C-STEAM embodied the nurturing value of cultivating students’ core literacy, the carrying value of passing on excellent traditional culture, and the social value of creating a culture with regional characteristics. On this basis, the researcher proposed the ETIC curriculum classification framework and 6 C implementation model, which provided a reference for promoting the construction and development of the regional C-STEAM curriculum. (Zhan et al. 2020 , 2021 ; Huo et al. 2020 ).
“Professional development” ranked fourth with 15.62%. The theme words related were “knowledge”, “professional development”, “attitudes”, “conceptions”, “beliefs”, “teacher preparation”, and “teacher collaboration”. Researchers have indicated that changing teachers to interdisciplinary teaching requires first developing the skills and attitudes of interdisciplinary teaching, and professional development (PD) was considered a key component to helping teachers through this transition process (Al Salami et al. 2017 ). The link between teacher preparation to teach STEM and student STEM achievement has motivated researchers to develop professional development programs to address teacher confidence, attitudes, knowledge, pedagogy, and other preparation issues (Nadelson et al. 2013 ). Understanding the beliefs held by educators was central to influencing change and improving instruction, so researchers needed to be able to design educational programs that address teachers’ beliefs and work to change them when appropriate (Nathan et al. 2010 ; Vossen et al. 2020 ).
Furthermore, there was still considerable uncertainty about “what STEM education is” and “what it means” in terms of curriculum and student achievement, research and discussion on the concept of STEM aimed to create a shared concept of STEM education to facilitate dialog between different stakeholders (Dare et al. 2019 ; Holmes et al. 2018 ). The above topics can all be categorized as preparations for STEM education, primarily referring to pre-service and in-service STEM teacher training. In addition to the mentioned content, this also included language training, relevant technical learning, and teaching methods. Furthermore, due to the interdisciplinary nature of STEM education, collaboration among teachers from multiple disciplines was necessary, especially when humanities and social sciences were involved (Park and Cho 2022 ). Therefore, teacher cooperation was also an important way for teachers’ professional development.
“Pedagogy” received the least attention (10.25%). The theme words related were “inquiry based learning”, “game based learning”, “project based learning”, and “self-regulated learning”. Game based learning demonstrated a close association with technology and computers. Nowadays, students are generally passionate about electronic games, however, they often lack sufficient computer programming knowledge and skills, which limits their development in the computer and technology fields. To address this issue, game based learning has received significant attention in the K-12 stage. The purpose of inquiry based learning was to cultivate students’ inquiry skills, which was also at the core of the science curriculum. In STEM education, this method was considered to have three components: data analysis, interpretive reflection, and critical reflection. Using inquiry based learning could integrate various disciplines, enhance educators’ attitudes, and it’s also suitable for the special needs of gifted students (Abdurrahman et al. 2019 ).
STEM PBL (STEM Project-Based Learning) is a student-centered teaching approach based on constructivism, characterized by clear outcomes and vaguely defined tasks (Capraro and Slough 2013 ). STEM PBL activities are fundamentally interdisciplinary, encouraging students to construct knowledge, identify problems independently, and collaborate to solve them (Han et al. 2015 ). Self-regulated learning (SRL) refers to an active, iterative process in which learners achieve their goals by controlling, monitoring, and adjusting their cognitive/metacognitive processes and learning behaviors. This approach was effective in activating and monitoring learners’ behaviors, cognitions, and emotions, which is crucial for task performance in the STEM field (Li et al. 2020 ).
Through the above analysis, it is evident that research topics in different disciplines have varying emphases. “Achievement” and “gender” were highly popular topics in the scientific community. Additionally, in the fields of math, physics, chemistry, and biology, there was a greater emphasis on “technological empowerment” and “pedagogy”. Technology placed the most emphasis on “modeling”, while computer science was concerned with “computational thinking”. Engineering exhibited a relatively even distribution of research topics. In contrast, the focus areas within humanities and social sciences were relatively scattered.
Subject theme evolution in higher education
In comparison to the K-12 level, research theme distribution in higher education appeared to be more concentrated. This was primarily manifested in the prevalence of research related to “learning outcomes” and “social relevance”, which collectively account for over three-quarters of the total research. Conversely, research areas focusing on “teachers’ professional development”, “technology empowerment”, and “pedagogy” were relatively scarce. However, from a disciplinary perspective, research topics in the humanities and social sciences at the higher education level exhibited greater diversity and richness.
“Social relevance” was the most popular theme in higher education research (47.31%). The research content could be broadly categorized into three types. The first category was educational equity and justice, including keywords “gender”, “identity”, “stereotype threat”, “race”, “equity”, “minority”, and “marginalized populations”. STEM identity is an expressed connection between one’s self and STEM, which depends on the individual’s beliefs about their abilities and their conceptual and practical knowledge of their particular STEM subject (Charleston et al. 2014 ). Enhancing the self-identity of minority groups and optimizing the experience of marginalized populations, especially females, contributed to their more active participation in STEM education. Stereotype threat is a risk experienced by individuals in which individuals fear that they will validate negative stereotypes of the group to which they belong (Spencer et al. 1999 ). Stereotype threat has been shown to have a significant impact on the likelihood of women, minorities, and white men leaving STEM professions (Beasley and Fischer 2012 ).
The second category was students’ career development, including the keywords “career” and “choice”. Career orientation was more prominent at the higher education level than at the K-12 level, with researchers focusing on career goals, career preparation, the position of STEM talent in the labor market, major selection, and attrition.
The third category was culture-related research, which, in higher education, connected with various humanities and social sciences disciplines such as psychology, philosophy, history, linguistics, and more. Research in this category focused on promoting educational equity and students’ full participation in STEM education by addressing the fair treatment of students from different sociocultural backgrounds and using “culturally responsive pedagogy”. This approach involved leveraging the cultural characteristics, experiences, and perspectives of ethnically diverse students to teach them more effectively, fostering educational equity and comprehensive engagement in STEM education (Gay 2003 ).
“Learning outcomes” was also a theme that received a lot of attention in higher education, with 30.47%. The related themes included “achievement”, “performance”, “self-efficacy”, “motivation”, “persistence”, “innovation”, “critical thinking”, “computational thinking”, “creativity”, and “digital skills”. It was evident from this that higher education was not only concerned with issues such as students’ achievement, performance, and computational thinking but also paid attention to influencing factors such as students’ self-efficacy and motivation. How to sustain students in STEM majors and reduce attrition of STEM majors, especially among minority and female populations, was a concern in studies related to “persistence” (Burt et al. 2019 ; Ong et al. 2018 ).
Compared to the K-12 stage, higher education placed less emphasis on computational thinking and creativity but focused more on innovation and critical thinking. Creativity refers to “the generation of novel and useful ideas by an individual or a small group of individuals” while innovation is “the successful implementation of creative ideas within an organization” (Amabile 1988 ). The distinction between creativity and innovation lies in the emphasis on products and outcomes in innovation. Higher education demands that students not only have creative ideas but also successfully transform these ideas into scalable products. In contrast, K-12 education placed more emphasis on encouraging students to generate new ideas. Besides, Critical thinking was another important developmental goal at the higher education level. It served as a method and tool for problem-solving, conceptualized as purposeful, self-regulated judgment involving various thinking skills such as analysis, evaluation, and reasoning (Gadot and Tsybulsky 2023 ).
Digital skill is a concept encompassing skills and specific techniques that are necessary for the use of effective digital technology (van Laar et al. 2019 ). In research, various terms were used to describe the ability to use digital technology effectively in learning activities, such as digital skills, technical skills, digital literacy, digital competence, digital tools, 21st-century skills, ICT literacy, and ICT skills. Studies have shown a positive correlation between students’ digital skills and their creative self-efficacy, and higher levels of digital skills were often predictive of higher levels of actual performance (Chonsalasin and Khampirat 2022 ).
“Technology empowerment” was ranked third with 11.53%, and the related themes were “modeling”, “robotics”, “programming”, “augmented reality” and “virtual reality”. Modeling is a useful tool to identify current problem situations, predict future societal changes, and identify possible solutions (Suh and Han 2019 ). Programming was considered to be related to problem-solving and the main pedagogical challenge was the lack of appropriate methods and tools as well as scaled and personalized instruction (Medeiros et al. 2019 ). Robots were often used in the classroom to develop students’ human-machine collaboration skills (Mathers et al. 2012 ).
Augmented Reality (AR) refers to the technology that enhances virtual information in the real environment through ongoing activities and user input, while “Virtual Reality (VR)” is the technology that immerses users in a purely virtual environment. The learning environments created by VR and AR technologies contributed to the formation of collaborative, interactive, and highly immersive learning experiences, thereby enhancing the efficiency of learning for learners (Zhong et al. 2021 ). Additionally, they demonstrated the potential to help students improve their cross-cultural communication skills (Akdere et al. 2021 ).
“Teachers’ Professional Development” was ranked fourth with 6.11% of the total, and related terms were “faculty training”, “professional development”, and “educational innovation”. Faculty training and professional development were broadly defined terms, and there was a significant degree of overlap in their research content. They encompassed research related to teacher development (such as teacher reflection and active learning), diversity and equity issues among the teaching staff, curriculum design, teaching methodologies, and pedagogical knowledge. Research related to educational innovation encompassed the introduction of new educational technologies, teaching methods, curriculum designs, and assessment approaches to address evolving learning needs and societal challenges.
“Pedagogy” was the least studied topic (4.58%), with related themes including “collaborative learning”, “active learning”, “experiential learning”, “game based learning”, and “positive learning”. Collaborative learning played a significant role in enhancing the likelihood of successful problem-solving. Additionally, collaborative skills are crucial for individuals pursuing STEM careers. Active learning is a method characterized by students taking control of their learning to some extent through metacognition, self-assessment, and reflection, within student-centered and inquiry based learning approaches (National Research Council et al. 2000 ; Kuh 2008 ). The American Association for the Advancement of Science encouraged university science educators to shift their teaching from traditional lectures to active learning (American Association for the Advancement of Science 2011 ).
Experiential Learning is an educational approach that emphasizes acquiring knowledge and skills through first-hand experiences, practice, and reflection, often in forms such as teaching, research, and internships. Experiential learning can facilitate the transfer of classroom learning to real-world practice and has the potential to enhance students’ learning, motivation, skill development, and graduation rates (Gong et al. 2022 ). Game based learning was not very common in higher education, and research in this area was quite scattered, covering topics such as computer-based learning and the creation of diverse and inclusive learning environments. The origins of positive learning can be traced back to the early days of the positive psychology movement, to promote students’ overall well-being, not just the imparting of knowledge and skills, but also the cultivation of their positive psychological traits and qualities (White 2016 ).
Undoubtedly, in higher education, almost all disciplines focused their research on “learning outcomes” and “social relevance”. Among these, the most emphasized areas included students’ performance, diversity, equity, and career development. Furthermore, engineering placed a significant emphasis on programming and robotics technology; mathematics and technology prioritized students’ self-efficacy, motivation, persistence, and programming skills. Chemistry, on the other hand, exhibited a unique pattern by showing less focus on learning outcomes but a greater emphasis on technology integration and pedagogy. The arts concentrated more on technology integration and social relevance. However, many other disciplines lacked a substantial focus on teacher professional development.
Comparing the evolution of subject themes at different educational levels
From the above analysis, it can be found that the distribution of research topics in K-12 education was relatively balanced, while in higher education, it was more concentrated. However, in higher education, research in the humanities and social sciences was more in-depth, and the distribution of themes was more extensive. The research hotspots at the two levels have shown the following differences.
Overall, in the K-12 stage, “learning outcomes” received the most attention, while career education for students was lacking. In higher education, “learning outcomes” and “social relevance” were the most emphasized aspects, while “teachers’ professional development” and “pedagogy” were relatively neglected.
Specifically, concerning “learning outcomes”, achievement, performance, and self-efficacy were common topics across different educational levels. K-12 education placed more emphasis on computational thinking, creativity, and design thinking, while higher education focused more on innovation and critical thinking. Regarding “teachers’ professional development”, higher education paid relatively less attention to teachers and their development, lacking a systematic body of research. In “technology empowerment”, technologies in the research were highly similar, but there was a greater volume of publications in K-12 education. The knowledge or tools learned were also more foundational and straightforward at this level. In the realm of “social relevance” research, gender, equity, and culture were common topics of interest, but higher education delved into students’ career choices and development, an area that lacked emphasis in K-12 education. In terms of “pedagogy” research, K-12 education primarily focused on inquiry based learning and game based learning, while higher education emphasized collaborative learning and active learning.
This study analyzed and compared the development of the STEM research field in two aspects: subject integration and subject themes distribution, to clarify the STEM subject orientation and the ecological map of subject integration in the STEM field.
Referring to RQ1, the subject time distribution maps were used to find out how subjects integrated into STEM education at the K-12 and higher education levels. From the above analysis, it is clear that subject integration followed the evolutionary path of science, technology, engineering, and mathematics to the addition of social sciences and humanities. The addition of the latter has qualitatively improved the connotation of STEM education and fundamentally changed the subject integration path. In other words, the field of STEM studies has expanded from science education to the whole education field, and the cross-fertilization of subjects has become its most fundamental feature. This conclusion has been corroborated by existing research and policies (Perignat and Katz-Buonincontro 2019 ; Zhan et al. 2022a ).
Referring to RQ2, the subject themes distribution maps at the K-12 and higher education levels reflected the main research content of STEM education. Research themes were not evenly distributed, especially since the research on “learning outcomes” was much more than the research on “teachers’ professional development” and “pedagogy”, which implied that the current attention to STEM teachers was insufficient. Previous research indicated that teacher education programs lack content related to interdisciplinary integration across different subject areas and do not provide suitable activities for integrating STEM education (Türk et al. 2018 ). In addition, although K-12 education started late, it has developed rapidly due to the promotion of policies and the future needs of society, but there is still much room for expansion of its research scope, especially career issues. In recent years, with the further development of globalization, student diversity has become evident not only in higher education but also in K-12 education. Research has shown that multicultural education and culturally supportive teaching contribute to addressing the persistent inequalities in the field of STEM education (Charity Hudley and Mallinson 2017 ).
STEM education has obvious interdisciplinary characteristics, in which different subjects play different roles, as shown in Table 1 . The essence of science subjects is to understand the objective laws of the world, and science education aims to help students understand the world through inquiry methods, knowledge is the key to its teaching. The essence of technology is the application of knowledge scenarios, and technology achieves the purpose of transforming the world by manipulating and optimizing the variables that affect the results (products), the key to its teaching is the acquisition of skills. Engineering is the integrated application of technology, and its purpose is also to transform the world, but unlike technology, engineering places more emphasis on the coordination of all elements within the system to find the optimal solution to the problem, and engineering operates and optimizes the variables that affect the system to achieve the purpose of system optimization. The essence of mathematics is measurement and calculation, which develops itself through abstract, non-empirical mathematical operations and heuristic logical deduction, and can provide the logical and calculative basis for other subjects, and the key to its teaching is calculation, measurement, and logical deduction.
Unlike the above subjects, the essence of humanities and social sciences is to feel, interpret, and create the man-made world. It contributes to the all-around development of human beings, the enhancement of moral values and cultural identity, and the development of creative and innovative thinking through the unity of awareness, expression, values, and emotions, the key to teaching is tasting, designing, and creating. In addition, there is a slight difference between the humanities and social sciences. The social sciences involved in STEM fields mainly reflect on the social issues that exist or are raised in STEM education from the perspective of research, but are less reflected in the teaching of the subjects, such as psychology. The involvement of the humanities is mainly reflected in the teaching of the subjects, and the educational goals are achieved through teaching students to appreciate the appeal and value of the arts.
The STEM education research ecosystem comprises two parts. The upper elliptical portion reveals the distribution of disciplines and research topics, while the lower timeline illustrates the timeline of interdisciplinary integration. The central part of the ellipse indicates the disciplinary composition of STEM education. Science, oriented towards exploration, forms the foundation of STEM education. Engineering, driven by creativity and innovation, plays a crucial role in fostering students’ creativity and innovation. Science and engineering mutually reinforce each other and progress together. Technology provides the tools and support for STEM education, while mathematics serves as the computational foundation, collectively facilitating STEM education activities.
STEM education, through interdisciplinary teaching, emphasizes the cultivation of students’ higher-order thinking skills, such as scientific thinking, design thinking, engineering thinking, and computational thinking. The outermost circle includes other disciplines involved in STEM education, such as arts, economics, history, political science, linguistics, psychology, philosophy, physics, biology, computer science, environmental studies, chemistry, and more. This demonstrates the trend in STEM education shifting from STEM to STEAM (Science, Technology, Engineering, Arts, and Mathematics) and the integration of science, technology, engineering, mathematics, and social sciences in education. The pink and blue sections represent the distribution of research topics in the K-12 and higher education stages.
From the above analysis, we could outline the ecological map of STEM subject integration in terms of subject integration and subject themes distribution, as shown in Fig. 4 , which demonstrates the subject integration and main research contents of STEM education.
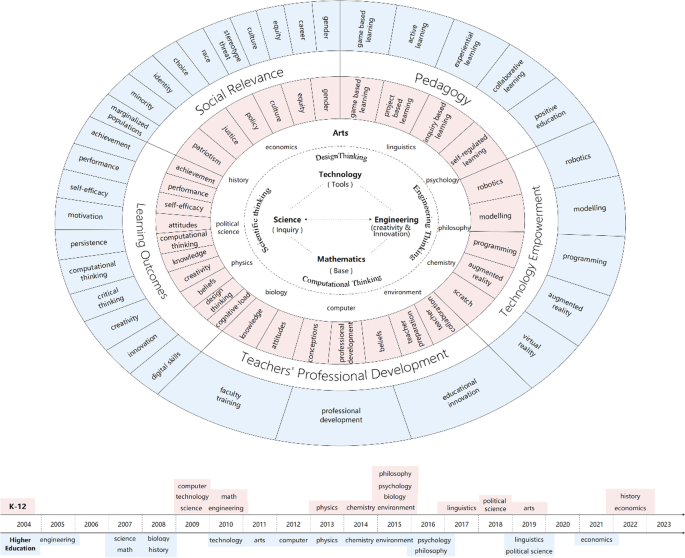
This figure is composed of two parts, with the upper part representing the content dimension, and the lower part representing the time dimension. The pink area within the ellipse illustrates the most prominent research themes in the K-12 stage, while the blue area illustrates the most prominent research themes in higher education.
Conclusion and future research
Based on the literature related to STEM education in the WOS database from 2004 to 2023, covering 903 papers at the K-12 level and 873 papers at the higher education level, this study conducted a bibliometric analysis from the perspective of subject evolution, including subject timeline evolution analysis and subject theme evolution analysis, to reveal the subject evolution trends and research hotspots in STEM education. The following conclusions were reached.
First, regarding subject integration, the interdisciplinary and cross-subject collaboration in STEM education was constantly expanding and deepening, forming a new situation in which science, engineering, humanities, and social sciences are integrated. Since 2004, a total of 16 subjects have been involved, among them, arts, physics, chemistry, biology, computer science, and environmental science were the main integrated subjects. Interdisciplinary integration promoted the innovation and development of STEM education research.
Second, regarding the research themes, humanism was more and more emphasized in STEM education. In the temporal evolution of subjects in STEM education, it was found that the research outputs of humanities and social science subjects such as arts, psychology, and philosophy kept increasing. The cultural themes have enriched the diversity of participants and the uniqueness of regions in STEM education research, viewed from perspectives such as theory, teaching methods, and regional development. “Social relevance” has garnered significant attention across different educational levels. In K-12 education, research topics were relatively balanced, but there was a lack of research on students’ career choices and development. In higher education, research topics in the humanities and social sciences were more diverse in their distribution.
To sum up, this study analyzed the developmental lineage of STEM education, focusing on the subject roles, and hot topics of research, and summing up potential guidance for subsequent subject integration research. Future work should prioritize the articulation of STEM subject integration between K-12 education and higher education. At the K-12 level, it is necessary to enhance vocational education appropriately, while in higher education, reducing the attrition rate of STEM majors may become a crucial issue. Additionally, attention to multi-discipline teacher collaboration and professional development, high-quality curricula design, and regional policy support should continue to be emphasized. Moreover, different countries present different characteristics in the development of STEM education due to their different cultural, political, and economic backgrounds. In future studies, we aim to conduct a comparative study on the development of STEM education on a country-by-country basis.
Data availability
The datasets generated during and/or analyzed during the current study are available in the supplementary file.
Abdurrahman A, Nurulsari N, Maulina H, Ariyani F (2019) Design and validation of inquiry-based STEM learning strategy as a powerful alternative solution to facilitate gift students facing 21st century challenging. J Educ Gifted Young Sci 7(1):33–56. https://doi.org/10.17478/jegys.513308
Article Google Scholar
Akdere M, Acheson K, Jiang Y (2021) An examination of the effectiveness of virtual reality technology for intercultural competence development. Int J Intercult Relat 82:109–120. https://doi.org/10.1016/j.ijintrel.2021.03.009
Al Salami MK, Makela CJ, de Miranda MA (2017) Assessing changes in teachers’ attitudes toward interdisciplinary STEM teaching. Int J Technol Des Educ 27(1):63–88. https://doi.org/10.1007/s10798-015-9341-0
Amabile TM (1988) A model of creativity and innovation in organizations. Res Organ Behav 10(1):123–167. https://web.mit.edu/curhan/www/docs/Articles/15341_Readings/Group_Performance/Amabile_A_Model_of_CreativityOrg.Beh_v10_pp123-167.pdf
Google Scholar
American Association for the Advancement of Science (2011) Vision and change in undergraduate biology education: a call to action. Washington, DC. https://www.aaas.org/sites/default/files/content_files/VC_report.pdf
Bandura A, Freeman WH, Lightsey R (1999) Self-efficacy: the exercise of control. J Cognit Psychother 13(2):158–166. https://doi.org/10.1891/0889-8391.13.2.158
Beasley MA, Fischer MJ (2012) Why they leave: the impact of stereotype threat on the attrition of women and minorities from science, math and engineering majors. Soc Psychol Educ 15(4):427–448. https://doi.org/10.1007/s11218-012-9185-3
Bermúdez A, Casado R, Fernández G, Guijarro M, Olivas P (2019) Drone challenge: a platform for promoting programming and robotics skills in K-12 education. Int J Adv Robot Syst 16(1):172988141882042. https://doi.org/10.1177/1729881418820425
Buckley J, Seery N, Canty D (2018) A heuristic framework of spatial ability: a review and synthesis of spatial factor literature to support its translation into STEM education. Educ Psychol Rev 30(3):947–972. https://doi.org/10.1007/s10648-018-9432-z
Burt BA, Williams KL, Palmer GJM (2019) It takes a village: the role of emic and etic adaptive strengths in the persistence of black men in engineering graduate programs. Am Educ Res J 56(1):39–74. https://doi.org/10.3102/0002831218789595
Capraro RM, Slough SW (2013) Why PBL? Why STEM? Why now? An introduction to STEM project-based learning: an integrated science, technology, engineering, and mathematics (STEM) approach. In STEM project-based learning. Brill, Rotterdam, p 1–5
Charity Hudley AH, Mallinson C (2017) “It’s worth our time”: a model of culturally and linguistically supportive professional development for K-12 STEM educators. Cult Stud Sci Educ 12:637–660. https://doi.org/10.1007/s11422-016-9743-7
Charleston LJ, George PL, Jackson JFL, Berhanu J, Amechi MH (2014) Navigating underrepresented STEM spaces: experiences of Black women in U.S. computing science higher education programs who actualize success. J Diversity Higher Educ 7(3):166–176. https://doi.org/10.1037/a0036632
Chonsalasin D, Khampirat B (2022) The impact of achievement goal orientation, learning strategies, and digital skill on engineering skill self-efficacy in Thailand. IEEE Access 10:11858–11870. https://doi.org/10.1109/ACCESS.2022.3146128
Conde MÁ, Rodríguez‐Sedano FJ, Fernández‐Llamas C, Gonçalves J, Lima J, García‐Peñalvo FJ (2021) Fostering STEAM through challenge‐based learning, robotics, and physical devices: a systematic mapping literature review. Comput Appl Eng Educ 29(1):46–65. https://doi.org/10.1002/cae.22354
Dare EA, Ring-Whalen EA, Roehrig GH (2019) Creating a continuum of STEM models: exploring how K-12 science teachers conceptualize STEM education. Int. J. Sci. Educ. 41(12):1701–1720. https://doi.org/10.1080/09500693.2019.1638531
Department of Education (2022) Science, Technology, Engineering, and Math, including Computer Science. https://www.ed.gov/stem
Eisenhart M, Allen CD (2020) Addressing underrepresentation of young women of color in engineering and computing through the lens of sociocultural theory. Cult Stud Sci Educ 15:793–824. https://doi.org/10.1007/s11422-020-09976-6
Gadot R, Tsybulsky D (2023) Digital curation as a pedagogical approach to promote critical thinking. J Sci Educ Technol. https://doi.org/10.1007/s10956-022-10016-x
Gay G (2003) Becoming multicultural educators: personal journey toward professional agency. Jossey-Bass Publishers, San Francisco, CA
Gilligan KA, Flouri E, Farran EK (2017) The contribution of spatial ability to mathematics achievement in middle childhood. J Exp Child Psychol 163:107–125. https://doi.org/10.1016/j.jecp.2017.04.016
Article PubMed Google Scholar
Gong HJ, Kwon J, Brock M (2022) Experiential learning through a peer learning assistant model in STEM. Educ Res 64(3):317–333. https://doi.org/10.1080/00131881.2022.2096092
Grover S, Pea R (2013) Computational thinking in K–12: a review of the state of the field. Educ Res 42(1):38–43. https://doi.org/10.3102/0013189X12463051
Han S, Yalvac B, Capraro MM, Capraro RM (2015) In-service teachers’ implementation and understanding of STEM project based learning. Eur J Math Sci Technol Educ 11(1):63–76. https://doi.org/10.12973/eurasia.2015.1306a
Hernandez PR, Bodin R, Elliott JW, Ibrahim B, Rambo-Hernandez KE, Chen TW, de Miranda MA (2014) Connecting the STEM dots: measuring the effect of an integrated engineering design intervention. Int J Technol Des Educ 24:107–120. https://doi.org/10.1007/s10798-013-9241-0
Holmes K, Gore J, Smith M, Lloyd A (2018) An integrated analysis of school students’ aspirations for STEM careers: which student and school factors are most predictive? Int J Sci Math Educ 16(4):655–675. https://doi.org/10.1007/s10763-016-9793-z
Huo L, Zhan Z, Mai Z, Yao X, Zheng Y (2020) A case study on C-STEAM education: Investigating the effects of students’ STEAM literacy and cultural inheritance literacy. In Technology in Education. Innovations for Online Teaching and Learning: 5th International Conference, ICTE 2020, Macau, China, August 19-22, 2020, Revised Selected Papers 5 (pp. 3–12). Springer Singapore, https://doi.org/10.1007/978-981-33-4594-2_1
Kao GYM, Ruan CA (2022) Designing and evaluating a high interactive augmented reality system for programming learning. Comput Hum Behav 132:107245. https://doi.org/10.1016/j.chb.2022.107245
Kayumova S, McGuire CJ, Cardello S (2019) From empowerment to response-ability: Rethinking socio-spatial, environmental justice, and nature-culture binaries in the context of STEM education. Cult Stud Sci Educ 14:205–229. https://doi.org/10.1007/s11422-018-9861-5
Kuh GD (2008) Excerpt from high-impact educational practices: what they are, who has access to them, and why they matter. Assoc Am Coll Univ, 14(3), 28–29. file:///C:/Users/lenovo/Downloads/High-Impact_Educational_Practices_What_They_Are_Who_Has_Access_to_Them_and_Why_They_Matter%20(2).pdf
Li KC, Wong BTM (2023) Personalisation in STE (A) M education: a review of literature from 2011 to 2020. J Comput Higher Educ 35(1):186–201. https://doi.org/10.1007/s12528-022-09341-2
Li S, Chen G, Xing W, Zheng J, Xie C (2020) Longitudinal clustering of students’ self-regulated learning behaviors in engineering design. Comput Educ 153:103899. https://doi.org/10.1016/j.compedu.2020.103899
Li T, Zhan Z (2022) A systematic review on design thinking Integrated Learning in K-12 education. Appl Sci 12(16):8077. https://doi.org/10.3390/app12168077
Article CAS Google Scholar
Liu CY, Wu CJ, Chien YH, Tzeng SY, Kuo HC (2021) Examining the quality of art in STEAM learning activities. Psychol Aesthet Creat Arts, https://doi.org/10.1037/aca0000404
Ludwig PM, Nagel JK, Lewis EJ (2017) Student learning outcomes from a pilot medical innovations course with nursing, engineering, and biology undergraduate students. Int J STEM Educ 4(1):1–14. https://doi.org/10.1186/s40594-017-0095-y
Lv L, Zhong B, Liu X (2023) A literature review on the empirical studies of the integration of mathematics and computational thinking. Educ Inform Technol 28(7):8171–8193. https://doi.org/10.1007/s10639-022-11518-2
Mallinson C, Hudley AHC (2018) Balancing the communication equation: An outreach and engagement model for using sociolinguistics to enhance culturally and linguistically sustaining K–12 STEM education. Language 94(3):e191–e215. https://doi.org/10.1353/lan.2018.0048
Martín-Páez T, Aguilera D, Perales-Palacios FJ, Vílchez-González JM (2019) What are we talking about when we talk about STEM education? A review of literature. Sci Educ 103(4):799–822. https://doi.org/10.1002/sce.21522
Mathers N, Goktogen A, Rankin J, Anderson M (2012) Robotic mission to mars: hands-on, minds-on, web-based learning. Acta Astronautica 80:124–131. https://doi.org/10.1016/j.actaastro.2012.06.003
Article ADS Google Scholar
Medeiros RP, Ramalho GL, Falcao TP (2019) A systematic literature review on teaching and learning introductory programming in higher education. IEEE Trans Educ 62(2):77–90. https://doi.org/10.1109/TE.2018.2864133
Morrison J (2006) Attributes of STEM education: the student, the school, the classroom. TIES Teach Inst Excellence STEM) 20:2–7. http://leadingpbl.pbworks.com/f/Jans%20pdf%20Attributes_of_STEM_Education-1.pdf
Nadelson LS, Callahan J, Pyke P, Hay A, Dance M, Pfiester J (2013) Teacher STEM perception and preparation: inquiry-based STEM professional development for elementary teachers. J Educ Res 106(2):157–168. https://doi.org/10.1080/00220671.2012.667014
National Research Council, Division of Behavioral and Social Sciences and Education, Board on Behavioral, Cognitive, and Sensory Sciences, & Committee on Developments in the Science of Learning with additional material from the Committee on Learning Research and Educational Practice (2000) How people learn: Brain, mind, experience, and school: Expanded edition. National Academies Press, Washington, DC
National Research Council (2013) Next Generation Science Standards, https://www.nextgenscience.org/
National Science Board (1986) Undergraduate Science, Mathematics and Engineering Education, https://www.nsf.gov/nsb/publications/1986/nsb0386.pdf
National Science Foundation (2014) NSF approved STEM fields, https://btaa.org/docs/default-source/diversity/nsf-approved-fields-of-studycac2.pdf?sfvrsn=642824bc_2
Nathan MJ, Tran NA, Atwood AK, Prevost A, Phelps LA (2010) Beliefs and expectations about engineering preparation exhibited by high school STEM teachers. J Eng Educ 99(4):409–426. https://doi.org/10.1002/j.2168-9830.2010.tb01071.x
Nelson CA (2014) Generating transferable skills in STEM through educational robotics. In K-12 education: concepts, methodologies, tools, and applications (pp. 433–444). Igi Global, https://doi.org/10.4018/978-1-4666-4502-8.ch026
Ong M, Smith JM, Ko LT (2018) Counterspaces for women of color in STEM higher education: marginal and central spaces for persistence and success: COUNTERSPACES FOR WOMEN OF COLOR IN STEM EDUCATION. J Res Sci Teach 55(2):206–245. https://doi.org/10.1002/tea.21417
Ortiz-Revilla J, Adúriz-Bravo A, Greca IM (2020) A framework for epistemological discussion on integrated STEM education. Sci Educ 29(4):857–880. https://doi.org/10.1007/s11191-020-00131-9
Park H, Byun SY, Sim J, Han HS, Baek YS (2016) Teachers’ perceptions and practices of STEAM education in South Korea. Eurasia J Math Sci Technol Educ 12(7):1739–1753. https://doi.org/10.12973/eurasia.2016.1531a
Park W, Cho H (2022) The interaction of history and STEM learning goals in teacher-developed curriculum materials: opportunities and challenges for STEAM education. Asia Pac Educ Rev 23(3):457–474. https://doi.org/10.1007/s12564-022-09741-0
Perignat E, Katz-Buonincontro J (2019) STEAM in practice and research: an integrative literature review. Think Skills Creat 31:31–43. https://doi.org/10.1016/j.tsc.2018.10.002
Salas-Pilco SZ, Xiao K, Oshima J (2022) Artificial intelligence and new technologies in inclusive education for minority students: a systematic review. Sustainability 14(20):13572. https://doi.org/10.3390/su142013572
Sharma J, Yarlagadda PK (2018) Perspectives of ‘STEM education and policies’ for the development of a skilled workforce in Australia and India. Int J Sci Educ 40(16):1999–2022. https://doi.org/10.1080/09500693.2018.1517239
Sırakaya M, Alsancak Sırakaya D (2022) Augmented reality in STEM education: a systematic review. Interact Learn Environ 30(8):1556–1569. https://doi.org/10.1080/10494820.2020.1722713
Spencer SJ, Steele CM, Quinn DM (1999) Stereotype threat and women’s math performance. J Exp Soc Psychol 35(1):4–28. https://doi.org/10.1006/jesp.1998.1373
Subotnik RF, Stoeger H, Olszewski-Kubilius P (2017) Talent development research, policy, and practice in Europe and the United States: outcomes from a summit of international researchers. Gifted Child Q 61(3):262–269. https://doi.org/10.1177/0016986217701839
Suh H, Han S (2019) Promoting sustainability in university classrooms using a STEM project with mathematical modeling. Sustainability 11(11):3080. https://doi.org/10.3390/su11113080
Sümen ÖÖ, Çalisici H (2016) Pre-service teachers’ mind maps and opinions on STEM education implemented in an environmental literacy course. Educ Sci Theory Pract 16(2):459–476. https://doi.org/10.12738/estp.2016.2.0166
Taylor HA, Hutton A (2013) Think3d!: training spatial thinking fundamental to STEM education. Cognit Instr 31(4):434–455. https://doi.org/10.1080/07370008.2013.828727
Türk N, Kalayci N, Yamak H (2018) New trends in higher education in the globalizing world: STEM in teacher education. Univ J Educ Res 6(6):1286–1304. https://doi.org/10.13189/ujer.2018.060620
Uskoković V (2023) Natural sciences and chess: a romantic relationship missing from higher education curricula. Heliyon. https://www.cell.com/heliyon/pdf/S2405-8440(23)02222-3.pdf
van Laar E, van Deursen AJ, van Dijk JA, de Haan J (2019) Determinants of 21st-century digital skills: a large-scale survey among working professionals. Comput Hum Behav 100:93–104. https://doi.org/10.1016/j.chb.2019.06.017
Vossen TE, Henze I, De Vries MJ, Van Driel JH (2020) Finding the connection between research and design: the knowledge development of STEM teachers in a professional learning community. Int J Technol Des Educ 30:295–320. https://doi.org/10.1007/s10798-019-09507-7
Weintrop D, Beheshti E, Horn M, Orton K, Jona K, Trouille L, Wilensky U (2016) Defining computational thinking for mathematics and science classrooms. J Sci Educ Technol 25:127–147. https://doi.org/10.1007/s10956-015-9581-5
White MA (2016) Why won’t it stick? Positive psychology and positive education. Psychol Well Being 6(1):1–16. https://doi.org/10.1186/s13612-016-0039-1
Article MathSciNet CAS Google Scholar
Wing JM (2006) Computational thinking. Commun ACM 49(3):33–35. https://www.microsoft.com/en-us/research/wp-content/uploads/2012/08/Jeannette_Wing.pdf
Yakman G (2008) STEAM education: an overview of creating a model of integrative education. https://www.researchgate.net/publication/327351326_STEAM_Education_an_overview_of_creating_a_model_of_integrative_education
Yakman G (2010) What is the point of STE@ M?–a brief overview. Steam: a framework for teaching across the disciplines. STEAM Educ 7(9):1–9. https://www.researchgate.net/profile/Georgette-Yakman-2/publication/327449281_What_is_the_point_of_STEAM-A_Brief_Overview/links/5b901b98a6fdcce8a4c2f290/What-is-the-point-of-STEAM-A-Brief-Overview.pdf
Zhan Z, He W, Yi X, Ma S (2022b) Effect of unplugged programming teaching aids on children’s computational thinking and classroom interaction: with respect to Piaget’s four stages theory. J Educ Comput Res 60(5):1277–1300. https://doi.org/10.1177/07356331211057143
Zhan Z, Ma S, Li W, Shen W, Huo L, Yao X (2021) Effect of “6C” instructional design model on students’ STEAM competency and cultural inheritance literacy in a Dragon Boat C-STEAM course. In Proceedings of the 2021 5th International Conference on Education and E-Learning (pp. 229–236). https://doi.org/10.1145/3502434.3502436
Zhan Z, Shen W, Xu Z, Niu S, You G (2022a) A bibliometric analysis of the global landscape on STEM education (2004-2021): towards global distribution, subject integration, and research trends. Asia Pac J Innov Entrepreneurship 16(2):171–203. https://doi.org/10.1108/APJIE-08-2022-0090
Zhan Z, Xiao Y, Li T (2023) Effects of association interventions on students’ creative thinking, aptitude, empathy, and design scheme in a STEAM course: considering remote and close association. Int J Technol Des Educ 33(5):1773–1795. https://doi.org/10.1007/s10798-022-09801-x
Zhan Z, Zhong B, Huo L, Huang M (2020) Transdisciplinary education for cultural inheritance (C-STEAM): value orientation and classification framework. China Educ Technol 3:69–76. https://doi.org/10.3969/j.issn.1006-9860.2020.03.010
Zhong B, Liu X, Huang Y (2023) Effects of pair learning on girls’ learning performance in robotics education. J Educ Comput Res 61(1):151–177. https://doi.org/10.1177/0735633122109266
Zhong B, Liu X, Zhan Z, Ke Q, Wang F (2022) What should a Chinese top-level design in STEM Education look like? Humanit Soc Sci Commun 9(1):1–8. https://doi.org/10.1057/s41599-022-01279-1
Zhong B, Su S, Liu X, Zhan Z (2021) A literature review on the empirical studies of technology-based embodied learning. Interact Learn Environ, 1–20. https://doi.org/10.1080/10494820.2021.1999274
Download references
Acknowledgements
This research was financially supported by the National Natural Science Foundation in China (62277018; 62237001), Ministry of Education in China Project of Humanities and Social Sciences (22YJC880106), the Major Project of Social Science in South China Normal University (ZDPY2208), the Degree and graduate education Reform research project in Guangdong (2023JGXM046).
Author information
Authors and affiliations.
School of Information Technology in Education, South China Normal University, Guangzhou, China
Zehui Zhan & Shijing Niu
Key Laboratory of Brain, Ministry of Education, Cognition and Education Sciences (South China Normal University), Guangzhou, China
You can also search for this author in PubMed Google Scholar
Contributions
ZZ identified the research idea, designed and facilitated the study, wrote the draft, and revised the work extensively. SN was involved in data processing (including collection, refinement, visualization, and analysis), writing, and revising the manuscript.
Corresponding author
Correspondence to Zehui Zhan .
Ethics declarations
Competing interests.
The authors declare no competing interests.
Ethical approval
This study does not involve human participants. All procedures performed in studies were in accordance with the ethical standards of the institutional and national research committee and with the 1964 Helsinki Declaration and its later amendments or comparable ethical standards.
Informed consent
This article does not contain any studies with human participants performed by any of the authors.
Additional information
Publisher’s note Springer Nature remains neutral with regard to jurisdictional claims in published maps and institutional affiliations.
Supplementary information
Rights and permissions.
Open Access This article is licensed under a Creative Commons Attribution 4.0 International License, which permits use, sharing, adaptation, distribution and reproduction in any medium or format, as long as you give appropriate credit to the original author(s) and the source, provide a link to the Creative Commons license, and indicate if changes were made. The images or other third party material in this article are included in the article’s Creative Commons license, unless indicated otherwise in a credit line to the material. If material is not included in the article’s Creative Commons license and your intended use is not permitted by statutory regulation or exceeds the permitted use, you will need to obtain permission directly from the copyright holder. To view a copy of this license, visit http://creativecommons.org/licenses/by/4.0/ .
Reprints and permissions
About this article
Cite this article.
Zhan, Z., Niu, S. Subject integration and theme evolution of STEM education in K-12 and higher education research. Humanit Soc Sci Commun 10 , 781 (2023). https://doi.org/10.1057/s41599-023-02303-8
Download citation
Received : 18 June 2023
Accepted : 23 October 2023
Published : 03 November 2023
DOI : https://doi.org/10.1057/s41599-023-02303-8
Share this article
Anyone you share the following link with will be able to read this content:
Sorry, a shareable link is not currently available for this article.
Provided by the Springer Nature SharedIt content-sharing initiative
Quick links
- Explore articles by subject
- Guide to authors
- Editorial policies

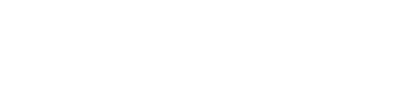
Along with Stanford news and stories, show me:
- Student information
- Faculty/Staff information
We want to provide announcements, events, leadership messages and resources that are relevant to you. Your selection is stored in a browser cookie which you can remove at any time using “Clear all personalization” below.
Image credit: Claire Scully
New advances in technology are upending education, from the recent debut of new artificial intelligence (AI) chatbots like ChatGPT to the growing accessibility of virtual-reality tools that expand the boundaries of the classroom. For educators, at the heart of it all is the hope that every learner gets an equal chance to develop the skills they need to succeed. But that promise is not without its pitfalls.
“Technology is a game-changer for education – it offers the prospect of universal access to high-quality learning experiences, and it creates fundamentally new ways of teaching,” said Dan Schwartz, dean of Stanford Graduate School of Education (GSE), who is also a professor of educational technology at the GSE and faculty director of the Stanford Accelerator for Learning . “But there are a lot of ways we teach that aren’t great, and a big fear with AI in particular is that we just get more efficient at teaching badly. This is a moment to pay attention, to do things differently.”
For K-12 schools, this year also marks the end of the Elementary and Secondary School Emergency Relief (ESSER) funding program, which has provided pandemic recovery funds that many districts used to invest in educational software and systems. With these funds running out in September 2024, schools are trying to determine their best use of technology as they face the prospect of diminishing resources.
Here, Schwartz and other Stanford education scholars weigh in on some of the technology trends taking center stage in the classroom this year.
AI in the classroom
In 2023, the big story in technology and education was generative AI, following the introduction of ChatGPT and other chatbots that produce text seemingly written by a human in response to a question or prompt. Educators immediately worried that students would use the chatbot to cheat by trying to pass its writing off as their own. As schools move to adopt policies around students’ use of the tool, many are also beginning to explore potential opportunities – for example, to generate reading assignments or coach students during the writing process.
AI can also help automate tasks like grading and lesson planning, freeing teachers to do the human work that drew them into the profession in the first place, said Victor Lee, an associate professor at the GSE and faculty lead for the AI + Education initiative at the Stanford Accelerator for Learning. “I’m heartened to see some movement toward creating AI tools that make teachers’ lives better – not to replace them, but to give them the time to do the work that only teachers are able to do,” he said. “I hope to see more on that front.”
He also emphasized the need to teach students now to begin questioning and critiquing the development and use of AI. “AI is not going away,” said Lee, who is also director of CRAFT (Classroom-Ready Resources about AI for Teaching), which provides free resources to help teach AI literacy to high school students across subject areas. “We need to teach students how to understand and think critically about this technology.”
Immersive environments
The use of immersive technologies like augmented reality, virtual reality, and mixed reality is also expected to surge in the classroom, especially as new high-profile devices integrating these realities hit the marketplace in 2024.
The educational possibilities now go beyond putting on a headset and experiencing life in a distant location. With new technologies, students can create their own local interactive 360-degree scenarios, using just a cell phone or inexpensive camera and simple online tools.
“This is an area that’s really going to explode over the next couple of years,” said Kristen Pilner Blair, director of research for the Digital Learning initiative at the Stanford Accelerator for Learning, which runs a program exploring the use of virtual field trips to promote learning. “Students can learn about the effects of climate change, say, by virtually experiencing the impact on a particular environment. But they can also become creators, documenting and sharing immersive media that shows the effects where they live.”
Integrating AI into virtual simulations could also soon take the experience to another level, Schwartz said. “If your VR experience brings me to a redwood tree, you could have a window pop up that allows me to ask questions about the tree, and AI can deliver the answers.”
Gamification
Another trend expected to intensify this year is the gamification of learning activities, often featuring dynamic videos with interactive elements to engage and hold students’ attention.
“Gamification is a good motivator, because one key aspect is reward, which is very powerful,” said Schwartz. The downside? Rewards are specific to the activity at hand, which may not extend to learning more generally. “If I get rewarded for doing math in a space-age video game, it doesn’t mean I’m going to be motivated to do math anywhere else.”
Gamification sometimes tries to make “chocolate-covered broccoli,” Schwartz said, by adding art and rewards to make speeded response tasks involving single-answer, factual questions more fun. He hopes to see more creative play patterns that give students points for rethinking an approach or adapting their strategy, rather than only rewarding them for quickly producing a correct response.
Data-gathering and analysis
The growing use of technology in schools is producing massive amounts of data on students’ activities in the classroom and online. “We’re now able to capture moment-to-moment data, every keystroke a kid makes,” said Schwartz – data that can reveal areas of struggle and different learning opportunities, from solving a math problem to approaching a writing assignment.
But outside of research settings, he said, that type of granular data – now owned by tech companies – is more likely used to refine the design of the software than to provide teachers with actionable information.
The promise of personalized learning is being able to generate content aligned with students’ interests and skill levels, and making lessons more accessible for multilingual learners and students with disabilities. Realizing that promise requires that educators can make sense of the data that’s being collected, said Schwartz – and while advances in AI are making it easier to identify patterns and findings, the data also needs to be in a system and form educators can access and analyze for decision-making. Developing a usable infrastructure for that data, Schwartz said, is an important next step.
With the accumulation of student data comes privacy concerns: How is the data being collected? Are there regulations or guidelines around its use in decision-making? What steps are being taken to prevent unauthorized access? In 2023 K-12 schools experienced a rise in cyberattacks, underscoring the need to implement strong systems to safeguard student data.
Technology is “requiring people to check their assumptions about education,” said Schwartz, noting that AI in particular is very efficient at replicating biases and automating the way things have been done in the past, including poor models of instruction. “But it’s also opening up new possibilities for students producing material, and for being able to identify children who are not average so we can customize toward them. It’s an opportunity to think of entirely new ways of teaching – this is the path I hope to see.”
ETS R&D
Quality assessments, groundbreaking research and measurement, and user-driven educational solutions
Learn more about ETS Research & Development.
K–12 Teaching, Learning and Assessment Research Center
K–12 assessments are an integral part of learning and teaching. The K–12 Teaching, Learning and Assessment Research Center at ETS aims to:
- improve K–12 teaching and learning
- align assessment with principles of learning and teaching
- inform innovations and operations in K–12 student and teacher assessment
- research and address inequities in the teacher pipeline
The center's work is supported through a combination of internally funded projects and external grants, which are primarily funded by the Institute of Education Sciences and the National Science Foundation. Learn more about ETS grants and alliances .
The center's work employs and contributes to the development of learning theories and models including cognitive, social and cultural dimensions of learning. It is also informed by new standards intended to prepare college- and career-ready young adults, including Next Generation Science Standards, and college- and career-ready standards in mathematics and English-language arts.
Supporting teachers and teacher education
The center staff recognize that teaching and teachers are among the most important school-related factors in student learning, and that assessment (formative and summative) plays an essential role of supporting teachers to make instructional decisions and evaluate student progress. The center remains at the forefront of engagement with teachers, students, school districts, and state and national stakeholders in order to best address the issues and challenges they face.
View the 2019 Simulations in Teacher Education Conference for short papers and presentations from the conference, which examines the role of simulations in K–12 science and mathematics teacher education.
Foundational and applied research
The center conducts foundational research to leverage modern technologies and methodologies to design and investigate the effectiveness of assessment, learning and instructional innovations. The center also conducts applied research that provides evidence to inform new product development related to student and teacher assessments.
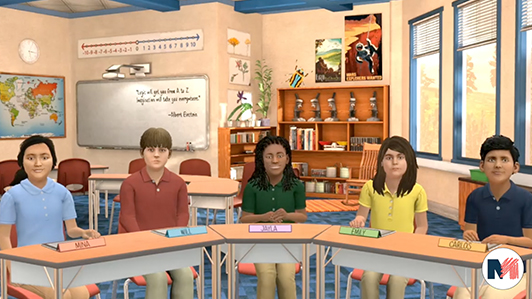
Focus areas
The center currently has the following major focus areas:
- defining and improving measurement of essential constructs in academic, social and emotional learning
- technology-supported assessment and scoring solutions
- development and validation of learning progressions
- diversification of the teaching population
- understanding the role of content knowledge in effective teaching
- supporting teacher learning throughout a teacher’s career
- increasing the depth and breadth of formative assessment
Correlates of K-12 Students’ Intertextual Integration
- REVIEW ARTICLE
- Open access
- Published: 07 May 2024
- Volume 36 , article number 48 , ( 2024 )
Cite this article
You have full access to this open access article
- Daniel R. Espinas 1 , 2 &
- Brennan W. Chandler 2
280 Accesses
8 Altmetric
Explore all metrics
We conducted a systematic review of research involving K-12 students that examined associations among individual differences factors (e.g., working memory) and intertextual integration. We identified 25 studies published in 23 peer-reviewed journal articles and two dissertations/theses. These examined a wide range of individual difference factors, which we organized into four categories: (a) language and literacy, (b) cognition and metacognition, (c) knowledge and beliefs, and (d) motivation, emotion, and personality. We found large variation in the participants, tasks, and document types, and little systematic replication across studies. Nonetheless, results generally showed that variation in literacy, cognition, metacognition, knowledge, beliefs, and motivation are positively and moderately associated with intertextual integration. We discuss the limitations of this work and offer four recommendations for future research.
Similar content being viewed by others
The use of cronbach’s alpha when developing and reporting research instruments in science education.
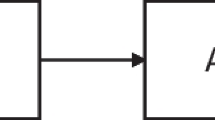
Theories of Motivation in Education: an Integrative Framework
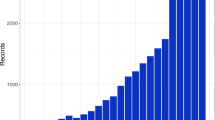
The Impact of Peer Assessment on Academic Performance: A Meta-analysis of Control Group Studies
Avoid common mistakes on your manuscript.
Reading is a social practice defined by one’s historical and cultural context (Gee, 2007 ). Indeed, from its on origins on clay pots, advances in writing technology (e.g., printing press) have radically changed the forms that texts can take; how they are shared and accessed; their roles in social, cultural, and economic practices; and expectations for who should be able to read and produce them (Manguel, 1996 ; Olson, 1994 ). This gradual evolution has seen rapid developments over the past 30 years, with advances in digital technology now offering near universal access to text documents, and in effect changing what readers read (Mackey, 2020 ), how they do it (Baron, 2021 ; Rouet, 2005 ; Wolf, 2018 ), and for what purposes (Coiro, 2021 ; Leu et al., 2019 ; Magliano et al., 2018 ). Consequently, what is now regarded as literate differs markedly from that of even several decades ago (Alexander & The Disciplined Reading and Learning Laboratory 2012 ; Britt et al., 2018 ). It is within this new context that we consider one aspect of literacy that has become increasingly common: intertextual integration.
The matter of integrating multiple information sources has been a topic of great interest in several fields (Alexander & The Disciplined Reading and Learning Research Laboratory, 2012 ; Braasch et al., 2018 ; Nelson & King, 2022 ). Given our interest in applications for K-12 literacy learning and instruction, we focus in this study on intertextual integration as it has been conceptualized within educational psychology. In this context, intertextual integration refers to the mental process of selecting, organizing, corroborating, and synthesizing information from multiple document sources for the purpose of constructing a coherent representation of the situation presented among them (Barzilai et al., 2018 ; Brand-Gruwel et al., 2009 ; Britt et al., 1999 ; Leu et al., 2015 ; Perfetti et al., 1999 ; Wineburg, 1991 ). We focus here primarily on connections among text documents made during reading and writing, rather than connections made between information presented in other types of media (e.g., pictures, videos) or in other forms of discourse (e.g., conversations). Note that this definition of intertextual integration overlaps with the concept of intertextuality (Allen, 2022 ; Bazerman, 2004 ; Lemke, 2004 ). The latter has been defined in a variety of ways but generally refers to connections a person makes between texts and their store of accumulated textual, contextual, and cultural knowledge.
The remainder of this introduction is organized into three parts. First, we discuss the importance of intertextual integration in K-12 educational contexts. Second, provide a capsule summary of how intertextual integration has been conceptualized in frameworks, theories, and models of multiple document use. Third, we discuss hypothesized predictors of intertextual integration, and previous efforts to review this literature.
Intertextual Integration in K-12 Education
Over the past century, reading research and practice have focused largely on processes involved in reading single, carefully curated documents for a small range of academic purposes (Anderson et al., 1985 ; Gibson & Levin, 1975 ; Huey, 1908 ; Perfetti, 1985 ; Reichle, 2021 ; Scammacca et al., 2016 ). This work has shown that reading depends on learning how oral and written language are structured and connected (Adams, 1990 ; Byrne, 1998 ; Castles et al., 2018 ; Ehri, 2014 ); acquiring rich, well-connected, and easily accessible stores of word, domain, disciplinary, and cultural knowledge (Goldman et al., 2016 ; Hwang et al., 2022 ; Lee, 2007 ; McCarthy & McNamara, 2021 ; Perfetti & Helder, 2022 ); and efficient use of cognitive and metacognitive strategies to monitor and construct meaning within a document (Garner, 1987 ; Graesser, 2007 ). A large body of work has also shown that reader characteristics (e.g., motivation) interact with features of the document (e.g., length, genre) and task (e.g., form an argument) to influence comprehension processes and outcomes (Afflerbach 2016 ; Snow, 2002 ).
Although skillful single document reading remains vital, present-day readers often encounter tasks involving multiple printed and digital text documents (Baron, 2021 ). Therefore, beyond the skills just outlined, readers must now also develop facility with a variety of multiple document use skills, to include searching for, selecting, navigating, evaluating, and integrating multiple printed and digital documents (Britt et al., 2018 ). Such skills are critical for academic achievement (Alexander & The Disciplined Reading and Learning Laboratory, 2012 ; National Assessment Governing Board, 2021 ; National Governors Association & Council of Chief State School Officers, 2010 ; National Research Council, 2013 ), to competitively participate in information-based economies (National Research Council, 2012a , 2012b ; Rouet et al., 2021 ), and to engage in informed civic discourse on matters of science, history, and culture (Goldman et al., 2016 ; Leinhardt & Young, 1996 ; List, 2023 ; Stadtler & Bromme, 2013 ).
Among the skills of multiple document use, intertextual integration plays a prominent role in current college- and career-readiness standards (National Council for the Social Studies, 2013 ; National Governors Association & Council of Chief State School Officers, 2010 ; National Research Council, 2013 ), in national and international assessment frameworks for K-12 students and adults (National Assessment Governing Board, 2021 ; Rouet et al., 2021 ; Sparks & Deane, 2015 ), and in conceptual frameworks for disciplinary and digital literacy (Coiro, 2021 ; Goldman et al., 2016 ; Leu et al., 2019 ). For example, the Common Core State Standards state that kindergarten students should be able to, “…identify similarities in and differences between two texts on the same topic…” (National Governors Association & Council of Chief State School Officers, 2010 ). Fifth graders are to “[d]raw on information from multiple print or digital sources…” and “[i]ntegrate information from several texts on the same topic…” And by 11th and 12th grade, students are to “[i]ntegrate and evaluate multiple sources of information presented in different media formats…” Similarly, it is expected in K-12 standards for social studies (National Council for the Social Studies, 2013 ) and science (National Research Council, 2013 ) that students develop competency with gathering, evaluating, and integrating information from multiple sources.
Tasks involving intertextual integration unfortunately prove difficult for many readers (Cho, 2013 ; Goldman et al., 2012 ; Many, 1996 ; McGrew et al., 2018 ; Raphael & Boyd, 1991 ; Rott & Gavin, 2015 ; Segev-Miller, 2007 ; Yang, 2002 ). Indeed, although children and adolescents regularly work with multiple documents in language arts, social studies, and science, they rarely form spontaneous intertextual connections (Cho et al., 2018 ; Many, 1996 ; Many et al., 1996 ; Stahl et al., 1996 ; VanSledright, 2002 ; VanSledright & Kelly, 1998 ; Wolfe & Goldman, 2005 ). This has been found even for college-level students (Greene, 1993 ; Kennedy, 1985 ; McGinley, 1992 ; Rott & Gavin, 2015 ; Segev-Miller, 2007 ; Yang, 2002 ). As we will discuss in the following sections, many factors have been proposed for why intertextual integration proves so difficult.
As with single document comprehension (Magliano et al., 2023 ; Wang et al., 2019 ), it is possible that intertextual integration skills cannot fully develop until foundational reading and writing skills are secured. However, evidence from at least one study suggests that intertextual integration is possible even with impaired word-level reading skills (Andresen, Anmarkrud, Salmerón, et al., 2019 ). Furthermore, even as children are developing word-level reading skills, they can be capable of performing complex comprehension tasks (Williams et al., 2016 ). Moreover, even by the age of six, children are able to monitor information sources (Drummey & Newcombe, 2002 ; Lindsay et al., 1991 ) and even critically evaluate their trustworthiness (Koenig & Harris, 2005 ). As reflected in current instructional standards (National Governors Association & Council of Chief State School Officers, 2010 ), it is not unreasonable to expect that even in kindergarten, and certainly by the upper elementary grades, children may be capable of integrating information from multiple text sources. In the following sections, we discuss what is known about intertextual integration and its predictors.
Frameworks, Theories, and Models of Intertextual Integration
Many psychological frameworks, theories, and models have been proposed to account for intertextual integration within the broader context of multiple document use (Afflerbach et al., 2014 ; Afflerbach & Cho, 2009 ; Bråten et al., 2020 ; Britt et al., 2018 ; Butterfuss & Kendeou, 2021 ; Cho & Afflerbach, 2017 ; Leu et al., 2019 ; List, 2020 ; List & Alexander, 2017a , 2019 ; Perfetti et al., 1999 ; Richter & Maier, 2017 ; Rouet et al., 2017 ; Segev-Miller, 2007 ; Stadtler & Bromme, 2014 ; van den Broek & Kendeou, 2022 ; Yang, 2002 ). In Table A1 , we have listed a selection of 12, ranging from generalized to specialized accounts. We have provided summaries in the table of the major points of each and have noted the cognitive and affective factors that are explicitly specified in them. As context for the rest of the article, we provide a detailed description of the Documents Model Framework (DMF; Britt et al., 1999 ; Perfetti et al., 1999 ) as it has provided the foundation for much of the theorizing and empirical work that has followed.
The DMF provided the first attempt at a psychological account of how meaning is constructed from multiple documents. It emerged from research on text-based history learning (Perfetti et al., 1995 ), in which it is common for readers to engage with multiple documents that present discrepant information and often vary in length, layout, format, structure, purpose, and reliability. Within the discipline of history, it is important to evaluate features of the document sources (i.e., sourcing) and consider how information presented among them is related (i.e., corroborate; Wineburg, 1991 ). Notably, intertextual integration proves important also in science and literature (Goldman et al., 2016 ).
It is proposed in the DMF that integration is accomplished by constructing two representations: an integrated situations model and an inter-text model (Britt et al., 1999 ; Perfetti et al., 1999 ). The integrated situations model contains from each document semantic information about the common topic or issue being discussed. The inter-text model comprises meta-document information, such as the source author, publication, date, and purpose. This information is captured in networks of source-document nodes (Perfetti et al., 1999 ).
The reader’s comprehension will depend on the forms of these two models (Britt et al., 1999 ). Following Britt et al.'s ( 1999 ) framework, three possible outcomes are illustrated in Fig. 1 : (a) a mush model, (b) a separate representation model, and (c) a document model. A mush model is one in which the reader constructs a well-formed integrated situation model but a undeveloped inter-text model. In this case, information from across the documents is well represented but not linked to the sources from which it originated. Moreover, information about the sources and links among them are not captured. The opposite pattern can be seen in the separate representations model , wherein the inter-text model is well developed but the situation model is unformed. In this case, content is recalled and linked to each source but is not cohesively integrated. With the documents model , both the situation model and the inter-text model are well-formed. Information is clearly linked to the documents from which it originated, relations among the documents are captured (e.g., agree, disagree), and a well-integrated representation of the situation is formed.
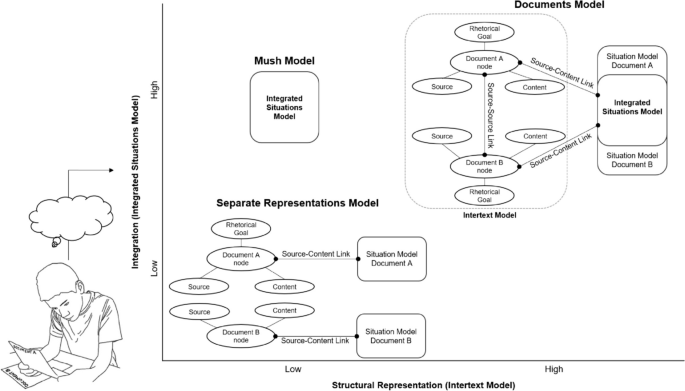
Adapted from Fig. 3 in List et al. ( 2019 ), Fig. 1 in Saux et al. ( 2021 ), and Fig. 4.2a in Perfetti et al. ( 1999 )
Multiple document representations.
Forming intertextual connections is highly effortful—requiring both bottom-up and top-down processes (Kurby et al., 2005 ; van den Broek & Kendeou, 2022 ). Accordingly, these connections may not be formed in cases where the reader views them as unnecessary or is unable to do so. In certain contexts (e.g., history class) and for certain tasks (e.g., research report), though, it may be important to construct well-formed intertextual representations (e.g., documents model). Certain tasks can also promote greater intertextual integration than others (e.g., arguments vs. summaries; McNamara et al., 2023 ; Wiley & Voss, 1999 ). However, in other contexts, and for other tasks, the high cognitive effort may outweigh the need. Therefore, the likelihood that a reader will construct a particular representational form (e.g., mush model) depends in part on the context and task at hand (Frederiksen, 1975 ; Rouet & Britt, 2011 ; Rouet et al., 2017 ; Spivey, 1995 ; Van Dijk, 1979 ). In the following section, we turn the role played by individual differences in shaping intertextual integration.
Individual Differences in Intertextual Integration
A sizeable literature has examined the role that individual differences play in intertextual integration (Barzilai & Strømsø, 2018 ). However, few of these studies have used intervention, longitudinal, or computational designs. This has greatly limited what can be concluded about the existence, direction, or magnitude of any potential causal relations. However, predictors of intertextual integration have been studied in many cross-sectional investigations. As an initial step toward synthesizing this literature, we conducted a systematic review of studies reporting concurrent associations among individual differences factors (e.g., working memory) and intertextual integration.
Previous efforts to review this literature have revealed many useful insights (Alexander & Disciplined Reading and Learning Research Laboratory, 2020 ; Anmarkrud et al., 2022 ; Barzilai & Strømsø, 2018 ; Bråten et al., 2011 ; List & Sun, 2023 ; Richter & Maier, 2017 ; Tarchi et al., 2021 ) but are limited by several factors. First, only several reviews have used systematic and transparent search procedures (Anmarkrud et al., 2022 ; List & Sun, 2023 ; Richter & Maier, 2017 ; Tarchi et al., 2021 ). This is critical for reducing sampling error and for ensuring replicability (Alexander, 2020 ; Cumming et al., 2023 ). Second, previous reviews have synthesized results from studies involving K-12, college, and adult readers (Anmarkrud et al., 2022 ; Barzilai & Strømsø, 2018 ). This approach has the benefit of comprehensively mapping the terrain. However, combining such a broad range of ages can obscure potential developmental differences. Moreover, it complicates interpretation for both K-12 and college educators. Finally, most previous reviews have examined a wide range of multiple document use processes (e.g., source selection, source evaluation, intertextual integration) without clearly distinguishing how each is associated with particular individual differences factors.
Despite these limitations, previous reviews have provided useful insights into the roles that individual differences play in intertextual integration. For example, Barzilai and Strømsø ( 2018 ) identified a broad range of individual differences factors (cognition, metacognition, motivation, affect, socio-culture) that are associated with searching for, selecting, evaluating, and integrating information from multiple documents. Furthermore, they found evidence that these individual difference factors interact with one other, the reading task, and the context to produce different comprehension outcomes. However, few sources were cited for most factors and there were often large differences in the populations, documents, and tasks that were studied. This may point to limitations within the literature.
Other reviews have adopted narrower approaches. For example, Richter and Maier ( 2017 ) examined how individual differences in cognition and affect influence how readers identify and resolve discrepancies encountered across multiple documents. Using systematic search procedures, they identified 18 studies involving adolescent and adult readers. They found that readers’ prior beliefs about a topic and epistemic monitoring will determine whether a reader detects belief-inconsistent information. Then, once an inconsistency is detected, the reader’s epistemic goals and beliefs, background knowledge, working memory resources, and store of metacognitive strategies will influence whether they simply form a belief-consistent representation of the controversy or a more balanced mental model. Bråten et al. ( 2011 ) also exampled how beliefs influence multiple document comprehension. With a focus on expository texts, they found that epistemological beliefs (i.e., simplicity, certainty, source, justification) play important roles in creating and updating a task model, assessing information from each source and its relevance for the task, processing source contents, and creating and updating intertextual representations.
Finally, Tarchi et al. ( 2021 ) examined how executive functions (e.g., inhibition) are associated with multiple document use and comprehension. However, by including a wide variety of executive functions (working memory span, working memory reading span, working memory updating, problem solving, strategic processing, regulation, fluid reasoning), a mix of multiple document comprehension processes (intertextual integration, sourcing), and a broad age range (secondary school, undergraduate, adults), they were unable to any find robust patterns of association.
Present Study
The present study advances upon previous reviews in four ways. First, to reduce sampling error and to ensure transparency, we have used systematic search, selection, and coding procedures (Alexander, 2020 ; Cumming et al., 2023 ). Furthermore, to reduce publication bias, we included both peer-reviewed journal articles and dissertation/theses. Second, whereas others have combined results for K-12, college, and adult populations, we focused only on K-12 students. As noted, although intertextual integration is a cognitive taxing processes, it is an integral component of K-12 education (National Governors Association & Council of Chief State School Officers, 2010 , 2010). However, in comparison to undergraduate and adult readers, K-12 students have been studied far less in research on multiple document use (Anmarkrud et al., 2022 ). By focusing exclusively on this age range, implications for K-12 educators may be more clearly understood.
Third, whereas most other reviews have examined the roles played by individual differences factors in several components of multiple document use (e.g., search, selection, evaluation, integration), we focused here only on intertextual integration. To this end, we included only studies that clearly measured intertextual integration; not, as others have, combinations of document searching, selecting, evaluating, and integrating sources. In doing so, we provide a clearer assessment of associations among individual differences and intertextual integration. We decided to focus exclusively on intertextual integration for several reasons. First, intertextual integration is clearly referenced in K-12 standards and assessment frameworks (National Assessment Governing Board, 2021 ; National Council for the Social Studies, 2013 ; National Governors Association & Council of Chief State School Officers, 2010 ; National Research Council, 2013 ). Therefore, there is clear practical importance for understanding the factors associated with this skill. Second, although individual differences in multiple document sourcing have been the subject of a recent and comprehensive systematic review (Anmarkrud et al., 2022 ), previous reviews of intertextual integration have either not used systematic search procedures or focused more narrowly on specific individual differences factors or task types.
Fourth, whereas previous reviews have combined findings from studies with markedly different designs (e.g., cross-sectional, longitudinal, intervention), we examine only concurrent associations among individual differences factors and intertextual integration. This provides for a clearer interpretation of the results. In these four ways, this systematic review provides a systematic and transparent assessment of the nature, features, and volume of research that has examined associations among individual differences factors and intertextual integration with K-12 students.
Research Questions
Our review was guided by four primary questions.
First, what are the characteristics of the participants involved in research on individual differences in intertextual integration? We predicted that more studies would involve secondary than elementary-level students. Furthermore, we predicted that studies would involve participants from a broad range of Western countries, language backgrounds, and socio-economic backgrounds. Finally, we predicted that few studies would involve participants with educational disabilities (e.g., dyslexia).
Second, what are the features of studies that have examined intertextual integration among K-12 students? Specifically, what kinds of tasks (e.g., form an argument, synthesize the information), documents (e.g., domain, genre, number of texts), and measures (e.g., essay, multiple choice) have been used? Based on previous reviews (Anmarkrud et al., 2022 ; Primor & Katzir, 2018 ), we predicted that a wide range of tasks, document types, and measures would be represented. Argumentation tasks, essay measures, and informational documents would be the most common, and narrative tasks, oral response measures, and narrative documents would be used infrequently.
Third, which types of individual differences factors have been studied? Based on previous reviews (Anmarkrud et al., 2022 ; Barzilai & Strømsø, 2018 ) and the frameworks, theories, and models of multiple document use and comprehension listed in Table A1 , we predicted that the following types of factors would be examined: language and literacy skills (e.g., word-level reading); cognition and metacognition; motivation, emotion, and personality; and knowledge and beliefs. Given the selected age range, we predicted that language and literacy skills would be studied the most and personality the least. Semantic knowledge (e.g., domain, topic) and cognitive processes (e.g., working memory, attention) would be well represented, whereas emotion and epistemic beliefs would not.
Fourth, what is the direction and strength of associations among individual differences factors and intertextual integration? We predicted that certain epistemic beliefs (e.g., belief in authority) would be negatively and moderately correlated with intertextual integration (Bråten et al., 2011 ). All other factors would be positively correlated with intertextual integration, with literacy and knowledge being strongly correlated and cognitive skills and emotions being moderately correlated (Barzilai & Strømsø, 2018 ).
We conducted two rounds of searches. The first was in May 2022 and the second in February 2024. For the first round, we used three methods: (1) an electronic database search, (2) a backward search, and (3) a forward search. For the second round, we used a snowballing approach.
Electronic Database Search
We consulted a university librarian to identify databases and search terms to capture relevant records. On 2 May 2022, we searched PsycInfo, ERIC, and ProQuest Dissertations and Theses Global databases for articles and theses/dissertations published prior to 2022. We used the following terms to search titles and abstracts: (noft("multiple text" OR "multiple texts" OR "multi-text" OR ("multiple source" OR "multiple sources") OR ("multiple document" OR "multiple documents") OR ("intertext" OR "intertexual")) AND ti,ab,su("read*" OR "write*" OR "process*" OR "navigat*" OR "integrat*" OR "teach*" OR "instruct*" OR "histor*" OR "scien*" OR "socioscientific") AND noft("elementary" OR "primary" OR "middle*" OR "high* " OR "secondary*")). These terms reflect the various ways multiple text use and comprehension have been defined (Goldman & Scardamalia, 2013 ), the disciplines in which this work has been conducted (Barzilai & Strømsø, 2018 ), and the targeted age range (i.e., K-12). The search yielded 4,667 sources.
Backward, Forward, and Snowballing Search
We also conducted a backward search of references from seven reviews and conceptual articles on multiple document comprehension (i.e., Anmarkrud et al., 2022 ; Barzilai et al., 2018 ; Bråten et al., 2020 ; List & Alexander, 2019 ; Nelson & King, 2022 ; Primor & Katzir, 2018 ; Saux et al., 2021 ). Then, using Google Scholar, we conducted an initial forward search for any records that had cited these reviews. Through these methods, we identified 294 sources.
In February 2024, we updated our search using the SnowGlobe application (McWeeny et al., 2021 ). SnowGlobe using a snowballing approach to search the references and citations of selected records. We used the 23 records identified through our initial searches. Using SnowGlobe, we identified 1115 records, of which 186 were duplicates. We used the inclusion and exclusion criteria described in the following section to screen studies in both rounds of searches.
Inclusion and Exclusion Criteria
We included only sources that met the following six criteria in this review:
The record was a peer-reviewed journal article, dissertation, or thesis. We excluded technical reports, conference abstracts, books, and book chapters. If the study was published as both a dissertation/thesis and journal article, we selected the journal version.
Study participants were enrolled in kindergarten through 12th grade (i.e., elementary, middle, or secondary/high school). We excluded studies that only involved undergraduate, graduate, or adult participants. We also excluded studies that did not disaggregate K-12 and adult populations. However, we did include studies involving participants attending prevocational programs.
The study reported original data from a cross-sectional, longitudinal, or group experimental design. We excluded studies that used qualitative or single-case designs.
The study included at least one measure of participants’ intertextual integration. We excluded studies that measured a dimension of multiple document use (e.g., search, selection, evaluation) but not intertextual integration and studies that only measured intertextual integration in combination with another skill (e.g., source evaluation).
The study reported at least one direct (i.e., not partial correlation) and concurrent correlation between an intertextual integration measure and an individual differences measure. If the study used an experimental or quasi-experimental design, measures had to be collected prior to the intervention. Data from any measures collected during or after the intervention were excluded.
The study was published in English prior to February 2024.
Screening and Data Extraction
We used covidence, a commercial web-based platform, for screening and data extraction. As illustrated in Fig. 2 , the process involved three successive stages: (a) title and abstract screening, (b) full text screening, and (c) data extraction.
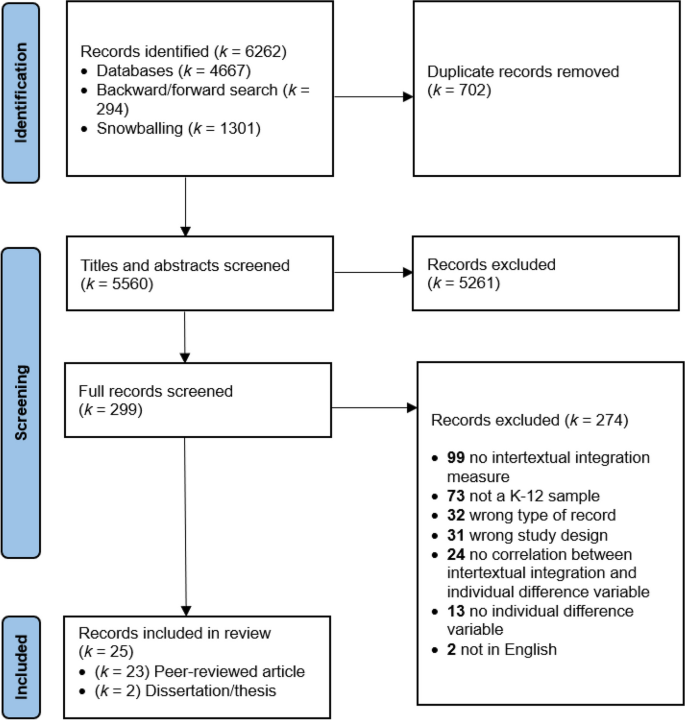
PRISMA diagram
Title/Abstract and Full-Text Screening
Results from the three search procedures produced 4961 records. After removing duplicates ( k = 512), we (the first and second author) independently screened the titles and abstracts of the 4449 records identified in the first round of screening. The first author independently screened the titles and abstracts of the 1115 records identified in the second round. For title and abstract screening, we used the above noted inclusion and exclusion criteria. When it was unclear whether a record met the inclusion criteria, we advanced it to full-text screening for further review. We addressed all discrepancies through discussions.
Based on the exclusion criteria, we deemed 5561 irrelevant. We independently reviewed the full texts of the remaining 293 from the first search and six from the second. For the first search, we met after coding the first 20 records and then again after the next 50 to discuss discrepancies. After coding the initial 70, we revised wording for several of the exclusion criteria to improve clarity. We met again after the next 100 and then once all the records had been screened to discuss discrepancies. Total agreement for title/abstract screening was 95%, and Cohen’s \(\kappa\) was 0.63. For full-text screening, total agreement was 94% and Cohen’s \(\kappa\) was 0.67. We excluded 274 for various reasons reported in Fig. 2 . This resulted in 25 records that met the full inclusion criteria. These are listed in the Appendix.
Data Extraction
We developed a codebook based on variables included in several previous reviews of single and multiple document comprehension (Anmarkrud et al., 2022 ; Barzilai & Strømsø, 2018 ; Primor & Katzir, 2018 ; Toste et al., 2020 ). The codebook included general information (study ID, publication year, country where the study was conducted, type of source, research question), information about participants (inclusion criteria, exclusion criteria, sample size, demographic information), documents information (number, length, format, mode, type, agreement, difficulty), the intertextual integration task (directions, genre, format), and individual differences in literacy skills (e.g., phonological awareness, rapid naming, decoding, single-text comprehension, written composition), cognition and metacognition (e.g., working memory, attention, processing speed, metacognition), motivation and emotion (e.g., self-efficacy, interest, emotional reactivity), personality (e.g., conscientiousness, need for cognition), knowledge and beliefs (e.g., content/topic knowledge, epistemic cognition), sourcing ability (e.g., trustworthiness), and demographics (e.g., age, sex/gender). We independently coded 24% ( k = 6) of the sources. Total agreement was 87%, and Cohen’s \(\kappa\) was 0.73. We handled all disagreements through discussion. We made several revisions to the codebook to capture the full range of individual differences that were studied. The first author then independently coded the remaining 19 sources. Coded studies are available through the Open Science Framework ( https://osf.io/xqb4h/ ).
Participant Characteristics
Records included 23 peer-reviewed journal articles (92%) and two dissertations/theses (8%), all published within a 13-year range from 2009 to 2022. Each record reported results from a single study with an independent sample. Across the studies, 5600 participants were included (median = 99, range = 44–1434). Twenty-two studies (96%) reported sex or gender, of which there 53% were female. Studies were conducted in five countries: Norway ( k = 9, 36%), Italy ( k = 6, 24%), the USA ( k = 5, 20%), the Netherlands ( k = 3, 12%), Israel ( k = 1, 4%), and Hong Kong ( k = 1, 4%). Mean participant age was reported in 21 studies (84%) and ranged from 9 years 7 months (Florit, Cain, Mason 2020 ) to 18 years 6 months (Strømsø et al., 2010 ). Grade levels ranged from fourth to twelfth, with five studies (20%) involving students at the elementary level (grades K-5) and (80%) at the secondary level (grades 6–12). Eight (32%) of the secondary-level studies involved students enrolled in college preparatory and prevocational programs. Although we consider these as part of the K-12 span, specific grade-level correspondences are unclear.
Authors in 19 (76%) and 20 (80%) of the studies did not report inclusionary or exclusionary criteria, respectively. Exclusionary criteria included having a disability ( k = 2, 8%), being a minority language speaker ( k = 1, 4%), and having poor eye movement registration ( k = 2, 8%). Twenty studies (80%) did not report the number of disabled participants included. The two (8%) that did included students with developmental dyslexia ( n = 27 across the two studies; Andresen et al., 2019a , 2019b ; de Ruyter, 2020 ). The number of multilingual participants was reported in 15 studies (60%), with percentages ranging from 0% (Florit, Cain, et al., 2020 ; Florit, De Carli, et al., 2020 ) to 72% (Davis et al., 2017 ). Sixteen studies (64%) reported participants’ socioeconomic status. Of these, 14 reported that their samples were largely homogenous and from middle-class families. In the other two studies, participants came from lower socioeconomic families.
Features of Tasks, Measures, and Documents
In each study, participants performed a task requiring them to independently read a set of multiple documents. The most common task ( k = 16, 64%) involved having participants read the documents for the purpose of writing an essay. These included writing arguments ( k = 9, 36%), opinions ( k = 1, 4%), or combinations of arguments and summaries/syntheses ( k = 5, 20%). In other studies, participants answered verification questions ( k = 6, 24%), oral open-ended questions ( k = 2, 8%), multiple-choice questions ( k = 1, 4%), or application questions ( k = 1, 4%). In 16 of the studies (64%), participants were provided with information about the document source (e.g., author, publication, date).
The number of documents per set ranged from 2 to 10 (mean = 4.72, SD = 1.86), with an average of 2.6 (SD = 0.54) at the elementary level and 5.25 (SD = 1.68) at the secondary. In several studies, participants read multiple sets of documents (e.g., Beker et al., 2019 ; Mason et al., 2020 ), bringing the total number of documents read between 2 and 40. In all the studies, the documents were informational rather than narrative. Most studies ( k = 23, 92%) involved document sets that addressed socioscientific topics (e.g., human impact on climate change) and presented a combination of complementary and contrasting viewpoints ( k = 18, 72%). Of the 20 (80%) studies that reported document format, nine (36%) used only digital documents, ten (40%) used only print documents, and one (4%) used both. Ten (40%) studies reported whether multimedia documents (e.g., text with image) were used. Of these, multimedia documents were used in six (24%) text only documents in four (16%). Seventeen studies (68%) reported efforts to evaluate text difficulty. In each case, a readability formula was used. Several also reported consulting with content-area experts in developing the texts.
Generally similar tasks, measures, and document formats were used at the elementary and secondary levels. For both levels, argumentative essays and inference verification questions were used to measure intertextual integration. However, application questions were used only at the elementary level and multiple-choice questions, open-ended questions, and summary essays were used only at the secondary level. At both levels, print and digital formatted documents were used. Finally, the average number of documents per set differed across these two developmental spans, with a mean 2.6 (SD = 0.55) at the elementary level and 5.25 (SD = 1.68) at the secondary level.
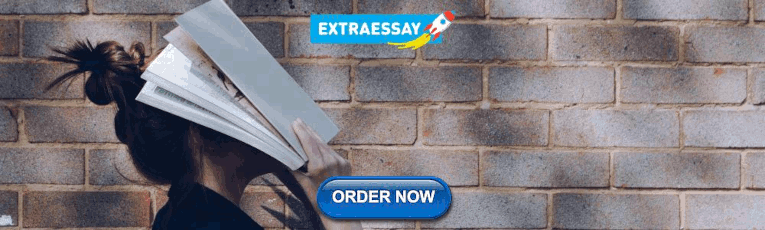
Associations with Individual Differences
Tables 2 – 4 report zero-order correlations between performance on measures of intertextual integration and measures of individual differences in language and literacy, cognition and metacognition, knowledge and beliefs, and motivation, emotion, and personality. Results are discussed in the following four sections. To avoid redundancy, we provide information about the participants (e.g., nationality, age, sample size) and measures only once for each study.
Language and Literacy
Eighteen studies (72%) examined associations among intertextual integration, language, and literacy (see Table 1 ). Single-document comprehension was the most studied ( k = 12, 48%), followed by word-level reading ( k = 6, 24%), and then a variety of other factors (e.g., strategy knowledge). In the following sections, we discuss results first for elementary- and then secondary-level participants.
Elementary-Level. Four studies (16%) examined associations between intertextual integration and literacy factors among elementary-level students. Three of these examined associations with single document comprehension. Beker et al., 2019 ) had fourth- and sixth-grade Dutch children ( n = 105) read 20 brief (i.e., about eight sentences) expository text pairs (40 individual texts in total). In each pair, the second text contained an internal inconsistency. Across pairs, they randomly varied whether the first text contained an explanation that could help to resolve the inconsistency presented in the second text. To assess intertextual integration, they asked participants open-ended questions after every fourth pair of texts. They assessed single document comprehension by having participants answer multiple-choice questions about several brief texts. They found an association of r = 0.39 ( p < 0.001) between these two factors.
In two studies, Florit and colleagues (Florit, Cain, Mason, 2020 ; Florit et al. 2020 ) had Italian participants complete two multiple document tasks that each involved reading three texts about a socioscientific issue (e.g., “Are videogames beneficial?”) and then writing an argumentative essay (Florit, Cain, et al., 2020 ; Florit et al., 2020 ). They assessed single-document comprehension by having students answer inferential and literal questions about an informational text. Florit, De Carli, et al. ( 2020 ) found associations of r = 0.06 ( p > 0.05) and r = 0.15 ( p < 0.05) between single document comprehension and intertextual integration for a sample of fourth- and fifth-grade students ( n = 184).
With a separate sample of fourth-grade students ( n = 94), Florit, Cain, et al. ( 2020 ) found associations of r = 0.23 ( p < 0.05) and r = 0.47 ( p < 0.01). In the same study, they also assessed students’ comprehension monitoring with a task that measured their ability to detect inconsistencies in 24 six-sentence texts. Correlations between comprehension monitoring and intertextual integration were r = 0.22 ( p < 0.05) and r = 0.35 ( p < 0.01).
Three studies involving Italian children also examined associations between word reading fluency and intertextual integration. Florit, Cain, et al. ( 2020 ) had fourth-grade students ( n = 94) read 112 words and 48 non-words as quickly as they could; for their analyses, they combined scores from these two tasks. Correlations between word-level reading and intertextual integration were r = 0.14 ( p > 0.05) and r = 0.36 ( p < 0.01). Florit, De Carli, et al. ( 2020 ) tested a separate sample of four- and fifth-grade students ( n = 184) with the same word-level reading and intertextual integration tasks, and found correlations of r = 0.10 ( p > 0.05) and r = 0.16 ( p < 0.05). Finally, Raccanello et al. ( 2022 ) found a correlation of r = 0.21 ( p < 0.001) between fourth- and fifth-grade students’ ( n = 334) word reading fluency and intertextual integration, as measured by an argumentative essay based on a document set.
Secondary-Level. Fourteen studies (56%) examined associations between intertextual integration and literacy factors among secondary-level students. Nine (36%) measured students’ single document comprehension, three of which were conducted by Bråten and colleagues and involved Norwegian students enrolled in college preparatory or prevocational courses. Bråten et al. ( 2018 ) had participants ( n = 127) complete a cloze comprehension measure involving narrative and expository texts. They randomly divided students into two groups, each assigned to read a document set of ten texts about either climate change or nuclear power. To measure intertextual integration, they had students in both conditions write argumentative essays based on the document sets. Correlations between single document comprehension and intertextual integration ranged from r = 0.20 ( p > 0.05) to r = 0.28 ( p < 0.05).
Strømsø and Bråten ( 2009 ) had participants ( n = 282) read a document set comprising seven texts on different aspects of climate change. After reading, they assessed students’ single document comprehension for each of texts using sentence and intratextual inference verification measures; scores were summed across texts for analyses. They also had students complete an intertextual inference verification task based on the document set that they had read. They found an association of r = 0.52 ( p < 0.001) between intertextual integration and sentence verification (single document comprehension), and a correlation of r = 0.54 ( p < 0.001) between intertextual integration and intratextual integration (single document comprehension). Finally, Strømsø et al. ( 2010 ) had participants ( n = 233) complete intratextual and intertextual inference verification tasks based on the same set of seven text documents and found a correlation of r = 0.57 ( p < 0.01) between them.
de Ruyter ( 2020 ) had Dutch prevocational students ( n = 83) write argumentative essays after reading a set of four digital documents. They measured students’ single document comprehension by having them read five texts, and after each, answering a series of multiple-choice and open-ended questions. They found a correlation of r = 0.28 ( p < 0.05) between these two variables. Finally, Wang et al. ( 2021 ) had US ninth-twelfth students’ ( n = 1107) read four digital text documents and measured their intertextual integration with a multiple-choice and yes/no questions. To measure single document comprehension, they had students read 10 short passages and answer questions about key ideas and details for each. The correlation between these two variables was r = 0.57 ( p < 0.01).
Four studies examined associations among single document comprehension and intertextual integration among seventh-grade students. Mason and colleagues conducted three with Italian students (samples ranged from 47 to 104). In each, they used a multiple-choice task to measure single document comprehension and an argumentative essay task to measure intertextual integration. Across these studies, correlations between these two variables ranged from r = 0.18 ( p > 0.05; Mason et al., 2017 ) to r = 0.48 ( p < 0.01; Mason et al., 2020 ). Mason et al. ( 2017 ) also measured single document comprehension with a sentence verification task and found a correlation of r = 0.13 ( p > 0.05) between it and intertextual integration. Forzani ( 2016 ) had US seventh-grade students’ ( n = 1434) complete an online research and comprehension assessment. As part of this, they assessed students’ ability to synthesize information (i.e., intertextual integration) by writing argumentative essays. They also assessed students’ single document comprehension by having them read several brief passages and answer multiple-choice questions about them. The correlation between single document comprehension and intertextual integration was r = 0.37 ( p < 0.01).
Six studies examined associations among word-level reading and intertextual integration. In each, researchers used measures that involved reading from word lists with accuracy and speed (i.e., fluency). Three involved Norwegian students and used a word chain task involving 360 words arranged in 30 rows. Across these studies, correlations between word chain reading (i.e., word-level reading) and intertextual integration ranged from r = 0.16 ( p > 0.05) to r = 0.43 ( p < 0.001). Bråten et al., ( 2013a , 2013b ) had tenth-grade students ( n = 65) read six digital text documents and assessed their intertextual integration by having them write brief essays. The correlation between word-level reading and intertextual integration was r = 0.43 ( p < 0.001). Andresen et al., ( 2019a , 2019b ) also examined the association between word-level reading and intertextual integration with tenth-grade students ( n = 44), half of whom were neurotypical in reading (i.e., > 20th percentile on a national standardized reading test) and half of whom had school-based diagnoses of developmental dyslexia. Participants viewed three webpages containing information presented through video, text, and pictures. Intertextual integration was assessed by having students orally respond to two open-ended questions about the document set. They found an association of r = 0.45 ( p < 0.01) between word reading and intertextual integration for the entire sample. Finally, Braasch et al. ( 2014 ) had college preparatory students ( n = 59) read six printed documents about assessed intertextual integration with an inference verification task. The correlation between this and word-level reading was r = 0.22 ( p > 0.05).
Beyond word level reading and single document comprehension, researchers also examined associations between intertextual and several additional language and literacy skills. For example, Davis et al. ( 2017 ) examined associations among US fifth- to seventh-grade students’ intertextual integration and receptive ( r = 0.47, p < 0.01) and productive syntax ( r = 0.24, p < 0.05). To measure intertextual integration, they had students read two informational texts about a scientific issue and then complete a sentence and inference verification task. Wang et al. ( 2021 ) found an association of r = 0.53 ( p < 0.01) between US ninth-twelfth grade students’ sentence processing and intertextual integration.
Finally, Cheong et al. ( 2019 ) examined the association between intertextual integration and written composition among secondary-level Hong Konger students ( n = 415). They presented students with two, parallel-structured multiple documents tasks in Chinese (L1) and English (L2). For both tasks, students read six documents. They measured intertextual integration and written composition in Chinese and English with inference verification and argumentative essay tasks, respectively. Associations between Chinese intertextual integration and written composition in Chinese ( r = 0.41, p < 0.01) and English ( r = 0.28, p < 0.05) were similar to associated between English intertextual integration and written composition in Chinese ( r = 0.30, p < 0.01) and English ( r = 0.41, p < . 01).
Cognition and Metacognition
Fourteen studies (56%) examined associations between intertextual integration, cognition, and metacognition (Table 2 ). Of these, five (20%) examined associations between verbal working memory and intertextual integration, with correlations ranging from r = 0.06 ( p > 0.05) to r = 0.538 ( p < 0.001). Two were conducted with elementary-level students. Beker et al. ( 2019 ) measured Dutch fourth- and sixth-grade children’s ( n = 105) verbal working memory with a task adapted from Daneman and Carpenter ( 1980 ). They had students listen to sets of unrelated sentences, answer a comprehension-related question about one of them, and then recall the last word of each sentence. Performance on this task correlated r = 0.13 ( p = 0.183) with intertextual integration, as measured by the open-ended questions task described in the preceding section (Beker et al., 2019 ). Florit, Cain, et al. ( 2020 ) measured fourth-grade Italian students’ ( n = 94) verbal working memory with a task involving six lists of nouns. For each list, students had to remember and write down the nouns representing the three smallest objects in the list, and in the order in which they were presented. Correlations between performance on this working memory task and the two intertextual integration measures described above were r = 0.18 ( p > 0.05) and r = 0.22 ( p < 0.05).
The three studies that examined associations between intertextual integration and verbal working memory with secondary-level students, all measured working memory with a version of a task developed by Daneman and Carpenter ( 1980 ). As described above for Beker et al. ( 2019 ), Andresen et al., ( 2019a , 2019b ), and Braasch et al. ( 2014 ) had students listen to sets of sentences, answer comprehension-related questions about them, and then recall the last word of each. Andresen et al., ( 2019a , 2019b ) found a correlation of r = 0.54 ( p < 0.001) between Norwegian tenth-grade students’ ( n = 44) working memory and intertextual integration, as measured with the oral opened-ended questions task described in the preceding section. Braasch et al. ( 2014 ) found a correlation of r = 0.29 ( p < 0.05) between Norwegian secondary-level students ( n = 59) working memory and intertextual integration, as measured by the inference verification task also described above. Mason et al. ( 2017 ) adapted the working memory task to have students read the sentences themselves. They found that performance on this task correlated r = 0.06 ( p > 0.05) with intertextual integration, as measured with the previously described argumentative essay task.
Twelve (48%) studies examined associations between metacognition and intertextual integration. Florit, Cain, et al. ( 2020 ) measured Italian fourth-grade children’s ( n = 94) comprehension monitoring by assessing their ability to detect inconsistencies in stories with and without inconsistent sentences. They found that comprehension monitoring was weakly but significantly associated with intertextual integration ( r s = 0.22–0.35, p < 0.05), as measured by two essay tasks. Braasch et al. ( 2022 ) examined the association between sixth-grade US students ( n = 54) metacognitive awareness and an argumentative essay task. They measured metacognitive awareness by having students rate items designed to measure their knowledge and regulation of cognition. They found that metacognitive scores were weakly correlated with students’ inclusion of belief-consistent ( r = 0.11 ( p > 0.05) and belief-inconsistent ( r = 0.10 ( p > 0.05) ideas in their writing. Davis et al. ( 2017 ) examined associations between intertextual integration and fifth- through seventh-grade US students’ ( n = 83) comprehension strategy knowledge (i.e., predicting and verifying predictions, previewing, purpose setting, self-questioning, using background knowledge, and summarizing; r = 0.23, p < 0.05) and strategy awareness/use ( r = 0.05, p > 0.05). Bråten et al. ( 2014 ) surveyed Norwegian students in the first year of secondary school ( n = 279) about their use of strategies for comparing, contrasting, and integrating multiple texts, finding a correlation of r = 0.36 ( p < 0.001) between this and intertextual integration. Cheong et al. ( 2019 ) surveyed students’ use of self-regulatory, discourse synthesis, and test-taking strategies before, during, and after writing. They found this to be weakly correlated with intertextual integration measured in Chinese ( r = 0.10, p < 0.05) and English ( r = 0.19, p < 0.01). Finally, Stang Lund et al. ( 2019 ) assessed secondary-level Norwegian students’ ( n = 86) knowledge and potential use of reading strategies, such as how they would deal with information about scientific issues presented in various media sources. They found a moderate association between students’ comprehension strategies and their intertextual integration ( r = 0.29, p < 0.05), as measured by a verification task.
Ten (40%) studies examined associations between intertextual integration and document sourcing skills. Only one involved students at the elementary level (Florit, Cain, et al., 2020 ). In that study, students’ essays were coded for intertextual integration and the inclusion of source-content links, with the correlation between them being r = 0.24 ( p < 0.05). A variety of sourcing dimensions were examined in nine (36%) studies involving secondary-level students. These included students’ source selection (Bråten et al., 2018 ; Forzani, 2016 ), source evaluation (Braasch et al., 2014 ; Forzani, 2016 ), links made between sources and their contents (Braasch et al., 2022 ; Mason et al., 2017 , 2018 ), and memory for sources (Stang Lund et al., 2019 ; Strømsø et al., 2010 ). Intertextual integration was measured with essay tasks in six of these studies and with verification tasks in three. Associations between intertextual integration and sourcing were all positive and small to moderate in magnitude (range = 0.05 to 41).
Knowledge and Beliefs
Nineteen studies (76%) examined associations among students’ knowledge, beliefs, and intertextual integration (Table 3 ). At the elementary level, Florit, De Carli, et al. ( 2020 ) assessed Italian fourth- and fifth-grade students’ topic knowledge, general vocabulary knowledge, and theory of mind. Correlations with their two measures of intertextual integration were as follows: topic knowledge = 0.08–0.15, vocabulary = 0.24–0.27, and theory of mind = 0.15–0.26. Davis et al. ( 2017 ) assessed US fifth- through seventh-grade students’ topic knowledge, general vocabulary knowledge, morphological knowledge, and two measures of epistemic beliefs: stability and structure of knowledge. Associations among intertextual integration and performance on these measures were as follows: topic knowledge = 0.42 ( p < 0.01), general vocabulary knowledge = 0.56 ( p < 0.01), morphological knowledge = 0.52 ( p < 0.01), stability of knowledge = − 0.10 ( p > 0.05), and structure of knowledge = 0.27 ( p < 0.05).
At the secondary level, researchers examined associations among intertextual integration and domain knowledge ( k = 1), topic knowledge ( k = 18), epistemic beliefs ( k = 7), and vocabulary and morphological knowledge ( k = 2). Wang et al. ( 2021 ) assessed US ninth-twelfth grade students’ domain knowledge with 25-item multiple-choice tests in history and science. Correlations with performance on an intertextual integration task about American football were r = 0.53 ( p < 0.01) for history and r = 0.57 ( p < 0.01) for science. They also assessed students’ knowledge of football, which was slightly less correlated with intertextual integration ( r = 0.50, p < 0.01). Topic knowledge was assessed in ten studies with multiple-choice tests (Andresen et al., 2019a , 2019b ; Bråten et al., 2014 , 2018 , 2013a , 2013b ; Stang Lund et al., 2017 ; Strømsø & Bråten, 2009 ; Strømsø et al., 2016 ; Wang et al., 2021 ), in five with open-ended questions (Barzilai & Ka’adan, 2017 ; Braasch et al., 2014 ; Mason, 2018 ; Mason et al., 2017 , 2020 ), in one with a verification task (Davis et al., 2017 ), and in two with rating scales (Braasch et al., 2022 ; Griffin et al., 2012 ). Correlations with intertextual integration ranged between − 0.03 (Strømsø et al., 2016 ) and 0.50 (Wang et al., 2021 ). Given the variation in how topic knowledge and intertextual integration were measured across these studies, patterns in the magnitude of these correlations are unclear. Moreover, the smallest and largest correlations were both found with secondary-level students and used multiple-choice tests to measure topic knowledge (Strømsø et al., 2016 ; Wang et al., 2021 ). However, these studies differed in the number (4 vs. 5) and format (digital vs. print) of the texts, the topic (American football vs. health), language (American English vs. Norwegian), and how intertextual integration was measured (multiple choice vs. essay).
Additionally, two studies (8%) examined associations among intertextual integration, vocabulary, and morphological knowledge. Results from Davis et al. ( 2017 ) were discussed above. Wang et al. ( 2021 ) found a correlation of r = 0.50 ( p < 0.01) between intertextual integration and morphology and r = 0.54 ( p > 0.05) with vocabulary.
Epistemic beliefs were assessed in eight studies (32%) with a variety of methods. For example, Barzilai and Ka’adan ( 2017 ) used a scenario-based approach to assess students’ topic-specific perspectives (i.e., absolutism, multiplism, evaluativism) about the nature, sources, certainty, validity, and justification of knowledge. In contrast, Strømsø et al. ( 2016 ) assessed students’ beliefs in the justification of knowledge by personal accounts, authority figures, or multiple sources. Across the studies, correlations with intertextual integration ranged from r = − 0.04 (Strømsø & Bråten, 2009 ) to r = − 0.43 (Bråten et al., 2013a , 2013b ). This wide range is expected, as the direction and magnitude of association is thought to vary by belief type (Bråten et al., 2011 ).
Motivation, Emotion, and Personality
Fourteen studies (56%) examined associations among students’ intertextual integration, motivation ( k = 11), emotion ( k = 4), and personality ( k = 2) (Table 4 ). At the elementary-level, Raccanello et al. ( 2022 ) examined how Italian fourth- and fifth-grade students’ performance on an intertextual integration task was associated with how they valued the task ( r = 0.18, p < 0.01) and their level of boredom with it ( r = − 0.05, p > 0.05). At the secondary level, studies examined associations between intertextual integration and several dimensions of motivation: self-efficacy (Bråten et al., 2013a , 2013b ; de Ruyter, 2020 ), task value (Bråten et al., 2013a , 2013b ), effort (de Ruyter, 2020 ), engagement (de Ruyter, 2020 ), and interest (Bråten et al., 2014 , 2018 ; Griffin et al., 2012 ; Stang Lund et al., 2017 ; Strømsø & Bråten, 2009 ; Strømsø et al., 2010 ; Wang et al., 2021 ). Correlations were all small and in the range of − 0.04 to 0.39.
Three studies (12%), all involving Italian seventh-grade students, examined associations between intertextual integration and emotion (Mason, 2018 ; Mason et al., 2017 , 2020 ). In each study, students’ emotional reactivity was assessed during reading with a heart rate monitor. Correlations with intertextual integration ranged from r = 0.02 ( p > 0.05; Mason et al., 2017 ) to r = 0.25 ( p > 0.05; Mason, 2018 ). Two studies, both with secondary-level Norwegian students, examined associations between intertextual integration and personality. Bråten et al. ( 2014 ) found an association of r = 0.14 between students’ need for cognition and their intertextual integration performance. Braasch et al. ( 2014 ) found an association of r = 0.02 ( p > 0.05) between intertextual integration and an entity theory of intelligence (i.e., fixed mindset); the correlation with an incremental theory of intelligence (i.e., growth mindset) was r = 0.19 ( p > 0.05).
We begin this section by discussing characteristics of the participants that have been studied (research question 1) and features of the tasks and materials that have been used (research question 2). We then discuss what types of individual differences factors have been studied (research question 3) and what has been learned about their associations with intertextual integration (research question 4). We will then conclude by offering four recommendations for future research.
Research Questions 1 and 2: Participant Characteristics and Materials
Given the many potential ways that multiple document comprehension has been studied, we were interested in first taking stock of the types of participants, tasks, measures, and documents that have been used. As predicted, there was wide variation in each of these parameters. However, certain grade levels, tasks, measures, and types of documents were examined more frequently than others.
As expected, we located more studies involving secondary- than elementary-level participants. At the secondary level, participants ranged from grade 6 to 12. Although it was encouraging to find that intertextual integration had been studied with elementary-level children, only five did so and none involved children below grade 4. Furthermore, few authors reported clear inclusion/exclusionary criteria and demographic information.
Nonetheless, several findings are important to discuss. First, individuals with disabilities were clearly included in only two studies, both of which were at the secondary level. Moreover, in several studies, disabled participants were purposely excluded. Second, in contrast to most other reading research (Share, 2008 ), relatively few studies involved English L1 participants. Finally, of the few studies that reported information about participants’ socioeconomic status, most were from middle-class backgrounds. We were limited, though, by our decision to include only studies reported in English. It may studies reported in other languages include more diverse populations. Nonetheless, we can draw two clear conclusions. First, improved demographic reporting is needed to better understand to whom these findings may apply. Second, efforts are needed to study more diverse populations, to include individuals with disabilities, those from different socioeconomic strata, individuals from non-Western societies, and those who speak multiple languages.
Tasks, Measures, and Documents
Consistent with Primor and Katzir’s ( 2018 ) findings, we found that intertextual integration was measured in a variety of ways, with essay tasks being the most widely used. The reason for this is unclear. It may be that essays are viewed as a particularly valid form of assessment. However, few studies clearly defined intertextual integration or provided a justification for why and how they selected their measure(s). Perhaps as a result, even with the stringent inclusion criteria that we set, we are not confident that the same construct was measured in each of the studies. For the field to advance, work is needed to better define intertextual integration and to develop more standardized and comparable methods for measuring it (Flake & Fried, 2020 ).
In contrast, there was consistency in the genres, structures, and topics of the documents. This allowed for much clearer comparisons across studies, particularly when considering associations with topic, domain, and disciplinary knowledge. Interestingly, the documents were nearly evenly split between print and digital formats. Although print documents still have a place in formal educational settings, they are quickly being replaced by digital ones. There is a pressing need, then, to better understand how young readers process and comprehend information distributed across multiple digital documents.
Research Questions 3 and 4: Individual Differences Factors and Intertextual Integration
In research questions 2 and 4, we asked what types of individual differences factors had been examined and the direction and strength of their associations with intertextual integration. As predicted, a wide range of individual differences factors had indeed been examined. However, few appeared in more than one study. Therefore, although nearly all were positively correlated with intertextual integration, variation in the samples, tasks, measures, and documents greatly limit what can be concluded about these relations.
Language and literacy were investigated in 72% ( k = 18) of the studies. This was somewhat unexpected; although language and literacy are acknowledged as an obviously important factor in intertextual integration, they have received less attention than other factors in in frameworks, theories, and models of multiple document use (see Table A1 ). This is likely because most theorizing has been based on secondary, undergraduate, and adult readers, for whom word-level reading and single document comprehension skills are likely assumed to be well developed. Nonetheless, it would appear safe to assume that intertextual integration depends on both skillsets.
Only six studies examined word-level reading skills. Results indicated small associations with intertextual integration (0.14 to 0.36) in the upper elementary grades and small to moderate associations (0.22 to 0.45) at the secondary level. Word-level reading has been clearly shown to affect single document comprehension performance (Perfetti & Helder, 2022 ). Although these six studies are not much to draw strong conclusions from, they do suggest a role for word-level reading in intertextual integration as well. Interestingly, the three elementary-level studies were conducted in Italian. In comparison to English, Italian has a relatively transparent alphabetic writing system (i.e., clear and consistent mappings between units of sound and print), which on average, proves easier for learning to read and write (Job et al., 2006 ). The three secondary-level studies were conducted in English and Norwegian, which in comparison to Italian, have considerably more opaque alphabetic systems (Hagtvet et al., 2006 ; Perfetti & Harris, 2017 ). Consequently, in addition to developmental differences, these writing system differences further complicate how we might interpret the results.
Across the 12 (48%) studies that examined single document comprehension, the magnitude of associations with intertextual integration ranged widely (0.06 to 0.57). A variety of factors undoubtedly account for this variation. Among them, the different ways that both constructs were measured likely played a role. For example, the smallest association was found in a study involving fourth- and fifth-grade Italian students (Florit, Cain, et al., 2020 ). Intertextual integration was assessed with an argumentative essay task and single document comprehension with a standardized measure of inferential and literal questions. In the two studies with the largest associations (Strømsø et al., 2010 ; Wang et al., 2021 ), there was much closer alignment between the single and multiple document comprehension measures. In both studies, for example, the single document comprehension and intertextual integration measures involved texts written about the same topics and used the same response formats. In one of the studies (Strømsø et al., 2010 ), the same texts were even used for both measures. Different approaches to measuring single document comprehension tap different underlying skills (Cutting & Scarborough, 2006 ; Keenan et al., 2008 ), and therefore, single indicators of single document comprehension should be not assumed to be interchangeable (Clemens & Fuchs, 2022 ). The same likely holds for measures of intertextual integration, which introduce even greater complexity. In sum, we can conclude from these results only that there is emerging evidence of associations between literacy skills—both word-level and comprehension focused—and intertextual integration.
Given the complexity of involved with intertextual integration, many cognitive and metacognitive skills have been hypothesized to play important roles (Follmer & Tise, 2022 ; Tarchi et al., 2021 ; see also Table A1 ). Cognition and metacognition were examined in a little over half (56%, k = 14) of the studies, with cognition examined in five (20%) and metacognition in nine (36%).
From among the multitude of cognitive variables (e.g., working memory, attention, intelligence) and operations (e.g., searching, monitoring, assembling, rehearsing, translating; Winne, 2018 ) that have been discussed within the multiple document literature, only verbal working memory was examined in these studies. Across the studies, associations between verbal working memory and intertextual ranged widely from r = 0.05 to r = 0.54. Although similar methods were used to measure verbal working memory, the number of studies was small and there was wide variation in the sampled populations, documents, and tasks. Accordingly, the results provide little insight into how individual differences in cognition—broadly or narrowly defined—are associated with intertextual integration. This stands in stark contrast to the extensive body of work that has examined associations between cognition, word-level reading, and single document comprehension (Butterfuss & Kendeou, 2018 ; Follmer, 2018 ; Peng et al., 2018 , 2022 ).
Metacognition was examined in 12 (48%) of the studies. In two of these, metacognitive strategy knowledge was assessed (Braasch et al., 2022 ; Davis et al., 2017 ), with results showing small associations with intertextual integration. Results were mixed, though, for the three that examined metacognitive strategy use. In one study, the association between strategy use and intertextual integration was small and insignificant (Davis et al., 2017 ), However, in the other two, the relations were both moderate and signification (Bråten et al., 2014 ; Florit, Cain, et al., 2020 ). These results suggest that while knowledge of metacognitive strategies is unrelated to intertextual integration, actual metacognitive strategy use may be. However, with only four studies—and large differences in the populations and materials used among them—any conclusions are at best tentative.
Sourcing skills were examined in ten studies (40%). As noted above, a variety of sourcing skills were examined, to include source selection, source evaluation, source-content links, and source memory. Results ranged from almost no association ( r = 0.05) to one of moderate magnitude ( r = 0.41). This wide range is likely due to variation in the dimensions of sourcing that were examined, the different ways intertextual integration was measured, and differences in the populations that were sampled. In sum, it appears that various dimensions of sourcing may be differentially associated with intertextual integration.
Knowledge and beliefs appeared in 19 studies (76%), the most frequent of the four individual differences categories. Correlations with intertextual integration ranged widely (− 0.39 to 0.57). The bulk of studies involved secondary-level participants and examined associations between topic/domain knowledge and intertextual integration. Vocabulary and morphological knowledge were examined in four studies (16%). Various dimensions of knowledge, spanning from word to disciplinary, have been discussed extensively in theories of single and multiple document comprehension (Alexander, 2005 ; Goldman et al., 2016 ; Kintsch, 1988 ; Perfetti & Helder, 2022 ; Perfetti et al., 1999 ). As Kintsch ( 1974 , p. 10) observed, for example, “understanding a text…consists of assimilating it with one’s general store of knowledge…[s]ince every person’s knowledge and experience is somewhat different…the way in which different people understand the same text may not always be the same.” Knowledge in its various forms is generally understood to facilitate comprehension and learning (Ackerman, 1991 ; Alexander et al., 1994 ; Cabell & Hwang, 2020 ; Hwang et al., 2022 ). However, in certain cases, it can be an impediment (Simonsmeier et al., 2022 ). For instance, one’s knowledge of a topic can stand stubbornly in the way of learning new concepts (Vosniadou, 1992 ). Furthermore, possessing relevant knowledge does not ensure that it will be effectively used to aid comprehension. Indeed, Wolfe and Goldman ( 2005 ) found that some adolescents sometimes use their background knowledge to make irrelevant elaborations that do help in building a coherent multiple document representation.
As many have noted, there is unfortunately little consistency in how knowledge is conceptualized, defined, and measured (Alexander et al., 1991 ; McCarthy & McNamara, 2021 ; Murphy et al., 2012 , 2018 ). This was certainly what we found. Although the bulk of studies examined topic knowledge, this was done in a variety of ways. Moreover, the specific topics that were examined varied across studies. It is unclear, then, how comparable scores from these different measures may be. Additionally, few studies examined more than one dimension of knowledge. In any study, it is perhaps impossible to measure the full extent of a single domain or discipline much less multiple. However, the narrow focus on topic knowledge in this literature provides little insight into how other dimensions (e.g., domain, disciplinary) may influence intertextual integration. Nonetheless, it appears from these studies that knowing something of the documents’ topic, domain, or discipline is associated with better intertextual integration.
Epistemic beliefs were examined in seven studies (28%). Much research has shown that epistemic beliefs are an important factor in learning (Mason, 2010 ) and that variation in them is associated with literacy performance (Bråten et al., 2016 ; Lee et al., 2016 ). This may be the case particularly when engaging with multiple documents of varying quality and perspectives (Bråten et al., 2011 ; Strømsø & Kammerer, 2016 ). Consistent with previous research (Bråten et al., 2011 ), results indicated that endorsing certain beliefs is associated with better intertextual integration than others. For example, Davis et al. ( 2017 ) found that the belief that knowledge is singular and absolute was more weakly associated with intertextual integration ( r = − 0.10) than the belief that there are multiple forms of knowledge but that certain forms are more valid than others ( r = 0.27). There were similar patterns in several of the other studies (Barzilai & Ka’adan, 2017 ; Bråten et al., 2013a , 2013b ; Strømsø & Bråten, 2009 ). Using a slightly different framework, Bråten et al., ( 2013a , 2013b ) found that intertextual integration was negatively associated with beliefs that knowledge is justified by personal knowledge (− 0.43) and authority (− 0.08), but positively associated with the belief that it is justified by multiple sources (0.17). In sum, whereas absolutist beliefs about knowledge (i.e., “knowledge come from an external source and is certain,” Kuhn, 1999 , p. 23) appear to be negatively associated with intertextual integration, multiplist or evaluative beliefs (“knowledge comes from human minds and is uncertain,” Kuhn, 1999 , p. 23) appear to be positively correlated. However, due to the variability in how epistemic beliefs were measured and considerable differences in the tasks, document sets, and participants involved, more research is needed to confirm the robustness of these findings. It is important to also note that in addition to individual differences, beliefs can vary across cultures (Buehl, 2008 ). Further research is therefore needed to systematically examine how epistemic beliefs may shape multiple document use and comprehension across different conditions, points in development, and cultural contexts.
The final category represents a broad mixture of affective processes and personality variables. Motivational factors (e.g., self-efficacy, interest, task value) appeared the most frequently ( k = 11, 44%). Whereas there is no evidence that self-efficacy is meaningfully associated with intertextual integration (Bråten et al., 2013a , 2013b ; de Ruyter, 2020 ), several studies reported small to moderate associations with interest and task value. As has become a common theme in this review, wide variation in the tasks, documents, and participants renders what can be claimed about any potential patterns unclear.
Emotion was examined in three studies (12%), all with Italian seventh-grade participants, representing a rare case of systematic replication. Correlations for these three studies ranged from − 0.28 to 0.02. Not much can be concluded from this other than that certain emotions, such as boredom, appear to be negatively associated with intertextual integration. Future work should examine how motivation and emotion interact with specific types of documents and tasks over microperiods (e.g., minutes, hours) and macroperiods (e.g., days, months, years) of development (e.g., Neugebauer & Gilmour, 2020 ).
Personality was examined in only two studies (8%), both of which involved Norwegian secondary-level participants. Given that they measured different facets of personality, not much can be said beyond what is reported in the individual studies. Although personality is a major branch of psychology (Burger, 2015 ), and has been shown be associated with a broad range of human functioning (Anglim et al., 2022 ; Komarraju et al., 2011 ), it has not be a topic of much interest in reading research. The small correlations observed in these two studies do not inspire much confidence that this is a promising area for much further investigation.
General Discussion
Much has been proposed about the roles that various individual differences factors play in intertextual integration (see Table A1 ). However, much of this theorizing has been based on undergraduate and adult readers. It is unclear, then, how existing conceptualizations may apply to younger readers. In this review, we did not attempt to answer that question. Rather, we examined the nature, features, and volume of research that has examined associations between individual differences and intertextual integration. These associations are shown in Fig. 3 with an evidence gap map (Polanin et al., 2022 ). The map reveals that many of the factors specified in frameworks, theories, and models listed in Table A1 have been examined alongside intertextual integration with K-12 students. Noticeably, though, the coverage is uneven and incomplete. This greatly limits what can be concluded about the reliability and generalizability of these findings. Indeed, even in the two-dimensional map, we can see that many cells are blank and that few are shaded darker than the lightest tone. Were dimensions added for contexts, tasks, documents, and additional participant characteristics, the map would reveal even sparser coverage. Although there are few fields for which such a map would be fully darkened, it is clear far more research is needed.
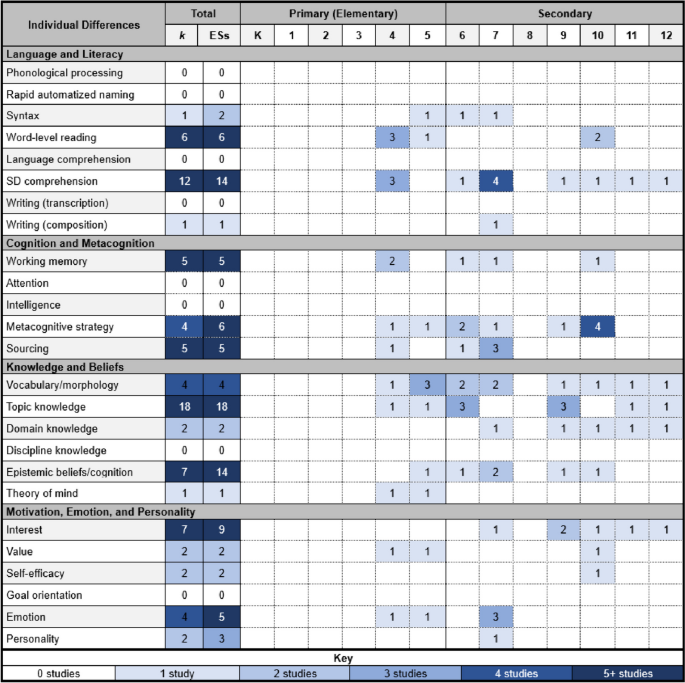
Evidence gap map of associations among individual difference factors and intertextual integration. Shading corresponds to the number of studies that have examined an association between a particular individual differences factor (listed along the vertical axis) and intertextual integration at particular grade level (listed along the horizontal axis). As the number of studies increases, the shading darkens. White cells indicate that no study has examined the association between that particular factor and intertextual integration at that particular grade level. Studies that involved unspecified secondary-level students are not represented. SD, single document. ESs, effect sizes (i.e., zero-order correlation coefficients)
Recommendations
As for how the field might productively advance, we offer four recommendations. First, as has been observed for the broader social sciences (Shrout & Rodgers, 2018 ), more replication is needed. Indeed, as we have noted throughout this review, no associations have been studied under similar enough conditions to discern reliable patterns. Moreover, none of the factors reviewed have been studied across the full span of K-12 development. To build a fuller and more reliable understanding of how individual differences are associated with intertextual integration, a program of replicated research is needed that carefully examines relations among specific factors across the K-12 developmental span with well specified populations and comparable tasks, measures, and document sets.
Our second recommendation is for greater attention is needed to how intertextual integration is measured. There are several examples in the literature of well-validated measures of intertextual integration and multiple document use more broadly (Goldman et al., 2013 ; Hastings et al., 2012 ; Leu et al., 2015 ). However, most of the studies in this review used unvalidated measures, with little attention matters of dimensionality, reliability, or validity. These issues are characteristic of the broader psychological field (Flake & Fried, 2020 ). Although developing a measure involves many considerations (Lane et al., 2016 ), we will focus here on four points that have gone largely unaddressed.
First, research is needed to examine the dimensionality of intertextual integration. The definition we supplied in the introduction indicates that multiple subprocesses may be involved. In all the studies we reviewed, though, intertextual integration was treated as unidimensional. However, efforts generally were not made to test this assumption. Moving forward, this should be a priority. Care should also be taken to ensure that measures are invariant across participant subgroups, to ensure that individual items are unbiased, and that tests and items are well calibrated for their targeted populations. Second, greater attention is needed to score reliability. Although many of the studies reported internal consistency estimates, few considered other types of reliability (American Educational Research Association et al., 2014 ). Moreover, some of the measures suffered from poor internal consistency. The implications of this are not trivial. For the simple bivariate relations examined in this review, measurement error can attenuate effects. In multivariate models, the effects are less predictable (Bollen, 1989 ). Accordingly, developing measures that produce reliable scores is imperative. Third, it will be important to consider the validity of different types of intertextual integration measures (e.g., inference verification, essays. That is, are certain measures better at capturing intertextual integration than others? Furthermore, how should performance on different measures be interpreted? Finally, and related to our third recommendation, research is needed to understand which measures are best suited for assessing intertextual integration at different points in development and for tracking short- and long-term growth.
Our third recommendation is for research examining the precursors and early development of intertextual integration. We found only five studies that involved elementary-level participants, and none with children below grade 4. It is possible that the complex reasoning involved in many multiple document tasks may be viewed as too challenging for young children. However, current instructional standards make it clear that beginning in kindergarten, children are expected to engage with multiple documents for a variety of purposes (National Governors Association & Council of Chief State School Officers, 2010 ). Although this initially involves being read multiple documents, the expectations quickly shift to children themselves reading, searching for, selecting, evaluating, and integrating multiple documents. Current theories and empirical findings provide little insight into how children first develop these skills and the factors that give rise to early and perhaps ongoing performance differences.
A large body of work has shown that even preschool-aged children can adopt a critical stance when presented with information from multiple informants (for reviews, see Harris et al., 2018 ; Mills, 2013 ). This work has yet to involve textual sources and often requires participants to select the testimony from one of several informants rather than to integrate information from among them. Nonetheless, the insights garnered from research on the early development of source memory and evaluation may provide an entry point into understanding how children later develop multiple document use skills.
Young children are already exposed to multiple text documents in many early literacy programs. Take for instance a first-grade lesson from a language arts curriculum (Author, 2013 ) used in approximately 20% of US schools (Kaufman et al., 2017 ). In the lesson, children are read different versions of the same fable and are then asked to compare them (Author, 2013 ). In other programs, young children are read multiple informational texts on the same topic (Kim et al., 2021 ; Language and Reading Research Consortium et al., 2014 ). There is not an explicit focus in these programs on sourcing or intertextual integration. However, attention to these skills could easily be incorporated. Such research could provide useful insights into the early development of intertextual integration, particularly if studied alongside other literacy, cognitive, and affective factors.
Our fourth and final recommendation is to develop more formalized theories Footnote 1 of intertextual integration. The proposals listed in Table A1 have proven useful for guiding and interpreting research on the role that individual differences play in shaping intertextual integration. Indeed, many have proposed how particular factors (e.g., working memory) are involved in forming intertextual representations, with some offering hypotheses about the direction and general magnitude of these relations. However, these verbal theories are best suited for significance testing, which alone cannot corroborate a theory (Meehl, 1978 ; Robinaugh et al., 2021 ). Greater specificity is needed for more risky tests that could falsify or advance a theory (Frankenhuis et al., 2023 ; Gershman, 2019 ; Meehl, 1978 ; Robinaugh et al., 2021 ).
Formal theories and models have been used extensively in research on word-level reading and to a lesser extent on single-document comprehension (Goldman et al., 2007 ; Reichle, 2021 ). This approach has proven critical for testing how specific factors (e.g., phonological processing, background knowledge) are associated with reading (Harm & Seidenberg, 1999 ; Van Den Broek et al., 1996 ) and for understanding psychological and inter-agent processes more generally (Sun et al., 2005 ). Similar advantages may be found in developing formalized theories of intertextual integration and multiple document use. For instance, a formalized theory of intertextual integration could be used to construct a computational model. It would then be possible to test the effects of particular individual differences factors through experimental manipulations with actual and simulated data (Goldman et al., 2007 ; J. A. Greene, 2022 ; Robinaugh et al., 2021 ). Such work might provide useful insights into key pressure points in the processes and development of intertextual integration that could be targeted for assessment and intervention. To these ends, we recommend supplementing the proposals listed in Table A1 with formalized theories that more clearly state their constraints and explicate the circumstances under which they may be falsified.
Limitations
As with any study, this review is limited by several factors. First, although we placed no restrictions on the types of individual differences included, we adopted a much narrower approach than has been used in most previous reviews by focusing on only one component of multiple document comprehension (i.e., intertextual integration). Second, we examined only concurrent associations and included only studies that involved K-12 participants. In doing so, we omitted work that has examined other components (e.g., sourcing) and involved other populations. Furthermore, by examining only concurrent associations, we were unable to consider matters of causality, which is critical for an explanatory theory. However, we believe our approach provided for a more precise and measured assessment of findings relevant for K-12 students than has been offered in the past. Even with this more circumscribed approach, though, limitations within the literature made it difficult to find clear and reliable patterns.
In theorizing about how readers use and make sense of multiple documents, much has been hypothesized about the roles played by a wide range of cognitive and affective factors (see Table A1 ). We found that many of these have been examined in the context of K-12 students’ intertextual integration. However, much remains to be learned about how variation in them gives rise to differences in multiple document processing across the span of K-12 development. Although associations were generally positive and small to medium in magnitude, design and measurement issues greatly limit the conclusions we can draw. As Underwood ( 1975 ) noted, individual differences stand as, “…a critical test of theories as they are being born” (p. 130). We agree and believe that to better understand and ultimately improve multiple document comprehension among K-12 students, research is needed that (a) more systematically examines individual differences factors, (b) places a greater focus on early development, and (c) builds more formalized and testable theories.
A distinction is often made between formal theories and models (Jaccard & Jacoby, 2020 ; Smaldino, 2023 ). A theory is generally regarded as more comprehensive than any specific model (Goldman et al., 2007 ). A formal differs from an informal theory in that it is implemented using formalisms such as mathematical equations or computer programs (Reichle, 2021 ; Smaldino, 2023 ).
References
Ackerman, J. M. (1991). Reading, writing, and knowing: The role of disciplinary knowledge in comprehension and composing. Research in the Teaching of English, 25 (2), 133–178.
Article Google Scholar
Adams, M. J. (1990). Beginning to read: Thinking and learning about print . The MIT Press.
Afflerbach, P. (Ed.). (2016). Handbook of individual differences in reading: Reader, text, and context . Routledge.
Afflerbach, P., & Cho, B.-Y. (2009). Identifying and describing constructively responsive comprehension strategies in new and traditional forms of reading. In S. E. Israel & G. G. Duffy (Eds.), Handbook of research on reading comprehension (pp. 69–90). Routledge.
Google Scholar
Afflerbach, P., Cho, B.-Y., & Kim, J.-Y. (2014). Inaccuracy and reading in multiple text and Internet/hypertext environments. In D. N. Rapp & J. L. G. Braasch (Eds.), Processing inaccurate information: Theoretical and applied perspectives from cognitive science and the educational sciences (pp. 403–424). The MIT Press. https://doi.org/10.7551/mitpress/9737.003.0024
Alexander, P. A. (2005). The path to competence: A lifespan developmental perspective on reading. Journal of Literacy Research, 37 (4), 413–436. https://doi.org/10.1207/s15548430jlr3704_1
Alexander, P. A. (2020). Methodological guidance paper: The art and science of quality systematic reviews. Review of Educational Research, 90 (1), 6–23. https://doi.org/10.3102/0034654319854352
Alexander, P. A., Schallert, D. L., & Hare, V. C. (1991). Coming to terms: How researchers in learning and literacy talk about knowledge. Review of Educational Research, 61 (3), 315–343. https://doi.org/10.3102/00346543061003315
Alexander, P. A., Kulikowich, J. M., & Jetton, T. L. (1994). The role of subject-matter knowledge and interest in the processing of linear and non-linear texts. Review of Educational Research, 64 (2), 201–252.
Alexander, P. A., & The Disciplined Reading and Learning Research Laboratory (2012). Reading into the future: Competence for the 21st century. Educational Psychologist, 47 (4), 259–280. https://doi.org/10.1080/00461520.2012.722511
Alexander, P., & Disciplined Reading and Learning Research Laboratory. (2020). Relational reasoning: The bedrock of integration within and across multiple representations, documents, and perspectives. In P. Van Meter, A. List, D. Lombardi, & P. Kendeou (Eds.), Handbook of learning from multiple representations and perspectives (pp. 401–424). Routledge.
Allen, G. (2022). Intertextuality (3rd ed.). Routledge.
Anderson, R. C., Hiebert, E. H., Scott, J. A., & Wilkinson, I. A. G. (1985). Becoming a national of readers: The report of the commission on reading . The national Academy of Education, The National Institute of Education, The Center for the Study of Reading.
Andresen, A., Anmarkrud, Ø., & Bråten, I. (2019). Investigating multiple source use among students with and without dyslexia. Reading and Writing, 32 (5), 1149–1174. https://doi.org/10.1007/s11145-018-9904-z
Andresen, A., Anmarkrud, Ø., Salmerón, L., & Bråten, I. (2019). Processing and learning from multiple sources: A comparative case study of students with dyslexia working in a multiple source multimedia context. Frontline Learning Research, 7 (3), 1–26. https://doi.org/10.14786/flr.v7i3.451
Anglim, J., Dunlop, P. D., Wee, S., Horwood, S., Wood, J. K., & Marty, A. (2022). Personality and intelligence: A meta-analysis. Psychological Bulletin, 148 (5–6), 301–336. https://doi.org/10.1037/bul0000373
Anmarkrud, Ø., Bråten, I., Florit, E., & Mason, L. (2022). The role of individual differences in sourcing: A systematic review. Educational Psychology Review, 34 (2), 749–792. https://doi.org/10.1007/s10648-021-09640-7
Author. (2013). EngageNY: Common core curriculum . Expeditionary Learning.
Baron, N. S. (2021). How We Read Now . Oxford University Press.
Book Google Scholar
Barzilai, S., & Ka’adan, I. (2017). Learning to integrate divergent information sources: The interplay of epistemic cognition and epistemic metacognition. Metacognition and Learning, 12 (2), 193–232. https://doi.org/10.1007/s11409-016-9165-7
Barzilai, S., & Strømsø, H. I. (2018). Individual differences in multiple document comprehension. In J. L. G. Braasch, I. Bråten, & M. T. McCrudden (Eds.), Handbook of multiple source use (pp. 99–116). Routledge.
Chapter Google Scholar
Barzilai, S., Zohar, A. R., & Mor-Hagani, S. (2018). Promoting integration of multiple texts: A review of instructional approaches and practices. Educational Psychology Review, 30 (3), 973–999. https://doi.org/10.1007/s10648-018-9436-8
Bazerman, C. (2004). Intertextualities: Volosinov, Bakhtin, literary theory, and literacy studies. In A. F. Ball & S. W. Freedman (Eds.), Bakhtinian perspectives on language, literacy, and learning (pp. 53–65). Cambridge University Press.
Beker, K., van den Broek, P., & Jolles, D. (2019). Children’s integration of information across texts: Reading processes and knowledge representations. Reading and Writing, 32 (3), 663–687. https://doi.org/10.1007/s11145-018-9879-9
Bollen, K. A. (1989). Structual equations with latent variables . John Wiley & Sons.
Braasch, J. L. G., & Bråten, I. (2017). The discrepancy-induced source comprehension (D-ISC) model: Basic assumptions and preliminary evidence. Educational Psychologist, 52 (3), 167–181. https://doi.org/10.1080/00461520.2017.1323219
Braasch, J. L. G., Bråten, I., Strømsø, H. I., & Anmarkrud, Ø. (2014). Incremental theories of intelligence predict multiple document comprehension. Learning and Individual Differences, 31 , 11–20. https://doi.org/10.1016/j.lindif.2013.12.012
Braasch, J. L. G., Haverkamp, Y. E., Latini, N., Shaw, S., Arshad, M. S., & Bråten, I. (2022). Belief bias when adolescents read to comprehend multiple conflicting texts. Reading and Writing, 35 (8), 1759–1785. https://doi.org/10.1007/s11145-022-10262-w
Braasch, J. L. G., Bråten, I., & McCrudden, M. T. (Eds.). (2018). Handbook of multiple source use . Routledge.
Brand-Gruwel, S., Wopereis, I., & Walraven, A. (2009). A descriptive model of information problem solving while using internet. Computers & Education, 53 (4), 1207–1217. https://doi.org/10.1016/j.compedu.2009.06.004
Bråten, I., Britt, M. A., Strømsø, H. I., & Rouet, J.-F. (2011). The role of epistemic beliefs in the comprehension of multiple expository texts: Toward an integrated model. Educational Psychologist, 46 (1), 48–70. https://doi.org/10.1080/00461520.2011.538647
Bråten, I., Ferguson, L. E., Anmarkrud, Ø., & Strømsø, H. I. (2013a). Prediction of learning and comprehension when adolescents read multiple texts: The roles of word-level processing, strategic approach, and reading motivation. Reading and Writing, 26 (3), 321–348. https://doi.org/10.1007/s11145-012-9371-x
Bråten, I., Ferguson, L. E., Strømsø, H. I., & Anmarkrud, Ø. (2013). Justification beliefs and multiple-documents comprehension. European Journal of Psychology of Education, 28 (3), 879–902. https://doi.org/10.1007/s10212-012-0145-2
Bråten, I., Anmarkrud, Ø., Brandmo, C., & Strømsø, H. I. (2014). Developing and testing a model of direct and indirect relationships between individual differences, processing, and multiple-text comprehension. Learning and Instruction, 30 , 9–24. https://doi.org/10.1016/j.learninstruc.2013.11.002
Bråten, I., Strømsø, H. I., & Ferguson, L. E. (2016). The role of epistemic beliefs in the comprehension of single and multiple texts. In P. P. Afflerbach (Ed.), Handbook of individual differences in reading: Reader, text, and context (pp. 67–79). Routledge.
Bråten, I., Brante, E. W., & Strømsø, H. I. (2018). What really matters: The role of behavioural engagement in multiple document literacy tasks. Journal of Research in Reading, 41 (4), 680–699. https://doi.org/10.1111/1467-9817.12247
Bråten, I., Braasch, J. L. G., & Salmerón, L. (2020). Reading multiple and non-traditional texts: New opportunities and new challenges. In E. B. Moje, P. Afflerbach, P. Enciso, & N. K. Lesaux (Eds.), Handbook of reading research 5: 79–98. Routledge.
Britt, M. A., Perfetti, C. A., Sandak, R., & Rouet, J. (1999). Content integration and source separation in learning from multiple texts. In S. R. Goldman, A. C. Graesser, & P. van den Broek (Eds.), Narrative comprehension, causality, and coherence (pp. 209–234). Lawrence Erlbaum Associates.
Britt, M. A., Rouet, J., & Durik, A. M. (2018). Literacy beyond text comprehension: A theory of purposeful reading . Routledge.
van den Broek, P., & Kendeou, P. (2022). Reading comprehension I: Discourse. In The science of reading: A handbook (2nd ed., pp. 239–260). Wiley Blackwell.
Buehl, M. M. (2008). Assessing the multidimensionality of students’ epistemic beliefs across diverse cultures. In M. S. Khine (Ed.), Knowing, knowledge and beliefs (pp. 65–112). Springer.
Burger, J. M. (2015). Personality (9th ed.). Cengage Learning.
Butterfuss, R., & Kendeou, P. (2018). The role of executive functions in reading comprehension. Educational Psychology Review, 30 (3), 801–826. https://doi.org/10.1007/s10648-017-9422-6
Butterfuss, R., & Kendeou, P. (2021). KReC-MD: Knowledge revision with multiple documents. Educational Psychology Review, 33 (4), 1475–1497. https://doi.org/10.1007/s10648-021-09603-y
Byrne, B. (1998). The foundation of literacy: The child’s acquisition of the alphabetic principle . Psychology Press.
Cabell, S. Q., & Hwang, H. (2020). Building content knowledge to boost comprehension in the primary grades. Reading Research Quarterly , 55 (S1). https://doi.org/10.1002/rrq.338
Castles, A., Rastle, K., & Nation, K. (2018). Ending the reading wars: Reading acquisition from novice to expert. Psychological Science in the Public Interest, 19 (1), 5–51. https://doi.org/10.1177/1529100618772271
Cheong, C. M., Zhu, X., Li, G. Y., & Wen, H. (2019). Effects of intertextual processing on L2 integrated writing. Journal of Second Language Writing, 44 , 63–75. https://doi.org/10.1016/j.jslw.2019.03.004
Cho, B.-Y. (2013). Adolescents’ constructively responsive reading strategy use in a critical Internet reading task. Reading Research Quarterly, 48 (4), 329–332. https://doi.org/10.1002/rrq.49
Cho, B.-Y., Han, H., & Kucan, L. L. (2018). An exploratory study of middle-school learners’ historical reading in an internet environment. Reading and Writing, 31 (7), 1525–1549. https://doi.org/10.1007/s11145-018-9847-4
Cho, B., & Afflerbach, P. (2017). An evolving perspective of constructively responsive reading comprehension strategies in multilayered digital text environments. In S. E. Israel (Ed.), Handbook of research on reading comprehension (2nd ed., pp. 109–134). The Guilford Press.
Clemens, N. H., & Fuchs, D. (2022). Commercially developed tests of reading comprehension: Gold standard or fool’s gold? Reading Research Quarterly, 57 (2), 385–397. https://doi.org/10.1002/rrq.415
Coiro, J. (2021). Toward a multifaceted heuristic of digital reading to inform assessment, research, practice, and policy. Reading Research Quarterly, 56 (1), 9–31. https://doi.org/10.1002/rrq.302
Cumming, M. M., Bettini, E., & Chow, J. C. (2023). High-quality systematic literature reviews in special education: Promoting coherence, contextualization, generativity, and transparency. Exceptional Children , 001440292211465. https://doi.org/10.1177/00144029221146576
Cutting, L. E., & Scarborough, H. S. (2006). Prediction of reading comprehension: Relative contributions of word recognition, language proficiency, and other cognitive skills can depend on how comprehension is measured. Scientific Studies of Reading, 10 (3), 277–299. https://doi.org/10.1207/s1532799xssr1003_5
Daneman, M., & Carpenter, P. A. (1980). Individual differences in working memory and reading. Journal of Verbal Learning and Verbal Behavior, 19 (4), 450–466. https://doi.org/10.1016/S0022-5371(80)90312-6
Davis, D. S., Huang, B., & Yi, T. (2017). Making sense of science texts: A mixed-methods examination of predictors and processes of multiple-text comprehension. Reading Research Quarterly, 52 (2), 227–252. https://doi.org/10.1002/rrq.162
Drummey, A. B., & Newcombe, N. S. (2002). Developmental changes in source memory. Developmental Science, 5 (4), 502–513. https://doi.org/10.1111/1467-7687.00243
Ehri, L. C. (2014). Orthographic mapping in the acquisition of sight word reading, spelling memory, and vocabulary learning. Scientific Studies of Reading, 18 (1), 5–21. https://doi.org/10.1080/10888438.2013.819356
Flake, J. K., & Fried, E. I. (2020). Measurement Schmeasurement: Questionable measurement practices and how to avoid them. Advances in Methods and Practices in Psychological Science, 3 (4), 456–465. https://doi.org/10.1177/2515245920952393
Florit, E., Cain, K., & Mason, L. (2020). Going beyond children’s single-text comprehension: The role of fundamental and higher-level skills in 4th graders’ multiple-document comprehension. British Journal of Educational Psychology, 90 (2), 449–472. https://doi.org/10.1111/bjep.12288
Florit, E., De Carli, P., Giunti, G., & Mason, L. (2020). Advanced theory of mind uniquely contributes to children’s multiple-text comprehension. Journal of Experimental Child Psychology, 189 , 104708. https://doi.org/10.1016/j.jecp.2019.104708
Follmer, D. J. (2018). Executive function and reading comprehension: A meta-analytic review. Educational Psychologist, 53 (1), 42–60. https://doi.org/10.1080/00461520.2017.1309295
Follmer, D. J., & Tise, J. (2022). Effects of an executive function-based text support on strategy use and comprehension–integration of conflicting informational texts. Reading and Writing . https://doi.org/10.1007/s11145-022-10257-7
Forzani, E. (2016). Individual differences in evaluating the credibility of online information in science: Contributions of prior knowledge, gender, socioeconomic status, and offline reading ability . University of Connecticut.
Frankenhuis, W. E., Borsboom, D., Nettle, D., & Roisman, G. I. (2023). Formalizing theories of child development: Introduction to the special section. Child Development , cdev.14020. https://doi.org/10.1111/cdev.14020
Frederiksen, C. H. (1975). Effects of context-induced processing operations on semantic information acquired from discourse. Cognitive Psychology, 7 (2), 139–166. https://doi.org/10.1016/0010-0285(75)90007-9
Garner, R. (1987). Metacognition and reading comprehension . Ablex Publishing Company.
Gee, J. P. (2007). Social linguistics and literacies: Ideology and discourse . Routledge.
Gershman, S. J. (2019). How to never be wrong. Psychonomic Bulletin & Review, 26 (1), 13–28. https://doi.org/10.3758/s13423-018-1488-8
Gibson, E. J., & Levin, H. (1975). The psychology of reading . The MIT Press.
Goldman, S. R., & Scardamalia, M. (2013). Managing, understanding, applying, and creating knowledge in the information age: Next-generation challenges and opportunities. Cognition and Instruction, 31 (2), 255–269. https://doi.org/10.1080/10824669.2013.773217
Goldman, S. R., Golden, R. M., & van den Broek, P. (2007). Why are computation models of text comprehension useful? In F. Schmalhofer & C. A. Perfetti (Eds.), Higher level language processes in the brain: Inference and comprehension processes (pp. 27–52). Lawrence Erlbaum Associates.
Goldman, S. R., Braasch, J. L. G., Wiley, J., Graesser, A. C., & Brodowinska, K. (2012). Comprehending and learning from internet sources: Processing patterns of better and poorer learners. Reading Research Quarterly, 47 (4), 356–381. https://doi.org/10.1002/RRQ.027
Goldman, S. R., Lawless, K., & Manning, F. (2013). Research and development of multiple source comprehension assessment. In M. A. Britt, S. R. Goldman, & J.-F. Rouet (Eds.), Reading-from words to multiple texts (pp. 180–199). Routledge.
Goldman, S. R., Britt, M. A., Brown, W., Cribb, G., George, M., Greenleaf, C., Lee, C. D., Shanahan, C., & Project READI. (2016). Disciplinary literacies and learning to read for understanding: A conceptual framework for disciplinary literacy. Educational Psychologist, 51 (2), 219–246. https://doi.org/10.1080/00461520.2016.1168741
Graesser, A. C. (2007). An introduction to strategic reading comprehension. In D. McNamara (Ed.), Reading comprehension strategies: Theories, interventions, and technologies (pp. 3–26). Lawrence Erlbaum Associates.
Greene, S. (1993). The role of task in the development of academic thinking through reading and writing in a college history course. Research in the Teaching of English, 27 (1), 46–75.
Greene, J. A. (2022). What can educational psychology learn from, and contribute to, theory development scholarship? Educational Psychology Review, 34 (4), 3011–3035. https://doi.org/10.1007/s10648-022-09682-5
Griffin, T. D., Wiley, J., Britt, M. A., & Salas, C. R. (2012). The Role of CLEAR Thinking in Learning Science from Multiple-Document Inquiry Tasks., 1 , 16.
Hagtvet, B. E., Helland, T., & Lyster, S.-A. H. (2006). Literacy acquisition in Norwegian. In R. Malatesha Joshi & P. G. Aaron (Eds.), Handbook of orthography and literacy (pp. 15–30). Lawrence Erlbaum Associates.
Harm, M. W., & Seidenberg, M. S. (1999). Phonology, reading acquisition, and dyslexia: Insights from connectionist models. Psychological Review, 106 (3), 491–528.
Harris, P. L., Koenig, M. A., Corriveau, K. H., & Jaswal, V. K. (2018). Cognitive foundations of learning from testimony. Annual Review of Psychology, 69 (1), 251–273. https://doi.org/10.1146/annurev-psych-122216-011710
Hastings, P., Hughes, S., Magliano, J. P., Goldman, S. R., & Lawless, K. (2012). Assessing the use of multiple sources in student essays. Behavior Research Methods, 44 (3), 622–633. https://doi.org/10.3758/s13428-012-0214-0
Huey, E. B. (1908). The psychology and pedagogy of reading . The MacMillan Company.
Hwang, H., Cabell, S. Q., & Joyner, R. E. (2022). Effects of integrated literacy and content-area instruction on vocabulary and comprehension in the elementary years: A meta-analysis. Scientific Studies of Reading, 26 (3), 223–249. https://doi.org/10.1080/10888438.2021.1954005
Jaccard, J., & Jacoby, J. (2020). Theory construction and model-building skills: A practical guide for social scientists (2nd ed.). Guilford Press.
Job, R., Peressotti, F., & Mulatti, C. (2006). The acquisition of literacy in Italian. In R. M. Joshi & P. G. Aaron (Eds.), Handbook of orthography and literacy (pp. 105–120). Lawrence Erlbaum Associates.
Kaufman, J., Davis, J., Wang, E., Thompson, L., Pane, J., Pfrommer, K., & Harris, M. (2017). Use of open educational resources in an era of common standards: A case study on the use of EngageNY. RAND Corporation . https://doi.org/10.7249/RR1773
Keenan, J. M., Betjemann, R. S., & Olson, R. K. (2008). Reading comprehension tests vary in the skills they assess: Differential dependence on decoding and oral comprehension. Scientific Studies of Reading, 12 (3), 281–300. https://doi.org/10.1080/10888430802132279
Kennedy, M. L. (1985). The composing process of college students writing from sources. Written Communication, 2 , 434–456.
Kim, J. S., Relyea, J. E., Burkhauser, M. A., Scherer, E., & Rich, P. (2021). Improving elementary grade students’ science and social studies vocabulary knowledge depth, reading comprehension, and argumentative writing: A conceptual replication. Educational Psychology Review, 33 (4), 1935–1964. https://doi.org/10.1007/s10648-021-09609-6
Kintsch, W. (1974). The representation of meaning in memory . Lawrence Erlbaum Associates.
Kintsch, W. (1988). The role of knowledge in discourse comprehension: A construction-integration model. Psychological Review, 95 (2), 163–182.
Koenig, M. A., & Harris, P. L. (2005). Preschoolers mistrust ignorant and inaccurate speakers. Child Development, 76 (6), 1261–1277. https://doi.org/10.1111/j.1467-8624.2005.00849.x
Komarraju, M., Karau, S. J., Schmeck, R. R., & Avdic, A. (2011). The Big Five personality traits, learning styles, and academic achievement. Personality and Individual Differences, 51 (4), 472–477. https://doi.org/10.1016/j.paid.2011.04.019
Kuhn, D. (1999). A developmental model of critical thinking. Educational Researcher, 28 (2), 16–46. https://doi.org/10.2307/1177186
Kurby, C. A., Britt, M. A., & Magliano, J. P. (2005). The role of top-down and bottom-up processes in between-text integration. Reading Psychology, 26 (4–5), 335–362. https://doi.org/10.1080/02702710500285870
Lane, S., Raymond, M. R., & Haladyna, T. M. (Eds.). (2016). Handbook of test development . Routledge.
Language and Reading Research Consortium, Pratt, A., & Logan, J. (2014). Improving language-focused comprehension instruction in primary-grade classrooms: Impacts of the let’s know! Experimental Curriculum. Educational Psychology Review, 26 (3), 357–377. https://doi.org/10.1007/s10648-014-9275-1
Lee, C. D., Goldman, S. R., Levine, S., & Magliano, J. P. (2016). Epistemic cognition in literary reasoning. In J. A. Greene, W. A. Sandoval, & I. Bråten (Eds.), Handbook of epistemic cognition (pp. 165–183). Routledge.
Lee, C. D. (2007). Culture, literacy, and learning: Taking bloom in the midst of the whirlwind . Teachers College Press.
Leinhardt, G., & Young, K. M. (1996). Two texts, three readers: Distance and expertise in reading history. Cognition and Instruction, 14 (4), 441–486. https://doi.org/10.1207/s1532690xci1404_2
Lemke, J. L. (2004). Intertextuality and educational research. In N. Shuart-Faris & D. Bloome (Eds.), Uses of intertextuality in classroom and educational research (pp. 3–14). Information Age Publishing.
Leu, D. J., Forzani, E., Rhoads, C., Maykel, C., Kennedy, C., & Timbrell, N. (2015). The new literacies of online research and comprehension: Rethinking the reading achievement gap. Reading Research Quarterly, 50 (1), 37–59. https://doi.org/10.1002/rrq.85
Leu, D. J., Kinzer, C. K., Coiro, J., Castek, J., & Henry, L. A. (2019). New literacies: A dual-level theory of the changing nature of literacy, instruction, and assessment. In D. E. Alvermann, N. J. Unrau, M. Sailors, & R. B. Ruddell (Eds.), Theoretical models and processes of literacy (7th ed., pp. 319–346). Routledge.
Lindsay, D. S., Johnson, M. K., & Kwon, P. (1991). Developmental changes in memory source monitoring. Journal of Experimental Child Psychology, 52 (3), 297–318. https://doi.org/10.1016/0022-0965(91)90065-Z
List, A. (2020). Six questions regarding strategy use when learning from multiple texts. In D. L. Dinsmore, L. K. Fryer, & M. M. Parkinson (Eds.), Handbook of strategies and strategic processing (pp. 119–140). Routledge.
List, A. (2023). Social justice reasoning when students learn about social issues using multiple texts. Discourse Processes, 60 (4–5), 244–272. https://doi.org/10.1080/0163853X.2023.2197692
List, A., & Alexander, P. A. (2017). Analyzing and integrating models of multiple text comprehension. Educational Psychologist, 52 (3), 143–147. https://doi.org/10.1080/00461520.2017.1328309
List, A., & Alexander, P. A. (2017). Cognitive affective engagement model of multiple source use. Educational Psychologist, 52 (3), 182–199. https://doi.org/10.1080/00461520.2017.1329014
List, A., & Alexander, P. A. (2019). Toward an integrated framework of multiple text use. Educational Psychologist, 54 (1), 20–39. https://doi.org/10.1080/00461520.2018.1505514
List, A., & Sun, Y. (2023). To clarity and beyond: Situating higher-order, critical, and critical-analytic thinking in the literature on learning from multiple texts. Educational Psychology Review, 35 (2), 40. https://doi.org/10.1007/s10648-023-09756-y
List, A., Du, H., Wang, Y., & Lee, H. Y. (2019). Toward a typology of integration: Examining the documents model framework. Contemporary Educational Psychology, 58 , 228–242. https://doi.org/10.1016/j.cedpsych.2019.03.003
Mackey, M. (2020). Who reads what, in which formats, and why? In E. B. Moje, P. P. Afflerbach, P. Enciso, & N. K. Lesaux (Eds.), Handbook of reading research 5: 99–115. Routledge.
Magliano, J. P., McCrudden, M. T., Rouet, J.-F., & Sabatini, J. (2018). The modern reader: Should changes to how we read affect research and theory? In M. F. Schober, D. N. Rapp, & M. A. Britt (Eds.), The Routledge handbook of discourse processes (pp. 343–361). Routledge.
Magliano, J. P., Talwar, A., Feller, D. P., Wang, Z., O’Reilly, T., & Sabatini, J. (2023). Exploring thresholds in the foundational skills for reading and comprehension outcomes in the context of postsecondary readers. Journal of Learning Disabilities, 56 (1), 43–57. https://doi.org/10.1177/00222194221087387
Manguel, A. (1996). A history of reading . Penguin Press.
Many, J. E. (1996). Patterns of selectivity in drawing on sources: Examining students’ use of intertextuality across literacy events. Reading Research and Instruction, 36 (1), 51–63. https://doi.org/10.1080/19388079609558227
Many, J. E., Fyfe, R., Lewis, G., & Mitchell, E. (1996). Traversing the topical landscape: Exploring students’ self-directed reading-writing-research processes. Reading Research Quarterly, 31 (1), 12–35. https://doi.org/10.1598/RRQ.31.1.2
Mason, L. (2010). Beliefs about knowledge and revision of knowledge: On the importance of epistemic beliefs for intentional conceptual change in elementary and middle school students. In L. D. Bendixen & F. C. Feucht (Eds.), Personal epistemology in the classroom: Theory, research, and implications for practice (pp. 258–291). Cambridge University Press.
Mason, L. (2018). Multiplicity in the digital era: Processing and learning from multiple sources and modalities of instructional presentations. Learning and Instruction, 57 , 76–81. https://doi.org/10.1016/j.learninstruc.2018.03.004
Mason, L., Scrimin, S., Tornatora, M. C., & Zaccoletti, S. (2017). Emotional reactivity and comprehension of multiple online texts. Learning and Individual Differences, 58 , 10–21. https://doi.org/10.1016/j.lindif.2017.07.002
Mason, L., Scrimin, S., Zaccoletti, S., Tornatora, M. C., & Goetz, T. (2018). Webpage reading: Psychophysiological correlates of emotional arousal and regulation predict multiple-text comprehension. Computers in Human Behavior, 87 , 317–326. https://doi.org/10.1016/j.chb.2018.05.020
Mason, L., Zaccoletti, S., Scrimin, S., Tornatora, M. C., Florit, E., & Goetz, T. (2020). Reading with the eyes and under the skin: Comprehending conflicting digital texts. Journal of Computer Assisted Learning, 36 (1), 89–101. https://doi.org/10.1111/jcal.12399
McCarthy, K. S., & McNamara, D. S. (2021). The multidimensional knowledge in text comprehension framework. Educational Psychologist, 56 (3), 196–214. https://doi.org/10.1080/00461520.2021.1872379
McGinley, W. (1992). The role of reading and writing while composing from sources. Reading Research Quarterly, 27 (3), 226. https://doi.org/10.2307/747793
McGrew, S., Breakstone, J., Ortega, T., Smith, M., & Wineburg, S. (2018). Can students evaluate online sources? Learning from assessments of civic online reasoning. Theory & Research in Social Education, 46 (2), 165–193. https://doi.org/10.1080/00933104.2017.1416320
McNamara, D. S., Watanabe, M., Huynh, L., McCarthy, K. S., Allen, L. K., & Magliano, J. P. (2023). Summarizing versus rereading multiple documents. Contemporary Educational Psychology , 102238. https://doi.org/10.1016/j.cedpsych.2023.102238
McWeeny, S., Choe, J., & Norton, E. (2021). SnowGlobe: An interactive search tool for systematic reviews and meta-analyses. [Computer software]. 10.17605/OSF.IO/U25RN
Meehl, P. E. (1978). Theoretical risks and tabular asterisks: Sir Karl, Sir Ronald, and the slow progress of soft psychology. Journal of Consulting and Clinical Psychology, 46 (4), 806–834. https://doi.org/10.1037/0022-006X.46.4.806
Mills, C. M. (2013). Knowing when to doubt: Developing a critical stance when learning from others. Developmental Psychology, 49 (3), 404–418. https://doi.org/10.1037/a0029500
Murphy, P. K., Firetto, C. M., & Li, M. (2018). Knowledge and the model of domain learning. In H. Fives & D. L. Dinsmore (Eds.), The model of domain learning: Understanding the development of expertise (pp. 19–36). Routledge.
Murphy, P. K., Alexander, P. A., & Muis, K. R. (2012). Knowledge and knowing: The journey from philosophy and psychology to human learning. In K. R. Harris, S. Graham, T. Urdan, C. B. McCormick, G. M. Sinatra, & J. Sweller (Eds.), APA educational psychology handbook, Vol 1: Theories, constructs, and critical issues. (pp. 189–226). American Psychological Association. https://doi.org/10.1037/13273-008
National Assessment Governing Board. (2021). Reading framework for the 2026 National Assessment of Educational Progress . U.S. Department of Education.
National Council for the Social Studies. (2013). The college, career, and civic life (C3) framework for social studies state standards: Guidance for enhancing the rigor of K-12 civics, economics, geography, and history . National Council for the Social Studies.
American Educational Research Association, American Psychological Association, & National Council on Measurement in Education. (2014). Standards for educational and psychological testing . American Educational Research Association, American Psychological Association, & National Council on Measurement in Education.
National Governors Association, & Council of Chief State School Officers. (2010). Common core state standards for English language arts & literacy in history/social studies, science, and technical subjects .
National Research Council. (2012a). Education for life and work: Developing transferable knowledge and skills in the 21st century . National Academies Press.
National Research Council. (2012b). Improving adult literacy instruction: Options for practice and research . National Academies Press.
National Research Council. (2013). Next generation science standards: For states, by states . National Academies Press.
Nelson, N., & King, J. R. (2022). Discourse synthesis: Textual transformations in writing from sources. Reading and Writing . https://doi.org/10.1007/s11145-021-10243-5
Neugebauer, S. R., & Gilmour, A. F. (2020). The ups and downs of reading across content areas: The association between instruction and fluctuations in reading motivation. Journal of Educational Psychology, 112 (2), 344–363. https://doi.org/10.1037/edu0000373
Olson, D. R. (1994). The world on paper . Cambridge University Press.
Peng, P., Barnes, M., Wang, C., Wang, W., Li, S., Swanson, H. L., Dardick, W., & Tao, S. (2018). A meta-analysis on the relation between reading and working memory. Psychological Bulletin, 144 (1), 48–76. https://doi.org/10.1037/bul0000124
Peng, P., Zhang, Z., Wang, W., Lee, K., Wang, T., Wang, C., Luo, J., & Lin, J. (2022). A meta-analytic review of cognition and reading difficulties: Individual differences, moderation, and language mediation mechanisms. Psychological Bulletin, 148 (3–4), 227–272. https://doi.org/10.1037/bul0000361
Perfetti, C. A. (1985). Reading ability . Oxford University Press.
Perfetti, C. A., & Harris, L. (2017). Learning to read in English. In L. Verhoeven & C. A. Perfetti (Eds.), Learning to read across languages and writing systems (pp. 347–370). Cambridge University Press.
Perfetti, C. A., Rouet, J., & Britt, M. A. (1999). Toward a theory of documents representation. In H. van Oostendorp & S. R. Goldman (Eds.), The construction of mental representations during reading (pp. 99–122). Lawrence Erlbaum Associates.
Perfetti, C., & Helder, A. (2022). Progress in reading science: Word identification, comprehension, and universal perspectives. In M. J. Snowling, C. Hulme, & K. Nation (Eds.), The science of reading: A handbook (2nd ed., pp. 5–35). Wiley. https://doi.org/10.1002/9781119705116.ch1
Perfetti, C. A., Britt, M. A., & Georgi, M. C. (1995). Text-based learning and reasoning: Studies in history . Lawrence Erlbaum Associates.
Polanin, J. R., Zhang, Q., Taylor, J. A., Williams, R. T., Joshi, M., & Burr, L. (2022). Evidence gap maps in education research. Journal of Research on Educational Effectiveness , 1–21. https://doi.org/10.1080/19345747.2022.2139312
Primor, L., & Katzir, T. (2018). Measuring multiple text integration: A review. Frontiers in Psychology, 9 , 2294. https://doi.org/10.3389/fpsyg.2018.02294
Raccanello, D., Florit, E., Brondino, M., Rodà, A., & Mason, L. (2022). Control and value appraisals and online multiple‐text comprehension in primary school: The mediating role of boredom and the moderating role of word‐reading fluency. British Journal of Educational Psychology , 92 (1). https://doi.org/10.1111/bjep.12448
Raphael, T. E., & Boyd, F. B. (1991). Synthesizing information from multiple sources: A descriptive study of elementary students’ perceptions and performance of discourse synthesis (Elementary Subjects Center 45). Center for the Learning and Teaching of Elementary Subjects.
Reichle, E. D. (2021). Computational models of reading: A handbook . Oxford University Press.
Richter, T., & Maier, J. (2017). Comprehension of multiple documents with conflicting information: A two-step model of validation. Educational Psychologist, 52 (3), 148–166. https://doi.org/10.1080/00461520.2017.1322968
Robinaugh, D. J., Haslbeck, J. M. B., Ryan, O., Fried, E. I., & Waldorp, L. J. (2021). Invisible hands and fine calipers: A call to use formal theory as a toolkit for theory construction. Perspectives on Psychological Science, 16 (4), 725–743. https://doi.org/10.1177/174569162097469
Rott, S., & Gavin, B. (2015). Comprehending and learning from Internet sources: A conceptual replication study of Goldman, Braasch, Wiley, Greasser and Brodowinska (2012). CALICO Journal, 32 (2), 323–354. https://doi.org/10.1558/cj.v32i2.25139
Rouet, J.-F., Britt, M. A., & Durik, A. M. (2017). RESOLV: Readers’ representation of reading contexts and tasks. Educational Psychologist, 52 (3), 200–215. https://doi.org/10.1080/00461520.2017.1329015
Rouet, J., & Britt, M. A. (2011). Relevance processes in multiple document comprehension. In M. T. McCrudden, J. P. Magliano, & G. Schraw (Eds.), Text relevance and learning from text (pp. 19–52).
Rouet, J.-F., Britt, M. A., Gabrielsen, E., Kaakinen, J., Richter, T., & Lennon, M. (2021). PIAAC Cycle 2 assessment framework: Literacy. In The assessment frameworks for cycle 2 of the programme for the international assessment of adult competencies . Organisation for Economic Co-Operation. https://doi.org/10.1787/4bc2342d-en
Rouet, J.-F. (2005). The skills of document use: From text comprehension to web-based learning . Lawrence Erlbaum Associates.
de Ruyter, S. (2020). The effect of the cooperative jigsaw method on multiple document reading comprehension for prevocational education [Master’s Thesis]. University of Twente.
Salmerón, L., Strømsø, H. I., Kammerer, Y., Stadtler, M., & van den Broek, P. (2018). Comprehension processes in digital reading. In M. Barzillai, J. Thomson, S. Schroeder, & P. van den Broek (Eds.), Studies in written language and literacy (Vol. 17, pp. 91–120). John Benjamins Publishing Company. https://doi.org/10.1075/swll.17.04sal
Saux, G., Britt, M. A., Vibert, N., & Rouet, J. (2021). Building mental models from multiple texts: How readers construct coherence from inconsistent sources. Language and Linguistics Compass , 15 (3). https://doi.org/10.1111/lnc3.12409
Scammacca, N. K., Roberts, G. J., Cho, E., Williams, K. J., Roberts, G., Vaughn, S. R., & Carroll, M. (2016). A century of progress: Reading interventions for students in grades 4–12, 1914–2014. Review of Educational Research, 86 (3), 756–800. https://doi.org/10.3102/0034654316652942
Segev-Miller, R. (2007). Cognitive processes in discourse synthesis: The case of intertextual processing strategies. In Torrance, L. Van Waes, & D. Galbraith (Eds.), Writing and cognition: Research and applications (Vol. 20, pp. 231–250).
Share, D. L. (2008). On the Anglocentricities of current reading research and practice: The perils of overreliance on an “outlier” orthography. Psychological Bulletin, 134 (4), 584–615. https://doi.org/10.1037/0033-2909.134.4.584
Shrout, P. E., & Rodgers, J. L. (2018). Psychology, science, and knowledge construction: Broadening perspectives from the replication crisis. Annual Review of Psychology, 69 (1), 487–510. https://doi.org/10.1146/annurev-psych-122216-011845
Simonsmeier, B. A., Flaig, M., Deiglmayr, A., Schalk, L., & Schneider, M. (2022). Domain-specific prior knowledge and learning: A meta-analysis. Educational Psychologist, 57 (1), 31–54. https://doi.org/10.1080/00461520.2021.1939700
Smaldino, P. E. (2023). Modeling social behavior: Mathematical and agent-based models of social dynamics and cultural evolution . Princeton University Press.
Snow, C. E. (2002). Reading for understanding: Toward an R&D program in reading comprehension . Rand.
Sparks, J. R., & Deane, P. (2015). Cognitively based assessment of research and inquiry skills: Defining a key practice in the English language arts: Cognitively based assessment of research and inquiry skills. ETS Research Report Series, 2015 (2), 1–55. https://doi.org/10.1002/ets2.12082
Spivey, N. N. (1995). Written discourse: A constructivist perspective. In L. P. Steffe & J. Gale (Eds.), Constructivism in education (pp. 313–329). Lawrence Erlbaum Associates.
Stadtler, M., & Bromme, R. (2013). Multiple document comprehension: An approach to public understanding of science. Cognition and Instruction, 31 (2), 122–129. https://doi.org/10.1080/07370008.2013.771106
Stadtler, M., & Bromme, R. (2014). The content–source integration model: A taxonomic description of how readers comprehend conflicting scientific information. In D. N. Rapp & J. L. G. Braasch (Eds.), Processing inaccurate information: Theoretical and applied perspectives from cognitive science and the educational sciences (pp. 379–402). MIT Press.
Stahl, S. A., Hynd, C. R., Britton, B. K., McNish, M. M., & Bosquet, D. (1996). What happens when students read multiple source documents in history? Reading Research Quarterly, 31 (4), 430–456. https://doi.org/10.1598/RRQ.31.4.5
Stang Lund, E., Bråten, I., Brante, E. W., & Strømsø, H. I. (2017). Memory for textual conflicts predicts sourcing when adolescents read multiple expository texts. Reading Psychology, 38 (4), 417–437. https://doi.org/10.1080/02702711.2016.1278417
Stang Lund, E., Bråten, I., Brandmo, C., Brante, E. W., & Strømsø, H. I. (2019). Direct and indirect effects of textual and individual factors on source-content integration when reading about a socio-scientific issue. Reading and Writing, 32 (2), 335–356. https://doi.org/10.1007/s11145-018-9868-z
Strømsø, H. I., & Bråten, I. (2009). Beliefs about knowledge and knowing and multiple-text comprehension among upper secondary students. Educational Psychology, 29 (4), 425–445. https://doi.org/10.1080/01443410903046864
Strømsø, H. I., & Kammerer, Y. (2016). Epistemic cognition and reading for understanding in the internet age. In J. A. Greene, W. A. Sandoval, & I. Bråten (Eds.), Handbook of epistemic cognition (pp. 230–246). Routledge.
Strømsø, H. I., Bråten, I., & Britt, M. A. (2010). Reading multiple texts about climate change: The relationship between memory for sources and text comprehension. Learning and Instruction, 20 (3), 192–204. https://doi.org/10.1016/j.learninstruc.2009.02.001
Strømsø, H. I., Bråten, I., Anmarkrud, Ø., & Ferguson, L. E. (2016). Beliefs about justification for knowing when ethnic majority and ethnic minority students read multiple conflicting documents. Educational Psychology, 36 (4), 638–657. https://doi.org/10.1080/01443410.2014.920080
Sun, R., Coward, L. A., & Zenzen, M. J. (2005). On levels of cognitive modeling. Philosophical Psychology, 18 (5), 613–637. https://doi.org/10.1080/09515080500264248
Tarchi, C., Ruffini, C., & Pecini, C. (2021). The contribution of executive functions when reading multiple texts: A systematic literature review. Frontiers in Psychology, 12 , 716463. https://doi.org/10.3389/fpsyg.2021.716463
Toste, J. R., Didion, L., Peng, P., Filderman, M. J., & McClelland, A. M. (2020). A meta-analytic review of the relations between motivation and reading achievement for K–12 students. Review of Educational Research, 90 (3), 420–456. https://doi.org/10.3102/0034654320919352
Underwood, B. J. (1975). Individual differences as a crucible in theory construction. American Psychologist, 30 (2), 128–134. https://doi.org/10.1037/h0076759
Van Den Broek, P., Risden, K., Fletcher, C. R., & Thurlow, R. (1996). A “landscape” view of reading: Fluctuating patterns of activation and construction of a stable memory representation. In B. K. Britton & A. C. Graesser (Eds.), Models of understanding text (pp. 165–188). Lawrence Erlbaum Associates.
Van Dijk, T. A. (1979). Relevance assignment in discourse comprehension. Discourse Processes, 2 (2), 113–126. https://doi.org/10.1080/01638537909544458
VanSledright, B. A. (2002). Fifth graders investigating history in the classroom: Results from a researcher-practitioner design experiment. The Elementary School Journal, 103 (2), 131–160. https://doi.org/10.1086/499720
vanSledright, B. A., & Kelly, C. (1998). Reading American history: The influence of multiple sources on six fifth graders. The Elementary School Journal, 98 (3), 239–265. https://doi.org/10.1086/461893
Vosniadou, S. (1992). Knowledge acquisition and conceptual change. Applied Psychology, 41 (4), 347–357. https://doi.org/10.1111/j.1464-0597.1992.tb00711.x
Wang, Z., Sabatini, J., O’Reilly, T., & Weeks, J. (2019). Decoding and reading comprehension: A test of the decoding threshold hypothesis. Journal of Educational Psychology, 111 (3), 387–401. https://doi.org/10.1037/edu0000302
Wang, Z., O’Reilly, T., Sabatini, J., McCarthy, K. S., & McNamara, D. S. (2021). A tale of two tests: The role of topic and general academic knowledge in traditional versus contemporary scenario-based reading. Learning and Instruction, 73 , 101462. https://doi.org/10.1016/j.learninstruc.2021.101462
Wiley, J., & Voss, J. F. (1999). Constructing arguments from multiple sources: Tasks that promote understanding and just memory for text. Journal of Educational Psychology, 2 , 301–311.
Williams, J. P., Kao, J. C., Pao, L. S., Ordynans, J. G., Atkins, J. G., Cheng, R., & DeBonis, D. (2016). Close analysis of texts with structure (CATS): An intervention to teach reading comprehension to at-risk second graders. Journal of Educational Psychology, 108 (8), 1061–1077. https://doi.org/10.1037/edu0000117
Wineburg, S. S. (1991). Historical problem solving: A study of the cognitive processes used in the evaluation of documentary and pictorial evidence. Journal of Educational Psychology, 83 (1), 73–87. https://doi.org/10.1037/0022-0663.83.1.73
Winne, P. H. (2018). Cognition and metacognition within self-regulated learning. In D. H. Schunk & J. A. Greene (Eds.), Handbook of self-regulation of learning and performance (pp. 36–48). Routledge.
Wolf, M. (2018). Reader, come home: The reading brain in the digital world . Harper Collins Publishers.
Wolfe, M. B. W., & Goldman, S. R. (2005). Relations between adolescents’ text processing and reasoning. Cognition and Instruction, 23 (4), 467–502. https://doi.org/10.1207/s1532690xci2304_2
Yang, S. C. (2002). Multidimensional taxonomy of learners cognitive processing in discourse synthesis with hypermedia. Computers in Human Behavior, 18 (1), 37–68. https://doi.org/10.1016/S0747-5632(01)00031-0
Download references
Daniel R. Espinas was supported in part by the Office of Special Education Programs (Grant H325D180086) in the US Department of Education to Vanderbilt University. Brennan W. Chandler was supported in part by the Office of Special Education Programs (Grant H325H190003) in the US Department of Education to The University of Texas at Austin.
Author information
Authors and affiliations.
Vanderbilt University, Nashville, USA
Daniel R. Espinas
The University of Texas at Austin, Austin, USA
Daniel R. Espinas & Brennan W. Chandler
You can also search for this author in PubMed Google Scholar
Corresponding author
Correspondence to Daniel R. Espinas .
Ethics declarations
The content is solely the responsibility of the authors and does not necessarily represent the official views of the Office of Special Education Programs or the US Department of Education.
Additional information
Publisher's note.
Springer Nature remains neutral with regard to jurisdictional claims in published maps and institutional affiliations.
Rights and permissions
Open Access This article is licensed under a Creative Commons Attribution 4.0 International License, which permits use, sharing, adaptation, distribution and reproduction in any medium or format, as long as you give appropriate credit to the original author(s) and the source, provide a link to the Creative Commons licence, and indicate if changes were made. The images or other third party material in this article are included in the article's Creative Commons licence, unless indicated otherwise in a credit line to the material. If material is not included in the article's Creative Commons licence and your intended use is not permitted by statutory regulation or exceeds the permitted use, you will need to obtain permission directly from the copyright holder. To view a copy of this licence, visit http://creativecommons.org/licenses/by/4.0/ .
Reprints and permissions
About this article
Espinas, D.R., Chandler, B.W. Correlates of K-12 Students’ Intertextual Integration. Educ Psychol Rev 36 , 48 (2024). https://doi.org/10.1007/s10648-024-09889-8
Download citation
Accepted : 23 April 2024
Published : 07 May 2024
DOI : https://doi.org/10.1007/s10648-024-09889-8
Share this article
Anyone you share the following link with will be able to read this content:
Sorry, a shareable link is not currently available for this article.
Provided by the Springer Nature SharedIt content-sharing initiative
- Multiple document comprehension
- Multiple source use
- Intertextual integration
- Find a journal
- Publish with us
- Track your research
- International Peace and Security
- Higher Education and Research in Africa
- Andrew Carnegie Fellows
- Great Immigrants
- Carnegie Medal of Philanthropy
- Reporting Requirements
- Modification Requests
- Communications FAQs
- Grants Database
- Philanthropic Resources
- Grantee FAQs
- Grantmaking Highlights
- Past Presidents
- The Gospel of Wealth
- Other Carnegie Organizations
- Andrew Carnegie’s Story
- Governance and Policies
- Media Center
K–12 Science Education in the United States: A Landscape Study for Improving the Field
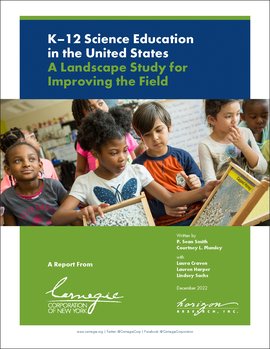
To better understand the state of K–12 science education today, Carnegie Corporation of New York commissioned Horizon Research to develop a landscape study. The report K–12 Science Education in the United States: A Landscape Study for Improving the Field assesses the progress over the last decade toward the vision of science instruction provided in 2012 by the Corporation-supported Framework for K–12 Science Education , published by the National Research Council.
K–12 Science Education in the United States: A Landscape Study for Improving the Field . Written by P. Sean Smith and Courtney L. Plumley; with Laura Craven, Lauren Harper, and Lindsey Sachs. New York: Carnegie Corporation of New York, December 2022. 51 pp.
Program: Education
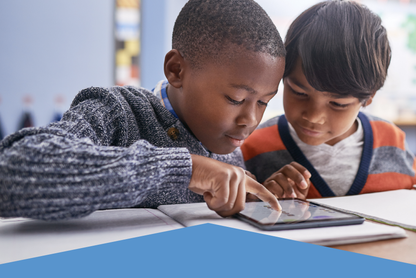
- Search for: Search
- Get Free Newsletters
New $90 Million Project to Create Digital Research Hub Focused on K-12 Education
- Click to share on Facebook (Opens in new window)
- Click to share on Twitter (Opens in new window)
- Click to share on LinkedIn (Opens in new window)
- Click to print (Opens in new window)

The National Science Foundation has awarded Rice University $90 million to build what is being described as a first-of-its-kind education research hub that will leverage data from an array of major digital learning platforms currently serving tens of millions of students.
The university recently announced the investment as its largest ever federal research grant. OpenStax at Rice, a major publisher of open education resources, will build the research and development hub known as SafeInsights .
The project will focus on producing “research-informed insights about teaching and learning for educators, institutions and learning platforms to use to create tailored programs, pedagogies and policies that will equip learners to thrive.”
“Just like there are bigger telescopes that let astronomers see deeper into the night, SafeInsights’ goal is to have this large student population that will enable researchers to see deeper into the student learning experience,” Slavinsky said in an interview.
The SafeInsights hub will take five years to build, he said, with early research projects b eginning in 16 to 18 months, and full-scale research operations starting in 2029.
School districts’ commitment to seeking and using research-based educational strategies is uneven at best. Many district officials complain that academic and other scientifically based research is too abstract, disconnected from their work, and outdated to be of practical use in their decision-making.
When surveyed recently by EdWeek Market Brief on what sorts of research they value most when choosing products and services, district and school leaders were much more likely to point to data on student outcomes, or product usage data than they were rigorous, experimental research.
Data Security in Focus
At $90 million, the award is NSF’s largest investment in research and development infrastructure for education at a national scale, the university said.
In the past, education research has been hampered by small study groups and short time frames, but the recent boom in digital learning can provide researchers with a plethora of data needed to better understand academic outcomes, Slavinsky said.
SafeInsights’ goal is to have this large student population that will enable researchers to see deeper into the student learning experience. J.P. Slavinsky, Executive Director, SafeInsights
And research will not be limited to only STEM (science, technology, engineering, and math) areas. Schools, education companies, and researchers participating in SafeInsights will bring their own research agenda, Slavinsky said.
Protecting student data is a major concern within school districts, particularly as schools’ and students’ reliance on technology has steadily grown.
The data collected as part of the new project will remain secure, Slavinsky said. No student information will be revealed to researchers.
Instead, researchers will submit their inquiries to SafeInsights, and the research hub will have the data in question analyzed where it is originally stored — by schools or on a digital platform — and provide researchers with aggregate results.
The research and development project will be a central hub for 80 partners and collaborating institutions. That number is expected to grow, Slavinsky said.
“One of the great things about SafeInsights is that it is very scalable,” he said. “And we want to build this community, so we can get a better and better picture of the students and the teachers we’re trying to help.”
Follow EdWeek Market Brief on Twitter @EdMarketBrief or connect with us on LinkedIn .
Image credit Stockyarder/DigitalVision/Getty
- When Districts Say They Value ‘Research,’ What Do They Mean?
- Need a Partner to Research Your Product? Here Are 5 Questions to Ask
- The Bar for Evidence That Education Companies Are Asked to Meet
- What’s the Value of Research to School Districts, and Education Companies?
Most Popular
- Where Instructional Materials (Still) Fall Short in Addressing Racial and Ethnic Diversity May 17, 2024
- “Building for Our Younger Selves”: How Teams With Learning Differences Shape Education Products May 17, 2024
- N.C. System Shops for Substitute Staffing; Calif. District to Buy Tutoring Services May 17, 2024
- K-12 Dealmaking: ETS Acquires Mastery Transcript Consortium; Elevate K-12 Secures $25M From Trinity Capital May 16, 2024
- New $90 Million Project to Create Digital Research Hub Focused on K-12 Education May 14, 2024
Related Posts
Commerce dept. uses iste to tout opportunities for u.s. ed-tech providers abroad, miami-dade schools to work with hp, lenovo, microsoft on 1-to-1 technology plan, how districts will pare out nonessential products, post-stimulus, schedule a tour.
Username or Email address *
Remember me
Lost your password?
- Open access
- Published: 19 April 2023
AI literacy in K-12: a systematic literature review
- Lorena Casal-Otero ORCID: orcid.org/0000-0002-0906-4321 1 ,
- Alejandro Catala ORCID: orcid.org/0000-0002-3677-672X 2 , 3 ,
- Carmen Fernández-Morante ORCID: orcid.org/0000-0003-4398-3361 1 ,
- Maria Taboada ORCID: orcid.org/0000-0002-2353-596X 2 ,
- Beatriz Cebreiro ORCID: orcid.org/0000-0003-2064-915X 1 &
- Senén Barro ORCID: orcid.org/0000-0001-6035-540X 3
International Journal of STEM Education volume 10 , Article number: 29 ( 2023 ) Cite this article
36k Accesses
37 Citations
28 Altmetric
Metrics details
The successful irruption of AI-based technology in our daily lives has led to a growing educational, social, and political interest in training citizens in AI. Education systems now need to train students at the K-12 level to live in a society where they must interact with AI. Thus, AI literacy is a pedagogical and cognitive challenge at the K-12 level. This study aimed to understand how AI is being integrated into K-12 education worldwide. We conducted a search process following the systematic literature review method using Scopus. 179 documents were reviewed, and two broad groups of AI literacy approaches were identified, namely learning experience and theoretical perspective. The first group covered experiences in learning technical, conceptual and applied skills in a particular domain of interest. The second group revealed that significant efforts are being made to design models that frame AI literacy proposals. There were hardly any experiences that assessed whether students understood AI concepts after the learning experience. Little attention has been paid to the undesirable consequences of an indiscriminate and insufficiently thought-out application of AI. A competency framework is required to guide the didactic proposals designed by educational institutions and define a curriculum reflecting the sequence and academic continuity, which should be modular, personalized and adjusted to the conditions of the schools. Finally, AI literacy can be leveraged to enhance the learning of disciplinary core subjects by integrating AI into the teaching process of those subjects, provided the curriculum is co-designed with teachers.
Introduction
In recent years, the convergence of huge computing power, massive amounts of data and improved machine learning algorithms have led to remarkable advances in Artificial Intelligence (AI) based technologies, which are set to be the most socially and economically disruptive technologies ever developed (Russell, 2021 ). The irruption of AI-based technology in our daily lives (e.g., robot vacuum cleaners, real-time location and search systems, virtual assistants, etc.) has generated a growing social and political interest in educating citizens about AI. The scientific community has also begun to engage in this education after detecting a significant gap in the understanding of AI, based on comments and fears expressed by citizens about this technology (West & Allen, 2018 ). Therefore, integrating AI into curricula is necessary to train citizens who must increasingly live and act in a world with a significant presence of AI.
It is worth noting that AI education addresses not only the learning of the scientific and technological foundations of AI, but also the knowledge and critical reflection on how a trustworthy AI should be developed and the consequences of not doing so. Hence, it is crucial to incorporate AI teaching from the earliest stages of education (Heintz, 2021 ). However, although some countries are making significant efforts to promote AI teaching in K-12 (Touretzky et al., 2019a ), this is being implemented through highly varied AI training experiences, such as data-driven design (Vartiainen et al., 2021 ), interactive data visualizations (Chittora & Baynes, 2020 ; von Wangenheim et al., 2021 ), virtual reality and robotics (Narahara & Kobayashi, 2018 ), games (Giannakos et al., 2020 ), or even based on combined workshop series (Lee et al., 2021 ). To date, there are very few methodological proposals on how to introduce the AI curriculum in K-12 education (Lee et al., 2020 ).
Since the development of a field requires prior research, we propose in this paper to identify and examine the way in which AI literacy is developing in K-12 around the world, to draw conclusions and guide teaching proposals for AI literacy in K-12. By highlighting and discussing the pros and cons of the different approaches and experiences in the literature, we aim to inspire new initiatives and guide the actors involved, from decisions-makers, for example in education policy, to teachers involved in their conception, design and implementation. We also hope to raise awareness of the importance of learning about AI from an early age, emphasizing the key aspects of this training and, hopefully, fueling the debate that needs to be fostered in our research community.
Integration of AI into the K12 curriculum
As a scientific-technological field, AI is just a few decades old. The name was coined in 1956, and since then different disciplines (such as computer science, mathematics, philosophy, neuroscience, or psychology) have contributed to its development from an interdisciplinary focus. AI is oriented to comprehend, model, and replicate human intelligence and cognitive processes into artificial systems. Currently, it covers a wide range of subfields such as machine learning, perception, natural language processing, knowledge representation and reasoning, computer vision, among many others (Russell & Norvig, 2021 ).
Starting in the 1970s, AI began to emerge in educational contexts through tools specifically designed to support learning, teaching, and the management of educational institutions. Since many jobs are now AI-related and will continue to increase in the coming years, some researchers believe that AI education should be considered as important as literacy in reading and writing (Kandlhofer et al., 2016 ). The highly interdisciplinary character is also another factor to consider. AI literacy can be defined as a set of skills that enable a solid understanding of AI through three priority axes: learning about AI, learning about how AI works, and learning for life with AI (Long & Magerko, 2020 ; Miao et al., 2021 ). The first axis focuses on understanding AI concepts and techniques to enable the recognition of which artifacts/platforms use AI and which do not. The second axis addresses the understanding of how AI works, to effectively interact with it. The third axis seeks to understand how AI can affect our lives, allowing us to critically evaluate its technology. Thus, AI literacy goes beyond the use of AI applications in education, such as Intelligent Tutoring Systems (ITS) (du Boulay, 2016 ).
The teaching of knowledge in AI has traditionally been carried out at the university level, focused on students who study disciplines closely related to computing and ICT in general. In recent years, AI learning has also started to be relevant both in university programs with diverse study backgrounds (Kong et al., 2021 ), as well as at the K-12 level (Kandlhofer & Steinbauer, 2021 ; Tedre et al., 2021 ). However, teaching AI at the K-12 level is not yet prevalent in formal settings and is considered challenging. Experts believe it is important to have some thought on what AI education should look like at the K-12 level so that future generations can become informed citizens who understand the technologies they interact with in their daily lives (Touretzky et al., 2019a ). Training children and teenagers will allow them to understand the basics of the science and technology that underpins AI, its possibilities, its limits and its potential social and economic impact. It also stimulates and better prepares them to pursue further studies related to AI or even to become creators and developers of AI themselves (Heintz, 2021 ).
Nowadays, research on AI teaching is still scarce (Chai et al., 2020a , 2020b ; Lee et al., 2020 ). The acquisition of knowledge in AI represents a great pedagogical challenge for both experts and teachers, and a cognitive challenge for students (Micheuz, 2020 ). Some countries are making significant efforts to promote AI education in K-12 (Touretzky et al., 2019b ), by developing relatively comprehensive curriculum guidelines (Yue et al., 2021 ). Through interviews with practitioners and policy makers from three different continents (America, Asia and Europe), some studies report on continuing works to introduce AI in K-12 education (He et al., 2020 ). Some other work focuses on examining and comparing AI curricula in several countries (Yue et al., 2021 ). In addition, there are a growing number of AI training experiences that explore pathways to optimize AI learning for K-12 students. However, most of them are somehow thematically limited as they do not adequately address key areas of AI, such as planning, knowledge representation and automated reasoning (Nisheva-Pavlova, 2021 ). Additionally, due to the rapid growth of AI, there is a need to understand how educators can best leverage AI techniques for the academic success of their students. Zhai et al. ( 2021 ) recommend that educators work together with AI experts to bridge the gap between technique and pedagogy.
Using a systematic review method, our research aims to present an overview of current approaches to understand how AI is taught worldwide. Several studies have conducted systematic reviews concerning applications of AI in education. Zhai et al. ( 2021 ) analyzed how AI was applied to the education domain from 2010 to 2020. Their review covers research on AI-based learning environments, from their construction to their application and integration in the educational environment. Guan et al. ( 2020 ) reviewed the main themes and trends in AI research in education over the past two decades. The authors found that research on the use of AI techniques to support teaching or learning has stood the test of time and that learner profiling models and learning analytics have proliferated in the last two decades. Ng et al. ( 2022 ) examined learner types, teaching tools and pedagogical approaches in AI teaching and learning, mainly in university computer science education. Chen et al. ( 2020 ) covered education enhanced by AI techniques aimed to back up teaching and learning. All these studies have focused on the main role that AI has played in educational applications over the last decades. However, in light of the recent need to consider how AI education should be approached at the K-12 level (Kandlhofer et al., 2016 ; Long & Magerko, 2020 ; Miao et al., 2021 ; Touretzky et al., 2019b ), it would be of great value to structure and characterize the different approaches used so far to develop AI literacy in K-12, as well as to identify research gaps to be explored. Recently, Yue et al. ( 2022 ) analyzed the main components of the pedagogical design in 32 empirical studies in K-12 AI education and Su et al. ( 2022 ) examined 14 learning experiences carried out in the Asian-Pacific region. These components included target audience, setting, duration, contents, pedagogical approaches to teaching, and assessment methods. Sanusi et al. ( 2022 ) reviewed research on teaching machine learning in K-12 from four perspectives: curriculum development, technology development, pedagogical development, and teacher training development. The findings of the study revealed that more studies are needed on how to integrate machine learning into subjects other than computer science. Crompton et al. ( 2022 ) carried out a systematic review on the use of AI as a supporting tool in K-12 teaching, which entails an interesting but narrower scope. Our study extends previous reviews on K-12 AI research by emphasizing how the current approaches are integrating AI literacy in K-12 education worldwide.
Research question
To begin the systematic review, a single research question (RQ) was formulated.
RQ: How are current approaches integrating AI literacy into K-12 education worldwide?
In essence, the RQ aims to investigate the characterization of the different approaches being employed to incorporate AI education in K-12. The following subsections in the methodology describe the search and the data collection process in such a way that an answer to the RQ can be provided in a replicable and objective fashion.
The research method chosen to conduct this research was the systematic literature review (SLR), following the guidelines posed by Kitchenham ( 2004 ). Accordingly, the following subsections summarize and document the key steps implemented in this research method.
Search process
We used Scopus to implement the search process. Scopus provides an integrated search facility to find relevant papers in its database based on curated metadata. It includes primary bibliographic sources published by Elsevier , Springer , ACM , and IEEE , among others. It provides a comprehensive coverage of journals and top-ranked conferences within fields of interest. We did not limit our search to specific journals or regular conference proceedings, as there is not yet a clearly established body of literature on the subject. All searches were performed based on title, keywords and abstract, and conducted between 21 October 2021 and 9 March 2023.
To decide the search string, we ran an initial search and found only a few papers focused on ‘literacy’ whereas the vast majority referred to the broader term ‘education’. Therefore, we decided to use both search terms (key issue 1 in Table 1 ). As some recent works combine the terms ‘Artificial Intelligence’ and ‘education’/’literacy’ into single terms such as ‘AI literacy’ or ‘AI education’, these were added to the search string (key issue 2 in Table 1 ). The educational stage was also included in the search string (key issue 3 in Table 1 ). As the search term ‘education’ also returns AI-based learning environments which are outside the scope of our review, we explicitly considered negated terms to leave out both computer-based learning and intelligent tutoring systems (key issue 4 in Table 1 ). A final decision was whether to use the term ‘Artificial Intelligence’ as a single umbrella term or to add narrower terms related to AI subfields (e.g., machine learning). After a preliminary inspection of a few relevant papers, we observed that such additional specific terms usually co-occur with the string ‘Artificial Intelligence’ in education, and they were therefore regarded as unnecessary. Thus, to capture the essence of our RQ and to build up the complete search string, we considered the search terms as shown in Table 1 . Eventually, this resulted in the following complete search string in Scopus:
TITLE-ABS-KEY ( ( ( ( literacy OR education) AND ( ( artificial AND intelligence))) OR ( "AI literacy" OR "AI education")) AND ( "primary school" OR "secondary school" OR k-12 OR "middle school"
OR "high school") AND NOT ( "computer-based learning") AND NOT ( "intelligent tutoring system")).
We included peer-reviewed papers published on topics related to literacy and education on AI at school. Then we excluded papers whose usage of AI was limited to 1) supporting computer-based learning only, with no focus on learning about AI; 2) supporting assessment/tutoring based on AI. We also excluded papers that targeted college students and those that were limited to K-12 programming/CS concepts as a prerequisite for learning about AI in the future. Following these inclusion and exclusion criteria, our search in Scopus returned an initial list of 750 documents. After we inspected the title, abstract, keywords and full-text screening, we obtained a final list of 179 documents.
Data collection extraction and synthesis strategy
Data collection extraction was performed, discussed, and coordinated through regular meetings. After inspecting and discussing 10% of the papers over multiple meetings, the authors agreed on the annotations presented in Table 2 . This process is important as it allowed us to build a data annotation scheme empirically emerging from the sampled papers. A copy of the papers was also kept for easy review in case of doubts or disagreements.
The data resulted in a spreadsheet with the metadata of the papers which passed the inclusion and exclusion criteria, and a document with the list of paper IDs together with the rest of annotations. Some Python scripts were used to further process metadata (e.g., counting participating countries, frequencies, etc.) and produce a more complete bibliographic report with histograms and overview counting. A more qualitative analysis was carried out to answer the research question based on paper reading and annotations.
The results were organized into two subsections. The first subsection is a bibliometric analysis of the reviewed studies, which is based on the metadata provided by Scopus. The second subsection provides a qualitative analysis of the studies, which is based on the extracted data annotations (see Table 2 ). Both analyses are complementary and together deliver a better understanding of the research articles retrieved.
Bibliometric analysis
Figure 1 shows that the annual scientific production has been modest. It gained traction in 2016 and increased sharply in 2020.
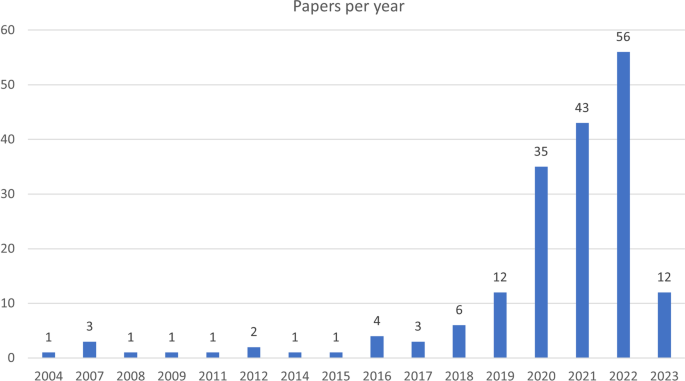
Annual scientific production: number of papers by year
Most of the contributions are conference publications (126 papers), while 52 are journal articles and one is a book chapter (Fig. 2 ).
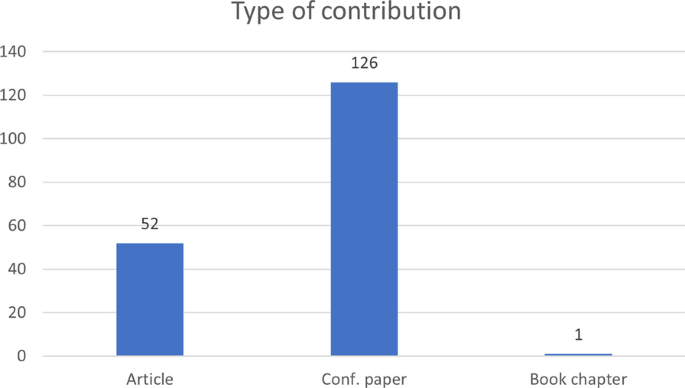
Type of contributions: number of papers by type
Eighty out of 179 papers have at least a citation in Scopus. There are 13 papers that have 10 or more citations, and the most cited papers are Long and Magerko ( 2020 ) and Touretzky et al. ( 2019b ). Figure 3 summarizes the number of contributions by publishers, where Springer, IEEE and ACM stand out, followed by Elsevier. As for journals, there are no single journals concentrating the publication of articles. Nevertheless, there are some journals that are especially relevant and well-known by the community such as the International Journal of Child-Computer Interaction, Computers and Education: Artificial Intelligence, International Journal of Artificial Intelligence in Education, or IEEE Transactions on Education.
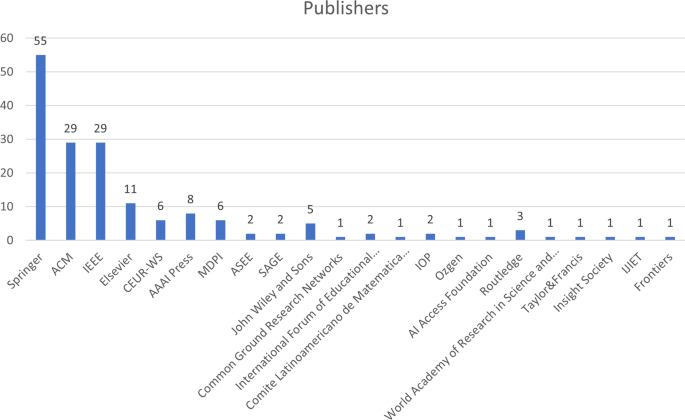
Frequency of publishers: number of papers by publisher
As for conferences, Fig. 4 summarizes the main conference events where papers are published. It includes flagship conferences Footnote 1 such as CHI and AAAI, top-ranked conferences such as HRI or SIGCSE and several noteworthy events (IDC, ICALT, ITiCSE, VL/HCC, to name a few). It is worth mentioning that AAAI is receiving contributions from recent years, which confirms the interest in the field in broadening the discussion to education. There are some additional publications associated with satellite AAAI events, such as workshops in CEUR-WS that deal with the issue under study. Although such contributions may sometimes be short, we decided to include them as they were relevant. For instance, the works published in (Herrero et al., 2020 ) and (Micheuz, 2020 ) include the German countrywide proposal for educating about AI, through a 6-module course focusing on explaining how AI works, the social discourse on AI and reducing existing misconceptions. On the other hand, Aguar et al. ( 2016 ) talk about teaching AI via an optional course which does not contribute to the final grades.
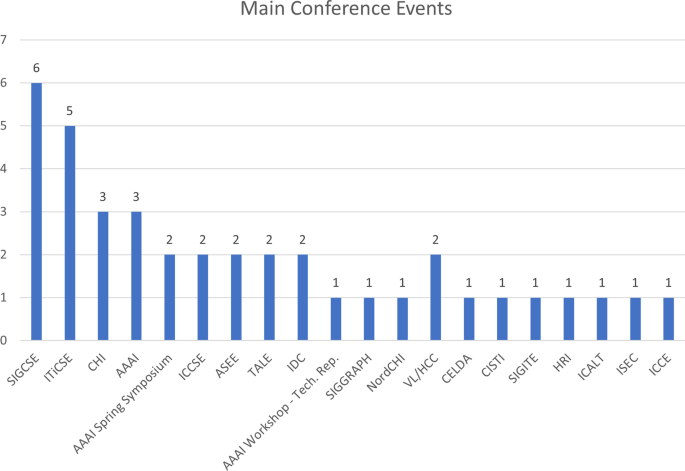
Main conference events: number of papers by conference
The analysis did not reveal particularly outstanding institutions (see Table 3 for a summary). Among the 299 affiliated institutions, we mostly find universities and research centers along with a few collaboration associations. The most active institutions are the Chinese University of Hong Kong, University of Eastern Finland and MIT, whose authors participated in a total of 19, 11 and 10 contributions, respectively.
Finally, the retrieved papers were co-authored by 643 different authors affiliated to research institutions from 42 countries. Figure 5 shows the histogram of participation by country. Of the 179 papers reviewed, most papers were written by authors affiliated with institutions in the same country. Only 32 papers involved authors from several countries. It is remarkable that in these cases at least one author is from the US, Hong Kong or China.
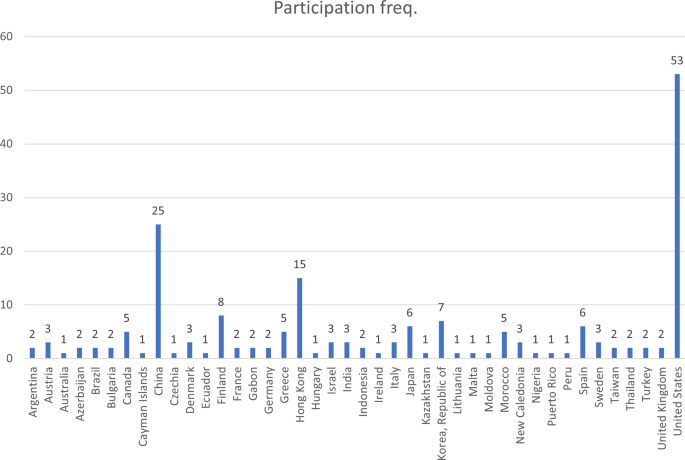
Country participation: number of papers by country
Literature analysis
By analyzing the data extracted, the papers were classified into two broad thematic categories according to the type of educational approach, namely, learning experience and theoretical perspective. The first category covers AI learning experiences focused on understanding a particular AI concept/technique or using specific tools/platforms to illustrate some AI concepts. The second category involves initiatives for the implementation of AI education for K-12 through the development of guidelines, curriculum design or teacher training, among others. Each main category was further subdivided into other subcategories to structure the field and characterize the different approaches used in developing AI literacy in K-12. Figure 6 shows all the identified categories and subcategories.
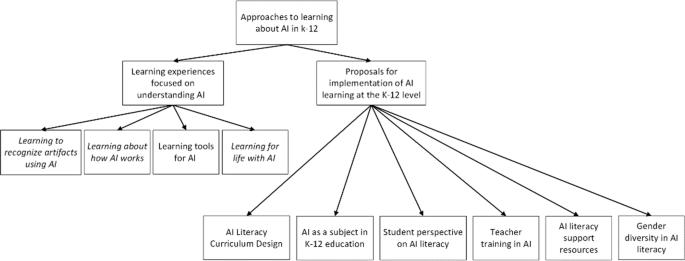
Taxonomy of approaches to AI learning in K-12
Learning experiences focused on understanding AI
This category covers learning experiences aimed at experimenting and becoming familiar with AI concepts and techniques. Based on the priority axes in AI literacy (Long & Magerko, 2020 ; Miao et al., 2021 ), we identified experiences aimed at acquiring basic AI knowledge to recognize artifacts using AI, learning how AI works, learning tools for AI and learning to live with AI.
Learning to recognize artifacts using AI
This subcategory refers to experiences that aim to understand AI concepts and techniques enabling the recognition of which artifacts/platforms use AI and which do not. Four studies were found in this subcategory. They are proposals aimed at helping young people to understand and demystify AI through different types of activities. These activities included conducting discussions after watching AI-related movies (Tims et al., 2012 ), carrying out computer-based simulations of human-like behaviors (Ho et al., 2019 ), experimenting as active users of social robots (Gonzalez et al., 2017 ) and programming AI-based conversational agents (Van Brummelen et al., 2021b ).
Learning about how AI works
This topic covers proposals designed to understand how AI works to make user interaction with AI easier and more effective. In this type of proposal, the focus is on methodology and learning is achieved through technology (Kim et al., 2023 ). The objective is to provide a better understanding of a particular aspect of reality in order to carry out a project or solve a problem (Lenoir & Hasni, 2016 ). The activities are supported by active experiences based on building and creating intelligent devices to achieve the understanding of AI concepts following the idea of Papert’s constructionism.
These experiences are mainly focused on teaching AI subfields such as ML or AI algorithms applied to robotics. Understanding the principles of ML, its workflows and its role in everyday practices to solve real-life problems has been the main objective of some studies (Burgsteiner et al., 2016 ; Evangelista et al., 2019 ; Lee et al., 2020 ; Sakulkueakulsuk et al., 2019 ; Vartiainen et al., 2021 ). In addition, there are also experiences focused on unplugged activities that simulate AI algorithms. For example, through classic games such as Mystery Hunt, one can learn how to traverse a graph without being able to see beyond the next path to be traversed (blind search) (Kandlhofer et al., 2016 ). Similarly, the AI4K12 initiative (Touretzky et al., 2019b ) collects a large set of activities and resources to simulate AI algorithms.
Learning tools for AI
This topic includes approaches that involve learning about AI support tools. The development of intelligent devices in the context of teaching AI requires specific programming languages or age-appropriate tools. Many of the tools currently available are focused on ML, with the aim of demystifying this learning in K-12 education (Wan et al., 2020 ). Some of them are integrated into block-based programming languages (such as Scratch or App Inventor) (Toivonen et al., 2020 ; von Wangenheim et al., 2021 ), enabling the deployment of the ML models built into games or mobile applications. Other approaches use data visualization and concepts of gamification to engage the student in the learning process (Reyes et al., 2020 ; Wan et al., 2020 ) or combine traditional programming activities with ML model building (Rodríguez-García et al., 2020 ).
This type of proposal aims to introduce AI through tools that enable the use of AI techniques. It is therefore an approach focused on learning by using AI-oriented tools. In this vein, different experiences have focused on learning programming tools for applications based on Machine Learning (Reyes et al., 2020 ; Toivonen et al., 2020 ; von Wangenheim et al., 2021 ; Wan et al., 2020 ), robotics (Chen et al., 2017 ; Eguchi, 2021 ; Eguchi & Okada, 2020 ; Holowka, 2020 ; Narahara & Kobayashi, 2018 ; Nurbekova et al., 2018 ; Verner et al., 2021 ), programming and the creation of applications (Chittora & Baynes, 2020 ; Giannakos et al., 2020 ; Kahn et al., 2018 ; Kelly et al., 2008 ; Park et al., 2021 ). Some of these tools use Scratch-based coding platforms to make AI-based programming attractive to children. In (Kahn et al., 2018 ), students play around with machine learning to classify self-captured images, using a block-based coding platform.
There are also experiences in which other types of environments are used to facilitate learning (Aung et al., 2022 ). In (Holowka, 2020 ; Verner et al., 2021 ), students can learn reinforcement learning through online simulation. In (Narahara & Kobayashi, 2018 ), a virtual environment helps students generate data in a playful setting, which is then used to train a neural network for the autonomous driving of a toy car-lab. In (Avanzato, 2009 ; Croxell et al., 2007 ), students experiment with different AI-based tasks through robotics-oriented competitions.
Learning for life with AI
This subcategory covers experiences aimed at understanding how AI can affect our lives thus providing us with skills to critically assess its technology. In (Vachovsky et al., 2016 ), technically rigorous AI concepts are contextualized through the impact on society. There are also experiences where students explore how a robot equipped with AI components can be used in society (Eguchi & Okada, 2018 ), program conversational agents (Van Brummelen et al., 2021b ), or learn to recognize credible but fake media products (video, photos), which have been generated using AI-based techniques ( 2021b ; Ali et al., 2021a ).
The ethical and philosophical implications of AI have also been addressed in some experiences ( 2021b ; Ali et al., 2021a ; Ellis et al., 2005 ), whereas others focus on training students to participate in present-day society and become critical consumers of AI (Alexandre et al., 2021 ; Cummings et al., 2021 ; Díaz et al., 2015 ; Kaspersen et al., 2022 ; Lee et al., 2021 ; Vartiainen et al., 2020 ).
Proposals for implementation of AI learning at the K-12 level
Some countries are making efforts to promote AI education in K-12. In the U.S., intense work is being carried out on the integration of AI in schools and among these schemes, AI4K12 stands out (Heintz, 2021 ). This scheme is especially interesting since it defines the national guidelines for future curricula, highlighting the essential collaborative work between developers, teachers and students (Touretzky et al., 2019a ). This idea of co-creation is also stressed in other schemes (Chiu, 2021 ). In the U.S. we can also mention the proposal made by the Massachusetts Institute of Technology, which is an AI curriculum that aims to engage students with its social and ethical implications (Touretzky et al., 2019a ). Although the United States is working intensively on the design of integrating this knowledge into the curriculum, so far AI is not widely offered in most K-12 schools (Heintz, 2021 ).
In China, the Ministry of Education has integrated AI into the compulsory secondary school curriculum (Ottenbreit-Leftwich et al., 2021 ; Xiao & Song, 2021 ). Among their schemes we can reference the AI4Future initiative of the Chinese University of Hong Kong (CUHK), which promotes the co-creation process to implement AI education (Chiu et al., 2021 ). In Singapore, a program for AI learning in schools has also been developed, where K-12 children learn AI interactively. However, the program is hindered by a lack of professionals (teachers) with adequate training (Heintz, 2021 ). In Germany, there are also several initiatives to pilot AI-related projects and studies (Micheuz, 2020 ), including the launch of a national initiative to teach a holistic view of AI. This initiative consists of a 6-module course aimed at explaining how AI works, stimulating a social discourse on AI and clarifying the abundant existing misconceptions (Micheuz, 2020 ). Canada has also designed an AI course for high schools. The course is intended to empower students with knowledge about AI, covering both its philosophical and conceptual underpinnings as well as its practical aspects. The latter are achieved by building AI projects that solve real-life problems (Nisheva-Pavlova, 2021 ).
The literature also highlights the different approaches that AI literacy should focus on: curriculum design, AI subject design, student perspective, teacher training, resource design and gender diversity. All these approaches are described in depth below.
AI literacy curriculum design
Approaches to curriculum development differ widely, ranging from the product-centered model (technical-scientific perspective) to the process-centered model (learner perspective) (Yue et al., 2021 ). AI literacy can be launched in primary and secondary education depending on the age and computer literacy of the students. To do this, it is necessary to define the core competencies for AI literacy according to three dimensions: AI concepts, AI applications and AI ethics and security (Long & Magerko, 2020 ; Wong et al., 2020 ). Research has focused on the understanding of the concepts, the functional roles of AI, and the development of problem-solving skills (Woo et al., 2020 ). This has led to proposing a redefinition of the curriculum (Han et al., 2019 ; Malach & Vicherková, 2020 ; Zhang et al., 2020 ) supported by different ideas that K-12 students should know (Chiu et al., 2021 ; Sabuncuoglu, 2020 ; Touretzky et al., 2019b ). Several countries have already made different curricular proposals (Alexandre et al., 2021 ; Micheuz, 2020 ; Nisheva-Pavlova, 2021 ; Ottenbreit-Leftwich et al., 2021 ; Touretzky et al., 2019b ; Xiao & Song, 2021 ), where they argue that the curricular design must include different elements such as content, product, process and praxis (Chiu, 2021 ). It is also convenient for learning in AI to follow the computational thinking model (Shin, 2021 ), contextualizing the proposed curriculum (Eguchi et al., 2021 ; Wang et al., 2020 ) and providing it with the necessary resources for teachers (Eguchi et al., 2021 ). In this sense, emerging initiatives highlight the need to involve teachers in the process of co-creating a curriculum associated to their context (Barlex et al., 2020 ; Chiu et al., 2021 ; Dai et al., 2023 ; Lin & Brummelen, 2021 ; Yau et al., 2022 ).
AI as a subject in K-12 education
Traditionally, including computer science or new technologies in the educational system has been carried out through a specific subject integrated into the curriculum or through the offer of extracurricular activities. In this sense, different proposals have suggested the integration of AI as a subject in K-12 education (Ellis et al., 2009 ; Knijnenburg et al., 2021 ; Micheuz, 2020 ; Sperling & Lickerman, 2012 ), in short-term courses (around 15 h) and divided into learning modules focused on classical and modern AI (Wong, 2020 ) or through MOOCs (Alexandre et al., 2021 ).
Student perspective on AI Literacy
Student-focused studies explore and analyze attitudes and previous knowledge to make didactic proposals adapted to the learner. Some of them measure their intention and interest in learning AI (Bollin et al., 2020 ; Chai et al., 2021 , 2020a , 2020b ; Gao & Wang, 2019 ; Harris et al., 2004 ; Sing, et al., 2022 ; Suh & Ahn, 2022 ), whereas others discuss their views on the integration of technologies in the education system (Sorensen & Koefoed, 2018 ) and on teaching–learning support tools in AI (Holstein et al., 2019 ).
Teacher training in AI
Teachers are key players for the integration of AI literacy in K-12, as proven by the numerous studies that examine this issue (An et al., 2022 ; Bai & Yang, 2019 ; Chiu & Chai, 2020 ; Chiu et al., 2021 ; Chounta et al., 2021 ; Judd, 2020 ; Kandlhofer et al., 2019 , 2021 ; Kim et al., 2021 ; Korenova, 2016 ; Lin et al., 2022 ; Lindner & Berges, 2020 ; Oh, 2020 ; Summers et al., 1995 ; Wei et al., 2020 ; Wu et al., 2020 ; Xia & Zheng, 2020 ). This approach places teachers at the center, bearing in mind what they need to know so as to integrate AI into K-12 (Itmazi & Khlaif, 2022 ; Kim et al., 2021 ). The literature analyzed reports on the factors that influence the knowledge of novice teachers (Wei, 2021 ) and focuses on teacher training in AI (Lindner & Berges, 2020 ; Olari & Romeike, 2021 ). Thus, AI training proposals can be found aimed at both teachers in training (Xia & Zheng, 2020 ) and practicing educators. Training schemes focus on their knowledge in technologies to facilitate their professional development (Wei et al., 2020 ) through the TPACK (Technological, Pedagogical and Content Knowledge) teaching knowledge model (Gutiérrez-Fallas & Henriques, 2020 ). Studies focusing on teachers’ opinions on curriculum development in AI are relevant (Chiu & Chai, 2020 ), as are their self-efficacy in relation to ICT (Wu et al., 2020 ), their opinions on the tools that support the teaching–learning process in AI (Holstein et al., 2019 ) and their teacher training in technologies (Cheung et al, 2018 ; Jaskie et al., 2021 ). These elements are central to the design of an AI literacy strategy in K-12. Both the co-design of ML curricula between AI researchers and K-12 teachers, and the assessment of the impact of these educational interventions on K-12 are important issues today. At present, there is a shortage of teachers with training in AI and working with teachers in training (Xia & Zheng, 2020 ) or with teachers in schools (Chiu et al., 2021 ) is proposed as an effective solution. One of the most interesting analyses of teacher competency proposes the acquisition of this skill for the teaching of AI in K-12, through the analysis of the curricula and resources of AI using TPACK. This model was formulated by (Mishra & Koehler, 2006 ) and aims to define the different types of knowledge that teachers need to integrate ICT effectively in the classroom. In this regard, it is suggested that teachers imparting AI to K-12 students require TPACK to build an environment and facilitate project-based classes that solve problems using AI technologies (Kim et al., 2021 ).
AI literacy support resources
Research using this approach focuses on presenting resources that support AI literacy (Kandlhofer & Steinbauer, 2021 ), considering that the creation of resources and repositories is a priority in supporting this teaching–learning process (Matarić et al., 2007 ; Mongan & Regli, 2008 ). However, these resources largely do not meet an interdisciplinary approach and do not embody a general approach to AI development (Sabuncuoglu, 2020 ).
Gender diversity in AI literacy
AI education, as a broad branch of computer science, also needs to address the issue of gender diversity. Lack of gender diversity can impact the lives of the people for whom AI-based systems are developed. The literature highlights the existence of proposals designed with a perspective toward gender, where the activities designed are specifically aimed at girls (Ellis et al., 2009 ; Jagannathan & Komives, 2019 ; Perlin et al., 2005 ; Summers et al., 1995 ; Vachovsky et al., 2016 ; Xia et al., 2022 ).
The huge impact that AI is having on our lives, at work and in every type of organization and business sector is easily recognizable today. No one doubts that AI is one of the most disruptive technologies in history, if not the most. In recent years, the expectations generated by AI, far from being deflated, have only grown. We are still a long way from general-purpose AI, but the application of AI to solve real problems has already taken hold for a wide range of purposes. It is therefore necessary for young people to know how AI works, as this learning will make it easier for them to use these technologies in their daily lives, both to learn and to interact with others.
Like any other technology, the potential uses and abuses of AI go hand in hand with its disruptive capacity. Many social groups and governments are expressing concern about the possible negative consequences of AI misuse. Although it is crucial to adequately regulate the use of AI, education is as important, if not more important, than regulation. Everything, whether good or bad, stems from the education received. Thus, education systems must prepare students for a society in which they will have to live and interact with AI. AI education will enable young people to discover how these tools work and, consequently, to act responsibly and critically. Therefore, AI literacy has become a relevant and strategic issue (Chiu & Chai, 2020 ).
This systematic review has focused on analyzing AI teaching–learning proposals in K-12 globally. The results confirm that the teaching of basic AI- related concepts and techniques at the K-12 level is scarce (Kandlhofer et al., 2016 ). Our work shows that there have been, on the one hand, different AI learning experiences and, on the other hand, proposals for the implementation of AI literacy, made at the political level and by different experts. The learning experiences described show that AI literacy in schools has focused on technical, conceptual, and applied skills in some domains of interest. Proposals for AI implementation, especially those defined by the US and China, reveal that significant efforts are being made to design models that frame AI literacy proposals.
We also found that there are hardly any AI learning experiences that have analyzed learning outcomes, e.g., through assessments of learners’ understanding of AI concepts. Obviously, this is a result of the infancy of these AI learning experiences at the K-12 level. However, it is important for learning experiences to be based on clearly defined competencies in a particular AI literacy framework, such as those proposed in the literature (Alexandre et al., 2021 ; Han et al., 2019 ; Long & Magerko, 2020 ; Malach & Vicherková, 2020 ; Micheuz, 2020 ; Ottenbreit-Leftwich et al., 2021 ; Touretzky et al., 2019a ; Wong et al., 2020 ; Xiao & Song, 2021 ; Zhang et al., 2020 ). Recently, Van Brummelen et al. ( 2021a ) designed a curriculum for a five-day online workshop based on the specific AI competencies proposed by Long and Magerko ( 2020 ). They used several types of questionnaires to assess the quality of the program through the knowledge acquired by the students in these competencies. Therefore, clearly defined competency-based learning experiences can provide a rigorous assessment of student learning outcomes.
The research shows that clear guidelines are needed on what students are expected to learn about AI in K-12 (Chiu, 2021 ; Chiu & Chai, 2020 ; Lee et al., 2020 ). These studies highlight the need for a competency framework to guide the design of didactic proposals for AI literacy in K-12 in educational institutions. This framework would provide a benchmark for describing the areas of competency that K-12 learners should develop and which specific educational projects can be designed. Furthermore, it would support the definition of a curriculum reflecting sequence and academic continuity (Woo et al., 2020 ). Such a curriculum should be modular and personalized (Gong et al., 2019 ) and adjusted to the conditions of the schools (Wang et al., 2020 ). In the teaching of AI, an exploratory education should be adopted, which integrates science, computer science and integral practice (Wang et al., 2020 ). It should also address issues related to the ethical dimension, which is fundamental to the literacy of K-12 students as it enables them to understand the basic principles of AI (Henry et al., 2021 ). This training facilitates the development of students’ critical capacity, and this is necessary to understand that technology is not neutral and to benefit from and make appropriate use of it. Ethics, complementary to legal norms, enhances the democratic quality of society by setting legitimate limits in the shaping of technological life. In this sense, different AI literacy proposals in K-12 already support the addressing of ethical, social and security issues linked to AI technologies (Eguchi et al., 2021 ; Micheuz, 2020 ; Wong et al., 2020 ). Moreover, considering designing for social good could foster or help to motivate learning about AI (Chai et al., 2021 ). Without a doubt, all this will impact on the achievement of a more democratic society. Due to the gender gap in issues related to computer science, it is also necessary to address the gender perspective. In this vein, the research proposes, among other strategies, to focus AI literacy on real-world elements since this approach favors the motivation of girls and greater involvement in learning (Jagannathan & Komives, 2019 ). However, little attention is paid to the undesirable consequences of an indiscriminate and insufficiently thought-out application of AI, both in higher education and especially in K-12. For example, the increase in socio-economic inequality between countries and within countries, resulting from the increasing automation of employment, is of particular concern. This is leading to growing inequality in wages and preservation of human employment, but it is not usually a subject of interest in education.
Currently, the challenges of this AI literacy require an interdisciplinary and critical approach (Henry et al., 2021 ). We believe that AI literacy can be leveraged to enhance the learning of disciplinary core subjects by integrating AI into the teaching process of those subjects. AI literacy should rely on transferring AI knowledge and methods to core subjects, allowing education to cross disciplinary boundaries, but staying within the framework of disciplinary core subjects. To achieve this change, educators need to take a closer look at the current capabilities of AI. This would enable them to identify all options to improve the core of educational practice and thus optimize the educational process. For example, understanding and using word clouds is a powerful educational strategy to enhance education in core subjects such as science (e.g., to facilitate object classification), language (e.g. to enable the matching of different topics or authors’ works), music (e.g., to support the analysis of song lyrics) or social sciences (e.g., to assist in comparing different discourses). Since AI is highly interdisciplinary in nature, it has a broad projection on multiple fields and problems that require a transversal and applied approach. For example, the basic algorithms of ML could be taught in Mathematics and related disciplines, the design of supervised classifiers could be performed for the study of taxonomies in Biology, natural language processing could be used to make the study of a language more attractive, or the ethical issues surrounding AI could be discussed in Philosophy and Social Sciences subjects.
Finally, for this meaningful learning to take place, AI teaching must be addressed through holistic, active, and collaborative pedagogical strategies in which real problem solving is the starting point of the learning process. An important gap regarding the integration of AI in K-12 concerns teachers, as it is unclear how to prepare and involve them in the process (Chiu & Chai, 2020 ). Teachers’ attitudes towards AI have a significant influence on the effectiveness of using AI in education. Teachers can swing between total resistance and overconfidence. The first could arise from inadequate, inappropriate, irrelevant, or outdated professional development. On the one hand, teachers must be digitally-competent enough to integrate AI into the teaching–learning processes of their subjects. Therefore, teacher training is also necessary following a framework of standard competencies. This should include new ways of organizing the professional role of teachers, as well as enhancing students’ attitudes towards these changes. On the other hand, research reveals that it is essential for didactic proposals to be co-designed and implemented by the teachers at those schools involved (Henry et al., 2021 ), to undergo training in the specific AI subjects and for this knowledge to be integrated into non-computer subjects (Lin & Brummelen, 2021 ). To this end, it is crucial to identify the perception and knowledge that teachers have about AI and involve them in the design of curricular proposals (Chiu, 2021 ; Chiu & Chai, 2020 ; Chiu et al., 2021 ).
This study aimed to understand how AI literacy is being integrated into K-12 education. To achieve this, we conducted a search process following the systematic literature review method and using Scopus. Two broad groups of AI literacy approaches were identified, namely learning experiences and theoretical perspective. The study revealed that learning experiences in schools have focused mainly on technical and applied skills limited to a specific domain without rigorously assessing student learning outcomes. In contrast, the US and China are leading the way in AI literacy implementation schemes which are broader in scope and involve a more ambitious approach. However, there is still a need to test these initiatives through comprehensive learning experiences that incorporate an analysis of learning outcomes. This work has allowed us to draw several conclusions that can be considered in the design of AI literacy proposals in K-12. Firstly, AI literacy should be based on an interdisciplinary and competency-based approach and integrated into the school curriculum. There is no need to include a new AI subject in the curriculum, but rather to build on the competencies and content of disciplinary subjects and then integrate AI literacy into those subjects. Given the interdisciplinary nature of AI, AI education can break disciplinary boundaries and adopt a global, practical, and active approach in which project-based and contextualized work plays an important role. Secondly, AI literacy should be leveraged to extend and enhance learning in curricular subjects. As a final point, AI literacy must prioritize the competency of teachers and their active participation in the co-design of didactic proposals, together with pedagogues and AI experts.
Availability of data and materials
Last revision round required update the review. Thus, Additional file 1 contains a.csv file with the listing of papers that are not cited but are part of the reviewed papers. The papers cited in text already appear in the Reference section and, therefore, not in the Additional file.
1 Conference categorization and ranking based on the GII-GRIN-SCIE (GGS) Conference Ratings: https://scie.lcc.uma.es/
Aguar, K., Arabnia, H. R., Gutierrez, J. B., Potter, W. D., & Taha, T. R. (2016). Making cs inclusive: An overview of efforts to expand and diversify cs education. In International Conference on Computational Science and Computational Intelligence (CSCI). (pp. 321–326). https://doi.org/10.1109/CSCI.2016.0067
Alexandre, F., Becker, J., Comte, M. H., Lagarrigue, A., Liblau, R., Romero, M., & Viéville, T. (2021). Why, What and How to help each citizen to understand artificial intelligence? KI Kunstliche Intelligenz, 35 (2), 191–199. https://doi.org/10.1007/s13218-021-00725-7
Article Google Scholar
Ali, S., DiPaola, D., Lee, I., Hong, J., & Breazeal, C. (2021a). Exploring generative models with middle school students. In Proceedings of the 2021a CHI Conference on Human Factors in Computing Systems. (pp. 1–13). https://doi.org/10.1145/3411764.3445226
Ali, S., DiPaola, D., Lee, I., Sindato, V., Kim, G., Blumofe, R., & Breazeal, C. (2021b). Children as creators, thinkers and citizens in an AI-driven future. Computers and Education Artificial Intelligence . https://doi.org/10.1016/j.caeai.2021.100040
An, X., Chai, C. S., Li, Y., Zhou, Y., Shen, X., Zheng, C., & Chen, M. (2022). Modeling English teachers’ behavioral intention to use artificial intelligence in middle schools. Education and Information Technologies . https://doi.org/10.1007/s10639-022-11286-z
Aung, Z. H., Sanium, S., Songsaksuppachok, C., Kusakunniran, W., Precharattana, M., Chuechote, S., & Ritthipravat, P. (2022). Designing a novel teaching platform for AI: A case study in a Thai school context. Journal of Computer Assisted Learning, 38 (6), 1714–1729. https://doi.org/10.1111/jcal.12706
Avanzato, R. L. (2009). Autonomous Outdoor Mobile Robot Challenge. Computer in Education Journal (July-September 2009).
Bai, H., & Yang, S. (2019, October). Research on the Sustainable Development Model of Information Technology Literacy of Normal Students Based on Deep Learning Recommendation System. In 2019 4th International Conference on Mechanical, Control and Computer Engineering (ICMCCE). (pp. 837–840). https://doi.org/10.1109/ICMCCE48743.2019.00192
Barlex, D., Steeg, T., & Givens, N. (2020). Teaching about disruption: A key feature of new and emerging technologies. Learning to Teach Design and Technology in the Secondary School, 4 , 137–154. https://doi.org/10.4324/9780429321191-9
Bollin, A., Kesselbacher, M., & Mößlacher, C. (2020). Ready for computing science? A closer look at personality, interests and self-concept of girls and boys at secondary level. In Informatics in Schools. Engaging Learners in Computational Thinking: 13th International Conference, ISSEP. (pp. 107–118). https://doi.org/10.1007/978-3-030-63212-0_9
Burgsteiner, H., Kandlhofer, M., & Steinbauer, G. (2016). IRobot: teaching the basics of artificial intelligence in high schools. Proceedings of the AAAI Conference on Artificial Intelligence . https://doi.org/10.1609/aaai.v30i1.9864
Chai, C.S., Lin, P.-Y., Jong, M.S.-Y., Dai, Y., Chiu, T.K., & Huang, B. (2020a). Factors influencing students' behavioral intention to continue artificial intelligence learning. In 2020a International Symposium on Educational Technology (ISET). (pp. 147–150). https://doi.org/10.1109/ISET49818.2020.00040
Chai, C. S., Wang, X., & Xu, C. (2020b). An extended theory of planned behavior for the modelling of chinese secondary school students’ intention to learn artificial intelligence. Mathematics, 8 (11), 1–18. https://doi.org/10.3390/math8112089
Chai, C.S., Lin, P.-Y., Jong, M.S.-Y., Dai, Y., Chiu, T.K., & Qin, J. (2021). Perceptions of and behavioral intentions towards learning artificial intelligence in primary school students. Educational Technology & Society , 24 (3), 89–101. Retrieved from https://www.jstor.org/stable/27032858
Chen, S., Qian, B., & Cheng, H. (2017). Voice recognition for STEM education using robotics. In Volume 9: 13th ASME/IEEE International Conference on Mechatronic and Embedded Systems and Applications. ASME . https://doi.org/10.1115/DETC2017-68368
Chen, M., Zhou, C., & Wu, Y. (2020). Research on the model and development status of information literacy self-improvement ability of primary and secondary school teachers. In Ninth International Conference of Educational Innovation through Technology (EITT). (pp. 87–91). https://doi.org/10.1109/EITT50754.2020.00021
Cheung, S. K., Lam, J., Li, K. C., Au, O., Ma, W. W., & Ho, W. S. (Eds.). (2018). Technology in Education. Innovative Solutions and Practices: Third International Conference, ICTE 2018. Springer.
Chittora, S., & Baynes, A. (2020, October). Interactive Visualizations to Introduce Data Science for High School Students. In Proceedings of the 21st Annual Conference on Information Technology Education. (pp. 236–241). https://doi.org/10.1145/3368308.3415360
Chiu, T. K. F. (2021). A holistic approach to the design of artificial intelligence (AI) education for k-12 schools. TechTrends, 65 (5), 796–807. https://doi.org/10.1007/s11528-021-00637-1
Chiu, T. K., & Chai, C.-S. (2020). Sustainable curriculum planning for artificial intelligence education: A self-determination theory perspective. Sustainability (switzerland) . https://doi.org/10.3390/su12145568
Chiu, T. K., Meng, H., Chai, C. S., King, I., Wong, S., & Yam, Y. (2021). Creation and evaluation of a pretertiary artificial intelligence (AI) curriculum. IEEE Transactions on Education, 65 (1), 30–39. https://doi.org/10.1109/TE.2021.3085878
Chounta, I.-A., Bardone, E., Raudsep, A., & Pedaste, M. (2021). Exploring teachers’ perceptions of artificial intelligence as a tool to support their practice in estonian k-12 education. International Journal of Artificial Intelligence in Education, 32 , 725–755. https://doi.org/10.1007/s40593-021-00243-5
Crompton, H., Jones, M. V., & Burke, D. (2022). Affordances and challenges of artificial intelligence in K-12 education: a systematic review. Journal of Research on Technology in Education . https://doi.org/10.1080/15391523.2022.2121344
Croxell, J., Mead, R., & Weinberg, J. (2007). Designing robot competitions that promote ai solutions: Lessons learned competing and designing. Technical Report of the 2007 American Association of Artificial Intelligence. Spring Symposia , SS-07–09. (pp. 29–34).
Cummings D., Anthony M., Watson C., Watson A., & Boone S. (2021). Combating social injustice and misinformation to engage minority youth in computing sciences. In Proceedings of the 52nd ACM Technical Symposium on Computer Science Education. (pp.1006–1012). https://doi.org/10.1145/3408877.3432452 .
Dai, Y., Liu, A., Qin, J., Guo, Y., Jong, M. S. Y., Chai, C. S., & Lin, Z. (2023). Collaborative construction of artificial intelligence curriculum in primary schools. Journal of Engineering Education, 112 (1), 23–42. https://doi.org/10.1002/jee.20503
Díaz, J., Queiruga, C., Tzancoff, C., Fava, L., & Harari, V. (2015). Educational robotics and videogames in the classroom. In 2015 10th Iberian Conference on Information Systems and Technologies (CISTI) . Aveiro, Portugal. (pp. 1–6). https://doi.org/10.1109/CISTI.2015.7170616
du Boulay, B. (2016). Artificial intelligence as an effective classroom assistant. IEEE Intelligent Systems, 31 (6), 76–81. https://doi.org/10.1109/MIS.2016.93
Eguchi, A. (2021). AI-robotics and ai literacy. Studies in Computational Intelligence, 982 , 75–85. https://doi.org/10.1007/978-3-030-77022-8
Eguchi, A., & Okada, H. (2018). If you give students a social robot? - world robot summit pilot study. In Companion of the 2018 ACM/IEEE International Conference on Human-Robot Interaction. (pp. 103–104). https://doi.org/10.1145/3173386.3177038
Eguchi, A., & Okada, H. (2020). Imagine the Future with Social Robots - World Robot Summit’s Approach: Preliminary Investigation. In M. Moro, D. Alimisis, & L. Iocchi, L. (Eds) Educational Robotics in the Context of the Maker Movement. Edurobotics 2018. Advances in Intelligent Systems and Computing, (p. 946). Springer. https://doi.org/10.1007/978-3-030-18141-3_10
Eguchi, A., Okada, H., & Muto, Y. (2021). Contextualizing AI education for k- 12 students to enhance their learning of ai literacy through culturally responsive approaches. KI Kunstliche Intelligenz, 35 (2), 153–161. https://doi.org/10.1007/s13218-021-00737-3
Ellis, G., Ory, E., Bhushan, N. (2005). Organizing a K-12 AI curriculum using philosophy of the mind. Engineering: Faculty Publications, Smith College. Retrieved from https://scholarworks.smith.edu/egr_facpubs/96
Ellis, G., Silva, K., Epstein, T., & Giammaria, N. (2009). Artificial intelligence in pre-college education: Learning within a philosophy of the mind framework. International Journal of Engineering Education, 25 (3), 511–522.
Google Scholar
Evangelista, I., Blesio, G., & Benatti, E. (2019). Why are we not teaching machine learning at high school? a proposal. In 2018 World Engineering Education Forum - Global Engineering Deans Council (WEEF-GEDC). (pp. 1–6). https://doi.org/10.1109/WEEF-GEDC.2018.8629750
Gao, J., & Wang, L. (2019). Reverse thinking teaching discussion in high school information technology under new curriculum standards. In 14th International Conference on Computer Science & Education (ICCSE). (pp. 222–226). https://doi.org/10.1109/ICCSE.2019.8845429
Giannakos, M., Voulgari, I., Papavlasopoulou, S., Papamitsiou, Z., & Yannakakis, G. (2020). Games for artificial intelligence and machine learning education: Review and perspectives. Lecture Notes in Educational Technology . https://doi.org/10.1007/978-981-15-6747-6_7
Gong, X., Zhao, L., Tang, R., Guo, Y., Liu, X., He, J., … Wang, X. (2019). AI education system for primary and secondary schools. In 2019 ASEE Annual Conference & Exposition .
Gonzalez, A. J., Hollister, J. R., DeMara, R. F., Leigh, J., Lanman, B., Lee, S. Y., & Wilder, B. (2017). AI in informal science education: bringing turing back to life to perform the turing test. International Journal of Artificial Intelligence in Education, 27 (2), 353–384. https://doi.org/10.1007/s40593-017-0144-1
Guan, C., Mou, J., & Jiang, Z. (2020). Artificial intelligence innovation in education: A twenty-year data-driven historical analysis. International Journal of Innovation Studies, 4 (4), 134–147. https://doi.org/10.1016/j.ijis.2020.09.001
Gutiérrez, L. F., & Henriques, A. (2020). Prospective mathematics teachers’ tpack in a context of a teacher education experiment. Revista Latinoamericana De Investigación En Matemática Educativa, 23 (2), 175–202. https://doi.org/10.12802/relime.20.2322
Han, X., Hu, F., Xiong, G., Liu, X., Gong, X., Niu, X., … Wang, X. (2019). Design of AI + curriculum for primary and secondary schools in Qingdao. In Chinese Automation Congress (CAC) . (pp. 4135–4140). https://doi.org/10.1109/CAC.2018.8623310
Harris, E., Lamonica, A., & Weinberg. JB. (2004) Interfacing the public and technology: a web controlled mobile robot. In Accessible hands-on artificial intelligence and robotics education: working papers of the 2004. AAAI spring symposium series. AAAI Press. (pp.106–110)
He, Y.-T., Guo, B.-J., Lu, J., Xu, Y.-P., & Gong, M. (2020). Research of scratch programming recommendation system based on med and knowledge graph. In 2020 5th International Conference on Mechanical, Control and Computer Engineering (ICMCCE) . (pp. 2158–2163). https://doi.org/10.1109/ICMCCE51767.2020.00469
Heintz, F. (2021). Three interviews about k-12 ai education in america, europe, and singapore. KI Kunstliche Intelligenz, 35 (2), 233–237. https://doi.org/10.1007/s13218-021-00730-w
Henry, J., Hernalesteen, A., & Collard, A.-S. (2021). Teaching artificial intelli- gence to k-12 through a role-playing game questioning the intelligence concept. KI Kunstliche Intelligenz, 35 (2), 171–179. https://doi.org/10.1007/s13218-021-00733-7
Herrero, Á., Cambra, C., Urda, D., Sedano, J., Quintián, H., & Corchado, E. (Eds) (2020). 11th International Conference on European Transnational Educational (ICEUTE 2020). ICEUTE 2020. Advances in Intelligent Systems and Computing, 1266 . Springer. https://doi.org/10.1007/978-3-030-57799-5_8
Ho, J. W., Scadding, M., Kong, S. C., Andone, D., Biswas, G., Hoppe, H. U., & Hsu, T. C. (2019). Classroom activities for teaching artificial intelligence to primary school students. In Proceedings of international conference on computational thinking education. The Education University of Hong Kong. (pp. 157–159).
Holowka, P. (2020). Teaching robotics during COVID-19: Machine learning, simulation, and aws deepracer. In 17th International Conference on Cognition and Exploratory Learning in Digital Age, CELDA .
Holstein, K., McLaren, B. M., & Aleven, V. (2019). Designing for complementarity: Teacher and student needs for orchestration support in ai-enhanced classrooms. In S. Isotani, E. Millán, A. Ogan, P. Hastings, B. McLaren, & R. Luckin (Eds.), Artificial intelligence in education. AIED 2019. Lecture notes in computer science (p. 11625). Springer. 10.1007/978-3-030-23204-7_14.
Itmazi, J., & Khlaif, Z. N. (2022). Science education in Palestine: Hope for a better future. Lecture Notes in Educational Technology . https://doi.org/10.1007/978-981-16-6955-2_9
Jagannathan, R. K., & Komives, C. (2019). Teaching by induction: Project- based learning for Silicon Valley. Journal of Engineering Education Transformations, 33 (1), 22–26. https://doi.org/10.16920/jeet/2019/v33i1/149003
Jaskie, K., Larson, J., Johnson, M., Turner, K., O’Donnell, M., Christen, J.B., & Spanias, A. (2021). Research experiences for teachers in machine learning. In IEEE Frontiers in Education Conference (FIE) . Lincoln, NE, USA. (pp. 1–5). https://doi.org/10.1109/FIE49875.2021.9637132
Judd, S. (2020). Activities for Building Understanding: How AI4ALL Teaches AI to Diverse High School Students. In Proceedings of the 51st ACM Technical Symposium on Computer Science Education. (pp. 633–634). https://doi.org/10.1145/3328778.3366990
Kahn, K., Megasari, R., Piantari, E., & Junaeti, E. (2018). AI programming by children using Snap! block programming in a developing country. In Thirteenth European Conference on Technology Enhanced Learning . (p. 11082). https://doi.org/10.1007/978-3-319-98572-5
Kandlhofer, M., Steinbauer, G., Hirschmugl-Gaisch, S., & Huber, P. (2016). Artificial intelligence and computer science in education: From kinder- garten to university. In IEEE Frontiers in Education Conference. (pp. 1–9). https://doi.org/10.1109/FIE.2016.7757570
Kandlhofer, M., Steinbauer, G., Lasnig, J.P., Baumann, W., Plomer, S., Ballagi, A., & Alfoldi, I. (2019). Enabling the creation of intelligent things: Bringing artificial intelligence and robotics to schools. In IEEE Frontiers in Education Conference (FIE) . (pp. 1–5). https://doi.org/10.1109/FIE43999.2019.9028537
Kandlhofer, M., & Steinbauer, G. (2021). AI k-12 education service. KI Kunstliche Intelligenz, 35 (2), 125–126. https://doi.org/10.1007/s13218-021-00715-9
Kandlhofer, M., Steinbauer, G., Lassnig, J., Menzinger, M., Baumann, W., Ehardt-Schmiederer, M., & Szalay, I. (2021). EDLRIS: A European driving license for robots and intelligent systems. KI Kunstliche Intelligenz, 35 (2), 221–232. https://doi.org/10.1007/s13218-021-00716-8
Kaspersen, M. H., Bilstrup, K. E. K., Van Mechelen, M., Hjort, A., Bouvin, N. O., & Petersen, M. G. (2022). High school students exploring machine learning and its societal implications Opportunities and challenges. International Journal of Child-Computer Interaction, 34 , 1–12. https://doi.org/10.1016/j.ijcci.2022.100539
Kelly, J., Binney, J., Pereira, A., Khan, O., & Sukhatme, G. (2008). Just add wheels: Leveraging commodity laptop hardware for robotics and ai education. In Proceedings of AAAI Education Colloquium , 22.
Kim, K., Kwon, K., Ottenbreit-Leftwich, A., Bae, H., & Glazewski, K. (2023). Exploring middle school students’ common naive conceptions of Artificial Intelligence concepts, and the evolution of these ideas. Education and Information Technologies . https://doi.org/10.1007/s10639-023-11600-3
Kim, S., Jang, Y., Choi, S., Kim, W., Jung, H., Kim, S., & Kim, H. (2021). Analyzing teacher competency with tpack for k-12 ai education. KI Kunstliche Intelligenz, 35 (2), 139–151. https://doi.org/10.1007/s13218-021-00731-9
Kitchenham, B. (2004). Procedures for performing systematic reviews (Vol. 33, pp. 1–26). Keele: Keele University.
Knijnenburg, B., Bannister, N., & Caine, K. (2021). Using mathematically- grounded metaphors to teach ai-related cybersecurity. In IJCAI-21 Workshop on Adverse Impacts and Collateral Effects of Artificial Intelligence Technologies (AIofAI) .
Kong, S. C., ManYinCheung, W., & Zhang, G. (2021). Evaluation of an artificial intelligence literacy course for university students with diverse study backgrounds. Computers and Education: Artificial Intelligence . https://doi.org/10.1016/j.caeai.2021.100026
Korenova, L. (2016). Digital technologies in teaching mathematics on the faculty of education of the Comenius University in Bratislava. In 15 Conference on Applied Mathematics. Slovak University of Technology in Bratislava. (p. 690–699).
Lee, S., Mott, B., Ottenbriet-Leftwich, A., Scribner, A., Taylor, S., Glazewski, K.,…Lester, J. (2020). Designing a collaborative game-based learning environment for ai-infused inquiry learning in elementary school class- rooms. In Proceedings of the 2020 ACM conference on innovation and technology in computer science education . (pp. 566–566). https://doi.org/10.1145/3341525.3393981
Lee, I., Ali, S., Zhang, H., Dipaola, D., & Breazeal, C. (2021). Developing middle school students’ ai literacy. In Association for Computing Machinery, Inc. (pp. 191–197). https://doi.org/10.1145/3408877.3432513
Lenoir, Y., & Hasni, A. (2016). Interdisciplinarity in primary and secondary school: Issues and perspectives. Creative Education, 7 (16), 2433–2458. https://doi.org/10.4236/ce.2016.716233
Lin, P., & Brummelen, J. (2021). Engaging teachers to co-design integrated ai curriculum for k-12 classrooms. In CHI '21: Proceedings of the 2021 CHI Conference on Human Factors in Computing Systems . (pp.1–12). https://doi.org/10.1145/3411764.3445377
Lin, X. F., Chen, L., Chan, K. K., Peng, S., Chen, X., Xie, S., & Hu, Q. (2022). Teachers’ perceptions of teaching sustainable artificial intelligence: A design frame perspective. Sustainability, 14 (13), 1–20. https://doi.org/10.3390/su14137811
Lindner, A., & Berges, M. (2020). Can you explain ai to me? teachers’ pre- concepts about artificial intelligence. In IEEE Frontiers in Education Conference (FIE). (pp. 1–9). https://doi.org/10.1109/FIE44824.2020.9274136
Long, D., & Magerko, B. (2020). What is AI literacy? Competencies and design considerations. In Proceedings of the 2020 chi conference on human factors in computing systems. (pp. 1–16). https://doi.org/10.1145/3313831.3376727
Malach, J., & Vicherková, D. (2020). Background of the Revision of the Secondary School Engineering Curriculum in the Context of the Society 4.0. In M. Auer, H. Hortsch & P. Sethakul (Eds). The Impact of the 4th Industrial Revolution on Engineering Education. ICL Advances in Intelligent Systems and Computing, vol 1135. Springer. https://doi.org/10.1007/978-3-030-40271-6_27
Matarić, M.J., Koenig, N., & Feil-Seifer, D. (2007). Materials for enabling hands- on robotics and stem education. In AAAI Spring Symposium: Semantic Scientific Knowledge Integration. (pp. 99–102). http://www.aaai.org/Papers/Symposia/Spring/2007/SS-07-09/SS07-09-022.pdf
Miao, F., Holmes, W., Huang, R., & Zhang, H. (2021). AI and education: A guidance for policymakers . UNESCO Publishing.
Micheuz, P. (2020). Approaches to Artificial Intelligence as a Subject in School Education. In T. Brinda, D. Passey, & T. Keane (Eds), Empowering Teaching for Digital Equity and Agency. OCCE 2020. IFIP Advances in Information and Communication Technology, 595. Springer. https://doi.org/10.1007/978-3-030-59847-1_1
Mishra, P., & Koehler, M. J. (2006). Technological pedagogical content knowledge: A framework for teacher knowledge. Teachers College Record, 108 (6), 1017–1054. https://doi.org/10.1111/j.1467-9620.2006.00684.x
Mongan, W.M., & Regli, W.C. (2008). A cyber-infrastructure for supporting k-12 engineering education through robotics, WS-08-02, 68–73.
Narahara, T., & Kobayashi, Y. (2018). Personalizing homemade bots with plug & play ai for steam education. In SIGGRAPH Asia 2018 technical briefs . (pp. 1–4). https://doi.org/10.1145/3283254.3283270
Ng, D. T. K., Lee, M., Tan, R. J. Y., Hu, X., Downie, J. S., & Chu, S. K. W. (2022). A review of AI teaching and learning from 2000 to 2020. Education and Information Technologies . https://doi.org/10.1007/s10639-022-11491-w
Nisheva-Pavlova, M.M. (2021). Ai courses for secondary and high school - comparative analysis and conclusions. In CEUR Workshop Proceedings, 3061. (pp. 9–16).
Nurbekova, Z., Mukhamediyeva, K., & Assainova, A. (2018). Educational robotics technologies in Kazakhstan and in the world: Comparative analysis, current state and perspectives. Astra Salvensis, 6 (1), 665–686.
Oh, W. (2020). Physics teachers’ perception of it convergence-based physics education. New Physics: Sae Mulli, 70 (8), 660–666. https://doi.org/10.3938/NPSM.70.660
Olari, V., & Romeike, R. (2021). Addressing ai and data literacy in teacher education: A review of existing educational frameworks. In WiPSCE '21: The 16th Workshop in Primary and Secondary Computing Education, 17. (pp. 1–2) https://doi.org/10.1145/3481312.3481351
Ottenbreit-Leftwich, A., Glazewski, K., Jeon, M., Hmelo-Silver, C., Mott, B., Lee, S., & Lester, J. (2021). How do elementary students conceptualize artificial intelligence? In SIGCSE '21: Proceedings of the 52nd ACM Technical Symposium on Computer Science Education . (pp. 1261). https://doi.org/10.1145/3408877.3439642
Park, K., Mott, B., Lee, S., Glazewski, K., Scribner, J., Ottenbreit-Leftwich, A., & Lester, J. (2021). Designing a visual interface for elementary students to formulate ai planning tasks. In IEEE Symposium on Visual Languages and Human-Centric Computing (VL/HCC). (pp. 1–9). https://doi.org/10.1109/VL/HCC51201.2021.9576163
Perlin, K., Flanagan, M., & Hollingshead, A. (2005). The Rapunsel Project. In Subsol, G. (Eds). Virtual Storytelling. Using Virtual Reality Technologies for Storytelling. ICVS 2005. Lecture Notes in Computer Science , 3805. Springer. https://doi.org/10.1007/11590361_29
Reyes, A., Elkin, C., Niyaz, Q., Yang, X., Paheding, S., & Devabhaktuni, V. (2020). A preliminary work on visualization-based education tool for high school machine learning education. In IEEE Integrated STEM Education Conference (ISEC). (pp. 1–5). https://doi.org/10.1109/ISEC49744.2020.9280629
Rodríguez-García, J., Moreno-León, J., Román-González, M., & Robles, G. (2020). Introducing artificial intelligence fundamentals with learning ML: Artificial intelligence made easy. In TEEM'20: Eighth International Conference on Technological Ecosystems for Enhancing Multiculturality . (pp. 18–20). https://doi.org/10.1145/3434780.3436705
Russell, S. (2021). The history and future of AI. Oxford Review of Economic Policy, 37 (3), 509–520. https://doi.org/10.1093/oxrep/grab013
Russell, S., & Norvig, P. (2021). Artificial Intelligence, global edition a modern approach . Pearson Deutschland.
Sabuncuoglu, A. (2020). Designing one year curriculum to teach artificial intelligence for middle school. In Proceedings of the 2020 ACM conference on innovation and technology in computer science education . (pp. 96–102). https://doi.org/10.1145/3341525.3387364
Sakulkueakulsuk, B., Witoon, S., Ngarmkajornwiwat, P., Pataranutapom, P., Surareungchai, W., Pataranutaporn, P., & Subsoontorn, P. (2019). Kids making ai: Integrating machine learning, gamification, and social context. In 2018 IEEE international conference on teaching, assessment, and learning for engineering (TALE) . (pp. 1005–1010). https://doi.org/10.1109/TALE.2018.8615249
Sanusi, I. T., Oyelere, S. S., Vartiainen, H., Suhonen, J., & Tukiainen, M. (2022). A systematic review of teaching and learning machine learning in K-12 education. Education and Information Technologies . https://doi.org/10.1007/s10639-022-11416-7
Shin, S. (2021). A study on the framework design of artificial intelligence thinking for artificial intelligence education. International Journal of Information and Education Technology, 11 (9), 392–397. https://doi.org/10.18178/ijiet.2021.11.9.1540
Sing, C. C., Teo, T., Huang, F., Chiu, T. K., & Xing Wei, W. (2022). Secondary school students’ intentions to learn AI: Testing moderation effects of readiness, social good and optimism. Educational Technology Research and Development, 70 (3), 765–782. https://doi.org/10.1007/s11423-022-10111-1
Sorensen, L., & Koefoed, N. (2018). The future of teaching—what are students’ expectations. In 2018 11th CMI International Conference: Prospects and Challenges Towards Developing a Digital Economy within the EU . (pp. 62–66). https://doi.org/10.1109/PCTDDE.2018.8624771
Sperling, A., & Lickerman, D. (2012). Integrating AI and machine learning in software engineering course for high school students. In Proceedings of the 17th ACM annual conference on Innovation and technology in computer science education . (pp. 244–249). https://doi.org/10.1145/2325296.2325354
Su, J., Zhong, Y., & Ng, D. T. K. (2022). A meta-review of literature on educational approaches for teaching AI at the K-12 levels in the Asia-Pacific region. Computers and Education: Artificial Intelligence, 3 , 1–18. https://doi.org/10.1016/j.caeai.2022.100065
Suh, W., & Ahn, S. (2022). Development and validation of a scale measuring student attitudes toward artificial intelligence. SAGE Open, 12 (2), 1–12. https://doi.org/10.1177/21582440221100463
Summers, B.G., Hicks, H., & Oliver, C. (1995). Reaching minority, female and disadvantaged students. In Proceedings Frontiers in Education 1995 25th Annual Conference. Engineering Education for the 21st Century , 1 . (992a4–16). https://doi.org/10.1109/FIE.1995.483030
Tedre, M., Toivonen, T., Kahila, J., Vartiainen, H., Valtonen, T., Jormanainen, I., & Pears, A. (2021). Teaching machine learning in k-12 classroom: Pedagogical and technological trajectories for artificial intelligence education. IEEE Access, 9 , 110558–110572. https://doi.org/10.1109/ACCESS.2021.3097962
Tims, H., Turner III, G., Cazes, G., & Marshall, J. (2012). Junior cyber discovery: Creating a vertically integrated middle school cyber camp. In 2012 ASEE Annual Conference & Exposition. (pp. 25–867). Retrieved from https://peer.asee.org/21624
Toivonen, T., Jormanainen, I., Kahila, J., Tedre, M., Valtonen, T., Vartiainen, H. (2020). Co-designing machine learning apps in k-12 with primary school children. In 2020 IEEE 20th International Conference on Advanced Learning Technologies (ICALT). IEEE. (pp. 308–310). https://doi.org/10.1109/ICALT49669.2020.00099
Touretzky, D., Gardner-McCune, C., Breazeal, C., Martin, F., & Seehorn, D. (2019a). A year in k-12 ai education. AI Magazine, 40 (4), 88–90. https://doi.org/10.1609/aimag.v40i4.5289
Touretzky, D., Gardner-McCune, C., Martin, F., & Seehorn, D. (2019b). Envisioning ai for k-12: What should every child know about ai? Proceedings of the AAAI Conference on Artificial Intelligence, 33 (01), 9795–9799. https://doi.org/10.1609/aaai.v33i01.33019795
Vachovsky, M., Wu, G., Chaturapruek, S., Russakovsky, O., Sommer, R., & Fei-Fei, L. (2016). Towards more gender diversity in cs through an arti- ficial intelligence summer program for high school girls. In Proceedings of the 47th ACM technical symposium on computing science education. (pp. 303–308). https://doi.org/10.1145/2839509.2844620
Van Brummelen, J., Heng, T., & Tabunshchyk, V. (2021a). Teaching tech to talk: K-12 conversational artificial intelligence literacy curriculum and development tools. Proceedings of the AAAI Conference on Artificial Intelligence, 35 (17), 15655–15663. https://doi.org/10.1609/aaai.v35i17.17844
Van Brummelen, J., Tabunshchyk, V., & Heng, T. (2021b). Alexa, can i program you? Student perceptions of conversational artificial intelligence before and after programming Alexa. In IDC '21: Interaction Design and ChildrenJune. (pp. 305–313) https://doi.org/10.1145/3459990.3460730
Vartiainen, H., Tedre, M., & Valtonen, T. (2020). Learning machine learning with very young children: Who is teaching whom? International Journal of Child-Computer Interaction, 25 , 1–11. https://doi.org/10.1016/j.ijcci.2020.100182
Vartiainen, H., Toivonen, T., Jormanainen, I., Kahila, J., Tedre, M., & Valtonen, T. (2021). Machine learning for middle schoolers: Learning through data- driven design. International Journal of Child-Computer Interaction, 29 , 1–12. https://doi.org/10.1016/j.ijcci.2021.100281
Verner, I., Cuperman, D., & Reitman, M. (2021). Exploring robot connectivity and collaborative sensing in a high-school enrichment program. Robotics, 10 (1), 1–19. https://doi.org/10.3390/robotics10010013
von Wangenheim, C. G., Hauck, J. C., Pacheco, F. S., & Bueno, M. F. B. (2021). Visual tools for teaching machine learning in K-12: A ten-year systematic mapping. Education and Information Technologies, 26 (5), 5733–5778. https://doi.org/10.1007/s10639-021-10570-8
Wan, X., Zhou, X., Ye, Z., Mortensen, C., & Bai, Z. (2020). Smileyclus- ter: Supporting accessible machine learning in k-12 scientific discovery. In proceedings of the Interaction Design and Children Conference. (pp. 23–35). https://doi.org/10.1145/3392063.3394440
Wang, H., Liu, Y., Han, Z., & Wu, J. (2020). Extension of media literacy from the perspective of artificial intelligence and implementation strategies of artificial intelligence courses in junior high schools. In 2020 International Conference on Artificial Intelligence and Education (ICAIE). (pp. 63–66). https://doi.org/10.1109/ICAIE50891.2020.00022
Wei, Y. (2021). Influence factors of using modern teaching technology in the classroom of junior middle school teachers under the background of artificial intelligence-analysis based on HLM. Advances in Intelligent Systems and Computing, 1282 , 110–118. https://doi.org/10.1007/978-3-030-62743-0_16
Wei, Q., Li, M., Xiang, K., & Qiu, X. (2020). Analysis and strategies of the professional development of information technology teachers under the vision of artificial intelligence. In 2020 15th International Conference on Computer Science & Education (ICCSE). (pp. 716–721). https://doi.org/10.1109/ICCSE49874.2020.9201652
West, D.M., & Allen, J.R. (2018). How artificial intelligence is transforming the world. Report. Retrieved April 24, 2018, f rom https://www.brookings.edu/research/how-artificial-intelligence-is-transforming-the-world/
Wong, K.-C. (2020). Computational thinking and artificial intelligence education: A balanced approach using both classical AI and modern AI. CoolThink@ JC , 108.
Wong, G. K., Ma, X., Dillenbourg, P., & Huen, J. (2020). Broadening artificial intelligence education in k-12: Where to start? ACM Inroads, 11 (1), 20–29. https://doi.org/10.1145/3381884
Woo, H., Kim, J., Kim, J., & Lee, W. (2020). Exploring the ai topic composition of k-12 using nmf-based topic modeling. International Journal on Advanced Science, Engineering and Information Technology, 10 (4), 1471–1476. https://doi.org/10.18517/ijaseit.10.4.12787
Wu, D., Zhou, C., Meng, C., & Chen, M. (2020). Identifying multilevel factors influencing ICT self-efficacy of k-12 teachers in China. In Blended Learning. Education in a Smart Learning Environment: 13th International Conference, ICBL 2020. (pp. 303–314). Springer International Publishing. https://doi.org/10.1007/978-3-030-51968-1
Xia, Q., Chiu, T. K., & Chai, C. S. (2022). The moderating effects of gender and need satisfaction on self-regulated learning through Artificial Intelligence (AI). Education and Information Technologies . https://doi.org/10.1007/s10639-022-11547-x
Xia, L., & Zheng, G. (2020). To meet the trend of AI: The ecology of developing ai talents for pre-service teachers in China. International Journal of Learning, 6 (3), 186–190. https://doi.org/10.18178/IJLT.6.3.186-190
Xiao, W., & Song, T. (2021). Current situation of artificial intelligence education in primary and secondary schools in China. In The Sixth International Conference on Information Management and Technology. (pp. 1–4). https://doi.org/10.1145/3465631.3465980
Yau, K. W., Chai, C. S., Chiu, T. K., Meng, H., King, I., Wong, S. W. H., & Yam, Y. (2022). Co-designing artificial intelligence curriculum for secondary schools: A grounded theory of teachers' experience. In 2022 International Symposium on Educational Technology (ISET). (pp. 58–62). https://doi.org/10.1109/ISET55194.2022.00020
Yue, M., Dai, Y., Siu-Yung, M., & Chai, C.-S. (2021). An analysis of k-12 artificial intelligence curricula in eight countries. In Proceedings of the 29th International Conference on Computers in Education. (pp. 22–26).
Yue, M., Jong, M. S. Y., & Dai, Y. (2022). Pedagogical design of K-12 artificial intelligence education: A systematic review. Sustainability, 14 (23), 15620. https://doi.org/10.3390/su142315620
Zhai, X., Chu, X., Chai, C. S., Jong, M. S. Y., Istenic, A., Spector, M., & Li, Y. (2021). A review of artificial intelligence (AI) in education from 2010 to 2020. Complexity . https://doi.org/10.1155/2021/8812542
Zhang, N., Biswas, G., McElhaney, K.W., Basu, S., McBride, E., & Chiu, J.L. (2020). Studying the interactions between science, engineering, and computational thinking in a learning-by-modeling environment. In International conference on artificial intelligence in education. (pp. 598–609). Springer.
Download references
Acknowledgements
Authors would like to thank the reviewers and editors, whose comments and feedback helped us to improve the original manuscript.
This work has partially been funded by the Spanish Ministry of Science, Innovation and Universities (PID2021-123152OB-C21), and the Consellería de Educación, Universidade e Formación Profesional (accreditation 2019–2022 ED431C2022/19 and reference competitive group, ED431G2019/04) and the European Regional Development Fund (ERDF), which acknowledges the CiTIUS—Centro Singular de Investigación en Tecnoloxías Intelixentes da Universidade de Santiago de Compostela as a Research Center of the Galician University System. This work also received support from the Educational Knowledge Transfer (EKT), the Erasmus + project (reference number 612414-EPP-1-2019-1-ES-EPPKA2-KA) and the Knowledge Alliances call (Call EAC/A03/2018).
Author information
Authors and affiliations.
Pedagogy and Didactics Department, University of Santiago de Compostela, 15782, Santiago de Compostela, Spain
Lorena Casal-Otero, Carmen Fernández-Morante & Beatriz Cebreiro
Departamento de Electrónica e Computación, Universidade de Santiago de Compostela, 15782, Santiago de Compostela, Spain
Alejandro Catala & Maria Taboada
Centro Singular de Investigación en Tecnoloxías Intelixentes (CiTIUS), Universidade de Santiago de Compostela, 15782, Santiago de Compostela, Spain
Alejandro Catala & Senén Barro
You can also search for this author in PubMed Google Scholar
Contributions
All authors have contributed significantly to the authorship of this work in all stages of conceptualization, discussions, definition of the methodology, carrying out the analysis as well as writing—review & editing. All authors read and approved the final manuscript.
Corresponding author
Correspondence to Alejandro Catala .
Ethics declarations
Ethics approval and consent to participate.
This research is carried out in accordance to ethics recommendations. As it focuses on a systematic literature review as a research method, ethics approval by the University ethics committee does not apply.
Competing interests
The authors declare that they have no competing interests. The authors have no other relevant financial or non-financial interests to disclose and no further competing interests to declare that are relevant to the content of this article.
Additional information
Publisher's note.
Springer Nature remains neutral with regard to jurisdictional claims in published maps and institutional affiliations.
Supplementary Information
Additional file 1..
Additional listing.
Rights and permissions
Open Access This article is licensed under a Creative Commons Attribution 4.0 International License, which permits use, sharing, adaptation, distribution and reproduction in any medium or format, as long as you give appropriate credit to the original author(s) and the source, provide a link to the Creative Commons licence, and indicate if changes were made. The images or other third party material in this article are included in the article's Creative Commons licence, unless indicated otherwise in a credit line to the material. If material is not included in the article's Creative Commons licence and your intended use is not permitted by statutory regulation or exceeds the permitted use, you will need to obtain permission directly from the copyright holder. To view a copy of this licence, visit http://creativecommons.org/licenses/by/4.0/ .
Reprints and permissions
About this article
Cite this article.
Casal-Otero, L., Catala, A., Fernández-Morante, C. et al. AI literacy in K-12: a systematic literature review. IJ STEM Ed 10 , 29 (2023). https://doi.org/10.1186/s40594-023-00418-7
Download citation
Received : 06 September 2022
Accepted : 30 March 2023
Published : 19 April 2023
DOI : https://doi.org/10.1186/s40594-023-00418-7
Share this article
Anyone you share the following link with will be able to read this content:
Sorry, a shareable link is not currently available for this article.
Provided by the Springer Nature SharedIt content-sharing initiative
- Secondary education
- Teaching/learning strategies
- Twenty-first century skills
- Cultural and social implications
Numbers, Facts and Trends Shaping Your World
Read our research on:
Full Topic List
Regions & Countries
- Publications
- Our Methods
- Short Reads
- Tools & Resources
Read Our Research On:
Partisan divides over K-12 education in 8 charts
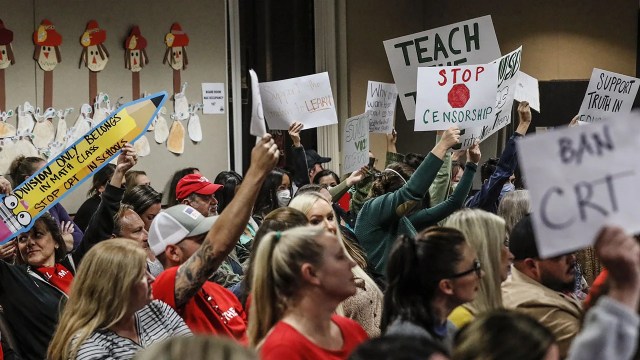
K-12 education is shaping up to be a key issue in the 2024 election cycle. Several prominent Republican leaders, including GOP presidential candidates, have sought to limit discussion of gender identity and race in schools , while the Biden administration has called for expanded protections for transgender students . The coronavirus pandemic also brought out partisan divides on many issues related to K-12 schools .
Today, the public is sharply divided along partisan lines on topics ranging from what should be taught in schools to how much influence parents should have over the curriculum. Here are eight charts that highlight partisan differences over K-12 education, based on recent surveys by Pew Research Center and external data.
Pew Research Center conducted this analysis to provide a snapshot of partisan divides in K-12 education in the run-up to the 2024 election. The analysis is based on data from various Center surveys and analyses conducted from 2021 to 2023, as well as survey data from Education Next, a research journal about education policy. Links to the methodology and questions for each survey or analysis can be found in the text of this analysis.
Most Democrats say K-12 schools are having a positive effect on the country , but a majority of Republicans say schools are having a negative effect, according to a Pew Research Center survey from October 2022. About seven-in-ten Democrats and Democratic-leaning independents (72%) said K-12 public schools were having a positive effect on the way things were going in the United States. About six-in-ten Republicans and GOP leaners (61%) said K-12 schools were having a negative effect.
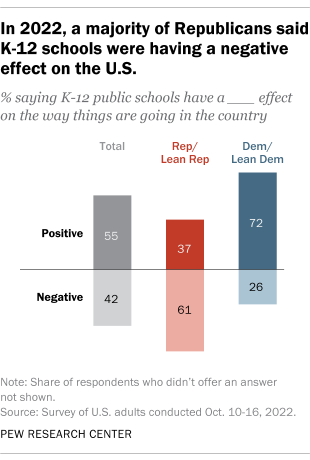
About six-in-ten Democrats (62%) have a favorable opinion of the U.S. Department of Education , while a similar share of Republicans (65%) see it negatively, according to a March 2023 survey by the Center. Democrats and Republicans were more divided over the Department of Education than most of the other 15 federal departments and agencies the Center asked about.
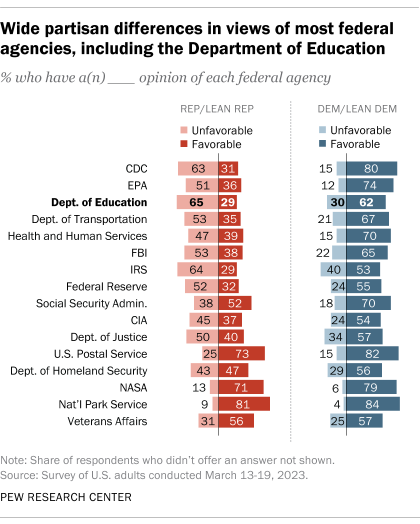
In May 2023, after the survey was conducted, Republican lawmakers scrutinized the Department of Education’s priorities during a House Committee on Education and the Workforce hearing. The lawmakers pressed U.S. Secretary of Education Miguel Cardona on topics including transgender students’ participation in sports and how race-related concepts are taught in schools, while Democratic lawmakers focused on school shootings.
Partisan opinions of K-12 principals have become more divided. In a December 2021 Center survey, about three-quarters of Democrats (76%) expressed a great deal or fair amount of confidence in K-12 principals to act in the best interests of the public. A much smaller share of Republicans (52%) said the same. And nearly half of Republicans (47%) had not too much or no confidence at all in principals, compared with about a quarter of Democrats (24%).
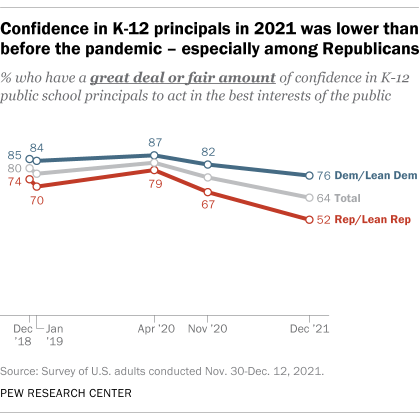
This divide grew between April 2020 and December 2021. While confidence in K-12 principals declined significantly among people in both parties during that span, it fell by 27 percentage points among Republicans, compared with an 11-point decline among Democrats.
Democrats are much more likely than Republicans to say teachers’ unions are having a positive effect on schools. In a May 2022 survey by Education Next , 60% of Democrats said this, compared with 22% of Republicans. Meanwhile, 53% of Republicans and 17% of Democrats said that teachers’ unions were having a negative effect on schools. (In this survey, too, Democrats and Republicans include independents who lean toward each party.)
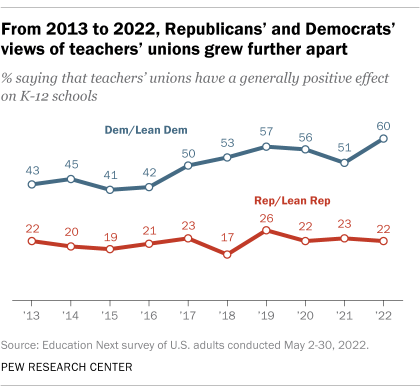
The 38-point difference between Democrats and Republicans on this question was the widest since Education Next first asked it in 2013. However, the gap has exceeded 30 points in four of the last five years for which data is available.
Republican and Democratic parents differ over how much influence they think governments, school boards and others should have on what K-12 schools teach. About half of Republican parents of K-12 students (52%) said in a fall 2022 Center survey that the federal government has too much influence on what their local public schools are teaching, compared with two-in-ten Democratic parents. Republican K-12 parents were also significantly more likely than their Democratic counterparts to say their state government (41% vs. 28%) and their local school board (30% vs. 17%) have too much influence.
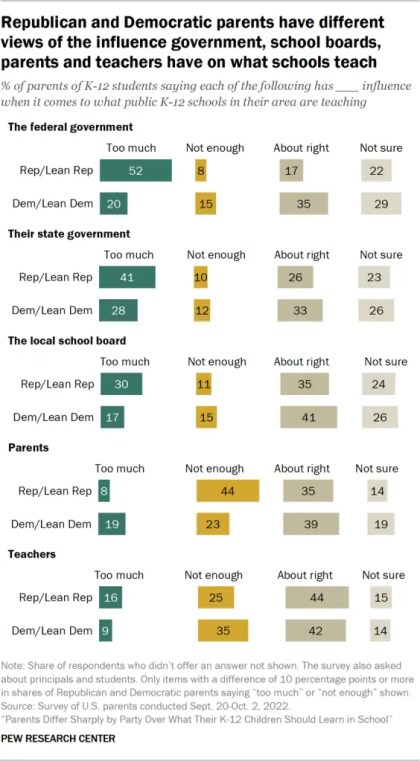
On the other hand, more than four-in-ten Republican parents (44%) said parents themselves don’t have enough influence on what their local K-12 schools teach, compared with roughly a quarter of Democratic parents (23%). A larger share of Democratic parents – about a third (35%) – said teachers don’t have enough influence on what their local schools teach, compared with a quarter of Republican parents who held this view.
Republican and Democratic parents don’t agree on what their children should learn in school about certain topics. Take slavery, for example: While about nine-in-ten parents of K-12 students overall agreed in the fall 2022 survey that their children should learn about it in school, they differed by party over the specifics. About two-thirds of Republican K-12 parents said they would prefer that their children learn that slavery is part of American history but does not affect the position of Black people in American society today. On the other hand, 70% of Democratic parents said they would prefer for their children to learn that the legacy of slavery still affects the position of Black people in American society today.
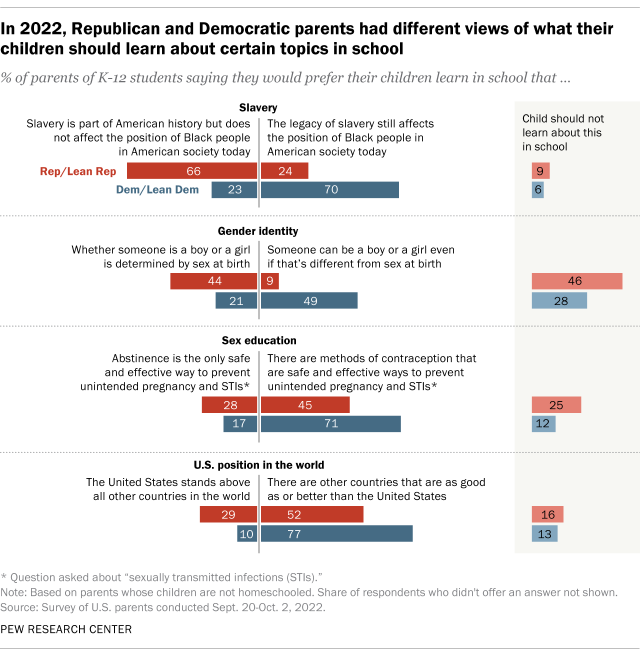
Parents are also divided along partisan lines on the topics of gender identity, sex education and America’s position relative to other countries. Notably, 46% of Republican K-12 parents said their children should not learn about gender identity at all in school, compared with 28% of Democratic parents. Those shares were much larger than the shares of Republican and Democratic parents who said that their children should not learn about the other two topics in school.
Many Republican parents see a place for religion in public schools , whereas a majority of Democratic parents do not. About six-in-ten Republican parents of K-12 students (59%) said in the same survey that public school teachers should be allowed to lead students in Christian prayers, including 29% who said this should be the case even if prayers from other religions are not offered. In contrast, 63% of Democratic parents said that public school teachers should not be allowed to lead students in any type of prayers.

In June 2022, before the Center conducted the survey, the Supreme Court ruled in favor of a football coach at a public high school who had prayed with players at midfield after games. More recently, Texas lawmakers introduced several bills in the 2023 legislative session that would expand the role of religion in K-12 public schools in the state. Those proposals included a bill that would require the Ten Commandments to be displayed in every classroom, a bill that would allow schools to replace guidance counselors with chaplains, and a bill that would allow districts to mandate time during the school day for staff and students to pray and study religious materials.
Mentions of diversity, social-emotional learning and related topics in school mission statements are more common in Democratic areas than in Republican areas. K-12 mission statements from public schools in areas where the majority of residents voted Democratic in the 2020 general election are at least twice as likely as those in Republican-voting areas to include the words “diversity,” “equity” or “inclusion,” according to an April 2023 Pew Research Center analysis .
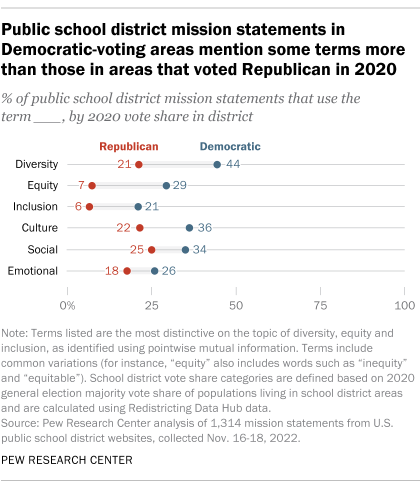
Also, about a third of mission statements in Democratic-voting areas (34%) use the word “social,” compared with a quarter of those in Republican-voting areas, and a similar gap exists for the word “emotional.” Like diversity, equity and inclusion, social-emotional learning is a contentious issue between Democrats and Republicans, even though most K-12 parents think it’s important for their children’s schools to teach these skills . Supporters argue that social-emotional learning helps address mental health needs and student well-being, but some critics consider it emotional manipulation and want it banned.
In contrast, there are broad similarities in school mission statements outside of these hot-button topics. Similar shares of mission statements in Democratic and Republican areas mention students’ future readiness, parent and community involvement, and providing a safe and healthy educational environment for students.
- Education & Politics
- Partisanship & Issues
- Politics & Policy
Jenn Hatfield is a writer/editor at Pew Research Center .
Half of Latinas Say Hispanic Women’s Situation Has Improved in the Past Decade and Expect More Gains
A quarter of u.s. teachers say ai tools do more harm than good in k-12 education, most americans think u.s. k-12 stem education isn’t above average, but test results paint a mixed picture, about 1 in 4 u.s. teachers say their school went into a gun-related lockdown in the last school year, about half of americans say public k-12 education is going in the wrong direction, most popular.
1615 L St. NW, Suite 800 Washington, DC 20036 USA (+1) 202-419-4300 | Main (+1) 202-857-8562 | Fax (+1) 202-419-4372 | Media Inquiries
Research Topics
- Age & Generations
- Coronavirus (COVID-19)
- Economy & Work
- Family & Relationships
- Gender & LGBTQ
- Immigration & Migration
- International Affairs
- Internet & Technology
- Methodological Research
- News Habits & Media
- Non-U.S. Governments
- Other Topics
- Race & Ethnicity
- Email Newsletters
ABOUT PEW RESEARCH CENTER Pew Research Center is a nonpartisan fact tank that informs the public about the issues, attitudes and trends shaping the world. It conducts public opinion polling, demographic research, media content analysis and other empirical social science research. Pew Research Center does not take policy positions. It is a subsidiary of The Pew Charitable Trusts .
Copyright 2024 Pew Research Center
Lessons in leadership: Transforming struggling US K–12 schools
In the early 2000s, Washington, DC, had the lowest math and reading achievement scores of any major metropolitan school district in the United States and had suffered years of declining enrollments. 1 Washington, DC, lagged every other large city that took the National Assessment of Educational Progress in 4th grade reading and math in 2003, 2005, and 2007. See “Mathematics and Reading Report Cards at Grades 4 and 8,” Nation’s Report Card, accessed March 2023; Total public school enrollment in Washington, DC, declined from the 1990s until the 2010s, even with the growing charter-school sector. As the charter-school sector grew, DCPS schools continued to shrink. See “Public school enrollment over time,” Office of the Deputy Mayor for Education, accessed March 2023; DCPS enrollment data prior to the mid-2000s is limited and counts may have been inaccurate; enrollment in the mid-1990s was recorded at between 72,000 and 81,000, down from a high of 150,000 in 1970. See C hildren in crisis: The failure of public education in the district , District of Columbia Financial Responsibility and Management Assistance Authority, posted by Washington Post , November 12, 1996. Fast-forward to 2017, and enrollments were increasing along with student performance. During that time, reforms were initiated under two school chancellors, Michelle Rhee and Kaya Henderson. Rhee was the first chancellor to serve District of Columbia Public Schools (DCPS) after the system was placed under mayoral control in 2007. Henderson joined as her deputy chancellor and went on to serve as chancellor from 2010 to 2016.
Under the leadership of both women, DCPS made significant improvements in basic operations, overhauled its talent strategy, implemented new curriculum that aligned to Common Core State Standards and spoke to the whole child, and streamlined accountability. Along the way, Henderson overcame common implementation challenges to successfully sustain momentum from Rhee’s tenure by motivating staff at all levels, engaging stakeholders throughout the city, and maintaining clarity of focus. In an interview with McKinsey’s Emma Dorn, Henderson shares her experiences with achieving lasting improvements in K–12 student outcomes (Exhibit 1). 2 Even with post-COVID-19 declines, academic achievement in Washington, DC, remains well above the early 2000 levels across all National Assessment of Educational Progress tests. See “District of Columbia overview,” Nation’s Report Card, accessed March 2023.
This interview has been edited for length and clarity.
Emma Dorn: What was the state of the DC public school system when you were appointed deputy chancellor under Chancellor Michelle Rhee back in June 2007, and what were your priorities as you came into the system?
Kaya Henderson: At the time, DC Public Schools was the lowest-performing urban school district in the country. 3 “Mathematics and Reading Report Cards at Grades 4 and 8,” accessed March 2023. We were losing thousands of students each year. Public education in DC was in a death spiral, partly because of poor performance but also because of a very robust charter market here in Washington. The public school system had not been innovating, and students were fleeing to charter schools.
There was only one priority in the early days, and that was changing every policy possible to allow us to get, grow, and keep great people.
We created the first office of human capital in any school system in the country. We understood that human resources does one set of things, which is transactional, but nobody in a school district was stepping back to look at the human-capital continuum: recruitment, selection, onboarding, induction, professional development, career ladders, and exits.
We negotiated a teachers’ union contract that completely changed how we were able to hire. We eliminated tenure and seniority, we created a pay-for-performance system, and we created a new teacher evaluation system, called IMPACT. 4 IMPACT: The DCPS Evaluation and Feedback System for School-Based Personnel, District of Columbia Public Schools, accessed March 2023.
Emma Dorn: Did you encounter opposition?
Kaya Henderson: We had a level of political cover that allowed us to be bold. Michelle did a really good job of communicating the narrative: why we were doing what we were doing, engaging in a broader conversation around teacher evaluation, getting national support for what we were doing—and raising money for it.
I was able to then work with our team within the district to hammer out the actual nuts and bolts of the policies and the union contract. Eighty percent of the teachers’ vote ratified the contract. People wanted change.
Eighty percent of the teachers’ vote ratified the contract. People wanted change.
We also had a leadership structure that allowed us to do all those things. I had been working with DC Public Schools for seven years before we came on. None of these people were new to me. I had positive working relationships with folks. In any other school district, it would have taken people a while to build the kinds of relationships that we had already, and that enabled us to just skate and go.
Emma Dorn: In 2010, you were named interim chancellor, then appointed permanently to the job in 2011. Was that a planned evolution?
Kaya Henderson: The interesting thing is, I refused the job, maybe fifty times. I said I would stay as interim chancellor, but my plan was not to be the chancellor of DC Public Schools. They were doing a national search.
Ultimately, I said yes, not because I wanted to, but because a lot of the great teachers and principals who I had spent three and a half years getting, growing, and retaining were literally telling me, “If you stay, we’ll stay.” So, I felt like it was really important for me to stay to maintain the work that we did and to keep our foot on the gas.
Emma Dorn: How did you maintain momentum from Michelle’s tenure as chancellor to yours, and what critical shifts did you make?
Kaya Henderson: When we got to DCPS in 2007, it was a mess and needed to be dismantled. We had 29 data systems and none of them talked to one another. We were paying people who were dead. We needed to break this whole thing apart and put it back together in a new way. And Michelle was a very good leader to help break it up. She’s a fighter and we needed a fighter at that point.
We needed to break this whole thing apart and put it back together in a new way.
The first thing that we did when I transitioned into the role was called the Hopes and Dreams campaign. 5 Kaya Henderson rolled out the “Hopes and Dreams” campaign in 2011 as acting chancellor. See “Collecting ‘Hopes and Dreams’ at DCPS,” blog post by Bill Turque, Washington Post , May 25, 2011. We asked people, “Ten years from now, what should DCPS look like? What are your hopes and your dreams for the district and for your students?” We had an online portal. We had boxes in barbershops, and beauty parlors, and laundromats. And 10,000 people told us what they wanted to see. We took that, crafted a strategic plan and then went back to the community and said, “Is this what you want to do?” And that’s how we did everything in my tenure, with community engagement at the center—even with hard decisions such as the school closings we decided to do in 2013.
The community understood the hard decisions that we had to make, in part because I spent a year communicating the mismatch of resources. After a year of helping people understand resource misalignment, some said, “Then, let’s just close schools.” At that point, I knew we were ready to do it. And we did it with the community. We had no protests, no drama. And we did not lose children that year. In fact, from 2010 to 2016, which was my tenure, we saw six consecutive years of enrollment growth, which hadn’t happened in 40 years in DC Public Schools (Exhibit 2).
Emma Dorn: You remained chancellor until 2016. How did your priorities shift and evolve across your tenure?
Kaya Henderson: My job was to listen to people, to be in constant communication with our teachers, our principals, and our central office staff to know what they needed to be successful.
While we continued to do human-capital work, it became very clear that we also needed to do curricular work. I characterize it as an equity floor. What is the least that we are going to guarantee to every single kid across the district? If people want to add to it, great. But it has to be at a high enough standard that you would be happy sending your kid to any school in the district.
Armed with the information from the evaluation system, which told us who our best teachers were, we pulled those people together and asked them to develop curriculum with us. We had some lofty goals. We wanted it to be rigorous. We wanted it to be joyful. We wanted our young people to see windows and mirrors, and see other communities, but also see themselves mightily reflected in the things that they were learning. And that was very different for us. We wanted to ensure that it wasn't just around the core subjects, but that we were creating an interdisciplinary curriculum. We were creating curriculum to develop the whole child. And our teachers were super on fire about it.
We were creating curriculum to develop the whole child. And our teachers were super on fire about it.
We engaged the city. We had a project called “City as our Classroom,” where after we released the curriculum, we then went to institutions in the city and asked, “Where does your content overlap with our curriculum, and how can we create field trips so that parents can take kids to places that align with what they’re learning?”
The third priority was, again, community engagement—co-creating solutions with our students, our parents, and our community members. So when people ask, “How did you do it at DC Public Schools?” it’s those three things: its human capital, its curriculum, and its family and community engagement. That was the cocktail.
Emma Dorn: How did you align different stakeholders around these initiatives?
Kaya Henderson: We spent a lot of time on alignment, making sure that every single person in the district knew what the priorities were as well as all the programs and initiatives that we were doing, and how they lined up to these goals. We set five big goals. We called it a “capital commitment.” It was our five-year strategic plan. And everybody knew what the five goals were. They could recite them. Everybody knew how their job contributed to one of the goals. Every single employee’s strategic plan, their department’s strategic plan, their division’s strategic plan all rolled up to those five goals.
We only chose two or three big things to do each year because you can’t do everything. But if you choose the right few things, and you do them well, and you continue to do them well, you create a flywheel effect. And we were able to catch that momentum and do a lot more than, I think, anybody expected.
Emma Dorn: How did you manage to motivate the department, to engage it in a new way, and attract top-caliber talent?
Kaya Henderson: I believe if you’re doing really hard work, you should do it with people who you’re inspired by and who challenge you.
I am no educational expert. I don’t have conviction about a whole lot of things. But I know how to find people who are experts. And I’m OK to let them do their thing. I’ve always been a collector of talented people. And I feel like, if we’re going to be doing really hard stuff, then we should have fun doing it. I think it’s important for you to help people remember why they are doing this stuff, to celebrate people when things are going well or when they do good things.
I know how to find people who are experts. And I’m okay to let them do their thing.
One of the things that we were noted for was our Standing Ovation Awards for DC teachers. We did a huge celebration at the Kennedy Center, honoring our best teachers.
Emma Dorn: Were there new capabilities or capacities that you discovered you needed at each level of the system?
Kaya Henderson: This was the beginning of publicly available data in school systems, so there was a level of transparency into school districts that you never had before. We needed to replace probably 80 to 90 percent of our principal corps, and I was like, “OK, let’s go find us some good principals.”
We scoured the school district websites of our surrounding jurisdictions to figure out which principals had really turned around a low-performing school. We called those people. We sent them emails saying, “Hi. I’m Kaya Henderson. I’m the deputy chancellor of DC Public Schools. We see you’re doing amazing work at your elementary school and wanted to say congratulations. We know how hard this is, and we’re sure you’re probably not even thinking about moving, but if you ever want to have a conversation about opportunities at DCPS, I personally would love to talk to you.”
Nobody ever says “congratulations” to principals. Nobody says “thank you.” The number of people who responded was astounding. Every single person that we reached out to said that they weren’t thinking about moving but would love to talk about positions at DC Public Schools.
The other thing that we did—and I can’t even tell you how much it paid off—was to create an internship program. We hired undergraduate students and graduate students to work for us for a semester, or a summer, or a year. And we gave them meaningful projects. We had interns from the best colleges and universities in America because we were the hotbed of education innovation, and everybody was looking at DC.
We were the hotbed of education innovation, and everybody was looking at DC.
Emma Dorn: Education leaders across the country are struggling right now with a host of challenges. What needs to happen to support them?
Kaya Henderson: What’s unfortunate to me is that many educational leaders and educators, in general, are so tired and so beaten down from the last three years that it is tough to rustle up the innovation. And nobody wants to come behind them and do this work because our narrative on public education is so terrible. I would love to reframe that narrative. This is a dynamic industry, where you are able to do amazing work. And we don’t sell it that way.
Emma Dorn: You’re currently CEO of Reconstruction, an education tech company that provides supplemental curricula for Black K–12 students. What inspired you to go in that direction, and what’s next for you?
Kaya Henderson: Reconstruction came directly out of my curricular work at DC Public Schools. We wanted kids to see themselves, and we built curriculum where kids’ communities were featured prominently. We watched kids attach to this stuff and engage in ways that I had never seen before.
I started thinking, what would a national Black curriculum look like? What are all the books that I want Black kids to read before they graduate from high school so that they know who they are and they know the history of Black excellence, resilience, and creativity in the United States?
We are just starting our third year at Reconstruction. We’ve served over 12,000 kids in the last two years and are in major school districts all across the country, working with community-based organizations, working with individuals. And it’s been a dream come true. I’ve got an amazing, talented team who has a lot of fun together. We built a product that is in tremendous demand. We’ve produced over 150 courses. And I cannot create content fast enough. Figuring out what the next phase of Reconstruction looks like is the big thing that I’m noodling on right now.
Kaya Henderson is the CEO of Reconstruction and the former chancellor of District of Columbia Public Schools. Emma Dorn is an associate partner in McKinsey’s Bay Area office.
The authors wish to thank Jake Bryant, Emmy Liss, Stephanie McBride, Jimmy Sarakatsannis, Doug Scott, and Laurie Sztejnberg for their contributions to this article.
The authors also wish to thank former District of Columbia Public Schools staff members for their contributions to this article, including Michelle Hudacsko, Jeanie Lee, Brian Pick, and Pete Weber.
Comments and opinions expressed by interviewees are their own and do not represent or reflect the opinions, policies, or positions of McKinsey & Company or have its endorsement.
Explore a career with us
Related articles.
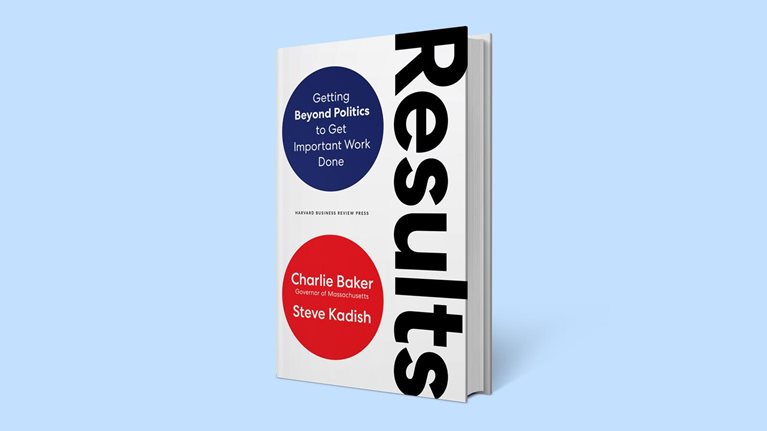
Lessons in leadership: Getting past politics to deliver for the people
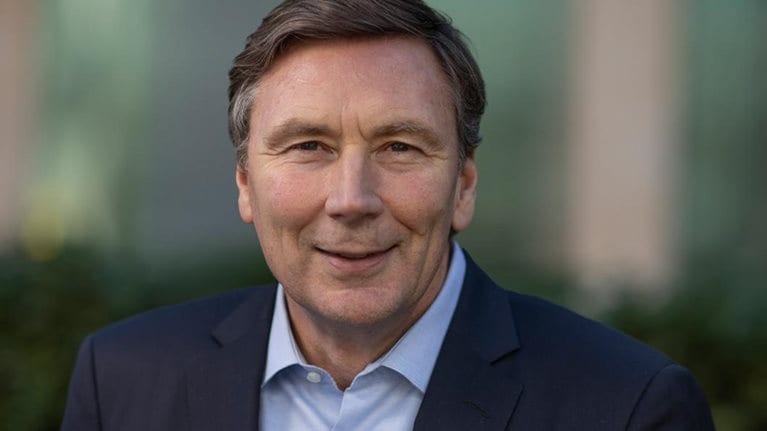
Lessons in leadership: Making government “a great place to work”

Lessons in leadership: Using data to drive public-health decisions
- Solutions Corporate Market Analysis Customer Experience Product Lifecycle Brand Strategy Research & Insights Higher Education Enrollment Management Academic Program Development Student Success Operations & Finance Advancement Marketing Grants Research & Insights K-12 Education Curriculum and Instruction Diversity, Equity, and Inclusion Academic Program Planning and Impact Strategic Planning Teacher Recruitment and Retention Operational Planning School Climate Research & Insights
- Data Analysis
- Qualitative Research
- Strategic Advising
- Benchmarking & Best Practices
- Market Modeling
- Research & Insights By Industry Corporate Higher Education K–12 Education By Type Insights Blog Reports & Briefs Case Studies Webinars All Research & Insights
- Client Testimonials
- Careers Overview
- Current Openings
- Recruitment Process
- Social Impact
- Careers in Research
- Careers in Sales and Account Management
- Diversity, Equity, and Inclusion
- Client Login
Using Data to Solve K–12 Challenges
- March 31, 2023
- Topic: K–12 Education , Operational Planning , Program Evaluation , Strategic Planning
- Resource type: Insights Blog
K–12 districts are data-rich, but often have limited capacity to turn it into actionable information. A continuous improvement mindset and data literacy tools help educators make decisions that improve outcomes.
In K–12 schools and districts, data can be found everywhere: in student records, test scores, learning management systems, budget spreadsheets, survey results, and teachers’ gradebooks. It’s a critical component of evaluating programs, establishing goals, monitoring changes over time, communicating with stakeholders, determining appropriate funding, and informing instructional decisions.
Yet despite a wealth of accessible data, K–12 districts don’t always have enough resources to interpret and act on that data in an ongoing, systematic way.
Educator and author Peter Drucker once said, “ You can’t improve what you don’t measure.” To make lasting improvements in classrooms, schools, and districts, it’s essential to use data to guide that work. Ultimately, using data should be a part of everyone’s regular practice, not just a handful of employees behind closed doors in a central district office.
Data Is Part of Everyone’s Job
In reality, school and district data are often stored in many different platforms. Employees may not have much experience working with data. Leaders may review some types of data (usually quantitative) and not enough of others, missing a fuller picture of their district or school. And busy teachers may lack the bandwidth to take on data-focused duties such as program evaluation .
Data literacy is the ability to read, interpret, and communicate data as information. It’s always been a critical skill for K–12 staff, but it’s not commonly included in teacher preparation or educational leadership coursework. With a continuous improvement mindset, however, and a few key data literacy tools and best practices , educators can learn how to use data to make more informed decisions that maximize student learning and success.
A Continuous Improvement Mindset
Plainly speaking, continuous improvement is a way of consistently reviewing and enhancing the quality of an organization’s programs and operations to ensure efficiency and effectiveness. The Carnegie Foundation defines it as “the act of integrating quality improvement into the daily work of individuals in the system.”
When it comes to using data, districts and schools that cultivate a continuous improvement mindset often share some similar traits:
- Frequent Use of Data: Using and interpreting data is part of everyday work, instead of being considered an add-on or an afterthought.
- Organization-Wide Data Literacy: Everyone at the district, school, and classroom levels understands how to interpret and use data to make informed decisions.
- Standard Processes: There is a central framework or structure in place that helps people follow a standard process for evaluating what’s going well and what to improve.
A continuous improvement mindset relies on a problem-solving learning process that invites questions, tests ideas, and uses results to adapt and adjust plans. It calls for flexible thinking and an openness to taking in new information, incorporating new skills, and modifying strategies based on where the facts take you. For this kind of mindset to take hold across an organization, the leadership must pave the way by not only modeling these behaviors, but also implementing a system-wide framework for improving work and providing professional learning opportunities to all staff.
Learn how district leaders are building their school system’s capacity to effectively evaluate programming. Watch Hanover’s webinar recording, Building System Capacity for Program Evaluation .
A system-wide approach to data use.
To guide your continuous improvement efforts, your organization needs a system-wide framework and process for using data (one example is the Carnegie Foundation’s improvement science protocol ).
Why is this important? A framework allows employees across a district or school to use data consistently. While some districts develop a framework on their own, others may want external support to get the ball rolling. Either way, making data part of your organization’s daily practice is key.
Here are a few tips as you begin to formalize a data collection and evaluation system in your district:
- Identify a handful of data evaluation goals and metrics related to your district’s priorities, aligned to your strategic plan. Start with fewer, specific goals focused on the areas with the greatest need and adjust over time to include other goals.
- Assign metrics or key performance indicators for each goal so you have something to evaluate your progress against.
- Look at both quantitative and qualitative data to get a view of the larger picture.
- Avoid making decisions based on just one snapshot or year of data. It’s better to look at trends over time to get a more comprehensive view, particularly in light of any disruptions to typical patterns due to the COVID-19 pandemic.
Learn more about how the s uperintendent of Danville Public Schools partnered with Hanover Research for system-wide support with quantitative and qualitative data tracking .
Building educators’ data literacy skills.
Data should be part of every decision in a district or school — from department meetings to professional learning communities across school sites. Getting everyone on board with system-wide data use often means people need some additional training. So, it’s important to develop professional learning opportunities that help leaders, teachers, and staff strengthen their data literacy skills. This is another area where you may want to lean on external support to build capacity initially. Once district and school-based administrators are comfortable with data use, they can begin to support teachers and diffuse new skills across the rest of the organization.
Learn a seven-step process for creating an engaging professional learning plan for your K–12 staff by downloading our District Leaders’ Guide for Developing a Professional Learning Plan .
Teachers, administrators, and staff alike can learn how to look for patterns or gaps in data, interpret trends, and apply data-driven insights to their decision making. When this happens, everyone — most of all, students — reap considerable benefits.
—Marriam Ewaida , PhD, Managing Director, K–12 Professional Services , Hanover Research
Build your district and school employees’ data literacy skills with our Data Literacy Checklist .
Author information.
Dr. Marriam Ewaida has almost two decades of practical and research experience in the K–12 sector. She has extensive training and experience working with culturally and linguistically diverse adolescent students, providing staff professional development, developing and aligning curriculum, and managing staff.
Dr. Ewaida currently manages a team that is intricately involved in supporting program evaluation, strategic planning, and equity initiatives for members across the country by facilitating workshops and providing regular consultations that support those initiatives. Dr. Ewaida also serves as an adjunct faculty at George Mason University and continues to teach, publish, and present on topics related to culturally sustaining pedagogy, critical literacy, teacher education and professional development, and curriculum implementation.

Related Content
- Reports & Briefs
K–12 Student Well-Being Program Evaluation Checklist
- Culture, Climate, & Family Engagement , K–12 Education , Program Evaluation , Student Success
AI Best Practices in K-12 Education
- Curriculum & Instruction , K–12 Education , Operational Planning
Related Tags
- Continuous Improvement , Data literacy , strategic planning
Related Research & Insights

- Case Studies
Dallas ISD Leverages K-12 Program Evaluation to Maximize Student Outcomes
- Academic Program Planning & Impact , K–12 Education , Program Evaluation , Student Success
Research & Insights
Receive industry-leading insights directly in your inbox.
If you have difficulty accessing any part of this website or the products or services offered by Hanover Research, please contact us at [email protected] for support.
Become a client
Access the best custom research to help hit your organization’s goals . Request your custom consult below and a member of our team will be in touch.
Have questions? Please visit our contact page .
Let us come to you!
Receive industry insights directly in your inbox.
Our newsletters are packed with helpful tips, industry guides, best practices, case studies, and more. Enter your email address below to opt in:
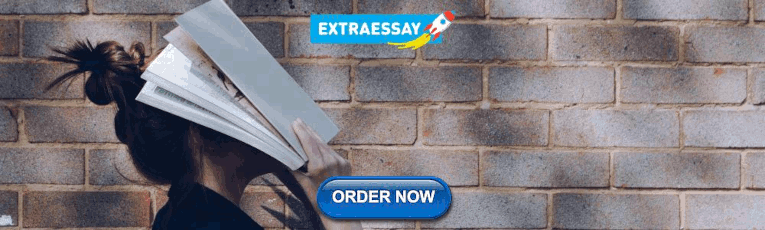
IMAGES
COMMENTS
With a focus on the K-12 context in the United States, research studies in this review included sample populations of elementary and secondary students, teachers, parents, and school leaders. ... Effects of the COVID-19 Pandemic on K-12 Education 56 . 2016). When examining the participation of students in remote learning environments, a growing
Download the 2023 Trends in K-12 Education report to find guidance for: "Even as they try to make sense of so many challenges and setbacks, district leaders are pushing forward and striving to turn the tide. Around the nation, districts are faced with tackling short-term hurdles while looking to the future with greater flexibility and empathy.
About 1 in 4 U.S. teachers say their school went into a gun-related lockdown in the last school year. 59% of public K-12 teachers say they are at least somewhat worried about the possibility of a shooting ever happening at their school. short readApr 4, 2024.
The field of research on K-12 online education delivery and outcomes is an emerging area is growing considerably in the wake of the COVID-19 pandemic. This systematic literature review was conducted in response to the need to better understand the evidence-based practices within the research base that could guide the design and implementation ...
October 2021. Each year, for the past four years, Education Week has produced a special report on big ideas in K-12 education. The reports focus on important and timely issues that schools have ...
A Framework for K-12 Science Education is the first step in a process that can inform state-level decisions and achieve a research-grounded basis for improving science instruction and learning across the country. The book will guide standards developers, teachers, curriculum designers, assessment developers, state and district science ...
All K-12 Education Research & Insights. Reports & Briefs; K-12 Student Well-Being Program Evaluation Checklist. 05/01/2024; Culture, Climate, & Family Engagement, K-12 Education, Program Evaluation, Student Success; Insights Blog; Developing K-12 AI Literacy for Your District. 04/26/2024;
For the past 20 years, I have taught research methods in education to students here in the U.S. and in other countries. While the purpose of the course is to show students how to do effective ...
The report reviews the evidence for the impact of integrated approaches on various student outcomes, and it proposes a set of priority research questions to advance the understanding of integrated STEM education. STEM Integration in K-12 Education proposes a framework to provide a common perspective and vocabulary for researchers, practitioners ...
Hanover's 2022 Trends in K-12 Education Report provides insights, recommendations, and case studies that can ease district leaders' 2022-23 planning, including guidance for: Reframing DEI initiatives. Strengthening teacher and administrator well-being. Supporting social, emotional, and academic recovery. Building consensus with communities.
The growth and effectiveness of online learning in K-12 education. Student enrollment in K-12 online programs has gradually increased over the last decade (Roy & Boboc, 2016; Zweig & Stafford, 2016).For the 2017-2018 school year, 297,712 students were enrolled in 501 full-time virtual schools, and 132,960 students were enrolled in 300 blended learning schools (Molnar et al., 2019).
In K-12 education, research topics were relatively balanced, but there was a lack of research on students' career choices and development. In higher education, research topics in the humanities ...
In 2023 K-12 schools experienced a rise in cyberattacks, underscoring the need to implement strong systems to safeguard student data. Technology is "requiring people to check their assumptions ...
K-12 Education. To support schools in improving K-12 teaching and learning, with a focus on math as the cornerstone skill for academic success and greater opportunities in the workforce. Students, like those in this 8th grade social studies class in Chicago, need safe, nurturing learning environments where they are equipped to meet high standards.
Most Americans think U.S. K-12 STEM education isn't above average, but test results paint a mixed picture. Just 28% of U.S. adults say America is the best in the world or above average in K-12 STEM education compared with other wealthy nations. short readApr 11, 2024.
For the most part, parents of K-12 students think their children's school spends about the right amount of time on core academic subjects like reading, math, science and social studies (73% say this). Still, one-in-five say their children's school doesn't spend enough time on these subjects, while 7% say too much time is spent on core ...
The K-12 Teaching, Learning and Assessment Research Center at ETS aims to: improve K-12 teaching and learning. align assessment with principles of learning and teaching. inform innovations and operations in K-12 student and teacher assessment. research and address inequities in the teacher pipeline. The center's work is supported through ...
The matter of integrating multiple information sources has been a topic of great interest in several fields (Alexander & The Disciplined Reading and Learning Research Laboratory, 2012; Braasch et al., 2018; Nelson & King, 2022).Given our interest in applications for K-12 literacy learning and instruction, we focus in this study on intertextual integration as it has been conceptualized within ...
The report K-12 Science Education in the United States: A Landscape Study for Improving the Field assesses the progress over the last decade toward the vision of science instruction provided in 2012 by the Corporation-supported Framework for K-12 Science Education, published by the National Research Council. Citation: K-12 Science ...
Hanover's 2021 Trends in K-12 Education Report summarizes what researchers and advisors observed in their work with hundreds of local, regional, and state education agencies across the country. Download Report. Find out what K-12 trends to expect in 2021 and get recommendations for how your district can innovate in the 2021-22 academic year.
Make decisions with confidence, not guesswork. Hanover Research provides high quality, custom research and analytics through a cost-effective model that helps clients in the corporate, education, and healthcare sectors make informed decisions, identify and seize opportunities, and heighten their effectiveness.
In recent years, a growing body of education research has documented neoliberal-driven policies that exacerbate what the racialization and racism communities of Color endure in K-12 education through issues such as testing, school choice, charter school development, and a divestment from public education (Buras, 2009; Gay, 2007; Prins, 2007).
Contributing Writer. The National Science Foundation has awarded Rice University $90 million to build what is being described as a first-of-its-kind education research hub that will leverage data from an array of major digital learning platforms currently serving tens of millions of students. The university recently announced the investment as ...
Similar shares of K-12 parents and adults who don't have a child in K-12 schools say the system is going in the wrong direction. A separate Center survey of public K-12 teachers found that 82% think the overall state of public K-12 education has gotten worse in the past five years. And many teachers are pessimistic about the future.
The successful irruption of AI-based technology in our daily lives has led to a growing educational, social, and political interest in training citizens in AI. Education systems now need to train students at the K-12 level to live in a society where they must interact with AI. Thus, AI literacy is a pedagogical and cognitive challenge at the K-12 level. This study aimed to understand how AI is ...
Pew Research Center conducted this analysis to provide a snapshot of partisan divides in K-12 education in the run-up to the 2024 election. The analysis is based on data from various Center surveys and analyses conducted from 2021 to 2023, as well as survey data from Education Next, a research journal about education policy.
In addition, the sponsorship of the study required that we focus our review on literature relevant to K-12 online instruction within the United States. An information database was constructed using the articles included in the systematic literature review. The database included general information (e.g., TABLE 1.
In an interview with McKinsey's Emma Dorn, Henderson shares her experiences with achieving lasting improvements in K-12 student outcomes (Exhibit 1). 2. 1. This interview has been edited for length and clarity. Emma Dorn: What was the state of the DC public school system when you were appointed deputy chancellor under Chancellor Michelle ...
Evaluating the Academic Performance of K-12 Students in the Philippines: A Standardized Evaluation Approach. ... Education Research International. V olume 2020, Article ID 8877712, 8 pa ges.
Using Data to Solve K-12 Challenges. By: Marriam Ewaida, PhD. March 31, 2023. Topic: K-12 Education, Operational Planning, Program Evaluation, Strategic Planning. Resource type: Insights Blog. K-12 districts are data-rich, but often have limited capacity to turn it into actionable information. A continuous improvement mindset and data ...