Unpacking in Python: Beyond Parallel Assignment
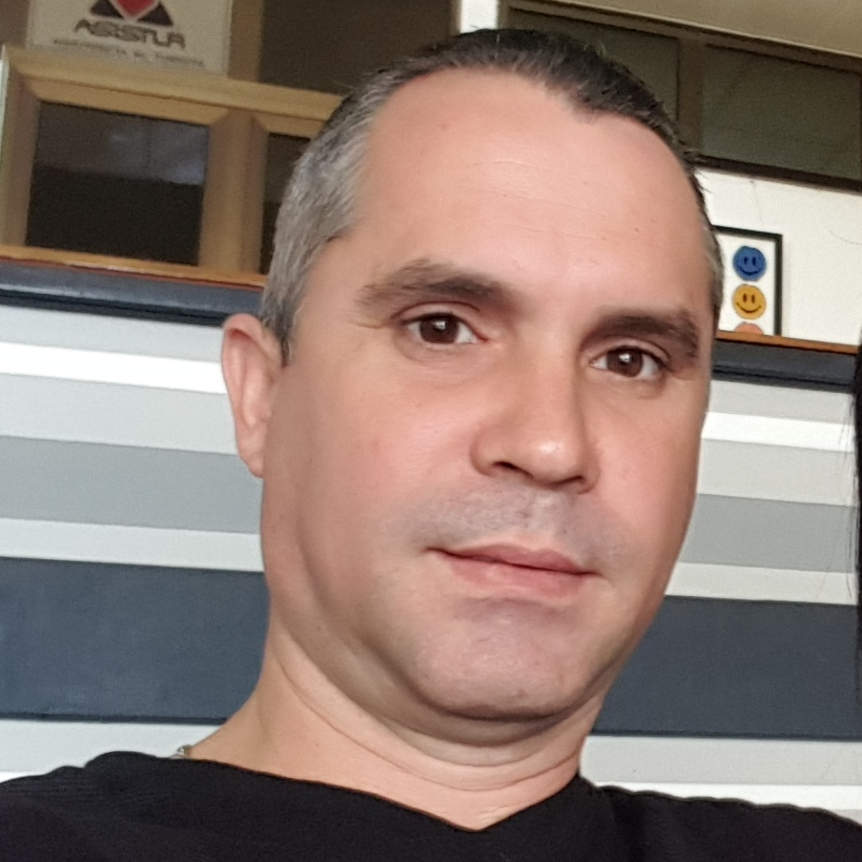
- Introduction
Unpacking in Python refers to an operation that consists of assigning an iterable of values to a tuple (or list ) of variables in a single assignment statement. As a complement, the term packing can be used when we collect several values in a single variable using the iterable unpacking operator, * .
Historically, Python developers have generically referred to this kind of operation as tuple unpacking . However, since this Python feature has turned out to be quite useful and popular, it's been generalized to all kinds of iterables. Nowadays, a more modern and accurate term would be iterable unpacking .
In this tutorial, we'll learn what iterable unpacking is and how we can take advantage of this Python feature to make our code more readable, maintainable, and pythonic.
Additionally, we'll also cover some practical examples of how to use the iterable unpacking feature in the context of assignments operations, for loops, function definitions, and function calls.
- Packing and Unpacking in Python
Python allows a tuple (or list ) of variables to appear on the left side of an assignment operation. Each variable in the tuple can receive one value (or more, if we use the * operator) from an iterable on the right side of the assignment.
For historical reasons, Python developers used to call this tuple unpacking . However, since this feature has been generalized to all kind of iterable, a more accurate term would be iterable unpacking and that's what we'll call it in this tutorial.
Unpacking operations have been quite popular among Python developers because they can make our code more readable, and elegant. Let's take a closer look to unpacking in Python and see how this feature can improve our code.
- Unpacking Tuples
In Python, we can put a tuple of variables on the left side of an assignment operator ( = ) and a tuple of values on the right side. The values on the right will be automatically assigned to the variables on the left according to their position in the tuple . This is commonly known as tuple unpacking in Python. Check out the following example:
When we put tuples on both sides of an assignment operator, a tuple unpacking operation takes place. The values on the right are assigned to the variables on the left according to their relative position in each tuple . As you can see in the above example, a will be 1 , b will be 2 , and c will be 3 .
To create a tuple object, we don't need to use a pair of parentheses () as delimiters. This also works for tuple unpacking, so the following syntaxes are equivalent:
Since all these variations are valid Python syntax, we can use any of them, depending on the situation. Arguably, the last syntax is more commonly used when it comes to unpacking in Python.
When we are unpacking values into variables using tuple unpacking, the number of variables on the left side tuple must exactly match the number of values on the right side tuple . Otherwise, we'll get a ValueError .
For example, in the following code, we use two variables on the left and three values on the right. This will raise a ValueError telling us that there are too many values to unpack:
Note: The only exception to this is when we use the * operator to pack several values in one variable as we'll see later on.
On the other hand, if we use more variables than values, then we'll get a ValueError but this time the message says that there are not enough values to unpack:
If we use a different number of variables and values in a tuple unpacking operation, then we'll get a ValueError . That's because Python needs to unambiguously know what value goes into what variable, so it can do the assignment accordingly.
- Unpacking Iterables
The tuple unpacking feature got so popular among Python developers that the syntax was extended to work with any iterable object. The only requirement is that the iterable yields exactly one item per variable in the receiving tuple (or list ).
Check out the following examples of how iterable unpacking works in Python:
When it comes to unpacking in Python, we can use any iterable on the right side of the assignment operator. The left side can be filled with a tuple or with a list of variables. Check out the following example in which we use a tuple on the right side of the assignment statement:
It works the same way if we use the range() iterator:
Even though this is a valid Python syntax, it's not commonly used in real code and maybe a little bit confusing for beginner Python developers.
Finally, we can also use set objects in unpacking operations. However, since sets are unordered collection, the order of the assignments can be sort of incoherent and can lead to subtle bugs. Check out the following example:
If we use sets in unpacking operations, then the final order of the assignments can be quite different from what we want and expect. So, it's best to avoid using sets in unpacking operations unless the order of assignment isn't important to our code.
- Packing With the * Operator
The * operator is known, in this context, as the tuple (or iterable) unpacking operator . It extends the unpacking functionality to allow us to collect or pack multiple values in a single variable. In the following example, we pack a tuple of values into a single variable by using the * operator:
For this code to work, the left side of the assignment must be a tuple (or a list ). That's why we use a trailing comma. This tuple can contain as many variables as we need. However, it can only contain one starred expression .
We can form a stared expression using the unpacking operator, * , along with a valid Python identifier, just like the *a in the above code. The rest of the variables in the left side tuple are called mandatory variables because they must be filled with concrete values, otherwise, we'll get an error. Here's how this works in practice.
Packing the trailing values in b :
Packing the starting values in a :
Packing one value in a because b and c are mandatory:
Packing no values in a ( a defaults to [] ) because b , c , and d are mandatory:
Supplying no value for a mandatory variable ( e ), so an error occurs:
Packing values in a variable with the * operator can be handy when we need to collect the elements of a generator in a single variable without using the list() function. In the following examples, we use the * operator to pack the elements of a generator expression and a range object to a individual variable:
In these examples, the * operator packs the elements in gen , and ran into g and r respectively. With his syntax, we avoid the need of calling list() to create a list of values from a range object, a generator expression, or a generator function.
Notice that we can't use the unpacking operator, * , to pack multiple values into one variable without adding a trailing comma to the variable on the left side of the assignment. So, the following code won't work:
If we try to use the * operator to pack several values into a single variable, then we need to use the singleton tuple syntax. For example, to make the above example works, we just need to add a comma after the variable r , like in *r, = range(10) .
- Using Packing and Unpacking in Practice
Packing and unpacking operations can be quite useful in practice. They can make your code clear, readable, and pythonic. Let's take a look at some common use-cases of packing and unpacking in Python.
- Assigning in Parallel
One of the most common use-cases of unpacking in Python is what we can call parallel assignment . Parallel assignment allows you to assign the values in an iterable to a tuple (or list ) of variables in a single and elegant statement.
For example, let's suppose we have a database about the employees in our company and we need to assign each item in the list to a descriptive variable. If we ignore how iterable unpacking works in Python, we can get ourself writing code like this:
Even though this code works, the index handling can be clumsy, hard to type, and confusing. A cleaner, more readable, and pythonic solution can be coded as follows:
Using unpacking in Python, we can solve the problem of the previous example with a single, straightforward, and elegant statement. This tiny change would make our code easier to read and understand for newcomers developers.
- Swapping Values Between Variables
Another elegant application of unpacking in Python is swapping values between variables without using a temporary or auxiliary variable. For example, let's suppose we need to swap the values of two variables a and b . To do this, we can stick to the traditional solution and use a temporary variable to store the value to be swapped as follows:
This procedure takes three steps and a new temporary variable. If we use unpacking in Python, then we can achieve the same result in a single and concise step:
In statement a, b = b, a , we're reassigning a to b and b to a in one line of code. This is a lot more readable and straightforward. Also, notice that with this technique, there is no need for a new temporary variable.
- Collecting Multiple Values With *
When we're working with some algorithms, there may be situations in which we need to split the values of an iterable or a sequence in chunks of values for further processing. The following example shows how to uses a list and slicing operations to do so:
Check out our hands-on, practical guide to learning Git, with best-practices, industry-accepted standards, and included cheat sheet. Stop Googling Git commands and actually learn it!
Even though this code works as we expect, dealing with indices and slices can be a little bit annoying, difficult to read, and confusing for beginners. It has also the drawback of making the code rigid and difficult to maintain. In this situation, the iterable unpacking operator, * , and its ability to pack several values in a single variable can be a great tool. Check out this refactoring of the above code:
The line first, *body, last = seq makes the magic here. The iterable unpacking operator, * , collects the elements in the middle of seq in body . This makes our code more readable, maintainable, and flexible. You may be thinking, why more flexible? Well, suppose that seq changes its length in the road and you still need to collect the middle elements in body . In this case, since we're using unpacking in Python, no changes are needed for our code to work. Check out this example:
If we were using sequence slicing instead of iterable unpacking in Python, then we would need to update our indices and slices to correctly catch the new values.
The use of the * operator to pack several values in a single variable can be applied in a variety of configurations, provided that Python can unambiguously determine what element (or elements) to assign to each variable. Take a look at the following examples:
We can move the * operator in the tuple (or list ) of variables to collect the values according to our needs. The only condition is that Python can determine to what variable assign each value.
It's important to note that we can't use more than one stared expression in the assignment If we do so, then we'll get a SyntaxError as follows:
If we use two or more * in an assignment expression, then we'll get a SyntaxError telling us that two-starred expression were found. This is that way because Python can't unambiguously determine what value (or values) we want to assign to each variable.
- Dropping Unneeded Values With *
Another common use-case of the * operator is to use it with a dummy variable name to drop some useless or unneeded values. Check out the following example:
For a more insightful example of this use-case, suppose we're developing a script that needs to determine the Python version we're using. To do this, we can use the sys.version_info attribute . This attribute returns a tuple containing the five components of the version number: major , minor , micro , releaselevel , and serial . But we just need major , minor , and micro for our script to work, so we can drop the rest. Here's an example:
Now, we have three new variables with the information we need. The rest of the information is stored in the dummy variable _ , which can be ignored by our program. This can make clear to newcomer developers that we don't want to (or need to) use the information stored in _ cause this character has no apparent meaning.
Note: By default, the underscore character _ is used by the Python interpreter to store the resulting value of the statements we run in an interactive session. So, in this context, the use of this character to identify dummy variables can be ambiguous.
- Returning Tuples in Functions
Python functions can return several values separated by commas. Since we can define tuple objects without using parentheses, this kind of operation can be interpreted as returning a tuple of values. If we code a function that returns multiple values, then we can perform iterable packing and unpacking operations with the returned values.
Check out the following example in which we define a function to calculate the square and cube of a given number:
If we define a function that returns comma-separated values, then we can do any packing or unpacking operation on these values.
- Merging Iterables With the * Operator
Another interesting use-case for the unpacking operator, * , is the ability to merge several iterables into a final sequence. This functionality works for lists, tuples, and sets. Take a look at the following examples:
We can use the iterable unpacking operator, * , when defining sequences to unpack the elements of a subsequence (or iterable) into the final sequence. This will allow us to create sequences on the fly from other existing sequences without calling methods like append() , insert() , and so on.
The last two examples show that this is also a more readable and efficient way to concatenate iterables. Instead of writing list(my_set) + my_list + list(my_tuple) + list(range(1, 4)) + list(my_str) we just write [*my_set, *my_list, *my_tuple, *range(1, 4), *my_str] .
- Unpacking Dictionaries With the ** Operator
In the context of unpacking in Python, the ** operator is called the dictionary unpacking operator . The use of this operator was extended by PEP 448 . Now, we can use it in function calls, in comprehensions and generator expressions, and in displays .
A basic use-case for the dictionary unpacking operator is to merge multiple dictionaries into one final dictionary with a single expression. Let's see how this works:
If we use the dictionary unpacking operator inside a dictionary display, then we can unpack dictionaries and combine them to create a final dictionary that includes the key-value pairs of the original dictionaries, just like we did in the above code.
An important point to note is that, if the dictionaries we're trying to merge have repeated or common keys, then the values of the right-most dictionary will override the values of the left-most dictionary. Here's an example:
Since the a key is present in both dictionaries, the value that prevail comes from vowels , which is the right-most dictionary. This happens because Python starts adding the key-value pairs from left to right. If, in the process, Python finds keys that already exit, then the interpreter updates that keys with the new value. That's why the value of the a key is lowercased in the above example.
- Unpacking in For-Loops
We can also use iterable unpacking in the context of for loops. When we run a for loop, the loop assigns one item of its iterable to the target variable in every iteration. If the item to be assigned is an iterable, then we can use a tuple of target variables. The loop will unpack the iterable at hand into the tuple of target variables.
As an example, let's suppose we have a file containing data about the sales of a company as follows:
From this table, we can build a list of two-elements tuples. Each tuple will contain the name of the product, the price, and the sold units. With this information, we want to calculate the income of each product. To do this, we can use a for loop like this:
This code works as expected. However, we're using indices to get access to individual elements of each tuple . This can be difficult to read and to understand by newcomer developers.
Let's take a look at an alternative implementation using unpacking in Python:
We're now using iterable unpacking in our for loop. This makes our code way more readable and maintainable because we're using descriptive names to identify the elements of each tuple . This tiny change will allow a newcomer developer to quickly understand the logic behind the code.
It's also possible to use the * operator in a for loop to pack several items in a single target variable:
In this for loop, we're catching the first element of each sequence in first . Then the * operator catches a list of values in its target variable rest .
Finally, the structure of the target variables must agree with the structure of the iterable. Otherwise, we'll get an error. Take a look at the following example:
In the first loop, the structure of the target variables, (a, b), c , agrees with the structure of the items in the iterable, ((1, 2), 2) . In this case, the loop works as expected. In contrast, the second loop uses a structure of target variables that don't agree with the structure of the items in the iterable, so the loop fails and raises a ValueError .
- Packing and Unpacking in Functions
We can also use Python's packing and unpacking features when defining and calling functions. This is a quite useful and popular use-case of packing and unpacking in Python.
In this section, we'll cover the basics of how to use packing and unpacking in Python functions either in the function definition or in the function call.
Note: For a more insightful and detailed material on these topics, check out Variable-Length Arguments in Python with *args and **kwargs .
- Defining Functions With * and **
We can use the * and ** operators in the signature of Python functions. This will allow us to call the function with a variable number of positional arguments ( * ) or with a variable number of keyword arguments, or both. Let's consider the following function:
The above function requires at least one argument called required . It can accept a variable number of positional and keyword arguments as well. In this case, the * operator collects or packs extra positional arguments in a tuple called args and the ** operator collects or packs extra keyword arguments in a dictionary called kwargs . Both, args and kwargs , are optional and automatically default to () and {} respectively.
Even though the names args and kwargs are widely used by the Python community, they're not a requirement for these techniques to work. The syntax just requires * or ** followed by a valid identifier. So, if you can give meaningful names to these arguments, then do it. That will certainly improve your code's readability.
- Calling Functions With * and **
When calling functions, we can also benefit from the use of the * and ** operator to unpack collections of arguments into separate positional or keyword arguments respectively. This is the inverse of using * and ** in the signature of a function. In the signature, the operators mean collect or pack a variable number of arguments in one identifier. In the call, they mean unpack an iterable into several arguments.
Here's a basic example of how this works:
Here, the * operator unpacks sequences like ["Welcome", "to"] into positional arguments. Similarly, the ** operator unpacks dictionaries into arguments whose names match the keys of the unpacked dictionary.
We can also combine this technique and the one covered in the previous section to write quite flexible functions. Here's an example:
The use of the * and ** operators, when defining and calling Python functions, will give them extra capabilities and make them more flexible and powerful.
Iterable unpacking turns out to be a pretty useful and popular feature in Python. This feature allows us to unpack an iterable into several variables. On the other hand, packing consists of catching several values into one variable using the unpacking operator, * .
In this tutorial, we've learned how to use iterable unpacking in Python to write more readable, maintainable, and pythonic code.
With this knowledge, we are now able to use iterable unpacking in Python to solve common problems like parallel assignment and swapping values between variables. We're also able to use this Python feature in other structures like for loops, function calls, and function definitions.
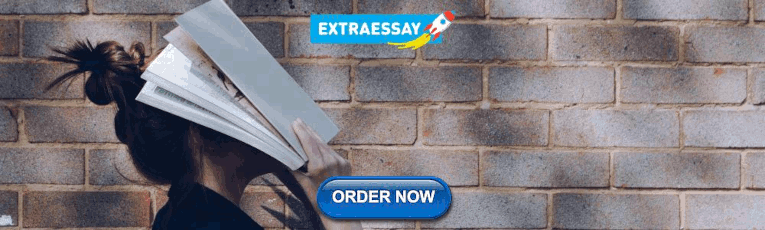
You might also like...
- Hidden Features of Python
- Python Docstrings
- Handling Unix Signals in Python
- The Best Machine Learning Libraries in Python
- Guide to Sending HTTP Requests in Python with urllib3
Improve your dev skills!
Get tutorials, guides, and dev jobs in your inbox.
No spam ever. Unsubscribe at any time. Read our Privacy Policy.
Leodanis is an industrial engineer who loves Python and software development. He is a self-taught Python programmer with 5+ years of experience building desktop applications with PyQt.
In this article
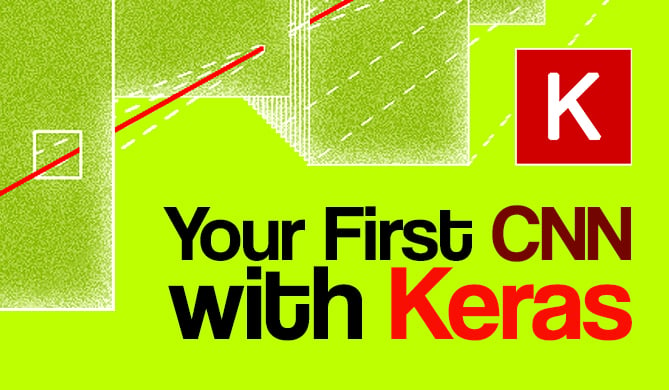
Building Your First Convolutional Neural Network With Keras
Most resources start with pristine datasets, start at importing and finish at validation. There's much more to know. Why was a class predicted? Where was...
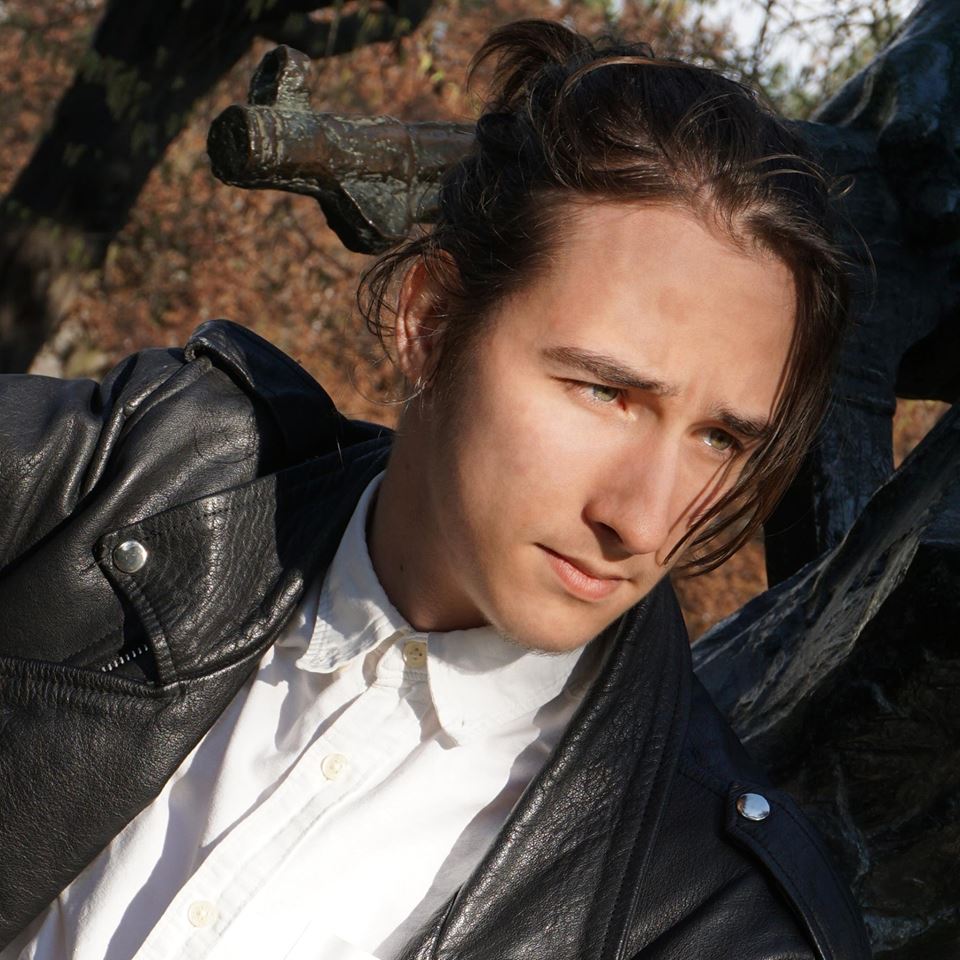
Data Visualization in Python with Matplotlib and Pandas
Data Visualization in Python with Matplotlib and Pandas is a course designed to take absolute beginners to Pandas and Matplotlib, with basic Python knowledge, and...
© 2013- 2024 Stack Abuse. All rights reserved.
Python Tutorial
File handling, python modules, python numpy, python pandas, python matplotlib, python scipy, machine learning, python mysql, python mongodb, python reference, module reference, python how to, python examples, python - unpack tuples, unpacking a tuple.
When we create a tuple, we normally assign values to it. This is called "packing" a tuple:
Packing a tuple:
But, in Python, we are also allowed to extract the values back into variables. This is called "unpacking":
Unpacking a tuple:
Note: The number of variables must match the number of values in the tuple, if not, you must use an asterisk to collect the remaining values as a list.
Advertisement
Using Asterisk *
If the number of variables is less than the number of values, you can add an * to the variable name and the values will be assigned to the variable as a list:
Assign the rest of the values as a list called "red":
If the asterisk is added to another variable name than the last, Python will assign values to the variable until the number of values left matches the number of variables left.
Add a list of values the "tropic" variable:

COLOR PICKER

Contact Sales
If you want to use W3Schools services as an educational institution, team or enterprise, send us an e-mail: [email protected]
Report Error
If you want to report an error, or if you want to make a suggestion, send us an e-mail: [email protected]
Top Tutorials
Top references, top examples, get certified.
Python Unpacking [Ultimate Guide]
In this article, you’ll learn about the following topics:
- List unpacking in Python
- Tuple unpacking in Python
- String unpacking in Python
ValueError: too many values to unpack (expected k)
Valueerror: not enough values to unpack (expected x, got y).
- Unpacking nested list or tuple
- Unpacking underscore
- Unpacking asterisk
Sequence Unpacking Basics
Python allows you to assign iterables such as lists and tuples and assign them to multiple variables.
💡 Iterable unpacking or sequence unpacking is the process of assigning the elements of an iterable (e.g., tuple , list , string ) to multiple variables. For it to work, you need to have enough variables to capture the number of elements in the iterable.
Python List Unpacking
List unpacking is the process of assigning k elements of a list to k different variables in a single line of code .
In the following example, you unpack the three elements in the list [1, 2, 3] into variables a , b , and c . Each variable captures one list element after the unpacking operation.
If the list has too many values to unpack — i.e., the number of elements in the list is larger than the variables to assign them to — Python will raise a ValueError: too many values to unpack (expected k) whereas k is the number of variables on the left-hand side of the assignment operation .
This can be seen in the following code snippet:
To resolve the ValueError: too many values to unpack (expected k) , make sure that the number of elements on the right and left-hand sides of the unpacking operation is the same.
Per convention, if you don’t need to store a certain list element in a variable, you can use the “throw-away” underscore variable name _ .
Here’s the same example resolved using the underscore name:
Alternatively, you can also use the asterisk operator on the left-hand side as will be explained at the end of this article—so keep reading! 💡
If the iterable has too few values to unpack — i.e., the number of elements in the iterable is larger than the variables to assign them to — Python will raise a ValueError: not enough values to unpack (expected x, got y) whereas x is the number of variables on the left-hand side of the assignment operation and y is the number of elements in the iterable.
To resolve the ValueError: not enough values to unpack (expected x, got y) , make sure that the number of elements on the right and left-hand sides of the unpacking operation is the same.
Python Tuple Unpacking
Tuple unpacking is the process of assigning k elements of a tuple to k different variables in a single line of code .
In the following example, you unpack the three elements in the tuple (1, 2, 3) into variables a , b , and c . Each variable captures one tuple element after the unpacking operation.
Note that the parentheses of tuples are optional, so you can omit them to obtain the same behavior with even fewer syntax overhead as shown in the following example. 😊
Python String Unpacking
String unpacking is the process of assigning k characters of a string to k different variables in a single line of code .
In the following example, you unpack the seven characters in the string 'finxter' into variables a , b , c , d , e , f , and g . Each variable captures one character from the string, in order, after the unpacking operation.
Python Unpacking Nested List or Tuple
You can also unpack a nested iterable (e.g., list of lists , or tuple of tuples ).
The simple case is where one variable (in our example c ) captures the whole inner list (in our example [3, 4, 5] ):
But how can you assign the elements of the inner list to variables as well?
This can be done by setting up a parallel structure on the left and right sides of the equation using parentheses (...) or square brackets [...] .
The simple heuristic to understand what is going on here is: parallel structures !
Python Unpacking Underscore
The underscore _ in Python behaves like a normal variable name. Per convention, it is used if you don’t actually care about the value stored in it but just use it to capture all values from an iterable in a syntactically correct way.
Here’s a simple example:
Python Unpacking Asterisk
You can use the asterisk operator * on the left-hand side of the equation to unpack multiple elements into a single variable.
This way, you can overcome the ValueError: too many values to unpack when there are more values in the iterable than there are variables to capture them.
Here’s an example where we capture two elements 2 and 3 in one variable b using the asterisk operator as a prefix in *b :
⚡ Note : This only works in Python 3 but not in Python 2. Here’s how to check your Python version .
Python will automatically assign the values in the iterable to the variables so that the asterisked’ variable captures all remaining elements.
In the following example, the asterisked variable *a captures all the remaining values that cannot be captured by the non-asterisked variables:
No matter how many elements are captured by the asterisked variable, they will always be stored as a list (even if a single variable would be enough):
However, you cannot use multiple asterisk operators in the same expression or Python wouldn’t know which iterable elements to assign to which variables.
The following screenshot shows how Python doesn’t compile with the warning “SyntaxError: multiple starred expressions in assignment” .
If you’re completely uninterested in all but a couple of elements of a large list, you can combine the throw-away underscore operator _ with the asterisk operator * like so *_ . Using this approach, the underscore will capture all the unnecessary elements from the iterable .
This can be seen in the following example:
How to Capture First and Last Element of an Iterable in Variable [Example]
Here’s an example of this:
All list elements 1 to 98 (included) are now stored in the throw-away variable _ .
Python assigns an empty list to the asterisked variable if no additional elements can be assigned to it because all are already assigned to other variables:
Where to Go From Here?
Enough theory. Let’s get some practice!
Coders get paid six figures and more because they can solve problems more effectively using machine intelligence and automation.
To become more successful in coding, solve more real problems for real people. That’s how you polish the skills you really need in practice. After all, what’s the use of learning theory that nobody ever needs?
You build high-value coding skills by working on practical coding projects!
Do you want to stop learning with toy projects and focus on practical code projects that earn you money and solve real problems for people?
🚀 If your answer is YES! , consider becoming a Python freelance developer ! It’s the best way of approaching the task of improving your Python skills—even if you are a complete beginner.
If you just want to learn about the freelancing opportunity, feel free to watch my free webinar “How to Build Your High-Income Skill Python” and learn how I grew my coding business online and how you can, too—from the comfort of your own home.
Join the free webinar now!
While working as a researcher in distributed systems, Dr. Christian Mayer found his love for teaching computer science students.
To help students reach higher levels of Python success, he founded the programming education website Finxter.com that has taught exponential skills to millions of coders worldwide. He’s the author of the best-selling programming books Python One-Liners (NoStarch 2020), The Art of Clean Code (NoStarch 2022), and The Book of Dash (NoStarch 2022). Chris also coauthored the Coffee Break Python series of self-published books. He’s a computer science enthusiast, freelancer , and owner of one of the top 10 largest Python blogs worldwide.
His passions are writing, reading, and coding. But his greatest passion is to serve aspiring coders through Finxter and help them to boost their skills. You can join his free email academy here.
Multiple Assignment Syntax in Python
- python-tricks
The multiple assignment syntax, often referred to as tuple unpacking or extended unpacking, is a powerful feature in Python. There are several ways to assign multiple values to variables at once.
Let's start with a first example that uses extended unpacking . This syntax is used to assign values from an iterable (in this case, a string) to multiple variables:
a : This variable will be assigned the first element of the iterable, which is 'D' in the case of the string 'Devlabs'.
*b : The asterisk (*) before b is used to collect the remaining elements of the iterable (the middle characters in the string 'Devlabs') into a list: ['e', 'v', 'l', 'a', 'b']
c : This variable will be assigned the last element of the iterable: 's'.
The multiple assignment syntax can also be used for numerous other tasks:
Swapping Values
This swaps the values of variables a and b without needing a temporary variable.
Splitting a List
first will be 1, and rest will be a list containing [2, 3, 4, 5] .
Assigning Multiple Values from a Function
This assigns the values returned by get_values() to x, y, and z.
Ignoring Values
Here, you're ignoring the first value with an underscore _ and assigning "Hello" to the important_value . In Python, the underscore is commonly used as a convention to indicate that a variable is being intentionally ignored or is a placeholder for a value that you don't intend to use.
Unpacking Nested Structures
This unpacks a nested structure (Tuple in this example) into separate variables. We can use similar syntax also for Dictionaries:
In this case, we first extract the 'person' dictionary from data, and then we use multiple assignment to further extract values from the nested dictionaries, making the code more concise.
Extended Unpacking with Slicing
first will be 1, middle will be a list containing [2, 3, 4], and last will be 5.
Split a String into a List
*split, is used for iterable unpacking. The asterisk (*) collects the remaining elements into a list variable named split . In this case, it collects all the characters from the string.
The comma , after *split is used to indicate that it's a single-element tuple assignment. It's a syntax requirement to ensure that split becomes a list containing the characters.
Trey Hunner
I help developers level-up their python skills, multiple assignment and tuple unpacking improve python code readability.
Mar 7 th , 2018 4:30 pm | Comments
Whether I’m teaching new Pythonistas or long-time Python programmers, I frequently find that Python programmers underutilize multiple assignment .
Multiple assignment (also known as tuple unpacking or iterable unpacking) allows you to assign multiple variables at the same time in one line of code. This feature often seems simple after you’ve learned about it, but it can be tricky to recall multiple assignment when you need it most .
In this article we’ll see what multiple assignment is, we’ll take a look at common uses of multiple assignment, and then we’ll look at a few uses for multiple assignment that are often overlooked.
Note that in this article I will be using f-strings which are a Python 3.6+ feature. If you’re on an older version of Python, you’ll need to mentally translate those to use the string format method.
How multiple assignment works
I’ll be using the words multiple assignment , tuple unpacking , and iterable unpacking interchangeably in this article. They’re all just different words for the same thing.
Python’s multiple assignment looks like this:
Here we’re setting x to 10 and y to 20 .
What’s happening at a lower level is that we’re creating a tuple of 10, 20 and then looping over that tuple and taking each of the two items we get from looping and assigning them to x and y in order.
This syntax might make that a bit more clear:
Parenthesis are optional around tuples in Python and they’re also optional in multiple assignment (which uses a tuple-like syntax). All of these are equivalent:
Multiple assignment is often called “tuple unpacking” because it’s frequently used with tuples. But we can use multiple assignment with any iterable, not just tuples. Here we’re using it with a list:
And with a string:
Anything that can be looped over can be “unpacked” with tuple unpacking / multiple assignment.
Here’s another example to demonstrate that multiple assignment works with any number of items and that it works with variables as well as objects we’ve just created:
Note that on that last line we’re actually swapping variable names, which is something multiple assignment allows us to do easily.
Alright, let’s talk about how multiple assignment can be used.
Unpacking in a for loop
You’ll commonly see multiple assignment used in for loops.
Let’s take a dictionary:
Instead of looping over our dictionary like this:
You’ll often see Python programmers use multiple assignment by writing this:
When you write the for X in Y line of a for loop, you’re telling Python that it should do an assignment to X for each iteration of your loop. Just like in an assignment using the = operator, we can use multiple assignment here.
Is essentially the same as this:
We’re just not doing an unnecessary extra assignment in the first example.
So multiple assignment is great for unpacking dictionary items into key-value pairs, but it’s helpful in many other places too.
It’s great when paired with the built-in enumerate function:
And the zip function:
If you’re unfamiliar with enumerate or zip , see my article on looping with indexes in Python .
Newer Pythonistas often see multiple assignment in the context of for loops and sometimes assume it’s tied to loops. Multiple assignment works for any assignment though, not just loop assignments.
An alternative to hard coded indexes
It’s not uncommon to see hard coded indexes (e.g. point[0] , items[1] , vals[-1] ) in code:
When you see Python code that uses hard coded indexes there’s often a way to use multiple assignment to make your code more readable .
Here’s some code that has three hard coded indexes:
We can make this code much more readable by using multiple assignment to assign separate month, day, and year variables:
Whenever you see hard coded indexes in your code, stop to consider whether you could use multiple assignment to make your code more readable.
Multiple assignment is very strict
Multiple assignment is actually fairly strict when it comes to unpacking the iterable we give to it.
If we try to unpack a larger iterable into a smaller number of variables, we’ll get an error:
If we try to unpack a smaller iterable into a larger number of variables, we’ll also get an error:
This strictness is pretty great. If we’re working with an item that has a different size than we expected, the multiple assignment will fail loudly and we’ll hopefully now know about a bug in our program that we weren’t yet aware of.
Let’s look at an example. Imagine that we have a short command line program that parses command-line arguments in a rudimentary way, like this:
Our program is supposed to accept 2 arguments, like this:
But if someone called our program with three arguments, they will not see an error:
There’s no error because we’re not validating that we’ve received exactly 2 arguments.
If we use multiple assignment instead of hard coded indexes, the assignment will verify that we receive exactly the expected number of arguments:
Note : we’re using the variable name _ to note that we don’t care about sys.argv[0] (the name of our program). Using _ for variables you don’t care about is just a convention.
An alternative to slicing
So multiple assignment can be used for avoiding hard coded indexes and it can be used to ensure we’re strict about the size of the tuples/iterables we’re working with.
Multiple assignment can be used to replace hard coded slices too!
Slicing is a handy way to grab a specific portion of the items in lists and other sequences.
Here are some slices that are “hard coded” in that they only use numeric indexes:
Whenever you see slices that don’t use any variables in their slice indexes, you can often use multiple assignment instead. To do this we have to talk about a feature that I haven’t mentioned yet: the * operator.
In Python 3.0, the * operator was added to the multiple assignment syntax, allowing us to capture remaining items after an unpacking into a list:
The * operator allows us to replace hard coded slices near the ends of sequences.
These two lines are equivalent:
These two lines are equivalent also:
With the * operator and multiple assignment you can replace things like this:
With more descriptive code, like this:
So if you see hard coded slice indexes in your code, consider whether you could use multiple assignment to clarify what those slices really represent.
Deep unpacking
This next feature is something that long-time Python programmers often overlook. It doesn’t come up quite as often as the other uses for multiple assignment that I’ve discussed, but it can be very handy to know about when you do need it.
We’ve seen multiple assignment for unpacking tuples and other iterables. We haven’t yet seen that this is can be done deeply .
I’d say that the following multiple assignment is shallow because it unpacks one level deep:
And I’d say that this multiple assignment is deep because it unpacks the previous point tuple further into x , y , and z variables:
If it seems confusing what’s going on above, maybe using parenthesis consistently on both sides of this assignment will help clarify things:
We’re unpacking one level deep to get two objects, but then we take the second object and unpack it also to get 3 more objects. Then we assign our first object and our thrice-unpacked second object to our new variables ( color , x , y , and z ).
Take these two lists:
Here’s an example of code that works with these lists by using shallow unpacking:
And here’s the same thing with deeper unpacking:
Note that in this second case, it’s much more clear what type of objects we’re working with. The deep unpacking makes it apparent that we’re receiving two 2-itemed tuples each time we loop.
Deep unpacking often comes up when nesting looping utilities that each provide multiple items. For example, you may see deep multiple assignments when using enumerate and zip together:
I said before that multiple assignment is strict about the size of our iterables as we unpack them. With deep unpacking we can also be strict about the shape of our iterables .
This works:
But this buggy code works too:
Whereas this works:
But this does not:
With multiple assignment we’re assigning variables while also making particular assertions about the size and shape of our iterables. Multiple assignment will help you clarify your code to both humans (for better code readability ) and to computers (for improved code correctness ).
Using a list-like syntax
I noted before that multiple assignment uses a tuple-like syntax, but it works on any iterable. That tuple-like syntax is the reason it’s commonly called “tuple unpacking” even though it might be more clear to say “iterable unpacking”.
I didn’t mention before that multiple assignment also works with a list-like syntax .
Here’s a multiple assignment with a list-like syntax:
This might seem really strange. What’s the point of allowing both list-like and tuple-like syntaxes?
I use this feature rarely, but I find it helpful for code clarity in specific circumstances.
Let’s say I have code that used to look like this:
And our well-intentioned coworker has decided to use deep multiple assignment to refactor our code to this:
See that trailing comma on the left-hand side of the assignment? It’s easy to miss and it makes this code look sort of weird. What is that comma even doing in this code?
That trailing comma is there to make a single item tuple. We’re doing deep unpacking here.
Here’s another way we could write the same code:
This might make that deep unpacking a little more obvious but I’d prefer to see this instead:
The list-syntax in our assignment makes it more clear that we’re unpacking a one-item iterable and then unpacking that single item into value and times_seen variables.
When I see this, I also think I bet we’re unpacking a single-item list . And that is in fact what we’re doing. We’re using a Counter object from the collections module here. The most_common method on Counter objects allows us to limit the length of the list returned to us. We’re limiting the list we’re getting back to just a single item.
When you’re unpacking structures that often hold lots of values (like lists) and structures that often hold a very specific number of values (like tuples) you may decide that your code appears more semantically accurate if you use a list-like syntax when unpacking those list-like structures.
If you’d like you might even decide to adopt a convention of always using a list-like syntax when unpacking list-like structures (frequently the case when using * in multiple assignment):
I don’t usually use this convention myself, mostly because I’m just not in the habit of using it. But if you find it helpful, you might consider using this convention in your own code.
When using multiple assignment in your code, consider when and where a list-like syntax might make your code more descriptive and more clear. This can sometimes improve readability.
Don’t forget about multiple assignment
Multiple assignment can improve both the readability of your code and the correctness of your code. It can make your code more descriptive while also making implicit assertions about the size and shape of the iterables you’re unpacking.
The use for multiple assignment that I often see forgotten is its ability to replace hard coded indexes , including replacing hard coded slices (using the * syntax). It’s also common to overlook the fact that multiple assignment works deeply and can be used with both a tuple-like syntax and a list-like syntax.
It’s tricky to recognize and remember all the cases that multiple assignment can come in handy. Please feel free to use this article as your personal reference guide to multiple assignment.
Get practice with multiple assignment
You don’t learn by reading articles like this one, you learn by writing code .
To get practice writing some readable code using tuple unpacking, sign up for Python Morsels using the form below. If you sign up to Python Morsels using this form, I’ll immediately send you an exercise that involves tuple unpacking.
Intro to Python courses often skip over some fundamental Python concepts .
Sign up below and I'll share ideas new Pythonistas often overlook .
You're nearly signed up. You just need to check your email and click the link there to set your password .
Right after you've set your password you'll receive your first Python Morsels exercise.
Tuple Unpacking
Tuple unpacking (aka "multiple assignment" or "iterable unpacking") is often underutilized by new Python programmers. It's tempting to reach for indexes when working with tuples, lists, and other sequences. But if we know the size/shape of the tuple (or other sequence ) we're working with, we can unpack it using
Tuple unpacking screencasts/articles
- Tuple unpacking
- Deep tuple unpacking
- Tuple unpacking isn't just for tuples
- Extended iterable unpacking
Recommended Resources
- Article: Multiple assignment and tuple unpacking improve Python code readability by Trey Hunner
A Python tip every week
Need to fill-in gaps in your Python skills?
Sign up for my Python newsletter where I share one of my favorite Python tips every week .
Need to fill-in gaps in your Python skills ? I send weekly emails designed to do just that.
Sign in to record your progress
Sign in to your Python Morsels account to track your progress.
Don't have an account yet? Sign up here .
Adventures in Machine Learning
Simplify your code with python’s unpacking assignment: a guide.
Unpacking Assignment in Python: A Guide to Simplifying Your Code
Python is a high-level, interpreted language that is known for its readability and simplicity. One of the great features of Python is its ability to work with sequences, like lists, tuples, and strings, which allow you to store multiple elements of data in a single variable.
However, working with these sequences can sometimes be cumbersome, especially if you need to extract specific elements to use elsewhere in your code. This is where unpacking assignment comes in handy.
Unpacking Assignment is the process of assigning elements of a sequence to multiple variables in a single statement. Its a concise and efficient way to extract data from a sequence and assign it to variables that can be used elsewhere in your code.
It is also alternatively known as destructuring assignment or multiple assignment. In this article, we will explore the basics of Unpacking Assignment and some of its benefits.
Index Notation vs. Unpacking Assignment
Before we dive into Unpacking Assignment, lets briefly talk about Index Notation.
Index Notation is used to access specific elements in a sequence using its index value. For example, if you have a list of fruit names and you want to access the third element, you would use the following code:
fruit = [“Apple”, “Banana”, “Cherry”, “Date”]
print(fruit[2])
This will output Cherry as the third element in the list is stored at index 2.
While Index Notation is a useful way to access specific elements in a sequence, it can become tedious if you need to assign multiple elements from a sequence to different variables. In such cases, Unpacking Assignment can greatly simplify your code.
Basic Unpacking Assignment
The basic syntax for Unpacking Assignment is as follows:
variable1, variable2, variable3, … = sequence
It is important to note that the number of variables on the left must match the number of elements in the sequence, otherwise, you will receive a ValueError: Too many/few values to unpack.
Lets take a look at an example:
a, b, c, d = fruit
print(a, b, c, d)
In this example, we are assigning each element of the list fruit to variables a, b, c, and d. When we print the variables, we get the output: Apple Banana Cherry Date.
This is an efficient and convenient way to extract elements from a sequence and assign them to different variables.
Discarding Elements using Underscore Variable
Sometimes, you may want to discard or ignore certain elements in a sequence. In such cases, you can use the Underscore Variable which is represented by an underscore _.
Lets consider an example where we have a list of fruit names, but we are only interested in the first and last elements. We can use the Underscore Variable to discard the middle elements:
first, _, _, last = fruit
print(first, last)
In this example, we are assigning the first and last elements of the list to the variables first and last respectively.
The middle elements are discarded using the Underscore Variable. When we print the variables, we get the output: Apple Date.
Unpacking Elements as a New List using Asterisk Operator
Another useful feature of Unpacking Assignment is the ability to unpack multiple elements from a sequence and pack them into a new list using the Asterisk Operator.
The Asterisk Operator is represented by the * symbol and can be used to unpack all remaining elements in a sequence.
For example:
first, *middle, last = fruit
print(first, middle, last)
In this example, we are assigning the first and last elements of the list to the variables first and last respectively. The middle elements are unpacked using the Asterisk Operator and assigned to the variable middle.
When we print the variables, we get the output: Apple [Banana, Cherry] Date. The middle elements are packed into a new list.
Benefits of Unpacking Assignment
Unpacking Assignment has several benefits that make it a valuable tool for working with sequences in Python.
Convenience and Efficiency
Unpacking Assignment is a simple and efficient way to extract elements from a sequence and assign them to variables. Its much faster and conveniences than having to use Index Notation to access each element separately.
This can be especially useful when dealing with large sequences.
Flexibility
Unpacking Assignment is compatible with various types of Python sequences, including lists, tuples, and strings. You can use it to assign elements from different types of sequences to variables in a single statement.
name = (“John”, “Doe”)
first, last = name
code = “ABC123”
a, b, c, d, e, f = code
In these examples, we are using Unpacking Assignment to assign elements from a tuple and a string to variables respectively.
Unpacking Assignment is a valuable feature of Python that simplifies working with sequences. It allows you to extract data from a sequence and assign it to multiple variables in a single statement, making your code cleaner, faster, and more efficient.
By discarding elements using Underscore Variables and unpacking elements as a new list using the Asterisk Operator, you can have greater flexibility when working with sequences. Its important to note that Unpacking Assignment is only compatible with sequences of equal length, and the order of variables matters.
So, when working with this feature, its important to pay attention to the details to avoid errors. In summary, the Unpacking Assignment feature in Python makes it easy and intuitive to extract data from a sequence and assign it to multiple variables in a single statement.
By discarding elements using Underscore Variables and unpacking elements as a new list using the Asterisk Operator, programmers can achieve greater flexibility when working with sequences of different lengths. The benefits of Unpacking Assignment include convenience and efficiency, and its compatibility with various types of Python sequences.
It is important to pay attention to details while working with this feature to avoid errors. As you continue to work with Python and sequences, incorporating Unpacking Assignment into your code will make it cleaner, faster and more efficient.
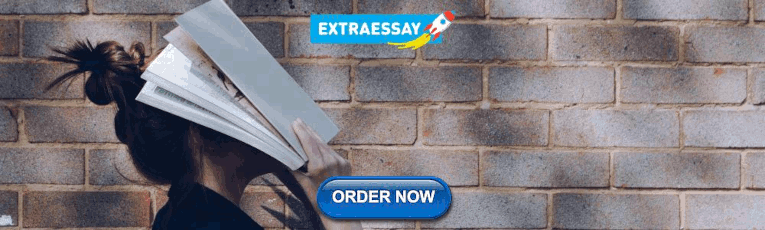
Popular Posts
Mastering sql server correlated subqueries: definition dependency and application, mastering pandas: dropping rows with multiple conditions, the role of cookies in modern web development.
- Terms & Conditions
- Privacy Policy
- Python Basics
- Interview Questions
- Python Quiz
- Popular Packages
- Python Projects
- Practice Python
- AI With Python
- Learn Python3
- Python Automation
- Python Web Dev
- DSA with Python
- Python OOPs
- Dictionaries
- Python Tuple Methods
- Python Tuple - max() Method
- Python Tuple - min() Method
- Python Tuple - index() Method
- Python Tuple - len() Method
- Python Tuple count() Method
- Python | Remove empty tuples from a list
- Python | Reversing a Tuple
- Python - Clearing a tuple
- Python - Create a List of Tuples
- Python | Convert a List into a Tuple
- Python | Unzip a list of tuples
Unpacking a Tuple in Python
- tuple() Function in Python
- Python | Sort a tuple by its float element
- Python | Accessing nth element from tuples in list
Python Tuples In python tuples are used to store immutable objects. Python Tuples are very similar to lists except to some situations. Python tuples are immutable means that they can not be modified in whole program.
Packing and Unpacking a Tuple: In Python, there is a very powerful tuple assignment feature that assigns the right-hand side of values into the left-hand side. In another way, it is called unpacking of a tuple of values into a variable. In packing, we put values into a new tuple while in unpacking we extract those values into a single variable.
Example 1
NOTE : In unpacking of tuple number of variables on left-hand side should be equal to number of values in given tuple a. Python uses a special syntax to pass optional arguments (*args) for tuple unpacking. This means that there can be many number of arguments in place of (*args) in python. All values will be assigned to every variable on the left-hand side and all remaining values will be assigned to *args .For better understanding consider the following code.
Example 2
In python tuples can be unpacked using a function in function tuple is passed and in function values are unpacked into normal variable. Consider the following code for better understanding.
Example 3 :
Please Login to comment...
Similar reads.
- python-tuple
Improve your Coding Skills with Practice
What kind of Experience do you want to share?
Unpacking And Multiple Assignment in
About unpacking and multiple assignment.
Unpacking refers to the act of extracting the elements of a collection, such as a list , tuple , or dict , using iteration. Unpacked values can then be assigned to variables within the same statement. A very common example of this behavior is for item in list , where item takes on the value of each list element in turn throughout the iteration.
Multiple assignment is the ability to assign multiple variables to unpacked values within one statement. This allows for code to be more concise and readable, and is done by separating the variables to be assigned with a comma such as first, second, third = (1,2,3) or for index, item in enumerate(iterable) .
The special operators * and ** are often used in unpacking contexts. * can be used to combine multiple lists / tuples into one list / tuple by unpacking each into a new common list / tuple . ** can be used to combine multiple dictionaries into one dictionary by unpacking each into a new common dict .
When the * operator is used without a collection, it packs a number of values into a list . This is often used in multiple assignment to group all "leftover" elements that do not have individual assignments into a single variable.
It is common in Python to also exploit this unpacking/packing behavior when using or defining functions that take an arbitrary number of positional or keyword arguments. You will often see these "special" parameters defined as def some_function(*args, **kwargs) and the "special" arguments used as some_function(*some_tuple, **some_dict) .
*<variable_name> and **<variable_name> should not be confused with * and ** . While * and ** are used for multiplication and exponentiation respectively, *<variable_name> and **<variable_name> are used as packing and unpacking operators.
Multiple assignment
In multiple assignment, the number of variables on the left side of the assignment operator ( = ) must match the number of values on the right side. To separate the values, use a comma , :
If the multiple assignment gets an incorrect number of variables for the values given, a ValueError will be thrown:
Multiple assignment is not limited to one data type:
Multiple assignment can be used to swap elements in lists . This practice is pretty common in sorting algorithms . For example:
Since tuples are immutable, you can't swap elements in a tuple .
The examples below use lists but the same concepts apply to tuples .
In Python, it is possible to unpack the elements of list / tuple / dictionary into distinct variables. Since values appear within lists / tuples in a specific order, they are unpacked into variables in the same order:
If there are values that are not needed then you can use _ to flag them:
Deep unpacking
Unpacking and assigning values from a list / tuple inside of a list or tuple ( also known as nested lists/tuples ), works in the same way a shallow unpacking does, but often needs qualifiers to clarify the values context or position:
You can also deeply unpack just a portion of a nested list / tuple :
If the unpacking has variables with incorrect placement and/or an incorrect number of values, you will get a ValueError :
Unpacking a list/tuple with *
When unpacking a list / tuple you can use the * operator to capture the "leftover" values. This is clearer than slicing the list / tuple ( which in some situations is less readable ). For example, we can extract the first element and then assign the remaining values into a new list without the first element:
We can also extract the values at the beginning and end of the list while grouping all the values in the middle:
We can also use * in deep unpacking:
Unpacking a dictionary
Unpacking a dictionary is a bit different than unpacking a list / tuple . Iteration over dictionaries defaults to the keys . So when unpacking a dict , you can only unpack the keys and not the values :
If you want to unpack the values then you can use the values() method:
If both keys and values are needed, use the items() method. Using items() will generate tuples with key-value pairs. This is because of dict.items() generates an iterable with key-value tuples .
Packing is the ability to group multiple values into one list that is assigned to a variable. This is useful when you want to unpack values, make changes, and then pack the results back into a variable. It also makes it possible to perform merges on 2 or more lists / tuples / dicts .
Packing a list/tuple with *
Packing a list / tuple can be done using the * operator. This will pack all the values into a list / tuple .
Packing a dictionary with **
Packing a dictionary is done by using the ** operator. This will pack all key - value pairs from one dictionary into another dictionary, or combine two dictionaries together.
Usage of * and ** with functions
Packing with function parameters.
When you create a function that accepts an arbitrary number of arguments, you can use *args or **kwargs in the function definition. *args is used to pack an arbitrary number of positional (non-keyworded) arguments and **kwargs is used to pack an arbitrary number of keyword arguments.
Usage of *args :
Usage of **kwargs :
*args and **kwargs can also be used in combination with one another:
You can also write parameters before *args to allow for specific positional arguments. Individual keyword arguments then have to appear before **kwargs .
Arguments have to be structured like this:
def my_function(<positional_args>, *args, <key-word_args>, **kwargs)
If you don't follow this order then you will get an error.
Writing arguments in an incorrect order will result in an error:
Unpacking into function calls
You can use * to unpack a list / tuple of arguments into a function call. This is very useful for functions that don't accept an iterable :
Using * unpacking with the zip() function is another common use case. Since zip() takes multiple iterables and returns a list of tuples with the values from each iterable grouped:
Learn Unpacking And Multiple Assignment
Unlock 3 more exercises to practice unpacking and multiple assignment.
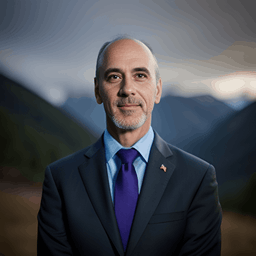
MachineLearningTutorials.org
Brief Machine Learning tutorials with examples.
Python Unpacking Argument Lists (With Examples)
Python is a versatile programming language known for its simplicity and readability. One of its powerful features is the ability to pass a sequence of arguments to a function using the unpacking syntax. This technique, often referred to as “unpacking argument lists,” allows you to pass individual elements of a sequence (such as a list or tuple) as separate arguments to a function. This tutorial will delve into the concept of unpacking argument lists, explore its various applications, and provide several real-world examples to solidify your understanding.
Table of Contents
- Introduction to Unpacking Argument Lists
- Basic Unpacking
Unpacking Lists
Unpacking tuples.
- Unpacking in Function Calls
Passing List Elements as Arguments
Passing tuple elements as arguments.
- Extended Unpacking
Unpacking into Variables
Ignoring certain values.
- Applications of Unpacking
Swapping Values
Multiple return values, iterating over sequences.
- Common Mistakes to Avoid
1. Introduction to Unpacking Argument Lists
Unpacking argument lists is a technique that lets you pass the elements of a sequence (like a list or tuple) as separate arguments to a function. This can be particularly useful when working with functions that expect a fixed number of arguments, but you have the values stored in a sequence.
Consider a scenario where you want to pass multiple values to a function, but these values are stored in a list or tuple. Instead of manually accessing each element and passing them one by one, you can use unpacking to simplify the process and enhance code readability.
2. Basic Unpacking
Let’s start by understanding how to unpack elements from a list and pass them as arguments to a function. Assume we have a function calculate_sum that takes three arguments and returns their sum:
If we have a list numbers containing three values, we can unpack them and pass them to the function using the * operator:
The *numbers syntax effectively unpacks the elements of the numbers list and passes them as separate arguments to the calculate_sum function.
Similarly, you can unpack elements from a tuple and pass them as arguments to a function. Let’s consider a function find_maximum that takes three arguments and returns the maximum of the three:
Suppose we have a tuple values containing the three values we want to find the maximum of:
The *values unpacks the tuple elements and passes them as separate arguments to the find_maximum function.
3. Unpacking in Function Calls
Unpacking isn’t limited to passing arguments to existing functions. You can also use it when defining your own functions to pass elements of a sequence as arguments. Let’s create a function calculate_product that takes three arguments and returns their product:
Suppose we have a list factors containing the values we want to multiply:
In this case, the *factors unpacks the list elements and passes them as separate arguments to the calculate_product function.
Unpacking argument lists is not limited to lists; you can use it with tuples as well. Let’s consider a function calculate_average that takes four arguments and returns their average:
If we have a tuple scores with the four values we want to calculate the average of:
The *scores unpacks the tuple elements and passes them as separate arguments to the calculate_average function.
4. Extended Unpacking
Unpacking argument lists can also be used to assign values from a sequence to individual variables. This is especially useful when you have a fixed number of values and want to avoid indexing the sequence multiple times. Let’s look at an example:
In this case, the tuple point is unpacked into the variables x and y , assigning the values 3 and 7 respectively.
Sometimes you might be interested in only a subset of the elements in a sequence. Python allows you to use an underscore _ as a placeholder for values you want to ignore during unpacking:
Here, we’re unpacking the tuple coordinates and assigning the first and third values to x and z , ignoring the second value using _ .
5. Applications of Unpacking
Unpacking argument lists can make swapping values between variables elegant and concise. Consider the traditional way of swapping two variables a and b :
Using unpacking, you can achieve the same result with fewer lines of code:
Functions in Python can return multiple values using tuples. With unpacking, you can easily capture and assign these returned values to variables. For example:
In this case, the function get_user_info returns a tuple, which is then unpacked and assigned to the variables user_name , user_age , and user_location .
Unpacking argument lists can be particularly useful when iterating over
sequences of tuples or lists. This makes the code more readable and reduces the need for indexing. Consider the following example:
Here, each tuple in the students list is unpacked into name and score , making it easy to iterate over and print the student names and their corresponding scores.
6. Common Mistakes to Avoid
While unpacking argument lists is a powerful technique, there are a few common mistakes you should be aware of:
- Incorrect Number of Elements: Ensure that the number of elements in the sequence matches the number of arguments expected by the function or variables you’re unpacking into.
- Using Unpacking in the Wrong Context: Unpacking can only be used in specific contexts, such as function calls or variable assignments. Trying to use it in unsupported situations will result in errors.
7. Conclusion
Unpacking argument lists is a valuable feature in Python that simplifies passing values to functions and working with sequences. It enhances code readability, reduces the need for explicit indexing, and allows for elegant solutions to common programming problems. By understanding the basics of unpacking and its various applications, you can make your Python code more concise and efficient. As you continue to explore the language, remember to leverage this powerful tool to write cleaner and more maintainable code.
In this tutorial, we covered the fundamental concepts of unpacking argument lists, demonstrated its usage with various examples, and discussed its applications in different scenarios. Armed with this knowledge, you’re well-equipped to take advantage of unpacking to enhance your Python programming skills.
Leave a Reply Cancel reply
Your email address will not be published. Required fields are marked *
Save my name, email, and website in this browser for the next time I comment.
- Python »
- 3.12.3 Documentation »
- The Python Language Reference »
- 7. Simple statements
- Theme Auto Light Dark |
7. Simple statements ¶
A simple statement is comprised within a single logical line. Several simple statements may occur on a single line separated by semicolons. The syntax for simple statements is:
7.1. Expression statements ¶
Expression statements are used (mostly interactively) to compute and write a value, or (usually) to call a procedure (a function that returns no meaningful result; in Python, procedures return the value None ). Other uses of expression statements are allowed and occasionally useful. The syntax for an expression statement is:
An expression statement evaluates the expression list (which may be a single expression).
In interactive mode, if the value is not None , it is converted to a string using the built-in repr() function and the resulting string is written to standard output on a line by itself (except if the result is None , so that procedure calls do not cause any output.)
7.2. Assignment statements ¶
Assignment statements are used to (re)bind names to values and to modify attributes or items of mutable objects:
(See section Primaries for the syntax definitions for attributeref , subscription , and slicing .)
An assignment statement evaluates the expression list (remember that this can be a single expression or a comma-separated list, the latter yielding a tuple) and assigns the single resulting object to each of the target lists, from left to right.
Assignment is defined recursively depending on the form of the target (list). When a target is part of a mutable object (an attribute reference, subscription or slicing), the mutable object must ultimately perform the assignment and decide about its validity, and may raise an exception if the assignment is unacceptable. The rules observed by various types and the exceptions raised are given with the definition of the object types (see section The standard type hierarchy ).
Assignment of an object to a target list, optionally enclosed in parentheses or square brackets, is recursively defined as follows.
If the target list is a single target with no trailing comma, optionally in parentheses, the object is assigned to that target.
If the target list contains one target prefixed with an asterisk, called a “starred” target: The object must be an iterable with at least as many items as there are targets in the target list, minus one. The first items of the iterable are assigned, from left to right, to the targets before the starred target. The final items of the iterable are assigned to the targets after the starred target. A list of the remaining items in the iterable is then assigned to the starred target (the list can be empty).
Else: The object must be an iterable with the same number of items as there are targets in the target list, and the items are assigned, from left to right, to the corresponding targets.
Assignment of an object to a single target is recursively defined as follows.
If the target is an identifier (name):
If the name does not occur in a global or nonlocal statement in the current code block: the name is bound to the object in the current local namespace.
Otherwise: the name is bound to the object in the global namespace or the outer namespace determined by nonlocal , respectively.
The name is rebound if it was already bound. This may cause the reference count for the object previously bound to the name to reach zero, causing the object to be deallocated and its destructor (if it has one) to be called.
If the target is an attribute reference: The primary expression in the reference is evaluated. It should yield an object with assignable attributes; if this is not the case, TypeError is raised. That object is then asked to assign the assigned object to the given attribute; if it cannot perform the assignment, it raises an exception (usually but not necessarily AttributeError ).
Note: If the object is a class instance and the attribute reference occurs on both sides of the assignment operator, the right-hand side expression, a.x can access either an instance attribute or (if no instance attribute exists) a class attribute. The left-hand side target a.x is always set as an instance attribute, creating it if necessary. Thus, the two occurrences of a.x do not necessarily refer to the same attribute: if the right-hand side expression refers to a class attribute, the left-hand side creates a new instance attribute as the target of the assignment:
This description does not necessarily apply to descriptor attributes, such as properties created with property() .
If the target is a subscription: The primary expression in the reference is evaluated. It should yield either a mutable sequence object (such as a list) or a mapping object (such as a dictionary). Next, the subscript expression is evaluated.
If the primary is a mutable sequence object (such as a list), the subscript must yield an integer. If it is negative, the sequence’s length is added to it. The resulting value must be a nonnegative integer less than the sequence’s length, and the sequence is asked to assign the assigned object to its item with that index. If the index is out of range, IndexError is raised (assignment to a subscripted sequence cannot add new items to a list).
If the primary is a mapping object (such as a dictionary), the subscript must have a type compatible with the mapping’s key type, and the mapping is then asked to create a key/value pair which maps the subscript to the assigned object. This can either replace an existing key/value pair with the same key value, or insert a new key/value pair (if no key with the same value existed).
For user-defined objects, the __setitem__() method is called with appropriate arguments.
If the target is a slicing: The primary expression in the reference is evaluated. It should yield a mutable sequence object (such as a list). The assigned object should be a sequence object of the same type. Next, the lower and upper bound expressions are evaluated, insofar they are present; defaults are zero and the sequence’s length. The bounds should evaluate to integers. If either bound is negative, the sequence’s length is added to it. The resulting bounds are clipped to lie between zero and the sequence’s length, inclusive. Finally, the sequence object is asked to replace the slice with the items of the assigned sequence. The length of the slice may be different from the length of the assigned sequence, thus changing the length of the target sequence, if the target sequence allows it.
CPython implementation detail: In the current implementation, the syntax for targets is taken to be the same as for expressions, and invalid syntax is rejected during the code generation phase, causing less detailed error messages.
Although the definition of assignment implies that overlaps between the left-hand side and the right-hand side are ‘simultaneous’ (for example a, b = b, a swaps two variables), overlaps within the collection of assigned-to variables occur left-to-right, sometimes resulting in confusion. For instance, the following program prints [0, 2] :
The specification for the *target feature.
7.2.1. Augmented assignment statements ¶
Augmented assignment is the combination, in a single statement, of a binary operation and an assignment statement:
(See section Primaries for the syntax definitions of the last three symbols.)
An augmented assignment evaluates the target (which, unlike normal assignment statements, cannot be an unpacking) and the expression list, performs the binary operation specific to the type of assignment on the two operands, and assigns the result to the original target. The target is only evaluated once.
An augmented assignment expression like x += 1 can be rewritten as x = x + 1 to achieve a similar, but not exactly equal effect. In the augmented version, x is only evaluated once. Also, when possible, the actual operation is performed in-place , meaning that rather than creating a new object and assigning that to the target, the old object is modified instead.
Unlike normal assignments, augmented assignments evaluate the left-hand side before evaluating the right-hand side. For example, a[i] += f(x) first looks-up a[i] , then it evaluates f(x) and performs the addition, and lastly, it writes the result back to a[i] .
With the exception of assigning to tuples and multiple targets in a single statement, the assignment done by augmented assignment statements is handled the same way as normal assignments. Similarly, with the exception of the possible in-place behavior, the binary operation performed by augmented assignment is the same as the normal binary operations.
For targets which are attribute references, the same caveat about class and instance attributes applies as for regular assignments.
7.2.2. Annotated assignment statements ¶
Annotation assignment is the combination, in a single statement, of a variable or attribute annotation and an optional assignment statement:
The difference from normal Assignment statements is that only a single target is allowed.
For simple names as assignment targets, if in class or module scope, the annotations are evaluated and stored in a special class or module attribute __annotations__ that is a dictionary mapping from variable names (mangled if private) to evaluated annotations. This attribute is writable and is automatically created at the start of class or module body execution, if annotations are found statically.
For expressions as assignment targets, the annotations are evaluated if in class or module scope, but not stored.
If a name is annotated in a function scope, then this name is local for that scope. Annotations are never evaluated and stored in function scopes.
If the right hand side is present, an annotated assignment performs the actual assignment before evaluating annotations (where applicable). If the right hand side is not present for an expression target, then the interpreter evaluates the target except for the last __setitem__() or __setattr__() call.
The proposal that added syntax for annotating the types of variables (including class variables and instance variables), instead of expressing them through comments.
The proposal that added the typing module to provide a standard syntax for type annotations that can be used in static analysis tools and IDEs.
Changed in version 3.8: Now annotated assignments allow the same expressions in the right hand side as regular assignments. Previously, some expressions (like un-parenthesized tuple expressions) caused a syntax error.
7.3. The assert statement ¶
Assert statements are a convenient way to insert debugging assertions into a program:
The simple form, assert expression , is equivalent to
The extended form, assert expression1, expression2 , is equivalent to
These equivalences assume that __debug__ and AssertionError refer to the built-in variables with those names. In the current implementation, the built-in variable __debug__ is True under normal circumstances, False when optimization is requested (command line option -O ). The current code generator emits no code for an assert statement when optimization is requested at compile time. Note that it is unnecessary to include the source code for the expression that failed in the error message; it will be displayed as part of the stack trace.
Assignments to __debug__ are illegal. The value for the built-in variable is determined when the interpreter starts.
7.4. The pass statement ¶
pass is a null operation — when it is executed, nothing happens. It is useful as a placeholder when a statement is required syntactically, but no code needs to be executed, for example:
7.5. The del statement ¶
Deletion is recursively defined very similar to the way assignment is defined. Rather than spelling it out in full details, here are some hints.
Deletion of a target list recursively deletes each target, from left to right.
Deletion of a name removes the binding of that name from the local or global namespace, depending on whether the name occurs in a global statement in the same code block. If the name is unbound, a NameError exception will be raised.
Deletion of attribute references, subscriptions and slicings is passed to the primary object involved; deletion of a slicing is in general equivalent to assignment of an empty slice of the right type (but even this is determined by the sliced object).
Changed in version 3.2: Previously it was illegal to delete a name from the local namespace if it occurs as a free variable in a nested block.
7.6. The return statement ¶
return may only occur syntactically nested in a function definition, not within a nested class definition.
If an expression list is present, it is evaluated, else None is substituted.
return leaves the current function call with the expression list (or None ) as return value.
When return passes control out of a try statement with a finally clause, that finally clause is executed before really leaving the function.
In a generator function, the return statement indicates that the generator is done and will cause StopIteration to be raised. The returned value (if any) is used as an argument to construct StopIteration and becomes the StopIteration.value attribute.
In an asynchronous generator function, an empty return statement indicates that the asynchronous generator is done and will cause StopAsyncIteration to be raised. A non-empty return statement is a syntax error in an asynchronous generator function.
7.7. The yield statement ¶
A yield statement is semantically equivalent to a yield expression . The yield statement can be used to omit the parentheses that would otherwise be required in the equivalent yield expression statement. For example, the yield statements
are equivalent to the yield expression statements
Yield expressions and statements are only used when defining a generator function, and are only used in the body of the generator function. Using yield in a function definition is sufficient to cause that definition to create a generator function instead of a normal function.
For full details of yield semantics, refer to the Yield expressions section.
7.8. The raise statement ¶
If no expressions are present, raise re-raises the exception that is currently being handled, which is also known as the active exception . If there isn’t currently an active exception, a RuntimeError exception is raised indicating that this is an error.
Otherwise, raise evaluates the first expression as the exception object. It must be either a subclass or an instance of BaseException . If it is a class, the exception instance will be obtained when needed by instantiating the class with no arguments.
The type of the exception is the exception instance’s class, the value is the instance itself.
A traceback object is normally created automatically when an exception is raised and attached to it as the __traceback__ attribute. You can create an exception and set your own traceback in one step using the with_traceback() exception method (which returns the same exception instance, with its traceback set to its argument), like so:
The from clause is used for exception chaining: if given, the second expression must be another exception class or instance. If the second expression is an exception instance, it will be attached to the raised exception as the __cause__ attribute (which is writable). If the expression is an exception class, the class will be instantiated and the resulting exception instance will be attached to the raised exception as the __cause__ attribute. If the raised exception is not handled, both exceptions will be printed:
A similar mechanism works implicitly if a new exception is raised when an exception is already being handled. An exception may be handled when an except or finally clause, or a with statement, is used. The previous exception is then attached as the new exception’s __context__ attribute:
Exception chaining can be explicitly suppressed by specifying None in the from clause:
Additional information on exceptions can be found in section Exceptions , and information about handling exceptions is in section The try statement .
Changed in version 3.3: None is now permitted as Y in raise X from Y .
Added the __suppress_context__ attribute to suppress automatic display of the exception context.
Changed in version 3.11: If the traceback of the active exception is modified in an except clause, a subsequent raise statement re-raises the exception with the modified traceback. Previously, the exception was re-raised with the traceback it had when it was caught.
7.9. The break statement ¶
break may only occur syntactically nested in a for or while loop, but not nested in a function or class definition within that loop.
It terminates the nearest enclosing loop, skipping the optional else clause if the loop has one.
If a for loop is terminated by break , the loop control target keeps its current value.
When break passes control out of a try statement with a finally clause, that finally clause is executed before really leaving the loop.
7.10. The continue statement ¶
continue may only occur syntactically nested in a for or while loop, but not nested in a function or class definition within that loop. It continues with the next cycle of the nearest enclosing loop.
When continue passes control out of a try statement with a finally clause, that finally clause is executed before really starting the next loop cycle.
7.11. The import statement ¶
The basic import statement (no from clause) is executed in two steps:
find a module, loading and initializing it if necessary
define a name or names in the local namespace for the scope where the import statement occurs.
When the statement contains multiple clauses (separated by commas) the two steps are carried out separately for each clause, just as though the clauses had been separated out into individual import statements.
The details of the first step, finding and loading modules, are described in greater detail in the section on the import system , which also describes the various types of packages and modules that can be imported, as well as all the hooks that can be used to customize the import system. Note that failures in this step may indicate either that the module could not be located, or that an error occurred while initializing the module, which includes execution of the module’s code.
If the requested module is retrieved successfully, it will be made available in the local namespace in one of three ways:
If the module name is followed by as , then the name following as is bound directly to the imported module.
If no other name is specified, and the module being imported is a top level module, the module’s name is bound in the local namespace as a reference to the imported module
If the module being imported is not a top level module, then the name of the top level package that contains the module is bound in the local namespace as a reference to the top level package. The imported module must be accessed using its full qualified name rather than directly
The from form uses a slightly more complex process:
find the module specified in the from clause, loading and initializing it if necessary;
for each of the identifiers specified in the import clauses:
check if the imported module has an attribute by that name
if not, attempt to import a submodule with that name and then check the imported module again for that attribute
if the attribute is not found, ImportError is raised.
otherwise, a reference to that value is stored in the local namespace, using the name in the as clause if it is present, otherwise using the attribute name
If the list of identifiers is replaced by a star ( '*' ), all public names defined in the module are bound in the local namespace for the scope where the import statement occurs.
The public names defined by a module are determined by checking the module’s namespace for a variable named __all__ ; if defined, it must be a sequence of strings which are names defined or imported by that module. The names given in __all__ are all considered public and are required to exist. If __all__ is not defined, the set of public names includes all names found in the module’s namespace which do not begin with an underscore character ( '_' ). __all__ should contain the entire public API. It is intended to avoid accidentally exporting items that are not part of the API (such as library modules which were imported and used within the module).
The wild card form of import — from module import * — is only allowed at the module level. Attempting to use it in class or function definitions will raise a SyntaxError .
When specifying what module to import you do not have to specify the absolute name of the module. When a module or package is contained within another package it is possible to make a relative import within the same top package without having to mention the package name. By using leading dots in the specified module or package after from you can specify how high to traverse up the current package hierarchy without specifying exact names. One leading dot means the current package where the module making the import exists. Two dots means up one package level. Three dots is up two levels, etc. So if you execute from . import mod from a module in the pkg package then you will end up importing pkg.mod . If you execute from ..subpkg2 import mod from within pkg.subpkg1 you will import pkg.subpkg2.mod . The specification for relative imports is contained in the Package Relative Imports section.
importlib.import_module() is provided to support applications that determine dynamically the modules to be loaded.
Raises an auditing event import with arguments module , filename , sys.path , sys.meta_path , sys.path_hooks .
7.11.1. Future statements ¶
A future statement is a directive to the compiler that a particular module should be compiled using syntax or semantics that will be available in a specified future release of Python where the feature becomes standard.
The future statement is intended to ease migration to future versions of Python that introduce incompatible changes to the language. It allows use of the new features on a per-module basis before the release in which the feature becomes standard.
A future statement must appear near the top of the module. The only lines that can appear before a future statement are:
the module docstring (if any),
blank lines, and
other future statements.
The only feature that requires using the future statement is annotations (see PEP 563 ).
All historical features enabled by the future statement are still recognized by Python 3. The list includes absolute_import , division , generators , generator_stop , unicode_literals , print_function , nested_scopes and with_statement . They are all redundant because they are always enabled, and only kept for backwards compatibility.
A future statement is recognized and treated specially at compile time: Changes to the semantics of core constructs are often implemented by generating different code. It may even be the case that a new feature introduces new incompatible syntax (such as a new reserved word), in which case the compiler may need to parse the module differently. Such decisions cannot be pushed off until runtime.
For any given release, the compiler knows which feature names have been defined, and raises a compile-time error if a future statement contains a feature not known to it.
The direct runtime semantics are the same as for any import statement: there is a standard module __future__ , described later, and it will be imported in the usual way at the time the future statement is executed.
The interesting runtime semantics depend on the specific feature enabled by the future statement.
Note that there is nothing special about the statement:
That is not a future statement; it’s an ordinary import statement with no special semantics or syntax restrictions.
Code compiled by calls to the built-in functions exec() and compile() that occur in a module M containing a future statement will, by default, use the new syntax or semantics associated with the future statement. This can be controlled by optional arguments to compile() — see the documentation of that function for details.
A future statement typed at an interactive interpreter prompt will take effect for the rest of the interpreter session. If an interpreter is started with the -i option, is passed a script name to execute, and the script includes a future statement, it will be in effect in the interactive session started after the script is executed.
The original proposal for the __future__ mechanism.
7.12. The global statement ¶
The global statement is a declaration which holds for the entire current code block. It means that the listed identifiers are to be interpreted as globals. It would be impossible to assign to a global variable without global , although free variables may refer to globals without being declared global.
Names listed in a global statement must not be used in the same code block textually preceding that global statement.
Names listed in a global statement must not be defined as formal parameters, or as targets in with statements or except clauses, or in a for target list, class definition, function definition, import statement, or variable annotation.
CPython implementation detail: The current implementation does not enforce some of these restrictions, but programs should not abuse this freedom, as future implementations may enforce them or silently change the meaning of the program.
Programmer’s note: global is a directive to the parser. It applies only to code parsed at the same time as the global statement. In particular, a global statement contained in a string or code object supplied to the built-in exec() function does not affect the code block containing the function call, and code contained in such a string is unaffected by global statements in the code containing the function call. The same applies to the eval() and compile() functions.
7.13. The nonlocal statement ¶
When the definition of a function or class is nested (enclosed) within the definitions of other functions, its nonlocal scopes are the local scopes of the enclosing functions. The nonlocal statement causes the listed identifiers to refer to names previously bound in nonlocal scopes. It allows encapsulated code to rebind such nonlocal identifiers. If a name is bound in more than one nonlocal scope, the nearest binding is used. If a name is not bound in any nonlocal scope, or if there is no nonlocal scope, a SyntaxError is raised.
The nonlocal statement applies to the entire scope of a function or class body. A SyntaxError is raised if a variable is used or assigned to prior to its nonlocal declaration in the scope.
The specification for the nonlocal statement.
Programmer’s note: nonlocal is a directive to the parser and applies only to code parsed along with it. See the note for the global statement.
7.14. The type statement ¶
The type statement declares a type alias, which is an instance of typing.TypeAliasType .
For example, the following statement creates a type alias:
This code is roughly equivalent to:
annotation-def indicates an annotation scope , which behaves mostly like a function, but with several small differences.
The value of the type alias is evaluated in the annotation scope. It is not evaluated when the type alias is created, but only when the value is accessed through the type alias’s __value__ attribute (see Lazy evaluation ). This allows the type alias to refer to names that are not yet defined.
Type aliases may be made generic by adding a type parameter list after the name. See Generic type aliases for more.
type is a soft keyword .
Added in version 3.12.
Introduced the type statement and syntax for generic classes and functions.
Table of Contents
- 7.1. Expression statements
- 7.2.1. Augmented assignment statements
- 7.2.2. Annotated assignment statements
- 7.3. The assert statement
- 7.4. The pass statement
- 7.5. The del statement
- 7.6. The return statement
- 7.7. The yield statement
- 7.8. The raise statement
- 7.9. The break statement
- 7.10. The continue statement
- 7.11.1. Future statements
- 7.12. The global statement
- 7.13. The nonlocal statement
- 7.14. The type statement
Previous topic
6. Expressions
8. Compound statements
- Report a Bug
- Show Source
Python Enhancement Proposals
- Python »
- PEP Index »
PEP 3132 – Extended Iterable Unpacking
Specification, grammar change, changes to the compiler, changes to the bytecode interpreter.
This PEP proposes a change to iterable unpacking syntax, allowing to specify a “catch-all” name which will be assigned a list of all items not assigned to a “regular” name.
An example says more than a thousand words:
Many algorithms require splitting a sequence in a “first, rest” pair. With the new syntax,
is replaced by the cleaner and probably more efficient:
For more complex unpacking patterns, the new syntax looks even cleaner, and the clumsy index handling is not necessary anymore.
Also, if the right-hand value is not a list, but an iterable, it has to be converted to a list before being able to do slicing; to avoid creating this temporary list, one has to resort to
A tuple (or list) on the left side of a simple assignment (unpacking is not defined for augmented assignment) may contain at most one expression prepended with a single asterisk (which is henceforth called a “starred” expression, while the other expressions in the list are called “mandatory”). This designates a subexpression that will be assigned a list of all items from the iterable being unpacked that are not assigned to any of the mandatory expressions, or an empty list if there are no such items.
For example, if seq is a sliceable sequence, all the following assignments are equivalent if seq has at least two elements:
It is an error (as it is currently) if the iterable doesn’t contain enough items to assign to all the mandatory expressions.
It is also an error to use the starred expression as a lone assignment target, as in
This, however, is valid syntax:
Note that this proposal also applies to tuples in implicit assignment context, such as in a for statement:
would print out
Starred expressions are only allowed as assignment targets, using them anywhere else (except for star-args in function calls, of course) is an error.
Implementation
This feature requires a new grammar rule:
In these two rules, expr is changed to star_expr :
A new ASDL expression type Starred is added which represents a starred expression. Note that the starred expression element introduced here is universal and could later be used for other purposes in non-assignment context, such as the yield *iterable proposal.
The compiler is changed to recognize all cases where a starred expression is invalid and flag them with syntax errors.
A new bytecode instruction, UNPACK_EX , is added, whose argument has the number of mandatory targets before the starred target in the lower 8 bits and the number of mandatory targets after the starred target in the upper 8 bits. For unpacking sequences without starred expressions, the old UNPACK_ITERABLE opcode is kept.
The function unpack_iterable() in ceval.c is changed to handle the extended unpacking, via an argcntafter parameter. In the UNPACK_EX case, the function will do the following:
- collect all items for mandatory targets before the starred one
- collect all remaining items from the iterable in a list
- pop items for mandatory targets after the starred one from the list
- push the single items and the resized list on the stack
Shortcuts for unpacking iterables of known types, such as lists or tuples, can be added.
The current implementation can be found at the SourceForge Patch tracker [SFPATCH] . It now includes a minimal test case.
After a short discussion on the python-3000 list [1] , the PEP was accepted by Guido in its current form. Possible changes discussed were:
- Only allow a starred expression as the last item in the exprlist. This would simplify the unpacking code a bit and allow for the starred expression to be assigned an iterator. This behavior was rejected because it would be too surprising.
- Try to give the starred target the same type as the source iterable, for example, b in a, *b = 'hello' would be assigned the string 'ello' . This may seem nice, but is impossible to get right consistently with all iterables.
- Make the starred target a tuple instead of a list. This would be consistent with a function’s *args , but make further processing of the result harder.
This document has been placed in the public domain.
Source: https://github.com/python/peps/blob/main/peps/pep-3132.rst
Last modified: 2023-09-09 17:39:29 GMT
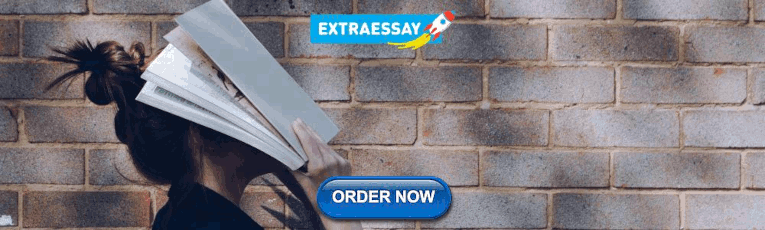
IMAGES
VIDEO
COMMENTS
Packing and Unpacking in Python. Python allows a tuple (or list) of variables to appear on the left side of an assignment operation. Each variable in the tuple can receive one value (or more, if we use the * operator) from an iterable on the right side of the assignment. For historical reasons, Python developers used to call this tuple unpacking.
Python idiomatic unpacking assignment or False. 2. Unpacking sequences in python. 1. Consecutive assignments in python. 1. Unpacking an unspecified amount of variables. 0. Assign fixed number of variables to elements in variable length list. 4. Unpack assignment vs chained assignment. 1.
In Python, unpacking is the process of extracting values from a sequence and assigning them to multiple variables. We achieve unpacking with the assignment operator on the left side and an iterable object on the left side. For example, we can unpack with a tuple like so: 1. 2. 3. myTuple = ( 1, 2, 3) a, b, c = myTuple.
Unpacking in Python is similar to unpack a box in real life. Let's translate this same example into code for a better understanding: >>> mybox = ['cables', 'headphones', 'USB'] >>> item1, item2, item3 = mybox ... item2, item2. This kind of variable assignment is the fundamental concept of unpacking in Python. If you try to get the value of ...
00:00 In this video, I'm going to show you tuple assignment through packing and unpacking. A literal tuple containing several items can be assigned to a single object, such as the example object here, t. 00:16 Assigning that packed object to a new tuple, unpacks the individual items into the objects in that new tuple. When unpacking, the number of variables on the left have to match the ...
The Python Logo from Python.org. Python has a cool feature that permits us to pack and unpack values from iterables in a single assignment. It initially started out as something that was only ...
W3Schools offers free online tutorials, references and exercises in all the major languages of the web. Covering popular subjects like HTML, CSS, JavaScript, Python, SQL, Java, and many, many more.
Python Tuple Unpacking. Tuple unpacking is the process of assigning k elements of a tuple to k different variables in a single line of code. In the following example, you unpack the three elements in the tuple (1, 2, 3) into variables a, b, and c. Each variable captures one tuple element after the unpacking operation.
The multiple assignment syntax, often referred to as tuple unpacking or extended unpacking, is a powerful feature in Python. There are several ways to assign multiple values to variables at once. Let's start with a first example that uses extended unpacking. This syntax is used to assign values from an iterable (in this case, a string) to ...
Python's multiple assignment looks like this: >>> x, y = 10, 20. Here we're setting x to 10 and y to 20. What's happening at a lower level is that we're creating a tuple of 10, 20 and then looping over that tuple and taking each of the two items we get from looping and assigning them to x and y in order.
Tuple unpacking (aka "multiple assignment" or "iterable unpacking") is often underutilized by new Python programmers. It's tempting to reach for indexes when working with tuples, lists, and other sequences. But if we know the size/shape of the tuple (or other sequence) we're working with, we can unpack it using. Tuple unpacking screencasts/articles
Unpacking Assignment in Python: A Guide to Simplifying Your Code . Python is a high-level, interpreted language that is known for its readability and simplicity. One of the great features of Python is its ability to work with sequences, like lists, tuples, and strings, which allow you to store multiple elements of data in a single variable. ...
Python tuples are immutable means that they can not be modified in whole program. Packing and Unpacking a Tuple: In Python, there is a very powerful tuple assignment feature that assigns the right-hand side of values into the left-hand side. In another way, it is called unpacking of a tuple of values into a variable.
About Unpacking And Multiple Assignment. Unpacking refers to the act of extracting the elements of a collection, such as a list, tuple, or dict, using iteration. Unpacked values can then be assigned to variables within the same statement. A very common example of this behavior is for item in list, where item takes on the value of each list ...
In Python, "Variable Unpacking" is a special assignment operation. It allows you to assign all members of an iterable object (such as list , set ) to multiple variables at once.
Using Unpacking in the Wrong Context: Unpacking can only be used in specific contexts, such as function calls or variable assignments. Trying to use it in unsupported situations will result in errors. 7. Conclusion. Unpacking argument lists is a valuable feature in Python that simplifies passing values to functions and working with sequences.
You can use assignment statements for iterable unpacking in Python. Unpacking an iterable means assigning its values to a series of variables one by one. The iterable must be the right operand in the assignment, while the variables must be the left operand. Like in parallel assignments, the variables must come as a tuple or list.
An augmented assignment evaluates the target (which, unlike normal assignment statements, cannot be an unpacking) and the expression list, performs the binary operation specific to the type of assignment on the two operands, and assigns the result to the original target. The target is only evaluated once.
As bool is a subclass of int in Python, the comparison won't raise an exception in either Python 2 or Python 3. ... Multiple Unpacking Assignment in Python when you don't know the sequence length. 0. Python idiomatic unpacking assignment or False. 90. Python conditional assignment operator. 3.
Python Enhancement Proposals (PEPs) Specification. A tuple (or list) on the left side of a simple assignment (unpacking is not defined for augmented assignment) may contain at most one expression prepended with a single asterisk (which is henceforth called a "starred" expression, while the other expressions in the list are called "mandatory").
There are 3 modules in this course. Our "Programming with Generative AI" course takes you on a practical journey, exploring how generative AI tools can transform your coding workflow. Whether you're a software developer, tech lead, or AI enthusiast, this hands-on program is designed for you. Learn by doing: - Dive deep into GitHub Copilot, the ...
14. In my case i use the typing.cast function to type hint an unpack operation. t: tuple[int, int] = (1, 2) a, b = t. # type hint of a -> Literal[1] # type hint of b -> Literal[2] By using the cast(new_type, old_type) you can cast those ugly literals into integers. from typing import cast.