If you're seeing this message, it means we're having trouble loading external resources on our website.
If you're behind a web filter, please make sure that the domains *.kastatic.org and *.kasandbox.org are unblocked.
To log in and use all the features of Khan Academy, please enable JavaScript in your browser.
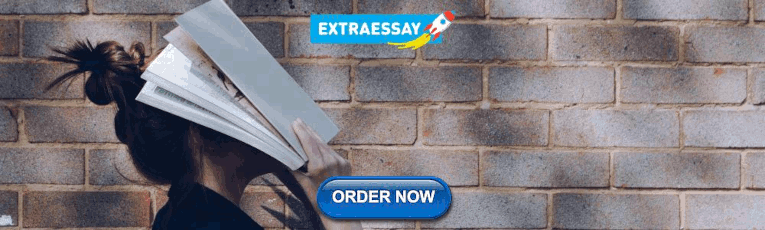
Biology library
Course: biology library > unit 1.
- The scientific method
Controlled experiments
- The scientific method and experimental design

Introduction
How are hypotheses tested.
- One pot of seeds gets watered every afternoon.
- The other pot of seeds doesn't get any water at all.
Control and experimental groups
Independent and dependent variables, independent variables, dependent variables, variability and repetition, controlled experiment case study: co 2 and coral bleaching.
- What your control and experimental groups would be
- What your independent and dependent variables would be
- What results you would predict in each group
Experimental setup
- Some corals were grown in tanks of normal seawater, which is not very acidic ( pH around 8.2 ). The corals in these tanks served as the control group .
- Other corals were grown in tanks of seawater that were more acidic than usual due to addition of CO 2 . One set of tanks was medium-acidity ( pH about 7.9 ), while another set was high-acidity ( pH about 7.65 ). Both the medium-acidity and high-acidity groups were experimental groups .
- In this experiment, the independent variable was the acidity ( pH ) of the seawater. The dependent variable was the degree of bleaching of the corals.
- The researchers used a large sample size and repeated their experiment. Each tank held 5 fragments of coral, and there were 5 identical tanks for each group (control, medium-acidity, and high-acidity). Experimental setup to test effects of water acidity on coral bleaching. Control group: Coral fragments are placed in a tank of normal seawater (pH 8.2). Experimental group 1: Coral fragments are placed in a tank of slightly acidified seawater (pH 7.9). Experimental group 2: Coral fragments are placed in a tank of more strongly acidified seawater (pH 7.65). The water acidity is the independent variable. 8 weeks are allowed to pass for each of the tanks... Control group: Corals are about 10% bleached on average. Experimental group 1 (medium acidity): Corals are about 20% bleached on average. Experimental group 2 (higher acidity): Corals are about 40% bleached on average. Degree of coral bleaching is the dependent variable. Note: None of these tanks was "acidic" on an absolute scale. That is, the pH values were all above the neutral pH of 7.0 . However, the two groups of experimental tanks were moderately and highly acidic to the corals , that is, relative to their natural habitat of plain seawater.
Analyzing the results
Non-experimental hypothesis tests, case study: coral bleaching and temperature, attribution:, works cited:.
- Hoegh-Guldberg, O. (1999). Climate change, coral bleaching, and the future of the world's coral reefs. Mar. Freshwater Res. , 50 , 839-866. Retrieved from www.reef.edu.au/climate/Hoegh-Guldberg%201999.pdf.
- Anthony, K. R. N., Kline, D. I., Diaz-Pulido, G., Dove, S., and Hoegh-Guldberg, O. (2008). Ocean acidification causes bleaching and productivity loss in coral reef builders. PNAS , 105 (45), 17442-17446. http://dx.doi.org/10.1073/pnas.0804478105 .
- University of California Museum of Paleontology. (2016). Misconceptions about science. In Understanding science . Retrieved from http://undsci.berkeley.edu/teaching/misconceptions.php .
- Hoegh-Guldberg, O. and Smith, G. J. (1989). The effect of sudden changes in temperature, light and salinity on the density and export of zooxanthellae from the reef corals Stylophora pistillata (Esper, 1797) and Seriatopora hystrix (Dana, 1846). J. Exp. Mar. Biol. Ecol. , 129 , 279-303. Retrieved from http://www.reef.edu.au/ohg/res-pic/HG%20papers/HG%20and%20Smith%201989%20BLEACH.pdf .
Additional references:
Want to join the conversation.
- Upvote Button navigates to signup page
- Downvote Button navigates to signup page
- Flag Button navigates to signup page

Chapter 6: Experimental Research
Conducting experiments, learning objectives.
- Describe several strategies for recruiting participants for an experiment.
- Explain why it is important to standardize the procedure of an experiment and several ways to do this.
- Explain what pilot testing is and why it is important.
The information presented so far in this chapter is enough to design a basic experiment. When it comes time to conduct that experiment, however, several additional practical issues arise. In this section, we consider some of these issues and how to deal with them. Much of this information applies to nonexperimental studies as well as experimental ones.
Recruiting Participants
Of course, at the start of any research project you should be thinking about how you will obtain your participants. Unless you have access to people with schizophrenia or incarcerated juvenile offenders, for example, then there is no point designing a study that focuses on these populations. But even if you plan to use a convenience sample, you will have to recruit participants for your study.
There are several approaches to recruiting participants. One is to use participants from a formal subject pool —an established group of people who have agreed to be contacted about participating in research studies. For example, at many colleges and universities, there is a subject pool consisting of students enrolled in introductory psychology courses who must participate in a certain number of studies to meet a course requirement. Researchers post descriptions of their studies and students sign up to participate, usually via an online system. Participants who are not in subject pools can also be recruited by posting or publishing advertisements or making personal appeals to groups that represent the population of interest. For example, a researcher interested in studying older adults could arrange to speak at a meeting of the residents at a retirement community to explain the study and ask for volunteers.
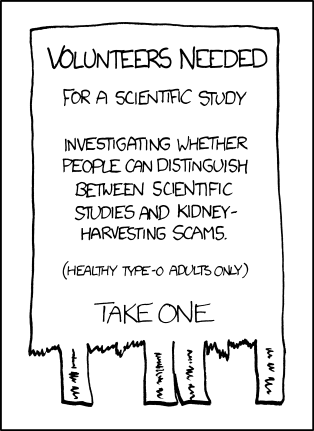
“Study” Retrieved from http://imgs.xkcd.com/comics/study.png (CC-BY-NC 2.5)
The Volunteer Subject
Even if the participants in a study receive compensation in the form of course credit, a small amount of money, or a chance at being treated for a psychological problem, they are still essentially volunteers. This is worth considering because people who volunteer to participate in psychological research have been shown to differ in predictable ways from those who do not volunteer. Specifically, there is good evidence that on average, volunteers have the following characteristics compared with nonvolunteers (Rosenthal & Rosnow, 1976) [1] :
- They are more interested in the topic of the research.
- They are more educated.
- They have a greater need for approval.
- They have higher intelligence quotients (IQs).
- They are more sociable.
- They are higher in social class.
This difference can be an issue of external validity if there is reason to believe that participants with these characteristics are likely to behave differently than the general population. For example, in testing different methods of persuading people, a rational argument might work better on volunteers than it does on the general population because of their generally higher educational level and IQ.
In many field experiments, the task is not recruiting participants but selecting them. For example, researchers Nicolas Guéguen and Marie-Agnès de Gail conducted a field experiment on the effect of being smiled at on helping, in which the participants were shoppers at a supermarket. A confederate walking down a stairway gazed directly at a shopper walking up the stairway and either smiled or did not smile. Shortly afterward, the shopper encountered another confederate, who dropped some computer diskettes on the ground. The dependent variable was whether or not the shopper stopped to help pick up the diskettes (Guéguen & de Gail, 2003) [2] . Notice that these participants were not “recruited,” but the researchers still had to select them from among all the shoppers taking the stairs that day. It is extremely important that this kind of selection be done according to a well-defined set of rules that is established before the data collection begins and can be explained clearly afterward. In this case, with each trip down the stairs, the confederate was instructed to gaze at the first person he encountered who appeared to be between the ages of 20 and 50. Only if the person gazed back did he or she become a participant in the study. The point of having a well-defined selection rule is to avoid bias in the selection of participants. For example, if the confederate was free to choose which shoppers he would gaze at, he might choose friendly-looking shoppers when he was set to smile and unfriendly-looking ones when he was not set to smile. As we will see shortly, such biases can be entirely unintentional.
Standardizing the Procedure
It is surprisingly easy to introduce extraneous variables during the procedure. For example, the same experimenter might give clear instructions to one participant but vague instructions to another. Or one experimenter might greet participants warmly while another barely makes eye contact with them. To the extent that such variables affect participants’ behaviour, they add noise to the data and make the effect of the independent variable more difficult to detect. If they vary across conditions, they become confounding variables and provide alternative explanations for the results. For example, if participants in a treatment group are tested by a warm and friendly experimenter and participants in a control group are tested by a cold and unfriendly one, then what appears to be an effect of the treatment might actually be an effect of experimenter demeanor. When there are multiple experimenters, the possibility for introducing extraneous variables is even greater, but is often necessary for practical reasons.
Experimenter’s Sex as an Extraneous Variable
It is well known that whether research participants are male or female can affect the results of a study. But what about whether the experimenter is male or female? There is plenty of evidence that this matters too. Male and female experimenters have slightly different ways of interacting with their participants, and of course participants also respond differently to male and female experimenters (Rosenthal, 1976) [3] .
For example, in a recent study on pain perception, participants immersed their hands in icy water for as long as they could (Ibolya, Brake, & Voss, 2004) [4] . Male participants tolerated the pain longer when the experimenter was a woman, and female participants tolerated it longer when the experimenter was a man.
Researcher Robert Rosenthal has spent much of his career showing that this kind of unintended variation in the procedure does, in fact, affect participants’ behaviour. Furthermore, one important source of such variation is the experimenter’s expectations about how participants “should” behave in the experiment. This outcome is referred to as an experimenter expectancy effect (Rosenthal, 1976) [5] . For example, if an experimenter expects participants in a treatment group to perform better on a task than participants in a control group, then he or she might unintentionally give the treatment group participants clearer instructions or more encouragement or allow them more time to complete the task. In a striking example, Rosenthal and Kermit Fode had several students in a laboratory course in psychology train rats to run through a maze. Although the rats were genetically similar, some of the students were told that they were working with “maze-bright” rats that had been bred to be good learners, and other students were told that they were working with “maze-dull” rats that had been bred to be poor learners. Sure enough, over five days of training, the “maze-bright” rats made more correct responses, made the correct response more quickly, and improved more steadily than the “maze-dull” rats (Rosenthal & Fode, 1963) [6] . Clearly it had to have been the students’ expectations about how the rats would perform that made the difference. But how? Some clues come from data gathered at the end of the study, which showed that students who expected their rats to learn quickly felt more positively about their animals and reported behaving toward them in a more friendly manner (e.g., handling them more).
The way to minimize unintended variation in the procedure is to standardize it as much as possible so that it is carried out in the same way for all participants regardless of the condition they are in. Here are several ways to do this:
- Create a written protocol that specifies everything that the experimenters are to do and say from the time they greet participants to the time they dismiss them.
- Create standard instructions that participants read themselves or that are read to them word for word by the experimenter.
- Automate the rest of the procedure as much as possible by using software packages for this purpose or even simple computer slide shows.
- Anticipate participants’ questions and either raise and answer them in the instructions or develop standard answers for them.
- Train multiple experimenters on the protocol together and have them practice on each other.
- Be sure that each experimenter tests participants in all conditions.
Another good practice is to arrange for the experimenters to be “blind” to the research question or to the condition that each participant is tested in. The idea is to minimize experimenter expectancy effects by minimizing the experimenters’ expectations. For example, in a drug study in which each participant receives the drug or a placebo, it is often the case that neither the participants nor the experimenter who interacts with the participants know which condition he or she has been assigned to. Because both the participants and the experimenters are blind to the condition, this technique is referred to as a double-blind study . (A single-blind study is one in which the participant, but not the experimenter, is blind to the condition.) Of course, there are many times this blinding is not possible. For example, if you are both the investigator and the only experimenter, it is not possible for you to remain blind to the research question. Also, in many studies the experimenter must know the condition because he or she must carry out the procedure in a different way in the different conditions.
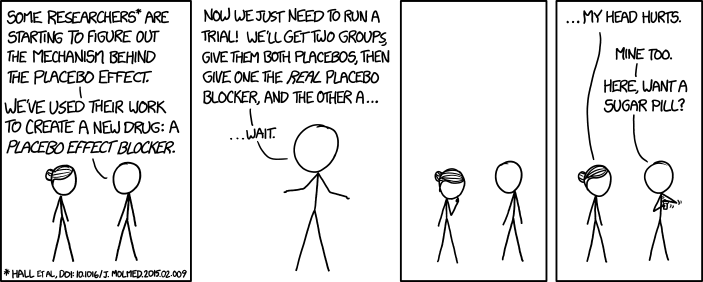
“Placebo Blocker” retrieved from http://imgs.xkcd.com/comics/placebo_blocker.png (CC-BY-NC 2.5)
Record Keeping
It is essential to keep good records when you conduct an experiment. As discussed earlier, it is typical for experimenters to generate a written sequence of conditions before the study begins and then to test each new participant in the next condition in the sequence. As you test them, it is a good idea to add to this list basic demographic information; the date, time, and place of testing; and the name of the experimenter who did the testing. It is also a good idea to have a place for the experimenter to write down comments about unusual occurrences (e.g., a confused or uncooperative participant) or questions that come up. This kind of information can be useful later if you decide to analy z e sex differences or effects of different experimenters, or if a question arises about a particular participant or testing session.
It can also be useful to assign an identification number to each participant as you test them. Simply numbering them consecutively beginning with 1 is usually sufficient. This number can then also be written on any response sheets or questionnaires that participants generate, making it easier to keep them together.
Pilot Testing
It is always a good idea to conduct a pilot test of your experiment. A pilot test is a small-scale study conducted to make sure that a new procedure works as planned. In a pilot test, you can recruit participants formally (e.g., from an established participant pool) or you can recruit them informally from among family, friends, classmates, and so on. The number of participants can be small, but it should be enough to give you confidence that your procedure works as planned. There are several important questions that you can answer by conducting a pilot test:
- Do participants understand the instructions?
- What kind of misunderstandings do participants have, what kind of mistakes do they make, and what kind of questions do they ask?
- Do participants become bored or frustrated?
- Is an indirect manipulation effective? (You will need to include a manipulation check.)
- Can participants guess the research question or hypothesis?
- How long does the procedure take?
- Are computer programs or other automated procedures working properly?
- Are data being recorded correctly?
Of course, to answer some of these questions you will need to observe participants carefully during the procedure and talk with them about it afterward. Participants are often hesitant to criticize a study in front of the researcher, so be sure they understand that their participation is part of a pilot test and you are genuinely interested in feedback that will help you improve the procedure. If the procedure works as planned, then you can proceed with the actual study. If there are problems to be solved, you can solve them, pilot test the new procedure, and continue with this process until you are ready to proceed.
Key Takeaways
- There are several effective methods you can use to recruit research participants for your experiment, including through formal subject pools, advertisements, and personal appeals. Field experiments require well-defined participant selection procedures.
- It is important to standardize experimental procedures to minimize extraneous variables, including experimenter expectancy effects.
- It is important to conduct one or more small-scale pilot tests of an experiment to be sure that the procedure works as planned.
- elderly adults
- unemployed people
- regular exercisers
- math majors
- Discussion: Imagine a study in which you will visually present participants with a list of 20 words, one at a time, wait for a short time, and then ask them to recall as many of the words as they can. In the stressed condition, they are told that they might also be chosen to give a short speech in front of a small audience. In the unstressed condition, they are not told that they might have to give a speech. What are several specific things that you could do to standardize the procedure?
- Rosenthal, R., & Rosnow, R. L. (1976). The volunteer subject . New York, NY: Wiley. ↵
- Guéguen, N., & de Gail, Marie-Agnès. (2003). The effect of smiling on helping behaviour: Smiling and good Samaritan behaviour. Communication Reports, 16 , 133–140. ↵
- Rosenthal, R. (1976). Experimenter effects in behavioural research (enlarged ed.). New York, NY: Wiley. ↵
- Ibolya, K., Brake, A., & Voss, U. (2004). The effect of experimenter characteristics on pain reports in women and men. Pain, 112 , 142–147. ↵
- Rosenthal, R., & Fode, K. (1963). The effect of experimenter bias on performance of the albino rat. Behavioural Science, 8 , 183-189. ↵
- Research Methods in Psychology. Authored by : Paul C. Price, Rajiv S. Jhangiani, and I-Chant A. Chiang. Provided by : BCCampus. Located at : https://opentextbc.ca/researchmethods/ . License : CC BY-NC-SA: Attribution-NonCommercial-ShareAlike
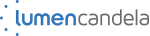
Privacy Policy
- Bipolar Disorder
- Therapy Center
- When To See a Therapist
- Types of Therapy
- Best Online Therapy
- Best Couples Therapy
- Best Family Therapy
- Managing Stress
- Sleep and Dreaming
- Understanding Emotions
- Self-Improvement
- Healthy Relationships
- Student Resources
- Personality Types
- Guided Meditations
- Verywell Mind Insights
- 2024 Verywell Mind 25
- Mental Health in the Classroom
- Editorial Process
- Meet Our Review Board
- Crisis Support
How to Conduct a Psychology Experiment
Kendra Cherry, MS, is a psychosocial rehabilitation specialist, psychology educator, and author of the "Everything Psychology Book."
:max_bytes(150000):strip_icc():format(webp)/IMG_9791-89504ab694d54b66bbd72cb84ffb860e.jpg)
Emily is a board-certified science editor who has worked with top digital publishing brands like Voices for Biodiversity, Study.com, GoodTherapy, Vox, and Verywell.
:max_bytes(150000):strip_icc():format(webp)/Emily-Swaim-1000-0f3197de18f74329aeffb690a177160c.jpg)
Conducting your first psychology experiment can be a long, complicated, and sometimes intimidating process. It can be especially confusing if you are not quite sure where to begin or which steps to take.
Like other sciences, psychology utilizes the scientific method and bases conclusions upon empirical evidence. When conducting an experiment, it is important to follow the seven basic steps of the scientific method:
- Ask a testable question
- Define your variables
- Conduct background research
- Design your experiment
- Perform the experiment
- Collect and analyze the data
- Draw conclusions
- Share the results with the scientific community
At a Glance
It's important to know the steps of the scientific method if you are conducting an experiment in psychology or other fields. The processes encompasses finding a problem you want to explore, learning what has already been discovered about the topic, determining your variables, and finally designing and performing your experiment. But the process doesn't end there! Once you've collected your data, it's time to analyze the numbers, determine what they mean, and share what you've found.
Find a Research Problem or Question
Picking a research problem can be one of the most challenging steps when you are conducting an experiment. After all, there are so many different topics you might choose to investigate.
Are you stuck for an idea? Consider some of the following:
Investigate a Commonly Held Belief
Folk knowledge is a good source of questions that can serve as the basis for psychological research. For example, many people believe that staying up all night to cram for a big exam can actually hurt test performance.
You could conduct a study to compare the test scores of students who stayed up all night with the scores of students who got a full night's sleep before the exam.
Review Psychology Literature
Published studies are a great source of unanswered research questions. In many cases, the authors will even note the need for further research. Find a published study that you find intriguing, and then come up with some questions that require further exploration.
Think About Everyday Problems
There are many practical applications for psychology research. Explore various problems that you or others face each day, and then consider how you could research potential solutions. For example, you might investigate different memorization strategies to determine which methods are most effective.
Define Your Variables
Variables are anything that might impact the outcome of your study. An operational definition describes exactly what the variables are and how they are measured within the context of your study.
For example, if you were doing a study on the impact of sleep deprivation on driving performance, you would need to operationally define sleep deprivation and driving performance .
An operational definition refers to a precise way that an abstract concept will be measured. For example, you cannot directly observe and measure something like test anxiety . You can, however, use an anxiety scale and assign values based on how many anxiety symptoms a person is experiencing.
In this example, you might define sleep deprivation as getting less than seven hours of sleep at night. You might define driving performance as how well a participant does on a driving test.
What is the purpose of operationally defining variables? The main purpose is control. By understanding what you are measuring, you can control for it by holding the variable constant between all groups or manipulating it as an independent variable .
Develop a Hypothesis
The next step is to develop a testable hypothesis that predicts how the operationally defined variables are related. In the recent example, the hypothesis might be: "Students who are sleep-deprived will perform worse than students who are not sleep-deprived on a test of driving performance."
Null Hypothesis
In order to determine if the results of the study are significant, it is essential to also have a null hypothesis. The null hypothesis is the prediction that one variable will have no association to the other variable.
In other words, the null hypothesis assumes that there will be no difference in the effects of the two treatments in our experimental and control groups .
The null hypothesis is assumed to be valid unless contradicted by the results. The experimenters can either reject the null hypothesis in favor of the alternative hypothesis or not reject the null hypothesis.
It is important to remember that not rejecting the null hypothesis does not mean that you are accepting the null hypothesis. To say that you are accepting the null hypothesis is to suggest that something is true simply because you did not find any evidence against it. This represents a logical fallacy that should be avoided in scientific research.
Conduct Background Research
Once you have developed a testable hypothesis, it is important to spend some time doing some background research. What do researchers already know about your topic? What questions remain unanswered?
You can learn about previous research on your topic by exploring books, journal articles, online databases, newspapers, and websites devoted to your subject.
Reading previous research helps you gain a better understanding of what you will encounter when conducting an experiment. Understanding the background of your topic provides a better basis for your own hypothesis.
After conducting a thorough review of the literature, you might choose to alter your own hypothesis. Background research also allows you to explain why you chose to investigate your particular hypothesis and articulate why the topic merits further exploration.
As you research the history of your topic, take careful notes and create a working bibliography of your sources. This information will be valuable when you begin to write up your experiment results.
Select an Experimental Design
After conducting background research and finalizing your hypothesis, your next step is to develop an experimental design. There are three basic types of designs that you might utilize. Each has its own strengths and weaknesses:
Pre-Experimental Design
A single group of participants is studied, and there is no comparison between a treatment group and a control group. Examples of pre-experimental designs include case studies (one group is given a treatment and the results are measured) and pre-test/post-test studies (one group is tested, given a treatment, and then retested).
Quasi-Experimental Design
This type of experimental design does include a control group but does not include randomization. This type of design is often used if it is not feasible or ethical to perform a randomized controlled trial.
True Experimental Design
A true experimental design, also known as a randomized controlled trial, includes both of the elements that pre-experimental designs and quasi-experimental designs lack—control groups and random assignment to groups.
Standardize Your Procedures
In order to arrive at legitimate conclusions, it is essential to compare apples to apples.
Each participant in each group must receive the same treatment under the same conditions.
For example, in our hypothetical study on the effects of sleep deprivation on driving performance, the driving test must be administered to each participant in the same way. The driving course must be the same, the obstacles faced must be the same, and the time given must be the same.
Choose Your Participants
In addition to making sure that the testing conditions are standardized, it is also essential to ensure that your pool of participants is the same.
If the individuals in your control group (those who are not sleep deprived) all happen to be amateur race car drivers while your experimental group (those that are sleep deprived) are all people who just recently earned their driver's licenses, your experiment will lack standardization.
When choosing subjects, there are some different techniques you can use.
Simple Random Sample
In a simple random sample, the participants are randomly selected from a group. A simple random sample can be used to represent the entire population from which the representative sample is drawn.
Drawing a simple random sample can be helpful when you don't know a lot about the characteristics of the population.
Stratified Random Sample
Participants must be randomly selected from different subsets of the population. These subsets might include characteristics such as geographic location, age, sex, race, or socioeconomic status.
Stratified random samples are more complex to carry out. However, you might opt for this method if there are key characteristics about the population that you want to explore in your research.
Conduct Tests and Collect Data
After you have selected participants, the next steps are to conduct your tests and collect the data. Before doing any testing, however, there are a few important concerns that need to be addressed.
Address Ethical Concerns
First, you need to be sure that your testing procedures are ethical . Generally, you will need to gain permission to conduct any type of testing with human participants by submitting the details of your experiment to your school's Institutional Review Board (IRB), sometimes referred to as the Human Subjects Committee.
Obtain Informed Consent
After you have gained approval from your institution's IRB, you will need to present informed consent forms to each participant. This form offers information on the study, the data that will be gathered, and how the results will be used. The form also gives participants the option to withdraw from the study at any point in time.
Once this step has been completed, you can begin administering your testing procedures and collecting the data.
Analyze the Results
After collecting your data, it is time to analyze the results of your experiment. Researchers use statistics to determine if the results of the study support the original hypothesis and if the results are statistically significant.
Statistical significance means that the study's results are unlikely to have occurred simply by chance.
The types of statistical methods you use to analyze your data depend largely on the type of data that you collected. If you are using a random sample of a larger population, you will need to utilize inferential statistics.
These statistical methods make inferences about how the results relate to the population at large.
Because you are making inferences based on a sample, it has to be assumed that there will be a certain margin of error. This refers to the amount of error in your results. A large margin of error means that there will be less confidence in your results, while a small margin of error means that you are more confident that your results are an accurate reflection of what exists in that population.
Share Your Results After Conducting an Experiment
Your final task in conducting an experiment is to communicate your results. By sharing your experiment with the scientific community, you are contributing to the knowledge base on that particular topic.
One of the most common ways to share research results is to publish the study in a peer-reviewed professional journal. Other methods include sharing results at conferences, in book chapters, or academic presentations.
In your case, it is likely that your class instructor will expect a formal write-up of your experiment in the same format required in a professional journal article or lab report :
- Introduction
- Tables and figures
What This Means For You
Designing and conducting a psychology experiment can be quite intimidating, but breaking the process down step-by-step can help. No matter what type of experiment you decide to perform, always check with your instructor and your school's institutional review board for permission before you begin.
NOAA SciJinks. What is the scientific method? .
Nestor, PG, Schutt, RK. Research Methods in Psychology . SAGE; 2015.
Andrade C. A student's guide to the classification and operationalization of variables in the conceptualization and eesign of a clinical study: Part 2 . Indian J Psychol Med . 2021;43(3):265-268. doi:10.1177/0253717621996151
Purna Singh A, Vadakedath S, Kandi V. Clinical research: A review of study designs, hypotheses, errors, sampling types, ethics, and informed consent . Cureus . 2023;15(1):e33374. doi:10.7759/cureus.33374
Colby College. The Experimental Method .
Leite DFB, Padilha MAS, Cecatti JG. Approaching literature review for academic purposes: The Literature Review Checklist . Clinics (Sao Paulo) . 2019;74:e1403. doi:10.6061/clinics/2019/e1403
Salkind NJ. Encyclopedia of Research Design . SAGE Publications, Inc.; 2010. doi:10.4135/9781412961288
Miller CJ, Smith SN, Pugatch M. Experimental and quasi-experimental designs in implementation research . Psychiatry Res . 2020;283:112452. doi:10.1016/j.psychres.2019.06.027
Nijhawan LP, Manthan D, Muddukrishna BS, et. al. Informed consent: Issues and challenges . J Adv Pharm Technol Rese . 2013;4(3):134-140. doi:10.4103/2231-4040.116779
Serdar CC, Cihan M, Yücel D, Serdar MA. Sample size, power and effect size revisited: simplified and practical approaches in pre-clinical, clinical and laboratory studies . Biochem Med (Zagreb) . 2021;31(1):010502. doi:10.11613/BM.2021.010502
American Psychological Association. Publication Manual of the American Psychological Association (7th ed.). Washington DC: The American Psychological Association; 2019.
By Kendra Cherry, MSEd Kendra Cherry, MS, is a psychosocial rehabilitation specialist, psychology educator, and author of the "Everything Psychology Book."
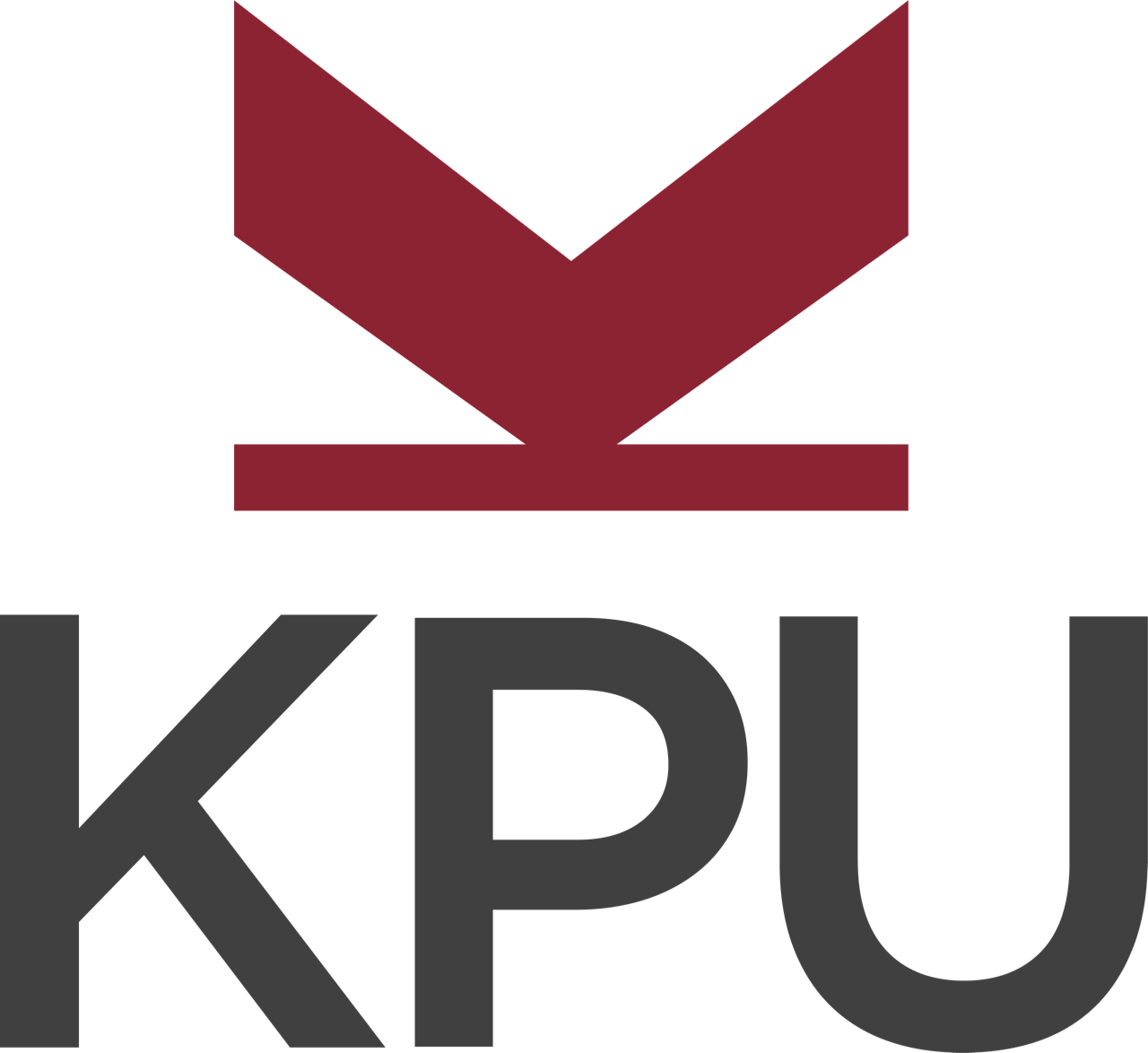
Want to create or adapt books like this? Learn more about how Pressbooks supports open publishing practices.
Experimental Research
23 Experiment Basics
Learning objectives.
- Explain what an experiment is and recognize examples of studies that are experiments and studies that are not experiments.
- Distinguish between the manipulation of the independent variable and control of extraneous variables and explain the importance of each.
- Recognize examples of confounding variables and explain how they affect the internal validity of a study.
- Define what a control condition is, explain its purpose in research on treatment effectiveness, and describe some alternative types of control conditions.
What Is an Experiment?
As we saw earlier in the book, an experiment is a type of study designed specifically to answer the question of whether there is a causal relationship between two variables. In other words, whether changes in one variable (referred to as an independent variable ) cause a change in another variable (referred to as a dependent variable ). Experiments have two fundamental features. The first is that the researchers manipulate, or systematically vary, the level of the independent variable. The different levels of the independent variable are called conditions . For example, in Darley and Latané’s experiment, the independent variable was the number of witnesses that participants believed to be present. The researchers manipulated this independent variable by telling participants that there were either one, two, or five other students involved in the discussion, thereby creating three conditions. For a new researcher, it is easy to confuse these terms by believing there are three independent variables in this situation: one, two, or five students involved in the discussion, but there is actually only one independent variable (number of witnesses) with three different levels or conditions (one, two or five students). The second fundamental feature of an experiment is that the researcher exerts control over, or minimizes the variability in, variables other than the independent and dependent variable. These other variables are called extraneous variables . Darley and Latané tested all their participants in the same room, exposed them to the same emergency situation, and so on. They also randomly assigned their participants to conditions so that the three groups would be similar to each other to begin with. Notice that although the words manipulation and control have similar meanings in everyday language, researchers make a clear distinction between them. They manipulate the independent variable by systematically changing its levels and control other variables by holding them constant.
Manipulation of the Independent Variable
Again, to manipulate an independent variable means to change its level systematically so that different groups of participants are exposed to different levels of that variable, or the same group of participants is exposed to different levels at different times. For example, to see whether expressive writing affects people’s health, a researcher might instruct some participants to write about traumatic experiences and others to write about neutral experiences. The different levels of the independent variable are referred to as conditions , and researchers often give the conditions short descriptive names to make it easy to talk and write about them. In this case, the conditions might be called the “traumatic condition” and the “neutral condition.”
Notice that the manipulation of an independent variable must involve the active intervention of the researcher. Comparing groups of people who differ on the independent variable before the study begins is not the same as manipulating that variable. For example, a researcher who compares the health of people who already keep a journal with the health of people who do not keep a journal has not manipulated this variable and therefore has not conducted an experiment. This distinction is important because groups that already differ in one way at the beginning of a study are likely to differ in other ways too. For example, people who choose to keep journals might also be more conscientious, more introverted, or less stressed than people who do not. Therefore, any observed difference between the two groups in terms of their health might have been caused by whether or not they keep a journal, or it might have been caused by any of the other differences between people who do and do not keep journals. Thus the active manipulation of the independent variable is crucial for eliminating potential alternative explanations for the results.
Of course, there are many situations in which the independent variable cannot be manipulated for practical or ethical reasons and therefore an experiment is not possible. For example, whether or not people have a significant early illness experience cannot be manipulated, making it impossible to conduct an experiment on the effect of early illness experiences on the development of hypochondriasis. This caveat does not mean it is impossible to study the relationship between early illness experiences and hypochondriasis—only that it must be done using nonexperimental approaches. We will discuss this type of methodology in detail later in the book.
Independent variables can be manipulated to create two conditions and experiments involving a single independent variable with two conditions are often referred to as a single factor two-level design . However, sometimes greater insights can be gained by adding more conditions to an experiment. When an experiment has one independent variable that is manipulated to produce more than two conditions it is referred to as a single factor multi level design . So rather than comparing a condition in which there was one witness to a condition in which there were five witnesses (which would represent a single-factor two-level design), Darley and Latané’s experiment used a single factor multi-level design, by manipulating the independent variable to produce three conditions (a one witness, a two witnesses, and a five witnesses condition).
Control of Extraneous Variables
As we have seen previously in the chapter, an extraneous variable is anything that varies in the context of a study other than the independent and dependent variables. In an experiment on the effect of expressive writing on health, for example, extraneous variables would include participant variables (individual differences) such as their writing ability, their diet, and their gender. They would also include situational or task variables such as the time of day when participants write, whether they write by hand or on a computer, and the weather. Extraneous variables pose a problem because many of them are likely to have some effect on the dependent variable. For example, participants’ health will be affected by many things other than whether or not they engage in expressive writing. This influencing factor can make it difficult to separate the effect of the independent variable from the effects of the extraneous variables, which is why it is important to control extraneous variables by holding them constant.
Extraneous Variables as “Noise”
Extraneous variables make it difficult to detect the effect of the independent variable in two ways. One is by adding variability or “noise” to the data. Imagine a simple experiment on the effect of mood (happy vs. sad) on the number of happy childhood events people are able to recall. Participants are put into a negative or positive mood (by showing them a happy or sad video clip) and then asked to recall as many happy childhood events as they can. The two leftmost columns of Table 5.1 show what the data might look like if there were no extraneous variables and the number of happy childhood events participants recalled was affected only by their moods. Every participant in the happy mood condition recalled exactly four happy childhood events, and every participant in the sad mood condition recalled exactly three. The effect of mood here is quite obvious. In reality, however, the data would probably look more like those in the two rightmost columns of Table 5.1 . Even in the happy mood condition, some participants would recall fewer happy memories because they have fewer to draw on, use less effective recall strategies, or are less motivated. And even in the sad mood condition, some participants would recall more happy childhood memories because they have more happy memories to draw on, they use more effective recall strategies, or they are more motivated. Although the mean difference between the two groups is the same as in the idealized data, this difference is much less obvious in the context of the greater variability in the data. Thus one reason researchers try to control extraneous variables is so their data look more like the idealized data in Table 5.1 , which makes the effect of the independent variable easier to detect (although real data never look quite that good).
One way to control extraneous variables is to hold them constant. This technique can mean holding situation or task variables constant by testing all participants in the same location, giving them identical instructions, treating them in the same way, and so on. It can also mean holding participant variables constant. For example, many studies of language limit participants to right-handed people, who generally have their language areas isolated in their left cerebral hemispheres [1] . Left-handed people are more likely to have their language areas isolated in their right cerebral hemispheres or distributed across both hemispheres, which can change the way they process language and thereby add noise to the data.
In principle, researchers can control extraneous variables by limiting participants to one very specific category of person, such as 20-year-old, heterosexual, female, right-handed psychology majors. The obvious downside to this approach is that it would lower the external validity of the study—in particular, the extent to which the results can be generalized beyond the people actually studied. For example, it might be unclear whether results obtained with a sample of younger lesbian women would apply to older gay men. In many situations, the advantages of a diverse sample (increased external validity) outweigh the reduction in noise achieved by a homogeneous one.
Extraneous Variables as Confounding Variables
The second way that extraneous variables can make it difficult to detect the effect of the independent variable is by becoming confounding variables. A confounding variable is an extraneous variable that differs on average across levels of the independent variable (i.e., it is an extraneous variable that varies systematically with the independent variable). For example, in almost all experiments, participants’ intelligence quotients (IQs) will be an extraneous variable. But as long as there are participants with lower and higher IQs in each condition so that the average IQ is roughly equal across the conditions, then this variation is probably acceptable (and may even be desirable). What would be bad, however, would be for participants in one condition to have substantially lower IQs on average and participants in another condition to have substantially higher IQs on average. In this case, IQ would be a confounding variable.
To confound means to confuse , and this effect is exactly why confounding variables are undesirable. Because they differ systematically across conditions—just like the independent variable—they provide an alternative explanation for any observed difference in the dependent variable. Figure 5.1 shows the results of a hypothetical study, in which participants in a positive mood condition scored higher on a memory task than participants in a negative mood condition. But if IQ is a confounding variable—with participants in the positive mood condition having higher IQs on average than participants in the negative mood condition—then it is unclear whether it was the positive moods or the higher IQs that caused participants in the first condition to score higher. One way to avoid confounding variables is by holding extraneous variables constant. For example, one could prevent IQ from becoming a confounding variable by limiting participants only to those with IQs of exactly 100. But this approach is not always desirable for reasons we have already discussed. A second and much more general approach—random assignment to conditions—will be discussed in detail shortly.
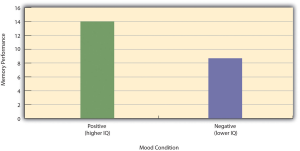
Treatment and Control Conditions
In psychological research, a treatment is any intervention meant to change people’s behavior for the better. This intervention includes psychotherapies and medical treatments for psychological disorders but also interventions designed to improve learning, promote conservation, reduce prejudice, and so on. To determine whether a treatment works, participants are randomly assigned to either a treatment condition , in which they receive the treatment, or a control condition , in which they do not receive the treatment. If participants in the treatment condition end up better off than participants in the control condition—for example, they are less depressed, learn faster, conserve more, express less prejudice—then the researcher can conclude that the treatment works. In research on the effectiveness of psychotherapies and medical treatments, this type of experiment is often called a randomized clinical trial .
There are different types of control conditions. In a no-treatment control condition , participants receive no treatment whatsoever. One problem with this approach, however, is the existence of placebo effects. A placebo is a simulated treatment that lacks any active ingredient or element that should make it effective, and a placebo effect is a positive effect of such a treatment. Many folk remedies that seem to work—such as eating chicken soup for a cold or placing soap under the bed sheets to stop nighttime leg cramps—are probably nothing more than placebos. Although placebo effects are not well understood, they are probably driven primarily by people’s expectations that they will improve. Having the expectation to improve can result in reduced stress, anxiety, and depression, which can alter perceptions and even improve immune system functioning (Price, Finniss, & Benedetti, 2008) [2] .
Placebo effects are interesting in their own right (see Note “The Powerful Placebo” ), but they also pose a serious problem for researchers who want to determine whether a treatment works. Figure 5.2 shows some hypothetical results in which participants in a treatment condition improved more on average than participants in a no-treatment control condition. If these conditions (the two leftmost bars in Figure 5.2 ) were the only conditions in this experiment, however, one could not conclude that the treatment worked. It could be instead that participants in the treatment group improved more because they expected to improve, while those in the no-treatment control condition did not.
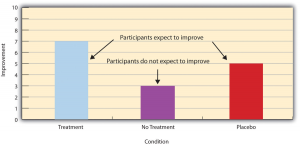
Fortunately, there are several solutions to this problem. One is to include a placebo control condition , in which participants receive a placebo that looks much like the treatment but lacks the active ingredient or element thought to be responsible for the treatment’s effectiveness. When participants in a treatment condition take a pill, for example, then those in a placebo control condition would take an identical-looking pill that lacks the active ingredient in the treatment (a “sugar pill”). In research on psychotherapy effectiveness, the placebo might involve going to a psychotherapist and talking in an unstructured way about one’s problems. The idea is that if participants in both the treatment and the placebo control groups expect to improve, then any improvement in the treatment group over and above that in the placebo control group must have been caused by the treatment and not by participants’ expectations. This difference is what is shown by a comparison of the two outer bars in Figure 5.4 .
Of course, the principle of informed consent requires that participants be told that they will be assigned to either a treatment or a placebo control condition—even though they cannot be told which until the experiment ends. In many cases the participants who had been in the control condition are then offered an opportunity to have the real treatment. An alternative approach is to use a wait-list control condition , in which participants are told that they will receive the treatment but must wait until the participants in the treatment condition have already received it. This disclosure allows researchers to compare participants who have received the treatment with participants who are not currently receiving it but who still expect to improve (eventually). A final solution to the problem of placebo effects is to leave out the control condition completely and compare any new treatment with the best available alternative treatment. For example, a new treatment for simple phobia could be compared with standard exposure therapy. Because participants in both conditions receive a treatment, their expectations about improvement should be similar. This approach also makes sense because once there is an effective treatment, the interesting question about a new treatment is not simply “Does it work?” but “Does it work better than what is already available?
The Powerful Placebo
Many people are not surprised that placebos can have a positive effect on disorders that seem fundamentally psychological, including depression, anxiety, and insomnia. However, placebos can also have a positive effect on disorders that most people think of as fundamentally physiological. These include asthma, ulcers, and warts (Shapiro & Shapiro, 1999) [3] . There is even evidence that placebo surgery—also called “sham surgery”—can be as effective as actual surgery.
Medical researcher J. Bruce Moseley and his colleagues conducted a study on the effectiveness of two arthroscopic surgery procedures for osteoarthritis of the knee (Moseley et al., 2002) [4] . The control participants in this study were prepped for surgery, received a tranquilizer, and even received three small incisions in their knees. But they did not receive the actual arthroscopic surgical procedure. Note that the IRB would have carefully considered the use of deception in this case and judged that the benefits of using it outweighed the risks and that there was no other way to answer the research question (about the effectiveness of a placebo procedure) without it. The surprising result was that all participants improved in terms of both knee pain and function, and the sham surgery group improved just as much as the treatment groups. According to the researchers, “This study provides strong evidence that arthroscopic lavage with or without débridement [the surgical procedures used] is not better than and appears to be equivalent to a placebo procedure in improving knee pain and self-reported function” (p. 85).
- Knecht, S., Dräger, B., Deppe, M., Bobe, L., Lohmann, H., Flöel, A., . . . Henningsen, H. (2000). Handedness and hemispheric language dominance in healthy humans. Brain: A Journal of Neurology, 123 (12), 2512-2518. http://dx.doi.org/10.1093/brain/123.12.2512 ↵
- Price, D. D., Finniss, D. G., & Benedetti, F. (2008). A comprehensive review of the placebo effect: Recent advances and current thought. Annual Review of Psychology, 59 , 565–590. ↵
- Shapiro, A. K., & Shapiro, E. (1999). The powerful placebo: From ancient priest to modern physician . Baltimore, MD: Johns Hopkins University Press. ↵
- Moseley, J. B., O’Malley, K., Petersen, N. J., Menke, T. J., Brody, B. A., Kuykendall, D. H., … Wray, N. P. (2002). A controlled trial of arthroscopic surgery for osteoarthritis of the knee. The New England Journal of Medicine, 347 , 81–88. ↵
A type of study designed specifically to answer the question of whether there is a causal relationship between two variables.
The variable the experimenter manipulates.
The variable the experimenter measures (it is the presumed effect).
The different levels of the independent variable to which participants are assigned.
Holding extraneous variables constant in order to separate the effect of the independent variable from the effect of the extraneous variables.
Any variable other than the dependent and independent variable.
Changing the level, or condition, of the independent variable systematically so that different groups of participants are exposed to different levels of that variable, or the same group of participants is exposed to different levels at different times.
An experiment design involving a single independent variable with two conditions.
When an experiment has one independent variable that is manipulated to produce more than two conditions.
An extraneous variable that varies systematically with the independent variable, and thus confuses the effect of the independent variable with the effect of the extraneous one.
Any intervention meant to change people’s behavior for the better.
The condition in which participants receive the treatment.
The condition in which participants do not receive the treatment.
An experiment that researches the effectiveness of psychotherapies and medical treatments.
The condition in which participants receive no treatment whatsoever.
A simulated treatment that lacks any active ingredient or element that is hypothesized to make the treatment effective, but is otherwise identical to the treatment.
An effect that is due to the placebo rather than the treatment.
Condition in which the participants receive a placebo rather than the treatment.
Condition in which participants are told that they will receive the treatment but must wait until the participants in the treatment condition have already received it.
Research Methods in Psychology Copyright © 2019 by Rajiv S. Jhangiani, I-Chant A. Chiang, Carrie Cuttler, & Dana C. Leighton is licensed under a Creative Commons Attribution-NonCommercial-ShareAlike 4.0 International License , except where otherwise noted.
Share This Book
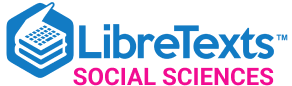
- school Campus Bookshelves
- menu_book Bookshelves
- perm_media Learning Objects
- login Login
- how_to_reg Request Instructor Account
- hub Instructor Commons
Margin Size
- Download Page (PDF)
- Download Full Book (PDF)
- Periodic Table
- Physics Constants
- Scientific Calculator
- Reference & Cite
- Tools expand_more
- Readability
selected template will load here
This action is not available.
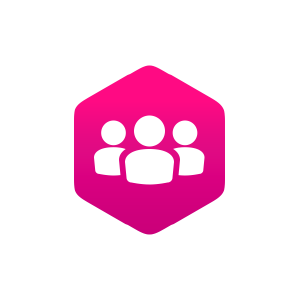
5.1: Experiment Basics
- Last updated
- Save as PDF
- Page ID 16114

\( \newcommand{\vecs}[1]{\overset { \scriptstyle \rightharpoonup} {\mathbf{#1}} } \)
\( \newcommand{\vecd}[1]{\overset{-\!-\!\rightharpoonup}{\vphantom{a}\smash {#1}}} \)
\( \newcommand{\id}{\mathrm{id}}\) \( \newcommand{\Span}{\mathrm{span}}\)
( \newcommand{\kernel}{\mathrm{null}\,}\) \( \newcommand{\range}{\mathrm{range}\,}\)
\( \newcommand{\RealPart}{\mathrm{Re}}\) \( \newcommand{\ImaginaryPart}{\mathrm{Im}}\)
\( \newcommand{\Argument}{\mathrm{Arg}}\) \( \newcommand{\norm}[1]{\| #1 \|}\)
\( \newcommand{\inner}[2]{\langle #1, #2 \rangle}\)
\( \newcommand{\Span}{\mathrm{span}}\)
\( \newcommand{\id}{\mathrm{id}}\)
\( \newcommand{\kernel}{\mathrm{null}\,}\)
\( \newcommand{\range}{\mathrm{range}\,}\)
\( \newcommand{\RealPart}{\mathrm{Re}}\)
\( \newcommand{\ImaginaryPart}{\mathrm{Im}}\)
\( \newcommand{\Argument}{\mathrm{Arg}}\)
\( \newcommand{\norm}[1]{\| #1 \|}\)
\( \newcommand{\Span}{\mathrm{span}}\) \( \newcommand{\AA}{\unicode[.8,0]{x212B}}\)
\( \newcommand{\vectorA}[1]{\vec{#1}} % arrow\)
\( \newcommand{\vectorAt}[1]{\vec{\text{#1}}} % arrow\)
\( \newcommand{\vectorB}[1]{\overset { \scriptstyle \rightharpoonup} {\mathbf{#1}} } \)
\( \newcommand{\vectorC}[1]{\textbf{#1}} \)
\( \newcommand{\vectorD}[1]{\overrightarrow{#1}} \)
\( \newcommand{\vectorDt}[1]{\overrightarrow{\text{#1}}} \)
\( \newcommand{\vectE}[1]{\overset{-\!-\!\rightharpoonup}{\vphantom{a}\smash{\mathbf {#1}}}} \)
Learning Objectives
- Explain what an experiment is and recognize examples of studies that are experiments and studies that are not experiments.
- Distinguish between the manipulation of the independent variable and control of extraneous variables and explain the importance of each.
- Recognize examples of confounding variables and explain how they affect the internal validity of a study.
What Is an Experiment?
As we saw earlier in the book, an experiment is a type of study designed specifically to answer the question of whether there is a causal relationship between two variables. In other words, whether changes in an independent variable cause a change in a dependent variable. Experiments have two fundamental features. The first is that the researchers manipulate, or systematically vary, the level of the independent variable. The different levels of the independent variable are called conditions . For example, in Darley and Latané’s experiment, the independent variable was the number of witnesses that participants believed to be present. The researchers manipulated this independent variable by telling participants that there were either one, two, or five other students involved in the discussion, thereby creating three conditions. For a new researcher, it is easy to confuse these terms by believing there are three independent variables in this situation: one, two, or five students involved in the discussion, but there is actually only one independent variable (number of witnesses) with three different levels or conditions (one, two or five students). The second fundamental feature of an experiment is that the researcher controls, or minimizes the variability in, variables other than the independent and dependent variable. These other variables are called extraneous variables . Darley and Latané tested all their participants in the same room, exposed them to the same emergency situation, and so on. They also randomly assigned their participants to conditions so that the three groups would be similar to each other to begin with. Notice that although the words manipulation and control have similar meanings in everyday language, researchers make a clear distinction between them. They manipulate the independent variable by systematically changing its levels and control other variables by holding them constant.
Manipulation of the Independent Variable
Again, to manipulate an independent variable means to change its level systematically so that different groups of participants are exposed to different levels of that variable, or the same group of participants is exposed to different levels at different times. For example, to see whether expressive writing affects people’s health, a researcher might instruct some participants to write about traumatic experiences and others to write about neutral experiences. As discussed earlier in this chapter, the different levels of the independent variable are referred to as conditions , and researchers often give the conditions short descriptive names to make it easy to talk and write about them. In this case, the conditions might be called the “traumatic condition” and the “neutral condition.”
Notice that the manipulation of an independent variable must involve the active intervention of the researcher. Comparing groups of people who differ on the independent variable before the study begins is not the same as manipulating that variable. For example, a researcher who compares the health of people who already keep a journal with the health of people who do not keep a journal has not manipulated this variable and therefore has not conducted an experiment. This distinction is important because groups that already differ in one way at the beginning of a study are likely to differ in other ways too. For example, people who choose to keep journals might also be more conscientious, more introverted, or less stressed than people who do not. Therefore, any observed difference between the two groups in terms of their health might have been caused by whether or not they keep a journal, or it might have been caused by any of the other differences between people who do and do not keep journals. Thus the active manipulation of the independent variable is crucial for eliminating potential alternative explanations for the results.
Of course, there are many situations in which the independent variable cannot be manipulated for practical or ethical reasons and therefore an experiment is not possible. For example, whether or not people have a significant early illness experience cannot be manipulated, making it impossible to conduct an experiment on the effect of early illness experiences on the development of hypochondriasis. This caveat does not mean it is impossible to study the relationship between early illness experiences and hypochondriasis—only that it must be done using nonexperimental approaches. We will discuss this type of methodology in detail later in the book.
Independent variables can be manipulated to create two conditions and experiments involving a single independent variable with two conditions is often referred to as a single factor two-level design. However, sometimes greater insights can be gained by adding more conditions to an experiment. When an experiment has one independent variable that is manipulated to produce more than two conditions it is referred to as a single factor multi level design. So rather than comparing a condition in which there was one witness to a condition in which there were five witnesses (which would represent a single-factor two-level design), Darley and Latané’s used a single factor multi-level design, by manipulating the independent variable to produce three conditions (a one witness, a two witnesses, and a five witnesses condition).
Control of Extraneous Variables
As we have seen previously in the chapter, an extraneous variable is anything that varies in the context of a study other than the independent and dependent variables. In an experiment on the effect of expressive writing on health, for example, extraneous variables would include participant variables (individual differences) such as their writing ability, their diet, and their gender. They would also include situational or task variables such as the time of day when participants write, whether they write by hand or on a computer, and the weather. Extraneous variables pose a problem because many of them are likely to have some effect on the dependent variable. For example, participants’ health will be affected by many things other than whether or not they engage in expressive writing. This influencing factor can make it difficult to separate the effect of the independent variable from the effects of the extraneous variables, which is why it is important to control extraneous variables by holding them constant.
Extraneous Variables as “Noise”
Extraneous variables make it difficult to detect the effect of the independent variable in two ways. One is by adding variability or “noise” to the data. Imagine a simple experiment on the effect of mood (happy vs. sad) on the number of happy childhood events people are able to recall. Participants are put into a negative or positive mood (by showing them a happy or sad video clip) and then asked to recall as many happy childhood events as they can. The two leftmost columns of Table \(\PageIndex{1}\) show what the data might look like if there were no extraneous variables and the number of happy childhood events participants recalled was affected only by their moods. Every participant in the happy mood condition recalled exactly four happy childhood events, and every participant in the sad mood condition recalled exactly three. The effect of mood here is quite obvious. In reality, however, the data would probably look more like those in the two rightmost columns of Table \(\PageIndex{1}\) . Even in the happy mood condition, some participants would recall fewer happy memories because they have fewer to draw on, use less effective recall strategies, or are less motivated. And even in the sad mood condition, some participants would recall more happy childhood memories because they have more happy memories to draw on, they use more effective recall strategies, or they are more motivated. Although the mean difference between the two groups is the same as in the idealized data, this difference is much less obvious in the context of the greater variability in the data. Thus one reason researchers try to control extraneous variables is so their data look more like the idealized data in Table \(\PageIndex{1}\) , which makes the effect of the independent variable easier to detect (although real data never look quite that good).
One way to control extraneous variables is to hold them constant. This technique can mean holding situation or task variables constant by testing all participants in the same location, giving them identical instructions, treating them in the same way, and so on. It can also mean holding participant variables constant. For example, many studies of language limit participants to right-handed people, who generally have their language areas isolated in their left cerebral hemispheres. Left-handed people are more likely to have their language areas isolated in their right cerebral hemispheres or distributed across both hemispheres, which can change the way they process language and thereby add noise to the data.
In principle, researchers can control extraneous variables by limiting participants to one very specific category of person, such as 20-year-old, heterosexual, female, right-handed psychology majors. The obvious downside to this approach is that it would lower the external validity of the study—in particular, the extent to which the results can be generalized beyond the people actually studied. For example, it might be unclear whether results obtained with a sample of younger heterosexual women would apply to older homosexual men. In many situations, the advantages of a diverse sample (increased external validity) outweigh the reduction in noise achieved by a homogeneous one.
Extraneous Variables as Confounding Variables
The second way that extraneous variables can make it difficult to detect the effect of the independent variable is by becoming confounding variables. A confounding variable is an extraneous variable that differs on average across levels of the independent variable (i.e., it is an extraneous variable that varies systematically with the independent variable). For example, in almost all experiments, participants’ intelligence quotients (IQs) will be an extraneous variable. But as long as there are participants with lower and higher IQs in each condition so that the average IQ is roughly equal across the conditions, then this variation is probably acceptable (and may even be desirable). What would be bad, however, would be for participants in one condition to have substantially lower IQs on average and participants in another condition to have substantially higher IQs on average. In this case, IQ would be a confounding variable.
To confound means to confuse , and this effect is exactly why confounding variables are undesirable. Because they differ systematically across conditions—just like the independent variable—they provide an alternative explanation for any observed difference in the dependent variable. Figure 5.1 shows the results of a hypothetical study, in which participants in a positive mood condition scored higher on a memory task than participants in a negative mood condition. But if IQ is a confounding variable—with participants in the positive mood condition having higher IQs on average than participants in the negative mood condition—then it is unclear whether it was the positive moods or the higher IQs that caused participants in the first condition to score higher. One way to avoid confounding variables is by holding extraneous variables constant. For example, one could prevent IQ from becoming a confounding variable by limiting participants only to those with IQs of exactly 100. But this approach is not always desirable for reasons we have already discussed. A second and much more general approach—random assignment to conditions—will be discussed in detail shortly.
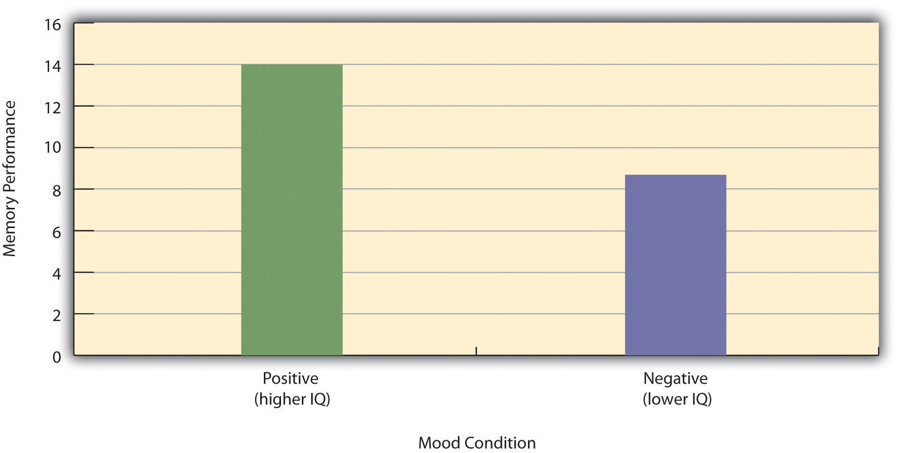
Key Takeaways
- An experiment is a type of empirical study that features the manipulation of an independent variable, the measurement of a dependent variable, and control of extraneous variables.
- An extraneous variable is any variable other than the independent and dependent variables. A confound is an extraneous variable that varies systematically with the independent variable.
- Practice: List five variables that can be manipulated by the researcher in an experiment. List five variables that cannot be manipulated by the researcher in an experiment.
- Effect of parietal lobe damage on people’s ability to do basic arithmetic.
- Effect of being clinically depressed on the number of close friendships people have.
- Effect of group training on the social skills of teenagers with Asperger’s syndrome.
- Effect of paying people to take an IQ test on their performance on that test.
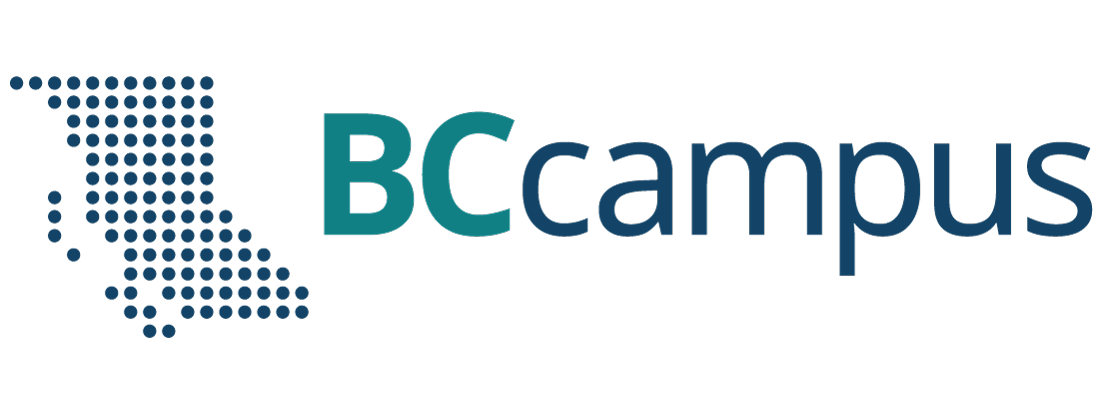
Want to create or adapt books like this? Learn more about how Pressbooks supports open publishing practices.
Chapter 6: Experimental Research
Conducting Experiments
Learning Objectives
- Describe several strategies for recruiting participants for an experiment.
- Explain why it is important to standardize the procedure of an experiment and several ways to do this.
- Explain what pilot testing is and why it is important.
The information presented so far in this chapter is enough to design a basic experiment. When it comes time to conduct that experiment, however, several additional practical issues arise. In this section, we consider some of these issues and how to deal with them. Much of this information applies to nonexperimental studies as well as experimental ones.
Recruiting Participants
Of course, at the start of any research project you should be thinking about how you will obtain your participants. Unless you have access to people with schizophrenia or incarcerated juvenile offenders, for example, then there is no point designing a study that focuses on these populations. But even if you plan to use a convenience sample, you will have to recruit participants for your study.
There are several approaches to recruiting participants. One is to use participants from a formal subject pool —an established group of people who have agreed to be contacted about participating in research studies. For example, at many colleges and universities, there is a subject pool consisting of students enrolled in introductory psychology courses who must participate in a certain number of studies to meet a course requirement. Researchers post descriptions of their studies and students sign up to participate, usually via an online system. Participants who are not in subject pools can also be recruited by posting or publishing advertisements or making personal appeals to groups that represent the population of interest. For example, a researcher interested in studying older adults could arrange to speak at a meeting of the residents at a retirement community to explain the study and ask for volunteers.
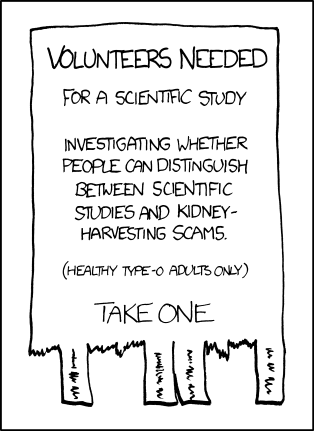
The Volunteer Subject
Even if the participants in a study receive compensation in the form of course credit, a small amount of money, or a chance at being treated for a psychological problem, they are still essentially volunteers. This is worth considering because people who volunteer to participate in psychological research have been shown to differ in predictable ways from those who do not volunteer. Specifically, there is good evidence that on average, volunteers have the following characteristics compared with nonvolunteers (Rosenthal & Rosnow, 1976) [1] :
- They are more interested in the topic of the research.
- They are more educated.
- They have a greater need for approval.
- They have higher intelligence quotients (IQs).
- They are more sociable.
- They are higher in social class.
This difference can be an issue of external validity if there is reason to believe that participants with these characteristics are likely to behave differently than the general population. For example, in testing different methods of persuading people, a rational argument might work better on volunteers than it does on the general population because of their generally higher educational level and IQ.
In many field experiments, the task is not recruiting participants but selecting them. For example, researchers Nicolas Guéguen and Marie-Agnès de Gail conducted a field experiment on the effect of being smiled at on helping, in which the participants were shoppers at a supermarket. A confederate walking down a stairway gazed directly at a shopper walking up the stairway and either smiled or did not smile. Shortly afterward, the shopper encountered another confederate, who dropped some computer diskettes on the ground. The dependent variable was whether or not the shopper stopped to help pick up the diskettes (Guéguen & de Gail, 2003) [2] . Notice that these participants were not “recruited,” but the researchers still had to select them from among all the shoppers taking the stairs that day. It is extremely important that this kind of selection be done according to a well-defined set of rules that is established before the data collection begins and can be explained clearly afterward. In this case, with each trip down the stairs, the confederate was instructed to gaze at the first person he encountered who appeared to be between the ages of 20 and 50. Only if the person gazed back did he or she become a participant in the study. The point of having a well-defined selection rule is to avoid bias in the selection of participants. For example, if the confederate was free to choose which shoppers he would gaze at, he might choose friendly-looking shoppers when he was set to smile and unfriendly-looking ones when he was not set to smile. As we will see shortly, such biases can be entirely unintentional.
Standardizing the Procedure
It is surprisingly easy to introduce extraneous variables during the procedure. For example, the same experimenter might give clear instructions to one participant but vague instructions to another. Or one experimenter might greet participants warmly while another barely makes eye contact with them. To the extent that such variables affect participants’ behaviour, they add noise to the data and make the effect of the independent variable more difficult to detect. If they vary across conditions, they become confounding variables and provide alternative explanations for the results. For example, if participants in a treatment group are tested by a warm and friendly experimenter and participants in a control group are tested by a cold and unfriendly one, then what appears to be an effect of the treatment might actually be an effect of experimenter demeanor. When there are multiple experimenters, the possibility for introducing extraneous variables is even greater, but is often necessary for practical reasons.
Experimenter’s Sex as an Extraneous Variable
It is well known that whether research participants are male or female can affect the results of a study. But what about whether the experimenter is male or female? There is plenty of evidence that this matters too. Male and female experimenters have slightly different ways of interacting with their participants, and of course participants also respond differently to male and female experimenters (Rosenthal, 1976) [3] .
For example, in a recent study on pain perception, participants immersed their hands in icy water for as long as they could (Ibolya, Brake, & Voss, 2004) [4] . Male participants tolerated the pain longer when the experimenter was a woman, and female participants tolerated it longer when the experimenter was a man.
Researcher Robert Rosenthal has spent much of his career showing that this kind of unintended variation in the procedure does, in fact, affect participants’ behaviour. Furthermore, one important source of such variation is the experimenter’s expectations about how participants “should” behave in the experiment. This outcome is referred to as an experimenter expectancy effect (Rosenthal, 1976) [5] . For example, if an experimenter expects participants in a treatment group to perform better on a task than participants in a control group, then he or she might unintentionally give the treatment group participants clearer instructions or more encouragement or allow them more time to complete the task. In a striking example, Rosenthal and Kermit Fode had several students in a laboratory course in psychology train rats to run through a maze. Although the rats were genetically similar, some of the students were told that they were working with “maze-bright” rats that had been bred to be good learners, and other students were told that they were working with “maze-dull” rats that had been bred to be poor learners. Sure enough, over five days of training, the “maze-bright” rats made more correct responses, made the correct response more quickly, and improved more steadily than the “maze-dull” rats (Rosenthal & Fode, 1963) [6] . Clearly it had to have been the students’ expectations about how the rats would perform that made the difference. But how? Some clues come from data gathered at the end of the study, which showed that students who expected their rats to learn quickly felt more positively about their animals and reported behaving toward them in a more friendly manner (e.g., handling them more).
The way to minimize unintended variation in the procedure is to standardize it as much as possible so that it is carried out in the same way for all participants regardless of the condition they are in. Here are several ways to do this:
- Create a written protocol that specifies everything that the experimenters are to do and say from the time they greet participants to the time they dismiss them.
- Create standard instructions that participants read themselves or that are read to them word for word by the experimenter.
- Automate the rest of the procedure as much as possible by using software packages for this purpose or even simple computer slide shows.
- Anticipate participants’ questions and either raise and answer them in the instructions or develop standard answers for them.
- Train multiple experimenters on the protocol together and have them practice on each other.
- Be sure that each experimenter tests participants in all conditions.
Another good practice is to arrange for the experimenters to be “blind” to the research question or to the condition that each participant is tested in. The idea is to minimize experimenter expectancy effects by minimizing the experimenters’ expectations. For example, in a drug study in which each participant receives the drug or a placebo, it is often the case that neither the participants nor the experimenter who interacts with the participants know which condition he or she has been assigned to. Because both the participants and the experimenters are blind to the condition, this technique is referred to as a double-blind study . (A single-blind study is one in which the participant, but not the experimenter, is blind to the condition.) Of course, there are many times this blinding is not possible. For example, if you are both the investigator and the only experimenter, it is not possible for you to remain blind to the research question. Also, in many studies the experimenter must know the condition because he or she must carry out the procedure in a different way in the different conditions.
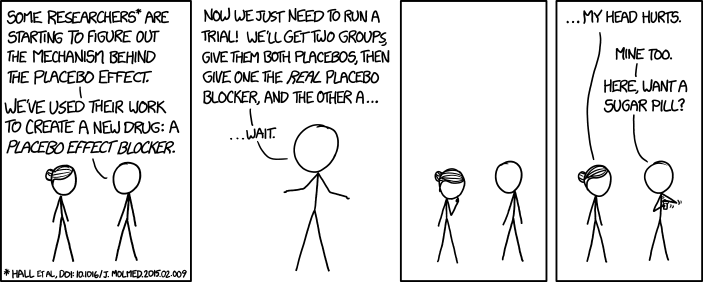
Record Keeping
It is essential to keep good records when you conduct an experiment. As discussed earlier, it is typical for experimenters to generate a written sequence of conditions before the study begins and then to test each new participant in the next condition in the sequence. As you test them, it is a good idea to add to this list basic demographic information; the date, time, and place of testing; and the name of the experimenter who did the testing. It is also a good idea to have a place for the experimenter to write down comments about unusual occurrences (e.g., a confused or uncooperative participant) or questions that come up. This kind of information can be useful later if you decide to analy z e sex differences or effects of different experimenters, or if a question arises about a particular participant or testing session.
It can also be useful to assign an identification number to each participant as you test them. Simply numbering them consecutively beginning with 1 is usually sufficient. This number can then also be written on any response sheets or questionnaires that participants generate, making it easier to keep them together.
Pilot Testing
It is always a good idea to conduct a pilot test of your experiment. A pilot test is a small-scale study conducted to make sure that a new procedure works as planned. In a pilot test, you can recruit participants formally (e.g., from an established participant pool) or you can recruit them informally from among family, friends, classmates, and so on. The number of participants can be small, but it should be enough to give you confidence that your procedure works as planned. There are several important questions that you can answer by conducting a pilot test:
- Do participants understand the instructions?
- What kind of misunderstandings do participants have, what kind of mistakes do they make, and what kind of questions do they ask?
- Do participants become bored or frustrated?
- Is an indirect manipulation effective? (You will need to include a manipulation check.)
- Can participants guess the research question or hypothesis?
- How long does the procedure take?
- Are computer programs or other automated procedures working properly?
- Are data being recorded correctly?
Of course, to answer some of these questions you will need to observe participants carefully during the procedure and talk with them about it afterward. Participants are often hesitant to criticize a study in front of the researcher, so be sure they understand that their participation is part of a pilot test and you are genuinely interested in feedback that will help you improve the procedure. If the procedure works as planned, then you can proceed with the actual study. If there are problems to be solved, you can solve them, pilot test the new procedure, and continue with this process until you are ready to proceed.
Key Takeaways
- There are several effective methods you can use to recruit research participants for your experiment, including through formal subject pools, advertisements, and personal appeals. Field experiments require well-defined participant selection procedures.
- It is important to standardize experimental procedures to minimize extraneous variables, including experimenter expectancy effects.
- It is important to conduct one or more small-scale pilot tests of an experiment to be sure that the procedure works as planned.
- elderly adults
- unemployed people
- regular exercisers
- math majors
- Discussion: Imagine a study in which you will visually present participants with a list of 20 words, one at a time, wait for a short time, and then ask them to recall as many of the words as they can. In the stressed condition, they are told that they might also be chosen to give a short speech in front of a small audience. In the unstressed condition, they are not told that they might have to give a speech. What are several specific things that you could do to standardize the procedure?
Image Descriptions
A comic of two stick figures talking.
Person 1: Some researchers are starting to figure out the mechanism behind the placebo effect. We’ve used their work to create a new drug: A placebo effect blocker. Now we just need to run a trial. We’ll get two groups, give them both placebos, then give one the REAL placebo blocker, and the other a…. wait.
[The two people scratch their heads]
Person 2: My head hurts.
Person 1: Mine too. Here, want a sugar pill?
[Return to Image]
Media Attributions
- Study by XKCD CC BY-NC (Attribution NonCommercial)
- Placebo blocker by XKCD CC BY-NC (Attribution NonCommercial)
- Rosenthal, R., & Rosnow, R. L. (1976). The volunteer subject . New York, NY: Wiley. ↵
- Guéguen, N., & de Gail, Marie-Agnès. (2003). The effect of smiling on helping behaviour: Smiling and good Samaritan behaviour. Communication Reports, 16 , 133–140. ↵
- Rosenthal, R. (1976). Experimenter effects in behavioural research (enlarged ed.). New York, NY: Wiley. ↵
- Ibolya, K., Brake, A., & Voss, U. (2004). The effect of experimenter characteristics on pain reports in women and men. Pain, 112 , 142–147. ↵
- Rosenthal, R., & Fode, K. (1963). The effect of experimenter bias on performance of the albino rat. Behavioural Science, 8 , 183-189. ↵
An established group of people who have agreed to be contacted about participating in research studies.
A source of variation in which the experimenter’s expectations about how participants “should” be have in the experiment.
An experiment in which both the participants and the experimenters are blind to which condition the participants have been assigned to.
A small-scale study conducted to make sure that a new procedure works as planned.
Research Methods in Psychology - 2nd Canadian Edition Copyright © 2015 by Paul C. Price, Rajiv Jhangiani, & I-Chant A. Chiang is licensed under a Creative Commons Attribution-NonCommercial-ShareAlike 4.0 International License , except where otherwise noted.
Share This Book
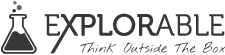
- Foundations
- Write Paper
Search form
- Experiments
- Anthropology
- Self-Esteem
- Social Anxiety

- Experiments >
Conducting an Experiment
Science revolves around experiments, and learning the best way of conducting an experiment is crucial to obtaining useful and valid results.
This article is a part of the guide:
- Experimental Research
- Pretest-Posttest
- Third Variable
- Research Bias
- Independent Variable
Browse Full Outline
- 1 Experimental Research
- 2.1 Independent Variable
- 2.2 Dependent Variable
- 2.3 Controlled Variables
- 2.4 Third Variable
- 3.1 Control Group
- 3.2 Research Bias
- 3.3.1 Placebo Effect
- 3.3.2 Double Blind Method
- 4.1 Randomized Controlled Trials
- 4.2 Pretest-Posttest
- 4.3 Solomon Four Group
- 4.4 Between Subjects
- 4.5 Within Subject
- 4.6 Repeated Measures
- 4.7 Counterbalanced Measures
- 4.8 Matched Subjects
When scientists speak of experiments, in the strictest sense of the word, they mean a true experiment , where the scientist controls all of the factors and conditions.
Real world observations, and case studies , should be referred to as observational research , rather than experiments.
For example, observing animals in the wild is not a true experiment, because it does not isolate and manipulate an independent variable .
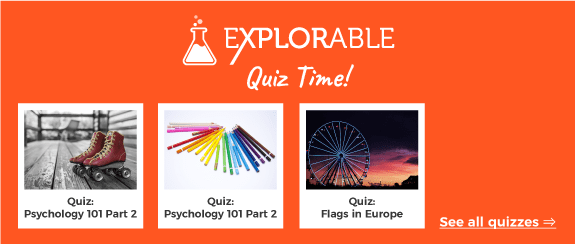
The Basis of Conducting an Experiment
With an experiment, the researcher is trying to learn something new about the world, an explanation of 'why' something happens.
The experiment must maintain internal and external validity, or the results will be useless.
When designing an experiment , a researcher must follow all of the steps of the scientific method , from making sure that the hypothesis is valid and testable , to using controls and statistical tests.
Whilst all scientists use reasoning , operationalization and the steps of the scientific process , it is not always a conscious process.
Experience and practice mean that many scientists follow an instinctive process of conducting an experiment, the 'streamlined' scientific process . Following the basic steps will usually generate valid results, but where experiments are complex and expensive, it is always advisable to follow the rigorous scientific protocols. Conducting an experiment has a number of stages, where the parameters and structure of the experiment are made clear.
Whilst it is rarely practical to follow each step strictly, any aberrations must be justified, whether they arise because of budget, impracticality or ethics .
After deciding upon a hypothesis , and making predictions, the first stage of conducting an experiment is to specify the sample groups. These should be large enough to give a statistically viable study, but small enough to be practical.
Ideally, groups should be selected at random , from a wide selection of the sample population. This allows results to be generalized to the population as a whole.
In the physical sciences, this is fairly easy, but the biological and behavioral sciences are often limited by other factors.
For example, medical trials often cannot find random groups. Such research often relies upon volunteers, so it is difficult to apply any realistic randomization . This is not a problem, as long as the process is justified, and the results are not applied to the population as a whole.
If a psychological researcher used volunteers who were male students, aged between 18 and 24, the findings can only be generalized to that specific demographic group within society.
The sample groups should be divided, into a control group and a test group, to reduce the possibility of confounding variables .
This, again, should be random, and the assigning of subjects to groups should be blind or double blind . This will reduce the chances of experimental error , or bias, when conducting an experiment.
Ethics are often a barrier to this process, because deliberately withholding treatment, as with the Tuskegee study , is not permitted.
Again, any deviations from this process must be explained in the conclusion. There is nothing wrong with compromising upon randomness, where necessary, as long as other scientists are aware of how, and why, the researcher selected groups on that basis.
Stage Three
This stage of conducting an experiment involves determining the time scale and frequency of sampling , to fit the type of experiment.
For example, researchers studying the effectiveness of a cure for colds would take frequent samples, over a period of days. Researchers testing a cure for Parkinson's disease would use less frequent tests, over a period of months or years.
The penultimate stage of the experiment involves performing the experiment according to the methods stipulated during the design phase.
The independent variable is manipulated, generating a usable data set for the dependent variable .
The raw data from the results should be gathered, and analyzed, by statistical means. This allows the researcher to establish if there is any relationship between the variables and accept, or reject, the null hypothesis .
These steps are essential to providing excellent results. Whilst many researchers do not want to become involved in the exact processes of inductive reasoning , deductive reasoning and operationalization , they all follow the basic steps of conducting an experiment. This ensures that their results are valid .
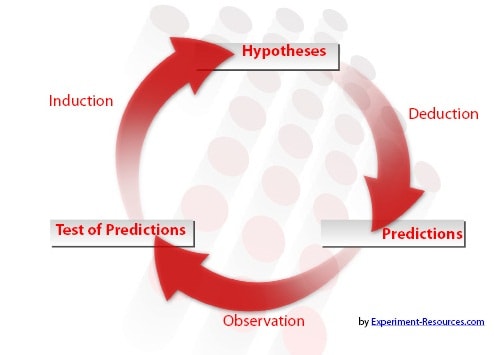
Preparing a Coordination Schema of the Whole Research Plan
Preparing a coordination schema of the research plan may be another useful tool in undertaking research planning. While preparing a coordination schema, one may have to identify the broad variable in the form of parameters, complex variables and disaggregate those in the form of simple variables. Coordination Schema: A Methodological Tool in Research Planning by Purnima Mohapatra is a very useful tool. Arranging everything in a schema not only makes the research more organised, it also saves a lot of valuable time for the researcher.
- Psychology 101
- Flags and Countries
- Capitals and Countries
Martyn Shuttleworth (May 24, 2008). Conducting an Experiment. Retrieved May 22, 2024 from Explorable.com: https://explorable.com/conducting-an-experiment
You Are Allowed To Copy The Text
The text in this article is licensed under the Creative Commons-License Attribution 4.0 International (CC BY 4.0) .
This means you're free to copy, share and adapt any parts (or all) of the text in the article, as long as you give appropriate credit and provide a link/reference to this page.
That is it. You don't need our permission to copy the article; just include a link/reference back to this page. You can use it freely (with some kind of link), and we're also okay with people reprinting in publications like books, blogs, newsletters, course-material, papers, wikipedia and presentations (with clear attribution).
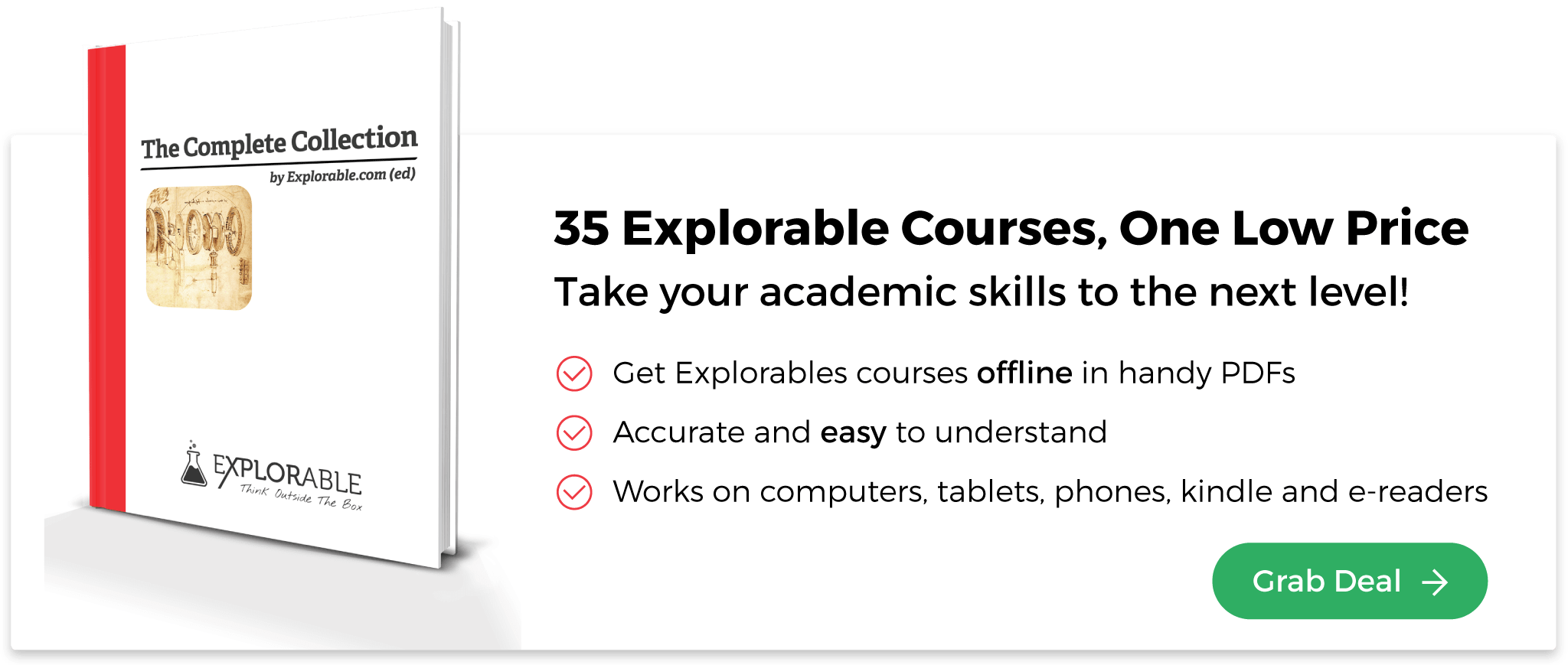
Related articles
Design of Experiment
Social Psychology Experiments
How to Conduct Science Experiments
True Experimental Design
Want to stay up to date? Follow us!
Get all these articles in 1 guide.
Want the full version to study at home, take to school or just scribble on?
Whether you are an academic novice, or you simply want to brush up your skills, this book will take your academic writing skills to the next level.
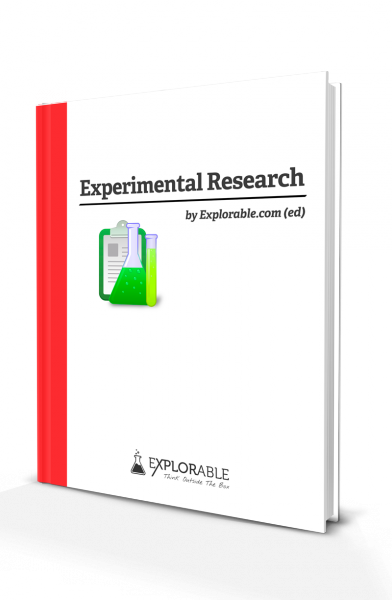
Download electronic versions: - Epub for mobiles and tablets - For Kindle here - For iBooks here - PDF version here
Save this course for later
Don't have time for it all now? No problem, save it as a course and come back to it later.
Footer bottom
- Privacy Policy
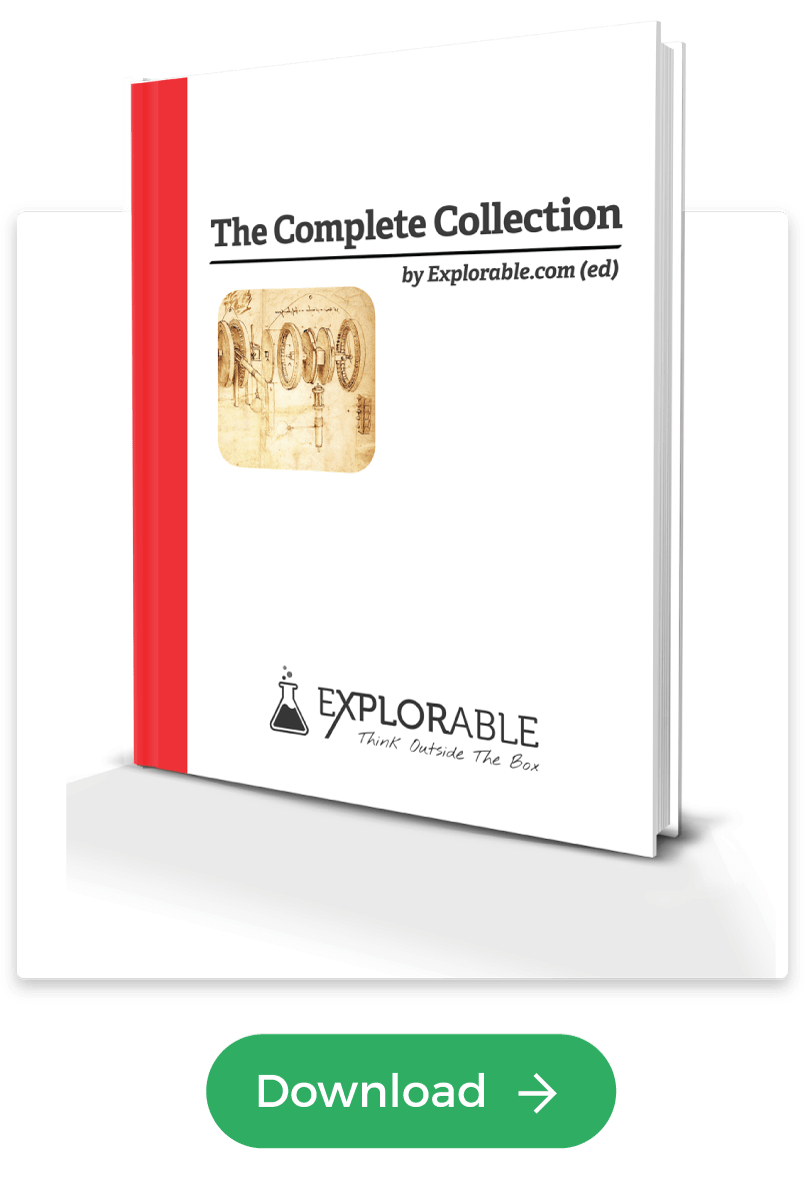
- Subscribe to our RSS Feed
- Like us on Facebook
- Follow us on Twitter
User Preferences
Content preview.
Arcu felis bibendum ut tristique et egestas quis:
- Ut enim ad minim veniam, quis nostrud exercitation ullamco laboris
- Duis aute irure dolor in reprehenderit in voluptate
- Excepteur sint occaecat cupidatat non proident
Keyboard Shortcuts
1.3 - steps for planning, conducting and analyzing an experiment.
The practical steps needed for planning and conducting an experiment include: recognizing the goal of the experiment, choice of factors, choice of response, choice of the design, analysis and then drawing conclusions. This pretty much covers the steps involved in the scientific method.
- Recognition and statement of the problem
- Choice of factors, levels, and ranges
- Selection of the response variable(s)
- Choice of design
- Conducting the experiment
- Statistical analysis
- Drawing conclusions, and making recommendations
What this course will deal with primarily is the choice of the design. This focus includes all the related issues about how we handle these factors in conducting our experiments.
Factors Section
We usually talk about "treatment" factors, which are the factors of primary interest to you. In addition to treatment factors, there are nuisance factors which are not your primary focus, but you have to deal with them. Sometimes these are called blocking factors, mainly because we will try to block on these factors to prevent them from influencing the results.
There are other ways that we can categorize factors:
Experimental vs. Classification Factors
Quantitative vs. qualitative factors, try it section .
Think about your own field of study and jot down several of the factors that are pertinent in your own research area? Into what categories do these fall?
Get statistical thinking involved early when you are preparing to design an experiment! Getting well into an experiment before you have considered these implications can be disastrous. Think and experiment sequentially. Experimentation is a process where what you know informs the design of the next experiment, and what you learn from it becomes the knowledge base to design the next.
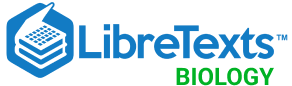
- school Campus Bookshelves
- menu_book Bookshelves
- perm_media Learning Objects
- login Login
- how_to_reg Request Instructor Account
- hub Instructor Commons
Margin Size
- Download Page (PDF)
- Download Full Book (PDF)
- Periodic Table
- Physics Constants
- Scientific Calculator
- Reference & Cite
- Tools expand_more
- Readability
selected template will load here
This action is not available.
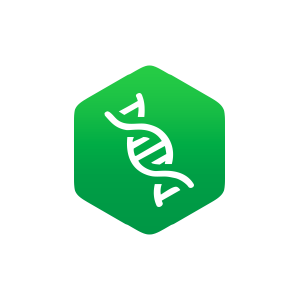
4.14: Experiments and Hypotheses
- Last updated
- Save as PDF
- Page ID 43806
\( \newcommand{\vecs}[1]{\overset { \scriptstyle \rightharpoonup} {\mathbf{#1}} } \)
\( \newcommand{\vecd}[1]{\overset{-\!-\!\rightharpoonup}{\vphantom{a}\smash {#1}}} \)
\( \newcommand{\id}{\mathrm{id}}\) \( \newcommand{\Span}{\mathrm{span}}\)
( \newcommand{\kernel}{\mathrm{null}\,}\) \( \newcommand{\range}{\mathrm{range}\,}\)
\( \newcommand{\RealPart}{\mathrm{Re}}\) \( \newcommand{\ImaginaryPart}{\mathrm{Im}}\)
\( \newcommand{\Argument}{\mathrm{Arg}}\) \( \newcommand{\norm}[1]{\| #1 \|}\)
\( \newcommand{\inner}[2]{\langle #1, #2 \rangle}\)
\( \newcommand{\Span}{\mathrm{span}}\)
\( \newcommand{\id}{\mathrm{id}}\)
\( \newcommand{\kernel}{\mathrm{null}\,}\)
\( \newcommand{\range}{\mathrm{range}\,}\)
\( \newcommand{\RealPart}{\mathrm{Re}}\)
\( \newcommand{\ImaginaryPart}{\mathrm{Im}}\)
\( \newcommand{\Argument}{\mathrm{Arg}}\)
\( \newcommand{\norm}[1]{\| #1 \|}\)
\( \newcommand{\Span}{\mathrm{span}}\) \( \newcommand{\AA}{\unicode[.8,0]{x212B}}\)
\( \newcommand{\vectorA}[1]{\vec{#1}} % arrow\)
\( \newcommand{\vectorAt}[1]{\vec{\text{#1}}} % arrow\)
\( \newcommand{\vectorB}[1]{\overset { \scriptstyle \rightharpoonup} {\mathbf{#1}} } \)
\( \newcommand{\vectorC}[1]{\textbf{#1}} \)
\( \newcommand{\vectorD}[1]{\overrightarrow{#1}} \)
\( \newcommand{\vectorDt}[1]{\overrightarrow{\text{#1}}} \)
\( \newcommand{\vectE}[1]{\overset{-\!-\!\rightharpoonup}{\vphantom{a}\smash{\mathbf {#1}}}} \)
Now we’ll focus on the methods of scientific inquiry. Science often involves making observations and developing hypotheses. Experiments and further observations are often used to test the hypotheses.
A scientific experiment is a carefully organized procedure in which the scientist intervenes in a system to change something, then observes the result of the change. Scientific inquiry often involves doing experiments, though not always. For example, a scientist studying the mating behaviors of ladybugs might begin with detailed observations of ladybugs mating in their natural habitats. While this research may not be experimental, it is scientific: it involves careful and verifiable observation of the natural world. The same scientist might then treat some of the ladybugs with a hormone hypothesized to trigger mating and observe whether these ladybugs mated sooner or more often than untreated ones. This would qualify as an experiment because the scientist is now making a change in the system and observing the effects.
Forming a Hypothesis
When conducting scientific experiments, researchers develop hypotheses to guide experimental design. A hypothesis is a suggested explanation that is both testable and falsifiable. You must be able to test your hypothesis, and it must be possible to prove your hypothesis true or false.
For example, Michael observes that maple trees lose their leaves in the fall. He might then propose a possible explanation for this observation: “cold weather causes maple trees to lose their leaves in the fall.” This statement is testable. He could grow maple trees in a warm enclosed environment such as a greenhouse and see if their leaves still dropped in the fall. The hypothesis is also falsifiable. If the leaves still dropped in the warm environment, then clearly temperature was not the main factor in causing maple leaves to drop in autumn.
In the Try It below, you can practice recognizing scientific hypotheses. As you consider each statement, try to think as a scientist would: can I test this hypothesis with observations or experiments? Is the statement falsifiable? If the answer to either of these questions is “no,” the statement is not a valid scientific hypothesis.
Practice Questions
Determine whether each following statement is a scientific hypothesis.
- No. This statement is not testable or falsifiable.
- No. This statement is not testable.
- No. This statement is not falsifiable.
- Yes. This statement is testable and falsifiable.
[reveal-answer q=”429550″] Show Answers [/reveal-answer] [hidden-answer a=”429550″]
- d: Yes. This statement is testable and falsifiable. This could be tested with a number of different kinds of observations and experiments, and it is possible to gather evidence that indicates that air pollution is not linked with asthma.
- a: No. This statement is not testable or falsifiable. “Bad thoughts and behaviors” are excessively vague and subjective variables that would be impossible to measure or agree upon in a reliable way. The statement might be “falsifiable” if you came up with a counterexample: a “wicked” place that was not punished by a natural disaster. But some would question whether the people in that place were really wicked, and others would continue to predict that a natural disaster was bound to strike that place at some point. There is no reason to suspect that people’s immoral behavior affects the weather unless you bring up the intervention of a supernatural being, making this idea even harder to test.
[/hidden-answer]
Testing a Vaccine
Let’s examine the scientific process by discussing an actual scientific experiment conducted by researchers at the University of Washington. These researchers investigated whether a vaccine may reduce the incidence of the human papillomavirus (HPV). The experimental process and results were published in an article titled, “ A controlled trial of a human papillomavirus type 16 vaccine .”
Preliminary observations made by the researchers who conducted the HPV experiment are listed below:
- Human papillomavirus (HPV) is the most common sexually transmitted virus in the United States.
- There are about 40 different types of HPV. A significant number of people that have HPV are unaware of it because many of these viruses cause no symptoms.
- Some types of HPV can cause cervical cancer.
- About 4,000 women a year die of cervical cancer in the United States.
Practice Question
Researchers have developed a potential vaccine against HPV and want to test it. What is the first testable hypothesis that the researchers should study?
- HPV causes cervical cancer.
- People should not have unprotected sex with many partners.
- People who get the vaccine will not get HPV.
- The HPV vaccine will protect people against cancer.
[reveal-answer q=”20917″] Show Answer [/reveal-answer] [hidden-answer a=”20917″]Hypothesis A is not the best choice because this information is already known from previous studies. Hypothesis B is not testable because scientific hypotheses are not value statements; they do not include judgments like “should,” “better than,” etc. Scientific evidence certainly might support this value judgment, but a hypothesis would take a different form: “Having unprotected sex with many partners increases a person’s risk for cervical cancer.” Before the researchers can test if the vaccine protects against cancer (hypothesis D), they want to test if it protects against the virus. This statement will make an excellent hypothesis for the next study. The researchers should first test hypothesis C—whether or not the new vaccine can prevent HPV.[/hidden-answer]
Experimental Design
You’ve successfully identified a hypothesis for the University of Washington’s study on HPV: People who get the HPV vaccine will not get HPV.
The next step is to design an experiment that will test this hypothesis. There are several important factors to consider when designing a scientific experiment. First, scientific experiments must have an experimental group. This is the group that receives the experimental treatment necessary to address the hypothesis.
The experimental group receives the vaccine, but how can we know if the vaccine made a difference? Many things may change HPV infection rates in a group of people over time. To clearly show that the vaccine was effective in helping the experimental group, we need to include in our study an otherwise similar control group that does not get the treatment. We can then compare the two groups and determine if the vaccine made a difference. The control group shows us what happens in the absence of the factor under study.
However, the control group cannot get “nothing.” Instead, the control group often receives a placebo. A placebo is a procedure that has no expected therapeutic effect—such as giving a person a sugar pill or a shot containing only plain saline solution with no drug. Scientific studies have shown that the “placebo effect” can alter experimental results because when individuals are told that they are or are not being treated, this knowledge can alter their actions or their emotions, which can then alter the results of the experiment.
Moreover, if the doctor knows which group a patient is in, this can also influence the results of the experiment. Without saying so directly, the doctor may show—through body language or other subtle cues—his or her views about whether the patient is likely to get well. These errors can then alter the patient’s experience and change the results of the experiment. Therefore, many clinical studies are “double blind.” In these studies, neither the doctor nor the patient knows which group the patient is in until all experimental results have been collected.
Both placebo treatments and double-blind procedures are designed to prevent bias. Bias is any systematic error that makes a particular experimental outcome more or less likely. Errors can happen in any experiment: people make mistakes in measurement, instruments fail, computer glitches can alter data. But most such errors are random and don’t favor one outcome over another. Patients’ belief in a treatment can make it more likely to appear to “work.” Placebos and double-blind procedures are used to level the playing field so that both groups of study subjects are treated equally and share similar beliefs about their treatment.
The scientists who are researching the effectiveness of the HPV vaccine will test their hypothesis by separating 2,392 young women into two groups: the control group and the experimental group. Answer the following questions about these two groups.
- This group is given a placebo.
- This group is deliberately infected with HPV.
- This group is given nothing.
- This group is given the HPV vaccine.
[reveal-answer q=”918962″] Show Answers [/reveal-answer] [hidden-answer a=”918962″]
- a: This group is given a placebo. A placebo will be a shot, just like the HPV vaccine, but it will have no active ingredient. It may change peoples’ thinking or behavior to have such a shot given to them, but it will not stimulate the immune systems of the subjects in the same way as predicted for the vaccine itself.
- d: This group is given the HPV vaccine. The experimental group will receive the HPV vaccine and researchers will then be able to see if it works, when compared to the control group.
Experimental Variables
A variable is a characteristic of a subject (in this case, of a person in the study) that can vary over time or among individuals. Sometimes a variable takes the form of a category, such as male or female; often a variable can be measured precisely, such as body height. Ideally, only one variable is different between the control group and the experimental group in a scientific experiment. Otherwise, the researchers will not be able to determine which variable caused any differences seen in the results. For example, imagine that the people in the control group were, on average, much more sexually active than the people in the experimental group. If, at the end of the experiment, the control group had a higher rate of HPV infection, could you confidently determine why? Maybe the experimental subjects were protected by the vaccine, but maybe they were protected by their low level of sexual contact.
To avoid this situation, experimenters make sure that their subject groups are as similar as possible in all variables except for the variable that is being tested in the experiment. This variable, or factor, will be deliberately changed in the experimental group. The one variable that is different between the two groups is called the independent variable. An independent variable is known or hypothesized to cause some outcome. Imagine an educational researcher investigating the effectiveness of a new teaching strategy in a classroom. The experimental group receives the new teaching strategy, while the control group receives the traditional strategy. It is the teaching strategy that is the independent variable in this scenario. In an experiment, the independent variable is the variable that the scientist deliberately changes or imposes on the subjects.
Dependent variables are known or hypothesized consequences; they are the effects that result from changes or differences in an independent variable. In an experiment, the dependent variables are those that the scientist measures before, during, and particularly at the end of the experiment to see if they have changed as expected. The dependent variable must be stated so that it is clear how it will be observed or measured. Rather than comparing “learning” among students (which is a vague and difficult to measure concept), an educational researcher might choose to compare test scores, which are very specific and easy to measure.
In any real-world example, many, many variables MIGHT affect the outcome of an experiment, yet only one or a few independent variables can be tested. Other variables must be kept as similar as possible between the study groups and are called control variables . For our educational research example, if the control group consisted only of people between the ages of 18 and 20 and the experimental group contained people between the ages of 30 and 35, we would not know if it was the teaching strategy or the students’ ages that played a larger role in the results. To avoid this problem, a good study will be set up so that each group contains students with a similar age profile. In a well-designed educational research study, student age will be a controlled variable, along with other possibly important factors like gender, past educational achievement, and pre-existing knowledge of the subject area.
What is the independent variable in this experiment?
- Sex (all of the subjects will be female)
- Presence or absence of the HPV vaccine
- Presence or absence of HPV (the virus)
[reveal-answer q=”68680″]Show Answer[/reveal-answer] [hidden-answer a=”68680″]Answer b. Presence or absence of the HPV vaccine. This is the variable that is different between the control and the experimental groups. All the subjects in this study are female, so this variable is the same in all groups. In a well-designed study, the two groups will be of similar age. The presence or absence of the virus is what the researchers will measure at the end of the experiment. Ideally the two groups will both be HPV-free at the start of the experiment.
List three control variables other than age.
[practice-area rows=”3″][/practice-area] [reveal-answer q=”903121″]Show Answer[/reveal-answer] [hidden-answer a=”903121″]Some possible control variables would be: general health of the women, sexual activity, lifestyle, diet, socioeconomic status, etc.
What is the dependent variable in this experiment?
- Sex (male or female)
- Rates of HPV infection
- Age (years)
[reveal-answer q=”907103″]Show Answer[/reveal-answer] [hidden-answer a=”907103″]Answer b. Rates of HPV infection. The researchers will measure how many individuals got infected with HPV after a given period of time.[/hidden-answer]
Contributors and Attributions
- Revision and adaptation. Authored by : Shelli Carter and Lumen Learning. Provided by : Lumen Learning. License : CC BY-NC-SA: Attribution-NonCommercial-ShareAlike
- Scientific Inquiry. Provided by : Open Learning Initiative. Located at : https://oli.cmu.edu/jcourse/workbook/activity/page?context=434a5c2680020ca6017c03488572e0f8 . Project : Introduction to Biology (Open + Free). License : CC BY-NC-SA: Attribution-NonCommercial-ShareAlike

An official website of the United States government
The .gov means it’s official. Federal government websites often end in .gov or .mil. Before sharing sensitive information, make sure you’re on a federal government site.
The site is secure. The https:// ensures that you are connecting to the official website and that any information you provide is encrypted and transmitted securely.
- Publications
- Account settings
Preview improvements coming to the PMC website in October 2024. Learn More or Try it out now .
- Advanced Search
- Journal List
- HHS Author Manuscripts

How to Conduct Responsible Research: A Guide for Graduate Students
Alison l. antes.
1 Department of Medicine, Division of General Medical Sciences, Washington University School of Medicine, St. Louis, Missouri, 314-362-6006
Leonard B. Maggi, Jr.
2 Department of Medicine, Division of Molecular Oncology, Siteman Cancer Center, Washington University School of Medicine, St. Louis, Missouri, 314-362-4102
Researchers must conduct research responsibly for it to have an impact and to safeguard trust in science. Essential responsibilities of researchers include using rigorous, reproducible research methods, reporting findings in a trustworthy manner, and giving the researchers who contributed appropriate authorship credit. This “how-to” guide covers strategies and practices for doing reproducible research and being a responsible author. The article also covers how to utilize decision-making strategies when uncertain about the best way to proceed in a challenging situation. The advice focuses especially on graduate students but is appropriate for undergraduates and experienced researchers. The article begins with an overview of the responsible conduct of research, research misconduct, and ethical behavior in the scientific workplace. The takeaway message is that responsible conduct of research requires a thoughtful approach to doing research to ensure trustworthy results and conclusions and that researchers receive fair credit.
INTRODUCTION
Doing research is stimulating and fulfilling work. Scientists make discoveries to build knowledge and solve problems, and they work with other dedicated researchers. Research is a highly complex activity, so it takes years for beginning researchers to learn everything they need to know to do science well. Part of this large body of knowledge is learning how to do research responsibly. Our purpose in this article is to provide graduate students a guide for how to perform responsible research. Our advice is also relevant to undergraduate researchers and for principal investigators (PIs), postdocs, or other researchers who mentor beginning researchers and wish to share our advice.
We begin by introducing some fundamentals about the responsible conduct of research (RCR), research misconduct, and ethical behavior. We focus on how to do reproducible science and be a responsible author. We provide practical advice for these topics and present scenarios to practice thinking through challenges in research. Our article concludes with decision-making strategies for addressing complex problems.
What is the responsible conduct of research?
To be committed to RCR means upholding the highest standards of honesty, accuracy, efficiency, and objectivity ( Steneck, 2007 ). Each day, RCR requires engaging in research in a conscientious, intentional fashion that yields the best science possible ( “Research Integrity is Much More Than Misconduct,” 2019 ). We adopt a practical, “how-to” approach, discussing the behaviors and habits that yield responsible research. However, some background knowledge about RCR is helpful to frame our discussion.
The scientific community uses many terms to refer to ethical and responsible behavior in research: responsible conduct of research, research integrity, scientific integrity, and research ethics ( National Academies of Science, 2009 ; National Academies of Sciences Engineering and Medicine, 2017 ; Steneck, 2007 ). A helpful way to think about these concepts is “doing good science in a good manner” ( DuBois & Antes, 2018 ). This means that the way researchers do their work, from experimental procedures to data analysis and interpretation, research reporting, and so on, leads to trustworthy research findings and conclusions. It also includes respectful interactions among researchers both within research teams (e.g., between peers, mentors and trainees, and collaborators) and with researchers external to the team (e.g., peer reviewers). We expand on trainee-mentor relationships and interpersonal dynamics with labmates in a companion article ( Antes & Maggi, 2021 ). When research involves human or animal research subjects, RCR includes protecting the well-being of research subjects.
We do not cover all potential RCR topics but focus on what we consider fundamentals for graduate students. Common topics covered in texts and courses on RCR include the following: authorship and publication; collaboration; conflicts of interest; data management, sharing, and ownership; intellectual property; mentor and trainee responsibilities; peer review; protecting human subjects; protecting animal subjects; research misconduct; the role of researchers in society; and laboratory safety. A number of topics prominently discussed among the scientific community in recent years are also relevant to RCR. These include the reproducibility of research ( Baker, 2016 ; Barba, 2016 ; Winchester, 2018 ), diversity and inclusion in science ( Asplund & Welle, 2018 ; Hofstra et al., 2020 ; Meyers, Brown, Moneta-Koehler, & Chalkley, 2018 ; National Academies of Sciences Engineering and Medicine, 2018a ; Roper, 2019 ), harassment and bullying ( Else, 2018 ; National Academies of Sciences Engineering and Medicine, 2018b ; “ No Place for Bullies in Science,” 2018 ), healthy research work environments ( Norris, Dirnagl, Zigmond, Thompson-Peer, & Chow, 2018 ; “ Research Institutions Must Put the Health of Labs First,” 2018 ), and the mental health of graduate students ( Evans, Bira, Gastelum, Weiss, & Vanderford, 2018 ).
The National Institutes of Health (NIH) ( National Institutes of Health, 2009 ) and the National Science Foundation ( National Science Foundation, 2017 ) have formal policies indicating research trainees must receive education in RCR. Researchers are accountable to these funding agencies and the public which supports research through billions in tax dollars annually. The public stands to benefit from, or be harmed by, research. For example, the public may be harmed if medical treatments or social policies are based on untrustworthy research findings. Funding for research, participation in research, and utilization of the fruits of research all rely on public trust ( Resnik, 2011 ). Trustworthy findings are also essential for good stewardship of scarce resources ( Emanuel, Wendler, & Grady, 2000 ). Researchers are further accountable to their peers, colleagues, and scientists more broadly. Trust in the work of other researchers is essential for science to advance. Finally, researchers are accountable for complying with the rules and policies of their universities or research institutions, such as rules about laboratory safety, bullying and harassment, and the treatment of animal research subjects.
What is research misconduct?
When researchers intentionally misrepresent or manipulate their results, these cases of scientific fraud often make the news headlines ( Chappell, 2019 ; O’Connor, 2018 ; Park, 2012 ), and they can seriously undermine public trust in research. These cases also harm trust within the scientific community.
The U.S. defines research misconduct as fabrication, falsification, and plagiarism (FFP) ( Department of Health and Human Services, 2005 ). FFP violate the fundamental ethical principle of honesty. Fabrication is making up data, and falsification is manipulating or changing data or results so they are no longer truthful. Plagiarism is a form of dishonesty because it includes using someone’s words or ideas and portraying them as your own. When brought to light, misconduct involves lengthy investigations and serious consequences, such as ineligibility to receive federal research funding, loss of employment, paper retractions, and, for students, withdrawal of graduate degrees.
One aspect of responsible behavior includes addressing misconduct if you observe it. We suggest a guide titled “Responding to Research Wrongdoing: A User-Friendly Guide” that provides advice for thinking about your options if you think you have observed misconduct ( Keith-Spiegel, Sieber, & Koocher, 2010 ). Your university will have written policies and procedures for investigating allegations of misconduct. Making an allegation is very serious. As Keith-Spiegel et al.’s guide indicates, it is important to know the evidence that supports your claim, and what to expect in the process. We encourage, if possible, talking to the persons involved first. For example, one of us knew of a graduate student who reported to a journal editor their suspicion of falsified data in a manuscript. It turned out that the student was incorrect. Going above the PI directly to the editor ultimately led to the PI leaving the university, and the student had a difficult time finding a new lab to complete their degree. If the student had first spoken to the PI and lab members, they could have learned that their assumptions about the data in the paper were wrong. In turn, they could have avoided accusing the PI of a serious form of scientific misconduct—making up data—and harming everyone’s scientific career.
What shapes ethical behavior in the scientific workplace?
Responsible conduct of research and research misconduct are two sides of a continuum of behavior—RCR upholds the ideals of research and research misconduct violates them. Problematic practices that fall in the middle but are not defined formally as research misconduct have been labeled as detrimental research practices ( National Academies of Sciences Engineering and Medicine, 2017 ). Researchers conducting misleading statistical analyses or PIs providing inadequate supervision are examples of the latter. Research suggests that characteristics of individual researchers and research environments explain (un)ethical behavior in the scientific workplace ( Antes et al., 2007 ; Antes, English, Baldwin, & DuBois, 2018 ; Davis, Riske-Morris, & Diaz, 2007 ; DuBois et al., 2013 ).
These two influences on ethical behavior are helpful to keep in mind when thinking about your behavior. When people think about their ethical behavior, they think about their personal values and integrity and tend to overlook the influence of their environment. While “being a good person” and having the right intentions are essential to ethical behavior, the environment also has an influence. In addition, knowledge of standards for ethical research is important for ethical behavior, and graduate students new to research do not yet know everything they need to. They also have not fully refined their ethical decision-making skills for solving professional problems. We discuss strategies for ethical decision-making in the final section of this article ( McIntosh, Antes, & DuBois, 2020 ).
The research environment influences ethical behavior in a number of ways. For example, if a research group explicitly discusses high standards for research, people will be more likely to prioritize these ideals in their behavior ( Plemmons et al., 2020 ). A mentor who sets a good example is another important factor ( Anderson et al., 2007 ). Research labs must also provide individuals with adequate training, supervision and feedback, opportunities to discuss data, and the psychological safety to feel comfortable communicating about problems, including mistakes ( Antes, Kuykendall, & DuBois, 2019a , 2019b ). On the other hand, unfair research environments, inadequate supervision, poor communication, and severe stress and anxiety may undermine ethical decision-making and behavior; particularly when many of these factors exist together. Thus, (un)ethical behavior is a complex interplay of individual factors (e.g., personality, stress, decision-making skills) and the environment.
For graduate students, it is important to attend to what you are learning and how the environment around you might influence your behavior. You do not know what you do not know, and you necessarily rely on others to teach you responsible practices. So, it is important to be aware. Ultimately, you are accountable for your behavior. You cannot just say “I didn’t know.” Rather, just like you are curious about your scientific questions, maintain a curiosity about responsible behavior as a researcher. If you feel uncomfortable with something, pay attention to that feeling, speak to someone you trust, and seek out information about how to handle the situation. In what follows, we cover key tips for responsible behavior in the areas of reproducibility and authorship that we hope will help you as you begin.
HOW TO DO REPRODUCIBLE SCIENCE
The foremost responsibility of scientists is to ensure they conduct research in such a manner that the findings are trustworthy. Reproducibility is the ability to duplicate results ( Goodman, Fanelli, & Ioannidis, 2016 ). The scientific community has called for greater openness, transparency, and rigor as key remedies for lack of reproducibility ( Munafò et al., 2017 ). As a graduate student, essential to fostering reproducibility is the rigor of your approach to doing experiments and handling data. We discuss how to utilize research protocols, document experiments in a lab notebook, and handle data responsibly.
Utilize research protocols
1. learn and utilize the lab’s protocols.
Research protocols describe the step-by-step procedures for doing an experiment. They are critical for the quality and reproducibility of experiments. Lab members must learn and follow the lab’s protocols with the understanding that they may need to make adjustments based on the requirements of a specific experiment.
Also, it is important to distinguish between the experiment you are performing and analyzing the data from that experiment. For example, the experiment you want to perform might be to determine if loss of a gene blocks cell growth. Several protocols, each with pros and cons, will allow you to examine “cell growth.” Using the wrong experimental protocol can produce data that leads to muddled conclusions. In this example, the gene does block cell growth, but the experiment used to produce the data that you analyze to understand cell growth is wrong, thus giving a result that is a false negative.
When first joining a lab, it is essential to commit to learning the protocols necessary for your assigned research project. Researchers must ensure they are proficient in executing a protocol and can perform their experiments reliably. If you do not feel confident with a protocol, you should do practice runs if possible. Repetition is the best way to work through difficulties with protocols. Often it takes several attempts to work through the steps of a protocol before you will be comfortable performing it. Asking to watch another lab member perform the protocol is also helpful. Be sure to watch closely how steps are performed, as often there are minor steps taken that are not written down. Also, experienced lab members may do things as second nature and not think to explicitly mention them when working through the protocol. Ask questions of other lab members so that you can improve your knowledge and gain confidence with a protocol. It is better to ask a question than potentially ruin a valuable or hard-to-get sample.
Be cautious of differences in the standing protocols in the lab and how you actually perform the experiment. Even the most minor deviations can seriously impact the results and reproducibility of an experiment. As mentioned above, often there are minor things that are done that might not be listed in the protocol. Paying attention and asking questions are the best ways to learn, in addition to adding notes to the protocol if you find minor details are missing.
2. Develop your own protocols
Often you will find that a project requires a protocol that has not been performed in the lab. If performing a new experiment in the lab and no protocol exists, find a protocol and try it. Protocols can be obtained from many different sources. A great source is other labs on campus, as you can speak directly to the person who performs the experiment. There are many journal sources as well, such as Current Protocols, Nature Protocols, Nature Methods, and Cell STAR Methods . These methods journals provide the most detailed protocols for experiments often with troubleshooting tips. Scientific papers are the most common source of protocols. However, keep in mind that due to the common brevity of methods sections, they often omit crucial details or reference other papers that may not contain a complete description of the protocol.
3. Handle mistakes or problems promptly
At some point, everyone encounters problems with a protocol, or realizes they made a mistake. You should be prepared to handle this situation by being able to detail exactly how you performed the experiment. Did you skip a step? Shorten or lengthen a time point? Did you have to make a new buffer or borrow a labmate’s buffer? There are too many ways an experiment can go wrong to list here but being able to recount all the steps you performed in detail will help you work through the problem. Keep in mind that often the best way to understand how to perform an experiment is learning from when something goes wrong. This situation requires you to critically think through what was done and understand the steps taken. When everything works perfectly, it is easy to pay less attention to the details, which can lead to problems down the line.
It is up to you to be attentive and meticulous in the lab. Paying attention to the details may feel like a pain at first, or even seem overwhelming. Practice and repetition will help this focus on details become a natural part of your lab work. Ultimately, this skill will be essential to being a responsible scientist.
Document experiments in a lab notebook
1. recognize the importance of a lab notebook.
Maintaining detailed documentation in a lab notebook allows researchers to keep track of their experiments and generation of data. This detailed documentation helps you communicate about your research with others in the lab, and serves as a basis for preparing publications. It also provides a lasting record for the lab that exists beyond your time in the lab. After graduate students leave the lab, sometimes it is necessary to go back to the results of older experiments. A complete and detailed notebook is essential, or all of the time, effort, and resources are lost.
2. Learn the note-keeping practices in your lab
When you enter a new lab, it is important to understand how the lab keeps notebooks and the expectations for documentation. Being conscientious about documentation will make you a better scientist. In some labs, the PI might routinely examine your notebook, while in other labs you may be expected to maintain a notebook, but it may not be regularly viewed by others. It is tempting to become relaxed in documentation if you think your notebook may not be reviewed. Avoid this temptation; documentation of your ideas and process will improve your ability to think critically about research. Further, even if the PI or lab members do not physically view your notebook, you will need to communicate with them about your experiments. This documentation is necessary to communicate effectively about your work.
3. Organize your lab notebook
Different labs use different formats; some use electronic notebooks while others handwritten notebooks. The contents of a good notebook include the purpose of the experiment, the details of the experimental procedure, the data, and thoughts about the results. To effectively document your experiment, there are 5 critical questions that the information you record should be able to answer.
- Why I am doing this experiment? (purpose)
- What did I do to perform the experiment? (protocol)
- What are the results of what I did? (data, graphs)
- What do I think about the results?
- What do I think are the next steps?
We also recommend a table of contents. It will make the information more useful to you and the lab in the future. The table of contents should list the title of the experiment, the date(s) it was performed, and the page numbers on which it is recorded. Also, make sure that you write clearly and provide a legend or explanation of any shorthand or non-standard abbreviation you use. Often labs will have a combination of written lab notebooks and electronic data. It is important to reference where electronic data are located that go with each experiment. The idea is to make it as easy as possible to understand what you did and where to find all the data (electronic and hard copy) that accompanies your experiment.
Keeping a lab notebook becomes easier with practice. It can be thought of almost like journaling about your experiment. Sometimes people think of it as just a place to paste their protocol and a graph or data. We strongly encourage you to include your thoughts about why you made the decisions you made when conducting the experiment and to document your thoughts about next steps.
4. Commit to doing it the right way
A common reason to become lax in documentation is feeling rushed for time. Although documentation takes time, it saves time in the long-run and fosters good science. Without good notes, you will waste time trying to recall precisely what you did, reproduce your findings, and remember what you thought would be important next steps. The lab notebook helps you think about your research critically and keep your thoughts together. It can also save you time later when writing up results for publication. Further, well-documented data will help you draft a cogent and rigorous dissertation.
Handle data responsibly
1. keep all data.
Data are the product of research. Data include raw data, processed data, analyzed data, figures, and tables. Many data today are electronic, but not all. Generating data requires a lot of time and resources and researchers must treat data with care. The first essential tip is to keep all data. Do not discard data just because the experiment did not turn out as expected. A lot of experiments do not turn out to yield publishable data, but the results are still important for informing next steps.
Always keep the original, raw data. That is, as you process and analyze data, always maintain an unprocessed version of the original data.
Universities and funding agencies have data retention policies. These policies specify the number of years beyond a grant that data must be kept. Some policies also indicate researchers need to retain original data that served as the basis for a publication for a certain number of years. Therefore, your data will be important well beyond your time in graduate school. Most labs require you to keep samples for reanalysis until a paper is published, then the analyzed data are enough. If you leave a lab before a paper is accepted for publication, you are responsible for ensuring your data and original samples are well documented for others to find and use.
2. Document all data
In addition to keeping all data, data must be well-organized and documented. This means that no matter the way you keep your data (e.g., electronic or in written lab notebooks), there is a clear guide—in your lab notebook, a binder, or on a lab hard drive—to finding the data for a particular experiment. For example, it must be clear which data produced a particular graph. Version control of data is also critical. Your documentation should include “metadata” (data about your data) that tracks versions of the data. For example, as you edit data for a table, you should save separate versions of the tables, name the files sequentially, and note the changes that were made to each version.
3. Backup your data
You should backup electronic data regularly. Ideally, your lab has a shared server or cloud storage to backup data. If you are supposed to put your data there, make sure you do it! When you leave the lab, it must be possible to find your data.
4. Perform data analysis honestly and competently
Inappropriate use of statistics is a major concern in the scientific community, as the results and conclusions will be misleading if done incorrectly ( DeMets, 1999 ). Some practices are clearly an abuse of statistics, while other inappropriate practices stem from lack of knowledge. For example, a practice called “p-hacking” describes when researchers “collect or select data or statistical analyses until nonsignificant results become significant” ( Head, Holman, Lanfear, Kahn, & Jennions, 2015 ). In addition to avoiding such misbehavior, it is essential to be proficient with statistics to ensure you do statistical procedures appropriately. Learning statistical procedures and analyzing data takes many years of practice, and your statistics courses may only cover the basics. You will need to know when to consult others for help. In addition to consulting members in your lab or your PI, your university may have statistical experts who can provide consultations.
5. Master pressure to obtain favored results
When you conduct an experiment, the results are the results. As a beginning researcher, it is important to be prepared to manage the frustration of experiments not turning out as expected. It is also important to manage the real or perceived pressure to produce favored results. Investigators can become wedded to a hypothesis, and they can have a difficult time accepting the results. Sometimes you may feel this pressure coming from yourself; for example, if you want to please your PI, or if you want to get results for a certain publication. It is important to always follow the data no matter where it leads.
If you do feel pressure, this situation can be uncomfortable and stressful. If you have been meticulous and followed the above recommendations, this can be one great safeguard. You will be better able to confidently communicate your results to the PI because of your detailed documentation, and you will be more confident in your procedures if the possibility of error is suggested. Typically, with enough evidence that the unexpected results are real, the PI will concede. We recommend seeking the support of friends or colleagues to vent and cope with stress. In the rare case that the PI does not relent, you could turn to an advisor outside the lab if you need advice about how to proceed. They can help you look at the data objectively and also help you think about the interpersonal aspects of navigating this situation.
6. Communicate about your data in the lab
A critical element of reproducible research is communication in the lab. Ideally, there are weekly or bi-weekly meetings to discuss data. You need to develop your communication skills for writing and speaking about data. Often you and your labmates will discuss experimental issues and results informally during the course of daily work. This is an excellent way to hone critical thinking and communication skills about data.
Scenario 1 – The Protocol is Not Working
At the beginning of a rotation during their first year, a graduate student is handed a lab notebook and a pen and is told to keep track of their work. There does not appear to be a specific format to follow. There are standard lab protocols that everyone follows, but minor tweaks to the protocols do not seem to be tracked from experiment to experiment in the standard lab protocol nor in other lab notebooks. After two weeks of trying to follow one of the standard lab protocols, the student still cannot get the experiment to work. The student has included the appropriate positive and negative controls which are failing, making the experiment uninterpretable. After asking others in the lab for help, the graduate student learns that no one currently in the lab has performed this particular experiment. The former lab member who had performed the experiment only lists the standard protocol in their lab notebook.
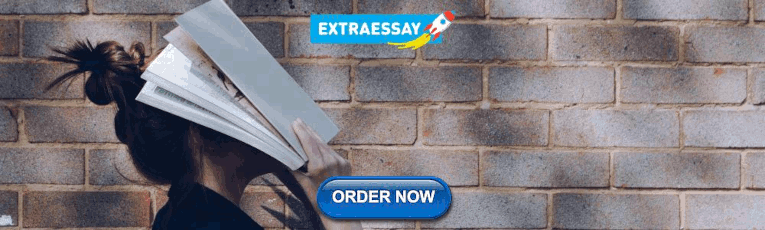
How should the graduate student start to solve the problem?
Speaking to the PI would be the next logical step. As a first-year student in a lab rotation, the PI should expect this type of situation and provide additional troubleshooting guidance. It is possible that the PI may want to see how the new graduate student thinks critically and handles adversity in the lab. Rather than giving an answer, the PI might ask the student to work through the problem. The PI should give guidance, but it may not be an immediate fix for the problem. If the PI’s suggestions fail to correct the problem, asking a labmate or the PI for the contact information of the former lab member who most recently performed the experiment would be a reasonable next step. The graduate student’s conversations with the PI and labmates in this situation will help them learn a lot about how the people in the lab interact.
Most of the answers for these types of problems will require you as a graduate student to take the initiative to answer. They will require your effort and ingenuity to talk to other lab members, other labs at the university, and even scour the literature for alternatives. While labs have standard protocols, there are multiple ways to do many experiments, and working out an alternative will teach you more than when everything works. Having to troubleshoot problems will result in better standard protocols in the lab and better science.
HOW TO BE A RESPONSIBLE AUTHOR
Researchers communicate their findings via peer-reviewed publications, and publications are important for advancing in a research career. Many graduate students will first author or co-author publications in graduate school. For good advice on how to write a research manuscript, consult the Current Protocols article “How to write a research manuscript” ( Frank, 2018 ). We focus on the issues of assigning authors and reporting your findings responsibly. First, we describe some important basics: journal impact factors, predatory journals, and peer review.
What are journal impact factors?
It is helpful to understand journal impact factors. There is criticism about an overemphasis on impact factors for evaluating the quality or importance of researchers’ work ( DePellegrin & Johnston, 2015 ), but they remain common for this purpose. Journal impact factors reflect the average number of times articles in a journal were cited in the last two years. Higher impact factors place journals at a higher rank. Approximately 2% of journals have an impact factor of 10 or higher. For example, Cell, Science, and Nature have impact factors of approximately 39, 42, and 43, respectively. Journals can be great journals but have lower impact factors; often this is because they focus on a smaller specialty field. For example, Journal of Immunology and Oncogene are respected journals, but their impact factors are about 4 and 7, respectively.
Research trainees often want to publish in journals with the highest possible impact factor because they expect this to be viewed favorably when applying to future positions. We encourage you to bear in mind that many different journals publish excellent science and focus on publishing where your work will reach the desired audience. Also, keep in mind that while a high impact factor can direct you to respectable, high-impact science, it does not guarantee that the science in the paper is good or even correct. You must critically evaluate all papers you read no matter the impact factor.
What are predatory journals?
Predatory journals have flourished over the past few years as publishing science has moved online. An international panel defined predatory journals as follows ( Grudniewicz et al., 2019 ):
Predatory journals and publishers are entities that prioritize self-interest at the expense of scholarship and are characterized by false or misleading information, deviation from best editorial and publication practices, a lack of transparency, and/or the use of aggressive and indiscriminate solicitation practices. (p. 211)
Often young researchers receive emails soliciting them to submit their work to a journal. There are typically small fees (around $99 US) requested but these fees will be much lower than open access fees of reputable journals (often around $2000 US). A warning sign of a predatory journal is outlandish promises, such as 24-hour peer review or immediate publication. You can find a list of predatory journals created by a postdoc in Europe at BeallsList.net ( “Beall’s List of Potential Predatory Journals and Publishers,” 2020 ).
What is peer review?
Peer reviewers are other scientists who have the expertise to evaluate a manuscript. Typically 2 or 3 reviewers evaluate a manuscript. First, an editor performs an initial screen of the manuscript to ensure its appropriateness for the journal and that it meets basic quality standards. At this stage, an editor can decide to reject the manuscript and not send it to review. Not sending a paper for peer review is common in the highest impact journals that receive more submissions per year than can be reviewed and published. For average-impact journals and specialty journals, typically your paper will be sent for peer review.
In general, peer review focuses on three aspects of a manuscript: research design and methods, validity of the data and conclusions, and significance. Peer reviewers assess the merit and rigor of the research design and methodology, and they evaluate the overall validity of the results, interpretations, and conclusions. Essentially, reviewers want to ensure that the data support the claims. Additionally, reviewers evaluate the overall significance, or contribution, of the findings, which involves the novelty of the research and the likelihood that the findings will advance the field. Significance standards vary between journals. Some journals are open to publishing findings that are incremental advancements in a field, while others want to publish only what they deem as major advancements. This feature can distinguish the highest impact journals which seek the most significant advancements and other journals that tend to consider a broader range of work as long as it is scientifically sound. It is important to keep in mind that determining at the stage of review and publication whether a paper is “high impact” is quite subjective. In reality, this can only really be determined in retrospect.
The key ethical issues in peer review are fairness, objectivity, and confidentiality ( Shamoo & Resnik, 2015 ). Peer reviewers are to evaluate the manuscript on its merits and not based on biases related to the authors or the science itself. If reviewers have a conflict of interest, this should be disclosed to the editor. Confidentiality of peer review means that the reviewers should keep private the information; they should not share the information with others or use it to their benefit. Reviewers can ultimately recommend that the manuscript is rejected, revised, and resubmitted (major or minor revisions), or accepted. The editor evaluates the reviewers’ feedback and makes a judgment about rejecting, accepting, or requesting a revision. Sometimes PIs will ask experienced graduate students to assist with peer reviewing a manuscript. This is a good learning opportunity. The PI should disclose to the editor that they included a trainee in preparing the review.
Assign authorship fairly
Authorship gives credit to the people who contributed to the research. This includes thinking of the ideas, designing and performing experiments, interpreting the results, and writing the paper. Two key questions regarding authorship include: 1 - Who will be an author? 2 - What will be the order in which authors are listed? These seem simple on the surface but can get quite complex.
1. Know authorship guidelines
Authorship guidelines published by journals, professional societies, and universities communicate key principles of authorship and standards for earning authorship. The core ethical principle of assigning authorship is fairness in who receives credit for the work. The people who contributed to the work should get credit for it. This seems simply enough, but determining authorship can (and often does) create conflict.
Many universities have authorship guidelines, and you should know the policies at your university. The International Committee of Medical Journal Editors (ICMJE) provides four criteria for determining who should be an author ( International Committee of Medical Journal Editors, 2020 ). These criteria indicate that an author should do all of the following: 1) make “substantial contributions” to the development of the idea or research design, or to acquiring, analyzing, or interpreting the data, 2) write the manuscript or revise it a substantive way, 3) give approval of the final manuscript (i.e., before it is submitted for review, and after it is revised, if necessary), and 4) agree to be responsible for any questions about the accuracy or integrity of the research.
Several types of authorship violate these guidelines and should be avoided. Guest authorship is when respected researchers are added out of appreciation, or to have the manuscript be perceived more favorably to get it published or increase its impact. Gift authorship is giving authorship to reward an individual, or as a favor. Ghost authorship is when someone made significant contributions to the paper but is not listed as an author. To increase transparency, some journals require authors to indicate how each individual contributed to the research and manuscript.
2. Apply the guidelines
Conflicts often arise from disagreements about how much people contributed to the research and whether those contributions merit authorship. The best approach is an open, honest, and ongoing discussion about authorship, which we discuss in #3 below. To have effective, informed conversations about authorship, you must understand how to apply the guidelines to your specific situation. The following is a simple rule of thumb that indicates there are three components of authorship. We do not list giving final approval of the manuscript and agreeing to be accountable, but we do consider these essentials of authorship.
- Thinking – this means contributing to the ideas leading to the hypothesis of the work, designing experiments to address the hypothesis, and/or analyzing the results in the larger context of the literature in the field.
- Doing – this means performing and analyzing the experiments.
- Writing – this means editing a draft, or writing the entire paper. The first author often writes the entire first draft.
In our experience, a first author would typically do all three. They also usually coordinate the writing and editing process. Co-authors are typically very involved in at least two of the three, and are somewhat involved in the other. The PI, who oversees and contributes to all three, is often the last, or “senior author.” The “senior author” is typically the “corresponding author”—the person listed as the individual to contact about the paper. The other co-authors are listed between the first and senior author either alphabetically, or more commonly, in order from the largest to smallest contribution.
Problems in assigning authorship typically arise due to people’s interpretations of #1 (thinking) and #2 (doing)—what and how much each individual contributed to a project’s design, execution, and analysis. Different fields or PIs may have their own slight variations on these guidelines. The potential conflicts associated with assigning authorship lead to the most common recommendation for responsibly assigning authorship: discuss authorship expectations early and revisit them during the project.
3. Discuss authorship with your collaborators
Publications are important for career advancement, so you can see why people might be worried about fairness in assigning authorship. If the problem arises from a lack of a shared understanding about contributions to the research, the only way to clarify this is an open discussion. This discussion should ideally take place very early at the beginning of a project, and should be ongoing. Hopefully you work in a laboratory that makes these discussions a natural part of the research process; this makes it much easier to understand the expectations upfront.
We encourage you to speak up about your interest in making a contribution that would merit authorship, especially if you want to earn first authorship. Sometimes norms about authoring papers in a lab make it clear you are expected to first and co-author publications, but it is best to communicate your interest in earning authorship. If the project is not yours, but you wish to collaborate, you can inquire what you may be able to contribute that would merit authorship.
If it is not a norm in your lab to discuss authorship throughout the life of projects, then as a graduate student you may feel reluctant to speak up. You could initiate a conversation with a more senior graduate student, a postdoc, or your PI, depending on the dynamics in the group. You could ask generally about how the lab approaches assignment of authorship, but discussing a specific project and paper may be best. It may feel awkward to ask, but asking early is less uncomfortable than waiting until the end of the project. If the group is already drafting a manuscript and you are told that your contribution is insufficient for authorship, this situation is much more discouraging than if you had asked earlier about what is expected to earn authorship.
How to report findings responsibly
The most significant responsibility of authors is to present their research accurately and honestly. Deliberately presenting misleading information is clearly unethical, but there are significant judgment calls about how to present your research findings. For example, an author can mislead by overstating the conclusions given what the data support.
1. Commit to presenting your findings honestly
Any good scientific manuscript writer will tell you that you need to “tell a good story.” This means that your paper is organized and framed to draw the reader into the research and convince them of the importance of the findings. But, this story must be sound and justified by the data. Other authors are presenting their findings in the best, most “publishable” light, so it is a balancing act to be persuasive but also responsible in presenting your findings in a trustworthy manner. To present your findings honestly, you must be conscious of how you interpret your data and present your conclusions so that they are accurate and not overstated.
One misbehavior known as “HARKing,” Hypothesis After the Results are Known, occurs when hypotheses are created after seeing the results of an experiment, but they are presented as if they were defined prior to collecting the data ( Munafò et al., 2017 ). This practice should be avoided. HARKing may be driven, in part, by a concern in scientific publishing known as publication bias. This bias is a preference that reviewers, editors, and researchers have for papers describing positive findings instead of negative findings ( Carroll, Toumpakari, Johnson, & Betts, 2017 ). This preference can lead to manipulating one’s practices, such as by HARKing, so that positive findings can be reported.
It is important to note that in addition to avoiding misbehaviors such as HARKing, all researchers are susceptible to a number of more subtle traps in judgment. Even the most well-intentioned researcher may jump to conclusions, discount alternative explanations, or accept results that seem correct without further scrutiny ( Nuzzo, 2015 ). Therefore, researchers must not only commit to presenting their findings honestly but consider how they can counteract such traps by slowing down and increasing their skepticism towards their findings.
2. Provide an appropriate amount of detail
Providing enough detail in a manuscript can be a challenge with the word limits imposed by most journals. Therefore, you will need to determine what details to include and which to exclude, or potentially include in the supplemental materials. Methods sections can be long and are often the first to be shortened, but complete methods are important for others to evaluate the research and to repeat the methods in other studies. Even more significant is making decisions about what experimental data to include and potentially exclude from the manuscript. Researchers must determine what data is required to create a complete scientific story that supports the central hypothesis of the paper. On the other hand, it is not necessary or helpful to include so much data in the manuscript, or in supplemental material, that the central point of the paper is difficult to discern. It is a tricky balance.
3. Follow proper citation practices
Of course, responsible authorship requires avoiding plagiarism. Many researchers think that plagiarism is not a concern for them because they assume it is always done intentionally by “copying and pasting” someone else’s words and claiming them as your own. Sometimes poor writing practices, such as taking notes from references without distinguishing between direct quotes and paraphrased material, can lead to including material that is not quoted properly. More broadly, proper citation practices include accurately and completely referencing prior studies to provide appropriate context for your manuscript.
4. Attend to the other important details
The journal will require several pieces of additional information, such as disclosure of sources of funding and potential conflicts of interest. Typically, graduate students do not have relationships that constitute conflicts of interest, but a PI who is a co-author may. In submitting a manuscript, also make sure to acknowledge individuals not listed as authors but who contributed to the work.
5. Share data and promote transparency
Data sharing is a key facet of promoting transparency in science ( Nosek et al., 2015 ). It will be important to know the expectations of the journals in which you wish to publish. Many top journals now require data sharing; for example, sharing your data files in an online repository so others have access to the data for secondary use. Funding agencies like NIH also increasingly require data sharing. To further foster transparency and public trust in research, researchers must deposit their final peer-reviewed manuscripts that report on research funded by NIH to PubMed Central. PubMed makes biomedical and life science research publicly accessible in a free, online database.
Scenario 2 – Authors In Conflict
To prepare a manuscript for publication, a postdoc’s data is added to a graduate student’s thesis project. After working together to combine the data and write the paper, the postdoc requests co-first authorship on the paper. The graduate student balks at this request on the basis that it is their thesis project. In a weekly meeting with the lab’s PI to discuss the status of the paper, the graduate student states that they should divide the data between the authors as a way to prove that the graduate student should be the sole first author. The PI agrees to this attempt to quantify how much data each person contributed to the manuscript. All parties agree the writing and thinking were equally shared between them. After this assessment, the graduate student sees that the postdoc actually contributed more than half of the data presented in the paper. The graduate student and a second graduate student contributed the remaining data; this means the graduate student contributed much less than half of the data in the paper. However, the graduate student is still adamant that they must be the sole first author of the paper because it is their thesis project.
Is the graduate student correct in insisting that it is their project, so they are entitled to be the sole first author?
Co-first authorship became popular about 10 years ago as a way to acknowledge shared contributions to a paper in which authors worked together and contributed equally. If the postdoc contributed half of the data and worked with the graduate student to combine their interpretations and write the first draft of the paper, then the postdoc did make a substantial contribution. If the graduate student wrote much of the first draft of the paper, contributed significantly to the second half of data, and played a major role in the thesis concept and design, this is also a major contribution. We summarized authorship requirements as contributing to thinking, doing, and writing, and we noted that a first author usually contributes to all of these. The graduate student has met all 3 elements to claim first authorship. However, it appears that the postdoc has also met these 3 requirements. Thus, it is at least reasonable for the postdoc to ask about co-first authorship.
The best way to move forward is to discuss their perspectives openly. Both the graduate student and postdoc want first authorship on papers to advance their careers. The postdoc feels they contributed more to the overall concept and design than the graduate student is recognizing, and the postdoc did contribute half of the data. This is likely frustrating and upsetting for the postdoc. On the other hand, perhaps the postdoc is forgetting how much a thesis becomes like “your baby,” so to speak. The work is the graduate student’s thesis, so it is easy to see why the graduate student would feel a sense of ownership of it. Given this fact, it may be hard for the graduate student to accept the idea that they would share first-author recognition for the work. Yet, the graduate student should consider that the manuscript would not be possible without the postdoc’s contribution. Further, if the postdoc was truly being unreasonable, then the postdoc could make the case for sole first authorship based on contributing the most data to the paper, in addition to contributing ideas and writing the paper. The graduate student should consider that the postdoc may be suggesting co-first authorship in good faith.
As with any interpersonal conflict, clear communication is key. While it might be temporarily uncomfortable to voice their views and address this disagreement, it is critical to avoiding permanent damage to their working relationship. The pair should consider each other’s perspectives and potential alternatives. For example, if the graduate student is first author and the postdoc second, at a minimum they could include an author note in the manuscript that describes the contribution of each author. This would make it clear the scope of the postdoc’s contribution, if they decided not to go with co-first authorship. Also, the graduate student should consider their assumptions about co-first authorship. Maybe they assume it makes it appear they contributed less, but instead, perhaps co-first authorship highlights their collaborative approach to science. Collaboration is a desirable quality many (although arguably not all) research organizations look for when they are hiring.
They will also need to speak with others for advice. The pair should definitely speak with the PI who could provide input about how these cases have been handled in the past. Ultimately, if they cannot reach an agreement, the PI, who is likely to be the last or “senior” author, may make the final decision. They should also speak to the other graduate student who is an author.
If either individual is upset with the situation, they will want to discuss it when they have had time to cool down. This might mean taking a day before discussing, or speaking with someone outside of the lab for support. Ideally, all authors on this paper would have initiated this conversation earlier, and the standards in the lab for first authorship would be discussed routinely. Clear communication may have avoided the conflict.
HOW TO USE DECISION-MAKING STRATEGIES TO NAVIGATE CHALLENGES
We have provided advice on some specific challenges you might encounter in research. This final section covers our overarching recommendation that you adopt a set of ethical decision-making strategies. These strategies help researchers address challenges by helping them think through a problem and possible alternatives ( McIntosh et al., 2020 ). The strategies encourage you to gather information, examine possible outcomes, consider your assumptions, and address emotional reactions before acting. They are especially helpful when you are uncertain how to proceed, face a new problem, or when the consequences of a decision could negatively impact you or others. The strategies also help people be honest with themselves, such as when they are discounting important factors or have competing goals, by encouraging them to identify outside perspectives and test their motivations. You can remember the strategies using the acronym SMART .
1. S eek Help
Obtain input from others who can be objective and that you trust. They can assist you with assessing the situation, predicting possible outcomes, and identifying potential options. They can also provide you with support. Individuals to consult may be peers, other faculty, or people in your personal life. It is important that you trust the people you talk with, but it is also good when they challenge your perspective, or encourage you to think in a new way about a problem. Keep in mind that people such as program directors and university ombudsmen are often available for confidential, objective advice.
2. M anage Emotions
Consider your emotional reaction to the situation and how it might influence your assessment of the situation, and your potential decisions and actions. In particular, identify negative emotions, like frustration, anxiety, fear, and anger, as they particularly tend to diminish decision-making and the quality of interactions with others. Take time to address these emotions before acting, for example, by exercising, listening to music, or simply taking a day before responding.
3. A nticipate Consequences
Think about how the situation could turn out. This includes for you, for the research team, and anyone else involved. Consider the short, middle-term, and longer-term impacts of the problem and your potential approach to addressing the situation. Ideally, it is possible to identify win-win outcomes. Often, however, in tough professional situations, you may need to select the best option from among several that are not ideal.
4. R ecognize Rules and Context
Determine if any ethical principles, professional policies, or rules apply that might help guide your choices. For instance, if the problem involves an authorship dispute, consider the authorship guidelines that apply. Recognizing the context means considering the situational factors that could impact your options and how you proceed. For example, factors such as the reality that ultimately the PI may have the final decision about authorship.
5. T est Assumptions and Motives
Examine your beliefs about the situation and whether any of your thoughts may not be justified. This includes critically examining the personal motivations and goals that are driving your interpretation of the problem and thoughts about how to resolve it.
These strategies do not have to be engaged in order, and they are interrelated. For example, seeking help can help you manage emotions, test assumptions, and anticipate consequences. Go back to the scenarios and our advice throughout this article, and you will see many of our suggestions align with these strategies. Practice applying SMART strategies when you encounter a problem and they will become more natural.
Learning practices for responsible research will be the foundation for your success in graduate school and your career. We encourage you to be reflective and intentional as you learn and hope that our advice helps you along the way.
ACKNOWLEDGEMENTS
This work was supported by the National Human Genome Research Institute (Antes, K01HG008990) and the National Center for Advancing Translational Sciences (UL1 TR002345).
LITERATURE CITED
- Anderson MS, Horn AS, Risbey KR, Ronning EA, De Vries R, & Martinson BC (2007). What Do Mentoring and Training in the Responsible Conduct of Research Have To Do with Scientists’ Misbehavior? Findings from a National Survey of NIH-Funded Scientists . Academic Medicine , 82 ( 9 ), 853–860. doi: 10.1097/ACM.0b013e31812f764c [ PubMed ] [ CrossRef ] [ Google Scholar ]
- Antes AL, Brown RP, Murphy ST, Waples EP, Mumford MD, Connelly S, & Devenport LD (2007). Personality and Ethical Decision-Making in Research: The Role of Perceptions of Self and Others . Journal of Empirical Research on Human Research Ethics , 2 ( 4 ), 15–34. doi: 10.1525/jer.2007.2.4.15 [ PubMed ] [ CrossRef ] [ Google Scholar ]
- Antes AL, English T, Baldwin KA, & DuBois JM (2018). The Role of Culture and Acculturation in Researchers’ Perceptions of Rules in Science . Science and Engineering Ethics , 24 ( 2 ), 361–391. doi: 10.1007/s11948-017-9876-4 [ PMC free article ] [ PubMed ] [ CrossRef ] [ Google Scholar ]
- Antes AL, Kuykendall A, & DuBois JM (2019a). The Lab Management Practices of “Research Exemplars” that Foster Research Rigor and Regulatory Compliance: A Qualitative Study of Successful Principal Investigators . PloS One , 14 ( 4 ), e0214595. doi: 10.1371/journal.pone.0214595 [ PMC free article ] [ PubMed ] [ CrossRef ] [ Google Scholar ]
- Antes AL, Kuykendall A, & DuBois JM (2019b). Leading for Research Excellence and Integrity: A Qualitative Investigation of the Relationship-Building Practices of Exemplary Principal Investigators . Accountability in Research , 26 ( 3 ), 198–226. doi: 10.1080/08989621.2019.1611429 [ PMC free article ] [ PubMed ] [ CrossRef ] [ Google Scholar ]
- Antes AL, & Maggi LB Jr. (2021). How to Navigate Trainee-Mentor Relationships and Interpersonal Dynamics in the Lab . Current Protocols Essential Laboratory Techniques. [ PMC free article ] [ PubMed ] [ Google Scholar ]
- Asplund M, & Welle CG (2018). Advancing Science: How Bias Holds Us Back . Neuron , 99 ( 4 ), 635–639. doi: 10.1016/j.neuron.2018.07.045 [ PubMed ] [ CrossRef ] [ Google Scholar ]
- Baker M (2016). Is There a Reproducibility Crisis? Nature , 533 , 452–454. doi: 10.1038/533452a [ PubMed ] [ CrossRef ] [ Google Scholar ]
- Barba LA (2016). The Hard Road to Reproducibility . Science , 354 ( 6308 ), 142. doi: 10.1126/science.354.6308.142 [ PubMed ] [ CrossRef ] [ Google Scholar ]
- Beall’s List of Potential Predatory Journals and Publishers . (2020). Retrieved from https://beallslist.net/#update [ Google Scholar ]
- Carroll HA, Toumpakari Z, Johnson L, & Betts JA (2017). The Perceived Feasibility of Methods to Reduce Publication Bias . PloS One , 12 ( 10 ), e0186472–e0186472. doi: 10.1371/journal.pone.0186472 [ PMC free article ] [ PubMed ] [ CrossRef ] [ Google Scholar ]
- Chappell B (2019). Duke Whistleblower Gets More Than $33 Million in Research Fraud Settlement . NPR. Retrieved from https://www.npr.org/2019/03/25/706604033/duke-whistleblower-gets-more-than-33-million-in-research-fraud-settlement [ Google Scholar ]
- Davis MS, Riske-Morris M, & Diaz SR (2007). Causal Factors Implicated in Research Misconduct: Evidence from ORI Case Files . Science and Engineering Ethics , 13 ( 4 ), 395–414. doi: 10.1007/s11948-007-9045-2 [ PubMed ] [ CrossRef ] [ Google Scholar ]
- DeMets DL (1999). Statistics and Ethics in Medical Research . Science and Engineering Ethics , 5 ( 1 ), 97–117. doi: 10.1007/s11948-999-0059-9 [ PubMed ] [ CrossRef ] [ Google Scholar ]
- Department of Health and Human Services. (2005). 42 CFR Parts 50 and 93 Public Health Service Policies on Research Misconduct; Final Rule. Retrieved from https://ori.hhs.gov/sites/default/files/42_cfr_parts_50_and_93_2005.pdf [ Google Scholar ]
- DePellegrin TA, & Johnston M (2015). An Arbitrary Line in the Sand: Rising Scientists Confront the Impact Factor . Genetics , 201 ( 3 ), 811–813. [ PMC free article ] [ PubMed ] [ Google Scholar ]
- DuBois JM, Anderson EE, Chibnall J, Carroll K, Gibb T, Ogbuka C, & Rubbelke T (2013). Understanding Research Misconduct: A Comparative Analysis of 120 Cases of Professional Wrongdoing . Account Res , 20 ( 5–6 ), 320–338. doi: 10.1080/08989621.2013.822248 [ PMC free article ] [ PubMed ] [ CrossRef ] [ Google Scholar ]
- DuBois JM, & Antes AL (2018). Five Dimensions of Research Ethics: A Stakeholder Framework for Creating a Climate of Research Integrity . Academic Medicine , 93 ( 4 ), 550–555. doi: 10.1097/ACM.0000000000001966 [ PMC free article ] [ PubMed ] [ CrossRef ] [ Google Scholar ]
- Else H (2018). Does Science have a Bullying Problem? Nature , 563 , 616–618. doi: 10.1038/d41586-018-07532-5 [ PubMed ] [ CrossRef ] [ Google Scholar ]
- Emanuel EJ, Wendler D, & Grady C (2000). What Makes Clinical Research Ethical ? Journal of the American Medical Association , 283 ( 20 ), 2701–2711. doi:jsc90374 [pii] [ PubMed ] [ Google Scholar ]
- Evans TM, Bira L, Gastelum JB, Weiss LT, & Vanderford NL (2018). Evidence for a Mental Health Crisis in Graduate Education . Nature Biotechnology , 36 ( 3 ), 282–284. doi: 10.1038/nbt.4089 [ PubMed ] [ CrossRef ] [ Google Scholar ]
- Frank DJ (2018). How to Write a Research Manuscript . Current Protocols Essential Laboratory Techniques , 16 ( 1 ), e20. doi: 10.1002/cpet.20 [ CrossRef ] [ Google Scholar ]
- Goodman SN, Fanelli D, & Ioannidis JPA (2016). What Does Research Reproducibility Mean? Science Translational Medicine , 8 ( 341 ), 341ps312. doi: 10.1126/scitranslmed.aaf5027 [ PubMed ] [ CrossRef ] [ Google Scholar ]
- Grudniewicz A, Moher D, Cobey KD, Bryson GL, Cukier S, Allen K, … Lalu MM (2019). Predatory journals: no definition, no defence . Nature , 576 ( 7786 ), 210–212. doi: 10.1038/d41586-019-03759-y [ PubMed ] [ CrossRef ] [ Google Scholar ]
- Head ML, Holman L, Lanfear R, Kahn AT, & Jennions MD (2015). The Extent and Consequences of P-Hacking in Science . PLoS Biology , 13 ( 3 ), e1002106. doi: 10.1371/journal.pbio.1002106 [ PMC free article ] [ PubMed ] [ CrossRef ] [ Google Scholar ]
- Hofstra B, Kulkarni VV, Munoz-Najar Galvez S, He B, Jurafsky D, & McFarland DA (2020). The Diversity–Innovation Paradox in Science . Proceedings of the National Academy of Sciences , 117 ( 17 ), 9284. doi: 10.1073/pnas.1915378117 [ PMC free article ] [ PubMed ] [ CrossRef ] [ Google Scholar ]
- International Committee of Medical Journal Editors. (2020). Defining the Role of Authors and Contributors . Retrieved from http://www.icmje.org/recommendations/browse/roles-and-responsibilities/defining-the-role-of-authors-and-contributors.html
- Keith-Spiegel P, Sieber J, & Koocher GP (2010). Responding to Research Wrongdoing: A User-Friendly Guide . Retrieved from http://users.neo.registeredsite.com/1/4/0/20883041/assets/RRW_11-10.pdf
- McIntosh T, Antes AL, & DuBois JM (2020). Navigating Complex, Ethical Problems in Professional Life: A Guide to Teaching SMART Strategies for Decision-Making . Journal of Academic Ethics . doi: 10.1007/s10805-020-09369-y [ PMC free article ] [ PubMed ] [ CrossRef ] [ Google Scholar ]
- Meyers LC, Brown AM, Moneta-Koehler L, & Chalkley R (2018). Survey of Checkpoints along the Pathway to Diverse Biomedical Research Faculty . PloS One , 13 ( 1 ), e0190606–e0190606. doi: 10.1371/journal.pone.0190606 [ PMC free article ] [ PubMed ] [ CrossRef ] [ Google Scholar ]
- Munafò MR, Nosek BA, Bishop DVM, Button KS, Chambers CD, Percie du Sert N, … Ioannidis JPA (2017). A manifesto for reproducible science . Nature Human Behaviour , 1 ( 1 ), 0021. doi: 10.1038/s41562-016-0021 [ PMC free article ] [ PubMed ] [ CrossRef ] [ Google Scholar ]
- National Academies of Science. (2009). On Being a Scientist: A Guide to Responsible Conduct in Research . Washington DC: National Academics Press. [ PubMed ] [ Google Scholar ]
- National Academies of Sciences Engineering and Medicine. (2017). Fostering Integrity in Research . Washington, DC: The National Academies Press [ PubMed ] [ Google Scholar ]
- National Academies of Sciences Engineering and Medicine. (2018a). An American Crisis: The Growing Absence of Black Men in Medicine and Science: Proceedings of a Joint Workshop . Washington, DC: The National Academies Press. [ PubMed ] [ Google Scholar ]
- National Academies of Sciences Engineering and Medicine. (2018b). Sexual harassment of women: climate, culture, and consequences in academic sciences, engineering, and medicine : National Academies Press. [ PubMed ] [ Google Scholar ]
- National Institutes of Health. (2009). Update on the Requirement for Instruction in the Responsible Conduct of Research . NOT-OD-10-019 . Retrieved from https://grants.nih.gov/grants/guide/notice-files/NOT-OD-10-019.html
- National Science Foundation. (2017). Important Notice No. 140 Training in Responsible Conduct of Research – A Reminder of the NSF Requirement . Retrieved from https://www.nsf.gov/pubs/issuances/in140.jsp
- No Place for Bullies in Science. (2018). Nature , 559 ( 7713 ), 151. doi: 10.1038/d41586-018-05683-z [ PubMed ] [ CrossRef ] [ Google Scholar ]
- Norris D, Dirnagl U, Zigmond MJ, Thompson-Peer K, & Chow TT (2018). Health Tips for Research Groups . Nature , 557 , 302–304. doi: 10.1038/d41586-018-05146-5 [ PubMed ] [ CrossRef ] [ Google Scholar ]
- Nosek BA, Alter G, Banks GC, Borsboom D, Bowman SD, Breckler SJ, … Yarkoni T (2015). Scientific Standards . Promoting an Open Research Culture. Science , 348 ( 6242 ), 1422–1425. doi: 10.1126/science.aab2374 [ PMC free article ] [ PubMed ] [ CrossRef ] [ Google Scholar ]
- Nuzzo R (2015). How Scientists Fool Themselves - and How They Can Stop . Nature , 526 , 182–185. [ PubMed ] [ Google Scholar ]
- O’Connor A (2018). More Evidence that Nutrition Studies Don’t Always Add Up . The New York Times. Retrieved from https://www.nytimes.com/2018/09/29/sunday-review/cornell-food-scientist-wansink-misconduct.html [ Google Scholar ]
- Park A (2012). Great Science Frauds . Time. Retrieved from https://healthland.time.com/2012/01/13/great-science-frauds/slide/the-baltimore-case/ [ Google Scholar ]
- Plemmons DK, Baranski EN, Harp K, Lo DD, Soderberg CK, Errington TM, … Esterling KM (2020). A Randomized Trial of a Lab-embedded Discourse Intervention to Improve Research Ethics . Proceedings of the National Academy of Sciences , 117 ( 3 ), 1389. doi: 10.1073/pnas.1917848117 [ PMC free article ] [ PubMed ] [ CrossRef ] [ Google Scholar ]
- Research Institutions Must Put the Health of Labs First. (2018). Nature , 557 ( 7705 ), 279–280. doi: 10.1038/d41586-018-05159-0 [ PubMed ] [ CrossRef ] [ Google Scholar ]
- Research Integrity is Much More Than Misconduct . (2019). ( 570 ). doi: 10.1038/d41586-019-01727-0 [ PubMed ] [ CrossRef ] [ Google Scholar ]
- Resnik DB (2011). Scientific Research and the Public Trust . Science and Engineering Ethics , 17 ( 3 ), 399–409. doi: 10.1007/s11948-010-9210-x [ PMC free article ] [ PubMed ] [ CrossRef ] [ Google Scholar ]
- Roper RL (2019). Does Gender Bias Still Affect Women in Science? Microbiology and Molecular Biology Reviews , 83 ( 3 ), e00018–00019. doi: 10.1128/MMBR.00018-19 [ PMC free article ] [ PubMed ] [ CrossRef ] [ Google Scholar ]
- Shamoo AE, & Resnik DB (2015). Responsible Conduct of Research (3rd ed.). New York: Oxford University Press. [ Google Scholar ]
- Steneck NH (2007). ORI Introduction to the Responsible Conduct of Research (Updated ed.). Washington, D.C.: U.S. Government Printing Office. [ Google Scholar ]
- Winchester C (2018). Give Every Paper a Read for Reproducibility . Nature , 557 , 281. doi: 10.1038/d41586-018-05140-x [ PubMed ] [ CrossRef ] [ Google Scholar ]
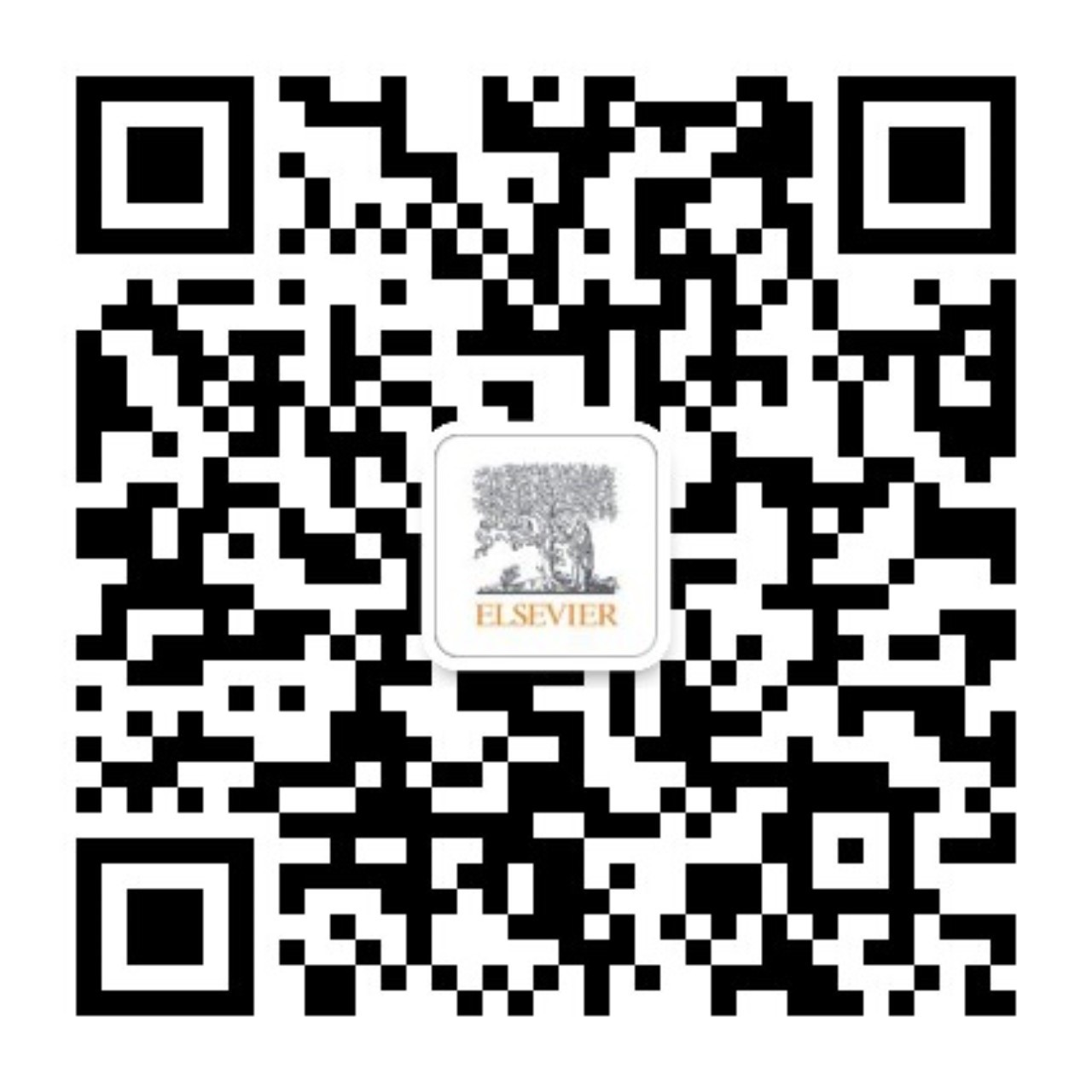
- Research Process
Research Team Structure
- 4 minute read
- 96.6K views
Table of Contents
A scientific research team is a group of individuals, working to complete a research project successfully. When run well, the research team members work closely, and have clearly defined roles. Every team member should know their role, and how it plays into the project as a whole. Ultimately, the principal investigator is responsible for every aspect of the project.
In this article, we’ll review research team roles and responsibilities, and the typical structure of a scientific research team. If you are forming a research team, or are part of one, this information can help you ensure smooth operations and effective teamwork.
Team Members
A group of individuals working toward a common goal: that’s what a research team is all about. In this case, the shared goal between team members is the successful research, data analysis, publication and dissemination of meaningful findings. There are key roles that must be laid out BEFORE the project is started, and the “CEO” of the team, namely the Principal Investigator, must provide all the resources and training necessary for the team to successfully complete its mission.
Every research team is structured differently. However, there are five key roles in each scientific research team.
1. Principal Investigator (PI):
this is the person ultimately responsible for the research and overall project. Their role is to ensure that the team members have the information, resources and training they need to conduct the research. They are also the final decision maker on any issues related to the project. Some projects have more than one PI, so the designated individuals are known as Co-Principal Investigators.
PIs are also typically responsible for writing proposals and grant requests, and selecting the team members. They report to their employer, the funding organization, and other key stakeholders, including all legal as well as academic regulations. The final product of the research is the article, and the PI oversees the writing and publishing of articles to disseminate findings.
2. Project or Research Director:
This is the individual who is in charge of the day-to-day functions of the research project, including protocol for how research and data collection activities are completed. The Research Director works very closely with the Principal Investigator, and both (or all, if there are multiple PIs) report on the research.
Specifically, this individual designs all guidelines, refines and redirects any protocol as needed, acts as the manager of the team in regards to time and budget, and evaluates the progress of the project. The Research Director also makes sure that the project is in compliance with all guidelines, including federal and institutional review board regulations. They also usually assist the PI in writing the research articles related to the project, and report directly to the PI.
3. Project Coordinator or Research Associate:
This individual, or often multiple individuals, carry out the research and data collection, as directed by the Research Director and/or the Principal Investigator. But their role is to also evaluate and assess the project protocol, and suggest any changes that might be needed.
Project Coordinators or Research Associates also need to be monitoring any experiments regarding compliance with regulations and protocols, and they often help in reporting the research. They report to the Principal Investigator, Research Director, and sometimes the Statistician (see below).
4. Research Assistant:
This individual, or individuals, perform the day-to-day tasks of the project, including collecting data, maintaining equipment, ordering supplies, general clerical work, etc. Typically, the research assistant has the least amount of experience among the team members. Research Assistants usually report to the Research Associate/Project Coordinator, and sometimes the Statistician.
5. Statistician:
This is the individual who analyzes any data collected during the project. Sometimes they just analyze and report the data, and other times they are more involved in the organization and analysis of the research throughout the entire study. Their primary role is to make sure that the project produces reliable and valid data, and significant data via analysis methodology, sample size, etc. The Statistician reports both to the Principal Investigator and the Research Director.
Research teams may include people with different roles, such as clinical research specialists, interns, student researchers, lab technicians, grant administrators, and general administrative support staff. As mentioned, every role should be clearly defined by the team’s Principal Investigator. Obviously, the more complex the project, the more team members may be required. In such cases, it may be necessary to appoint several Principal Administrators and Research Directors to the research team.
Elsevier Author Services
At every stage of your project, Elsevier Author Services is here to help. Whether it’s translation services, done by an expert in your field, or document review, graphics and illustrations, and editing, you can count on us to get your manuscript ready for publishing. Get started today!
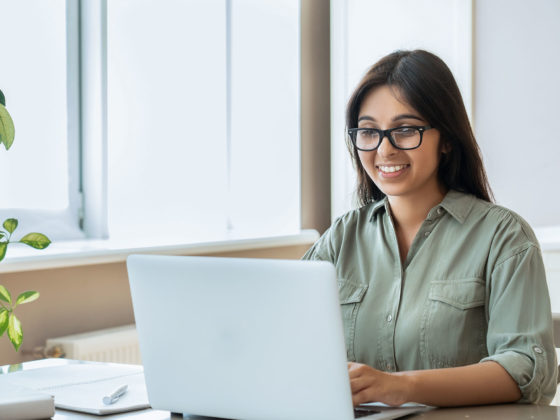
Writing a Scientific Research Project Proposal
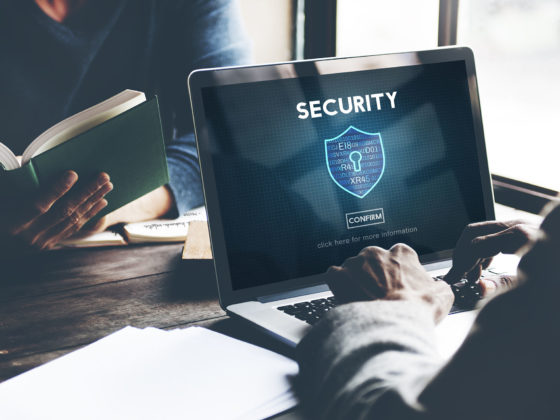
Confidentiality and Data Protection in Research
You may also like.
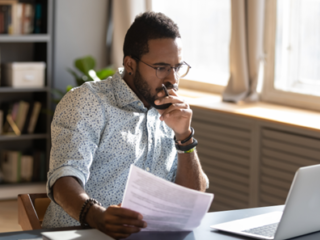
Descriptive Research Design and Its Myriad Uses
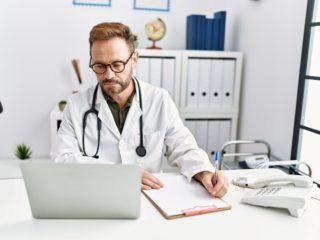
Five Common Mistakes to Avoid When Writing a Biomedical Research Paper
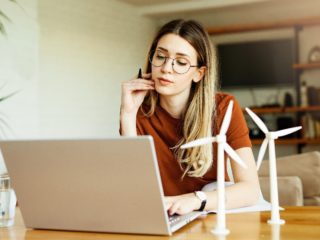
Making Technical Writing in Environmental Engineering Accessible
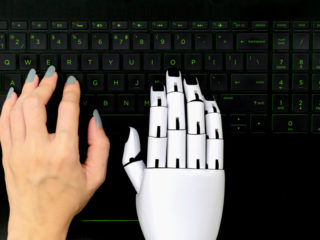
To Err is Not Human: The Dangers of AI-assisted Academic Writing
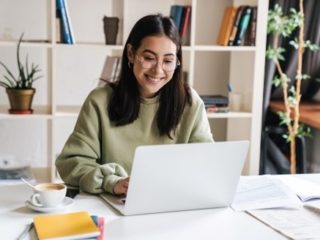
When Data Speak, Listen: Importance of Data Collection and Analysis Methods
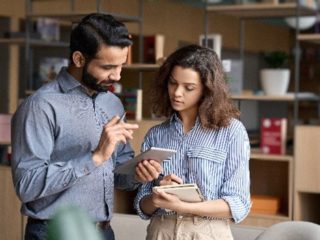
Choosing the Right Research Methodology: A Guide for Researchers
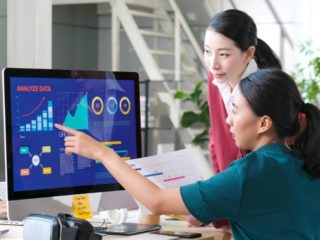
Why is data validation important in research?
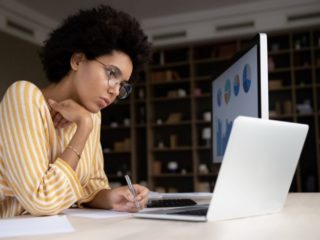
Writing a good review article
Input your search keywords and press Enter.
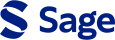
- Request new password
- Create a new account
Research Methods and Statistics in Psychology
Student resources, chapter 4: experimental design.
1. What is a manipulation check and what is its purpose? [TY4.1]
- A dependent measure used to check that manipulation of an independent variable has been successful.
- An independent variable used to check that measurement of a dependent variable has been successful.
- A measure used to check that an experiment has an independent variable.
- An independent measure used to check that the operationalization is relevant.
- A dependent measure used to check that an independent variable is sufficiently relevant.
2. Which of the following statements is true? [TY4.2]
- Dependent variables that do not measure the most relevant theoretical variable are pointless.
- A study that employs dependent variables that are sensitive enough to detect variation in the independent variable is a quasi-experiment.
- Unless dependent variables are sufficiently sensitive they will not reveal the effects of manipulating an independent variable.
- Unless dependent variables are sufficiently relevant they will not reveal the effects of manipulating an independent variable.
- None of the above.
3. A team of researchers is interested in conducting an experiment in order to test an important theory. In order to draw appropriate conclusions from any experiment they conduct, which of the following statements is true? [TY4.3]
- The experimental sample must be representative of the population to which they want to generalize the research on dimensions of age, sex and intelligence.
- The experimental sample must be representative of the population to which they want to generalize the research on all dimensions.
- The experimental sample must be representative of the population to which they want to generalize the research on all dimensions that can be measured in that population.
- The experimental sample must be representative of the population to which they want to generalize the research on all dimensions relevant to the process being studied.
4. An experimenter conducts a study examining the effects of television violence on children’s aggressiveness. To do this she asks 40 schoolboys who display normal levels of aggressiveness to watch one violent video a week for 40 weeks. On a standard measure of aggressiveness, which she administers both before and after each video over the 40-week treatment, she finds that the boys are much more aggressive in the last 10 weeks of the study. Without knowing anything more about this study, which of the following can be ruled out as a threat to the internal validity of any conclusions she may seek to draw? [TY4.4]
- History effects.
- Maturation effects.
- Mortality effects.
- Regression to the mean.
- Testing effects.
5. Which of the following can increase a researcher’s ability to generalize findings from a particular piece of research? [TY4.5]
- Experimenter bias.
- Deception and concealment.
- Participants’ sensitivity to demand characteristics.
- The use of unrepresentative samples.
6. A researcher conducts an experiment to investigate the effects of positive mood on memory. Mood is manipulated by giving participants a gift. In the experiment, before they are given a memory test, half of the participants are randomly assigned to a condition in which they are given a box of chocolates, and the other half are given nothing. Which of the following statements is not true? [TY4.6]
- Mood is a between-subjects variable.
- The experiment has two conditions.
- The experiment includes a control condition.
- The independent variable is manipulated within subjects.
- Experimental control eliminates potential threats to the internal validity of the experiment.
7. Which of the following is a researcher’s overall objective in using matching? [TY4.7]
- To control for extraneous variables in quasi-experimental designs.
- To increase participants’ enjoyment of correlational research.
- To ensure that participants are randomly assigned to conditions.
- To ensure that groups of participants do not differ in age and sex.
- To ensure that groups of participants do not differ in intelligence.
8. Which of the following threats to validity is the most difficult to control by improving experimental design? [TY4.8]
- Cheating by experimenters.
- Sensitivity to demand characteristics.
9. Some researchers decided to conduct an experiment to investigate the effects of a new psychological therapy on people’s self-esteem. To do this they asked all their clients who were currently receiving treatment for low self-esteem to continue using an old therapy but treated all their new clients with the new therapy. A year later they found that clients subjected to the new therapy had much higher self-esteem. Which of the following statements is true? [TY4.9]
- The greater self-esteem of the clients exposed to the new therapy resulted from the superiority of that therapy.
- The greater self-esteem of the clients exposed to the new therapy resulted from the fact that the new clients were more optimistic than those who were previously receiving treatment.
- The greater self-esteem of the clients exposed to the new therapy resulted from the fact that the new clients were less disillusioned with therapy than those who were previously receiving treatment.
- The greater self-esteem of the clients exposed to the new therapy resulted from the fact that the new clients were more intelligent than those who were previously receiving treatment.
- It is impossible to establish the validity of any of the above statements based on the results of this study.
10. An experimenter conducts an experiment to see whether people's reaction time is affected by their consumption of alcohol. To do this, she conducts a study in which students from University A describe symbols as ‘red’ ‘green’ or ‘blue’ before they consume two glasses of wine and students from University B describe symbols as ‘red’ ‘green’ or ‘blue’ after they consume two glasses of wine. She hypothesizes that reaction times will be slower and that there will be more errors in the responses of students who consume alcohol before reacting to the symbols. Which of the following statements is false ?
- It is appropriate to analyse the results using independent sample t -tests
- Type of University is a potential experimental confound
- The experiment has two dependent variables
- The experiment has three independent variables
- The experiment has a between-subjects design.
11. “Both (a) the process of constructing experiments and (b) the resulting structure of those experiments.” What research feature is this a glossary definition of?
- Experimental design.
- Constructive design.
- Experimental structuration.
- Solidification.
- Experimental procedure.
12. “A system for deciding how to arrange objects or events in a progressive series. These are used to assign relative magnitude to psychological and behavioural phenomena (e.g. intelligence or political attitudes).” What is this a glossary definition of?
- A measurement.
- A callibration.
13. “The principle that the more relevant a dependent variable is to the issue in which a researcher is interested, the less sensitive it may be to variation in the independent variable.” What is this a glossary definition of?
- Systematic desensitization.
- Random variation.
- Meaurement error.
- Relevance–sensitivity trade-off.
- Inferential uncertainty.
14. “The extent to which a research finding can be generalized to other situations.” What is this a glossary definition of?
- External validity
- Generalization.
- Extendability.
- Empirical applicability.
15. “Systematic change to an independent variable where the same participants are exposed to different levels of that variable by the experimenter.” What procedure is this a glossary definition of?
- Random assignment.
- Within-subjects manipulation.
- Between-subjects manipulation.
- Variable assignment.
- Experimenter manipulation.
Cultivating an Effective Research Team Through Application of Team Science Principles
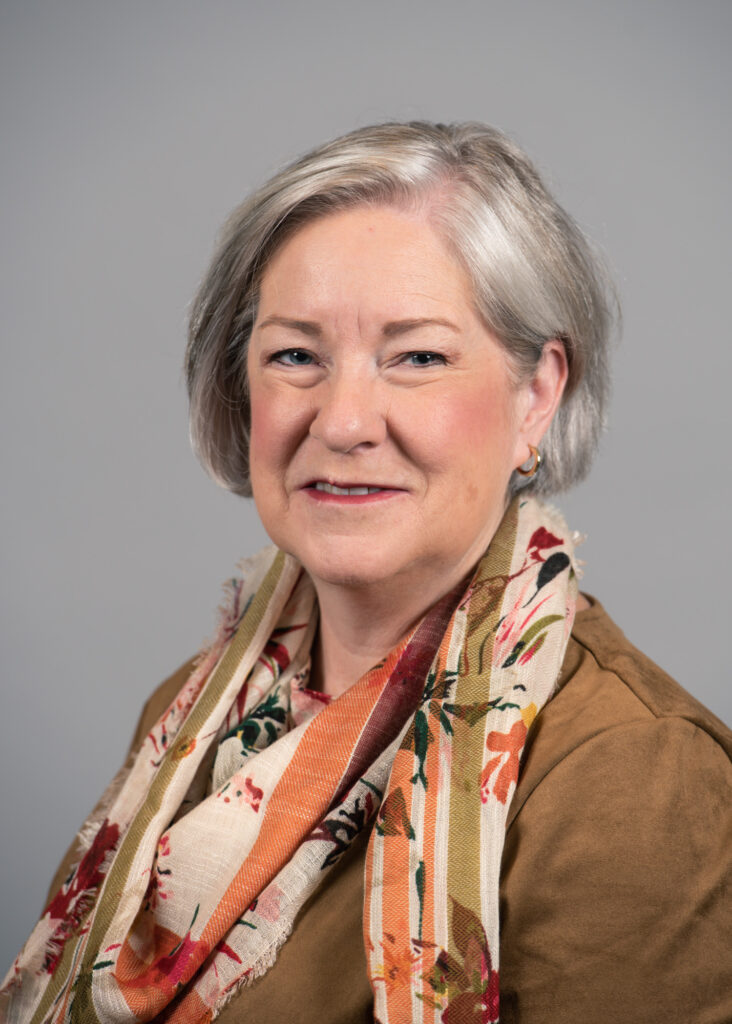
Shirley L.T. Helm, MS, CCRP Senior Administrator for Network Capacity & Workforce Strategies
C. Kenneth & Dianne Wright Center for Clinical and Translational Research
Virginia Commonwealth University
Abstract: The practice of team science allows clinical research professionals to draw from theory-driven principles to build an effective and efficient research team. Inherent in these principles are recognizing team member differences and welcoming diversity in an effort to integrate knowledge to solve complex problems. This article describes the basics of team science and how it can be applied to creating a highly-productive research team across the study continuum, including research administrators, budget developers, investigators, and research coordinators. The development of mutual trust, a shared vision, and open communication are crucial elements of a successful research team and research project. A case study illustrates the team science approach.
Introduction
Each research team is a community that requires trust, understanding, listening, and engagement. Stokols, Hall, Taylor, Moser, & Syme said that:
“There are many types of research teams, each one as dynamic as its team members. Research teams may comprise investigators from the same or different fields. Research teams also vary by size, organizational complexity, and geographic scope, ranging from as few as two individuals working together to a vast network of interdependent researchers across many institutions. Research teams have diverse goals spanning scientific discovery, training, clinical translation, public health, and health policy.” 1 1 Stokols D, Hall KL, Taylor BK, Moser RP. The science of team science: overview of the field and introduction to the supplement. Am J Prev Med. 2008 Aug;35(2 Suppl):S77-89. Accessed 8/10/20.
Team science arose from the National Science Foundation and the National Institutes of Health, which fund the work of researchers attempting to solve some of the most complex problems that require a multi-disciplinary approach, such as childhood obesity. 2 Team science is bringing in elements from various disciplines to solve these major problems. 3, 4 This article covers the intersection of team science with effective operationalizing of research teams and how teaming principles can be applied to the functioning of research teams.
Salas and colleagues state that, “a team consists of two or more individuals, who have specific roles, perform interdependent tasks, are adaptable, and share a common goal. . . team members must possess individual and team Knowledge, Skills, Attitudes ….” 5 Great teams have a plan for how people act and work together. There are three elements that must be aligned to ensure success: the individual, the team, and the task. Individuals have their own goals. These goals must align, and not compete with, goals of other individuals and team goals. Task goals are the nuts and bolts of clinical research. Like individuals, the team has an identity. It is necessary to provide feedback both as a team and as individuals.
In a typical clinical research team, the clinical investigator is at the center surrounded by the clinical research coordinators. The coordinator is the person who makes the team function. Other members of the typical clinical research team are:
· Research participant/family
· Financial/administrative staff
· Regulatory body (institutional review board)
· Study staff
· Ancillary services such as radiology or pathology
· Sponsor/monitor.
The Teaming Principles
Bruce Tuckman developed the teaming principles in 1965 and revised them in 1977 (Table 1). 6 Using the teaming principles is not a linear process. These principles start with establishing the team. The team leader does not have to establish the team. Any team member can use teaming principles to provide a framework and structure and systematically determine what the project needs. Storming is establishing roles and responsibilities, communications, and processes. The storming phase, when everybody has been brought together and is on board with the same goal, is a honeymoon period.
Norming is the heavy lifting of the team’s work. This involves working together effectively and efficiently. Team members must develop trust and comfort with each other. Performing focuses on working together efficiently, and satisfaction for team members and the research participants and their families.
Tuckman added adjourning or transforming to the teaming principles in 1977. The team might end or start working on a new project (study) with a new shared goal. Adjourning or transforming involves determining which processes can be transferred from one research study to another research study.
While the teaming principles seem intuitive and like common sense, people are not raised to be fully cooperative. Using the teaming principles provides framework and structure and takes the emotion out of teamwork. The teaming principles empower team members and provide the structure that is necessary for teams, which are constantly evolving and changing.
The shared goal at the center of the teaming principles provides a sense of purpose. This provides commitment, responsibility, and accountability, along with a clear understanding of roles, responsibilities, competencies, expectations, and contributions. In Dare to lead: Brave work. Tough conversations. Whole hearts, Brené Brown coined the phrase, “clear is kind, unclear is unkind.” 7 It is extremely important to define roles and ensure that each team member knows what the other team members are doing. This prevents duplication of effort and ensures that tasks do not fall through the cracks.
How to Use Teaming Principles
Table 2 briefly describes each of the five teaming principles. Forming begins with gathering the team members and involves determining who is needed on the team to ensure success. Each team member must be valued. The team may vary depending upon the study, project, and timelines. During the research study, team members may enter and exit from the team. Forming the team may mean working across boundaries with people and departments that team members do not know. It is also necessary to establish the required competencies and knowledge, skills, and attitudes of team members, and to recognize and celebrate differences. The team must have a shared goal and vision.
Storming the team involves establishing roles, responsibilities, and tasks. This includes determining who has the required competencies to perform tasks such as completing pre-screening logs or consenting research participants. Also, storming involves defining processes, including communication pathways and expectations. Simply sending an email is not an effective way to communicate. Team members need to know whether an email is providing information or requires a response. Expectations for responding to emails should be described and agreed upon by all team members. Emails might be color coded to show whether an email is informational or requires a response. If clinical research sites utilize a clinical trial management system, the process for updating it must be determined and clearly communicated.
Norming is how team members work together. The shared goal is re-visited often under norming. Team members are mutually dependent upon each other and must meet their commitments and established deadlines.
Trust lies at the heart of the team. Building trust takes work and does not come naturally. It is helpful to understand that there are several types of trust. Identity-based trust is based on personal understanding and is usually seen in relationships between partners, spouses, siblings, or best friends. This type of trust does not usually occur in the workplace.
Workplace trust resides in calculus-based trust and competence-based trust. Calculus-based trust is about keeping commitments, meeting deadlines, and meeting expectations. There are some people who can be counted upon to always do what they are supposed to do. These people have earned calculus-based trust. Competence-based trust is confidence in another person’s skills or competencies.
Swift trust is immediate and necessary during extreme situations where there is not time to develop deeper connections with individuals. It relies on personal experiences, stereotypes, and biases. Some people are naturally more trusting than other people.
The teaming principle of performing involves satisfaction in progressing toward the goal and being proactive in preventing issues from arising. There will always be issues; however, the most effective teams learn from issues and have processes for resolving them. This makes a team efficient. Performing also includes revisiting the shared goal, embracing diversity and differences, and continually improving knowledge, skills, and attitudes.
Adjourning/transforming is the completion of tasks and identification of lessons learned. Team members need to circle back and determine what worked well and can be applied to the next study. Celebrating successes and acknowledging the contributions of all team members are also an aspect of adjourning/transforming. When the author was managing a core laboratory, she performed tests for an oncology investigator’s study. Months later, the investigator gave her a thank-you card for her contribution to the study that was unexpected but greatly appreciated.
Strengthening the Team
Without a framework and structure, team dysfunction is likely. In The five dysfunctions of a team: A leadership fable , Lencioni presented team dysfunction as a pyramid. 8 Absence of trust is at the bottom of the pyramid. Absence of trust results in questioning everything people do and results in team members unwilling to share or to ask for help. Without asking for help, mistakes will be made.
Absence of trust leads to a fear of conflict and an inability to resolve issues or improve efficiencies. Fear of conflict leads to lack of commitment. Doubt prevails, team members lack confidence, and the goal is diminished. Team dysfunction leads to avoidance of accountability. Follow-through is poor and mediocrity is accepted, breeding resentment among team members.
At the top of the team dysfunction pyramid is inattention to results, which leads to loss of team members and future research studies. There are some teams where people are constantly moving in and out. This is
a symptom of team dysfunction. Loss of respect and reputation of the team, department, and individual team members is another consequence of inattention to results.
Table 3 highlights ways to strengthen the team. Recognizing the strengths of each team member starts with self-awareness. For example, the author had to understand her communication and learning style and how this is similar to and different than that of other team members. The VIA Institute of Character offers a free assessment that could be a fun activity for research teams.
There is no one road to self-awareness; however, each team member must recognize that other team members do not necessarily share their understanding or perceptions. There are many options and possibilities for how others may understand or perceive an experience, none of which are right or wrong. Each team member should appreciate that different understanding and perceptions of experiences do not have to threaten their identity or relationships.
One quick way to show this is through ambiguous images, in which people see entirely different things in the same image. Once they are aware that there are different ways of seeing the same thing, they can appreciate other perspectives. As Pablo Picasso said, “There is only one way to see things, until someone shows us how to look at them with different eyes.” Strengthening the team requires embracing demographic, educational, and personality diversity.
Open and honest communication should be encouraged. Team members should give and receive constructive feedback. This is a learned skill that is often difficult. However, tools are available for assessing communication and listening styles. Many institutions and human resource departments utilize the Crucial Conversations program by VitalSmarts, LC. One member of the team can participate in Crucial Conversations and bring the knowledge back to the team. Communication must include managing conflict and an awareness of cultural differences.
Opportunities for education and training to acquire new knowledge, skills, and attitudes/competencies should be provided. Education may be transportable across teams or may be study specific. Team members should be cross-trained, which may be accomplished through several methods. Positional clarification is where one person is told what another person is doing, which is primarily for information transfer. Positional modeling is receiving the information but also shadowing the other person while they perform the task/skill. Positional rotation is performing another person’s job. This is best for back-up positions, which are necessary for research teams.
Team success is facilitated by recognizing individual successes and commitment to shared goals. Recognizing individual successes reflects team success. For example, if a team member becomes a certified clinical research professional, this is a success for both the individual and the team. Also, the team must have a shared understanding of the goal or purpose. This shared goal must be linked to the individual goal of each team member.
Teamwork needs constant attention and annual evaluations, and team meetings are not sufficient. It is extremely important to regularly check in with people. Team members can check in with other team members simply to ask how things are going. Misunderstandings should be dealt with immediately. Clear direction, accountability, and rewards are necessary.
The author has a bell on her desk that team members ring when they have a success. This sounds cheesy, however, it is fun and team members really enjoy it. For example, when the author finished her slides for the SOCRA annual conference on time, she rang the bell. Her team members asked what happened, and they had a mini celebration. This small item helps to build and strengthen a team with small successes leading to larger successes.
Case Study Using the Teaming Principles
The following case study illustrates the application of the teaming principles to a team involving four major players. Olivia is a clinician with three clinic days and teaching duties who is a sought-after speaker for international conferences. In addition, Olivia is the clinical investigator for four clinical research studies: two studies are active, one is in long-term follow up, and one is in closeout. The studies are a blend of industry sponsored and investigator initiated. Olivia is also a co-clinical investigator on two additional studies and relies heavily upon Ansh for coordination of all studies and management of two research assistants.
Ansh is the lead research coordinator with seven years of experience in critical care research. Ansh is very detail-oriented and takes pride in error-free case report forms, coordinates with external monitors, and manages two research assistants as well as the day-to-day operations of Olivia’s research studies.
Bernita is a research assistant with six months of work experience in obtaining informed consents, scheduling study visits, and coordinating with ancillary services. Bernita is responsible for contacting participants for scheduled visits and providing participant payments. Bernita is developing coordinating skills, seeks out training and educational opportunities, and is a real people person.
Delroy is the regulatory affairs specialist for the Critical Care Department, which consists of eight clinicians (not all of whom are engaged in research). Studies include one multi-site clinical trial for which the clinical research site is the coordinating site, and one faculty-held Investigational New Drug/Investigational Device Exemption study. The department’s studies are a mixture of federal- and industry-funded studies. Delroy has been with the department for five years in this capacity. However, Delroy’s coworker recently and unexpectedly took family and medical leave, leaving Delroy to manage all regulatory issues for the department. Also, the department chair recently made growing the department’s industry-sponsored study portfolio a priority.
Olivia has received an invitation to be added as a clinical research site for a highly sought-after ongoing Phase II, multisite, industry-sponsored study comparing two asthma medications in an adult outpatient setting. The study uses a central institutional review board (IRB) and has competitive enrollment. It will require the following ancillary services: investigational pharmacy, radiology, and outpatient asthma clinic nursing. For the purposes of this case study, all contracts have been negotiated and all of the regulatory documents are available (e.g., FDA Form 1572, informed consent template, and the current protocol). The institution utilizes a clinical trial management system.
Oliva shares the study information and study enrollment goals with Ansh with the charge of getting this study activated and enrolling within 40 days. What are the potential barriers that might affect this outcome? One potential barrier to the study activation timeline is Delroy’s heavy workload. To ensure that the timeline is met, Ansh might contact Delroy and explain the situation, asking what Ansh can do to help facilitate study start-up to ensure that the timeline is met. Ansh should be clear in determining what Delroy needs for study activation, the deadlines for each item, and assist in facilitation of communicating to other members of the study activation team (e.g., ancillary services, IRB) what is needed. Priorities include the regulatory work and staff training. Barriers include managing the regulatory issues on time. This might be a good opportunity to connect with Bernita for providing Delroy some assistance, as Bernita is knowledgeable and eager to acquire additional skills and training. The shared goal of starting the study on time should be shared with all team members in order to meet the 40 day study activation and enrollment goal.
Nuggets for Success as a Team Member or Leader
Members of a research team must know the other team members and available resources. They need to know who is needed for a particular study. This will change during studies and across studies. Roles and responsibilities among the broader team should be identified.
Table 4 outlines nuggets of success as a team member or leader, starting with using the framework of the teaming principles. Next, the team member or leader should build and create networks for knowledge and access. A knowledge network enables team members to know who to contact to provide an answer to specific questions. Each team member is a knowledge network for someone else. Also, each team member should find a person who they admire to serve as a mentor, even informally.
Team members should take advantage of available training. LinkedIn has many free training programs, and the institution’s human resources department also offers training. Meeting times should be scheduled to set aside time for reflection. Team members should check in often with the team as a whole and individual team members, set realistic boundaries, and establish priorities. Team members should avoid making assumptions, and instead, communicate clearly and often. Other keys to team success are to be respectful and present, participate, and practice humanity.
This work was supported by CTSA award No. UL1TR002649 from the National Center for Advancing Translational Sciences. Its contents are solely the responsibility of the authors and do not necessarily represent official views of the National Center for Advancing Translational Sciences or the National Institutes of Health.
Overview of the Teaming Principles
- Establish team (top-down and bottom-up)
- Establish roles and responsibilities, communications, and processes
- Working together effectively and efficiently
- Individuals develop trust and comfort
- Work together efficiently
- Focus on a shared vision
- Resolves issues
- Natural end:dissolution
- New project (study) with a new shared goal
Description of the Teaming Principles
- Team members may vary depending upon the study, project, and timelines
- Work across boundaries
- Appropriate competencies and knowledge, skills, and attitudes
- Recognize and celebrate differences
- Shared goal and vision
- Determining who has the competencies for specific study tasks
- Communication pathways and expectation
- Completing clinical trial management systems updates
- Revisit the shared goal often
- Requires mutual dependence
- Identity-based: personal understanding
- Calculus-based: keep commitments, meet deadlines, meet expectations
- Competence-based: confidence in skills, competencies of another
- Satisfaction in progressing toward goal
- Proactive in preventing issues from arising
- Revisit the shared goal
- Embrace diversity and differences
- Continuous improvement in knowledge, skills, and attitudes
- Completion of tasks
- Identify lessons learned
- Celebrate success and acknowledge the contributions of all
- Self-awareness and assessments
- Demographic
- Educational
- Personality
- Give and receive constructive feedback
- Acquire new knowledge, skills, and attitudes/competencies
- Cross-train
- Recognize individual success, which reflects team success
- Commit to shared goals
Nuggets of Success as a Team Member of Leader
- Use the teaming principles as a framework
- Build and create networks for knowledge and access
- Find a mentor
- Take advantage of training
- Schedule meeting times for reflection
- Check in with the team and team members
- Set boundaries and priorities
- Never make assumptions
- Be respectful and present
- Participate
- Practice humanity
1 Stokols D, Hall KL, Taylor BK, Moser RP. The science of team science: overview of the field and introduction to the supplement. Am J Prev Med. 2008 Aug;35(2 Suppl):S77-89. Accessed 8/10/20.
2 Bennett LM, Gadlin H, Marchand C. Team Collaboration Field Guide. Publication No. 18-7660, 2nd ed., National Institutes of Health; 2018. Accessed 8/10/20.
3 National Research Council. Enhancing the Effectiveness of Team Science. Washington, DC: The National Academies Press; 2015. Accessed 8/10/20.
4 Teambuilding 1: How to build effective teams in healthcare. Nursing Times. Accessed 8/10/20.
5 Salas E, Dickinson TL, Converse SA. Toward an Understanding of Team Performance and Training. In: Swezey R W, Salas E, editors. Teams: Their Training and Performance. Norwood, NJ: Ablex; 1992. pp. 3–29.
6 Tuckman, BW, Jensen MA. Stages of small-group development revisited. Group and Organization Studies, 2. 1977: 419-427.
7 Brown B. Dare to lead: Brave work. Tough conversations. Whole hearts. New York: Random House, 2018.
8 Lencioni P. The five dysfunctions of a team: A leadership fable. San Francisco: Jossey-Bass: 2002.
One thought on “Cultivating an Effective Research Team Through Application of Team Science Principles”
Hey there! I just finished reading your article on cultivating an effective research team through the application of team science principles, and I couldn’t help but drop a comment. First off, kudos to you for sharing such valuable insights. Your article was not only informative but also highly engaging, making it a pleasure to read.
I particularly resonated with your emphasis on the importance of clear communication and collaboration within research teams. It’s incredible how these seemingly simple principles can make such a significant difference in the success of a research project. Your practical tips on fostering trust and encouraging diversity of thought were spot-on. I’ve had my fair share of experiences in research teams, and I can attest that when everyone is on the same page and feels heard, the results are remarkable. Your article has given me a fresh perspective on how to approach team dynamics in my future research endeavors, and I’ll definitely be sharing these insights with my colleagues. Thanks again for sharing your wisdom! Looking forward to more of your articles in the future.
Keep up the fantastic work, and please continue to share your expertise. Your writing style is not only informative but also very relatable, making complex topics like team science principles easy to grasp. I’ll be eagerly awaiting your next piece. Until then, wishing you all the best in your research and writing endeavors! 😊📚
Leave a Reply Cancel reply
Your email address will not be published. Required fields are marked *
Save my name, email, and website in this browser for the next time I comment.
This site uses Akismet to reduce spam. Learn how your comment data is processed .
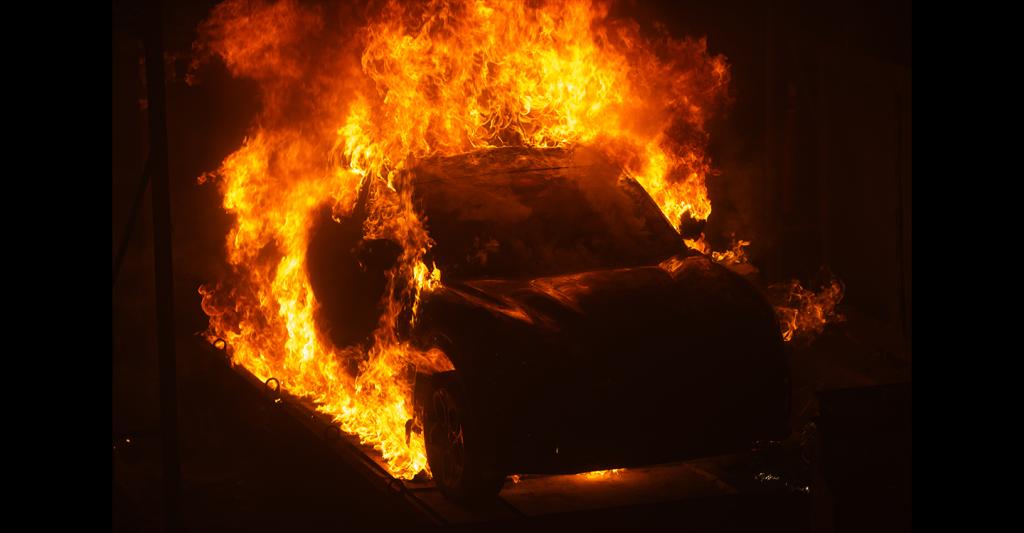
Experiments Enabling Analysis of Electric Vehicle Fire Behavior are Completed
The Fire Safety Research Institute (FSRI), part of UL Research Institutes , completed experiments this winter to study the burning characteristics of electric vehicles (EV). Experiments—the first of two phases in the Fire Safety of Batteries and Electric Vehicles research project—were conducted at UL’s indoor laboratory in Northbrook, IL. The objective of this phase is to obtain full-scale freeburn data without suppression and compare this data with internal combustion engine vehicle (ICEV) fires to identify and understand observed differences with fire behavior and toxic gas exposures.
Among the fire service, there are concerns about EV fire behavior and occupational exposure hazards. There is not yet sufficient data to characterize EV fire dynamics to develop efficient, effective, and safe size-up and fire control strategies. The fire service wants to understand which fire control strategies (i.e. allowing the EV to burn out and consume the battery, to initiate suppression tactics, or modification to existing ICEV approaches) may be most effective at controlling EV fires. Additionally, first responders want to understand occupational thermal and chemical exposure risks to individuals who may be present during an EV fire.
About the EV Fire Behavior Experiments
In this first phase of experiments, a total of six (6) popular EV models were used to understand the differences vehicle construction may have on EV fire behavior. Each test was conducted as a freeburn experiment allowing the vehicle to burn to completion and to simulate an approach of non-intervention with regard to suppression.
During each EV test, a propane burner was used to heat the lithium-ion battery pack and left to operate until thermal runaway was confirmed. Following thermal runaway, the burner was turned off and the vehicles continued to burn uninterrupted while measurements were collected. Once ignited, the vehicles typically burned out within approximately one hour. Data was collected to understand the fire growth rate, peak size, and duration. Measurements also included thermal and chemical exposure hazards to firefighters, and the ignition potential of nearby vehicles or structures.
EV Fire Behavior Measurement Data Collection
Both routine and innovative fire measurement instrumentation was used to collect data related to heat release rate, heat flux, and occupational exposure hazards to combustion products.
- EVs were positioned on top of a metal pan constructed to measure vehicle weight during the freeburn and determine its heat release rate.
- Heat flux gauges were positioned 9–15 feet from the vehicle to measure radiant heat flux.
- Sheet metal panels were placed on each side of the EV with infrared cameras positioned 11 feet from the unexposed sides to enable calculation of incident radiant heat flux to nearby vehicles or structures.
- Two stations containing turnout gear swatches were positioned at 10 and 15 feet from the EVs to collect chemical samples.
- Examination of deposition of contaminants on turnout gear composites and quantification of cleaning effectiveness following exposure to products of combustion were conducted with collaborators at North Carolina State University
- Fourier-transform infrared spectroscopy (FTIR), gas chromatography (GC), sorbent tubes, and solid particulate sample capturing methods were employed to enable measurement of gas, vapor, and particulate emissions in both the fire plume and locations where firefighters might work with partners at the National Institute for Occupational Safety and Health (NIOSH) and Duke University .
- Novel techniques were utilized for particulate chemistry and toxicity analysis of combustion products with partners at NIOSH and the U.S. Environmental Protection Agency (EPA).
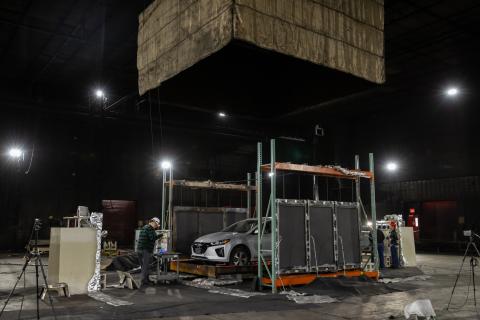
Next Steps and Planning for Phase Two Experiments
Following completion of the EV fire behavior experiments, the research team will conduct data analysis with input from the research project’s technical panel . Following data analysis, a technical report will be published later this year, and a series of suppression strategies will be selected for testing in the second phase of experiments next year.
“As we analyze the data to understand how EVs burn, we will work with the understanding that approximately 34,000 fire departments in North America need better information on EV fire control, and our colleagues overseas are working with the same urgency. It is imperative we improve the understanding of how EVs burn compared to the ICEVs we know, what risks they present to people, first responders and the built environment. Our recommendations must consider whether existing firefighting tools can be used to achieve fire control, as the resources of first responders must be considered with utmost responsibility.” —Adam Barowy, lead research engineer, FSRI
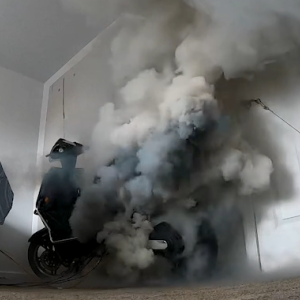
Fire Safety Hazards of e-Mobility Devices in Homes
Learn about the research focused on understanding battery failures in e-Mobility devices.
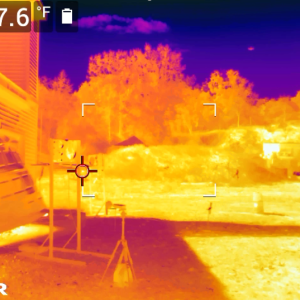
The Impact of Batteries on Fire Dynamics
Learn about the research focused on understanding fire and explosion hazards associated with lithium-ion batteries.
- Social Media Hub
- Apply for a Job
This paper is in the following e-collection/theme issue:
Published on 22.5.2024 in Vol 26 (2024)
The Power of Rapid Reviews for Bridging the Knowledge-to-Action Gap in Evidence-Based Virtual Health Care
Authors of this article:

- Megan MacPherson, PhD ;
- Sarah Rourke, MSN
Fraser Health, Surrey, BC, Canada
Corresponding Author:
Megan MacPherson, PhD
Fraser Health
400-13450 102nd Avenue
Surrey, BC, V3T 0H1
Phone: 1 6045616605
Email: [email protected]
Despite the surge in popularity of virtual health care services as a means of delivering health care through technology, the integration of research evidence into practice remains a challenge. Rapid reviews, a type of time-efficient evidence synthesis, offer a potential solution to bridge the gap between knowledge and action. This paper aims to highlight the experiences of the Fraser Health Authority’s Virtual Health team in conducting rapid reviews. This paper discusses the experiences of the Virtual Health team in conducting 15 rapid reviews over the course of 1.5 years and the benefit of involving diverse stakeholders including researchers, project and clinical leads, and students for the creation of user-friendly knowledge products to summarize results. The Virtual Health team found rapid reviews to be a valuable tool for evidence-informed decision-making in virtual health care. Involving stakeholders and focusing on implementation considerations are crucial for maximizing the impact of rapid reviews. Health care decision makers are encouraged to consider implementing rapid review processes to improve the translation of research evidence into practice, ultimately enhancing patient outcomes and promoting a culture of evidence-informed care.
Introduction
Virtual health care services, which involve the delivery of health care through information and communication technologies, have gained popularity among health care providers, patients, and organizations. In recent decades, several initiatives have been undertaken to implement virtual care and improve the access, quality, and safety of health care delivery in Canada [ 1 ]; however, technological advancement and a rapidly expanding evidence base make supporting virtual care with research evidence challenging. Specifically, to adequately support virtual care, health care decision makers are expected to keep up with available technologies, their applications, and evidence of their effectiveness among a variety of health conditions.
Despite decision makers recognizing the need to consider research evidence in the context of public health problems [ 2 , 3 ], there is still a knowledge-to-action (KTA) gap between what is known and what is put into practice clinically [ 4 - 6 ], with health care professionals worldwide demonstrating suboptimal use of research evidence within clinical practice [ 7 - 14 ]. Further, it has been estimated that one-third of patients do not receive treatments that have proven efficacious, one-quarter receive treatments that are potentially harmful, and up to three-quarters of patients and half of clinicians do not receive the information necessary for research-informed decision-making [ 15 ]. Clearly, there is a need to improve the translation of research evidence into practice, particularly in the case of virtual care where technological innovations and research evidence are rapidly expanding.
Knowledge Translation
The field of knowledge translation (KT) strives to enhance the usefulness of research evidence through the design and conduct of stakeholder-informed, patient-oriented studies as well as the dissemination and implementation of research findings into practice [ 16 ]. The Canadian Institutes for Health Research defines KT as the ethical exchange, synthesis, and application of knowledge among researchers and users to accelerate the benefits of research for Canadian people [ 17 ]. The ultimate goal of KT has been further described as the facilitation of evidence-informed decision-making [ 18 ] and the integration of various forms of evidence into public health practice and policy.
The Canadian Institutes for Health Research describes 2 “Death Valleys” on the continuum from research to action, which contributes to the KTA gap [ 19 ]. Valley 1 refers to the reduced ability to translate basic biomedical research discoveries from the laboratory to the bedside and to effectively commercialize health innovations. Valley 2 refers to the reduced ability to synthesize, disseminate, and integrate research findings more broadly into clinical practice and clinical decision-making. To improve the utility of biomedical and clinical research, enhance health outcomes, and ensure an evidence-based and sustainable health care system, strategic attempts to bridge these valleys must be made.
Rapid Reviews
One way to help overcome the second valley is through evidence syntheses such as systematic, scoping, and rapid reviews [ 20 ]. Evidence syntheses have emerged as valuable methods for KT as they can compile large bodies of evidence into a single knowledge product, making them an essential tool for decision makers to enhance evidence-informed decision-making [ 21 , 22 ]. Systematic reviews offer a comprehensive synthesis of available evidence on a particular topic, playing an ever-expanding role in informing policy making and practice [ 23 , 24 ]; however, the resource-intensive nature of conducting systematic reviews, in terms of both time and cost, presents a significant obstacle to facilitating prompt and efficient decision-making [ 25 ].
Given the time constraints health care practitioners and policy makers often face [ 26 ], rapid reviews provide a more resource- and time-efficient means to conduct evidence syntheses that offer actionable evidence in a more relevant manner compared to other types of evidence syntheses such as systematic or scoping reviews [ 20 , 26 - 34 ]. Specifically, rapid reviews are a form of evidence synthesis in which systematic review steps are streamlined to generate actionable evidence within a condensed time frame [ 35 ]. To expedite the review process, rapid reviews often compromise on the rigor typically associated with systematic reviews, resulting in a less precise and robust evaluation in comparison [ 32 ]. That being said, rapid reviews have gained traction in health systems’ policy making, health-related intervention development, and health technology assessment [ 34 - 36 ]. This paper outlines the experiences of the Fraser Health (FH) Authority Virtual Health team in rapidly producing and disseminating rapid review results to date. Rapid reviews were chosen as they are often highly driven by end-user demands [ 37 ] and have been highlighted as a viable tool to disseminate knowledge within the rapidly growing field of virtual health [ 33 ].
FH Authority Context
As the largest regional health authority in British Columbia, Canada, FH serves more than 1.9 million people in Canada [ 38 ]. In recent years, FH has prioritized the expansion of virtual care [ 39 ], conducting over 1.9 million virtual visits between January 2019 and 2023 (roughly 27% of all visits). Within the Virtual Health department at FH, the “research and evaluation team” aims to improve the translation of research into practice while engaging in ongoing collaborative evaluation of existing Virtual Health programming. During Virtual Health strategic planning, rapid reviews have emerged as a central tool for knowledge dissemination and have been used to inform the development of frameworks, services, and program scale-up. This paper highlights FH’s experience in conducting 15 rapid reviews over the course of 1.5 years. This paper is meant to serve as an overview on the utility and feasibility of rapid reviews within a health authority; for more information on rapid review methods to aid in conducting reviews within a team-based setting, see MacPherson et al [ 33 ].
Rapid reviews are used within the Virtual Health team to provide an overview of available evidence addressing a research question related to a single topic produced within a short time frame (typically 1 week to 4 months). From October 2022 until March 2024, the Virtual Health team conducted 15 rapid reviews following published recommendations [ 33 ]. Questions posed to date include the following:
- What are the perspectives on virtual care among immigrant, refugee, and Indigenous people in Canada [ 40 ]?
- What virtual care solutions exist for people with heart failure [ 41 ]?
- What virtual care solutions exist for people with diabetes [ 41 ]?
- What virtual care solutions exist for people with chronic obstructive pulmonary disease (COPD) [ 41 ]?
- What are currently used decision guides or algorithms to inform escalation within remote patient monitoring services for people with heart failure?
- What barriers, facilitators, and recommendations exist for remote patient monitoring services within the context of respiratory care [ 42 ]?
- What virtual care or digital innovations are used by physicians in acute care [ 43 ]?
- What barriers and facilitators exist for patient-to-provider virtual messaging (eg, SMS text messaging) [ 44 ]?
- What is the existing evidence for centralized remote patient monitoring services [ 45 ]?
- What domains are included within virtual care frameworks targeting appropriateness and safety?
- What are patient and provider barriers to virtual care [ 46 ]?
- What is the evidence for virtual hospital programs [ 47 ]?
- What KT strategies exist that could be used by the Virtual Health research and evaluation team in their efforts to translate research findings into practice?
- What is the available evidence on virtual decision-making and clinical judgment?
- What is the available evidence for, and are there existing validated assessment criteria for nursing assessment frameworks?
Team members assisting with the rapid reviews included researchers, project leads, clinical leads, and students previously unfamiliar with the review process. Knowledge users within the Virtual Health team (eg, clinical leads and clinical directors) were involved throughout the entirety of the review process from developing the research questions to the presentation of research findings in Virtual Health team meetings and the implementation of findings into Virtual Health practice.
Similar to other rapid reviews [ 20 ], results were collated and narratively or visually summarized (eg, through infographics) and presented to Virtual Health team members. The final knowledge products were created to offer a high-level overview of the evidence arranged in a user-friendly manner, aiming to provide VH team members with a high-level understanding of the available evidence [ 41 ].
Experiences and Lessons Learned
The Virtual Health team’s journey in conducting 15 rapid reviews over the course of 1.5 years has provided valuable insights into the feasibility and utility of rapid reviews within a health authority setting. These lessons learned are from the perspectives of the authors of this paper. MM is the research and KT lead of the Virtual Health department at the FH Authority. Prior to creating the rapid review program within the Virtual Health department, she has prior experience conducting systematic, scoping, and rapid reviews. SR is a clinical nurse specialist within the Virtual Health department at FH. As a system-level leader, SR leverages evidence to informed clinical and service model changes to optimize patient care and outcomes and support strategic priorities. Prior to her involvement in the Virtual Health rapid review program, SR had no previous experience with conducting evidence reviews.
Importance of Defining a Clear and Actionable Research Question
Throughout this journey, one of the key lessons learned was about the importance of the research question being actionable to ensure that the results of rapid reviews can be readily integrated into practice. Initially, our reviews had broader scopes aimed at informing future Virtual Health service implementations across various populations such as COPD, diabetes, and heart failure. While these reviews were informative, they did not lead to immediate changes in Virtual Health practice and required strategic efforts to disseminate findings and integrate results into practice. Subsequently, we learned that focusing on specific programs or initiatives within the Virtual Health setting yields more actionable results. For instance, a review focused on identifying patient and provider barriers to virtual care was conducted with the explicit purpose of informing the development of a framework to improve video visit uptake among primary care providers. This targeted approach enabled us to directly address the identified barriers through the development of a framework focused on the uptake of safe and appropriate video visits within primary care.
Benefits and Challenges Involving Knowledge Users
The involvement of knowledge users such as clinical leads and directors in the rapid review process proved to be invaluable. First, they helped focus the scope of reviews by providing insights into the practical needs and priorities within the FH context. For example, the reviews focusing on virtual care solutions for patients with heart failure, COPD, and diabetes were initiated by 1 of the directors within Virtual Health and included an occupational therapist and clinical nurse specialist on the review team. The diverse insights offered by clinician team members helped shape the review questions, search strategy, and analysis, ensuring it addressed the practical needs in delivering virtual care to this specific patient population.
Second, the engagement of nonresearchers, students, and health care professionals in the review process not only enhanced the quality and relevance of the rapid reviews but also provided an opportunity for experiential learning and professional development. By participating in the rapid review process, students and other team members developed essential skills such as critical appraisal, evidence synthesis, and scientific communication. This approach has the potential to bridge the gap between research and practice by building a generation of clinicians who are well versed in evidence-based practice and can effectively translate research findings into clinical decision-making. For example, a team of nursing students participated in a rapid review focused on algorithms for care escalation within remote patient monitoring services for patients with heart failure. While they lacked prior review experience, their fresh perspectives and familiarity with health care practice as it relates to heart failure brought unique insights helping to shape the clinician-oriented KT efforts.
While involving knowledge users throughout the review process offers numerous benefits, it can also extend the time required to complete a review. This is often due to the necessity for these individuals to familiarize themselves with new software while simultaneously mastering the intricacies of conducting reviews and adhering to all associated steps. For instance, several Virtual Health team members have observed that during their initial and subsequent reviews, they encountered difficulties in efficiently navigating the study screening phase. The abundance of potentially relevant literature posed a challenge, with concerns arising about potentially overlooking papers containing valuable insights or “hidden gems.” This underscores the importance of establishing clear eligibility criteria and providing comprehensive training from the outset to ensure reviewers feel empowered to exclude papers confidently, even those that may initially appear intriguing.
Resources and Staff Time Involved
Readers interested in starting a rapid review program in their own health systems may find it helpful to understand the resources and staff time involved in our process. As the research and KT lead within the Virtual Health team, MM has been responsible for building the rapid review program, training team members, and leading rapid reviews. Her full-time role allows for dedicated focus on these as well as other research and KT-related activities, ensuring the smooth operation of the rapid review process.
Additionally, strong leadership support within the Virtual Health team has been instrumental in fostering a culture of evidence-informed decision-making and facilitating the integration of research evidence into practice. While we do not have a core team with a dedicated full-time equivalent specifically for rapid reviews, a call is put out to the Virtual Health department at the beginning of a review to identify who has the capacity to assist in a review. A testament to the value of these reviews is that VH team members have begun autonomously conducting rapid reviews with the research and KT lead acting as an advisor, not a lead on the reviews. For example, a nurse who was tasked with creating a framework for a virtual nursing assessment requested assistance in running a search for her team to complete a rapid review, to ensure that the resulting framework did not miss any key components seen in the literature.
Rapid Review Process
The overall process map for our team (an adaptation of MacPherson et al [ 33 , 48 ]) can be found in Figure 1 . Our journey in conducting rapid reviews has been accompanied by several challenges and the implementation of quality assurance measures to ensure the integrity of our findings. The overall process of reviews within the Virtual Health team includes Virtual Health team members submitting a request or having an informal meeting with the research and KT lead outlining the scope and purpose of the review, which is then refined to ensure that it will result in actionable evidence relevant to the Virtual Health team and is in alignment with organizational priorities.
Challenges or obstacles encountered during the rapid review process have included resource constraints. When there are not enough people to assist with a review, either the time to complete the review needs to be extended or additional constraints must be placed on the review question. Time limitations have also been a factor, especially when there is an urgent request. Clear communication on how the results will be used is needed to refine the review topic and search strategy to quickly produce actionable evidence. Given the wealth of research, we have started all reviews by first exploring if our questions can be answered by conducting a review of reviews. This has allowed for the timely synthesis of evidence instead of relying on individual studies. We have also found that decision makers value the most up-to-date evidence (especially regarding virtual health care technologies); as such, many of our reviews have imposed limitations to the past 5-10 years to ensure their relevance to decision makers. Additionally, difficulties in accessing relevant literature have been noted, as health authorities often do not have access to the same resources as academic institutions. This results in increased time to secure papers through interlibrary loans, which can be overcome by collaborating with academics.

Another strength of the Virtual Health team’s rapid review approach was the development of easily digestible knowledge products highlighting key data synthesized in the review. Rather than providing end users with lengthy reports that often go unread, clinicians within the Virtual Health team helped to create brief summaries and infographics highlighting the main findings and recommendations. This approach was aimed at improving the uptake of research evidence into practice by presenting the information in a format that was easily accessible and understandable for clinicians and other stakeholders. By creating visually appealing and user-friendly knowledge products, the Virtual Health team was able to efficiently communicate key takeaways from the rapid reviews, thus facilitating their dissemination and implementation within the FH context. This approach also helped to overcome a common challenge of KT, where research evidence can be difficult to access, understand, and apply in practice. By presenting the information in a format that was relevant and easily digestible, the Virtual Health team was able to enhance the applicability of the rapid reviews, thereby building clinician capacity and increasing their potential impact on patient outcomes.
Leveraging Rapid Reviews for Clinically Based Tools
Our most recent reviews were focused on developing a virtual nursing assessment and virtual nursing decision-making framework. Unlike traditional KT efforts used within other reviews, where the focus often lies on creating user-friendly summaries and infographics, our approach took a slightly different path. We aimed to directly inform the development of clinical decision support tools (DSTs).
Rather than developing traditional KT products, the raw data extracted from these reviews served as a foundational resource for the development of the clinical DSTs. Each piece of information was carefully referenced and integrated into the tool, providing evidence-based support for specific components and functionalities. This direct integration of research evidence into the tool development process not only strengthened the validity and credibility of the tool but also facilitated the transparent communication of the evidence behind each recommendation or feature.
Within these reviews, the active participation of those who were responsible for the development of the DSTs proved invaluable. Their involvement was crucial in ensuring understanding and confidence in the information as well as in merging research evidence with their own clinical expertise. By involving end users in the review process, we could tailor the outcomes to their specific needs and preferences, ultimately enhancing the relevance and applicability of the extracted evidence. This collaborative approach ensured that the resulting DSTs were not only evidence based but also resonated effectively with the clinical context they were intended for.
Principal Findings
The Virtual Health team’s experience with conducting 15 rapid reviews over the course of 1.5 years highlights the potential of rapid reviews as a time-efficient tool for improving the translation and uptake of research evidence into Virtual Health programming. Compared to more traditional review types (eg, systematic or scoping), which can take more than a year to complete [ 49 ], rapid reviews provide a practical way of synthesizing available evidence to inform clinical decision-making. The ability to produce a high-quality evidence summary in a shorter time frame can be particularly valuable in rapidly evolving areas of health care, such as virtual health. While rapid reviews are not new, our program offers insights into their application in a dynamic and rapidly evolving field such as virtual health. The lessons learned from FH’s rapid review program have important implications for evidence-based decision-making and KT within health care settings.
One of our primary lessons learned underscores the importance of establishing clear and actionable research questions. By outlining precise objectives, rapid reviews can ensure the relevance and applicability of their results, thus facilitating their seamless integration into clinical practice. Moreover, our experiences highlight the transformative impact of involving knowledge users throughout the review process. This collaborative approach not only enhances the quality and relevance of the evidence synthesized but also fosters a culture of evidence-informed decision-making within the organization. This type of early and continued engagement of knowledge users in research endeavors has been increasingly recognized as pivotal for establishing research priorities and enhancing the utility of research findings in real-world health care contexts [ 50 , 51 ]. In line with this, the overarching goal of knowledge-user engagement in health research is to coproduce knowledge that directly addresses the needs of decision makers. By involving knowledge users from the outset, research priorities can be aligned with the practical requirements of health care delivery, thereby increasing the relevance and utility of research outputs [ 52 - 54 ].
Limitations of Rapid Reviews
Despite its benefits, the rapid review approach is not without limitations. Loss of rigor, as mentioned earlier in this paper, remains a concern. The rapid nature of the process may compromise the depth and comprehensiveness of the literature search and synthesis, potentially leading to oversights or biases in the evidence presented. Furthermore, within the context of virtual health, the rapid pace of technological advancements poses a challenge. New technologies may outpace the generation of peer-reviewed literature, resulting in a lag between their implementation and the availability of robust evidence.
In response to the challenge posed by rapidly evolving technologies, FH’s Virtual Health department has used creative solutions to capture relevant evidence. While peer-reviewed literature remains a primary source, we have also incorporated gray literature, such as news articles, trade publications, and reports, from other health care authorities or departments within the review processes when applicable. Additionally, to supplement reviews and provide more contextual evidence, additional research and evaluation methodologies are used (time permitting) to inform Virtual Health service development such as consulting Patient and Family Advisory Councils within FH, conducting interviews with patient and clinician partners, and conducting analyses on existing data within FH.
Next Steps for FH’s Rapid Review Program
We remain committed to advancing the rapid review program to meet the evolving needs of the Virtual Health department at FH. While we have heard anecdotally that knowledge users value the user-friendly knowledge products developed for rapid reviews, the next steps of this program include an evaluation of our knowledge dissemination to assess the reach and impact the reviews are having within the Virtual Health department.
Conclusions
Rapid reviews are a valuable tool for the timely synthesis of available research evidence to inform health care decision-making. The Virtual Health team’s experience with conducting rapid reviews highlights the importance of involving a diverse range of knowledge users in the review process and the need to focus on implementation considerations. By engaging knowledge users beyond designated researchers, and particularly by involving clinicians across the research process, rapid reviews become more robust, applicable, and aligned with the practical needs of health care providers and organizations, which can help to bridge the KTA gap.
Conflicts of Interest
None declared.
- Goodridge D, Marciniuk D. Rural and remote care: overcoming the challenges of distance. Chron Respir Dis. 2016;13(2):192-203. [ FREE Full text ] [ CrossRef ] [ Medline ]
- Bowen S, Zwi AB. Pathways to "evidence-informed" policy and practice: a framework for action. PLoS Med. 2005;2(7):e166. [ FREE Full text ] [ CrossRef ] [ Medline ]
- Jacobs JA, Jones E, Gabella BA, Spring B, Brownson RC. Tools for implementing an evidence-based approach in public health practice. Prev Chronic Dis. 2012;9:E116. [ FREE Full text ] [ CrossRef ] [ Medline ]
- Davis D, Evans M, Jadad A, Perrier L, Rath D, Ryan D, et al. The case for knowledge translation: shortening the journey from evidence to effect. BMJ. 2003;327(7405):33-35. [ FREE Full text ] [ CrossRef ] [ Medline ]
- Grol R, Grimshaw J. From best evidence to best practice: effective implementation of change in patients' care. Lancet. 2003;362(9391):1225-1230. [ CrossRef ] [ Medline ]
- Grol R, Jones R. Twenty years of implementation research. Fam Pract. 2000;17(Suppl 1):S32-S35. [ FREE Full text ] [ CrossRef ] [ Medline ]
- Straus SE, McAlister FA. Evidence-based medicine: a commentary on common criticisms. CMAJ. 2000;163(7):837-841. [ FREE Full text ] [ Medline ]
- Mellis C. Evidence-based medicine: what has happened in the past 50 years? J Paediatr Child Health. 2015;51(1):65-68. [ FREE Full text ] [ CrossRef ] [ Medline ]
- Villar J, Carroli G, Gülmezoglu AM. The gap between evidence and practice in maternal healthcare. Int J Gynaecol Obstet. 2001;75(Suppl 1):S47-S54. [ Medline ]
- Grol R. Successes and failures in the implementation of evidence-based guidelines for clinical practice. Med Care. 2001;39(8 Suppl 2):II46-II54. [ CrossRef ] [ Medline ]
- Schuster MA, McGlynn EA, Brook RH. How good is the quality of health care in the United States? Milbank Q. 1998;76(4):517-563. [ FREE Full text ] [ CrossRef ] [ Medline ]
- McGlynn EA, Asch SM, Adams J, Keesey J, Hicks J, DeCristofaro A, et al. The quality of health care delivered to adults in the United States. N Engl J Med. 2003;348(26):2635-2645. [ FREE Full text ] [ CrossRef ] [ Medline ]
- Lauer MS, Skarlatos S. Translational research for cardiovascular diseases at the National Heart, Lung, and Blood Institute: moving from bench to bedside and from bedside to community. Circulation. 2010;121(7):929-933. [ FREE Full text ] [ CrossRef ] [ Medline ]
- Lang ES, Wyer PC, Haynes RB. Knowledge translation: closing the evidence-to-practice gap. Ann Emerg Med. 2007;49(3):355-363. [ CrossRef ] [ Medline ]
- Kitson AL, Straus SE. Identifying knowledge to action gaps. Knowledge Transl Health Care. 2013:97-109. [ FREE Full text ] [ CrossRef ]
- Graham ID, Logan JL, Harrison MB, Straus SE, Tetroe J, Caswell W, et al. Lost in knowledge translation: time for a map? J Contin Educ Health Prof. 2006;26(1):13-24. [ FREE Full text ] [ CrossRef ] [ Medline ]
- Knowledge translation strategy 2004-2009: innovation in action. Canadian Institutes of Health Research. 2004. URL: https://cihr-irsc.gc.ca/e/26574.html [accessed 2024-04-25]
- Ciliska D, Thomas H, Buffett C. A compendium of critical appraisal tools for public health practice. National Collaborating Centre for Methods and Tools. 2008. URL: https://www.nccmt.ca/uploads/media/media/0001/01/b331668f85bc6357f262944f0aca38c14c89c5a4.pdf [accessed 2024-04-25]
- Canada's strategy for patient-oriented research. Government of Canada. Canadian Institutes of Health Research. 2011. URL: https://cihr-irsc.gc.ca/e/44000.html#a1.1 [accessed 2023-04-06]
- Khangura S, Konnyu K, Cushman R, Grimshaw J, Moher D. Evidence summaries: the evolution of a rapid review approach. Syst Rev. 2012;1(1):10. [ FREE Full text ] [ CrossRef ] [ Medline ]
- Chambers D, Wilson PM, Thompson CA, Hanbury A, Farley K, Light K. Maximizing the impact of systematic reviews in health care decision making: a systematic scoping review of knowledge-translation resources. Milbank Q. 2011;89(1):131-156. [ FREE Full text ] [ CrossRef ] [ Medline ]
- Grimshaw JM, Eccles MP, Lavis JN, Hill SJ, Squires JE. Knowledge translation of research findings. Implement Sci. 2012;7(1):50. [ FREE Full text ] [ CrossRef ] [ Medline ]
- Bosch-Capblanch X, Lavis JN, Lewin S, Atun R, Røttingen JA, Dröschel D, et al. Guidance for evidence-informed policies about health systems: rationale for and challenges of guidance development. PLoS Med. 2012;9(3):e1001185. [ FREE Full text ] [ CrossRef ] [ Medline ]
- Oxman AD, Lavis JN, Lewin S, Fretheim A. SUPPORT tools for evidence-informed health policymaking (STP). Norwegian Knowledge Centre for the Health Services. 2010. URL: https://fhi.brage.unit.no/fhi-xmlui/bitstream/handle/11250/2378076/NOKCrapport4_2010.pdf?sequence=1 [accessed 2023-11-22]
- Oliver K, Innvar S, Lorenc T, Woodman J, Thomas J. A systematic review of barriers to and facilitators of the use of evidence by policymakers. BMC Health Serv Res. 2014;14(1):2. [ FREE Full text ] [ CrossRef ] [ Medline ]
- Ganann R, Ciliska D, Thomas H. Expediting systematic reviews: methods and implications of rapid reviews. Implement Sci. 2010;5(1):56. [ FREE Full text ] [ CrossRef ] [ Medline ]
- Moore G, Redman S, Rudge S, Haynes A. Do policy-makers find commissioned rapid reviews useful? Health Res Policy Syst. 2018;16(1):17. [ FREE Full text ] [ CrossRef ] [ Medline ]
- Flores EJ, Jue JJ, Giradi G, Schoelles K, Mull NK, Umscheid CA. AHRQ EPC Series on improving translation of evidence: use of a clinical pathway for C. Difficile treatment to facilitate the translation of research findings into practice. Jt Comm J Qual Patient Saf. 2019;45(12):822-828. [ CrossRef ] [ Medline ]
- Hartling L, Guise J, Kato E, Anderson J, Belinson S, Berliner E, et al. A taxonomy of rapid reviews links report types and methods to specific decision-making contexts. J Clin Epidemiol. 2015;68(12):1451-1462.e3. [ CrossRef ] [ Medline ]
- Hartling L, Guise JM, Kato E, Anderson J, Aronson N, Belinson S, et al. EPC Methods: An Exploration of Methods and Context for the Production of Rapid Reviews. Rockville, MD. Agency for Healthcare Research and Quality; 2015.
- Hartling L, Guise JM, Hempel S, Featherstone R, Mitchell MD, Motu'apuaka ML, et al. Fit for purpose: perspectives on rapid reviews from end-user interviews. Syst Rev. 2017;6(1):32. [ FREE Full text ] [ CrossRef ] [ Medline ]
- Featherstone RM, Dryden DM, Foisy M, Guise JM, Mitchell MD, Paynter RA, et al. Advancing knowledge of rapid reviews: an analysis of results, conclusions and recommendations from published review articles examining rapid reviews. Syst Rev. 2015;4(1):50. [ FREE Full text ] [ CrossRef ] [ Medline ]
- MacPherson MM, Wang RH, Smith EM, Sithamparanathan G, Sadiq CA, Braunizer AR. Rapid reviews to support practice: a guide for professional organization practice networks. Can J Occup Ther. 2023;90(3):269-279. [ FREE Full text ] [ CrossRef ] [ Medline ]
- Watt A, Cameron A, Sturm L, Lathlean T, Babidge W, Blamey S, et al. Rapid reviews versus full systematic reviews: an inventory of current methods and practice in health technology assessment. Int J Technol Assess Health Care. 2008;24(2):133-139. [ CrossRef ] [ Medline ]
- Polisena J, Garritty C, Kamel C, Stevens A, Abou-Setta AM. Rapid review programs to support health care and policy decision making: a descriptive analysis of processes and methods. Syst Rev. 2015;4(1):26. [ FREE Full text ] [ CrossRef ] [ Medline ]
- Harker J, Kleijnen J. What is a rapid review? A methodological exploration of rapid reviews in health technology assessments. Int J Evid Based Healthc. 2012;10(4):397-410. [ FREE Full text ] [ CrossRef ] [ Medline ]
- Garritty C, Gartlehner G, Nussbaumer-Streit B, King VJ, Hamel C, Kamel C, et al. Cochrane Rapid Reviews Methods Group offers evidence-informed guidance to conduct rapid reviews. J Clin Epidemiol. 2021;130:13-22. [ FREE Full text ] [ CrossRef ] [ Medline ]
- Fraser Health. 2023. URL: https://www.fraserhealth.ca/ [accessed 2023-04-06]
- Virtual Health. Fraser Health. URL: https://www.fraserhealth.ca/patients-and-visitors/virtual-health [accessed 2023-04-06]
- MacPherson M. Immigrant, refugee, and Indigenous Canadians' experiences with virtual health care services: rapid review. JMIR Hum Factors. 09, 2023;10:e47288. [ FREE Full text ] [ CrossRef ] [ Medline ]
- MacPherson M. Virtual care in heart failure, chronic obstructive pulmonary disease, and diabetes: a rapid review protocol. OSF Registries. 2023. URL: https://osf.io/xn2pe [accessed 2023-09-11]
- MacPherson M. Barriers, facilitators, and recommendations to inform the expansion of remote patient monitoring services for respiratory care: a rapid review. OSF Registries. 2022. URL: https://osf.io/asf2v/ [accessed 2024-04-25]
- MacPherson M. Virtual health services in the context of acute care: a rapid review. OSF Registries. 2023. URL: https://osf.io/ub2d8/ [accessed 2024-04-25]
- MacPherson MM, Kapadia S. Barriers and facilitators to patient-to-provider messaging using the COM-B model and theoretical domains framework: a rapid umbrella review. BMC Digit Health. 2023;1(1):33. [ FREE Full text ] [ CrossRef ]
- Chan L, MacPherson M. Remote patient monitoring: an evidence synthesis. OSF Registries. 2023. URL: https://osf.io/7wqb8/ [accessed 2024-04-25]
- Montenegro M, MacPherson M. Barriers to virtual care experienced by patients and healthcare providers: a rapid umbrella review. OSF Registries. 2023. URL: https://osf.io/nufg4/ [accessed 2024-04-25]
- Montenegro M, MacPherson M. Virtual hospitals: a rapid review. OSF Registries. 2023. URL: https://osf.io/m3a4b/ [accessed 2024-04-25]
- Attribution 4.0 International (CC BY 4.0). Creative Commons. URL: https://creativecommons.org/licenses/by/4.0/ [accessed 2024-05-13]
- Borah R, Brown AW, Capers PL, Kaiser KA. Analysis of the time and workers needed to conduct systematic reviews of medical interventions using data from the PROSPERO registry. BMJ Open. 2017;7(2):e012545. [ FREE Full text ] [ CrossRef ] [ Medline ]
- Deverka PA, Lavallee DC, Desai PJ, Esmail LC, Ramsey SD, Veenstra DL, et al. Stakeholder participation in comparative effectiveness research: defining a framework for effective engagement. J Comp Eff Res. 2012;1(2):181-194. [ FREE Full text ] [ CrossRef ] [ Medline ]
- Bragge P, Clavisi O, Turner T, Tavender E, Collie A, Gruen RL. The Global Evidence Mapping Initiative: scoping research in broad topic areas. BMC Med Res Methodol. 2011;11(1):92. [ FREE Full text ] [ CrossRef ] [ Medline ]
- Langlois EV, Montekio VB, Young T, Song K, Alcalde-Rabanal J, Tran N. Enhancing evidence informed policymaking in complex health systems: lessons from multi-site collaborative approaches. Health Res Policy Syst. 2016;14(1):20. [ FREE Full text ] [ CrossRef ] [ Medline ]
- Vindrola-Padros C, Pape T, Utley M, Fulop NJ. The role of embedded research in quality improvement: a narrative review. BMJ Qual Saf. 2017;26(1):70-80. [ FREE Full text ] [ CrossRef ] [ Medline ]
- Ghaffar A, Langlois EV, Rasanathan K, Peterson S, Adedokun L, Tran NT. Strengthening health systems through embedded research. Bull World Health Organ. 2017;95(2):87. [ FREE Full text ] [ CrossRef ] [ Medline ]
Abbreviations
Edited by Z Yin; submitted 22.11.23; peer-reviewed by W LaMendola, M Willenbring, Y Zhang, P Blasi; comments to author 10.03.24; revised version received 15.03.24; accepted 13.04.24; published 22.05.24.
©Megan MacPherson, Sarah Rourke. Originally published in the Journal of Medical Internet Research (https://www.jmir.org), 22.05.2024.
This is an open-access article distributed under the terms of the Creative Commons Attribution License (https://creativecommons.org/licenses/by/4.0/), which permits unrestricted use, distribution, and reproduction in any medium, provided the original work, first published in the Journal of Medical Internet Research, is properly cited. The complete bibliographic information, a link to the original publication on https://www.jmir.org/, as well as this copyright and license information must be included.
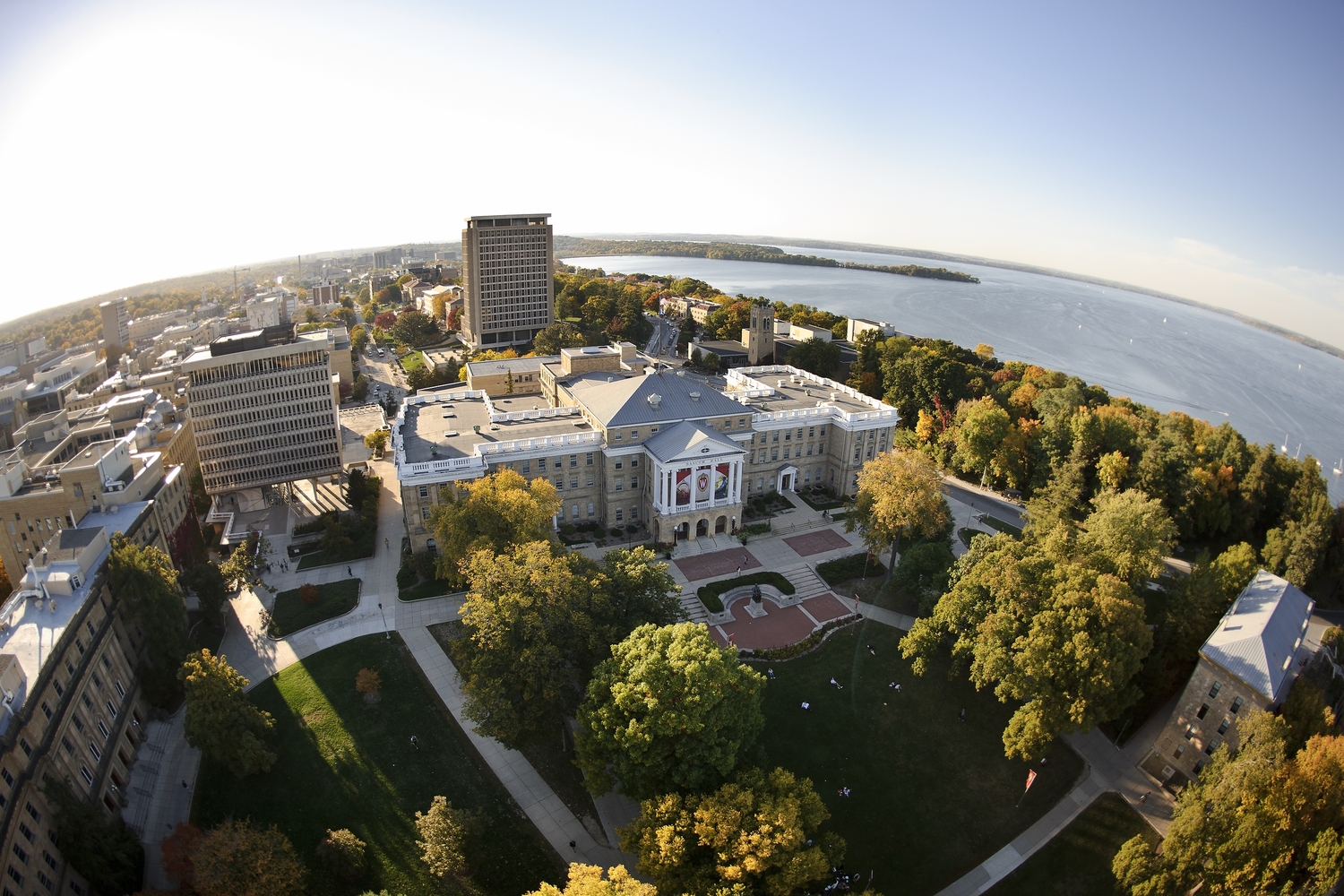
Research Technician
- Madison, Wisconsin
- SCHOOL OF MEDICINE AND PUBLIC HEALTH/DEPARTMENT OF SURGERY
- Staff-Full Time
- Staff-Part Time
- Opening at: May 21 2024 at 13:55 CDT
- Closing at: Jun 5 2024 at 23:55 CDT
Job Summary:
The Department of Surgery is seeking a highly motivated and self-directed Lab Technician to join an exciting research team studying the mechanisms of wound healing and therapies to improve healing using cell, animal and human wound models. This individual will perform general lab management duties, including tracking and replenishing supplies, maintaining equipment, updating protocols and records, as well as assisting in experiments. These duties require a presence in the lab during daytime weekday hours. There may be occasional evening or weekend hours if experimental support is needed. This individual may also work closely with students, residents and other researchers in different departments on a variety of exciting and challenging projects on the cutting edge of translational wound healing research. Work in this laboratory has the potential to lead to discoveries with direct translation to burn and wound patients. The University of Wisconsin School of Medicine and Public Health (SMPH) is recognized as an international, national, and statewide leader in education, research, and service. SMPH is committed to being a diverse, equitable, inclusive and anti-racist workplace and is an Equal Employment Opportunity, Affirmative Action employer.
Responsibilities:
- 25% Prepares lab and/or field test materials and samples according to established research requirements and specifications. Performs lab or field tests.
- 30% Inspects and maintains equipment, supplies, inventory, and facility spaces
- 25% Collects data and monitors test results according to established research requirements and specifications
- 20% Assist or work alongside with team members to conduct experiments, including cell, tissue and animals studies
Institutional Statement on Diversity:
Diversity is a source of strength, creativity, and innovation for UW-Madison. We value the contributions of each person and respect the profound ways their identity, culture, background, experience, status, abilities, and opinion enrich the university community. We commit ourselves to the pursuit of excellence in teaching, research, outreach, and diversity as inextricably linked goals. The University of Wisconsin-Madison fulfills its public mission by creating a welcoming and inclusive community for people from every background - people who as students, faculty, and staff serve Wisconsin and the world. For more information on diversity and inclusion on campus, please visit: Diversity and Inclusion
Preferred Bachelor's Degree Preferred focus in molecular biology or related field
Qualifications:
Preferred: -Experience in a basic science lab. -Experience with Microsoft Excel and Word. -A minimum of one year experience in molecular biology techniques including RNA, DNA extraction and amplification, PCR, Western Blot, cell culture, immunohistochemistry, ELISA, protocol and assay development. -Specific experience with animal work.
Work Schedule:
The regular work schedule will be Monday to Friday between the hours of 8:00am and 5:00pm (exact schedule to be set at the time of hire).
Full or Part Time: 80% - 100% It is anticipated this position requires work be performed in-person, onsite, at a designated campus work location.
Appointment Type, Duration:
Ongoing/Renewable
Minimum $18.00 HOURLY Depending on Qualifications The starting salary for the position is $18.00/hr but is negotiable based on experience and qualifications. Employees in this position can expect to receive benefits such as generous vacation, holidays, and paid time off; competitive insurances and savings accounts; retirement benefits. Benefits information can be found at ( https://hr.wisc.edu/benefits/ ) - SMPH University Staff Benefits flyer: ( https://uwmadison.box.com/s/656no0fcpy2tjg86s4v0chtxx25s3vsm )
Additional Information:
University sponsorship is not available for this position, including transfers of sponsorship. The selected applicant will be responsible for ensuring their continuous eligibility to work in the United States (i.e. a citizen or national of the United States, a lawful permanent resident, a foreign national authorized to work in the United States without the need of an employer sponsorship) on or before the effective date of appointment. This position is an ongoing position that will require continuous work eligibility. UW-Madison is not an E-Verify employer, and therefore, is not eligible to employ F1-OPT STEM Extension participants. If you are selected for this position you must provide proof of work authorization and eligibility to work.
How to Apply:
To apply for this position, please click on the "Apply Now" button. You will be asked to upload a current resume/CV and a cover letter briefly describing your qualifications and experience. You will also be asked to provide contact information for three (3) references, including your current/most recent supervisor during the application process. References will not be contacted without prior notice.
Robyn Dunkerley [email protected] 608-262-7227 Relay Access (WTRS): 7-1-1. See RELAY_SERVICE for further information.
Official Title:
Research Technician(RE038)
Department(s):
A53-MEDICAL SCHOOL/SURGERY/TRAUMA
Employment Class:
University Staff-Ongoing
Job Number:
The university of wisconsin-madison is an equal opportunity and affirmative action employer..
You will be redirected to the application to launch your career momentarily. Thank you!
Frequently Asked Questions
Applicant Tutorial
Disability Accommodations
Pay Transparency Policy Statement
Refer a Friend
You've sent this job to a friend!
Website feedback, questions or accessibility issues: [email protected] .
Learn more about accessibility at UW–Madison .
© 2016–2024 Board of Regents of the University of Wisconsin System • Privacy Statement
Facility for Rare Isotope Beams
At michigan state university, international research team uses wavefunction matching to solve quantum many-body problems, new approach makes calculations with realistic interactions possible.
FRIB researchers are part of an international research team solving challenging computational problems in quantum physics using a new method called wavefunction matching. The new approach has applications to fields such as nuclear physics, where it is enabling theoretical calculations of atomic nuclei that were previously not possible. The details are published in Nature (“Wavefunction matching for solving quantum many-body problems”) .
Ab initio methods and their computational challenges
An ab initio method describes a complex system by starting from a description of its elementary components and their interactions. For the case of nuclear physics, the elementary components are protons and neutrons. Some key questions that ab initio calculations can help address are the binding energies and properties of atomic nuclei not yet observed and linking nuclear structure to the underlying interactions among protons and neutrons.
Yet, some ab initio methods struggle to produce reliable calculations for systems with complex interactions. One such method is quantum Monte Carlo simulations. In quantum Monte Carlo simulations, quantities are computed using random or stochastic processes. While quantum Monte Carlo simulations can be efficient and powerful, they have a significant weakness: the sign problem. The sign problem develops when positive and negative weight contributions cancel each other out. This cancellation results in inaccurate final predictions. It is often the case that quantum Monte Carlo simulations can be performed for an approximate or simplified interaction, but the corresponding simulations for realistic interactions produce severe sign problems and are therefore not possible.
Using ‘plastic surgery’ to make calculations possible
The new wavefunction-matching approach is designed to solve such computational problems. The research team—from Gaziantep Islam Science and Technology University in Turkey; University of Bonn, Ruhr University Bochum, and Forschungszentrum Jülich in Germany; Institute for Basic Science in South Korea; South China Normal University, Sun Yat-Sen University, and Graduate School of China Academy of Engineering Physics in China; Tbilisi State University in Georgia; CEA Paris-Saclay and Université Paris-Saclay in France; and Mississippi State University and the Facility for Rare Isotope Beams (FRIB) at Michigan State University (MSU)—includes Dean Lee , professor of physics at FRIB and in MSU’s Department of Physics and Astronomy and head of the Theoretical Nuclear Science department at FRIB, and Yuan-Zhuo Ma , postdoctoral research associate at FRIB.
“We are often faced with the situation that we can perform calculations using a simple approximate interaction, but realistic high-fidelity interactions cause severe computational problems,” said Lee. “Wavefunction matching solves this problem by doing plastic surgery. It removes the short-distance part of the high-fidelity interaction, and replaces it with the short-distance part of an easily computable interaction.”
This transformation is done in a way that preserves all of the important properties of the original realistic interaction. Since the new wavefunctions look similar to that of the easily computable interaction, researchers can now perform calculations using the easily computable interaction and apply a standard procedure for handling small corrections called perturbation theory. A team effort
The research team applied this new method to lattice quantum Monte Carlo simulations for light nuclei, medium-mass nuclei, neutron matter, and nuclear matter. Using precise ab initio calculations, the results closely matched real-world data on nuclear properties such as size, structure, and binding energies. Calculations that were once impossible due to the sign problem can now be performed using wavefunction matching.
“It is a fantastic project and an excellent opportunity to work with the brightest nuclear scientist s in FRIB and around the globe,” said Ma. “As a theorist , I'm also very excited about programming and conducting research on the world's most powerful exascale supercomputers, such as Frontier , which allows us to implement wavefunction matching to explore the mysteries of nuclear physics.”
While the research team focused solely on quantum Monte Carlo simulations, wavefunction matching should be useful for many different ab initio approaches, including both classical and quantum computing calculations. The researchers at FRIB worked with collaborators at institutions in China, France, Germany, South Korea, Turkey, and United States.
“The work is the culmination of effort over many years to handle the computational problems associated with realistic high-fidelity nuclear interactions,” said Lee. “It is very satisfying to see that the computational problems are cleanly resolved with this new approach. We are grateful to all of the collaboration members who contributed to this project, in particular, the lead author, Serdar Elhatisari.”
This material is based upon work supported by the U.S. Department of Energy, the U.S. National Science Foundation, the German Research Foundation, the National Natural Science Foundation of China, the Chinese Academy of Sciences President’s International Fellowship Initiative, Volkswagen Stiftung, the European Research Council, the Scientific and Technological Research Council of Turkey, the National Natural Science Foundation of China, the National Security Academic Fund, the Rare Isotope Science Project of the Institute for Basic Science, the National Research Foundation of Korea, the Institute for Basic Science, and the Espace de Structure et de réactions Nucléaires Théorique.
Michigan State University operates the Facility for Rare Isotope Beams (FRIB) as a user facility for the U.S. Department of Energy Office of Science (DOE-SC), supporting the mission of the DOE-SC Office of Nuclear Physics. Hosting what is designed to be the most powerful heavy-ion accelerator, FRIB enables scientists to make discoveries about the properties of rare isotopes in order to better understand the physics of nuclei, nuclear astrophysics, fundamental interactions, and applications for society, including in medicine, homeland security, and industry.
The U.S. Department of Energy Office of Science is the single largest supporter of basic research in the physical sciences in the United States and is working to address some of today’s most pressing challenges. For more information, visit energy.gov/science.
research news
Instrument Machine Shop makes research, discovery possible

College of Arts and Sciences Instrument Machine Shop staff (from left) Thomas Brachmann, Kevin Cullinan and Gary Nottingham plan, design, fabricate and repair the precision devices UB scientists need to conduct their research. Photo: Douglas Levere
By JACKIE HAUSLER
Published May 13, 2024
As members of an R1 research institution, UB’s faculty, staff and students are determined to solve the world’s most complex problems. But who’s behind the curtain making the mechanics of these research discoveries and innovations possible?
Meet Thomas Brachmann, Kevin Cullinan and Gary Nottingham, the team in the College of Arts and Sciences’ Instrument Machine Shop. With more than 90 years of combined machining experience, these staff members tirelessly plan, design, fabricate and repair precision devices from start to finish.
They are the quintessential “jack of all trades” using the shop’s lathes, mills, saws, welding equipment and a complete woodworking shop on the first floor of Fronczak Hall to get their jobs done. And when the tools they need for a specific task don’t exist, they make them. Whether it’s custom fabrication of a thread for a machine, or outsmarting the computerized settings to optimize the outcome, they can do it all.
“Every single day the work is rewarding, and we never ever stop learning,” says Cullinan, instructional support technician in the Instrument Machine Shop. “When faculty come into the shop and explain their complex research to us, we work together to solve the other half — the mechanics of what they want — to help their vision come to life and build things accordingly.”
The team’s modesty competes with its workmanship. Not only are they extraordinary mechanics and technicians, but they are also expert communicators. The team has the experience to know the right questions to ask, be acute listeners and communicate plans effectively so everyone is on the same page with the extraordinarily precise details required.
“When we feel like we’ve asked a lot of questions, then we ask more and interject with our knowledge on the process,” says Nottingham, instructional support technician.
These details are vital to their success, as they are always working on a wide range of projects. They have built everything from lab and laser tables, to optic adapters, to recirculating flumes, including one that is 35-feet long.

Kevin Cullinan, instructional support technician, with the 35-foot recirculating flume constructed in the CAS Instrument Machine Shop. Photo: Douglas Levere
Yes, that’s right. A 35-foot recirculating flume that can perfectly mimic the environment of a small stream, recirculating water and sediment. The flume, housed in Wilkenson Quad in the Ellicott Complex, is managed by Sean Bennett, associate dean for social sciences in the college and professor in the Department of Geography.
“As an experimental geomorphologist, hydraulic flumes are the backbone of my entire research program,” says Bennett. Since arriving at UB, he has studied a variety of topics with flumes constructed, modified and maintained by Cullinan in the Instrument Machine Shop. The flumes help him investigate erosion and rill development on a soil-mantled landscape; the effect of large woody debris on streambank stability and local scour; fish movement and behavior in complex turbulent flows; the hydrodynamics of impinging jets and soil erosion; the effect of aquatic mussels on open channel flow; fish swimming capability and fatigue; mechanics of sediment suspension in a zero-mean shear flow; and the effect of vegetation on near-bank flow in rivers, among other topics.
Bigger projects like these not only greatly benefit faculty and their research efforts, but many students utilize the facilities as well. “Dozens of students have used the flumes for educational purposes and nearly all of the research was supported by external grants,” says Bennett. “The machine shop is an unrivaled resource for any faculty member or student whose work involves anything related to materials,” he adds.
While the influx of requests at the Instrument Machine Shop involves builds for nationally funded research and scientific study, it also supports creativity in the college in unique ways.

The team in the machine shop also helped design and build these tanks used by art professor Paul Vanouse in his artwork, "Labor." Photo: Douglas Levere
For faculty members like Paul Vanouse, SUNY Distinguished Professor in the Department of Art and director of Coalesce, the shop is vital to his artistry. His bio-media artwork often employs molecular biology techniques to challenge entrenched notions of individual, racial and national identity.
“I’ve been working with the college’s Instrument Machine Shop since I arrived at UB about 25 years ago,” says Vanouse. “I was told about their work by a representative from Fisher Scientific when I was working on my first large-scale bio-art project, “The Relative Velocity Inscription Device.” Gary Nottingham helped me realize a giant DNA gel electrophoresis rig out of plexiglass, which was the centerpiece of the project,” he adds.
Vanouse’s artwork, “Labor,” used bacteria to recreate the smell of human sweat inside 25-gallon industrial fermenters, also made by the Instrument Machine Shop. “With ‘Labor’ Kevin Cullinan and Tom Gruenauer (since retired) suggested key hardware, materials and design solutions — from how to fabricate concentric rings with minimal material to the perfect hardware connectors for the works aesthetic,” says Vanouse. In 2019, “Labor” was on exhibit at the Burchfield Penney Art Center and later received the “Golden Nica ,” the top prize from nearly 1,000 nominations and submissions at the 2020 Prix Ars Electronica Festival in Austria.
“The CAS Instrument Machine Shop is a fantastic resource for anyone who fabricates their own parts or devices, whether you are an artist, physicist, biologist, geologist, engineer or some combination of these,” says Vanouse.
Sambandamurthy Ganapathy, associate dean for research and professor in the Department of Physics, agrees.
“When I arrived in 2016, the first major task was to install gas recovery pipelines in my laboratory spaces,” says Ganapathy. “This is not a trivial task, and the team designed the recovery lines and flow meters connecting to the central helium liquefaction facility and then installed the pipelines successfully, which was critical for the successful operation of cryogenic instruments in my research group.
“The machine shop staff are not there only to complete the tasks requested, they are extremely collaborative in the projects from the designing stages all the way to fabrication and provide technical support and valuable inputs based on their expertise and experience,” added Ganapathy. “Often this has resulted in a product better than what was originally planned.”
Faculty and graduate students within the college interested in working with the Instrument Machine Shop can complete the online form to schedule a consultation . To learn more about the CAS Instrument Machine Shop, visit the website .

Want to create or adapt books like this? Learn more about how Pressbooks supports open publishing practices.
6.1 Experiment Basics
Learning objectives.
- Explain what an experiment is and recognize examples of studies that are experiments and studies that are not experiments.
- Explain what internal validity is and why experiments are considered to be high in internal validity.
- Explain what external validity is and evaluate studies in terms of their external validity.
- Distinguish between the manipulation of the independent variable and control of extraneous variables and explain the importance of each.
- Recognize examples of confounding variables and explain how they affect the internal validity of a study.
What Is an Experiment?
As we saw earlier in the book, an experiment is a type of study designed specifically to answer the question of whether there is a causal relationship between two variables. Do changes in an independent variable cause changes in a dependent variable? Experiments have two fundamental features. The first is that the researchers manipulate, or systematically vary, the level of the independent variable. The different levels of the independent variable are called conditions. For example, in Darley and Latané’s experiment, the independent variable was the number of witnesses that participants believed to be present. The researchers manipulated this independent variable by telling participants that there were either one, two, or five other students involved in the discussion, thereby creating three conditions. The second fundamental feature of an experiment is that the researcher controls, or minimizes the variability in, variables other than the independent and dependent variable. These other variables are called extraneous variables. Darley and Latané tested all their participants in the same room, exposed them to the same emergency situation, and so on. They also randomly assigned their participants to conditions so that the three groups would be similar to each other to begin with. Notice that although the words manipulation and control have similar meanings in everyday language, researchers make a clear distinction between them. They manipulate the independent variable by systematically changing its levels and control other variables by holding them constant.
Internal and External Validity
Internal validity.
Recall that the fact that two variables are statistically related does not necessarily mean that one causes the other. “Correlation does not imply causation.” For example, if it were the case that people who exercise regularly are happier than people who do not exercise regularly, this would not necessarily mean that exercising increases people’s happiness. It could mean instead that greater happiness causes people to exercise (the directionality problem) or that something like better physical health causes people to exercise and be happier (the third-variable problem).
The purpose of an experiment, however, is to show that two variables are statistically related and to do so in a way that supports the conclusion that the independent variable caused any observed differences in the dependent variable. The basic logic is this: If the researcher creates two or more highly similar conditions and then manipulates the independent variable to produce just one difference between them, then any later difference between the conditions must have been caused by the independent variable. For example, because the only difference between Darley and Latané’s conditions was the number of students that participants believed to be involved in the discussion, this must have been responsible for differences in helping between the conditions.
An empirical study is said to be high in internal validity if the way it was conducted supports the conclusion that the independent variable caused any observed differences in the dependent variable. Thus experiments are high in internal validity because the way they are conducted—with the manipulation of the independent variable and the control of extraneous variables—provides strong support for causal conclusions.
External Validity
At the same time, the way that experiments are conducted sometimes leads to a different kind of criticism. Specifically, the need to manipulate the independent variable and control extraneous variables means that experiments are often conducted under conditions that seem artificial or unlike “real life” (Stanovich, 2010). In many psychology experiments, the participants are all college undergraduates and come to a classroom or laboratory to fill out a series of paper-and-pencil questionnaires or to perform a carefully designed computerized task. Consider, for example, an experiment in which researcher Barbara Fredrickson and her colleagues had college students come to a laboratory on campus and complete a math test while wearing a swimsuit (Fredrickson, Roberts, Noll, Quinn, & Twenge, 1998). At first, this might seem silly. When will college students ever have to complete math tests in their swimsuits outside of this experiment?
The issue we are confronting is that of external validity. An empirical study is high in external validity if the way it was conducted supports generalizing the results to people and situations beyond those actually studied. As a general rule, studies are higher in external validity when the participants and the situation studied are similar to those that the researchers want to generalize to. Imagine, for example, that a group of researchers is interested in how shoppers in large grocery stores are affected by whether breakfast cereal is packaged in yellow or purple boxes. Their study would be high in external validity if they studied the decisions of ordinary people doing their weekly shopping in a real grocery store. If the shoppers bought much more cereal in purple boxes, the researchers would be fairly confident that this would be true for other shoppers in other stores. Their study would be relatively low in external validity, however, if they studied a sample of college students in a laboratory at a selective college who merely judged the appeal of various colors presented on a computer screen. If the students judged purple to be more appealing than yellow, the researchers would not be very confident that this is relevant to grocery shoppers’ cereal-buying decisions.
We should be careful, however, not to draw the blanket conclusion that experiments are low in external validity. One reason is that experiments need not seem artificial. Consider that Darley and Latané’s experiment provided a reasonably good simulation of a real emergency situation. Or consider field experiments that are conducted entirely outside the laboratory. In one such experiment, Robert Cialdini and his colleagues studied whether hotel guests choose to reuse their towels for a second day as opposed to having them washed as a way of conserving water and energy (Cialdini, 2005). These researchers manipulated the message on a card left in a large sample of hotel rooms. One version of the message emphasized showing respect for the environment, another emphasized that the hotel would donate a portion of their savings to an environmental cause, and a third emphasized that most hotel guests choose to reuse their towels. The result was that guests who received the message that most hotel guests choose to reuse their towels reused their own towels substantially more often than guests receiving either of the other two messages. Given the way they conducted their study, it seems very likely that their result would hold true for other guests in other hotels.
A second reason not to draw the blanket conclusion that experiments are low in external validity is that they are often conducted to learn about psychological processes that are likely to operate in a variety of people and situations. Let us return to the experiment by Fredrickson and colleagues. They found that the women in their study, but not the men, performed worse on the math test when they were wearing swimsuits. They argued that this was due to women’s greater tendency to objectify themselves—to think about themselves from the perspective of an outside observer—which diverts their attention away from other tasks. They argued, furthermore, that this process of self-objectification and its effect on attention is likely to operate in a variety of women and situations—even if none of them ever finds herself taking a math test in her swimsuit.
Manipulation of the Independent Variable
Again, to manipulate an independent variable means to change its level systematically so that different groups of participants are exposed to different levels of that variable, or the same group of participants is exposed to different levels at different times. For example, to see whether expressive writing affects people’s health, a researcher might instruct some participants to write about traumatic experiences and others to write about neutral experiences. The different levels of the independent variable are referred to as conditions , and researchers often give the conditions short descriptive names to make it easy to talk and write about them. In this case, the conditions might be called the “traumatic condition” and the “neutral condition.”
Notice that the manipulation of an independent variable must involve the active intervention of the researcher. Comparing groups of people who differ on the independent variable before the study begins is not the same as manipulating that variable. For example, a researcher who compares the health of people who already keep a journal with the health of people who do not keep a journal has not manipulated this variable and therefore not conducted an experiment. This is important because groups that already differ in one way at the beginning of a study are likely to differ in other ways too. For example, people who choose to keep journals might also be more conscientious, more introverted, or less stressed than people who do not. Therefore, any observed difference between the two groups in terms of their health might have been caused by whether or not they keep a journal, or it might have been caused by any of the other differences between people who do and do not keep journals. Thus the active manipulation of the independent variable is crucial for eliminating the third-variable problem.
Of course, there are many situations in which the independent variable cannot be manipulated for practical or ethical reasons and therefore an experiment is not possible. For example, whether or not people have a significant early illness experience cannot be manipulated, making it impossible to do an experiment on the effect of early illness experiences on the development of hypochondriasis. This does not mean it is impossible to study the relationship between early illness experiences and hypochondriasis—only that it must be done using nonexperimental approaches. We will discuss this in detail later in the book.
In many experiments, the independent variable is a construct that can only be manipulated indirectly. For example, a researcher might try to manipulate participants’ stress levels indirectly by telling some of them that they have five minutes to prepare a short speech that they will then have to give to an audience of other participants. In such situations, researchers often include a manipulation check in their procedure. A manipulation check is a separate measure of the construct the researcher is trying to manipulate. For example, researchers trying to manipulate participants’ stress levels might give them a paper-and-pencil stress questionnaire or take their blood pressure—perhaps right after the manipulation or at the end of the procedure—to verify that they successfully manipulated this variable.
Control of Extraneous Variables
An extraneous variable is anything that varies in the context of a study other than the independent and dependent variables. In an experiment on the effect of expressive writing on health, for example, extraneous variables would include participant variables (individual differences) such as their writing ability, their diet, and their shoe size. They would also include situation or task variables such as the time of day when participants write, whether they write by hand or on a computer, and the weather. Extraneous variables pose a problem because many of them are likely to have some effect on the dependent variable. For example, participants’ health will be affected by many things other than whether or not they engage in expressive writing. This can make it difficult to separate the effect of the independent variable from the effects of the extraneous variables, which is why it is important to control extraneous variables by holding them constant.
Extraneous Variables as “Noise”
Extraneous variables make it difficult to detect the effect of the independent variable in two ways. One is by adding variability or “noise” to the data. Imagine a simple experiment on the effect of mood (happy vs. sad) on the number of happy childhood events people are able to recall. Participants are put into a negative or positive mood (by showing them a happy or sad video clip) and then asked to recall as many happy childhood events as they can. The two leftmost columns of Table 6.1 “Hypothetical Noiseless Data and Realistic Noisy Data” show what the data might look like if there were no extraneous variables and the number of happy childhood events participants recalled was affected only by their moods. Every participant in the happy mood condition recalled exactly four happy childhood events, and every participant in the sad mood condition recalled exactly three. The effect of mood here is quite obvious. In reality, however, the data would probably look more like those in the two rightmost columns of Table 6.1 “Hypothetical Noiseless Data and Realistic Noisy Data” . Even in the happy mood condition, some participants would recall fewer happy memories because they have fewer to draw on, use less effective strategies, or are less motivated. And even in the sad mood condition, some participants would recall more happy childhood memories because they have more happy memories to draw on, they use more effective recall strategies, or they are more motivated. Although the mean difference between the two groups is the same as in the idealized data, this difference is much less obvious in the context of the greater variability in the data. Thus one reason researchers try to control extraneous variables is so their data look more like the idealized data in Table 6.1 “Hypothetical Noiseless Data and Realistic Noisy Data” , which makes the effect of the independent variable is easier to detect (although real data never look quite that good).
Table 6.1 Hypothetical Noiseless Data and Realistic Noisy Data
One way to control extraneous variables is to hold them constant. This can mean holding situation or task variables constant by testing all participants in the same location, giving them identical instructions, treating them in the same way, and so on. It can also mean holding participant variables constant. For example, many studies of language limit participants to right-handed people, who generally have their language areas isolated in their left cerebral hemispheres. Left-handed people are more likely to have their language areas isolated in their right cerebral hemispheres or distributed across both hemispheres, which can change the way they process language and thereby add noise to the data.
In principle, researchers can control extraneous variables by limiting participants to one very specific category of person, such as 20-year-old, straight, female, right-handed, sophomore psychology majors. The obvious downside to this approach is that it would lower the external validity of the study—in particular, the extent to which the results can be generalized beyond the people actually studied. For example, it might be unclear whether results obtained with a sample of younger straight women would apply to older gay men. In many situations, the advantages of a diverse sample outweigh the reduction in noise achieved by a homogeneous one.
Extraneous Variables as Confounding Variables
The second way that extraneous variables can make it difficult to detect the effect of the independent variable is by becoming confounding variables. A confounding variable is an extraneous variable that differs on average across levels of the independent variable. For example, in almost all experiments, participants’ intelligence quotients (IQs) will be an extraneous variable. But as long as there are participants with lower and higher IQs at each level of the independent variable so that the average IQ is roughly equal, then this variation is probably acceptable (and may even be desirable). What would be bad, however, would be for participants at one level of the independent variable to have substantially lower IQs on average and participants at another level to have substantially higher IQs on average. In this case, IQ would be a confounding variable.
To confound means to confuse, and this is exactly what confounding variables do. Because they differ across conditions—just like the independent variable—they provide an alternative explanation for any observed difference in the dependent variable. Figure 6.1 “Hypothetical Results From a Study on the Effect of Mood on Memory” shows the results of a hypothetical study, in which participants in a positive mood condition scored higher on a memory task than participants in a negative mood condition. But if IQ is a confounding variable—with participants in the positive mood condition having higher IQs on average than participants in the negative mood condition—then it is unclear whether it was the positive moods or the higher IQs that caused participants in the first condition to score higher. One way to avoid confounding variables is by holding extraneous variables constant. For example, one could prevent IQ from becoming a confounding variable by limiting participants only to those with IQs of exactly 100. But this approach is not always desirable for reasons we have already discussed. A second and much more general approach—random assignment to conditions—will be discussed in detail shortly.
Figure 6.1 Hypothetical Results From a Study on the Effect of Mood on Memory

Because IQ also differs across conditions, it is a confounding variable.
Key Takeaways
- An experiment is a type of empirical study that features the manipulation of an independent variable, the measurement of a dependent variable, and control of extraneous variables.
- Studies are high in internal validity to the extent that the way they are conducted supports the conclusion that the independent variable caused any observed differences in the dependent variable. Experiments are generally high in internal validity because of the manipulation of the independent variable and control of extraneous variables.
- Studies are high in external validity to the extent that the result can be generalized to people and situations beyond those actually studied. Although experiments can seem “artificial”—and low in external validity—it is important to consider whether the psychological processes under study are likely to operate in other people and situations.
- Practice: List five variables that can be manipulated by the researcher in an experiment. List five variables that cannot be manipulated by the researcher in an experiment.
Practice: For each of the following topics, decide whether that topic could be studied using an experimental research design and explain why or why not.
- Effect of parietal lobe damage on people’s ability to do basic arithmetic.
- Effect of being clinically depressed on the number of close friendships people have.
- Effect of group training on the social skills of teenagers with Asperger’s syndrome.
- Effect of paying people to take an IQ test on their performance on that test.
Cialdini, R. (2005, April). Don’t throw in the towel: Use social influence research. APS Observer . Retrieved from http://www.psychologicalscience.org/observer/getArticle.cfm?id=1762 .
Fredrickson, B. L., Roberts, T.-A., Noll, S. M., Quinn, D. M., & Twenge, J. M. (1998). The swimsuit becomes you: Sex differences in self-objectification, restrained eating, and math performance. Journal of Personality and Social Psychology, 75 , 269–284.
Stanovich, K. E. (2010). How to think straight about psychology (9th ed.). Boston, MA: Allyn & Bacon.
Research Methods in Psychology Copyright © 2016 by University of Minnesota is licensed under a Creative Commons Attribution-NonCommercial-ShareAlike 4.0 International License , except where otherwise noted.
No (sigh) HAARP didn't cause the May auroras; that's a solar storm | Fact check

The claim: HAARP caused May 2024 geomagnetic storms because solar flares aren’t real
A May 10 Facebook post ( direct link , archive link ) claims the northern lights that captivated much of the globe in May had nothing to do with radiation from the sun.
“Solar flares don't exist because the Sun isn't a ball of fire in space that throws off violent bursts of energy,” the post’s caption reads. “The Sun is just a light. The 'geomagnetic storms’ are caused by HAARP. Stop falling for the nonsense.”
The post was shared more than 800 times in four days.
More from the Fact-Check Team: How we pick and research claims | Email newsletter | Facebook page
Our rating: False
Solar flares exist, as established by more than 150 years of scientific research. Multiple experts said the auroras widely visible across the globe in May were caused by solar storms – not HAARP, which does not produce nearly enough energy to create one.
Claim ‘total and utter nonsense’
For three nights in mid-May, nighttime skies as far south as Florida and the Bahamas lit up in the colorful green and purple hues of auroras.
But the Facebook post gets their cause wrong. They were the product of geomagnetic storms stemming from the strongest solar storm in more than two decades . They were not a creation of the High-frequency Active Auroral Research Program, a program at the University of Alaska Fairbanks that researches Earth’s ionosphere and is the subject of widespread debunked conspiracy theories .
Fact check : Solar flares weren't strong enough, happened at wrong time to cause AT&T outage
The claim is “total and utter nonsense,” Scott McIntosh , the deputy director of the National Center for Atmospheric Research, told USA TODAY in an email.
One type of solar storm is a solar flare, an intense burst of high-energy radiation emitted from the sun at the speed of light that takes about eight minutes to reach the Earth. Those flares were first observed in 18 59 when British astronomer Richard Carrington timed the transit of sunspots across the solar surface. The Carrington Event helped other scientists connect flares to communications disruptions and the aurora borealis.
Those flares can cause the sun to shoot bursts of billions of tons of plasma along with charged particles in what are known as coronal mass ejections . If they reach Earth, they can interact with the atmosphere to cause geomagnetic storms and create auroras like those viewed across the globe in mid-May.
“Think of a cannon. When the cannon shoots off, there’s a great flash. That's the solar flare," said Bryan Brasher , a project manager at the National Oceanic and Atmospheric Administration’s Space Weather Prediction Center.
"The flash goes out 360 degrees in all directions," he added, comparing a coronal mass ejection to a cannonball that is "very directional."
Auroras ‘in no way linked’ to HAARP experiments
The auroral display first appeared during the final day that HAARP conducted experiments studying space debris in orbit.
Program officials said that was a coincidence, noting the study was scheduled March 16 , more than a month before the Space Weather Prediction Center issued its first storm watch .
“The HAARP scientific experiments were in no way linked to the solar storm or high auroral activity seen around the globe,” HAARP director Jessica Matthews said in a statement.
A previous HAARP project resulted in an artificial airglow visible 300 miles from its facility in Gakona, Alaska. But that’s not the same thing as an aurora. The project mimicked one by using high-frequency radio pulses to interact with electrons in the ionosphere. HAARP does not generate nearly enough energy to produce a real aurora, which is created when solar emissions interact with Earth’s magnetic field . Officials say it would take 10 billion years to generate enough power for that.
The post also includes an image consisting of two photos of the sun, each split in half and placed side-by-side, with one purported to be a fabrication. Both are authentic images that show different wavelengths of electromagnetic radiation , experts said.
The photo on the left shows the visible light emitted by the sun’s surface. The fiery image wrongly identified as "fake" captures the ultraviolet radiation emitted from a different layer of the sun’s atmosphere called the chromosphere, said Ryan French , a solar physicist from the National Science Foundation’s National Solar Observatory who has written a book about the sun .
“The chromosphere is not CGI,” he told USA TODAY in an email.
French said the post does get one thing right: the sun is not a ball of fire. Rather, it consists of super-hot ionized gas called plasma .
USA TODAY reached out to the Facebook user who shared the post but did not immediately receive a response.
PolitiFact debunked a version of the claim.
Our fact-check sources:
- Scott McIntosh , May 13, Email exchange with USA TODAY
- Bryan Brasher , May 13, Phone interview with USA TODAY
- Ryan French , May 13, Email exchange with USA TODAY
- National Oceanic and Atmospheric Administration, Feb. 23, 2012, The serendipitous discovery of solar flares
- Space.com, July 6, 2022, Solar flares: What are they and how do they affect Earth?
- Space.com, June 24, 2022, The Carrington Event: History’s greatest solar storm
- University of Alaska Fairbanks, May 13, May 13, 2024 Space Weather FAQ
- University of Alaska Fairbanks, May 13, Solar storm — not HAARP — creates intense auroral display
- NASA, March 16, 2020, Aurora, Meet Airglow
- NASA, accessed May 14, Solar Science
- Coe College, accessed May 14, The Sun
- University Corporation for Atmospheric Research, accessed May 14, Three Years of the Sun
Thank you for supporting our journalism. You can subscribe to our print edition, ad-free app or e-newspaper here .
USA TODAY is a verified signatory of the International Fact-Checking Network, which requires a demonstrated commitment to nonpartisanship, fairness and transparency. Our fact-check work is supported in part by a grant from Meta .
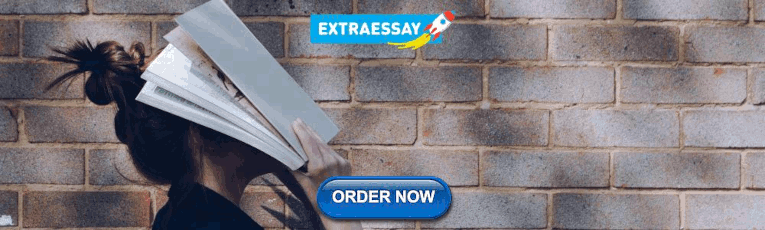
IMAGES
VIDEO
COMMENTS
Table of contents. Step 1: Define your variables. Step 2: Write your hypothesis. Step 3: Design your experimental treatments. Step 4: Assign your subjects to treatment groups. Step 5: Measure your dependent variable. Other interesting articles. Frequently asked questions about experiments.
Experiments have two fundamental features. The first is that the researchers manipulate, or systematically vary, the level of the independent variable. The different levels of the independent variable are called conditions. For example, in Darley and Latané's experiment, the independent variable was the number of witnesses that participants ...
A lot of research on the cause of bleaching has focused on water temperature 1 . However, a team of Australian researchers hypothesized that other factors might be important too. Specifically, they tested the hypothesis that high CO 2 levels, which make ocean waters more acidic, might also promote bleaching 2 .
Random assignment is a method for assigning participants in a sample to the different conditions, and it is an important element of all experimental research in psychology and other fields too. In its strictest sense, random assignment should meet two criteria. One is that each participant has an equal chance of being assigned to each condition ...
Record Keeping. It is essential to keep good records when you conduct an experiment. As discussed earlier, it is typical for experimenters to generate a written sequence of conditions before the study begins and then to test each new participant in the next condition in the sequence. As you test them, it is a good idea to add to this list basic ...
When conducting an experiment, it is important to follow the seven basic steps of the scientific method: Ask a testable question. Define your variables. Conduct background research. Design your experiment. Perform the experiment. Collect and analyze the data. Draw conclusions.
Experiments have two fundamental features. The first is that the researchers manipulate, or systematically vary, the level of the independent variable. The different levels of the independent variable are called conditions. For example, in Darley and Latané's experiment, the independent variable was the number of witnesses that participants ...
In an experiment, researchers manipulate, or cause changes, in the independent variable and observe or mea- sure any impact of those changes in the dependent variable. The independent variable is the one under the experimenter's control, or the variable that is intentionally altered between groups. In the case of Dunn's experiment, the ...
As we saw earlier in the book, an experiment is a type of study designed specifically to answer the question of whether there is a causal relationship between two variables. In other words, whether changes in an independent variable cause a change in a dependent variable. Experiments have two fundamental features.
Conducting Experiments. Learning Objectives. Describe several strategies for recruiting participants for an experiment. Explain why it is important to standardize the procedure of an experiment and several ways to do this. Explain what pilot testing is and why it is important. The information presented so far in this chapter is enough to design ...
This stage of conducting an experiment involves determining the time scale and frequency of sampling, to fit the type of experiment. For example, researchers studying the effectiveness of a cure for colds would take frequent samples, over a period of days. Researchers testing a cure for Parkinson's disease would use less frequent tests, over a ...
The practical steps needed for planning and conducting an experiment include: recognizing the goal of the experiment, choice of factors, choice of response, choice of the design, analysis and then drawing conclusions. This pretty much covers the steps involved in the scientific method. What this course will deal with primarily is the choice of ...
Abstract. If a research team conducts an experiment using a point value of .05 and gets statistically significant results, there is still a 5% possibility that their results are due to chance. This, combined with ________, are two main contributing factors to the reproducibility crisis. publication bias.
When conducting scientific experiments, researchers develop hypotheses to guide experimental design. A hypothesis is a suggested explanation that is both testable and falsifiable. You must be able to test your hypothesis, and it must be possible to prove your hypothesis true or false. ... For our educational research example, if the control ...
Abstract. Researchers must conduct research responsibly for it to have an impact and to safeguard trust in science. Essential responsibilities of researchers include using rigorous, reproducible research methods, reporting findings in a trustworthy manner, and giving the researchers who contributed appropriate authorship credit.
Every research team is structured differently. However, there are five key roles in each scientific research team. 1. Principal Investigator (PI): this is the person ultimately responsible for the research and overall project. Their role is to ensure that the team members have the information, resources and training they need to conduct the ...
A team of researchers is interested in conducting an experiment in order to test an important theory. ... The experimental sample must be representative of the population to which they want to generalize the research on dimensions of age, sex and intelligence. ... An experimenter conducts an experiment to see whether people's reaction time is ...
If a research team conducts an experiment using a point value of .05 and gets statistically significant results, there is still a 5% possibility that their results are due to chance. This, combined with _____, are two main contributing factors to the reproducibility crisis. experimenter bias publication bias time constraints methodology errors
Shirley L.T. Helm, MS, CCRP Senior Administrator for Network Capacity & Workforce Strategies C. Kenneth & Dianne Wright Center for Clinical and Translational Research Virginia Commonwealth University Abstract: The practice of team science allows clinical research professionals to draw from theory-driven principles to build an effective and efficient research team. Inherent in these principles are
Study with Quizlet and memorize flashcards containing terms like McCabe and Castel conducted experiments to assess whether a brain image influenced the way students perceived the quality of a scientific article. Across three experiments, they found that students rated the quality of scientific reasoning higher when a brain image was present. Their research would indicate that the study ...
FSRI research team completes the instrumentation and setup for one of the six EV fire behavior experiments. Next Steps and Planning for Phase Two Experiments. Following completion of the EV fire behavior experiments, the research team will conduct data analysis with input from the research project's technical panel. Following data analysis, a ...
Despite the surge in popularity of virtual health care services as a means of delivering health care through technology, the integration of research evidence into practice remains a challenge. Rapid reviews, a type of time-efficient evidence synthesis, offer a potential solution to bridge the gap between knowledge and action. This paper aims to highlight the experiences of the Fraser Health ...
25% Collects data and monitors test results according to established research requirements and specifications; 20% Assist or work alongside with team members to conduct experiments, including cell, tissue and animals studies; Institutional Statement on Diversity: Diversity is a source of strength, creativity, and innovation for UW-Madison.
New approach makes calculations with realistic interactions possibleFRIB researchers are part of an international research team solving challenging computational problems in quantum physics using a new method called wavefunction matching. The new approach has applications to fields such as nuclear physics, where it is enabling theoretical calculations of atomic nuclei that were previously not ...
The team's modesty competes with its workmanship. Not only are they extraordinary mechanics and technicians, but they are also expert communicators. The team has the experience to know the right questions to ask, be acute listeners and communicate plans effectively so everyone is on the same page with the extraordinarily precise details required.
This study aims to investigate the relationships of narrative role, empathy, host identification, spatial distance, and purchase intention. This study conducts three experiments with 460 participants to analyze the influencing mechanism of narrative role (in-group vs. out-group) on consumers' purchase intentions from the perspective of empathy and host identification through three ...
LLNL's Matthew Hill explains the experiment. Video courtesy of ELI ERIC. More Information: "LLNL's New Diffraction Gratings Will Enable the World's Most Powerful Laser," NIF & Photon Science News, Sept. 7, 2022 "Livermore Researchers Collect Three Awards Among the Top 100 Industrial Inventions," LLNL News, Sept. 7, 2022 "Researchers Design a Compact High-Power Laser Using ...
Experiments have two fundamental features. The first is that the researchers manipulate, or systematically vary, the level of the independent variable. The different levels of the independent variable are called conditions. For example, in Darley and Latané's experiment, the independent variable was the number of witnesses that participants ...
Study with Quizlet and memorize flashcards containing terms like Jane thinks that a longer wait time at the doctor's office will result in more negative reviews for the office, no matter the expertise or quality of the doctor. What steps should Jane follow to conduct a study consistent with the scientific method? a. make an observation, do an experiment, analyze the results, make a hypothesis ...
Auroras 'in no way linked' to HAARP experiments. The auroral display first appeared during the final day that HAARP conducted experiments studying space debris in orbit. Program officials said ...