Automatic Speech Recognition: Systematic Literature Review
Ieee account.
- Change Username/Password
- Update Address
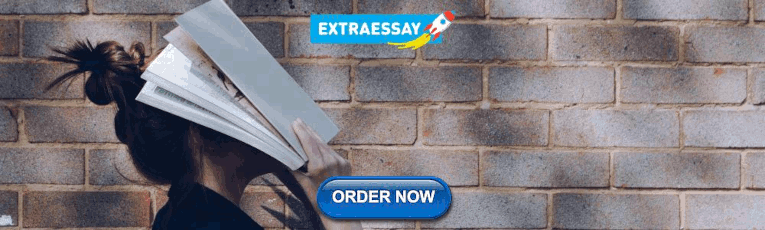
Purchase Details
- Payment Options
- Order History
- View Purchased Documents
Profile Information
- Communications Preferences
- Profession and Education
- Technical Interests
- US & Canada: +1 800 678 4333
- Worldwide: +1 732 981 0060
- Contact & Support
- About IEEE Xplore
- Accessibility
- Terms of Use
- Nondiscrimination Policy
- Privacy & Opting Out of Cookies
A not-for-profit organization, IEEE is the world's largest technical professional organization dedicated to advancing technology for the benefit of humanity. © Copyright 2024 IEEE - All rights reserved. Use of this web site signifies your agreement to the terms and conditions.
Automatic speech recognition: a survey
- Published: 10 November 2020
- Volume 80 , pages 9411–9457, ( 2021 )
Cite this article
- Mishaim Malik ORCID: orcid.org/0000-0002-4917-7144 1 ,
- Muhammad Kamran Malik 2 ,
- Khawar Mehmood 3 &
- Imran Makhdoom 4
10k Accesses
130 Citations
8 Altmetric
Explore all metrics
Recently great strides have been made in the field of automatic speech recognition (ASR) by using various deep learning techniques. In this study, we present a thorough comparison between cutting-edged techniques currently being used in this area, with a special focus on the various deep learning methods. This study explores different feature extraction methods, state-of-the-art classification models, and vis-a-vis their impact on an ASR. As deep learning techniques are very data-dependent different speech datasets that are available online are also discussed in detail. In the end, the various online toolkits, resources, and language models that can be helpful in the formulation of an ASR are also proffered. In this study, we captured every aspect that can impact the performance of an ASR. Hence, we speculate that this work is a good starting point for academics interested in ASR research.
This is a preview of subscription content, log in via an institution to check access.
Access this article
Price includes VAT (Russian Federation)
Instant access to the full article PDF.
Rent this article via DeepDyve
Institutional subscriptions
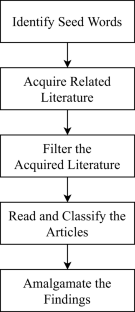
Similar content being viewed by others
Applications of Deep Learning Approaches in Speech Recognition: A Survey
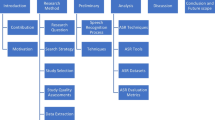
A comprehensive survey on automatic speech recognition using neural networks
Toolkits for Robust Speech Processing
Abdulla W H, Kasabov N (1999) The concepts of hidden Markov model in speech recognition.
Abe S (2003) Analysis of multiclass support vector machines. Thyroid 21(3):3772
Google Scholar
Alkhaldi W, Fakhr W, Hamdy N (2002) Automatic speech/speaker recognition in noisy environments using wavelet transform, The 2002 45th Midwest Symposium on Circuits and Systems, 2002. MWSCAS-2002., Tulsa, OK, USA, pp. I-463, doi: https://doi.org/10.1109/MWSCAS.2002.1187258 .
Anusuya MA, Katti SK (2011) Front end analysis of speech recognition: a review. Int J Speech Technol 14(2):99–145
Anusuya MA, Katti SK (2011) Comparison of different speech feature extraction techniques with and without wavelet transform to Kannada speech recognition. Int J Comput Appl 26(4):19–24
Atmaja BT, Akagi M (2020) Deep multilayer Perceptrons for dimensional speech emotion recognition. arXiv preprint arXiv:2004.02355.
Bahl LR, Brown PF, de Souza PV, Mercer RL (1989) A tree-based statistical language model for natural language speech recognition. IEEE Trans Acoust Speech Signal Process 37(7):1001–1008
Barker J, Watanabe S, Vincent E, Trmal J (2018) The fifth’CHiME’speech separation and recognition challenge: dataset, task and baselines. arXiv preprint arXiv:1803.10609.
Batuwita R, Palade V (2010) FSVM-CIL: fuzzy support vector machines for class imbalance learning. IEEE Trans Fuzzy Syst 18(3):558–571
Baum LE, Eagon JA (1967) An inequality with applications to statistical estimation for probabilistic functions of Markov processes and to a model for ecology. Bull Am Math Soc 73(3):360–363
MathSciNet MATH Google Scholar
Bernardo JM, Bayarri MJ, Berger JO, Dawid AP, Heckerman D, Smith AFM, West M (2007) Generative or discriminative? Getting the best of both worlds. Bayesian stat 8(3):3–24
MathSciNet Google Scholar
Besacier L, Barnard E, Karpov A, Schultz T (2014) Automatic speech recognition for under-resourced languages: a survey. Speech Comm 56:85–100
Birkenes O, Matsui T, Tanabe K, Siniscalchi SM, Myrvoll TA, Johnsen MH (2009) Penalized logistic regression with HMM log-likelihood regressors for speech recognition. IEEE Trans Audio Speech Lang Process 18(6):1440–1454
Bourlard H A, Morgan N (2012). Connectionist speech recognition: a hybrid approach (Vol. 247). Springer Science & Business Media.
Bu H, Du J, Na X, Wu B, Zheng H (2017). Aishell-1: an open-source mandarin speech corpus and a speech recognition baseline. In 2017 20th conference of the oriental chapter of the international coordinating committee on speech databases and speech I/O systems and assessment (O-COCOSDA) (pp. 1-5). IEEE.
Busso C, Bulut M, Lee CC, Kazemzadeh A, Mower E, Kim S, Chang JN, Lee S, Narayanan SS (2008) IEMOCAP: interactive emotional dyadic motion capture database. Lang Resour Eval 42(4):335–359
Campos MM, Carpenter GA (1998) WSOM: building adaptive wavelets with self-organizing maps. In 1998 IEEE international joint conference on neural networks proceedings. IEEE world congress on computational intelligence (cat. No. 98CH36227) (Vol. 1, pp. 763-767). IEEE
Chan W, Jaitly N, Le Q, Vinyals O (2016) Listen, attend and spell: a neural network for large vocabulary conversational speech recognition. In 2016 IEEE international conference on acoustics, speech and signal processing (ICASSP) (pp. 4960-4964). IEEE.
Chang T H, Luo Z Q, Deng L, Chi C Y (2008) A convex optimization method for joint mean and variance parameter estimation of large-margin CDHMM. In 2008 IEEE international conference on acoustics, speech and signal processing (pp. 4053-4056). IEEE.
Chen C P, Bilmes J, Ellis D P (2005) Speech feature smoothing for robust ASR. In proceedings.(ICASSP'05). IEEE international conference on acoustics, speech, and signal processing, 2005. (Vol. 1, pp. I-525). IEEE.
Cheng O, Abdulla W, Salcic Z (2005) Performance evaluation of front-end processing for speech recognition systems. The University of Auckland.
Chiu, C. C., Sainath, T. N., Wu, Y., Prabhavalkar, R., Nguyen, P., Chen, Z., ..., Jaitly, N. (2018) State-of-the-art speech recognition with sequence-to-sequence models. In 2018 IEEE International Conference on Acoustics, Speech and Signal Processing (ICASSP) (pp. 4774–4778). IEEE.
Chow Y, Dunham M, Kimball O, Krasner M, Kubala G, Makhoul J, ..., Schwartz R (1987) BYBLOS: The BBN continuous speech recognition system. In ICASSP'87. IEEE International Conference on Acoustics, Speech, and Signal Processing (Vol. 12, pp. 89–92). IEEE
Chow YL, Schwartz R (1989) The n-best algorithm: an efficient procedure for finding top n sentence hypotheses. In proceedings of the workshop on speech and natural language (pp. 199-202). Association for Computational Linguistics
Clarkson P, Moreno PJ (1999) On the use of support vector machines for phonetic classification. In 1999 IEEE international conference on acoustics, speech, and signal processing. Proceedings. ICASSP99 (cat. No. 99CH36258) (Vol. 2, pp. 585-588). IEEE
Coifman R R, Meyer Y, Wickerhauser V (1992) Wavelet analysis and signal processing. In In Wavelets and their applications.
Collobert R, Puhrsch C, Synnaeve G (2016) Wav2letter: an end-to-end convnet-based speech recognition system. arXiv preprint arXiv:1609.03193.
Cortes C, Vapnik V (1995) Support-vector networks. Mach Learn 20(3):273–297
MATH Google Scholar
Crouse MS, Nowak RD, Baraniuk RG (1998) Wavelet-based statistical signal processing using hidden Markov models. IEEE Trans Signal Process 46(4):886–902
Cutajar M, Gatt E, Grech I, Casha O, Micallef J (2013) Comparative study of automatic speech recognition techniques. IET Signal Proc 7(1):25–46
Cutajar M, Gatt E, Micallef J, Grech I, Casha O (2010) Digital hardware implementation of self-organising maps. In Melecon 2010-2010 15th IEEE Mediterranean Electrotechnical conference (pp. 1123-1128). IEEE
Dansena D K, Rathore Y A Survey Paper on Automatic Speech Recognition by Machine
Davis KH, Biddulph R, Balashek S (1952) Automatic recognition of spoken digits. J Acoust Soc Am 24(6):637–642
Deshmukh N, Picone J (1995) Methodologies for language modeling and search in continuous speech recognition. In proceedings IEEE Southeastcon’95. Visualize the future (pp. 192-198). IEEE
Du X P, He P L (2006) The clustering solution of speech recognition models with SOM. In international symposium on neural networks (pp. 150-157). Springer, Berlin, Heidelberg.
Duan KB, Keerthi SS (2005) Which is the best multiclass SVM method? An empirical study. In international workshop on multiple classifier systems (pp. 278-285). Springer, Berlin, Heidelberg
Dumitru C O, Gavat I (2006) A comparative study of feature extraction methods applied to continuous speech recognition in romanian language. In proceedings ELMAR 2006 (pp. 115-118). IEEE.
Fontaine V, Ris C, Leich H (1996) Nonlinear discriminant analysis with neural networks for speech recognition. In 1996 8th European signal processing conference (EUSIPCO 1996) (pp. 1-4). IEEE.
Forgie JW, Forgie CD (1959) Results obtained from a vowel recognition computer program. J Acoust Soc Am 31(11):1480–1489
Forsberg M (2003) Why is speech recognition difficult. Chalmers University of Technology.
Friedman JH (1996) Another approach to polychotomous classification. Statistics Department, Stanford University, Technical Report
Gaikwad SK, Gawali BW, Yannawar P (2010) A review on speech recognition technique. Int J Comput Appl 10(3):16–24
Gamulkiewicz B, Weeks M (2003) Wavelet based speech recognition. In 2003 46th Midwest symposium on circuits and systems (Vol. 2, pp. 678-681). IEEE.
Ganapathy S, Thomas S, Hermansky H (2009) Modulation frequency features for phoneme recognition in noisy speech. J Acoust Soc Am 125(1):EL8–EL12
Garofolo JS (1993) TIMIT acoustic phonetic continuous speech corpus. Linguist Data Consortium 1993
Graves A, Fernández S, Gomez F, Schmidhuber J (2006) Connectionist temporal classification: labelling unsegmented sequence data with recurrent neural networks. In proceedings of the 23rd international conference on machine learning (pp. 369-376)
Gupta M, Gilbert A (2001) Robust speech recognition using wavelet coefficient features. In IEEE workshop on automatic speech recognition and understanding, 2001. ASRU'01. (pp. 445-448). IEEE.
Hai J, Joo E M (2003) Improved linear predictive coding method for speech recognition. In fourth international conference on information, communications and signal processing, 2003 and the fourth Pacific rim conference on multimedia. Proceedings of the 2003 joint (Vol. 3, pp. 1614-1618). IEEE.
Halabi N (2016) Modern standard arabic phonetics for speech synthesis (Doctoral dissertation, University of Southampton).
Hannun A, Case C, Casper J, Catanzaro B, Diamos G, Elsen E, ..., Ng A Y (2014) Deep speech: Scaling up end-to-end speech recognition. arXiv preprint arXiv:1412.5567.
Hardy RL (1971) Multiquadric equations of topography and other irregular surfaces. J Geophys Res 76(8):1905–1915
Helmi N, Helmi BH (2008) Speech recognition with fuzzy neural network for discrete words. In 2008 fourth international conference on natural computation (Vol. 7, pp. 265-269). IEEE
Hemakumar G, Punitha P (2013) Speech recognition technology: a survey on Indian languages. Int J Inf Sci Intell Syst 2(4):1–38
Hennebert J, Hasler M, Dedieu H (1994) Neural networks in speech recognition. Department of Electrical Engineering, Swiss Federal Institute of Technology, 1015.
Hermansky H (1990) Perceptual linear predictive (PLP) analysis of speech. The. J Acoust Soc Am 87(4):1738–1752
Hermansky H, Morgan N (1994) RASTA processing of speech. IEEE Trans Speech Audio Process 2(4):578–589
Hermansky H, Morgan N, Bayya A, Kohn P (1991) RASTA-PLP speech analysis. In Proc. IEEE Int’l Conf. Acoustics, speech and signal processing (Vol. 1, pp. 121-124).
Hou X (2009) Noise robust speech recognition based on wavelet-RBF neural network. In PIAGENG 2009: intelligent information, control, and communication Technology for Agricultural Engineering (Vol. 7490, p. 74902O). International Society for Optics and Photonics
Hsu CW, Lin CJ (2002) A comparison of methods for multiclass support vector machines. IEEE Trans Neural Netw 13(2):415–425
Hu X, Zhan L, Xue Y, Zhou W, Zhang L (2011) Spoken arabic digits recognition based on wavelet neural networks. In 2011 IEEE international conference on systems, man, and cybernetics (pp. 1481-1485). IEEE.
Huang X, Alleva F, Hon HW, Hwang MY, Lee KF, Rosenfeld R (1993) The SPHINX-II speech recognition system: an overview. Comput Speech Lang 7(2):137–148
Huang X, Baker J, Reddy R (2014) A historical perspective of speech recognition. Commun ACM 57(1):94–103
Hung JW, Fan HT (2009) Subband feature statistics normalization techniques based on a discrete wavelet transform for robust speech recognition. IEEE Signal Process Lett 16(9):806–809
Hunt A, Favero R (1994) Using principal component analysis with wavelets in speech recognition. In SST Conf., ASSTA Inc., Perth (pp. 296-301).
Illina I, Gong Y (1996) Improvement in N-best search for continuous speech recognition. In proceeding of fourth international conference on spoken language processing. ICSLP'96 (Vol. 4, pp. 2147-2150). IEEE
Islam J, Mubassira M, Islam MR, Das AK (2019) A speech recognition system for Bengali language using recurrent neural network. In 2019 IEEE 4th international conference on computer and communication systems (ICCCS) (pp. 73-76). IEEE
Jiang H, Li X, Liu C (2006) Large margin hidden Markov models for speech recognition. IEEE Trans Audio Speech Lang Process 14(5):1584–1595
Juang BH, Rabiner LR (1991) Hidden Markov models for speech recognition. Technometrics 33(3):251–272
Juang B H, Rabiner L R (2005) Automatic speech recognition–a brief history of the technology development. Georgia Institute of Technology. Atlanta Rutgers University and the University of California. Santa Barbara, 1, 67.
Jung S, Son J, Bae K (2004) Feature extraction based on wavelet domain hidden Markov tree model for robust speech recognition. In Australasian joint conference on artificial intelligence (pp. 1154-1159). Springer, Berlin, Heidelberg.
Kaur P, Singh P, Garg V (2012) Speech recognition system; challenges and techniques. Int J Comput Sci Inf Technol 3(3):3989–3992
Kesarkar M P (2003) Feature extraction for speech recognition. Electronic systems, EE. Dept., IIT Bombay.
Khan A, Sohail A, Zahoora U, Qureshi AS (2020) A survey of the recent architectures of deep convolutional neural networks. Artif Intell Rev, 1–62
Köhn A, Stegen F, Baumann T (2016) Mining the spoken wikipedia for speech data and beyond. In proceedings of the tenth international conference on language resources and evaluation (LREC’16) (pp. 4644-4647).
Kohonen T (1982) Self-organized formation of topologically correct feature maps. Biol Cybern 43(1):59–69
Korba M C A, Messadeg D, Djemili R, Bourouba H (2008) Robust speech recognition using perceptual wavelet denoising and mel-frequency product spectrum cepstral coefficient features. Informatica, 32(3).
Kriman S, Beliaev S, Ginsburg B, Huang J, Kuchaiev O, Lavrukhin V, ..., Zhang Y (2020) Quartznet: Deep automatic speech recognition with 1d time-channel separable convolutions. In ICASSP 2020–2020 IEEE International Conference on Acoustics, Speech and Signal Processing (ICASSP) (pp. 6124–6128). IEEE
Krishnan VV, Anto PB (2009) Features of wavelet packet decomposition and discrete wavelet transform for malayalam speech recognition. Int J Recent Trends Eng 1(2):93
Krüger SE, Schafföner M, Katz M, Andelic E, Wendemuth A (2005) Speech recognition with support vector machines in a hybrid system. In Ninth European Conference on Speech Communication and Technology
Kupiec J (1989) Probabilistic models of short and long distance word dependencies in running text. In Speech and Natural Language: Proceedings of a Workshop Held at Philadelphia, Pennsylvania, February 21-23, 1989
Lamere P, Kwok P, Gouvea E, Raj B, Singh R, Walker W, ..., Wolf P (2003) The CMU SPHINX-4 speech recognition system. In IEEE Intl. Conf. on Acoustics, Speech and Signal Processing (ICASSP 2003), Hong Kong (Vol. 1, pp. 2–5)
Lawrence R (2008) Fundamentals of speech recognition. Pearson Education India.
Lazli L, Sellami M (2003) Connectionist probability estimators in HMM arabic speech recognition using fuzzy logic. In international workshop on machine learning and data Mining in Pattern Recognition (pp. 379-388). Springer, Berlin, Heidelberg.
Lee J Y, Hung J W (2011) Exploiting principal component analysis in modulation spectrum enhancement for robust speech recognition. In 2011 eighth international conference on fuzzy systems and knowledge discovery (FSKD) (Vol. 3, pp. 1947-1951). IEEE.
Lee A, Kawahara T, Shikano K (2001) Julius---an open source real-time large vocabulary recognition engine
Lekshmi KR, Elizabeth S (2016) Automatic speech recognition using different neural network architectures – a survey. Int J Comput Sci Inf Technol 7(6):2422–2427
Leung K F, Leung F H, Lam H K, Tam P K S (2003) Recognition of speech commands using a modified neural fuzzy network and an improved GA. In the 12th IEEE international conference on fuzzy systems, 2003. FUZZ’03. (Vol. 1, pp. 190-195). IEEE.
Li T F, Chang S C (2007) Speech recognition of mandarin syllables using both linear predict coding cepstra and Mel frequency cepstra. In ROCLING 2007 poster papers (pp. 379-390).
Lin CT (1996) Neural fuzzy systems: a neuro-fuzzy synergism to intelligent systems. Prentice hall PTR
Lin CF, Wang SD (2002) Fuzzy support vector machines. IEEE Trans Neural Netw 13(2):464–471
Liu X (2009) A new wavelet threshold denoising algorithm in speech recognition. In 2009 Asia-Pacific conference on information processing (Vol. 2, pp. 310-313). IEEE.
Lowerre BT (1976) The HARPY speech recognition system. CARNEGIE-MELLON UNIV PITTSBURGH PA DEPT OF COMPUTER SCIENCE
Maheswari NU, Kabilan AP, Venkatesh R (2010) A hybrid model of neural network approach for speaker independent word recognition. Int J Comput Theory Eng 2(6):912
Makino T, Liao H, Assael Y, Shillingford B, Garcia B, Braga O, Siohan O (2019) Recurrent neural network transducer for audio-visual speech recognition. In 2019 IEEE automatic speech recognition and understanding workshop (ASRU) (pp. 905-912). IEEE
Malekzadeh S, Gholizadeh M H, Razavi S N (2018). Persian vowel recognition with MFCC and ANN on PCVC speech dataset. arXiv preprint arXiv:1812.06953.
Mallat SG (1989) A theory for multiresolution signal decomposition: the wavelet representation. IEEE Trans Pattern Anal Mach Intell 11(7):674–693
Mehla R, Aggarwal R (2014) Automatic speech recognition: a survey. Int J Adv Res Comput Sci Electron Eng (IJARCSEE) 3(1):45–53
Messaoud Z B, Hamida A B (2010) CDHMM parameters selection for speaker-independent phone recognition in continuous speech system. In MELECON 2010-2010 15th IEEE Mediterranean Electrotechnical conference (pp. 253-258). IEEE.
Meyer Y (1993) Wavelets: Algorithms and Applications, SIAM, Philadelphia, 1993. MR 95f, 94005.
Milone DH, Di Persia LE (2008) Learning hidden Markov models with hidden Markov trees as observation distributions. Inteligencia artificial. Revista Iberoamericana de Inteligencia Artificial 12(37):7–13
Modic R, Lindberg B, Petek B (2003) Comparative wavelet and mfcc speech recognition experiments on the slovenian and english speechdat2. In ISCA tutorial and research workshop on non-linear speech processing
Mohamadpour M, Farokhi F (2009) A new approach for Persian speech recognition. In 2009 IEEE international advance computing conference (pp. 153-158). IEEE
Molau S, Pitz M, Schluter R, Ney H (2001) Computing mel-frequency cepstral coefficients on the power spectrum. In 2001 IEEE international conference on acoustics, speech, and signal processing. Proceedings (cat. No. 01CH37221) (Vol. 1, pp. 73-76). IEEE.
Morgan N, Bourlard H (1990). Continuous speech recognition using multilayer perceptrons with hidden Markov models. In international conference on acoustics, speech, and signal processing (pp. 413-416). IEEE
Mporas I, Ganchev T, Siafarikas M, Fakotakis N (2007) Comparison of speech features on the speech recognition task. J Comput Sci 3(8):608–616
Muller D N, De Siqueira M L, Navaux P O A (2006) A connectionist approach to speech understanding. In the 2006 IEEE international joint conference on neural network proceedings (pp. 3790-3797). IEEE.
Nataraj K S, Pandey P C, Shah M S (2011) Improving the consistency of vocal tract shape estimation. In 2011 National Conference on communications (NCC) (pp. 1-5). IEEE.
Nehe NS, Holambe RS (2009) New feature extraction techniques for Marathi digit recognition. Int J Recent Trends Eng 2(2):22
Nehe NS, Holambe RS (2012) DWT and LPC based feature extraction methods for isolated word recognition. EURASIP J Audio Speech Music Process 2012(1):7
Nguyen P, Heigold G, Zweig G (2010) Speech recognition with flat direct models. IEEE J Sel Top Sign Proces 4(6):994–1006
Nouza J, Zdansky J, Cerva P (2010) System for automatic collection, annotation and indexing of Czech broadcast speech with full-text search. In MELECON 2010–2010 15th IEEE Mediterranean Electrotechnical Conference (pp. 202–205). IEEE
O’Shaughnessy D (2008) Automatic speech recognition: history, methods and challenges. Pattern Recogn 41(10):2965–2979
O'Shaughnessy D (1988) Linear predictive coding. IEEE potentials 7(1):29–32
O'Shaughnessy D (2003) Interacting with computers by voice: automatic speech recognition and synthesis. Proc IEEE 91(9):1272–1305
Pallett DS, Fiscus JG, Garofolo JS (1990) DARPA resource management. In speech and natural language: proceedings of a workshop held at Hidden Valley, Pennsylvania, June 24-27, 1990 (p. 298). Morgan Kaufmann pub
Panayotov V, Chen G, Povey D, Khudanpur S (2015) Librispeech: an asr corpus based on public domain audio books. In 2015 IEEE international conference on acoustics, speech and signal processing (ICASSP) (pp. 5206-5210). IEEE.
Paul AK, Das D, Kamal MM (2009) Bangla speech recognition system using LPC and ANN. In 2009 seventh international conference on advances in pattern recognition (pp. 171-174). IEEE
Paulson LD (2006) Speech recognition moves from software to hardware. Computer 39(11):15–18
Picone JW (1993) Signal modeling techniques in speech recognition. Proc IEEE 81(9):1215–1247
Ping Z, Li-Zhen T, Dong-Feng X (2009) Speech recognition algorithm of parallel subband HMM based on wavelet analysis and neural network. Inf Technol J 8(5):796–800
Polikar R (1996) The wavelet tutorial.
Povey D, Ghoshal A, Boulianne G, Burget L, Glembek O, Goel N, ..., Silovsky J (2011) The Kaldi speech recognition toolkit. In IEEE 2011 workshop on automatic speech recognition and understanding (No. CONF). IEEE Signal Process Soc
Rabiner LR (1989) A tutorial on hidden Markov models and selected applications in speech recognition. Proc IEEE 77(2):257–286
Rabiner L, Juang B H (1993) Fundamental of speech recognition prentice-hall international.
Rabiner L, Levinson S (1981) Isolated and connected word recognition-theory and selected applications. IEEE Trans Commun 29(5):621–659
Radha V, Vimala C (2012) A review on speech recognition challenges and approaches. Doaj Org 2(1):1–7
Ranjan S (2010) A discrete wavelet transform based approach to Hindi speech recognition. In 2010 international conference on signal acquisition and processing (pp. 345-348). IEEE.
Rosenblatt F (1961). Principles of neurodynamics. Perceptrons and the theory of brain mechanisms (no. VG-1196-G-8). Cornell aeronautical lab Inc Buffalo NY
Rosenfeld R (1994) A hybrid approach to adaptive statistical language modeling. CARNEGIE-MELLON UNIV PITTSBURGH PA SCHOOL OF COMPUTER SCIENCE
Rosenfeld R, Huang X (1992) Improvements in stochastic language modeling. In Speech and Natural Language: Proceedings of a Workshop Held at Harriman, New York, February 23-26, 1992
Rousseau A, Deléglise P, Esteve Y (2012) TED-LIUM: an automatic speech recognition dedicated corpus. In LREC (pp. 125-129).
Rybach D, Gollan C, Heigold G, Hoffmeister B, Lööf J, Schlüter R, Ney H (2009) The RWTH Aachen University open source speech recognition system. In Tenth Annual Conference of the International Speech Communication Association
Sabah R, Ainon RN (2009) Isolated digit speech recognition in Malay language using neuro-fuzzy approach. In 2009 third Asia international conference on Modelling & Simulation (pp. 336-340). IEEE
Saeed TR, Salman J, Ali AH (2019) Classification improvement of spoken arabic language based on radial basis function. Int J Electr Comput Eng 9(1):2088–8708
Saha G, Chakroborty S, Senapati S (2005) A new silence removal and endpoint detection algorithm for speech and speaker recognition applications. In proceedings of the NCC (pp. 56-61).
Sainath TN, Pang R, Rybach D, He Y, Prabhavalkar R, Li W, ..., McGraw I (2019) Two-pass end-to-end speech recognition. arXiv preprint arXiv:1908.10992
Sak H, Senior A, Rao K, Beaufays F (2015) Fast and accurate recurrent neural network acoustic models for speech recognition. arXiv preprint arXiv:1507.06947.
Sakoe H, Chiba S (1978) Dynamic programming algorithm optimization for spoken word recognition. IEEE Trans Acoust Speech Signal Process 26(1):43–49
Sárosi G, Mozsáry M, Mihajlik P, Fegyó T (2011) Comparison of feature extraction methods for speech recognition in noise-free and in traffic noise environment. In 2011 6th conference on speech technology and human-computer dialogue (SpeD) (pp. 1-8). IEEE.
Sayers C (1991). Self organizing feature maps and their applications to robotics
Sha F, Saul LK (2007) Large margin hidden Markov models for automatic speech recognition. In advances in neural information processing systems (pp. 1249-1256)
Shanthi TS, Lingam C (2013) Review of feature extraction techniques in automatic speech recognition. Int J Sci Eng Technol 2(6):479–484
Shewalkar A, Nyavanandi D, Ludwig SA (2019) Performance evaluation of deep neural networks applied to speech recognition: RNN, LSTM and GRU. J Artif Intel Soft Comput Res 9(4):235–245
Singh MT, Fayjie AR, Kachari B (2015) A survey report on speech recognition system. Int J Comput Appl 121(11)
Sivaram GS, Hermansky H (2011) Multilayer perceptron with sparse hidden outputs for phoneme recognition. In 2011 IEEE international conference on acoustics, speech and signal processing (ICASSP) (pp. 5336-5339). IEEE
Sivaram GS, Hermansky H (2011) Sparse multilayer perceptron for phoneme recognition. IEEE Trans Audio Speech Lang Process 20(1):23–29
Smaragdis P, Radhakrishnan R, Wilson K W (2009) Context extraction through audio signal analysis. In multimedia content analysis (pp. 1–34). Springer, Boston, MA
Solera-Ureña R, Padrell-Sendra J, Martín-Iglesias D, Gallardo-Antolín A, Peláez-Moreno C, Díaz-de-María F (2007) Svms for automatic speech recognition: a survey. In Progress in nonlinear speech processing (pp. 190–216). Springer, Berlin, Heidelberg
Sonkamble BA, Doye DD, Sonkamble S, PICT P, MMCOE P (2009) An efficient use of support vector machines for speech signal classification. In Proc eighth WSEAS Int Conf computational intelligence., man-machine systems and cybernetics (pp. 117-120)
Sukumar AR, Shah AF, Anto PB (2010) Isolated question words recognition from speech queries by using artificial neural networks. In 2010 second international conference on computing, communication and networking technologies (pp. 1-4). IEEE.
Tang X (2009) Hybrid hidden Markov model and artificial neural network for automatic speech recognition. In 2009 Pacific-Asia conference on circuits, communications and systems (pp. 682-685). IEEE.
Tang H, Meng CH, Lee LS (2010) An initial attempt for phoneme recognition using structured support vector machine (SVM). In 2010 IEEE international conference on acoustics, speech and signal processing (pp. 4926-4929). IEEE
Tavanaei A, Manzuri M T, Sameti H (2011) Mel-scaled discrete wavelet transform and dynamic features for the Persian phoneme recognition. In 2011 international symposium on artificial intelligence and signal processing (AISP) (pp. 138-140). IEEE.
Thubthong N, Kijsirikul B (2001) Support vector machines for Thai phoneme recognition. Int J Uncertainty Fuzziness Knowledge Based Syst 9(06):803–813
Toshniwal S, Sainath T N, Weiss R J, Li B, Moreno P, Weinstein E, Rao K (2018) Multilingual speech recognition with a single end-to-end model. In 2018 IEEE international conference on acoustics, speech and signal processing (ICASSP) (pp. 4904-4908). IEEE.
Tóth L (2011) A hierarchical, context-dependent neural network architecture for improved phone recognition. In 2011 IEEE International Conference on Acoustics, Speech and Signal Processing (ICASSP) (pp. 5040–5043). IEEE
Trentin E, Gori M (2001) A survey of hybrid ANN/HMM models for automatic speech recognition. Neurocomputing 37(1–4):91–126
Trentin E, Gori M (2003) Robust combination of neural networks and hidden Markov models for speech recognition. IEEE Trans Neural Netw 14(6):1519–1531
Umarani SD, Raviram P, Wahidabanu RSD (2009) Implementation of HMM and radial basis function for speech recognition. In 2009 international conference on Intelligent Agent & Multi-Agent Systems (pp. 1-4). IEEE
Vadwala AY, Suthar KA, Karmakar YA, Pandya N (2017) Survey paper on different speech recognition algorithm: challenges and techniques. Int J Comput Appl 175(1):31–36
Vapnik V (2013) The nature of statistical learning theory. Springer science & business media
Veaux C, Yamagishi J, MacDonald K (2016) Superseded-cstr vctk corpus: English multi-speaker corpus for cstr voice cloning toolkit.
Veisi H, Sameti H (2011) The integration of principal component analysis and cepstral mean subtraction in parallel model combination for robust speech recognition. Digital Signal Process 21(1):36–53
Velichko VM, Zagoruyko NG (1970) Automatic recognition of 200 words. Int J Man Mach Stud 2(3):223–234
Venkateswarlu R L K, Kumari R V (2011) Novel approach for speech recognition by using self—organized maps. In 2011 international conference on emerging trends in networks and computer communications (ETNCC) (pp. 215-222). IEEE.
Venkateswarlu RLK, Kumari RV, Jayasri GV (2011) Speech recognition using radial basis function neural network. In 2011 3rd international conference on electronics computer technology (Vol. 3, pp. 441-445). IEEE
Walker SL, Foo SY (2003) Optimal wavelets for speech signal representations. J Syst Cybern Inform 1(4):44–46
Wang Y, Han K, Wang D (2012) Exploring monaural features for classification-based speech segregation. IEEE Trans Audio Speech Lang Process 21(2):270–279
Wang Y, Wang S, Lai KK (2005) A new fuzzy support vector machine to evaluate credit risk. IEEE Trans Fuzzy Syst 13(6):820–831
Wang D, Wang X, Lv S (2019) End-to-end mandarin speech recognition combining CNN and BLSTM. Symmetry 11(5):644
Wang B, Yin Y, Lin H (2020) Attention-based transducer for online speech recognition. arXiv preprint arXiv:2005.08497
Weston J, Watkins C (1998) Multi-class support vector machines (pp. 98-04). Technical report CSD-TR-98-04, Department of Computer Science, Royal Holloway, University of London, may
Weston J, Watkins C (1999) Support vector machines for multi-class pattern recognition. In Esann (Vol. 99, pp. 219-224)
Wijoyo S, Wijoyo S (2011) Speech recognition using linear predictive coding and artificial neural network for controlling movement of mobile robot. In proceedings of 2011 international conference on information and electronics engineering (ICIEE 2011) (pp. 28-29).
Woodland PC, Leggetter CJ, Odell JJ, Valtchev V, Young SJ (1995) The 1994 HTK large vocabulary speech recognition system. In 1995 international conference on acoustics, speech, and signal processing (Vol. 1, pp. 73-76). IEEE
Yegnanarayana B, Veldhuis RN (1998) Extraction of vocal-tract system characteristics from speech signals. IEEE Trans Speech Audio Process 6(4):313–327
Yu H, Xie T, Paszczynski S, Wilamowski BM (2011) Advantages of radial basis function networks for dynamic system design. IEEE Trans Ind Electron 58(12):5438–5450
Zamani B, Akbari A, Nasersharif B, Jalalvand A (2011) Optimized discriminative transformations for speech features based on minimum classification error. Pattern Recogn Lett 32(7):948–955
Zhao Y, Wakita H, Zhuang X (1991) An HMM based speaker-independent continuous speech recognition system with experiments on the TIMIT DATABASE. In acoustics, speech, and signal processing, IEEE international conference on (pp. 333-336). IEEE computer society
Download references
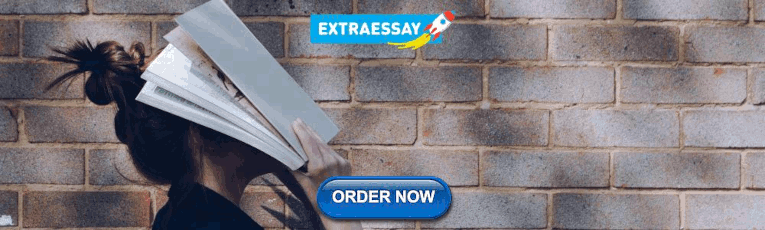
Author information
Authors and affiliations.
Punjab University College of Information Technology (PUCIT), Lahore, Pakistan
Mishaim Malik
Faculty of Punjab University College of Information Technology (PUCIT), Lahore, Pakistan
Muhammad Kamran Malik
School of Engineering and Information Technology, University of New South Wales (UNSW) Canberra at ADFA, Canberra, Australia
Khawar Mehmood
Faculty of Engineering and IT, University of Technology Sydney, Ultimo, Australia
Imran Makhdoom
You can also search for this author in PubMed Google Scholar
Corresponding author
Correspondence to Mishaim Malik .
Ethics declarations
Conflict of interest, declarations.
Not applicable.
Additional information
Publisher’s note.
Springer Nature remains neutral with regard to jurisdictional claims in published maps and institutional affiliations.
Rights and permissions
Reprints and permissions
About this article
Malik, M., Malik, M.K., Mehmood, K. et al. Automatic speech recognition: a survey. Multimed Tools Appl 80 , 9411–9457 (2021). https://doi.org/10.1007/s11042-020-10073-7
Download citation
Received : 31 May 2020
Revised : 04 September 2020
Accepted : 13 October 2020
Published : 10 November 2020
Issue Date : March 2021
DOI : https://doi.org/10.1007/s11042-020-10073-7
Share this article
Anyone you share the following link with will be able to read this content:
Sorry, a shareable link is not currently available for this article.
Provided by the Springer Nature SharedIt content-sharing initiative
- Speech recognition
- Automatic speech recognition
- Feature extraction
- Classification models
- Language models
- Find a journal
- Publish with us
- Track your research
Academia.edu no longer supports Internet Explorer.
To browse Academia.edu and the wider internet faster and more securely, please take a few seconds to upgrade your browser .
Enter the email address you signed up with and we'll email you a reset link.
- We're Hiring!
- Help Center
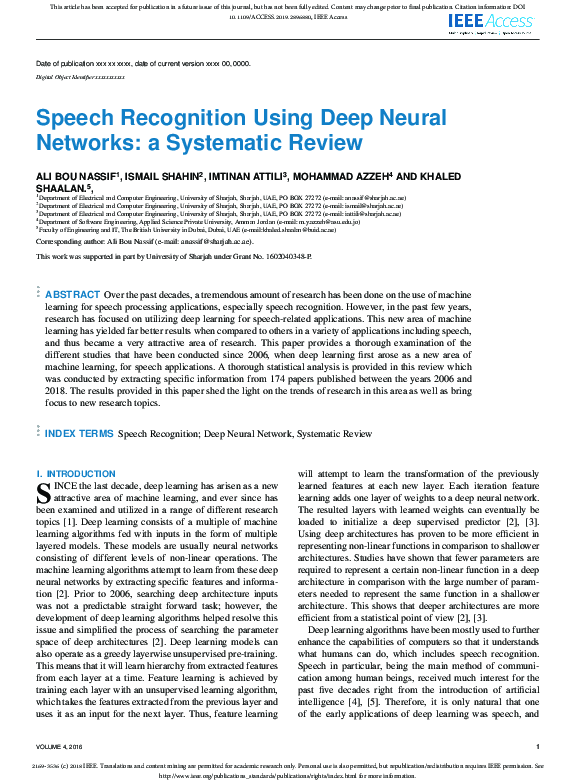
Speech Recognition Using Deep Neural Networks: a Systematic Review

IEEE Access
Related Papers
International journal for research in applied science and engineering technology ijraset
IJRASET Publication
Speech recognition is the method of translating spoken words into text. The speech recognition process digitizes the sound waves into basic language units. Speech recognition is one of the most used technologies in today’s life. This technology can be seen everywhere around a person, for example in phones, games, etc. The main purpose of the paper is to know the knowledge and the technology behind this superb invention.
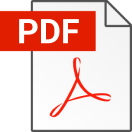
International Journal of Advanced Research in Computer Science and Software Engineering
Banumathi Manickaraj
International Journal for Research in Applied Science and Engineering Technology IJRASET
Speech is the essential method of communication between human beings. Several researches are done on the use of machine learning for speech recognition. Speech recognition mechanisms of converting the recorded speech signals into the text are one of the challenging task. In this paper a framework for speech recognition is proposed.
Rama Krishna Dyava
International Journal of Advanced Computer Science and Applications
Ramzan Talib
It is a difficult task of continuous automatic speech recognition, translating of spoken words into text due to the excessive viability in speech signals. In recent years speech recognition has been accomplishing pinnacle of success however it still has few limitations to overcome. Deep learning also known as representation learning or sometimes referred as unsupervised feature learning, is a subset of machine learning. Deep learning is becoming a conventional technology for speech recognition and has efficiently replaced Gaussian mixtures for speech recognition on a global scale. The predominant goal of this undertaking is to apply deep learning algorithms, together with Deep Neural Networks (DNN) and Deep Belief Networks (DBN), for automatic non-stop speech recognition. Keywords: Gaussian Mixture Model (GMM), Hidden Markov Models (HMMs), Deep Neural Networks (DNN), Deep Belief Networks (DBN).
Elizabeth Sherly
Speech is the vocalized form of communication based on lexical syntax. Each spoken word is a phonetic combination of vowels and consonants. Automatic Speech Recognition can be defined as computer-driven transcriptions of speech into human readable text. As it is an emerging technique many researchers are attracted to this and achieved progress to a certain extent in recent years. This survey paper aims at explaining the architecture of Deep Neural Network, Convolutional Neural Network and Recurrent Neural Network and their performance in the field of Automatic Speech Recognition. We also summarise main contributions of various researchers during 2010-2016 on Acoustic Modeling and Language Modeling (main components of Automatic Speech Recognition) using these architectures and pointing out their impact in ASR. We conclude this paper with a comparative study regarding the advantages of the architectures discussed during the survey with respect to Word Error Rate (WER), Phone Error Rat...
Süleyman Demirel Üniversitesi Fen Bilimleri Enstitüsü Dergisi
Ussen Kimanuka
IEEE Signal Processing Magazine
Abdelrahman Mohamed
IEEE/CAA J. Autom. Sinica
In this paper, we summarize recent progresses made in deep learning based acoustic models and the motivation and insights behind the surveyed techniques. We first discuss models such as recurrent neural networks (RNNs) and convolutional neural networks (CNNs) that can effectively exploit variablelength contextual information, and their various combination with other models. We then describe models that are optimized end-to-end and emphasize on feature representations learned jointly with the rest of the system, the connectionist temporal classification (CTC) criterion, and the attention-based sequenceto-sequence translation model. We further illustrate robustness issues in speech recognition systems, and discuss acoustic model adaptation, speech enhancement and separation, and robust training strategies. We also cover modeling techniques that lead to more efficient decoding and discuss possible future directions in acoustic model research.
RELATED PAPERS
Belva Delvara
Linda Lundmark
AIDS Research and Human Retroviruses
Kevin Ewans
Polymer Science Series A
Halina pawlak kruczek
Anita Čančar
Noel Constantino
Education and Information Technologies
Muhammad Raees
Veterinary microbiology
JOBIN THOMAS
İslam Ekonomisi ve Finansı Dergisi (İEFD)
Ibrahim Güran Yumuşak
Campo Territorio Revista De Geografia Agraria
Virginia E Etges
Brain Research
Amnon Sintov
Yıldıray Sipahi
Current Pharmaceutical Design
Pierre Legrain
Jurnal Plastik Rekonstruksi
fory fortuna
Jurnal Kepariwisataan
Andre Hanoo
Journal of Clinical and Translational Science
Ningyan Zhang
Journal of Medical Internet Research
Harneel Kaur
Scientific Reports
Hamzah Sakidin
SSRN Electronic Journal
Orlando Ferreira
- We're Hiring!
- Help Center
- Find new research papers in:
- Health Sciences
- Earth Sciences
- Cognitive Science
- Mathematics
- Computer Science
- Academia ©2024
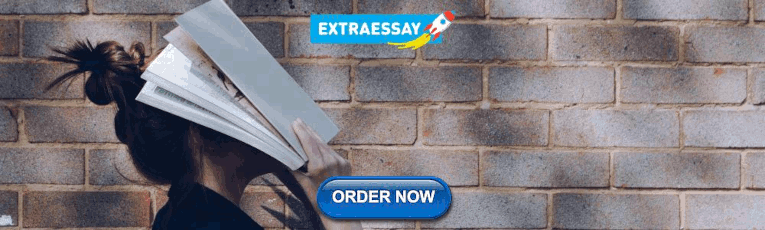
COMMENTS
A huge amount of research has been done in the field of speech signal processing in recent years. In particular, there has been increasing interest in the automatic speech recognition (ASR) technology field. ASR began with simple systems that responded to a limited number of sounds and has evolved into sophisticated systems that respond fluently to natural language. This systematic review of ...
Recently, the speech community is seeing a significant trend of moving from deep neural network based hybrid modeling to end-to-end (E2E) modeling for automatic speech recognition (ASR). While E2E models achieve the state-of-the-art results in most benchmarks in terms of ASR accuracy, hybrid models are still used in a large proportion of commercial ASR systems at the current time. There are ...
Title: End-to-End Speech Recognition: A Survey Authors: Rohit Prabhavalkar , Takaaki Hori , Tara N. Sainath , Ralf Schlüter , Shinji Watanabe View a PDF of the paper titled End-to-End Speech Recognition: A Survey, by Rohit Prabhavalkar and 4 other authors
ASR can be defined as the process of deriving the. transcription of speech, known as a word sequence, in which. the focus is on the shape of the speech wave [1]. In actuality, speech recognition ...
Before researching this topic, a literature review is performed to determine the cutting edge technologies in this field. In this regard, IEEE, arxiv.org, Microsoft Academic, and Google Scholar were used to search and obtain the papers relevant to the research domain.Most of the relevant scientific seed words were first identified using the generic words and their synonyms related to the domain.
ABSTRACT Over the past decades, a tremendous amount of research has been done on the use of machine. learning for speech processing applications, especially speech recognition. However, in the ...
A. Definition of speech recognition: Speech Recognition (is also known as Automatic Speech Recognition (ASR), or computer speech recognition) is the process of converting a speech signal to a sequence of words, by means of an algorithm implemented as a computer program. 1.2 Basic Model of Speech Recognition: Research in speech processing and ...
A key source of emotional information is the spoken expression, which may be part of the interaction between the human and the machine. Speech emotion recognition (SER) is a very active area of research that involves the application of current machine learning and neural networks tools. This ongoing review covers recent and classical approaches ...
Conference Call for Papers The ASRU Workshop is a flagship event of the IEEE Speech and Language Processing Technical Committee. The workshop is held every two years and has a tradition of bringing together researchers from academia and industry in an intimate and collegial setting to discuss problems of common interest in automatic speech recognition and understanding.
Also, 20% of the speech recognition papers were classified as other since they include sub areas that have a less than 1% publication percentage. C. RESEARCH QUESTION 3 To train and test algorithms, several databases were used in the research papers. Some were private while the majority of the databases, at 83%, were public and available on the ...
In this paper, we propose a simple, yet efficient, method for speech to text recognition based on a machine learning approach, using a Romanian speech corpus. View full-text Article
The research work presented in this paper describes an easy and effective method for speech recognition. ... IEEE-International Conference on ... Speech recognition is the process by which ...
2. Automatic Speech Recognition. Automatic speech recognition is one of the most automatic speech processing areas, allowing the machine to understand the. user's speech and convert it into a ...