Standard Oil as a Technological Innovator
- Published: 27 February 2011
- Volume 38 , pages 225–233, ( 2011 )
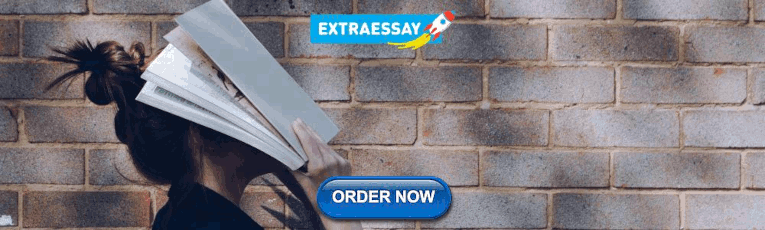
Cite this article
- F. M. Scherer 1
299 Accesses
2 Citations
Explore all metrics
A century ago, in 1911, the U.S. Supreme Court issued its path-breaking decision in the monopolization case against the Standard Oil Companies. Standard pleaded inter alia that its near-monopoly position was the result of superior innovation, citing in particular the Frasch-Burton process for refining the high-sulfur oil found around Lima, Ohio. This paper examines the role of Hermann Frasch in inventing and developing the desulfurization process, showing that Standard failed to recognize his inventive genius when he was its employee and purchased his rights and services only after he had applied it in his own Canadian company.
This is a preview of subscription content, log in via an institution to check access.
Access this article
Price includes VAT (Russian Federation)
Instant access to the full article PDF.
Rent this article via DeepDyve
Institutional subscriptions
Similar content being viewed by others
Technology Use in Traditional Industries: Catalysts for Petroleum Refining
Insights into the Fundamentals and Principles of the Oil and Gas Industry: The Impact of Nanotechnology
Homo Energeticus: Technological Rationality in the Alberta Tar Sands
Chandler A. D. (1990) Scale and scope: The dynamics of industrial capitalism. Harvard University Press, Cambridge
Google Scholar
de Chazeau M. G., Kahn A. (1959) Integration and competition in the petroleum industry. Yale University Press, New York
Dictionary of American biography. (1931). New York: Scribner.
Enos J. L. (1962) Petroleum progress and profits: A history of process innovations. MIT Press, Cambridge
Frasch H. (1912) Address of acceptance. Journal of Industrial and Engineering Chemistry 4: 134–140
Article Google Scholar
Gibb G. S., Knowlton E. (1956) History of Standard Oil Company: The resurgent years 1911–1927. Harper, New York
Hawthorne R. (1999) Biography of Herman Frasch. In: Garraty J., Carnes M. (eds) American national biography. Oxford University Press, New York
Heiss L. (1942) Der Schwefelkoenig von Louisiana (The Sulfur King of Louisiana). Ensslin & Laiblin, Reutlingen, Germany
Hidy R., Hidy M. (1955) Pioneering in big business. Harper, New York
Maclaurin W. R. (1949) Invention and innovation in the radio industry. Macmillan, New York
May C., Sell S. (2006) Intellectual property rights: A critical history. Lynne Rienner, Boulder, CO
Moody J. (1904) The truth about the trusts. Moody Publishing Co., New York
Nevins A. (1953) Study in power: John D. Rockefeller, industrialist and philanthropist (Vol. 2). Scribner, New York
Scherer F. M. (1996) Industry structure, strategy, and public policy. HarperCollins, New York
Scherer, F. M. (2008). Technological innovation and monopolization. In W. D. Collins (Ed.), Issues in competition law and policy (Vol. 2, pp. 1033–1069). Chicago: American Bar Association.
Schmookler, J. (undated). Statistics of patents classified by industry, United States, 1837–1957 . Privately published at the University of Minnesota.
U.S. Bureau of Corporations. (1907). Report of the Commissioner of Corporations on the petroleum industry . Washington.
U.S. Bureau of the Census. (1960). Historical statistics of the United States: Colonial times to 1957 . Washington.
U.S. Patent and Trademark Office. (1875–1899). Index of Patents . Washington, annually.
Williamson H. F., Daum A. (1959) The American petroleum industry: The age of illumination. Northwestern University Press, Evanston
Yergin D. (1991) The prize. Simon & Schuster, New York
Download references
Author information
Authors and affiliations.
John F. Kennedy School of Government, Harvard University, Cambridge, MA, 02138, USA
F. M. Scherer
You can also search for this author in PubMed Google Scholar
Corresponding author
Correspondence to F. M. Scherer .
Additional information
This paper is derived with extensive augmentation and amendment from Scherer ( 2008 ).
Rights and permissions
Reprints and permissions
About this article
Scherer, F.M. Standard Oil as a Technological Innovator. Rev Ind Organ 38 , 225–233 (2011). https://doi.org/10.1007/s11151-011-9283-y
Download citation
Published : 27 February 2011
Issue Date : May 2011
DOI : https://doi.org/10.1007/s11151-011-9283-y
Share this article
Anyone you share the following link with will be able to read this content:
Sorry, a shareable link is not currently available for this article.
Provided by the Springer Nature SharedIt content-sharing initiative
- Monopolization
- Standard Oil
- Find a journal
- Publish with us
- Track your research
Standard Oil as a Technological Innovator
In this section.
- Faculty Publications
- Publications by Centers & Initiatives
- Student Publications
HKS Authors
See citation below for complete author information.

- Harvard Kennedy School
- HKS Faculty Scholarship
- Communities & Collections
- By Issue Date
- FAS Department
- Quick submit
- Waiver Generator
- DASH Stories
- Accessibility
- COVID-related Research
- Terms of Use
- Privacy Policy
- By Collections
- By Departments
Show simple item record
Standard Oil as a Technological Innovator
Files in this item.
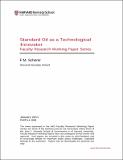
This item appears in the following Collection(s)
- HKS Faculty Scholarship [762]
Volume 4 Supplement 2
Proceedings of the Energy Informatics.Academy Conference Asia 2021
- Open access
- Published: 24 September 2021
Analysis and forecasting of crude oil price based on the variable selection-LSTM integrated model
- Quanying Lu 1 ,
- Shaolong Sun 2 ,
- Hongbo Duan 3 &
- Shouyang Wang 1 , 3
Energy Informatics volume 4 , Article number: 47 ( 2021 ) Cite this article
22k Accesses
14 Citations
2 Altmetric
Metrics details
In recent years, the crude oil market has entered a new period of development and the core influence factors of crude oil have also been a change. Thus, we develop a new research framework for core influence factors selection and forecasting. Firstly, this paper assesses and selects core influence factors with the elastic-net regularized generalized linear Model (GLMNET), spike-slab lasso method, and Bayesian model average (BMA). Secondly, the new machine learning method long short-term Memory Network (LSTM) is developed for crude oil price forecasting. Then six different forecasting techniques, random walk (RW), autoregressive integrated moving average models (ARMA), elman neural Networks (ENN), ELM Neural Networks (EL), walvet neural networks (WNN) and generalized regression neural network Models (GRNN) were used to forecast the price. Finally, we compare and analyze the different results with root mean squared error (RMSE), mean absolute percentage error (MAPE), directional symmetry (DS). Our empirical results show that the variable selection-LSTM method outperforms the benchmark methods in both level and directional forecasting accuracy.
Introduction
Since 2014, the international crude oil price has experienced the most significant volatility since the 2018 financial crisis. The oil market has taken on new features that affect the development of the global economy, national strategic security, and investor sentiment significantly. Especially as the primary alternative energy resources, the US tight oil production has been significant macroeconomic effects on the oil price (Kilian, 2017 ). In 2014, US shale oil producers plundered market share, leading to a change in global crude oil supply and demand balance. According to EIA, US shale oil production increased from 4.96 million barrels per day in 2017 to 5.59 million barrels per day in 2022. In addition, there are geopolitical events, trade frictions, and OPEC’s agreement have occurred in recent years, causing the volatility of oil price. The internal and external environment of the oil market is changing, and the influencing factors have become diverse and complex. As the factors affecting international oil prices become more and more complex, it becomes difficult to capture practical factors and predict oil prices. Many past kinds of literature about crude oil price forecasting show that the forecasting results are sensitive to the modeling sample data frequency and data interval selection (Yu et al., 2019 ; Yu et al., 2008a ; Zhang et al., 2015 ). As a strategic resource, crude oil plays a vital role in national energy security.
Meanwhile, with the financial properties of crude oil strengthened gradually, the volatility of crude oil prices is bound to affect oil companies’ earnings and investor behavior. Therefore, systematic analysis of the characteristics of complex international oil markets and accurate capture of the new trend in international oil prices are critical. However, as the linkage between the markets, the uncertainty of the world economy and energy, the influence factors of oil price have become complex. It is difficult to point out which factors have the dominant effect on the oil price. If all possible oil price factors are added into the existing forecast model, it may lead to over-fitting problems, which will affect the forecast results. How to forecast crude oil prices in a new and effective method is one problem that academics and practitioners are very concerned about all the time. It can provide reference and theoretical support for the formulation of national energy security strategy and enterprise avoidance of market risks. To better analyze the changing trend of the crude oil market, it is necessary to determine the main factors affecting the price, determine the impact of each factor on price, and establish a forecasting model finally.
The research on the prediction of international oil price has always been a hot topic. A large number of papers with theoretical and practical application value have appeared. We make a simple review from two aspects of influencing factors and forecasting methods as follow:
Influencing factor
Most of the research has divided the influence factors of crude oil price into supply and demand, finance factor, technology (Hamilton, 2009a ; Kilian & Murphy, 2014 ; Zhang et al., 2017 ; Wang et al., 2015 ; Tang et al., 2012 ).
Supply and demand
As the fundamental factor, supply and demand have been the main factors affecting oil prices. Supply and demand changes have always been the fundamental factors affecting the long-term trend of oil prices. (Hamilton, 2009b ) analyzed the drivers of oil prices and argued that the main reason for the rise in oil prices in 2007–2008 was the global demand for production. (Kilian, 2009 ) developed a structural VAR model to explain the global crude oil price fluctuation and understand the reaction of the US economy associated with oil price volatility. The crude oil price was decomposed into three components: crude oil supply shock, the shocks to the global demand for all industrial commodities, and the demand shock to the global crude oil market. However, in recent years, with the development of alternative energy sources, the worldwide supply and demand structure of crude oil has changed. (Kilian, 2017 ) reported the increased U.S. tight oil production not only reduced demand for oil in the rest of the world and lowering the Brent oil price but also caused other countries to cut back on their oil imports, lowering global oil demand.
Global economic development
Global economic development is a manifestation of supply and demand (Doroodian & Boyd, 2003 ; Sadorsky, 1999 ; Barsky & Kilian, 2001 ). (Kilian & Hicks, 2013 ) measured the global demand shock directly by correcting the real gross domestic product (GDP) growth forecast. The results showed that the forecast was associated with unexpected growth in emerging economies during the 2003 to 2008 period. These surprises were associated with a hump-shaped response of the real price of oil that reaches its peak after 12–16 months. The global real economic activity has always been considered to impact the changes in oil price significantly. (Özbek & Özlale, 2010 ) researched the relationship between global economic and oil prices with trend and cycle decomposition. They found that economic shock has a lasting effect on oil prices, which were considered mainly to be supply-side driven.
Financial factor
In addition to commodity attributes, crude oil also has financial attributes. The long-term trend of crude oil price is determined by the commodity attributes, which are affected by the supply and demand factors generated by the real economy; the short-term fluctuations of crude oil price are determined by the financial attributes, which are influenced by market expectations and speculative transactions. The financial factor mainly includes speculation factor, exchange rate and some other financial index, which connect the stock market and monetary market with the crude oil price (Narayan et al., 2010 ; Zhang, 2013 ; Reboredo, 2012 ; Cifarelli & Paladino, 2010 ). (Kilian & Murphy, 2014 ) developed a structural model to estimate the speculative component of oil price through the inventory data and found it played an important role during earlier oil price shock periods, including 1979, 1986 and 1990. (Sari et al., 2010 ) estimated the co-movement and information transmission among oil price, exchange rate and the spot prices of four precious metals (gold, silver, platinum, and palladium). Investors could diversify their investment risk by investing in precious metals, oil, and euros.
Technology factor
The Crack spread is defined as the price difference between crude oil and its refined oil, reflecting the supply and demand relationship between the crude oil market and its refined product market (Wang et al., 2015 ). (Murat & Tokat, 2009 ) used the random walk model (RWM) as a benchmark to compare the crack spread futures and crude oil futures and found the crack future could forecast the movements of oil spot price as reasonable as the crude oil futures. (Baumeister et al., 2013 ) selected crack spread as one of the variables to forecast crude oil prices, and the studies suggested it was an influential prediction factor.
Forecast method
Except for the influence factors, researchers are also very concerned about the forecast methods for improving forecast accuracy. The four main forecast method categories: time series models, econometric models, qualitative methods and artificial intelligence techniques are used in oil price modeling and forecasting (Wang et al., 2016 ; Charles & Darné, 2017 ; Yu et al., 2015 ; Sun et al., 2019 ; Suganthi & Samuel, 2012 ; Zhang et al., 2008 ; Valgaev et al., 2020 ). The autoregressive integrated moving average (ARIMA) and exponential smoothing (ETS) are the most widely used time series forecasting model, and they are usually used as the benchmark models (Wang et al., 2018 ; Chai et al., 2018 ; Zhu et al., 2017 ). In addition, the econometric models and qualitative methods like the generalized autoregressive conditional heteroskedastic model (GARCH), the vector autoregression model (VAR), the state-space models and the threshold models are also widely used (Kilian, 2010 ; Wang & Sun, 2017 ; Zhang & Wei, 2011 ; Ji & Fan, 2016 ; Drachal, 2016 ).
However, with the increasing of the data volume and influence factors complex, traditional models failed in predicting accurately. The machine learning forecasting method presents its superiority and mostly outperform traditional approaches when tested with empirical results, especially in dealing with the nonlinear problem and short-term prediction. Such as support vector machines (SVM), artificial neural networks (ANNs), genetic algorithms (GA) and wavelet analysis are introduced into oil price forecasting in recent years. For example, (Zhao et al., 2017 ) proposed the stacked denoising autoencoders model (SDAE) for oil price forecasting. Empirical results of the proposed method proved the effectiveness and robustness statistically. (Xiong et al., 2013 ) developed an integrated model EMD-FNN-SBN, which is the empirical mode decomposition (EMD) based on the feed-forward neural network (FNN) modeling framework incorporating the slope-based method (SBM). The results indicate this model using the (multiple-input multiple-output) MIMO strategy is the best in prediction accuracy.
During the last decades, more and more factors and models have been introduced, estimated and validated. Several different factors can address the oil price forecasting problem from the empirical and theoretical vision. Many researchers always select general factors and models directly, regardless of which indicators are the actual core variables. Especially with the expansion of data and quantitative indicators, variable selection becomes more and more critical. In recent years, there are some papers begin to extract core factors before forecasting. Even though there are some variable selection processes in some machine learning methods, they are all nested in the forecasting and just for the robustness of the model (Drezga & Rahman, 1998 ; May et al., 2008 ; Korobilis, 2013 ; Huang et al., 2014 ). There are fewer papers devoted to variable selection before predicting. For example, (Chai et al., 2018 ) used the Bayesian model average method for influence variable selection before establishing the oil price forecasting model. (Zhang et al., 2019 ) accurately screen out the main factors affecting oil price by using an elastic network model and LASSO shrinkage in the case of many predictive variables but relatively few data. The main factors influencing oil price forecast are studied from the Angle of variable selection. Secondly, the accuracy and robustness of the elastic network model and the LASSO contraction method in predicting oil prices are comprehensively verified using a variety of robustness tests. The results show that the LASSO contraction and elastic network model outperforms other standard oil price forecasting models. An investor who allocates assets based on the predictions of these two methods can achieve a more substantial return than other oil price forecasting models.
In this paper, we develop an integrated model with a new machine learning method for crude oil price forecasting based on core factor selection. This paper contributes to the variable selection and machine learning method in oil price forecasting. In the process of variable selection, we introduce three approaches with different advantages for comparison analysis and forecasting, elastic-net regularized generalized linear model, Bayesian model average and spike-slab lasso method. In addition, we combine them with a new machine learning method long short-term memory (LSTM) model for oil price forecasting. Finally, random walk (RW), autoregressive integrated moving average models (ARMA), elman neural Networks (ENN), ELM Neural Networks (EL), Walvet Neural Networks (WNN) and generalized regression neural network (GRNN) Models were used to forecast the price. Finally, we compare and analyze the different results with root mean squared error (RMSE), mean absolute percentage error (MAPE), directional symmetry (DS). The research framework is shown in Fig. 1 .
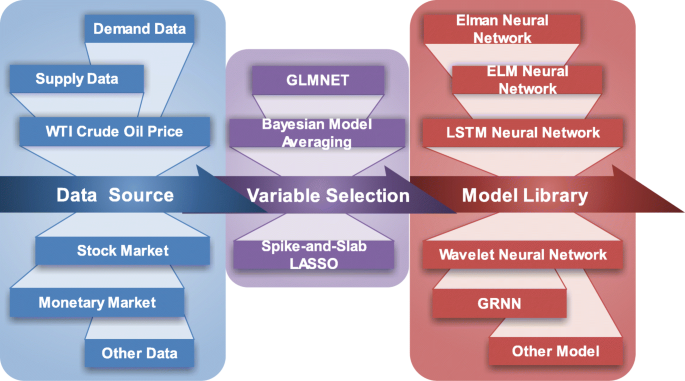
the flowchart of the variable selection-LSTM research framework
The structure of the paper is as follows: Section 1 reviews the related literature including influencing factors and forecast methods. Section 2 introduces the data. Section 3 develops the technique. Section 4 presents the empirical analysis. Finally, Section 5 concludes the paper and outlines future work.
According to the above literature, we selected 30 variables from supply and demand, inventory, financial market, technical aspects as the initially chosen variables (Hamilton, 2009a ; Kilian & Murphy, 2014 ; Zhang et al., 2017 ; Wang et al., 2015 ; Tang et al., 2012 ), and we choose the real monthly West Texas Intermediate (WTI) crude oil price as the dependent variable. The interval of the data is from January 2000 to December 2017. For data stability, we used the return rate of data. The crude oil production, consumption structure and replacement cost as the related variables measure the supply index. The demand index includes crude oil consumption and global economic development, a measure of real global economic activity.
Meanwhile, we select the total OECD petroleum inventory, U.S. crude Oil inventory (total, SPR, and Non-SPR) as the inventory index. In addition, the related variables are selected from speculation, monetary, stock, commodity market as the financial factor index. Finally, we calculated the WTI-Brent spot price spread, actual value of the WTI crack spread and Brent crack spread: actual value as the technical indicators. In Table 1 , we describe each variable and its corresponding data sources.
Theoretical background
As the crude oil market is very complex and has various uncertain determinants, we must select the core influence factors first before establishing forecasting models. The main four variable selection methods are significance test (forward and backward stepwise regression), information criteria (AIC BIC), principal component factor analysis model, lasso regression, ridge regression, and other punitive models (Castle et al., 2009 ). It is hard to tell which the best is because each has its own strong and weak points. Thus, we introduce three different methods to select core influence factors of crude oil price, which are elastic-net regularized generalized linear Models (GlMNET), spike-slab lasso method (SSL) and Bayesian model averaging (BMA). These three methods are effective variable selection methods and they are all improvements on the existing mature models (LASSO, Ridge regression). Thus, we use these new methods for variable selection (Zou & Hastie, 2005 ; Friedman et al., 2010 ).
Variable selection
The elastic-net regularized generalized linear models (glmnet).
Zou and Hastie (2005) (Zou & Hastie, 2005 ) proposed the elastic-net method for variable selection, which is considered to be the best contraction method for handling multicollinearity and variable screening, and its loss precision is not too great. Their simulation results showed that the elastic net outperformed the Lasso in terms of prediction accuracy. Like the Lasso, the elastic net simultaneously does automatic variable selection and continuous shrinkage, and it can select groups of correlated variables. On the one hand, it achieves the purpose of ridge regression to select essential features; on the other hand, it removes features that have little influence on dependent variables, like Lasso regression, and achieves good results, especially when the sample size n is smaller than the number of predictors. (The specific formula refers to (Zou & Hastie, 2005 )) In this paper, we choose the Elastic-Net Regularization Linear Model (GlMNET), which is a package that fits the Generalized Linear model by punishing maximum likelihood (Friedman et al., 2010 ). The regularization path of the Lasso or elastic net penalty is calculated on the value grid of the regularization parameter. The algorithm is high-speed, can make full use of the sparsity of input matrix X, and is suitable for linear, logic, polynomial, poisson and Cox regression models. It can also be applied to multi-response linear regression. These algorithms can process vast datasets and can make use of sparsity in the feature set.
Wherein the values of the grid λ cover the entire range, l ( y i , η i ) is the negative logarithmic likelihood distribution of the contribution to the observed value i . For example, it is \( \frac{1}{2}{\left(y-\eta \right)}^2 \) for Gaussian. The elastic mesh penalty is controlled by α . (The specific formula refer to Friedman et al. ( 2010 )).
Bayesian model averaging
The basic idea of the Bayesian model averaging approach (BMA) can comprehend. Each model is not fully accepted or not entirely negative (Leamer, 1978 ). The prior probability of each model should be assumed firstly. The posterior probability can be gain by extracting the dataset contains information as well as the reception of models to the dependent variables. The excellence of the BMA approach is not only that it can sort the influence factors according to their importance but also can calculate the posterior mean, standard deviation and other indicators of the corresponding coefficients. With the help of the Markov chain Monte Carlo method (MCMC), the weight distribution of the model according to the prior information could be estimated (Godsill et al., 2001 ; Green, 1995 ). The MCMC method can overcome the shortcoming of BIC, AIC and EM methods. (The specific formula refer to (Leamer, 1978 ; Merlise, 1999 ; Raftery et al., 1997 )).
It has the following three advantages: First, under different conditional probability distributions, there is no need to change the algorithm. Second, the posterior distribution of the weight and variance of BMA is considered comprehensively. Third, it can handle parameters with high BMA correlation.
- Spike-slab lasso
Although model averaging can be considered a method of handling the variable selection and hypothesis testing task, only in a Bayesian context since model probabilities are required. Recently, Ročková, V. and George, E. I. introduce a new class of self-adaptive penalty functions that moving beyond the separable penalty framework based on a fully Bayes spike-and-slab formulation (Ročková & George, 2018 ). Spike-slab Lasso (SSL) can borrow strength across coordinates, adapt to ensemble sparsity information and exert multiplicity adjustment by non-separable Bayes penalties. Meanwhile, it is using a sequence of Laplace mixtures with an increasing spike penalty λ 0 , and keeping λ 1 fixed to a small constant. It is different from Lasso, which a sequence of single Laplace priors with an increasing penalty λ . Furthermore, it revisits deployed the EMVS procedure (an efficient EM algorithm for Bayesian model exploration with a Gaussian spike-and-slab mixture (Ročková & George, 2018 )) for SSL priors, automatic variable selection through thresholding, diminished bias in the estimation, and provably faster convergence. (The specific formula refer to (Ročková & George, 2018 )).
Long short-term memory network
Long short-term memory (LSTM) neural networks are a special kind of recurrent neural network (RNN). LSTM was initially introduced by Hochreiter and Schmidhuber (1997) (Hochreiter & Schmidhuber, 1997 ), and the primary objectives of LSTM were to model long-term dependencies and determine the optimal time lag for time series issues. In this subsection, the architecture of RNN and its LSTM for forecasting crude oil prices are introduced. We start with the primary recurrent neural network and then proceed to the LSTM neural network.
The RNN is a type of deep neural network architecture with a deep structure in the temporal dimension. It has been widely used in time series modeling. A traditional neural network assumes that all units of the input vectors are independent of each other. As a result, the conventional neural network cannot make use of sequential information. In contrast, the RNN model adds a hidden state generated by the sequential information of a time series, with the output dependent on the hidden state. Figure 2 shows an RNN model being unfolded into a full network. The mathematical symbols in Fig. 2 are as follows:
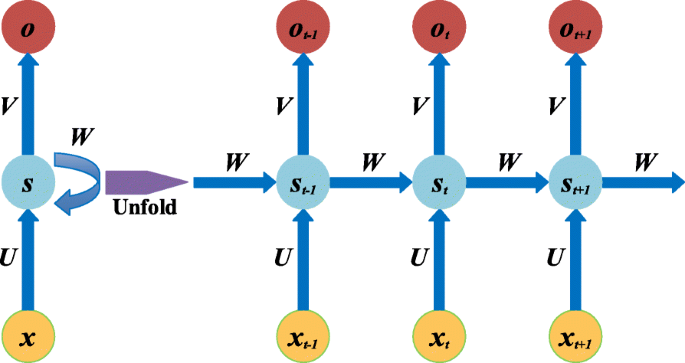
An unrolled recurrent neural network
1. x t denotes the input vector at time t .
2. s t denotes the hidden state at time t , which is determined relayed on the input vector x t and the previous hidden state. Then the hidden state s t is determined as follows:
Where f (·) is the activation function, it has many alternatives such as sigmoid function and ReLU. The initial hidden state s 0 is usually initialized to zero.
3. o t denotes the output vector at time t . It can be calculated by:
4. U and V denote the weights of the hidden layer and the output layer respectively. W denotes transition weights of the hidden state.
Although RNNs simulate time series data well, these are difficult to learn long-term dependence because of the vanishing gradient issue. LSTM is an effective way to solve the vanishing gradient by using memory cells. A memory cell is consists of four units: input gate, forget gate, output gate and a self-recurrent neuron, it is shown in Fig. 3 . The gate controls the interactions between the adjacent memory cells and the memory cell itself. Whether the input signal can change the state of the memory cell is controlled by the input gate. On the other hand, the output gate can control the state of the memory cell to decide whether it can change the state of other memory cells. Additionally, the forget gate can choose to remember or forget its previous state.
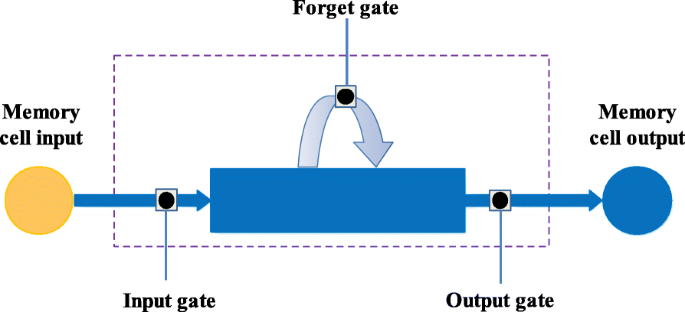
The architecture of an LSTM memory cell
Figure 4 illustrates the unrolled module in an LSTM network, which describes how the values of each gate are updated. The mathematical symbols in Fig. 4 are as follows:
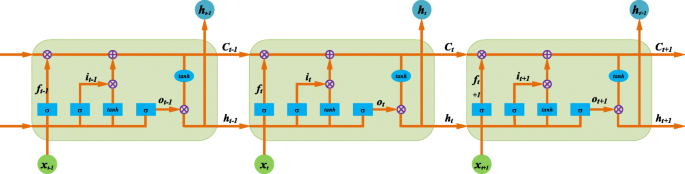
The repeating module in an LSTM contains four interacting layers
1. x t is the input vector of the memory cell at time t .
2. W i , W f , W c , W o , U i , U f , U c , U o and V o are weight matrices.
3. b i , b f , b c and b o are bias vectors.
4. h t is the value of the memory cell at time t .
5. i t and \( {\tilde{C}}_t \) are values of the input gate and the candidate state of the memory cell at time t , respectively. i t and \( {\tilde{C}}_t \) can be calculated by:
6. f t and C t are values of the forget gate and the state of the memory cell at time t , respectively. f t and C t can be formulated as follows:
7. o t and h t are values of the output gate and the value of the memory cell at time t , respectively. o t and h t can be calculated as follows:
The architecture of the LSTM neural network includes the number of hidden layers and the number of delays, which is the number of past data that account for training and testing. Currently, there is no rule of thumb to select the number of delays and hidden layers. In this work, the number of hidden layers and delays are set to 5 and 4 by trial and error. The back-propagation algorithm is employed to train the LSTM network and MLP neural network. The learning rate, batch size and number of epochs are 0.05, 60 and 1000, respectively. The speed of convergence is controlled by the learning rate, which is a decreasing function of time. Setting the number of epochs and the learning rate to 1000 and 0.05 can achieve the convergence of the training. The empirical result will become stable once convergence is achieved through the combinations of parameters are varied. Interesting readers may refer to (Hochreiter & Schmidhuber, 1997 ) for more information.
Empirical study
In this section, we compare the variable selection-LSTM integrated learning approach to the predictive performance of some benchmark models. First, section 4.1 analyzes the core influencing factors for the screening of the three feature extraction methods, and 4.2 describes the evaluation criteria and statistical tests to compare prediction accuracy. Second, section 4.3 provides an input selection and reference model parameter settings. Finally, Section 4.4 for the discussion.
We can see from Table 2 , the elastic-net selects the most number (18 variables), followed by the SSL method (11 variables), and the BMA method includes the least number (8 variables). Meanwhile, the variable of the SSL method is a subset of the BMA method, and the BMA method is a subset of the elastic-net. Non-OPEC production, shale oil (tight oil) production, Fed Fund effective, Kilian Index, USA CPI, USA PPI: Energy, Euro PPI, USA: PMI, OECD inventory, USA SPR inventory, USA Non-SPR inventory, Crude oil non-commercial net long ratio, Real dollar index: generalized, COMEX: Gold: Future closing price, LME: Copper: Future closing price, WTI-Brent spot price spread, WTI crack spread: actual value and Brent crack spread: real value. Firstly, we can see Non- OPEC production and tight oil production were selected from the supply aspect. It suggested that with the reduction of OPEC production, the production capacity of Non-OPEC countries is increasing gradually. In particular, the US tight oil production has become an essential factor affecting the trend of oil prices in recent years. According to the IEA report, the global oil production is expected to increase by 6.4 million barrels to 107 million barrels by 2023. The US tight oil production growth will account for 60 of the global growth. Meanwhile, in 2017, the US was the world’s largest producer.
From the demand aspect, global economic development is still the main driver of crude oil price. The USA PPI: energy, Euro PPI and USA: PMI factors are selected by all three methods. Crude oil is an important raw material for industry, agriculture, and transportation. It is also the main parent product of energy and chemical products in the middle and lower reaches. Therefore, crude oil plays a critical role in the price of domestic production and living materials. For example, when the oil price continued to fall sharply, which is bound to lower the overall price level of the country. Moreover, it will have a heavier negative effect on domestic currency fluctuations, which will shift the currency situation from expansion to contraction.
From the inventory aspect, the OECD inventory, the USA SPR inventory and the USA Non-SPR inventory are chosen into the elastic-net model. The inventor is an indicator of the balance of the supply and demand for crude oil. Furthermore, the impact of commercial inventory on oil prices is much more substantial. When the future price is much higher than the spot price, the oil companies tend to increase the commercial inventory, which stimulates the spot price to rise and reduces the price difference. When the future price is lower than spot prices, oil companies tend to reduce their commercial inventories, and spot prices fall, which will form a reasonable spread with futures prices.
From the speculate aspect, there is a positive correlation between the non-commercial net long ratio and oil price (Sanders et al., 2004 ). With the crude oil market and the stock market relation gradually strengthened, hedging plays a more important role in driving market trading (Coleman, 2012 ).
From the exchange rate market, the real dollar index: generalized was selected. The dollar index is used to measure the degree of change in the dollar exchange rate against a basket of currencies. If the dollar keeps falling, real revenues from oil products priced in dollars will fall, which will lead to the crude price high.
Form the commodity market, the LME: Copper: Future closing price factor is picked up. Copper has the function of resisting inflation, while the international crude oil price is closely related to the inflation level. There is an interaction between them from the long-term trend.
From the technical aspect, the WTI-Brent spread serves as an indicator of the tightening in the crude oil market. As the spread widens, which suggests the global supply and demand may be reached a tight equilibrium. The trend of price spread showed a significant difference around 2015 because of the US shale oil revolution. US shale production has surged substantially since 2014. However, due to the oil embargo, the excess US crude oil could not be exported, resulting in a significant increase in US Cushing crude oil inventories. The spread at this stage was mainly affected by the WTI price, and the correlation between the price difference and the oil price trend was not strong. After the United States lifted the ban on oil exports at the end of 2015, the correlation between the price spread and the oil price trend increased significantly. After the lifting of the oil export ban, the WTI oil price was no longer solely affected by the internal supply and demand of the United States. During this period, Brent and WTI oil prices were more consistent, resulting in a narrower spread. In addition, after 2015, the consistency of them increased significantly.
In summary, the factors selected by all three models are USA PPI: Energy, Euro PPI, USA: PMI (global economic development), Crude oil non-commercial net long ratio (speculate factor), Real dollar index: generalized (exchange rate market), LME: Copper: Future closing price (commodity market), WTI crack spread: actual value and Brent crack spread: actual value (technology factor).
Evaluation criteria and statistic test
To compare the forecasting performance of our proposed approach with some other benchmark models from level forecasting and directional forecasting, three main evaluation criteria, i.e., root mean squared error (RMSE), mean absolute percentage error (MAPE), directional symmetry (DS), which have been frequently used in recent years (Chiroma et al., 2015 ; Mostafa & El-Masry, 2016 ; Yu et al., 2008b ; Wang et al., 2017 ), are selected to evaluate the in-sample and out-of-sample forecasting performance. Three indicators are defined as follows:
where x t and \( {\hat{x}}_t \) denote the actual value and forecasted value at time t , respectively, d i = 1 if \( \left({x}_i-{x}_{i-1}\right)\left({\hat{x}}_i-{x}_{i-1}\right)\ge 0 \) or d i = 0 otherwise, and N is total number of observations.
To provide statistical evidence of the forecasting performance of our proposed ensemble learning approach, three tests, i.e., the Diebold-Mariano (DM) (Diebold & Mariano, 1995 ) test, the Superior Predictive Ability (Hansen, 2005 ) test and Pesaran-Timmermann (PT) (Pesaran & Timmermann, 1992 ) test, are performed. The DM test checks the null hypothesis of equal predictive accuracy. In this study, the Mean Squared Error is applied as DM loss function and each model is compared against a random walk. As for performance measurement, we use the RMSE and MAPE. The PT test examines whether the directional movements of the real and forecast values are the same. In other words, it checks how well rises and falls in the forecasted value follow the actual rises and falls of the time series. The null hypothesis is that the model under study has no power in forecasting the oil prices.
Forecasting performance evaluation
To verify the predictive power of the variable Select-machine learning integrated approach, we selected 8 benchmark models, including 6 multivariate models (MLP, RBFNN, GRNN, ELMAN, WNN, ELM) and 2 univariate models (RW and ARMA). In this paper, the number of MLP output layer neurons is 1, the number of iterations in the training stage is 10,000, and the number of hidden layer neurons is determined by the trial and error method to be 11. Similarly, the number of LSTM hidden layers and the number of delays were set as 5 and 4, respectively, and the number of output layer neurons was set as 1. The structure of the LSTM neural network was trained by backpropagation algorithm (BP), and the learning rate, batch size and the number of the epoch were set as 0.05, 60 and 1000 respectively. The convergence rate is controlled by the learning rate, which is a function of decreasing time. When the number and learning rate of epochs is set at 1000 and 0.05, the convergence of the training set is realized, and the empirical results tend to be stable, which can recognize the convergence of the training set data. When the parameter combination changes, once it converges, the experimental results tend to be stable. All models are implemented using Matlab 2017B software.
According to the forecast results of Table 3 , we can find some interesting conclusions: (1) no matter in the sample inside or outside the sample prediction, this chapter proposed variable selection - machine learning approach to integration in the training set and test set level precision (RMSE and MAPE) and direction (DS) were better than that of the single variable precision model and the core factors extracted model. (2) Among the variable selection-machine learning integration models, the BMA-LSTM integration model performs best, followed by Spike and Slab LASSO-LSTM and GLMNET-LSTM. For example, the RMSE, MAPE and DS predicted in the SAMPLE of rhe BMA-LSTM integrated module were 1.12%, 0.74% and 84.88%, respectively, which were 3.77%, 3.28% and 35.46% higher than RW, and 1.09%, 0.61% and 12.21% higher than the LSTM model without variable selection. (3) When predicted one step in advance, RMSE, MAPE and DS of the BMA-LSTM integrated model were 2.04%, 0.83% and 81.40%, respectively, 3.7%, 4.36% and 32.56% higher than that of the RW model, and 1.13%, 0.73% and 11.63% higher than that of the variable free LSTM model. It shows that the prediction accuracy of the variable selection-machine learning integrated model is significantly improved compared with that of the univariate model and the univariate model. Secondly, the number of core variables selected by BMA is neither the most nor the least among the three variable selection models, indicating that the number of core variables will also affect the prediction results.
Statistic tests
According to Table 4 statistical test results can be seen that (1) the step ahead prediction samples, variable selection - machine learning integration model of DM test results are less than 7.195, this means that the performance of the proposed method under the confidence level of 99% is better than all the other benchmark model, the possible reason is the variable selection - machine learning integration model significantly improves the prediction performance of the model. (2) In the out-of-sample prediction 1 step in advance, when the LR model is used as the test model, the DM test results of other test models are far less than − 7.015, indicating that the predictive performance of the three integrated methods and the other three single models is better than that of the machine learning model without variable selection at the 99% confidence level. (3) samples within 1 step ahead prediction and sample 1 step ahead prediction of the performance of the three variables extraction method was compared, the BMA-LSTM integration model to predict performance is the best, the next step is the Spike and slab LASSO-LSTM and GLMNET-LSTM, suggesting that this chapter puts forward the integrated research framework based on the variable selection-machine learning significantly improves the performance of the integrated machine learning approach.
PT test results also give three interesting points: (1) In the one step forward in-sample forecasting, the PT test results of the proposed variable selection-machine learning integration approach are all rejected the movement direction of the actual independence assumption under 99% confidence level. This also means that the variable selection-machine learning method is the best direction prediction performance and also can be seen that the direction of the ARIMA predicts performance is the worst. (2) In the out-of-sample one-step forecasting, the PT test value of the predicted results of the integrated method is significantly greater than that of the single model, which means that the direction prediction ability of the integrated method is better than that of the single model, mainly because the variable selection-machine learning integration idea significantly improves the direction prediction performance of the single model. (3) In the prediction in and out of sample 1 step in advance, it can be seen from the PT test results of variable Selection-machine learning integration method that the direction prediction accuracy of BMA-LSTM is the highest, followed by Spike-Slab Lasso-LSTM, which is mainly attributed to the direction prediction ability of variable Selection-machine learning method.
Conclusions and future work
In this paper, we proposed a variable selection and machine learning framework that combines the variable selection (BMA) and forecasting method (LSTM) to forecast the oil price and compared its forecasting performance with other primary and new variable selection methods (elastic-net and spike and slab Lasso). Moreover, compared to other popular benchmark forecast methods (RW, ARMA, MLP, RBFNN, GRNN, ElMAN, WNN, ELM). Specifically, our contributions are as follow:
Introduce the variable selection before forecasting. In this process, we compare three different methods and analyze core influencing factors based on the literature review from supply and demand, global economic development, financial market, and technology aspects. The results showed that the variable of the SSL method is a subset of the BMA method, and the BMA method is a subset of the elastic-net.
Testing the performance of the proposed variable selection and machine learning framework based on 3 variable selections and 8 individual forecasts. Comparing with the 8 individual forecasts without variable selection, the combinations forecasting reduces the errors. The results showed that (1) the variable choice-machine learning integration method proposed in this chapter is superior to the univariate model and the model without core factor extraction in both training set and test set level accuracy (RMSE, MAPE) and direction symmetric (DS). (2) Among the variable selection-machine learning integration models, The BMA-LSTM integration model performs best, followed by Spike and Slab LASSO-LSTM and GLMNET-LSTM. It shows that the prediction accuracy of the variable selection-machine learning integrated model is significantly improved compared with that of the univariate model and the univariate model. Secondly, the number of core variables selected by BMA is neither the most nor the least among the three variable selection models, indicating that the number of core variables will also affect the prediction results. (3) The statistical test results show that the prediction of 1 step in advance in-sample and 1 step in advance in out of sample. Compared with the prediction performance of the three variable extraction methods, the directional prediction accuracy and horizontal prediction accuracy of the BMA-LSTM integrated model are the best, followed by Spike and Slab-LASSO-LSTM and GLMNET-LSTM. This indicates that the variable selection-based machine learning integrated research framework proposed in this chapter significantly improves the forecasting performance of oil prices. In future research, we may introduce more independent variables with the help of internet search data, test our framework performance. Moreover, investor sentiment can be quantified in this process. In addition, different variable selection methods can be introduced more.
Availability of data and materials
Not applicable.
Abbreviations
artificial neural networks
autoregressive moving average
autoregressive integrated moving average
Diebold-Mariano
directional symmetry
empirical mode decomposition
elman neural Networks
exponential smoothing
feed-forward neural network
genetic algorithms
generalized autoregressive conditional heteroskedastic model
elastic-net regularized generalized linear Model
generalized regression neural network Models
long short-term memory
multiple-input multiple-output
mean absolute percentage error
Markov chain Monte Carlo method
Pesaran-Timmermann
root mean squared error
recurrent neural network
random walk
random walk model
slope-based method
stacked denoising autoencoders model
Spike-slab Lasso
support vector machines
vector autoregression model
Walvet Neural Networks
West Texas Intermediate
Barsky RB, Kilian L (2001) Do we really know that oil caused the great stagflation? A monetary alternative. NBER Macroecon Annu 16:137–183. https://doi.org/10.1086/654439
Article Google Scholar
Baumeister C, Kilian L, Zhou X (2013) Are product spreads useful for forecasting? An empirical evaluation of the verleger hypothesis. Available at SSRN DP9572
Castle JL, Qin X, Reed WR (2009) How to pick the best regression equation: a review and comparison of model selection algorithms. Working Papers in Economics 32(5):979–986
Google Scholar
Chai J, Xing LM, Zhou XY, Zhang ZG, Li JX (2018) Forecasting the WTI crude oil price by a hybrid-refined method. Energy Econ 71:114–127. https://doi.org/10.1016/j.eneco.2018.02.004
Charles A, Darné O (2017) Forecasting crude-oil market volatility: further evidence with jumps. Energy Econ 67:508–519. https://doi.org/10.1016/j.eneco.2017.09.002
Chiroma H, Abdulkareem S, Herawan T (2015) Evolutionary neural network model for West Texas intermediate crude oil price prediction. Appl Energy 142:266–273. https://doi.org/10.1016/j.apenergy.2014.12.045
Cifarelli G, Paladino G (2010) Oil price dynamics and speculation: a multivariate financial approach. Energy Econ 32(2):363–372. https://doi.org/10.1016/j.eneco.2009.08.014
Coleman L (2012) Explaining crude oil prices using fundamental measures. Energy Policy 40:318–324. https://doi.org/10.1016/j.enpol.2011.10.012
Diebold FX, Mariano RS (1995) Comparing predictive accuracy. J Bus Econ Stat 20(1):134–144
Article MathSciNet Google Scholar
Doroodian K, Boyd R (2003) The linkage between oil price shocks and economic growth with inflation in the presence of technological advances: a CGE model. Energy Policy 31(10):989–1006. https://doi.org/10.1016/S0301-4215(02)00141-6
Drachal K (2016) Forecasting spot oil price in a dynamic model averaging framework-have the determinants changed over time? Energy Econ 60:35–46. https://doi.org/10.1016/j.eneco.2016.09.020
Drezga I, Rahman S (1998) Input variable selection for ANN-based short-term load forecasting. IEEE Transactions on Power Systems Pwrs 13(4):1238–1244
Friedman J, Hastie T, Tibshirani R (2010) Regularization paths for generalized linear models via coordinate descent. J Stat Softw 33(1):1–22
Godsill S, Doucet A, West M (2001) Maximum a posteriori sequence estimation using Monte Carlo particle filters. Ann Inst Stat Math 53(1):82–96. https://doi.org/10.1023/A:1017968404964
Article MathSciNet MATH Google Scholar
Green PJ (1995) Reversible jump Markov chain Monte Carlo computation and Bayesian model determination. Biometrika 82(4):711–732. https://doi.org/10.1093/biomet/82.4.711
Hamilton JD (2009a) Understanding crude oil prices. Energy J 30(2):179–207
Hamilton JD (2009b) Causes and consequences of the oil shock of 2007-08 (no. w15002). National Bureau of economic research
Hansen PR (2005) A test for superior predictive ability. J Bus Econ Stat 23(4):365–380. https://doi.org/10.1198/073500105000000063
Hochreiter S, Schmidhuber J (1997) LSTM can solve hard long time lag problems. Adv Neural Inf Proces Syst:473–479
Huang T, Fildes R, Soopramanien D (2014) The value of competitive information in forecasting FMCG retail product sales and the variable selection problem. Eur J Oper Res 237(2):738–748. https://doi.org/10.1016/j.ejor.2014.02.022
Ji Q, Fan Y (2016) Evolution of the world crude oil market integration: a graph theory analysis. Energy Econ 53:90–100. https://doi.org/10.1016/j.eneco.2014.12.003
Kilian L (2009) Not all oil price shocks are alike: disentangling demand and supply shocks in the crude oil market. Am Econ Rev 99(3):1053–1069. https://doi.org/10.1257/aer.99.3.1053
Kilian L (2010) Explaining fluctuations in gasoline prices: a joint model of the global crude oil market and the US retail gasoline market. Energy J:87–112
Kilian L (2017) How the tight oil boom has changed oil and gasoline markets Social Science Electronic Publishing Available at SSRN No:6380
Kilian L, Hicks B (2013) Did unexpectedly strong economic growth cause the oil price shock of 2003–2008? J Forecast 32(5):385–394. https://doi.org/10.1002/for.2243
Kilian L, Murphy DP (2014) The role of inventories and speculative trading in the global market for crude oil. J Appl Econ 29(3):454–478. https://doi.org/10.1002/jae.2322
Korobilis D (2013) VAR forecasting using Bayesian variable selection. J Appl Econ 28(2):204–230. https://doi.org/10.1002/jae.1271
Leamer EE (1978) Specification searches. Wiley, New York
MATH Google Scholar
May RJ, Dandy GC, Maier HR, Nixon JB (2008) Application of partial mutual information variable selection to ANN forecasting of water quality in water distribution systems. Environ Model Softw 23(10–11):1289–1299. https://doi.org/10.1016/j.envsoft.2008.03.008
Merlise A (1999) Bayesian model averaging and model search strategies. Bayesian Statistics 6:157
MathSciNet MATH Google Scholar
Mostafa MM, El-Masry AA (2016) Oil price forecasting using gene expression programming and artificial neural networks. Econ Model 54:40–53. https://doi.org/10.1016/j.econmod.2015.12.014
Murat A, Tokat E (2009) Forecasting oil price movements with crack spread futures. Energy Econ 31(1):85–90. https://doi.org/10.1016/j.eneco.2008.07.008
Narayan PK, Narayan S, Zheng X (2010) Gold and oil futures markets: are markets efficient? Appl Energy 87(10):3299–3303. https://doi.org/10.1016/j.apenergy.2010.03.020
Özbek L, Özlale Ü (2010) Analysis of real oil prices via trend-cycle decomposition. Energy Policy 38(7):3676–3683. https://doi.org/10.1016/j.enpol.2010.02.045
Pesaran MH, Timmermann A (1992) A simple nonparametric test of predictive performance. J Bus Econ Stat 10(4):461–465
Raftery AE, Madigan D, Hoeting JA (1997) Bayesian model averaging for linear regression models. J Am Stat Assoc 92(437):179–191. https://doi.org/10.1080/01621459.1997.10473615
Reboredo JC (2012) Modelling oil price and exchange rate co-movements. J Policy Model 34(3):419–440. https://doi.org/10.1016/j.jpolmod.2011.10.005
Ročková V, George EI (2018) The spike-and-slab lasso. J Am Stat Assoc 113(521):431–444. https://doi.org/10.1080/01621459.2016.1260469
Sadorsky P (1999) Oil price shocks and stock market activity. Energy Econ 21(5):449–469. https://doi.org/10.1016/S0140-9883(99)00020-1
Sanders DR, Boris K, Manfredo M (2004) Hedgers, funds, and small speculators in the energy futures markets: an analysis of the CFTC's commitments of traders reports. Energy Econ 26(3):425–445. https://doi.org/10.1016/j.eneco.2004.04.010
Sari R, Hammoudeh S, Soytas U (2010) Dynamics of oil price, precious metal prices, and exchange rate. Energy Econ 32(2):351–362. https://doi.org/10.1016/j.eneco.2009.08.010
Suganthi L, Samuel AA (2012) Energy models for demand forecasting-a review. Renew Sust Energ Rev 16(2):1223–1240. https://doi.org/10.1016/j.rser.2011.08.014
Sun S, Wei Y, Tsui KL, Wang S (2019) Forecasting tourist arrivals with machine learning and internet search index. Tour Manag 70:1–10. https://doi.org/10.1016/j.tourman.2018.07.010
Tang L, Yu L, Wang S, Li J, Wang S (2012) A novel hybrid ensemble learning paradigm for nuclear energy consumption forecasting. Appl Energy 93:432–443. https://doi.org/10.1016/j.apenergy.2011.12.030
Valgaev O, Kupzog F, Schmeck H (2020) Adequacy of neural networks for wide-scale day-ahead load forecasts on buildings and distribution systems using smart meter data. Energy Informatics 3(1):1–17
Wang J, Li X, Hong T, Wang S (2018) A semi-heterogeneous approach to combining crude oil price forecasts. Inf Sci 460:279–292
Wang Q, Sun X (2017) Crude oil price: demand, supply, economic activity, economic policy uncertainty and wars–from the perspective of structural equation modelling (SEM). Energy 133:483–490. https://doi.org/10.1016/j.energy.2017.05.147
Wang Y, Liu L, Wu C (2017) Forecasting the real prices of crude oil using forecast combinations over time-varying parameter models. Energy Econ 66:337–348. https://doi.org/10.1016/j.eneco.2017.07.007
Wang Y, Wu C, Yang L (2015) Forecasting the real prices of crude oil: a dynamic model averaging approach. Available at SSRN 2590195
Wang Y, Wu C, Yang L (2016) Forecasting crude oil market volatility: a Markov switching multifractal volatility approach. Int J Forecast 32(1):1–9. https://doi.org/10.1016/j.ijforecast.2015.02.006
Xiong T, Bao Y, Hu Z (2013) Beyond one-step-ahead forecasting: evaluation of alternative multi-step-ahead forecasting models for crude oil prices. Energy Econ 40(2):405–415. https://doi.org/10.1016/j.eneco.2013.07.028
Yu L, Wang S, Lai KK (2008a) Forecasting crude oil price with an EMD-based neural network ensemble learning paradigm. Energy Econ 30(5):2623–2635. https://doi.org/10.1016/j.eneco.2008.05.003
Article MATH Google Scholar
Yu L, Wang S, Lai KK (2008b) Forecasting crude oil price with an EMD-based neural network ensemble learning paradigm. Energy Econ 30(5):2623–2635. https://doi.org/10.1016/j.eneco.2008.05.003
Yu L, Wang Z, Tang L (2015) A decomposition-ensemble model with data-characteristic-driven reconstruction for crude oil price forecasting. Appl Energy 156:251–267. https://doi.org/10.1016/j.apenergy.2015.07.025
Yu L, Zhao Y, Tang L, Yang Z (2019) Online big data-driven oil consumption forecasting with Google trends. Int J Forecast 35(1):213–223. https://doi.org/10.1016/j.ijforecast.2017.11.005
Zhang JL, Zhang YJ, Zhang L (2015) A novel hybrid method for crude oil price forecasting. Energy Econ 49:649–659. https://doi.org/10.1016/j.eneco.2015.02.018
Zhang X, Lai KK, Wang SY (2008) A new approach for crude oil price analysis based on empirical mode decomposition. Energy Econ 30(3):905–918. https://doi.org/10.1016/j.eneco.2007.02.012
Zhang Y, Ma F, Wang Y (2019) Forecasting crude oil prices with a large set of predictors: can LASSO select powerful predictors? J Empir Financ 54:97–117. https://doi.org/10.1016/j.jempfin.2019.08.007
Zhang YJ (2013) Speculative trading and WTI crude oil futures price movement: an empirical analysis. Appl Energy 107:394–402. https://doi.org/10.1016/j.apenergy.2013.02.060
Zhang YJ, Chevallier J, Guesmi K (2017) “De-financialization” of commodities? Evidence from stock, crude oil and natural gas markets. Energy Econ 68:228–239
Zhang YJ, Wei YM (2011) The dynamic influence of advanced stock market risk on international crude oil returns: an empirical analysis. Quantitative Finance 11(7):967–978. https://doi.org/10.1080/14697688.2010.538712
Zhao Y, Li J, Yu L (2017) A deep learning ensemble approach for crude oil price forecasting. Energy Econ 66:9–16. https://doi.org/10.1016/j.eneco.2017.05.023
Zhu B, Han D, Wang P, Wu Z, Zhang T, Wei YM (2017) Forecasting carbon price using empirical mode decomposition and evolutionary least squares support vector regression. Appl Energy 191:521–530. https://doi.org/10.1016/j.apenergy.2017.01.076
Zou H, Hastie T (2005) Regularization and variable selection via the elastic net. Journal of the Royal Statistical Society: Series B (Statistical Methodology) 67(2):301–320. https://doi.org/10.1111/j.1467-9868.2005.00503.x
Download references
About this supplement
This article has been published as part of Energy Informatics Volume 4, Supplement 2 2021: Proceedings of the Energy Informatics.Academy Conference Asia 2021. The full contents of the supplement are available at https://energyinformatics.springeropen.com/articles/supplements/volume-4-supplement-2 .
This research is supported by the National Natural Science Foundation of China (71988101; 71874177 and 72022019), China Postdoctoral Science Foundation (2020 M68719), and the University of Chinese Academy of Sciences.
Author information
Authors and affiliations.
Institute of Systems Science, Academy of Mathematics and Systems Science, Chinese Academy of Sciences, Beijing, 100190, China
Quanying Lu & Shouyang Wang
The School of Management, Xi’an Jiaotong University, Xi’an, 710049, China
Shaolong Sun
School of Economics and Management, University of Chinese Academy of Sciences, Beijing, 100190, China
Hongbo Duan & Shouyang Wang
You can also search for this author in PubMed Google Scholar
Contributions
Q. L.: Data curation, Conceptualization, Methodology, Software, Writing - original draft, Funding acquisition; S. S.: Methodology, Writing - review& editing; H. D.: Writing - review& editing, Funding acquisition.; S. W.: Conceptualization, Supervision,Validation, Funding acquisition. All co-authors have read and approved the final manuscript.
Corresponding author
Correspondence to Hongbo Duan .
Ethics declarations
Ethics approval and consent to participate, consent for publication, competing interests.
The authors declare that they have no competing interests.
Additional information
Publisher’s note.
Springer Nature remains neutral with regard to jurisdictional claims in published maps and institutional affiliations.
Supplementary Information
Additional file 1..
Neural networks training characteristics. Table A.1. Neural networks design and training characteristics
Rights and permissions
Open Access This article is licensed under a Creative Commons Attribution 4.0 International License, which permits use, sharing, adaptation, distribution and reproduction in any medium or format, as long as you give appropriate credit to the original author(s) and the source, provide a link to the Creative Commons licence, and indicate if changes were made. The images or other third party material in this article are included in the article's Creative Commons licence, unless indicated otherwise in a credit line to the material. If material is not included in the article's Creative Commons licence and your intended use is not permitted by statutory regulation or exceeds the permitted use, you will need to obtain permission directly from the copyright holder. To view a copy of this licence, visit http://creativecommons.org/licenses/by/4.0/ .
Reprints and permissions
About this article
Cite this article.
Lu, Q., Sun, S., Duan, H. et al. Analysis and forecasting of crude oil price based on the variable selection-LSTM integrated model. Energy Inform 4 (Suppl 2), 47 (2021). https://doi.org/10.1186/s42162-021-00166-4
Download citation
Published : 24 September 2021
DOI : https://doi.org/10.1186/s42162-021-00166-4
Share this article
Anyone you share the following link with will be able to read this content:
Sorry, a shareable link is not currently available for this article.
Provided by the Springer Nature SharedIt content-sharing initiative
- Crude oil price
Click through the PLOS taxonomy to find articles in your field.
For more information about PLOS Subject Areas, click here .
Loading metrics
Open Access
Peer-reviewed
Research Article
An analysis of crude oil prices in the last decade (2011-2020): With deep learning approach
Roles Conceptualization, Data curation, Formal analysis, Methodology, Software, Validation, Visualization, Writing – original draft, Writing – review & editing
* E-mail: [email protected]
Affiliation Department of Mathematics, Assam University, Dargakona, Assam, India

Roles Supervision
Affiliation Department of Mathematics, Institute of Technology,Nirma University, Gujarat, India
- Abhibasu Sen,
- Karabi Dutta Choudhury,
- Tapan Kumar Datta
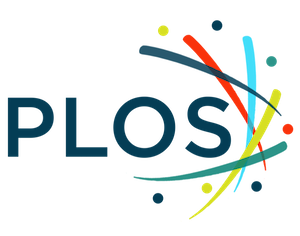
- Published: March 9, 2023
- https://doi.org/10.1371/journal.pone.0268996
- Reader Comments
Crude Oil is one of the most important commodities in this world. We have studied the effects of Crude Oil inventories on crude oil prices over the last ten years (2011 to 2020). We tried to figure out how the Crude Oil price variance responds to inventory announcements. We then introduced several other financial instruments to study the relation of these instruments with Crude Oil variation. To undertake this task, we took the help of several mathematical tools including machine learning tools such as Long Short Term Memory(LSTM) methods, etc. The previous researches in this area primarily focussed on statistical methods such as GARCH (1,1) etc. (Bu (2014)). Various researches on the price of crude oil have been undertaken with the help of LSTM. But the variation of crude oil price has not yet been studied. In this research, we studied the variance of crude oil prices with the help of LSTM. This research will be beneficial for the options traders who would like to get benefit from the variance of the underlying instrument.
Citation: Sen A, Dutta Choudhury K, Kumar Datta T (2023) An analysis of crude oil prices in the last decade (2011-2020): With deep learning approach. PLoS ONE 18(3): e0268996. https://doi.org/10.1371/journal.pone.0268996
Editor: Petre Caraiani, Institute for Economic Forecasting, Romanian Academy, ROMANIA
Received: August 20, 2021; Accepted: May 13, 2022; Published: March 9, 2023
Copyright: © 2023 Sen et al. This is an open access article distributed under the terms of the Creative Commons Attribution License , which permits unrestricted use, distribution, and reproduction in any medium, provided the original author and source are credited.
Data Availability: Data is available in https://www.investing.com/ .
Funding: The author(s) received no specific funding for this work.
Competing interests: The authors have declared that no competing interests exist.
1 Introduction
The price of a commodity generally depends on the demand and supply of the underlying commodity. That is why the amount of inventory of the commodity is an important aspect. Lots of research work have been done to predict commodity prices. Zhang and Na [ 1 ] formulated a hybrid MEA-SVM method which has higher predictive power for agro-based commodities. Xiong et al. [ 2 ] devised a novel hybrid forecasting method on the backdrop of seasonality in vegetable prices for the Chinese markets. Ouyang et al. [ 3 ] devised a Long-Short Time Series Network to predict global agricultural commodity futures price, which is found to be better performing than other methods. Mustaffa et al. [ 4 ] used the Swarm Intelligence approach to optimize parameters of least squares support vector machines. They found that their strategy performed better than the Back Propagation Neural Network and Genetic Algorithm . Kohzadi et al. [ 5 ] made a comparison of time series models with artificial neural network (ANN) , which could make better forecast of the commodity price. The data was monthly live cattle and wheat prices from 1950 to 1990. They found that the neural network model is a better performing one in the above context. Gunawan et al. [ 6 ] devised a neural network predicting method for Indonesian Palm Oil. They concluded that by increasing the number of iterations, it could increase accuracy but it comes at the cost of computational overheads. Gargano and Timmermann [ 7 ] forecasted the commodity price indices using macroeconomic and financial indicators. They found that commodity prices could better predict at monthly or quarterly time scale while macroeconomic indicators could better predict at a yearly time scale. They found that commodity prices could be best predicted during economic recessions. Claussen and Uhrig [ 8 ] found that neural networks could better forecast the directional changes of the price of cash soybean oil compared to other statistical tests. Chen and Wang [ 9 ] forecasted natural rubber prices using Genetic Fuzzy logic and Fuzzy logic . They found out that Genetic Fuzzy logic gives better results than Fuzzy logic. Cash Soybean Oil is an agricultural product being actively traded in Chicago Mercantile Exchange (CME). Mahajan [ 10 ] found that the Hybrid Quantum Neural Network method is better than the Classical Neural Network method. Chen et al. [ 11 ] devised an application for an automated commodity price prediction system in Malaysia. They compared ARIMA, SVR, Prophet, XGBoost, and LSTM and finally found that the LSTM method is the most reliable one. Kulkarni and Haidar [ 12 ] formulated an ANN model to forecast spot crude oil prices using futures crude oil prices successfully. Parida et al. [ 13 ] devised models for forecasting prices of Chana and Barley. The main aim of their research was to determine optimized weights in neural networks using a Kernel-based Extreme Learning Machine (KELM) . They compared between Genetic Algorithm (GA) based KELM (GA-KELM), Particle Swarm Optimization (PSO) based KELM (PSO-KELM), and Grey Wolf Optimization-based multi quadratic kernel KELM (GWO-KELM) . They found that GWO-KELM produces the best result. Yu et al. [ 14 ] forecasted the price of crude oil using a novel decomposition ensemble method. To begin with, they divided the whole data by the Extended Extreme Learning Machine (EELM) method. They then predicted the data using machine learning tools. In the end, the predicted results were ensembled to produce the final results.
2 Literature review
Hacer et al. [ 15 ] tried to forecast the monthly crude oil price using the bagging ensemble models and found that classification and regression trees perform better than artificial neural network-based models. With the idea of bagging being introduced, they claimed to have gained better accuracy in both the models. Abdollahi [ 16 ] devised a hybrid model for oil price forecasting and it is found to be better performing than other conventional models. Yu et al. [ 17 ] made a comparative analysis of five different prediction models and found that Support Vector Machine (SVM) is most suitable for crude oil price prediction. In the above work, the SVM model was compared with five other models namely, feed-forward neural networks (FNN), auto-regressive integrated moving average (ARIMA) model, fractional integrated ARIMA (ARFIMA) model, Markov-switching ARFIMA (MS-ARFIMA) model, and random walk (RW) model . The directional prediction is highest when the SVM method was used and found to be 74.42%. Sun et al. [ 18 ] predicted the interval-valued price of crude oil using the Interval Decomposition Ensemble method and found it to be performing better than other predictions. Gao and Lei [ 19 ] devised a novel approach to forecast the price of crude oil with a new ‘Machine Learning’ method known as Stream Learning . He et al. [ 20 ] devised a wavelet-based Decomposed Ensemble model , which takes care of the non-stationarity and dynamic changing nature of crude oil prices. Huang and Wang [ 21 ] devised a method which combined the Wavelet Neural Network (WNN) with random effective function to capture the nonlinearity in the data. They compared it with Back Propagation Neural Network, SVM, and WNN and finally found the hybrid model to be more effective than others. Shin et al. [ 22 ] predicted the monthly price of crude oil from January 1992 to June 2008, using the Semi-Supervised Learning (SSL) method. Chen et al. [ 23 ] made a hybrid model based on the deep learning method to forecast the price of crude oil, which they claimed performs better than others. Yu et al. [ 24 ] proposed a novel method to forecast the price of crude oil. They at first used Compressed Sensing based Denoising (CSD) to filter out the noises therein the data. Then they used the Artificial Neural Network (ANN) and Least Square Support Vector Regression (LSSVR) methods to forecast the price of crude oil which is better-performing than other benchmark models. Cao et al. [ 25 ] proposed two hybrid models for stock market prices forecasting. They used two kinds of Empirical Mode Decomposition (EMD) with LSTM for ensembling. They found greater forecasting power with the ensembled method when compared with other methods. Bristone et al. [ 26 ] proposed a hybrid model using Complex Network Analysis (CNN) and LSTM . Gupta and Pandey [ 27 ] studied the movements of Crude Oil, Gold and Indian stock index (NIFTY) based on LSTM technique. The prices of Crude Oil and Gold have been taken from Commodity Exchange of India. They could make several successsful predictions based on their work.
Many other research work have also been carried out on forecasting the prices of oil from inventory levels. Hui [ 28 ] found out that, inventory information shocks negatively affect the crude oil returns on the days of inventory announcements by EIA. However, inventory shocks do not influence the daily conditional variance. They have also found out that the results of inventory shocks do not hold good during rapid growth or decline stage. Miao et al. [ 29 ] tried to test the significance of six factors, namely; supply, demand, financial market, commodities market, speculative, and geopolitical; for various forecasting models. These models are used to forecast the price of crude oil. They found that Least Absolute Shrinkage and Selection Operator (LASSO) provides the best possible forecast as compared to other methods. Mikhaylov and Moiseev [ 30 ] proposed a Machine Learning approach to predict the prices of crude oil by analysing various factors such as US key rate, US dollar index, S&P 500 index, variance index, and US consumer price index. They predicted that the prices of crude oil would have a slight uptrend during 2019-2022. Later on, we can see that the price of crude oil; at the time of writing this article, maintained a steady price level. Ye et al. [ 31 ] studied the changing relationships between the prices of crude oil and several other factors. Their study covered the period from January 1992 to December 2007. They found out that prior to 2004, crude oil prices mainly depended on the inventory levels of OECD and excess production capacity of OPEC. They introduced a ratchet variable to indicate the changes in the relationships. The model they formulated had worked well during post-Gulf War I time. For future studies, in the last paragraph they mentioned:
“Several areas emerge for future studies. First, the changing role of short-term interest rates should be explored in future efforts to improve the model. Second, recent literature,14 as well as market practitioners, also speculates about impacts of exchange rates on crude oil prices, particularly since crude oil is traded in U.S. dollars, which has depreciated significantly against other major currencies since late 2007. Third, OECD inventories had been assumed to be representative of world crude oil inventories in the previous modeling efforts for crude oil markets in the 1990’s. This assumption may no longer be valid, given the rapidly growing economic activity and associated increase in petroleum consumption in the Asia-Pacific region. Finally, the interaction role of the financial market and commodity traders with physical markets needs to be studied to determine any effects on petroleum prices . ”
Many other research work have been carried out based on the US-bond yield too. Narayan et al. [ 32 ] conducted research comparing the lagged interactions between several economic segments like bonds, equities, commodities, inflations, etc. They found that bond prices negatively granger cause crude oil prices. Saenong et al. [ 33 ] studied the effect of crude oil prices and exchange rates on the 10-Year bond yields of Indonesia. They found out that in the long run both the crude oil prices and the exchange rate do not affect bond yields, but in the short term they do. Bhar and Lee [ 34 ] found out that risk premiums in equity and bond markets can explain the risk premiums in crude oil prices. Coleman [ 35 ] investigated the drivers of crude oil price and found out that OPEC market share, corporate bond yields (which are Aaa credited having the highest degree of creditworthiness), size of the oil futures market, global GDP, the number of US troops in the Middle East, and the frequency of fatal terrorist attacks in the Middle East are important driving forces. Turhan et al. [ 36 ] studied the long-run dynamic relationships between crude oil and stock markets, bond markets, gold, and the dollar index. They found that the correlation coefficient between crude oil and 10-year bond yield was hovering around zero before 2008 but after 2008, it is found to be around 0.25.
Crude Oil has a relationship with several other important financial instruments. Chen and Xu [ 37 ] developed a novel Generalized Auto-Regressive Score model to forecast the correlation between Crude Oil and Gold. Kumar [ 38 ] found non linear relationships between Crude Oil and Gold prices from an Indian perspective. We also studied in past the co-movement of Crude Oil and Gold prices with Indian stock index [ 39 ]. Wang and Chueh [ 40 ] found out that in the short term, Gold prices and Crude oil prices have a positive influence on each other; while in long term the interest rates have a negative influence on future Gold prices and a positive influence on future Crude Oil prices. They also found a long-run relationship, when interest rates influence the US dollar and in turn, the US dollar influences Crude Oil prices.Almost no relationships have been observed between Bitcoin and Crude Oil markets ([ 41 – 43 ]). Balcilar et al. [ 44 ] found a bidirectional return and volatility spillover for the Crude Oil, Gold, and S&P 500 index. Wu and Zhang [ 45 ] studied the role of China on Crude Oil prices. They did not find any significant role of the Chinese economy on Crude Oil prices, and on the contrary, they found that the contribution of Chinese economy is even less than the US Dollar Index effect on Crude Oil prices. But the research has been conducted on the data sets from October 2005 to November 2013. The Chinese economy has changed a lot since 2013. So, we need to again examine the effect of the Chinese economy on Crude Oil prices. Filippidis et al. [ 46 ] found out that the European Economic and Monetary Union (EMU) being the largest importer of Crude Oil, has an influence on Crude Oil prices. The correlation between 10 Year Yield spread and crude oil prices is time-varying which is again influenced during specific economic and geopolitical events. It is worth mentioning here that the spread is between the 10-year government bond yield issued by an EMU member country and the German Bund 10-year bond yield. The EMU countries are Austria, Belgium, Finland, France, Germany, Greece, Ireland, Italy, Netherlands, Portugal, and Spain.
3 Aims and objectives
Crude Oil is the most liquid commodity in Chicago Merchantile Exchange (CME) ([ 47 – 49 ]). Therefore, there is a huge number of options traders trading in the market. By Options , we mean Call and Put options and their various combinations. The options traders trade the volatility of the underlying, with the help of various strategies like the Butterfly strategy, Strangle strategy, etc ([ 50 , 51 ]). As a result, the variance of Crude Oil and its forecasting is very important for the traders. Moreover, options are also very important for hedging purposes.
Recently, Urolagin et al. [ 49 ] forecasted the price of Crude Oil using Multivariate LSTM. They used the Gold Prices, S&P Index, Dollar Index, US 10 Year Bond yield to forecast the Crude Oil prices. Though they attained a whooping R-squared value of 95.4%, it comes at the cost of filtering the data to 19.25% of the original size for Mahalanobis distance, and 9.62% for Z-score based outlier detection. Mahalanobis distance, in short, can be defined as some metrics that measures the distance between a point and a distribution. In easy language, Z-score can be said as some metrics which can give us the knowledge about how much far a point is from the mean. In their own language “Even though the outlier elimination has reduced the size of the data set nominally, but there is a significant improvement in the performance of both these models.”. Now as an example, during the recent Oil crash on April 20, 2020; many investors earned huge money, while many lost their everything ([ 52 – 55 ]). So, keeping this in mind, we did not filter out the data and used the data as it is.
They also mentioned in their paper, “During preprocessing, the missing values for the attributes were adjusted by taking the previous day’s values. Since data are not in a normal distribution, therefore mean cannot be used to replace the missing values”. That is a major shortcoming in their paper because oil is the most volatile commodity. As an example, let us take three consecutive days. 17 April 2020, 20 April 2020, and 21 April 2020 (18 April 2020, and 19 April 2020 are Saturday and Sunday respectively). The closing prices of Crude Oil on 17, 20, 21 April were 18.27$, -37.63$, and 10.01$. Now if we are having missing values on 20 April and if we take the value of 17 April for the missing value of 20 April, we shall get the wrong interpretation.
In the present paper, we are influenced by Hui’s paper [ 28 ]. She used the dataset from 2006 to 2011. Similar to her findings, we tried to study the effect of inventory information shock on crude oil price variance. There is a small difference between our work and Hui. Hui studied the crude oil volatility while we studied the daily crude oil price variance.
In her research, she studied the inventory information shock and its effects on Crude Oil prices’ conditional variance with the help of the GARCH (1,1) method. In our research, we incorporate non-linear deep learning algorithms like LSTM for the first time to study the inventory information shock and its effects on Crude Oil price variance.
In this paper, we study the variance of Crude Oil prices and try to forecast it with the help of Multivariate LSTM methods. At first, we take the variances of Gold, US 10 Year Bond Yield, German 10 Year Bund Yield, Bitcoin, S&P 500 Index, Shanghai Stock Exchange Index, Dollar Index, and previous day Crude Oil prices; and the Inventory levels. We then try to verify the results of Inventory with Hui [ 28 ] and examine whether her results are still valid in these changing times? The various factors are selected based on their ability to Granger Cause Crude Oil variance. The selected factors are used to predict the variance of Crude Oil prices. Various hyperparameters of LSTM are tuned to get the best predicted results. In our research, we did not filter out extreme values as big profits and losses are made on that very days.
4 Methodology: Description of analysing tools
4.1 data description.
For this study, the data has been collected for the end-of-day Crude Oil price variance, 10-Year US Bond yield price variance, Bitcoin Price variance, S&PIndex variance, Gold Price variance, Shanghai Stock Exchange Index variance, Dollar Index variance, German Bund Yield variance, inventory levels, and inventory levels estimations. The data was collected from investing.com . The period of the data is from November 2010 to June 2020, on both a daily and weekly basis. The markets in which the instruments are traded along with their symbols are given in Table 1 .
- PPT PowerPoint slide
- PNG larger image
- TIFF original image
https://doi.org/10.1371/journal.pone.0268996.t001
We take the rolling variance of the last ten days and try to forecast the variance of Crude Oil prices with the help of several other variances and the inventory factors. We have deliberately chosen the time frame from November 2010 to June 2020 to portray the events of the last decade.
Several economic incidents took place in the last decade. It all started with the earthquake in Japan causing damage of 18000 lives and property damage of 220 billion dollars [ 56 ]. In 2015 we saw the Greek debt crisis. The Greek economy almost became bankrupt [ 57 ]. It is said that the Greek rescue is the biggest rescue of any country in history. In the same year, based on the purchasing power parity , China became the first on the globe [ 58 ]. Moreover, China is also the second-largest holder of US debt [ 59 ]. In June 2017, United Kingdom voted to move out of European Union [ 60 ]. This act was popularly known as “Brexit”. Though it was assumed that it will hamper the economic growth of Britain but UK’s output rose to pre-Brexit times by 2020 [ 61 ]. On March 11, 2020, WHO declared COVID 19 a pandemic [ 62 ]. Thereafter, major economic downturns started to take place around the globe due to the spread of this virus. These are the major economic events that took place around the globe in the last decade.
4.1.1 Inventory shock.
Here,Δ Inv t −1 signifies the change of inventory levels in one week. The estimated values,E(Δ Inv t −1 ), and the original values, Δ Inv t −1 , are taken from investing.com.
Inventory Information shock is the difference between actual inventory changes and expected inventory changes. As a matter of fact, every Tuesday we get an expected value of inventory change, and on Wednesday, at about 8 a.m. E.T. the actual inventory change is announced. The expected inventory change is given by Reuters and the actual inventory change is announced by the U.S. Energy Information Administration.
Hui [ 49 ] introduced several dummy variables such as D EIA , D EIA , pos andD EIA , neg which were used to identify the days of inventory announcements, the days of positive inventory shocks, and the days of negative shocks respectively. D EIA | Inv EIA , tau | is the absolute shock term. The values related to inventory remained the same from one announcement to the next announcement.
4.2 Granger causality tests
We perform the Granger Causality test to examine whether a particular parametre has any effect on the variance of crude prices or not? We will choose only those parametre(s) for our analysis, which Granger cause Crude Oil price variance.
4.3 Long short-term memory
In 1997, Hochreiter and Schmidhuber [ 64 ] brought out a new method known as Long Short-Term Memory (LSTM) which solves complex, artificial long-time-lag tasks that have never been solved by previous recurrent network algorithms. LSTM has the inherent quality of remembering information for long periods. LSTM has a chain of repeating modules where each module has four neural networks.
In the Fig 1 , we can see an LSTM structure. We can see the cell state at the top of the figure.
Credits: Christopher Olah [ 65 ].
https://doi.org/10.1371/journal.pone.0268996.g001
After that, we will optimize the lookback period, the epoch and the batch size. An epoch is just an hyperparametre that is the number of times the learning algorithm will work through the entire data set. While the batch size is a hyperparametre which gives the number of samples to work with before updating the internal model parameters.
We will use the Mean Squared Error as the loss function and R-squared to measure the accuracy.
4.4 Optimization
The figure shows incremental steps on how the algorithm comes to a point of minimum error.
https://doi.org/10.1371/journal.pone.0268996.g002
4.4.1 Gradient descent optimization algorithms.
We compare the two Gradient Descent Optimization Algorithms namely: Adam and Nadam . In a nutshell, these are discussed below:
4.4.2 Adaptive moment estimation(Adam).
4.4.3 Nadam.
4.5 Shapley values
All the features of the model do not have the same amount of influence on prediction. To study this, the features can be examined individually. But again, this method won’t allow us to study the interdependence between features. To study the interdependence between features, we study the influence of each possible set of different features on prediction separately. These changes are then combined to present the contributions we get from each feature. That is, feature values work together in a model to change its predictive power with respect to the model’s output, and the Shapley values divide this total change in prediction among the features so that the process is fair among all the subsets of features.
To explain the concept of Shapley values [ 68 , 69 ], we have to define and explain some terms. First and foremost, we have to define the concept of Cooperative game theory.
4.5.1 Cooperative game.
There are four axioms which take the responsibility of fairness for a game. These are listed below.
- Symmetry: If for every set S, S ⊂ {1, … p }, there exist two players; i and j; such that i , j ∉ S , and the following equality holds, ν ( S ∪ { i }) = ν ( S ∪ { j }), then the following equality ϕ i ( ν ) = ϕ j ( ν ) is satisfied.
- Dummy: For every set S , where S ⊂ {1, …, p }, i ∉ S and ν ( S ∪ { i }) = ν ( S ), we have ϕ i ( ν ) = 0.
- Additivity: It ensures that for any pair of games, where ν , w ;( ν + w ) S = ν ( S ) + w ( S ), we have the equality ϕ ( ν + w ) = ϕ ( ν ) + ϕ ( w ).
The Shapley Value is used to distribute the total gains to the p players in an impartial way. The total gains is defined as v(1, …, p). Shapley Value ensures that the above four axioms are satisfied.
4.5.1.1 Method for computing Shapley Values. We are having X = X 1 × X 2 × X 3 × …. × X p as the feature space of p features, with f being the model that is to be explained. Here, let us take y = ( y 1 , y 2 , y 3 , …., y p ) ∈ X be any instance from the feature space. We want to explain the prediction of y . Now as per Strumbelj and Kononenko, Prediction Difference of a subset of feature values, gives us the change in expectation caused by those feature values [ 70 ]. Mathematically, Prediction Difference is given as:
The pseudocode for Shapley Value is given below:
Initialize Model f , instance y , and number of samples N
for j in range(1, N):
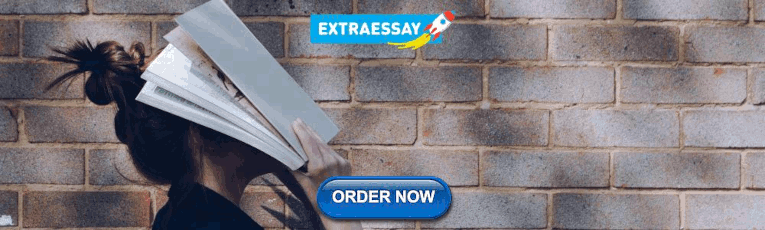
5 Results and discussions
In this research, we begin the process with the collection of data from investing.com. The data collected is for the period December 7, 2010, to June 9, 2020. Thus the data covers all important events like the Greek crisis, Brexit, Covid meltdown, etc. The inventory data is changed once every week. The actual inventory and the shock changes on Wednesday. After it is changed, the values remains the same for the whole week. The forecasted inventory value changes on Tuesday, and it remains the same for the whole week. We studied the effects of inventory on the variance of crude oil prices. This study will benefit the options trader who wants to benefit from the volatility of crude oil prices on the days of inventory announcements. We then tried to forecast the crude oil daily price variance.
We studied the granger causality of the different factors on the crude oil variance. We did not take the actual inventory as it has a correlation of above 0.5 with forecasted crude oil.
The factors which satisfy the granger causality tests are then taken into account for LSTM tests. In the LSTM tests, we optimized the lookback period and the gradient descent optimizer. The lookback period taken for studying is from 1 to 10 days. These 10 days will take care of 2 weeks on average. We did not take longer time periods as we have seen from Granger causality tests that variances of Gold and Dollar index influence even after 8 to 9 days. And the factors which do not influence crude oil daily variance even after ten days, are not taken seriously even if they show causality after 10 days. This is because it may be due to some spurious relationships. There may be some relationships between them in a weekly or monthly time frame, but in a fast-moving time frame, we neglect the relationships between these instruments after 10 days.
The LSTM and its optimization are then performed. The various statistical information of the factors is shown in the Fig 3 . The various statistical information of the factors is shown in the Table 2 . Except for Crude Oil Daily price volatility, other factors have 2218 data points from December 2010 to June 2020.
https://doi.org/10.1371/journal.pone.0268996.g003
https://doi.org/10.1371/journal.pone.0268996.t002
5.1 Inventory information shock
In the story of Crude Oil for the last decade (2011-2020), inventory plays a major role. We verified whether anything changed in the last decade compared to the previous decade (2006-2011) as found out by Hui [ 28 ]. To begin with, Hui compared the EIA reports with the Reuters’ value. Hui found out that the correlation between them was 0.4412 whereas in our case we found it to be 0.5871. Hui had several concerns over the Reuters’ data. She mentioned in her paper, “We have two main concerns regarding Reuters’ data. First, although these data have been used, we prefer to provide some direct evidence of their accuracy. Second, there is the possibility that Reuters’ forecasts may not capture all information available immediately before the EIA announcement. New information may reach the market between the time the survey is conducted and the time when the actual value is realized, and accordingly, such information may alter the expectations…..”
We reverified the second equation with the data for this decade (2011-2020). This is shown in Fig 4 . By comparing the results for the period (2006-2011) with Hui [ 28 ], we see that their results are quite comparable with ours (2011-2020). Her Adjusted R-squared is 0.1926 whereas ours is about 0.343. Her DW test result is 1.9589; whereas ours is 2.085. This implies that nothing, as such, is changed in this time. On the contrary, we can say that we have got better results over the course of time.
https://doi.org/10.1371/journal.pone.0268996.g004
In Table 3 we present the correlation between the inventory factors and daily Crude Oil price variance.
https://doi.org/10.1371/journal.pone.0268996.t003
Hui [ 28 ] mentioned in her paper, “To better understand the empirical results, we substitute actual inventory changes for inventory information shocks into the GARCH model. To do so, we incorporate D EIA Inv EIA , tau into the mean equation and D EIA | Inv EIA , tau | into the conditional variance equation. We then compare the results of the models with inventory information shocks and with actual inventory changes to identify which part of the inventory information has the greatest impact on crude oil futures daily returns and volatility……”. She also mentioned “…while inventory shocks can significantly affect the mean of returns, such shocks do not significantly affect conditional variance…..”. As seen from our correlation and regression Tables 3 and 4 , the conditional variance of daily Crude Price does not depend on Inventory Shock. The only difference between us and Hui is that she used conditional variance of daily returns while we used conditional variance of daily crude oil prices.
https://doi.org/10.1371/journal.pone.0268996.t004
So we could see that forecasted inventory and actual inventory are the major contributing factors for the variance of Crude Oil prices. But the correlation coefficient between forecasted inventory and actual inventory is 0.6057. So in order to avoid serial correlation, we only take forecasted inventory as one of the independent variables for forecasting crude oil variances. A diagram comparing actual inventory and forecasted inventory is given in Fig 5 .
https://doi.org/10.1371/journal.pone.0268996.g005
5.2 Granger causality tests
We performed the Granger Causality test for a lag term of 10 days, and we see that the forecasted inventory effects the crude oil price variance. So we have to accept our alternative hypothesis and claim that they are related.
Next we examine whether any of the following factors Bitcoin variance, S&P Index variance, Gold Price variance, Shanghai Stock Exchange Index variance, Dollar Index variance, German Bund Yield variance Granger cause crude oil price (variance) for a lag of 10 days or not?
Our results suggest that Bitcoin variance, Shanghai Stock Exchange variance, German 10-Year Bund Yield variance, US 10-Year Bond Yield variance do not Granger cause Crude Oil variance.
But the forecasted crude oil inventory, S&P Index variance and previous day Crude Oil Price variance Granger cause Crude Oil Price variance. Gold Price variance Granger causes Crude Oil variance after five days; while Dollar Index variance Granger causes Crude Oil variance after nine days.
So we choose forecasted crude oil inventory, S&P Index variance, Gold Price variance, and Dollar Index variance to be used as independent variable in order to predict Crude Oil price variance.
The situations are shown in Figs 6 and 13 .
Here, the correlation between two data is 0.3785.
https://doi.org/10.1371/journal.pone.0268996.g006
Here, the correlation between two data is 0.0188.
https://doi.org/10.1371/journal.pone.0268996.g007
Here, the correlation between two data is 0.0653.
https://doi.org/10.1371/journal.pone.0268996.g008
Here, the correlation between two data is 0.1589.
https://doi.org/10.1371/journal.pone.0268996.g009
Here, the correlation between two data is -0.0019.
https://doi.org/10.1371/journal.pone.0268996.g010
Here, the correlation between two data is 0.0388.
https://doi.org/10.1371/journal.pone.0268996.g011
Here, the correlation between two data is 0.0433.
https://doi.org/10.1371/journal.pone.0268996.g012
Here, the correlation between two data is 0.0241.
https://doi.org/10.1371/journal.pone.0268996.g013
5.3 Long Short-Term Memory (LSTM) test results
Crude Oil is the most volatile commodity traded in the market. As such, forecasting the variance of crude oil prices is one of the toughest and most important works. After performing the Granger causality tests, we are left with four variables. These are 1. Forecasted inventory, 2. S&P 500 index daily variance, 3. Gold daily price variance, and 4. Dollar index daily variance. We also used the previous day’s crude oil price variance as one of the deciding factors to forecast crude oil variances. One of the most important aspects of our research as compared to Urolagin et al. [ 49 ] is that we did not filter out the extreme values as those extreme values are very important for wealth creation and wealth destruction.
We use one LSTM layer, one dense layer, with the activation function of “tanh” and the loss was measured as “Mean Squared Error”. The training data and the test data are divided in 68:32 ratio. In Long Short-Term Method, the data have been scaled to a value between 0 and 1 using the min-max scalar value. We began with epoch = 70 and compared the lookback days from 0 to 10. This is done for both the “ Adam ” and “ Nadam ” optimizers. The results are shown in the Table 5 . A question may arise as to why did we choose only Adam and Nadam to compare with. The answer lies in the fact that in this case, the predictive power when the LSTM is performed with other gradient descent optimizers is way below the Adam and Nadam optimizers. So we chose the Adam and Nadam optimizers. The graphs are shown in the Fig 14 . We got a maximum R-squared corresponding to a lookback period of 1 day in the case of Adam and a lookback period of 8 days in the case of Nadam . In the case of Adam , it is 80.04% while in the case of Nadam it is 80.13%. Thereafter, we made a constant lookback period of 1 for Adam and 8 for Nadam , for our next optimization with respect to epochs.
https://doi.org/10.1371/journal.pone.0268996.t005
We can see that the best R-squared is found corresponding to a lookback period of 1 day for the “ Adam ” optimizer and a lookback period of 8 days for the “ Nadam ” optimizer.
https://doi.org/10.1371/journal.pone.0268996.g014
Now, corresponding to a lookback period of 1 for Adam optimizer, we made a comparative analysis for epochs from 50 to 100. Similarly, corresponding to a lookback period of 8 for Nadam optimizer, we made a comparative analysis for epochs from 50 to 100. The results are shown in the Tables 6 and 7 . The R squarred for the training data for Adam is 0.8944 while the same for Nadam is 0.8978. Coincidently, the best cases for both Adam and Nadam are found corresponding to an epoch of 68. We plotted a graph for the predicted value and the corresponding actual value for Crude Oil variances. The plots are given in Figs 15 and 16 .
The lookback period is 1 day. The number of epoch is 68. The activation function is ‘tanh’. and the gradient descent optimizer is “ Adam ”. It gives a R-squared of 80.18%. We can see that our model rightly predict the crude oil April 20, 2020, high volatility phase.
https://doi.org/10.1371/journal.pone.0268996.g015
The lookback period is 8 days. The number of epoch is 68. The activation function is ‘tanh’. and the gradient descent optimizer is “ Nadam ”. It gives a R-squared of 80.74%. We can see that our model rightly predict the crude oil April 20, 2020, high volatility phase.
https://doi.org/10.1371/journal.pone.0268996.g016
In the plots, we can see that the algorithm almost correctly predicted the actual values of variance. One of the most spectacular parts is that it could rightly predict price variance during the covid crisis. As a result, the options traders could use this algorithm to trade various crude oil options during these tumultuous times. Moreover, crude oil being important energy for human civilization, the governments could rightly make use of this algorithm to predict crude oil daily variance and make decisions. Besides that, we also found several new factors which influence crude oil daily variance and many other factors which do not influence the crude oil daily variance.
https://doi.org/10.1371/journal.pone.0268996.t006
https://doi.org/10.1371/journal.pone.0268996.t007
5.3.1 Shapley values.
We have calculated the Shapley Values of all the features for all the previous days, both for Adam and Nadam . The results are shown in Figs 17 – 25 .
https://doi.org/10.1371/journal.pone.0268996.g017
https://doi.org/10.1371/journal.pone.0268996.g018
https://doi.org/10.1371/journal.pone.0268996.g019
https://doi.org/10.1371/journal.pone.0268996.g020
https://doi.org/10.1371/journal.pone.0268996.g021
https://doi.org/10.1371/journal.pone.0268996.g022
https://doi.org/10.1371/journal.pone.0268996.g023
https://doi.org/10.1371/journal.pone.0268996.g024
https://doi.org/10.1371/journal.pone.0268996.g025
A lot of research has been done to forecast the volatility of crude oil using several classical methods. We have made a comparative study regarding some of the latest research in this context. In some of them, Mean Squared Error (MSE) is given. For that, we have converted the MSE to Root Mean Square Error (RMSE). The values are given in Table 8 below. We can see that our model has the least RMSE.
https://doi.org/10.1371/journal.pone.0268996.t008
6 Conclusions
The variance of daily crude oil prices has significance on several factors. On a smaller scale, it is directly beneficial for the options traders who trade on the volatility of the underlying instrument. But on a larger scale, it is beneficial for the oil-importing countries, the oil-exporting countries, producers, oil miners, consumers, etc. Oil is one of the major energies for human civilization, and it is known to be highly fluctuating. So a thorough study of its prices is important.
The inventory level of any commodity is an important deciding factor for the price of that commodity. We studied the actual inventory, forecasted inventory, inventory shocks, etc., of crude oil, and studied how these factors could help us in forecasting the variance of daily oil price.
We then studied the influence of several factors on the crude oil variance. The volatility of Gold is famous for being related to Crude Oil volatility. Likewise, US bond yield and German Bund yield are also studied as they signify the risk-free interest. Bitcoin is one of the newest forms of digital currencies and it has several complications. It is banned in countries like China while it is accepted as a legal tender in countries like El Salvador. So we studied its price variance and its predictive power for crude oil variance. The US dollar plays a significant role as far as transactions in crude oil are concerned. For international transactions in Crude Oil, the US dollar is the medium of exchange. As such the variance of the Dollar index is also studied. S&P 500 is the stock index of the leading stock market in the USA, while Shanghai Stock Exchange is the leading stock market in China. As the USA and China are the two major economies, their stock indices hold an important position in determining global trade and commerce. So the variance of S&P 500 and SSE are also included.
With the help of Granger causality, we studied the effects of the variances of several factors on the variance of crude oil. We chose a few factors from among them which will help us to forecast the variance of crude oil prices. For forecasting, we used the LSTM method, which is one of the newly developed methods for time series analysis. After optimizing the hyperparameters we got an R-squared value of 80.74%.
We can derive several important conclusions from our present work. To begin with, compared to 2006-2011, in the last decade 2011-2020, the forecasted inventory still has substantial predictive power over actual inventory. The forecasted inventory value has a huge impact on the variance of the daily crude oil prices, while the absolute value of the inventory information shock has no influence over the daily crude oil variance. We found out that past values of crude oil, forecasted inventory, variances of S&P 500, Dollar index, and Gold Granger cause crude oil. Thereafter, we found out that these values can explain the variation of the variances of daily crude oil prices to the extent of 80.74%. We could see that our method could successfully predict the variance in the oil crash during COVID, for the month of April 2020.
The results derived based on our present work can be presented pointwise below:
- Forecasted inventory can still regress actual inventory with an adjusted R-squared value of 0.343 for the time period of 2011-2020. Hui found that forecasted inventory regressed actual inventory for the time period of 2006-2011 with an R-squared value of 0.1926. The DW test result found by Hui is 1.9589 and while that of ours is 2.085. The correlation coefficient between forecasted inventory and actual inventory found by Hui is 0.4412 whereas the value found by us is 0.5871.
- The difference between Hui and our research is that Hui studied the conditional volatility of daily returns, whereas we studied the variance of daily Crude Oil prices. Another difference is that Hui primarily focussed on statistical methods to do the analysis, while we focussed on deep learning methods like LSTM for the same analysis.
- We found out that daily variance of Bitcoin, Shanghai Stock Exchange, German 10 Year Bund yield, and US 10 Year bond yield do not granger cause variance of daily crude oil prices.
- Likewise the daily variance of Bitcoin, Shanghai Stock Exchange, German 10 Year Bund yield, and US 10 Year bond yield do not granger cause variance of daily crude oil prices.
- The daily variance of Bitcoin, Shanghai Stock Exchange, German 10 Year Bund yield, and US 10 Year bond yield do not granger cause variance of daily crude oil prices.
- The forecasted inventory, and variance of S&P 500 granger cause variance of daily crude oil prices. Variances of gold and Dollar index granger cause variance of crude oil after several days.
- We also found that an LSTM strategy of one LSTM layer and one dense layer can forecast the variance of crude oil when forecasted inventory, variances of previous day crude oil, S&P 500, the Dollar index, and gold are taken as independent variables.
- After optimizing the various hyperparameters, we got that the maximum accuracy is found for the Adam optimizer when we take a lookback of 1 day, epochs = 68, activation function = tanh. The R- squared value is equal to 80.18%. This strategy could predict the high variance of oil prices during the COVID crisis of crude oil.
- After optimizing the various hyperparameters, we got that the maximum accuracy is found for the Nadam optimizer when we take a lookback of 8 days, epochs = 68, activation function = tanh. The R- squared value is equal to 80.74%. This strategy could predict the high variance of oil prices during the COVID crisis.
Supporting information
https://doi.org/10.1371/journal.pone.0268996.s001
- View Article
- Google Scholar
- 4. Mustaffa, Z., Yusof, Y. and Kamaruddin, S., 2021. Enhanced artificial bee colony for training least squares support vector machines in commodity price forecasting.
- 6. Gunawan, R., Khodra, M. and Harlili, 2013. Commodity price prediction using neural network case study: Crude palm oil price. 2013 International Conference on Computer, Control, Informatics and Its Applications (IC3INA),.
- 8. Claussen, K. L., and Dr. J. W. Uhrig. 1994. “Cash Soybean Price Prediction with Neural Networks.” Proceedings of the NCR-134 Conference on Applied Commodity Price Analysis, Forecasting, and Market Risk Management. Chicago, IL. [ http://www.farmdoc.uiuc.edu/nccc134 ].
- 9. Chen, J. and Wang, X., 2017. Development of fuzzy logic and genetic fuzzy commodity price prediction systems—An industrial case study. 2017 IEEE International Conference on Industrial Engineering and Engineering Management (IEEM),.
- 10. R. Mahajan, “Hybrid quantum inspired neural model for commodity price prediction,” 13th International Conference on Advanced Communication Technology (ICACT2011), 2011. pp. 1353–1357.
- 11. Chen, Z., Goh, H., Sin, K., Lim, K., Chung, N. and Liew, X., 2021. Automated Agriculture Commodity Price Prediction System with Machine Learning Techniques. arxiv, [online] Available at: https://arxiv.org/abs/2106.12747 [Accessed 11 July 2021].
- 20. He, K., Yu, L. and Lai, K., 2021. Crude oil price analysis and forecasting using wavelet decomposed ensemble model.
- 22. Shin, H., Hou, T., Park, K., Park, C. and Choi, S., 2021. Prediction of movement direction in crude oil prices based on semi-supervised learning.
- 36. Turhan, M., Sensoy, A., Ozturk, K. and Hacihasanoglu, E., 2021. A view to the long-run dynamic relationship between crude oil and the major asset classes.
- 41. Zhang, J. and He, Q., 2021. Dynamic Cross-Market Volatility Spillover Based on MSV Model: Evidence from Bitcoin, Gold, Crude Oil, and Stock Markets. Complexity, 2021. pp. 1–8.
- 42. Gkillas, K., Bouri, E., Gupta, R. and Roubaud, D., 2020. Spillovers in Higher-Order Moments of Crude Oil, Gold, and Bitcoin. The Quarterly Review of Economics and Finance,.
- 50. Liverance, E., 2010. Butterfly. Encyclopedia of Quantitative Finance,.
- 52. 2021. [online] Available at: https://www.cnbc.com/2020/06/16/how-negative-oil-prices-revealed-the-dangers-of-futures-trading.html [Accessed 5 August 2021].
- 53. Bloomberg.com. 2021. Bloomberg—Are you a robot?. [online] Available at: https://www.bloomberg.com/news/features/2020-12-10/stock-market-when-oil-when-negative-these-essex-traders-pounced [Accessed 5 August 2021].
- 54. Hedgeweek. 2021. Energy trader Andurand warns of “crazy losses” as US WTI oil prices turn negative. [online] Available at: https://www.hedgeweek.com/2020/04/21/284889/energy-trader-andurand-warns-crazy-losses-us-wti-oil-prices-turn-negative [Accessed 5 August 2021].
- 55. The Economic Times. 2021. Oil crash busted broker’s computers and inflicted big losses. [online] Available at: https://economictimes.indiatimes.com/markets/commodities/news/oil-crash-busted-brokers-computers-and-inflicted-big-losses/articleshow/75642150.cms?from=mdr [Accessed 5 August 2021].
- 56. National Centers for Environmental Information (NCEI). 2021. On This Day: 2011 Tohoku Earthquake and Tsunami. [online] Available at: https://www.ncei.noaa.gov/news/day-2011-japan-earthquake-and-tsunami [Accessed 11 August 2021].
- 57. Council on Foreign Relations. 2021. Greece’s Debt Crisis Timeline. [online] Available at: https://www.cfr.org/timeline/greeces-debt-crisis-timeline [Accessed 11 August 2021].
- 58. 2021. [online] Available at: https://www.imf.org/external///datamapper/PPPSH@WEO/OEMDC/ADVEC/WEOWORLD [Accessed 11 August 2021].
- 59. Ticdata.treasury.gov. 2021. [online] Available at: https://ticdata.treasury.gov/Publish/mfh.txt [Accessed 11 August 2021].
- 60. Walker, N., 2021. Brexit timeline: events leading to the UK’s exit from the European Union. [online] House of Commons Library. Available at: https://commonslibrary.parliament.uk/research-briefings/cbp-7960/ [Accessed 11 August 2021].
- 61. Ons.gov.uk. 2021. Gross Domestic Product: chained volume measures: Seasonally adjusted £m—Office for National Statistics. [online] Available at: https://www.ons.gov.uk/economy/grossdomesticproductgdp/timeseries/abmi/ukea [Accessed 11 August 2021].
- 62. Who.int. 2021. WHO Director-General’s opening remarks at the media briefing on COVID-19—11 March 2020. [online] Available at: https://www.who.int/director-general/speeches/detail/who-director-general-s-opening-remarks-at-the-media-briefing-on-covid-19--11-march-2020 [Accessed 11 August 2021].
- PubMed/NCBI
- 65. Colah.github.io. 2021. Understanding LSTM Networks—colah’s blog. [online] Available at: http://colah.github.io/posts/2015-08-Understanding-LSTMs/ [Accessed 7 August 2021].
- 66. P. Kingma, D. and Lei Ba, J., 2017. ADAM: A METHOD FOR STOCHASTIC OPTIMIZATION. arXiv:1412.6980, [online] Available at: https://arxiv.org/abs/1412.6980 [Accessed 12 August 2021].
- 67. Dozat, T., 2016. INCORPORATING NESTEROV MOMENTUM INTO ADAM. Workshop track—ICLR 2016, [online] Available at: https://openreview.net/forum?id=OM0jvwB8jIp57ZJjtNEZ [Accessed 12 August 2021].
- 68. Shapley, Lloyd S. (August 21, 1951). “Notes on the n-Person Game—II: The Value of an n-Person Game”. Santa Monica, Calif.: RAND Corporation.
- 69. Roth A. (1988) The Shapley Value: Essays in honor of Lloyd S. Shapley. Cambridge: Cambridge University Press.
- Research note
- Open access
- Published: 04 December 2019
Assessment of quality of edible vegetable oils accessed in Gondar City, Northwest Ethiopia
- Yonnas Adugna Negash 1 ,
- Dagnachew Eyachew Amare ORCID: orcid.org/0000-0003-1314-5586 1 ,
- Bikes Destaw Bitew 1 &
- Henok Dagne 1
BMC Research Notes volume 12 , Article number: 793 ( 2019 ) Cite this article
44k Accesses
47 Citations
Metrics details
Edible vegetable oils are prone to quality deterioration through oxidation and microbial degradation resulting in nutritional loss and off-flavors. Quality deterioration may contribute in the formation of oxidation products that are reactive and toxic, which ultimately pose health risks including cancer and inflammation. The objective of this study was to assess quality of both imported and locally made edible vegetable oils accessed in Gondar City, Ethiopia. Cross-sectional study design was used to collect 60 samples randomly; 30 from locally made (Niger seed at market 14, Niger seed at production center 11, sunflower at the market 5) and 30 from imported palm oil brands (Avena 11, Hayat 4, Jersey 5 and Chef 10).
The mean value for: moisture content (%) (0.333 ± 0.08 while 0.089 ± 0.11), specific-gravity (0.823 ± 0.14 and 0.807 ± 0.115), peroxide value (15.09 ± 1.61 and 7.05 ± 0.102 mill-equivalents of oxygen/kg), acid value (2.43 ± 0.9 and 0.98 ± 0.23 mg KOH/g oil) and iodine value (115.63 ± 6.77 and 21.8 ± 3.4 g I 2 /100 g oil) for local and imported edible oils, respectively. The results highlight that all rancidity quality parameters of the locally made oil samples were not within the joint WHO/FAO standards whilst the imported oils showed a greater fatty acid saturation.
Introduction
Edible vegetable oils are triglycerides of plant origin that include olive, palm, soybean, canola, and sunflower oil [ 1 , 2 ]. Oil and fat are important nutritional components with variety of functions in our body as an energy source, membrane structures, regulating body temperature and insulate organs [ 3 , 4 ].
Vegetable oils may rancid and hence lose its nutritional values and flavor upon improper extraction process, handling and storage [ 5 , 6 ]. Moisture, microbes, air, anti-oxidants and exposure to sunlight are among factors determining the oils rancidity or deterioration time [ 7 , 8 , 9 ].
In quality control, several parameters such as iodine value (degree of unsaturation), peroxide value (formation of primary oxidation products), moisture content, specific gravity (purity), and acid value (free fatty acids formation because of rancidity) are key parameters of interest as they determine the shelf-life quality and hence the economic value of oils [ 3 , 10 ]. Rancidity of vegetable oils may pose health risks including cancer and inflammation because of the formation of toxic and reactive oxidation products [ 2 , 11 , 12 ]. For healthy consumption, unsaturated oils are better than the saturated. Consumption of palmitic oil (highly saturated) is associated with an increased risk of developing cardiovascular diseases [ 12 , 13 ]. In contrast, edible vegetable oils such as sunflower, olive, canola and Niger-seed oils contains high levels of polyunsaturated fats [ 2 , 14 ] which make them susceptible for rancidity.
Unlike in developing countries like Ethiopia, the developed countries have a strict food safety regulation [ 5 ]. Studies showed that developed countries society have greater awareness compared to developing countries in edible oil purchasing choice [ 15 , 16 , 17 ]. WHO/FAO has outlined quality standards for various edible vegetable oils constituents; heavy metals, fatty acids composition, antioxidants, micronutrients and other physicochemical parameters [ 18 ]. The WHO/FAO guideline sets the maximum allowable limit for edible oils quality parameters including moisture (0.2%), acid value (0.6 mg potassium hydroxide/g oil) and peroxide value (10 mill-equivalents oxygen/kg oil) [ 19 ].
Due to limited published researches and its public health importance, periodic oil quality analysis is required. Therefore, the aim of the study was to assess qualities of edible vegetable oils accessed in Gondar city, Ethiopia regarding rancidity and level of fatty-acid saturation.
Study design and sample size
A cross-sectional study was conducted on selected edible vegetable oils in the local market of Gondar city in 2019. Sixty samples; 30 from locally made (Niger seed at the market 14, Niger seed at the production center 11, sunflower at the market 5) and 30 from imported palm oil brands (Avena 11, Hayat 4, Jersey 5 and Chef 10) were taken.
Experimental procedure
Purchased oil brands in the local market were transported to the Department of Biology laboratory, University of Gondar for analysis. Care was taken to avoid air contact during analysis to keep-away oxidation reactions.
All the parameters analysis were performed using the standard methods of oil analysis by Paquot [ 20 ].
Moisture content
Ten g of oil sample was placed in a weighed crucible. The samples were dried for 1 h to constant weights in an oven set at 105 °C and then allowed to cool in desiccators for 15 min and finally, the difference was calculated using the following equation.
where, W1 = weight loss (g) upon drying, W2 = weight (g) of the oil sample.
Specific gravity
Dry pycnometer was used to determine specific gravity. Specific gravity were measured by Relative Density of oil to water. Distilled water was added into the pycnometer followed by measurement using electronic balance. Similarly, oil weight was measured. Care was taken to avoid leakage of air into the pycometer. The specific gravity value was calculated as follows:
Peroxide value
Ten mL of oil sample was dissolved in acetic-acid/chloroform (3: 2 ratios) solvents. This solution was further reacted with 0.5 mL of 15% potassium iodide (KI). The liberated iodine was titrated with 0.1 N sodium-thiosulphate using 0.5 mL starch as indicator. Blank titration was performed. The peroxide value was calculated as follows:
where, S = volume of sodium-thiosulphate consumed by the oil sample, B = volume of sodium-thiosulphate used for blank, W = weight of oil sample, N = the normality of sodium-thiosulphate.
Mixture of 10 mL of oil sample and 100 mL of ethyl-alcohol was heated until the content started boiling. The hot content was cooled and titrated with 15% KOH solution using phenolphthalein as endpoint indicator. Acid value was calculated as follows:
where, V = volume of standard KOH solution in mL, N = normality of standard KOH solution, W = weight of oil sample in grams, M.wt (molecular weight) of KOH = 56.1 g/mol.
Iodine value
Mixture of 0.5 mL of oil sample and 10 mL chloroform was added into 25 ml of iodine solution, stayed for 30 min for a complete reaction between iodine and the unsaturated bonds of oils. The flask was covered by aluminum foil to avoid light exposure. Then, 20 mL of 15% aqueous KI and 100 mL of water was added to transform leftover iodine to iodide. The final content was titrated with 0.1 N sodium-thiosulphate (Na 2 S 2 O 3 ) solutions using starch as an indicator. Iodine value was calculated as follows:
where, A = mL of 0.1 N Na 2 S 2 O 3 required by oil sample, B = mL of 0.1 N Na 2 S 2 O 3 required by the blank, N = normality of Na 2 S 2 O 3, W = weight of oil in gram, 1 mL 1 N Na 2 S 2 O 3 = 0.127 g I 2.
Quality assurance
For quality assurance, instrument calibration and pretest for functionality of instruments were conducted before the laboratory analysis. Analysis involved blank measurements and all the measurements were done triplicate wise. Standard analysis methods were followed.
Data processing and analysis
Data were entered in Epi-Info-version-7.2.10 and exported to SPSS-version-20 for statistical analysis. Mean values and standard deviations were calculated. ANOVA was used for the analysis of variance among the oil brands for the respective parameters, and independent t-test was used for comparison between locally made and imported oils.
Quality analysis results
From the 60 samples analyzed, moisture content 9 (30%) and 25 (83.3%), specific gravity 8 (26.7%) and 7 (23.3%), peroxide value 5 (16.7%) and 20 (66.7%), acid value 4 (13.3%) and 2 (6.7%) and iodine value 8 (26.7%) and 2 (6.7%) were within the limits of WHO/FAO standards for locally made and imported edible vegetable oil, respectively (Table 1 ).
The mean moisture value for locally made and imported oils were found to be 0.333 ± 0.08 and 0.089 ± 0.11, respectively. There is a significant difference in moisture content between local made and imported edible vegetable oils having p-value 0.016 of 95% confidence interval (CI). However, there is no significant difference within oil brands.
The mean specific gravity value for the locally made and imported oils were 0.823 ± 0.14 and 0.807 ± 0.115, respectively. There is a significant difference on specific gravity between locally made and imported edible oils with p-value < 0.001 of 95% CI. Whereas, there was no significant difference among the various brands.
The mean peroxide value for the local products and imported were 15.09 ± 1.61 and 7.05 ± 0.102, respectively. There is a significant difference being local and imported edible oil with p-value < 0.003 of 95% CI.
The mean acid value for the locally made and imported oils were 2.43 ± 0.9 and 0.98 ± 0.23, respectively. The acid value between the locally made and imported edible oils significantly differ with p-value < 0.001 of 95% of the CI. The locally made edible oils have shown a greater deviation from the WHO/FAO standard value (0.6 mg KOH/g oil). Niger seed edible oil has shown a significant difference with avena, Chef, and jersey brands with p-value < 0.001, 0.002 and 0.001, respectively of 95% CI.
The iodine value was significantly differ between locally made and imported edible oils with p-value < 0.001 of 95% CI. The iodine value for the local products and imported were 115.63 ± 6.77 and 21.8 ± 3.4, respectively. It was observed that the iodine value significantly different among the brands with a p-value < 0.001 of 95% CI.
Discussions
Iodine value of oils was determined mainly to determine which oil types are more saturated. The results depicted that the locally made oils (sunflower and Niger seed) have displayed a higher iodine value, proportional to the unsaturated fatty acids when compared to the imported. Unsaturated fatty-acids is recommended for a healthy consumption over highly saturated fatty-acids containing oil.
Previous studies depicted that sunflower and Niger seed oils have a higher unsaturated fatty-acids compared to palm oils [ 21 ]. Hence, the observed higher iodine value in the locally made oils indicated that they are likely to be healthier for consumption. Studies have recommended to switch from saturated to unsaturated fats because of the risk of cardiovascular disease associated with high consumption of saturated fatty-acids [ 22 , 23 , 24 , 25 ]. However, highly unsaturated oils undertake oxidation degradation because of their double bonds [ 7 ] unless sufficient antioxidant is added [ 26 , 27 , 28 ].
The mean peroxide value in this study was 2.73 and 15.03 mill-equivalents of active oxygen/kg oil for imported oils and locally made oils, respectively. Peroxide values of the locally made edible oils were deviated significantly (P < 0.05) from the WHO/FAO limits (10 mill-equivalents of active oxygen/kg oil). The lower peroxide value for the imported oils is likely attributed to their higher saturation, which resists oxidation. Moreover, palm oils are known for having antioxidants (vitamin E) that extend its shelf-life [ 10 , 29 ].
When moisture content ranges from 0.05 to 0.3 in edible oils, it shows that rancidity likely to occur [ 29 ]. The maximum allowed moisture content in edible oils is 0.2% [ 14 ]. The higher moisture content observed in the local products could be the poor moisture refining process as the companies are using low technology for oil production [ 30 ]. The other reason could be that those locally made oils require extra heating for reducing the moisture content kept inside the seed [ 30 , 31 ] before production. Previous studies have found that oils which were produced using low technology displayed a higher moisture [ 31 , 32 ]. Therefore, the higher moisture content in the locally made oils indicated that they are likely to undergo rancidity. This is because the presence of sufficient amount of moisture favors microbial growth [ 31 ]. Previous studies have shown that fungus species such as Aspergillus niger and Mucor species survive and reproduce when the moisture content value is higher than 0.2% [ 29 ].
The acid values obtained in this study were 2.728 mg KOH/g for the locally made oils and 0.999 mg KOH/g for the imported oils which showed that both were above the permissible level (0.6 mg KOH/g) [ 18 ]. The local edible oils have displayed a higher acid value than the imported products where the formers higher fatty unsaturation could be responsible for the deviation. Consuming rancid edible oil is unlikely to cause immediate health impact, but can reduce significantly the nutritional value of foods by degrading the essential fatty-acids and nutrients [ 22 , 33 ]. The acid value of palm oil brands in this study is in line with the previous study [ 34 ].
In this study, the mean value (0.823) of specific gravity for locally made oils was not in line with the WHO limit (Niger seed 0.917–0.92; Sunflower 0.919–0.923) [ 18 ]. The specific gravity value which was significantly deviated from the standards could be related to the poor refining and upgrading process in the local production facilities. Insufficient refining process may lead for a higher impure oil grade [ 14 , 29 ]. Furthermore, the specific gravity values of the locally made oils were below the standard ranges make them susceptible to adulteration [ 30 ].
Conclusions
The results showed that locally made edible oils displayed higher degree of rancidity compared to the imported. The higher iodine value in the locally made oils indicated that these oil types are better for public consumption with respect to health risks.
Limitation of the study
Comparison of oil qualities between oils taken from the production facility and oils taken in the market were only performed for the locally made oil types.
Availability of data and materials
The dataset is accessible at the corresponding author upon request.
Abbreviations
analysis of variance
confidence interval
Food and Agriculture Organization
potassium iodine
potassium hydroxide
standard deviation
sodium thiosulfate
World Health Organization
Ramadhas A, Jayaraj S, Muraleedharan C. Use of vegetable oils as IC engine fuels—a review. Renew Energy. 2004;29(5):727–42.
Article CAS Google Scholar
Ekwu F, Nwagu A. Effect of processing on the quality of cashew nut oils. J Sci Agric Food Tech Environ. 2004;2004(4):105–10.
Google Scholar
Endo Y. Analytical methods to evaluate the quality of edible fats and oils: the JOCS standard methods for analysis of fats, oils and related materials (2013) and advanced methods. J Oleo Sci. 2018;67(1):1–10.
Lawson-Wood K, Seer Green U, Bohman A, Shelton C, Way K. Determination of quality parameters of crude palm oil using near-infrared spectroscopy and multivariate analysis.
Okparanta S, Daminabo V, Solomon L. Assessment of rancidity and other physicochemical properties of edible oils (mustard and corn oils) stored at room temperature. J Food Nutr Sci. 2018;6(3):70–5.
CAS Google Scholar
Abulude F, Ogunkoya M, Ogunleye R. Storage properties of oils of two Nigerian oil seeds Jatropha curcas (physic nut) and Helianthus annuus (sunflower). Am J Food Technol. 2007;2(3):207–11.
Adetola O, Alabi O, Abdulrauf I. Investigating storage duration and packaging materials on quality of fresh palm oil.
Kaleem A, Aziz S, Iqtedar M. Investigating changes and effect of peroxide values in cooking oils subject to light and heat. FUUAST J Biol. 2015;5(2):191–6.
Sherwin E. Oxidation and antioxidants in fat and oil processing. J Am Oil Chem Soc. 1978;55(11):809–14.
Decker EA, Elias RJ, McClements DJ. Oxidation in foods and beverages and antioxidant applications: management in different industry sectors. Amsterdam: Elsevier; 2010.
Book Google Scholar
Mehmood T, Ahmad A, Ahmed A, Khalid N. Quality evaluation and safety assessment of different cooking oils available in Pakistan. J Chem Soc Pak. 2012;34(3):518–25.
Mukherjee S, Mitra A. Health effects of palm oil. J Hum Ecol. 2009;26(3):197–203.
Article Google Scholar
Mozaffarian D, Clarke R. Quantitative effects on cardiovascular risk factors and coronary heart disease risk of replacing partially hydrogenated vegetable oils with other fats and oils. Eur J Clin Nutr. 2009;63(S2):S22.
Federation AO. Section 1: Quality Standards, Technical Information & Typical Analysis. Australian Oilseeds Federation, Australia Square. 2011, pp 40–45.
Melesse MB, van den Berg M, de Brauw A, Abate GT. Understanding Urban Consumers’ Food Choice Behavior in Ethiopia.
Thompson KE, Haziris N, Alekos PJ. Attitudes and food choice behaviour. Br Food J. 1994;96(11):9–13.
Ali J, Kapoor S, Moorthy J. Buying behaviour of consumers for food products in an emerging economy. Br Food J. 2010;112(2):109–24.
Alimentarius C. Codex standard for named vegetable oils.
Alimentarius C. Codex standards for fats and oils from vegetable sources. ALIMENTARIUM, C. 1999.
Paquot C. Standard methods for the analysis of oils, fats and derivatives. Amsterdam: Elsevier; 2013.
He L, Guoying Z, Huaiyun Z, Yuanhao H. Chemical constituents and biological activities of saponin from the seed of Camellia oleifera . Sci Res Essays. 2010;5(25):4088–92.
De Souza RJ, Mente A, Maroleanu A, Cozma AI, Ha V, Kishibe T, et al. Intake of saturated and trans unsaturated fatty acids and risk of all cause mortality, cardiovascular disease, and type 2 diabetes: systematic review and meta-analysis of observational studies. BMJ. 2015;351:h3978.
Li Y, Hruby A, Bernstein AM, Ley SH, Wang DD, Chiuve SE, et al. Saturated fats compared with unsaturated fats and sources of carbohydrates in relation to risk of coronary heart disease: a prospective cohort study. J Am Coll Cardiol. 2015;66(14):1538–48.
German JB, Dillard CJ. Saturated fats: what dietary intake? Am J Clin Nutr. 2004;80(3):550–9.
Nettleton JA. Omega-3 fatty acids and health. Omega-3 fatty acids and health. Berlin: Springer; 1995. p. 64–76.
Xiu-Qin L, Chao J, Yan-Yan S, Min-Li Y, Xiao-Gang C. Analysis of synthetic antioxidants and preservatives in edible vegetable oil by HPLC/TOF-MS. Food Chem. 2009;113(2):692–700.
Cheung SCM, Szeto YT, Benzie IF. Antioxidant protection of edible oils. Plant Foods Hum Nutr. 2007;62(1):39–42.
Dziedzic SZ, Hudson BJ. Polyhydroxy chalcones and flavanones as antioxidants for edible oils. Food Chem. 1983;12(3):205–12.
Commission CA. Codex standard for named vegetable oils codex stan 210-1999. Report of the 17th session of the codex committee on fats and oils, London; 2001, pp 19–23.
Orji MU, Mbata TI. Effect of extraction methods on the quality and spoilage of Nigerian palm oil. Afr J Biochem Res. 2008;2(9):192–6.
Okechalu JN, Dashen MM, Lar PM, Okechalu B, Gushop T. Microbiological quality and chemical characteristics of palm oil sold within Jos Metropolis. Nigeria: Plateau State; 2011.
Abbas NM, Al-Fatlawi AM. Investigating peroxides and acid value in used edible vegetable oils. Iraqi J Agric Sci. 2010;41(4):123–32.
Shahidi F, Wanasundara UN. Methods for measuring oxidative rancidity in fats and oils. Food Lipids Chem Nutr Biotechnol. 2002;17:387–403.
Hasan M, Rownok Jahan M, Alam A, Khatun MK, Al-Reza SM. Study on physicochemical properties of edible oils available in Bangladeshi local market. Arch Curr Res Int. 2016;6(1):1–6.
Download references
Acknowledgements
The authors’ would like to thank the Gondar trade administration officials for the information about the types of oils were available at the market.
No funding agent.
Author information
Authors and affiliations.
Department of Environmental and Occupational Health & Safety, Institute of Public Health, College of Medicine and Health Sciences, University of Gondar, Gondar, Ethiopia
Yonnas Adugna Negash, Dagnachew Eyachew Amare, Bikes Destaw Bitew & Henok Dagne
You can also search for this author in PubMed Google Scholar
Contributions
YAN involved in the proposal development, lab works and report writing; DEA and BDB involved in guiding the work, data analysis and manuscript writing; HD involved in data analysis and manuscript writing. All the authors read and approved the manuscript.
Corresponding author
Correspondence to Dagnachew Eyachew Amare .
Ethics declarations
Ethical approval and consent to participate.
Ethical approval was obtained from Institutional ethical review board of the Institute of public health, College of Medicine and health sciences, University of Gondar. Support letter was obtained from Gondar town trade and industry office.
Consent for publication
Not applicable.
Competing interests
The authors declare that they have no competing interests.
Additional information
Publisher's note.
Springer Nature remains neutral with regard to jurisdictional claims in published maps and institutional affiliations.
Rights and permissions
Open Access This article is distributed under the terms of the Creative Commons Attribution 4.0 International License ( http://creativecommons.org/licenses/by/4.0/ ), which permits unrestricted use, distribution, and reproduction in any medium, provided you give appropriate credit to the original author(s) and the source, provide a link to the Creative Commons license, and indicate if changes were made. The Creative Commons Public Domain Dedication waiver (http://creativecommons.org/publicdomain/zero/1.0/) applies to the data made available in this article, unless otherwise stated.
Reprints and permissions
About this article
Cite this article.
Negash, Y.A., Amare, D.E., Bitew, B.D. et al. Assessment of quality of edible vegetable oils accessed in Gondar City, Northwest Ethiopia. BMC Res Notes 12 , 793 (2019). https://doi.org/10.1186/s13104-019-4831-x
Download citation
Received : 30 October 2019
Accepted : 27 November 2019
Published : 04 December 2019
DOI : https://doi.org/10.1186/s13104-019-4831-x
Share this article
Anyone you share the following link with will be able to read this content:
Sorry, a shareable link is not currently available for this article.
Provided by the Springer Nature SharedIt content-sharing initiative
- Edible vegetable oil
- Locally made
- Quality parameters
BMC Research Notes
ISSN: 1756-0500
- Submission enquiries: [email protected]
- General enquiries: [email protected]
Thank you for visiting nature.com. You are using a browser version with limited support for CSS. To obtain the best experience, we recommend you use a more up to date browser (or turn off compatibility mode in Internet Explorer). In the meantime, to ensure continued support, we are displaying the site without styles and JavaScript.
- View all journals
Crude oil articles from across Nature Portfolio
Latest research and reviews.
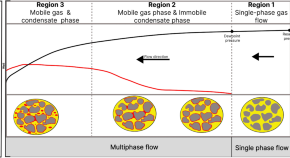
A Microfluidic and Numerical Analysis of Non-equilibrium Phase Behavior of Gas Condensates
- Desmond Batsa Dorhjie
- Dmitrii Pereponov
- Alexey Cheremisin
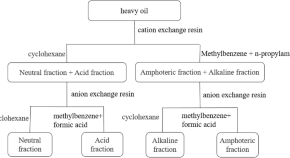
Study on the kinetics of formation process of emulsion of heavy oil and its functional group components
- Chengdi Sun
- Pujiang Yang
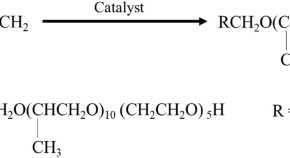
Preparation and performance evaluation of a novel temperature-resistant anionic/nonionic surfactant
- Yongwei Duan
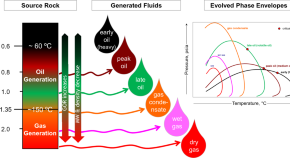
Phase envelopes in reservoir fill analysis: Two contrasting scenarios
- Khaled R. Arouri
- Carlos G. Herrera
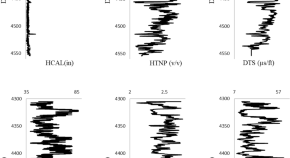
Comparing the performance of machine learning methods in estimating the shear wave transit time in one of the reservoirs in southwest of Iran
- MohammadRasool Dehghani
- Shahryar Jahani
- Ali Ranjbar
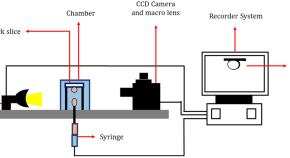
Experimental investigation of the sequence injection effect of sea water and smart water into an offshore carbonate reservoir for enhanced oil recovery
- Amir Hossein Saeedi Dehaghani
- Reza Daneshfar
News and Comment
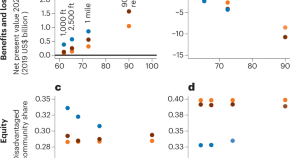
Well setbacks limit California’s oil supply with larger health benefits and employment losses than excise and carbon taxes
Compared to excise taxes and carbon taxes, setback restrictions on new oil wells have larger health benefits and worker compensation losses, but are more equitable by bringing greater benefits and lower losses to disadvantaged communities in California. For California to meet green gas emissions (GHG) targets, larger setbacks than currently proposed or additional supply-side policies are needed.
- Ranjit Deshmukh
- Paige Weber
- Kyle C. Meng
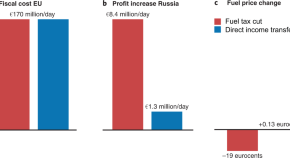
European fuel tax cuts increase Russian oil profits
European fuel tax cuts will lead to significant income transfers to Russia, thus undermining the Union’s sanction efforts against the country. EU politicians should instead consider alternative policies, such as direct income transfers to households, if they want to shield citizens from increased fuel prices without benefiting Russia.
- Daniel Spiro
- Henrik Wachtmeister
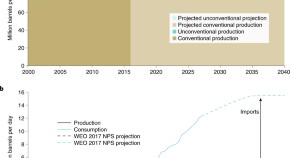
Mapping Chinese supply
Documenting the emissions and net energy of a crude supply could be essential to meeting national emission and energy security targets. Using data from hundreds of fields worldwide, a well-to-refinery study presents a high-granularity profile of China’s crude oil supply in terms of emissions and energy return on input.
- Mikael Höök
Oil production: Impact of age
- James Gallagher
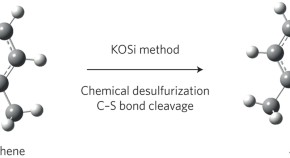
Transportation fuels: Desulfurizing diesel
Transportation fuels such as diesel contain organosulfur molecules that, when combusted, form sulfur oxides that are toxic and poison vehicles' catalytic convertors. Now, a method is demonstrated that can reduce the sulfur concentration of diesel fuel to very low levels at low temperatures and pressures.
- Carole Lamonier
Quick links
- Explore articles by subject
- Guide to authors
- Editorial policies


An official website of the United States government
The .gov means it’s official. Federal government websites often end in .gov or .mil. Before sharing sensitive information, make sure you’re on a federal government site.
The site is secure. The https:// ensures that you are connecting to the official website and that any information you provide is encrypted and transmitted securely.
- Publications
- Account settings
Preview improvements coming to the PMC website in October 2024. Learn More or Try it out now .
- Advanced Search
- Journal List
- Indian Heart J
- v.68(4); Jul-Aug 2016
Selecting healthy edible oil in the Indian context
Coronary heart disease (CHD) is the leading cause of mortality all over the world; its incidence is rising rapidly, especially in developing countries, including India. Dietary factors, particularly the edible oils, play an important role in the causation, treatment, management, and prevention of CHD. Cooking oils form an integral part of Indian diets; however, one is confronted with an array of commonly marketed edible oils asserting host of health claims. Therefore, right selection of edible oil is extremely important, especially in the Indian context, where cooking methods are different then in the west. Numerous clinical trials and observational/metabolic studies among diverse populations indicate a consistent association between quality/quantity of fat intake and the CHD risk. 1 , 2 The effect of dietary fats on plasma lipids constitutes a key link in the causal pathway that connects diet to CVD.
Edible oils have several fatty acids, which can be grouped into three classes–saturated fatty acids (SFA) (which have 3 groups, short-chain, medium-chain, and long-chain SFA), monosaturated (MUFA), and polyunsaturated (PUFA) (further subdivided into linolenic (LC or n6), alpha-linolenic (ALNA or n3) acid, and transfatty acids (TFA)), which are produced by hydrogenation of vegetable oils (Vanaspati ghee) or marine oils. Table 1 shows the approximate fatty acid composition of various edible oils.
Approximate fatty acid composition of visible fats (g/100 g).
nd, not detected; SFA, saturated fatty acids; MUFA, mono saturated fatty acid; LA, linolenic acid; ALNA, alpha linolenic acid.
In addition, edible oils contain several antioxidants (like tocopherols, oryzanol, carotenes, tocotrienols, etc.), phytosterols, and micronutrients.
SFA have been considered harmful, as they can increase total cholesterol (Tc) and LDL cholesterol – a risk factor for atherosclerosis. 2 A meta-analysis of randomized trials suggested a 17% reduction in risk of CHD in studies that reduced SFA from about 17% to about 9% of energy (RR 0.83, 95% CI 0.72–0.98). 3 However, short- and medium-chain SFA are not harmful, as they do not affect the serum lipids. 4 A randomized study in this issue of the journal has also indicated that even after 2 years of follow-up, serum lipids were not altered by coconut oil (which is rich in SFA) as compared to sunflower oil. 5 A recent systemic review also suggests that SFA may not be harmful as considered earlier. 6 PUFA and MUFA are other types of fatty acids that can lower LDLc and are cardioprotective. 2 Jakobsen et al. 7 have reported that substitution of SFA with PUFA can significantly reduce the CHD risk in a pooled analysis of 11 cohort studies. N6 (linolenic acid) and N3 (alpha-linolenic acid) are essential fatty acids required for proper functioning of the body. N6 PUFA lowers not only LDLc but can also decreases HDL, whereas N3 PUFA may lower triglycerides, blood pressure, inflammation, improve vascular function, and sudden death. 8 , 9 N6 and N3 PUFA should be present in adequate and balanced proportion in the body because both compete for the enzymes that convert them into more active compounds. Several dietary recommendations suggest that the ratio of n6:n3 PUFA should be 5–10:1 or lower to prevent heart disease. 10 , 11 There is evidence that in humans when omega-6 intake is kept low, plant-based omega-3 can be converted to long-chain n3 fatty acids as found in fish oils (eicosapentaenoic acid) in limited amounts. On the other hand, transfatty acids (TFA) produced by hydrogenation of vegetable fat (Vanaspati ghee) due to the undesirable effects on serum lipids are associated with an elevated risk of CHD and are considered even worse than the saturated fats. 12 , 13 Several reviews have demonstrated that high intake of TFA was associated with increased CHD events and mortality and also possibly other chronic diseases like Alzhiemer's disease, cancer, diabetes, obesity, inflammation, depression, etc. Antioxidants present in several oils (like tocotrienols, tocopherols, oryzanol, and phytosteroles) have favorable effects on lipids and oxidative stress and can prevent heart disease. 14 , 15 , 16
Studies indicate that olive oil intake can confer various health benefits in addition to reduced CHD risk. 17 A randomized controlled intervention by Covas et al. 18 has demonstrated that in olive oil, apart from monounsaturated fatty acids, its polyphenolic compounds confer beneficial effects on plasma lipid concentrations and bring about linear reduction in oxidative stress markers. However, the main limitation is that olive oil does not have ideal N6 N3 ratio and may not be suitable for Indian cooking. Mustard oil is considered healthy edible oil because it is low in SFA, high in MUFA and PUFA, specially alpha-linolenic acid, and a good n6:n3 ratio (6:5). It is also available in nonrefined (cold compressed) form and is relatively stable during cooking at high temperatures. Several studies also suggest that mustard oil may be associated with lower CHD risk as compared to other oils. A multicentre epidemiologic study by Rastogi et al. 19 reported 71% reduction in CHD risk among individuals using mustard oil for frying as compared to sunflower oil (RR 0.29, 95% CI 0.13–0.64). Another double-blind RCT has demonstrated that in acute MI patients using mustard oil, there was reduction in arrhythmias, heart failure, and angina. 20 Based on earlier studies in rats, there was a concern regarding high erucic acid content of mustard oil 21 ; however, later studies showed that in rats there is an inefficient activation of erucic acid to erucyl-CoA coupled with lowered activity of triglyceride lipase and enzymes associated with β-oxidation of erucic acid, which possibly contribute to the accumulation and retention of cardiac lipids. Other species, including humans, have not demonstrated to have such toxic effects. Low erucic acid rapeseed oil (canola), by virtue of its ideal LA/ALNA ratio, has also been found to exert cardioprotective effects. 22 Flaxseed oil , though a rich source of ALNA, is not commonly consumed; however, blending it with other edible oils is a good strategy to increase ALNA intakes.
1. Indian cooking conditions
Indian cooking conditions subject oil to very high temperatures, like in deep frying during which the oil temperatures can go above 170 °C.
It has been demonstrated that certain oils, especially refined oils with high PUFA, can degrade easily to toxic components like free radicals, transfats, melondialdehyde (MDA), etc., which are potentially mutagenic and atherogenic. 23 Repeated frying of the oil can further damage the oil and produce more toxic components that are highly harmful to the heart. An Indian study has demonstrated that TFA content of oil samples drawn for the halwais, who use same oil for repeated frying, have high TFA. 24 It is also preferable to avoid refined oils and use cold-pressed or extra virgin oils. Refined oils are purified oils from oil cakes using highly intense mechanical and chemical (solvent extraction) processes to extract the oil from the seeds and vegetables products. The crushed seeds are heated repeatedly to high temperatures up to 270 °C in a steam bath for deodorization and to start the oil extraction process. These high temperatures can result in loss of antioxidants (like tocopherols) and sterols, produce free radicals and TFA, and polymeric components, which are potentially atherogenic and mutagenic. Oils high in saturated fats like ghee/coconut are ideal for deep-frying, as they are more stable.
Blending of oils combines the potency of two/more edible oils; it offers a balance of fatty acids and antioxidants, and this approach is used to enhance the oxidative and thermal stability of oils. A blend of rice bran oil and safflower oil (70:30) with added antioxidants reportedly improved several lipid parameters and certain inflammatory markers. 25 Study by Gillingham et al. 26 has indicated that canola, or in blend with flaxseed oil, effectively reduced serum TC and LDL-c. Moreover, the canola–flaxseed oil blend further reduced plasma E-selectin by targeting the inflammation and atherogenic pathways. Therefore, replacement of commonly consumed fats with canola–flaxseed oil or similar blends is a viable option to achieve dietary recommendations, as well as target the CVD risk factors.
2. Conclusions
In the global context, Indian cooking conditions differ greatly, since the oils are often subjected to rather high temperatures, as stir-frying is a routine process in every curry or other similar preparations. As a result, exposure to high temperatures not only destroys antioxidants like vitamin E and β-carotene but also produces toxic compounds that may potentially be mutagenic and atherogenic. It is advisable to avoid refined oils, since during the refining process, oils are heated to high temperatures resulting in their degradation and generation of toxic substances. Refined oils, particularly high in PUFAs, degrade easily and therefore, should be avoided for frying. On the contrary, oils high in saturated fats (like ghee /coconut oil) can be used for Indian cooking, as they are comparatively stable during frying. Earlier, oils high in SFA were considered harmful since they increase LDL-c but recent studies indicate that oils high in short/medium-chain SFA (like coconut oil) have not demonstrated adverse health effects. Mustard and rapeseed oils – due to their favorable LA/ALA ratio, low SFA, and high MUFA content along with their relative stability during cooking – can be a preferred choice, particularly mustard oil in its nonrefined (cold-pressed) form. In fact epidemiologic studies among Indians do suggest that mustard oil consumption can reduce the risk of CHD. Further, appropriate blending of edible oils (such as rice bran and safflower oil; coconut and sesame oil; canola and flaxseed oil) also appears to be a good option to reduce the plasma lipids, inflammation and, thus, the CHD risk.
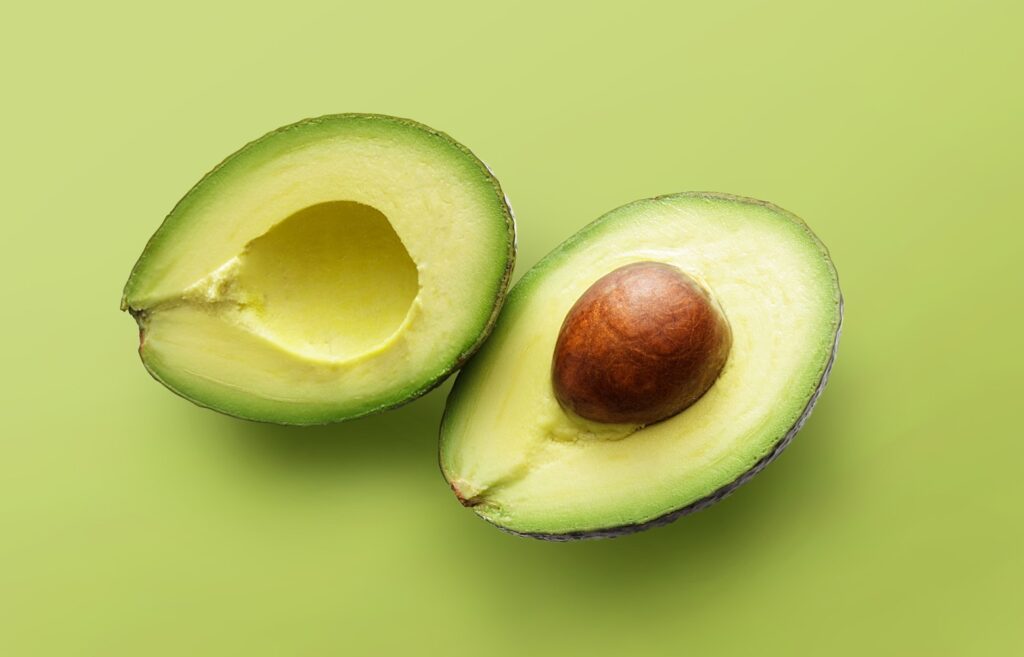
Avocados or “alligator pears” are known for their creamy smooth flesh and bumpy skin. They are a popular food across many cultures. Perhaps best known as the star ingredient in guacamole, they are versatile and prepared in an array of dishes, or simply eaten plain with a spoon. Although not sweet, avocados are botanically classified as a fruit with a large berry and single center pit, grown from the Persea americana tree. They are believed to have originated in Mexico or Central America, with Mexico being the leading producer worldwide. [1]
Their nutrition profile makes them a staple in various healthful meal plans. Avocados are a good source of fiber, and contain more fat (the good kind) than carbohydrate, so are popular on lower-carbohydrate diets such as with diabetes. Their heart-friendly fats do not increase blood cholesterol, which can provide satisfaction on a traditional cholesterol-lowering diet that is often low in fat and cholesterol. It is one the highest-fat plant foods, making it a popular inclusion in vegan and vegetarian diets. The slightly earthy but neutral flavor of avocados works well in sauces, salad dressings, sandwiches, baked goods, salads, and grain dishes to add richness.
- Fat (mostly monounsaturated 67%)
- Fiber (mostly insoluble but also soluble)
- Carotenoids (lutein, zeaxanthin)
A whole medium avocado contains about 240 calories, 13 grams carbohydrate, 3 grams protein, 22 grams fat (15 grams monounsaturated, 4 grams polyunsaturated, 3 grams saturated), 10 grams fiber, and 11 milligrams sodium. Along with their low sodium levels, avocados contain no cholesterol.
Avocados and Health
Avocados contain several nutrients including carotenoids, monounsaturated fats , potassium , and fiber that have been associated with a reduced risk of chronic diseases, especially when included as part of a balanced nutritious diet . The nutritional profile of avocados fits well with healthful dietary patterns such as the Mediterranean and DASH diets. Published health research on avocados is largely funded by avocado industry groups; the research cited below attempts to include non-industry-funded studies.
The primary type of monounsaturated fat in avocados is from oleic acid, the main fatty acid in olive oil. Some studies show a reduction in LDL cholesterol when replacing other types of fat in the diet with avocados. [2] As with all plant foods, avocados are cholesterol-free. However, they contain phytosterols, or plant sterols, that have a similar chemical structure to cholesterol but are poorly absorbed in the intestines and therefore may interfere with cholesterol absorption. According to the American Heart Association, phytosterols have been found to reduce total and LDL cholesterol levels in the body by lowering cholesterol absorption. [3] Avocados are also rich in potassium, a mineral that helps to regulate blood pressure by maintaining normal levels of fluid inside of cells and helping muscles to contract. Potassium also works as an electrolyte that sends electrical signals in the heart to create a steady heartbeat.
A large cohort of almost 69,000 women and 42,000 men were followed for 30 years to see if long-term avocado intake affected risk of cardiovascular disease (CVD). [4] The authors adjusted for diet and lifestyle factors associated with CVD to pinpoint the specific effect of avocados. Compared with those who didn’t eat avocados, those who ate two or more servings a week (1 serving = ½ avocado) had a 16% lower risk of CVD and a 21% lower risk of heart disease. The benefit was especially strong when swapping fat-filled foods like whole-milk dairy (butter, cheese, yogurt), processed meats (hot dogs, bacon, sausage), and eggs with an equal serving of avocado. However, there were similar heart benefits when swapping high-fat plant foods like nuts or olive oil with avocado.
Avocados are a good source of fiber, low in total carbohydrate, and rich in monounsaturated fats. They have a low glycemic index (GI) of about 40; low glycemic foods have a rating of 55 or less. Low GI foods are less likely to cause surges in blood glucose. Large epidemiological studies have found that replacing saturated fats with unsaturated (monounsaturated and polyunsaturated) fats can improve insulin sensitivity and reduce the risk of type 2 diabetes. [5] These nutritional components are favorable for the prevention and control of type 2 diabetes .
Avocados contain plant chemicals called carotenoids. Two types of these fat-soluble carotenoids, lutein and zeaxanthin, are found in the human eye and protect the eye from ultraviolet light damage from the sun. A diet rich in these carotenoids is associated with a lower risk of macular degeneration and cataracts. [6]
The fibers in avocados act as prebiotics (food for beneficial bacteria in the intestines) and have been found to improve the diversity of microflora in the colon. [1] These bacteria digest and break down fibers into short chain fatty acids , which are actively researched for their role in chronic disease prevention.
Depending on the variety, avocados may be round or pear-shaped, green or black, and small or large. The skin is typically bumpy. The flesh when ripe is smooth and buttery. They are a climacteric fruit, which continues to ripen after harvesting. The Hass avocado is the most common type, available year-round.
If you are planning to use an avocado immediately after purchase, choose a ripe one with dark green or almost black skin. It should yield to pressure when squeezed. Avocados with light green skin that are very firm are unripe and will need to sit a few days before eating. If the avocado has dark shriveled skin, dents, or spots of mushy flesh, it may be overripe and unpleasant to eat.
Avocado oil is extracted from the flesh of pressed avocados. It can replace other liquid cooking oils and has a very high smoke point of almost 500°F. Avocado oil is often compared with olive oil because they are both rich in the fatty acid, oleic acid, but avocado oil has a more neutral flavor. [7] It can also be used to make a homemade salad dressing: whisk or blend together ¼ cup avocado oil, 2 tablespoons Dijon mustard, and 4 teaspoons balsamic or apple cider vinegar; add additional low-sodium spices like black pepper or garlic powder as desired.
Avocados are often sold with hard, unripe flesh, which will ripen in 2-3 days. You can leave the fruit at room temperature, or place in direct sunlight to speed ripening. You can also place the avocado sealed in a paper bag with a banana ; the ethylene gases in the banana will speed ripening. When ripe, avocados will feel slightly soft when squeezed. The flesh of avocados is infamous for quickly turning brown once exposed to air, called enzymatic browning. Although unappetizing to see, the brown flesh is perfectly edible. Still, there are tips to slow or reduce browning after cutting into an avocado:
- Cover the flesh with lemon or lime juice.
- Wrap tightly with plastic wrap or place in a sealed airtight container and store in the refrigerator to reduce oxygen exposure.
- Store an avocado half with some sliced onion in a sealed airtight container; the sulfur compounds in the onion help preserve the avocado.
Removing an avocado pit isn’t as challenging as you might think. Although a popular method is to stab a knife into the pit of an avocado half and cleanly remove it, this carries the potential danger of stabbing your hand! Instead, place your index and middle finger on the flesh on each side of the pit, placing your thumb behind the avocado on the skin; push into the center with your thumb until the pit pops out. From there, slice, dice, or mash the flesh as desired to be used in recipes.
The monounsaturated fat in avocados is stable in high heat and can be used not only in cooking but also in baking. Pureed avocado can be substituted for butter or oil in baking recipes, using a 1:1 ratio (1 cup butter = 1 cup avocado).
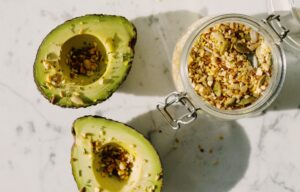
- Diced and sprinkled into salads, soups, tacos, or whole grains
- Blended into smoothies to thicken and add richness
- Mashed as a spread on sandwiches and crackers
- Mashed onto whole grain breakfast toast, sprinkled with blueberries and ground flaxseeds or hemp seeds
- Sliced and rolled into maki sushi
- Cut in half, drizzled with a squeeze of lemon or lime juice, and eaten with a spoon as a snack
Did You Know?
- One serving of a medium avocado (half the fruit) has more potassium than a medium banana , 487 mg potassium versus 422 mg potassium, respectively.
- A ripe mashed avocado is sometimes used as a facial mask due its high content of hydrating oils and vitamin E .
- Bhuyan DJ, Alsherbiny MA, Perera S, Low M, Basu A, Devi OA, Barooah MS, Li CG, Papoutsis K. The odyssey of bioactive compounds in avocado (Persea americana) and their health benefits. Antioxidants . 2019 Oct;8(10):426.
- Schoeneck M, Iggman D. The effects of foods on LDL cholesterol levels: A systematic review of the accumulated evidence from systematic reviews and meta-analyses of randomized controlled trials. Nutrition, Metabolism and Cardiovascular Diseases . 2021 May 6;31(5):1325-38.
- Lichtenstein AH, Deckelbaum RJ. Stanol/sterol ester–containing foods and blood cholesterol levels: a statement for healthcare professionals from the nutrition committee of the council on nutrition, physical activity, and metabolism of the American Heart Association. Circulation . 2001 Feb 27;103(8):1177-9.
- Pacheco LS, Li Y, Rimm EB, Manson JE, Sun Q, Rexrode K, Hu FB, Guasch-Ferré M. Avocado Consumption and Risk of Cardiovascular Disease in US Adults. Journal of the American Heart Association . 2022 Mar 30:e024014. Author disclosure: Dr. Pacheco collaborated in the Hass Avocado Board-funded trial Effects of Avocado Intake on the Nutritional Status of Families during 2016 to 2019 as a graduate student researcher, but the present study was not supported or endorsed by the Hass Avocado Board.
- Risérus U, Willett WC, Hu FB. Dietary fats and prevention of type 2 diabetes. Progress in lipid research . 2009 Jan 1;48(1):44-51.
- Wu J, Cho E, Willett WC, Sastry SM, Schaumberg DA. Intakes of lutein, zeaxanthin, and other carotenoids and age-related macular degeneration during 2 decades of prospective follow-up. JAMA ophthalmology . 2015 Dec 1;133(12):1415-24.
- Cervantes-Paz B, Yahia EM. Avocado oil: Production and market demand, bioactive components, implications in health, and tendencies and potential uses. Comprehensive Reviews in Food Science and Food Safety . 2021 Jul;20(4):4120-58.
Last reviewed April 2022
Terms of Use
The contents of this website are for educational purposes and are not intended to offer personal medical advice. You should seek the advice of your physician or other qualified health provider with any questions you may have regarding a medical condition. Never disregard professional medical advice or delay in seeking it because of something you have read on this website. The Nutrition Source does not recommend or endorse any products.

by Michael Friedrich
As part of a new series profiling participants in SSRC’s Criminal Justice Innovation Fellowship program, Romaine Campbell talks about his research on police and prison policies. This is a cross-posting with Arnold Ventures .
Recently, the Social Science Research Council (SSRC), with support from Arnold Ventures (AV), launched the Criminal Justice Innovation (CJI) Fellowship program , which supports early-career researchers who are exploring what works to make communities safer and the criminal justice system fairer and more effective.
“These CJI fellows will spend the next three years investing in their own policy-relevant research, as well as conducting policy analyses for AV that will directly inform our work,” Jennifer Doleac , executive vice president of criminal justice at AV, says. “We are eager to know if particular policies and programs are working, and this group of researchers will figure that out. I’m thrilled to get to work with these brilliant, talented scholars.”
According to Anna Harvey , president of the SSRC, this new fellowship program will uniquely foster innovative and rigorous causal research on criminal justice policies. “By supporting ‘people, not projects,’ the CJI fellowships will give these exceptional young researchers the time and freedom to pursue novel and creative approaches to evaluating criminal justice policies and practices. We can’t wait to see what they produce,” she says.
In part one of a new series profiling the CJI fellows, AV spoke with Romaine Campbell, a Ph.D candidate in economics at Harvard University whose work addresses racial disparities in the criminal justice system.
Romaine Campbell: Police Behavior and Community Safety
A labor economist by training, Campbell will produce research as a fellow through the CJI fellowship program over the next two years before joining the faculty at Cornell University’s Brooks School of Public Policy. His research will focus on how federal scrutiny impacts police behavior and community safety, as well as the effects of higher education in prison on the outcomes of people who are incarcerated, among other topics.
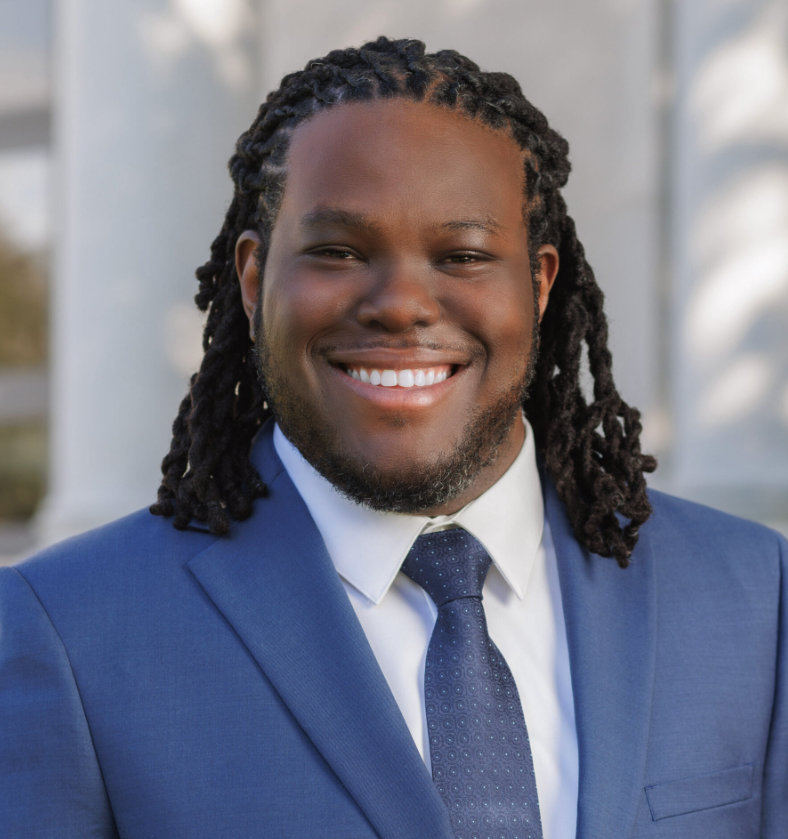
Campbell, who is originally from the Caribbean, says that he has seen how rigorous empirical research can help to explain the things that are important for his community. “A lot of my work looks at how we can improve law enforcement in the United States,” he says. “Policing serves an important role in ensuring the public safety of communities, but increasingly we’re aware of the social costs that can sometimes come with policing. My work examines policies that can help balance the important work that officers do with trying to mitigate the harms that come out of the excesses of policing.”
In 2023, Campbell published a working paper on the results of federal oversight of policing in Seattle. Using administrative data from the Seattle Police Department, the paper found that federal oversight resulted in a 26% reduction in police stops in the city — mostly by reducing stop-and-frisk style stops. Importantly, that reduction had no impact on the rates of serious crime or other community safety measures.
As part of the new fellowship, Campbell expects to expand his work on the impacts of police oversight. By working with other police departments across the country, he will explore how officers respond to federal investigations, how it affects their behavior, and what types of policing are actually effective for crime reduction. Some policymakers, Campbell notes, have expressed concerns that adding oversight to police departments causes them to pull back from policing, which can damage community safety. As such, policies are needed that reduce the harms of policing while also allowing officers to address serious crime and build trust with the communities they serve. “As our society considers the best ways to improve policing,” he says, “it’s going to be important to document the types of policies that can achieve this without having deleterious effects for communities.”
Additionally, working in partnership with the Philadelphia District Attorney’s Office, Campbell and colleagues intend to explore the impact of Brady Lists — public-facing records of information about police misconduct, decertification, use-of-force reports, and other metrics — to understand how prosecutors use such information in charging decisions in their cases.
Separately, Campbell and colleagues plan to launch a project to understand how the provision of higher education in prison affects short- and long-term outcomes of people who are incarcerated, especially their social and economic mobility. He will focus on Iowa, where agreements with the state’s department of corrections, department of education, and workforce development agency will provide him with the necessary data.
Campbell says that rigorous research is important for decision-making about public policy in the criminal justice system. “When you operate in public policy spaces, you really want to build out evidence-based policy,” he explains. “We can all have our feelings and intuitions about what will happen when a policy goes into effect, but the gold standard should be to implement policies that are supported by data.”
Privacy Overview

- Personal Finance
- Today's Paper
- Partner Content
- Entertainment
- Social Viral
- Pro Kabaddi League
Jayant Agro Organics consolidated net profit rises 22.06% in the March 2024 quarter

Disclaimer: No Business Standard Journalist was involved in creation of this content
FMCG stocks edge lower
Tcs, cipla, jio financial services, manappuram finance in action, jayant agro organics consolidated net profit rises 108.77% in the december 2023 quarter, fmcg shares fall, rvnl emerges as l-1 bidder for 187 cr metro project, ahlada engineers standalone net profit rises 108.92% in the march 2024 quarter, devhari exports (india) reports standalone net loss of rs 0.03 crore in the march 2024 quarter, shree karthik papers reports standalone net loss of rs 0.37 crore in the march 2024 quarter, sakthi finance standalone net profit rises 21.45% in the march 2024 quarter.
Don't miss the most important news and views of the day. Get them on our Telegram channel
First Published: May 27 2024 | 8:06 AM IST
Explore News
- Suzlon Energy Share Price Adani Enterprises Share Price Adani Power Share Price IRFC Share Price Tata Motors Share Price Tata Steel Share Price Yes Bank Share Price Infosys Share Price SBI Share Price Tata Power Share Price
- Latest News Company News Market News India News Politics News Cricket News Personal Finance Technology News World News Industry News Education News Opinion Shows Economy News Lifestyle News Health News
- Today's Paper About Us T&C Privacy Policy Cookie Policy Disclaimer Investor Communication GST registration number List Compliance Contact Us Advertise with Us Sitemap Subscribe Careers BS Apps
- ICC T20 World Cup 2024 T20 World Cup 2024 Schedule Budget 2024 Lok Sabha Election 2024 IPL Points Table 2024 T20 World Cup 2024 Points Table

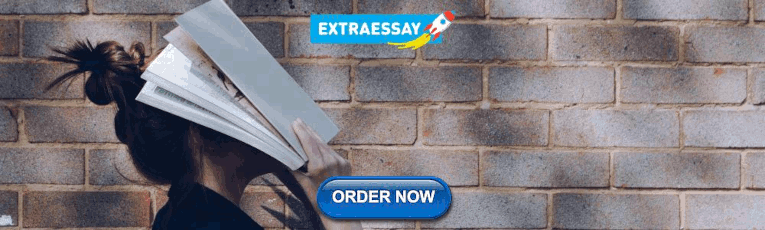
IMAGES
VIDEO
COMMENTS
The publication of Ida Tarbell's muckraking classic, "The History of the Standard Oil. Company," in McClure's Magazine from 1902 to 1903 created a public firestorm, prompting John D. Rockefeller and his associates' turn to the infant field of public rela tions. Despite their efforts, historians largely ignore, marginalize, or criticize Standard.
Faculty Research Working Papers have not undergone formal review and approval. Such papers are included in this series to elicit feedback and ... Standard Oil generated only a small share of the 443 refining patents identified in Schmookler's tabulation for the years 1875-1899. A search was conducted in the Patent Office's Annual .
Abstract. Reconstructs the entrepreneurial journey of John D. Rockefeller, founder of Standard Oil and one of the most famous business figures in history. Traces his early career as a retailer in Cleveland through his decision to enter the oil industry in the early 1860s to his creation of Standard Oil. Pays particular attention to the critical ...
Published/Created: 1975. This work explores how seven companies came to dominate the oil industry by the 1940s, after anti-trust laws forced Standard Oil into 34 different companies. Short histories of each of Seven Sisters are located in chapters three and four. Standard Oil by Scott Benjamin; Wayne Henderson.
A century ago, in 1911, the U.S. Supreme Court issued its path-breaking decision in the monopolization case against the Standard Oil Companies. Standard pleaded inter alia that its near-monopoly position was the result of superior innovation, citing in particular the Frasch-Burton process for refining the high-sulfur oil found around Lima, Ohio. This paper examines the role of Hermann Frasch ...
pricing practices observed in the Standard Oil case.15 In explaining the history of Section 3, the Court has cited Standard Oil for the fact ―[t]hat sales below cost without a justifying business reason may come within the proscriptions of the Sherman Act has long been established.‖16 In short, Standard Oil was a predatory pricing case ...
This paper challenges that explanation, claiming that there were attempts to cartelize at all levels of the oil industry - producers, gathering pipelines, refiners, and the railroads. There are good economic reasons that explain why Standard Oil, a refiner and at the remote western location of Cleveland, secured a monopoly as against the ...
In the early 1900s, Standard Oil Co., chaired by John Rockefeller, was a powerful monopoly dissolved by SCOTUS. ... important dates related to this topic and a section of this guide provides some suggested search strategies for further research in the collection. Timeline. 1901-1904: Standard Oil gets control of railroads along east coast.
Scherer, F.M. "Standard Oil as a Technological Innovator." HKS Faculty Research Working Paper Series RWP11-008, January 2011. A century ago, in 1911, the U.S. Supreme Court issued its path-breaking decision in the monopolization case against the Standard Oil Companies. Standard pleaded inter alia that its near-monopoly position was the result ...
A century ago, in 1911, the U.S. Supreme Court issued its path-breaking decision in the monopolization case against the Standard Oil Companies. Standard pleaded inter alia that its near-monopoly position was the result of superior innovation, citing in particular the Frasch-Burton process for refining the high-sulphur oil found around Lima, Ohio.
The Long Shadow of Standard Oil: Policy, Petroleum, and Politics at the Federal Trade Commission. Southern California Law Review, Vol. 85, No. 4, May 2012, pp. 843-915 ... Law & Economics Research Paper Series. Subscribe to this free journal for more curated articles on this topic FOLLOWERS. 4,852. PAPERS. 1,172. This Journal is curated by:
In recent years, the crude oil market has entered a new period of development and the core influence factors of crude oil have also been a change. Thus, we develop a new research framework for core influence factors selection and forecasting. Firstly, this paper assesses and selects core influence factors with the elastic-net regularized generalized linear Model (GLMNET), spike-slab lasso ...
Crude Oil is one of the most important commodities in this world. We have studied the effects of Crude Oil inventories on crude oil prices over the last ten years (2011 to 2020). We tried to figure out how the Crude Oil price variance responds to inventory announcements. We then introduced several other financial instruments to study the relation of these instruments with Crude Oil variation.
Introduction. In the last decade, structural vector autoregressive (VAR) models of the global oil market have. become the standard tool for understanding the evolution of the real price of oil and its effect on. the macro economy. These models have helped create a consensus among researchers and.
Edible vegetable oils are triglycerides of plant origin that include olive, palm, soybean, canola, and sunflower oil [1, 2].Oil and fat are important nutritional components with variety of functions in our body as an energy source, membrane structures, regulating body temperature and insulate organs [3, 4].Vegetable oils may rancid and hence lose its nutritional values and flavor upon improper ...
This paper studies the ability of manufacturing-specific shocks to explain oil prices. In an estimated three-region model (US, OPEC, rest of world) incorporating two sectors (manufacturing and services) in the oil-importing economies and featuring cross-border supply chains, such shocks rationalize the observed pattern of a positive comovement between oil prices and the global cyclical gap ...
The complex composition of the EOs justifies the constant search for new stationary phases characterized by different selectivity and at the same time with good chromatographic properties (i.e., ionic liquids). Table 2 lists the most significant published papers in the essential oil field.
Using data from hundreds of fields worldwide, a well-to-refinery study presents a high-granularity profile of China's crude oil supply in terms of emissions and energy return on input. Mikael ...
Standard Glossy coated wood-free paper Uncoated white paper; Grammage (g/m 2) ISO 536: 115.0: 120.0: Bulk (cm 3 /g) ISO 534:1995: ... the effect of oil on color changes decreased on the white paper as oil in the ink penetrated the paper. When the oil is lower on to the paper surface, the oxidative polymerization is low and penetration of oil is ...
The Standard Oil case continues to inform many aspects of current antitrust policy. Part of Standard Oil's significance, however, has been lost over time. The S ... Irvine School of Law & Legal Studies Research Paper Series. Subscribe to this free journal for more curated articles on this topic FOLLOWERS. 5,323. PAPERS. 1,027. This Journal is ...
Therefore, right selection of edible oil is extremely important, especially in the Indian context, where cooking methods are different then in the west. ... A Report of the Expert Group of the Indian Council of Medical Research; pp. 90-111. [Google Scholar] 11. AO/WHO . FAO; 2010. Fats and Fatty Acids in Human Nutrition Report of an Expert ...
Significance of Transformer Oil Testing. Dr. P K Maiti. Central Power Research Institute, Sector V, Salt Lake, Kolkata 700 091. Abstract: Transformer is one of the vital components in power ...
Therefore, a multi-level quality grading standard for CO needs to be established in order to better serve human health, similar to the standard for olive oil. Comprehensive physicochemical, biological and clinical research of CO should be carried out to promote its application in functional foods, cosmetics, and healthcare products in the ...
Source Of. Coconut oil is 100% fat, 80-90% of which is saturated fat. This gives it a firm texture at cold or room temperatures. Fat is made up of smaller molecules called fatty acids, and there are several types of saturated fatty acids in coconut oil. The predominant type is lauric acid (47%), with myristic and palmitic acids present in ...
Potassium. Magnesium. Carotenoids (lutein, zeaxanthin) A whole medium avocado contains about 240 calories, 13 grams carbohydrate, 3 grams protein, 22 grams fat (15 grams monounsaturated, 4 grams polyunsaturated, 3 grams saturated), 10 grams fiber, and 11 milligrams sodium. Along with their low sodium levels, avocados contain no cholesterol.
A substantial discussion regarding the applications of chemical enhanced oil recovery and CO 2 injection procedures for sustainable production of energy is presented to show the paradigm shift in enhanced oil recovery methods. This paper reviews the previous works done by the researchers on various enhanced oil recovery (EOR) methods and tries ...
Indian Oil Corporation acquired a 100% stake in Mercator Petroleum for Rs 154 crore. TVS Motor Company announced the launch of the TVS HLX 125 with 5-gears in Tanzania. Godrej Properties clarified on the reports on the Mumbai project that it has not received communication from any concerned authority citing non-compliance.
The Social Science Research Council fosters innovative research, nurtures new generations of social scientists, deepens how inquiry is practiced within and across disciplines, and mobilizes necessary knowledge on important public issues. ... the paper found that federal oversight resulted in a 26% reduction in police stops in the city ...
Net profit of Jayant Agro Organics rose 22.06% to Rs 16.82 crore in the quarter ended March 2024 as against Rs 13.78 crore during the previous quarter ended March 2023. Sales rose 3.20% to Rs 626.05 crore in the quarter ended March 2024 as against Rs 606.66 crore during the previous quarter ended ...