82 Data Mining Essay Topic Ideas & Examples
🏆 best data mining topic ideas & essay examples, 💡 good essay topics on data mining, ✅ most interesting data mining topics to write about.
- Disadvantages of Using Web 2.0 for Data Mining Applications This data can be confusing to the readers and may not be reliable. Lastly, with the use of Web 2.
- Data Warehouse and Data Mining in Business The circumstances leading to the establishment and development of the concept of data warehousing was attributed to the fact that failure to have a data warehouse led to the need of putting in place large […]
- The Data Mining Method in Healthcare and Education Thus, I would use data mining in both cases; however, before that, I would discover a way to improve the algorithms used for it.
- Data Mining Tools and Data Mining Myths The first problem is correlated with keeping the identity of the person evolved in data mining secret. One of the major myths regarding data mining is that it can replace domain knowledge.
- Hybrid Data Mining Approach in Healthcare One of the healthcare projects that will call for the use of data mining is treatment evaluation. In this case, it is essential to realize that the main aim of health data mining is to […]
- Terrorism and Data Mining Algorithms However, this is a necessary evil as the nation’s security has to be prioritized since these attacks lead to harm to a larger population compared to the infringements.
- Data Mining and Its Major Advantages Thus, it is possible to conclude that data mining is a convenient and effective way of processing information, which has many advantages.
- Transforming Coded and Text Data Before Data Mining However, to complete data mining, it is necessary to transform the data according to the techniques that are to be used in the process.
- Data Mining and Machine Learning Algorithms The shortest distance of string between two instances defines the distance of measure. However, this is also not very clear as to which transformations are summed, and thus it aims to a probability with the […]
- Summary of C4.5 Algorithm: Data Mining 5 algorism: Each record from set of data should be associated with one of the offered classes, it means that one of the attributes of the class should be considered as a class mark.
- Data Mining in Social Networks: Linkedin.com One of the ways to achieve the aim is to understand how users view data mining of their data on LinkedIn.
- Ethnography and Data Mining in Anthropology The study of cultures is of great importance under normal circumstances to enhance the understanding of the same. Data mining is the success secret of ethnography.
- Issues With Data Mining It is necessary to note that the usage of data mining helps FBI to have access to the necessary information for terrorism and crime tracking.
- Large Volume Data Handling: An Efficient Data Mining Solution Data mining is the process of sorting huge amount of data and finding out the relevant data. Data mining is widely used for the maintenance of data which helps a lot to an organization in […]
- Data Mining and Analytical Developments In this era where there is a lot of information to be handled at ago and actually with little available time, it is necessarily useful and wise to analyze data from different viewpoints and summarize […]
- Levi’s Company’s Data Mining & Customer Analytics Levi, the renowned name in jeans is feeling the heat of competition from a number of other brands, which have come upon the scene well after Levi’s but today appear to be approaching Levi’s market […]
- Cryptocurrency Exchange Market Prediction and Analysis Using Data Mining and Artificial Intelligence This paper aims to review the application of A.I.in the context of blockchain finance by examining scholarly articles to determine whether the A.I.algorithm can be used to analyze this financial market.
- Data Mining in Healthcare: Applications and Big Data Analyze Big data analysis is among the most influential modern trends in informatics and it has applications in virtually every sphere of human life.
- “Data Mining and Customer Relationship Marketing in the Banking Industry“ by Chye & Gerry First of all, the article generally elaborates on the notion of customer relationship management, which is defined as “the process of predicting customer behavior and selecting actions to influence that behavior to benefit the company”.
- Data Mining Techniques and Applications The use of data mining to detect disturbances in the ecosystem can help to avert problems that are destructive to the environment and to society.
- Ethical Data Mining in the UAE Traffic Department The research question identified in the assignment two is considered to be the following, namely whether the implementation of the business intelligence into the working process will beneficially influence the work of the Traffic Department […]
- Canadian University Dubai and Data Mining The aim of mining data in the education environment is to enhance the quality of education for the mass through proactive and knowledge-based decision-making approaches.
- Data Mining and Customer Relationship Management As such, CRM not only entails the integration of marketing, sales, customer service, and supply chain capabilities of the firm to attain elevated efficiencies and effectiveness in conveying customer value, but it obliges the organization […]
- E-Commerce: Mining Data for Better Business Intelligence The method allowed the use of Intel and an example to build the study and the literature on data mining for business intelligence to analyze the findings.
- Ethical Implications of Data Mining by Government Institutions Critics of personal data mining insist that it infringes on the rights of an individual and result to the loss of sensitive information.
- Data Mining Role in Companies The increasing adoption of data mining in various sectors illustrates the potential of the technology regarding the analysis of data by entities that seek information crucial to their operations.
- Data Mining: Concepts and Methods Speed of data mining process is important as it has a role to play in the relevance of the data mined. The accuracy of data is also another factor that can be used to measure […]
- Data Mining Technologies According to Han & Kamber, data mining is the process of discovering correlations, patterns, trends or relationships by searching through a large amount of data that in most circumstances is stored in repositories, business databases […]
- Data Mining: A Critical Discussion In recent times, the relatively new discipline of data mining has been a subject of widely published debate in mainstream forums and academic discourses, not only due to the fact that it forms a critical […]
- Commercial Uses of Data Mining Data mining process entails the use of large relational database to identify the correlation that exists in a given data. The principal role of the applications is to sift the data to identify correlations.
- A Discussion on the Acceptability of Data Mining Today, more than ever before, individuals, organizations and governments have access to seemingly endless amounts of data that has been stored electronically on the World Wide Web and the Internet, and thus it makes much […]
- Applying Data Mining Technology for Insurance Rate Making: Automobile Insurance Example
- Applebee’s, Travelocity and Others: Data Mining for Business Decisions
- Applying Data Mining Procedures to a Customer Relationship
- Business Intelligence as Competitive Tool of Data Mining
- Overview of Accounting Information System Data Mining
- Applying Data Mining Technique to Disassembly Sequence Planning
- Approach for Image Data Mining Cultural Studies
- Apriori Algorithm for the Data Mining of Global Cyberspace Security Issues
- Database Data Mining: The Silent Invasion of Privacy
- Data Management: Data Warehousing and Data Mining
- Constructive Data Mining: Modeling Consumers’ Expenditure in Venezuela
- Data Mining and Its Impact on Healthcare
- Innovations and Perspectives in Data Mining and Knowledge Discovery
- Data Mining and Machine Learning Methods for Cyber Security Intrusion Detection
- Linking Data Mining and Anomaly Detection Techniques
- Data Mining and Pattern Recognition Models for Identifying Inherited Diseases
- Credit Card Fraud Detection Through Data Mining
- Data Mining Approach for Direct Marketing of Banking Products
- Constructive Data Mining: Modeling Argentine Broad Money Demand
- Data Mining-Based Dispatching System for Solving the Pickup and Delivery Problem
- Commercially Available Data Mining Tools Used in the Economic Environment
- Data Mining Climate Variability as an Indicator of U.S. Natural Gas
- Analysis of Data Mining in the Pharmaceutical Industry
- Data Mining-Driven Analysis and Decomposition in Agent Supply Chain Management Networks
- Credit Evaluation Model for Banks Using Data Mining
- Data Mining for Business Intelligence: Multiple Linear Regression
- Cluster Analysis for Diabetic Retinopathy Prediction Using Data Mining Techniques
- Data Mining for Fraud Detection Using Invoicing Data
- Jaeger Uses Data Mining to Reduce Losses From Crime and Waste
- Data Mining for Industrial Engineering and Management
- Business Intelligence and Data Mining – Decision Trees
- Data Mining for Traffic Prediction and Intelligent Traffic Management System
- Building Data Mining Applications for CRM
- Data Mining Optimization Algorithms Based on the Swarm Intelligence
- Big Data Mining: Challenges, Technologies, Tools, and Applications
- Data Mining Solutions for the Business Environment
- Overview of Big Data Mining and Business Intelligence Trends
- Data Mining Techniques for Customer Relationship Management
- Classification-Based Data Mining Approach for Quality Control in Wine Production
- Data Mining With Local Model Specification Uncertainty
- Employing Data Mining Techniques in Testing the Effectiveness of Modernization Theory
- Enhancing Information Management Through Data Mining Analytics
- Evaluating Feature Selection Methods for Learning in Data Mining Applications
- Extracting Formations From Long Financial Time Series Using Data Mining
- Financial and Banking Markets and Data Mining Techniques
- Fraudulent Financial Statements and Detection Through Techniques of Data Mining
- Harmful Impact Internet and Data Mining Have on Society
- Informatics, Data Mining, Econometrics, and Financial Economics: A Connection
- Integrating Data Mining Techniques Into Telemedicine Systems
- Investigating Tobacco Usage Habits Using Data Mining Approach
- Chicago (A-D)
- Chicago (N-B)
IvyPanda. (2024, March 2). 82 Data Mining Essay Topic Ideas & Examples. https://ivypanda.com/essays/topic/data-mining-essay-topics/
"82 Data Mining Essay Topic Ideas & Examples." IvyPanda , 2 Mar. 2024, ivypanda.com/essays/topic/data-mining-essay-topics/.
IvyPanda . (2024) '82 Data Mining Essay Topic Ideas & Examples'. 2 March.
IvyPanda . 2024. "82 Data Mining Essay Topic Ideas & Examples." March 2, 2024. https://ivypanda.com/essays/topic/data-mining-essay-topics/.
1. IvyPanda . "82 Data Mining Essay Topic Ideas & Examples." March 2, 2024. https://ivypanda.com/essays/topic/data-mining-essay-topics/.
Bibliography
IvyPanda . "82 Data Mining Essay Topic Ideas & Examples." March 2, 2024. https://ivypanda.com/essays/topic/data-mining-essay-topics/.
- Electronics Engineering Paper Topics
- Cyber Security Topics
- Google Paper Topics
- Hacking Essay Topics
- Identity Theft Essay Ideas
- Internet Research Ideas
- Microsoft Topics
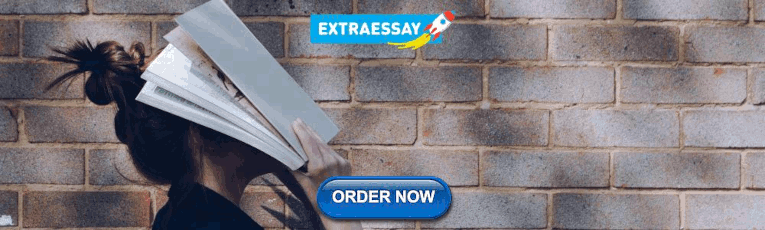
data mining Recently Published Documents
Total documents.
- Latest Documents
- Most Cited Documents
- Contributed Authors
- Related Sources
- Related Keywords
Distance Based Pattern Driven Mining for Outlier Detection in High Dimensional Big Dataset
Detection of outliers or anomalies is one of the vital issues in pattern-driven data mining. Outlier detection detects the inconsistent behavior of individual objects. It is an important sector in the data mining field with several different applications such as detecting credit card fraud, hacking discovery and discovering criminal activities. It is necessary to develop tools used to uncover the critical information established in the extensive data. This paper investigated a novel method for detecting cluster outliers in a multidimensional dataset, capable of identifying the clusters and outliers for datasets containing noise. The proposed method can detect the groups and outliers left by the clustering process, like instant irregular sets of clusters (C) and outliers (O), to boost the results. The results obtained after applying the algorithm to the dataset improved in terms of several parameters. For the comparative analysis, the accurate average value and the recall value parameters are computed. The accurate average value is 74.05% of the existing COID algorithm, and our proposed algorithm has 77.21%. The average recall value is 81.19% and 89.51% of the existing and proposed algorithm, which shows that the proposed work efficiency is better than the existing COID algorithm.
Implementation of Data Mining Technology in Bonded Warehouse Inbound and Outbound Goods Trade
For the taxed goods, the actual freight is generally determined by multiplying the allocated freight for each KG and actual outgoing weight based on the outgoing order number on the outgoing bill. Considering the conventional logistics is insufficient to cope with the rapid response of e-commerce orders to logistics requirements, this work discussed the implementation of data mining technology in bonded warehouse inbound and outbound goods trade. Specifically, a bonded warehouse decision-making system with data warehouse, conceptual model, online analytical processing system, human-computer interaction module and WEB data sharing platform was developed. The statistical query module can be used to perform statistics and queries on warehousing operations. After the optimization of the whole warehousing business process, it only takes 19.1 hours to get the actual freight, which is nearly one third less than the time before optimization. This study could create a better environment for the development of China's processing trade.
Multi-objective economic load dispatch method based on data mining technology for large coal-fired power plants
User activity classification and domain-wise ranking through social interactions.
Twitter has gained a significant prevalence among the users across the numerous domains, in the majority of the countries, and among different age groups. It servers a real-time micro-blogging service for communication and opinion sharing. Twitter is sharing its data for research and study purposes by exposing open APIs that make it the most suitable source of data for social media analytics. Applying data mining and machine learning techniques on tweets is gaining more and more interest. The most prominent enigma in social media analytics is to automatically identify and rank influencers. This research is aimed to detect the user's topics of interest in social media and rank them based on specific topics, domains, etc. Few hybrid parameters are also distinguished in this research based on the post's content, post’s metadata, user’s profile, and user's network feature to capture different aspects of being influential and used in the ranking algorithm. Results concluded that the proposed approach is well effective in both the classification and ranking of individuals in a cluster.
A data mining analysis of COVID-19 cases in states of United States of America
Epidemic diseases can be extremely dangerous with its hazarding influences. They may have negative effects on economies, businesses, environment, humans, and workforce. In this paper, some of the factors that are interrelated with COVID-19 pandemic have been examined using data mining methodologies and approaches. As a result of the analysis some rules and insights have been discovered and performances of the data mining algorithms have been evaluated. According to the analysis results, JRip algorithmic technique had the most correct classification rate and the lowest root mean squared error (RMSE). Considering classification rate and RMSE measure, JRip can be considered as an effective method in understanding factors that are related with corona virus caused deaths.
Exploring distributed energy generation for sustainable development: A data mining approach
A comprehensive guideline for bengali sentiment annotation.
Sentiment Analysis (SA) is a Natural Language Processing (NLP) and an Information Extraction (IE) task that primarily aims to obtain the writer’s feelings expressed in positive or negative by analyzing a large number of documents. SA is also widely studied in the fields of data mining, web mining, text mining, and information retrieval. The fundamental task in sentiment analysis is to classify the polarity of a given content as Positive, Negative, or Neutral . Although extensive research has been conducted in this area of computational linguistics, most of the research work has been carried out in the context of English language. However, Bengali sentiment expression has varying degree of sentiment labels, which can be plausibly distinct from English language. Therefore, sentiment assessment of Bengali language is undeniably important to be developed and executed properly. In sentiment analysis, the prediction potential of an automatic modeling is completely dependent on the quality of dataset annotation. Bengali sentiment annotation is a challenging task due to diversified structures (syntax) of the language and its different degrees of innate sentiments (i.e., weakly and strongly positive/negative sentiments). Thus, in this article, we propose a novel and precise guideline for the researchers, linguistic experts, and referees to annotate Bengali sentences immaculately with a view to building effective datasets for automatic sentiment prediction efficiently.
Capturing Dynamics of Information Diffusion in SNS: A Survey of Methodology and Techniques
Studying information diffusion in SNS (Social Networks Service) has remarkable significance in both academia and industry. Theoretically, it boosts the development of other subjects such as statistics, sociology, and data mining. Practically, diffusion modeling provides fundamental support for many downstream applications (e.g., public opinion monitoring, rumor source identification, and viral marketing). Tremendous efforts have been devoted to this area to understand and quantify information diffusion dynamics. This survey investigates and summarizes the emerging distinguished works in diffusion modeling. We first put forward a unified information diffusion concept in terms of three components: information, user decision, and social vectors, followed by a detailed introduction of the methodologies for diffusion modeling. And then, a new taxonomy adopting hybrid philosophy (i.e., granularity and techniques) is proposed, and we made a series of comparative studies on elementary diffusion models under our taxonomy from the aspects of assumptions, methods, and pros and cons. We further summarized representative diffusion modeling in special scenarios and significant downstream tasks based on these elementary models. Finally, open issues in this field following the methodology of diffusion modeling are discussed.
The Influence of E-book Teaching on the Motivation and Effectiveness of Learning Law by Using Data Mining Analysis
This paper studies the motivation of learning law, compares the teaching effectiveness of two different teaching methods, e-book teaching and traditional teaching, and analyses the influence of e-book teaching on the effectiveness of law by using big data analysis. From the perspective of law student psychology, e-book teaching can attract students' attention, stimulate students' interest in learning, deepen knowledge impression while learning, expand knowledge, and ultimately improve the performance of practical assessment. With a small sample size, there may be some deficiencies in the research results' representativeness. To stimulate the learning motivation of law as well as some other theoretical disciplines in colleges and universities has particular referential significance and provides ideas for the reform of teaching mode at colleges and universities. This paper uses a decision tree algorithm in data mining for the analysis and finds out the influencing factors of law students' learning motivation and effectiveness in the learning process from students' perspective.
Intelligent Data Mining based Method for Efficient English Teaching and Cultural Analysis
The emergence of online education helps improving the traditional English teaching quality greatly. However, it only moves the teaching process from offline to online, which does not really change the essence of traditional English teaching. In this work, we mainly study an intelligent English teaching method to further improve the quality of English teaching. Specifically, the random forest is firstly used to analyze and excavate the grammatical and syntactic features of the English text. Then, the decision tree based method is proposed to make a prediction about the English text in terms of its grammar or syntax issues. The evaluation results indicate that the proposed method can effectively improve the accuracy of English grammar or syntax recognition.
Export Citation Format
Share document.
Thank you for visiting nature.com. You are using a browser version with limited support for CSS. To obtain the best experience, we recommend you use a more up to date browser (or turn off compatibility mode in Internet Explorer). In the meantime, to ensure continued support, we are displaying the site without styles and JavaScript.
- View all journals
- Explore content
- About the journal
- Publish with us
- Sign up for alerts
Data mining articles within Scientific Reports
Article 06 May 2024 | Open Access
Joint extraction of wheat germplasm information entity relationship based on deep character and word fusion
- Xiaoxiao Jia
- , Guang Zheng
- & Lei Xi
Article 25 April 2024 | Open Access
Low ACADM expression predicts poor prognosis and suppressive tumor microenvironment in clear cell renal cell carcinoma
- & Huimin Long
Article 19 April 2024 | Open Access
Automatic inference of ICD-10 codes from German ophthalmologic physicians’ letters using natural language processing
- D. Böhringer
- , P. Angelova
- & T. Reinhard
Robust identification of interactions between heat-stress responsive genes in the chicken brain using Bayesian networks and augmented expression data
- E. A. Videla Rodriguez
- , John B. O. Mitchell
- & V. Anne Smith
Article 16 April 2024 | Open Access
Potential routes of plastics biotransformation involving novel plastizymes revealed by global multi-omic analysis of plastic associated microbes
- Rodney S. Ridley Jr
- , Roth E. Conrad
- & Konstantinos T. Konstantinidis
Article 13 April 2024 | Open Access
Identifying and overcoming COVID-19 vaccination impediments using Bayesian data mining techniques
- , Arvind Mahajan
- & Bani Mallick
Article 10 April 2024 | Open Access
A decision support system based on recurrent neural networks to predict medication dosage for patients with Parkinson's disease
- Atiye Riasi
- , Mehdi Delrobaei
- & Mehri Salari
Article 03 April 2024 | Open Access
A distributed feature selection pipeline for survival analysis using radiomics in non-small cell lung cancer patients
- Benedetta Gottardelli
- , Varsha Gouthamchand
- & Andrea Damiani
Article 02 April 2024 | Open Access
Characterization of a putative orexin receptor in Ciona intestinalis sheds light on the evolution of the orexin/hypocretin system in chordates
- Maiju K. Rinne
- , Lauri Urvas
- & Henri Xhaard
Multiomics analysis to explore blood metabolite biomarkers in an Alzheimer’s Disease Neuroimaging Initiative cohort
- , Yuki Matsuzawa
- & Balebail Ashok Raj
Article 01 April 2024 | Open Access
Information heterogeneity between progress notes by physicians and nurses for inpatients with digestive system diseases
- Yukinori Mashima
- , Masatoshi Tanigawa
- & Hideto Yokoi
Article 25 March 2024 | Open Access
Integrated image and location analysis for wound classification: a deep learning approach
- , Tirth Shah
- & Zeyun Yu
Article 19 March 2024 | Open Access
Persistence of collective memory of corporate bankruptcy events discussed on X (Twitter) is influenced by pre-bankruptcy public attention
- Kathleen M. Jagodnik
- , Sharon Dekel
- & Alon Bartal
Article 18 March 2024 | Open Access
Clustering analysis for the evolutionary relationships of SARS-CoV-2 strains
- Xiangzhong Chen
- , Mingzhao Wang
- & Juanying Xie
Article 15 March 2024 | Open Access
Development of phenotyping algorithms for hypertensive disorders of pregnancy (HDP) and their application in more than 22,000 pregnant women
- Satoshi Mizuno
- , Maiko Wagata
- & Soichi Ogishima
Article 13 March 2024 | Open Access
Predicting early Alzheimer’s with blood biomarkers and clinical features
- Muaath Ebrahim AlMansoori
- , Sherlyn Jemimah
- & Aamna AlShehhi
Article 09 March 2024 | Open Access
Sentiment analysis of video danmakus based on MIBE-RoBERTa-FF-BiLSTM
- Jianbo Zhao
- , Huailiang Liu
- & Shanzhuang Zhang
Article 05 March 2024 | Open Access
A new R package to parse plant species occurrence records into unique collection events efficiently reduces data redundancy
- Pablo Hendrigo Alves de Melo
- , Nadia Bystriakova
- & Alexandre K. Monro
Article 02 March 2024 | Open Access
Prediction of lncRNA and disease associations based on residual graph convolutional networks with attention mechanism
- Shengchang Wang
- , Jiaqing Qiao
- & Shou Feng
Article 01 March 2024 | Open Access
Analysis and visualisation of electronic health records data to identify undiagnosed patients with rare genetic diseases
- Daniel Moynihan
- , Sean Monaco
- & Saumya Shekhar Jamuar
Article 21 February 2024 | Open Access
Tuning attention based long-short term memory neural networks for Parkinson’s disease detection using modified metaheuristics
- , Timea Bezdan
- & Nebojsa Bacanin
Article 19 February 2024 | Open Access
Effects of different KRAS mutants and Ki67 expression on diagnosis and prognosis in lung adenocarcinoma
- , Liwen Dong
- & Pan Li
Article 15 February 2024 | Open Access
Identification of SLC40A1, LCN2, CREB5, and SLC7A11 as ferroptosis-related biomarkers in alopecia areata through machine learning
- , Dongfan Wei
- & Xiuzu Song
Article 07 February 2024 | Open Access
Unsupervised analysis of whole transcriptome data from human pluripotent stem cells cardiac differentiation
- Sofia P. Agostinho
- , Mariana A. Branco
- & Carlos A. V. Rodrigues
Article 03 February 2024 | Open Access
AI models for automated segmentation of engineered polycystic kidney tubules
- Simone Monaco
- , Nicole Bussola
- & Daniele Apiletti
Article 02 February 2024 | Open Access
Development and validation of a cuproptosis-related prognostic model for acute myeloid leukemia patients using machine learning with stacking
- Xichao Wang
- & Suning Chen
Article 30 January 2024 | Open Access
Assessing the feasibility of applying machine learning to diagnosing non-effusive feline infectious peritonitis
- Dawn Dunbar
- , Simon A. Babayan
- & William Weir
Article 29 January 2024 | Open Access
Survival prediction of glioblastoma patients using modern deep learning and machine learning techniques
- Samin Babaei Rikan
- , Amir Sorayaie Azar
- & Uffe Kock Wiil
Article 25 January 2024 | Open Access
Identification of gene signatures and molecular mechanisms underlying the mutual exclusion between psoriasis and leprosy
- You-Wang Lu
- , Rong-Jing Dong
- & Yu-Ye Li
Article 24 January 2024 | Open Access
Identification of shared pathogenetic mechanisms between COVID-19 and IC through bioinformatics and system biology
- Zhenpeng Sun
- & Jiangang Gao
Article 18 January 2024 | Open Access
Integrated image and sensor-based food intake detection in free-living
- Tonmoy Ghosh
- & Edward Sazonov
Article 17 January 2024 | Open Access
Global characterization of biosynthetic gene clusters in non-model eukaryotes using domain architectures
- Taehyung Kwon
- & Blake T. Hovde
Article 16 January 2024 | Open Access
Parkinson’s disease detection based on features refinement through L1 regularized SVM and deep neural network
- , Ashir Javeed
- & Amir H. Gandomi
Article 12 January 2024 | Open Access
Identification of important genes related to HVSMC proliferation and migration in graft restenosis based on WGCNA
- Xiankun Liu
- , Mingzhen Qin
- & Zhigang Guo
Article 06 January 2024 | Open Access
Acute ischemic stroke prediction and predictive factors analysis using hematological indicators in elderly hypertensives post-transient ischemic attack
- , Chenguang Zheng
- & Le Ge
Article 04 January 2024 | Open Access
Immune, metabolic landscapes of prognostic signatures for lung adenocarcinoma based on a novel deep learning framework
- , Shibin Sun
- & Lina Chen
Article 02 January 2024 | Open Access
Integrated whole transcriptome profiling revealed a convoluted circular RNA-based competing endogenous RNAs regulatory network in colorectal cancer
- Hasan Mollanoori
- , Yaser Ghelmani
- & Mohammadreza Dehghani
Article 28 December 2023 | Open Access
Quantitative gait analysis and prediction using artificial intelligence for patients with gait disorders
- Nawel Ben Chaabane
- , Pierre-Henri Conze
- & Mathieu Lamard
Article 27 December 2023 | Open Access
Statistical analysis of synonymous and stop codons in pseudo-random and real sequences as a function of GC content
- Valentin Wesp
- , Günter Theißen
- & Stefan Schuster
StackER: a novel SMILES-based stacked approach for the accelerated and efficient discovery of ERα and ERβ antagonists
- Nalini Schaduangrat
- , Nutta Homdee
- & Watshara Shoombuatong
Article 18 December 2023 | Open Access
Analyzing the impact of feature selection methods on machine learning algorithms for heart disease prediction
- Zeinab Noroozi
- , Azam Orooji
- & Leila Erfannia
Article 13 December 2023 | Open Access
surviveR: a flexible shiny application for patient survival analysis
- Tamas Sessler
- , Gerard P. Quinn
- & Simon S. McDade
Article 08 December 2023 | Open Access
Dictionary-based matching graph network for biomedical named entity recognition
- & Kai Tan
Article 23 November 2023 | Open Access
Computer-aided diagnosis of keratoconus through VAE-augmented images using deep learning
- Zhila Agharezaei
- , Reza Firouzi
- & Saeid Eslami
Article 14 November 2023 | Open Access
Node embedding-based graph autoencoder outlier detection for adverse pregnancy outcomes
- , Nazar Zaki
- & Luai A. Ahmed
Article 13 November 2023 | Open Access
Emerging infectious disease surveillance using a hierarchical diagnosis model and the Knox algorithm
- Mengying Wang
- , Bingqing Yang
- & Cheng Yang
Article 10 November 2023 | Open Access
Toward MR protocol-agnostic, unbiased brain age predicted from clinical-grade MRIs
- Pedro A. Valdes-Hernandez
- , Chavier Laffitte Nodarse
- & Yenisel Cruz-Almeida
Article 06 November 2023 | Open Access
Prognostic and immunotherapeutic significance of immunogenic cell death-related genes in colon adenocarcinoma patients
- & Jian Wang
Article 05 November 2023 | Open Access
Predicting dengue transmission rates by comparing different machine learning models with vector indices and meteorological data
- Song Quan Ong
- , Pradeep Isawasan
- & Gomesh Nair
Article 02 November 2023 | Open Access
Anoikis-related genes signature development for clear cell renal cell carcinoma prognosis and tumor microenvironment
- Yinglei Jiang
- , Ying Wang
- & Xukai Wang
Browse broader subjects
- Computational biology and bioinformatics
Quick links
- Explore articles by subject
- Guide to authors
- Editorial policies

Recent advances in domain-driven data mining
- Published: 27 December 2022
- Volume 15 , pages 1–7, ( 2023 )
Cite this article
- Chuanren Liu 1 ,
- Ehsan Fakharizadi 2 ,
- Tong Xu 3 &
- Philip S. Yu 4
2630 Accesses
1 Altmetric
Explore all metrics
Data mining research has been significantly motivated by and benefited from real-world applications in novel domains. This special issue was proposed and edited to draw attention to domain-driven data mining and disseminate research in foundations, frameworks, and applications for data-driven and actionable knowledge discovery. Along with this special issue, we also organized a related workshop to continue the previous efforts on promoting advances in domain-driven data mining. This editorial report will first summarize the selected papers in the special issue, then discuss various industrial trends in the context of the selected papers, and finally document the keynote talks presented by the workshop. Although many scholars have made prominent contributions with the theme of domain-driven data mining, there are still various new research problems and challenges calling for more research investigations in the future. We hope this special issue is helpful for scholars working along this critically important line of research.
Avoid common mistakes on your manuscript.
1 Summary of research contributions
Data mining has been a trending research area with contributions from diverse communities including computer scientists, statisticians, mathematicians, as well as other researchers and engineers working on data-intensive problems. While many researchers focus on general data mining methodologies for standardized problem settings, such as unsupervised learning and supervised learning, applying general solutions to specific problems may still be a nontrivial challenge. This is mainly due to the need to incorporate domain knowledge in implementing data mining solutions for novel real-world applications. Oftentimes standardized solutions must be significantly revised to accommodate unique characteristics of input data and deliver actionable results in novel application domains. Essentially, data mining research is highly applied. Many classic research problems are motivated by real-world applications and results of data mining research are expected to provide practical implications to business managers, government agencies, and all members of our society.
1.1 Overview of domain-driven data mining
Domain-driven data mining aims to bridge the gaps between theoretical research and practical applications in data mining and transform data intelligence to business value and impact [ 11 , 12 ]. Domain-driven data mining has been proposed as a research framework for discovering actionable knowledge and intelligence in a complex environment to directly transform data to decisions or enable decision-making actions [ 3 , 16 ].
Domain-driven data mining handles ubiquitous X-complexities and X-intelligences surrounding domain-driven actionable intelligence discovery. Examples of X-complexities and X-intelligences are related to domain complexity and intelligence, data complexity and intelligence, behavior complexity and intelligence, network complexity and intelligence, social complexity and intelligence, organizational complexity and intelligence, human complexity and intelligence, and their integration and meta-synthesis [ 8 , 16 ]. Analyzing and learning X-complexities and X-intelligences result in X-analytics [ 8 ] in various domains and on specific purposes. Examples are business analytics, behavior analytics, social analytics, operational analytics, risk analytics, customer analytics, insurance analytics, learning analytics, cybersecurity analytics, and financial analytics [ 15 , 21 , 24 , 26 , 28 , 29 , 31 , 38 , 40 , 41 , 42 , 43 , 51 ]. One prominent example of learning data complexities for in-depth data intelligence is the research on non-IID learning, which learns interactions and couplings (including correlation and dependency) involved in heterogeneous data, behaviors, and systems. Non-IID learning is applicable to many real-world applications such as non-IID outlier detection, non-IID recommendation, non-IID multimedia and multimodal analytics, and non-IID federated learning [ 5 , 6 , 17 ].
Domain-driven data mining also handles typical research issues and gaps in existing body of knowledge for domain-driven and actionable intelligence delivery. The research on domain-driven actionable intelligence discovery includes but is not limited to: quantifying knowledge actionability (rather than just interestingness) of data mining results [ 14 ], domain knowledge representation and domain generalization [ 30 ], domain-driven actionable knowledge discovery process [ 3 , 16 ], context-aware analytics and learning [ 46 ], discovering actionable patterns by combined mining [ 4 , 54 ] and high-utility mining [ 27 ], pattern relation analysis [ 4 ], cross-domain and transfer learning [ 24 , 36 , 45 , 51 ], data-to-decision transformation [ 8 ], personalized learning and recommendation [ 49 ], next-best action learning and recommendation [ 13 , 23 ], reflective learning with explicit and implicit feedback [ 32 , 50 ], explainable and interpretable analytics and learning [ 18 ], unbiased and fair analytics and learning [ 1 , 25 , 32 ], privacy and security-preserved analytics [ 52 ], and ethical analytics [ 34 ].
To better understand the challenges, recent advances, and new opportunities in domain-driven data mining, this special issue, along with other related activities, was proposed to call for the latest theoretical and practical developments, expert opinions on the open challenges, lessons learned, and best practices in domain-driven data mining. The special issue received submissions from researchers with different backgrounds, but all focusing on data-intensive research topics with novel applications. The papers accepted in this special issue explored novel factors and challenges such as socioeconomic, organizational, human-centered, and cultural aspects in different data mining tasks. In the following, we first provide a summary of the selected papers in the special issue.
1.2 Applied and flexible deep learning
Deep representation learning has attracted much attention in recent years. For chronic disease diagnosis, Zhang et al. [ 48 ] designed an unsupervised representation learning method to obtain informative correlation-aware signals from multivariate time series data. The key idea was a contrastive learning framework with a graph neural network (GNN) encoder to capture inter- and intra-correlation of multiple longitudinal variables. The work also considered modeling uncertainty quantification with evidential theory to assist the decision-making process in detecting chronic diseases. Also based on deep learning models, Sun et al. [ 37 ] adopted the sequential long short-term memory (LSTM) models in the domain of sports analytics for the baseball industry. With the numbers of home runs as the predictive target, the authors applied their models on the data from Major league Baseball (MLB) to support important decisions in managing players and teams. The results showed that deep learning model could perform better and bring valuable information to meet users’ needs. Focusing on more fundamental deep learning techniques, Zhao et al. [ 53 ] developed a flexible approach to compact architecture search for deep multitask learning (MTL) problems. Though sharing model architectures is a popular method for MTL problems, identifying the appropriate components to be shared by multiple tasks is still a challenge. Based on the expressive reinforcement learning framework, this paper proposed to discover flexible and compact MTL architectures with efficient search space and cost.
1.3 Interpretable and actionable predictions
A critical challenge facing data mining research is to discover actionable knowledge that can directly support decision-making tasks. In the domain of agricultural business and ecosystem management, Basak et al. [ 2 ] applied machine learning methods for a novel problem of soil moisture forecasting. The two modeling challenges were accurate long-term prediction and interpretable hydrological parameters. The proposed domain-driven solution was rooted in deterministic and physically based hydrological redistribution processes of gravity and suction.
As another example of actionable knowledge discovery, Dey et al. [ 19 ] proposed a systematic approach for fire station location planning. As urban fires could adversely affect the socioeconomic growth and ecosystem health of our communities, the authors applied various data mining and machine learning models in working with the Victoria Fire Department to make important decisions for selecting location of a new fire station. The key idea in their approach was to develop effective models for demand prediction and utilize the models to define a generalized index to measure quality of fire service in urban settings. The paper integrated multiple data sources and important domain knowledge/requirements in the modeling process. The final decision task was formulated as an integer programming problem to select the optimal location with maximum service coverage.
For sequential e-commerce product recommendation, Nasir and Ezeife [ 33 ] proposed the Semantic Enabled Markov Model Recommendation system to address long-standing challenges such as model complexity, data sparsity, and ambiguous predictions. Their system was proposed to extract and integrate sequential and semantic knowledge as well as contextual features. The new system showed improved recommendation performance for multiple e-commerce recommendation tasks.
1.4 Unsupervised learning with domain knowledge
Incorporating domain knowledge for unsupervised learning is particularly challenging due to the lack of clearly defined learning target. In the domain of health care, Jasinska-Piadlo et al. [ 22 ] explored the advantages and the challenges of a “domain-led” approach versus a data-driven approach to K -means clustering analysis. The authors compared expert opinions and principal component analysis for selecting the most useful variables to be used for the K -means clustering. The paper discussed comparative advantages of each approach and illustrated that domain knowledge played an important role at the interpretation stage of the clustering results. The authors developed a practical checklist guiding how to enable the integration of domain knowledge into a data mining project.
Similarly, text mining and natural language process are important research tools in many areas. However, many state-of-the-art text and language models are developed for general context, and careful adaption is often needed in applying such techniques on domain-specific data. In this special issue, Villanes and Healey [ 39 ] investigated the use of sentiment dictionaries to estimate sentiment for large document collections. The authors presented a semiautomatic method for extending general sentiment dictionary for a specific target domain. To minimize manual effort, the authors combined statistical term identification and term evaluation using Amazon Mechanical Turk in a study on dengue fever. The same approach could be potentially applied for constructing similar term-based sentiment dictionary in other target domains.
2 New trends from the industry perspective
A continuing trend in the data mining field has been the proliferation of its applications to new domains. This is partly due to the advancements in machine learning technologies evidenced by and promoted through frequent reports of new performance records on benchmark tasks. Another contributor to this proliferation is the increase in the quantity of data collected, stored, and appropriately documented for mining since the benefits of leveraging this data has become more apparent. Some of the works in this special issue demonstrated how data mining techniques can be applied in agriculture [ 2 ], health care and medicine [ 22 , 48 ], and city planning [ 19 ].
One aspect of data quality at the core of this expansion is the growing use of rich data formats. Image, audio, video, and raw text can now be almost directly fed into models that process them to extract meaningful features, patterns, and insights. These formats now often supplement the tabular data structures of the past as shown by Nasir and Ezeife [ 33 ]. To accommodate using these new formats, data mining and machine learning models have adapted to support multi-channel, multimodal, and sequential inputs [ 33 , 37 ].
As more domains employ novel data mining techniques, there have been more opportunities for cross-domain spillovers. We now see more examples of transfer learning, where models trained on one (source) domain are applied in another (target) domain suffering from data scarcity. However, learning generalized models that perform well on multiple tasks could be a challenging process [ 53 ]. These models are often trained with self-supervision on large data and contain millions or billions of learned parameters, such as models for language processing (e.g., BERT, GPT-3, XLNet) and image classification (ResNet, EfficientNet, Inception). A fundamental property of many generalized models is their ability to encode the input data into a vectorized representation, as evidenced by Zhang et al. [ 48 ].
Another recent challenge in data mining, one that is especially amplified in the case of transfer learning involving large models, is the issue of compactness. In many domains, where there is a need for scalable low-latency inferences and when the cost of training new models and deploying them could get high, it becomes necessary to restrict the model size. There are several techniques to accomplish these objectives including pruning, distilling, and training with constraints as Zhao et al. [ 53 ] demonstrated in this special issue.
Along with these trends, there have been several key developments in the structures used for data mining. One that has drastically improved the ability to digest sequential data is the invention of transformer structures. Transformers have effectively revolutionized the deep learning field by enabling models to understand the internal relationship between interdependent data points. These structures are the primary building blocks of some of the large generalized models mentioned above. Another recent progress is the improved ability of the generative models that learn not to score or classify but to create rich outputs such as images, texts, or audio. We also continue seeing more expansion in the field of graph neural network, where models learn and reproduce attributes of a graph data structure [ 48 ].
The sophistication of data mining methods has resulted in improved performance but comes at a cost. Models that use larger and richer input data, capture complex interaction between data points, and map the inputs to abstract representation spaces are very hard if not impossible to interpret. In many domains, it is important for the model outputs to be explainable to decision makers. Explainability matters for three reasons. First, explainable results are more powerful at both convincing decision makers and educating them with insights from the data [ 2 ]. Explainability is also a safeguard against models learning human biases and learning to discriminate. Finally, in some applications, it is necessary to understand not just the predicted value, but also the uncertainty of the predictions. Uncertainty modeling and quantification may be necessary in order to know when to rely on the machine and when to rely on the human. A recently popularized concept in this area is the human-in-the-loop approach, where models continuously receive and learn input from human experts and human decision makers, and meanwhile, experts use model predictions in their decision making on regular basis. Our authors in this special issue have demonstrated great potential of domain-driven data mining in addressing the aforementioned challenges, and more work is needed in the future with the collaboration between academia and industry.
3 Domain-driven data mining workshop
To facilitate the exchange of recent advances in domain-driven data mining, the Domain-Driven Data Mining Workshop was organized as a part of the 2021 SIAM International Conference on Data Mining. The workshop invited three keynote speakers and received paper submissions from multiple institutions. The papers accepted by the workshop were later invited for potential publication in this special issue. In the following, we review the invited keynote talks at the Domain-Driven Data Mining Workshop.
3.1 Actionable intelligence discovery
We first invited Dr. Longbing Cao for his keynote talk, “Domain-Driven and Actionable Intelligence Discovery.” In 2004, Dr. Cao proposed the concept “domain-driven data mining” and has led to implement many large enterprise data science projects for actionable knowledge discovery for governments and businesses, involving over 10 domains including capital markets, banking, insurance, telecommunication, transport, education, smart cities, online business, and public sectors (e.g., financial service, taxation, social welfare, IP, regulation, immigration).
Dr. Cao led a series of activities and proposed “domain-driven data mining” for “actionable knowledge discovery” in complex domains and problems, when discovering “actionable intelligence” was not a trivial task. The significant developments of data science, new-generation AI, and deep neural learning make domain-driven actionable intelligent discovery possible with progress made such as in representing and learning various complexities and intelligences in complex systems, data, and behaviors. In his talk, Dr. Cao first reviewed the aims, progresses, and gaps of conventional data mining/knowledge discovery and machine learning, domain-driven actionable knowledge discovery, and challenges and opportunities in domain-driven actionable intelligence discovery. Then, Dr. Cao discussed related strategic issues in data science thinking [ 8 ], new-generation AI [ 9 ], and actionable deep learning. Dr. Cao shared many thought-provoking illustrations, case studies, and theoretical and practical challenges in industry and government data sciences.
Particularly, Dr. Cao has made broad and in-depth contribution in understanding data complexities and data intelligence. One of his recent foci is learning from non-IID data, forming the research on non-IID learning [ 10 , 17 ]. Non-IID learning goes beyond the classic analytical and learning systems based on the common independent and identically distributed (IID) assumption widely taken in existing science, technology, and engineering. It studies the comprehensive non-IIDnesses [ 5 ], i.e., coupling relationships and interactions (including but beyond correlation and dependency) [ 6 ], and heterogeneities (including but beyond nonidentical distribution) in data, behaviors, and systems. The research on non-IID learning has evolved to almost all areas in data mining, analytics, and learning [ 17 ], such as non-IID data preparation, non-IID feature engineering, non-IID representation learning, non-IID similarity and metric learning, non-IID statistical learning, non-IID learning architecture, non-IID ensemble learning, non-IID federated learning, non-IID transfer learning, non-IID evaluation and validation, and various non-IID learning applications, such as non-IID recommender systems, non-IID outlier detection, non-IID information retrieval, and non-IID image and vision learning [ 5 , 20 , 35 , 47 , 55 ].
For instance, Cao [ 7 ] emphasized the critical issues of the intrinsic assumption that IID users and items in existing recommender systems, leading to false, misleading or incorrect recommendation, and poor performance in cold-start, sparse, and dynamic recommendations. Therefore, a non-IID theoretical framework is needed in order to build a deep and comprehensive understanding of the intrinsic nature of recommendation problems, from the perspective of both couplings and heterogeneities. Such research investigations led by Dr. Cao have triggered the paradigm shift from IID to non-IID recommendation research and can hopefully deliver informed, relevant, personalized, and actionable recommendations. All together, these contributions led to exciting new directions and fundamental solutions to address various challenges including cold-start, sparse data-based, cross-domain, group-based, and shilling attack-related issues in recommender systems.
3.2 A deep learning framework
We invited Dr. Balaji Padmanabhan for his keynote talk titled “Domain-Driven Data Mining: Examples and a Deep Learning Framework.” Dr. Padmanabhan is the Anderson Professor of Global Management and Professor of Information Systems at the University of South Florida’s Muma College of Business, where he is also the director of the Center for Analytics and Creativity. He has worked in data science, AI/machine learning, and business analytics for over two decades in the areas of research, teaching, business management, mentoring graduate students, and designing academic programs. He has also worked with over twenty firms on machine learning and data science initiatives in a variety of sectors. He has published extensively in data science and related areas at premier journals and conferences in the field and has served on the editorial board of leading journals including Management Science, MIS Quarterly, INFORMS Journal on Computing, Information Systems Research, Big Data, ACM Transactions on MIS, and the Journal of Business Analytics.
Dr. Padmanabhan witnessed and led the development of data mining. “I did my PhD at that time when the term of data mining first came up,” he shared with the audience of the workshop audience and reviewed the history of domain-driven data mining research. Then he presented a series of examples over the last two decades of his work. In generalizing from these examples, he emphasized that there are often different extents to which “domain” matters in different data mining endeavors. Dr. Padmanabhan encouraged the workshop audience to “think domain-driven,” which often motivates novel domain-driven methods that can meanwhile be applied more broadly (or “domain free”). Dr. Padmanabhan also shared a general framework for domain-driven deep learning in business research and used this framework to show how researchers can highlight significant contributions and position their own papers and ideas. Dr. Padmanabhan’s insightful cases and valuable research advice were greatly appreciated by the workshop audience from research communities of both computer science and management information systems.
In his talk, Dr. Padmanabhan also shared that his department has completed 100 projects in 7 years with about 30 companies, and funded postdoctoral research in analytics. His department has several outreach initiatives such as Economic Analytics Initiative and Florida Business Analytics Forum. Dr. Padmanabhan highlighted that such industrial collaborations and initiatives have greatly rewarded research activities particularly in domain-driven data mining projects. Dr. Padmanabhan encouraged researchers to actively reach out to industry not only when finding data but also to ask for new research questions.
3.3 Human resource management
We invited Dr. Hui Xiong for his keynote talk, “Artificial Intelligence in Human Resource Management.” Dr. Hui Xiong is a Distinguished Professor at the Rutgers, the State University of New Jersey. He also served as the Smart City Chief Scientist and the Deputy Dean of Baidu Research Institute in charge of several research laboratories. He is a co-Editor-in-Chief of Encyclopedia of GIS, an Associate Editor of IEEE Transactions on Big Data (TBD), ACM Transactions on Knowledge Discovery from Data (TKDD), and ACM Transactions on Management Information Systems (TMIS). Dr. Xiong has chaired for many international conferences in data mining, including a Program Co-Chair (2013) and a General Co-Chair (2015) for the IEEE International Conference on Data Mining (ICDM), and a Program Co-Chair of the Research Track (2018) and the Industry Track (2012) for the ACM SIGKDD International Conference on Knowledge Discovery and Data Mining (KDD). Dr. Xiong’s research has generated substantive impact beyond academia. He is an ACM distinguished scientist and has been honored by the ICDM-2011 Best Research Paper Award, the 2017 IEEE ICDM Outstanding Service Award, and the 2018 Ram Charan Management Practice Award as the Grand Prix winner from the Harvard Business Review. In 2020, he was named as an AAAS Fellow and an IEEE Fellow.
Dr. Xiong shared a successful story in leveraging big data technology for human resource management. Indeed, the availability of large-scale human resource (HR) data has enabled unparalleled opportunities for business leaders to understand talent behaviors and generate useful talent knowledge, which in turn deliver intelligence for real-time decision making and effective people management at work. In his talk, Dr. Xiong introduced a powerful set of innovative Artificial Intelligence (AI) techniques developed for intelligent human resource management, such as recruiting, performance evaluation, talent retention, talent development, job matching, team management, leadership development, and organization culture analysis. With his rich experiences and close collaborations with the industry, Dr. Xiong demonstrated how the results of talent analytics can be used for other business applications, such as market trend analysis and financial investment.
4 Concluding remarks
This special issue was proposed and edited to draw attention to domain-driven data mining and disseminate research in foundations, frameworks, and applications for data-driven and actionable knowledge discovery. This special issue and related activities on recent advances in domain-driven data mining continued the previous efforts including the workshop series on the same topic during 2007–2014 with the IEEE International Conference on Data Mining and a special issue published by the IEEE Transactions on Knowledge and Data Engineering [ 44 ]. Although many scholars have made significant contributions with the theme of domain-driven data mining, there are still various new research problems and challenges calling for more research investigations in the coming years. We hope this special issue is helpful for scholars working along this critically important line of research.
Alves, G., Amblard, M., Bernier, F., Couceiro, M., Napoli, A.: Reducing unintended bias of ML models on tabular and textual data. In: DSAA, pp. 1–10 (2021)
Basak, A., Schmidt, K.M., Mengshoel, O.J.: From data to interpretable models: machine learning for soil moisture forecasting. Int. J. Data Sci. Anal. (2022). https://doi.org/10.1007/s41060-022-00347-8
Cao, L.: Domain-driven data mining: challenges and prospects. IEEE Trans. Knowl. Data Eng. 22 (6), 755–769 (2010)
Article Google Scholar
Cao, L.: Combined mining: analyzing object and pattern relations for discovering and constructing complex yet actionable patterns. Wiley Interdiscip. Rev. Data Min. Knowl. Discov. 3 (2), 140–155 (2013)
Cao, L.: Non-iidness learning in behavioral and social data. Comput. J. 57 (9), 1358–1370 (2014)
Cao, L.: Coupling learning of complex interactions. Inf. Process. Manag. 51 (2), 167–186 (2015)
Cao, L.: Non-iid recommender systems: a review and framework of recommendation paradigm shifting. Engineering 2 (2), 212–224 (2016)
Cao, L.: Data Science Thinking: The Next Scientific, Technological and Economic Revolution. Data Analytics. Springer, Berlin (2018)
Book Google Scholar
Cao, L.: A new age of AI: features and futures. IEEE Intell. Syst. 37 (1), 25–37 (2022)
Cao, L.: Beyond i.i.d.: non-iid thinking, informatics, and learning. IEEE Intell. Syst. 37 (04), 5–17 (2022)
Cao, L., Zhang, C.: Domain-driven actionable knowledge discovery in the real world. In: PAKDD 2006, pp. 821–830 (2006)
Cao, L., Zhang, C.: The evolution of kdd: towards domain-driven data mining. IJPRAI 21 (4), 677–692 (2007)
Google Scholar
Cao, L., Zhu, C.: Personalized next-best action recommendation with multi-party interaction learning for automated decision-making. PLoS ONE 17 , 1–22 (2022)
Cao, L., Luo, D., Zhang, C.: Knowledge actionability: satisfying technical and business interestingness. IJBIDM 2 (4), 496–514 (2007)
Cao, L., Zhang, C., Yang, Q., Bell, D.A., Vlachos, M., Taneri, B., Keogh, E.J., Yu, P.S., Zhong, N., Ashrafi, M.Z., Taniar, D., Dubossarsky, E., Graco, W.: Domain-driven, actionable knowledge discovery. IEEE Intell. Syst. 22 (4), 78–88 (2007)
Cao, L., Yu, P.S., Zhang, C., Zhao, Y.: Domain Driven Data Mining. Springer, Berlin (2010)
Book MATH Google Scholar
Cao, L., Philip, S.Y., Zhao, Z.: Shallow and deep non-iid learning on complex data. In: Proceedings of the ACM SIGKDD International Conference on Knowledge Discovery and Data Mining, (2022)
Carlevaro, A., Mongelli, M.: A new SVDD approach to reliable and explainable AI. IEEE Intell. Syst. 37 (2), 55–68 (2022)
Dey, A., Heger, A., England, D.: Urban fire station location planning using predicted demand and service quality index. Int. J. Data Sci. Anal. (2022). https://doi.org/10.1007/s41060-022-00328-x
Do, T.D.T., Cao, L.: Gamma-Poisson dynamic matrix factorization embedded with metadata influence. In: NeurIPS 2018, pp. 5829–5840 (2018)
He, F., Li, Y., Xu, T., Yin, L., Zhang, W., Zhang, X.: A data-analytics approach for risk evaluation in peer-to-peer lending platforms. IEEE Intell. Syst. 35 (3), 85–95 (2020)
Jasinska-Piadlo, A., Bond, R., Biglarbeigi, P., Brisk, R., Campbell, P., Browne, F., McEneaneny, D.: Data-driven versus a domain-led approach to k-means clustering on an open heart failure dataset. Int. J. Data Sci. Anal. (2022). https://doi.org/10.1007/s41060-022-00346-9
Jin, B., Yang, H., Sun, L., Liu, C., Qu, Y., Tong, J.: A treatment engine by predicting next-period prescriptions. In: KDD, pp. 1608–1616 (2018)
Kanter, J.M., Gillespie, O., Veeramachaneni, K.: Label, segment, featurize: a cross domain framework for prediction engineering. In: DSAA, pp. 430–439 (2016)
Ke, W., Liu, C., Shi, X., Dai, Y., Yu, P.S., Zhu, X.: Addressing exposure bias in uplift modeling for large-scale online advertising. In: ICDM, pp. 1156–1161 (2021)
Kompan, M., Gaspar, P., Macina, J., Cimerman, M., Bieliková, M.: Exploring customer price preference and product profit role in recommender systems. IEEE Intell. Syst. 37 (1), 89–98 (2022)
Lin, J.C.-W., Gan, W., Fournier-Viger, P., Hong, T.-P., Tseng, V.S.: Mining high-utility itemsets with various discount strategies. In: DSAA, pp. 1–10 (2015)
Liu, C., Zhu, W.: Precision coupon targeting with dynamic customer triage. In: DSAA, pp. 420–428 (2020)
Liu, Q., Zeng, X., Liu, C., Zhu, H., Chen, E., Xiong, H., Xie, X.: Mining indecisiveness in customer behaviors. In: ICDM, pp. 281–290 (2015)
Long, M., Wang, J., Sun, J.-G., Yu, P.S.: Domain invariant transfer kernel learning. IEEE Trans. Knowl. Data Eng. 27 (6), 1519–1532 (2015)
Ma, D., Narayanan, V.K., Liu, C., Fakharizadi, E.: Boundary salience: the interactive effect of organizational status distance and geographical proximity on coauthorship tie formation. Soc. Netw. 63 , 162–173 (2020)
Melucci, M.: Investigating sample selection bias in the relevance feedback algorithm of the vector space model for information retrieval. In: DSAA, pp. 83–89 (2014)
Nasir, M., Ezeife, C.I.: Semantic enhanced Markov model for sequential e-commerce product recommendation. Int. J. Data Sci. Anal., (2022) https://doi.org/10.1007/s41060-022-00343-y
O’Leary, D.E.: Ethics for big data and analytics. IEEE Intell. Syst. 31 (4), 81–84 (2016)
Pang, G., Cao, L., Chen, L.: Homophily outlier detection in non-iid categorical data. Data Min. Knowl. Discov. 35 (4), 1163–1224 (2021)
Article MATH Google Scholar
Ruiz-Dolz, R., Alemany, J., Barberá, S.H., García-Fornes, A.: Transformer-based models for automatic identification of argument relations: a cross-domain evaluation. IEEE Intell. Syst. 36 (6), 62–70 (2021)
Sun, H.-C., Lin, T.-Y., Tsai, Y.-L.: Performance prediction in major league baseball by long short-term memory networks. Int. J. Data Sci. Anal. (2022). https://doi.org/10.1007/s41060-022-00313-4
Teng, M., Zhu, H., Liu, C., Xiong, H.: Exploiting network fusion for organizational turnover prediction. ACM Trans. Manag. Inf. Syst. 12 (2), 16:1-16:18 (2021)
Villanes, A., Healey, C.G.: Domain-specific text dictionaries for text analytics. Int. J. Data Sci. Analy., Special Issue on Domain-Driven Data Mining (2022)
Xiang, H., Lin, J., Chen, C.-H., Kong, Y.: Asymptotic meta learning for cross validation of models for financial data. IEEE Intell. Syst. 35 (2), 16–24 (2020)
Xu, L., Wei, X., Cao, J., Yu, P.S.: Multiple social role embedding. In: DSAA, pp. 581–589. IEEE (2017)
Yang, D., Bingqing, Q., Cudré-Mauroux, P.: Location-centric social media analytics: challenges and opportunities for smart cities. IEEE Intell. Syst. 36 (5), 3–10 (2021)
Yang, J., Liu, C., Teng, M., Xiong, H., Liao, M., Zhu, V.: Exploiting temporal and social factors for B2B marketing campaign recommendations. In: ICDM, pp. 499–508 (2015)
Zhang, C., Yu, P., Bell, D.: Introduction to the domain-drive data mining special section. IEEE Trans. Knowl. Data Eng. 22 (6), 753–754 (2010)
Zhang, J., He, M.: CRTL: context restoration transfer learning for cross-domain recommendations. IEEE Intell. Syst. 36 (4), 65–72 (2021)
Zhang, K., Chen, E., Liu, Q., Liu, C., Lv, G.: A context-enriched neural network method for recognizing lexical entailment. In: AAAI, pp. 3127–3134 (2017)
Zhang, Q., Cao, L., Zhu, C., Li, Z., Sun, J.: Coupledcf: learning explicit and implicit user-item couplings in recommendation for deep collaborative filtering. In: IJCAI 2018, pp. 3662–3668 (2018)
Zhang, X., Wang, Y., Zhang, L., Jin, B., Zhang, H.: Exploring unsupervised multivariate time series representation learning for chronic disease diagnosis. Int. J. Data Sci. Anal. (2022). https://doi.org/10.1007/s41060-021-00290-0
Zhang, Y., Liu, G., Liu, A., Zhang, Y., Li, Z., Zhang, X., Li, Q.: Personalized geographical influence modeling for POI recommendation. IEEE Intell. Syst. 35 (5), 18–27 (2020)
Zhang, Y., Bai, G., Zhong, M., Li, X., Ryan, K.L.K.: Differentially private collaborative coupling learning for recommender systems. IEEE Intell. Syst. 36 (1), 16–24 (2021)
Zhang, Y., Zhang, X., Shen, T., Zhou, Y., Wang, Z.: Feature-option-action: a domain adaption transfer reinforcement learning framework. In: DSAA, pp. 1–12 (2021)
Zhang, Z., Liu, Q., Huang, Z., Wang, H., Lu, C., Liu, C., Chen, E.: Graphmi: extracting private graph data from graph neural networks. In: IJCAI, pp. 3749–3755 (2021)
Zhao, J., Lv, W., Du, B., Ye, J., Sun, L., Xiong, G.: Deep multi-task learning with flexible and compact architecture search. Int. J. Data Sci. Anal., Special Issue on Domain-Driven Data Mining (2022)
Zhao, Y., Zhang, H., Cao, L., Zhang, C., Bohlscheid, H.: Combined pattern mining: from learned rules to actionable knowledge. In: AI 2008, pp. 393–403 (2008)
Zhu, C., Cao, L., Yin, J.: Unsupervised heterogeneous coupling learning for categorical representation. IEEE Trans. Pattern Anal. Mach. Intell. 44 (1), 533–549 (2022)
Download references
Author information
Authors and affiliations.
The University of Tennessee, Knoxville, USA
Chuanren Liu
Snap Inc., Seattle, WA, USA
Ehsan Fakharizadi
University of Science and Technology of China, Hefei, China
University of Illinois Chicago, Chicago, USA
Philip S. Yu
You can also search for this author in PubMed Google Scholar
Corresponding author
Correspondence to Chuanren Liu .
Additional information
Publisher's note.
Springer Nature remains neutral with regard to jurisdictional claims in published maps and institutional affiliations.
Rights and permissions
Reprints and permissions
About this article
Liu, C., Fakharizadi, E., Xu, T. et al. Recent advances in domain-driven data mining. Int J Data Sci Anal 15 , 1–7 (2023). https://doi.org/10.1007/s41060-022-00378-1
Download citation
Published : 27 December 2022
Issue Date : January 2023
DOI : https://doi.org/10.1007/s41060-022-00378-1
Share this article
Anyone you share the following link with will be able to read this content:
Sorry, a shareable link is not currently available for this article.
Provided by the Springer Nature SharedIt content-sharing initiative
- Find a journal
- Publish with us
- Track your research
Home » Blog » Dissertation » Topics » Information Technology » 80 Data Mining Research Topics
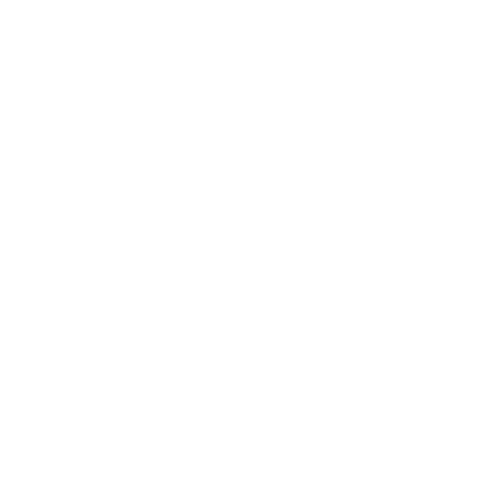
80 Data Mining Research Topics
FacebookXEmailWhatsAppRedditPinterestLinkedInAre you a student embarking on a research journey in the field of data mining, searching for that perfect set of research topics to catalyze your undergraduate, master’s, or doctoral thesis or dissertation? Well, you’ve arrived at the right place! The world of data mining is a captivating realm that offers a plethora of opportunities […]
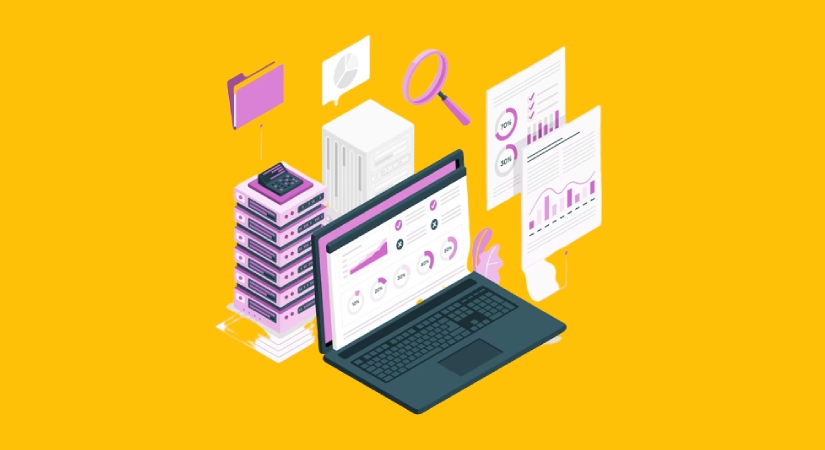
Are you a student embarking on a research journey in the field of data mining, searching for that perfect set of research topics to catalyze your undergraduate, master’s, or doctoral thesis or dissertation? Well, you’ve arrived at the right place! The world of data mining is a captivating realm that offers a plethora of opportunities for exploration and innovation. Your research journey begins with the careful selection of research topics, a dec All Posts ision that will set the course for your academic pursuits. In this comprehensive guide, we will delve into an array of intriguing data mining research topics, spanning various complexity levels and domains, to help you navigate the fascinating landscape of data-driven discovery.
Data Mining, often referred to as”data analysis,” “data exploration,” “pattern recognition,” and “predictive modeling”, is the process of extracting valuable patterns, insights, and knowledge from large datasets.
A List Of Potential Research Topics In Data Mining:
- Assessing the impact of data mining in UK law enforcement for crime prevention.
- Analyzing the use of data mining in predicting and mitigating the impact of Brexit on UK businesses.
- A review of data mining in personalized education and adaptive learning systems.
- A comprehensive analysis of data mining for image and video analysis in computer vision.
- Assessing the role of data mining in identifying and mitigating insider threats in organizations.
- A systematic review of data mining applications in the healthcare industry.
- Investigating the effectiveness of data mining in predicting stock market trends.
- A review of data mining approaches for text and sentiment analysis.
- A review of data mining applications in the automotive industry for predictive maintenance.
- Evaluating the impact of the COVID-19 pandemic on data mining techniques in healthcare analytics.
- Exploring the application of data mining in optimizing energy consumption in smart homes.
- A critical examination of data mining methods for social network analysis and community detection.
- A critical assessment of data mining tools and software for beginners.
- Examining the use of data mining in optimizing resource allocation in cloud computing.
- Analyzing the application of data mining in improving personalized healthcare recommendations.
- Investigating the use of data mining in natural language processing for sentiment analysis.
- Investigating the application of data mining in predicting and preventing cyberattacks.
- Investigating the challenges and opportunities of data mining in UK higher education institutions.
- Investigating the effectiveness of data mining techniques in identifying rare events in medical data.
- Analyzing the use of data mining in optimizing manufacturing processes for quality control.
- Assessing the use of data mining in predicting urban traffic congestion.
- Data mining techniques for detecting cybersecurity threats.
- A systematic review of data mining approaches for predicting customer churn in telecommunications.
- Investigating the use of data mining in cybersecurity for threat detection and prevention.
- Exploring the application of data mining in predicting disease outbreaks using epidemiological data.
- Exploring the role of data mining in credit scoring and risk assessment for lending institutions.
- Analyzing the application of data mining in enhancing energy efficiency in UK homes.
- Assessing the role of data mining in remote monitoring and telehealth during and post-COVID-19.
- Analyzing the use of data mining in tracking and predicting the spread of infectious diseases.
- Assessing the application of data mining in optimizing agricultural practices for crop yield prediction.
- Analyzing the impact of data mining in improving personalized healthcare interventions.
- Assessing the role of data mining in personalized recommendation systems for e-commerce.
- An assessment of data mining in optimizing energy consumption in smart cities.
- Exploring the application of data mining in optimizing public transportation systems in the UK.
- A comparative analysis of data mining techniques for fraud detection in the banking sector.
- Investigating the role of data mining in analyzing social media data for political campaigns in the UK.
- Investigating the use of data mining in optimizing inventory management for e-commerce businesses.
- A comprehensive review of data preprocessing techniques in data mining.
- Exploring the ethical implications of data mining in online privacy and data protection.
- Analyzing the impact of data mining in sentiment analysis of customer reviews in the hospitality industry.
- Assessing the impact of data mining in analyzing social network data for marketing strategies.
- Exploring the role of data mining in enhancing recommendation systems for online learning platforms.
- Analyzing the ethical implications of data mining in the collection and use of personal data.
- Investigating the impact of deep learning techniques on sentiment analysis in social media data.
- Investigating the role of data mining in identifying patterns of criminal behavior for law enforcement.
- An in-depth review of data mining techniques for anomaly detection in cybersecurity.
- A critical review of data mining algorithms for imbalanced datasets.
- Exploring the role of data mining in analyzing cultural trends and social behaviors.
- Analyzing the application of data mining in recommendation systems for streaming platforms.
- Leveraging artificial intelligenc e in data mining for enhanced insights.
- Examining the ethical implications of data mining in public policy decision-making.
- Assessing the effectiveness of data mining in predicting customer churn in telecommunications.
- Investigating the challenges and opportunities of data mining in analyzing pandemic-related data.
- Investigating the challenges and opportunities of data mining in educational data analytics.
- A review of data mining applications in environmental science and climate modeling.
- Assessing the effectiveness of clustering algorithms in customer segmentation for marketing.
- Investigating the challenges and opportunities of data mining in analyzing geospatial data.
- A review of data mining algorithms for recommendation systems in e-commerce.
- Analyzing the effectiveness of data mining in addressing climate change challenges in the UK.
- Assessing the effectiveness of data mining techniques in early detection of diseases from medical images.
- A comparative review of clustering algorithms in data mining.
- Examining the use of data mining in improving personalized healthcare services in the UK.
- A comprehensive review of data mining in the context of big data analytics.
- Evaluating the adoption and impact of data mining in the UK’s National Health Service (NHS).
- A survey of data mining applications in the financial sector.
- Assessing the impact of COVID-19 on data privacy and ethical considerations in data mining.
- Analyzing the impact of data mining in predicting disease outbreaks in developing countries.
- Examining the use of data mining in analyzing vaccine distribution and uptake data.
- Assessing the role of data mining in analyzing COVID-19 data for policy decisions in the UK.
- Investigating the ethical considerations in data mining for personalized advertising.
- Assessing the impact of data mining on sentiment analysis in political discourse.
- Analyzing the use of data mining in optimizing energy consumption in smart grid systems.
- Exploring the role of data mining in predicting student academic performance in online education.
- Analyzing the effectiveness of data mining in understanding changes in consumer behavior during the pandemic.
- Examining the impact of data preprocessing techniques on the performance of classification models.
- Assessing the ethical considerations in data mining for social media content analysis.
- Analyzing the utilization of blockchain technology for secure and transparent data sharing in healthcare.
- Examining the effectiveness of data mining techniques in analyzing environmental data for climate studies.
- An extensive review of data mining techniques for time-series data analysis.
- Investigating the role of data mining in predicting and preventing traffic accidents.
In the exhilarating world of data mining research, the possibilities are as limitless as the data itself. Whether you’re pursuing an undergraduate, master’s, or doctoral degree, we’ve provided you with a diverse spectrum of research topics to ignite your academic journey. Now equipped with this list of thought-provoking themes, it’s time to dive headfirst into the depths of data mining, where research, topics, and innovation converge to shape the future of data-driven discovery. Happy exploring!
Order Data Mining Dissertation Now!
External Links:
- Download Data Mining Dissertation Sample For Your Perusal
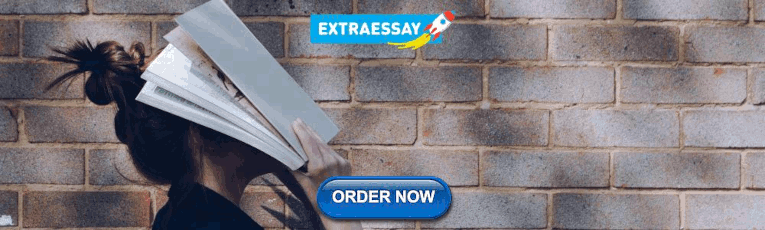
Research Topic Help Service
Get unique research topics exactly as per your requirements. We will send you a mini proposal on the chosen topic which includes;
- Research Statement
- Research Questions
- Key Literature Highlights
- Proposed Methodology
- View a Sample of Service
Ensure Your Good Grades With Our Writing Help
- Talk to the assigned writer before payment
- Get topic if you don't have one
- Multiple draft submissions to have supervisor's feedback
- Free revisions
- Complete privacy
- Plagiarism Free work
- Guaranteed 2:1 (With help of your supervisor's feedback)
- 2 Installments plan
- Special discounts
Other Posts
- 80 Artificial Intelligence Research Topics September 10, 2023 -->
- 80 Cyber Security Research Topics September 10, 2023 -->
- 80 Data Science Research Topics September 10, 2023 -->
- 80 Information Technology Research Topics July 30, 2023 -->
WhatsApp us
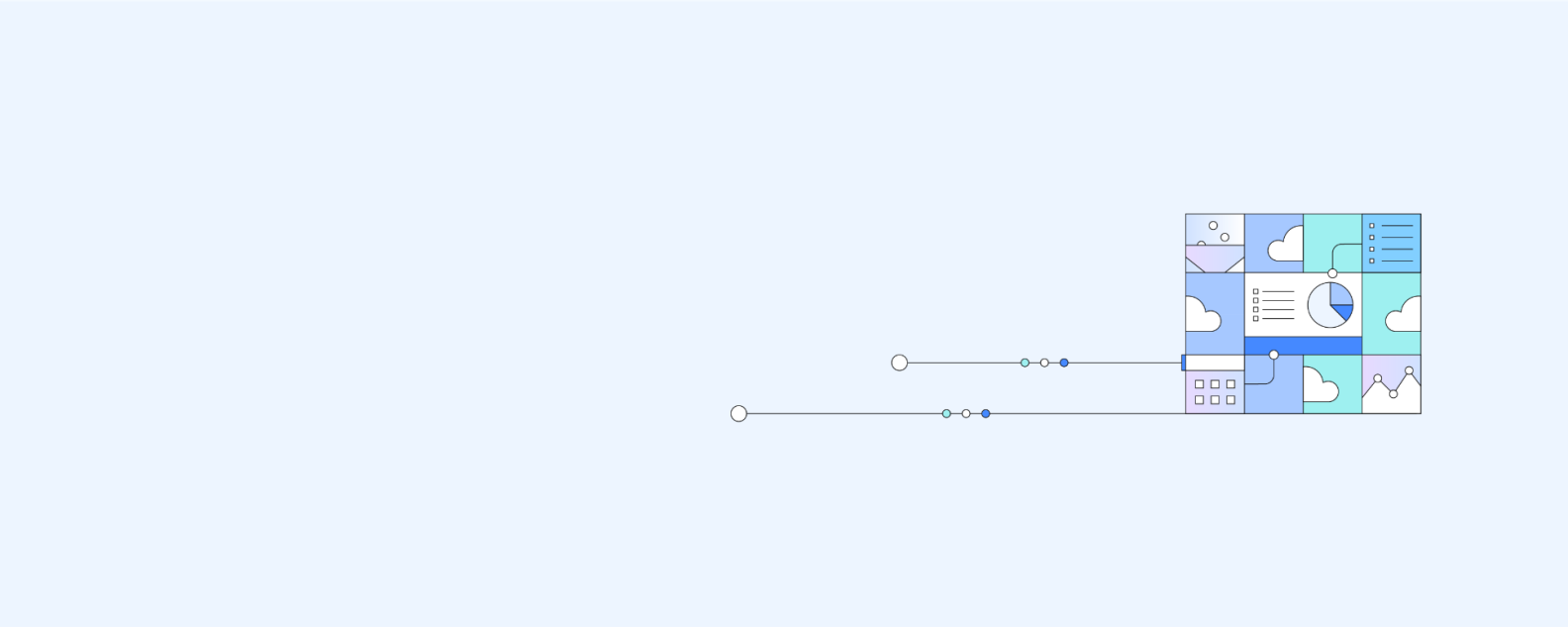
Data mining, also known as knowledge discovery in data (KDD), is the process of uncovering patterns and other valuable information from large data sets.
Given the evolution of data warehousing technology and the growth of big data, adoption of data mining techniques has rapidly accelerated over the last couple of decades, assisting companies by transforming their raw data into useful knowledge. However, despite the fact that that technology continuously evolves to handle data at a large scale, leaders still face challenges with scalability and automation.
Data mining has improved organizational decision-making through insightful data analyses. The data mining techniques that underpin these analyses can be divided into two main purposes; they can either describe the target dataset or they can predict outcomes through the use of machine learning algorithms. These methods are used to organize and filter data, surfacing the most interesting information, from fraud detection to user behaviors, bottlenecks and even security breaches.
When combined with data analytics and visualization tools, like Apache Spark , delving into the world of data mining has never been easier and extracting relevant insights has never been faster. Advances within artificial intelligence only continue to expedite adoption across industries.
Learn how to leverage the right databases for applications, analytics and generative AI.
Register for the ebook on generative AI
Data mining, also known as knowledge discovery in data (KDD), is the process of uncovering patterns and other valuable information from large data sets. Given the evolution of data warehousing technology and the growth of big data, adoption of data mining techniques has rapidly accelerated over the last couple of decades, assisting companies by transforming their raw data into useful knowledge. However, despite the fact that that technology continuously evolves to handle data at a large scale, leaders still face challenges with scalability and automation.
When combined with data analytics and visualization tools, like Apache Spark , delving into the world of data mining has never been easier and extracting relevant insights has never been faster. Advances within artificial intelligence only continue to expedite adoption across industries.
Scale AI workloads for all your data anywhere.
The data mining process involves a number of steps from data collection to visualization to extract valuable information from large data sets. As mentioned above, data mining techniques are used to generate descriptions and predictions about a target data set. Data scientists describe data through their observations of patterns, associations and correlations. They also classify and cluster data through classification and regression methods, and identify outliers for use cases, like spam detection.
Data mining usually consists of four main steps: setting objectives, data gathering and preparation, applying data mining algorithms and evaluating results.
1. Set the business objectives: This can be the hardest part of the data mining process, and many organizations spend too little time on this important step. Data scientists and business stakeholders need to work together to define the business problem, which helps inform the data questions and parameters for a given project. Analysts may also need to do additional research to understand the business context appropriately.
2. Data preparation: Once the scope of the problem is defined, it is easier for data scientists to identify which set of data will help answer the pertinent questions to the business. Once they collect the relevant data, it will be cleaned, removing any noise, such as duplicates, missing values and outliers. Depending on the dataset, an additional step may be taken to reduce the number of dimensions as too many features can slow down any subsequent computation. Data scientists will look to retain the most important predictors to ensure optimal accuracy within any models.
3. Model building and pattern mining: Depending on the type of analysis, data scientists may investigate any interesting data relationships, such as sequential patterns, association rules or correlations. While high-frequency patterns have broader applications, sometimes the deviations in the data can be more interesting, highlighting areas of potential fraud.
Deep learning algorithms may also be applied to classify or cluster a data set depending on the available data. If the input data is labelled (i.e. supervised learning ), a classification model may be used to categorize data, or alternatively, a regression may be applied to predict the likelihood of a particular assignment. If the dataset isn’t labelled (i.e. unsupervised learning ), the individual data points in the training set are compared with one another to discover underlying similarities, clustering them based on those characteristics.
4. Evaluation of results and implementation of knowledge: Once the data is aggregated, the results need to be evaluated and interpreted. When finalizing results, they should be valid, novel, useful and understandable. When this criteria is met, organizations can use this knowledge to implement new strategies, achieving their intended objectives.
Data mining works by using various algorithms and techniques to turn large volumes of data into useful information. Here are some of the most common ones:
Association rules: An association rule is a rule-based method for finding relationships between variables in a given dataset. These methods are frequently used for market basket analysis, allowing companies to better understand relationships between different products. Understanding consumption habits of customers enables businesses to develop better cross-selling strategies and recommendation engines.
Neural networks: Primarily leveraged for deep learning algorithms, neural networks process training data by mimicking the interconnectivity of the human brain through layers of nodes. Each node is made up of inputs, weights, a bias (or threshold) and an output. If that output value exceeds a given threshold, it “fires” or activates the node, passing data to the next layer in the network. Neural networks learn this mapping function through supervised learning, adjusting based on the loss function through the process of gradient descent. When the cost function is at or near zero, we can be confident in the model’s accuracy to yield the correct answer.
Decision tree: This data mining technique uses classification or regression methods to classify or predict potential outcomes based on a set of decisions. As the name suggests, it uses a tree-like visualization to represent the potential outcomes of these decisions.
K- nearest neighbor (KNN): K-nearest neighbor, also known as the KNN algorithm, is a non-parametric algorithm that classifies data points based on their proximity and association to other available data. This algorithm assumes that similar data points can be found near each other. As a result, it seeks to calculate the distance between data points, usually through Euclidean distance, and then it assigns a category based on the most frequent category or average.
Data mining techniques are widely adopted among business intelligence and data analytics teams, helping them extract knowledge for their organization and industry. Some data mining use cases include:
Sales and marketing
Companies collect a massive amount of data about their customers and prospects. By observing consumer demographics and online user behavior, companies can use data to optimize their marketing campaigns, improving segmentation, cross-sell offers and customer loyalty programs, yielding higher ROI on marketing efforts. Predictive analyses can also help teams to set expectations with their stakeholders, providing yield estimates from any increases or decreases in marketing investment.
Education
Educational institutions have started to collect data to understand their student populations as well as which environments are conducive to success. As courses continue to transfer to online platforms, they can use a variety of dimensions and metrics to observe and evaluate performance, such as keystroke, student profiles, classes, universities, time spent, etc.
Operational optimization
Process mining leverages data mining techniques to reduce costs across operational functions, enabling organizations to run more efficiently. This practice has helped to identify costly bottlenecks and improve decision-making among business leaders.
Fraud detection
While frequently occurring patterns in data can provide teams with valuable insight, observing data anomalies is also beneficial, assisting companies in detecting fraud. While this is a well-known use case within banking and other financial institutions, SaaS-based companies have also started to adopt these practices to eliminate fake user accounts from their datasets.
Find critical answers and insights from your business data using AI-powered enterprise search technology.
A fully managed, elastic cloud data warehouse built for high-performance analytics and AI.
Build and scale trusted AI on any cloud, and automate the AI lifecycle for ModelOps.
Identify patterns and trends with predictive analytics and key techniques.
Explore how to mitigate your own biases when creating machine learning models.
Train, validate, tune and deploy generative AI, foundation models and machine learning capabilities with IBM watsonx.ai, a next-generation enterprise studio for AI builders. Build AI applications in a fraction of the time with a fraction of the data.
Trending Data Mining Thesis Topics
Data mining seems to be the act of analyzing large amounts of data in order to uncover business insights that can assist firms in fixing issues, reducing risks, and embracing new possibilities . This article provides a complete picture on data mining thesis topics where you can get all information regarding data mining research
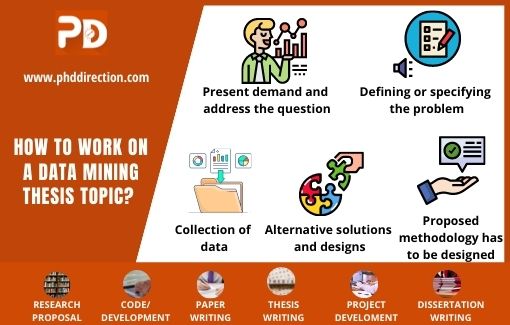
How does data mining work?
- A standard data mining design begins with the appropriate business statement in the questionnaire, the appropriate data is collected to tackle it, and the data is prepared for the examination.
- What happens in the earlier stages determines how successful the later versions are.
- Data miners should assure the data quality they utilize as input for research because bad data quality results in poor outcomes.
- Establishing a detailed understanding of the design factors, such as the present business scenario, the project’s main business goal, and the performance objectives.
- Identifying the data required to address the problem as well as collecting this from all sorts of sources.
- Addressing any errors and bugs, like incomplete or duplicate data, and processing the data in a suitable format to solve the research questions.
- Algorithms are used to find patterns from data.
- Identifying if or how another model’s output will contribute to the achievement of a business objective.
- In order to acquire the optimum outcome, an iterative process is frequently used to identify the best method.
- Getting the project’s findings suitable for making decisions in real-time
The techniques and actions listed above are repeated until the best outcomes are achieved. Our engineers and developers have extensive knowledge of the tools, techniques, and approaches used in the processes described above. We guarantee that we will provide the best research advice w.r.t to data mining thesis topics and complete your project on schedule. What are the important data mining tasks?
Data Mining Tasks
- Data mining finds application in many ways including description, Analysis, summarization of data, and clarifying the conceptual understanding by data description
- And also prediction, classification, dependency analysis, segmentation, and case-based reasoning are some of the important data mining tasks
- Regression – numerical data prediction (stock prices, temperatures, and total sales)
- Data warehousing – business decision making and large-scale data mining
- Classification – accurate prediction of target classes and their categorization
- Association rule learning – market-based analytical tools that were involved in establishing variable data set relationship
- Machine learning – statistical probability-based decision making method without complicated programming
- Data analytics – digital data evaluation for business purposes
- Clustering – dataset partitioning into clusters and subclasses for analyzing natural data structure and format
- Artificial intelligence – human-based Data analytics for reasoning, solving problems, learning, and planning
- Data preparation and cleansing – conversion of raw data into a processed form for identification and removal of errors
You can look at our website for a more in-depth look at all of these operations. We supply you with the needed data, as well as any additional data you may need for your data mining thesis topics . We supply non-plagiarized data mining thesis assistance in any fresh idea of your choice. Let us now discuss the stages in data mining that are to be included in your thesis topics
How to work on a data mining thesis topic?
The following are the important stages or phases in developing data mining thesis topics.
- First of all, you need to identify the present demand and address the question
- The next step is defining or specifying the problem
- Collection of data is the third step
- Alternative solutions and designs have to be analyzed in the next step
- The proposed methodology has to be designed
- The system is then to be implemented
Usually, our experts help in writing codes and implementing them successfully without hassles . By consistently following the above steps you can develop one of the best data mining thesis topics of recent days. Furthermore, technically it is important for you to have a better idea of all the tasks and techniques involved in data mining about which we have discussed below
- Data visualization
- Neural networks
- Statistical modeling
- Genetic algorithms and neural networks
- Decision trees and induction
- Discriminant analysis
- Induction techniques
- Association rules and data visualization
- Bayesian networks
- Correlation
- Regression analysis
- Regression analysis and regression trees
If you are looking forward to selecting the best tool for your data mining project then evaluating its consistency and efficiency stands first. For this, you need to gain enough technical data from real-time executed projects for which you can directly contact us. Since we have delivered an ample number of data mining thesis topics successfully we can help you in finding better solutions to all your research issues. What are the points to be remembered about the data mining strategy?
- Furthermore, data mining strategies must be picked before instruments in order to prevent using strategies that do not align with the article’s true purposes.
- The typical data mining strategy has always been to evaluate a variety of methodologies in order to select one which best fits the situation.
- As previously said, there are some principles that may be used to choose effective strategies for data mining projects.
- Since they are easy to handle and comprehend
- They could indeed collaborate with definitional and parametric data
- Tare unaffected by critical values, they could perhaps function with incomplete information
- They could also expose various interrelationships and an absence of linear combinations
- They could indeed handle noise in records
- They can process huge amounts of data.
- Decision trees, on the other hand, have significant drawbacks.
- Many rules are frequently necessary for dependent variables or numerous regressions, and tiny changes in the data can result in very different tree architectures.
All such pros and cons of various data mining aspects are discussed on our website. We will provide you with high-quality research assistance and thesis writing assistance . You may see proof of our skill and the unique approach that we generated in the field by looking at the samples of the thesis that we produced on our website. We also offer an internal review to help you feel more confident. Let us now discuss the recent data mining methodologies
Current methods in Data Mining
- Prediction of data (time series data mining)
- Discriminant and cluster analysis
- Logistic regression and segmentation
Our technical specialists and technicians usually give adequate accurate data, a thorough and detailed explanation, and technical notes for all of these processes and algorithms. As a result, you can get all of your questions answered in one spot. Our technical team is also well-versed in current trends, allowing us to provide realistic explanations for all new developments. We will now talk about the latest data mining trends
Latest Trending Data Mining Thesis Topics
- Visual data mining and data mining software engineering
- Interaction and scalability in data mining
- Exploring applications of data mining
- Biological and visual data mining
- Cloud computing and big data integration
- Data security and protecting privacy in data mining
- Novel methodologies in complex data mining
- Data mining in multiple databases and rationalities
- Query language standardization in data mining
- Integration of MapReduce, Amazon EC2, S3, Apache Spark, and Hadoop into data mining
These are the recent trends in data mining. We insist that you choose one of the topics that interest you the most. Having an appropriate content structure or template is essential while writing a thesis . We design the plan in a chronological order relevant to the study assessment with this in mind. The incorporation of citations is one of the most important aspects of the thesis. We focus not only on authoring but also on citing essential sources in the text. Students frequently struggle to deal with appropriate proposals when commencing their thesis. We have years of experience in providing the greatest study and data mining thesis writing services to the scientific community, which are promptly and widely acknowledged. We will now talk about future research directions of research in various data mining thesis topics
Future Research Directions of Data Mining
- The potential of data mining and data science seems promising, as the volume of data continues to grow.
- It is expected that the total amount of data in our digital cosmos will have grown from 4.4 zettabytes to 44 zettabytes.
- We’ll also generate 1.7 gigabytes of new data for every human being on this planet each second.
- Mining algorithms have completely transformed as technology has advanced, and thus have tools for obtaining useful insights from data.
- Only corporations like NASA could utilize their powerful computers to examine data once upon a time because the cost of producing and processing data was simply too high.
- Organizations are now using cloud-based data warehouses to accomplish any kinds of great activities with machine learning, artificial intelligence, and deep learning.
The Internet of Things as well as wearable electronics, for instance, has transformed devices to be connected into data-generating engines which provide limitless perspectives into people and organizations if firms can gather, store, and analyze the data quickly enough. What are the aspects to be remembered for choosing the best data mining thesis topics?
- An excellent thesis topic is a broad concept that has to be developed, verified, or refuted.
- Your thesis topic must capture your curiosity, as well as the involvement of both the supervisor and the academicians.
- Your thesis topic must be relevant to your studies and should be able to withstand examination.
Our engineers and experts can provide you with any type of research assistance on any of these data mining development tools . We satisfy the criteria of your universities by ensuring several revisions, appropriate formatting and editing of your thesis, comprehensive grammar check, and so on . As a result, you can contact us with confidence for complete assistance with your data mining thesis. What are the important data mining thesis topics?
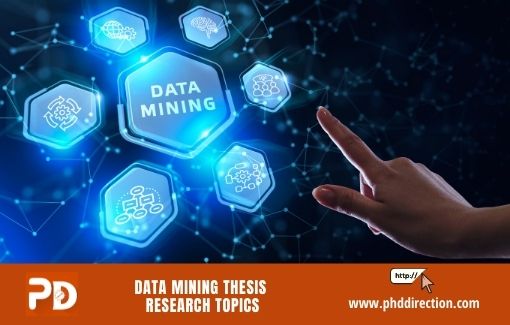
Research Topics in Data Mining
- Handling cost-effective, unbalanced non-static data
- Issues related to data mining and their solutions
- Network settings in data mining and ensuring privacy, security, and integrity of data
- Environmental and biological issues in data mining
- Complex data mining and sequential data mining (time series data)
- Data mining at higher dimensions
- Multi-agent data mining and distributed data mining
- High-speed data mining
- Development of unified data mining theory
We currently provide full support for all parts of research study, development, investigation, including project planning, technical advice, legitimate scientific data, thesis writing, paper publication, assignments and project planning, internal review, and many other services. As a result, you can contact us for any kind of help with your data mining thesis topics.
Why Work With Us ?
Senior research member, research experience, journal member, book publisher, research ethics, business ethics, valid references, explanations, paper publication, 9 big reasons to select us.
Our Editor-in-Chief has Website Ownership who control and deliver all aspects of PhD Direction to scholars and students and also keep the look to fully manage all our clients.
Our world-class certified experts have 18+years of experience in Research & Development programs (Industrial Research) who absolutely immersed as many scholars as possible in developing strong PhD research projects.
We associated with 200+reputed SCI and SCOPUS indexed journals (SJR ranking) for getting research work to be published in standard journals (Your first-choice journal).
PhDdirection.com is world’s largest book publishing platform that predominantly work subject-wise categories for scholars/students to assist their books writing and takes out into the University Library.
Our researchers provide required research ethics such as Confidentiality & Privacy, Novelty (valuable research), Plagiarism-Free, and Timely Delivery. Our customers have freedom to examine their current specific research activities.
Our organization take into consideration of customer satisfaction, online, offline support and professional works deliver since these are the actual inspiring business factors.
Solid works delivering by young qualified global research team. "References" is the key to evaluating works easier because we carefully assess scholars findings.
Detailed Videos, Readme files, Screenshots are provided for all research projects. We provide Teamviewer support and other online channels for project explanation.
Worthy journal publication is our main thing like IEEE, ACM, Springer, IET, Elsevier, etc. We substantially reduces scholars burden in publication side. We carry scholars from initial submission to final acceptance.
Related Pages
Our benefits, throughout reference, confidential agreement, research no way resale, plagiarism-free, publication guarantee, customize support, fair revisions, business professionalism, domains & tools, we generally use, wireless communication (4g lte, and 5g), ad hoc networks (vanet, manet, etc.), wireless sensor networks, software defined networks, network security, internet of things (mqtt, coap), internet of vehicles, cloud computing, fog computing, edge computing, mobile computing, mobile cloud computing, ubiquitous computing, digital image processing, medical image processing, pattern analysis and machine intelligence, geoscience and remote sensing, big data analytics, data mining, power electronics, web of things, digital forensics, natural language processing, automation systems, artificial intelligence, mininet 2.1.0, matlab (r2018b/r2019a), matlab and simulink, apache hadoop, apache spark mlib, apache mahout, apache flink, apache storm, apache cassandra, pig and hive, rapid miner, support 24/7, call us @ any time, +91 9444829042, [email protected].
Questions ?
Click here to chat with us
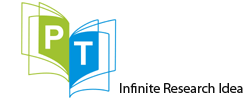
Data Mining Research Topics
Data Mining Research Topics is a service with monumental benefits for any scholars, who aspire to reach the pinnacle of success. Data mining technologies are also offered, which obtains the needed information from a pool of information. We live in a world that recently undergoes a digital revolution. The base and source for the digital world are abundant data. As data is the base of everything, mining research topics becomes a universal field, which also never goes out of style. Our service also extends over a decade of innovation, constant updation, and satisfaction.
We also offer you a service, a product of critical thinking and pioneering ideas of our team of experts and professionals. The mining research topics are your one-stop also for attaining all the information regarding design methodology and fast-growing technologies in the field of data mining. We are also the powerhouse of research topics……..
Mining Research Topics
Data Mining Research Topics is our research package where we offer thousands of research topics for students and research scholars. Scholars always seek perfect guidance also for their project completion. They want to make sure that they came in safe hands when it comes to framing their thesis. We tell you that you can also cease your search for perfect guidance as we offer you the best guidance anyone can ask for.
Grab our hand, and we will lead you straightaway towards your success. If you are also in need of any prior assistance regarding mining research topics, you make use of our online service today and also get all the information you want. Our online service is also available 24X7 to lessen your burden. Here we also offer you the importance of data mining for your reference.
—-“ Data Mining is also defined as the extraction of huge amounts of data, which is previously known yet possesses monumental importance for the current scenario. Discovery of these data does also lead to framing new patterns and trends”. Let’s view our current interest also in data mining,
Platforms We Support
- Ubuntu Linux
- Microsoft Windows XP
- Redhat Enterprise Linux
- Cent OS Linux
- KDnuggets Poll
- Windows Vista
Key Research Application Fields
- Data warehouse
- Domain driven data mining
- Behavior also in informatics
- Bioinformatics
- Predictive analytics
- Business intelligence
- Big data also in analytics
- Decision support also in system
- Drug discovery
- Image Processing
- Text mining
- Named Entity Recognition
- Opinion mining
Major Algorithms as We Use in Data Mining Projects
Decision tree algorithms.
- MARS algorithm
- Conditional decision tree
- Regression and also in Classification tree
- Iterative also in Dicnotomister 3
- C4.5 and C5.0
- Chi-squared automatics also in interaction detection
- Assistant Decision tree learning algorithm
- Hunt’s Algorithm
- SPRINT and also SLIQ Algorithm
Clustering Algorithms
- K-means and also in K-means++
- Hierarchical Clustering
- Expectation maximization
- Spectral and also Canopy Algorithms
- Fuzzy K-means
- Streaming K-Means
Association Rule Learning Algorithms
- Apriori algorithm
- Eclat algorithm
- FP-tree /FP-growth also in algorithm
- DIC algorithm
- H-Mine algorithm
Regularization Algorithms
- Ridge regression
- Least Angle Regression
- Elastic Net
- Least absolute shrinkage and also Selection operations
- Modular Regularization algorithm
- Machine Learning algorithm (supervised and also unsupervised)
Bayesian Algorithms
- Naïve Bayes
- Multinomial Naïve Bayes
- Gaussian waive Bayes
- K-dependence Bayesian Network also in Classifiers
- Hybrid Bayesian algorithm
- Complementary Naïve Bayes
Ensemble Algorithms
- Gradient Boosting Machines
- Gradient Boosted Regression also in trees
- Boot Strapped Aggregation
- Random forest
- Stacked Generalization
- BootStrap Sampling
- Bayesian Averaging
- Error correcting output coding
- Random subspace method
Artificial Neural Network Algorithms
- Hopfield Network
- Radial Basis Function also in Network
- Back Propagation also Neural Network
- Perceptron also in Neural Network
- Convolutional also in Neural Network
- Single layer and also multi-layer perceptron
- SOM (Kohonen’s) algorithm
- Bayesian Regularized also in Neural Network
Dimensionality Reduction Algorithms
- Sammon Mapping
- Discriminant Analysis (LDA, also PDA)
- Multidimensional Scaling
- Projection Pursuit
- Quadratic Discriminant Analysis
- Principal component also in Regression
- Partial Least Square also in Regression
- Mixture Discriminant Analysis
- Singular and also stochastic value decomposition
- Latent Dirichlet allocation
- Lanczos algorithm
Deep Learning Algorithms
- Deep-Convolutional also in Neural Network
- Deep Boltzmann machine
- Stacked Auto-Encodes
- Deep Belief also in Networks
- Deep-Q-Network
- Double Deep-Q-Network
Support for GUI Interfacing and Database
Gui interface:.
- Orange (version 3.3.6)
- Rapid Miner (version – Rapid Miner Studio 7.1)
- Oracle Data miner GUI (version 4.0)
- Weka ( version 3.6.8)
- Rattle GUI (version 2.6.25) [Latest beta Version 5.0.12]
- Matlab based GUI
- KNIME GUI (version R2011b, also R2013a etc.)
Database used:
- Oracle Database RC
- Apache Mahout
- Apache-Spark
- Apache Hadoop
- Cassandra DB
- Amazon Web Services
Prominent Data Mining Research Topics
- Data integrity, privacy and also security issues in data mining
- Mining of Multi-agent data also using data mining Concepts
- For data mining a unifying theory can also be created
- For network setting data mining can be also used
- High dimensional data and high speed data can be also streamed
- Mining of sequence information and also time unbalanced data
- Distributed data mining applications
We also hope that the information provided regarding data mining is adequate also for you to attain firsthand information about data mining. If not satisfied with the given data, you can also contact us directly or seek our online guidance through Mail / Team viewer / Skype. Trust us completely with your project, and also, you will not go disappointed. Ordinary minds will benefit extraordinarily from our service. . . . . . .
Related Pages
Services we offer.
Mathematical proof
Pseudo code
Conference Paper
Research Proposal
System Design
Literature Survey
Data Collection
Thesis Writing
Data Analysis
Rough Draft
Paper Collection
Code and Programs
Paper Writing
Course Work
M.Tech/Ph.D Thesis Help in Chandigarh | Thesis Guidance in Chandigarh
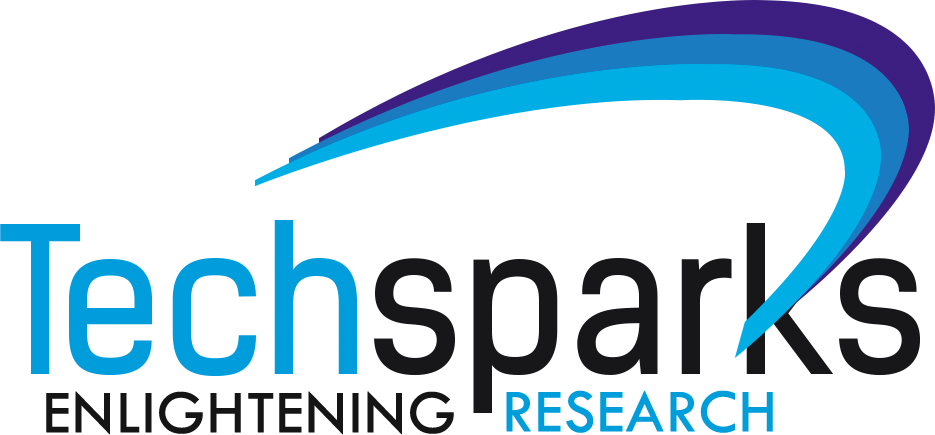

+91-9465330425
Data Mining

Best Data Mining Tools in 2024
What is data mining.
Data mining , also known as Knowledge Discovery in Data (KDD), is a powerful technique that analyzes and unlocks hidden insights from vast amounts of information and datasets. Data mining goes beyond simple analysis—leveraging extensive data processing and complex mathematical algorithms to detect underlying trends or calculate the probability of future events.
What Are Data Mining Tools?
Data mining tools are software that assist users in discovering patterns, trends, and relationships within vast amounts of data. They come in various forms, from simple to complex, catering to different needs.
Why Are Data Mining Tools Important?
Data mining allows businesses to analyze historical data, helping them predict future outcomes, identify risks, and optimize processes. Data mining tools help organizations solve problems, predict trends, mitigate risks, reduce costs, and discover new opportunities. Whether it’s choosing the right marketing strategy, pricing a product, or managing supply chains, data mining impacts businesses in various ways:
- Finance : Banks use predictive models to assess credit risk, detect fraudulent transactions, and optimize investment portfolios. These tools enhance financial stability and customer satisfaction.
- Healthcare : Medical researchers analyze patient data to discover disease patterns, predict outbreaks, and personalize treatment plans. Data mining tools aid early diagnosis, drug discovery, and patient management.
- Marketing : Marketers rely on customer segmentation, recommendation engines, and sentiment analysis. These tools enhance targeted advertising, customer retention, and campaign effectiveness.
- Customer Insights: Data mining tools enable users to analyze customer interactions, preferences, and feedback. This helps them understand customer behavior and pinpoint buying patterns, allowing them to tailor offerings, improve customer experiences, and build brand loyalty.
- Process Optimization: Data mining tools help identify bottlenecks, inefficiencies, and gaps in business processes. Whether it’s supply chain logistics, manufacturing, or service delivery, these tools optimize operations, reduce costs, and enhance productivity.
- Competitive Advantage: Data mining tools help businesses harness data effectively, revealing market trends, competitor strategies, and emerging opportunities.
Top 8 Data Mining Tools
1. apache mahout.
Apache Mahout is a linear algebra framework that supports scalable machine learning and data mining. It offers several algorithms and tools tailored for developing machine learning models capable of processing large datasets.
With its distributed architecture, Apache Mahout allows scalability over machine clusters. It also allows mathematicians and data scientists to create and execute custom algorithms for various machine-learning models.
Key Features:
- Mathematically expressive Scala DSL
- Support for multiple distributed backends (including Apache Spark)
- Integration with Hadoop and Spark
- Scalability
- Algorithm support
- Can handle large datasets.
- Offers fast model training and prediction times.
- Supports a wide range of machine-learning algorithms.
- Integrates with platforms like Hadoop.
- There’s a high learning curve for using Apache Mahout.
Implementing custom machine learning algorithms.
2. MonkeyLearn:
MonkeyLearn is a machine-learning-based text analysis platform. It utilizes artificial intelligence to analyze and understand textual data. Therefore, it can help businesses extract insights from text-based sources such as social media posts, customer reviews, articles, and more.
- Text Mining Specialization
- Custom Machine Learning Models
- Integration Capabilities
- Easy to use and integrate with other platforms.
- Can handle large volumes of data.
- Sometimes the segregation is generic based on the email content and needs more examples to learn.
- Financial category is not easily segregated/tagged.
- Challenging to have Monkey Learn bucket support tickets into distinct user-readable buckets based on ticket text.
Businesses that need to process large volumes of data quickly and easily integrate their data mining models with other platforms.
3. Oracle Data Mining:
Oracle Data Miner is an extension to Oracle SQL Developer for data scientists and analysts. It enables users to leverage Oracle databases for building, evaluating, and comparing machine learning models directly within the database environment.
Oracle Data Miner provides access to advanced algorithms for data mining and machine learning. Users can integrate these algorithms into their SQL queries, allowing efficient model-building and evaluation processes within the familiar Oracle SQL Developer interface.
- Interactive Workflow Tool
- Explore and Graph nodes for visualizing data
- Automated Model Building features
- Integration with RWorks with Big Data SQL
- Seamless integration with the Oracle Database Enterprise Edition.
- Offers a graphical user interface for easy data mining.
- Multiple data mining algorithms and techniques are available.
- Requires more technical knowledge to use effectively.
- Microsoft Excel is required to decrypt data.
- Integration failures can occur due to complexity in the system across other platforms.
- Dependence on Oracle Database.
Businesses that require a wide range of data mining algorithms and techniques and are working directly with data inside Oracle databases.
Sisense is a data analytics platform emphasizing flexibility in handling diverse data architectures. It offers the ability to connect with various data sources, which benefits businesses with complex data structures.
The data mining platform offers features such as data preparation, exploration, and the creation of machine learning models, all aimed at optimizing performance and quality.
- Ad-hoc Analysis
- Centralized Data Hub
- Data Connectors
- Scalable Data Handling
- Interactive Dashboards
- Limited to certain types of models (e.g., classification, regression, and clustering).
- May not be suitable for businesses with complex data mining needs.
Businesses that require a user-friendly interface for creating and deploying predictive models.
5. SAS Enterprise Miner
SAS Enterprise Miner is a data mining tool offering offers various predictive modeling, data mining, and analytics capabilities. The data mining tool provides users access to various statistical, data mining, and machine learning algorithms.
- Interactive GUI and batch processing
- Data preparation and exploration
- Model building and evaluation
- Multithreaded high-performance procedures
- Self-sufficiency for business users
- Users expressed their dissatisfaction with the software’s interface.
- Several users have found the software difficult to learn.
KNIME is an open-source analytics platform. It’s notable for its adaptable and modular design. It equips users with the capability to conduct extensive data transformations, explorations, and analyses, all facilitated by a user-friendly graphical interface.
Knime’s modular structure allows for the straightforward assembly and personalization of data workflows. It also connects to an array of pre-designed nodes and components.
- Drag-and-drop workflow creation
- Integration with R
- Open-source nature
- Customizable workflows
- Community support
- Accessible and customizable due to its open-source nature.
- Some users have reported issues integrating Knime with specific platforms, such as Jupyter notebooks.
Businesses that require robust data analytics capabilities without the complexity of more intricate data mining systems.
Orange is an open-source tool for data mining, visualization, and analysis, crafted to support exploratory tasks and interactive visualizations.
The tool comes equipped with an extensive array of visualization instruments and widgets, enabling the examination and analysis of various datasets.
- Visual programming
- Machine learning widgets
- Customizable machine learning models
- Pre-trained classifiers and extractors
- No coding required
- Versatility
- Offers various machine learning algorithms.
- Integrates with platforms like Python.
- Manual Troubleshooting.
- Advance analysis is not so easy.
- Support isn’t always reliable.
- A high learning curve.
Businesses that need to visually program custom machine learning models.
8. RapidMiner
RapidMiner is an open-source platform widely recognized in the field of data science. It offers several tools that help in various stages of the data analysis process, including data mining, text mining, and predictive analytics. The data mining tool is designed to assist users in extracting insights from data.
- Distributed Algebraic optimizer
- R-Like DSL Scala API
- linear algebra operations
- Text analysis and sentiment detection
- No coding skills needed
- Easy to set up
- Dashboard is clean
- Performance issues with large datasets
- Software stability
- Data output limitations
How to Choose the Right Data Mining Tool
Selecting the appropriate data mining tool can significantly influence the outcomes of data analysis efforts. To assist users in navigating this choice, the following guide outlines the essential considerations for choosing a data mining tool that aligns with their specific needs:
1. Understanding Data Requirements
Before diving into the selection process, users must have a clear understanding of their data:
- Data Types : It’s imperative to ensure that the chosen tool is adept at handling the particular types of data users work with, be it structured or unstructured.
- Data Volume : The tool’s capacity to efficiently process the amount of data users plan to analyze should not be overlooked.
2. Define Your Requirements
Clarifying requirements upfront can streamline the selection process:
- Analytical Needs : Users should pinpoint the types of analysis they aim to conduct, such as predictive modeling, clustering, or regression.
- User Expertise : The tool should correspond to the proficiency level of its users, catering to environments ranging from code-intensive for data scientists to graphical user interfaces for business analysts.
3. Evaluate Tool Capabilities
A thorough evaluation of the tool’s capabilities is crucial:
- Functionality : Seek out tools that boast a comprehensive feature set in line with the analytical tasks users intend to perform.
- Performance : The tool’s capability to manage complex computations and sizable datasets is a key performance indicator.
- Scalability : The chosen tool should accomodate the growth of user data needs and remain relevant as their organization develops.
4. Integration and Compatibility
The tool’s ability to integrate and coexist with existing systems is vital:
- Data Sources : Confirm that the tool offers support for the data sources that users employ.
- Software Ecosystem : The degree to which the tool integrates with other software in the user’s tech stack, such as databases, BI platforms, or cloud services, should be considered.
5. Support and Documentation
The level of support and resources available can greatly affect user experience:
- Vendor Support : Opt for tools that are supported by dependable vendor assistance or a strong user community.
- Documentation and Training : Adequate learning materials and troubleshooting guides are essential for mastering the tool and resolving potential issues.
6. Trial and Testing
Hands-on experience with the tool can provide valuable insights:
- Free Trials : Users are encouraged to utilize free trials or community editions to gauge the data mining tool’s capabilities firsthand.
Weighing these factors can help users choose a data mining tool that satisfies their immediate requirements. It’s important to remember that the most suitable tool is the one that best harmonizes with the users’ data, objectives, and available resources.
The Prerequisite to Data Mining: Astera
Data mining requires meticulous data preparation and processing. This is where Astera , a leading end-to-end data management platform , comes into play.
Astera offers a comprehensive suite of features that swiftly prepares data for analysis. It empowers users to construct end-to-end data pipelines, leveraging sophisticated ETL features and a robust enterprise-grade integration engine.
A key aspect of data preparation is the extraction of large datasets from a variety of data sources. Astera excels in this area, offering automated and bulk extraction from disparate sources, including unstructured sources, databases, data warehouses, cloud data providers, file systems, transfer protocols, web services, and various file formats.
Transformation and conversion capabilities are another crucial component of data preparation. Astera provides users with advanced tools for reformatting data to meet specific analysis requirements or converting data from one format to another, ensuring both flexibility and efficiency.
Data quality is a priority for Astera. It incorporates built-in features for data cleansing and scrubbing, and its rule-based data quality verification ensures the accuracy and integrity of data.
Finally, Astera’s user-centric design simplifies complex tasks. Its intuitive drag-and-drop or single-click operations eliminate the need for extensive coding, significantly boosting productivity and efficiency in data mapping, validation, and cleansing tasks. In essence, Astera provides a comprehensive solution for making data analytics-ready, thereby facilitating efficient data mining.
- AI-Driven Data Management : Streamlines unstructured data extraction, preparation, and data processing through AI and automated workflows.
- Enterprise-Grade Integration Engine : Offers comprehensive tools for integrating diverse data sources and native connectors for easy mapping.
- Interactive, Automated Data Preparation : Ensures data quality using data health monitors, interactive grids, and robust quality checks.
- Advanced Data Transformation : Offers a vast library of transformations for preparing analysis-ready data.
- Dynamic Process Orchestration : Automates data processing tasks, allowing for execution based on time-based schedules or event triggers.
- User-Centric Design : With its no-code, drag-and-drop interface, Astera makes data management accessible to users of all technical backgrounds.
- Seamless Integration : Integrating with a wide array of data sources, both on-premises and cloud-based, ensures a smooth data management experience.
- Comprehensive Data Handling : Offers a unified platform for all data-related tasks, from extraction to insights, backed by a vast library of data operations.
How Astera Enables Robust Data Mining Workflows
Data mining helps organizations extract valuable insights from their data. However, without automated data pipelines, it’s difficult for organizations to ensure the integrity and usefulness of data throughout the analysis process.
Astera empowers organizations to create data pipelines with minimal effort, leveraging automation to streamline the data mining process. Data pipelines play a pivotal role in processing data from disparate sources. They seamlessly integrate data from various origins and transform it into a format that is ready for analysis. This transformation process, which includes data cleaning, normalization, aggregation, and conversion, ensures a consistent and unified view of data.
Furthermore, data pipelines offer the advantage of real-time processing, providing up-to-date information that is crucial for prompt decision-making. Automated data pipelines also save time and resources by reducing manual errors in the extraction, transformation, and loading (ETL) process.
As organizations grow, their data grows correspondingly. Data pipelines, designed to scale, accommodate this growth, ensuring the data infrastructure keeps pace with organizational needs.
Lastly, data pipelines prioritize maintaining high data quality. They ensure data consistency, identify and correct errors, and remove duplicates through built-in features for data cleansing, validation, and verification.
Here’s how Astera achieves this:
- AI-Powered Document Extraction: Astera’s advanced AI technology enables users to capture data fields from unstructured files .
- Data Transformation and Conversion: Users can easily transform and prepare datasets for analysis using built-in transformations.
- Automated Rule-Based Data Quality: Users can ensure data extracted is accurately and reliably through rule-based verification and correction.
- No-Code Data Integration: Allows business users to manage complex data processes with minimal IT intervention, thanks to its no-code platform.
- Automation : With Astera, much of the data pipeline process is automated. Users can extract, transform, validate, and load data seamlessly, which significantly reduces manual effort and the potential for errors.
- Scalability : Astera’s solution is capable of handling growing data volumes and complexity without a drop in performance.
Ready to transform your data mining processes with unparalleled efficiency and ease? Download your free 14-day trial and experience the power of seamless data integration or schedule a personalized demo to see Astera in action.
You MAY ALSO LIKE
What is a business glossary definition, components & benefits.
A solid understanding of internal technical and business terms is essential to manage and use data. Business glossaries are pivotal...
What is Online Transaction Processing (OLTP)?
OLTP is a transaction-centric data processing that follows a three-tier architecture. Every day, businesses worldwide perform millions of financial transactions....
Data Warehouse Testing: Process, Importance & Challenges
The success of data warehouse solutions depends on how well organizations implement test cases to guarantee data integrity. As organizations...
Considering Astera For Your Data Management Needs?
Establish code-free connectivity with your enterprise applications, databases, and cloud applications to integrate all your data.

Videos, Software, Training, etc. Data & Statistics MSHA Data Files NIOSH Mining en Español
Mining Safety and Health Topics News & Articles Mining Links Publications
Mining Program Projects Contracts Strategic Plan Funding Opportunities
About Us Contact NIOSH Mining Employment Visitor Information Technology Innovations Awards Partnerships
- Workplace Safety & Health Topics
- Publications and Products
Exit Notification / Disclaimer Policy
- The Centers for Disease Control and Prevention (CDC) cannot attest to the accuracy of a non-federal website.
- Linking to a non-federal website does not constitute an endorsement by CDC or any of its employees of the sponsors or the information and products presented on the website.
- You will be subject to the destination website's privacy policy when you follow the link.
- CDC is not responsible for Section 508 compliance (accessibility) on other federal or private website.
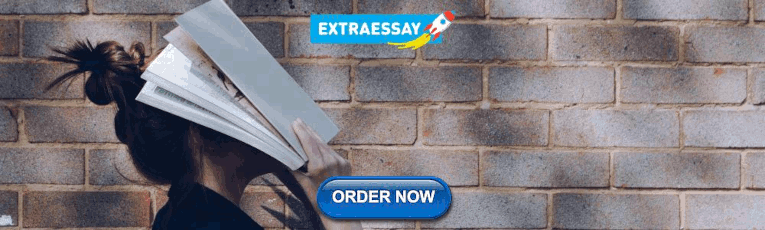
IMAGES
VIDEO
COMMENTS
Commercial Uses of Data Mining. Data mining process entails the use of large relational database to identify the correlation that exists in a given data. The principal role of the applications is to sift the data to identify correlations. A Discussion on the Acceptability of Data Mining.
Data mining is the process of extracting potentially useful information from data sets. It uses a suite of methods to organise, examine and combine large data sets, including machine learning ...
Mar 2024. Nadella Sunil. G. Narsimha. The privacy and security of big data have become a major concern in recent years, necessitating privacy-preserving data mining strategies to preserve the ...
Find the latest published documents for data mining, Related hot topics, top authors, the most cited documents, and related journals. ScienceGate; Advanced Search; Author Search; Journal Finder; Blog; ... This research is aimed to detect the user's topics of interest in social media and rank them based on specific topics, domains, etc. Few ...
Data mining is the procedure of identifying valid, potentially suitable, and understandable information; detecting patterns; building knowledge graphs; and finding anomalies and relationships in big data with Artificial-Intelligence-enabled IoT (AIoT). This process is essential for advancing knowledge in various fields dealing with raw data ...
Identifying and overcoming COVID-19 vaccination impediments using Bayesian data mining techniques. Bowen Lei. , Arvind Mahajan. & Bani Mallick. Article. 10 April 2024 | Open Access.
Data mining research has been significantly motivated by and benefited from real-world applications in novel domains. This special issue was proposed and edited to draw attention to domain-driven data mining and disseminate research in foundations, frameworks, and applications for data-driven and actionable knowledge discovery. Along with this special issue, we also organized a related ...
The research direction related to practical Applications of data mining also shows a tendency to grow. The last two topics, Text Mining and Data Streams have attracted steady interest from ...
Nowadays, big data is a hot topic for data mining and IoT; we also discuss the new characteristics of big data and analyze the challenges in data extracting, data mining algorithms, and data mining system area. Based on the survey of the current research, a suggested big data mining system is proposed.
The goal of this research topic is to bring together theories and applications of efficient deep learning techniques to big-data mining problems. The proposed research theme will focus on efficient deep learning techniques for big data mining. The topics of interest include but are not limited to the following areas: • Neural Network Pruning.
Data mining is an interdisciplinary research area spanning severals disciplines such as database systems, machine learning, intelligent information systems, statistics, and expert systems.
John S Kimball. Nitesh V Chawla. Murat Kantarcioglu. Elena Ferrari. Dongwon Lee. Jean-Roch Vlimant. 19,637 views. 4 articles. Part of an innovative multidisciplinary journal, exploring a wide range of topics, such as intelligent data management, information retrieval, privacy-preserving data mining, and data visual analyt...
Therefore, data mining has unique advantages in clinical big-data research, especially in large-scale medical public databases. This article introduced the main medical public database and described the steps, tasks, and models of data mining in simple language. Additionally, we described data-mining methods along with their practical applications.
A List Of Potential Research Topics In Data Mining: Assessing the impact of data mining in UK law enforcement for crime prevention. Analyzing the use of data mining in predicting and mitigating the impact of Brexit on UK businesses. A review of data mining in personalized education and adaptive learning systems.
This Research Topic on "Medical Data Mining and Medical Intelligence Services" is dedicated to exploring the multifaceted landscape where advanced data mining techniques meet the evolving needs of modern healthcare. This Research Topic serves as a platform to unite researchers, healthcare practitioners, data scientists, and industry experts to ...
Data mining research has led to the development of useful techniques for analyzing time series data, including dynamic time warping [10] and Discrete Fourier Transforms (DFT) in combination with spatial queries [ 5 ]. To date, this work has paid little attention to query specification or interactive systems.
The main research objective of this article is to study how data mining methodologies are applied by researchers and practitioners. To this end, we use systematic literature review (SLR) as scientific method for two reasons. Firstly, systematic review is based on trustworthy, rigorous, and auditable methodology.
What is data mining? Data mining, also known as knowledge discovery in data (KDD), is the process of uncovering patterns and other valuable information from large data sets. Given the evolution of data warehousing technology and the growth of big data, adoption of data mining techniques has rapidly accelerated over the last couple of decades ...
Explore 100+ world-class research topics on data mining with creative and innovative ideas from 120+ branches. Learn about the latest trends, new machine learning techniques, and real-time applications of data mining in various domains and domains.
Integration of MapReduce, Amazon EC2, S3, Apache Spark, and Hadoop into data mining. These are the recent trends in data mining. We insist that you choose one of the topics that interest you the most. Having an appropriate content structure or template is essential while writing a thesis.
Data Mining Research Topics Data Mining Research Topics is a service with monumental benefits for any scholars, who aspire to reach the pinnacle of success. Data mining technologies are also offered, which obtains the needed information from a pool of information. We live in a world that recently undergoes a digital revolution.
Topics to study in data mining. Data mining is a relatively new thing and many are not aware of this technology. This can also be a good topic for M.Tech thesis and for presentations. Following are the topics under data mining to study: Fraud Detection. Crime Rate Prediction.
The goal of this Research Topic is to present the latest research regarding reliable innovative solutions that are applied to healthcare to enhance the quality of life, as well as related issues and challenges. ... • Recent advancements of machine learning and/or data mining methods to facilitate medical informatics and health data analytics ...
7. Orange. Orange is an open-source tool for data mining, visualization, and analysis, crafted to support exploratory tasks and interactive visualizations. The tool comes equipped with an extensive array of visualization instruments and widgets, enabling the examination and analysis of various datasets.
Use EXAMiner to practice and teach hazard recognition skills for mining operations in any sector. Browse the Mining site by subject. Tools You Can Use. Videos, Software, Training, etc. Data & Statistics MSHA Data Files NIOSH Mining en Español. Information Resources. Mining Safety and Health Topics