- How it works
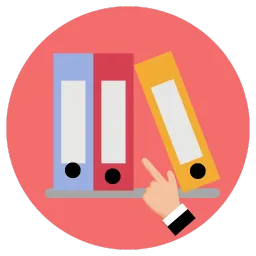
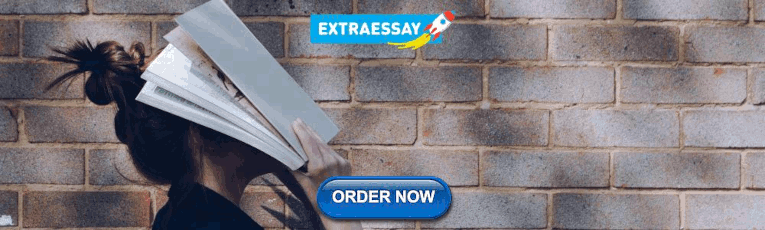
Reliability and Validity – Definitions, Types & Examples
Published by Alvin Nicolas at August 16th, 2021 , Revised On October 26, 2023
A researcher must test the collected data before making any conclusion. Every research design needs to be concerned with reliability and validity to measure the quality of the research.
What is Reliability?
Reliability refers to the consistency of the measurement. Reliability shows how trustworthy is the score of the test. If the collected data shows the same results after being tested using various methods and sample groups, the information is reliable. If your method has reliability, the results will be valid.
Example: If you weigh yourself on a weighing scale throughout the day, you’ll get the same results. These are considered reliable results obtained through repeated measures.
Example: If a teacher conducts the same math test of students and repeats it next week with the same questions. If she gets the same score, then the reliability of the test is high.
What is the Validity?
Validity refers to the accuracy of the measurement. Validity shows how a specific test is suitable for a particular situation. If the results are accurate according to the researcher’s situation, explanation, and prediction, then the research is valid.
If the method of measuring is accurate, then it’ll produce accurate results. If a method is reliable, then it’s valid. In contrast, if a method is not reliable, it’s not valid.
Example: Your weighing scale shows different results each time you weigh yourself within a day even after handling it carefully, and weighing before and after meals. Your weighing machine might be malfunctioning. It means your method had low reliability. Hence you are getting inaccurate or inconsistent results that are not valid.
Example: Suppose a questionnaire is distributed among a group of people to check the quality of a skincare product and repeated the same questionnaire with many groups. If you get the same response from various participants, it means the validity of the questionnaire and product is high as it has high reliability.
Most of the time, validity is difficult to measure even though the process of measurement is reliable. It isn’t easy to interpret the real situation.
Example: If the weighing scale shows the same result, let’s say 70 kg each time, even if your actual weight is 55 kg, then it means the weighing scale is malfunctioning. However, it was showing consistent results, but it cannot be considered as reliable. It means the method has low reliability.
Internal Vs. External Validity
One of the key features of randomised designs is that they have significantly high internal and external validity.
Internal validity is the ability to draw a causal link between your treatment and the dependent variable of interest. It means the observed changes should be due to the experiment conducted, and any external factor should not influence the variables .
Example: age, level, height, and grade.
External validity is the ability to identify and generalise your study outcomes to the population at large. The relationship between the study’s situation and the situations outside the study is considered external validity.
Also, read about Inductive vs Deductive reasoning in this article.
Looking for reliable dissertation support?
We hear you.
- Whether you want a full dissertation written or need help forming a dissertation proposal, we can help you with both.
- Get different dissertation services at ResearchProspect and score amazing grades!
Threats to Interval Validity
Threats of external validity, how to assess reliability and validity.
Reliability can be measured by comparing the consistency of the procedure and its results. There are various methods to measure validity and reliability. Reliability can be measured through various statistical methods depending on the types of validity, as explained below:
Types of Reliability
Types of validity.
As we discussed above, the reliability of the measurement alone cannot determine its validity. Validity is difficult to be measured even if the method is reliable. The following type of tests is conducted for measuring validity.
Does your Research Methodology Have the Following?
- Great Research/Sources
- Perfect Language
- Accurate Sources
If not, we can help. Our panel of experts makes sure to keep the 3 pillars of Research Methodology strong.
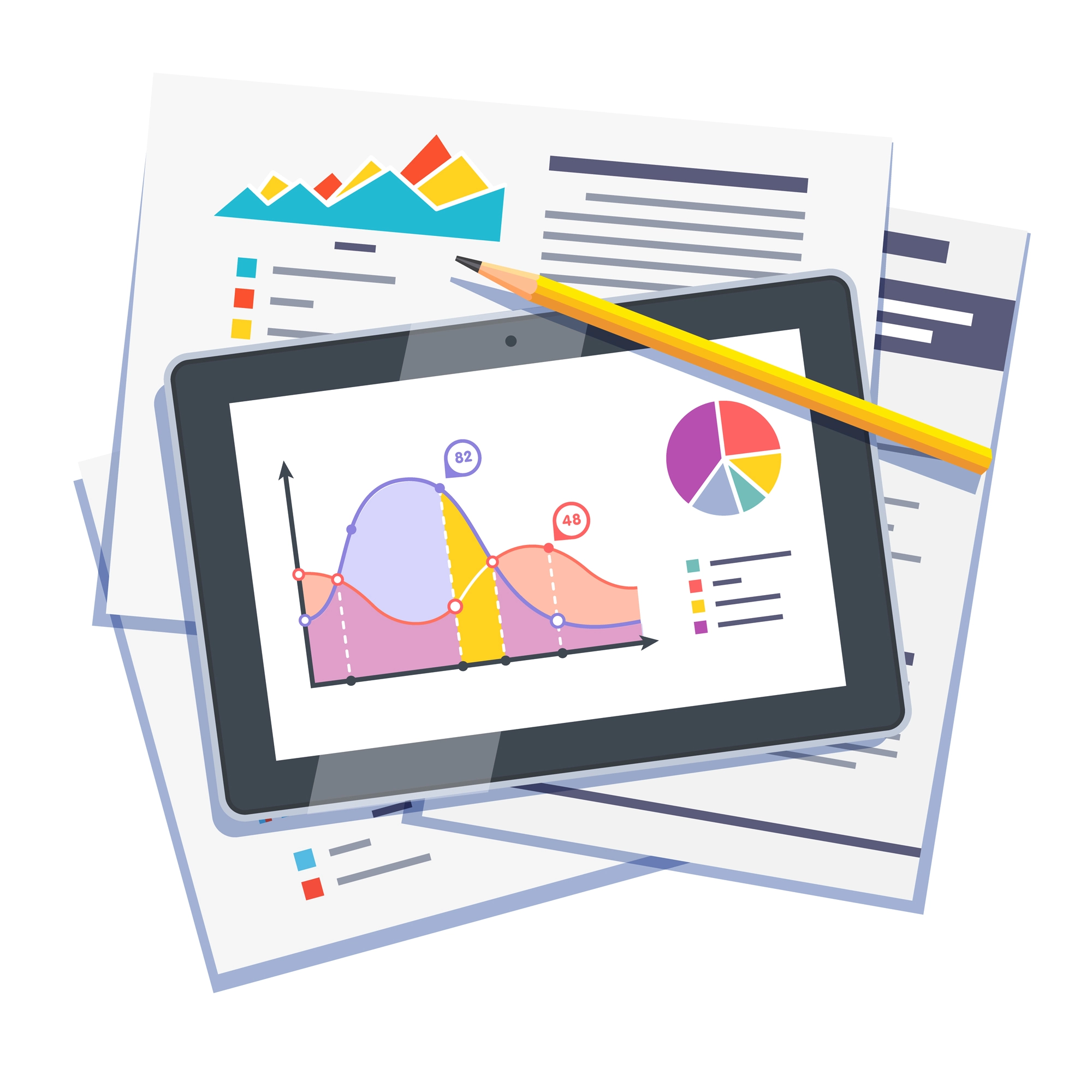
How to Increase Reliability?
- Use an appropriate questionnaire to measure the competency level.
- Ensure a consistent environment for participants
- Make the participants familiar with the criteria of assessment.
- Train the participants appropriately.
- Analyse the research items regularly to avoid poor performance.
How to Increase Validity?
Ensuring Validity is also not an easy job. A proper functioning method to ensure validity is given below:
- The reactivity should be minimised at the first concern.
- The Hawthorne effect should be reduced.
- The respondents should be motivated.
- The intervals between the pre-test and post-test should not be lengthy.
- Dropout rates should be avoided.
- The inter-rater reliability should be ensured.
- Control and experimental groups should be matched with each other.
How to Implement Reliability and Validity in your Thesis?
According to the experts, it is helpful if to implement the concept of reliability and Validity. Especially, in the thesis and the dissertation, these concepts are adopted much. The method for implementation given below:
Frequently Asked Questions
What is reliability and validity in research.
Reliability in research refers to the consistency and stability of measurements or findings. Validity relates to the accuracy and truthfulness of results, measuring what the study intends to. Both are crucial for trustworthy and credible research outcomes.
What is validity?
Validity in research refers to the extent to which a study accurately measures what it intends to measure. It ensures that the results are truly representative of the phenomena under investigation. Without validity, research findings may be irrelevant, misleading, or incorrect, limiting their applicability and credibility.
What is reliability?
Reliability in research refers to the consistency and stability of measurements over time. If a study is reliable, repeating the experiment or test under the same conditions should produce similar results. Without reliability, findings become unpredictable and lack dependability, potentially undermining the study’s credibility and generalisability.
What is reliability in psychology?
In psychology, reliability refers to the consistency of a measurement tool or test. A reliable psychological assessment produces stable and consistent results across different times, situations, or raters. It ensures that an instrument’s scores are not due to random error, making the findings dependable and reproducible in similar conditions.
What is test retest reliability?
Test-retest reliability assesses the consistency of measurements taken by a test over time. It involves administering the same test to the same participants at two different points in time and comparing the results. A high correlation between the scores indicates that the test produces stable and consistent results over time.
How to improve reliability of an experiment?
- Standardise procedures and instructions.
- Use consistent and precise measurement tools.
- Train observers or raters to reduce subjective judgments.
- Increase sample size to reduce random errors.
- Conduct pilot studies to refine methods.
- Repeat measurements or use multiple methods.
- Address potential sources of variability.
What is the difference between reliability and validity?
Reliability refers to the consistency and repeatability of measurements, ensuring results are stable over time. Validity indicates how well an instrument measures what it’s intended to measure, ensuring accuracy and relevance. While a test can be reliable without being valid, a valid test must inherently be reliable. Both are essential for credible research.
Are interviews reliable and valid?
Interviews can be both reliable and valid, but they are susceptible to biases. The reliability and validity depend on the design, structure, and execution of the interview. Structured interviews with standardised questions improve reliability. Validity is enhanced when questions accurately capture the intended construct and when interviewer biases are minimised.
Are IQ tests valid and reliable?
IQ tests are generally considered reliable, producing consistent scores over time. Their validity, however, is a subject of debate. While they effectively measure certain cognitive skills, whether they capture the entirety of “intelligence” or predict success in all life areas is contested. Cultural bias and over-reliance on tests are also concerns.
Are questionnaires reliable and valid?
Questionnaires can be both reliable and valid if well-designed. Reliability is achieved when they produce consistent results over time or across similar populations. Validity is ensured when questions accurately measure the intended construct. However, factors like poorly phrased questions, respondent bias, and lack of standardisation can compromise their reliability and validity.
You May Also Like
Inductive and deductive reasoning takes into account assumptions and incidents. Here is all you need to know about inductive vs deductive reasoning.
This article provides the key advantages of primary research over secondary research so you can make an informed decision.
You can transcribe an interview by converting a conversation into a written format including question-answer recording sessions between two or more people.
USEFUL LINKS
LEARNING RESOURCES
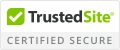
COMPANY DETAILS
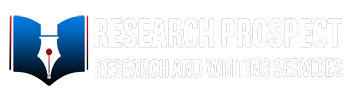
- How It Works
- Privacy Policy

Home » Reliability – Types, Examples and Guide
Reliability – Types, Examples and Guide
Table of Contents

Reliability
Definition:
Reliability refers to the consistency, dependability, and trustworthiness of a system, process, or measurement to perform its intended function or produce consistent results over time. It is a desirable characteristic in various domains, including engineering, manufacturing, software development, and data analysis.
Reliability In Engineering
In engineering and manufacturing, reliability refers to the ability of a product, equipment, or system to function without failure or breakdown under normal operating conditions for a specified period. A reliable system consistently performs its intended functions, meets performance requirements, and withstands various environmental factors, stress, or wear and tear.
Reliability In Software Development
In software development, reliability relates to the stability and consistency of software applications or systems. A reliable software program operates consistently without crashing, produces accurate results, and handles errors or exceptions gracefully. Reliability is often measured by metrics such as mean time between failures (MTBF) and mean time to repair (MTTR).
Reliability In Data Analysis and Statistics
In data analysis and statistics, reliability refers to the consistency and repeatability of measurements or assessments. For example, if a measurement instrument consistently produces similar results when measuring the same quantity or if multiple raters consistently agree on the same assessment, it is considered reliable. Reliability is often assessed using statistical measures such as test-retest reliability, inter-rater reliability, or internal consistency.
Research Reliability
Research reliability refers to the consistency, stability, and repeatability of research findings . It indicates the extent to which a research study produces consistent and dependable results when conducted under similar conditions. In other words, research reliability assesses whether the same results would be obtained if the study were replicated with the same methodology, sample, and context.
What Affects Reliability in Research
Several factors can affect the reliability of research measurements and assessments. Here are some common factors that can impact reliability:
Measurement Error
Measurement error refers to the variability or inconsistency in the measurements that is not due to the construct being measured. It can arise from various sources, such as the limitations of the measurement instrument, environmental factors, or the characteristics of the participants. Measurement error reduces the reliability of the measure by introducing random variability into the data.
Rater/Observer Bias
In studies involving subjective assessments or ratings, the biases or subjective judgments of the raters or observers can affect reliability. If different raters interpret and evaluate the same phenomenon differently, it can lead to inconsistencies in the ratings, resulting in lower inter-rater reliability.
Participant Factors
Characteristics or factors related to the participants themselves can influence reliability. For example, factors such as fatigue, motivation, attention, or mood can introduce variability in responses, affecting the reliability of self-report measures or performance assessments.
Instrumentation
The quality and characteristics of the measurement instrument can impact reliability. If the instrument lacks clarity, has ambiguous items or instructions, or is prone to measurement errors, it can decrease the reliability of the measure. Poorly designed or unreliable instruments can introduce measurement error and decrease the consistency of the measurements.
Sample Size
Sample size can affect reliability, especially in studies where the reliability coefficient is based on correlations or variability within the sample. A larger sample size generally provides more stable estimates of reliability, while smaller samples can yield less precise estimates.
Time Interval
The time interval between test administrations can impact test-retest reliability. If the time interval is too short, participants may recall their previous responses and answer in a similar manner, artificially inflating the reliability coefficient. On the other hand, if the time interval is too long, true changes in the construct being measured may occur, leading to lower test-retest reliability.
Content Sampling
The specific items or questions included in a measure can affect reliability. If the measure does not adequately sample the full range of the construct being measured or if the items are too similar or redundant, it can result in lower internal consistency reliability.
Scoring and Data Handling
Errors in scoring, data entry, or data handling can introduce variability and impact reliability. Inaccurate or inconsistent scoring procedures, data entry mistakes, or mishandling of missing data can affect the reliability of the measurements.
Context and Environment
The context and environment in which measurements are obtained can influence reliability. Factors such as noise, distractions, lighting conditions, or the presence of others can introduce variability and affect the consistency of the measurements.
Types of Reliability
There are several types of reliability that are commonly discussed in research and measurement contexts. Here are some of the main types of reliability:
Test-Retest Reliability
This type of reliability assesses the consistency of a measure over time. It involves administering the same test or measure to the same group of individuals on two separate occasions and then comparing the results. If the scores are similar or highly correlated across the two testing points, it indicates good test-retest reliability.
Inter-Rater Reliability
Inter-rater reliability examines the degree of agreement or consistency between different raters or observers who are assessing the same phenomenon. It is commonly used in subjective evaluations or assessments where judgments are made by multiple individuals. High inter-rater reliability suggests that different observers are likely to reach the same conclusions or make consistent assessments.
Internal Consistency Reliability
Internal consistency reliability assesses the extent to which the items or questions within a measure are consistent with each other. It is commonly measured using techniques such as Cronbach’s alpha. High internal consistency reliability indicates that the items within a measure are measuring the same construct or concept consistently.
Parallel Forms Reliability
Parallel forms reliability assesses the consistency of different versions or forms of a test that are intended to measure the same construct. Two equivalent versions of a test are administered to the same group of individuals, and the scores are compared to determine the level of agreement between the forms.
Split-Half Reliability
Split-half reliability involves splitting a measure into two halves and examining the consistency between the two halves. It can be done by dividing the items into odd-even pairs or by randomly splitting the items. The scores from the two halves are then compared to assess the degree of consistency.
Alternate Forms Reliability
Alternate forms reliability is similar to parallel forms reliability, but it involves administering two different versions of a test to the same group of individuals. The two forms should be equivalent and measure the same construct. The scores from the two forms are then compared to assess the level of agreement.
Applications of Reliability
Reliability has several important applications across various fields and disciplines. Here are some common applications of reliability:
Psychological and Educational Testing
Reliability is crucial in psychological and educational testing to ensure that the scores obtained from assessments are consistent and dependable. It helps to determine the accuracy and stability of measures such as intelligence tests, personality assessments, academic exams, and aptitude tests.
Market Research
In market research, reliability is important for ensuring consistent and dependable data collection. Surveys, questionnaires, and other data collection instruments need to have high reliability to obtain accurate and consistent responses from participants. Reliability analysis helps researchers identify and address any issues that may affect the consistency of the data.
Health and Medical Research
Reliability is essential in health and medical research to ensure that measurements and assessments used in studies are consistent and trustworthy. This includes the reliability of diagnostic tests, patient-reported outcome measures, observational measures, and psychometric scales. High reliability is crucial for making valid inferences and drawing reliable conclusions from research findings.
Quality Control and Manufacturing
Reliability analysis is widely used in industries such as manufacturing and quality control to assess the reliability of products and processes. It helps to identify and address sources of variation and inconsistency, ensuring that products meet the required standards and specifications consistently.
Social Science Research
Reliability plays a vital role in social science research, including fields such as sociology, anthropology, and political science. It is used to assess the consistency of measurement tools, such as surveys or observational protocols, to ensure that the data collected is reliable and can be trusted for analysis and interpretation.
Performance Evaluation
Reliability is important in performance evaluation systems used in organizations and workplaces. Whether it’s assessing employee performance, evaluating the reliability of scoring rubrics, or measuring the consistency of ratings by supervisors, reliability analysis helps ensure fairness and consistency in the evaluation process.
Psychometrics and Scale Development
Reliability analysis is a fundamental step in psychometrics, which involves developing and validating measurement scales. Researchers assess the reliability of items and subscales to ensure that the scale measures the intended construct consistently and accurately.
Examples of Reliability
Here are some examples of reliability in different contexts:
Test-Retest Reliability Example: A researcher administers a personality questionnaire to a group of participants and then administers the same questionnaire to the same participants after a certain period, such as two weeks. The scores obtained from the two administrations are highly correlated, indicating good test-retest reliability.
Inter-Rater Reliability Example: Multiple teachers assess the essays of a group of students using a standardized grading rubric. The ratings assigned by the teachers show a high level of agreement or correlation, indicating good inter-rater reliability.
Internal Consistency Reliability Example: A researcher develops a questionnaire to measure job satisfaction. The researcher administers the questionnaire to a group of employees and calculates Cronbach’s alpha to assess internal consistency. The calculated value of Cronbach’s alpha is high (e.g., above 0.8), indicating good internal consistency reliability.
Parallel Forms Reliability Example: Two versions of a mathematics exam are created, which are designed to measure the same mathematical skills. Both versions of the exam are administered to the same group of students, and the scores from the two versions are highly correlated, indicating good parallel forms reliability.
Split-Half Reliability Example: A researcher develops a survey to measure self-esteem. The survey consists of 20 items, and the researcher randomly divides the items into two halves. The scores obtained from each half of the survey show a high level of agreement or correlation, indicating good split-half reliability.
Alternate Forms Reliability Example: A researcher develops two versions of a language proficiency test, which are designed to measure the same language skills. Both versions of the test are administered to the same group of participants, and the scores from the two versions are highly correlated, indicating good alternate forms reliability.
Where to Write About Reliability in A Thesis
When writing about reliability in a thesis, there are several sections where you can address this topic. Here are some common sections in a thesis where you can discuss reliability:
Introduction :
In the introduction section of your thesis, you can provide an overview of the study and briefly introduce the concept of reliability. Explain why reliability is important in your research field and how it relates to your study objectives.
Theoretical Framework:
If your thesis includes a theoretical framework or a literature review, this is a suitable section to discuss reliability. Provide an overview of the relevant theories, models, or concepts related to reliability in your field. Discuss how other researchers have measured and assessed reliability in similar studies.
Methodology:
The methodology section is crucial for addressing reliability. Describe the research design, data collection methods, and measurement instruments used in your study. Explain how you ensured the reliability of your measurements or data collection procedures. This may involve discussing pilot studies, inter-rater reliability, test-retest reliability, or other techniques used to assess and improve reliability.
Data Analysis:
In the data analysis section, you can discuss the statistical techniques employed to assess the reliability of your data. This might include measures such as Cronbach’s alpha, Cohen’s kappa, or intraclass correlation coefficients (ICC), depending on the nature of your data and research design. Present the results of reliability analyses and interpret their implications for your study.
Discussion:
In the discussion section, analyze and interpret the reliability results in relation to your research findings and objectives. Discuss any limitations or challenges encountered in establishing or maintaining reliability in your study. Consider the implications of reliability for the validity and generalizability of your results.
Conclusion:
In the conclusion section, summarize the main points discussed in your thesis regarding reliability. Emphasize the importance of reliability in research and highlight any recommendations or suggestions for future studies to enhance reliability.
Importance of Reliability
Reliability is of utmost importance in research, measurement, and various practical applications. Here are some key reasons why reliability is important:
- Consistency : Reliability ensures consistency in measurements and assessments. Consistent results indicate that the measure or instrument is stable and produces similar outcomes when applied repeatedly. This consistency allows researchers and practitioners to have confidence in the reliability of the data collected and the conclusions drawn from it.
- Accuracy : Reliability is closely linked to accuracy. A reliable measure produces results that are close to the true value or state of the phenomenon being measured. When a measure is unreliable, it introduces error and uncertainty into the data, which can lead to incorrect interpretations and flawed decision-making.
- Trustworthiness : Reliability enhances the trustworthiness of measurements and assessments. When a measure is reliable, it indicates that it is dependable and can be trusted to provide consistent and accurate results. This is particularly important in fields where decisions and actions are based on the data collected, such as education, healthcare, and market research.
- Comparability : Reliability enables meaningful comparisons between different groups, individuals, or time points. When measures are reliable, differences or changes observed can be attributed to true differences in the underlying construct, rather than measurement error. This allows for valid comparisons and evaluations, both within a study and across different studies.
- Validity : Reliability is a prerequisite for validity. Validity refers to the extent to which a measure or assessment accurately captures the construct it is intended to measure. If a measure is unreliable, it cannot be valid, as it does not consistently reflect the construct of interest. Establishing reliability is an important step in establishing the validity of a measure.
- Decision-making : Reliability is crucial for making informed decisions based on data. Whether it’s evaluating employee performance, diagnosing medical conditions, or conducting research studies, reliable measurements and assessments provide a solid foundation for decision-making processes. They help to reduce uncertainty and increase confidence in the conclusions drawn from the data.
- Quality Assurance : Reliability is essential for maintaining quality assurance in various fields. It allows organizations to assess and monitor the consistency and dependability of their processes, products, and services. By ensuring reliability, organizations can identify areas of improvement, address sources of variation, and deliver consistent and high-quality outcomes.
Limitations of Reliability
Here are some limitations of reliability:
- Limited to consistency: Reliability primarily focuses on the consistency of measurements and findings. However, it does not guarantee the accuracy or validity of the measurements. A measurement can be consistent but still systematically biased or flawed, leading to inaccurate results. Reliability alone cannot address validity concerns.
- Context-dependent: Reliability can be influenced by the specific context, conditions, or population under study. A measurement or instrument that demonstrates high reliability in one context may not necessarily exhibit the same level of reliability in a different context. Researchers need to consider the specific characteristics and limitations of their study context when interpreting reliability.
- Inadequate for complex constructs: Reliability is often based on the assumption of unidimensionality, which means that a measurement instrument is designed to capture a single construct. However, many real-world phenomena are complex and multidimensional, making it challenging to assess reliability accurately. Reliability measures may not adequately capture the full complexity of such constructs.
- Susceptible to systematic errors: Reliability focuses on minimizing random errors, but it may not detect or address systematic errors or biases in measurements. Systematic errors can arise from flaws in the measurement instrument, data collection procedures, or sample selection. Reliability assessments may not fully capture or address these systematic errors, leading to biased or inaccurate results.
- Relies on assumptions: Reliability assessments often rely on certain assumptions, such as the assumption of measurement invariance or the assumption of stable conditions over time. These assumptions may not always hold true in real-world research settings, particularly when studying dynamic or evolving phenomena. Failure to meet these assumptions can compromise the reliability of the research.
- Limited to quantitative measures: Reliability is typically applied to quantitative measures and instruments, which can be problematic when studying qualitative or subjective phenomena. Reliability measures may not fully capture the richness and complexity of qualitative data, limiting their applicability in certain research domains.
Also see Reliability Vs Validity
About the author
Muhammad Hassan
Researcher, Academic Writer, Web developer
You may also like

Validity – Types, Examples and Guide

Alternate Forms Reliability – Methods, Examples...

Construct Validity – Types, Threats and Examples

Internal Validity – Threats, Examples and Guide

Reliability Vs Validity

Internal Consistency Reliability – Methods...
Have a language expert improve your writing
Run a free plagiarism check in 10 minutes, automatically generate references for free.
- Knowledge Base
- Methodology
- The 4 Types of Reliability in Research | Definitions & Examples
The 4 Types of Reliability in Research | Definitions & Examples
Published on 3 May 2022 by Fiona Middleton . Revised on 26 August 2022.
Reliability tells you how consistently a method measures something. When you apply the same method to the same sample under the same conditions, you should get the same results. If not, the method of measurement may be unreliable.
There are four main types of reliability. Each can be estimated by comparing different sets of results produced by the same method.
Table of contents
Test-retest reliability, interrater reliability, parallel forms reliability, internal consistency, which type of reliability applies to my research.
Test-retest reliability measures the consistency of results when you repeat the same test on the same sample at a different point in time. You use it when you are measuring something that you expect to stay constant in your sample.
Why test-retest reliability is important
Many factors can influence your results at different points in time: for example, respondents might experience different moods, or external conditions might affect their ability to respond accurately.
Test-retest reliability can be used to assess how well a method resists these factors over time. The smaller the difference between the two sets of results, the higher the test-retest reliability.
How to measure test-retest reliability
To measure test-retest reliability, you conduct the same test on the same group of people at two different points in time. Then you calculate the correlation between the two sets of results.
Improving test-retest reliability
- When designing tests or questionnaires , try to formulate questions, statements, and tasks in a way that won’t be influenced by the mood or concentration of participants.
- When planning your methods of data collection , try to minimise the influence of external factors, and make sure all samples are tested under the same conditions.
- Remember that changes can be expected to occur in the participants over time, and take these into account.
Prevent plagiarism, run a free check.
Inter-rater reliability (also called inter-observer reliability) measures the degree of agreement between different people observing or assessing the same thing. You use it when data is collected by researchers assigning ratings, scores or categories to one or more variables .
Why inter-rater reliability is important
People are subjective, so different observers’ perceptions of situations and phenomena naturally differ. Reliable research aims to minimise subjectivity as much as possible so that a different researcher could replicate the same results.
When designing the scale and criteria for data collection, it’s important to make sure that different people will rate the same variable consistently with minimal bias. This is especially important when there are multiple researchers involved in data collection or analysis.
How to measure inter-rater reliability
To measure inter-rater reliability, different researchers conduct the same measurement or observation on the same sample. Then you calculate the correlation between their different sets of results. If all the researchers give similar ratings, the test has high inter-rater reliability.
Improving inter-rater reliability
- Clearly define your variables and the methods that will be used to measure them.
- Develop detailed, objective criteria for how the variables will be rated, counted, or categorised.
- If multiple researchers are involved, ensure that they all have exactly the same information and training.
Parallel forms reliability measures the correlation between two equivalent versions of a test. You use it when you have two different assessment tools or sets of questions designed to measure the same thing.
Why parallel forms reliability is important
If you want to use multiple different versions of a test (for example, to avoid respondents repeating the same answers from memory), you first need to make sure that all the sets of questions or measurements give reliable results.
How to measure parallel forms reliability
The most common way to measure parallel forms reliability is to produce a large set of questions to evaluate the same thing, then divide these randomly into two question sets.
The same group of respondents answers both sets, and you calculate the correlation between the results. High correlation between the two indicates high parallel forms reliability.
Improving parallel forms reliability
- Ensure that all questions or test items are based on the same theory and formulated to measure the same thing.
Internal consistency assesses the correlation between multiple items in a test that are intended to measure the same construct.
You can calculate internal consistency without repeating the test or involving other researchers, so it’s a good way of assessing reliability when you only have one dataset.
Why internal consistency is important
When you devise a set of questions or ratings that will be combined into an overall score, you have to make sure that all of the items really do reflect the same thing. If responses to different items contradict one another, the test might be unreliable.
How to measure internal consistency
Two common methods are used to measure internal consistency.
- Average inter-item correlation : For a set of measures designed to assess the same construct, you calculate the correlation between the results of all possible pairs of items and then calculate the average.
- Split-half reliability : You randomly split a set of measures into two sets. After testing the entire set on the respondents, you calculate the correlation between the two sets of responses.
Improving internal consistency
- Take care when devising questions or measures: those intended to reflect the same concept should be based on the same theory and carefully formulated.
It’s important to consider reliability when planning your research design , collecting and analysing your data, and writing up your research. The type of reliability you should calculate depends on the type of research and your methodology .
If possible and relevant, you should statistically calculate reliability and state this alongside your results .
Cite this Scribbr article
If you want to cite this source, you can copy and paste the citation or click the ‘Cite this Scribbr article’ button to automatically add the citation to our free Reference Generator.
Middleton, F. (2022, August 26). The 4 Types of Reliability in Research | Definitions & Examples. Scribbr. Retrieved 14 May 2024, from https://www.scribbr.co.uk/research-methods/reliability-explained/
Is this article helpful?
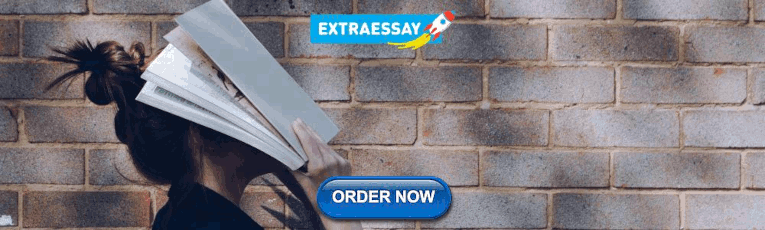
Fiona Middleton
Other students also liked, reliability vs validity in research | differences, types & examples, the 4 types of validity | types, definitions & examples, a quick guide to experimental design | 5 steps & examples.
Reliability In Psychology Research: Definitions & Examples
Saul Mcleod, PhD
Editor-in-Chief for Simply Psychology
BSc (Hons) Psychology, MRes, PhD, University of Manchester
Saul Mcleod, PhD., is a qualified psychology teacher with over 18 years of experience in further and higher education. He has been published in peer-reviewed journals, including the Journal of Clinical Psychology.
Learn about our Editorial Process
Olivia Guy-Evans, MSc
Associate Editor for Simply Psychology
BSc (Hons) Psychology, MSc Psychology of Education
Olivia Guy-Evans is a writer and associate editor for Simply Psychology. She has previously worked in healthcare and educational sectors.
On This Page:
Reliability in psychology research refers to the reproducibility or consistency of measurements. Specifically, it is the degree to which a measurement instrument or procedure yields the same results on repeated trials. A measure is considered reliable if it produces consistent scores across different instances when the underlying thing being measured has not changed.
Reliability ensures that responses are consistent across times and occasions for instruments like questionnaires . Multiple forms of reliability exist, including test-retest, inter-rater, and internal consistency.
For example, if people weigh themselves during the day, they would expect to see a similar reading. Scales that measured weight differently each time would be of little use.
The same analogy could be applied to a tape measure that measures inches differently each time it is used. It would not be considered reliable.
If findings from research are replicated consistently, they are reliable. A correlation coefficient can be used to assess the degree of reliability. If a test is reliable, it should show a high positive correlation.
Of course, it is unlikely the same results will be obtained each time as participants and situations vary. Still, a strong positive correlation between the same test results indicates reliability.
Reliability is important because unreliable measures introduce random error that attenuates correlations and makes it harder to detect real relationships.
Ensuring high reliability for key measures in psychology research helps boost the sensitivity, validity, and replicability of studies. Estimating and reporting reliable evidence is considered an important methodological practice.
There are two types of reliability: internal and external.
- Internal reliability refers to how consistently different items within a single test measure the same concept or construct. It ensures that a test is stable across its components.
- External reliability measures how consistently a test produces similar results over repeated administrations or under different conditions. It ensures that a test is stable over time and situations.
Some key aspects of reliability in psychology research include:
- Test-retest reliability : The consistency of scores for the same person across two or more separate administrations of the same measurement procedure over time. High test-retest reliability suggests the measure provides a stable, reproducible score.
- Interrater reliability : The level of agreement in scores on a measure between different raters or observers rating the same target. High interrater reliability suggests the ratings are objective and not overly influenced by rater subjectivity or bias.
- Internal consistency reliability : The degree to which different test items or parts of an instrument that measure the same construct yield similar results. Analyzed statistically using Cronbach’s alpha, a high value suggests the items measure the same underlying concept.
Test-Retest Reliability
The test-retest method assesses the external consistency of a test. Examples of appropriate tests include questionnaires and psychometric tests. It measures the stability of a test over time.
A typical assessment would involve giving participants the same test on two separate occasions. If the same or similar results are obtained, then external reliability is established.
Here’s how it works:
- A test or measurement is administered to participants at one point in time.
- After a certain period, the same test is administered again to the same participants without any intervention or treatment in between.
- The scores from the two administrations are then correlated using a statistical method, often Pearson’s correlation.
- A high correlation between the scores from the two test administrations indicates good test-retest reliability, suggesting the test yields consistent results over time.
This method is especially useful for tests that measure stable traits or characteristics that aren’t expected to change over short periods.
The disadvantage of the test-retest method is that it takes a long time for results to be obtained. The reliability can be influenced by the time interval between tests and any events that might affect participants’ responses during this interval.
Beck et al. (1996) studied the responses of 26 outpatients on two separate therapy sessions one week apart, they found a correlation of .93 therefore demonstrating high test-restest reliability of the depression inventory.
This is an example of why reliability in psychological research is necessary, if it wasn’t for the reliability of such tests some individuals may not be successfully diagnosed with disorders such as depression and consequently will not be given appropriate therapy.
The timing of the test is important; if the duration is too brief, then participants may recall information from the first test, which could bias the results.
Alternatively, if the duration is too long, it is feasible that the participants could have changed in some important way which could also bias the results.
The test-retest method assesses the external consistency of a test. This refers to the degree to which different raters give consistent estimates of the same behavior. Inter-rater reliability can be used for interviews.
Inter-Rater Reliability
Inter-rater reliability, often termed inter-observer reliability, refers to the extent to which different raters or evaluators agree in assessing a particular phenomenon, behavior, or characteristic. It’s a measure of consistency and agreement between individuals scoring or evaluating the same items or behaviors.
High inter-rater reliability indicates that the findings or measurements are consistent across different raters, suggesting the results are not due to random chance or subjective biases of individual raters.
Statistical measures, such as Cohen’s Kappa or the Intraclass Correlation Coefficient (ICC), are often employed to quantify the level of agreement between raters, helping to ensure that findings are objective and reproducible.
Ensuring high inter-rater reliability is essential, especially in studies involving subjective judgment or observations, as it provides confidence that the findings are replicable and not heavily influenced by individual rater biases.
Note it can also be called inter-observer reliability when referring to observational research. Here, researchers observe the same behavior independently (to avoid bias) and compare their data. If the data is similar, then it is reliable.
Where observer scores do not significantly correlate, then reliability can be improved by:
- Train observers in the observation techniques and ensure everyone agrees with them.
- Ensuring behavior categories have been operationalized. This means that they have been objectively defined.
For example, if two researchers are observing ‘aggressive behavior’ of children at nursery they would both have their own subjective opinion regarding what aggression comprises.
In this scenario, they would be unlikely to record aggressive behavior the same, and the data would be unreliable.
However, if they were to operationalize the behavior category of aggression, this would be more objective and make it easier to identify when a specific behavior occurs.
For example, while “aggressive behavior” is subjective and not operationalized, “pushing” is objective and operationalized. Thus, researchers could count how many times children push each other over a certain duration of time.
Internal Consistency Reliability
Internal consistency reliability refers to how well different items on a test or survey that are intended to measure the same construct produce similar scores.
For example, a questionnaire measuring depression may have multiple questions tapping issues like sadness, changes in sleep and appetite, fatigue, and loss of interest. The assumption is that people’s responses across these different symptom items should be fairly consistent.
Cronbach’s alpha is a common statistic used to quantify internal consistency reliability. It calculates the average inter-item correlations among the test items. Values range from 0 to 1, with higher values indicating greater internal consistency. A good rule of thumb is that alpha should generally be above .70 to suggest adequate reliability.
An alpha of .90 for a depression questionnaire, for example, means there is a high average correlation between respondents’ scores on the different symptom items.
This suggests all the items are measuring the same underlying construct (depression) in a consistent manner. It taps the unidimensionality of the scale – evidence it is measuring one thing.
If some items were unrelated to others, the average inter-item correlations would be lower, resulting in a lower alpha. This would indicate the presence of multiple dimensions in the scale, rather than a unified single concept.
So, in summary, high internal consistency reliability evidenced through high Cronbach’s alpha provides support for the fact that various test items successfully tap into the same latent variable the researcher intends to measure. It suggests the items meaningfully cohere together to reliably measure that construct.
Split-Half Method
The split-half method assesses the internal consistency of a test, such as psychometric tests and questionnaires.
There, it measures the extent to which all parts of the test contribute equally to what is being measured.
The split-half approach provides another method of quantifying internal consistency by taking advantage of the natural variation when a single test is divided in half.
It’s somewhat cumbersome to implement but avoids limitations associated with Cronbach’s alpha. However, alpha remains much more widely used in practice due to its relative ease of calculation.
- A test or questionnaire is split into two halves, typically by separating even-numbered items from odd-numbered items, or first-half items vs. second-half.
- Each half is scored separately, and the scores are correlated using a statistical method, often Pearson’s correlation.
- The correlation between the two halves gives an indication of the test’s reliability. A higher correlation suggests better reliability.
- To adjust for the test’s shortened length (because we’ve split it in half), the Spearman-Brown prophecy formula is often applied to estimate the reliability of the full test based on the split-half reliability.
The reliability of a test could be improved by using this method. For example, any items on separate halves of a test with a low correlation (e.g., r = .25) should either be removed or rewritten.
The split-half method is a quick and easy way to establish reliability. However, it can only be effective with large questionnaires in which all questions measure the same construct. This means it would not be appropriate for tests that measure different constructs.
For example, the Minnesota Multiphasic Personality Inventory has sub scales measuring differently behaviors such as depression, schizophrenia, social introversion. Therefore the split-half method was not be an appropriate method to assess reliability for this personality test.
Validity vs. Reliability In Psychology
In psychology, validity and reliability are fundamental concepts that assess the quality of measurements.
- Validity refers to the degree to which a measure accurately assesses the specific concept, trait, or construct that it claims to be assessing. It refers to the truthfulness of the measure.
- Reliability refers to the overall consistency, stability, and repeatability of a measurement. It is concerned with how much random error might be distorting scores or introducing unwanted “noise” into the data.
A key difference is that validity refers to what’s being measured, while reliability refers to how consistently it’s being measured.
An unreliable measure cannot be truly valid because if a measure gives inconsistent, unpredictable scores, it clearly isn’t measuring the trait or quality it aims to measure in a truthful, systematic manner. Establishing reliability provides the foundation for determining the measure’s validity.
A pivotal understanding is that reliability is a necessary but not sufficient condition for validity.
It means a test can be reliable, consistently producing the same results, without being valid, or accurately measuring the intended attribute.
However, a valid test, one that truly measures what it purports to, must be reliable. In the pursuit of rigorous psychological research, both validity and reliability are indispensable.
Ideally, researchers strive for high scores on both -Validity to make sure you’re measuring the correct construct and reliability to make sure you’re measuring it accurately and precisely. The two qualities are independent but both crucial elements of strong measurement procedures.
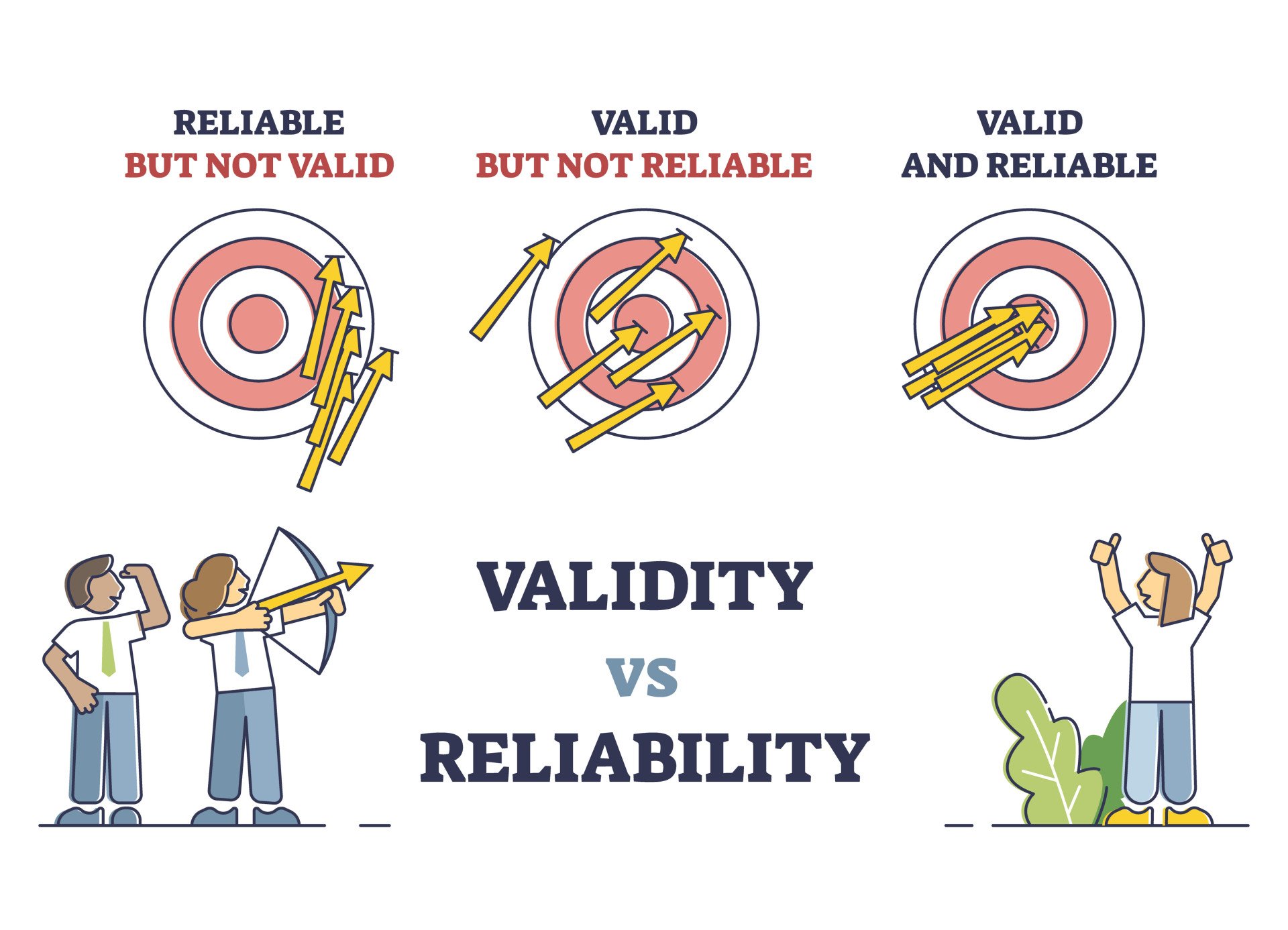
Beck, A. T., Steer, R. A., & Brown, G. K. (1996). Manual for the beck depression inventory The Psychological Corporation. San Antonio , TX.
Clifton, J. D. W. (2020). Managing validity versus reliability trade-offs in scale-building decisions. Psychological Methods, 25 (3), 259–270. https:// doi.org/10.1037/met0000236
Guttman, L. (1945). A basis for analyzing test-retest reliability. Psychometrika, 10 (4), 255–282. https://doi.org/10.1007/BF02288892
Hathaway, S. R., & McKinley, J. C. (1943). Manual for the Minnesota Multiphasic Personality Inventory . New York: Psychological Corporation.
Jannarone, R. J., Macera, C. A., & Garrison, C. Z. (1987). Evaluating interrater agreement through “case-control” sampling. Biometrics, 43 (2), 433–437. https://doi.org/10.2307/2531825
LeBreton, J. M., & Senter, J. L. (2008). Answers to 20 questions about interrater reliability and interrater agreement. Organizational Research Methods, 11 (4), 815–852. https://doi.org/10.1177/1094428106296642
Watkins, M. W., & Pacheco, M. (2000). Interobserver agreement in behavioral research: Importance and calculation. Journal of Behavioral Education, 10 , 205–212

Related Articles
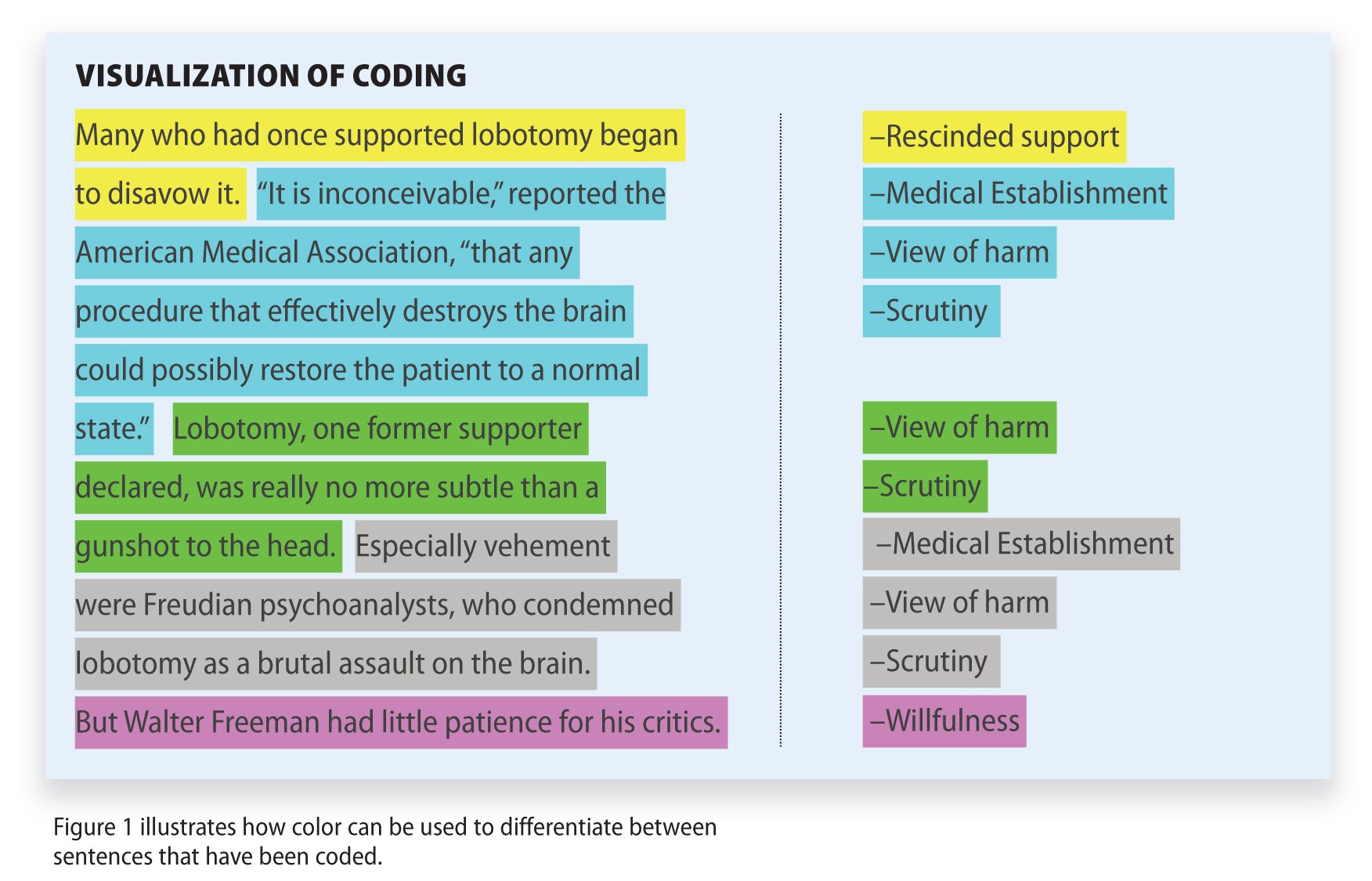
Research Methodology
Qualitative Data Coding
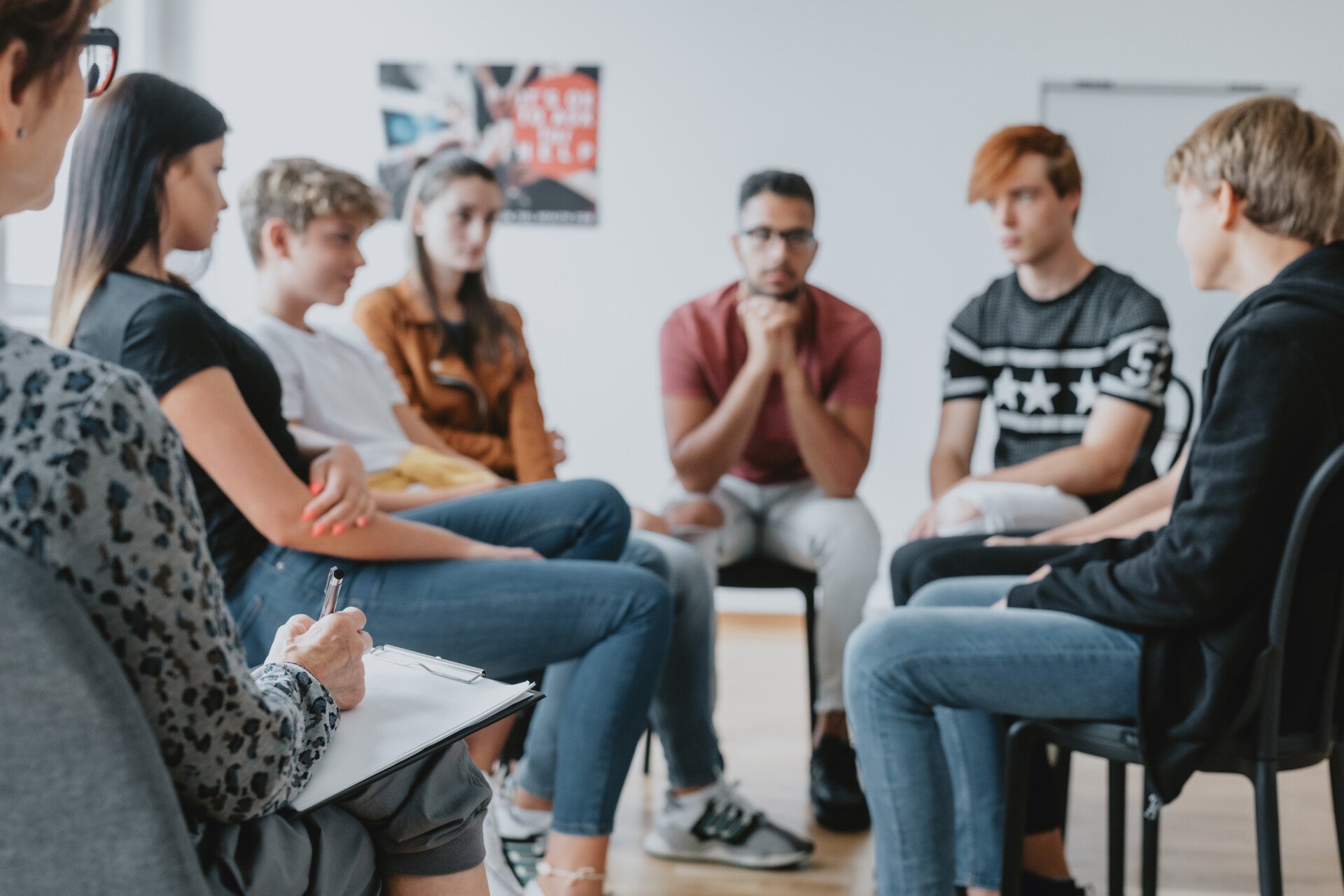
What Is a Focus Group?
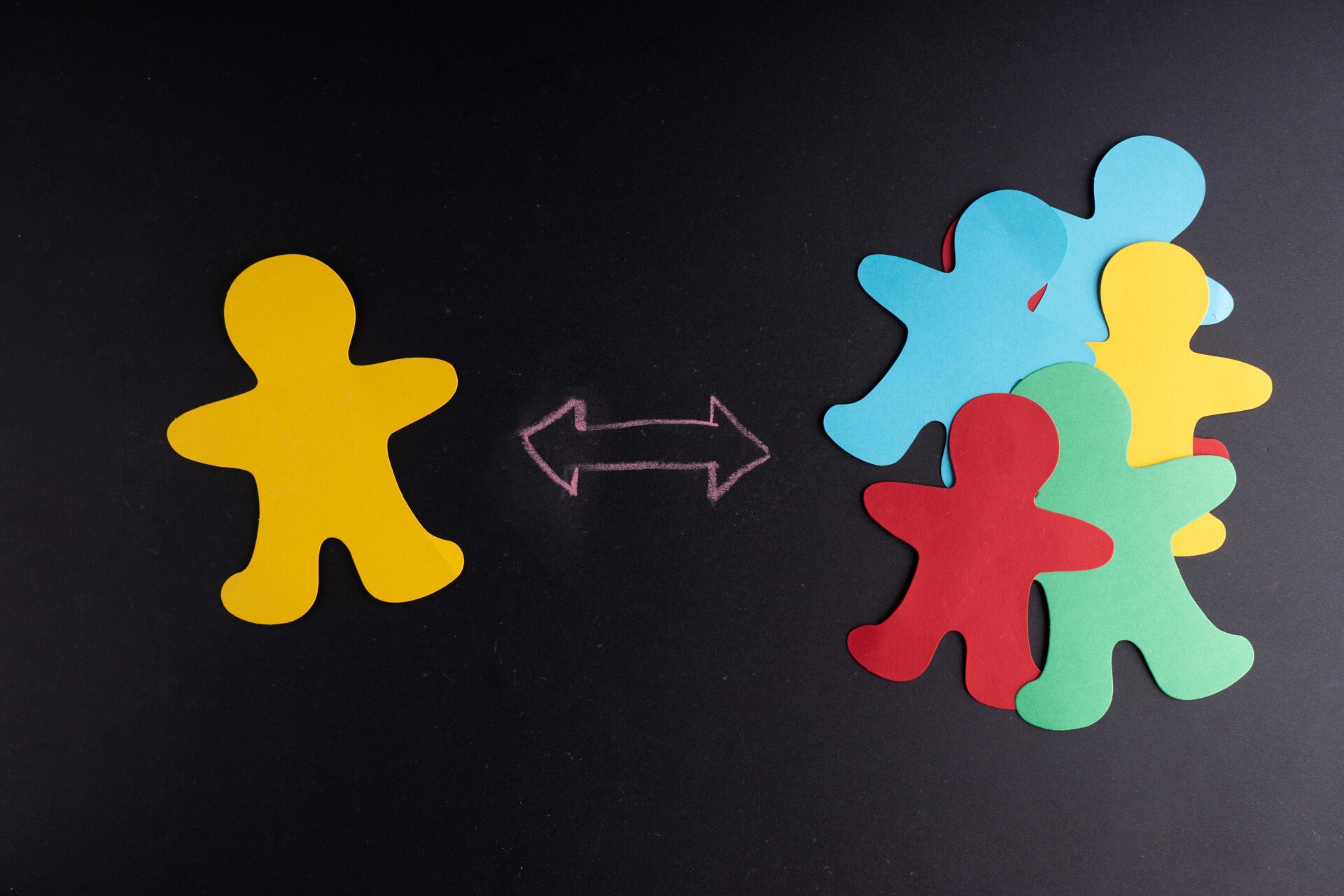
Cross-Cultural Research Methodology In Psychology
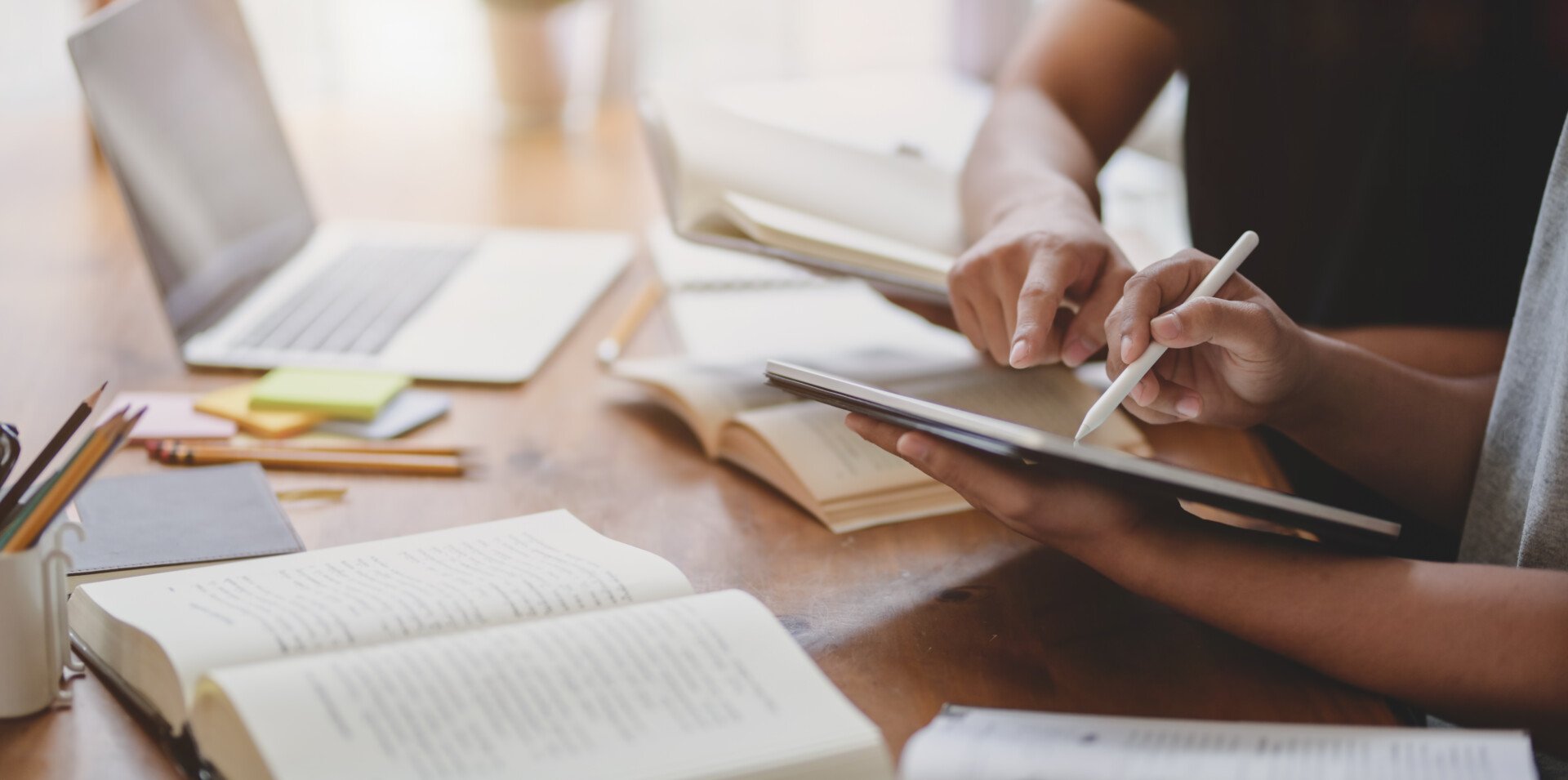
What Is Internal Validity In Research?
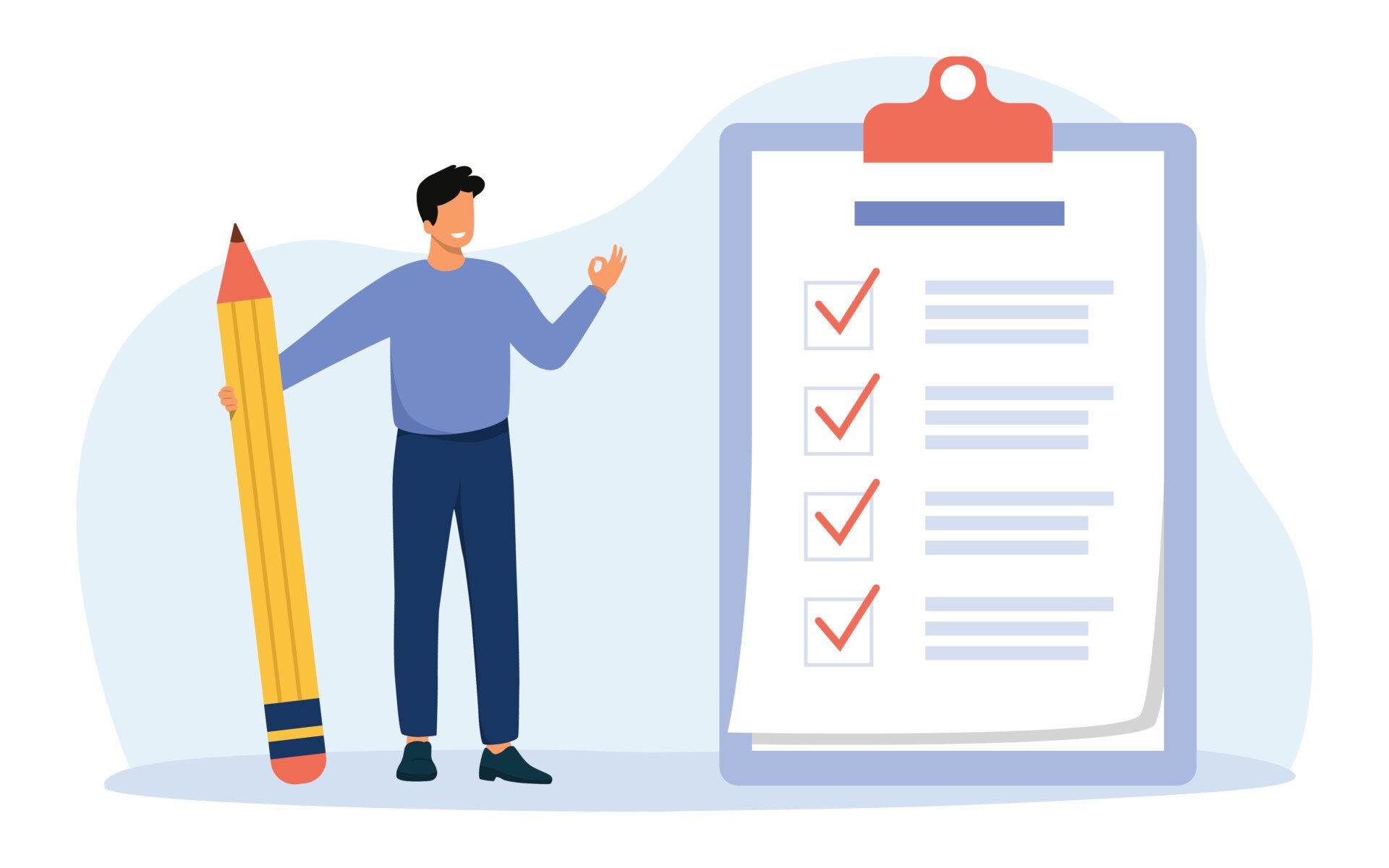
Research Methodology , Statistics
What Is Face Validity In Research? Importance & How To Measure
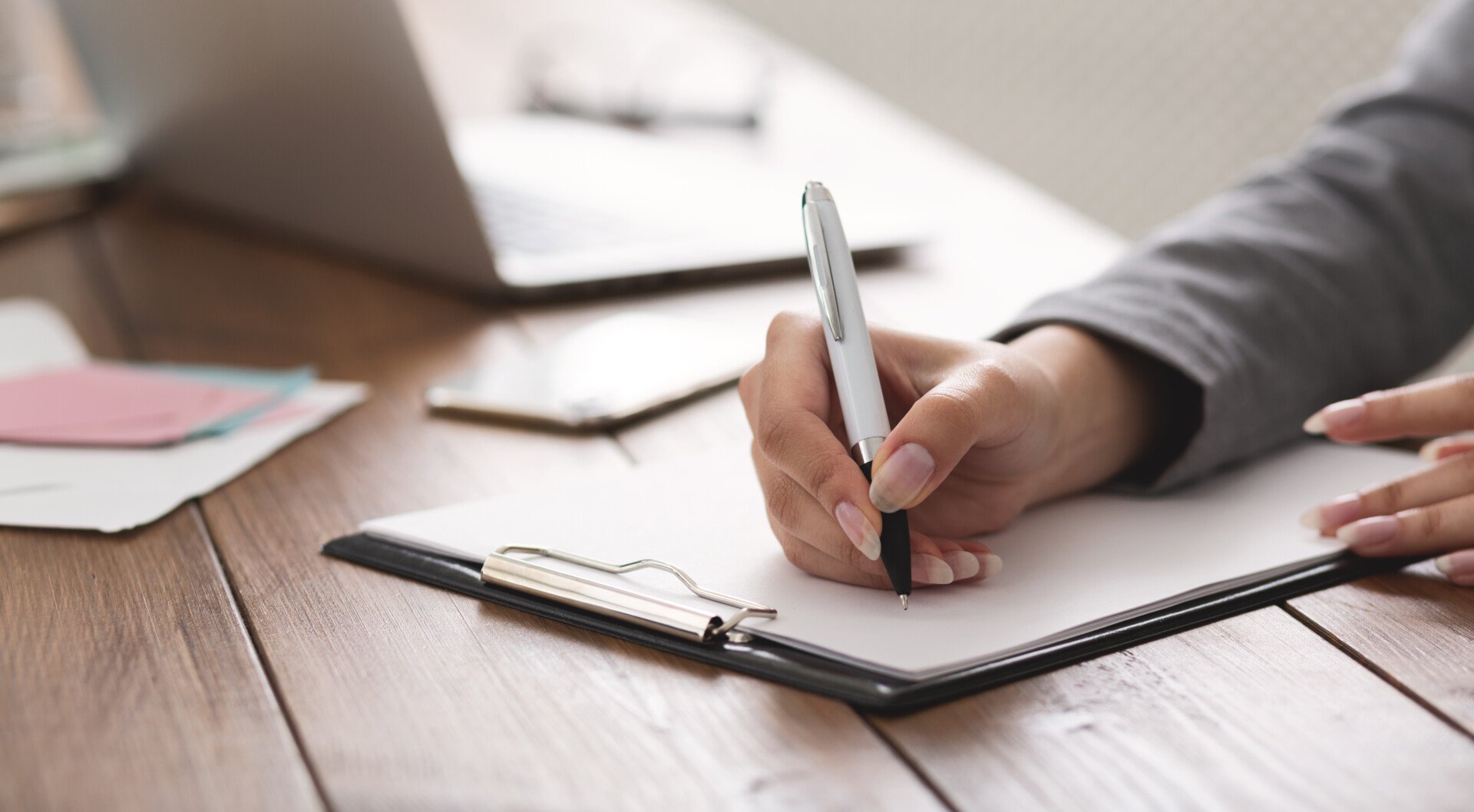
Criterion Validity: Definition & Examples

Researching and Writing a Paper: Reliability of Sources
- Outline Note-Taking
- Summarizing
- Bibliography / Annotated Bibliography
- Thesis Sentences
- Ideas for Topics
- The Big List of Databases and Resource Sources
- Keywords and Controlled Vocabulary
- Full Text Advice
- Database Searching Videos!
- How to Read a Scholarly Article.
- Citation Styles
- Citation Videos!
- Citation Tips & Tricks
- Videos about Evaluating Sources!
- Unreliable Sources and 'Fake News'
- An Outline for Writing!
- Formatting your paper!
Techniques for Evaluating Resources
No matter how good the database you search in is, or how reliable an information website has been in the past, you need to evaluate the sources you want to use for credibility and bias before you use them*. (You may also want to spot fake news as you browse the Internet or other media - not all fake news is online).
This page discusses eight different tools for evaluating sources (there are so many different tools because evaluating the reliability or quality of information is an important topic, because there are many ways to look at the topic, and every librarian wants to help you succeed). Look through these approaches and use the approaches or combinations of approaches that work for you. The tools are:
5Ws (and an H)
A.S.P.E.C.T.
- Evaluating Research Articles
- Lateral Reading ("what do other sources say?")
The CRAAP Test
We also have a variety of videos about evaluating sources available for your learning and entertainment: Click Here !
* Note: a biased source - and technically most sources are biased - can be a useful source as long as you understand what the bias or biases are. A source that is trying to be reliable will often identify some or all of its biases. (Every person has a limited perspective on the events they observe or participate in, and most of the time their perceptions are influenced by assumptions they may not be aware of. So, even when you have some really solid reasons to trust a source as 100% reliable and accurate, be alert for 'what is not mentioned' and for what biases there might be [this is particularly tricky when you share those biases, and why reviewing your research/paper/presentation with someone else is always a good idea]).
The 5Ws and an H.
Back to the top of the page
- Who are the authors or creators?
- What are their credentials? Can you find something out about them in another place?
- Who is the publisher or sponsor?
- Are they reputable?
- What is the publisher’s interest (if any) in this information?
- If it's from a website, does it have advertisements?
- Is this fact or opinion?
- Is it biased? Can you still use the information, even if you know there is bias?
- Is the the site trying to sell you something, convert you to something, or make you vote for someone?
- What kind of information is included in the resource?
- Is content of the resource primarily opinion? Is is balanced?
- Is it provided for a hobbiest, for entertainment, or for a serious audience?
- Does the creator provide references or sources for data or quotations?
- How recent is the information?
- Is it current enough for your topic?
- If the information is from a website, when was the site last updated?
Authority Information resources are a product of their creator's expertise and reliability, and are evaluated based on the information need and the context in which the information will be used. Authority is constructed: various communities often recognize different types of authority (knowledge, accuracy). Authority is contextual because you may need additional information to help determine the accuracy or comprehensiveness, and the sort of authority the source contains. (Writing a paper about ' urban myths ' requires different sorts of authority than writing a paper disproving an urban myth.)
Using this concept means you have to identify the different types of authority that might be relevant, and why the author considers themselves reliable, as well as why their community considers them reliable. An author can be a person, journalist, scholar, organization, website, etc. Author is different from authority, authority is the quality that gives an author trustworthiness....and not all authors have the same trustworthiness.
Evaluating research articles
Evaluating research articles: Evaluating evidence-based research articles in scholarly journals requires deep knowledge of the discipline, which you might not acquire until you are deeper into your education. These guiding questions can help you evaluate a research report, even if you are not an expert in the field. Questions include:
- Why was the study undertaken? The aim of the research may be intended to generate income, lobby for policy changes, evaluate the impact of a program, or create a new theory. These variations in intent influence the research questions, the data collection, the analysis, and how the results are presented. To make best use of the findings for your purposes, you should keep the intent of the study in mind.
- Who conducted the study? It is important to look at who conducted the research, and if the organization or individual in question has the expertise required for conducting research on the topic. Looking to see if the organization is interested in a specific research outcome is also a good practice. The research should be clear about how the different stages of the study were conducted to guarantee its objectivity.
- Who funded the research? It is equally important to look at who sponsored or funded the study because this sometimes affects the objectivity or accuracy of the study. (If, for example, a soap-maker sponsors a study on the efficiency of different soaps, you should be critical of the results, particularly if their brand of soap is the best at cleaning.)
- How was the data collected? In the social sciences, structured interviews and self-completion questionnaires are perhaps the two most common ways of collecting quantitative data. How the people in the study were recruited is essential for determining how representative the results are. (There are two main types of samples, probability and non-probability samples. A probability sample is one in which every individual in the population has the same chance of being included in the study. It is also a prerequisite for being able to generalize the findings to the population. Pretend you survey first-year students by asking student clubs to share the survey on their social media. This non-probability snowball sample is more likely to reach students active in the clubs, therefore the results will not be representative of, or generalizable to, all students.)
- Is the sample size and response rate sufficient? The bigger the sample size the greater the chance that the results are accurate. After a sample size of around 1000, gains in accuracy become less significant. However, limited time and money often make such a large sample not practical. The similarity of the population also affects the desired sample size; a more diverse population requires a larger sample to sufficiently include the different parts of the population. The response rate is a complementary measure to the sample size, showing how many of the suitable individuals in the sample have provided a usable response. (In web surveys, response rates tend to be lower than in other types of surveys, and are therefore less accurate.)
- Does the research make use of secondary data? Data can be collected for the purposes of the study or existing data gathered for a different study can be used. If existing data sets collected for another study are used, reflecting on how usable that data is for the newer study is important.
- Does the research measure what it claims to measure? A commonly used word in statistics to describe the trustworthiness of research is ‘validity’. Validity refers to the extent to which an assumption or measurement is consistent with reality. Does it measure what it intends to measure? For example, a study investigates gender discrimination of faculty and looks at the number of cases of discrimination presented by female faculty. But, if the study does not look at the reason for these discrimination complaints (gender, ethnicity, religion, age, sexual orientation, etc.) it cannot be assumed that gender discrimination either increased or decreased.
- Can the findings be generalized to my situation? There is often a tendency to generalize research findings. Two key standards have to be met to do this. First, results apply only to the population of the study. Second, data must be collected via a probability sample, i.e. everyone eligible to be in the study has the same chance of being included in the study. Too often papers do not discuss many of the aspects of the data collection and analysis. Transparently and clearly describing how the research was conducted is essential for the reader to understand the trustworthiness of the research paper in their hands.
Lateral Reading
The Internet has democratized access to information, but the Internet has also been filled with a flood of misinformation, fake news, propaganda, and idiocy, presented as objective analysis. Since any single source is suspect, fact checkers read laterally. They leave a site in its tab after a quick look around and open up new browser tabs in order to judge the credibility of the original site.
Lateral reading is the process of verifying what you are reading while you are reading it. It allows you to read deeply and broadly while gaining a fuller understanding of an issue or topic and determining whether, or how much, to trust the content as presented.
Vertical reading occurs when the reader simply reads the article or site without going further, assuming that if it ‘looks reliable’ it is reliable. The reader may use some superficial evaluation strategies to determine if the site is credible, such as reading the ‘about’ page, looking at its URL extension (.edu, .org, .com, .gov, etc.), or assessing its advertising. A good start, but there is much more to look at:
- Determine the author's reliability, intents, and biases, by searching for articles by other writers on the same topic (and also looking for other articles by that same author).
- Understand the perspective of the site's analyses. (What are they assuming, what do they want you to assume?)
- Determine whether the site has an editorial process or expert reputation supporting the reliability and accuracy of its content.
Use the strategies and ask the questions that professional fact-checkers use:
- Go beyond the "about" page of the site you are reading.
- Search for articles by other writers on the same topic.
- Search for articles about the site/publication you are reading (and/or articles about the authors featured on the site).
Ask the following:
- Who funds or sponsors the site where the original piece was published? (And who funds/sponsors the site you found the article at?)
- What do other authoritative sources have to say about that site and that topic?
- When you do a search on the topic of the original piece, are the initial results from fact-checking organizations? (If so, what do they say?)
- Have questions been raised about other articles the author has written or that have appeared on that site?
- Does what you are finding elsewhere contradict the original piece? (If there are contradictions, what is the reliability of those contradicting sites?)
Are reliable news outlets reporting on (or perhaps more important, not reporting on) what you are reading? (Does why reliable news outlets are or are-not reporting on the topic increase or decrease the reliability of the site you are assessing?)
Sometimes the 'good answer' to the above questions is a 'yes', sometimes a 'no', and sometimes 'it's complicated'. Reliable and unreliable sources are everywhere in the information we have access to - some sources are rarely reliable, but even the most 'consistently reliable sources' are sometimes unreliable (everyone has blind spots and biases, and everyone is able to make mistakes). There are no consistent rules for which questions must be answered which way. However, if you ask these questions and find out what the answers seem to be you will have a better understanding of how reliable or unreliable a particular source is.
S.I.F.T. Method
SIFT (The Four Moves)
Use the SIFT method to separate fact from fake when reading websites and other media.
- What is its reputation?
- For deeper research, verify the information.
- Know what you're reading.
- Where is it from? Biases, point of view?
- Understand the context of the information.
- Find the best source on the subject.
- Trace claims, quotes or media back to its original context.
- Was the source you read/viewed an accurate depiction of the original?
More Information about the SIFT method , and a free 3-hour online course (five easy lessons) that will seriously improve your information evaluation skills!
!!! Do you have questions, confusions, or opinons about anything on this page, in this LibGuide, or anything else? We are happy to Listen to and Answer Your Questions, Concerns, and more ! !!!
- << Previous: Citation Tips & Tricks
- Next: Videos about Evaluating Sources! >>
- Last Updated: May 16, 2024 6:11 PM
- URL: https://libguides.rtc.edu/researching_and_writing

An official website of the United States government
The .gov means it’s official. Federal government websites often end in .gov or .mil. Before sharing sensitive information, make sure you’re on a federal government site.
The site is secure. The https:// ensures that you are connecting to the official website and that any information you provide is encrypted and transmitted securely.
- Publications
- Account settings
Preview improvements coming to the PMC website in October 2024. Learn More or Try it out now .
- Advanced Search
- Journal List
- Int J Med Educ
Making sense of Cronbach's alpha
Medical educators attempt to create reliable and valid tests and questionnaires in order to enhance the accuracy of their assessment and evaluations. Validity and reliability are two fundamental elements in the evaluation of a measurement instrument. Instruments can be conventional knowledge, skill or attitude tests, clinical simulations or survey questionnaires. Instruments can measure concepts, psychomotor skills or affective values. Validity is concerned with the extent to which an instrument measures what it is intended to measure. Reliability is concerned with the ability of an instrument to measure consistently. 1 It should be noted that the reliability of an instrument is closely associated with its validity. An instrument cannot be valid unless it is reliable. However, the reliability of an instrument does not depend on its validity. 2 It is possible to objectively measure the reliability of an instrument and in this paper we explain the meaning of Cronbach’s alpha, the most widely used objective measure of reliability.
Calculating alpha has become common practice in medical education research when multiple-item measures of a concept or construct are employed. This is because it is easier to use in comparison to other estimates (e.g. test-retest reliability estimates) 3 as it only requires one test administration. However, in spite of the widespread use of alpha in the literature the meaning, proper use and interpretation of alpha is not clearly understood. 2 , 4 , 5 We feel it is important, therefore, to further explain the underlying assumptions behind alpha in order to promote its more effective use. It should be emphasised that the purpose of this brief overview is just to focus on Cronbach’s alpha as an index of reliability. Alternative methods of measuring reliability based on other psychometric methods, such as generalisability theory or item-response theory, can be used for monitoring and improving the quality of OSCE examinations 6 - 10 , but will not be discussed here.
Alpha was developed by Lee Cronbach in 1951 11 to provide a measure of the internal consistency of a test or scale; it is expressed as a number between 0 and 1. Internal consistency describes the extent to which all the items in a test measure the same concept or construct and hence it is connected to the inter-relatedness of the items within the test. Internal consistency should be determined before a test can be employed for research or examination purposes to ensure validity. In addition, reliability estimates show the amount of measurement error in a test. Put simply, this interpretation of reliability is the correlation of test with itself. Squaring this correlation and subtracting from 1.00 produces the index of measurement error. For example, if a test has a reliability of 0.80, there is 0.36 error variance (random error) in the scores (0.80×0.80 = 0.64; 1.00 – 0.64 = 0.36). 12 As the estimate of reliability increases, the fraction of a test score that is attributable to error will decrease. 2 It is of note that the reliability of a test reveals the effect of measurement error on the observed score of a student cohort rather than on an individual student. To calculate the effect of measurement error on the observed score of an individual student, the standard error of measurement must be calculated (SEM). 13
If the items in a test are correlated to each other, the value of alpha is increased. However, a high coefficient alpha does not always mean a high degree of internal consistency. This is because alpha is also affected by the length of the test. If the test length is too short, the value of alpha is reduced. 2 , 14 Thus, to increase alpha, more related items testing the same concept should be added to the test. It is also important to note that alpha is a property of the scores on a test from a specific sample of testees. Therefore investigators should not rely on published alpha estimates and should measure alpha each time the test is administered. 14
Use of Cronbach’s alpha
Improper use of alpha can lead to situations in which either a test or scale is wrongly discarded or the test is criticised for not generating trustworthy results. To avoid this situation an understanding of the associated concepts of internal consistency, homogeneity or unidimensionality can help to improve the use of alpha. Internal consistency is concerned with the interrelatedness of a sample of test items, whereas homogeneity refers to unidimensionality. A measure is said to be unidimensional if its items measure a single latent trait or construct. Internal consistency is a necessary but not sufficient condition for measuring homogeneity or unidimensionality in a sample of test items. 5 , 15 Fundamentally, the concept of reliability assumes that unidimensionality exists in a sample of test items 16 and if this assumption is violated it does cause a major underestimate of reliability. It has been well documented that a multidimensional test does not necessary have a lower alpha than a unidimensional test. Thus a more rigorous view of alpha is that it cannot simply be interpreted as an index for the internal consistency of a test. 5 , 15 , 17
Factor Analysis can be used to identify the dimensions of a test. 18 Other reliable techniques have been used and we encourage the reader to consult the paper “Applied Dimensionality and Test Structure Assessment with the START-M Mathematics Test” and to compare methods for assessing the dimensionality and underlying structure of a test. 19
Alpha, therefore, does not simply measure the unidimensionality of a set of items, but can be used to confirm whether or not a sample of items is actually unidimensional. 5 On the other hand if a test has more than one concept or construct, it may not make sense to report alpha for the test as a whole as the larger number of questions will inevitable inflate the value of alpha. In principle therefore, alpha should be calculated for each of the concepts rather than for the entire test or scale. 2 , 3 The implication for a summative examination containing heterogeneous, case-based questions is that alpha should be calculated for each case.
More importantly, alpha is grounded in the ‘tau equivalent model’ which assumes that each test item measures the same latent trait on the same scale. Therefore, if multiple factors/traits underlie the items on a scale, as revealed by Factor Analysis, this assumption is violated and alpha underestimates the reliability of the test. 17 If the number of test items is too small it will also violate the assumption of tau-equivalence and will underestimate reliability. 20 When test items meet the assumptions of the tau-equivalent model, alpha approaches a better estimate of reliability. In practice, Cronbach’s alpha is a lower-bound estimate of reliability because heterogeneous test items would violate the assumptions of the tau-equivalent model. 5 If the calculation of “standardised item alpha” in SPSS is higher than “Cronbach’s alpha”, a further examination of the tau-equivalent measurement in the data may be essential.
As pointed out earlier, the number of test items, item inter-relatedness and dimensionality affect the value of alpha. 5 There are different reports about the acceptable values of alpha, ranging from 0.70 to 0.95. 2 , 21 , 22 A low value of alpha could be due to a low number of questions, poor inter-relatedness between items or heterogeneous constructs. For example if a low alpha is due to poor correlation between items then some should be revised or discarded. The easiest method to find them is to compute the correlation of each test item with the total score test; items with low correlations (approaching zero) are deleted. If alpha is too high it may suggest that some items are redundant as they are testing the same question but in a different guise. A maximum alpha value of 0.90 has been recommended. 14
High quality tests are important to evaluate the reliability of data supplied in an examination or a research study. Alpha is a commonly employed index of test reliability. Alpha is affected by the test length and dimensionality. Alpha as an index of reliability should follow the assumptions of the essentially tau-equivalent approach. A low alpha appears if these assumptions are not meet. Alpha does not simply measure test homogeneity or unidimensionality as test reliability is a function of test length. A longer test increases the reliability of a test regardless of whether the test is homogenous or not. A high value of alpha (> 0.90) may suggest redundancies and show that the test length should be shortened.
Conclusions
Alpha is an important concept in the evaluation of assessments and questionnaires. It is mandatory that assessors and researchers should estimate this quantity to add validity and accuracy to the interpretation of their data. Nevertheless alpha has frequently been reported in an uncritical way and without adequate understanding and interpretation. In this editorial we have attempted to explain the assumptions underlying the calculation of alpha, the factors influencing its magnitude and the ways in which its value can be interpreted. We hope that investigators in future will be more critical when reporting values of alpha in their studies.
Reliability Analysis—A Critical Review
- Conference paper
- First Online: 24 July 2021
- Cite this conference paper
- Janender Kumar ORCID: orcid.org/0000-0002-3323-2620 12 ,
- Suneev Anil Bansal ORCID: orcid.org/0000-0001-7644-3113 12 &
- Munish Mehta ORCID: orcid.org/0000-0002-1380-2809 13
Part of the book series: Lecture Notes in Mechanical Engineering ((LNME))
664 Accesses
1 Citations
Present article discusses the problem of lesser availability of the operating systems in process industries. Complex systems, continuous breakdowns and unplanned maintenance strategies are the major reasons for poor performance of the plants. The concept of reliability plays a significant role in improving the system availability. Uninterrupted service and long-run availability are the basic needs of complex systems as in the sugar industry, thermal power plants, milk industry, mining, petroleum industries, etc. Different approaches are utilized by researchers in various fields to check the performance of the operating equipment. These approaches are genetic algorithm (GA), Petri nets (PN), fault tree analysis (FTA), supplementary variable technique (SVT), fuzzy Lambda-Tau technique, reliability, availability and maintainability (RAM) analysis, reliability, availability, maintainability, dependability (RAMD) analysis, failure mode and effect analysis (FMEA) and degradation modelling techniques, etc. The improvement has been seen in the performance of the systems based on mathematical data using the above techniques. Article also proposes new areas where research can be carried out.
This is a preview of subscription content, log in via an institution to check access.
Access this chapter
- Available as PDF
- Read on any device
- Instant download
- Own it forever
- Available as EPUB and PDF
- Compact, lightweight edition
- Dispatched in 3 to 5 business days
- Free shipping worldwide - see info
Tax calculation will be finalised at checkout
Purchases are for personal use only
Institutional subscriptions
Gupta P, Tiwari PC, Sharma AK (2008) Performance modeling and decision support system of feed water unit. South African J Industr Eng 19(2):125–134. ISSN: 2224-7890
Google Scholar
Mehta M, Kumar B, Kumar S (2012) Performance evaluation of butteroil production system of a milk plant. In: International conference on advancement and futuristic trends in mechanical engineering. PTU, Jalandhar, pp 230–235
Garg D, Kumar K (2010) Availability optimization for screw plant based on Genetic Algorithm. Int J Eng Sci Technol 2(4):658–668
Sharma SP, Vishwakarma Y (2014) Application of Markov process in performance analysis of feeding system of sugar industry. J Industr Mathe 2014:1–9. https://doi.org/10.1155/2014/593176
Article Google Scholar
Mehta M, Singh J, Sharma S (2018) Availability analysis of an industrial system using supplementary variable technique. Jordan J Mech Industr Eng 12(4):245–251. ISSN: 1995-6665
Sharma RK, Kumar S (2008) Performance modeling in critical engineering systems using RAM analysis. Reliab Eng Syst Saf 93(6):913–919. https://doi.org/10.1016/j.ress.2007.03.039
Article MathSciNet Google Scholar
Aggarwal AK, Kumar S, Singh V (2016) Reliability and availability analysis of the serial processes in skim milk powder system of a dairy plant: a case study. Int J Industr Syst 22(1):36–62
Aggarwal AK, Kumar S, Singh V (2015) Performance modeling of the skim milk powder production system of a dairy plant using RAMD analysis. Int J Qual Reliab Manage 32(2):167–181. https://doi.org/10.1108/IJQRM-01-2014-0007
Gorjian N, Ma L, Mittinty M, Yarlagadda P, Sun Y (2009) A review on degradation models in reliability analysis. In: Proceedings of the 4th World Congress on engineering asset management, pp 369–384, Greece
Waghmode LY, Patil RB (2016) Reliability analysis and life cycle cost optimization: a case study from Indian industry. Int J Qual Reliab Manage 33(3):414–429. https://doi.org/10.1108/IJQRM-11-2014-0184 .
Yang K, Yang G (1998) Degradation reliability assessment using severe critical values. Int J Reliab Qual Safety Eng 5(1):85–95
Gerbec M (2010) A reliability analysis of a natural-gas pressure-regulating installation. Reliab Eng Syst Saf 95(11):1154–1163. https://doi.org/10.1016/j.ress.2010.06.022
Ding F, He Z (2011) Cutting tool wear monitoring for reliability analysis using proportional hazards model. Int J Adv Manuf Technol 57(5–8):565–574. https://doi.org/10.1007/s00170-011-3316-4 .
Furuly S, Barabadi A, Barabady J (2013) Reliability analysis of mining equipment considering operational environments-a case study. Int J Performab Eng 9:287–294
Barends DM, Oldenhof MT, Vredenbregt MJ, Nauta MJ (2012) Risk analysis of analytical validations by probabilistic modification of FMEA. J Pharm Biomed Anal 64–65, 82–86. https://doi.org/10.1016/j.jpba.2012.02.009
Rahbi YAL, Rizwan SM, Alkali BM, Cowel A, Taneja G (2017) Reliability analysis of a subsystem in aluminum industry plant. In: 6th international conference on reliability, infocom technologies and optimization (ICRITO), pp 199–203. AU, Noida
Yuan L, Meng XY (2011) Reliability analysis of a warm standby repairable system with priority in use. Appl Math Modell 35(9):4295–4303. https://doi.org/10.1016/j.apm.2011.03.002
Yunusa-Kaltungo A, Kermani MM, Labib A (2017) Investigation of critical failures using root cause analysis methods: case study of ASH cement PLC engineering. Fail Anal 73:25–45. https://doi.org/10.1016/j.engfailanal.2016.11.016
Piadeh F, Ahmadi M, Behzadian K (2018) Reliability assessment for hybrid systems of advanced treatment units of industrial wastewater reuse using combined event tree and fuzzy fault tree analyses. J Clean Prod 201:958–973. https://doi.org/10.1016/j.jclepro.2018.08.052
Badida P, Balasubramaniam Y, Jayaprakash J (2019) Risk evaluation of oil and natural gas pipelines due to natural hazards using fuzzy fault tree analysis. J Nat Gas Sci Eng 66:284–292. https://doi.org/10.1016/j.jngse.2019.04.010
Manoharan P, Selvam Dennsion M, Ganesan V, Palanisamy S (2019) Reliability enhancement of steel rolling mill using fault tree analysis. U.P.B. Sci Bull Series D 81(1):165–178
Uniyal N, Pant S, Kumar A (2020) An overview of few nature inspired optimization techniques and its reliability applications. Int J Math Eng Manage Sci 5(4):732–743. https://doi.org/10.33889/ijmems.2020.5.4.058
Gowid S, Dixon R, Ghani S (2014) Optimization of reliability and maintenance of liquefaction system on FLNG terminals using Markov modelling. Int J Qual Reliab Manage 31(3):293–310. https://doi.org/10.1108/IJQRM-12-2012-0156
DuS ZengZ, CuiL KR (2017) Reliability analysis of Markov history-dependent repairable systems with neglected failures. Reliab Eng Syst Saf 159:134–142. https://doi.org/10.1016/j.ress.2016.10.030
Zhang X, Mahadevan S, Deng X (2017) Reliability analysis with linguistic data: an evidential network approach. Reliab Eng Syst Saf 162:111–121. https://doi.org/10.1016/j.ress.2017.01.009
Zhang CW, Zhang T, Chen N, Jin T (2013) Reliability modeling and analysis for a novel design of modular converter system of wind turbines. Reliab Eng Syst Safety 111:86–94. https://doi.org/10.1016/j.ress.2012.10.005
Dharmaraja S, Vinayak R, Trivedi KS (2016) Reliability and survivability of vehicular ad hoc networks: an analytical approach. Reliab Eng Syst Saf 153:28–38. https://doi.org/10.1016/j.ress.2016.04.004
Rashid HSJ (2015) Reliability model for helicopter main gearbox lubrication system using influence diagrams. Reliab Eng Syst Safety 139:50–57. https://doi.org/10.1016/j.ress.2015.01.021
Kim H, Kim P (2017) Reliability–redundancy allocation problem considering optimal redundancy strategy using parallel genetic algorithm. Reliab Eng Syst Saf 159:153–160. https://doi.org/10.1016/j.ress.2016.10.033
Kong X, Gao L, Ouyang H, Li S (2015) Solving the redundancy allocation problem with multiple strategy choices using a new simplified particle swarm optimization. Reliab Eng Syst Safety 144:147–158. https://doi.org/10.1016/j.ress.2015.07.019
Byun JE, Noh HM, Song J (2017) Reliability growth analysis of k-out-of-N systems using matrix-based system reliability method. Reliab Eng Syst Saf 165:410–421. https://doi.org/10.1016/j.ress.2017.05.001
Endrenyi J, Andrew GJ (2006) Aging, maintenance and reliability- approaches to preserving equipment health and extending equipment life. Power Energy Maga 4(3):59–67
Liu X, Zhang T (2011) Reliability engineering work flow and application in product development process. In: International conference on quality, reliability, risk, maintenance & reliability. IEEE, China, pp 1–4
Schoenig R, Aubry JF, Cambois T, Hutinet T (2006) An aggregation method of Markov graphs for the reliability analysis of hybrid systems. Reliab Eng Syst Saf 91(2):137–148
Kim MC, Seong PH (2006) An analytic model for situation assessment of nuclear power plant operators based on Bayesian inference. Reliab Eng Syst Saf 91(3):270–282
Rajpal PS, Shishodia KS, Sekhon GS (2006) An artificial neural network for modeling reliability, availability and maintainability of a repairable system. Reliab Eng Syst Saf 91(7):809–819
Davis P, Burn S, Moglia M, Gould S (2007) A physical probabilistic model to predict failure rates in buried PVC pipelines. Reliab Eng Syst Saf 92(9):1258–1266
Zhang YL (2008) A geometrical process repair model for a repairable system with delayed repair. Comput Math with Appl 55(8):1629–1643
Haarla L, Pulkkinen U, Koskinen M, Jyrinsalo J (2008) A method for analysing the reliability of a transmission grid. Reliab Eng Syst Saf 93(2):277–287
Leicester RH, Wang CH, Cookson LJ (2008) A reliability model for assessing the risk of termite attack on housing in Australia. Reliab Eng Syst Saf 93(3):468–475
Galante G, Passannanti G (2009) An exact algorithm for preventive maintenance planning of series-parallel systems. Reliab Eng Syst Saf 94(10):1517–1525
Garg S, Singh J, Singh DV (2010) Mathematical modeling and performance analysis of combed yarn production system: Based on few data. Appl Math Model 34(11):3300–3308
Kumar N, Tiwari PC, Sachdeva A (2020) Petri Nets modelling and analysis of the Veneer Layup system of plywood manufacturing plant. Int J Eng Model 33(1–2):95–107. https://doi.org/10.31534/engmod.2020.1-2.ri.07v
Download references
Author information
Authors and affiliations.
Department of Mechanical Engineering, MAIT, Maharaja Agrasen University, Solan, Himachal Pradesh, 174103, India
Janender Kumar & Suneev Anil Bansal
School of Mechanical Engineering, Lovely Professional University, Phagwara, Punjab, India
Munish Mehta
You can also search for this author in PubMed Google Scholar
Editor information
Editors and affiliations.
Department of Mechanical Engineering, MNNIT Allahabad, Prayagraj, India
Avanish Kumar Dubey
Department of Industrial and Production Engineering, Dr. B. R. Ambedkar National Institute of Technology Jalandhar, Jalandhar, Punjab, India
Anish Sachdeva
School of Mechanical Engineering, Lovely Professional University, Phagwara, India
Rights and permissions
Reprints and permissions
Copyright information
© 2022 The Author(s), under exclusive license to Springer Nature Singapore Pte Ltd.
About this paper
Cite this paper.
Kumar, J., Bansal, S.A., Mehta, M. (2022). Reliability Analysis—A Critical Review. In: Dubey, A.K., Sachdeva, A., Mehta, M. (eds) Recent Trends in Industrial and Production Engineering. Lecture Notes in Mechanical Engineering. Springer, Singapore. https://doi.org/10.1007/978-981-16-3135-1_22
Download citation
DOI : https://doi.org/10.1007/978-981-16-3135-1_22
Published : 24 July 2021
Publisher Name : Springer, Singapore
Print ISBN : 978-981-16-3134-4
Online ISBN : 978-981-16-3135-1
eBook Packages : Engineering Engineering (R0)
Share this paper
Anyone you share the following link with will be able to read this content:
Sorry, a shareable link is not currently available for this article.
Provided by the Springer Nature SharedIt content-sharing initiative
- Publish with us
Policies and ethics
- Find a journal
- Track your research
Suggestions or feedback?
MIT News | Massachusetts Institute of Technology
- Machine learning
- Social justice
- Black holes
- Classes and programs
Departments
- Aeronautics and Astronautics
- Brain and Cognitive Sciences
- Architecture
- Political Science
- Mechanical Engineering
Centers, Labs, & Programs
- Abdul Latif Jameel Poverty Action Lab (J-PAL)
- Picower Institute for Learning and Memory
- Lincoln Laboratory
- School of Architecture + Planning
- School of Engineering
- School of Humanities, Arts, and Social Sciences
- Sloan School of Management
- School of Science
- MIT Schwarzman College of Computing
Using ideas from game theory to improve the reliability of language models
Press contact :.
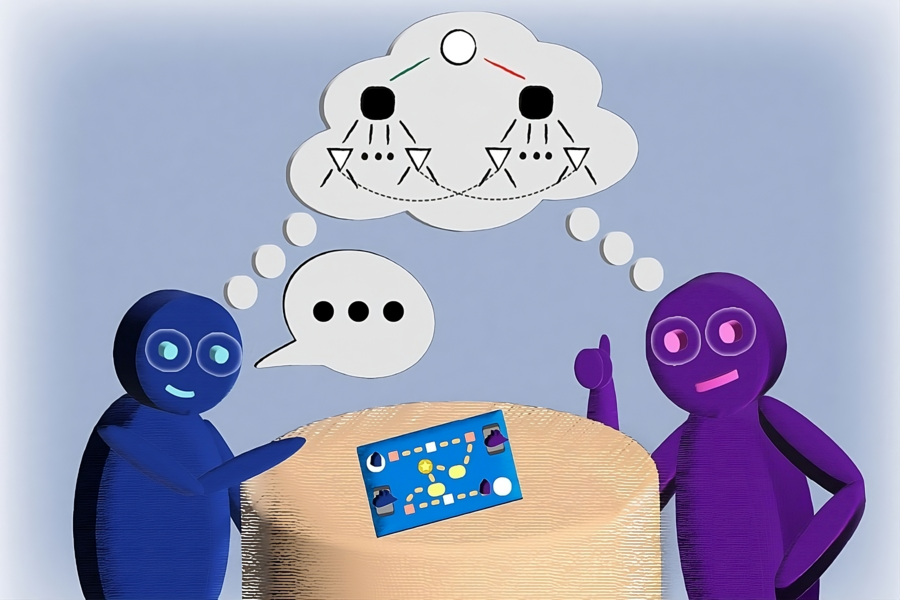
Previous image Next image
Imagine you and a friend are playing a game where your goal is to communicate secret messages to each other using only cryptic sentences. Your friend's job is to guess the secret message behind your sentences. Sometimes, you give clues directly, and other times, your friend has to guess the message by asking yes-or-no questions about the clues you've given. The challenge is that both of you want to make sure you're understanding each other correctly and agreeing on the secret message.
MIT Computer Science and Artificial Intelligence Laboratory (CSAIL) researchers have created a similar "game" to help improve how AI understands and generates text. It is known as a “consensus game” and it involves two parts of an AI system — one part tries to generate sentences (like giving clues), and the other part tries to understand and evaluate those sentences (like guessing the secret message).
The researchers discovered that by treating this interaction as a game, where both parts of the AI work together under specific rules to agree on the right message, they could significantly improve the AI's ability to give correct and coherent answers to questions. They tested this new game-like approach on a variety of tasks, such as reading comprehension, solving math problems, and carrying on conversations, and found that it helped the AI perform better across the board.
Traditionally, large language models answer one of two ways: generating answers directly from the model (generative querying) or using the model to score a set of predefined answers (discriminative querying), which can lead to differing and sometimes incompatible results. With the generative approach, "Who is the president of the United States?" might yield a straightforward answer like "Joe Biden." However, a discriminative query could incorrectly dispute this fact when evaluating the same answer, such as "Barack Obama."
So, how do we reconcile mutually incompatible scoring procedures to achieve coherent, efficient predictions?
"Imagine a new way to help language models understand and generate text, like a game. We've developed a training-free, game-theoretic method that treats the whole process as a complex game of clues and signals, where a generator tries to send the right message to a discriminator using natural language. Instead of chess pieces, they're using words and sentences," says Athul Jacob, an MIT PhD student in electrical engineering and computer science and CSAIL affiliate. "Our way to navigate this game is finding the 'approximate equilibria,' leading to a new decoding algorithm called 'equilibrium ranking.' It's a pretty exciting demonstration of how bringing game-theoretic strategies into the mix can tackle some big challenges in making language models more reliable and consistent."
When tested across many tasks, like reading comprehension, commonsense reasoning, math problem-solving, and dialogue, the team's algorithm consistently improved how well these models performed. Using the ER algorithm with the LLaMA-7B model even outshone the results from much larger models. "Given that they are already competitive, that people have been working on it for a while, but the level of improvements we saw being able to outperform a model that's 10 times the size was a pleasant surprise," says Jacob.
"Diplomacy," a strategic board game set in pre-World War I Europe, where players negotiate alliances, betray friends, and conquer territories without the use of dice — relying purely on skill, strategy, and interpersonal manipulation — recently had a second coming. In November 2022, computer scientists, including Jacob, developed “Cicero,” an AI agent that achieves human-level capabilities in the mixed-motive seven-player game, which requires the same aforementioned skills, but with natural language. The math behind this partially inspired the Consensus Game.
While the history of AI agents long predates when OpenAI's software entered the chat in November 2022, it's well documented that they can still cosplay as your well-meaning, yet pathological friend.
The consensus game system reaches equilibrium as an agreement, ensuring accuracy and fidelity to the model's original insights. To achieve this, the method iteratively adjusts the interactions between the generative and discriminative components until they reach a consensus on an answer that accurately reflects reality and aligns with their initial beliefs. This approach effectively bridges the gap between the two querying methods.
In practice, implementing the consensus game approach to language model querying, especially for question-answering tasks, does involve significant computational challenges. For example, when using datasets like MMLU, which have thousands of questions and multiple-choice answers, the model must apply the mechanism to each query. Then, it must reach a consensus between the generative and discriminative components for every question and its possible answers.
The system did struggle with a grade school right of passage: math word problems. It couldn't generate wrong answers, which is a critical component of understanding the process of coming up with the right one.
“The last few years have seen really impressive progress in both strategic decision-making and language generation from AI systems, but we’re just starting to figure out how to put the two together. Equilibrium ranking is a first step in this direction, but I think there’s a lot we’ll be able to do to scale this up to more complex problems,” says Jacob.
An avenue of future work involves enhancing the base model by integrating the outputs of the current method. This is particularly promising since it can yield more factual and consistent answers across various tasks, including factuality and open-ended generation. The potential for such a method to significantly improve the base model's performance is high, which could result in more reliable and factual outputs from ChatGPT and similar language models that people use daily.
"Even though modern language models, such as ChatGPT and Gemini, have led to solving various tasks through chat interfaces, the statistical decoding process that generates a response from such models has remained unchanged for decades," says Google Research Scientist Ahmad Beirami, who was not involved in the work. "The proposal by the MIT researchers is an innovative game-theoretic framework for decoding from language models through solving the equilibrium of a consensus game. The significant performance gains reported in the research paper are promising, opening the door to a potential paradigm shift in language model decoding that may fuel a flurry of new applications."
Jacob wrote the paper with MIT-IBM Watson Lab researcher Yikang Shen and MIT Department of Electrical Engineering and Computer Science assistant professors Gabriele Farina and Jacob Andreas, who is also a CSAIL member. They presented their work at the International Conference on Learning Representations (ICLR) earlier this month, where it was highlighted as a "spotlight paper." The research also received a “best paper award” at the NeurIPS R0-FoMo Workshop in December 2023.
Share this news article on:
Press mentions, quanta magazine.
MIT researchers have developed a new procedure that uses game theory to improve the accuracy and consistency of large language models (LLMs), reports Steve Nadis for Quanta Magazine . “The new work, which uses games to improve AI, stands in contrast to past approaches, which measured an AI program’s success via its mastery of games,” explains Nadis.
Previous item Next item
Related Links
- Article: "Game Theory Can Make AI More Correct and Efficient"
- Jacob Andreas
- Athul Paul Jacob
- Language & Intelligence @ MIT
- Computer Science and Artificial Intelligence Laboratory (CSAIL)
- Department of Electrical Engineering and Computer Science
- MIT-IBM Watson AI Lab
Related Topics
- Computer science and technology
- Artificial intelligence
- Human-computer interaction
- Natural language processing
- Game theory
- Electrical Engineering & Computer Science (eecs)
Related Articles
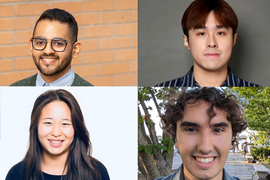
Reasoning and reliability in AI
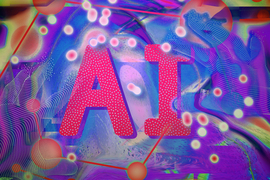
Explained: Generative AI
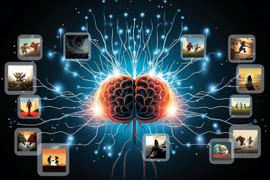
Synthetic imagery sets new bar in AI training efficiency
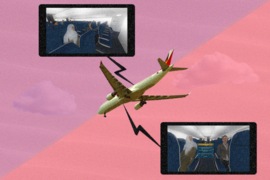
Simulating discrimination in virtual reality
More mit news.
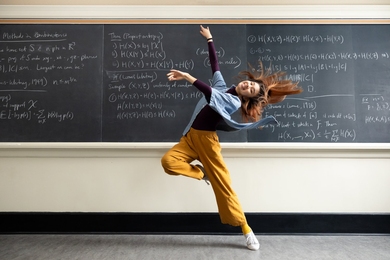
Janabel Xia: Algorithms, dance rhythms, and the drive to succeed
Read full story →
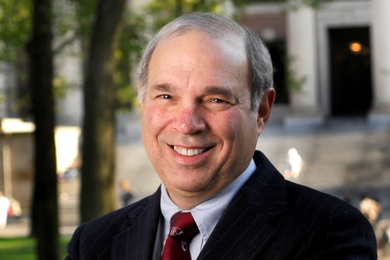
Jonathan Byrnes, MIT Center for Transportation and Logistics senior lecturer and visionary in supply chain management, dies at 75
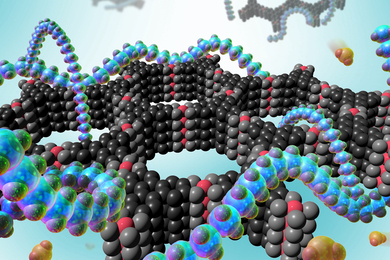
Researchers develop a detector for continuously monitoring toxic gases
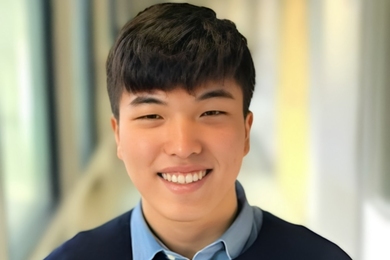
The beauty of biology
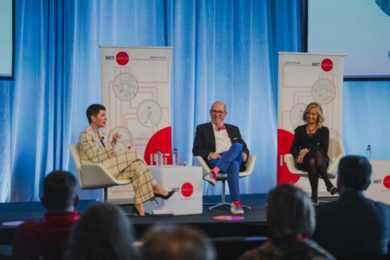
Navigating longevity with industry leaders at MIT AgeLab PLAN Forum
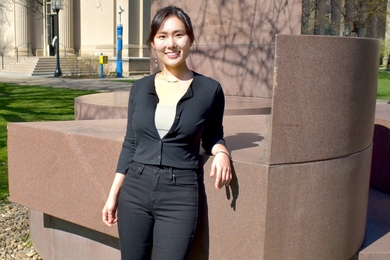
Jeong Min Park earns 2024 Schmidt Science Fellowship
- More news on MIT News homepage →
Massachusetts Institute of Technology 77 Massachusetts Avenue, Cambridge, MA, USA
- Map (opens in new window)
- Events (opens in new window)
- People (opens in new window)
- Careers (opens in new window)
- Accessibility
- Social Media Hub
- MIT on Facebook
- MIT on YouTube
- MIT on Instagram
Peter A Allard School of Law
Audience selector.
- Current Students
- Future Students
- Faculty & Staff
Vancouver is Awesome: Critics slam Paper Excellence review that found no APP links
May 17, 2024
Source: Vancouver is Awesome
Other Recent News

It’s time to expand what we think of as "work," says Allard Law’s Supriya Routh
Allard Law Professor Supriya Routh has been awarded a Canada Research Chair in Labour Law and Social Justice to examine how the law can more effectively support workers.
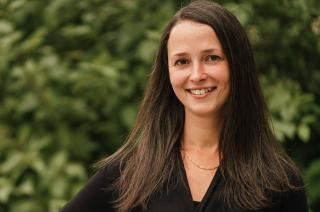
Professor Bethany Hastie awarded UBC Killam Accelerator Research Fellowship
Professor Hastie hopes to shed light on the challenges that new technologies are raising for workers – and the gaps in current labour law and policy in Canada.
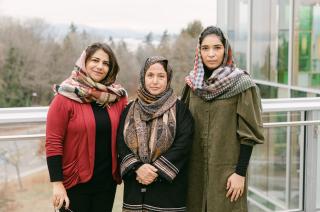
Celebrating 1 year, Allard Law program at UBC gives Afghan women judges a new professional home
The Peter A. Allard School of Law celebrated the one-year anniversary of the law school's Afghan Women Judges Program on February 9 with an event at Allard Hall.
Main Navigation
- Contact NeurIPS
- Code of Ethics
- Code of Conduct
- Create Profile
- Journal To Conference Track
- Diversity & Inclusion
- Proceedings
- Future Meetings
- Exhibitor Information
- Privacy Policy
Call for High School Projects
Machine learning for social impact .
The Thirty-Eighth Annual Conference on Neural Information Processing Systems (NeurIPS 2024) is an interdisciplinary conference that brings together researchers in machine learning, neuroscience, statistics, optimization, computer vision, natural language processing, life sciences, natural sciences, social sciences, and other adjacent fields.
This year, we invite high school students to submit research papers on the topic of machine learning for social impact. A subset of finalists will be selected to present their projects virtually and will have their work spotlighted on the NeurIPS homepage. In addition, the leading authors of up to five winning projects will be invited to attend an award ceremony at NeurIPS 2024 in Vancouver.
Each submission must describe independent work wholly performed by the high school student authors. We expect each submission to highlight either demonstrated positive social impact or the potential for positive social impact using machine learning. Application areas may include but are not limited to the following:
- Agriculture
- Climate change
- Homelessness
- Food security
- Mental health
- Water quality
Authors will be asked to confirm that their submissions accord with the NeurIPS code of conduct and the NeurIPS code of ethics .
Submission deadline: All submissions must be made by June 27th, 4pm EDT. The system will close after this time, and no further submissions will be possible.
We are using OpenReview to manage submissions. Papers should be submitted here . Submission will open June 1st. Submissions under review will be visible only to their assigned program committee. We will not be soliciting comments from the general public during the reviewing process. Anyone who plans to submit a paper as an author or a co-author will need to create (or update) their OpenReview profile by the full paper submission deadline.
Formatting instructions: All submissions must be in PDF format. Submissions are limited to four content pages , including all figures and tables; additional pages containing only references are allowed. You must format your submission using the NeurIPS 2024 LaTeX style file using the “preprint” option for non-anonymous submission. The maximum file size for submissions is 50MB. Submissions that violate the NeurIPS style (e.g., by decreasing margins or font sizes) or page limits may be rejected without further review. Papers may be rejected without consideration of their merits if they fail to meet the submission requirements, as described in this document.
Mentorship and collaboration: The submitted research can be a component of a larger research endeavor involving external collaborators, but the submission should describe only the authors’ contributions. The authors can also have external mentors but must disclose the nature of the mentorship. At the time of submission, the authors will be asked to describe the involvement of any mentors or external collaborators and to distinguish mentor and collaborator contributions from those of the authors. In addition, the authors may (optionally) to include an acknowledgements section acknowledging the contributions of others following the content sections of the submission. The acknowledgements section will not count toward the submission page limit.
Proof of high school attendance: Submitting authors will also be asked to upload a signed letter, on school letterhead, from each author’s high school confirming that the author was enrolled in high school during the 2023-2024 academic year.
Supplementary artifacts: In their submission, authors may link to supplementary artifacts including videos, working demonstrations, digital posters, websites, or source code. Please do not link to additional text. All such supplementary material should be wholly created by the authors and should directly support the submission content.
Review process: Each submission will be reviewed by anonymous referees. The authors, however, should not be anonymous. No written feedback will be provided to the authors.
Use of Large Language Models (LLMs): We welcome authors to use any tool that is suitable for preparing high-quality papers and research. However, we ask authors to keep in mind two important criteria. First, we expect papers to fully describe their methodology. Any tool that is important to that methodology, including the use of LLMs, should be described also. For example, authors should mention tools (including LLMs) that were used for data processing or filtering, visualization, facilitating or running experiments, or proving theorems. It may also be advisable to describe the use of LLMs in implementing the method (if this corresponds to an important, original, or non-standard component of the approach). Second, authors are responsible for the entire content of the paper, including all text and figures, so while authors are welcome to use any tool they wish for writing the paper, they must ensure that all text is correct and original.
Dual submissions: Submissions that are substantially similar to papers that the authors have previously published or submitted in parallel to other peer-reviewed venues with proceedings or journals may not be submitted to NeurIPS. Papers previously presented at workshops or science fairs are permitted, so long as they did not appear in a conference proceedings (e.g., CVPRW proceedings), a journal, or a book. However, submissions will not be published in formal proceedings, so work submitted to this call may be published elsewhere in the future. Plagiarism is prohibited by the NeurIPS Code of Conduct .
Paper checklist: In order to improve the rigor and transparency of research submitted to and published at NeurIPS, authors are required to complete a paper checklist . The paper checklist is intended to help authors reflect on a wide variety of issues relating to responsible machine learning research, including reproducibility, transparency, research ethics, and societal impact. The checklist does not count towards the page limit and will be entered in OpenReview.
Contact: [email protected]
Thank you for visiting nature.com. You are using a browser version with limited support for CSS. To obtain the best experience, we recommend you use a more up to date browser (or turn off compatibility mode in Internet Explorer). In the meantime, to ensure continued support, we are displaying the site without styles and JavaScript.
- View all journals
- Explore content
- About the journal
- Publish with us
- Sign up for alerts
- Published: 14 May 2024
2023 summer warmth unparalleled over the past 2,000 years
- Jan Esper ORCID: orcid.org/0000-0003-3919-014X 1 , 2 ,
- Max Torbenson ORCID: orcid.org/0000-0003-2720-2238 1 &
- Ulf Büntgen 2 , 3 , 4
Nature ( 2024 ) Cite this article
4952 Accesses
3363 Altmetric
Metrics details
We are providing an unedited version of this manuscript to give early access to its findings. Before final publication, the manuscript will undergo further editing. Please note there may be errors present which affect the content, and all legal disclaimers apply.
- Climate change
- Palaeoclimate
Including an exceptionally warm Northern Hemisphere (NH) summer 1 ,2 , 2023 has been reported as the hottest year on record 3-5 . Contextualizing recent anthropogenic warming against past natural variability is nontrivial, however, because the sparse 19 th century meteorological records tend to be too warm 6 . Here, we combine observed and reconstructed June-August (JJA) surface air temperatures to show that 2023 was the warmest NH extra-tropical summer over the past 2000 years exceeding the 95% confidence range of natural climate variability by more than half a degree Celsius. Comparison of the 2023 JJA warming against the coldest reconstructed summer in 536 CE reveals a maximum range of pre-Anthropocene-to-2023 temperatures of 3.93°C. Although 2023 is consistent with a greenhouse gases-induced warming trend 7 that is amplified by an unfolding El Niño event 8 , this extreme emphasizes the urgency to implement international agreements for carbon emission reduction.
This is a preview of subscription content, access via your institution
Access options
Access Nature and 54 other Nature Portfolio journals
Get Nature+, our best-value online-access subscription
24,99 € / 30 days
cancel any time
Subscribe to this journal
Receive 51 print issues and online access
185,98 € per year
only 3,65 € per issue
Rent or buy this article
Prices vary by article type
Prices may be subject to local taxes which are calculated during checkout
Similar content being viewed by others
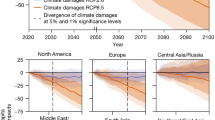
The economic commitment of climate change
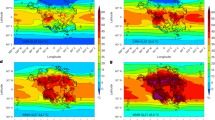
Climate extremes likely to drive land mammal extinction during next supercontinent assembly
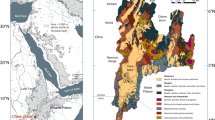
Early warning signals of the termination of the African Humid Period(s)
Author information, authors and affiliations.
Department of Geography, Johannes Gutenberg University, Mainz, Germany
Jan Esper & Max Torbenson
Global Change Research Institute of the Czech Academy of Sciences, Brno, Czech Republic
Jan Esper & Ulf Büntgen
Department of Geography, University of Cambridge, Cambridge, United Kingdom
Ulf Büntgen
Department of Geography, Masaryk University, Brno, Czech Republic
You can also search for this author in PubMed Google Scholar
Corresponding author
Correspondence to Jan Esper .
Rights and permissions
Reprints and permissions
About this article
Cite this article.
Esper, J., Torbenson, M. & Büntgen, U. 2023 summer warmth unparalleled over the past 2,000 years. Nature (2024). https://doi.org/10.1038/s41586-024-07512-y
Download citation
Received : 16 January 2024
Accepted : 02 May 2024
Published : 14 May 2024
DOI : https://doi.org/10.1038/s41586-024-07512-y
Share this article
Anyone you share the following link with will be able to read this content:
Sorry, a shareable link is not currently available for this article.
Provided by the Springer Nature SharedIt content-sharing initiative
By submitting a comment you agree to abide by our Terms and Community Guidelines . If you find something abusive or that does not comply with our terms or guidelines please flag it as inappropriate.
Quick links
- Explore articles by subject
- Guide to authors
- Editorial policies
Sign up for the Nature Briefing newsletter — what matters in science, free to your inbox daily.

- College of Engineering and Computing
- Location Location
- Contact Contact
- Colleges and Schools
- News and Events
- 2024 News Archive
Jamshidi earns recognition for most influential paper
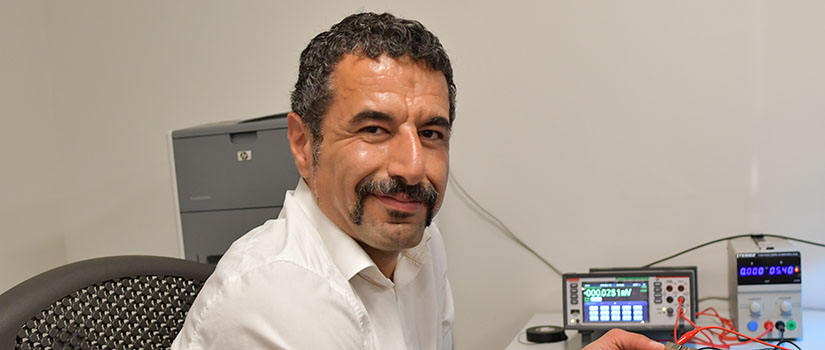
When someone in academia publishes a research paper, one of the goals is to have the paper cited by other professors and researchers. A paper published 10 years ago by Computer Science and Engineering Assistant Professor Pooyan Jamshidi was recently recognized for its significant impact.
Jamshidi received the Most Influential Paper Award in April at the 19th International Conference on Software Engineering for Adaptive and Self-Managing Systems (SEAMS) in Lisbon, Portugal. Jamshidi’s paper, “ Autonomic Resource Provision for Cloud-based Software ,” was submitted, accepted and published just prior to earning his Ph.D. from Dublin City University in Ireland in 2014. It was presented at the 2014 SEAMS Conference in India.
For the most influential paper award, a select committee considers conference publications published approximately 10 years previously and selects those that have made the most impact according to several criteria, including the number of citations, practical applications and industry adoption, and influence on subsequent research. The most influential award is selected from this short list.
“I wanted to publish the most important part of my Ph.D. research at SEAMS because it was a special community, and their work was close to mine,” Jamshidi says. “Receiving this award is important because this was my first paper with the community. I kept publishing with SEAMS and remained engaged.”
The paper’s title referred to a groundbreaking approach to fundamentally transform how resources are managed and allocated in cloud environments. The key innovation was to enable multiple tenants to describe their adaptation rules for cloud and multi-cloud resource provisioning using a specific language that enables the incorporation of reasoning, inference and resolution of conflicting adaptation rules.
Since the paper was published, it has received 188 citations according to Google Scholar . In addition, the autonomic resource provision technique has been integrated with Microsoft Azure and OpenStack . The concepts and methods introduced in the paper have also led to follow-up research in cloud autoscaling, Edge-and-Internet of Things resource scaling, and networking and autonomous driving.
The paper has impacted the field of software engineering, especially in the context of adaptive and self-managing systems in the cloud, research, industry practices and the broader technological landscape.
While Jamshidi admits that autonomous autoscaling system for cloud-based software is not as a hot topic as it was when his paper was published, it is still a relevant research area that is leading to new ideas, methods, and approaches.
“The most exciting direction in cloud auto-scaling and resource provisioning overall is sustainability-aware approaches to enable sustainable computer usage for modern applications, such as AI systems,” Jamshidi says. “We plan to continue this line of research. For example, thanks to funds provided by the National Science Foundation and collaborators from Carnegie Mellon University and Rochester Institute of Technology, we are investigating software-driven sustainability.”
Challenge the conventional. Create the exceptional. No Limits.
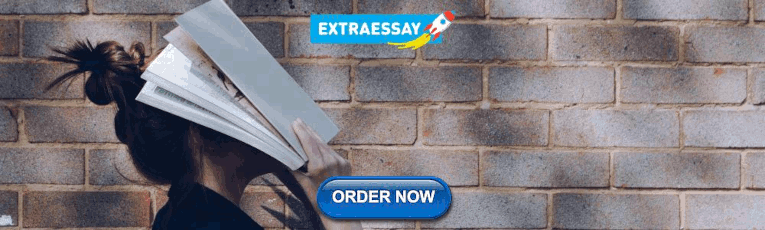
IMAGES
VIDEO
COMMENTS
Reliability is about the consistency of a measure, and validity is about the accuracy of a measure.opt. It's important to consider reliability and validity when you are creating your research design, planning your methods, and writing up your results, especially in quantitative research. Failing to do so can lead to several types of research ...
Reliability refers to the consistency of the measurement. Reliability shows how trustworthy is the score of the test. If the collected data shows the same results after being tested using various methods and sample groups, the information is reliable. If your method has reliability, the results will be valid. Example: If you weigh yourself on a ...
Revised on 10 October 2022. Reliability and validity are concepts used to evaluate the quality of research. They indicate how well a method, technique, or test measures something. Reliability is about the consistency of a measure, and validity is about the accuracy of a measure. It's important to consider reliability and validity when you are ...
Reliability and validity are among the most important and fundamental domains in the assessment of any measuring methodology for data-collection in a good research. Validity is about what an instrument measures and how well it does so, whereas reliability concerns the truthfulness in the data obtain …
Hence, the essence of reliability for qualitative research lies with consistency.[24,28] A margin of variability for results is tolerated in qualitative research provided the methodology and epistemological logistics consistently yield data that are ontologically similar but may differ in richness and ambience within similar dimensions.
Reliability refers to the consistency, dependability, and trustworthiness of a system, process, or measurement to perform its intended function or produce consistent results over time. It is a desirable characteristic in various domains, including engineering, manufacturing, software development, and data analysis. Reliability In Engineering.
Interrater reliability. Inter-rater reliability (also called inter-observer reliability) measures the degree of agreement between different people observing or assessing the same thing. You use it when data is collected by researchers assigning ratings, scores or categories to one or more variables.. Example: Inter-rater reliability In an observational study where a team of researchers collect ...
Validity. Validity is defined as the extent to which a concept is accurately measured in a quantitative study. For example, a survey designed to explore depression but which actually measures anxiety would not be considered valid. The second measure of quality in a quantitative study is reliability, or the accuracy of an instrument.In other words, the extent to which a research instrument ...
Reliability in psychology research refers to the reproducibility or consistency of measurements. Specifically, it is the degree to which a measurement instrument or procedure yields the same results on repeated trials. A measure is considered reliable if it produces consistent scores across different instances when the underlying thing being measured has not changed.
Abstract. This article examines reliability and validity as ways to demonstrate the rigour and trustworthiness of quantitative and qualitative research. The authors discuss the basic principles of ...
Despite the differences across methods for exploring reliability, the findings mostly converge, identifying similar respondent and question characteristics as major determinants of reliability. The next section of the paper looks at the correlations among estimates of reliability derived from the different methods; it finds some support for the ...
There is often a tendency to generalize research findings. Two key standards have to be met to do this. First, results apply only to the population of the study. Second, data must be collected via a probability sample, i.e. everyone eligible to be in the study has the same chance of being included in the study.
Abstract and Figures. The validity and reliability of the scales used in research are important factors that enable the research to yield healthy results. For this reason, it is useful to ...
Although the tests and measures used to establish the validity and reliability of quantitative research cannot be applied to qualitative research, there are ongoing debates about whether terms such as validity, reliability and generalisability are appropriate to evaluate qualitative research.2-4 In the broadest context these terms are applicable, with validity referring to the integrity and ...
High quality tests are important to evaluate the reliability of data supplied in an examination or a research study. Alpha is a commonly employed index of test reliability. Alpha is affected by the test length and dimensionality. Alpha as an index of reliability should follow the assumptions of the essentially tau-equivalent approach.
Learn how to estimate, test, and plan sample size for Cronbach's alpha, a widely used measure of reliability in social and organizational sciences.
Table 3 shows the profound implications of misreporting the reliability of edTPA scores. If the reliability is around 0.90, as reported in the documentation, classification consistency will be appropriate across the board. But if the actual reliability of the scores is lower, classification consistency will be substantially reduced.
Reliability analysis concept helps to compute the system availability and also increasing the mean time between failures, as different research papers are the evidence of its success. 2. The literature revealed that the number of reliability techniques has been utilized in different industries, and positive results are observed in terms of ...
The main goal of this paper is to provide statistical perspectives on the reliability of AI systems. In particular, we introduce a so-called "SMART" statistical framework for AI reliability research, including Structure of the system, Metrics of reliability, Analysis of failure causes, Reliability assessment, and Test planning.
MIT researchers' "consensus game" is a game-theoretic approach for language model decoding. The equilibrium-ranking algorithm harmonizes generative and discriminative querying to enhance prediction accuracy across various tasks, outperforming larger models and demonstrating the potential of game theory in improving language model consistency and truthfulness.
A Feature Paper should be a substantial original Article that involves several techniques or approaches, provides an outlook for future research directions and describes possible research applications. Feature papers are submitted upon individual invitation or recommendation by the scientific editors and must receive positive feedback from the ...
The research plan proposed in this paper aimed to identify the necessary components for designing a machine monitoring system in an industrial setting. 13 To achieve this, ... The decline in reliability could stem from factors such as degradation (wear), suboptimal quality control, or inadequate maintenance. ...
The research paper proposes a solution called CuMo, which stands for Co-upcycled Top-K sparsely-gated Mixture-of-experts. ... CHECKEREVAL: The third module is an extensible solution for gauging the reliability of automatic fact-checkers' verification results using human-annotated datasets.
Professor Bethany Hastie awarded UBC Killam Accelerator Research Fellowship. Professor Hastie hopes to shed light on the challenges that new technologies are raising for workers - and the gaps in current labour law and policy in Canada.
Explore the latest full-text research PDFs, articles, conference papers, preprints and more on RELIABILITY ANALYSIS. Find methods information, sources, references or conduct a literature review on ...
Papers may be rejected without consideration of their merits if they fail to meet the submission requirements, as described in this document. Mentorship and collaboration: The submitted research can be a component of a larger research endeavor involving external collaborators, but the submission should describe only the authors' contributions ...
Global Change Research Institute of the Czech Academy of Sciences, Brno, Czech Republic. Jan Esper & Ulf Büntgen. Department of Geography, University of Cambridge, Cambridge, United Kingdom. Ulf ...
According to the existing research gaps, the contributions of this research are presented as follows: (i) This study presents the operation of 33-bus and a real 59-bus distribution network allocation and scheduling using the multi-HMG structure of energy with network reconfiguration to improve reliability while taking self-healing into account ...
The concepts and methods introduced in the paper have also led to follow-up research in cloud autoscaling, Edge-and-Internet of Things resource scaling, and networking and autonomous driving. The paper has impacted the field of software engineering, especially in the context of adaptive and self-managing systems in the cloud, research, industry ...