- Open access
- Published: 08 February 2024
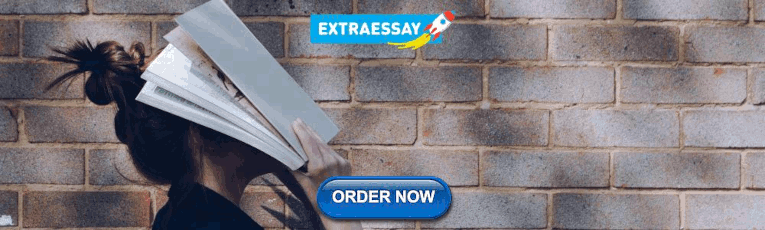
The utility of behavioral biometrics in user authentication and demographic characteristic detection: a scoping review
- O. L. Finnegan ORCID: orcid.org/0000-0003-0625-1479 1 ,
- J. W. White III 1 ,
- B. Armstrong 1 ,
- E. L. Adams 1 ,
- S. Burkart 1 ,
- M. W. Beets 1 ,
- S. Nelakuditi 2 ,
- E. A. Willis 3 ,
- L. von Klinggraeff 1 ,
- H. Parker 1 ,
- M. Bastyr 1 ,
- Z. Zhong 2 &
- R. G. Weaver 1
Systematic Reviews volume 13 , Article number: 61 ( 2024 ) Cite this article
1381 Accesses
8 Altmetric
Metrics details
Objective measures of screen time are necessary to better understand the complex relationship between screen time and health outcomes. However, current objective measures of screen time (e.g., passive sensing applications) are limited in identifying the user of the mobile device, a critical limitation in children’s screen time research where devices are often shared across a family. Behavioral biometrics, a technology that uses embedded sensors on modern mobile devices to continuously authenticate users, could be used to address this limitation.
The purpose of this scoping review was to summarize the current state of behavioral biometric authentication and synthesize these findings within the scope of applying behavioral biometric technology to screen time measurement.
We systematically searched five databases (Web of Science Core Collection, Inspec in Engineering Village, Applied Science & Technology Source, IEEE Xplore, PubMed), with the last search in September of 2022. Eligible studies were on the authentication of the user or the detection of demographic characteristics (age, gender) using built-in sensors on mobile devices (e.g., smartphone, tablet). Studies were required to use the following methods for authentication: motion behavior, touch, keystroke dynamics, and/or behavior profiling. We extracted study characteristics (sample size, age, gender), data collection methods, data stream, model evaluation metrics, and performance of models, and additionally performed a study quality assessment. Summary characteristics were tabulated and compiled in Excel. We synthesized the extracted information using a narrative approach.
Of the 14,179 articles screened, 122 were included in this scoping review. Of the 122 included studies, the most highly used biometric methods were touch gestures ( n = 76) and movement ( n = 63), with 30 studies using keystroke dynamics and 6 studies using behavior profiling. Of the studies that reported age (47), most were performed exclusively in adult populations ( n = 34). The overall study quality was low, with an average score of 5.5/14.
The field of behavioral biometrics is limited by the low overall quality of studies. Behavioral biometric technology has the potential to be used in a public health context to address the limitations of current measures of screen time; however, more rigorous research must be performed in child populations first.
Systematic review registration
The protocol has been pre-registered in the Open Science Framework database ( https://doi.org/10.17605/OSF.IO/92YCT ).
Peer Review reports
Introduction
Screen time is a critical health behavior related to a variety of health outcomes in children [ 1 , 2 , 3 , 4 , 5 , 6 ]. Historically, measuring screen time has been reliant on self-report or proxy-report measures [ 7 ], due in part to the nature of digital media consumption (e.g., in-home computer and TV use). The introduction of mobile devices (tablets, smartphones) has substantially altered the landscape of digital media consumption, and these devices have become the favored media choice for children due to their portability, interactivity, and capacity to stream a wide range of content [ 8 , 9 , 10 , 11 ]. Self-report measures are of limited validity in assessing mobile screen use due to the intermittent and on-demand use of mobile devices, which hamper one’s ability to retrospectively report screen time [ 7 , 12 , 13 ]. In addition to not being sensitive enough to sufficiently capture all mobile screen use, self-report measures are also subject to recall bias and social desirability bias [ 14 , 15 ]. Given the proliferation of mobile devices [ 8 , 10 ], there has been a growing demand to advance our current screen time measures to more effectively capture mobile screen use [ 16 ], specifically using objective measures [ 17 ].
Researchers have begun to use passive sensing applications (e.g., Chronicle) to overcome the limitations of subjective reports and which unobtrusively monitor mobile screen use on mobile devices [ 13 , 18 ]. Chronicle is an Android passive sensing application that tracks the duration, frequency, and timing of data, general application type, and application status (foreground vs. background) using Google API every 15 s [ 13 ]. Benefits of passive sensing applications include a reduced researcher and participant burden compared to self-report measures and lower cost for researchers to employ. However, while this data can be relevant for tracking the duration of use and the context of use, these passive sensing applications are not able to capture who specifically is using the device. For child screen time research, this limitation in identifying the user of a device is of particular concern as mobile devices are often shared between siblings or between the parent and the child [ 12 , 19 ]. Therefore, identifying the user of the device is critical to optimizing the potential for passive sensing methods in tracking objective screen use metrics in children.
Behavioral biometrics could be used to address this shortcoming of objective screen time measurement by identifying users of mobile devices. Modern mobile devices contain a variety of sensors (e.g., accelerometer, gyroscope, magnetometer, touch) that collect multiple data streams and can provide characteristic information about the user. These sensors provide the basis for behavioral biometric authentication [ 20 , 21 , 22 ]. Unlike physiological biometrics (e.g., fingerprint, iris, facial recognition), behavioral biometrics do not require additional hardware in modern mobile devices [ 23 , 24 ], making it a feasible research tactic for screen time measurement. Additionally, behavioral biometrics can provide continuous user authentication, whereas physiological biometrics are typically a one-time authentication for gaining access to a device [ 23 ]. There are several types of behavioral biometrics used for authentication, including behavior profiling, keystroke dynamics (typing dynamics), touch dynamics, and motion behavior [ 23 ]. Behavior profiling uses data such as the type of applications being used and battery life (host-based approach) as well as calls, texts, and GPS location (network-based approach) for user authentication [ 21 ]. This type of authentication has been used for fraud detection systems, in which unusual activities (e.g., calls, texts) and a new location can identify device theft and subsequently initiate a fraud protection mechanism [ 25 ]. Keystroke dynamics involves the characteristic way in which an individual types, specifically identifying the habitual typing pattern [ 21 ]. There are two types of keystroke dynamics, including static text, which analyzes a fixed text (e.g., a password), and dynamic text, which analyzes free-living text from participants [ 26 ]. Keystroke dynamics have largely been used for fraud detection and for authentication into computers or applications [ 26 ]. Touch dynamics, or touch gestures, evaluates touch strokes (size, length, speed, pressure, direction) and their corresponding coordinates on the touchscreen of a phone. Authentication using touch dynamics began as mobile devices were developed without a physical keyboard and rather a touchscreen [ 20 ]. Lastly, motion behavior authentication relies on the distinct movement patterns of individuals holding and interacting with a mobile device [ 27 , 28 ].
Data produced by these sensors can be harnessed without additional hardware, evidenced by the growing body of research in the field of behavioral biometric authentication [ 21 , 24 , 29 ]. In child screen time research, employing continuous user identification may prove useful, especially when the device is being shared among a child and their family. Furthermore, applying behavioral biometric technology to screen time may be a relatively inexpensive solution, as it leverages built-in technology [ 24 ]. These benefits of behavioral biometrics are important attributes to consider when applying this technology to other contexts.
Behavioral biometric authentication is a highly established field of literature within cybersecurity; however, this technology has not yet been applied to objective screen time measurement research, to continuously identify the user of the mobile device [ 21 , 30 ]. In order to begin applying this technology to screen time measurement, it is important to have an updated understanding of behavioral biometric technology and fit this updated understanding within the perspective of screen time research. The purpose of this scoping review was to first summarize the current state of behavioral biometric authentication, including identifying the behavioral biometric methods and data streams used, the characteristics predicted, and the model evaluation metrics used. This review also sought to characterize these findings within the scope of applying behavioral biometric technology to address the critical limitations of current measures of screen time to provide future directions for applying this technology to a public health context.
This systematic review was conducted in accordance with the Preferred Reporting Items for Systematic Reviews and Meta-Analyses extension for Scoping Reviews (PRISMA-ScR) Checklist [ 31 ] and was pre-registered in the Open Science Framework database ( https://doi.org/10.17605/OSF.IO/92YCT ).
Information sources, search, and screening
Literature searches were conducted in Web of Science Core Collection, Inspec in Engineering Village, Applied Science & Technology Source, IEEE Xplore, and PubMed, all of which were selected for their relevance to the topic and database size. The final database search was conducted on September 19, 2022. All authors and collaborators discussed the search strategy and the query strings specific to each database. Searches used keywords: smart device, tablet, phone, smartphone, handphone, mobile, Android, iOS, sensor, accelerometer, gyroscope, magnetometer, touch, biometric, hand, motion, move, swipe, keystroke, detect, verify, authenticate, infer, predict, determine, and classify, with Boolean operators, wildcard, and truncation used. The comprehensive list of search terms with notation specific to each database can be found in Additional file 2 : Supplementary Table 2. The primary author (OF) performed the initial search. The search yielded 6,161 results from Web of Science Core Collection, 11,181 results from Inspec, 787 from Applied Science & Technology Source, 3584 from IEEE Xplore, and 823 from PubMed, for a total of 22,537 studies. References were exported to EndNote (Clarivate, London, UK), where an initial duplicate screen was completed using the “remove duplicates” function. Following this, references were exported to Covidence (Melbourne, Australia) for title and abstract screening, where duplicates were also removed, bringing the total studies for title and abstract screening down to 14,179. The primary author (OF) and an additional research assistant screened the titles and abstracts of the 14,179 studies on Covidence. Both reviewers established quality control of their screening process prior to independently screening the articles. This was done by screening 600 of the same articles independently and ensuring reviewers had consistency above 80%. Consistency between reviewers was met (99.9%) and then reviewers divided the remaining articles and independently screened the title and abstracts of those articles. Following title and abstract screening, 13,972 articles were excluded, and 207 articles were left for full text retrieval and screening. Four articles were not able to be located using the Interlibrary Loan (ILL) service; therefore, 203 articles were retrieved for eligibility assessment. The primary author (OF) reviewed the full texts of the 203 articles to assess whether these articles fully met the predefined inclusion and exclusion criteria. Of the 203 articles, 122 articles were considered eligible for inclusion and were extracted (Fig. 1 ).
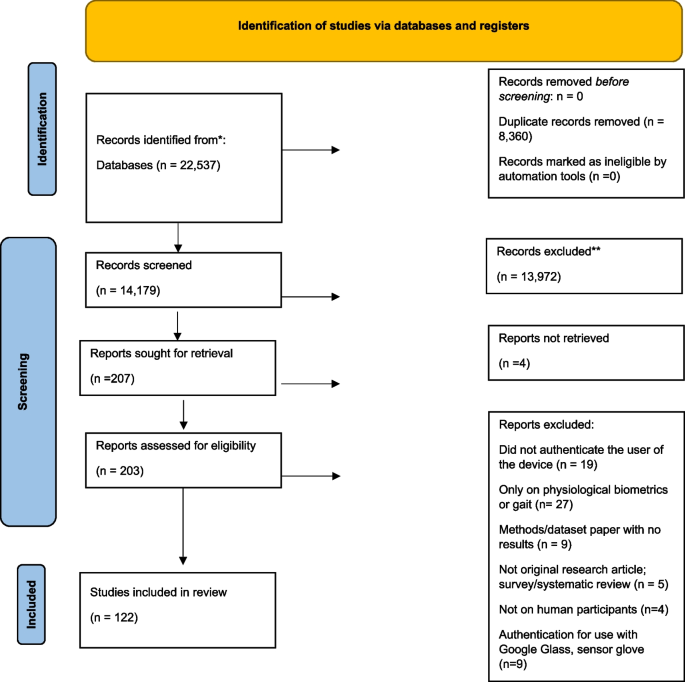
PRISMA flowchart
Eligibility criteria
Studies were required to focus on the sensors of mobile devices, defined as tablets or smartphones [ 32 ]. These sensors needed to be built-in to the device, including but not limited to motion sensors, accelerometer, gyroscope, magnetometer, and touch. Studies were eligible if they used these sensors for verification, detection, and/or authentication of the device user. Using an adapted version of the Meng 2015 [ 23 ] framework of biometric authentication, articles were required to focus on behavior profiling, keystroke dynamics (typing dynamics), touch dynamics, or motion behavior. Because the first smartphone (i.e., iPhone) was released in 2007 [ 33 ], and modern mobile tablets were developed after this in 2010, only articles after 2007 were included. Articles in a peer-reviewed academic source and published in English were eligible for inclusion. Articles that simulated data and did not collect data on human participants were excluded. Studies were excluded if they used other technology and/or required additional equipment beyond the mobile device (e.g., sensor glove, stylus) for verification. These articles were excluded because of their limited applicability to screen time measurement, as the goal of applying this technology would be to capture the typical way in which the child is interacting with their shared device. Articles that evaluated smart watches, fitness tracking devices, or wearable sensors were excluded. These articles were excluded because the tablet and smartphone are the preferred choice for digital media consumption in children [ 34 ] and are more closely related to health outcomes (e.g., sleep) [ 35 ]. Lastly, while the purpose of this review was to characterize these findings within the lens of child screen time measurement, we did not limit our search to only include studies on children. We included studies on adult populations since it is a relatively newer field and area of application to children and to inform future research on child populations from the current literature on adult biometric authentication.
Data extraction
The primary author (OLF) extracted study characteristics (sample size, age, gender), data collection methods, data stream, model evaluation metrics, and performance of models from the 122 studies. All extracted data was reviewed by a second author (RGW).
Study characteristics
The extraction of study characteristics included details on the sample population, including sample size, gender distribution (number of female participants), and age (mean, standard deviation, and range). There were studies in this review that used freely available dataset(s) for their sample ( n = 27), with several studies compiling data from more than one dataset ( n = 8). Studies that used publicly available datasets for their sample are presented with a superscript letter in Table 1 . Each superscript letter refers to a specific database, with several repeating databases used across studies, as depicted in Table 1 . For studies using more than one dataset for their sample, sample sizes of the datasets were pooled, and number of female participants (gender distribution) were pooled. Additionally, for studies that used more than one dataset, we compiled the age ranges into one comprehensive age range across all included datasets. Device brand (iOS or Android) and outcome predicted (identity, age, or gender) were also extracted from the studies. The protocol of each study included was evaluated to determine whether it was a free-living or in-lab protocol. We defined in-lab protocols as those completed in a researcher-supervised controlled setting, while free-living refers to protocols in which participants use the device in their typical environment (e.g., home, work). Furthermore, we extracted whether the protocol was structured or free-use. We defined structured protocols as those in which the researchers give the participant a specific task to complete on the device, such as a questionnaire, a game, or using a particular application. Free-use protocols refer to protocols in which the participant can interact with the device in their normal manner and select which applications they use, with no restrictions from the researchers.
Extraction of study characteristics also included identifying the biometric method(s) employed for authentication of the user and/or detection of demographic characteristics. We first recorded the biometric method described by each study in the precise language used by the authors. Given the lack of standardized terminology in the field of biometric authentication, these methods needed to be condensed into broader categories. The categories for biometric methods were consolidated into four categories, with agreement from all authors. These categories included movement (encompassing hand movement, arm gesture, hand gesture, and posture), behavior profiling , keystroke dynamics , and touch gestures .
Data stream
Extraction of study characteristics also included the identification of the specific data stream(s), or sensors, used for biometric authentication. We first recorded the data stream(s) used in each study using the precise language used by the authors. Similar to categorizing biometric methods, the categories for data streams also needed to be condensed to broader categories of similar characteristics. These categories included accelerometer (gravity, linear acceleration), orientation , gyroscope (rotation, angular velocity), touch , location , magnetometer , and other (ambient light, Bluetooth, temperature, proximity, application usage, power).
Model evaluation metrics
Extraction of study characteristics also included identifying the model evaluation metric(s) used in each study. We first identified the evaluation metric described in each study using the precise language used by the authors. Metrics were condensed into broader categories given the lack of consistent terminology in machine learning model performance metrics. These categories included area under the curve (receiver operating characteristic), equal error rate (EER), precision , recall (sensitivity, true acceptance rate, true positive rate), false rejection rate (FRR, false negative rate), false acceptance rate (FAR, false positive rate, “false alarm rate”), accuracy (correct recognition rate, mean recognition rate, success rate), F1 score (F-measure), and other (kappa, root mean square error H-mean, detection error tradeoff curve, specificity/true rejection rate, average match rate, mean square error rate, average number of impostor actions, and average number of genuine actions).
Quality assessment
The quality of the included studies was assessed using an adapted framework from Papi 2017 [ 158 ], which is a research quality scale specific to the field of engineering with a focus on sensor technology (Additional file 1 : Supplementary Table 1). The primary author (OLF) assessed the study quality of all 122 studies. Each question was scored as either 1, meeting the criteria, or 0, not meeting the criteria. Composite quality assessment scores were calculated by adding together the number of criteria met, with a score of 14 meaning that the study was of highest quality and a score of 0 meaning that the study was of lowest quality.
Data analysis
The characteristics of the included studies were tabulated in Excel (Microsoft, Version 2304). We then compiled summary statistics in Excel to describe our findings. Means and standard deviations were calculated for sample size and gender distribution across all studies.
Across all 122 studies, sample sizes ranged from 1 to 2221 participants, with an average of 89 participants per study (± 224.2). Android was the most common operating system, with 89 studies (73%) using Android devices for their protocol(s). The iOS operating system was used in 5 (4%) of protocols and the remaining 28 studies (23%) did not report the operating system used. Most of the studies ( n = 112, 92%) identified the specific user of the device, while 5 (4%) studies aimed to detect the gender of the user and 7 studies (6%) aimed to detect the age/age group of the user. For the study setting, most study protocols were conducted in a lab setting ( n = 99, 81%), while fewer studies were carried out in a free-living environment ( n = 17, 14%), one study used both lab and free-living settings, and 5 studies (4%) did not provide sufficient information to determine study setting. Most protocols were structured ( n = 96, 79%), with specific guidance and directions given to the participants on how to interact with the device (e.g., playing a game, watching a specific video). Few studies ( n = 19, 16%) allowed participants to interact with the device in their normal manner, considered “free use” of the device, one study had both structured and free-use, and 6 studies (5%) did not provide sufficient information to determine protocol format. Many studies did not report demographic characteristics of the sample; 75 (61%) did not report gender, 70 (57%) did not report age, and none reported race/ethnicity. Of those that did report gender, on average, the distribution of female participants was 39% of the sample. Of the 122 studies, 75 studies (61%) did not report an age range, 34 studies (28%) had a sample of adults, and 13 studies (11%) had age ranges that included children (< 18 years).
Of the 122 studies included in this review, 63 (52%) used movement (e.g., hand movement, hand or arm gesture and posture) as their biometric method for authentication. Thirty studies (25%) used keystroke dynamics for biometric authentication, while 76 studies (62%) used touch gestures. Behavior profiling, such as app usage, battery, and WiFi, was used in 7 studies (6%) for biometric authentication.
Touch was the most extensively used data stream, with 93 studies using touch behavior for biometric authentication. The accelerometer sensor was the second most frequently used sensor of this review, with n = 68 studies (56%). Other data streams employed include gyroscope ( n = 46 studies, 38%), orientation ( n = 9 studies, 7%), location ( n = 8 studies, 7%), and magnetometer ( n = 22 studies, 18%). As depicted in Additional file 3 : Supplementary Table 3, all other data streams that were used in less than 3 studies were combined into an “Other” category. These included ambient light ( n = 3, 2%), Bluetooth ( n = 3, 2%), temperature ( n = 1), proximity ( n = 3, 2%), application usage ( n = 1), power/battery level ( n = 2), motion quaternion ( n = 1), directional heading ( n = 1), and heat map ( n = 1).
When evaluating the performance of their models, the included studies used a wide range of evaluation metrics. Equal error rate (EER) and accuracy were the most highly used evaluation metrics, with 57 studies (47%) using EER and 56 studies (46%) using accuracy. Following EER and accuracy, false rejection rate (FRR) ( n = 42 studies, 34%) and false acceptance rate (FAR) ( n = 47 studies, 39%) were also highly used to evaluate model performance. Area under the curve (AUC) and the receiver operating characteristic curve (ROC) were used in 20 studies (16%). Recall/sensitivity ( n = 20, 16%), F1 score ( n = 14, 11%), and precision ( n = 10, 8%) were also frequently used among the included studies. As depicted in Additional file 3 : Supplementary Table 3, all other model evaluation metrics that were used in less than 4 studies were combined into an “Other” category. These included kappa ( n = 2), root mean square error (RMSE) ( n = 1), H-mean ( n = 1), detection error tradeoff (DET) curve ( n = 4, 3%), specificity/true rejection rate ( n = 3), average match rate ( n = 1), mean square error rate ( n = 1), average number of impostor actions (ANIA) ( n = 2), and average number of genuine actions (ANGIA/ANGA) ( n = 2).
Quality of the included studies
The average quality score of the included studies was 5.5 out of 14, with a high score of 11 and a low score of 3. The most commonly met criteria were #11, reporting main outcomes, with 122 out of 122 studies meeting this criterion, and #1, clearly stating research objectives, with 121 out of 122 studies meeting this criterion. Most studies also met the criteria for #12, reporting the main findings ( n = 119 studies), and for #13, clearly describing and justifying the statistical tests ( n = 118 studies). The selection of sensors (#9) was appropriately justified in 65 studies, while data handling was clearly described (#10) in 35 studies. Only some studies met the criteria for #14, clearly describing the limitations ( n = 33), or met the criteria for #8, clearly describing the equipment design ( n = 23). Few studies of this review met the criteria for #3, adequately describing the study population ( n = 19), as many did not report demographic characteristics such as age and gender. Only 8 out of the 122 studies met the criteria for #5, appropriately describing the sampling methodology, and only 7 out of the 122 studies met the criteria for #7, providing detailed methods that could be replicated. None of the included studies met the criteria for #4, specifying eligibility criteria, and for #6, providing a rationale for the sample size.
Behavioral biometrics have the potential to improve screen time measurement because researchers can capitalize on built-in mobile device sensors to determine who is using the device at specific time points to address a critical limitation in child screen time research. This scoping review sought to summarize the current state of behavioral biometric authentication, including identifying the behavioral biometric methods used, the data streams used, the characteristics predicted, and the model evaluation metrics used. On a larger scale, this updated understanding of the methodology of behavioral biometric studies can inform future research applying this technology to a public health context.
Overall, in the 122 included studies, the most highly used behavioral biometric methods were touch gestures and movement. The most highly used data streams for behavioral biometric authentication were touch and accelerometry. Motion sensors, such as accelerometer, gyroscope, and magnetometer, are straightforward to access and record with a sensor tracking application (e.g., Sensor Log) on mobile devices. Using touch sensors presents more challenges, both in terms of accessing this sensor stream as well as the privacy and security concerns of participants. Several of the studies using touch in this review used their own gaming application that only tracked touch behavior while the participant was using the application, which has limited applicability to screen time measurement, as it only records touch behavior during the use of one application. In addition to challenges in accessing this sensor stream, there are privacy concerns, as research participants may not feel comfortable with sensor data from their devices being collected continuously. While collecting motion behavior may not be as much of a concern, there may be a particular concern in tracking touch sensor data when using banking applications or typing passwords (e.g., concerns in researchers deciphering passwords). Therefore, while touch is a highly used behavioral biometric method, it may have more limited applicability to screen time measurement when compared to motion sensors (e.g., accelerometer, gyroscope, magnetometer).
Most behavioral biometric authentication studies in this review aimed to identify the user of the device, with fewer studies aiming to detect demographic characteristics, such as age and gender. Studies that used behavioral biometrics to detect age were designed to tailor technology interfaces towards children (e.g., widget layout) and to improve parental control options. Similarly, in studies examining the ability of behavioral biometrics to determine gender, their objective was to adapt interfaces to be more relevant for the user. Based on current evidence, behavioral biometrics are less accurate at detecting demographic characteristics compared to detecting a unique user [ 159 ]. It is likely more challenging to identify similar characteristics in user behavior across a group of individuals, as user interaction can vary substantially on an inter-individual level [ 60 , 159 ]. Relative to applied screen time measurement in a public health context, detecting the age of the user may be a relevant finding to distinguish between the parent and the child when they are sharing the device. However, the ability to detect only the age of a user would not be as useful when a child shares a device with a sibling of a similar age. Thus, determining the unique identity of a user of the device rather than demographic characteristics would be more relevant for research purposes.
Furthermore, of the included studies, a majority of studies used samples of adult participants, with fewer studies tested on samples of children. The lack of research on children highlights a gap in the literature, as there are inherent behavioral differences in the ways in which children interact with mobile devices compared to adults [ 159 ] (e.g., children are more active), and findings from adult studies cannot be universally applied to children. Therefore, we need additional research on biometrics among children before applying this technology to measure children’s screen time.
The most popular model evaluation metrics used in the included studies were equal error rate (EER), accuracy, false acceptance rate (FAR), and false rejection rate (FRR). There were a wide range of model evaluation metrics used across studies, with several reporting the same metric under different terms. For example, several studies used the term “Correct Recognition Rate,” instead of accuracy and “False Positive Rate” instead of false acceptance rate. This highlights a lack of standardization in terminology that is consistent across the field of behavioral biometric authentication, which limits our ability to compare findings across studies.
Of the studies included in this review, the average study quality was low (5.5/14), highlighting the lack of proper reporting in many of the studies in the field of behavioral biometric authentication. Overall, most authors did not provide sufficient information on equipment design, study population, sampling methodology, and eligibility criteria. Very few authors provided adequate justification for their sample size. The insufficiency in reporting key elements of study design limits the ability to replicate these findings in other samples and contexts. Furthermore, the lack of standardization in the terminology used across studies hampers the ability to make larger conclusions on the efficacy of behavioral biometrics and their application in the measurement of children’s screen time.
Behavioral biometric tools and innovative directions for future research
Though the purpose of this review is to examine the current scope of literature on behavioral biometrics through the lens of its application to public health (i.e., screen time measurement), it is necessary to also distinguish this from the domain of behavioral biometrics research for security. Given the vast amount of sensitive information stored on mobile devices, secure user authentication has become a prominent concern and a highly studied concept over recent years [ 22 , 160 , 161 ]. User authentication has shifted from “what you know,” such as an ID, PIN, or password, to “what you are,” or biometric authentication, with behavioral biometrics referring to the specific user-device interaction. A specific framework developed by Bo and colleagues in 2013, SilentSense, provides a touch-based biometrics model that leverages touch events from the system API [ 162 , 163 ]. This tool additionally integrated movement into its scheme, presenting a multi-modal authentication method. Another more recent development in behavioral biometrics is the generation of behavioral biometric datasets using engaging tools [ 164 , 165 ]. There have been challenges in collecting biometric data on participants due to the long protocols necessary to capture sufficient amount of data [ 164 ]. Therefore, researchers have developed gaming applications that collect a variety of behavioral biometric data (e.g., keystroke dynamics, touch gestures, motion) [ 164 , 165 ]. BrainRun, developed by Papamichail and colleagues, is a cognitive skills gaming application that collects touch data. BioGames, developed by Stylios and colleagues, is also a gaming application that collects touch, motion, and keystroke data [ 164 ]. These applications are important tools in the feasible generation of large-scale behavioral biometric data. Lastly, a challenge within behavioral biometrics research is the power usage concerns on mobile devices, particularly for continuous authentication methods. In future behavioral biometrics research, power consumption of individual applications should be monitored to ensure that the authentication application is not substantially impacting the device battery. Power consumption of individual applications can be monitored using a method from Murmuria and colleagues that uses per-subsystem time shares from the operating system, which can provide clarity on the feasibility of deploying behavioral biometric methods in a larger research context [ 166 ].
Advantages and disadvantages of the behavioral biometric methods
As these behavioral biometric methods have been highly studied and applied for use within the field of cybersecurity, this work has highlighted some of the advantages and disadvantages associated with each of these methods. While all methods are subject to privacy concerns [ 30 ], behavior profiling in particular has been scrutinized for its reliance on sensitive and private data (e.g., calls, texts, location). However, an advantage of behavior profiling is that unlike other methods (e.g., keystroke dynamics), it does not require the user to perform a specific activity for authentication [ 25 ]. A disadvantage of keystroke dynamics is that its accuracy for user authentication can be impacted by factors including injury, psychological state (e.g., stress), and distraction [ 167 ]. Additionally, the way in which an individual types on a keyboard is considered less permanent than other traits, such as physiological biometrics (e.g., facial and fingerprint recognition) [ 167 ]. However, relative to other authentication methods, keystroke dynamics is relatively low cost and does not rely on external hardware. Additionally, the way in which an individual types is challenging to replicate; therefore, this method can detect impostors more effectively [ 167 ]. An advantage of touch dynamics authentication is that the user does not need to complete a specific task for authentication; rather, this method works continuously in the background [ 20 ]. However, a disadvantage could be identifying the most salient features for user authentication, as using a large number of touch features increases data size and subsequently can slow down authentication speed [ 23 ]. Lastly, motion authentication can be impacted by behavioral variability, as this type of authentication is reliant on the user to interact with the device similarly over time [ 168 ]. However, similar to other methods, motion authentication can be an unobtrusive authentication method [ 168 ], and there may be less privacy concerns compared to touch-based authentication.
Methodological considerations and implications for future research
Subsequent research should examine the effectiveness of behavioral biometrics to determine the user of the device among children across development. Most of the studies included in this review exclusively used adult samples, which has limited applicability to child screen time research. The present review also highlighted the lack of studies being done on iOS devices (iPhone, iPad) in the field of behavioral biometrics. This is a limitation of the field because iOS use is highly prevalent, as 55% of tablets in the USA are iPads [ 169 ]. In 2022, over 50% of smartphone owners in the USA used an iPhone, surpassing Android for the first time in history [ 170 ]. A majority of the studies ( n = 85) tested Android devices, with only 5 studies using an iOS operating system, warranting further testing on a diversity of devices, including both iOS and Android.
When applying this technology to objective screen time measurement, participants may be apprehensive about researchers tracking mobile device usage data. However, there are practices in place to reduce concerns with tracking technology. Specifically with the passive-sensing application Chronicle, data are not associated with IP addresses or phone numbers and only indicate the type of application used (e.g., educational, social media), not the information on websites visited or the content of messages and emails. Parents are comfortable with using passive sensing technology when participating in a research study, as indicated by a feasibility study reporting no dropouts due to privacy concerns in using this technology [ 171 ]. While passive sensing applications have been shown to be accepted for use by families, future research can examine the extent to which families are comfortable with sensor tracking technology (e.g., accelerometer, gyroscope, touch) continuously monitoring user behavior on shared mobile devices. Prior to employing this technology in screen time measurement on a large scale, a necessary first step is to determine the feasibility and acceptability of this technology for families participating in research.
Additionally, research using this technology to measure screen time should consider the storage and battery life concerns inherent to using mobile device usage data. The computational burden of running applications to track sensor data may impact the feasibility of longitudinally monitoring screen time behavior in children [ 30 ]. Selecting the appropriate sensor tracking application and sampling frequency to use, as well as only recording sensor data when the device is unlocked must be a priority for researchers using behavioral biometric technology for screen time research [ 159 ].
Lastly, within the field of behavioral biometric authentication, there is a necessity to standardize the terminology used to describe various elements of behavioral biometrics. The lack of uniform language needs to be addressed to apply this technology on a larger scale. A way in which the field of behavioral biometrics can move towards more cohesive language is by adopting best-practice guidelines for reporting performance metrics, similar to the fields of physical activity measurement [ 172 ] and sleep measurement [ 173 ].
The present review has several strengths, including a comprehensive review of the current state of behavioral biometric authentication. This provided an updated evaluation of the most highly used behavioral biometric methods, data streams, and model evaluation metrics. The current review is limited by the low quality of the included studies and the lack of consistency in the terminology used across studies. Given the lack of standardization in model evaluation metrics, we were unable to sum results across studies and use meta-analytic methods to evaluate the overall efficacy of behavioral biometrics in identifying the user of a device. Furthermore, a limitation of the current review is the narrow focus on behavioral biometrics (touch, accelerometry, behavioral profiling) and not including studies on physiological biometrics. While physiological biometrics presents an important tool in authentication, these sensors (e.g., camera, video) are not freely available and feasible to use in public health research. Despite these limitations, behavioral biometric technology highlights a window of opportunity, as it shows the initial potential to harness sensor data to identify the user of a device. This review can inform future research applying behavioral biometric technology to contexts outside of cybersecurity and to address the limitations of objective measures of screen time.
Availability of data and materials
Not applicable; no datasets were generated or analyzed during the current study.
Domingues-Montanari S. Clinical and psychological effects of excessive screen time on children: effects of screen time on children. J Paediatr Child Health. 2017;53(4):333–8. https://doi.org/10.1111/jpc.13462 .
Article PubMed Google Scholar
Liu W, Wu X, Huang K, et al. Early childhood screen time as a predictor of emotional and behavioral problems in children at 4 years: a birth cohort study in China. Environ Health Prev Med. 2021;26(1):3. https://doi.org/10.1186/s12199-020-00926-w .
Article PubMed PubMed Central Google Scholar
Saunders TJ, Vallance JK. Screen time and health indicators among children and youth: current evidence, limitations and future directions. Appl Health Econ Health Policy. 2017;15(3):323–31. https://doi.org/10.1007/s40258-016-0289-3 .
Tezol O, Yildiz D, Yalcin S, et al. Excessive screen time and lower psychosocial well-being among preschool children. Arch Pediatr. 2022;29(1):61–6. https://doi.org/10.1016/j.arcped.2021.10.003 .
Article CAS PubMed Google Scholar
Webster EK, Martin CK, Staiano AE. Fundamental motor skills, screen-time, and physical activity in preschoolers. J Sport Health Sci. 2019;8(2):114–21. https://doi.org/10.1016/j.jshs.2018.11.006 .
Muppalla SK, Vuppalapati S, Reddy Pulliahgaru A, Sreenivasulu H. Effects of excessive screen time on child development: an updated review and strategies for management. Cureus. https://doi.org/10.7759/cureus.40608 . Published online June 18, 2023.
K. Kaye L, Orben A, A. Ellis D, C. Hunter S, Houghton S. The conceptual and methodological mayhem of “screen time.” IJERPH. 2020;17(10):3661. https://doi.org/10.3390/ijerph17103661 .
Kabali HK, Irigoyen MM, Nunez-Davis R, et al. Exposure and use of mobile media devices by young children. Pediatrics. 2015;136(6):1044–50. https://doi.org/10.1542/peds.2015-2151 .
Radesky JS, Schumacher J, Zuckerman B. Mobile and interactive media use by young children: the good, the bad, and the unknown. Pediatrics. 2015;135(1):1–3. https://doi.org/10.1542/peds.2014-2251 .
Computer and Internet Use in the United States: 2018. Published online April 21, 2021. https://www.census.gov/newsroom/press-releases/2021/computer-internet-use.html#:~:text=Smartphones%20were%20present%20in%2084,ownership%20fell%20behind%20at%2063%25 .
Auxier B, Anderson M, Turner E. Children’s engagement with digital devices, screen time. Published online July 28, 2020.
Radesky JS, Weeks HM, Ball R, et al. Young children’s use of smartphones and tablets. Pediatrics. 2020;146(1):e20193518. https://doi.org/10.1542/peds.2019-3518 .
Barr R, Kirkorian H, Radesky J, et al. Beyond screen time: a synergistic approach to a more comprehensive assessment of family media exposure during early childhood. Front Psychol. 2020;11:1283. https://doi.org/10.3389/fpsyg.2020.01283 .
Guo N, Luk TT, Wang MP, et al. Self-reported screen time on social networking sites associated with problematic smartphone use in Chinese adults: a population-based study. Front Psychiatry. 2021;11:614061. https://doi.org/10.3389/fpsyt.2020.614061 .
Sewall CJR, Bear TM, Merranko J, Rosen D. How psychosocial well-being and usage amount predict inaccuracies in retrospective estimates of digital technology use. Mob Media Commun. 2020;8(3):379–99. https://doi.org/10.1177/2050157920902830 .
Article Google Scholar
Understanding how digital media affects child development. NIH Eunice Kennedy shriver national institute of child health and human development. 2023. https://www.nichd.nih.gov/about/org/od/directors_corner/prev_updates/digital-media-child-development-feb2023 .
Perez O, Garza T, Hindera O, et al. Validated assessment tools for screen media use: a systematic review. Karakulah AS, ed. PLoS One. 2023;18(4):e0283714. https://doi.org/10.1371/journal.pone.0283714 .
Article CAS PubMed PubMed Central Google Scholar
Domoff SE, Banga CA, Borgen AL, et al. Use of passive sensing to quantify adolescent mobile device usage: feasibility, acceptability, and preliminary validation of the eMoodie application. Human Behav Emerg Tech. 2021;3(1):63–74. https://doi.org/10.1002/hbe2.247 .
Livingstone S, Mascheroni G, Dreier M, Chaudron S, Lagae K. How parents of young children manage digital devices at home: the role of income, education, and parental style. London: EU Kids Online, LSE; 2015. ISSN 2045-256X.
Teh PS, Zhang N, Teoh ABJ, Chen K. A survey on touch dynamics authentication in mobile devices. Comput Secur. 2016;59:210–35. https://doi.org/10.1016/j.cose.2016.03.003 .
Mahfouz A, Mahmoud TM, Eldin AS. A survey on behavioral biometric authentication on smartphones. J Inf Secur Applic. 2017;37:28–37. https://doi.org/10.1016/j.jisa.2017.10.002 .
Ibrahim TM, Abdulhamid SM, Alarood AA, et al. Recent advances in mobile touch screen security authentication methods: a systematic literature review. Comput Secur. 2019;85:1–24. https://doi.org/10.1016/j.cose.2019.04.008 .
Meng W, Wong DS, Furnell S, Zhou J. Surveying the development of biometric user authentication on mobile phones. IEEE Commun Surv Tutorials. 2015;17(3):1268–93. https://doi.org/10.1109/COMST.2014.2386915 .
Abdulhak SA, Abdulaziz AA. A systematic review of features identification and extraction for behavioral biometrie authentication in touchscreen mobile devices. In: 2018 20th International Conference on Advanced Communication Technology (ICACT). New York: IEEE; 2018. p. 68–73. https://doi.org/10.23919/ICACT.2018.8323648 .
Li F, Clarke N, Papadaki M, Dowland P. Active authentication for mobile devices utilising behaviour profiling. Int J Inf Secur. 2014;13(3):229–44. https://doi.org/10.1007/s10207-013-0209-6 .
Pisani PH, Lorena AC. A systematic review on keystroke dynamics. J Braz Comput Soc. 2013;19(4):573–87. https://doi.org/10.1007/s13173-013-0117-7 .
Kumar R, Kundu PP, Shukla D, Phoha VV. Continuous user authentication via unlabeled phone movement patterns. In: 2017 IEEE International Joint Conference on Biometrics (IJCB). New York: IEEE; 2017. p. 177–184. https://doi.org/10.1109/BTAS.2017.8272696 .
Davarci E, Anarim E. User identification on smartphones with motion sensors and touching behaviors. In: 2022 30th Signal Processing and Communications Applications Conference (SIU). New York: IEEE; 2022. p. 1–4. https://doi.org/10.1109/SIU55565.2022.9864837 .
Alzubaidi A, Kalita J. Authentication of smartphone users using behavioral biometrics. IEEE Commun Surv Tutorials. 2016;18(3):1998–2026. https://doi.org/10.1109/COMST.2016.2537748 .
Stylios I, Kokolakis S, Thanou O, Chatzis S. Behavioral biometrics & continuous user authentication on mobile devices: a survey. Inf Fusion. 2021;66:76–99. https://doi.org/10.1016/j.inffus.2020.08.021 .
Tricco AC, Lillie E, Zarin W, et al. PRISMA Extension for Scoping Reviews (PRISMA-ScR): checklist and explanation. Ann Intern Med. 2018;169(7):467–73. https://doi.org/10.7326/M18-0850 .
Silverio-Fernández M, Renukappa S, Suresh S. What is a smart device? - a conceptualisation within the paradigm of the Internet of Things. Vis in Eng. 2018;6(1):3. https://doi.org/10.1186/s40327-018-0063-8 .
Goggin G. Adapting the mobile phone: the iPhone and its consumption. Continuum. 2009;23(2):231–44. https://doi.org/10.1080/10304310802710546 .
Papadakis S, Alexandraki F, Zaranis N. Mobile device use among preschool-aged children in Greece. Educ Inf Technol. 2022;27(2):2717–50. https://doi.org/10.1007/s10639-021-10718-6 .
Domoff SE, Borgen AL, Foley RP, Maffett A. Excessive use of mobile devices and children’s physical health. Hum Behav Emerg Tech. 2019;1(2):169–75. https://doi.org/10.1002/hbe2.145 .
Davis SP, Ashayer A, Tabrizi N. Predicting sex and age using swipe-gesture data from a mobile device. In: 2020 IEEE Intl Conf on Parallel & Distributed Processing with Applications, Big Data & Cloud Computing, Sustainable Computing & Communications, Social Computing & Networking (ISPA/BDCloud/SocialCom/SustainCom). IEEE; 2020. p. 1136-1143. https://doi.org/10.1109/ISPA-BDCloud-SocialCom-SustainCom51426.2020.00169 .
Sun Z, Wang Y, Qu G, Zhou Z. A 3-D hand gesture signature based biometric authentication system for smartphones. Secur Comm Networks. 2016;9(11):1359–73. https://doi.org/10.1002/sec.1422 .
Liu Q, Wang M, Zhao P, Yan C, Ding Z. A behavioral authentication method for mobile gesture against resilient user posture. In: 2016 3rd International Conference on Systems and Informatics (ICSAI). IEEE; 2016. p. 324–331. https://doi.org/10.1109/ICSAI.2016.7810976 .
Maghsoudi J, Tappert CC. A behavioral biometrics user authentication study using motion data from android smartphones. In: 2016 European Intelligence and Security Informatics Conference (EISIC). IEEE; 2016. p. 184-187. https://doi.org/10.1109/EISIC.2016.047 .
Putri AN, Asnar YDW, Akbar S. A continuous fusion authentication for Android based on keystroke dynamics and touch gesture. In: 2016 International Conference on Data and Software Engineering (ICoDSE). New York: IEEE; 2016. p. 1–6. https://doi.org/10.1109/ICODSE.2016.7936146 .
Lamiche I, Bin G, Jing Y, Yu Z, Hadid A. A continuous smartphone authentication method based on gait patterns and keystroke dynamics. J Ambient Intell Human Comput. 2019;10(11):4417–30. https://doi.org/10.1007/s12652-018-1123-6 .
Smith-Creasey M, Rajarajan M. A continuous user authentication scheme for mobile devices. In: 2016 14th Annual Conference on Privacy, Security and Trust (PST). New York: IEEE; 2016. p .104–113. https://doi.org/10.1109/PST.2016.7906944 .
Shih DH, Lu CM, Shih MH. A flick biometric authentication mechanism on mobile devices. In: 2015 Internationalonal Conference on Informative and Cybernetics for Computational Social Systems (ICCSS). New York: IEEE; 2015. p. 31–33. https://doi.org/10.1109/ICCSS.2015.7281144 .
Zaidi AZ, Chong CY, Parthiban R, Sadiq AS. A framework of dynamic selection method for user classification in touch-based continuous mobile device authentication. J Inform Secur Applic. 2022;67:103217. https://doi.org/10.1016/j.jisa.2022.103217 .
Soni D, Hanmandlu M, Saini HC. A Machine learning approach for user authentication using touchstroke dynamics. In: Somani AK, Srivastava S, Mundra A, Rawat S, eds. Proceedings of First International Conference on Smart System, Innovations and Computing. Vol 79. Smart Innovation, Systems and Technologies. Singapore: Springer Singapore; 2018. p. 391–410. https://doi.org/10.1007/978-981-10-5828-8_38 .
Lin CC, Chang CC, Liang D, Yang CH. A new non-intrusive authentication method based on the orientation sensor for smartphone users. In: 2012 IEEE Sixth International Conference on Sofware Security and Reliability. New York: IEEE; 2012. p. 245–252. https://doi.org/10.1109/SERE.2012.37 .
Li G, Bours P. A novel mobilephone application authentication approach based on accelerometer and gyroscope data. In: 2018 International Conference of the Biometrics Special Interest Group (BIOSIG). New York City: IEEE; 2018. p. 1–4. https://doi.org/10.23919/BIOSIG.2018.8553503 .
Smith-Creasey M, Rajarajan M. A novel scheme to address the fusion uncertainty in multi-modal continuous authentication schemes on mobile devices. In: 2019 International Conference on Biometrics (ICB). New York City; IEEE; 2019. p. 1–8. https://doi.org/10.1109/ICB45273.2019.8987390 .
Salem A, Obaidat MS. A novel security scheme for behavioral authentication systems based on keystroke dynamics. Secur Priv. 2019;2(2):e64. https://doi.org/10.1002/spy2.64 .
Zhao S, Guo Z, Zhong C, Xian L, Liu Y. A novel smartphone identity authentication mechanism. In: Proceedings of the ACM Turing Celebration Conference - China. New York: ACM; 2020. p. 157–161. https://doi.org/10.1145/3393527.3393554 .
Qiao M, Zhang S, Sung AH, Liu Q. A novel touchscreen-based authentication scheme using static and dynamic hand biometrics. In: 2015 IEEE 39th Annual Computer Software and Applications Conference. New York: IEEE; 2015. p. 494–503. https://doi.org/10.1109/COMPSAC.2015.133 .
Smith-Creasey M, Rajarajan M. A novel word-independent gesture-typing continuous authentication scheme for mobile devices. Comput Secur. 2019;83:140–50. https://doi.org/10.1016/j.cose.2019.02.001 .
Alariki AA, Bt Abdul Manaf A, Khan S. A study of touching behavior for authentication in touch screen smart devices. In: 2016 International Conference on Intelligent Systems Engineering (ICISE). New York: IEEE; 2016. p. 216–221. https://doi.org/10.1109/INTELSE.2016.7475123 .
Lee SH, Roh JH, Kim S, Jin SH. A study on feature of keystroke dynamics for improving accuracy in mobile environment. In: Choi D, Guilley S, eds. Information Security Applications. Vol 10144. Lecture Notes in Computer Science. New York: Springer International Publishing; 2017. p. 366–375. https://doi.org/10.1007/978-3-31956549-1_31 .
Li W, Tan J, Meng W, Wang Y. A swipe-based unlocking mechanism with supervised learning on smartphones: design and evaluation. J Netw Comput Applic. 2020;165:102687. https://doi.org/10.1016/j.jnca.2020.102687 .
Saini BS, Singh P, Nayyar A, et al. A three-step authentication model for mobile phone user using keystroke dynamics. IEEE Access. 2020;8:125909125922. https://doi.org/10.1109/ACCESS.2020.3008019 .
Takahashi H, Ogura K, Bista BB, Takata T. A user authentication scheme using keystrokes for smartphones while moving. New York: IEEE; 2016.
Deb D, Ross A, Jain AK, Prakah-Asante K, Prasad KV. Actions speak louder than (Pass)words: passive authentication of smartphone users via deep temporal features. In: 2019 International Conference on Biometrics (ICB). IEEE; 2019. p. 1–8. https://doi.org/10.1109/ICB45273.2019.8987433 .
Leingang W, Gunn D, Kim JH, Yuan X, Roy K. Active authentication using touch dynamics. In: SoutheastCon 2018. New York: IEEE; 2018.p. 1–5. https://doi.org/10.1109/SECON.2018.8479298 .
Acien A, Morales A, Fierrez J, Vera-Rodriguez R, Hernandez-Ortega J. Active detection of age groups based on touch interaction. IET Biom. 2019;8(1):101–8. https://doi.org/10.1049/iet-bmt.2018.5003 .
Mahbub U, Sarkar S, Patel VM, Chellappa R. Active user authentication for smartphones: A challenge data set and benchmark results. In: 2016 IEEE 8th International Conference on Biometrics Theory, Applications and Systems (BTAS). New York: IEEE; 2016. p. 1–8. https://doi.org/10.1109/BTAS.2016.7791155 .
Guarino A, Lemeri N, Malandrino D, Zaccagnino R, Capo C. Adam or Eve? Automatic users’ gender classification via gestures analysis on touch devices. Neural Comput Applic. 2022;34(21):18473–95. https://doi.org/10.1007/s00521-022-07454-4 .
Wang S, Yuan J, Wen J. Adaptive phone orientation method for continuous authentication based on mobile motion sensors. In: 2019 IEEE 31st International Conference on Tools with Artificial Intelligence (ICTAI). New York: IEEE; 2019. p. 1623–1627. https://doi.org/10.1109/ICTAI.2019.00236 .
Davarci E, Soysal B, Erguler I, Aydin SO, Dincer O, Anarim E. Age group detection using smartphone motion sensors. In: 2017 25th European Signal Processing Conference (EUSIPCO). New York: IEEE; 2017. p. 2201–2205. https://doi.org/10.23919/EUSIPCO.2017.8081600 .
Chakraborty B, Nakano K, Tokoi Y, Hashimoto T. An approach for designing low cost deep neural network based biometric authentication model for smartphone user. In: TENCON 2019 - 2019 IEEE Region 10 Conference (TENCON). New York: IEEE; 2019. p. 772–777. https://doi.org/10.1109/TENCON.2019.8929241 .
Antal M, Szabo LZ. An Evaluation of One-class and two-class classification algorithms for keystroke dynamics authentication on mobile devices. In: 2015 20th International Conference on Control Systems and Computer Science. New York: IEEE; 2015. p. 343–350. https://doi.org/10.1109/CSCS.2015.16 .
Roy A, Halevi T, Memon N. An HMM-based multi-sensor approach for continuous mobile authentication. In: MILCOM 2015 - 2015 IEEE Military Communications Conference. New York: IEEE; 2015. p. 1311–1316. https://doi.org/10.1109/MILCOM.2015.7357626 .
Salem A, Zaidan D, Swidan A, Saifan R. Analysis of strong password using keystroke dynamics authentication in touch screen devices. In: 2016 Cybersecurity and Cyberforensics Conference (CCC). New York: IEEE; 2016. p. 15–21. https://doi.org/10.1109/CCC.2016.11 .
Roy S, Roy U, Sinha DD. Analysis of typing pabern in identifying sop biometric information and its impact in user recognition. In: Chandra P, Giri D, Li F, Kar S, Jana DK, eds. Information Technology and Applied Mathematics. Vol 699. Advances in Intelligent Systems and Computing. Singapore: Springer Singapore; 2019. p. 69–83. https://doi.org/10.1007/978-981-10-7590-2_5 .
Lee W. Analyzing motion of touching screen for inferring user characteristics. In: 2021 Twelfth International Conference on Ubiquitous and Future Networks (ICUFN). New York: IEEE; 2021. p. 78–80. https://doi.org/10.1109/ICUFN49451.2021.9528699 .
Buriro A, Crispo B, Conti M. AnswerAuth: a bimodal behavioral biometric-based user authentication scheme for smartphones. J Inform Secur Applic. 2019;44:89–103. https://doi.org/10.1016/j.jisa.2018.11.008 .
Praher C, Sonntag M. Applicability of keystroke dynamics as a biometric security feature for mobile touchscreen devices with virtualised keyboards. IJICS. 2016;8(1):72. https://doi.org/10.1504/IJICS.2016.075311 .
Baran M, Siwik L, Rzecki K. Application of elastic principal component analysis to person recognition based on screen gestures. In: Rutkowski L, Scherer R, Korytkowski M, Pedrycz W, Tadeusiewicz R, Zurada JM, eds. Artificial Intelligence and Soft Computing. Vol 11508. Lecture Notes in Computer Science. New York: Springer International Publishing; 2019. p. 553–560. https://doi.org/10.1007/978-3-030-20912-4_50 .
Ali Z, Payton J, Sritapan V. At your fingertips: considering finger distinctness in continuous touch-based authentication for mobile devices. In: 2016 IEEE Security and Privacy Workshops (SPW). New York: IEEE; 2016. p. 272–275. https://doi.org/10.1109/SPW.2016.29 .
Guerra-Casanova J, Sánchez-Ávila C, Bailador G, de Santos SA. Authentication in mobile devices through hand gesture recognition. Int J Inf Secur. 2012;11(2):65–83. https://doi.org/10.1007/s10207-012-0154-9 .
Primo A. Keystroke-based continuous authentication while listening to music on your smart-phone. In: 2017 IEEE 8th Annual Ubiquitous Computing, Electronics and Mobile Communication Conference (UEMCON). New York: IEEE; 2017. p. 217–225. https://doi.org/10.1109/UEMCON.2017.8249029 .
Yang Y, Guo B, Wang Z, Li M, Yu Z, Zhou X. BehaveSense: continuous authentication for security-sensitive mobile apps using behavioral biometrics. Ad Hoc Netw. 2019;84:9–18. https://doi.org/10.1016/j.adhoc.2018.09.015 .
Wolff M. Behavioral biometric identification on mobile devices. In: Schmorrow DD, Fidopiastis CM, eds. Foundations of Augmented Cognition. Vol 8027. Lecture Notes in Computer Science. Berlin: Springer Berlin Heidelberg; 2013. p. 783–791. https://doi.org/10.1007/978-3-642-39454-6_84 .
Tse KW, Hung K. Behavioral biometrics scheme with keystroke and swipe dynamics for user authentication on mobile platform. In: 2019 IEEE 9th Symposium on Computer Applications & Industrial Electronics (ISCAIE). New York: IEEE; 2019. p. 125–130. https://doi.org/10.1109/ISCAIE.2019.8743995 .
Antal M, Szabó LZ. Biometric authentication based on touchscreen swipe patterns. Proc Technol. 2016;22:862–9. https://doi.org/10.1016/j.protcy.2016.01.061 .
Laghari A, Waheed-ur-Rehman, Memon ZA. Biometric authentication technique using smartphone sensor. In: 2016 13th International Bhurban Conference on Applied Sciences and Technology (IBCAST). New York: IEEE; 2016. p. 381–384. https://doi.org/10.1109/IBCAST.2016.7429906 .
Tolosana R, Vera-Rodriguez R, Fierrez J, Morales A. BioTouchPass demo: handwriben passwords for touchscreen biometrics. In: Proceedings of the 27th ACM International Conference on Multimedia. New York: ACM; 2019. p. 1023–1025. https://doi.org/10.1145/3343031.3350578 .
Ray A, Hou D, Schuckers S, Barbir A. Continuous authentication based on hand micro-movement during smartphone form filling by seated human subjects: In: Proceedings of the 7th International Conference on Information Systems Security and Privacy. Setubal, Portugal: SCITEPRESS - Science and Technology Publications; 2021. p. 424–431. https://doi.org/10.5220/0010225804240431 .
Ambol S, Rashad S. Continuous authentication of smartphone users using machine learning. In: 2020 11th IEEE Annual Ubiquitous Computing, Electronics & Mobile Communication Conference (UEMCON). New York: IEEE; 2020. p. 0056–0062. https://doi.org/10.1109/UEMCON51285.2020.9298040 .
Garbuz A, Epishkina A, Kogos K. Continuous authentication of smartphone users via swipes and taps analysis. In: 2019 European Intelligence and Security Informatics Conference (EISIC). New York: IEEE; 2019. p. 48–53. https://doi.org/10.1109/EISIC49498.2019.9108780 .
Dybczak J, Nawrocki P. Continuous authentication on mobile devices using behavioral biometrics. In: 2022 22nd IEEE International Symposium on Cluster, Cloud and Internet Computing (CCGrid). New York: IEEE; 2022. p. 1028–1035. https://doi.org/10.1109/CCGrid54584.2022.00125 .
Murmuria R, Stavrou A, Barbará D, Fleck D. Continuous authentication on mobile devices using power consumption, touch gestures and physical movement of users. In: Bos H, Monrose F, Blanc G, eds. Research in Attacks, Intrusions, and Defenses. Vol 9404. Lecture Notes in Computer Science. New York: Springer International Publishing; 2015. p. 405–424. https://doi.org/10.1007/978-3-319-26362-5_19 .
Karanikiotis T, Papamichail MD, Chatzidimitriou KC, Oikonomou NCI, Symeonidis AL, Saripalle SK. Continuous implicit authentication through touch traces modelling. In: 2020 IEEE 20th International Conference on Software Quality, Reliability and Security (QRS). New York: IEEE; 2020. p. 111–120. https://doi.org/10.1109/QRS51102.2020.00026 .
Zhao X, Feng T, Shi W. Continuous mobile authentication using a novel graphic touch gesture feature. In: 2013 IEEE Sixth International Conference on Biometrics: Theory, Applications and Systems (BTAS). IEEE; 2013. p. 1–6. https://doi.org/10.1109/BTAS.2013.6712747 .
Zhao X, Feng T, Lu X, Shi W, Kakadiaris IA. Continuous mobile authentication using user-phone interaction. Inst Eng Technol. 2017;3:209–33. https://doi.org/10.1049/PBSE003E_ch8 .
Leyfer K, Spivak A. Continuous user authentication by the classification method based on the dynamic touchscreen biometrics. In: 2019 24th Conference of Open Innovations Association (FRUCT). New York City IEEE; 2019. p. 228–234. https://doi.org/10.23919/FRUCT.2019.8711941 .
Herath HMCKB, Dulanga KGC, Tharindu NVD, Ganegoda GU. Continuous user authentication using keystroke dynamics for touch devices. In: 2022 2nd International Conference on Image Processing and Robotics (ICIPRob). New York: IEEE; 2022. p. 1–6. https://doi.org/10.1109/ICIPRob54042.2022.9798728 .
Kumar R, Kundu PP, Shukla D, Phoha VV. Continuous user authentication via unlabeled phone movement patterns. In: 2017 IEEE International Joint Conference on Biometrics (IJCB). New York: IEEE; 2017. p. 177–184. https://doi.org/10.1109/BTAS.2017.8272696 .
Barlas Y, Basar OE, Akan Y, Isbilen M, Alptekin GI, Incel OD. DAKOTA: continuous authentication with behavioral biometrics in a mobile banking application. In: 2020 5th International Conference on Computer Science and Engineering (UBMK). New York: IEEE; 2020. p. 1–6. https://doi.org/10.1109/UBMK50275.2020.9219365 .
Incel OD, Gunay S, Akan Y, et al. DAKOTA: sensor and touch screen-based continuous authentication on a mobile banking application. IEEE Access. 2021;9:38943–60. https://doi.org/10.1109/ACCESS.2021.3063424 .
Hernandez-Ortega J, Morales A, Fierrez J, Acien A. Detecting age groups using touch interaction based on neuromotor characteristics. Electron Lea. 2017;53(20):1349–50. https://doi.org/10.1049/el.2017.0492 .
Article ADS Google Scholar
Nguyen TV, Sae-Bae N, Memon N. DRAW-A-PIN: authentication using finger-drawn PIN on touch devices. Comput Secur. 2017;66:115–28. https://doi.org/10.1016/j.cose.2017.01.008 .
Al-Showarah SA. Dynamic recognition for user age-group classification using hand-writing based finger on smartphones. In: 2019 10th International Conference on Information and Communication Systems (ICICS). New York: IEEE; 2019. p. 140–146. https://doi.org/10.1109/IACS.2019.8809083 .
Ng’ang’a A, Musuva PMW. Enhancing accuracy in a touch operation biometric system: a case on the android pabern lock scheme. Mobile Inform Syst. 2020;2020:1–12. https://doi.org/10.1155/2020/4165457 .
Ray-Dowling A, Hou D, Schuckers S, Barbir A. Evaluating multi-modal mobile behavioral biometrics using public datasets. Comput Secur. 2022;121:102868. https://doi.org/10.1016/j.cose.2022.102868 .
Buriro A, Gupta S, Crispo B. Evaluation of motion-based touch-typing biometrics for online banking. In: 2017 International Conference of the Biometrics Special Interest Group (BIOSIG). New York: IEEE; 2017. p. 1–5. https://doi.org/10.23919/BIOSIG.2017.8053504 .
Ouadjer Y, Adnane M, Bouadjenek N. Feature importance evaluation of smartphone touch gestures for biometric authentication. In: 2020 2nd International Workshop on Human-Centric Smart Environments for Health and Well-Being (IHSH). New York: IEEE; 2021. p. 103–107. https://doi.org/10.1109/IHSH51661.2021.9378750 .
Suharsono A, Liang D. Hand stability based features for touch behavior smartphone authentication. In: 2020 3rd IEEE International Conference on Knowledge Innovation and Invention (ICKII). New York: IEEE; 2020. p. 167–170. https://doi.org/10.1109/ICKII50300.2020.9318982 .
Barra S, Fenu G, De Marsico M, Castiglione A, Nappi M. Have you permission to answer this phone? In: 2018 International Workshop on Biometrics and Forensics (IWBF). New York: IEEE; 2018. p. 1–7. https://doi.org/10.1109/IWBF.2018.8401563 .
Mallet J, Pryor L, Dave R, Seliya N, Vanamala M, Sowells-Boone E. Hold on and swipe: a touch-movement based continuous authentication schema based on machine learning. In: 2022 Asia Conference on Algorithms, Computing and Machine Learning (CACML). New York: IEEE; 2022. p. 442–447. https://doi.org/10.1109/CACML55074.2022.00081 .
Abate AF, Nappi M, Ricciardi S. I-Am: implicitly authenticate me—person authentication on mobile devices through ear shape and arm gesture. IEEE Trans Syst Man Cybern, Syst. 2019;49(3):469–81. https://doi.org/10.1109/TSMC.2017.2698258 .
Cheng Y, Ji X, Li X, et al. Identifying child users via touchscreen interactions. ACM Trans Sen Netw. 2020;16(4):1–25. https://doi.org/10.1145/3403574 .
Alqarni MA, Chauhdary SH, Malik MN, Ehatisham-ul-Haq M, Azam MA. Identifying smartphone users based on how they interact with their phones. Hum Cent Comput Inf Sci. 2020;10(1):7. https://doi.org/10.1186/s13673-020-0212-7 .
Rao KR, Anne VPK, Sai Chand U, Alakananda V, Navya Rachana K. Inclination and pressure based authentication for touch devices. In: Satapathy SC, Avadhani PS, Udgata SK, Lakshminarayana S, eds. ICT and Critical Infrastructure: Proceedings of the 48th Annual Convention of Computer Society of India- Vol I. Vol 248. Advances in intelligent systems and computing. New York: Springer International Publishing; 2014. p. 781–788. https://doi.org/10.1007/978-3-319-03107-1_86 .
Coakley MJ, Monaco JV, Tappert CC. Keystroke biometric studies with short numeric input on smartphones. In: 2016 IEEE 8th International Conference on Biometrics Theory, Applications and Systems (BTAS). New York: IEEE; 2016. p. 1–6. https://doi.org/10.1109/BTAS.2016.7791181 .
Gautam P, Dawadi PR. Keystroke biometric system for touch screen text input on android devices optimization of equal error rate based on medians vector proximity. In: 2017 11th International Conference on Software, Knowledge, Information Management and Applications (SKIMA). New York: IEEE; 2017. p. 1–7. https://doi.org/10.1109/SKIMA.2017.8294136 .
Deng Y, Zhong Y. Keystroke dynamics advances for mobile devices using deep neural network. In: Zhong Y, Deng Y, eds. Gate to computer science and research. Vol 2. 1st ed. Thrace, Greece: Science Gate Publishing P.C.; 2015. p. 59–70. https://doi.org/10.15579/gcsr.vol2.ch4 .
Roh J hyuk, Lee SH, Kim S. Keystroke dynamics for authentication in smartphone. In: 2016 International Conference on Information and Communication Technology Convergence (ICTC). New York: IEEE; 2016. p. 1155–1159. https://doi.org/10.1109/ICTC.2016.7763394 .
Acien A, Morales A, Vera-Rodriguez R, Fierrez J. Keystroke mobile authentication: performance of long-term approaches and fusion with behavioral profiling. In: Morales A, Fierrez J, Sánchez JS, Ribeiro B, eds. Paaern recognition and image analysis. Vol 11868. Lecture Notes in Computer Science. New York: Springer International Publishing; 2019. p. 12–24. https://doi.org/10.1007/978-3-030-31321-0_2 .
Sun L, Cao B, Wang J, et al. Kollector: detecting fraudulent activities on mobile devices using deep learning. IEEE Trans on Mobile Comput. 2021;20(4):1465–76. https://doi.org/10.1109/TMC.2020.2964226 .
Peralta RT, Rebguns A, Fasel IR, Barnard K. Learning a policy for gesture-based active multi-touch authentication. In: Marinos L, Askoxylakis I, eds. Human Aspects of information security, privacy, and trust. Vol 8030. Lecture notes in computer science. Berlin: Springer Berlin Heidelberg; 2013. p. 59–68. https://doi.org/10.1007/978-3642-39345-7_7 .
Stragapede G, Vera-Rodriguez R, Tolosana R, Morales A, Acien A, Le Lan G. Mobile behavioral biometrics for passive authentication. Paaern Recog Leaers. 2022;157:35–41. https://doi.org/10.1016/j.patrec.2022.03.014 .
Liang X, Zou F, Li L, Yi P. Mobile terminal identity authentication system based on behavioral characteristics. Int J Distrib Sens Netw. 2020;16(1):155014771989937. https://doi.org/10.1177/1550147719899371 .
Li C, Jing J, Liu Y. Mobile user authentication-turn it to unlock. In: 2021 6th International Conference on Mathematics and Artificial Intelligence. New York: ACM; 2021. p. 101–107. https://doi.org/10.1145/3460569.3460577 .
Corpus KR, Gonzales RJDL, Morada AS, Vea LA. Mobile user identification through authentication using keystroke dynamics and accelerometer biometrics. In: Proceedings of the international conference on mobile Software engineering and systems. New York: ACM; 2016. p. 11–12. https://doi.org/10.1145/2897073.2897111 .
Akhtar Z, Buriro A, Crispo B, Falk TH. Multimodal smartphone user authentication using touchstroke, phone-movement and face patterns. In: 2017 IEEE Global Conference on Signal and Information Processing (GlobalSIP). New York: IEEE; 2017. p. 1368–1372. https://doi.org/10.1109/GlobalSIP.2017.8309185 .
Song Y, Cai Z, Zhang ZL. Multi-touch authentication using hand geometry and behavioral information. In: 2017 IEEE Symposium on Security and Privacy (SP). New York: IEEE; 2017. p. 357–372. https://doi.org/10.1109/SP.2017.54 .
Primo A, Phoha VV. Music and images as contexts in a context-aware touch-based authentication system. In: 2015 IEEE 7th International Conference on Biometrics Theory, Applications and Systems (BTAS). New York: IEEE; 2015. p. 1–7. https://doi.org/10.1109/BTAS.2015.7358779 .
Phillips ME, Stepp ND, Cruz-Albrecht J, De Sapio V, Lu TC, Sritapan V. Neuromorphic and early warning behavior-based authentication for mobile devices. In: 2016 IEEE Symposium on Technologies for Homeland Security (HST). New York: IEEE; 2016. p. 1–5. https://doi.org/10.1109/THS.2016.7568965 .
Li N, Liu J, Li Q, Luo X, Duan J. Online signature verification based on biometric features. In: 2016 49th Hawaii International Conference on System Sciences (HICSS). New York: IEEE; 2016. p. 5527–5534. https://doi.org/10.1109/HICSS.2016.683 .
Haberfeld C, Hossain MS, Lancor L. Open code biometric tap pad for smartphones. J Inform Secur Applic. 2021;57:102688. https://doi.org/10.1016/j.jisa.2020.102688 .
Tharwat A, Ibrahim A, Gaber T, Hassanien AE. Personal Identification based on mobile-based keystroke dynamics. In: Hassanien AE, Tolba MF, Shaalan K, Azar AT, eds. Proceedings of the international conference on advanced intelligent systems and informatics 2018. Vol 845. Advances in intelligent systems and computing. New York: Springer International Publishing; 2019. p. 457–466. https://doi.org/10.1007/978-3-319-99010-1_42 .
Tang C, Cui Z, Chu M, Lu Y, Zhou F, Gao S. Piezoelectric and machine learning based keystroke dynamics for highly secure user authentication. IEEE Sensors J. 2022:1–1. https://doi.org/10.1109/JSEN.2022.3141872 .
Mahfouz A, Mahmoud TM, Sharaf Eldin A. A behavioral biometric authentication framework on smartphones. In: Proceedings of the 2017 ACM on Asia Conference on Computer and Communications Security. New York: ACM; 2017. p. 923–925. https://doi.org/10.1145/3052973.3055160 .
Hernandez-Ortega J, Morales A, Fierrez J, Acien A. Predicting age groups from touch patterns based on neuromotor models. In: 8th International Conference of Pattern Recognition Systems (ICPRS 2017). Institution of Engineering and Technology; Stevenage, United Kingdom, 2017. https://doi.org/10.1049/cp.2017.0135 .
Miguel-Hurtado O, Stevenage SV, Bevan C, Guest R. Predicting sex as a sop-biometrics from device interaction swipe gestures. Pattern Recog Leaers. 2016;79:44–51. https://doi.org/10.1016/j.patrec.2016.04.024 .
Wang S, Yuan J, Chen S. Quality-based score level fusion for continuous authentication with motion sensor and face. In: Proceedings of the 2020 4th International Conference on Cryptography, Security and Privacy. New York: ACM; 2020. p. 58–62. https://doi.org/10.1145/3377644.3377647 .
Inguanez F, Ahmadi S. Securing smartphones via typing heat maps. In: 2016 IEEE 6th International Conference on Consumer Electronics - Berlin (ICCEBerlin). New York: IEEE; 2016. p. 193–197. https://doi.org/10.1109/ICCE-Berlin.2016.7684753 .
Zhu H, Hu J, Chang S, Lu L. ShakeIn: secure user authentication of smartphones with single-handed shakes. IEEE Trans Mobile Comput. 2017;16(10):2901–12. https://doi.org/10.1109/TMC.2017.2651820 .
Bo C, Zhang L, Li XY, Huang Q, Wang Y. SilentSense: silent user identification via touch and movement behavioral biometrics. In: Proceedings of the 19th Annual International Conference on Mobile Computing & Networking - MobiCom ’13. New York: ACM Press; 2013. p. 187. https://doi.org/10.1145/2500423.2504572 .
Gunn DJ, Roy K, Bryant K. Simulated cloud authentication based on touch dynamics with SVM. In: 2018 IEEE Symposium Series on Computational Intelligence (SSCI). New York: IEEE; 2018. p. 639-644. https://doi.org/10.1109/SSCI.2018.8628762 .
Wang Z, Zhou N, Chen F, et al. Smart_Auth: user identity authentication based on smartphone motion sensors. In: 2021 6th International Conference on Image, Vision and Computing (ICIVC). New York: IEEE; 2021. p. 480–485. https://doi.org/10.1109/ICIVC52351.2021.9526964 .
Abate AF, Nappi M, Ricciardi S. Smartphone enabled person authentication based on ear biometrics and arm gesture. In: 2016 IEEE International Conference on Systems, Man, and Cybernetics (SMC). New York: IEEE; 2016. p. 003719–003724. https://doi.org/10.1109/SMC.2016.7844812 .
Acien A, Morales A, Vera-Rodriguez R, Fierrez J. Smartphone sensors for modeling human-computer interaction: general outlook and research datasets for user authentication. In: 2020 IEEE 44th Annual Computers, Software, and Applications Conference (COMPSAC). New York: IEEE; 2020. p. 1273–1278. https://doi.org/10.1109/COMPSAC48688.2020.00-81 .
Anusas-amornkul T. Strengthening password authentication using keystroke dynamics and smartphone sensors. In: Proceedings of the 9th International Conference on Information Communication and Management. New York: ACM; 2019. p. 70–74. https://doi.org/10.1145/3357419.3357425 .
Temper M, Tjoa S. The applicability of fuzzy rough classifier for continuous person authentication. In: 2016 International Conference on Software Security and Assurance (ICSSA). New York: IEEE; 2016. p. 17–23. https://doi.org/10.1109/ICSSA.2016.10 .
Roy S, Roy U, Sinha DD. The probability of predicting personality traits by the way user types on touch screen. Innov Syst Softw Eng. 2019;15(1):27–34. https://doi.org/10.1007/s11334-018-0317-6 .
Shrestha B, Mohamed M, Tamrakar S, Saxena N. Theft-resilient mobile wallets: transparently authenticating NFC users with tapping gesture biometrics. In: Proceedings of the 32nd Annual Conference on Computer Security Applications. New York: ACM; 2016 p. 265–276. https://doi.org/10.1145/2991079.2991097 .
Cascone L, Nappi M, Narducci F, Pero C. Touch keystroke dynamics for demographic classification. Pattern Recog Leaers. 2022;158:63–70. https://doi.org/10.1016/j.patrec.2022.04.023 .
Temper M, Tjoa S, Kaiser M. Touch to authenticate — continuous biometric authentication on mobile devices. In: 2015 1st International Conference on Software Security and Assurance (ICSSA). New York: IEEE; 2015. p. 30–35. https://doi.org/10.1109/ICSSA.2015.016 .
Frank M, Biedert R, Ma E, Martinovic I, Song D. Touchalytics: on the applicability of touchscreen input as a behavioral biometric for continuous authentication. New York: IEEE; 2012. https://doi.org/10.48550/ARXIV.1207.6231 .
Watanabe Y, Houryu A, Fujita T. Toward introduction of immunity-based model to continuous behavior-based user authentication on smart phone. Proc Comput Sci. 2013;22:1319–27.
Volaka HC, Alptekin G, Basar OE, Isbilen M, Incel OD. Towards continuous authentication on mobile phones using deep learning models. Proc Comput Sci. 2019;155:177–84. https://doi.org/10.1016/j.procs.2019.08.027 .
Brown J, Raval A, Anwar M. Towards passive authentication using inertia variations: an experimental study on smartphones. In: 2020 Second Internationalonal Conference on Transdisciplinary AI (TransAI). New York: IEEE; 2020. p. 88–91. https://doi.org/10.1109/TransAI49837.2020.00019 .
Sharma V, Enbody R. User authentication and identification from user interface interactions on touch-enabled devices. In: Proceedings of the 10th ACM Conference on Security and Privacy in Wireless and Mobile Networks. New York: ACM; 2017. p. 1–11. https://doi.org/10.1145/3098243.3098262 .
Kroeze CJ, Malan KM. User authentication based on continuous touch biometrics. SACJ. Cape Town, South Africa. 2016;28(2). https://doi.org/10.18489/sacj.v28i2.374 .
Filippov AI, Iuzbashev AV, Kurnev AS. User authentication via touch pattern recognition based on isolation forest. In: 2018 IEEE Conference of Russian Young Researchers in Electrical and Electronic Engineering (EIConRus). New York: IEEE; 2018. p. 1485–1489. https://doi.org/10.1109/EIConRus.2018.8317378 .
Karakaya N, Alptekin GI, İncel ÖD. Using behavioral biometric sensors of mobile phones for user authentication. Proc Comput Sci. 2019;159:475–84. https://doi.org/10.1016/j.procs.2019.09.202 .
Serwadda A, Phoha VV, Wang Z. Which verifiers work?: A benchmark evaluation of touch-based authentication algorithms. In: 2013 IEEE Sixth International Conference on Biometrics: Theory, Applications and Systems (BTAS). New York: IEEE; 2013. p. 1–8. https://doi.org/10.1109/BTAS.2013.6712758 .
Buriro A, Crispo B, Delfrari F, Wrona K. Hold and sign: a novel behavioral biometrics for smartphone user authentication. In: 2016 IEEE Security and Privacy Workshops (SPW). IEEE; 2016. p. 276–285. https://doi.org/10.1109/SPW.2016.20 .
Shen C, Yu T, Yuan S, Li Y, Guan X. Performance analysis of motion-sensor behavior for user authentication on smartphones. Sensors. 2016;16(3):345. https://doi.org/10.3390/s16030345 .
Article PubMed PubMed Central ADS Google Scholar
Stylios I, Skalkos A, Kokolakis S, Karyda M. BioPrivacy: a behavioral biometrics continuous authentication system based on keystroke dynamics and touch gestures. ICS. 2022;30(5):687–704. https://doi.org/10.1108/ICS-12-2021-0212 .
Papi E, Koh WS, McGregor AH. Wearable technology for spine movement assessment: a systematic review. J Biomech. 2017;64:186–97. https://doi.org/10.1016/j.jbiomech.2017.09.037 .
Nguyen T, Roy A, Memon N. Kid on the phone! Toward automatic detection of children on mobile devices. Comput Secur. 2019;84:334–48. https://doi.org/10.1016/j.cose.2019.04.001 .
Wang Z, Chen F, Zhou N, et al. Identity authentication based on dynamic touch behavior on smartphone. In: 2021 6th International Conference on Image, Vision and Computing (ICIVC). IEEE; 2021. p. 469–474. https://doi.org/10.1109/ICIVC52351.2021.9527023 .
Thompson N, McGill TJ, Wang X. “Security begins at home”: determinants of home computer and mobile device security behavior. Comput Secur. 2017;70:376–91. https://doi.org/10.1016/j.cose.2017.07.003 .
Bo C, Zhang L, Jung T, Han J, Li XY, Wang Y. Continuous user identification via touch and movement behavioral biometrics. In: 2014 IEEE 33rd International Performance Computing and Communications Conference (IPCCC). IEEE; 2014. p. 1-8. https://doi.org/10.1109/PCCC.2014.7017067 .
Bo C, Zhang L, Li XY, Huang Q, Wang Y. SilentSense: silent user identification via touch and movement behavioral biometrics. In: Proceedings of the 19th Annual International Conference on Mobile Computing & Networking - MobiCom ’13. ACM Press; 2013. p. 187. https://doi.org/10.1145/2500423.2504572 .
Stylios I, Kokolakis S, Skalkos A, Chatzis S. BioGames: a new paradigm and a behavioral biometrics collection tool for research purposes. ICS. 2022;30(2):243–54. https://doi.org/10.1108/ICS-12-2020-0196 .
Papamichail MD, Chatzidimitriou KC, Karanikiotis T, Oikonomou NCI, Symeonidis AL, Saripalle SK. Behavioral biometrics dataset towards continuous implicit authentication. https://doi.org/10.5281/ZENODO.2598135 . Published online March 19, 2019.
Murmuria R, Stavrou A, Barbará D, Fleck D. Continuous authentication on mobile devices using power consumption, touch gestures and physical movement of users. In: Bos H, Monrose F, Blanc G, eds. Research in Attacks, Intrusions, and Defenses. Vol 9404. Lecture Notes in Computer Science. Springer International Publishing; 2015. p. 405–424. https://doi.org/10.1007/978-3-319-26362-5_19 .
Teh PS, Teoh ABJ, Yue S. A survey of keystroke dynamics biometrics. ScientificWorldJournal. 2013;2013:1–24. https://doi.org/10.1155/2013/408280 .
Shen C, Li Y, Chen Y, Guan X, Maxion RA. Performance analysis of multi-motion sensor behavior for active smartphone authentication. IEEE Trans Inform Forensic Secur. 2018;13(1):48–62. https://doi.org/10.1109/TIFS.2017.2737969 .
Tablets in the U.S. - statistics and facts. Statista. Published online May 23, 2023. https://www.statista.com/topics/2927/tablets-in-the-us/#topicOverview .
Counterpoint Quarterly. Global smartphone shipments market data (Q4 2021 - Q3 2023). https://www.counterpointresearch.com/insights/global-smartphone-share/ .
Parker H, Burkart S, Reesor-Oyer L, et al. Feasibility of measuring screen time, activity, and context among families with preschoolers: intensive longitudinal pilot study. JMIR Form Res. 2022;6(9):e40572. https://doi.org/10.2196/40572 .
Welk GJ, Bai Y, Lee JM, Godino J, Saint-Maurice PF, Carr L. Standardizing analytic methods and reporting in activity monitor validation studies. Med Sci Sports Exerc. 2019;51(8):1767–80. https://doi.org/10.1249/MSS.0000000000001966 .
Menghini L, Cellini N, Goldstone A, Baker FC, de Zambotti M. A standardized framework for testing the performance of sleep-tracking technology: step-by-step guidelines and open-source code. Sleep. 2021;44(2):zsaa170. https://doi.org/10.1093/sleep/zsaa170 .
Download references
Acknowledgements
This publication was made possible in part by Grant Number T32-GM081740 from NIH-NIGMS. Research reported in this publication was also supported in part by the National Institute of Diabetes and Digestive and Kidney Diseases Award Number R01DK129215. Co-author White was supported in part by the National Institute of Diabetes and Digestive and Kidney Diseases Award Number F31DK136205. Its contents are solely the responsibility of the authors and do not necessarily represent the official views of the NIGMS or NIH.
Author information
Authors and affiliations.
Department of Exercise Science, University of South Carolina, Columbia, USA
O. L. Finnegan, J. W. White III, B. Armstrong, E. L. Adams, S. Burkart, M. W. Beets, L. von Klinggraeff, H. Parker, M. Bastyr, X. Zhu & R. G. Weaver
Department of Computer Science and Engineering, University of South Carolina, Columbia, USA
S. Nelakuditi & Z. Zhong
Center for Health Promotion and Disease Prevention, University of North Carolina Chapel Hill, Chapel Hill, USA
E. A. Willis
You can also search for this author in PubMed Google Scholar
Contributions
RGW secured funding. OLF, RGW, and BA conceptualized the idea. OLF secured articles and completed data extraction. All authors assisted with data interpretation. OLF and RGW drafted the manuscript. All authors read, provided substantive feedback, and approved the final manuscript.
Corresponding author
Correspondence to O. L. Finnegan .
Ethics declarations
Ethics approval and consent to participate.
Not applicable.
Consent for publication
Competing interests.
The authors declare that they have no competing interests.
Additional information
Publisher’s note.
Springer Nature remains neutral with regard to jurisdictional claims in published maps and institutional affiliations.
Supplementary Information
Additional file 1: supplementary table 1..
Quality Assessment Framework.
Additional file 2: Supplementary Table 2.
List of Search Terms.
Additional file 3: Supplementary Table 3.
Data Stream, Biometric Methods & Model Evaluation Metrics of Included Studies.
Additional file 4: Table 2.
Quality Assessment of Included Studies.
Rights and permissions
Open Access This article is licensed under a Creative Commons Attribution 4.0 International License, which permits use, sharing, adaptation, distribution and reproduction in any medium or format, as long as you give appropriate credit to the original author(s) and the source, provide a link to the Creative Commons licence, and indicate if changes were made. The images or other third party material in this article are included in the article's Creative Commons licence, unless indicated otherwise in a credit line to the material. If material is not included in the article's Creative Commons licence and your intended use is not permitted by statutory regulation or exceeds the permitted use, you will need to obtain permission directly from the copyright holder. To view a copy of this licence, visit http://creativecommons.org/licenses/by/4.0/ . The Creative Commons Public Domain Dedication waiver ( http://creativecommons.org/publicdomain/zero/1.0/ ) applies to the data made available in this article, unless otherwise stated in a credit line to the data.
Reprints and permissions
About this article
Cite this article.
Finnegan, O.L., White, J.W., Armstrong, B. et al. The utility of behavioral biometrics in user authentication and demographic characteristic detection: a scoping review. Syst Rev 13 , 61 (2024). https://doi.org/10.1186/s13643-024-02451-1
Download citation
Received : 22 August 2023
Accepted : 03 January 2024
Published : 08 February 2024
DOI : https://doi.org/10.1186/s13643-024-02451-1
Share this article
Anyone you share the following link with will be able to read this content:
Sorry, a shareable link is not currently available for this article.
Provided by the Springer Nature SharedIt content-sharing initiative
Systematic Reviews
ISSN: 2046-4053
- Submission enquiries: Access here and click Contact Us
- General enquiries: [email protected]
Biometric Technology and Ethics: Beyond Security Applications
- Review Paper
- Published: 08 March 2019
- Volume 167 , pages 433–450, ( 2020 )
Cite this article
- Andrea North-Samardzic 1
7048 Accesses
25 Citations
6 Altmetric
Explore all metrics
Biometric technology was once the purview of security, with face recognition and fingerprint scans used for identification and law enforcement. This is no longer the case; biometrics is increasingly used for commercial and civil applications. Due to the widespread diffusion of biometrics, it is important to address the ethical issues inherent to the development and deployment of the technology. This article explores the burgeoning research on biometrics for non-security purposes and the ethical implications for organizations. This will be achieved by reviewing the literature on biometrics and business ethics and drawing from disciplines such as computer ethics to inform a more robust discussion of key themes. Although there are many ethical concerns, privacy is the key issue, with associated themes. These include definitions of privacy, the privacy paradox, informed consent, regulatory frameworks and guidelines, and discrimination. Despite the proliferation of biometric technology, there is little empirical research on applied biometrics and business ethics. As such, there are several avenues for research to improve understanding of the ethical implications of using this technology.
This is a preview of subscription content, log in via an institution to check access.
Access this article
Price includes VAT (Russian Federation)
Instant access to the full article PDF.
Rent this article via DeepDyve
Institutional subscriptions
Similar content being viewed by others
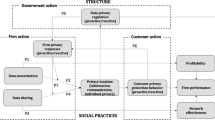
Digital technologies: tensions in privacy and data
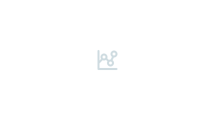
The ethical application of biometric facial recognition technology
The ethics of facial recognition technologies, surveillance, and accountability in an age of artificial intelligence: a comparative analysis of us, eu, and uk regulatory frameworks.
This review was updated in October 2018.
Albrecht, J. P. (2016). How the GDPR will change the world. European Data Protection Law Review, 2 , 287–289.
Google Scholar
Alterman, A. (2003). “A piece of yourself”: Ethical issues in biometric identification. Ethics and Information Technology, 5 (3), 139–150.
Ball, K. (2005). Organization, surveillance and the body: Towards a politics of resistance. Organization, 12 (1), 89–108.
Bhattacharyya, D., Ranjan, R., Alisherov, F., & Choi, M. (2009). Biometric authentication: A review. International Journal of u-and e-Service, Science and Technology, 2 (3), 13–28.
Breland, A. (2017). How white engineers built racist code—and why it’s dangerous for black people. https://www.theguardian.com/technology/2017/dec/04/racist-facial-recognition-white-coders-black-people-police . Accessed 1 Nov 2018.
Brown, W. S. (1996). Technology, workplace privacy and personhood. Journal of Business Ethics, 15 (11), 1237–1248.
Brusoni, S., & Vaccaro, A. (2017). Ethics, technology and organizational innovation. Journal of Business Ethics, 143 (2), 223–226.
Buchholz, R. A., & Rosenthal, S. B. (2002). Technology and business: Rethinking the moral dilemma. Journal of Business Ethics, 41 (1–2), 45–50.
Campisi, P. (2013). Security and privacy in biometrics (Vol. 24). New York: Springer.
Carpenter, D., McLeod, A., Hicks, C., & Maasberg, M. (2018). Privacy and biometrics: An empirical examination of employee concerns. Information Systems Frontiers, 20 (1), 91–110.
Cavoukian, A., Chibba, M., & Stoianov, A. (2012). Advances in biometric encryption: Taking privacy by design from academic research to deployment. Review of Policy Research, 29 (1), 37–61.
Corcoran, P., & Costache, C. (2016). Smartphones, biometrics, and a brave new world. IEEE Technology and Society Magazine, 35 (3), 59–66.
Custers, B., Dechesne, F., Sears, A. M., Tani, T., & van der Hof, S. (2018). A comparison of data protection legislation and policies across the EU. Computer Law and Security Review, 34 (2), 234–243.
D’Mello, S. K., Craig, S. D., & Graesser, A. C. (2009). Multimethod assessment of affective experience and expression during deep learning. International Journal of Learning Technology, 4 (3–4), 165–187.
D’Mello, S. K., & Graesser, A. (2010). Multimodal semi-automated affect detection from conversational cues, gross body language, and facial features. User Modeling and User-Adapted Interaction, 20 (2), 147–187.
Davis, J., & Nathan, L. P. (2015). Value sensitive design: Applications, adaptations, and critiques. In Handbook of ethics, values, and technological design (pp. 11–40). New York: Springer.
de Vries, R. E., & van Gelder, J.-L. (2015). Explaining workplace delinquency: The role of Honesty–Humility, ethical culture, and employee surveillance. Personality and Individual Differences, 86 , 112–116.
Dhanani, L. Y., Beus, J. M., & Joseph, D. L. (2018). Workplace discrimination: A meta-analytic extension, critique, and future research agenda. Personnel Psychology, 71 (2), 147–179.
Dierksmeier, C., & Seele, P. (2018). Cryptocurrencies and business ethics. Journal of Business Ethics, 152 (1), 1–14.
Dixon, P. (2008). Ethical issues implicit in library authentication and access management: Risks and best practices. Journal of Library Administration, 47 (3–4), 141–162.
Economist, T. (2017). Advances in AI are used to spot signs of sexuality. https://www.economist.com/news/science-and-technology/21728614-machines-read-faces-are-coming-advances-ai-are-used-spot-signs . Accessed 1 Nov 2018.
European Commission. (2018a). What does the General Data Protection Regulation (GDPR) govern? https://ec.europa.eu/info/law/law-topic/data-protection/reform/what-does-general-data-protection-regulation-gdpr-govern_en . Accessed 28 Dec.
European Commission. (2018b). What data can we process and under what conditions? https://ec.europa.eu/info/law/law-topic/data-protection/reform/rules-business-and-organisations/principles-gdpr/what-data-can-we-process-and-under-which-conditions_en . Accessed 29 Dec.
Evans, R., McNamee, M., & Owen, G. (2017). Ethics, nanobiosensors and elite sport: The need for a new governance framework. Science and Engineering Ethics, 23 (6), 1487–1505.
Eyers, J. (2017). Westpac testing AI to monitor staff and customers. Australian Financial Review. November 14. https://www.afr.com/technology/westpac-testing-ai-to-monitor-staff-and-customers-20171114-gzks7h . Accessed 29 Dec 2018.
Fairweather, N. B. (1999). Surveillance in employment: The case of teleworking. Journal of Business Ethics, 22 (1), 39–49.
Grafsgaard, J., Wiggins, J. B., Boyer, K. E., Wiebe, E. N., & Lester, J. (2013). Automatically recognizing facial expression: Predicting engagement and frustration. In Proceedings of the 6th International Conference on Educational Data Mining (pp. 43–50).
Greenwood, M., & Van Buren, I. I. I., H. J (2010). Trust and stakeholder theory: Trustworthiness in the organisation–stakeholder relationship. Journal of Business Ethics, 95 (3), 425–438.
Gregersen, A., Langkjær, B., Heiselberg, L., & Wieland, J. L. (2017). Following the viewers: Investigating television drama engagement through skin conductance measurements. Poetics, 64 , 1–13.
Gurdus, E. (2017). UnitedHealthcare and Fitbit to pay users up to $1,500 to use devices, Fitbit co-founder says. https://www.cnbc.com/2017/01/05/unitedhealthcare-and-fitbit-to-pay-users-up-to-1500-to-use-devices.html . Accessed 4 Nov 2018.
Hassib, M., Schneegass, S., Eiglsperger, P., Henze, N., Schmidt, A., & Alt, F., EngageMeter: A system for implicit audience engagement sensing using electroencephalography. In Proceedings of the 2017 CHI Conference on Human Factors in Computing Systems, 2017 (pp. 5114–5119): ACM.
Henschke, A. (2017). Ethics in an age of surveillance: Personal information and virtual identities . Cambridge: Cambridge University Press.
Herschel, R., & Miori, V. M. (2017). Ethics and big data. Technology in Society, 49 , 31–36.
Holland, P. J., Cooper, B., & Hecker, R. (2015). Electronic monitoring and surveillance in the workplace: The effects on trust in management, and the moderating role of occupational type. Personnel Review, 44 (1), 161–175.
Hoofnagle, C. J., King, Jennifer, Li, Su, & Turow, J. (2010). How different are young adults from older adults when it comes to information privacy attitudes and policies?. Available at https://doi.org/10.2139/ssrn.1589864 .
Hou, R. (2017). Neoliberal governance or digitalized autocracy? The rising market for online opinion surveillance in China. Surveillance and Society, 15 (3/4), 418–424.
Introna, L., & Nissenbaum, H. (2010). Facial recognition technology a survey of policy and implementation issues. Lancaster University Management School Working Paper (Vol. 2010/030): Lancaster University.
Jain, A. K., Hong, L., & Pankanti, S. (2000). Biometric identification. Communications of the ACM, 43 (2), 90–98.
Jain, A. K., & Kumar, A. (2012). Biometric recognition: an overview. In Second generation biometrics: The ethical, legal and social context (pp. 49–79). New York: Springer.
Jain, A. K., Ross, A. A., & Nandakumar, K. (2011). Introduction. In Introduction to biometrics (pp. 1–49). New York: Springer.
Karkazis, K., & Fishman, J. R. (2017). Tracking US professional athletes: The ethics of biometric technologies. The American Journal of Bioethics, 17 (1), 45–60.
Kirchberg, V., & Tröndle, M. (2012). Experiencing exhibitions: A review of studies on visitor experiences in museums. Curator, 55 (4), 435–452.
Kirchberg, V., & Tröndle, M. (2015). The museum experience: Mapping the experience of fine art. Curator, 58 (2), 169–193.
Klare, B. F., Burge, M. J., Klontz, J. C., Bruegge, R. W. V., & Jain, A. K. (2012). Face recognition performance: Role of demographic information. IEEE Transactions on Information Forensics and Security, 7 (6), 1789–1801.
Kokolakis, S. (2017). Privacy attitudes and privacy behaviour: A review of current research on the privacy paradox phenomenon. Computers and Security, 64 , 122–134.
Li, Y., Kobsa, A., Knijnenburg, B. P., & Nguyen, M. C. (2017). Cross-cultural privacy prediction. Proceedings on Privacy Enhancing Technologies , 2017(2), 113–132.
Loch, K. D., Conger, S., & Oz, E. (1998). Ownership, privacy and monitoring in the workplace: A debate on technology and ethics. Journal of Business Ethics, 17 (6), 653–663.
Lodge, J. (2006). Ethical EU eJustice: Elusive or illusionary? Journal of Information, Communication and Ethics in Society, 4 (3), 131–144.
Lodge, J. (2012). The dark side of the moon: Accountability, ethics and new biometrics. In Second generation biometrics: The ethical, legal and social context (pp. 305–328). New York: Springer.
Lupton, D. (2013). Quantifying the body: Monitoring and measuring health in the age of mHealth technologies. Critical Public Health, 23 (4), 393–403.
Lupton, D. (2016a). The diverse domains of quantified selves: Self-tracking modes and dataveillance. Economy and Society, 45 (1), 101–122.
Lupton, D. (2016b). Personal data practices in the age of lively data. Digital sociologies, 335–350.
Lupton, D. (2016c). You are your data: Self-tracking practices and concepts of data. In Lifelogging (pp. 61–79): Springer.
Lupton, D. (2017). Feeling your data: Touch and making sense of personal digital data. New Media and Society, 19 (10), 1599–1614.
Malsch, I. (2013). The just war theory and the ethical governance of research. Science and Engineering Ethics, 19 (2), 461–486.
Maltseva, K., & Lutz, C. (2018). A quantum of self: A study of self-quantification and self-disclosure. Computers in Human Behavior, 81 , 102–114.
Martella, C., Gedik, E., Cabrera-Quiros, L., Englebienne, G., & Hung, H. (2015). How was it?: Exploiting smartphone sensing to measure implicit audience responses to live performances. In Proceedings of the 23rd ACM International conference on Multimedia (pp. 201–210). Brisbane Australia.
Martella, C., Miraglia, A., Frost, J., Cattani, M., & van Steen, M. (2017). Visualizing, clustering, and predicting the behavior of museum visitors. Pervasive and Mobile Computing, 38 , 430–443.
Martin, A. J., Wellen, J. M., & Grimmer, M. R. (2016). An eye on your work: How empowerment affects the relationship between electronic surveillance and counterproductive work behaviours. The International Journal of Human Resource Management, 27 (21), 2635–2651.
Martin, K. (2016). Understanding privacy online: Development of a social contract approach to privacy. Journal of Business Ethics, 137 (3), 551–569.
Martin, K. (2018). Ethical implications and accountability of algorithms. Journal of Business Ethics , 1–16.
Martin, K., & Freeman, R. E. (2003). Some problems with employee monitoring. Journal of Business Ethics, 43 (4), 353–361.
Martin, K. E., & Freeman, R. E. (2004). The separation of technology and ethics in business ethics. Journal of Business Ethics, 53 (4), 353–364.
McDaniel, B., D’Mello, S., King, B., Chipman, P., Tapp, K., & Graesser, A. (2007). Facial features for affective state detection in learning environments. In D.S. McNamara and J.G. Trafton (Eds.) Proceedings of the 29th Annual Cognitive Science Society , (pp. 467–472), Austin.
McStay, A. (2014). Privacy and philosophy: New media and affective protocol . New York, Peter Lang.
McStay, A. (2016). Empathic media and advertising: Industry, policy, legal and citizen perspectives (the case for intimacy). Big Data and Society, 3 (2), 1–11.
McStay, A. (2018). Emotional AI: The rise of empathic media . Bangor: Sage.
Milligan, C. S. (1999). Facial recognition technology, video surveillance, and privacy. Southern California Interdisciplinary Law Journal, 9 , 295.
Miltgen, C. L., & Peyrat-Guillard, D. (2014). Cultural and generational influences on privacy concerns: A qualitative study in seven European countries. European Journal of Information Systems, 23 (2), 103–125.
Mingers, J., & Walsham, G. (2010). Toward ethical information systems: The contribution of discourse ethics. MIS Quarterly, 34 (4), 833.
Moore, P., & Piwek, L. (2017). Regulating wellbeing in the brave new quantified workplace. Employee Relations, 39 (3), 308–316.
Murry, W. D., Wimbush, J. C., & Dalton, D. R. (2001). Genetic screening in the workplace: Legislative and ethical implications. Journal of Business Ethics, 29 (4), 365–378.
Naker, S., & Greenbaum, D. (2017). Now you see me: Now you still do: Facial recognition technology and the growing lack of privacy. Boston University Journal of Science & Technology Law, 23 , 88.
Nissenbaum, H. (2004). Privacy as contextual integrity. Washington Law Review, 79 , 119–158.
Nissenbaum, H. (2009). Privacy in context: Technology, policy, and the integrity of social life . Stanford: Stanford University Press.
Nissenbaum, H. (2011). A contextual approach to privacy online. Daedalus, 140 (4), 32–48.
NIST (2018). National Institute of Standards and Technology Face Recognition Vendor Test. https://www.nist.gov/programs-projects/face-recognition-vendor-test-frvt . Accessed 4 Nov.
Norval, A., & Prasopoulou, E. (2017). Public faces? A critical exploration of the diffusion of face recognition technologies in online social networks. New Media and Society, 19 (4), 637–654.
Nunan, D., & Di Domenico, M. (2017). Big data: A normal accident waiting to happen? Journal of Business Ethics, 145 (3), 481–491.
Odoherty, K. C., Christofides, E., Yen, J., Bentzen, H. B., Burke, W., Koenig, N. H. A., et al. (2016). If you build it, they will come: Unintended future uses of organised health data collections. BMC Medical Ethics, 17 (54), 1–16.
Park, Y. J. (2013). Digital literacy and privacy behavior online. Communication Research, 40 (2), 215–236.
Park, Y. J., & Skoric, M. (2017). Personalized ad in your Google Glass? Wearable technology, hands-off data collection, and new policy imperative. Journal of Business Ethics, 142 (1), 71–82.
Pentina, I., Zhang, L., Bata, H., & Chen, Y. (2016). Exploring privacy paradox in information-sensitive mobile app adoption: A cross-cultural comparison. Computers in Human Behavior, 65 , 409–419.
Prabhakar, S., Pankanti, S., & Jain, A. K. (2003). Biometric recognition: Security and privacy concerns. IEEE Security and Privacy, 99 (2), 33–42.
Royakkers, L., Timmer, J., Kool, L., & Rinie van, E. (2018). Societal and ethical issues of digitization. Ethics and Information Technology, 20 (2), 127–142.
Schuelke-Leech, B. A. (2018). A model for understanding the orders of magnitude of disruptive technologies. Technological Forecasting and Social Change, 129 , 261–274.
Schumacher, G. (2012). Behavioural biometrics: Emerging trends and ethical risks. In Second Generation Biometrics: The Ethical, Legal and Social Context (pp. 215–227). New York: Springer.
Sheehy, B. (2015). Defining CSR: Problems and solutions. Journal of Business Ethics, 131 (3), 625–648.
Shi, X., & Wu, X. (2017). An overview of human genetic privacy. Annals of the New York Academy of Sciences, 1387 (1), 61–72.
Smith, G. J. D., San Roque, M., Westcott, H., & Marks, P. (2013). Surveillance texts and textualism: Truthtelling and trustmaking in an uncertain world. Surveillance and Society, 11 (3), 215–221.
Soleymani, M., Asghari-Esfeden, S., Pantic, M., & Fu, Y. Continuous emotion detection using EEG signals and facial expressions. In Multimedia and Expo (ICME), 2014 IEEE International Conference on, 2014 (pp. 1–6): IEEE**.
Sprokkereef, A., and de Hert, P. (2012). Biometrics, privacy and agency. In Second generation biometrics: The ethical, legal and social context (pp. 81–101). New York: Springer.
Sud, M., & VanSandt, C. (2015). Identity rights: A structural void in inclusive growth. Journal of Business Ethics, 132 (3), 589–601.
Sutrop, M., & Laas-Mikko, K. (2012). From identity verification to behavior prediction: Ethical implications of second generation biometrics. Review of Policy Research, 29 (1), 21–36.
Taddicken, M. (2014). The ‘privacy paradox’in the social web: The impact of privacy concerns, individual characteristics, and the perceived social relevance on different forms of self-disclosure. Journal of Computer-Mediated Communication, 19 (2), 248–273.
Trocchia, P. J., & Ainscough, T. L. (2006). Characterizing consumer concerns about identification technology. International Journal of Retail and Distribution Management, 34 (8), 609–620.
Ulman, Y. I., Cakar, T., & Yildiz, G. (2015). Ethical issues in neuromarketing: “I Consume, Therefore I am!”. Science and Engineering Ethics, 21 (5), 1271–1284.
Unar, J., Seng, W. C., & Abbasi, A. (2014). A review of biometric technology along with trends and prospects. Pattern Recognition, 47 (8), 2673–2688.
Van der Ploeg, I. (2003). Biometrics and privacy: A note on the politics of theorizing technology. Information, Communication and Society, 6 (1), 85–104.
Wang, C., & Cesar, P. Do we react in the same manner?: Comparing GSR patterns across scenarios. In Proceedings of the 8th Nordic Conference on Human-Computer Interaction: Fun, Fast, Foundational , 2014 (pp. 501–510): ACM.
Wang, C., & Cesar, P. The play is a hit: But how can you tell? In Proceedings of the 2017 ACM SIGCHI Conference on Creativity and Cognition , 2017 (pp. 336–347): ACM.
Wang, C., Geelhoed, E. N., Stenton, P. P., & Cesar, P. Sensing a live audience. In Proceedings of the 32nd annual ACM conference on Human factors in computing systems , 2014 (pp. 1909–1912): ACM.
Wang, C., Wong, J., Zhu, X., Röggla, T., Jansen, J., & Cesar, P. Quantifying audience experience in the wild: Heuristics for developing and deploying a biosensor infrastructure in theaters. In Quality of Multimedia Experience (QoMEX), 2016 Eighth International Conference on IEEE , 2016 (pp. 1–6).
Webb, A. M., Wang, C., Kerne, A., & Cesar, P. Distributed liveness: Understanding how new technologies transform performance experiences. In Proceedings of the 19th ACM Conference on Computer-Supported Cooperative Work and Social Computing , 2016 (pp. 432–437): ACM.
West, J. P., & Bowman, J. S. (2016). Electronic surveillance at work: An ethical analysis. Administration and Society, 48 (5), 628–651.
Whitehill, J., Serpell, Z., Lin, Y.-C., Foster, A., & Movellan, J. R. (2014). The faces of engagement: Automatic recognition of student engagementfrom facial expressions. IEEE Transactions on Affective Computing, 5 (1), 86–98.
Winter, J. S. (2014). Surveillance in ubiquitous network societies: Normative conflicts related to the consumer in-store supermarket experience in the context of the Internet of Things. Ethics and Information Technology, 16 (1), 27–41.
Winter, S. J., Stylianou, A. C., & Giacalone, R. A. (2004). Individual differences in the acceptability of unethical information technology practices: The case of Machiavellianism and ethical ideology. Journal of Business Ethics, 54 (3), 273–301.
Zarsky, T. Z. (2017). Incompatible: The GDPR in the age of big data. Seton Hall Law Review, 47 (4), 995–1020.
Zhao, W., Chellappa, R., Phillips, P. J., & Rosenfeld, A. (2003). Face recognition: A literature survey. ACM Computing Surveys, 35 (4), 399–458.
Zwitter, A. (2014). Big data ethics. Big Data and Society, 1 (2), 1–6.
Wickins, J. (2007). The ethics of biometrics: the risk of social exclusion from the widespread use of electronic identification. Science and Engineering Ethics, 13 (1), 45–54.
Finn, R. L., Wright, D., & Friedewald, M. (2013). Seven types of privacy. In European data protection: coming of age (pp. 3–32). Dordrecht: Springer.
Jain, A. K., Ross, A., & Prabhakar, S. (2004). An introduction to biometric recognition. IEEE Transactions on circuits and systems for video technology, 14 (1), 1–29.
Download references
Acknowledgements
Feedback from the anonymous reviewers informed this updated version; I greatly appreciate their efforts and useful suggestions in improving the manuscript. I would also like to acknowledge the research assistance of Ishan Senarathna. I would also like to thank Nicholas Patterson and Matthew Warren for their feedback and guidance in the early stages.
Funding for this research was provided by the Deakin Business School, Centre for Sustainable and Responsible Organisations (CSaRO).
Author information
Authors and affiliations.
Department of Management, Deakin Business School, Deakin University, 70 Elgar Road, Burwood, VIC, 3125, Australia
Andrea North-Samardzic
You can also search for this author in PubMed Google Scholar
Corresponding author
Correspondence to Andrea North-Samardzic .
Ethics declarations
Conflict of interest.
I declare that I have no conflict of interest.
Additional information
Publisher’s note.
Springer Nature remains neutral with regard to jurisdictional claims in published maps and institutional affiliations.
An earlier version of this paper was accepted for the 77th Academy of Management Meeting.
Rights and permissions
Reprints and permissions
About this article
North-Samardzic, A. Biometric Technology and Ethics: Beyond Security Applications. J Bus Ethics 167 , 433–450 (2020). https://doi.org/10.1007/s10551-019-04143-6
Download citation
Received : 14 July 2018
Accepted : 04 March 2019
Published : 08 March 2019
Issue Date : December 2020
DOI : https://doi.org/10.1007/s10551-019-04143-6
Share this article
Anyone you share the following link with will be able to read this content:
Sorry, a shareable link is not currently available for this article.
Provided by the Springer Nature SharedIt content-sharing initiative
- Biometric technology
- Find a journal
- Publish with us
- Track your research
Services on Demand
Related links.

South African Journal of Information Management
On-line version issn 1560-683x print version issn 2078-1865, sajim (online) vol.23 n.1 cape town 2021, http://dx.doi.org/10.4102/sajim.v23i1.1407 .
ORIGINAL RESEARCH
Biometric technology in banking institutions: 'The customers' perspectives'
Abraham Morake; Lucas T. Khoza; Tebogo Bokaba
Department of Applied Information Systems, College of Business and Economics, University of Johannesburg, Auckland Park, South Africa
Correspondence
BACKGROUND : Over the years, attention has been focused on digital banking and financial technology with little or no attention being paid to biometric banking technology. OBJECTIVE : The study aimed to investigate the need for security and simplicity in the authentication of retail payments, digital banking and financial technology through the application of biometric systems. METHOD : The study employed quantitative research methodology and a response rate of 52% was achieved. A set of questionnaires was distributed for data collection. RESULTS : The study's findings indicated it is imperative for all businesses that participate in financial businesses to fully implement the best possible security measures or systems to ensure or enhance security for financial business activities CONCLUSION : Based on the findings of the study, it is recommended that businesses must adopt the new innovative and secured mechanisms of financial dealings to enhance innovation, security and flexibility.
Keywords : biometrics; financial technology; security; authentication methods; digital banking.
Introduction
There are increasing concerns related to the security in authenticity and integrity of banking systems (De Souza Faria & Kim 2013; Petrlic & Sorge 2013). The weakness of the current verification or authentication methods such as pin numbers and passwords contributes significantly towards information leakage stored in Automated Teller Machine (ATM) smartcard which results in loss of money in bank account (Jaiswal & Bartere 2014). The word biometrics originates from ancient Greek and implies measures - bios mean life, whilst metrics mean measuring, therefore in full it means measuring life (Prabhakar, Pankanti & Jain 2003). It can be described as the process of identifying human uniqueness employing physical traits that include the face, fingerprint, iris and behavioural traits (Jain, Flynn & Ross 2007). There are various biometrics classifications: fingerprint scrutiny, face examination, hand geometry, iris observation, voice recognition, and signature acknowledgement (Clodfelter 2010).
The birth of biometrics can be traced back to the 19th century where it mainly focused on gaining knowledge of people's physical traits to secure their identity (Maguire 2009). Earlier biometrics was mainly applied within high-security applications. However, it is currently applied within a wider variety of public-facing applications, for example, in prisons, by police for drivers' license verification, canteen administration, payment systems, in the borders for verification control, including electoral system (Ashbourn 1999). Since the late 1990s, there have been changes in biometrics as a primary security replacement technology from an older form of identification such as passwords and security pin-codes (Maguire 2009). Biometrics initially was used to measure the physical and behavioural features of a person (Galton 1901). Upcoming biometric verification applications comprise ATM use, workplace authentication, network access, travel and tourism, world wide web connections, and mobile connections (Ashbourn 1999).
Over the last few years, more studies have been done on digital banking, financial technology and other areas rather than the impact of biometrics within banking and retailing in South Africa. Digital is the separation of information from physical data storage to the technical potential or digital (Legner et al. 2017). There are various characteristics of digitisation, namely collaboration, sharing, co-creation, connectivity, communication, mobility, and flexibility (Syler & Baker 2016). Digital networks started to join retailers together with traders, clients and customers to develop the identified needs for the first online connected catalogues and inventory software systems (Kelman 2016). Digital banking refers to the process of shifting into online banking and the digitisation of the entire outdated banking activities, including plans that were historically offered to the bank customers and required customers to physically visit the bank to do specific activities such as money deposit, withdrawals, money transfers and account management (Coetzee 2018). Digital banking has facilitated customers to overcome controlled time banking and local area operations (Das 2018). Digital banks use advanced banking systems that can swiftly implement new services allowing for seamless mobility for bank users (Varga & David 2017).
The main problem with this research is that there is a demand for more innovative and secured banking systems that will enable customers to access their money at any given time and location. In this fourth industrial revolution (4IR) era and the need for transformation within the banking sectors, technological advancement has provided better opportunities for financial institutes to tap. In contrast, many financial institutions have conformed to the traditional digital banking platforms as a mode of operation. This digital banking platform enables customers to make money deposits, withdrawals, transfers and account management without physically visiting the bank. However, none of these banking sectors have been able to take full advantage of the capacity and possibilities of the 4IR for a more innovative and simplified banking platform.
There are only few studies which have studies and covered biometric banking and payment systems. To bridge the gap, this study seeks to evaluate the innovative and secured methods of paying for items at retail stores and accessing money without physically having a bank card and hard cash through the application of biometrics. The study focuses on biometrics digital banking financial technology as an alternative means of authentication for mobile baking transactions such as payments and bank transfers. Current authentication methods still use traditional password authentication. In addition, this article seeks to create awareness in banks and retailers on the significant role of biometrics as an essential mechanism in providing speedy, secured, flexible and innovative authentication process to protect the funds/money of customers and the organisation, which can result in crime being lowered or prevented.
This article is structured as follows: section literature review, discusses the current knowledge and findings around biometric technology in the banking system. Section Challenges of biometrics covers the research problem that this research study attempts to address. Section Research method and design discusses the research methodology. Lastly, sections Results and analysis through 11 is the data analysis.
Literature review: Biometric technology in the banking system
As submitted by Ateba et al. (2013), for banks to remain relevant, successful and competitive in today's competitive world, they must provide innovative and best-secured services to their customers.
Customer and organisational perspectives
A customer can be described as a stakeholder of an organisation who provides payment in exchange for products or services (Ateba et al. 2013). In addition, a customer cannot only be described as a person but also an organisation (e.g. university, bank, construction company, school, legal firm and hospital) that buys goods and services from other retailers (Rahman & Safeena 2016). Organisations (banks and retail) need to understand that customers come from various occupations (Rahman & Safeena 2016). More banking and other financial transactions are being done online by customers and fraudsters have followed suit, initiating ever-more sophisticated attacks. With the risk of digital fraud and theft increasing many organisations have searched for solutions to stop fraudsters from launching ever-more sophisticated attacks. Banks cannot stop or limit the high rate of transaction scams and security breaks by using traditional security systems such as password/pin and identification cards; therefore, digital banking solutions appear to be a perfect mechanism to defeat these threats (Hosseini & Mohammadi 2012). Pin code verification alone cannot be regarded as a strong defence mechanism against security breaches. Using digital banking solutions, the operator's data or information is securely kept in an encrypted container or sandbox (Johnson 2019).
Digital perspective
Digital banking solutions have proven to be more innovative for end-users, who appreciate replacing a complicated password with a fingerprint or face scan, which features biometric technologies (Agidi 2018). By applying biometrics, traditional passwords are becoming a thing of the past; biometrics is taking over banking security. To achieve safeguarding of operations and customer transactions, one solution is to secure banking using a consistent authentication method such as biometric (Hosseini & Mohammadi 2012). Biometrics characteristics include fingerprints, veins, palm veins, iris, retina, face, voice, and handwritten signature. The patterns of blood vessels in the palm finger are so different that no two or more individuals possess the same, and this can serve as a trusted security system (Ahmad, Ali & Adnan 2012). Biometrics is still in its early stages in developing countries, but it has been developed and adopted by businesses to increase the security and efficiency of the adopter's operations (Agidi 2018).
Usage of biometrics in banking institutions is popular in developed countries thus, the adoption rate of biometrics is growing significantly (Venkatraman & Delpachitra 2008). There is no hesitation that biometrics are escalating for banking security, to an extant identifying authentication through biometric application is highly secured compared to password authentication (Liang, Samtani, Guo & Yu 2020). Biometric authentication is also coming to physical payments cards; biometrics are progressively being used for account access, even replacing debit cards at the ATMs (Lee 2016). Biometrics provides a much more reliable and efficient method of verification than relying only on human agents. The security and efficiency principles of biometrics make the adoption of biometrics an attractive prospect to banking institutions across the world (Agidi 2018). With the average banking customer managing a broader range of financial transactions online through desktop and mobile devices, the need for simple and secured access to their banking data is becoming a top priority for banking service providers intending to differentiate themselves from the direct rivals. As the digital age expands, banks need to balance security and accessibility (Varga 2018).
Major South African banks include: ABSA, FNB, Nedbank, Standard Bank, and Capitec (Coetzee 2018). This is based on their revenue generation, large base of customers, services and products they offer and marketing strategies they deliver. Without the successful implementation and adaption of e-banking by the South African banking industry, most banks will struggle to perform optimally through the adaption of the 4IR and FinTech (Abukhzam & Lee 2010). Businesses have realised the increasing value digitisation provides towards the growth of businesses (Neumeier et al. 2017).
It is important that digital payment service providers (banks) have a comprehensive cybersecurity strategy aided by a robust framework to assist all stakeholders participating in the ecosystem (Kristensen & Solvoll 2019). There is a demand for managing service interface and customisation of products and services influenced by the input of technology offered in business settings (De Farias et al. 2014). Advanced biometric payment methods enhance the convenience, choice of payments and alternative payment methods for customers. Payment methods allow customers to conduct business and commercial activities with ease and flexibility at any given time (Kristensen & Solvoll 2019). Payment experts concur that electronic payment techniques are efficient, convenient and fast (Crowe, Schuh & Stavins 2006).
Biometrics application in automated teller machines
Biometrics in banking for ATM authentication provides both the banks and the customers with an outstanding benefit through providing customers with the flexibility to do transactions without physically having their bank cards; thus, banks can avoid the costs and liabilities of customer problems because of lost and/or stolen bank cards (Vernkatraman & Delpachitra 2008). Using biometrics in banking, ATMs are popular in developed countries; thus, the adoption rate of biometrics is growing significantly (Venkatraman & Delpachitra 2008). There is no doubt that biometrics is escalating in banking security, but it might be a while before identifying authentication without passwords is completely secure (Furnell & Evangelatos 2007). Biometric authentication is also coming to physical payment cards; thus, biometrics is progressively being used for account access, even replacing debit cards at the ATMs (Choi et al. 2007).
Challenges of biometrics
Biometric challenges can negatively impact people and businesses or customers and organisations. Bank crises and failures can be attributed to the growing extent to which scammers and fraudsters operate (Bhasin 2015). Fraud is considered a global phenomenon that negatively challenges all sectors of the economy (Bhasin 2015). A rapid increase in security cracks and transactional breaches within traditional security systems such as pin codes and passwords is speedily influencing the evolution of a strong biometric authentication method (Hosseini & Mohammadi 2012).
In addition, a factor that can contribute towards the challenges of adopting biometrics is too much time and money spent to educate people who are technologically and biometrically illiterate (Ahmad et al. 2012). New deployments or the premature phase of biometric technology are quite similar to the introduction of any other system, since it might take a while for general users to accept it, depending on the system's impact on them (Wayman et al. 2005).
Any form of change in the customers finger (a user cuts him-/herself by mistake) may lead to the users being denied access to their respective systems that has been created by the users with their normal fingerprint (Ahmad et al. 2012).
Another significant challenge of biometrics includes a scenario whereby, should the user be involved in an accident and lose an eye, finger or facial changes occur because of scratches or cuts, the biometric system will not recognise the user and will reject the user as a result of the physical changes or damages (Aly et al. 2008; Buddharaju, Pavlidis & Manohar 2008).
Biometrics has difficult challenges that may impact the human rights of a person negatively, for example, when a thief decides to cut off a victim's finger to gain access to their information and the system (Choi et al. 2007; Chetty & Wagner 2009; Jin, Kim & Elliott 2007; Pacut & Czaika 2006; Tan et al. 2010; Toth 2005). Dust and grime on the fingerprint scanner may impact the quality of the system negatively, which may result in a situation where the system does not recognise the user (Ahmad et al. 2012).
There are various issues that characterise the challenges of biometrics in problems such as signature authentication forgery, the high cost of implementing liveness detection, dust dropped on scanners, poor quality of the scanner to recognise the user, a time-consuming system, poor human machine interaction, lack of guidance for interacting with the system and a lack of proper information security policies and procedures (Brooks 2010; Jain & Kumar 2010; Koppenhaver 2007; Park 2008).
The main contributing factors to the challenges of biometric information usage is the misuses, negative interpretation, and failure to complying to the Protection of Personal Information Act (POPIA). The purpose of the Act is to protect people from harm by protecting their personal information, to stop their money being stolen, to stop their identity being stolen, and generally to protect their privacy, which is a fundamental human right (POPIA Act 2021). In South Africa, a person's fingerprints and blood type are considered personal information under the Electronic Communications and Transactions Act (ECTA 2002).
Since early 2020, the COVID-19 pandemic has impacted on and disrupted many aspects of peoples daily life. Touch-based technologies such as fingerprint and facial recognition scanners can be considered as indirect contributing factors for COVID-19, because they are used by many people for authentication and verification purposes at ATMs, stores and banks (Gomez-Barrero et al. 2021). Hygiene related fears have increased the societal resistance towards the use of touch-based biometrics sensors (Priesnitz et al. 2021). In addition, it is important to note that such fears have in turn fuelled research efforts in 2D or 3D touchless fingerprint recognition systems (Gomez-Barrero et al. 2021).
Benefits of biometrics
Biometric benefits can impact both people and businesses or customers and organisations. Moreover, biometrics can be considered a quicker information tracer and recovery method than manual or traditional verification methods carried out at the counter (Ahmad et al. 2012; Jain & Kumar 2010; Jain, Ross & Pankanti 2006).
Biometric security can be considered a method that contributes significantly towards ensuring the integrity, confidentiality and availability of information (Ahmad et al. 2012). Biometrics protects both logical and physical access controls. Logical access controls include the protection of network facilities, computers and information systems against unauthorised admission (Jain et al. 2006), whilst physical access controls ensure that only authorised people have access to IT infrastructures and document filing (Jain et al. 2006).
Forensic accounting is a requirement for banks to decrease the speedy growth of financial frauds (Bhasin 2015). In addition, biometric authentication methods offer a natural, unforgettable and rarely breached verification (Hosseini & Mohammadi 2012). Password, pin and code word authentication can be forgotten, cracked and guessed by hackers or scammers (Jain et al. 2006). In addition, fingerprint authentication is more secure, as it provides users with quicker verification and is impossible to forget compared to a password (Johnson 2019). Smartcards are also at risk of being lost, stolen and duplicated (Jain et al. 2006). Therefore, biometrics can be considered a solution for enhanced security, as the authentication relies on a person's physical traits (Jain et al. 2006). Physiological biometric features include retina, fingerprint, hand vein, iris, hand geometry facial recognition, and ear shape. These features are unique, and no one in the world shares them (Ahmad et al. 2012). Behavioural biometric features include voice recognition and signature verification (Ahmad et al. 2012; Jain & Kumar 2010).
Biometric security systems can assist banks with various benefits such as forensic application, criminal identification, border control and surveillance (Rhodes 2003). Various impacts may characterise the benefits of biometrics, for example, human signature authentication, being user-friendly, convenient and flexible, maintaining accuracy, faster information retrieval, strong matching algorithm and speaker recognition (Koppenhaver 2007; Park 2008; Wang et al. 2011).
Multi-factor authentication methods
The use of a password (or a PIN) to confirm the ownership of the user ID could be considered as a single-factor authentication (SFA) method (Ometov et al. 2018). Evidently, this is the weakest level of authentication (Dasgupta, Roy & Nag 2016; Bonneau et al. 2015).
Authentication with just a single-factor method is not reliable to provide enough protection because of several security threats such as rainbow table and dictionary attacks (Gunson et al. 2011). Two-factor authentication (2FA) methods consist of something the user has, such as cards, smartphones, or other tokens (Sun et al. 2014; Bruun, Jensen & Kristensen 2014). Multi-factor authentication (MFA) methods consist of something the user/customer is, specifically, biometric data or behaviour patterns such as fingerprint, face recognition, behaviour recognition and others (Ometov et al. 2018).
The need for reliable user authentication method has increased in the wake of intensified concerns about security and rapid advancements in communication, mobility, and networking (Yadav & Gothwal 2011). Frequently, MFA is based on biometrics, which is automated recognition of individuals based on their behavioural and biological characteristics (Frank, Biedert, Ma, Martinovic & Song 2012). Biometrics challenges and benefits will be further discussed in detail, because the term can be considered as a key technique of MFA. Figure 1 shows the evolution from SFA factor to MFA.
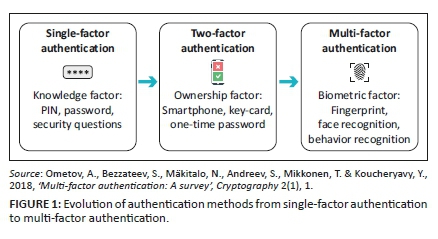
Research method and design
The research design that was used in this study was quantitative. Quantitative research refers to a numerical illustration of explanations of the phenomena (Sukamolson 2000). Throughout the study, data have been collected by means of:
A literature review and comparing a list of similar work done over the years.
Conducting an online survey to evaluate the use of biometrics to authenticate payment and day-to-day personal banking transactions.
Consulting with shoppers, bank users, financial institutions such as banks and general societies (students, employed and unemployed community members) with bank accounts.
A questionnaire survey was conducted on a sample population of respondents who have knowledge on biometrics, digital banking, financial technology, retail and customers. The overall number of the questionnaires shared received 336 responses. Out of the 336 responses, only 173 respondents submitted fully completed questionnaire, the remaining 162 respondents did not complete the survey. This process gave the questionnaire a successful completion response rate of 52%. The questionnaire was designed into four sections which are: A, B, C and D. Section A gathered the background information of the respondents, Section B collected the challenges of biometric, Section C collected benefits of biometric, and the final Section D gathered biometric solutions to enhance secured and innovative means of accessing, transferring and sharing money. The survey was distributed electronically via different social media platforms. The selected sample technique for this study is the probability sampling technique which facilitates study of a large population, and therefore was relevant for this study as its targeted sample size was 300 responses. Furthermore, quantitative research is commonly aligned with the probability sampling technique to enhance generalisability (Saunders et al. 2019). The reason for the study to employ students is because financial decision-making is very important for the success of students in their lives and careers; therefore, it is critical for students to understand funds management (Sachitra, Wijesinghe & Gunasena 2019). Another contributing reason for the study to use bank members such as managers is because they value financial information and have key financial knowledge (Akhtar & Liu 2018).
The study employed the random sampling technique in preference of the systematic, stratified and cluster random sampling techniques. The inclusion criterion for the study was shoppers with one or more bank accounts. The study mainly focused on the city of Johannesburg in Gauteng province. Johannesburg has an estimated population of 5 782 747. Out of this population, about 30% are below the standard age of owning a bank account (Department of Statistics South Africa 2019), totalling 1 734 824. From the remaining 4 047 923 shoppers with bank accounts, the sample size of the research was limited to 300 respondents because of issues such as time and resource constraints. The study only targeted the age group of 18-60. The study also targeted the population using payment mechanisms such as:
Electronic Fund Transfers (EFTs)
Credit and cheque cards
Internet banking transfers
Card-based payments
Debit cards
Visa Checkout
Samsung Pay/ Mobile Pay
Validity of the data collection tool used
The validity of the collected data was demonstrated through questionnaires and surveys. Content validity will be determined based on the reliably collected data provided by respondents (bank managers, retail managers and customers). Thus, constructive validity will be determined through evaluating the views of customers, bank managers and retail managers using biometrics authentication for payments and other activities. Both Cronbach's alpha and Statistical Package for the Social Sciences (SPSS Version 26) were used to ensure that the collected data is accurate, logical and factual (Scherbaum & Shockley 2015).
Using the Cronbach's alpha analytical tool on SPSS, it was found that the validity of the response regarding 'usage of biometrics in terms of financial sector' is 0.857. Table 1 shows the Cronbach's alpha values.
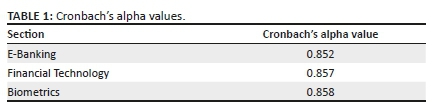
Ethical considerations
Approval to conduct the study was obtained from the College of Business and Economics, the University of Johannesburg. During data collection, personal information was not requested and participation in this research work was voluntary, and participants were allowed to withdraw upon completing the questionnaires.
Results and analysis
This section of the study presents findings of the study obtained during questionnaire distribution.
Descriptive statistics
Figure 2 describes the sector or occupation in which respondents are involved. In a practical example, the people belonging to academic and education sectors visit retail stores to purchase books, laptops and other academic or education-related merchandise. In construction sector, there must be a purchase of building or construction materials; the same applies to other occupations. This shows that data supplied by these categories of respondents are reliable.
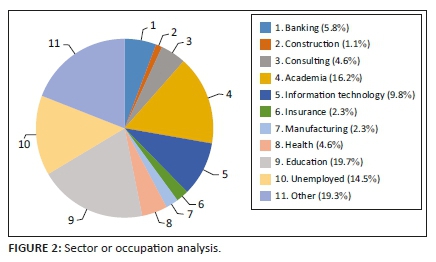
The education sector has the highest percentage of 19.7%, followed by Other with 19.1%. Academia is the third-ranked sector with 16.2%, followed by the unemployed respondents with 14.5%. The information technology sector is ranked fifth with 9.8%, followed by the banking sector with 5.8%. Both the health and consulting sectors have a percentage of 4.6%. The manufacturing and insurance sectors both have a percentage of 2.3%. The construction sector has the lowest percentage of 1.1%. Table 2 shows the sector or occupation values to clarify values in Figure 2 .
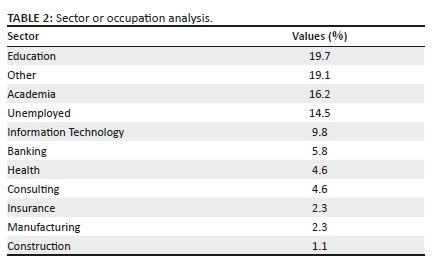
The results show that the study has covered various organisational sectors. Academia, construction, consulting, education, health, information technology, insurance, manufacturing and other sectors, including the unemployed individuals, are all represented. This concludes that most organisational sectors have been represented in the study.
Highest qualification
Figure 3 describes the highest qualification of respondents to evaluate their potential level of understanding new topics that impact their daily lives and activities in this modern era of the 4IR. The results are arranged from the highest to the lowest percentage. Results reveal that 31.8% of the respondents have obtained a bachelor's degree, 16.8% matric or Grade 12, 15.0% an honours degree, another 13.3% a university diploma, 11.0% a Master's degree, 6.4% college diplomas, 4.0% other qualifications, 1.2% with a Ph.D. degree and 0.5% without matric.
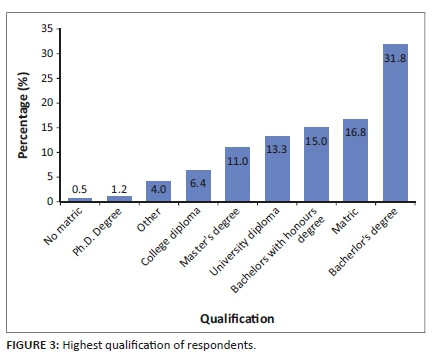
The results indicate that a large percentage of the respondents have obtained a bachelor's degree. This indicates that most respondents have a good education and are more knowledgeable (Bosupeng 2017). A question on rating the educational level of the respondents has been included to evaluate their level of understanding new topics impacting their daily lives and activities in this modern era of the fourth industrial revolution.
It is important to be educated, well-informed and technologically exposed because education contributes significantly to developing a person's opinions, character, trading with others and preparing one for life experiences (Al-Shuaibi 2014). Additional literature aligned with the study provides that promising stages to prevent fraud activities are educating customers with various processes of avoiding being a victim of fraudsters (Bhasin 2015).
Correlation statistics
The purpose of this section is to describe the relationship between variables. Thus, extensive literature was used to analyse other sections of the article; the Pearson's correlation was conducted to explore statistical relationships amongst variables. Moreover, data analysis was conducted through matching and comparing the benefits variables together with the challenge variables. The Pearson's correlation was used because it works with the raw data values of the variables, whereas Spearman works with rank-ordered variables. Moreover, the Pearson's correlation evaluates the linear relationship between two continuous variables, whilst the Spearman correlation coefficient is based on the ranked values for each variable rather than the raw data (De Winter, Gosling & Potter 2016).
The data analysis technique used to analyse the data was correlation to predict the strength and direction between two variables. The strength of correlation between the variables is shown under the Pearson's correlation, whilst Sig. (2-tailed) represents the significance of the influence amongst the variables. Sig. (2-tailed) below 0.05 indicates that the relationship between the variables is significant, whilst Sig. (2-tailed) above 0.05 indicates that there is no significant relationship between the variables (Pallant 2020). Table 3 illustrates the Sig. (2-tailed) declaration, whilst Table 4 demonstrates the Pearson's correlation declaration.
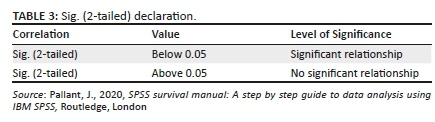
Biometric challenges and benefits analysis
The Pearson's correlation was conducted to examine the relationship between biometric challenges and biometric benefits.
Appendix 1 shows the Pearson's correlation for enhanced surveillance with involvement in an accident, sensitivity of sensor performance and biometric characteristics. There is a weak positive significant relationship between enhanced surveillance and involvement in an accident ( r = 0.004; p = 0.216), enhanced surveillance and sensitivity of sensor performance ( r = 0.008; p = 0.202), and enhanced surveillance and biometric characteristics ( r = 0.004; p = 0.220).
These findings indicate that should the user be involved in an accident and have cuts on their biometric features such as fingers, face or iris, it will be difficult for a biometric reader to fully recognise the authorised user to gain access to a system. The biometric security system can assist banks and retailers with a wide range of benefits such as surveillance, as reported by Rhodes (2003).
Appendix 1 also shows the Pearson's correlation for enhanced border control with involvement in an accident, sensitivity of sensor performance and biometric characteristics. Statistical results indicate that there is a weak positive significant relationship between enhanced border control and involvement in accident ( r = 0.001; p = 0.253), enhanced border control and sensitivity of sensor performance ( r = 0.007; p = 0.205), and enhanced border control and biometric characteristics ( r = 0.048; p = 0.150).
These findings indicate that enhanced border control can be challenged by the fact that biometric characteristics such as face, fingerprint and voice recognition can be copied. The biometric security system can assist banks and retailers with a wide range of benefits such as forensic application, criminal identification, border control and surveillance (Rhodes 2003).
Appendix 1 next shows the Pearson's correlation for criminal identification with sensitivity of sensor performance and biometric characteristics. Statistical results indicate that there is a weak positive significant relationship between criminal identification and sensitivity of sensor performance ( r = 0.017; p = 0.181) and criminal identification and biometric characteristics ( r = 0.032; p = 0.163).
These findings imply that the process of effortlessly providing information about the criminal record of the individual can be challenged by a sensitivity of sensor performance. The biometric security system can assist banks and retailers with a wide range of benefits such as forensic applications, criminal identification, border control and surveillance (Rhodes 2003).
From Appendix 1 , the Pearson's correlation for ease of information retrieval with involvement in an accident and biometric characteristics can be observed. Statistical results show that there is a weak positive significant relationship between ease of information retrieval and being involved in an accident ( r = 0.014; p = 0.166) and ease of information retrieval and biometric characteristics ( r = 0.003; p = 0.224).
These findings indicate that the process of providing users with quicker verification can be hindered by damages or changes to the users' physical biometric features such as face, eyes and fingers caused by accidents. The biometric security system can assist banks with features maintaining accuracy, convenience, faster information retrieval, strong matching algorithm and speaker recognition (Koppenhaver 2007; Park 2008; Wang et al. 2011).
Appendix 1 shows the Pearson's correlation for strong matching algorithm with involvement in an accident, sensitivity of sensor performance and non-technologically inclined individuals. Moreover, statistical results indicate that there is a weak positive significant relationship between strong matching algorithm and being involved in an accident ( r = 0.017; p = 0.161), strong matching algorithm and sensitivity of sensor performance ( r = 0.007; p = 0.204), and strong matching algorithm and non-technologically inclined individuals ( r = 0.008; p = 0.200).
These findings indicate that biometric systems, which can easily differentiate between two or more biometric traits such as hands, eyes and face, can also be hindered by damages or changes to the user's physical biometric features such as the face, eyes and fingers caused by accidents. Pin code verification alone cannot be regarded as a strong defence mechanism against security breaches. However, by using biometric verification, the operator is secured to their data or information which is securely kept in an encrypted container or sandbox (Johnson 2019).
Appendix 1 shows the Pearson's correlation for lost or stolen smartcards and mobile devices with scammers, fraudsters and non-technologically inclined individuals. There is a weak positive significant relationship between lost or stolen smartcards and mobile devices and scammers ( r = 0.013; p = 0.188), lost or stolen smartcards and mobile devices and fraudsters ( r = 0.003; p = 0.224), and lost or stolen smartcards and mobile devices and non-technologically inclined individuals ( r = 0.005; p = 0.214).
These findings indicate that individuals, such as scammers, who participate in dishonest schemes by committing fraudulent activities may intend to exploit lost or stolen smartcards and mobile devices and steal funds of individuals. Biometrics in banking for ATM authentication provides both banks and customers with an outstanding benefit through providing customers with the flexibility to do transactions without physically having their bank cards; thus, banks can avoid the costs and liabilities of customer problems because of lost and/or stolen bank cards (Vernkatraman & Delpachitra 2008).
Appendix 1 also shows the Pearson's correlation for impossible to forget fingerprint authentication, non-technologically inclined individuals and biometric characteristics. There is a weak positive significant relationship between impossible to forget fingerprint authentication and non-technologically inclined individuals ( r = 0.014; p = 0.187) and impossible to forget fingerprint authentication and biometric characteristics ( r = 0.041; p = 0.155).
These findings indicate that fingerprint authentication is impossible to forget compared to a password. Moreover, non-technologically inclined individuals still trust that the pin code or password authentication method is the best technique for security authorisation (Bhasin 2015).
Appendix 1 further shows the Pearson's correlation for uniqueness, involvement in accident, sensitivity of sensor performance, non-technologically inclined individuals and biometric characteristics. Statistical results reveal that there is a weak positive significant relationship between uniqueness and being involved in an accident ( r = 0.011; p = 0.194), uniqueness and sensitivity of sensor performance ( r = 0.042; p = 0.155), uniqueness and non-technologically inclined individuals ( r = 0.001; p = 0.247), and uniqueness and biometric characteristics ( r = 0.002; p = 0.253).
These findings indicate that the uniqueness and benefits of the biometric authentication systems are supported by variables such as being involved in an accident, sensitivity of sensor performance, non-technologically inclined individuals and biometric characteristics such as the face, fingerprint and voice recognition (Hosseini & Mohammadi 2012). Physiological biometric features include retina, fingerprint, hand vein, iris, hand geometry, facial recognition and ear shape. These features are unique and no one in the world shares them (Ahmad et al. 2012).
Appendix 1 shows the Pearson's correlation for forensic application and dust dropped on the fingerprint scanner, involvement in an accident, sensitivity of sensor performance, non-technologically inclined individuals, and fake fingerprint forgery. There is a weak positive significant relationship between forensic application and dust dropped on the fingerprint scanner ( r = 0.015; p = 0.185), forensic application and involvement in an accident ( r = 0.002; p = 0.236), forensic application and sensitivity of sensor performance ( r = 0.000; p = 0.278), forensic application and non-technologically inclined individuals ( r = 0.000; p = 0.273), and lastly forensic application and fake fingerprint forgery ( r = 0.045; p = 0.153).
These findings indicate that because of physical biometric changes acquired by the users through an accident, it will be difficult for a biometric scanner system to easily recognise the user in a system. Forensic accounting is a requirement for banks to decrease the speedy growth of financial fraud (Bhasin 2015). In addition, the biometric authentication method offers natural, unforgettable, and hardly breached verification (Hosseini & Mohammadi 2012).
Biometric connections as solutions to deliver secured and innovative means of accessing, transferring and sharing money
Table 5 represents biometrics connections, including the level of agreeing and disagreeing by the respondents that the above-mentioned biometrics connections can be labelled as solutions that can assist banks and retailers in delivering secured and more innovative means of accessing, transferring and sharing money. From the 173 surveyed respondents, 93.1% of the respondents agreed that advanced authentications systems/single authentication that a user shares with no one (such as fingerprint compared to the old traditional authentication such as pins and passwords that can be guessed or traced) could be labelled as one of the solutions that can assist banks and retailers in delivering secured and innovative means of accessing, transferring and sharing money, whilst 6.9% disagreed on the statement. A majority (94.2%) of the respondents agreed that simple and secured access (ability to manage a broader range of financial transactions online) can be labelled as one of the solutions that can assist banks and retailers to deliver secured and innovative means of accessing, transferring and sharing money. In comparison, 5.8% disagreed with the statement. Whilst, 97.1% of the respondents agreed that enhanced convenience could be labelled as one solution that can assist banks and retailers in delivering secured and innovative means of accessing, transferring and sharing money, 2.9% disagreed with the statement. A higher percentage (91.9%) of the respondents agreed that increased security could be labelled as one of the solutions that can assist banks and retailers in delivering secured and innovative means of accessing, transferring and sharing money, whilst 8.1% disagreed with the statement. Regarding the final connection, 92.5% of the respondents agreed that reliable and efficient verification relying only on human agents could be labelled as one of the solutions that can assist banks and retailers in delivering secured and innovative means of accessing, transferring and sharing money, whilst 7.5% disagreed on the statement.
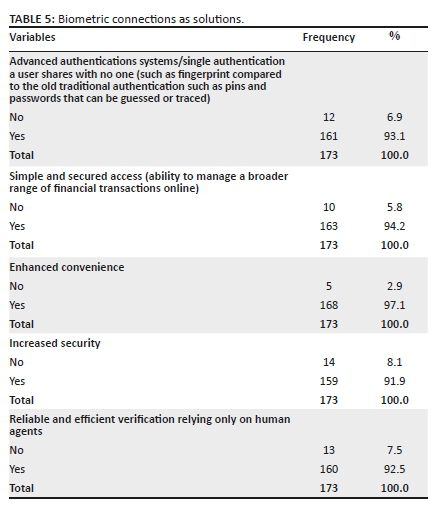
Literature postulates that banks must provide customers with more innovative and secured banking services (Hosseini & Mohammadi 2012). Biometric authentication or verification method that includes face and fingerprint recognition is considered a precise security solution for accessing, transferring and sharing money (Hosseini & Mohammadi 2012).
Pearson's correlation for enhanced surveillance indicates that should the user be involved in an accident and have cuts on biometric features such as fingerprint, face, and iris, it will be difficult for a biometric reader to fully recognise the authorised user to gain access into a system. Biometric security systems can assist banks and retailers with a wide range of benefits such as surveillance, as reported by Rhodes (2003).
Pearson's correlation for enhanced border control indicates that enhanced border control can be challenged by the fact that biometric characteristics such as face recognition, fingerprint and voice can be copied none are 100%. Biometric security systems can assist banks and retailers with a wide range of benefits such as forensic application, criminal identification, border control and surveillance (Rhodes 2003).
Pearson's correlation for criminal identification shows that the process of effortlessly providing information about the criminal record of the individual can also be challenged by the sensitivity of sensor performance. A biometric security system can assist banks and retailers with a wide range of benefits such as forensic application, criminal identification, border control and surveillance (Rhodes 2003).
Pearson's correlation for ease of information retrieval indicates that the process of providing users with quicker verification can be hindered by damages or changes to the user's physical biometric features such as face, eyes and fingers caused by accidents. Biometric security systems can assist banks with the following features maintaining accuracy, convenience, faster information retrieval, strong matching algorithm and speaker recognition (Koppenhaver 2007; Park 2008; Wang et al. 2011).
Pearson's correlation for strong matching algorithm findings indicate that biometric systems that can easily differentiate between two or more biometric traits such as hands, eyes and iris, can also be hindered by damages or changes to the user's physical biometric features such as face, eyes and fingers caused by accidents. Pin code verification alone cannot be regarded as a strong defence mechanism against security breaches, using biometric verification, the operator is secured to their data or information which is securely kept in an encrypted container or sandbox (Johnson 2019).
Pearson's correlation for lost or stolen smartcards and mobile devices findings indicate that individuals who participate in dishonest schemes through committing fraudulent activities such as scammers may intend to exploit lost or stolen smartcards and mobile devices of other users and steal funds of other individuals. Biometrics in banking for ATMs authentication provides both banks and customers with an outstanding benefit through providing customers with the flexibility to make transactions without physically having their bank cards. Thus, banks can avoid the costs and liabilities of customer's problems because of lost and stolen bank cards (Vernkatraman & Delpachitra 2008).
Pearson's correlation for impossible to forget fingerprint authentication indicates that fingerprint authentication is impossible to forget as compared to a password. Moreover, non-technologically inclined individuals still trust that pin code or password authentication methods are the best security authorisation techniques (Bhasin 2015).
Findings for the Pearson's correlation for uniqueness indicate that the uniqueness and benefits of the biometric authentication systems can be astounded by matters such as, involved in an accident, sensitivity of sensor performance, non-technologically inclined individuals and biometric characteristics such as face recognition, fingerprint and voice can be copied none are 100% (Hosseini & Mohammadi 2012). Physiological biometric features include retina, fingerprint, hand vein, iris, hand geometry, facial recognition and ear shape, these features are unique and no one in the world shares them or have the same (Ahmad et al. 2012).
Finally, Pearson's correlation for forensic application findings indicate that, because of physical biometric changes acquired by the users through an accident, it will be difficult for a biometric scanner system to recognise the user in a system easily. Forensic accounting is a requirement for banks to decrease financial fraud's speedy growth (Bhasin 2015). In addition, the biometric authentication method offers a natural, unforgettable and hardly breached verification (Hosseini & Mohammadi 2012).
This study was carried out to investigate the need for security and simplicity in the authentication of retail payments, digital banking and financial technology through the application of the biometric system. Furthermore, the study assessed the possible challenges, benefits and solutions to the biometrics authentication payment system. From the findings, the study further elaborated and discussed the biometric solutions that can assist banks and retailers in enhancing secured and innovative means of accessing, transferring, and sharing money. It is concluded that biometric technology is the innovative technology that different banking institutions can use to enhance security and innovation, protect the funds of their customers against scammers, fraudsters, hackers, and other constraints. Therefore, further studies can focus on the combined relationship amongst biometrics, digital banking and financial technology.
Acknowledgements
My genuine gratitude to Alpha and Omega, Creator of heaven and earth. Thank you to my supervisor, Mr Lucas Khoza and co-supervisor Mrs Tebogo Bokaba, for their patience, guidance, and continuous support towards completing this Journal.
Competing interests
The authors have declared that no competing interest exist.
Authors' contributions
All authors contributed equally to this work.
Funding information
This study received no specific funding from any agency in public, commercial or non-profit sectors. Because of the budget and time constraints, the study sampled only 173 respondents. The authors acknowledge that this could have impacted the ability to generalise the results of the study. It is therefore recommended that future studies should look at larger sample size. In addition, because of the limited number of individuals who are technologically inclined in the South African society, it was difficult to find respondents who fitted the criteria used to select respondents for the study. The study has not been extended to other provinces, as it is limited to the Gauteng province of South Africa.
Data availability
Data that support the findings of the study can be obtained from the corresponding author L.T.K.
The views and opinions expressed in this article are those of the authors and do not necessarily reflect the official policy or position of any affiliated agency of the authors.
Adewole, K.S., Abdulsalam, S.O., Babatunde, R.S., Shittu, T.M. & Oloyede, M.O., 2014, 'Development of fingerprint biometric attendance system for non-academic staff in a tertiary institution', Development 5(2), 62-70. [ Links ]
Agidi, R.C., 2018, 'Using biometric in solving terrorism and crime activities in Nigeria', Techsplend Journal of Technology 1(12), 91-105. [ Links ]
Ahmad, S.M.S., Ali, B.M. & Adnan, W.A.W., 2012, 'Technical issues and challenges of biometric applications as access control tools of information security', International Journal of Innovative Computing, Information and Control 8(11), 7983-7999. [ Links ]
Akhtar, S. & Liu, Y., 2018, 'SMEs' use of financial statements for decision making: Evidence from Pakistan', Journal of Applied Business Research (JABR) 34(2), 381-392. [ Links ]
Aly, S., Sagheer, A., Tsuruta, N., & R.I. Taniguchi, 2008, 'Face recognition across illumination', Artificial Life and Robotics 12(1-2), 33-37. [ Links ]
Ashbourn, J., 1999, The biometric white paper , viewed 30 July 2021, from http://homepage.ntlworld.com/avanti/whitepaper.htm .
Ateba, B.B., Maredza, A., Ohei, K., Deka, P. & Schutte, D., 2015, 'Marketing mix: it's role in customer satisfaction in the South African banking retailing', Banks and Bank Systems (open-access) 10(1), 82-91. [ Links ]
Bhasin, M.L., 2015, 'An empirical study of frauds in the banks', European Journal of Business and Social Sciences 4(7), 1-12. [ Links ]
Brooks, D.J., 2010, 'Assessing vulnerabilities of biometric readers using an applied defeat evaluation methodology', Paper presented at the Proceedings of the 3rd Australian Security and Intelligence Conference, Perth, November 30, 2010.
Board of Governors of the Federal Reserve System, Consumers and Mobile Financial Services, 2016, Board of Governors of the Federal Reserve System Washington, DC, pp. 1-86.
Bonneau, J., Herley, C., Van Oorschot, P.C. & Stajano, F., 2015, 'Passwords and the evolution of imperfect authentication', Communications of the ACM 58(7), 78-87. [ Links ]
Bosupeng, M., 2017, 'How Relevant Are Academic Degrees In The Workplace?', Munich Personal RePEc Archive, MPRA Paper No. 77914. pp. 2-7.
Bruun, A., Jensen, K. and Kristensen, D., 2014, 'Usability of single-and multi-factor authentication methods on tabletops: a comparative study', in International Conference on Human-Centred Software Engineering, Springer, Berlin, Heidelberg, pp. 299-306.
Buddharaju, P., Pavlidis, I. & Manohar, C., 2008, 'Face recognition beyond the visible spectrum', in Advances in Biometrics , pp. 157-180, Springer, London.
Chandran, R., 2014, 'Pros and cons of mobile banking', International Journal of Scientific and Research Publications 4(10), 1-5. [ Links ]
Chetty, G. & Wagner, M., 2009, 'Biometric person authentication with liveness detection based on audio-visual fusion', International Journal of Biometrics 1(4), 463-478. [ Links ]
Choi, H., Kang, R., Choi, K. & Kim, J., 2007, 'Aliveness detection of fingerprints using multiple static features', in Proc. of World Academy of Science, International Journal of Biological and Medical Sciences , vol. 2, pp. 200-205, Engineering and Technology.
Crowe, M.D., Schuh, S.D. & Stavins, J., 2006, 'Consumer behavior and payment choice: A conference summary', FRB of Boston Public Policy Discussion Paper, (06-1).
Das, S.S., 2018, 'A study of digital banking facilities: With reference to Guwahati in kamrup (metro) district of assam', Journal of Management 5(1), 6-13. [ Links ]
Dasgupta, D., Roy, A. & Nag, A., 2016, 'Toward the design of adaptive selection strategies for multi-factor authentication', Computers & Security 63, 85-116. [ Links ]
De Farias, S.A., Aguiar, E.C. & Melo, F.V.S., 2014, 'Store atmospherics and experiential marketing: A conceptual framework and research propositions for an extraordinary customer experience', International Business Research 7(2), 87-99. [ Links ]
De Winter, J.C., Gosling, S.D. & Potter, J., 2016, 'Comparing the Pearson and Spearman correlation coefficients across distributions and sample sizes: A tutorial using simulations and empirical data', Psychological Methods 21(3), 273-290. [ Links ]
Electronic Communications and Transactions Act (ECTA), 2002, South Africa Government Gazette 446(23708), 1-41.
Eze, S.G. & Chijioke, E.O., 2016, 'Public enlightenment education on the acceptance of fingerprint biometric technologies for administration in academic institutions and other organisations', Journal of Education and Practice 7(28), 158-163. [ Links ]
Frank, M., Biedert, R., Ma, E., Martinovic, I. & Song, D., 2012, 'Touchalytics: On the applicability of touchscreen input as a behavioral biometric for continuous authentication', IEEE transactions on information forensics and security 8(1), 136-148. [ Links ]
Gomez-Barrero, M., Drozdowski, P., Rathgeb, C., Patino, J., Todisco, M., Nautsch, A. et al., 2021, 'Biometrics in the era of COVID-19: Challenges and opportunities', arXiv preprint arXiv:2102.09258 , 1-14.
Gunson, N., Marshall, D., Morton, H. & Jack, M., 2011, 'User perceptions of security and usability of single-factor and two-factor authentication in automated telephone banking', Computers & Security 30(4), 208-220. [ Links ]
Hosseini, S.S. & Mohammadi, S., 2012, 'Review banking on biometric in the world's banks and introducing a biometric model for Iran's banking system', Journal of Basic and Applied Scientific Research 2(9), 9152-9160. [ Links ]
Jain, A.K., Flynn, P. & Ross, A., 2007, Handbook of biometrics , pp. 1-556, Springer Science & Business Media, New York.
Jain, A.K. & Kumar, A., 2010, 'Biometrics of next generation: An overview', Second Generation Biometrics 12(1), 2-3. [ Links ]
Jain, A.K., Ross, A. & Pankanti, S., 2006, 'Biometrics: A tool for information security', IEEE Transactions on Information Forensics and Security 1(2), 125-143. [ Links ]
Jaiswal, A.M. & Bartere, M., 2014, 'Enhancing ATM security using fingerprint and GSM technology', International Journal of Computer Science and Mobile Computing (IJCSMC) 3(4), 28-32. [ Links ]
Jin, C., Kim, H. & Elliott, S., 2007, 'Liveness detection of fingerprint based on band-selective Fourier spectrum', in International Conference on Information Security and Cryptology, Springer, Berlin, Heidelberg, pp. 168-179.
Kelman, J., 2016, The history of banking: A comprehensive reference source & guide , pp. 1-384, CreateSpace Independent Publishing Platform, California,
Kim, S., 2007, 'Governance of information security: New paradigm of security management', in Computational intelligence in information assurance and security , pp. 235-254, Springer, Berlin.
Koppenhaver, K.M., 2007, Forensic document examination: Principles and practice , pp. 1-389, Springer Science & Business Media, New Jersey.
Kristensen, L.B.K. & Solvoll, M., 2019, 'Digital payments for a digital generation', Nordic Journal of Media Studies 1(1), 125-136. [ Links ]
Lee, T., 2016, Biometrics and disability rights: legal compliance in biometric identification programs , U. Ill. JL Tech. & Pol'y, p. 209.
Neumeier, A., Wolf, T., & Oesterle, S. 2017, 'The Manifold Fruits of Digitalization - Determining the Literal Value Behind', in St. Gallen, J.M. Leimeister & W. Brenner (eds.), Proceedings der 13 , Internationalen Tagung Wirtschaftsinformatik (WI 2017), St. Gallen, pp. 484-498.
Pacut, A. & Czajka, A., 2006, 'Aliveness detection for iris biometrics', in Proceedings 40th annual 2006 international carnahan conference on security technology, IEEE, pp. 122-129.
Pallant, J., 2020, SPSS survival manual: A step by step guide to data analysis using IBM SPSS , Routledge, London.
Park, R.C., 2008, 'Signature identification in the light of science and experience', Hastings LJ 59, 1101. [ Links ]
Rahman, M.R. and Safeena, P.K., 2016, 'Customer Needs and Customer Satisfaction', In Ramees Rahman (eds.), book: Theeranaipunya - A Capacity Building Training Programme Equipping the Fisher women Youth for the Future , Central Marine Fisheries Research Institute, pp. 259-262, Kochi, India.
Rattani, A. & Derakhshani, R., 2018, 'A survey of mobile face biometrics', Computers & Electrical Engineering 72, 39-52. [ Links ]
Rhodes, K.A., 2003, Information security: Challenges in using biometrics , General Accounting OfficeTechnical Report # GAO-03-1137T.
Saunders, M., Lewis, P. & Thornhill, A. 2019, Research methods for business students , 8th edn., Pearson Education Limited, Harlow.
Sukamolson, S., 2000, Conducting and developing a multimedia computer-assisted instruction program for teaching Foundation English IIII , Chulalongkorn University, Language Institute.
Sun, Y., Zhang, M., Sun, Z. & Tan, T., 2017, 'Demographic analysis from biometric data: Achievements, challenges, and new frontiers', IEEE transactions on pattern analysis and machine intelligence 40(2), 332-351. [ Links ]
Syler, R. & Baker, E., 2016, Building a framework for the influence of digital content on student course engagement .
Tan, X., Li, Y., Liu, J. & Jiang, L., 2010, 'Face liveness detection from a single image with sparse low rank bilinear discriminative model', in European Conference on Computer Vision, Springer, Berlin, Heidelberg, pp. 504-517.
Ten Have, H. & Gordijn, B. (eds.), 2014, Handbook of global bioethics , vol. 4, Springer, New York, NY.
Toth, B., 2005, 'Biometrics. Biometric Liveness Detection', Information Security Bulletin 10, 291-297. [ Links ]
World Health Organization, n.d., Coronavirus disease (COVID-19) pandemic , viewed 16 September 2021, from https://www.who.int/emergencies/diseases/novel-coronavirus-2019 .
Wayman, J.L., Jain, A.K., Maltoni, D. & Maio, D. (eds.), 2005, Biometric systems: Technology, design and performance evaluation , Springer Science & Business Media, London, United Kingdom.
Zhu, Y., Tan, T. & Wang, Y., 2000, 'Biometric personal identification based on iris patterns', in Proceedings 15th International conference on pattern recognition, ICPR-2000, vol. 2, pp. 801-804, IEEE.

Received: 13 May 2021 Accepted: 28 Sept. 2021 Published: 10 Dec. 2021
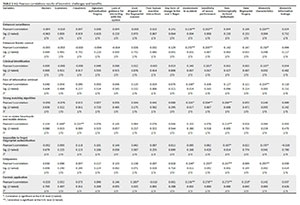

An official website of the United States government
The .gov means it’s official. Federal government websites often end in .gov or .mil. Before sharing sensitive information, make sure you’re on a federal government site.
The site is secure. The https:// ensures that you are connecting to the official website and that any information you provide is encrypted and transmitted securely.
- Publications
- Account settings
Preview improvements coming to the PMC website in October 2024. Learn More or Try it out now .
- Advanced Search
- Journal List
- Micromachines (Basel)
- PMC10305017

A Review of Fingerprint Sensors: Mechanism, Characteristics, and Applications
1 School of Optoelectronic Engineering, Xi’an Technological University, Xi’an 710032, China; moc.duolci@uuygnoriy (Y.Y.); nc.ude.utax@gnayuxil (X.L.); moc.361@uilgw (W.L.)
Jianshe Xue
2 BOE Display Technology Co., Ltd., Beijing 100176, China; nc.moc.eob@ehsnaijeux
Identification technology based on biometrics is a branch of research that employs the unique individual traits of humans to authenticate identity, which is the most secure method of identification based on its exceptional high dependability and stability of human biometrics. Common biometric identifiers include fingerprints, irises, and facial sounds, among others. In the realm of biometric recognition, fingerprint recognition has gained success with its convenient operation and fast identif ication speed. Different fingerprint collecting techniques, which supply fingerprint information for fingerprint identification systems, have attracted a significant deal of interest in authentication technology regarding fingerprint identification systems. This work presents several fingerprint acquisition techniques, such as optical capacitive and ultrasonic, and analyzes acquisition types and structures. In addition, the pros and drawbacks of various sensor types, as well as the limits and benefits of optical, capacitive, and ultrasonic kinds, are discussed. It is the necessary stage for the application of the Internet of Things (IoT).
1. Introduction
The biometric traits of individuals include a wealth of information and are the basis for a variety of well-established biometric technologies. As a new information authentication technique, biometric identification based on human features may significantly improve the quality of life for individuals and foster economic growth. Recently, biometric identification technology has been utilized not only by government agencies to identify persons and protect public safety but also by businesses and social groups for a variety of commercial purposes. As a result of the development of biometric identification technology, biological traits provide major benefits, such as passwords, and human biometric characteristics for identity authentication have attracted considerable attention. Biometric identification mainly includes iris recognition [ 1 , 2 , 3 ], facial recognition [ 4 ], voice recognition [ 5 ], retina recognition [ 6 ], palm print recognition [ 7 ], vein recognition [ 8 , 9 ], fingerprint recognition [ 10 , 11 , 12 ], and so on. Biometric technology has vast applicability and business potential. Physiological and behavioral features are constant and distinct, and the likelihood of two individuals having identical physiological characteristics is quite remote.
The fingerprint is a pattern created by the ridged skin at the tip of a human finger [ 13 ]. The fingerprint is formed before birth, and its shape does not alter as the individual matures [ 14 ]. There are several methods for acquiring fingerprint pictures, which is the initial stage of fingerprint identification [ 15 ]. Many fingerprint acquisition methods exist: optical, capacitive, temperature [ 16 ], ultrasonic, and electromagnetic wave [ 17 ] fingerprint acquisition technology. After fingerprint photos are accepted, pre-processing is undertaken to simplify the extraction of fingerprint features, classifying fingerprints according to several characteristic points, then comparing them to those stored in the database to determine if they are the same.
Early fingerprint identification technology was mainly developed in cell phones. After Siemens demonstrated the feasibility of fingerprint identification technology in cell phones in 1998, companies such as Fuji, Motorola, and Apple began to produce fingerprint identification technology in cell phones, and so far, under-screen fingerprint technology is a major trend in cell phones. Fingerprint identification technology has developed particularly rapidly due to its great portability and high-cost performance, gradually becoming one of the more common biometric identification methods than others in life, e.g., attendance systems at work, smart door locks for family, identity determination in society, etc.
In recent years, fingerprint identification technology, from early ink identification to optical identification and capacitive identification, in particular the ultrasonic fingerprint identification system, has been gradually discovered by everyone. Ultrasonic fingerprint identification can reduce the error rate of fingerprint identification technology and improve the accuracy of fingerprint identification technology in the case of pollution identification. As the Figure 1 shows, the ultrasonic fingerprint sensor has high integration, which can effectively solve problems such as the size and accuracy of the fingerprint sensor. Fingerprint recognition technology is currently a convenient, highly reliable, and low-priced biometric technology with great potential for large-scale applications.

An illustration showing a summary of characteristics, physical principles, and technologies for the fabrication of fingerprint sensors in different applications. TFT (thin film transistor), MEMS (micro electromechanical system), and OCT (optical coherence tomography).
In this review, recent progress on fingerprints is summarized from research all over the world in more than 250 references, with 40% being published in the last five years. This work reviews the mechanism, characteristics, and industry application of fingerprint sensors. The first section outlines modern biometric technologies and describes the development and application of specific biometric technologies, which is helpful in understanding the background of fingerprint recognition technology. The second section discusses the mechanism of optical fingerprint sensors and details the structure of various types of optical fingerprint sensors, enumerating the characteristics and application fields of various types of sensors. The third section discusses the characteristics and application fields of various types of optical fingerprint sensors. The fourth section describes the structure and characteristics of fingerprint sensors based on self-capacitance and mutual-capacitance. The fifth section provides ultrasonic fingerprint identification technology based on various sensors.
2. Biometric Recognition Mechanism
Recently, biometrics as a security technique has emerged [ 18 ]. Combining the physiological properties of the human body with computers, optics, and acoustics [ 19 ] led to the development of a range of biometric technologies, including iris, voice, fingerprint, face, etc., technology. Their comparison is shown in Table 1 . Such features can be identified using advanced technologies to clarify personal identifying information [ 20 ]. Such physiological traits can provide stronger assurance for information security and lower the danger of data loss, leakage, etc. Moreover, biological features are a kind of characteristic information that accompany human beings, have high anti-counterfeiting performance, are difficult to fabricate or steal, are portable, and can be accessed at all times [ 21 ]. Nowadays, the research of biometric identification technology has made remarkable achievements in many fields [ 22 ]. For example, biometric identification technology plays a positive role in criminal identification and confirmation in the field of public security [ 23 ]. It can realize the encryption and decryption of relevant parts of the computer information system [ 24 ]. The use of biometric identification technology in the process of electronic commerce and monetary transactions can ensure the circulation of funds in social life [ 25 ]. Furthermore, it can provide remote medical monitoring [ 26 ] and other medical fields [ 27 ].
Comparison of different biometrics.
2.1. Iris Recognition
Iris recognition is a biometric system that identifies an individual based on the iris tissue in the human eye [ 28 ]. Iris fiber tissue is very intricate. The composition of its quantitative characteristic points may reach 266, which is an order of magnitude greater than comparable biometric technologies. To attain maturity, fiber tissue is created during the fetal stage, and the development process is highly unpredictable. Once generated, it cannot be altered, hence providing security, uniqueness, stability, unforgeability, and non-falsifiability [ 29 ]. According to the study, the iris can still be used for detection within 5–7 h to 21 days after human death [ 30 ]. The market for iris recognition technology is quite modest, although studies have demonstrated its viability on mobile phones [ 31 ] and in access control systems [ 32 ]. IOM (iris on the move) technology is a high-speed, easy, and high-throughput long-distance iris recognition security solution. It can reach a distance of 3 m to capture and analyze the iris photographs of several individuals.
Malgheet [ 33 ] has provided a summary of the many techniques for iris recognition research. Lei [ 2 ] proposed a model-agnostic meta-learning (MAML)-based several-shot learning method (new-shot learning) for iris recognition to solve the problem of a limited number of samples in deep learning for iris recognition technology; Sun [ 25 ] proposed an open-set iris recognition method based on deep learning that can effectively differentiate iris samples of location classes without impacting the known iris recognition capability. Wang [ 34 ] introduced a cross-spectral iris identification algorithm based on convolutional neural networks (CNNs) and supervised discrete hashing, which not only achieves superior performance than previously examined CNN [ 35 ] designs but also greatly reduces the template size.
2.2. Facial Recognition
The face is the most straightforward identifier of private information. Currently, facial recognition is prevalent, less expensive than other biometric technologies, does not require direct touch, and can be performed at a distance [ 3 , 36 ]. Multiples with a similar appearance, face occlusion, and facial photographs and videos might affect the accuracy of facial recognition. Fourati [ 37 ] distinguished faces in videos and images by image quality assessment and motion cues; Afaneh [ 38 ] provided matching decisions for multiples with a correct rate of more than 95% by constructing a biometric system; Kute [ 39 ] discovered local correlations in faces to achieve recognition and confirmation of obscured faces; Zeng [ 40 ] proposed a simultaneous occlusion invariant depth network (SOIDN) to simultaneously recognize and match unobscured and synthetically obscured faces and make full use of the relationship between the two; Zhang [ 41 ] proposed hierarchical feature fusion, which can be used in strong illumination and occlusion situations to improve recognition accuracy, especially to achieve good recognition results, and can be applied to smart cities; Madarkar [ 42 ] extracted the facial occlusion part to establish non-coherent samples, and improved the recognition of occluded faces through a non-coherent dictionary.
Expression recognition has a significant influence on the field of pattern recognition in facial recognition. Approximately 55% of human-to-human communication is transmitted by facial expressions, according to studies [ 43 ], and facial expressions are the best tool for identifying human emotions and intentions [ 44 ]. The identification of facial expressions is important to artificial intelligence and has enormous promise in psychological research, driver fatigue monitoring, interactive game creation, virtual reality [ 45 ], intelligent education [ 46 ], and medical fields [ 47 ]. After recognizing facial expressions, Wu comprehended the emotional content of images and generated image captions using the Face-Cap model [ 48 ]; Cha used surface electromyography (sEMG) around the eyes [ 49 ] (sEMG reference) to react to the user’s facial expressions [ 50 ], thereby performing expression recognition.
2.3. Finger Vein Recognition
Vein technology uses the veins beneath the epidermis, such as finger veins, hand veins, foot veins, and palm veins, for identification authentication [ 51 ]. Veins are fundamental properties that are exclusive to living beings [ 52 ] and are difficult to duplicate and forge [ 53 ]. Compared to fingerprint recognition, blood must be flowing in vivo to be detected; to a certain extent, the security degree is high. Additionally, the vein pattern is distinct even after several deliveries. Vein pictures of the hand are typically obtained under transmission illumination utilizing near-infrared (700–900 nm) [ 54 ], and the images can vary significantly according to the hand’s size, thickness, and placement angle. Compared to the same camera capture approach, Prommegger [ 55 ] utilized numerous cameras to acquire veins from different angles to increase the identification rate around the fingers. Despite its rarity, vein recognition offers a multitude of applications: Immanuel [ 56 ] used biometric authentication protection of finger veins for ATM network security. Su [ 57 ] integrated finger veins with ECG signals for personal identification, and the method was significantly better than separate systems in terms of recognition accuracy and security. Yang [ 58 ] encrypted medical data by vein recognition, and information was stored on a card to achieve convenience and privacy of information.
2.4. Voice Recognition
Voice recognition comprises speech and non-speech recognition, and voice recognition systems typically sample at a rate of 8000 Hz or more, with a frame size of 256 or 512 samples. It has several uses, including audio monitoring, sound event detection, and ambient sound recognition [ 59 ]. Hu [ 60 ] used pathological speech recognition to detect various vocal cord diseases while reducing laryngoscopy, which can be applied to medical facilities lacking laryngoscopy during telemedicine. Wijers [ 61 ] used a bio recorder to associate African lions with their vocals, find features, and locate and distinguish the vocal information transmitted to the lion’s roar. Nakamura [ 62 ] developed the ability to match and record an individual’s voice through accurate recognition of vocal features to improve the efficiency of the recordings. Beritelli [ 63 ] conducted identity verification based on the measurement of heartbeat frequency with an accuracy of up to 90%.
2.5. Fingerprint Recognition
Fingerprints are phenotypic genetic features that people are born with and form at the end of their fingers. Under normal circumstances, fingerprints vary, mainly in the detailed features of fingerprint ridges and valleys [ 64 ]. These detailed features are the key to the fingerprint recognition process. The fingerprint recognition process relies mainly on the bifurcation points and nodes in Figure 2 . When the fingerprint is taken on the scanner surface, the ridge skin area is shown as black (or dark gray value), while the valley area and the background area are white (or light gray value).

( a ) Basic fingerprint feature points: bifurcation and node. ( b ) Arch, loop, and whorl patterns on adult fingertips. Tr., triradius; Cr., crease [ 13 ].
Each individual has a distinct and stable fingerprint, recognizable by the irregular lines on the finger belly. Figure 2 depicts the different categories of fingerprint characteristics [ 65 , 66 ]: sweat holes, patterns of lines, early lines, scars, etc., on fingerprint lines. The earliest fingerprint recognition was collected with ink and paper, and the emergence of electronic computers spurred the development of fingerprint collection methods that enhanced the resolution and clarity of fingerprint images and were conducive to improving the processing efficiency of computers. Fingerprint sensors can employ the biometric code for a variety of purposes, including mobile phone unlocking [ 67 ], attendance systems [ 68 ], mobile payment [ 69 ], access control unlocking [ 70 ], ID cards [ 71 ], medical information [ 72 ], driver’s licenses, and so on. Its primary function is to identify personal data [ 73 ].
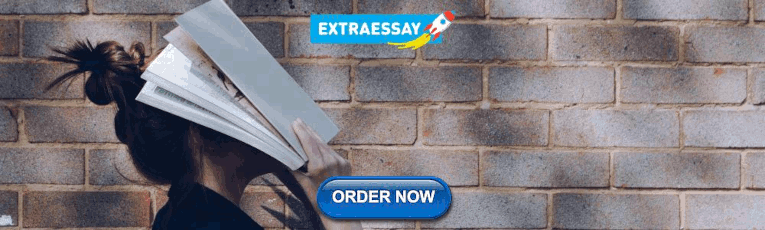
3. Optical Fingerprint Recognition
Optical fingerprint sensors, which use the principles of light refraction and reflection to produce pictures, have replaced ink for obtaining fingerprints since the invention of computers [ 74 ]. Charge-coupled devices are the central elements of optical scanning systems (CCD and CMOS). CMOS and CCD image sensor development began concurrently [ 75 ]. Due to the constraints of the process level at the time, CMOS resolution was low, there was a great deal of noise, light sensitivity was low [ 76 ], and the quality was poor; it also acheived little improvement. In contrast, CCD image sensors have dominated the market for two or three decades due to their broad effective light sensitivity area, uniform acquisition, low noise, and other advantages. Due to advancements in integrated circuit design technology and process levels over the past decade, CMOS’s previous problems have been substantially eliminated. Intra-pixel amplification, column-parallel structure, and random reading are incomparable to CCD [ 77 ]. CMOS sensors are smaller in size (to achieve the same image effect), consume less energy, and have improved integration and electronic-voltage conversion efficiencies [ 78 , 79 , 80 , 81 , 82 ]. Active CMOS image sensors (CIS) have developed rapidly in recent years [ 83 , 84 , 85 ] and have gradually become the primary choice for most imaging fields. Omni Vision, Micron, Panasonic, Toshiba, etc., have made significant breakthroughs in CIS pixels, making CIS development a later trend.
With the continuous development of optical fingerprint sensors, from single prism optical total reflection technology to optical thin film transistor (TFT) technology, and then to optical coherence tomography type technology, the resolution and clarity of the scanned image have been greatly enhanced, as has the success rate of fingerprint identification.
3.1. Single Prism Recognition Method
In 1971, optical fingerprint capture machines began obtaining pictures by scanning the fingerprint left on the surface of the finger, a process subject to interference. Various designs of optical fingerprint sensors emerged thereafter. Early optical fingerprint scanners typically required a separate light source and prism. Figure 3 a is a typical total reflection principle, and this total reflection identification is affected by finger moisture and folds [ 86 ]. Figure 3 b shows the imaging device placed beyond the critical angle, and only the light reflected on the ridge beyond the critical angle reaches the imaging device. Figure 3 c employs the dispersion method inside the finger [ 87 ], wherein the light source penetrates inside the finger, scatters, and passes through the fingerprint layer to reach the transparent plate of the sensor and must have a refractive index near that of the human tissue. Figure 3 d depicts a multispectral light source for detecting fingerprint pictures on the surface and within the fingerprint [ 88 ]. Because the transmission qualities of biological tissue depend on wavelength, various wavelengths penetrate to different depths, necessitating multiple light sources and two polarizers for this sensor [ 89 ]. In 2016, Baek created a fingerprint sensor based on the modification of the optical path [ 90 ] that enables the detection of wet fingerprints. Due to the sensor’s utilization of numerous optical components, its size is considerable. The necessity for minimal size has led to the development of linear scanning sensors [ 91 ], which suit both the need for mobile device integration and the demand for big fingerprint pictures. Most linear scanning sensors are sliding sensors, which introduce degrees of freedom as a result of changes in sliding speed and direction. Existing fingerprint sliding sensors can be categorized as capacitive or optical [ 92 ]. The optical type has a smaller size(4 × 0.9 mm 2 ) and a greater resolution (1000(H) 625(V)), is made up of an optical binder with the same refractive index as the overlay glass, and has a single sliding direction [ 93 ].

Many optical fingerprint sensor designs. ( a ) The principle of the whole internal reflection technique; ( b ) the principle of the light-path separation method; ( c ) the principle of the in-finger light dispersion method; ( d ) the principle of the multispectral method [ 89 ].
3.2. Identification Method through TFT Technology
Thin-film transistors (TFTs) have been developed for over 40 years, replacing traditional semiconductor sensors with light-sensitive TFT panels. In 1961, Weimer created the first thin-film transistor (TFT) [ 94 ]. Using amorphous silicon (a-Si:H) as the active layer, Street created a TFT device in 1979, and it was discovered that amorphous thin-film transistors (a-Si TFT) might be employed as switching devices for active array liquid crystal displays (AMLCD) [ 95 ]. TFTs are produced by sputtering and chemical deposition methods on non-monocrystalline substrates such as glass or plastic wafers, and large-scale semiconductor integrated circuits (LSICs) are produced by treating the films. A standard TFT optical sensing device is comprised of a grid of pixels on glass and an operational amplifier (OPAMP) in an external readout integrated circuit (IC) [ 96 ]. This technique may be utilized to create large-area sensing arrays using optical fingerprint sensors. Table 2 lists the particular pairings for the four primary types of TFT technology: no crystalline silicon TFT, polycrystalline silicon TFT, organic TFT, and amorphous oxide TFT.
Different types of TFT.
3.2.1. Based on Amorphous Silicon TFT Technology
Hydrogenated amorphous silicon (a-Si:H) thin-film transistors (TFTs) are the predominant thin-film transistor technology used in flat panel displays today [ 97 ]. A-Si:H TFTs offer the benefits of low process temperature (<350 °C), good large-area uniformity, cheap cost, and low leakage current; they are the predominant technology for commercial LCD flat panel displays now. However, the electron field-effect mobility of a-Si:H TFTs is only 0.4–1.5 cm 2 /(Vs), and the hole field-effect mobility is considerably lower, which prevents their use in high-definition and current-driven displays. In addition, low mobility a-Si:H TFTs must be accomplished by extending the channel width to obtain adequate drive current. However, big-size a-Si:H TFT devices lower the display openness, resolution, and display brightness [ 98 ].
The absorption spectra and quantum efficiencies of a-Si:H and Si have been compared. In the range of 0.4–0.75 µm, the material absorbs lighter than the Si layer, but the Si layer absorbs more light at longer wavelengths (>0.75 m) than the a-Si:H film. In visible light, the a-Si:H-based diode generates a greater photocurrent than the Si-based diode, and a-Si:H pin diodes have a greater QE output than Si p+/n diodes over the majority of the visible spectrum [ 99 ].
Based on amorphous silicon TFT technology, optical fingerprint sensors may achieve slimness, compactness, and wide areas [ 100 ]. The PPS structure enables the creation of A-Si:H field-effect devices [ 101 ]. Thin optical touch (TOT), hidden optical touch (HOT), and hidden display (HUD) fingerprint sensors based on hydrogenated amorphous silicon are already available for mass production [ 99 ]. The sensor sizes range from tiny (4.0 mm × 8.0 mm) for smartphone user identification to the size of four fingers (3.2″ × 3.0″) for public safety inspection. Table 3 and Figure 4 provide a comparison of the three fingerprint sensors.

( a ) the structure of TOT, ( b ) the structure of HOT, and ( c ) the structure of HUD [ 99 ].
The structure of TOT, HOT, and HUD.
3.2.2. Based on Polycrystalline Silicon TFT Technology
Low-temperature poly-Si technology refers to the process method for producing high-quality poly-Si films and thin-film transistors (TFTs) at temperatures below 600 °C [ 102 ]. On the same glass substrate, the pixel array and the driver circuit of a poly-Si TFT-LCD may be merged to accomplish the integration of the peripheral driver and display. Compared to a-Si:H TFT, LTPS TFT offers the benefits of high mobility (often two orders of magnitude greater) and big drive current, as well as reduced power consumption and a smaller device area; it also addresses the problem of high density to increase yield and decrease manufacturing costs [ 103 ]. Moreover, p-Si is resistant to light interference, and the leakage current does not rise under light circumstances, allowing the light-shielding layer to be omitted. It is possible to produce a high-definition display system on a panel (system on panel, SOP) that merges a display matrix and peripheral drivers, considerably enhancing the system’s dependability. LTPS TFTs have already replaced OLEDs in tiny commercial displays.
When amorphous silicon thin-film transistors shift to high capacity, high brightness, and high resolution, LTPS technology compensates for the smaller pixel size and shorter unit pixel charging time and also solves the problems of difficult high-density leads and integration of the display area with the surrounding driver circuit [ 104 ]. There are two ways to obtain poly-Si films: direct methods and indirect approaches. The direct methods are low-pressure chemical vapor deposition (LPCVD) [ 105 ] and catalytic chemical vapor deposition (cat-CVD) [ 106 ], and the indirect methods use amorphous silicon film recrystallization, whose crystallization methods primarily consist of solid-phase crystallization (SPC) [ 107 ], rapidly cooked annealing (RTA) [ 108 ], metal-induced lateral crystallization (MILC) [ 109 ], microwave crystallization [ 110 ], and laser crystallization (LC).
As the sensing material, a new photosensitive material is employed. The sensor’s metal/sensing material/ITO structure is incorporated into the LTPS TFT process [ 111 , 112 , 113 ]. Using an active pixel sensor circuit (APS), optical intracellular fingerprint sensor (iFP) technology that integrates display, touch, and FPS capabilities is created [ 114 ]. Meanwhile, amorphous silicon can be placed above polysilicon in a manner similar to Figure 5 to generate a vertical hybrid PIN photodiode (HPAS-PIN) [ 115 ]. The fingerprints that may be effectively acquired by this optical image sensor array are depicted in Figure 6 a. However, this array cannot capture the fingerprint picture when a color filter is present. An LTPS image sensor (LIS) can be operated using an LTPS backplane-based active pixel sensor (APS) circuit [ 116 ] shown in Figure 6 c. A 400PPI LTPS-TFT LCD with in-cell touch and in-cell screen fingerprint scanning capabilities has been designed, capable of fingerprint image capture even when the finger is at the height of 1.0 mm on the LIS array, with a fingerprint scanning area resolution of 256 × 256 and a sensor with enhanced sensitivity and interference resistance.

( a ) Structure of optical image sensor array and fingerprint image captured by it. ( b ) Deposit a 300 nm oxide silicon buffer layer and a 50 nm intrinsic a-Si layer sequentially, then transform a-Si to p-Si by using Excimer-Laser Annealing. After that, phosphorus ions are doped into the p-Si to form an N+ p-Si layer. ( c ) Deposit a 230 nm insulating layer and form a 20 × 10 µm first via hole pattern by photolithography and dry etching. ( d ) A 600 nm intrinsic a-Si and 50 nm oxide silicon is deposited to cover the first via hole and insulating layer, followed by boron ions implanted to the surface of the intrinsic a-Si layer, turning part of the upper intrinsic a-Si layer into P+ a-Si layer. As ion concentration distribution is Gaussian distribution, no clear boundary between P+ a-Si and intrinsic a-Si exists. In this step, intrinsic a-Si in the first via contacts the N+ p-Si, together with the upper P+ a-Si layer, to form a hybrid p-Si and a-Si PIN photo-diode. After that, define the a-Si to a 25 × 15µm island by photolithography and dry etching. ( e ) Form a second via hole of insulating layer next to the first via hole using the same photolithography and dry etching. ( f ) Deposit a metal layer on the second via hole to serve as the cathode contactor of the photo-diode. ( g ) Coat an organic flat layer and form a third via a hole above the first hole. In this step, the oxide silicon is removed to expose the surface of P+ a-Si at the same time. ( h ) Deposit a transparent conductor layer ITO to serve as anode contactor of the photo-diode [ 115 ].

( a ) Structure of optical image sensor array and ( b ) fingerprint image captured by it [ 115 ]; ( c ) cross-structure of in-cell FPS. ( d ) Structure of LCD with in-cell FPS; ( e ) the fingerprint image [ 116 ]. ( f ) Field of view diagram of the micro-lens array, ( g ) a collimating optical path based on microlens array, ( h ) the fingerprint image captured by it [ 117 ], ( i ) Stack structure of the fingerprint under OLED, and ( j ) diagram of a collimating system on the photo sensor. ( k ) Influence of collimating system on SNR [ 118 ], and ( l ) fingerprint on display (FoD Module). Assembly stack of the FoD module, ( m ) construction of organic image sensor, ( n ) the fingerprint capture by it [ 119 ], ( o ) structure of fingerprint sensor, and ( p ) the fingerprint capture by it [ 120 ].
When the LCD is used as a light source, the LCD and its opaque backlight unit obstruct visible light, and there is an unavoidable air gap between the LCD and the backlight unit. An optical image sensor array can be built directly into the LCD with the backlight unit or an extra LED as the light source and the LIS array on a TFT substrate that eliminates the backlight as the light source. It is also feasible to position the IR CIS and IR light source under the backlight, with the IR light emitted by the IR light source passing through the LCD module and being received by the IR CIS upon fingerprint reflection.
Ye [ 117 ] proposed a collimating optical route based on a microlens array with an IR light source of 940 nm wavelength inserted beneath the cover’s lower plate. By regulating the exit angle and luminescence angle of the light source, the entire reflection of all oblique incident light on the top and lower surfaces of the CG can be achieved. As shown in Figure 6 f,g,h the beam conveying the fingerprint information passes successively through each film layer before being caught by the sensor array beneath the display. Due to the size of the sensor, this lens unit must be constrained to a tiny region.
LCD in-finger detection versus OLED under-screen detection: OLED uses self-luminescence as its light source and employs micro-collimators to prevent the overlapping of light signals. Due to the under-screen design and self-luminous property, the depth-to-width ratio of the micro-collimation aperture can be chosen freely to achieve adequate collimation without allowing an excessive amount of stray light to enter the CIS.
Additionally, the researchers created optical fingerprint recognition [ 118 ] that can be integrated into LTPS image display technology beneath the OLED display and can cover the entire display’s dimensions. OLED uses its own self-illuminating light as its light source and employs micro-collimators to prevent overlapping light signals. The signal variation rate is improved by 50 percent by refining the process and circuit architecture. By improving the collimation technology, the signal-to-noise ratio is virtually doubled.
As depicted in the illustration, the collimation system consists of a collimation hole and a microlens. One approach involves installing the collimation system above the photo sensor, as depicted in Figure 6 f,g, while another technique involves preparing the collimation system directly above the photo sensor, as depicted in Figure 6 i,j,k, showing the SNR result of the collimation system. A collimation hole and a microlens are included in the collimation system. The light-receiving capabilities of the sensor can be enhanced by exposing and then immediately aligning the sensor’s collimation mechanism.
3.2.3. Oxide TFT-Based Technology
Thin film transistors (MOS TFTS): Due to the benefits of good uniformity, high mobility, and strong process compatibility with a-Si TFT, the metal oxide TFT line drive circuit is often concerned with the following performance aspects, speed [ 121 ], power consumption [ 122 ], and reliability [ 123 , 124 ].
Traditional amorphous silicon (a-Si:H) TFT and low-temperature poly-Si (low-temperature poly-Si, LTPS) TFT have been proved challenging to employ to fulfill the need for flat panel displays with huge sizes and high resolutions. The semiconductor industry has reached an epoch in which material limitations restrict device size. Since 2003, transparent TFTs fabricated from amorphous oxide semiconductors have been a focus of worldwide research [ 125 ]. Compared to silicon materials, oxide semiconductor films exemplified by a-IGZO have superior low process temperature, long service life, high transmittance, large forbidden bandwidth (transparency), and high carrier mobility [ 126 , 127 , 128 , 129 , 130 ], and are widely applicable in liquid crystal displays, memory [ 131 , 132 ], and the Internet of Things [ 133 ]. Therefore, AOS TFTs are anticipated to replace a-Si:H TFTs as the primary devices for the subsequent generation of flat panel displays (flexible displays, transparent displays, etc.).
Qin [ 134 ] presented a near-infrared optical fingerprint sensor based on an array of passive pixel sensors (PPS) and oxide (IGZO) TFT technology. This optical sensor has an organic photodiode as its sensing element (OPD). This organic–inorganic hybrid thin-film photodetector is able to capture a crisp picture of a fingerprint. Additionally accomplished was a flexible fingerprint sensor based on a PI substrate. Due to the strong penetrability of near-infrared light, some of it can travel through the finger and land on the sensing element when the finger hits the sensor surface. In addition, the fingerprint’s ridges and troughs represent distinct optical routes. In the region of the ridge, a portion of the NIR light is reflected at the surface, while the remaining light falls on the detecting pixel. In the valley region, only a portion of the NIR light is transmitted into the air and subsequently reaches the detecting pixel. Through the ridge and valley sections of the fingerprint, different quantities of light are permitted to reach the surface of the sensor pixel accordingly. Therefore, fingerprint recognition is accomplished. Each pixel has a size of 50.8µm, equivalent to a resolution of 500 PPI.
3.2.4. Organic TFT
The transition from TFT to OTFT is not easy. It is commonly accepted that Heilmeier [ 135 ] discovered the OTFT field effect phenomena on copper phthalocyanine thin film. In 1986, the first organic thin-film transistor was invented by Tsumura, who electrochemically synthesized polythiophene and employed it as the primary OTFT material; however, the final mobility was only 10 −5 cm 2 /(Vs) [ 136 ]. On the other hand, because OTFT has good pliability, low-cost advantages, and the characteristics of organic materials themselves, different institutions at different times conduct in-depth research on thin film morphology and preparation techniques, and the application in the field of display devices will become increasingly widespread and significant.
Optical fingerprint biometrics based on organic photodiode (OPD) technology [ 119 ] may be embedded under the smartphone display to obtain complete coverage and capture up to four fingerprints [ 120 ]. As Figure 6 o shows, the full-screen optical fingerprint module is readily integrated into mobile devices (attached to an OLED panel or with an air gap). It effectively prevents spoofing when applied to visible light (540 nm) and near-infrared light (850 nm and 940 nm). Figure 6 l demonstrates that an Android application has been built for this purpose, allowing the user to register and validate the fingerprint with a matching time kept below 500 ms and a contrast-rich picture resolution of 5 Cy/mm.
Faced with the difficulty of full-screen TFT technology, Tai [ 137 ] opted for a gap-type TFT as the optical array system in Figure 6 n. For fingerprint recognition applications, the high photocurrent of gap-type TFTs results in a big signal and rapid readout, which is a major benefit. Between the OLED screen and the gap-type TFT sensor array lies the collimator. Algorithms are used to the fingerprint picture for background removal and image enhancement. The fingerprint’s ridges and troughs are plainly apparent.
3.3. Identification Method by Optical Coherent Layer Scanning Technology
Modern civilization is becoming great interested in the development of fingerprint recognition systems with high security and resilience in the fingerprint identification process. The capacity of optical coherence tomography (OCT) to capture the depth information of the skin layers [ 16 ] has created a new study field for fingerprint recognition [ 138 , 139 ]. OCT was initially introduced to the field of fingerprint recognition for anti-spoofing [ 140 ], including automatic spoofing and live detection [ 141 , 142 , 143 , 144 , 145 , 146 ], internal fingerprint (i.e., subcutaneous fingerprint corresponding to live epidermis) reconstruction [ 147 , 148 , 149 , 150 , 151 , 152 ], and fingerprint identification/recognition [ 153 , 154 , 155 , 156 ]. Currently, confocal scanning OCT offers the greatest image depth and is much costly due to the inclusion of additional components such as lasers. Using a low-cost light source, such as a light-emitting diode (LED) or a heat source, it is feasible to perform OCT variant-full-field optical coherence tomography (FF-OCT). FF-OCT utilizes a camera with virtually instantaneous access to fingerprints and point-by-point image scanning to produce axial pictures, as opposed to typical confocal scanning OCT. The absence of pinholes in the detection path of confocal OCT and the shallow image depth are drawbacks of FF-OCT. However, FF-OCT has been shown to be effective in a variety of biological applications, including imaging of skin, brain tissue, the gastrointestinal wall, and the cornea.
Imaging methods based on optical coherence layers account for the effects of wetness, folds, and lack of contact. Using the varying transmittance of ridges and valleys, a red LED illuminates the nail side of the finger to form a picture of the fingerprint [ 87 ]. FF-OCT may also make use of an imaging Michelson interferometer and a silicon camera [ 157 ]. It comprises a small, lightweight LINOS opt mechanical system covered by a 30 cm × 30 cm × 1 cm Plexiglas panel. The sensor produces 1.7 cm × 1.7 cm pictures with a spatial sampling rate of 2116 dots per inch (dpi). The fingerprint is an exterior picture captured at 15 m. The picture displayed between 33–103 µm is the cuticle, and the sweat duct has white dots. The presented photos between 121–210 µm match the live epidermis. The 121–156 µm images are the ridges of the internal fingerprints, whilst the 191–210 µm depth images are the valleys, allowing fingerprint pictures to be produced [ 158 ]. Figure 7 shows a scanning picture of common fingerprints, moist fingerprints, and fingerprints based on an optical coherence layer.

( a ) Construction of a novel fingerprint sensor using scattered transmission light. ( b ) Normal fingerprint and the OCT images. ( c ) Fingerprint images of a wrinkled finger and the OCT image. ( d ) Almost flat finger image and the optical coherence tomography (OTC) image [ 89 ]. ( e ) Construction of equipment, ( f ) structure of subsurface fingerprints, ( g ) different layers of subsurface fingerprint, and ( g – k ) different fingerprint images. The images from left to right correspond to the cornea, internal, papillary, fusion, and traditional 2D fingerprints [ 159 ].
Liu [ 159 ] suggested the optical coherence tomography (OCT)-based fingerprint identification system shown in Figure 7 e–g. This device’s SD-OCT approach utilizes a light source centered at 840 nm; the light source light is generated by a super light emitting diode (SLED), and two identical telephoto lenses serve as a focusing lens and a scanning lens, respectively [ 160 ]. The gadget is designed for capturing by touch. During fingerprint capture, it is necessary to place the finger on a fixed cover glass. In contactless OCT, the use of a fixed cover glass eliminates the depth-dependent roll-off issue. The SD-OCT device’s axial and lateral resolutions are 8 µm and 12 μm, respectively. The apparatus records a 15 mm × 15 mm picture with an average scatter size of 24 μm. Each acquisition records a fingertip region of 1.8 mm × 15 mm × 15 mm with a spatial dimension of 500 × 1500 × 400 pixels.
4. Capacitive Fingerprint Recognition
As semiconductor [ 161 ] technology progresses and authentication devices in mobile devices such as smartphones and integrated circuit cards demand compact, low-cost packaging, capacitive fingerprint sensors are appearing. In principle, capacitive and inductive fingerprint sensors share a “flat” plate with hundreds of integrated semiconductor devices and a surface layer that is generally a few microns thick. The unevenness of the fingertip’s fingerprint, the actual distance between the bump and the bump touching the plate varies when the finger is placed on the capacitive sensor’s surface, results in different capacitance values [ 162 , 163 ]. The capacitance values are converted into current or voltage values, which are then converted into clerk data by an ADC. The greater the contrast of the fingerprint impression, the closer the fingertip is to the surface. The device completes the fingerprint-gathering process by averaging the gathered results.
The optical under-display fingerprint sensor exploits the difference in light reflected from finger ridges and valley areas to recognize fingerprints, but it has difficulty distinguishing dry fingers, which do not create regular and consistent contact with the sensor cover layer [ 86 , 164 ]. In terms of identification time, recognition of dry fingers, and manufacturing yields, ultrasonic under-display fingerprint sensors have the potential for development. In contrast, optical and ultrasonic screen sensors are exclusively compatible with OLED panels. On the screen, a reciprocal capacitive can function. Authenticity can be determined by detecting the impedance of fingerprints, which has different impedance frequencies [ 165 ] and different amounts of eddy currents due to the impedance turbo effect [ 166 ], as well as by a temperature sensor [ 167 ]. Capacitive fingerprint sensors still need great improvement in fingerprint detection.
The breadth of the ridge is between 100 m and 400 m, the depth of the valley is between 60 µm and 220 µm, and the width of the valley is between 75 µm and 200 µm [ 157 , 168 ]. When the ridge and valley are neighboring electrodes, the mutual capacitance differential can exceed 400 aF [ 169 ]. Through cost-sharing [ 92 ], charge transfer, feedback capacitive [ 170 ], and sample-and-hold schemes, capacitive sensors [ 171 ] may detect weak signals.
4.1. Fingerprint Sensor Based on Self-Capacitance
The capacitance between the measuring pin and the power source is monitored in the self-capacitance fingerprint sensor. When a finger is put on the sensor, its capacitance increases and the measured voltage increases and changes, enabling the detection of finger contact. In self-capacitive sensing, the unit cell typically consists of a sensor circuit based on Si technology [ 92 , 165 , 172 ], and the sensor’s capacitance may be directly and independently sensed and detected. Si-based fingerprint sensors can only be manufactured on opaque, brittle, and inflexible silicon wafers and thus can only be implemented in rigid devices, such as smartphone buttons. Self-capacitance is not compatible with multitouch functionality.
In the self-capacitance sensing approach, the cell typically comprises a Si-based sensing plate and a readout circuit [ 165 , 173 ]. High sensitivity may be achieved because the controller can be individually addressed, and the sensing electrodes can be directly and independently recognized. However, Si technology sensors can only be manufactured on opaque, brittle, and inflexible Si wafers, limiting their use to rigid devices.
Self-capacitance is not favorable to multi-touch and is also susceptible to ghosting issues. Oxide TFT is an advantageous option due to its medium mobility, high on/off ratio, cheap process cost, and suitability for transparent and/or flexible substrates [ 174 , 175 , 176 , 177 , 178 ]. To increase the safety level of self-capacitance frames per second (FPS), even at tiny scales, great resolution is necessary while keeping high sensitivity [ 179 ]. To guarantee the high resolution and sensitivity of FPS, oxide TFTs are therefore indispensable.
Oxide TFTs predominantly employ amorphous indium gallium zinc oxide (aIGZO) thin-film transistors (TFTs), which have an optical band gap of 3.05 eV compared to typical a-Si semiconductors (1.6 eV) and ensure up to 75 percent transparency. By eliminating the opaque fingerprint sensor array and accompanying control electronics, the display bezel’s size is drastically decreased. The results of the measurements reveal a distinct fingerprint picture, including the aperture. Depicted in Figure 8 a, using only one a-IGZO TFT, a capacitive touch fingerprint sensor may be incorporated into a display, achieving a tiny sensor size, removing two bus lines, decreasing noise, and enhancing sensitivity while keeping the same amplification performance [ 180 ]. Based on a touch sensor, the capacitive fingerprint sensor (CFS) uses an amorphous indium gallium zinc oxide (aIGZO) thin film transistor (TFT) passive matrix [ 181 ]. Due to their high sensitivity, visual clarity, durability, and exceptional performance, such capacitive touch sensor (CTS) sensing systems (TSSs) are utilized in a variety of applications, including mobile devices, automotive, military, and industrial equipment [ 182 , 183 , 184 , 185 , 186 , 187 ]. This sensor minimizes the number of sensing lines in an active matrix, decreasing expenses. This sensor’s construction is depicted in Figure 8 f: 2.8 mm cover glass, 0.1 mm top optically clear adhesive (OCA), 0.005 mm RX electrode, 0.21 mm top film, 0.05 mm bottom optically clear adhesive (OCA), 0.005 mm TX electrode, and 0.21 mm bottom film pixel; 500 PPI resolution and 50.8 µm × 50.8 µm unit pixel size. AFE IC compensates for CSTRAY in sensing mode, boosting the dynamic range to 40.78 dB with an SNR of 47.8 dB and 25.0 dB for CTS and CFS, respectively. Figure 8 h demonstrates that readout integrated circuits (ROICs) with a pixel size of less than 44 µm × 44 µm per pixel and a resolution path of more than 500 DPI may be employed.

( a ) Principle of capacitive touch-fingerprint sensor. ( b ) Simulation results for the capacitive sensor for touch-fingerprint circuits [ 180 ]. ( c ) Simulation results for the capacitive sensor for the proposed circuit, ( d ) top view of the proposed sensor pixel, ( e ) the circuit of the sensor, ( f ) cross-sectional view of the proposed sensor pixel, ( g ) the assembled module of the transparent fingerprint sensing system, and ( h ) the image of the fingerprint [ 188 ].
4.2. Fingerprint Sensor Based on Mutual Capacitance
In the smartphone market, all-screen devices with thin bezels and no physical buttons have become the norm. On smartphones, fingerprint unlocking positioned on the back or side of the device is significantly less successful than front-mounted unlocking. In the mutual capacitance fingerprint sensor, two electrodes are utilized: the transmitting electrode and the receiving electrode. The TX pin provides the digital voltage and measures the charge received on the RX pin; the charge received on the RX electrode is proportional to the mutual capacitance between the two electrodes, and when a finger is placed between the TX and RX electrodes, the mutual capacitance decreases, and the charge received on the RX electrode also decreases. By detecting the charge on the RX electrode, the touch/non-touch condition may be determined.
Mutual capacitance is easily applicable to fingerprint sensors with two electrodes and an insulator structure, one for driving and the other for sensing. Mutual capacitance makes multi-touch functionality possible [ 189 , 190 , 191 ]. Mutual capacitance sensors can operate on OLED (organic light emitting diode) and LCD (liquid crystal display) displays with detecting distances typically ≤ 300 µm [ 192 , 193 ]. On glass substrates or by adhering polymer films on window glass, these sensors can be manufactured [ 194 ]. Transparent capacitive on-screen fingerprint sensors created utilizing mass-producible materials and techniques are ideally suited for smartphones and other mobile devices and can also be utilized to create large-area fingerprint touch combination sensors and sensors for flexible/stretchable electronics.
Five varieties (thickness of these layers: 100 m) of transparent sensor films are available: PET, cellulose nanofiber (CNF) films, and CNF films embedded with BaTiO 3 . Three nanoparticles (Chinese fibers + BaTiO 3 , banknote iron content two nanoparticles: 1 wt%, average size: 25 nm) and CNF films are embedded with AgNF (CNF+AgNF, silver fluorine content: 1.2 wt%, average length of AgNF: 200 ± 20 µm, average diameter: 380 ± 35 nm) [ 195 , 196 , 197 ]. CNF films have advantages due to their high transparency and good mechanical flexibility and durability; however, the low dielectric constant of the original CNF films (k = 1.4–3.0) restricts their usage as overlays for fingerprint sensor arrays [ 198 , 199 , 200 , 201 ]. CNF films, including AgNF (CNF+AgNF), have a k value of 9.2 and a transmittance of at least 90%.
Transparent electrodes with high conductivity and optical transmittance (T) are required for the operation of capacitive fingerprint sensors in the high-frequency region in the transparent form. Thin layers of resistance (Rs) of standard transparent electrode materials prevent high-frequency signals from driving capacitive fingerprint sensors when mobile devices emit interference. To achieve great transparency with metals as electrodes, the width of the electrode lines must be restricted due to their opacity, according to the data in Table 4 .
Fingerprint sensor based on mutual capacitance.
When the finger hits the contact surface, capacitive fingerprint sensors can also generate a potential difference through the piezoelectric capabilities of the material, in which the sensor is bent by stress. It is reported that zinc oxide nanoarrays, which gather the charge caused by the piezoelectric potential generated by each nano, can reconstitute the fingerprint’s three-dimensional deformation field [ 206 ]. For the sensor surface to be encased with a chemically inert UV-cross-linkable polymer [ 207 ], the encapsulation layer must have a thickness between 1.5 µm and 10 µm and possess excellent hydrophobicity and oleophobicity [ 208 ], as shown in Figure 9 . Hsiung [ 209 ] proposed an 8 × 32 pressure sensor array, with a cell size of 65 µm × 65 µm, a sensitivity of 0.39 fF/MPa, a maximum capacitance variation of 16%, and a resolution of 390 DPI, with no further film processing needed. Sugiyama [ 210 ] presented a 32 × 32 silicon pressure sensor array with a sensor chip size of 10 mm × 10 mm and an array element spacing of 250 µm, capable of displaying stable 2D or 3D images.

Schematic representation of polymer-encapsulated multi-NWs pressure-based fingerprint sensor [ 208 ].
5. Ultrasonic Fingerprint Recognition
The ultrasonic fingerprint sensor [ 211 ] is the most precise and accurate instrument for acquiring fingerprint images. There are two primary imaging techniques: pulse-echo imaging and impedance imaging. Ultrasonic fingerprint imaging is based on the reflection effect of ultrasound as it propagates across various impedance mediums [ 212 ]. When a finger is placed on a mobile phone’s touch panel, the pressure sensor detects the pressure and transmits an electrical pulse to activate the ultrasonic fingerprint sensor, which emits an electrical pulse wave. Due to the difference in acoustic impedance between human tissue and air, the echo amplitude of human tissue is larger than that of air; hence, the woven pattern can be determined by determining the echo amplitude at each point. The intensity of the sensor’s ultrasonic waves is comparable to medical diagnosis, which is safe for the human body [ 213 ]. Due to the fact that ultrasonic waves have a high penetrating power [ 214 ], they can be identified even when there is a small amount of dirt or moisture on the finger, and they can penetrate materials such as glass, aluminum, stainless steel, sapphire, and plastic, which increases the device’s applicability and success rate. It is also feasible to place the sensor into the gadget in order to increase its durability.
Ultrasound imaging relies on the detection of ultrasound pulse echoes, with hills and valleys providing distinct echo signals. It is also possible to study acoustic impedance approaches by measuring the ring drop of the pulse, which measures the damping of the contact region (i.e., the fingertip) [ 215 ]. Acoustic impedance-based fingerprint recognition relies on the direct touch method with air-filled valleys of 430 Rayl and ridges of about 1.5 MRayl of human tissue, and the difference in amplitude and phase of the impedance helps to differentiate between ridges and valleys, thereby generating a fingerprint image.
The primary component of the ultrasonic fingerprint sensor is the ultrasonic transducer, which captures the ultrasonic signal impulse response and completes the graphic reconstruction using the reflection and diffraction properties of ultrasonic waves. Ultrasonic fingerprint recognition technology is unaffected by surface clutter and can penetrate the dead skin layer to reflect the fingerprint pattern architecture in the dermis, capturing not only the fingerprints visible on the surface but also obtaining credible information from within the tissue.
The earliest ultrasonic fingerprint sensor is a type of ultrasonic probe detection (US5224174A) jointly proposed by Ultrasonic Scan and Niagara and is a probe ultrasonic detection system [ 216 ]. The probe emits ultrasonic energy and scans twice from two directions at right angles; after the finger reflects, the pulse receiver absorbs the reflected signal and translates it into frequency data, and a processing circuit creates the fingerprint image. With the introduction of micro electromechanical system (MEMS) technology, the MEMS ultrasonic transducer stage into CMOS has made ultrasonic fingerprint recognition applicable to smartphones [ 217 ]. Capacitive micromechanical ultrasonic transducers (CMUT) and piezoelectric micromechanical ultrasonic transducers are typical transducers (PMUT).
5.1. Fingerprint Sensor Based on Capacitive Ultrasonic Transducer
A capacitive micromechanical ultrasonic transducer (CMUT) has a simple design, a compact size, low noise, high sensitivity, high resolution, and excellent matching between silicon material and dielectric impedance [ 218 , 219 , 220 ]. CMUT can be used to manufacture conventional semiconductor processes due to its simpler structure and developed technology, and it entered the market before PMUT. Metal uppers are a standard component of CMUT’s fundamental structure. Metal upper electrode, vibrating film, edge support, vacuum cavity, insulating layer, bottom electrode, and base constitute the standard CMUT construction (North Central University thesis) [ 221 ]. The lower electrode is attached to the base, whereas the upper electrode is capable of vibrating with the membrane. In the transmitting mode, the alternating electric field between the upper and lower electrodes causes the vibrating membrane to bend and vibrate, thereby generating sound waves; in the receiving mode, the vibrating membrane vibrates under the action of external sound pressure, causing the electric capacity between the upper and lower electrodes to change, and the electric signal corresponding to the magnitude of the sound pressure can be obtained through it.
Figure 10 a depicts a CMUT composed of several tiny vibration cells (cells) connected in parallel, and multiple microelements are connected in parallel in a certain configuration to form a CMUT in order to obtain a greater output sound pressure. It can be designed in a variety of shapes, including circular, square, hexagonal, etc., depending on the specific requirements.

( a ) The structure of CMUT; ( b ) the structure of PMUT. ( c ) Optical images of the 24 × 8 PMUT array after de-bonding to remove the CMOS wafer, ( d ) cross-sectional SEM images of a single PMUT after partial de-bonding to remove the MEMS wafer, and ( e , f ) 2D pulse-echo ultrasonic image of the PDMS fingerprint phantom [ 222 ].
The first capacitive micromachined ultrasonic transducers (CMUTs)-based fingerprint sensor could only collect 2D fingerprint images via mechanical scanning. Savoia [ 223 ] avoided the requirement for mechanical scanning and provided a linear array of probes for a fingerprint sensor. The transducer is comprised of 192 rectangular elements with 112 µm element spacing and a total aperture of 21.5 mm, which corresponds to the average width of a human fingertip. This aluminum-encased, probe-type fingerprint sensor is utilized in medical therapy. Grayscale intensity representation of obtained 3D objects and 2D display of flat sections are also viable. However, the resolution of the photographs it captures must be enhanced. Pulse-echo measurements are not possible due to the array’s restricted bandwidth. The interface between this huge sensor array and the signal processing circuits is too complicated to meet the size requirements. Kwak [ 224 ] utilized waveguide obstacle mapping techniques for fingerprint imaging. It is possible to obtain fingerprint images from hard materials such as glass, which can also be used for fingerprint imaging under glass. Adjusting the waveguide’s height and width, as well as the medium’s composition, can enhance the resolution of a fingerprint image.
The waveguide method provides a higher resolution than the direct contact approach for detecting phase shifts of 0.6 degrees at ridges and valleys at 2.4 MHz. The enhanced fingerprint protrusion resolution safeguards and stabilizes the CMUT impedance signal.
5.2. Manufacture of Capacitive Ultrasonic Transducer
CMUT fabrication usually uses a silicon wafer as the base, aluminum material as the metal electrode, SiO 2 as the edge support, and the vibrating film can be made of silicon, SiN, etc. On the silicon wafer substrate, a thin layer of metal is precipitated as the lower electrode pole plate of the vibrating unit. The insulation layer plays a protective role during etching and fabrication and also ensures the insulation of electrical characteristics between the upper and lower electrodes to prevent short circuits due to accidental joining of the upper and lower electrodes during operation. On the upper surface of the vibration film, a thin layer of metal is deposited, which is the upper electrode pole plate of the sensor. The edge support acts as a support for the vibration film [ 225 ].
The leads of the array are connected to the flexible part of the PCB, and a preformed feeler is cast onto the custom acoustic and mechanical backing of the CMUT chip to fix the rigid part of the PCB to the die. The silicon substrate of the chip is removed using the HNA wet etching process. CMUT is based on the localized oxide of silicon (LOCOS) direct wafer bonding. It consists of a silicon top plate, a vacuum gap for silicon nitride, and silicon oxide at the bottom. The vibrating top plate consists of a single crystal of silicon with a radius of 24 µm and a thickness of 1.5 µm below the flat plate with a vacuum gap of 200 nm. Fixed with epoxy resin to solder the 64-channel CMUT to the chip carrier, and the lead bonding end is also fixed with epoxy resin to prevent dislodgement.
5.3. Fingerprint Sensor Based on Piezoelectric Ultrasonic Transducer
CMUT is based on a capacitive electrode separated by a submicron vacuum gap, whereas PMUT is composed of a solid piezoelectric capacitor [ 226 ], which simplifies the production process and enhances mechanical stability. As depicted in Figure 10 b, the PMUT consists of a piezoelectric membrane, upper and lower electrodes, and a membrane that vibrates. In the emission mode, a certain voltage is supplied between the upper and lower electrodes of the piezoelectric membrane, and the pressure generated by the membrane’s inverse piezoelectric effect forces the membrane structure to bend, causing the vibrating membrane to deform. When the alternating voltage is applied, the membrane vibrates and emits sound pressure, thereby converting electrical energy into sound energy. In the receiving mode, the vibrating membrane deforms due to the external acoustic pressure and causes the piezoelectric membrane to deform, thereby generating a corresponding charge due to the piezoelectric effect and enabling the conversion of acoustic energy to electrical energy and the reception of acoustic signals via the receiving circuit [ 227 ].
As illustrated in Figure 10 c, the early arrays of AlN-based PMUTs for ultrasonic fingerprint sensors were only 24 × 8 [ 222 ]. Each PMUT is directly connected to a dedicated CMOS receiver amplifier, avoiding electrical parasitic and eliminating the need to pass via silicon vias. Fluoride FC-70 is utilized as the coupling layer between the PMUT and the finger, and a 100 µm PVC cover seals the fluoride and preserves the sensor [ 228 ]. To preserve the scratch resistance of the surface and reduce the impact of scratches and deformation on the sensor surface on the image, a protective layer can be added to the material surface [ 229 ]. Typically, a 1µm thick coating of Al 2 O 3 , a material with known hardness and scratch resistance [ 230 ], can be selected. It can be placed on plastic and glass, among other substrates. Thin enough layers of this material have little effect on the transmission of sound. In 2016, Horsley [ 231 ] proposed a monolithic 110 × 56-cell ultrasonic pulse-echo fingerprint sensor based on piezoelectric micromachined ultrasonic transducers (PMUTs) that were directly linked to a CMOS readout ASIC [ 232 ]. The array’s fill factor is 51.7%, which is three times greater than the 24 × 8 array. The ultrasonic fingerprint sensor captures not just surface-visible fingerprints but also information from within the tissue, demonstrating three-dimensional information with significant security enhancement [ 233 ]. Compared to the 110 × 56 array, the 65 × 42 array has a larger pitch to decrease crosstalk. The sensor is capable of multi-channel TX beamforming, which increases the signal-to-noise ratio by 7 dB and permits a little improvement in lateral picture resolution. With a five-column TX beam, peak pressure, RX voltage, and image contrast are all improved by a factor of 1.5. In the unsupported zone, the array’s amplitude is −17 dB, compared to −2.7 dB for 110 × 56. The sensor surface is initially covered with a 215µm-thick polydimethylsiloxane layer (PDMS). It is possible to create a 4.6 mm × 3.2 mm fingerprint image [ 234 ].
Typically, the piezoelectric coefficient of lead zirconate titanate (PZT) is two orders of magnitude greater than that of aluminum nitride (AlN). The PZT-based fingerprint sensor (50 × 50 PMUT array) employs a single pixel and a mechanical scanning mode of operation, resulting in a large size, poor frame rate, and high cost [ 235 ]. In Table 5 , the various arrays are compared.
Structure of ultrasonic fingerprint.
5.4. Fingerprint Sensors Based on Other Sensors
Typically, ultrasonic waves are produced by a transducer that converts electrical or other sources of energy into acoustic energy. Employing the inverse piezoelectric effect, the majority of ultrasonic transducers generate sound waves from an alternating electric field using piezoelectric materials. The same transducer can also receive ultrasonic waves through the direct piezoelectric effect. For the conversion of acoustic energy to electrical energy, piezoelectric nanomaterials are composed of lead zirconate titanate (PZT) and polyvinylidene fluoride (PVDF) nanofibers. The g 33 value of PZT nanofibers (0.079 Vm/N) is significantly greater than that of bulk PZT (0.025 Vm/N) and PZT microfibers (0.059 Vm/N), resulting in an increased sensitivity for acoustic detection. Due to their high electrical coefficients and energy conversion efficiency, nanostructures based on PVDF are also commonly used as piezoelectric active materials. The combination of nanotechnology and ultrasonic technologies can alter standard ultrasound procedures. PZT-5 piezoelectric active material and P(VDF-TrFE) film were used to create ultrasonic fingerprint sensors by the Peng team [ 236 , 237 ]. The PZT-5H-based sensor effectively captured electronic images of the surface fingerprint pattern and the inner vascular simulation channel by simulating skin with two layers of polydimethylsiloxane (PDMS) and simulating blood vessels with bovine blood in the inner vascular simulation channel. The performance of the various sensors is compared in Table 6 , where it can be seen that the PZT-based sensor structure has an order of magnitude higher emission sensitivity than the 110 × 56 PMUT-based sensor and is two orders of magnitude higher than the CMUT-based sensor and that the PZT-5 based sensor has the highest loop sensitivity of all designs. P(VDF-TrFE) films, unlike piezoelectric ceramics (e.g., PZT), are CMOS-compatible and can be directly integrated with supporting electronics [ 238 ]. Schmitt [ 239 ] suggested a new fingerprint sensor comprised of 1–3 piezoelectric ceramics that transfer the acoustic impedance of fingerprint patterns to the electrical impedance of the sensor element. The cost-effective sensor substrate delivers a resolution of up to 50 µm over a 25 × 25 mm 2 area. It offers better electromechanical coupling, broader bandwidth, and lower acoustic impedance than piezoelectric ceramics, the traditional principal material (PZT).
Performance comparison of different piezoelectric materials.
Qualcomm released the ultrasonic fingerprint sensor that is currently prevalent in cell phones; the sensor is laminated directly on the OLED display to allow quick single-touch authentication with high speed, superior security, and low battery consumption. It occupies little space between the battery and the display, as its thickness is less than 150 µm. Strohmann [ 240 ] proposed the notion of contact gestures utilizing the Qualcomm fingerprint sensor system, and in addition to its authentication function, the same sensor can be used to recognize finger contact gestures and movement directions, such as light/heavy touch and finger navigation. Xu [ 241 ] presents a large-area (20 × 30 mm 2 ), multifunctional, highly integrated ultrasonic sensor beneath a thick (>1 mm) mobile display made of organic light-emitting diodes (OLEDs) and protective cover glass (CG). Dual fingerprint verification enhances the sensor’s dependability and security by enabling simultaneous and rapid finger scanning to identify finger contact pressure levels and non-electromagnetic hard stylus position and tracking [ 242 ].
5.5. Manufactur of Piezoelectric Ultrasonic Transducer
Common PMUT diaphragm shapes include round, square, rectangular, shell, cylinder, and dome; piezoelectric membrane materials are shown in Table 7 , including PZT [ 243 ], ZnO [ 244 , 245 ], AlN [ 246 ], PMN-PT [ 247 , 248 , 249 , 250 ], and PVDF [ 251 ], among others. The following table illustrates a comparison of various piezoelectric materials. Vibrating membrane materials are frequently silicon, silicon dioxide [ 252 , 253 ], silicon nitride, polysilicon, etc.; boundary conditions are approximate solid support, approximate simple support, approximate free vibration, etc.; vibration modes are double stacked bending vibration modes, multi-layer stacked bending vibration modes, thickness vibration modes, etc.; operating frequencies can range from tens of kHz to gigahertz, from the development of a single PMUT to the development of an array of PMUTs.
Piezoelectric membrane materials.
PMUT is generally based on silicon or SOI wafer-bonded piezoelectric materials. AlN is generally carried out by magnetron sputtering. AlN is often patterned by wet etching, using TMAH (C₄H 13 NO, tetramethylammonium hydroxide) solution as the wet etchant. AlN can also be patterned by dry etching, and the etching gas system can be BC l3 (boron trichloride)/C l2 (chlorine)/Ar (argon). Compared to wet etching, the steepness of dry etching is very high, and the surface roughness after etching is better.
In contrast, the PZT films fabricated by the magnetron sputtering method still have better film density and consistency at 6″ and 8″ sizes. Magnetron sputter-grown PZT films have better orientation growth characteristics, and despite their polycrystalline orientation, their internal domain orientation is highly uniform, such that the film-forming PZT can no longer be subjected to post-polarization. Of course, the problem with magnetron-sputtered PZT films is the higher cost, and the control of the growth environment and process conditions is much more complicated than that of magnetron-sputtered AlN [ 254 ].
After generating y piezoelectric material, it is necessary to pattern the piezoelectric material and the upper electrode. AlN is often patterned by wet etching, using TMAH (C₄H 13 NO, tetramethylammonium hydroxide) solution as the wet etching agent. AlN can also be patterned by dry etching, and the etching gas system can be BC l3 (boron trichloride)/C l2 . Of course, the problem of magnetron sputtering PZT films is the high cost, and the control of the growth environment and process conditions is much more complicated than magnetron sputtering AlN. The wet corrosion of PZT is complicated, and the corrosion environment is mainly various acidic solutions. The dry etching of PZT, ICP (inductive coupled plasma), and RIE (reactive ion etching) are mainly mixed solutions. Wet etching can also be carried out. The choice of etching gases is also relatively large. SF 6 (sulfur hexafluoride)/Ar, CF 4 (carbon tetrafluoride)/Ar, and CHF 3 (trifluoromethane)/Ar can be used for dry etching of PZT [ 255 ].
The ultrasonic fingerprint sensor is a current identification method with high accuracy. The advantages and disadvantages of different fingerprint identification principles are compared in Table 8 . It can be seen from the table that ultrasonic fingerprint identification technology is a major development trend of fingerprint identification technology in the future. Wet or unclean fingerprints can be read by ultrasonic fingerprint sensors, and the ultrasonic type of flexible piezoelectric materials can be used in a variety of wearable sensors [ 256 ].
Compare that with the different types of fingerprint sensors.
6. Conclusions
This work focuses on the current development status of fingerprint sensors, mainly describes three types of technologies currently available on the market, presents the structure and evolution of three distinct fingerprint sensor principles, and introduces the production methods for ultrasonic fingerprints.
Optical fingerprint sensors are mature and use in fingerprint sensors with large sizes, such as attendance systems, etc. The capacitive fingerprint sensor is further integrated on the basis of the optical type, which reduces the size to a certain extent and can be applied in portable devices such as cell phones. Recently, further research has been conducted on ultrasonic fingerprint recognition technology in order to reduce the error rate of fingerprint recognition and, at the same time, improve the integration of fingerprint recognition systems. For ultrasonic fingerprint recognition devices, ultrasonic transducers are mainly used to send and receive ultrasonic signals, and there are already ultrasonic transducers made of PZT, PVDF, and AlN. AlN was earlier used in piezoelectric ultrasonic transducers, PZT has cost advantages, and PVDF is mainly for flexible devices. For various applications of fingerprint transducers, different materials of piezoelectric materials can be selected.
Fingerprint recognition technology still has several shortcomings and directions for improvement:
- The accuracy of the algorithm is not sufficient to prevent recognition errors due to the proximity of fingerprints between relatives and needs to be improved;
- The fingerprint information left when touching an object is easily accessible, and the security is poor. Therefore, the detection of location authenticity must be enhanced to prevent the harmful effects of fingerprint theft;
- With the emergence of wearable devices such as mobile fingerprint unlock bracelets and car fingerprint locks, the integration of fingerprint recognition technology into flexible wearable devices has become a major challenge, which will drive the development of small, ultra-thin fingerprint capture chips;
- Fingerprint capture is easily affected by posture and angle, and the problem of finger pressure can be solved using a contactless fingerprint sensor.
Fingerprint recognition is a convenient and widely applicable biometric technology. A variety of different capture methods are committed to transferring fingerprint recognition devices from high-end devices to low-cost, miniaturized devices. In particular, fingerprint recognition technology can also be greatly applied to information security and medical testing of wearable devices. At present, ultrasonic fingerprint recognition technology is still a challenge to be widely used in life as one of the fingerprint recognition methods with better recognition effects. However, there is still lots of work to be carried out to achieve robust, interoperable, secure, privacy-preserving, and user-friendly systems.
Funding Statement
This research received no external funding.
Author Contributions
Y.Y. was responsible for sections concerning optical, capacitive, and ultrasonic devices. Q.N. contributed to sections of the introduction. All authors were involved in the writing and refinement of the manuscript under the direction of D.L., who revised the manuscript. All authors have read and agreed to publish a version of the manuscript.
Conflicts of Interest
The authors declare no conflict of interest.
Disclaimer/Publisher’s Note: The statements, opinions and data contained in all publications are solely those of the individual author(s) and contributor(s) and not of MDPI and/or the editor(s). MDPI and/or the editor(s) disclaim responsibility for any injury to people or property resulting from any ideas, methods, instructions or products referred to in the content.
A Survey on Biometric Authentication: Toward Secure and Privacy-Preserving Identification
Ieee account.
- Change Username/Password
- Update Address
Purchase Details
- Payment Options
- Order History
- View Purchased Documents
Profile Information
- Communications Preferences
- Profession and Education
- Technical Interests
- US & Canada: +1 800 678 4333
- Worldwide: +1 732 981 0060
- Contact & Support
- About IEEE Xplore
- Accessibility
- Terms of Use
- Nondiscrimination Policy
- Privacy & Opting Out of Cookies
A not-for-profit organization, IEEE is the world's largest technical professional organization dedicated to advancing technology for the benefit of humanity. © Copyright 2024 IEEE - All rights reserved. Use of this web site signifies your agreement to the terms and conditions.
Academia.edu no longer supports Internet Explorer.
To browse Academia.edu and the wider internet faster and more securely, please take a few seconds to upgrade your browser .
Enter the email address you signed up with and we'll email you a reset link.
- We're Hiring!
- Help Center
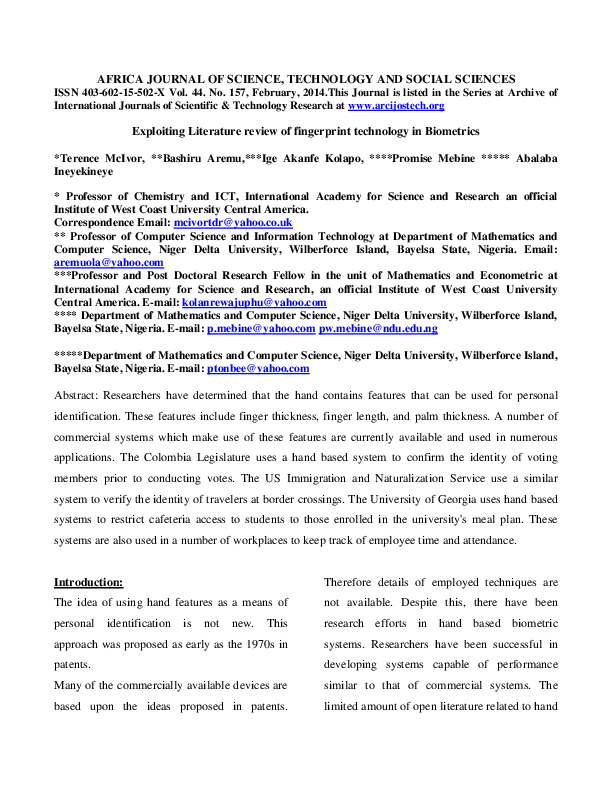
Exploiting Literature review of fingerprint technology in Biometrics

Related Papers
Kolapo A Ige ( Professor) , Prof. Sir Bashiru Aremu
Biometrics can provide a greater degree of security than traditional authentication methods, meaning that resources are accessible only to authorized users and are kept protected from unauthorized users in theory a password is memorized by a single person, it's hard guess it's never written down, and it's never shared. They can offer much greater convenience than systems based on remembering multiple passwords or on keeping possession of an authentication token for PC application in which a user must access multiple resources, biometrics can greatly simple.
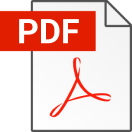
Prof. Sir Bashiru Aremu
Prof. Sir Bashiru Aremu , Promise Mebine
RELATED PAPERS
Pattern Recognition
Xudong Jiang , Setsuko Katoh
shiva prasad
Prasad Banoth
Aythami Morales
IEEE Signal Processing Magazine
J. Ortega-garcia
Computer Vision and Image Understanding
Recent Advances in Signal Processing
Gonzalo Bailador-del-pozo
Information Sciences
J. Ortega-garcia , Aythami Morales
Chhaya Methani
Pattern Recognition Letters
Aparrnaa Raghuraman
IJAET Journal
Jordanian Journal of Computers and Information Technology JJCIT , Ezdihar Bifari
Proceedings of the IEEE
Rebecca Heyer
Expert Systems with Applications
Shahrel Suandi
Proceedings 1999 International Conference on Image Processing (Cat. 99CH36348)
IJERA Journal
IEEE Transactions on Information Forensics and Security
IEEE Transactions on Pattern …
Sushmita Chauhan
IEEE Transactions on Signal Processing
Xudong Jiang
Rogerio Enriquez-Caldera
Md Jan Nordin
Andrew Beng Jin Teoh
jayavani kumar
Serge Belongie
neha dahiya
Rex Journal
Rafael Marcos Luque Baena , Ezequiel López-Rubio
International Journal of Biometrics
Mita Nasipuri
Saroj Panigrahy
In proc. of Third COST 275 Workshop (COST 275)
Florian Evéquoz , Catherine Pugin , Jean Hennebert , Dijana Petrovska-delacrétaz
American Journal of Applied Sciences
Associate Professor Dr. Fatma Susilawati Mohamad
- We're Hiring!
- Help Center
- Find new research papers in:
- Health Sciences
- Earth Sciences
- Cognitive Science
- Mathematics
- Computer Science
- Academia ©2024
- Open access
- Published: 29 May 2024
High-standard predictive equations for estimating body composition using bioelectrical impedance analysis: a systematic review
- Francesco Campa ORCID: orcid.org/0000-0002-3028-7802 1 na1 ,
- Giuseppe Coratella 2 na1 ,
- Giuseppe Cerullo 1 ,
- Zeasseska Noriega 3 ,
- Rubén Francisco 4 ,
- Davide Charrier 1 ,
- Alfredo Irurtia 3 ,
- Henry Lukaski 5 ,
- Analiza Mónica Silva 4 &
- Antonio Paoli 1
Journal of Translational Medicine volume 22 , Article number: 515 ( 2024 ) Cite this article
Metrics details
The appropriate use of predictive equations in estimating body composition through bioelectrical impedance analysis (BIA) depends on the device used and the subject’s age, geographical ancestry, healthy status, physical activity level and sex. However, the presence of many isolated predictive equations in the literature makes the correct choice challenging, since the user may not distinguish its appropriateness. Therefore, the present systematic review aimed to classify each predictive equation in accordance with the independent parameters used. Sixty-four studies published between 1988 and 2023 were identified through a systematic search of international electronic databases. We included studies providing predictive equations derived from criterion methods, such as multi-compartment models for fat, fat-free and lean soft mass, dilution techniques for total-body water and extracellular water, total-body potassium for body cell mass, and magnetic resonance imaging or computerized tomography for skeletal muscle mass. The studies were excluded if non-criterion methods were employed or if the developed predictive equations involved mixed populations without specific codes or variables in the regression model. A total of 106 predictive equations were retrieved; 86 predictive equations were based on foot-to-hand and 20 on segmental technology, with no equations used the hand-to-hand and leg-to-leg. Classifying the subject’s characteristics, 19 were for underaged, 26 for adults, 19 for athletes, 26 for elderly and 16 for individuals with diseases, encompassing both sexes. Practitioners now have an updated list of predictive equations for assessing body composition using BIA. Researchers are encouraged to generate novel predictive equations for scenarios not covered by the current literature.
Registration code in PROSPERO : CRD42023467894.
Introduction
The determination of body composition is a common practice for evaluating health and nutritional status, as well as monitoring the effects of training or diet strategies [ 1 , 2 ]. While a simplistic approach involves assessing body composition based on body mass and its changes over time, breaking it down into different components enables a more meticulous and in-depth evaluation [ 3 , 4 ]. At the molecular level of body composition analysis [ 4 ] initial attempts to break down body mass were based on a two-component model, categorizing it into fat (FM) and fat-free mass (FFM), also known as lean mass [ 5 ]. It is worth noting that FFM is often also referred to by synonyms such as lean mass or lean body mass [ 6 ]. However, monitoring FFM alone can obscure meaningful changes in body composition since its composition comprises several components (e.g., water, protein, and minerals) not considered in the assessment [ 5 , 6 ].
To overcome the limitations of the two-component model and enhance the accuracy of body composition analysis, various multi-component models have been developed over time [ 2 ]. These models necessitate the use of one or more methods, including dual-energy X-ray absorptiometry (DXA) for bone mineral assessment, dilution techniques for total-body water, hydrostatic weighing or air displacement plethysmography for body volume, total-body potassium for body cell mass, and magnetic resonance imaging (MRI) and computed tomography for skeletal muscle or other tissues and organs [ 5 ]. For example, Wang et al. [ 3 ] presented a procedure based on a four-component model that is currently considered the state-of-art method for determining FFM and FM. Additionally, the use of multi-components models allows for the breakdown of FFM into different parts such as total body water (TBW), intra (ICW) and extracellular water (ECW), body cell mass (BCM), lean soft mass (LSM), skeletal muscle mass (SMM), and bone mass [ 7 , 8 ]. With these procedures available to researchers, predicting body mass components by assuming constant hydration of FFM (i.e., TBW/FFM = 0.73) or neglecting the potential variation in FFM and FM density [ 6 ] cannot be considered as a valid approach for assessing body composition. Indeed, several dated studies have employed coefficients such as this to derive FFM and subsequently FM based on TBW predictions, or they have relied on density assumptions to derive FFM and FM using only densitometry techniques (i.e., hydrostatic weighing or air displacement plethysmography) [ 9 , 10 , 11 ]. In particular, the practice of deriving FFM by assuming that TBW represents a constant fraction of it has led to BIA being incorrectly identified as a hydration-dependent method. Therefore, a comprehensive determination of body mass components using multi-components models enables a valid assessment of body composition in different populations, especially those with non-conventional hydration or density properties (e.g., children, athletes, or older individuals) [ 6 , 12 ].
Considering the limitations of densitometric, hydrometric, or imaging techniques for quantifying body composition due to high costs, lengthy procedures, and non-user-friendly processes, alternative methods such as bioelectrical impedance analysis (BIA) or surface anthropometry are often employed for routine assessments [ 13 , 14 , 15 , 16 ]. The theoretical basis of BIA revolves around the conductivity properties of biological tissues, quantified as bioelectrical resistance and reactance, deriving by conductor volumes (i.e., lean soft components) [ 17 ]. Bioelectrical resistance represents the opposition offered by the body to the flow of an alternating electrical current and is inversely related to the water and electrolyte content of tissues [ 17 ]. Bioelectrical reactance is related to the capacitance properties of the cell membrane and variations that can occur depending on its integrity, function, and composition [ 17 ]. Notably, these properties can be attributed to theoretical models, according to which biological tissues are traditionally conceived as electrical circuits arranged in series with each other [ 18 ]. Starting from the relationships between resistance and reactance with TBW [ 19 ], numerous BIA-based predictive equations have been developed over the years [ 20 ]. Previous studies have demonstrated that the use of different BIA technologies (i.e., hand to hand, leg to leg, foot to hand, and segmental) and sampling frequencies results in different outputs, so that these equations cannot be interchangeable between different devices [ 21 , 22 , 23 , 24 ]. Additionally, the choice of the equation should be made considering the subjects' characteristics, such as chronological and biological age, geographical provenance, sex, health status, and level of physical activity [ 25 , 26 , 27 ]. Therefore, the choice of the appropriate predictive equation is crucial to ensure the validity of the body composition estimation. A further question is that various studies including those providing reference data based on BIA do not disclose the procedures used [ 24 , 28 ]. Many of these studies merely mention the type of software employed, making it impossible to discern which formulas were used to convert raw bioelectrical parameters into components of body mass. Furthermore, such data may no longer be representative over time because companies producing such software can alter the equations without notifying users [ 29 ]. While this approach is undoubtedly quicker than a systematic and accurate selection of the most appropriate equation among those available in the literature, does not guarantee a high-standard validity.
The literature is now replete with predictive equations developed for estimating body composition using BIA. However, several limitations may question the validity of some equations. First, the use of the two-component model instead of a multi-component model approach. Second, predicting FFM and FM starting from hydration or density assumptions. Third, the development of predictive models without using criterion methods. Fourth, mixing different populations without including specific factors (e.g., age, maturity status, sex, physical activity level) as independent variables in the predictive models [ 30 ]. Therefore, the present review aimed to examine the relevant literature to extract all the predictive equations currently available, and list only those free of the aforementioned limitations. In addition, we provided a clear organization of the predictive equations based on the BIA technology, sampling frequency, and population characteristics. Such a list will help scientists and practitioners select the most appropriate predictive equations in accordance with the BIA devices and population peculiarities. Summarizing the state-of-the-art of BIA-based prediction of body composition will also be helpful to optimize the development of new predictive equations considering what is currently lacking in the literature. Obtaining accurate results would also allow for utilizing the data to evaluate other health-related parameters through estimating basal metabolism or the quantity of macronutrients based on body composition data [ 31 ]. Figure 1 depicts the premise and the problem at the basis of the present study, presenting the aims and future perspectives.
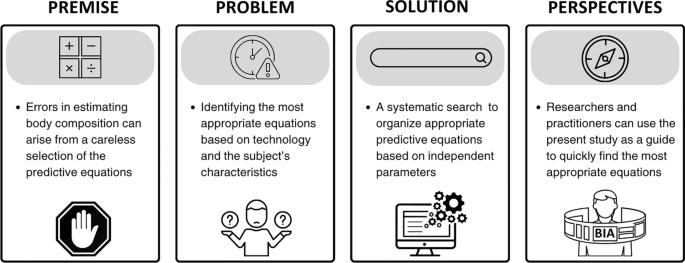
A schematization of the background and objectives set for the current study
Search strategy and eligibility criteria
The present study was carried out following the Preferred Reporting Items for Systematic reviews and Meta-Analyses (PRISMA) guidelines [ 32 ]. The two independent researchers (F.C. and Z.N.) conducted systematic searches in Scopus, and PubMed on December 22th, 2023, identifying potential eligible studies without any restriction related to year of publication. A search query included combinations of at least one of the terms identifying BIA, with at least one of the terms regarding the study design, a term regarding reference techniques, and a term referring to body composition. We composed different query strings depending on the variability of the databases functioning, using the following terms: (bioelectrical impedance analysis) OR (bioimpedance) OR (BIA) AND (development) OR (new predictive equation) OR (prediction) OR (estimation) OR (Dual Energy X-ray absorptiometry) OR (dilution techniques) OR (deuterium dilution) OR (tritium dilution) OR (bromide dilution) OR (magnetic resonance imaging) OR (computerized tomography) OR (total-body potassium) OR (DXA) OR (MRI) AND (fat mass) OR (fat-free mass) OR (total body water) OR (body fluids) OR (extracellular water) OR (intracellular water) OR (body cell mass) OR (skeletal muscle mass) OR (lean soft mass) OR (lean soft tissue) OR (FM) OR (FFM) OR (TBW) OR (ECW) OR (ICW) OR (BCM) OR (SMM) OR (LSM) OR (LST) AND (three-compartment model) OR (four-compartment model) OR (3C model) OR (4C model).
The inclusion criteria were as follows:
Peer-reviewed articles that developed at least one predictive equation for estimating body mass components (i.e., FM, FFM, TBW, BCM, LSM, and SMM) using BIA.
Accessible in English in full text.
The exclusion criteria were as follows:
Research protocols, theses/dissertations, abstracts, letters to the editor, case reports, book chapters, guidelines, position papers, and unpublished works.
Articles aimed at developing predictive equations without recognized reference techniques or considering a body composition assessment based on a two-component model for FM and FFM.
Articles aimed at validating predictive equations.
Articles where the characteristics of the BIA devices (e.g., technology and sampling frequency) were not available.
Articles where predictive equations were developed by mixing different populations without including variables such as sex, maturity status, or age in the development of regression models. For example, if an equation included participants of both sexes and a sex code was included among the independent variables, the equation can be considered eligible. On the contrary, an equation including participants of both sexes without including a sex code in the predictive model cannot be considered eligible. Similarly, an equation including participants aged from 15 to 90 years old that does not require the age as independent variable was excluded from the review. Moreover, studies developing predictive equations where participants of different health status were mixed were not considered eligible.
Study selection and data processing
Based on the initial titles retrieved, duplicates were removed. After concluding the search, all records were compiled into the Endnote for Windows version X9, 2018 (Clarivate, Philadelphia, USA) software to delete all duplicates showing the same: (a) title, authors and year of publication and (b) title, authors, and journal title. The records remaining after the deletion of duplicates were exported to an Excel file for Windows version 16.75.2 (Microsoft, Washinton, EUA) organized based on essential information for screening, such as authors’ names, publication year, journal title, digital object identification (DOI), article title and abstract. Abstracts identified from the literature searches were screened for potential inclusion by two authors (F.C. and Z.N.) and a third author (G.C.) when there was a disagreement between the first two. Data extraction included information about each article, such as: authors, year, reference methods, participants’ information (gender, age, sports, diseases, geographical ancestry), bioelectrical impedance techniques and devices, predictive equations, and their characteristics. The selected studies were grouped in five categories, such as under 18 years old subjects, adults, athletes, elderly, and people with disease.
Quality assessment
Two reviewers independently assessed the quality of the included studies with the Quality Assessment of Diagnostic Accuracy Studies (Quadas-2) [ 33 ]. This scale has two dimensions (risk of bias and applicability concerns) and four domains (patient selection, index test, reference standard and flow and timing) that are scored with unclear risk, low risk, or high risk. Differences were resolved through discussions and consultations with a third researcher.
Study selection
A total of 106 BIA-based predictive equations resulted from the 64 studies included in the review. The PRISMA flow chart is shown in Fig. 2 .
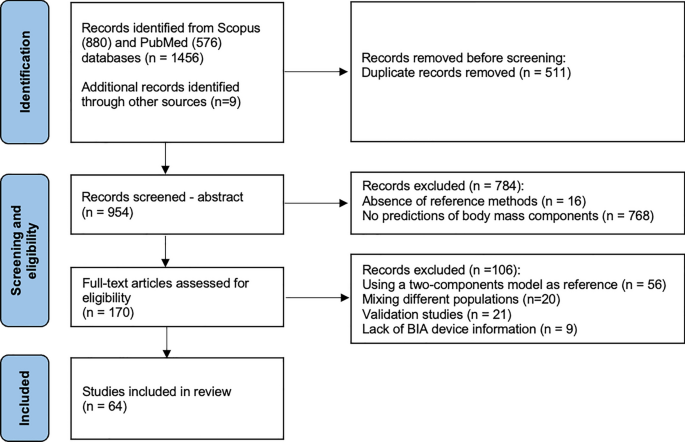
PRISMA Flow chart of the studies’ selection
Risk of bias
A detailed view of the quality assessment is presented in Supplementary Table 1. Overall, the quality of studies was good. Risk of bias was high in two [ 34 , 35 ] and unclear in six [ 36 , 37 , 38 , 39 , 40 , 41 ] studies due to the low to moderate (R 2 < 80%) coefficient of determination (R 2 ) resulted from the multiple regression models. Concerns with applicability were high in 19 studies [ 37 , 42 , 43 , 44 , 45 , 46 , 47 , 48 , 49 , 50 , 51 , 52 , 53 , 54 , 55 , 56 , 57 , 58 , 59 ] and unclear [ 41 , 50 , 60 , 61 , 62 , 63 , 64 , 65 , 66 , 67 , 68 , 69 ] in 12 studies due to the restricted sample size.
Participants
A total of 40,626 individuals were involved in the present systematic review. Out of the total number, 18,417 participants were males and 21,414 females. Three studies involving 186 [ 70 ], 72 [ 52 ], 462 [ 71 ], and 75 [ 65 ] subjects did not specify the sex of the participants. A total of 793 males, 908 females, and 186 subjects without sex info were included in 14 studies [ 37 , 43 , 44 , 45 , 47 , 55 , 57 , 58 , 59 , 72 , 73 , 74 , 75 , 76 ] aimed to develop predictive equations for subjects aged < 18 years. A total of 14,912 males, 17,362 females, and 534 subjects without any sex designated were included in 14 studies [ 34 , 42 , 47 , 50 , 52 , 54 , 61 , 66 , 71 , 77 , 78 , 79 , 80 , 81 , 82 ] aimed to develop predictive equations for adults (from 18 to 65 years old) from the general population. A total of 885 males and 396 females were included in 11 studies [ 31 , 39 , 48 , 51 , 62 , 69 , 83 , 84 , 85 , 86 , 87 , 88 ] aimed to develop predictive equations for athletes. A total of 1469 males and N = 2111 females were included in 17 studies [ 36 , 38 , 46 , 53 , 60 , 63 , 67 , 68 , 89 , 90 , 91 , 92 , 93 , 94 , 95 , 96 , 97 ] aimed to develop predictive equations for elderly people. A total of 358 males, 637 females, and 75 subjects without sex designated were included in 8 studies [ 35 , 40 , 41 , 56 , 64 , 65 , 98 , 99 ] aimed to develop predictive equations for people with diseases. The number of the studies for each geographical area us depicted in Fig. 2 for each of the considered populations (Fig. 3 ).
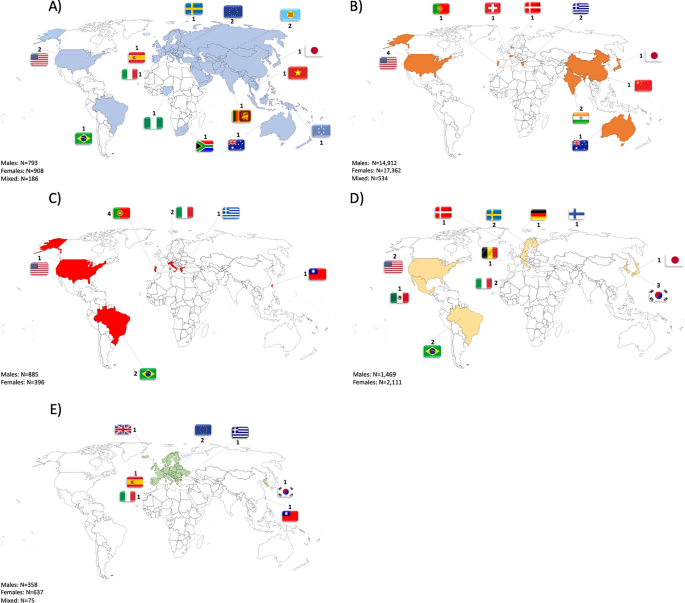
World map with number of included studies for under 18 years old subjects ( A ), adults ( B ), athletes ( C ), elderly ( D ), and people with disease ( E )
Bioelectrical impedance-based predictive models according to gender, populations, and technologies
Figure 4 schematizes the number of equations available for each BIA technology (e.g., hand to hand, leg to leg, foot to hand, and segmental) according to sex and population.
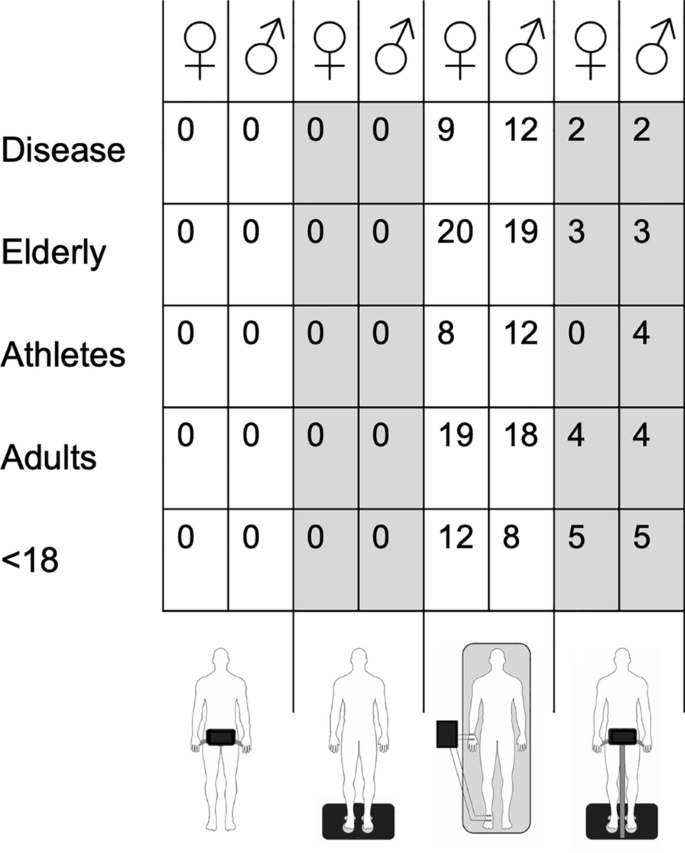
Number of predictive equations available for each BIA technology (e.g., hand to hand, leg to leg, foot to hand, and segmental) according to sex and population
The number of the predictive equations for each estimable body mass component, according to sex and population are shown in Figs. 5 and 6 , for the foot to hand and segmental technologies, respectively.
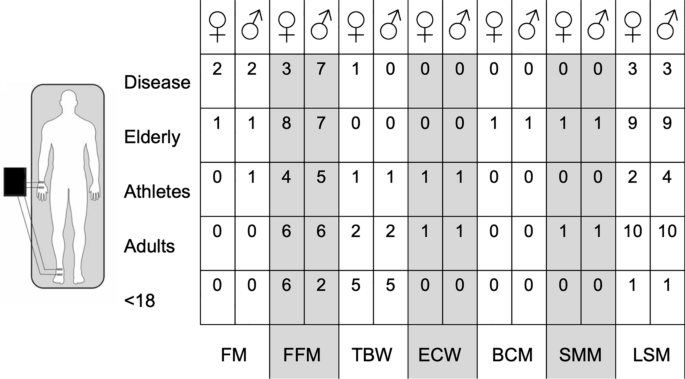
Number of the predictive equations for each estimable body mass component, according to sex and population for the foot to hand technology. FM fat mass, FFM fat-free mass, TBW total body water, ECW extracellular water, ECW extracellular water, BCM body cell mass, LST lean soft mass, SMM skeletal muscle mass
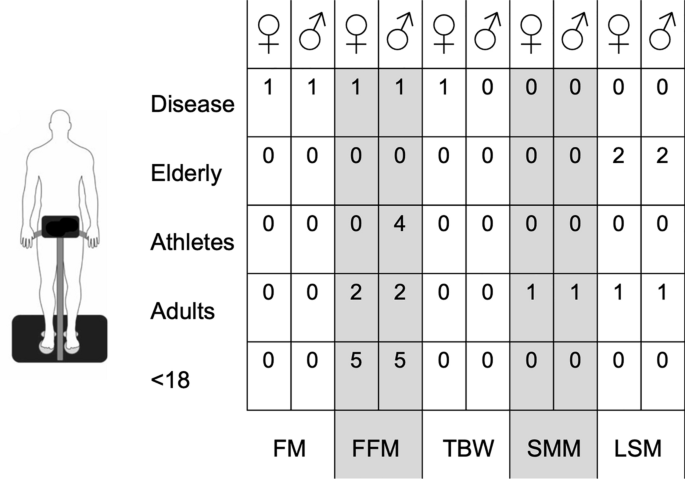
Number of the predictive equations for each estimable body mass component, according to sex and population for the segmental technology. FM fat mass, FFM fat-free mass, TBW total body water, LST lean soft mass, SMM skeletal muscle mass
Bioelectrical impedance-based equations for under 18 years old people
Table 1 reports the characteristics of the 19 predictive equations developed in 14 studies on subjects < 18 years old from the general populations. Fifteen predictive equations from 11 studies were developed with a SF-BIA, while four predictive equations from three studies were developed with MF-BIA using bioelectrical parameters measured at 50 kHz.
Bioelectrical impedance-based equations for adults from the general population
Table 2 reports the characteristics of the 26 predictive equations developed in 14 studies on subjects from the general populations. Twenty-two predictive equations from 12 studies were developed with a SF-BIA, while four predictive equations from two studies were developed with MF-BIA using bioelectrical parameters measured at 5, 50, and 250 kHz. Only one study provided a predictive equation using BIS at a frequency of 50 kHz.
Bioelectrical impedance-based equations for athletes
Table 3 reports the characteristics of the 19 predictive equations developed in 11 studies on athletes. Fifteen predictive equations from 10 studies were developed with a SF-BIA, while four predictive equations from one study were developed with MF-BIA using bioelectrical parameters measured at 50 kHz.
Bioelectrical impedance-based equations for elderly
Table 4 reports the characteristics of the 26 predictive equations developed in 17 studies on elderly subjects. Seventeen predictive equations from 11 studies were developed with a SF-BIA, while eight predictive equations from seven studies were developed with MF-BIA using bioelectrical parameters measured at 2, 50, and 250 kHz. One study provided a predictive equations using bioelectrical parameters measured with BIS using at a frequency of 5 kHz.
Bioelectrical impedance-based equations for people with diseases
Table 5 reports the characteristics of the 16 predictive equations developed in 8 studies on subjects with diseases. Three studies presented seven predictive equations using SF-BIA, while six predictive equations from two studies were developed with MF-BIA using bioelectrical parameters measured at 50 and 100 kHz. Three studies developed three predictive equation using BIS at a frequency of 50 kHz.
The present systematic review was designed to compile a list of BIA-based predictive equations for estimating body mass components in target populations. Specifically, we included only articles that developed new predictive models and excluded experimental designs that validated existing equations, mixed different populations, or used the two-component model as a reference for developing predictive equations. This resulted in 106 predictive equations that met high-standard procedures, organized based on the four BIA technologies (i.e., hand-to-hand, leg-to-leg, foot-to-hand, and segmental), the sampling frequency (i.e., single and multi-frequency) and five population categories (i.e., under 18 years old, adults, athletes, elderly, and people with diseases). Additionally, the geographical areas of the participants were identified. The present findings provide an updated starting point for researchers to identify potential gaps in the literature and develop further predictive equations. Moreover, this updated list facilitates the easy identification of the most accurate equations for specific populations and technologies, aiding practitioners in implementing best practices.
Nineteen predictive equations developed in 14 studies on subjects under 18 years old from general populations were included. Two of these studies were conducted in the USA, where no female participants were involved [ 55 , 76 ], while the remaining 12 studies were performed in different countries around the world with both sexes included [ 37 , 43 , 44 , 45 , 57 , 58 , 59 , 72 , 73 , 74 , 75 , 100 ]. Out of the 19 equations, 12 were developed using foot to hand technology [ 37 , 55 , 58 , 59 , 72 , 73 , 75 , 76 , 100 ] and seven equations were developed using segmental technology [ 43 , 44 , 45 , 57 , 74 ]. Concerning foot-to-hand technology, four equations are suitable only for females [ 55 , 76 ], while eight are suitable for both sexes [ 37 , 58 , 59 , 72 , 73 , 75 , 100 ]. Regarding segmental technology, five are suitable for both sexes [ 43 , 44 , 45 , 57 , 74 ]. Concerning foot-to-hand technology, four equations were found for estimating FFM in females only [ 55 , 76 ] and two for both sexes [ 59 , 73 ]. As for TBW, five equations are suitable for both sexes [ 37 , 58 , 72 , 75 , 100 ]. Considering segmental technology, five equations are available for estimating FFM in both sexes [ 43 , 44 , 45 , 57 , 74 ]. All 19 equations were developed at a single frequency of 50 kHz, although four predictive equations were developed with multifrequency devices but at the frequency of 50 kHz [ 43 , 44 , 57 ].
Bioelectrical impedance-based equations for adults
Twenty-six predictive equations developed in 14 studies on adults were reviewed. Although most of the studies (n = 4) were conducted in the USA [ 34 , 42 , 54 , 82 ], the largest participant number occurred in one Chinese study [ 81 ]. The remaining studies involved European [ 50 , 52 , 71 , 77 , 101 ], Eastern Asian [ 47 , 78 ], and Australian [ 66 ] populations with a balanced number of male and female participants. Out of the 26 available equations, 22 were developed with foot to hand technology [ 34 , 42 , 47 , 50 , 52 , 54 , 61 , 66 , 71 , 77 , 78 , 102 ], and four with segmental technology Concerning foot to hand technology, 17 equations are available for both sexes [ 42 , 47 , 52 , 54 , 61 , 66 , 71 , 77 , 102 ], while one is suitable for males [ 78 ] and two are suitable for females only [ 34 , 50 ]. As for segmental technology, four equations are suitable for both sexes [ 80 , 81 ]. Regarding foot-to-hand technology, five equations are suitable for estimating FFM in males [ 47 , 52 , 66 , 71 , 78 ] and six in females [ 34 , 47 , 50 , 52 , 66 , 71 ]. Two equations are suitable for estimating TBW [ 42 , 54 ], ten for estimating LSM [ 61 , 77 ], and one for estimating SMM [ 82 ] in both sexes. Particularly, the equations predicting LSM were developed for the appendicular [ 61 , 77 ] and trunk [ 61 ] body segments. Considering segmental technology, two equations for estimating FFM [ 81 ], one equation for estimating SMM [ 80 ], and one equation for estimating LSM [ 81 ] are available for males and females. Out of the equations for predicting FFM or LSM, both estimate the appendicular mass only [ 81 ]. All these equations were developed at a single frequency of 50 kHz, with the exception of four equations [ 66 , 81 ] developed with a multifrequency devices that used bioelectrical parameters measured only at 50 kHz [ 66 ], as well as mixing frequencies sampled at 50 and 250 kHz [ 81 ].
Nineteen predictive equations developed in 11 studies on athletes were included. Four studies involved Portuguese male and female participants from different sport disciplines [ 39 , 69 , 84 , 87 ], while two studies recruited male Brazilian army cadets [ 85 ] considered as very active individuals [ 103 ] and adolescents from various sport disciplines [ 104 ]. One study was conducted on USA collegiate female athletes from different sports [ 62 ], one on Greek elite female dancers [ 51 ], and two studies on elite male soccer players in Taiwan [ 88 ] and Italy [ 31 ]. One study con considered Italian paddle players [ 48 ]. Out of the 19 available equations, 15 were developed with foot to hand technology [ 31 , 39 , 48 , 51 , 62 , 69 , 84 , 85 , 87 , 104 ], while four equations were developed using segmental technology [ 88 ]. Considering the foot to hand technology, 12 equations are suitable for male [ 31 , 39 , 48 , 69 , 84 , 85 , 87 , 104 ] and eight for female people only [ 39 , 51 , 62 , 69 , 87 , 104 ]. As for segmental technology, four equations are specific for males [ 88 ], and no equation is available for females. Regarding foot to hand technology, we found an equation for estimating FM in males [ 48 ], five equations for estimating FFM in males [ 31 , 69 , 84 , 85 , 104 ], and four in females only [ 51 , 62 , 69 , 104 ]. Moreover, we retrieved one equation for estimating TBW [ 39 ], one for estimating ECW [ 39 ] and five for estimating LSM [ 31 , 87 ], where three of them are suitable for both sexes [ 39 , 87 ], and two in male only [ 31 ]. As for segmental technology, four equations for estimating whole-body, upper-limb, lower-limb, and trunk FFM are available for males only [ 88 ]. All these equations were developed at a single frequency of 50 kHz, even those developed using a multifrequency device [ 88 ].
Twenty-six predictive equations developed in 17 studies on the elderly were included. The selected studies encompassed Central[ 36 , 38 , 49 ] and Southern American [ 46 , 67 ], European [ 53 , 63 , 89 , 90 , 91 , 93 , 95 , 97 ], and Asian [ 68 , 94 ] male and female participants. Out of the 26 available equations, 23 were developed with foot to hand technology [ 36 , 38 , 46 , 49 , 53 , 63 , 67 , 89 , 90 , 91 , 92 , 93 , 95 , 97 ], while three equations were developed using segmental technology [ 68 , 94 ]. Concerning foot-to-hand technology, three predictive equations were suitable for males [ 36 , 49 , 53 ] and four for females only [ 36 , 49 , 53 , 97 ], while thirteen equations suitable for both sexes [ 38 , 46 , 63 , 67 , 89 , 90 , 91 , 92 , 93 , 95 ]. Two different equations are available to determine FM in males and females [ 53 ], while there are two equations for assessing FFM in males [ 36 , 49 ], three in females only [ 36 , 49 , 97 ], and five suitable for both sexes [ 89 , 91 ]. There is one equation for estimating BCM [ 63 ], four equations for assessing appendicular LSM [ 46 , 67 , 92 , 93 ], and one equation for assessing SMM [ 95 ] suitable for males and females. As for the segmental technology, only the appendicular LSM is currently possible to be determined using two equations suitable for both sexes [ 68 , 94 ]. Sixteen equations were developed using single frequency devices at 50 kHz [ 36 , 38 , 46 , 49 , 53 , 63 , 67 , 89 , 90 , 91 , 97 ], while the equations developed with multifrequency devices were obtained measuring the bioelectrical parameters at 5 kHz [ 95 ], 50 kHz [ 93 , 94 ], 250 kHz [ 92 ], or 50 and 250 kHz [ 68 ].
Sixteen predictive equations developed in eight studies on people with diseases were included. The selected studies included European [ 35 , 40 , 56 , 64 , 65 , 98 ] and Asian [ 41 , 99 ] male and female participants. Out of the 16 available equations, 14 were developed with foot to hand technology [ 40 , 64 , 98 ] and eight using segmental technology [ 40 , 41 , 56 , 64 , 65 , 98 , 99 ]. Regarding the foot to hand technology, there is one equation suitable for assessing FFM in overweight and obese postmenopausal females [ 98 ], four for appendicular FM and FFM in functionally limited male and female individuals [ 40 ], four for assessing FFM in amputee males [ 41 ], one for predicting TBW in anorexic females [ 56 ], and three for appendicular LSM in frail [ 64 ], chronic kidney [ 65 ], or hemodialysis male and female subjects [ 99 ]. Seven equations were developed using single frequency devices at 50 kHz [ 35 , 40 , 98 ], while 9 equations were made with multifrequency devices involving measures obtained at 50 [ 41 , 64 , 65 , 99 ] and 100 kHz [ 56 ]. However, the use of BIA in individuals with underlying medical conditions necessitates a thorough consideration of the reliability features inherent in the measurements. This is imperative due to the heightened likelihood of fluctuations in water content, which may manifest more frequently in this cohort compared to other populations [ 105 ].
Limitations of the review and future perspectives
One limitation of the current manuscript may concern the grouping of predictive equations based on the BIA technology, since different devices using the same technology may yield different outcomes due to potential between-device inconsistencies [ 106 ], even though agreement has been observed [ 21 ]. Therefore, new comparisons between different devices of the same technology should be conducted. Other considerations could be made, not merely as limitations of the present manuscript but intrinsic to BIA. First, considering the lack of agreement between bioelectrical values obtained at different frequencies [ 21 , 107 ], the sampling frequency information has been reported for each of the included studies. In this regard, future studies should clarify the advantages of using multi-frequency devices given that most predictive equations predominantly include measures obtained at a single frequency of 50 kHz. Second, the procedures for BIA were updated in 2004 [ 15 ] and further in 2020 for athletes [ 2 ]. Therefore, inconsistencies in methodological procedures (e.g., the standardization of food and beverage intake before the assessment, electrode placement, skin cleansing) may have impacted the final outcomes for each study [ 108 ]. Third, the standard error is often reported as absolute values of body mass, and this does not take into account how much each error is related to the total body mass. For example, a standard error of 1.5 kg in a female population with a mean FFM equal to 50 kg is less precise than in men with a mean FFM equal to 80 kg. Fourth, even though some variables are not directly estimated by the BIA (e.g., FM and ICW), these can be easily derived as the difference between the body mass and the FFM (for FM) or the total water and the ECW (for ICW). Lastly, the utilization of multicomponent models to estimate body composition may be susceptible to error propagation, stemming from the use of multiple methods for reference body composition values. A procedure for identifying the most valid equation for predicting body composition is illustrated in Fig. 7 . There is reason to believe that such procedures will be considered in the development of AI-based software systems in the future.
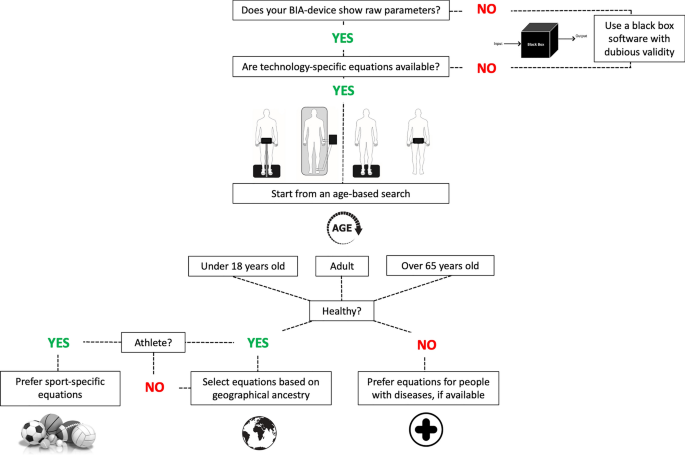
Procedures for accurately selecting predictive equations for assessing body composition using the bioelectrical impedance analysis
Conclusions
Numerous predictive equations for quantifying body composition with BIA have been developed using high-standard procedures. However, upon categorizing these equations based on BIA technology, target population, and estimable body mass components, the overall availability of predictive equations seems limited. Notably, no predictive equations for hand to hand and leg to leg technology are included here, implying that these BIA devices predominantly employ procedures of low quality, often unknown, and lacking scrutiny, with equations typically owned by manufacturers. In contrast, there are several predictive equations available for foot to hand and segmental technology. However, the accuracy of estimating certain body components is compromised due to the absence of population-specific predictive equations. Given the necessity for reference values and BIA-based predictive equations to be population- and technology-specific, the current findings underscore the urgency of developing new predictive equations tailored to specific BIA technology, population characteristics, and body mass components. Nevertheless, the foot to hand technology stands out with the highest number of predictive equations, offering the most accurate estimation of body composition. That said, the predictive equations available so far do not cover all possible combinations of technology, population and body mass component. Hence, the present manuscript may be helpful to pinpoint what has been done so far and what is currently lacking, so to generate novel predictive equations in cases not covered by the present literature. This may help to increase the trust of the practitioners in the BIA, often doubtful about the goodness of the results provided by inaccurate equations included in the device. Practitioners now possess an updated list of predictive equations for assessing body composition.
Availability of data and materials
No new data were created or analyzed in this study. Data sharing is not applicable to this article.
Hume PA, Ackland T. Chapter 3—physical and clinical assessment of nutritional status. In: Coulston AM, Boushey CJ, Ferruzzi MG, Delahanty E, editors. LMBT-N in the P and T of D, Academic Press; 2017. p. 71–84. https://www.sciencedirect.com/science/article/pii/B9780128029282000035 .
Campa F, Toselli S, Mazzilli M, Gobbo LA, Coratella G. Assessment of body composition in athletes: a narrative review of available methods with special reference to quantitative and qualitative bioimpedance analysis. Nutrients. 2021;13:1620.
Article PubMed PubMed Central Google Scholar
Wang Z, Pi-Sunyer FX, Kotler DP, Wielopolski L, Withers RT, Pierson RNJ, et al. Multicomponent methods: evaluation of new and traditional soft tissue mineral models by in vivo neutron activation analysis. Am J Clin Nutr. 2002;76:968–74.
Article CAS PubMed Google Scholar
Wang ZM, Pierson RNJ, Heymsfield SB. The five-level model: a new approach to organizing body-composition research. Am J Clin Nutr. 1992;56:19–28.
Heymsfield S, Lohman T, Wang Z, Going S. Human body composition, 2nd edn. Leeds in Champaign, IL: Human Kinetics; 2005.
Book Google Scholar
Lohman TG, Milliken LA. ACSM’s body composition assessment. Human Kinetics: Leeds; 2020.
Google Scholar
Heymsfield SB, Ebbeling CB, Zheng J, Pietrobelli A, Strauss BJ, Silva AM, et al. Multi-component molecular-level body composition reference methods: evolving concepts and future directions. Obes Rev England. 2015;16:282–94.
Article CAS Google Scholar
Pietrobelli A, Heymsfield SB, Wang ZM, Gallagher D. Multi-component body composition models: recent advances and future directions. Eur J Clin Nutr. 2001. https://doi.org/10.1038/sj.ejcn.1601105 .
Article PubMed Google Scholar
Vazquez JA, Janosky JE. Validity of bioelectrical-impedance analysis in measuring changes in lean body mass during weight reduction. Am J Clin Nutr. 1991;54:970–5.
Segal KR, Van Loan M, Fitzgerald PI, Hodgdon JA, Van Itallie TB. Lean body mass estimation by bioelectrical impedance analysis: a four-site cross-validation study. Am J Clin Nutr. 1988;47:7–14.
Deurenberg P, Kusters CS, Smit HE. Assessment of body composition by bioelectrical impedance in children and young adults is strongly age-dependent. Eur J Clin Nutr. 1990;44:261–8.
CAS PubMed Google Scholar
Francisco R, Jesus F, Nunes CL, Cioffi I, Alvim M, Mendonca GV, et al. Athletes with different habitual fluid intakes differ in hydration status but not in body water compartments. Scand J Med Sci Sports. 2023;33:1072–8.
Campa F, Coratella G, Cerullo G, Stagi S, Paoli S, Marini S, et al. New bioelectrical impedance vector references and phase angle centile curves in 4,367 adults: the need for an urgent update after 30 years. Clin Nutr. 2023;42:1749–58.
Lukaski H, Raymond-Pope CJ. New frontiers of body composition in sport. Int J Sports Med. 2021;42(07):588–601. https://doi.org/10.1055/a-1373-5881 .
Kyle UG, Bosaeus I, De Lorenzo AD, Deurenberg P, Elia M, Manuel Gomez J, et al. Bioelectrical impedance analysis-part II: utilization in clinical practice. Clin Nutr. 2004;23:1430–53.
Petri C, Campa F, Holway F, Pengue L, Arrones LS. ISAK-based anthropometric standards for elite male and female soccer players. Sports. 2024;12(3):69. https://doi.org/10.3390/sports12030069 .
Kyle UG, Bosaeus I, De Lorenzo AD, Deurenberg P, Elia M, Gomez JM, et al. Bioelectrical impedance analysis–part I: review of principles and methods. Clin Nutr. 2004;23:1226–43.
Francisco R, Jesus F, Nunes CL, Carvalho A, Alvim M, Campa F, et al. Prediction of body water compartments by raw bioelectrical impedance parameters in athletes: comparison between series and parallel measurements. Scand J Med Sci Sports. 2023;33:1998–2008.
Lukaski H, Bolonchuk W. Theory and validation of the tetrapolar bioelectrical impedance method to assess human body composition. Med Sci Sports Exerc. 1987. https://doi.org/10.1249/00005768-198604001-00079 .
Article Google Scholar
Moon JR. Body composition in athletes and sports nutrition: an examination of the bioimpedance analysis technique. Eur J Clin Nutr. 2013;67(S1):S54–9. https://doi.org/10.1038/ejcn.2012.165 .
Dellinger JR, Johnson BA, Benavides ML, Moore ML, Stratton MT, Harty PS, et al. Agreement of bioelectrical resistance, reactance, and phase angle values from supine and standing bioimpedance analyzers. Physiol Meas. 2021. https://doi.org/10.1088/1361-6579/abe6fa .
Stratton MT, Smith RW, Harty PS, Rodriguez C, Johnson BA, Dellinger JR, et al. Longitudinal agreement of four bioimpedance analyzers for detecting changes in raw bioimpedance during purposeful weight gain with resistance training. Eur J Clin Nutr. 2021;75:1060–8.
Tinsley GM, Moore ML, Silva AM, Sardinha LB. Cross-sectional and longitudinal agreement between two multifrequency bioimpedance devices for resistance, reactance, and phase angle values. Eur J Clin Nutr. 2020;74:900–11.
Campa F, Gobbo LA, Stagi S, Cyrino LT, Toselli S, Marini E, Coratella G. Bioelectrical impedance analysis versus reference methods in the assessment of body composition in athletes. Eur J Appl Physiol. 2022;122(3):561–89. https://doi.org/10.1007/s00421-021-04879-y .
Campa F, Matias CN, Moro T, Cerullo G, Casolo A, Teixeira FJ, Paoli A. Methods over materials: the need for sport-specific equations to accurately predict fat mass using bioimpedance analysis or anthropometry. Nutrients. 2023;15(2):278. https://doi.org/10.3390/nu15020278 .
Coratella G, Campa F, Matias C, Toselli S, Koury J, Andreoli A, et al. Generalized bioelectric impedance-based equations underestimate body fluids in athletes. Scand J Med Sci Sport. 2021;31:2123–32.
Campa F, Matias CN, Teixeira FJ, Reis JF, Valamatos MJ, Coratella G, et al. Comparison of generalized and athletic bioimpedance-based predictive equations for estimating fat-free mass in resistance-trained exercisers. Nutrition. 2022;102: 111694.
Amaral MA, Mundstock E, Scarpatto CH, Cañon-Montañez W, Mattiello R. Reference percentiles for bioimpedance body composition parameters of healthy individuals: a cross-sectional study. Clinics (Sao Paulo). 2022;77: 100078.
Siedler MR, Rodriguez C, Stratton MT, Harty PS, Keith DS, Green JJ, et al. Assessing the reliability and cross-sectional and longitudinal validity of fifteen bioelectrical impedance analysis devices. Br J Nutr. 2023;130:827–40.
Toselli S, Campa F, Latessa PM, Greco G, Loi A, Grigoletto A, Zaccagni L. Differences in maturity and anthropometric and morphological characteristics among young male basketball and soccer players and non-players. Int J Environ Res Public Health. 2021;18(8):3902. https://doi.org/10.3390/ijerph18083902 .
Campa F, Bongiovanni T, Rossi A, Cerullo G, Casolo A, Martera G, et al. Athletic bioimpedance-based equations underestimate fat free mass components in male elite soccer players: development and validation of new soccer-specific predictive models. J Transl Med. 2023;21:912.
Page MJ, McKenzie JE, Bossuyt PM, Boutron I, Hoffmann TC, Mulrow CD, et al. The PRISMA 2020 statement: an updated guideline for reporting systematic reviews. BMJ. 2021;372: n71.
Whiting PF. QUADAS-2: a revised tool for the quality assessment of diagnostic accuracy studies. Ann Internal Med. 2011;155(8):529. https://doi.org/10.7326/0003-4819-155-8-201110180-00009 .
Jakicic JM, Wing RR, Lang W. Bioelectrical impedance analysis to assess body composition in obese adult women: the effect of ethnicity. Int J Obes Relat Metab Disord J Int Assoc Study Obes. 1998;22:243–9.
Jiménez A, Omaña W, Flores L, Coves MJ, Bellido D, Perea V, et al. Prediction of whole-body and segmental body composition by bioelectrical impedance in morbidly obese subjects. Obes Surg. 2012;22:587–93.
Roubenoff R, Baumgartner RN, Harris TB, Dallal GE, Hannan MT, Economos CD, et al. Application of bioelectrical impedance analysis to elderly populations. J Gerontol A Biol Sci Med Sci. 1997;52:M129–36.
Leman CR, Adeyemo AA, Schoeller DA, Cooper RS, Luke A. Body composition of children in south-western Nigeria: validation of bio-electrical impedance analysis. Ann Trop Paediatr. 2003;23:61–7.
Rangel Peniche DB, Raya Giorguli G, Alemán-Mateo H. Accuracy of a predictive bioelectrical impedance analysis equation for estimating appendicular skeletal muscle mass in a non-Caucasian sample of older people. Arch Gerontol Geriatr. 2015;61:39–43.
Matias CN, Santos DA, Júdice PB, Magalhães JP, Minderico CS, Fields DA, et al. Estimation of total body water and extracellular water with bioimpedance in athletes: a need for athlete-specific prediction models. Clin Nutr. 2016;35:468–74.
Scafoglieri A, Clarys JP, Bauer JM, Verlaan S, Van Malderen L, Vantieghem S, et al. Predicting appendicular lean and fat mass with bioelectrical impedance analysis in older adults with physical function decline—The PROVIDE study. Clin Nutr. 2017;36:869–75.
Choi H-J, Ko C-Y, Chang Y, Kim G-S, Choi K, Kim C-H. Development and validation of bioimpedance prediction equations for fat-free mass in unilateral male amputees. PeerJ. 2021;9: e10970.
Lukaski HC, Bolonchuk WW. Estimation of body fluid volumes using tetrapolar bioelectrical impedance measurements. Aviat Space Environ Med. 1988;59:1163–9.
van Zyl A, White Z, Ferreira J, Wenhold FAM. Developing an impedance based equation for fat-free mass of black preadolescent south African children. Nutrients. 2019;11(9):2021. https://doi.org/10.3390/nu11092021 .
Article CAS PubMed PubMed Central Google Scholar
Nguyen PH, Young MF, Khuong LQ, Ramakrishnan U, Martorell R, Hoffman DJ. Development of population-specific prediction equations for bioelectrical impedance analyses in Vietnamese children. Br J Nutr. 2020;124:1345–52.
Gutiérrez-Marín D, Escribano J, Closa-Monasterolo R, Ferré N, Venables M, Singh P, et al. Validation of bioelectrical impedance analysis for body composition assessment in children with obesity aged 8–14y. Clin Nutr. 2021;40:4132–9.
Toselli S, Campa F, Matias CN, de Alencar Silva BS, Dos Santos VR, Maietta Latessa P, et al. Predictive equation for assessing appendicular lean soft tissue mass using bioelectric impedance analysis in older adults: effect of body fat distribution. Exp Gerontol. 2021;150: 111393.
Rush EC, Chandu V, Plank LD. Prediction of fat-free mass by bioimpedance analysis in migrant Asian Indian men and women: a cross validation study. Int J Obes (Lond). 2006;30:1125–31.
Mauro M, Toselli S, De Giorgi S, Heinrich KM, Di Gioia G, Moretti B, et al. New regression models to predict fat mass in intermediate-level male padel players. Heliyon. 2023;9: e18719.
Williams DP, Going SB, Milliken LA, Hall MC, Lohman TG. Practical techniques for assessing body composition in middle-aged and older adults. Med Sci Sports Exerc. 1995;27:776–83.
Kontogianni MD, Panagiotakos DB, Skopouli FN. Does body mass index reflect adequately the body fat content in perimenopausal women? Maturitas. 2005;51:307–13.
Yannakoulia M, Keramopoulos A, Tsakalakos N, Matalas AL. Body composition in dancers: the bioelectrical impedance method. Med Sci Sports Exerc. 2000;32:228–34.
Heitmann BL. Evaluation of body fat estimated from body mass index, skinfolds and impedance. A comparative study. Eur J Clin Nutr. 1990;44:831–7.
Svendsen OL, Haarbo J, Heitmann BL, Gotfredsen A, Christiansen C. Measurement of body fat in elderly subjects by dual-energy x-ray absorptiometry, bioelectrical impedance, and anthropometry. Am J Clin Nutr. 1991;53(5):1117–23. https://doi.org/10.1093/ajcn/53.5.1117 .
Zillikens MC, Conway JM. Estimation of total body water by bioelectrical impedance analysis in blacks. Am J Hum Biol. 1991;3:25–32.
Guo SS, Khoury PR, Specker B, Heubi J, Chumlea WC, Siervogel RM, et al. Prediction of fat-free mass in black and white pre-adolescent and adolescent girls from anthropometry and impedance. Am J Hum Biol. 1993;5:735–45.
Scalfi L, Bedogni G, Marra M, Di Biase G, Caldara A, Severi S, et al. The prediction of total body water from bioelectrical impedance in patients with anorexia nervosa. Br J Nutr. 1997;78:357–65.
Pietrobelli A, Andreoli A, Cervelli V, Carbonelli MG, Peroni DG, De Lorenzo A. Predicting fat-free mass in children using bioimpedance analysis. Acta Diabetol. 2003;40(Suppl 1):S212–5.
Masuda T, Komiya S. A prediction equation for total body water from bioelectrical impedance in Japanese children. J Physiol Anthropol Appl Human Sci. 2004;23:35–9.
Nielsen BM, Dencker M, Ward L, Linden C, Thorsson O, Karlsson MK, et al. Prediction of fat-free body mass from bioelectrical impedance among 9- to 11-year-old Swedish children. Diabetes Obes Metab. 2007;9:521–39.
Kim H, Song K-H, Ambegaonkar JP, Chung S, Jeon K, Jiang FL, et al. Two-megahertz impedance index prediction equation for appendicular lean mass in Korean older people. BMC Geriatr. 2022;22:385.
Sardinha LB, Rosa GB, Hetherington-Rauth M, Correia IR, Magalhães JP, Silva AM, et al. Development and validation of bioelectrical impedance prediction equations estimating regional lean soft tissue mass in middle-aged adults. Eur J Clin Nutr. 2023;77:202–11. https://doi.org/10.1038/s41430-022-01224-0 .
Fornetti WC, Pivarnik JM, Foley JM, Fiechtner JJ. Reliability and validity of body composition measures in female athletes. J Appl Physiol. 1999;87:1114–22.
Dittmar M, Reber H. New equations for estimating body cell mass from bioimpedance parallel models in healthy older Germans. Am J Physiol Endocrinol Metab. 2001;281:E1005–14.
van Baar H, Hulshof PJM, Tieland M, de Groot CPGM. Bio-impedance analysis for appendicular skeletal muscle mass assessment in (pre-) frail elderly people. Clin Nutr ESPEN. 2015;10:e147–53.
Macdonald JH, Marcora SM, Jibani M, Roberts G, Kumwenda MJ, Glover R, et al. Bioelectrical impedance can be used to predict muscle mass and hence improve estimation of glomerular filtration rate in non-diabetic patients with chronic kidney disease. Nephrol Dial Transplant. 2006;21:3481–7.
Hughes JT, Maple-Brown LJ, Piers LS, Meerkin J, O’Dea K, Ward LC. Development of a single-frequency bioimpedance prediction equation for fat-free mass in an adult Indigenous Australian population. Eur J Clin Nutr. 2015;69:28–33.
Barbosa-Silva TG, Gonzalez MC, Bielemann RM, Santos LP, Menezes AMB. Think globally, act locally: the importance of population-specific bioelectrical impedance analysis prediction equations for muscle mass assessment. JPEN J Parenter Enteral Nutr. 2020;44:1338–46.
Jeon KC, Kim S-Y, Jiang FL, Chung S, Ambegaonkar JP, Park J-H, Kim Y-J, Kim C-H. Prediction equations of the multifrequency standing and supine bioimpedance for appendicular skeletal muscle mass in Korean older people. Int J Environ Res Public Health. 2020;17(16):5847. https://doi.org/10.3390/ijerph17165847 .
Matias CN, Campa F, Santos DA, Lukaski H, Sardinha LB, Silva AM. Fat-free mass bioelectrical impedance analysis predictive equation for athletes using a 4-compartment model. Int J Sports Med. 2021;42:27–32.
Kourkoumelis N, Grujic VR, Grabez M, Vidic A, Siksna I, Lazda I, et al. New bioelectrical impedance analysis equations for children and adolescents based on the deuterium dilution technique. Clin Nutr. 2021;44:402–9.
Kanellakis S, Skoufas E, Karaglani E, Ziogos G, Koutroulaki A, Loukianou F, et al. Development and validation of a bioelectrical impedance prediction equation estimating fat free mass in Greek - Caucasian adult population. Clin Nutr ESPEN. 2020;36:166–70.
Wickramasinghe VP, Lamabadusuriya SP, Cleghorn GJ, Davies PSW. Assessment of body composition in Sri Lankan children: validation of a bioelectrical impedance prediction equation. Eur J Clin Nutr. 2008;62:1170–7. https://doi.org/10.1038/sj.ejcn.1602835 .
da Costa RF, Silva AM, Masset KVSB, Cesário TM, Cabral BGAT, Ferrari G, et al. Development and cross-validation of a predictive equation for fat-free mass in Brazilian adolescents by bioelectrical impedance. Front Nutr. 2022;9: 820736.
Sluyter JD, Schaaf D, Scragg RKR, Plank LD. Prediction of fatness by standing 8-electrode bioimpedance: a multiethnic adolescent population. Obesity (Silver Spring). 2010;18:183–9.
Kourkoumelis N, Grujic VR, Grabez M, Vidic A, Siksna I, Lazda I, et al. New bioelectrical impedance analysis equations for children and adolescents based on the deuterium dilution technique. Clin Nutr ESPEN. 2021;44:402–9.
Morrison JA, Guo SS, Specker B, Chumlea WC, Yanovski SZ, Yanovski JA. Assessing the body composition of 6–17-year-old Black and White girls in field studies. Am J Hum Biol. 2001;13:249–54.
Kyle UG, Genton L, Hans D, Pichard C. Validation of a bioelectrical impedance analysis equation to predict appendicular skeletal muscle mass (ASMM). Clin Nutr. 2003;22:537–43.
Dasgupta R, Anoop S, Samuel P, Kurian ME, Inbakumari M, Finney G, et al. Bioimpedance analysis with a novel predictive equation—a reliable technique to estimate fat free mass in birth weight based cohorts of Asian Indian males. Diabetes Metab Syndr. 2019;13:738–42.
López-Taylor JR, González-Mendoza RG, Gaytán-González A, Jiménez-Alvarado JA, Villegas-Balcázar M, Jáuregui-Ulloa EE, et al. Accuracy of anthropometric equations for estimating body fat in professional male soccer players compared with DXA. J Sport Med. 2018;2018:6843792.
Oshima Y, Shiga T, Namba H, Kuno S. Estimation of whole-body skeletal muscle mass by bioelectrical impedance analysis in the standing position. Obes Res Clin Pract. 2010;4:e1-82.
Xu H-Q, Liu J-M, Zhang X, Xue Y-T, Shi J-P, Chen W, et al. Estimation of skeletal muscle mass by bioimpedance and differences among skeletal muscle mass indices for assessing sarcopenia. Clin Nutr. 2021;40:2308–18.
Janssen I, Heymsfield SB, Wang ZM, Ross R. Skeletal muscle mass and distribution in 468 men and women aged 18–88 yr. J Appl Physiol. 2000;89:81–8.
Silva AM, Campa F, Stagi S, et al. The bioelectrical impedance analysis (BIA) international database: aims, scope, and call for data. Eur J Clin Nutr. 2023;77(12):1143-1150. https://doi.org/10.1038/s41430-023-01310-x
Matias CN, Campa F, Cavaca M, Paoli A, Teixeira FJ. Fat-free mass estimation in male elite futsal players: Development and validation of a new bioelectrical impedance-based predictive equation. Nutrition. 2023;107:111931. https://doi.org/10.1016/j.nut.2022.111931
Langer RD, Borges JH, Pascoa MA, Cirolini VX, Guerra-Júnior G, Gonçalves EM. Validity of bioelectrical impedance analysis to estimation fat-free mass in the army cadets. Nutrients. 2016;8:121.
Koury JC, de Oliveira-Junior AV, Portugal MRC, de Oliveira KJF, Donangelo CM. Bioimpedance parameters in adolescent athletes in relation to bone maturity and biochemical zinc indices. J Trace Elem Med Biol. 2018;46:26–31.
Sardinha LB, Correia IR, Magalhães JP, Júdice PB, Silva AM, Hetherington-Rauth M. Development and validation of BIA prediction equations of upper and lower limb lean soft tissue in athletes. Eur J Clin Nutr. 2020;74:1646–52.
Chao J-J, Kao M-F, Chuang C-L, Lu H-K, Wu MC, Chen Y-Y, et al. The bioelectrical impedance analysis with newly predictive equations for measuring segments body composition of elite male football players in Taiwan. 2011. Available from: https://api.semanticscholar.org/CorpusID:110303696
Dey DK, Bosaeus I, Lissner L, Steen B. Body composition estimated by bioelectrical impedance in the Swedish elderly. Development of population-based prediction equation and reference values of fat-free mass and body fat for 70- and 75-y olds. Eur J Clin Nutr. 2003;57(8):909–16. https://doi.org/10.1038/sj.ejcn.1601625 .
Sergi G, De Rui M, Veronese N, Bolzetta F, Berton L, Carraro S, et al. Assessing appendicular skeletal muscle mass with bioelectrical impedance analysis in free-living Caucasian older adults. Clin Nutr. 2015;34:667–73.
De Rui M, Veronese N, Bolzetta F, Berton L, Carraro S, Bano G, et al. Validation of bioelectrical impedance analysis for estimating limb lean mass in free-living Caucasian elderly people. Clin Nutr. 2017;36:577–84.
Kim JH, Choi SH, Lim S, Kim KW, Lim JY, Cho NH, et al. Assessment of appendicular skeletal muscle mass by bioimpedance in older community-dwelling Korean adults. Arch Gerontol Geriatr. 2014;58:303–7.
Vermeiren S, Beckwée D, Vella-Azzopardi R, Beyer I, Knoop V, Jansen B, et al. Evaluation of appendicular lean mass using bio impedance in persons aged 80+: a new equation based on the BUTTERFLY-study. Clin Nutr. 2019;38:1756–64.
Yoshida D, Shimada H, Park H, Anan Y, Ito T, Harada A, et al. Development of an equation for estimating appendicular skeletal muscle mass in Japanese older adults using bioelectrical impedance analysis. Geriatr Gerontol Int. 2014;14:851–7.
Tengvall M, Ellegård L, Malmros V, Bosaeus N, Lissner L, Bosaeus I. Body composition in the elderly: reference values and bioelectrical impedance spectroscopy to predict total body skeletal muscle mass. Clin Nutr. 2009;28:52–8.
Williams CA, Bale P. Bias and limits of agreement between hydrodensitometry, bioelectrical impedance and skinfold calipers measures of percentage body fat. Eur J Appl Physiol Occup Physiol. 1998;77:271–7.
Haapala I, Hirvonen A, Niskanen L, Uusitupa M, Kröger H, Alhava E, et al. Anthropometry, bioelectrical impedance and dual-energy X-ray absorptiometry in the assessment of body composition in elderly Finnish women. Clin Physiol Funct Imaging. 2002;22:383–91.
Kanellakis S, Kourlaba G, Moschonis G, Vandorou A, Manios Y. Development and validation of two equations estimating body composition for overweight and obese postmenopausal women. Maturitas. 2010;65:64–8.
Lin T-Y, Wu M-Y, Chen H-S, Hung S-C, Lim P-S. Development and validation of a multifrequency bioimpedance spectroscopy equation to predict appendicular skeletal muscle mass in hemodialysis patients. Clin Nutr. 2021;40:3288–95.
Liu A, Byrne NM, Ma G, Nasreddine L, Trinidad TP, Kijboonchoo K, et al. Validation of bioelectrical impedance analysis for total body water assessment against the deuterium dilution technique in Asian children. Eur J Clin Nutr. 2011;65:1321–7.
Sardinha LB, Rosa GB, Hetherington-Rauth M, Correia IR, Magalhães JP, Silva AM, et al. Development and validation of bioelectrical impedance prediction equations estimating regional lean soft tissue mass in middle-aged adults. Eur J Clin Nutr. 2023;77:202–11.
Janssen I, Heymsfield SB, Baumgartner RN, Ross R. Estimation of skeletal muscle mass by bioelectrical impedance analysis. J Appl Physiol. 2000;89:465–71.
Campa F, Toselli S, Mazzilli M, Gobbo LA, Coratella G. Assessment of Body Composition in Athletes: A Narrative Review of Available Methods with Special Reference to Quantitative and Qualitative Bioimpedance Analysis. Nutrients. 2021;13(5):1620. https://doi.org/10.3390/nu13051620
Koury JC, Ribeiro MA, Massarani FA, Vieira F, Marini E. Fat-free mass in adolescent athletes: accuracy of bioimpedance equations and identification of new predictive equations. Nutrition. 2019;60:59–65.
Piccoli A, Codognotto M, Piasentin P, Naso A. Combined evaluation of nutrition and hydration in dialysis patients with bioelectrical impedance vector analysis (BIVA). Clin Nutr. 2014;33:673–7.
Ræder H, Kværner AS, Henriksen C, Florholmen G, Henriksen HB, Bøhn SK, et al. Validity of bioelectrical impedance analysis in estimation of fat-free mass in colorectal cancer patients. Clin Nutr. 2018;37:292–300.
Silva AM, Matias CN, Nunes CL, Santos DA, Marini E, Lukaski HC, et al. Lack of agreement of in vivo raw bioimpedance measurements obtained from two single and multi-frequency bioelectrical impedance devices. Eur J Clin Nutr. 2019;73:1077–83.
Campa F, Gatterer H, Lukaski H, Toselli S. Stabilizing bioimpedance-vector-analysis measures with a 10-minute cold shower after running exercise to enable assessment of body hydration. Int J Sports Physiol Performance. 2019;14(7):1006–9. https://doi.org/10.1123/ijspp.2018-0676 .
Download references
Acknowledgements
We thank the corresponding authors of the studies included in the systematic review for providing the data where not directly available.
Open access funding provided by Università degli Studi di Padova. No funding was received to assist with the preparation of this manuscript.
Author information
F. Campa and G. Coratella contributed equally to this work.
Authors and Affiliations
Department of Biomedical Sciences, University of Padua, Padua, Italy
Francesco Campa, Giuseppe Cerullo, Davide Charrier & Antonio Paoli
Department of Biomedical Sciences for Health, Università degli Studi di Milano, Milan, Italy
Giuseppe Coratella
NEFC-Barcelona Sports Sciences Research Group, Institut Nacional d’Educació Física de Catalunya (INEFC), Universitat de Barcelona (UB), 08038, Barcelona, Spain
Zeasseska Noriega & Alfredo Irurtia
Exercise and Health Laboratory, CIPER, Faculdade de Motricidade Humana, Universidade de Lisboa, Cruz-Quebrada, Portugal
Rubén Francisco & Analiza Mónica Silva
Department of Kinesiology and Public Health Education, Hyslop Sports Center, University of North Dakota, Grand Forks, USA
Henry Lukaski
You can also search for this author in PubMed Google Scholar
Contributions
FC, GC, ZN, GC, DC, and AP: Conceptualization and Methodology; FC, GC, ZN, RF, AI, AMS, GC, and AP: Writing—Original draft preparation; HL and AP: Supervision. All authors: Writing—Reviewing and Editing.
Corresponding author
Correspondence to Francesco Campa .
Ethics declarations
Consent for publication.
Not applicable.
Competing interests
The authors declare that they have no competing interests.
Additional information
Publisher's note.
Springer Nature remains neutral with regard to jurisdictional claims in published maps and institutional affiliations.
Supplementary Information
Additional file1 (docx 40 kb), rights and permissions.
Open Access This article is licensed under a Creative Commons Attribution 4.0 International License, which permits use, sharing, adaptation, distribution and reproduction in any medium or format, as long as you give appropriate credit to the original author(s) and the source, provide a link to the Creative Commons licence, and indicate if changes were made. The images or other third party material in this article are included in the article's Creative Commons licence, unless indicated otherwise in a credit line to the material. If material is not included in the article's Creative Commons licence and your intended use is not permitted by statutory regulation or exceeds the permitted use, you will need to obtain permission directly from the copyright holder. To view a copy of this licence, visit http://creativecommons.org/licenses/by/4.0/ . The Creative Commons Public Domain Dedication waiver ( http://creativecommons.org/publicdomain/zero/1.0/ ) applies to the data made available in this article, unless otherwise stated in a credit line to the data.
Reprints and permissions
About this article
Cite this article.
Campa, F., Coratella, G., Cerullo, G. et al. High-standard predictive equations for estimating body composition using bioelectrical impedance analysis: a systematic review. J Transl Med 22 , 515 (2024). https://doi.org/10.1186/s12967-024-05272-x
Download citation
Received : 23 January 2024
Accepted : 04 May 2024
Published : 29 May 2024
DOI : https://doi.org/10.1186/s12967-024-05272-x
Share this article
Anyone you share the following link with will be able to read this content:
Sorry, a shareable link is not currently available for this article.
Provided by the Springer Nature SharedIt content-sharing initiative
- Fat-free mass
- Total body water
- Resistance training
Journal of Translational Medicine
ISSN: 1479-5876
- Submission enquiries: Access here and click Contact Us
- General enquiries: [email protected]
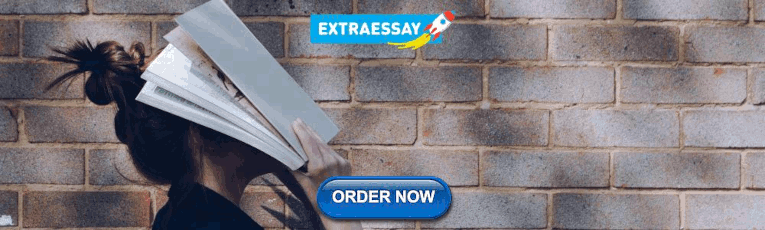
IMAGES
VIDEO
COMMENTS
A Comparative Analysis of Biometrics Types: Literature Review. December 2020; Journal of Computer Science 16(12):1778-1788; ... technology is the same as most finger ridge ide ntification .
Biometric technology, founded on recognizing distinctive individual features, enhances identification and automation, yielding personal and institutional benefits. This literature review employs ...
A literature review is performed to present an overview of biometric technology applications for educational purposes, challenges that must overcome to implement biometric technology, and potentially foreshadowing trends effectively. ... In 2001 the MIT Technology Review considered biometrics a world-changing emerging technology . Biometric ...
An extensive review of biometric technology is presented here. Besides the mono-modal systems, the article also discusses multi-modal biometric systems along with their architecture and information fusion levels. The paper along with the exemplary evidences highlights the potential for biometric technology, market value and prospects. Previous.
Behavioral biometric technology has the potential to be used in a public health context to address the limitations of current measures of screen time; however, more rigorous research must be performed in child populations first. ... Recent advances in mobile touch screen security authentication methods: a systematic literature review. Comput ...
Biometric technology is fast gaining pace as a veritable developmental tool. So far, biometric procedures have been predominantly used to ensure identity and ear recognition techniques continue to provide very robust research prospects. This paper proposes to identify and review present techniques for ear biometrics using certain parameters: machine learning methods, and procedures and provide ...
Biometric deals with the verification and identification of a person based on behavioural and physiological traits. This article presents recent advances in physiological-based biometric multimodalities, where we focused on finger vein, palm vein, fingerprint, face, lips, iris, and retina-based processing methods. The authors also evaluated the architecture, operational mode, and performance ...
Abstract - Biometric technology, founded on recognizing distinctive individual features, enhances identification and automation, yielding personal and institutional benefits. This literature review employs the Kitchenham and Charters methodology to analyze recent perspectives on biometric technology in educational time and attendance.
This literature review employs the Kitchenham and Charters methodology to analyze recent perspectives on biometric technology in educational time and attendance. The analysis identifies benefits, such as preventing impersonation and enhancing data security, but emphasizes concerns about biometric alterations and hardware/software failures ...
With an insight into the state-of-the-art research in biometrics for IoT security, this review paper helps advance the study in the field and assists researchers in gaining a good understanding of forward-looking issues and future research directions. Keywords: biometrics, IoT, security, access control, authentication, encryption.
Identity management through biometrics offer potential advantages over knowledge and possession based methods. A wide variety of biometric modalities have been tested so far but several factors paralyze the accuracy of mono-modal biometric systems. Usually, the analysis of multiple modalities offers better accuracy. An extensive review of biometric technology is presented here.
This study is a literature survey of multimodal biometric systems (MBS) that present the most important publications in this research area. We first provide an overview of important concepts in multimodal biometric recogntion. Then we provide a snapshot of the current state of research in multimodal biometrics with regards to modalities, fusion functions and remaining challenges. Lastly, we ...
Literature Review History and Importance of Biometrics As an early form of recognition, people have used sight to identify each other based on facial images ... biometric technology faces many problems, such as noisy data, intra-class variations and a limited degree of freedom-anti-universality, spoof attacks and an
A 2003 literature review of the applications of FRT (Zhao et al. 2003) identified four main uses of the technology: entertainment ... A review of biometric technology along with trends and prospects. Pattern Recognition, 47(8), 2673-2688. Google Scholar Van der Ploeg, I. (2003). Biometrics and privacy: A note on the politics of theorizing ...
Literature review: Biometric technology in the banking system. As submitted by Ateba et al. (2013), for banks to remain relevant, successful and competitive in today's competitive world, they must provide innovative and best-secured services to their customers. Customer and organisational perspectives
A literature review is performed to present an overview of biometric technology applications for educational purposes. The paper outline is as follows: Sect. 2 describes the applications biometrics offers in the academic field. Section 3 presents the challenges that must be overcome to implement the technology under consideration effectively.
technology implementation. The literature review helped authors to explore and solve different ambiguities in authors' minds, related to work flow, methods and procedures for different tasks. In our research, we selected five different interviewees from biometric solution supplier companies in Sweden and Denmark, Blekinge healthcare staff ...
This paper presents a literature overview of the recent. works on automated and smart attendance tracking systems. Conc erning technology, application domain, and key findings, our critical ...
Keywords: biometric, identification, system, technology. Suggested Citation: Suggested Citation. jindal, udit and Kaur, Harshdeep and das, subarna, Literature Review on Security Issues and Limitations in Biometric Applications (April 1, 2020). Proceedings of the International Conference on Innovative Computing & Communications ...
This review presents the broad range of methods used for face recognition and attempts to discuss their advantages and disadvantages. Initially, we present the basics of face-recognition technology, its standard workflow, background and problems, and the potential applications.
Objective: The study aimed to investigate the need for security and simplicity in the. authentication of retail payments, digital banking and financial technology through the. application of ...
2. Biometric Recognition Mechanism. Recently, biometrics as a security technique has emerged [].Combining the physiological properties of the human body with computers, optics, and acoustics [] led to the development of a range of biometric technologies, including iris, voice, fingerprint, face, etc., technology.Their comparison is shown in Table 1.
The literature still lacks a thorough review on the recent advances of biometric authentication for the purpose of secure and privacy-preserving identification. In this paper, we classify and thoroughly review the existing biometric authentication systems by focusing on the security and privacy solutions.
AFRICA JOURNAL OF SCIENCE, TECHNOLOGY AND SOCIAL SCIENCES ISSN 403-602-15-502-X Vol. 44. No. 157, February, 2014.This Journal is listed in the Series at Archive of International Journals of Scientific & Technology Research at www.arcijostech.org Exploiting Literature review of fingerprint technology in Biometrics *Terence McIvor, **Bashiru Aremu,***Ige Akanfe Kolapo, ****Promise Mebine ...
Here we present literature survey for some of the biometrics o f the two categories. 2.1. ... technique and is currently the leadin g biometric technology [Jain et al. 2004].
The appropriate use of predictive equations in estimating body composition through bioelectrical impedance analysis (BIA) depends on the device used and the subject's age, geographical ancestry, healthy status, physical activity level and sex. However, the presence of many isolated predictive equations in the literature makes the correct choice challenging, since the user may not distinguish ...