- Skip to main content
- Skip to primary sidebar
- Skip to footer
- QuestionPro

- Solutions Industries Gaming Automotive Sports and events Education Government Travel & Hospitality Financial Services Healthcare Cannabis Technology Use Case NPS+ Communities Audience Contactless surveys Mobile LivePolls Member Experience GDPR Positive People Science 360 Feedback Surveys
- Resources Blog eBooks Survey Templates Case Studies Training Help center

Home Market Research
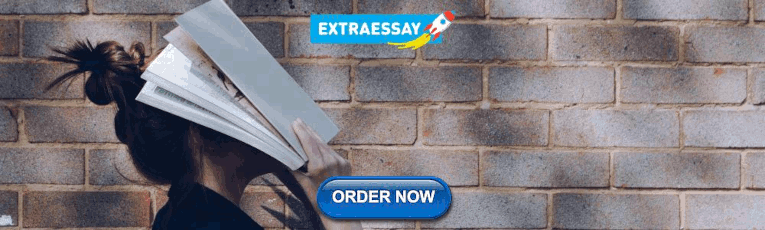
Data Analysis in Research: Types & Methods
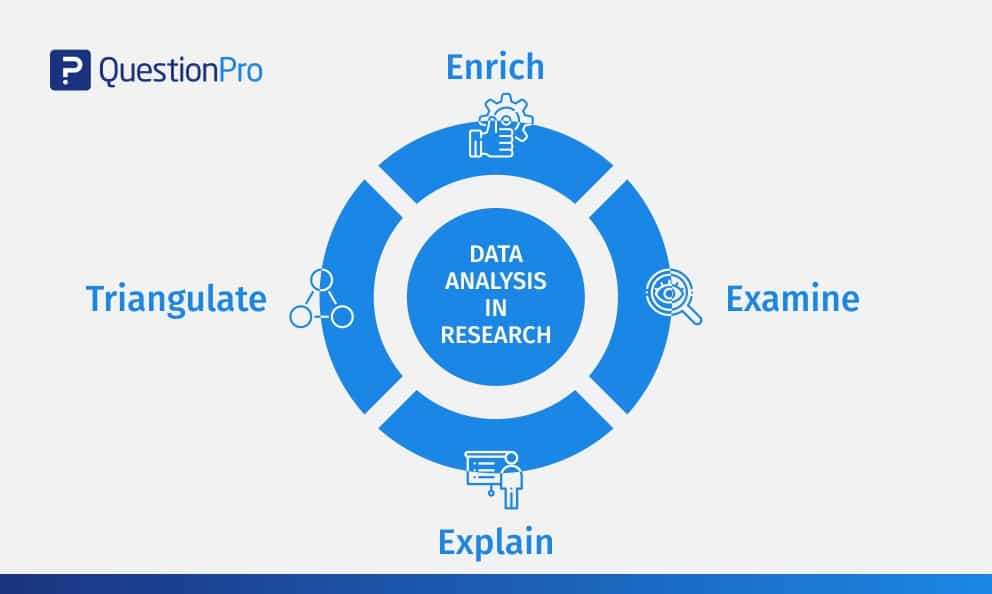
Content Index
Why analyze data in research?
Types of data in research, finding patterns in the qualitative data, methods used for data analysis in qualitative research, preparing data for analysis, methods used for data analysis in quantitative research, considerations in research data analysis, what is data analysis in research.
Definition of research in data analysis: According to LeCompte and Schensul, research data analysis is a process used by researchers to reduce data to a story and interpret it to derive insights. The data analysis process helps reduce a large chunk of data into smaller fragments, which makes sense.
Three essential things occur during the data analysis process — the first is data organization . Summarization and categorization together contribute to becoming the second known method used for data reduction. It helps find patterns and themes in the data for easy identification and linking. The third and last way is data analysis – researchers do it in both top-down and bottom-up fashion.
LEARN ABOUT: Research Process Steps
On the other hand, Marshall and Rossman describe data analysis as a messy, ambiguous, and time-consuming but creative and fascinating process through which a mass of collected data is brought to order, structure and meaning.
We can say that “the data analysis and data interpretation is a process representing the application of deductive and inductive logic to the research and data analysis.”
Researchers rely heavily on data as they have a story to tell or research problems to solve. It starts with a question, and data is nothing but an answer to that question. But, what if there is no question to ask? Well! It is possible to explore data even without a problem – we call it ‘Data Mining’, which often reveals some interesting patterns within the data that are worth exploring.
Irrelevant to the type of data researchers explore, their mission and audiences’ vision guide them to find the patterns to shape the story they want to tell. One of the essential things expected from researchers while analyzing data is to stay open and remain unbiased toward unexpected patterns, expressions, and results. Remember, sometimes, data analysis tells the most unforeseen yet exciting stories that were not expected when initiating data analysis. Therefore, rely on the data you have at hand and enjoy the journey of exploratory research.
Create a Free Account
Every kind of data has a rare quality of describing things after assigning a specific value to it. For analysis, you need to organize these values, processed and presented in a given context, to make it useful. Data can be in different forms; here are the primary data types.
- Qualitative data: When the data presented has words and descriptions, then we call it qualitative data . Although you can observe this data, it is subjective and harder to analyze data in research, especially for comparison. Example: Quality data represents everything describing taste, experience, texture, or an opinion that is considered quality data. This type of data is usually collected through focus groups, personal qualitative interviews , qualitative observation or using open-ended questions in surveys.
- Quantitative data: Any data expressed in numbers of numerical figures are called quantitative data . This type of data can be distinguished into categories, grouped, measured, calculated, or ranked. Example: questions such as age, rank, cost, length, weight, scores, etc. everything comes under this type of data. You can present such data in graphical format, charts, or apply statistical analysis methods to this data. The (Outcomes Measurement Systems) OMS questionnaires in surveys are a significant source of collecting numeric data.
- Categorical data: It is data presented in groups. However, an item included in the categorical data cannot belong to more than one group. Example: A person responding to a survey by telling his living style, marital status, smoking habit, or drinking habit comes under the categorical data. A chi-square test is a standard method used to analyze this data.
Learn More : Examples of Qualitative Data in Education
Data analysis in qualitative research
Data analysis and qualitative data research work a little differently from the numerical data as the quality data is made up of words, descriptions, images, objects, and sometimes symbols. Getting insight from such complicated information is a complicated process. Hence it is typically used for exploratory research and data analysis .
Although there are several ways to find patterns in the textual information, a word-based method is the most relied and widely used global technique for research and data analysis. Notably, the data analysis process in qualitative research is manual. Here the researchers usually read the available data and find repetitive or commonly used words.
For example, while studying data collected from African countries to understand the most pressing issues people face, researchers might find “food” and “hunger” are the most commonly used words and will highlight them for further analysis.
LEARN ABOUT: Level of Analysis
The keyword context is another widely used word-based technique. In this method, the researcher tries to understand the concept by analyzing the context in which the participants use a particular keyword.
For example , researchers conducting research and data analysis for studying the concept of ‘diabetes’ amongst respondents might analyze the context of when and how the respondent has used or referred to the word ‘diabetes.’
The scrutiny-based technique is also one of the highly recommended text analysis methods used to identify a quality data pattern. Compare and contrast is the widely used method under this technique to differentiate how a specific text is similar or different from each other.
For example: To find out the “importance of resident doctor in a company,” the collected data is divided into people who think it is necessary to hire a resident doctor and those who think it is unnecessary. Compare and contrast is the best method that can be used to analyze the polls having single-answer questions types .
Metaphors can be used to reduce the data pile and find patterns in it so that it becomes easier to connect data with theory.
Variable Partitioning is another technique used to split variables so that researchers can find more coherent descriptions and explanations from the enormous data.
LEARN ABOUT: Qualitative Research Questions and Questionnaires
There are several techniques to analyze the data in qualitative research, but here are some commonly used methods,
- Content Analysis: It is widely accepted and the most frequently employed technique for data analysis in research methodology. It can be used to analyze the documented information from text, images, and sometimes from the physical items. It depends on the research questions to predict when and where to use this method.
- Narrative Analysis: This method is used to analyze content gathered from various sources such as personal interviews, field observation, and surveys . The majority of times, stories, or opinions shared by people are focused on finding answers to the research questions.
- Discourse Analysis: Similar to narrative analysis, discourse analysis is used to analyze the interactions with people. Nevertheless, this particular method considers the social context under which or within which the communication between the researcher and respondent takes place. In addition to that, discourse analysis also focuses on the lifestyle and day-to-day environment while deriving any conclusion.
- Grounded Theory: When you want to explain why a particular phenomenon happened, then using grounded theory for analyzing quality data is the best resort. Grounded theory is applied to study data about the host of similar cases occurring in different settings. When researchers are using this method, they might alter explanations or produce new ones until they arrive at some conclusion.
LEARN ABOUT: 12 Best Tools for Researchers
Data analysis in quantitative research
The first stage in research and data analysis is to make it for the analysis so that the nominal data can be converted into something meaningful. Data preparation consists of the below phases.
Phase I: Data Validation
Data validation is done to understand if the collected data sample is per the pre-set standards, or it is a biased data sample again divided into four different stages
- Fraud: To ensure an actual human being records each response to the survey or the questionnaire
- Screening: To make sure each participant or respondent is selected or chosen in compliance with the research criteria
- Procedure: To ensure ethical standards were maintained while collecting the data sample
- Completeness: To ensure that the respondent has answered all the questions in an online survey. Else, the interviewer had asked all the questions devised in the questionnaire.
Phase II: Data Editing
More often, an extensive research data sample comes loaded with errors. Respondents sometimes fill in some fields incorrectly or sometimes skip them accidentally. Data editing is a process wherein the researchers have to confirm that the provided data is free of such errors. They need to conduct necessary checks and outlier checks to edit the raw edit and make it ready for analysis.
Phase III: Data Coding
Out of all three, this is the most critical phase of data preparation associated with grouping and assigning values to the survey responses . If a survey is completed with a 1000 sample size, the researcher will create an age bracket to distinguish the respondents based on their age. Thus, it becomes easier to analyze small data buckets rather than deal with the massive data pile.
LEARN ABOUT: Steps in Qualitative Research
After the data is prepared for analysis, researchers are open to using different research and data analysis methods to derive meaningful insights. For sure, statistical analysis plans are the most favored to analyze numerical data. In statistical analysis, distinguishing between categorical data and numerical data is essential, as categorical data involves distinct categories or labels, while numerical data consists of measurable quantities. The method is again classified into two groups. First, ‘Descriptive Statistics’ used to describe data. Second, ‘Inferential statistics’ that helps in comparing the data .
Descriptive statistics
This method is used to describe the basic features of versatile types of data in research. It presents the data in such a meaningful way that pattern in the data starts making sense. Nevertheless, the descriptive analysis does not go beyond making conclusions. The conclusions are again based on the hypothesis researchers have formulated so far. Here are a few major types of descriptive analysis methods.
Measures of Frequency
- Count, Percent, Frequency
- It is used to denote home often a particular event occurs.
- Researchers use it when they want to showcase how often a response is given.
Measures of Central Tendency
- Mean, Median, Mode
- The method is widely used to demonstrate distribution by various points.
- Researchers use this method when they want to showcase the most commonly or averagely indicated response.
Measures of Dispersion or Variation
- Range, Variance, Standard deviation
- Here the field equals high/low points.
- Variance standard deviation = difference between the observed score and mean
- It is used to identify the spread of scores by stating intervals.
- Researchers use this method to showcase data spread out. It helps them identify the depth until which the data is spread out that it directly affects the mean.
Measures of Position
- Percentile ranks, Quartile ranks
- It relies on standardized scores helping researchers to identify the relationship between different scores.
- It is often used when researchers want to compare scores with the average count.
For quantitative research use of descriptive analysis often give absolute numbers, but the in-depth analysis is never sufficient to demonstrate the rationale behind those numbers. Nevertheless, it is necessary to think of the best method for research and data analysis suiting your survey questionnaire and what story researchers want to tell. For example, the mean is the best way to demonstrate the students’ average scores in schools. It is better to rely on the descriptive statistics when the researchers intend to keep the research or outcome limited to the provided sample without generalizing it. For example, when you want to compare average voting done in two different cities, differential statistics are enough.
Descriptive analysis is also called a ‘univariate analysis’ since it is commonly used to analyze a single variable.
Inferential statistics
Inferential statistics are used to make predictions about a larger population after research and data analysis of the representing population’s collected sample. For example, you can ask some odd 100 audiences at a movie theater if they like the movie they are watching. Researchers then use inferential statistics on the collected sample to reason that about 80-90% of people like the movie.
Here are two significant areas of inferential statistics.
- Estimating parameters: It takes statistics from the sample research data and demonstrates something about the population parameter.
- Hypothesis test: I t’s about sampling research data to answer the survey research questions. For example, researchers might be interested to understand if the new shade of lipstick recently launched is good or not, or if the multivitamin capsules help children to perform better at games.
These are sophisticated analysis methods used to showcase the relationship between different variables instead of describing a single variable. It is often used when researchers want something beyond absolute numbers to understand the relationship between variables.
Here are some of the commonly used methods for data analysis in research.
- Correlation: When researchers are not conducting experimental research or quasi-experimental research wherein the researchers are interested to understand the relationship between two or more variables, they opt for correlational research methods.
- Cross-tabulation: Also called contingency tables, cross-tabulation is used to analyze the relationship between multiple variables. Suppose provided data has age and gender categories presented in rows and columns. A two-dimensional cross-tabulation helps for seamless data analysis and research by showing the number of males and females in each age category.
- Regression analysis: For understanding the strong relationship between two variables, researchers do not look beyond the primary and commonly used regression analysis method, which is also a type of predictive analysis used. In this method, you have an essential factor called the dependent variable. You also have multiple independent variables in regression analysis. You undertake efforts to find out the impact of independent variables on the dependent variable. The values of both independent and dependent variables are assumed as being ascertained in an error-free random manner.
- Frequency tables: The statistical procedure is used for testing the degree to which two or more vary or differ in an experiment. A considerable degree of variation means research findings were significant. In many contexts, ANOVA testing and variance analysis are similar.
- Analysis of variance: The statistical procedure is used for testing the degree to which two or more vary or differ in an experiment. A considerable degree of variation means research findings were significant. In many contexts, ANOVA testing and variance analysis are similar.
- Researchers must have the necessary research skills to analyze and manipulation the data , Getting trained to demonstrate a high standard of research practice. Ideally, researchers must possess more than a basic understanding of the rationale of selecting one statistical method over the other to obtain better data insights.
- Usually, research and data analytics projects differ by scientific discipline; therefore, getting statistical advice at the beginning of analysis helps design a survey questionnaire, select data collection methods , and choose samples.
LEARN ABOUT: Best Data Collection Tools
- The primary aim of data research and analysis is to derive ultimate insights that are unbiased. Any mistake in or keeping a biased mind to collect data, selecting an analysis method, or choosing audience sample il to draw a biased inference.
- Irrelevant to the sophistication used in research data and analysis is enough to rectify the poorly defined objective outcome measurements. It does not matter if the design is at fault or intentions are not clear, but lack of clarity might mislead readers, so avoid the practice.
- The motive behind data analysis in research is to present accurate and reliable data. As far as possible, avoid statistical errors, and find a way to deal with everyday challenges like outliers, missing data, data altering, data mining , or developing graphical representation.
LEARN MORE: Descriptive Research vs Correlational Research The sheer amount of data generated daily is frightening. Especially when data analysis has taken center stage. in 2018. In last year, the total data supply amounted to 2.8 trillion gigabytes. Hence, it is clear that the enterprises willing to survive in the hypercompetitive world must possess an excellent capability to analyze complex research data, derive actionable insights, and adapt to the new market needs.
LEARN ABOUT: Average Order Value
QuestionPro is an online survey platform that empowers organizations in data analysis and research and provides them a medium to collect data by creating appealing surveys.
MORE LIKE THIS
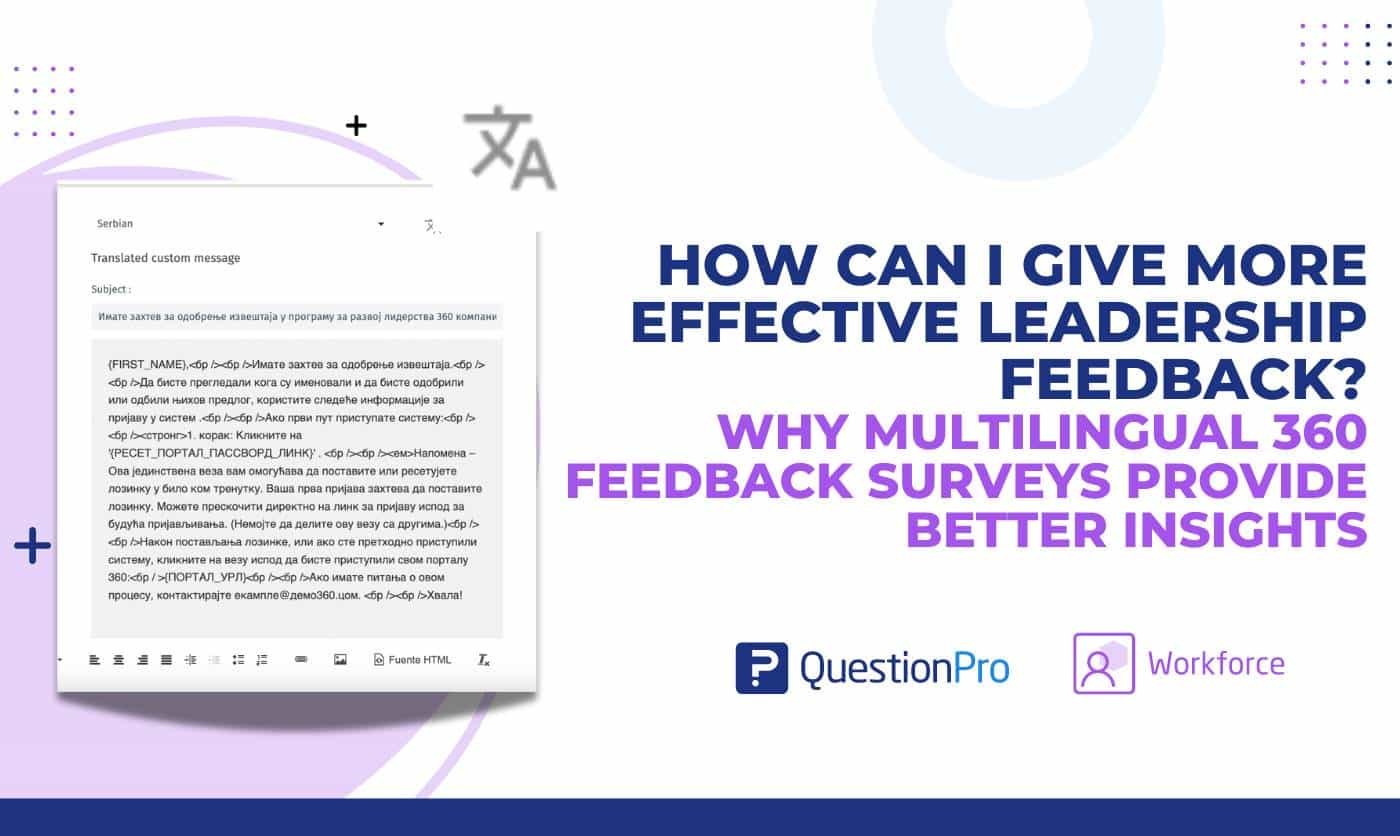
Why Multilingual 360 Feedback Surveys Provide Better Insights
Jun 3, 2024
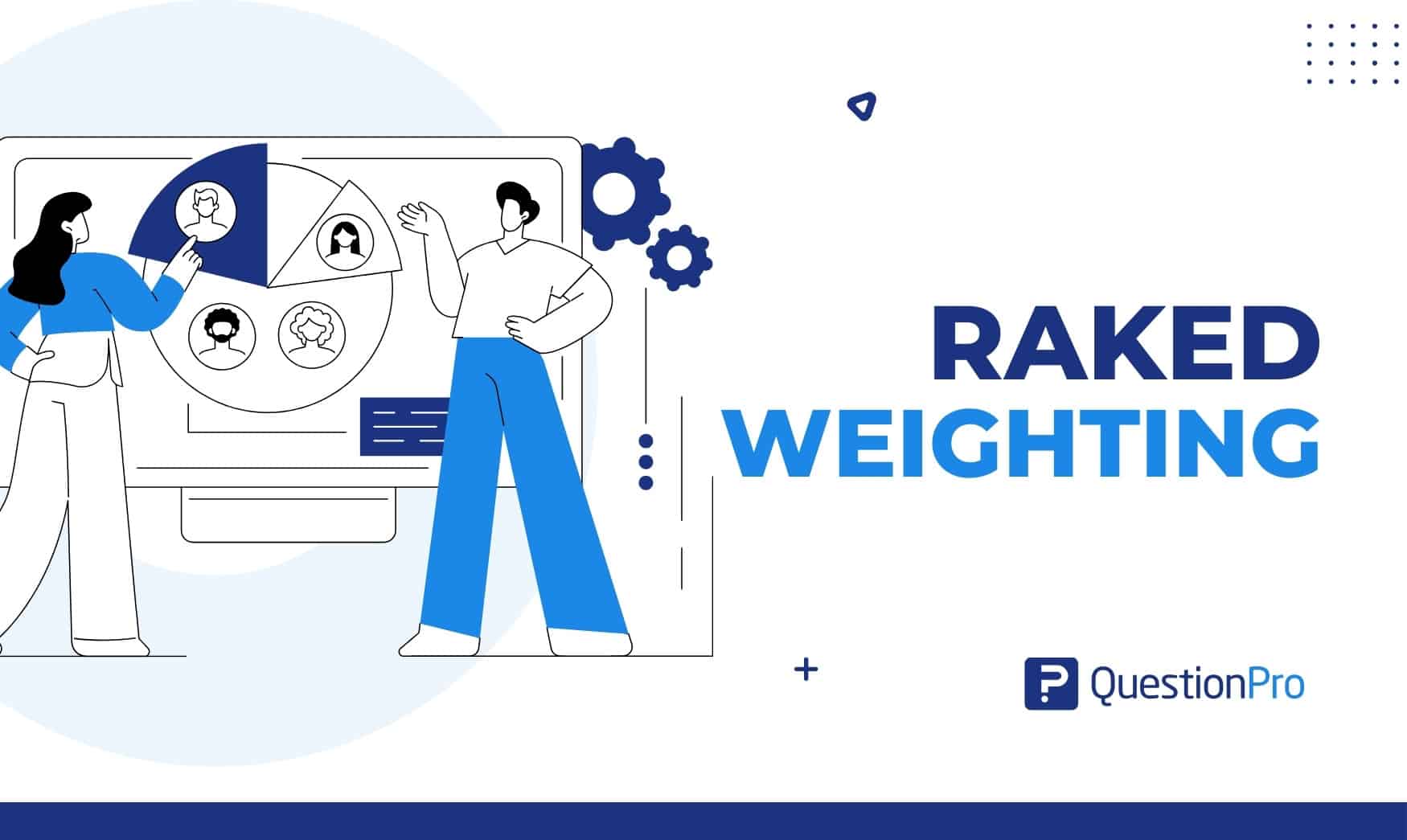
Raked Weighting: A Key Tool for Accurate Survey Results
May 31, 2024
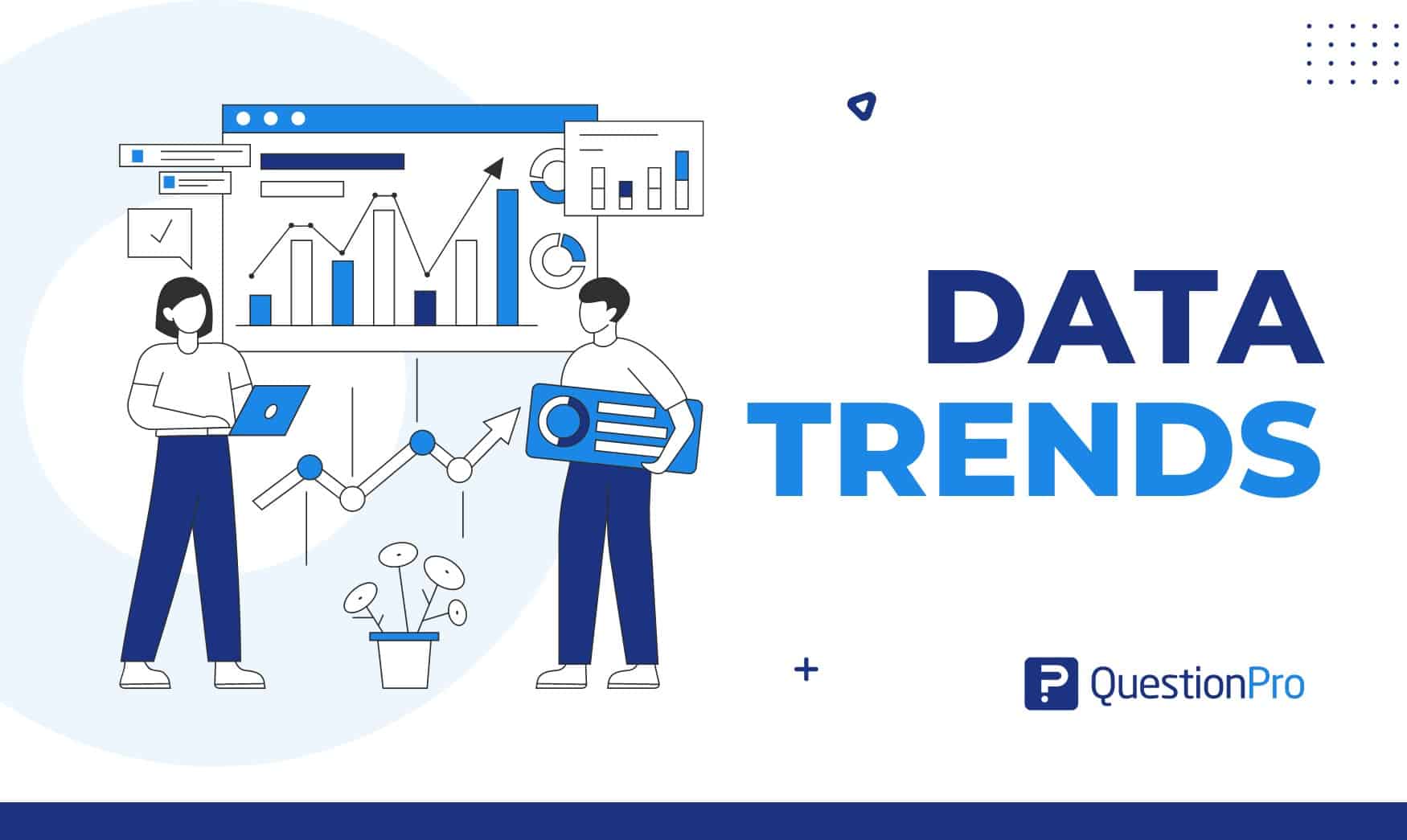
Top 8 Data Trends to Understand the Future of Data
May 30, 2024
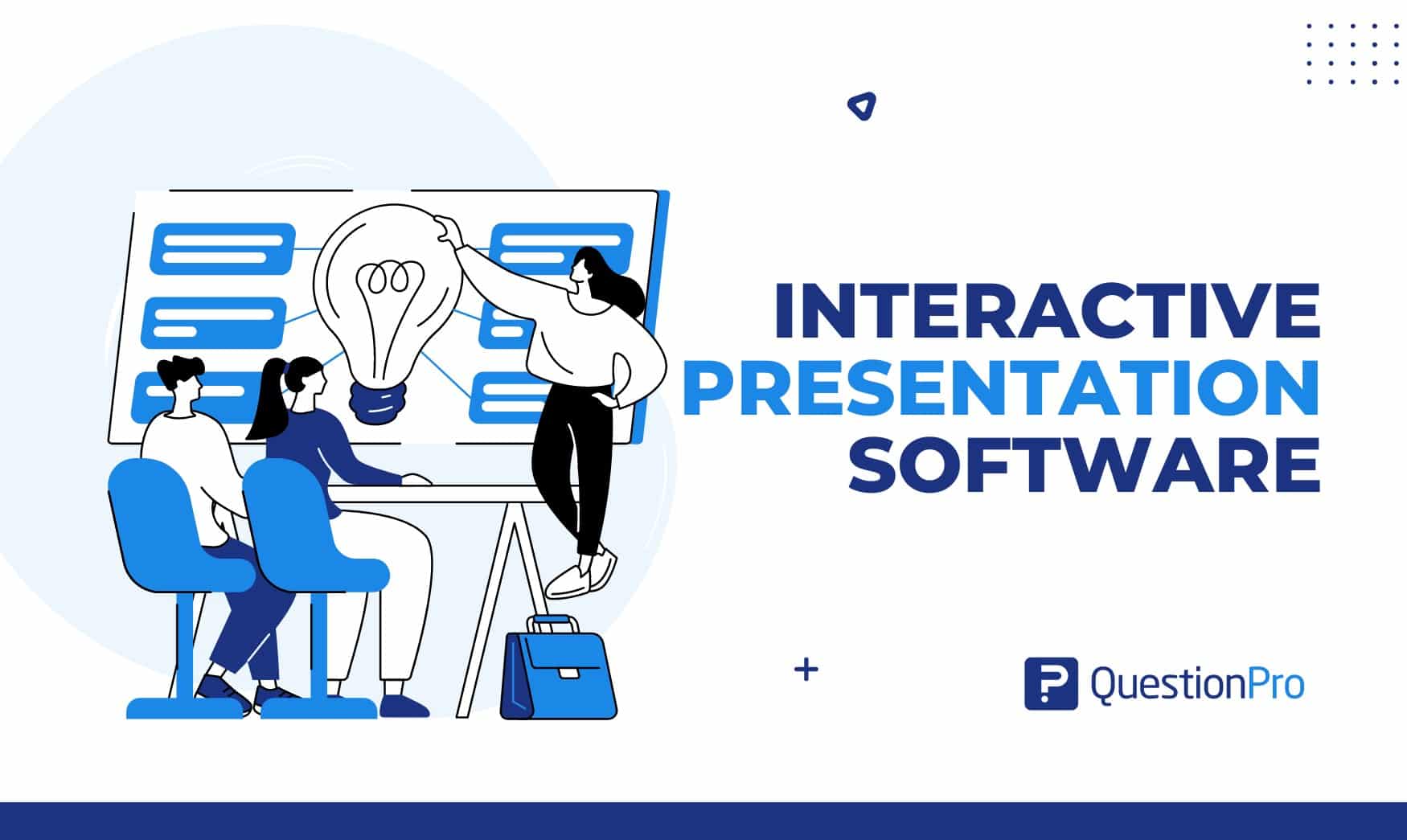
Top 12 Interactive Presentation Software to Engage Your User
May 29, 2024
Other categories
- Academic Research
- Artificial Intelligence
- Assessments
- Brand Awareness
- Case Studies
- Communities
- Consumer Insights
- Customer effort score
- Customer Engagement
- Customer Experience
- Customer Loyalty
- Customer Research
- Customer Satisfaction
- Employee Benefits
- Employee Engagement
- Employee Retention
- Friday Five
- General Data Protection Regulation
- Insights Hub
- Life@QuestionPro
- Market Research
- Mobile diaries
- Mobile Surveys
- New Features
- Online Communities
- Question Types
- Questionnaire
- QuestionPro Products
- Release Notes
- Research Tools and Apps
- Revenue at Risk
- Survey Templates
- Training Tips
- Uncategorized
- Video Learning Series
- What’s Coming Up
- Workforce Intelligence

An official website of the United States government
The .gov means it’s official. Federal government websites often end in .gov or .mil. Before sharing sensitive information, make sure you’re on a federal government site.
The site is secure. The https:// ensures that you are connecting to the official website and that any information you provide is encrypted and transmitted securely.
- Publications
- Account settings
Preview improvements coming to the PMC website in October 2024. Learn More or Try it out now .
- Advanced Search
- Journal List
- HCA Healthc J Med
- v.1(2); 2020
- PMC10324782

Introduction to Research Statistical Analysis: An Overview of the Basics
Christian vandever.
1 HCA Healthcare Graduate Medical Education
Description
This article covers many statistical ideas essential to research statistical analysis. Sample size is explained through the concepts of statistical significance level and power. Variable types and definitions are included to clarify necessities for how the analysis will be interpreted. Categorical and quantitative variable types are defined, as well as response and predictor variables. Statistical tests described include t-tests, ANOVA and chi-square tests. Multiple regression is also explored for both logistic and linear regression. Finally, the most common statistics produced by these methods are explored.
Introduction
Statistical analysis is necessary for any research project seeking to make quantitative conclusions. The following is a primer for research-based statistical analysis. It is intended to be a high-level overview of appropriate statistical testing, while not diving too deep into any specific methodology. Some of the information is more applicable to retrospective projects, where analysis is performed on data that has already been collected, but most of it will be suitable to any type of research. This primer will help the reader understand research results in coordination with a statistician, not to perform the actual analysis. Analysis is commonly performed using statistical programming software such as R, SAS or SPSS. These allow for analysis to be replicated while minimizing the risk for an error. Resources are listed later for those working on analysis without a statistician.
After coming up with a hypothesis for a study, including any variables to be used, one of the first steps is to think about the patient population to apply the question. Results are only relevant to the population that the underlying data represents. Since it is impractical to include everyone with a certain condition, a subset of the population of interest should be taken. This subset should be large enough to have power, which means there is enough data to deliver significant results and accurately reflect the study’s population.
The first statistics of interest are related to significance level and power, alpha and beta. Alpha (α) is the significance level and probability of a type I error, the rejection of the null hypothesis when it is true. The null hypothesis is generally that there is no difference between the groups compared. A type I error is also known as a false positive. An example would be an analysis that finds one medication statistically better than another, when in reality there is no difference in efficacy between the two. Beta (β) is the probability of a type II error, the failure to reject the null hypothesis when it is actually false. A type II error is also known as a false negative. This occurs when the analysis finds there is no difference in two medications when in reality one works better than the other. Power is defined as 1-β and should be calculated prior to running any sort of statistical testing. Ideally, alpha should be as small as possible while power should be as large as possible. Power generally increases with a larger sample size, but so does cost and the effect of any bias in the study design. Additionally, as the sample size gets bigger, the chance for a statistically significant result goes up even though these results can be small differences that do not matter practically. Power calculators include the magnitude of the effect in order to combat the potential for exaggeration and only give significant results that have an actual impact. The calculators take inputs like the mean, effect size and desired power, and output the required minimum sample size for analysis. Effect size is calculated using statistical information on the variables of interest. If that information is not available, most tests have commonly used values for small, medium or large effect sizes.
When the desired patient population is decided, the next step is to define the variables previously chosen to be included. Variables come in different types that determine which statistical methods are appropriate and useful. One way variables can be split is into categorical and quantitative variables. ( Table 1 ) Categorical variables place patients into groups, such as gender, race and smoking status. Quantitative variables measure or count some quantity of interest. Common quantitative variables in research include age and weight. An important note is that there can often be a choice for whether to treat a variable as quantitative or categorical. For example, in a study looking at body mass index (BMI), BMI could be defined as a quantitative variable or as a categorical variable, with each patient’s BMI listed as a category (underweight, normal, overweight, and obese) rather than the discrete value. The decision whether a variable is quantitative or categorical will affect what conclusions can be made when interpreting results from statistical tests. Keep in mind that since quantitative variables are treated on a continuous scale it would be inappropriate to transform a variable like which medication was given into a quantitative variable with values 1, 2 and 3.
Categorical vs. Quantitative Variables
Both of these types of variables can also be split into response and predictor variables. ( Table 2 ) Predictor variables are explanatory, or independent, variables that help explain changes in a response variable. Conversely, response variables are outcome, or dependent, variables whose changes can be partially explained by the predictor variables.
Response vs. Predictor Variables
Choosing the correct statistical test depends on the types of variables defined and the question being answered. The appropriate test is determined by the variables being compared. Some common statistical tests include t-tests, ANOVA and chi-square tests.
T-tests compare whether there are differences in a quantitative variable between two values of a categorical variable. For example, a t-test could be useful to compare the length of stay for knee replacement surgery patients between those that took apixaban and those that took rivaroxaban. A t-test could examine whether there is a statistically significant difference in the length of stay between the two groups. The t-test will output a p-value, a number between zero and one, which represents the probability that the two groups could be as different as they are in the data, if they were actually the same. A value closer to zero suggests that the difference, in this case for length of stay, is more statistically significant than a number closer to one. Prior to collecting the data, set a significance level, the previously defined alpha. Alpha is typically set at 0.05, but is commonly reduced in order to limit the chance of a type I error, or false positive. Going back to the example above, if alpha is set at 0.05 and the analysis gives a p-value of 0.039, then a statistically significant difference in length of stay is observed between apixaban and rivaroxaban patients. If the analysis gives a p-value of 0.91, then there was no statistical evidence of a difference in length of stay between the two medications. Other statistical summaries or methods examine how big of a difference that might be. These other summaries are known as post-hoc analysis since they are performed after the original test to provide additional context to the results.
Analysis of variance, or ANOVA, tests can observe mean differences in a quantitative variable between values of a categorical variable, typically with three or more values to distinguish from a t-test. ANOVA could add patients given dabigatran to the previous population and evaluate whether the length of stay was significantly different across the three medications. If the p-value is lower than the designated significance level then the hypothesis that length of stay was the same across the three medications is rejected. Summaries and post-hoc tests also could be performed to look at the differences between length of stay and which individual medications may have observed statistically significant differences in length of stay from the other medications. A chi-square test examines the association between two categorical variables. An example would be to consider whether the rate of having a post-operative bleed is the same across patients provided with apixaban, rivaroxaban and dabigatran. A chi-square test can compute a p-value determining whether the bleeding rates were significantly different or not. Post-hoc tests could then give the bleeding rate for each medication, as well as a breakdown as to which specific medications may have a significantly different bleeding rate from each other.
A slightly more advanced way of examining a question can come through multiple regression. Regression allows more predictor variables to be analyzed and can act as a control when looking at associations between variables. Common control variables are age, sex and any comorbidities likely to affect the outcome variable that are not closely related to the other explanatory variables. Control variables can be especially important in reducing the effect of bias in a retrospective population. Since retrospective data was not built with the research question in mind, it is important to eliminate threats to the validity of the analysis. Testing that controls for confounding variables, such as regression, is often more valuable with retrospective data because it can ease these concerns. The two main types of regression are linear and logistic. Linear regression is used to predict differences in a quantitative, continuous response variable, such as length of stay. Logistic regression predicts differences in a dichotomous, categorical response variable, such as 90-day readmission. So whether the outcome variable is categorical or quantitative, regression can be appropriate. An example for each of these types could be found in two similar cases. For both examples define the predictor variables as age, gender and anticoagulant usage. In the first, use the predictor variables in a linear regression to evaluate their individual effects on length of stay, a quantitative variable. For the second, use the same predictor variables in a logistic regression to evaluate their individual effects on whether the patient had a 90-day readmission, a dichotomous categorical variable. Analysis can compute a p-value for each included predictor variable to determine whether they are significantly associated. The statistical tests in this article generate an associated test statistic which determines the probability the results could be acquired given that there is no association between the compared variables. These results often come with coefficients which can give the degree of the association and the degree to which one variable changes with another. Most tests, including all listed in this article, also have confidence intervals, which give a range for the correlation with a specified level of confidence. Even if these tests do not give statistically significant results, the results are still important. Not reporting statistically insignificant findings creates a bias in research. Ideas can be repeated enough times that eventually statistically significant results are reached, even though there is no true significance. In some cases with very large sample sizes, p-values will almost always be significant. In this case the effect size is critical as even the smallest, meaningless differences can be found to be statistically significant.
These variables and tests are just some things to keep in mind before, during and after the analysis process in order to make sure that the statistical reports are supporting the questions being answered. The patient population, types of variables and statistical tests are all important things to consider in the process of statistical analysis. Any results are only as useful as the process used to obtain them. This primer can be used as a reference to help ensure appropriate statistical analysis.
Funding Statement
This research was supported (in whole or in part) by HCA Healthcare and/or an HCA Healthcare affiliated entity.
Conflicts of Interest
The author declares he has no conflicts of interest.
Christian Vandever is an employee of HCA Healthcare Graduate Medical Education, an organization affiliated with the journal’s publisher.
This research was supported (in whole or in part) by HCA Healthcare and/or an HCA Healthcare affiliated entity. The views expressed in this publication represent those of the author(s) and do not necessarily represent the official views of HCA Healthcare or any of its affiliated entities.

Quantitative Data Analysis 101
The lingo, methods and techniques, explained simply.
By: Derek Jansen (MBA) and Kerryn Warren (PhD) | December 2020
Quantitative data analysis is one of those things that often strikes fear in students. It’s totally understandable – quantitative analysis is a complex topic, full of daunting lingo , like medians, modes, correlation and regression. Suddenly we’re all wishing we’d paid a little more attention in math class…
The good news is that while quantitative data analysis is a mammoth topic, gaining a working understanding of the basics isn’t that hard , even for those of us who avoid numbers and math . In this post, we’ll break quantitative analysis down into simple , bite-sized chunks so you can approach your research with confidence.
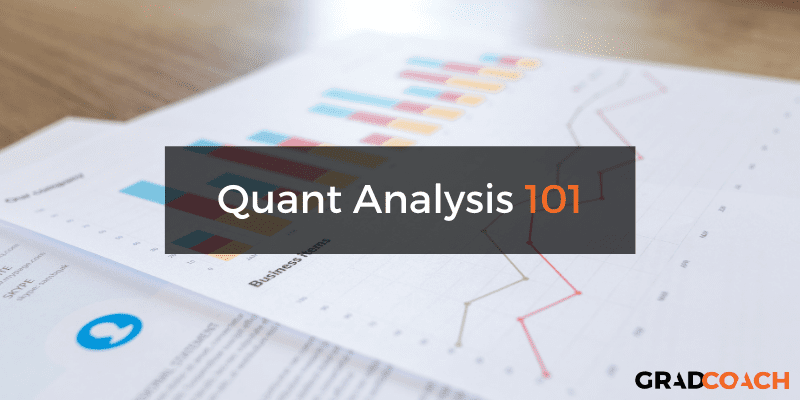
Overview: Quantitative Data Analysis 101
- What (exactly) is quantitative data analysis?
- When to use quantitative analysis
- How quantitative analysis works
The two “branches” of quantitative analysis
- Descriptive statistics 101
- Inferential statistics 101
- How to choose the right quantitative methods
- Recap & summary
What is quantitative data analysis?
Despite being a mouthful, quantitative data analysis simply means analysing data that is numbers-based – or data that can be easily “converted” into numbers without losing any meaning.
For example, category-based variables like gender, ethnicity, or native language could all be “converted” into numbers without losing meaning – for example, English could equal 1, French 2, etc.
This contrasts against qualitative data analysis, where the focus is on words, phrases and expressions that can’t be reduced to numbers. If you’re interested in learning about qualitative analysis, check out our post and video here .
What is quantitative analysis used for?
Quantitative analysis is generally used for three purposes.
- Firstly, it’s used to measure differences between groups . For example, the popularity of different clothing colours or brands.
- Secondly, it’s used to assess relationships between variables . For example, the relationship between weather temperature and voter turnout.
- And third, it’s used to test hypotheses in a scientifically rigorous way. For example, a hypothesis about the impact of a certain vaccine.
Again, this contrasts with qualitative analysis , which can be used to analyse people’s perceptions and feelings about an event or situation. In other words, things that can’t be reduced to numbers.
How does quantitative analysis work?
Well, since quantitative data analysis is all about analysing numbers , it’s no surprise that it involves statistics . Statistical analysis methods form the engine that powers quantitative analysis, and these methods can vary from pretty basic calculations (for example, averages and medians) to more sophisticated analyses (for example, correlations and regressions).
Sounds like gibberish? Don’t worry. We’ll explain all of that in this post. Importantly, you don’t need to be a statistician or math wiz to pull off a good quantitative analysis. We’ll break down all the technical mumbo jumbo in this post.
Need a helping hand?
As I mentioned, quantitative analysis is powered by statistical analysis methods . There are two main “branches” of statistical methods that are used – descriptive statistics and inferential statistics . In your research, you might only use descriptive statistics, or you might use a mix of both , depending on what you’re trying to figure out. In other words, depending on your research questions, aims and objectives . I’ll explain how to choose your methods later.
So, what are descriptive and inferential statistics?
Well, before I can explain that, we need to take a quick detour to explain some lingo. To understand the difference between these two branches of statistics, you need to understand two important words. These words are population and sample .
First up, population . In statistics, the population is the entire group of people (or animals or organisations or whatever) that you’re interested in researching. For example, if you were interested in researching Tesla owners in the US, then the population would be all Tesla owners in the US.
However, it’s extremely unlikely that you’re going to be able to interview or survey every single Tesla owner in the US. Realistically, you’ll likely only get access to a few hundred, or maybe a few thousand owners using an online survey. This smaller group of accessible people whose data you actually collect is called your sample .
So, to recap – the population is the entire group of people you’re interested in, and the sample is the subset of the population that you can actually get access to. In other words, the population is the full chocolate cake , whereas the sample is a slice of that cake.
So, why is this sample-population thing important?
Well, descriptive statistics focus on describing the sample , while inferential statistics aim to make predictions about the population, based on the findings within the sample. In other words, we use one group of statistical methods – descriptive statistics – to investigate the slice of cake, and another group of methods – inferential statistics – to draw conclusions about the entire cake. There I go with the cake analogy again…
With that out the way, let’s take a closer look at each of these branches in more detail.
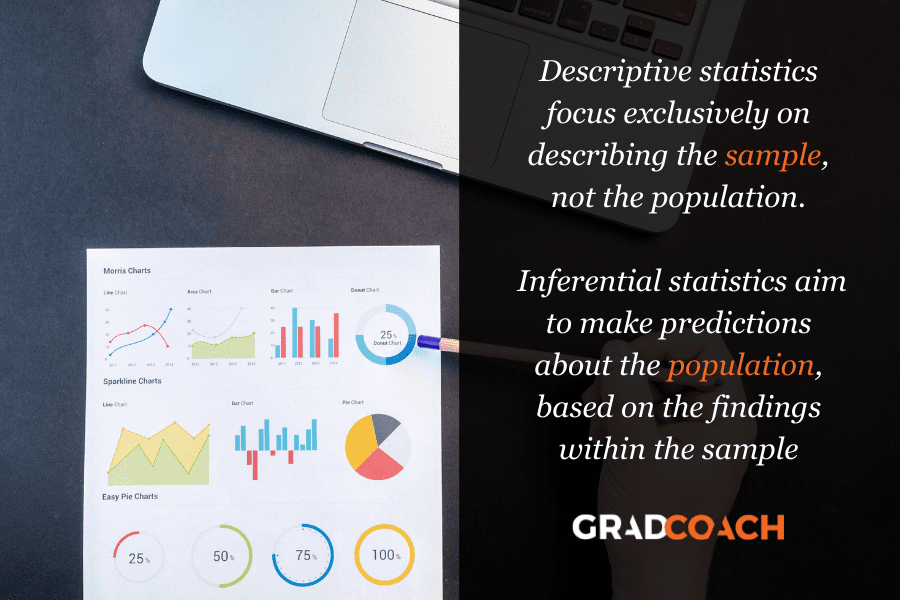
Branch 1: Descriptive Statistics
Descriptive statistics serve a simple but critically important role in your research – to describe your data set – hence the name. In other words, they help you understand the details of your sample . Unlike inferential statistics (which we’ll get to soon), descriptive statistics don’t aim to make inferences or predictions about the entire population – they’re purely interested in the details of your specific sample .
When you’re writing up your analysis, descriptive statistics are the first set of stats you’ll cover, before moving on to inferential statistics. But, that said, depending on your research objectives and research questions , they may be the only type of statistics you use. We’ll explore that a little later.
So, what kind of statistics are usually covered in this section?
Some common statistical tests used in this branch include the following:
- Mean – this is simply the mathematical average of a range of numbers.
- Median – this is the midpoint in a range of numbers when the numbers are arranged in numerical order. If the data set makes up an odd number, then the median is the number right in the middle of the set. If the data set makes up an even number, then the median is the midpoint between the two middle numbers.
- Mode – this is simply the most commonly occurring number in the data set.
- In cases where most of the numbers are quite close to the average, the standard deviation will be relatively low.
- Conversely, in cases where the numbers are scattered all over the place, the standard deviation will be relatively high.
- Skewness . As the name suggests, skewness indicates how symmetrical a range of numbers is. In other words, do they tend to cluster into a smooth bell curve shape in the middle of the graph, or do they skew to the left or right?
Feeling a bit confused? Let’s look at a practical example using a small data set.
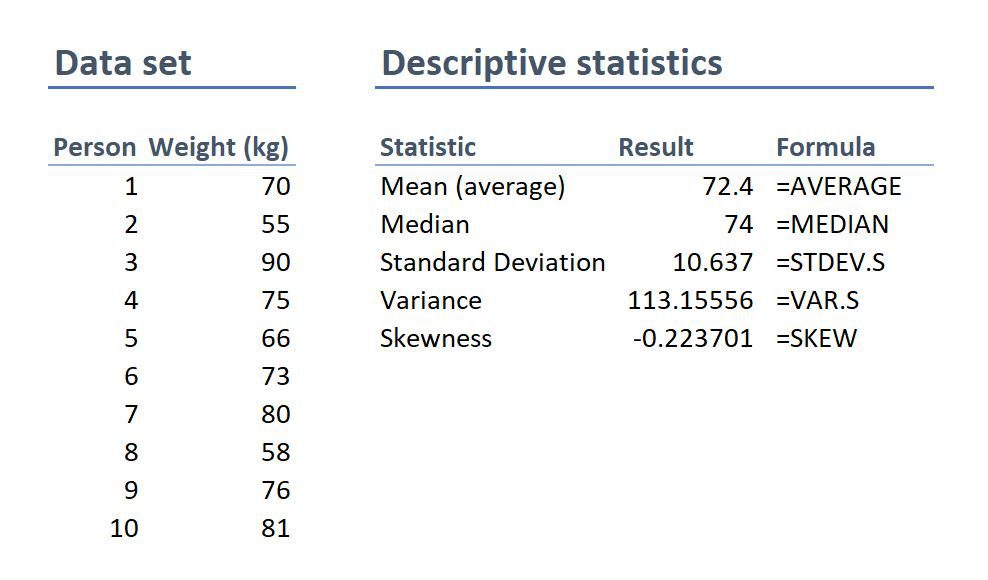
On the left-hand side is the data set. This details the bodyweight of a sample of 10 people. On the right-hand side, we have the descriptive statistics. Let’s take a look at each of them.
First, we can see that the mean weight is 72.4 kilograms. In other words, the average weight across the sample is 72.4 kilograms. Straightforward.
Next, we can see that the median is very similar to the mean (the average). This suggests that this data set has a reasonably symmetrical distribution (in other words, a relatively smooth, centred distribution of weights, clustered towards the centre).
In terms of the mode , there is no mode in this data set. This is because each number is present only once and so there cannot be a “most common number”. If there were two people who were both 65 kilograms, for example, then the mode would be 65.
Next up is the standard deviation . 10.6 indicates that there’s quite a wide spread of numbers. We can see this quite easily by looking at the numbers themselves, which range from 55 to 90, which is quite a stretch from the mean of 72.4.
And lastly, the skewness of -0.2 tells us that the data is very slightly negatively skewed. This makes sense since the mean and the median are slightly different.
As you can see, these descriptive statistics give us some useful insight into the data set. Of course, this is a very small data set (only 10 records), so we can’t read into these statistics too much. Also, keep in mind that this is not a list of all possible descriptive statistics – just the most common ones.
But why do all of these numbers matter?
While these descriptive statistics are all fairly basic, they’re important for a few reasons:
- Firstly, they help you get both a macro and micro-level view of your data. In other words, they help you understand both the big picture and the finer details.
- Secondly, they help you spot potential errors in the data – for example, if an average is way higher than you’d expect, or responses to a question are highly varied, this can act as a warning sign that you need to double-check the data.
- And lastly, these descriptive statistics help inform which inferential statistical techniques you can use, as those techniques depend on the skewness (in other words, the symmetry and normality) of the data.
Simply put, descriptive statistics are really important , even though the statistical techniques used are fairly basic. All too often at Grad Coach, we see students skimming over the descriptives in their eagerness to get to the more exciting inferential methods, and then landing up with some very flawed results.
Don’t be a sucker – give your descriptive statistics the love and attention they deserve!
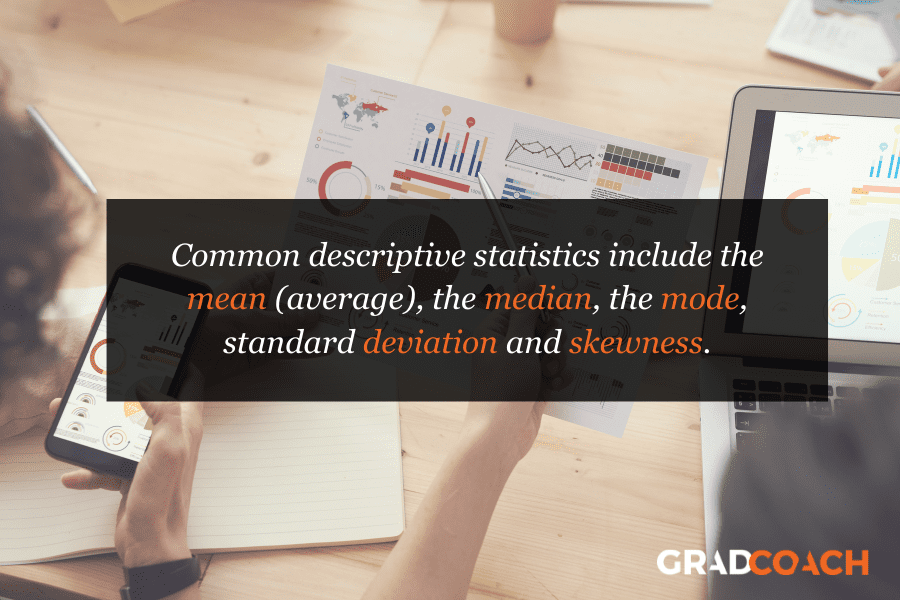
Branch 2: Inferential Statistics
As I mentioned, while descriptive statistics are all about the details of your specific data set – your sample – inferential statistics aim to make inferences about the population . In other words, you’ll use inferential statistics to make predictions about what you’d expect to find in the full population.
What kind of predictions, you ask? Well, there are two common types of predictions that researchers try to make using inferential stats:
- Firstly, predictions about differences between groups – for example, height differences between children grouped by their favourite meal or gender.
- And secondly, relationships between variables – for example, the relationship between body weight and the number of hours a week a person does yoga.
In other words, inferential statistics (when done correctly), allow you to connect the dots and make predictions about what you expect to see in the real world population, based on what you observe in your sample data. For this reason, inferential statistics are used for hypothesis testing – in other words, to test hypotheses that predict changes or differences.
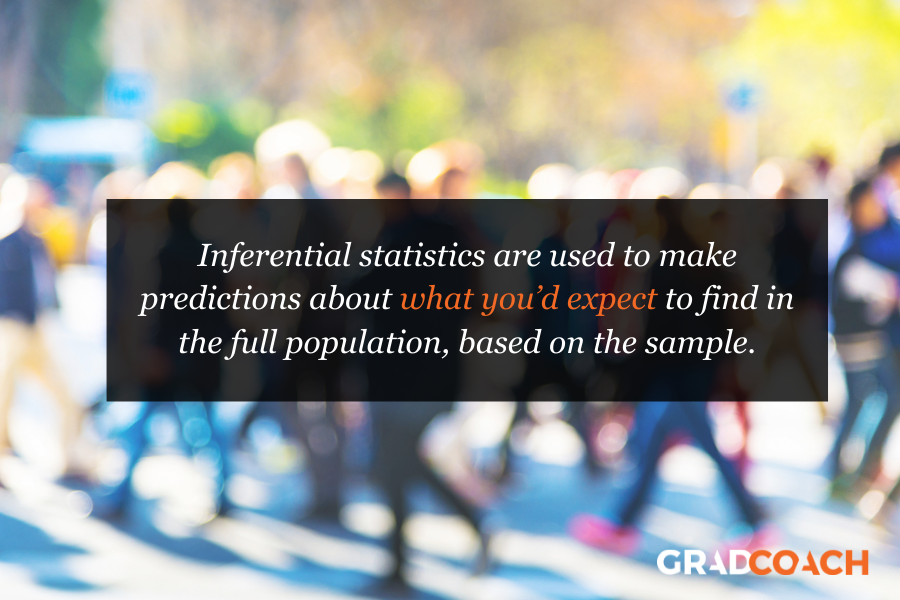
Of course, when you’re working with inferential statistics, the composition of your sample is really important. In other words, if your sample doesn’t accurately represent the population you’re researching, then your findings won’t necessarily be very useful.
For example, if your population of interest is a mix of 50% male and 50% female , but your sample is 80% male , you can’t make inferences about the population based on your sample, since it’s not representative. This area of statistics is called sampling, but we won’t go down that rabbit hole here (it’s a deep one!) – we’ll save that for another post .
What statistics are usually used in this branch?
There are many, many different statistical analysis methods within the inferential branch and it’d be impossible for us to discuss them all here. So we’ll just take a look at some of the most common inferential statistical methods so that you have a solid starting point.
First up are T-Tests . T-tests compare the means (the averages) of two groups of data to assess whether they’re statistically significantly different. In other words, do they have significantly different means, standard deviations and skewness.
This type of testing is very useful for understanding just how similar or different two groups of data are. For example, you might want to compare the mean blood pressure between two groups of people – one that has taken a new medication and one that hasn’t – to assess whether they are significantly different.
Kicking things up a level, we have ANOVA, which stands for “analysis of variance”. This test is similar to a T-test in that it compares the means of various groups, but ANOVA allows you to analyse multiple groups , not just two groups So it’s basically a t-test on steroids…
Next, we have correlation analysis . This type of analysis assesses the relationship between two variables. In other words, if one variable increases, does the other variable also increase, decrease or stay the same. For example, if the average temperature goes up, do average ice creams sales increase too? We’d expect some sort of relationship between these two variables intuitively , but correlation analysis allows us to measure that relationship scientifically .
Lastly, we have regression analysis – this is quite similar to correlation in that it assesses the relationship between variables, but it goes a step further to understand cause and effect between variables, not just whether they move together. In other words, does the one variable actually cause the other one to move, or do they just happen to move together naturally thanks to another force? Just because two variables correlate doesn’t necessarily mean that one causes the other.
Stats overload…
I hear you. To make this all a little more tangible, let’s take a look at an example of a correlation in action.
Here’s a scatter plot demonstrating the correlation (relationship) between weight and height. Intuitively, we’d expect there to be some relationship between these two variables, which is what we see in this scatter plot. In other words, the results tend to cluster together in a diagonal line from bottom left to top right.
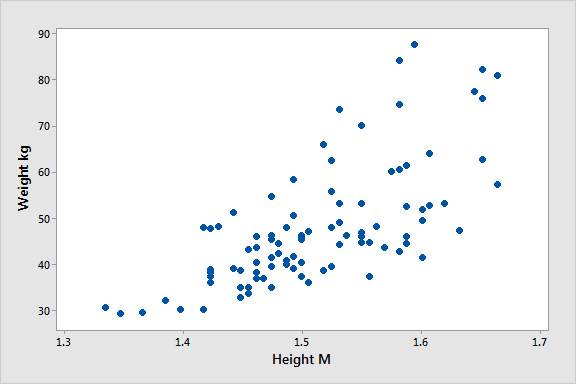
As I mentioned, these are are just a handful of inferential techniques – there are many, many more. Importantly, each statistical method has its own assumptions and limitations .
For example, some methods only work with normally distributed (parametric) data, while other methods are designed specifically for non-parametric data. And that’s exactly why descriptive statistics are so important – they’re the first step to knowing which inferential techniques you can and can’t use.
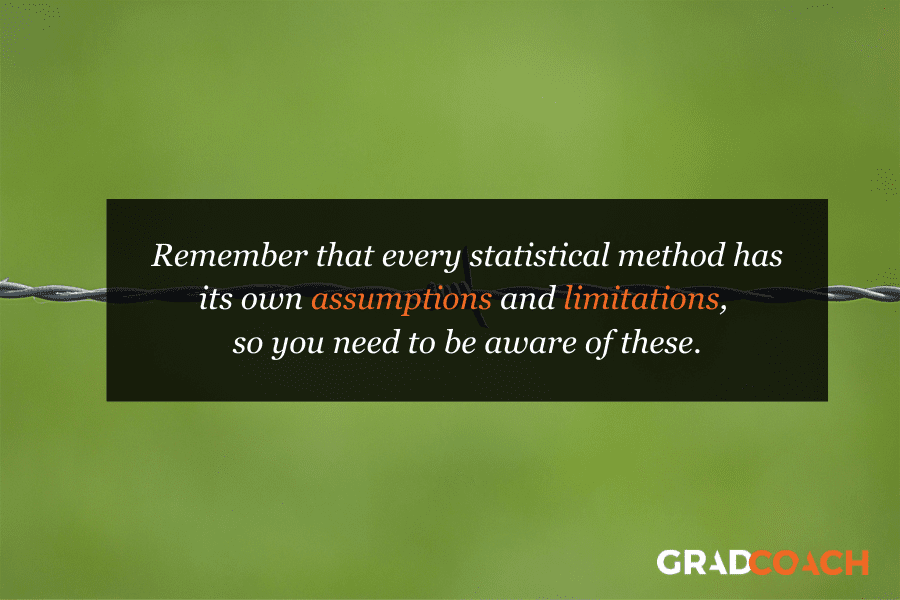
How to choose the right analysis method
To choose the right statistical methods, you need to think about two important factors :
- The type of quantitative data you have (specifically, level of measurement and the shape of the data). And,
- Your research questions and hypotheses
Let’s take a closer look at each of these.
Factor 1 – Data type
The first thing you need to consider is the type of data you’ve collected (or the type of data you will collect). By data types, I’m referring to the four levels of measurement – namely, nominal, ordinal, interval and ratio. If you’re not familiar with this lingo, check out the video below.
Why does this matter?
Well, because different statistical methods and techniques require different types of data. This is one of the “assumptions” I mentioned earlier – every method has its assumptions regarding the type of data.
For example, some techniques work with categorical data (for example, yes/no type questions, or gender or ethnicity), while others work with continuous numerical data (for example, age, weight or income) – and, of course, some work with multiple data types.
If you try to use a statistical method that doesn’t support the data type you have, your results will be largely meaningless . So, make sure that you have a clear understanding of what types of data you’ve collected (or will collect). Once you have this, you can then check which statistical methods would support your data types here .
If you haven’t collected your data yet, you can work in reverse and look at which statistical method would give you the most useful insights, and then design your data collection strategy to collect the correct data types.
Another important factor to consider is the shape of your data . Specifically, does it have a normal distribution (in other words, is it a bell-shaped curve, centred in the middle) or is it very skewed to the left or the right? Again, different statistical techniques work for different shapes of data – some are designed for symmetrical data while others are designed for skewed data.
This is another reminder of why descriptive statistics are so important – they tell you all about the shape of your data.
Factor 2: Your research questions
The next thing you need to consider is your specific research questions, as well as your hypotheses (if you have some). The nature of your research questions and research hypotheses will heavily influence which statistical methods and techniques you should use.
If you’re just interested in understanding the attributes of your sample (as opposed to the entire population), then descriptive statistics are probably all you need. For example, if you just want to assess the means (averages) and medians (centre points) of variables in a group of people.
On the other hand, if you aim to understand differences between groups or relationships between variables and to infer or predict outcomes in the population, then you’ll likely need both descriptive statistics and inferential statistics.
So, it’s really important to get very clear about your research aims and research questions, as well your hypotheses – before you start looking at which statistical techniques to use.
Never shoehorn a specific statistical technique into your research just because you like it or have some experience with it. Your choice of methods must align with all the factors we’ve covered here.
Time to recap…
You’re still with me? That’s impressive. We’ve covered a lot of ground here, so let’s recap on the key points:
- Quantitative data analysis is all about analysing number-based data (which includes categorical and numerical data) using various statistical techniques.
- The two main branches of statistics are descriptive statistics and inferential statistics . Descriptives describe your sample, whereas inferentials make predictions about what you’ll find in the population.
- Common descriptive statistical methods include mean (average), median , standard deviation and skewness .
- Common inferential statistical methods include t-tests , ANOVA , correlation and regression analysis.
- To choose the right statistical methods and techniques, you need to consider the type of data you’re working with , as well as your research questions and hypotheses.
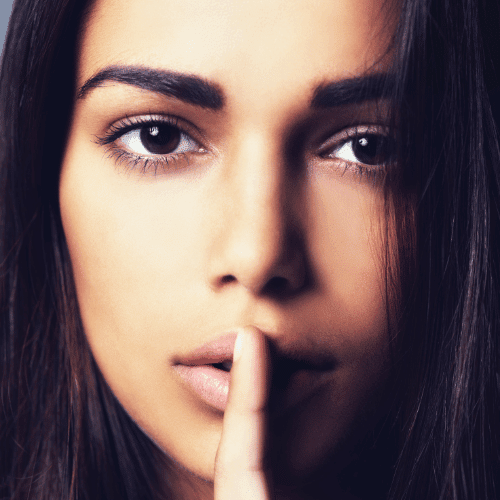
Psst... there’s more!
This post was based on one of our popular Research Bootcamps . If you're working on a research project, you'll definitely want to check this out ...
You Might Also Like:
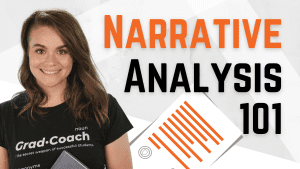
76 Comments
Hi, I have read your article. Such a brilliant post you have created.
Thank you for the feedback. Good luck with your quantitative analysis.
Thank you so much.
Thank you so much. I learnt much well. I love your summaries of the concepts. I had love you to explain how to input data using SPSS
Amazing and simple way of breaking down quantitative methods.
This is beautiful….especially for non-statisticians. I have skimmed through but I wish to read again. and please include me in other articles of the same nature when you do post. I am interested. I am sure, I could easily learn from you and get off the fear that I have had in the past. Thank you sincerely.
Send me every new information you might have.
i need every new information
Thank you for the blog. It is quite informative. Dr Peter Nemaenzhe PhD
It is wonderful. l’ve understood some of the concepts in a more compréhensive manner
Your article is so good! However, I am still a bit lost. I am doing a secondary research on Gun control in the US and increase in crime rates and I am not sure which analysis method I should use?
Based on the given learning points, this is inferential analysis, thus, use ‘t-tests, ANOVA, correlation and regression analysis’
Well explained notes. Am an MPH student and currently working on my thesis proposal, this has really helped me understand some of the things I didn’t know.
I like your page..helpful
wonderful i got my concept crystal clear. thankyou!!
This is really helpful , thank you
Thank you so much this helped
Wonderfully explained
thank u so much, it was so informative
THANKYOU, this was very informative and very helpful
This is great GRADACOACH I am not a statistician but I require more of this in my thesis
Include me in your posts.
This is so great and fully useful. I would like to thank you again and again.
Glad to read this article. I’ve read lot of articles but this article is clear on all concepts. Thanks for sharing.
Thank you so much. This is a very good foundation and intro into quantitative data analysis. Appreciate!
You have a very impressive, simple but concise explanation of data analysis for Quantitative Research here. This is a God-send link for me to appreciate research more. Thank you so much!
Avery good presentation followed by the write up. yes you simplified statistics to make sense even to a layman like me. Thank so much keep it up. The presenter did ell too. i would like more of this for Qualitative and exhaust more of the test example like the Anova.
This is a very helpful article, couldn’t have been clearer. Thank you.
Awesome and phenomenal information.Well done
The video with the accompanying article is super helpful to demystify this topic. Very well done. Thank you so much.
thank you so much, your presentation helped me a lot
I don’t know how should I express that ur article is saviour for me 🥺😍
It is well defined information and thanks for sharing. It helps me a lot in understanding the statistical data.
I gain a lot and thanks for sharing brilliant ideas, so wish to be linked on your email update.
Very helpful and clear .Thank you Gradcoach.
Thank for sharing this article, well organized and information presented are very clear.
VERY INTERESTING AND SUPPORTIVE TO NEW RESEARCHERS LIKE ME. AT LEAST SOME BASICS ABOUT QUANTITATIVE.
An outstanding, well explained and helpful article. This will help me so much with my data analysis for my research project. Thank you!
wow this has just simplified everything i was scared of how i am gonna analyse my data but thanks to you i will be able to do so
simple and constant direction to research. thanks
This is helpful
Great writing!! Comprehensive and very helpful.
Do you provide any assistance for other steps of research methodology like making research problem testing hypothesis report and thesis writing?
Thank you so much for such useful article!
Amazing article. So nicely explained. Wow
Very insightfull. Thanks
I am doing a quality improvement project to determine if the implementation of a protocol will change prescribing habits. Would this be a t-test?
The is a very helpful blog, however, I’m still not sure how to analyze my data collected. I’m doing a research on “Free Education at the University of Guyana”
tnx. fruitful blog!
So I am writing exams and would like to know how do establish which method of data analysis to use from the below research questions: I am a bit lost as to how I determine the data analysis method from the research questions.
Do female employees report higher job satisfaction than male employees with similar job descriptions across the South African telecommunications sector? – I though that maybe Chi Square could be used here. – Is there a gender difference in talented employees’ actual turnover decisions across the South African telecommunications sector? T-tests or Correlation in this one. – Is there a gender difference in the cost of actual turnover decisions across the South African telecommunications sector? T-tests or Correlation in this one. – What practical recommendations can be made to the management of South African telecommunications companies on leveraging gender to mitigate employee turnover decisions?
Your assistance will be appreciated if I could get a response as early as possible tomorrow
This was quite helpful. Thank you so much.
wow I got a lot from this article, thank you very much, keep it up
Thanks for yhe guidance. Can you send me this guidance on my email? To enable offline reading?
Thank you very much, this service is very helpful.
Every novice researcher needs to read this article as it puts things so clear and easy to follow. Its been very helpful.
Wonderful!!!! you explained everything in a way that anyone can learn. Thank you!!
I really enjoyed reading though this. Very easy to follow. Thank you
Many thanks for your useful lecture, I would be really appreciated if you could possibly share with me the PPT of presentation related to Data type?
Thank you very much for sharing, I got much from this article
This is a very informative write-up. Kindly include me in your latest posts.
Very interesting mostly for social scientists
Thank you so much, very helpfull
You’re welcome 🙂
woow, its great, its very informative and well understood because of your way of writing like teaching in front of me in simple languages.
I have been struggling to understand a lot of these concepts. Thank you for the informative piece which is written with outstanding clarity.
very informative article. Easy to understand
Beautiful read, much needed.
Always greet intro and summary. I learn so much from GradCoach
Quite informative. Simple and clear summary.
I thoroughly enjoyed reading your informative and inspiring piece. Your profound insights into this topic truly provide a better understanding of its complexity. I agree with the points you raised, especially when you delved into the specifics of the article. In my opinion, that aspect is often overlooked and deserves further attention.
Absolutely!!! Thank you
Thank you very much for this post. It made me to understand how to do my data analysis.
its nice work and excellent job ,you have made my work easier
Wow! So explicit. Well done.
Submit a Comment Cancel reply
Your email address will not be published. Required fields are marked *
Save my name, email, and website in this browser for the next time I comment.
- Print Friendly
A Step-by-Step Guide to the Data Analysis Process
Like any scientific discipline, data analysis follows a rigorous step-by-step process. Each stage requires different skills and know-how. To get meaningful insights, though, it’s important to understand the process as a whole. An underlying framework is invaluable for producing results that stand up to scrutiny.
In this post, we’ll explore the main steps in the data analysis process. This will cover how to define your goal, collect data, and carry out an analysis. Where applicable, we’ll also use examples and highlight a few tools to make the journey easier. When you’re done, you’ll have a much better understanding of the basics. This will help you tweak the process to fit your own needs.
Here are the steps we’ll take you through:
- Defining the question
- Collecting the data
- Cleaning the data
- Analyzing the data
- Sharing your results
- Embracing failure
On popular request, we’ve also developed a video based on this article. Scroll further along this article to watch that.
Ready? Let’s get started with step one.
1. Step one: Defining the question
The first step in any data analysis process is to define your objective. In data analytics jargon, this is sometimes called the ‘problem statement’.
Defining your objective means coming up with a hypothesis and figuring how to test it. Start by asking: What business problem am I trying to solve? While this might sound straightforward, it can be trickier than it seems. For instance, your organization’s senior management might pose an issue, such as: “Why are we losing customers?” It’s possible, though, that this doesn’t get to the core of the problem. A data analyst’s job is to understand the business and its goals in enough depth that they can frame the problem the right way.
Let’s say you work for a fictional company called TopNotch Learning. TopNotch creates custom training software for its clients. While it is excellent at securing new clients, it has much lower repeat business. As such, your question might not be, “Why are we losing customers?” but, “Which factors are negatively impacting the customer experience?” or better yet: “How can we boost customer retention while minimizing costs?”
Now you’ve defined a problem, you need to determine which sources of data will best help you solve it. This is where your business acumen comes in again. For instance, perhaps you’ve noticed that the sales process for new clients is very slick, but that the production team is inefficient. Knowing this, you could hypothesize that the sales process wins lots of new clients, but the subsequent customer experience is lacking. Could this be why customers don’t come back? Which sources of data will help you answer this question?
Tools to help define your objective
Defining your objective is mostly about soft skills, business knowledge, and lateral thinking. But you’ll also need to keep track of business metrics and key performance indicators (KPIs). Monthly reports can allow you to track problem points in the business. Some KPI dashboards come with a fee, like Databox and DashThis . However, you’ll also find open-source software like Grafana , Freeboard , and Dashbuilder . These are great for producing simple dashboards, both at the beginning and the end of the data analysis process.
2. Step two: Collecting the data
Once you’ve established your objective, you’ll need to create a strategy for collecting and aggregating the appropriate data. A key part of this is determining which data you need. This might be quantitative (numeric) data, e.g. sales figures, or qualitative (descriptive) data, such as customer reviews. All data fit into one of three categories: first-party, second-party, and third-party data. Let’s explore each one.
What is first-party data?
First-party data are data that you, or your company, have directly collected from customers. It might come in the form of transactional tracking data or information from your company’s customer relationship management (CRM) system. Whatever its source, first-party data is usually structured and organized in a clear, defined way. Other sources of first-party data might include customer satisfaction surveys, focus groups, interviews, or direct observation.
What is second-party data?
To enrich your analysis, you might want to secure a secondary data source. Second-party data is the first-party data of other organizations. This might be available directly from the company or through a private marketplace. The main benefit of second-party data is that they are usually structured, and although they will be less relevant than first-party data, they also tend to be quite reliable. Examples of second-party data include website, app or social media activity, like online purchase histories, or shipping data.
What is third-party data?
Third-party data is data that has been collected and aggregated from numerous sources by a third-party organization. Often (though not always) third-party data contains a vast amount of unstructured data points (big data). Many organizations collect big data to create industry reports or to conduct market research. The research and advisory firm Gartner is a good real-world example of an organization that collects big data and sells it on to other companies. Open data repositories and government portals are also sources of third-party data .
Tools to help you collect data
Once you’ve devised a data strategy (i.e. you’ve identified which data you need, and how best to go about collecting them) there are many tools you can use to help you. One thing you’ll need, regardless of industry or area of expertise, is a data management platform (DMP). A DMP is a piece of software that allows you to identify and aggregate data from numerous sources, before manipulating them, segmenting them, and so on. There are many DMPs available. Some well-known enterprise DMPs include Salesforce DMP , SAS , and the data integration platform, Xplenty . If you want to play around, you can also try some open-source platforms like Pimcore or D:Swarm .
Want to learn more about what data analytics is and the process a data analyst follows? We cover this topic (and more) in our free introductory short course for beginners. Check out tutorial one: An introduction to data analytics .
3. Step three: Cleaning the data
Once you’ve collected your data, the next step is to get it ready for analysis. This means cleaning, or ‘scrubbing’ it, and is crucial in making sure that you’re working with high-quality data . Key data cleaning tasks include:
- Removing major errors, duplicates, and outliers —all of which are inevitable problems when aggregating data from numerous sources.
- Removing unwanted data points —extracting irrelevant observations that have no bearing on your intended analysis.
- Bringing structure to your data —general ‘housekeeping’, i.e. fixing typos or layout issues, which will help you map and manipulate your data more easily.
- Filling in major gaps —as you’re tidying up, you might notice that important data are missing. Once you’ve identified gaps, you can go about filling them.
A good data analyst will spend around 70-90% of their time cleaning their data. This might sound excessive. But focusing on the wrong data points (or analyzing erroneous data) will severely impact your results. It might even send you back to square one…so don’t rush it! You’ll find a step-by-step guide to data cleaning here . You may be interested in this introductory tutorial to data cleaning, hosted by Dr. Humera Noor Minhas.
Carrying out an exploratory analysis
Another thing many data analysts do (alongside cleaning data) is to carry out an exploratory analysis. This helps identify initial trends and characteristics, and can even refine your hypothesis. Let’s use our fictional learning company as an example again. Carrying out an exploratory analysis, perhaps you notice a correlation between how much TopNotch Learning’s clients pay and how quickly they move on to new suppliers. This might suggest that a low-quality customer experience (the assumption in your initial hypothesis) is actually less of an issue than cost. You might, therefore, take this into account.
Tools to help you clean your data
Cleaning datasets manually—especially large ones—can be daunting. Luckily, there are many tools available to streamline the process. Open-source tools, such as OpenRefine , are excellent for basic data cleaning, as well as high-level exploration. However, free tools offer limited functionality for very large datasets. Python libraries (e.g. Pandas) and some R packages are better suited for heavy data scrubbing. You will, of course, need to be familiar with the languages. Alternatively, enterprise tools are also available. For example, Data Ladder , which is one of the highest-rated data-matching tools in the industry. There are many more. Why not see which free data cleaning tools you can find to play around with?
4. Step four: Analyzing the data
Finally, you’ve cleaned your data. Now comes the fun bit—analyzing it! The type of data analysis you carry out largely depends on what your goal is. But there are many techniques available. Univariate or bivariate analysis, time-series analysis, and regression analysis are just a few you might have heard of. More important than the different types, though, is how you apply them. This depends on what insights you’re hoping to gain. Broadly speaking, all types of data analysis fit into one of the following four categories.
Descriptive analysis
Descriptive analysis identifies what has already happened . It is a common first step that companies carry out before proceeding with deeper explorations. As an example, let’s refer back to our fictional learning provider once more. TopNotch Learning might use descriptive analytics to analyze course completion rates for their customers. Or they might identify how many users access their products during a particular period. Perhaps they’ll use it to measure sales figures over the last five years. While the company might not draw firm conclusions from any of these insights, summarizing and describing the data will help them to determine how to proceed.
Learn more: What is descriptive analytics?
Diagnostic analysis
Diagnostic analytics focuses on understanding why something has happened . It is literally the diagnosis of a problem, just as a doctor uses a patient’s symptoms to diagnose a disease. Remember TopNotch Learning’s business problem? ‘Which factors are negatively impacting the customer experience?’ A diagnostic analysis would help answer this. For instance, it could help the company draw correlations between the issue (struggling to gain repeat business) and factors that might be causing it (e.g. project costs, speed of delivery, customer sector, etc.) Let’s imagine that, using diagnostic analytics, TopNotch realizes its clients in the retail sector are departing at a faster rate than other clients. This might suggest that they’re losing customers because they lack expertise in this sector. And that’s a useful insight!
Predictive analysis
Predictive analysis allows you to identify future trends based on historical data . In business, predictive analysis is commonly used to forecast future growth, for example. But it doesn’t stop there. Predictive analysis has grown increasingly sophisticated in recent years. The speedy evolution of machine learning allows organizations to make surprisingly accurate forecasts. Take the insurance industry. Insurance providers commonly use past data to predict which customer groups are more likely to get into accidents. As a result, they’ll hike up customer insurance premiums for those groups. Likewise, the retail industry often uses transaction data to predict where future trends lie, or to determine seasonal buying habits to inform their strategies. These are just a few simple examples, but the untapped potential of predictive analysis is pretty compelling.
Prescriptive analysis
Prescriptive analysis allows you to make recommendations for the future. This is the final step in the analytics part of the process. It’s also the most complex. This is because it incorporates aspects of all the other analyses we’ve described. A great example of prescriptive analytics is the algorithms that guide Google’s self-driving cars. Every second, these algorithms make countless decisions based on past and present data, ensuring a smooth, safe ride. Prescriptive analytics also helps companies decide on new products or areas of business to invest in.
Learn more: What are the different types of data analysis?
5. Step five: Sharing your results
You’ve finished carrying out your analyses. You have your insights. The final step of the data analytics process is to share these insights with the wider world (or at least with your organization’s stakeholders!) This is more complex than simply sharing the raw results of your work—it involves interpreting the outcomes, and presenting them in a manner that’s digestible for all types of audiences. Since you’ll often present information to decision-makers, it’s very important that the insights you present are 100% clear and unambiguous. For this reason, data analysts commonly use reports, dashboards, and interactive visualizations to support their findings.
How you interpret and present results will often influence the direction of a business. Depending on what you share, your organization might decide to restructure, to launch a high-risk product, or even to close an entire division. That’s why it’s very important to provide all the evidence that you’ve gathered, and not to cherry-pick data. Ensuring that you cover everything in a clear, concise way will prove that your conclusions are scientifically sound and based on the facts. On the flip side, it’s important to highlight any gaps in the data or to flag any insights that might be open to interpretation. Honest communication is the most important part of the process. It will help the business, while also helping you to excel at your job!
Tools for interpreting and sharing your findings
There are tons of data visualization tools available, suited to different experience levels. Popular tools requiring little or no coding skills include Google Charts , Tableau , Datawrapper , and Infogram . If you’re familiar with Python and R, there are also many data visualization libraries and packages available. For instance, check out the Python libraries Plotly , Seaborn , and Matplotlib . Whichever data visualization tools you use, make sure you polish up your presentation skills, too. Remember: Visualization is great, but communication is key!
You can learn more about storytelling with data in this free, hands-on tutorial . We show you how to craft a compelling narrative for a real dataset, resulting in a presentation to share with key stakeholders. This is an excellent insight into what it’s really like to work as a data analyst!
6. Step six: Embrace your failures
The last ‘step’ in the data analytics process is to embrace your failures. The path we’ve described above is more of an iterative process than a one-way street. Data analytics is inherently messy, and the process you follow will be different for every project. For instance, while cleaning data, you might spot patterns that spark a whole new set of questions. This could send you back to step one (to redefine your objective). Equally, an exploratory analysis might highlight a set of data points you’d never considered using before. Or maybe you find that the results of your core analyses are misleading or erroneous. This might be caused by mistakes in the data, or human error earlier in the process.
While these pitfalls can feel like failures, don’t be disheartened if they happen. Data analysis is inherently chaotic, and mistakes occur. What’s important is to hone your ability to spot and rectify errors. If data analytics was straightforward, it might be easier, but it certainly wouldn’t be as interesting. Use the steps we’ve outlined as a framework, stay open-minded, and be creative. If you lose your way, you can refer back to the process to keep yourself on track.
In this post, we’ve covered the main steps of the data analytics process. These core steps can be amended, re-ordered and re-used as you deem fit, but they underpin every data analyst’s work:
- Define the question —What business problem are you trying to solve? Frame it as a question to help you focus on finding a clear answer.
- Collect data —Create a strategy for collecting data. Which data sources are most likely to help you solve your business problem?
- Clean the data —Explore, scrub, tidy, de-dupe, and structure your data as needed. Do whatever you have to! But don’t rush…take your time!
- Analyze the data —Carry out various analyses to obtain insights. Focus on the four types of data analysis: descriptive, diagnostic, predictive, and prescriptive.
- Share your results —How best can you share your insights and recommendations? A combination of visualization tools and communication is key.
- Embrace your mistakes —Mistakes happen. Learn from them. This is what transforms a good data analyst into a great one.
What next? From here, we strongly encourage you to explore the topic on your own. Get creative with the steps in the data analysis process, and see what tools you can find. As long as you stick to the core principles we’ve described, you can create a tailored technique that works for you.
To learn more, check out our free, 5-day data analytics short course . You might also be interested in the following:
- These are the top 9 data analytics tools
- 10 great places to find free datasets for your next project
- How to build a data analytics portfolio
- AI & NLP
- Churn & Loyalty
- Customer Experience
- Customer Journeys
- Customer Metrics
- Feedback Analysis
- Product Experience
- Product Updates
- Sentiment Analysis
- Surveys & Feedback Collection
- Try Thematic
Welcome to the community
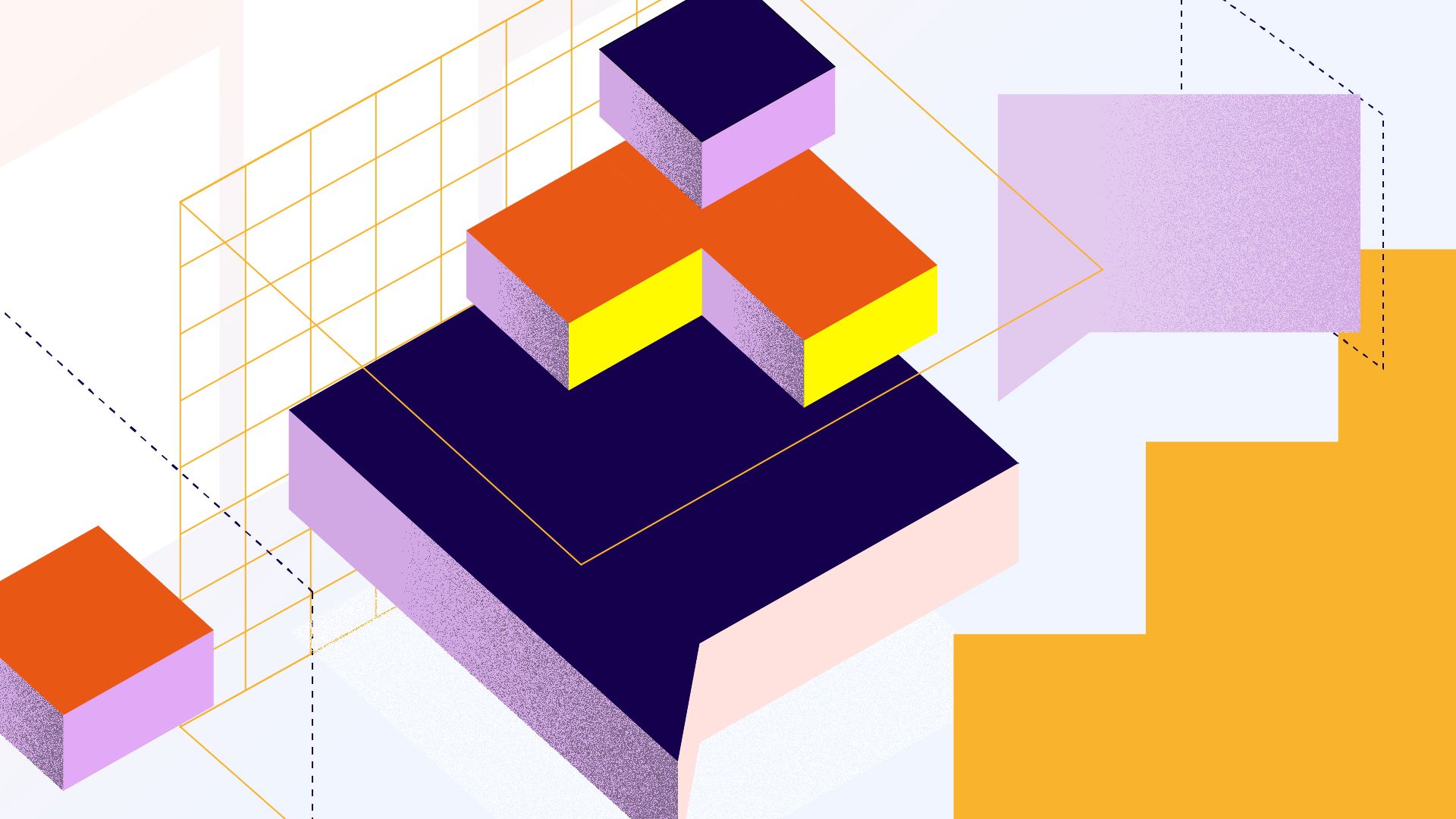
Qualitative Data Analysis: Step-by-Step Guide (Manual vs. Automatic)
When we conduct qualitative methods of research, need to explain changes in metrics or understand people's opinions, we always turn to qualitative data. Qualitative data is typically generated through:
- Interview transcripts
- Surveys with open-ended questions
- Contact center transcripts
- Texts and documents
- Audio and video recordings
- Observational notes
Compared to quantitative data, which captures structured information, qualitative data is unstructured and has more depth. It can answer our questions, can help formulate hypotheses and build understanding.
It's important to understand the differences between quantitative data & qualitative data . But unfortunately, analyzing qualitative data is difficult. While tools like Excel, Tableau and PowerBI crunch and visualize quantitative data with ease, there are a limited number of mainstream tools for analyzing qualitative data . The majority of qualitative data analysis still happens manually.
That said, there are two new trends that are changing this. First, there are advances in natural language processing (NLP) which is focused on understanding human language. Second, there is an explosion of user-friendly software designed for both researchers and businesses. Both help automate the qualitative data analysis process.
In this post we want to teach you how to conduct a successful qualitative data analysis. There are two primary qualitative data analysis methods; manual & automatic. We will teach you how to conduct the analysis manually, and also, automatically using software solutions powered by NLP. We’ll guide you through the steps to conduct a manual analysis, and look at what is involved and the role technology can play in automating this process.
More businesses are switching to fully-automated analysis of qualitative customer data because it is cheaper, faster, and just as accurate. Primarily, businesses purchase subscriptions to feedback analytics platforms so that they can understand customer pain points and sentiment.
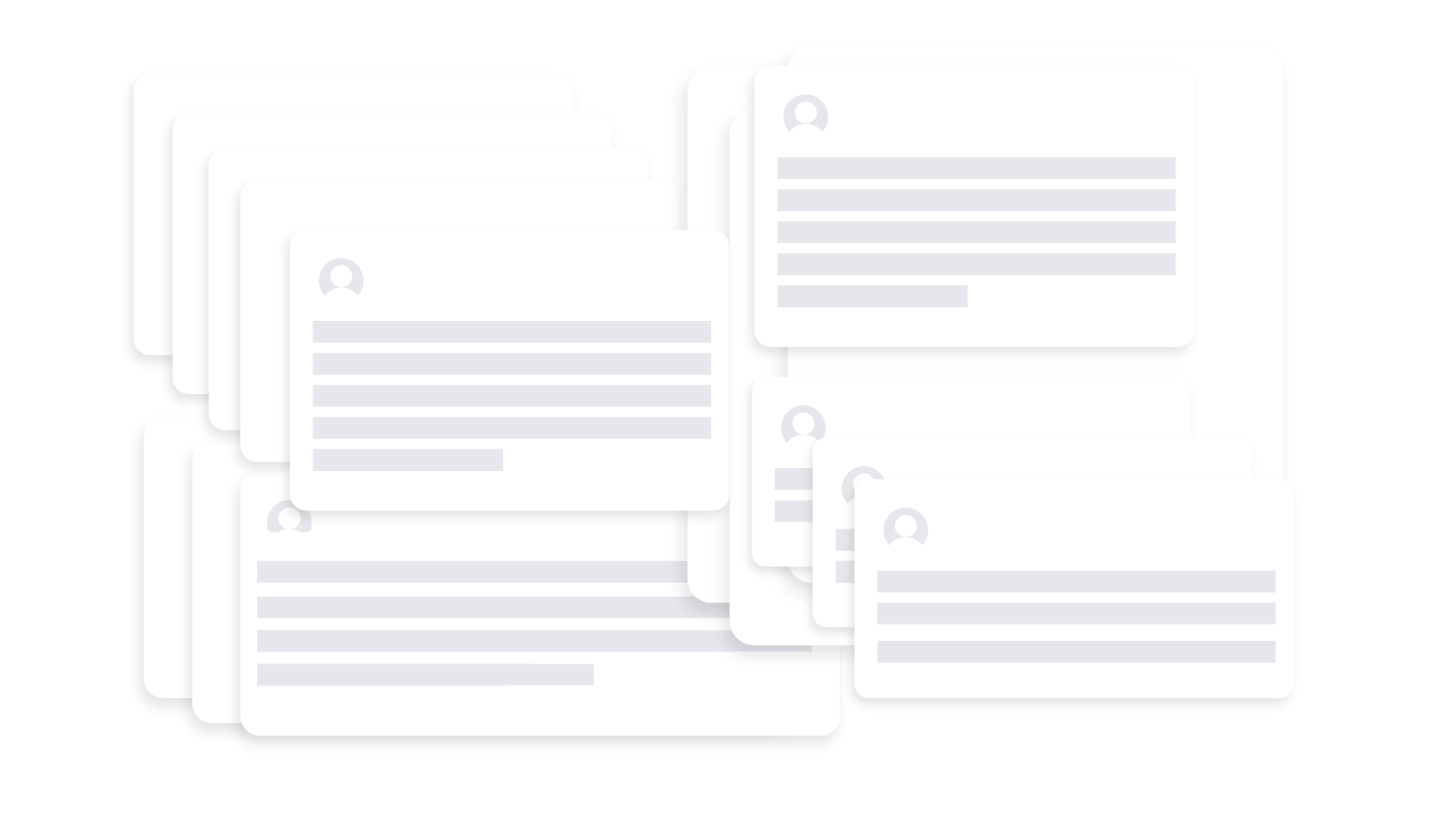
We’ll take you through 5 steps to conduct a successful qualitative data analysis. Within each step we will highlight the key difference between the manual, and automated approach of qualitative researchers. Here's an overview of the steps:
The 5 steps to doing qualitative data analysis
- Gathering and collecting your qualitative data
- Organizing and connecting into your qualitative data
- Coding your qualitative data
- Analyzing the qualitative data for insights
- Reporting on the insights derived from your analysis
What is Qualitative Data Analysis?
Qualitative data analysis is a process of gathering, structuring and interpreting qualitative data to understand what it represents.
Qualitative data is non-numerical and unstructured. Qualitative data generally refers to text, such as open-ended responses to survey questions or user interviews, but also includes audio, photos and video.
Businesses often perform qualitative data analysis on customer feedback. And within this context, qualitative data generally refers to verbatim text data collected from sources such as reviews, complaints, chat messages, support centre interactions, customer interviews, case notes or social media comments.
How is qualitative data analysis different from quantitative data analysis?
Understanding the differences between quantitative & qualitative data is important. When it comes to analyzing data, Qualitative Data Analysis serves a very different role to Quantitative Data Analysis. But what sets them apart?
Qualitative Data Analysis dives into the stories hidden in non-numerical data such as interviews, open-ended survey answers, or notes from observations. It uncovers the ‘whys’ and ‘hows’ giving a deep understanding of people’s experiences and emotions.
Quantitative Data Analysis on the other hand deals with numerical data, using statistics to measure differences, identify preferred options, and pinpoint root causes of issues. It steps back to address questions like "how many" or "what percentage" to offer broad insights we can apply to larger groups.
In short, Qualitative Data Analysis is like a microscope, helping us understand specific detail. Quantitative Data Analysis is like the telescope, giving us a broader perspective. Both are important, working together to decode data for different objectives.
Qualitative Data Analysis methods
Once all the data has been captured, there are a variety of analysis techniques available and the choice is determined by your specific research objectives and the kind of data you’ve gathered. Common qualitative data analysis methods include:
Content Analysis
This is a popular approach to qualitative data analysis. Other qualitative analysis techniques may fit within the broad scope of content analysis. Thematic analysis is a part of the content analysis. Content analysis is used to identify the patterns that emerge from text, by grouping content into words, concepts, and themes. Content analysis is useful to quantify the relationship between all of the grouped content. The Columbia School of Public Health has a detailed breakdown of content analysis .
Narrative Analysis
Narrative analysis focuses on the stories people tell and the language they use to make sense of them. It is particularly useful in qualitative research methods where customer stories are used to get a deep understanding of customers’ perspectives on a specific issue. A narrative analysis might enable us to summarize the outcomes of a focused case study.
Discourse Analysis
Discourse analysis is used to get a thorough understanding of the political, cultural and power dynamics that exist in specific situations. The focus of discourse analysis here is on the way people express themselves in different social contexts. Discourse analysis is commonly used by brand strategists who hope to understand why a group of people feel the way they do about a brand or product.
Thematic Analysis
Thematic analysis is used to deduce the meaning behind the words people use. This is accomplished by discovering repeating themes in text. These meaningful themes reveal key insights into data and can be quantified, particularly when paired with sentiment analysis . Often, the outcome of thematic analysis is a code frame that captures themes in terms of codes, also called categories. So the process of thematic analysis is also referred to as “coding”. A common use-case for thematic analysis in companies is analysis of customer feedback.
Grounded Theory
Grounded theory is a useful approach when little is known about a subject. Grounded theory starts by formulating a theory around a single data case. This means that the theory is “grounded”. Grounded theory analysis is based on actual data, and not entirely speculative. Then additional cases can be examined to see if they are relevant and can add to the original grounded theory.
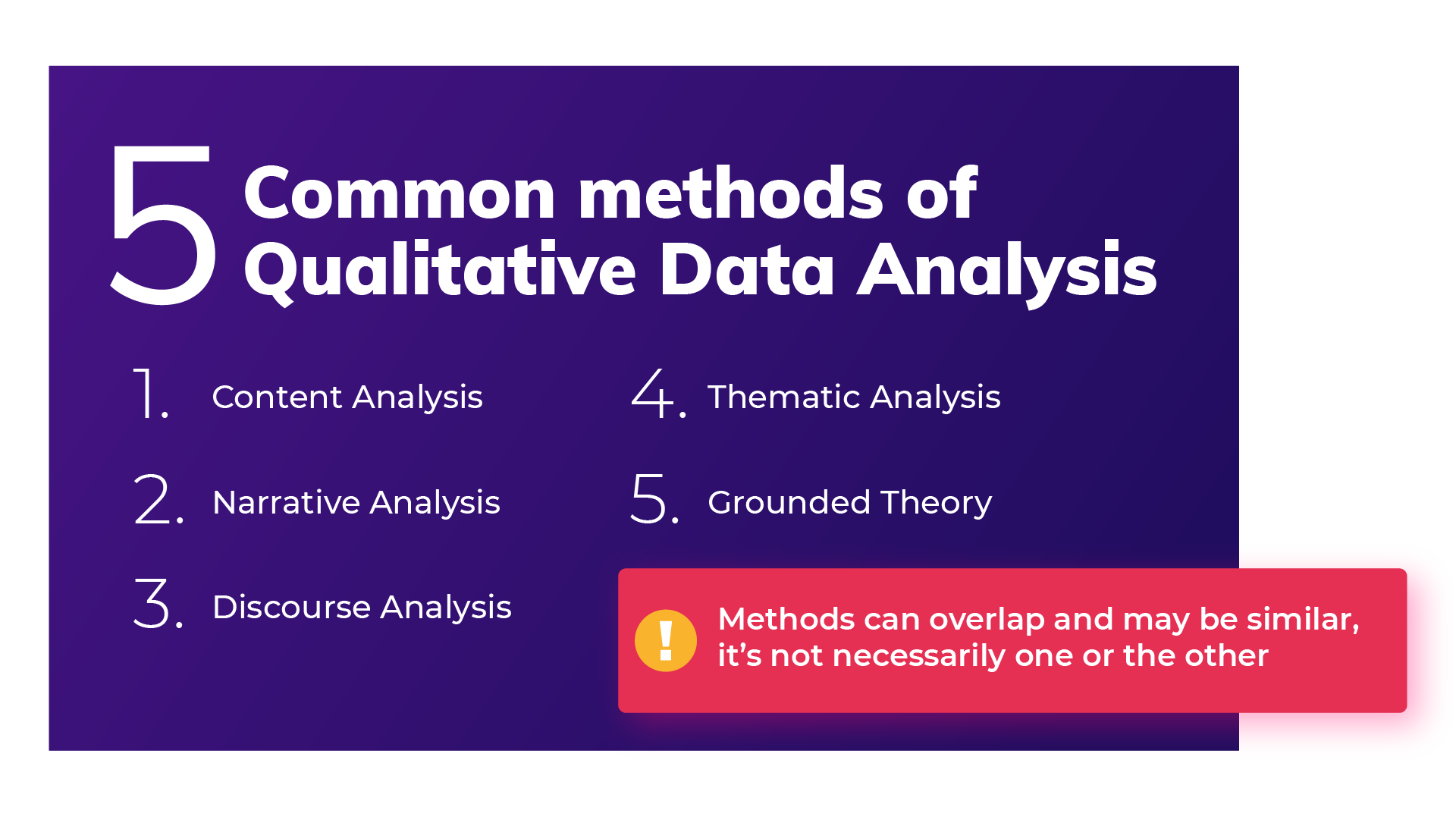
Challenges of Qualitative Data Analysis
While Qualitative Data Analysis offers rich insights, it comes with its challenges. Each unique QDA method has its unique hurdles. Let’s take a look at the challenges researchers and analysts might face, depending on the chosen method.
- Time and Effort (Narrative Analysis): Narrative analysis, which focuses on personal stories, demands patience. Sifting through lengthy narratives to find meaningful insights can be time-consuming, requires dedicated effort.
- Being Objective (Grounded Theory): Grounded theory, building theories from data, faces the challenges of personal biases. Staying objective while interpreting data is crucial, ensuring conclusions are rooted in the data itself.
- Complexity (Thematic Analysis): Thematic analysis involves identifying themes within data, a process that can be intricate. Categorizing and understanding themes can be complex, especially when each piece of data varies in context and structure. Thematic Analysis software can simplify this process.
- Generalizing Findings (Narrative Analysis): Narrative analysis, dealing with individual stories, makes drawing broad challenging. Extending findings from a single narrative to a broader context requires careful consideration.
- Managing Data (Thematic Analysis): Thematic analysis involves organizing and managing vast amounts of unstructured data, like interview transcripts. Managing this can be a hefty task, requiring effective data management strategies.
- Skill Level (Grounded Theory): Grounded theory demands specific skills to build theories from the ground up. Finding or training analysts with these skills poses a challenge, requiring investment in building expertise.
Benefits of qualitative data analysis
Qualitative Data Analysis (QDA) is like a versatile toolkit, offering a tailored approach to understanding your data. The benefits it offers are as diverse as the methods. Let’s explore why choosing the right method matters.
- Tailored Methods for Specific Needs: QDA isn't one-size-fits-all. Depending on your research objectives and the type of data at hand, different methods offer unique benefits. If you want emotive customer stories, narrative analysis paints a strong picture. When you want to explain a score, thematic analysis reveals insightful patterns
- Flexibility with Thematic Analysis: thematic analysis is like a chameleon in the toolkit of QDA. It adapts well to different types of data and research objectives, making it a top choice for any qualitative analysis.
- Deeper Understanding, Better Products: QDA helps you dive into people's thoughts and feelings. This deep understanding helps you build products and services that truly matches what people want, ensuring satisfied customers
- Finding the Unexpected: Qualitative data often reveals surprises that we miss in quantitative data. QDA offers us new ideas and perspectives, for insights we might otherwise miss.
- Building Effective Strategies: Insights from QDA are like strategic guides. They help businesses in crafting plans that match people’s desires.
- Creating Genuine Connections: Understanding people’s experiences lets businesses connect on a real level. This genuine connection helps build trust and loyalty, priceless for any business.
How to do Qualitative Data Analysis: 5 steps
Now we are going to show how you can do your own qualitative data analysis. We will guide you through this process step by step. As mentioned earlier, you will learn how to do qualitative data analysis manually , and also automatically using modern qualitative data and thematic analysis software.
To get best value from the analysis process and research process, it’s important to be super clear about the nature and scope of the question that’s being researched. This will help you select the research collection channels that are most likely to help you answer your question.
Depending on if you are a business looking to understand customer sentiment, or an academic surveying a school, your approach to qualitative data analysis will be unique.
Once you’re clear, there’s a sequence to follow. And, though there are differences in the manual and automatic approaches, the process steps are mostly the same.
The use case for our step-by-step guide is a company looking to collect data (customer feedback data), and analyze the customer feedback - in order to improve customer experience. By analyzing the customer feedback the company derives insights about their business and their customers. You can follow these same steps regardless of the nature of your research. Let’s get started.
Step 1: Gather your qualitative data and conduct research (Conduct qualitative research)
The first step of qualitative research is to do data collection. Put simply, data collection is gathering all of your data for analysis. A common situation is when qualitative data is spread across various sources.
Classic methods of gathering qualitative data
Most companies use traditional methods for gathering qualitative data: conducting interviews with research participants, running surveys, and running focus groups. This data is typically stored in documents, CRMs, databases and knowledge bases. It’s important to examine which data is available and needs to be included in your research project, based on its scope.
Using your existing qualitative feedback
As it becomes easier for customers to engage across a range of different channels, companies are gathering increasingly large amounts of both solicited and unsolicited qualitative feedback.
Most organizations have now invested in Voice of Customer programs , support ticketing systems, chatbot and support conversations, emails and even customer Slack chats.
These new channels provide companies with new ways of getting feedback, and also allow the collection of unstructured feedback data at scale.
The great thing about this data is that it contains a wealth of valubale insights and that it’s already there! When you have a new question about user behavior or your customers, you don’t need to create a new research study or set up a focus group. You can find most answers in the data you already have.
Typically, this data is stored in third-party solutions or a central database, but there are ways to export it or connect to a feedback analysis solution through integrations or an API.
Utilize untapped qualitative data channels
There are many online qualitative data sources you may not have considered. For example, you can find useful qualitative data in social media channels like Twitter or Facebook. Online forums, review sites, and online communities such as Discourse or Reddit also contain valuable data about your customers, or research questions.
If you are considering performing a qualitative benchmark analysis against competitors - the internet is your best friend, and review analysis is a great place to start. Gathering feedback in competitor reviews on sites like Trustpilot, G2, Capterra, Better Business Bureau or on app stores is a great way to perform a competitor benchmark analysis.
Customer feedback analysis software often has integrations into social media and review sites, or you could use a solution like DataMiner to scrape the reviews.
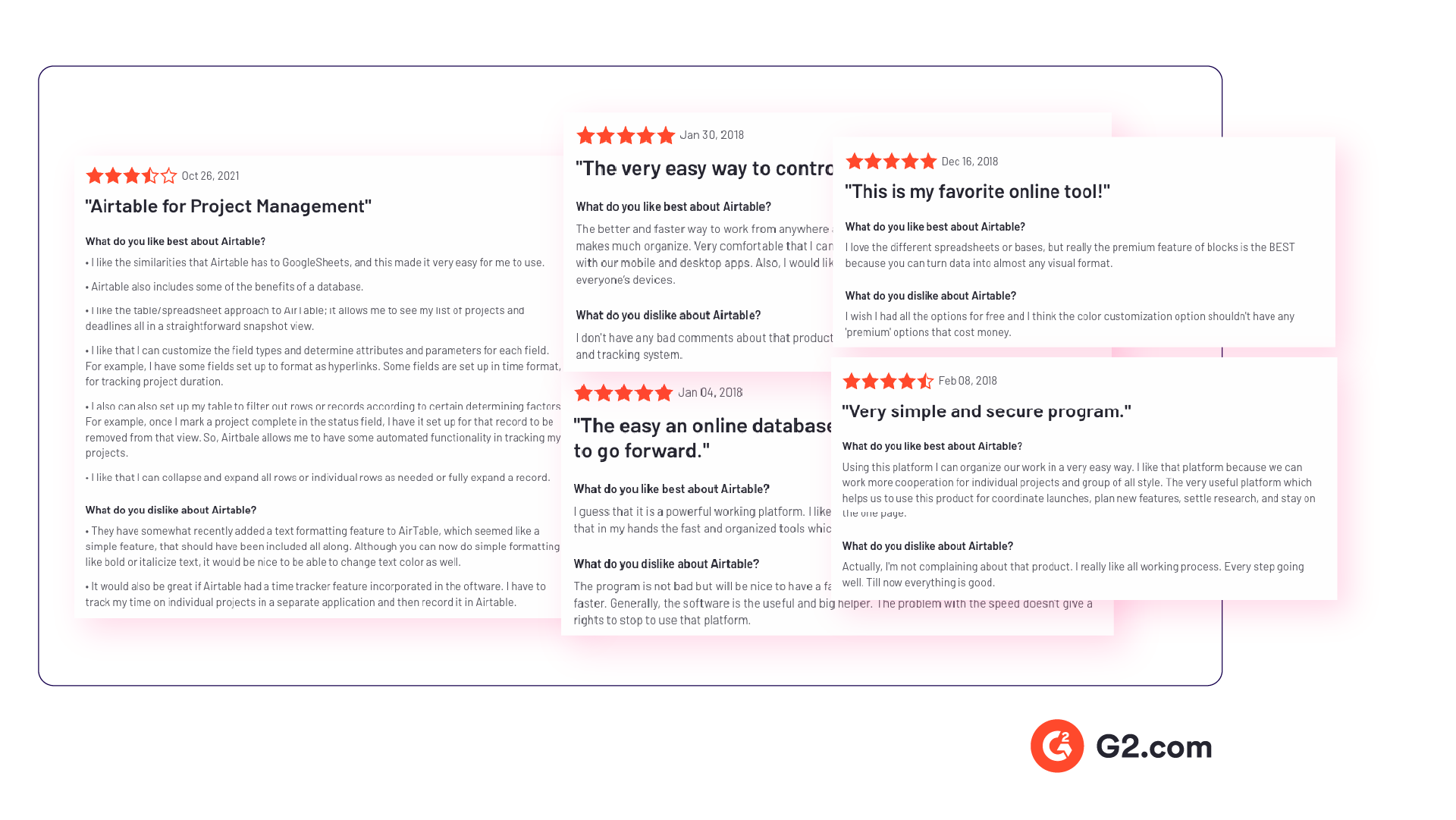
Step 2: Connect & organize all your qualitative data
Now you all have this qualitative data but there’s a problem, the data is unstructured. Before feedback can be analyzed and assigned any value, it needs to be organized in a single place. Why is this important? Consistency!
If all data is easily accessible in one place and analyzed in a consistent manner, you will have an easier time summarizing and making decisions based on this data.
The manual approach to organizing your data
The classic method of structuring qualitative data is to plot all the raw data you’ve gathered into a spreadsheet.
Typically, research and support teams would share large Excel sheets and different business units would make sense of the qualitative feedback data on their own. Each team collects and organizes the data in a way that best suits them, which means the feedback tends to be kept in separate silos.
An alternative and a more robust solution is to store feedback in a central database, like Snowflake or Amazon Redshift .
Keep in mind that when you organize your data in this way, you are often preparing it to be imported into another software. If you go the route of a database, you would need to use an API to push the feedback into a third-party software.
Computer-assisted qualitative data analysis software (CAQDAS)
Traditionally within the manual analysis approach (but not always), qualitative data is imported into CAQDAS software for coding.
In the early 2000s, CAQDAS software was popularised by developers such as ATLAS.ti, NVivo and MAXQDA and eagerly adopted by researchers to assist with the organizing and coding of data.
The benefits of using computer-assisted qualitative data analysis software:
- Assists in the organizing of your data
- Opens you up to exploring different interpretations of your data analysis
- Allows you to share your dataset easier and allows group collaboration (allows for secondary analysis)
However you still need to code the data, uncover the themes and do the analysis yourself. Therefore it is still a manual approach.
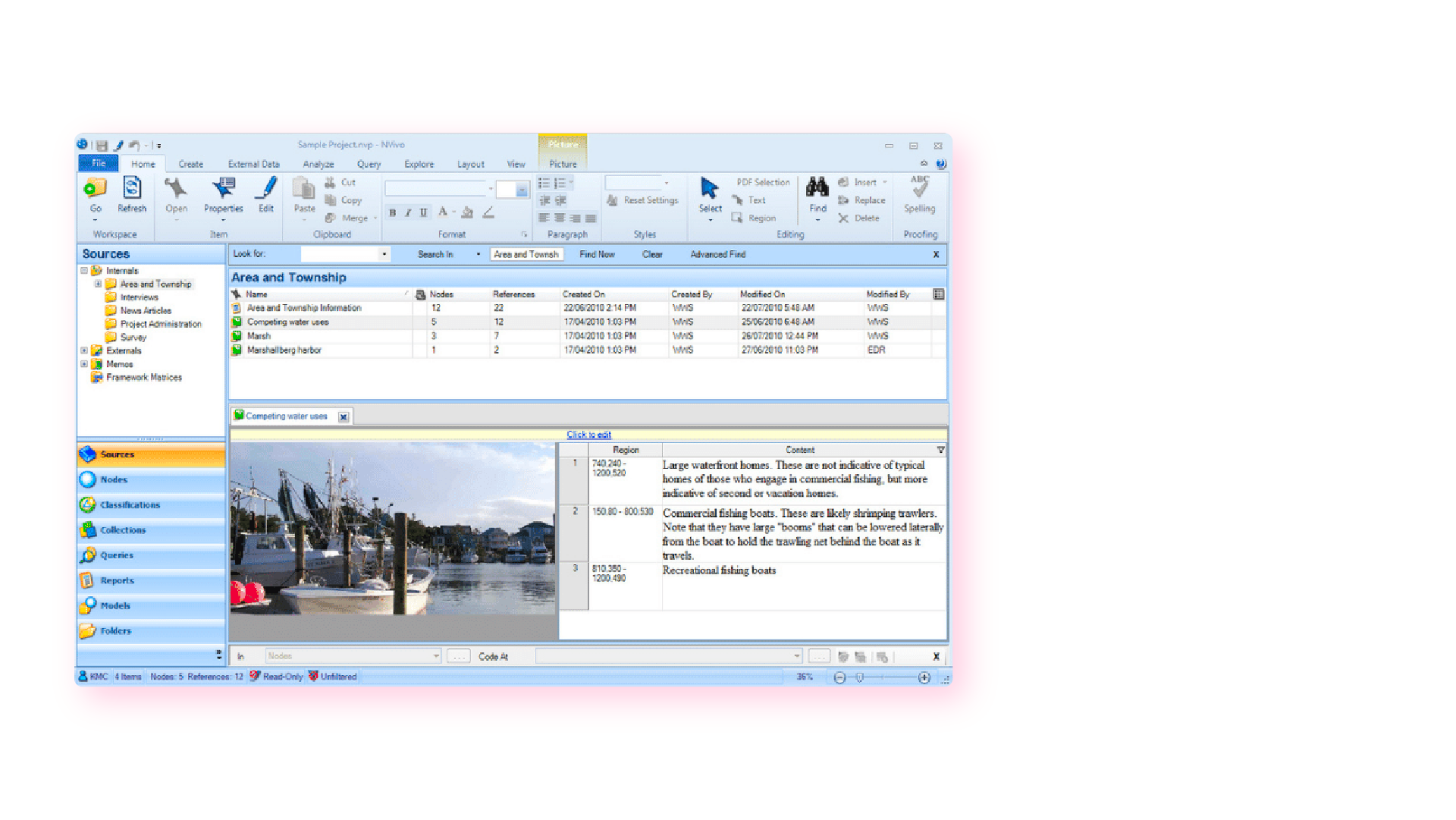
Organizing your qualitative data in a feedback repository
Another solution to organizing your qualitative data is to upload it into a feedback repository where it can be unified with your other data , and easily searchable and taggable. There are a number of software solutions that act as a central repository for your qualitative research data. Here are a couple solutions that you could investigate:
- Dovetail: Dovetail is a research repository with a focus on video and audio transcriptions. You can tag your transcriptions within the platform for theme analysis. You can also upload your other qualitative data such as research reports, survey responses, support conversations, and customer interviews. Dovetail acts as a single, searchable repository. And makes it easier to collaborate with other people around your qualitative research.
- EnjoyHQ: EnjoyHQ is another research repository with similar functionality to Dovetail. It boasts a more sophisticated search engine, but it has a higher starting subscription cost.
Organizing your qualitative data in a feedback analytics platform
If you have a lot of qualitative customer or employee feedback, from the likes of customer surveys or employee surveys, you will benefit from a feedback analytics platform. A feedback analytics platform is a software that automates the process of both sentiment analysis and thematic analysis . Companies use the integrations offered by these platforms to directly tap into their qualitative data sources (review sites, social media, survey responses, etc.). The data collected is then organized and analyzed consistently within the platform.
If you have data prepared in a spreadsheet, it can also be imported into feedback analytics platforms.
Once all this rich data has been organized within the feedback analytics platform, it is ready to be coded and themed, within the same platform. Thematic is a feedback analytics platform that offers one of the largest libraries of integrations with qualitative data sources.
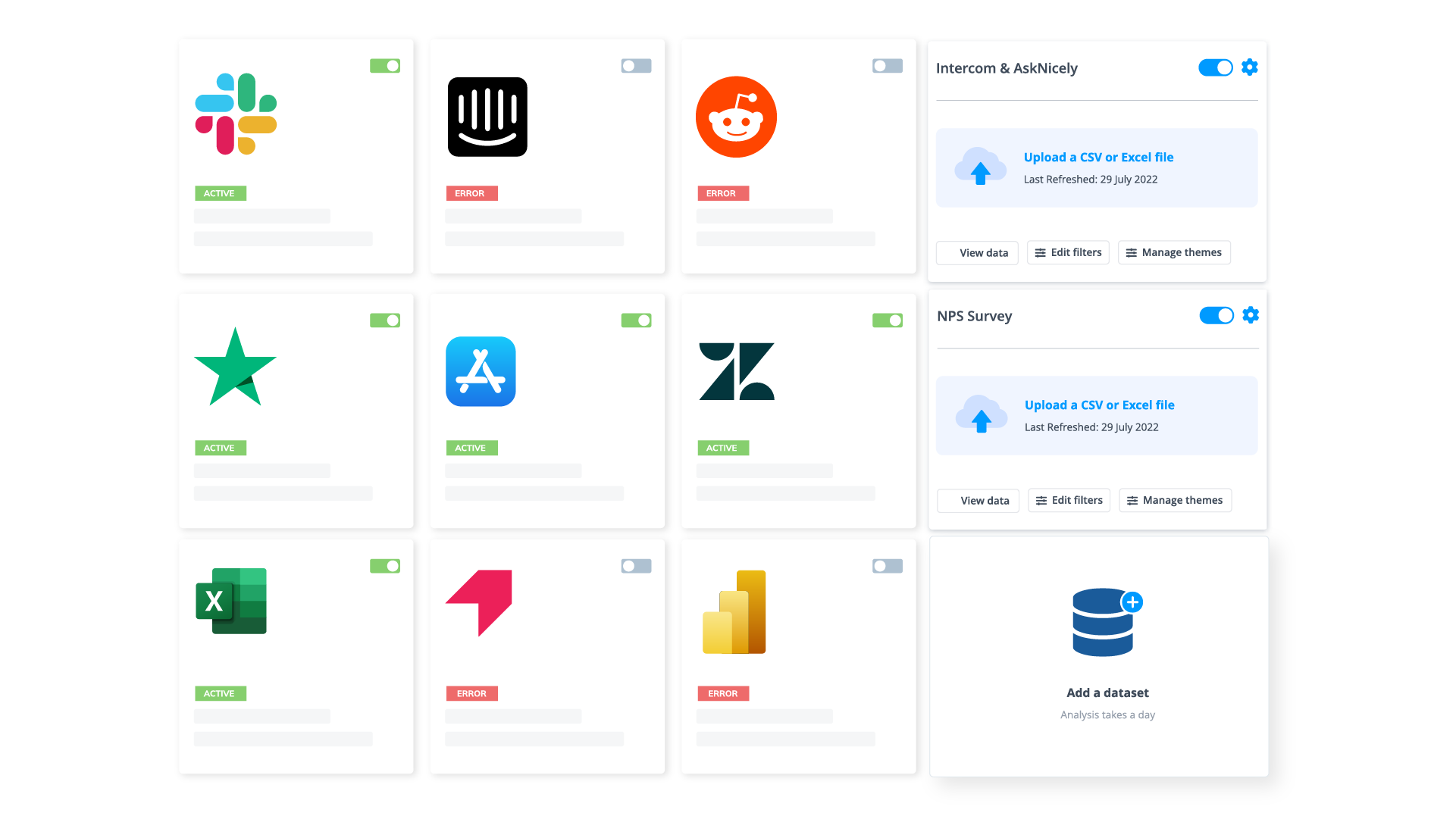
Step 3: Coding your qualitative data
Your feedback data is now organized in one place. Either within your spreadsheet, CAQDAS, feedback repository or within your feedback analytics platform. The next step is to code your feedback data so we can extract meaningful insights in the next step.
Coding is the process of labelling and organizing your data in such a way that you can then identify themes in the data, and the relationships between these themes.
To simplify the coding process, you will take small samples of your customer feedback data, come up with a set of codes, or categories capturing themes, and label each piece of feedback, systematically, for patterns and meaning. Then you will take a larger sample of data, revising and refining the codes for greater accuracy and consistency as you go.
If you choose to use a feedback analytics platform, much of this process will be automated and accomplished for you.
The terms to describe different categories of meaning (‘theme’, ‘code’, ‘tag’, ‘category’ etc) can be confusing as they are often used interchangeably. For clarity, this article will use the term ‘code’.
To code means to identify key words or phrases and assign them to a category of meaning. “I really hate the customer service of this computer software company” would be coded as “poor customer service”.
How to manually code your qualitative data
- Decide whether you will use deductive or inductive coding. Deductive coding is when you create a list of predefined codes, and then assign them to the qualitative data. Inductive coding is the opposite of this, you create codes based on the data itself. Codes arise directly from the data and you label them as you go. You need to weigh up the pros and cons of each coding method and select the most appropriate.
- Read through the feedback data to get a broad sense of what it reveals. Now it’s time to start assigning your first set of codes to statements and sections of text.
- Keep repeating step 2, adding new codes and revising the code description as often as necessary. Once it has all been coded, go through everything again, to be sure there are no inconsistencies and that nothing has been overlooked.
- Create a code frame to group your codes. The coding frame is the organizational structure of all your codes. And there are two commonly used types of coding frames, flat, or hierarchical. A hierarchical code frame will make it easier for you to derive insights from your analysis.
- Based on the number of times a particular code occurs, you can now see the common themes in your feedback data. This is insightful! If ‘bad customer service’ is a common code, it’s time to take action.
We have a detailed guide dedicated to manually coding your qualitative data .
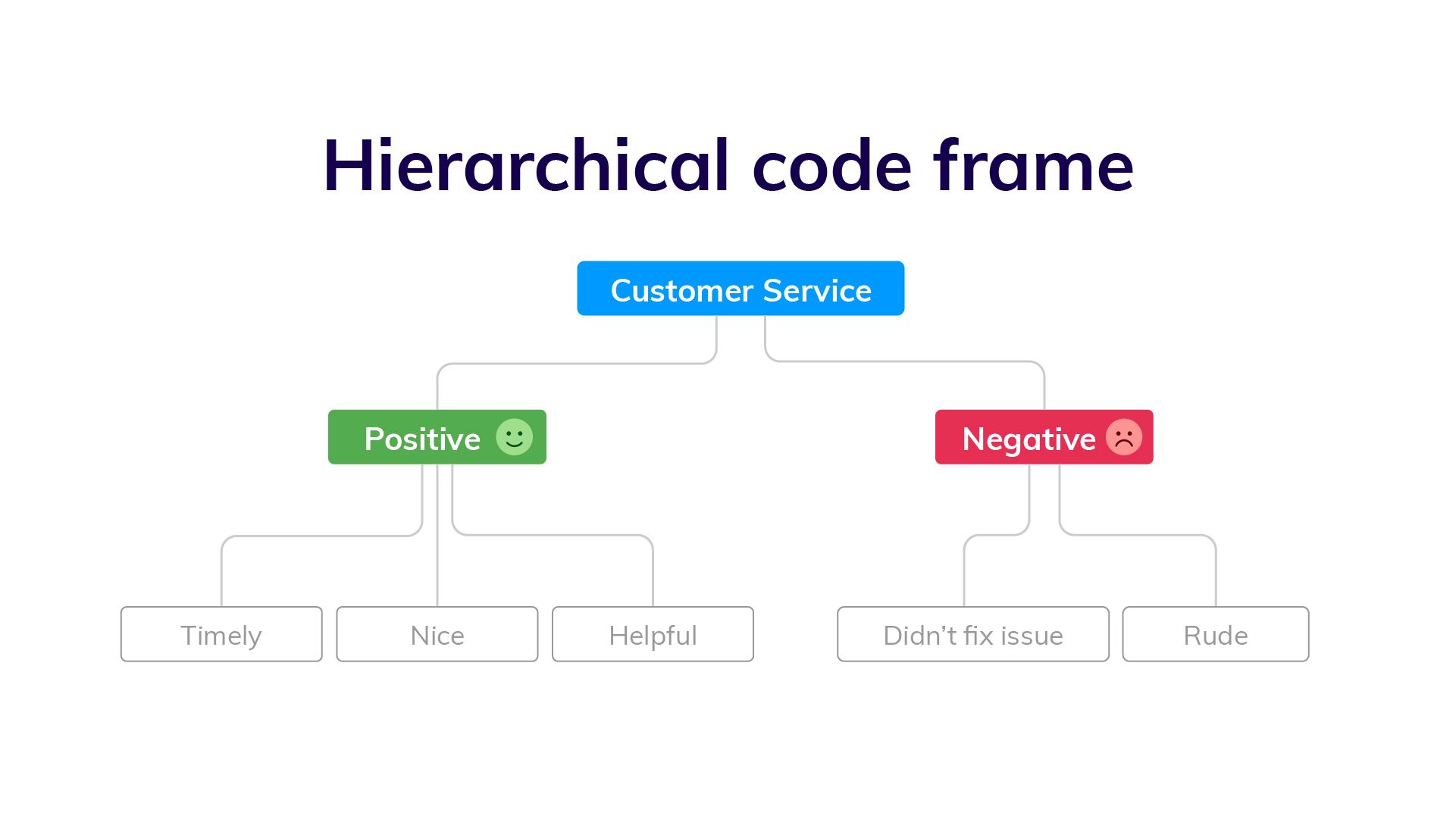
Using software to speed up manual coding of qualitative data
An Excel spreadsheet is still a popular method for coding. But various software solutions can help speed up this process. Here are some examples.
- CAQDAS / NVivo - CAQDAS software has built-in functionality that allows you to code text within their software. You may find the interface the software offers easier for managing codes than a spreadsheet.
- Dovetail/EnjoyHQ - You can tag transcripts and other textual data within these solutions. As they are also repositories you may find it simpler to keep the coding in one platform.
- IBM SPSS - SPSS is a statistical analysis software that may make coding easier than in a spreadsheet.
- Ascribe - Ascribe’s ‘Coder’ is a coding management system. Its user interface will make it easier for you to manage your codes.
Automating the qualitative coding process using thematic analysis software
In solutions which speed up the manual coding process, you still have to come up with valid codes and often apply codes manually to pieces of feedback. But there are also solutions that automate both the discovery and the application of codes.
Advances in machine learning have now made it possible to read, code and structure qualitative data automatically. This type of automated coding is offered by thematic analysis software .
Automation makes it far simpler and faster to code the feedback and group it into themes. By incorporating natural language processing (NLP) into the software, the AI looks across sentences and phrases to identify common themes meaningful statements. Some automated solutions detect repeating patterns and assign codes to them, others make you train the AI by providing examples. You could say that the AI learns the meaning of the feedback on its own.
Thematic automates the coding of qualitative feedback regardless of source. There’s no need to set up themes or categories in advance. Simply upload your data and wait a few minutes. You can also manually edit the codes to further refine their accuracy. Experiments conducted indicate that Thematic’s automated coding is just as accurate as manual coding .
Paired with sentiment analysis and advanced text analytics - these automated solutions become powerful for deriving quality business or research insights.
You could also build your own , if you have the resources!
The key benefits of using an automated coding solution
Automated analysis can often be set up fast and there’s the potential to uncover things that would never have been revealed if you had given the software a prescribed list of themes to look for.
Because the model applies a consistent rule to the data, it captures phrases or statements that a human eye might have missed.
Complete and consistent analysis of customer feedback enables more meaningful findings. Leading us into step 4.
Step 4: Analyze your data: Find meaningful insights
Now we are going to analyze our data to find insights. This is where we start to answer our research questions. Keep in mind that step 4 and step 5 (tell the story) have some overlap . This is because creating visualizations is both part of analysis process and reporting.
The task of uncovering insights is to scour through the codes that emerge from the data and draw meaningful correlations from them. It is also about making sure each insight is distinct and has enough data to support it.
Part of the analysis is to establish how much each code relates to different demographics and customer profiles, and identify whether there’s any relationship between these data points.
Manually create sub-codes to improve the quality of insights
If your code frame only has one level, you may find that your codes are too broad to be able to extract meaningful insights. This is where it is valuable to create sub-codes to your primary codes. This process is sometimes referred to as meta coding.
Note: If you take an inductive coding approach, you can create sub-codes as you are reading through your feedback data and coding it.
While time-consuming, this exercise will improve the quality of your analysis. Here is an example of what sub-codes could look like.
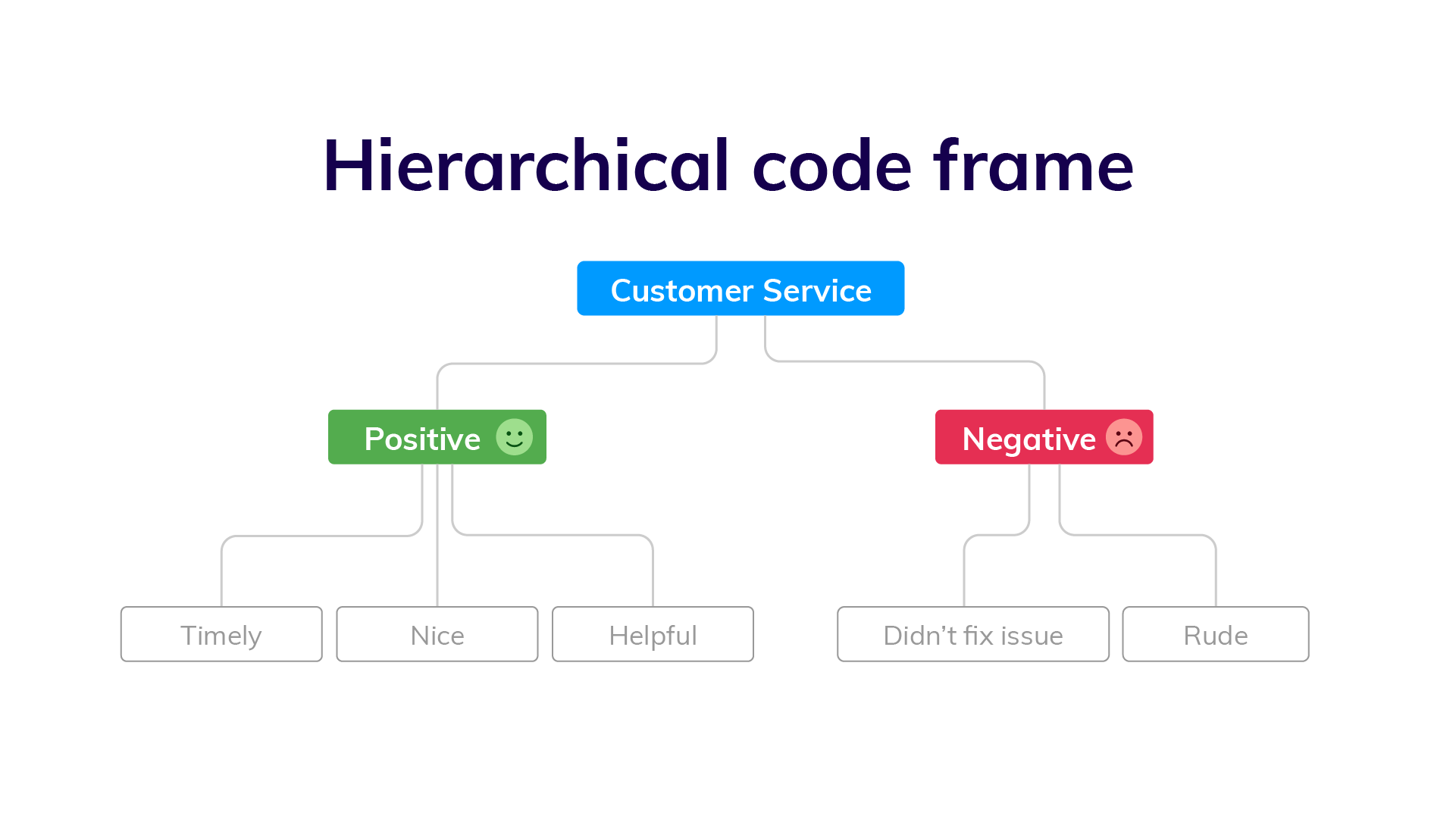
You need to carefully read your qualitative data to create quality sub-codes. But as you can see, the depth of analysis is greatly improved. By calculating the frequency of these sub-codes you can get insight into which customer service problems you can immediately address.
Correlate the frequency of codes to customer segments
Many businesses use customer segmentation . And you may have your own respondent segments that you can apply to your qualitative analysis. Segmentation is the practise of dividing customers or research respondents into subgroups.
Segments can be based on:
- Demographic
- And any other data type that you care to segment by
It is particularly useful to see the occurrence of codes within your segments. If one of your customer segments is considered unimportant to your business, but they are the cause of nearly all customer service complaints, it may be in your best interest to focus attention elsewhere. This is a useful insight!
Manually visualizing coded qualitative data
There are formulas you can use to visualize key insights in your data. The formulas we will suggest are imperative if you are measuring a score alongside your feedback.
If you are collecting a metric alongside your qualitative data this is a key visualization. Impact answers the question: “What’s the impact of a code on my overall score?”. Using Net Promoter Score (NPS) as an example, first you need to:
- Calculate overall NPS
- Calculate NPS in the subset of responses that do not contain that theme
- Subtract B from A
Then you can use this simple formula to calculate code impact on NPS .
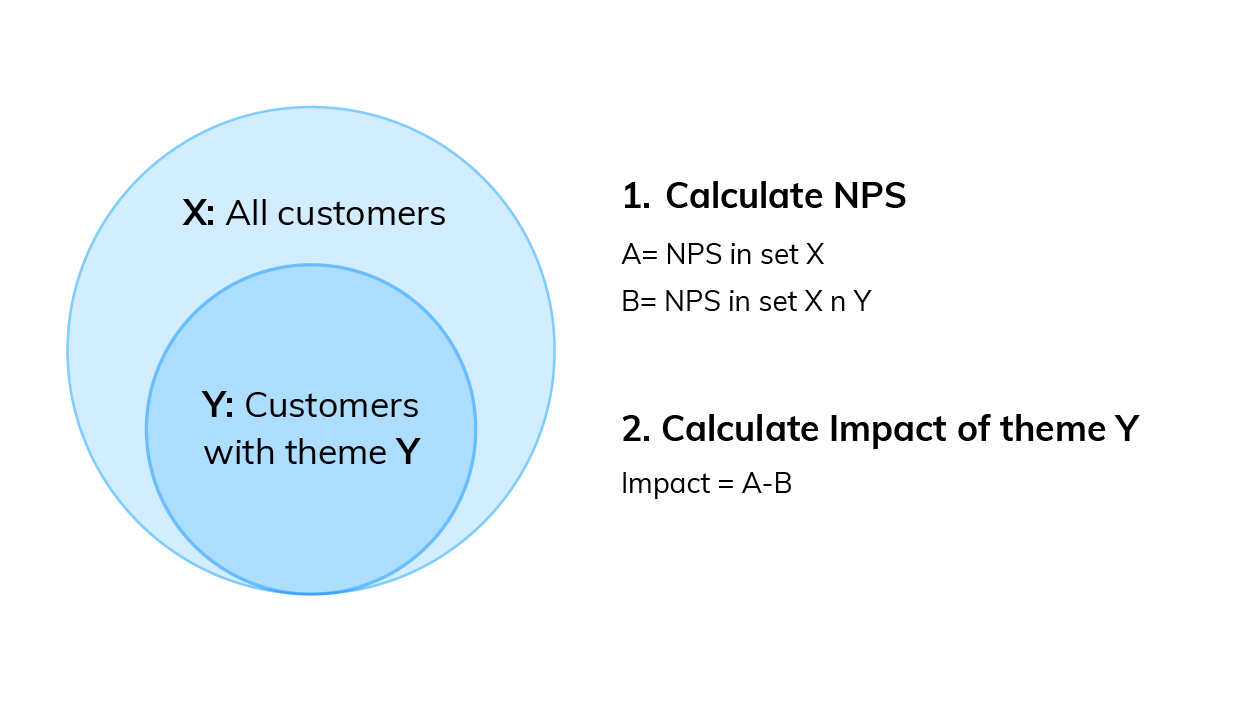
You can then visualize this data using a bar chart.
You can download our CX toolkit - it includes a template to recreate this.
Trends over time
This analysis can help you answer questions like: “Which codes are linked to decreases or increases in my score over time?”
We need to compare two sequences of numbers: NPS over time and code frequency over time . Using Excel, calculate the correlation between the two sequences, which can be either positive (the more codes the higher the NPS, see picture below), or negative (the more codes the lower the NPS).
Now you need to plot code frequency against the absolute value of code correlation with NPS. Here is the formula:
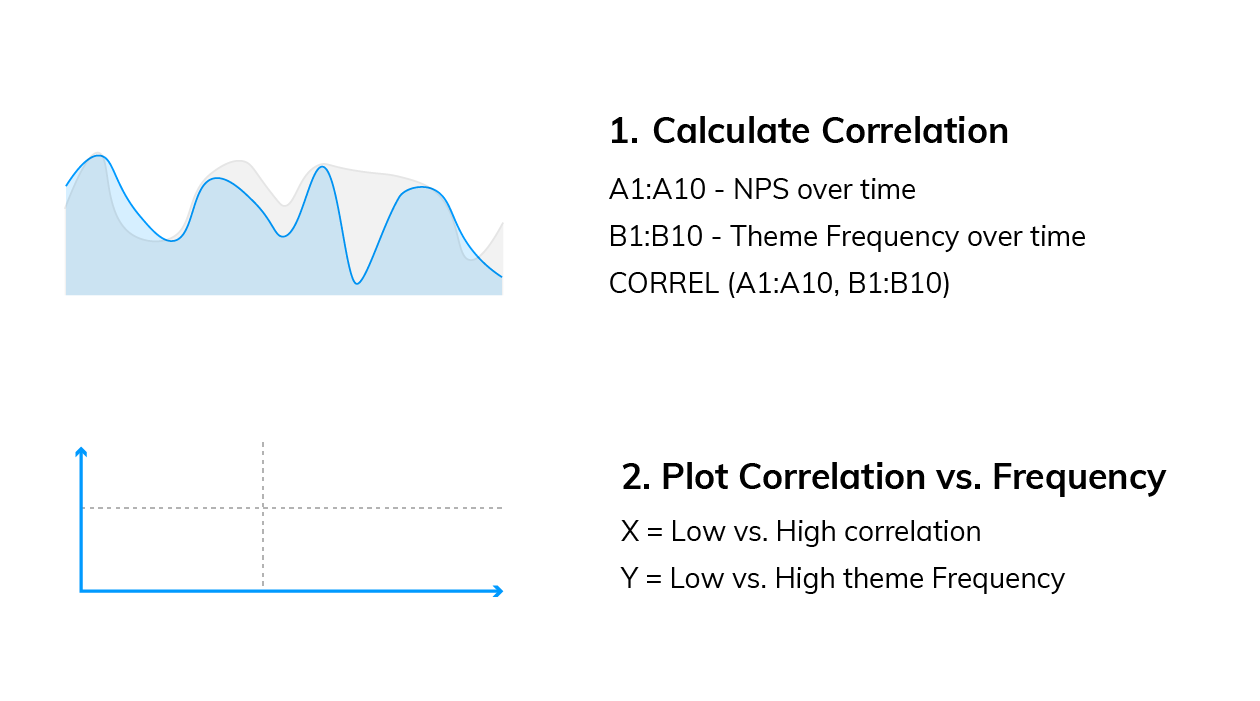
The visualization could look like this:
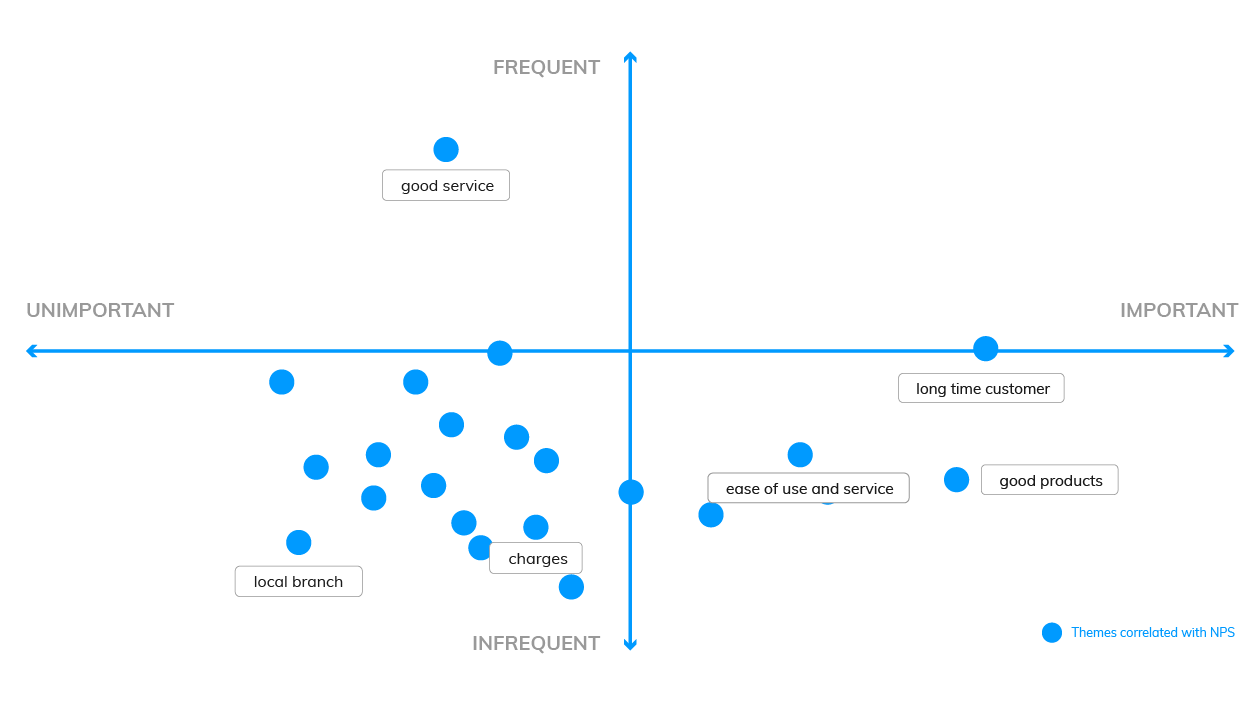
These are two examples, but there are more. For a third manual formula, and to learn why word clouds are not an insightful form of analysis, read our visualizations article .
Using a text analytics solution to automate analysis
Automated text analytics solutions enable codes and sub-codes to be pulled out of the data automatically. This makes it far faster and easier to identify what’s driving negative or positive results. And to pick up emerging trends and find all manner of rich insights in the data.
Another benefit of AI-driven text analytics software is its built-in capability for sentiment analysis, which provides the emotive context behind your feedback and other qualitative textual data therein.
Thematic provides text analytics that goes further by allowing users to apply their expertise on business context to edit or augment the AI-generated outputs.
Since the move away from manual research is generally about reducing the human element, adding human input to the technology might sound counter-intuitive. However, this is mostly to make sure important business nuances in the feedback aren’t missed during coding. The result is a higher accuracy of analysis. This is sometimes referred to as augmented intelligence .
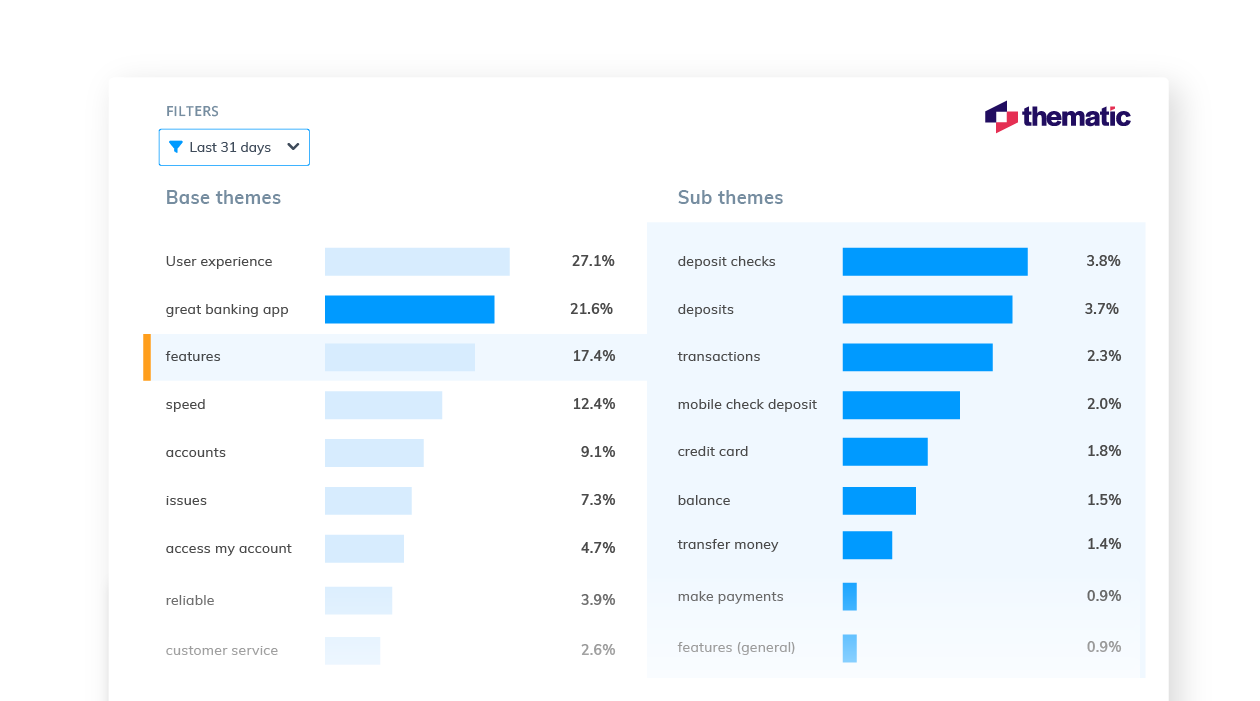
Step 5: Report on your data: Tell the story
The last step of analyzing your qualitative data is to report on it, to tell the story. At this point, the codes are fully developed and the focus is on communicating the narrative to the audience.
A coherent outline of the qualitative research, the findings and the insights is vital for stakeholders to discuss and debate before they can devise a meaningful course of action.
Creating graphs and reporting in Powerpoint
Typically, qualitative researchers take the tried and tested approach of distilling their report into a series of charts, tables and other visuals which are woven into a narrative for presentation in Powerpoint.
Using visualization software for reporting
With data transformation and APIs, the analyzed data can be shared with data visualisation software, such as Power BI or Tableau , Google Studio or Looker. Power BI and Tableau are among the most preferred options.
Visualizing your insights inside a feedback analytics platform
Feedback analytics platforms, like Thematic, incorporate visualisation tools that intuitively turn key data and insights into graphs. This removes the time consuming work of constructing charts to visually identify patterns and creates more time to focus on building a compelling narrative that highlights the insights, in bite-size chunks, for executive teams to review.
Using a feedback analytics platform with visualization tools means you don’t have to use a separate product for visualizations. You can export graphs into Powerpoints straight from the platforms.
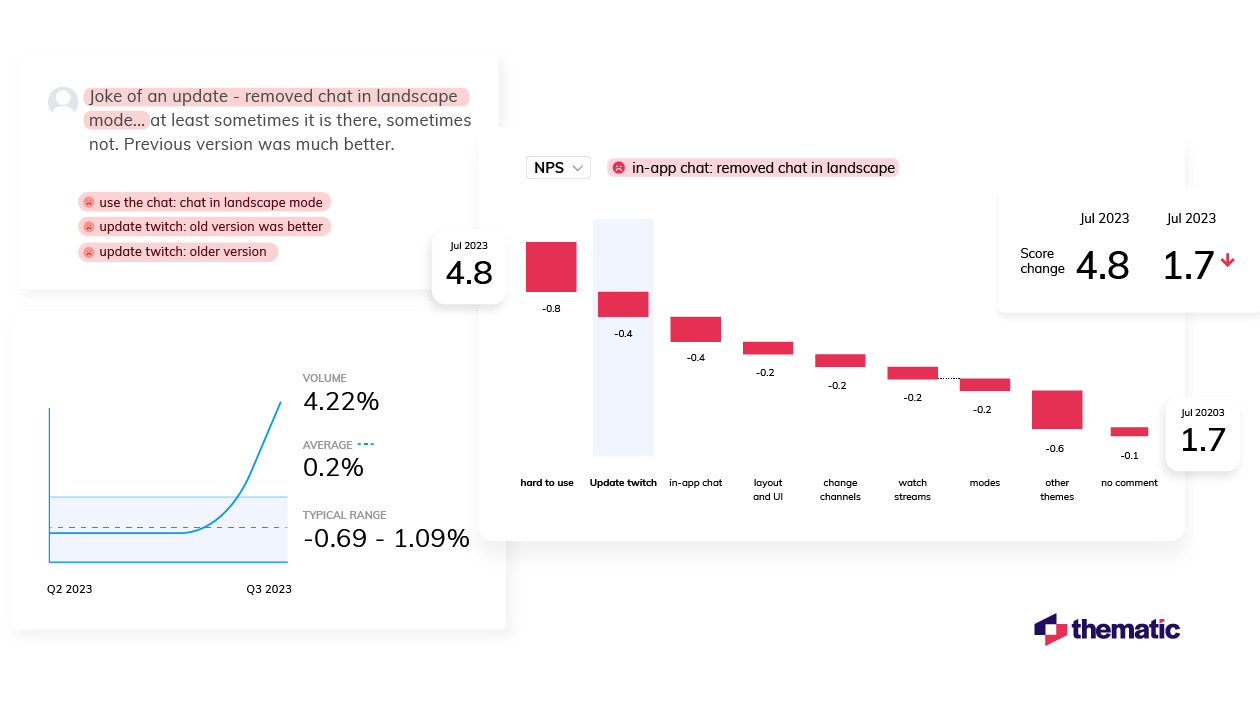
Conclusion - Manual or Automated?
There are those who remain deeply invested in the manual approach - because it’s familiar, because they’re reluctant to spend money and time learning new software, or because they’ve been burned by the overpromises of AI.
For projects that involve small datasets, manual analysis makes sense. For example, if the objective is simply to quantify a simple question like “Do customers prefer X concepts to Y?”. If the findings are being extracted from a small set of focus groups and interviews, sometimes it’s easier to just read them
However, as new generations come into the workplace, it’s technology-driven solutions that feel more comfortable and practical. And the merits are undeniable. Especially if the objective is to go deeper and understand the ‘why’ behind customers’ preference for X or Y. And even more especially if time and money are considerations.
The ability to collect a free flow of qualitative feedback data at the same time as the metric means AI can cost-effectively scan, crunch, score and analyze a ton of feedback from one system in one go. And time-intensive processes like focus groups, or coding, that used to take weeks, can now be completed in a matter of hours or days.
But aside from the ever-present business case to speed things up and keep costs down, there are also powerful research imperatives for automated analysis of qualitative data: namely, accuracy and consistency.
Finding insights hidden in feedback requires consistency, especially in coding. Not to mention catching all the ‘unknown unknowns’ that can skew research findings and steering clear of cognitive bias.
Some say without manual data analysis researchers won’t get an accurate “feel” for the insights. However, the larger data sets are, the harder it is to sort through the feedback and organize feedback that has been pulled from different places. And, the more difficult it is to stay on course, the greater the risk of drawing incorrect, or incomplete, conclusions grows.
Though the process steps for qualitative data analysis have remained pretty much unchanged since psychologist Paul Felix Lazarsfeld paved the path a hundred years ago, the impact digital technology has had on types of qualitative feedback data and the approach to the analysis are profound.
If you want to try an automated feedback analysis solution on your own qualitative data, you can get started with Thematic .
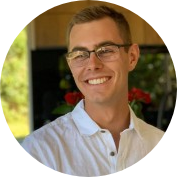
Community & Marketing
Tyler manages our community of CX, insights & analytics professionals. Tyler's goal is to help unite insights professionals around common challenges.
We make it easy to discover the customer and product issues that matter.
Unlock the value of feedback at scale, in one platform. Try it for free now!
- Questions to ask your Feedback Analytics vendor
- How to end customer churn for good
- Scalable analysis of NPS verbatims
- 5 Text analytics approaches
- How to calculate the ROI of CX
Our experts will show you how Thematic works, how to discover pain points and track the ROI of decisions. To access your free trial, book a personal demo today.
Recent posts
When two major storms wreaked havoc on Auckland and Watercare’s infrastructurem the utility went through a CX crisis. With a massive influx of calls to their support center, Thematic helped them get inisghts from this data to forge a new approach to restore services and satisfaction levels.
Become a qualitative theming pro! Creating a perfect code frame is hard, but thematic analysis software makes the process much easier.
Qualtrics is one of the most well-known and powerful Customer Feedback Management platforms. But even so, it has limitations. We recently hosted a live panel where data analysts from two well-known brands shared their experiences with Qualtrics, and how they extended this platform’s capabilities. Below, we’ll share the
How to conduct a meta-analysis in eight steps: a practical guide
- Open access
- Published: 30 November 2021
- Volume 72 , pages 1–19, ( 2022 )
Cite this article
You have full access to this open access article
- Christopher Hansen 1 ,
- Holger Steinmetz 2 &
- Jörn Block 3 , 4 , 5
153k Accesses
50 Citations
158 Altmetric
Explore all metrics
Avoid common mistakes on your manuscript.
1 Introduction
“Scientists have known for centuries that a single study will not resolve a major issue. Indeed, a small sample study will not even resolve a minor issue. Thus, the foundation of science is the cumulation of knowledge from the results of many studies.” (Hunter et al. 1982 , p. 10)
Meta-analysis is a central method for knowledge accumulation in many scientific fields (Aguinis et al. 2011c ; Kepes et al. 2013 ). Similar to a narrative review, it serves as a synopsis of a research question or field. However, going beyond a narrative summary of key findings, a meta-analysis adds value in providing a quantitative assessment of the relationship between two target variables or the effectiveness of an intervention (Gurevitch et al. 2018 ). Also, it can be used to test competing theoretical assumptions against each other or to identify important moderators where the results of different primary studies differ from each other (Aguinis et al. 2011b ; Bergh et al. 2016 ). Rooted in the synthesis of the effectiveness of medical and psychological interventions in the 1970s (Glass 2015 ; Gurevitch et al. 2018 ), meta-analysis is nowadays also an established method in management research and related fields.
The increasing importance of meta-analysis in management research has resulted in the publication of guidelines in recent years that discuss the merits and best practices in various fields, such as general management (Bergh et al. 2016 ; Combs et al. 2019 ; Gonzalez-Mulé and Aguinis 2018 ), international business (Steel et al. 2021 ), economics and finance (Geyer-Klingeberg et al. 2020 ; Havranek et al. 2020 ), marketing (Eisend 2017 ; Grewal et al. 2018 ), and organizational studies (DeSimone et al. 2020 ; Rudolph et al. 2020 ). These articles discuss existing and trending methods and propose solutions for often experienced problems. This editorial briefly summarizes the insights of these papers; provides a workflow of the essential steps in conducting a meta-analysis; suggests state-of-the art methodological procedures; and points to other articles for in-depth investigation. Thus, this article has two goals: (1) based on the findings of previous editorials and methodological articles, it defines methodological recommendations for meta-analyses submitted to Management Review Quarterly (MRQ); and (2) it serves as a practical guide for researchers who have little experience with meta-analysis as a method but plan to conduct one in the future.
2 Eight steps in conducting a meta-analysis
2.1 step 1: defining the research question.
The first step in conducting a meta-analysis, as with any other empirical study, is the definition of the research question. Most importantly, the research question determines the realm of constructs to be considered or the type of interventions whose effects shall be analyzed. When defining the research question, two hurdles might develop. First, when defining an adequate study scope, researchers must consider that the number of publications has grown exponentially in many fields of research in recent decades (Fortunato et al. 2018 ). On the one hand, a larger number of studies increases the potentially relevant literature basis and enables researchers to conduct meta-analyses. Conversely, scanning a large amount of studies that could be potentially relevant for the meta-analysis results in a perhaps unmanageable workload. Thus, Steel et al. ( 2021 ) highlight the importance of balancing manageability and relevance when defining the research question. Second, similar to the number of primary studies also the number of meta-analyses in management research has grown strongly in recent years (Geyer-Klingeberg et al. 2020 ; Rauch 2020 ; Schwab 2015 ). Therefore, it is likely that one or several meta-analyses for many topics of high scholarly interest already exist. However, this should not deter researchers from investigating their research questions. One possibility is to consider moderators or mediators of a relationship that have previously been ignored. For example, a meta-analysis about startup performance could investigate the impact of different ways to measure the performance construct (e.g., growth vs. profitability vs. survival time) or certain characteristics of the founders as moderators. Another possibility is to replicate previous meta-analyses and test whether their findings can be confirmed with an updated sample of primary studies or newly developed methods. Frequent replications and updates of meta-analyses are important contributions to cumulative science and are increasingly called for by the research community (Anderson & Kichkha 2017 ; Steel et al. 2021 ). Consistent with its focus on replication studies (Block and Kuckertz 2018 ), MRQ therefore also invites authors to submit replication meta-analyses.
2.2 Step 2: literature search
2.2.1 search strategies.
Similar to conducting a literature review, the search process of a meta-analysis should be systematic, reproducible, and transparent, resulting in a sample that includes all relevant studies (Fisch and Block 2018 ; Gusenbauer and Haddaway 2020 ). There are several identification strategies for relevant primary studies when compiling meta-analytical datasets (Harari et al. 2020 ). First, previous meta-analyses on the same or a related topic may provide lists of included studies that offer a good starting point to identify and become familiar with the relevant literature. This practice is also applicable to topic-related literature reviews, which often summarize the central findings of the reviewed articles in systematic tables. Both article types likely include the most prominent studies of a research field. The most common and important search strategy, however, is a keyword search in electronic databases (Harari et al. 2020 ). This strategy will probably yield the largest number of relevant studies, particularly so-called ‘grey literature’, which may not be considered by literature reviews. Gusenbauer and Haddaway ( 2020 ) provide a detailed overview of 34 scientific databases, of which 18 are multidisciplinary or have a focus on management sciences, along with their suitability for literature synthesis. To prevent biased results due to the scope or journal coverage of one database, researchers should use at least two different databases (DeSimone et al. 2020 ; Martín-Martín et al. 2021 ; Mongeon & Paul-Hus 2016 ). However, a database search can easily lead to an overload of potentially relevant studies. For example, key term searches in Google Scholar for “entrepreneurial intention” and “firm diversification” resulted in more than 660,000 and 810,000 hits, respectively. Footnote 1 Therefore, a precise research question and precise search terms using Boolean operators are advisable (Gusenbauer and Haddaway 2020 ). Addressing the challenge of identifying relevant articles in the growing number of database publications, (semi)automated approaches using text mining and machine learning (Bosco et al. 2017 ; O’Mara-Eves et al. 2015 ; Ouzzani et al. 2016 ; Thomas et al. 2017 ) can also be promising and time-saving search tools in the future. Also, some electronic databases offer the possibility to track forward citations of influential studies and thereby identify further relevant articles. Finally, collecting unpublished or undetected studies through conferences, personal contact with (leading) scholars, or listservs can be strategies to increase the study sample size (Grewal et al. 2018 ; Harari et al. 2020 ; Pigott and Polanin 2020 ).
2.2.2 Study inclusion criteria and sample composition
Next, researchers must decide which studies to include in the meta-analysis. Some guidelines for literature reviews recommend limiting the sample to studies published in renowned academic journals to ensure the quality of findings (e.g., Kraus et al. 2020 ). For meta-analysis, however, Steel et al. ( 2021 ) advocate for the inclusion of all available studies, including grey literature, to prevent selection biases based on availability, cost, familiarity, and language (Rothstein et al. 2005 ), or the “Matthew effect”, which denotes the phenomenon that highly cited articles are found faster than less cited articles (Merton 1968 ). Harrison et al. ( 2017 ) find that the effects of published studies in management are inflated on average by 30% compared to unpublished studies. This so-called publication bias or “file drawer problem” (Rosenthal 1979 ) results from the preference of academia to publish more statistically significant and less statistically insignificant study results. Owen and Li ( 2020 ) showed that publication bias is particularly severe when variables of interest are used as key variables rather than control variables. To consider the true effect size of a target variable or relationship, the inclusion of all types of research outputs is therefore recommended (Polanin et al. 2016 ). Different test procedures to identify publication bias are discussed subsequently in Step 7.
In addition to the decision of whether to include certain study types (i.e., published vs. unpublished studies), there can be other reasons to exclude studies that are identified in the search process. These reasons can be manifold and are primarily related to the specific research question and methodological peculiarities. For example, studies identified by keyword search might not qualify thematically after all, may use unsuitable variable measurements, or may not report usable effect sizes. Furthermore, there might be multiple studies by the same authors using similar datasets. If they do not differ sufficiently in terms of their sample characteristics or variables used, only one of these studies should be included to prevent bias from duplicates (Wood 2008 ; see this article for a detection heuristic).
In general, the screening process should be conducted stepwise, beginning with a removal of duplicate citations from different databases, followed by abstract screening to exclude clearly unsuitable studies and a final full-text screening of the remaining articles (Pigott and Polanin 2020 ). A graphical tool to systematically document the sample selection process is the PRISMA flow diagram (Moher et al. 2009 ). Page et al. ( 2021 ) recently presented an updated version of the PRISMA statement, including an extended item checklist and flow diagram to report the study process and findings.
2.3 Step 3: choice of the effect size measure
2.3.1 types of effect sizes.
The two most common meta-analytical effect size measures in management studies are (z-transformed) correlation coefficients and standardized mean differences (Aguinis et al. 2011a ; Geyskens et al. 2009 ). However, meta-analyses in management science and related fields may not be limited to those two effect size measures but rather depend on the subfield of investigation (Borenstein 2009 ; Stanley and Doucouliagos 2012 ). In economics and finance, researchers are more interested in the examination of elasticities and marginal effects extracted from regression models than in pure bivariate correlations (Stanley and Doucouliagos 2012 ). Regression coefficients can also be converted to partial correlation coefficients based on their t-statistics to make regression results comparable across studies (Stanley and Doucouliagos 2012 ). Although some meta-analyses in management research have combined bivariate and partial correlations in their study samples, Aloe ( 2015 ) and Combs et al. ( 2019 ) advise researchers not to use this practice. Most importantly, they argue that the effect size strength of partial correlations depends on the other variables included in the regression model and is therefore incomparable to bivariate correlations (Schmidt and Hunter 2015 ), resulting in a possible bias of the meta-analytic results (Roth et al. 2018 ). We endorse this opinion. If at all, we recommend separate analyses for each measure. In addition to these measures, survival rates, risk ratios or odds ratios, which are common measures in medical research (Borenstein 2009 ), can be suitable effect sizes for specific management research questions, such as understanding the determinants of the survival of startup companies. To summarize, the choice of a suitable effect size is often taken away from the researcher because it is typically dependent on the investigated research question as well as the conventions of the specific research field (Cheung and Vijayakumar 2016 ).
2.3.2 Conversion of effect sizes to a common measure
After having defined the primary effect size measure for the meta-analysis, it might become necessary in the later coding process to convert study findings that are reported in effect sizes that are different from the chosen primary effect size. For example, a study might report only descriptive statistics for two study groups but no correlation coefficient, which is used as the primary effect size measure in the meta-analysis. Different effect size measures can be harmonized using conversion formulae, which are provided by standard method books such as Borenstein et al. ( 2009 ) or Lipsey and Wilson ( 2001 ). There also exist online effect size calculators for meta-analysis. Footnote 2
2.4 Step 4: choice of the analytical method used
Choosing which meta-analytical method to use is directly connected to the research question of the meta-analysis. Research questions in meta-analyses can address a relationship between constructs or an effect of an intervention in a general manner, or they can focus on moderating or mediating effects. There are four meta-analytical methods that are primarily used in contemporary management research (Combs et al. 2019 ; Geyer-Klingeberg et al. 2020 ), which allow the investigation of these different types of research questions: traditional univariate meta-analysis, meta-regression, meta-analytic structural equation modeling, and qualitative meta-analysis (Hoon 2013 ). While the first three are quantitative, the latter summarizes qualitative findings. Table 1 summarizes the key characteristics of the three quantitative methods.
2.4.1 Univariate meta-analysis
In its traditional form, a meta-analysis reports a weighted mean effect size for the relationship or intervention of investigation and provides information on the magnitude of variance among primary studies (Aguinis et al. 2011c ; Borenstein et al. 2009 ). Accordingly, it serves as a quantitative synthesis of a research field (Borenstein et al. 2009 ; Geyskens et al. 2009 ). Prominent traditional approaches have been developed, for example, by Hedges and Olkin ( 1985 ) or Hunter and Schmidt ( 1990 , 2004 ). However, going beyond its simple summary function, the traditional approach has limitations in explaining the observed variance among findings (Gonzalez-Mulé and Aguinis 2018 ). To identify moderators (or boundary conditions) of the relationship of interest, meta-analysts can create subgroups and investigate differences between those groups (Borenstein and Higgins 2013 ; Hunter and Schmidt 2004 ). Potential moderators can be study characteristics (e.g., whether a study is published vs. unpublished), sample characteristics (e.g., study country, industry focus, or type of survey/experiment participants), or measurement artifacts (e.g., different types of variable measurements). The univariate approach is thus suitable to identify the overall direction of a relationship and can serve as a good starting point for additional analyses. However, due to its limitations in examining boundary conditions and developing theory, the univariate approach on its own is currently oftentimes viewed as not sufficient (Rauch 2020 ; Shaw and Ertug 2017 ).
2.4.2 Meta-regression analysis
Meta-regression analysis (Hedges and Olkin 1985 ; Lipsey and Wilson 2001 ; Stanley and Jarrell 1989 ) aims to investigate the heterogeneity among observed effect sizes by testing multiple potential moderators simultaneously. In meta-regression, the coded effect size is used as the dependent variable and is regressed on a list of moderator variables. These moderator variables can be categorical variables as described previously in the traditional univariate approach or (semi)continuous variables such as country scores that are merged with the meta-analytical data. Thus, meta-regression analysis overcomes the disadvantages of the traditional approach, which only allows us to investigate moderators singularly using dichotomized subgroups (Combs et al. 2019 ; Gonzalez-Mulé and Aguinis 2018 ). These possibilities allow a more fine-grained analysis of research questions that are related to moderating effects. However, Schmidt ( 2017 ) critically notes that the number of effect sizes in the meta-analytical sample must be sufficiently large to produce reliable results when investigating multiple moderators simultaneously in a meta-regression. For further reading, Tipton et al. ( 2019 ) outline the technical, conceptual, and practical developments of meta-regression over the last decades. Gonzalez-Mulé and Aguinis ( 2018 ) provide an overview of methodological choices and develop evidence-based best practices for future meta-analyses in management using meta-regression.
2.4.3 Meta-analytic structural equation modeling (MASEM)
MASEM is a combination of meta-analysis and structural equation modeling and allows to simultaneously investigate the relationships among several constructs in a path model. Researchers can use MASEM to test several competing theoretical models against each other or to identify mediation mechanisms in a chain of relationships (Bergh et al. 2016 ). This method is typically performed in two steps (Cheung and Chan 2005 ): In Step 1, a pooled correlation matrix is derived, which includes the meta-analytical mean effect sizes for all variable combinations; Step 2 then uses this matrix to fit the path model. While MASEM was based primarily on traditional univariate meta-analysis to derive the pooled correlation matrix in its early years (Viswesvaran and Ones 1995 ), more advanced methods, such as the GLS approach (Becker 1992 , 1995 ) or the TSSEM approach (Cheung and Chan 2005 ), have been subsequently developed. Cheung ( 2015a ) and Jak ( 2015 ) provide an overview of these approaches in their books with exemplary code. For datasets with more complex data structures, Wilson et al. ( 2016 ) also developed a multilevel approach that is related to the TSSEM approach in the second step. Bergh et al. ( 2016 ) discuss nine decision points and develop best practices for MASEM studies.
2.4.4 Qualitative meta-analysis
While the approaches explained above focus on quantitative outcomes of empirical studies, qualitative meta-analysis aims to synthesize qualitative findings from case studies (Hoon 2013 ; Rauch et al. 2014 ). The distinctive feature of qualitative case studies is their potential to provide in-depth information about specific contextual factors or to shed light on reasons for certain phenomena that cannot usually be investigated by quantitative studies (Rauch 2020 ; Rauch et al. 2014 ). In a qualitative meta-analysis, the identified case studies are systematically coded in a meta-synthesis protocol, which is then used to identify influential variables or patterns and to derive a meta-causal network (Hoon 2013 ). Thus, the insights of contextualized and typically nongeneralizable single studies are aggregated to a larger, more generalizable picture (Habersang et al. 2019 ). Although still the exception, this method can thus provide important contributions for academics in terms of theory development (Combs et al., 2019 ; Hoon 2013 ) and for practitioners in terms of evidence-based management or entrepreneurship (Rauch et al. 2014 ). Levitt ( 2018 ) provides a guide and discusses conceptual issues for conducting qualitative meta-analysis in psychology, which is also useful for management researchers.
2.5 Step 5: choice of software
Software solutions to perform meta-analyses range from built-in functions or additional packages of statistical software to software purely focused on meta-analyses and from commercial to open-source solutions. However, in addition to personal preferences, the choice of the most suitable software depends on the complexity of the methods used and the dataset itself (Cheung and Vijayakumar 2016 ). Meta-analysts therefore must carefully check if their preferred software is capable of performing the intended analysis.
Among commercial software providers, Stata (from version 16 on) offers built-in functions to perform various meta-analytical analyses or to produce various plots (Palmer and Sterne 2016 ). For SPSS and SAS, there exist several macros for meta-analyses provided by scholars, such as David B. Wilson or Andy P. Field and Raphael Gillet (Field and Gillett 2010 ). Footnote 3 Footnote 4 For researchers using the open-source software R (R Core Team 2021 ), Polanin et al. ( 2017 ) provide an overview of 63 meta-analysis packages and their functionalities. For new users, they recommend the package metafor (Viechtbauer 2010 ), which includes most necessary functions and for which the author Wolfgang Viechtbauer provides tutorials on his project website. Footnote 5 Footnote 6 In addition to packages and macros for statistical software, templates for Microsoft Excel have also been developed to conduct simple meta-analyses, such as Meta-Essentials by Suurmond et al. ( 2017 ). Footnote 7 Finally, programs purely dedicated to meta-analysis also exist, such as Comprehensive Meta-Analysis (Borenstein et al. 2013 ) or RevMan by The Cochrane Collaboration ( 2020 ).
2.6 Step 6: coding of effect sizes
2.6.1 coding sheet.
The first step in the coding process is the design of the coding sheet. A universal template does not exist because the design of the coding sheet depends on the methods used, the respective software, and the complexity of the research design. For univariate meta-analysis or meta-regression, data are typically coded in wide format. In its simplest form, when investigating a correlational relationship between two variables using the univariate approach, the coding sheet would contain a column for the study name or identifier, the effect size coded from the primary study, and the study sample size. However, such simple relationships are unlikely in management research because the included studies are typically not identical but differ in several respects. With more complex data structures or moderator variables being investigated, additional columns are added to the coding sheet to reflect the data characteristics. These variables can be coded as dummy, factor, or (semi)continuous variables and later used to perform a subgroup analysis or meta regression. For MASEM, the required data input format can deviate depending on the method used (e.g., TSSEM requires a list of correlation matrices as data input). For qualitative meta-analysis, the coding scheme typically summarizes the key qualitative findings and important contextual and conceptual information (see Hoon ( 2013 ) for a coding scheme for qualitative meta-analysis). Figure 1 shows an exemplary coding scheme for a quantitative meta-analysis on the correlational relationship between top-management team diversity and profitability. In addition to effect and sample sizes, information about the study country, firm type, and variable operationalizations are coded. The list could be extended by further study and sample characteristics.

Exemplary coding sheet for a meta-analysis on the relationship (correlation) between top-management team diversity and profitability
2.6.2 Inclusion of moderator or control variables
It is generally important to consider the intended research model and relevant nontarget variables before coding a meta-analytic dataset. For example, study characteristics can be important moderators or function as control variables in a meta-regression model. Similarly, control variables may be relevant in a MASEM approach to reduce confounding bias. Coding additional variables or constructs subsequently can be arduous if the sample of primary studies is large. However, the decision to include respective moderator or control variables, as in any empirical analysis, should always be based on strong (theoretical) rationales about how these variables can impact the investigated effect (Bernerth and Aguinis 2016 ; Bernerth et al. 2018 ; Thompson and Higgins 2002 ). While substantive moderators refer to theoretical constructs that act as buffers or enhancers of a supposed causal process, methodological moderators are features of the respective research designs that denote the methodological context of the observations and are important to control for systematic statistical particularities (Rudolph et al. 2020 ). Havranek et al. ( 2020 ) provide a list of recommended variables to code as potential moderators. While researchers may have clear expectations about the effects for some of these moderators, the concerns for other moderators may be tentative, and moderator analysis may be approached in a rather exploratory fashion. Thus, we argue that researchers should make full use of the meta-analytical design to obtain insights about potential context dependence that a primary study cannot achieve.
2.6.3 Treatment of multiple effect sizes in a study
A long-debated issue in conducting meta-analyses is whether to use only one or all available effect sizes for the same construct within a single primary study. For meta-analyses in management research, this question is fundamental because many empirical studies, particularly those relying on company databases, use multiple variables for the same construct to perform sensitivity analyses, resulting in multiple relevant effect sizes. In this case, researchers can either (randomly) select a single value, calculate a study average, or use the complete set of effect sizes (Bijmolt and Pieters 2001 ; López-López et al. 2018 ). Multiple effect sizes from the same study enrich the meta-analytic dataset and allow us to investigate the heterogeneity of the relationship of interest, such as different variable operationalizations (López-López et al. 2018 ; Moeyaert et al. 2017 ). However, including more than one effect size from the same study violates the independency assumption of observations (Cheung 2019 ; López-López et al. 2018 ), which can lead to biased results and erroneous conclusions (Gooty et al. 2021 ). We follow the recommendation of current best practice guides to take advantage of using all available effect size observations but to carefully consider interdependencies using appropriate methods such as multilevel models, panel regression models, or robust variance estimation (Cheung 2019 ; Geyer-Klingeberg et al. 2020 ; Gooty et al. 2021 ; López-López et al. 2018 ; Moeyaert et al. 2017 ).
2.7 Step 7: analysis
2.7.1 outlier analysis and tests for publication bias.
Before conducting the primary analysis, some preliminary sensitivity analyses might be necessary, which should ensure the robustness of the meta-analytical findings (Rudolph et al. 2020 ). First, influential outlier observations could potentially bias the observed results, particularly if the number of total effect sizes is small. Several statistical methods can be used to identify outliers in meta-analytical datasets (Aguinis et al. 2013 ; Viechtbauer and Cheung 2010 ). However, there is a debate about whether to keep or omit these observations. Anyhow, relevant studies should be closely inspected to infer an explanation about their deviating results. As in any other primary study, outliers can be a valid representation, albeit representing a different population, measure, construct, design or procedure. Thus, inferences about outliers can provide the basis to infer potential moderators (Aguinis et al. 2013 ; Steel et al. 2021 ). On the other hand, outliers can indicate invalid research, for instance, when unrealistically strong correlations are due to construct overlap (i.e., lack of a clear demarcation between independent and dependent variables), invalid measures, or simply typing errors when coding effect sizes. An advisable step is therefore to compare the results both with and without outliers and base the decision on whether to exclude outlier observations with careful consideration (Geyskens et al. 2009 ; Grewal et al. 2018 ; Kepes et al. 2013 ). However, instead of simply focusing on the size of the outlier, its leverage should be considered. Thus, Viechtbauer and Cheung ( 2010 ) propose considering a combination of standardized deviation and a study’s leverage.
Second, as mentioned in the context of a literature search, potential publication bias may be an issue. Publication bias can be examined in multiple ways (Rothstein et al. 2005 ). First, the funnel plot is a simple graphical tool that can provide an overview of the effect size distribution and help to detect publication bias (Stanley and Doucouliagos 2010 ). A funnel plot can also support in identifying potential outliers. As mentioned above, a graphical display of deviation (e.g., studentized residuals) and leverage (Cook’s distance) can help detect the presence of outliers and evaluate their influence (Viechtbauer and Cheung 2010 ). Moreover, several statistical procedures can be used to test for publication bias (Harrison et al. 2017 ; Kepes et al. 2012 ), including subgroup comparisons between published and unpublished studies, Begg and Mazumdar’s ( 1994 ) rank correlation test, cumulative meta-analysis (Borenstein et al. 2009 ), the trim and fill method (Duval and Tweedie 2000a , b ), Egger et al.’s ( 1997 ) regression test, failsafe N (Rosenthal 1979 ), or selection models (Hedges and Vevea 2005 ; Vevea and Woods 2005 ). In examining potential publication bias, Kepes et al. ( 2012 ) and Harrison et al. ( 2017 ) both recommend not relying only on a single test but rather using multiple conceptionally different test procedures (i.e., the so-called “triangulation approach”).
2.7.2 Model choice
After controlling and correcting for the potential presence of impactful outliers or publication bias, the next step in meta-analysis is the primary analysis, where meta-analysts must decide between two different types of models that are based on different assumptions: fixed-effects and random-effects (Borenstein et al. 2010 ). Fixed-effects models assume that all observations share a common mean effect size, which means that differences are only due to sampling error, while random-effects models assume heterogeneity and allow for a variation of the true effect sizes across studies (Borenstein et al. 2010 ; Cheung and Vijayakumar 2016 ; Hunter and Schmidt 2004 ). Both models are explained in detail in standard textbooks (e.g., Borenstein et al. 2009 ; Hunter and Schmidt 2004 ; Lipsey and Wilson 2001 ).
In general, the presence of heterogeneity is likely in management meta-analyses because most studies do not have identical empirical settings, which can yield different effect size strengths or directions for the same investigated phenomenon. For example, the identified studies have been conducted in different countries with different institutional settings, or the type of study participants varies (e.g., students vs. employees, blue-collar vs. white-collar workers, or manufacturing vs. service firms). Thus, the vast majority of meta-analyses in management research and related fields use random-effects models (Aguinis et al. 2011a ). In a meta-regression, the random-effects model turns into a so-called mixed-effects model because moderator variables are added as fixed effects to explain the impact of observed study characteristics on effect size variations (Raudenbush 2009 ).
2.8 Step 8: reporting results
2.8.1 reporting in the article.
The final step in performing a meta-analysis is reporting its results. Most importantly, all steps and methodological decisions should be comprehensible to the reader. DeSimone et al. ( 2020 ) provide an extensive checklist for journal reviewers of meta-analytical studies. This checklist can also be used by authors when performing their analyses and reporting their results to ensure that all important aspects have been addressed. Alternative checklists are provided, for example, by Appelbaum et al. ( 2018 ) or Page et al. ( 2021 ). Similarly, Levitt et al. ( 2018 ) provide a detailed guide for qualitative meta-analysis reporting standards.
For quantitative meta-analyses, tables reporting results should include all important information and test statistics, including mean effect sizes; standard errors and confidence intervals; the number of observations and study samples included; and heterogeneity measures. If the meta-analytic sample is rather small, a forest plot provides a good overview of the different findings and their accuracy. However, this figure will be less feasible for meta-analyses with several hundred effect sizes included. Also, results displayed in the tables and figures must be explained verbally in the results and discussion sections. Most importantly, authors must answer the primary research question, i.e., whether there is a positive, negative, or no relationship between the variables of interest, or whether the examined intervention has a certain effect. These results should be interpreted with regard to their magnitude (or significance), both economically and statistically. However, when discussing meta-analytical results, authors must describe the complexity of the results, including the identified heterogeneity and important moderators, future research directions, and theoretical relevance (DeSimone et al. 2019 ). In particular, the discussion of identified heterogeneity and underlying moderator effects is critical; not including this information can lead to false conclusions among readers, who interpret the reported mean effect size as universal for all included primary studies and ignore the variability of findings when citing the meta-analytic results in their research (Aytug et al. 2012 ; DeSimone et al. 2019 ).
2.8.2 Open-science practices
Another increasingly important topic is the public provision of meta-analytical datasets and statistical codes via open-source repositories. Open-science practices allow for results validation and for the use of coded data in subsequent meta-analyses ( Polanin et al. 2020 ), contributing to the development of cumulative science. Steel et al. ( 2021 ) refer to open science meta-analyses as a step towards “living systematic reviews” (Elliott et al. 2017 ) with continuous updates in real time. MRQ supports this development and encourages authors to make their datasets publicly available. Moreau and Gamble ( 2020 ), for example, provide various templates and video tutorials to conduct open science meta-analyses. There exist several open science repositories, such as the Open Science Foundation (OSF; for a tutorial, see Soderberg 2018 ), to preregister and make documents publicly available. Furthermore, several initiatives in the social sciences have been established to develop dynamic meta-analyses, such as metaBUS (Bosco et al. 2015 , 2017 ), MetaLab (Bergmann et al. 2018 ), or PsychOpen CAMA (Burgard et al. 2021 ).
3 Conclusion
This editorial provides a comprehensive overview of the essential steps in conducting and reporting a meta-analysis with references to more in-depth methodological articles. It also serves as a guide for meta-analyses submitted to MRQ and other management journals. MRQ welcomes all types of meta-analyses from all subfields and disciplines of management research.
Gusenbauer and Haddaway ( 2020 ), however, point out that Google Scholar is not appropriate as a primary search engine due to a lack of reproducibility of search results.
One effect size calculator by David B. Wilson is accessible via: https://www.campbellcollaboration.org/escalc/html/EffectSizeCalculator-Home.php .
The macros of David B. Wilson can be downloaded from: http://mason.gmu.edu/~dwilsonb/ .
The macros of Field and Gillet ( 2010 ) can be downloaded from: https://www.discoveringstatistics.com/repository/fieldgillett/how_to_do_a_meta_analysis.html .
The tutorials can be found via: https://www.metafor-project.org/doku.php .
Metafor does currently not provide functions to conduct MASEM. For MASEM, users can, for instance, use the package metaSEM (Cheung 2015b ).
The workbooks can be downloaded from: https://www.erim.eur.nl/research-support/meta-essentials/ .
Aguinis H, Dalton DR, Bosco FA, Pierce CA, Dalton CM (2011a) Meta-analytic choices and judgment calls: Implications for theory building and testing, obtained effect sizes, and scholarly impact. J Manag 37(1):5–38
Google Scholar
Aguinis H, Gottfredson RK, Joo H (2013) Best-practice recommendations for defining, identifying, and handling outliers. Organ Res Methods 16(2):270–301
Article Google Scholar
Aguinis H, Gottfredson RK, Wright TA (2011b) Best-practice recommendations for estimating interaction effects using meta-analysis. J Organ Behav 32(8):1033–1043
Aguinis H, Pierce CA, Bosco FA, Dalton DR, Dalton CM (2011c) Debunking myths and urban legends about meta-analysis. Organ Res Methods 14(2):306–331
Aloe AM (2015) Inaccuracy of regression results in replacing bivariate correlations. Res Synth Methods 6(1):21–27
Anderson RG, Kichkha A (2017) Replication, meta-analysis, and research synthesis in economics. Am Econ Rev 107(5):56–59
Appelbaum M, Cooper H, Kline RB, Mayo-Wilson E, Nezu AM, Rao SM (2018) Journal article reporting standards for quantitative research in psychology: the APA publications and communications BOARD task force report. Am Psychol 73(1):3–25
Aytug ZG, Rothstein HR, Zhou W, Kern MC (2012) Revealed or concealed? Transparency of procedures, decisions, and judgment calls in meta-analyses. Organ Res Methods 15(1):103–133
Begg CB, Mazumdar M (1994) Operating characteristics of a rank correlation test for publication bias. Biometrics 50(4):1088–1101. https://doi.org/10.2307/2533446
Bergh DD, Aguinis H, Heavey C, Ketchen DJ, Boyd BK, Su P, Lau CLL, Joo H (2016) Using meta-analytic structural equation modeling to advance strategic management research: Guidelines and an empirical illustration via the strategic leadership-performance relationship. Strateg Manag J 37(3):477–497
Becker BJ (1992) Using results from replicated studies to estimate linear models. J Educ Stat 17(4):341–362
Becker BJ (1995) Corrections to “Using results from replicated studies to estimate linear models.” J Edu Behav Stat 20(1):100–102
Bergmann C, Tsuji S, Piccinini PE, Lewis ML, Braginsky M, Frank MC, Cristia A (2018) Promoting replicability in developmental research through meta-analyses: Insights from language acquisition research. Child Dev 89(6):1996–2009
Bernerth JB, Aguinis H (2016) A critical review and best-practice recommendations for control variable usage. Pers Psychol 69(1):229–283
Bernerth JB, Cole MS, Taylor EC, Walker HJ (2018) Control variables in leadership research: A qualitative and quantitative review. J Manag 44(1):131–160
Bijmolt TH, Pieters RG (2001) Meta-analysis in marketing when studies contain multiple measurements. Mark Lett 12(2):157–169
Block J, Kuckertz A (2018) Seven principles of effective replication studies: Strengthening the evidence base of management research. Manag Rev Quart 68:355–359
Borenstein M (2009) Effect sizes for continuous data. In: Cooper H, Hedges LV, Valentine JC (eds) The handbook of research synthesis and meta-analysis. Russell Sage Foundation, pp 221–235
Borenstein M, Hedges LV, Higgins JPT, Rothstein HR (2009) Introduction to meta-analysis. John Wiley, Chichester
Book Google Scholar
Borenstein M, Hedges LV, Higgins JPT, Rothstein HR (2010) A basic introduction to fixed-effect and random-effects models for meta-analysis. Res Synth Methods 1(2):97–111
Borenstein M, Hedges L, Higgins J, Rothstein H (2013) Comprehensive meta-analysis (version 3). Biostat, Englewood, NJ
Borenstein M, Higgins JP (2013) Meta-analysis and subgroups. Prev Sci 14(2):134–143
Bosco FA, Steel P, Oswald FL, Uggerslev K, Field JG (2015) Cloud-based meta-analysis to bridge science and practice: Welcome to metaBUS. Person Assess Decis 1(1):3–17
Bosco FA, Uggerslev KL, Steel P (2017) MetaBUS as a vehicle for facilitating meta-analysis. Hum Resour Manag Rev 27(1):237–254
Burgard T, Bošnjak M, Studtrucker R (2021) Community-augmented meta-analyses (CAMAs) in psychology: potentials and current systems. Zeitschrift Für Psychologie 229(1):15–23
Cheung MWL (2015a) Meta-analysis: A structural equation modeling approach. John Wiley & Sons, Chichester
Cheung MWL (2015b) metaSEM: An R package for meta-analysis using structural equation modeling. Front Psychol 5:1521
Cheung MWL (2019) A guide to conducting a meta-analysis with non-independent effect sizes. Neuropsychol Rev 29(4):387–396
Cheung MWL, Chan W (2005) Meta-analytic structural equation modeling: a two-stage approach. Psychol Methods 10(1):40–64
Cheung MWL, Vijayakumar R (2016) A guide to conducting a meta-analysis. Neuropsychol Rev 26(2):121–128
Combs JG, Crook TR, Rauch A (2019) Meta-analytic research in management: contemporary approaches unresolved controversies and rising standards. J Manag Stud 56(1):1–18. https://doi.org/10.1111/joms.12427
DeSimone JA, Köhler T, Schoen JL (2019) If it were only that easy: the use of meta-analytic research by organizational scholars. Organ Res Methods 22(4):867–891. https://doi.org/10.1177/1094428118756743
DeSimone JA, Brannick MT, O’Boyle EH, Ryu JW (2020) Recommendations for reviewing meta-analyses in organizational research. Organ Res Methods 56:455–463
Duval S, Tweedie R (2000a) Trim and fill: a simple funnel-plot–based method of testing and adjusting for publication bias in meta-analysis. Biometrics 56(2):455–463
Duval S, Tweedie R (2000b) A nonparametric “trim and fill” method of accounting for publication bias in meta-analysis. J Am Stat Assoc 95(449):89–98
Egger M, Smith GD, Schneider M, Minder C (1997) Bias in meta-analysis detected by a simple, graphical test. BMJ 315(7109):629–634
Eisend M (2017) Meta-Analysis in advertising research. J Advert 46(1):21–35
Elliott JH, Synnot A, Turner T, Simmons M, Akl EA, McDonald S, Salanti G, Meerpohl J, MacLehose H, Hilton J, Tovey D, Shemilt I, Thomas J (2017) Living systematic review: 1. Introduction—the why, what, when, and how. J Clin Epidemiol 91:2330. https://doi.org/10.1016/j.jclinepi.2017.08.010
Field AP, Gillett R (2010) How to do a meta-analysis. Br J Math Stat Psychol 63(3):665–694
Fisch C, Block J (2018) Six tips for your (systematic) literature review in business and management research. Manag Rev Quart 68:103–106
Fortunato S, Bergstrom CT, Börner K, Evans JA, Helbing D, Milojević S, Petersen AM, Radicchi F, Sinatra R, Uzzi B, Vespignani A (2018) Science of science. Science 359(6379). https://doi.org/10.1126/science.aao0185
Geyer-Klingeberg J, Hang M, Rathgeber A (2020) Meta-analysis in finance research: Opportunities, challenges, and contemporary applications. Int Rev Finan Anal 71:101524
Geyskens I, Krishnan R, Steenkamp JBE, Cunha PV (2009) A review and evaluation of meta-analysis practices in management research. J Manag 35(2):393–419
Glass GV (2015) Meta-analysis at middle age: a personal history. Res Synth Methods 6(3):221–231
Gonzalez-Mulé E, Aguinis H (2018) Advancing theory by assessing boundary conditions with metaregression: a critical review and best-practice recommendations. J Manag 44(6):2246–2273
Gooty J, Banks GC, Loignon AC, Tonidandel S, Williams CE (2021) Meta-analyses as a multi-level model. Organ Res Methods 24(2):389–411. https://doi.org/10.1177/1094428119857471
Grewal D, Puccinelli N, Monroe KB (2018) Meta-analysis: integrating accumulated knowledge. J Acad Mark Sci 46(1):9–30
Gurevitch J, Koricheva J, Nakagawa S, Stewart G (2018) Meta-analysis and the science of research synthesis. Nature 555(7695):175–182
Gusenbauer M, Haddaway NR (2020) Which academic search systems are suitable for systematic reviews or meta-analyses? Evaluating retrieval qualities of Google Scholar, PubMed, and 26 other resources. Res Synth Methods 11(2):181–217
Habersang S, Küberling-Jost J, Reihlen M, Seckler C (2019) A process perspective on organizational failure: a qualitative meta-analysis. J Manage Stud 56(1):19–56
Harari MB, Parola HR, Hartwell CJ, Riegelman A (2020) Literature searches in systematic reviews and meta-analyses: A review, evaluation, and recommendations. J Vocat Behav 118:103377
Harrison JS, Banks GC, Pollack JM, O’Boyle EH, Short J (2017) Publication bias in strategic management research. J Manag 43(2):400–425
Havránek T, Stanley TD, Doucouliagos H, Bom P, Geyer-Klingeberg J, Iwasaki I, Reed WR, Rost K, Van Aert RCM (2020) Reporting guidelines for meta-analysis in economics. J Econ Surveys 34(3):469–475
Hedges LV, Olkin I (1985) Statistical methods for meta-analysis. Academic Press, Orlando
Hedges LV, Vevea JL (2005) Selection methods approaches. In: Rothstein HR, Sutton A, Borenstein M (eds) Publication bias in meta-analysis: prevention, assessment, and adjustments. Wiley, Chichester, pp 145–174
Hoon C (2013) Meta-synthesis of qualitative case studies: an approach to theory building. Organ Res Methods 16(4):522–556
Hunter JE, Schmidt FL (1990) Methods of meta-analysis: correcting error and bias in research findings. Sage, Newbury Park
Hunter JE, Schmidt FL (2004) Methods of meta-analysis: correcting error and bias in research findings, 2nd edn. Sage, Thousand Oaks
Hunter JE, Schmidt FL, Jackson GB (1982) Meta-analysis: cumulating research findings across studies. Sage Publications, Beverly Hills
Jak S (2015) Meta-analytic structural equation modelling. Springer, New York, NY
Kepes S, Banks GC, McDaniel M, Whetzel DL (2012) Publication bias in the organizational sciences. Organ Res Methods 15(4):624–662
Kepes S, McDaniel MA, Brannick MT, Banks GC (2013) Meta-analytic reviews in the organizational sciences: Two meta-analytic schools on the way to MARS (the Meta-Analytic Reporting Standards). J Bus Psychol 28(2):123–143
Kraus S, Breier M, Dasí-Rodríguez S (2020) The art of crafting a systematic literature review in entrepreneurship research. Int Entrepreneur Manag J 16(3):1023–1042
Levitt HM (2018) How to conduct a qualitative meta-analysis: tailoring methods to enhance methodological integrity. Psychother Res 28(3):367–378
Levitt HM, Bamberg M, Creswell JW, Frost DM, Josselson R, Suárez-Orozco C (2018) Journal article reporting standards for qualitative primary, qualitative meta-analytic, and mixed methods research in psychology: the APA publications and communications board task force report. Am Psychol 73(1):26
Lipsey MW, Wilson DB (2001) Practical meta-analysis. Sage Publications, Inc.
López-López JA, Page MJ, Lipsey MW, Higgins JP (2018) Dealing with effect size multiplicity in systematic reviews and meta-analyses. Res Synth Methods 9(3):336–351
Martín-Martín A, Thelwall M, Orduna-Malea E, López-Cózar ED (2021) Google Scholar, Microsoft Academic, Scopus, Dimensions, Web of Science, and OpenCitations’ COCI: a multidisciplinary comparison of coverage via citations. Scientometrics 126(1):871–906
Merton RK (1968) The Matthew effect in science: the reward and communication systems of science are considered. Science 159(3810):56–63
Moeyaert M, Ugille M, Natasha Beretvas S, Ferron J, Bunuan R, Van den Noortgate W (2017) Methods for dealing with multiple outcomes in meta-analysis: a comparison between averaging effect sizes, robust variance estimation and multilevel meta-analysis. Int J Soc Res Methodol 20(6):559–572
Moher D, Liberati A, Tetzlaff J, Altman DG, Prisma Group (2009) Preferred reporting items for systematic reviews and meta-analyses: the PRISMA statement. PLoS medicine. 6(7):e1000097
Mongeon P, Paul-Hus A (2016) The journal coverage of Web of Science and Scopus: a comparative analysis. Scientometrics 106(1):213–228
Moreau D, Gamble B (2020) Conducting a meta-analysis in the age of open science: Tools, tips, and practical recommendations. Psychol Methods. https://doi.org/10.1037/met0000351
O’Mara-Eves A, Thomas J, McNaught J, Miwa M, Ananiadou S (2015) Using text mining for study identification in systematic reviews: a systematic review of current approaches. Syst Rev 4(1):1–22
Ouzzani M, Hammady H, Fedorowicz Z, Elmagarmid A (2016) Rayyan—a web and mobile app for systematic reviews. Syst Rev 5(1):1–10
Owen E, Li Q (2021) The conditional nature of publication bias: a meta-regression analysis. Polit Sci Res Methods 9(4):867–877
Page MJ, McKenzie JE, Bossuyt PM, Boutron I, Hoffmann TC, Mulrow CD, Shamseer L, Tetzlaff JM, Akl EA, Brennan SE, Chou R, Glanville J, Grimshaw JM, Hróbjartsson A, Lalu MM, Li T, Loder EW, Mayo-Wilson E,McDonald S,McGuinness LA, Stewart LA, Thomas J, Tricco AC, Welch VA, Whiting P, Moher D (2021) The PRISMA 2020 statement: an updated guideline for reporting systematic reviews. BMJ 372. https://doi.org/10.1136/bmj.n71
Palmer TM, Sterne JAC (eds) (2016) Meta-analysis in stata: an updated collection from the stata journal, 2nd edn. Stata Press, College Station, TX
Pigott TD, Polanin JR (2020) Methodological guidance paper: High-quality meta-analysis in a systematic review. Rev Educ Res 90(1):24–46
Polanin JR, Tanner-Smith EE, Hennessy EA (2016) Estimating the difference between published and unpublished effect sizes: a meta-review. Rev Educ Res 86(1):207–236
Polanin JR, Hennessy EA, Tanner-Smith EE (2017) A review of meta-analysis packages in R. J Edu Behav Stat 42(2):206–242
Polanin JR, Hennessy EA, Tsuji S (2020) Transparency and reproducibility of meta-analyses in psychology: a meta-review. Perspect Psychol Sci 15(4):1026–1041. https://doi.org/10.1177/17456916209064
R Core Team (2021). R: A language and environment for statistical computing . R Foundation for Statistical Computing, Vienna, Austria. URL https://www.R-project.org/ .
Rauch A (2020) Opportunities and threats in reviewing entrepreneurship theory and practice. Entrep Theory Pract 44(5):847–860
Rauch A, van Doorn R, Hulsink W (2014) A qualitative approach to evidence–based entrepreneurship: theoretical considerations and an example involving business clusters. Entrep Theory Pract 38(2):333–368
Raudenbush SW (2009) Analyzing effect sizes: Random-effects models. In: Cooper H, Hedges LV, Valentine JC (eds) The handbook of research synthesis and meta-analysis, 2nd edn. Russell Sage Foundation, New York, NY, pp 295–315
Rosenthal R (1979) The file drawer problem and tolerance for null results. Psychol Bull 86(3):638
Rothstein HR, Sutton AJ, Borenstein M (2005) Publication bias in meta-analysis: prevention, assessment and adjustments. Wiley, Chichester
Roth PL, Le H, Oh I-S, Van Iddekinge CH, Bobko P (2018) Using beta coefficients to impute missing correlations in meta-analysis research: Reasons for caution. J Appl Psychol 103(6):644–658. https://doi.org/10.1037/apl0000293
Rudolph CW, Chang CK, Rauvola RS, Zacher H (2020) Meta-analysis in vocational behavior: a systematic review and recommendations for best practices. J Vocat Behav 118:103397
Schmidt FL (2017) Statistical and measurement pitfalls in the use of meta-regression in meta-analysis. Career Dev Int 22(5):469–476
Schmidt FL, Hunter JE (2015) Methods of meta-analysis: correcting error and bias in research findings. Sage, Thousand Oaks
Schwab A (2015) Why all researchers should report effect sizes and their confidence intervals: Paving the way for meta–analysis and evidence–based management practices. Entrepreneurship Theory Pract 39(4):719–725. https://doi.org/10.1111/etap.12158
Shaw JD, Ertug G (2017) The suitability of simulations and meta-analyses for submissions to Academy of Management Journal. Acad Manag J 60(6):2045–2049
Soderberg CK (2018) Using OSF to share data: A step-by-step guide. Adv Methods Pract Psychol Sci 1(1):115–120
Stanley TD, Doucouliagos H (2010) Picture this: a simple graph that reveals much ado about research. J Econ Surveys 24(1):170–191
Stanley TD, Doucouliagos H (2012) Meta-regression analysis in economics and business. Routledge, London
Stanley TD, Jarrell SB (1989) Meta-regression analysis: a quantitative method of literature surveys. J Econ Surveys 3:54–67
Steel P, Beugelsdijk S, Aguinis H (2021) The anatomy of an award-winning meta-analysis: Recommendations for authors, reviewers, and readers of meta-analytic reviews. J Int Bus Stud 52(1):23–44
Suurmond R, van Rhee H, Hak T (2017) Introduction, comparison, and validation of Meta-Essentials: a free and simple tool for meta-analysis. Res Synth Methods 8(4):537–553
The Cochrane Collaboration (2020). Review Manager (RevMan) [Computer program] (Version 5.4).
Thomas J, Noel-Storr A, Marshall I, Wallace B, McDonald S, Mavergames C, Glasziou P, Shemilt I, Synnot A, Turner T, Elliot J (2017) Living systematic reviews: 2. Combining human and machine effort. J Clin Epidemiol 91:31–37
Thompson SG, Higgins JP (2002) How should meta-regression analyses be undertaken and interpreted? Stat Med 21(11):1559–1573
Tipton E, Pustejovsky JE, Ahmadi H (2019) A history of meta-regression: technical, conceptual, and practical developments between 1974 and 2018. Res Synth Methods 10(2):161–179
Vevea JL, Woods CM (2005) Publication bias in research synthesis: Sensitivity analysis using a priori weight functions. Psychol Methods 10(4):428–443
Viechtbauer W (2010) Conducting meta-analyses in R with the metafor package. J Stat Softw 36(3):1–48
Viechtbauer W, Cheung MWL (2010) Outlier and influence diagnostics for meta-analysis. Res Synth Methods 1(2):112–125
Viswesvaran C, Ones DS (1995) Theory testing: combining psychometric meta-analysis and structural equations modeling. Pers Psychol 48(4):865–885
Wilson SJ, Polanin JR, Lipsey MW (2016) Fitting meta-analytic structural equation models with complex datasets. Res Synth Methods 7(2):121–139. https://doi.org/10.1002/jrsm.1199
Wood JA (2008) Methodology for dealing with duplicate study effects in a meta-analysis. Organ Res Methods 11(1):79–95
Download references
Open Access funding enabled and organized by Projekt DEAL. No funding was received to assist with the preparation of this manuscript.
Author information
Authors and affiliations.
University of Luxembourg, Luxembourg, Luxembourg
Christopher Hansen
Leibniz Institute for Psychology (ZPID), Trier, Germany
Holger Steinmetz
Trier University, Trier, Germany
Erasmus University Rotterdam, Rotterdam, The Netherlands
Wittener Institut Für Familienunternehmen, Universität Witten/Herdecke, Witten, Germany
You can also search for this author in PubMed Google Scholar
Corresponding author
Correspondence to Jörn Block .
Ethics declarations
Conflict of interest.
The authors have no relevant financial or non-financial interests to disclose.
Additional information
Publisher's note.
Springer Nature remains neutral with regard to jurisdictional claims in published maps and institutional affiliations.
See Table 1 .
Rights and permissions
Open Access This article is licensed under a Creative Commons Attribution 4.0 International License, which permits use, sharing, adaptation, distribution and reproduction in any medium or format, as long as you give appropriate credit to the original author(s) and the source, provide a link to the Creative Commons licence, and indicate if changes were made. The images or other third party material in this article are included in the article's Creative Commons licence, unless indicated otherwise in a credit line to the material. If material is not included in the article's Creative Commons licence and your intended use is not permitted by statutory regulation or exceeds the permitted use, you will need to obtain permission directly from the copyright holder. To view a copy of this licence, visit http://creativecommons.org/licenses/by/4.0/ .
Reprints and permissions
About this article
Hansen, C., Steinmetz, H. & Block, J. How to conduct a meta-analysis in eight steps: a practical guide. Manag Rev Q 72 , 1–19 (2022). https://doi.org/10.1007/s11301-021-00247-4
Download citation
Published : 30 November 2021
Issue Date : February 2022
DOI : https://doi.org/10.1007/s11301-021-00247-4
Share this article
Anyone you share the following link with will be able to read this content:
Sorry, a shareable link is not currently available for this article.
Provided by the Springer Nature SharedIt content-sharing initiative
- Find a journal
- Publish with us
- Track your research
- PRO Courses Guides New Tech Help Pro Expert Videos About wikiHow Pro Upgrade Sign In
- EDIT Edit this Article
- EXPLORE Tech Help Pro About Us Random Article Quizzes Request a New Article Community Dashboard This Or That Game Popular Categories Arts and Entertainment Artwork Books Movies Computers and Electronics Computers Phone Skills Technology Hacks Health Men's Health Mental Health Women's Health Relationships Dating Love Relationship Issues Hobbies and Crafts Crafts Drawing Games Education & Communication Communication Skills Personal Development Studying Personal Care and Style Fashion Hair Care Personal Hygiene Youth Personal Care School Stuff Dating All Categories Arts and Entertainment Finance and Business Home and Garden Relationship Quizzes Cars & Other Vehicles Food and Entertaining Personal Care and Style Sports and Fitness Computers and Electronics Health Pets and Animals Travel Education & Communication Hobbies and Crafts Philosophy and Religion Work World Family Life Holidays and Traditions Relationships Youth
- Browse Articles
- Learn Something New
- Quizzes Hot
- This Or That Game
- Train Your Brain
- Explore More
- Support wikiHow
- About wikiHow
- Log in / Sign up
- Finance and Business
- Business Skills
- Business Writing
How to Write an Analysis
Last Updated: April 3, 2024 Fact Checked
This article was co-authored by Christopher Taylor, PhD and by wikiHow staff writer, Megaera Lorenz, PhD . Christopher Taylor is an Adjunct Assistant Professor of English at Austin Community College in Texas. He received his PhD in English Literature and Medieval Studies from the University of Texas at Austin in 2014. There are 14 references cited in this article, which can be found at the bottom of the page. This article has been fact-checked, ensuring the accuracy of any cited facts and confirming the authority of its sources. This article has been viewed 295,671 times.
An analysis is a piece of writing that looks at some aspect of a document in detail. To write a good analysis, you’ll need to ask yourself questions that focus on how and why the document works the way it does. You can start the process by gathering information about the subject of your analysis and defining the questions your analysis will answer. Once you’ve outlined your main arguments, look for specific evidence to support them. You can then work on putting your analysis together into a coherent piece of writing.
Gathering Information and Building Your Argument

- If your analysis is supposed to answer a specific question or focus on a particular aspect of the document you are analyzing.
- If there are any length or formatting requirements for the analysis.
- The citation style your instructor wants you to use.
- On what criteria your instructor will evaluate your analysis (e.g., organization, originality, good use of references and quotations, or correct spelling and grammar).

- The title of the document (if it has one).
- The name of the creator of the document. For example, depending on the type of document you’re working with, this could be the author, artist, director, performer, or photographer.
- The form and medium of the document (e.g., “Painting, oil on canvas”).
- When and where the document was created.
- The historical and cultural context of the work.

- Who you believe the intended audience is for the advertisement.
- What rhetorical choices the author made to persuade the audience of their main point.
- What product is being advertised.
- How the poster uses images to make the product look appealing.
- Whether there is any text in the poster, and, if so, how it works together with the images to reinforce the message of the ad.
- What the purpose of the ad is or what its main point is.

- For example, if you’re analyzing an advertisement poster, you might focus on the question: “How does this poster use colors to symbolize the problem that the product is intended to fix? Does it also use color to represent the beneficial results of using the product?”

- For example, you might write, “This poster uses the color red to symbolize the pain of a headache. The blue elements in the design represent the relief brought by the product.”
- You could develop the argument further by saying, “The colors used in the text reinforce the use of colors in the graphic elements of the poster, helping the viewer make a direct connection between the words and images.”

- For example, if you’re arguing that the advertisement poster uses red to represent pain, you might point out that the figure of the headache sufferer is red, while everyone around them is blue. Another piece of evidence might be the use of red lettering for the words “HEADACHE” and “PAIN” in the text of the poster.
- You could also draw on outside evidence to support your claims. For example, you might point out that in the country where the advertisement was produced, the color red is often symbolically associated with warnings or danger.
Tip: If you’re analyzing a text, make sure to properly cite any quotations that you use to support your arguments. Put any direct quotations in quotation marks (“”) and be sure to give location information, such as the page number where the quote appears. Additionally, follow the citation requirements for the style guide assigned by your instructor or one that's commonly used for the subject matter you're writing about.
Organizing and Drafting Your Analysis

- For example, “The poster ‘Say! What a relief,’ created in 1932 by designer Dorothy Plotzky, uses contrasting colors to symbolize the pain of a headache and the relief brought by Miss Burnham’s Pep-Em-Up Pills. The red elements denote pain, while blue ones indicate soothing relief.”
Tip: Your instructor might have specific directions about which information to include in your thesis statement (e.g., the title, author, and date of the document you are analyzing). If you’re not sure how to format your thesis statement or topic sentence, don’t hesitate to ask.

- a. Background
- ii. Analysis/Explanation
- iii. Example
- iv. Analysis/Explanation
- III. Conclusion

- For example, “In the late 1920s, Kansas City schoolteacher Ethel Burnham developed a patent headache medication that quickly achieved commercial success throughout the American Midwest. The popularity of the medicine was largely due to a series of simple but eye-catching advertising posters that were created over the next decade. The poster ‘Say! What a relief,’ created in 1932 by designer Dorothy Plotzky, uses contrasting colors to symbolize the pain of a headache and the relief brought by Miss Burnham’s Pep-Em-Up Pills.”

- Make sure to include clear transitions between each argument and each paragraph. Use transitional words and phrases, such as “Furthermore,” “Additionally,” “For example,” “Likewise,” or “In contrast . . .”
- The best way to organize your arguments will vary based on the individual topic and the specific points you are trying to make. For example, in your analysis of the poster, you might start with arguments about the red visual elements and then move on to a discussion about how the red text fits in.

- For example, you might end your essay with a few sentences about how other advertisements at the time might have been influenced by Dorothy Plotzky’s use of colors.

- For example, in your discussion of the advertisement, avoid stating that you think the art is “beautiful” or that the advertisement is “boring.” Instead, focus on what the poster was supposed to accomplish and how the designer attempted to achieve those goals.
Polishing Your Analysis

- For example, if your essay currently skips around between discussions of the red and blue elements of the poster, consider reorganizing it so that you discuss all the red elements first, then focus on the blue ones.

- For example, you might look for places where you could provide additional examples to support one of your major arguments.

- For example, if you included a paragraph about Dorothy Plotzky’s previous work as a children’s book illustrator, you may want to cut it if it doesn’t somehow relate to her use of color in advertising.
- Cutting material out of your analysis may be difficult, especially if you put a lot of thought into each sentence or found the additional material really interesting. Your analysis will be stronger if you keep it concise and to the point, however.

- You may find it helpful to have someone else go over your essay and look for any mistakes you might have missed.
Tip: When you’re reading silently, it’s easy to miss typos and other small errors because your brain corrects them automatically. Reading your work out loud can make problems easier to spot.
Sample Analysis Outline and Conclusion

Expert Q&A

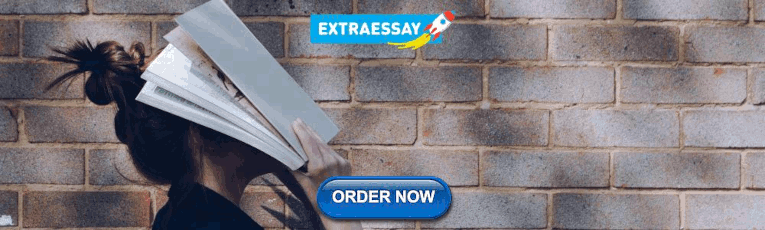
You Might Also Like

- ↑ https://lsa.umich.edu/sweetland/undergraduates/writing-guides/how-do-i-make-sure-i-understand-an-assignment-.html
- ↑ https://www.bucks.edu/media/bcccmedialibrary/pdf/HOWTOWRITEALITERARYANALYSISESSAY_10.15.07_001.pdf
- ↑ https://owl.purdue.edu/owl/general_writing/visual_rhetoric/analyzing_visual_documents/elements_of_analysis.html
- ↑ https://lsa.umich.edu/sweetland/undergraduates/writing-guides/how-can-i-create-stronger-analysis-.html
- ↑ https://lsa.umich.edu/sweetland/undergraduates/writing-guides/how-do-i-decide-what-i-should-argue-.html
- ↑ https://lsa.umich.edu/sweetland/undergraduates/writing-guides/how-do-i-effectively-integrate-textual-evidence-.html
- ↑ https://writingcenter.uagc.edu/writing-a-thesis
- ↑ https://owl.purdue.edu/owl/general_writing/visual_rhetoric/analyzing_visual_documents/organizing_your_analysis.html
- ↑ https://lsa.umich.edu/sweetland/undergraduates/writing-guides/how-do-i-write-an-intro--conclusion----body-paragraph.html
- ↑ http://utminers.utep.edu/omwilliamson/engl0310/Textanalysis.htm
- ↑ https://owl.purdue.edu/owl/graduate_writing/graduate_writing_topics/graduate_writing_organization_structure_new.html
- ↑ https://owl.purdue.edu/owl/general_writing/mechanics/sentence_clarity.html
- ↑ https://writingcenter.unc.edu/tips-and-tools/conciseness-handout/
- ↑ https://writingcenter.unc.edu/tips-and-tools/editing-and-proofreading/
About This Article

If you need to write an analysis, first look closely at your assignment to make sure you understand the requirements. Then, gather background information about the document you’ll be analyzing and do a close read so that you’re thoroughly familiar with the subject matter. If it’s not already specified in your assignment, come up with one or more specific question’s you’d like your analysis to answer, then outline your main arguments. Finally, gather evidence and examples to support your arguments. Read on to learn how to organize, draft, and polish your analysis! Did this summary help you? Yes No
- Send fan mail to authors
Did this article help you?

Featured Articles

Trending Articles

Watch Articles

- Terms of Use
- Privacy Policy
- Do Not Sell or Share My Info
- Not Selling Info
Don’t miss out! Sign up for
wikiHow’s newsletter
How to Do Market Research: The Complete Guide
Learn how to do market research with this step-by-step guide, complete with templates, tools and real-world examples.
Access best-in-class company data
Get trusted first-party funding data, revenue data and firmographics
What are your customers’ needs? How does your product compare to the competition? What are the emerging trends and opportunities in your industry? If these questions keep you up at night, it’s time to conduct market research.
Market research plays a pivotal role in your ability to stay competitive and relevant, helping you anticipate shifts in consumer behavior and industry dynamics. It involves gathering these insights using a wide range of techniques, from surveys and interviews to data analysis and observational studies.
In this guide, we’ll explore why market research is crucial, the various types of market research, the methods used in data collection, and how to effectively conduct market research to drive informed decision-making and success.
What is market research?
Market research is the systematic process of gathering, analyzing and interpreting information about a specific market or industry. The purpose of market research is to offer valuable insight into the preferences and behaviors of your target audience, and anticipate shifts in market trends and the competitive landscape. This information helps you make data-driven decisions, develop effective strategies for your business, and maximize your chances of long-term growth.
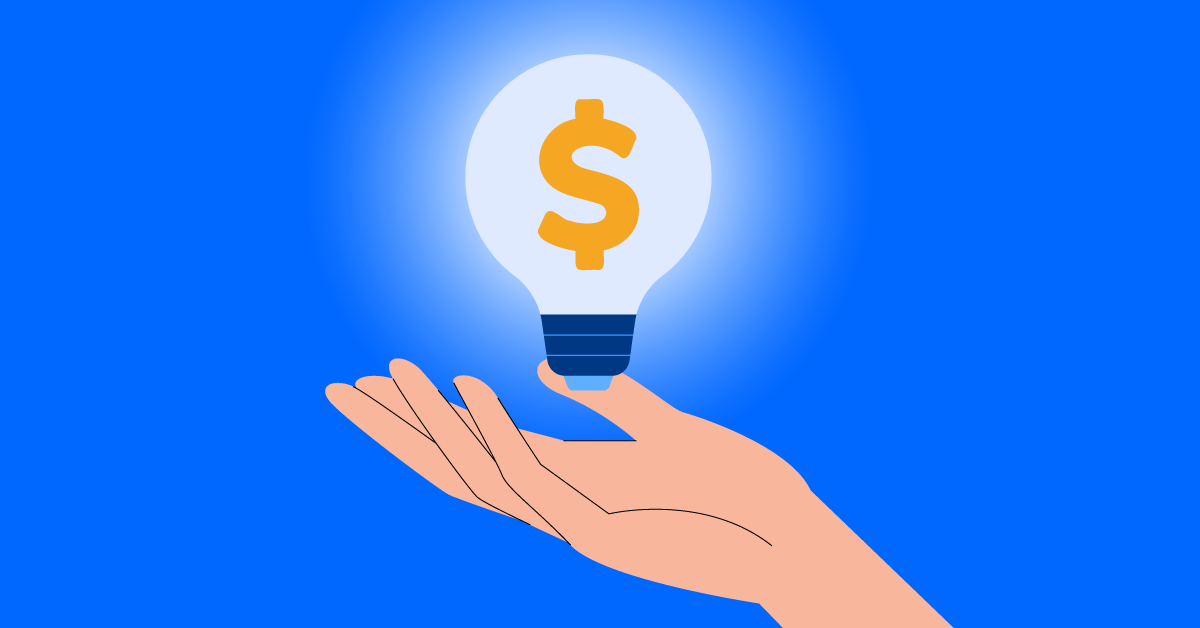
Why is market research important?
By understanding the significance of market research, you can make sure you’re asking the right questions and using the process to your advantage. Some of the benefits of market research include:
- Informed decision-making: Market research provides you with the data and insights you need to make smart decisions for your business. It helps you identify opportunities, assess risks and tailor your strategies to meet the demands of the market. Without market research, decisions are often based on assumptions or guesswork, leading to costly mistakes.
- Customer-centric approach: A cornerstone of market research involves developing a deep understanding of customer needs and preferences. This gives you valuable insights into your target audience, helping you develop products, services and marketing campaigns that resonate with your customers.
- Competitive advantage: By conducting market research, you’ll gain a competitive edge. You’ll be able to identify gaps in the market, analyze competitor strengths and weaknesses, and position your business strategically. This enables you to create unique value propositions, differentiate yourself from competitors, and seize opportunities that others may overlook.
- Risk mitigation: Market research helps you anticipate market shifts and potential challenges. By identifying threats early, you can proactively adjust their strategies to mitigate risks and respond effectively to changing circumstances. This proactive approach is particularly valuable in volatile industries.
- Resource optimization: Conducting market research allows organizations to allocate their time, money and resources more efficiently. It ensures that investments are made in areas with the highest potential return on investment, reducing wasted resources and improving overall business performance.
- Adaptation to market trends: Markets evolve rapidly, driven by technological advancements, cultural shifts and changing consumer attitudes. Market research ensures that you stay ahead of these trends and adapt your offerings accordingly so you can avoid becoming obsolete.
As you can see, market research empowers businesses to make data-driven decisions, cater to customer needs, outperform competitors, mitigate risks, optimize resources and stay agile in a dynamic marketplace. These benefits make it a huge industry; the global market research services market is expected to grow from $76.37 billion in 2021 to $108.57 billion in 2026 . Now, let’s dig into the different types of market research that can help you achieve these benefits.
Types of market research
- Qualitative research
- Quantitative research
- Exploratory research
- Descriptive research
- Causal research
- Cross-sectional research
- Longitudinal research
Despite its advantages, 23% of organizations don’t have a clear market research strategy. Part of developing a strategy involves choosing the right type of market research for your business goals. The most commonly used approaches include:
1. Qualitative research
Qualitative research focuses on understanding the underlying motivations, attitudes and perceptions of individuals or groups. It is typically conducted through techniques like in-depth interviews, focus groups and content analysis — methods we’ll discuss further in the sections below. Qualitative research provides rich, nuanced insights that can inform product development, marketing strategies and brand positioning.
2. Quantitative research
Quantitative research, in contrast to qualitative research, involves the collection and analysis of numerical data, often through surveys, experiments and structured questionnaires. This approach allows for statistical analysis and the measurement of trends, making it suitable for large-scale market studies and hypothesis testing. While it’s worthwhile using a mix of qualitative and quantitative research, most businesses prioritize the latter because it is scientific, measurable and easily replicated across different experiments.
3. Exploratory research
Whether you’re conducting qualitative or quantitative research or a mix of both, exploratory research is often the first step. Its primary goal is to help you understand a market or problem so you can gain insights and identify potential issues or opportunities. This type of market research is less structured and is typically conducted through open-ended interviews, focus groups or secondary data analysis. Exploratory research is valuable when entering new markets or exploring new product ideas.
4. Descriptive research
As its name implies, descriptive research seeks to describe a market, population or phenomenon in detail. It involves collecting and summarizing data to answer questions about audience demographics and behaviors, market size, and current trends. Surveys, observational studies and content analysis are common methods used in descriptive research.
5. Causal research
Causal research aims to establish cause-and-effect relationships between variables. It investigates whether changes in one variable result in changes in another. Experimental designs, A/B testing and regression analysis are common causal research methods. This sheds light on how specific marketing strategies or product changes impact consumer behavior.
6. Cross-sectional research
Cross-sectional market research involves collecting data from a sample of the population at a single point in time. It is used to analyze differences, relationships or trends among various groups within a population. Cross-sectional studies are helpful for market segmentation, identifying target audiences and assessing market trends at a specific moment.
7. Longitudinal research
Longitudinal research, in contrast to cross-sectional research, collects data from the same subjects over an extended period. This allows for the analysis of trends, changes and developments over time. Longitudinal studies are useful for tracking long-term developments in consumer preferences, brand loyalty and market dynamics.
Each type of market research has its strengths and weaknesses, and the method you choose depends on your specific research goals and the depth of understanding you’re aiming to achieve. In the following sections, we’ll delve into primary and secondary research approaches and specific research methods.
Primary vs. secondary market research
Market research of all types can be broadly categorized into two main approaches: primary research and secondary research. By understanding the differences between these approaches, you can better determine the most appropriate research method for your specific goals.
Primary market research
Primary research involves the collection of original data straight from the source. Typically, this involves communicating directly with your target audience — through surveys, interviews, focus groups and more — to gather information. Here are some key attributes of primary market research:
- Customized data: Primary research provides data that is tailored to your research needs. You design a custom research study and gather information specific to your goals.
- Up-to-date insights: Because primary research involves communicating with customers, the data you collect reflects the most current market conditions and consumer behaviors.
- Time-consuming and resource-intensive: Despite its advantages, primary research can be labor-intensive and costly, especially when dealing with large sample sizes or complex study designs. Whether you hire a market research consultant, agency or use an in-house team, primary research studies consume a large amount of resources and time.
Secondary market research
Secondary research, on the other hand, involves analyzing data that has already been compiled by third-party sources, such as online research tools, databases, news sites, industry reports and academic studies.
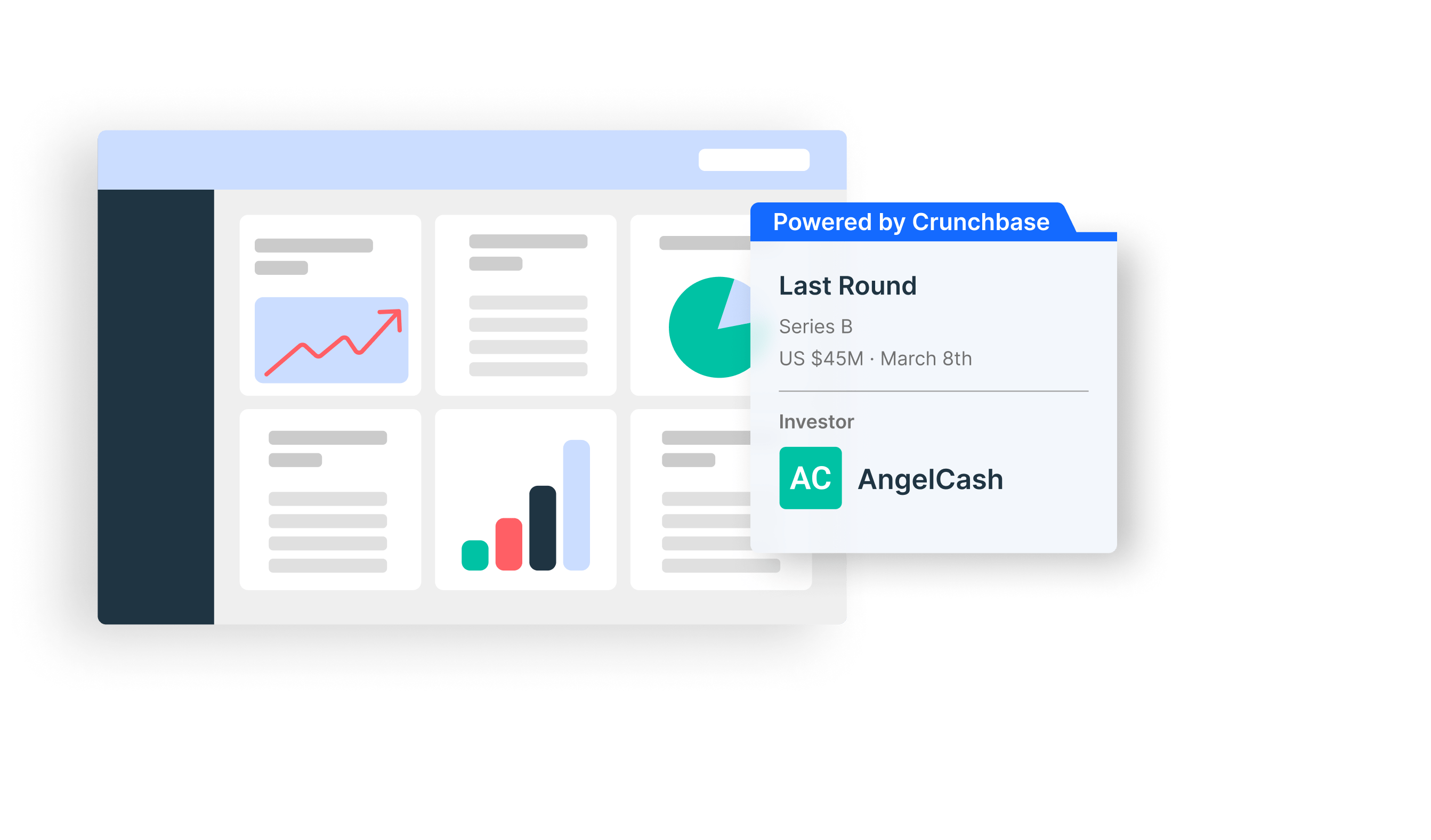
Here are the main characteristics of secondary market research:
- Cost-effective: Secondary research is generally more cost-effective than primary research since it doesn’t require building a research plan from scratch. You and your team can look at databases, websites and publications on an ongoing basis, without needing to design a custom experiment or hire a consultant.
- Leverages multiple sources: Data tools and software extract data from multiple places across the web, and then consolidate that information within a single platform. This means you’ll get a greater amount of data and a wider scope from secondary research.
- Quick to access: You can access a wide range of information rapidly — often in seconds — if you’re using online research tools and databases. Because of this, you can act on insights sooner, rather than taking the time to develop an experiment.
So, when should you use primary vs. secondary research? In practice, many market research projects incorporate both primary and secondary research to take advantage of the strengths of each approach.
One rule of thumb is to focus on secondary research to obtain background information, market trends or industry benchmarks. It is especially valuable for conducting preliminary research, competitor analysis, or when time and budget constraints are tight. Then, if you still have knowledge gaps or need to answer specific questions unique to your business model, use primary research to create a custom experiment.
Market research methods
- Surveys and questionnaires
- Focus groups
- Observational research
- Online research tools
- Experiments
- Content analysis
- Ethnographic research
How do primary and secondary research approaches translate into specific research methods? Let’s take a look at the different ways you can gather data:
1. Surveys and questionnaires
Surveys and questionnaires are popular methods for collecting structured data from a large number of respondents. They involve a set of predetermined questions that participants answer. Surveys can be conducted through various channels, including online tools, telephone interviews and in-person or online questionnaires. They are useful for gathering quantitative data and assessing customer demographics, opinions, preferences and needs. On average, customer surveys have a 33% response rate , so keep that in mind as you consider your sample size.
2. Interviews
Interviews are in-depth conversations with individuals or groups to gather qualitative insights. They can be structured (with predefined questions) or unstructured (with open-ended discussions). Interviews are valuable for exploring complex topics, uncovering motivations and obtaining detailed feedback.
3. Focus groups
The most common primary research methods are in-depth webcam interviews and focus groups. Focus groups are a small gathering of participants who discuss a specific topic or product under the guidance of a moderator. These discussions are valuable for primary market research because they reveal insights into consumer attitudes, perceptions and emotions. Focus groups are especially useful for idea generation, concept testing and understanding group dynamics within your target audience.
4. Observational research
Observational research involves observing and recording participant behavior in a natural setting. This method is particularly valuable when studying consumer behavior in physical spaces, such as retail stores or public places. In some types of observational research, participants are aware you’re watching them; in other cases, you discreetly watch consumers without their knowledge, as they use your product. Either way, observational research provides firsthand insights into how people interact with products or environments.
5. Online research tools
You and your team can do your own secondary market research using online tools. These tools include data prospecting platforms and databases, as well as online surveys, social media listening, web analytics and sentiment analysis platforms. They help you gather data from online sources, monitor industry trends, track competitors, understand consumer preferences and keep tabs on online behavior. We’ll talk more about choosing the right market research tools in the sections that follow.
6. Experiments
Market research experiments are controlled tests of variables to determine causal relationships. While experiments are often associated with scientific research, they are also used in market research to assess the impact of specific marketing strategies, product features, or pricing and packaging changes.
7. Content analysis
Content analysis involves the systematic examination of textual, visual or audio content to identify patterns, themes and trends. It’s commonly applied to customer reviews, social media posts and other forms of online content to analyze consumer opinions and sentiments.
8. Ethnographic research
Ethnographic research immerses researchers into the daily lives of consumers to understand their behavior and culture. This method is particularly valuable when studying niche markets or exploring the cultural context of consumer choices.
How to do market research
- Set clear objectives
- Identify your target audience
- Choose your research methods
- Use the right market research tools
- Collect data
- Analyze data
- Interpret your findings
- Identify opportunities and challenges
- Make informed business decisions
- Monitor and adapt
Now that you have gained insights into the various market research methods at your disposal, let’s delve into the practical aspects of how to conduct market research effectively. Here’s a quick step-by-step overview, from defining objectives to monitoring market shifts.
1. Set clear objectives
When you set clear and specific goals, you’re essentially creating a compass to guide your research questions and methodology. Start by precisely defining what you want to achieve. Are you launching a new product and want to understand its viability in the market? Are you evaluating customer satisfaction with a product redesign?
Start by creating SMART goals — objectives that are specific, measurable, achievable, relevant and time-bound. Not only will this clarify your research focus from the outset, but it will also help you track progress and benchmark your success throughout the process.
You should also consult with key stakeholders and team members to ensure alignment on your research objectives before diving into data collecting. This will help you gain diverse perspectives and insights that will shape your research approach.
2. Identify your target audience
Next, you’ll need to pinpoint your target audience to determine who should be included in your research. Begin by creating detailed buyer personas or stakeholder profiles. Consider demographic factors like age, gender, income and location, but also delve into psychographics, such as interests, values and pain points.
The more specific your target audience, the more accurate and actionable your research will be. Additionally, segment your audience if your research objectives involve studying different groups, such as current customers and potential leads.
If you already have existing customers, you can also hold conversations with them to better understand your target market. From there, you can refine your buyer personas and tailor your research methods accordingly.
3. Choose your research methods
Selecting the right research methods is crucial for gathering high-quality data. Start by considering the nature of your research objectives. If you’re exploring consumer preferences, surveys and interviews can provide valuable insights. For in-depth understanding, focus groups or observational research might be suitable. Consider using a mix of quantitative and qualitative methods to gain a well-rounded perspective.
You’ll also need to consider your budget. Think about what you can realistically achieve using the time and resources available to you. If you have a fairly generous budget, you may want to try a mix of primary and secondary research approaches. If you’re doing market research for a startup , on the other hand, chances are your budget is somewhat limited. If that’s the case, try addressing your goals with secondary research tools before investing time and effort in a primary research study.
4. Use the right market research tools
Whether you’re conducting primary or secondary research, you’ll need to choose the right tools. These can help you do anything from sending surveys to customers to monitoring trends and analyzing data. Here are some examples of popular market research tools:
- Market research software: Crunchbase is a platform that provides best-in-class company data, making it valuable for market research on growing companies and industries. You can use Crunchbase to access trusted, first-party funding data, revenue data, news and firmographics, enabling you to monitor industry trends and understand customer needs.
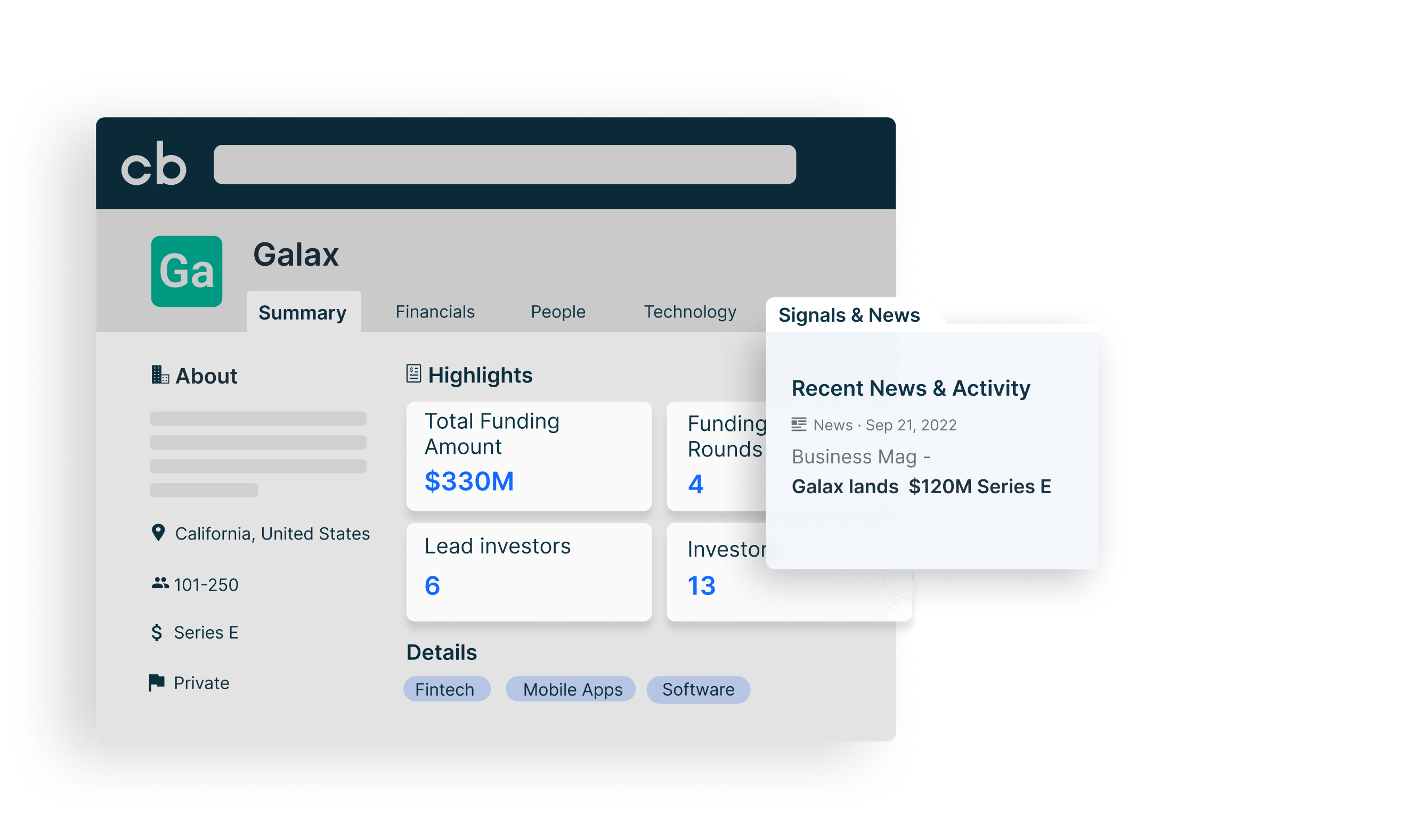
- Survey and questionnaire tools: SurveyMonkey is a widely used online survey platform that allows you to create, distribute and analyze surveys. Google Forms is a free tool that lets you create surveys and collect responses through Google Drive.
- Data analysis software: Microsoft Excel and Google Sheets are useful for conducting statistical analyses. SPSS is a powerful statistical analysis software used for data processing, analysis and reporting.
- Social listening tools: Brandwatch is a social listening and analytics platform that helps you monitor social media conversations, track sentiment and analyze trends. Mention is a media monitoring tool that allows you to track mentions of your brand, competitors and keywords across various online sources.
- Data visualization platforms: Tableau is a data visualization tool that helps you create interactive and shareable dashboards and reports. Power BI by Microsoft is a business analytics tool for creating interactive visualizations and reports.
5. Collect data
There’s an infinite amount of data you could be collecting using these tools, so you’ll need to be intentional about going after the data that aligns with your research goals. Implement your chosen research methods, whether it’s distributing surveys, conducting interviews or pulling from secondary research platforms. Pay close attention to data quality and accuracy, and stick to a standardized process to streamline data capture and reduce errors.
6. Analyze data
Once data is collected, you’ll need to analyze it systematically. Use statistical software or analysis tools to identify patterns, trends and correlations. For qualitative data, employ thematic analysis to extract common themes and insights. Visualize your findings with charts, graphs and tables to make complex data more understandable.
If you’re not proficient in data analysis, consider outsourcing or collaborating with a data analyst who can assist in processing and interpreting your data accurately.
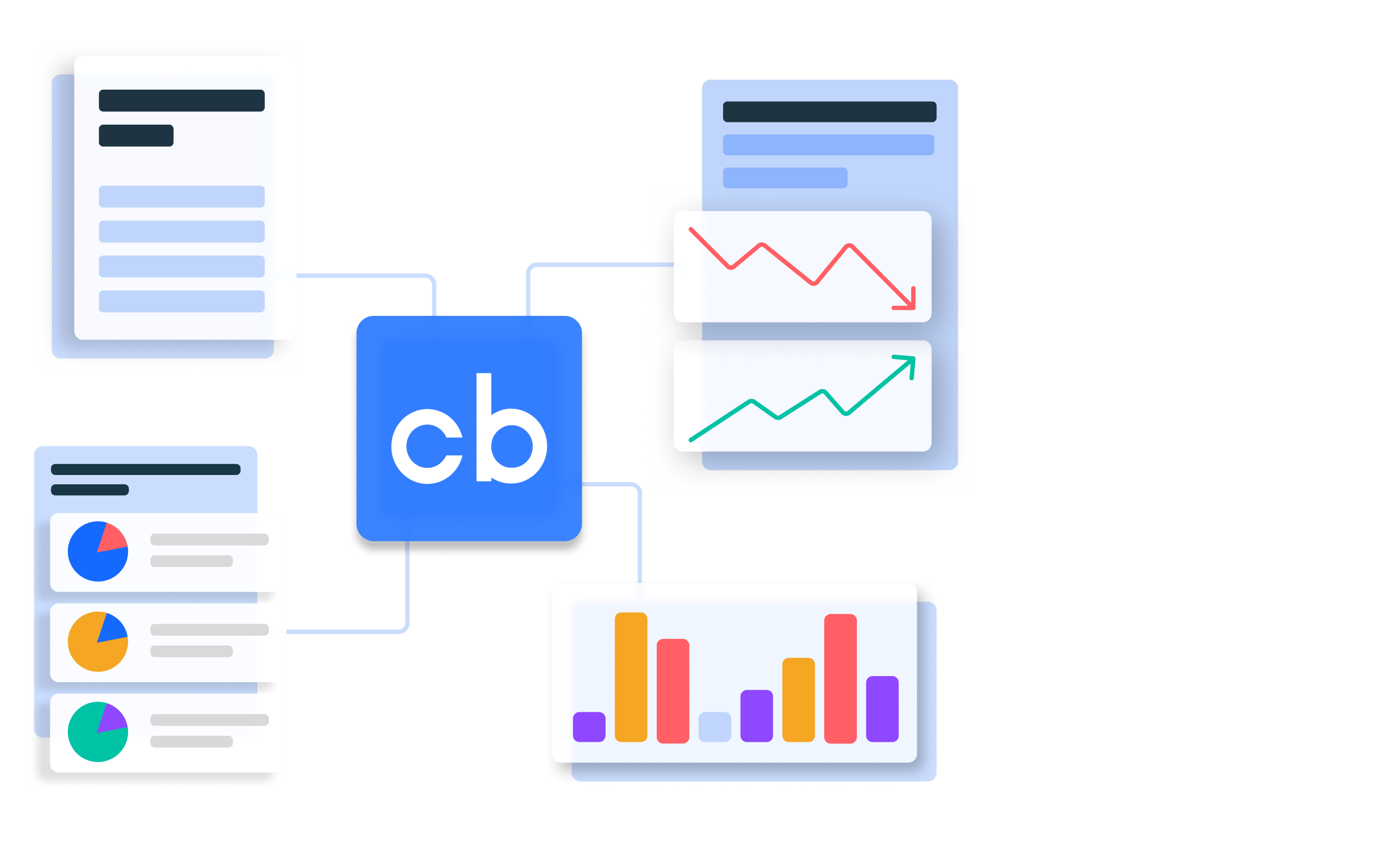
7. Interpret your findings
Interpreting your market research findings involves understanding what the data means in the context of your objectives. Are there significant trends that uncover the answers to your initial research questions? Consider the implications of your findings on your business strategy. It’s essential to move beyond raw data and extract actionable insights that inform decision-making.
Hold a cross-functional meeting or workshop with relevant team members to collectively interpret the findings. Different perspectives can lead to more comprehensive insights and innovative solutions.
8. Identify opportunities and challenges
Use your research findings to identify potential growth opportunities and challenges within your market. What segments of your audience are underserved or overlooked? Are there emerging trends you can capitalize on? Conversely, what obstacles or competitors could hinder your progress?
Lay out this information in a clear and organized way by conducting a SWOT analysis, which stands for strengths, weaknesses, opportunities and threats. Jot down notes for each of these areas to provide a structured overview of gaps and hurdles in the market.
9. Make informed business decisions
Market research is only valuable if it leads to informed decisions for your company. Based on your insights, devise actionable strategies and initiatives that align with your research objectives. Whether it’s refining your product, targeting new customer segments or adjusting pricing, ensure your decisions are rooted in the data.
At this point, it’s also crucial to keep your team aligned and accountable. Create an action plan that outlines specific steps, responsibilities and timelines for implementing the recommendations derived from your research.
10. Monitor and adapt
Market research isn’t a one-time activity; it’s an ongoing process. Continuously monitor market conditions, customer behaviors and industry trends. Set up mechanisms to collect real-time data and feedback. As you gather new information, be prepared to adapt your strategies and tactics accordingly. Regularly revisiting your research ensures your business remains agile and reflects changing market dynamics and consumer preferences.
Online market research sources
As you go through the steps above, you’ll want to turn to trusted, reputable sources to gather your data. Here’s a list to get you started:
- Crunchbase: As mentioned above, Crunchbase is an online platform with an extensive dataset, allowing you to access in-depth insights on market trends, consumer behavior and competitive analysis. You can also customize your search options to tailor your research to specific industries, geographic regions or customer personas.
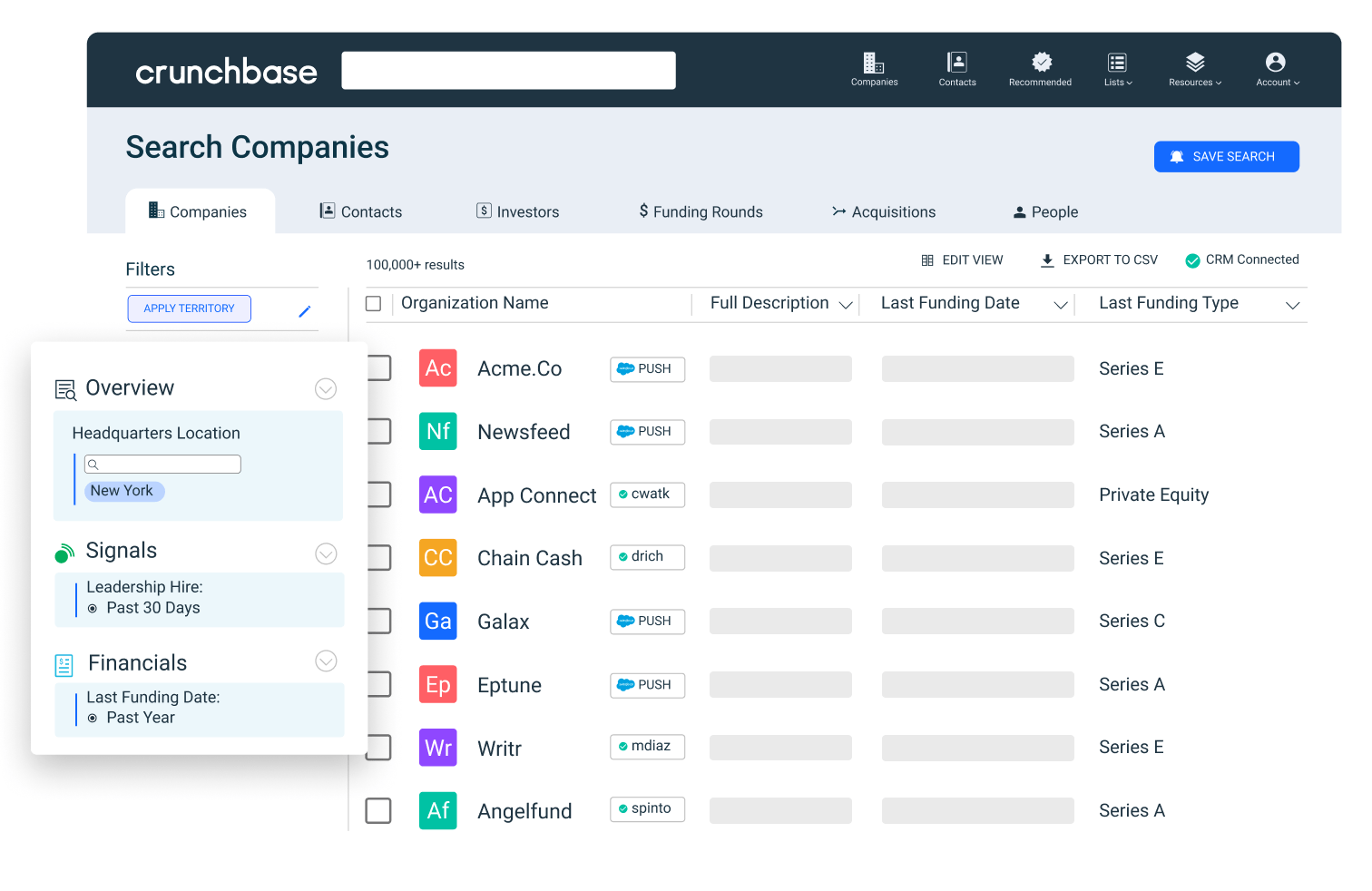
- Academic databases: Academic databases, such as ProQuest and JSTOR , are treasure troves of scholarly research papers, studies and academic journals. They offer in-depth analyses of various subjects, including market trends, consumer preferences and industry-specific insights. Researchers can access a wealth of peer-reviewed publications to gain a deeper understanding of their research topics.
- Government and NGO databases: Government agencies, nongovernmental organizations and other institutions frequently maintain databases containing valuable economic, demographic and industry-related data. These sources offer credible statistics and reports on a wide range of topics, making them essential for market researchers. Examples include the U.S. Census Bureau , the Bureau of Labor Statistics and the Pew Research Center .
- Industry reports: Industry reports and market studies are comprehensive documents prepared by research firms, industry associations and consulting companies. They provide in-depth insights into specific markets, including market size, trends, competitive analysis and consumer behavior. You can find this information by looking at relevant industry association databases; examples include the American Marketing Association and the National Retail Federation .
- Social media and online communities: Social media platforms like LinkedIn or Twitter (X) , forums such as Reddit and Quora , and review platforms such as G2 can provide real-time insights into consumer sentiment, opinions and trends.
Market research examples
At this point, you have market research tools and data sources — but how do you act on the data you gather? Let’s go over some real-world examples that illustrate the practical application of market research across various industries. These examples showcase how market research can lead to smart decision-making and successful business decisions.
Example 1: Apple’s iPhone launch
Apple ’s iconic iPhone launch in 2007 serves as a prime example of market research driving product innovation in tech. Before the iPhone’s release, Apple conducted extensive market research to understand consumer preferences, pain points and unmet needs in the mobile phone industry. This research led to the development of a touchscreen smartphone with a user-friendly interface, addressing consumer demands for a more intuitive and versatile device. The result was a revolutionary product that disrupted the market and redefined the smartphone industry.
Example 2: McDonald’s global expansion
McDonald’s successful global expansion strategy demonstrates the importance of market research when expanding into new territories. Before entering a new market, McDonald’s conducts thorough research to understand local tastes, preferences and cultural nuances. This research informs menu customization, marketing strategies and store design. For instance, in India, McDonald’s offers a menu tailored to local preferences, including vegetarian options. This market-specific approach has enabled McDonald’s to adapt and thrive in diverse global markets.
Example 3: Organic and sustainable farming
The shift toward organic and sustainable farming practices in the food industry is driven by market research that indicates increased consumer demand for healthier and environmentally friendly food options. As a result, food producers and retailers invest in sustainable sourcing and organic product lines — such as with these sustainable seafood startups — to align with this shift in consumer values.
The bottom line? Market research has multiple use cases and is a critical practice for any industry. Whether it’s launching groundbreaking products, entering new markets or responding to changing consumer preferences, you can use market research to shape successful strategies and outcomes.
Market research templates
You finally have a strong understanding of how to do market research and apply it in the real world. Before we wrap up, here are some market research templates that you can use as a starting point for your projects:
- Smartsheet competitive analysis templates : These spreadsheets can serve as a framework for gathering information about the competitive landscape and obtaining valuable lessons to apply to your business strategy.
- SurveyMonkey product survey template : Customize the questions on this survey based on what you want to learn from your target customers.
- HubSpot templates : HubSpot offers a wide range of free templates you can use for market research, business planning and more.
- SCORE templates : SCORE is a nonprofit organization that provides templates for business plans, market analysis and financial projections.
- SBA.gov : The U.S. Small Business Administration offers templates for every aspect of your business, including market research, and is particularly valuable for new startups.
Strengthen your business with market research
When conducted effectively, market research is like a guiding star. Equipped with the right tools and techniques, you can uncover valuable insights, stay competitive, foster innovation and navigate the complexities of your industry.
Throughout this guide, we’ve discussed the definition of market research, different research methods, and how to conduct it effectively. We’ve also explored various types of market research and shared practical insights and templates for getting started.
Now, it’s time to start the research process. Trust in data, listen to the market and make informed decisions that guide your company toward lasting success.
Related Articles
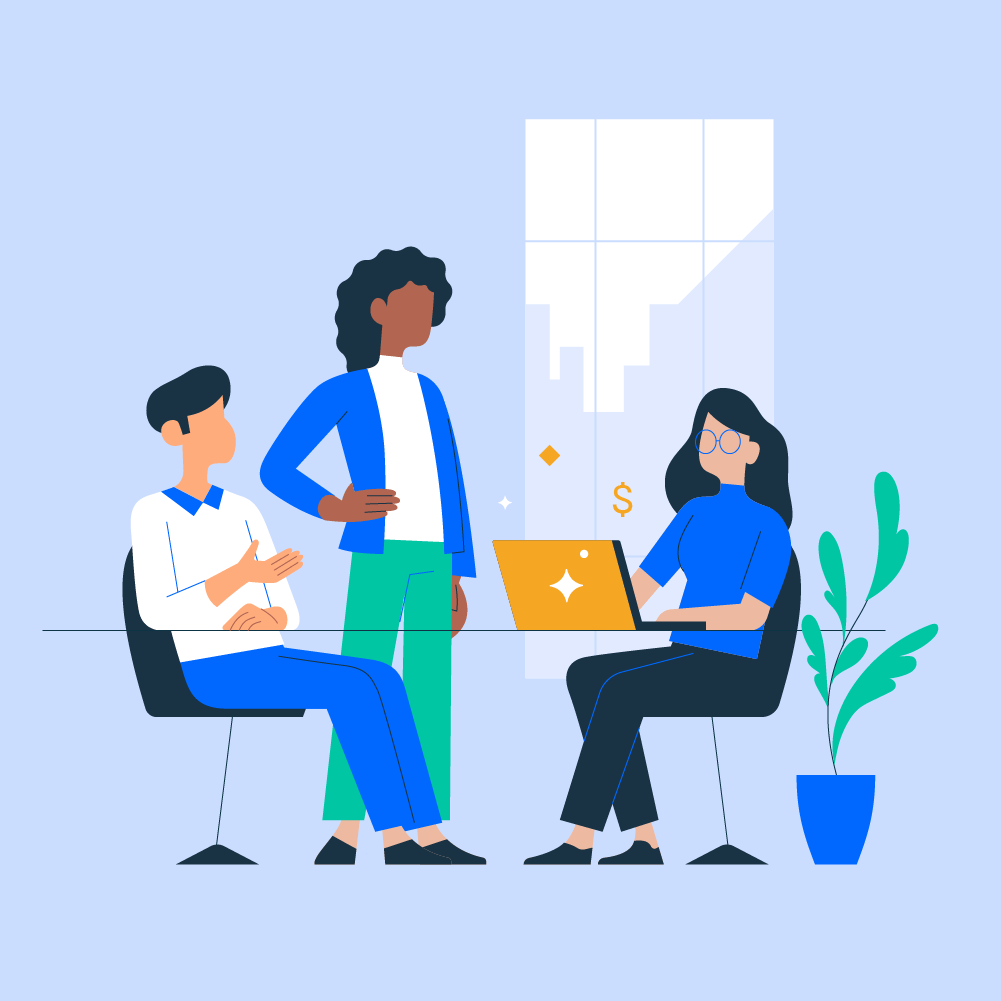
- Entrepreneurs
- 15 min read
What Is Competitive Analysis and How to Do It Effectively
Rebecca Strehlow, Copywriter at Crunchbase
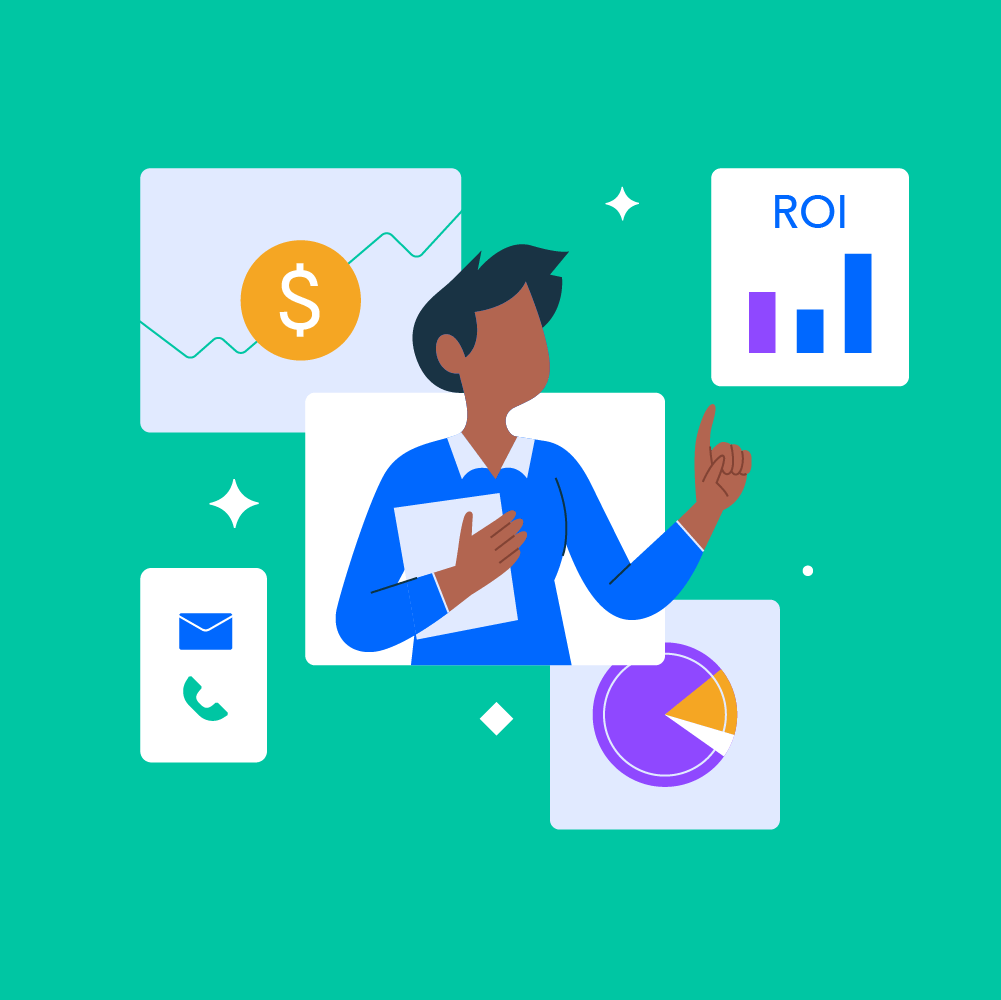
17 Best Sales Intelligence Tools for 2024
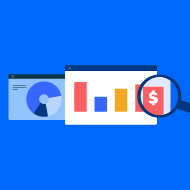
- Market research
- 10 min read
How to Do Market Research for a Startup: Tips for Success
Jaclyn Robinson, Senior Manager of Content Marketing at Crunchbase
Search less. Close more.
Grow your revenue with Crunchbase, the all-in-one prospecting solution. Start your free trial.
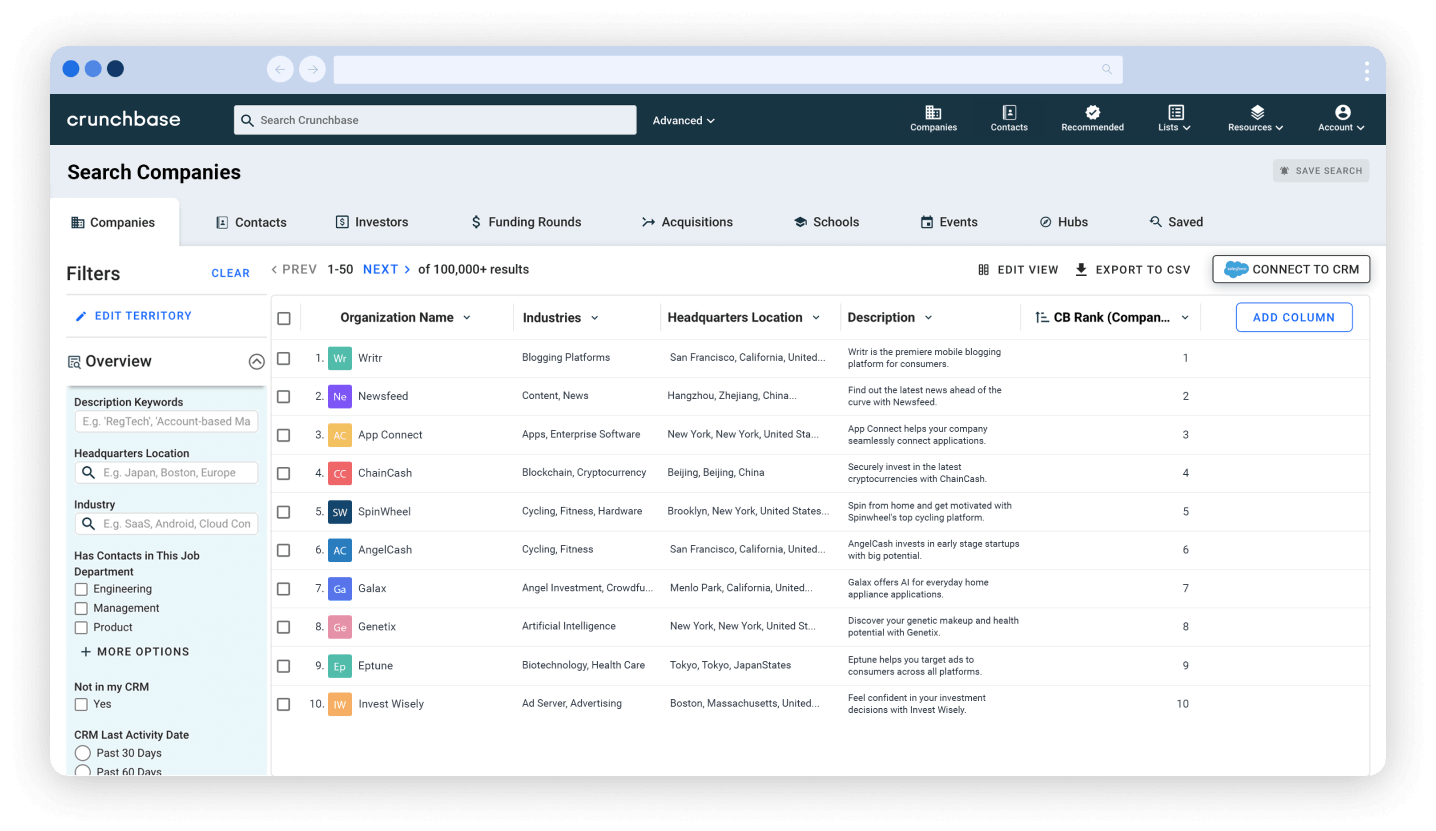
Have a thesis expert improve your writing
Check your thesis for plagiarism in 10 minutes, generate your apa citations for free.
- Knowledge Base
The Beginner's Guide to Statistical Analysis | 5 Steps & Examples
Statistical analysis means investigating trends, patterns, and relationships using quantitative data . It is an important research tool used by scientists, governments, businesses, and other organisations.
To draw valid conclusions, statistical analysis requires careful planning from the very start of the research process . You need to specify your hypotheses and make decisions about your research design, sample size, and sampling procedure.
After collecting data from your sample, you can organise and summarise the data using descriptive statistics . Then, you can use inferential statistics to formally test hypotheses and make estimates about the population. Finally, you can interpret and generalise your findings.
This article is a practical introduction to statistical analysis for students and researchers. We’ll walk you through the steps using two research examples. The first investigates a potential cause-and-effect relationship, while the second investigates a potential correlation between variables.
Table of contents
Step 1: write your hypotheses and plan your research design, step 2: collect data from a sample, step 3: summarise your data with descriptive statistics, step 4: test hypotheses or make estimates with inferential statistics, step 5: interpret your results, frequently asked questions about statistics.
To collect valid data for statistical analysis, you first need to specify your hypotheses and plan out your research design.
Writing statistical hypotheses
The goal of research is often to investigate a relationship between variables within a population . You start with a prediction, and use statistical analysis to test that prediction.
A statistical hypothesis is a formal way of writing a prediction about a population. Every research prediction is rephrased into null and alternative hypotheses that can be tested using sample data.
While the null hypothesis always predicts no effect or no relationship between variables, the alternative hypothesis states your research prediction of an effect or relationship.
- Null hypothesis: A 5-minute meditation exercise will have no effect on math test scores in teenagers.
- Alternative hypothesis: A 5-minute meditation exercise will improve math test scores in teenagers.
- Null hypothesis: Parental income and GPA have no relationship with each other in college students.
- Alternative hypothesis: Parental income and GPA are positively correlated in college students.
Planning your research design
A research design is your overall strategy for data collection and analysis. It determines the statistical tests you can use to test your hypothesis later on.
First, decide whether your research will use a descriptive, correlational, or experimental design. Experiments directly influence variables, whereas descriptive and correlational studies only measure variables.
- In an experimental design , you can assess a cause-and-effect relationship (e.g., the effect of meditation on test scores) using statistical tests of comparison or regression.
- In a correlational design , you can explore relationships between variables (e.g., parental income and GPA) without any assumption of causality using correlation coefficients and significance tests.
- In a descriptive design , you can study the characteristics of a population or phenomenon (e.g., the prevalence of anxiety in U.S. college students) using statistical tests to draw inferences from sample data.
Your research design also concerns whether you’ll compare participants at the group level or individual level, or both.
- In a between-subjects design , you compare the group-level outcomes of participants who have been exposed to different treatments (e.g., those who performed a meditation exercise vs those who didn’t).
- In a within-subjects design , you compare repeated measures from participants who have participated in all treatments of a study (e.g., scores from before and after performing a meditation exercise).
- In a mixed (factorial) design , one variable is altered between subjects and another is altered within subjects (e.g., pretest and posttest scores from participants who either did or didn’t do a meditation exercise).
- Experimental
- Correlational
First, you’ll take baseline test scores from participants. Then, your participants will undergo a 5-minute meditation exercise. Finally, you’ll record participants’ scores from a second math test.
In this experiment, the independent variable is the 5-minute meditation exercise, and the dependent variable is the math test score from before and after the intervention. Example: Correlational research design In a correlational study, you test whether there is a relationship between parental income and GPA in graduating college students. To collect your data, you will ask participants to fill in a survey and self-report their parents’ incomes and their own GPA.
Measuring variables
When planning a research design, you should operationalise your variables and decide exactly how you will measure them.
For statistical analysis, it’s important to consider the level of measurement of your variables, which tells you what kind of data they contain:
- Categorical data represents groupings. These may be nominal (e.g., gender) or ordinal (e.g. level of language ability).
- Quantitative data represents amounts. These may be on an interval scale (e.g. test score) or a ratio scale (e.g. age).
Many variables can be measured at different levels of precision. For example, age data can be quantitative (8 years old) or categorical (young). If a variable is coded numerically (e.g., level of agreement from 1–5), it doesn’t automatically mean that it’s quantitative instead of categorical.
Identifying the measurement level is important for choosing appropriate statistics and hypothesis tests. For example, you can calculate a mean score with quantitative data, but not with categorical data.
In a research study, along with measures of your variables of interest, you’ll often collect data on relevant participant characteristics.
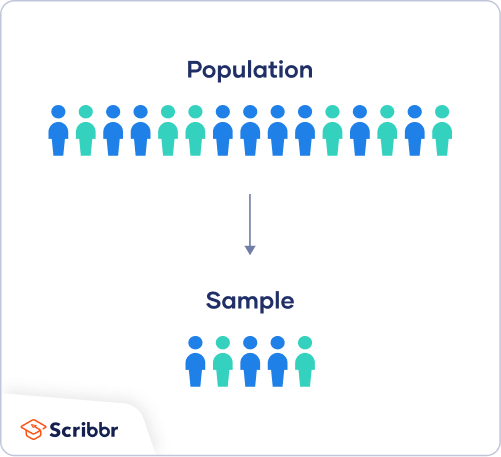
In most cases, it’s too difficult or expensive to collect data from every member of the population you’re interested in studying. Instead, you’ll collect data from a sample.
Statistical analysis allows you to apply your findings beyond your own sample as long as you use appropriate sampling procedures . You should aim for a sample that is representative of the population.
Sampling for statistical analysis
There are two main approaches to selecting a sample.
- Probability sampling: every member of the population has a chance of being selected for the study through random selection.
- Non-probability sampling: some members of the population are more likely than others to be selected for the study because of criteria such as convenience or voluntary self-selection.
In theory, for highly generalisable findings, you should use a probability sampling method. Random selection reduces sampling bias and ensures that data from your sample is actually typical of the population. Parametric tests can be used to make strong statistical inferences when data are collected using probability sampling.
But in practice, it’s rarely possible to gather the ideal sample. While non-probability samples are more likely to be biased, they are much easier to recruit and collect data from. Non-parametric tests are more appropriate for non-probability samples, but they result in weaker inferences about the population.
If you want to use parametric tests for non-probability samples, you have to make the case that:
- your sample is representative of the population you’re generalising your findings to.
- your sample lacks systematic bias.
Keep in mind that external validity means that you can only generalise your conclusions to others who share the characteristics of your sample. For instance, results from Western, Educated, Industrialised, Rich and Democratic samples (e.g., college students in the US) aren’t automatically applicable to all non-WEIRD populations.
If you apply parametric tests to data from non-probability samples, be sure to elaborate on the limitations of how far your results can be generalised in your discussion section .
Create an appropriate sampling procedure
Based on the resources available for your research, decide on how you’ll recruit participants.
- Will you have resources to advertise your study widely, including outside of your university setting?
- Will you have the means to recruit a diverse sample that represents a broad population?
- Do you have time to contact and follow up with members of hard-to-reach groups?
Your participants are self-selected by their schools. Although you’re using a non-probability sample, you aim for a diverse and representative sample. Example: Sampling (correlational study) Your main population of interest is male college students in the US. Using social media advertising, you recruit senior-year male college students from a smaller subpopulation: seven universities in the Boston area.
Calculate sufficient sample size
Before recruiting participants, decide on your sample size either by looking at other studies in your field or using statistics. A sample that’s too small may be unrepresentative of the sample, while a sample that’s too large will be more costly than necessary.
There are many sample size calculators online. Different formulas are used depending on whether you have subgroups or how rigorous your study should be (e.g., in clinical research). As a rule of thumb, a minimum of 30 units or more per subgroup is necessary.
To use these calculators, you have to understand and input these key components:
- Significance level (alpha): the risk of rejecting a true null hypothesis that you are willing to take, usually set at 5%.
- Statistical power : the probability of your study detecting an effect of a certain size if there is one, usually 80% or higher.
- Expected effect size : a standardised indication of how large the expected result of your study will be, usually based on other similar studies.
- Population standard deviation: an estimate of the population parameter based on a previous study or a pilot study of your own.
Once you’ve collected all of your data, you can inspect them and calculate descriptive statistics that summarise them.
Inspect your data
There are various ways to inspect your data, including the following:
- Organising data from each variable in frequency distribution tables .
- Displaying data from a key variable in a bar chart to view the distribution of responses.
- Visualising the relationship between two variables using a scatter plot .
By visualising your data in tables and graphs, you can assess whether your data follow a skewed or normal distribution and whether there are any outliers or missing data.
A normal distribution means that your data are symmetrically distributed around a center where most values lie, with the values tapering off at the tail ends.
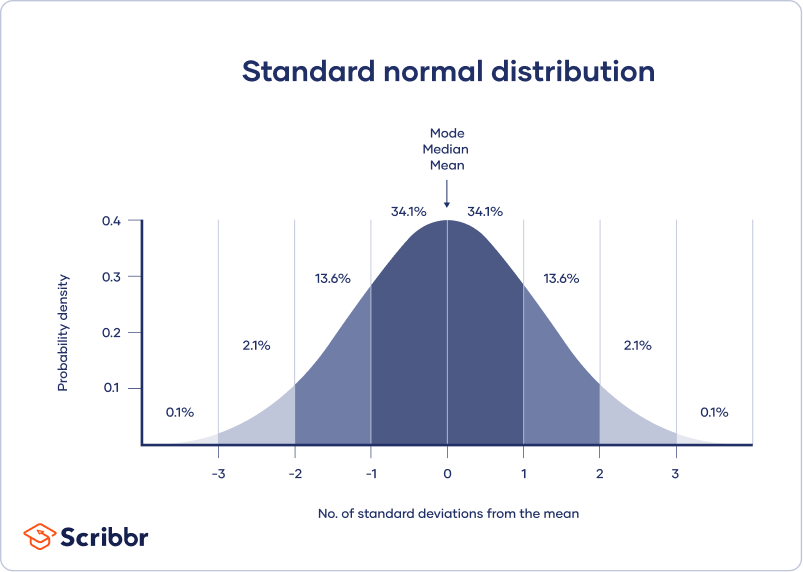
In contrast, a skewed distribution is asymmetric and has more values on one end than the other. The shape of the distribution is important to keep in mind because only some descriptive statistics should be used with skewed distributions.
Extreme outliers can also produce misleading statistics, so you may need a systematic approach to dealing with these values.
Calculate measures of central tendency
Measures of central tendency describe where most of the values in a data set lie. Three main measures of central tendency are often reported:
- Mode : the most popular response or value in the data set.
- Median : the value in the exact middle of the data set when ordered from low to high.
- Mean : the sum of all values divided by the number of values.
However, depending on the shape of the distribution and level of measurement, only one or two of these measures may be appropriate. For example, many demographic characteristics can only be described using the mode or proportions, while a variable like reaction time may not have a mode at all.
Calculate measures of variability
Measures of variability tell you how spread out the values in a data set are. Four main measures of variability are often reported:
- Range : the highest value minus the lowest value of the data set.
- Interquartile range : the range of the middle half of the data set.
- Standard deviation : the average distance between each value in your data set and the mean.
- Variance : the square of the standard deviation.
Once again, the shape of the distribution and level of measurement should guide your choice of variability statistics. The interquartile range is the best measure for skewed distributions, while standard deviation and variance provide the best information for normal distributions.
Using your table, you should check whether the units of the descriptive statistics are comparable for pretest and posttest scores. For example, are the variance levels similar across the groups? Are there any extreme values? If there are, you may need to identify and remove extreme outliers in your data set or transform your data before performing a statistical test.
From this table, we can see that the mean score increased after the meditation exercise, and the variances of the two scores are comparable. Next, we can perform a statistical test to find out if this improvement in test scores is statistically significant in the population. Example: Descriptive statistics (correlational study) After collecting data from 653 students, you tabulate descriptive statistics for annual parental income and GPA.
It’s important to check whether you have a broad range of data points. If you don’t, your data may be skewed towards some groups more than others (e.g., high academic achievers), and only limited inferences can be made about a relationship.
A number that describes a sample is called a statistic , while a number describing a population is called a parameter . Using inferential statistics , you can make conclusions about population parameters based on sample statistics.
Researchers often use two main methods (simultaneously) to make inferences in statistics.
- Estimation: calculating population parameters based on sample statistics.
- Hypothesis testing: a formal process for testing research predictions about the population using samples.
You can make two types of estimates of population parameters from sample statistics:
- A point estimate : a value that represents your best guess of the exact parameter.
- An interval estimate : a range of values that represent your best guess of where the parameter lies.
If your aim is to infer and report population characteristics from sample data, it’s best to use both point and interval estimates in your paper.
You can consider a sample statistic a point estimate for the population parameter when you have a representative sample (e.g., in a wide public opinion poll, the proportion of a sample that supports the current government is taken as the population proportion of government supporters).
There’s always error involved in estimation, so you should also provide a confidence interval as an interval estimate to show the variability around a point estimate.
A confidence interval uses the standard error and the z score from the standard normal distribution to convey where you’d generally expect to find the population parameter most of the time.
Hypothesis testing
Using data from a sample, you can test hypotheses about relationships between variables in the population. Hypothesis testing starts with the assumption that the null hypothesis is true in the population, and you use statistical tests to assess whether the null hypothesis can be rejected or not.
Statistical tests determine where your sample data would lie on an expected distribution of sample data if the null hypothesis were true. These tests give two main outputs:
- A test statistic tells you how much your data differs from the null hypothesis of the test.
- A p value tells you the likelihood of obtaining your results if the null hypothesis is actually true in the population.
Statistical tests come in three main varieties:
- Comparison tests assess group differences in outcomes.
- Regression tests assess cause-and-effect relationships between variables.
- Correlation tests assess relationships between variables without assuming causation.
Your choice of statistical test depends on your research questions, research design, sampling method, and data characteristics.
Parametric tests
Parametric tests make powerful inferences about the population based on sample data. But to use them, some assumptions must be met, and only some types of variables can be used. If your data violate these assumptions, you can perform appropriate data transformations or use alternative non-parametric tests instead.
A regression models the extent to which changes in a predictor variable results in changes in outcome variable(s).
- A simple linear regression includes one predictor variable and one outcome variable.
- A multiple linear regression includes two or more predictor variables and one outcome variable.
Comparison tests usually compare the means of groups. These may be the means of different groups within a sample (e.g., a treatment and control group), the means of one sample group taken at different times (e.g., pretest and posttest scores), or a sample mean and a population mean.
- A t test is for exactly 1 or 2 groups when the sample is small (30 or less).
- A z test is for exactly 1 or 2 groups when the sample is large.
- An ANOVA is for 3 or more groups.
The z and t tests have subtypes based on the number and types of samples and the hypotheses:
- If you have only one sample that you want to compare to a population mean, use a one-sample test .
- If you have paired measurements (within-subjects design), use a dependent (paired) samples test .
- If you have completely separate measurements from two unmatched groups (between-subjects design), use an independent (unpaired) samples test .
- If you expect a difference between groups in a specific direction, use a one-tailed test .
- If you don’t have any expectations for the direction of a difference between groups, use a two-tailed test .
The only parametric correlation test is Pearson’s r . The correlation coefficient ( r ) tells you the strength of a linear relationship between two quantitative variables.
However, to test whether the correlation in the sample is strong enough to be important in the population, you also need to perform a significance test of the correlation coefficient, usually a t test, to obtain a p value. This test uses your sample size to calculate how much the correlation coefficient differs from zero in the population.
You use a dependent-samples, one-tailed t test to assess whether the meditation exercise significantly improved math test scores. The test gives you:
- a t value (test statistic) of 3.00
- a p value of 0.0028
Although Pearson’s r is a test statistic, it doesn’t tell you anything about how significant the correlation is in the population. You also need to test whether this sample correlation coefficient is large enough to demonstrate a correlation in the population.
A t test can also determine how significantly a correlation coefficient differs from zero based on sample size. Since you expect a positive correlation between parental income and GPA, you use a one-sample, one-tailed t test. The t test gives you:
- a t value of 3.08
- a p value of 0.001
The final step of statistical analysis is interpreting your results.
Statistical significance
In hypothesis testing, statistical significance is the main criterion for forming conclusions. You compare your p value to a set significance level (usually 0.05) to decide whether your results are statistically significant or non-significant.
Statistically significant results are considered unlikely to have arisen solely due to chance. There is only a very low chance of such a result occurring if the null hypothesis is true in the population.
This means that you believe the meditation intervention, rather than random factors, directly caused the increase in test scores. Example: Interpret your results (correlational study) You compare your p value of 0.001 to your significance threshold of 0.05. With a p value under this threshold, you can reject the null hypothesis. This indicates a statistically significant correlation between parental income and GPA in male college students.
Note that correlation doesn’t always mean causation, because there are often many underlying factors contributing to a complex variable like GPA. Even if one variable is related to another, this may be because of a third variable influencing both of them, or indirect links between the two variables.
Effect size
A statistically significant result doesn’t necessarily mean that there are important real life applications or clinical outcomes for a finding.
In contrast, the effect size indicates the practical significance of your results. It’s important to report effect sizes along with your inferential statistics for a complete picture of your results. You should also report interval estimates of effect sizes if you’re writing an APA style paper .
With a Cohen’s d of 0.72, there’s medium to high practical significance to your finding that the meditation exercise improved test scores. Example: Effect size (correlational study) To determine the effect size of the correlation coefficient, you compare your Pearson’s r value to Cohen’s effect size criteria.
Decision errors
Type I and Type II errors are mistakes made in research conclusions. A Type I error means rejecting the null hypothesis when it’s actually true, while a Type II error means failing to reject the null hypothesis when it’s false.
You can aim to minimise the risk of these errors by selecting an optimal significance level and ensuring high power . However, there’s a trade-off between the two errors, so a fine balance is necessary.
Frequentist versus Bayesian statistics
Traditionally, frequentist statistics emphasises null hypothesis significance testing and always starts with the assumption of a true null hypothesis.
However, Bayesian statistics has grown in popularity as an alternative approach in the last few decades. In this approach, you use previous research to continually update your hypotheses based on your expectations and observations.
Bayes factor compares the relative strength of evidence for the null versus the alternative hypothesis rather than making a conclusion about rejecting the null hypothesis or not.
Hypothesis testing is a formal procedure for investigating our ideas about the world using statistics. It is used by scientists to test specific predictions, called hypotheses , by calculating how likely it is that a pattern or relationship between variables could have arisen by chance.
The research methods you use depend on the type of data you need to answer your research question .
- If you want to measure something or test a hypothesis , use quantitative methods . If you want to explore ideas, thoughts, and meanings, use qualitative methods .
- If you want to analyse a large amount of readily available data, use secondary data. If you want data specific to your purposes with control over how they are generated, collect primary data.
- If you want to establish cause-and-effect relationships between variables , use experimental methods. If you want to understand the characteristics of a research subject, use descriptive methods.
Statistical analysis is the main method for analyzing quantitative research data . It uses probabilities and models to test predictions about a population from sample data.
Is this article helpful?
Other students also liked, a quick guide to experimental design | 5 steps & examples, controlled experiments | methods & examples of control, between-subjects design | examples, pros & cons, more interesting articles.
- Central Limit Theorem | Formula, Definition & Examples
- Central Tendency | Understanding the Mean, Median & Mode
- Correlation Coefficient | Types, Formulas & Examples
- Descriptive Statistics | Definitions, Types, Examples
- How to Calculate Standard Deviation (Guide) | Calculator & Examples
- How to Calculate Variance | Calculator, Analysis & Examples
- How to Find Degrees of Freedom | Definition & Formula
- How to Find Interquartile Range (IQR) | Calculator & Examples
- How to Find Outliers | Meaning, Formula & Examples
- How to Find the Geometric Mean | Calculator & Formula
- How to Find the Mean | Definition, Examples & Calculator
- How to Find the Median | Definition, Examples & Calculator
- How to Find the Range of a Data Set | Calculator & Formula
- Inferential Statistics | An Easy Introduction & Examples
- Levels of measurement: Nominal, ordinal, interval, ratio
- Missing Data | Types, Explanation, & Imputation
- Normal Distribution | Examples, Formulas, & Uses
- Null and Alternative Hypotheses | Definitions & Examples
- Poisson Distributions | Definition, Formula & Examples
- Skewness | Definition, Examples & Formula
- T-Distribution | What It Is and How To Use It (With Examples)
- The Standard Normal Distribution | Calculator, Examples & Uses
- Type I & Type II Errors | Differences, Examples, Visualizations
- Understanding Confidence Intervals | Easy Examples & Formulas
- Variability | Calculating Range, IQR, Variance, Standard Deviation
- What is Effect Size and Why Does It Matter? (Examples)
- What Is Interval Data? | Examples & Definition
- What Is Nominal Data? | Examples & Definition
- What Is Ordinal Data? | Examples & Definition
- What Is Ratio Data? | Examples & Definition
- What Is the Mode in Statistics? | Definition, Examples & Calculator
8 Types of Data Analysis

Data analysis is an aspect of data science and data analytics that is all about analyzing data for different kinds of purposes. The data analysis process involves inspecting, cleaning, transforming and modeling data to draw useful insights from it.
What Are the Different Types of Data Analysis?
- Descriptive analysis
- Diagnostic analysis
- Exploratory analysis
- Inferential analysis
- Predictive analysis
- Causal analysis
- Mechanistic analysis
- Prescriptive analysis
With its multiple facets, methodologies and techniques, data analysis is used in a variety of fields, including business, science and social science, among others. As businesses thrive under the influence of technological advancements in data analytics, data analysis plays a huge role in decision-making , providing a better, faster and more efficacious system that minimizes risks and reduces human biases .
That said, there are different kinds of data analysis catered with different goals. We’ll examine each one below.
Two Camps of Data Analysis
Data analysis can be divided into two camps, according to the book R for Data Science :
- Hypothesis Generation — This involves looking deeply at the data and combining your domain knowledge to generate hypotheses about why the data behaves the way it does.
- Hypothesis Confirmation — This involves using a precise mathematical model to generate falsifiable predictions with statistical sophistication to confirm your prior hypotheses.
Types of Data Analysis
Data analysis can be separated and organized into types, arranged in an increasing order of complexity.
1. Descriptive Analysis
The goal of descriptive analysis is to describe or summarize a set of data. Here’s what you need to know:
- Descriptive analysis is the very first analysis performed in the data analysis process.
- It generates simple summaries about samples and measurements.
- It involves common, descriptive statistics like measures of central tendency, variability, frequency and position.
Descriptive Analysis Example
Take the Covid-19 statistics page on Google, for example. The line graph is a pure summary of the cases/deaths, a presentation and description of the population of a particular country infected by the virus.
Descriptive analysis is the first step in analysis where you summarize and describe the data you have using descriptive statistics, and the result is a simple presentation of your data.
More on Data Analysis: Data Analyst vs. Data Scientist: Similarities and Differences Explained
2. Diagnostic Analysis
Diagnostic analysis seeks to answer the question “Why did this happen?” by taking a more in-depth look at data to uncover subtle patterns. Here’s what you need to know:
- Diagnostic analysis typically comes after descriptive analysis, taking initial findings and investigating why certain patterns in data happen.
- Diagnostic analysis may involve analyzing other related data sources, including past data, to reveal more insights into current data trends.
- Diagnostic analysis is ideal for further exploring patterns in data to explain anomalies.
Diagnostic Analysis Example
A footwear store wants to review its website traffic levels over the previous 12 months. Upon compiling and assessing the data, the company’s marketing team finds that June experienced above-average levels of traffic while July and August witnessed slightly lower levels of traffic.
To find out why this difference occurred, the marketing team takes a deeper look. Team members break down the data to focus on specific categories of footwear. In the month of June, they discovered that pages featuring sandals and other beach-related footwear received a high number of views while these numbers dropped in July and August.
Marketers may also review other factors like seasonal changes and company sales events to see if other variables could have contributed to this trend.
3. Exploratory Analysis (EDA)
Exploratory analysis involves examining or exploring data and finding relationships between variables that were previously unknown. Here’s what you need to know:
- EDA helps you discover relationships between measures in your data, which are not evidence for the existence of the correlation, as denoted by the phrase, “ Correlation doesn’t imply causation .”
- It’s useful for discovering new connections and forming hypotheses. It drives design planning and data collection.
Exploratory Analysis Example
Climate change is an increasingly important topic as the global temperature has gradually risen over the years. One example of an exploratory data analysis on climate change involves taking the rise in temperature over the years from 1950 to 2020 and the increase of human activities and industrialization to find relationships from the data. For example, you may increase the number of factories, cars on the road and airplane flights to see how that correlates with the rise in temperature.
Exploratory analysis explores data to find relationships between measures without identifying the cause. It’s most useful when formulating hypotheses.
4. Inferential Analysis
Inferential analysis involves using a small sample of data to infer information about a larger population of data.
The goal of statistical modeling itself is all about using a small amount of information to extrapolate and generalize information to a larger group. Here’s what you need to know:
- Inferential analysis involves using estimated data that is representative of a population and gives a measure of uncertainty or standard deviation to your estimation.
- The accuracy of inference depends heavily on your sampling scheme. If the sample isn’t representative of the population, the generalization will be inaccurate. This is known as the central limit theorem .
Inferential Analysis Example
The idea of drawing an inference about the population at large with a smaller sample size is intuitive. Many statistics you see on the media and the internet are inferential; a prediction of an event based on a small sample. For example, a psychological study on the benefits of sleep might have a total of 500 people involved. When they followed up with the candidates, the candidates reported to have better overall attention spans and well-being with seven-to-nine hours of sleep, while those with less sleep and more sleep than the given range suffered from reduced attention spans and energy. This study drawn from 500 people was just a tiny portion of the 7 billion people in the world, and is thus an inference of the larger population.
Inferential analysis extrapolates and generalizes the information of the larger group with a smaller sample to generate analysis and predictions.
5. Predictive Analysis
Predictive analysis involves using historical or current data to find patterns and make predictions about the future. Here’s what you need to know:
- The accuracy of the predictions depends on the input variables.
- Accuracy also depends on the types of models. A linear model might work well in some cases, and in other cases it might not.
- Using a variable to predict another one doesn’t denote a causal relationship.
Predictive Analysis Example
The 2020 US election is a popular topic and many prediction models are built to predict the winning candidate. FiveThirtyEight did this to forecast the 2016 and 2020 elections. Prediction analysis for an election would require input variables such as historical polling data, trends and current polling data in order to return a good prediction. Something as large as an election wouldn’t just be using a linear model, but a complex model with certain tunings to best serve its purpose.
Predictive analysis takes data from the past and present to make predictions about the future.
More on Data: Explaining the Empirical for Normal Distribution
6. Causal Analysis
Causal analysis looks at the cause and effect of relationships between variables and is focused on finding the cause of a correlation. Here’s what you need to know:
- To find the cause, you have to question whether the observed correlations driving your conclusion are valid. Just looking at the surface data won’t help you discover the hidden mechanisms underlying the correlations.
- Causal analysis is applied in randomized studies focused on identifying causation.
- Causal analysis is the gold standard in data analysis and scientific studies where the cause of phenomenon is to be extracted and singled out, like separating wheat from chaff.
- Good data is hard to find and requires expensive research and studies. These studies are analyzed in aggregate (multiple groups), and the observed relationships are just average effects (mean) of the whole population. This means the results might not apply to everyone.
Causal Analysis Example
Say you want to test out whether a new drug improves human strength and focus. To do that, you perform randomized control trials for the drug to test its effect. You compare the sample of candidates for your new drug against the candidates receiving a mock control drug through a few tests focused on strength and overall focus and attention. This will allow you to observe how the drug affects the outcome.
Causal analysis is about finding out the causal relationship between variables, and examining how a change in one variable affects another.
7. Mechanistic Analysis
Mechanistic analysis is used to understand exact changes in variables that lead to other changes in other variables. Here’s what you need to know:
- It’s applied in physical or engineering sciences, situations that require high precision and little room for error, only noise in data is measurement error.
- It’s designed to understand a biological or behavioral process, the pathophysiology of a disease or the mechanism of action of an intervention.
Mechanistic Analysis Example
Many graduate-level research and complex topics are suitable examples, but to put it in simple terms, let’s say an experiment is done to simulate safe and effective nuclear fusion to power the world. A mechanistic analysis of the study would entail a precise balance of controlling and manipulating variables with highly accurate measures of both variables and the desired outcomes. It’s this intricate and meticulous modus operandi toward these big topics that allows for scientific breakthroughs and advancement of society.
Mechanistic analysis is in some ways a predictive analysis, but modified to tackle studies that require high precision and meticulous methodologies for physical or engineering science .
8. Prescriptive Analysis
Prescriptive analysis compiles insights from other previous data analyses and determines actions that teams or companies can take to prepare for predicted trends. Here’s what you need to know:
- Prescriptive analysis may come right after predictive analysis, but it may involve combining many different data analyses.
- Companies need advanced technology and plenty of resources to conduct prescriptive analysis. AI systems that process data and adjust automated tasks are an example of the technology required to perform prescriptive analysis.
Prescriptive Analysis Example
Prescriptive analysis is pervasive in everyday life, driving the curated content users consume on social media. On platforms like TikTok and Instagram, algorithms can apply prescriptive analysis to review past content a user has engaged with and the kinds of behaviors they exhibited with specific posts. Based on these factors, an algorithm seeks out similar content that is likely to elicit the same response and recommends it on a user’s personal feed.
When to Use the Different Types of Data Analysis
- Descriptive analysis summarizes the data at hand and presents your data in a comprehensible way.
- Diagnostic analysis takes a more detailed look at data to reveal why certain patterns occur, making it a good method for explaining anomalies.
- Exploratory data analysis helps you discover correlations and relationships between variables in your data.
- Inferential analysis is for generalizing the larger population with a smaller sample size of data.
- Predictive analysis helps you make predictions about the future with data.
- Causal analysis emphasizes finding the cause of a correlation between variables.
- Mechanistic analysis is for measuring the exact changes in variables that lead to other changes in other variables.
- Prescriptive analysis combines insights from different data analyses to develop a course of action teams and companies can take to capitalize on predicted outcomes.
A few important tips to remember about data analysis include:
- Correlation doesn’t imply causation.
- EDA helps discover new connections and form hypotheses.
- Accuracy of inference depends on the sampling scheme.
- A good prediction depends on the right input variables.
- A simple linear model with enough data usually does the trick.
- Using a variable to predict another doesn’t denote causal relationships.
- Good data is hard to find, and to produce it requires expensive research.
- Results from studies are done in aggregate and are average effects and might not apply to everyone.
Built In’s expert contributor network publishes thoughtful, solutions-oriented stories written by innovative tech professionals. It is the tech industry’s definitive destination for sharing compelling, first-person accounts of problem-solving on the road to innovation.
Great Companies Need Great People. That's Where We Come In.
Thrive On: The Campaign for Utica University → Delays Due to Weather → -->
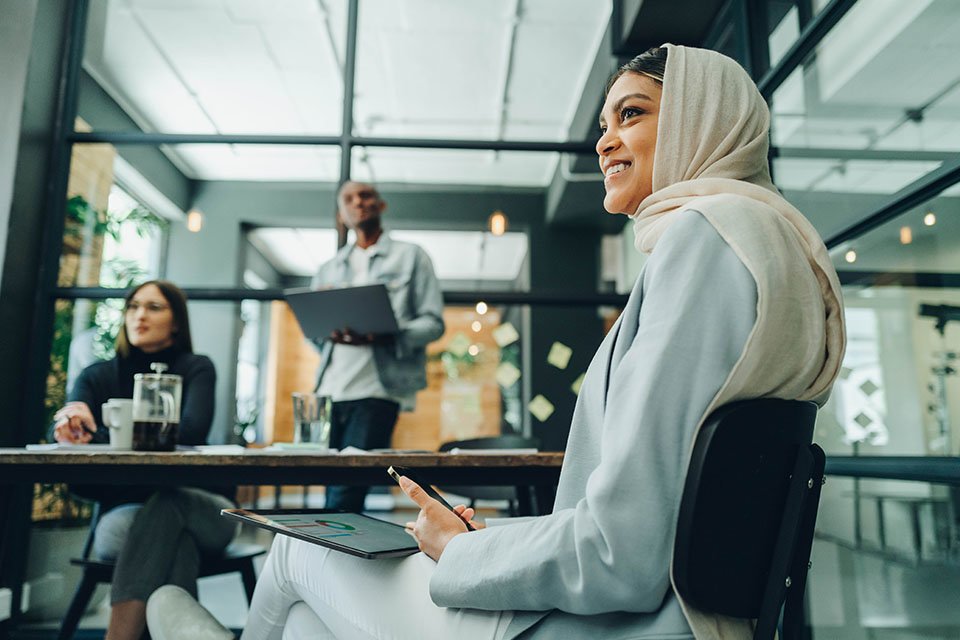
What Do You Learn in an M.B.A. Program?
April 18, 2024
In today’s competitive business environment, professionals are constantly seeking ways to advance their careers and stand out from others. The Master of Business Administration (M.B.A.) degree is a popular educational choice because of its ability to shape well-rounded business leaders, offering a wealth of knowledge and experiences that are crucial for success across industries.
Whether you’re a seasoned professional hoping to climb to executive ranks or a recent graduate eager to make your mark in the business world, a high-quality M.B.A. can help transform your professional prospects. Keep reading as we explore the components of an M.B.A. curriculum, the essential skills you’ll acquire, and the profound impact they could have on your career trajectory.
What to expect from an M.B.A. curriculum
Earning an M.B.A. should be a multifaceted journey – one designed to equip you with the tools necessary to navigate complex business challenges and seize opportunities with confidence. From teaching you how to master core business concepts to how to hone your strategic skills, an M.B.A. program can serve as a cornerstone for both personal and professional development.
A typical M.B.A. curriculum should allow you to delve into an extensive range of subjects designed to provide a holistic understanding of business operations. Core courses should cover fundamental principles like management, finance, marketing, and leadership, laying the groundwork for specialized studies.
Through interactive lectures, case analyses, and collaborative projects, M.B.A. students typically engage in dynamic learning experiences that combine theoretical concepts with practical application. Many M.B.A. programs incorporate experiential learning, offering opportunities for internships and consulting projects. These hands-on experiences will enable you to gain valuable insights into real-world business challenges and develop essential skills in leadership, communication, and problem-solving.
8 competencies you can gain from an M.B.A. degree
By working through the M.B.A. curriculum and embracing experiential opportunities, you should emerge with a versatile skill set and a strategic mindset. This process will equip you to excel in diverse roles across various industries.
But what do you learn in an M.B.A. program, exactly? Consider the following examples of what you can expect:
1. Strategic problem-solving
In an M.B.A. program, strategic problem-solving takes center stage as students learn to dissect complex challenges, identify underlying issues, and formulate innovative solutions. Strategic problem-solving helps foster adaptability and resilience as a business professional, empowering graduates to navigate uncertainty and capitalize on opportunities in dynamic corporate environments.
Students exit their M.B.A. programs with the confidence and agility needed to drive sustainable growth in their organizations.
2. Collaborative leadership
Throughout the typical M.B.A. curriculum, students learn to harness the collective intelligence of teams. Through group projects and leadership seminars, they’ll gain important interpersonal skills and communication strategies essential for guiding diverse teams toward shared goals.
Equipped with this competency, M.B.A. grads emerge as effective leaders capable of driving organizational success through synergy and collective action.
3. In-depth data analysis
M.B.A. students delve into the realm of data analysis, mastering techniques needed to extract actionable insights from complex datasets. Through coursework in business statistics, data mining, and quantitative methods, students acquire the skills needed to interpret data effectively and make data-driven business decisions.
This skill helps business professionals leverage data-driven approaches to problem-solving and strategic decision-making. This enables them to confidently navigate the intricacies of today’s data-rich business environment.
4. Efficient project management
In M.B.A. programs, students become adept at the principles and techniques that are essential for overseeing initiatives from conception to completion. Through coursework and hands-on projects, students learn to define project scope, allocate resources effectively, and manage timelines and budgets.
By implementing efficient project management practices, business professionals can drive project success, enhance organizational efficiency, and deliver value to stakeholders, positioning themselves as effective leaders in project-driven environments.
5. Methodical market research
As an M.B.A. student, you’ll explore the complexities of market research, honing the skills necessary to gather, analyze, and interpret data to inform strategic business decisions. Through coursework and practical exercises, students learn to conduct market segmentation, assess competitive landscapes nationally and internationally, and identify consumer preferences and trends.
Proficiency in market research techniques like surveys, focus groups, and data analytics equips business professionals to gather valuable insights and validate hypotheses with precision.
6. Precise financial forecasting
M.B.A. students can expect to study the details of precise financial forecasting, mastering techniques to predict future financial performance. Through coursework in financial modeling, quantitative analysis, and risk management, students learn to analyze historical financial data, identify key trends, and develop accurate forecasts for revenue, expenses, and cash flow.
This skill empowers business leaders to assess the financial health of organizations, anticipate market fluctuations, and allocate resources effectively.
7. Comprehensive global business perspective
When working through the rigorous curriculum of a typical M.B.A. program, students develop a comprehensive global business perspective. They’ll gain insights into the interconnectedness of economies, cultures, and markets around the world. Exposure to international trade dynamics, cross-cultural communication strategies, and global market trends equips students with the knowledge and skills needed to conduct business in diverse geopolitical contexts.
By understanding the implications of globalization on business strategy, market expansion, and supply chain management, business professionals can identify opportunities for international expansion, mitigate risks associated with global operations, and lead cross-functional teams with cultural sensitivity and awareness.
8. Advanced networking
In addition to the range of impressive skills listed above, M.B.A. students also have the opportunity to practice the art of effective networking. They’ll learn to recognize the importance of building and nurturing professional relationships to foster career growth and business success. Proficiency in networking strategies like informational interviews, networking pitches, and online networking platforms empowers business professionals to establish meaningful connections and leverage them for opportunities.
Some M.B.A. programs, like the one at Utica University, allow students to begin this practice during their courses. By building group work into the curriculum, they’ll build their networking skills while collaborating with other likeminded professionals from a wide range of industries. Not only does this offer valuable perspective, but it could also unlock doors to career advancement and entrepreneurial endeavors down the road.
Level up your business skills with an M.B.A.
A high-quality M.B.A. program offers a comprehensive educational journey that will provide you with an array of in-demand skills that are critical for success in the modern business landscape. Each piece of the M.B.A. curriculum is strategically crafted to guide students like you to become adaptable and effective business leaders.
If you’re eager to unlock your full professional potential, a Master of Business Administration degree can help you get there. Utica University offers a range of specialized programs that can be tailored to your career goals or lifestyle needs. Explore our M.B.A. opportunities below:
- M.B.A. in Management (on-campus)
- M.B.A. in Management (online)
- M.B.A. in Professional Accounting
Ready to Take the Next Step?
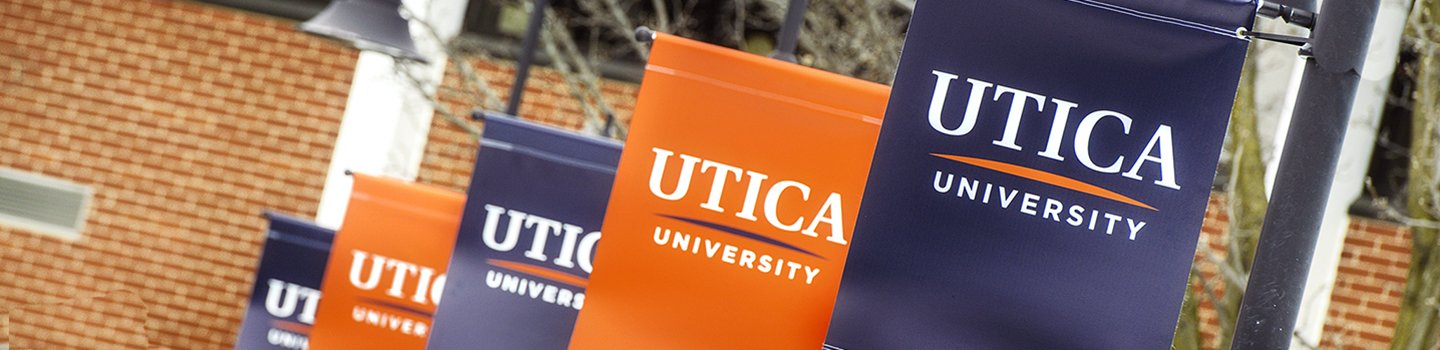
Request Information
We're excited you're interested in learning more about Utica University. Please fill out and submit the form, and we'll be in touch shortly!
I authorize Utica University and its representatives to contact me via SMS or phone. By submitting this form, I am providing my consent. Message and data rates may apply.
I would like to see logins and resources for:
For a general list of frequently used logins, you can also visit our logins page .
- Scientific Visualization Studio Galleries Help
5000 Years of Total Solar Eclipses
For more information, technical support, release date, papers used in this visualization, datasets used in this visualization, five millennium canon of solar eclipses (five millennium canon of solar eclipses).
This dataset can be found at: https://eclipse.gsfc.nasa.gov/SEpubs/5MCSE.html
DE441 (DE441)
This dataset can be found at: https://naif.jpl.nasa.gov/naif/data_generic.html
5000 Years of Total Solar Eclipses: The Movie
You may also like..., no results., an error occurred. please reload this page and try again..
Cancer patients often do better with less intensive treatment, research shows
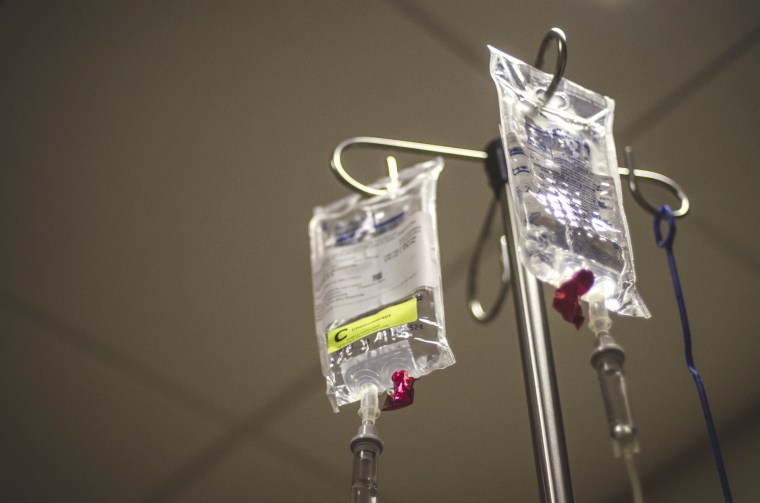
Scaling back treatment for three kinds of cancer can make life easier for patients without compromising outcomes, doctors reported at the world’s largest cancer conference .
It’s part of a long-term trend toward studying whether doing less — less surgery, less chemotherapy or less radiation — can help patients live longer and feel better. The latest studies involved ovarian and esophageal cancer and Hodgkin lymphoma.
Thirty years ago, cancer research was about doing more, not less. In one sobering example, women with advanced breast cancer were pushed to the brink of death with massive doses of chemotherapy and bone marrow transplants. The approach didn’t work any better than chemotherapy and patients suffered.
Now, in a quest to optimize cancer care, researchers are asking: “Do we need all that treatment that we have used in the past?”
It’s a question, “that should be asked over and over again,” said Dr. Tatjana Kolevska, medical director for the Kaiser Permanente National Cancer Excellence Program, who was not involved in the new research.
Often, doing less works because of improved drugs.
“The good news is that cancer treatment is not only becoming more effective, it’s becoming easier to tolerate and associated with less short-term and long-term complications,” said Dr. William G. Nelson of Johns Hopkins School of Medicine, who was also not involved in the new research.
Latest news on cancer treatment
- Cancer-fighting antibodies inject chemo directly into tumor cells, upping effectiveness.
- Long-term study shows 'remarkable' treatment helps patients with deadly nonsmoking-related lung cancer.
- FDA approves groundbreaking treatment for advanced melanoma.
Studies demonstrating the trend were discussed over the weekend at an American Society of Clinical Oncology conference in Chicago. Here are the highlights:
Ovarian cancer
French researchers found that it’s safe to avoid removing lymph nodes that appear healthy during surgery for advanced ovarian cancer. The study compared the results for 379 patients — half had their lymph nodes removed and half did not. After nine years, there was no difference in how long the patients lived and those with less-extreme surgery had fewer complications, such as the need for blood transfusions. The research was funded by the National Institute of Cancer in France.
Esophageal cancer
This German study looked at 438 people with a type of cancer of the esophagus that can be treated with surgery. Half received a common treatment plan that included chemotherapy and surgery on the esophagus, the tube that carries food from the throat to the stomach. Half got another approach that includes radiation too. Both techniques are considered standard. Which one patients get can depend on where they get treatment.
After three years, 57% of those who got chemo and surgery were alive, compared to 51% of those who got chemo, surgery and radiation. The German Research Foundation funded the study.
Hodgkin lymphoma
A comparison of two chemotherapy regimens for advanced Hodgkin lymphoma found the less intensive treatment was more effective for the blood cancer and caused fewer side effects.
After four years, the less harsh chemo kept the disease in check in 94% of people, compared to 91% of those who had the more intense treatment. The trial included 1,482 people in nine countries — Germany, Austria, Switzerland, the Netherlands, Denmark, Sweden, Norway, Australia and New Zealand — and was funded by Takeda Oncology, the maker of one of the drugs used in the gentler chemo that was studied.

The Associated Press
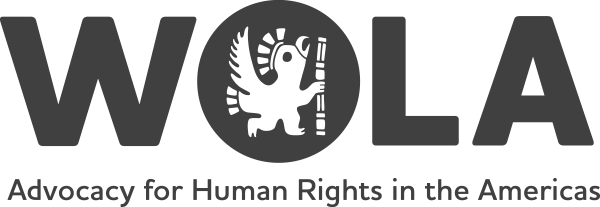
Mario Tama/Getty Images
The Futility of “Shutting Down Asylum” by Executive Action at the U.S.-Mexico Border
Today the Biden administration published a proclamation and an interim final rule curtailing the right to seek asylum at the U.S.-Mexico border. A White House fact sheet states that it “will make it easier for immigration officers to remove those without a lawful basis to remain and reduce the burden on our Border Patrol agents.”
What does the executive action do?
This “asylum shutdown” will deny, in most cases, the right to seek asylum for migrants apprehended on the U.S. side of the border with Mexico. It goes into effect at 12:01 AM on June 5, and will remain in effect until two weeks after Border Patrol’s weekly average of migrant apprehensions drops below 1,500 per day. That hasn’t happened since July 2000, in the early days of the COVID-19 pandemic; in fact, 58 percent of all months this century (172 of 296) have seen daily averages above 1,500.

Even then, the “asylum shutdown” would resume should the daily average again exceed 2,500 per day. It is over 3,500 per day right now; in fact, the U.S.-Mexico border has crossed that threshold in 110 of the past 296 months: 37 percent of this century.
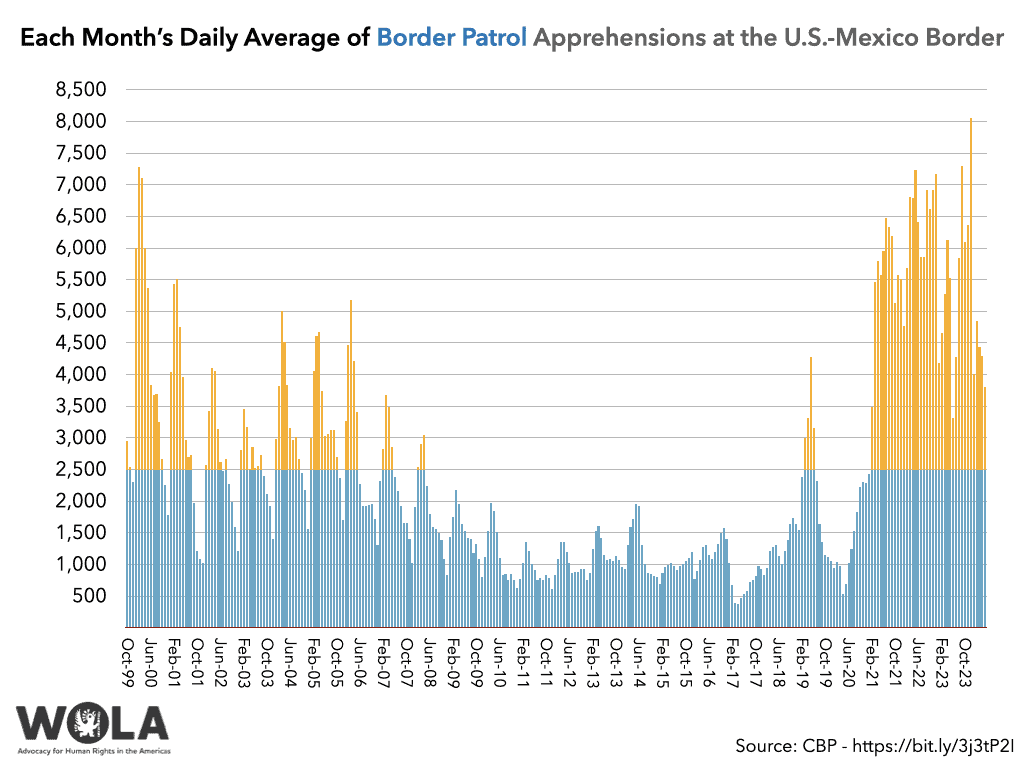
By creating such difficult-to-meet numerical thresholds, and very narrow exceptions, this new “asylum shutdown” resembles the Trump administration’s 2018 attempt to ban asylum access between ports of entry with no numerical limits. Courts struck that provision down because the law states clearly that people on U.S. soil may request asylum without regard to how they arrived.
The executive action calls on U.S. authorities to deport asylum seekers from U.S. soil without affording them a chance to seek protection, making exceptions for unaccompanied children, severe trafficking victims, and those who can prove a very high threshold of fear of return. Since January 2023, as well as its own citizens, Mexico has agreed to accept a combined total of up to 30,000 cross-border returns of citizens of Cuba, Haiti, Nicaragua, and Venezuela every month. At the time of publishing, we have seen no mention yet of whether the government of Mexico will allow any returns beyond that.
Doesn’t this violate U.S. law?
Yes. The Refugee Act of 1980 (enshrined as Section 208 of the Immigration and Nationality Act , INA) states that any non-citizen on U.S. soil has the right to request asylum if they fear for their life or freedom “on account of race, religion, nationality, membership in a particular social group, or political opinion.” They must receive due process for their asylum request regardless of how they arrived in the United States. This law placed the United States in compliance with the Refugee Convention of 1951, which emerged after World War II when nations pledged never to repeat that era’s tragic turnbacks of people fleeing extermination campaigns. The executive action shuts down this legal right, based not on fleeing migrants’ protection needs but on a daily number. Like the Supreme Court’s 2022 Dobbs decision, this is a rollback of a human right granted during a previous generation.
Is this a new “Title 42?”
The proclamation and interim final rule are similar to the Title 42 expulsion authority that the Trump and Biden administrations carried out during the COVID-19 pandemic, between March 2020 and May 2023. Both administrations interpreted an old public health law as permitting U.S. border authorities to expel migrants, regardless of asylum needs, from the U.S.-Mexico border, which they did 2,912,200 times . Like Title 42, the executive action will remove asylum seekers without due process. It is certain to endanger many of them : during the Title 42 period, Human Rights First and border-area groups documented 13,480 violent crimes and abuses committed against migrants stranded in northern Mexican border cities. As confirmed recently by alarming reports from the border, crimes and abuse have persisted for many migrants waiting in Mexico for appointments scheduled in the U.S through the CBP One app. As with Title 42, the executive action’s authority to remove asylum seekers rests on shaky legal ground: a federal judge struck down the expulsions program in 2022, though appeals were not yet resolved when the authority expired following the end of the COVID-19 public health emergency. Unlike Title 42, migrants will not be expelled: they will be processed and deported, which means that they would face harsher penalties , including a five-year ban on re-entry to the United States and possible criminal prosecution.
The Senate failed twice to pass a law trying to do this. How is this executive action legal?
This “asylum shutdown” was part of a “ border deal ” that emerged from negotiations between Democratic and Republican senators between November 2023 and February 2024. Faced with opposition from Republicans who felt it did not go far enough, and from some Democrats who objected to truncating asylum, this legislation failed twice to pass the Senate, in February and May 2024. Without this legislative underpinning, the proclamation and interim final rule do not have a clear basis in law. The administration is justifying the new authority by citing Section 212(f) of the INA, which allows the President to bar the entry of entire classes of non-citizens considered “detrimental to the interests of the United States.” However, courts have cast doubt on whether 212(f) can in fact be used to remove an asylum seeker already on U.S. soil and asking for protection , who is protected by Section 208 of the INA.
Doesn’t this rely heavily on Mexico’s cooperation?
Yes, and the timing of the announcement followed Mexico’s national elections on June 2, where voters chose Claudia Sheinbaum, from the ruling Morena party, as the next president; she will take office on October 1. It is impossible for the Biden administration to deport large numbers of non-Mexican citizens to their home countries. Immigration and Customs Enforcement (ICE) operates an average of about 130 deportation flights per month, 100 of them to nearby nations in Mexico, Central America, and the Caribbean. Flights to more distant nations are costly, and some countries prohibit or strictly limit the number of U.S. deportation flights that they receive. Instead of aerial deportations, policies that rapidly reject asylum seekers—like Title 42 and this new executive action—would rely on the Mexican government’s willingness to accept people expelled across the United States’ southern border, which is cheaper. As of early afternoon on June 4, we have seen no announcement from either the U.S. or the Mexican government about any change in Mexico’s posture toward deported non-Mexican migrants. We can expect the migrant population in Mexico’s violent border cities to swell again.
Will this, in fact, deter migrants?
Not only is it illegal and wrong to “deter” people who might qualify for protection, it doesn’t work. Any change in border and migration policy brings a short-term drop in the number of migrants encountered at the U.S.-Mexico border. Migration fell, for instance, when Title 42 began and every time it was expanded; it also fell after Title 42 ended.
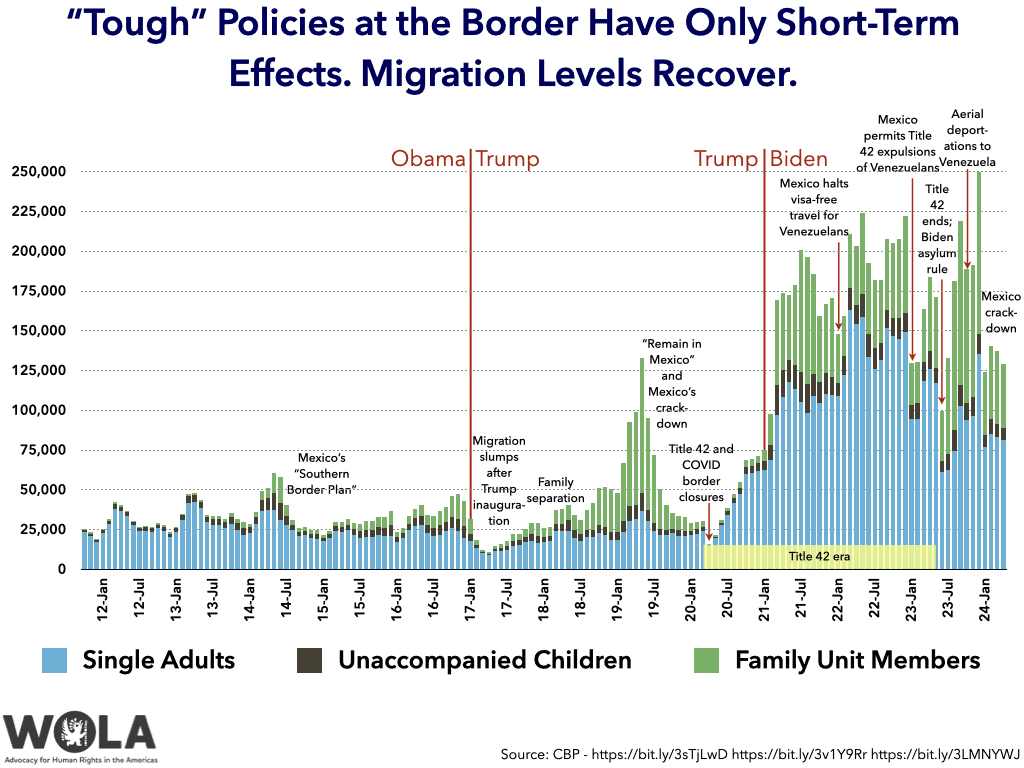
Changes trigger a “wait and see” effect: numbers plummet temporarily as migrants (and smugglers) evaluate how a new policy will deliver consequences, and in particular, which profiles of migrants it affects the most. This “wait and see” period fades quickly as migrants adapt to the new policy, and numbers recover. In the case of Title 42, migration numbers not only recovered, they spiked to historic levels: expulsions enabled repeat attempts to cross with no consequences, while migrants from distant countries realized that their chances of expulsion were minimal. Although harsher penalties for re-entry may deter some migrants, we have seen time and time again that people fleeing for their lives will continue to seek protection in the United States, regardless of the risks.
Instead of “shutting down” asylum, is there a better way to manage large numbers of arriving asylum seekers?
There is a better way, and the U.S. government should have been building it since the spring of 2014, the first time asylum-seeking children and families from Central America started turning themselves in to U.S. border authorities. WOLA and like-minded organizations have been pointing to this better way for years.
Solutions must address the reasons why people migrate, the response from governments in the region, and the shortcomings and lack of resources in the U.S. immigration system , rather than try to stop people from seeking asylum in legally dubious ways. A better policy rests on having the capacity to give asylum seekers meaningful due process in less time than our current, badly backlogged system takes. That means vastly improving the adjudication capacity of a system that today has over 4,500 immigration cases per judge. It means vastly expanding the ability to process asylum seekers who arrive at ports of entry, a function that does not need to be filled by armed, uniformed law-enforcement officers. And it means effective case-management programs to keep asylum seekers on track within the byzantine U.S. immigration system, along with expanded access to legal counsel. Had such policies been put in place years ago, when the need was already evident, by now we wouldn’t be seeing panicked officials—people who should know better—proposing executive actions to “shut down” the human right to seek asylum at the border.
Related Content
Weekly u.s.-mexico border update: asylum executive order, mexico crackdown, border patrol centennial, south america migration, new country of origin research materials for u.s asylum attorneys, weekly u.s.-mexico border update: border act fails, migration keeps dropping, texas updates, weekly u.s.-mexico border update: april dip in migration, drug seizure data, investigations published, weekly u.s.-mexico border update: mexico blocks migration, u.s. legislation, migrant removals, nationalities.
Numbers, Facts and Trends Shaping Your World
Read our research on:
Full Topic List
Regions & Countries
- Publications
- Our Methods
- Short Reads
- Tools & Resources
Read Our Research On:
How Americans and Israelis view one another and the U.S. role in the Israel-Hamas war
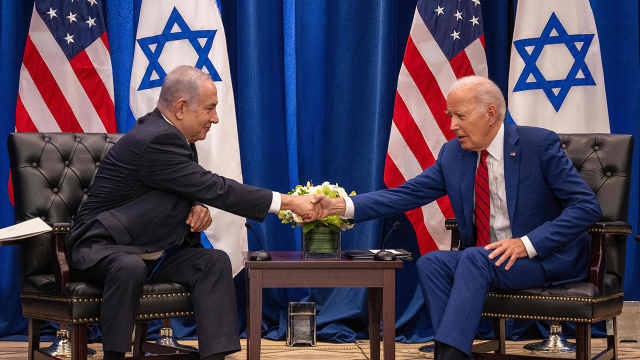
The United States continues to support Israel in its war against Hamas, but relations appear increasingly strained between the two countries and their leaders . The war also seems to have affected public opinion in both countries: Americans and Israelis now see one another’s leaders more negatively than in the recent past, and other key views have shifted as well.
Below, we explore American and Israeli public opinion in detail. This analysis is based on two Pew Research Center surveys of U.S. adults – one conducted in February and one in early April – as well as a survey of Israeli adults conducted in March and early April.
Pew Research Center analyzed Americans’ and Israelis’ attitudes on a variety of questions related to the Israel-Hamas war. The recent U.S. data is from two polls: a survey of 12,693 U.S. adults conducted Feb. 13-25, 2024, and a survey of 3,600 U.S. adults conducted April 1-7, 2024. The data for Israel is from a survey of 1,001 Israeli adults conducted March 3-April 4, 2024.
Most of the respondents in the February survey of U.S. adults (10,642) are members of the Center’s American Trends Panel (ATP). The ATP is an online survey panel that is recruited through national, random sampling of residential addresses, which gives nearly all U.S. adults a chance of selection. Read more about the ATP’s methodology .
The remaining 2,051 respondents are members of three other survey panels – Ipsos’ KnowledgePanel, SSRS’s Opinion Panel, and NORC at the University of Chicago’s AmeriSpeak Panel – who were interviewed because they identify as Jewish or Muslim.
The February survey “oversampled” (i.e., interviewed a disproportionately large number of) Jews and Muslims to provide more reliable estimates of their views. But these groups are not overrepresented in the national figures reported, because we adjusted for the oversampling in the weighting of the data.
Although the February survey included questions about where U.S. adults were born and whether they identify as Arab or of Arab origin, there was insufficient sample size to analyze Arab Americans or people of Palestinian descent separately. In that survey , only about a quarter of U.S. Muslims identified as Arab or of Arab ancestry. As a result, in our U.S. analysis, we describe differences between religious groups, rather than differences in opinion between Arab Americans and Jewish Americans. In contrast, our surveys in Israel have sufficient samples to allow comparisons between Israeli Arabs and Israeli Jews.
Both U.S. surveys are weighted to be representative of the U.S. adult population by gender, race, ethnicity, partisan affiliation, education, religious affiliation and other categories.
The survey of Israeli adults was conducted face-to-face. Interviews were conducted in Hebrew and Arabic, and the survey is representative of the adult population ages 18 and older, excluding those in East Jerusalem and non-sanctioned outposts. (The survey also does not include the West Bank or Gaza.) The survey included an oversample of Arabs in Israel. It was subsequently weighted to be representative of the Israeli adult population with the following variables: gender by ethnicity, age by ethnicity, education, region, urbanicity and probability of selection of respondent.
Here are the February and April questions and responses for the United States used for this analysis, and the questions and responses for Israel . Here are the February and April survey methodologies for the U.S. , and the survey methodology for Israel .
Americans have less confidence in Israeli Prime Minister Benjamin Netanyahu than Israelis have in U.S. President Joe Biden. Only 30% of U.S. adults express some or a lot of confidence in Netanyahu, while around half (53%) have not too much or no confidence in him. An additional 15% say they have not heard of him.
By contrast, more than half of Israeli adults (57%) have some or a great deal of confidence in Biden to do the right thing regarding world affairs, whereas 42% have little or no confidence in him.
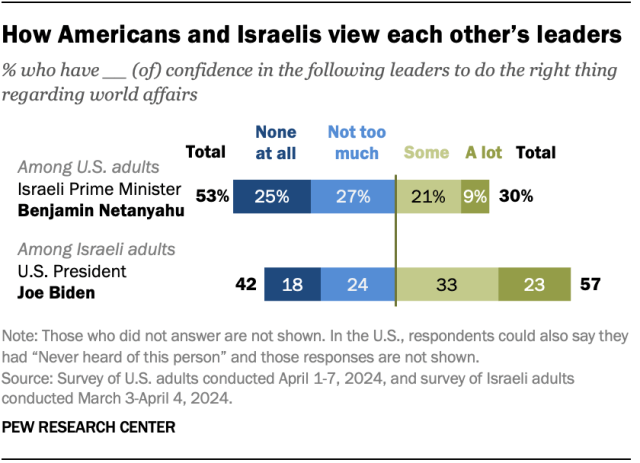
Americans’ views of Netanyahu have turned more negative over the past year. The share of Americans with little or no confidence in Netanyahu to do the right thing regarding world affairs is up 11 percentage points since March 2023 (42% then, 53% today).
Democrats are more likely than Republicans to have a negative view of Netanyahu. Approximately seven-in-ten Democrats and Democratic-leaning independents (71%) have little or no confidence in him, up from 56% in 2023. Around a third of Republicans and GOP leaners (34%) now take this view – up from 29% in 2023.
Related: A growing share of Americans have little or no confidence in Netanyahu
Israelis’ opinions of Biden have also turned more negative. While 57% of Israelis express a lot or some confidence in Biden, that’s down from 68% last year . The share of Israelis who have no confidence at all in Biden has doubled, from 9% to 18%.
Israeli Jews and Arabs differ widely in their views of the U.S. president: 66% of Jews have confidence in Biden, but only 21% of Arabs agree. Among both groups, overall confidence in Biden has decreased by 10 points since last year.
Older Israelis, as well as those in the ideological center or on the right, have more confidence in Biden than younger and left-leaning Israelis do.
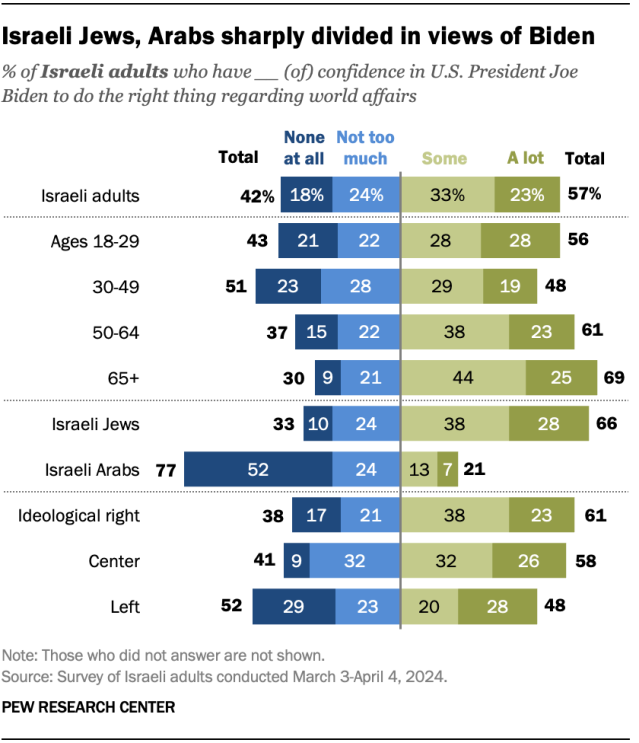
The share of Israelis with a favorable opinion of the U.S. has declined. While a broad majority of Israelis (77%) continue to see the U.S. favorably, this is down 10 points since last year. This marks the lowest overall rating of the U.S. in Israeli public opinion since 2011, based on our nearly two-decade trend .
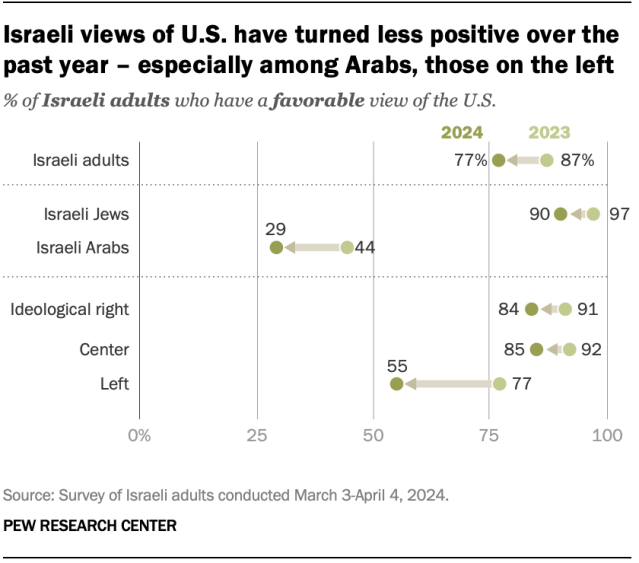
Views of the U.S. are significantly more positive among Jewish Israelis (90%) than Arab Israelis (29%). They are also much more positive among Israelis who place themselves on the ideological right (85%) or in the center (84%) than on the left (55%).
Since last year, views of the U.S. have soured among most demographic groups in Israel, but particularly among Arab Israelis and people on the ideological left.
Americans’ views of the Israeli people are broadly positive, but far fewer express favorable views of the Israeli government . In our recent U.S. polling, we have asked separately about views of the Israeli government and the Israeli people , rather than ask a single question about views of Israel. ( Past research suggests that views of the country tend to fall somewhere between views of its people and its government.)
In our survey this past February, 64% of Americans had a favorable view of the Israeli people, while 41% voiced a favorable view of the Israeli government. Both of these figures were down modestly from 2022. Views of the Israeli people have ticked downward more among younger Americans than among older ones.
Americans and Israelis alike are divided over whether Biden is favoring one side too much in the Israel-Hamas war. In our February survey, Americans were about evenly split as to whether Biden was favoring the Israelis too much (22%), favoring the Palestinians too much (16%) or striking the right balance (21%). However, the largest share of all – 40% – was not sure.
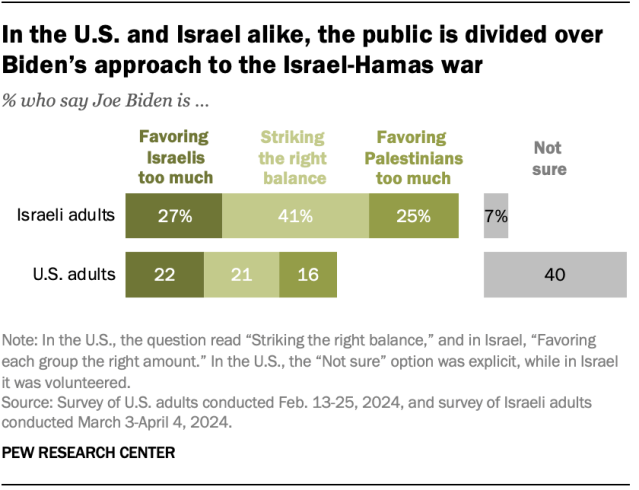
In Israel, 41% of adults say Biden is striking the right balance, but nearly equal shares say the U.S. president favors the Israelis too much (27%) or favors Palestinians too much (25%). Just 7% of Israelis aren’t sure or did not answer the question. (It’s important to note that U.S. respondents took the survey online and were given an explicit “Don’t know” option. Israeli respondents, interviewed in person, were not. Previous research shows that offering an explicit “Don’t know” option in online surveys is a better comparison with face-to-face survey data.)
In both the U.S. and Israel , people on the ideological left are more likely than those on the right to say Biden is favoring Israelis too much (in the U.S., ideology was measured as liberal, moderate and conservative).
In the U.S., Muslim Americans are far more likely than Jewish Americans to say Biden favors the Israelis too much (60% vs. 13%). In Israel, Arabs (86%) are much more likely than Jews to say the same (86% vs. 11%). (In the U.S., we “oversampled” Muslim and Jewish Americans but did not have sufficient sample size to analyze Arab Americans. For more details on the sampling, read the “How we did this” box.)
Around seven-in-ten Israelis (72%) want to see the U.S. play a major diplomatic role in resolving the Israel-Hamas war, but Americans are more divided. In the U.S., a majority of Americans (55%) also say the U.S. should play a role in ending the war, but more favor a minor role than a major one (35% vs. 20%). Another 27% want the U.S. to play no role at all – a view shared by only 10% of Israelis.
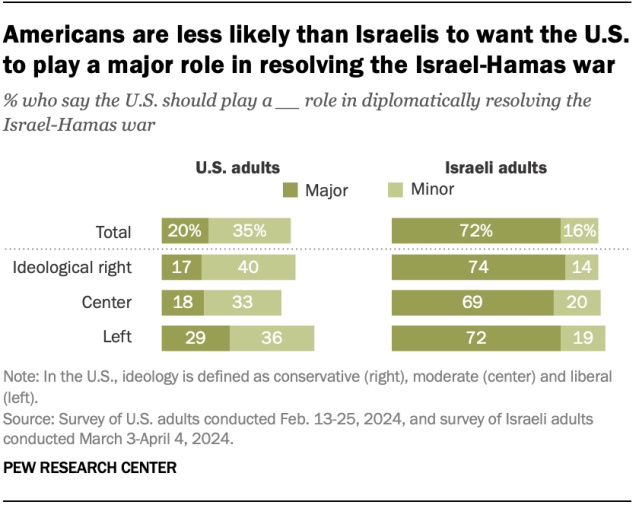
Americans on the ideological left are more supportive of the U.S. playing a major role than are those in the center or on the right. In Israel, U.S. involvement is broadly popular across the ideological spectrum.
In the U.S., 45% of Jews think the U.S. should play a major role in resolving the war, compared with 27% of Muslims. In Israel, Jews and Arabs are both generally supportive of the U.S. playing a major role (74% and 62%, respectively).
Note: Here are the February and April questions and responses for the United States used for this analysis, and the questions and responses for Israel . Here are the February and April survey methodologies for the U.S. , and the survey methodology for Israel .
- Israel Global Image
- U.S. Global Image
- War & International Conflict
- World Leaders
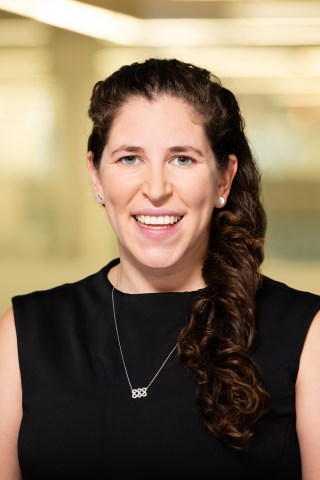
Laura Silver is an associate director focusing on global attitudes at Pew Research Center .
In East Asia, many people see China’s power and influence as a major threat
How views of the u.s., china and their leaders have changed over time, comparing views of the u.s. and china in 24 countries, poles and hungarians differ over views of russia and the u.s., 6 facts about how mexicans view the u.s. and their own country, most popular.
1615 L St. NW, Suite 800 Washington, DC 20036 USA (+1) 202-419-4300 | Main (+1) 202-857-8562 | Fax (+1) 202-419-4372 | Media Inquiries
Research Topics
- Email Newsletters
ABOUT PEW RESEARCH CENTER Pew Research Center is a nonpartisan fact tank that informs the public about the issues, attitudes and trends shaping the world. It conducts public opinion polling, demographic research, media content analysis and other empirical social science research. Pew Research Center does not take policy positions. It is a subsidiary of The Pew Charitable Trusts .
© 2024 Pew Research Center
Uncertainty about young voters stems from the age group’s complexity
Young Americans are definitionally the country’s future. That’s particularly true in the realm of politics.

Barack Obama’s election in 2008 heralded a new era in American politics. Younger Americans turned out heavily for the popular Democrat, facilitating his easy win over Republican John McCain and ripping open a partisan divide between their voting patterns and those of older Americans.
But that was 16 years ago, and voters who were under 30 in 2008 are now between 34 and 45 years old. America’s young voters today hold different views than young voters used to — and look different from the Obama-coalition young voters who have driven so much analysis in the ensuing years. Those differences, in fact, are central to understanding why there’s so much uncertainty about how young voters will vote in November — if they do at all.
Sign up for the How to Read This Chart newsletter
For one thing, we often talk about President Biden ’s weak polling with young voters and his weak polling with non-White voters as separate things. But they are related, given that young voters are more likely to be non-White.
Using data from the two most recent General Social Surveys (national polls completed in 2021 and 2022), we get a sense of how the U.S. adult population breaks down. Among Americans 18-30, there were about twice as many White people as non-White people. Among Americans ages 65 and older, there were about six White respondents for every non-White respondent. Many of the younger non-White respondents were Hispanic; part of the reason that older populations are more heavily White is that immigration was restricted during the mid-20th century baby boom.
At the same time, younger Americans are more likely to identify as independent than older Americans. That’s particularly true of young non-White people. In the GSS, about half of Whites under 30 were independent or independents who lean to one party or the other. Six in 10 non-Whites were. Among the oldest respondents, about a third of Whites were independents but only 3 in 10 non-Whites.
This is a central issue. As I wrote in November , the fact that younger Americans are often not actually Democrats means there isn’t a sense of institutional loyalty to the party or its candidates. That they are independents who often vote for Democrats has been good enough for a lot of Democrats in a lot of elections, but when the question at hand centers on a particular person — in November, President Biden — that is disadvantageous. In statewide races, younger voters (like lots of voters) will recognize the (D) or (R) before the name. They know Biden’s name and that of his opponent … which we’ll come back to in a moment.
We can see how this question of institutional association manifests in participation. Earlier this year, Pew Research Center published data showing that there was still a wide gap between younger and older Americans in their political views.
This overlaps with race, too. Non-White respondents in Pew’s data were much more likely to identify as Democrats or Democratic-leaning independents and, as noted above, younger Americans are more likely to be non-White. The GSS data also shows that 4 in 5 of those under 30 who identify as Republican or Republican-leaning independents are White.
The Pew analysis, though, looked only at registered voters — people who were participating in the political process to at least some extent. Gallup data released in February considered the political views of adults regardless of registration status. It found, unlike Pew, that there was a significant shift toward Republican identification among younger and non-White Americans, even if they didn’t register as Republicans.
There is some indication that younger voters are more frequently registering as Republicans than in the past. That my be a reflection of Biden’s increased unpopularity and its corollary: Donald Trump’s decreased unpopularity.
Earlier this year, I used YouGov data to show that views of Trump now are less negative among several groups than during his presidency. Compare the percentage of respondents each month who say they view him unfavorably with the average during his presidency.
Democratic, Black and Hispanic respondents still generally view Trump negatively, but less than they used to.
That analysis didn’t break down age, so I asked YouGov for data by age and gender. In part, this was a function of recent Brookings analysis documenting the divergence in values between young men and young women. What the YouGov polling shows is that, in every age group, men view Trump less negatively than women. But notice the annual averages broken out at the bottom of the chart below: Views among younger Americans have grown more positive since he first took office while views among older Americans sank before rebounding slightly.
In 2024, young men view Trump about as negatively as older women do. The 2017 annual average showed a nearly 50-point gap.
The silver lining for Biden is that he fares much better among those more-engaged voters. The best encapsulation of this phenomenon comes from the Harvard Youth Poll released earlier this year. Among all respondents under 30, Biden gets about 45 percent. Among those most likely to vote, he’s closer to 60 percent. Support for Trump, meanwhile, doesn’t change.
Historically, of course, younger people are less likely to vote anyway. That was one reason the Obama result was so striking: Young people actually turned out! Siena College polling conducted for the New York Times and Philadelphia Inquirer in six battleground states found that younger people were less likely to say they were likely to vote than respondents overall.
That same poll also showed that younger people were a bit more skeptical about the political and economic system than older Americans. More than three-quarters said the system needs to be torn down completely or changed significantly. Such sentiments turn up elsewhere , too — and in states where young people were more likely to view Trump’s interest in upending the system as a positive, Trump fared better.
Here we could segue into other areas in which younger Americans diverge from older Americans, like media consumption. New research from Pew shows that younger Americans are less likely to get news about the election from journalists and news organizations than older Americans, while they are more likely than older people to get it from friends, celebrities, social media personalities and other ordinary people they don’t know.
But this is probably to a significant extent a function of whether those groups seek out election news. If you aren’t trying to stay up to speed on the election, it makes sense that you would get more information passively from non-news sources. Consider that the Harvard Youth Poll found that younger Americans had the same general concerns about the election as older Americans, suggesting that the differences in consumption (or reception) of political news didn’t lead to a divergence in priorities.
So what do we have? A less engaged, more diverse population that isn’t as hostile to Trump as it used to be even as it has grown more skeptical of Biden — and lacks the institutional ties to the Democratic Party that might incline them to vote for the incumbent president anyway. There are a lot of fringes and asterisks that can modify those descriptors, but that appears to be the important distillation.
What will matter in November, then, is who turns out to vote. Which, of course, is what matters every November.
Election 2024
Get the latest news on the 2024 election from our reporters on the campaign trail and in Washington.
Who is running?: President Biden and Donald Trump secured their parties’ nominations for the presidency . Here’s how we ended up with a Trump-Biden rematch .
Presidential debates: Biden and Trump agreed to a June 27 debate on CNN and a Sept. 10 debate broadcast by ABC News.
Key dates and events: From January to June, voters in all states and U.S. territories will pick their party’s nominee for president ahead of the summer conventions. Here are key dates and events on the 2024 election calendar .
Abortion and the election: Voters in about a dozen states could decide the fate of abortion rights with constitutional amendments on the ballot in a pivotal election year. Biden supports legal access to abortion , and he has encouraged Congress to pass a law that would codify abortion rights nationwide. After months of mixed signals about his position, Trump said the issue should be left to states . Here’s how Biden’s and Trump’s abortion stances have shifted over the years.

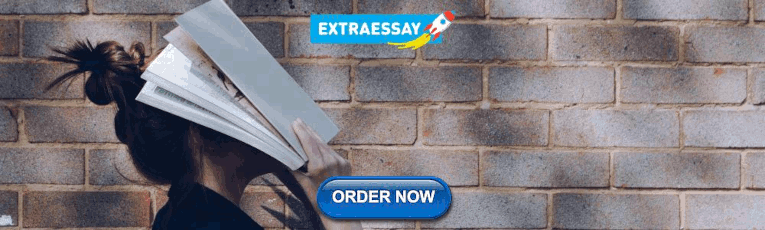
IMAGES
VIDEO
COMMENTS
Table of contents. Step 1: Write your hypotheses and plan your research design. Step 2: Collect data from a sample. Step 3: Summarize your data with descriptive statistics. Step 4: Test hypotheses or make estimates with inferential statistics.
Definition of research in data analysis: According to LeCompte and Schensul, research data analysis is a process used by researchers to reduce data to a story and interpret it to derive insights. The data analysis process helps reduce a large chunk of data into smaller fragments, which makes sense. Three essential things occur during the data ...
Writing an analysis requires a particular structure and key components to create a compelling argument. The following steps can help you format and write your analysis: Choose your argument. Define your thesis. Write the introduction. Write the body paragraphs. Add a conclusion. 1. Choose your argument.
For many researchers unfamiliar with qualitative research, determining how to conduct qualitative analyses is often quite challenging. Part of this challenge is due to the seemingly limitless approaches that a qualitative researcher might leverage, as well as simply learning to think like a qualitative researcher when analyzing data. From framework analysis (Ritchie & Spencer, 1994) to content ...
Introduction. Statistical analysis is necessary for any research project seeking to make quantitative conclusions. The following is a primer for research-based statistical analysis. It is intended to be a high-level overview of appropriate statistical testing, while not diving too deep into any specific methodology.
Analyzing Research Articles: A Guide for Readers and Writers1 Sam Mathews, Ph.D. Department of Psychology The University of West Florida The critical reader of a research report expects the writer to provide logical and coherent rationales for conducting the study, concrete descriptions of methods, procedures, design, and analyses, accurate and clear reports of the findings, and plausible ...
The two "branches" of quantitative analysis. As I mentioned, quantitative analysis is powered by statistical analysis methods.There are two main "branches" of statistical methods that are used - descriptive statistics and inferential statistics.In your research, you might only use descriptive statistics, or you might use a mix of both, depending on what you're trying to figure out.
1. Step one: Defining the question. The first step in any data analysis process is to define your objective. In data analytics jargon, this is sometimes called the 'problem statement'. Defining your objective means coming up with a hypothesis and figuring how to test it.
Content analysis is a research method used to identify patterns in recorded communication. To conduct content analysis, you systematically collect data from a set of texts, which can be written, oral, or visual: Books, newspapers and magazines. Speeches and interviews. Web content and social media posts. Photographs and films.
Step 1: Gather your qualitative data and conduct research (Conduct qualitative research) The first step of qualitative research is to do data collection. Put simply, data collection is gathering all of your data for analysis. A common situation is when qualitative data is spread across various sources.
2.1 Step 1: defining the research question. The first step in conducting a meta-analysis, as with any other empirical study, is the definition of the research question. Most importantly, the research question determines the realm of constructs to be considered or the type of interventions whose effects shall be analyzed.
2. Create an outline for your analysis. Building on your thesis and the arguments you sketched out while doing your close read of the document, create a brief outline. Make sure to include the main arguments you would like to make as well as the evidence you will use to support each argument.
How to Do Thematic Analysis | Step-by-Step Guide & Examples. Published on September 6, 2019 by Jack Caulfield.Revised on June 22, 2023. Thematic analysis is a method of analyzing qualitative data.It is usually applied to a set of texts, such as an interview or transcripts.The researcher closely examines the data to identify common themes - topics, ideas and patterns of meaning that come up ...
Step 1: Select the content you will analyse. Based on your research question, choose the texts that you will analyse. You need to decide: The medium (e.g., newspapers, speeches, or websites) and genre (e.g., opinion pieces, political campaign speeches, or marketing copy)
Monitor and adapt. Now that you have gained insights into the various market research methods at your disposal, let's delve into the practical aspects of how to conduct market research effectively. Here's a quick step-by-step overview, from defining objectives to monitoring market shifts. 1. Set clear objectives.
Organize your data. 4. Use your tools. 5. Report your results. Be the first to add your personal experience. 6. Review your analysis. Be the first to add your personal experience.
There are various approaches to conducting thematic analysis, but the most common form follows a six-step process: Familiarisation. Coding. Generating themes. Reviewing themes. Defining and naming themes. Writing up. This process was originally developed for psychology research by Virginia Braun and Victoria Clarke.
Step 1: Study for a degree. A bachelor's degree in a business-related subject, math, economics, or social science is typically the entry point to work as a research analyst, with some employers asking for a master's degree. According to Zippia, 70 percent of research analysts have a bachelor's degree, with a further 18 percent going on to ...
Measuring variables. When planning a research design, you should operationalise your variables and decide exactly how you will measure them.. For statistical analysis, it's important to consider the level of measurement of your variables, which tells you what kind of data they contain:. Categorical data represents groupings. These may be nominal (e.g., gender) or ordinal (e.g. level of ...
Exploratory analysis. Inferential analysis. Predictive analysis. Causal analysis. Mechanistic analysis. Prescriptive analysis. With its multiple facets, methodologies and techniques, data analysis is used in a variety of fields, including business, science and social science, among others. As businesses thrive under the influence of ...
5. Methodical market research. As an M.B.A. student, you'll explore the complexities of market research, honing the skills necessary to gather, analyze, and interpret data to inform strategic business decisions. Through coursework and practical exercises, students learn to conduct market segmentation, assess competitive landscapes nationally ...
Work on the "double empathy problem" (DEP) is rapidly growing in academic and applied settings (e.g., clinical practice). It is most popular in research on conditions, like autism, which are characterized by social cognitive difficulties. Drawing from this literature, we propose that, while research on the DEP has the potential to improve understanding of both typical and atypical social ...
32,000. 65,000. 100,000. According to a 2022 FISH Safety Foundation study commissioned by Pew, more than 100,000 people die annually while engaged in fishing, legal or illegal. Although fishing is inherently risky, the study highlights how climate change, overfishing and IUU fishing contribute to these deaths by increasing risks at sea.
Download. A grayscale version of the heatmap showing the frequency of total solar eclipses over the 5000 years from 2000 BCE to 3000 CE. The floating-point image contains the actual count of eclipse paths crossing each pixel. Fractional counts indicate the edge of a path passed through only part of the pixel. The 8-bit image stretches the count ...
Textual analysis is a broad term for various research methods used to describe, interpret and understand texts. All kinds of information can be gleaned from a text - from its literal meaning to the subtext, symbolism, assumptions, and values it reveals. The methods used to conduct textual analysis depend on the field and the aims of the ...
The research was funded by the National Institute of Cancer in France. Esophageal cancer . This German study looked at 438 people with a type of cancer of the esophagus that can be treated with ...
This "asylum shutdown" will deny, in most cases, the right to seek asylum for migrants apprehended on the U.S. side of the border with Mexico. It goes into effect at 12:01 AM on June 5, and will remain in effect until two weeks after Border Patrol's weekly average of migrant apprehensions drops below 1,500 per day.
Around seven-in-ten Israelis (72%) want to see the U.S. play a major diplomatic role in resolving the Israel-Hamas war, but Americans are more divided. In the U.S., a majority of Americans (55%) also say the U.S. should play a role in ending the war, but more favor a minor role than a major one (35% vs. 20%).
Albuquerque, New Mexico. Typical Rent: $1,583. Average Recent College Graduate Income: $65,727. Share of Estimated Entry-Level Income Spent on Rent: 28.9%. Also situated in a desert climate, approximately 400 miles east of Phoenix, lies Zillow's second-best market for college graduates, Albuquerque.
Using data from the two most recent General Social Surveys (national polls completed in 2021 and 2022), we get a sense of how the U.S. adult population breaks down.