- Open access
- Published: 21 March 2023
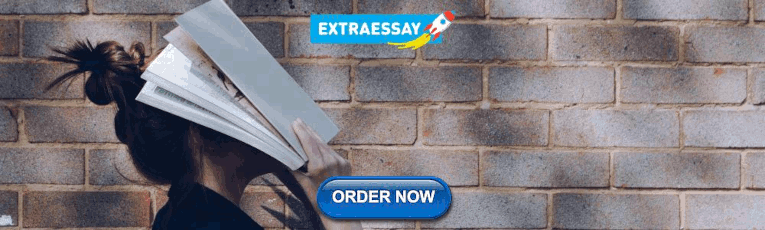
Changing positive and negative affects through music experiences: a study with university students
- José Salvador Blasco-Magraner 1 ,
- Gloria Bernabé-Valero 2 ,
- Pablo Marín-Liébana 1 &
- Ana María Botella-Nicolás 1
BMC Psychology volume 11 , Article number: 76 ( 2023 ) Cite this article
10k Accesses
1 Citations
4 Altmetric
Metrics details
Currently, there are few empirical studies that demonstrate the effects of music on specific emotions, especially in the educational context. For this reason, this study was carried out to examine the impact of music to identify affective changes after exposure to three musical stimuli.
The participants were 71 university students engaged in a music education course and none of them were musicians. Changes in the affective state of non-musical student teachers were studied after listening to three pieces of music. An inter-subject repeated measures ANOVA test was carried out using the Positive and Negative Affect Schedule (PANAS) to measure their affective state.
The results revealed that: (i) the three musical experiences were beneficial in increasing positive affects and reducing negative affects, with significant differences between the interaction of Music Experiences × Moment (pre-post); (ii) listening to Mahler’s sad fifth symphony reduced more negative affects than the other experimental conditions; (iii) performing the blues had the highest positive effects.
Conclusions
These findings provide applied keys aspects for music education and research, as they show empirical evidence on how music can modify specific affects of personal experience.
Peer Review reports
Introduction
The studies published on the benefits of music have been on the increase in the last two decades [ 1 , 2 , 3 ] and have branched out into different areas of research such as psychology [ 4 , 5 , 6 , 7 , 8 ], education [ 1 , 9 , 10 ] and health [ 11 , 12 ] providing ways of using music as a resource for people’s improvement.
The publication in 1996 of the famous report “Education Hides a Treasure” submitted to the UNESCO by the International Commission was an important landmark in the educational field. This report pointed out the four basic pillars of twenty-first century education: learning to know, learning to do, learning to live together, and learning to be [ 13 ]. The two last ones clearly refer to emotional education. This document posed a challenge to Education in terms of both academically and emotionally development at all levels from kindergarten to university. In this regard, there has been a notable increase in the number of studies that have shown the strong impact of music on the emotions in the different stages of education and our lives. For example, from childhood to adolescence, involving primary, secondary and university education, music is especially relevant for its beneficial effects on developing students’ emotional intelligence and prosocial skills [ 1 , 14 ]. In adults, music benefits emotional self-regulation [ 15 ], while in old age it helps to maintain emotional welfare and to experience and express spirituality [ 16 ]. This underlines the importance of providing empirical evidence on the emotional influence of music.
Influence of music on positive affects
Numerous studies have used the Positive and Negative Affect Schedule (PANAS) to evaluate the emotional impact of music [ 17 ]. This scale is valid and effective for measuring the influence of positive and negative effects of music on listeners and performers [ 10 , 18 , 19 ]. Thus, for example, empirical evidence shows that exposure to a musical stimulus favours the increase of positive affects [ 20 , 21 ] found a significant increase in three positive affects in secondary school students after listening to music, and the same results has been found after listening to diverse musical styles. These results are consistent with Schubert [ 22 ], who demonstrated that music seems to improve or maintain well-being by means of positive valence emotions (e. g. happiness, joy and calm). Other research studied extreme metal fans aged between 18 and 34 years old and found statements of physiological excitement together with increased positive affects [ 21 ]. Positive outcomes after listening to sad music have also been found [ 23 ], who played Samuel Barbers’ Adagio for Strings , described by the BBC as the world’s saddest piece of classical music, to 20 advanced music students and 20 advanced psychology students with no musical background and subsequently found that the music only had positive affects on both groups.
Several experimental designs that used sad music on university students noticed that they experienced both sadness and positive affects [ 24 , 25 ] and also found that music labeled as “happy” increased positive affects while the one labeled as “sad” reduced both positive and negative affects [ 26 ]. For other authors the strongest and most pleasant responses to sad music are associated with empathy [ 27 ]. Moreover, listening to sad music had benefits since attributes of empathy were intensified [ 27 , 28 ]. In relation to musical performances, empirical evidence found a significant increase in positive affects [ 29 ]. Thus, music induces listeners to experience positive affects, which could turn music into an instrument for personal development.
Following on from Fredrickson’s ‘broaden‐and‐build’ framework of positive emotions [ 30 ], positive affects cause changes in cognitive activities which, in turn, can cause behaviour changes. They can also expand the possibilities for action and improve physical resources. According to Fredrickson [ 30 ], positive affects trigger three sequential effects: (1) amplification of the scope for thought and action; (2) construction of personal resources to deal with difficult simplifications; (3) personal transformation by making one more creative, with a better understanding of situations, better able to face up to difficulties and better socially integrated. This leads to an “upward spiral” in which even more positive affects are experienced. A resource such as music that can increase positive affects, can therefore be considered as a step forward in personal transformation. Thus, music teachers could have a powerful tool to help students enhance their personal development.
Influence of music on negative affects
There is a great deal of controversy as regards the influence of music on negative affects. Blasco and Calatrava [ 20 ] found a significant reduction of five negative affects in secondary school students after listening to Arturo Marquez’s typically happy Danzón N O 2. Different results were found in an experiment in which the change in participants ‘affects was assessed after listening the happy "Eye of the Tiger" by Survivor and the sad "Everybody Hurts" by REM [ 26 ]. They found that the happy piece only increased the positive affects but did not reduce the negative ones, while the sad piece reduced both positive and negative affects. However, neither of these findings agree with Miller and Au [ 31 ], who carried out an experiment to compare the influence of sad and happy music on undergraduates ‘mood arousal and found that listening to both types had no significant changes on negative affects. Shulte [ 32 ] conducted a study with 30 university students to examine the impact that nostalgic music has on affects, and found that after listening to different songs, negative affects decreased. Matsumoto [ 33 ] found that sad music reduced sad feelings in deeply sad university students, while Vuoskoski and Eerola [ 34 ] showed that sad music could produce changes in memory and emotional judgements related to emotions and that experiencing music-induced sadness is intrinsically more pleasant than sad memories. It therefore seems that reducing negative affects has mostly been studied with sad and nostalgic musical stimuli. In this way, if music can reduce negative affects, it can also be involved in educational and psychological interventions focused on improving the emotional-affective sphere. Thus, for example, one study examined the effects of a wide range of music activities and found that it would be necessary to specify exactly what types of music activity lead to what types of outcomes [ 2 ]. Moore [ 3 ] also found that certain music experiences and characteristics had both desirable and undesirable effects on the neural activation patterns involved in emotion regulation. Furthermore, recent research on university students shows that music could be used to assess mood congruence effects, since these effects are reactions to the emotions evoked by music [ 35 ].
These studies demonstrate that emotional experience can be actively driven by music. Moreover, they synthesize the efforts to find ways in which music can enhance affective emotional experience by increasing positive affects and reducing the negative ones (e. g. hostility, nervousness and irritability). Although negative emotions have a great value for personal development and are necessary for psychological adjustment, coping with them and self-regulation capacities are issues that have concerned psychology. For example, Emotional Intelligence [ 36 ], which has currently been established in the educational field, constitutes a fundamental conceptual framework to increase well-being when facing negative emotions, providing keys for greater control and management of emotional reactions. It also establishes how to decrease the intensity and frequency of negative emotional states [ 37 ], providing techniques such as mindfulness meditation that have proven their effectiveness in reducing negative emotional experiences and increasing the positive ones [ 38 ]. The purpose of this research is to find whether music can be part of the varied set of resources that can be used by a teacher to modify students’ emotional experience.
Thus, although empirical evidence of the effects of music on the emotional sphere is still incipient. It seems that they can increase positive effects, but it is not clear their impact on the negative ones, since diverse and contradictory results (no change and reduction of negative affects after listening to music) were found. In addition, the effects of the type of musical piece (e.g. happy or sad music) need further investigation as different effects were found. Moreover, previous studies do not compare between the effects of listening to versus performing music. Such an approach could provide keys to highlight the importance of performing within music education. Therefore, this study aims to contribute to this scientific field, providing experimental evidence on the effects of listening to music as compared to performing music, as well as determining the effects of different types of music on positive and negative affects.
To this end, the effects of three different types of music experiences were compared: (1) listening to a sad piece, (2) listening to an epic and solemn piece, and (3) performing of a rhythm and a blues piece, to determine whether positive and negative affects were modified after exposure to these experimental situations. In particular, two hypotheses guided this study: (1) After exposure to each musical experience (listening to a sad piece; listening to a solemn piece and playing a blues), all participants will improve their emotional experience, increasing their positive affects and reducing their negative ones; and (2) the music performance will induce a greater change as compared to the listening conditions.
Participants
A total of 71 students were involved in this study, 6 men and 65 women between the ages of 20 and 40, who were studying a Teaching Grade. These students were enrolled in the "Music Education" program as part of their university degree’s syllabus. None of them had special music studies from conservatories, academies or were self-taught; thus, all had similar musical knowledge. None of them had previously listened to music in an instructional context nor had performed music with their fellow students. In addition, none of them had listening before to the musical pieces selected for this experiment.
All signed an informed consent form before participating and no payment was given for taking part in the study. As the experiment was carried out in the context of a university course, they were assured that their participation and responses would be anonymous and would have no impact on their qualifications. The research was approved by the ethical committee at the Universidad Católica de Valencia San Vicente Mártir: UCV2017- 18-28 code.
Questionnaire
To assess emotional states, the Positive and Negative Affective States scales (PANAS), was administered [ 39 ]. In particular, the Spanish version of the scale [ 17 ], whose study shows a high degree of internal consistency; in males 0.89 in positive affects and 0.91 in negative affects; in women 0.87 in positive affects and 0.89 in negative affects. In this study, good reliability level in each experimental condition was obtained (0.836–0.913 for positive affects and 0.805–0.917 for negative affects (see Table 1 for more information on Cronbach’s α for each experimental condition).
The PANAS consists of 20 items which describe different dimensions of emotional experience. Participants must answer them regarding to their current affective state. The scale is composed of 20 items; 10 positive affects (PA) and 10 negative affects (NA). Answers are graded in a 5-options (Likert scale), with reversed items, ranging from extremely (1) to very slightly or not at all (5).
Musical pieces
The musical pieces choice stemmed from the analysis of some of the music elements that most influence the perception of emotions: mode, melody and intervals. Within the melody, range and melodic direction were distinguished. The range or amplitude of the melodic line is commonly divided into wide or narrow, while the melodic direction is often classified as ascending or descending. Chang and Hoffman [ 10 ] associated narrow amplitude melodies with sadness, while Schimmark and Grob [ 40 ] related melodic amplitude with highly activated emotions. Regarding the melodic direction, Gerardi and Gerken [ 41 ] found a relationship between ascending direction and happiness and heroism, and between descending direction and sadness.
In relation to the mode, Tizón [ 42 ] stated that the major one is completely happy, while the minor one represents sadness. Thompson and Robitaille [ 43 ] considered that, in order to cause emotions such as happiness, solemnity or joy, composers use tonal melodies, while to obtain negative emotions, they use atonality and chromaticism.
In this research, the selected pieces (“Adagietto” from Gustav Mahler's Fifth Symphony, MML; and “Titans” from Alexander The Great from Vangelis, VML) are representative examples of the melodic, intervallic and modal characteristics previously exposed. Mahler's and Vangelis's pieces completely differ in modes and melodic amplitude (sad vs. heroism). Likewise, Mahler's piece is much more chromatic than Vangelis' one, which has a broader melody made up of third, fourth and fifth intervals, often representative of heroism. Those features justify the fact that they have been used as soundtracks in two films belonging to the epic genre (Alexander The Great, 2004) and drama (Death in Venice, 1971).
The musical piece that was performed by the students was chosen in order to be easy to learn in a few sessions, since they were not musicians. So, three musical pieces were used for the experimental conditions, the first two musical pieces were recordings in a CD, while the third one was performed by the subjects.
The three chosen pieces are described below:
Condition 1 (MML): “Adagietto” from Gustav Mahler’s Fifth Symphony (9:01 min), performed by the Berlin Philharmonic conducted by Claudio Abbado [ 44 ]. This is a sad, melancholic and dramatic piece that Luchino Visconti used in the film Death in Venice, made in 1971 and based on the book by Thomas Mann.
Condition 2 (VML): “Titans Theme” from Alexander the Great (3:59 min), directed by Oliver Stone and premiered in 2004, whose music was composed, produced and performed by Vangelis [ 45 ]. It has a markedly epic character with large doses of heroism and solemnity.
Condition 3 (BP): “Rhythm’s Blues” composed and played by Ana Bort (4:00 min). This is a popular African-American piece of music with an insistent rhythm and harmonically sustained by tonal degrees. This piece was performed by the participants using percussion instruments (carillons and a range of xylophones and metallophones).
The sample was divided into two groups (N 1 = 36 and N 2 = 35) that participated separately in all the phases of the study. The first two conditions (MML and VML) were carried out in each group's classroom, while the performance (BP) was developed in the musical instruments room. This room had 52 percussion instruments, including different types of chimes, xylophones and metallophones (soprano, alto and bass). It is a large space where there are only chairs and musical instruments and stands. The first group was distributed as follows: 6 chimes (3 soprano and 3 alto), 5 soprano xylophones, 5 alto xylophones, 5 bass xylophones, 5 soprano metallophones, 5 alto metallophones and 5 bass metallophones. The distribution of the second group was similar, but with one less alto metallophone.
Prior to the experiment, participants received two practical lessons in order to learn how to collectively perform the music score (third experimental condition). After the two practical lessons, during the next three sessions (leaving two weeks between each session), the experiment was carried out. In each session, an experimental condition was applied and PANAS was on-line administered online beforehand and afterwards (Pre-Post design). All participants were exposed to the three experimental conditions and completed the scale before and after listening to music.
In each of these three sessions, a different music condition was applied: MML in the first one, VML in the second one and BP in the third one.
As conditions VML and MML were listening to pieces of music, the instructions received by the subjects were: “You are going to listen to a musical piece, you ought to listen actively, avoiding distractions. You can close your eyes if you feel like to”. For the BP condition, they were said to play the musical sheet all together.
The aim of the study was to examine the effect of the music experience variable (with three levels: MML, VML and BP) in the Positive and Negative Affects subscales from the PANAS scale. The variable Moment was also studied to control biases and to analyze differences between the Pre and Post conditions.
The experiment was designed as a two-way repeated measure (RM) ANOVA with two dependent variables: Positive Affects and Negative Affects, one for each PANAS’ subscales.
The two repeated measures used in the experiment were the variables Musical Experience (ME), with three levels (MML, VML and BP) and the variable Moment, with two levels (PRE and POST). All participants were exposed to the three experimental conditions.
The design did not include a control group, similar to many other studies in the field of music psychology [ 27 , 30 ]. The control was carried out from the intra-subject pre-post measurement of all the participants. The rationale for this design lies in the complexity of the control condition (or placebo) design in psychology [ 46 ]. While placebos in pharmacological trials are sugar pills, in psychology it is difficult to establish an equivalent period of time similar to the musical pieces (e. g. 9 min) without activity, so that cognitive activity occurred during this period of time (e. g. daydreaming, reading a story, etc.) could bias and limit the generalization of results.
Additionally, one of the goals of this study was to compare the effects of listening to music compared to performance on affects. For this reason, two music listening experiences (MML and VML) and a musical performance experience (BP) were designed. In order to control potential biases, participants did not know the musical pieces in the experimental conditions and they had a low level of musical performance competence (musicians were excluded).
It was used SPSS statistics v.26 for the statistical analyzes.
Two ANOVA were performed. The first one, analyzed two dependent variables at the same time: Positive Affects (PA) and Negative Affects (NA).
In the second ANOVA, the 20 items of the PANAS scale were taken as dependent variables. The rest of the experimental design was similar to the first one, a two-way RM ANOVA with variables Musical Experience (ME) and Moment as repeated measures.
Examination of frequency distributions, histograms, and tests of homogeneity of variance and normality for the criterion measures indicated that the assumptions for the use of parametric statistics were met. Normality was met in all tests except for one, but the ANOVA is robust against this assumption violation. All the analyses presented were performed with the significance level (alpha) set at 0.05, two-tailed tests. Means and standard deviations for the 6 experimental conditions for both subscales, Positive Affects and Negative Affects, are presented in Table 1 .
Mauchly’s test of sphericity was statistically significant for Musical Experience and Musical Experience*Moment focusing on NA as the dependent variable ( p < 0.05). The test only was significant for Musical Experience for PA as dependent variable ( p < 0.05). The rest of the W’s Mauchly were not significant ( p > 0.05), so we assumed sphericity for the non-mentioned variables and worked with the assumed sphericity univariate solution. For the variables which the W’s Mauchly was significant, the univariate solution was also taken, but choosing the corrected Greenhouse–Geisser epsilon approximation due to its conservativeness.
A significant principal effect of the Musical Experience variable F(1.710,119.691) = 22.505, p < 0.05, η 2 = 0.243; the Moment variable F(1,70) = 45.291, p < 0.05, η 2 = 0.393; and the Musical Experience*Moment interaction F(2,140) = 32.502, p < 0.05, η 2 = 0.317 were found for PA.
Statistically significance was found for Moment F(1, 70) = 70.729, p < 0.05, η 2 = 0.503 and Musical Experience*Moment interaction F(1.822, 127.555) = 8.594, p < 0.05, η 2 = 0.109, but not for Musical Experience F(1.593, 111.540) = 2.713, p < 0.05, η 2 = 0.037, for the other dependent variable, NA.
Table 2 shows pairwise comparisons between Musical Experience levels. Bonferroni’s correction was applied in order to control type I error. We only interpret the results for the Positive Affects because the Musical Experience effect was not statistically significant for Negative Affects. Results show that condition VML presents a significant higher punctuation in Positive Affects than the other two conditions ( p < 0.05). It also shows that the musical condition MML is significantly above BP in Positive Affects ( p < 0.05).
As regards Moment variable (Table 3 ), all but one Pre-Post differences were statistically significant ( p < 0.05) for all the three conditions for both Positive and Negative Affects dependent variables. The Pre-Post difference found in Positive Affects for the VML Musical Experience did not reach the statistical level ( p = 0.319).
Focusing on these statistically significant differences, we observe that conditions MML and BP, for PA, decreased from Pre to Post condition, indicating that positive emotions increased significantly between pre and post measures. On the other hand, for NA, all conditions increased from Pre to Post conditions, indicating that negative affects were decreased between pre and post conditions. Once again, one should bear in mind that items were reversed, thus, a higher scores in NA means a decrease in affects.
In order to measure the interaction effect, significant differences between simple effects were analysed.
The simple effect of Moment (level2-level1) in the first Music Experience condition (MML) in PA was compared with the simple effect of Moment (level2-level1) in the second Musical Experience condition (VML). Music Experience conditions 2–3 (VML-BP) and 1–3 (MML-BP) were compared in the same way. Thus, taking into account PA and NA variables, a total of 6 comparisons, 3 per dependent variable, were made.
The results of these comparisons are shown in Table 4 . Comparisons for PA range from T1 to T3 and comparisons for NA range from T4 to T6. All of them are significant ( p < 0.05) which means that there are statistically significant differences between all the Musical Experience conditions when comparing the Moment (pre/post) simple effects.
In Table 5 , we can look at the differences’ values. As we said before the differences between Pre and Post conditions are significant when comparing the three musical conditions. The biggest difference for positive affects is between MML and BP (T3 = 8.443), and between VML and MML (T4 = − 6.887) for negative affects.
In this second part, the results obtained from the second two-way RM ANOVA with the 20 items as dependent variables are considered. Results of the descriptive analysis of each item: Interested, Excited, Strong, Enthusiastic, Proud, Alert, Inspired, Determined, Attentive, Active, Distressed, Upset, Guilty, Afraid, Hostile, Irritable, Ashamed, Nervous, Jittery, Scared ; in each musical condition: MML, VML and BP; and for the PRE and POST measurements, can be found in the Additional file 1 (Appendix A).
As regards the ANOVA test that compares the three experimental conditions in each mood, Mauchly’s Sphericity Test indicates that sphericity cannot be assumed for the musical experience in most of the variables of the items of effects, except for Interested, Alert, Inspired, Active and Irritable . For these items, the highest observed power index among Greenhouse–Geisser, Huynh–Feldt and Lower-bound epsilon corrections was taken for each variable. For the interaction Musical Experience*Moment, sphericity was not assumed for Distressed, Guilty, Hostile and Scared . For these items, the same above-cited criterion was followed.
Musical experience has a principal effect on all the positive affects, but only has it for 5 negative affects ( Nervous, Jittery, Scared, Hostile and Upset ) ( p < 0.05). For more detail see Table S1 from Additional file 1 : Appendix B.
The principal effect of Moment is also statistically significant ( p < 0.05) for all (positive and negative), but two items: Guilty ( p = 0.073) and Hostile ( p = 0.123). All the differences between Pre and Post for positive affects are positive, which means that scores in conditions Pre were significantly higher than in condition Post. The other way around occurs for negative affects, all the differences Pre-Post are negative, meaning that the Post condition is significantly higher than the Pre condition. For more detail, see Table S2 from Additional file 1 : Appendix B. In this way, Pre-post changes (Moment) improve affective states; the positive affects increase while the negative are reduced, except for Guilty ( p = 0.073) and Hostile ( p = 0.123).
Comparing the proportion of variance explained by the musical experienced and Moment (Tables s1 and s2 from the Additional file 1 : Appendix B), it is observed that most of the η 2 scores in musical experience are below 0.170, except Active and Alert , which are higher. On the other hand, the η 2 scores for Moment are close to 0.300. From these results we can state that, taking only one of the variables at a time, the proportion of the dependent variable’s variance explained by Moment is higher than the proportion of the dependent variable’s variance explained by Musical Experience.
The effect of interaction, shown in Table S3 from the Additional file 1 : Appendix B is significant in 7 positive moods ( Interested, Excited, Enthusiastic, Alert, Determined, Active and Proud ) and 4 negative moods ( Hostile , Irritable, Nervous , and Jittery ).
The pairwise comparisons of Musical Experience’s levels show a wide variety of patterns. Looking at Positive Affects, there is only one item ( Active ) which present significant differences between the three musical conditions. Items Concentrated and Decided do not present any significant difference between any musical conditions. The rest of the Positive items show at least one significant difference between conditions VML and BP. All differences are positive when comparing VML-MML, VML-BP MML-BP, except for Alert and Proud. So, in general, scores are higher for the first two conditions in relation to the third one, meaning that third musical condition presents the biggest increase for Positive Affects (remember items where reversed). For more detail see Additional file 1 : Appendix C.
As regard pairwise comparisons of Musical Experience’s for negative affects, only the items which had a significant principal effect of the variable Musical Experience are shown here. There is a significant difference between conditions VML and MML in item Nervous ; between VML and BP for Scared ( p < 0.05). For Jittery ; all three conditions differed significantly from each other ( p < 0.05). Conditions MML and BP differed significantly for Hostile ( p < 0.05) and conditions VML and BP almost differed significantly for Upset item, but null hypothesis cannot be rejected as p = 0.056. For more detail see Additional file 1 : Appendix C. All differences were negative when comparing VML-MML, VML-BP MML-BP, except for Nervous and Jittery . So, in general, scores are lower for the first and second condition in relation to the third one.
Positive effects increased significantly during the post phase of all the music experiences, showing that exposure to any of the three music stimuli improved positive affectivity. There were also significant differences between the three experiences in this phase, according to the following order of improvements in positive affectivity: (1) the rhythm and blues performance (BP), (2) listening to Mahler (MML) and (3) listening to Vangelis (VML). As regards the effects of the musical experience x Moment interaction , all the comparisons were significant, with bigger differences in the interpretation of the blues (BP) than in listening to Mahler (MML) and Vangelis (VML). However, the comparison between both experiences, although significant, was smaller. These results indicate that performing music is significantly effective in increasing positive effects. We will explain these results in greater detail below as regards the specific affective states.
As regards Negative Affects, the comparison of the simple effects showed that these decreased after the musical experiences, although in this first analysis the VML musical experience did not differ from the other two. However, the results of the effects of the interaction between musical experiencie x Moment showed that all the comparisons were significant, with a larger difference between MML and VML than the one between BP and each of the other experiences. Listening to Mahler (MML) was more effective in reducing negative affects, compared to both listening to Vangelis and interpreting the blues (BP). These results agree with previous studies [ 26 , 32 ], in which listening to sad music helped to reduce negative affectivity. In this study, it was the most effective condition, although exposure to all three musical experiences reduced negative affects.
The analysis of the specific affective states shows that most items that belong to Positive Affect scale are the most sensitive ones to the PRE-POST change, the different musical conditions and the interpretation of both effects. However, some items of the Negative Affect scale did not differ in the different music conditions or in the music experience × Moment interaction . For example, there were two items (Guilty and Hostile) that did not obtain significance. These results are consistent with the fact that music has certain limits as regards its impact on people’s affects and does not influence all equally. For example, Guilty has profound psychological implications that cannot be affected by simple exposure to certain musical experiences. This means we should be cautious in inferring that music alone can have therapeutical effects on complex emotional states whose treatment should include empirically validated methods. Also, emotional experiences are widely diverse so that any instrument used to measure them is limited as regards the affective/emotional state under study. These results suggest the importance of reviewing the items that compose the PANAS scale in musical studies to adapt it in order to include affective states more sensitive to musical experiences and eliminate the least relevant items.
The analysis of the results in the specific affective states, allows us to delve deeper into each experimental condition. Thus, regarding the results obtained in the complete scale of PANAS, listening to Mahler (MML), causes desirable changes by raising two positive affects ( Inspired and Attentive ) and reducing 10 negative affects ( Distressed, Upset, Afraid, Hostile, Irritable, Ashamed, Nervous, Jittery, and Scared ). This shows that this music condition had a greater effect on the negative affects than the other ones. These results agree with previous studies [ 26 , 32 ], which found that sad music could effectively reduce negative affects, although other studies came to the opposite conclusion. For instance, Miller and Au [ 31 ] found that sad music did not significantly change negative affects. Some authors [ 47 , 48 ] have argued that adults prefer to listen to sad music to regulate their feelings after a negative psychological experience in order to feel better. Taruffi and Koelsch [ 49 ] concluded that sad music could induce listeners to a wide range of positive effects, after a study with 772 participants. In order to contribute to this debate. It would be interesting to control personality variables that might explain these differences on the specific emotions evoked by sad music. In this study, it has been shown that a sad piece of music can be more effective in reducing negative affects than in increasing positive ones. Although the results come from undergraduate students, similar outcomes could be obtained from children and adolescents, although further research is required. In fact, Borella et al. [ 50 ] studied the influence of age on the effects of music and found that the emotional effects influenced cognitive performance (working memory) in such a way that the type of music (Mozart vs. Albinoni) had a stronger influence on young people than on adults. Kawakami and Hatahira [ 28 ], in a study on 84 primary schoolchildren, also found that exposure to sad music pleased them and their level of empathy correlated with their taste for sad music.
Listening to Vangelis (VML) increased 3 positive affects ( Excited, Inspired and Attentive ) and reduced 8 negative affects ( Distressed, Upset, Afraid, Irritable, Ashamed, Nervous, Jittery , and Scared ). Surprisingly, two positive affects were reduced in this experimental condition ( Alert and Attentive ). It could be explained due to the characteristic ostinato rhythm of this piece of music. It was found a similar effect in the study by Campbell et al., [ 26 ] in which sad music reduced both positive and negative affects. This musical condition also managed to modify negative affects more than positive ones.
Performing the blues (BP) increased all 10 positive affects, indicating that performing is more effective in increasing positive affects than listening. These results agree with the study by Dunbar et al. [ 29 ], who found that music performance significantly increased positive affects.
Performing the blues (BP) reduced 6 negative affects, although it was more effective in increasing positive affective states. Vigorous rhythmic music was also found to be positively associated with the use of all the forms of regulating emotions, which suggests that this type of music is especially useful for emotion modulation [ 51 ]. It was found an exception, since Jittery increased after the blues performance. It could be explained by the negative experience that is sometimes associated with music performance. Therefore, it should be taken into account that music performance could increase some negative effects. For example, Dimsdale et al. [ 52 ] found that a strong negative emotional response to a certain type of music in adolescents was related to risk behaviour, indicating that research into the repertory of music experiences needs to be broadened to diverse styles in different age groups to identify all the types of emotional response and their psychological consequences. However, this result should be taken with caution and further research should focus on whether the effect of increased agitation is usual after music performances.
To sum up, this study contributes to the scientific field on the following points: (1) all the musical experiences had significant effects on improving emotional states, increasing positive affects and decreasing the negative ones, which shows the importance of musical experiences on improving the affective sphere; (2) the specific affects that increased, decreased or did not change for each musical experience were identified, providing specific and useful keys for the design of future interventions; and (3) the differences between various types of musical experiences were analyzed, finding more improvements in the performing conditions than in the listening ones.
Limitations and future directions
Limitations.
The sample, made up of university students with a very homogeneous profile in terms of age and sociodemographic characteristics, could limit the generalization of the results. In addition, the low percentage of men in the sample could also affect the generalizability of the results, although no previous studies have reported gender-based differential effects on the positive and negative affects after musical experiences.
Besides, the choice of the pieces of music was based on theoretical criteria and students’ music preferences were not taken into account. This will be included in future research, since the specific choice of the pieces could affect the positive or negative valence of participants’ emotions. However, the goal of using pieces of music not chosen by participants was to elicit new musical experiences for them. Furthermore, no participant was a musician and none of them had previous knowledge of any of the pieces, which may lead to a bias in the results.
In relation to this, the huge amount of available pieces of music, all of them influenced by their cultural and historical context, make it difficult to generalize that certain music parameters correlate with specific emotions. It would be necessary a cross-cultural approach to reach that conclusion.
Future directions
It is recommended to introduce the variables of music preferences and music history to control their effect on the results and to be able to compare the different musical parameters of the pieces together with participants’ preferences.
Likewise, it would be interesting to identify the affects with a greater or lesser degree of influence by music, to adjust the psychological evaluation instrument to the characteristics of the experiment, including items of emotions that can be modified after exposure to a music experience.
The PANAS manual [ 39 ] indicates that a wide variety of affective states (60) and eight different temporal instructions were included in its construction, showing its great versatility. In further research, this instrument should be adapted to for a more specific application to music studies. For instance, by including other emotional states that could be related with the influence of music (e.g. Tranquility , Gratitude , Elevation ), in order to measure more exactly the effects of music on people’s affective experiences.
Accordingly, it would be interesting to evaluate participants' affective traits to establish a baseline and control personality variables, helping to delve into the different levels of the hierarchical structure of affectivity and its relationship with the various music parameters.
Finally, it is recommended that the psychology of music include objective psychophysiological measurements together with self-report evaluations, so that conclusions arising from the experiments have greater robustness and can increase the impact of the contribution to the scientific community.
This study have shown how different music experiences, such as listening and performing, influence the changes in positive and negative affects in student teachers. The results show that the three musical experiences studied are effective in improving the affects by comparing the emotional states before and after the music experiences. It was also showed that there are differences between the effects obtained in each of the music experiences. Besides, improving both types of affects will depend largely on the selected music for the purpose. Although further evidence is required, the results support the importance of music in education, since it provides tools to increase positive affects and to decrease the negative ones, which is important for emotional intelligence development [ 53 , 54 ].
The three music experiences studied are more effective in reducing negative emotional states than in increasing the positive ones. This finding provides useful clues for music teachers to provide strategies that favor emotional regulation. For instance, in order to reduce hostility, irritability and nervousness, students could be exposed to musical auditions of both sad and solemn pieces, choosing musical pieces with similar characteristics to those described in this study. These auditions will be a resource for stress management in the classroom, as well as a tool that students can adopt and generalize to other contexts. Moreover, it is highly likely that students have not heard this type of music before and this experience could increase their repertoire of musical preferences, enhancing their emotional regulation.
The blues performance had a greater impact on participants' positive affects than listening to the other two pieces so, if any teacher wants to increase them (e.g., enthusiasm, interest, etc.), students could be asked to perform simple pieces such as Rhythm's Blues. In this way, musical performance could increase students' resources, contributing to higher levels of motivation, concentration and interest, which promotes learning [ 55 , 56 , 57 , 58 ]. Likewise, it could be very useful for elementary and secondary music teachers, who will be able to contribute to socio-emotional improvement and personal development of their students. Particularly, musical experiences could be a valuable resource for secondary teachers, since music is important in adolescents' lives and can be an interesting tool for meeting their emotional needs [ 59 ]. This is supported by Kokotsaki and Hallam [ 60 ], who consider that performing music helps students feel like active agents of a group, develop a strong sense of belonging, gain popularity, make "like-minded" relationships, improve their social skills and foster a strong sense of self-esteem and satisfaction.
This study shows that experiencing with various unknown musical pieces can have positive effects on emotions. According to this finding, university professors of Teaching grade in music education should encourage future teachers to experience various musical styles, rhythms and tonalities, avoiding prejudices. Thereby, future music teachers will be able to use a diversity of musical experiences that broaden the emotional effects and fulfill the socio-emotional function of music education. In relation to Fredrickson's 'broaden‐and‐build' framework of positive emotions [ 30 ], music can become a mean of widening other positive emotional states, constructing personal resources and transforming people, and contribute to an upward spiral of positive emotions. Taking into account the underlying psychological mechanisms of the impact of music on the emotional states it will be possible to use it to improve emotional area and other aspects of the personal sphere, as Chang et al., [ 10 ] maintain. Therefore, music education is an important resource to improve the emotional development of students.
Availability of data and materials
The datasets generated during and/or analyzed during the current study are available from the corresponding author on reasonable request.
Blasco-Magraner JS, Bernabe-Valero G, Marín-Liébana P, Moret-Tatay C. Effects of the educational use of music on 3-to 12-year-old children’s emotional development: a systematic review. Int J Environ Res Public Health. 2021;18(7):1–29. https://doi.org/10.3390/ijerph18073668 .
Article Google Scholar
Fancourt D, Ockelford A, Belai A. Brain, behavior, and immunity the psychoneuroimmunological effects of music: a systematic review and a new model. BRAIN Behav Immun. 2013;36:15–26. https://doi.org/10.1016/j.bbi.2013.10.014 .
Article PubMed Google Scholar
Moore KS. A systematic review on the neural effects of music on emotion regulation: implications for music therapy practice. J Music Therapy. 2013;50(3):198–242. https://doi.org/10.1093/jmt/50.3.198 .
Bonde LO, Beck BD. Imagining nature during music listening. An exploration of the meaning, sharing and therapeutic potential of nature imagery in guided imagery and music. In: Pfeifer E, editor. Natur in Psychotherapie und Künstlerischer Therapie: Theoretische, methodische und praktische Grundlagen (2 Bände). Psychosozial-Verlag; 2019. p. 147–68.
Google Scholar
Cespedes-Guevara J, Eerola T. Music communicates affects, not basic emotions: a constructionist account of attribution of emotional meanings to music. Front Psychol. 2018;9(1):1–19. https://doi.org/10.3389/fpsyg.2018.00215 .
Cotter KN, Silvia PJ, Fayn K. What does feeling like crying when listening to music feel like? Psychol Aesth Creat Arts. 2018;2018(12):216–27. https://doi.org/10.1037/aca0000108 .
Sakka LS, Juslin PN. Emotional reactions to music in depressed individuals. Psychol Music. 2017;46(6):1–19. https://doi.org/10.1177/0305735617730425 .
Ter Bogt T, Canale N, Lenzi M, Vieno A, van den Eijnden R. Sad music depresses sad adolescents: a listener’s profile. Psychol Music. 2021;49(2):257–72. https://doi.org/10.1177/0305735619849622 .
Akkermans J, Schapiro R, Müllensiefen D, Jakubowski K, Shanahan D, Baker D, et al. Decoding emotions in expressive music performances: a multi-lab replication and extension study. Cogn Emot. 2019;33(6):1099–118. https://doi.org/10.1080/02699931.2018.1541312 .
Chang J, Lin P, Hoffman E. Music major, affects, and positive music listening experience. Psychol Music. 2021;49(4):841–54. https://doi.org/10.1177/0305735619901151 .
Kreuth G. Music students’ health problems and health-promoting behaviours. Med Probl Perform Art. 2008;23(1):3–11.
MacDonald R, Kreutz G, Mitchell L. Music, health, and wellbeing. Oxford: Oxford University Press; 2013.
Delors J. La Educación encierra un tesoro. Informe a la UNESCO de la Comisión Internacional sobre la Educación para el siglo XXI. Santillana. 1996.
Requena SO. Música y adolescencia: usos, funciones y consideraciones educativas. UT: Revista de Ciències de l’Educació. 2015;2:28–45.
Saarikallio S. Music as emotional self-regulation throughout adulthood. Psychol Music. 2011;39(3):307–27. https://doi.org/10.1177/0305735610374894 .
Hays T, Minichiello V. The meaning of music in the lives of older people: a qualitative study. Psychol Music. 2005;33(4):437–51. https://doi.org/10.1177/0305735605056160 .
Sandín B, Chorot P, Lostao L, Joiner TE, Santed MA, Valiente RM. Escalas PANAS de afecto positivo y negativo: validacion factorial y convergencia transcultural. Psicothema. 1999;11:37–51.
Arjmand HA, Hohagen J, Paton B, Rickard NS. Emotional responses to music: shifts in frontal brain asymmetry mark periods of musical change. Front Psychol. 2017;8(1):1–13. https://doi.org/10.3389/fpsyg.2017.02044 .
Miu AC, Baltes FR. Empathy manipulation impacts music-induced emotions: a psychophysiological study on opera. PLoS ONE. 2012;7(1):1–6. https://doi.org/10.1371/journal.pone.0030618 .
Blasco JS, Calatrava C. Influencia de la música en las emociones percibidas en el alumnado de educación secundaria y bachillerato. Espiral Cuad del Profr. 2020;13(27):180–91. https://doi.org/10.25115/ecp.v13i27.2909 .
Sharman L, Dingle GA. Extreme metal music and anger. Front Hum Neurosci. 2015;9(1):1–11. https://doi.org/10.3389/fnhum.2015.00272 .
Schubert E. A special class of experience: positive affect evoked by music and the arts. Int J Environ Res Public Health. 2022;19(8):4735. https://doi.org/10.3390/ijerph19084735 .
Article PubMed PubMed Central Google Scholar
Tasso F. Influencia de la empatía y la instrucción musical en el reconocimiento de emociones y estimación temporal en la música [Internet]. 2019. Available from: https://repositorio.uca.edu.ar/bitstream/123456789/10189/1/influencia-empatia-instruccion-musical.pdf .
Brattico E, Bogert B, Alluri V, Tervaniemi M, Eerola T, Jacobsen T. It’s sad but i like it: THE neural dissociation between musical emotions and liking in experts and laypersons. Front Hum Neurosci. 2016;9(JAN2016):1–21. https://doi.org/10.3389/fnhum.2015.00676 .
Kawakami A, Furukawa K, Katahira K, Okanoya K. Sad music induces pleasant emotion. Front Psychol. 2013;4(June):1–15. https://doi.org/10.3389/fpsyg.2013.00311 .
Campbell EA, Berezina E, Gill CMHD. The effects of music induction on mood and affect in an Asian context. Psychol Music. 2021;49(5):1132–44. https://doi.org/10.1177/0305735620928 .
Vuoskoski JK, Eerola T. The pleasure evoked by sad music is mediated by feelings of being moved. Front Psychol. 2017;8(March):1–11. https://doi.org/10.3389/fpsyg.2017.00439 .
Kawakami A, Katahira K. Influence of trait empathy on the emotion evoked by sad music and on the preference for it. Front Psychol. 2015;6(OCT):1–9. https://doi.org/10.3389/fpsyg.2015.01541 .
Dunbar RIM, Kaskatis K, MacDonald I, Barra V. Performance of music elevates pain threshold and positive affect: implications for the evolutionary function of music. Evol Psychol. 2012;10(4):688–702. https://doi.org/10.1177/147470491201000 .
Fredrickson BL, Tugade MM, Waugh CE, Larkin GR. What good are positive emotions in crises? A prospective study of resilience and emotions following the terrorist attacks on the United States on September 11th, 2001. J Personal Soc Psychol. 2003;84(2):365–76.
Miller S, Au A. The comparison of happy and sad music on mood and task-switching. In: Proceedings of the 37th Australasian Experimental Psychology Conference [Internet]. 2010. p. 8–10. Available from: https://www.psychology.org.au/Assets/Files/2010-Combined-Abstracts.pdf .
Schulte B, ScholarWorks at WMU Music Evoked Nostalgia and Mood States Music Evoked Nostalgia and Mood States. West Mich Uni 2018; https://scholarworks.wmich.edu/honors_theses/3085 .
Matsumoto J. Why people listen to sad music: effects of music on sad moods. Japanese J Educ Psychol. 2002;50(1):23–32. https://doi.org/10.5926/jjep1953.50.1_23 .
Vuoskoski JK, Eerola T. Can sad music really make you sad? Indirect measures of affective states induced by music and autobiographical memories. Front Aesth Arts. 2012;6(3):204–13. https://doi.org/10.1037/a0026937 .
Talamini F, Eller G, Vigl J, Zentner M. Musical emotions affect memory for emotional pictures. Sci Rep. 2022;12(1):1–8. https://doi.org/10.1038/s41598-022-15032-w .
Mayer JD, Salovey P, Caruso D. Models of emotional intelligence. In: Sternberg RJ, editor. The handbook of intelligence. New York: Cambridge University Press; 2000. p. 396–420.
Chapter Google Scholar
Fernández-Berrocal P, Extremera N, Ruiz-Aranda D, Cabello R. Inteligencia emocional, estilos de respuesta y depresión. Ansiedad y Estrés. 2006;12(3):191–205.
Eberth J, Sedlmeier P. The effects of mindfulness meditation: a meta-analysis. Mindfulness. 2012;3(3):174–89. https://doi.org/10.1007/s12671-012-0101-x .
Watson D, Clark LA, Tellegen A. Development and validation of brief measures of positive and negative affect: the PANAS scales. J Personal Soc Psychol. 1988;54(6):1063–70. https://doi.org/10.1037/0022-3514.54.6.1063 .
Schimmack U, Grob A. Dimensional models of core affect: A quantitative comparison by means of structural equation modeling. Eur J Pers. 2000;14(4):325–45.
Gerardi GM, Gerken L. The development of affective responses to modality and melodic contour. Music Percept. 1995;12(3):279–90.
Tizón Díaz MA. La influencia del estilo musical en la emoción percibida. 2015; Available from: https://burjcdigital.urjc.es/handle/10115/13620#.YtizDsdrafw.mendeley .
Thompson WF, Robitaille B. Can composers express emotions through music? Empir Stud Arts. 1992;10(1):79–89. https://doi.org/10.2190/NBNY-AKDK-GW58-M .
Mahler, G. Symphony nº 5. [CDROM recorded by Berliner Philharmoniker/Claudio Abbado]. Berlin: Deutsche Grammophon, 1993.
Vangelis, E. Alexander. [CDROM recorded by Vangelis]. Sony Classical, 2004.
Locher C, Gaab J, Blease C. When a placebo is not a placebo: Problems and solutions to the gold standard in psychotherapy research. Front Psychol. 2018;9(NOV):1–4. https://doi.org/10.3389/fpsyg.2018.02317 .
Saarikallio S, Erkkilä J. The role of music in adolescents’ mood regulation. Psychol Music. 2007;35(1):88–109. https://doi.org/10.1177/0305735607068889 .
Van Den Tol AJM, Edwards J. Exploring a rationale for choosing to listen to sad music when feeling sad. Psychol Music. 2013;41(4):440–65. https://doi.org/10.1177/0305735611430433 .
Taruffi L, Koelsch S. The paradox of music-evoked sadness: an online survey. PLoS ONE. 2014;9(10):1–17. https://doi.org/10.1371/journal.pone.0110490 .
Borella E, Carretti B, Grassi M, Nucci M, Sciore R. Are age-related differences between young and older adults in an affective working memory test sensitive to the music effects? Front Aging Neurosci. 2014;6(OCT):1–9. https://doi.org/10.3389/fnagi.2014.00298 .
Cook T, Roy ARK, Welker KM. Music as an emotion regulation strategy: an examination of genres of music and their roles in emotion regulation. Psychol Music. 2019;47(1):144–54. https://doi.org/10.1177/03057356177346 .
Roberts KR, Dimsdale J, East P, Ph D, Friedman L. Adolescent emotional response to music and its relationship to risk-taking behaviors. J Adolesc Health. 1998;1:49–54. https://doi.org/10.1016/S1054-139X(97)00267-X .
Park N, Peterson C, Seligman M. Strengths of character and well-being. J Social Clin Psychol. 2004;23(5):603–19. https://doi.org/10.1521/jscp.23.5.603.50748 .
Zysberg L, Raz S. Personality and Individual Differences Emotional intelligence and emotion regulation in self-induced emotional states: physiological evidence. Personal Individ Differ. 2019;139:202–7. https://doi.org/10.1016/j.paid.2018.11.027 .
Brown ED, Sax KL. Arts enrichment and preschool emotions for low-income children at risk. Early Child Res Q. 2013;28:337–46. https://doi.org/10.1016/j.ecresq.2012.08.002 .
Ramdane T, Souad M, Marusin R, Sidek, SS. The usefullness of music as a tool of teaching islamic education: Teachers’ perspective. Al-Shajarah J. Isl. 2018, 267–286.
Pimenta MA, Trevisan VL. Música e psicologia na escola: Mobilizando afetos na classe de recuperação. Psicol Esc Educ. 2018;22:17–25. https://doi.org/10.1590/2175-35392018019065 .
Rauduvaite A. The educational aspects of integrating popular music into lessons. Rural Environ Educ Personal. 2018;11:94–100. https://doi.org/10.22616/reep.2018.01153 .
North AC, Hargreaves DJ, Neill SAO. The importance of music to adolescents. British J Educ Psychol. 2000;70(2):255–72. https://doi.org/10.1348/000709900158083d .
Kokotsaki D, Hallam S. Higher education music students’ perceptions of the benefits of participative music making. Music Educ Res. 2007;9(1):93–109. https://doi.org/10.1080/14613800601127577 .
Download references
Acknowledgements
We should like to express our gratitude to the Valencia University student teachers for their disinterested and valuable contribution to this study.
Not applicable.
Author information
Authors and affiliations.
Department of Music Education, University of Valencia, Av. Dels Tarongers, 4, 46022, Valencia, Spain
José Salvador Blasco-Magraner, Pablo Marín-Liébana & Ana María Botella-Nicolás
Department of Occupational Sciences, Speech Therapy, Evolutionary and Educational Psychology, Catholic University of Valencia San Vicente Mártir, Av. De La Ilustración, 2, 46100, Burjassot, Valencia, Spain
Gloria Bernabé-Valero
You can also search for this author in PubMed Google Scholar
Contributions
JSBM and GBV contributed to the study conception and design. Material preparation, data collection and analysis were performed by JSBM and GBV. The first draft of the manuscript was written by JSBM, GBV and PML. PML and ABN review, translate and editing the manuscript. All authors read and approved the final manuscript.
Corresponding author
Correspondence to José Salvador Blasco-Magraner .
Ethics declarations
Ethics and consent to participate.
The study protocol was according to the declaration of Helsinki. The research was approved by the ethical committee at the Catholic University of Valencia San Vicente Mártir: UCV2017-18-28 code. Informed written consents were obtained from all participants in the present study.
Consent for publication
Competing interests.
The authors indicate that they have no conflict of interests that impacted this study.
Additional information
Publisher's note.
Springer Nature remains neutral with regard to jurisdictional claims in published maps and institutional affiliations.
Supplementary Information
Additional file 1.
. Results obtained from item analyses.
Rights and permissions
Open Access This article is licensed under a Creative Commons Attribution 4.0 International License, which permits use, sharing, adaptation, distribution and reproduction in any medium or format, as long as you give appropriate credit to the original author(s) and the source, provide a link to the Creative Commons licence, and indicate if changes were made. The images or other third party material in this article are included in the article's Creative Commons licence, unless indicated otherwise in a credit line to the material. If material is not included in the article's Creative Commons licence and your intended use is not permitted by statutory regulation or exceeds the permitted use, you will need to obtain permission directly from the copyright holder. To view a copy of this licence, visit http://creativecommons.org/licenses/by/4.0/ . The Creative Commons Public Domain Dedication waiver ( http://creativecommons.org/publicdomain/zero/1.0/ ) applies to the data made available in this article, unless otherwise stated in a credit line to the data.
Reprints and permissions
About this article
Cite this article.
Blasco-Magraner, J.S., Bernabé-Valero, G., Marín-Liébana, P. et al. Changing positive and negative affects through music experiences: a study with university students. BMC Psychol 11 , 76 (2023). https://doi.org/10.1186/s40359-023-01110-9
Download citation
Received : 12 November 2022
Accepted : 06 March 2023
Published : 21 March 2023
DOI : https://doi.org/10.1186/s40359-023-01110-9
Share this article
Anyone you share the following link with will be able to read this content:
Sorry, a shareable link is not currently available for this article.
Provided by the Springer Nature SharedIt content-sharing initiative
- Positive affects
- Negative affects
- Music experiences
- University students
BMC Psychology
ISSN: 2050-7283
- General enquiries: [email protected]
Thank you for visiting nature.com. You are using a browser version with limited support for CSS. To obtain the best experience, we recommend you use a more up to date browser (or turn off compatibility mode in Internet Explorer). In the meantime, to ensure continued support, we are displaying the site without styles and JavaScript.
- View all journals
- Explore content
- About the journal
- Publish with us
- Sign up for alerts
- Review Article
- Published: 29 March 2022
Music in the brain
- Peter Vuust ORCID: orcid.org/0000-0002-4908-735X 1 ,
- Ole A. Heggli ORCID: orcid.org/0000-0002-7461-0309 1 ,
- Karl J. Friston ORCID: orcid.org/0000-0001-7984-8909 2 &
- Morten L. Kringelbach ORCID: orcid.org/0000-0002-3908-6898 1 , 3 , 4
Nature Reviews Neuroscience volume 23 , pages 287–305 ( 2022 ) Cite this article
25k Accesses
96 Citations
278 Altmetric
Metrics details
- Neuroscience
Music is ubiquitous across human cultures — as a source of affective and pleasurable experience, moving us both physically and emotionally — and learning to play music shapes both brain structure and brain function. Music processing in the brain — namely, the perception of melody, harmony and rhythm — has traditionally been studied as an auditory phenomenon using passive listening paradigms. However, when listening to music, we actively generate predictions about what is likely to happen next. This enactive aspect has led to a more comprehensive understanding of music processing involving brain structures implicated in action, emotion and learning. Here we review the cognitive neuroscience literature of music perception. We show that music perception, action, emotion and learning all rest on the human brain’s fundamental capacity for prediction — as formulated by the predictive coding of music model. This Review elucidates how this formulation of music perception and expertise in individuals can be extended to account for the dynamics and underlying brain mechanisms of collective music making. This in turn has important implications for human creativity as evinced by music improvisation. These recent advances shed new light on what makes music meaningful from a neuroscientific perspective.
This is a preview of subscription content, access via your institution
Access options
Access Nature and 54 other Nature Portfolio journals
Get Nature+, our best-value online-access subscription
24,99 € / 30 days
cancel any time
Subscribe to this journal
Receive 12 print issues and online access
176,64 € per year
only 14,72 € per issue
Buy this article
- Purchase on Springer Link
- Instant access to full article PDF
Prices may be subject to local taxes which are calculated during checkout
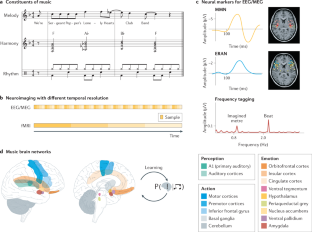
Similar content being viewed by others
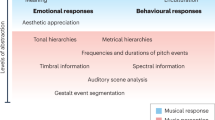
Universality, domain-specificity and development of psychological responses to music
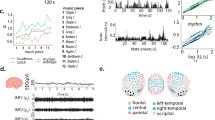
Scaling behaviour in music and cortical dynamics interplay to mediate music listening pleasure
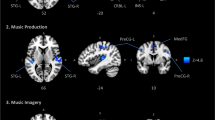
An ALE meta-analytic review of top-down and bottom-up processing of music in the brain
Zatorre, R. J., Chen, J. L. & Penhune, V. B. When the brain plays music: auditory–motor interactions in music perception and production. Nat. Rev. Neurosci. 8 , 547–558 (2007). A seminal review of auditory–motor coupling in music .
Article CAS PubMed Google Scholar
Koelsch, S. Toward a neural basis of music perception–a review and updated model. Front. Psychol. 2 , 110 (2011).
Article PubMed PubMed Central Google Scholar
Maes, P. J., Leman, M., Palmer, C. & Wanderley, M. M. Action-based effects on music perception. Front. Psychol. 4 , 1008 (2014).
Koelsch, S. Brain correlates of music-evoked emotions. Nat. Rev. Neurosci. 15 , 170–180 (2014). In this review, the author shows how music engages phylogenetically old reward networks in the brain to evoke emotions, and not merely subjective feelings .
Vuust, P. & Witek, M. A. Rhythmic complexity and predictive coding: a novel approach to modeling rhythm and meter perception in music. Front. Psychol. 5 , 1111 (2014).
Friston, K. The free-energy principle: a unified brain theory? Nat. Rev. Neurosci. 11 , 127–138 (2010). This review posits that several global brain theories may be unified by the free-energy principle .
Koelsch, S., Vuust, P. & Friston, K. Predictive processes and the peculiar case of music. Trends Cogn. Sci. 23 , 63–77 (2019). This review focuses specifically on predictive coding in music .
Article PubMed Google Scholar
Meyer, L. Emotion and Meaning in Music (Univ. of Chicago Press, 1956).
Lerdahl, F. & Jackendoff, R. A Generative Theory of Music (MIT Press, 1999).
Huron, D. Sweet Anticipation (MIT Press, 2006). In this book, Huron draws on evolutionary theory and statistical learning to propose a general theory of musical expectation .
Hansen, N. C. & Pearce, M. T. Predictive uncertainty in auditory sequence processing. Front. Psychol. https://doi.org/10.3389/fpsyg.2013.01008 (2014).
Vuust, P., Brattico, E., Seppanen, M., Naatanen, R. & Tervaniemi, M. The sound of music: differentiating musicians using a fast, musical multi-feature mismatch negativity paradigm. Neuropsychologia 50 , 1432–1443 (2012).
Altenmüller, E. O. How many music centers are in the brain? Ann. N. Y. Acad. Sci. 930 , 273–280 (2001).
Monelle, R. Linguistics and Semiotics in Music (Harwood Academic Publishers, 1992).
Rohrmeier, M. A. & Koelsch, S. Predictive information processing in music cognition. A critical review. Int. J. Psychophysiol. 83 , 164–175 (2012).
Vuust, P., Dietz, M. J., Witek, M. & Kringelbach, M. L. Now you hear it: a predictive coding model for understanding rhythmic incongruity. Ann. N. Y. Acad. Sci. https://doi.org/10.1111/nyas.13622 (2018).
Vuust, P., Ostergaard, L., Pallesen, K. J., Bailey, C. & Roepstorff, A. Predictive coding of music–brain responses to rhythmic incongruity. Cortex 45 , 80–92 (2009).
Vuust, P. & Frith, C. Anticipation is the key to understanding music and the effects of music on emotion. Behav. Brain Res. 31 , 599–600 (2008). This is the foundation for the PCM model used in this Review .
Google Scholar
Garrido, M. I., Sahani, M. & Dolan, R. J. Outlier responses reflect sensitivity to statistical structure in the human brain. PLoS Comput. Biol. 9 , e1002999 (2013).
Article CAS PubMed PubMed Central Google Scholar
Lumaca, M., Baggio, G., Brattico, E., Haumann, N. T. & Vuust, P. From random to regular: neural constraints on the emergence of isochronous rhythm during cultural transmission. Soc. Cogn. Affect. Neurosci. 13 , 877–888 (2018).
Quiroga-Martinez, D. R. et al. Musical prediction error responses similarly reduced by predictive uncertainty in musicians and non-musicians. Eur. J. Neurosci. https://doi.org/10.1111/ejn.14667 (2019).
Article Google Scholar
Koelsch, S., Schröger, E. & Gunter, T. C. Music matters: preattentive musicality of the human brain. Psychophysiology 39 , 38–48 (2002).
Koelsch, S., Schmidt, B.-h & Kansok, J. Effects of musical expertise on the early right anterior negativity: an event-related brain potential study. Psychophysiology 39 , 657–663 (2002).
Lumaca, M., Dietz, M. J., Hansen, N. C., Quiroga-Martinez, D. R. & Vuust, P. Perceptual learning of tone patterns changes the effective connectivity between Heschl’s gyrus and planum temporale. Hum. Brain Mapp. 42 , 941–952 (2020).
Lieder, F., Daunizeau, J., Garrido, M. I., Friston, K. J. & Stephan, K. E. Modelling trial-by-trial changes in the mismatch negativity. PLoS Comput. Biol. 9 , e1002911 (2013).
Wacongne, C., Changeux, J. P. & Dehaene, S. A neuronal model of predictive coding accounting for the mismatch negativity. J. Neurosci. 32 , 3665–3678 (2012).
Kiebel, S. J., Garrido, M. I. & Friston, K. J. Dynamic causal modelling of evoked responses: the role of intrinsic connections. Neuroimage 36 , 332–345 (2007).
Feldman, H. & Friston, K. J. Attention, uncertainty, and free-energy. Front. Hum. Neurosci. 4 , 215 (2010).
Cheung, V. K. M. et al. Uncertainty and surprise jointly predict musical pleasure and amygdala, hippocampus, and auditory cortex activity. Curr. Biol. 29 , 4084–4092 e4084 (2019). This fMRI study ties uncertainty and surprise to musical pleasure .
McDermott, J. H. & Oxenham, A. J. Music perception, pitch, and the auditory system. Curr. Opin. Neurobiol. 18 , 452–463 (2008).
Thoret, E., Caramiaux, B., Depalle, P. & McAdams, S. Learning metrics on spectrotemporal modulations reveals the perception of musical instrument timbre. Nat. Hum. Behav. 5 , 369–377 (2020).
Siedenburg, K. & McAdams, S. Four distinctions for the auditory “wastebasket” of timbre. Front. Psychol. 8 , 1747 (2017).
Bendor, D. & Wang, X. The neuronal representation of pitch in primate auditory cortex. Nature 436 , 1161–1165 (2005).
Zatorre, R. J. Pitch perception of complex tones and human temporal-lobe function. J. Acoustical Soc. Am. 84 , 566–572 (1988).
Article CAS Google Scholar
Warren, J. D., Uppenkamp, S., Patterson, R. D. & Griffiths, T. D. Separating pitch chroma and pitch height in the human brain. Proc. Natl Acad. Sci. USA 100 , 10038–10042 (2003). Using fMRI data, this study shows that pitch chroma is represented anterior to the primary auditory cortex, and pitch height is represented posterior to the primary auditory cortex .
Rauschecker, J. P. & Scott, S. K. Maps and streams in the auditory cortex: nonhuman primates illuminate human speech processing. Nat. Neurosci. 12 , 718–724 (2009).
Leaver, A. M., Van Lare, J., Zielinski, B., Halpern, A. R. & Rauschecker, J. P. Brain activation during anticipation of sound sequences. J. Neurosci. 29 , 2477–2485 (2009).
Houde, J. F. & Chang, E. F. The cortical computations underlying feedback control in vocal production. Curr. Opin. Neurobiol. 33 , 174–181 (2015).
Lee, Y. S., Janata, P., Frost, C., Hanke, M. & Granger, R. Investigation of melodic contour processing in the brain using multivariate pattern-based fMRI. Neuroimage 57 , 293–300 (2011).
Janata, P. et al. The cortical topography of tonal structures underlying Western music. Science 298 , 2167–2170 (2002).
Saffran, J. R., Aslin, R. N. & Newport, E. L. Statistical learning by 8-month-old infants. Science 274 , 1926–1928 (1996).
Saffran, J. R., Johnson, E. K., Aslin, R. N. & Newport, E. L. Statistical learning of tone sequences by human infants and adults. Cognition 70 , 27–52 (1999).
Krumhansl, C. L. Perceptual structures for tonal music. Music. Percept. 1 , 28–62 (1983).
Margulis, E. H. A model of melodic expectation. Music. Percept. 22 , 663–714 (2005).
Temperley, D. A probabilistic model of melody perception. Cogn. Sci. 32 , 418–444 (2008).
Pearce, M. T. & Wiggins, G. A. Auditory expectation: the information dynamics of music perception and cognition. Top. Cogn. Sci. 4 , 625–652 (2012).
Sears, D. R. W., Pearce, M. T., Caplin, W. E. & McAdams, S. Simulating melodic and harmonic expectations for tonal cadences using probabilistic models. J. N. Music. Res. 47 , 29–52 (2018).
Näätänen, R., Gaillard, A. W. & Mäntysalo, S. Early selective-attention effect on evoked potential reinterpreted. Acta Psychol. 42 , 313–329 (1978).
Näätänen, R., Paavilainen, P., Rinne, T. & Alho, K. The mismatch negativity (MMN) in basic research of central auditory processing: a review. Clin. Neurophysiol. 118 , 2544–2590 (2007). This classic review covers three decades of MMN research to understand auditory perception .
Wallentin, M., Nielsen, A. H., Friis-Olivarius, M., Vuust, C. & Vuust, P. The Musical Ear Test, a new reliable test for measuring musical competence. Learn. Individ. Differ. 20 , 188–196 (2010).
Tervaniemi, M. et al. Top-down modulation of auditory processing: effects of sound context, musical expertise and attentional focus. Eur. J. Neurosci. 30 , 1636–1642 (2009).
Burunat, I. et al. The reliability of continuous brain responses during naturalistic listening to music. Neuroimage 124 , 224–231 (2016).
Burunat, I. et al. Action in perception: prominent visuo-motor functional symmetry in musicians during music listening. PLoS ONE 10 , e0138238 (2015).
Article PubMed PubMed Central CAS Google Scholar
Alluri, V. et al. Large-scale brain networks emerge from dynamic processing of musical timbre, key and rhythm. Neuroimage 59 , 3677–3689 (2012). A free-listening fMRI study showing brain networks involved in perception of distinct acoustical features of music .
Halpern, A. R. & Zatorre, R. J. When that tune runs through your head: a PET investigation of auditory imagery for familiar melodies. Cereb. Cortex 9 , 697–704 (1999).
Herholz, S. C., Halpern, A. R. & Zatorre, R. J. Neuronal correlates of perception, imagery, and memory for familiar tunes. J. Cogn. Neurosci. 24 , 1382–1397 (2012).
Pallesen, K. J. et al. Emotion processing of major, minor, and dissonant chords: a functional magnetic resonance imaging study. Ann. N. Y. Acad. Sci. 1060 , 450–453 (2005).
McPherson, M. J. et al. Perceptual fusion of musical notes by native Amazonians suggests universal representations of musical intervals. Nat. Commun. 11 , 2786 (2020).
Helmholtz H. L. F. On the Sensations of Tone as a Physiological Basis for the Theory of Music (Cambridge Univ. Press, 1954).
Vassilakis, P. N. & Kendall, R. A. in Human Vision and Electronic Imaging XV . 75270O (International Society for Optics and Photonics, 2010).
Plomp, R. & Levelt, W. J. M. Tonal consonance and critical bandwidth. J. Acoustical Soc. Am. 38 , 548–560 (1965).
McDermott, J. H., Schultz, A. F., Undurraga, E. A. & Godoy, R. A. Indifference to dissonance in native Amazonians reveals cultural variation in music perception. Nature 535 , 547–550 (2016). An ethnomusicology study showing that consonance preference may be absent in people with minimal exposure to Western music .
Mehr, S. A. et al. Universality and diversity in human song. Science https://doi.org/10.1126/science.aax0868 (2019).
Patel, A. D., Gibson, E., Ratner, J., Besson, M. & Holcomb, P. J. Processing syntactic relations in language and music: an event-related potential study. J. Cogn. Neurosci. 10 , 717–733 (1998). This classic study compares responses to syntactic incongruities in both language and Western tonal music .
Janata, P. The neural architecture of music-evoked autobiographical memories. Cereb. Cortex 19 , 2579–2594 (2009).
Maess, B., Koelsch, S., Gunter, T. C. & Friederici, A. D. Musical syntax is processed in Broca’s area: an MEG study. Nat. Neurosci. 4 , 540–545 (2001).
Koelsch, S. et al. Differentiating ERAN and MMN: an ERP study. Neuroreport 12 , 1385–1389 (2001). Using EEG, the authors show that ERAN and MMN reflect different cognitive mechanisms .
Loui, P., Grent-‘t-Jong, T., Torpey, D. & Woldorff, M. Effects of attention on the neural processing of harmonic syntax in Western music. Cogn. Brain Res. 25 , 678–687 (2005).
Koelsch, S., Fritz, T., Schulze, K., Alsop, D. & Schlaug, G. Adults and children processing music: an fMRI study. Neuroimage 25 , 1068–1076 (2005).
Tillmann, B., Janata, P. & Bharucha, J. J. Activation of the inferior frontal cortex in musical priming. Ann. N. Y. Acad. Sci. 999 , 209–211 (2003).
Garza-Villarreal, E. A., Brattico, E., Leino, S., Ostergaard, L. & Vuust, P. Distinct neural responses to chord violations: a multiple source analysis study. Brain Res. 1389 , 103–114 (2011).
Leino, S., Brattico, E., Tervaniemi, M. & Vuust, P. Representation of harmony rules in the human brain: further evidence from event-related potentials. Brain Res. 1142 , 169–177 (2007).
Sammler, D. et al. Co-localizing linguistic and musical syntax with intracranial EEG. Neuroimage 64 , 134–146 (2013).
Loui, P., Wessel, D. L. & Hudson Kam, C. L. Humans rapidly learn grammatical structure in a new musical scale. Music. Percept. 27 , 377–388 (2010).
Loui, P., Wu, E. H., Wessel, D. L. & Knight, R. T. A generalized mechanism for perception of pitch patterns. J. Neurosci. 29 , 454–459 (2009).
Cheung, V. K. M., Meyer, L., Friederici, A. D. & Koelsch, S. The right inferior frontal gyrus processes nested non-local dependencies in music. Sci. Rep. 8 , 3822 (2018).
Haueisen, J. & Knosche, T. R. Involuntary motor activity in pianists evoked by music perception. J. Cogn. Neurosci. 13 , 786–792 (2001).
Bangert, M. et al. Shared networks for auditory and motor processing in professional pianists: evidence from fMRI conjunction. Neuroimage 30 , 917–926 (2006).
Baumann, S. et al. A network for audio-motor coordination in skilled pianists and non-musicians. Brain Res. 1161 , 65–78 (2007).
Lahav, A., Saltzman, E. & Schlaug, G. Action representation of sound: audiomotor recognition network while listening to newly acquired actions. J. Neurosci. 27 , 308–314 (2007).
Bianco, R. et al. Neural networks for harmonic structure in music perception and action. Neuroimage 142 , 454–464 (2016).
Eerola, T., Vuoskoski, J. K., Peltola, H.-R., Putkinen, V. & Schäfer, K. An integrative review of the enjoyment of sadness associated with music. Phys. Life Rev. 25 , 100–121 (2018).
Huron, D. M. D. The harmonic minor scale provides an optimum way of reducing average melodic interval size, consistent with sad affect cues. Empir. Musicol. Rev. 7 , 15 (2012).
Huron, D. A comparison of average pitch height and interval size in major-and minor-key themes: evidence consistent with affect-related pitch prosody. 3 , 59-63 (2008).
Juslin, P. N. & Laukka, P. Communication of emotions in vocal expression and music performance: different channels, same code? Psychol. Bull. 129 , 770 (2003).
Fritz, T. et al. Universal recognition of three basic emotions in music. Curr. Biol. 19 , 573–576 (2009).
London, J. Hearing in Time: Psychological Aspects of Musical Meter (Oxford Univ. Press, 2012).
Honing, H. Without it no music: beat induction as a fundamental musical trait. Ann. N. Y. Acad. Sci. 1252 , 85–91 (2012).
Hickok, G., Farahbod, H. & Saberi, K. The rhythm of perception: entrainment to acoustic rhythms induces subsequent perceptual oscillation. Psychol. Sci. 26 , 1006–1013 (2015).
Yabe, H., Tervaniemi, M., Reinikainen, K. & Näätänen, R. Temporal window of integration revealed by MMN to sound omission. Neuroreport 8 , 1971–1974 (1997).
Andreou, L.-V., Griffiths, T. D. & Chait, M. Sensitivity to the temporal structure of rapid sound sequences — an MEG study. Neuroimage 110 , 194–204 (2015).
Jongsma, M. L., Meeuwissen, E., Vos, P. G. & Maes, R. Rhythm perception: speeding up or slowing down affects different subcomponents of the ERP P3 complex. Biol. Psychol. 75 , 219–228 (2007).
Graber, E. & Fujioka, T. Endogenous expectations for sequence continuation after auditory beat accelerations and decelerations revealed by P3a and induced beta-band responses. Neuroscience 413 , 11–21 (2019).
Brochard, R., Abecasis, D., Potter, D., Ragot, R. & Drake, C. The “ticktock” of our internal clock: direct brain evidence of subjective accents in isochronous sequences. Psychol. Sci. 14 , 362–366 (2003).
Lerdahl, F. & Jackendoff, R. An overview of hierarchical structure in music. Music. Percept. 1 , 229–252 (1983).
Large, E. W. & Kolen, J. F. Resonance and the perception of musical meter. Connect. Sci. 6 , 177–208 (1994).
Large, E. W. & Jones, M. R. The dynamics of attending: how people track time-varying events. Psychol. Rev. 106 , 119–159 (1999).
Cutietta, R. A. & Booth, G. D. The influence of metre, mode, interval type and contour in repeated melodic free-recall. Psychol. Music 24 , 222–236 (1996).
Smith, K. C. & Cuddy, L. L. Effects of metric and harmonic rhythm on the detection of pitch alterations in melodic sequences. J. Exp. Psychol. 15 , 457–471 (1989).
CAS Google Scholar
Palmer, C. & Krumhansl, C. L. Mental representations for musical meter. J. Exp. Psychol. 16 , 728–741 (1990).
Einarson, K. M. & Trainor, L. J. Hearing the beat: young children’s perceptual sensitivity to beat alignment varies according to metric structure. Music. Percept. 34 , 56–70 (2016).
Large, E. W., Herrera, J. A. & Velasco, M. J. Neural networks for beat perception in musical rhythm. Front. Syst. Neurosci. 9 , 159 (2015).
Nozaradan, S., Peretz, I., Missal, M. & Mouraux, A. Tagging the neuronal entrainment to beat and meter. J. Neurosci. 31 , 10234–10240 (2011).
Nozaradan, S., Peretz, I. & Mouraux, A. Selective neuronal entrainment to the beat and meter embedded in a musical rhythm. J. Neurosci. 32 , 17572–17581 (2012).
Nozaradan, S., Schonwiesner, M., Keller, P. E., Lenc, T. & Lehmann, A. Neural bases of rhythmic entrainment in humans: critical transformation between cortical and lower-level representations of auditory rhythm. Eur. J. Neurosci. 47 , 321–332 (2018).
Lenc, T., Keller, P. E., Varlet, M. & Nozaradan, S. Neural and behavioral evidence for frequency-selective context effects in rhythm processing in humans. Cereb. Cortex Commun. https://doi.org/10.1093/texcom/tgaa037 (2020).
Jacoby, N. & McDermott, J. H. Integer ratio priors on musical rhythm revealed cross-culturally by iterated reproduction. Curr. Biol. 27 , 359–370 (2017).
Hannon, E. E. & Trehub, S. E. Metrical categories in infancy and adulthood. Psychol. Sci. 16 , 48–55 (2005).
Hannon, E. E. & Trehub, S. E. Tuning in to musical rhythms: infants learn more readily than adults. Proc. Natl Acad. Sci. USA 102 , 12639–12643 (2005).
Vuust, P. et al. To musicians, the message is in the meter pre-attentive neuronal responses to incongruent rhythm are left-lateralized in musicians. Neuroimage 24 , 560–564 (2005).
Grahn, J. A. & Brett, M. Rhythm and beat perception in motor areas of the brain. J. Cogn. Neurosci. 19 , 893–906 (2007). This fMRI study investigates participants listening to rhythms of varied complexity .
Toiviainen, P., Burunat, I., Brattico, E., Vuust, P. & Alluri, V. The chronnectome of musical beat. Neuroimage 216 , 116191 (2019).
Chen, J. L., Penhune, V. B. & Zatorre, R. J. Moving on time: brain network for auditory-motor synchronization is modulated by rhythm complexity and musical training. J. Cogn. Neurosci. 20 , 226–239 (2008).
Levitin, D. J., Grahn, J. A. & London, J. The psychology of music: rhythm and movement. Annu. Rev. Psychol. 69 , 51–75 (2018).
Winkler, I., Haden, G. P., Ladinig, O., Sziller, I. & Honing, H. Newborn infants detect the beat in music. Proc. Natl Acad. Sci. USA 106 , 2468–2471 (2009).
Phillips-Silver, J. & Trainor, L. J. Feeling the beat: movement influences infant rhythm perception. Science 308 , 1430–1430 (2005).
Cirelli, L. K., Trehub, S. E. & Trainor, L. J. Rhythm and melody as social signals for infants. Ann. N. Y. Acad. Sci. https://doi.org/10.1111/nyas.13580 (2018).
Cirelli, L. K., Einarson, K. M. & Trainor, L. J. Interpersonal synchrony increases prosocial behavior in infants. Dev. Sci. 17 , 1003–1011 (2014).
Repp, B. H. Sensorimotor synchronization: a review of the tapping literature. Psychon. Bull. Rev. 12 , 969–992 (2005).
Repp, B. H. & Su, Y. H. Sensorimotor synchronization: a review of recent research (2006-2012). Psychon. Bull. Rev. 20 , 403–452 (2013). This review, and Repp (2005), succinctly covers the field of sensorimotor synchronization .
Zarco, W., Merchant, H., Prado, L. & Mendez, J. C. Subsecond timing in primates: comparison of interval production between human subjects and rhesus monkeys. J. Neurophysiol. 102 , 3191–3202 (2009).
Honing, H., Bouwer, F. L., Prado, L. & Merchant, H. Rhesus monkeys ( Macaca mulatta ) sense isochrony in rhythm, but not the beat: additional support for the gradual audiomotor evolution hypothesis. Front. Neurosci. 12 , 475 (2018).
Hattori, Y. & Tomonaga, M. Rhythmic swaying induced by sound in chimpanzees (Pan troglodytes). Proc. Natl Acad. Sci. USA 117 , 936–942 (2020).
Danielsen, A. Presence and Pleasure. The Funk Grooves of James Brown and Parliament (Wesleyan Univ. Press, 2006).
Madison, G., Gouyon, F., Ullen, F. & Hornstrom, K. Modeling the tendency for music to induce movement in humans: first correlations with low-level audio descriptors across music genres. J. Exp. Psychol. Hum. Percept. Perform. 37 , 1578–1594 (2011).
Stupacher, J., Hove, M. J., Novembre, G., Schutz-Bosbach, S. & Keller, P. E. Musical groove modulates motor cortex excitability: a TMS investigation. Brain Cogn. 82 , 127–136 (2013).
Janata, P., Tomic, S. T. & Haberman, J. M. Sensorimotor coupling in music and the psychology of the groove. J. Exp. Psychol. 141 , 54 (2012). Using a systematic approach, this multiple-studies article shows that the concept of groove can be widely understood as a pleasurable drive towards action .
Witek, M. A. et al. A critical cross-cultural study of sensorimotor and groove responses to syncopation among Ghanaian and American university students and staff. Music. Percept. 37 , 278–297 (2020).
Friston, K., Mattout, J. & Kilner, J. Action understanding and active inference. Biol. Cybern. 104 , 137–160 (2011).
Longuet-Higgins, H. C. & Lee, C. S. The rhythmic interpretation of monophonic music. Music. Percept. 1 , 18 (1984).
Sioros, G., Miron, M., Davies, M., Gouyon, F. & Madison, G. Syncopation creates the sensation of groove in synthesized music examples. Front. Psychol. 5 , 1036 (2014).
Witek, M. A., Clarke, E. F., Wallentin, M., Kringelbach, M. L. & Vuust, P. Syncopation, body-movement and pleasure in groove music. PLoS ONE 9 , e94446 (2014).
Kowalewski, D. A., Kratzer, T. M. & Friedman, R. S. Social music: investigating the link between personal liking and perceived groove. Music. Percept. 37 , 339–346 (2020).
Bowling, D. L., Ancochea, P. G., Hove, M. J. & Tecumseh Fitch, W. Pupillometry of groove: evidence for noradrenergic arousal in the link between music and movement. Front. Neurosci. 13 , 1039 (2019).
Matthews, T. E., Witek, M. A. G., Heggli, O. A., Penhune, V. B. & Vuust, P. The sensation of groove is affected by the interaction of rhythmic and harmonic complexity. PLoS ONE 14 , e0204539 (2019).
Matthews, T. E., Witek, M. A., Lund, T., Vuust, P. & Penhune, V. B. The sensation of groove engages motor and reward networks. Neuroimage 214 , 116768 (2020). This fMRI study shows that the sensation of groove engages both motor and reward networks in the brain .
Vaquero, L., Ramos-Escobar, N., François, C., Penhune, V. & Rodríguez-Fornells, A. White-matter structural connectivity predicts short-term melody and rhythm learning in non-musicians. Neuroimage 181 , 252–262 (2018).
Zatorre, R. J., Halpern, A. R., Perry, D. W., Meyer, E. & Evans, A. C. Hearing in the mind’s ear: a PET investigation of musical imagery and perception. J. Cogn. Neurosci. 8 , 29–46 (1996).
Benadon, F. Meter isn’t everything: the case of a timeline-oriented Cuban polyrhythm. N. Ideas Psychol. 56 , 100735 (2020).
London, J., Polak, R. & Jacoby, N. Rhythm histograms and musical meter: a corpus study of Malian percussion music. Psychon. Bull. Rev. 24 , 474–480 (2017).
Huron, D. Is music an evolutionary adaptation? Ann. N. Y. Acad. Sci. 930 , 43–61 (2001).
Koelsch, S. Towards a neural basis of music-evoked emotions. Trends Cogn. Sci. 14 , 131–137 (2010).
Eerola, T. & Vuoskoski, J. K. A comparison of the discrete and dimensional models of emotion in music. Psychol. Music. 39 , 18–49 (2010).
Lonsdale, A. J. & North, A. C. Why do we listen to music? A uses and gratifications analysis. Br. J. Psychol. 102 , 108–134 (2011).
Juslin, P. N. & Laukka, P. Expression, perception, and induction of musical emotions: a review and a questionnaire study of everyday listening. J. N. Music. Res. 33 , 217–238 (2004).
Huron, D. Why is sad music pleasurable? A possible role for prolactin. Music. Sci. 15 , 146–158 (2011).
Brattico, E. et al. It’s sad but I like it: the neural dissociation between musical emotions and liking in experts and laypersons. Front. Hum. Neurosci. 9 , 676 (2015).
PubMed Google Scholar
Sachs, M. E., Damasio, A. & Habibi, A. Unique personality profiles predict when and why sad music is enjoyed. Psychol. Music https://doi.org/10.1177/0305735620932660 (2020).
Sachs, M. E., Habibi, A., Damasio, A. & Kaplan, J. T. Dynamic intersubject neural synchronization reflects affective responses to sad music. Neuroimage 218 , 116512 (2020).
Juslin, P. N. & Vastfjall, D. Emotional responses to music: the need to consider underlying mechanisms. Behav. Brain Sci. 31 , 559–575 (2008). Using a novel theoretical framework, the authors propose that the mechanisms that evoke emotions from music are not unique to music .
Rickard, N. S. Intense emotional responses to music: a test of the physiological arousal hypothesis. Psychol. Music. 32 , 371–388 (2004).
Cowen, A. S., Fang, X., Sauter, D. & Keltner, D. What music makes us feel: at least 13 dimensions organize subjective experiences associated with music across different cultures. Proc. Natl Acad. Sci. USA 117 , 1924–1934 (2020).
Argstatter, H. Perception of basic emotions in music: culture-specific or multicultural? Psychol. Music. 44 , 674–690 (2016).
Stevens, C. J. Music perception and cognition: a review of recent cross-cultural research. Top. Cogn. Sci. 4 , 653–667 (2012).
Pearce, M. Cultural distance: a computational approach to exploring cultural influences on music cognition. in Oxford Handbook of Music and the Brain Vol. 31 (Oxford Univ. Press, 2018).
van der Weij, B., Pearce, M. T. & Honing, H. A probabilistic model of meter perception: simulating enculturation. Front. Psychol. 8 , 824 (2017).
Kringelbach, M. L. & Berridge, K. C. Towards a functional neuroanatomy of pleasure and happiness. Trends Cogn. Sci. 13 , 479–487 (2009).
Blood, A. J. & Zatorre, R. J. Intensely pleasurable responses to music correlate with activity in brain regions implicated in reward and emotion. Proc. Natl Acad. Sci. USA 98 , 11818–11823 (2001). This seminal positron emission tomography study shows that the experience of musical chills correlates with activity in the reward system .
Salimpoor, V. N. & Zatorre, R. J. Complex cognitive functions underlie aesthetic emotions: comment on “From everyday emotions to aesthetic emotions: towards a unified theory of musical emotions” by Patrik N. Juslin. Phys. Life Rev. 10 , 279–280 (2013).
Salimpoor, V. N. et al. Interactions between the nucleus accumbens and auditory cortices predict music reward value. Science 340 , 216–219 (2013).
Salimpoor, V. N., Benovoy, M., Larcher, K., Dagher, A. & Zatorre, R. J. Anatomically distinct dopamine release during anticipation and experience of peak emotion to music. Nat. Neurosci. 14 , 257–262 (2011).
Salimpoor, V. N., Benovoy, M., Longo, G., Cooperstock, J. R. & Zatorre, R. J. The rewarding aspects of music listening are related to degree of emotional arousal. PLoS ONE 4 , e7487 (2009).
Mas-Herrero, E., Zatorre, R. J., Rodriguez-Fornells, A. & Marco-Pallares, J. Dissociation between musical and monetary reward responses in specific musical anhedonia. Curr. Biol. 24 , 699–704 (2014).
Martinez-Molina, N., Mas-Herrero, E., Rodriguez-Fornells, A., Zatorre, R. J. & Marco-Pallares, J. Neural correlates of specific musical anhedonia. Proc. Natl Acad. Sci. USA 113 , E7337–E7345 (2016).
Gebauer, L. K., M., L. & Vuust, P. Musical pleasure cycles: the role of anticipation and dopamine. Psychomusicology 22 , 16 (2012).
Shany, O. et al. Surprise-related activation in the nucleus accumbens interacts with music-induced pleasantness. Soc. Cogn. Affect. Neurosci. 14 , 459–470 (2019).
Gold, B. P., Pearce, M. T., Mas-Herrero, E., Dagher, A. & Zatorre, R. J. Predictability and uncertainty in the pleasure of music: a reward for learning? J. Neurosci. 39 , 9397–9409 (2019).
Swaminathan, S. & Schellenberg, E. G. Current emotion research in music psychology. Emot. Rev. 7 , 189–197 (2015).
Madison, G. & Schiölde, G. Repeated listening increases the liking for music regardless of its complexity: implications for the appreciation and aesthetics of music. Front. Neurosci. 11 , 147 (2017).
Corrigall, K. A. & Schellenberg, E. G. Liking music: genres, contextual factors, and individual differences. in Art, Aesthetics, and the Brain (Oxford Univ. Press, 2015).
Zentner, A. Measuring the effect of file sharing on music purchases. J. Law Econ. 49 , 63–90 (2006).
Rentfrow, P. J. & Gosling, S. D. The do re mi’s of everyday life: the structure and personality correlates of music preferences. J. Pers. Soc. Psychol. 84 , 1236–1256 (2003).
Vuust, P. et al. Personality influences career choice: sensation seeking in professional musicians. Music. Educ. Res. 12 , 219–230 (2010).
Rohrmeier, M. & Rebuschat, P. Implicit learning and acquisition of music. Top. Cogn. Sci. 4 , 525–553 (2012).
Münthe, T. F., Altenmüller, E. & Jäncke, L. The musician’s brain as a model of neuroplasticity. Nat. Rev. Neurosci. 3 , 1–6 (2002). This review highlights how professional musicians represent an ideal model for investigating neuroplasticity .
Habibi, A. et al. Childhood music training induces change in micro and macroscopic brain structure: results from a longitudinal study. Cereb. Cortex 28 , 4336–4347 (2018).
Schlaug, G., Jancke, L., Huang, Y., Staiger, J. F. & Steinmetz, H. Increased corpus callosum size in musicians. Neuropsychologia 33 , 1047–1055 (1995).
Baer, L. H. et al. Regional cerebellar volumes are related to early musical training and finger tapping performance. Neuroimage 109 , 130–139 (2015).
Kleber, B. et al. Voxel-based morphometry in opera singers: increased gray-matter volume in right somatosensory and auditory cortices. Neuroimage 133 , 477–483 (2016).
Gaser, C. & Schlaug, G. Brain structures differ between musicians and non-musicians. J. Neurosci. 23 , 9240–9245 (2003). Using a morphometric technique, this study shows a grey matter volume difference in multiple brain regions between professional musicians and a matched control group of amateur musicians and non-musicians .
Sluming, V. et al. Voxel-based morphometry reveals increased gray matter density in Broca’s area in male symphony orchestra musicians. Neuroimage 17 , 1613–1622 (2002).
Palomar-García, M.-Á., Zatorre, R. J., Ventura-Campos, N., Bueichekú, E. & Ávila, C. Modulation of functional connectivity in auditory–motor networks in musicians compared with nonmusicians. Cereb. Cortex 27 , 2768–2778 (2017).
Schneider, P. et al. Morphology of Heschl’s gyrus reflects enhanced activation in the auditory cortex of musicians. Nat. Neurosci. 5 , 688–694 (2002).
Bengtsson, S. L. et al. Extensive piano practicing has regionally specific effects on white matter development. Nat. Neurosci. 8 , 1148–1150 (2005).
Zamorano, A. M., Cifre, I., Montoya, P., Riquelme, I. & Kleber, B. Insula-based networks in professional musicians: evidence for increased functional connectivity during resting state fMRI. Hum. Brain Mapp. 38 , 4834–4849 (2017).
Kraus, N. & Chandrasekaran, B. Music training for the development of auditory skills. Nat. Rev. Neurosci. 11 , 599–605 (2010).
Koelsch, S., Schröger, E. & Tervaniemi, M. Superior pre-attentive auditory processing in musicians. Neuroreport 10 , 1309–1313 (1999).
Münte, T. F., Kohlmetz, C., Nager, W. & Altenmüller, E. Superior auditory spatial tuning in conductors. Nature 409 , 580 (2001).
Seppänen, M., Brattico, E. & Tervaniemi, M. Practice strategies of musicians modulate neural processing and the learning of sound-patterns. Neurobiol. Learn. Mem. 87 , 236–247 (2007).
Guillot, A. et al. Functional neuroanatomical networks associated with expertise in motor imagery. Neuroimage 41 , 1471–1483 (2008).
Bianco, R., Novembre, G., Keller, P. E., Villringer, A. & Sammler, D. Musical genre-dependent behavioural and EEG signatures of action planning. a comparison between classical and jazz pianists. Neuroimage 169 , 383–394 (2018).
Vuust, P., Brattico, E., Seppänen, M., Näätänen, R. & Tervaniemi, M. Practiced musical style shapes auditory skills. Ann. N. Y. Acad. Sci. 1252 , 139–146 (2012).
Bangert, M. & Altenmüller, E. O. Mapping perception to action in piano practice: a longitudinal DC-EEG study. BMC Neurosci. 4 , 26 (2003).
Li, Q. et al. Musical training induces functional and structural auditory-motor network plasticity in young adults. Hum. Brain Mapp. 39 , 2098–2110 (2018).
Herholz, S. C., Coffey, E. B. J., Pantev, C. & Zatorre, R. J. Dissociation of neural networks for predisposition and for training-related plasticity in auditory-motor learning. Cereb. Cortex 26 , 3125–3134 (2016).
Putkinen, V., Tervaniemi, M. & Huotilainen, M. Musical playschool activities are linked to faster auditory development during preschool-age: a longitudinal ERP study. Sci. Rep. 9 , 11310–11310 (2019).
Putkinen, V., Tervaniemi, M., Saarikivi, K., Ojala, P. & Huotilainen, M. Enhanced development of auditory change detection in musically trained school-aged children: a longitudinal event-related potential study. Dev. Sci. 17 , 282–297 (2014).
Jentschke, S. & Koelsch, S. Musical training modulates the development of syntax processing in children. Neuroimage 47 , 735–744 (2009).
Chobert, J., François, C., Velay, J. L. & Besson, M. Twelve months of active musical training in 8-to 10-year-old children enhances the preattentive processing of syllabic duration and voice onset time. Cereb. Cortex 24 , 956–967 (2014).
Moreno, S. et al. Musical training influences linguistic abilities in 8-year-old children: more evidence for brain plasticity. Cereb. Cortex 19 , 712–723 (2009).
Putkinen, V., Huotilainen, M. & Tervaniemi, M. Neural encoding of pitch direction is enhanced in musically trained children and is related to reading skills. Front. Psychol. 10 , 1475 (2019).
Wong, P. C., Skoe, E., Russo, N. M., Dees, T. & Kraus, N. Musical experience shapes human brainstem encoding of linguistic pitch patterns. Nat. Neurosci. 10 , 420–422 (2007).
Virtala, P. & Partanen, E. Can very early music interventions promote at-risk infants’ development? Ann. N. Y. Acad. Sci. 1423 , 92–101 (2018).
Flaugnacco, E. et al. Music training increases phonological awareness and reading skills in developmental dyslexia: a randomized control trial. PLoS ONE 10 , e0138715 (2015).
Fiveash, A. et al. A stimulus-brain coupling analysis of regular and irregular rhythms in adults with dyslexia and controls. Brain Cogn. 140 , 105531 (2020).
Schellenberg, E. G. Correlation = causation? music training, psychology, and neuroscience. Psychol. Aesthet. Creat. Arts 14 , 475–480 (2019).
Sala, G. & Gobet, F. Cognitive and academic benefits of music training with children: a multilevel meta-analysis. Mem. Cogn. 48 , 1429–1441 (2020).
Saffran, J. R. Musical learning and language development. Ann. N. Y. Acad. Sci. 999 , 397–401 (2003).
Friston, K. The free-energy principle: a rough guide to the brain? Trends Cogn. Sci. 13 , 293–301 (2009).
Pearce, M. T. Statistical learning and probabilistic prediction in music cognition: mechanisms of stylistic enculturation. Ann. N. Y. Acad. Sci. 1423 , 378–395 (2018).
Article PubMed Central Google Scholar
Novembre, G., Knoblich, G., Dunne, L. & Keller, P. E. Interpersonal synchrony enhanced through 20 Hz phase-coupled dual brain stimulation. Soc. Cogn. Affect. Neurosci. 12 , 662–670 (2017).
Konvalinka, I. et al. Frontal alpha oscillations distinguish leaders from followers: multivariate decoding of mutually interacting brains. Neuroimage 94C , 79–88 (2014).
Novembre, G., Mitsopoulos, Z. & Keller, P. E. Empathic perspective taking promotes interpersonal coordination through music. Sci. Rep. 9 , 12255 (2019).
Wolpert, D. M., Ghahramani, Z. & Jordan, M. I. An internal model for sensorimotor integration. Science 269 , 1880–1882 (1995).
Patel, A. D. & Iversen, J. R. The evolutionary neuroscience of musical beat perception: the action simulation for auditory prediction (ASAP) hypothesis. Front. Syst. Neurosci. 8 , 57 (2014).
Sebanz, N. & Knoblich, G. Prediction in joint action: what, when, and where. Top. Cogn. Sci. 1 , 353–367 (2009).
Friston, K. J. & Frith, C. D. Active inference, communication and hermeneutics. Cortex 68 , 129–143 (2015). This article proposes a link between active inference, communication and hermeneutics .
Konvalinka, I., Vuust, P., Roepstorff, A. & Frith, C. D. Follow you, follow me: continuous mutual prediction and adaptation in joint tapping. Q. J. Exp. Psychol. 63 , 2220–2230 (2010).
Wing, A. M. & Kristofferson, A. B. Response delays and the timing of discrete motor responses. Percept. Psychophys. 14 , 5–12 (1973).
Repp, B. H. & Keller, P. E. Sensorimotor synchronization with adaptively timed sequences. Hum. Mov. Sci. 27 , 423–456 (2008).
Vorberg, D. & Schulze, H.-H. Linear phase-correction in synchronization: predictions, parameter estimation, and simulations. J. Math. Psychol. 46 , 56–87 (2002).
Novembre, G., Sammler, D. & Keller, P. E. Neural alpha oscillations index the balance between self-other integration and segregation in real-time joint action. Neuropsychologia 89 , 414–425 (2016). Using dual-EEG, the authors propose alpha oscillations as a candidate for regulating the balance between internal and external information in joint action .
Keller, P. E., Knoblich, G. & Repp, B. H. Pianists duet better when they play with themselves: on the possible role of action simulation in synchronization. Conscious. Cogn. 16 , 102–111 (2007).
Fairhurst, M. T., Janata, P. & Keller, P. E. Leading the follower: an fMRI investigation of dynamic cooperativity and leader-follower strategies in synchronization with an adaptive virtual partner. Neuroimage 84 , 688–697 (2014).
Heggli, O. A., Konvalinka, I., Kringelbach, M. L. & Vuust, P. Musical interaction is influenced by underlying predictive models and musical expertise. Sci. Rep. 9 , 1–13 (2019).
Heggli, O. A., Cabral, J., Konvalinka, I., Vuust, P. & Kringelbach, M. L. A Kuramoto model of self-other integration across interpersonal synchronization strategies. PLoS Comput. Biol. 15 , e1007422 (2019).
Heggli, O. A. et al. Transient brain networks underlying interpersonal strategies during synchronized action. Soc. Cogn. Affect. Neurosci. 16 , 19–30 (2020). This EEG study shows that differences in interpersonal synchronization are reflected by activity in a temporoparietal network .
Patel, A. D. Music, Language, and the Brain (Oxford Univ. Press, 2006).
Molnar-Szakacs, I. & Overy, K. Music and mirror neurons: from motion to ‘e’motion. Soc. Cogn. Affect. Neurosci. 1 , 235–241 (2006).
Beaty, R. E., Benedek, M., Silvia, P. J. & Schacter, D. L. Creative cognition and brain network dynamics. Trends Cogn. Sci. 20 , 87–95 (2016).
Limb, C. J. & Braun, A. R. Neural substrates of spontaneous musical performance: an FMRI study of jazz improvisation. PLoS ONE 3 , e1679 (2008).
Liu, S. et al. Neural correlates of lyrical improvisation: an FMRI study of freestyle rap. Sci. Rep. 2 , 834 (2012).
Rosen, D. S. et al. Dual-process contributions to creativity in jazz improvisations: an SPM-EEG study. Neuroimage 213 , 116632 (2020).
Boasen, J., Takeshita, Y., Kuriki, S. & Yokosawa, K. Spectral-spatial differentiation of brain activity during mental imagery of improvisational music performance using MEG. Front. Hum. Neurosci. 12 , 156 (2018).
Berkowitz, A. L. & Ansari, D. Generation of novel motor sequences: the neural correlates of musical improvisation. Neuroimage 41 , 535–543 (2008).
Loui, P. Rapid and flexible creativity in musical improvisation: review and a model. Ann. N. Y. Acad. Sci. 1423 , 138–145 (2018).
Beaty, R. E. The neuroscience of musical improvisation. Neurosci. Biobehav. Rev. 51 , 108–117 (2015).
Vuust, P. & Kringelbach, M. L. Music improvisation: a challenge for empirical research. in Routledge Companion to Music Cognition (Routledge, 2017).
Norgaard, M. Descriptions of improvisational thinking by artist-level jazz musicians. J. Res. Music. Educ. 59 , 109–127 (2011).
Kringelbach, M. L. & Deco, G. Brain states and transitions: insights from computational neuroscience. Cell Rep. 32 , 108128 (2020).
Deco, G. & Kringelbach, M. L. Hierarchy of information processing in the brain: a novel ‘intrinsic ignition’ framework. Neuron 94 , 961–968 (2017).
Pinho, A. L., de Manzano, O., Fransson, P., Eriksson, H. & Ullen, F. Connecting to create: expertise in musical improvisation is associated with increased functional connectivity between premotor and prefrontal areas. J. Neurosci. 34 , 6156–6163 (2014).
Pinho, A. L., Ullen, F., Castelo-Branco, M., Fransson, P. & de Manzano, O. Addressing a paradox: dual strategies for creative performance in introspective and extrospective networks. Cereb. Cortex 26 , 3052–3063 (2016).
de Manzano, O. & Ullen, F. Activation and connectivity patterns of the presupplementary and dorsal premotor areas during free improvisation of melodies and rhythms. Neuroimage 63 , 272–280 (2012).
Beaty, R. E. et al. Robust prediction of individual creative ability from brain functional connectivity. Proc. Natl Acad. Sci. USA 115 , 1087–1092 (2018).
Daikoku, T. Entropy, uncertainty, and the depth of implicit knowledge on musical creativity: computational study of improvisation in melody and rhythm. Front. Comput. Neurosci. 12 , 97 (2018).
Belden, A. et al. Improvising at rest: differentiating jazz and classical music training with resting state functional connectivity. Neuroimage 207 , 116384 (2020).
Arkin, C., Przysinda, E., Pfeifer, C. W., Zeng, T. & Loui, P. Gray matter correlates of creativity in musical improvisation. Front. Hum. Neurosci. 13 , 169 (2019).
Bashwiner, D. M., Wertz, C. J., Flores, R. A. & Jung, R. E. Musical creativity “revealed” in brain structure: interplay between motor, default mode, and limbic networks. Sci. Rep. 6 , 20482 (2016).
Przysinda, E., Zeng, T., Maves, K., Arkin, C. & Loui, P. Jazz musicians reveal role of expectancy in human creativity. Brain Cogn. 119 , 45–53 (2017).
Large, E. W., Kim, J. C., Flaig, N. K., Bharucha, J. J. & Krumhansl, C. L. A neurodynamic account of musical tonality. Music. Percept. 33 , 319–331 (2016).
Large, E. W. & Palmer, C. Perceiving temporal regularity in music. Cogn. Sci. 26 , 1–37 (2002). This article proposes an oscillator-based approach for the perception of temporal regularity in music .
Cannon, J. J. & Patel, A. D. How beat perception co-opts motor neurophysiology. Trends Cogn. Sci. 25 , 137–150 (2020). The authors propose that cyclic time-keeping activity in the supplementary motor area, termed ‘proto-actions’, is organized by the dorsal striatum to support hierarchical metrical structures .
Keller, P. E., Novembre, G. & Loehr, J. Musical ensemble performance: representing self, other and joint action outcomes. in Shared Representations: Sensorimotor Foundations of Social Life Cambridge Social Neuroscience (eds Cross, E. S. & Obhi, S. S.) 280-310 (Cambridge Univ. Press, 2016).
Rao, R. P. & Ballard, D. H. Predictive coding in the visual cortex: a functional interpretation of some extra-classical receptive-field effects. Nat. Neurosci. 2 , 79–87 (1999).
Clark, A. Whatever next? Predictive brains, situated agents, and the future of cognitive science. Behav. Brain Sci. 36 , 181–204 (2013).
Kahl, R. Selected Writings of Hermann Helmholtz (Wesleyan Univ. Press, 1878).
Gregory, R. L. Perceptions as hypotheses. Philos. Trans. R. Soc. Lond. B Biol. Sci. 290 , 181–197 (1980).
Gibson, J. J. The Ecological Approach to Visual Perception (Houghton Mifflin, 1979).
Fuster, J. The Prefrontal Cortex Anatomy, Physiology and Neuropsychology of the Frontal Lobe (Lippincott-Raven, 1997).
Neisser, U. Cognition and Reality: Principles and Implications of Cognitive Psychology (W H Freeman/Times Books/ Henry Holt & Co, 1976).
Arbib, M. A. & Hesse, M. B. The Construction of Reality (Cambridge Univ. Press, 1986).
Cisek, P. & Kalaska, J. F. Neural mechanisms for interacting with a world full of action choices. Annu. Rev. Neurosci. 33 , 269–298 (2010).
Isomura, T., Parr, T. & Friston, K. Bayesian filtering with multiple internal models: toward a theory of social intelligence. Neural Comput. 31 , 2390–2431 (2019).
Friston, K. & Frith, C. A duet for one. Conscious. Cogn. 36 , 390–405 (2015).
Hunt, B. R., Ott, E. & Yorke, J. A. Differentiable generalized synchronization of chaos. Phys. Rev. E 55 , 4029–4034 (1997).
Ghazanfar, A. A. & Takahashi, D. Y. The evolution of speech: vision, rhythm, cooperation. Trends Cogn. Sci. 18 , 543–553 (2014).
Wilson, M. & Wilson, T. P. An oscillator model of the timing of turn-taking. Psychon. Bull. Rev. 12 , 957–968 (2005).
Download references
Acknowledgements
Funding was provided by The Danish National Research Foundation (DNRF117). The authors thank E. Altenmüller and D. Huron for comments on early versions of the manuscript.
Author information
Authors and affiliations.
Center for Music in the Brain, Aarhus University and The Royal Academy of Music (Det Jyske Musikkonservatorium), Aarhus, Denmark
Peter Vuust, Ole A. Heggli & Morten L. Kringelbach
Wellcome Centre for Human Neuroimaging, University College London, London, UK
Karl J. Friston
Department of Psychiatry, University of Oxford, Oxford, UK
Morten L. Kringelbach
Centre for Eudaimonia and Human Flourishing, Linacre College, University of Oxford, Oxford, UK
You can also search for this author in PubMed Google Scholar
Contributions
The authors contributed equally to all aspects of this article.
Corresponding author
Correspondence to Peter Vuust .
Ethics declarations
Competing interests.
The authors declare no competing interests.
Peer review
Peer review information.
Nature Reviews Neuroscience thanks D. Sammler and the other, anonymous, reviewer(s) for their contribution to the peer review of this work.
Additional information
Publisher’s note.
Springer Nature remains neutral with regard to jurisdictional claims in published maps and institutional affiliations.
Patterns of pitched sounds unfolding over time, in accordance with cultural conventions and constraints.
The combination of multiple, simultaneously pitched sounds to form a chord, and subsequent chord progressions, a fundamental building block of Western music. The rules of harmony are the hierarchically organized expectations for chord progressions.
The structured arrangement of successive sound events over time, a primary parameter of musical structure. Rhythm perception is based on the perception of duration and grouping of these events and can be achieved even if sounds are not discrete, such as amplitude-modulated sounds.
Mathematically, the expected values or means of random variables.
The ability to extract statistical regularities from the world to learn about the environment.
In Western music, the organization of melody and harmony in a hierarchy of relations, often pointing towards a referential pitch (the tonal centre or the tonic).
A predictive framework governing the interpretation of regularly recurring patterns and accents in rhythm.
The output of a model generating outcomes from their causes. In predictive coding, the prediction is generated from expected states of the world and compared with observed outcomes to form a prediction error.
The subjective experience accompanying a strong expectation that a particular event will occur.
An enactive generalization of predictive coding that casts both action and perception as minimizing surprise or prediction error (active inference is considered a corollary of the free-energy principle).
A quantity used in predictive coding to denote the difference between an observation or point estimate and its predicted value. Predictive coding uses precision-weighted prediction errors to update expectations that generate predictions.
Expectations of musical events based on prior knowledge of regularities and patterns in musical sequences, such as melodies and chords.
Expectations of specific events or patterns in a familiar musical sequence.
Short-lived expectations that dynamically shift owing to the ongoing musical context, such as when a repeated musical phrase causes the listener to expect similar phrases as the work continues.
The inverse variance or negative entropy of a random variable. It corresponds to a second-order statistic (for example, a second-order moment) of the variable’s probability distribution or density. This can be contrasted with the mean or expectation, which constitutes a first-order statistic (for example, a first-order moment).
(MMN). A component of the auditory event-related potential recorded with electroencephalography or magnetoencephalography related to a change in different sound features such as pitch, timbre, location of the sound source, intensity and rhythm. It peaks approximately 110–250 ms after change onset and is typically recorded while participants’ attention is distracted from the stimulus, usually by watching a silent film or reading a book. The amplitude and latency of the MMN depends on the deviation magnitude, such that larger deviations in the same context yield larger and faster MMN responses.
(fMRI). A neuroimaging technique that images rapid changes in blood oxygenation levels in the brain.
In the realm of contemporary music, a persistently repeated pattern played by the rhythm section (usually drums, percussion, bass, guitar and/or piano). In music psychology, the pleasurable sensation of wanting to move.
The perceptual correlate of periodicity in sounds that allows their ordering on a frequency-related musical scale.
Also known as tone colour or tone quality, the perceived sound quality of a sound, including its spectral composition and its additional noise characteristics.
The pitch class containing all pitches separated by an integer number of octaves. Humans perceive a similarity between notes having the same chroma.
The contextual unexpectedness or surprise associated with an event.
In the Shannon sense, the expected surprise or information content (self-information). In other words, it is the uncertainty or unpredictability of a random variable (for example, an event in the future).
(MEG). A neuroimaging technique that measures the magnetic fields produced by naturally occurring electrical activity in the brain.
A very small electrical voltage generated in the brain structures in response to specific events or stimuli.
Psychologically, consonance is when two or more notes sound together with an absence of perceived roughness. Dissonance is the antonym of consonance. Western listeners consider intervals produced by frequency ratios such as 1:2 (octave), 3:2 (fifth) or 4:3 (fourth) as consonant. Dissonances are intervals produced by frequency ratios formed from numbers greater than 4.
Stereotypical patterns consisting of two or more chords that conclude a phrase, section or piece of music. They are often used to establish a sense of tonality.
(EEG). An electrophysiological method that measures electrical activity of the brain.
A method of analysing steady-state evoked potentials arising from stimulation or aspects of stimulation repeated at a fixed rate. An example of frequency tagging analysis is shown in Fig. 1c .
A shift of rhythmic emphasis from metrically strong accents to weak accents, a characteristic of multiple musical genres, such as funk, jazz and hip hop.
In Aristotelian ethics, refers to a life well lived or human flourishing, and in affective neuroscience, it is often used to describe meaningful pleasure.
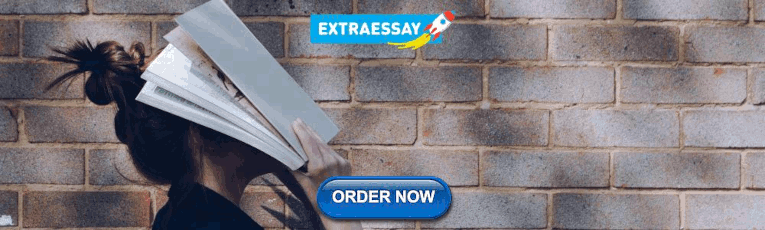
Rights and permissions
Springer Nature or its licensor holds exclusive rights to this article under a publishing agreement with the author(s) or other rightsholder(s); author self-archiving of the accepted manuscript version of this article is solely governed by the terms of such publishing agreement and applicable law.
Reprints and permissions
About this article
Cite this article.
Vuust, P., Heggli, O.A., Friston, K.J. et al. Music in the brain. Nat Rev Neurosci 23 , 287–305 (2022). https://doi.org/10.1038/s41583-022-00578-5
Download citation
Accepted : 22 February 2022
Published : 29 March 2022
Issue Date : May 2022
DOI : https://doi.org/10.1038/s41583-022-00578-5
Share this article
Anyone you share the following link with will be able to read this content:
Sorry, a shareable link is not currently available for this article.
Provided by the Springer Nature SharedIt content-sharing initiative
This article is cited by
Improved emotion differentiation under reduced acoustic variability of speech in autism.
- Mathilde Marie Duville
- Luz María Alonso-Valerdi
- David I. Ibarra-Zarate
BMC Medicine (2024)
Exploring the neural underpinnings of chord prediction uncertainty: an electroencephalography (EEG) study
- Kentaro Ono
- Ryohei Mizuochi
- Shigeto Ymawaki
Scientific Reports (2024)
Effekte von klassischer Musik oder von Heavy Metal bei Mensch und Tier: Implikationen für die Intensivmedizin
- Hans-Joachim Trappe
- Eva-Maria Völkel
- Gerald Reiner
Medizinische Klinik - Intensivmedizin und Notfallmedizin (2024)
Frontal and cerebellar contributions to pitch and rhythm processing: a TMS study
- Silvia Picazio
- Barbara Magnani
- Laura Petrosini
Brain Structure and Function (2024)
Care intervention on psychological outcomes among patients admitted to intensive care unit: an umbrella review of systematic reviews and meta-analyses
- Yafang Zheng
- Lijuan Zhang
- Jingxia Zheng
Systematic Reviews (2023)
Quick links
- Explore articles by subject
- Guide to authors
- Editorial policies
Sign up for the Nature Briefing newsletter — what matters in science, free to your inbox daily.

Music mood and human emotion recognition based on physiological signals: a systematic review
- Regular Paper
- Published: 22 April 2021
- Volume 28 , pages 21–44, ( 2022 )
Cite this article
- Vybhav Chaturvedi 1 ,
- Arman Beer Kaur 1 ,
- Vedansh Varshney 1 ,
- Anupam Garg 1 ,
- Gurpal Singh Chhabra 1 &
- Munish Kumar 2
4398 Accesses
30 Citations
24 Altmetric
Explore all metrics
Scientists and researchers have tried to establish a bond between the emotions conveyed and the subsequent mood perceived in a person. Emotions play a major role in terms of our choices, preferences, and decision-making. Emotions appear whenever a person perceives a change in their surroundings or within their body. Since early times, a considerable amount of effort has been made in the field of emotion detection and mood estimation. Listening to music forms a major part of our daily life. The music we listen to, the emotions it induces, and the resulting mood are all interrelated in ways we are unbeknownst to, and our survey is entirely based on these two areas of research. Differing viewpoints on this issue have led to the proposal of different ways of emotion annotation, model training, and result visualization. This paper provides a detailed review of the methods proposed in music mood recognition. It also discusses the different sensors that have been utilized to acquire various physiological signals. This paper will focus upon the datasets created and reused, different classifiers employed to obtain results with higher accuracy, features extracted from the acquired signals, and music along with an attempt to determine the exact features and parameters that will help in improving the classification process. It will also investigate several techniques to detect emotions and the different music models used to assess the music mood. This review intends to answer the questions and research issues in identifying human emotions and music mood to provide a greater insight into this field of interest and develop a better understanding to comprehend and answer the perplexing problems that surround us.
This is a preview of subscription content, log in via an institution to check access.
Access this article
Price includes VAT (Russian Federation)
Instant access to the full article PDF.
Rent this article via DeepDyve
Institutional subscriptions
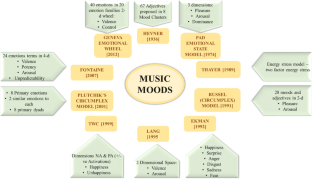
Similar content being viewed by others
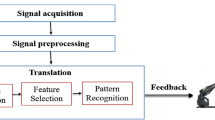
Human emotion recognition from EEG-based brain–computer interface using machine learning: a comprehensive review
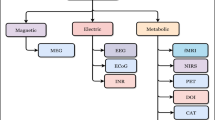
Role of machine learning and deep learning techniques in EEG-based BCI emotion recognition system: a review
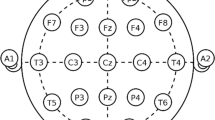
Emotion recognition with EEG-based brain-computer interfaces: a systematic literature review
Available at https://www.nielsen.com/in/en/insights/report/2018/india-music-360-report , The Nielsen Company (US) (Last Checked—6-Jan-2020) .
Available at https://indianmi.org/?id=12060&t=Digital%20Music%20Study,%202019 (Last Checked—6-Jan-2020).
Available at https://github.com/tyiannak/pyAudioAnalysis (Last Checked—6-Jan-2020).
Available at https://librosa.github.io/librosa (Last Checked—6-Jan-2020).
Abadi, M.K., Subramanian, R., Kia, S.M., Avesani, P., Patras, I., Sebe, N.: DECAF: MEG-based multimodal database for decoding affective physiological responses. IEEE Trans. Affect. Comput. 6 (3), 209–222 (2015). https://doi.org/10.1109/TAFFC.2015.2392932
Article Google Scholar
Acharya, U.R., Hagiwara, Y., Deshpande, S.N., Suren, S., Koh, J.E., Oh, S.L., Arunkumar, N., Ciaccio, E.J., Lim, C.M.: Characterization of focal EEG signals: a review. Future Gener. Comput. Syst. 91 (2019), 290–299 (2018). https://doi.org/10.1016/j.future.2018.08.044
Alakuş T.B., Turkoglu I. (2018). Determination of accuracies from different wavelet methods in emotion estimation based on EEG signals by applying KNN classifier. In: 3rd International Conference on Engineering Technology and Applied Science, pp 250–254 (2018)
Alarcão, S.M., Fonseca, M.J.: Emotions recognition using EEG signals: a survey. IEEE Trans. Affective Comput. 10 (3), 374–393 (2019). https://doi.org/10.1109/TAFFC.2017.2714671
Aljanaki, A., Yang, Y.H., Soleymani, M.: Developing a benchmark for emotional analysis of music. PLoS ONE 12 (3), 2017 (2017). https://doi.org/10.1371/journal.pone.0173392
Aljanaki, F.W., Veltkamp, R.C.: (2015) Studying emotion induced by music through a crowdsourcing game. Inf. Process. Manag. 52 (1), 115–128 (2015)
Andersson, P.K., Kristensson, P., Wastlund, E., Gustafsson, A.: Let the music play or not: the influence of background music on consumer behaviour. J. Retail. Consumer Behavior 19 (6), 553–560 (2012)
Andrzejak, R.G., Schindler, K., Rummel, C.: Non-Randomness, nonlinear dependence, and non-stationarity of electroencephalographic recordings from epilepsy patients. Phys. Rev. Am. Phys. Soc. E 86 , 2012 (2012). https://doi.org/10.1103/PhysRevE.86.046206
Arnrich, B., Marca, R.L., Ehlert, U.: Self-Organizing Maps for Affective State Detection. 2010 , 1–8 (2010)
Google Scholar
Atkinson, J., Campos, D.: Improving BCI-based emotion recognition by combining EEG feature selection and kernel classifiers. Expert Syst. Appl. 47 (C), 35–41 (2016). https://doi.org/10.1016/j.eswa.2015.10.049
Besson, M., Faïta, F., Peretz, I., Bonnel, A., Requin, J.: Singing in the brain: independence of lyrics and tunes. Psychol. Sci. 9 (6), 494–498 (1998)
Bhatti, A.M., Majid, M., Anwar, S.M., Khan, B.: Human emotion recognition and analysis in response to audio music using brain signals. Comput. Hum. Behav. 65 (2), 267–275 (2016). https://doi.org/10.1016/j.chb.2016.08.029
Bittner R., Salamon J, Tierney M., Mauch M., Cannam C., Bello J.P.: MedleyDB: a multitrack dataset for annotation-intensive MIR research. In: 15th International Society for Music Information Retrieval Conference, ISMIR, pp 155–160 (2014)
Bojorquez, G.R., Jackson, K.E., Andrews, A.K.: Music therapy for surgical patients. Crit. Care Nurs. Q. 43 (1), 81–85 (2020). https://doi.org/10.1097/cnq.0000000000000294
Bos D.O.: EEG-based Emotion Recognition. The Influence of Visual and Auditory Stimuli (2006)
Brown L., Grundlehner B., Penders J.: Towards wireless emotional valence detection from EEG. In: Annual International Conference of the IEEE Engineering in Medicine and Biology Society, pp. 2188–2191 (2011). https://doi.org/10.1109/IEMBS.2011.6090412
Chanel G., Ansari-Asl K., Pun T.: Valence-arousal evaluation using physiological signals in an emotion recall paradigm. In: IEEE International Conference on Systems, Man and Cybernetics, Montreal, pp. 2662–2667 (2007)
Chen, Y.-A., Yang Y-H., Wang J-C., Chen H.: The AMG1608 dataset for music emotion recognition. In: 2015 IEEE International Conference on Acoustics, Speech and Signal Processing (ICASSP). QLD, pp. 693–697 (2015). https://doi.org/10.1109/icassp.2015.7178058
Defferrard M., Benzi K., Vandergheynst P., Bresson X.: FMA: a dataset for music analysis. In: 18th International Society for Music Information Retrieval Conference 2017 (2017). arxiv.org/abs/1612.01840
Duan R., Zhu J., Lu B. Differential entropy feature for EEG-based emotion classification. In: 6th International IEEE/EMBS Conference on Neural Engineering (NER), pp. 81–84 (2013). https://doi.org/10.1109/NER.2013.6695876
Eerola, T., Vuoskoski, J.K.: A comparison of the discrete and dimensional models of emotion in music. Psychol. Music 39 (1), 18–49 (2010). https://doi.org/10.1177/0305735610362821
Ekman, P.: An Argument for Basic Emotions. Cognition Emotion 6 (3–4), 169–200 (1992). https://doi.org/10.1080/02699939208411068
Fernández-Aguilar, L., Martínez-Rodrigo, A., Moncho-Bogani, J., Fernández-Caballero, A., Latorre, J.M.: Emotion detection in aging adults through continuous monitoring of electro-dermal activity and heart-rate variability. IWINAC (2019). https://doi.org/10.1007/978-3-030-19591-5_26
Fernández-Sotos, A., Fernández-Caballero, A., Latorre, J.M.: Influence of tempo and rhythmic unit in musical emotion regulation. Front. Comput. Neurosci. 10 (80), 1–13 (2016)
Foote, J.: Content-based retrieval of music and audio. Multimed. Storage Arch. Syst. II 3229 , 1997 (1997). https://doi.org/10.1117/12.290336
Fu, Z., Lu, G., Ting, K.M., Zhang, D.: A survey of audio-based music classification and annotation. IEEE Trans. Multimed. 13 (2), 303–319 (2011)
Gemmeke J.F., Ellis D., Freedman D., Jansen A., Lawrence W., Moore R.C., Plakal M., Ritter M.: Audio Set: an ontology and human-labeled dataset for audio events. In: 2017 IEEE International Conference on Acoustics, Speech and Signal Processing (ICASSP), pp 776–780 (2017). https://doi.org/10.1109/ICASSP.2017.7952261 .
Hadjidimitriou, S., Hadjileontiadis, L.J.: EEG-based classification of music appraisal responses using time-frequency analysis and familiarity ratings. IEEE Trans. Affect. Comput. 4 (2), 161–172 (2013). https://doi.org/10.1109/T-AFFC.2013.6
Hadjidimitriou, S.K., Hadjileontiadis, L.J.: Towards an EEG-based recognition of music liking using time-frequency analysis. IEEE Trans. Biomed. Eng. 59 (2012), 3498–3510 (2012). https://doi.org/10.1109/TBME.2012.2217495
Harati A., Lopez S., Obeid I., Picone J., Jacobson M., Tobochnik S.: The TUH EEG CORPUS: a big data resource for automated EEG interpretation. In: IEEE Signal Processing in Medicine and Biology Symposium, IEEE SPMB (2014). https://doi.org/10.1109/SPMB.2014.7002953
Hevner, K.: Experimental studies of the elements of expression in music. Am. J. Psychol. 48 (2), 246–268 (1936)
Homburg, H., Mierswa, I., Moller, B., Morik, K., Wurst, M.: A benchmark dataset for audio classification and clustering. Int. Symp. Music Inf. Retrieval 2005 , 528–531 (2005)
Hosseini, S.A., Naghibi-Sistani, M.B., Rahati-quchani, S.: Dissection and analysis of psychophysiological and EEG signals for emotional stress evaluation. J. Biol. Syst. 18 (spec01), 101–114 (2010)
Hu X., Downie J. S., Laurier C., Bay M., Ehmann A.F.: The 2007 Mirex audio mood classification task: Lessons learned. In: ISMIR 9th International Conference on Music Information Retrieval, pp. 462–467 (2008). https://doi.org/10.5281/zenodo.1416380
Hu X., Sanghvi V.R., Vong B., On P.J., Leong C.N., Angelica J.: Moody: a web-based music mood classification and recommendation system. In: Proceedings of the International Conference on Music Information Retrieval (2008)
Huron D.: Perceptual and cognitive applications in music information retrieval. In: ISMIR, 1st International Symposium on Music Information Retrieval (2000)
Ito, S., Mitsukura, Y., Fukumi, M., Akamatsu, N.: A feature extraction of the EEG during listening to the music using the factor analysis and neural networks. Proc. Int. Jt. Conf. Neural Netw. 3 , 2263–2267 (2003). https://doi.org/10.1109/IJCNN.2003.1223763
Ito S., Mitsukura Y., Fukumi M., Cao J. (2007). Detecting method of music to match the user's mood in prefrontal cortex EEG activity using the GA. In: International Conference on Control, Automation and Systems, Seoul, South Korea, (2007). https://doi.org/10.1109/ICCAS.2007.4406685
Jain, R., Bagdare, S.: Music and consumption experience: a review. Int. J. Retail Distrib. Manag. 39 (4), 289–302 (2011). https://doi.org/10.1108/09590551111117554
Jaques N., Rudovic O., Taylor S., Sano A., Picard R.: Predicting tomorrow’s mood, health, and stress level using personalized multitask learning and domain adaptation. In: IJCAI 2017 Workshop on Artificial Intelligence in Affective Computing, Journal of Machine Learning Research Vol. 66, pp. 17–33 (2017)
Jatupaiboon, N., Pan-Ngum, S., Israsena, P.: Real-time EEG-based happiness detection system. Sci. World J. (2013). https://doi.org/10.1155/2013/618649
Jerritta S., Murugappan M., Nagarajan R., Wan K.: Physiological signals based human emotion recognition: a review. In: IEEE 7th International Colloquium on Signal Processing and its Applications, pp. 410–415 (2011)
Jiang, D., Lu, L., Zhang, H., Tao, J., Cai, L.: Music type classification by spectral contrast feature. IEEE Int. Conf. Multimed. Expo 1 (2002), 113–116 (2002)
Juslin, P.N., Laukka, P.: Expression, perception, and induction of musical emotions: a review and a questionnaire study of everyday listening. J. New Music Res. 33 (2004), 217–238 (2004). https://doi.org/10.1080/0929821042000317813
Khosrowabadi R., Quek H.C., Wahab A., Ang K.K.: EEG-based emotion recognition using self-organizing map for boundary detection. In: 20th International Conference on Pattern Recognition, ICPR’10. IEEE Computer Society, pp. 4242–4245 (2010). https://doi.org/10.1109/ICPR.2010.1031
Khosrowabadi R., Wahab A., Ang K.K., Baniasad M.H.: Affective computation on EEG correlates of emotion from musical and vocal stimuli. In: International Joint Conference on Neural Networks, Atlanta, GA, USA (2009). https://doi.org/10.1109/IJCNN.2009.5178748
Kim, D., Koo, D.: Analysis of pre-processing methods for music information retrieval in noisy environments using mobile devices. Int. J. Contents 8 (2), 1–6 (2012). https://doi.org/10.5392/IJOC.2012.8.2.001
Kim, K.H., Bang, S.W., Kim, S.R.: Emotion recognition system using short-term monitoring of physiological signals. Med. Biol. Eng. Comput. 42 (2004), 419–427 (2004). https://doi.org/10.1007/BF02344719
Kim Y.E., Schmidt E.M., Migneco R., Morton B.G., Richardson P., Scott J.J., Speck J.A., Turnbull D.: State of the art report: music emotion recognition: a state-of-the-art review. In: International Society for Music Information Retrieval Conference ISMIR (2010)
Kim Y.E., Schmidt E.M., Emelle L.: MoodSwings: a collaborative game for music mood label collection. In: ISMIR, pp. 231–236 (2008)
Koelstra, S., Mühl, C., Soleymani, M., Jong-Seok, L., Yazdani, A., Ebrahimi, T., Pun, T., Nijholt, A., Patras, I.: DEAP: a database for emotion analysis using physiological signals. IEEE Trans. Affective Comput. 3 (1), 18–31 (2011). https://doi.org/10.1109/T-AFFC.2011.15
Lahane, P.U., Thirugnanam, M.: Human emotion detection and stress analysis using EEG signal. Int. J. Innov. Technol. Explor. Eng. (IJITEE) 8 (42), 96–100 (2019)
Lang, P.J.: The emotion probe: studies of motivation and attention. Am. Psychol. Assoc. 50 (5), 372–385 (1995)
Laurier C., Grivolla, N., Herrera P.: Multimodal music mood classification using audio and lyrics. In: 7th International Conference on Machine Learning and Applications, pp. 688–693 (2008). https://doi.org/10.1109/ICMLA.2008.96
Law E., Ahn L.V., Dannenberg R.B., Crawford M.J.: TagATune: a game for music and sound annotation. In: 8th International Conference on Music Information Retrieval ISMIR (2007)
Lehmberg, L.J., Fung, C.V.: Benefits of music participation for senior citizens: a review of the literature. Music Educ. Res. Int. 4 (2010), 19–30 (2010)
Li T., Ogihara M.: Content-based music similarity search and emotion detection. In: ICASSP, pp. 705–708 (1988). https://doi.org/10.1109/ICASSP.2004.1327208
Lin, Y.P., Wang, C.H., Jung, T.P., Wu, T.L., Jeng, S.K., Duann, J.R., Chen, J.H.: EEG-Based Emotion Recognition in Music Listening. IEEE Trans. Biomed. Eng. 57 (2010), 1798–1806 (2010). https://doi.org/10.1109/TBME.2010.2048568
Liu Y., Yan N., Hu D.: Chorlody: a music learning game. In: CHI Extended Abstracts on Human Factors in Computing Systems, pp. 277–280 (2014). https://doi.org/10.1145/2559206.2580098
Liu, Y.J., Yu, M., Zhao, G., Song, J., Ge, Y., Shi, Y.: Real-time movie-induced discrete emotion recognition from EEG signals. IEEE Trans. Affect. Comput. 9 (4), 550–562 (2018). https://doi.org/10.1109/TAFFC.2017.2660485
Losorelli S., Nguyen D.C., Dmochowski J.P., Kaneshiro B.: NMED-T: a tempo-focused dataset of cortical and behavioral responses to naturalistic music. In: 18th International Society for Music Information Retrieval Conference, pp. 339–346 (2017). https://doi.org/10.5281/zenodo.1417917
Lotte, F., Bougrain, L., Cichocki, A., Clerc, M., Congedo, M., Rakotomamonjy, A., Yger, F.: A review of classification algorithms for EEG-based brain–computer interfaces: a 10-year update. J. Neural Eng. 15 (3), 2018 (2018). https://doi.org/10.1088/1741-2552/aab2f2
Lu, L., Liu, D., Zhang, H.J.: Automatic Mood Detection and Tracking of Music Audio Signals. IEEE Trans. Audio Speech Lang. Process. 14 (2006), 5–18 (2005). https://doi.org/10.1109/TSA.2005.860344
Luo, S., Yu, Y., Liu, S., Qiao, H., Liu, Y., Feng, L.: Deep attention-based music genre classification. Neurocomputing 372 (2020), 84–91 (2020). https://doi.org/10.1016/j.neucom.2019.09.054
Ma K., Wang X., Yang X., Zhang M., Girard J.M., Morency L.P.: ElderReact: a multimodal dataset for recognizing emotional response in aging adults. In: 2019 International Conference on Multimodal Interaction, ICMI, pp. 349–357 (2019). https://doi.org/10.1145/3340555.3353747
Mandel, M.I., Ellis, D.P.: A web-based game for collecting music metadata. J. New Music Res. 37 , 365–366 (2007). https://doi.org/10.1080/09298210802479300
McCraty, R., Barrios-Choplin, B., Atkinson, M., Tomasino, D.: The effects of different types of music on mood, tension, and mental clarity. Altern. Ther. Health Med. 4 (1), 75–84 (1998)
McKeown, G., Valstar, M., Cowie, R., Pantic, M., Schroder, M.: The SEMAINE database: annotated multimodal records of emotionally colored conversations between a person and a limited agent. IEEE Trans. Affect. Comput. 3 (1), 5–17 (2012). https://doi.org/10.1109/T-AFFC.2011.20
Mehrabian, A., Russell, J.A.: An Approach to Environmental Psychology. MIT Press, Cambridge (1974)
Miranda, J., Abadi, M.K., Nicu, S., Patras, I.: AMIGOS: a dataset for mood, personality and affect research on individuals and groups. IEEE Trans. Affect. Comput. 2017 , 1–14 (2017). https://doi.org/10.1109/TAFFC.2018.2884461
Moody, G.B., Mark, R.G.: The impact of the MIT-BIH arrhythmia database. IEEE Eng. Med. Biomed. 20 (3), 45–50 (2001)
Murthy, Y.V.S., Koolagudi, S.G.: Content-based music information retrieval (CB-MIR) and its applications towards the music industry: a review. ACM Comput. Surv. 51 (3), 1–46 (2018)
Naji, M., Firoozabadi, M., Azadfallah, P.: Classification of music-induced emotions based on information fusion of forehead biosignals and electrocardiogram. Cognit. Comput. 6 (2), 241–252 (2013). https://doi.org/10.1007/s12559-013-9239-7
Naji, M., Firoozabadi, M., Azadfallah, P.: Emotion classification during music listening from forehead biosignals. Signal Image Video Process. 9 (6), 1365–1375 (2015). https://doi.org/10.1007/s11760-013-0591-6
Nasehi, S., Pourghassem, H.: An optimal EEG-based emotion recognition algorithm using Gabor features. WSEAS Trans. Signal Process. 8 , 87–99 (2012)
Naser, D.S., Saha, G.: Recognition of emotions induced by music videos using DT-CWPT. Indian Conf. Med. Inf. Telemed. (ICMIT) (2013). https://doi.org/10.1109/IndianCMIT.2013.6529408
Nasoz, F., Lisetti, C.L., Alvarez, K., Finkelstein, N.: Emotion recognition from physiological signals for user modelling of affect. Cognit. Technol. Work 6 (1), 4–14 (2003). https://doi.org/10.1007/s10111-003-0143-x
North, A.C., Hargreaves, D.J.: (2009) Music and consumer behavior. In: Hallam, S., Cross, I., Thaut, M. (eds.) The Oxford Handbook of Music Psychology, pp. 481–490. Oxford University Press, Oxford (2009)
Oh S., Hahn M., Kim J.: Music mood classification using intro and refrain parts of lyrics. In: 2013 International Conference on Information Science and Applications (ICISA), pp. 1–3 (2013). https://doi.org/10.1109/ICISA.2013.6579495
Pandey P., Seeja K.R.: Subject-independent emotion detection from EEG signals using deep neural network. In: Proceedings of ICICC 2018, Vol. 2, pp. 41–46 (2018). https://doi.org/10.1007/978-981-13-2354-6_5
Park, H.S., Yoo, J.O., Cho, S.B.: A Context-aware music recommendation system using fuzzy Bayesian networks with utility theory. In: Fuzzy Systems and Knowledge Discovery. FSKD 2006. Lecture Notes in Computer Science, vol. 4223. Springer, New York (2006). https://doi.org/10.1007/11881599_121
Chapter Google Scholar
Paul, D.J., Kundu, S.: A survey of music recommendation systems with a proposed music recommendation system. In: Emerging Technology in Modelling and Graphics. Advances in Intelligent Systems and Computing, vol. 937. Springer, New York (2020). https://doi.org/10.1007/978-981-13-7403-6_26
Petrantonakis, P.C., Hadjileontiadis, L.J.: Emotion recognition from EEG using higher order crossing. IEEE Trans. Inf Technol. Biomed. 14 (2010), 186–197 (2009). https://doi.org/10.1109/TITB.2009.2034649
Petrantonakis, P.C., Hadjileontiadis, L.J.: Emotion recognition from brain signals using hybrid adaptive filtering and higher order crossing analysis. IEEE Trans. Affect. Comput. 1 (2010), 81–97 (2010). https://doi.org/10.1109/T-AFFC.2010.7
Plutchik, R.: The Nature of Emotions: Human emotions have deep evolutionary roots, a fact that may explain their complexity and provide tools for clinical practice. Am. Sci. 89 (4), 344–350 (2001)
Russell, J.A.: Culture and the categorization of emotions. Psychol. Bull. 110 (3), 425–450 (1991)
Ruvolo P., Fasel I., Movellan J.: Auditory mood detection for social and educational robots. In: 2008 IEEE International Conference on Robotics and Automation, Pasadena, CA, USA (2008). https://doi.org/10.1109/ROBOT.2008.4543754
Scaringella, N., Zoia, G., Mlynek, D.: Automatic genre classification of music content: a survey. IEEE Signal Process. Mag. 23 (2), 133–141 (2006)
Schalk, G., McFarland, D.J., Hinterberger, T., Birbaumer, N., Wolpaw, J.R.: BCI2000: a general-purpose brain-computer interface (BCI) system. IEEE Trans. Biomed. Eng. 51 (6), 1034–1043 (2004)
Shu, L., Xie, J., Yang, M., Li, Z., Li, Z., Liao, D., Xu, X., Yang, X.: A review of emotion recognition using physiological signals. Sensors 18 , 2074 (2018). https://doi.org/10.3390/s18072074
Shuman, V., Schlegel, K., Scherer, K.: Geneva Emotion Wheel rating study (Report). Swiss Centre for Affective Sciences, Geneva (2015)
Silverman, M.J.: Effects of live music in oncology waiting rooms: two mixed methods pilot studies. Int. J. Music Perform. Arts 3 (1), 1–15 (2015)
Skowronek J., McKinney M.F., Par S.V.: Ground truth for automatic music mood classification. In: Proceedings of the International Conference on Music Information Retrieval, pp. 395–396 (2006)
Snyder D., Chen G., & Povey D.: MUSAN: A Music, Speech, and Noise Corpus. ArXiv, abs/1510.08484 (2015)
Soleymani M., Asghari-Esfeden S., Pantic M., Fu Y. Continuous emotion detection using EEG signals and facial expressions. In: IEEE International Conference on Multimedia and Expo (ICME), pp. 1–6 (2014). https://doi.org/10.1109/ICME.2014.6890301
Soleymani M., Caro M.N., Schmidt E.M., Sha C.Y., Yang YH.: 1000 songs for emotional analysis of music. In: 2nd ACM international workshop on Crowdsourcing for multimedia (CrowdMM'13). ACM, pp. 1–6 (2013). https://doi.org/10.1145/2506364.2506365
Soleymani, M., Lichtenauer, J., Pun, T., Pantic, M.: A multimodal database for affect recognition and implicit tagging. IEEE Trans. Affect. Comput. 3 (1), 42–55 (2012). https://doi.org/10.1109/T-AFFC.2011.25
Song Y., Dixon S., Pearce M.T.: A survey of music recommendation systems and future perspectives. In: 9th International Symposium on Computer Music Modeling and Retrieval (CMMR’12) (2012)
Sourina O., Liu Y.: A fractal-based algorithm of emotion recognition from EEG using arousal-valence model. BIOSIGNALS. In: Proceedings of the International Conference on Bio-Inspired Systems and Signal Processing, pp. 209–214 (2011)
Sourina, O., Wang, Q., Liu, Y., Nguyen, M.K.: A real-time fractal-based brain state recognition from EEG and its applications. In: International Joint Conference on Biomedical Engineering Systems and Technologies BIOSTEC, pp. 258–272 (2011)
Stober S., Sternin A., Owen A.M., Grahn J.A.: Deep Feature Learning for EEG Recordings. ArXiv, abs/1511.04306 (2015)
Subramanian, R., Wache, J., Abadi, M.K., Vieriu, R.L., Winkler, S., Sebe, N.: ASCERTAIN: emotion and personality recognition using commercial sensors. IEEE Trans. Affect. Comput. 9 (2), 147–160 (2018). https://doi.org/10.1109/TAFFC.2016.2625250
Takahashi K.: Remarks on emotion recognition from bio-potential signals. In: 2nd International Conference on Autonomous Robots and Agents, pp. 186–191 (2004)
Thaut, M.H.: Rhythm, Music, and the Brain: Scientific Foundations and Clinical Applications. Routledge, New York (2005).. ( ISBN 0415973708 )
Bertin-Mahieux, T., Ellis, D.P.W., Whitman, B., Lamere, P. The million song dataset. In: 12th International Society for Music Information Retrieval Conference ISMIR, pp. 591–596 (2011)
Tseng K.C., Lin B.S., Han C.M., Wang P.S. Emotion recognition of EEG underlying favourite music by support vector machine. In: 1st International Conference on Orange Technologies (ICOT), pp. 155–158 (2013). https://doi.org/10.1109/ICOT.2013.6521181
Turnbull D., Liu R., Barrington L., Lanckriet, G.R. A game-based approach for collecting semantic annotations of music. In: International Society on Music Information Retrieval ISMIR, pp. 535–538 (2007)
Tzanetakis, G., Cook, P.: Musical genre classification of audio signals. IEEE Trans. Speech Audio Process. 10 (5), 293–302 (2002)
Van Der Zwaag, M.D., Dijksterhuis, C., De Waard, D., Mulder, B.L.J.M., Westerink, J.H.D.M., Brookhuis, K.A.: The influence of music on mood and performance while driving. Ergonomics 55 (1), 12–22 (2012). https://doi.org/10.1080/00140139.2011.638403
Vijayan A.E., Sen D., Sudheer A.P.: EEG-based emotion recognition using statistical measures and auto-regressive modelling. In: IEEE International Conference on Computational Intelligence & Communication Technology, pp. 587–591 (2015). https://doi.org/10.1109/CICT.2015.24
Wagner J., Kim J., Andre E.: From physiological signals to emotions: implementing and comparing selected methods for feature extraction and classification. In: 2005 IEEE International Conference on Multimedia and Expo, pp. 940–943 (2005)
Weihs C., Ligges U., Mörchen F., Müllensiefen D.: Classification in music research. Adv. Data Anal. Classification, 1(3): 255–291 (2007). 1111 S. (2013). Using Physiological Signals for Emotion Recognition. In: 6th International Conference on Human System Interactions. 2013: 556–561. DOI: https://doi.org/10.1109/HSI.2013.6577880
Yang, Y., Chen, H.H.: Machine recognition of music emotion: a review. ACM Trans. Intell. Syst. Technol. (TIST) 3 (3), 40:1-40:30 (2012). https://doi.org/10.1145/2168752.2168754
Yang, Y., Lin, Y., Su, Y., Chen, H.H.: A regression approach to music emotion recognition. IEEE Trans. Audio Speech Lang. Process. 16 (2), 448–457 (2008). https://doi.org/10.1109/TASL.2007.911513
Yang Y., Liu C.C., Chen H.H.: Music emotion classification: a fuzzy approach. In: Proceedings of the ACM International Conference on Multimedia, pp. 81–84 (2006). https://doi.org/10.1145/1180639.1180665
Yıldırım, Ö., Baloglu, U.B., Acharya, U.R.: A deep convolutional neural network model for automated identification of abnormal EEG signals. Neural Comput. Appl. (2018). https://doi.org/10.1007/s00521-018-3889-z
Zheng, W., Lu, B.: A Multimodal approach to estimating vigilance using EEG and forehead EOG. J. Neural Eng. 14 (2), 026017 (2016). https://doi.org/10.1088/1741-2552/aa5a98
Zheng, W., Lu, B.: Investigating critical frequency bands and channels for EEG-based emotion recognition with deep neural networks. IEEE Trans. Auton. Mental Dev. 7 (3), 162–175 (2015). https://doi.org/10.1109/TAMD.2015.2431497
Zheng, W., Zhu, J., Lu, B.: Identifying stable patterns over time for emotion recognition from EEG. IEEE Trans. Affect. Comput. 10 (3), 417–429 (2016). https://doi.org/10.1109/TAFFC.2017.2712143
Zong C., Chetouani M.: Hilbert-Huang transform based physiological signals analysis for emotion recognition. In: IEEE International Symposium on Signal Processing and Information Technology (ISSPIT), pp. 334–339 (2009). https://doi.org/10.1109/ISSPIT.2009.5407547
Thayer, R.E.: The Biopsychology of Mood and Arousal. Oxford University Press, New York (1989).. ( ISBN0-19-506827-0 )
Fontaine, J.R.J., Scherer, K.R., Roesch, E.B., Ellsworth, P.C.: The world of emotions is not two-dimensional. Psychol. Sci. 18 (12), 1050–1057 (2007). https://doi.org/10.1111/j.1467-9280.2007.02024.x
Stober S., Sternin A., Owen A.M., & Grahn, J.A.: Towards Music Imagery Information Retrieval: Introducing the OpenMIIR Dataset of EEG Recordings from Music Perception and Imagination. In: 16th International Society for Music Information Retrieval Conference, pp. 763–769 (2015). https://doi.org/10.5281/zenodo.1416270
Alakuş, T.B., Türkoglu, I.: Feature selection with sequential forward selection algorithm from emotion estimation based on EEG signals. Sakarya Univ. J. Sci. 23 (6), 1096–1105 (2019). https://doi.org/10.16984/saufenbilder.501799
Hosseini, M., Hosseini, A., Ahi, K.: A review on machine learning for EEG signal processing in bioengineering. IEEE Rev. Biomed. Eng. (2020). https://doi.org/10.1109/RBME.2020.2969915
Zhang, K., Zhang, H.B., Li, S., Yang, C., Sun, L.: The PMEmo dataset for music emotion recognition. Int. Conf. Multimed. Retrieval. (2018). https://doi.org/10.1145/3206025.3206037
Warrenburg, L.A.: Choosing the right tune: a review of music stimuli used in emotion research. Music Perception Interdiscip. J. 37 (3), 240–258 (2020). https://doi.org/10.1525/mp.2020.37.3.240
Su, K., Hairston, W., Robbins, K.: EEG-annotate: automated identification and labeling of events in continuous signals with applications to EEG. J. Neurosci. Methods 293 , 359–374 (2018)
Amin, H.U., Mumtaz, W., Subhani, A., Saad, M., & Malik, A.: Classification of EEG signals based on pattern recognition approach. Front. Comput. Neurosci. 11 , 103 (2017). https://doi.org/10.3389/fncom.2017.00103 . eCollection 2017
Delorme, A., Makeig, S.: EEGLAB: an open-source toolbox for analysis of single-trial EEG dynamics including independent component analysis. J. Neurosci. Methods 134 , 9–21 (2004)
Ghosh, A., Danieli, M., & Riccardi, G.: Annotation and prediction of stress and workload from physiological and inertial signals. In: 2015 37th Annual International Conference of the IEEE Engineering in Medicine and Biology Society (EMBC), pp. 1621–1624 (2015)
Wu, S., Xu, X., Shu, L., Hu, B.: Estimation of valence of emotion using two frontal EEG channels. In: Proceedings of the IEEE International Conference on Bioinformatics and Biomedicine, Kansas City, MO, USA, 13–16 November 2017; pp. 1127–1130 (2017)
Lan, Z., Sourina, O., Wang, L., Liu, Y.: Real-time EEG-based emotion monitoring using stable features. Vis. Comput. 32 , 347–358 (2016)
Dror, O.E.: The cannon-bard thalamic theory of emotions: a brief genealogy and reappraisal. Emot. Rev. 6 (1), 13–20 (2014). https://doi.org/10.1177/1754073913494898
Download references
Author information
Authors and affiliations.
Computer Science and Engineering Department, Thapar Institute of Engineering and Technology, Patiala, Punjab, India
Vybhav Chaturvedi, Arman Beer Kaur, Vedansh Varshney, Anupam Garg & Gurpal Singh Chhabra
Department of Computational Sciences, Maharaja Ranjit Singh Punjab Technical University, Bathinda, Punjab, India
Munish Kumar
You can also search for this author in PubMed Google Scholar
Corresponding author
Correspondence to Munish Kumar .
Additional information
Communicated by I. Bartolini.
Publisher's Note
Springer Nature remains neutral with regard to jurisdictional claims in published maps and institutional affiliations.
Rights and permissions
Reprints and permissions
About this article
Chaturvedi, V., Kaur, A.B., Varshney, V. et al. Music mood and human emotion recognition based on physiological signals: a systematic review. Multimedia Systems 28 , 21–44 (2022). https://doi.org/10.1007/s00530-021-00786-6
Download citation
Received : 20 September 2020
Accepted : 24 March 2021
Published : 22 April 2021
Issue Date : February 2022
DOI : https://doi.org/10.1007/s00530-021-00786-6
Share this article
Anyone you share the following link with will be able to read this content:
Sorry, a shareable link is not currently available for this article.
Provided by the Springer Nature SharedIt content-sharing initiative
- Emotion recognition
- Music mood classification
- Physiological signals
- EEG feature extraction
- Random forest
- Find a journal
- Publish with us
- Track your research
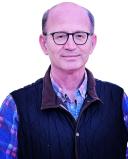
How Listening to Music Affects Your Mood
Music can be seen as a coping resource that enhances internal abilities..
Posted November 8, 2022 | Reviewed by Abigail Fagan
- Music is a tool that can ease negative emotions and enhance positive emotions.
- Music can help people cope with stress or distract them from a bad mood.
- Many people use music to trigger their memory and remind themselves of important past events.
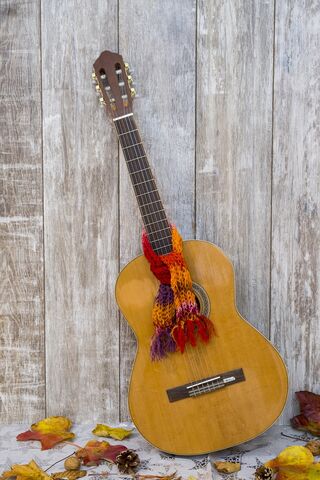
Music makes life better. Music is used across cultures and ages as a powerful mood regulator. We regularly use music to soothe our souls and comfort our pain. The emotional power of music is one of the main motivations why people devote so much time, energy, and money to it. People use music to achieve various goals, such as feeling more energized, maintaining focus on tasks, and reducing boredom . For example, we rely on music to help us power through workouts and tackle tasks we’d rather ignore, and we manipulate our moods with melodies.
So, how does music produce such a powerful effect on the mind?
1. Coping with stress
Music offers a resource for emotion regulation . For instance, sad music enables the listener to disengage from the distressing situations (breakup, death, etc.), and focus instead on the beauty of the music. Further, lyrics that resonate with the listener’s personal experience can give voice to feelings or experiences that one might not be able to express oneself. Listening to soft and slow music, such as classical music, is better for managing negative emotions compared to hard or heavy music. Listening to happy music can influence the way one perceives the world in a stressful situation. For example, upbeat tunes can give you an optimistic outlook and make you feel better.
2. Music and memory
Listening to a piece of music that was played a lot during a significant life event (e.g., a family celebration) many years ago can trigger a deeply nostalgic emotional experience. The feeling is not in the music, but in what it reminds us. Many listeners use music to remind themselves of valued past events, becoming nostalgic. For example, nostalgia is a powerful force around Christmas ('White Christmas'). Some people may have a fond memory associated with Elgar (Pomp and Circumstance) for graduation ceremonies, and Pachelbel’s “Canon in D Major,” (or Mendelssohn’s “Wedding March” in C major) for weddings.
3. Pleasure in sad music
For some, sad music intensifies the feelings of sorrow and loss connected to personal events and memories. Listening to a sad song while in a sad mood is like someone (a friend) empathizing with your experience. The reward could be purely biochemical. We have all experienced the feeling of relief and serenity after a good cry. At the biological level, sad music is linked to the hormone prolactin (associated with crying), a chemical that helps to curb grief (Huron, 2011). Prolactin produces feelings of calmness to counteract mental pain.
4. The feeling of being moved
Music often makes us feel like crying because we experience a sense of awe and admiration. The feeling is a kind of wonder at realizing what other minds can create. In response to these emotions, we may experience goosebumps and motivation for the improvement of self and society. The experience is often intense and pleasurable.
5. Music and time perception
Music shows that time perception is essentially subjective — music can distort “clock time”. Music is a powerful emotional stimulus that changes our relationship with time. Time does indeed seem to fly when listening to pleasant music. Hearing pleasant music seems to divert attention away from time processing. Moreover, this attention-related shortening effect appears to be greater in the case of low-arousing (calm) music with a slow tempo. For example, music is used in waiting rooms to reduce the subjective duration of time spent waiting or in supermarkets to encourage people to stay for longer and buy more. Consumers spend more time in the grocery store when the background music is slow. Music keeps workers happy when doing repetitive and otherwise boring work.
In sum, music can alter our moods, emotions, and motivation. We can use music to validate or challenge our moods. Music can also reflect the mood on a national level. For example, the protest song “Baraye,” (the anthem of Iran’s “Woman, Life, Liberty” protest movement) expresses Iranians’ painful grievances.
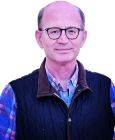
Shahram Heshmat, Ph.D., is an associate professor emeritus of health economics of addiction at the University of Illinois at Springfield.
- Find a Therapist
- Find a Treatment Center
- Find a Psychiatrist
- Find a Support Group
- Find Teletherapy
- United States
- Brooklyn, NY
- Chicago, IL
- Houston, TX
- Los Angeles, CA
- New York, NY
- Portland, OR
- San Diego, CA
- San Francisco, CA
- Seattle, WA
- Washington, DC
- Asperger's
- Bipolar Disorder
- Chronic Pain
- Eating Disorders
- Passive Aggression
- Personality
- Goal Setting
- Positive Psychology
- Stopping Smoking
- Low Sexual Desire
- Relationships
- Child Development
- Therapy Center NEW
- Diagnosis Dictionary
- Types of Therapy
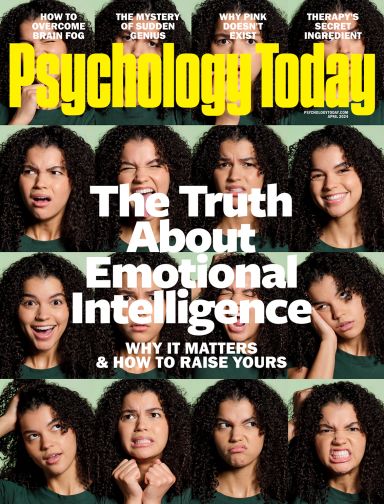
Understanding what emotional intelligence looks like and the steps needed to improve it could light a path to a more emotionally adept world.
- Coronavirus Disease 2019
- Affective Forecasting
- Neuroscience

An official website of the United States government
The .gov means it’s official. Federal government websites often end in .gov or .mil. Before sharing sensitive information, make sure you’re on a federal government site.
The site is secure. The https:// ensures that you are connecting to the official website and that any information you provide is encrypted and transmitted securely.
- Publications
- Account settings
Preview improvements coming to the PMC website in October 2024. Learn More or Try it out now .
- Advanced Search
- Journal List
- World J Psychiatry
- v.5(1); 2015 Mar 22
Effects of music and music therapy on mood in neurological patients
Correspondence to: Alfredo Raglio, MT, PhD, Department of Public Health, Experimental and Forensic Medicine, University of Pavia, Via S. Boezio 24, 27100 Pavia, Italy. [email protected]
Telephone: +39-0382-593797 Fax: +39-0382-593797
Mood disorder and depressive syndromes represent a common comorbid condition in neurological disorders with a prevalence rate that ranges between 20% and 50% of patients with stroke, epilepsy, multiple sclerosis, and Parkinson’s disease. Notwithstanding, these conditions are often under-diagnosed and under-treated in the clinical practice and negatively affect the functional recovery, the adherence to treatment, the quality of life, and even the mortality risk. In addition, a bidirectional association between depression and neurological disorders may be possible being that depressive syndromes may be considered as a risk factor for certain neurological diseases. Despite the large amount of evidence regarding the effects of music therapy (MT) and other musical interventions on different aspects of neurological disorders, no updated article reviewing outcomes such as mood, emotions, depression, activity of daily living and so on is actually available; for this reason, little is known about the effectiveness of music and MT on these important outcomes in neurological patients. The aim of this article is to provide a narrative review of the current literature on musical interventions and their effects on mood and depression in patients with neurological disorders. Searching on PubMed and PsycInfo databases, 25 studies corresponding to the inclusion criteria have been selected; 11 of them assess the effects of music or MT in Dementia, 9 explore the efficacy on patients with Stroke, and 5 regard other neurological diseases like Multiple Sclerosis, Amyotrophic Lateral Sclerosis/motor neuron disease, Chronic quadriplegia, Parkinson’s Disease, and Acquired Brain dysfunctions. Selected studies are based on relational and rehabilitative music therapy approaches or concern music listening interventions. Most of the studies support the efficacy of MT and other musical interventions on mood, depressive syndromes, and quality of life on neurological patients.
Core tip: We conducted a search on PubMed and PsychInfo databases identifying 25 Randomized Controlled Trials or Clinical Controlled Trials regarding the effects of Music Therapy and other musical interventions on mood disorders in neurological patients. Although the Jadad score evaluation revealed a generally poor methodological quality of the research protocols, we found that almost all studies supported the effectiveness of musical interventions in improving mood, depression, quality of life, functional recovery, and neuromotor performances. Therefore Music Therapy and other musical approaches seem to be effective, inexpensive and non-invasive, being that no adverse side-effects were observed.
INTRODUCTION
Neurology and psychiatry.
Neurological diseases are often associated with several behavioral and psychological symptoms that are usually overlooked by neurologists because require diagnostic methods that differ from those used for classical somatic symptoms and are more suitable to the field of psychiatry. On the other hand, psychiatrists do not seem to give an adequate attention to these symptoms considering them as a consequence of a cerebral damage and more pertinent to neurologists. This clinical attitude is historically based on the obsolete and reductive distinction between “organic” and “functional” behavioral disorders introduced by the phrenologist George Combe in 19 th century. According to Combe, cerebral diseases were respectively classified depending on the presence or the absence of cerebral lesions and from that time on this terminology has been used to indicate that some behavioral disorders are linked to a neurological damage while others are not. However, the reductionism of Combe’s distinction clearly emerges from the clinical observation given that a wide range of nervous system’s illnesses with different etiology shows both neurological and psychiatric symptoms. Emotional and behavioral disturbances with a polymorphic symptomatology are often connected to neurological disorders such as Multiple Sclerosis (MS)[ 1 - 3 ], Parkinson’s Disease (PD)[ 4 ], stroke[ 5 ], dementia[ 6 ], traumatic brain injury[ 7 ], epilepsy[ 8 , 9 ], Amyotrophic Lateral Sclerosis (ALS) and others Motor Neuron Diseases (MND)[ 10 , 11 ], pain syndromes (like headaches) and can be observed even with or without “organic” neurological diseases, thus miming in some cases an idiopathic psychiatric disorder.
Most common psychiatric disorders in neurology are depression, anxiety, maniacal states, and thought and perception disorders. Other psychiatric syndromes that can be seen in persons with neurological disorders are alexithymia, worry, and locus of control[ 12 ]. For example, mood disorders are often associated with acute or chronic cerebrovascular pathologies where the most common complications is certainly depression, usually defined post-stroke depression (PSD). The frequency of this syndrome is variable accordingly to different studies with a mean of 40% of the cases[ 13 ] and data obtained by numerous studies seem to indicate the presence of multiple etiological factors, both structural-endogenous and environmental-external, that may change depending on the early or late onset of the depressive disorder. In addition, a bidirectional association between depression and neurological disorder may be possible being that depressive syndromes may be considered as a risk factor for certain neurological disorders. As sustained by two recent meta-analysis, depressive syndromes, particularly major depressive disorder (MDD), are associated with a significantly increased risk of stroke[ 14 , 15 ]. On the other hand, lower rates of depression in equally impaired orthopedic patients suggest that PSD may even result from a stroke-specific neurobiological change and not only from a consequence of the psychological distress or the related impairments[ 16 - 18 ].
The second most common neurodegenerative disorder is represented by PD, with a prevalence of 1% of the elderly worldwide population. About 30% of PD patients show clinically significant depressive syndromes and, again, it appears to be also an increased risk for depressed patients to develop PD[ 19 - 23 ].
As far as regard MS, depressive syndromes are psychiatric most common disorders associated to the illness. Among individuals with MS, relative to the general population, lifetime prevalence rates are elevated for MDD (36%-54%), bipolar disorder (13%), anxiety disorders (35.7%), adjustment disorders (22%), and psychotic disorders (2%-3%). Suicide may be at least twice as common[ 1 ].
Many reports of depression and its correlation with numerous variables in clinical samples of people with MS have been published. The few population-based studies have reported a high prevalence of depression, despite using different methods of data collection. The lifetime risk of major depression in people with MS has been estimated to be as high as 50% compared to 10% to 15% in the general population[ 24 , 25 ].
In a recent cross-sectional, population-based study conducted in Stockholm county, the authors reported a prevalence rate of depression of 19% [Beck Depression Inventory (BDI) > 13] among patients suffering of MS. It’s interesting to note how depressive symptoms were associated with worse self-reported functioning, with poor memory function and with weak sense of coherence (SOC) (referring to ‘‘general resistance resources’’ - capacities that facilitate coping with stressors). Moreover, the authors suggested to incorporate depressive symptoms or mental health as a standard parameter for assessment and follow-up in clinical MS management[ 26 ].
Data from the United Kingdom MS Register, those obtained directly from MS patients, confirmed a high rate of anxiety and depression: over half of the respondents (54.1%) reported anxiety and 46.9% reported a variable level of depression[ 27 ]. From this registry data were recently examined about the positive relationships between physical disability, anxiety and depression[ 28 ].
Other reports confirmed the need to recognize and treat, having widely effective treatments, several emotional disorders which may worsen functioning and quality of life, decrease treatment adherence, and increase risk of suicide[ 1 ].
The prevalence of depressive disorders is higher in MS patients than patients with other chronic disease, suggesting a possible direct effect of the illness on the pathogenesis of the depressive syndromes in addition to the reactive disorder. Some evidences suggested that depression in MS is largely biologically mediated by some of the same processes involved in the immunopathogenesis of this neurologic disease. In particular, the increase in proinflammatory cytokines, the activation of the hypothalamic-pituitary-adrenal axis, and the reduction in neurotrophic factors. Notwithstanding, depression and mood disorders still remain under-diagnosed and under-treated in neurological patients claiming for a bio-psychosocial model be used[ 29 - 32 ].
Music therapy
In last decades, a growing body of evidence in the use of musical intervention in clinical setting have been seen, concerning singing, music listening, musical improvisation, and other musical activities, as long as more structured music therapy (MT) treatments. Given that music engages a variety of brain areas involved in emotion, motivation, cognition, and motor functions, musical interventions have been used to increase socialization and cognitive, emotional, and neuromotor functioning[ 33 - 38 ]. Although the debate on what the boundaries of MT is still going on, different approaches of musical intervention are actually available referring to three principal domains: relational approaches, rehabilitative approaches and music listening.
Relational approaches refer to psychological models and involve both active and receptive techniques[ 39 , 40 ]. The former consist of different musical activities such as free or structured musical improvisation by means of simple musical instruments, singing, songwriting etc . that allow patient and therapist to directly interact building a musical relationship[ 41 ]. In receptive approaches music imagery and music listening are used to induce psychological beneficial effects and even to evoke and process emotions and thoughts[ 40 ].
Rehabilitative approaches, such as Neurologic Music Therapy (NMT)[ 42 ] refer to neuroscientific models and use primarily the potential of musical stimuli to activate perception and production areas in the human brain, providing a series of therapeutic applications to sensory, cognitive, and motor dysfunctions resulting from neurological disorders. Using directive approach based on a series of exercises, NMT may be used, for example, to improve gait and movements in post-stroke and PD patients[ 43 - 47 ] and language in persons with aphasia[ 48 , 49 ].
On the other hand, simple music listening interventions don’t require neither a specifically trained therapist nor a direct therapeutic relationship with the patient being that beneficial effects are induced by the content of the musical stimuli and by the activity of listening itself. For these reasons, this practice is sometimes defined with the term “Music Medicine” rather than “MT”[ 41 , 50 , 51 ]. Notwithstanding, listening interventions seem to be quite common in clinical literature, usually based on self-selected or other-selected music proposed individually[ 52 , 53 ] or in group, as in the case of background music[ 54 , 55 ].
As far as regard neurological disorders, MT may promote functional recovery and also improve social and psychological outcomes such as socialization, motivation, mood, and depression[ 56 ]. Literature in this field shows that most of the musical interventions are currently used in clinical practice, being that the majority of the interventions are based on a combination of rehabilitative and relational techniques. Also music listening seems to be a common practice in neurological rehabilitation. Due to the possible side effects of pharmacological treatment of depressive syndromes following neurological disease, music and MT may represent a valid support in reducing depressive symptoms, improving mood and adherence to treatment while contributing to the functional recovery at the same time.
PubMed and PsychInfo databases were considered for articles to include in the current narrative review. The research has been conducted by three independent reviewers using the following search terms: (“Music” OR “MT”) AND (name of pathology) AND (“Mood” OR “Depression”). Names of pathologies where used alone or in combination with “OR” Boolean operator and included: “Stroke”, “Parkinson”, “Dementia”, “Epilepsy”, “ALS”, “MS”, “Cerebral palsy”, “Neurological disease”, and “Acquired brain injury”.
We included only Randomized Controlled Trials (RCTs) or Clinical Controlled Trials (CCTs) studies in English language published in peer-reviewed journals between 1 st January 1997 and 31 st May 2014. Importantly, we considered only trials including outcomes concerning mood or depression where experimental conditions were clearly stated and consisted only or primarily of musical activities.
Assessments of methodological quality of selected studies have been provided using Jadad score[ 57 ]. Jadad scale is based on 7 items that evaluate three main characteristics of a clinical trial: the random assignment, the double-blinding of assessments, and the flow of participants. Scoring ranges from a minimum of 0 to a maximum of 5 points where a score of 3 indicates a good quality study. Being that 2 points on 5 are scored for double-blinding and none of the included studies had double-blinding assessment, the maximum possible score was 3. Even if it doesn’t take into account allocation concealment and has been criticized for placing too much emphasis on blinding[ 58 ], Jadad scale represents a simple, easy and common way to evaluate the methodological quality of a clinical trial with good validity and reliability[ 59 ]. Due to the heterogeneity of the outcomes, no meta-analysis was carried out.
DESCRIPTION OF SELECTED STUDIES
A total of 464 records resulted from the search of which 301 from PubMed and 163 from PsychInfo. Twenty-five articles that met the inclusion criteria have been found and were included in the current review. Most of the selected studies are related to dementia (44%) and stroke (36%) while others regard MS, ALS/motor neuron disease, PD, Chronic quadriplegia, and acquired brain dysfunctions (20%). Fourteen studies (56%) employed a relational approach including both active and receptive techniques, six studies (24%) adopted a rehabilitative approach, and five (20%) concerned music listening interventions. Activities were conducted by trained music therapists in the most part of the experimental interventions. As far as regard the methodological quality of included studies, our analysis showed that only nine on twenty-five (36%) of the included studies received a Jadad score of 3 and thus can be considered of good quality. Five studies (20%) had a Jadad score of 2, three studies (12%) a score 1, and eight studies (32%) were evaluated with a score of 0. Results of the methodological assessment pointed out a general poor rigor in research protocols.
In the following subsections, results are presented through a subdivision of the selected studies by pathology (Table (Table1, 1 , Table Table2, 2 , Table Table3 3 ).
Characteristics of the included studies concerning effects on dementia
ADAS-Cog: Alzheimer's Disease Assessment Scale-Cognitive Subscale; ADL: Activities of daily living; BEHAVE-AD: Behavioral Pathology in Alzheimer’s Disease Scale; CCT: Controlled Clinical Trial; CMAI: Cohen-Mansfield Agitation Inventory; CSDD: Cornell Scale for Depression in Dementia; C-CSDD: Chinese Cornell Scale for Depression in Dementia; DQOL: Dementia Quality of Life; ECG Holter: Electrocardiography Holter; GDS: Geriatric Depression Scale; GMP: Good Manufacturing Practice; GQoL: Geriatric Quality of Life; HRSD: Hamilton Rating Scale for Depression; IADL: Instrumental Activities of Dailiy Living; ICEA-D: Inventory to Asses Communication, Emotional Expression and Activity in Dementia; MMSE: Mini-Mental State Examination; NPI: Neuropsychiatric Inventory; NPI-Q: Neuropsychiatric Inventory Questionnaire; RCT: Randomized Controlled Trial; SVAM: Metacognition Assessment Scale.
Characteristics of the included studies concerning effects on stroke
Characteristics of the included studies concerning effects on other neurological disorders
HM: Happiness Measure; MS; Motor Subscale; PDQL: Parkinson's Disease Quality of Life Questionnaire; UPDRS: Unified Parkinson’s Disease Rating Scale; BDI: Beck Depression Inventory; HADS: Hospital Anxiety and Depression Scale; SESA: Self-Acceptance Scale; HAQUAMS: Hamburg Quality of Life Questionnaire in Multiple Sclerosis; MSFC: Multiple Sclerosis Functional Composite; WAIS-III: Wechsler Adult Intelligence Scale-III; AVLT: Auditory Verbal Learning Test; TMT-B: Trial Making Test Part B; BSI-18: Brief Symptoms Inventory-18; MAACL: Multiple Affect Adjective Check List; SEQ: Self Efficacy Questionnaire; HADS: Hospital Anxiety and Depression Scale; ESAS: Edmonton Symptom Assessment System; HR: Heart Rate; EMG: Electromyogram; PVP: Perceptual Voice Profile; POMS: Profile of Mood State; AQoL: Assessment of Quality of Life.
Effects on dementia
Eleven studies assessed the effects of music and MT on dementia[ 60 - 70 ]. Eight studies employed a relational approach[ 60 - 61 , 63 - 65 , 68 - 70 ] based either on active or receptive techniques or a combination of both of them. Two studies concerned music listening interventions[ 62 , 67 ] and one study adopted a rehabilitative approach[ 66 ]. In most cases the results show a positive effect on mood, depression, and anxiety. Two studies revealed no significant effect of musical intervention[ 64 , 66 ] while in three studies both experimental and control group improved emotional and behavioral functioning in the same way[ 65 , 67 , 69 ]. Characteristics of the studies and main results have been summarized in Table Table1 1 .
Effects on stroke
Nine studies assessed the effects of music or MT on post-stroke patients[ 71 - 79 ]. Four of them were based on a relational approach[ 71 , 72 , 76 , 77 ], three regarded music listening interventions[ 74 , 75 , 78 ], and two used a rehabilitative approach[ 73 , 79 ]. All studies show a positive effect of music or MT on mood in patients with Stroke. For a synthesis of studies and results please see Table Table2 2 .
Effects on other neurological disorder
Five studies concerning other neurological disorders such as MS, ALS/motor neuron disease, PD, Chronic quadriplegia, and Acquired Brain dysfunctions, have been found[ 80 - 84 ]. Three studies concerned a rehabilitative approach[ 80 , 82 , 84 ] and two studies adopted a relational approach using an active technique[ 81 ] or both active and receptive techniques depending on what the therapist deemed appropriate in consultation with the patient[ 83 ]. All studies but one[ 83 ] reported positive effects of music and MT on outcomes as mood, depression, anxiety, and quality of life. Characteristics of the studies and main results have been summarized in Table Table3 3 .
In the last few decades, the development of neuroscience demonstrated that the brain isn't a static structure only influenced by genetic determinants but it is a plastic organ that continuously reorganizes synaptic connections under the influence of inner and outer factors such as genetic programs, environmental stimulation, learning and expertise[ 85 - 87 ].
Neurological illnesses that provoke behavioral disturbances might originate from both endogenous and external causal factors thus determining, depending on the circumstances, a more “structural” or a more “environmental” etiology. The mutual interaction between these factors occurs in the brain and gives rise to a variety of psychiatric disorders that can be distributed upon a continuum, on one end of which are behavioral disturbances clearly linked to neuroanatomic and neurochemical alterations while on the opposite those more associated to the environment.
Synaptic functions and neuroanatomic structures are proper “organic” factors that determine those alterations that are usually treated by neuropsychiatry and biological psychiatry. Behavioral disorders resulting from these factors include psychiatric syndromes that are linked to alterations of the neural transmission caused by receptor’s abnormalities and by modifications of the synaptic concentrations of one or more neurotransmitters. Given that neurotransmitters regulate the neural impulse transmission processes into neurotransmitter systems, with a widespread projection in the brain, the whole emotional, motivational, and affective state of the person will be altered[ 88 , 89 ].
External causal factors related to the environment may promote and characterize those behavioral disorders that are commonly counted accordingly to a bio-psychosocial model and interfere with the cognitive and emotional state of the person thus inducing an important change in the quality of the inter-individual relationships. These disorders may be considered as a reaction to the physical disability and the psycho-social difficulties produced by the disease but also as an adjustment disorder if we consider the impact of the diagnosis on patient’s life, or the weight of a chronic illness and all the other factors that may affect patient’s quality of life[ 90 - 92 ].
Depressive syndromes in chronic neurological illness are common and disabling. Their etiology is complex and may be multifactorial. Good history taking and detailed examination of physical and mental state (including cognitive function) will usually reveal the diagnosis and the formulation.
Providing a correct diagnosis of an emotional disorder and starting an appropriate treatment may help physicians to increases in function and quality of life of their neurological patients[ 93 ].
The current review showed how MT and musical interventions can improve mood and psychological well-being in neurological patients. These clinical results are in accordance with the literature that highlights the effects that music listening and music making have on brain structures of emotion regulation[ 36 ], on various neurochemical systems[ 38 ], and on neural plasticity[ 94 , 95 ]. However, the strength of this review’s findings is limited due to a generally poor methodological quality of the studies and the restricted size of samples. Moreover, the heterogeneity of the outcomes prevented any meta-analysis. Notwithstanding, the analysis of the 25 RCTs or CCTs included in this work points out a positive effect of interventions with music on psychosocial outcomes such as mood, depression, and quality of life when compared to standard care or other treatments.
Music-based activities can represent a valid and without side effects intervention for reducing psychological and behavioral disturbances related to neurological disorders and also for promoting the functional recovery. Specifically, the most significant results of the music interventions on the psychological side can be identified in the aspects more closely related to mood, especially in the reduction of the depressive and anxiety's component, and in the improvement of the emotional expression, communication and interpersonal skills, self esteem and quality of life. As revealed in advance, the efficacy of music and MT interventions could be explained by different points of view. From the neurochemistry point of view we know that music can activate limbic and paralimbic structures, such as the amygdala, the hippocampus, the nucleus accumbens, etc. that function abnormally in patients with a high depressive component. At the psychological level music can engage several social functions, can increase communication and social cohesion and can promote empathetic relationships, especially in the active MT approaches. Finally, from the rehabilitative point of view, making music can involve and influence motor areas functioning and regulation. This effect appears to be connected to the pleasure and thereby can positively affect the mood and consequently the rehabilitative process[ 36 - 38 ].
In conclusion, a more methodological rigor and a clearer definition of music approaches are needed to improve the quality of MT research and to focus on the specific role of music-based interventions in psychological symptoms in the field of neurology.
P- Reviewer: Boehm K, Mishra J S- Editor: Ji FF L- Editor: A E- Editor: Lu YJ
Conflict-of-interest: The Authors have not any competing interests to declare and no funding was received for this research.
Open-Access: This article is an open-access article which was selected by an in-house editor and fully peer-reviewed by external reviewers. It is distributed in accordance with the Creative Commons Attribution Non Commercial (CC BY-NC 4.0) license, which permits others to distribute, remix, adapt, build upon this work non-commercially, and license their derivative works on different terms, provided the original work is properly cited and the use is non-commercial. See: http://creativecommons.org/licenses/by-nc/4.0/
Peer-review started: September 28, 2014
First decision: December 17, 2014
Article in press: February 11, 2015
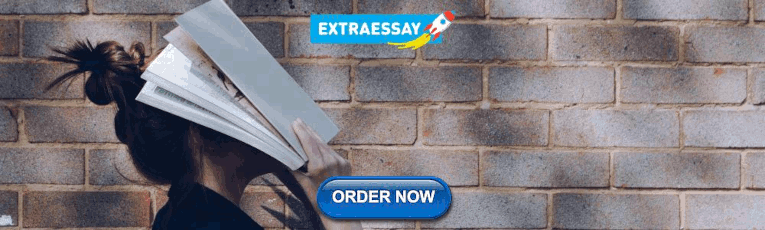
IMAGES
VIDEO
COMMENTS
Depression and Media Use. Access to online media has increased exponentially with the onset of digitisation and technological advancement (Brown and Bobkowski, 2011).Research has demonstrated that young people are even more likely to turn to media when they are in a negative mood (Dillman Carpentier et al., 2008).In fact, withdrawal from socialization and normal daily activity has been ...
Music has the pote ntial to influence mood, feelings, and thoughts; it has the abili ty to change the emotional and. physical status of people, whether they are in b ad, good, or sad moods. Music ...
This research aims to explore the impact of music on mood and mental health, with the goal of guiding future research and deepening our understanding of the connections between music and human ...
The majority of reviews conclude that music interventions have a positive effect on pain, mood, and anxious or depressive symptoms in both children and adults in clinical settings. This suggests ...
The studies published on the benefits of music have been on the increase in the last two decades [1,2,3] and have branched out into different areas of research such as psychology [4,5,6,7,8], education [1, 9, 10] and health [11, 12] providing ways of using music as a resource for people's improvement.The publication in 1996 of the famous report "Education Hides a Treasure" submitted to ...
Abstract. Music is ubiquitous across human cultures — as a source of affective and pleasurable experience, moving us both physically and emotionally — and learning to play music shapes both ...
In contrast, after listening to the designer music (music designed to have specific effects on the listener), significant increases in caring, relaxation, mental clarity, and vigor were measured ...
Listening to music as a transcendent experience. In their most recent work, scholars in the field of positive media psychology have identified research designed to investigate and understand self-transcendent media experiences as essential to moving the field forward (Oliver et al., Citation 2018; Raney et al., Citation 2018).Self-transcendence refers to "a motivational state in which the ...
Scientists and researchers have tried to establish a bond between the emotions conveyed and the subsequent mood perceived in a person. Emotions play a major role in terms of our choices, preferences, and decision-making. Emotions appear whenever a person perceives a change in their surroundings or within their body. Since early times, a considerable amount of effort has been made in the field ...
One potential mechanism that may explain how background music influences attention is that background music modulates the listener's internal mood and arousal levels, which may then enhance performance (Aquino & Arnell, 2007; Husain et al., 2002; Jefferies et al., 2008).Mood refers to a positive or negative emotional state (e.g., happy and sad), whereas arousal is related to the level of ...
Med. Sci. 2021;1 (1 ):101-106. INTRODUCTION: Music affects our brain at different levels. Our mood changes with. different types of music. H owever, at a very deep level, its effect is similar to ...
With the growth in the accessibility, exposure, and consumption of music in everyday life, people engage with music listening in a wide variety of situations and contexts (Bull, 2006; North et al., 2004).Interestingly, amongst these music listening behaviors, research shows that on most occasions people listen to music when they are engaged with other tasks like studying or working, exercising ...
5. Music and time perception. Music shows that time perception is essentially subjective — music can distort "clock time". Music is a powerful emotional stimulus that changes our ...
Negative emotions can affect the HR and BP through the link between the nervous system and the cardiovascular system. Our study demonstrates the positive effect of classical music on HR, BP parameters, and mood states. Keywords: autonomic nervous system, mood and anxiety, blood pressure variability, heart rate recovery, classical music.
In a psychological survey conducted in 2013 examining the reasons why people listen to music, the analysis found that people listen to music to: Regulate mood and stress (arousal). Achieve self-awareness. Express social relatedness. The first two reasons in this list were found to be more important than the third.
A number of studies have revealed that music affects moods, attitudes and behaviours. Gardner. (1985) defined mood as a fleeting, temporary fee ling state, usually not intense, a nd not tied to a ...
Core tip: We conducted a search on PubMed and PsychInfo databases identifying 25 Randomized Controlled Trials or Clinical Controlled Trials regarding the effects of Music Therapy and other musical interventions on mood disorders in neurological patients. Although the Jadad score evaluation revealed a generally poor methodological quality of the research protocols, we found that almost all ...
Thus, we believe that one of the factors that can have an effect on short-term. memory is music. Music is a play of tones, which is fixed and is usually perceived as satisfying. In other words, it ...