- Open access
- Published: 23 July 2022
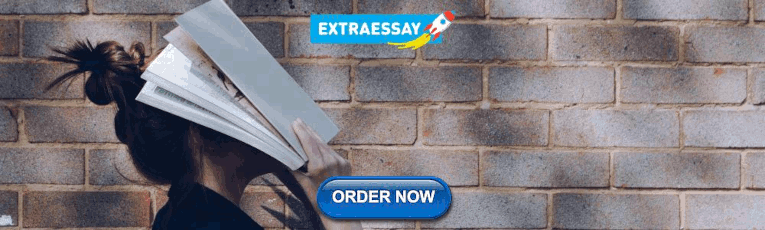
Recent advances in proteomics and metabolomics in plants
- Shijuan Yan 1 ,
- Ruchika Bhawal 2 ,
- Zhibin Yin 1 ,
- Theodore W. Thannhauser 3 &
- Sheng Zhang ORCID: orcid.org/0000-0001-8206-1007 2
Molecular Horticulture volume 2 , Article number: 17 ( 2022 ) Cite this article
9682 Accesses
24 Citations
Metrics details
Over the past decade, systems biology and plant-omics have increasingly become the main stream in plant biology research. New developments in mass spectrometry and bioinformatics tools, and methodological schema to integrate multi-omics data have leveraged recent advances in proteomics and metabolomics. These progresses are driving a rapid evolution in the field of plant research, greatly facilitating our understanding of the mechanistic aspects of plant metabolisms and the interactions of plants with their external environment. Here, we review the recent progresses in MS-based proteomics and metabolomics tools and workflows with a special focus on their applications to plant biology research using several case studies related to mechanistic understanding of stress response, gene/protein function characterization, metabolic and signaling pathways exploration, and natural product discovery. We also present a projection concerning future perspectives in MS-based proteomics and metabolomics development including their applications to and challenges for system biology. This review is intended to provide readers with an overview of how advanced MS technology, and integrated application of proteomics and metabolomics can be used to advance plant system biology research.
Introduction
Plant research includes a wide range of scientific disciplines that involves all aspects of plant biology. It’s importance is becoming more apparent as we recognize how broadly plants impact human life, through nutrition, food security, medicine, biofuels and environmental sustainability (Gemperline et al. 2016a ). Plant research is essential to address key issues in environmental science, agriculture and medicine that are closely associated with human health and wellbeing. Over the past decade, systems biology studies have increasingly become the main stream in plant research (Sheth and Thaker 2014 ), leveraging the development of omics technologies through multi-omics integration and data processing (Feussner and Polle 2015 ; Ramalingam et al. 2015 ; Pazhamala et al. 2021 ).
The proteome is a time-dependent expression of an organism’s genome that is characterized with regard to protein localization, interactions, modification and turnover. Proteomics is the systematic identification and quantification of an organism’s proteome at a given time. It is a useful approach to discover biomarkers for specific stimuli, or for determining relevant biological pathways, molecular mechanisms and functional networks at the levels of biological organization (i.e., cell, tissue, organ etc.). Over the last two decades, comprehensive genomic sequence information has become available for an ever-increasing number of species. The development of next-generation sequencing and single molecule, real-time sequencing technologies for RNA sequencing has permitted genome-wide expression analysis in response to various stimuli, providing unparalleled opportunities for biomarker discovery by transcriptomics. However, mRNA levels do not provide a complete picture of cellular function. Most cellular functions such as plant stress tolerance involve multiple interactions of proteins and their metabolites. Furthermore, protein expression levels are dependent not only on transcript levels but also on translational efficiency and regulated degradation (Batelli et al. 2007 ; Liu et al. 2016b ). Finally, proteins function at specific sub-cellular localizations and are susceptible to post-translational modifications (PTMs, often required to enable function) in ways that cannot be predicted from transcript expression levels or from the genomic sequence. Therefore, it is essential to supplement transcriptomics data with direct measurement of protein abundance.
Metabolomics is another important component of “omics” lexicon, providing a global identification and profile of all metabolites (the metabolome) in a given biological system (Dettmer et al. 2007 ; Alseekh and Fernie 2018 ; Pinu et al. 2019 ). It is a rapidly evolving field of research in plant research as changes in metabolite abundance represent the chemical flux generated from various biochemical reactions, molecular mechanisms and biological pathways. Their proximity to phenotype is thought to make them more representative of the cell/organism’s physiological state, more directly reflecting the cascading effects of the environment, gene expression and regulatory processes (Astarita and Langridge 2013 ; Guijas et al. 2018a ). Thus, metabolomics is becoming a powerful tool to study plant molecular phenotypes for plant growth and development, and stress response.
Proteomics and metabolomics both rely on three basic technological cornerstones that include a method of fractionation to simplify complex mixtures; mass spectrometry (MS) to selectively acquire the data needed to identify and quantify individual peptides and metabolites, and bioinformatics analyses to correlate the empirical mass data with genomic or metabolite databases. In the past decade, the advent of high mass accuracy/resolution MS coupled with liquid/gas-chromatography, the development of new bioinformatics tools, and methodological schema for multi-omics integration, have provided not only high-throughput and high quality data generation but also significantly contributed to both biomarker discovery and mechanistic studies in plant research (Feussner and Polle 2015 ; Ramalingam et al. 2015 ; Gao et al. 2017 ; Tang et al. 2020 ; Pazhamala et al. 2021 ). Recently, omics research communities have begun to develop MS-based proteomics and metabolomics approaches applicable to the single cell-type and single cell levels. These new developments seek to unravel the unique functions of distinct cell types and/or single cells, despite the tremendous technical challenges involved, including the sensitivity limitations related to the nature of the sample, miniaturization and presence of cell wall in plant single cell analysis (Labib and Kelley 2020 ; Hu et al. 2021a ; Taylor et al. 2021 ).
In this article, we focus on new advancements in proteomics and metabolomics technologies including discovery proteomics, quantitative PTMs, protein interactions, untargeted metabolomics, fluxomics, targeted metabolomic and their applications in plant biology research. We also highlight the latest developments in single-cell-type and single-cell proteomics and metabolomics in plants as outlined in Fig. 1 . We present an intensive review on how these applications are leveraged by the advanced MS technologies and the development of reoptimized workflows that enable omics research in plants.
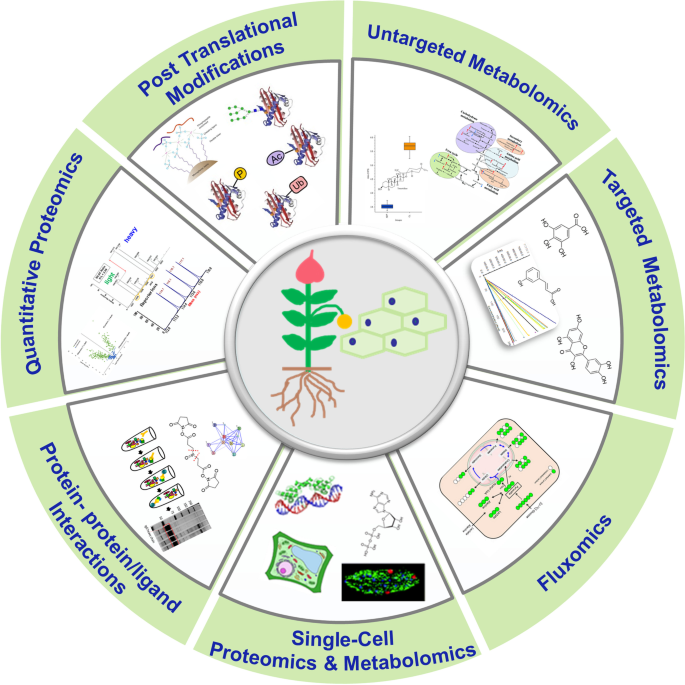
Major types of mass spectrometric based proteomics and metabolomics techniques used for global proteins and metabolites study that are applicable to plant research
Advances in proteomics technologies
Experimental design, sample preparation, and separation technologies.
Over the last two decades, proteomics has been grown in prominence as a strategy to study plant biology (Agrawal et al. 2013 ; Liu et al. 2019 ; Jorrin Novo 2021 ). Similar to other fields (Bhawal et al. 2020 ; Nakayasu et al. 2021 ) a rigorous experimental design is essential for meaningful biological interpretation. This requirement includes all phases of the experiment including plant cultivation, application of treatments, choice of plant tissues, etc. (Rose et al. 2004 ; Atwell 2016 ). The most critical steps in any proteomics study are protein extraction and sample preparation (Rose et al. 2004 ; Komatsu and Jorrin-Novo 2021 ). Given the complexity and diversity of proteins with respect to molecular weight, charge state, hydrophobicity, dynamic range, modification state and cellular distribution, any single extraction protocol will incorporate biases with respect to particular classes of proteins, particularly in whole body or organ extracts. An effective way to minimize this bias is to focus on a subfraction of the proteome by targeting a particular tissue, cell type or subcellular structure (Zhang et al. 2011 ; Bouchnak et al. 2019 ). A variety of sample preparation strategies incorporating many separation technologies have been developed for an array of applications. There are two main approaches: an electrophoretic-based approach (also known as a protein-based approach) and a gel-free approach (also called a peptide-based approach). The electrophoretic approach has been largely abandoned as a viable experimental strategy, although it still finds occasional use in a number of niche applications (Jorrin-Novo et al. 2019 ), it is generally considered an archaic technique putting it beyond the scope of the current work.
Since the first decade of this century chromatographic separation technologies (ultra-high performance liquid chromatography (UHPLC) and associated columns) and MS hardware have made dramatic advances and these have enabled the shift from gel-based to chromatography-based proteomics employing both label-free or label-assisted techniques for quantitation. Most current strategies involve separation/quantification at the peptide level. Complex protein samples are denatured and enzymatically digested, and then the resulting peptide mixture is separated using one or two dimensions of LC (2D-LC). The choice between these two approaches hinges on 1), the complexity of the proteome being investigated and 2), the depth of coverage required. One-dimension approaches have the advantage of speed and utilize a minimum of instrument time but at the cost of reduce proteome coverage and a bias in favor of the largest and most abundant proteins. Two dimensional approaches utilize significantly larger amounts of instrument time and increase turnaround but dramatically increase proteome coverage and minimize the bias for large and abundant proteins. There are several standard approaches to achieve the first dimension of separation that include high pH reversed-phase LC (RPLC), strong cation ion exchange (SCX), hydrophilic-interaction chromatography (HILIC), and affinity chromatography. The second dimension of chromatography is almost always low-pH RPLC coupled directly with tandem mass spectrometry. These approaches have emerged as the preferred tools for protein profiling and PTMs characterization (Ceballos-Laita et al. 2020 ).
At the whole protein level, affinity purification MS (AP-MS) was a major breakthrough in plant research and is used to investigate protein degradation, PTM identification and localization as well as protein-protein interactions (Bontinck et al. 2018 ; Zhang et al. 2019 ). It works on the basic principle of reversible interaction between the affinity ligand and the targeted proteins or specific PTMs. While used less frequently, this approach has been successfully applied at the peptide level as well. A related approach called Immunoprecipitation is a single-step purification that uses an antibody specific for the bait protein or a generic antibody against an exogenous affinity tag covalently linked to the bait protein. This single-step AP can also be performed without the need for specific antibodies such as the streptavidin-binding peptide-tag and His tag, which enable trapping with streptavidin and Ni 2+ resins, respectively. Hence this technique is also referred as pull-down. Due to its efficiency and simplicity, this approach has gained a growing popularity in plant research, but development of specific antibodies against targeted proteins is expensive and time consuming. As a result, generic antibodies for specific affinity tags including florescent proteins like GFP are most commonly used for purification. One of the major limitations of this approach is the requirement for constructing a recombinant clone expressed in an appropriate host cell. Other limitation includes tedious experimental procedures and non-specific binding that can result in false positive results. Affinity binding is also commonly used as an enrichment method of PTMs like phosphorylation with immobilized metal affinity chromatography (Bontinck et al. 2018 ). Laser capture microdissection (LCM) is a technique by which cells of a single-type can be harvested from tissue sections visualized under microscope (Chen et al. 2020b ). Harvested cells can provide DNA, RNA, and protein for the profiling of genomic characteristics, gene expression, and protein abundance from single-type of cell. Earlier, proteomic analysis of LCM tissues required a larger number of cells, however this problem is dramatically improved with the advent of more powerful separation technologies and highly sensitive mass spectrometers.
Advanced MS technologies for proteomics
The growing list of applications that are amenable to proteomics has been driven by the rapid advancement of MS technologies over the last decade. The latest Orbitrap Eclipse Tribrid mass spectrometer with advanced quadrupole mass filter, dual-pressure linear ion trap and Orbitrap mass analyzers, is an excellent example (Yu et al. 2020 ). The system provides maximum analytical capability and flexibility for both top-down proteomics (Kelleher 2004 ; Cleland et al. 2017 ) in direct analysis of intact proteins, and bottom-up proteomics for analysis of peptides resulting from the digestion of complex protein mixtures. Bottom-up proteomics has demonstrated broad applications to plant research and is the focus of this review. Another excellent example is the advancement of ion mobility spectrometry (IMS)-based MS such as trapped IMS in timsTOF Pro (Meier et al. 2021 ), Twave IMS in SYNAPT G2-Si (Hernandez-Mesa et al. 2020 ) and field asymmetric IMS (FAIMS) in Orbitrap mass spectrometers (Hebert et al. 2018 ). IMS, as a gas phase “electrophoresis” technique offers rapid structural separation with measured collision cross-section (CCS) values, providing an additional dimension of separation for isobaric molecules and isomers in complex samples (Burnum-Johnson et al. 2019 ). Thus, IMS-based MS greatly facilitates fast, sensitive and robust proteomics and metabolomics profiling, allowing proteomics for a true high-throughput era. Furthermore, IMS-MS has been demonstrated in contributing greatly to the recent success of single-cell proteomics for increased selectivity by removing singly charged species (Kelly 2020 ).
Effective MS-based proteomics strategies have been developed to address the different biological and analytical challenges depicted in Fig. 2 . MS data acquisition for most labeled or label-free proteomics protocols the experiments are carried out in a data dependent acquisition (DDA) mode with dynamic exclusion to minimize the collection of redundant MS spectra (Hart-Smith et al. 2017 ). DDA defines the maximal scan rate at which mass spectrometers can acquire MS/MS data for near co-eluting peptides. Another data acquisition strategy is data-independent acquisition (DIA) (Zhang et al. 2020a ), which has been gaining acceptance in recent years. DIA involves parallel MS/MS analysis of multiple precursor ions simultaneously, allowing for improvements in quantitative reproducibility, depth of proteome coverage while allowing for a post hoc targeted interrogation of the data using either in-silico fasta database or specially constructed spectral libraries.
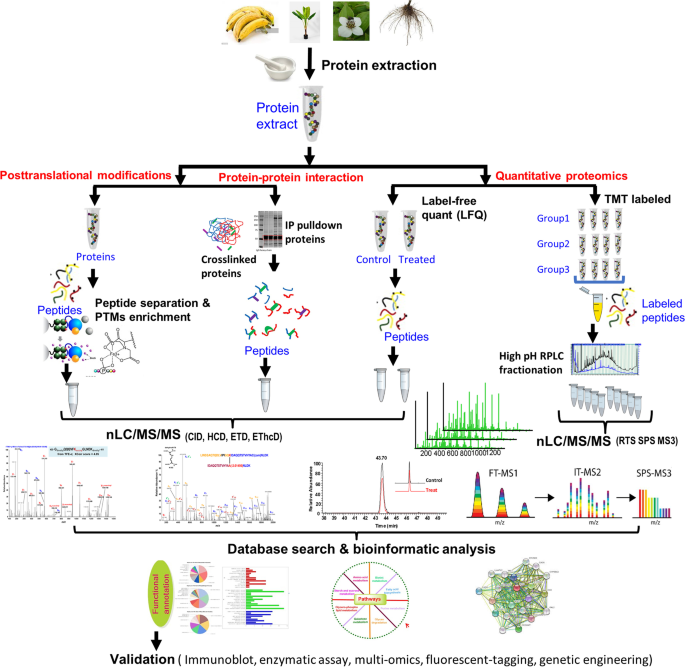
Schematic diagram represents general plant proteomics workflows. Proteins extracted from plant materials are fractionated using either gel-based or gel-free techniques. Three major types of proteomics analysis for identification and quantitation of posttranslational modifications, protein-protein interaction and quantitative proteomics were presented. The MS raw data generated from each workflow using different mass spectrometric techniques are carried out by database search, statistical analysis and bioinformatics analysis. Finally, the outcomes are to be further validated for either generating biological hypothesis or validation of initial mechanistic hypothesis
Quantitative proteomics
One of the advantages of MS-based proteomics is the ability to systematically highlight changes in protein abundance between biological samples through quantitative analysis. Protein abundance difference reflects different regulated states of the cells, a disease state or other biological perturbations such as stress from biotic or abiotic factors or experimental manipulation. Quantitative proteomics for protein abundance profiling is widely-used technique to establish a chemical phenotype associated with a given biological states and to identify biomarkers associated with specific biological state such as disease, abiotic stress. However, it is extremely important to evaluate the replicate datasets to dissect and distinguish biological variations from technical variations in pilot experiments whenever possible. So far either stable isotope-labeling methods or label-free techniques have been frequently applied in quantitative proteomics studies.
The isotopically coded chemical label-based approaches remain most popular in quantitative proteomics due to their multiplexing capabilities which couple high throughput, quantitative accuracy and compatibility with 2D liquid chromatography tandem mass spectrometry (LC-MS/MS) analysis. Chemical labeling using isotope coded isobaric reagents like tandem mass tag (TMT) (Thompson et al. 2003 ) and Isobaric tags for relative and absolute quantification (iTRAQ) (Ross et al. 2004 ) represent a high-throughput and robust technique that requires further discussion. They have emerged as one of the most widely-used techniques in quantitative shotgun analysis that is particularly useful for global proteome profiling and associated functional changes in plants. The isobaric tag consists of an amine-specific reactive group for labeling free amine groups of peptides, a balance group and a reporter group released under MS2 or MS3 fragmentation that provides mass signature for relative quantitation. The major advantage of TMT labeling is that it can afford a high degree of multiplexing (up to 18 plex) since it is able to monitor up to 18 samples in a single analysis (Li et al. 2021b ). The main disadvantage of MS2-based quantitation is the co-isolation and co-fragmentation of near isobaric labeled ions, which causes a ratio distortion problem (Ting et al. 2011 ). This distorted ratio issue can be dramatically reduced using synchronous precursor selection (SPS) MS3 method (McAlister et al. 2014 ) in which multiple MS2 fragment ions (acquired for peptide identification) are further fragmented in MS3 yielding a reporter ion population without the interfering signals. However, due to the extended cycle time associated with the inclusion of an MS3 scan, the SPS MS3 approach results in a decreased spectral acquisition rate and a reduction in the number of proteins quantified of ≈ 30% compared to traditional MS2-DDA analysis. To maintain in-depth proteome coverage with accurate and precise quantitative profiling, a real-time search (RTS) SPS MS3 method has been developed recently (Erickson et al. 2019 ). RTS SPS MS3 approach carries out MS2 spectral identifications in real time within milliseconds so that only identified peptides can trigger quantitative MS3 scan, which increases the number of productive precursors that are subject to MS3 offsetting the longer cycle time and resulting in enhanced proteome coverage and accurate TMT quantitation (Fu et al. 2021 ).
Another popular labeling approach is stable-isotope labelling by amino acids in cell culture (SILAC). This has been covered in literally many excellent prior reviews of this subject (Gruhler et al. 2005 ; Matthes et al., 2014 ). Few studies have been done with SILAC labeling in plant research, due to the requirement for metabolically labeling under culture conditions. So far, most SILAC-based labeling proteomics studies have been done to study the formation of signal-dependent protein complexes, and modification-dependent protein-protein interactions in Arabidopsis thaliana seedlings by SILAC, but suboptimal labeling efficiency have been known to compromise quantitation (Gruhler et al. 2005 ; Thelen and Peck 2007 ; Schutz et al. 2011 ).
Despite the popularity of label-based methods, the main limitations of the label methods include cost of isotopic labeling and increased complexity of the experimental procedures which can lead to sample loss and add experimental variations. As a result, label-free quantitation (LFQ) approaches have recently been widely used as alternatives. In LFQ analysis, the intensities of identical peptides from two or more samples can be compared directly by traditional DDA method. However, recent developments in bioinformatics software allows DIA to be comparable with DDA in the number of peptide identifications for label-free samples while still allowing precise quantification (Hu et al. 2016 ). The improved protein coverage by DIA makes it possible to carry out label-free quantitative analysis of very complex samples (Rosenberger et al., 2014 ).
Protein PTMs: plant phosphoproteomics
PTMs are important chemical changes of proteins that increase proteome diversity tremendously and allow for effective regulation of cellular processes. More than 400 distinct types of PTMs have been found which have been shown to impact protein function (Khoury et al. 2011 ). Therefore, the identification and mapping of PTMs are important activities because they help to define the proteome in terms of “proteoforms”. These PTM studies typically require an enrichment step due to the low stoichiometry of most PTMs. The presence of PTMs is implied by specific mass shifts, thus the specific site of the modification can be deduced through the analysis of fragmentation data (MS 2 -MS n ). Historically, CID or HCD fragmentation has been used to analyze PTM peptides. However, several non-ergodic fragmentation strategies (ETD, ECD, etc.) have proven valuable for the analysis of labile PTMs (Chi et al. 2007 ).
The most extensively studied PTM types are phosphorylation (on S/T and Y residues), acylation (acetylation, succinylation and malonylation on K), ubiquitination (K) and glycosylation (N, S/T and hydroxyproline) (Ramazi and Zahiri 2021 ). Phosphorylation is one of the most important and well-studied reversible PTMs in plants. Kinases catalyze protein phosphorylation by transferring a phosphoryl group typically from ATP or ADP to the hydroxyl group of S/T/Y residues, but phosphorylation on several unusual residues such as His, Asp, Cys, Arg and Lys has been reported (Hardman et al. 2019 ). Phosphatases are responsible for removing the phosphor group from the modified residues. Phosphorylation in plants regulates a wide range of cellular processes such as transmembrane signaling, intracellular amplification of signals, and cell-cycle control including hormone sensing and environmental stress responses (Schulze 2010 ; Ingelsson and Vener 2012 ). It often leads to protein structural changes that can directly modulate protein activity, and induce changes in interaction partners or subcellular localization (Li et al. 2015b ). Plant genomes encode twice the number and diversity of kinases compared with mammalian genome, indicating the importance of the plant phosphoproteome in regulating responses to both abiotic and biotic stresses (Zulawski et al. 2013 ; Silva-Sanchez et al. 2015 ).
Considerable analytical challenges remain for the study of PTM in plants particularly in phosphoproteomics due to a number of factors including the high dynamic range and complexity of the plant proteomes, the unique challenges associated with the plant cell walls, and the interference from chlorophyll and secondary metabolites. A universal protocol incorporating optimized protein extraction procedures involving guanidine hydrochloride, methanol-chloroform precipitation, and phase-transfer surfactant assisted tryptic digestion has been reported recently. This new approach was found to increase the coverage of tomato phosphoproteome more than 2-fold compared with the standard protocol (Hsu et al. 2018 ). In the past decade, many phospho-enrichment strategies have been developed to enhance the identification of low abundant phosphopeptides and phosphoproteins (Batalha et al. 2012 ; Silva-Sanchez et al. 2015 ). Immobilized metal affinity chromatography (IMAC) is often coupled with strong cation exchange (SCX) for two-step phosphopeptide enrichment in large-scale phosphoproteomic studies. However, more recently the use of metal dioxide affinity chromatography such as titanium dioxide (TiO 2 ) and zirconium dioxide (ZrO 2 ) has become more common. A serial enrichment procedure with both TiO 2 and ZrO 2 has been shown to increase the efficiency of capturing phosphopeptides (Gates et al. 2010 ). A combination of TiO 2 enrichment and HILIC fractionation for subsequent LC-MS/MS analysis resulted in the identification of 1500 phosphopeptides from 685 phosphoproteins in the leaves of two varieties of wheat that suggested differential regulation of the phosphorylation status of signaling proteins, transcription and translation regulators, and membrane-associated proteins (Yang et al. 2013a ). Given the required enrichment steps, most phosphoproteomics studies are conducted using label-free LC-MS/MS workflows (Engelsberger and Schulze 2012 ; Wang et al. 2013a ; Qiu et al. 2016 ; Ford et al. 2020 ; Li et al. 2021c ). However, iTRAQ/TMT labeling has also become popular in plant phosphoproteomics (Yang et al. 2013a ; Fan et al. 2014 ) due to their shared capability to multiplex up to 18 samples in a single experiment for both enrichment and LC-MS/MS analysis. Furthermore, this approach allows for parallel global proteome analysis using either a TiO 2 flow-through fraction (Yang et al. 2013a ) or a small aliquot of the sample for the quantitative work while reserving the majority of the sample for enrichment and phosphopeptide analysis (Yang et al. 2018 ). Parallel proteomics and phosphoproteomics analyses allow one to distinguish the changes for each of the phosphorylation sites of a given protein from the change of that proteins general abundance and is particularly useful for determining the biologically important sites of proteins that contain multiple phosphorylated sites with different degrees of change.
It should be pointed out that identifications, localizations, and quantifications of different combinations of PTMs on the same protein (various proteoforms) are generally difficult by traditional bottom-up proteomics. However, both top-down proteomics for direct analysis of whole proteins and middle-down proteomics for analysis of large peptides by limited proteolysis can be used to detect multiple co-occurring PTMs in a specific proteoform and a large peptide, respectively (Leutert et al. 2021 ). Top-down proteomics for characterization of proteoforms has been well covered by several recent review articles (Schaffer et al. 2019 ; Carbonara et al. 2021 ; Melby et al. 2021 ). We will omit this topic and direct the interested reader to these.
Protein-protein interactions and protein complexes
Protein–protein interactions (PPIs) are fundamental to all biological processes (Cusick et al. 2005 ). Vital cellular functions such as DNA replication, transcription and mRNA translation, require the coordinated action of many proteins that are assembled into an array of multi-protein complexes of distinct composition and structure. Many important biological processes in plants such as organ formation, homeostasis control, plant defense, signal transduction and stress response are comprised of, and regulated by, dynamic signaling networks of interacting proteins that directly or indirectly respond to specific effector molecules (Bontinck et al. 2018 ; Struk et al. 2019 ). Since almost all proteins interact with other molecules a comprehensive determination of PPIs within an organism is an essential aspect of systems biology that is used to uncover unknown functions and to gain insight into complex cellular networks. However, understanding the dynamic nature of protein complexes with respect to composition and stability and cellular state presents a significant challenge. Many different methods for determining PPIs have been developed and the topic has been well-reviewed (Struk et al. 2019 ). These include the yeast two hybrid (YTH) system, the first technique used for large-scale interactome maps (Uetz et al. 2000 ), affinity purification coupled to MS (AP-MS) (Gingras et al. 2007 ), proximity labeling coupled to MS (PL-MS) (Kerbler et al. 2021 ) and bimolecular fluorescence complementation (Miller et al. 2015 ). Substantial advances in determining composition, regulation and function of molecular complexes have been obtained by MS-based proteomics (called interaction proteomics or interactomics) leading to a greater understanding of the molecular basis of complex biological processes (Aebersold and Mann 2016 ).
Affinity purification and mass spectrometry (AP-MS) is one of the enabling developments for PPI studies. Targeted proteins complexes are isolated from plants using antibodies against either the protein of interest or a tagged protein, which is often called co-immunoprecipitation (Co-IP). It has the great advantage of capturing the physiological state, abundance, and interactions of the targeted protein without the need for cloning or overexpression. The protein complexes isolated by Co-IP are then eluted and analyzed by LC-MS/MS (Fukao 2012 ). These affinity-based methods have improved greatly because of the development of highly sensitive MS instrumentation and novel bioinformatics approaches (Armean et al. 2013 ; Qu et al. 2017 ). To minimize the impact of non-specific binding, a second purification step has been introduced by means of a double affinity tag. One of the most frequently applied tandem affinity purification tags in plant research is the GS tag and its derivatives (Van Leene et al. 2007 ). The GS tag consists of two immunoglobulin domains of protein G and a streptavidin-binding peptide separated by a cleavage site. AP-MS is used to study plant growth and development in the relevant biological contexts, such as specific plant organs, for example, flowers, leaves and roots and provides an enhanced view of the protein complex composition (Batelli et al. 2007 ; Chang et al. 2009 ). A combined AP-MS with an LFQ method has been developed (Keilhauer et al. 2015 ) and well described in a recent review article (Kerbler et al. 2021 ). This has become a common approach to differentiate true interactors from the background. In this method, the increased amounts of unspecific binding proteins can be advantageous, because they are used in the postprocessing pipeline for a more exact normalization and as a kind of quality control. The LFQ combined with AP-MS can also be used to assess the dynamics of PPIs during cellular signaling or after cellular perturbations, because protein complexes copurified with the same bait under two different conditions can be compared in a quantitative manner. The Co-IP allows for the identification of PPIs in certain tissues or during specific developmental stages and in different genetic backgrounds and is also considered one of the standard methods for PPI validation. However, this AP-MS technique does not provide information about the direct interaction between proteins but rather about their coexistence in a higher order protein complex (Xing et al. 2016 ). The topology of the protein interactome is not achievable from the AP-MS strategy. PL-MS uses enzymes that produce reactive molecules for covalently interacting with proteins in close proximity. Although the use of PL-MS in plant still remains its infancy, recent development of new proximity labeling enzymes TurboID in planta (Zhang et al. 2020b ), pupylation-based interaction tagging (Pup-IT) for PPIs at membranes (Siva Sankar and Dengjel 2021 ) and limited proteolysis-MS (LiP-MS) (Pepelnjak et al. 2020 ) for protein-small molecule interactions has considerably expanded its applications in plants.
Another development for the identification of PPIs in plants involves cross-linking mass spectrometry (XL-MS) (Zhu et al. 2016a ; Liu et al. 2018 ). Chemical cross-linking followed by mass spectrometry analysis enables identification of proximal amino acid residues within protein complexes, providing vital insights into the structure and interactions of proteins/protein complexes (Chavez and Bruce 2019 ). Notably, the recent development of a MS-cleavable cross linkers such as disuccinimidyl sulfoxide (Kao et al. 2011 ) and disuccinimidyl dibutyric urea (DSBU) (Ihling et al. 2020 ) allows to cleave cross-linked peptides during MS/MS for subsequent MS3 acquisition of cleaved peptides, which facilitates peptide identification using traditional database based approaches (Liu et al., 2015 ) and allows multiplexed quantitative XL-MS (Yu et al. 2016 ). In addition, hydrogen–deuterium exchange mass spectrometry (HDX–MS) is able to determine the interaction surfaces and solvent-exposed regions and is emerging as a powerful methodology to study protein dynamics, protein folding, protein-protein interactions, and protein-small molecule interactions (Masson et al. 2019 ; Li et al. 2020d ; Gutkowska et al. 2021 ).
The latest development of thermal proteome profiling (TPP) technology can also be used for interrogating protein-protein interactions (Mateus et al. 2020 ). This TPP was initially developed for drug discovery in screening of the targeted proteins by the known ligands (drugs) under a more physiologically relevant environment such as intact live cells level (Savitski et al. 2014 ). The basic concept of TPP is that proteins become more resistant to heat-induced unfolding when complexed with a ligand or other macromolecules. Combining the principle of the cellular thermal shift assay (TSA) with multiplexed quantitative MS such as TMT10-plex compared to the lysate TSA, TPP allows for detecting protein thermal stability (melting temperature, Tm ) on a proteome-wide scale (Franken et al. 2015 ). TPP is becoming a powerful tool for detecting a wide range of physiological changes in protein state: protein-metabolite interactions, post-translational modifications, protein-protein and protein-DNA interactions. (Mateus et al. 2020 ) and an example in plant has been reported in Arabidopsis thaliana (Volkening et al. 2019 ). However, its limitations include: 1) requirement of substantially changed percentage of the population of any single protein sequence to be reflected in its altered T m, which will lead the induced change of T m by most PTMs with low stoichiometry difficult to be detected; 2) no information on domain change and what amino acids responsible for identified proteins with altered Tm . Therefore, TPP is best used in conjunction with other structural proteomics methods (Blackburn et al. 2022 ).
MS data processing, assembly and bioinformatics
A key advancement in MS-based proteomics was the development of algorithms in database search software for peptide identifications (which infer protein identity) by matching the observed masses of precursor and fragment ions with those predicted from a sequence database. The algorithms allow for automated interrogation of genomic databases with acquired large MS and MS/MS datasets using predetermined parameters and other search criteria to generate lists of putative peptide spectrum matches. As genome-wide next-generation sequencing and RNA sequencing technologies continued to advance, the number of species with fully sequenced genomes has exponentially increased, including 341 plant genomes ( https://www.ncbi.nlm.nih.gov/genome ) and 181 horticultural species (Chen et al. 2019 ). Thus, the lack of databases is no longer a major bottleneck in most plant proteomics research. Most protein sequence databases derived from plant genomic sequences can be downloaded from NCBI Viridiplantae, GenBank, DDBJ, and UniProt. Several specific sequence retrievals can also be performed from databases dedicated to plants such as Phytozome, plaBi, and Gramene database, which are subsets of the Ensembl Plants database and PlantGDB. However, large proteomics datasets acquired by rapidly evolving MS technology with different acquisition workflows employing multiple fragmentation methods present a number of challenges to determine the correct peptide assignments to MS/MS spectra. These challenges require that extremely powerful search algorithms be constantly enhanced and developed to take full advantage of the data acquisition technology. Table 1 shows a partial list of commonly used database search software having the search engines tools for DDA proteomics raw files from five major mass spectrometer vendors (Thermo, ABSciex, Waters, Bruker and Agilent) and 10 public available search tools such as MaxQuant and Mascot etc.. The search engines and software tools specifically for DIA proteomics datasets were extensively described in a recent review paper (Zhang et al. 2020a ). To properly interpret the protein identification in particularly large shotgun proteomics dataset against a large database, it is necessary to have a reliable estimate of the false discovery rate (FDR), which is a measure of the percentage of putative protein identifications that are likely to be false. Almost all database search algorithms have integrated a target/decoy strategy for determining the FDR with Benjamini–Hochberg procedure. Even with the high quality of MS and MS/MS spectra acquired by high mass accuracy/resolution instruments, it is necessary to set up an FDR threshold in effectively controlling the number of false positives in proteomic data (Choi and Nesvizhskii 2008 ).
The diversity in data-analysis strategies from different types of mass spectrometers including various outputs leads to big challenges for the computational analysis of MS data that often leads to substantial differences between results obtained with different software tools. Therefore, some “3rd party” software tools were developed enabling analysis of raw data files from multiple vendors’ platforms to address some of these challenges. Among them, MASCOT (Perkins et al. 1999 ) and MaxQuant/Andromeda (Cox and Mann 2008 ) are the most widely used database searching tools for large-scale proteomics data. Mascot used an additional Distiller algorithm for label-free proteomics. The universal free software, MaxQuant using its own Andromeda search engine for peptide identification (Cox et al. 2011 ; Valikangas et al. 2018 ) is applicable for mostly label-free and labeled quantifications from high resolution data files, OpenMS, an open-source software platform is another tool providing a highly flexible and professional software environment equally suited for end users (Rost et al. 2016 ). Peptide identifications were performed within PEAKS software, another vendor-neutral tool also using its own search engine PEAKS DB combined with PEAKS de novo sequencing (Zhang et al. 2012 ). An additional Peaks Q module allows for relative protein abundance changes across a set of samples simultaneously. For quantitative proteomics, due to different strategies or workflows used for data acquisition, more suitable tools were developed, such as PyQuant and SILVER for stable isotope labeling quantification, RIPPER and LFQuant for label-free quantification (Chang et al. 2014 ; Mitchell et al. 2016 ; Van Riper et al. 2016 ). Most recently, another efficient quantitative software PANDA was developed that supports both label free and labeled quantitation with existing peptide identification tools and accurate quantitation (Chang et al. 2019 ).
Following data processing, database search and statistical analysis for discovery of candidate proteins and/or their modifications or interacting complexes, further bioinformatics analyses are required for functional annotation of those protein candidates. The most widely used functional annotation is ‘Gene Ontology’ (GO) having three separate GO terms as biological process, cellular component, and molecular function respectively, along with pathway enrichment analysis. A biological pathway is a series of reactions within the cell that exert a specific biological function. The proteins that are directly involved in reactions plus those that regulates the pathways belong to pathway databases. Some resources and databases available for the protein pathways such as KEGG, Ingenuity and Pathway Knowledge Base Reactome are the most often used pathway databases with a comprehensive data for protein metabolism, signaling and interactions. Perhaps the best-known software for automated functional annotation pipeline is BLAST2GO (Conesa and Gotz 2008 ) that also incorporates InterProScan for protein family classification and KEGG data. In functional analysis involved in cellular signaling, plant phosphorylation site databases including PhosPhAt (Durek et al. 2010 ) for Arabidopsis , Plant Protein Phosphorylation DataBase (P3DB) (Gao et al. 2009 ) for 45 plant species ( https://www.p3db.org/home ) and PHOSIDA (Gnad et al. 2011 ) can be used for predicting phosphorylation sites with an average predicting accuracy of 82.4% for pSer, 78.6% for pThr, and 89.0% for predicting pTyr by PlantPhos tool (Lee et al. 2011 ). For protein complex studies, STRING is not only a widely used database with wealthy protein interaction data, but also it connects to various other resources for literature mining. Protein networks can be acquired based on the list of proteins/genes provided and the available interactions using the STRING database ( https://string-db.org/ ). In addition, Biological General Repository for Interaction Datasets (BioGRID) contains a large collection of protein–protein interactions for all major model organism species and humans (Chatr-Aryamontri et al. 2017 ). Another popular tool is Skyline, an open source software developed for targeted proteomics data analysis (Pino et al. 2020 ) over the past decade, but recently it becomes available for targeted metabolomics data analysis (Adams et al. 2020 ). Notably, Skyline enables to support almost all of data analysis workflows such as SRM/MRM, PRM, DIA and targeted DDA.
Proteomic applications in plant research
Mechanistic understanding of plant stress tolerance.
Plants are constantly affected by abiotic and biotic stresses during growth, development and adaptation to their environment. Plant proteins and metabolites play an important role in the maintenance of cellular homeostasis, and regulate physiological changes to better adapt to prevailing environmental stresses. The plant immune system responds to biotic stress as a complex system with interactions and crosstalk between multiple signaling pathways characterized by various signaling proteins and with a diverse set of stress-related proteins. Therefore, protein profiling under various stress conditions has been extensively investigated (Kosova et al. 2018 ; Liu et al. 2019 ). Quantitative proteomics provides comprehensive analysis of proteins allowing for the identification of key metabolic pathways affected by biotic or abiotic stress. iTRAQ labeling in proteomics enables to analyze and quantify up to eight phenotypes with high resolution (Pierce et al. 2008 ), and is widely used in model plants such as Arabidopsis (Lan et al. 2011 ) and rice (Wang et al. 2014b ) but also has provided a platform to profile and understand the non-model species through comparative proteomics (Yang et al. 2011 ; Zhou et al. 2016a ). We are one of the earlier groups applying iTRAQ-based quantitative proteomics to investigate the temporal responses of plantain ( Musa spp. Dajiao; ABB Group) proteome to identify the proteins related to the cold stress as Dajiao has superior cold tolerance compared with Cavendish Banana ( Musa spp. Cavendish; AAA Group), an important tropical fruit with high economic value (Yang et al. 2012 ). The global proteome results suggest that an increase in antioxidant capacity via adapted ROS scavenging capability, reduced production of ROS and lipid peroxidation contributes to molecular mechanisms for the increased cold tolerance in plantain. Proteomic profiling and identification of some membrane proteins has great potential value for developing cold tolerant banana cultivars. Further iTRAQ analysis of the membrane proteomes of both Daojiao and Cavendish Banana under cold stress showed membrane-bound proteins such as peroxidases and aquaporins that were consistently induced at an early stage of cold stress (He et al. 2018 ). After cross-verification by qRT-PCR and MRM-targeted quantitation, and fluorescent-based subcellular localization analysis, the authors concluded that 2 peroxidases, and 5 aquaporins are mainly involved in decreased lipid peroxidation and maintaining leaf cell water potential, which appear the key cellular adaptations contributing to the cold tolerance of Dajiao (He et al. 2018 ). These proteomics findings provided a good complement to the transcriptomics datasets for Dajiao’s high cold tolerance and its mechanisms (Yang et al. 2015 ). Meanwhile, a similar iTRAQ approach was used for discovery of the key ergosterol biosynthesis pathway to the conidial germination of the soilborne fungus Fusarium oxysporum f. sp. cubense tropical race 4 ( Foc TR4), a most important lethal disease of Cavendish banana (Deng et al. 2015 ). This finding led to the successful development of transgenic bananas with superior resistance by host-induced gene silencing of two ergosterol biosynthesis genes ( ERG6/ERG11 ) in Foc TR4 (Dou et al. 2020 ), which lays the groundwork for disease-resistance breeding in bananas and possible other crops.
Since poor correlation is often found between gene expression levels and protein abundances in organisms under abiotic and biotic stresses, proteomics has thus become the preferable strategy to identify underlying key factors and metabolic pathways (Feussner and Polle 2015 ), which is proven as an effective approach to identify candidate proteins in response to cold stress in plant species (Zhang et al. 2016 ; Gao et al. 2019 ). Jiang et al. conducted a TMT10plex-based global proteome analysis for the leaves of Citrus junos seedling under the cold stress. These authors identified over 400 proteins accumulated in seeding leaves that are mainly related to the starch and sucrose metabolism as well as secondary metabolism. After physiological analysis, the authors propose that enhanced sugar and secondary metabolisms are the potential factors underlying the response of citrus rootstock to cold stress (Jiang et al. 2021 ). Qin et.al. performed root proteome analysis by the TMT-based quantitative methods for the characterization of proteins in rapeseed to identify the mechanisms underlying rapeseed root adaptions to nitrogen deficiency (Qin et al. 2019 ). Proteins involved in cell wall organization or biogenesis were observed in high abundance, while most identified peroxidases were reduced in the N-deficient roots. Peroxidase activities were found decreased, which might promote root elongation while lowering the solidity of N-deficient roots. In recent plant proteomics analysis, LFQ approach particularly based on DIA method has been increasingly used for global quantitative profiling that provides insights into many processes like stress response and tolerance, nutrient sensing and development. Several proteomic studies have reported in-depth identification of differentially expressed transcription factors responsible for the development of fruits or other organs of tissues at various stages of development. One such study was performed using DIA for two winter rapeseed cultivars, one with cold tolerance and another with cold sensitive, and these authors discovered that the cold tolerance is related to reactive oxygen species (ROS) scavenging, possibly through metabolic pathways including flavonoid and ubiquinone biosynthesis, and other terpenoid-quinone biosynthesis (Mi et al. 2021 ). Li et al. conducted a global proteomics analysis for Morus alba leaves under high level ultraviolet-B (UV-B) radiation and dark incubation (UVD) by SWATH-based DIA quantitative analysis. Other than significantly increased photorespiration in UVB group and phenolic compounds in UVD group, the abundances of proteins involved in the ubiquitin-proteasome system (UPS) and antioxidative enzyme activities were significantly increased in both UVB and UVD groups, suggesting UPS related proteins participated in the resistance to UV-B radiation through abscisic acid (ABA) signaling and protein degradation (Li et al. 2022b ). Another DIA-based LFQ investigation of the global proteomes of WT tomato fruit and its cd2 mutant was conducted to identify tomato proteins regulated by the CUTIN DEFICIENT2 transcription factor and involved in cutin biosynthesis (Martin et al. 2016 ).
Quantitative proteomics for global PTM analysis is a fast-growing field that provides new insights into the regulatory roles of protein PTMs in cellular metabolic networks and has been widely used for probing stress tolerance in plants (Liu et al. 2019 ). Protein phosphorylation is an important signaling mechanism underlying the plant response to biotic and abiotic stress (Rampitsch 2017 ; Liu et al. 2019 ). Most studies in plants have been focused on protein kinases and identification of the phosphorylated substrates. For example, many plant kinases are activated and positively regulate plant frost tolerance at the post-translational level. The mitogen-activated protein kinases (MAPK) constitute one of the most important signaling mechanisms in plants, and plays essential roles in enhanced frost tolerance (Furuya et al. 2013 ; Gao et al. 2017 ). In a TMT-based comparative phosphoproteomics analysis of Daojiao and Cavendish bananas under cold stress, the phosphorylation level of Thr31 on MAPK kinase 2 (MKK2) was increased significantly in the cold-tolerant Dajiao cultivar along with decreased MKK2 abundance for a time course of cold stress. Meanwhile, no detectable T31 phosphorylation with increased abundance of MKK2 protein was found in the cold-sensitive cultivar, Cavendish (Gao et al. 2017 ). These findings provide new evidence that the signaling pathway of cellular MKK2 phosphorylation is associated with the molecular mechanisms of high tolerance to cold stress in Dajiao. Tan et al. reported a parallel proteome and phospoproteome profiling of Arabidopsis seedlings under short-time cold stress using a DIA-LFQ analysis. These authors found a rapid (within 2 h of cold stress) and broad change of phosphorylated peptides from >1200 proteins that includes >140 kinases, >40 transcriptional factors and >40 E3 ligases. Those early response proteins to cold stress were linked to phospholipid signaling, cytoskeleton reorganization, calcium signaling, and MAPK cascades (Tan et al. 2021 ). In the plant target of rapamycin (TOR) kinase, a conserved serine/threonine protein kinase was found to play an essential role in maintaining cellular homeostasis. A combined quantitative phosphoproteomics analysis involving a targeted TOR complex in Arabidopsis thaliana has been recently reported to not only detect TOR-regulated phosphoproteins linked to the TOR signaling network but also enabled the identification of candidate TOR substrates (Van Leene et al. 2019 ). Phosphorylation is not only responsible for many biological processes in plants, but also often functions in coordination with other PTMs, resulting in crosstalk between PTMs on the same protein. O-GlcNAcylation and phosphorylation are examples that occur at the same amino acid sites/residues and are involved in the regulation of several cellular processes such as transcription, cell signaling, hormone sensing and others (van der Laarse et al. 2018 ).
Signaling pathways exploration by protein interactomics
Mapping protein-protein interaction (PPI) networks and their dynamics is fundamental in understanding protein function and signaling transduction in cellular activities. Arabidopsis thaliana is the well-studied model plant for PPI with 95,382 PPIs being published for 12,617 proteins (approximately 46% of Arabidopsis genes coding for proteins) and deposited in databases being used as the basis for a Cytoscape network (Yilmaz et al. 2022 ). AP-MS is one of the popular approaches to study many aspects of plant cellular processes including plant growth and development. Nee et al. used an AP-MS approach for uncovering the role of GERMINATION 1 (DOG1) and its regulatory mechanisms underlying Arabidopsis seed germination. The GFP tagged DELAY OF GERMINATION 1 (DOG1) transgenic lines were constructed and used for IP pulldown of DOG1 interacting complexes of native seed protein extracts that were subjected to subsequent protein identifications by LFQ-MS analysis (Nee et al. 2017 ). Four phosphatases: AHG1, AHG3, RDO5 and PDF1 were found among the proteins that intact with DOG1 in seeds while two of them: AHG1 and AHG3 are Clade A type 2C protein phosphatases (PP2Cs) and essential for DOG1-dependent control of seed dormancy. In combination with genetic analysis, the authors found that the interaction of DOG1 with AHG1 and AHG3 can negatively affect the function of these PP2Cs rather than that these phosphatases control DOG1 activity by phosphorylation (Nee et al. 2017 ).
In planta chemical cross-linking MS (XL-MS) has emerged as an alternative approach for mapping PPIs and studying protein complexes (Zhu et al. 2016a ; Liu et al. 2018 ). Liu et al. developed a chemical cross-linker, azide-tag-modified disuccinimidyl pimelate that was used in planta for chemical cross-linking within Arabidopsis tissue, followed by streptavidin enrichment of the biotin-tagged cross-linked peptides, LC-MS/MS analysis, and the use of specialized software (ECL2 and SQUA-D) to identify and quantify cross-linked peptides (Liu et al. 2018 ). A total of 354 unique cross-linked peptides were identified with 61 representing the inter-protein crosslinks including a conserved protein family: prohibitins (PHBs) that are related to cell proliferation, saline and oxidative stress (Wang et al. 2021a ). The PHB3–PHB6 protein interaction was confirmed by Co-IP and super-resolution microscopy experiments (Liu et al. 2018 ). Recently, a MS-cleavable cross linkers DSSO has been applied for intermolecular and intramolecular interactions of the Arabidopsis plasma membrane proton pump (H + -ATPase), an essential enzyme for cell surface energetics, regulation of cell elongation and response to abiotic and biotic stimuli (Nguyen et al. 2020 ). The strep-HA-tagged Arabidopsis H + -ATPase 2 (AHA2) expressed in yeast under both N14 and N15 media was purified on streptactin resin prior to DSSO crosslinking reaction, and the C-terminal domain of AHA2 was found to be extensively crosslinked to other domains in intramolecular monomer as well as intermolecular interactions through observed mixed-isotope cross-linking pairs. The results not only suggest the regulatory role of C-terminal domain dynamic interaction in the catalytic activity of AHA2, but also support an Interface structure between monomers of AHA2 based on many intermolecular crosslinks found in the cytoplasmic domain (Nguyen et al. 2020 ). Another recent development for plant PPI application is the combination of crosslinking and tandem affinity purification coupled to MS (XL–TAP–MS) to address the big analytical challenge for detection of low-abundance protein complexes and in vivo protein–protein interactions in complex biological samples. Leissing et al. used an in vivo-biotinylated protein domain flanked by two hexahistidine sequences for affinity isolation of formaldehyde–crosslinked protein complexes of the MKK2-MPK4 signaling module in Arabidopsis (Leissing et al. 2021 ). Out of 107 proteins identified as putative interactors of the MKK2–MPK4 module, 9 are interacted specifically with MKK2, 47 with MPK4, and 51 interactors are co-purified with both bait proteins. More importantly, many of the module-interacting proteins are involved in abiotic stress signaling and various biotic responsive pathways in Arabidopsis (Leissing et al. 2021 ).
Functional characterization of cell-specific proteins by single-cell-type proteomics
Studies conducted using standard bottom-up protocols generally involve the use of bulked tissue or organ samples containing uncharacterized mixtures of diverse and intermingled cell types, each with unique proteomes optimized for specific sets of biological functions. Studies of these bulked samples capture only the weighted population mean of protein expression and obscure important information concerning intercellular heterogeneity as well as all spatial effects. It is becoming increasingly clear that a very granular sampling strategy is required to provide the high spatial resolution and cell specific proteomic information required to facilitate the disambiguation of the biological complexity that underlie a plants response to biological stimuli.
Considerations of this kind have given rise to a growing effort to develop methods to carry out comprehensive, quantitative proteomics studies on single or small numbers of cells and make these accessible to all researchers. While other non-MS centric methods have been utilized (Seyfferth et al. 2021 ; Shaw et al. 2021 ; Cuperus 2022 ), single cell proteomics by MS seems positioned to revolutionize our understanding of cellular functions and regulatory networks. In the past decade proteomics workflows have been developed to capture samples consisting of small numbers of plant cells (hundreds to thousands) collected either by laser capture microdissection (LCM) or fluorescent activated cell sorting (FACS) and microfluidic nanodroplet-based sample preparation followed by nanoLC-MS/MS analysis (Balasubramanian et al. 2021 ).
Studies of this type are termed single-cell-type experiments as the specimens analyzed consist of a highly reduced (but still relatively large) number of cells of a specific type (i.e., epithelial, endothelial, cortical etc.). The advantages of this approach are that it preserves the information concerning the distinct nature of the subject cell-type’s proteome. However, they do not produce high-resolution spatial information nor do they provide information concerning cell-to-cell variation. Nevertheless, this approach has proven successful in certain cases including reproductive cells (pollen grains and egg cells), mesophyll cells, and specialized epidermic cells (root hairs, guard cells and trichomes) (Dai and Chen 2012 ). Zhu et al. reported an application of single-cell-type proteomics using LCM in tomato seedlings grown in hydroponic tanks containing a 14.5 μM Al 3+ solution to simulate the effects of aluminum toxicity in soil (Zhu et al. 2016b ). Epidermal and cortical cells (5,000–7,000 cells per tissue type) of roots in 10-day old seedlings were collected by LCM for subsequent. protein extraction, in-gel tryptic digestion and nanoLC-MS/MS analysis. In this study, they found that a significant portion of each proteome contain proteins unique to the individual cell types, and identified several important proteins related to Al-induced morphological characteristics of roots that were not found in studies of the bulked tissue (Zhu et al. 2016b ). The same groups expanded their studies to include heat-induced proteomes in meiotic pollen cells of tomato (Li et al. 2018b ; Li et al. 2022a ) and Al-induced proteomes of tomato root epidermal and outer cortical cells (Yang et al. 2020 ; Potts et al. 2022 ) by integrating LCM-based single-cell-type approach with TMT labeled quantitative proteomics. As shown in Fig. 3 A, Al-treated outer layer cells (Type I) and interior tissues (Type II) along with control outer layer cells (Type III) in the apical meristem/cell division regions of tomato root-tips were collected by LCM for TMT10-plex quantitative proteomics analysis. Out of 6,000 quantified proteins, 313 were found to differ in abundance between the different cell types compared. These differential abundance proteins (DAPs) were used to categorize them as Al-responsive proteins (Potts et al. 2022 ). Figure 3 B shows the volcano plot and heatmap were created between type I and type II cellular proteomes used to designate the DAPs. The complete set of DAPs identified were used to construct an association network in STRING ( https://string-db.org/ ) using the tomato ( Solanum lycopersicum ) database (Fig. 3 C). A total of 17 protein clusters and interactions were found, one of which is characterized as significant due to the increases in MATE and anti-oxidation proteins in the outer layer cells compared to those found in the interior cells (Potts et al. 2022 ). This data demonstrates that the single-cell-type approach is a useful strategy for the specific case of identifying novel Al tolerance mechanisms in plants, but also for the more general case of proteomics analysis of spatially resolved cells in complex tissues (Potts et al. 2022 ).
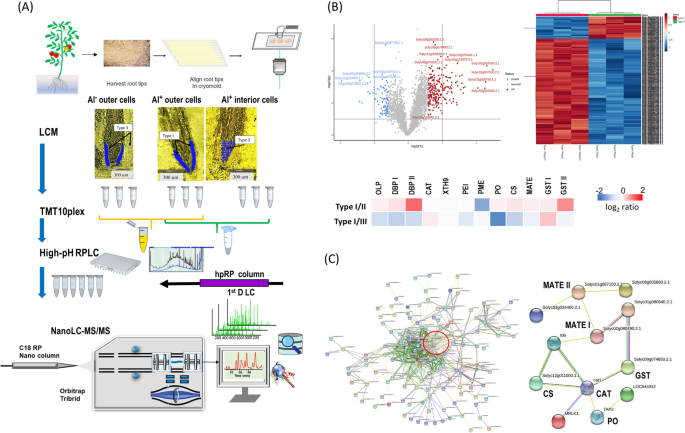
Identification of Al-induced proteomes in outer layer cells and interior of tomato root-tips. A TMT comparative proteomics analysis of differentially abundance proteins (DAPs) on tomato root-tips samples using laser capture microdissection (LCM); B Heatmap analysis based on ANOVA and Volcano plot for the DAPs between type I and type II tissues; C Protein network analysis by STRING ( https://string-db.org/ ) for the DAPs found between type I and type II tissues
Recent advances in protoplasting and sequencing technologies with single-cell whole-genome amplification and single-cell RNA sequencing have allowed for direct single-cell genomic, epigenomic and transcriptomic studies in plants (Luo et al. 2020 ; Shaw et al. 2021 ). In the furtherance of the single-cell system biology strategy, this progress has stimulated efforts to develop true single-cell proteomics methods (Kelly 2020 ; Labib and Kelley 2020 ) despite the considerable technical challenges that remain, such as low sensitivity and throughput that apply to all single-cell proteomics applications, independent of phylogenetic concerns. Furthermore, plants bring additional challenges associated with the cell wall. As with all proteomic applications, the dynamic range of protein abundance can vary from a single copy to a few million copies per cell. This restricts detection to the most abundant proteins (Labib and Kelley 2020 ). Since the initial reports in 2018 on profiling hundreds of proteins from single mammalian cells (Budnik et al. 2018 ; Zhu et al. 2018b ), the field has advanced steadily, albeit mostly in animal systems. Over 1,000 protein groups can now be reliably quantified by label-free analyses (Cong et al. 2020 ; Brunner et al. 2022 ) from single HeLa cells, and by isobaric labeling workflows (Dou et al. 2019 ; Tsai et al. 2020 ; Specht et al. 2021 ) from other mammalian cells. These successes were attributed to the recent innovations including an automated platform (nanoPOTs) for miniaturized sample preparation to reduce sample loss (Kelly 2020 ; Liang et al. 2021b ), optimized gradients with very low flow-rates (<100 nL/min) and the introduction of next generation ion mobility MS sources which provides a >10-fold improvement sensitivity for peptides (Cong et al. 2020 ; Brunner et al. 2022 ). The development of a TMT-based multiplexed single-cell proteomics strategy incorporating a carrier proteome into one of the quantitation channels, enhances sensitivity, and improves throughput through multiplexing (Budnik et al. 2018 ; Dou et al. 2019 ). With the recent improvements allowing for the direct deposition of single cells on 96/384-well plates after cell sorting and integration of automated sample handling using the Minimal ProteOmic sample Preparation (mPOP) method, Specht et al. have reported quantifying >3,000 proteins from a single-cell proteomes using 1,490 single monocytes and macrophages in just 10-days of instrument time employing both TMT11-plex and TMT16-plex reagents for two biological replicate single-cell samples (Specht et al. 2021 ). It should be noted that the use of higher concentrations of carrier in TMT has been shown to compromise quantitation accuracy in the single-cell channels. To overcome this issue, optimal carrier proteome amounts and optimized MS instrument settings are required.
In addition, mass spectrometry imaging (MSI) technology has been incorporated with LCM for spatially resolved proteomics study. Compared to smaller metabolites in plant tissues, MSI for plant peptides and proteins possesses more challenges due to larger molecular weights with decreased ionization efficiency (Bjarnholt et al. 2014 ). For example, Cavatorta et al. demonstrated the localization of the major peach allergen, Prup3, in three different varieties of peach (Cavatorta et al. 2009 ) while Prup3 was present only in the outer skin of peaches that give allergic sensitivities to peach peels. Bencivenni et al. reported that non-specific lipid-transfer proteins, are one of the major human allergens in various plants that are located in tomato seeds instead of peels and pulps (Bencivenni et al. 2014 ). Gemperline et al. found that different distributions of endogenous peptides and protein fragments can be observed between seedlings and mature of M. truncatula (Gemperline et al. 2016b ).
Emerging MS based metabolomics techniques
Sample preparation and separation technologies.
Sample preparation is an essential step in plant metabolomics workflow (Fig. 4 ). An efficient extraction procedure for endogenous metabolites is the most critical step for achieving high quality plant metabolomics data. Notably, lipidomics analysis is not included in this section. The rapid turnover rate of metabolites, both primary and secondary metabolites, can occur during the extraction process (Heise et al. 2014 ; Rampler et al. 2021 ). Therefore, simple, rapid, and reproducible extraction methods are required for sample preparation. Due to the difficulties associated with extraction through cell wall, it is important to grind the plant material to a homogeneous powder before extraction. To achieve this, several strategies, such as a vibration mill (Jonsson et al. 2004 ), ball mill (Weckwerth et al. 2004 ), and Ultra Turrax (Roessner et al. 2000 ), have been utilized to disrupt cell walls and homogenize the sample. It is recommended that these homogenization procedures be performed in liquid nitrogen in order to avoid degradation. After homogenization, several selective metabolite extraction methods, including microwave-assisted extraction (Teo et al. 2013 ; Gemperline et al. 2016b ; Wei et al. 2016 ) , ultrasound-assisted extraction (Chemat et al. 2017 ), high voltage electric discharge extraction (Li et al. 2019 ), supercritical fluid extraction (SFE) (Gallego et al. 2019 ), enzyme-assisted extraction (Puri et al. 2012 ), and solid-phase extraction (SPE) (Reyes-Garcés and Gionfriddo 2019 ) are commonly used in combination or sequentially. Notably, given the fact that SFE affords various advantages such as chemically stable, environmentally friendly, low toxicity, and not flammable, it has been chosen as a good extraction strategy for volatile compounds (e.g., terpenes and aromatic compounds) from plant samples (Naz et al. 2017 ). In recent years, a wide range of novel sorbents that are selective for the extraction of metabolites have been developed (Li et al. 2018c ; Faraji et al. 2019 ; Rocío-Bautista and Termopoli 2019 ; Li et al. 2020a ), such as molecularly imprinted polymers (MIPs), multiwalled carbon nanotubes (MWCNTs), metal-organic frameworks (MOFs), and covalent organic frameworks (COFs). More specifically, Li et al. prepared the cellulose magnetic molecularly imprinted polymer micro-spheres (CMMIPs) for efficient extraction and determination of plant hormone (e.g., indole-3-acetic acid) in plant tissues (Li et al. 2018d ). Alireza et al. developed a MWCNT-polyaniline nanocomposite-coated platinized stainless-steel fiber for the extraction of thymol and carvacrol in medicinal plants (Ghiasvand et al. 2015 ). Liu et al. developed a zirconium (IV)-based MOF (UIO-67) as efficient sorbent for enrichment of eight plant growth regulators in fruit samples (Liu et al. 2016a ). Recently, Li et al. reported a novel magnetic COF nanomaterial (Fe 3 O 4 @COF(TpDA)) as an adsorbent for SPE of plant growth regulators from fruits and vegetables (Li et al. 2020b ). Taken together, these emerging nanostructured materials result in a reduction in sorbent amounts and higher extraction recoveries, and automation of SPE methods might facilitate the development of greener sample preparation methods. In addition, solid-phase microextraction (SPME) has been currently re-explored for metabolomics, which is a non-exhaustive extraction way particularly attractive for time-resolved or spatially metabolomics. Recently, the developments and applications of SPME as a sample preparation tool for GC-MS- and LC-MS-based metabolomics have been demonstrated and summarized (Reyes-Garcés and Gionfriddo 2019 ).
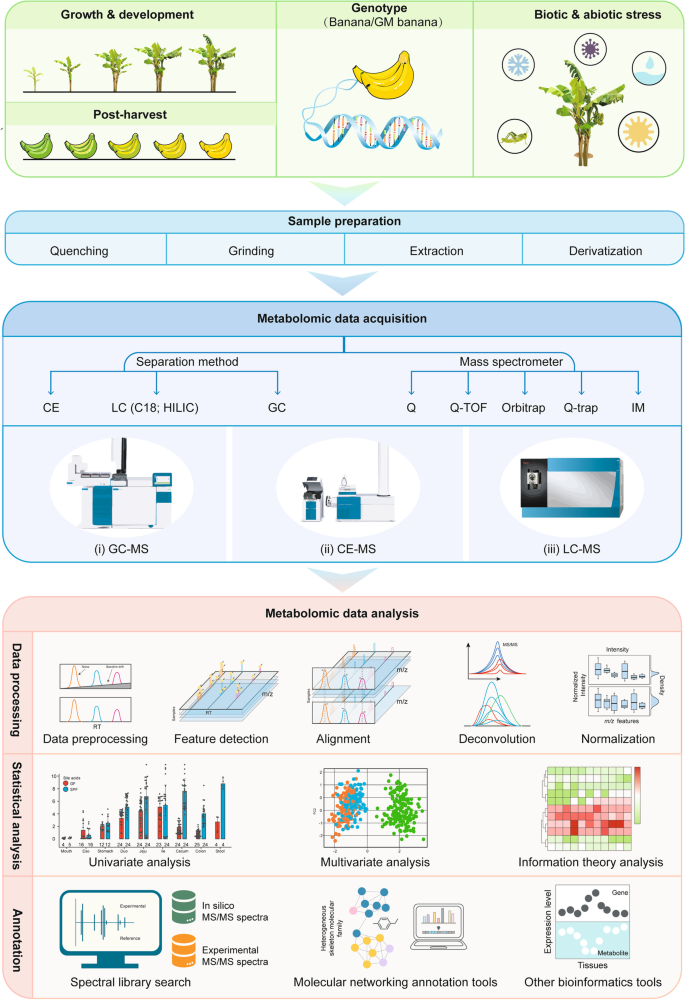
A general workflow for MS-based plant metabolomics study, including experimental design, sample preparation, metabolomic data acquisition, and metabolomic data analysis
Separation method
As shown in Fig. 4 , gas chromatography (GC), LC, and capillary electrophoresis (CE) are the common metabolite separation methods, which can be tandem with MS for metabolomics study. Since 2000, GC-MS has become a central platform in targeted metabolomics for volatile and nonpolar metabolites, which are major classes of primary and secondary compounds in plant science (Roessner et al. 2000 ). Although GC-MS has significantly advanced, two-dimensional GC (2D-GC) demonstrates superior chromatographic capabilities, including resolving power, peak capacity, and sensitivity, especially for the separation of low molecular weight (Balasubramanian et al. 2021 ) plant metabolites in complex samples (Tranchida et al. 2016 ). Recently, Gavin Sacks’ group developed solid-phase mesh-enhanced sorption from headspace (SPMESH) coupled to GC–MS for the quantitation of linalool and 3-isobutyl-2-methoxypyrazine in real grape samples (Jastrzembski and Sacks 2016 ). This SPMESH strategy afforded greater loading capability and was more cost-effective.
However, due to involvement of derivatization steps and other challenges in GC-MS, LC-MS has become the most valuable analytical tool for the analysis of polar and nonpolar metabolites with greater selectively and reduced ion-suppression and matrix effects. For example, Gray et al. demonstrated that a compact 1 mm i.d. column was able to reduce solvent consumption by 75% and increased sensitivity by 2-3-fold compared to the standard 2.1 mm i.d. column (Gray et al. 2015 ). Coelution and resistance to retention of low polarity compounds makes RPLC as a more suitable technique for separation. Thus, mobile-phase modifiers, including formic acid, ammonium formate/acetate, or ammonium hydroxide can be used (Cajka and Fiehn 2014 ). Tufi et al. found that the zwitterionic phase based on silica gel columns provides the optimal performance compared to four others commercial HILIC packing materials (Tufi et al. 2015 ). Currently, multidimensional liquid chromatography is emerging to resolve this issue, which will be summarized in the below subsection.
CE-MS has also proven to be a promising platform in metabolomics. For the separation of cations and anions, bare capillaries are typically used for CE-MS with conditions of pH < 2 and surface coated fused-silica is used when pH > 8. Tanaka et al. revealed that polymer-based fused-silica capillaries can be used to better control the EOF and lower ion adsorption (Tanaka et al. 2008 ). Additionally, a modification to the liquid junction in CE-MS interfaces has recently been conducted based on sheath flow or sheathless electrospray designs. Recently, a new liquid junction-based electrospray interface has been developed for automated CE-MS analysis through computer modeling of transport conditions (Krenkova et al. 2019 ). Using this liquid junction interface, small peptides, proteins and oligosaccharides can be well separated. Beyond that, recent decades have witnessed great improvements of CE-MS in single-cell and subcellular analyses due to its compatibility with low volume sample requirements (DeLaney et al. 2019 ; Kristoff et al. 2020 ). Williams et al. used a CE-MS method for metabolic profiling of amino acids of Medicago truncatula liquid suspension cell cultures in response to stress (Williams et al. 2007 ). Huang et al. developed an online single-cell CE-MS platform for single-cell metabolomics from a red onion ( Allium cepa ) cell, and hundreds of metabolites were successfully separated and putatively identified (Huang et al. 2021 ). Taken together, these results strongly suggest that CE-MS is expected to be more commonly used in single-cell metabolomics research.
In addition, super critical fluid chromatography (SFC), which utilizes liquid CO2 as a solvent, is a complementary method to GC, LC and CE. The review papers have described the advances in GC, LC, and SFC methods to improve the metabolome (Haggarty and Burgess 2017 ), and compared different LC and SFC strategies in terms of efficiency versus throughout, showing the performance from each method to readers (Fekete et al. 2015 ). With the development of new robust column and instrumentation, SFC method has been improved and applied in targeted metabolomics (West et al. 2016 ).
Combination of orthogonal chromatography techniques
Another way to reduce the coelution of metabolites and improved separation efficiency is to combine two or more separation techniques in a single analysis, such as serial combination of two different column (Alvarez-Segura et al. 2016 ), two-dimensional LC (2D-LC) (Stoll et al. 2007 ; Sandra and Sandra 2013 ), 2D-GC (Kouremenos et al. 2010 ), and 2D-SFC (Zhou et al. 2014 ). Among them, 2D-LC has been widely applied in untargeted metabolomics studies. An online 2D-LC can provide high-throughput and automated analysis, but short separation time might compromise the chromatographic resolution of the second LC separation (Holčapek et al. 2015 ). Xu’ s group recently established 2D-LC-MS and parallel column based 2D-LC (PC-2DLC)-MS approach to simultaneously perform metabolomics and lipidomics (Wang et al. 2017 ; Lv et al. 2020 ). Using orthogonal HILIC and RPLC chromatography with distinct retention mechanisms, HILIC × RPLC is capable of simultaneous separation and detection of hydrophilic and hydrophobic compounds in complex samples in a single injection, which significantly increases peak capacity and separation flux. For example, Wang et al. used stop-flow HILIC × RPLC to identify 372 lipids from 13 different classes of compounds in positive mode (Wang et al. 2013b ). Zhou et al. developed a HILIC × RPLC system to separate a total of 896 peaks from G. jasminoides Ellis (GJE) and identify 16 active ingredients (Zhou et al. 2016b ). Dang et al. demonstrated an orthogonal 2D HILIC/RPLC system for the isolation of 18 flavonoids from S. tangutica , which was quantitatively evaluated based on the construction of normalized 2D plots (Dang et al. 2018 ). Specifically, normalized 2D plots were divided into 9×10 bins, and distinct flavonoids with fractions 1–9 occupied 55 bins. Navarro-Reig and his colleagues used an HILIC × RPLC system with chemometric tools to acquire a lipidomic assessment of the effect of arsenic pollution on rice ( Oryza sativa L.) growth (Navarro-Reig et al. 2018 ). Lisa et al. achieved fractionation of total lipid extracts of soya tissues into individual lipid classes using HILIC as the first separation dimension, followed by RPLC-MS separation and identification of individual species (Lísa et al. 2011 ).
Advanced MS technologies for metabolomics data acquisition
Untargeted metabolomics.
Metabolomics has rapidly grown as the major methodologies for systems biology studies, driven by new developments in MS that provides high sensitivity and high throughput coverage of the metabolome. Untargeted metabolomics analysis involves identification of global metabolites having peak intensities in a mass chromatogram processed in an unbiased way. Ideally, the MS platform instrument should provide precise mass measurements with superior mass accuracy and mass resolution for untargeted metabolomics (Treviño et al. 2015 ). The lack of mass resolving power will undoubtedly lead to overlap of co-eluting isobaric metabolites and will result in false positive results. To this end, untargeted metabolomics studies have been dominated by the high-resolution mass spectrometers, such as time-of-flight (TOF), Orbitrap, and Fourier transfer ion cyclotron resonance (FT-ICR) mass analyzers, which greatly improve the chemical specificity and provide high confidence in analyte identification. However, some compromises must be considered in terms of desired detection sensitivity, dynamic linearity range, and acquisition rate. Up to date, the majority of published plant metabolomics studies use Orbitrap or TOF equipment considering coverage, selectivity, and throughput (Maia et al. 2021 ). Recently several reviews covered well the topic of GC/LC-MS- and FT-ICR-based plant metabolomics studies (Alseekh et al. 2021 ; Alvarez and Naldrett 2021 ; Maia et al. 2021 ; Perez de Souza et al. 2021 ), so we will not cover too much in detail about the progress in this field over the past decade. In this section, we will focus on the newest technological advancements in untargeted metabolomics, such as DIA, IMS, fluxomics.
To achieve optimal analyte identification confidence, tandem MS is required to obtain characteristic fragments. Among these techniques, classic DDA remains the standard for untargeted metabolomics. However, DIA is a rapidly emerging and robust method for improved coverage of low-abundance metabolites (Tsugawa et al. 2015 ; Li et al. 2020c ). Specifically, DIA provides more robust data than DDA acquisition technique because all fragment ions from all precursor ions are acquired simultaneously. Thus, DIA allows for increased chemical coverage of metabolites and reduced identification artifacts. One issue to note is that the molecular identification with the DIA method can be compromised due to the decoupling of precursor ions and their fragments, which can be exacerbated when large mass windows or all-fragment-ion mode are selected. Thus, these challenges promote the development of deconvolution algorithms software that matches precursor ions and fragment ions based on retention-time alignment (Tsugawa et al. 2015 ; Perez de Souza et al. 2021 ). To better distinguish metabolites of interest from background contaminants (e.g., polyethylene glycol, polypropylene glycol, siloxanes) commonly found in LC-MS/MS and enhance the coverage of metabolome, the automated intelligent workflow AcquireX (Thermo Fisher) has been developed to differentiate metabolite signals (Cho et al. 2021 ; Schwaiger-Haber et al. 2021 ). The AcquireX workflow enables which precursors to select for enhanced fragmentation (MS/MS or MS n ) in real time by automated exclusion/inclusion list generation and updating in five consecutive LC-MS runs, allowing not only for background exclusion, but also for digging deeper into the low abundance metabolites.
For plant untargeted metabolomics, many factors still limit metabolite annotation. Ion mobility spectrometry (IMS) has been proposed for improved identification capabilities by providing an additional separation dimension within the millisecond time-window (Hofmann and Pagel 2017 ; Wu et al. 2021 ). To date, there are several available IMS platforms, such as traveling wave IMS, drift-time IMS, high field asymmetric waveform IMS, and trapped IMS, Open-loop IMS (González-Riano et al. 2020 ), which have been used with GC-/LC-MS for untargeted metabolomics. Given that IMS enables the CCS measurement of a wide range of metabolites and protein complexes, four-dimensional (4D) information, including retention time, CCS value, accurate molecular mass, and MS/MS fragmentation, can be obtained simultaneously. This significant increases in improving the identification confidence and prediction of unknown molecular structures. Additionally, the incorporation of IMS offers improved separation of coeluting and isobaric compounds, lower background noise, and higher mass resolution (Szykuła et al. 2019 ). Specifically, Jia et al. used UPLC-IMS-QTOFMS to reveal the chemical diagnostic markers for the differentiation of three Panax species ( P. ginseng , P. quinquefolius , and P. notoginseng ), which are easily misidentified and widely consumed as healthcare products (Jia et al. 2019 ). More than 162 compounds from fungicide-infected potato samples ( S. tuberosum ) were able to be distinguished by UPLC-IMS-QTOFMS due to its ability to produce confident compound annotations from elemental composition determination, retention times, specific CCS-values, and MS fragment spectra (Claassen et al. 2019 ). McCullagh et al. adopted IMS coupled to MS for profiling 6- C and 8- C -glycosylflavone isomer pairs in medicinal plants (e.g., Passiflora species) (McCullagh et al. 2019 ). Recently, Zhu’s group developed an ion mobility CCS atlas, AIICCS, to further improve annotation of both known and unknown metabolites acquired in IM-MS-based metabolomics (Zhou et al. 2020 ). Given the fact that CCS values are a unique physiochemical property of detected metabolites, this method will expand the chemical coverage and assessment of annotated metabolites in metabolic pathways and biological processes (Li et al. 2021a ).
Fluxomics, as a new untargeted metabolomics approach to monitor the dynamic changes of metabolites in metabolic pathways, thereby provides an overview of the global regulation network with regard to transcriptional, translational, and metabolic processes (Cascante and Marin 2008 ). Fluxomics that combines 13 C isotope or 15 N labelling and computational approaches provides a deep insight into the correlation between genotype and metabolic phenotype. This technique also provides a measure of the flux through each reaction in the network, which can be considered a direct measure of the phenotype (Heux et al. 2017 ). Several methods have been proposed to decipher complex plant metabolic pathways. One method, metabolic flux analysis (MFA), has been widely adopted for heterotrophic tissues that lie in a metabolic and isotopic steady state (Crown and Antoniewicz 2013 ; Salon et al. 2017 ). For systems in a metabolic steady state that are isotopically dynamic, isotopically non-stationary MFA (INST-MFA) has been developed to quantify flux variations, dynamically (Wiechert and Nöh 2013 ; Salon et al. 2017 ; Wieloch 2021 ). Flux balance analysis (FBA) was invented as a complementary tool for deciphering genome-scale metabolic models (Shi and Schwender 2016 ). Recently, a machine learning-based framework has been developed to circumvent computational limitations of traditional MFA algorithms, facilitating high-throughput phenotyping and advances of synthetic biology (Wu et al. 2016 ; Millard et al. 2021 ; Wu et al. 2022 ). To date, fluxomics has been widely applied to determine exchange rates of a given element between various organs and nutrient use efficiency (Kichey et al. 2007 ) at the plant level. Specifically, fluxomics applications in the assessment of C and N use have been conducted in a range of plant species. 15 N-based MFA methods have been applied in maize (Gallais et al. 2006 ), rice, wheat (Kichey et al. 2007 ), pea (Schiltz et al. 2005 ), and B. napus (Malagoli et al. 2005 ). Recently, Ma et al. and Xu et al. used 13 C-labeling INST-MFA to estimate C fluxes in central metabolism during photosynthesis (Ma et al. 2014a ; Xu et al. 2021 ). Cocuron et al. used isotope-labeled fluxomics to compare the metabolism of two different maize lines through 13 C-MFA (Cocuron et al. 2019 ). In addition, the labeling-based fluxomics strategy has been used to determine endogenous and exogenous N and S fluxes at the whole-plant level to evaluate nutrient use efficiency (NUE) (Salon et al. 2014 ), as well as the N harvest index, nutrient uptake efficiency, and nutrient remobilization efficiency in Arabidopsis (Guiboileau et al. 2012 ) and maize (Li et al. 2015a ) during development. MFA and INST-MFA are thus promising synergistic toolsets for uncovering the basis of C and energy conversion efficiencies in plant systems (Chen and Shachar-Hill 2012 ; Kruger and Ratcliffe 2021 ).
Targeted metabolomics
While untargeted metabolomics is devoted to maximizing coverage of metabolites, targeted metabolomics is concerned with the quantitative analysis or rapid profiling of a very specific set of compounds using highly selective techniques. The standardization of chromatographic conditions in GC together with reproducible spectral databases resulting from electron ionization results in a bias in favor of the use of GC-MS for targeted applications. GC-MS methods provide quantitative data for large-scale analysis of metabolites involved in central metabolism. Hence, GC-MS is the method of choice for targeted profiling and quantitation of various plant primary metabolites, with low MW, low polarity, and low boiling points. Chemical derivatization strategies are commonly required to improve ionization efficiency of non- or semi-volatile metabolites (e.g., nucleotides, fatty acids, and amino acids). Incomplete derivation not only affects the quantification of peaks, but increases the spectral complexity. Therefore, two-dimensional GC has proven to be a robust method with improved separation capacity, peak resolution, and spectral reproducibility (Almstetter et al. 2012 ). Targeted GC-MS methods normally adopt specific conditions for known compounds, such as select ion monitoring (SIM) and multiple reaction monitoring (MRM). Recently, Xu’s group proposed the “quasi-targeted” SIM or MRM methods instead of untargeted full-scan (Zheng et al. 2020 ). Yuan et al. reported a widely targeted volatilomics method based on GC-MS using MRM to profile the volatilome of rice grains (Yuan et al. 2022 ). To improve the quantitative capability of targeted metabolomics, use of adequate (isotope labeled) internal standards is common practice, which are ionized under nearly identical conditions as the targeted metabolites of interest (Chen et al. 2013 ). For targeted metabolic profiling and quantitation, triple quadrupole mass spectrometry (QqQ-MS) and quadrupole-linear ion trap mass spectrometry (QLIT-MS) are most widely used (Dettmer et al. 2007 ). Both SIM and MRM represent routine operation modes for QqQ-MS and QLIT-MS, which provide richer structural information, and more precise quantification analysis. Recently, a high-coverage and quantitative LC-MS/MS method using both positive and negative-mode MRM was reported for targeted 206 primary and secondary plant metabolites (Zheng et al. 2021 ). In past decades, LC-MS/MS for targeted metabolomics profiling and quantitation has been gaining the popularity for the broad application of plant metabolites including plant hormones such as cytokinins (Hu et al. 2021b ; Pino et al. 2022 ), salicylic acid and glycolytic metabolites (Wang et al. 2022 ; Yang et al. 2022 ).
- Spatially resolved metabolomics
The ability to visualize metabolite distribution within plant tissues with high spatial resolution is crucial for a detailed understanding of the synthesis, accumulation, and cross-regulation of metabolites in plants (Gemperline et al. 2016a ). Metabolomic analysis of bulked tissues focuses yield information concerning the mean concentrations in the bulked tissue which is of limited biological value. Thus, mass spectrometry imaging (MSI) was developed that possesses the advantages of label-free, non-specific, and visualized detection, and simultaneous analysis of hundreds of compounds in a single analysis. MSI has recently proven to be a robust technique for characterizing the spatial distributions of a wide range of metabolites within plant tissues and single cells (Yin et al. 2019 ; Meng et al. 2020 ; Li et al. 2021a ; Samarah et al. 2021 ). Several ion sources are available for plant MSI, such as matrix-assisted laser desorption ionization (MALDI), secondary ion mass spectrometry (Wang et al. 2016 ), desorption electrospray ionization (Li et al. 2013 ), and laser ablation electrospray ionization (LAESI). The choice of ion source is dependent on metabolite species, its spatial resolution, the pressure regime, and scanning speed (Liang et al. 2016 ; Wang et al. 2020b ). Among the available techniques, MALDI-MSI has been the most widely used technique for plant tissue imaging. As shown in Fig. 5 (unpublished data), we can observe the dynamic spatial distribution of disaccharides, dopamine, and lysine within banana pulps at different postharvest ripening stage, and the dynamic spatial distribution of disaccharides, glucose-6-phosphate, and TAG (52:3) within maize kernels at different development stages using MALDI-MSI. Sample preparation is the most crucial step for MSI to provide high-quality ion images. Improper sample preparation can damage the original sample by altering the analyte distribution, and abundance and can therefore lead to degraded detection sensitivity and spatial resolution (Dong et al. 2016b ). Thus, histologic sectioning is normally adopted in MSI for roots and stems of plants (Li et al. 2016a ), whereas imprinting strategies are preferred for leaves and petals due to fluctuating surfaces, wax-layer protection, and their inability to be sliced (Wu et al. 2020 ; Qin et al. 2021 ).
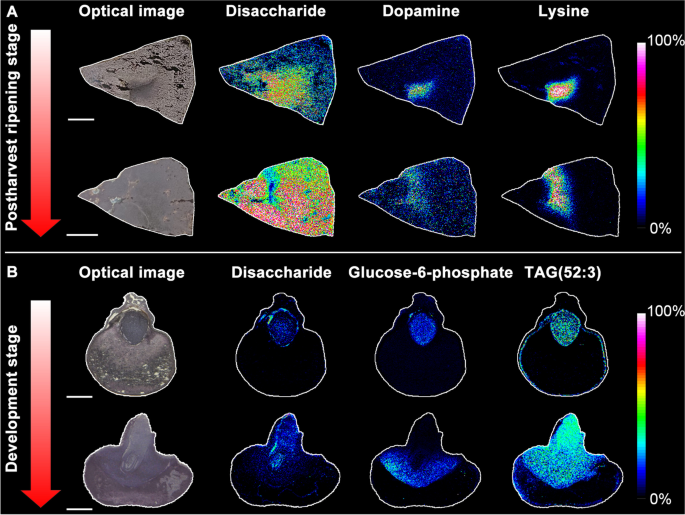
Matrix-assisted laser desorption/ionization mass spectrometry imaging (MALDI-MSI) as a molecular exploratory tool in plant science. A The dynamic spatial distribution of disaccharides, dopamine, and lysine within banana pulps at different postharvest ripening stage. Scale bar, 5 mm. B The dynamic spatial distribution of disaccharides, glucose-6-phosphate, and TAG (52:3) within maize kernels at different development stages. Scale bars, 2.5 mm
Recently, spatially resolved metabolomics, which integrates MSI and metabolomics methods, has been proposed for accurate determination of types, contents, and spatial differentiation of primary and secondary metabolites within plant tissues (Sumner et al. 2011 ; Etalo et al. 2015 ; Dong et al. 2016a ). This integrated method has been applied in various emerging applications, such as precise localization of metabolites, biosynthesis and translocation pathways of functional metabolites, and functional gene verification. Specifically, Li et al. used MADLI-MSI to visualize the tissue-specific distribution of free flavonoids, flavonoid glycosides, and saponins, which provided deep insights into their biosynthetic pathway in legumes (Li et al. 2014 ). Germination and maturation are two highly active metabolic stages in seed growth and development. Bhandari et al. found that spermidine and cyclic spermidine conjugate can move from the hypocotyl to the young root of oilseed rape during seed germination, facilitating an understanding of the dynamic changes of plant metabolites at different development stages (Bhandari et al. 2015 ). Furthermore, organ- and tissue-specific distributions of various metabolites (e.g., flavonoid glycosides, biflavonoids, ginkgolides, and phenolic lipids) can be visualized in the root, young stem, and leaf of ginkgo (Li et al. 2018a ). Dong et al. used the integrated LC-MS and MSI to visualize the steroidal glycoalkaloid (Villas-Bôas et al. 2007 ) pathway in wild-type and GAME25 silenced-construct tomato fruits, revealing accurate gene-metabolite relationships and novel gene-associated metabolites (Dong et al. 2020 ). Stopka et al. utilized an optical fiber-based laser ablation electrospray ionization mass spectrometry (f-LAESI-MS) technique to analyze individual Egeria densa leaf blade cells, revealing the metabolic differences between different cell types (Stopka et al. 2018 ).
However, despite its advantages, spatially resolved metabolomics has several challenges including limited detection sensitivity, spatial resolution, and molecular identification capability. Continuing efforts have been made to allow sub-micrometer resolution imaging, especially for near-field based techniques (Liang et al. 2016 ; Yin et al. 2019 ; Cheng et al. 2020 ). Additionally, laser post-ionization has recently been implemented in commercial MS instruments (known as MALDI-2), which significantly improves ion yields for numerous lipid classes, vitamins, and saccharides by up to two orders of magnitude (Soltwisch et al. 2015 ; Niehaus et al. 2019 ).
Taken together, spatially resolved metabolomics is still under rapid development and will become a robust strategy for plant science in near future.
MS data analysis
The handling of MS raw data is now a mature technique for untargeted metabolomics. Several review papers have summarized the characteristics of available software for metabolomic data processing, metabolite annotation, statistics, and data interpretation, as well as the level of programing skills required to exploit their basic functions (Perez de Souza et al. 2017 ; Tsugawa 2018 ; Chaleckis et al. 2019 ; Misra 2021 ). In the subsection, we will describe the advances of MS data analysis.
MS data pre- or post-processing
After MS-based metabolomic data acquisition (Fig. 4 ), and MS data format conversion to the NetCDF, mzXML, or mzML standardized file formats, the metabolomics datasets will be processed by different computational solutions. Until now, many powerful tools have been reported to facilitate mass spectral data pre- and post-processing, such as AMDIS (Stein 1999 ), MetaboliteDetector (Hiller et al. 2009 ), MET-COFEA (Zhang et al. 2014 ), MetaboQC (Leonid Brodsky 2010 ), xMSanalyzer (Uppal et al. 2013 ), TracMass 2(Tengstrand et al. 2014 ), DecoMetDIA (Yin et al. 2019a ), MET-XAlign (Lommen 2009 ), Normalyzer (Chawade et al. 2014 ), TargetSearch (Cuadros-Inostroza et al. 2009 ), and NOREVA (Fu et al. 2022 ). They have basic functions for GC-MS or LC-MS data processing, including spectral deconvolution, baseline correction, peak picking, peak annotation, alignment, and normalization (Tsugawa 2018 ). However, XCMS (Smith et al. 2006 ), Mzmine2 (Pluskal et al. 2010 ), OpenMS (Rost et al. 2016 ), and MS-DIAL (Tsugawa et al. 2015 ) have been widely used for different metabolomics datasets, which works based on almost similar principles. MS-DIAL, OpenSWATH (Rost et al. 2014 ), and MetDIA (Li et al. 2016b ) are specifically used to deconvolulate the MS/MS spectra for comprehensive DIA data. Additionally, AMDIS is widely used for GC-MS based metabolomics datasets (Stein 1999 ). There are still several challenges in MS data processing with high false positives that are attributed to background noise, contamination, and in-source fragmentation. For example, CAMERA can perform better peak picking based on several parameters (Kuhl et al. 2012 ), and in-source fragmentation can be obtained both by CAMERA or RAMClust (Broeckling et al. 2014 ). CAMERA and other open-source software tools can cluster mass features belonging to the same metabolite, which is an important step aiming for reducing data redundancy and facilitating further statistical analyse and metabolite annotation (Broeckling et al. 2014 ; Uppal et al. 2017 ). In addition, NormAE, a deep learning model, has been developed to remove batch effects of large-scale untargeted metabolomics data (Fernández-Albert et al. 2014 ; Rong et al. 2020 ). IntCor is focused on drift removal and data normalization for LC-MS metabolomics data (Fernández-Albert et al. 2014 ). SPICA is built to extract relevant information from noisy datasets by analyzing ion pairs instead of individual ions (Mak et al. 2015 ). Several other software tools, such as MIA (Weindl et al. 2016 ), ICT (Jungreuthmayer et al. 2015 ), geoRge (Capellades et al. 2015 ), Massifquant (Conley et al. 2014 ), Allocator (Lisacek et al. 2014 ), Corrector (Huege et al. 2014 ), and X 13 CMS (Huang et al. 2014 ), have been developed to determine, correct, visualize and analyze mass isotope distributions in isotope labeling experiments.
Statistical analysis
Processed MS data, which consists of high dimensional data matrices, can be analyzed statistically by multivariate and univariate analysis. There are numerous software packages reported for statistical analyses of metabolomic data, as well as useful pipelines to facilitate statistical analysis, including MetaboLyzer (Mak et al. 2014 ), IPM4 (Liang et al. 2020 ), Pathomx (Fitzpatrick et al., 2014 ), MetabR (Ernest et al. 2012 ), COVAIN (Sun and Weckwerth 2012 ), metaP-server (Kastenmüller et al. 2021 ), OpenMS (Rost et al. 2016 ), MathDAMP (Baran et al. 2006 ) , RepExplore (Glaab and Schneider 2015 ), Metabomxtr (Nodzenski et al. 2014 ). Recently, other more specialized tools, including LipidSuite (Mohamed and Hill 2021 ), MSEA (Xia and Wishart 2010 ), MPA-RF (Huang et al. 2013 ), and probabilistic principal component analysis (PPCA) have been developed (Nyamundanda et al. 2010 ). Specifically, LipidSuite offers a step-by-step workflow for MS data processing, differential analysis and enrichment analysis of lipidomics data (Mohamed and Hill 2021 ). MSEA offers three different enrichment analyses for metabolomic data (Xia and Wishart 2010 ). PPCA addresses some of the limitations of PCA, and probabilistic principal component and covariates analysis provides a flexible approach to jointly model metabolomic data and additional covariate information (Nyamundanda et al. 2010 ). MPA-RF helps to identify the informative biomarkers in complex metabolic datasets (Huang et al. 2013 ). Subpathway-GM is a method for pathway analysis that integrates information from genes and metabolites, as well as their positions and cascade regions within the given pathway (Li et al. 2013 ).
Metabolite annotation
Accurate metabolite annotation is very vital for data interpretation. But it is still considered as the most challenging step for MS-based metabolomics study, which largely depends on retention time, accurate mass, mass spectra, MS/MS fragmentation pattern, and other sample-related information. The guide for metabolite annotation by current MS-based cheminformatics is reviewed by Tsugawa et al. (Tsugawa 2018 ). As a guideline for annotation, the Metabolomics Standards Initiative has recommended four confidence levels: from level 1 to level 4 (Sumner et al. 2007 ), and metabolites identified using authentic standards compounds are considered as level 1. Once a metabolite is identified, its MS/MS spectra can be deposited in databases. Therefore, it is important to establish a spectral database covering accurate mass with retention time using authentic standards compounds for leveraging metabolite annotation, although generation of spectral library will be expensive with the high cost of commercial standard compounds. MS/MS spectra are currently the starting point for most of MS-based metabolite annotation approaches to search through different experimentally derived databases, including experimentally derived databases, including NIST, METLIN, MoNA, MassBank, European MassBank, mzCloud, GMD, GNPS, ReSpect and LipidMaps, which are covered in other comprehensive review papers about a complete databases (Vinaixa et al. 2016 ; Perez de Souza et al. 2017 ; Blazenovic et al. 2018 ). Some in silico generated MS/MS spectra are also included in popular compound databases for metabolite annotation, such as PubChem, Chemspider, HMDB, KEGG, ChEBI, ChemBank, and universal natural product database (UNPD) (Wang et al. 2016 ; Blazenovic et al. 2018 ). Accordingly, MetaboSearch (Fernandez-Fuentes et al. 2012 ), PUTMEDID-LCMS (Brown et al. 2011 ), MFSearcher (Sakurai et al. 2013 ), MetAssign (Daly et al. 2014 ), MS-FINDER (Tsugawa et al. 2016 ), HR3 (Lommen 2014 ), SIRIUS (Böcker et al. 2009 ), and MS2Analyzer (Ma et al. 2014b ), are developed for interrogating and matching unannotated mass spectra from those databases. Recently, METLIN, as the largest individual collection of MS/MS experimental data acquired in multiple collision energies, has been directly integrated with XCMS online processing platform (Guijas et al. 2018b ).
One of the major obstacles for proper MS-based metabolite annotation is the limited number of characterized authentic standards for the majority of the different compounds derived from plants. Thus, several alternatives have been developed to support metabolite annotation, some large databases expand the coverage by including in silico generated MS/MS spectra of known compounds based on machine learning or quantum mechanics calculations. MetFrag (Ruttkies et al. 2016 ), MolFind (Menikarachchi et al. 2012 ), CFM-ID (Allen et al. 2014 ), MAGMa (Ridder et al. 2014 ), CSI: FingerID (Dührkop et al. 2015 ), MetFusion (Gerlich and Neumann 2013 ), and MetFrag (Ruttkies et al. 2016 ), can be used to predict molecular properties of unknown compounds by their tandem mass spectra based on the integration of machine learning model and known mass spectra. CANOPUS uses a deep neural network to predict 2,497 compound classes from fragmentation spectra, including all biologically relevant classes (Dührkop et al. 2020 ). DLEMMA is a valuable tool and is analogous to DNA arrays in that it enables the identification and relative quantification of differential metabolites in a single sample (Feldberg et al. 2009 ). ChemDistiller combines automated large-scale annotation of metabolites using tandem MS data with a compiled database containing tens of millions of compounds with pre-calculated ‘fingerprints’ and fragmentation patterns (Laponogov et al. 2018 ). One issue to note is that, molecular networking has been widely used in the last half decade, which relies on the idea that structurally related compounds have similar MS/MS fragmentation, and thus generate spectra similarity networks (Yang et al. 2013b ). The GNPS provides an open-source platform for sharing mass spectral data and data analysis based on molecular networking (Wang et al. 2016 ). As shown in Table 2 , we summarized the function characterization of the advanced molecular networking tools for metabolite annotation of LC-MS data, such as IIMN (Schmid et al. 2021 ), NetID (Chen et al. 2021 ), FBMN (Nothias et al. 2020 ), SIRIUS 4 (Duhrkop et al. 2019 ), NAP (da Silva et al. 2018 ), MSHub/GNPS (Aksenov et al. 2021 ), MetDNA (Shen et al. 2019 ), Metwork, MolNetEnhancer (Ernst et al. 2019 ), Qemistree (Tripathi et al. 2021 ), DEREPLICATOR+ (Mohimani et al. 2018 ), DEREPLICATOR (Mohimani et al. 2017 ), ISDB (Allard et al. 2016 ), MS2LDA (van Der Hooft et al. 2016 ) etc. To date, the strategy based on molecular networking has shown outstanding potential to increase the number of signals assigned to a putative chemical structure in MS based metabolomics, which has been comprehensively reviewed (Fox Ramos et al. 2019 ; Perez De Souza et al. 2020 ; Beniddir et al. 2021 ).
Metabolomics applications to plant research
Gene function characterization and metabolic pathway exploration.
Plant endogenous metabolites are indispensable for human being and the plant itself. Hence, comparative metabolomics has been very powerful to highlight the metabolic differences in different plant species (Wang et al. 2014a ) and in the cross-comparison of metabolite quantitative trait loci (mQTL) in populations of the same plant species (Wen et al. 2014 ). Metabolomics also allows the determination of metabolites in plants with vital roles in tolerance to abiotic or resistance to biotic stress. Integrating metabolomics with other-omics technologies is used to pinpoint the causal genes and further exploration of metabolic pathway, which becomes more and more important for marker-assisted breeding and metabolic engineering to target key pathways of plants. Previous studies show that multi-omics analysis using near isogenic lines and natural variants of a given plant could be used to identify new metabolites (Tsugawa et al. 2021 ).
Firstly, most traits of crops are polygenic, therefore, their genetic basis can be readily elucidated with the strategies of quantitative genetics. With the rapid development of metabolomics- and genomics-related technologies, mQTL and metabolite genome-wide association studies (mGWAS) have been commonly used to dissect the genetic architectures underlying the varied metabolites in plants in the last 15 years, which will generate the linkages or associations between chromosomal locations and metabolite contents. Subsequently, the candidate genes included within the genomic interval or adjacent to the associated marker loci are identified in many plant species with annotated genomes, which have been comprehensively reviewed (Fang and Luo 2019 ). Recently, by integrating comparative metabolomics and mGWAS analyse, Liang et al. identify 10 candidate genes significantly associated with the abundances of 37 metabolites, which are biomarkers related to salt stress tolerance (Liang et al. 2021a ). Chen et al. identify 26 candidate genes and validate two genes involved in the flavonoid decoration pathway of wheat kernel through wheat mGWAS study (Chen et al. 2020a ). And numerous studies have been reported to characterize genes and dissect the genetics of metabolic traits in other crops using a similar multi-omics integration approach, such as in cucumber (Shang et al. 2014 ), in maize (Wen et al. 2014 ; Wen et al. 2016 ), in tomato (Sauvage et al. 2014 ; Alseekh et al. 2015 ; Zhu et al. 2018a ), and in rice (Chen et al. 2014 ; Chen et al. 2016 ). We also conducted a study on integrated metabolomics, genomics, and other technologies to validate the function of several candidate genes in rice (Liu et al. 2020 ).
Secondly, the strategy of integrating metabolomics with transcriptomics also help to unveil the relationship between the genotype and phenotype of an organism and identify the function of core genes involved in specific metabolic pathways, such as proanthocyanidin biosynthesis in Arabidopsis thaliana (Kitamura et al. 2010 ), noscapine (Winzer et al. 2012 ), tanshione biosynthesis in Salvia miltiorrhiza (Gao et al. 2014 ), flavonoids and amino acids biosynthesis in Camellia sinensis L. (Huang et al. 2018 ), anthocyanin biosynthesis in Triticum aestivum L. (Wang et al. 2021b ). For example, we have integrated metabolomics with transcriptomics to demonstrate the carbon metabolism and plant hormones regulation in Vigna radiata during post-germination seedling growth (Wang et al. 2020a ). Many previous studies used the same multi-omics strategy to reveal the metabolic shifts or metabolic regulation occurred during plant development (Rohrmann et al. 2011 ; Asfaw Degu et al. 2014 ), or to understand the global metabolic responses to different kinds of stresses (Hirai et al. 2004 ; Oates et al. 2015 ; Agarrwal et al. 2016 ).
Thirdly, the strategy of integrating metabolomics with proteomics has been also used to demonstrate the alterations in the metabolic and protein composition of a cell required to manifest phenotypic plasticity by plants, such as responses to perturbation of glucosinolate biosynthesis (Chen et al. 2012 ), and to different stress (Kushalappa and Gunnaiah 2013 ). Amiour et al. have integrated metabolomics, transcriptomics, and proteomics to identify key steps involved in nitrogen metabolism in maize (Amiour et al., 2012 ). Recently, we have conducted comparative metabolomics, proteomics, and transcriptomics analyses between the winter and spring tender shoots of a novel ever-growing tea tree, and observed phytohormone, amino acid and energy metabolism response to winter adaptation (Dai et al. 2021 ). By integrating metabolomics with proteomics datasets for banana carotenoid study, we found that increased abundance of carotenogenesis-associated proteins alongside elevated carbohydrate accumulation contribute to high carotenoid content in banana pulp during its development, implying that a multi-target approach is necessary in order to improve carotenoid content in banana (Heng et al. 2019 ).
Safety assessment of genetic modified (GM) plants
Metabolic engineering is the use of particular metabolites for plant improvement strategy, that involves the transgenic expression and RNAi silencing of targeted genes encoding enzymes involved in the specific metabolic pathway to modulate the metabolite biosynthesis. However, beyond the intended changes, there are certain unintended changes that occur in these plants. Therefore, untargeted metabolomics technology has long been suggested and applied for safety assessment of the GM plants (Cellini et al. 2004 ; Heinemann et al. 2011 ; Stewart and Shepherd 2013 ; Guijas et al. 2018b ). Metabolic profiling of transgenic rice, which has a mutated anthranilate synthase gene for feedback inhibition-insensitive synthesis of tryptophan, reveal the elevated levels of free tryptophan and only minor changes in levels of other free amino acids (Wakasa et al. 2006 ). Several other crops such as soy (Padgette et al. 1996 ), potatoes (Hellwege et al. 2000 ), wheat (Obert et al. 2004 ), and alfalfa (McCann et al. 2006 ) have also been analyzed at the metabolic level to establish substantial equivalence between their GM and non-GM counterparts. Knock-out mutants of the phenylalanine ammonia lyase involved in the phenylpropanoid pathway in Arabidopsis accumulate higher levels of phenylalanine and also show perturbed metabolisms of other aromatic amino acids (Rohde et al. 2004 ). Recently, the unintended metabolic consequences of the BAR gene, which encodes a bacterial acetyltransferase that has N-acetylation activity towards the herbicidal amino acid phosphinothricin, expressed in transgenic crops is another illustration of the utility of untargeted metabolomics in assessing new genetically engineered traits in crops (Christ et al. 2017 ). Until now, numerous studies have illustrated the utility of untargeted metabolomics to improve GM crop safety assessment (Catchpole et al. 2005 ; Shepherd et al. 2015 ).
Natural products chemistry
Plant natural products (NPs) represent a large family of diverse chemical entities with a wide variety of biological activities that have been found with multiple uses, notably in human and veterinary medicine and in agriculture (Katz and Baltz 2016 ). For NP discovery, the separation of metabolites is usually performed using GC or HPLC columns, and the eluants are usually analyzed directly by MS detection. Several MS-based databases and software tools are now applied for NPs identification, such as ReSpect (Sawada et al. 2012 ) and GNPS (Wang et al. 2016 ). Elucidation of the metabolic pathways of NPs would help to determine their efficacy and safety. Integrating metabolomics and next-generation sequencing data help to elucidate the pathways of NP metabolism in medicinal plants. For example, the metabolic pathways of benzoisoquinoline and monoterpenoid indole alkaloids, cannabinoids, caffeine, ginsenosides, with anolides, artemisinin, and taxol are elucidated (Scossa et al. 2018 ). Furthermore, the labelling approach in metabolomics such as 13 C-based metabolomics studies, can also be applied for discovering novel secondary metabolism (Creek et al. 2012 ; Ellis and Goodacre 2012 ).
Using metabolomics technology, the metabolic maps of many bioactive NPs have been reported, such as myrislignan (Yang et al. 2017 ), triptonide (Hu et al. 2018 ), osthole (Zhao et al. 2018 ), dehydrodiisoeugenol (Lv et al. 2017 ) and so on. Biological activities of NPs can also be evaluated and their pharmacological effects can be predicted by MS-based metabolomics, which has been extensively reviewed (Zhao et al. 2018 ). Furthermore, metabolomics has also been employed in the quality control of NPs, being used to monitor the variation of metabolic profiles among individuals, environmental alterations during growth and harvesting, post harvesting treatment, extraction, and method of isolation (Salem et al. 2020 ). Metabolomics, combined with unsupervised principal component analysis and supervised partial least square analysis/partial least square analysis with discriminant analysis, are the common methods used in the quality control. For example, Wang et al. used this method to study the effect of location on the percentage of various constituents of chamomile ( Matricaria recutita L.) (Wang et al. 2004 ).
Challenges and future perspectives
Over the past decade, technological advances have led to significant improvements for in-depth proteomics and metabolomics analyses and have enabled the development of true single-cell proteomics and metabolomics technologies. However, despite all the progress, significant technical challenges remain mainly due to the inherent nature of the complex molecules involved. This is particularly true for single-cell proteomics and metabolomics given the limited amounts of materials available. Foremost among these challenges is sample preparation. To maximize proteome or metabolome coverage, one must develop an efficient and unbiased solubilization method for all cellular proteins and metabolites. When dealing with plant tissues, the cell wall poses an additional challenge in preparation of cells for FACS from plant tissue. In order to render the cells mobile, plant cells will have to be reduced to protoplasts by the removal cell wall through enzymatic degradation. This is a slow process that can, by itself, alter the composition of the cell’s proteome creating a highly artificial sample. A sufficient number of controls must be included to demonstrate that the proteomics information obtained after this process is at all relevant to biological question being asked and not just a consequence of the stress introduced by the removal of the cell wall.
Continuous developments concerning the technical aspects of high throughput sampling with miniaturization, fast and high resolution/accuracy MS with superior sensitivity, and machine learning-based data analysis are expected to push the boundaries enabling new capabilities allowing for single-cell measurement of PTMs, and improved dynamic range ultimately leading towards full coverage of both proteomes and metabolomes with quantitative accuracy. These front-end sample processing developments have propelled MS to become the central analytical tool. An integration of post-ionization gas-phase fractionation with advanced MS instruments is seeing increased use as an additional and complementary orthogonal separation to column chromatography. This development is of particular benefit to single-cell proteomics (Clark et al. 2021 ; Brunner et al. 2022 ). Therefore, the use of IMS is expected to expand particularly for single cell proteomics and metabolomics in future.
Further development of MSI in both proteomics and metabolomics is highly anticipated. In recent years, MSI is becoming more widely used for plant-omics as it provides molecular analysis of tissue with the spatial distribution of the different analytes in the tissue sample. To date, MSI has been the most impactful tool for metabolomic applications including single cell metabolomics. One advantage of MSI-MALDI is that matrix application and ionization are conducted directly on tissue, which is superior to cell isolation for metabolome integrity while retaining the relative localization of cells and allowing for assessment of the intercellular space (Korte et al. 2015 ). However, there are many challenges for MSI analysis that include data analysis, reproducibility, low throughput, poor quantitative accuracy and low ionization efficiency for plant peptides and proteins. Nevertheless, it is anticipated that further refinement and development of MSI platforms and associated data analysis software are necessary for reliable in situ analysis of proteins/peptides and metabolites reflecting specific plant phenotypes.
Many effective and intelligent MS analysis strategies with real-time instrument control and decision making have emerged as a means for improving the quality of acquired spectra and maximizing confident identification and quantitative accuracy. A recently developed RTS MS3 acquisition method on the Orbitrap Tribrid MS instrument is a good example for enhanced TMT-based quantitative proteomics (Erickson et al. 2019 ). Another is the use of the AcquireX intelligent data acquisition workflow for in-depth MS n analysis of numerous low abundance metabolites in untargeted metabolomics.
Interpreting the tens of thousands of MS/MS spectra, including chimeric spectra from multiple co-isolated peptides, and translating them into biological information is another hurdle. Automated MS/MS-based peptide identifications and metabolite annotations have relied on database search engines with statistically based scoring algorithms. Thus, we expect that improved database search engines, particularly deep learning-based algorithms will be developed for enhanced search outcome. In untargeted metabolomics and DIA-based proteomics, spectral library databases are required for confident identities (Tada et al. 2019 ; Zhang et al. 2020a ). However, building these reference libraries from chemical standards or DDA of peptide fractions is limited in size relative to known chemicals or initial DDA identities. Recently, development of algorithms predicting in silico MS/MS spectra has shown great promise (McEachran et al. 2019 ; Chao et al. 2020 ) for DIA in-depth proteome (Hu et al. 2016 ). Thus, we anticipate the current search engines and algorithms of in silico MS/MS spectra annotation will be further improved.
Perhaps the biggest challenge for future proteomics and metabolomics is to interrogate the acquired data with multi-omics datasets in system biology studies. We have reached a stage where high-throughput sequencing and MS cover all the large-scale disciplines we can define: genomics, transcriptomics, proteomics, metabolomics, and lipidomics. With this amount of information, a systematic multi-omics integration (MOI) of the large data sets from all those techniques remains a need (Gomez-Cabrero et al. 2014 ). Despite the unique challenges in plants, three levels of MOI were summarized covering element-based, pathway-based and mathematical-based integration (Jamil et al. 2020 ). PaintOmics, a web server for the integrated analysis and visualization of multiple omic data has been continually developed in the past decade, allowing researchers for interactive exploration of their multi-omics datasets including transcriptomics, proteomics and metabolomics (Hernandez-de-Diego et al. 2018 ; Liu et al. 2022 ). Since machine learning has been demonstrated to aid in integrating the multi-omics platforms for plant-environment interaction (Moore et al. 2019 ) and precision breeding (Weckwerth et al. 2020 ), we anticipate the development of effective machine learning algorithms will be one of the focuses in facilitating plant MOI research.
Availability of data and materials
All data discussed in this review are associated with the supporting primary research papers except of Fig. 5 (unpublished data) which will be available upon request.
Abbreviations
Affinity purification coupled to mass spectrometry
Capillary electrophoresis tandem mass spectrometry
Chemical entities of biological interest
Co-immunoprecipitation
Collision cross-section
Collision induced dissociation
Covalent organic frameworks
Data dependent acquisition
Data independent acquisition
Differential abundance proteins
Electron capture dissociation
Electron transfer dissociation
Electroosmotic flow
False discovery rate
Fluorescent activated cell sorting
Fourier transfer ion cyclotron resonance
Full width at half maximum
Gas chromatography tandem mass spectrometry
Gene Ontology
Genetic modified
Global natural products social molecular networking resource
Golm metabolome database
Headspace-solid-phase microextraction
Higher energy collisional dissociation
Human metabolome database
Hydrophilic interaction chromatography
Immobilized metal affinity chromatography
Ion mobility spectrometry
Isobaric tags for relative and absolute quantification
Isotopically non-stationary metabolic flux analysis
Kyoto Encyclopedia of Genes and Genomes
Label free quantitation
Laser capture microdissection
Liquid chromatography tandem mass spectrometry
- Mass spectrometry imaging
Massbank of north america
Matrix-assisted laser desorption ionization
Metabolic flux analysis
Metabolite genome-wide association studies
Metabolite link
Metabolite quantitative trait loci
Metal-organic frameworks
Minimal ProteOmic sample Preparation
Molecularly imprinted polymers
Multi-omics integration
Multiple reaction monitoring
Multiwalled carbon nanotubes
Nanodroplet processing in one pot for trace samples
National institute of standards and technology
Natural products
Post translational modifications
Protein–protein interactions
Quadrupole-linear ion trap mass spectrometry
Real-time search
Reversed phase liquid chromatography
RIKEN MSn spectral database for phytochemicals
Select ion monitoring
Single-pot solid-phase-enhanced sample preparation
Solid-phase extraction
Solid-phase mesh-enhanced sorption from headspace
Solid-phase microextraction
Stable-isotope labelling by amino acids in cell culture
Strong cation exchange
Super critical fluid chromatography
Synchronous precursor selection
- Tandem mass tag
Target of rapamycin
Thermal proteome profiling
Titanium dioxide
Triple quadrupole mass spectrometry
Two-dimensional liquid chromatography
Ultra performance liquid chromatography
Ultraviolet photodissociation
Universal natural product database
Yeast two hybrid
Zirconium dioxide
Adams KJ, Pratt B, Bose N, Dubois LG, St John-Williams L, Perrott KM, et al. Skyline for Small Molecules: A Unifying Software Package for Quantitative Metabolomics. J Proteome Res. 2020;19:1447–58.
Article CAS PubMed PubMed Central Google Scholar
Aebersold R, Mann M. Mass-spectrometric exploration of proteome structure and function. Nature. 2016;537:347–55.
Article CAS PubMed Google Scholar
Agarrwal R, Padmakumari AP, Bentur JS, Nair S. Metabolic and transcriptomic changes induced in host during hypersensitive response mediated resistance in rice against the Asian rice gall midge. Rice. 2016;9:5.
Article PubMed PubMed Central Google Scholar
Agrawal GK, Sarkar A, Righetti PG, Pedreschi R, Carpentier S, Wang T, et al. A decade of plant proteomics and mass spectrometry: translation of technical advancements to food security and safety issues. Mass Spectrom Rev. 2013;32:335–65.
Aksenov AA, Laponogov I, Zhang Z, et al. Auto-deconvolution and molecular networking of gas chromatography–mass spectrometry data. Nat Biotechnol. 2021;39:169–73.
Allard PM, Peresse T, Bisson J, Gindro K, Marcourt L, Pham VC, et al. Integration of Molecular Networking and In-Silico MS/MS Fragmentation for Natural Products Dereplication. Anal Chem. 2016;88:3317–23.
Almstetter MF, Oefner PJ, Dettmer K. Comprehensive two-dimensional gas chromatography in metabolomics. Anal Bioanal Chem. 2012;402:1993–2013.
Alseekh S, Fernie AR. Metabolomics 20 years on: what have we learned and what hurdles remain? Plant J. 2018;94:933–42.
Alseekh S, Tohge T, Wendenberg R, Scossa F, Omranian N, Li J, et al. Identification and mode of inheritance of quantitative trait loci for secondary metabolite abundance in tomato. Plant Cell. 2015;27:485–512.
Alseekh S, Kostova D, Bulut M, Fernie AR. Genome-wide association studies: assessing trait characteristics in model and crop plants. CMLS. 2021;78:5743–54.
Alvarez S, Naldrett MJ. Mass spectrometry based untargeted metabolomics for plant systems biology. Emerg Top Life Sci. 2021;5:189–201.
Alvarez-Segura T, Torres-Lapasio JR, Ortiz-Bolsico C, Garcia-Alvarez-Coque MC. Stationary phase modulation in liquid chromatography through the serial coupling of columns: A review. Anal Chim Acta. 2016;923:1–23.
Amiour N, Imbaud S, Clément G, Agier N, Zivy M, Valot B, et al. The use of metabolomics integrated with transcriptomic and proteomic studies for identifying key steps involved in the control of nitrogen metabolism in crops such as maize. J Exp Bot. 2012;63(2):695–709.
CAS Google Scholar
Armean IM, Lilley KS, Trotter MW. Popular computational methods to assess multiprotein complexes derived from label-free affinity purification and mass spectrometry (AP-MS) experiments. Mol Cell Proteomics. 2013;12:1–13.
Article PubMed CAS Google Scholar
Asfaw Degu UH, Sikron N, Venturini L, Buson G, Ghan R, Plaschkes I, et al. Metabolite and transcript profiling of berry skin during fruit development elucidates differential regulation between Cabernet Sauvignon and Shiraz cultivars at branching points in the polyphenol pathway. BMC Plant Biol. 2014;14:188.
Article PubMed PubMed Central CAS Google Scholar
Astarita G, Langridge J. An emerging role for metabolomics in nutrition science. J Nutrigenet Nutrigenomics. 2013;6:181–200.
CAS PubMed Google Scholar
Atwell BJ. Well-Designed Experiments Make Proteomic Studies on Stressed Plants Meaningful. In: Salekdeh GH, editor. Agricultural Proteomics Volume 2: Environmental Stresses. Cham: Springer International Publishing; 2016. p. 1–18.
Google Scholar
Balasubramanian VK, Purvine SO, Liang Y, Kelly RT, Pasa-Tolic L, Chrisler WB, et al. Cell-Type-Specific Proteomics Analysis of a Small Number of Plant Cells by Integrating Laser Capture Microdissection with a Nanodroplet Sample Processing Platform. Curr Protoc. 2021;1:e153.
Baran R, Kochi H, Saito N, Suematsu M, Soga T, Nishioka T, Robert M, Tomita M. MathDAMP: a package for differential analysis of metabolite profiles. BMC bioinformatics. 2006;7:1–9.
Article CAS Google Scholar
Batalha IL, Lowe CR, Roque AC. Platforms for enrichment of phosphorylated proteins and peptides in proteomics. Trends Biotechnol. 2012;30:100–10.
Batelli G, Verslues PE, Agius F, Qiu Q, Fujii H, Pan S, et al. SOS2 promotes salt tolerance in part by interacting with the vacuolar H+-ATPase and upregulating its transport activity. Mol Cell Biol. 2007;27:7781–90.
Beauxis Y, Genta-Jouve G. MetWork: a web server for natural products anticipation. Bioinformatics. 2019;35(10):1795–6. https://doi.org/10.1093/bioinformatics/bty864 . https://pubmed.ncbi.nlm.nih.gov/30295702/ .
Bencivenni M, Faccini A, Zecchi R, Boscaro F, Moneti G, Dossena A, et al. Electrospray MS and MALDI imaging show that non-specific lipid-transfer proteins (LTPs) in tomato are present as several isoforms and are concentrated in seeds. J Mass Spectrom. 2014;49:1264–71.
Beniddir MA, Kang KB, Genta-Jouve G, Huber F, Rogers S, van der Hooft JJJ. Advances in decomposing complex metabolite mixtures using substructure- and network-based computational metabolomics approaches. Nat Prod Rep. 2021;38:1967–93.
Bern M, Cai Y, Goldberg D. Lookup peaks: a hybrid of de novo sequencing and database search for protein identification by tandem mass spectrometry. Anal Chem. 2007;79(4):1393–400. https://doi.org/10.1021/ac0617013 . https://pubmed.ncbi.nlm.nih.gov/17243770/ .
Bhandari DR, Wang Q, Friedt W, Spengler B, Gottwald S, Rompp A. High Resolution Mass Spectrometry Imaging of Plant Tissues: Towards a Plant Metabolite Atlas. Analyst. 2015;140:7696–709.
Bhawal R, Oberg AL, Zhang S, Kohli M. Challenges and Opportunities in Clinical Applications of Blood-Based Proteomics in Cancer. Cancers. 2020;12.
Bjarnholt N, Li B, D'Alvise J, Janfelt C. Mass spectrometry imaging of plant metabolites – principles and possibilities. Nat Prod Rep. 2014;31:818–37.
Blackburn MR, Minkoff BB, Sussman MR. Mass spectrometry-based technologies for probing the 3D world of plant proteins. Plant Physiol. 2022;189:12–22.
Article PubMed Google Scholar
Blazenovic I, Kind T, Ji J, Fiehn O. Software Tools and Approaches for Compound Identification of LC-MS/MS Data in Metabolomics. Metabolites. 2018;8.
Böcker S, Letzel MC, Lipták Z, Pervukhin A. SIRIUS: decomposing isotope patterns for metabolite identification†. Bioinformatics. 2009;25:218–24.
Bontinck M, Van Leene J, Gadeyne A, De Rybel B, Eeckhout D, Nelissen H, et al. Recent Trends in Plant Protein Complex Analysis in a Developmental Context. Front Plant Sci. 2018;9:640.
Bouchnak I, Brugiere S, Moyet L, Le Gall S, Salvi D, Kuntz M, et al. Unraveling Hidden Components of the Chloroplast Envelope Proteome: Opportunities and Limits of Better MS Sensitivity. Mol Cell Proteomics. 2019;18:1285–306.
Broeckling CD, Afsar FA, Neumann S, Ben-Hur A, Prenni JE. RAMClust: a novel feature clustering method enables spectral-matching-based annotation for metabolomics data. Anal Chem. 2014;86:6812–7.
Brown M, Wedge DC, Goodacre R, Kell DB, Baker PN, Kenny LC, et al. Automated workflows for accurate mass-based putative metabolite identification in LC_MS-derived metabolomic datasets. Bioinformatics. 2011;27.
Brunner A, Thielert M, Vasilopoulou CG, Mann M. Ultra-high sensitivity mass spectrometry quantifies single-cell proteome changes upon perturbation. bioRxiv. 2022;(22 Dec 2020).
Budnik B, Levy E, Harmange G, Slavov N. SCoPE-MS: mass spectrometry of single mammalian cells quantifies proteome heterogeneity during cell differentiation. Genome Biol. 2018;19:161.
Burnum-Johnson KE, Zheng X, Dodds JN, Ash J, Fourches D, Nicora CD, et al. Ion Mobility Spectrometry and the Omics: Distinguishing Isomers, Molecular Classes and Contaminant Ions in Complex Samples. Trends Anal Chem. 2019;116:292–9.
Cajka T, Fiehn O. Comprehensive analysis of lipids in biological systems by liquid chromatography-mass spectrometry. TrAC Trends Anal Chem. 2014;61:192–206.
Capellades J, Navarro M, Samino S, Garcia-Ramirez M, Hernandez C, Simo R, et al. geoRge: A Computational Tool To Detect the Presence of Stable Isotope Labeling in LC/MS-Based Untargeted Metabolomics. Anal Chem. 2015;88:621–8.
Carbonara K, Andonovski M, Coorssen JR. Proteomes Are of Proteoforms: Embracing the Complexity. Proteomes. 2021;9.
Cascante M, Marin S. Metabolomics and fluxomics approaches. Essays Biochem. 2008;45:67–82.
Catchpole GS, Beckmann M, Enot DP, Mondhe M, Zywicki B, Taylor J, et al. Hierarchical metabolomics demonstrates substantial compositional similarity between genetically modified and conventional potato crops. Proc Natl Acad Sci U S A. 2005;102:14458–62.
Cavatorta V, Sforza S, Mastrobuoni G, Pieraccini G, Francese S, Moneti G, et al. Unambiguous characterization and tissue localization of Pru P 3 peach allergen by electrospray mass spectrometry and MALDI imaging. J Mass Spectrom. 2009;44:891–7.
Ceballos-Laita L, Gutierrez-Carbonell E, Takahashi D, Lonsdale A, Abadia A, Doblin MS, Bacic A, Uemura M, Abadia J, Lopez-Millan AF. Effects of Excess Manganese on the Xylem Sap Protein Profile of Tomato ( Solanum lycopersicum ) as Revealed by Shotgun Proteomic Analysis. International journal of molecular sciences. 2020;21(22):8863.
Article CAS PubMed Central Google Scholar
Cellini F, Chesson A, Colquhoun I, Constable A, Davies HV, Engel KH, et al. Unintended effects and their detection in genetically modified crops. Food Chem Toxicol. 2004;42:1089–125.
Chaleckis R, Meister I, Zhang P, Wheelock CE. Challenges, progress and promises of metabolite annotation for LC-MS-based metabolomics. Curr Opin Biotechnol. 2019;55:44–50.
Chalkley RJ, Baker PR, Huang L, Hansen KC, Allen NP, Rexach M, et al. Comprehensive analysis of a multidimensional liquid chromatography mass spectrometry dataset acquired on a quadrupole selecting, quadrupole collision cell, time-of-flight mass spectrometer: II. New developments in Protein Prospector allow for reliable and comprehensive automatic analysis of large datasets. Mol Cell Proteomics. 2005;4(8):1194–204. https://doi.org/10.1074/mcp.D500002-MCP200 . https://pubmed.ncbi.nlm.nih.gov/15937296/ .
Chang IF, Curran A, Woolsey R, Quilici D, Cushman JC, Mittler R, et al. Proteomic profiling of tandem affinity purified 14-3-3 protein complexes in Arabidopsis thaliana. Proteomics. 2009;9:2967–85.
Chang C, Zhang J, Han M, Ma J, Zhang W, Wu S, et al. SILVER: an efficient tool for stable isotope labeling LC-MS data quantitative analysis with quality control methods. Bioinformatics. 2014;30:586–7.
Chang C, Li M, Guo C, Ding Y, Xu K, Han M, et al. PANDA: A comprehensive and flexible tool for quantitative proteomics data analysis. Bioinformatics. 2019;35:898–900.
Chao A, Al-Ghoul H, McEachran AD, Balabin I, Transue T, Cathey T, et al. In silico MS/MS spectra for identifying unknowns: a critical examination using CFM-ID algorithms and ENTACT mixture samples. Anal Bioanal Chem. 2020;412:1303–15.
Chatr-Aryamontri A, Oughtred R, Boucher L, Rust J, Chang C, Kolas NK, et al. The BioGRID interaction database: 2017 update. Nucleic Acids Res. 2017;45:D369–79.
Chavez JD, Bruce JE. Chemical cross-linking with mass spectrometry: a tool for systems structural biology. Curr Opin Chem Biol. 2019;48:8–18.
Chawade A, Alexandersson E, Levander F. Normalyzer: A Tool for Rapid Evaluation of Normalization Methods for Omics Data Sets. J Proteome Res. 2014;13:3114–20.
Chemat F, Rombaut N, Sicaire A-G, Meullemiestre A, Fabiano-Tixier A-S, Abert-Vian M. Ultrasound assisted extraction of food and natural products. Mechanisms, techniques, combinations, protocols and applications. A review. Ultrason Sonochem. 2017;34:540–60.
Chen X, Shachar-Hill Y. Insights into metabolic efficiency from flux analysis. J Exp Bot. 2012;63:2343–51.
Chen YZ, Pang QY, He Y, Zhu N, Branstrom I, Yan XF, et al. Proteomics and metabolomics of Arabidopsis responses to perturbation of glucosinolate biosynthesis. Mol Plant. 2012;5:1138–50.
Chen S, Hoene M, Li J, Li Y, Zhao X, Häring H-U, et al. Simultaneous extraction of metabolome and lipidome with methyl tert-butyl ether from a single small tissue sample for ultra-high performance liquid chromatography/mass spectrometry. J Chromatogr A. 2013;1298:9–16.
Chen W, Gao Y, Xie W, Gong L, Lu K, Wang W, et al. Genome-wide association analyses provide genetic and biochemical insights into natural variation in rice metabolism. Nat Genet. 2014;46:714–21.
Chen W, Wang W, Peng M, Gong L, Gao Y, Wan J, et al. Comparative and parallel genome-wide association studies for metabolic and agronomic traits in cereals. Nat Commun. 2016;7:12767.
Chen F, Song Y, Li X, Chen J, Mo L, Zhang X, et al. Genome sequences of horticultural plants: past, present, and future. Hortic Res. 2019;6:112.
Chen Q, Huang R, Xu Z, Zhang Y, Li L, Fu J, Wang G, Wang J, Du X, Gu R. Label-Free Comparative Proteomic Analysis Combined with Laser-Capture Microdissection Suggests Important Roles of Stress Responses in the Black Layer of Maize Kernels. International journal of molecular sciences. 2020;21:1369.
Chen J, Hu X, Shi T, Yin H, Sun D, Hao Y, et al. Metabolite-based genome-wide association study enables dissection of the flavonoid decoration pathway of wheat kernels. Plant Biotechnol J. 2020a;18:1722–35.
Chen L, Lu W, Wang L, Xing X, Chen Z, Teng X, et al. Metabolite discovery through global annotation of untargeted metabolomics data. Nat Methods. 2021;18:1377–85.
Cheng X, Yin Z, Rong L, Hang W. Subcellular chemical imaging of structurally similar acridine drugs by near-field laser desorption/laser postionization mass spectrometry. Nano Res. 2020;13:745–51.
Chi A, Huttenhower C, Geer LY, Coon JJ, Syka JE, Bai DL, et al. Analysis of phosphorylation sites on proteins from Saccharomyces cerevisiae by electron transfer dissociation (ETD) mass spectrometry. Proc Natl Acad Sci U S A. 2007;104:2193–8.
Cho K, Schwaiger-Haber M, Naser FJ, Stancliffe E, Sindelar M, Patti GJ. Targeting unique biological signals on the fly to improve MS/MS coverage and identification efficiency in metabolomics. Anal Chim Acta. 2021;1149:338210.
Choi H, Nesvizhskii AI. False discovery rates and related statistical concepts in mass spectrometry-based proteomics. J Proteome Res. 2008;7:47–50.
Christ B, Hochstrasser R, Guyer L, Francisco R, Aubry S, Hortensteiner S, et al. Non-specific activities of the major herbicide-resistance gene BAR. Nat Plants. 2017;3:937–45.
Claassen C, Ebel E, Kuballa J, Rohn S. Impacts of Fungicide Treatment and Conventional Fertilization Management on the Potato Metabolome (Solanum tuberosum L.) Evaluated with UPLC-IMS-QToF. J Agric Food Chem. 2019;67:11542–52.
Clark NM, Elmore JM, Walley JW. To the proteome and beyond: advances in single-cell omics profiling for plant systems. Plant Physiol. 2021;188:726–37.
Article PubMed Central CAS Google Scholar
Cleland TP, DeHart CJ, Fellers RT, VanNispen AJ, Greer JB, LeDuc RD, et al. High-Throughput Analysis of Intact Human Proteins Using UVPD and HCD on an Orbitrap Mass Spectrometer. J Proteome Res. 2017;16:2072–9.
Cocuron JC, Koubaa M, Kimmelfield R, Ross Z, Alonso AP. A Combined Metabolomics and Fluxomics Analysis Identifies Steps Limiting Oil Synthesis in Maize Embryos. Plant Physiol. 2019;181:961–75.
Colinge J, Masselot A, Giron M, Dessingy T, Magnin J. OLAV: towards high-throughput tandem mass spectrometry data identification. Proteomics. 2003;3(8):1454–63. https://doi.org/10.1002/pmic.200300485 . https://pubmed.ncbi.nlm.nih.gov/12923771/ .
Conesa A, Gotz S. Blast2GO: A comprehensive suite for functional analysis in plant genomics. Int J Plant Genomics. 2008;2008:619832.
Cong Y, Motamedchaboki K, Misal SA, Liang Y, Guise AJ, Truong T, et al. Ultrasensitive single-cell proteomics workflow identifies >1000 protein groups per mammalian cell. Chem Sci. 2020;12:1001–6.
Conley CJ, Smith R, Torgrip RJ, Taylor RM, Tautenhahn R, Prince JT. Massifquant: open-source Kalman filter based XC-MS isotope trace feature detection. Bioinformatics. 2014;18:2636–43.
Cox J, Mann M. MaxQuant enables high peptide identification rates, individualized p.p.b.-range mass accuracies and proteome-wide protein quantification. Nat Biotechnol. 2008;26:1367–72.
Cox J, Neuhauser N, Michalski A, Scheltema RA, Olsen JV, Mann M. Andromeda: a peptide search engine integrated into the MaxQuant environment. J Proteome Res. 2011;10:1794–805.
Creek DJ, Chokkathukalam A, Jankevics A, Burgess KE, Breitling R, Barrett MP. Stable isotope-assisted metabolomics for network-wide metabolic pathway elucidation. Anal Chem. 2012;84:8442–7.
Crown SB, Antoniewicz MR. Publishing 13C metabolic flux analysis studies: A review and future perspectives. Metab Eng. 2013;20:42–8.
Cuadros-Inostroza Á, Caldana C, Redestig H, Kusano M, Lisec J, Peña-Cortés H, et al. TargetSearch - a Bioconductor package for the efficient preprocessing of GC-MS metabolite profiling data. BMC Bioinformatics. 2009;10:1–2.
Cuperus JT. Single-cell genomics in plants: current state, future directions, and hurdles to overcome. Plant Physiol. 2022;188:749–55.
Cusick ME, Klitgord N, Vidal M, Hill DE Interactome: gateway into systems biology. Hum Mol Genet . 2005;14 Spec No 2:R171–181.
da Silva RR, Wang M, Nothias LF, van der Hooft JJJ, Caraballo-Rodriguez AM, Fox E, et al. Propagating annotations of molecular networks using in silico fragmentation. PLoS Comput Biol. 2018;14:e1006089.
Dai S, Chen S. Single-cell-type proteomics: toward a holistic understanding of plant function. Mol Cell Proteomics. 2012;11:1622–30.
Dai Z, Huang H, Zhang Q, Bei J, Chen Z, Liu Q, et al. Comparative Multi-Omics of Tender Shoots from a Novel Evergrowing Tea Cultivar Provide Insight into the Winter Adaptation Mechanism. Plant Cell Physiol. 2021;62:366–77.
Daly R, Rogers S, Wandy J, Jankevics A, Burgess KEV, Breitling R. MetAssign: probabilistic annotation of metabolites from LC–MS data using a Bayesian clustering approach. Bioinformatics. 2014;30:2764–71.
Dang J, Zhang L, Wang Q, Mei L, Yue H, Liu Z, et al. Target separation of flavonoids from Saxifraga tangutica using two-dimensional hydrophilic interaction chromatography/reversed-phase liquid chromatography. J Sep Sci. 2018;41:4419–29.
DeLaney K, Sauer CS, Vu NQ, Li L. Recent Advances and New Perspectives in Capillary Electrophoresis-Mass Spectrometry for Single Cell “Omics”. Molecules. 2019;24:42.
Deng GM, Yang QS, He WD, Li CY, Yang J, Zuo CW, et al. Proteomic analysis of conidia germination in Fusarium oxysporum f. sp. cubense tropical race 4 reveals new targets in ergosterol biosynthesis pathway for controlling Fusarium wilt of banana. Appl Microbiol Biotechnol. 2015;99:7189–207.
Dettmer K, Aronov PA, Hammock BD. Mass spectrometry-based metabolomics. Mass Spectrom Rev. 2007;26:51–78.
Dong Y, Li B, Malitsky S, Rogachev I, Aharoni A, Kaftan F, et al. Sample Preparation for Mass Spectrometry Imaging of Plant Tissues: A Review. Frontiers in plant science 7; 2016b.
Book Google Scholar
Dong Y, Li B, Aharoni A. More than Pictures: When MS Imaging Meets Histology. Trends Plant Sci. 2016a;21:686–98.
Dong Y, Sonawane P, Cohen H, Polturak G, Avivi S, Rogachev I, et al. High Mass Resolution, Spatial Metabolite Mapping Enhances the Current Plant Gene and Pathway Discovery Toolbox. New Phytol. 2020;228:1986–2002.
Dou M, Clair G, Tsai CF, Xu K, Chrisler WB, Sontag RL, et al. High-Throughput Single Cell Proteomics Enabled by Multiplex Isobaric Labeling in a Nanodroplet Sample Preparation Platform. Anal Chem. 2019;91:13119–27.
Dou T, Shao X, Hu C, Liu S, Sheng O, Bi F, et al. Host-induced gene silencing of Foc TR4 ERG6/11 genes exhibits superior resistance to Fusarium wilt of banana. Plant Biotechnol J. 2020;18:11–3.
Dührkop K, Shen H, Meusel M, Rousu J, Böcker S. Searching molecular structure databases with tandem mass spectra using CSI: FingerID. PNAS. 2015;112:12580–5.
Duhrkop K, Fleischauer M, Ludwig M, Aksenov AA, Melnik AV, Meusel M, et al. SIRIUS 4: a rapid tool for turning tandem mass spectra into metabolite structure information. Nat Methods. 2019;16:299–302.
Dührkop K, Nothias L-F, Fleischauer M, Reher R, Ludwig M, Hoffmann MA, et al. Systematic classification of unknown metabolites using high-resolution fragmentation mass spectra. Nat Biotechnol. 2020;39:462–71.
Durek P, Schmidt R, Heazlewood JL, Jones A, MacLean D, Nagel A, et al. PhosPhAt: the Arabidopsis thaliana phosphorylation site database. An update. Nucleic Acids Res. 2010;38:D828–34.
Ellis DI, Goodacre R. Metabolomics-assisted synthetic biology. Curr Opin Biotechnol. 2012;23:22–8.
Engelsberger WR, Schulze WX. Nitrate and ammonium lead to distinct global dynamic phosphorylation patterns when resupplied to nitrogen-starved Arabidopsis seedlings. Plant J. 2012;69:978–95.
Erickson BK, Mintseris J, Schweppe DK, Navarrete-Perea J, Erickson AR, Nusinow DP, et al. Active Instrument Engagement Combined with a Real-Time Database Search for Improved Performance of Sample Multiplexing Workflows. J Proteome Res. 2019;18:1299–306.
Ernest B, Gooding JR, Campagna SR, Saxton AM, Voy BH. MetabR_ an R script for linear model analysis of quantitative metabolomic data. BMC Res Notes. 2012;5:596.
Ernst M, Kang KB, Caraballo-Rodriguez AM, Nothias LF, Wandy J, Chen C, et al. MolNetEnhancer: Enhanced Molecular Networks by Integrating Metabolome Mining and Annotation Tools. Metabolites. 2019;9:144.
Etalo DW, De Vos RCH, Joosten MHAJ, Hall RD. Spatially Resolved Plant Metabolomics: Some Potentials and Limitations of Laser-Ablation Electrospray Ionization Mass Spectrometry Metabolite Imaging. Plant Physiol. 2015;169:1424–35.
Fan S, Meng Y, Song M, Pang C, Wei H, Liu J, et al. Quantitative phosphoproteomics analysis of nitric oxide-responsive phosphoproteins in cotton leaf. PLoS One. 2014;9:e94261.
Fang C, Luo J. Metabolic GWAS-based dissection of genetic bases underlying the diversity of plant metabolism. Plant J. 2019;97:91–100.
Faraji M, Yamini Y, Gholami M. Recent Advances and Trends in Applications of Solid-Phase Extraction Techniques in Food and Environmental Analysis. Chromatographia. 2019;82:1207–49.
Fekete S, Veuthey JL, Guillarme D. Comparison of the most recent chromatographic approaches applied for fast and high resolution separations: Theory and practice. J Chromatogr A. 2015;1408:1–14.
Feldberg L, Venger I, Malitsky S, Rogachev I, Aharoni A. Dual Labeling of Metabolites for Metabolome Analysis (DLEMMA). Anal Chem. 2009;81:9257–66.
Felicity Allen AP, Wilson M, Greiner R, DavidWishart. CFM-ID: a web server__for annotation, spectrum prediction and metabolite identification__from tandem mass spectra. Nucleic Acids Res. 2014;42:W94–9.
Fernández-Albert F, Llorach R, Garcia-Aloy M, Ziyatdinov A, Andres-Lacueva C, Perera A. Intensity drift removal in LC/MS metabolomics by common variance compensation. Bioinformatics. 2014;30:2899–905.
Fernandez-Fuentes N, Zhou B, Wang J, Ressom HW. MetaboSearch: Tool for Mass-Based Metabolite Identification Using Multiple Databases. PLoS One. 2012;7:e40096.
Feussner I, Polle A. What the transcriptome does not tell - proteomics and metabolomics are closer to the plants' patho-phenotype. Curr Opin Plant Biol. 2015;26:26–31.
Fitzpatrick MA, Mcgrath CM, Young SP. Pathomx: an interactive workflow-based tool for the analysis of metabolomic data. BMC Bioinformatics. 2014;15:396.
Ford MM, Lawrence SR 2nd, Werth EG, McConnell EW, Hicks LM. Label-Free Quantitative Phosphoproteomics for Algae. Methods Mol Biol. 2020;2139:197–211.
Fox Ramos AE, Evanno L, Poupon E, Champy P, Beniddir MA. Natural products targeting strategies involving molecular networking: different manners, one goal. Nat Prod Rep. 2019;36:960–80.
Franken H, Mathieson T, Childs D, Sweetman GM, Werner T, Togel I, et al. Thermal proteome profiling for unbiased identification of direct and indirect drug targets using multiplexed quantitative mass spectrometry. Nat Protoc. 2015;10:1567–93.
Fu Q, Liu Z, Bhawal R, Anderson ET, Sherwood RW, Yang Y, et al. Comparison of MS(2), synchronous precursor selection MS(3), and real-time search MS(3) methodologies for lung proteomes of hydrogen sulfide treated swine. Anal Bioanal Chem. 2021;413:419–29.
Fu J, Zhang Y, Wang Y, Zhang H, Liu J, Tang J, et al. Optimization of metabolomic data processing using NOREVA. Nat Protoc. 2022;17:129–51.
Fukao Y. Protein-protein interactions in plants. Plant Cell Physiol. 2012;53:617–25.
Furuya T, Matsuoka D, Nanmori T. Phosphorylation of Arabidopsis thaliana MEKK1 via Ca(2+) signaling as a part of the cold stress response. J Plant Res. 2013;126:833–40.
Gallais A, Coque M, Quilléré I, Prioul J-L, Hirel B. Modelling postsilking nitrogen fluxes in maize (Zea mays) using 15N-labelling field experiments. New Phytol. 2006;172:696–707.
Gallego R, Bueno M, Herrero M. Sub- and supercritical fluid extraction of bioactive compounds from plants, food-by-products, seaweeds and microalgae – An update. TrAC Trends Anal Chem. 2019;116:198–213.
Gao J, Agrawal GK, Thelen JJ, Xu D. P3DB: a plant protein phosphorylation database. Nucleic Acids Res. 2009;37:D960–2.
Gao W, Sun HX, Xiao H, Cui G, Hillwig ML, Jackson A, et al. Combining metabolomics and transcriptomics to characterize tanshinone biosynthesis in Salvia miltiorrhiza. BMC Genomics. 2014;15:73.
Gao J, Zhang S, He WD, Shao XH, Li CY, Wei YR, et al. Comparative Phosphoproteomics Reveals an Important Role of MKK2 in Banana (Musa spp.) Cold Signal Network. Sci Rep. 2017;7:40852.
Gao F, Ma P, Wu Y, Zhou Y, Zhang G. Quantitative Proteomic Analysis of the Response to Cold Stress in Jojoba, a Tropical Woody Crop. International journal of molecular sciences. 2019;20:243.
Geer LY, Markey SP, Kowalak JA, Wagner L, Xu M, Maynard DM, et al. Open mass spectrometry search algorithm. J Proteome Res. 2004;3(5):958–64. https://doi.org/10.1021/pr0499491 . https://pubmed.ncbi.nlm.nih.gov/15473683/ .
Gates MB, Tomer KB, Deterding LJ. Comparison of metal and metal oxide media for phosphopeptide enrichment prior to mass spectrometric analyses. J Am Soc Mass Spectrom. 2010;21:1649–59.
Gemperline E, Keller C, Jayaraman D, Maeda J, Sussman MR, Ané J-M, et al. Examination of Endogenous Peptides in Medicago truncatula Using Mass Spectrometry Imaging. J Proteome Res. 2016b;15:4403–11.
Gemperline E, Keller C, Li L. Mass Spectrometry in Plant-omics. Anal Chem. 2016a;88:3422–34.
Gerlich M, Neumann S. MetFusion: integration of compound identification strategies. J Mass Spectrom. 2013;48:291–8.
Ghiasvand A, Dowlatshah S, Nouraei N, Heidari N, Yazdankhah F. A solid-phase microextraction platinized stainless steel fiber coated with a multiwalled carbon nanotube-polyaniline nanocomposite film for the extraction of thymol and carvacrol in medicinal plants and honey. J Chromatogr A. 2015;1406:87–93.
Gingras AC, Gstaiger M, Raught B, Aebersold R. Analysis of protein complexes using mass spectrometry. Nat Rev Mol Cell Biol. 2007;8:645–54.
Glaab E, Schneider R. RepExplore: addressing technical replicate variance in proteomics and metabolomics data analysis. Bioinformatics. 2015;31:2235–7.
Gnad F, Gunawardena J, Mann M. PHOSIDA 2011: the posttranslational modification database. Nucleic Acids Res. 2011;39:D253–60.
Gomez-Cabrero D, Abugessaisa I, Maier D, Teschendorff A, Merkenschlager M, Gisel A, et al. Data integration in the era of omics: current and future challenges. BMC Syst Biol. 2014;8(Suppl 2):I1.
González-Riano C, Dudzik D, Garcia A, Gil-de-la-Fuente A, Gradillas A, Godzien J, et al. Recent Developments along the Analytical Process for Metabolomics Workflows. Anal Chem. 2020;92:203–26.
Gray N, Lewis MR, Plumb RS, Wilson ID, Nicholson JK. High-Throughput Microbore UPLC–MS Metabolic Phenotyping of Urine for Large-Scale Epidemiology Studies. J Proteome Res. 2015;14:2714–21.
Gruhler A, Schulze WX, Matthiesen R, Mann M, Jensen ON. Stable isotope labeling of Arabidopsis thaliana cells and quantitative proteomics by mass spectrometry. Mol Cell Proteomics. 2005;4:1697–709.
Guiboileau A, Yoshimoto K, Soulay F, Bataillé M-P, Avice J-C, Masclaux-Daubresse C. Autophagy machinery controls nitrogen remobilization at the whole-plant level under both limiting and ample nitrate conditions in Arabidopsis. New Phytol. 2012;194:732–40.
Guijas C, Montenegro-Burke JR, Domingo-Almenara X, Palermo A, Warth B, Hermann G, et al. METLIN: A Technology Platform for Identifying Knowns and Unknowns. Anal Chem. 2018b;90:3156–64.
Guijas C, Montenegro-Burke JR, Warth B, Spilker ME, Siuzdak G. Metabolomics activity screening for identifying metabolites that modulate phenotype. Nat Biotechnol. 2018a;36:316–20.
Gutkowska M, Kaus-Drobek M, Hoffman-Sommer M, Malgorzata Pamula M, Daria Leja A, Perycz M, et al. Impact of C-terminal truncations in the Arabidopsis Rab escort protein (REP) on REP-Rab interaction and plant fertility. Plant J. 2021;108:1400–21.
Haggarty J, Burgess KE. Recent advances in liquid and gas chromatography methodology for extending coverage of the metabolome. Curr Opin Biotechnol. 2017;43:77–85.
Hardman G, Perkins S, Brownridge PJ, Clarke CJ, Byrne DP, Campbell AE, et al. Strong anion exchange-mediated phosphoproteomics reveals extensive human non-canonical phosphorylation. EMBO J. 2019;38:e100847.
Hart-Smith G, Reis RS, Waterhouse PM, Wilkins MR. Improved Quantitative Plant Proteomics via the Combination of Targeted and Untargeted Data Acquisition. Front Plant Sci. 2017;8:1669.
He WD, Gao J, Dou TX, Shao XH, Bi FC, Sheng O, et al. Early Cold-Induced Peroxidases and Aquaporins Are Associated With High Cold Tolerance in Dajiao (Musa spp. 'Dajiao'). Front Plant Sci. 2018;9:282.
Hebert AS, Prasad S, Belford MW, Bailey DJ, McAlister GC, Abbatiello SE, et al. Comprehensive Single-Shot Proteomics with FAIMS on a Hybrid Orbitrap Mass Spectrometer. Anal Chem. 2018;90:9529–37.
Heinemann JA, Kurenbach B, Quist D. Molecular profiling--a tool for addressing emerging gaps in the comparative risk assessment of GMOs. Environ Int. 2011;37:1285–93.
Heise R, Arrivault S, Szecowka M, Tohge T, Nunes-Nesi A, Stitt M, et al. Flux profiling of photosynthetic carbon metabolism in intact plants. Nat Protoc. 2014;9:1803–24.
Hellwege EM, Czapla S, Jahnke A, Willmitzer L, Heyer AG. Transgenic potato (Solanum tuberosum) tubers synthesize the full spectrum of inulin molecules naturally occurring in globe artichoke (Cynara scolymus) roots. Proc Natl Acad Sci U S A. 2000;97:8699–704.
Heng Z, Sheng O, Huang W, Zhang S, Fernie AR, Motorykin I, et al. Integrated proteomic and metabolomic analysis suggests high rates of glycolysis are likely required to support high carotenoid accumulation in banana pulp. Food Chem. 2019;297:125016.
Hernandez-de-Diego R, Tarazona S, Martinez-Mira C, Balzano-Nogueira L, Furio-Tari P, Pappas GJ Jr, et al. PaintOmics 3: a web resource for the pathway analysis and visualization of multi-omics data. Nucleic Acids Res. 2018;46:W503–9.
Hernandez-Mesa M, D'Atri V, Barknowitz G, Fanuel M, Pezzatti J, Dreolin N, et al. Interlaboratory and Interplatform Study of Steroids Collision Cross Section by Traveling Wave Ion Mobility Spectrometry. Anal Chem. 2020;92:5013–22.
Heux S, Bergès C, Millard P, Portais J-C, Létisse F. Recent advances in high-throughput 13C-fluxomics. Curr Opin Biotechnol. 2017;43:104–9.
Hiller K, Hangebrauk J, Jäger C, Spura J, Schreiber K, Schomburg D. MetaboliteDetector: Comprehensive Analysis Tool for Targeted and Nontargeted GC_MS Based Metabolome Analysis. Anal Chem. 2009;81:3429–39.
Hirai MY, Yano M, Goodenowe DB, Kanaya S, Kimura T, Awazuhara M, et al. Integration of transcriptomics and metabolomics for understanding of global responses to nutritional stresses in Arabidopsis thaliana. PNAS. 2004;101:10205–10.
Hofmann J, Pagel K. Glycan Analysis by Ion Mobility-Mass Spectrometry. Angew Chem Int Ed. 2017;56:8342–9.
Holčapek M, Ovčačíková M, Lísa M, Cífková E, Hájek T. Continuous comprehensive two-dimensional liquid chromatography–electrospray ionization mass spectrometry of complex lipidomic samples. Anal Bioanal Chem. 2015;407:5033–43.
Hsu CC, Zhu Y, Arrington JV, Paez JS, Wang P, Zhu P, et al. Universal Plant Phosphoproteomics Workflow and Its Application to Tomato Signaling in Response to Cold Stress. Mol Cell Proteomics. 2018;17:2068–80.
Hu A, Noble WS, Wolf-Yadlin A. Technical advances in proteomics: new developments in data-independent acquisition. F1000Res 2016;5.
Hu DD, Chen XL, Xiao XR, Wang YK, Liu F, Zhao Q, et al. Comparative metabolism of tripolide and triptonide using metabolomics. Food Chem Toxicol. 2018;115:98–108.
Hu Y, Liu B, Ren H, Chen L, Watkins CB, Gan S-S. The leaf senescence-promoting transcription factor AtNAP activates its direct target gene CYTOKININ OXIDASE 3 to facilitate senescence processes by degrading cytokinins. Molecular Horticulture. 2021b;1:12.
Hu R, Li Y, Yang Y, Liu M. Mass spectrometry-based strategies for single-cell metabolomics. Mass Spectrom Rev. 2021. https://doi.org/10.1002/mas.21704 .
Huang J-H, Yan J, Wu Q-H, Duarte Ferro M, Yi L-Z, Lu H-M, et al. Selective of informative metabolites using random forests based on model population analysis. Talanta. 2013;117:549–55.
Huang X, Chen Y Jr, Cho K, Nikolskiy I, Crawford PA, Patti GJ. X13CMS: Global Tracking of Isotopic Labels in Untargeted Metabolomics. Anal Chem. 2014;86:1632–9.
Huang H, Yao Q, Xia E, Gao L. Metabolomics and Transcriptomics Analyses Reveal Nitrogen Influences on the Accumulation of Flavonoids and Amino Acids in Young Shoots of Tea Plant ( Camellia sinensis L.) Associated with Tea Flavor. J Agric Food Chem. 2018;66:9828–38.
Huang L, Fang M, Cupp-Sutton KA, Wang Z, Smith K, Wu S. Spray-Capillary-Based Capillary Electrophoresis Mass Spectrometry for Metabolite Analysis in Single Cells. Anal Chem. 2021;93:4479–87.
Huege J, Goetze J, Dethloff F, Junker B, Kopka J. Corrector_Quantifi cation of Stable Isotope Label in Metabolites via Mass Spectrometry. Methods Mol Biol. 2014;1056:213–23.
Ihling CH, Springorum P, Iacobucci C, Hage C, Gotze M, Schafer M, et al. The Isotope-Labeled, MS-Cleavable Cross-Linker Disuccinimidyl Dibutyric Urea for Improved Cross-Linking/Mass Spectrometry Studies. J Am Soc Mass Spectrom. 2020;31:183–9.
Ingelsson B, Vener AV. Phosphoproteomics of Arabidopsis chloroplasts reveals involvement of the STN7 kinase in phosphorylation of nucleoid protein pTAC16. FEBS Lett. 2012;586:1265–71.
Jamil IN, Remali J, Azizan KA, Nor Muhammad NA, Arita M, Goh HH, et al. Systematic Multi-Omics Integration (MOI) Approach in Plant Systems Biology. Front Plant Sci. 2020;11:944.
Jastrzembski JA, Sacks GL. Solid Phase Mesh Enhanced Sorption from Headspace (SPMESH) Coupled to DART-MS for Rapid Quantification of Trace-Level Volatiles. Anal Chem. 2016;88:8617–23.
Jia L, Zuo T, Zhang C, Li W, Wang H, Hu Y, et al. Simultaneous Profiling and Holistic Comparison of the Metabolomes among the Flower Buds of Panax ginseng, Panax quinquefolius, and Panax notoginseng by UHPLC/IM-QTOF-HDMSE-Based Metabolomics Analysis. Molecules. 2019;24:2188.
Jiang J, Hou R, Yang N, Li L, Deng J, Qin G, et al. Physiological and TMT-labeled proteomic analyses reveal important roles of sugar and secondary metabolism in Citrus junos under cold stress. J Proteomics. 2021;237:104145.
Jonsson P, Gullberg J, Nordström A, Kusano M, Kowalczyk M, Sjöström M, et al. A Strategy for Identifying Differences in Large Series of Metabolomic Samples Analyzed by GC/MS. Anal Chem. 2004;76:1738–45.
Jorrin Novo JV. Proteomics and plant biology: contributions to date and a look towards the next decade. Expert Rev Proteomics. 2021;18:93–103.
Jorrin-Novo JV, Komatsu S, Sanchez-Lucas R, Rodriguez de Francisco LE. Gel electrophoresis-based plant proteomics: Past, present, and future. Happy 10th anniversary Journal of Proteomics! J Proteomics. 2019;198:1–10.
Jungreuthmayer C, Neubauer S, Mairinger T, Zanghellini J, Hann S. ICT: isotope correction toolbox. Bioinformatics. 2016;32(1):154–6.
Kao A, Chiu CL, Vellucci D, Yang Y, Patel VR, Guan S, et al. Development of a novel cross-linking strategy for fast and accurate identification of cross-linked peptides of protein complexes. Mol Cell Proteomics. 2011;10(M110):002212.
PubMed Google Scholar
Kastenmüller G, Römisch-Margl W, Wägele B, Altmaier E, Suhre K. metaP-Server: A Web-BasedMetabolomics Data Analysis Tool. J Biomed Biotechnol. 2021;2011:1–7.
Katz L, Baltz RH. Natural product discovery: past, present, and future. J Ind Microbiol Biotechnol. 2016;43:155–76.
Keilhauer EC, Hein MY, Mann M. Accurate protein complex retrieval by affinity enrichment mass spectrometry (AE-MS) rather than affinity purification mass spectrometry (AP-MS). Mol Cell Proteomics. 2015;14:120–35.
Kelleher NL. Top-down proteomics. Anal Chem. 2004;76:197A–203A.
Kelly RT. Single-cell Proteomics: Progress and Prospects. Mol Cell Proteomics. 2020;19:1739–48.
Kerbler SM, Natale R, Fernie AR, Zhang Y. From Affinity to Proximity Techniques to Investigate Protein Complexes in Plants. International journal of molecular sciences. 2021;22:7101.
Khoury GA, Baliban RC, Floudas CA. Proteome-wide post-translational modification statistics: frequency analysis and curation of the swiss-prot database. Sci Rep. 2011;1:1–5.
Kichey T, Hirel B, Heumez E, Dubois F, Le Gouis J. In winter wheat (Triticum aestivum L.), post-anthesis nitrogen uptake and remobilisation to the grain correlates with agronomic traits and nitrogen physiological markers. Field Crop Res. 2007;102:22–32.
Article Google Scholar
Kitamura S, Matsuda F, Tohge T, Yonekura-Sakakibara K, Yamazaki M, Saito K, et al. Metabolic profiling and cytological analysis of proanthocyanidins in immature seeds of Arabidopsis thaliana flavonoid accumulation mutants. Plant J. 2010;62:549–59.
Komatsu S, Jorrin-Novo, JV. Plant Proteomic Research 3.0: Challenges and Perspectives. Int J Mol Sci. 2021;22.
Kong AT, Leprevost FV, Avtonomov DM, Mellacheruvu D, Nesvizhskii AI. MSFragger: ultrafast and comprehensive peptide identification in mass spectrometry-based proteomics. Nat Methods. 2017;14(5):513–20. https://doi.org/10.1038/nmeth.4256 . https://pubmed.ncbi.nlm.nih.gov/28394336/ .
Korte AR, Yandeau-Nelson MD, Nikolau BJ, Lee YJ. Subcellular-level resolution MALDI-MS imaging of maize leaf metabolites by MALDI-linear ion trap-Orbitrap mass spectrometer. Anal Bioanal Chem. 2015;407:2301–9.
Kosova K, Vitamvas P, Urban MO, Prasil IT, Renaut J. Plant Abiotic Stress Proteomics: The Major Factors Determining Alterations in Cellular Proteome. Front Plant Sci. 2018;9:122.
Kouremenos KA, Pitt J, Marriott PJ. Metabolic profiling of infant urine using comprehensive two-dimensional gas chromatography: Application to the diagnosis of organic acidurias and biomarker discovery. J Chromatogr A. 2010;1217:104–11.
Krenkova J, Kleparnik K, Luksch J, Foret F. Microfabricated liquid junction hybrid capillary electrophoresis-mass spectrometry interface for fully automated operation. Electrophoresis. 2019;40:2263–70.
Kristoff CJ, Bwanali L, Veltri LM, Gautam GP, Rutto PK, Newton EO, et al. Challenging Bioanalyses with Capillary Electrophoresis. Anal Chem. 2020;92:49–66.
Kruger NJ, Ratcliffe RG. Whither metabolic flux analysis in plants? J Exp Bot. 2021;72:7653–7.
Kuhl C, Tautenhahn R, Bottcher C, Larson TR, Neumann S. CAMERA: an integrated strategy for compound spectra extraction and annotation of liquid chromatography/mass spectrometry data sets. Anal Chem. 2012;84:283–9.
Kushalappa AC, Gunnaiah R. Metabolo-proteomics to discover plant biotic stress resistance genes. Trends Plant Sci. 2013;18:522–31.
Labib M, Kelley SO. Single- cell analysis targeting the proteome. Nat Rev Chem. 2020;4:143–58.
Lan P, Li W, Wen TN, Shiau JY, Wu YC, Lin W, et al. iTRAQ protein profile analysis of Arabidopsis roots reveals new aspects critical for iron homeostasis. Plant Physiol. 2011;155:821–34.
Laponogov I, Sadawi N, Galea D, Mirnezami R, Veselkov KA. ChemDistiller: an engine for metabolite annotation in mass spectrometry. Bioinformatics. 2018;34:2096–102.
Lee TY, Bretana NA, Lu CT. PlantPhos: using maximal dependence decomposition to identify plant phosphorylation sites with substrate site specificity. BMC Bioinformatics. 2011;12:261.
Leissing F, Misch NV, Wang X, Werner L, Huang L, Conrath U, et al. Purification of MAP-kinase protein complexes and identification of candidate components by XL-TAP-MS. Plant Physiol. 2021;187:2381–92.
Leonid Brodsky AM, Shahaf N, Aharoni A, Rogachev I. Evaluation of Peak Picking Quality in LC-MS Metabolomics Data. Anal Chem. 2010;82:9177–87.
Leutert M, Entwisle SW, Villen J. Decoding Post-Translational Modification Crosstalk With Proteomics. Mol Cell Proteomics. 2021;20:100129.
Li C, Han J, Yao Q, Zou C, Xu Y, Zhang C, et al. Subpathway-GM: identification of metabolic subpathways via joint power of interesting genes and metabolites and their topologies within pathways. Nucleic Acids Res. 2013;41:e101.
Li B, Bhandari DR, Janfelt C, Römpp A, Spengler B. Natural products in Glycyrrhiza glabra (licorice) rhizome imaged at the cellular level by atmospheric pressure matrix-assisted laser desorption/ionization tandem mass spectrometry imaging. Plant J. 2014;80:161–71.
Li J, Silva-Sanchez C, Zhang T, Chen S, Li H. Phosphoproteomics technologies and applications in plant biology research. Front Plant Sci. 2015b;6:430.
PubMed PubMed Central Google Scholar
Li F, Chung T, Pennington JG, Federico ML, Kaeppler HF, Kaeppler SM, et al. Autophagic Recycling Plays a Central Role in Maize Nitrogen Remobilization. Plant Cell. 2015a;27:1389–408.
Li H, Cai Y, Guo Y, Chen F, Zhu ZJ. MetDIA: Targeted Metabolite Extraction of Multiplexed MS/MS Spectra Generated by Data-Independent Acquisition. Anal Chem. 2016b;88:8757–64.
Li B, Bhandari DR, Römpp A, Spengler B. High-resolution MALDI mass spectrometry imaging of gallotannins and monoterpene glucosides in the root of Paeonia lactiflora. Sci Rep. 2016a;6:36074.
Li B, Neumann EK, Ge J, Gao W, Yang H, Li P, et al. Interrogation of spatial metabolome of Ginkgo biloba with high-resolution matrix-assisted laser desorption/ionization and laser desorption/ionization mass spectrometry imaging. Plant Cell Environ. 2018a;41:2693–703.
Li H, Zhu Y, Rangu M, Wu X, Bhatti S, Zhou S, et al. Identification of Heat-Induced Proteomes in Tomato Microspores Using LCM-Proteomics Analysis. Single Cell Biol. 2018b;7:9.
Li N, Du J, Wu D, Liu J, Li N, Sun Z, et al. Recent advances in facile synthesis and applications of covalent organic framework materials as superior adsorbents in sample pretreatment. TrAC Trends Anal Chem. 2018c;108:154–66.
Li Z, Lu S, Jin J, Wang T. Preparation of a new cellulose magnetic molecularly imprinted polymer micro-spheres to extract and analyze the indole-3-acetic acid in plant tissues. J Chromatogr B Analyt Technol Biomed Life Sci. 2018d;1092:343–9.
Li Z, Fan Y, Xi J. Recent advances in high voltage electric discharge extraction of bioactive ingredients from plant materials. Food Chem. 2019;277:246–60.
Li L, Chen Y, Yang L, Wang Z, Liu H. Recent advances in applications of metal–organic frameworks for sample preparation in pharmaceutical analysis. Coord Chem Rev. 2020a;411:213235.
Li N, Wu D, Li X, Zhou X, Fan G, Li G, et al. Effective enrichment and detection of plant growth regulators in fruits and vegetables using a novel magnetic covalent organic framework material as the adsorbents. Food Chem. 2020b;306:125455.
Li W, Lin L, Yan D, Jin Y, Xu Y, Li Y, et al. Application of a Pseudotargeted MS Method for the Quantification of Glycated Hemoglobin for the Improved Diagnosis of Diabetes Mellitus. Anal Chem. 2020c;92:3237–45.
Li YW, Chi Q, Feng T, Xiao H, Li L, Wang X. Interactions of indole alkaloids with myoglobin: A mass spectrometry based spectrometric and computational method. Rapid Commun Mass Spectrom. 2020d;34:e8656.
Li B, Ge J, Liu W, Hu D, Li P. Unveiling spatial metabolome of Paeonia suffruticosa and Paeonia lactiflora roots using MALDI MS imaging. New Phytol. 2021a;231:892–902.
Li J, Cai Z, Bomgarden RD, Pike I, Kuhn K, Rogers JC, et al. TMTpro-18plex: The Expanded and Complete Set of TMTpro Reagents for Sample Multiplexing. J Proteome Res. 2021b;20:2964–72.
Li S, Zan H, Zhu Z, Lu D, Krall L. Plant Phosphopeptide Identification and Label-Free Quantification by MaxQuant and Proteome Discovery Software. Methods Mol Biol. 2021c;2358:179–87.
Li H, Qin Y, Wu X, O'Hair J, Potts J, Zhou S, et al. Identification of heat-induced proteomes in meiotic pollen mother cells of tomato 'Maxifort' using single-cell-type tandem mass tag (TMT) proteomics. Vegetable Res. 2022a;2:14.
Li Y, Liu S, Shawky E, Tao M, Liu A, Sulaiman K, et al. SWATH-based quantitative proteomic analysis of Morus alba L. leaves after exposure to ultraviolet-B radiation and incubation in the dark. J Photochem Photobiol B. 2022b;230:112443.
Liang Z, Yin Z, Yang H, Xiao Y, Hang W, Li J. Nanoscale surface analysis that combines scanning probe microscopy and mass spectrometry: A critical review. TrAC Trends Anal Chem. 2016;75:24–34.
Liang D, Liu Q, Zhou K, Jia W, Xie G, Chen T. IP4M: an integrated platform for mass spectrometry-based metabolomics data mining. BMC Bioinformatics. 2020;21:1–6.
Liang X, Liu S, Wang T, Li F, Cheng J, Lai J, et al. Metabolomics-driven gene mining and genetic improvement of tolerance to salt-induced osmotic stress in maize. New Phytol. 2021a;230:2355–70.
Liang Y, Acor H, McCown MA, Nwosu AJ, Boekweg H, Axtell NB, et al. Fully Automated Sample Processing and Analysis Workflow for Low-Input Proteome Profiling. Anal Chem. 2021b;93:1658–66.
Lísa M, Cífková E, Holčapek M. Lipidomic profiling of biological tissues using off-line two-dimensional high-performance liquid chromatography–mass spectrometry. J Chromatogr A. 2011;1218:5146–56.
Lisacek F, Kessler N, Walter F, Persicke M, Albaum SP, Kalinowski J, et al. ALLocator: An Interactive Web Platform for the Analysis of Metabolomic LC-ESI-MS Datasets, Enabling Semi-Automated, User-Revised Compound Annotation and Mass Isotopomer Ratio Analysis. PLoS One. 2014;9:e113909.
Liu F, Rijkers DT, Post H, Heck AJ. Proteome-wide profiling of protein assemblies by cross-linking mass spectrometry. Nat Methods. 2015;12(12):1179–84. https://doi.org/10.1038/nmeth.3603 . https://pubmed.ncbi.nlm.nih.gov/26414014/ .
Liu Y, Beyer A, Aebersold R. On the Dependency of Cellular Protein Levels on mRNA Abundance. Cell. 2016b;165:535–50.
Liu L, Xia L, Wu C, Qu F, Li G, Sun Z, et al. Zirconium (IV)-based metal organic framework (UIO-67) as efficient sorbent in dispersive solid phase extraction of plant growth regulator from fruits coupled with HPLC fluorescence detection. Talanta. 2016a;154:23–30.
Liu S, Yu F, Hu Q, Wang T, Yu L, Du S, et al. Development of in Planta Chemical Cross-Linking-Based Quantitative Interactomics in Arabidopsis. J Proteome Res. 2018;17:3195–213.
Liu Y, Lu S, Liu K, Wang S, Huang L, Guo L. Proteomics: a powerful tool to study plant responses to biotic stress. Plant Methods. 2019;15:135.
Liu X, Qin D, Piersanti A, Zhang Q, Miceli C, Wang P. Genome-wide association study identifies candidate genes related to oleic acid content in soybean seeds. BMC Plant Biol. 2020;20:399.
Liu T, Salguero P, Petek M, Martinez-Mira C, Balzano-Nogueira L, Ramsak Z, et al. PaintOmics 4: new tools for the integrative analysis of multi-omics datasets supported by multiple pathway databases. Nucleic Acids Res. 2022.
Lommen A. MetAlign: Interface-Driven, Versatile Metabolomics Tool for Hyphenated Full-Scan Mass Spectrometry Data Preprocessing. Anal Chem. 2009;81:3079–86.
Lommen A. Ultrafast PubChem Searching Combined with Improved Filtering Rules for Elemental Composition Analysis. Anal Chem. 2014;86:5463–9.
Luo C, Fernie AR, Yan J. Single-Cell Genomics and Epigenomics: Technologies and Applications in Plants. Trends Plant Sci. 2020;25:1030–40.
Lv QQ, Yang XN, Yan DM, Liang WQ, Liu HN, Yang XW, et al. Metabolic profiling of dehydrodiisoeugenol using xenobiotic metabolomics. J Pharm Biomed Anal. 2017;145:725–33.
Lv W, Wang L, Xuan Q, Zhao X, Liu X, Shi X, et al. Pseudotargeted Method Based on Parallel Column Two-Dimensional Liquid Chromatography-Mass Spectrometry for Broad Coverage of Metabolome and Lipidome. Anal Chem. 2020;92:6043–50.
Ma F, Jazmin LJ, Young JD, Allen DK. Isotopically nonstationary 13 C flux analysis of changes in Arabidopsis thaliana leaf metabolism due to high light acclimation. Proc Natl Acad Sci. 2014a;111:16967–72.
Ma Y, Kind T, Yang D, Leon C, Fiehn O. MS2Analyzer: A Software for Small Molecule Substructure Annotations from Accurate Tandem Mass Spectra. Anal Chem. 2014b;86:10724–31.
Maia M, Figueiredo A, Cordeiro C, Sousa Silva M. FT-ICR-MS-based metabolomics: A deep dive into plant metabolism. Mass Spectrom Rev. 2021. https://doi.org/10.1002/mas.21731 .
Mak TD, Laiakis EC, Goudarzi M, Fornace AJ. MetaboLyzer: A Novel Statistical Workflow for Analyzing Postprocessed LC–MS Metabolomics Data. Anal Chem. 2014;86:506–13.
Mak TD, Laiakis EC, Goudarzi M, Fornace AJ. Selective Paired Ion Contrast Analysis: A Novel Algorithm for Analyzing Postprocessed LC-MS Metabolomics Data Possessing High Experimental Noise. Anal Chem. 2015;87:3177–86.
Malagoli P, Laine P, Rossato L, Ourry A. Dynamics of Nitrogen Uptake and Mobilization in Field-grown Winter Oilseed Rape (Brassica napus) From Stem Extension to Harvest. II. An 15N-labelling-based Simulation Model of N Partitioning Between Vegetative and Reproductive Tissues. Ann Bot. 2005;95:1187–98.
Martin LB, Sherwood RW, Nicklay JJ, Yang Y, Muratore-Schroeder TL, Anderson ET, et al. Application of wide selected-ion monitoring data-independent acquisition to identify tomato fruit proteins regulated by the CUTIN DEFICIENT2 transcription factor. Proteomics. 2016;16(15–16):2081–94.
Masson GR, Burke JE, Ahn NG, Anand GS, Borchers C, Brier S, et al. Recommendations for performing, interpreting and reporting hydrogen deuterium exchange mass spectrometry (HDX-MS) experiments. Nat Methods. 2019;16:595–602.
Mateus A, Kurzawa N, Becher I, Sridharan S, Helm D, Stein F, et al. Thermal proteome profiling for interrogating protein interactions. Mol Syst Biol. 2020;16:e9232.
Matthes A, Köhl K, Schulze WX. SILAC and alternatives in studying cellular proteomes of plants. Methods Mol Biol. 2014;1188:65–83. https://doi.org/10.1007/978-1-4939-1142-4_6 . https://pubmed.ncbi.nlm.nih.gov/25059605/ .
McAlister GC, Nusinow DP, Jedrychowski MP, Wuhr M, Huttlin EL, Erickson BK, et al. MultiNotch MS3 enables accurate, sensitive, and multiplexed detection of differential expression across cancer cell line proteomes. Anal Chem. 2014;86:7150–8.
McCann MC, Rogan GJ, Fitzpatrick S, Trujillo WA, Sorbet R, Hartnell GF, et al. Glyphosate-Tolerant Alfalfa Is Compositionally Equivalent to Conventional Alfalfa (Medicago sativa L.). J Agric Food Chem. 2006;54:7187–92.
McCullagh M, Pereira CAM, Yariwake JH. Use of ion mobility mass spectrometry to enhance cumulative analytical specificity and separation to profile 6-C/8-C-glycosylflavone critical isomer pairs and known-unknowns in medicinal plants. Phytochem Anal. 2019;30:424–36.
McEachran AD, Balabin I, Cathey T, Transue TR, Al-Ghoul H, Grulke C, et al. Linking in silico MS/MS spectra with chemistry data to improve identification of unknowns. Scientific Data. 2019;6:141.
Meier F, Park MA, Mann M. Trapped Ion Mobility Spectrometry and Parallel Accumulation-Serial Fragmentation in Proteomics. Mol Cell Proteomics. 2021;20:100138.
Melby JA, Roberts DS, Larson EJ, Brown KA, Bayne EF, Jin S, et al. Novel Strategies to Address the Challenges in Top-Down Proteomics. J Am Soc Mass Spectrom. 2021;32:1278–94.
Meng Y, Cheng X, Wang T, Hang W, Li X, Nie W, et al. Micro-LensedFiber Laser Desorption Mass Spectrometry Imaging Reveals Subcellular DistributionofDrugs within Single Cells. Angew Chem Int Ed. 2020;59:17864–71.
Menikarachchi LC, Cawley S, Hill DW, Hall LM, Hall L, Lai S, et al. MolFind: A Software Package Enabling HPLC/MS-Based Identification of Unknown Chemical Structures. Anal Chem. 2012;84:9388–94.
Mi W, Liu Z, Jin J, Dong X, Xu C, Zou Y, et al. Comparative proteomics analysis reveals the molecular mechanism of enhanced cold tolerance through ROS scavenging in winter rapeseed (Brassica napus L.). PLoS One. 2021;16:e0243292.
Millard P, Sokol S, Kohlstedt M, Wittmann C, Létisse F, Lippens G, et al. IsoSolve: An Integrative Framework to Improve Isotopic Coverage and Consolidate Isotopic Measurements by Mass Spectrometry and/or Nuclear Magnetic Resonance. Anal Chem. 2021;93:9428–36.
Miller KE, Kim Y, Huh WK, Park HO. Bimolecular Fluorescence Complementation (BiFC) Analysis: Advances and Recent Applications for Genome-Wide Interaction Studies. J Mol Biol. 2015;427:2039–55.
Misra BB. New software tools, databases, and resources in metabolomics: updates from 2020. Metabolomics. 2021;17:49.
Mitchell CJ, Kim MS, Na CH, Pandey A. PyQuant: A Versatile Framework for Analysis of Quantitative Mass Spectrometry Data. Mol Cell Proteomics. 2016;15:2829–38.
Mohamed A, Hill MM. LipidSuite: interactive web server for lipidomics differential and enrichment analysis. Nucleic Acids Res. 2021;49:W346–51.
Mohimani H, Gurevich A, Mikheenko A, Garg N, Nothias LF, Ninomiya A, et al. Dereplication of peptidic natural products through database search of mass spectra. Nat Chem Biol. 2017;13:30–7.
Mohimani H, Gurevich A, Shlemov A, Mikheenko A, Korobeynikov A, Cao L, et al. Dereplication of microbial metabolites through database search of mass spectra. Nat Commun. 2018;9:4035.
Moore BM, Wang P, Fan P, Leong B, Schenck CA, Lloyd JP, et al. Robust predictions of specialized metabolism genes through machine learning. Proc Natl Acad Sci U S A. 2019;116:2344–53.
Muth T, Vaudel M, Barsnes H, Martens L, Sickmann A. XTandem Parser: an open-source library to parse and analyse X!Tandem MS/MS search results. Proteomics. 2010;10(7):1522–4. https://doi.org/10.1002/pmic.200900759 . Erratum in: Proteomics. 2010;10(13):2560. https://pubmed.ncbi.nlm.nih.gov/20140905/ .
Nakayasu ES, Gritsenko M, Piehowski PD, Gao Y, Orton DJ, Schepmoes AA, et al. Tutorial: best practices and considerations for mass-spectrometry-based protein biomarker discovery and validation. Nat Protoc. 2021;16:3737–60.
Navarro-Reig M, Jaumot J, Tauler R. An untargeted lipidomic strategy combining comprehensive two-dimensional liquid chromatography and chemometric analysis. J Chromatogr A. 2018;1568:80–90.
Naz S, Hanif MA, Bhatti HN, Ansari TM. Impact of Supercritical Fluid Extraction and Traditional Distillation on the Isolation of Aromatic Compounds from Cannabis indica and Cannabis sativa. J Essential Oil Bearing Plants. 2017;20:175–84.
Nee G, Kramer K, Nakabayashi K, Yuan B, Xiang Y, Miatton E, et al. DELAY OF GERMINATION1 requires PP2C phosphatases of the ABA signalling pathway to control seed dormancy. Nat Commun. 2017;8:72.
Nguyen TT, Blackburn MR, Sussman MR. Intermolecular and Intramolecular Interactions of the Arabidopsis Plasma Membrane Proton Pump Revealed Using a Mass Spectrometry Cleavable Cross-Linker. Biochemistry. 2020;59:2210–25.
Niehaus M, Soltwisch J, Belov M, Dreisewerd K. Transmission-mode MALDI-2 mass spectrometry imaging of cells and tissues at subcellular resolution. Nat Methods. 2019;16:925–31.
Nodzenski M, Muehlbauer MJ, Bain JR, Reisetter AC, Lowe WL, Scholtens DM. Metabomxtr: an R package for mixture-model analysis of non-targeted metabolomics data. Bioinformatics. 2014;30:3287–8.
Nothias LF, Petras D, Schmid R, Duhrkop K, Rainer J, Sarvepalli A, et al. Feature-based molecular networking in the GNPS analysis environment. Nat Methods. 2020;17:905–8.
Nyamundanda G, Brennan L, Gormley IC. Probabilistic principal component analysis for metabolomic data. BMC Bioinformatics. 2010;11:1–11.
Oates CN, Kulheim C, Myburg AA, Slippers B, Naidoo S. The Transcriptome and Terpene Profile of Eucalyptus grandis Reveals Mechanisms of Defense Against the Insect Pest, Leptocybe invasa. Plant Cell Physiol. 2015;56:1418–28.
Obert JC, Ridley WP, Schneider RW, Riordan SG, Nemeth MA, Trujillo WA, et al. The Composition of Grain and Forage from Glyphosate Tolerant Wheat MON 71800 Is Equivalent to That of Conventional Wheat (Triticum aestivum L.). J Agric Food Chem. 2004;52:1375–84.
Padgette SR, Taylor NB, Nida DL, Bailey MR, MacDonald J, Holden LR, et al. The Composition of Glyphosate-Tolerant Soybean Seeds Is Equivalent to That of Conventional Soybeans. J Nutr. 1996;126:702–16.
Pazhamala LT, Kudapa H, Weckwerth W, Millar AH, Varshney RK. Systems biology for crop improvement. The plant genome; 2021. p. e20098.
Pepelnjak M, de Souza N, Picotti P. Detecting Protein-Small Molecule Interactions Using Limited Proteolysis-Mass Spectrometry (LiP-MS). Trends Biochem Sci. 2020;45:919–20.
Perez de Souza L, Naake T, Tohge T, Fernie AR. From chromatogram to analyte to metabolite. How to pick horses for courses from the massive web resources for mass spectral plant metabolomics. GigaScience. 2017;6.
Perez De Souza L, Alseekh S, Brotman Y, Fernie AR. Network-based strategies in metabolomics data analysis and interpretation: from molecular networking to biological interpretation. Expert Rev Proteomics. 2020;17:243–55.
Perez de Souza L, Alseekh S, Scossa F, Fernie AR. Ultra-high-performance liquid chromatography high-resolution mass spectrometry variants for metabolomics research. Nat Methods. 2021;18:733–46.
Perkins DN, Pappin DJ, Creasy DM, Cottrell JS. Probability-based protein identification by searching sequence databases using mass spectrometry data. Electrophoresis. 1999;20:3551–67.
Pierce A, Unwin RD, Evans CA, Griffiths S, Carney L, Zhang L, et al. Eight-channel iTRAQ enables comparison of the activity of six leukemogenic tyrosine kinases. Mol Cell Proteomics. 2008;7:853–63.
Pino LK, Searle BC, Bollinger JG, Nunn B, MacLean B, MacCoss MJ. The Skyline ecosystem: Informatics for quantitative mass spectrometry proteomics. Mass Spectrom Rev. 2020;39:229–44.
Pino LE, Lima JE, Vicente MH, de Sá AFL, Pérez-Alfocea F, Albacete A, et al. Increased branching independent of strigolactone in cytokinin oxidase 2-overexpressing tomato is mediated by reduced auxin transport. Molecular Horticulture. 2022;2:12.
Pinu, F.R., Goldansaz, S.A., and Jaine, J. (2019). Translational Metabolomics: Current Challenges and Future Opportunities. Metabolites 9.
Pluskal T, Castillo S, Villar-Briones A, Orešič M. MZmine 2: Modular framework for processing, visualizing, and analyzing mass spectrometry-based molecular profile data. BMC Bioinformatics. 2010;11:1.
Potts J, Li H, Qin Y, Wu X, Hui D, Nasr KA, et al. Using single cell type proteomics to identify Al-induced proteomes in outer layer cells and interior tissues in the apical meristem/cell division regions of tomato root-tips. J Proteomics. 2022;255:104486.
Puri M, Sharma D, Barrow CJ. Enzyme-assisted extraction of bioactives from plants. Trends Biotechnol. 2012;30:37–44.
Qin L, Walk TC, Han P, Chen L, Zhang S, Li Y, et al. Adaption of Roots to Nitrogen Deficiency Revealed by 3D Quantification and Proteomic Analysis. Plant Physiol. 2019;179:329–47.
Qin R, Li P, Du M, Ma L, Huang Y, Yin Z, et al. Spatiotemporal Visualization of Insecticides and Fungicides within Fruits and Vegetables Using Gold Nanoparticle-Immersed Paper Imprinting Mass Spectrometry Imaging. Nanomaterials. 2021;11:1327.
Qiu J, Hou Y, Tong X, Wang Y, Lin H, Liu Q, et al. Quantitative phosphoproteomic analysis of early seed development in rice (Oryza sativa L.). Plant Mol Biol. 2016;90:249–65.
Qu M, An B, Shen S, Zhang M, Shen X, Duan X, et al. Qualitative and quantitative characterization of protein biotherapeutics with liquid chromatography mass spectrometry. Mass Spectrom Rev. 2017;36:734–54.
Ramalingam A, Kudapa H, Pazhamala LT, Weckwerth W, Varshney RK. Proteomics and Metabolomics: Two Emerging Areas for Legume Improvement. Front Plant Sci. 2015;6:1116.
Ramazi S, Zahiri J. Posttranslational modifications in proteins: resources, tools and prediction methods. Database (Oxford). 2021.
Rampitsch C. Phosphoproteomics Analysis for Probing Plant Stress Tolerance. Methods Mol Biol. 2017;1631:181–93.
Rampler E, Abiead YE, Schoeny H, Rusz M, Hildebrand F, Fitz V, et al. Recurrent Topics in Mass Spectrometry-Based Metabolomics and Lipidomics—Standardization, Coverage, and Throughput. Anal Chem. 2021;93:519–45.
Reyes-Garcés N, Gionfriddo E. Recent developments and applications of solid phase microextraction as a sample preparation approach for mass-spectrometry-based metabolomics and lipidomics. TrAC Trends Anal Chem. 2019;113:172–81.
Ridder L, van der Hooft JJ, Verhoeven S. Automatic compound annotation from mass spectrometry data using MAGMa. Mass Spectrometry. 2014;3:S0033.
Rocío-Bautista P, Termopoli V. Metal–Organic Frameworks in Solid-Phase Extraction Procedures for Environmental and Food Analyses. Chromatographia. 2019;82:1191–205.
Roessner U, Wagner C, Kopka J, Trethewey RN, Willmitzer L. Simultaneous analysis of metabolites in potato tuber by gas chromatography–mass spectrometry. Plant J. 2000;23:131–42.
Rohde A, Morreel K, Ralph J, Goeminne G, Hostyn V, De Rycke R, et al. Molecular phenotyping of the pal1 and pal2 mutants of Arabidopsis thaliana reveals far-reaching consequences on phenylpropanoid, amino acid, and carbohydrate metabolism. Plant Cell. 2004;16:2749–71.
Rohrmann J, Tohge T, Alba R, Osorio S, Caldana C, McQuinn R, et al. Combined transcription factor profiling, microarray analysis and metabolite profiling reveals the transcriptional control of metabolic shifts occurring during tomato fruit development. Plant J. 2011;68:999–1013.
Rong Z, Tan Q, Cao L, Zhang L, Deng K, Huang Y, et al. NormAE: Deep Adversarial Learning Model to Remove Batch Effects in Liquid Chromatography Mass Spectrometry-Based Metabolomics Data. Anal Chem. 2020;92:5082–90.
Rose JK, Bashir S, Giovannoni JJ, Jahn MM, Saravanan RS. Tackling the plant proteome: practical approaches, hurdles and experimental tools. Plant J. 2004;39:715–33.
Rosenberger G, Koh CC, Guo T, Röst HL, Kouvonen P, Collins BC, et al. A repository of assays to quantify 10,000 human proteins by SWATH-MS. Sci Data. 2014;1:140031. https://doi.org/10.1038/sdata.2014.31 . https://pubmed.ncbi.nlm.nih.gov/25977788/ .
Ross PL, Huang YN, Marchese JN, Williamson B, Parker K, Hattan S, et al. Multiplexed protein quantitation in Saccharomyces cerevisiae using amine-reactive isobaric tagging reagents. Mol Cell Proteomics. 2004;3:1154–69.
Rost HL, Rosenberger G, Navarro P, Gillet L, Miladinovic SM, Schubert OT, et al. OpenSWATH enables automated, targeted analysis of data-independent acquisition MS data. Nat Biotechnol. 2014;32:219–23.
Rost HL, Sachsenberg T, Aiche S, Bielow C, Weisser H, Aicheler F, et al. OpenMS: a flexible open-source software platform for mass spectrometry data analysis. Nat Methods. 2016;13:741–8.
Ruttkies C, Schymanski EL, Wolf S, Hollender J, Neumann S. MetFrag relaunched: incorporating strategies beyond in silico fragmentation. Journal of Cheminformatics. 2016;8:3. https://doi.org/10.1186/s13321-016-0115-9 .
Sakurai N, Ara T, Kanaya S, Nakamura Y, Iijima Y, Enomoto M, et al. An application of a relational database system for high-throughput prediction of elemental compositions from accurate mass values. Bioinformatics. 2013;29:290–1.
Salem MA, Perez de Souza L, Serag A, Fernie AR, Farag MA, Ezzat SM, et al. Metabolomics in the Context of Plant Natural Products Research: From Sample Preparation to Metabolite Analysis. Metabolites. 2020;10:37.
Salon C, Bataillé M-P, Gallardo K, Jeudy C, Santoni A-L, Trouverie J, et al. 34S and 15N Labelling to Model S and N Flux in Plants and Determine the Different Components of N and S Use Efficiency. In: Dieuaide-Noubhani M, Alonso AP, editors. Plant Metabolic Flux Analysis: Methods and Protocols. Totowa: Humana Press; 2014. p. 335–46.
Chapter Google Scholar
Salon C, Avice J-C, Colombié S, Dieuaide-Noubhani M, Gallardo K, Jeudy C, et al. Fluxomics links cellular functional analyses to whole-plant phenotyping. J Exp Bot. 2017;68:2083–98.
Samarah LZ, Tran TH, Stacey G, Vertes A. Mass Spectrometry Imaging of Bio-oligomer Polydispersity in Plant Tissues by Laser Desorption Ionization from Silicon Nanopost Arrays. Angew Chem Int Ed. 2021;60:9071–7.
Sandra K, Sandra P. Lipidomics from an analytical perspective. Curr Opin Chem Biol. 2013;17:847–53.
Sauvage C, Segura V, Bauchet G, Stevens R, Do PT, Nikoloski Z, et al. Genome-Wide Association in Tomato Reveals 44 Candidate Loci for Fruit Metabolic Traits. Plant Physiol. 2014;165:1120–32.
Savitski MM, Reinhard FB, Franken H, Werner T, Savitski MF, Eberhard D, et al. Tracking cancer drugs in living cells by thermal profiling of the proteome. Science. 2014;346:1255784.
Sawada Y, Nakabayashi R, Yamada Y, Suzuki M, Sato M, Sakata A, et al. RIKEN tandem mass spectral database (ReSpect) for phytochemicals: a plant-specific MS/MS-based data resource and database. Phytochemistry. 2012;82:38–45.
Schaffer LV, Millikin RJ, Miller RM, Anderson LC, Fellers RT, Ge Y, et al. Identification and Quantification of Proteoforms by Mass Spectrometry. Proteomics. 2019;19:e1800361.
Schiltz S, Munier-Jolain N, Jeudy C, Burstin J, Salon C. Dynamics of Exogenous Nitrogen Partitioning and Nitrogen Remobilization from Vegetative Organs in Pea Revealed by 15N in Vivo Labeling throughout Seed Filling. Plant Physiol. 2005;137:1463–73.
Schmid R, Petras D, Nothias LF, Wang M, Aron AT, Jagels A, et al. Ion identity molecular networking for mass spectrometry-based metabolomics in the GNPS environment. Nat Commun. 2021;12:3832.
Schulze WX. Proteomics approaches to understand protein phosphorylation in pathway modulation. Curr Opin Plant Biol. 2010;13:280–7.
Schutz W, Hausmann N, Krug K, Hampp R, Macek B. Extending SILAC to proteomics of plant cell lines. Plant Cell. 2011;23:1701–5.
Schwaiger-Haber M, Stancliffe E, Arends V, Thyagarajan B, Sindelar M, Patti GJ. A Workflow to Perform Targeted Metabolomics at the Untargeted Scale on a Triple Quadrupole Mass Spectrometer. ACS Measurement Sci Au. 2021;1:35–45.
Scossa F, Benina M, Alseekh S, Zhang Y, Fernie AR. The Integration of Metabolomics and Next-Generation Sequencing Data to Elucidate the Pathways of Natural Product Metabolism in Medicinal Plants. Planta Med. 2018;84:855–73.
Searle BC. Scaffold: a bioinformatic tool for validating MS/MS-based proteomic studies. Proteomics. 2010;10(6):1265–9. https://doi.org/10.1002/pmic.200900437 . https://pubmed.ncbi.nlm.nih.gov/20077414/ .
Senan O, Aguilar-Mogas A, Navarro M, Capellades J, Noon L, Burks D, et al. CliqueMS: a computational tool for annotating in-source metabolite ions from LC-MS untargeted metabolomics data based on a coelution similarity network. Bioinformatics. 2019;35(20):4089–97. https://doi.org/10.1093/bioinformatics/btz207 . https://pubmed.ncbi.nlm.nih.gov/30903689/ .
Seyfferth C, Renema J, Wendrich JR, Eekhout T, Seurinck R, Vandamme N, et al. Advances and Opportunities in Single-Cell Transcriptomics for Plant Research. Annu Rev Plant Biol. 2021;72:847–66.
Shang Y, Ma Y, Zhou Y, Zhang H, Duan L, Chen H, et al. Plant science. Biosynthesis, regulation, and domestication of bitterness in cucumber. Science. 2014;346:1084–8.
Shaw R, Tian X, Xu J. Single-Cell Transcriptome Analysis in Plants: Advances and Challenges. Mol Plant. 2021;14:115–26.
Shen X, Wang R, Xiong X, Yin Y, Cai Y, Ma Z, et al. Metabolic reaction network-based recursive metabolite annotation for untargeted metabolomics. Nature Communications. 2019;10:1–4.
Shepherd LV, Hackett CA, Alexander CJ, McNicol JW, Sungurtas JA, Stewart D, et al. Modifying glycoalkaloid content in transgenic potato--Metabolome impacts. Food Chem. 2015;187:437–43.
Sheth BP, Thaker VS. Plant systems biology: insights, advances and challenges. Planta. 2014;240:33–54.
Shi H, Schwender J. Mathematical models of plant metabolism. Curr Opin Biotechnol. 2016;37:143–52.
Silva-Sanchez C, Li H, Chen S. Recent advances and challenges in plant phosphoproteomics. Proteomics. 2015;15:1127–41.
Siva Sankar D, Dengjel J. Protein complexes and neighborhoods driving autophagy. Autophagy. 2021;17:2689–705.
Smith CA, Want EJ, O’Maille G, Abagyan R, Siuzdak G. XCMS: processing mass spectrometry data for metabolite profiling using nonlinear peak alignment, matching, and identification. Anal Chem. 2006;78:779–87.
Soltwisch J, Kettling H, Vens-Cappell S, Wiegelmann M, Müthing J, Dreisewerd K. Mass Spectrometry Imaging with Laser-Induced Postionization. Science. 2015;348:211–5.
Specht H, Emmott E, Petelski AA, Huffman RG, Perlman DH, Serra M, et al. Single-cell proteomic and transcriptomic analysis of macrophage heterogeneity using SCoPE2. Genome Biol. 2021;22:50.
Stein SE. An Integrated Method for Spectrum Extraction and Compound Identification from Gas Chromatography/Mass Spectrometry Data. J Am Soc Mass Spectrom. 1999;10:770–81.
Stewart D, Shepherd LVT. Metabolomics for the safety assessment of genetically modified (GM) crops, 192-216; 2013.
Stoll DR, Li X, Wang X, Carr PW, Porter SEG, Rutan SC. Fast, comprehensive two-dimensional liquid chromatography. J Chromatogr A. 2007;1168:3–43.
Stopka SA, Khattar R, Agtuca BJ, Anderton CR, Pasa-Tolic L, Stacey G, et al. Metabolic Noise and Distinct Subpopulations Observed by Single Cell LAESI Mass Spectrometry of Plant Cells in situ. Front Plant Sci. 2018;9:1646.
Struk S, Jacobs A, Sanchez Martin-Fontecha E, Gevaert K, Cubas P, Goormachtig S. Exploring the protein-protein interaction landscape in plants. Plant Cell Environ. 2019;42:387–409.
Sumner LW, Amberg A, Barrett D, Beale MH, Beger R, Daykin CA, et al. Proposed minimum reporting standards for chemical analysis Chemical Analysis Working Group (CAWG) Metabolomics Standards Initiative (MSI). Metabolomics. 2007;3(3):211–21. https://doi.org/10.1007/s11306-007-0082-2 . https://pubmed.ncbi.nlm.nih.gov/24039616/ .
Sumner LW, Yang DS, Bench BJ, Watson BS, Li C, Jones AD. Spatially Resolved Plant Metabolomics. Ann Plant Rev. 2011;43:343–66.
Sun X, Weckwerth W. COVAIN: a toolbox for uni- and multivariate statistics, timeseries and correlation network analysis and inverse estimation of the differential Jacobian from metabolomics covariance data. Metabolomics. 2012;8:S81–93.
Szykuła KM, Meurs J, Turner MA, Creaser CS, Reynolds JC. Combined hydrophilic interaction liquid chromatography-scanning field asymmetric waveform ion mobility spectrometry-time-of-flight mass spectrometry for untargeted metabolomics. Anal Bioanal Chem. 2019;411:6309–17.
Tada I, Tsugawa H, Meister I, Zhang P, Shu R, Katsumi R, et al. Creating a Reliable Mass Spectral-Retention Time Library for All Ion Fragmentation-Based Metabolomics. Metabolites. 2019;9:251.
Tan J, Zhou Z, Feng H, Xing J, Niu Y, Deng Z. Data-Independent Acquisition-Based Proteome and Phosphoproteome Profiling Reveals Early Protein Phosphorylation and Dephosphorylation Events in Arabidopsis Seedlings upon Cold Exposure. Int J Mol Sci. 2021;22:12856.
Tanaka Y, Higashi T, Rakwal R, Wakida SI, Iwahashi H. Development of a capillary electrophoresis-mass spectrometry method using polymer capillaries for metabolomic analysis of yeast. Electrophoresis. 2008;29:2016–23.
Tang H, Zhang X, Gong B, Yan Y, Shi Q. Proteomics and metabolomics analysis of tomato fruit at different maturity stages and under salt treatment. Food Chem. 2020;311:126009.
Taylor MJ, Lukowski JK, Anderton CR. Spatially Resolved Mass Spectrometry at the Single Cell: Recent Innovations in Proteomics and Metabolomics. J Am Soc Mass Spectrom. 2021;32:872–94.
Tengstrand E, Lindberg J, Åberg KM. TracMass 2—A Modular Suite of Tools for Processing Chromatography-Full Scan Mass Spectrometry Data. Anal Chem. 2014;86:3435–42.
Teo CC, Chong WPK, Ho YS. Development and application of microwave-assisted extraction technique in biological sample preparation for small molecule analysis. Metabolomics. 2013;9:1109–28.
Thelen JJ, Peck SC. Quantitative proteomics in plants: choices in abundance. Plant Cell. 2007;19:3339–46.
Thompson A, Schafer J, Kuhn K, Kienle S, Schwarz J, Schmidt G, et al. Tandem mass tags: a novel quantification strategy for comparative analysis of complex protein mixtures by MS/MS. Anal Chem. 2003;75:1895–904.
Ting L, Rad R, Gygi SP, Haas W. MS3 eliminates ratio distortion in isobaric multiplexed quantitative proteomics. Nat Methods. 2011;8:937–40.
Tranchida PQ, Franchina FA, Dugo P, Mondello L. Comprehensive two-dimensional gas chromatography-mass spectrometry: Recent evolution and current trends. Mass Spectrom Rev. 2016;35:524–34.
Treviño V, Yañez-Garza I-L, Rodriguez-López CE, Urrea-López R, Garza-Rodriguez M-L, Barrera-Saldaña H-A, et al. GridMass: a fast two-dimensional feature detection method for LC/MS. J Mass Spectrom. 2015;50:165–74.
Tripathi A, Vázquez-Baeza Y, Gauglitz JM, Wang M, Dührkop K, Nothias-Esposito M, et al. Chemically informed analyses of metabolomics mass spectrometry data with Qemistree. Nat Chem Biol. 2021;17:1–6.
Tsai CF, Zhao R, Williams SM, Moore RJ, Schultz K, Chrisler WB, et al. An Improved Boosting to Amplify Signal with Isobaric Labeling (iBASIL) Strategy for Precise Quantitative Single-cell Proteomics. Mol Cell Proteomics. 2020;19:828–38.
Tsugawa H. Advances in computational metabolomics and databases deepen the understanding of metabolisms. Curr Opin Biotechnol. 2018;54:10–7.
Tsugawa H, Cajka T, Kind T, Ma Y, Higgins B, Ikeda K, et al. MS-DIAL: data-independent MS/MS deconvolution for comprehensive metabolome analysis. Nat Methods. 2015;12:523–6.
Tsugawa H, Kind T, Nakabayashi R, Yukihira D, Tanaka W, Cajka T, et al. Hydrogen Rearrangement Rules: Computational MS/MS Fragmentation and Structure Elucidation Using MS-FINDER Software. Anal Chem. 2016;88:7946–58.
Tsugawa H, Rai A, Saito K, Nakabayashi R. Metabolomics and complementary techniques to investigate the plant phytochemical cosmos. Nat Prod Rep. 2021;38:1729–59.
Tufi S, Lamoree M, de Boer J, Leonards P. Simultaneous analysis of multiple neurotransmitters by hydrophilic interaction liquid chromatography coupled to tandem mass spectrometry. J Chromatogr A. 2015;1395:79–87.
Uetz P, Giot L, Cagney G, Mansfield TA, Judson RS, Knight JR, et al. A comprehensive analysis of protein-protein interactions in Saccharomyces cerevisiae. Nature. 2000;403:623–7.
Uppal K, Soltow QA, Strobel FH, Pittard WS, Gernert KM, Yu T, et al. xMSanalyzer: automated pipeline for improved feature detection and downstream analysis of large-scale, non-targeted metabolomics data. BMC Bioinformatics. 2013;14:1–2.
Uppal K, Walker DI, Jones DP. xMSannotator: An R Package for Network-Based Annotation of High-Resolution Metabolomics Data. Anal Chem. 2017;89:1063–7.
Valikangas T, Suomi T, Elo LL. A comprehensive evaluation of popular proteomics software workflows for label-free proteome quantification and imputation. Brief Bioinform. 2018;19:1344–55.
van Der Hooft JJ, Wandy J, Barrett MP, Burgess KE, Rogers S. Topic modeling for untargeted substructure exploration in metabolomics. PNAS. 2016;113:13738–43.
van der Laarse SAM, Leney AC, Heck AJR. Crosstalk between phosphorylation and O-GlcNAcylation: friend or foe. FEBS J. 2018;285:3152–67.
Van Leene J, Stals H, Eeckhout D, Persiau G, Van De Slijke E, Van Isterdael G, et al. A tandem affinity purification-based technology platform to study the cell cycle interactome in Arabidopsis thaliana. Mol Cell Proteomics. 2007;6:1226–38.
Van Leene J, Han C, Gadeyne A, Eeckhout D, Matthijs C, Cannoot B, et al. Capturing the phosphorylation and protein interaction landscape of the plant TOR kinase. Nat Plants. 2019;5:316–27.
Van Riper SK, Higgins L, Carlis JV, Griffin TJ. RIPPER: a framework for MS1 only metabolomics and proteomics label-free relative quantification. Bioinformatics. 2016;32:2035–7.
Villas-Bôas SG, Roessner U, Hansen MAE, Smedsgaard J, Nielsen J. Metabolome Analysis: An Introduction. In: Metabolome Analysis: An Introduction. New York: Wiley-Interscience Series in Mass Spectrometry; 2007. p. 15–38.
Vinaixa M, Schymanski EL, Neumann S, Navarro M, Salek RM, Yanes O. Mass spectral databases for LC/MS- and GC/MS-based metabolomics: State of the field and future prospects. TrAC Trends Anal Chem. 2016;78:23–35.
Volkening JD, Stecker KE, Sussman MR. Proteome-wide Analysis of Protein Thermal Stability in the Model Higher Plant Arabidopsis thaliana. Mol Cell Proteomics. 2019;18:308–19.
Wakasa K, Hasegawa H, Nemoto H, Matsuda F, Miyazawa H, Tozawa Y, et al. High-level tryptophan accumulation in seeds of transgenic rice and its limited effects on agronomic traits and seed metabolite profile. J Exp Bot. 2006;57:3069–78.
Wang Y, Tang H, Nicholson JK, Hylands PJ, Sampson J, Whitcombe I, et al. Metabolomic Strategy for the Classification andQuality Control of Phytomedicine: A Case Study ofChamomile Flower MatricariarecutitaL. Planta Med. 2004;70:250–5.
Wang P, Xue L, Batelli G, Lee S, Hou YJ, Van Oosten MJ, et al. Quantitative phosphoproteomics identifies SnRK2 protein kinase substrates and reveals the effectors of abscisic acid action. Proc Natl Acad Sci U S A. 2013a;110:11205–10.
Wang S, Li J, Shi X, Qiao L, Lu X, Xu G. A novel stop-flow two-dimensional liquid chromatography–mass spectrometry method for lipid analysis. J Chromatogr A. 2013b;1321:65–72.
Wang L, Czedik-Eysenberg A, Mertz RA, Si Y, Tohge T, Nunes-Nesi A, et al. Comparative analyses of C(4) and C(3) photosynthesis in developing leaves of maize and rice. Nat Biotechnol. 2014a;32:1158–65.
Wang ZQ, Xu XY, Gong QQ, Xie C, Fan W, Yang JL, et al. Root proteome of rice studied by iTRAQ provides integrated insight into aluminum stress tolerance mechanisms in plants. J Proteomics. 2014b;98:189–205.
Wang M, Carver JJ, Phelan VV, Sanchez LM, Garg N, Peng Y, et al. Sharing and community curation of mass spectrometry data with Global Natural Products Social Molecular Networking. Nat Biotechnol. 2016;34:828–37.
Wang S, Zhou L, Wang Z, Shi X, Xu G. Simultaneous metabolomics and lipidomics analysis based on novel heart-cutting two-dimensional liquid chromatography-mass spectrometry. Anal Chim Acta. 2017;966:34–40.
Wang H, Guo X, Li Q, Lu Y, Huang W, Zhang F, et al. Integrated Transcriptomic and Metabolic Framework for Carbon Metabolism and Plant Hormones Regulation in Vigna radiata during Post-Germination Seedling Growth. Sci Rep. 2020a;10:3745.
Wang T, Cheng X, Xu H, Meng Y, Yin Z, Li X, et al. Perspective on Advances in Laser-Based High-Resolution Mass Spectrometry Imaging. Anal Chem. 2020b;92:543–53.
Wang X, Jin S, Chang X, Li G, Zhang L, Jin S. Two interaction proteins between AtPHB6 and AtSOT12 regulate plant salt resistance through ROS signaling. Plant Physiol Biochem. 2021a;169:70–80.
Wang X, Yao X, Zhao A, Yang M, Zhao W, LeTourneau MK, et al. Phosphoinositide-specific phospholipase C gene involved in heat and drought tolerance in wheat (Triticum aestivum L.). Genes Genomics. 2021b;43:1167–77.
Wang Y, Liu B, Hu Y, Gan S-S. A positive feedback regulatory loop, SA-AtNAP-SAG202/SARD1-ICS1-SA, in SA biosynthesis involved in leaf senescence but not defense response. Molecular Horticulture. 2022;2:15.
Weckwerth W, Wenzel K, Fiehn O. Process for the integrated extraction, identification and quantification of metabolites, proteins and RNA to reveal their co-regulation in biochemical networks. Proteomics. 2004;4:78–83.
Weckwerth W, Ghatak A, Bellaire A, Chaturvedi P, Varshney RK. PANOMICS meets germplasm. Plant Biotechnol J. 2020;18:1507–25.
Wei J, Chen J, Liang X, Guo X. Microwave-assisted extraction in combination with HPLC-UV for quantitative analysis of six bioactive oxoisoaporphine alkaloids in Menispermum dauricum DC. Biomed Chromatography. 2016;30:241–8.
Weindl D, Wegner A, Hiller K. MIA: non-targeted mass isotopolome analysis. Bioinformatics. 2016;32:2875–6.
Wen W, Li D, Li X, Gao Y, Li W, Li H, et al. Metabolome-based genome-wide association study of maize kernel leads to novel biochemical insights. Nat Commun. 2014;5:3438.
Wen W, Liu H, Zhou Y, Jin M, Yang N, Li D, et al. Combining Quantitative Genetics Approaches with Regulatory Network Analysis to Dissect the Complex Metabolism of the Maize Kernel. Plant Physiol. 2016;170:136–46.
West C, Lemasson E, Bertin S, Hennig P, Lesellier E. An improved classification of stationary phases for ultra-high performance supercritical fluid chromatography. J Chromatogr A. 2016;1440:212–28.
Wiechert W, Nöh K. Isotopically non-stationary metabolic flux analysis: complex yet highly informative. Curr Opin Biotechnol. 2013;24:979–86.
Wieloch T. The next phase in the development of 13C isotopically non-stationary metabolic flux analysis. J Exp Bot. 2021;72:6087–90.
Williams BJ, Cameron CJ, Workman R, Broeckling CD, Sumner LW, Smith JT. Amino acid profiling in plant cell cultures: an inter-laboratory comparison of CE-MS and GC-MS. Electrophoresis. 2007;28:1371–9.
Winzer T, Gazda V, He Z, Kaminski F, Kern M, Larson TR, et al. A Papaver somniferum 10-gene cluster for synthesis of the anticancer alkaloid noscapine. Science. 2012;336:1704–8.
Wu SG, Wang Y, Jiang W, Oyetunde T, Yao R, Zhang X, et al. Rapid Prediction of Bacterial Heterotrophic Fluxomics Using Machine Learning and Constraint Programming. PLoS Comput Biol. 2016;12(4):e1004838.
Wu X, Qin R, Wu H, Yao G, Zhang Y, Li P, et al. Nanoparticle-immersed paper imprinting mass spectrometry imaging reveals uptake and translocation mechanism of pesticides in plants. Nano Res. 2020;13:611–20.
Wu X, Zhang Y, Qin R, Li P, Wen Y, Yin Z, et al. Discrimination of isomeric monosaccharide derivatives using collision-induced fingerprinting coupled to ion mobility mass spectrometry. Talanta. 2021;224:121901.
Wu C, Yu J, Guarnieri M, Xiong W. Computational Framework for Machine-Learning-Enabled 13C Fluxomics. ACS Synthetic Biol. 2022;11:103–15.
Xia J, Wishart DS. MSEA: a web-based tool to identify biologically meaningful patterns in quantitative metabolomic data. Nucleic Acids Res. 2010;38:W71–7.
Xing S, Wallmeroth N, Berendzen KW, Grefen C. Techniques for the Analysis of Protein-Protein Interactions in Vivo. Plant Physiol. 2016;171:727–58.
CAS PubMed PubMed Central Google Scholar
Xu Y, Fu X, Sharkey TD, Shachar-Hill Y, Walker, and J, B. The metabolic origins of non-photorespiratory CO2 release during photosynthesis: a metabolic flux analysis. Plant Physiol. 2021;186:297–314.
Yang Y, Qiang X, Owsiany K, Zhang S, Thannhauser TW, Li L. Evaluation of different multidimensional LC-MS/MS pipelines for isobaric tags for relative and absolute quantitation (iTRAQ)-based proteomic analysis of potato tubers in response to cold storage. J Proteome Res. 2011;10:4647–60.
Yang QS, Wu JH, Li CY, Wei YR, Sheng O, Hu CH, et al. Quantitative proteomic analysis reveals that antioxidation mechanisms contribute to cold tolerance in plantain (Musa paradisiaca L.; ABB Group) seedlings. Mol Cell Proteomics. 2012;11:1853–69.
Yang F, Melo-Braga MN, Larsen MR, Jorgensen HJ, Palmisano G. Battle through signaling between wheat and the fungal pathogen Septoria tritici revealed by proteomics and phosphoproteomics. Mol Cell Proteomics. 2013a;12:2497–508.
Yang JY, Sanchez LM, Rath CM, Liu X, Boudreau PD, Bruns N, et al. Molecular networking as a dereplication strategy. J Nat Prod. 2013b;76:1686–99.
Yang QS, Gao J, He WD, Dou TX, Ding LJ, Wu JH, et al. Comparative transcriptomics analysis reveals difference of key gene expression between banana and plantain in response to cold stress. BMC Genomics. 2015;16:446.
Yang X-N, Lv Q-Q, Zhao Q, Li X-M, Yan D-M, Yang X-W, et al. Metabolic profiling of myrislignan by UPLC-ESI-QTOFMS-based metabolomics. RSC Adv. 2017;7:40131–40.
Yang S, Li X, Liu X, Ding X, Xin X, Jin C, et al. Parallel comparative proteomics and phosphoproteomics reveal that cattle myostatin regulates phosphorylation of key enzymes in glycogen metabolism and glycolysis pathway. Oncotarget. 2018;9:11352–70.
Yang S, Li H, Bhatti S, Zhou S, Yang Y, Fish T, et al. The Al-induced proteomes of epidermal and outer cortical cells in root apex of cherry tomato 'LA 2710'. J Proteomics. 2020;211:103560.
Yang L, Wang Z, Zhang A, Bhawal R, Li C, Zhang S, et al. Reduction of the canonical function of a glycolytic enzyme enolase triggers immune responses that further affect metabolism and growth in Arabidopsis. Plant Cell. 2022;34:1745–67.
Yilmaz M, Paulic M, Seidel T. Interactome of Arabidopsis Thaliana. Plants (Basel). 2022;11:350.
Yin Y, Wang R, Cai Y, Wang Z, Zhu ZJ. DecoMetDIA_ Deconvolution of Multiplexed MS/MS Spectra for Metabolite Identification in SWATH-MS-Based Untargeted Metabolomics. Anal Chem. 2019;91:11897–904.
Yin Z, Cheng X, Liu R, Li X, Hang L, Hang W, et al. Chemical and Topographical Single-Cell Imaging by Near-Field Desorption Mass Spectrometry. Angew Chem Int Ed. 2019;58:4541–6.
Yu C, Huszagh A, Viner R, Novitsky EJ, Rychnovsky SD, Huang L. Developing a Multiplexed Quantitative Cross-Linking Mass Spectrometry Platform for Comparative Structural Analysis of Protein Complexes. Anal Chem. 2016;88(20):10301–8. https://doi.org/10.1021/acs.analchem.6b03148 . https://pubmed.ncbi.nlm.nih.gov/27626298/ .
Yu Q, Paulo JA, Naverrete-Perea J, McAlister GC, Canterbury JD, Bailey DJ, et al. Benchmarking the Orbitrap Tribrid Eclipse for Next Generation Multiplexed Proteomics. Anal Chem. 2020;92:6478–85.
Yuan H, Cao G, Hou X, Huang M, Du P, Tan T, et al. Development of a widely targeted volatilomics method for profiling volatilomes in plants. Mol Plant. 2022;15:189–202.
Zhang S, Yang Y, Thannhauser TW. Impact of Whole Genome Protein Analysis on Gene Discovery of Disease Models. In: Gu W, Wang Y, editors. Gene Discovery for Disease Models: Wiley; 2011. p. 471–530. https://doi.org/10.1002/9780470933947.ch22 . https://onlinelibrary.wiley.com/doi/10.1002/9780470933947.ch22 .
Zhang J, Xin L, Shan B, Chen W, Xie M, Yuen D, et al. PEAKS DB: de novo sequencing assisted database search for sensitive and accurate peptide identification. Mol Cell Proteomics. 2012;11(M111):010587.
Zhang W, Chang J, Lei Z, Huhman D, Sumner LW, Zhao PX. MET-COFEA: A Liquid Chromatography/Mass Spectrometry Data Processing Platform for Metabolite Compound Feature Extraction and Annotation. Anal Chem. 2014;86:6245–53.
Zhang W, Zhang H, Ning L, Li B, Bao M. Quantitative Proteomic Analysis Provides Novel Insights into Cold Stress Responses in Petunia Seedlings. Front Plant Sci. 2016;7:136.
Zhang Y, Natale R, Domingues APJ, Toleco MR, Siemiatkowska B, Fabregas N, et al. Rapid Identification of Protein-Protein Interactions in Plants. Curr Protoc Plant Biol. 2019;4:e20099.
Zhang F, Ge W, Ruan G, Cai X, Guo T. Data-Independent Acquisition Mass Spectrometry-Based Proteomics and Software Tools: A Glimpse in 2020. Proteomics. 2020a;20:e1900276.
Zhang Y, Li Y, Yang X, Wen Z, Nagalakshmi U, Dinesh-Kumar SP. TurboID-Based Proximity Labeling for In Planta Identification of Protein-Protein Interaction Networks. J Vis Exp. 2020. https://doi.org/10.3791/60728 .
Zhao Q, Li XM, Liu HN, Gonzalez FJ, Li F. Metabolic map of osthole and its effect on lipids. Xenobiotica. 2018;48:285–99.
Zheng F, Zhao X, Zeng Z, Wang L, Lv W, Wang Q, et al. Development of a plasma pseudotargeted metabolomics method based on ultra-high-performance liquid chromatography–mass spectrometry. Nat Protoc. 2020;15:2519–37.
Zheng J, Johnson M, Mandal R, Wishart DS. A Comprehensive Targeted Metabolomics Assay for Crop Plant Sample Analysis. Metabolites. 2021;11.
Zhou Q, Gao B, Zhang X, Xu Y, Shi H, Yu LL. Chemical profiling of triacylglycerols and diacylglycerols in cow milk fat by ultra-performance convergence chromatography combined with a quadrupole time-of-flight mass spectrometry. Food Chem. 2014;143:199–204.
Zhou X, Chen C, Ye X, Song F, Fan G, Wu F. Analysis of lignans in Magnoliae Flos by turbulent flow chromatography with online solid-phase extraction and high-performance liquid chromatography with tandem mass spectrometry. J Sep Sci. 2016b;39:1266–72.
Zhou D, Yang Y, Zhang J, Jiang F, Craft E, Thannhauser TW, et al. Quantitative iTRAQ Proteomics Revealed Possible Roles for Antioxidant Proteins in Sorghum Aluminum Tolerance. Front Plant Sci. 2016a;7:2043.
Zhou Z, Luo M, Chen X, Yin Y, Xiong X, Wang R, et al. Ion mobility collision cross-section atlas for known and unknown metabolite annotation in untargeted metabolomics. Nat Commun. 2020;11:4334.
Zhu X, Yu F, Yang Z, Liu S, Dai C, Lu X, et al. In planta chemical cross-linking and mass spectrometry analysis of protein structure and interaction in Arabidopsis. Proteomics. 2016a;16:1915–27.
Zhu Y, Li H, Bhatti S, Zhou S, Yang Y, Fish T, et al. Development of a laser capture microscope-based single-cell-type proteomics tool for studying proteomes of individual cell layers of plant roots. Hortic Res. 2016b;3:16026.
Zhu G, Wang S, Huang Z, Zhang S, Liao Q, Zhang C, et al. Rewiring of the Fruit Metabolome in Tomato Breeding. Cell. 2018a;172:249–261 e212.
Zhu Y, Piehowski PD, Zhao R, Chen J, Shen Y, Moore RJ, et al. Nanodroplet processing platform for deep and quantitative proteome profiling of 10-100 mammalian cells. Nat Commun. 2018b;9:882.
Zulawski M, Braginets R, Schulze WX. PhosPhAt goes kinases--searchable protein kinase target information in the plant phosphorylation site database PhosPhAt. Nucleic Acids Res. 2013;41:D1176–84.
Download references
Acknowledgement
We thank Professor Suping Zhou at College of Agriculture, Tennessee State University; Drs. Qiaosong Yang and Wenjie Huang at Guangdong Academic of Agricultural Sciences, and Dr. Qin Fu, Ms Elizabeth Anderson and Mr. Robert Sherwood at Proteomics and Metabolomics Facility of Cornell University for helpful discussions during drafting the manuscript.
This research was supported by the Key Realm R&D Program of Guangdong Province (No. 2020B0202090005), the Science and Technology Program of Guangdong Province (2021A0505030050), the Project of Collaborative Innovation Center of Guangdong Academy of Agricultural Sciences (XTXM202203), the Special Fund for Scientific Innovation Strategy-construction of High-Level Academy of Agriculture Science (No. R2020PY-JX019; R2021YJ-QG004), and two USDA grants (No. 8062-21000-046-00D and No. 8062-21000-047-00D)
Author information
Authors and affiliations.
Guangdong Key Laboratory for Crop Germplasm Resources Preservation and Utilization, Agro-biological Gene Research Center, Guangdong Academy of Agricultural Sciences, Guangzhou, China
Shijuan Yan & Zhibin Yin
Proteomics and Metabolomics Facility, Institute of Biotechnology, Cornell University, 139 Biotechnology Building, 526 Campus Road, Ithaca, NY, 14853, USA
Ruchika Bhawal & Sheng Zhang
R. W. Holley Center for Agriculture & Health, USDA/ARS, Ithaca, NY, USA
Theodore W. Thannhauser
You can also search for this author in PubMed Google Scholar
Contributions
S.Z. wrote the sections on Abstract, Introduction, Proteomic applications in plant research, Challenge and future perspectives; RB and SZ. wrote the section on Advances in proteomics technologies, and prepared for Figs. 1 , 2 , 3 and Table 1 . TWT and SZ drafted the subsection of “Functional characterization of cell-specific proteins by single-cell-type proteomics” and edited the entire manuscript. SY wrote the majority section of Emerging MS-based metabolomics and all sections on Metabolomics application to plant research, prepared for Figs. 4 , 5 and Table 2 , as well as edited the entire manuscsript. ZY contributed to drafting the subsections of “Spatially resolved metabolomics” and “flux metabolic analysis”. All the authors read, provided inputs and approved the final version of the manuscript.
Corresponding author
Correspondence to Sheng Zhang .
Ethics declarations
Ethics approval and consent to participate.
Not applicable
Consent for publication
All authors hereby consent to publication of the work.
Competing interests
The authors declare no competing financial interests.
Additional information
Publisher’s note.
Springer Nature remains neutral with regard to jurisdictional claims in published maps and institutional affiliations.
Rights and permissions
Open Access This article is licensed under a Creative Commons Attribution 4.0 International License, which permits use, sharing, adaptation, distribution and reproduction in any medium or format, as long as you give appropriate credit to the original author(s) and the source, provide a link to the Creative Commons licence, and indicate if changes were made. The images or other third party material in this article are included in the article's Creative Commons licence, unless indicated otherwise in a credit line to the material. If material is not included in the article's Creative Commons licence and your intended use is not permitted by statutory regulation or exceeds the permitted use, you will need to obtain permission directly from the copyright holder. To view a copy of this licence, visit http://creativecommons.org/licenses/by/4.0/ . The Creative Commons Public Domain Dedication waiver ( http://creativecommons.org/publicdomain/zero/1.0/ ) applies to the data made available in this article, unless otherwise stated in a credit line to the data.
Reprints and permissions
About this article
Cite this article.
Yan, S., Bhawal, R., Yin, Z. et al. Recent advances in proteomics and metabolomics in plants. Mol Horticulture 2 , 17 (2022). https://doi.org/10.1186/s43897-022-00038-9
Download citation
Received : 24 April 2022
Accepted : 20 June 2022
Published : 23 July 2022
DOI : https://doi.org/10.1186/s43897-022-00038-9
Share this article
Anyone you share the following link with will be able to read this content:
Sorry, a shareable link is not currently available for this article.
Provided by the Springer Nature SharedIt content-sharing initiative
- Single cell proteomics
- Phosphoproteomics
Molecular Horticulture
ISSN: 2730-9401
- Submission enquiries: [email protected]
Thank you for visiting nature.com. You are using a browser version with limited support for CSS. To obtain the best experience, we recommend you use a more up to date browser (or turn off compatibility mode in Internet Explorer). In the meantime, to ensure continued support, we are displaying the site without styles and JavaScript.
- View all journals
- Explore content
- About the journal
- Publish with us
- Sign up for alerts
- Published: 10 January 2007
Innovations
Towards the plant metabolome and beyond
- Robert L. Last 1 ,
- A. Daniel Jones 2 &
- Yair Shachar-Hill 3
Nature Reviews Molecular Cell Biology volume 8 , pages 167–174 ( 2007 ) Cite this article
2140 Accesses
91 Citations
Metrics details
Methods for network-wide analysis are increasingly showing that the textbook view of the regulation of plant metabolism is often incomplete and misleading. Recent innovations in small-molecule analysis have created the ability to rapidly identify and quantify numerous compounds, and these data are creating new opportunities for understanding plant metabolism and for plant metabolic engineering.
This is a preview of subscription content, access via your institution
Access options
Subscribe to this journal
Receive 12 print issues and online access
176,64 € per year
only 14,72 € per issue
Buy this article
- Purchase on Springer Link
- Instant access to full article PDF
Prices may be subject to local taxes which are calculated during checkout
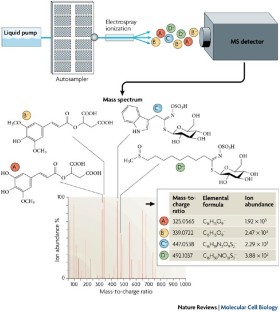
Similar content being viewed by others
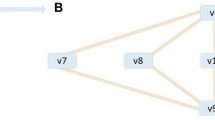
Identification of flux trade-offs in metabolic networks
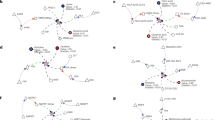
Metaboverse enables automated discovery and visualization of diverse metabolic regulatory patterns
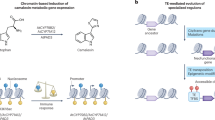
Emerging mechanistic insights into the regulation of specialized metabolism in plants
Fiehn, O. Metabolomics — the link between genotypes and phenotypes. Plant Mol. Biol. 48 , 155–171 (2002).
Article CAS PubMed Google Scholar
Dunn, W. B. & Ellis, D. I. Metabolomics: current analytical platforms and methodologies. Trends Anal. Chem. 24 , 285–294 (2005).
Article CAS Google Scholar
Sweetlove, L. J., Last, R. L. & Fernie, A. R. Predictive metabolic engineering: a goal for systems biology. Plant Physiol. 132 , 420–425 (2003).
Article CAS PubMed PubMed Central Google Scholar
Ward, J. L., Harris, C., Lewis, J. & Beale, M. H. Assessment of 1 H NMR spectroscopy and multivariate analysis as a technique for metabolite fingerprinting of Arabidopsis thaliana . Phytochemistry 62 , 949–957 (2003).
Krishnan, P., Kruger, N. J. & Ratcliffe, R. G. Metabolite fingerprinting and profiling in plants using NMR. J. Exp. Bot. 56 , 255–265 (2005).
Ratcliffe, R. G. & Shachar-Hill, Y. Probing plant metabolism with NMR. Annu. Rev. Plant Physiol. Plant Mol. Biol. 52 , 499–526 (2001).
Ratcliffe, R. G., Roscher, A. & Shachar-Hill, Y. Plant NMR spectroscopy. Prog. Nucl. Magn. Reson. Spectros. 39 , 267–300 (2001).
Article Google Scholar
Glinski, M. & Weckwerth, W. The role of mass spectrometry in plant systems biology. Mass Spectrom. Rev. 25 , 173–214 (2006).
Villas-Bôas, S. G., Mas, S., Åkesson, M., Smedsgaard, J. & Nielsen, J. Mass spectrometry in metabolome analysis. Mass Spectrom. Rev. 24 , 613–646 (2005).
Article PubMed Google Scholar
Aharoni, A. et al. Nontargeted metabolome analysis by use of fourier transform ion cyclotron mass spectrometry. OMICS 6 , 217–234 (2002).
Tohge, T. et al. Functional genomics by integrated analysis of metabolome and transcriptome of Arabidopsis plants over-expressing an MYB transcription factor. Plant J. 42 , 218–235 (2005).
Catchpole, G. S. et al. Hierarchical metabolomics demonstrates substantial compositional similarity between genetically modified and conventional potato crops. Proc. Natl Acad. Sci. USA 102 , 14458–14462 (2005).
Hu, Q. et al. The Orbitrap: a new mass spectrometer. J. Mass. Spectrom. 40 , 430–443 (2005).
Weckwerth, W., Loureiro, M. E., Wenzel, K. & Fiehn, O. Differential metabolic networks unravel the effects of silent plant phenotypes. Proc. Natl Acad. Sci. USA 101 , 7809–7814 (2004).
Ratcliffe, R. G. & Shachar-Hill, Y. Revealing metabolic phenotypes in plants: inputs from NMR analysis. Biol. Rev. 80 , 27–43 (2005).
Janes, K. A. & Yaffe, M. B. Data-driven modelling of signal-transduction networks. Nature Rev. Mol. Cell Biol. 7 , 820–828 (2006).
Fan, T. W. M. et al. Comprehensive chemical profiling of gramineous plant root exudates using high-resolution NMR and MS. Phytochemistry 57 , 209–221 (2001).
Shachar-Hill, Y. & Pfeffer, P. Nuclear Magnetic Resonance in Plant Biology (American Society of Plant Biologists, Rockville, 1996).
Google Scholar
Bailey, N. J., Stanley, P. D., Hadfield, S. T., Lindon, J. C. & Nicholson, J. K. Mass spectrometrically detected directly coupled high performance liquid chromatography/nuclear magnetic resonance spectroscopy/mass spectrometry for the identification of xenobiotic metabolites in maize plants. Rapid Commun. Mass Spectrom. 14 , 679–684 (2000).
Fan, T. W. M., Higashi, R. M., Lane, A. N. & Jardetzky, O. Combined use of 1 H-NMR and GC–MS for metabolite monitoring and in vivo 1 H-NMR assignments. Biochim. Biophys. Acta 882 , 154–167 (1986).
Morgenthal, K., Weckwerth, W. & Steuer, R. Metabolic networks in plants: transitions from pattern recognition to biological interpretation. BioSystems 83 , 108–117 (2006).
Pettersson, G. & Ryde-Pettersson, U. A mathematical model of the Calvin photosynthesis cycle. Eur. J. Biochem. 175 , 661–672 (1988).
Tikunov, Y. et al. A novel approach for nontargeted data analysis for metabolomics. Large-scale profiling of tomato fruit volatiles. Plant Physiol. 139 , 1125–1137 (2005).
Broeckling, C. D., Reddy, I. R., Duran, A. L., Zhao, X. & Sumner, L. W. MET-IDEA: data extraction tool for mass spectrometry-based metabolomics. Anal. Chem. 78 , 4334–4341 (2006).
Jonsson, P. et al. High-throughput data analysis for detecting and identifying differences between samples in GC–MS-based metabolomic analyses. Anal. Chem. 77 , 5635–5642 (2005).
Scholz, M., Gatzek, S., Sterling, A., Fiehn, O. & Selbig, J. Metabolite fingerprinting: detecting biological features by independent component analysis. Bioinformatics 20 , 2447–2454 (2004).
Goodacre, R., York, E. V., Heald, J. K. & Scott, I. M. Chemometric discrimination of unfractionated plant extracts analyzed by electrospray mass spectrometry. Phytochemistry 62 , 859–863 (2003).
Hirai, M. Y. et al. Integration of transcriptomics and metabolomics for understanding of global responses to nutritional stresses in Arabidopsis thaliana . Proc. Natl Acad. Sci. USA 101 , 10205–10210 (2004).
Wurtele, E. S. et al. MetNet: software to build and model the biogenetic lattice of Arabidopsis . Comp. Funct. Genomics 4 , 239–245 (2003).
Thimm, O. et al. MAPMAN: a user-driven tool to display genomics data sets onto diagrams of metabolic pathways and other biological processes. Plant J. 37 , 914–939 (2004).
Zimmermann, P., Hirsch-Hoffmann, M., Hennig, L. & Gruissem, W. GENEVESTIGATOR. Arabidopsis microarray database and analysis toolbox. Plant Physiol. 136 , 2621–2632 (2004).
Cook, D., Fowler, S., Fiehn, O. & Thomashow, M. F. A prominent role for the CBF cold response pathway in configuring the low-temperature metabolome of Arabidopsis . Proc. Natl Acad. Sci. USA 101 , 15243–15248 (2004).
Van Eenennaam, A. L. et al. Engineering vitamin E content: from Arabidopsis mutant to soy oil. Plant Cell 15 , 3007–3019 (2003).
Charlton, A. et al. NMR profiling of transgenic peas. Plant Biotech. J. 2 , 27–35 (2004).
Le Gall, G., Colquhoun, I. J. & Defernez, M. Metabolite profiling using 1 H NMR spectroscopy for quality assessment of green tea, Camellia sinensis (L.). J. Agric. Food Chem. 52 , 692–700 (2004).
Noteborn, H. P., Lommen, A., van der Jagt, R. C. & Weseman, J. M. Chemical fingerprinting for the evaluation of unintended secondary metabolic changes in transgenic food crops. J. Biotechnol. 77 , 103–114 (2000).
Manetti, C. et al. A metabonomic study of transgenic maize ( Zea mays ) seeds revealed variations in osmolytes and branched amino acids. J. Exp. Bot. 57 , 2613–2625 (2006).
Benning, C. Genetic mutant screening by direct metabolite analysis. Anal. Biochem. 332 , 1–9 (2004).
Rose, A. B., Li, J. & Last, R. L. An allelic series of blue fluorescent trp1 mutants of Arabidopsis thaliana . Genetics 145 , 197–205 (1997).
CAS PubMed PubMed Central Google Scholar
Winkel-Shirley, B. Flavonoid biosynthesis. A colorful model for genetics, biochemistry, cell biology, and biotechnology. Plant Physiol. 126 , 485–493 (2001).
Chapple, C. C., Vogt, T., Ellis, B. E. & Somerville, C. R. An Arabidopsis mutant defective in the general phenylpropanoid pathway. Plant Cell 4 , 1413–1424 (1992).
Somerville, C. & Browse, J. Plant lipids: metabolism, mutants and membranes. Science 252 , 80–87 (1991).
Cheng, Z. et al. Highly divergent methyltransferases catalyze a conserved reaction in tocopherol and plastoquinone synthesis in cyanobacteria and photosynthetic eukaryotes. Plant Cell 15 , 2343–2356 (2003).
Valentin, H. E. et al. The Arabidopsis vitamin E pathway gene 5–1 mutant reveals a critical role for phytol kinase in seed tocopherol biosynthesis. Plant Cell 18 , 212–224 (2006).
Conklin, P. L., Saracco, S. A., Norris, S. R. & Last, R. L. Identification of ascorbic acid-deficient Arabidopsis thaliana mutants. Genetics 154 , 847–856 (2000).
Jander, G. et al. Application of a high-throughput HPLC–MS/MS assay to Arabidopsis mutant screening; evidence that threonine aldolase plays a role in seed nutritional quality. Plant J. 39 , 465–475 (2004).
Broeckling, C. D. et al. Metabolic profiling of Medicago truncatula cell cultures reveals the effects of biotic and abiotic elicitors on metabolism. J. Exp. Bot. 56 , 323–336 (2005).
Schauer, N. et al. Comprehensive metabolic profiling and phenotyping of interspecific introgression lines for tomato improvement. Nature Biotechnol. 24 , 447–454 (2006).
Eshed, Y. & Zamir, D. An introgression line population of Lycopersicon pennellii in the cultivated tomato enables the identification and fine mapping of yield-associated QTL. Genetics 141 , 1147–1162 (1995).
Keurentjes, J. J. et al. The genetics of plant metabolism. Nature Genet. 38 , 842–849 (2006).
Fell, D. A. Enzymes, metabolites and fluxes. J. Exp. Bot. 56 , 267–272 (2005).
Tomos, A. D. & Leigh, R. A. The pressure probe: a versatile tool in plant cell physiology. Annu. Rev. Plant Physiol. Plant Mol. Biol. 50 , 447–472 (1999).
Lalonde, S., Ehrhardt, D. W. & Frommer, W. B. Shining light on signaling and metabolic networks by genetically encoded biosensors. Curr. Opin. Plant Biol. 8 , 574–581 (2005).
Ratcliffe, R. G. & Shachar-Hill, Y. Measuring multiple fluxes through plant metabolic networks. Plant J. 45 , 490–511 (2006).
Raamsdonk, L. M. et al. A functional genomics strategy that uses metabolome data to reveal the phenotype of silent mutations. Nature Biotechnol. 19 , 45–50 (2001).
Download references
Acknowledgements
Research in the Jones, Last and Shachar-Hill laboratories is funded by grants from the National Science Foundation, United States Department of Agriculture Competitive Grants Program, the National Institutes of Health and Michigan State University.
Author information
Authors and affiliations.
Robert L. Last is at the Department of Biochemistry and Molecular Biology and the Department of Plant Biology, Michigan State University, East Lansing, Michigan 48824, USA.,
Robert L. Last
A. Daniel Jones is at the Department of Biochemistry and Molecular Biology and the Department of Chemistry, Michigan State University, East Lansing, Michigan 48824, USA.,
A. Daniel Jones
Yair Shachar-Hill is at the Department of Plant Biology, Michigan State University, East Lansing, Michigan 48824, USA.,
Yair Shachar-Hill
You can also search for this author in PubMed Google Scholar
Corresponding author
Correspondence to Robert L. Last .
Ethics declarations
Competing interests.
The authors declare no competing financial interests.
Supplementary information
Supplementary information s1 (box).
Statistical methods used in the analysis of metabolomics data. (PDF 594 kb)
Related links
Further information.
Robert L. Last's homepage
A. Daniel Jones's homepage
Yair Shachar-Hill's homepage
AraCyc Arabidopsis metabolic pathway annotations
Genevestigator
Iowa Gene Expression Toolkit
KEGG Pathway Database
L. penellii Introgression Lines
MapMan tool
Metabolic modeling
Metabolic Ontology Working Group
MetAlign tool for GC– or LC–MS data analysis
Pathexplore tool
Plant metabolites database
Solcyc Solanaceae metabolic pathway annotations
The Golm Metabolome Database
WTEC Panel Report on International Research and Development in Systems Biology
The known or postulated ability of a plant-produced chemical to modify the physiology of a nearby plant, which constitutes a form of chemical communication between plants.
Separation of compounds by the different times that they take to traverse a narrow column under the influence of an electric field.
(ESI). A gentle method for ionizing compounds that applies high voltage to a solution of compounds that are sprayed through a capillary.
A large family of structurally diverse molecules that are produced by plants. They confer common colours to leaves, flowers and fruits, absorb ultraviolet light and have other known and postulated roles in plant growth and development.
(FIA). Introduction of a sample into a flowing medium that transports the sample components to a detector, such as a mass spectrometer, without chromatographic separation.
Spectroscopy that enables the identification of classes of compound by analysing their interactions with infrared light. Mass resolution is the ability to distinguish ions of different masses, usually expressed as a ratio of the ion mass to the difference in mass that the mass spectrometer can reliably distinguish.
A method for obtaining accurate measurements of the mass-to-charge ratio of ions in a complex mixture, allowing the identification and measurement of the molecules.
A measure of the ability of an organism to survive when exposed to below-freezing temperatures.
(GC). Method for the separation of volatile compounds in a gas phase through differential binding to a column at elevated temperatures.
(HPLC). Method for separating compounds in solution through their differential interactions with a column.
Method for the separation of metabolites by HPLC followed by the detection of the metabolites based on their redox behaviour or spectral absorption properties.
(m/z). The ratio of the mass of an ion (in Daltons, or atomic mass units) to the number of charges on the ion.
The mass of a molecule, rounded off to the nearest integer value. Two compounds with different elemental formulas can have identical nominal masses, although their exact masses might differ by a small fraction of a Dalton (or atomic mass unit).
A high-resolution mass spectrometer that traps ions in an electric field and measures their abundances and mass-to-charge ratios based on their orbital motions in the ion trap.
Quantitative analysis of a pre-selected list of metabolites.
Method for the separation of molecules through their differential interactions with a matrix on a glass or plastic plate.
Rights and permissions
Reprints and permissions
About this article
Cite this article.
Last, R., Jones, A. & Shachar-Hill, Y. Towards the plant metabolome and beyond. Nat Rev Mol Cell Biol 8 , 167–174 (2007). https://doi.org/10.1038/nrm2098
Download citation
Published : 10 January 2007
Issue Date : February 2007
DOI : https://doi.org/10.1038/nrm2098
Share this article
Anyone you share the following link with will be able to read this content:
Sorry, a shareable link is not currently available for this article.
Provided by the Springer Nature SharedIt content-sharing initiative
This article is cited by
Metabolomics: a systems biology approach for enhancing heat stress tolerance in plants.
Plant Cell Reports (2022)
Infrastructures of systems biology that facilitate functional genomic study in rice
- Woo-Jong Hong
- Ki-Hong Jung
Rice (2019)
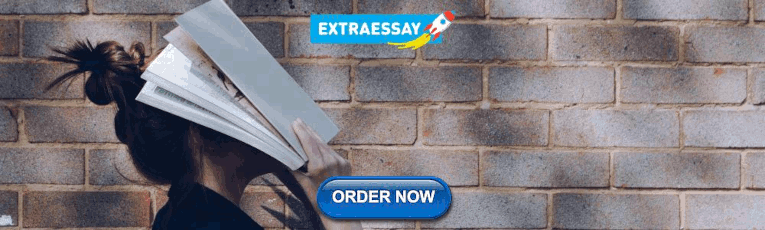
β-Aminobutyric acid increases drought tolerance and reorganizes solute content and water homeostasis in flax (Linum usitatissimum)
- Anthony Quéro
- Ophélie Fliniaux
- François Mesnard
Metabolomics (2015)
Computational mass spectrometry for small molecules
- Kerstin Scheubert
- Franziska Hufsky
- Sebastian Böcker
Journal of Cheminformatics (2013)
Physiological and metabolomic analysis of a knockout mutant suggests a critical role of MtP5CS3 gene in osmotic stress tolerance of Medicago truncatula
- Minh Luan Nguyen
- Goon-Bo Kim
- Young-Woo Nam
Euphytica (2013)
Quick links
- Explore articles by subject
- Guide to authors
- Editorial policies
Sign up for the Nature Briefing newsletter — what matters in science, free to your inbox daily.

PMhub 1.0: a comprehensive plant metabolome database
Affiliations.
- 1 National Key Laboratory of Crop Genetic Improvement and National Center of Plant Gene Research (Wuhan), Huazhong Agricultural University, Wuhan 430070, China.
- 2 Hubei Hongshan Laboratory, Wuhan 430070, China.
- 3 Spice and Beverage Research Institute, Chinese Academy of Tropical Agricultural Sciences, Wanning, Hainan 571533, China.
- 4 BioBioPha Co., Ltd., Kunming 650201, China.
- PMID: 37819039
- PMCID: PMC10767808
- DOI: 10.1093/nar/gkad811
The Plant Metabolome Hub (PMhub), available at https://pmhub.org.cn, is a valuable resource designed to provide scientists with comprehensive information on plant metabolites. It offers extensive details about their reference spectra, genetic foundations, chemical reactions, metabolic pathways and biological functions. The PMhub contains chemical data for 188 837 plant metabolites gathered from various sources, with 1 467 041 standard/in-silico high-resolution tandem mass-spectrometry (HRMS/MS) spectra corresponding to these metabolites. Beyond its extensive literature-derived data, PMhub also boasts a sizable collection of experimental metabolites; it contains 144 366 detected features in 10 typical plant species, with 16 423 successfully annotated by using standard/in-silico HRMS/MS data, this collection is further supplemented with thousands of features gathered from reference metabolites. For each metabolite, the PMhub enables the reconstructed of a simulated network based on structural similarities and existing metabolic pathways. Unlike previous plant-specific metabolome databases, PMhub not only contains a vast amount of metabolic data but also assembles the corresponding genomic and/or transcriptomic information, incorporating multiple methods for the comprehensive genetic analysis of metabolites. To validate the practicality, we verified a synthetic pathway for N-p-coumaroyltyramine by in vitro enzymatic activity experiments. In summary, the robust functionality provided by the PMhub will make it an indispensable tool for studying plant metabolomics.
© The Author(s) 2023. Published by Oxford University Press on behalf of Nucleic Acids Research.
- Databases, Factual*
- Metabolic Networks and Pathways
- Metabolome* / genetics
- Metabolomics / methods
- Plants* / chemistry
- Plants* / metabolism
- Tandem Mass Spectrometry
Grants and funding
- 2021YFF1000204/National Key Research and Development Program of China
- 2021CFA058/Natural Science Foundation for Distinguished Young Scientists of Hubei Province
- Young Top-notch Talent Cultivation Program of Hubei Province
- 2023ZKPY00/Fundamental Research Funds for the Central Universities
ORIGINAL RESEARCH article
Metabolomics and physio-chemical analyses of mulberry plants leaves response to manganese deficiency and toxicity reveal key metabolites and their pathways in manganese tolerance.

- 1 Jiangsu University of Science and Technology, Zhenjiang, Jiangsu Province, China
- 2 Institute for Plant Nutrition and Soil Science, Faculty of Agricultural and Nutritional Sciences, University of Kiel, Kiel, Germany
The final, formatted version of the article will be published soon.
Select one of your emails
You have multiple emails registered with Frontiers:
Notify me on publication
Please enter your email address:
If you already have an account, please login
You don't have a Frontiers account ? You can register here
Manganese (Mn) plays a pivotal role in plant growth and development. Aside aiding in plant growth and development, Mn as heavy metal (HM) can be toxic in soil when applied in excess.Morus alba is an economically significant plant, capable of adapting to a range of environmental conditions and possessing the potential for phytoremediation of contaminated soil by HMs. The mechanism by which M. alba tolerates Mn stresses remains obscure. In this study, Mn concentrations comprising sufficiency (0.15 mM), higher regimes (1.5 mM and 3 mM), and deficiency (0 mM and 0.03 mM), were applied to M. alba in pot treatment for 21 days to understand M. alba Mn tolerance.Mn stress effects on the net photosynthetic rate (Pn), stomatal conductance (Gs), transpiration rate (Tr), intercellular CO2 concentration (Ci), chlorophyll content, plant morphological traits, enzymatic and non-enzymatic parameters were analyzed as well as metabolome signatures via non-targeted LC-MS technique. Mn deficiency and toxicity decrease plant biomass, Pn, Ci, Gs, Tr, and chlorophyll content and plant biomass. Mn stresses induced a decline in the activities of catalase (CAT) and superoxide dismutase (SOD), while peroxidase (POD) activity, and leaf Mn content, increased. Soluble sugars, soluble proteins, malondialdehyde (MDA) and proline exhibited an elevation in Mn deficiency and toxicity concentrations. Metabolomic analysis indicates that Mn concentrations induced 1031 differentially expressed metabolites (DEMs), particularly amino acids, lipids, carbohydrates, benzene and derivatives and secondary metabolites. The DEMs are significantly enriched in alpha-linolenic acid metabolism, biosynthesis of unsaturated fatty acids, galactose metabolism, pantothenate and CoA biosynthesis, pentose phosphate pathway, carbon metabolism, etc.The upregulation of Galactinol, Myo-inositol, Jasmonic acid, L-aspartic acid, Coproporphyrin I, Trigonelline, Pantothenol, and Pantothenate and their significance in the metabolic pathways makes them Mn stress tolerance metabolites in M. alba. Our findings reveal the fundamental understanding of DEMs in M. alba's response to Mn nutrition and the metabolic mechanisms involved, which may hold potential significance for the advancement of M. alba genetic improvement initiatives and phytoremediation programs.
Keywords: mulberry, Manganese stress, physio-chemical, enzymatic activities, LC-MS, metabolomics, metabolic pathways
Received: 04 Dec 2023; Accepted: 10 May 2024.
Copyright: © 2024 Li, Ackah, Amoako, Cui, Sun, Li, Tsigbey and Zhao. This is an open-access article distributed under the terms of the Creative Commons Attribution License (CC BY) . The use, distribution or reproduction in other forums is permitted, provided the original author(s) or licensor are credited and that the original publication in this journal is cited, in accordance with accepted academic practice. No use, distribution or reproduction is permitted which does not comply with these terms.
* Correspondence: Michael Ackah, Jiangsu University of Science and Technology, Zhenjiang, Jiangsu Province, China Weiguo Zhao, Jiangsu University of Science and Technology, Zhenjiang, Jiangsu Province, China
Disclaimer: All claims expressed in this article are solely those of the authors and do not necessarily represent those of their affiliated organizations, or those of the publisher, the editors and the reviewers. Any product that may be evaluated in this article or claim that may be made by its manufacturer is not guaranteed or endorsed by the publisher.
- Search Menu
- Advance Access
- Collections
- Author Guidelines
- Submission Site
- Open Access Policy
- Self-Archiving Policy
- Why Submit?
- About Horticulture Research
- About Nanjing Agricultural University
- Editorial Board
- Advertising & Corporate Services
- Journals on Oxford Academic
- Books on Oxford Academic

Article Contents
Introduction, conclusions, materials and methods, acknowledgements, author contributions, data availability, conflict of interest statement.
- < Previous
Multi-omics analysis reveals key regulatory defense pathways and genes involved in salt tolerance of rose plants
These authors contributed equally to this work.
- Article contents
- Figures & tables
- Supplementary Data
Haoran Ren, Wenjing Yang, Weikun Jing, Muhammad Owais Shahid, Yuming Liu, Xianhan Qiu, Patrick Choisy, Tao Xu, Nan Ma, Junping Gao, Xiaofeng Zhou, Multi-omics analysis reveals key regulatory defense pathways and genes involved in salt tolerance of rose plants, Horticulture Research , Volume 11, Issue 5, May 2024, uhae068, https://doi.org/10.1093/hr/uhae068
- Permissions Icon Permissions
Salinity stress causes serious damage to crops worldwide, limiting plant production. However, the metabolic and molecular mechanisms underlying the response to salt stress in rose ( Rosa spp.) remain poorly studied. We therefore performed a multi-omics investigation of Rosa hybrida cv. Jardin de Granville (JDG) and Rosa damascena Mill. (DMS) under salt stress to determine the mechanisms underlying rose adaptability to salinity stress. Salt treatment of both JDG and DMS led to the buildup of reactive oxygen species (H 2 O 2 ). Palisade tissue was more severely damaged in DMS than in JDG, while the relative electrolyte permeability was lower and the soluble protein content was higher in JDG than in DMS. Metabolome profiling revealed significant alterations in phenolic acid, lipids, and flavonoid metabolite levels in JDG and DMS under salt stress. Proteome analysis identified enrichment of flavone and flavonol pathways in JDG under salt stress. RNA sequencing showed that salt stress influenced primary metabolism in DMS, whereas it substantially affected secondary metabolism in JDG. Integrating these datasets revealed that the phenylpropane pathway, especially the flavonoid pathway, is strongly enhanced in rose under salt stress. Consistent with this, weighted gene coexpression network analysis (WGCNA) identified the key regulatory gene chalcone synthase 1 ( CHS1 ), which is important in the phenylpropane pathway. Moreover, luciferase assays indicated that the bHLH74 transcription factor binds to the CHS1 promoter to block its transcription. These results clarify the role of the phenylpropane pathway, especially flavonoid and flavonol metabolism, in the response to salt stress in rose.
Rose ( Rosa spp.) is a popular ornamental crop that is also used in the cosmetics, perfume and medicine. Rose plants contains various bioactive substances, including flavonoids, fragrant components, and hydrolysable and condensed tannins, which have high value and market potential [ 1 ]. However, soil salinization is common in many rose-growing regions, and high salt concentrations in soil can severely inhibit rose plant growth, reduce flower quality, and cause significant economic losses [ 2 ]. Additionally, salt stress can enhance the secondary metabolites of roses such as citronellol, geraniol, and phenyl ethyl alcohol [ 3 , 4 ]. Such alterations in secondary metabolites may help to regulate the salt tolerance of rose. Research on roses has focused mainly on flower quality, petal development, and flower bloom [ 5–7 ], and there are limited data available regarding signaling pathways linking plant development and secondary metabolites associated with salt stress.
In plants, salt stress induces osmotic imbalances, which lead to the closure of leaf stomata, limit photosynthesis, and affect plant growth and metabolism [ 8 ]. To alleviate osmotic stress and protect themselves from its adverse effects, plants accumulate numerous compatible solutes (such as soluble proteins, soluble sugars, and proline), known collectively as osmoprotectants [ 9 ]. Moreover, plants generate reactive oxygen species (ROS) to cope with salt stress [ 10 ]. Nevertheless, excessive ROS accumulation can lead to oxidative DNA damage, affect protein biosynthesis, and ultimately result in cell damage and death [ 11 , 12 ]. Plant cells utilize both enzymatic and nonenzymatic antioxidant mechanisms to diminish ROS levels and prevent oxidative damage. Superoxide dismutase (SOD), peroxidase (POD), ascorbate peroxidase (APX), catalase (CAT), and glutathione peroxidase (GPX) are antioxidant enzymes that work as O 2− and H 2 O 2 scavengers [ 13 , 14 ]. Nonenzymatic antioxidants, such as ascorbate, glutathione, phenols, and flavonoids, also play vital roles in ROS scavenging [ 15 , 16 ].
Flavonoids are naturally occurring bioactive substances found in fruits, vegetables, tea, and medicinal plants [ 17 ]. Flavonoids comprise more than 9000 compounds and constitute a substantial category of plant secondary metabolites [ 18 ]. They have diverse biological functions in the growth and development of plants, including improving pollen fertility, imparting color, and influencing seed dormancy and germination [ 19 , 20 ]. In addition, flavonoids have protective roles against biotic and abiotic stresses, such as pathogen infections, ultraviolet (UV)-B, cold, drought, and salinity [ 21–23 ]. Flavonoids have also received widespread attention due to their possible benefits for human health [ 24 ].
The molecular mechanism of flavonoid biosynthesis has been elucidated in many plants [ 25 ]. Chalcone synthase (CHS) mediates the first step in flavonoid production, catalyzing the formation of naringenin chalcone from three molecules of malonyl CoA and one molecule of 4-coumaroyl CoA. Chalcone isomerase (CHI) then quickly converts naringenin chalcone into naringenin (flavanone), which is further biosynthesized into different flavonoids by the subsequent enzymes in this pathway [ 26 ]. Although the biosynthesis of flavonoids has attracted increasing attention from scholars, current research does not fully explain the effects of regulatory factors on the transcription and activity of the major enzymes in flavonoid metabolism. Therefore, further research on the signaling molecules and regulatory pathways associated with flavonoids, as well as their regulatory mechanisms, is needed to elucidate the physiological activity of flavonoids.
Rosa hybrida cv. Jardin de Granville (JDG) is a new hybrid rose developed by 'Les Roses Anciennes André Eve' for the Prestige range of Christian Dior skin care products. JDG possesses twice the vitality of a traditional rose and grows and blooms vigorously in the salty air and harsh winds of coastal climates. JDG is also rich in beneficial bioactive substances that are mainly used in cosmetics and anti-aging skin care creams [ 27 , 28 ]. Rosa damascena Mill. (DMS) is one of the most common fragrant roses in the Rosaceae family. Its essential oils and aromatic compounds are used extensively in the cosmetic and food industries worldwide [ 29 ]. DMS is considered an excellent rose throughout the world due to its high resistance to abiotic stress and abundance of beneficial secondary metabolites [ 30 ].
Here, we conducted an integrated analysis on the transcriptomes, proteomes, and metabolomes of JDG and DMS to explore the relationship between plant development and secondary metabolites of rose under salt stress. We used WGCNA and Cytoscape software to decipher the similarities and differences in the complex metabolic pathways and regulatory genes of JDG and DMS under salt stress. These results provide comprehensive information on the metabolic and molecular mechanisms of the response to salt stress in rose, promoting the cultivation of excellent new rose varieties that are both salt tolerant and rich in beneficial secondary metabolites.
JDG is more tolerant than DMS to salt stress
To explore the salt tolerance of rose, plants of JDG and DMS were treated with 400 mM NaCl for 2 weeks. DMS plants showed typical damage with yellowing and death of leaves, while JDG leaves only exhibited slight wilting ( Fig. 1A ). Additionally, detached rose leaves were treated with salt for 4 days; DMS leaves showed significantly more necrosis than JDG leaves ( Fig. 1B ). In order to quickly observe the response of rose cultivars to salt stress and convenience sampling, subsequent experiments mainly used detached rose leaves. To examine the overall anatomy and morphology of leaves treated for 2 days with NaCl, we stained treated and control leaves with toluidine blue and prepared thin sections. Palisade tissue damage in response to salt treatment was more severe in DMS than in JDG (indicated by red arrowheads in Fig. 1C ). To investigate ROS accumulation in response to salt stress, we performed 3, 3'-diaminobenzidine (DAB) staining. DMS leaves accumulated substantially more ROS (deeper staining) than JDG plants after salt stress, whereas there was no difference in ROS content between these two cultivars under normal conditions ( Fig. 1D, E ). Soluble protein content was higher in JDG leaves after 4 days of salt stress than after 2 days of salt stress, while the soluble protein content of DMS leaves was much higher than that of before treatment leaves after 2 days and decreased by 4 days of salt treatment ( Fig. 1F ). The relative electrolyte permeability of JDG leaves was increased slightly after 2 days of salt treatment and more substantially after 4 days of treatment, while relative electrolyte permeability was much higher in DMS than in JDG on both days after salt treatment ( Fig. 1G ). Phenotypic and physiological analyses indicated that JDG is more salt tolerant than DMS.

Phenotypes of JDG and DMS under salt stress. (A) Phenotypes of JDG and DMS plants after 2 weeks of treatment with 400 mM NaCl. Left, phenotype of the whole plant; right, enlarged image of the protruding part indicated by the red circle. Bars, 3 cm. (B) Detached leaves of rose on different days after onset of salt stress (400 mM NaCl). (C) Anatomical analysis of leaves in (B). Red arrowheads represent the palisade tissue. Mock (0 mM NaCl); NaCl (400 mM NaCl). Bars, 50 μm. (D) Tissue staining of rose leaves under salt stress using DAB. (E) Quantitative statistics of the relative staining intensity in (D). Brown staining area and total leaf area were measured using ImageJ software, their ratio is the relative staining intensity. (F) Soluble protein content of rose leaves at different days under salt treatment. (G) Relative electrolyte permeability of rose leaves at different days under salt treatment. Data are based on the mean ± SE of at least three repeated biological experiments.
Flavonoid metabolites play an important role in the salinity tolerance of rose
To better understand how salt stress affects rose metabolites, we performed a comprehensive untargeted analysis of metabolites using ultra-performance liquid chromatography/mass spectrometry (UPLC/MS). Fig. S1A shows the different metabolites detected, and Fig. S1B shows the curves of the quality control samples, indicating that the mass spectral data were highly reproducible and reliable. Principal component analysis (PCA) was used to reduce the data dimensions and clarify the relationships among the samples. The two principal components PC1, and PC2 could explain 50.07% and 23.36% of the variance, respectively. Moreover, PC1 revealed variance in genotypes, while PC2 revealed differences in time of exposure to salt stress. Thus, the metabolite-based PCA revealed obvious differences in salt tolerance between the two cultivars ( Fig. S2A ).
Our screening for differentially accumulated metabolites (DAMs) identified hundreds of metabolites with significantly altered accumulation under salt stress ( Fig. 2A , Table S1 ). Preliminary analysis indicated that DAMs included amino acids and their derivatives, nucleotides and their derivatives, phenolic acids, flavonoids, lipids, tannins, lignans and coumarins, organic acids, alkaloids, and terpenoids, and most of the DAMs were upregulated under salt stress ( Fig. 2B ). Phenolic acids, lipids, and flavonoid metabolites showed significantly altered accumulation under salt stress in both JDG and DMS. Compared with their levels in DMS, flavonoid metabolites, phenolic acid metabolites, and lipids were differentially accumulated in JDG leaves under both control conditions and salt stress ( Table S1 ). These results indicate that flavonoid metabolites, phenolic acid metabolites, and lipids may play important roles in the salt tolerance of rose.

Metabolomic analysis of JDG and DMS under salt stress. (A) Number of DAMs in different comparison groups. (B) Classification of DAMs in each comparison. (C) Classification of DAMs upregulated in both JDG and DMS under salt treatment. (D) Classification of DAMs upregulated in JDG compared with DMS under both control and salt treatments. (E, F) KEGG pathway enrichment of DAMs under salt stress: (E) JDG-NaCl vs JDG-Mock and (F) DMS-NaCl vs DMS-Mock.
To determine how metabolites differ between JDG and DMS, we summarized the differences in metabolite accumulation in the different comparison groups using Venn diagrams. Groups JDG-NaCl vs JDG-Mock and DMS-NaCl vs DMS-Mock shared 109 of the same metabolite changes, of which 79 were increases and 15 were decreases. Among the upregulated metabolites, phenolic acids and flavonoids accounted for 21.52% and 7.59%, respectively. These metabolites included ferulic acid, coniferaldehyde, pinocembrin (dihydrochrysin), naringin, eucalyptin (5-hydroxy-7,4'-dimethoxy-6,8-dimethylflavone), patuletin (quercetagetin-6-methyl ether), naringenin-7- O -rutinoside-4'- O -glucoside, naringin (naringenin-7- O -neohesperidoside), and sudachitin ( Fig. 2C , Fig. S2B–D , Table S1 ). Notably, 5,7,8,4'-tetramethoxyflavone, vanillic acid-4- O -glucoside, and 3',4',5',5,7-pentamethoxyflavone were upregulated in JDG and downregulated in DMS under salt stress, while kaempferol-3- O -arabinoside-7- O -rhamnoside was upregulated in DMS and downregulated in JDG. Groups JDG-Mock vs DMS-Mock and JDG-NaCl vs DMS-NaCl shared 408 metabolites showing the same tendency in alteration, of which accumulation of 188 was increased and 202 was decreased. Among the upregulated metabolites, phenolic acids and flavonoids accounted for 29.26% and 33.51%, respectively ( Fig. 2D ). Notably, the genkwanin (apigenin 7-methyl ether) content was 12.74-fold higher, the 5,7-dihydroxy-6,3′,4′,5′-tetramethoxyflavone (arteanoflavone) content was 15.64-fold higher, the naringenin-4′,7-dimethyl ether content was 13-fold higher, and the naringin dihydrochalcone content was 13.30-fold in JDG compared with DMS under control conditions; all of these are flavonoid metabolites. Venn analysis also showed that many metabolites displaying changes under salt stress were genotype specific, indicating that the cultivars have different mechanisms of response to salinity. There were 77 metabolites that specifically accumulated in JDG under salt stress, which may represent the major metabolites in the salt stress response of JDG. Notably, four metabolites—ethylsalicylate (a phenolic acid), salidroside (a phenolic acid), L-ornithine (amino acids and derivatives), and epiafzelechin (a flavonoid)—accumulated specifically in JDG after salt treatment and were also highly accumulated under control conditions in JDG compared with DMS ( Fig. S2B–D , Table S1 ).
All DAMs were analyzed using Kyoto Encyclopedia of Genes and Genomes (KEGG) pathway enrichment ( Fig. 2E, F , Fig. S3A, B ). In JDG (JDG-NaCl vs JDG-Mock group), salt stress induced changes in metabolites mainly involved 'purine metabolism,' 'phenylpropanoid biosynthesis,' 'linoleic acid metabolism,' and 'alpha-linolenic acid metabolism' ( Fig. 2E ). In DMS (DMS-NaCl vs DMS-Mock group), the DAMs in leaves under salt stress were mainly associated with 'phenylpropanoid biosynthesis,' 'alpha-linolenic acid metabolism,' 'linoleic acid metabolism,' and 'pentose and glucuronate interconversions' ( Fig. 2F ). In the JDG-Mock vs DMS-Mock group, DAMs between leaves of DMS and JDG were mostly associated with 'flavonoid biosynthesis,' 'flavone and flavonol biosynthesis,' and 'phenylpropanoid biosynthesis' ( Fig. S3A ). Meanwhile, in the JDG-NaCl vs DMS-NaCl group, DAMs were largely involved in 'flavonoid biosynthesis,' 'flavone and flavonol biosynthesis,' and 'linoleic acid metabolism' ( Fig. S3B ). KEGG enrichment analysis showed that 'linolenic acid/α-linolenic acid metabolism' and 'phenylpropanoid biosynthesis' were significantly enriched under salt stress in both cultivars, indicating that these two pathways play important roles under salt stress in rose. Regardless of the presence of salt stress, DAMs between DMS and JDG were concentrated in the flavone, flavonoid, and flavonol biosynthetic pathways, indicating that differential accumulation of these metabolites may be the main reason for different salt sensitivities among rose cultivars. Notably, 'caffeine metabolism' was enriched in JDG, while 'starch and sucrose metabolism' was significantly increased in DMS.
Salt stress causes dynamic changes in distinct sets of proteins
To delve deeper into the molecular mechanisms of the salt stress response in rose plants, we performed a proteome profiling analysis under the same salt treatment and control conditions as the metabolome analysis and characterized proteins on the basis of fold changes in their accumulation level. We identified 119 (87 upregulated and 32 downregulated) and 163 (83 downregulated and 80 upregulated) proteins with significantly differential accumulation under salt stress in JDG and DMS, respectively ( Fig. 3A, B ). Only 18 differentially accumulated proteins (DAPs) overlapped between the two cultivars, of which 13 were upregulated and 4 were downregulated in both JDG and DMS, while one DUF1279 domain–containing protein was upregulated in JDG and downregulated in DMS. Moreover, 101 DAPs were unique to JDG, whereas 145 DAPs were unique to DMS ( Table S2 ).

Proteomic analysis of rose under salt stress. (A) Number of DAPs in JDG and DMS. (B) Venn diagram of the DAPs in JDG and DMS. (C) Localizations of DAPs identified in JDG. (D) Functional categorization of DAPs unique to JDG. (E, F) KEGG enrichment analysis of DAPs in JDG (upregulated, E) and DMS (upregulated, F).
We predicted that most of the DAPs are located in chloroplasts in rose, according to the WoLFPSORT database ( Fig. 3C , Fig. S4A ). Gene Ontology (GO) and KEGG analyses were performed to analyze and annotate protein functions. The 20 most highly enriched GO terms associated with the DAPs are depicted in a circle diagram ( Fig. S5A, B , Table S2 ). Among them, GO:0046658 (anchored component of plasma membrane), GO:0051554 (flavonol metabolic process), GO:0047893 (flavonol 3- O -glucosyltransferase activity), and GO:0051555 (flavonol biosynthetic process) were highly enriched in JDG under salt stress. In DMS, GO:0006720 (isoprenoid catabolic process), GO:0005764 (lysosome), and GO:0004602 (glutathione peroxidase activity) were the most enriched among all GO terms. In addition, the GO data indicated that the DAPs specific to JDG were highly involved in the 'icosanoid metabolic process,' 'diterpenoid metabolic process,' and 'diterpenoid biosynthetic process' ( Fig. 3D ), whereas the DAPs specific to DMS were enriched in 'cellular hyperosmotic salinity response,' 'monocarboxylic acid catabolic process,' 'terpenoid catabolic process,' 'sesquiterpenoid catabolic process,' and 'apocarotenoid catabolic process' functions ( Fig. S4B ). DAPs shared by JDG and DMS included Q2VA35 (xyloglucan endotransglucosylase/hydrolase) and A0A2P6P708 (glutathione peroxidase), which are present only in extracellular regions ( Table S2 ). The DAPs in different comparison groups were classified and then clustered according to enrichment of their associated GO terms ( Fig. S4C ). We determined that salinity mainly influences flavone and flavonol metabolism pathways in JDG. Flavones and flavonols are antioxidants and bioactive reagents [ 24 ]. In DMS, salt mainly influences the osmotic response, water stimulus response, and salt stress response pathways, most of which are stress related [ 31 ]. We used KEGG enrichment to determine the metabolic pathways associated with the DAPs in JDG and DMS under salt stress ( Fig. 3E, F ). Many DAPs in JDG were associated with phenylpropanoid biosynthesis and alpha-linolenic acid metabolism, with examples including lipoxygenase (A0A2P6S713), 12-oxophytodienoate reductase (A0A2P6PFD8), peroxidase (A0A2P6R8H8), and flavone 3′- O -methyltransferase (A0A2P6RK21). The DAPs upregulated in DMS under salt stress were frequently associated with alpha-linolenic acid metabolism and glutathione metabolism, whereas the DAPs that were downregulated were associated with ribosomes ( Table S2 ). Notably, alpha-linolenic acid metabolism was significantly upregulated in both JDG and DMS under salt stress. Collectively, the GO and KEGG enrichment results show that salt stress causes dynamic changes in distinct sets of proteins in rose.
Salt stress differentially alters the transcriptomes of JDG and DMS
To identify the genes involved in salt stress and explore the molecular mechanisms of salt tolerance in DMS and JDG, we sequenced the transcriptomes of JDG and DMS leaves by RNA sequencing (RNA-seq). We obtained high-quality reads for transcriptome analysis ( Table S3 ). PCA showed a distinct difference between the two cultivars along PC1, and PC2 separated the treatment from the control. The three biological replicates in the ordination space were mostly clustered together, suggesting an acceptable correlation between replicates ( Fig. 4A ).

Transcriptomic analysis of JDG and DMS under salt stress. (A) PCA score plot of transcriptomic profiles from different cultivars. (B) Number of DEGs in JDG and DMS. (C–E) Venn diagrams of DEGs in JDG and DMS: (C) total DEGs, (D) upregulated DEGs, and (E) downregulated DEGs. (F, G) KEGG enrichment analysis of DEGs in JDG (F) and DMS (G).

Correlation analysis of transcriptome, proteome, and metabolomics data. (A, B) KEGG enrichment analysis of combined transcriptome, proteome, and metabolome data: (A) JDG-NaCl vs JDG-Mock, and (B) DMS-NaCl vs DMS-Mock. The x-axis shows the enrichment factor of the pathway in different omics, and the y-axis shows the name of the KEGG pathway; the color from red to green represents the significance of enrichment from high to low (indicated by the P value). The size of bubbles indicates the number of DEGs, DAPs, or DAMs; the larger the number, the larger the symbol. The shape of bubbles illustrates the various omics: circles represent genes omics, triangles represent metabolites omics, and squares represent proteins omics. (C) Co-expression network of major genes, proteins, and metabolites in the phenylpropanoid pathway. Different colors indicate the value of log 2 Fold Change (NaCl/Mock), with red for upregulated and blue for downregulated genes, proteins, or metabolites.
We analyzed differentially expressed genes (DEGs) in JDG and DMS under control and salt stress conditions. We detected 10,662 DEGs in DMS under salt stress, of which 4651 were upregulated and 6011 were downregulated. However, only 1990 genes were differentially expressed in JDG: 1102 upregulated and 888 downregulated ( Fig. 4B ). The smaller number of DEGs in JDG than in DMS under salt stress implies that JDG is less affected by salt stress. We used a Venn diagram to display the differences between various genes in DMS and JDG under salt stress. Group DMS-NaCl vs DMS-Mock and group JDG-NaCl vs JDG-Mock shared 1120 DEGs under salt stress, with 577 upregulated genes and 433 downregulated genes ( Fig. 4C–E ).
Next, we performed GO analysis of DEGs in the categories cellular component (CC), biological process (BP), and molecular function (MF). The top 21 most enriched GO terms associated with DEGs of JDG-NaCl vs JDG-Mock and DMS-NaCl vs DMS-Mock are presented in circle diagrams ( Fig. S6 , Table S4 ). Seven GO terms associated with the JDG-NaCl vs JDG-Mock group were highly involved in the BP category, among which GO:0016052 (carbohydrate catabolic process), GO:0009813 (flavonoid biosynthetic process), and GO:0009812 (flavonoid metabolic process) contained the most DEGs (43, 26, and 27, respectively), and most of these enriched genes were upregulated. Thirteen GO terms were highly involved in the MF category, among which GO:0010427 (abscisic acid binding), GO:0016832 (aldehyde-lyase activity), and GO:0019840 (isoprenoid binding) were highly significant. One GO term was highly involved in the CC category: GO:0031226 (intrinsic component of plasma membrane). Moreover, 19 GO terms associated with the DMS-NaCl vs DMS-Mock group were enriched in the BP category, among which GO:0036294 (cellular response to decreased oxygen levels), GO:0048511 (rhythmic process), and GO:0048585 (negative regulation of response to stimulus) contained the most DEGs (85, 95, and 146, respectively), and most of these enriched genes were downregulated. One GO term was enriched in the MF category: GO:0016854 (racemase and epimerase activity). Similarly, one GO term was enriched in the CC category: GO:0009501 (amyloplast). KEGG pathway enrichment analysis for JDG-NaCl vs JDG-Mock revealed that the DEGs were mainly involved in metabolic pathways, plant hormone signal transduction, biosynthesis of secondary metabolites, and glycolysis/gluconeogenesis ( Fig. 4F , Table S4 ). In the DMS-NaCl vs DMS-Mock group, the DEGs were chiefly enriched in metabolic pathways, plant hormone signal transduction, the MAPK signaling pathway, biosynthesis of cofactors, and ubiquitin-mediated proteolysis ( Fig. 4G , Table S4 ). These findings indicate that the biosynthesis of secondary metabolites is substantially enhanced under salt stress in JDG, but not in DMS. However, the biosynthesis of cofactors associated with primary metabolism is enhanced under salt stress in DMS. Therefore, we speculate that salinity results in large changes in primary metabolism in DMS, while it influences secondary metabolism in JDG.
Transcription factors (TFs) are essential for regulating the expression of stress response genes. Among the DEGs, we identified 114 TFs in JDG and 491 TFs in DMS, covering 39 TF families ( Table S4 ). The most abundant genes belonged to the AP2/ERF-ERF, MYB, NAC, bHLH, and C2C2 families ( Fig. S7A, B ). Moreover, 64 TFs were differentially expressed in both cultivars in response to salinity. We speculate that these TFs form a highly complex transcriptional regulatory network and could perform critical functions in the mechanism of salt tolerance in rose.
Expression of phenylpropanoid-related genes is correlated with proteins and metabolites affected by salt stress
Integrated analysis of multi-omics data provides a powerful tool for identifying significantly different pathways and crucial metabolites in biological processes. Here, we integrated our transcriptome, proteome, and metabolome data to determine the performance of the two rose cultivars under salt stress. Pathways associated with alpha-linolenic acid metabolism, phenylpropanoid biosynthesis, and starch and sucrose metabolism were significantly enriched in JDG under salt stress ( Fig. 5A ), while the pathways enriched in DMS were involved in starch and sucrose metabolism, cyanoamino acid metabolism, and phenylpropanoid biosynthesis ( Fig. 5B ). Starch and sucrose metabolism represent primary metabolic functions common to different cultivars [ 32 ], while alpha-linolenic acid metabolism is related to the biosynthesis of jasmonic acid, which is a phytohormone involved in fungal invasion and senescence [ 7 ]. The phenylpropanoid biosynthesis pathway comprises multiple secondary metabolites, which confer a range of colors, flavors, nutritional components, and bioactivities in plants. Flavonoids are an important type of phenylpropanoid that play key roles in resistance against biotic and abiotic stresses [ 24 ]. Thus, we focused on the phenylpropanoid pathway.
Gene–protein–metabolite correlation networks can be used to elucidate functional relationships and identify regulatory factors. Therefore, we analyzed the regulatory networks of the DEGs, DAPs, and DAMs related to phenylpropanoid metabolism. We identified 14 DEGs that were strongly correlated with one DAP and six DAMs in JDG under salt stress. Similarly, 25 DEGs were strongly correlated with one DAP and eight DAMs in DMS under salt stress ( Table S5 ). For example, in JDG, there was a strong correlation between the expression of one gene (RchiOBHmChr4g0430951) and the abundance of one protein (A0A2P6PM56) and two metabolites [coniferyl alcohol (mws0093) and sinapyl alcohol (mws0853)]. Epiafzelechin (mws1422) was also significantly associated with the expression of the gene RchiOBHmChr2g0092641. In DMS, there was a close association between the expression of three genes (RchiOBHmChr2g0092671, RchiOBHmChr3g0480401, and RchiOBHmChr5g0041231) and the abundance of one protein (A0A2P6QM41) and one metabolite [L-tyrosine (mws0250)]. The strong association of particular genes with phenylpropanoid proteins or metabolites suggests that these genes play a major role in phenylpropanoid biosynthesis under salt stress.
We selected 20 important genes in the biosynthetic pathway of phenylpropanoid and compared their expression between rose cultivars ( Table S6 ). The transcript levels of many genes ( 4CL1 , CCR1 , HCT1 , HCT2 , HCT3 , HCT4 , CHS1 , CHS2 , CHI , DFR , F3H , and ANR ) were higher in JDG than in DMS, which may be valuable for salt tolerance by stimulating JDG to produce more flavonoids. Our multi-omics analysis revealed that ferulic acid, sinapic acid, and coniferaldehyde accumulated to high levels in JDG under salt stress ( Fig. 5C , Table S1 ). We also compared the flavonoid compounds in the two cultivars. Quercetin-3,3′-dimethyl ether, 5,7-dihydroxy-6,3′,4′,5′-tetramethoxyflavone (arteanoflavone), naringenin-4′,7-dimethyl ether, naringin dihydrochalcone, genkwanin (apigenin 7-methyl ether), and mearnsetin accumulated to greater levels in JDG than in DMS under control conditions. Correspondingly, the flavonoids brickellin, 3- O -methylquercetin, 5,2′,5′-trihydroxy-3,7,4′-trimethoxyflavone-2′- O -glucoside, and kaempferol-3- O -(6′′-acetyl)glucosyl-(1→3)-galactoside were more abundant in JDG than in DMS under salt stress. By contrast, naringenin-4′,7-dimethyl ether, aromadendrin (dihydrokaempferol), pinocembrin-7- O -(6′′- O -malonyl)glucoside, Quercetin-3- O -(2”- O -glucosyl)glucuronide, were specifically accumulated in DMS. Moreover, 3′,4′,5′,5,7-pentamethoxyflavone, 3,5,7,3′4′-pentamethoxyflavone, and 5,7,8,4′-tetramethoxyflavone were abundant in JDG under salt stress but were decreased in DMS ( Table S7 ). Overall, the integration of the three omics datasets indicated that the phenylpropane pathway, especially the flavonoid pathway, is strongly enhanced under salinity conditions and that this contributes to salt tolerance in roses, especially in the JDG genotype.
Networks of co-expressed genes associated with phenylpropanoid biosynthesis are involved in the salt stress response
To identify candidate genes associated with phenylpropanoid biosynthesis, we constructed co-expression gene network modules via weighted gene correlation network analysis (WGCNA). We constructed a cluster tree based on correlation between expression levels (indicated by fragments per kilobase of script per million fragments mapped, FPKM), which partitioned the genes into 11 different gene modules ( Fig. 6A, B ). To identify candidate genes that play significant roles within the gene networks, we extracted annotation information for all these genes from the Rosa chinensis 'Old Blush' reference genome annotation database. We selected 16 genes contributing to phenylpropanoid biosynthesis and four genes associated with flavonoid biosynthesis. Table S8 lists the annotated genes participating in flavonoid-related pathways in JDG. Among the 11 modules, the green module contained 10 of these genes: CHS1 , CHS2 , CCR1 , HCT3 , HCT4 , CCoAOMT , F3H , DFR , ANR , and CHI . The turquoise module contained three genes: CCR2 , HCT1 , and CAD2 . The blue module contained three genes: PRDX1 , 4CL1 , and ANS . The red, yellow, brown, and black modules each contained one gene: CAD1 , PRDX2 , HCT2 , and 4CL2 , respectively ( Table S8 ). After combining certain genes in modules and comparing them with the DEGs, we checked and confirmed these results using reverse-transcription quantitative PCR (RT-qPCR). The expression trends of eight DEGs from phenylpropanoid and flavonoid biosynthesis pathways matched the results of RNA-seq ( Fig. S8 ).

Co-expression network related to flavonoid biosynthesis. (A) Clustering tree based on the correlation between gene expression levels. (B) Module–sample relationships. Each row represents a gene module, with the same color in as (A); each column represents a sample; the boxes within the chart contain corresponding correlations and P values. (C–E) Networks built from correlations among structural genes and TFs. Circles represent genes, and the size of the circle represents the number of relationships between genes in the network and surrounding genes. Lines represent regulatory relationships between genes, and different colored lines represent different connection strengths: red, strong connections; green, weak connections. (F) Heat map depicting the expression profiles of 15 TF genes. The scale bar denotes the Fold change/(mean expression levels across the three treatment groups). The color indicates relative levels of gene expression, horizontal rows represent the different treatments in JDG, and vertical columns show the TFs. (G) Representative images of transient expression of bHLH74 and LUC driven by the CHS1 promoter in Nicotiana benthamiana leaves. The color scale represents the signal level. High represents a strong signal, and low represents a weak signal. (H) Relative value of LUC/REN. Data are based on the mean ± SE of at least three repeated biological experiments. Significance determined using Student’s t -test ( ** P < 0.01).
To determine the regulatory genes involved in phenylpropanoid biosynthesis in JDG, we constructed three subnetworks from the different modules using the 20 phenylpropanoid biosynthesis–related DEGs as the nodes ( Table S9 ). In the regulatory networks of phenylpropanoid biosynthesis, we identified 15 TF genes from seven TF families: AP2/ERF-ERF (5 unigenes), bHLH (3 unigenes), MYB (3 unigenes), Alfin-like (1 unigene), SBP (1 unigene), C2C2-GATA (1 unigene), and TCP (1 unigene). bHLH62 and bHLH74 were strongly associated with CHS1 , CHS2 , CHI , CCR1 , and F3H ; ERF81 was strongly associated with 4CL1 ; and ERF110 and MYB-related were strongly associated with 4CL2 ( Fig. 6C–E ), indicating that CHS and 4CL are the major target genes in phenylpropanoid biosynthesis. Therefore, we speculated that the abundance of flavonoids is increased by enhancing the expression of upstream flavonoid biosynthesis genes. Fig. 6F shows a heat map of expression of the 15 TF genes after NaCl treatment. The green module contained a substantial number of phenylpropanoid biosynthesis genes, among which CHS1 was closely related to the TFs bHLH74 and bHLH62. Therefore, dual-luciferase reporter assays were conducted to determine their regulatory relationship ( Fig. 6G, H ). We used bHLH74 and bHLH62 driven by the CaMV35S promoter as effectors in a transient expression system, with the CHS1 promoter fused with LUC as a reporter. When we cotransformed Nicotiana benthamiana leaves with the effectors and the reporter, the LUC/REN ratio of CHS1 was 0.3/1, which was drastically lower than those of the controls ( Fig. 6G, H , Fig. S9A, B ). These results indicate that bHLH74, but not bHLH62, inhibits the expression of CHS1 .
Salt stress damages the structure and osmotic potential of rose leaves
Roses belong to the Rosaceae family and are one of the most important commercial flower crops. Extracts from various parts of the rose plant have also been shown to have excellent biological activity and are used in industries such as cosmetics, perfume and medicine [ 1 ]. Meanwhile, an increasing number of wild rose varieties with significant health benefits are being domesticated and brought into mainstream cultivation [ 33 ]. Salt stress is one of the most widespread abiotic constraints for rose cultivation. Salt stress threatens plant survival and growth but can stimulate an increase in the biosynthesis of secondary metabolites [ 34 ]. Previous studies have shown that optimal coordination between leaf structure and photosynthetic processes is essential for enabling plants to tolerate salt stress [ 35 ]. When exposed to salt treatment, leaves become thicker and smaller while the palisade tissue and spongy tissue become loose and jumbled and the intercellular space of the mesophyll becomes thinner [ 36–39 ]. We observed that the palisade tissue of DMS was loose, disordered, and severely damaged compared with that in JDG under salt stress ( Fig. 1C ). This indicates that DMS is more sensitive to salt stress than JDG. Typically, excessive ROS accumulate under stress conditions, which can lead to membrane oxidative damage (lipid peroxidation) [ 40 ]. Silencing of the gene GmNAC06 in soybean ( Glycine max ) leads to accumulation of ROS under salt stress, which in turn leads to significant losses in soybean production [ 41 ]. In Arabidopsis , the sibp1 mutant accumulates more ROS than wild-type plants or AtSIBP1-overexpressing plants, resulting in a lower survival rate under salt treatment [ 42 ]. In this study, salinity led to a greater accumulation of ROS in DMS compared with JDG, as detected by DAB staining ( Fig. 1D, E ). This indicates that DMS suffers greater damage under salinity stress. Excessive accumulation of ROS in cells can lead to membrane oxidative damage and trigger the production of enzyme systems or non-enzyme free radical scavengers to cope with oxidative damage [ 10 ]. Here, antioxidant enzyme activities such as peroxidase (A0A2P6R8H8) and glutathione peroxidase (A0A2P6P708) were upregulated in roses under salt treatment ( Table S2 ). This suggests that rose plants maintain lower ROS levels by upregulating the activity of antioxidant enzymes, thereby protecting photosynthetic mechanisms and maintaining plant growth under salt stress. Among the nonenzymatic antioxidants, phenols and flavonoids accumulate in various tissues and contribute to free radical scavenging that enhances plant salt tolerance [ 43 ]. Indeed, we identified significant differences in the contents of phenolic acids, lipids, and flavonoid metabolites in JDG and DMS under control and salt stress conditions ( Table S1 ). Moreover, our transcriptomic and proteomic analysis revealed the activation of genes and proteins within the phenylpropanoid and flavonol pathways. This activation results in the accumulation of various phenolic compounds, potentially enhancing their capacity for scavenging ROS.
Flavonoids are beneficial for improving salt stress in rose
Phenolic compounds, such as flavonoids, are among the most widespread secondary metabolites observed throughout the plant kingdom [ 44 ]. These compounds fulfill various biochemical and molecular functions within plants, encompassing roles in plant defense, signal transduction, antioxidant action, and the scavenging of free radicals [ 45 ]. Environmental changes commonly trigger the flavonoid pathway, which aids in shielding plants from the harmful effects of ultraviolet radiation, salt, heat, and drought [ 23 , 46 , 47 ]. Moreover, flavonoids demonstrate potent biological activity and serve as significant antioxidants [ 48 ]. Recently, researchers and consumers have been interested in plant-based polyphenols and flavonoids for their antioxidant potential, their dietary accessibility, and their role in preventing fatal diseases such as cardiovascular disease and cancer [ 49 ]. Our transcriptomics analysis showed that salinity causes significant alterations in the secondary metabolism of JDG, while affecting the primary metabolism of DMS. Proteomics showed that phenylpropanoid biosynthesis is significantly enhanced in JDG under salt stress, especially through the flavonoid pathway. In DMS, glutathione metabolism is significantly enhanced under salt stress, indicating differences in salt tolerance pathways between the two cultivars. Our metabolome data indicated that the abundance of phenolic acid and flavonoid metabolites was significantly altered in both JDG and DMS under salt stress. Furthermore, by comparing their contents in leaves under salt stress and control conditions, we found that more flavonoids accumulated in DMS than in JDG under salt stress. This evidence suggests that DMS requires an increased presence of flavones to withstand the damage caused by salinity. By contrast, salinity stress did not trigger a substantial buildup of flavonoids in JDG, possibly due to the adequate levels of flavonoids already present under normal conditions, which provided ample tolerance to salt-induced stress. This observation could also explain the higher tolerance of JDG to salt stress ( Table S1 ). When we compared the flavonoid metabolites of the phenylpropanoid pathway to identify flavonoid metabolites associated with salt tolerance, we found that 17 phenolic acid metabolites and 6 flavonoid metabolites were significantly differentially accumulated in both genotypes. Of these compounds, ferulic acid serves as a free radical scavenger, while simultaneously serving as an inhibitor for enzymes engaged in generating free radicals and boosting the activity of scavenger enzymes [ 49 ]. Sinapic acid is a bioactive phenolic acid with anti-inflammatory and anti-anxiety effects [ 50 ]. Pinocembrin, a naturally occurring flavonoid found in fruits, vegetables, nuts, seeds, flowers, and tea, is an anti-inflammatory, antimicrobial, and antioxidant agent [ 51 ]. This indicates that these two rose cultivars contain beneficial metabolites with some economic value. We investigated the possible effects of these metabolites in conferring salt tolerance in rose by comparing specific DAMs between JDG and DMS. Among these DAMs, eight metabolites were upregulated and six metabolites were downregulated under salt treatment in JDG compared to DMS. Among these eight upregulated DAMs, the contents of 3- O -methylquercetin, brickellin, 5,2′,5′-trihydroxy-3,7,4′-trimethoxyflavone-2′- O -glucoside, and kaempferol-3- O -(6′′-acetyl)glucosyl-(1→3)-galactoside accumulated significantly with salinity ( Table S7 ). These metabolites have important functions. For example, 3- O -methylquercetin has potent anticancer, antioxidant, antiallergy, and antimicrobial activities and shows strong antiviral activity against tomato ringspot virus [ 52 ]. Kaempferol, a biologically active compound found in numerous fruits, vegetables, and herbs, demonstrates various pharmacological benefits, such as antimicrobial, antioxidant, and anticancer properties [ 53 ]. This indicates that JDG is an excellent rose cultivar that is both salt tolerant and rich in beneficial bioactive substances.
bHLHL74 regulates flavonoid biosynthesis
The biosynthesis of flavonoids is initiated from the amino acid phenylalanine, giving rise to phenylpropanoids that subsequently enter the flavonoid-anthocyanin pathway [ 25 ]. The CHS enzyme is situated at a crucial regulatory position preceding the flavonoid biosynthetic pathway, directing the flow of the phenylpropanoid pathway towards flavonoid production, which has been extensively documented in many plant species [ 54 , 55 ]. In rice ( Oryza sativa ), defects in the flavonoid biosynthesis gene CHS can alter the distribution of flavonoids and lignin [ 56 ]. In eggplant ( Solanum melongena L.), CHS regulates the content of anthocyanins in eggplant skin under heat stress [ 57 ]. In apple ( Malus domestica ), overexpression of CHS increases the accumulation of flavonoids and enhances nitrogen absorption [ 58 ]. We identified a positive correlation between flavonoid accumulation and the expression of CHS genes, in agreement with previous reports. The bHLH TFs involved in regulating flavonoid biosynthesis work in a MYB-dependent or -independent manner. For example, DvIVS, a bHLH transcription factor in dahlia ( Dahlia variabilis ), activates flavonoid biosynthesis by regulating the expression of Chalcone synthase 1 ( CHS1 ) [ 59 ]. The Arabidopsis bHLH proteins TRANSPARENT TESTA 8 (AtTT8) and ENHANCER OF GLABRA 3 (AtEGL3) are all involved in the biosynthesis of various flavonoids [ 60–62 ]. In Chrysanthemum ( Chrysanthemum morifolium ), CmbHLH2 significantly activates CmDFR transcription, leading to anthocyanin accumulation, especially when in coordination with CmMYB6 [ 63 ]. In blueberry ( Vaccinium sect. Cyanococcus ), the bHLH25 and bHLH74 TFs potentially engage with MYB or directly hinder the expression of genes responsible for flavonoid biosynthesis, thereby regulating flavonoid accumulation [ 64 ]. In apple ( Malus domestica ), expression of bHLH62, bHLH74, and bHLH162 is significantly negatively correlated with anthocyanin content and has been shown to inhibit anthocyanin biosynthesis [ 65 ]. In apple fruit skin, hypermethylation of bHLH74 in the mCG context leads to transcriptional inhibition of downstream anthocyanin biosynthesis genes [ 66 ]. In rose, our co-expression network revealed a strong correlation between CHS and genes encoding TFs such as bHLH74 and bHLH62 in the key gene network. bHLH proteins can bind to the promoter regions of pivotal genes encoding enzymes, playing important roles in regulating DAMs under salt stress. Dual-luciferase reporter assays showed that LUC bioluminescence was suppressed well below background levels in Nicotiana benthamiana leaves infiltrated with pCHS1:LUC plus 35S:bHLH74, but not 35S:bHLH62 ( Fig. 6G, H , Fig. S9A, B ). Thus, we conclude that bHLHL74 TFs negatively regulate flavonoid biosynthesis by directly inhibiting the expression of CHS1 , which is involved in the flavonoid biosynthetic pathway.
We examined the morphological phenotypes, transcriptomes, proteomes, and widely targeted metabolomes of JDG and DMS under salt stress. Multi-omics analysis revealed that the phenylpropane pathway, especially the flavonoid pathway, contributes strongly to salt tolerance in rose, particularly JDG. Meanwhile, the bHLHL74 TF negatively regulates flavonoid biosynthesis by repressing the expression of the CHS1 gene involved in the flavonoid biosynthetic pathway. This research facilitates our understanding of the regulatory mechanisms of plant development and secondary metabolites underlying salt stress responses in rose, offering valuable insights that could be used to develop new strategies for improving plant tolerance to salinity.
Plant materials and growth conditions
Rosa hybrida cv. Jardin de Granville (JDG) and Rosa damascena Mill. (DMS) were planted in the Science and Technology Park of China Agricultural University (40°03′N, 116°29′E). Rose plants were propagated by cutting culture. Rose shoots with at least two nodes and approximately 6 cm in length were used as cuttings and inserted into square flowerpots (diameter 8 cm) containing a mixture of vermiculite and peat soil [1:1 (v/v)]. Cuttings were soaked in 0.15% (v/v) indole-3-butytric acid (IBA) before insertion into pots and then grown in a growth chamber at 25°C with 50% relative humidity and a cycle of 8 hours of darkness/16 hours of light for 1 month until rooting [ 67 ].
Nicotiana benthamiana plants were used for measurement of transient expression. Seeds were sown in square flowerpots (diameter 8 cm); after 1 week, seedlings were transplanted into different pots. The soil and cultivation conditions for N. benthamiana cultivation were the same as those for roses.
Salt treatment
Twenty JDG and 20 DMS rose cuttings displaying good rooting and uniform appearance were selected for salt treatment experiments. JDG or DMS plants were randomly divided into two groups watered with either 0 or 400 mM NaCl. Phenotypes were recorded after 2 weeks. This process was repeated three times [ 68 ].
Salt treatment of rose leaves was described previously [ 68 ]. Thirty JDG and 30 DMS rose cuttings with good rooting and uniform appearance were selected, and mature leaves of similar size were collected. The leaves were divided into two treatment groups, each containing 30 leaves: group A, immersed in deionized water treatment, and group B, immersed in 400 mM NaCl treatment. Phenotypes were observed after 0, 2, and 4 days. On the second day of treatment, leaves showed obvious differences. By the fourth day of treatment, the leaves had become soft or had died. Therefore, sequencing data from the second day were used. Three independent biological replicates were assayed.
Relative electrolyte permeability
Determination of relative electrolyte permeability was as previously reported [ 69 ] with the following modifications. Salt-treated leaves (0.1 g) were weighed, placed in a 50-ml centrifuge tube, and covered with 20 ml deionized water. The conductivity of the distilled water was measured and defined as EC0. After shaking for 20 minutes at 60 rpm on an orbital shaker, the conductivity at room temperature was measured and defined as EC1. The centrifuge tube was then placed in boiling water for 10 minutes and cooled to room temperature, and the conductivity of the solution was measured as EC2. The relative permeability of the electrolytes (as a percentage) was determined as (EC1-EC0) / (EC2-EC0) × 100%.
Soluble protein content
Soluble protein content was determined following the method of Bradford (1976) [ 70 ]. Leaf samples (0.5 g) were placed in a mortar with 8 ml distilled water and a small amount of quartz sand, crushed thoroughly, and incubated at room temperature for 0.5 hours. After centrifugation at 3,000 g for 20 minutes at 4 °C, the supernatant was transferred to a 10-ml volumetric flask and the volume was adjusted to 10 ml with distilled water. Two 1.0-ml aliquots of this sample extraction solution (or distilled water as a control) were transferred to clean test tubes, 5 ml of Coomassie Brilliant Blue reagent was added, and the tubes were shaken well. After 2 minutes, when the reaction was complete, the absorbance and chromaticity at 595 nm were measured, and the protein content was determined using a standard curve.
Leaf anatomical structure
Paraffin sections were prepared as described previously with some modifications [ 71 ]. Leaves from the control and NaCl treatments were collected, washed slowly with deionized water at normal room temperature, and stored at 4°C until further use. A 3-mm × 5-mm sample was cut from the same part of each leaf, and these leaf samples were fixed in 2.5% (v/v) glutaraldehyde. Samples were dehydrated using acetone through a concentration gradient of 30%, 50%, 70%, 80%, 95%, and 100% (v/v) and then embedded in paraffin. The embedded tissues (3-μm sections) were sectioned using a Leica RM2265 rotary slicer (Leica Microsystems, Wetzlar, Germany). Slides were stained with 0.02% (v/v) toluidine blue for 5 minutes, and the residual toluidine blue was removed using distilled water. Slides were allowed to dry and then observed under a microscope (OLYMPUS BH-2, Tokyo, Japan). Three independent biological replicates were examined.
DAB (3,3′-diaminobenzidine) staining for H 2 O 2
H 2 O 2 content was detected using the DAB staining method [ 72 ]. Leaves treated with NaCl or control leaves were rinsed clean with distilled water, immersed in DAB solution (1 mg/ml, pH 3.8), and placed under vacuum at approximately 0.8 Mpa for 5 minutes; this process was repeated three to six times until the leaves were completely infiltrated. Leaves were then incubated in a box in the dark for 8 hours until a brown sediment was observed. Chlorophyll was removed by repeatedly washing with eluent (ethanol:lactic acid:glycerol, 3:1:1, v/v/v). Decolorized leaves were photographed to record their phenotypes. ImageJ was used to quantify the stained areas.
UPLC-QQQ-based widely targeted metabolome analysis
Metabolomics analysis was performed on four groups of samples: JDG-Mock, JDG-NaCl, DMS-Mock, and DMS-NaCl. Extraction and determination of metabolites were performed with the assistance of Wuhan Metware Biotechnology Co., Ltd. Samples were crushed using a stirrer containing zirconia beads (MM 400, Retsch). Freeze-dried samples (0.1 g) were incubated overnight with 1.2 ml 70% (v/v) methanol solution at 4 °C, then centrifuged at 13,400 g for 10 minutes. The extracts were filtered and subjected to LC-MS/MS analysis [ 73 ]. A previously described procedure [ 74 ] was followed for analyzing the conditions and quantifying metabolites using an LC-ESI-Q TRAP-MS/MS in multi-reaction monitoring (MRM) mode. The prcomp function was used for PCA, significantly different metabolites were determined by |log 2 Fold Change| ≥ 1, and annotated metabolites were mapped to the KEGG pathway database ( http://www.kegg.jp/kegg/pathway.html ). Comparisons are described as follows: e.g., JDG-NaCl vs JDG-Mock, indicating that the treated sample is being compared with the untreated sample and that metabolites are upregulated or downregulated in the NaCl sample compared with the Mock sample.
Tandem mass tag-based proteomic analysis
Experiments were carried out with the assistance of Hangzhou Jingjie Biotechnology Co., Ltd. Samples were thoroughly ground into powder using liquid nitrogen, and protein extraction was performed using the phenol extraction method. The protein was added to trypsin for enzymolysis overnight, and then the peptide segments were labeled with TMT tags. LC-MS/MS analysis was performed using an EASY-nLC 1200 UPLC system (ThermoFisher Scientific) and a Q Active TM HF-X (ThermoFisher Scientific) [ 75 ]. An absolute value of 1.3 was used as the threshold for significant changes. GO ( http://www.ebi.ac.uk/GOA/ ) and KEGG categories were used to annotate DAPs; WoLFPSORT software was used to predict subcellular localization ( https://wolfpsort.hgc.jp/ ).
Transcriptome sequencing
We constructed 12 cDNA libraries (three biological replicates for each of JDG and DMS under each treatment) for RNA-seq. Transcriptome sequencing was completed at Wuhan Metware Biotechnology Co., Ltd. RNA purity and RNA integrity were determined using a nanophotometer spectrophotometer and an Agilent 2100 bioanalyzer, respectively. The RNA library was then sequenced on the Illumina Hiseq platform. Raw data were filtered using fastp v 0.19.3 and compared with the reference genome ( https://lipm-browsers.toulouse.inra.fr/pub/RchiOBHm-V2/ ). FPKM (fragments per kilobase of script per million fragments mapped) was used as an indicator to measure gene expression levels, with the threshold for significant differential expression being an absolute |log 2 Fold Change| ≥ 1 and False Discovery Rate < 0.05. GO and KEGG categories were used to annotate DEGs [ 76 ].
To identify modules with high gene correlation, co-expression network analysis was performed using the R-based WGCNA package (v.1.69) with default parameters [ 77 ]. The varFilter function of the R language genefilter package was used to remove genes with low or stable expression levels in all samples. Modules based on the correlation between gene expression levels were identified, and a correlation matrix between each module and the sample was calculated using the R-based WGCNA software package. The module network was visualized using Cytoscape software (v.3.7.2).
RT-qPCR was performed on eight DEGs in the phenylpropanoid pathway to verify the accuracy of the data obtained from high-throughput sequencing. Total RNA was extracted using the hot borate method [ 72 ] and reverse transcribed using HiScript III All-in-one RT SuperMix (R333-01, Vazyme Biotech Co., Ltd., Nanjing, China). Subsequently, 2 × ChamQ SYBR qPCR Master Mix (Q331, Vazyme Biotech Co., Ltd., Nanjing, China) was used for quantitative detection of gene expression. The relative expression of genes was calculated using the 2 −ΔΔCt method [ 76 ]. GAPDH was used as an endogenous control, and primers for RT-qPCR are listed in Table S10 .
Dual-LUC reporter assay
A transactivation assay was designed to evaluate the effect of BHLH74/BHLH62 on the CHS1 promoter using methods described previously [ 78 ]. Initially, a 2000-bp segment of the CHS1 promoter was cloned into the pGreenII 0800-LUC vector, generating the ProCHS1:LUC reporter plasmid. Concurrently, the coding sequences of BHLH74/BHLH62 were inserted into the pGreenII0029 62-SK vector, resulting in the construction of Pro35S: BHLH74/BHLH62 effector plasmids. pGreenII 0800-LUC vector containing REN under control of the 35S promoter was used as a positive control.
Following plasmid construction, these constructs were introduced into Agrobacterium tumefaciens strain GV3101, which harbored the pSoup plasmid. Subsequently, A. tumefaciens containing different combinations of effector and reporter plasmids was infiltrated into N. benthamiana plants with six to eight young leaves. After a 3-day incubation period, the ratios of LUC to REN were quantified using the Bio-Lite Luciferase Assay System (DD1201, Vazyme Biotech Co., Ltd., Nanjing, China). Images capturing LUC signals were acquired using a CCD camera (Night Shade LB 985, Germany). Primer sequences are listed in Table S10 .
Statistical analysis
Statistical analyses of data were conducted using IBM SPSS Statistics, while graphical representations were created using GraphPad Prism 8.0.1. Paired data comparisons were assessed through Student's t -tests ( * P < 0.05, ** P < 0.01, *** P < 0.001). Each experiment was performed using a minimum of three biological replicates, and error bars depicted on graphs denote the standard error (SE) of the mean value. The NetWare Cloud platform ( https://cloud.metware.cn ) and OmicShare tools ( https://www.chiplot.online/ ) were used for bioinformatics analyses and mapping.
This work was supported by the Consult of Flower Industry of Jinning District (202204BI090022), General Project of Shenzhen Science and Technology and Innovation Commission (Grant No. 6020330006K0).
ZX, MN conceived and designed the experiments. RH and YW conducted the experiments. RH, YW, ZX analyzed the data. LY, JW, QX, CP, XT, GJ and MN performed the research. RH, SM and ZX wrote the manuscript. All authors read and approved the manuscript. RH and YW contributed equally to this work.
The datasets generated and analyzed during the current study are available in the Biological Research Project Data (BioProject), National Center for Biotechnology Information (NCBI) repository, accession: PRJNA1030783.
The authors declare that they have no competing interests.
Mileva M , Ilieva Y , Jovtchev G . et al. Rose flowers—a delicate perfume or a natural healer? Biomol Ther . 2021 ; 11 : 127
Google Scholar
Katsoulas N , Kittas C , Dimokas G . et al. Effect of irrigation frequency on rose flower production and quality . Biosyst Eng . 2006 ; 93 : 237 – 44
Isah T . Stress and defense responses in plant secondary metabolites production . Biol Res . 2019 ; 52 : 39
Feng D , Zhang H , Qiu X . et al. Comparative transcriptomic and metabonomic analysis revealed the relationships between biosynthesis of volatiles and flavonoid metabolites in Rosa rugosa . Ornam Plant Res . 2021 ; 1 : 1 – 10
Wang X , Zhao F , Wu Q . et al. Physiological and transcriptome analyses to infer regulatory networks in flowering transition of Rosa rugosa . Ornam Plant Res . 2023 ; 3 : 1 – 12
Jia Y , Chen C , Gong F . et al. An aux/IAA family member, RhIAA14 , involved in ethylene-inhibited petal expansion in rose ( Rosa hybrida ) . Genes . 2022 ; 13 : 1041
Ren H , Bai M , Sun J . et al. RcMYB84 and RcMYB123 mediate jasmonate-induced defense responses against Botrytis cinerea in rose ( Rosa chinensis ) . Plant J . 2020 ; 103 : 1839 – 49
Chaves MM , Flexas J , Pinheiro C . Photosynthesis under drought and salt stress: regulation mechanisms from whole plant to cell . Ann Bot . 2009 ; 103 : 551 – 60
Askari Kelestani A , Ramezanpour S , Borzouei A . et al. Application of gamma rays on salinity tolerance of wheat ( Triticum aestivum L.) and expression of genes related to biosynthesis of proline, glycine betaine and antioxidant enzymes . Physiol Mol Biol Plants . 2021 ; 27 : 2533 – 47
Qi S , Wang X , Wu Q . et al. Morphological, physiological and transcriptomic analyses reveal potential candidate genes responsible for salt stress in Rosa rugosa . Ornam Plant Res . 2023 ; 3 :21
Gill SS , Tuteja N . Reactive oxygen species and antioxidant machinery in abiotic stress tolerance in crop plants . Plant Physiol Biochem . 2010 ; 48 : 909 – 30
Ye C , Zheng S , Jiang D . et al. Initiation and execution of programmed cell death and regulation of reactive oxygen species in plants . Int J Mol Sci . 2021 ; 22 : 12942
He L , He T , Farrar S . et al. Antioxidants maintain cellular redox homeostasis by elimination of reactive oxygen species . Cell Physiol Biochem . 2017 ; 44 : 532 – 53
Challabathula D , Analin B , Mohanan A . et al. Differential modulation of photosynthesis, ROS and antioxidant enzyme activities in stress-sensitive and -tolerant rice cultivars during salinity and drought upon restriction of COX and AOX pathways of mitochondrial oxidative electron transport . J Plant Physiol . 2022 ; 268 :153583
Li C , Mur LAJ , Wang Q . et al. ROS scavenging and ion homeostasis is required for the adaptation of halophyte Karelinia caspia to high salinity . Front Plant Sci . 2022 ; 13 :
Ren G , Yang P , Cui J . et al. Multiomics analyses of two sorghum cultivars reveal the molecular mechanism of salt tolerance . Front Plant Sci . 2022 ; 13 :
Petrussa E , Braidot E , Zancani M . et al. Plant Flavonoids--Biosynthesis, Transport and Involvement in Stress Responses . Int J Mol Sci . 2013 ; 14 : 14950 – 73
Das S , Rosazza JPN . Microbial and enzymatic transformations of flavonoids . J Nat Prod . 2006 ; 69 : 499 – 508
Gao Y , Liu J , Chen Y . et al. Tomato SlAN11 regulates flavonoid biosynthesis and seed dormancy by interaction with bHLH proteins but not with MYB proteins . Hortic Res . 2018 ; 5 :
Zhang Z , Liu Y , Yuan Q . et al. The bHLH1-DTX35/DFR module regulates pollen fertility by promoting flavonoid biosynthesis in Capsicum annuum L . Hortic Res . 2022 ; 9 :
Ramaroson M , Koutouan C , Helesbeux JJ . et al. Role of Phenylpropanoids and flavonoids in plant resistance to pests and diseases . Molecules . 2022 ; 27 : 8371
Schulz E , Tohge T , Winkler JB . et al. Natural variation among Arabidopsis accessions in the regulation of flavonoid metabolism and stress gene expression by combined UV radiation and cold . Plant Cell Physiol . 2021 ; 62 : 502 – 14
Wang F , Zhu H , Kong W . et al. The antirrhinum AmDEL gene enhances flavonoids accumulation and salt and drought tolerance in transgenic Arabidopsis . Planta . 2016 ; 244 : 59 – 73
Shen N , Wang T , Gan Q . et al. Plant flavonoids: classification, distribution, biosynthesis, and antioxidant activity . Food Chem . 2022 ; 383 :132531
Liu W , Feng Y , Yu S . et al. The flavonoid biosynthesis network in plants . Int J Mol Sci . 2021 ; 22 : 12824
Zhang X , Abrahan C , Colquhoun TA . et al. A proteolytic regulator controlling chalcone synthase stability and flavonoid biosynthesis in Arabidopsis . Plant Cell . 2017 ; 29 : 1157 – 74
Riffault-Valois L , Blanchot L , Colas C . et al. Molecular fingerprint comparison of closely related rose varieties based on UHPLC-HRMS analysis and chemometrics . Phytochem Anal . 2017 ; 28 : 42 – 9
Riffault L , Destandau E , Pasquier L . et al. Phytochemical analysis of Rosa hybrida cv. ‘Jardin de Granville' by HPTLC, HPLC-DAD and HPLC-ESI-HRMS: polyphenolic fingerprints of six plant organs . Phytochemistry . 2014 ; 99 : 127 – 34
Omidi M , Khandan-Mirkohi A , Kafi M . et al. Biochemical and molecular responses of Rosa damascena mill. cv. Kashan to salicylic acid under salinity stress . BMC Plant Biol . 2022 ; 22 : 373
Azizi S , Seyed Hajizadeh H , Aghaee A . et al. In vitro assessment of physiological traits and ROS detoxification pathways involved in tolerance of damask rose genotypes under salt stress . Sci Rep . 2023 ; 13 : 17795
Zhao S , Zhang Q , Liu M . et al. Regulation of plant responses to salt stress . Int J Mol Sci . 2021 ; 22 : 4609
Zhang C , Zhang H , Zhan Z . et al. Transcriptome analysis of sucrose metabolism during bulb swelling and development in onion ( Allium cepa L.) . Front Plant Sci . 2016 ; 7 :1425
Kumari P , Raju DVS , Prasad KV . et al. Characterization of anthocyanins and their antioxidant activities in Indian rose varieties ( Rosa × hybrida ) using HPLC . Antioxidants . 2022 ; 11 : 2032
Akula R , Ravishankar GA . Influence of abiotic stress signals on secondary metabolites in plants . Plant Signal Behav . 2011 ; 6 : 1720 – 31
Barhoumi Z , Djebali W , Chaïbi W . et al. Salt impact on photosynthesis and leaf ultrastructure of Aeluropus littoralis . J Plant Res . 2007 ; 120 : 529 – 37
Jiang D , Lu B , Liu L . et al. Exogenous melatonin improves the salt tolerance of cotton by removing active oxygen and protecting photosynthetic organs . BMC Plant Biol . 2021 ; 21 : 331
Liu D , Dong S , Miao H . et al. A large-scale genomic association analysis identifies the candidate genes regulating salt tolerance in cucumber ( Cucumis sativus L.) seedlings . Int J Mol Sci . 2022 ; 23 : 8260
Garrido Y , Tudela JA , Marín A . et al. Physiological, phytochemical and structural changes of multi-leaf lettuce caused by salt stress . J Sci Food Agric . 2014 ; 94 : 1592 – 9
Yao X , Meng L , Zhao W . et al. Changes in the morphology traits, anatomical structure of the leaves and transcriptome in Lycium barbarum L. under salt stress . Front Plant Sci . 2023 ; 14 :1090366
Tan Y , Duan Y , Chi Q . et al. The role of reactive oxygen species in plant response to radiation . Int J Mol Sci . 2023 ; 24 : 3346
Li M , Chen R , Jiang Q . et al. GmNAC06 , a NAC domain transcription factor enhances salt stress tolerance in soybean . Plant Mol Biol . 2021 ; 105 : 333 – 45
Wan X , Peng L , Xiong J . et al. AtSIBP1 , a novel BTB domain-containing protein, positively regulates salt signaling in Arabidopsis thaliana . Plan Theory . 2019 ; 8 : 573
Rezayian M , Niknam V , Ebrahimzadeh H . Oxidative damage and antioxidative system in algae . Toxicol Rep . 2019 ; 6 : 1309 – 13
Liu X , Cheng X , Cao J . et al. GOLDEN 2-LIKE transcription factors regulate chlorophyll biosynthesis and flavonoid accumulation in response to UV-B in tea plants . Hortic Plant J . 2023 ; 9 : 1055 – 66
Barreca D , Gattuso G , Bellocco E . et al. Flavanones: citrus phytochemical with health-promoting properties . Biofactors . 2017 ; 43 : 495 – 506
Zhang F , Huang J , Guo H . et al. OsRLCK160 contributes to flavonoid accumulation and UV-B tolerance by regulating OsbZIP48 in rice . Sci China Life Sci . 2022 ; 65 : 1380 – 94
Cui M , Liang Z , Liu Y . et al. Flavonoid profile of Anoectochilus roxburghii (wall.) Lindl. Under short-term heat stress revealed by integrated metabolome, transcriptome, and biochemical analyses . Plant Physiol Biochem . 2023 ; 201 :107896
Dias MC , Pinto DCGA , Silva AMS . Plant flavonoids: chemical characteristics and biological activity . Molecules . 2021 ; 26 : 5377
Kumar S , Pandey AK . Chemistry and biological activities of flavonoids: an overview . Sci World J . 2013 ; 2013 : 1 – 16
Chen C . Sinapic acid and its derivatives as medicine in oxidative stress-induced diseases and aging . Oxidative Med Cell Longev . 2016 ; 2016 : 1 – 10
Rasul A , Millimouno FM , Ali Eltayb W . et al. Pinocembrin: a novel natural compound with versatile pharmacological and biological activities . Biomed Res Int . 2013 ; 2013 : 1 – 9
Doneda E , Bianchi SE , Pittol V . et al. 3-O-methylquercetin from Achyrocline satureioides -cytotoxic activity against A375-derived human melanoma cell lines and its incorporation into cyclodextrins-hydrogels for topical administration . Drug Deliv Transl Res . 2021 ; 11 : 2151 – 68
Alam W , Khan H , Shah MA . et al. Kaempferol as a dietary anti-inflammatory agent: current therapeutic standing . Molecules . 2020 ; 25 : 4073
Chen Y , Mao Y , Liu H . et al. Transcriptome analysis of differentially expressed genes relevant to variegation in peach flowers . PLoS One . 2014 ; 9 :e90842
Duan B , Tan X , Long J . et al. Integrated transcriptomic-metabolomic analysis reveals that cinnamaldehyde exposure positively regulates the phenylpropanoid pathway in postharvest Satsuma mandarin ( Citrus unshiu ) . Pestic Biochem Physiol . 2023 ; 189 :105312
Lam PY , Wang L , Lui ACW . et al. Deficiency in flavonoid biosynthesis genes CHS , CHI , and CHIL alters rice flavonoid and lignin profiles . Plant Physiol . 2022 ; 188 : 1993 – 2011
Wu X , Zhang S , Liu X . et al. Chalcone synthase (CHS) family members analysis from eggplant ( Solanum melongena L.) in the flavonoid biosynthetic pathway and expression patterns in response to heat stress . PLoS One . 2020 ; 15 :e0226537
Wang X , Chai X , Gao B . et al. Multi-omics analysis reveals the mechanism of bHLH130 responding to low-nitrogen stress of apple rootstock . Plant Physiol . 2023 ; 191 : 1305 – 23
Ohno S , Hosokawa M , Hoshino A . et al. A bHLH transcription factor, DvIVS , is involved in regulation of anthocyanin synthesis in dahlia ( Dahlia variabilis ) . J Exp Bot . 2011 ; 62 : 5105 – 16
Baudry A , Caboche M , Lepiniec L . TT8 controls its own expression in a feedback regulation involving TTG1 and homologous MYB and bHLH factors, allowing a strong and cell-specific accumulation of flavonoids in Arabidopsis thaliana . Plant J . 2006 ; 46 : 768 – 79
Gao C , Guo Y , Wang J . et al. Brassica napus GLABRA3-1 promotes anthocyanin biosynthesis and trichome formation in true leaves when expressed in Arabidopsis thaliana . Plant Biol (Stuttg) . 2018 ; 20 : 3 – 9
Feyissa DN , Løvdal T , Olsen KM . et al. The endogenous GL3 , but not EGL3 , gene is necessary for anthocyanin accumulation as induced by nitrogen depletion in Arabidopsis rosette stage leaves . Planta . 2009 ; 230 : 747 – 54
Lim S , Kim D , Jung J . et al. Alternative splicing of the basic helix-loop-helix transcription factor gene CmbHLH2 affects anthocyanin biosynthesis in ray florets of chrysanthemum ( Chrysanthemum morifolium ) . Front Plant Sci . 2021 ; 12 :
Song Y , Ma B , Guo Q . et al. UV-B induces the expression of flavonoid biosynthetic pathways in blueberry ( Vaccinium corymbosum ) calli . Front Plant Sci . 2022 ; 13 :
Li W , Mao J , Yang SJ . et al. Anthocyanin accumulation correlates with hormones in the fruit skin of 'Red Delicious' and its four generation bud sport mutants . BMC Plant Biol . 2018 ; 18 : 363
Li W , Ning GX , Mao J . et al. Whole-genome DNA methylation patterns and complex associations with gene expression associated with anthocyanin biosynthesis in apple fruit skin . Planta . 2019 ; 250 : 1833 – 47
Sun J , Lu J , Bai M . et al. Phytochrome-interacting factors interact with transcription factor CONSTANS to suppress flowering in rose . Plant Physiol . 2021 ; 186 : 1186 – 201
Su L , Zhang Y , Yu S . et al. RcbHLH59-RcPRs module enhances salinity stress tolerance by balancing Na+/K+ through callose deposition in rose ( Rosa chinensis ) . Hortic Res . 2023 ; 10 :
Liu W , Zhang R , Xiang C . et al. Transcriptomic and physiological analysis reveal that α-linolenic acid biosynthesis responds to early chilling tolerance in pumpkin rootstock varieties . Front Plant Sci . 2021 ; 12 :
Bradford MM . A rapid and sensitive method for the quantitation of microgram quantities of protein utilizing the principle of protein-dye binding . Anal Biochem . 1976 ; 72 : 248 – 54
Cheng C , Yu Q , Wang Y . et al. Ethylene-regulated asymmetric growth of the petal base promotes flower opening in rose ( Rosa hybrida ) . Plant Cell . 2021 ; 33 : 1229 – 51
Zhang Y , Wu Z , Feng M . et al. The circadian-controlled PIF8-BBX28 module regulates petal senescence in rose flowers by governing mitochondrial ROS homeostasis at night . Plant Cell . 2021 ; 33 : 2716 – 35
Meng Y , Zhang H , Fan Y . et al. Anthocyanins accumulation analysis of correlated genes by metabolome and transcriptome in green and purple peppers ( Capsicum annuum ) . BMC Plant Biol . 2022 ; 22 : 358
Deng H , Wu G , Zhang R . et al. Comparative nutritional and metabolic analysis reveals the taste variations during yellow rambutan fruit maturation . Food Chem X . 2023 ; 17 :100580
Liu D , Pan Y , Li K . et al. Proteomics reveals the mechanism underlying the inhibition of Phytophthora sojae by propyl gallate . J Agric Food Chem . 2020 ; 68 : 8151 – 62
Yang B , He S , Liu Y . et al. Transcriptomics integrated with metabolomics reveals the effect of regulated deficit irrigation on anthocyanin biosynthesis in cabernet sauvignon grape berries . Food Chem . 2020 ; 314 :126170
Umer MJ , Bin Safdar L , Gebremeskel H . et al. Identification of key gene networks controlling organic acid and sugar metabolism during watermelon fruit development by integrating metabolic phenotypes and gene expression profiles . Hortic Res . 2020 ; 7 : 193
Liang Y , Jiang C , Liu Y . et al. Auxin regulates sucrose transport to repress petal abscission in rose ( Rosa hybrida ) . Plant Cell . 2020 ; 32 : 3485 – 99
Author notes
Supplementary data, email alerts, citing articles via.
- International Horticulture Research Conference
- Advertising & Corporate Services
Affiliations
- Online ISSN 2052-7276
- Print ISSN 2662-6810
- Copyright © 2024 Nanjing Agricultural University
- About Oxford Academic
- Publish journals with us
- University press partners
- What we publish
- New features
- Open access
- Institutional account management
- Rights and permissions
- Get help with access
- Accessibility
- Advertising
- Media enquiries
- Oxford University Press
- Oxford Languages
- University of Oxford
Oxford University Press is a department of the University of Oxford. It furthers the University's objective of excellence in research, scholarship, and education by publishing worldwide
- Copyright © 2024 Oxford University Press
- Cookie settings
- Cookie policy
- Privacy policy
- Legal notice
This Feature Is Available To Subscribers Only
Sign In or Create an Account
This PDF is available to Subscribers Only
For full access to this pdf, sign in to an existing account, or purchase an annual subscription.
- Skip to main content
- Keyboard shortcuts for audio player
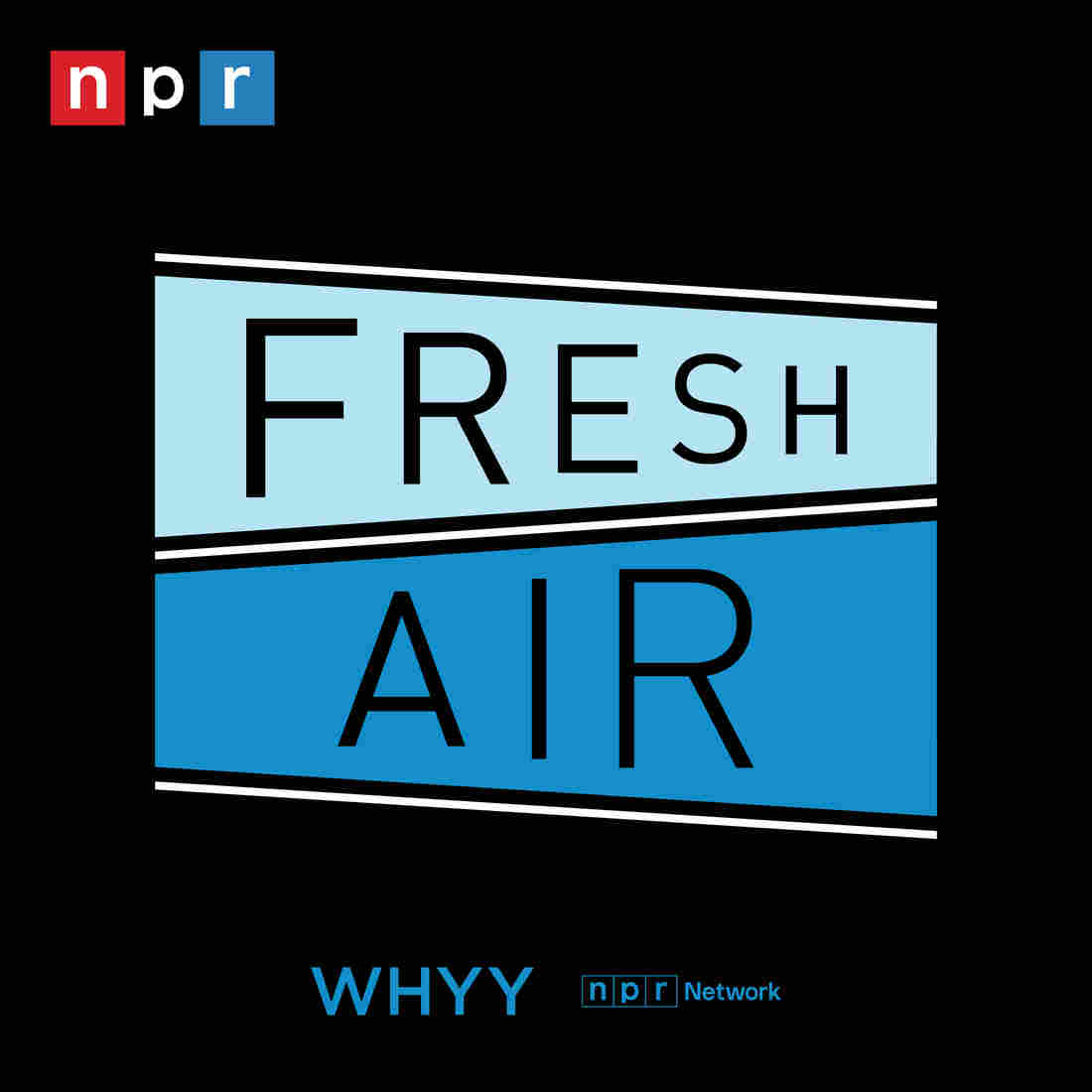
Author Interviews
- LISTEN & FOLLOW
- Apple Podcasts
- Google Podcasts
- Amazon Music
Your support helps make our show possible and unlocks access to our sponsor-free feed.
Plants can communicate and respond to touch. Does that mean they're intelligent?

Tonya Mosley
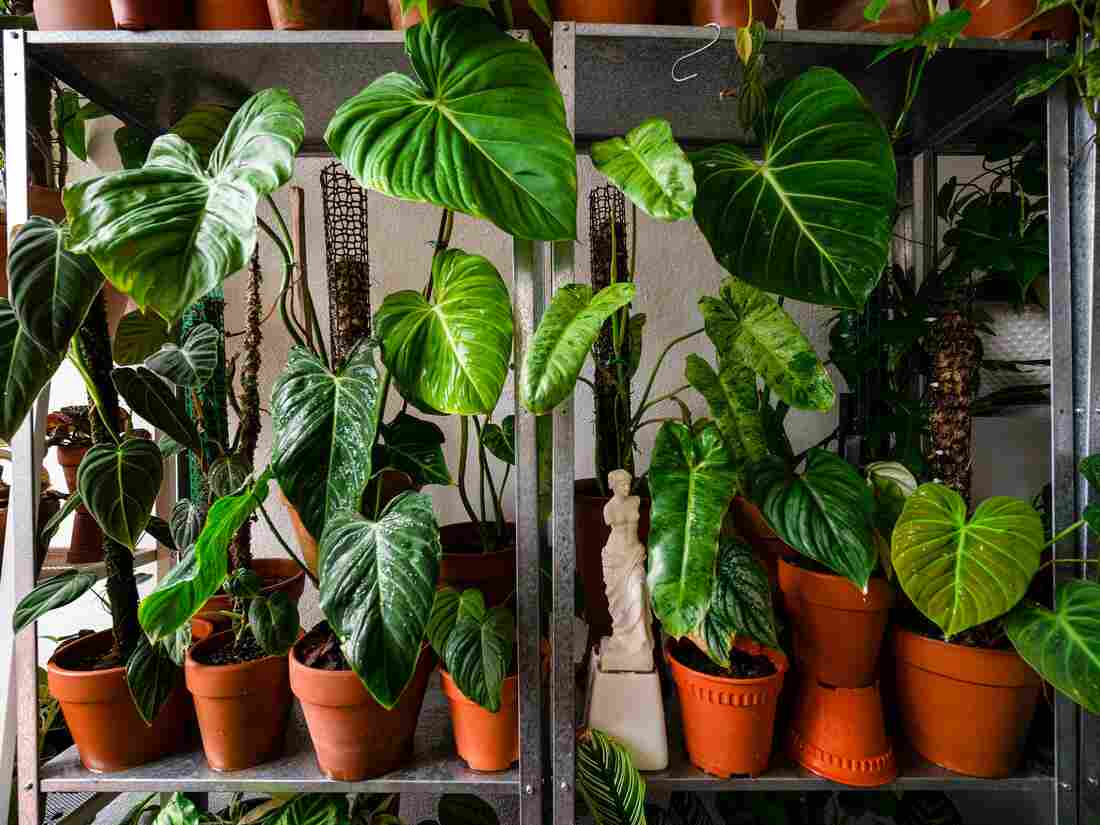
"The primary way plants communicate with each other is through a language, so to speak, of chemical gasses," journalist Zoë Schlanger says. Mohd Rasfan/AFP via Getty Images hide caption
"The primary way plants communicate with each other is through a language, so to speak, of chemical gasses," journalist Zoë Schlanger says.
In the 1960s and '70s, a series of questionable experiments claimed to prove that plants could behave like humans, that they had feelings, responded to music and could even take a polygraph test .
Though most of those claims have since been debunked, climate journalist Zoë Schlanger says a new wave of research suggests that plants are indeed "intelligent" in complex ways that challenge our understanding of agency and consciousness.
"Agency is this effect of having ... an active stake in the outcome of your life," Schlanger says. "And when I was looking at plants and speaking to botanists, it became very clear to me that plants have this."
In her new book, The Light Eaters: How the Unseen World of Plant Intelligence Offers a New Understanding of Life on Earth , Schlanger, a staff reporter at The Atlantic, writes about how plants use information from the environment, and from the past, to make "choices" for the future.

Happy Arbor Day! These 20 books will change the way you think about trees
Schlanger notes that some tomato plants, when being eaten by caterpillars, fill their leaves with a chemical that makes them so unappetizing that the caterpillars start eating each other instead. Corn plants have been known to sample the saliva of predator caterpillars — and then use that information to emit a chemical to attract a parasitic wasp that will attack the caterpillar.
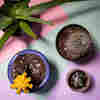
Stop overwatering your houseplants, and other things plant experts want you to know
Schlanger acknowledges that our understanding of plants is still developing — as are the definitions of "intelligence" and "consciousness." "Science is there [for] observation and to experiment, but it can't answer questions about this ineffable, squishy concept of intelligence and consciousness," she says.
But, she adds, "part of me feels like it almost doesn't matter, because what we see plants doing — what we now understand they can do — simply brings them into this realm of alert, active processing beings, which is a huge step from how many of us were raised to view them, which is more like ornaments in our world or this decorative backdrop for our our lives."
Interview highlights
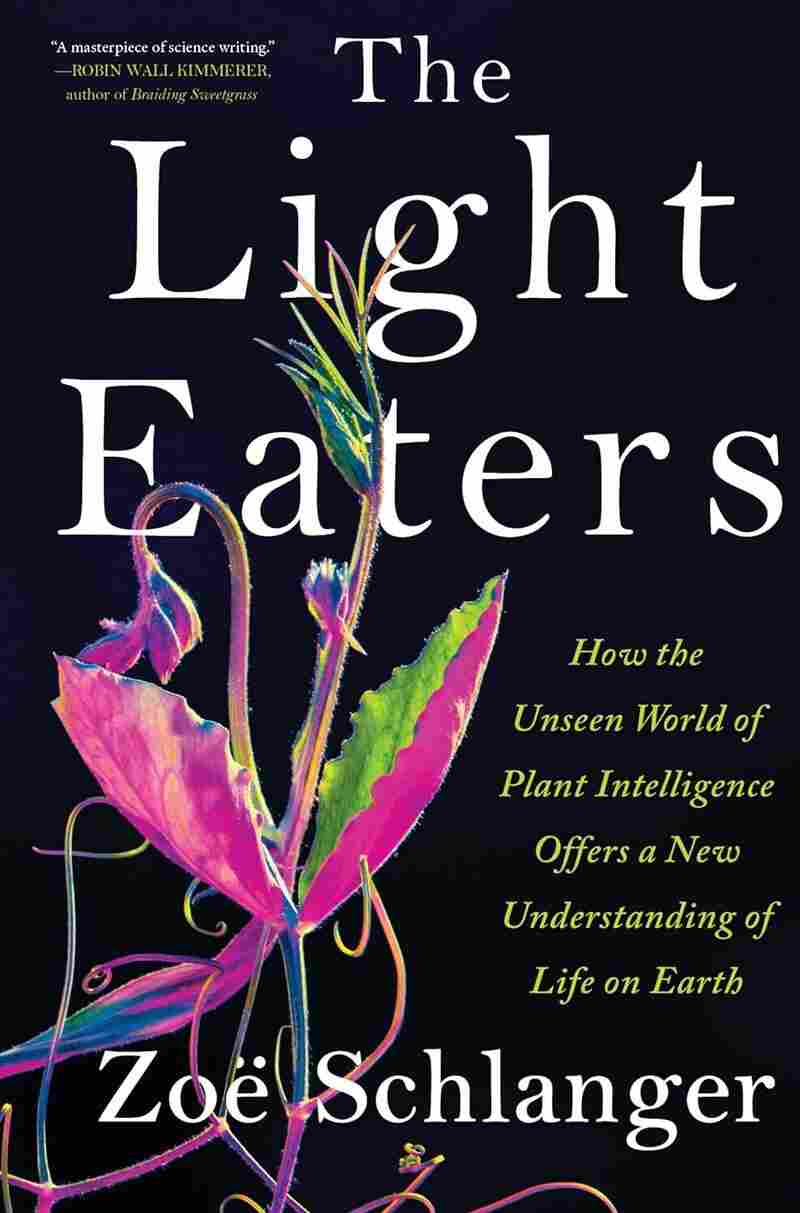
On the concept of plant "intelligence"
Intelligence is this thing that's loaded with so much human meaning. It's too muddled up sometimes with academic notions of intelligence. ... Is this even something we want to layer on to plants? And that's something that I hear a lot of plant scientists talk about. They recognize more than anyone that plants are not little humans. They don't want their subjects to be reduced in a way to human tropes or human standards of either of those things.
On the debate over if plants have nervous systems
I was able to go to a lab in Wisconsin where there [were] plants that had ... been engineered to glow, but only to glow when they've been touched. So I used tweezers to pinch a plant on its vein, ... the kind of mid-rib of a leaf. And I got to watch this glowing green signal emanate from the point where I pinch the plant out to the whole rest of the plant. Within two minutes, the whole plant had received a signal of my touch, of my "assault," so to speak, with these tweezers. And research like that is leading people within the plant sciences, but also people who work on neurobiology in people to question whether or not it's time to expand the notion of a nervous system.
On if plants feel pain
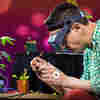
TED Radio Hour
Plants don't have brains — but they sure act smart.
We have nothing at the moment to suggest that plants feel pain, but do they sense being touched, or sense being eaten, and respond with a flurry of defensive chemicals that suggest that they really want to prevent whatever's going on from continuing? Absolutely. So this is where we get into tricky territory. Do we ascribe human concepts like pain ... to a plant, even though it has no brain? And we can't ask it if it feels pain. We have not found pain receptors in a plant. But then again, I mean, the devil's advocate view here is that we only found the mechanoreceptors for pain in humans, like, fairly recently. But we do know plants are receiving inputs all the time. They know when a caterpillar is chewing on them, and they will respond with aggressive defensiveness. They will do wild things to keep that caterpillar from destroying them further.
On how plants communicate with each other
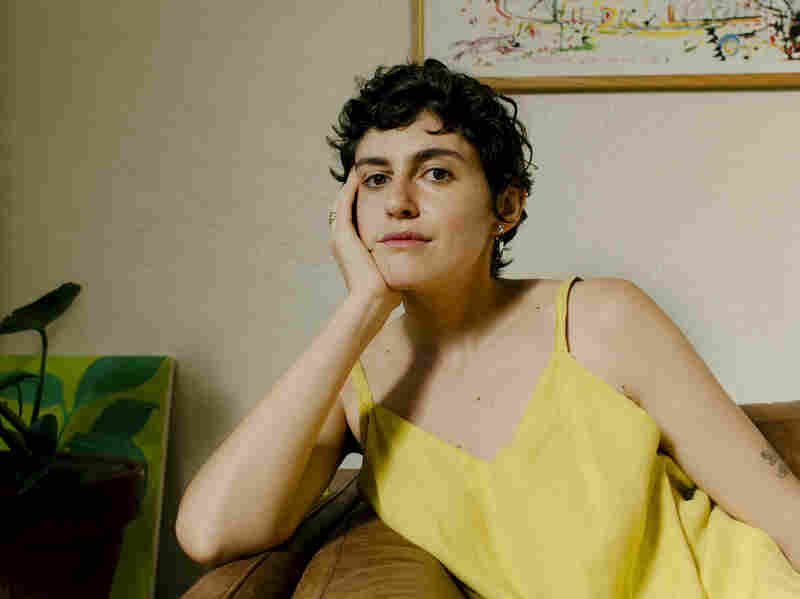
Zoë Schlanger is a staff writer at The Atlantic. Heather Sten/Harper Collins hide caption
Zoë Schlanger is a staff writer at The Atlantic.
The primary way plants communicate with each other is through a language, so to speak, of chemical gasses. ... And there's little pores on plants that are microscopic. And under the microscope, they look like little fish lips. ... And they open to release these gasses. And those gasses contain information. So when a plant is being eaten or knocked over by an animal or hit by wind too hard, it will release an alarm call that other plants in the area can pick up on. And this alarm call can travel pretty long distances, and the plants that receive it will prime their immune systems and their defense systems to be ready for this invasion, for this group of chewing animals before they even arrive. So it's a way of saving themselves, and it makes evolutionary sense. If you're a plant, you don't want to be standing out in a field alone, so to speak. It's not good for reproductive fitness. It's not good for attracting pollinators. It's often in the interest of plants to warn their neighbors of attacks like this.
On plant "memory"
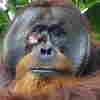
Research News
Orangutan in the wild applied medicinal plant to heal its own injury, biologists say.
There's one concept that I think is very beautiful, called the "memory of winter." And that's this thing where many plants, most of our fruit trees, for example, have to have the "memory," so to speak, of a certain number of days of cold in the winter in order to bloom in the spring. It's not enough that the warm weather comes. They have to get this profound cold period as well, which means to some extent they're counting. They're counting the elapsed days of cold and then the elapsed days of warmth to make sure they're also not necessarily emerging in a freak warm spell in February. This does sometimes happen, of course. We hear stories about farmers losing their crops to freak warm spells. But there is evidence to suggest there's parts of plants physiology that helps them record this information. But much like in people, we don't quite know the substrate of that memory. We can't quite locate where or how it's possibly being recorded.
On not anthropomorphizing plants
What's interesting is that scientists and botany journals will do somersaults to avoid using human language for plants. And I totally get why. But when you go meet them in their labs, they are willing to anthropomorphize the heck out of their study subjects. They'll say things like, "Oh, the plants hate when I do that." Or, "They really like this when I do this or they like this treatment." I once heard a scientist talk about, "We're going to go torture the plant again." So they're perfectly willing to do that in private. And the reason for that is not because they're holding some secret about how plants are actually just little humans. It's that they've already resolved that complexity in their mind. They trust themselves to not be reducing their subjects to human, simplistic human tropes. And that's going to be a task for all of us to somehow come to that place.
It's a real challenge for me. So much of what I was learning while doing research for this book was super intangible. You can't see a plant communicating, you can't watch a plant priming its immune system or manipulating an insect. A lot of these things are happening in invisible ways. ... Now when I go into a park, I feel totally surrounded by little aliens. I know that there is immense plant drama happening all over the place around me.
Sam Briger and Susan Nyakundi produced and edited this interview for broadcast. Bridget Bentz and Molly Seavy-Nesper adapted it for the web.
Systems biology of plant metabolic interactions
- Published: 07 May 2024
- Volume 49 , article number 56 , ( 2024 )
Cite this article
- Devlina Sarkar 1 &
- Sudip Kundu ORCID: orcid.org/0000-0003-1235-2540 1
39 Accesses
Explore all metrics
Metabolism is the key cellular process of plant physiology. Understanding metabolism and its dynamical behavior under different conditions may help plant biotechnologists to design new cultivars with desired goals. Computational systems biochemistry and incorporation of different omics data unravelled active metabolism and its variations in plants. In this review, we mainly focus on the basics of flux balance analysis (FBA), elementary flux mode analysis (EFMA), and some advanced computational tools. We describe some important results that were obtained using these tools. Limitations and challenges are also discussed.
This is a preview of subscription content, log in via an institution to check access.
Access this article
Price includes VAT (Russian Federation)
Instant access to the full article PDF.
Rent this article via DeepDyve
Institutional subscriptions
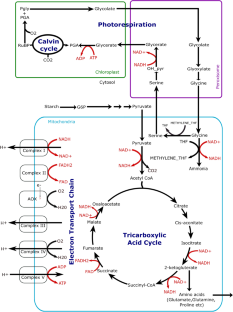
Similar content being viewed by others
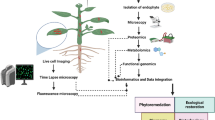
From Microscopy to Omics: A Comprehensive Review of Tools and Techniques in Studying Endophytic Adaptation Under Abiotic and Biotic Stress
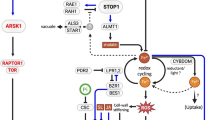
Recent advances in research on phosphate starvation signaling in plants
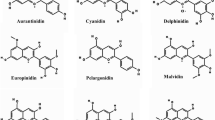
Multifaceted Regulation of Anthocyanin Biosynthesis in Plants: A Comprehensive Review
Affourtit C, Krab K, Leach GR, et al . 2001 New insights into the regulation of plant succinate dehydrogenase: on the role of the protonmotive force. J. Biol. Chem. 276 32567–32574
Article CAS PubMed Google Scholar
Åkesson M, Förster J and Nielsen J 2004 Integration of gene expression data into genome-scale metabolic models. Metab. Eng. 6 285–293
Article PubMed Google Scholar
Alejandre M, Segovia J, Zafra M, et al . 1979 Characteristics of citrate synthase from Agave americana L. leaves. Z. Pflanzenphysiol. 94 85–93
Article CAS Google Scholar
Araujo WL, Nunes-Nesi A, Nikoloski Z, et al . 2012 Metabolic control and regulation of the tricarboxylic acid cycle in photosynthetic and heterotrophic plant tissues. Plant Cell Environ. 35 1–21
Armstrong AF, Badger MR, Day DA, et al . 2008 Dynamic changes in the mitochondrial electron transport chain underpinning cold acclimation of leaf respiration. Plant Cell Environ. 31 1156–1169
Arnon DI 1971 The light reactions of photosynthesis. Proc. Natl. Acad. Sci. USA 68 2883–2892
Article CAS PubMed PubMed Central Google Scholar
Barbareschi D, Longo GP, Servettaz O, et al . 1974 Citrate synthetase in mitochondria and glyoxysomes of maize scutellum. Plant Physiol. 53 802–807
Bartoli CG, Gomez F, Gergoff G, et al . 2005 Up-regulation of the mitochondrial alternative oxidase pathway enhances photosynthetic electron transport under drought conditions. J. Exp. Bot. 56 1269–1276
Becker SA and Palsson BO 2008 Context-specific metabolic networks are consistent with experiments. PLoS Comput. Biol. 4 e1000082
Article PubMed PubMed Central Google Scholar
Behal RH and Oliver DJ 1997 Biochemical and molecular characterization of fumarase from plants: purification and characterization of the enzyme-cloning, sequencing, and expression of the gene. Arch. Biochem. Biophys. 348 65–74
Bocobza SE, Malitsky S, Araújo WL, et al . 2013 Orchestration of thiamin biosynthesis and central metabolism by combined action of the thiamin pyrophosphate riboswitch and the circadian clock in Arabidopsis . Plant Cell 25 288–307
Bogart E and Myers CR 2016 Multiscale metabolic modeling of C4 plants: connecting nonlinear genome-scale models to leaf-scale metabolism in developing maize leaves. PLoS One 11 e0151722
Bourguignon J, Neuburger M and Douce R 1988 Resolution and characterization of the glycinecleavage reaction in pea leaf mitochondria. Properties of the forward reaction catalysed by glycine decarboxylase and serine hydroxymethyltransferase. Biochem. J. 255 169–178
Burgard AP, Nikolaev EV, Schilling CH, et al . 2004 Flux coupling analysis of genome scale metabolic network reconstructions. Genome Res. 14 301–312
Bunik VI and Fernie AR 2009 Metabolic control exerted by the 2-oxoglutarate dehydrogenase reaction: a cross-kingdom comparison of the crossroad between energy production and nitrogen assimilation. Biochem. J. 422 405–421
Campbell C, Atkinson L, Zaragoza-Castells J, et al . 2007 Acclimation of photosynthesis and respiration is asynchronous in response to changes in temperature regardless of plant functional group. New Phytol. 176 375–389
Chandrasekaran S and Price ND 2010 Probabilistic integrative modeling of genome-scale metabolic and regulatory networks in Escherichia coli and mycobacterium tuberculosis. Proc. Natl. Acad. Sci. USA 107 17845–17850
Chatterjee A, Huma B, Shaw R, et al . 2017 Reconstruction of Oryza sativa indica genome scale metabolic model and its responses to varying RuBisCO activity, light intensity, and enzymatic cost conditions. Front. Plant Sci. 8 2060
Chavali AK, Whittemore JD, Eddy JA, et al . 2008 Systems analysis of metabolism in the pathogenic trypanosomatid Leishmania major . Mol. Syst. Biol. 4 177
Cheung CM, Poolman MG, Fell DA, et al . 2014 A diel flux balance model captures interactions between light and dark metabolism during day-night cycles in c3 and crassulacean acid metabolism leaves. Plant Physiol. 165 917–929
Cheung CM, Ratcliffe RG and Sweetlove LJ 2015 A method of accounting for enzyme costs in flux balance analysis reveals alternative pathways and metabolite stores in an illuminated Arabidopsis leaf. Plant Physiol. 169 1671–1682
Cheung CM, Williams TC, Poolman MG, et al . 2013 A method for accounting for maintenance costs in flux balance analysis improves the prediction of plant cell metabolic phenotypes under stress conditions. Plant J. 75 1050–1061
Colijn C, Brandes A, Zucker J, et al . 2009 Interpreting expression data with metabolic flux models: predicting Mycobacterium tuberculosis mycolic acid production. PLoS Comput. Biol. 5 e1000489
Cooper CE and Brown GC 2008 The inhibition of mitochondrial cytochrome oxidase by the gases carbon monoxide, nitric oxide, hydrogen cyanide and hydrogen sulfide: chemical mechanism and physiological significance. J. Bioenerg. Biomembr. 40 533–539
Covert MW, Knight EM, Reed JL, et al . 2004 Integrating highthroughput and computational data elucidates bacterial networks. Nature 429 92–96
Covert MW and Palsson BØ 2002 Transcriptional regulation in constraints-based metabolic models of Escherichia coli *210. J. Biol. Chem. 277 28058–28064
Covert MW and Palsson BØ 2003 Constraints-based models: regulation of gene expression reduces the steady-state solution space. J. Theor. Biol. 221 309–325
Covert MW, Xiao N, Chen TJ, et al . 2008 Integrating metabolic, transcriptional regulatory and signal transduction models in Escherichia coli . Bioinformatics 24 2044–2050
Covert MW, Schilling CH and Palsson BØ 2001 Regulation of gene expression in flux balance models of metabolism. J. Theor. Biol. 213 73–88
Cox G and Davies D 1969 The effects of pH and citrate on the activity of nicotinamide–adenine dinucleotide-specific isocitrate dehydrogenase from pea mitochondria. Biochem. J. 113 813–820
da Fonseca-Pereira P, Souza PV, Hou LY, et al . 2020 Thioredoxin h2 contributes to the redox regulation of mitochondrial photorespiratory metabolism. Plant Cell Environ. 43 188–208
Daloso DM, Müller K, Obata T, et al . 2015 Thioredoxin, a master regulator of the tricarboxylic acid cycle in plant mitochondria. Proc. Natl. Acad. Sci. USA 112 E1392–E1400
De RK, Das M and Mukhopadhyay S 2008 Incorporation of enzyme concentrations into fba and identification of optimal metabolic pathways. BMC Syst. Biol. 2 1–16
Article Google Scholar
de Oliveira Dal’Molin CG, Quek LE, Palfreyman RW, et al . 2010 AraGEM, a genome-scale reconstruction of the primary metabolic network in Arabidopsis . Plant Physiol. 152 579–589
de Oliveira Dal’Molin CG, Quek LE, Palfreyman RW, et al . 2010 C4GEM, a genome-scale metabolic model to study c4 plant metabolism. Plant Physiol. 154 , 1871–1885
Deb A and Kundu S 2015 Deciphering cis-regulatory element mediated combinatorial regulation in rice under blast infected condition. PLoS One 10 e0137295
Ederli L, Morettini R, Borgogni A, et al . 2006 Interaction between nitric oxide and ethylene in the induction of alternative oxidase in ozone-treated tobacco plants. Plant Physiol. 142 595–608
Eprintsev AT, Fedorin DN, Nikitina MV, et al . 2015 Expression and properties of the mitochondrial and cytosolic forms of aconitase in maize scutellum. J. Plant Physiol. 181 14–19
Eprintsev AT, Fedorin DN, Sazonova OV, et al . 2018 Expression and properties of the mitochondrial and cytosolic forms of fumarase in sunflower cotyledons. Plant Physiol. Biochem. 129 305–309
Fiorani F, Umbach AL and Siedow JN 2005 The alternative oxidase of plant mitochondria is involved in the acclimation of shoot growth at low temperature. A study of Arabidopsis AOX1a transgenic plants. Plant Physiol. 139 1795–1805
Flügel F, Timm S, Arrivault S, et al . 2017 The photorespiratory metabolite 2-phosphoglycolate regulates photosynthesis and starch accumulation in Arabidopsis . Plant Cell 29 2537–2551
Gupta KJ, Shah JK, Brotman Y, et al . 2012 Inhibition of aconitase by nitric oxide leads to induction of the alternative oxidase and to a shift of metabolism towards biosynthesis of amino acids. J. Exp. Bot. 63 1773–1784
Hanning I, Baumgarten K, Schott K, et al . 1999 Oxaloacetate transport into plant mitochondria. Plant Physiol. 119 1025–1032
Herrgård MJ, Lee BS, Portnoy V, et al . 2006 Integrated analysis of regulatory and metabolic networks reveals novel regulatory mechanisms in Saccharomyces cerevisiae . Genome Res. 16 627–635
Hodges M, Flesch V, Gálvez S, et al . 2003 Higher plant NADP+-dependent isocitrate dehydrogenases, ammonium assimilation and NADPH production. Plant Physiol. Biochem. 41 577–585
Hoppe A, Hoffmann S and Holzhütter HG 2007 Including metabolite concentrations into flux balance analysis: thermodynamic realizability as a constraint on flux distributions in metabolic networks. BMC Syst. Biol. 1 1–12
Huang W, Hu H and Zhang SB 2015 Photorespiration plays an important role in the regulation of photosynthetic electron flow under fluctuating light in tobacco plants grown under full sunlight. Front. Plant Sci. 6 621
Huma B, Kundu S, Poolman MG, et al . 2018 Stoichiometric analysis of the energetics and metabolic impact of photorespiration in c3 plants. Plant J. 96 1228–1241
Igamberdiev AU and Gardeström P 2003 Regulation of NAD-and NADP-dependent isocitrate dehydrogenases by reduction levels of pyridine nucleotides in mitochondria and cytosol of pea leaves. Biochim. Biophys. Acta 1606 117–125
Jacoby RP, Li L, Huang S, et al . 2012 Mitochondrial composition, function, and stress response in plants. J. Integr. Plant Biol. 54 887–906
Jensen PA and Papin JA 2011 Functional integration of a metabolic network model and expression data without arbitrary thresholding. Bioinformatics 27 541–547
Jerby L, Shlomi T and Ruppin E 2010 Computational reconstruction of tissue-specific metabolic models: application to human liver metabolism. Mol. Syst. Biol. 6 401
Kurata H, Zhao Q, Okuda R, et al . 2007 Integration of enzyme activities into metabolic flux distributions by elementary mode analysis. BMC Syst. Biol. 1 1–14
Lakshmanan M, Lim SH, Mohanty B, et al . 2015 Unraveling the light-specific metabolic and regulatory signatures of rice through combined in silico modeling and multiomics analysis. Plant Physiol. 169 3002–3020
CAS PubMed PubMed Central Google Scholar
Lancien M, Gadal P and Hodges M 1998 Molecular characterization of higher plant NAD-dependent isocitrate dehydrogenase: evidence for a heteromeric structure by the complementation of yeast mutants. Plant J. 16 325–333
Le XH, Lee CP, Monachello D, et al . 2022 Metabolic evidence for distinct pyruvate pools inside plant mitochondria. Nat. Plants 8 694–705
Leegood RC, Lea PJ, Adcock MD, et al . 1995 The regulation and control of photorespiration. J. Exp. Bot. 1397–1414
Lee JM, Gianchandani EP, Eddy JA, et al . 2008 Dynamic analysis of integrated signaling, metabolic, and regulatory networks. PLoS Comput. Biol. 4 e1000086
Lewis NE, Hixson KK, Conrad TM, et al . 2010 Omic data from evolved E. coli are consistent with computed optimal growth from genome-scale models. Mol. Syst. Biol. 6 390
Long CP, Gonzalez JE, Feist AM, et al . 2017 Fast growth phenotype of E. coli k-12 from adaptive laboratory evolution does not require intracellular flux rewiring. Metab. Eng. 44 100–107
Lotz K, Hartmann A, Grafahrend-Belau E, et al . 2014 Elementary flux modes, flux balance analysis, and their application to plant metabolism. Methods Mol. Biol. 1083 231–252
Machado D and Herrgård M 2014 Systematic evaluation of methods for integration of transcriptomic data into constraint-based models of metabolism. PLoS Comput. Biol. 10 e1003580
Mahadevan R and Schilling CH 2003 The effects of alternate optimal solutions in constraint-based genome-scale metabolic models. Metab. Eng. 5 264–276
Maiti R, Shaw R, Cheung CM, et al . 2023 Metabolic modelling revealed metabolic interactions between four segments of Setaria viridis leaves. J. Biosci. 48 26
McIntosh CA and Oliver DJ 1992 NAD+-linked isocitrate dehydrogenase: isolation, purification, and characterization of the protein from pea mitochondria. Plant Physiol. 100 69–75
Millar AH, Whelan J, Soole KL, et al . 2011 Organization and regulation of mitochondrial respiration in plants. Annu. Rev. Plant Biol. 62 79–104
Mintz-Oron S, Meir S, Malitsky S, et al . 2012 Reconstruction of Arabidopsis metabolic network models accounting for subcellular compartmentalization and tissue specificity. Proc. Natl. Acad. Sci. USA 109 339–344
Moore AL and Siedow JN 1991 The regulation and nature of the cyanide-resistant alternative oxidase of plant mitochondria. Biochim. Biophys. Acta 1059 121–140
Moore AL, Umbach AL and Siedow JN 1995 Structure-function relationships of the alternative oxidase of plant mitochondria: a model of the active site. J. Bioenerg. Biomembr. 27 367–377
Moreira TB, Shaw R, Luo X, et al . 2019 A genome-scale metabolic model of soybean ( Glycine max ) highlights metabolic fluxes in seedlings. Plant Physiol. 180 1912–1929
Morgan MJ, Lehmann M, Schwarzlander M, et al . 2008 Decrease in manganese superoxide dismutase leads to reduced root growth and affects tricarboxylic acid cycle flux and mitochondrial redox homeostasis. Plant Physiol. 147 101–114
Neuburger M, Bourguignon J and Douce R 1986 Isolation of a large complex from the matrix of pea leaf mitochondria involved in the rapid transformation of glycine into serine. FEBS Lett. 207 18–22
Nishio K and Mizushima T 2020 Structural and biochemical characterization of mitochondrial citrate synthase 4 from Arabidopsis thaliana . Acta Crystallogr. F Struct. Biol. Commun. 76 109–115
Nunes-Nesi A, Araújo WL, Obata T, et al . 2013 Regulation of the mitochondrial tricarboxylic acid cycle. Curr. Opin. Plant Biol. 16 335–343
Ogren WL 1984 Photorespiration: pathways, regulation, and modification. Ann. Rev. Plant Physiol. 35 415–442
Oikawa K, Hayashi M, Hayashi Y, et al . 2019 Re-evaluation of physical interaction between plant peroxisomes and other organelles using live-cell imaging techniques. J. Integr. Plant Biol. 61 836–852
Orth JD, Thiele I and Palsson BØ 2010 What is flux balance analysis? Nat. Biotechnol. 28 245–248
Palmer J and Wedding R 1966 Purification and properties of succinyl-CoA synthetase from jerusalem artichoke mitochondria. Biochim. Biophys. Acta. 113 167–174
Pastore D, Trono D, Laus MN, et al . 2001 Alternative oxidase in durum wheat mitochondria. activation by pyruvate, hydroxypyruvate and glyoxylate and physiological role. Plant Cell Physiol. 42 1373–1382
Pfau T, Christian N, Masakapalli SK, et al . 2018 The intertwined metabolism during symbiotic nitrogen fixation elucidated by metabolic modelling. Sci. Rep. 8 12504
Poolman MG, Kundu S, Shaw R, et al . 2013 Responses to light intensity in a genome-scale model of rice metabolism. Plant Physiol. 162 1060–1072
Poolman MG, Miguet L, Sweetlove LJ, et al . 2009 A genome-scale metabolic model of Arabidopsis and some of its properties. Plant Physiol. 151 1570–1581
Popova TN and de Carvalho MAAP 1998 Citrate and isocitrate in plant metabolism. Biochim. Biophys. Acta 1364 307–325
Reinholdt O, Bauwe H, Hagemann M, et al . 2019 Redox-regulation of mitochondrial metabolism through thioredoxin o1 facilitates light induction of photosynthesis. Plant Signal. Behav. 14 1674607
Rohwer JM 2012 Kinetic modelling of plant metabolic pathways. J. Exp. Bot. 63 2275–2292
Rhoads DM and Subbaiah CC 2007 Mitochondrial retrograde regulation in plants. Mitochondrion 7 177–194
Ribas-Carbo M, Aroca R, Gonzalez-Meler MA, et al . 2000 The electron partitioning between the cytochrome and alternative respiratory pathways during chilling recovery in two cultivars of maize differing in chilling sensitivity. Plant Physiol. 122 199–204
Rohwer JM and Botha FC 2001 Analysis of sucrose accumulation in the sugar cane culm on the basis of in vitro kinetic data. Biochem. J. 358 437–445
Rustin P, Moreau F and Lance C 1980 Malate oxidation in plant mitochondria via malic enzyme and the cyanide-insensitive electron transport pathway. Plant Physiol. 66 457–462
Saha B, Borovskii G and Panda SK 2016 Alternative oxidase and plant stress tolerance. Plant Signal. Behav. 11 e1256530
Saha R, Suthers PF and Maranas CD 2011 Zea mays iRS1563: a comprehensive genome-scale metabolic reconstruction of maize metabolism. PLoS One 6 e21784
Schuster S and Fell D 2007 Modeling and simulating metabolic networks; in Bioinformatics – From genomes to therapies (Ed.) T Lengauer (Weinheim: Wiley-VCH) pp 755–805
Seelert H and Dencher NA 2011 ATP synthase superassemblies in animals and plants: two or more are better. Biochim. Biophys. Acta 1807 1185–1197
Schilling CH, Edwards JS, Letscher D, et al . 2000 Combining pathway analysis with flux balance analysis for the comprehensive study of metabolic systems. Biotechnol. Bioeng. 71 286–306
Schmidtmann E, König AC, Orwat A, et al . 2014 Redox regulation of Arabidopsis mitochondrial citrate synthase. Mol Plant. 7 156–169
Schuster S, Dandekar T and Fell DA 1999 Detection of elementary flux modes in biochemical networks: a promising tool for pathway analysis and metabolic engineering. Trends Biotechnol. 17 53–60
Searle SY, Thomas S, Griffin KL, et al . 2011 Leaf respiration and alternative oxidase in field-grown alpine grasses respond to natural changes in temperature and light. New Phytol. 189 1027–1039
Sharkey TD 2023 The discovery of rubisco. J. Exp. Bot. 74 510–519
Shaw R and Cheung CM 2019 A mass and charge balanced metabolic model of setaria viridis revealed mechanisms of proton balancing in c4 plants. BMC Bioinform. 20 1–11
Shaw R and Kundu S 2013 Random weighting through linear programming into intracellular transporters of rice metabolic network; in Pattern recognition and machine intelligence (Eds.) P Maji, A Ghosh, MN Murty, et al. (Berlin: Springer) pp. 662–728
Shaw R and Kundu S 2015 Metabolic plasticity and inter-compartmental interactions in rice metabolism: an analysis from reaction deletion study. PLoS One 10 e0133899
Shlomi T, Cabili MN, Herrgård MJ, et al . 2008 Network-based prediction of human tissue-specific metabolism. Nat. Biotechnol. 26 1003–1010
Shlomi T, Eisenberg Y, Sharan R, et al . 2007 A genome-scale computational study of the interplay between transcriptional regulation and metabolism. Mol. Syst. Biol. 3 101
Shi K, Fu LJ, Zhang S, et al . 2013 Flexible change and cooperation between mitochondrial electron transport and cytosolic glycolysis as the basis for chilling tolerance in tomato plants. Planta 237 589–601
Siedow JN and Day DA 2000 Respiration and photorespiration; in Biochemistry and molecular biology of plants 1st edition (Eds.) BB Buchanan, W Gruissem and RL Jones (Rockville, MD: American Soceity of Plant Physiologists) pp 676–728
Simonin V and Galina A 2013 Nitric oxide inhibits succinate dehydrogenase-driven oxygen consumption in potato tuber mitochondria in an oxygen tension-independent manner. Biochem. J. 449 263–273
Sipari N, Lihavainen J, Shapiguzov A, et al . 2020 Primary metabolite responses to oxidative stress in early-senescing and paraquat resistant Arabidopsis thaliana rcd1 (radical-induced cell death 1). Front. Plant Sci. 11 194
Sluse F and Jarmuszkiewicz W 1998 Alternative oxidase in the branched mitochondrial respiratory network: an overview on structure, function, regulation, and role. Braz. J. Med. Biol. Res. 31 733–747
Stevens FJ, Dong Li A, Salman Lateef S, et al . 1997 Identification of potential interdomain disulfides in three higher plant mitochondrial citrate synthases: paradoxical differences in redox-sensitivity as compared with the animal enzyme. Photosynth. Res. 54 185–197
Strumilo S 2005 Short-term regulation of the α-ketoglutarate dehydrogenase complex by energylinked and some other effectors. Biochemistry 70 726–729
CAS PubMed Google Scholar
Studart-Guimarães C, Gibon Y, Frankel N, et al . 2005 Identification and characterisation of the α and β subunits of succinyl CoA ligase of tomato. Plant Mol. Biol. 59 781–791
Sweetlove LJ, Beard KF, Nunes-Nesi A, et al . 2010 Not just a circle: flux modes in the plant TCA cycle. Trends Plant Sci. 15 462–470
Tan XJ and Cheung CM 2020 A multiphase flux balance model reveals flexibility of central carbon metabolism in guard cells of c3 plants. Plant J. 104 1648–1656
Timm S, Florian A, Arrivault S, et al . 2012 Glycine decarboxylase controls photosynthesis and plant growth. FEBS Lett. 586 3692–3697
Timm S and Hagemann M 2020 Photorespiration—how is it regulated and how does it regulate overall plant metabolism? J. Exp. Bot. 71 3955–3965
Tovar-Méndez A, Miernyk JA and Randall DD 2003 Regulation of pyruvate dehydrogenase complex activity in plant cells. Eur. J. Biochem. 270 1043–1049
Tretter L and Adam-Vizi V 2000 Inhibition of krebs cycle enzymes by hydrogen peroxide: a key role of α-ketoglutarate dehydrogenase in limiting nadh production under oxidative stress. J. Neurosci. 20 8972–8979
Umbach AL and Siedow JN 1993 Covalent and noncovalent dimers of the cyanide-resistant alternative oxidase protein in higher plant mitochondria and their relationship to enzyme activity. Plant Physiol. 103 845–854
Unger EA and Vasconcelos AC 1989 Purification and characterization of mitochondrial citrate synthase. Plant Physiol. 89 719–723
Varma A and Palsson BO 1994 Stoichiometric flux balance models quantitatively predict growth and metabolic by-product secretion in wild-type Escherichia coli w3110. Appl. Environ. Microbiol. 60 3724–3731
van Berlo RJ, de Ridder D, Daran JM, et al . 2009 Predicting metabolic fluxes using gene expression differences as constraints. IEEE/ACM Trans. Comput. Biol. Bioinform. 8 206–216
Vanlerberghe GC and McIntosh L 1997 Alternative oxidase: from gene to function. Annu. Rev. Plant Physiol. Plant Mol. Biol. 48 703–734
Vanlerberghe GC 2013 Alternative oxidase: a mitochondrial respiratory pathway to maintain metabolic and signaling homeostasis during abiotic and biotic stress in plants. Int. J. Mol. Sci. 14 6805–6847
Verniquet F, Gaillard J, Neuburger M, et al . 1991 Rapid inactivation of plant aconitase by hydrogen peroxide. Biochem. J. 276 643–648
Wang H, Huang J, Liang X, et al . 2012 Involvement of hydrogen peroxide, calcium, and ethylene in the induction of the alternative pathway in chilling-stressed Arabidopsis callus. Planta 235 53–67
Wedding RT and Black MK 1971 Nucleotide activation of cauliflower α-ketoglutarate dehydrogenase. J. Biol. Chem. 246 1638–1643
Wedding RT, Black MK and Pap D 1976 Malate dehydrogenase and NAD malic enzyme in the oxidation of malate by sweet potato mitochondria. Plant Physiol. 58 740–743
Yuan H, Cheung CM, Poolman MG, et al . 2016 A genome-scale metabolic network reconstruction of tomato ( Solanum lycopersicum L. ) and its application to photorespiratory metabolism. Plant J. 85 289–304
Zoglowek C, Kromer S and Heldt HW 1988 Oxaloacetate and malate transport by plant mitochondria. Plant Physiol. 87 109–115
Download references
Author information
Authors and affiliations.
Department of Biophysics, Molecular Biology and Bioinformatics, University of Calcutta, Kolkata, India
Devlina Sarkar & Sudip Kundu
You can also search for this author in PubMed Google Scholar
Corresponding author
Correspondence to Sudip Kundu .
Additional information
Corresponding editor: A gepati R aghavendra
This article is part of the Topical Collection: Plant Mitochondria: Properties and Interactions with Other Organelles.
Rights and permissions
Springer Nature or its licensor (e.g. a society or other partner) holds exclusive rights to this article under a publishing agreement with the author(s) or other rightsholder(s); author self-archiving of the accepted manuscript version of this article is solely governed by the terms of such publishing agreement and applicable law.
Reprints and permissions
About this article
Sarkar, D., Kundu, S. Systems biology of plant metabolic interactions. J Biosci 49 , 56 (2024). https://doi.org/10.1007/s12038-023-00416-5
Download citation
Received : 31 May 2023
Accepted : 03 October 2023
Published : 07 May 2024
DOI : https://doi.org/10.1007/s12038-023-00416-5
Share this article
Anyone you share the following link with will be able to read this content:
Sorry, a shareable link is not currently available for this article.
Provided by the Springer Nature SharedIt content-sharing initiative
- Elementary flux mode analysis
- flux balance analysis
- metabolic interactions
- mitochondria
- regulations
- systems biochemistry
Advertisement
- Find a journal
- Publish with us
- Track your research
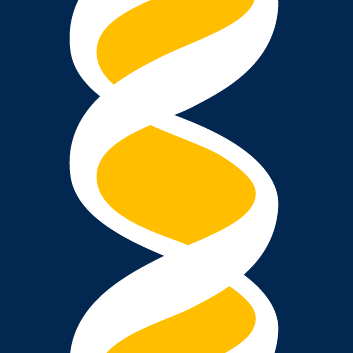
College of Biological Sciences
Bits & bites # 05: using ms-dial to generate accurate comprehensive lc-ms/ms metabolomics datasets.
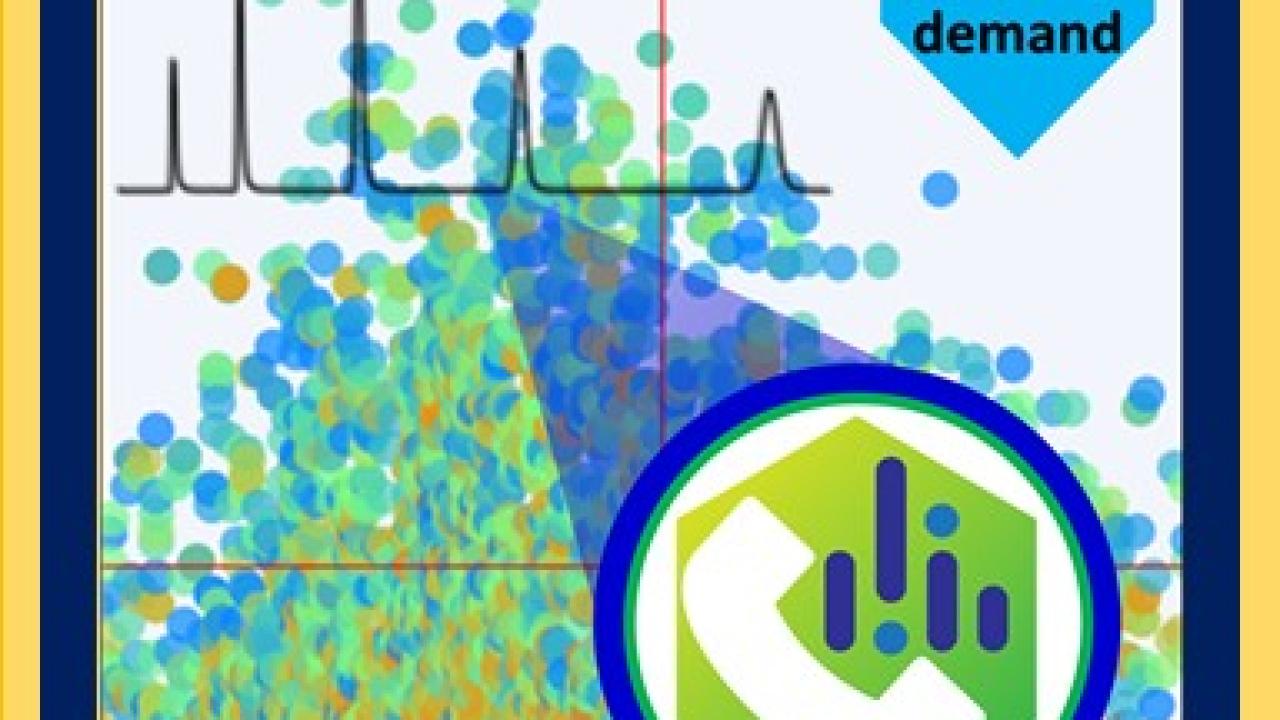
Event Date Thu, May 23, 2024 @ 9:00am - 1:30pm
Date : May 23, 2024 Instructor : Dr. Jake Folz, ETH Zurich Required Software : MS-DIAL for Windows. Versions of the tool to be used will be announced closer to the course date. Participant prerequisites : Basic understanding of LC-MS and understanding of how MS/MS spectra are used in metabolite identification. Level : Intermediate Description : Metabolomics demands meticulous data processing, especially with large complex datasets (i.e., 1000s of samples, different shipping batches, longitudinal studies). Using hands-on examples, we will provide best practices on how to perform fine-tuned curation of LC-MS/MS data generated through MS-DIAL a freely-available data processing tool for untargeted metabolomics data and other tips for quality assessment and reducing unknown features to ensure accuracy.
Learn more here!
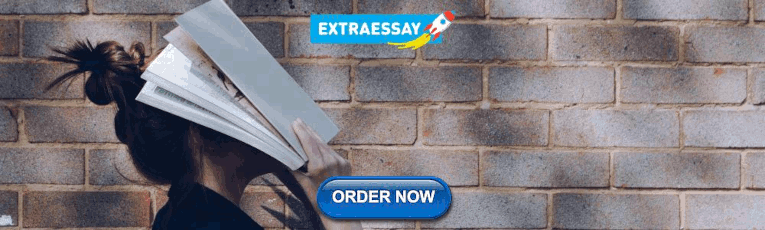
IMAGES
VIDEO
COMMENTS
New technology and approach. Although studies on metabolism and metabolites have a long history in plant science, this research focus has reached the systems level of study, namely metabolomics, owing to the technological development of analytical instruments that enabled high-throughput analysis of a large number of compounds with high sensitivity and accuracy.
Metabolomics has achieved a prominent role in plant science research. It has wide applications ranging from investigating the stress-specific metabolites for different climatic stresses, evaluating candidate metabolic gene functions to analyzing the biological mechanism in plant cells, and dissecting the genotype-phenotype relationship in ...
Metabolomics has occupied a prominent place in plant stress physiology and biology research. Metabolic change due to abiotic stress is complex to describe the variability between different plant species. Nevertheless, metabolomics needs more extensive research in data annotation, assessment, processing, and evaluation.
Plant metabolomics is a rapidly advancing field of plant sciences and systems biology. It involves comprehensive analyses of small molecules (metabolites) in plant tissues and cells. These metabolites include a wide range of compounds, such as sugars, amino acids, organic acids, secondary metabolites (e.g., alkaloids and flavonoids), lipids, and more. Metabolomics allows an understanding of ...
A similar recommendation was made to the plant research community in 2011 ... From models to crop species: caveats and solutions for translational metabolomics. Front. Plant Sci. 2, 61 (2011).
This Biology Special Issue "Recent Advances in Plant Metabolomics: From Metabolic Pathways to Health Impact" addresses cutting-edge knowledge on plants' metabolism, underlying stress responses, plant natural product chemistry and benefits and the role of plant metabolites in human nutrition and health. It comprises 4 articles from 26 ...
Abiotic and biotic stresses affect plant physiology and growth. The development of metabolomics, along with other -omics technologies, allowed in depth analysis of the reactive processes characterizing plant stress as the result of the alteration of metabolites and gene expressions. Here, we organize and interpret data from 151 studies to provide an overview about metabolomic shift after ...
Advances in plant metabolomics in recent time has allowed the precise selection of desirable traits along with offering opportunities to undertake metabolic engineered plants. ... C., Butelli, E., Petroni, K., and Tonelli, C. (2011). How can research on plants contribute to promoting human health? Plant Cell 23, 1685-1699. doi: 10.1105/tpc ...
Over the past decade, systems biology and plant-omics have increasingly become the main stream in plant biology research. New developments in mass spectrometry and bioinformatics tools, and methodological schema to integrate multi-omics data have leveraged recent advances in proteomics and metabolomics. These progresses are driving a rapid evolution in the field of plant research, greatly ...
The Metabolomics Standards Initiative (MSI Board Members:, 2007) has developed MIS for different metabolomics types (e.g. LC-MS, GC-MS, NMR) and sources (e.g. plant, environmental…) and should be used as a reference for metabolomics metadata. In general, MIS ensures that data can be verified, analyzed and clearly interpreted by the broader ...
Plant metabolomics — the high-throughput analysis of plant compounds — is an invaluable tool for understanding plant metabolism. ... an important tool in metabolomics research. Metabolites are ...
Plant Research International, Wageningen University and Research Centre (Wageningen-UR), PO Box 16, Wageningen, AA, 6700 The Netherlands ... Moving on from the many and wide-ranging hopes, enthused upon in a multitude of early reviews, metabolomics for plant research has already proved itself despite the technology still experiencing certain ...
The flexibility of metabolomics in plant research is incredibly essential within the disclosure of biomarkers and in the improvement of harvest yield and quality. Unambiguous traceability of a crop ensures its origin, quality, and security. Untargeted metabolomics approaches have been extremely useful in the search for quality traits within crops.
Metabolomics plays a crucial role in molecular networking to bridge the gaps between genotypes and phenotypes. However, the greater complexity of metabolites with diverse chemical and physical properties has limited the advances in plant metabolomics. For several years, applications of liquid/gas chromatography (LC/GC)-mass spectrometry (MS ...
Plant metabolomics deals with the interpretation of various metabolic pathways in contrast to other -omics technologies applied in systems biology. Metabolomics is a highly challenging field where the metabolite analysis is done by high-end technologies for proposing metabolic pathways. The high-throughput technologies utilized for these ...
This review summarizes the state of the art of methods and techniques in plant metabolomics research and presents different metabolic profiling scenarios in plant sciences. In addition, the latest studies combining metabolomics and other omics approaches to understand the metabolic regulatory network are discussed. Finally, the authors propose key challenges and future directions in the use of ...
As genomes of many plant species have been sequenced, demand for functional genomics has dramatically accelerated the improvement of other omics including metabolomics. Despite a large amount of metabolites still remaining to be identified, metabolomics has contributed significantly not only to the understanding of plant physiology and biology from the view of small chemical molecules that ...
Combining more analytical techniques in the future will likely facilitate metabolomics and the basic research behind it. In plant metabolism research, metabolite analysis has progressed from the analysis of a single or small number of metabolites into the study of a massive amount metabolic data on different levels of plant organs, tissues and ...
Plant metabolomics with a huge impetus to comprehensively characterize the metabolites and clarify the complexity and integrity of CMM, has been widely used in the research of CMM. This article comprehensively reviewed the application of plant metabolomics in the quality control of CMM. It introduced the concept, technique, and application ...
This detailed book aims to address the need for standardizing key steps in plant metabolomics research that is still considered a critical issue in the field. The collection unites diverse mass spectrometry (MS)-based protocols, from sample preparation and extraction of plant primary and secondary metabolites (e.g. a range of sugars, lipids ...
The Plant Metabolome Hub (PMhub), available at https://pmhub.org.cn, is a valuable resource designed to provide scientists with comprehensive information on plant metabolites. It offers extensive details about their reference spectra, genetic foundations, chemical reactions, metabolic pathways and b …
Plant metabolomics, alone or combined with functional genomics, has been applied in many fields. Even though it has some limitations currently, it is no doubt an important tool that is revolutionizing plant biology and crop breeding. ... (2016ZX08012-002) and China Innovative Research Team, Ministry of Education, and the Programme of ...
Research studies in Arabidopsis thaliana showed that metabolites of root exudates including both primary metabolites (PMs) and SMs of plants account for ~10% of photosynthetical carbon and ~15% of total plant nitrogen compounds (Walker et al., 2003). In addition, the root exudates can significantly shape the diversities and mediate mutual ...
Manganese (Mn) plays a pivotal role in plant growth and development. Aside aiding in plant growth and development, Mn as heavy metal (HM) can be toxic in soil when applied in excess.Morus alba is an economically significant plant, capable of adapting to a range of environmental conditions and possessing the potential for phytoremediation of contaminated soil by HMs.
Abstract. Salinity stress causes serious damage to crops worldwide, limiting plant production. However, the metabolic and molecular mechanisms underlying the response to salt stress in rose (Rosa spp.) remain poorly studied.We therefore performed a multi-omics investigation of Rosa hybrida cv. Jardin de Granville (JDG) and Rosa damascena Mill. (DMS) under salt stress to determine the ...
Bupleuri Radix is an important medicinal plant, which has been used in China and other Asian countries for thousands of years. Cultivated Bupleurum chinense DC. (B. chinense) is the main commodity of Bupleuri Radix.The benefits of intercropping with various crops for B. chinense have been recognized; however, the influence of intercropping on the chemical composition of B. chinense is still ...
Utilizing transcriptomics and metabolomics reveal drought tolerance mechanism in Nicotiana tabacum Quanyu Yin a, Zhao Feng a, Zhichao Ren a, Hui Wang b, Dongling Wu b, Amit Jaisi c,d, Mengquan Yang a,* a National Tobacco Cultivation, Physiology and Biochemistry Research Center, College of Tobacco Science, Henan Agricultural University, Zhengzhou, Henan,
Climate journalist Zoë Schlanger says research suggests that plants are indeed "intelligent" in complex ways that challenge our understanding of agency and consciousness. Her book is The Light ...
Metabolism is the key cellular process of plant physiology. Understanding metabolism and its dynamical behavior under different conditions may help plant biotechnologists to design new cultivars with desired goals. Computational systems biochemistry and incorporation of different omics data unravelled active metabolism and its variations in plants. In this review, we mainly focus on the basics ...
Description: Metabolomics demands meticulous data processing, especially with large complex datasets (i.e., 1000s of samples, different shipping batches, longitudinal studies). Using hands-on examples, we will provide best practices on how to perform fine-tuned curation of LC-MS/MS data generated through MS-DIAL a freely-available data ...