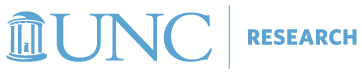
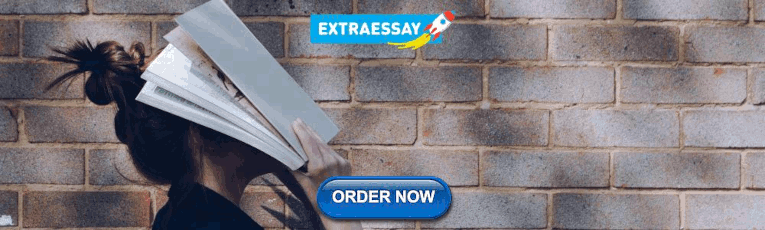
RCR Module Descriptions from CITI
Authorship (rcr-basic) (id 16597).
Describes the important issues relating to publishing research results and working with authors. It also discusses the potential pitfalls and errors that can lead to research misconduct and authorship disputes, as well as strategies for avoiding and resolving authorship disputes. Go back.
Collaborative Research (RCR-Basic) (ID 16598)
Discusses styles of collaboration and potential trade-offs relating to collaborative research. It also identifies some of the complexities of managing and assessing collaborations, and special considerations involved in collaborative work, including working with industry, across disciplinary and international boundaries, and with students. Go back.
Communicating with the Public (ID 19270)
Researchers have a range of ethical obligations when designing and conducting their research activities. These obligations also pertain to how researchers share their findings with others. Module provides an in-depth discussion on communicating research results to the public, including approaches for making results more understandable to difference audiences. Go back.
Conflicts of Interest (RCR-Basic) (ID 16599)
Explains why conflicts of interest can be ethically problematic and how organizations can have a conflict of interest. Go back.
Consent and Cultural Competence (ID 17263)
Focuses on cultural competence, as it applies to developing consent processes, obtaining consent, and evaluating the appropriateness of the consent processes. Describes strategies for enhancing understanding of research among diverse populations and communities during the consent process. Go back.
Data Management (RCR-Basic) (ID 20896)
Identifies the steps, concepts, and importance of data management throughout a research study. The module also discusses institutional support services that can help manage research data; methodological, technological, and regulatory considerations that affect data management practices; documentation needed to facilitate the accessibility and reproducibility of research findings; and ethical and compliance issues relating to data ownership, sharing, and protection. Go back.
Environmental and Social Dimensions of Engineering Research (ID 12835)
Provides an overview of the responsibilities that engineering researchers have when it comes to environmental and social considerations. It includes a discussion of moral development, risk, and sustainability. Go back.
Ethical Issues in Public Health Research (ID 17640)
Summarizes the application of ethical principles to public health research, identifies additional ethical challenges unique to public health research, and provides a six-step framework for application to public health problems. Go back.
Introduction to Public Health Research (ID 17637)
Describes the roles, responsibilities, and activities of public health systems as relevant to research. Discusses characteristics of international public health systems and identifies public health services and their interrelationships with core public health functions. Go back.
Mentoring and Healthy Research Environments (RCR-Basic) (ID 20983)
Discusses mentoring and why it is essential to research, factors that can make mentoring relationships challenging, and practices and strategies mentors and mentees can use to establish and navigate mentor-mentee relationships successfully. The module also describes a psychologically healthy and supportive research environment and the leadership behaviors mentors can use to create positive research environments. Go back.
Peer Review (RCR-Basic) (ID 16603)
Discusses the history and evolution of peer review, value of peer review as it relates to publications and grant awards, ethical issues associated with peer review, and ethical duties of a peer reviewer. Go back.
Plagiarism (RCR-Basic) (ID 15156)
Discusses the definition of plagiarism, appropriate summarization of material, and strategies for properly citing information sources. Go back.
Presentation of Research Findings (ID 19355)
Researchers have a range of ethical obligations when designing and conducting their research activities. These obligations also pertain to how researchers share their findings with others. Module discusses how to present research findings accurately for publication, including strategies for preparing figures, graphs, and digital images. Go back.
Records-Based Research (ID 5)
Records-based research has its own risks, and researchers who propose to conduct such research must have an understanding of those risks and how to minimize them. Learners will be presented with an overview of the risks associated with and the types of review required for records-based research. They will also learn about privacy and confidentiality, certificates of confidentiality, and the federal privacy law. Go back.
Research, Ethics, and Society (ID 15198)
Introduces social responsibilities of researchers. The discussion includes the meaning of social responsibility, why researchers have social responsibilities, and how researchers can act on their social responsibilities. Go back.
Research Involving Human Subjects (RCR-Basic) (ID 13566)
Provides an introduction to ethical and regulatory issues relating to the participation of human beings in research. It includes a description of the informed consent process and the Common Rule—a set of regulations adopted by a number of U.S. federal agencies. Go back.
Research Misconduct (RCR-Basic) (ID 16604)
Describes the three practices (fabrication, falsification, and plagiarism) that constitute research misconduct and the steps that can be taken to handle allegations of research misconduct. Go back.
Reproducibility of Research Results (ID 17756)
Discusses factors that contribute to the lack of reproducibility and the resulting problems that can emerge. The module also describes the stakeholders affected by reproducibility problems, a collection of reproducibility initiatives, and strategies that can mitigate or prevent irreproducibility. Go back.
Using Animal Subjects in Research (RCR-Basic) (ID 13301)
Provides an introduction to ethical and regulatory issues relating to the use of animal subjects in research. It includes a description of federal regulations governing how research with animal subjects should be conducted. Go back.
RCR Course Information
I completed the CITI RCR (Basic Course) at my former institution. Can I receive credit for the RCR (Basic Course) at UK?
What modules are required in the UK CITI RCR course?
How long does it take to complete the CITI RCR course?
Am I able to quit the CITI RCR course and return to complete it later?
Are there rcr optional modules pertaining to my discipline that i can complete, will the rcr optional modules effect my overall grade, is there a paper-based alternative for the uk citi rcr course if i do not have access to a computer, i completed the citi rcr (basic course) at my former institution. can i receive credit for the rcr (basic course) at uk.
The training must have been taken within the last 12 months and must contain the same modules as the UK CITI RCR (Basic Course). For approval, send the "Completion Report" to [email protected] .
Instructions on how to retrieve the Completion Report [ HTML ]
Back to Getting Started | Top of page
- Responsible Conduct of Research Special Instructions;
- Peer Review;
- Research Misconduct;
- Conflicts of Interest;
- Data Management; and
- Reproducibility of Research Results.
How long will it take to complete the RCR Course?
The average learner spends approximately 3 hours to complete the RCR (Basic) Course.
Yes. CITI allows you to complete the course at your own pace. You may quit and return to the course later. However, you must quit after taking the quiz for a particular module, not DURING the taking of that quiz, or your answers will not be recorded.
- Go to your UK Courses page;
- Scroll to "Active Courses" and find the RCR course;
- Click the button to continue.
You will be able to start at either the next module or, if you did not complete a module and need to take the quiz, at the module that you were viewing previously.
Yes, they are referred to as "Supplemental Modules". You may complete any module(s) you choose under "Supplemental Modules".
Optional modules available:
- Communicating with the Public;
- Presentation of Research Findings;
- Financial Responsibility;
- Introduction to RCR;
- Environmental and Social Dimensions of Engineering Research;
- Using Animal Subjects in Research;
- Plagiarism;
- Research, Ethics, and Society;
- Authorship; and
- Collaborative Research.
No, the RCR optional (Supplemental) modules will not effect your overall course percentage.
If extenuating circumstances prohibit access to a computer, contact Jennifer Hill ( [email protected] , 859-257-2978) to obtain an alternate paper-based course and exam.
UK CITI Access
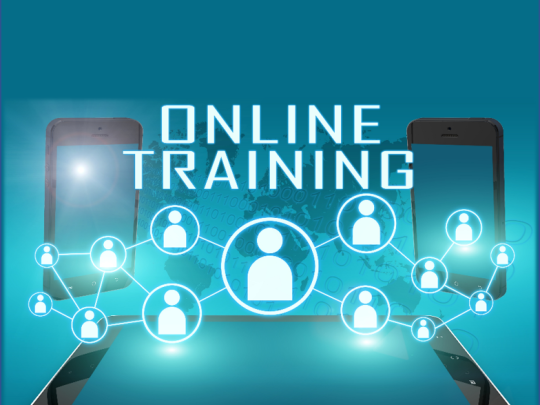
(HSP & RCR Training Platform)
Log in using your link blue ID and password
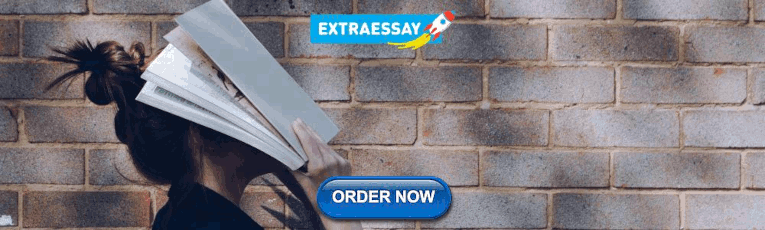
HSP or RCR? Interactive Tool
Choose your path to find the correct training for the University mandate.
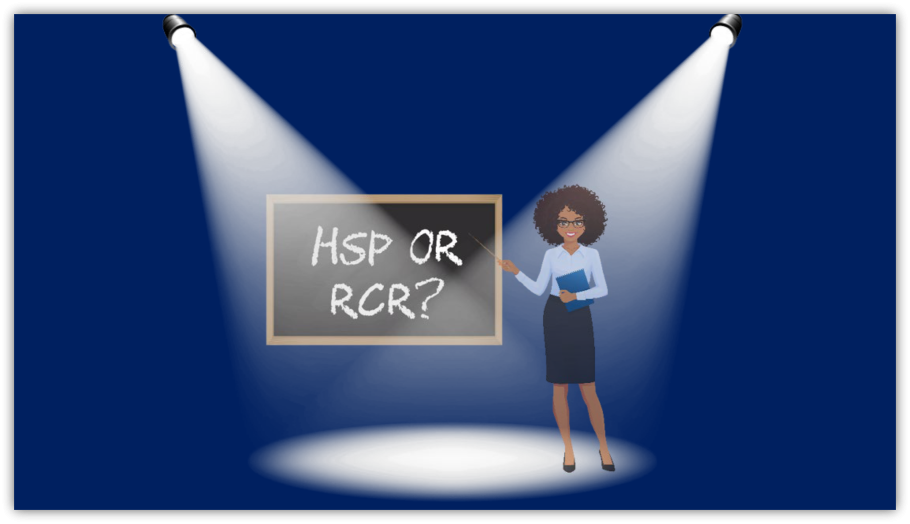
Loading metrics
Open Access
Perspective
The Perspective section provides experts with a forum to comment on topical or controversial issues of broad interest.
See all article types »
The Economics of Reproducibility in Preclinical Research
* E-mail: [email protected]
Affiliation Global Biological Standards Institute, Washington, D.C., United States of America
Affiliation Boston University School of Management, Boston, Massachusetts, United States of America
Affiliations Boston University School of Management, Boston, Massachusetts, United States of America, Council of Economic Advisers, Washington, D.C., United States of America
- Leonard P. Freedman,
- Iain M. Cockburn,
- Timothy S. Simcoe
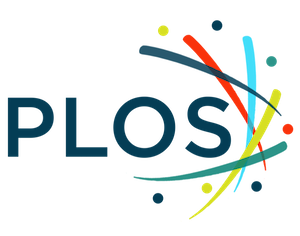
Published: June 9, 2015
- https://doi.org/10.1371/journal.pbio.1002165
- Reader Comments
10 Apr 2018: The PLOS Biology Staff (2018) Correction: The Economics of Reproducibility in Preclinical Research. PLOS Biology 16(4): e1002626. https://doi.org/10.1371/journal.pbio.1002626 View correction
Low reproducibility rates within life science research undermine cumulative knowledge production and contribute to both delays and costs of therapeutic drug development. An analysis of past studies indicates that the cumulative (total) prevalence of irreproducible preclinical research exceeds 50%, resulting in approximately US$28,000,000,000 (US$28B)/year spent on preclinical research that is not reproducible—in the United States alone. We outline a framework for solutions and a plan for long-term improvements in reproducibility rates that will help to accelerate the discovery of life-saving therapies and cures.
Citation: Freedman LP, Cockburn IM, Simcoe TS (2015) The Economics of Reproducibility in Preclinical Research. PLoS Biol 13(6): e1002165. https://doi.org/10.1371/journal.pbio.1002165
Copyright: © 2015 Freedman et al. This is an open access article distributed under the terms of the Creative Commons Attribution License , which permits unrestricted use, distribution, and reproduction in any medium, provided the original author and source are credited
Funding: The authors received no specific funding for this work.
Competing interests: Dr. Simcoe currently works as a Senior Economist for the Council of Economic Advisers (CEA). The CEA disclaims responsibility for any of the views expressed herein and these views do not necessarily represent the views of the CEA or the United States.
Abbreviations: AAAS, American Association for the Advancement of Science; FDA, US Food and Drug Association; GBSI, Global Biological Standards Institute; IETF, Internet Engineering Task Force; NIH, National Institutes of Health; PI, principal investigator; STR, short tandem repeat; W3C, World Wide Web Consortium
Introduction
Much has been written about the alarming number of preclinical studies that were later found to be irreproducible [ 1 , 2 ]. Flawed preclinical studies create false hope for patients waiting for lifesaving cures; moreover, they point to systemic and costly inefficiencies in the way preclinical studies are designed, conducted, and reported. Because replication and cumulative knowledge production are cornerstones of the scientific process, these widespread accounts are scientifically troubling. Such concerns are further complicated by questions about the effectiveness of the peer review process itself [ 3 ], as well as the rapid growth of postpublication peer review (e.g., PubMed Commons, PubPeer), data sharing, and open access publishing that accelerate the identification of irreproducible studies [ 4 ]. Indeed, there are many different perspectives on the size of this problem, and published estimates of irreproducibility range from 51% [ 5 ] to 89% [ 6 ] ( Fig 1 ). Our primary goal here is not to pinpoint the exact irreproducibility rate, but rather to identify root causes of the problem, estimate the direct costs of irreproducible research, and to develop a framework to address the highest priorities. Based on examples from within life sciences, application of economic theory, and reviewing lessons learned from other industries, we conclude that community-developed best practices and standards must play a central role in improving reproducibility going forward.
- PPT PowerPoint slide
- PNG larger image
- TIFF original image
Source: Begley and Ellis [ 6 ], Prinz et al. [ 7 ], Vasilevsky [ 8 ], Hartshorne and Schachner [ 5 ], and Glasziou et al. [ 9 ].
https://doi.org/10.1371/journal.pbio.1002165.g001
Defining Reproducibility
Studies of reproducibility define the phenomenon in a number of ways [ 10 ]. For example, some studies define reproducibility as the ability to replicate the same results demonstrated in a particular study using precisely the same methods and materials [ 11 ]; others evaluate whether the study’s methodology and results were presented in sufficient detail to allow replication or reanalysis [ 8 ]. The definition of reproducibility may also vary depending upon whether a particular study is confirmatory (designed to test basic theories through rigorous study design and analysis) or exploratory (primarily aimed at developing theories and frameworks for further study) [ 12 ]. For this paper, we adopt an inclusive definition of irreproducibility that encompasses the existence and propagation of one or more errors, flaws, inadequacies, or omissions (collectively referred to as errors) that prevent replication of results. Clearly, perfect reproducibility across all preclinical research is neither possible nor desirable. Attempting to achieve total reproducibility would dramatically increase the cost of such studies and radically curb their volume. Our assumption that current irreproducibility rates exceed a theoretically (and perhaps indeterminable) optimal level is based on the tremendous gap between the conventional 5% false positive rate (i.e., statistical significance level of 0.05) and the estimates reported below and elsewhere (see S1 Text and Fig 1 ). Although the optimal statistical power of each study will depend on its objectives, this large gap suggests that published preclinical study results are often less reliable than claimed. From an economic perspective, the system is highly inefficient. While there are several root causes, one overarching source of inefficiency is the continued emphasis on placing responsibility with the researcher—despite the fact that a significant portion of the costs of irreproducibility are ultimately borne by downstream parties in the translation of bench discoveries to bedside therapies [ 13 ].
Analysis of Four Categories of Irreproducibility
Many studies have concluded that the prevalence of irreproducible biomedical research is substantial [ 1 ]. The wide range of published estimates reflects the challenges of accurately quantifying and subsequently addressing the problem. Multiple systemic causes contribute to irreproducibility and many can ultimately be traced to an underlying lack of a standards and best practices framework [ 13 ]. However, it is reasonable to state that cumulative errors in the following broad categories—as well as underlying biases that could contribute to each problem area [ 14 ] or even result in entire studies never being published or reported [ 15 ]—are the primary causes of irreproducibility [ 16 ]: (1) study design, (2) biological reagents and reference materials, (3) laboratory protocols, and (4) data analysis and reporting. Fig 2 , S1 Text , S1 and S2 Datasets show the results of our analysis, which estimates the prevalence (low, high, and midpoint estimates) of errors in each category and builds up to a cumulative (total) irreproducibility rate that exceeds 50%. Using a highly conservative probability bounds approach [ 17 ], we estimate that the cumulative rate of preclinical irreproducibility lies between 18% (the maximum of the low estimates, assuming maximum overlap between categories), and 88.5% (the sum of the high estimates, assuming minimal overlap). A natural point estimate of the cumulative irreproducibility rate is the midpoint of the upper and lower bounds, or 53.3%.
Note that the percentage value of error for each category is the midpoint of the high and low prevalence estimates for that category divided (weighted) by the sum of all midpoint error rates (see S1 Dataset ). Source: Chakma et al. [ 18 ] and the American Association for the Advancement of Science (AAAS) [ 19 ].
https://doi.org/10.1371/journal.pbio.1002165.g002
Limitations of the Analysis
This analysis is subject to a number of important limitations, including (1) the small number of studies we were able to identify that provide or support the determination of low, high, and midpoint estimates of prevalence rates for one or more categories of irreproducibility; (2) the lack of consistency as to how reproducibility and irreproducibility are defined across studies; and (3) in some cases, extrapolating from a clinical environment to the preclinical setting when no suitable preclinical studies were available. For these reasons, a rigorous meta-analysis or systematic review was also not feasible. To estimate a theoretically optimal baseline rate of irreproducibility, we would also need data on the financial and opportunity costs of irreproducibility and how these costs (and benefits) vary within the population of preclinical studies. Nonetheless, even simple calculations of direct costs can show that irreproducible preclinical research is a significant problem in terms of lost dollars and lost opportunities for scientific discovery.
Economic Impact of Irreproducibility
Extrapolating from 2012 data, an estimated US$114.8B in the United States [ 18 ] is spent annually on life sciences research, with the pharmaceutical industry being the largest funder at 61.8%, followed by the federal government (31.5%), nonprofits (3.8%), and academia (3.0%) [ 20 ]. Of this amount, an estimated US$56.4B (49%) is spent on preclinical research, with government sources providing the majority of funding (roughly US$38B) [ 19 ]. Using a conservative cumulative irreproducibility rate of 50% means that approximately US$28B/year is spent on research that cannot be replicated (see Fig 2 and S2 Dataset ). Of course, uncertainty remains about the precise magnitude of the direct economic costs—the conservative probability bounds approach reported above suggest that these costs could plausibly be much smaller or much larger than US$28B. Nevertheless, we believe a 50% irreproducibility rate, leading to direct costs of approximately US$28B/year, provides a reasonable starting point for further debate. To be clear, this does not imply that there was no return on that investment. As noted in a recent paper by Stern et al. [ 21 ], even in cases of retracted publications due to scientific misconduct, which is not a major source of irreproducibility [ 13 , 22 ], “it is conceivable that some of the research resulting in a retracted article still provides useful information for other nonretracted studies.” However, it does suggest that, even under our relatively conservative assumptions, the impact of the reproducibility problem is economically significant.
Irreproducibility also has downstream impacts in the drug development pipeline. Academic research studies with potential clinical applications are typically replicated within the pharmaceutical industry before clinical studies are begun, with each study replication requiring between 3 and 24 months and between US$500,000 to US$2,000,000 investment [ 23 ]. While industry will continue to replicate external studies for their own drug discovery process, a substantially improved preclinical reproducibility rate would derisk or result in an increased hit rate on such investments, both increasing the productivity of life science research and improving the speed and efficiency of the therapeutic drug development processes. The annual value added to the return on investment from taxpayer dollars would be in the billions in the US alone.
The Role of Best Practices and Standards
Many key stakeholder groups are developing and piloting a range of solutions to help increase reproducibility in preclinical research. For example, the National Institutes of Health (NIH) have recently announced a list of Principles and Guidelines for Reporting Preclinical Research [ 24 ], which over 100 journals have joined as cosignatories and that builds on previous recommendations by Landis et al. [ 25 ] to improve methodological reporting of animal studies in grant applications and publications. Despite the emergence of a wide variety of reporting guidelines to improve reporting of biomedical research methods and results, to date, compliance levels and their impact to improve reproducibility have been disappointing [ 26 ]. Given the size, scale, and complexity of the challenge of reproducibility in preclinical research, there is no single magic bullet solution to the problem. However, one issue that has shown demonstrable impact on similar challenges in other settings is the expanded development and adoption of standards and best practices [ 13 ].
In the information and communication technology industries, several standard development organizations have moved beyond simply defining technical interfaces to assume the role of a governing body for critical pieces of shared infrastructure. The Internet is a prime example. The evolution of the Web has been messy; constrained by patent claims, the financial benefit of controlling standards, and confusion over the evolutionary model. However, two organizations, the World Wide Web Consortium (W3C) and the Internet Engineering Task Force (IETF) emerged to develop Web standards and maintain its interoperability as a universal space. The W3C is an excellent example of a successful, internally driven and self-regulating international consortium comprising a public and private partnership working together. Similarly, the IETF operates as a noncommercial/not-for-profit/nongovernmental organization and operates a large number of work groups and informal discussion groups, working on specific, timely issues, then disbanding once these issues are addressed. In the early days of the Internet, both groups successfully steered major global players toward common standards requiring each to compromise and adapt in the short term, but ultimately gain tremendous benefits over the longer horizon.
Although neither example focuses directly on reproducibility, they highlight the importance for the life sciences to engage all stakeholders in a dynamic, collaborative effort to standardize common scientific processes. In the clinical research arena, where the stakes are high and oversight by the US Food and Drug Administration (FDA) is stringent, irreproducibility has been reduced to rates that are generally considered to be scientifically and commercially appropriate [ 1 ]. However, this level of stringent oversight often precludes the direct application of clinical methods, practices, and procedures to preclinical research [ 27 ]. Furthermore, in a clinical setting, the number of assays and interventions is tightly controlled, which is not typically possible in a basic or preclinical research environment without incurring a significant increase in time and cost. Nonetheless, economic research also has shown that standardization and auditing of biological materials—through biological resource centers—can enhance cumulative production of scientific knowledge by improving both availability and reliability of research inputs [ 28 ].
An illustrative example is the use and misuse of cancer cell lines. The history of cell lines used in biomedical research is riddled with misidentification and cross-contamination events [ 29 ], which have been estimated to range from 15% to 36% [ 30 ]. Yet despite the availability of the short tandem repeat (STR) analysis as an accepted standard to authenticate cell lines, and its relatively low cost (approximately US$200 per assay), only one-third of labs typically test their cell lines for identity [ 31 ]. For an NIH-funded academic researcher receiving an average US$450,000, four-year grant, purchasing cell lines from a reputable vendor (or validating their own stock) and then authenticating annually will only cost about US$1,000 or 0.2% of the award. A search of NIH Reporter for projects using “cell line” or “cell culture” suggests that NIH currently funds about US$3.7B annually on research using cell lines. Given that a quarter of these research projects apparently use misidentified or contaminated cell lines, reducing this to even 10% through a broader application of the STR standard—a very realistic goal—would ensure a more effective use of nearly three-quarters of a billion dollars and ultimately speed the progress of research and the development of new treatments for disease.
The economics literature on standardization posits that unless there is a clearly dominant platform leader willing to impose a solution, complex challenges such as irreproducibility that require a coordinated response are best solved by internally organized and driven, dynamic, and self-regulating collaborations of key stakeholders who establish and enforce their respective rules of engagement [ 32 , 33 ]. What is needed is not another list of unfunded mandates, but rather community consensus on priorities for improvement and commitment for the additional funding for implementation. This includes training that focuses specifically on the importance of standards and best practices in basic research in graduate and postdoctoral programs, as well as quality management systems to ensure that best practices are implemented throughout the research process. No doubt that improving training and increasing quality control measures will add costs to the preclinical research enterprise. One estimate in a clinical setting suggests the adoption of mandated quality control procedures would increase costs to 15% to 25% above current spending levels [ 34 ]. However, the societal benefits garnered from an increase in reproducible life science research far outweigh the cost. Assuming that we could recover even half of the approximately US$28 billion annually spent on irreproducible preclinical research in the US alone by applying best practices and standards, the savings would be roughly US$14B/year. Moreover, because our analysis indicates that errors in study design and biological reagents and materials contribute to a majority of this spend (see Fig 2 ), implementing steps to improve preclinical reproducibility should be a priority in these two areas (see Box 1 ).
Box 1. Investing in Practical Solutions
Taking immediate steps in two areas where there will be significant return on investment—study design and biological reagents and reference materials—will yield substantial improvements in preclinical reproducibility rates.
Study Design
- Improve training programs at academic institutions to ensure that best practices are reinforced in the areas of core skills, methods, technology, and tools.
- Establish targeted training, coaching, and certification of established principal investigators (PIs) to reinforce application of best practices throughout the research process.
- Establish research funder policies, including funders such as NIH and leading disease foundations, requiring successful completion of training courses at all levels.
Biological Reagents and Reference Materials
- Promote broad adoption by vendors to offer only validated reagents (e.g., antibodies and cell lines) and broad utilization of these reagents by PIs as a documented best practice in the research process.
- Ensure that research funder policies require documented use of validated and noncontaminated reagents, annual reagent authentication throughout the research study, and adequate funding to cover these additional costs.
- Ensure that procedures to document reagent validation and lack of contamination are required by publishers.
- Incentivize the continued development of tools for reagent validation using improved genomics data.
- Define standard operating procedures for biological materials handling throughout the material’s lifecycle.
In order to change practices throughout the preclinical research community, all invested stakeholders (academia, journals, industry, and government) must work in partnership to develop, institutionalize, and reward (or even sanction) behaviors, working within a mutually agreed upon set of rules and guiding principles. Such dynamic collaborations could more efficiently represent the needs of all stakeholders and provide unifying guidance and funding suggestions to facilitate meaningful change. Establishing effective collaborative efforts is no simple feat, but we can look to other industries that have been successful in the past as models for the life science community.
Conclusions
Although differing perspectives on the irreproducibility rate in preclinical research may persist, one fact remains clear: the challenge of increasing reproducibility and addressing the costs associated with the lack of reproducibility in life science research is simply too important and costly to ignore. Lifesaving therapies are being delayed, research budgets face increasing pressure, and drug development and treatment costs are rising. Improving reproducibility remains a critical cornerstone to solving each of these challenges. There are no easy answers to this problem. Real solutions, such as addressing errors in study design and using high quality biological reagents and reference materials, will require time, resources, and collaboration between diverse stakeholders that will be a key precursor to change. Millions of patients are waiting for therapies and cures that must first survive preclinical challenges. Although any effort to improve reproducibility levels will require a measured investment in capital and time, the long-term benefits to society that are derived from increased scientific fidelity will greatly exceed the upfront costs.
Supporting Information
S1 text. analysis to determine irreproducibility rate of preclinical research..
https://doi.org/10.1371/journal.pbio.1002165.s001
S1 Dataset. Analysis.
https://doi.org/10.1371/journal.pbio.1002165.s002
S2 Dataset. Economic impact.
https://doi.org/10.1371/journal.pbio.1002165.s003
Acknowledgments
We thank A. Gerstein and S. Rosenfield of Genesis Revenue Partners and the staff of the Global Biological Standards Institute (GBSI) for their support of this project.
- View Article
- PubMed/NCBI
- Google Scholar
- 13. GBSI (2013) The Case for Standards in Life Science Research: Seizing Opportunities at a Time of Critical Need. Washington, D.C.: Global Biological Standards Institute (GBSI). 41 p.
- 17. Manski CF (2003) Partial Identification of Probability Distributions. In: Manski CF, editor. Springer Series in Statistics New York, New York, USA: Springer International Publishing AG. pp. 178.
- 19. AAAS (2013) AAAS Report XXXVIII: Research and Development FY 2014. Washington, DC, USA: American Association for the Advancement of Science (AAAS). 315 p.
- 20. Battelle (2013) 2014 Global R&D Funding Forecast. Columbus, Ohio, USA: Battelle. 36 p.
- 23. PhRMA (2013) 2013 Biopharmaceutical Research Industry Profile. Washington, DC, USA: Pharmaceutical Research and Manufacturers of America (PhRMA). 78 p.
- 32. Farrell J, Simcoe T (2012) Four Paths to Compatibility. In: Peitz M, Waldfogel J, editors. The Oxford Handbook of the Digital Economy. New York, New York, USA: Oxford University Press. pp. 34–58.
- 33. Ostrom E (1990) Governing the Commons: The Evolution of Institutions for Collective Action. New York, New York, USA: Oxford University Press. 298 p.
- 34. Berte LM, Daley AT (2014) Understanding the Cost of Quality in the Laboratory; A Report. Wayne, Pennsylvania, USA: Clinical Laboratory Standards Institute (CLSI). QMS20-R QMS20-R 94 p.
- Technical Help
- CE/CME Help
- Billing Help
- Sales Inquiries
- CE Certificates
- Billing Inquiries
- Purchase Inquiries
The Importance of Rigor and Reproducibility in Research
The National Institutes of Health (NIH) emphasizes rigor in designing and performing scientific research and the ability to reproduce biomedical research findings as two of the cornerstones of science advancement. See Rigor and Reproducibility to learn more about NIH’s initiatives.
Research Study Design Training
The Research Study Design course provides you with:
- An introduction to research study design
- A detailed overview of scientific inquiry
- Examples of various research designs
- A discussion of data management methods
- An introduction to statistical analysis
- Sound approaches to optimize the reproducibility of research results
View Course | Add Course
Responsible Conduct of Research (RCR) Training
CITI Program’s RCR Basic course covers the core norms, principles, regulations, and best practices for the conduct of ethical research. A module on “Reproducibility of Research Results” discusses factors that contribute to the lack of reproducibility and the effects non-reproducibility has on the scientific community and society in general. The module also describes the stakeholders affected by non-reproducible science, initiatives to enhance reproducibility, and strategies that you can employ to enhance reproducibility in your research.
View Courses | Add Course | Add Module
Back to Newsletter
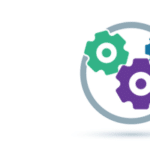
- Dr. Paul Braunschweiger Scholarship Awards its 2023 Recipient
- New Webinar – I’ve Been Funded, Now What?
- New Biotility Course – The Foundations of Lean Manufacturing
- New Webinar – Expanding Our Concept of Access: Universal Design in Online Course Design
Privacy Overview

An official website of the United States government
The .gov means it's official. Federal government websites often end in .gov or .mil. Before sharing sensitive information, make sure you're on a federal government site.
The site is secure. The https:// ensures that you are connecting to the official website and that any information you provide is encrypted and transmitted securely.
- Publications
- Account settings
- Browse Titles
NCBI Bookshelf. A service of the National Library of Medicine, National Institutes of Health.
National Academies of Sciences, Engineering, and Medicine; Policy and Global Affairs; Committee on Science, Engineering, Medicine, and Public Policy; Board on Research Data and Information; Division on Engineering and Physical Sciences; Committee on Applied and Theoretical Statistics; Board on Mathematical Sciences and Analytics; Division on Earth and Life Studies; Nuclear and Radiation Studies Board; Division of Behavioral and Social Sciences and Education; Committee on National Statistics; Board on Behavioral, Cognitive, and Sensory Sciences; Committee on Reproducibility and Replicability in Science. Reproducibility and Replicability in Science. Washington (DC): National Academies Press (US); 2019 May 7.

Reproducibility and Replicability in Science.
- Hardcopy Version at National Academies Press
3 Understanding Reproducibility and Replicability
In 2013, the cover story of The Economist, “How Science Goes Wrong,” brought public attention to issues of reproducibility and replicability across science and engineering. In this chapter, we discuss how the practice of science has evolved and how these changes have introduced challenges to reproducibility and replicability. Because the terms reproducibility and replicability are used differently across different scientific disciplines, introducing confusion to a complicated set of challenges and solutions, the committee also details its definitions and highlights the scope and expression of the problems of non-reproducibility and non-replicability across science and engineering research.
- THE EVOLVING PRACTICES OF SCIENCE
Scientific research has evolved from an activity mainly undertaken by individuals operating in a few locations to many teams, large communities, and complex organizations involving hundreds to thousands of individuals worldwide. In the 17th century, scientists would communicate through letters and were able to understand and assimilate major developments across all the emerging major disciplines. In 2016—the most recent year for which data are available—more than 2,295,000 scientific and engineering research articles were published worldwide ( National Science Foundation, 2018e ). In addition, the number of scientific and engineering fields and subfields of research is large and has greatly expanded in recent years, especially in fields that intersect disciplines (e.g., biophysics); more than 230 distinct fields and subfields can now be identified. The published literature is so voluminous and specialized that some researchers look to information retrieval, machine learning, and artificial intelligence techniques to track and apprehend the important work in their own fields.
Another major revolution in science came with the recent explosion of the availability of large amounts of data in combination with widely available and affordable computing resources. These changes have transformed many disciplines, enabled important scientific discoveries, and led to major shifts in science. In addition, the use of statistical analysis of data has expanded, and many disciplines have come to rely on complex and expensive instrumentation that generates and can automate analysis of large digital datasets.
Large-scale computation has been adopted in fields as diverse as astronomy, genetics, geoscience, particle physics, and social science, and has added scope to fields such as artificial intelligence. The democratization of data and computation has created new ways to conduct research; in particular, large-scale computation allows researchers to do research that was not possible a few decades ago. For example, public health researchers mine large databases and social media, searching for patterns, while earth scientists run massive simulations of complex systems to learn about the past, which can offer insight into possible future events.
Another change in science is an increased pressure to publish new scientific discoveries in prestigious and what some consider high-impact journals, such as Nature and Science. 1 This pressure is felt worldwide, across disciplines, and by researchers at all levels but is perhaps most acute for researchers at the beginning of their scientific careers who are trying to establish a strong scientific record to increase their chances of obtaining tenure at an academic institution and grants for future work. Tenure decisions have traditionally been made on the basis of the scientific record (i.e., published articles of important new results in a field) and have given added weight to publications in more prestigious journals. Competition for federal grants, a large source of academic research funding, is intense as the number of applicants grows at a rate higher than the increase in federal research budgets. These multiple factors create incentives for researchers to overstate the importance of their results and increase the risk of bias—either conscious or unconscious—in data collection, analysis, and reporting.
In the context of these dynamic changes, the questions and issues related to reproducibility and replicability remain central to the development and evolution of science. How should studies and other research approaches be designed to efficiently generate reliable knowledge? How might hypotheses and results be better communicated to allow others to confirm, refute, or build on them? How can the potential biases of scientists themselves be understood, identified, and exposed in order to improve accuracy in the generation and interpretation of research results? How can intentional misrepresentation and fraud be detected and eliminated? 2
Researchers have proposed approaches to answering some of the questions over the past decades. As early as the 1960s, Jacob Cohen surveyed psychology articles from the perspective of statistical power to detect effect sizes, an approach that launched many subsequent power surveys (also known as meta-analyses) in the social sciences in subsequent years ( Cohen, 1988 ).
Researchers in biomedicine have been focused on threats to validity of results since at least the 1970s. In response to the threat, biomedical researchers developed a wide variety of approaches to address the concern, including an emphasis on randomized experiments with masking (also known as blinding), reliance on meta-analytic summaries over individual trial results, proper sizing and power of experiments, and the introduction of trial registration and detailed experimental protocols. Many of the same approaches have been proposed to counter shortcomings in reproducibility and replicability.
Reproducibility and replicability as they relate to data and computation-intensive scientific work received attention as the use of computational tools expanded. In the 1990s, Jon Claerbout launched the “reproducible research movement,” brought on by the growing use of computational workflows for analyzing data across a range of disciplines ( Claerbout and Karrenbach, 1992 ). Minor mistakes in code can lead to serious errors in interpretation and in reported results; Claerbout's proposed solution was to establish an expectation that data and code will be openly shared so that results could be reproduced. The assumption was that reanalysis of the same data using the same methods would produce the same results.
In the 2000s and 2010s, several high-profile journal and general media publications focused on concerns about reproducibility and replicability (see, e.g., Ioannidis, 2005 ; Baker, 2016 ), including the cover story in The Economist ( “How Science Goes Wrong,” 2013 ) noted above. These articles introduced new concerns about the availability of data and code and highlighted problems of publication bias, selective reporting, and misaligned incentives that cause positive results to be favored for publication over negative or nonconfirmatory results. 3 Some news articles focused on issues in biomedical research and clinical trials, which were discussed in the general media partly as a result of lawsuits and settlements over widely used drugs ( Fugh-Berman, 2010 ).
Many publications about reproducibility and replicability have focused on the lack of data, code, and detailed description of methods in individual studies or a set of studies. Several attempts have been made to assess non-reproducibility or non-replicability within a field, particularly in social sciences (e.g., Camerer et al., 2018 ; Open Science Collaboration, 2015 ). In Chapters 4 , 5 , and 6 , we review in more detail the studies, analyses, efforts to improve, and factors that affect the lack of reproducibility and replicability. Before that discussion, we must clearly define these terms.
- DEFINING REPRODUCIBILITY AND REPLICABILITY
Different scientific disciplines and institutions use the words reproducibility and replicability in inconsistent or even contradictory ways: What one group means by one word, the other group means by the other word. 4 These terms—and others, such as repeatability—have long been used in relation to the general concept of one experiment or study confirming the results of another. Within this general concept, however, no terminologically consistent way of drawing distinctions has emerged; instead, conflicting and inconsistent terms have flourished. The difficulties in assessing reproducibility and replicability are complicated by this absence of standard definitions for these terms.
In some fields, one term has been used to cover all related concepts: for example, “replication” historically covered all concerns in political science ( King, 1995 ). In many settings, the terms reproducible and replicable have distinct meanings, but different communities adopted opposing definitions ( Claerbout and Karrenbach, 1992 ; Peng et al., 2006 ; Association for Computing Machinery, 2018 ). Some have added qualifying terms, such as methods reproducibility, results reproducibility, and inferential reproducibility to the lexicon ( Goodman et al., 2016 ). In particular, tension has emerged between the usage recently adopted in computer science and the way that researchers in other scientific disciplines have described these ideas for years ( Heroux et al., 2018 ).
In the early 1990s, investigators began using the term “reproducible research” for studies that provided a complete digital compendium of data and code to reproduce their analyses, particularly in the processing of seismic wave recordings ( Claerbout and Karrenbach, 1992 ; Buckheit and Donoho, 1995 ). The emphasis was on ensuring that a computational analysis was transparent and documented so that it could be verified by other researchers. While this notion of reproducibility is quite different from situations in which a researcher gathers new data in the hopes of independently verifying previous results or a scientific inference, some scientific fields use the term reproducibility to refer to this practice. Peng et al. (2006 , p. 783) referred to this scenario as “replicability,” noting: “Scientific evidence is strengthened when important results are replicated by multiple independent investigators using independent data, analytical methods, laboratories, and instruments.” Despite efforts to coalesce around the use of these terms, lack of consensus persists across disciplines. The resulting confusion is an obstacle in moving forward to improve reproducibility and replicability ( Barba, 2018 ).
In a review paper on the use of the terms reproducibility and replicability, Barba (2018) outlined three categories of usage, which she characterized as A, B1, and B2:
A: The terms are used with no distinction between them. B1: “Reproducibility” refers to instances in which the original researcher's data and computer codes are used to regenerate the results, while “replicability” refers to instances in which a researcher collects new data to arrive at the same scientific findings as a previous study. B2: “Reproducibility” refers to independent researchers arriving at the same results using their own data and methods, while “replicability” refers to a different team arriving at the same results using the original author's artifacts.
B1 and B2 are in opposition of each other with respect to which term involves reusing the original authors' digital artifacts of research (“research compendium”) and which involves independently created digital artifacts. Barba (2018) collected data on the usage of these terms across a variety of disciplines (see Table 3-1 ). 5
Usage of the Terms Reproducibility and Replicability by Scientific Discipline.
The terminology adopted by the Association for Computing Machinery (ACM) for computer science was published in 2016 as a system for badges attached to articles published by the society. The ACM declared that its definitions were inspired by the metrology vocabulary, and it associated using an original author's digital artifacts to “replicability,” and developing completely new digital artifacts to “reproducibility.” These terminological distinctions contradict the usage in computational science, where reproducibility is associated with transparency and access to the author's digital artifacts, and also with social sciences, economics, clinical studies, and other domains, where replication studies collect new data to verify the original findings.
Regardless of the specific terms used, the underlying concepts have long played essential roles in all scientific disciplines. These concepts are closely connected to the following general questions about scientific results:
- Are the data and analysis laid out with sufficient transparency and clarity that the results can be checked ?
- If checked, do the data and analysis offered in support of the result in fact support that result?
- If the data and analysis are shown to support the original result, can the result reported be found again in the specific study context investigated?
- Finally, can the result reported or the inference drawn be found again in a broader set of study contexts ?
Computational scientists generally use the term reproducibility to answer just the first question—that is, reproducible research is research that is capable of being checked because the data, code, and methods of analysis are available to other researchers. The term reproducibility can also be used in the context of the second question: research is reproducible if another researcher actually uses the available data and code and obtains the same results. The difference between the first and the second questions is one of action by another researcher; the first refers to the availability of the data, code, and methods of analysis, while the second refers to the act of recomputing the results using the available data, code, and methods of analysis.
In order to answer the first and second questions, a second researcher uses data and code from the first; no new data or code are created by the second researcher. Reproducibility depends only on whether the methods of the computational analysis were transparently and accurately reported and whether that data, code, or other materials were used to reproduce the original results. In contrast, to answer question three, a researcher must redo the study, following the original methods as closely as possible and collecting new data. To answer question four, a researcher could take a variety of paths: choose a new condition of analysis, conduct the same study in a new context, or conduct a new study aimed at the same or similar research question.
For the purposes of this report and with the aim of defining these terms in ways that apply across multiple scientific disciplines, the committee has chosen to draw the distinction between reproducibility and replicability between the second and third questions. Thus, reproducibility includes the act of a second researcher recomputing the original results, and it can be satisfied with the availability of data, code, and methods that makes that recomputation possible. This definition of reproducibility refers to the transparency and reproducibility of computations: that is, it is synonymous with “computational reproducibility,” and we use the terms interchangeably in this report.
When a new study is conducted and new data are collected, aimed at the same or a similar scientific question as a previous one, we define it as a replication. A replication attempt might be conducted by the same investigators in the same lab in order to verify the original result, or it might be conducted by new investigators in a new lab or context, using the same or different methods and conditions of analysis. If this second study, aimed at the same scientific question but collecting new data, finds consistent results or can draw consistent conclusions, the research is replicable. If a second study explores a similar scientific question but in other contexts or populations that differ from the original one and finds consistent results, the research is “generalizable.” 6
In summary, after extensive review of the ways these terms are used by different scientific communities, the committee adopted specific definitions for this report.
CONCLUSION 3-1: For this report, reproducibility is obtaining consistent results using the same input data; computational steps, methods, and code; and conditions of analysis. This definition is synonymous with “computational reproducibility,” and the terms are used interchangeably in this report.
Replicability is obtaining consistent results across studies aimed at answering the same scientific question, each of which has obtained its own data.
Two studies may be considered to have replicated if they obtain consistent results given the level of uncertainty inherent in the system under study. In studies that measure a physical entity (i.e., a measurand), the results may be the sets of measurements of the same measurand obtained by different laboratories. In studies aimed at detecting an effect of an intentional intervention or a natural event, the results may be the type and size of effects found in different studies aimed at answering the same question. In general, whenever new data are obtained that constitute the results of a study aimed at answering the same scientific question as another study, the degree of consistency of the results from the two studies constitutes their degree of replication.
Two important constraints on the replicability of scientific results rest in limits to the precision of measurement and the potential for altered results due to sometimes subtle variation in the methods and steps performed in a scientific study. We expressly consider both here, as they can each have a profound influence on the replicability of scientific studies.
- PRECISION OF MEASUREMENT
Virtually all scientific observations involve counts, measurements, or both. Scientific measurements may be of many different kinds: spatial dimensions (e.g., size, distance, and location), time, temperature, brightness, colorimetric properties, electromagnetic properties, electric current, material properties, acidity, and concentration, to name a few from the natural sciences. The social sciences are similarly replete with counts and measures. With each measurement comes a characterization of the margin of doubt, or an assessment of uncertainty ( Possolo and Iyer, 2017 ). Indeed, it may be said that measurement, quantification, and uncertainties are core features of scientific studies.
One mark of progress in science and engineering has been the ability to make increasingly exact measurements on a widening array of objects and phenomena. Many of the things taken for granted in the modern world, from mechanical engines to interchangeable parts to smartphones, are possible only because of advances in the precision of measurement over time ( Winchester, 2018 ).
The concept of precision refers to the degree of closeness in measurements. As the unit used to measure distance, for example, shrinks from meter to centimeter to millimeter and so on down to micron, nanometer, and angstrom, the measurement unit becomes more exact and the proximity of one measurand to a second can be determined more precisely.
Even when scientists believe a quantity of interest is constant, they recognize that repeated measurement of that quantity may vary because of limits in the precision of measurement technology. It is useful to note that precision is different from the accuracy of a measurement system, as shown in Figure 3-1 , demonstrating the differences using an archery target containing three arrows.
Accuracy and precision of a measurement. NOTE: See text for discussion. SOURCE: Chemistry LibreTexts. Available: https://chem.libretexts.org/Bookshelves/Introductory_Chemistry/Book%3A_IntroductoryChemistry_(CK-12)/03%3A_Measurements/3.12%3A_Accuracy_and_Precision. (more...)
In Figure 3-1 , A, the three arrows are in the outer ring, not close together and not close to the bull's eye, illustrating low accuracy and low precision (i.e., the shots have not been accurate and are not highly precise). In B, the arrows are clustered in a tight band in an outer ring, illustrating low accuracy and high precision (i.e., the shots have been more precise, but not accurate). The other two figures similarly illustrate high accuracy and low precision (C) and high accuracy and high precision (D).
It is critical to keep in mind that the accuracy of a measurement can be judged only in relation to a known standard of truth. If the exact location of the bull's eye is unknown, one must not presume that a more precise set of measures is necessarily more accurate; the results may simply be subject to a more consistent bias, moving them in a consistent way in a particular direction and distance from the true target.
It is often useful in science to describe quantitatively the central tendency and degree of dispersion among a set of repeated measurements of the same entity and to compare one set of measurements with a second set. When a set of measurements is repeated by the same operator using the same equipment under constant conditions and close in time, metrologists refer to the proximity of these measurements to one another as measurement repeatability (see Box 3-1 ). When one is interested in comparing the degree to which the set of measurements obtained in one study are consistent with the set of measurements obtained in a second study, the committee characterizes this as a test of replicability because it entails the comparison of two studies aimed at the same scientific question where each obtained its own data.
Terms Used in Metrology and How They Differ from the Committee's Definitions.
Consider, for example, the set of measurements of the physical constant obtained over time by a number of laboratories (see Figure 3-2 ). For each laboratory's results, the figure depicts the mean observation (i.e., the central tendency) and standard error of the mean, indicated by the error bars. The standard error is an indicator of the precision of the obtained measurements, where a smaller standard error represents higher precision. In comparing the measurements obtained by the different laboratories, notice that both the mean values and the degrees of precision (as indicated by the width of the error bars) may differ from one set of measurements to another.
Evolution of scientific understanding of the fine structure constant over time. NOTES: Error bars indicate the experimental uncertainty of each measurement. See text for discussion. SOURCE: Reprinted figure with permission from Peter J. Mohr, David B. (more...)
We may now ask what is a central question for this study: How well does a second set of measurements (or results) replicate a first set of measurements (or results)? Answering this question, we suggest, may involve three components:
proximity of the mean value (central tendency) of the second set relative to the mean value of the first set, measured both in physical units and relative to the standard error of the estimate
similitude in the degree of dispersion in observed values about the mean in the second set relative to the first set
likelihood that the second set of values and the first set of values could have been drawn from the same underlying distribution
Depending on circumstances, one or another of these components could be more salient for a particular purpose. For example, two sets of measures could have means that are very close to one another in physical units, yet each were sufficiently precisely measured as to be very unlikely to be different by chance. A second comparison may find means are further apart, yet derived from more widely dispersed sets of observations, so that there is a higher likelihood that the difference in means could have been observed by chance. In terms of physical proximity, the first comparison is more closely replicated. In terms of the likelihood of being derived from the same underlying distribution, the second set is more highly replicated.
A simple visual inspection of the means and standard errors for measurements obtained by different laboratories may be sufficient for a judgment about their replicability. For example, in Figure 3-2 , it is evident that the bottom two measurement results have relatively tight precision and means that are nearly identical, so it seems reasonable these can be considered to have replicated one another. It is similarly evident that results from LAMPF (second from the top of reported measurements with a mean value and error bars in Figure 3-2 ) are better replicated by results from LNE-01 (fourth from top) than by measurements from NIST-89 (sixth from top). More subtle may be judging the degree of replication when, for example, one set of measurements has a relatively wide range of uncertainty compared to another. In Figure 3-2 , the uncertainty range from NPL-88 (third from top) is relatively wide and includes the mean of NIST-97 (seventh from top); however, the narrower uncertainty range for NIST-97 does not include the mean from NPL-88. Especially in such cases, it is valuable to have a systematic, quantitative indicator of the extent to which one set of measurements may be said to have replicated a second set of measurements, and a consistent means of quantifying the extent of replication can be useful in all cases.
- VARIATIONS IN METHODS EMPLOYED IN A STUDY
When closely scrutinized, a scientific study or experiment may be seen to entail hundreds or thousands of choices, many of which are barely conscious or taken for granted. In the laboratory, exactly what size of Erlenmeyer flask is used to mix a set of reagents? At what exact temperature were the reagents stored? Was a drying agent such as acetone used on the glassware? Which agent and in what amount and exact concentration? Within what tolerance of error are the ingredients measured? When ingredient A was combined with ingredient B, was the flask shaken or stirred? How vigorously and for how long? What manufacturer of porcelain filter was used? If conducting a field survey, how exactly, were the subjects selected? Are the interviews conducted by computer or over the phone or in person? Are the interviews conducted by female or male, young or old, the same or different race as the interviewee? What is the exact wording of a question? If spoken, with what inflection? What is the exact sequence of questions? Without belaboring the point, we can say that many of the exact methods employed in a scientific study may or may not be described in the methods section of a publication. An investigator may or may not realize when a possible variation could be consequential to the replicability of results.
In a later section, we will deal more generally with sources of non-replicability in science (see Chapter 5 and Box 5-2 ). Here, we wish to emphasize that countless subtle variations in the methods, techniques, sequences, procedures, and tools employed in a study may contribute in unexpected ways to differences in the obtained results (see Box 3-2 ).
Data Collection, Cleaning, and Curation.
Finally, note that a single scientific study may entail elements of the several concepts introduced and defined in this chapter, including computational reproducibility, precision in measurement, replicability, and generalizability or any combination of these. For example, a large epidemiological survey of air pollution may entail portable, personal devices to measure various concentrations in the air (subject to precision of measurement), very large datasets to analyze (subject to computational reproducibility), and a large number of choices in research design, methods, and study population (subject to replicability and generalizability).
- RIGOR AND TRANSPARENCY
The committee was asked to “make recommendations for improving rigor and transparency in scientific and engineering research” (refer to Box 1-1 in Chapter 1 ). In response to this part of our charge, we briefly discuss the meanings of rigor and of transparency below and relate them to our topic of reproducibility and replicability.
Rigor is defined as “the strict application of the scientific method to ensure robust and unbiased experimental design” ( National Institutes of Health, 2018e ). Rigor does not guarantee that a study will be replicated, but conducting a study with rigor—with a well-thought-out plan and strict adherence to methodological best practices—makes it more likely. One of the assumptions of the scientific process is that rigorously conducted studies “and accurate reporting of the results will enable the soundest decisions” and that a series of rigorous studies aimed at the same research question “will offer successively ever-better approximations to the truth” ( Wood et al., 2019 , p. 311). Practices that indicate a lack of rigor, including poor study design, errors or sloppiness, and poor analysis and reporting, contribute to avoidable sources of non-replicability (see Chapter 5 ). Rigor affects both reproducibility and replicability.
Transparency has a long tradition in science. Since the advent of scientific reports and technical conferences, scientists have shared details about their research, including study design, materials used, details of the system under study, operationalization of variables, measurement techniques, uncertainties in measurement in the system under study, and how data were collected and analyzed. A transparent scientific report makes clear whether the study was exploratory or confirmatory, shares information about what measurements were collected and how the data were prepared, which analyses were planned and which were not, and communicates the level of uncertainty in the result (e.g., through an error bar, sensitivity analysis, or p- value). Only by sharing all this information might it be possible for other researchers to confirm and check the correctness of the computations, attempt to replicate the study, and understand the full context of how to interpret the results. Transparency of data, code, and computational methods is directly linked to reproducibility, and it also applies to replicability. The clarity, accuracy, specificity, and completeness in the description of study methods directly affects replicability.
FINDING 3-1: In general, when a researcher transparently reports a study and makes available the underlying digital artifacts, such as data and code, the results should be computationally reproducible. In contrast, even when a study was rigorously conducted according to best practices, correctly analyzed, and transparently reported, it may fail to be replicated.
“High-impact” journals are viewed by some as those which possess high scores according to one of the several journal impact indicators such as Citescore, Scimago Journal Ranking (SJR), Source Normalized Impact per Paper (SNIP)—which are available in Scopus—and Journal Impact Factor (IF), Eigenfactor (EF), and Article Influence Score (AIC)—which can be obtained from the Journal Citation Report (JCR).
See Chapter 5 , Fraud and Misconduct, which further discusses the association between misconduct as a source of non-replicability, its frequency, and reporting by the media.
One such outcome became known as the “file drawer problem”: see Chapter 5 ; also see Rosenthal (1979) .
For the negative case, both “non-reproducible” and “irreproducible” are used in scientific work and are synonymous.
See also Heroux et al. (2018) for a discussion of the competing taxonomies between computational sciences (B1) and new definitions adopted in computer science (B2) and proposals for resolving the differences.
The committee definitions of reproducibility, replicability, and generalizability are consistent with the National Science Foundation's Social, Behavioral, and Economic Sciences Perspectives on Robust and Reliable Science ( Bollen et al., 2015 ).
- Cite this Page National Academies of Sciences, Engineering, and Medicine; Policy and Global Affairs; Committee on Science, Engineering, Medicine, and Public Policy; Board on Research Data and Information; Division on Engineering and Physical Sciences; Committee on Applied and Theoretical Statistics; Board on Mathematical Sciences and Analytics; Division on Earth and Life Studies; Nuclear and Radiation Studies Board; Division of Behavioral and Social Sciences and Education; Committee on National Statistics; Board on Behavioral, Cognitive, and Sensory Sciences; Committee on Reproducibility and Replicability in Science. Reproducibility and Replicability in Science. Washington (DC): National Academies Press (US); 2019 May 7. 3, Understanding Reproducibility and Replicability.
- PDF version of this title (2.9M)
In this Page
Recent activity.
- Understanding Reproducibility and Replicability - Reproducibility and Replicabil... Understanding Reproducibility and Replicability - Reproducibility and Replicability in Science
Your browsing activity is empty.
Activity recording is turned off.
Turn recording back on
Connect with NLM
National Library of Medicine 8600 Rockville Pike Bethesda, MD 20894
Web Policies FOIA HHS Vulnerability Disclosure
Help Accessibility Careers
14. CE Certified Modules & Instructions RCR Refresher
Ce certified modules for the rcr refresher course.
- Optional Modules
- Elective Modules
- Supplemental Modules
Instructions and Requirements
- Complete 7 modules from the above list
- 70% passing score on each module
Trending Articles
- Planned Maintenance
- Cookie Listing
- Guide to Getting Started
- How do I access my Completion Report/Certificate?
- 1. CE certified Modules & Instructions - HSR Basic
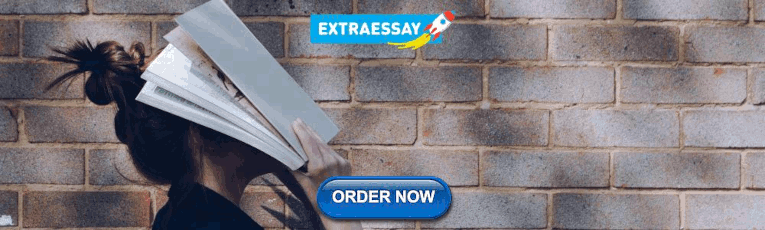
IMAGES
VIDEO
COMMENTS
Terms in this set (113) Within the context of RCR, social responsibility primarily refers to: The obligation to design technology or acquire information in ways that take into account what effects those actions may have on society. Within the context of RCR, compliance primarily refers to: The obligation to follow specific directives, such as ...
95% confidence interval. quantifies precision. if excludes the null value, then we can reject Hnot at an alpha of 0.05, assuming the study was well conducted. -Point estimate +/- measure CI precision. Point estimate= value of statistic in sample. -Measure of how confident we want to be. Study with Quizlet and memorize flashcards containing ...
repeatedly randomly sampling observations with replacement from the data set to compute desired statistic in each resample, where one can assess the the significance or nonsignificance of a mediation effect, if 0 doesn't fall between the confidence intervals, once can confidently conclude that there is a significant mediation effect
At the beginning of the study, the subject's pain scores are at ten out of a possible ten. After four weeks in the study, the subject's pain scores are consistently down to three. In their review of the study conduct, the researchers found that the lack of blinding of the researchers had an effect. Because researchers knew to which groups ...
Report and Reproducibility. The research process from design to publication. (DRAR PI) Click the card to flip 👆. 1. [THE RESEARCHER] Design (based on past theories) 2. run (gathering data) 3. analyze (significant test, null hypothesis procedures, etc.) 4. report (describes study from motivation to results that can be clearly replicated)
Study with Quizlet and memorize flashcards containing terms like Introduction to RCR (RCR-Basic) Introduction This module introduces concepts and principles related to what has come to be known as the Responsible Conduct of Research (RCR), and provides an overview of the subject matter covered in CITI Program's RCR courses. The term RCR is sometimes used interchangeably with research integrity ...
The Research Study Design course provides learners with an introduction to research study design, a detailed overview of scientific inquiry, examples of various research designs, a discussion of data management methods, an introduction to statistical analysis, and sound approaches to optimize the reproducibility of research results.
CITI Program is pleased to announce the availability of a new "Reproducibility of Research Results" module. The module, written by Dr. Teri A. Hamill from Nova Southeastern University, is designed to complement the topical offerings in the Responsible Conduct of Research (RCR) Basic Course.
Reproducibility of Research Results (ID 17756) Discusses factors that contribute to the lack of reproducibility and the resulting problems that can emerge. The module also describes the stakeholders affected by reproducibility problems, a collection of reproducibility initiatives, and strategies that can mitigate or prevent irreproducibility.
The module also describes the stakeholders affected by reproducibility problems, a collection of reproducibility initiatives, and strategies that can mitigate or prevent irreproducibility. *Note, this module is part of the CITI Program's Responsible Conduct of Research (RCR) Basic course, but is also included in this course.
Reproducibility of Research Results Discusses factors that contribute to the lack of reproducibility and the resulting problems that can emerge. The module also describes the stakeholders affected by reproducibility problems, a collection of reproducibility initiatives, and strategies that can mitigate or prevent irreproducibility.
Reproducibility of Research Results. Back to Getting Started | Top of page. ... CITI allows you to complete the course at your own pace. You may quit and return to the course later. However, you must quit after taking the quiz for a particular module, not DURING the taking of that quiz, or your answers will not be recorded.
Reviews proposed research in order to assess that the research is in keeping with ethical guidelines and regulatory requirements in order to protect human subjects. The alternative hypothesis states that the connection between key factors of interest and any observed connection is most likely:
Low reproducibility rates within life science research undermine cumulative knowledge production and contribute to both delays and costs of therapeutic drug development. An analysis of past studies indicates that the cumulative (total) prevalence of irreproducible preclinical research exceeds 50%, resulting in approximately US$28,000,000,000 ...
Reproducibility—the ability of independent researchers to obtain the same (or similar) results when repeating an experiment or test—is one of the hallmarks of good science ( Popper 1959 ). Reproducibility provides scientists with evidence that research results are objective and reliable and not due to bias or chance ( Rooney et al 2016 ).
Improving substandard research practices—including poor study design, failure to report details, and inadequate data analysis—has the potential to improve reproducibility and replicability by ensuring that research is more rigorous, thoughtful, and dependable. Rigorous research practices were important long before reproducibility and replicability emerged as notable issues in science, but ...
CITI Program's RCR Basic course covers the core norms, principles, regulations, and best practices for the conduct of ethical research. A module on "Reproducibility of Research Results" discusses factors that contribute to the lack of reproducibility and the effects non-reproducibility has on the scientific community and society in general.
Reproducibility and replicability are often cited as hallmarks of good science. Being able to reproduce the computational results of another researcher starting with the same data and replicate a previous study to test its results or inferences both facilitate the self-correcting nature of science. A newly reported discovery may prompt retesting and confirmation, examination of the limits of ...
o Reproducibility of Research Results (ID 17756) ... (RCR-Basic) (ID 15156) o Research Involving Human Subjects (RCR-Basic) (ID 13566) o Using Animal Subjects in Research (RCR-Basic) (ID 13301) o Research Misconduct (RCR-Basic) (ID 16604) ... Individual CITI members can locate their completion records by logging into their CITI account ...
The term reproducibility can also be used in the context of the second question: research is reproducible if another researcher actually uses the available data and code and obtains the same results. The difference between the first and the second questions is one of action by another researcher; the first refers to the availability of the data ...
Research Misconduct. Refresher Course* - for Researchers who have previously completed. the Basic Course - RCR Training *This course is for researchers paid from certain research grants from federal agencies that require periodic RCR re-training/update (e.g., every 4 years) • Plagiarism • Authorship • Collaborative Research
Collaborative Research (RCR-Refresher) 15662: 3: Conflicts of Interest (RCR-Refresher) 15663: 4: ... 15669: 10: Reproducibility of Research Results: 17756: 11: Plagiarism (RCR-Refresher) 19267: Be aware that modules assigned to you may not all be posted in "Required Modules" section of your grade book. ... CITI Courses Certified for CME Credits;
Office of Research Integrity. Undergraduate, graduate students and postdoctoral researchers who participate in NSF-funded projects must complete the Responsible Conduct of Research module through CITI. Modules required to satisfy the National Science Foundation training requirement for all undergraduate, graduate students and postdoctoral ...