Thank you for visiting nature.com. You are using a browser version with limited support for CSS. To obtain the best experience, we recommend you use a more up to date browser (or turn off compatibility mode in Internet Explorer). In the meantime, to ensure continued support, we are displaying the site without styles and JavaScript.
- View all journals
- Explore content
- About the journal
- Publish with us
- Sign up for alerts
- Published: 31 January 2022
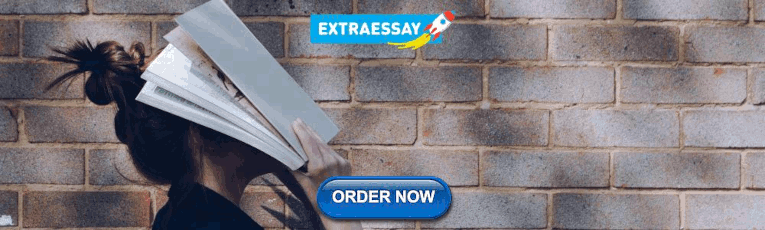
The clinician’s guide to interpreting a regression analysis
- Sofia Bzovsky 1 ,
- Mark R. Phillips ORCID: orcid.org/0000-0003-0923-261X 2 ,
- Robyn H. Guymer ORCID: orcid.org/0000-0002-9441-4356 3 , 4 ,
- Charles C. Wykoff 5 , 6 ,
- Lehana Thabane ORCID: orcid.org/0000-0003-0355-9734 2 , 7 ,
- Mohit Bhandari ORCID: orcid.org/0000-0001-9608-4808 1 , 2 &
- Varun Chaudhary ORCID: orcid.org/0000-0002-9988-4146 1 , 2
on behalf of the R.E.T.I.N.A. study group
Eye volume 36 , pages 1715–1717 ( 2022 ) Cite this article
19k Accesses
9 Citations
1 Altmetric
Metrics details
- Outcomes research
Introduction
When researchers are conducting clinical studies to investigate factors associated with, or treatments for disease and conditions to improve patient care and clinical practice, statistical evaluation of the data is often necessary. Regression analysis is an important statistical method that is commonly used to determine the relationship between several factors and disease outcomes or to identify relevant prognostic factors for diseases [ 1 ].
This editorial will acquaint readers with the basic principles of and an approach to interpreting results from two types of regression analyses widely used in ophthalmology: linear, and logistic regression.
Linear regression analysis
Linear regression is used to quantify a linear relationship or association between a continuous response/outcome variable or dependent variable with at least one independent or explanatory variable by fitting a linear equation to observed data [ 1 ]. The variable that the equation solves for, which is the outcome or response of interest, is called the dependent variable [ 1 ]. The variable that is used to explain the value of the dependent variable is called the predictor, explanatory, or independent variable [ 1 ].
In a linear regression model, the dependent variable must be continuous (e.g. intraocular pressure or visual acuity), whereas, the independent variable may be either continuous (e.g. age), binary (e.g. sex), categorical (e.g. age-related macular degeneration stage or diabetic retinopathy severity scale score), or a combination of these [ 1 ].
When investigating the effect or association of a single independent variable on a continuous dependent variable, this type of analysis is called a simple linear regression [ 2 ]. In many circumstances though, a single independent variable may not be enough to adequately explain the dependent variable. Often it is necessary to control for confounders and in these situations, one can perform a multivariable linear regression to study the effect or association with multiple independent variables on the dependent variable [ 1 , 2 ]. When incorporating numerous independent variables, the regression model estimates the effect or contribution of each independent variable while holding the values of all other independent variables constant [ 3 ].
When interpreting the results of a linear regression, there are a few key outputs for each independent variable included in the model:
Estimated regression coefficient—The estimated regression coefficient indicates the direction and strength of the relationship or association between the independent and dependent variables [ 4 ]. Specifically, the regression coefficient describes the change in the dependent variable for each one-unit change in the independent variable, if continuous [ 4 ]. For instance, if examining the relationship between a continuous predictor variable and intra-ocular pressure (dependent variable), a regression coefficient of 2 means that for every one-unit increase in the predictor, there is a two-unit increase in intra-ocular pressure. If the independent variable is binary or categorical, then the one-unit change represents switching from one category to the reference category [ 4 ]. For instance, if examining the relationship between a binary predictor variable, such as sex, where ‘female’ is set as the reference category, and intra-ocular pressure (dependent variable), a regression coefficient of 2 means that, on average, males have an intra-ocular pressure that is 2 mm Hg higher than females.
Confidence Interval (CI)—The CI, typically set at 95%, is a measure of the precision of the coefficient estimate of the independent variable [ 4 ]. A large CI indicates a low level of precision, whereas a small CI indicates a higher precision [ 5 ].
P value—The p value for the regression coefficient indicates whether the relationship between the independent and dependent variables is statistically significant [ 6 ].
Logistic regression analysis
As with linear regression, logistic regression is used to estimate the association between one or more independent variables with a dependent variable [ 7 ]. However, the distinguishing feature in logistic regression is that the dependent variable (outcome) must be binary (or dichotomous), meaning that the variable can only take two different values or levels, such as ‘1 versus 0’ or ‘yes versus no’ [ 2 , 7 ]. The effect size of predictor variables on the dependent variable is best explained using an odds ratio (OR) [ 2 ]. ORs are used to compare the relative odds of the occurrence of the outcome of interest, given exposure to the variable of interest [ 5 ]. An OR equal to 1 means that the odds of the event in one group are the same as the odds of the event in another group; there is no difference [ 8 ]. An OR > 1 implies that one group has a higher odds of having the event compared with the reference group, whereas an OR < 1 means that one group has a lower odds of having an event compared with the reference group [ 8 ]. When interpreting the results of a logistic regression, the key outputs include the OR, CI, and p-value for each independent variable included in the model.
Clinical example
Sen et al. investigated the association between several factors (independent variables) and visual acuity outcomes (dependent variable) in patients receiving anti-vascular endothelial growth factor therapy for macular oedema (DMO) by means of both linear and logistic regression [ 9 ]. Multivariable linear regression demonstrated that age (Estimate −0.33, 95% CI − 0.48 to −0.19, p < 0.001) was significantly associated with best-corrected visual acuity (BCVA) at 100 weeks at alpha = 0.05 significance level [ 9 ]. The regression coefficient of −0.33 means that the BCVA at 100 weeks decreases by 0.33 with each additional year of older age.
Multivariable logistic regression also demonstrated that age and ellipsoid zone status were statistically significant associated with achieving a BCVA letter score >70 letters at 100 weeks at the alpha = 0.05 significance level. Patients ≥75 years of age were at a decreased odds of achieving a BCVA letter score >70 letters at 100 weeks compared to those <50 years of age, since the OR is less than 1 (OR 0.96, 95% CI 0.94 to 0.98, p = 0.001) [ 9 ]. Similarly, patients between the ages of 50–74 years were also at a decreased odds of achieving a BCVA letter score >70 letters at 100 weeks compared to those <50 years of age, since the OR is less than 1 (OR 0.15, 95% CI 0.04 to 0.48, p = 0.001) [ 9 ]. As well, those with a not intact ellipsoid zone were at a decreased odds of achieving a BCVA letter score >70 letters at 100 weeks compared to those with an intact ellipsoid zone (OR 0.20, 95% CI 0.07 to 0.56; p = 0.002). On the other hand, patients with an ungradable/questionable ellipsoid zone were at an increased odds of achieving a BCVA letter score >70 letters at 100 weeks compared to those with an intact ellipsoid zone, since the OR is greater than 1 (OR 2.26, 95% CI 1.14 to 4.48; p = 0.02) [ 9 ].
The narrower the CI, the more precise the estimate is; and the smaller the p value (relative to alpha = 0.05), the greater the evidence against the null hypothesis of no effect or association.
Simply put, linear and logistic regression are useful tools for appreciating the relationship between predictor/explanatory and outcome variables for continuous and dichotomous outcomes, respectively, that can be applied in clinical practice, such as to gain an understanding of risk factors associated with a disease of interest.
Schneider A, Hommel G, Blettner M. Linear Regression. Anal Dtsch Ärztebl Int. 2010;107:776–82.
Google Scholar
Bender R. Introduction to the use of regression models in epidemiology. In: Verma M, editor. Cancer epidemiology. Methods in molecular biology. Humana Press; 2009:179–95.
Schober P, Vetter TR. Confounding in observational research. Anesth Analg. 2020;130:635.
Article Google Scholar
Schober P, Vetter TR. Linear regression in medical research. Anesth Analg. 2021;132:108–9.
Szumilas M. Explaining odds ratios. J Can Acad Child Adolesc Psychiatry. 2010;19:227–9.
Thiese MS, Ronna B, Ott U. P value interpretations and considerations. J Thorac Dis. 2016;8:E928–31.
Schober P, Vetter TR. Logistic regression in medical research. Anesth Analg. 2021;132:365–6.
Zabor EC, Reddy CA, Tendulkar RD, Patil S. Logistic regression in clinical studies. Int J Radiat Oncol Biol Phys. 2022;112:271–7.
Sen P, Gurudas S, Ramu J, Patrao N, Chandra S, Rasheed R, et al. Predictors of visual acuity outcomes after anti-vascular endothelial growth factor treatment for macular edema secondary to central retinal vein occlusion. Ophthalmol Retin. 2021;5:1115–24.
Download references
R.E.T.I.N.A. study group
Varun Chaudhary 1,2 , Mohit Bhandari 1,2 , Charles C. Wykoff 5,6 , Sobha Sivaprasad 8 , Lehana Thabane 2,7 , Peter Kaiser 9 , David Sarraf 10 , Sophie J. Bakri 11 , Sunir J. Garg 12 , Rishi P. Singh 13,14 , Frank G. Holz 15 , Tien Y. Wong 16,17 , and Robyn H. Guymer 3,4
Author information
Authors and affiliations.
Department of Surgery, McMaster University, Hamilton, ON, Canada
Sofia Bzovsky, Mohit Bhandari & Varun Chaudhary
Department of Health Research Methods, Evidence & Impact, McMaster University, Hamilton, ON, Canada
Mark R. Phillips, Lehana Thabane, Mohit Bhandari & Varun Chaudhary
Centre for Eye Research Australia, Royal Victorian Eye and Ear Hospital, East Melbourne, VIC, Australia
Robyn H. Guymer
Department of Surgery, (Ophthalmology), The University of Melbourne, Melbourne, VIC, Australia
Retina Consultants of Texas (Retina Consultants of America), Houston, TX, USA
Charles C. Wykoff
Blanton Eye Institute, Houston Methodist Hospital, Houston, TX, USA
Biostatistics Unit, St. Joseph’s Healthcare Hamilton, Hamilton, ON, Canada
Lehana Thabane
NIHR Moorfields Biomedical Research Centre, Moorfields Eye Hospital, London, UK
Sobha Sivaprasad
Cole Eye Institute, Cleveland Clinic, Cleveland, OH, USA
Peter Kaiser
Retinal Disorders and Ophthalmic Genetics, Stein Eye Institute, University of California, Los Angeles, CA, USA
David Sarraf
Department of Ophthalmology, Mayo Clinic, Rochester, MN, USA
Sophie J. Bakri
The Retina Service at Wills Eye Hospital, Philadelphia, PA, USA
Sunir J. Garg
Center for Ophthalmic Bioinformatics, Cole Eye Institute, Cleveland Clinic, Cleveland, OH, USA
Rishi P. Singh
Cleveland Clinic Lerner College of Medicine, Cleveland, OH, USA
Department of Ophthalmology, University of Bonn, Bonn, Germany
Frank G. Holz
Singapore Eye Research Institute, Singapore, Singapore
Tien Y. Wong
Singapore National Eye Centre, Duke-NUD Medical School, Singapore, Singapore
You can also search for this author in PubMed Google Scholar
- Varun Chaudhary
- , Mohit Bhandari
- , Charles C. Wykoff
- , Sobha Sivaprasad
- , Lehana Thabane
- , Peter Kaiser
- , David Sarraf
- , Sophie J. Bakri
- , Sunir J. Garg
- , Rishi P. Singh
- , Frank G. Holz
- , Tien Y. Wong
- & Robyn H. Guymer
Contributions
SB was responsible for writing, critical review and feedback on manuscript. MRP was responsible for conception of idea, critical review and feedback on manuscript. RHG was responsible for critical review and feedback on manuscript. CCW was responsible for critical review and feedback on manuscript. LT was responsible for critical review and feedback on manuscript. MB was responsible for conception of idea, critical review and feedback on manuscript. VC was responsible for conception of idea, critical review and feedback on manuscript.
Corresponding author
Correspondence to Varun Chaudhary .
Ethics declarations
Competing interests.
SB: Nothing to disclose. MRP: Nothing to disclose. RHG: Advisory boards: Bayer, Novartis, Apellis, Roche, Genentech Inc.—unrelated to this study. CCW: Consultant: Acuela, Adverum Biotechnologies, Inc, Aerpio, Alimera Sciences, Allegro Ophthalmics, LLC, Allergan, Apellis Pharmaceuticals, Bayer AG, Chengdu Kanghong Pharmaceuticals Group Co, Ltd, Clearside Biomedical, DORC (Dutch Ophthalmic Research Center), EyePoint Pharmaceuticals, Gentech/Roche, GyroscopeTx, IVERIC bio, Kodiak Sciences Inc, Novartis AG, ONL Therapeutics, Oxurion NV, PolyPhotonix, Recens Medical, Regeron Pharmaceuticals, Inc, REGENXBIO Inc, Santen Pharmaceutical Co, Ltd, and Takeda Pharmaceutical Company Limited; Research funds: Adverum Biotechnologies, Inc, Aerie Pharmaceuticals, Inc, Aerpio, Alimera Sciences, Allergan, Apellis Pharmaceuticals, Chengdu Kanghong Pharmaceutical Group Co, Ltd, Clearside Biomedical, Gemini Therapeutics, Genentech/Roche, Graybug Vision, Inc, GyroscopeTx, Ionis Pharmaceuticals, IVERIC bio, Kodiak Sciences Inc, Neurotech LLC, Novartis AG, Opthea, Outlook Therapeutics, Inc, Recens Medical, Regeneron Pharmaceuticals, Inc, REGENXBIO Inc, Samsung Pharm Co, Ltd, Santen Pharmaceutical Co, Ltd, and Xbrane Biopharma AB—unrelated to this study. LT: Nothing to disclose. MB: Research funds: Pendopharm, Bioventus, Acumed—unrelated to this study. VC: Advisory Board Member: Alcon, Roche, Bayer, Novartis; Grants: Bayer, Novartis—unrelated to this study.
Additional information
Publisher’s note Springer Nature remains neutral with regard to jurisdictional claims in published maps and institutional affiliations.
Rights and permissions
Reprints and permissions
About this article
Cite this article.
Bzovsky, S., Phillips, M.R., Guymer, R.H. et al. The clinician’s guide to interpreting a regression analysis. Eye 36 , 1715–1717 (2022). https://doi.org/10.1038/s41433-022-01949-z
Download citation
Received : 08 January 2022
Revised : 17 January 2022
Accepted : 18 January 2022
Published : 31 January 2022
Issue Date : September 2022
DOI : https://doi.org/10.1038/s41433-022-01949-z
Share this article
Anyone you share the following link with will be able to read this content:
Sorry, a shareable link is not currently available for this article.
Provided by the Springer Nature SharedIt content-sharing initiative
This article is cited by
Factors affecting patient satisfaction at a plastic surgery outpatient department at a tertiary centre in south africa.
- Chrysis Sofianos
BMC Health Services Research (2023)
Quick links
- Explore articles by subject
- Guide to authors
- Editorial policies

Quantitative Research Methods for Political Science, Public Policy and Public Administration for Undergraduates: 1st Edition With Applications in R
14 topics in multiple regression.
Thus far we have developed the basis for multiple OLS reression using matrix algebra, delved into the meaning of the estimated partial regression coefficient, and revisited the basis for hypothesis testing in OLS. In this chapter we turn to one of the key strengths of OLS: the robust flexibility of OLS for model specification. First we will discuss how to include binary variables (referred to as ``dummy variables") as IVs in an OLS model. Next we will show you how to build on dummy variables to model their interactions with other variables in your model. Finally, we will address an alternative way to express the partial regression coefficients – using standardized coefficients – that permit you to compare the magnitudes of the estimated effects of your IVs even when they are measured on different scales. As has been our custom, the examples in this chapter are based on variables from the class data set.
14.1 Dummy Variables
Thus far, we have considered OLS models that include variables measured on interval level scales (or, in a pinch and with caution, ordinal scales). That is fine when we have variables for which we can develop valid and reliable interval (or ordinal) measures. But in the policy and social science worlds, we often want to include in our analysis concepts that do not readily admit to interval measure – including many cases in which a variable has an “on - off”, or “present - absent” quality. In other cases we want to include a concept that is essentially nominal in nature, such that an observation can be categorized as a subset but not measured on a “high-low” or “more-less” type of scale. In these instances we can utilize what is generally known as a dummy variable, but are also referred to as indicator variables, Boolean variables, or categorical variables.
What the Heck are “Dummy Variables”?
- A dichotomous variable, with values of 0 and 1;
- A value of 1 represents the presence of some quality, a zero its absence;
- The 1s are compared to the 0s, who are known as the ``referent group";
- Dummy variables are often thought of as a proxy for a qualitative variable.
Dummy variables allow for tests of the differences in overall value of the \(Y\) for different nominal groups in the data. They are akin to a difference of means test for the groups identified by the dummy variable. Dummy variables allow for comparisons between an included (the 1s) and an omitted (the 0s) group. Therefore, it is important to be clear about which group is omitted and serving as the ``comparison category."
It is often the case that there are more than two groups represented by a set of nominal categories. In that case, the variable will consist of two or more dummy variables, with 0/1 codes for each category except the referent group (which is omitted). Several examples of categorical variables that can be represented in multiple regression with dummy variables include:
- Experimental treatment and control groups (treatment=1, control=0)
- Gender (male=1, female=0 or vice versa)
- Race and ethnicity (a dummy for each group, with one omitted referent group)
- Region of residence (dummy for each region with one omitted reference region)
- Type of education (dummy for each type with omitted reference type)
- Religious affiliation (dummy for each religious denomination with omitted reference)
The value of the dummy coefficient represents the estimated difference in \(Y\) between the dummy group and the reference group. Because the estimated difference is the average over all of the \(Y\) observations, the dummy is best understood as a change in the value of the intercept ( \(A\) ) for the ``dummied" group. This is illustrated in Figure 14.1 . In this illustration, the value of \(Y\) is a function of \(X_1\) (a continuous variable) and \(X_2\) (a dummy variable). When \(X_2\) is equal to 0 (the referent case) the top regression line applies. When \(X_2 = 1\) , the value of \(Y\) is reduced to the bottom line. In short, \(X_2\) has a negative estimated partial regression coefficient represented by the difference in height between the two regression lines.

Figure 14.1: Dummy Intercept Variables
For a case with multiple nominal categories (e.g., region) the procedure is as follows: (a) determine which category will be assigned as the referent group; (b) create a dummy variable for each of the other categories. For example, if you are coding a dummy for four regions (North, South, East and West), you could designate the South as the referent group. Then you would create dummies for the other three regions. Then, all observations from the North would get a value of 1 in the North dummy, and zeros in all others. Similarly, East and West observations would receive a 1 in their respective dummy category and zeros elsewhere. The observations from the South region would be given values of zero in all three categories. The interpretation of the partial regression coefficients for each of the three dummies would then be the estimated difference in \(Y\) between observations from the North, East and West and those from the South.
Now let’s walk through an example of an \(R\) model with a dummy variable and the interpretation of that model. We will predict climate change risk using age, education, income, ideology, and “gend”, a dummy variable for gender for which 1 = male and 0 = female.
First note that the inclusion of the dummy variables does not change the manner in which you interpret the other (non-dummy) variables in the model; the estimated partial regression coefficients for age, education, income and ideology should all be interpreted as described in the prior chapter. Note that the estimated partial regression coefficient for ``gender" is negative and statistically significant, indicating that males are less likely to be concerned about the environment than are females. The estimate indicates that, all else being equal, the average difference between men and women on the climate change risk scale is -0.2221178.
14.2 Interaction Effects
Dummy variables can also be used to estimate the ways in which the effect of a variable differs across subsets of cases. These kinds of effects are generally called ``interactions." When an interaction occurs, the effect of one \(X\) is dependent on the value of another. Typically, an OLS model is additive, where the \(B\) ’s are added together to predict \(Y\) ;
\(Y_i = A + BX_1 + BX_2 + BX_3 + BX_4 + E_i\) .
However, an interaction model has a multiplicative effect where two of the IVs are multiplied;
\(Y_i = A + BX_1 + BX_2 + BX_3 * BX_4 + E_i\) .
A ``slope dummy" is a special kind of interaction in which a dummy variable is interacted with (multiplied by) a scale (ordinal or higher) variable. Suppose, for example, that you hypothesized that the effects of political of ideology on perceived risks of climate change were different for men and women. Perhaps men are more likely than women to consistently integrate ideology into climate change risk perceptions. In such a case, a dummy variable (0=women, 1=men) could be interacted with ideology (1=strong liberal, 7=strong conservative) to predict levels of perceived risk of climate change (0=no risk, 10=extreme risk). If your hypothesized interaction was correct, you would observe the kind of pattern as shown in Figure 14.2 .

Figure 14.2: Illustration of Slope Interaction
We can test our hypothesized interaction in R , controlling for the effects of age and income.
The results indicate a negative and significant interaction effect for gender and ideology. Consistent with our hypothesis, this means that the effect of ideology on climate change risk is more pronounced for males than females. Put differently, the slope of ideology is steeper for males than it is for females. This is shown in Figure 14.3 .

Figure 14.3: Interaction of Ideology and Gender
In sum, dummy variables add greatly to the flexibility of OLS model specification. They permit the inclusion of categorical variables, and they allow for testing hypotheses about interactions of groups with other IVs within the model. This kind of flexibility is one reason that OLS models are widely used by social scientists and policy analysts.
14.3 Standardized Regression Coefficients
In most cases, the various IVs in a model are represented on different measurement scales. For example, ideology ranges from 1 to 7, while age ranges from 18 to over 90 years old. These different scales make comparing the effects of the various IVs difficult. If we want to directly compare the magnitudes of the effects of ideology and age on levels of environmental concern, we would need to standardize the variables.
One way to standardized variables is to create a \(Z\) -score based on each variable. Variables are standardized in this way as follows:
\[\begin{equation} Z_i = \frac{X_i-\bar{X}}{s_x} \tag{14.1} \end{equation}\]
where \(s_x\) is the s.d. of \(X\) . Standardizing the variables by creating \(Z\) -scores re-scales them so that each variables has a mean of \(0\) and a s.d. of \(1\) . Therefore, all variables have the same mean and s.d. It is important to realize (and it is somewhat counter-intuitive) that the standardized variables retain all of the variation that was in the original measure.
A second way to standardize variables converts the unstandardized \(B\) , into a standardized \(B'\) .
\[\begin{equation} B'_k = B_k\frac{s_k}{s_Y} \tag{14.2} \end{equation}\]
where \(B_k\) is the unstandardized coefficient of \(X_k\) , \(s_k\) is the s.d. of \(X_k\) , and \(s_y\) is the s.d. of \(Y\) . Standardized regression coefficients, also known as beta weights or “betas”, are those we would get if we regress a standardized \(Y\) onto standardized \(X\) ’s.
Interpreting Standardized Betas
- The standard deviation change in \(Y\) for a one-standard deviation change in \(X\)
- All \(X\) ’ss on an equal footing, so one can compare the strength of the effects of the \(X\) ’s
- Variances will differ across different samples
We can use the scale function in R to calculate a \(Z\) score for each of our variables, and then re-run our model.
In addition, we can convert the original unstandardized coefficient for ideology, to a standardized coefficient.
Using either approach, standardized coefficients allow us to compare the magnitudes of the effects of each of the IVs on \(Y\) .
14.4 Summary
This chapter has focused on options in designing and using OLS models. We first covered the use of dummy variables to capture the effects of group differences on estimates of \(Y\) . We then explained how dummy variables, when interacted with scale variables, can provide estimates of the differences in how the scale variable affects \(Y\) across the different subgroups represented by the dummy variable. Finally, we introduced the use of standardized regression coefficients as a means to compare the effects of different \(Xs\) on \(Y\) when the scales of the \(Xs\) differ. Overall, these refinements in the use of OLS permit great flexibility in the application of regression models to estimation and hypothesis testing in policy analysis and social science research.
14.5 Study Questions
- What is a dummy variable? When should we use it? How do you interpret coefficients on dummy variables?
- What is an interaction effect? When should you include an interacton effect in your model?
- What is the primary benefit of standardizing regression coefficients?
Regression Analysis
- Reference work entry
- First Online: 03 December 2021
- Cite this reference work entry
- Bernd Skiera 4 ,
- Jochen Reiner 4 &
- Sönke Albers 5
7502 Accesses
3 Citations
Linear regression analysis is one of the most important statistical methods. It examines the linear relationship between a metric-scaled dependent variable (also called endogenous, explained, response, or predicted variable) and one or more metric-scaled independent variables (also called exogenous, explanatory, control, or predictor variable). We illustrate how regression analysis work and how it supports marketing decisions, e.g., the derivation of an optimal marketing mix. We also outline how to use linear regression analysis to estimate nonlinear functions such as a multiplicative sales response function. Furthermore, we show how to use the results of a regression to calculate elasticities and to identify outliers and discuss in details the problems that occur in case of autocorrelation, multicollinearity and heteroscedasticity. We use a numerical example to illustrate in detail all calculations and use this numerical example to outline the problems that occur in case of endogeneity.
This is a preview of subscription content, log in via an institution to check access.
Access this chapter
- Available as PDF
- Read on any device
- Instant download
- Own it forever
- Available as EPUB and PDF
- Durable hardcover edition
- Dispatched in 3 to 5 business days
- Free shipping worldwide - see info
Tax calculation will be finalised at checkout
Purchases are for personal use only
Institutional subscriptions
Albers, S. (2012). Optimizable and implementable aggregate response modeling for marketing decision support. International Journal of Research in Marketing, 29 (2), 111–122.
Article Google Scholar
Albers, S., Mantrala, M. K., & Sridhar, S. (2010). Personal selling elasticities: A meta-analysis. Journal of Marketing Research, 47 (5), 840–853.
Assmus, G., Farley, J. W., & Lehmann, D. R. (1984). How advertising affects sales: A meta-analysis of econometric results. Journal of Marketing Research, 21 (1), 65–74.
Bijmolt, T. H. A., van Heerde, H., & Pieters, R. G. M. (2005). New empirical generalizations on the determinants of price elasticity. Journal of Marketing Research, 42 (2), 141–156.
Chatterjee, S., & Hadi, A. S. (1986). Influential observations, high leverage points, and outliers in linear regressions. Statistical Science, 1 (3), 379–416.
Google Scholar
Greene, W. H. (2008). Econometric analysis (6th ed.). Upper Saddle River: Pearson.
Gujarati, D. N. (2003). Basic econometrics (4th ed.). New York: McGraw Hill.
Hair, J. F., Black, W. C., Babin, J. B., & Anderson, R. E. (2014). Multivariate data analysis (7th ed.). Upper Saddle River: Pearson.
Hair, J. F., Hult, G. T. M., Ringle, C. M., & Sarstedt, M. (2017). A primer on partial least squares structural equation modeling (PLS-SEM) (2nd ed.). Thousand Oaks: Sage.
Hanssens, D. M., Parsons, L. J., & Schultz, R. L. (1990). Market response models: Econometric and time series analysis . Boston: Springer.
Hsiao, C. (2014). Analysis of panel data (3rd ed.). Cambridge: Cambridge University Press.
Book Google Scholar
Irwin, J. R., & McClelland, G. H. (2001). Misleading heuristics and moderated multiple regression models. Journal of Marketing Research, 38 (1), 100–109.
Koutsoyiannis, A. (1977). Theory of econometrics (2nd ed.). Houndmills: MacMillan.
Laurent, G. (2013). EMAC distinguished marketing scholar 2012: Respect the data! International Journal of Research in Marketing, 30 (4), 323–334.
Leeflang, P. S. H., Wittink, D. R., Wedel, M., & Neart, P. A. (2000). Building models for marketing decisions . Berlin: Kluwer.
Lodish, L. L., Abraham, M. M., Kalmenson, S., Livelsberger, J., Lubetkin, B., Richardson, B., & Stevens, M. E. (1995). How TV advertising works: A meta-analysis of 389 real world split cable T. V. advertising experiments. Journal of Marketing Research, 32 (2), 125–139.
Pindyck, R. S., & Rubenfeld, D. (1998). Econometric models and econometric forecasts (4th ed.). New York: McGraw-Hill.
Sethuraman, R., Tellis, G. J., & Briesch, R. A. (2011). How well does advertising work? Generalizations from meta-analysis of brand advertising elasticities. Journal of Marketing Research, 48 (3), 457–471.
Snijders, T. A. B., & Bosker, R. J. (2012). Multilevel analysis: An introduction to basic and advanced multilevel modeling (2nd ed.). London: Sage.
Stock, J., & Watson, M. (2015). Introduction to econometrics (3rd ed.). Upper Saddle River: Pearson.
Tellis, G. J. (1988). The price sensitivity of selective demand: A meta-analysis of econometric models of sales. Journal of Marketing Research, 25 (4), 391–404.
White, H. (1980). A heteroskedasticity-consistent covariance matrix estimator and a direct test for heteroskedasticity. Econometrica, 48 (4), 817–838.
Wooldridge, J. M. (2009). Introductory econometrics: A modern approach (4th ed.). Mason: South-Western Cengage.
Download references
Author information
Authors and affiliations.
Goethe University Frankfurt, Frankfurt, Germany
Bernd Skiera & Jochen Reiner
Kuehne Logistics University, Hamburg, Germany
Sönke Albers
You can also search for this author in PubMed Google Scholar
Corresponding author
Correspondence to Bernd Skiera .
Editor information
Editors and affiliations.
Department of Business-to-Business Marketing, Sales, and Pricing, University of Mannheim, Mannheim, Germany
Christian Homburg
Department of Marketing & Sales Research Group, Karlsruhe Institute of Technology (KIT), Karlsruhe, Germany
Martin Klarmann
Marketing & Sales Department, University of Mannheim, Mannheim, Germany
Arnd Vomberg
Rights and permissions
Reprints and permissions
Copyright information
© 2022 Springer Nature Switzerland AG
About this entry
Cite this entry.
Skiera, B., Reiner, J., Albers, S. (2022). Regression Analysis. In: Homburg, C., Klarmann, M., Vomberg, A. (eds) Handbook of Market Research. Springer, Cham. https://doi.org/10.1007/978-3-319-57413-4_17
Download citation
DOI : https://doi.org/10.1007/978-3-319-57413-4_17
Published : 03 December 2021
Publisher Name : Springer, Cham
Print ISBN : 978-3-319-57411-0
Online ISBN : 978-3-319-57413-4
eBook Packages : Business and Management Reference Module Humanities and Social Sciences Reference Module Business, Economics and Social Sciences
Share this entry
Anyone you share the following link with will be able to read this content:
Sorry, a shareable link is not currently available for this article.
Provided by the Springer Nature SharedIt content-sharing initiative
- Publish with us
Policies and ethics
- Find a journal
- Track your research
A Comprehensive Study of Regression Analysis and the Existing Techniques
Ieee account.
- Change Username/Password
- Update Address
Purchase Details
- Payment Options
- Order History
- View Purchased Documents
Profile Information
- Communications Preferences
- Profession and Education
- Technical Interests
- US & Canada: +1 800 678 4333
- Worldwide: +1 732 981 0060
- Contact & Support
- About IEEE Xplore
- Accessibility
- Terms of Use
- Nondiscrimination Policy
- Privacy & Opting Out of Cookies
A not-for-profit organization, IEEE is the world's largest technical professional organization dedicated to advancing technology for the benefit of humanity. © Copyright 2024 IEEE - All rights reserved. Use of this web site signifies your agreement to the terms and conditions.
Click through the PLOS taxonomy to find articles in your field.
For more information about PLOS Subject Areas, click here .
Loading metrics
Open Access
Peer-reviewed
Research Article
Anxiety, Affect, Self-Esteem, and Stress: Mediation and Moderation Effects on Depression
Affiliations Department of Psychology, University of Gothenburg, Gothenburg, Sweden, Network for Empowerment and Well-Being, University of Gothenburg, Gothenburg, Sweden
Affiliation Network for Empowerment and Well-Being, University of Gothenburg, Gothenburg, Sweden
Affiliations Department of Psychology, University of Gothenburg, Gothenburg, Sweden, Network for Empowerment and Well-Being, University of Gothenburg, Gothenburg, Sweden, Department of Psychology, Education and Sport Science, Linneaus University, Kalmar, Sweden
* E-mail: [email protected]
Affiliations Network for Empowerment and Well-Being, University of Gothenburg, Gothenburg, Sweden, Center for Ethics, Law, and Mental Health (CELAM), University of Gothenburg, Gothenburg, Sweden, Institute of Neuroscience and Physiology, The Sahlgrenska Academy, University of Gothenburg, Gothenburg, Sweden
- Ali Al Nima,
- Patricia Rosenberg,
- Trevor Archer,
- Danilo Garcia
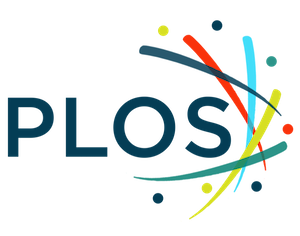
- Published: September 9, 2013
- https://doi.org/10.1371/journal.pone.0073265
- Reader Comments
23 Sep 2013: Nima AA, Rosenberg P, Archer T, Garcia D (2013) Correction: Anxiety, Affect, Self-Esteem, and Stress: Mediation and Moderation Effects on Depression. PLOS ONE 8(9): 10.1371/annotation/49e2c5c8-e8a8-4011-80fc-02c6724b2acc. https://doi.org/10.1371/annotation/49e2c5c8-e8a8-4011-80fc-02c6724b2acc View correction
Mediation analysis investigates whether a variable (i.e., mediator) changes in regard to an independent variable, in turn, affecting a dependent variable. Moderation analysis, on the other hand, investigates whether the statistical interaction between independent variables predict a dependent variable. Although this difference between these two types of analysis is explicit in current literature, there is still confusion with regard to the mediating and moderating effects of different variables on depression. The purpose of this study was to assess the mediating and moderating effects of anxiety, stress, positive affect, and negative affect on depression.
Two hundred and two university students (males = 93, females = 113) completed questionnaires assessing anxiety, stress, self-esteem, positive and negative affect, and depression. Mediation and moderation analyses were conducted using techniques based on standard multiple regression and hierarchical regression analyses.
Main Findings
The results indicated that (i) anxiety partially mediated the effects of both stress and self-esteem upon depression, (ii) that stress partially mediated the effects of anxiety and positive affect upon depression, (iii) that stress completely mediated the effects of self-esteem on depression, and (iv) that there was a significant interaction between stress and negative affect, and between positive affect and negative affect upon depression.
The study highlights different research questions that can be investigated depending on whether researchers decide to use the same variables as mediators and/or moderators.
Citation: Nima AA, Rosenberg P, Archer T, Garcia D (2013) Anxiety, Affect, Self-Esteem, and Stress: Mediation and Moderation Effects on Depression. PLoS ONE 8(9): e73265. https://doi.org/10.1371/journal.pone.0073265
Editor: Ben J. Harrison, The University of Melbourne, Australia
Received: February 21, 2013; Accepted: July 22, 2013; Published: September 9, 2013
Copyright: © 2013 Nima et al. This is an open-access article distributed under the terms of the Creative Commons Attribution License, which permits unrestricted use, distribution, and reproduction in any medium, provided the original author and source are credited.
Funding: The authors have no support or funding to report.
Competing interests: The authors have declared that no competing interests exist.
Introduction
Mediation refers to the covariance relationships among three variables: an independent variable (1), an assumed mediating variable (2), and a dependent variable (3). Mediation analysis investigates whether the mediating variable accounts for a significant amount of the shared variance between the independent and the dependent variables–the mediator changes in regard to the independent variable, in turn, affecting the dependent one [1] , [2] . On the other hand, moderation refers to the examination of the statistical interaction between independent variables in predicting a dependent variable [1] , [3] . In contrast to the mediator, the moderator is not expected to be correlated with both the independent and the dependent variable–Baron and Kenny [1] actually recommend that it is best if the moderator is not correlated with the independent variable and if the moderator is relatively stable, like a demographic variable (e.g., gender, socio-economic status) or a personality trait (e.g., affectivity).
Although both types of analysis lead to different conclusions [3] and the distinction between statistical procedures is part of the current literature [2] , there is still confusion about the use of moderation and mediation analyses using data pertaining to the prediction of depression. There are, for example, contradictions among studies that investigate mediating and moderating effects of anxiety, stress, self-esteem, and affect on depression. Depression, anxiety and stress are suggested to influence individuals' social relations and activities, work, and studies, as well as compromising decision-making and coping strategies [4] , [5] , [6] . Successfully coping with anxiety, depressiveness, and stressful situations may contribute to high levels of self-esteem and self-confidence, in addition increasing well-being, and psychological and physical health [6] . Thus, it is important to disentangle how these variables are related to each other. However, while some researchers perform mediation analysis with some of the variables mentioned here, other researchers conduct moderation analysis with the same variables. Seldom are both moderation and mediation performed on the same dataset. Before disentangling mediation and moderation effects on depression in the current literature, we briefly present the methodology behind the analysis performed in this study.
Mediation and moderation
Baron and Kenny [1] postulated several criteria for the analysis of a mediating effect: a significant correlation between the independent and the dependent variable, the independent variable must be significantly associated with the mediator, the mediator predicts the dependent variable even when the independent variable is controlled for, and the correlation between the independent and the dependent variable must be eliminated or reduced when the mediator is controlled for. All the criteria is then tested using the Sobel test which shows whether indirect effects are significant or not [1] , [7] . A complete mediating effect occurs when the correlation between the independent and the dependent variable are eliminated when the mediator is controlled for [8] . Analyses of mediation can, for example, help researchers to move beyond answering if high levels of stress lead to high levels of depression. With mediation analysis researchers might instead answer how stress is related to depression.
In contrast to mediation, moderation investigates the unique conditions under which two variables are related [3] . The third variable here, the moderator, is not an intermediate variable in the causal sequence from the independent to the dependent variable. For the analysis of moderation effects, the relation between the independent and dependent variable must be different at different levels of the moderator [3] . Moderators are included in the statistical analysis as an interaction term [1] . When analyzing moderating effects the variables should first be centered (i.e., calculating the mean to become 0 and the standard deviation to become 1) in order to avoid problems with multi-colinearity [8] . Moderating effects can be calculated using multiple hierarchical linear regressions whereby main effects are presented in the first step and interactions in the second step [1] . Analysis of moderation, for example, helps researchers to answer when or under which conditions stress is related to depression.
Mediation and moderation effects on depression
Cognitive vulnerability models suggest that maladaptive self-schema mirroring helplessness and low self-esteem explain the development and maintenance of depression (for a review see [9] ). These cognitive vulnerability factors become activated by negative life events or negative moods [10] and are suggested to interact with environmental stressors to increase risk for depression and other emotional disorders [11] , [10] . In this line of thinking, the experience of stress, low self-esteem, and negative emotions can cause depression, but also be used to explain how (i.e., mediation) and under which conditions (i.e., moderation) specific variables influence depression.
Using mediational analyses to investigate how cognitive therapy intervations reduced depression, researchers have showed that the intervention reduced anxiety, which in turn was responsible for 91% of the reduction in depression [12] . In the same study, reductions in depression, by the intervention, accounted only for 6% of the reduction in anxiety. Thus, anxiety seems to affect depression more than depression affects anxiety and, together with stress, is both a cause of and a powerful mediator influencing depression (See also [13] ). Indeed, there are positive relationships between depression, anxiety and stress in different cultures [14] . Moreover, while some studies show that stress (independent variable) increases anxiety (mediator), which in turn increased depression (dependent variable) [14] , other studies show that stress (moderator) interacts with maladaptive self-schemata (dependent variable) to increase depression (independent variable) [15] , [16] .
The present study
In order to illustrate how mediation and moderation can be used to address different research questions we first focus our attention to anxiety and stress as mediators of different variables that earlier have been shown to be related to depression. Secondly, we use all variables to find which of these variables moderate the effects on depression.
The specific aims of the present study were:
- To investigate if anxiety mediated the effect of stress, self-esteem, and affect on depression.
- To investigate if stress mediated the effects of anxiety, self-esteem, and affect on depression.
- To examine moderation effects between anxiety, stress, self-esteem, and affect on depression.
Ethics statement
This research protocol was approved by the Ethics Committee of the University of Gothenburg and written informed consent was obtained from all the study participants.
Participants
The present study was based upon a sample of 206 participants (males = 93, females = 113). All the participants were first year students in different disciplines at two universities in South Sweden. The mean age for the male students was 25.93 years ( SD = 6.66), and 25.30 years ( SD = 5.83) for the female students.
In total, 206 questionnaires were distributed to the students. Together 202 questionnaires were responded to leaving a total dropout of 1.94%. This dropout concerned three sections that the participants chose not to respond to at all, and one section that was completed incorrectly. None of these four questionnaires was included in the analyses.
Instruments
Hospital anxiety and depression scale [17] ..
The Swedish translation of this instrument [18] was used to measure anxiety and depression. The instrument consists of 14 statements (7 of which measure depression and 7 measure anxiety) to which participants are asked to respond grade of agreement on a Likert scale (0 to 3). The utility, reliability and validity of the instrument has been shown in multiple studies (e.g., [19] ).
Perceived Stress Scale [20] .
The Swedish version [21] of this instrument was used to measures individuals' experience of stress. The instrument consist of 14 statements to which participants rate on a Likert scale (0 = never , 4 = very often ). High values indicate that the individual expresses a high degree of stress.
Rosenberg's Self-Esteem Scale [22] .
The Rosenberg's Self-Esteem Scale (Swedish version by Lindwall [23] ) consists of 10 statements focusing on general feelings toward the self. Participants are asked to report grade of agreement in a four-point Likert scale (1 = agree not at all, 4 = agree completely ). This is the most widely used instrument for estimation of self-esteem with high levels of reliability and validity (e.g., [24] , [25] ).
Positive Affect and Negative Affect Schedule [26] .
This is a widely applied instrument for measuring individuals' self-reported mood and feelings. The Swedish version has been used among participants of different ages and occupations (e.g., [27] , [28] , [29] ). The instrument consists of 20 adjectives, 10 positive affect (e.g., proud, strong) and 10 negative affect (e.g., afraid, irritable). The adjectives are rated on a five-point Likert scale (1 = not at all , 5 = very much ). The instrument is a reliable, valid, and effective self-report instrument for estimating these two important and independent aspects of mood [26] .
Questionnaires were distributed to the participants on several different locations within the university, including the library and lecture halls. Participants were asked to complete the questionnaire after being informed about the purpose and duration (10–15 minutes) of the study. Participants were also ensured complete anonymity and informed that they could end their participation whenever they liked.
Correlational analysis
Depression showed positive, significant relationships with anxiety, stress and negative affect. Table 1 presents the correlation coefficients, mean values and standard deviations ( sd ), as well as Cronbach ' s α for all the variables in the study.
- PPT PowerPoint slide
- PNG larger image
- TIFF original image
https://doi.org/10.1371/journal.pone.0073265.t001
Mediation analysis
Regression analyses were performed in order to investigate if anxiety mediated the effect of stress, self-esteem, and affect on depression (aim 1). The first regression showed that stress ( B = .03, 95% CI [.02,.05], β = .36, t = 4.32, p <.001), self-esteem ( B = −.03, 95% CI [−.05, −.01], β = −.24, t = −3.20, p <.001), and positive affect ( B = −.02, 95% CI [−.05, −.01], β = −.19, t = −2.93, p = .004) had each an unique effect on depression. Surprisingly, negative affect did not predict depression ( p = 0.77) and was therefore removed from the mediation model, thus not included in further analysis.
The second regression tested whether stress, self-esteem and positive affect uniquely predicted the mediator (i.e., anxiety). Stress was found to be positively associated ( B = .21, 95% CI [.15,.27], β = .47, t = 7.35, p <.001), whereas self-esteem was negatively associated ( B = −.29, 95% CI [−.38, −.21], β = −.42, t = −6.48, p <.001) to anxiety. Positive affect, however, was not associated to anxiety ( p = .50) and was therefore removed from further analysis.
A hierarchical regression analysis using depression as the outcome variable was performed using stress and self-esteem as predictors in the first step, and anxiety as predictor in the second step. This analysis allows the examination of whether stress and self-esteem predict depression and if this relation is weaken in the presence of anxiety as the mediator. The result indicated that, in the first step, both stress ( B = .04, 95% CI [.03,.05], β = .45, t = 6.43, p <.001) and self-esteem ( B = .04, 95% CI [.03,.05], β = .45, t = 6.43, p <.001) predicted depression. When anxiety (i.e., the mediator) was controlled for predictability was reduced somewhat but was still significant for stress ( B = .03, 95% CI [.02,.04], β = .33, t = 4.29, p <.001) and for self-esteem ( B = −.03, 95% CI [−.05, −.01], β = −.20, t = −2.62, p = .009). Anxiety, as a mediator, predicted depression even when both stress and self-esteem were controlled for ( B = .05, 95% CI [.02,.08], β = .26, t = 3.17, p = .002). Anxiety improved the prediction of depression over-and-above the independent variables (i.e., stress and self-esteem) (Δ R 2 = .03, F (1, 198) = 10.06, p = .002). See Table 2 for the details.
https://doi.org/10.1371/journal.pone.0073265.t002
A Sobel test was conducted to test the mediating criteria and to assess whether indirect effects were significant or not. The result showed that the complete pathway from stress (independent variable) to anxiety (mediator) to depression (dependent variable) was significant ( z = 2.89, p = .003). The complete pathway from self-esteem (independent variable) to anxiety (mediator) to depression (dependent variable) was also significant ( z = 2.82, p = .004). Thus, indicating that anxiety partially mediates the effects of both stress and self-esteem on depression. This result may indicate also that both stress and self-esteem contribute directly to explain the variation in depression and indirectly via experienced level of anxiety (see Figure 1 ).
Changes in Beta weights when the mediator is present are highlighted in red.
https://doi.org/10.1371/journal.pone.0073265.g001
For the second aim, regression analyses were performed in order to test if stress mediated the effect of anxiety, self-esteem, and affect on depression. The first regression showed that anxiety ( B = .07, 95% CI [.04,.10], β = .37, t = 4.57, p <.001), self-esteem ( B = −.02, 95% CI [−.05, −.01], β = −.18, t = −2.23, p = .03), and positive affect ( B = −.03, 95% CI [−.04, −.02], β = −.27, t = −4.35, p <.001) predicted depression independently of each other. Negative affect did not predict depression ( p = 0.74) and was therefore removed from further analysis.
The second regression investigated if anxiety, self-esteem and positive affect uniquely predicted the mediator (i.e., stress). Stress was positively associated to anxiety ( B = 1.01, 95% CI [.75, 1.30], β = .46, t = 7.35, p <.001), negatively associated to self-esteem ( B = −.30, 95% CI [−.50, −.01], β = −.19, t = −2.90, p = .004), and a negatively associated to positive affect ( B = −.33, 95% CI [−.46, −.20], β = −.27, t = −5.02, p <.001).
A hierarchical regression analysis using depression as the outcome and anxiety, self-esteem, and positive affect as the predictors in the first step, and stress as the predictor in the second step, allowed the examination of whether anxiety, self-esteem and positive affect predicted depression and if this association would weaken when stress (i.e., the mediator) was present. In the first step of the regression anxiety ( B = .07, 95% CI [.05,.10], β = .38, t = 5.31, p = .02), self-esteem ( B = −.03, 95% CI [−.05, −.01], β = −.18, t = −2.41, p = .02), and positive affect ( B = −.03, 95% CI [−.04, −.02], β = −.27, t = −4.36, p <.001) significantly explained depression. When stress (i.e., the mediator) was controlled for, predictability was reduced somewhat but was still significant for anxiety ( B = .05, 95% CI [.02,.08], β = .05, t = 4.29, p <.001) and for positive affect ( B = −.02, 95% CI [−.04, −.01], β = −.20, t = −3.16, p = .002), whereas self-esteem did not reach significance ( p < = .08). In the second step, the mediator (i.e., stress) predicted depression even when anxiety, self-esteem, and positive affect were controlled for ( B = .02, 95% CI [.08,.04], β = .25, t = 3.07, p = .002). Stress improved the prediction of depression over-and-above the independent variables (i.e., anxiety, self-esteem and positive affect) (Δ R 2 = .02, F (1, 197) = 9.40, p = .002). See Table 3 for the details.
https://doi.org/10.1371/journal.pone.0073265.t003
Furthermore, the Sobel test indicated that the complete pathways from the independent variables (anxiety: z = 2.81, p = .004; self-esteem: z = 2.05, p = .04; positive affect: z = 2.58, p <.01) to the mediator (i.e., stress), to the outcome (i.e., depression) were significant. These specific results might be explained on the basis that stress partially mediated the effects of both anxiety and positive affect on depression while stress completely mediated the effects of self-esteem on depression. In other words, anxiety and positive affect contributed directly to explain the variation in depression and indirectly via the experienced level of stress. Self-esteem contributed only indirectly via the experienced level of stress to explain the variation in depression. In other words, stress effects on depression originate from “its own power” and explained more of the variation in depression than self-esteem (see Figure 2 ).
https://doi.org/10.1371/journal.pone.0073265.g002
Moderation analysis
Multiple linear regression analyses were used in order to examine moderation effects between anxiety, stress, self-esteem and affect on depression. The analysis indicated that about 52% of the variation in the dependent variable (i.e., depression) could be explained by the main effects and the interaction effects ( R 2 = .55, adjusted R 2 = .51, F (55, 186) = 14.87, p <.001). When the variables (dependent and independent) were standardized, both the standardized regression coefficients beta (β) and the unstandardized regression coefficients beta (B) became the same value with regard to the main effects. Three of the main effects were significant and contributed uniquely to high levels of depression: anxiety ( B = .26, t = 3.12, p = .002), stress ( B = .25, t = 2.86, p = .005), and self-esteem ( B = −.17, t = −2.17, p = .03). The main effect of positive affect was also significant and contributed to low levels of depression ( B = −.16, t = −2.027, p = .02) (see Figure 3 ). Furthermore, the results indicated that two moderator effects were significant. These were the interaction between stress and negative affect ( B = −.28, β = −.39, t = −2.36, p = .02) (see Figure 4 ) and the interaction between positive affect and negative affect ( B = −.21, β = −.29, t = −2.30, p = .02) ( Figure 5 ).
https://doi.org/10.1371/journal.pone.0073265.g003
Low stress and low negative affect leads to lower levels of depression compared to high stress and high negative affect.
https://doi.org/10.1371/journal.pone.0073265.g004
High positive affect and low negative affect lead to lower levels of depression compared to low positive affect and high negative affect.
https://doi.org/10.1371/journal.pone.0073265.g005
The results in the present study show that (i) anxiety partially mediated the effects of both stress and self-esteem on depression, (ii) that stress partially mediated the effects of anxiety and positive affect on depression, (iii) that stress completely mediated the effects of self-esteem on depression, and (iv) that there was a significant interaction between stress and negative affect, and positive affect and negative affect on depression.
Mediating effects
The study suggests that anxiety contributes directly to explaining the variance in depression while stress and self-esteem might contribute directly to explaining the variance in depression and indirectly by increasing feelings of anxiety. Indeed, individuals who experience stress over a long period of time are susceptible to increased anxiety and depression [30] , [31] and previous research shows that high self-esteem seems to buffer against anxiety and depression [32] , [33] . The study also showed that stress partially mediated the effects of both anxiety and positive affect on depression and that stress completely mediated the effects of self-esteem on depression. Anxiety and positive affect contributed directly to explain the variation in depression and indirectly to the experienced level of stress. Self-esteem contributed only indirectly via the experienced level of stress to explain the variation in depression, i.e. stress affects depression on the basis of ‘its own power’ and explains much more of the variation in depressive experiences than self-esteem. In general, individuals who experience low anxiety and frequently experience positive affect seem to experience low stress, which might reduce their levels of depression. Academic stress, for instance, may increase the risk for experiencing depression among students [34] . Although self-esteem did not emerged as an important variable here, under circumstances in which difficulties in life become chronic, some researchers suggest that low self-esteem facilitates the experience of stress [35] .
Moderator effects/interaction effects
The present study showed that the interaction between stress and negative affect and between positive and negative affect influenced self-reported depression symptoms. Moderation effects between stress and negative affect imply that the students experiencing low levels of stress and low negative affect reported lower levels of depression than those who experience high levels of stress and high negative affect. This result confirms earlier findings that underline the strong positive association between negative affect and both stress and depression [36] , [37] . Nevertheless, negative affect by itself did not predicted depression. In this regard, it is important to point out that the absence of positive emotions is a better predictor of morbidity than the presence of negative emotions [38] , [39] . A modification to this statement, as illustrated by the results discussed next, could be that the presence of negative emotions in conjunction with the absence of positive emotions increases morbidity.
The moderating effects between positive and negative affect on the experience of depression imply that the students experiencing high levels of positive affect and low levels of negative affect reported lower levels of depression than those who experience low levels of positive affect and high levels of negative affect. This result fits previous observations indicating that different combinations of these affect dimensions are related to different measures of physical and mental health and well-being, such as, blood pressure, depression, quality of sleep, anxiety, life satisfaction, psychological well-being, and self-regulation [40] – [51] .
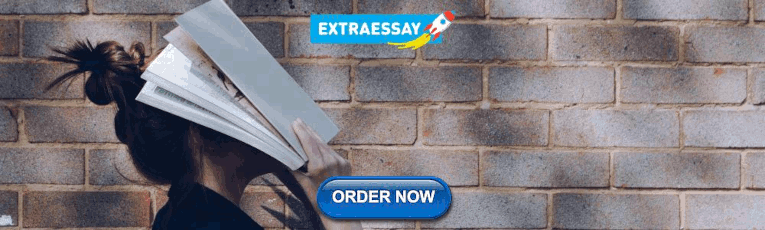
Limitations
The result indicated a relatively low mean value for depression ( M = 3.69), perhaps because the studied population was university students. These might limit the generalization power of the results and might also explain why negative affect, commonly associated to depression, was not related to depression in the present study. Moreover, there is a potential influence of single source/single method variance on the findings, especially given the high correlation between all the variables under examination.
Conclusions
The present study highlights different results that could be arrived depending on whether researchers decide to use variables as mediators or moderators. For example, when using meditational analyses, anxiety and stress seem to be important factors that explain how the different variables used here influence depression–increases in anxiety and stress by any other factor seem to lead to increases in depression. In contrast, when moderation analyses were used, the interaction of stress and affect predicted depression and the interaction of both affectivity dimensions (i.e., positive and negative affect) also predicted depression–stress might increase depression under the condition that the individual is high in negative affectivity, in turn, negative affectivity might increase depression under the condition that the individual experiences low positive affectivity.
Acknowledgments
The authors would like to thank the reviewers for their openness and suggestions, which significantly improved the article.
Author Contributions
Conceived and designed the experiments: AAN TA. Performed the experiments: AAN. Analyzed the data: AAN DG. Contributed reagents/materials/analysis tools: AAN TA DG. Wrote the paper: AAN PR TA DG.
- View Article
- Google Scholar
- 3. MacKinnon DP, Luecken LJ (2008) How and for Whom? Mediation and Moderation in Health Psychology. Health Psychol 27 (2 Suppl.): s99–s102.
- 4. Aaroe R (2006) Vinn över din depression [Defeat depression]. Stockholm: Liber.
- 5. Agerberg M (1998) Ut ur mörkret [Out from the Darkness]. Stockholm: Nordstedt.
- 6. Gilbert P (2005) Hantera din depression [Cope with your Depression]. Stockholm: Bokförlaget Prisma.
- 8. Tabachnick BG, Fidell LS (2007) Using Multivariate Statistics, Fifth Edition. Boston: Pearson Education, Inc.
- 10. Beck AT (1967) Depression: Causes and treatment. Philadelphia: University of Pennsylvania Press.
- 21. Eskin M, Parr D (1996) Introducing a Swedish version of an instrument measuring mental stress. Stockholm: Psykologiska institutionen Stockholms Universitet.
- 22. Rosenberg M (1965) Society and the Adolescent Self-Image. Princeton, NJ: Princeton University Press.
- 23. Lindwall M (2011) Självkänsla – Bortom populärpsykologi & enkla sanningar [Self-Esteem – Beyond Popular Psychology and Simple Truths]. Lund:Studentlitteratur.
- 25. Blascovich J, Tomaka J (1991) Measures of self-esteem. In: Robinson JP, Shaver PR, Wrightsman LS (Red.) Measures of personality and social psychological attitudes San Diego: Academic Press. 161–194.
- 30. Eysenck M (Ed.) (2000) Psychology: an integrated approach. New York: Oxford University Press.
- 31. Lazarus RS, Folkman S (1984) Stress, Appraisal, and Coping. New York: Springer.
- 32. Johnson M (2003) Självkänsla och anpassning [Self-esteem and Adaptation]. Lund: Studentlitteratur.
- 33. Cullberg Weston M (2005) Ditt inre centrum – Om självkänsla, självbild och konturen av ditt själv [Your Inner Centre – About Self-esteem, Self-image and the Contours of Yourself]. Stockholm: Natur och Kultur.
- 34. Lindén M (1997) Studentens livssituation. Frihet, sårbarhet, kris och utveckling [Students' Life Situation. Freedom, Vulnerability, Crisis and Development]. Uppsala: Studenthälsan.
- 35. Williams S (1995) Press utan stress ger maximal prestation [Pressure without Stress gives Maximal Performance]. Malmö: Richters förlag.
- 37. Garcia D, Kerekes N, Andersson-Arntén A–C, Archer T (2012) Temperament, Character, and Adolescents' Depressive Symptoms: Focusing on Affect. Depress Res Treat. DOI:10.1155/2012/925372.
- 40. Garcia D, Ghiabi B, Moradi S, Siddiqui A, Archer T (2013) The Happy Personality: A Tale of Two Philosophies. In Morris EF, Jackson M-A editors. Psychology of Personality. New York: Nova Science Publishers. 41–59.
- 41. Schütz E, Nima AA, Sailer U, Andersson-Arntén A–C, Archer T, Garcia D (2013) The affective profiles in the USA: Happiness, depression, life satisfaction, and happiness-increasing strategies. In press.
- 43. Garcia D, Nima AA, Archer T (2013) Temperament and Character's Relationship to Subjective Well- Being in Salvadorian Adolescents and Young Adults. In press.
- 44. Garcia D (2013) La vie en Rose: High Levels of Well-Being and Events Inside and Outside Autobiographical Memory. J Happiness Stud. DOI: 10.1007/s10902-013-9443-x.
- 48. Adrianson L, Djumaludin A, Neila R, Archer T (2013) Cultural influences upon health, affect, self-esteem and impulsiveness: An Indonesian-Swedish comparison. Int J Res Stud Psychol. DOI: 10.5861/ijrsp.2013.228.
- Privacy Policy

Home » Regression Analysis – Methods, Types and Examples
Regression Analysis – Methods, Types and Examples
Table of Contents

Regression Analysis
Regression analysis is a set of statistical processes for estimating the relationships among variables . It includes many techniques for modeling and analyzing several variables when the focus is on the relationship between a dependent variable and one or more independent variables (or ‘predictors’).
Regression Analysis Methodology
Here is a general methodology for performing regression analysis:
- Define the research question: Clearly state the research question or hypothesis you want to investigate. Identify the dependent variable (also called the response variable or outcome variable) and the independent variables (also called predictor variables or explanatory variables) that you believe are related to the dependent variable.
- Collect data: Gather the data for the dependent variable and independent variables. Ensure that the data is relevant, accurate, and representative of the population or phenomenon you are studying.
- Explore the data: Perform exploratory data analysis to understand the characteristics of the data, identify any missing values or outliers, and assess the relationships between variables through scatter plots, histograms, or summary statistics.
- Choose the regression model: Select an appropriate regression model based on the nature of the variables and the research question. Common regression models include linear regression, multiple regression, logistic regression, polynomial regression, and time series regression, among others.
- Assess assumptions: Check the assumptions of the regression model. Some common assumptions include linearity (the relationship between variables is linear), independence of errors, homoscedasticity (constant variance of errors), and normality of errors. Violation of these assumptions may require additional steps or alternative models.
- Estimate the model: Use a suitable method to estimate the parameters of the regression model. The most common method is ordinary least squares (OLS), which minimizes the sum of squared differences between the observed and predicted values of the dependent variable.
- I nterpret the results: Analyze the estimated coefficients, p-values, confidence intervals, and goodness-of-fit measures (e.g., R-squared) to interpret the results. Determine the significance and direction of the relationships between the independent variables and the dependent variable.
- Evaluate model performance: Assess the overall performance of the regression model using appropriate measures, such as R-squared, adjusted R-squared, and root mean squared error (RMSE). These measures indicate how well the model fits the data and how much of the variation in the dependent variable is explained by the independent variables.
- Test assumptions and diagnose problems: Check the residuals (the differences between observed and predicted values) for any patterns or deviations from assumptions. Conduct diagnostic tests, such as examining residual plots, testing for multicollinearity among independent variables, and assessing heteroscedasticity or autocorrelation, if applicable.
- Make predictions and draw conclusions: Once you have a satisfactory model, use it to make predictions on new or unseen data. Draw conclusions based on the results of the analysis, considering the limitations and potential implications of the findings.
Types of Regression Analysis
Types of Regression Analysis are as follows:
Linear Regression
Linear regression is the most basic and widely used form of regression analysis. It models the linear relationship between a dependent variable and one or more independent variables. The goal is to find the best-fitting line that minimizes the sum of squared differences between observed and predicted values.
Multiple Regression
Multiple regression extends linear regression by incorporating two or more independent variables to predict the dependent variable. It allows for examining the simultaneous effects of multiple predictors on the outcome variable.
Polynomial Regression
Polynomial regression models non-linear relationships between variables by adding polynomial terms (e.g., squared or cubic terms) to the regression equation. It can capture curved or nonlinear patterns in the data.
Logistic Regression
Logistic regression is used when the dependent variable is binary or categorical. It models the probability of the occurrence of a certain event or outcome based on the independent variables. Logistic regression estimates the coefficients using the logistic function, which transforms the linear combination of predictors into a probability.
Ridge Regression and Lasso Regression
Ridge regression and Lasso regression are techniques used for addressing multicollinearity (high correlation between independent variables) and variable selection. Both methods introduce a penalty term to the regression equation to shrink or eliminate less important variables. Ridge regression uses L2 regularization, while Lasso regression uses L1 regularization.
Time Series Regression
Time series regression analyzes the relationship between a dependent variable and independent variables when the data is collected over time. It accounts for autocorrelation and trends in the data and is used in forecasting and studying temporal relationships.
Nonlinear Regression
Nonlinear regression models are used when the relationship between the dependent variable and independent variables is not linear. These models can take various functional forms and require estimation techniques different from those used in linear regression.
Poisson Regression
Poisson regression is employed when the dependent variable represents count data. It models the relationship between the independent variables and the expected count, assuming a Poisson distribution for the dependent variable.
Generalized Linear Models (GLM)
GLMs are a flexible class of regression models that extend the linear regression framework to handle different types of dependent variables, including binary, count, and continuous variables. GLMs incorporate various probability distributions and link functions.
Regression Analysis Formulas
Regression analysis involves estimating the parameters of a regression model to describe the relationship between the dependent variable (Y) and one or more independent variables (X). Here are the basic formulas for linear regression, multiple regression, and logistic regression:
Linear Regression:
Simple Linear Regression Model: Y = β0 + β1X + ε
Multiple Linear Regression Model: Y = β0 + β1X1 + β2X2 + … + βnXn + ε
In both formulas:
- Y represents the dependent variable (response variable).
- X represents the independent variable(s) (predictor variable(s)).
- β0, β1, β2, …, βn are the regression coefficients or parameters that need to be estimated.
- ε represents the error term or residual (the difference between the observed and predicted values).
Multiple Regression:
Multiple regression extends the concept of simple linear regression by including multiple independent variables.
Multiple Regression Model: Y = β0 + β1X1 + β2X2 + … + βnXn + ε
The formulas are similar to those in linear regression, with the addition of more independent variables.
Logistic Regression:
Logistic regression is used when the dependent variable is binary or categorical. The logistic regression model applies a logistic or sigmoid function to the linear combination of the independent variables.
Logistic Regression Model: p = 1 / (1 + e^-(β0 + β1X1 + β2X2 + … + βnXn))
In the formula:
- p represents the probability of the event occurring (e.g., the probability of success or belonging to a certain category).
- X1, X2, …, Xn represent the independent variables.
- e is the base of the natural logarithm.
The logistic function ensures that the predicted probabilities lie between 0 and 1, allowing for binary classification.
Regression Analysis Examples
Regression Analysis Examples are as follows:
- Stock Market Prediction: Regression analysis can be used to predict stock prices based on various factors such as historical prices, trading volume, news sentiment, and economic indicators. Traders and investors can use this analysis to make informed decisions about buying or selling stocks.
- Demand Forecasting: In retail and e-commerce, real-time It can help forecast demand for products. By analyzing historical sales data along with real-time data such as website traffic, promotional activities, and market trends, businesses can adjust their inventory levels and production schedules to meet customer demand more effectively.
- Energy Load Forecasting: Utility companies often use real-time regression analysis to forecast electricity demand. By analyzing historical energy consumption data, weather conditions, and other relevant factors, they can predict future energy loads. This information helps them optimize power generation and distribution, ensuring a stable and efficient energy supply.
- Online Advertising Performance: It can be used to assess the performance of online advertising campaigns. By analyzing real-time data on ad impressions, click-through rates, conversion rates, and other metrics, advertisers can adjust their targeting, messaging, and ad placement strategies to maximize their return on investment.
- Predictive Maintenance: Regression analysis can be applied to predict equipment failures or maintenance needs. By continuously monitoring sensor data from machines or vehicles, regression models can identify patterns or anomalies that indicate potential failures. This enables proactive maintenance, reducing downtime and optimizing maintenance schedules.
- Financial Risk Assessment: Real-time regression analysis can help financial institutions assess the risk associated with lending or investment decisions. By analyzing real-time data on factors such as borrower financials, market conditions, and macroeconomic indicators, regression models can estimate the likelihood of default or assess the risk-return tradeoff for investment portfolios.
Importance of Regression Analysis
Importance of Regression Analysis is as follows:
- Relationship Identification: Regression analysis helps in identifying and quantifying the relationship between a dependent variable and one or more independent variables. It allows us to determine how changes in independent variables impact the dependent variable. This information is crucial for decision-making, planning, and forecasting.
- Prediction and Forecasting: Regression analysis enables us to make predictions and forecasts based on the relationships identified. By estimating the values of the dependent variable using known values of independent variables, regression models can provide valuable insights into future outcomes. This is particularly useful in business, economics, finance, and other fields where forecasting is vital for planning and strategy development.
- Causality Assessment: While correlation does not imply causation, regression analysis provides a framework for assessing causality by considering the direction and strength of the relationship between variables. It allows researchers to control for other factors and assess the impact of a specific independent variable on the dependent variable. This helps in determining the causal effect and identifying significant factors that influence outcomes.
- Model Building and Variable Selection: Regression analysis aids in model building by determining the most appropriate functional form of the relationship between variables. It helps researchers select relevant independent variables and eliminate irrelevant ones, reducing complexity and improving model accuracy. This process is crucial for creating robust and interpretable models.
- Hypothesis Testing: Regression analysis provides a statistical framework for hypothesis testing. Researchers can test the significance of individual coefficients, assess the overall model fit, and determine if the relationship between variables is statistically significant. This allows for rigorous analysis and validation of research hypotheses.
- Policy Evaluation and Decision-Making: Regression analysis plays a vital role in policy evaluation and decision-making processes. By analyzing historical data, researchers can evaluate the effectiveness of policy interventions and identify the key factors contributing to certain outcomes. This information helps policymakers make informed decisions, allocate resources effectively, and optimize policy implementation.
- Risk Assessment and Control: Regression analysis can be used for risk assessment and control purposes. By analyzing historical data, organizations can identify risk factors and develop models that predict the likelihood of certain outcomes, such as defaults, accidents, or failures. This enables proactive risk management, allowing organizations to take preventive measures and mitigate potential risks.
When to Use Regression Analysis
- Prediction : Regression analysis is often employed to predict the value of the dependent variable based on the values of independent variables. For example, you might use regression to predict sales based on advertising expenditure, or to predict a student’s academic performance based on variables like study time, attendance, and previous grades.
- Relationship analysis: Regression can help determine the strength and direction of the relationship between variables. It can be used to examine whether there is a linear association between variables, identify which independent variables have a significant impact on the dependent variable, and quantify the magnitude of those effects.
- Causal inference: Regression analysis can be used to explore cause-and-effect relationships by controlling for other variables. For example, in a medical study, you might use regression to determine the impact of a specific treatment while accounting for other factors like age, gender, and lifestyle.
- Forecasting : Regression models can be utilized to forecast future trends or outcomes. By fitting a regression model to historical data, you can make predictions about future values of the dependent variable based on changes in the independent variables.
- Model evaluation: Regression analysis can be used to evaluate the performance of a model or test the significance of variables. You can assess how well the model fits the data, determine if additional variables improve the model’s predictive power, or test the statistical significance of coefficients.
- Data exploration : Regression analysis can help uncover patterns and insights in the data. By examining the relationships between variables, you can gain a deeper understanding of the data set and identify potential patterns, outliers, or influential observations.
Applications of Regression Analysis
Here are some common applications of regression analysis:
- Economic Forecasting: Regression analysis is frequently employed in economics to forecast variables such as GDP growth, inflation rates, or stock market performance. By analyzing historical data and identifying the underlying relationships, economists can make predictions about future economic conditions.
- Financial Analysis: Regression analysis plays a crucial role in financial analysis, such as predicting stock prices or evaluating the impact of financial factors on company performance. It helps analysts understand how variables like interest rates, company earnings, or market indices influence financial outcomes.
- Marketing Research: Regression analysis helps marketers understand consumer behavior and make data-driven decisions. It can be used to predict sales based on advertising expenditures, pricing strategies, or demographic variables. Regression models provide insights into which marketing efforts are most effective and help optimize marketing campaigns.
- Health Sciences: Regression analysis is extensively used in medical research and public health studies. It helps examine the relationship between risk factors and health outcomes, such as the impact of smoking on lung cancer or the relationship between diet and heart disease. Regression analysis also helps in predicting health outcomes based on various factors like age, genetic markers, or lifestyle choices.
- Social Sciences: Regression analysis is widely used in social sciences like sociology, psychology, and education research. Researchers can investigate the impact of variables like income, education level, or social factors on various outcomes such as crime rates, academic performance, or job satisfaction.
- Operations Research: Regression analysis is applied in operations research to optimize processes and improve efficiency. For example, it can be used to predict demand based on historical sales data, determine the factors influencing production output, or optimize supply chain logistics.
- Environmental Studies: Regression analysis helps in understanding and predicting environmental phenomena. It can be used to analyze the impact of factors like temperature, pollution levels, or land use patterns on phenomena such as species diversity, water quality, or climate change.
- Sports Analytics: Regression analysis is increasingly used in sports analytics to gain insights into player performance, team strategies, and game outcomes. It helps analyze the relationship between various factors like player statistics, coaching strategies, or environmental conditions and their impact on game outcomes.
Advantages and Disadvantages of Regression Analysis
About the author.
Muhammad Hassan
Researcher, Academic Writer, Web developer
You may also like

Cluster Analysis – Types, Methods and Examples

Discriminant Analysis – Methods, Types and...

MANOVA (Multivariate Analysis of Variance) –...

Documentary Analysis – Methods, Applications and...

ANOVA (Analysis of variance) – Formulas, Types...

Graphical Methods – Types, Examples and Guide
Root out friction in every digital experience, super-charge conversion rates, and optimize digital self-service
Uncover insights from any interaction, deliver AI-powered agent coaching, and reduce cost to serve
Increase revenue and loyalty with real-time insights and recommendations delivered to teams on the ground
Know how your people feel and empower managers to improve employee engagement, productivity, and retention
Take action in the moments that matter most along the employee journey and drive bottom line growth
Whatever they’re are saying, wherever they’re saying it, know exactly what’s going on with your people
Get faster, richer insights with qual and quant tools that make powerful market research available to everyone
Run concept tests, pricing studies, prototyping + more with fast, powerful studies designed by UX research experts
Track your brand performance 24/7 and act quickly to respond to opportunities and challenges in your market
Explore the platform powering Experience Management
- Free Account
- For Digital
- For Customer Care
- For Human Resources
- For Researchers
- Financial Services
- All Industries
Popular Use Cases
- Customer Experience
- Employee Experience
- Net Promoter Score
- Voice of Customer
- Customer Success Hub
- Product Documentation
- Training & Certification
- XM Institute
- Popular Resources
- Customer Stories
- Artificial Intelligence
- Market Research
- Partnerships
- Marketplace
The annual gathering of the experience leaders at the world’s iconic brands building breakthrough business results, live in Salt Lake City.
- English/AU & NZ
- Español/Europa
- Español/América Latina
- Português Brasileiro
- REQUEST DEMO
- Experience Management
- Survey Data Analysis & Reporting
- Regression Analysis
Try Qualtrics for free
The complete guide to regression analysis.
19 min read What is regression analysis and why is it useful? While most of us have heard the term, understanding regression analysis in detail may be something you need to brush up on. Here’s what you need to know about this popular method of analysis.
When you rely on data to drive and guide business decisions, as well as predict market trends, just gathering and analyzing what you find isn’t enough — you need to ensure it’s relevant and valuable.
The challenge, however, is that so many variables can influence business data: market conditions, economic disruption, even the weather! As such, it’s essential you know which variables are affecting your data and forecasts, and what data you can discard.
And one of the most effective ways to determine data value and monitor trends (and the relationships between them) is to use regression analysis, a set of statistical methods used for the estimation of relationships between independent and dependent variables.
In this guide, we’ll cover the fundamentals of regression analysis, from what it is and how it works to its benefits and practical applications.
Free eBook: 2024 global market research trends report
What is regression analysis?
Regression analysis is a statistical method. It’s used for analyzing different factors that might influence an objective – such as the success of a product launch, business growth, a new marketing campaign – and determining which factors are important and which ones can be ignored.
Regression analysis can also help leaders understand how different variables impact each other and what the outcomes are. For example, when forecasting financial performance, regression analysis can help leaders determine how changes in the business can influence revenue or expenses in the future.
Running an analysis of this kind, you might find that there’s a high correlation between the number of marketers employed by the company, the leads generated, and the opportunities closed.
This seems to suggest that a high number of marketers and a high number of leads generated influences sales success. But do you need both factors to close those sales? By analyzing the effects of these variables on your outcome, you might learn that when leads increase but the number of marketers employed stays constant, there is no impact on the number of opportunities closed, but if the number of marketers increases, leads and closed opportunities both rise.
Regression analysis can help you tease out these complex relationships so you can determine which areas you need to focus on in order to get your desired results, and avoid wasting time with those that have little or no impact. In this example, that might mean hiring more marketers rather than trying to increase leads generated.
How does regression analysis work?
Regression analysis starts with variables that are categorized into two types: dependent and independent variables. The variables you select depend on the outcomes you’re analyzing.
Understanding variables:
1. dependent variable.
This is the main variable that you want to analyze and predict. For example, operational (O) data such as your quarterly or annual sales, or experience (X) data such as your net promoter score (NPS) or customer satisfaction score (CSAT) .
These variables are also called response variables, outcome variables, or left-hand-side variables (because they appear on the left-hand side of a regression equation).
There are three easy ways to identify them:
- Is the variable measured as an outcome of the study?
- Does the variable depend on another in the study?
- Do you measure the variable only after other variables are altered?
2. Independent variable
Independent variables are the factors that could affect your dependent variables. For example, a price rise in the second quarter could make an impact on your sales figures.
You can identify independent variables with the following list of questions:
- Is the variable manipulated, controlled, or used as a subject grouping method by the researcher?
- Does this variable come before the other variable in time?
- Are you trying to understand whether or how this variable affects another?
Independent variables are often referred to differently in regression depending on the purpose of the analysis. You might hear them called:
Explanatory variables
Explanatory variables are those which explain an event or an outcome in your study. For example, explaining why your sales dropped or increased.
Predictor variables
Predictor variables are used to predict the value of the dependent variable. For example, predicting how much sales will increase when new product features are rolled out .
Experimental variables
These are variables that can be manipulated or changed directly by researchers to assess the impact. For example, assessing how different product pricing ($10 vs $15 vs $20) will impact the likelihood to purchase.
Subject variables (also called fixed effects)
Subject variables can’t be changed directly, but vary across the sample. For example, age, gender, or income of consumers.
Unlike experimental variables, you can’t randomly assign or change subject variables, but you can design your regression analysis to determine the different outcomes of groups of participants with the same characteristics. For example, ‘how do price rises impact sales based on income?’
Carrying out regression analysis
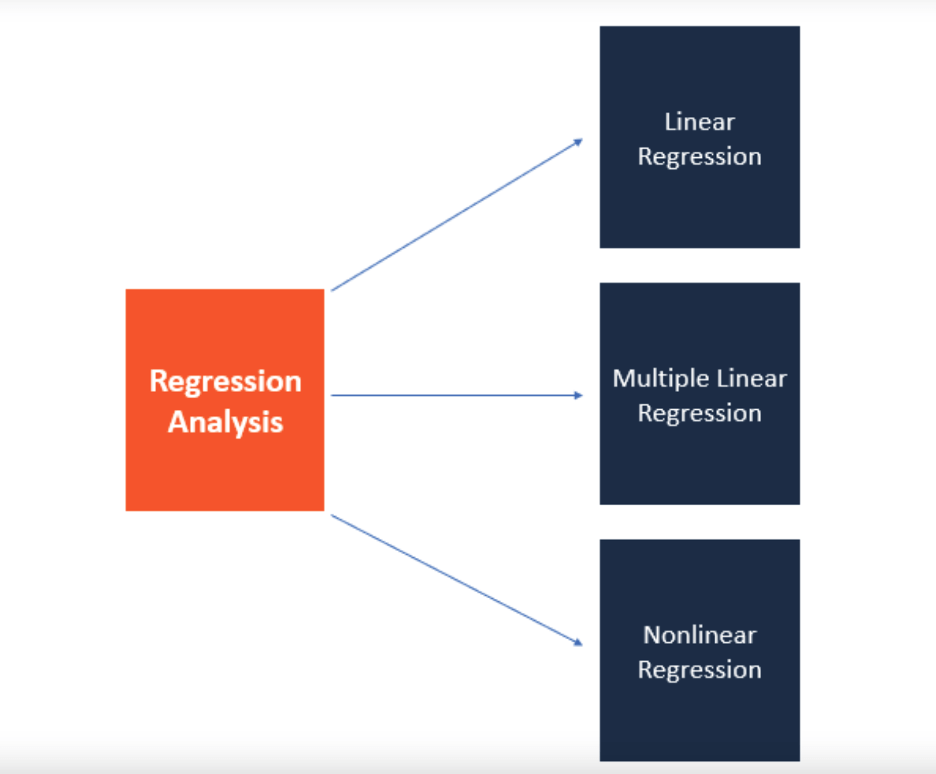
So regression is about the relationships between dependent and independent variables. But how exactly do you do it?
Assuming you have your data collection done already, the first and foremost thing you need to do is plot your results on a graph. Doing this makes interpreting regression analysis results much easier as you can clearly see the correlations between dependent and independent variables.
Let’s say you want to carry out a regression analysis to understand the relationship between the number of ads placed and revenue generated.
On the Y-axis, you place the revenue generated. On the X-axis, the number of digital ads. By plotting the information on the graph, and drawing a line (called the regression line) through the middle of the data, you can see the relationship between the number of digital ads placed and revenue generated.
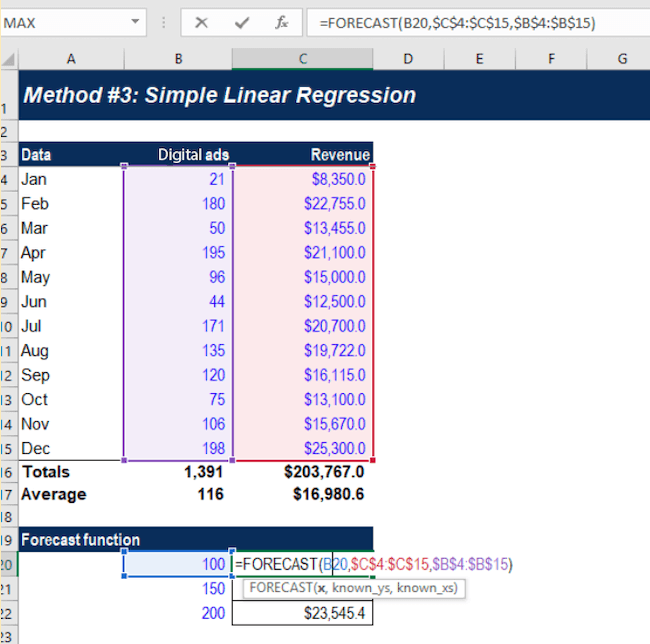
This regression line is the line that provides the best description of the relationship between your independent variables and your dependent variable. In this example, we’ve used a simple linear regression model.
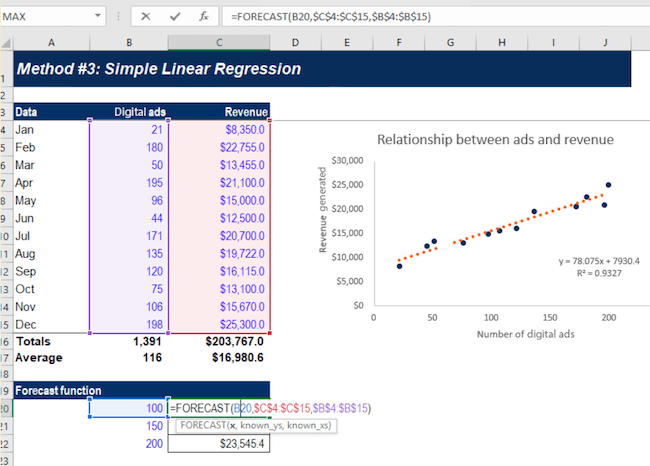
Statistical analysis software can draw this line for you and precisely calculate the regression line. The software then provides a formula for the slope of the line, adding further context to the relationship between your dependent and independent variables.
Simple linear regression analysis
A simple linear model uses a single straight line to determine the relationship between a single independent variable and a dependent variable.
This regression model is mostly used when you want to determine the relationship between two variables (like price increases and sales) or the value of the dependent variable at certain points of the independent variable (for example the sales levels at a certain price rise).
While linear regression is useful, it does require you to make some assumptions.
For example, it requires you to assume that:
- the data was collected using a statistically valid sample collection method that is representative of the target population
- The observed relationship between the variables can’t be explained by a ‘hidden’ third variable – in other words, there are no spurious correlations.
- the relationship between the independent variable and dependent variable is linear – meaning that the best fit along the data points is a straight line and not a curved one
Multiple regression analysis
As the name suggests, multiple regression analysis is a type of regression that uses multiple variables. It uses multiple independent variables to predict the outcome of a single dependent variable. Of the various kinds of multiple regression, multiple linear regression is one of the best-known.
Multiple linear regression is a close relative of the simple linear regression model in that it looks at the impact of several independent variables on one dependent variable. However, like simple linear regression, multiple regression analysis also requires you to make some basic assumptions.
For example, you will be assuming that:
- there is a linear relationship between the dependent and independent variables (it creates a straight line and not a curve through the data points)
- the independent variables aren’t highly correlated in their own right
An example of multiple linear regression would be an analysis of how marketing spend, revenue growth, and general market sentiment affect the share price of a company.
With multiple linear regression models you can estimate how these variables will influence the share price, and to what extent.
Multivariate linear regression
Multivariate linear regression involves more than one dependent variable as well as multiple independent variables, making it more complicated than linear or multiple linear regressions. However, this also makes it much more powerful and capable of making predictions about complex real-world situations.
For example, if an organization wants to establish or estimate how the COVID-19 pandemic has affected employees in its different markets, it can use multivariate linear regression, with the different geographical regions as dependent variables and the different facets of the pandemic as independent variables (such as mental health self-rating scores, proportion of employees working at home, lockdown durations and employee sick days).
Through multivariate linear regression, you can look at relationships between variables in a holistic way and quantify the relationships between them. As you can clearly visualize those relationships, you can make adjustments to dependent and independent variables to see which conditions influence them. Overall, multivariate linear regression provides a more realistic picture than looking at a single variable.
However, because multivariate techniques are complex, they involve high-level mathematics that require a statistical program to analyze the data.
Logistic regression
Logistic regression models the probability of a binary outcome based on independent variables.
So, what is a binary outcome? It’s when there are only two possible scenarios, either the event happens (1) or it doesn’t (0). e.g. yes/no outcomes, pass/fail outcomes, and so on. In other words, if the outcome can be described as being in either one of two categories.
Logistic regression makes predictions based on independent variables that are assumed or known to have an influence on the outcome. For example, the probability of a sports team winning their game might be affected by independent variables like weather, day of the week, whether they are playing at home or away and how they fared in previous matches.
What are some common mistakes with regression analysis?
Across the globe, businesses are increasingly relying on quality data and insights to drive decision-making — but to make accurate decisions, it’s important that the data collected and statistical methods used to analyze it are reliable and accurate.
Using the wrong data or the wrong assumptions can result in poor decision-making, lead to missed opportunities to improve efficiency and savings, and — ultimately — damage your business long term.
- Assumptions
When running regression analysis, be it a simple linear or multiple regression, it’s really important to check that the assumptions your chosen method requires have been met. If your data points don’t conform to a straight line of best fit, for example, you need to apply additional statistical modifications to accommodate the non-linear data. For example, if you are looking at income data, which scales on a logarithmic distribution, you should take the Natural Log of Income as your variable then adjust the outcome after the model is created.
- Correlation vs. causation
It’s a well-worn phrase that bears repeating – correlation does not equal causation. While variables that are linked by causality will always show correlation, the reverse is not always true. Moreover, there is no statistic that can determine causality (although the design of your study overall can).
If you observe a correlation in your results, such as in the first example we gave in this article where there was a correlation between leads and sales, you can’t assume that one thing has influenced the other. Instead, you should use it as a starting point for investigating the relationship between the variables in more depth.
- Choosing the wrong variables to analyze
Before you use any kind of statistical method, it’s important to understand the subject you’re researching in detail. Doing so means you’re making informed choices of variables and you’re not overlooking something important that might have a significant bearing on your dependent variable.
- Model building The variables you include in your analysis are just as important as the variables you choose to exclude. That’s because the strength of each independent variable is influenced by the other variables in the model. Other techniques, such as Key Drivers Analysis, are able to account for these variable interdependencies.
Benefits of using regression analysis
There are several benefits to using regression analysis to judge how changing variables will affect your business and to ensure you focus on the right things when forecasting.
Here are just a few of those benefits:
Make accurate predictions
Regression analysis is commonly used when forecasting and forward planning for a business. For example, when predicting sales for the year ahead, a number of different variables will come into play to determine the eventual result.
Regression analysis can help you determine which of these variables are likely to have the biggest impact based on previous events and help you make more accurate forecasts and predictions.
Identify inefficiencies
Using a regression equation a business can identify areas for improvement when it comes to efficiency, either in terms of people, processes, or equipment.
For example, regression analysis can help a car manufacturer determine order numbers based on external factors like the economy or environment.
Using the initial regression equation, they can use it to determine how many members of staff and how much equipment they need to meet orders.
Drive better decisions
Improving processes or business outcomes is always on the minds of owners and business leaders, but without actionable data, they’re simply relying on instinct, and this doesn’t always work out.
This is particularly true when it comes to issues of price. For example, to what extent will raising the price (and to what level) affect next quarter’s sales?
There’s no way to know this without data analysis. Regression analysis can help provide insights into the correlation between price rises and sales based on historical data.
How do businesses use regression? A real-life example
Marketing and advertising spending are common topics for regression analysis. Companies use regression when trying to assess the value of ad spend and marketing spend on revenue.
A typical example is using a regression equation to assess the correlation between ad costs and conversions of new customers. In this instance,
- our dependent variable (the factor we’re trying to assess the outcomes of) will be our conversions
- the independent variable (the factor we’ll change to assess how it changes the outcome) will be the daily ad spend
- the regression equation will try to determine whether an increase in ad spend has a direct correlation with the number of conversions we have
The analysis is relatively straightforward — using historical data from an ad account, we can use daily data to judge ad spend vs conversions and how changes to the spend alter the conversions.
By assessing this data over time, we can make predictions not only on whether increasing ad spend will lead to increased conversions but also what level of spending will lead to what increase in conversions. This can help to optimize campaign spend and ensure marketing delivers good ROI.
This is an example of a simple linear model. If you wanted to carry out a more complex regression equation, we could also factor in other independent variables such as seasonality, GDP, and the current reach of our chosen advertising networks.
By increasing the number of independent variables, we can get a better understanding of whether ad spend is resulting in an increase in conversions, whether it’s exerting an influence in combination with another set of variables, or if we’re dealing with a correlation with no causal impact – which might be useful for predictions anyway, but isn’t a lever we can use to increase sales.
Using this predicted value of each independent variable, we can more accurately predict how spend will change the conversion rate of advertising.
Regression analysis tools
Regression analysis is an important tool when it comes to better decision-making and improved business outcomes. To get the best out of it, you need to invest in the right kind of statistical analysis software.
The best option is likely to be one that sits at the intersection of powerful statistical analysis and intuitive ease of use, as this will empower everyone from beginners to expert analysts to uncover meaning from data, identify hidden trends and produce predictive models without statistical training being required.
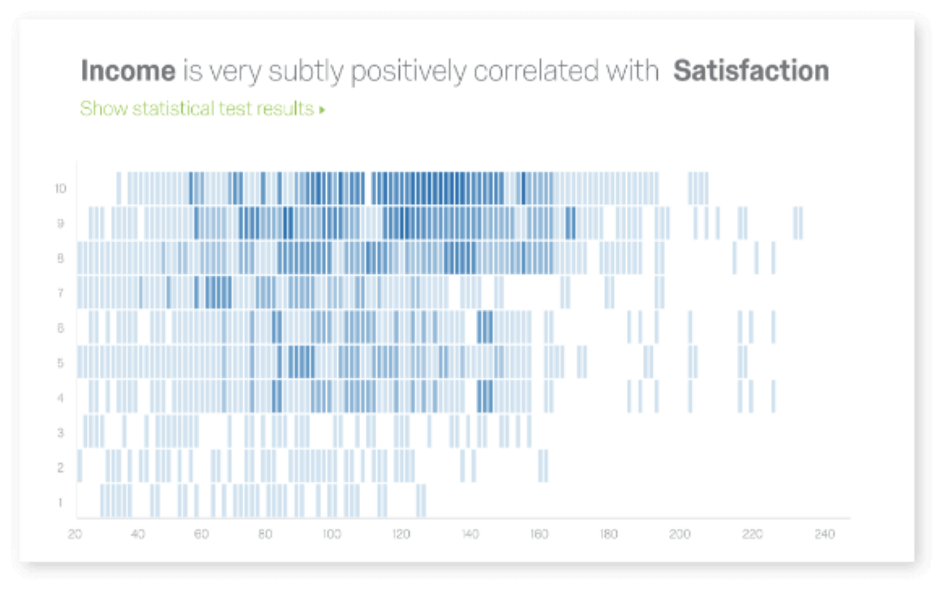
To help prevent costly errors, choose a tool that automatically runs the right statistical tests and visualizations and then translates the results into simple language that anyone can put into action.
With software that’s both powerful and user-friendly, you can isolate key experience drivers, understand what influences the business, apply the most appropriate regression methods, identify data issues, and much more.
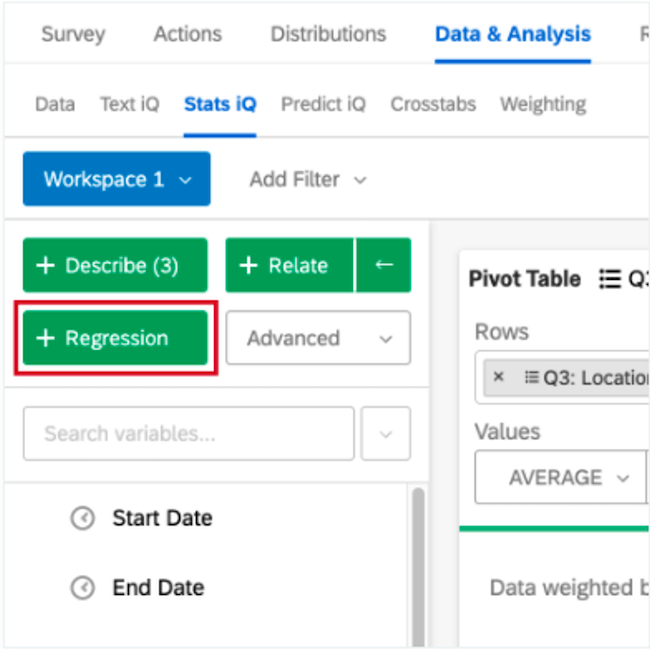
With Qualtrics’ Stats iQ™, you don’t have to worry about the regression equation because our statistical software will run the appropriate equation for you automatically based on the variable type you want to monitor. You can also use several equations, including linear regression and logistic regression, to gain deeper insights into business outcomes and make more accurate, data-driven decisions.
Related resources
Analysis & Reporting
Data Analysis 31 min read
Social media analytics 13 min read, kano analysis 21 min read, margin of error 11 min read, data saturation in qualitative research 8 min read, thematic analysis 11 min read, behavioral analytics 12 min read, request demo.
Ready to learn more about Qualtrics?
Top Articles Related to Regression Analysis Research
Learn all about Regression Analysis Research. Quirks.com is the largest source of marketing research information.
Search Results
More Filters
Loading filters...
Article A new approach to key driver analysis for CX research Dominic Nieto, Michael Garver, Richard Divine | October 1, 2017
Article Meta-analysis offers research on research for marketing research Kevin Gray | May 1, 2014
Article Analyzing a pharmaceutical product’s key drivers Michael Latta | March 1, 2014
Article Best practices for key driver analysis Kevin Gray | February 1, 2014
Article By the Numbers: The top five mistakes in marketing statistics William Briggs | December 1, 2013
Article Data Use: To progress you must first regress Joseph Kreitzer | January 1, 1990
Article Data Use: Hitting the target: Effective techniques for market share and response rate modeling using demographics Charles Schwartz | June 1, 1991
Article Data Use: Regression regression Gary Mullet | October 1, 1994
Article Data Use: Have you ever wondered... Gary Mullet | June 1, 1998
Article Data Use: What mother never told you about linear regression George Butler | October 1, 2000
Article Data Use: Steering the vote: the case of the Oglala Sioux casino Michael Lieberman | July 1, 2013
Article Data Use: Comments on Mullet's regression article Albert Madansky, Gary Mullet | March 1, 1995
Article A comparison of missing value options in regression analysis Gary Mullet | December 1, 1995
Article Regression analysis is a key to actionable results in CSM Eleonora Malpa, Patrick McPhillips | October 1, 1995
Article Surviving the competitive warfare Arup Sen | April 1, 1992
linear regression Recently Published Documents
Total documents.
- Latest Documents
- Most Cited Documents
- Contributed Authors
- Related Sources
- Related Keywords
Modeling the impact of some independent parameters on the syngas characteristics during plasma gasification of municipal solid waste using artificial neural network and stepwise linear regression methods
The effect of conflict and termination of employment on employee's work spirit.
This study aims to find out the conflict and termination of employment both partially and simultaneously have a significant effect on the morale of employees at PT. The benefits of Medan Technique and how much it affects. The method used in this research is quantitative method with several tests namely reliability analysis, classical assumption deviation test and linear regression. Based on the results of primary data regression processed using SPSS 20, multiple linear regression equations were obtained as follows: Y = 1,031 + 0.329 X1+ 0.712 X2.In part, the conflict variable (X1)has a significant effect on the employee's work spirit (Y) at PT. Medan Technical Benefits. This means that the hypothesis in this study was accepted, proven from the value of t calculate > t table (3,952 < 2,052). While the variable termination of employment (X2) has a significant influence on the work spirit of employees (Y) in PT. Medan Technical Benefits. This means that the hypothesis in this study was accepted, proven from the value of t calculate > t table (7,681 > 2,052). Simultaneously, variable conflict (X1) and termination of employment (X2) have a significant influence on the morale of employees (Y) in PT. Medan Technical Benefits. This means that the hypothesis in this study was accepted, as evidenced by the calculated F value > F table (221,992 > 3.35). Conflict variables (X1) and termination of employment (X2) were able to contribute an influence on employee morale variables (Y) of 94.3% while the remaining 5.7% was influenced by other variables not studied in this study. From the above conclusions, the author advises that employees and leaders should reduce prolonged conflict so that the spirit of work can increase. Leaders should be more selective in severing employment relationships so that decent employees are not dismissed unilaterally. Employees should work in a high spirit so that the company can see the quality that employees have.
Truncated $L^1$ Regularized Linear Regression: Theory and Algorithm
Hypothesis testing in high-dimensional linear regression: a normal reference scale-invariant test, new tests for high-dimensional linear regression based on random projection, asymptotic optimality of cp-type criteria in high-dimensional multivariate linear regression models, scaled partial envelope model in multivariate linear regression, implementation of a non-linear regression model in rolling bearing diagnostics, factors affecting employees’ job satisfaction at vinh long radio and television station.
The study’s objective is to determine factors impacting employees’ job satisfaction at Vinh Long Radio and Television (TV) Station. The authors used convenient sampling to collect data from 233 employees working at Vinh Long Radio and TV Station. The exploratory factor analysis and multivariable linear regression help the study find seven factors affecting job satisfaction. They include nature of work, training and promotion, income, leadership, colleagues, working environment, work pressure, and work autonomy. In which, work pressure has the most negative influence on job satisfaction of employees working at Vinh Long Radio and TV Station.
Pengaruh Produk Domestik Regional Bruto (PDRB) terhadap Tingkat Kemiskinan Kota Medan
This study has the benefit of analyzing the effect of regional gross domestic product on poverty in the city of Medan in 2010-2020. The research method used is a quantitative method with reference to a descriptive approach. The data used is time series data on economic growth and poverty at the Central Statistics Agency (BPS) of Medan City in 2010-2020. Data collection techniques used are journals, book documentation, and previous reports. The technique of analyzing the data uses simple linear regression analysis which is carried out to determine whether the model used is free from deviations from the classical assumption test. The equations obtained from the simple linear regression analysis test Y = 24576.325 – 0.365X and have the understanding that the GRDP variable (X) has a significant effect on Poverty (Y). Obtained a value of R2 (R square) of 0.556 with the understanding that the independent variable, namely GRDP, affects the variable of the poverty level in Medan City by 55.6%. Meanwhile, the remaining 44.4% are influenced by different independent variables and are not included in this study. For this reason, it can be concluded that when GRDP increases, it will have an impact on decreasing the value of Poverty in Medan City, and vice versa. Keywords: Gross Regional Domestic Product; Poverty; Medan city
Export Citation Format
Share document.
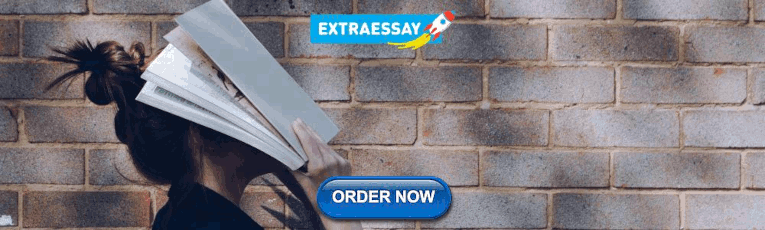
IMAGES
VIDEO
COMMENTS
These ideas for linear regression projects in python help students learn various aspects of linear regression that help them in their careers. Types of Linear Regression: Linear regression is commonly divided into two types i.e., Simple linear regression and multiple linear regression. Let's discuss these types in detail. 1. Simple Linear ...
Regression analysis allows researchers to understand the relationship between two or more variables by estimating the mathematical relationship between them (Sarstedt & Mooi, 2014). In this case ...
Regression analysis is an important statistical method that is commonly used to determine the relationship between ... Schober P, Vetter TR. Linear regression in medical research. Anesth Analg ...
Complex Survey Design. Raghunath Arnab, in Survey Sampling Theory and Applications, 2017. 20.1 Introduction. In regression analysis we describe the relationship between a response (dependent) variable and a number of explanatory (independent) variables. We also predict the future value of the dependent variable using the established relationship. The relationships are explained through ...
Abstract. Multiple regression is one of the most significant forms of regression and has a wide range. of applications. The study of the implementation of multiple regression analysis in different ...
In short, \(X_2\) has a negative estimated partial regression coefficient represented by the difference in height between the two regression lines. Figure 14.1: Dummy Intercept Variables For a case with multiple nominal categories (e.g., region) the procedure is as follows: (a) determine which category will be assigned as the referent group; (b ...
The aim of linear regression analysis is to estimate the coefficients of the regression equation b 0 and b k (k∈K) so that the sum of the squared residuals (i.e., the sum over all squared differences between the observed values of the i th observation of y i and the corresponding predicted values \( {\hat{y}}_i \)) is minimized.The lower part of Fig. 1 illustrates this approach, which is ...
Linear regression is used to study the linear relationship between a dependent variable Y (blood pressure) and one or more independent variables X (age, weight, sex). The dependent variable Y must be continuous, while the independent variables may be either continuous (age), binary (sex), or categorical (social status).
Regression analysis is a data analysis and prediction method to express the relationship between variables that affect a phenomenon and factors. The regression analysis assumes that the more the data samples, the more the number converges on the mean response E ( y ). The analysis is not limited to a specific field but is used in various ways.
This chapter covers the specialized topics within the area of regression analysis; using categorical and dummy variables in regression models, constrained least squares, and estimation using the method of moments and its generalization. Categorical variables are variables that represent group membership. If the two categories are investment ...
Alan O. Sykes, "An Introduction to Regression Analysis" (Coase-Sandor Institute for Law & Economics Working Paper No. 20, 1993). This Working Paper is brought to you for free and open access by the Coase-Sandor Institute for Law and Economics at Chicago Unbound. It has been accepted for inclusion in Coase-Sandor Working Paper Series in Law and ...
In many different sciences, including medicine, engineering, and observational studies, the investigation of the relationship between variables, i.e., dependents, and independents, is defined as research objectives. Employing statistical methods to achieve the relationship between variables is very time-consuming or costly in many scenarios and does not provide practical application. Therefore ...
1. Introduction. An outbreak is announced as a pandemic when it spreads in a large geographical area, infects, and results in mortality for a high number of people, and all of that is caused by a virus that is a subtype of a current virus [].The first pandemic recorded was in 1580 [].Before 1889, pandemics' patterns show a 50-60-year cycle, while, after 1889, a 10-40-year cycle is shown ...
Background Mediation analysis investigates whether a variable (i.e., mediator) changes in regard to an independent variable, in turn, affecting a dependent variable. Moderation analysis, on the other hand, investigates whether the statistical interaction between independent variables predict a dependent variable. Although this difference between these two types of analysis is explicit in ...
Home environment and reading achievement research has been largely dominated by a focus on early reading acquisition, while research on the relationship between home environments and reading success with preadolescents (Grades 4-6) has been largely overlooked. There are other limitations as well. Clarke and Kurtz-Costes (1997) argued that prior ...
Abstract. Although regression models play a central role in the analysis of medical research projects, there still exist many misconceptions on various aspects of modeling leading to faulty analyses. Indeed, the rapidly developing statistical methodology and its recent advances in regression modeling do not seem to be adequately reflected in ...
Logistic Regression: Logistic regression is used when the dependent variable is binary or categorical. The logistic regression model applies a logistic or sigmoid function to the linear combination of the independent variables. Logistic Regression Model: p = 1 / (1 + e^- (β0 + β1X1 + β2X2 + … + βnXn)) In the formula: p represents the ...
Regression analysis is a statistical method. It's used for analyzing different factors that might influence an objective - such as the success of a product launch, business growth, a new marketing campaign - and determining which factors are important and which ones can be ignored.
Regression models with one dependent variable and more than one independent variables are called multilinear regression. In this study, data for multilinear regression analysis is occur from Sakarya University Education Faculty student's lesson (measurement and evaluation, educational psychology, program development, counseling and ...
Find the latest published documents for multiple linear regression, Related hot topics, top authors, the most cited documents, and related journals ... The three methods used in this research are Multiple Linear Regression, Artificial Neural Network, and Deep Learning. ... A multiple linear regression analysis showed that screen time (β = −0 ...
Top Articles Related to Regression Analysis Research. Learn all about Regression Analysis Research. Quirks.com is the largest source of marketing research information. ... Search above or browse by research topic, market focus and content type below. Search Results. Sort By: More Filters. Loading filters... Showing: Regression Analysis . Total ...
The method used in this research is quantitative method with several tests namely reliability analysis, classical assumption deviation test and linear regression. Based on the results of primary data regression processed using SPSS 20, multiple linear regression equations were obtained as follows: Y = 1,031 + 0.329 X1+ 0.712 X2.In part, the ...
This article provides an overview of some of these topics, primarily focused on stratification, regression adjustment, and cluster randomization. The past two decades have witnessed a surge of new research in the analysis of randomized experiments. The emergence of this literature may seem surprising given the widespread use and long history of ...
Regression Analysis Is the Technique. PAGES 2 WORDS 567. Its name tells us the criterion used to select the best fitting line, namely that the sum of the squares of the residuals should be least. In other words, the least squares regression equation is the line for which the sum of squared residuals is a minimum (Dallal, 2008).