
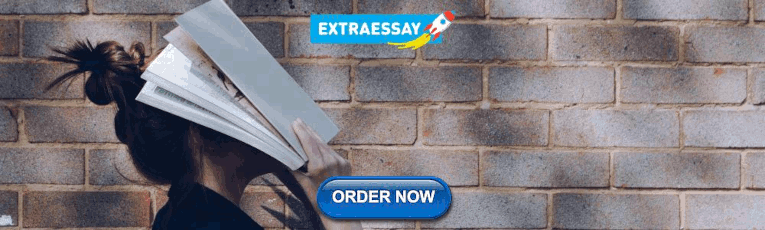
Responsible Thesis-Writing Process
- Information searching
Research data management and the FAIR principles
Stages of data management, data protection (gdpr).
- Interview and Survey Data
- Research ethics
- Research notification
- Research permission
- Business collaboration
- Accessibility
- Publishing thesis
- Save in Osuva
- More useful information
Research data refers to any data with which the analysis and results of a study can be repeated and validated. The data may have been collected by the researcher, generated during the study or consist of pre-existing archival data, and include various measurement results, survey and interview data, notes, research diaries, software or source codes.
Data management refers to the systematic collection, processing, storing and description of research data. Students are encouraged to learn about data management early in their studies, because good data management skills are beneficial to study progress and to adopting suitable data management practices during the thesis-writing process.
Data management practices should seek to comply with the FAIR principles, ensuring that the data is
- Accessible,
- Interoperable and
This is achieved, for example, through the use of open file formats, clear definition of ownership, terms and conditions, as well as licenses, versatile description of the content, structure and rights of use of the data (rich metadata), and the use of persistent identifiers (e.g., DOI, URN, ORCID). Learn more about the FAIR principles and the policy component for open access to research data .

1. Planning
Before you collect any data, record the most suitable data practices in a Data Management Plan (DMP) that can be supplemented as the work progresses and plans become more accurate. Formulating a plan will help you identify potential data protection risks, as well as solutions suitable for storing and describing your data. Careful data management also allows you to make the data accessible for potential reuse and thus improve the reliability of your research and the repeatability of the results.
Planning can be done using the DMPTuuli tool that is accessible with your HAKA credentials. DMPTuuli contains templates and instructions that can be applied to the data management plan of a thesis.

2. Storing and Organising Data
Choose a secure storage solution for your data, based on the demand for the data and its confidentiality level. Secure storage, version control and backup help prevent any unintentional deletion of data. Open file formats, logical file naming and folder structure, as well as rich content descriptions facilitate the findability, intelligibility and sharing of data. Consider the following questions when choosing the storage solution:
- Ensure secure access control so that only authorised parties have access to the data.
- Utilise the university’s cloud storage solutions (e.g., Owncloud, SharePoint) suitable for data sharing.
- Protect confidential data with a password or encryption.
- Confidential data should not be stored in commercial cloud services, such as Dropbox or Google Drive.
- If you store data on your personal devices, ensure backups, device password protection and anti-virus protection.
- If you use services provided by the university, using automatic backup solutions is recommended.
NB: External storage media, such as flash drives, are not recommended as primary storage solutions, because data stored on them is susceptible to becoming lost, deleted and unintentionally shared with outsiders.
Backing up helps you decrease the risk of irreparable damage to or deletion of data. Always keep separate working and backup copies of the research data. Choose storage solutions that include automatic backup. Backing up should be based on the 3-2-1 Rule, meaning that data is stored in the following way:
- in at least three copies
- on two different types of storage media
- one of which is kept physically separate from the others.
File formats
To ensure the usability of your data on a variety of devices and software, using open, non-commercial file formats is recommended. Most software supports the following common file formats:
- Text: txt, .odt., .rtf, .csv, PDF/A, .html,.xml
- Images: jpeg, tiff, png, dng
- Video: MPEG-4 (.mp4), dpx
- Sound: FLAC, aif, aac.
File naming and Folder structure
Systematic file naming practices and folder structures ensure the identifiability and findability of your data, even when there are time lapses in processing it. Clear file naming also simplifies file sharing. When you name a file:
- Choose a descriptive name
- Avoid names that are too long or short
- Avoid special characters and spaces
- To separate parts of the name, use the underscore (_), hyphen (-) or initial capital letters
- Add dates, version numbers and/or modifier initials to distinguish between different file versions
- Avoid overlap in folder and file names
NB: If you use abbreviations, remember to define them in writing so that they can be understood.
Documenting
Document the basics of your data during the thesis writing process to ensure the findability and usability of your data. Documenting makes it easy to check the contents of your data, how it has been processed and where it is stored. The simplest option is to record the descriptive data (or metadata) related to your data in a text file (a.k.a. README file) that you save as a separate file along with your data. Metadata may also be published according to the description guidelines of the particular publishing service. Record at least the following information in the file:
- Data name, size and file format
- Data content and descriptions of variables (abbreviations, measuring scales, coding)
- Data collection (who, where, when, how)
- Data processing (who, how, when)
- Storing data and terms and conditions
Read more about storing, file naming , recommended file formats and documenting in the Data Management Guidelines of the Finnish Social Science Data Archive.
3. Publishing, archiving or deleting
Take care of your research data even after the completion of the thesis. Electronic data requires further measures to stay up-to-date, and not all data needs to be archived for long periods of time. Based on the reuse value of your data, choose appropriate measures, such as data archiving, publishing or deletion. Keep in mind that your right to use the University of Vaasa IT services expires after graduation, unless you continue in another university role, such as a position of doctoral researcher or employee. If the data is stored in the University of Vaasa systems, remember to transfer or delete it before your access rights expire.
If your data contains personal details, it is usually deleted after the thesis has been accepted. Keep in mind that moving a file to the recycle bin does not sufficiently delete the data. More thorough measures, such as overwriting a drive or mechanically destroying a flash drive, are needed. Further information on deleting the data: Office of the Data Protection Ombudsman or Data Management Guidelines of the Finnish Social Science Data Archive .
If the data has reuse value and you have permission to reuse or publish the data, you may publish or archive your data in a chosen data archive. Keep in mind that you may need permission for data reuse or publishing from your research subjects or potential customer, and that data anonymisation may be a condition for publishing. For example, the Finnish Social Science Data Archive , The Language Bank of Finland , and Fairdata’s IDA and Qvain offer domestic solutions for publishing data and related metadata, while Zenodo or EUDAT B2Share are some of the international service provider options.
Data protection refers to the safeguarding of personal data. The notion of personal data is broad, and what qualifies as personal data is any information that either directly or indirectly enables the identification of a person, for example by connecting an individual piece of information to another piece of information. More information on personal data: Office of the Data Protection Ombudsman . Personal data processing related to studies must adhere to the principles of the University of Vaasa data protection policy: University of Vaasa Information Security Policy .
Before collecting and processing personal data:
- Discuss the issue with your thesis supervisor or the teacher in charge of the course
- Familiarise yourself with the University of Vaasa personal data processing instructions: Data Processing Instructions (NB: The instructions can only be accessed by university personnel) and University of Vaasa Data Protection Statement (NB: currently available only in Finnish).
- Be sure to provide the research participants with a privacy notice. Further reading available in this guide under Privacy Notice .
While collecting and processing personal data:
- Limit the processing of personal data to what is necessary to achieve the aims of the thesis. Do not collect personal data “just in case”!
- Store personal data in a secure way and ensure that third parties do not accidentally or intentionally gain access to personal data.
The student collecting personal data acts as the data controller.
Useful links

- << Previous: Information searching
- Next: Interview and Survey Data >>
- Last Updated: Jan 31, 2024 11:56 AM
- URL: https://uva.libguides.com/responsible-thesis
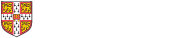
Study at Cambridge
About the university, research at cambridge.
- Undergraduate courses
- Events and open days
- Fees and finance
- Postgraduate courses
- How to apply
- Postgraduate events
- Fees and funding
- International students
- Continuing education
- Executive and professional education
- Courses in education
- How the University and Colleges work
- Term dates and calendars
- Visiting the University
- Annual reports
- Equality and diversity
- A global university
- Public engagement
- Give to Cambridge
- For Cambridge students
- For our researchers
- Business and enterprise
- Colleges & departments
- Email & phone search
- Museums & collections
- Open Research
- Share Your Research
- Open Research overview
- Share Your Research overview
- Open Research Position Statement
- Scholarly Communication overview
- Join the discussion overview
- Author tools overview
- Publishing Schol Comm research overview
- Open Access overview
- Open Access policies overview
- Places to find OA content
- Open Access Monographs overview
- Open Access Infrastructure
- Repository overview
- How to Deposit overview
- Digital Object Identifiers (DOI)
- Request a Copy
- Copyright overview
- Third party copyright
- Licensing options
- Creative Commons
- Authorship and IP
- Copyright and VLE
- Copyright resources
- Events overview
- Training overview
- Contact overview
- Governance overview
Data and your thesis
- Scholarly Communication
- Open Access
- Training and Events
What is research data?
Research data are the evidence that underpins the answer to your research question and can support the findings or outputs of your research. Research data takes many different forms. They may include for example, statistics, digital images, sound recordings, films, transcripts of interviews, survey data, artworks, published texts or manuscripts, or fieldwork observations. The term 'data' is more familiar to researchers in Science, Technology, Engineering and Mathematics (STEM), but any outputs from research could be considered data. For example, Humanities, Arts and Social Sciences (HASS) researchers might create data in the form of presentations, spreadsheets, documents, images, works of art, or musical scores. The Research Data Management Team in the University Library aim to help you plan, create, organise, share, and look after your research materials, whatever form they take. For more information about the Research data Management Team, visit their website .
Data Management Plans
Research Data Management is a complex issue, but if done correctly from the start, could save you a lot of time and hassle when you are writing up your thesis. We advise all students to consider data management as early as possible and create a Data Management Plan (DMP). The Research Data Management Team offer help in creating your DMP and can offer advice and training on how to do this. There are some departments that have joined a pilot project to include Data Management Plans in the registration reviews of PhD students. As part of the pilot, students are asked to complete a brief Data Management Plan (DMP) and supervisors and assessors ensure that the student has thought about all the issues and their responses are reasonable. If your department is taking part in the pilot or would like to, see the Data Management Plans for Pilot for Cambridge PhD Students page. The Research Data Management Team will provide support for any students, supervisors or assessors that are in need.
Submitting your digital thesis and depositing your data
If you have created data that is connected to your thesis and the data is in a format separate to the thesis file itself, we recommend that you deposit it in the data repository and make it open access to improve discoverability. We will accept data that either does not contain third party copyright, or contains third party copyright that has been cleared and is data of the following types:
- computer code written by the researcher
- software written by the researcher
- statistical data
- raw data from experiments
If you have created a research output which is not one of those listed above, please contact us on the [email protected] address and we will advise whether you should deposit this with your thesis, or separately in the data repository. If you are ready to deposit your data in the data repository, please do so via symplectic elements. More information on how to deposit can be found on the Research Data Management pages . If you wish to cite your data in your thesis, we can arranged for placeholder DOIs to be created in the data repository before your thesis is submitted. For further information, please email: [email protected]
Third party copyright in your data
For an explanation of what is third party copyright, please see the OSC third party copyright page . If your data is based on, or contains third party copyright you will need to obtain clearance to make your data open access in the data repository. It is possible to apply a 12 month embargo to datasets while clearance is obtained if you need extra time to do this. However, if it is not possible to clear the third party copyrighted material, it is not possible to deposit your data in the data repository. In these cases, it might be preferable to deposit your data with your thesis instead, under controlled access, but this can be complicated if you wish to deposit the thesis itself under a different access level. Please email [email protected] with any queries and we can advise on the best solution.
Open Research Newsletter sign-up
Please contact us at [email protected] to be added to the mailing list to receive our quarterly e-Newsletter.
The Office of Scholarly Communication sends this Newsletter to its subscribers in order to disseminate information relevant to open access, research data management, scholarly communication and open research topics. For details on how the personal information you enter here is used, please see our privacy policy .
Privacy Policy
© 2024 University of Cambridge
- Contact the University
- Accessibility
- Freedom of information
- Privacy policy and cookies
- Statement on Modern Slavery
- Terms and conditions
- University A-Z
- Undergraduate
- Postgraduate
- Research news
- About research at Cambridge
- Spotlight on...
Research data management
Creating a data management plan.
This video outlines how to create a Data Management Plan (DMP) using the Curtin Data Management Planning Tool (DMP Tool).
Data management plans
A DMP is a document outlining how you intend to handle your data as you perform your research.
A DMP may ask you to consider things such as:
- What type of data you will collect
- How and where you’ll store that data
- Who will have access to it
- How you’ll address any legislative requirements
- Any agreements about ownership of the data between collaborators
- How, where and when you’ll share your data at the end of the project
Managing the data over the whole project is very important for the success of your research and having a plan on how you’ll manage that data is equally important. Having a good DMP will also help ensure all of the elements of research data FAIRness are present - Findability, Accessibility, Interoperability and Reusability.
Curtin considers this data management plan process to be so important that completing a DMP is a required for anyone seeking to:
- Commence a higher degree by research (HDR)
- Obtain ethics approval for human or animal research
- Obtain access to the Research Drive (R: drive)
The DMP Tool linked below will help you create a DMP and update it when needed; it will also allow supervisors to review and check the plan and request the creation of R: drives for students.
Creating a Data Management Plan [00:25:19] This video covers how to create a DMP at Curtin.
Curtin Research Data Management Planning Tool The Curtin DMP tool guides the process of creating a research data management plan and ensures that important aspects of research data management are explored at the start of a research project.
Data Management Plan workshop This 1 hour hands-on session will help researchers create their data management plan using the Curtin DMP tool. It is particularly aimed at research staff and students who are preparing for their candidacy or ethics applications and is relevant for all disciplines and fields.
DMP Advice Tool This tool outlines areas of possible concern that may arise in your research that should be addressed in a DMP.
DMP Tool Help
Below are instructions for researchers and supervisors on how to resolve common issues when using the Curtin DMP Tool .
Students completing a DMP
Once you have completed all the fields, you should see this message:
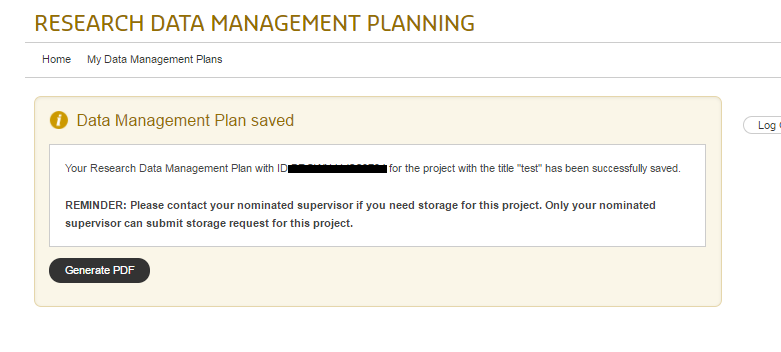
This means the DMP has been submitted to your nominated supervisor. You should notify them that your DMP has been created and that they have been nominated as the supervisor.
They will need to log on to the DMP Tool with their own details and complete the steps below before Curtin Digital & Technology Solutions (DTS) will provision your Research Drive access.
DMP Process Flowchart
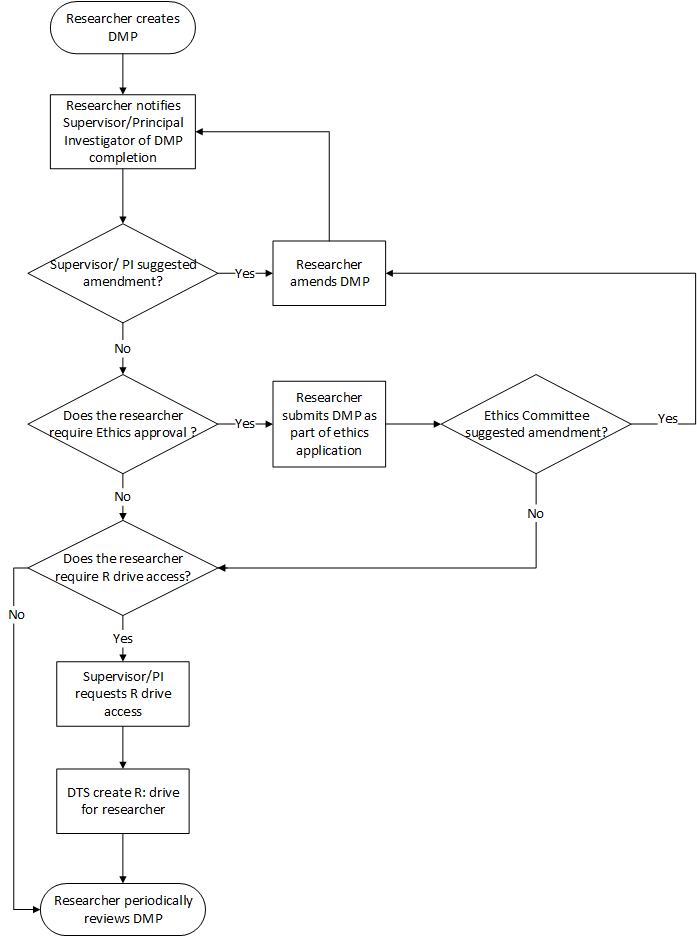
Student FAQs
Q. How do I setup my R: drive?
A. Once you’ve completed your plan, you should notify your supervisor that your plan is complete.
They can then check your plan and follow the steps as noted in the DMP Tool Help > Help for staff> Steps for Supervisors to complete Students’ Research Drive requests and have DTS create the storage space.
Q. I understand the questions, but I don’t know the answers to the questions the DMP Tool is asking me. Who can I talk to?
A. Your supervisor should be your first point of contact, as they will have the best knowledge of your individual research project and the common research processes and ethics issues in your discipline.
Q. I need more space on my Research Drive. How do I get it?
A. Please contact DTS as described in Student Oasis / SupportU - “Request additional storage for existing research project folders”.
Q. I need to give access to my Research Drive so my Curtin collaborators can access my data. How can I add them to my drive?
A. Please contact DTS as described in Student Oasis / SupportU - “How to request an access change for the R drive”.
Q. I still am having problems. Who can help?
A. Contact the Research Data Management team who will try to help you.
Steps for Supervisors to complete Students’ Research Drive requests
- Go to My Students’ Plans and click Generate PDF from the drop down menu and click Select .
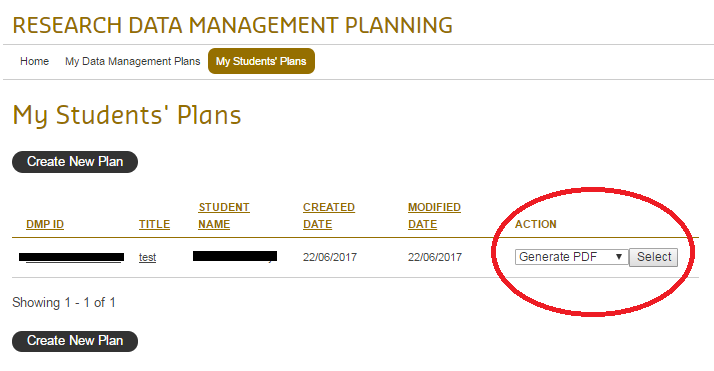
You will need some of the information in the generated PDF to approve the request.
From the same drop down menu, click Request Storage and then click Select .
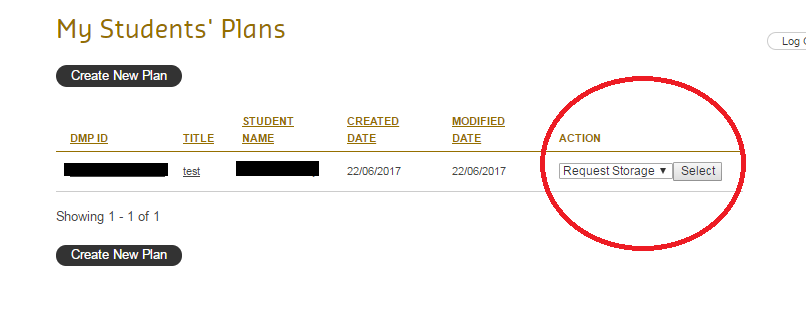
You will then be asked to provide some details about the DMP submitted by the researcher. These should be included in the generated PDF (N.B. – If the project has a finite timeframe, enter “0” at Projected Yearly Growth ).
Once the details have been entered, select Review . Check the information is correct then select Submit .
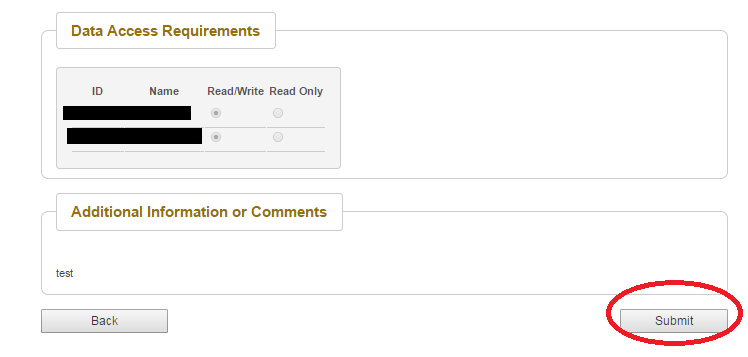
- You should then get this message indicating the researchers new DMP has been approved by you and a request will now be sent to Curtin IT for them to provision the R: drive space.
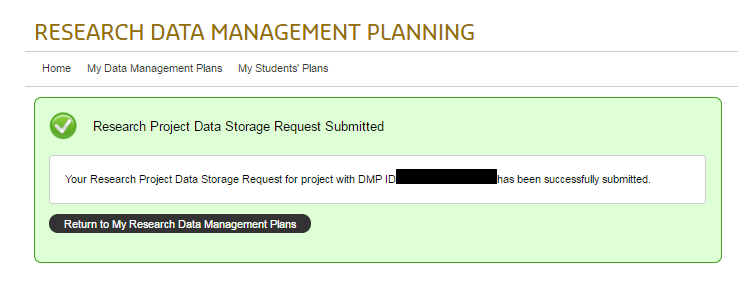
Steps for Staff to complete Staff Research Drive requests
- Go to My Data Management Plans and click Generate PDF from the drop down menu and click Select .
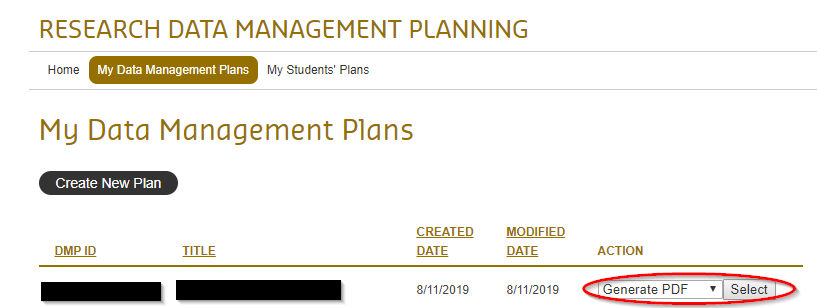
You will then be asked to some details about the submitted DMP. These should be included in the generated PDF (N.B. – If the project has a finite timeframe, enter “0” at Projected Yearly Growth ).
- You should then get this message. The request will now be sent to DTS for them to provision the R: drive space.
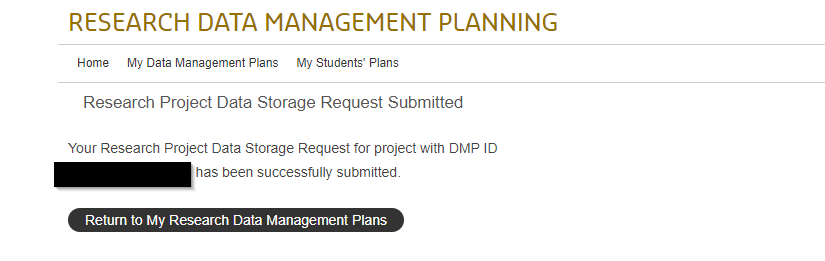
A. Please contact DTS as described in their Data and Storage - Shared Drives self-help portal (requires Curtin staff login).
Q. I need to give access to my Research Drive so my Curtin collaborators or students can access my data. How can I add them to my drive?
A. Please contact DTS as described in their Data and Storage - Research Drive self-help portal (requires Curtin staff login).
Example DMPs
These examples are fictitious DMPs in the faculties of Science and Engineering, Health Sciences, Business & Law, Humanities, Centre for Aboriginal Studies and the Vice-Chancellory, which may provide assistance.
Science and Engineering example data management plan [PDF, 80kB]
Health Sciences example data management plan [PDF, 60kB]
Business & Law example data management plan [PDF, 61kB]
Humanities example data management plan [PDF, 60kB]
Centre for Aboriginal Studies example data management plan [PDF, 59kB]
Vice Chancellory example data management plan [PDF, 59kB]
Funder requirements
Research funding bodies are concerned with obtaining the best outcomes for the research they fund. One of the ways they do this is by ensuring that researchers have a plan for their data throughout the whole research process.
An example of this is the Australian Research Council (ARC) - a key funding body of fundamental and applied research in Australia. Since 2020, the ARC has required funding applications for National Competitive Grants to have a completed data management plan before the project starts - this is to ensure that researchers are addressing the responsibilities as outlined in the Australian Code for the Responsible Conduct of Research 2018 .
It’s important to note that for most grants the ARC does not require you to submit a full, detailed DMP for assessment. However, you will need to have one in place before the start of the project and provide it to the ARC when requested.
ARC - Research Data Management Information about the ARC’s requirements around RDM
The Australian Code for the Responsible Conduct of Research 2018 The Code is a principles-based document that articulates the broad principles and responsibilities that underpin the conduct of Australian research.
More resources
Data management plans Provides information about managing and sharing research data.
The what, why and how of data management planning [00:05:30] A useful background on data management planning from Research Data Netherlands.
AIATSIS Code of Ethics for Aboriginal and Torres Strait Islander Research Researchers engaging in research relating to or involving indigenous participants should be aware of the guidance provided by the AIATSIS Code around research data.
Reference management. Clean and simple.
How to collect data for your thesis
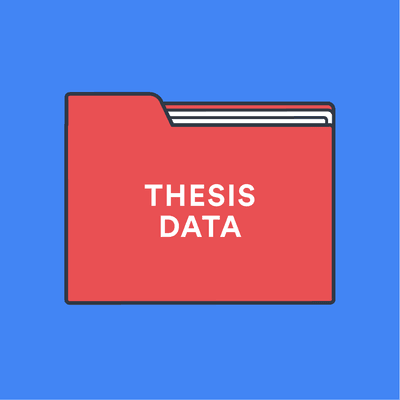
Collecting theoretical data
Search for theses on your topic, use content-sharing platforms, collecting empirical data, qualitative vs. quantitative data, frequently asked questions about gathering data for your thesis, related articles.
After choosing a topic for your thesis , you’ll need to start gathering data. In this article, we focus on how to effectively collect theoretical and empirical data.
Empirical data : unique research that may be quantitative, qualitative, or mixed.
Theoretical data : secondary, scholarly sources like books and journal articles that provide theoretical context for your research.
Thesis : the culminating, multi-chapter project for a bachelor’s, master’s, or doctoral degree.
Qualitative data : info that cannot be measured, like observations and interviews .
Quantitative data : info that can be measured and written with numbers.
At this point in your academic life, you are already acquainted with the ways of finding potential references. Some obvious sources of theoretical material are:
- edited volumes
- conference proceedings
- online databases like Google Scholar , ERIC , or Scopus
You can also take a look at the top list of academic search engines .
Looking at other theses on your topic can help you see what approaches have been taken and what aspects other writers have focused on. Pay close attention to the list of references and follow the bread-crumbs back to the original theories and specialized authors.
Another method for gathering theoretical data is to read through content-sharing platforms. Many people share their papers and writings on these sites. You can either hunt sources, get some inspiration for your own work or even learn new angles of your topic.
Some popular content sharing sites are:
With these sites, you have to check the credibility of the sources. You can usually rely on the content, but we recommend double-checking just to be sure. Take a look at our guide on what are credible sources?
The more you know, the better. The guide, " How to undertake a literature search and review for dissertations and final year projects ," will give you all the tools needed for finding literature .
In order to successfully collect empirical data, you have to choose first what type of data you want as an outcome. There are essentially two options, qualitative or quantitative data. Many people mistake one term with the other, so it’s important to understand the differences between qualitative and quantitative research .
Boiled down, qualitative data means words and quantitative means numbers. Both types are considered primary sources . Whichever one adapts best to your research will define the type of methodology to carry out, so choose wisely.
In the end, having in mind what type of outcome you intend and how much time you count on will lead you to choose the best type of empirical data for your research. For a detailed description of each methodology type mentioned above, read more about collecting data .
Once you gather enough theoretical and empirical data, you will need to start writing. But before the actual writing part, you have to structure your thesis to avoid getting lost in the sea of information. Take a look at our guide on how to structure your thesis for some tips and tricks.
The key to knowing what type of data you should collect for your thesis is knowing in advance the type of outcome you intend to have, and the amount of time you count with.
Some obvious sources of theoretical material are journals, libraries and online databases like Google Scholar , ERIC or Scopus , or take a look at the top list of academic search engines . You can also search for theses on your topic or read content sharing platforms, like Medium , Issuu , or Slideshare .
To gather empirical data, you have to choose first what type of data you want. There are two options, qualitative or quantitative data. You can gather data through observations, interviews, focus groups, or with surveys, tests, and existing databases.
Qualitative data means words, information that cannot be measured. It may involve multimedia material or non-textual data. This type of data claims to be detailed, nuanced and contextual.
Quantitative data means numbers, information that can be measured and written with numbers. This type of data claims to be credible, scientific and exact.
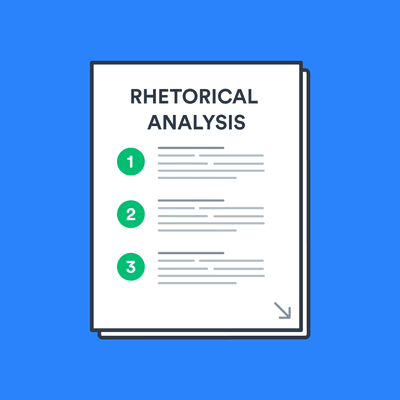

Dissertations and major projects
- Planning your dissertation
- Researching your dissertation
- Introduction
Before collecting your data
During your data collection, after your project.
- Writing up your dissertation
Useful links for dissertations and major projects
- Study Advice Helping students to achieve study success with guides, video tutorials, seminars and one-to-one advice sessions.
- Maths Support A guide to Maths Support resources which may help if you're finding any mathematical or statistical topic difficult during the transition to University study.
- Academic writing LibGuide Expert guidance on punctuation, grammar, writing style and proof-reading.
- Guide to citing references Includes guidance on why, when and how to use references correctly in your academic writing.
- The Final Chapter An excellent guide from the University of Leeds on all aspects of research projects
- Royal Literary Fund: Writing a Literature Review A guide to writing literature reviews from the Royal Literary Fund
- Academic Phrasebank Use this site for examples of linking phrases and ways to refer to sources.

But it is also really important to consider how you will organise , store , and keep track of your data as you collect it. Good data management strategies:
- Prevent you from losing data
- Increase your efficiency when analysing the data
- Show trends, patterns, and themes more clearly
- Ensure your findings are based on robust, comprehensive results
- Demonstrate that you are a rigorous researcher
What do I need to collect?
Good data management starts by collecting suitable data to answer your research questions. Gathering data that is fit for purpose means your analysis will be more efficient, and prevents you from becoming overwhelmed by having to process a lot of irrelevant information. When designing your data collection methods, look back at your research question(s) and keep asking yourself: How will the information I plan to collect help me answer these questions?
For further information about different research approaches and how to write the method chapter, have a look at our methodologies video below
Ethics forms
If you are gathering data that involves human subjects, it is likely you'll need to fill in an ethics form which will ask you to consider issues such as the confidentiality of your participants. Your project supervisor or department should be able to advise you on the type of ethics form you need to complete. Plan ahead to complete the ethics form in good time as it may need to be approved by a departmental committee, and you won't be able to start collecting your data without it.
- Research approaches and the methodology chapter Study Advice video on research approaches and the methodology chapter to help you navigate this important stage. Log in with your student account to watch
Keep your electronic files on the University network (N drive) as it is reliable and backed up.
If you are storing data directly on your own laptop or PC outside the University network, make sure you have a rigorous backup system in case your device crashes, or is lost or stolen. Use an external hard drive or USB stick and save your data regularly. Have a safe place to keep your USB stick or hard drive and remember to take it with you when you leave the library!

Collect the minimum amount of personal data necessary and avoid collecting any personal information that you don't need.
Store any personal data in an appropriate, secure location, e.g. a locked filing cabinet, or password-protected or encrypted online files.
Avoid sending or storing personal data over unsecure networks such as via email or in cloud services like Dropbox.
Process and safely destroy any personal data as soon as they are no longer needed, for example promptly downloading and saving interview recordings from your phone or recording device into a password protected file.
If you have said on your ethics form that you will be annonymising data (e.g. interview responses) to protect participants' confidentiality, make sure you do this. Have a system for anonymously labelling each response such as assigning a letter, number, or changing their name (Participant A, Interviewee 1, 'Johnny').
Organisation
Have a systematic and clear way of naming your online files and, most importantly, stick to it!
You should be able to tell what's in a file without opening it. Including a date formatted like YYYY-MM-DD means you can sort files chronologically Having a version control number means you can easily distinguish between your 1st, 2nd, or 10th draft!
Store your electronic files in a logical folder structure to make them easier to locate and manage, e.g. creating folders to group files according to content type, activity, or date. For further examples see guidance from the UK Data Service (link below).
Also have a system for safely storing any field notes. You don't want to lose vital parts of your research on site or in an unfamiliar library that you won't be returning to. Simple systems are the best, for example putting things in box files is easier than having to find a hole-punch and ring binders.
Documentation
As well as making good notes from the books and journal articles you read (including the full bibliographic details for your references) it is also important to keep clear records of other parts of your research process:
- Record your search strategy: Note down the combinations of keywords you use and the library databases you have searched to avoid duplication and confusion later.
- Keep your lab book up to date: If you are doing primary scientific research, a good lab book helps you record what you did whilst it is fresh in your mind; it makes writing your methods and results much easier.
- Label your equipment and any work in progress: If you are using a shared research space, clearly identify your work, as you don't want people accidentally moving it or throwing it away!
- UK Data Service: organising data Guidance on file formats and organisation.

If you have the opportunity to continue with similar research, for example in a postgraduate degree, or present it to a public audience, such as at a conference or in a journal paper, it is good practice to keep your data in case fellow researchers want to access it; your project supervisor can help advise you about this.
In most cases, though, for undergraduate research projects it is very unlikely you will have to store your data after you have graduated. However, before you rush off to burn your notes, it is a good idea to keep everything safely until you have your final marks, just in case!
Advice adapted from the University of Reading's Research Data Management pages.
- Research data management website (University of Reading) Information about what you need to consider when collecting and storing data.
- << Previous: Researching your dissertation
- Next: Writing up your dissertation >>
- Last Updated: May 14, 2024 8:59 AM
- URL: https://libguides.reading.ac.uk/dissertations

- Cambridge Libraries
Study Skills
Research skills.
- Searching the literature
- Note making for dissertations
Research Data Management
- Copyright and licenses
- Publishing in journals
- Publishing academic books
- Depositing your thesis
- Research metrics
- Build your online profile
- Finding support
Welcome to this module, where we will cover all the main aspects of looking after your research data, including:
- how to store and backup up data
- how to organise data
- what to do with protected data (personal or commercially sensitive)
- why sharing data is important and how to do it
- writing Data Management Plans
Data can take many forms: not only spreadsheets, but also images, interview recordings and transcripts, old texts, survey results, protocols... the list goes on.
To complete this section, you will need:

- Approximately 60 minutes.
- Access to the internet. All the resources used here are available freely.
- Some equipment for jotting down your thoughts, a pen and paper will do, or your phone or another electronic device.
Where did it all go wrong?
Lack of planning at the start of a project can cause problems (and much more work!) later on. Think of data management as a time investment to make sure that the data you collect is used effectively and remains usable over time.
Watch this video by the NYU Health Sciences Library as an example of poor data management and take some brief notes on any mistakes you spot. When you’re done, compare your notes with our answers underneath.
Check your answers
What did this researcher do wrong?
Here are all the mistakes we spotted: -he did not consider how others may want to reuse his data -he did not share the data in a repository -he was not aware of his funder and publisher requirements -he did not have multiple backups -he did not keep the data in a safe place -data on a USB stick is easy to lose -he did not use a safe way to share data (the post could have been lost) -he did not save the data in a common format -he did not save instructions on how to open the data -he did not plan for long-term preservation -he did not give variables intuitive names -he did not save metadata on what the variable names mean -he relied on knowledge found only in the brain of one person, rather than writing metadata
Keeping your data safe and up to date
Ensuring your data are safe is crucial to any research project. A good storage and backup strategy will help prevent potential data loss. Explore this scenario to see if your choices align with good research practice. Click on the link below to begin
Note: scenario opens in new window. Please view the scenario in full-screen. Return to this window to continue with the module, or if you wish to restart the scenario
Data storage and backup - why bother?
- Please visit the UK Data Service for more detailed tips on Storage and Backup of research data
- Branching scenario built using YoScenario
- All photos are CC-0 from Pexels
Organising data
Once you are sure that your data is safe from accidental loss, you should be thinking about how to organise it. Are your computer files ‘an amorphous plethora of objects’? In this video by the University of Edinburgh Data Library, Professor Jeff Haywood talks about his experiences of organising data.
If you want to read more about organising your data, including folder structures and file naming, there is a detailed guide on the Cambridge data website.
If you are at the start of a project, spend some time now preparing an organisational structure for your data. Create all the folders you are likely to need and a few named placeholders for files you will create. If you would like some feedback on it, email me .
Actvity - What should a PhD student do with her data?
Follow Martha in our scenario and help her make the best choices!
Sharing your data
Take a look a this video of Cambridge researchers talking about their experience of sharing data.
Using repositories
So what does it mean in practice to share your data? All you have to do is upload your dataset and information about it on a repository, either a subject-specific one, an institutional one like Apollo, or a general one. The repository then lets people find and download the data. Find out more in the video below.
Useful resources related to the video:
- Link to upload in Apollo
- Cornell metadata guide
- Funders policies on data website
- License Selector Tool
- Re3data
- Blog post by Blair Fix
- Bioinformatics Training Facility courses
- Slides for Sharing your data in repositories
Protected data
If your research data is of a personal or sensitive nature, you must make sure you understand and respect the additional requirements associated with managing it. If possible, get in touch with your department’s ethics committee, or your industrial sponsor to check what they expect of you. Additional help can be sought from the Research Data team , the Research Integrity team , and the Information Compliance Office .

What are personal and sensitive data?
Personal data is data relating to a living individual, which allows the individual to be identified from the information itself or from the information plus any other information held by the 'data controller' (or from information available in the public domain). The University of Cambridge as a whole is the data controller. Sensitive data is personal data about: racial or ethnic origin, political opinions, religious beliefs, Trade Union membership, physical and mental health, sexual life, or criminal offences and court proceedings about these.
What are the legal requirements for data protection?
The The EU General Data Protection Regulation (GDPR), coupled with the UK Data Protection Act 2018 (DPA 2018) gives individuals certain rights and imposes obligations on those who record and use personal information to be open about how information is used and to follow eight data protection principles. Personal data must be: processed fairly, lawfully and transparently; obtained for specified, explicit and lawful purposes; adequate, relevant and not excessive; accurate and, where necessary, kept up-to-date; not kept for longer than necessary; processed in accordance with the subject's rights; kept secure; not transferred abroad without adequate protection
How should I store my sensitive or confidential data?
You should limit physical access to sensitive data or encrypt it (speak with your local IT/Computing Officer or the University Information Services Help Desk for help in doing this). To avoid accidentally compromising the data at some future date, you should always store information about the data's sensitivity and any available information on participants' consent or use agreements from your data provider with the data itself (i.e. put information about lawful and ethical data use in your data documentation or metadata description).
Data supporting my research is personal or sensitive. How do I share these data?
There can be a potential conflict between abiding by data protection legislation and ethical guidelines, whilst at the same time fulfilling funder's and individual's requirements to make research results available. Consult your ethics committee before deciding to share participants’ data. Your plans for research data processing, storage and sharing should be considered at the start of each project and reflected in both your data management plan and consent form. For example, you can inform your participants that anonymised data will be shared via the University of Cambridge data repository. There is good guidance on consent forms at the UK Data Archive (www.ukdataservice.ac.uk). The UK Data Archive also provides a sample consent form. Your Department’s Ethics Committee may also provide sample consent forms.
If you would like to learn more about personal and sensitive data and do some practical exercises on identifying these data types, the University of Cambridge offers short 30-mins long online courses on personal and sensitive data .
You should also consider whether your data is commercially sensitive: do you or a sponsor plan to profit from the research in the future? There should be a collaboration agreement in place from the start to clarify the terms of any commercial collaboration. The Research Operations Office can help with this. If you are working with both public funders and commercial partners, clarify early what data can be shared and what can’t, so you can make this clear to all parties.
Data Management Plans
Throughout this module we have seen how important it is to plan the way you will manage your data right at the start of a project. A Data Management Plan (DMP) is a document that captures that process.

To end this module and pull together everything you have learnt, we recommend you write your own DMP for a project you are about to start or have recently started. Use these instuctions as a guide.
- DMP activity
Did you know?

How did you find this Research Skills module

- << Previous: Note making for dissertations
- Next: Copyright and licenses >>
- Last Updated: Apr 11, 2024 9:35 AM
- URL: https://libguides.cam.ac.uk/research-skills
© Cambridge University Libraries | Accessibility | Privacy policy | Log into LibApps

Library Support Services for RDI
- Open RDI and Library Support Services
- Data and project preparation
- Data Management Plan
- Legislation, Agreements, and Ethics
- Identifiable Data and Anonymisation
- Use of Open Data
- Storing Data
- Data Description, Documentation and Metadata
- When RDI project ends
- Opening Research Data
- Training videos and Self-study Materials
- Data Management Planning
- Research Ethics
- Data Protection in the Thesis
- Data Security and Storage
- Data Description and Documentation
- When the Thesis is Completed
- Publishing Data Collection
- Self-archiving and Open Access Publishing
- Open educational resources
- Persistent identifiers (e.g. DOI and ORCID)

What is data management?
In the thesis, data management refers to the research data management of the thesis.
Then the research data is:
- created, stored and organized so that the material remains usable and reliable
- data protection, information security and research ethics have been ensured
What is research data in a thesis?
Research data in a thesis refers to the data that is collected or generated, analyzed, and used specifically for the purpose of the thesis. The data is used to support the findings and results presented in the thesis.
In the process of conducting a thesis, various types and quantities of research data can be generated. Some examples of research data in a thesis may include:
- Measurement results
- Surveys and interviews
- Recordings and videos
- Research diaries and notes
- Drawings, photographs, text samples, and other collected materials
- Newly created data based on existing datasets
- Self-developed software and source codes
Since the research data strengthens the findings of the thesis, attention should be given to the quality and handling of the data. If the data used as the basis for the results is not reliable, the results of the thesis will also be unreliable.
In some cases, research data may not exist in a digital format or may not be converted into a digital format for various reasons. This can include physical samples, tangible objects, or paper-based materials. Responsible data management practices also apply to physical research data.
Note the conceptual differences:
Research methodology = how you acquire and analyze research data Research data = the data that you analyze Source literature / research literature = the theoretical framework of your research, such as articles or books that you use as sources
Why is data management important?
- Data management is an essential part of good scientific practice , which applies not only to scientific research but also to theses, teaching, and guidance. Metropolia, like other higher education institutions, is committed to adhering to good scientific practice.
- Planning and implementing data management are forms of risk management. Laws, agreements, and research ethics also apply to the data used in theses. Planning becomes particularly crucial when collecting personal data or other sensitive information, such as trade secrets.
- Properly managed data remains reliable , enabling the generation of trustworthy results.
- Good data management significantly facilitates the process of conducting a thesis. When files are systematically stored, they are easy to find. Backing up data prevents accidental loss. Documentation makes the data understandable to both thesis supervisors and the author, even if some time has passed since the data collection.
- Practicing data management principles during thesis work brings benefits in the professional world . Therefore, it is worth familiarizing oneself with these practices while working on a thesis.
- If there is a desire to share or reuse the data after completing the thesis, the data must be of high quality and well-managed. In such cases, the life cycle of the data does not end with the thesis; instead, it can provide broader societal benefits.
Stages of data management in a thesis
Data management always begins with planning , just like the entire thesis project. The data management plan is part of the thesis plan and covers all relevant aspects of data management. The plan can be seen as a checklist or risk management tool: Are all necessary aspects considered, such as agreements, research permits, data protection, or the need for ethical pre-assessment?
During the implementation phase , data is collected or produced, stored, and processed while taking into account data security, data protection, and research ethics. It is also important to describe or document the data and its processing procedures.
When the data is no longer being processed , it is advisable to "package it up." If the data contains personal information or sensitive data such as trade secrets, this information should either be removed or anonymised as soon as it is no longer needed, but no later than the end of the thesis project.
The life cycle of the data may continue even after graduating, for example, if the data is made openly accessible or transferred for further use to a specific party. Agreements and the consent of participants may limit the possibilities for further use, so this should be considered in the data management plan.
- << Previous: Training videos and Self-study Materials
- Next: Data Management Planning >>
- Last Update: Apr 5, 2024 1:24 PM
- URL: https://libguides.metropolia.fi/RDI-support-services
Metropolia Library and Information Services | Accessibility Statement

Research Data Management: Data Plan for your PhD
- Sensitive Data & Data Protection (GDPR)
- PhD DMP supervisors guide
- Support and Training
DMP for your PhD research
All first year post graduate researchers should complete a data management plan for their research and include it as part of their first three month review. There is also a Blackboard course Data Management Plans for Doctoral Students - mandatory for all new doctoral students - to introduce you to research data management and help you complete the plan. Log into Blackboard using your university username and password.
A data management plan or DMP is a living document that helps you consider how you will organise your data, files, research notes and other supporting documentation throughout the length of the project. The aim is to help you find these easily, keep them safe and have sufficient documentation to be able to re-use throughout your research and beyond.
You will need to complete a preliminary data management plan in your first three months, along with your Academic Needs Analysis. Your DMP will continue to develop as your research progresses and you will need to update and review your DMP at every progression review. ( Code of Practice for Research Degree Candidature and Supervision, )

All researchers will have data. Data can be broadly defined as 'Material intended for analysis'. This covers many forms and formats, and is not just about digital data.
For example,
Art History - high resolution reproductions of photographs, notebook describing context
English literature - research notes on text, textual analysis
Engineering - experimental measurements on the physical properties of liquid metals
The University also has a definition for “Research Data” in its Research Data Management Policy that you should consider.
A PhD DMP template and guidance on how to complete your Data Management Plan is available ( see below ). All new doctoral students should complete the Data Management Plans for Doctoral Students module on Blackboard. Contact us if you need further information or have feedback via [email protected]
Guidance on depositing your research data at the end of your doctorate can be found on the Thesis Data Deposit guide. Please also see our depositing research data videos at https://library.soton.ac.uk/researchdata/datasetvideos
Creating your DMP
- Introduction
- DMP and Project Overview
- About your Project Data
- Making Data Findable
- Making Data Accessible
- Making Data Reusable
- Making Data Secure
- Implementing the Plan
- Example Plans
What are data management plans? A data management plan is a document that describes:
- What data will be created
- What policies will apply to the data
- Who will own and have access to the data
- What data management practices will be used
- What facilities and equipment will be required
- Who will be responsible for each of these activities
Your data management plan should be written specifically for the research that you will be doing. Our template is a guide to help you identify the key areas that you need to consider, but not all sections will apply to everyone. You may need to seek further guidance from your supervisor, colleagues in your department or other sources on best practice in your discipline. We provide some details of guidance available in our training section and on our general research data management pages.
Each of the tabs looks at the different topics that can be included in a data management plan. You can move through the tabs in any order.
Describing your Project
At the start of your data management plan (DMP) it is useful to include some basic information about the research you are planning to do. This may already exist in other documents in more detail, but for the purposes of the DMP try to summarise in as few sentences as possible.
What policies will apply?
It is important that you think about who is funding your research and whether there are any requirements that you need to meet. Are you funded by a UK Research Council? What policies do they have on research data - see Funder Guidance . What does our University Research Data Management policy and Code for Conduct for Research state is required?
Does the type of data you will be creating, using, collecting mean that you have to meet certain legal conditions? Will you be collecting any form of personal data, (see ICO Personal Data Definition ), special category data (see ICS Special Category definition ) or is it commercially sensitive? For example, if you are involved in population health and clinical studies research data and records minimum retention could be 20-25 years for certain types of data - see the MRC Retention framework for research data and records for further details.
Do you need Ethics Approval?
Anyone who is dealing with human subjects or cultural heritage (see University policies ) will require to obtain ethics approval and this must be done prior to collecting any data. Your DMP should inform what you say in your ethics application about how you will collect, store and re-use your data. It is important that your DMP and your ethics application are in agreement and you provide your participants with the correct information. Once you receive your ethics approval, review your data management plan and update as necessary.
Reviewing your Data Management Plan
A DMP should be a living document and should be updated as your research develops. It should be reviewed on a regular basis and good practice would encourage that the dates of review are included in the plan itself. Use of a version table in any document can be helpful.
What data will be created?
In your data management plan you need to provide some detail about the material you will be collecting to support your research. This should cover how you will collect notes, supporting documentation and bibliographic management as well as your primary data. Will all your data be held electronically or will you require to maintain a print notebook to collect your observations?
Are you using Secondary Data?
Not everyone has to collect their own data, it may already have been collected and made available. This data is known as secondary data. Some secondary data are freely available, but other data are released with terms and conditions that you need to meet. In some cases this may influence where you can store and analyse the data. You need to be aware of this as you plan the work you intend to do.
How are you collecting or creating your data?
How you collect or gather the material for your research will influence what you need to do to manage them. The way you do this may alter as your research progresses and you should update your plan as required. Will you be collecting data by observing, note-taking in an archive, carrying out experiments or a mixture of these?
How much data are you likely to have?
Knowing how much data you might create is important as it will dictate where you can store your data and whether you need to ask for additional storage from iSolutions. It is unlikely that you can say exactly what volume of data you might create, but you will have an idea of individual file sizes. If you will be working with word, excel documents and a reference management software library then you are likely to be dealing with megabytes or gigabytes of data. If you will be collecting high resolution images then you may end up needing to store terabytes. Estimate as early as possible and if you think you may need additional space you should discuss this with your supervisor.
What formats will you be using?
A crucial factor in being able to share data is that it is in an open format or collected using disciplinary standard software that allow export to open formats. Consider how open the format of your data will be when selecting the software, instruments, word processing packages that you use. See the Data formats section in Introducing Research Data Part III for points to consider.
Who will own the data?
If you have been sponsored by a research council, government, industry or commercial body the agreement you signed may cover ownership of the data that you create. Being aware of this early is useful as it will influence what you are able to do when you come to writing papers, sharing and depositing your data when your finish. It may also impact on where you can store your data.
How will you make your data findable?
Using standards to capture the essential metadata is a good way to help create data that will be easy to find. It will also make preparing for deposit in the future more straightforward. The Research Data Alliance has a helpful list of disciplinary metadata and use case examples. You can make reference to these in your plan once you know what will be most appropriate to use.
Where will you store the data during your PhD?
Where you store your data will depend on things such as the type and size of data you are collecting. Certain types of data, such as personal , special category data (formerly referred to as sensitive data) or commercially confidential data, will require to be stored more securely than others. This type of data generally requires to be stored on University network drives that have additional protection and not on personal computers or cloud storage (for example, Office 365, One Drive). Where you are collecting less sensitive data your choice of storage is wider. For all storage it should in a location with good back-up procedures in place. Consult iSolutions knowledge base for further information.
How will you name your files and folders?
It can be helpful to think about creating a procedure on how you will name your files. This is a basic step where it is useful to consider how easy it will be to interpret the name in the future. Abbreviations can be good, but ask yourself how someone else might understand the file name should you need to share it with them. What would make it easy to know what each file contains? While it is possible to have quite longer file names this can cause problems when you zip files.
How will you tell one version of a file from another?
How will you be able to tell whether you are dealing with the latest version of a file? How will you manage major versus minor changes? What if you want to return to an earlier version? Use the data management plan to investigate what would be the optimum method for you and establish a good procedure from the beginning. Generally the use of 'draft', 'latest' or 'final' should be avoided. Instead consider using the data (YYYY-MM-DD) or a version number, for example, v.1.0 where the nominal value increases with major changes and decimal for minor ones. Adding a version table at the end of a document can also be helpful.
How can you share your data?
To make data accessible is not about doing something at the end of the project, but needs to be planned for from the beginning. During your research you are likely to have colleagues or collaborators who will need to be able to access the data - how will you do this? Will you need a collaborative space and if so what can you use? Does it need to be is a protected location with restricted access due to the type of data you are using? By establishing good procedures on documentation, metadata collection, file-naming and using disciplinary standards this will assist you throughout your research, as well as helping at the end.
How do you handle personal, sensitive or commercially confidential data?
If the data you are collecting contains personal , special category data (formerly referred to as sensitive data) or commercially confidential data then sharing or transferring the files needs to be carried out in a way that does not make the data vulnerable. Data should be anonymised or pseudo-anonymised as early as possible after collection, seek disciplinary guidance prior to collection.
The medium of transfer must be secure and where necessary encryption should be used. You may want to consider one of the following:
There may be other software available and you should check if there is a standard in your discipline.
Transferring data via USB or external drives is not recommended, but where required these should be encrypted. Avoid using email to send files and instead use our University SafeSend service. This offers transfer of files up to 50GB and your files can be encrypted by ticking "Encrypt every file" when creating a new drop-off - see ' How secure is SaveSend'
What data do you need to keep and what do you need to destroy?
Not all the data from a project needs to be kept and the data you collect should be reviewed regularly. The Digital Curation Centre (2014) guide ' Five steps to decide what data to keep: a checklist for appraising research data v.1 ' may help you to decide what to retain. It is important that you retain or discard data in line with your ethics approval.
You also need to consider what data needs to be destroyed, how you will mark the data for destruction and when this needs to happen. Destroying paper based records is relatively easy through our confidential waste system. Destroying digital data is less so as it may need to be done so that it cannot be forensically recovered. Guidance on destroying your data is available or contact iSolutions for advice.
Why do you need to consider the long-term storage now?
At the end of your PhD you will be encouraged to share your data as openly as possible, and as closed as necessary. To do this safely consider what you need to do to enable your data to be accessible in the future. Knowing where the best place to store your data may inform what you need to plan for in its creation or collection. Are you aware of any disciplinary data repositories that hold similar data? Examples are:
- Archaeology - Archaeology Data Service
- ESRC - UK Data Archive
- STFC - eData
- NERC - data centres
- Biology - GenBank
- General repository - Zenodo
Investigate what requirements these repositories have on formats, documentation etc and incorporate these into your plan. Otherwise you should plan to deposit in the University Institutional Repository .
There are currently no costs for depositing most dataset in our Institutional Repository unless the data requires specialist archive storage or is in excess of 1TB. External repositories may have charges for depositing data.
Who will be creating the archive?
Generally as a PhD the job of drawing together your data into a dataset ready for deposit will fall to you as the researcher. It is not the responsibility of your supervisor, although they may be able to advise on what needs to be done. If you are part of a larger project there may be someone designated to curate the project data. For further assistance contact [email protected] .
How long should the data be kept?
This will depend on a number of factors. Your funder may have a policy that requires the data to be held for a minimum of 10 years from last use. If you are working in certain medical areas the data may need to be held for 25 years. There may be some restrictions on how long you can retain personal data relating to Data Protection Act 2018 (GDPR). Significant data that has been given a persistent identifier (DOI) will be kept permanently.
What documentation or additional information needs to accompany the data?
Keeping a record of what changes you have made, when data was collected, where data was collected from, observations, definitions of what has been collected are all crucial to allowing data to be used safely and with integrity. How do you plan to do this? How will you make sure that you can match up your notes with the files they refer to? Some programming languages such as Python and R allow you to make notes in the files about what you are doing which is really helpful. Where this is not an option then you will need to develop your own method to make sure that processes applied to the data are recorded and available to you to refer back to later. Creating a register of your files by type using an excel spreadsheet may be worth considering, but it should be manageable and importantly kept up-to-date.
In order for data to be reusable it requires data provenance. Data provenance is used to document where a piece of data comes from and the process and methodology by which it is produced. It is important to confirm the authenticity of data enabling trust, credibility and reproducibility. This is becoming increasingly important, especially in the eScience community where research is data intensive and often involves complex data transformations and procedures.
- Research Data Management and Sharing - Documentation The importance of systematically documenting your research data. more... less... From the Coursera Research Data Management and Sharing course https://www.coursera.org/learn/data-management
What restrictions will need to apply?
Not all data can be made openly available. Some data may only be shared once a data sharing agreement has been signed, while other data may not be suitable for sharing. Funding councils encourage all data to be as open as possible and as closed as necessary. Where will your data fit with this? What agreements do you need to be able to share your data?
When can data be made available?
Data can be deposited in our Institutional Repository and kept as an 'entry in progress' until it is ready for publication.
Not all data needs to be made immediately available at the end of your PhD. It is possible to add an embargo to give yourself some additional time to find funding to continue your work and re-use your own data. See Regulations on embargoes.
However, it is not always necessary for you to wait until the end of your PhD before depositing data. If you write a conference or journal paper it is likely that you will be asked to make the underpinning data available.
How will you keep your data safe?
What would happen if your files became corrupted or your laptop was stolen, would you be able to restore them? What would happen if someone was able to access your data without your knowledge or approval? If you are holding personal or special category data (formerly referred to as sensitive data) and these became public this would be a data breach with potentially serious consequences.
Dr Fitzgerald Loss of seven years of Ebola research
Consider carefully the impact to you and your research if these were to happen and what procedures you may need to put into place to reduce the risk of these happening.
- Research Data Management and Sharing - Data Security Ensuring your research data are kept safe from corruption, and that access is suitably controlled more... less... From the Coursera Research Data Management and Sharing course https://www.coursera.org/learn/data-management
How will you back up your data?
Good housing keeping of your data is important and this includes doing regular back ups of your data. University storage is backed up regularly but it is important to have your own 'back up' folders, kept separately from your working files. Back up should be done on as regular a basis as required. This can be defined by the length of time you are prepared to repeat work lost. You may need to back up daily, weekly or monthly depending on the nature of your research.
- Research Data Management and Sharing - Backup Effective backup strategies for your research data. more... less... From the Coursera Research Data Management and Sharing course https://www.coursera.org/learn/data-management
As well as establishing a process for backing up your files, you should check the process of restoring your files. You will need to check that the files restore correctly. Having good documentation on what your files contain, what transformations or analysis has been carried out will be invaluable for this process.
How can you safely destroy data?
Destroying data, especially personal , special category data (formerly referred to as sensitive data) or commercially confidential data , is not as straightforward as just deleting the file. Further action is required otherwise the data could be recovered. Please read our guidance on destruction of data and GDPR regulations .
- Data Disposal Essential guidance from the UK Data Archive on data disposal
An important part of research data management is that your plan is implemented and part of your everyday good research practice. The plan should be a living document and reflect your practice. You may find that some parts become redundant or that there is a better way to carry out a process so your plan should be updated. As a PhD researcher it is likely that you will be the person responsible for implementing the plan. If your research is part of a wider research project there may be someone in the team who has been given the role and you should discuss your data management plan with them.
Having written your plan consider what actions do you need to take in order to carry it out? What further information do you need to find? Investigate what training or briefing sessions are available via PGR Manager. If you want to enhance your data analysis skills check out material on Linked in Learning
Over time we will add plans to this section as we get permission to share them.
- PhD DMP Example (Web Science) This is an example PhD Data Management Plan for a research project looking at learner engagement and peer support in digital environments.
- Arts and Humanities
- Science, Medicine and Engineering
- Social Sciences
- Further Reading
Courses offered by the University:
Data Management Plans for Doctoral Students - mandatory course on for all new doctoral students. Log into Blackboard using your university username and password.
Data Management Plan: Q&A Clinic - as a follow up to the compulsary online course, the Library is running twice weekly clinics to answer your DMP queries. Book PGR Development Hub .
Data Management Plan: Why Plan? 45 minute briefing. A Panopto recording of this course is available
Research Data Management: What you need to know from the start . 45 minute briefing. Book via Gradbook
Research Data Management Workshop .180 minute workshop Book via Gradbook

- Introduction to research data (visual arts) Introduction to research data in the visual arts, wirtten by Marie-Therese Gramstadt as part of the Kultur project
- Manage, improve and open up your research and data PARTHENOS training module on various aspect on data management
- VADS4R Data Management Planning A toolkit developed by the Visual Arts Data Skills for Researchers (vads4R)
- Cross-Linguistic Data Formats, advancing data sharing and re-use in comparative linguistics The Cross-Linguistic Data Formats initiative proposes new standards for two basic types of data in historical and typological language comparison (word lists, structural datasets) and a framework to incorporate more data types (e.g. parallel texts, and dictionaries). The new specification for cross-linguistic data formats comes along with a software package for validation and manipulation, a basic ontology which links to more general frameworks, and usage examples of best practices. [article]
- EMBL-EBI Training EMBL-EBI train scientists at all levels to get the most out of publicly available biological data.
- Datatree - Data Training A free online course, aimed at PhD and early career researchers, with all you need to know for research data management, along with ways to engage and share data with business, policymakers, media and the wider public. more... less... The course is for any scientist, whether you look after your own data or are guided by an organisation.
- Expert Tour Guide on Data Management A guide for social science researchers who are in an early stage of practising research data management.
- CESSDA ERIC RDM User Guides Brief guides on important topics in data management and a helpful checklist
- Guide to Social Science Data Preparation and Archiving An important guide covering the different stages of data management to enable the sharing and preserving of data in the Social Sciences
- Managing your dissertation data : Thinking ahead Maureen Haaker and Scott Summers from the UK Data Service gave this presentation. The session sought to help the students ensure transparency in the collection and writing up of their dissertation, whilst also ensuring that good practices in data management were followed. more... less... Although aimed at undergraduate dissertation it provides useful information for everyone.
- UK Data Service Prepare and Manage Data Good data management practices are essential in research, to make sure that research data are of high quality, are well organised, documented, preserved and accessible and their validity controlled at all times. This results in efficient and excelling research.
- FAIR Principlies Guidelines to improve the findability, accessibility, interoperability, and reuse of digital assets
- How to Develop a Data Management and Sharing Plan Jones, S. (2011). ‘How to Develop a Data Management and Sharing Plan’. DCC How-to Guides. Edinburgh: Digital Curation Centre. Available online: http://www.dcc.ac.uk/resources/how-guides
- MRC Retention framework for research data and records guidance on retention of research data an records resulting from population health and clinical studies
- Open Data Handbook Handbook that discusses the why, what and how of open data – why to go open, what open is, and the how to ‘open’ data.
- Open Research Data and Materials Open Science Training Handbook section on research data
Key Documents
- DMP Templates
- Deposit Guide
The template below has been provided to assist you in writing your data management plan. Not all sections will be relevant, but you should consider carefully each section.
- Template for PhD DMP (pdf)
- Template for PhD DMP (Word)
- Code of Conduct for Research University of Southampton policy - October 2017
- Data Protection Policy University of Southampton policy May 2018
- Data Sharing Protocol University of Southampton protocol - May 2018. [Login required]
- Ethics - Human participant policy University of Southampton policy - March 2012
- Ethics - Policy on Cultural Heritage University of Southampton policy - October 2018
- Research Data Management Policy University of Southampton policy - 2015
When the time comes to deposit your data, follow the advice in our Thesis Data Deposit guide .

Email us on: [email protected]
Who's Who in the Research Engagement Team
Research Support Guide
research support.
- << Previous: Sensitive Data & Data Protection (GDPR)
- Next: PhD DMP supervisors guide >>
- Last Updated: May 14, 2024 10:30 AM
- URL: https://library.soton.ac.uk/researchdata

Data Science Journal
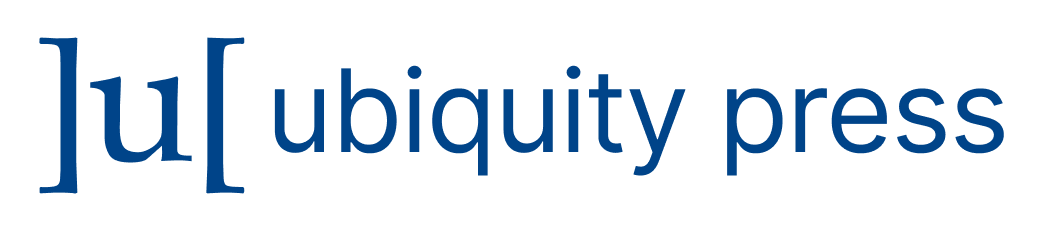
- Download PDF (English) XML (English)
- Alt. Display
Research Papers
Research data management for master’s students: from awareness to action.
- Daen Adriaan Ben Smits
- Marta Teperek
This article provides an analysis of how sixteen recently graduated master’s students from the Netherlands perceive research data management. It is important to study the master’s students’ attitudes towards this, as students in this phase prepare themselves for their career. Some of them might become future academics or policymakers, thus, potentially, the future advocates of good data management and reproducible science.
In general, students were rather unsure what ‘data management’ meant and would often confuse it with data analysis, study design or methodology, or ethics and privacy. When students defined the concept, they focussed on privacy aspects. Concepts such as open data and the ‘FAIR’ principles were rarely mentioned, even though these are the cornerstones of contemporary data management efforts. In practice, the students managed their own data in an ad hoc way, and only a few of them worked with a clear data management plan. Illustrative of this is that half of the interviewees did not know where to find their data anymore. Furthermore, their study programmes had diverse approaches to data management education. Most of the classes offered were limited in scope. Nevertheless, the students seemed to be aware of the importance of data management and were willing to learn more about good data management practices.
This report helps to catch an important first glimpse of how master’s students (from different scientific backgrounds) think about research data management. Only by knowing this, accurate measures can be taken to improve data management awareness and skills. The article also provides some useful recommendations on what such measures might be, and introduces some of the steps already taken by the Delft University of Technology (TU Delft).
- Research Data Management
- Master Students
- Reproducibility crisis
- FAIR Data Principles
I. Introduction
The adequate management of research data has always been an indispensable element of trustworthy scientific research, but the interest for research data management practices, skills and experiences has flourished in the last decade. This increased recognition for data management and the sense of urgency attached to it ( Feijen 2011 ), is partly fuelled by questions about research reproducibility and the perceived existence of a reproducibility crisis in research. Monya Baker’s survey of 1,500 researchers in Nature ( 2016 ), revealed that 90% of researchers felt that there was a reproducibility crisis ( Baker 2016 ). It was found that, in some disciplines, over 80% of respondents experienced problems when reproducing other people’s results. The survey also investigated the reasons behind such irreproducible research. In addition to a toxic publication culture (‘selective reporting’, ‘pressure to publish’ etc.), respondents also referred to (a lack of) data availability, indicating that ‘methods and code were unavailable’ and that ‘the raw data from the original lab was not available’. The fact that poor data management practices contribute to irreproducible research, was re-emphasised in a recent study in Molecular Brain ( Miyakawa 2020 ). The author noted that ‘more than 97% of the 41 manuscripts did not present the raw data supporting their results, when requested by an editor’. To prevent such flaws and to increase the reproducibility of scientific studies, reliable data management throughout the entire research cycle is essential.
Not surprisingly, multiple stakeholders intervened with policies to improve research data management practices. National (e.g. the Dutch NWO ( n.d. ) and ZonMw ( n.d. )) and international funders, for example, the European Commission ( n.d. ), require their grantees to submit a data management plan at the beginning of the research project. Moreover, grantees need to share research data, generated during the project, as openly as possible. In line with these measures, publishers also reacted with updated policies. Science was called to strengthen its data management policies for data reporting and publication ( Science, n.d. ) after a high profile paper retraction in May 2017 ( Berg 2017 ). In a commentary on this matter, Roche ( 2017 ) wrote: ‘The only computer containing the study’s raw data was allegedly stolen and no backups existed on another machine or an online repository. Many are left wondering how this could happen in an era of cloud computing and open data’. Many other publishers, for example PLOS ( Bloom, Ganley & Winker 2014 ) and Springer Nature ( n.d. ), are similarly committed to better data reporting. They increasingly require that the research data, which supports findings described in an academic article, is made available.
Governments also took the initiative to improve research data management practices at national levels. In the Netherlands, a revised Netherlands Code of Conduct for Research Integrity has been published in 2018. That research data and its management play an important role in the revised document, is illustrated by the fact that the code mentions the word ‘data’ 49 times. The code obliges researchers to ‘manage the collected data carefully’ and to ‘contribute, where appropriate, towards making data findable, accessible, interoperable and reusable’ ( FAIR, KNAW et al. 2018: 17 ). Here, the code of conduct refers to the FAIR principles ( Wilkinson et al. 2016 ), a concept that has been widely adopted by various stakeholders who are interested in good data management across Europe ( European Commission 2018 ; Wittenburg et al. 2019 ), but also on other continents ( Van Reisen et al. 2020 ; Sales et al. 2020 ). Hence, the new standard for researchers, according to the code of conduct, is to ‘make research findings and research data public subsequent to the completion of the research’ ( KNAW et al. 2018: 18 ). However, institutions also have their ‘duties of care’ towards data management. It is made explicit that the institutions have to ‘provide a research infrastructure’, ‘ensure that all data, software codes and research materials, published or unpublished, are managed and securely stored’ and ‘that, in accordance with the FAIR principles, data is open and accessible to the extent possible and remains confidential to the extent necessary’ ( KNAW et al. 2018: 20–21 ).
In parallel, research institutions have also been developing their own policies aimed at improving research data practices. For example, the Delft University of Technology (TU Delft) has a policy framework for research data management ( Dunning, 2018 ). This document determines the roles and responsibilities of various stakeholders within the university. It addresses both central university support units (such as IT, legal, library) and introduces discipline-specific faculty-policies (e.g. TU Delft faculty of Mechanical, Maritime, and Materials Engineering 2019 ). Some smaller departments within TU Delft, also defined their own, even more specific policies on how to manage their research data assets ( Akhmerov & Steele 2019 ). To further stimulate such initiatives on institutional level, the European Commission funded a project called the Leaders Activating Research Networks ( 2017 ), which defines a framework for a research data management policy for research performing institutions ( LEARN, 2017 ).
The paragraphs above underline that research data management has attracted the attention of policymakers. However, these policies are only effective when they stay close to the daily research practice ( Cruz et al. 2019 ) and adhere to the researcher’s perceptions of good research data management. When the policies are not truly embedded and do not appeal to the intrinsic motivation of researchers, behavioural change at a university level might be difficult to achieve ( Carlson & Stowell-Bracke 2013 ). Maria Cruz ( 2019 ) highlighted that this gap between policy and practice needs to be addressed, before good research data management can be properly implemented across various research domains. Feijen ( 2011: 28 ) agrees: ‘There is a growing awareness that there should be more focus [on research data management], but it seems that this awareness is stronger in circles outside the research groups’.
Illustrative in this regard is that researchers are, in contrast to policymakers in this field, not very aware of the FAIR data principles. This is visible on smaller and larger scales. A survey from TU Delft, conducted in 2017 and 2018 (almost 700 respondents), revealed that researchers were largely unaware of these FAIR principles. Moreover, more than 50% of the respondents at each faculty were not ‘aware’ or were ‘not sure’ of the funders expectations related to FAIR data ( Mancilla et al. 2019 ). In the State of Open Data Report, Fane et al. ( 2019 ) found something similar, as they reported that more than 50% of the 8,500 researchers that responded to their questionnaire never heard of the FAIR principles.
Interestingly, most research on research data management practices tends to focus on practices of research staff (including PhD candidates). The experiences of master’s students are often overlooked. The lack of particular attention for master’s students is at least partially explained by the fact that, most of the time, master’s students are not the leading authors on research papers and grant proposals. In addition, the way intellectual property rights apply to master’s students is complex. For example, master’s students in the Netherlands retain ownership of the research data and any intellectual property they create. Therefore, it is unclear to what extent the research data management policies of universities, publishers or funders actually apply to them. Despite all this, data literacy is very important for this group of students. As Carlson et al. ( 2011: 629 ) noted: ‘Researchers increasingly need to integrate the disposition, management and curation of their data into their current workflows, but it is not yet clear to what extent faculty and students are sufficiently prepared to take on these responsibilities’. This causes an interesting friction, because it was learned that most data management-related curricula are not openly accessible and are not targeted on students outside of information science programs ( Piorun et al. 2012: 47 ).
In this light, it is interesting to gain insight into how university master’s students perceive research data management. They form an important group to study, as they conduct their first major research project but do not receive the same amount of research data training as PhD students often do. Moreover, the master’s students might be the future academics or policymakers and, thus, potentially, the future advocates of good research data management and reproducible science. Carlson & Stowell-Bracke ( 2013: 5 ), who interviewed master’s students in a water field station, argue that: ‘A significant gap in efforts to understand the practices of researchers through case studies, surveys or other means of investigation, is the overall lack of attention given to the role of graduate students and their work in generating, processing, analysing and managing data’.
This exploratory study addresses the gap in the understanding of data management perceptions and practices by master’s students. It presents the qualitative outcome of sixteen interviews with master student’s in the Netherlands. In the next section, the methodology of the study is explained. The findings will be discussed in section III. In the discussion (section IV), suggestions for follow up actions are included, together with some concrete steps already undertaken by TU Delft.
II. Methodology
To learn more about the data management attitudes and perceptions of master’s students, sixteen semi-structured interviews were conducted in September and October 2019. All interviewees have obtained a master’s diploma (all after 2015) from a Dutch university. In the Netherlands, master’s programmes have a minimum length of one year. For admission to a master’s programme, a bachelor’s degree (or a recognised equivalent) is mandatory ( NUFFIC, 2018 ). Most disciplines offer one-year master programmes, but two-year and three-year programmes are common in applied disciplines, such as physics, applied sciences or medicine. Master’s programmes offer the students further academic specialisation. In a master’s curriculum, a lot of emphasis is put on the education and the acquiring of academic research skills. In the vast majority of programmes, the completion of a master thesis research is obligatory ( NUFFIC, 2018 ), which generally comprises at least 25% of the length of the programme. All interviewees included in this study have performed such a master’s thesis research.
To invite the interviewees, the purposive sampling method was used, meaning that the researcher used own judgments to select the participants. According to Tongco ( 2007: 147 ), ‘the purposive sampling technique, also called judgment sampling, is the deliberate choice of an informant due to the qualities the informant possesses’. In this case, the informants are all persons with a master’s diploma and they are member of a strategic alliance of three universities in the Netherlands. The interviewees had diverse study backgrounds, although most of them studied social sciences. Interviewing students from different disciplines and different universities allows for diverse views and experiences to be included. The results of these interviews help to catch an important first glimpse of how diversely educated master’s students think about research data management. The participant’s university backgrounds (master’s studies only) and their fields of study are included in a table in the supplementary material of this article.
To select the interviewees, twenty ex-students were emailed in advance (in early September 2019), and were asked if they wanted to participate. Four participants decided not to do so, or did not reply to the email. All others consented via email to participate in the study. Before starting the interviews, the ex-students were informed about the procedure and they were orally asked for their consent again. They were told that the interviews were not recorded, but that detailed notes were taken. Recording was considered but was eventually decided against. It was believed that this approach would lead to better exploratory information on the students’ real attitude towards data management and that this would ensure that the interviewees felt more comfortable and at ease when answering personal questions about their own data management. All participants gave the researcher permission to use direct quotes in the publication, given that all interview notes would be anonymised. The interviews were conducted in Dutch, so the notes were also taken in Dutch. The open and axial codes (method will be explained in the next paragraph) in the data file and the direct quotes used in the report were translated into English. At TU Delft, where this study was conducted, MSc procedures regarding ethics are overseen by the faculties/units where the research is conducted. Only if these units deem it appropriate, applications are made to the central TU Delft Human Research Ethics Committee. In this case, following a discussion with the traineeship supervisor, the supervisor’s decision was that the ethical risks to the study participants are negligible, therefore a formal application to the TU Delft Human Research Ethics Committee was deemed not essential.
The participants all answered the same eleven questions (see Table 1 below) about research data management, in an interview that took between thirty and forty-five minutes. The interview questions were divided in three categories: a) the participant’s experiences with data management, b) attention for data management during the study curriculum and c) data in today’s world.
Interview questions asked to participants.
To analyse the interviews, open and axial coding methods were used. Coding helps to distinguish patterns, repeated concepts and categories in interview data ( Given 2008 ). Open coding is the act of closely interpreting interview data, in order to summarize the main idea of the text and to be able to make a first selection of concepts ( Given 2008 ). In the axial coding phase, the data is structured in such a way that it becomes possible to make theoretical connections between categories, questions, answers and concepts ( Kolb 2012 ). Due to the semi-structured interviews, the axial coding is very valuable to distinguish patterns and concepts across interview questions and participants. The full interview notes, the open coding (with English translations) and the axial coding tags are openly accessible and downloadable from the 4TU.ResearchData repository ( Smits, 2020 ). The full supplementary material includes tables with the participants’ study backgrounds, the (anonymised) distribution of universities, the translations of the full quotes used in this article and an extensive table that shows how many times an axial code was applied by the researcher. For privacy reasons, the question about the interviewees’ studies and thesis topics was left out.
Limitations of the study
This study was carried out to gain a better understanding of how graduated master’s students in the Netherlands perceive research data management and how they put data management into practice during their master’s studies. The interviewees studied at different universities and within different fields. Due to the exploratory nature of the study, the semi-structured interview approach and the fact that the students have shared their own opinions, the results should be interpreted with this specific context in mind and care should be taken with any potential generalisations. Nevertheless, the results outline how diversely educated master’s students think about research data management. Only by knowing (and further exploring) this, accurate measures can be taken to improve data management awareness and skills. For a more university-specific view, it is important to carry out this study within a more focused group.
III. The interview findings
In this section, the findings of the study are presented. In their interviews, the students defined data management, they talked about their experiences, their attitudes and how they learnt (or not) about data management during their curricula. The participants also indicated what they needed to become better in data management and how they thought about ‘data’ in the current day and age.
All the interviewed students have dealt with data during their research, whether quantitative or qualitative in nature. Five of them indicated to have used only qualitative methods, nine of them solely utilised quantitative methods. Two of the participants mixed these approaches. In their research, the students processed (pre-existing) datasets in SPSS, 1 collected longitudinal patient data, held interviews, analysed documents or conducted surveys. The findings, and their possible relation to the data management literature identified in the introduction, have been grouped under the five main subheadings to come.
Confusion about the definition of ‘research data management’
Before diving into more specific questions about research data management, the students were asked to define the concept. The Technical University Eindhoven ( TU/e, n.d. ), uses the following definition:
‘Research data management (RDM) is the careful handling and organization of research data during the entire research cycle, with the aim of making the research process as efficient as possible and to facilitate cooperation with others. More specifically, RDM helps to protect data, it facilitates in sharing the data with others and it ensures that research data is findable, accessible and (re)usable’.
Even though the participants, right at the start of the interviews, did not give such an extensive and comprehensive answer, some of the aforementioned factors surfaced in their definitions too. One participant, who studied languages, answered: ‘I believe that data is just a modern word for information. Everything around you can be data. So data management is the processing of all the information that you find relevant for a certain purpose’. All the interviewees actually believed that data management describes how researchers handle and safely store their data, during but also after the research. The ‘FAIR’ principles, which some members of the data management community see as the cornerstone of data management, were only mentioned by one interviewee. This is in line with the earlier reported findings by the State of Open Data Report ( Fane et al. 2019 ) and Mancilla et al. ( 2019 ). Only one interviewee, who studied educational sciences, said that data sharing was an inherent part of the definition of good data management: ‘It would be very valuable if data is stored in a standardised manner, so that it is very easy to combine and compare different datasets. Also, it is important to make the data visible to the outside world’.
That said, many interviewees also confused data management with other aspects of good research practice, such as methodology or study design. For example, a psychology student commented:
‘My data and the management of it was a mess. The survey questions did not correlate well with one another, and as too many participants did not answer to a question, or filled in ‘not applicable’, I got into trouble with my sample. My sample was too small so it could not lead to a significant test. I should have asked better questions, on which the answer ‘not applicable’ was not a possibility. After all these problems, I asked for external help and I wrote a longer discussion because I had so little data’.
Other students, when asked about their data management experiences, often spoke about being unsure how to code data, what kind of statistical test to run, how to hold scientific interviews or when to use consent forms.
Students are aware of privacy issues associated with data processing
When the students recalled their data management experiences, they often referred to privacy issues. In fact, twelve students predominantly focussed on the privacy concerns that came along with their data collection. Privacy-related issues have been addressed fifty-eight times throughout all these interviews about data management, by fifteen different interviewees. Only one interviewee did not explicitly mention privacy aspects. In general, students asked for consent, they anonymised their data and destroyed it when the sensitive information was no longer essential. Thus, the students who worked with personal data were very conscious about its sensitivity and the importance of anonymising what they found. The overwhelming attention for privacy is not surprising, due to the relatively recent introduction of the GDPR 2 and the attention this has generated.
The students also came back to these privacy concerns when answering questions about how they felt about the role of data in today’s world. Eleven students were concerned about the data gathering practices of both technology companies and the government. They were unified in the opinion that big data and privacy play an important role in our society. Generally, however, the students’ opinions on data collection seemed to depend on instinctive feelings, rather than on facts. One interviewee used the analogy that your personal data belongs to you as much as your hair or DNA, so you should have the right to sell it yourself if you wish. Five students realised that big data collection also offers chances to solve societal problems and improve services and technologies. Moreover, four students believed that collecting (personal) data for the sake of collecting data had to be minimised. This is where the link to their own research data came in, because two students also collected more data than they actually needed. While doing surveys, they collected all the data that could potentially be of relevance to their research questions, without knowing whether they would actually need these additional data.
An ad hoc approach to data management
When facing problems or challenges with their data, the students dealt with them intuitively, whether or not with the help of their thesis supervisor. Most of the students (13/16) could not rely on a data management plan, as only three interviewees within the sample mentioned that they developed such a plan. In fact, nine diversely educated interviewees were explicit that they managed their data in an ad hoc way. The students also did not always foresee the implications of the data choices they made in the beginning of their project.
One psychology student reflected on the lack of proper documentation during the data processing: ‘The data that we stored in the digital environment did not show up as we wanted in SPSS. We used certain formulas which changed something in the data, but we could not find the error. We knew it was not something substantial to our dataset, so we continued with the mistake still in there’. A sociology student also mentioned that she never knew how reliable the data she used actually were: ‘One researcher gave me the results of a quantitative study related to my topic. I used these results, which were already processed and analysed in SPSS, without questioning anything. I have never seen the raw dataset. Who knows these data were actually reliable?’
Illustrative of this ad-hoc approach to data management is the fact that half (8/16) of the 16 interviewees did not know how to access their data anymore. This lack of raw data availability makes their studies irreproducible, a flaw that Miyakawa ( 2020 ) found among researchers too. When thinking about their own research, the sixteen participants did not see their data as a research output on its own, or a valuable set of information that could help science forward. The students did not directly see the point of publishing their own data to provide evidence for the work they had done. Carlson & Stowell-Bracke ( 2013: 19 ) also underlined this, as in their sample ‘none of the students had really given the long term maintenance of their digital datasets much thought or taken action to ensure long term access to their data’. The interviewed students were only concerned about finishing their thesis and the dataset was merely an instrument to achieve this.
One of the students stated: ‘I did not have a plan what to do with my data. Of course I anonymised my interviews, but I have no clue what I did with the transcripts. I also don’t know where the data are anymore. You use the data for your analysis, but that’s it. Then it does not feel like your own problem anymore. Nobody is interested in the process after publishing the report’. In addition, a pedagogy student reflected: ‘It was clear that fast graduation was a big concern for our study. My master thesis felt as an obligatory goal to reach.’
Feijen ( 2011: 27 ) found a similar pattern. He wrote: ‘In most cases, data from the previous project will stay where it was at the end of the research project – where, more often than not, the storage situation is unreliable and the data is likely to deteriorate over time. Although all who were involved know that data will probably be lost forever, there is no time to take protective measures. Researchers have the feeling that they are not in a position to solve this problem, nor do they tend to accept responsibility for it. Their willingness to take responsibility is not highly developed’.
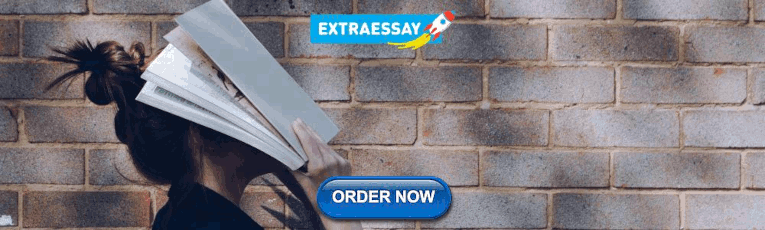
Students are aware of the importance of data sharing for research reproducibility, but they do not publish their data
There was unanimity among the students that good data management during research is important for research reproducibility. Eleven participants stressed that good data management and sharing is essential to reproduce studies. However, they did not always translate these overall principles into practical actions. A languages student said: ‘I was quite aware of the importance of reproducibility. However, during the coding of my data, I realised that nobody would actually be interested in my data ever again. As a result, I did not manage my data with utmost care, which could have harmed the credibility of my research’.
None of the interviewed students published their data. Two interviewees suggested the creation of a data archive for master’s students, to facilitate data sharing and knowledge flow. 3 One of the international relations students stated:
‘My data was not published anywhere. For an outsider, it is impossible to retrieve my data. I have the feeling that many other students did research on similar issues, so many fished in the same pool of information. But none of this data was findable for me to work with. That is a missed chance. It would be good to have a data archive in which master’s students can store their data.’
When discussing data sharing, similarly to the study conducted by Carlson & Stowell-Bracke ( 2013 ), some interviewees underlined the importance of openly accessible research data, while others pointed out the potential risks. They thought that open data can foster new research, help increase transparency and improve the reliability of scientific claims. One student stated, for instance, that when certain issues get sensationalised in the media, the underlying dataset helps to retrieve the exact context in which things happened, so that a more balanced picture could be put forward.
A sociology student also mentioned the positive role open data could play in the competitive research climate: ‘It is good to be more transparent about research data. Research is also a lot about raising funds and getting grants. That is why I think data checks are so important. I think the market has a bad influence on research’. The moral duty to openly publish data also plays a role. An art history student reflected: ‘why does society need to pay twice, by subsidising the research first and then paying again for access to the results?’
Students also discussed the risks associated with data sharing. Some were concerned about the abuse of sensitive data, or that others might not be skilled enough to interpret the data in the way it was intended, leading to unreliable new results. There was a feeling that caution was needed when interpreting data, because it could be hard to reproduce the exact same circumstances as when the data were gathered, even when rich metadata is available. Some also stressed that the data re-users could have a different social background than the creator of the content, leading to other assumptions or beliefs. One student, who studied educational science, reflected on her own data: ‘it is crucial to know what your data really means. After some time, I realised that my quantitative data had to be interpreted in a different way than I thought. If I wouldn’t have found this, I would have come to the wrong conclusions’.
Gaps in data management training for Master’s students
The students also answered questions on whether there was enough attention for data management during their curriculum, what their biggest challenges were and what they needed to become better at data management. In essence, students did not receive dedicated training on data management. Various studies had elements of data management incorporated into the curricula, but the focus was typically on other topics, such as ethics, statistical analysis or research methods. Sometimes, data documentation or safe data handling was discussed in thesis groups, during seminars or in specific classes.
A comment from a pedagogy student nicely illustrates the issue that data management was confused with other topics, as she mentioned that ‘data management education was all about analysing in SPSS.’ During the interviews, thirteen out of the sixteen interviewees declared that their study did not have enough attention for data management in general, and that they wanted to learn more about the subject. A similar observation was made in the study by Piorun et al. ( 2012 ), in which their students also confirmed their demand for data management education. One student, who studied an international relations specialisation, said: ‘I wish there was more attention for data management. It was all quite unclear to us and that caused a lot of unrest and confusion. It would have been good if the instructors would have shown us why and how to do thorough data management. Now I just kept doing my own thing’.
Remarkably, there was a media studies student who questioned the increased attention for data management in science and education: ‘Data management is a trend. If it was so important, why wasn’t it such a hot topic earlier? It seems that universities now put so much effort into it, only to ensure that they are not responsible anymore when something goes wrong. Then the university is innocent because they have taught us about the risks. I don’t think that data were not well managed before the attention increased, it was just more inherent to the research and we trusted more on common sense’.
IV. Discussion and final remarks
This preliminary study captured the attitudes of sixteen master’s students towards data management. Overall, the results suggest that, with the exception of awareness of data privacy issues and GDPR, the students had a rather fragmented knowledge of data management. Interestingly, many of them were confused about what data management meant, and seemed to associate data management issues with other research topics, such as data analysis, methodology and study design. Consequently, most of the students managed their data in an ad hoc fashion, without any dedicated planning upfront. This intuitive approach also surfaced in their attitudes towards data sharing. While students were aware that data availability is essential for reproducibility, none of them shared their own research data, knew how to do it, or felt this was important for their study. Given the relatively low awareness about data management among the students, it was not surprising to note that none of them has received comprehensive training on this matter. Data management education, if any, seemed to be added to existing courses and study discussions, but without a coherent approach. Nevertheless, almost all students understood the importance of data management and wished they had received better data management training.
The results presented in this exploratory study suggest that academic institutions could invest more resources into the data management education of master’s students. While approaches to data management can differ in different research fields, a comprehensive overview of research data management is needed for master’s students, regardless of scientific discipline. This is particularly important given that, as indicated in this study, students tend to process sensitive data that needs to be managed responsibly. Good data management and data sharing could also enhance the visibility of the students’ work, improve the rigour of the research and improve the overall transparency.
Even though these findings are exploratory in nature, they already prompted TU Delft to embark on several initiatives aimed at improving data management awareness among master’s students. At the Faculty of Architecture and the Built Environment, the faculty data steward is now running a pilot to provide data management education in one of the master’s courses. The data steward teaches the students about data management, just before the students start their thesis projects. The advantage of partnering with an experienced faculty data steward guarantees that all key aspects of data management education are addressed in a coherent fashion. The results of this pilot are preliminary – the data steward only joined one course so far – but the feedback received from the students and the course coordinator was positive. The data steward was asked to regularly present to the students participating in this course. This faculty data steward is now also initiating discussions with the coordinators of other MSc courses. Pending the outcomes of this pilot at the Faculty of Architecture and the Built Environment, similar approaches might be adapted at other faculties at TU Delft.
The DelftOpenHardware community 4 is also a promising development in this field. This community is a bottom-up, community driven initiative by TU Delft researchers, to encourage collaboration on Open Hardware projects. Hardware and design projects are quite common at TU Delft (e.g. the designs of new machines, equipment, tools etc.), especially among master’s students who conduct such efforts for their thesis projects. One of the core missions of the DelftOpenHardware community is to teach good documentation and to promote the sharing of data in hardware designs. The majority of its members are master’s students, who value the informal support they receive through the DelftOpenHardware community. The community is meeting every week and students regularly join the drop-in sessions, to receive support on data management and documentation. Data management help is offered directly by experts in the field, while administrative support (such as venue booking) is offered by library staff. That way, students receive very practical, hands-on data management support in their projects.
Finally, this study also touched upon the importance of strict privacy measures when processing sensitive and personal research data. At TU Delft, the compliance of research data with GDPR is achieved by following a dedicated workflow, which starts with a data management plan (DMP). Every project that processes personal information needs to have a DMP ( TU Delft, n.d. ). These DMPs are created in a dedicated tool, called DMPonline. 5 Whenever a new DMP is created, the faculty data steward is notified about it and then reviews the plan. Following the review, the data steward advises the researcher on appropriate data management steps, such as an ethical review or a data protection impact assessment. So far, however, the focus of this service was on researchers and PhD candidates. The outcomes of this study highlight that the data processing of master’s students also needs to be addressed, in particular because most master’s students have been following very ad hoc data management procedures. However, given the sheer number of master’s students, it is impossible to ask all master’s students to follow the aforementioned workflow. That is why the ‘GDPR Research Data Working Group’ at TU Delft is currently conducting community consultations to explore the best possible solution for master’s students.
Overall, the findings of this exploratory study provided important insights on data management practices among master’s students. It highlighted that data management awareness among master’s students is rather low. Therefore, research institutions need to invest in more thorough education on this matter. TU Delft has already undertaken some preliminary steps in the right direction, but more work needs to be done, including extended research on this topic.
Supplementary material
All research material is openly available and accessible for any interested reader. The supplementary material includes tables with the participants’ study backgrounds, the involved universities, the translations of the full quotes used in this article and an extensive table that indicates how many times a certain code, to label the interview answers, was applied by the researchers. An explanation on how to interpret the coding is also included in the supplementary material. Please click this link to access the full dataset: http://doi.org/10.4121/uuid:ee978f4b-4b2a-4fb1-aeed-829f773eb316 .
SPSS (Statistical Package for the Social Sciences) is a computer programme for statistical analyses, particularly used in social sciences.
The General Data Protection Regulation came into effect as of May 2018. For more information please visit the website of the European Commission: https://ec.europa.eu .
The 4TU Centre for Research Data actually hosts a section in which also master’s students can submit their data. For more information, please visit: https://data.4tu.nl/repository/collection:masterthesis_data .
For more information, please visit: https://delftopenhardware.nl/
The DMP online portal is accessible through https://dmponline.tudelft.nl/?perform_check=false .
Acknowledgements
We would especially like to thank Alastair Dunning (Head of Research Data Services at TU Delft) for his help, comments and feedback. We are also grateful to the sixteen interviewees, who reserved time for us and provided us with enlightening comments, opinions and insights.
Competing Interests
The authors have no competing interests to declare.
Akhmerov, A and Steele, G. 2019. TU Delft Open Data Policy of the Quantum Nanoscience Department. TU Delft . DOI: https://doi.org/10.5281/zenodo.2556949
Baker, M. 2016. 1,500 Scientists Lift the Lid on Reproducibility, 25 May 2016. Nature News . [Last accessed 30 April 2020]. DOI: https://doi.org/10.1038/533452a
Berg, J. 2017. Editorial Retraction. Science , 356(6340): 812. DOI: https://doi.org/10.1126/science.aan5763
Bloom, T, Ganley, E and Winker, M. 2014. Data Access for the Open Access Literature: PLOS’s Data Policy. PLOS Biology , 12(2): 1–3. DOI: https://doi.org/10.1371/journal.pbio.1001797
Carlson, J, Fosmire, M, Miller, CC and Nelson, MS. 2011. Determining Data Information Literacy Needs: A Study of Students and Research Faculty. Portal: Libraries and the Academy , 11(2): 629–657. DOI: https://doi.org/10.1353/pla.2011.0022
Carlson, J and Stowell-Bracke, M. 2013. Data Management and Sharing from the Perspective of Graduate Students: An Examination of the Culture and Practice at the Water Quality Field Station. Portal: Libraries and the Academy , 13(4): 343–361. DOI: https://doi.org/10.1353/pla.2013.0034
Cruz, M. 2019. Bringing Researchers Along on the Road to FAIR data. Zenodo . DOI: https://doi.org/10.5281/zenodo.3249802
Cruz, M, Dintzner, N, Dunning, A, Kuil, A. van der, Plomp, E, Teperek, M, der Velden, YT and Versteeg, A. 2019. Policy Needs to Go Hand in Hand with Practice: The Learning and Listening Approach to Data Management. Data Science Journal , 18(1): 45. DOI: https://doi.org/10.5334/dsj-2019-045
Dunning, A. 2018. TU Delft Research Data Framework Policy. TU Delft. Available at: https://www.tudelft.nl/en/library/current-topics/research-data-management/r/policies/tu-delft-faculty-policies/ . [Last accessed 1 May 2020].
European Commission. 2018. Turning FAIR into Reality. Brussels, Belgium: European Commission. Available at: http://op.europa.eu/en/publication-detail/-/publication/7769a148-f1f6-11e8-9982-01aa75ed71a1/language-en/format-PDF [Last accessed 30 April 2020].
European Commission. n.d. Data management – H2020 Online Manual. Belgium: European Commission. Available at: https://ec.europa.eu/research/participants/docs/h2020-funding-guide/cross-cutting-issues/open-access-data-management/data-management_en.htm [Last accessed 30 April 2020].
Fane, B, Ayris, P, Hahnel, M, Hrynaszkiewicz, I, Baynes, G and Farrell, E. 2019. The State of Open Data Report 2019. Digital Science . DOI: https://doi.org/10.6084/m9.figshare.9980783.v2
Feijen, M. 2011. What Researchers Want. SURF Foundation . Available at: https://www.bvekennis.nl/wp-content/uploads/documents/11-0198-What-researchers-want.pdf [Last accessed 30 April 2020].
Given, LM. 2008. The SAGE Encyclopedia of Qualitative Research Methods. Thousand Oaks: SAGE Publications. DOI: https://doi.org/10.4135/9781412963909
KNAW; NFU; NWO; TO2-federatie; Vereniging Hogescholen; VSNU. 2018. Wetenschappelijke Gedragscode Integriteit [Netherlands Code of Conduct for Research Integrity]. The Hague, Netherlands. Available at: https://www.vsnu.nl/files/documents/Netherlands%20Code%20of%20Conduct%20for%20Research%20Integrity%202018.pdf [Last accessed 1 May 2020].
Kolb, SM. 2012. Grounded Theory and the Constant Comparative Method: Valid Research Strategies for Educators. Journal of Emerging Trends in Educational Research and Policy Studies , 3(1): 83–86. Available at: http://jeteraps.scholarlinkresearch.com/articles/Grounded%20Theory%20and%20the%20Constant%20Comparative%20Method.pdf [Last accessed 1 May 2020].
Leaders Activating Research Networks. 2017. Model Policy for Research Data Management (RDM) at Research Institutions/Institutes: 133–136. Available at: https://discovery.ucl.ac.uk/id/eprint/1546606/1/25_Learn_Model%20Policy_133-136.pdf [Last accessed 1 May 2020].
Mancilla, HA, Teperek, M, van Dijck, J., den Heijer, K, Eggermont, R, Plomp, E, der Velden, YT and Kurapati, S. 2019. On a Quest for Cultural Change – Surveying Research Data Management Practices at Delft University of Technology. LIBER Quarterly , 29(1): 1–27. DOI: https://doi.org/10.18352/lq.10287
Miyakawa, T. 2020. No Raw Data, No Science: Another Possible Source of the Reproducibility Crisis. Molecular Brain , 13(24). DOI: https://doi.org/10.1186/s13041-020-0552-2
NUFFIC. 2018. Onderwijssysteem Nederland [Education system in The Netherlands]. The Hague, Netherlands: Nuffic. Available at: https://www.nuffic.nl/publicaties/onderwijssysteem-nederland/ [Last accessed 1 May 2020].
NWO. n.d. Open (FAIR) data. The Hague, The Netherlands: NWO. Available at: https://www.nwo.nl/en/policies/open+science/data+management [Last accessed 1 May 2020].
Piorun, M, Kafel, D, Leger-Hornby, T, Najafi, S, Martin, E, Colombo, P and LaPelle, N. 2012. Teaching Research Data Management: An Undergraduate/Graduate Curriculum. JESLIB , 1(1): 46–50. DOI: https://doi.org/10.7191/jeslib.2012.1003
Roche, DG. 2017. Evaluating Science’s Open-data Policy. Science , 357(6352): 654. DOI: https://doi.org/10.1126/science.aan8158
Sales, L, Henning, P, Veiga, V, Costa, MM, Sayão, LF, da Silva Santos, LOB and Pires, LF. 2020. GO FAIR Brazil: A Challenge for Brazilian Data Science. Data Intelligence , 2(1): 238–245. DOI: https://doi.org/10.1162/dint_a_00046
Science. n.d. Science Journals: editorial policies. Washington, DC, United States of America: Science. Available at: https://www.sciencemag.org/authors/science-journals-editorial-policies [Last accessed 1 May 2020].
Smits, DAB. 2020. Research Data Attitudes of Recently Graduated Master Students (interview notes and code) [Dataset]. DOI: https://doi.org/10.4121/uuid:ee978f4b-4b2a-4fb1-aeed-829f773eb316
Springer Nature. n.d. Research Data Policies. Berlin, Germany: Springer Nature. Available at: https://www.springernature.com/gp/authors/research-data-policy [Last accessed 1 May 2020].
Technische Universiteit Eindhoven. n.d. Wat is Research Data Management? Eindhoven, The Netherlands: TUE. Available at: https://www.tue.nl/universiteit/bibliotheek/ondersteuning-onderwijs-onderzoek/wetenschappelijk-publiceren/data-coach/begrippen-en-achtergrond/wat-is-research-data-management/ [Last accessed 1 May 2020].
Tongco, M. 2007. Purposive Sampling as a Tool for Informant Selection. Ethnobotany Research and Applications , 5(1): 147–158. Available at: Ethnobotany Research Journal [Last accessed 1 May 2020]. DOI: https://doi.org/10.17348/era.5.0.147-158
TU Delft. n.d. Personal Data: Personal Research Data Workflow. Delft, The Netherlands: TUD. Available at: https://www.tudelft.nl/en/library/current-topics/research-data-management/r/manage/confidential-data/personal-data/ [Last accessed 1 May 2020].
TU Delft Faculty of Mechanical Maritime and Materials Engineering. 2019. The Faculty of Mechanical, Maritime and Materials Engineering Research Data Management Policy. Delft, The Netherlands: TU Delft. DOI: https://doi.org/10.5281/zenodo.3524106
Van Reisen, M, Stokmans, M, Mawere, M, Basajja, M, Ong’ayo, AO, Nakazibwe, P, Kirkpatrick, C and Chindoza, K. 2020. FAIR Practices in Africa. Data Intelligence , 2(1): 246–256. DOI: https://doi.org/10.1162/dint_a_00047
Wilkinson, MD, Dumontier, M, Aalbersberg, IJ, Appleton, G, Axton, M, Baak, A, Bouwman, J, et al. 2016. The FAIR Guiding Principles for Scientific Data Management and Stewardship. Scientific Data , 3(160018). DOI: https://doi.org/10.1038/sdata.2016.18
Wittenburg, P, Lautenschlager, M, Thiemann, H, Baldauf, C and Trilsbeek, P. 2019. FAIR Practices in Europe. Data Intelligence , 2(1): 257–263. DOI: https://doi.org/10.1162/dint_a_00048
ZonMw. n.d. ZonMw-procedure Datamanagement. The Hague, The Netherlands: ZonMW. Available at: https://www.zonmw.nl/nl/over-zonmw/toegang-tot-data/zonmw-procedure-datamanagement/ [Last accessed 1 May 2020].
- Switch language, Suomi fi
- Current language, English en
5 Data management plan guidelines for thesis
At Jamk, a data management plan (dmp) is prepared as an appendix to the thesis plan. It is a concrete plan for handling (saving, sharing, archiving, destroying) the data in the different phases of the thesis. The content complements the thesis plan.
- Managing research data and preparing a data management plan are part of good scientific practice.
- The data management plan is an indication of a competence as a higher education student.
- With the help of the plan, the student prepares operating instructions for him/herself, how to handle the data during the thesis process. The student must solve these things in any case, the plan is tool for that!
- A data management plan drawn up in advance reduces the risk of loss or destruction of materials. The plan helps to identify risks related to data protection, for example.
- With the help of the plan, the student is able to anticipate and control the details related to ownership and usage rights, if the thesis is done for a commissioner or project.
- A plan drawn up in advance enables the utilization of data in further use.
Instructions and template for the data management plan
The structure of the data management plan (see below) follows the national model. Create a data management plan according to the student’s choice using either the Word template or with the DPM Tuuli data management planning tool. They both also contain instructions on how to prepare the plan and what is described in it.
The DMP Tuuli planning tool is used with Haka login. The first time, the ID must be registered, and Jamk University of Applied Sciences must be selected as the organization. In the future, the user can log in directly through Haka under Sign in with your institutional credentials. This is how you find the Jamk’s template: select Create a new plan, write Jamk University of Applied Sciences as a research organization, check the box No funder associated, and finally select the template of Jamk. The plan created in the program can be shared in different file formats and shared with other users, for example to the thesis tutor or another thesis author.
Structure of the data management plan
- General description of data
- Personal data, ethical principles and legal compliance
- Documentation and metadata
- Storage and backup during the thesis project
- Archiving and opening, destroying or storing the data after the thesis project
- Data management responsibilities and resources
Best practices for the thesis tutor
- The plan must be concrete and describe the solutions to the questions asked specifically for the thesis in question. The plan is an operating manual for the student.
- The instructions found in the DMP template are also to support the thesis tutor. Check there what should be described in the plan. The questions will be answered in the applicable parts. The intention is not to repeat the contents of the thesis plan.
- Processing personal data : Pay special attention to the fact that the student recognizes whether he/she is processing personal data in some form in the thesis. This results in the need for a privacy statement and adequate Informing research participants about the processing. The type of personal data also affects how the data can be stored and shared, for example.
- Consider the role of the commissioner , for example as the owner, user or archiver of the data. Have the research participants been informed correctly, who has the rights to the data and what will be done with it after the thesis is completed. Does the organization require a research permit?
- Master’s degree data storage for two years: The data of master’s degree theses must be stored securely for two years from the completion of the thesis. The data will not be anonymized or destroyed before this. This enables the data to be checked if necessary if there is reason to suspect fraud. The storage location is indicated in the data management plan.
- Data storage options are described in the thesis guide (chapter 4.4.5). What is important is a data-secure way of storing and sharing the data, as well as disposing of or storing the data properly after the thesis is completed. When saving, it is recommended to use the services offered by the organization instead of private user accounts.
- Basically, the data must always be anonymized if it is saved for further use after the work is completed. Note! The data of master thesis must be stored for a possible review for two years after the completion of the thesis in a data-secure manner. If there is still a need to store the data after that, anonymization is done at this stage. If the master thesis data is further used in other research, it is recommended to make an anonymized version for this purpose. Jamk is not responsible for archiving the thesis data.
- The length of the plan is approximately 1-3 pages.
Data management plan and processing of research data (Thesis guide for students)
DMPTuuli (Data management planning tool)
Data Management Guidelines (The Finnish Social Science Data Archive , FSD): Detailed instructions for all stages of data management, e.g. informing the research subject, storage and disposal of material, rights, anonymization
What is persona data? (Data Protection Ombudsman)
Research permit at Jamk (jamk.fi)
Updated 24.10.2022 Elina Kirjalainen
Thank you for visiting nature.com. You are using a browser version with limited support for CSS. To obtain the best experience, we recommend you use a more up to date browser (or turn off compatibility mode in Internet Explorer). In the meantime, to ensure continued support, we are displaying the site without styles and JavaScript.
- View all journals
- My Account Login
- Explore content
- About the journal
- Publish with us
- Sign up for alerts
- Data Descriptor
- Open access
- Published: 03 May 2024
A dataset for measuring the impact of research data and their curation
- Libby Hemphill ORCID: orcid.org/0000-0002-3793-7281 1 , 2 ,
- Andrea Thomer 3 ,
- Sara Lafia 1 ,
- Lizhou Fan 2 ,
- David Bleckley ORCID: orcid.org/0000-0001-7715-4348 1 &
- Elizabeth Moss 1
Scientific Data volume 11 , Article number: 442 ( 2024 ) Cite this article
- Research data
- Social sciences
Science funders, publishers, and data archives make decisions about how to responsibly allocate resources to maximize the reuse potential of research data. This paper introduces a dataset developed to measure the impact of archival and data curation decisions on data reuse. The dataset describes 10,605 social science research datasets, their curation histories, and reuse contexts in 94,755 publications that cover 59 years from 1963 to 2022. The dataset was constructed from study-level metadata, citing publications, and curation records available through the Inter-university Consortium for Political and Social Research (ICPSR) at the University of Michigan. The dataset includes information about study-level attributes (e.g., PIs, funders, subject terms); usage statistics (e.g., downloads, citations); archiving decisions (e.g., curation activities, data transformations); and bibliometric attributes (e.g., journals, authors) for citing publications. This dataset provides information on factors that contribute to long-term data reuse, which can inform the design of effective evidence-based recommendations to support high-impact research data curation decisions.
Similar content being viewed by others
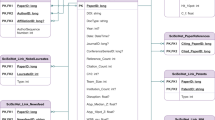
SciSciNet: A large-scale open data lake for the science of science research
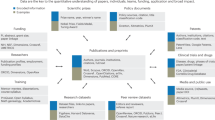
Data, measurement and empirical methods in the science of science
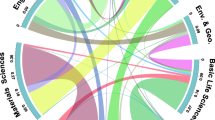
Interdisciplinarity revisited: evidence for research impact and dynamism
Background & summary.
Recent policy changes in funding agencies and academic journals have increased data sharing among researchers and between researchers and the public. Data sharing advances science and provides the transparency necessary for evaluating, replicating, and verifying results. However, many data-sharing policies do not explain what constitutes an appropriate dataset for archiving or how to determine the value of datasets to secondary users 1 , 2 , 3 . Questions about how to allocate data-sharing resources efficiently and responsibly have gone unanswered 4 , 5 , 6 . For instance, data-sharing policies recognize that not all data should be curated and preserved, but they do not articulate metrics or guidelines for determining what data are most worthy of investment.
Despite the potential for innovation and advancement that data sharing holds, the best strategies to prioritize datasets for preparation and archiving are often unclear. Some datasets are likely to have more downstream potential than others, and data curation policies and workflows should prioritize high-value data instead of being one-size-fits-all. Though prior research in library and information science has shown that the “analytic potential” of a dataset is key to its reuse value 7 , work is needed to implement conceptual data reuse frameworks 8 , 9 , 10 , 11 , 12 , 13 , 14 . In addition, publishers and data archives need guidance to develop metrics and evaluation strategies to assess the impact of datasets.
Several existing resources have been compiled to study the relationship between the reuse of scholarly products, such as datasets (Table 1 ); however, none of these resources include explicit information on how curation processes are applied to data to increase their value, maximize their accessibility, and ensure their long-term preservation. The CCex (Curation Costs Exchange) provides models of curation services along with cost-related datasets shared by contributors but does not make explicit connections between them or include reuse information 15 . Analyses on platforms such as DataCite 16 have focused on metadata completeness and record usage, but have not included related curation-level information. Analyses of GenBank 17 and FigShare 18 , 19 citation networks do not include curation information. Related studies of Github repository reuse 20 and Softcite software citation 21 reveal significant factors that impact the reuse of secondary research products but do not focus on research data. RD-Switchboard 22 and DSKG 23 are scholarly knowledge graphs linking research data to articles, patents, and grants, but largely omit social science research data and do not include curation-level factors. To our knowledge, other studies of curation work in organizations similar to ICPSR – such as GESIS 24 , Dataverse 25 , and DANS 26 – have not made their underlying data available for analysis.
This paper describes a dataset 27 compiled for the MICA project (Measuring the Impact of Curation Actions) led by investigators at ICPSR, a large social science data archive at the University of Michigan. The dataset was originally developed to study the impacts of data curation and archiving on data reuse. The MICA dataset has supported several previous publications investigating the intensity of data curation actions 28 , the relationship between data curation actions and data reuse 29 , and the structures of research communities in a data citation network 30 . Collectively, these studies help explain the return on various types of curatorial investments. The dataset that we introduce in this paper, which we refer to as the MICA dataset, has the potential to address research questions in the areas of science (e.g., knowledge production), library and information science (e.g., scholarly communication), and data archiving (e.g., reproducible workflows).
We constructed the MICA dataset 27 using records available at ICPSR, a large social science data archive at the University of Michigan. Data set creation involved: collecting and enriching metadata for articles indexed in the ICPSR Bibliography of Data-related Literature against the Dimensions AI bibliometric database; gathering usage statistics for studies from ICPSR’s administrative database; processing data curation work logs from ICPSR’s project tracking platform, Jira; and linking data in social science studies and series to citing analysis papers (Fig. 1 ).
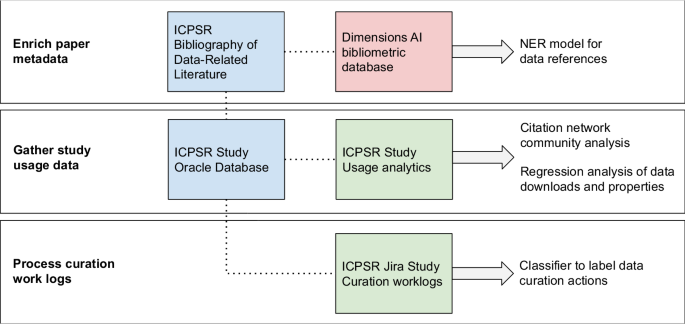
Steps to prepare MICA dataset for analysis - external sources are red, primary internal sources are blue, and internal linked sources are green.
Enrich paper metadata
The ICPSR Bibliography of Data-related Literature is a growing database of literature in which data from ICPSR studies have been used. Its creation was funded by the National Science Foundation (Award 9977984), and for the past 20 years it has been supported by ICPSR membership and multiple US federally-funded and foundation-funded topical archives at ICPSR. The Bibliography was originally launched in the year 2000 to aid in data discovery by providing a searchable database linking publications to the study data used in them. The Bibliography collects the universe of output based on the data shared in each study through, which is made available through each ICPSR study’s webpage. The Bibliography contains both peer-reviewed and grey literature, which provides evidence for measuring the impact of research data. For an item to be included in the ICPSR Bibliography, it must contain an analysis of data archived by ICPSR or contain a discussion or critique of the data collection process, study design, or methodology 31 . The Bibliography is manually curated by a team of librarians and information specialists at ICPSR who enter and validate entries. Some publications are supplied to the Bibliography by data depositors, and some citations are submitted to the Bibliography by authors who abide by ICPSR’s terms of use requiring them to submit citations to works in which they analyzed data retrieved from ICPSR. Most of the Bibliography is populated by Bibliography team members, who create custom queries for ICPSR studies performed across numerous sources, including Google Scholar, ProQuest, SSRN, and others. Each record in the Bibliography is one publication that has used one or more ICPSR studies. The version we used was captured on 2021-11-16 and included 94,755 publications.
To expand the coverage of the ICPSR Bibliography, we searched exhaustively for all ICPSR study names, unique numbers assigned to ICPSR studies, and DOIs 32 using a full-text index available through the Dimensions AI database 33 . We accessed Dimensions through a license agreement with the University of Michigan. ICPSR Bibliography librarians and information specialists manually reviewed and validated new entries that matched one or more search criteria. We then used Dimensions to gather enriched metadata and full-text links for items in the Bibliography with DOIs. We matched 43% of the items in the Bibliography to enriched Dimensions metadata including abstracts, field of research codes, concepts, and authors’ institutional information; we also obtained links to full text for 16% of Bibliography items. Based on licensing agreements, we included Dimensions identifiers and links to full text so that users with valid publisher and database access can construct an enriched publication dataset.
Gather study usage data
ICPSR maintains a relational administrative database, DBInfo, that organizes study-level metadata and information on data reuse across separate tables. Studies at ICPSR consist of one or more files collected at a single time or for a single purpose; studies in which the same variables are observed over time are grouped into series. Each study at ICPSR is assigned a DOI, and its metadata are stored in DBInfo. Study metadata follows the Data Documentation Initiative (DDI) Codebook 2.5 standard. DDI elements included in our dataset are title, ICPSR study identification number, DOI, authoring entities, description (abstract), funding agencies, subject terms assigned to the study during curation, and geographic coverage. We also created variables based on DDI elements: total variable count, the presence of survey question text in the metadata, the number of author entities, and whether an author entity was an institution. We gathered metadata for ICPSR’s 10,605 unrestricted public-use studies available as of 2021-11-16 ( https://www.icpsr.umich.edu/web/pages/membership/or/metadata/oai.html ).
To link study usage data with study-level metadata records, we joined study metadata from DBinfo on study usage information, which included total study downloads (data and documentation), individual data file downloads, and cumulative citations from the ICPSR Bibliography. We also gathered descriptive metadata for each study and its variables, which allowed us to summarize and append recoded fields onto the study-level metadata such as curation level, number and type of principle investigators, total variable count, and binary variables indicating whether the study data were made available for online analysis, whether survey question text was made searchable online, and whether the study variables were indexed for search. These characteristics describe aspects of the discoverability of the data to compare with other characteristics of the study. We used the study and series numbers included in the ICPSR Bibliography as unique identifiers to link papers to metadata and analyze the community structure of dataset co-citations in the ICPSR Bibliography 32 .
Process curation work logs
Researchers deposit data at ICPSR for curation and long-term preservation. Between 2016 and 2020, more than 3,000 research studies were deposited with ICPSR. Since 2017, ICPSR has organized curation work into a central unit that provides varied levels of curation that vary in the intensity and complexity of data enhancement that they provide. While the levels of curation are standardized as to effort (level one = less effort, level three = most effort), the specific curatorial actions undertaken for each dataset vary. The specific curation actions are captured in Jira, a work tracking program, which data curators at ICPSR use to collaborate and communicate their progress through tickets. We obtained access to a corpus of 669 completed Jira tickets corresponding to the curation of 566 unique studies between February 2017 and December 2019 28 .
To process the tickets, we focused only on their work log portions, which contained free text descriptions of work that data curators had performed on a deposited study, along with the curators’ identifiers, and timestamps. To protect the confidentiality of the data curators and the processing steps they performed, we collaborated with ICPSR’s curation unit to propose a classification scheme, which we used to train a Naive Bayes classifier and label curation actions in each work log sentence. The eight curation action labels we proposed 28 were: (1) initial review and planning, (2) data transformation, (3) metadata, (4) documentation, (5) quality checks, (6) communication, (7) other, and (8) non-curation work. We note that these categories of curation work are very specific to the curatorial processes and types of data stored at ICPSR, and may not match the curation activities at other repositories. After applying the classifier to the work log sentences, we obtained summary-level curation actions for a subset of all ICPSR studies (5%), along with the total number of hours spent on data curation for each study, and the proportion of time associated with each action during curation.
Data Records
The MICA dataset 27 connects records for each of ICPSR’s archived research studies to the research publications that use them and related curation activities available for a subset of studies (Fig. 2 ). Each of the three tables published in the dataset is available as a study archived at ICPSR. The data tables are distributed as statistical files available for use in SAS, SPSS, Stata, and R as well as delimited and ASCII text files. The dataset is organized around studies and papers as primary entities. The studies table lists ICPSR studies, their metadata attributes, and usage information; the papers table was constructed using the ICPSR Bibliography and Dimensions database; and the curation logs table summarizes the data curation steps performed on a subset of ICPSR studies.
Studies (“ICPSR_STUDIES”): 10,605 social science research datasets available through ICPSR up to 2021-11-16 with variables for ICPSR study number, digital object identifier, study name, series number, series title, authoring entities, full-text description, release date, funding agency, geographic coverage, subject terms, topical archive, curation level, single principal investigator (PI), institutional PI, the total number of PIs, total variables in data files, question text availability, study variable indexing, level of restriction, total unique users downloading study data files and codebooks, total unique users downloading data only, and total unique papers citing data through November 2021. Studies map to the papers and curation logs table through ICPSR study numbers as “STUDY”. However, not every study in this table will have records in the papers and curation logs tables.
Papers (“ICPSR_PAPERS”): 94,755 publications collected from 2000-08-11 to 2021-11-16 in the ICPSR Bibliography and enriched with metadata from the Dimensions database with variables for paper number, identifier, title, authors, publication venue, item type, publication date, input date, ICPSR series numbers used in the paper, ICPSR study numbers used in the paper, the Dimension identifier, and the Dimensions link to the publication’s full text. Papers map to the studies table through ICPSR study numbers in the “STUDY_NUMS” field. Each record represents a single publication, and because a researcher can use multiple datasets when creating a publication, each record may list multiple studies or series.
Curation logs (“ICPSR_CURATION_LOGS”): 649 curation logs for 563 ICPSR studies (although most studies in the subset had one curation log, some studies were associated with multiple logs, with a maximum of 10) curated between February 2017 and December 2019 with variables for study number, action labels assigned to work description sentences using a classifier trained on ICPSR curation logs, hours of work associated with a single log entry, and total hours of work logged for the curation ticket. Curation logs map to the study and paper tables through ICPSR study numbers as “STUDY”. Each record represents a single logged action, and future users may wish to aggregate actions to the study level before joining tables.
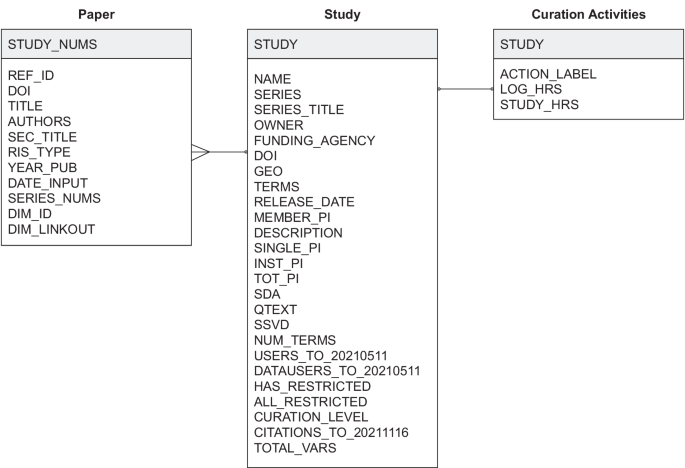
Entity-relation diagram.
Technical Validation
We report on the reliability of the dataset’s metadata in the following subsections. To support future reuse of the dataset, curation services provided through ICPSR improved data quality by checking for missing values, adding variable labels, and creating a codebook.
All 10,605 studies available through ICPSR have a DOI and a full-text description summarizing what the study is about, the purpose of the study, the main topics covered, and the questions the PIs attempted to answer when they conducted the study. Personal names (i.e., principal investigators) and organizational names (i.e., funding agencies) are standardized against an authority list maintained by ICPSR; geographic names and subject terms are also standardized and hierarchically indexed in the ICPSR Thesaurus 34 . Many of ICPSR’s studies (63%) are in a series and are distributed through the ICPSR General Archive (56%), a non-topical archive that accepts any social or behavioral science data. While study data have been available through ICPSR since 1962, the earliest digital release date recorded for a study was 1984-03-18, when ICPSR’s database was first employed, and the most recent date is 2021-10-28 when the dataset was collected.
Curation level information was recorded starting in 2017 and is available for 1,125 studies (11%); approximately 80% of studies with assigned curation levels received curation services, equally distributed between Levels 1 (least intensive), 2 (moderately intensive), and 3 (most intensive) (Fig. 3 ). Detailed descriptions of ICPSR’s curation levels are available online 35 . Additional metadata are available for a subset of 421 studies (4%), including information about whether the study has a single PI, an institutional PI, the total number of PIs involved, total variables recorded is available for online analysis, has searchable question text, has variables that are indexed for search, contains one or more restricted files, and whether the study is completely restricted. We provided additional metadata for this subset of ICPSR studies because they were released within the past five years and detailed curation and usage information were available for them. Usage statistics including total downloads and data file downloads are available for this subset of studies as well; citation statistics are available for 8,030 studies (76%). Most ICPSR studies have fewer than 500 users, as indicated by total downloads, or citations (Fig. 4 ).
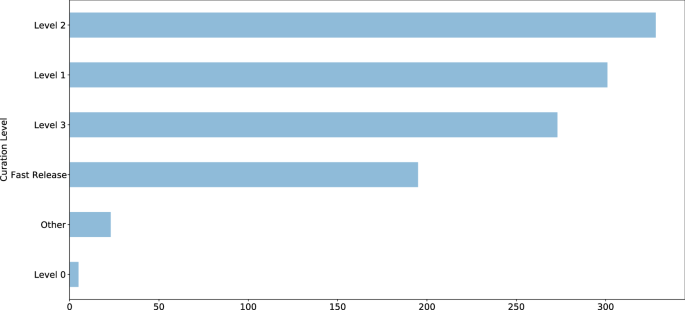
ICPSR study curation levels.
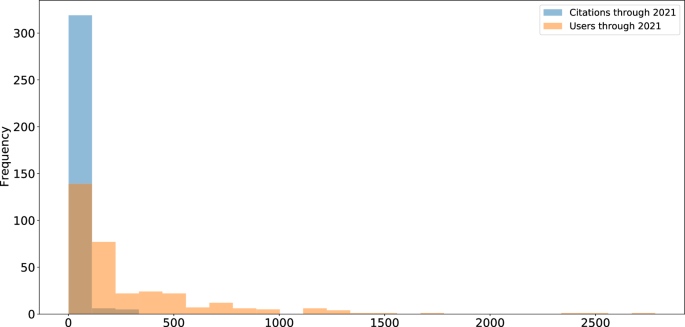
ICPSR study usage.
A subset of 43,102 publications (45%) available in the ICPSR Bibliography had a DOI. Author metadata were entered as free text, meaning that variations may exist and require additional normalization and pre-processing prior to analysis. While author information is standardized for each publication, individual names may appear in different sort orders (e.g., “Earls, Felton J.” and “Stephen W. Raudenbush”). Most of the items in the ICPSR Bibliography as of 2021-11-16 were journal articles (59%), reports (14%), conference presentations (9%), or theses (8%) (Fig. 5 ). The number of publications collected in the Bibliography has increased each decade since the inception of ICPSR in 1962 (Fig. 6 ). Most ICPSR studies (76%) have one or more citations in a publication.
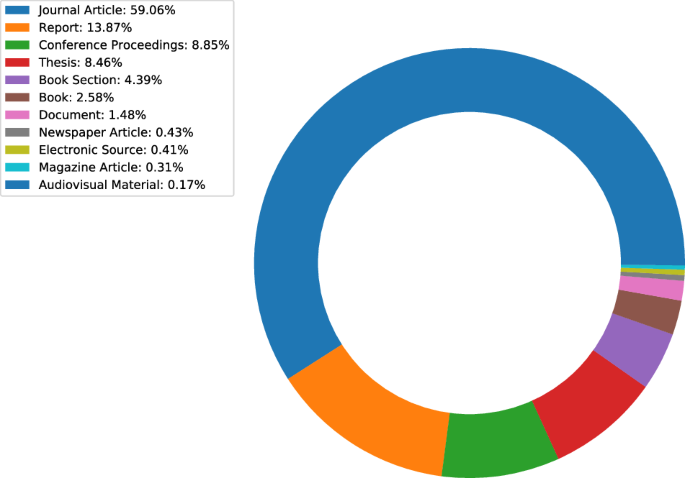
ICPSR Bibliography citation types.
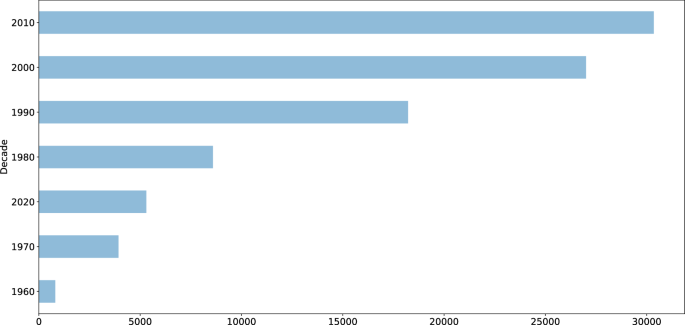
ICPSR citations by decade.
Usage Notes
The dataset consists of three tables that can be joined using the “STUDY” key as shown in Fig. 2 . The “ICPSR_PAPERS” table contains one row per paper with one or more cited studies in the “STUDY_NUMS” column. We manipulated and analyzed the tables as CSV files with the Pandas library 36 in Python and the Tidyverse packages 37 in R.
The present MICA dataset can be used independently to study the relationship between curation decisions and data reuse. Evidence of reuse for specific studies is available in several forms: usage information, including downloads and citation counts; and citation contexts within papers that cite data. Analysis may also be performed on the citation network formed between datasets and papers that use them. Finally, curation actions can be associated with properties of studies and usage histories.
This dataset has several limitations of which users should be aware. First, Jira tickets can only be used to represent the intensiveness of curation for activities undertaken since 2017, when ICPSR started using both Curation Levels and Jira. Studies published before 2017 were all curated, but documentation of the extent of that curation was not standardized and therefore could not be included in these analyses. Second, the measure of publications relies upon the authors’ clarity of data citation and the ICPSR Bibliography staff’s ability to discover citations with varying formality and clarity. Thus, there is always a chance that some secondary-data-citing publications have been left out of the bibliography. Finally, there may be some cases in which a paper in the ICSPSR bibliography did not actually obtain data from ICPSR. For example, PIs have often written about or even distributed their data prior to their archival in ICSPR. Therefore, those publications would not have cited ICPSR but they are still collected in the Bibliography as being directly related to the data that were eventually deposited at ICPSR.
In summary, the MICA dataset contains relationships between two main types of entities – papers and studies – which can be mined. The tables in the MICA dataset have supported network analysis (community structure and clique detection) 30 ; natural language processing (NER for dataset reference detection) 32 ; visualizing citation networks (to search for datasets) 38 ; and regression analysis (on curation decisions and data downloads) 29 . The data are currently being used to develop research metrics and recommendation systems for research data. Given that DOIs are provided for ICPSR studies and articles in the ICPSR Bibliography, the MICA dataset can also be used with other bibliometric databases, including DataCite, Crossref, OpenAlex, and related indexes. Subscription-based services, such as Dimensions AI, are also compatible with the MICA dataset. In some cases, these services provide abstracts or full text for papers from which data citation contexts can be extracted for semantic content analysis.
Code availability
The code 27 used to produce the MICA project dataset is available on GitHub at https://github.com/ICPSR/mica-data-descriptor and through Zenodo with the identifier https://doi.org/10.5281/zenodo.8432666 . Data manipulation and pre-processing were performed in Python. Data curation for distribution was performed in SPSS.
He, L. & Han, Z. Do usage counts of scientific data make sense? An investigation of the Dryad repository. Library Hi Tech 35 , 332–342 (2017).
Article Google Scholar
Brickley, D., Burgess, M. & Noy, N. Google dataset search: Building a search engine for datasets in an open web ecosystem. In The World Wide Web Conference - WWW ‘19 , 1365–1375 (ACM Press, San Francisco, CA, USA, 2019).
Buneman, P., Dosso, D., Lissandrini, M. & Silvello, G. Data citation and the citation graph. Quantitative Science Studies 2 , 1399–1422 (2022).
Chao, T. C. Disciplinary reach: Investigating the impact of dataset reuse in the earth sciences. Proceedings of the American Society for Information Science and Technology 48 , 1–8 (2011).
Article ADS Google Scholar
Parr, C. et al . A discussion of value metrics for data repositories in earth and environmental sciences. Data Science Journal 18 , 58 (2019).
Eschenfelder, K. R., Shankar, K. & Downey, G. The financial maintenance of social science data archives: Four case studies of long–term infrastructure work. J. Assoc. Inf. Sci. Technol. 73 , 1723–1740 (2022).
Palmer, C. L., Weber, N. M. & Cragin, M. H. The analytic potential of scientific data: Understanding re-use value. Proceedings of the American Society for Information Science and Technology 48 , 1–10 (2011).
Zimmerman, A. S. New knowledge from old data: The role of standards in the sharing and reuse of ecological data. Sci. Technol. Human Values 33 , 631–652 (2008).
Cragin, M. H., Palmer, C. L., Carlson, J. R. & Witt, M. Data sharing, small science and institutional repositories. Philosophical Transactions of the Royal Society A: Mathematical, Physical and Engineering Sciences 368 , 4023–4038 (2010).
Article ADS CAS Google Scholar
Fear, K. M. Measuring and Anticipating the Impact of Data Reuse . Ph.D. thesis, University of Michigan (2013).
Borgman, C. L., Van de Sompel, H., Scharnhorst, A., van den Berg, H. & Treloar, A. Who uses the digital data archive? An exploratory study of DANS. Proceedings of the Association for Information Science and Technology 52 , 1–4 (2015).
Pasquetto, I. V., Borgman, C. L. & Wofford, M. F. Uses and reuses of scientific data: The data creators’ advantage. Harvard Data Science Review 1 (2019).
Gregory, K., Groth, P., Scharnhorst, A. & Wyatt, S. Lost or found? Discovering data needed for research. Harvard Data Science Review (2020).
York, J. Seeking equilibrium in data reuse: A study of knowledge satisficing . Ph.D. thesis, University of Michigan (2022).
Kilbride, W. & Norris, S. Collaborating to clarify the cost of curation. New Review of Information Networking 19 , 44–48 (2014).
Robinson-Garcia, N., Mongeon, P., Jeng, W. & Costas, R. DataCite as a novel bibliometric source: Coverage, strengths and limitations. Journal of Informetrics 11 , 841–854 (2017).
Qin, J., Hemsley, J. & Bratt, S. E. The structural shift and collaboration capacity in GenBank networks: A longitudinal study. Quantitative Science Studies 3 , 174–193 (2022).
Article PubMed PubMed Central Google Scholar
Acuna, D. E., Yi, Z., Liang, L. & Zhuang, H. Predicting the usage of scientific datasets based on article, author, institution, and journal bibliometrics. In Smits, M. (ed.) Information for a Better World: Shaping the Global Future. iConference 2022 ., 42–52 (Springer International Publishing, Cham, 2022).
Zeng, T., Wu, L., Bratt, S. & Acuna, D. E. Assigning credit to scientific datasets using article citation networks. Journal of Informetrics 14 , 101013 (2020).
Koesten, L., Vougiouklis, P., Simperl, E. & Groth, P. Dataset reuse: Toward translating principles to practice. Patterns 1 , 100136 (2020).
Du, C., Cohoon, J., Lopez, P. & Howison, J. Softcite dataset: A dataset of software mentions in biomedical and economic research publications. J. Assoc. Inf. Sci. Technol. 72 , 870–884 (2021).
Aryani, A. et al . A research graph dataset for connecting research data repositories using RD-Switchboard. Sci Data 5 , 180099 (2018).
Färber, M. & Lamprecht, D. The data set knowledge graph: Creating a linked open data source for data sets. Quantitative Science Studies 2 , 1324–1355 (2021).
Perry, A. & Netscher, S. Measuring the time spent on data curation. Journal of Documentation 78 , 282–304 (2022).
Trisovic, A. et al . Advancing computational reproducibility in the Dataverse data repository platform. In Proceedings of the 3rd International Workshop on Practical Reproducible Evaluation of Computer Systems , P-RECS ‘20, 15–20, https://doi.org/10.1145/3391800.3398173 (Association for Computing Machinery, New York, NY, USA, 2020).
Borgman, C. L., Scharnhorst, A. & Golshan, M. S. Digital data archives as knowledge infrastructures: Mediating data sharing and reuse. Journal of the Association for Information Science and Technology 70 , 888–904, https://doi.org/10.1002/asi.24172 (2019).
Lafia, S. et al . MICA Data Descriptor. Zenodo https://doi.org/10.5281/zenodo.8432666 (2023).
Lafia, S., Thomer, A., Bleckley, D., Akmon, D. & Hemphill, L. Leveraging machine learning to detect data curation activities. In 2021 IEEE 17th International Conference on eScience (eScience) , 149–158, https://doi.org/10.1109/eScience51609.2021.00025 (2021).
Hemphill, L., Pienta, A., Lafia, S., Akmon, D. & Bleckley, D. How do properties of data, their curation, and their funding relate to reuse? J. Assoc. Inf. Sci. Technol. 73 , 1432–44, https://doi.org/10.1002/asi.24646 (2021).
Lafia, S., Fan, L., Thomer, A. & Hemphill, L. Subdivisions and crossroads: Identifying hidden community structures in a data archive’s citation network. Quantitative Science Studies 3 , 694–714, https://doi.org/10.1162/qss_a_00209 (2022).
ICPSR. ICPSR Bibliography of Data-related Literature: Collection Criteria. https://www.icpsr.umich.edu/web/pages/ICPSR/citations/collection-criteria.html (2023).
Lafia, S., Fan, L. & Hemphill, L. A natural language processing pipeline for detecting informal data references in academic literature. Proc. Assoc. Inf. Sci. Technol. 59 , 169–178, https://doi.org/10.1002/pra2.614 (2022).
Hook, D. W., Porter, S. J. & Herzog, C. Dimensions: Building context for search and evaluation. Frontiers in Research Metrics and Analytics 3 , 23, https://doi.org/10.3389/frma.2018.00023 (2018).
https://www.icpsr.umich.edu/web/ICPSR/thesaurus (2002). ICPSR. ICPSR Thesaurus.
https://www.icpsr.umich.edu/files/datamanagement/icpsr-curation-levels.pdf (2020). ICPSR. ICPSR Curation Levels.
McKinney, W. Data Structures for Statistical Computing in Python. In van der Walt, S. & Millman, J. (eds.) Proceedings of the 9th Python in Science Conference , 56–61 (2010).
Wickham, H. et al . Welcome to the Tidyverse. Journal of Open Source Software 4 , 1686 (2019).
Fan, L., Lafia, S., Li, L., Yang, F. & Hemphill, L. DataChat: Prototyping a conversational agent for dataset search and visualization. Proc. Assoc. Inf. Sci. Technol. 60 , 586–591 (2023).
Download references
Acknowledgements
We thank the ICPSR Bibliography staff, the ICPSR Data Curation Unit, and the ICPSR Data Stewardship Committee for their support of this research. This material is based upon work supported by the National Science Foundation under grant 1930645. This project was made possible in part by the Institute of Museum and Library Services LG-37-19-0134-19.
Author information
Authors and affiliations.
Inter-university Consortium for Political and Social Research, University of Michigan, Ann Arbor, MI, 48104, USA
Libby Hemphill, Sara Lafia, David Bleckley & Elizabeth Moss
School of Information, University of Michigan, Ann Arbor, MI, 48104, USA
Libby Hemphill & Lizhou Fan
School of Information, University of Arizona, Tucson, AZ, 85721, USA
Andrea Thomer
You can also search for this author in PubMed Google Scholar
Contributions
L.H. and A.T. conceptualized the study design, D.B., E.M., and S.L. prepared the data, S.L., L.F., and L.H. analyzed the data, and D.B. validated the data. All authors reviewed and edited the manuscript.
Corresponding author
Correspondence to Libby Hemphill .
Ethics declarations
Competing interests.
The authors declare no competing interests.
Additional information
Publisher’s note Springer Nature remains neutral with regard to jurisdictional claims in published maps and institutional affiliations.
Rights and permissions
Open Access This article is licensed under a Creative Commons Attribution 4.0 International License, which permits use, sharing, adaptation, distribution and reproduction in any medium or format, as long as you give appropriate credit to the original author(s) and the source, provide a link to the Creative Commons licence, and indicate if changes were made. The images or other third party material in this article are included in the article’s Creative Commons licence, unless indicated otherwise in a credit line to the material. If material is not included in the article’s Creative Commons licence and your intended use is not permitted by statutory regulation or exceeds the permitted use, you will need to obtain permission directly from the copyright holder. To view a copy of this licence, visit http://creativecommons.org/licenses/by/4.0/ .
Reprints and permissions
About this article
Cite this article.
Hemphill, L., Thomer, A., Lafia, S. et al. A dataset for measuring the impact of research data and their curation. Sci Data 11 , 442 (2024). https://doi.org/10.1038/s41597-024-03303-2
Download citation
Received : 16 November 2023
Accepted : 24 April 2024
Published : 03 May 2024
DOI : https://doi.org/10.1038/s41597-024-03303-2
Share this article
Anyone you share the following link with will be able to read this content:
Sorry, a shareable link is not currently available for this article.
Provided by the Springer Nature SharedIt content-sharing initiative
Quick links
- Explore articles by subject
- Guide to authors
- Editorial policies
Sign up for the Nature Briefing newsletter — what matters in science, free to your inbox daily.

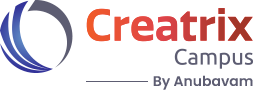
Thesis Management System
Easy-to-use and effective software that easily manages thesis and dissertation processes by minimizing challenges faced by scholars, advisors and committee members.
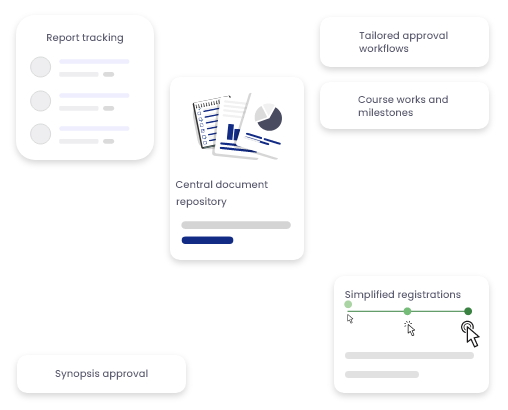
Online Thesis Management System that caters to everyone's needs
Research scholar.
No more frustration - right from initial application, guide selection to DC invite, course works, publications, synopsis, and final thesis submission and viva, everything is automated.
Research Guide
Constructs a bridge between scholars and guides with easy communication channels. Easy acceptance and approval of the coursework, with immediate review processes.
Research Committee Coordinator
Keep track of the entire thesis process in a centralized place. Facilitates research interviews, coordination with external reviewers, and final approvals/rejection in just clicks.
Thesis Management System, for on-the-go thesis, approvals, and submissions
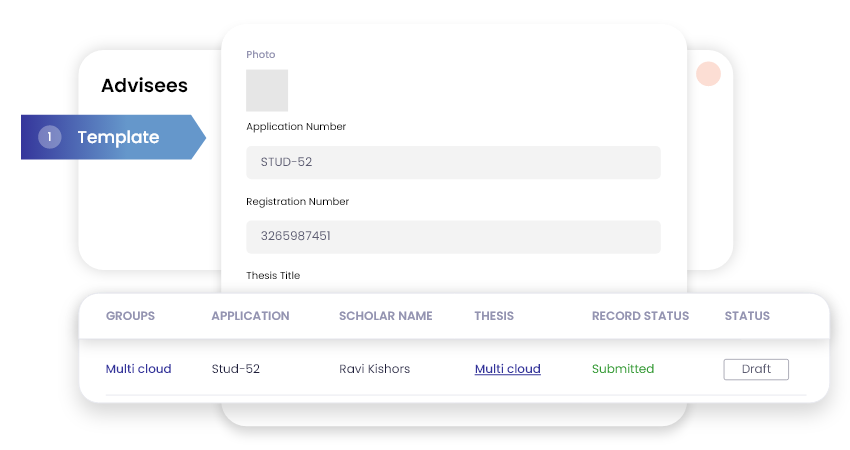
Make thesis registration effortless
Reduce long wait lines of your thesis registration process - from the applications, verification, PET score updation, and interviews to shortlisting and guide allocation, our thesis management system puts your admission committee in charge.
The system is intelligent enough to handle multiple statuses just in a few clicks. With automated processes and reporting tools, your admission team can stay empowered throughout their thesis registration cycle.
Do more with Creatrix:
- Reminders to speed up registrations
- Online thesis registration forms
- Status management for submissions
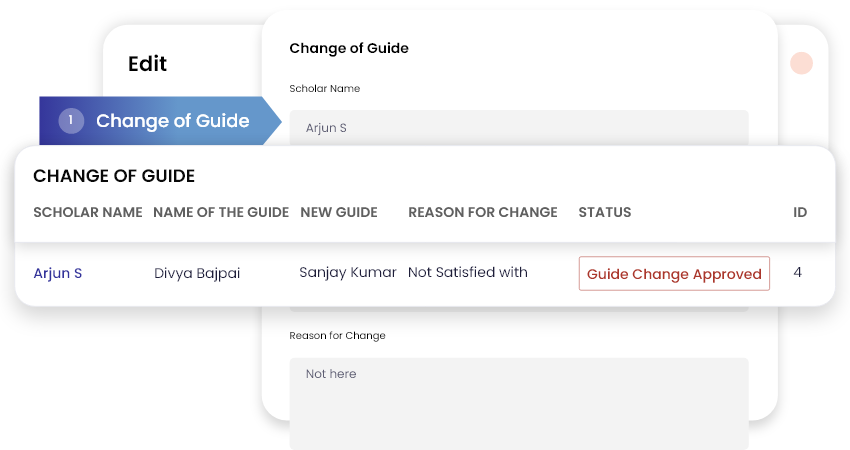
Handle a range of coursework
Supercharge your coursework registrations with an end-to-end thesis management system from Creatrix. Let researchers start any number of coursework related to their thesis, and maintain, and track it to completion.
Get on discussions with the guide, initiate meetings, send emails, create tasks, and follow-up notes. Get a clear understanding of the coursework done, sent for approval, and submitted, all in one view!
- Add/Edit/ Manage courses associated
- Manage documents related to coursework
- Coursework mapping with credit breakup
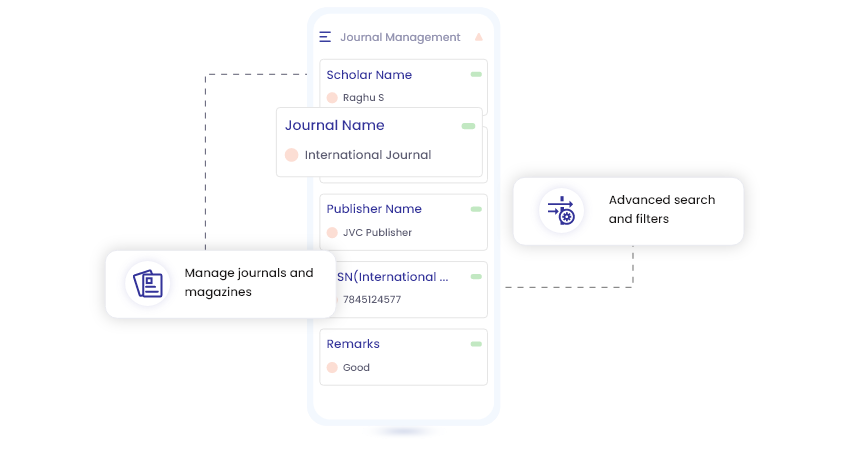
Keep publications on track
Compile your thesis publication process with a high-quality repository that tracks all research publications. Input all details associated with the publication along with remarks, so your scholarly efforts are in one place.
Configure filters to quickly sort out the right publication made from the list. View, edit, and trash them whenever needed. Facilitate effective connections between research scholars and their guides.
- Add/manage journals and magazines online
- Various tools to take action
- Faster communication channels for completion
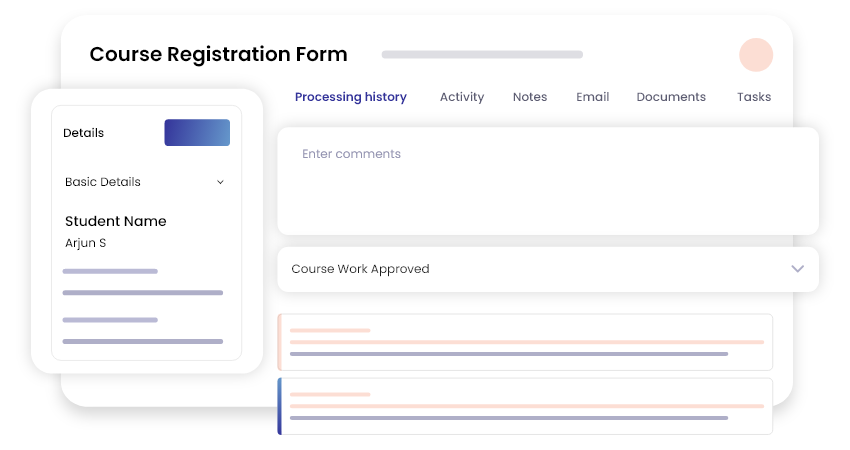
On-the-go approvals
Whether it is topic, thesis, or synopsis approval processes, handle them easily using Creatrix’s configurable workflows. Custom design multiple roles and permissions for RRC, RAC, VC, Referee, and much more.
Expedite thesis submission with individual portal logins, multiple statuses, automatic scheduling, alerts, reports, proper coordination, progress monitoring, and proactive support.
- Integration with third-party fee systems
- Guided thesis support with counsel
- No-code approval workflows for your unique needs

Configure topic approvals
Obtain quicker thesis approvals from your adviser, departmental thesis reviewer, and other committee members at the touch of a button, with our intuitive interface!

Progress report tracking
The progress reporting feature in our thesis management software allows you to track the status of the project, and plan for upcoming milestones in your thesis submission journey.

Extension requests in a snap
Initiate dissertation extension to your thesis panel with all supporting documents with the change of date with our flexible thesis platform

Change of guide requests
Request a change of guide anywhere in the middle of your thesis journey and seek approval through a series of customized steps.

No more extension delays
By inputting new guide details with the old one and the reason, get underway to apply for a change of guide within the specific time using our flexible thesis software.

Enjoy simplicity and efficiency
Empower every one part of the thesis committee with a more functional, easy-to-use, and less code-driven thesis software that dynamically changes as per your needs.
Frequently Asked Questions
What is a thesis management system.
A Thesis Management System is a software solution designed to support the management and administration of theses or dissertations in academic institutions. It provides tools and features to streamline the entire thesis process, including proposal submission, review and approval workflows, document management, and tracking of progress.
What are the key features of a Thesis Management System?
Common features of a Thesis Management System include:
- Online submission of thesis proposals
- Centralized repository for document management
- Review and approval workflows
- Document management and version control
- Communication tools for supervisors and students
- Progress tracking and milestone management
- Plagiarism detection
- Secure storage and access control for thesis documents
- Collaboration tools for co-authors and committee members Integration with other modules or systems
- Customizable templates and forms for thesis management
- Reporting and analytics capabilities for monitoring thesis progress
- User-friendly interface for easy navigation and use.
How does a Thesis Management System benefit academic institutions?
A Thesis Management System offers several benefits to academic institutions, including:
- Streamlined thesis submission and approval processes, reducing paperwork and administrative overhead.
- Reduces administrative burden. Improves efficiency and saves time.
- Improved communication and collaboration between students, supervisors, and committee members.
- Enhanced document management and version control, ensuring proper organization and access to thesis materials.
- Efficient progress tracking, enabling timely feedback and intervention when needed.
- Plagiarism detection tools, ensuring academic integrity in thesis submissions.
- Reporting and analytics for evaluating thesis progress and outcomes.
Can a Thesis Management System handle different types of theses?
Yes, a Thesis Management System can handle different types of theses, including master's theses, doctoral dissertations, research papers, and other scholarly works. The system allows for customization in defining thesis categories and associated workflows.
Is it possible to integrate a Thesis Management System with other systems?
Integration with other systems is possible depending on the capabilities and compatibility of the Thesis Management System. Integration with student information systems, library databases, or plagiarism detection tools can streamline data exchange and provide a seamless experience for users.
How secure is the data in a Thesis Management System?
Data security is crucial for any software system, including a Thesis Management System. These systems employ security measures such as encryption, user authentication, access controls, and regular backups to ensure data protection. It's important to choose a reputable system provider that prioritizes data security and complies with relevant privacy regulations.
Can a Thesis Management System facilitate collaboration among co-authors and committee members?
Yes, many Thesis Management Systems provide collaboration tools to facilitate communication and collaboration among co-authors and committee members. These tools enable online discussions, document sharing, and feedback exchange, ensuring smooth collaboration throughout the thesis process.
Can a Thesis Management System handle multiple reviewers and approval workflows?
Yes, a Thesis Management System can handle multiple reviewers and approval workflows. The system allows for the customization of review and approval processes based on the specific requirements of the academic institution. Different roles and permissions can be assigned to reviewers and approvers to ensure appropriate access and control.
Is it possible to customize a Thesis Management System?
Customization options may vary depending on the system provider. Some providers offer flexibility to customize certain aspects of the system, such as thesis categories, approval workflows, or reporting formats. It's recommended to discuss customization requirements with the system provider to understand the available options and tailor the system to specific institutional needs.
Checkout our more innovative solutions for higher education
Higher education strategic.
Place your long-term and short-term institutional goals efficiently and track them to…
Learning Management System
Most intuitive, user-friendly Learning Management System that augments teaching and…
Student Portfolio
An enriching student ePortfolio that improves and empowers today’s modern students.
Student Assessment Management
An LMS integrated, OBE, CBE, flipped learning supported Assessment platform for modern…
Placement Management System
An integrated campus placement management system that manages everything from student…
Student Advising System
A student planning and advising platform that empowers students to take charge of their…
Student Information System
A comprehensive, mobile-first student information management system that takes care of…
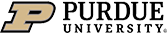
Comparison of Soil Carbon Dynamics Between Restored Prairie and Agricultural Soils in the U.S. Midwest
Globally, soils hold more carbon than both the atmosphere and aboveground terrestrial biosphere combined. Changes in land use and land cover have the potential to alter soil carbon cycling throughout the soil profile, from the surface to meters deep, yet most studies focus only on the near surface impact ( 3 and C 4 photosynthetic pathway plant community composition. Comparative analysis of edaphic properties and soil carbon suggests that deep loess deposits in Nebraska permit enhanced water infiltration and SOC deposition to depths of ~100 cm in 60 years of prairie restoration. In Illinois, poorly drained, clay/lime rich soils on glacial till and a younger restored prairie age (15 years) restricted the influence of prairie restoration to the upper 30 cm. Comparing the δ 13 C values of SOC and SIC in each system demonstrated that SIC at each site is likely of lithogenic origin. This work indicates that the magnitude of influence of restoration management is dependent on edaphic properties inherited from geological and geomorphological controls. Future work should quantify root structures and redox properties to better understand the influence of rooting depth on soil carbon concentrations. Fast-cycling C dynamics can be assessed using continuous, in-situ CO 2 and O 2 soil gas concentration changes. The secondary objective of my thesis was to determine if manual, low temporal resolution gas sampling and analysis are a low cost and effective means of measuring soil O 2 and CO 2 , by comparing it with data from in-situ continuous (hourly) sensors. Manual analysis of soil CO 2 and O 2 from field replicates of buried gas collection cups resulted in measurement differences from the continuous sensors. Measuring CO2 concentration with manual methods often resulted in higher concentrations than hourly, continuous measurements across all sites. Additionally, O 2 concentrations measured by manual methods were higher than hourly values in the restored prairie and less in agricultural sites. A variety of spatial variability, pressure perturbations, calibration offsets, and system leakage influences on both analysis methods could cause the discrepancy.
NSF Grant 1331906
Degree type.
- Master of Science
- Earth, Atmospheric and Planetary Sciences
Campus location
- West Lafayette
Advisor/Supervisor/Committee Chair
Additional committee member 2, additional committee member 3, additional committee member 4, additional committee member 5, usage metrics.
- Environmental biogeochemistry
- Soil chemistry and soil carbon sequestration (excl. carbon sequestration science)

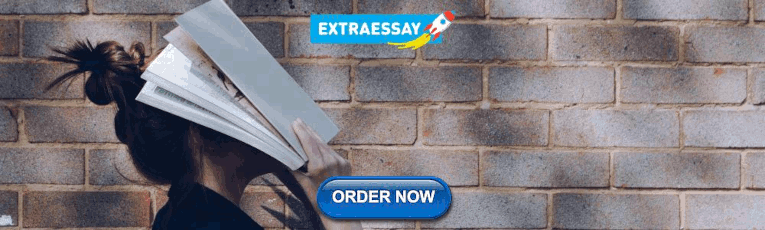
IMAGES
VIDEO
COMMENTS
Data management refers to the systematic collection, processing, storing and description of research data. Students are encouraged to learn about data management early in their studies, because good data management skills are beneficial to study progress and to adopting suitable data management practices during the thesis-writing process.
The Research Data Management Team will provide support for any students, supervisors or assessors that are in need. Submitting your digital thesis and depositing your data. If you have created data that is connected to your thesis and the data is in a format separate to the thesis file itself, we recommend that you deposit it in the data ...
The Curtin DMP tool guides the process of creating a research data management plan and ensures that important aspects of research data management are explored at the start of a research project. Data Management Plan workshop. This 1 hour hands-on session will help researchers create their data management plan using the Curtin DMP tool.
Empirical data: unique research that may be quantitative, qualitative, or mixed.. Theoretical data: secondary, scholarly sources like books and journal articles that provide theoretical context for your research.. Thesis: the culminating, multi-chapter project for a bachelor's, master's, or doctoral degree.. Qualitative data: info that cannot be measured, like observations and interviews.
Data management made simple. Keeping your research data freely available is crucial for open science — and your funding could depend on it. When Marjorie Etique learnt that she had to create a ...
Good data management strategies: Prevent you from losing data. Increase your efficiency when analysing the data. Show trends, patterns, and themes more clearly. Ensure your findings are based on robust, comprehensive results. Demonstrate that you are a rigorous researcher.
Research Data Management. Welcome to this module, where we will cover all the main aspects of looking after your research data, including: how to store and backup up data. how to organise data. what to do with protected data (personal or commercially sensitive) why sharing data is important and how to do it. writing Data Management Plans.
Practicing data management principles during thesis work brings benefits in the professional world. Therefore, it is worth familiarizing oneself with these practices while working on a thesis. If there is a desire to share or reuse the data after completing the thesis, the data must be of high quality and well-managed. In such cases, the life ...
What are data management plans? A data management plan is a document that describes: What data will be created; What policies will apply to the data ; Who will own and have access to the data; What data management practices will be used ; What facilities and equipment will be required ; Who will be responsible for each of these activities
Some courses at Radboud University ask you to fill in a Data Management Plan (DMP) for your thesis or other research project via RIS for Students, which you can do here, otherwise see the template below: Data Management Plan template. The following DMP structure may also help you as a checklist for how to manage your data: nature of research data.
The implementation of this thesis data management used SQLite. SQLite is a relational database management system (DBMS) built by using the C programming language. SQLite is not included in the ...
Revised on April 16, 2024. A thesis is a type of research paper based on your original research. It is usually submitted as the final step of a master's program or a capstone to a bachelor's degree. Writing a thesis can be a daunting experience. Other than a dissertation, it is one of the longest pieces of writing students typically complete.
The CODATA Data Science Journal is a peer-reviewed, open access, electronic journal, publishing papers on the management, dissemination, use and reuse of research data and databases across all research domains, including science, technology, the humanities and the arts. The scope of the journal includes descriptions of data systems, their implementations and their publication, applications ...
At Jamk, a data management plan (dmp) is prepared as an appendix to the thesis plan. It is a concrete plan for handling (saving, sharing, archiving, destroying) the data in the different phases of the thesis. The content complements the thesis plan. Managing research data and preparing a data management plan are part of good scientific practice.
The data management plan is a description of how you collect, use and process research material (data) in your thesis. Create the data management plan using the accompanying questions during the planning phase of the thesis and save it on Wihi. Publish the completed data management plan as an appendix to the thesis: APPENDIX x thesis data ...
The documented data archive of the study should be deposited with the promotor upon handing in the final manuscript for the manuscript committee. The promotor will only sign the approval form of the PhD thesis when the data archive of the study has been handed in. The promotor deposits the data archive of the thesis in the repositorye within 1 ...
Comprehensive Review. Subaveerapandiyan A. Former Chief Librarian. Department of Library and Information Science. DMI-St. Eugene University, Lusaka, Zambia. Email: [email protected] ...
Research data. managem ent (RDM) is about "the organization of data, from its entry to the research cycle through. to the dissemination and archiving of valuable results" (Whyte and Tedds ...
OATD.org provides open access graduate theses and dissertations published around the world. Metadata (information about the theses) comes from over 1100 colleges, universities, and research institutions. OATD currently indexes 6,654,285 theses and dissertations.
Science funders, publishers, and data archives make decisions about how to responsibly allocate resources to maximize the reuse potential of research data. This paper introduces a dataset ...
A Thesis Management System is a software solution designed to support the management and administration of theses or dissertations in academic institutions. It provides tools and features to streamline the entire thesis process, including proposal submission, review and approval workflows, document management, and tracking of progress.
Influence of big data and analytics on management control Why changes in management control by means of big data and analytics are not achieved yet Luuk Vloet - s4493435 Accounting and Control Master thesis - Economics Supervisor: Drs. R.H.R.M. Aernoudts Nijmegen School of Management Radboud University June 27th, 2016
Theses/Dissertations from 2021. PDF. The IS Social Continuance Model: Using Conversational Agents to Support Co-creation, Naif Alawi. PDF. The Use of Data Analytic Visualizations to Inform the Audit Risk Assessment: The Impact of Initial Visualization Form and Documentation Focus, Rebecca N. Baaske (Becca) PDF.
describe and apply foundational knowledge to set up new databases in accordance with FAIR data principles, ensuring accessibility and interoperability of stored biological and medical data, use relational database management skills to design and implement databases, and carry out queries to retrieve specific instances, demonstrating proficiency ...
creating a manag ement sy stem w ith the concep t of a web-based Thesis Management information system. that focuses on submitting titles to the final trial of student thesis. With several ...
Latanya Sweeney's Finding §Massachusetts: GIG* is responsible for health insurance of state emps; public data §Sweeney paid $20 and bought voter registration list
The primary objective of my thesis research is to evaluate the factors controlling the impact of deep-rooting perennial grass on soil carbon cycling during prairie restoration of soil following long term, row crop agriculture. ... This work indicates that the magnitude of influence of restoration management is dependent on edaphic properties ...