
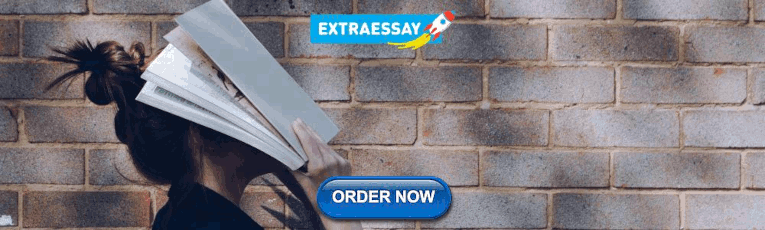
Research Variables 101
Independent variables, dependent variables, control variables and more
By: Derek Jansen (MBA) | Expert Reviewed By: Kerryn Warren (PhD) | January 2023
If you’re new to the world of research, especially scientific research, you’re bound to run into the concept of variables , sooner or later. If you’re feeling a little confused, don’t worry – you’re not the only one! Independent variables, dependent variables, confounding variables – it’s a lot of jargon. In this post, we’ll unpack the terminology surrounding research variables using straightforward language and loads of examples .
Overview: Variables In Research
What (exactly) is a variable.
The simplest way to understand a variable is as any characteristic or attribute that can experience change or vary over time or context – hence the name “variable”. For example, the dosage of a particular medicine could be classified as a variable, as the amount can vary (i.e., a higher dose or a lower dose). Similarly, gender, age or ethnicity could be considered demographic variables, because each person varies in these respects.
Within research, especially scientific research, variables form the foundation of studies, as researchers are often interested in how one variable impacts another, and the relationships between different variables. For example:
- How someone’s age impacts their sleep quality
- How different teaching methods impact learning outcomes
- How diet impacts weight (gain or loss)
As you can see, variables are often used to explain relationships between different elements and phenomena. In scientific studies, especially experimental studies, the objective is often to understand the causal relationships between variables. In other words, the role of cause and effect between variables. This is achieved by manipulating certain variables while controlling others – and then observing the outcome. But, we’ll get into that a little later…
The “Big 3” Variables
Variables can be a little intimidating for new researchers because there are a wide variety of variables, and oftentimes, there are multiple labels for the same thing. To lay a firm foundation, we’ll first look at the three main types of variables, namely:
- Independent variables (IV)
- Dependant variables (DV)
- Control variables
What is an independent variable?
Simply put, the independent variable is the “ cause ” in the relationship between two (or more) variables. In other words, when the independent variable changes, it has an impact on another variable.
For example:
- Increasing the dosage of a medication (Variable A) could result in better (or worse) health outcomes for a patient (Variable B)
- Changing a teaching method (Variable A) could impact the test scores that students earn in a standardised test (Variable B)
- Varying one’s diet (Variable A) could result in weight loss or gain (Variable B).
It’s useful to know that independent variables can go by a few different names, including, explanatory variables (because they explain an event or outcome) and predictor variables (because they predict the value of another variable). Terminology aside though, the most important takeaway is that independent variables are assumed to be the “cause” in any cause-effect relationship. As you can imagine, these types of variables are of major interest to researchers, as many studies seek to understand the causal factors behind a phenomenon.
Need a helping hand?
What is a dependent variable?
While the independent variable is the “ cause ”, the dependent variable is the “ effect ” – or rather, the affected variable . In other words, the dependent variable is the variable that is assumed to change as a result of a change in the independent variable.
Keeping with the previous example, let’s look at some dependent variables in action:
- Health outcomes (DV) could be impacted by dosage changes of a medication (IV)
- Students’ scores (DV) could be impacted by teaching methods (IV)
- Weight gain or loss (DV) could be impacted by diet (IV)
In scientific studies, researchers will typically pay very close attention to the dependent variable (or variables), carefully measuring any changes in response to hypothesised independent variables. This can be tricky in practice, as it’s not always easy to reliably measure specific phenomena or outcomes – or to be certain that the actual cause of the change is in fact the independent variable.
As the adage goes, correlation is not causation . In other words, just because two variables have a relationship doesn’t mean that it’s a causal relationship – they may just happen to vary together. For example, you could find a correlation between the number of people who own a certain brand of car and the number of people who have a certain type of job. Just because the number of people who own that brand of car and the number of people who have that type of job is correlated, it doesn’t mean that owning that brand of car causes someone to have that type of job or vice versa. The correlation could, for example, be caused by another factor such as income level or age group, which would affect both car ownership and job type.
To confidently establish a causal relationship between an independent variable and a dependent variable (i.e., X causes Y), you’ll typically need an experimental design , where you have complete control over the environmen t and the variables of interest. But even so, this doesn’t always translate into the “real world”. Simply put, what happens in the lab sometimes stays in the lab!
As an alternative to pure experimental research, correlational or “ quasi-experimental ” research (where the researcher cannot manipulate or change variables) can be done on a much larger scale more easily, allowing one to understand specific relationships in the real world. These types of studies also assume some causality between independent and dependent variables, but it’s not always clear. So, if you go this route, you need to be cautious in terms of how you describe the impact and causality between variables and be sure to acknowledge any limitations in your own research.
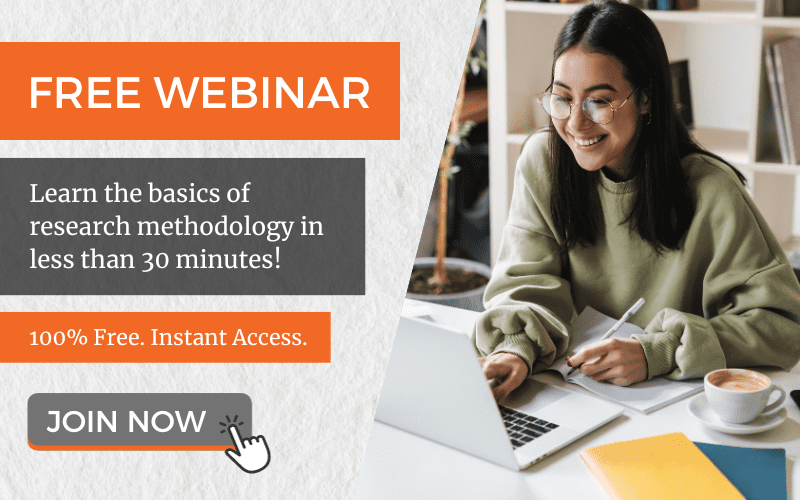
What is a control variable?
In an experimental design, a control variable (or controlled variable) is a variable that is intentionally held constant to ensure it doesn’t have an influence on any other variables. As a result, this variable remains unchanged throughout the course of the study. In other words, it’s a variable that’s not allowed to vary – tough life 🙂
As we mentioned earlier, one of the major challenges in identifying and measuring causal relationships is that it’s difficult to isolate the impact of variables other than the independent variable. Simply put, there’s always a risk that there are factors beyond the ones you’re specifically looking at that might be impacting the results of your study. So, to minimise the risk of this, researchers will attempt (as best possible) to hold other variables constant . These factors are then considered control variables.
Some examples of variables that you may need to control include:
- Temperature
- Time of day
- Noise or distractions
Which specific variables need to be controlled for will vary tremendously depending on the research project at hand, so there’s no generic list of control variables to consult. As a researcher, you’ll need to think carefully about all the factors that could vary within your research context and then consider how you’ll go about controlling them. A good starting point is to look at previous studies similar to yours and pay close attention to which variables they controlled for.
Of course, you won’t always be able to control every possible variable, and so, in many cases, you’ll just have to acknowledge their potential impact and account for them in the conclusions you draw. Every study has its limitations, so don’t get fixated or discouraged by troublesome variables. Nevertheless, always think carefully about the factors beyond what you’re focusing on – don’t make assumptions!
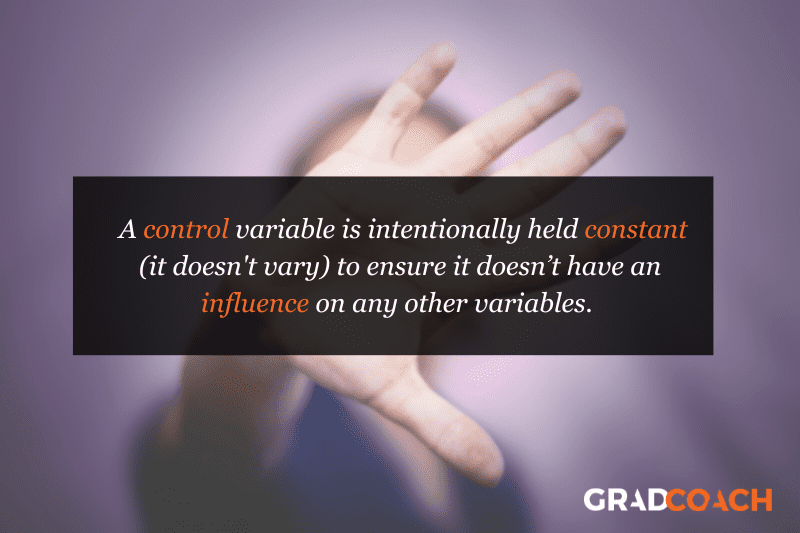
Other types of variables
As we mentioned, independent, dependent and control variables are the most common variables you’ll come across in your research, but they’re certainly not the only ones you need to be aware of. Next, we’ll look at a few “secondary” variables that you need to keep in mind as you design your research.
- Moderating variables
- Mediating variables
- Confounding variables
- Latent variables
Let’s jump into it…
What is a moderating variable?
A moderating variable is a variable that influences the strength or direction of the relationship between an independent variable and a dependent variable. In other words, moderating variables affect how much (or how little) the IV affects the DV, or whether the IV has a positive or negative relationship with the DV (i.e., moves in the same or opposite direction).
For example, in a study about the effects of sleep deprivation on academic performance, gender could be used as a moderating variable to see if there are any differences in how men and women respond to a lack of sleep. In such a case, one may find that gender has an influence on how much students’ scores suffer when they’re deprived of sleep.
It’s important to note that while moderators can have an influence on outcomes , they don’t necessarily cause them ; rather they modify or “moderate” existing relationships between other variables. This means that it’s possible for two different groups with similar characteristics, but different levels of moderation, to experience very different results from the same experiment or study design.
What is a mediating variable?
Mediating variables are often used to explain the relationship between the independent and dependent variable (s). For example, if you were researching the effects of age on job satisfaction, then education level could be considered a mediating variable, as it may explain why older people have higher job satisfaction than younger people – they may have more experience or better qualifications, which lead to greater job satisfaction.
Mediating variables also help researchers understand how different factors interact with each other to influence outcomes. For instance, if you wanted to study the effect of stress on academic performance, then coping strategies might act as a mediating factor by influencing both stress levels and academic performance simultaneously. For example, students who use effective coping strategies might be less stressed but also perform better academically due to their improved mental state.
In addition, mediating variables can provide insight into causal relationships between two variables by helping researchers determine whether changes in one factor directly cause changes in another – or whether there is an indirect relationship between them mediated by some third factor(s). For instance, if you wanted to investigate the impact of parental involvement on student achievement, you would need to consider family dynamics as a potential mediator, since it could influence both parental involvement and student achievement simultaneously.
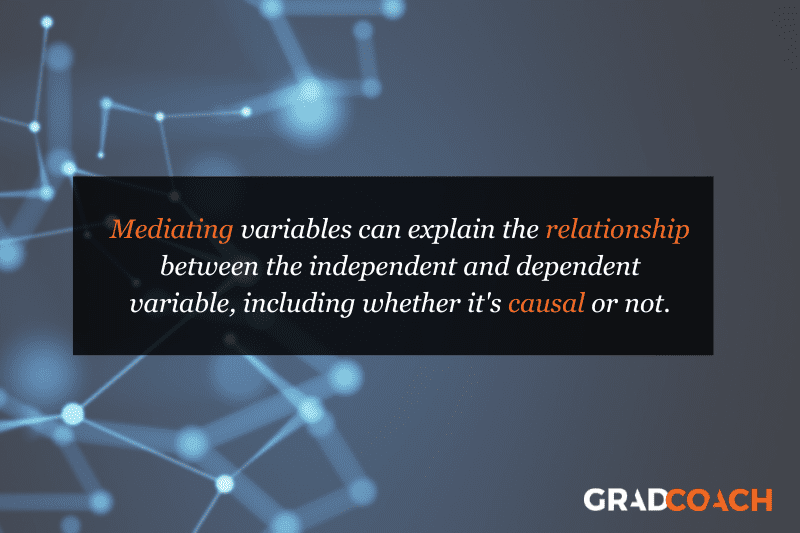
What is a confounding variable?
A confounding variable (also known as a third variable or lurking variable ) is an extraneous factor that can influence the relationship between two variables being studied. Specifically, for a variable to be considered a confounding variable, it needs to meet two criteria:
- It must be correlated with the independent variable (this can be causal or not)
- It must have a causal impact on the dependent variable (i.e., influence the DV)
Some common examples of confounding variables include demographic factors such as gender, ethnicity, socioeconomic status, age, education level, and health status. In addition to these, there are also environmental factors to consider. For example, air pollution could confound the impact of the variables of interest in a study investigating health outcomes.
Naturally, it’s important to identify as many confounding variables as possible when conducting your research, as they can heavily distort the results and lead you to draw incorrect conclusions . So, always think carefully about what factors may have a confounding effect on your variables of interest and try to manage these as best you can.
What is a latent variable?
Latent variables are unobservable factors that can influence the behaviour of individuals and explain certain outcomes within a study. They’re also known as hidden or underlying variables , and what makes them rather tricky is that they can’t be directly observed or measured . Instead, latent variables must be inferred from other observable data points such as responses to surveys or experiments.
For example, in a study of mental health, the variable “resilience” could be considered a latent variable. It can’t be directly measured , but it can be inferred from measures of mental health symptoms, stress, and coping mechanisms. The same applies to a lot of concepts we encounter every day – for example:
- Emotional intelligence
- Quality of life
- Business confidence
- Ease of use
One way in which we overcome the challenge of measuring the immeasurable is latent variable models (LVMs). An LVM is a type of statistical model that describes a relationship between observed variables and one or more unobserved (latent) variables. These models allow researchers to uncover patterns in their data which may not have been visible before, thanks to their complexity and interrelatedness with other variables. Those patterns can then inform hypotheses about cause-and-effect relationships among those same variables which were previously unknown prior to running the LVM. Powerful stuff, we say!
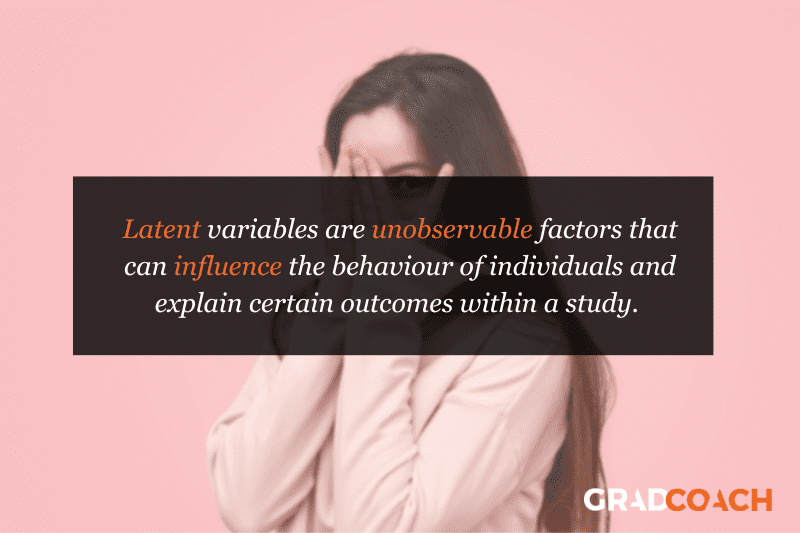
Let’s recap
In the world of scientific research, there’s no shortage of variable types, some of which have multiple names and some of which overlap with each other. In this post, we’ve covered some of the popular ones, but remember that this is not an exhaustive list .
To recap, we’ve explored:
- Independent variables (the “cause”)
- Dependent variables (the “effect”)
- Control variables (the variable that’s not allowed to vary)
If you’re still feeling a bit lost and need a helping hand with your research project, check out our 1-on-1 coaching service , where we guide you through each step of the research journey. Also, be sure to check out our free dissertation writing course and our collection of free, fully-editable chapter templates .
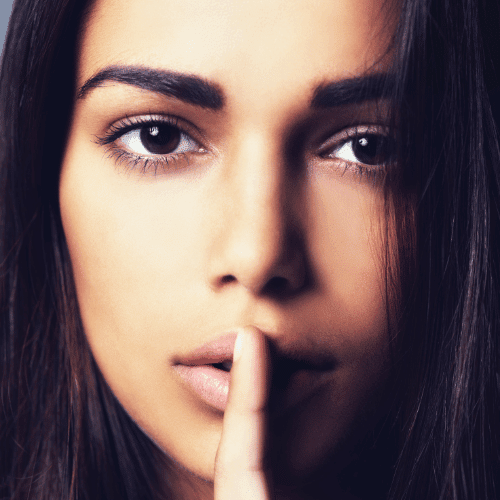
Psst... there’s more!
This post was based on one of our popular Research Bootcamps . If you're working on a research project, you'll definitely want to check this out ...
You Might Also Like:
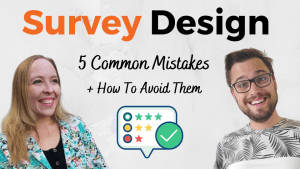
Very informative, concise and helpful. Thank you
Helping information.Thanks
practical and well-demonstrated
Very helpful and insightful
Submit a Comment Cancel reply
Your email address will not be published. Required fields are marked *
Save my name, email, and website in this browser for the next time I comment.
- Print Friendly
- Privacy Policy

Home » Control Variable – Definition, Types and Examples
Control Variable – Definition, Types and Examples
Table of Contents

Control Variable
Definition :
Control variable, also known as a “constant variable,” is a variable that is held constant or fixed during an experiment or study to prevent it from affecting the outcome. In other words, a control variable is a variable that is kept the same or held constant to isolate the effects of the independent variable on the dependent variable.
For example, if you were conducting an experiment to test how temperature affects plant growth, you might want to control variables such as the amount of water, the amount of sunlight, and the type of soil to ensure that these variables do not interfere with the results. By controlling these variables, you can isolate the effect of temperature on plant growth and draw more accurate conclusions from the experiment.
Types of Control Variables
Types of Control Variables are as follows:
Environmental Control Variables
These are variables related to the physical environment in which the experiment is conducted, such as temperature, humidity, light, and sound.
Participant Control Variables
These are variables related to the participants in the experiment, such as age, gender, prior knowledge, or experience.
Experimental Control Variables
These are variables that the researcher manipulates or controls to ensure that they do not affect the outcome of the experiment. For example, in a study on the effects of a new medication, the researcher might control the dosage, frequency, or duration of the treatment.
Procedural Control Variables
These are variables related to the procedures or methods used in the experiment, such as the order in which tasks are completed, the timing of measurements, or the instructions given to participants.
Equipment Control Variables
These are variables related to the equipment or instruments used in the experiment, such as calibration, maintenance, or proper functioning.
How to Control a Variable
To control a variable in a scientific experiment, you need to ensure that it is kept constant or unchanged throughout the experiment. Here are some steps to help you control a variable:
Identify the Variable
Start by identifying the variable that you want to control. This can be an environmental, subject, procedural, or instrumentation variable.
Determine the Level of Control Needed
Depending on the variable, you may need to exert varying levels of control. For example, environmental variables may require you to control the temperature, humidity, and lighting in your experiment, while subject variables may require you to select a specific group of participants that meet certain criteria.
Establish a Standard Level
Determine the standard level or value of the variable that you want to control. For example, if you are controlling the temperature, you may set the temperature to a specific degree and ensure that it is maintained at that level throughout the experiment.
Monitor the Variable
Throughout the experiment, monitor the variable to ensure that it remains constant. Use appropriate equipment or instruments to measure the variable and make adjustments as necessary to maintain the desired level.
Document the Process
Document the process of controlling the variable to ensure that the experiment is replicable. This includes documenting the standard level, monitoring procedures, and any adjustments made during the experiment.
Examples of Control Variables
Here are some examples of control variables in Scientific Experiments and Research:
- Environmental Control Variables Example: Suppose you are conducting an experiment to study the effect of light on plant growth. You would want to control environmental factors such as temperature, humidity, and soil nutrients. In this case, you might keep the temperature and humidity constant and use the same type and amount of soil for all the plants.
- Subject Control Variables Example : If you are conducting an experiment to study the effect of a new medication on blood pressure, you would want to control subject variables such as age, gender, and health status. In this case, you might select a group of participants with similar ages, genders, and health conditions to ensure that these variables do not affect the results.
- Procedural Control Variables Example : Suppose you are conducting an experiment to study the effect of distraction on reaction time. You would want to control procedural variables such as the time of day, the order of the tasks, and the instructions given to the participants. In this case, you might ensure that all participants perform the tasks in the same order, at the same time of day, and receive the same instructions.
- Instrumentation Control Variables Example : If you are conducting an experiment to study the effect of a new measurement device on the accuracy of readings, you would want to control instrumentation variables such as the type and calibration of the device. In this case, you might use the same type and model of the device and ensure that it is calibrated before each use.
Applications of Control Variable
Control variables are widely used in scientific research across various fields, including physics, biology, psychology, and engineering. Here are some applications of control variables:
- In medical research , control variables are used to ensure that any observed effects of a new treatment or medication are due to the treatment and not some other variable. By controlling subject variables such as age, gender, and health status, researchers can isolate the effects of the treatment and determine its effectiveness.
- In environmental research , control variables are used to study the effects of changes in the environment on various species or ecosystems. By controlling environmental variables such as temperature, humidity, and lighting, researchers can determine how different species adapt to changes in the environment.
- In psychology research, control variables are used to study the effects of different interventions or therapies on cognitive or behavioral outcomes. By controlling procedural variables such as the order of tasks, the length of time allotted for each task, and the instructions given to participants, researchers can isolate the effects of the intervention and determine its effectiveness.
- In engineering research, control variables are used to study the effects of different design parameters on the performance of a system or device. By controlling instrumentation variables such as the type of measurement device used and the calibration of instruments, researchers can ensure that the measurements are accurate and reliable.
Purpose of Control Variable
The purpose of a control variable in an experiment is to ensure that any observed changes or effects are a result of the manipulation of the independent variable and not some other variable. By keeping certain variables constant, researchers can isolate the effects of the independent variable and determine whether it has a significant effect on the dependent variable.
Control variables are important because they help to increase the reliability and validity of the experiment. Reliability refers to the consistency and reproducibility of the results, while validity refers to the accuracy and truthfulness of the results. By controlling variables, researchers can reduce the potential for extraneous or confounding variables that can affect the outcome of the experiment and increase the likelihood that the results accurately reflect the effect of the independent variable on the dependent variable.
Characteristics of Control Variable
Control variables have the following characteristics:
- Constant : Control variables are kept constant or unchanged throughout the experiment. This means that their values do not vary or change during the experiment. Keeping control variables constant helps to ensure that any observed effects or changes are due to the manipulation of the independent variable and not some other variable.
- Independent : Control variables are independent of the independent variable being studied. This means that they do not affect the relationship between the independent and dependent variables. By controlling for independent variables, researchers can isolate the effect of the independent variable and determine its impact on the dependent variable.
- Documented: Control variables are documented in the experiment. This means that their values and methods of control are recorded and reported in the results section of the research paper. By documenting control variables, researchers can demonstrate the rigor and transparency of their study and allow other researchers to replicate their methods.
- Relevant: Control variables are relevant to the research question. This means that they are chosen based on their potential to affect the outcome of the experiment. By selecting relevant control variables, researchers can reduce the potential for extraneous or confounding variables that can affect the outcome of the experiment and increase the reliability and validity of the results.
- Varied : Control variables can be varied across different conditions or groups. This means that different levels of control may be needed depending on the research question or hypothesis being tested. By varying control variables, researchers can test different hypotheses and determine the factors that affect the outcome of the experiment.
Advantages of Control Variable
The advantages of using control variables in an experiment are:
- Increased accuracy : Control variables help to increase the accuracy of the results by reducing the potential for extraneous or confounding variables that can affect the outcome of the experiment. By controlling for these variables, researchers can isolate the effect of the independent variable on the dependent variable and determine whether it has a significant impact.
- Increased reliability : Control variables help to increase the reliability of the results by reducing the variability in the experiment. By keeping certain variables constant, researchers can ensure that any observed changes or effects are due to the manipulation of the independent variable and not some other variable.
- Reproducibility: Control variables help to increase the reproducibility of the results by ensuring that the same results can be obtained when the experiment is repeated. By documenting and reporting control variables, researchers can demonstrate the rigor and transparency of their study and allow other researchers to replicate their methods.
- Generalizability : Control variables help to increase the generalizability of the results by reducing the potential for bias and increasing the external validity of the experiment. By controlling for relevant variables, researchers can ensure that their findings are applicable to a broader population or context.
- Causality : Control variables help to establish causality by ensuring that any observed changes or effects are due to the manipulation of the independent variable and not some other variable. By controlling for confounding variables, researchers can increase the internal validity of the experiment and establish a cause-and-effect relationship between the independent and dependent variables.
Disadvantages of Control Variable
There are some potential disadvantages or limitations of using control variables in an experiment:
- Complexity : Controlling for multiple variables can make an experiment more complex and time-consuming. This can increase the likelihood of errors and reduce the feasibility of the experiment, especially if the control variables require a lot of resources or are difficult to measure.
- Artificiality : Controlling for variables can make the experimental conditions artificial and not reflective of real-world situations. This can reduce the external validity of the experiment and limit the generalizability of the findings to real-world settings.
- Limited scope : Controlling for specific variables can limit the scope of the experiment and make it difficult to generalize the results to other situations or populations. This can reduce the external validity of the experiment and limit its practical applications.
- Assumptions: Controlling for variables requires making assumptions about which variables are relevant and how they should be controlled. These assumptions may not be valid or accurate, and the results of the experiment may be affected by uncontrolled variables that were not considered.
- Cost : Controlling for variables can be costly, especially if the control variables require additional resources or equipment. This can limit the feasibility of the experiment, especially for researchers with limited funding or resources.
Limitations of Control Variable
There are several limitations of using control variables in an experiment, including:
- Not all variables can be controlled : There may be some variables that cannot be controlled or manipulated in an experiment. For example, some variables may be too difficult or expensive to measure or control, or they may be affected by factors outside of the researcher’s control.
- Interaction effects : Control variables can interact with each other, which can lead to unexpected results. For example, controlling for one variable may have a different effect when another variable is also controlled, or when the two variables interact with each other. These interaction effects can be difficult to predict or control for.
- Over-reliance on statistical significance: Controlling for variables can increase the statistical significance of the results, but this may not always translate to practical significance or real-world significance. Researchers should interpret the results of an experiment in light of the practical significance, not just the statistical significance.
- Limited generalizability : Controlling for variables can limit the generalizability of the results to other populations or situations. If the control variables are not representative of other populations or situations, the results of the experiment may not be applicable to those contexts.
- May mask important effects : Controlling for variables can mask important effects that are related to the independent variable. By controlling for certain variables, researchers may miss important interactions between the independent variable and the controlled variable, which can limit the understanding of the causal relationship between the two.
About the author
Muhammad Hassan
Researcher, Academic Writer, Web developer
You may also like

Moderating Variable – Definition, Analysis...

Qualitative Variable – Types and Examples

Variables in Research – Definition, Types and...

Categorical Variable – Definition, Types and...

Independent Variable – Definition, Types and...

Ratio Variable – Definition, Purpose and Examples
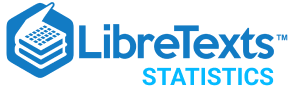
- school Campus Bookshelves
- menu_book Bookshelves
- perm_media Learning Objects
- login Login
- how_to_reg Request Instructor Account
- hub Instructor Commons
- Download Page (PDF)
- Download Full Book (PDF)
- Periodic Table
- Physics Constants
- Scientific Calculator
- Reference & Cite
- Tools expand_more
- Readability
selected template will load here
This action is not available.
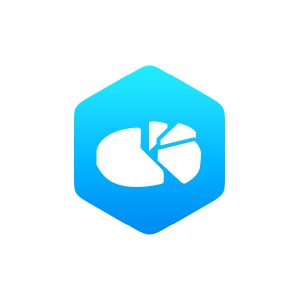
4.1: Overview of the Control Variable
- Last updated
- Save as PDF
- Page ID 32930
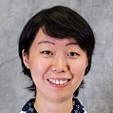
- Yang Lydia Yang
- Kansas State University
In the first chapter, we talked about many different threats to internal validity of a research design and one of the control techniques is to build the extraneous variable into our research design. In this chapter, we will extend between-subjects design by looking at different ways to add in an extraneous or a control variable. Why do we need to add control variables? And what criteria should we use when selecting control variables? The main reason we want to include control variables is that the control variables are having an effect on the dependent variable we are studying. Since control variables are not the independent variables in our research, they could potentially confound the results of the study if left unattended. In other words, they can impose threats to the internal validity of the research design. By taking some measures to include the control variables, we are minimizing their effect on the dependent variable, which gives us more confidence to claim it is the independent variable, not the control variable, that causes changes in the dependent variable.
Using the example from the previous chapter, let's say we are conducting an experiment on the effect of cell phone use (yes vs. no) on driving ability. The independent variable is cell phone use with two treatment conditions (yes or no) and the dependent variable is driving ability. A potential control variable would be driving experience as driving experience is most likely to have an impact on driving ability. In order to reduce the potential threat driving experience has on driving ability, we can add it into our study as a control variable. Although it is not the focus of the study, control variable IS a part of your study as we know it influences the outcome variable. By including driving experience into our study, we can minimizing its effect on our research design, and be more confident it is the cell phone use, not driving experience, that leads to changes in driving ability. Therefore adding control variables can increase the internal validity of the research design.
How do we select control variables? Any variables can be potential control variables as long as there is good theoretical or empirical evidence(s) to show they influence the outcome variables. The nature of the variable is not a concern. The control variable can be categorical or continuous. Using the same example above, to measure driving experience, we can ask participants to identify which following level of driving experience represents them the best - seasoned, intermediate, or inexperienced. Or we can ask participants to identify how many months they have driven. Or if you are concerned about the accuracy of participants' own estimation, you can ask participants the age they received their driving license and do the calculation yourself. Regardless how you measure it, as long as the control variable is solid, as in it indeed influences the outcome variable, it can be included in the research study.
Then how do we use different types of control variables? There are two major ways to use control variables. One is randomized block design, which uses control variables at the design stage when we actively set up the experiment. Randomized block design typically uses categorical control variables. The other one is analysis of covariance, which uses control variables at the data analysis stage when we analyze the statistical data. Analysis of covariance typically uses continuous variables. We will look at each of them closely in the following sections.
- How it works
Published by Nicolas at March 17th, 2024 , Revised On March 12, 2024
A Practical Guide to Choosing the Right Control Variables for Your Research
Research is a dynamic process, where scientists strive to unravel the mysteries of the world through systematic inquiry. In this pursuit, control variables play a crucial role in shaping the reliability and validity of research findings. This blog serves as a practical guide to aid researchers in the thoughtful selection of control variables.
Table of Contents
“Control variables, often referred to as covariates, are elements in a study that are intentionally held constant or systematically manipulated to examine their impact on the relationship between independent and dependent variables. These variables act as safeguards against confounding factors, ensuring that the observed effects can be attributed more accurately to the independent variable under investigation.
Importance of Selecting the Right Control Variables
The choice of control variables is not arbitrary; it demands careful consideration and a deep understanding of the research context. The significance of selecting the right control variables cannot be overstated, as these elements serve as the bedrock for establishing the internal validity of a study.
Internal validity refers to the accuracy of causal inferences within an experiment – the extent to which changes in the dependent variable can be confidently attributed to manipulating the independent variable.
By meticulously selecting control variables, researchers can minimize the risk of alternative explanations, ensuring that observed effects are more likely to reflect true causal relationships.
How Control Variables Enhance Research Validity
Research validity is a multifaceted concept that encompasses various dimensions, including internal, external, construct, and statistical validity. Control variables primarily enhance internal validity by minimizing the influence of extraneous variables that could introduce bias or confound the results.
Researchers create a more controlled and precise experimental environment by strategically incorporating control variables. This, in turn, allows for a clearer understanding of the relationship between the independent and dependent variables, bolstering the overall validity of the research findings.
In essence, control variables act as gatekeepers, fortifying the integrity of the research process and paving the way for more robust and trustworthy scientific conclusions.
Understanding Control Variables
Control variables, also known as covariates, are integral components of experimental design and statistical analysis in research. Their primary purpose is to add precision to investigations by accounting for potential confounding factors that might otherwise distort the interpretation of results.
For instance, imagine a study examining the impact of a new drug on patients’ recovery time after surgery. The type of anesthesia used, the patient’s age, and pre-existing health conditions are all factors that could influence the recovery time.
By identifying and controlling for these variables, researchers can more confidently attribute any observed changes in recovery time to the specific effects of the drug being studied.
How Control Variables Differ from Independent and Dependent Variables
To grasp the role of control variables, it is essential to differentiate them from independent and dependent variables. The researcher manipulates or selects independent variables to observe their effect on the dependent variable.
On the other hand, dependent variables are the outcomes or responses measured in the experiment, dependent on the changes in the independent variable.
Control variables, however, are not the variables of primary interest. Instead, they are chosen to minimize the influence of extraneous variables that might interfere with the relationship between the independent and dependent variables. While independent and dependent variables are central to the research question, control variables act as safeguards to ensure the integrity and validity of the study.
Examples of Control Variables
Control variables are versatile and their selection depends on the specifics of each study.
In social science research, control variables may include demographic factors like age, gender, and socioeconomic status.
In experimental studies in the physical sciences, factors such as temperature, humidity, or pressure might be controlled to isolate the effects of the manipulated variables.
Consider a psychological study exploring the impact of a new therapy on reducing anxiety levels. Control variables in this scenario could include the participants’ previous experiences with therapy, baseline anxiety levels, or even the time of day the therapy sessions are conducted.
These variables, when controlled, allow the researcher to attribute any observed changes in anxiety levels more confidently to the therapeutic intervention.
Criteria for Selecting Control Variables
The following are the criteria for selecting the right control variables.
Relevance to the Research Question
One of the foremost considerations when selecting control variables is their relevance to the research question or thesis statement . The chosen control variables should have a logical and theoretical connection to the study, aligning with the overarching objectives.
Researchers must carefully evaluate whether the control variables are likely to influence the relationship between the independent and dependent variables. A judicious selection based on relevance ensures that the controlled factors contribute meaningfully to the study’s internal validity.
Potential Confounding Factors
Control variables act as a shield against confounding factors—variables that might distort the observed relationship between the independent and dependent variables. Identifying potential confounding factors requires an understanding of the subject and a thorough literature review.
Researchers must anticipate variables that could muddy the waters and strategically incorporate them as control variables to isolate the effects of the independent variable accurately.
Feasibility and Practicality
While researchers aim for inclusivity in control variable selection, practical considerations cannot be ignored. Feasibility and practicality play a pivotal role in the decision-making process.
Researchers must assess whether the chosen control variables are measurable, obtainable, and manageable within the constraints of the study. Pragmatic decisions ensure that the research remains feasible without compromising the overall quality and validity.
Balance Between Inclusivity and Specificity
Achieving a delicate balance between inclusivity and specificity is crucial in control variable selection. Including too few control variables may leave the study vulnerable to lurking confounders, while an overly exhaustive list may complicate the analysis and risk diluting the primary focus.
Researchers must strike a balance, aiming for inclusivity without sacrificing the specificity necessary to draw meaningful and precise conclusions from the data.
Common Pitfalls in Control Variable Selection
Here are some common pitfalls in control variable selection.
Overlooking Relevant Variables
One common pitfall in control variable selection is overlooking variables that could significantly impact the study’s outcomes. Researchers may inadvertently omit relevant factors that, when unaccounted for, introduce bias or confound the results.
Rigorous literature reviews and a comprehensive understanding of the research domain are crucial in avoiding this oversight.
Including Unnecessary Variables
Conversely, the inclusion of unnecessary variables poses another challenge. Researchers may be tempted to incorporate a multitude of control variables without clear theoretical or empirical justification.
This not only complicates the study unnecessarily but can also lead to overfitting models, reducing the generalizability of findings. Prudent selection is key to avoiding this pitfall.
Confusing Control Variables with Mediators or Moderators
Control variables should not be confused with mediators or moderators . Mediators explain how an independent variable affects a dependent variable, while moderators influence the strength or direction of the relationship between the independent and dependent variables.
Confusing these concepts can lead to misinterpretation of results and compromise the overall integrity of the study. Researchers must delineate between control variables, mediators, and moderators to ensure accurate analyses.
Strategies for Identifying Control Variables
You can identify control variables with the help of the following strategies.
Literature Review and Prior Research
A robust literature review is a cornerstone for identifying relevant control variables. Existing research provides valuable insights into potential factors that could confound or influence the relationships under investigation.
By examining similar studies and drawing on the collective knowledge within the field, researchers can identify common control variables used by peers and gain a better understanding of the variables that warrant consideration in their own work.
Preliminary Data Analysis
Conducting preliminary data analysis can unearth patterns and relationships that may guide the selection of control variables. Exploratory data analysis allows researchers to identify potential confounding factors by examining correlations, patterns, and outliers.
By scrutinizing the data before formal analysis, researchers can make informed decisions about which variables to control for, refine their study design, and ensure a more robust research paper approach.
Expert Consultation and Peer Feedback
Seeking input from experts in the field and obtaining peer feedback can provide valuable perspectives on control variable selection. Collaborating with colleagues who have expertise in the subject or statistical methods can offer fresh insights and help researchers consider variables they might have overlooked.
Peer review processes also serve as a checkpoint, allowing external experts to assess the validity and appropriateness of chosen control variables.
Documentation and Transparency
Thorough documentation of control variable choices is essential for the transparency and replicability of research. Researchers should meticulously record the rationale behind each control variable selection, detailing the theoretical or empirical basis for inclusion.
This documentation serves as a critical reference point for both internal and external stakeholders, aiding in the understanding and evaluation of the study’s design and validity.
Case Studies
Here are some case studies to help you better understand control variables.
Examining real-world examples of well-selected control variables can provide valuable insights into effective research practices. In a study investigating the impact of a nutritional intervention on weight loss, well-chosen control variables might include participants’ baseline body mass index (BMI), exercise habits, and pre-existing medical conditions.
These control variables help ensure that observed changes in weight can be confidently attributed to the nutritional intervention, minimizing the influence of extraneous factors.
In another example, a social science study exploring the effects of a community development program may appropriately control for demographic factors such as income, education level, and employment status. By doing so, the researchers can isolate the specific impact of the intervention on community outcomes without the interference of socioeconomic disparities.
Analysis of Studies with Inadequate Control Variable Selection
Conversely, inadequate control variable selection can compromise the validity of study findings. For instance, a study examining the effectiveness of a new teaching method in improving student performance may fall short if it fails to control for factors like students’ prior academic achievement, socio-economic background, or teacher-student ratios.
In such cases, the observed improvements in student performance may be confounded by these uncontrolled variables, making it challenging to attribute the effects solely to the teaching method.
Similarly, a health-related study investigating the impact of a wellness program may encounter issues if it neglects to control for participants’ pre-existing health conditions or lifestyle factors. Without proper controls, the study risks drawing inaccurate conclusions about the program’s effectiveness.
Lessons Learned from Real-World Examples
Analyzing case studies with both effective and inadequate control variable selection provides valuable lessons for researchers. It underscores the importance of understanding the research context and the critical role that control variables play in ensuring the internal validity of a study.
Researchers can learn to anticipate potential confounding factors, appreciate the complexity of real-world scenarios, and recognize the significance of meticulous control variable selection in generating trustworthy research outcomes.
The research paper we write have:
- Precision and Clarity
- Zero Plagiarism
- High-level Encryption
- Authentic Sources
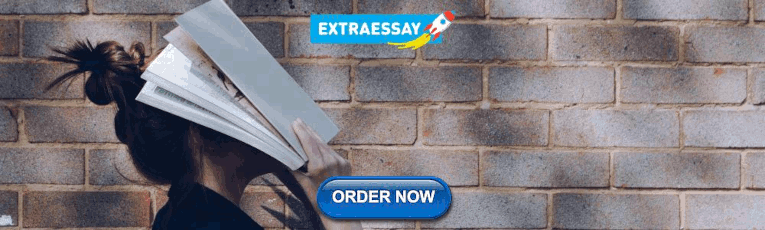
Practical Tips for Implementing Control Variables
With the help of these tips, you can implement control variables.
Tip 1: Monitoring and Adjusting Control Variables During the Research Process
The research process is dynamic, and unforeseen variables may emerge. Researchers should adopt a proactive approach to monitor and adjust control variables as necessary throughout the study.
Regularly assessing the relevance and impact of control variables allows researchers to adapt to changing circumstances, ensuring that the study remains robust and that unexpected confounding factors are addressed promptly.
Tip 2: Using Statistical Techniques to Assess the Impact of Control Variables
Statistical techniques can aid researchers in assessing the impact of control variables on study outcomes. Regression analysis, for example, allows researchers to examine how changes in the independent variable relate to changes in the dependent variable while holding control variables constant.
This analysis helps quantify the contribution of each variable and ensures that control variables are appropriately considered in the interpretation of results.
Tip 3: Considerations for Longitudinal or Experimental Studies
Longitudinal or experimental studies present unique challenges in control variable selection. In longitudinal studies, where data is collected over an extended period, researchers must carefully choose control variables that account for changes over time.
In experimental studies, the manipulation of variables introduces complexities that require strategic control variable selection. Researchers should be attuned to their study design, ensuring that control variables are relevant and measurable, and effectively mitigate potential confounding factors specific to their experimental or longitudinal context.
Frequently Asked Questions
What are the examples of variable control.
Examples of variable control include maintaining consistent temperature in a scientific experiment, controlling for participants’ age and gender in social research, or standardizing testing conditions to isolate the impact of an independent variable on a dependent variable.
What are 3 controlled variables?
- Temperature: Ensuring a constant temperature in an experiment to isolate the effects of other variables.
- Time: Controlling the duration of an experiment to prevent time-related influences on the dependent variable.
- Light: Standardizing light conditions to eliminate its impact on experimental outcomes.
What are system control variables?
System control variables are parameters or factors intentionally regulated or kept constant in a system to observe the impact of independent variables. By controlling these elements, researchers can isolate and assess the effects of specific variables on the system’s behaviour or outcomes.
You May Also Like
The central idea of this excerpt revolves around the exploration of key themes, offering insights that illuminate the concepts within the text.
Don’t know how to write a hypothesis? Follow our simple guide with hypothesis examples, null hypothesis and alternative hypothesis.
A preliminary literature review is an initial exploration of existing research on a topic, setting the foundation for in-depth study.
Ready to place an order?
USEFUL LINKS
Learning resources, company details.
- How It Works
Automated page speed optimizations for fast site performance
Have a language expert improve your writing
Run a free plagiarism check in 10 minutes, automatically generate references for free.
- Knowledge Base
- Methodology
- Controlled Experiments | Methods & Examples of Control
Controlled Experiments | Methods & Examples of Control
Published on 19 April 2022 by Pritha Bhandari . Revised on 10 October 2022.
In experiments , researchers manipulate independent variables to test their effects on dependent variables. In a controlled experiment , all variables other than the independent variable are controlled or held constant so they don’t influence the dependent variable.
Controlling variables can involve:
- Holding variables at a constant or restricted level (e.g., keeping room temperature fixed)
- Measuring variables to statistically control for them in your analyses
- Balancing variables across your experiment through randomisation (e.g., using a random order of tasks)
Table of contents
Why does control matter in experiments, methods of control, problems with controlled experiments, frequently asked questions about controlled experiments.
Control in experiments is critical for internal validity , which allows you to establish a cause-and-effect relationship between variables.
- Your independent variable is the colour used in advertising.
- Your dependent variable is the price that participants are willing to pay for a standard fast food meal.
Extraneous variables are factors that you’re not interested in studying, but that can still influence the dependent variable. For strong internal validity, you need to remove their effects from your experiment.
- Design and description of the meal
- Study environment (e.g., temperature or lighting)
- Participant’s frequency of buying fast food
- Participant’s familiarity with the specific fast food brand
- Participant’s socioeconomic status
Prevent plagiarism, run a free check.
You can control some variables by standardising your data collection procedures. All participants should be tested in the same environment with identical materials. Only the independent variable (e.g., advert colour) should be systematically changed between groups.
Other extraneous variables can be controlled through your sampling procedures . Ideally, you’ll select a sample that’s representative of your target population by using relevant inclusion and exclusion criteria (e.g., including participants from a specific income bracket, and not including participants with colour blindness).
By measuring extraneous participant variables (e.g., age or gender) that may affect your experimental results, you can also include them in later analyses.
After gathering your participants, you’ll need to place them into groups to test different independent variable treatments. The types of groups and method of assigning participants to groups will help you implement control in your experiment.
Control groups
Controlled experiments require control groups . Control groups allow you to test a comparable treatment, no treatment, or a fake treatment, and compare the outcome with your experimental treatment.
You can assess whether it’s your treatment specifically that caused the outcomes, or whether time or any other treatment might have resulted in the same effects.
- A control group that’s presented with red advertisements for a fast food meal
- An experimental group that’s presented with green advertisements for the same fast food meal
Random assignment
To avoid systematic differences between the participants in your control and treatment groups, you should use random assignment .
This helps ensure that any extraneous participant variables are evenly distributed, allowing for a valid comparison between groups .
Random assignment is a hallmark of a ‘true experiment’ – it differentiates true experiments from quasi-experiments .
Masking (blinding)
Masking in experiments means hiding condition assignment from participants or researchers – or, in a double-blind study , from both. It’s often used in clinical studies that test new treatments or drugs.
Sometimes, researchers may unintentionally encourage participants to behave in ways that support their hypotheses. In other cases, cues in the study environment may signal the goal of the experiment to participants and influence their responses.
Using masking means that participants don’t know whether they’re in the control group or the experimental group. This helps you control biases from participants or researchers that could influence your study results.
Although controlled experiments are the strongest way to test causal relationships, they also involve some challenges.
Difficult to control all variables
Especially in research with human participants, it’s impossible to hold all extraneous variables constant, because every individual has different experiences that may influence their perception, attitudes, or behaviors.
But measuring or restricting extraneous variables allows you to limit their influence or statistically control for them in your study.
Risk of low external validity
Controlled experiments have disadvantages when it comes to external validity – the extent to which your results can be generalised to broad populations and settings.
The more controlled your experiment is, the less it resembles real world contexts. That makes it harder to apply your findings outside of a controlled setting.
There’s always a tradeoff between internal and external validity . It’s important to consider your research aims when deciding whether to prioritise control or generalisability in your experiment.
Experimental designs are a set of procedures that you plan in order to examine the relationship between variables that interest you.
To design a successful experiment, first identify:
- A testable hypothesis
- One or more independent variables that you will manipulate
- One or more dependent variables that you will measure
When designing the experiment, first decide:
- How your variable(s) will be manipulated
- How you will control for any potential confounding or lurking variables
- How many subjects you will include
- How you will assign treatments to your subjects
Cite this Scribbr article
If you want to cite this source, you can copy and paste the citation or click the ‘Cite this Scribbr article’ button to automatically add the citation to our free Reference Generator.
Bhandari, P. (2022, October 10). Controlled Experiments | Methods & Examples of Control. Scribbr. Retrieved 29 April 2024, from https://www.scribbr.co.uk/research-methods/controlled-experiments/
Is this article helpful?
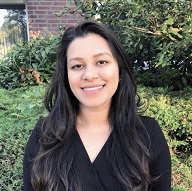
Pritha Bhandari

- Science Notes Posts
- Contact Science Notes
- Todd Helmenstine Biography
- Anne Helmenstine Biography
- Free Printable Periodic Tables (PDF and PNG)
- Periodic Table Wallpapers
- Interactive Periodic Table
- Periodic Table Posters
- How to Grow Crystals
- Chemistry Projects
- Fire and Flames Projects
- Holiday Science
- Chemistry Problems With Answers
- Physics Problems
- Unit Conversion Example Problems
- Chemistry Worksheets
- Biology Worksheets
- Periodic Table Worksheets
- Physical Science Worksheets
- Science Lab Worksheets
- My Amazon Books
What Is a Control Variable? Definition and Examples

A control variable is any factor that is controlled or held constant during an experiment . For this reason, it’s also known as a controlled variable or a constant variable. A single experiment may contain many control variables . Unlike the independent and dependent variables , control variables aren’t a part of the experiment, but they are important because they could affect the outcome. Take a look at the difference between a control variable and control group and see examples of control variables.
Importance of Control Variables
Remember, the independent variable is the one you change, the dependent variable is the one you measure in response to this change, and the control variables are any other factors you control or hold constant so that they can’t influence the experiment. Control variables are important because:
- They make it easier to reproduce the experiment.
- The increase confidence in the outcome of the experiment.
For example, if you conducted an experiment examining the effect of the color of light on plant growth, but you didn’t control temperature, it might affect the outcome. One light source might be hotter than the other, affecting plant growth. This could lead you to incorrectly accept or reject your hypothesis. As another example, say you did control the temperature. If you did not report this temperature in your “methods” section, another researcher might have trouble reproducing your results. What if you conducted your experiment at 15 °C. Would you expect the same results at 5 °C or 35 5 °C? Sometimes the potential effect of a control variable can lead to a new experiment!
Sometimes you think you have controlled everything except the independent variable, but still get strange results. This could be due to what is called a “ confounding variable .” Examples of confounding variables could be humidity, magnetism, and vibration. Sometimes you can identify a confounding variable and turn it into a control variable. Other times, confounding variables cannot be detected or controlled.
Control Variable vs Control Group
A control group is different from a control variable. You expose a control group to all the same conditions as the experimental group, except you change the independent variable in the experimental group. Both the control group and experimental group should have the same control variables.
Control Variable Examples
Anything you can measure or control that is not the independent variable or dependent variable has potential to be a control variable. Examples of common control variables include:
- Duration of the experiment
- Size and composition of containers
- Temperature
- Sample volume
- Experimental technique
- Chemical purity or manufacturer
- Species (in biological experiments)
For example, consider an experiment testing whether a certain supplement affects cattle weight gain. The independent variable is the supplement, while the dependent variable is cattle weight. A typical control group would consist of cattle not given the supplement, while the cattle in the experimental group would receive the supplement. Examples of control variables in this experiment could include the age of the cattle, their breed, whether they are male or female, the amount of supplement, the way the supplement is administered, how often the supplement is administered, the type of feed given to the cattle, the temperature, the water supply, the time of year, and the method used to record weight. There may be other control variables, too. Sometimes you can’t actually control a control variable, but conditions should be the same for both the control and experimental groups. For example, if the cattle are free-range, weather might change from day to day, but both groups have the same experience. When you take data, be sure to record control variables along with the independent and dependent variable.
- Box, George E.P.; Hunter, William G.; Hunter, J. Stuart (1978). Statistics for Experimenters : An Introduction to Design, Data Analysis, and Model Building . New York: Wiley. ISBN 978-0-471-09315-2.
- Giri, Narayan C.; Das, M. N. (1979). Design and Analysis of Experiments . New York, N.Y: Wiley. ISBN 9780852269145.
- Stigler, Stephen M. (November 1992). “A Historical View of Statistical Concepts in Psychology and Educational Research”. American Journal of Education . 101 (1): 60–70. doi: 10.1086/444032
Related Posts
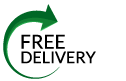
The Plagiarism Checker Online For Your Academic Work
Start Plagiarism Check
Editing & Proofreading for Your Research Paper
Get it proofread now
Online Printing & Binding with Free Express Delivery
Configure binding now
- Academic essay overview
- The writing process
- Structuring academic essays
- Types of academic essays
- Academic writing overview
- Sentence structure
- Academic writing process
- Improving your academic writing
- Titles and headings
- APA style overview
- APA citation & referencing
- APA structure & sections
- Citation & referencing
- Structure and sections
- APA examples overview
- Commonly used citations
- Other examples
- British English vs. American English
- Chicago style overview
- Chicago citation & referencing
- Chicago structure & sections
- Chicago style examples
- Citing sources overview
- Citation format
- Citation examples
- College essay overview
- Application
- How to write a college essay
- Types of college essays
- Commonly confused words
- Definitions
- Dissertation overview
- Dissertation structure & sections
- Dissertation writing process
- Graduate school overview
- Application & admission
- Study abroad
- Master degree
- Harvard referencing overview
- Language rules overview
- Grammatical rules & structures
- Parts of speech
- Punctuation
- Methodology overview
- Analyzing data
- Experiments
- Observations
- Inductive vs. Deductive
- Qualitative vs. Quantitative
- Types of validity
- Types of reliability
- Sampling methods
- Theories & Concepts
- Types of research studies
- Types of variables
- MLA style overview
- MLA examples
- MLA citation & referencing
- MLA structure & sections
- Plagiarism overview
- Plagiarism checker
- Types of plagiarism
- Printing production overview
- Research bias overview
- Types of research bias
- Example sections
- Types of research papers
- Research process overview
- Problem statement
- Research proposal
- Research topic
- Statistics overview
- Levels of measurment
- Frequency distribution
- Measures of central tendency
- Measures of variability
- Hypothesis testing
- Parameters & test statistics
- Types of distributions
- Correlation
- Effect size
- Hypothesis testing assumptions
- Types of ANOVAs
- Types of chi-square
- Statistical data
- Statistical models
- Spelling mistakes
- Tips overview
- Academic writing tips
- Dissertation tips
- Sources tips
- Working with sources overview
- Evaluating sources
- Finding sources
- Including sources
- Types of sources
Your Step to Success
Plagiarism Check within 10min
Printing & Binding with 3D Live Preview
Control Variables in Statistical Studies
How do you like this article cancel reply.
Save my name, email, and website in this browser for the next time I comment.
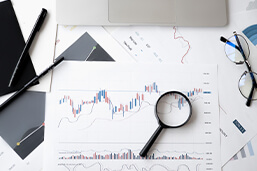
Control variables play a pivotal role in scientific methodologies , ensuring the integrity of research outcomes. These are specific variables that researchers keep constant throughout an experiment to minimize external influence and ensure that the results accurately reflect the relationship between independent vs. dependent variables .
Inhaltsverzeichnis
- 1 Control Variables – In a Nutshell
- 2 Definition: Control variables
- 3 The importance of control variables
- 4 How to control variables
- 5 Control variables vs. Control groups
Control Variables – In a Nutshell
- Control variables let us control an experiment’s conditions via set criteria by improving statistical repeatability, validity, and applicability.
- Control variables protect the independent and dependent variables of the study from undue bias (e.g. confounding causative variables).
- How and which variables are selected depends on the topic and mode of study.
- Control variables are expressed as simple quantities (numerical) or qualitative statements.
- Experimental variables govern ‘test lab’ conditions via stabilization.
- Non-experimental variables help eliminate bias in active (wild) observation, where complete control is impossible.
Definition: Control variables
Control variables are essential empirical tools. They help us create replicable, verifiable data (i.e. statistics) from direct experimentation, observation, and sampling by setting hard limits. They also allow us to bypass ‘false’ causatives in observational studies.
Variables may be expressed as a qualitative or quantitative statement and may be limited (i.e. within a set range) or total. Integers (i.e. whole numbers) are usual for statistical controls. A single study may use many different control variables.

The importance of control variables
Control variables are crucial, as they greatly enhance an experiment’s internal validity. When you’re assessing a study’s statistical power, internal validity plays an important role.
Why? Quality, empirical statistics must reflect ‘control’ conditions relevant to that topic (i.e. model reality) to their absolute best. Improving internal validity means a study becomes more reliable, repeatable, broadly applicable, and likely to survive intense peer review.
Using control variables in experiments
Experimental control values let us isolate the dependent and independent variables in a closed environment. They’re the ‘lab’ variety.
In research, to see if adding a different mineral to soil stimulates houseplant growth, set control variables must detail how much sunlight, water, and air each plant should receive. As these factors are recognized as growth causatives (i.e. positive and negative confounders), quantitive nominal amounts may be required.
To do so, the scientists may read past papers, search through data about chronic conditions and rainfall, and examine similar nearby plants in the wild. Afterwards, they may agree that the plants should all receive 8.0 hours of daily light and 500 ml of daily water in a closed, single-fan-circulated shed. As a result, they may reach a consensus on their chosen brand of compost, pot diameter and volume, and the species and variety of plant seed.
Due to control variables, the experiment’s active and passive constants and constraints may now be ready and the effects of the independent variable (i.e. mineral type) on the dependent variable (i.e. plant growth rate) may now be safely observable.
Using control variables in non-experimental studies
Non-experimental control variables are similar. However, they’re tailored much more towards validating observations of natural phenomena, particularly human behavior.
Non-experimental variables are helpful when potential confounding causative factors (i.e. income, age, gender) may not be removed entirely from samples for ethical, legal, or practical reasons. Instead, they monitor or neutralize data on known causatives.
Suppose a freak lightning strike incinerates the carefully planned plant lab. The paper is due for submission in two weeks. There’s no money left. How will the researchers create useable statistics now?
One generous scientist suggests they may borrow their garden and spare (uniform) compost bags as a ‘real’ test bed. However, a few non-experimental design changes are needed first so that this new, natural approach works.
It’s impossible to control the rainfall and sunlight each day outdoors. However, they may be tracked instead by converting the set of experimental variables into monitored, non-experimental categories. Statistical reasoning (i.e. mean value calculation) allows the scientists to work out expected ‘real’ growth ranges for the plants they study, limiting bias.
How to control variables
Three advanced techniques that use control variables help remove bias from sample sets – if applied correctly. Here’s how they work.
Control variable methodology – Random assignment
If you have a set prone to outliers or clusters with wildly different behavior, you may want to make sure ‘lucky’ or ‘unlucky’ samples don’t skew your findings.
Researchers may use variables to ‘scramble’ set populations containing biased sub-sets, offsetting sampling bias. Random assignment ensures that sub-samples, with a balanced demographic ratio, occur. Pure luck determines which sample points are selected.
Here’s another example.
The unfortunate scientists double-check their equipment to find they have accidentally purchased three different varieties of tomato seed packet plant – ‘Medium-Mato’, ‘Mini-Mato’, and ‘Mega-Mato’ (x 100). A conundrum!
Luckily, the scientists also find past papers indicating these three varieties map comfortably onto a standard natural distribution. The researchers quickly pour the seeds into a container and shake it thoroughly for ten minutes before selecting exactly 100 samples (c.33%). Here, randomization guards against confounding influence.
Modern statistical studies may use a digital database to calculate random samples rather than a simple glass jar. Demographic weighting (i.e. stratification) might also be applied to better model divided random populations.
Control variable methodology – Standardized procedures
Do you have a repetitive daily routine that governs your time? Experiments often do. The exact time and way something must be delivered may have surprising, unforeseen effects on a subject.
It’s therefore critical that all manual test procedures remain uniform. We may set variables as timed and listed instructions to ensure this happens. They may also exclude any eccentric behavior that might skew the tests.
Let’s look at our final practical example.
Forward-thinking researchers set an additional control rule that outside plants should be watered at 10 AM and 5 PM if it hasn’t rained for two consecutive days. They also specify that no researchers should substitute cups of tea for rain-trough water as an exclusionary control.
Control variable methodology – Statistical controls
If everything else fails? You can apply statistical control methods to limit bias via final analysis.
Sometimes, removing all traces of extraneous influence is impossible. By applying modeling, weighting, and averaging based on what’s known about the factors you’re trying to account for, a more realistic statistical picture may emerge.
Applying multiple linear regression may help. By using averaged predictors as hypotheticals to weight and limit your values, trends and correlations may be better isolated.
Control variables vs. Control groups
Control variables shouldn’t be confused with control groups ! Control groups are governed by control variables, allowing the creation of a ‘neutral’ sub-sample.
What's a control variable?
A scientific safeguard that details a factor in a study that should (ideally) be kept the same. Control variables can also set out what factors should be accounted for and excluded from causative arguments.
Why do we use control variables?
Control variables add immense statistical power and validity. They’re an easy-to-use, effective way to guard against confounding factors that might warp our understanding of a complex topic.
What can control variables do?
A good set of variables may create an unchanging, dependable ‘test chamber’. Within, researchers may modify an independent variable to see how it affects a dependent one without risk.
We use cookies on our website. Some of them are essential, while others help us to improve this website and your experience.
- External Media
Individual Privacy Preferences
Cookie Details Privacy Policy Imprint
Here you will find an overview of all cookies used. You can give your consent to whole categories or display further information and select certain cookies.
Accept all Save
Essential cookies enable basic functions and are necessary for the proper function of the website.
Show Cookie Information Hide Cookie Information
Statistics cookies collect information anonymously. This information helps us to understand how our visitors use our website.
Content from video platforms and social media platforms is blocked by default. If External Media cookies are accepted, access to those contents no longer requires manual consent.
Privacy Policy Imprint
Controlled Experiment
Saul Mcleod, PhD
Editor-in-Chief for Simply Psychology
BSc (Hons) Psychology, MRes, PhD, University of Manchester
Saul Mcleod, PhD., is a qualified psychology teacher with over 18 years of experience in further and higher education. He has been published in peer-reviewed journals, including the Journal of Clinical Psychology.
Learn about our Editorial Process
Olivia Guy-Evans, MSc
Associate Editor for Simply Psychology
BSc (Hons) Psychology, MSc Psychology of Education
Olivia Guy-Evans is a writer and associate editor for Simply Psychology. She has previously worked in healthcare and educational sectors.
This is when a hypothesis is scientifically tested.
In a controlled experiment, an independent variable (the cause) is systematically manipulated, and the dependent variable (the effect) is measured; any extraneous variables are controlled.
The researcher can operationalize (i.e., define) the studied variables so they can be objectively measured. The quantitative data can be analyzed to see if there is a difference between the experimental and control groups.
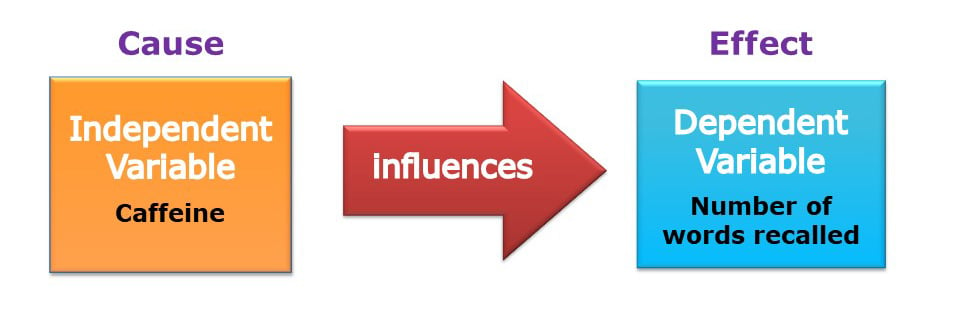
What is the control group?
In experiments scientists compare a control group and an experimental group that are identical in all respects, except for one difference – experimental manipulation.
Unlike the experimental group, the control group is not exposed to the independent variable under investigation and so provides a baseline against which any changes in the experimental group can be compared.
Since experimental manipulation is the only difference between the experimental and control groups, we can be sure that any differences between the two are due to experimental manipulation rather than chance.
Randomly allocating participants to independent variable groups means that all participants should have an equal chance of participating in each condition.
The principle of random allocation is to avoid bias in how the experiment is carried out and limit the effects of participant variables.
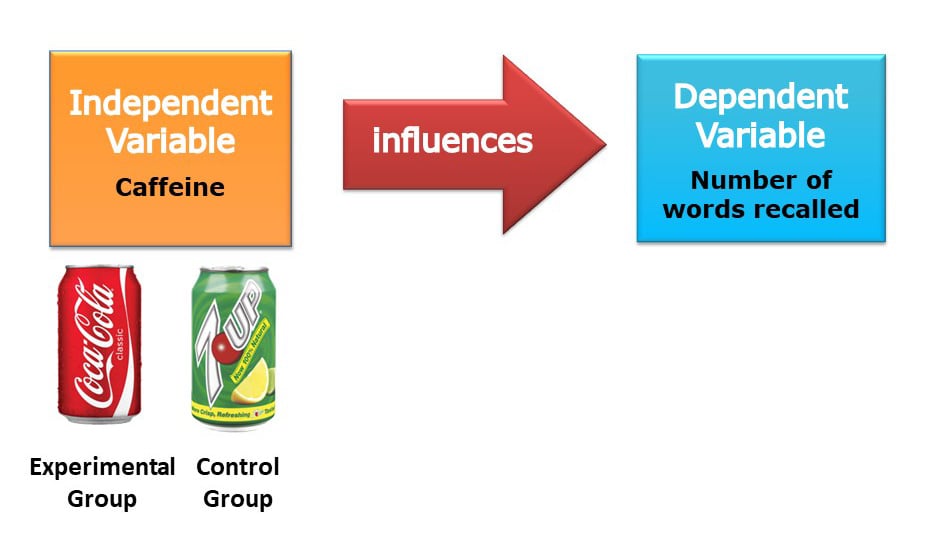
What are extraneous variables?
The researcher wants to ensure that the manipulation of the independent variable has changed the changes in the dependent variable.
Hence, all the other variables that could affect the dependent variable to change must be controlled. These other variables are called extraneous or confounding variables.
Extraneous variables should be controlled were possible, as they might be important enough to provide alternative explanations for the effects.
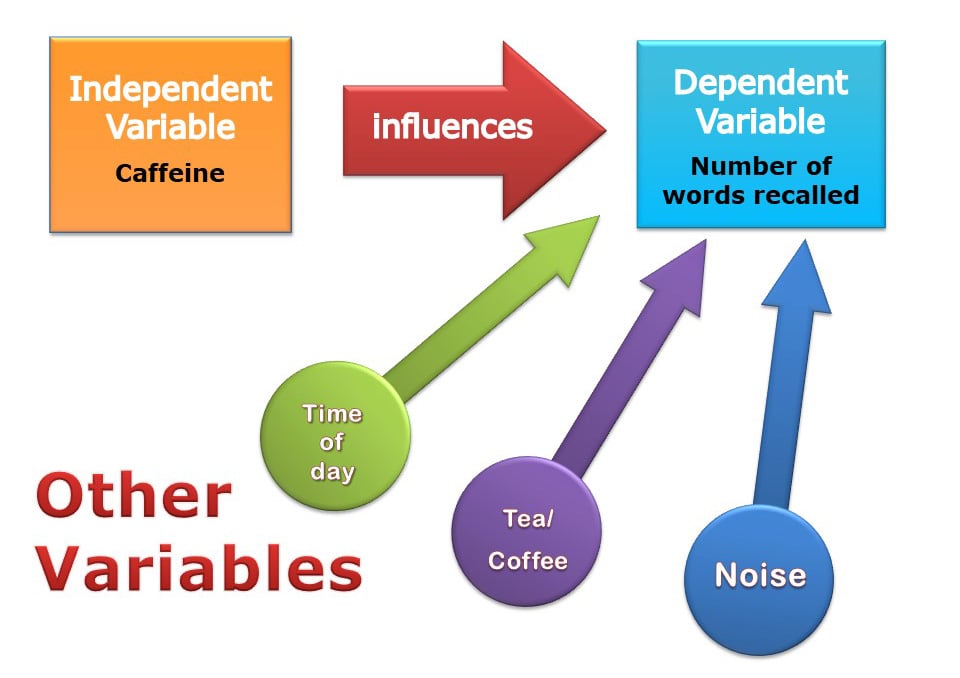
In practice, it would be difficult to control all the variables in a child’s educational achievement. For example, it would be difficult to control variables that have happened in the past.
A researcher can only control the current environment of participants, such as time of day and noise levels.
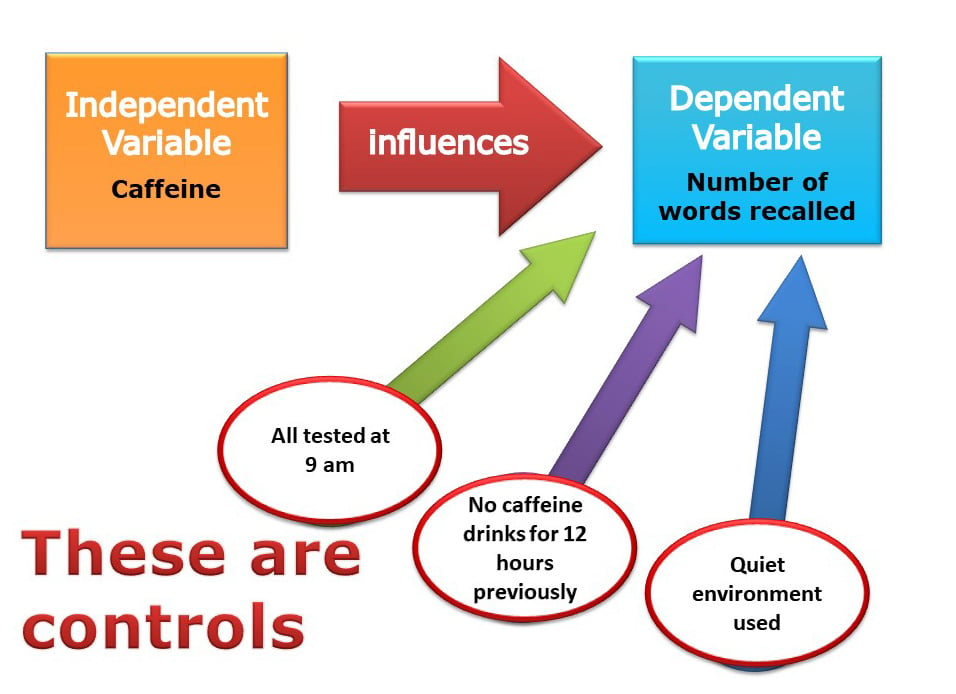
Why conduct controlled experiments?
Scientists use controlled experiments because they allow for precise control of extraneous and independent variables. This allows a cause-and-effect relationship to be established.
Controlled experiments also follow a standardized step-by-step procedure. This makes it easy for another researcher to replicate the study.
Key Terminology
Experimental group.
The group being treated or otherwise manipulated for the sake of the experiment.
Control Group
They receive no treatment and are used as a comparison group.
Ecological validity
The degree to which an investigation represents real-life experiences.
Experimenter effects
These are the ways that the experimenter can accidentally influence the participant through their appearance or behavior.
Demand characteristics
The clues in an experiment lead the participants to think they know what the researcher is looking for (e.g., the experimenter’s body language).
Independent variable (IV)
The variable the experimenter manipulates (i.e., changes) – is assumed to have a direct effect on the dependent variable.
Dependent variable (DV)
Variable the experimenter measures. This is the outcome (i.e., the result) of a study.
Extraneous variables (EV)
All variables that are not independent variables but could affect the results (DV) of the experiment. Extraneous variables should be controlled where possible.
Confounding variables
Variable(s) that have affected the results (DV), apart from the IV. A confounding variable could be an extraneous variable that has not been controlled.
Random Allocation
Randomly allocating participants to independent variable conditions means that all participants should have an equal chance of participating in each condition.
Order effects
Changes in participants’ performance due to their repeating the same or similar test more than once. Examples of order effects include:
(i) practice effect: an improvement in performance on a task due to repetition, for example, because of familiarity with the task;
(ii) fatigue effect: a decrease in performance of a task due to repetition, for example, because of boredom or tiredness.
What is the control in an experiment?
In an experiment , the control is a standard or baseline group not exposed to the experimental treatment or manipulation. It serves as a comparison group to the experimental group, which does receive the treatment or manipulation.
The control group helps to account for other variables that might influence the outcome, allowing researchers to attribute differences in results more confidently to the experimental treatment.
Establishing a cause-and-effect relationship between the manipulated variable (independent variable) and the outcome (dependent variable) is critical in establishing a cause-and-effect relationship between the manipulated variable.
What is the purpose of controlling the environment when testing a hypothesis?
Controlling the environment when testing a hypothesis aims to eliminate or minimize the influence of extraneous variables. These variables other than the independent variable might affect the dependent variable, potentially confounding the results.
By controlling the environment, researchers can ensure that any observed changes in the dependent variable are likely due to the manipulation of the independent variable, not other factors.
This enhances the experiment’s validity, allowing for more accurate conclusions about cause-and-effect relationships.
It also improves the experiment’s replicability, meaning other researchers can repeat the experiment under the same conditions to verify the results.
Why are hypotheses important to controlled experiments?
Hypotheses are crucial to controlled experiments because they provide a clear focus and direction for the research. A hypothesis is a testable prediction about the relationship between variables.
It guides the design of the experiment, including what variables to manipulate (independent variables) and what outcomes to measure (dependent variables).
The experiment is then conducted to test the validity of the hypothesis. If the results align with the hypothesis, they provide evidence supporting it.
The hypothesis may be revised or rejected if the results do not align. Thus, hypotheses are central to the scientific method, driving the iterative inquiry, experimentation, and knowledge advancement process.
What is the experimental method?
The experimental method is a systematic approach in scientific research where an independent variable is manipulated to observe its effect on a dependent variable, under controlled conditions.

Regression analysis with control variables ¶
Why do we need control variables ¶.
A major strength of regression analysis is that we can control relationships for alternative explanations. You've probably heard the expression "correlation is not causation." It means that just because we can see that two variables are related, one did not necessarily cause the other.
No statistical method can really prove that causality is present. However, we can make it more or less likely. And at the very least, we can investigate whether a relationship is spurious, that is, caused by other variables.
To take a simple example. Imagine that we want to investigate the effect of a persons height on running speed. For data we take all the times in the finals of the 100 meters in the Olympics 2016. We will then find that taller persons ran faster, on average. It is actually a quite strong relationship.
If this was a causal relationship - for instance because you can run faster if you have long legs - we could encourage tall youth to get into track and field.
But it would be unwise, without taking other relevant variables into account; variables that can affect both height and running speed. The obvious variable is gender. On average, men are taller than women, and they also have other physiological properties that make them run faster. If we don't account for the runners' gender, we would not pick that up.
To "control" for the variable gender in principle means that we compare men with men, and women with women. What we are looking at is whether tall women run faster than short women, and whether tall men run faster than short men. And if we actually run this analysis (which I have!) we will see that no relationship between height and time remains. The relationship was spurious.
Had there been a relationship between height and speed even under control for gender, this would still not have implied that the relationship was causal, but it would at least have made it more less unlikely.
In this guide I will show how to do a regression analysis with control variables in Stata.
A more relevant example: Democracy and life expectancy ¶
Let's start by loading the data, which in this case is the QoG Basic dataset, with information about the world's countries. The unit of analysis is country, and information about the countries are stored in the variables. The data can be downloaded here.
In the command, you need to write in the adress to the file on the computer, for instance "/Users/anders/data/qog_bas_cs_jan18.dta", otherwise it won't work.
The dataset has a lot of different variables. We are going to look at the relationship between democracy and life expectancy. Do people in more democratic countries live longer, and if so, is it because the countries are democratic, or is it due to something else? A causal interpretation would for instance be that the state takes better care of its citizens in democratic countries.
Our dependent variable is life expectancy, wdi_lifexp , and as our independent variable we use the degree of democracy, as measured by the Polity project, p_polity2 . First, we look at some descriptive statistics by writing:
We can see that we have information about 185 countries, and that life expectancy (at birth) on average is 71.25 years. However, we only have information about democracy for 165 countries. Our analyses will only be based on the countries for which we have information on all variables.
The democracy variable runs from -10 (max dictatorship) to +10 (max democracy), with a mean value of 4.07.
Running the regression analysis ¶
Now it is time to do the first regression analysis, which we do by writing:
Here we can see a lot of interesting stuff, but the most important is the b-coefficient for the democracy variable, which we find in the column "Coef." It is 0.39, which means that for each step up we take on the democracy variable, life expectancy increases by 0.39 years. The relationship is statistically significant, which we see in the column "P>|t", since the p-value is below 0.050.
Up to the right, we see that "R-squared = 0.0844". This means that the variables in the model - only democracy in this case - explain 8.4% of the variation in the dependent variable. Not a lot, but something. We have no thresholds by which to judge whether the value is large or small - it completely depends on the context.
If we want to look at the relationship graphically with a scatterplot we write:
The red regression line slopes upward slightly, which the regression analysis also showed (the b-coefficient was positive). But we can also see that the line is not a great fit to the dots - there is considerable spread around the line. This explains the low R squared value.
But does this positive relationship mean that democracy causes life expectancy to increase? Not necessarily. There might be other factors that lead to both democracy and high life expectancy. Democracy and life expectancy might be two symptoms, rather than cause and effect.
Controlling for a variable ¶
An obvious suspect is the level of economic development. Democracy research shows that countries with more economic prosperity are more likely to both democratize and keep democracy, once attained. Richer countries can also invest more in health care and disease prevention, for instance through better water supply and waste management.
To test the hypothesis that democracy leads to longer life expectancy, we will control for economic development. A standard measure of that is GDP per capita:
The variable gle_rgdpc shows a country's GDP per capita in US dollars. The mean is 12596, but the poorest country (Kongo-Kinshasa) only has a meager 286, while the richest (Monaco) has a whopping 95697.
To make sure that it is a relevant control variable, and that are assumptions are right, we look at the bivariate correlations between the control variable, democracy, and life expectancy. We do this by writing:
In this matrix we find three relationships, standardized according to the Pearson's R measure, which runs from -1 (perfect negative relationship) to +1 (perfect positive relationship), via 0 (no relationship). The relationship between democracy p_polity2 and GDP gle_rgdpc is 0.15. More GDP per capita is associated with more democracy, and and more democracy is associated with more GDP. Democratic countries are thus richer, on average.
High GDP per capita is also associated with higher life expectancy. This relationship is very strong, 0.63, considerably more than the relationship between democracy and life expectancy (0.29).
When we control for variables that have a postive correlation with both the independent and the dependent variable, the original relationship will be pushed down, and become more negative. The same is true if we control for a variable that has a negative correlation with both independent and dependent. It is thus likely that the relationship between democracy and life expectancy will weaken under control for GDP per capita.
Conversely, if we control for a variable that has a positive correlation with the dependent, and a negative correlation with the independent, the original relationship will become more positive. The main relationship will also become more positive if we control for a variable that has a negative correlation with the dependent variable, and a positive correlation with the independent.
Regression analysis with a control variable ¶
By running a regression analysis where both democracy and GDP per capita are included, we can, simply put, compare rich democracies with rich nondemocracies, and poor democracies with poor nondemocracies. This comparison is more fair. But will there remain a relationship between democracy and life expectancy?
When we run the analysis, we reuse the previous regression command, we just add gle_rgdpc after p_polity2 . If we want to add more variables, we just list them after. The order of the independent variables does not matter (but the dependent must always be first).
What happened with the original relationship? The coefficient sank from 0.39 to 0.26. But it is still positive, and statistically significant (the p-value is lower than 0.05). But a part of the original association was due to the democratic countries on average being richer. When we hold the level of economic development constant, the relationship is no longer as clear.
The coefficient for GDP per capita is, as expected, positive. An increase of GDP per capita with one dollar (holding the level of democracy constant) is associated with an increase of life expectancy of 0.00037 years. It might not sound much, but neither is an increase of GDP per capita of one dollar. If we instead increase GDP per capita with 10,000 dollars, life expectancy would increase 3.7 years, which is substantial.
R2 also increased markedly compared to the model with only democracy in it. Together, democracy and GDP per capita explain 45.7% of the variation in the dependent variable. Primarily, it is due to the strong explanatory power of the GDP variable. People live much longer in richer countries.
The main conclusion is that a relationship between democracy and life expectancy remains. This does however not imply that we now have showed that there is a causal effect. There is still a lot of other relevant variables to control for, and in a thesis you should definitely do. But the principle is the same, we would only add more variables to the regression analysis.
Conclusion ¶
To prove that a relationship is causal is extremely hard. It is a shame, since proving causality is usually what we need in order to make recommendations, regardless if it is about health care or policy.
But regression analysis with control variables at the very least help us to avoid the most common pitfalls. In this example, we could see that the relationship between democracy and life expectancy was not completely due to democratic countries being richer, and non-democratic countries poorer. But by doing so, we have accounted for one alternative explanation for the original relationship.
It is however important to think through which control variables that should be included. The analysis is not better or more sofisticated just because more control variables are included. We should for example not control for variables that come after the independent variable in the causal chain. That is, if democracy causes something that in turn causes longer life expectancy, we should not control for it. To rule out alternative explanations we should only control for variables that come before both independent and dependent variables.
It might also be a good idea to run the analyses stepwise, adding one control variable at a time, to see how the main relationship changes (see here how to present the results in a nice table , or here how to visualize the coefficients ). This helps us to get a better sense of what is going on, and to think theoretically about. How we eventually present the results for a wider audience is another question, and we might not then need to show all the steps.
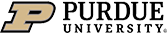
DEVELOPMENT OF PASSIVE VISION BASED RELATIVE STATION KEEPING FOR UNMANNED SURFACE VEHICLES
Unmanned surface vehicles (USVs) offer a versatile platform for various maritime applications, including research, surveillance, and search-and-rescue operations. A critical capability for USVs is maintaining position (station keeping) in dynamic environments and coordinating movement with other USVs (formation control) for collaborative missions. This thesis investigates control strategies for USVs operating in challenging conditions.
The initial focus is on evaluating traditional control methods like Backstepping and Sliding Mode controllers for station keeping in simulated environments with disturbances. The results from these tests pointed towards the need for a more robust control technique, like deep-learning based control for enhanced performance.
The thesis then explores formation control, a crucial aspect of cooperative USV missions. A vision-based passive control strategy utilizing a virtual leader concept is proposed. This approach leverages onboard cameras to detect markers on other USVs, eliminating the need for direct communication and potentially improving scalability and resilience.
Then the thesis presents vision-based formation control architecture and the station keeping controller evaluations. Simulation results are presented, analyzed, and used to draw conclusions about the effectiveness of the proposed approaches. Finally, the thesis discusses the implications of the findings and proposes potential future research directions
Degree Type
- Master of Science
- Mechanical Engineering
Campus location
- West Lafayette
Advisor/Supervisor/Committee Chair
Advisor/supervisor/committee co-chair, additional committee member 2, usage metrics.
- Autonomous vehicle systems
- Control engineering
- Mechatronics hardware design and architecture
- Field robotics

Anti-Israel protesters take over Columbia University academic building in latest campus chaos
A huge mob of masked anti-Israel protesters broke into an academic building at Columbia University and seized it early Tuesday – hours after the school finally began suspending students who refused to vacate the tent encampment that brought campus life to a halt.
Dozens stormed into Hamilton Hall and sealed themselves in shortly before 1 a.m. – with some using metal barricades, chairs and tables to block others from getting inside, footage posted on social media shows.
One shocking video captured a violent, hammer-wielding demonstrator smashing two glass windows of a door and placing what appeared to be a bike lock around the door’s handles.
Others covered security cameras inside the building with tape and black trash bags, according to the student-led Columbia Daily Spectator.
As of 6:30 a.m., the rogue protesters were still occupying the South Lawn building, which has been the scene of the school’s anti-Israel encampment for over a week, the paper reported.
Students at Columbia University have occupied Hamilton hall. Today the university president explicitly said to students the school would not be divesting and threatened to suspend students protesting. The last time this building was taken over by student protesters was in 1968 pic.twitter.com/IhoWVYlocB
אירוע מטורף בדקות האלה באוניברסיטת קולומביה: פרו פלסטינים מנפצים שמשות ומשתלטים על בניין המילטון בקמפוס.
אמרתי בשידורים בשבוע האחרון שהאירוע יצא משליטה. צפו ותבינו למה התכוונתי.
השעה 1:30 בלילה במנהטן. מתרחשת כאן נקודת מפנה. pic.twitter.com/6DYLKpLzDf
Meanwhile, hundreds more swarmed outside the campus building, with some linking arms to form a human chain blocking the entrance.
“Hey hey, ho ho, the occupation has got to go,” protesters outside the building could be heard chanting during the wild scenes, according to footage posted to X by an independent news blog.
“We will not leave until Columbia meets every one of our demands,” others raged.
When the group initially barged into the building in the early hours, several campus facilities workers were still inside. The protesters removed some of the barricades to let them out.
One of the workers yelled, “They held me hostage” as he left the building and smacked somebody’s camera, the student newspaper reported.
. @Columbia University — Organized rioters dressed in black bloc broke and entered into a campus building in Manhattan. Hamilton Hall had had been smashed up and occupied by masked far-left extremists saying they're doing it for Palestine. pic.twitter.com/ffBXmPCKxa
Four protesters wearing masks over their heads then lowered a banner reading “Hind’s Hall” from a window overlooking the cheering crowd.
“An autonomous group reclaimed Hind’s Hall, previously known as ‘Hamilton Hall,’ in honor of Hind Rajab , a martyr murdered at the hands of the genocidal Israeli state at the age of six years old,” CU Apartheid Divest, an student-led anti-Israel group, posted on X.
Hind Rajab, a 6-year-old Palestinian girl, was killed in Gaza alongside the two paramedics from the Palestine Red Crescent Society who tried to rescue her in January when the car she was in was allegedly struck by Israeli gunfire.
The ambulance was bombed as it was “just meters” from reaching the vehicle, the Red Crescent said in a statement at the time. The little girl’s body was found 12 days later in the car riddled with bullet holes.
After the banner was unraveled, the group at Columbia then broke out into chants of “Free Palestine,” “From the river to the sea, Palestine will be free,” and “Columbia, you will see – Palestine is almost free.”
Columbia officials haven’t publicly addressed the latest wave of violence.
The wild escalation erupted nearly 12 hours after hundreds of Columbia students defied the Ivy League’s 2 p.m. ultimatum to vacate their sprawling tent encampment.
That followed the deadline repeatedly being pushed back at the school, which has already been forced to hold all classes virtually for the rest of the semester because of the safety fears.
The school’s embattled president Minouche Shafik had warned students would be suspended if they didn’t clear out – but it still took several hours past deadline for the punishments to be doled out.
“We have begun suspending students as part of this next phase of our efforts to ensure safety on our campus,” school Vice President of Public Affairs Ben Chang said at a 5.30 p.m. press conference.
Still, hordes of defiant and pampered protesters – including some who were munching protein bars and beating tambourines — dug their heels in by refusing to vacate.
NYPD officers stationed nearby were not called in to clear them.
The dramatic escalation at Columbia’s campus comes as universities across the US are grappling with how to clear out similar encampments.
Unlike at Columbia, dozens of people were cuffed and hauled away by cops Monday during protests at universities in Texas, Utah and Virginia.
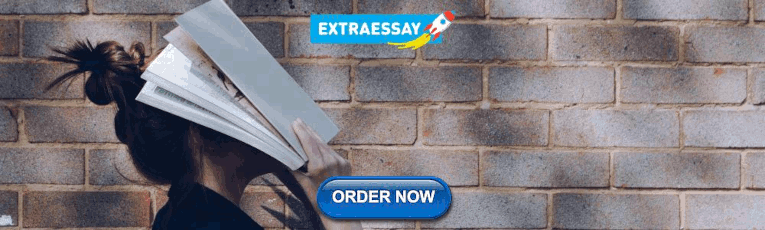
IMAGES
VIDEO
COMMENTS
A control variable is anything that is held constant or limited in a research study. It's a variable that is not of interest to the study's objectives, but is controlled because it could influence the outcomes. Variables may be controlled directly by holding them constant throughout a study (e.g., by controlling the room temperature in an ...
A control variable is anything that is held constant or limited in a research study. It's a variable that is not of interest to the study's aims but is controlled because it could influence the outcomes. Variables may be controlled directly by holding them constant throughout a study (e.g., by controlling the room temperature in an ...
What is a control variable? In an experimental design, a control variable (or controlled variable) is a variable that is intentionally held constant to ensure it doesn't have an influence on any other variables. As a result, this variable remains unchanged throughout the course of the study. In other words, it's a variable that's not allowed to vary - tough life 🙂
Definition: Control variable, also known as a "constant variable," is a variable that is held constant or fixed during an experiment or study to prevent it from affecting the outcome. In other words, a control variable is a variable that is kept the same or held constant to isolate the effects of the independent variable on the dependent ...
4.1: Overview of the Control Variable. In the first chapter, we talked about many different threats to internal validity of a research design and one of the control techniques is to build the extraneous variable into our research design. In this chapter, we will extend between-subjects design by looking at different ways to add in an extraneous ...
You can start conceptualizing this as you determine your relevant paper, thesis, or dissertation topic. Keep reading for a step-by-step guide to help you construct your own conceptual framework. ... Some common variables to include are moderating, mediating, and control variables. Moderating variables. Moderating variable (or moderators) alter ...
The choice of control variables is not arbitrary; it demands careful consideration and a deep understanding of the research context. The significance of selecting the right control variables cannot be overstated, as these elements serve as the bedrock for establishing the internal validity of a study. Internal validity refers to the accuracy of ...
Control variables, also known as controlled variables, are properties that researchers hold constant for all observations in an experiment. While these variables are not the primary focus of the research, keeping their values consistent helps the study establish the true relationships between the independent and dependent variables. The ...
A control variable is any variable that's held constant in a research study. It's not a variable of interest in the study, but it's controlled because it could influence the outcomes. ... Defining a scope can be very useful in any research project, from a research proposal to a thesis or dissertation. A scope is needed for all types of ...
Control in experiments is critical for internal validity, which allows you to establish a cause-and-effect relationship between variables. Example: Experiment. You're studying the effects of colours in advertising. You want to test whether using green for advertising fast food chains increases the value of their products.
Control Variable Examples. Anything you can measure or control that is not the independent variable or dependent variable has potential to be a control variable. Examples of common control variables include: Duration of the experiment. Size and composition of containers. Temperature.
What is a control variable? A control variable is any variable that's held constant in a research study. It's not a variable of interest in the study, but it's controlled because it could influence the outcomes. ... In a longer or more complex research project, such as a thesis or dissertation, ...
Control variables protect the independent and dependent variables of the study from undue bias (e.g. confounding causative variables). How and which variables are selected depends on the topic and mode of study. Control variables are expressed as simple quantities (numerical) or qualitative statements. Experimental variables govern 'test lab ...
In an experiment, the control is a standard or baseline group not exposed to the experimental treatment or manipulation.It serves as a comparison group to the experimental group, which does receive the treatment or manipulation. The control group helps to account for other variables that might influence the outcome, allowing researchers to attribute differences in results more confidently to ...
1. Introduction. Control variables (CVs) constitute a central element of the research design of any empirical study. Confounding variables are likely to covary with the hypothesized focal independent variables thus limiting both the elucidation of causal inference as well as the explanatory power of the model (Pehazur & Schmelkin, 1991; Stone-Romero, 2009).
Regression analysis with a control variable ¶. By running a regression analysis where both democracy and GDP per capita are included, we can, simply put, compare rich democracies with rich nondemocracies, and poor democracies with poor nondemocracies. This comparison is more fair.
Published on April 19, 2021 by Pritha Bhandari . Revised on June 22, 2023. In experiments, researchers manipulate independent variables to test their effects on dependent variables. In a controlled experiment, all variables other than the independent variable are controlled or held constant so they don't influence the dependent variable.
independent variable(s) ___ will be defined generally as ___ (provide a general definition). The dependent variable(s) will be defined generally as (provide a general definition), and the intervening variable(s), ___(identify the intervening variables) will be statistically controlled in the study." Actual Example of a Purpose Statement
The purpose of research is to describe and explain variance in the world, that is, variance that. occurs naturally in the world or chang e that we create due to manipulation. Variables are ...
Variables that represent the outcome of the experiment. Any measurement of plant health and growth: in this case, plant height and wilting. Control variables: Variables that are held constant throughout the experiment. The temperature and light in the room the plants are kept in, and the volume of water given to each plant.
Serkan Dolma. Pamukkale University. From a statistical analysis point of view, control variables can usually be treated like independent variables. In your regression model, you can just include ...
The thesis then explores formation control, a crucial aspect of cooperative USV missions. A vision-based passive control strategy utilizing a virtual leader concept is proposed. This approach leverages onboard cameras to detect markers on other USVs, eliminating the need for direct communication and potentially improving scalability and ...
As of 6:30 a.m., the rogue protesters were still occupying the South Lawn building, which has been the scene of the school's anti-Israel encampment for over a week, the paper reported.
The independent variable is the cause. Its value is independent of other variables in your study. The dependent variable is the effect. Its value depends on changes in the independent variable. Example: Independent and dependent variables. You design a study to test whether changes in room temperature have an effect on math test scores.