An Introduction to Structural Equation Modeling
- Open Access
- First Online: 04 November 2021
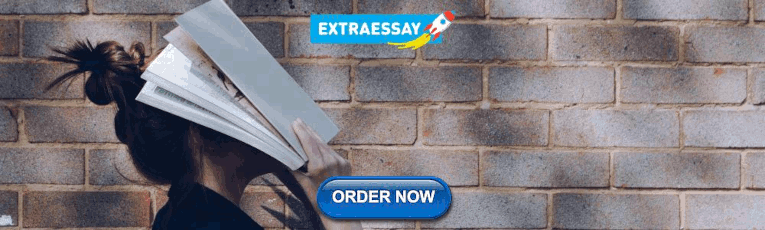
Cite this chapter
You have full access to this open access chapter
- Joseph F. Hair Jr. 8 ,
- G. Tomas M. Hult ORCID: orcid.org/0000-0003-1728-7856 9 ,
- Christian M. Ringle ORCID: orcid.org/0000-0002-7027-8804 10 ,
- Marko Sarstedt ORCID: orcid.org/0000-0002-5424-4268 11 , 12 ,
- Nicholas P. Danks ORCID: orcid.org/0000-0001-6902-2708 13 &
- Soumya Ray ORCID: orcid.org/0000-0002-7497-3281 14
Part of the book series: Classroom Companion: Business ((CCB))
120k Accesses
129 Citations
11 Altmetric
Structural equation modeling is a multivariate data analysis method for analyzing complex relationships among constructs and indicators. To estimate structural equation models, researchers generally draw on two methods: covariance-based SEM (CB-SEM) and partial least squares SEM (PLS-SEM). Whereas CB-SEM is primarily used to confirm theories, PLS represents a causal–predictive approach to SEM that emphasizes prediction in estimating models, whose structures are designed to provide causal explanations. PLS-SEM is also useful for confirming measurement models. This chapter offers a concise overview of PLS-SEM’s key characteristics and discusses the main differences compared to CB-SEM. The chapter also describes considerations when using PLS-SEM and highlights situations that favor its use compared to CB-SEM.
You have full access to this open access chapter, Download chapter PDF
- Common factor-based SEM
- Composite-based SEM
- Construct scores
- Covariance-based SEM (CB-SEM)
- Endogenous latent variables
- Error terms
- Exogenous latent variables
- Factor (score) indeterminacy
- Formative measurement model
- Inner model
- Inverse square root method
- Latent variables
- Measurement error
- Measurement model
- Measurement theory
- Metric scale
- Metrological uncertainty
- Minimum sample size requirements
- Missing value treatment
- Outer model
- Partial least squares structural equation modeling (PLS-SEM)
- PLS path modeling
- PLS regression
- PLS-SEM bias
- Ratio scale
- Reflective measurement model
- Secondary data
- Second-generation techniques
- Statistical power
- Structural equation modeling (SEM)
- Structural model
- Structural theory
- Variance-based SEM
After reading this chapter, you should:
Understand the principles of structural equation modeling (SEM)
Describe the basic elements of a structural equation model
Comprehend the basic concepts of partial least squares structural equation modeling (PLS-SEM)
Explain the differences between covariance-based structural equation modeling (CB-SEM) and PLS-SEM and when to use each of the approaches
1.1 What Is Structural Equation Modeling?
First-generation multivariate data analysis techniques, such as multiple regression, logistic regression, and analysis of variance, belong to the core set of statistical methods employed by researchers to empirically test hypothesized relationships between variables of interest. Numerous researchers in various scientific disciplines have applied these methods to generate findings that have significantly shaped the way we see the world today. These techniques have three important limitations in common, namely (1) the postulation of a simple model structure, (2) requiring that all variables can be considered observable, and (3) the assumption that all variables are measured without error (Haenlein & Kaplan, 2004 ).
With regard to the first limitation, multiple regression analysis and its extensions postulate a simple model structure involving one layer of dependent and independent variables. Causal chains, such as “A leads to B leads to C” or more complex nomological networks involving a large number of intervening variables, can only be estimated piecewise with these methods rather than simultaneously, which can have severe consequences for the quality of the results (Sarstedt, Hair, Nitzl, Ringle, & Howard, 2020 ).
With regard to the second limitation, regression-type methods are restricted to processing observable variables, such as age or sales (in units or dollars). Theoretical concepts, which are “abstract, unobservable properties or attributes of a social unit of entity” (Bagozzi & Philipps, 1982 , p. 465), can only be considered after prior stand-alone validation by means of, for example, a confirmatory factor analysis (CFA). The ex post inclusion of measures of theoretical concepts, however, comes with various shortcomings.
With regard to the third limitation and related to the previous point, one has to bear in mind that each observation of the real world is accompanied by a certain degree of measurement error , which can be systematic or random. First-generation techniques are, strictly speaking, only applicable when measured variables contain neither systematic nor random error. This situation is, however, rarely encountered in reality, particularly when the aim is to estimate relationships among measures of theoretical concepts. Since the social sciences, and many other fields of scientific inquiry, routinely deal with theoretical concepts, such as perceptions, attitudes, and intentions, these limitations of first-generation techniques are fundamental.
To overcome these limitations, researchers have increasingly been turning to second-generation techniques . These methods, referred to as structural equation modeling (SEM) , enable researchers to simultaneously model and estimate complex relationships among multiple dependent and independent variables. The concepts under consideration are typically unobservable and measured indirectly by multiple indicators. In estimating the relationships, SEM accounts for measurement error in observed variables. As a result, the method obtains a more precise measurement of the theoretical concepts of interest (Cole & Preacher, 2014 ). We will discuss these aspects in the following sections in greater detail.
Two popular methods dominate SEM in practice: covariance-based SEM (CB-SEM) and partial least squares SEM ( PLS-SEM , also called PLS path modeling ). CB-SEM is primarily used to confirm (or reject) theories and their underlying hypotheses. This approach confirms/rejects hypotheses by determining how closely a proposed theoretical model can reproduce the covariance matrix for an observed sample dataset. In contrast, PLS has been introduced as a “causal–predictive” approach to SEM (Jöreskog & Wold, 1982 , p. 270), which focuses on explaining the variance in the model’s dependent variables (Chin et al., 2020 ).
PLS-SEM is evolving rapidly as a statistical modeling technique. Over the last few decades, there have been numerous introductory articles on this methodology (e.g., Chin, 1998 ; Haenlein & Kaplan, 2004 ; Hair et al., 2020 ; Hair, Howard, & Nitzl, 2020 ; Hair, Risher, Sarstedt, & Ringle, 2019 ; Nitzl & Chin, 2017 ; Rigdon, 2013 ; Roldán & Sánchez-Franco, 2012 ; Tenenhaus, Esposito Vinzi, Chatelin, & Lauro, 2005 ; Wold, 1985 ) as well as review articles examining how researchers across different disciplines have used the method (◘ Table 1.1 ). In light of the increasing maturation of the field, researchers have also started exploring the knowledge infrastructure of methodological research on PLS-SEM by analyzing the structures of authors, countries, and co-citation networks (Hwang, Sarstedt, Cheah, & Ringle, 2020 ; Khan et al., 2019 ).
The remainder of this chapter first provides a brief introduction of measurement and structural theory as a basis for presenting the PLS-SEM method. In describing the PLS-SEM method’s characteristics, we also discuss distinguishing features vis-à-vis CB-SEM. Finally, we outline considerations when using PLS-SEM and highlight situations that favor its use compared to CB-SEM.
1.2 Principles of Structural Equation Modeling
1.2.1 path models with latent variables.
Path models are diagrams used to visually display the hypotheses and variable relationships that are examined when SEM is applied (Hair, Page, & Brunsveld, 2020 ; Hair, Ringle, & Sarstedt, 2011 ). An example of a path model is shown in ◘ Fig. 1.1 .
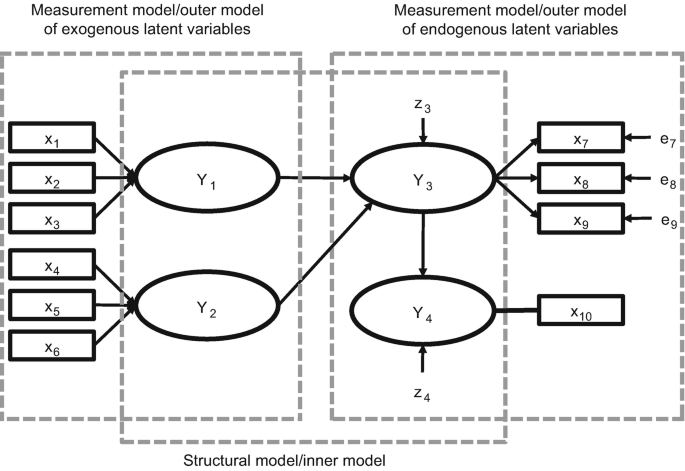
A simple path model. (Source: Hair et al., 2022 , Chap. 1; used with permission by Sage)
Constructs (i.e., variables that are not directly measurable), also referred to as latent variables , are represented in path models as circles or ovals ( Y 1 to Y 4 ). The indicators , also called items or manifest variables, are the directly measured variables that contain the raw data. They are represented in path models as rectangles ( x 1 to x 10 ). Relationships between constructs, as well as between constructs and their assigned indicators, are depicted as arrows. In PLS-SEM, the arrows are always single headed, thus representing directional relationships. Single-headed arrows are considered predictive relationships and, with strong theoretical support, can be interpreted as causal relationships.
A PLS path model consists of two elements. First, there is a structural model (also called the inner model in the context of PLS-SEM) that links together the constructs (circles or ovals). The structural model also displays the relationships (paths) between the constructs. Second, there are the measurement models (also referred to as the outer models in PLS-SEM) of the constructs that display the relationships between the constructs and the indicator variables (rectangles). In ◘ Fig. 1.1 , there are two types of measurement models: one for the exogenous latent variables (i.e., those constructs that only explain other constructs in the model) and one for the endogenous latent variables (i.e., those constructs that are being explained in the model). Rather than referring to measurement models of exogenous and endogenous latent variables, researchers often refer to the measurement model of one specific latent variable. For example, x 1 to x 3 are the indicators used in the measurement model of Y 1 , while Y 4 only includes the x 10 indicator in the measurement model.
The error terms (e.g., e 7 or e 8 ; ◘ Fig. 1.1 ) are connected to the (endogenous) constructs and (reflectively) measured variables by single-headed arrows. Error terms represent the unexplained variance when path models are estimated (i.e., the difference between the model’s in-sample prediction of a value and an observed value of a manifest or latent variable). Error terms e 7 to e 9 in ◘ Fig. 1.1 are connected to those indicators whose relationships point from the construct ( Y 3 ) to the indicators (i.e., reflectively measured indicators).
In contrast, the formatively measured indicators x 1 to x 6 , where the relationship goes from the indicator to the construct ( Y 1 and Y 2 ), do not have error terms (Sarstedt, Hair, Ringle, Thiele, & Gudergan, 2016 ). Finally, for the single-item construct Y 4 , the direction of the relationships between the construct and the indicator is not relevant, as construct and item are equivalent. For the same reason, there is no error term connected to x 10 . The structural model also contains error terms. In ◘ Fig. 1.1 , z 3 and z 4 are associated with the endogenous latent variables Y 3 and Y 4 (note that error terms on constructs and measured variables are labeled differently). In contrast, the exogenous latent variables ( Y 1 and Y 2 ) that only explain other latent variables in the structural model do not have an error term, regardless of whether they are specified reflectively or formatively.
1.2.2 Testing Theoretical Relationships
Path models are developed based on theory and are often used to test theoretical relationships. Theory is a set of systematically related hypotheses developed following the scientific method that can be used to explain and predict outcomes. Thus, hypotheses are individual conjectures, whereas theories are multiple hypotheses that are logically linked together and can be tested empirically. Two types of theory are required to develop path models: measurement theory and structural theory. Measurement theory specifies which indicators and how these are used to measure a certain theoretical concept. In contrast, structural theory specifies how the constructs are related to one another in the structural model.
Testing theory using PLS-SEM follows a two-step process (Hair, Black, Babin, & Anderson, 2019 ). We first test the measurement theory to confirm the reliability and validity of the measurement models. After the measurement models are confirmed, we move on to testing the structural theory. The logic is that we must first confirm the measurement theory before testing the structural theory, because structural theory cannot be confirmed if the measures are unreliable or invalid.
1.2.3 Measurement Theory
Measurement theory specifies how the latent variables (constructs) are measured. Generally, there are two different ways to measure unobservable variables. One approach is referred to as reflective measurement, and the other is formative measurement. Constructs Y 1 and Y 2 in ◘ Fig. 1.1 are modeled based on a formative measurement model . Note that the directional arrows are pointing from the indicator variables ( x 1 to x 3 for Y 1 and x 4 to x 6 for Y 2 ) to the construct, indicating a predictive (causal) relationship in that direction.
In contrast, Y 3 in ◘ Fig. 1.1 is modeled based on a reflective measurement model . With reflective indicators, the direction of the arrows is from the construct to the indicator variables, indicating the assumption that the construct “causes” the measurement (more precisely, the covariation) of the indicator variables. As indicated in ◘ Fig. 1.1 , reflective measures have an error term associated with each indicator, which is not the case with formative measures. The latter are assumed to be error-free (Diamantopoulos, 2006 ). Finally, note that Y 4 is measured using a single item rather than multi-item measures. Therefore, the relationship between construct and indicator is undirected.
Deciding whether to measure the constructs reflectively versus formatively and whether to use multiple items or a single-item measure is fundamental when developing path models. Hair, Hult, Ringle, and Sarstedt ( 2022 ; Chap. 2) explain these approaches to modeling constructs in more detail.
1.2.4 Structural Theory
Structural theory shows how the latent variables are related to one another (i.e., it shows the constructs and their path relationships in the structural model). The location and sequence of the constructs are either based on theory or the researcher’s experience and accumulated knowledge or both. When path models are developed, the sequence is from left to right. The variables on the left side of the path model are independent variables, and any variable on the right side is a dependent variable. Moreover, variables on the left are shown as sequentially preceding and predicting the variables on the right. However, when variables are in the middle of the path model (between the variables that serve only as independent or dependent variables – Y 3 ; ◘ Fig. 1.1 ), they serve as both independent and dependent variables in the structural model.
When latent variables only serve as independent variables, they are called exogenous latent variables ( Y 1 and Y 2 ). When latent variables only serve as dependent variables ( Y 4 ) or as both independent and dependent variables ( Y 3 ), they are called endogenous latent variables (◘ Fig. 1.1 ). Any latent variable that has only single-headed arrows going out of it is an exogenous latent variable. In contrast, endogenous latent variables can have either single-headed arrows going both into and out of them ( Y 3 ) or only going into them ( Y 4 ). Note that the exogenous latent variables Y 1 and Y 2 do not have error terms, since these constructs are the entities (independent variables) that are explaining the dependent variables in the path model.
1.3 PLS-SEM and CB-SEM
There are two main approaches to estimating the relationships in a structural equation model (Hair et al., 2011 ; Hair, Black, et al., 2019 ). One is CB-SEM, and the other is PLS-SEM, the latter being the focus of this book. Each is appropriate for a different research context, and researchers need to understand the differences in order to apply the correct method (Marcoulides & Chin, 2013 ; Rigdon, Sarstedt, & Ringle, 2017 ). Finally, some researchers have argued for using regressions based on sum scores, instead of some type of indicator weighting as is done by PLS-SEM. The sum scores approach offers practically no value compared to the PLS-SEM weighted approach and in fact can produce erroneous results (Hair et al., 2017 ). For this reason, in the following sections, we only briefly discuss sum scores and instead focus on the PLS-SEM and CB-SEM methods.
A crucial conceptual difference between PLS-SEM and CB-SEM relates to the way each method treats the latent variables included in the model. CB-SEM represents a common factor-based SEM method that considers the constructs as common factors that explain the covariation between its associated indicators. This approach is consistent with the measurement philosophy underlying reflective measurement, in which the indicators and their covariations are regarded as manifestations of the underlying construct. In principle, CB-SEM can also accommodate formative measurement models, even though the method follows a common factor model estimation approach. To estimate this model type, however, researchers must follow rules that require specific constraints on the model to ensure model identification (Bollen & Davies, 2009 ; Diamantopoulos & Riefler, 2011 ), which means that the method can calculate estimates for all model parameters. As Hair, Sarstedt, Ringle, and Mena ( 2012 , p. 420) note, “[t]hese constraints often contradict theoretical considerations, and the question arises whether model design should guide theory or vice versa.”
PLS-SEM, on the other hand, assumes the concepts of interest can be measured as composites (Jöreskog & Wold, 1982 ), which is why PLS is considered a composite-based SEM method (Hwang et al., 2020 ). Model estimation in PLS-SEM involves linearly combining the indicators of a measurement model to form composite variables. The composite variables are assumed to be comprehensive representations of the constructs, and, therefore, valid proxies of the conceptual variables being examined (e.g., Hair & Sarstedt, 2019 ). The composite-based approach is consistent with the measurement philosophy underlying formative measurement, but this does not imply that PLS-SEM is only capable of estimating formatively specified constructs. The reason is that the estimation perspective (i.e., forming composites to represent conceptual variables) should not be confused with the measurement theory perspective (i.e., specifying measurement models as reflective or formative). The way a method like PLS-SEM estimates the model parameters needs to be clearly distinguished from any measurement theoretical considerations on how to operationalize constructs (Sarstedt et al., 2016 ). Researchers can include reflectively and formatively specified measurement models that PLS-SEM can straightforwardly estimate.
In following a composite-based approach to SEM, PLS relaxes the strong assumption of CB-SEM that all of the covariation between the sets of indicators is explained by a common factor (Henseler et al., 2014 ; Rigdon, 2012 ; Rigdon et al., 2014 ). At the same time, using weighted composites of indicator variables facilitates accounting for measurement error, thus making PLS-SEM superior compared to multiple regression using sum scores , where each indicator is weighted equally.
It is important to note that the composites produced by PLS-SEM are not assumed to be identical to the theoretical concepts, which they represent. They are explicitly recognized as approximations (Rigdon, 2012 ). As a consequence, some scholars view CB-SEM as a more direct and precise method to empirically measure theoretical concepts (e.g., Rönkkö, McIntosh, & Antonakis, 2015 ), while PLS-SEM provides approximations. Other scholars contend, however, that such a view is quite shortsighted, since common factors derived in CB-SEM are also not necessarily equivalent to the theoretical concepts that are the focus of the research (Rigdon, 2012 ; Rigdon et al., 2017 ; Rossiter, 2011 ; Sarstedt et al., 2016 ). Rigdon, Becker, and Sarstedt ( 2019a ) show that common factor models can be subject to considerable degrees of metrological uncertainty. Metrological uncertainty refers to the dispersion of the measurement values that can be attributed to the object or concept being measured (JCGM/WG1, 2008 ). Numerous sources contribute to metrological uncertainty such as definitional uncertainty or limitations related to the measurement scale design, which go well beyond the simple standard errors produced by CB-SEM analyses (Hair & Sarstedt, 2019 ). As such, uncertainty is a validity threat to measurement and has adverse consequences for the replicability of study findings (Rigdon, Sarstedt, & Becker, 2020 ). While uncertainty also applies to composite-based SEM, the way researchers treat models in CB-SEM analyses typically leads to a pronounced increase in uncertainty (Rigdon & Sarstedt, 2021 ). More precisely, in an effort to improve model fit, researchers typically reduce the number of indicators per construct, which in turn increases uncertainty (Hair, Matthews, Matthews, & Sarstedt, 2017 ; Rigdon et al., 2019a ). These issues do not necessarily imply that composite models are superior, but they cast considerable doubt on the assumption of some researchers that CB-SEM constitutes the gold standard when measuring unobservable concepts. In fact, researchers in various fields of science show increasing appreciation that common factors may not always be the right approach to measure concepts (e.g., Rhemtulla, van Bork, & Borsboom, 2020 ; Rigdon, 2016 ). Similarly, Rigdon, Becker, and Sarstedt ( 2019b ) show that using sum scores can significantly increase the degree of metrological uncertainty, which questions this measurement practice.
Apart from differences in the philosophy of measurement, the differing treatment of latent variables and, more specifically, the availability of construct scores also have consequences for the methods’ areas of application. Specifically, while it is possible to estimate latent variable scores within a CB-SEM framework, these estimated scores are not unique. That is, an infinite number of different sets of latent variable scores that will fit the model equally well are possible. A crucial consequence of this factor (score) indeterminacy is that the correlations between a common factor and any variable outside the factor model are themselves indeterminate (Guttman, 1955 ). That is, they may be high or low, depending on which set of factor scores one chooses. As a result, this limitation makes CB-SEM grossly unsuitable for prediction (e.g., Dijkstra, 2014 ; Hair & Sarstedt, 2021 ). In contrast, a major advantage of PLS-SEM is that it always produces a single specific (i.e., determinate) score for each composite of each observation, once the indicator weights/loadings are established. These determinate scores are proxies of the theoretical concepts being measured, just as factors are proxies for the conceptual variables in CB-SEM (Rigdon et al., 2017 ; Sarstedt et al., 2016 ).
Using these proxies as input, PLS-SEM applies ordinary least squares regression with the objective of minimizing the error terms (i.e., the residual variance) of the endogenous constructs. In short, PLS-SEM estimates coefficients (i.e., path model relationships) with the goal of maximizing the R 2 values of the endogenous (target) constructs. This feature achieves the (in-sample) prediction objective of PLS-SEM (Hair & Sarstedt, 2021 ), which is therefore the preferred method when the research objective is theory development and explanation of variance (prediction of the constructs). For this reason, PLS-SEM is also regarded a variance-based SEM approach. Specifically, the logic of the PLS-SEM approach is that all of the indicators’ variance should be used to estimate the model relationships, with particular focus on prediction of the dependent variables (e.g., McDonald, 1996 ). In contrast, CB-SEM divides the total variance into three types – common, unique, and error variance – but utilizes only common variance (i.e., the variance shared with other indicators in the same measurement model) for the model estimation (Hair, Black, et al., 2019 ). That is, CB-SEM only explains the covariation between measurement and structural model indicators (Jöreskog, 1973 ) and does not focus on predicting dependent variables (Hair, Matthews, et al., 2017 ).
PLS-SEM is similar but not equivalent to PLS regression , another popular multivariate data analysis technique (Abdi, 2010 ; Wold, Sjöström, & Eriksson, 2001 ). PLS regression is a regression-based approach that explores the linear relationships between multiple independent variables and a single or multiple dependent variable(s). PLS regression differs from regular regression, however, because, in developing the regression model, the method derives composite factors from the multiple independent variables by means of principal component analysis. PLS-SEM, on the other hand, relies on prespecified networks of relationships between constructs as well as between constructs and their measures (see Mateos-Aparicio, 2011 , for a more detailed comparison between PLS-SEM and PLS regression).
1.4 Considerations When Applying PLS-SEM
1.4.1 key characteristics of the pls-sem method.
Several considerations are important when deciding whether or not to apply PLS-SEM. These considerations also have their roots in the method’s characteristics. The statistical properties of the PLS-SEM algorithm have important features associated with the characteristics of the data and model used. Moreover, the properties of the PLS-SEM method affect the evaluation of the results. Four critical issues are relevant to the application of PLS-SEM (Hair et al., 2011 ; Hair, Risher, et al., 2019 ): (1) data characteristics, (2) model characteristics, (3) model estimation, and (4) model evaluation. ◘ Table 1.2 summarizes the key characteristics of the PLS-SEM method. An initial overview of these issues is provided in this chapter. For a more detailed explanation, see Hair et al. ( 2022 ).
PLS-SEM works efficiently with small sample sizes and complex models (Cassel, Hackl, & Westlund, 1999 ; Hair, Sarstedt, & Ringle, 2019 ). In addition, different from maximum likelihood-based CB-SEM, which requires normally distributed data, PLS-SEM makes no distributional assumptions (i.e., it is nonparametric). PLS-SEM can easily handle reflective and formative measurement models, as well as single-item constructs, with no identification problems. It can therefore be applied in a wide variety of research situations. When applying PLS-SEM, researchers also benefit from high efficiency in parameter estimation, which is manifested in the method’s greater statistical power in comparison to that of CB-SEM. Greater statistical power means that PLS-SEM is more likely to render a specific relationship significant when it is in fact present in the population. The same holds for the comparison with regression using sum score data, which is also characterized by lower statistical power than PLS-SEM (Hair, Hollingsworth, et al., 2017 ).
There are, however, several limitations of PLS-SEM. In its basic form, the technique cannot be applied when structural models contain causal loops or circular relationships between the latent variables (i.e., non-recursive models). Early extensions of the basic PLS-SEM algorithm that have not yet been implemented in standard PLS-SEM software packages, however, enable the handling of circular relationships (Lohmöller, 1989 ). Furthermore, since PLS-SEM does not have an established global goodness-of-fit measure, its use for theory testing and confirmation is more limited in certain situations. Recent research has attempted to promote common goodness-of-fit measures within a PLS-SEM framework (Schuberth, Henseler, & Dijkstra, 2018 ) but with very limited success. The concept of model fit – as defined in CB-SEM – is not applicable to PLS-SEM because of the methods’ different functioning principles (Hair, Sarstedt, & Ringle, 2019 ). Instead, PLS-SEM-based model estimation and assessment follow a causal–predictive paradigm, in which the objective is to test the predictive power of a model, derived from theory and logic. As such, the method strikes a balance between machine learning methods, which are fully predictive in nature and CB-SEM, which focuses on confirmation and model fit (Richter, Cepeda Carrión, Roldán, & Ringle, 2016 ). Its causal–predictive nature makes PLS-SEM particularly appealing for research in fields that aim to derive recommendations for practice. For example, recommendations in managerial implication sections in business research journals always include predictive statements (“our results suggest that managers should…”). Making such statements requires a prediction focus on model estimation and evaluation (Sarstedt & Danks, 2021 ). PLS-SEM perfectly emphasizes this need as the method sheds light on the mechanisms (i.e., the structural model relationships) through which the predictions are generated (Hair, 2020 ; Hair & Sarstedt, 2019 , 2021 ).
In early writing, researchers noted that PLS estimation is “deliberately approximate” to factor-based SEM (Hui & Wold, 1982 , p. 127), a characteristic previously referred to as the PLS-SEM bias (e.g., Chin, Marcolin, & Newsted, 2003 ). A number of studies have used simulations to demonstrate the alleged PLS-SEM bias (e.g., Goodhue, Lewis, & Thompson, 2012 ; McDonald, 1996 ; Rönkkö & Evermann, 2013 ). According to prior research on the PLS-SEM bias, measurement model estimates are biased upward, whereas structural model estimates are biased downward compared to CB-SEM results. The studies conclude that parameter estimates will approach what has been labeled the “true” parameter values when both the number of indicators per construct and sample size increase (Hui & Wold, 1982 ). However, all the abovementioned simulation studies used CB-SEM as the benchmark against which the PLS-SEM estimates were evaluated with the assumption that they should be the same. Because PLS-SEM is a composite-based approach, which uses the total variance to estimate parameters, differences can be expected in such an assessment (Lohmöller, 1989 ; Schneeweiß, 1991 ). Not surprisingly, the very same issues apply when composite models are used to estimate CB-SEM results. In fact, Sarstedt et al. ( 2016 ) show that the bias produced by CB-SEM is far more severe than that of PLS-SEM, when applying the method to the wrong type of model (i.e., estimating composite models with CB-SEM versus estimating common factor models with PLS-SEM). Apart from these conceptual concerns, simulation studies show that the differences between PLS-SEM and CB-SEM estimates, when assuming the latter as a standard of comparison, are very small, provided that measurement models meet minimum recommended standards in terms of measurement quality (i.e., reliability and validity). Specifically, when the measurement models have four or more indicators and indicator loadings meet the common standards (≥ 0.70), there are practically no differences between the two methods in terms of parameter accuracy (e.g., Reinartz, Haenlein, & Henseler, 2009 ; Sarstedt et al., 2016 ). Thus, the extensively discussed PLS-SEM bias is of no practical relevance for the vast majority of applications (e.g., Binz Astrachan, Patel, & Wanzenried, 2014 ).
Finally, methodological research has substantially extended the original PLS-SEM method by introducing advanced modeling, assessment, and analysis procedures. Examples include different types of robustness checks (Sarstedt et al., 2020 ), higher-order constructs (Sarstedt, Hair, Cheah, Becker, & Ringle, 2019 ), discrete choice modeling (Hair, Sarstedt, & Ringle, 2019 ), necessary condition analysis and related methods (Rasoolimanesh, Ringle, Sarstedt, & Olya, 2021 ; Richter, Schubring, Hauff, Ringle, & Sarstedt, 2020 ), and out-of-sample prediction metrics (Hair, 2020 ). Hair, Sarstedt, Ringle, and Gudergan ( 2018 ) offer an introduction into several of these advanced topics.
In the following, we discuss aspects related to data characteristics (e.g., minimum sample size requirements) and model characteristics (e.g., model complexity).
1.4.2 Data Characteristics
Data characteristics, such as minimum sample size requirements, non-normal data, and scales of measurement (i.e., the use of different scale types), are among the most often stated reasons for applying PLS-SEM across numerous disciplines (e.g., Ghasemy, Teeroovengadum, Becker, & Ringle, 2020 ; Hair, Sarstedt, Ringle, & Mena, 2012 ; Ringle et al., 2020 ). While some of the arguments are consistent with the method’s capabilities, others are not. In the following sections, we discuss these and also aspects related data characteristics.
1.4.2.1 Minimum Sample Size Requirements
Small sample size is likely the most often abused reason stated for using PLS-SEM, with some researchers obtaining model solutions with unacceptably low sample sizes (Goodhue et al., 2012 ; Marcoulides & Saunders, 2006 ). These researchers oftentimes believe there is some “magic” in the PLS-SEM approach that allows them to use a very small sample to obtain results representing the effects that exist in large populations of several million elements or individuals. No multivariate analysis technique, including PLS-SEM, has this kind of “magic” inferential capability (Petter, 2018 ).
PLS-SEM can certainly obtain solutions with smaller samples, but the population’s nature determines the situations in which small sample sizes are acceptable (Rigdon, 2016 ). For example, in business-to-business research, populations are often restricted in size. Assuming that other situational characteristics are equal, the more heterogeneous the population, the larger the sample size needed to achieve an acceptable accuracy (Cochran, 1977 ). If basic sampling theory guidelines are not considered (Sarstedt, Bengart, Shaltoni, & Lehmann, 2018 ), questionable results are produced.
In addition, when applying multivariate analysis techniques, the technical dimension of the sample size becomes relevant. Adhering to the minimum sample size guidelines ensures the results of a statistical method, such as PLS-SEM, have adequate statistical power. In these regards, an analysis based on an insufficient sample size may not reveal an effect that exists in the underlying population (which results in committing a type II error). Moreover, executing statistical analyses based on minimum sample size guidelines will ensure the results of the statistical method are robust and the model is generalizable to another sample from that same population. Thus, an insufficient sample size may lead to PLS-SEM results that differ from those of another larger sample. In the following, we focus on the PLS-SEM method and its technical requirements of the minimum sample size.
The overall complexity of a structural model has little influence on the minimum sample size requirements for PLS-SEM. The reason is the PLS-SEM algorithm does not compute all relationships in the structural model at the same time. Instead, it uses ordinary least squares regressions to estimate the model’s partial regression relationships. Two early studies systematically evaluated the performance of PLS-SEM with small sample sizes and concluded that the method performed well (e.g., Chin & Newsted, 1999 ; Hui & Wold, 1982 ). More recently, simulation studies by Hair et al. (2017) and Reinartz et al. ( 2009 ) indicate that PLS-SEM is the method of choice when the sample size is small. Moreover, compared with its covariance-based counterpart, PLS-SEM has higher levels of statistical power in situations with complex model structures and smaller sample sizes. Similarly, Henseler et al. ( 2014 ) show that results can be obtained with PLS-SEM when other methods do not converge or provide inadmissible solutions. For example, problems often are encountered when using CB-SEM on complex models, especially when the sample size is limited. Finally, CB-SEM encounters identification and convergence issues when formative measures are involved (e.g., Diamantopoulos & Riefler, 2011 ).
Unfortunately, some researchers believe sample size considerations do not play a role in the application of PLS-SEM. This idea has been fostered by the often-cited 10-time rule (Barclay, Higgins, & Thompson, 1995 ), which suggests the sample size should be equal to 10 times the number of independent variables in the most complex regression in the PLS path model (i.e., considering both measurement and structural models). This rule of thumb is equivalent to saying the minimum sample size should be 10 times the maximum number of arrowheads pointing at a latent variable anywhere in the PLS path model. While this rule offers a rough guideline, the minimum sample size requirement should consider the statistical power of the estimates. To assess statistical power, researchers can consider power tables (Cohen, 1992 ) or power analyses using programs, such as G*Power (Faul, Erdfelder, Buchner, & Lang, 2009 ), which is available free of charge at ► http://www.gpower.hhu.de/ . These approaches do not explicitly consider the entire model but instead use the most complex regression in the (formative) measurement models and structural model of a PLS path model as a point of reference for assessing the statistical power. In doing so, researchers typically aim at achieving a power level of 80%. However, the minimum sample size resulting from these calculations may still be too small (Kock & Hadaya, 2018 ).
Addressing these concerns, Kock and Hadaya ( 2018 ) proposed the inverse square root method , which considers the probability that the ratio of a path coefficient and its standard error will be greater than the critical value of a test statistic for a specific significance level. The results depend, therefore, on only one path coefficient and are dependent neither on the size of the most complex regression in the (formative) models nor on the size of the overall model. Assuming a common power level of 80% and significance levels of 1%, 5%, and 10%, the minimum sample size (n min ) is given by the following equations, respectively, where p min is the value of the path coefficient with the minimum magnitude in the PLS path model:
Significance level = 1%: \( {n}_{\mathrm{min}}>{\left(\frac{3.168}{\left|{p}_{\mathrm{min}}\right|}\right)}^2 \) .
Significance level = 5%: \( {n}_{\mathrm{min}}>{\left(\frac{2.486}{\left|{p}_{\mathrm{min}}\right|}\right)}^2 \) .
Significance level = 10%: \( {n}_{\mathrm{min}}>{\left(\frac{2.123}{\left|{p}_{\mathrm{min}}\right|}\right)}^2 \) .
For example, assuming a significance level of 5% and a minimum path coefficient of 0.2, the minimum sample size is given by
This result needs to be rounded to the next integer, so the minimum sample size is 155.
The inverse square root method is rather conservative, in that it slightly overestimates the sample size required to render an effect significant at a given power level. Most importantly, the method is characterized by its ease of use, since it can be readily implemented.
Nevertheless, two considerations are important when using the inverse square root method. First, by using the smallest statistical path coefficient as the point of reference, the method can be misleading as researchers will not expect marginal effects to be significant. For example, assuming a 5% significance level and a minimum path coefficient of 0.01 would require a sample size of 61,802! Hence, researchers should choose a higher path coefficient as input, depending on whether the model produces either overall weak or strong effects or the smallest relevant (to be detected) effect.
Second, by relying on model estimates, the inverse square root method follows a retrospective approach. As a result, this assessment approach can be used as a basis for additional data collection or adjustments in the model. If possible, however, researchers should follow a prospective approach by trying to derive the minimum expected effect size prior to data analysis. To do so, researchers can draw on prior research involving a comparable conceptual background or models with similar complexity or, preferably, the results of a pilot study, which tested the hypothesized model using a smaller sample of respondents from the same population. For example, if the pilot study produced a minimum path coefficient of 0.15, this value should be chosen as input for computing the required sample size for the main study.
In most cases, however, researchers have only limited information regarding the expected effect sizes, even if a pilot study has been conducted. Hence, it is reasonable to consider ranges of effect sizes rather than specific values to determine the sample size required for a specific study. ◘ Table 1.3 shows the minimum sample size requirement for different significance levels and varying ranges of p min . In deriving the minimum sample size, it is reasonable to consider the upper boundary of the effect range as reference, since the inverse square root method is rather conservative. For example, when assuming that the minimum path coefficient expected to be significant is between 0.11 and 0.20, one would need approximately 155 observations to render the corresponding effect significant at 5%. Similarly, if the minimum path coefficient expected to be significant is between 0.31 and 0.40, then the recommended sample size would be 39.
1.4.2.2 Missing Value Treatment
As with other statistical analyses, missing values should be dealt with when using PLS-SEM. For reasonable limits (i.e., less than 5% values missing per indicator), missing value treatment options, such as mean replacement, the EM (expectation–maximization) algorithm, and nearest neighbor (e.g., Hair, Black, et al., 2019 ), generally result in only slightly different PLS-SEM estimates (Grimm & Wagner, 2020 ). Alternatively, researchers can opt for deleting all observations with missing values, which decreases variation in the data and may introduce biases when certain groups of observations have been deleted systematically.
1.4.2.3 Non-normal Data
The use of PLS-SEM has two other key advantages associated with data characteristics (i.e., distribution and scales). In situations where it is difficult or impossible to meet the stricter requirements of more traditional multivariate techniques (e.g., normal data distribution), PLS-SEM is always the preferred method. PLS-SEM’s greater flexibility is described by the label “soft modeling,” coined by Wold ( 1982 ), who developed the method. It should be noted, however, that “soft” is attributed only to the distributional assumptions and not to the concepts, models, or estimation techniques (Lohmöller, 1989 ). PLS-SEM’s statistical properties provide very robust model estimations with data that have normal as well as extremely non-normal (i.e., skewness and/or kurtosis) distributional properties (Hair, Hollingsworth, et al., 2017 ; Hair, Matthews, et al., 2017 ; Reinartz et al., 2009 ). It must be remembered, however, that influential observations, outliers, and collinearity do influence the ordinary least squares regressions in PLS-SEM and researchers should evaluate the data and results for these issues (Hair, Black, et al., 2019 ).
1.4.2.4 Scales of Measurement
The PLS-SEM algorithm generally requires variables to be measured on a metric scale ( ratio scale or interval scale ) for the measurement model indicators. But the method also works well with ordinal scales with equidistant data points (i.e., quasi-metric scales; Sarstedt & Mooi, 2019 ; Chap. 3.6) and with binary-coded data. The use of binary-coded data is often a means of including categorical control variables (Hair et al., 2022 ) or moderators in PLS-SEM models. In short, binary indicators can be included in PLS-SEM models but require special attention. For example, using PLS-SEM in discrete choice experiments, where the aim is to explain or to predict a binary dependent variable, requires specific designs and estimation routines (Hair, Ringle, Gudergan, Fischer, Nitzl, & Menictas, 2019 ).
1.4.2.5 Secondary Data
Secondary data are data that have already been gathered, often for a different research purpose some time ago (Sarstedt & Mooi, 2019 ; Chap. 3.2.1). Secondary data are increasingly available to explore real-world phenomena. Research based on secondary data typically focuses on a different objective than in a standard CB-SEM analysis, which is strictly confirmatory in nature. More precisely, secondary data are mainly used in exploratory research to propose causal–predictive relationships in situations that have little clearly defined theory (Hair, Matthews, et al., 2017 ; Hair, Hollingsworth, et al., 2017 ). Such settings require researchers to place greater emphasis on examining all possible relationships rather than achieving model fit (Nitzl, 2016 ). By its nature, this process creates large, complex models that can hardly be analyzed with the CB-SEM method. In contrast, due to its less stringent data requirements, PLS-SEM offers the flexibility needed for the interplay between theory and data (Nitzl, 2016 ). Or, as Wold ( 1982 , p. 29) notes, “soft modeling is primarily designed for research contexts that are simultaneously data-rich and theory-skeletal.” Furthermore, the increasing popularity of secondary data analysis (e.g., by using data that stem from company databases, social media, customer tracking, national statistical bureaus, or publicly available survey data) shifts the research focus from strictly confirmatory to predictive and causal–predictive modeling. Such research settings are a perfect fit for the prediction-oriented PLS-SEM approach and even more so when assessing out-of-sample prediction (Shmueli, et al., 2019).
PLS-SEM also proves valuable for analyzing secondary data from a measurement theory perspective. First, unlike survey measures, which are usually crafted to confirm a well-developed theory, measures used in secondary data sources are typically not created and refined over time for confirmatory analyses. Thus, achieving model fit is very unlikely with secondary data measures in most research situations when using CB-SEM. Second, researchers who use secondary data do not have the opportunity to revise or refine the measurement model to achieve fit. Third, a major advantage of PLS-SEM when using secondary data is that it permits the unrestricted use of single-item and formative measures. This is extremely valuable for research involving secondary data, because many measures included in corporate databases are artifacts, such as financial ratios and other firm-fixed factors (Henseler, 2017 ). Such artifacts typically are reported in the form of formative indices whose estimation dictates the use of PLS-SEM.
◘ Table 1.4 summarizes key considerations related to data characteristics.
1.4.3 Model Characteristics
PLS-SEM is very flexible in its modeling properties. In its basic form, the PLS-SEM algorithm requires all models to not include circular relationships or loops of relationships between the latent variables in the structural model. Although causal loops are sometimes specified in business research, this characteristic does not limit the applicability of PLS-SEM, if such models are required as Lohmöller’s ( 1989 ) extensions of the basic PLS-SEM algorithm allow for handling such model types. Other model specification requirements that constrain the use of CB-SEM, such as distribution and identification assumptions, are generally not relevant with PLS-SEM.
Measurement model difficulties are one of the major obstacles to obtaining a solution with CB-SEM. For instance, estimation of complex models with many latent variables and/or indicators is often impossible with CB-SEM. In contrast, PLS-SEM can easily be applied in such situations, since this method is not constrained by identification and other technical issues. Consideration of reflective and formative measurement models is a key issue in the application of SEM (Bollen & Diamantopoulos, 2017 ). PLS-SEM can easily handle both formative and reflective measurement models and is (therefore) considered the primary approach when the hypothesized model incorporates formative measures. CB-SEM can accommodate formative indicators, but to ensure model identification, they must follow distinct specification rules (Diamantopoulos & Riefler, 2011 ). In fact, the requirements often prevent running the analysis as originally planned. In contrast, PLS-SEM does not have such requirements and handles formative measurement models without any limitation. This also applies to model settings in which endogenous constructs are measured formatively. The applicability of CB-SEM to such model settings has been subject to considerable debate (Cadogan & Lee, 2013 ; Rigdon, 2014 ), but due to PLS-SEM’s multistage estimation process (Tenenhaus et al., 2005 ), which separates measurement from structural model estimation, the inclusion of formatively measured endogenous constructs is not an issue in PLS-SEM (Rigdon et al., 2014 ). The only problematic issue is when high levels of collinearity exist between the indicator variables of a formative measurement model.
Different from CB-SEM, PLS-SEM facilitates easy specification of interaction terms to map moderation effects in a path model. This makes PLS-SEM the method of choice in simple moderation models and more complex conditional process models, which combine moderation and mediation effects (Sarstedt, Hair, et al., 2020 ). Similarly, higher-order constructs, which allow specifying a construct simultaneously on different levels of abstraction (Sarstedt et al., 2019 ), can readily be implemented in PLS-SEM.
Finally, PLS-SEM is capable of estimating very complex models. For example, if theoretical or conceptual assumptions support large models and sufficient data are available (i.e., meeting minimum sample size requirements), PLS-SEM can handle models of almost any size, including those with dozens of constructs and hundreds of indicator variables. As noted by Wold ( 1985 ), PLS-SEM is virtually without competition when path models with latent variables are complex in their structural relationships. ◘ Table 1.5 summarizes rules of thumb for PLS-SEM model considerations.
1.5 Guidelines for Choosing Between PLS-SEM and CB-SEM
Summarizing the previous discussions and drawing on Hair, Risher, et al. ( 2019 ), ◘ Table 1.6 displays the rules of thumb applied when deciding whether to use CB-SEM or PLS-SEM. As can be seen, PLS-SEM is not recommended as a universal alternative to CB-SEM. Both methods differ from a statistical point of view, are designed to achieve different objectives, and rely on different measurement philosophies. Neither of the techniques is generally superior to the other, and neither of them is appropriate for all situations (Petter, 2018 ). Hence, to answer the question of when to use PLS-SEM versus CB-SEM, researchers should focus on the characteristics and objectives that distinguish the two methods (Hair, Sarstedt, Ringle, & Mena, 2012 ). Broadly speaking, with its strong focus on model fit and in light of its extensive data requirements, CB-SEM is particularly suitable for testing a theory in the confinement of a concise theoretical model. However, if the primary research objective is prediction and explanation of target constructs (Rigdon, 2012 ), PLS-SEM should be given preference (Hair, Sarstedt, & Ringle, 2019 ; Hair, Hollingsworth, Randolph, & Chong, 2017 ).
In general, the strengths of PLS-SEM are CB-SEM’s limitations and vice versa, although PLS-SEM is increasingly being applied for scale development and confirmation (Hair, Howard, & Nitzl, 2020 ). It is important that researchers understand the different applications each approach was developed for and use them accordingly. Researchers need to apply the SEM technique that best suits their research objective, data characteristics, and model setup (Roldán & Sánchez-Franco, 2012 ).
SEM is a second-generation multivariate data analysis method, which facilitates analyzing the relationships among constructs, each measured by one or more indicator variables. The primary advantage of SEM is its ability to measure complex model relationships while accounting for measurement error inherent in the indicators. There are two types of SEM methods – CB-SEM and PLS-SEM. The two method types differ in the way they estimate the model parameters and their assumptions regarding the nature of measurement. Compared to CB-SEM, PLS-SEM emphasizes prediction, while simultaneously relaxing the demands regarding the data and specification of relationships. PLS-SEM aims at maximizing the endogenous latent variables’ explained variance by estimating partial model relationships in an iterative sequence of ordinary least squares regressions. In contrast, CB-SEM estimates model parameters, such that the discrepancy between the estimated and sample covariance matrices is minimized. Instead of following a common factor model logic in estimating concept proxies as CB-SEM does, PLS-SEM calculates composites of indicators that serve as proxies for the concepts under research. The method is not constrained by identification issues, even if the model becomes complex – a situation that typically restricts CB-SEM use – and does not rely on distributional assumptions. Moreover, PLS-SEM can better handle formative measurement models and has advantages when sample sizes are relatively small as well as when analyzing secondary data. Researchers should consider the two SEM approaches as complementary and apply the SEM technique that best suits their research objective, data characteristics, and model setup.
Please answer the following questions:
When would SEM methods be more advantageous than first-generation techniques in understanding relationships between variables?
Why should social science researchers consider using SEM instead of multiple regression?
What are the most important considerations in deciding whether to use CB-SEM or PLS-SEM?
Under what circumstances is PLS-SEM the preferred method over CB-SEM?
Why is an understanding of theory important when deciding whether to use PLS-SEM or CB-SEM?
Why is PLS-SEM’s prediction focus a major advantage of the method?
Abdi, H. (2010). Partial least squares regression and projection on latent structure regression (PLS-Regression). WIREs Computational Statistics, 2 (1), 97–106.
Article Google Scholar
Ali, F., Rasoolimanesh, S. M., Sarstedt, M., Ringle, C. M., & Ryu, K. (2018). An assessment of the use of partial least squares structural equation modeling (PLS-SEM) in hospitality research. The International Journal of Contemporary Hospitality Management, 30 (1), 514–538.
Bagozzi, R. P., & Philipps, L. W. (1982). Representing and testing organizational theories: A holistic construal. Administrative Science Quarterly, 27 (3), 459–489.
Barclay, D. W., Higgins, C. A., & Thompson, R. (1995). The partial least squares approach to causal modeling: Personal computer adoption and use as illustration. Technology Studies, 2 (2), 285–309.
Google Scholar
Bayonne, E., Marin-Garcia, J. A., & Alfalla-Luque, R. (2020). Partial least squares (PLS) in operations management research: Insights from a systematic literature review. Journal of Industrial Engineering and Management, 13 (3), 565–597.
Binz Astrachan, C. B., Patel, V. K., & Wanzenried, G. (2014). A comparative study of CB-SEM and PLS-SEM for theory development in family firm research. Journal of Family Business Strategy, 5 (1), 116–128.
Bollen, K. A., & Davies, W. R. (2009). Causal indicator models: Identification, estimation, and testing. Structural Equation Modeling, 16 (3), 498–522.
Bollen, K. A., & Diamantopoulos, A. (2017). In defense of causal-formative indicators: A minority report. Psychological Methods, 22 (3), 581–596.
Cadogan, J. W., & Lee, N. (2013). Improper use of endogenous formative variables. Journal of Business Research, 66 (2), 233–241.
Cassel, C., Hackl, P., & Westlund, A. H. (1999). Robustness of partial least squares method for estimating latent variable quality structures. Journal of Applied Statistics, 26 (4), 435–446.
Cepeda Carrión, G., Cegarra-Navarro, J.-G., & Cillo, V. (2019). Tips to use partial least squares structural equation modelling (PLS-SEM) in knowledge management. Journal of Knowledge Management, 23 (1), 67–89.
Chin, W. W. (1998). The partial least squares approach to structural equation modeling. In G. A. Marcoulides (Ed.), Modern methods for business research (pp. 295–358). Mahwah, NJ: Erlbaum.
Chin, W. W., Cheah, J.-H., Liu, Y., Ting, H., Lim, X.-J., & Cham, T. H. (2020). Demystifying the role of causal-predictive modeling using partial least squares structural equation modeling in information systems research. Industrial Management & Data Systems, 120 (12), 2161–2209.
Chin, W. W., Marcolin, B. L., & Newsted, P. R. (2003). A partial least squares latent variable modeling approach for measuring interaction effects: Results from a Monte Carlo simulation study and an electronic-mail emotion/adoption study. Information Systems Research, 14 (2), 189–217.
Chin, W. W., & Newsted, P. R. (1999). Structural equation modeling analysis with small samples using partial least squares. In R. H. Hoyle (Ed.), Statistical strategies for small sample research (pp. 307–341). Thousand Oaks, CA: Sage.
Cochran, W. G. (1977). Sampling techniques . New York, NY: Wiley.
Cohen, J. (1992). A power primer. Psychological Bulletin, 112 (1), 155–159.
Cole, D. A., & Preacher, K. J. (2014). Manifest variable path analysis: Potentially serious and misleading consequences due to uncorrected measurement error. Psychological Methods, 19 (2), 300–315.
Diamantopoulos, A. (2006). The error term in formative measurement models: Interpretation and modeling implications. Journal of Modelling in Management, 1 (1), 7–17.
Diamantopoulos, A., & Riefler, P. (2011). Using formative measures in international marketing models: A cautionary tale using consumer animosity as an example. In M. Sarstedt, M. Schwaiger, & C. R. Taylor (Eds.), Measurement and research methods in international marketing (Advances in International Marketing, 22) (pp. 11–30). Bingley: Emerald.
Dijkstra, T. K. (2014). PLS’ Janus face—Response to Professor Rigdon’s “Rethinking partial least squares modeling: In praise of simple methods”. Long Range Planning, 47 (3), 146–153.
do Valle, P. O., & Assaker, G. (2016). Using partial least squares structural equation modeling in tourism research: A review of past research and recommendations for future applications. Journal of Travel Research, 55 (6), 695–708.
Faul, F., Erdfelder, E., Buchner, A., & Lang, A.-G. (2009). Statistical power analyses using G*Power 3.1: Tests for correlation and regression analyses. Behavior Research Methods, 41 (4), 1149–1160.
Ghasemy, M., Teeroovengadum, V., Becker, J.-M., & Ringle, C. M. (2020). This fast car can move faster: A review of PLS-SEM application in higher education research. Higher Education, 80 , 1121–1152.
Goodhue, D. L., Lewis, W., & Thompson, R. (2012). Does PLS have advantages for small sample size or non-normal data? MIS Quarterly, 36 (3), 981–1001.
Grimm, M. S., & Wagner, R. (2020). The impact of missing values on PLS, ML and FIML model fit. Archives of Data Science, Series A , 6 (1), 04.
Guttman, L. (1955). The determinacy of factor score matrices with implications for five other basic problems of common-factor theory. British Journal of Statistical Psychology, 8 (2), 65–81.
Haenlein, M., & Kaplan, A. M. (2004). A beginner’s guide to partial least squares analysis. Understanding Statistics, 3 (4), 283–297.
Hair, J. F. (2020). Next generation prediction metrics for composite-based PLS-SEM. Industrial Management & Data Systems, 121 (1), 5–11.
Hair, J. F., Binz Astrachan, C., Moisescu, O. I., Radomir, L., Sarstedt, M., Vaithilingam, S., & Ringle, C. M. (2020). Executing and interpreting applications of PLS-SEM: Updates for family business researchers. Journal of Family Business Strategy, 12 (3), 100392.
Hair, J. F., Black, W. C., Babin, B. J., & Anderson, R. E. (2019). Multivariate data analysis (8 th ed.). London: Cengage Learning.
Hair, J. F., Hollingsworth, C. L., Randolph, A. B., & Chong, A. Y. L. (2017). An updated and expanded assessment of PLS-SEM in information systems research. Industrial Management & Data Systems, 117 (3), 442–458.
Hair, J. F., Howard, M. C., & Nitzl, C. (2020). Assessing measurement model quality in PLS-SEM using confirmatory composite analysis. Journal of Business Research, 109 , 101–110.
Hair, J. F., Hult, G. T. M., Ringle, C. M., & Sarstedt, M. (2022). A primer on partial least squares structural equation modeling (PLS-SEM) (3rd ed.). Thousand Oaks, CA: Sage.
Hair, J. F., Matthews, L., Matthews, R., & Sarstedt, M. (2017). PLS-SEM or CB-SEM: Updated guidelines on which method to use. International Journal of Multivariate Data Analysis, 1 (2), 107–123.
Hair, J. F., Page, M. J., & Brunsveld, N. (2020). Essentials of business research methods (4th ed.). New York, NY: Routledge.
Hair, J. F., Ringle, C. M., Gudergan, S. P., Fischer, A., Nitzl, C., & Menictas, C. (2019). Partial least squares structural equation modeling-based discrete choice modeling: An illustration in modeling retailer choice. Business Research, 12 (1), 115–142.
Hair, J. F., Ringle, C. M., & Sarstedt, M. (2011). PLS-SEM: Indeed a silver bullet. Journal of Marketing Theory and Practice, 19 (2), 139–151.
Hair, J. F., Risher, J. J., Sarstedt, M., & Ringle, C. M. (2019). When to use and how to report the results of PLS-SEM. European Business Review, 31 (1), 2–24.
Hair, J. F., & Sarstedt, M. (2019). Composites vs. factors: Implications for choosing the right SEM method. Project Management Journal, 50 (6), 1–6.
Hair, J. F., & Sarstedt, M. (2021). Explanation plus prediction – The logical focus of project management research. Project Management Journal, 52 (4), 319–322.
Hair, J. F., Sarstedt, M., Pieper, T., & Ringle, C. M. (2012). The use of partial least squares structural equation modeling in strategic management research: A review of past practices and recommendations for future applications. Long Range Planning, 45 (5–6), 320–340.
Hair, J. F., Sarstedt, M., & Ringle, C. M. (2019). Rethinking some of the rethinking of partial least squares. European Journal of Marketing, 53 (4), 566–584.
Hair, J. F., Sarstedt, M., Ringle, C. M., & Gudergan, S. P. (2018). Advanced issues in partial least squares structural equation modeling (PLS-SEM) . Thousand Oaks, CA: Sage.
Hair, J. F., Sarstedt, M., Ringle, C. M., & Mena, J. A. (2012). An assessment of the use of partial least squares structural equation modeling in marketing research. Journal of the Academy of Marketing Science, 40 (3), 414–433.
Henseler, J. (2017). Bridging design and behavioral research with variance-based structural equation modeling. Journal of Advertising, 46 (1), 178–192.
Henseler, J., Dijkstra, T. K., Sarstedt, M., Ringle, C. M., Diamantopoulos, A., Straub, D. W., Ketchen, D. J., Hair, J. F., Hult, G. T. M., & Calantone, R. J. (2014). Common beliefs and reality about partial least squares: Comments on Rönkkö & Evermann (2013). Organizational Research Methods, 17 (1), 182–209.
Hui, B. S., & Wold, H. (1982). Consistency and consistency at large of partial least squares estimates. In K. G. Jöreskog & H. Wold (Eds.), Systems under indirect observation, part II (pp. 119–130). Amsterdam: North-Holland.
Hwang, H., Sarstedt, M., Cheah, J.-H., & Ringle, C. M. (2020). A concept analysis of methodological research on composite-based structural equation modeling: Bridging PLSPM and GSCA. Behaviormetrika, 47 (1), 219–241.
JCGM/WG1 (2008). Joint committee for guides in metrology/working group on the expression of uncertainty in measurement (JCGM/WG1): Evaluation of measurement data - guide to the expression of uncertainty in measurement. Retrieved from https://www.bipm.org/utils/common/documents/jcgm/JCGM_100_2008_E.pdf . Access date: 26 Feb 2021
Jöreskog, K. G. (1973). A general method for estimating a linear structural equation system. In A. S. Goldberger & O. D. Duncan (Eds.), Structural equation models in the social sciences (pp. 255–284). New York, NJ: Seminar Press.
Jöreskog, K. G., & Wold, H. (1982). The ML and PLS techniques for modeling with latent variables: Historical and comparative aspects. In H. Wold & K. G. Jöreskog (Eds.), Systems under indirect observation, part I (pp. 263–270). Amsterdam: North-Holland.
Kaufmann, L., & Gaeckler, J. (2015). A structured review of partial least squares in supply chain management research. Journal of Purchasing and Supply Management, 21 (4), 259–272.
Khan, G., Sarstedt, M., Shiau, W.-L., Hair, J. F., Ringle, C. M., & Fritze, M. (2019). Methodological research on partial least squares structural equation modeling (PLS-SEM): A social network analysis. Internet Research, 29 (3), 407–429.
Kock, N., & Hadaya, P. (2018). Minimum sample size estimation in PLS-SEM: The inverse square root and gamma-exponential methods. Information Systems Journal, 28 (1), 227–261.
Lee, L., Petter, S., Fayard, D., & Robinson, S. (2011). On the use of partial least squares path modeling in accounting research. International Journal of Accounting Information Systems, 12 (4), 305–328.
Lohmöller, J.-B. (1989). Latent variable path modeling with partial least squares . Heidelberg: Physica.
Book Google Scholar
Manley, S. C., Hair, J. F., Williams, R. I., & McDowell, W. C. (2020). Essential new PLS-SEM analysis methods for your entrepreneurship analytical toolbox. International Entrepreneurship and Management Journal, forthcoming.
Marcoulides, G. A., & Chin, W. W. (2013). You write but others read: Common methodological misunderstandings in PLS and related methods. In H. Abdi, W. W. Chin, V. Esposito Vinzi, G. Russolillo, & Trinchera (Eds.), New perspectives in partial least squares and related methods (pp. 31–64). New York, NY: Springer.
Chapter Google Scholar
Marcoulides, G. A., & Saunders, C. (2006). PLS: A silver bullet? MIS Quarterly, 30 (2), iii–ix.
Mateos-Aparicio, G. (2011). Partial least squares (PLS) methods: Origins, evolution, and application to social sciences. Communications in Statistics–Theory and Methods, 40 (13), 2305–2317.
McDonald, R. P. (1996). Path analysis with composite variables. Multivariate Behavioral Research, 31 (2), 239–270.
Nitzl, C. (2016). The use of partial least squares structural equation modelling (PLS-SEM) in management accounting research: Directions for future theory development. Journal of Accounting Literature, 37 (December), 19–35.
Nitzl, C., & Chin, W. W. (2017). The case of partial least squares (PLS) path modeling in managerial accounting research. Journal of Management Control, 28 , 137–156.
Peng, D. X., & Lai, F. (2012). Using partial least squares in operations management research: A practical guideline and summary of past research. Journal of Operations Management, 30 (6), 467–480.
Petter, S. (2018). "Haters gonna hate": PLS and information systems research. ACM SIGMIS Database: the DATABASE for Advances in Information Systems, 49 (2), 10–13.
Rasoolimanesh, S. M., Ringle, C. M., Sarstedt, M., & Olya, H. (2021). The combined use of prediction-oriented approaches: Partial least squares-structural equation modeling and fuzzyset qualitative comparative analysis. International Journal of Contemporary Hospitality Management , 33(5), 1571–1592.
Reinartz, W., Haenlein, M., & Henseler, J. (2009). An empirical comparison of the efficacy of covariance-based and variance-based SEM. International Journal of Research in Marketing, 26 (4), 332–344.
Rhemtulla, M., van Bork, R., & Borsboom, D. (2020). Worse than measurement error: Consequences of inappropriate latent variable measurement models. Psychological Methods, 25 (1), 30–45.
Richter, N. F., Cepeda Carrión, G., Roldán, J. L., & Ringle, C. M. (2016). European management research using partial least squares structural equation modeling (PLS-SEM): Editorial. European Management Journal, 34 (6), 589–597.
Richter, N. F., Schubring, S., Hauff, S., Ringle, C. M., & Sarstedt, M. (2020). When predictors of outcomes are necessary: Guidelines for the combined use of PLS-SEM and NCA. Industrial Management & Data Systems, 120 (12), 2243–2267.
Richter, N. F., Sinkovics, R. R., Ringle, C. M., & Schlägel, C. (2016). A critical look at the use of SEM in International Business Research. International Marketing Review, 33 (3), 376–404.
Rigdon, E. E. (2012). Rethinking partial least squares path modeling: In praise of simple methods. Long Range Planning, 45 (5–6), 341–358.
Rigdon, E. E. (2013). Partial least squares path modeling. In G. R. Hancock & R. D. Mueller (Eds.), Structural equation modeling: A second course (2nd ed., pp. 81–116). Charlotte, NC: Information Age.
Rigdon, E. E. (2014). Rethinking partial least squares path modeling: Breaking chains and forging ahead. Long Range Planning, 47 (3), 161–167.
Rigdon, E. E. (2016). Choosing PLS path modeling as analytical method in European management research: A realist perspective. European Management Journal, 34 (6), 598–605.
Rigdon, E. E., Becker, J.-M., Rai, A., Ringle, C. M., Diamantopoulos, A., Karahanna, E., Straub, D. W., & Dijkstra, T. K. (2014). Conflating antecedents and formative indicators: A comment on Aguirre-Urreta and Marakas. Information Systems Research, 25 (4), 780–784.
Rigdon, E. E., Becker, J.-M., & Sarstedt, M. (2019a). Factor indeterminacy as metrological uncertainty: Implications for advancing psychological measurement. Multivariate Behavioral Research, 54 (3), 429–443.
Rigdon, E. E., Becker, J.-M., & Sarstedt, M. (2019b). Parceling cannot reduce factor indeterminacy in factor analysis: A research note. Psychometrika, 84 (3), 772–780.
Rigdon, E. E., Sarstedt, M., & Becker, J.-M. (2020). Quantify uncertainty in behavioral research. Nature Human Behaviour, 4 (4), 329–331.
Rigdon, E. E., & Sarstedt, M. (2021). Accounting for uncertainty in the measurement of unobservable marketing phenomena. In H. Baumgartner & B. Weijters (Eds.), Review of marketing research , forthcoming.
Rigdon, E. E., Sarstedt, M., & Ringle, C. M. (2017). On comparing results from CB-SEM and PLS-SEM: Five perspectives and five recommendations. Marketing ZFP, 39 (3), 4–16.
Ringle, C. M., Sarstedt, M., Mitchell, R., & Gudergan, S. P. (2020). Partial least squares structural equation modeling in HRM research. International Journal of Human Resource Management, 31 (12), 1617–1643.
Ringle, C. M., Sarstedt, M., & Straub, D. W. (2012). A critical look at the use of PLS-SEM in MIS Quarterly . MIS Quarterly, 36 (1), iii–xiv.
Roldán, J. L., & Sánchez-Franco, M. J. (2012). Variance-based structural equation modeling: Guidelines for using partial least squares in information systems research. In M. Mora, O. Gelman, A. L. Steenkamp, & M. Raisinghani (Eds.), Research methodologies, innovations and philosophies in software systems engineering and information systems (pp. 193–221). Hershey, PA: IGI Global.
Rönkkö, M., & Evermann, J. (2013). A critical examination of common beliefs about partial least squares path modeling. Organizational Research Methods, 16 (3), 425–448.
Rönkkö, M., McIntosh, C. N., & Antonakis, J. (2015). On the adoption of partial least squares in psychological research: Caveat emptor. Personality and Individual Differences, 87 (December), 76–84.
Rossiter, J. R. (2011). Measurement for the social sciences: The C-OAR-SE method and why it must replace psychometrics . Berlin: Springer.
Russo, D., & Stol, K.-J. (2021). PLS-SEM for software engineering research: An introduction and survey. ACM Computing Surveys , 54(4), Article 78.
Sarstedt, M., Bengart, P., Shaltoni, A. M., & Lehmann, S. (2018). The use of sampling methods in advertising research: A gap between theory and practice. International Journal of Advertising, 37 (4), 650–663.
Sarstedt, M., Hair, J. F., Cheah, J.-H., Becker, J.-M., & Ringle, C. M. (2019). How to specify, estimate, and validate higher-order models. Australasian Marketing Journal, 27 (3), 197–211.
Sarstedt, M., Hair, J. F., Nitzl, C., Ringle, C. M., & Howard, M. C. (2020). Beyond a tandem analysis of SEM and PROCESS: Use PLS-SEM for mediation analyses! International Journal of Market Research, 62 (3), 288–299.
Sarstedt, M., Hair, J. F., Ringle, C. M., Thiele, K. O., & Gudergan, S. P. (2016). Estimation issues with PLS and CB-SEM: Where the bias lies! Journal of Business Research, 69 (10), 3998–4010.
Sarstedt, M., & Mooi, E. A. (2019). A concise guide to market research: The process, data, and methods using IBM SPSS statistics (3rd ed.). Berlin: Springer.
Sarstedt, M., Ringle, C. M., Cheah, J.-H., Ting, H., Moisescu, O. I., & Radomir, L. (2020). Structural model robustness checks in PLS-SEM. Tourism Economics, 26 (4), 531–554.
Sarstedt, M., & Danks, N. (2021). Prediction in HRM research—A gap between rhetoric and reality. Human Resource Management Journal , forthcoming.
Sarstedt, M., Ringle, C. M., Smith, D., Reams, R., & Hair, J. F. (2014). Partial least squares structural equation modeling (PLS-SEM): A useful tool for family business researchers. Journal of Family Business Strategy, 5 (1), 105–115.
Schneeweiß, H. (1991). Models with latent variables: LISREL versus PLS. Statistica Neerlandica, 45 (2), 145–157.
Schuberth, F., Henseler, J., & Dijkstra, T. K. (2018). Confirmatory composite analysis. Frontiers in Psychology, 9 , 2541.
Tenenhaus, M., Esposito Vinzi, V., Chatelin, Y.-M., & Lauro, C. (2005). PLS path modeling. Computational Statistics & Data Analysis, 48 (1), 159–205.
Usakli, A., & Kucukergin, K. G. (2018). Using partial least squares structural equation modeling in hospitality and tourism: Do researchers follow practical guidelines? International Journal of Contemporary Hospitality Management, 30 (11), 3462–3512.
Willaby, H., Costa, D., Burns, B., MacCann, C., & Roberts, R. (2015). Testing complex models with small sample sizes: A historical overview and empirical demonstration of what partial least squares (PLS) can offer differential psychology. Personality and Individual Differences, 84 , 73–78.
Wold, H. (1982). Soft modeling: The basic design and some extensions. In K. G. Jöreskog & H. Wold (Eds.), Systems under indirect observations, part II (pp. 1–54). Amsterdam: North-Holland.
Wold, H. (1985). Partial least squares. In S. Kotz & N. L. Johnson (Eds.), Encyclopedia of statistical sciences (pp. 581–591). New York, NY: John Wiley.
Wold, S., Sjöström, M., & Eriksson, L. (2001). PLS-regression: A basic tool of chemometrics. Chemometrics and Intelligent Laboratory Systems, 58 (2), 109–130.
Zeng, N., Liu, Y., Gong, P., Hertogh, M., & König, M. (2021). Do right PLS and do PLS right: A critical review of the application on PLS in construction management research. Frontiers of Engineering Management , 8 (3), 356–369.
Suggested Readings
Sarstedt, M., Hair, J. F., Ringle, C. M., Thiele, K. O., & Gudergan, S. P. (2016). Estimation issues with PLS and CBSEM: Where the bias lies! Journal of Business Research, 69 (10), 3998–4010.
Download references
Author information
Authors and affiliations.
Mitchell College of Business, University of South Alabama, Mobile, AL, USA
Joseph F. Hair Jr.
Broad College of Business, Michigan State University, East Lansing, MI, USA
G. Tomas M. Hult
Department of Management Science and Technology, Hamburg University of Technology, Hamburg, Germany
Christian M. Ringle
Otto-von-Guericke University Magdeburg, Magdeburg, Germany
Marko Sarstedt
Babeș-Bolyai University, Faculty of Economics and Business Administration, Cluj, Romania
Trinity Business School, Trinity College, Dublin, Ireland
Nicholas P. Danks
National Tsing Hua University, Hsinchu, Taiwan
You can also search for this author in PubMed Google Scholar
Rights and permissions
Open Access This chapter is licensed under the terms of the Creative Commons Attribution 4.0 International License ( http://creativecommons.org/licenses/by/4.0/ ), which permits use, sharing, adaptation, distribution and reproduction in any medium or format, as long as you give appropriate credit to the original author(s) and the source, provide a link to the Creative Commons license and indicate if changes were made.
The images or other third party material in this chapter are included in the chapter’s Creative Commons license, unless indicated otherwise in a credit line to the material. If material is not included in the chapter’s Creative Commons license and your intended use is not permitted by statutory regulation or exceeds the permitted use, you will need to obtain permission directly from the copyright holder.
Reprints and permissions
Copyright information
© 2021 The Author(s)
About this chapter
Hair, J.F., Hult, G.T.M., Ringle, C.M., Sarstedt, M., Danks, N.P., Ray, S. (2021). An Introduction to Structural Equation Modeling. In: Partial Least Squares Structural Equation Modeling (PLS-SEM) Using R. Classroom Companion: Business. Springer, Cham. https://doi.org/10.1007/978-3-030-80519-7_1
Download citation
DOI : https://doi.org/10.1007/978-3-030-80519-7_1
Published : 04 November 2021
Publisher Name : Springer, Cham
Print ISBN : 978-3-030-80518-0
Online ISBN : 978-3-030-80519-7
eBook Packages : Business and Management Business and Management (R0)
Share this chapter
Anyone you share the following link with will be able to read this content:
Sorry, a shareable link is not currently available for this article.
Provided by the Springer Nature SharedIt content-sharing initiative
- Publish with us
Policies and ethics
- Find a journal
- Track your research
- Skip to primary navigation
- Skip to main content
- Skip to primary sidebar
Institute for Digital Research and Education
Introduction to Structural Equation Modeling (SEM) in R with lavaan
This seminar will introduce basic concepts of structural equation modeling using lavaan in the R statistical programming language. Its emphasis is on identifying various manifestations of SEM models and interpreting the output rather than a thorough mathematical treatment or a comprehensive list of syntax options in lavaan . Since SEM is a broad topic, only the most fundamental topics such as matrix notation, identification and model fit will be introduced in this seminar. Topics such as estimation, multiple groups, measurement invariance and latent growth modeling will not be covered in this seminar. The assumption is that all variables are continuous and normally distributed.
This seminar is the second in a three-part series on latent variable modeling. The first seminar of this seminar introduces CFA:
- Confirmatory Factor Analysis (CFA) in R with lavaan
The third seminar introdues intermediate topics in CFA including latent growth modeling and measurement invariance:
- Latent Growth Models (LGM) and Measurement Invariance with R in lavaan
For exploratory factor analysis (EFA), please refer to A Practical Introduction to Factor Analysis: Exploratory Factor Analysis .
In addition to the pre-requisites above, a rudimentary knowledge of linear regression is required to understand some of the material in this seminar.
Proceed through the seminar in order or click on the hyperlinks below to go to a particular section:
Introduction
Motivating example, definitions, the path diagram, quick reference of lavaan syntax.
Regression and Path analysis
Simple regression (Model 1A)
Maximum likelihood vs. least squares, multiple regression (model 2), multivariate regression with default covariance (model 3a), multivariate regression removing default covariances (model 3d), fully saturated multivariate regression (model 3e), known values, parameters, and degrees of freedom, path analysis (model 4a), modification index, path analysis after modification (model 4b), model fit statistics, model chi-square, a note on sample size, the baseline model, cfi (comparative fit index), tli (tucker lewis index).
Measurement Model
Exogenous factor analysis (Model 5A)
A note on endogenous measurement models (model 5b).
Structural Model
Structural Regression Model
Structural regression with one endogenous variable (model 6a), structural regression with two endogenous variables (model 6b).
- (Optional) Structural regression with an observed endogenous variable (Model 6C)
Requirements
Before beginning the seminar, please make sure you have R and RStudio installed.
Please also make sure to have the following R package installed, and if not, run these commands in R (RStudio).
Once you’ve installed the package, you can load lavaan via the following
Download the complete R code
You may download the complete R code here: sem.r
After clicking on the link, you can copy and paste the entire code into R or RStudio.
Structural equation modeling is a linear model framework that models both simultaneous regression equations with latent variables. Models such as linear regression, multivariate regression, path analysis, confirmatory factor analysis, and structural regression can be thought of as special cases of SEM. The following relationships are possible in SEM:
- observed to observed variables ($\gamma$, e.g., regression)
- latent to observed variables ($\lambda$, e.g., confirmatory factor analysis)
- latent to latent variables ($\gamma,\beta$ e.g., structural regression)
SEM uniquely encompasses both measurement and structural models . The measurement model relates observed to latent variables and the structural model relates latent to latent variables. Various software programs currently handle SEM models including Mplus, EQS, SAS PROC CALIS, Stata’s sem and more recently, R’s lavaan . The benefit of lavaan is that it is open source, freely available, and is relatively easy to use.
This seminar will introduce the most common models that fall under the SEM framework including
- simple regression
- multiple regression
- multivariate regression
- path analysis
- confirmatory factor analysis
- structural regression
The purpose of the seminar will be to introduce within each model its
- matrix formulation
- path diagram
- lavaan syntax
- interpretation of parameters and output
By the end of this training, you should be able to understand enough of these concepts to correctly identify the model, recognize each parameter in the matrix formulation and interpret the output of each model in lavaan . Finally, for those of you who are interested, the technical underpinnings of all these models are based on classic LISREL (linear structural relations) notation in honor of Karl Joreskög (1969, 1973). Although critics of LISREL note its complexity and sharp learning curve for the causal user, all modern implementations of SEM are derivations of LISREL and serve either to expand its capabilities or whittle down its complexity. Thus understanding LISREL is foundational to understanding more modern SEM frameworks and programs.
Suppose you are a researcher studying the effects of student background on academic achievement. The lab recently finished collecting and uploading the dataset ( worland5.csv ) of $N=500$ students each with 9 observed variables: Motivation, Harmony, Stability, Negative Parental Psychology, SES, Verbal IQ, Reading, Arithmetic and Spelling. The principal investigator hypothesizes three latent constructs Adjustment, Risk, Achievement measured with its corresponding to the following codebook mapping:
- motiv Motivation
- harm Harmony
- stabi Stability
- ppsych (Negative) Parental Psychology
- verbal Verbal IQ
Achievement
- read Reading
- arith Arithmetic
- spell Spelling
Due to budget constraints, your lab uses the freely available R statistical programming language, and lavaan as the structural equation modeling (SEM) package of choice. You can download the file from the link here worland5.csv or load the file directly into R with the following command
The most essential component of a structural equation model is covariance or the statistical relationship between items. The true population covariance, denoted $\Sigma$, is called the variance-covariance matrix . Since we do not know $\Sigma$ we can estimate it with our sample, and call it $\hat{\Sigma}=S$, or the sample variance-covariance matrix . The function cov specifies that we want to obtain the covariance matrix $S$ from the data.
Both $\Sigma$ and $S$ share similar properties. The diagonals constitute the variances of the item and the off-diagonals the covariances. Notice that the variance of all variables in our study happens to be 100 (down the diagonals). Looking at the relationships between variables (the off-diagonal elements), recall that a positive covariance means that as one item increases the other increases, a negative covariance means that as one item increases the other item decreases. The covariance of motiv and ppsych is -25 which means that as negative parental psychology increases student motivation decreases. Notice that the covariances in the upper right triangle are the same as those in the lower right triangle, meaning the covariance of Motivation and Harmony is the same as the covariance of Harmony and Motivation. This property is known as symmetry and will be important later on. The variance-covariance matrix $\Sigma$ should not be confused with $\Sigma{(\theta)}$ which is the model-implied covariance matrix. The purpose of SEM is to reproduce the variance-covariance matrix using parameters $\theta$ we hypothesize will account for either the measurement or structural relations between variables. If the model perfectly reproduces the variance-covariance matrix then $\Sigma=\Sigma{(\theta)}$.
True or False. Running the cov command in R allows us to obtain the population variance-covariance matrix $\Sigma$
Answer: False, since we are running our analysis on a sample we are obtaining $S$.
True or False. $S=\hat{\Sigma}$ means that it is an estimate of the population variance-covariance matrix.
Answer: True, the hat symbol indicates an estimate
True or False. $\Sigma{(\theta)}$ is the variance-covariance matrix reproduced from model parameters
Answer: True, $\theta$ denotes model parameters. The goal of SEM is to reproduce $\Sigma$ with $\Sigma{(\theta)}$.
- observed variable: a variable that exists in the data, a.k.a item or manifest variable
- latent variable: a variable that is constructed and does not exist in the data
- exogenous variable: an independent variable either observed (x) or latent ($\xi$) that explains an endogenous variable
- endogenous variable: a dependent variable, either observed (y) or latent ($\eta$) that has a causal path leading to it
- measurement model: a model that links observed variables with latent variables
- indicator: an observed variable in a measurement model (can be exogenous or endogenous)
- factor : a latent variable defined by its indicators (can be exogenous or endogenous)
- loading: a path between an indicator and a factor
- structural model: a model that specifies causal relationships among exogenous variables to endogenous variables (can be observed or latent)
- regression path: a path between exogenous and endogenous variables (can be observed or latent)
There is subtlety to the definition of an indicator. Although $x$ variables are typically seen as independent variables in a linear regression, the $x$ or $y$ label of an indicator in a measurement model depends on the factor it belongs to. An indicator is an $x$-side indicator if it depends on an exo genous factor and a $y$-side indicator if it depends on an endo genous factor.
True or False. If an observed $x$-variable is being predicted by an endogenous factor, then it is called an $x$-side indicator.
Answer: False. If an indicator is being predicted by an endogenous factor, it is a $y$-side indicator and should be relabeled $y$.
True or False. Because an $x$-variable is being predicted by a factor, it is a dependent variable and should be relabeled $y$.
Answer: False. Both $x$-side and $y$-side variables are dependent variables, but whether it is $x$-side or $y$-side depends on the endogenity of its factor.
To facilitate understanding of the matrix equations (which can be a bit intimidating), a path diagram will be presented with every matrix formulation as it is a symbolic one-to-one visualization. Before we present the actual path diagram, the table below defines the symbols we will be using. Circles represent latent variables, squares represent observed indicators, triangles represent intercepts or means, one-way arrows represent paths and two-way arrows represent either variances or covariances.
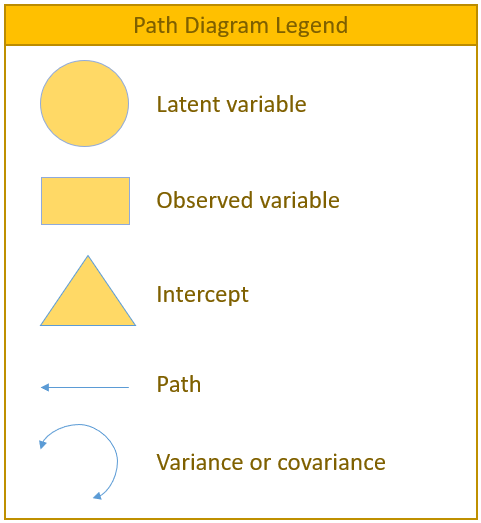
For example in the figure below, the diagram on the left depicts the regression of a factor on an item (contained in a measurement model) and the diagram on the right depicts the variance of the factor (a two-way arrow pointing to a the factor itself).

The following is a path diagram of all the types of variables and relationships you will see in this seminar.
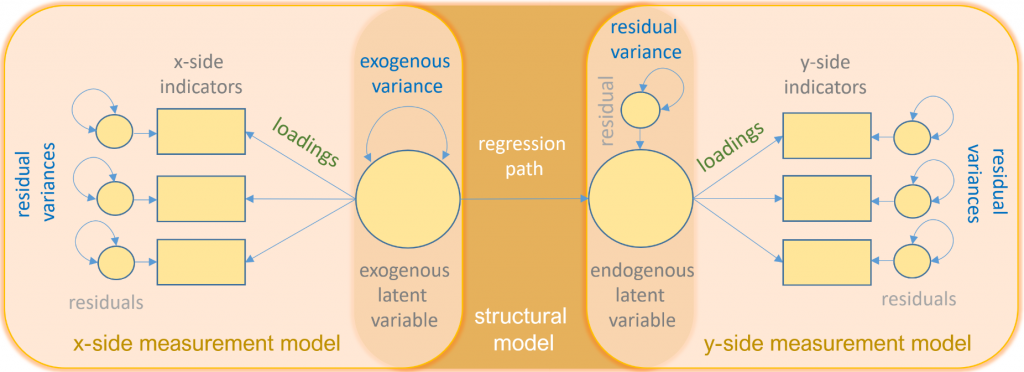
Before running our first regression analysis, let us introduce some of the most frequently used syntax in lavaan
- ~ predict , used for regression of observed outcome to observed predictors (e.g., y ~ x )
- =~ indicator , used for latent variable to observed indicator in factor analysis measurement models (e.g., f =~ q + r + s )
- ~~ covariance (e.g., x ~~ x )
- ~1 intercept or mean (e.g., x ~ 1 estimates the mean of variable x )
- 1* fixes parameter or loading to one (e.g., f =~ 1*q )
- NA* frees parameter or loading (useful to override default marker method, (e.g., f =~ NA*q )
- a* labels the parameter ‘a’, used for model constraints (e.g., f =~ a*q )
Regression and Path Analysis
Simple regression models the relationship of an observed exogenous variable on a single observed endogenous variable. For a single subject, the simple linear regression equation is most commonly defined as:
$$y_1 = b_0 + b_1 x_1 + \epsilon_1$$
where $b_0$ is the intercept and $b_1$ is the coefficient and $x$ is an observed predictor and $\epsilon$ is the residual. Karl Joreskög, the originator of LISREL (linear structural relations), developed a special notation for the exact same model for a single observation:
$$y_1 = \alpha + \gamma x_1 + \zeta_1$$
- $x_1$ single exogenous variable
- $y_1$ single endogenous variable
- $b_0, \alpha_1$ intercept of $y_1$, “alpha”
- $b_1, \gamma_1$ regression coefficient, “gamma”
- $\epsilon_1, \zeta_1$ residual of $y_1$, “epsilon” and “zeta”
- $\phi$, variance or covariance of the exogenous variable, “phi”
- $\psi$ residual variance or covariance of the endogenous variable, “psi”
True or False. In Model 1A above, there is one endogenous outcome and one exogenous predictor.
Answer: True.
True or False. In Model 1A above, $y_1$ means that I have 1 observation (i.e., student) in my sample
Answer: False. $y_1$ indicates that there is one endogenous variable with $N$ samples.
To see the matrix visually, we can use a path diagram (Model 1A):
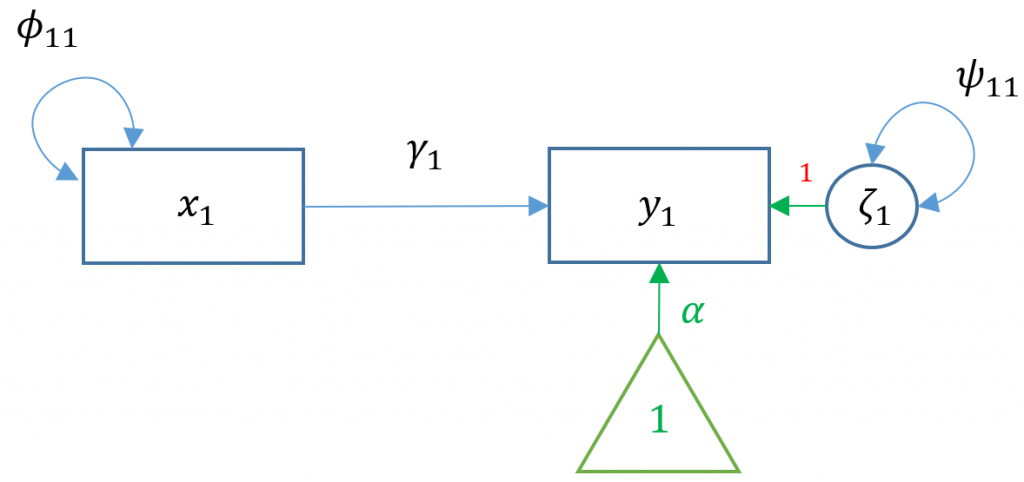
In R, the most basic way to run a linear regression is to use the lm() function which is available in base R.
The output of lm() appears as below:
The predicted mean of read is 0 for a student with motiv =0 and for a one unit increase in motivation, the reading score improves 0.530 points. Make special note of the residual standard error which is $8.488$. The square of that $8.488^2=72.04$ is the residual variance, which we will see later in lavaan() . We can run the equivalent code in lavaan. The syntax is very similar to lm() in that read ~ motiv specifies the predictor motiv on the outcome read . However, by default the intercept is not included in the output but is implied. If we want to add an intercept, we need to include read ~ 1 + motiv . Optionally, you can request the variance of motiv using motiv ~~ motiv . If this syntax is not provided, the parameter is still estimated but just implied.
The output appears as below:
The intercept of .read (-0.000) and the regression coefficient of read ~ motiv (0.530) matches the output of lm() with small rounding errors. Note that the . in front of the parameter denotes an endogenous variable under Intercepts and a residual variance if under Variances or Covariances. The intercept for motiv (0.000) does not have a . nor does its variance ( 99.800 ) signifying that it is an exogenous mean and variance. The exogenous mean and variance $\phi_{11}$ should closely match the univariate mean (0) and variance (100) as shown below:
Match every parameter estimate to its corresponding path diagram shown above. Why is the intercept of motiv zero? What is the parameter that is not shown by default in lavaan ?
Answer: : $y$= read , $x$= motiv , $\gamma_1=0.53$, $\alpha=0$, $\psi_{11}=71.766$. The intercept is zero because the researcher decided to center all variables at zero. The exogenous variance $\phi_{11}$ is not shown but is implicitly estimated, but we specified motiv ~~ motiv to explicitly model it.
Try running the simple regression without the 1+ . What do you notice about the number of free parameters and degrees of freedom?
Answer: : The number of free parameters decreases by 1 because we were estimating an additional intercept but this does not change the degrees of freedom because we are simply subtracting another term to the total parameters. Linear regression models are always saturated.
The important output to note is that the estimator is ML for Maximum Likelihood. Linear regression by default uses the least square estimator . As we will see below the coefficients will be the same but the residual variances will be slightly different. Additionally, there is default output for Variances, which is $71.766$.
The estimates of the regression coefficients are equivalent between the two methods but the variance differs. For least squares, the estimate of the residual variance is: $$ \hat{\sigma}^2_{LS} = \frac{\sum_{i=1}^{N} \hat{\zeta_i}^2}{N-k} $$ where $N$ is the sample size, and $k$ is the number of predictors + 1 (intercept) For maximum likelihood the estimate of the residual variance is: $$ \hat{\sigma}^2_{ML} = \frac{\sum_{i=1}^{N} \hat{\zeta_i}^2}{N} $$
To convert from the least squares residual variance to maximum likelihood:
$$ \hat{\sigma}^2_{ML} = \left(\frac{N-k}{n}\right) \hat{\sigma}^2_{LS} $$
Going back to the lm() output we see that
So the least square variance is $8.488^2=72.046$. To convert the variance to maximum likelihood, since $k=2$ we have $498/500(72.046)=71.76$.
This matches the lavaan output.
True or False. The lm() function in R uses least squares estimation and lavaan uses maximum likelihood.
Answer: : True. These are the default estimation methods for each function; lavaan allows the user to change the estimation method such as generalized least squares (GLS) by specifying estimator=GLS . Refer to the lavaan documentation on Estimators for more information.
True or False. The fixed effects of an ordinary least squares regression is equivalent to maximum likelihood but the residual variance differs between the two.
Answer: : True.
Simple regression is limited to just a single exogenous variable. In practice, a researcher may be interested in how a group of exogenous variable predict an outcome. Suppose we still have one endogenous outcome but two exogenous predictors; this is known as multiple regression (not to be confused with multivariate regression). Matrix form allows us to concisely represent the equation for all observations
$$y_1 = \alpha_1 + \mathbf{x \gamma} + \zeta_1$$
- $\alpha_1$ intercept for $y_1$
- $\mathbf{x}$ vector ($1 \times q$) of exogenous variables
- $\mathbf{\gamma}$ vector ($q \times 1$) of regression coefficients where $q$ is the total number of exogenous variables
- $\zeta_1$ residual of $y_1$, pronounced “zeta”.
- $\phi$, variance or covariance of the exogenous variable
- $\psi$ residual variance or covariance of the endogenous variable
Assumptions
- $E(\zeta)=0$ the mean of the residuals is zero
- $\zeta$ is uncorrelated with $\mathbf{x}$
Suppose we have two exogenous variables $x_1, x_2$ predicting a single endogenous variable $y_1$. The path diagram for this multiple regression (Model 2) is:
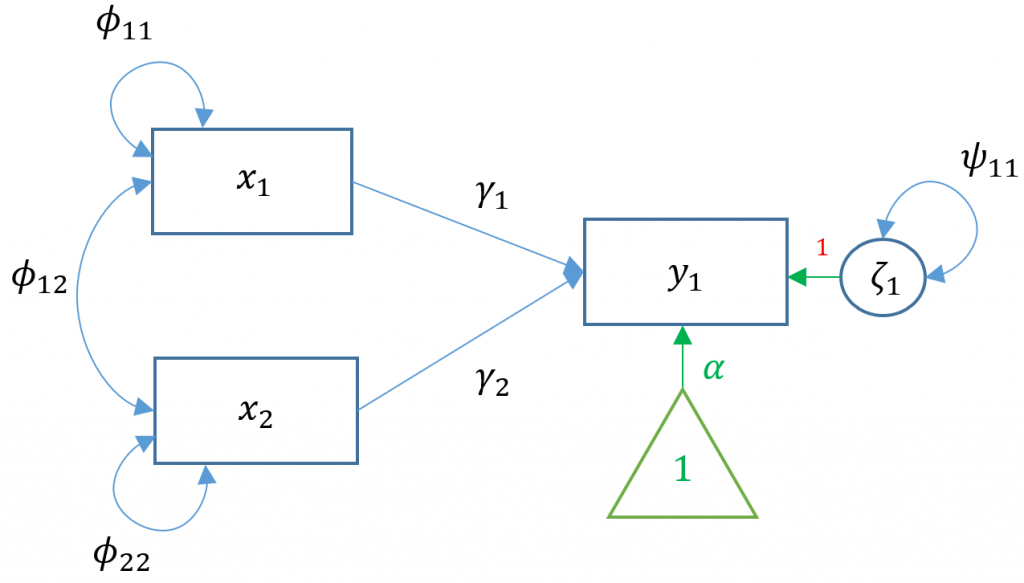
The corresponding output is as follows. Compare the number of free parameters and degrees of freedom to the simple regression.
True or False. In Model 2, the vector $\alpha$ can be replaced with $\alpha_1$ as in the univariate regression model (Model 1A).
Answer: False. Unlike the univariate regression model (Model 1A) which has only one intercept, in multivariate regression, every outcome has its own intercept.
True or False. In Model 2 above, there are two $\gamma$’s because there are two exogenous variables. Given the same model, this would not change if we increased the sample size.
Answer: True, each endogenous variable gets its own regression model and can have as many exogenous variables as desired (theoretically). This is independent of the sample size.
Re-run the analysis above without specifying ppsych ~~ motiv . Note the number of free parameters and degrees of freedom. Do the coefficients change? What does this imply?
Answer: : Degrees of freedom is still zero even though number of free parameters is 4. The extra parameters are the intercepts and variances of ppsych and motiv corresponding to $\kappa_{1}$ and $\kappa_{2}$ as well as $\phi_{11}$ and $\phi_{22}$. The covariance of ppsych and motiv $\phi_{12}$ which makes a total of five extra parameters. The total parameters is thus $4+5=9$.
Even though we increased our number of free parameters our degrees of freedom is still zero! We will explore why in the following section.
Multivariate regression (Models 3A-E)
Simple and multiple regression model one outcome ($y$) at a time. In multivariate or simultaneous linear regression, multiple outcomes $y_1,y_2,…,y_p$ are modeled simultaneously, where $q$ is the number of outcomes. The General Multivariate Linear Model is defined as
$$\mathbf{y} = \mathbf{\alpha} + \mathbf{\Gamma} \mathbf{x} + \mathbf{\zeta}$$
To see the matrix formulation more clearly, consider two (i.e., bivariate) endogenous variables ($y_1, y_2$) predicted by two exogenous predictors $x_1, x_2$.
$$ \begin{pmatrix} y_{1} \\ y_{2} \end{pmatrix} = \begin{pmatrix} \alpha_1 \\ \alpha_2 \end{pmatrix} + \begin{pmatrix} \gamma_{11} & \gamma_{12}\\ 0 & \gamma_{22} \end{pmatrix} \begin{pmatrix} x_1 \\ x_2 \end{pmatrix} + \begin{pmatrix} \zeta_{1}\\ \zeta_{2} \end{pmatrix} $$
$\Gamma$ here is known as a structural parameter and defines the relationship of exogenous to endogenous variables.
- $\mathbf{y} = (y_1, \cdots, y_p)’$ vector of $p$ endogenous variables (not the number of observations!)
- $\mathbf{x}= (x_1, \cdots, x_q)’$ vector of $q$ exogenous variables
- $\mathbf{\alpha}$ vector of $p$ intercepts
- $\Gamma$ matrix of regression coefficients ($p \times q$) linking endogenous to exogenous variables whose $i$-th row indicates the endogenous variable and $j$-th column indicates the exogenous variable
- $\mathbf{\zeta}= ( \zeta_1, \cdots, \zeta_p)’$ vector of $p$ residuals (for the number of endogenous variables not observations)
True or False. The size of the vector of residuals $\zeta$ in the model above is determined by the number of observations.
Answer: False. The size of $\zeta$ is determined by the number of endogenous variables $p$. This does not mean there is only one observation however. In reality there are $N \cdot p$ residuals but it’s not depicted in the model above.
True or False. $\gamma_{12}$ represents a regression coefficient of the second exogenous variable $x_2$ on the first outcome $y_1$.
Answer: True. The $i$-th row indicates the endogenous variable and $j$-th column indicates the exogenous variable.
True or False. $\Gamma$ is always a square matrix meaning the number of rows equals the number of columns
Answer: False, the rows of $\Gamma$ indicate the number of endogenous variables and the columns of $\Gamma$ indicate the number of exogenous variables. This matrix would only be square if the number of exogenous variables equals the number of endogenous variables.
Due to the added complexity of this and the following multivariate models, we will explicitly model intercepts in lavaan code but exclude them from the path diagrams. With that said, the path diagram for a multivariate regression model (Model 3A) is depicted as:
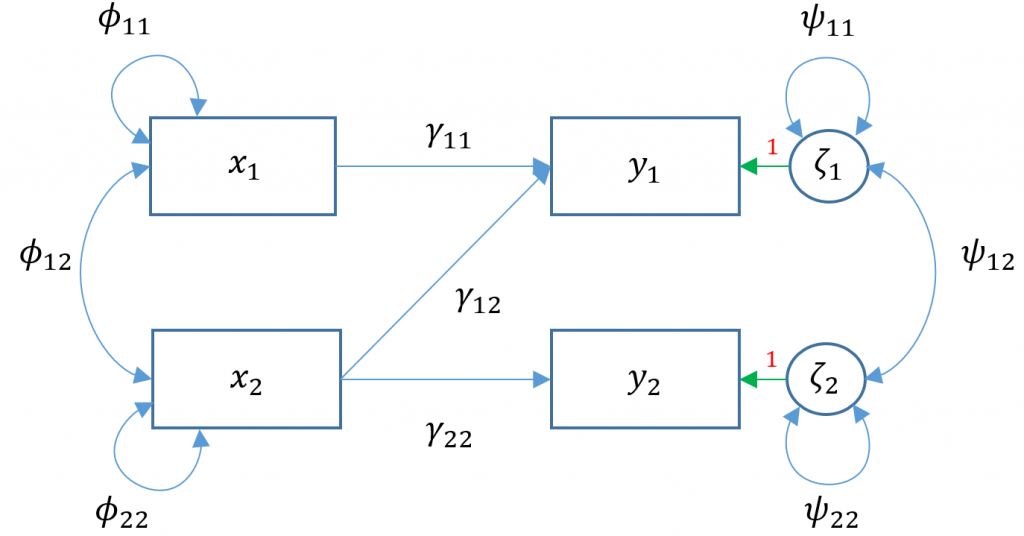
True or False. The $y_1$ in the simple and multiple regression index one endogenous variable and in the multivariate outcome $y_1, y_2$ indexes two endogenous variables , not two samples.
Answer: True. If we had to index the number of observations in the multivariate regression, we would need two indexes for $y$, namely $y_{ij}$ where $i$ is the observation and $j$ the endogenous variable. For simplicity, we remove the index $i$ for the observations and simply focus on $j$.
True or False. Looking at Model 3A, $\psi_{11},\psi_{22}$ are the variances of $\zeta_1, \zeta_2$
Answer: True, they are the residual variances.
The output is as follows:
As expected, the intercepts of read and arith are zero and its residual variances are 65.032 and 63.872. For multivariate regressions, the covariance of the residuals is given by default in lavaan and here estimated to be 39.179 (positive association between the variance of read and arith not accounted for by the exogenous variables. The relationship of read on ppsych is -0.216. For every one unit increase in Negative Parental Psychology, student Reading scores drop by 0.216 points controlling for the effects of student Motivation. The relationship of read on motiv is 0.476 meaning as student Motivation increases by one unit, Reading scores increase by 0.476 points controlling for the effects of Negative Parental Psychology. Finally arith on motiv is 0.6; an increase of one point in student Motivation results in a 0.6 increase in Arithmetic scores.
Is multivariate regression the same as running two separate linear regressions? Let’s run lm() to find out. First let’s run model of read on ppsych and motiv .
Now let’s run the model of arith on motiv in lm()
We see that the coefficients read ~~ ppsych is -0.216 in lavaan but -0.2747. Could this be rounding error? Furthermore, looking at the residual standard errors we see that the residual variance of arith is $8.008^2=64.12$ which is different from lavaan ‘s estimate of $63.872$. Knowing what you know from the previous section, what could be the cause of this difference? We explore these questions in the following section.
There are two facets to the question above. We already know previously that lm() uses the least squares estimator but lavaan uses maximum likelihood, hence the difference in residual variance estimates. However, regardless of which of the two estimators used, the regression coefficients should be unbiased so there must be something else accounting for the difference in coefficients. The answer lies in the implicit fact that lavaan by default will covary residual variances of endogenous variables .read ~~ .arith . Removing the default residual covariances, we see in the path diagram (Model 3D)
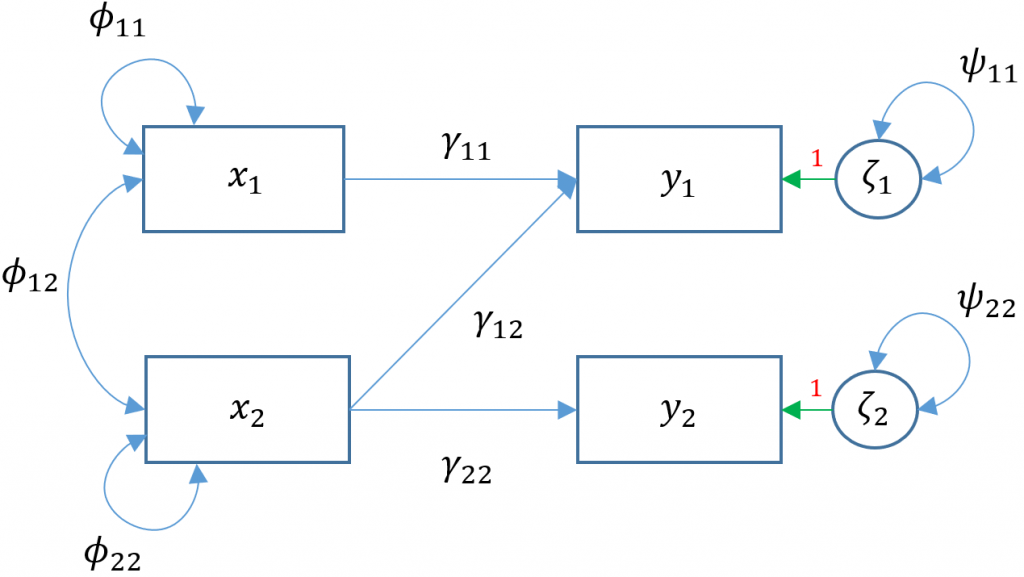
Notice now the coefficient of read on ppsych is -0.275 and read on motiv is 0.461. Compare now to the m3b
Compare the arith ~ motiv coefficient from m3c . Why do you think the coefficients are the same between lm() and lavaan ?
Answer: The residual variance is the variance that is not explained by all exogenous predictors in the model. The coefficients change between covariance on and off models if the additional exogenous variable is correlated with other endogenous variables. If the covariance is turned on then the residual variance will account for the effect of ppsych on arith that was not modeled. Any coefficients that are not modeled in the other regressions will appear in the residual covariances, hence the difference in the coefficients when covariances are turned off. Notice that motiv appears in both read ~ motiv and arith ~ ppsych + motiv which means that turning the covariance on or off will result in the same coefficients.
Re-run m3a and m3d with ppsych and motiv predicting both read and arith with covariances on and off. What do you notice about the coefficients?
Answer: Add the following syntax
The coefficients for both models are the same
True or False. In lavaan , multivariate regression is equivalent to running to separate regressions.
Answer: : False. By default, lavaan correlates the residual variance of the endogenous variables. To make it equivalent, constrain this covariance to zero.
True or False. The reason the residual variances differ between Model 3D versus Models 3B,C is due to maximum likelihood vs. least squares estimation
True or False. The variance term .arith in Model 3D is the variance of arithmetic itself. Compare this value to the variance calculated from Model 3C.
Answer: False. It is the residual variance. Note that even though we turned off the covariance, the estimate 63.872 differs from Model 3C’s estimate of the variance, which is the square of the residual standard error: 8.008^2 = 64.128 .
Both simple regression and multiple regression are saturated models which means that all parameters are fully estimated and there are no degrees of freedom. This is not necessarily true for multivariate regression models as Models 3A and 3D had degrees of freedom of 1 and 2 respectively. Let’s understand how the degrees of freedom is calculated. To begin, first count the number of known values in your observed population variance-covariance matrix $\Sigma$, given by the formula $p(p+1)/2$ where $p$ is the number of items in your survey. To see why this is true refer to the optional section Degrees of freedom with means from Confirmatory Factor Analysis (CFA) in R with lavaan for the more technically accurate explanation. Looking at the variables in the multivariate regression, we would like to obtain the variance-covariance matrix for Reading, Arithmetic, Parental Psychology and Motivation. To obtain the sample covariance matrix $S=\hat{\Sigma}$, which is an estimate of the population covariance matrix $\Sigma$, use the command cov and reference the column index of the dataset by name dat[,c("read","arith","ppsych","motiv")] .
The off-diagonal cells in $S$ correspond to bivariate sample covariances between two pairs of items; and the diagonal cells in $S$ correspond to the sample variance of each item (hence the term “ variance-covariance matrix “). Reading is positively related to Arithmetic, but Reading has a negative relationship with Parental Psychology. Just as in the larger covariance matrix we calculated before, the lower triangular elements in the covariance matrix are duplicated with the upper triangular elements. For example, the covariance of Reading with Arithmetic is 73, which is the same as the covariance of Arithmetic with Reading (recall the property of symmetry). Because the covariances are duplicated, the number of free parameters in SEM is determined by the number of unique variances and covariances. With 4 items, the number of known values is $p(p+1)/2 =4(5)/2=10$. The known values serve as the upper limit of the number of parameters you can possibly estimate in your model. The parameters coming from the model are called model parameters . Recall the multivariate model:
$$\mathbf{y = \alpha + \Gamma x + \zeta}$$
Since we care about the covariance of $y$, then by the assumption that $\alpha, x, \zeta$ are independent. Recall that covariance of a constant vector is zero since $Cov(\alpha)=E[(\alpha-E(\alpha))(\alpha-E(\alpha)]=E[0]=0$:
$$\Sigma(\theta)=Cov(\mathbf{y}) = Cov(\mathbf{\alpha} + \mathbf{\Gamma x} + \mathbf{\zeta}) = Cov(\mathbf{\alpha}) + Cov(\mathbf{\Gamma x}) + Cov(\mathbf{\zeta}) = \mathbf{\Gamma} Cov(\mathbf{x}) \mathbf{\Gamma}’ + \mathbf{\Psi} = \mathbf{\Gamma} \mathbf{\Phi} \mathbf{\Gamma}’ + \mathbf{\Psi}$$
This is a $2 \times 2$ matrix which serves as the upper left block of $\Sigma(\theta)$. Note that that full matrix of $\Sigma(\theta)$ here is $4 \times 4$. Since there are exactly two exogenous variables and two endogenous variables, each block is a $2 \times 2$ matrix.
$$ \Sigma(\theta) = \begin{pmatrix} \mathbf{\Gamma \Phi \Gamma’ + \Psi} & \mathbf{\Gamma \Phi}\\ \mathbf{\Phi \Gamma}’ & \mathbf{\Phi} \end{pmatrix} $$
However, since model parameters can be completely determined by elements of $y$, we do not consider parameters of $\Phi$ or $\Phi \Gamma’$. Spelling out the covariance matrix of $y$,
$$ \mathbf{\Gamma \Phi \Gamma’ + \Psi} = \begin{pmatrix} \gamma_{11} & \gamma_{12} \\ \gamma_{21} & \gamma_{22} \end{pmatrix} \begin{pmatrix} \phi_{11} & \phi_{12}\\ & \phi_{22} \end{pmatrix} \begin{pmatrix} \gamma_{11} & \gamma_{21} \\ \gamma_{12} & \gamma_{22} \end{pmatrix} + \begin{pmatrix} \psi_{11} & \psi_{12}\\ & \psi_{22} \end{pmatrix} $$
Notice that the $\alpha$ does not appear in covariance model. First consider the dimensions of each parameter in Model 3A and 3D. Since there are two endogenous variables and exogenous variables $\Gamma$ is $2\times 2$ for a total of 4 parameters. For two exogenous variables, the dimension of $\Phi$ is $2 \times 2$ but by symmetry, $\phi_{12}=\phi_{21}$ which means we have 3 $\phi$’s. For two endogenous variables the dimension of $\Psi$ is also $2 \times 2$ but since $\psi_{12}=\psi_{21}$ there are a total of 3 $\psi$’s.
Then the total number of (unique) parameters here is $4 + 3 + 3 = 10$ parameters. It’s not a coincidence then that the number of model parameters matches the number of known values. If we estimate every possible parameter then this is by definition a saturated model (see Model 3E below).
True or False. $\Gamma$ is always a symmetric matrix.
Answer : False $\gamma_{12} \ne \gamma_{21}$. The first is the regression of $y_1$ on $x_1$ and the second is the regression of $y_2$ on $x_2$.
Example using Model 3A
For edification purposes, we will modify the syntax of Model 3A slightly (without going too deeply in technical details, this syntax models implied parameters explicitly and removes intercept parameters). You should now be able to match every term in this output to the path diagram.
The output is as follows
Use the output generated above to calculate the number of free parameters.
Answer: The number of unique model parameters is 10 and the number of fixed parameters is 1, namely we fixed $\gamma_{21}=0$
Fixed versus free parameters
However we know from the output of Model 3A that the number of free parameters is 9 rather than 10. This is due to the fact that we have allowed for fixed parameters which are parameters that are not estimated and pre-determined to have a specific value. Which parameter have we fixed (see Exercise above)?
The number of free parameters is defined as
$$\mbox{number of free parameters} = \mbox{number of (unique) model parameters } – \mbox{number of fixed parameters}.$$
The goal is to maximize the degrees of freedom (df) which is defined as
$$\mbox{df} = \mbox{number of known values } – \mbox{ number of free parameters}$$
How many degrees of freedom do we have now?
Answer: $10 – 9 = 1$
Models that are just-identified or saturated have df = 0, which means that the number of free parameters equals the number of known values in $\Sigma$. An under-identified model means that the number known values is less than the number of free parameters and an over-identified model means that the number of known values is greater than the number of free parameters. To summarize
- known < free implies degrees of freedom < 0 ( under-identified , bad)
- known = free implies degrees of freedom = 0 ( just identified or saturated , neither bad nor good)
- known > free implies degrees of freedom > 0 ( over-identified , good)
True or False. Over-identified models are good because you have extra degrees of freedom to work with
Answer : True. Over-identified models are the reason we can assess model fit (to be discussed later).
True or False. Linear regression and multiple regression models are saturated models.
Answer : True. Calculate the degrees of freedom and you will see it is zero.
Using what you now know, explain why the degrees of freedom in simple regression is zero (exclude the intercept).
Answer: There three model parameters without the intercept, $\gamma_1, \phi_{11}$ and $\psi_{11}$ and no fixed values. So the number of free parameters is $3-0=3$. Since there are $2(3)/2=3$ known values, the degrees of freedom is $3-3=0$.
Why are there 5 free parameters in the output of m1b ?
Answer: We included the intercept term of the endogenous variable, so the free parameters are $\alpha_1, \gamma_1, \phi_{11}$ and $\psi_{11}$. Although we did not discuss this, lavaan implicitly models the intercept of the exogenous variable $\kappa_1$ which makes a total of five free parameters.
Now that we know how to distinguish between over-identified and just-identified models, we understand that adding a single path of $\gamma_{21}$ turns Model 3A into a just-identified or fully saturated model which we call Model 3E.
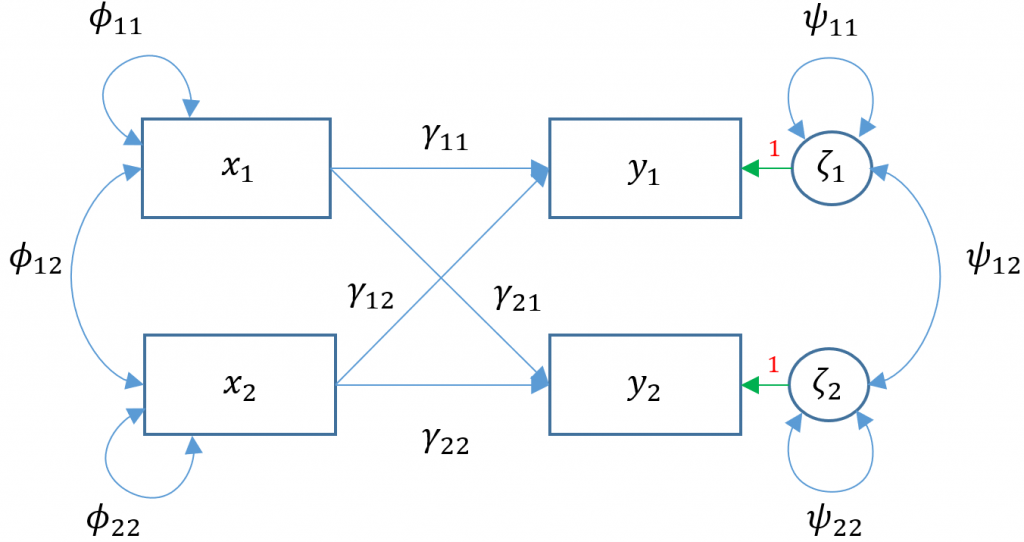
In lavaan , this is easy as adding the additional path of arith on ppsych but remembering that lavaan also by default models the covariance of $\psi_{12}$ which is the residual covariance between read and arith .
We see that Negative Parental Psychology has a negative relationship with Arithmetic: for every one unit increase in the exogenous variable ppsych , the endogenous variable read decreases by 0.275.
Up to this point, we have explored multiple regression where one endogenous variable is predicted by two or more exogenous variables, multivariate regression where multiple exogenous variables can predict multiple endogenous variables. Finally, in the section to follow, we will see how path analysis allows endogenous variables to predict each other.
Multivariate regression is a special case of path analysis where only exogenous variables predict endogenous variables. Path analysis is a more general model where all variables are still manifest but endogenous variables are allowed to explain other endogenous variables. Since $\Gamma$ specifies relations between an endogenous ($y$) and exogenous ($x$) variable, we need to create a new matrix $B$ that specifies the relationship between two endogenous ($y$) variables.
$$\mathbf{y = \alpha + \Gamma x + By + \zeta}$$
The matrix $B$ is a $p \times p$ matrix that is not necessarily symmetric. The rows of this matrix specify which $y$ variable is being predicted and the columns specify which $y$’s are predicting. For example, $\beta_{21}$ is in the second row meaning $y_2$ is being predicted and first column meaning $y_1$ is predicting .
True or False. $\beta_{21}$ is the same as $\beta_{12}$
Answer : False. In $\beta_{21}$ the endogenous variable $y_2$ is being predicted whereas $\beta_{12}$ means $y_1$ is being predicted.
Let’s extend our previous multivariate regression Model 3A so that we believe read which is an endogenous variable also predicts arith . Then the path diagram for Model 4A is shown below. What is the only path that is different between Model 3A and 4A?
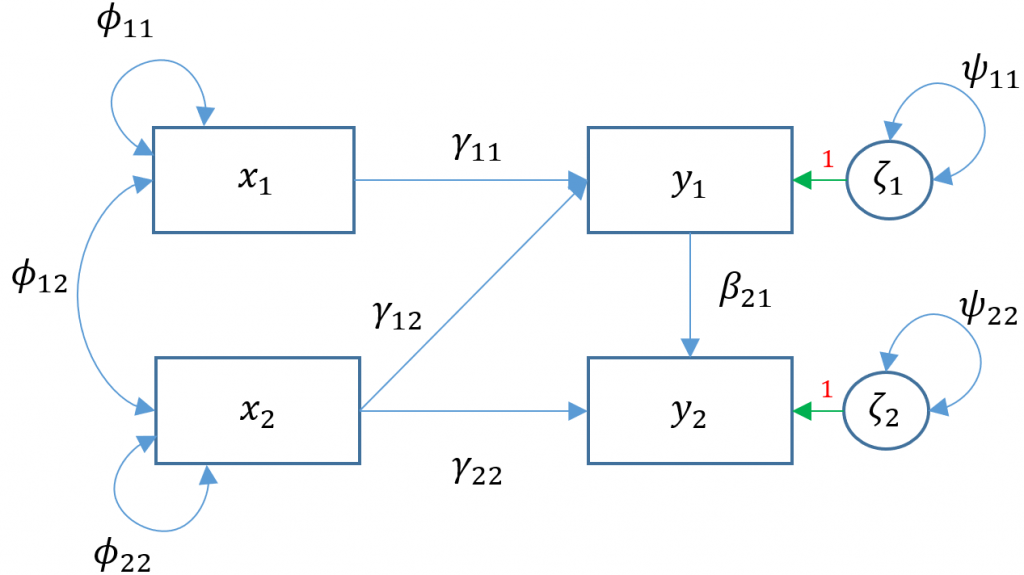
To see the matrix formulation for Model 4A, we have:
$$ \begin{pmatrix} y_{1} \\ y_{2} \end{pmatrix} = \begin{pmatrix} \alpha_1 \\ \alpha_2 \end{pmatrix} + \begin{pmatrix} \gamma_{11} & \gamma_{12}\\ 0 & \gamma_{22} \end{pmatrix} \begin{pmatrix} x_1 \\ x_2 \end{pmatrix} + \begin{pmatrix} 0 & 0\\ \beta_{21} & 0 \end{pmatrix} \begin{pmatrix} y_1 \\ y_2 \end{pmatrix} + \begin{pmatrix} \zeta_{1}\\ \zeta_{2} \end{pmatrix} $$
- $\mathbf{y} = (y_1, \cdots, y_p)’$ vector of $p$ endogenous variables
- $\mathbf{x} =(x_1, \cdots, x_q)’$ vector of $q$ exogenous variables
- $\mathbf{\Gamma}$ matrix of regression coefficients ($p \times q$) of exogenous to endogenous variables whose $i$-th row indicates the endogenous variable and $j$-th column indicates the exogenous variable
- $\mathbf{B}$ matrix of regression coefficients ($p \times p$) of endogenous to endogenous variables whose $i$-th row indicates the source variable and $j$-th column indicates the target variable
- $\mathbf{\zeta}= ( \zeta_1, \cdots, \zeta_p)’$ vector of residuals
- $\zeta$ is uncorrelated with $x$
- $(I-B)$ is invertible (for example $B \ne I$)
True or False. The assumption of path analysis states that I cannot predict $y_1$ only to itself.
Answer: True. This would mean $B=I$.
True or False. $B=0$ means that there are no endogenous variable that predict another and equates to the multivariate regression model.
Answer: True. See Multivariate Regression model above.
True or False. $\beta_{21}$ represents a regression coefficient from the second endogenous variable $y_2$ to the first $y_1$.
Answer: False. $\beta_{21}$ is the regression coefficient from the first endogenous variable $y_1$ to the second $y_2$. The $i$-th row indicates the target endogenous variable and $j$-th column indicates the source endogenous variable.
Modeling a path analysis in lavaan
Just as in Model 3A, we hypothesize that Negative Parental Psychology ppsych and Motivation motiv predict Reading scores read and Motivation predicts Arithmetic. Additionally, suppose we believe also that students who have higher reading ability (Reading read ) are able to read word problems more effectively which can positively predict math scores (Arithmetic arith ). The syntax is as easy in lavaan as adding read as a predictor.
We see that Reading positively predicts Arithmetic over and above the effects of Motivation. So for every one unit increase in Reading scores, Arithmetic is predicted to increase by 0.573 points. Note also that unlike Model 3A, the residual covariance of .arith and .read are turned off by default. This is because by adding a regression path from Reading to Arithmetic, we presume that Reading accounts for all the variance in Arithmetic hence a residual covariance is unnecessary.
Try making the path analysis model above a saturated model by adding a covariance between the residuals of the endogenous variables. How do you interpret this covariance?
Answer : Add the covariance read ~~ arith ; the interpretation is the residual covariance of read and arith accounting for the effect of all exogenous and endogenous predictors.
We see that the path analysis Model 4A as well as the multivariate regressions (Models 3A and 3D) are over-identified models which means that their degrees of freedom is greater than zero. Over-identified models allow flexibility in modeling the remaining degrees of freedom. For example in Model 4A, we can add an additional path between ppsych and read but we can also add a covariance between .read and .arith . Adding either of these parameters results in a fully saturated model. Without a strong a priori hypothesis, it may be difficult ascertain the best parameter to estimate. One solution is to use the modification index, which is a one degree of freedom chi-square test that assesses how the model chi-square will change as a result of including the parameter in the model. The higher the chi-square change, the bigger the impact of adding the additional parameter. To implement the modification index in lavaan , we must input into the modindices function a previously estimated lavaan model, which in this case is fit4a . The option sort=TRUE requests the most impactful parameters be placed first based on the change in chi-square.
The columns represent the
- left-hand side ( lhs )
- operation ( op )
- right-hand side of the equation ( rhs )
- the modification index ( mi ) which is a 1-degree chi-square statistic
- expected parameter change ( epc ) represents how much the parameter is expected to change
- sepec.lv standardizes the epc by the latent variable (in this case there are none)
- sepec.all standardizes by both Y and X
- sepc.nox standardizes all but X variables (in this case Y only)
We see that by far the most impactful parameter is motiv ~ arith with a chi-square change of 68.073. The modification index is suggesting that we regard Motivation as an endogenous predictor and Arithmetic as its exogenous predictor. The expected change (from zero) in this regression coefficient would be 8.874. Although this sounds promosing, not all modification index suggestions make sense. Recall that the chi-square for Model 4A is 4.870 and subtracting 68.073 = -63.20 makes the resulting chi-square negative! Additionally, Rows 17, 20, 11, 19, 10 and 22 have a chi-square change of zero which would make their modifications unnecessary. For this example, we decide to look at the second row which suggests that we maintain Arithmetic as an endogenous variable but add Negative Parental Psychology as an exogenous variable. Based on our hypothesis, it makes sense that Negative Parental Psychology predicts both Arithmetic and Reading. As suggested, we add arith ~ ppsych into our path analysis Model 4A and rename it Model 4B. As an exercise, let’s manually re-run Model 4B and see how closely the expected parameter change epc matches 0.068 and the expected chi-square change of 4.847.
For more information see Modification indices from lavaan ‘s website.
True or False. You should always use modification indexes to improve model fit.
Answer: False. Not all modifications to the model make sense. For example, the covariance of residuals is often times seen as an “easy” way to improve fit without changing the model. However, by adding residual covariances, you are modeling unexplained covariance between variables that by definition are not modeled by your hypothesized model. Although modeling these covariances artificially improves fit, they say nothing about the causal mechanisms your model hypothesizes.
True or False. Each modification index tests one parameter at a time.
Answer: True. Each modification index is a 1 degree of freedom chi-square difference test which means that it only tests one parameter at a time, although the algorithm simultaneously estimates all parameter changes.
True or False. Two fully saturated models must have exactly the same estimated parameters.
Answer: False. There are many ways to specify a saturated model that results in the same zero degrees of freedom. Just because a model is saturated does not mean it is the best model because there may be many more equivalently saturated models.
Based on the modification index above we decide to add arith ~ ppsych to Model 4A and call it Model 4B.
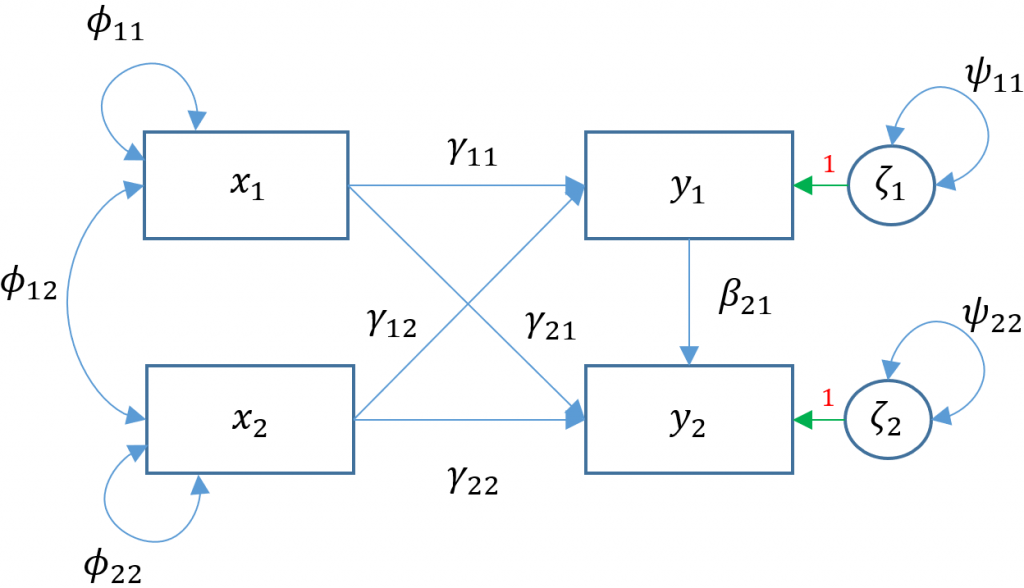
The modification index is as follows:
Why is there no modification index left in fit4b ? Is the chi-square you obtain the same or different from the modification index?
Answer: There is no modification index because it is a saturated model. The modification index is close to the Test User chi-square but slightly bigger since it is an approximation.
In Model 4B, we see that the degrees of freedom is zero which is what we expected from the modification index of 4.847. Recall that for Model 4A, the chi-square was 4.870 which is very close to our approximation. Additionally, the coefficient estimate is 0.068 which matches our modification index output exactly! In general, the modification index is just an approximation of the chi-square based on the Lagrange multiplier and may not match the results of actually re-running the model and obtaining the difference of the chi-square values. The degrees of freedom however, match what we expect, which is a one degree of freedom change resulting in our saturated path analysis model (Model 4B).
Caveats of using modification indexes
Just because modification indexes present us with suggestions for improving our model fit does not mean as a researcher we can freely alter our model. Note that Type I error is the probability of finding a false positive, and altering our model based on modification indexes can have a grave impact on our Type I error. This means that you may be finding many statistically significant relationships that fail to be replicated in another sample. See our page on Retiring Statistical Significance for more information about the consequences of tweaking your hypothesis after your hypothesis has been made.
Modification indexes gives suggestions about ways to improve model fit, but it is helpful to assess the model fit of your current model to see if improvements are necessary. As we have seen, multivariate regression and path analysis models are not always saturated, meaning the degrees of freedom is not zero. This allows us to look at what are called Model Fit Statistics, which measure how closely the (population) model-implied covariance matrix $\Sigma{(\theta)}$ matches the (population) observed covariance matrix $\Sigma$. SEM is also known as covariance structure analysis, which means the hypothesis of interest is regarding the covariance matrix. The null and alternative hypotheses in an SEM model are
$$H_0: \Sigma{(\theta)}=\Sigma$$
$$H_1: \Sigma{(\theta)} \ne \Sigma$$
Typically, rejecting the null hypothesis is a good thing, but if we reject the SEM null hypothesis then we would reject our user model (which is bad). Failing to reject the model is good for our model because we have failed to disprove that our model is bad. Note that based on the logic of hypothesis testing, failing to reject the null hypothesis does not prove that our model is the true model, nor can we say it is the best model, as there may be many other competing models that can also fail to reject the null hypothesis. However, we can certainly say it isn’t a bad model, and it is the best model we can find at the moment. Think of a jury where it has failed to prove the criminal guilty, but it doesn’t necessarily mean he is innocent. Can you think of a famous person from the 90’s who fits this criteria?
Since we don’t have the population covariances to evaluate, they are estimated by the sample model-implied covariance $\Sigma(\hat{\theta})$ and sample covariance $S$. Then the difference $S-\Sigma(\hat{\theta})$ is a proxy for the fit of the model, and is defined as the residual covariance with values close to zero indicating that there is a relatively good fit.
True or False. The residual covariance is defined as $\Sigma -\Sigma{(\theta)}$ and the closer this difference is to zero the better the fit.
Answer : False, the residual covariance uses sample estimates $S-\Sigma(\hat{\theta})$. Note that $\Sigma -\Sigma{(\theta)}=0$ is always true under the null hypothesis.
By default, lavaan outputs the model chi-square a.k.a Model Test User Model . To request additional fit statistics you add the fit.measures=TRUE option to summary , passing in the lavaan object fit4a .
When fit measures are requested, lavaan outputs a plethora of statistics, but we will focus on the four commonly used ones:
- Model chi-square is the chi-square statistic we obtain from the maximum likelihood statistic (in lavaan, this is known as the Test Statistic for the Model Test User Model)
- CFI is the Comparative Fit Index – values can range between 0 and 1 (values greater than 0.90, conservatively 0.95 indicate good fit)
- TLI Tucker Lewis Index which also ranges between 0 and 1 (if it’s greater than 1 it should be rounded to 1) with values greater than 0.90 indicating good fit. If the CFI and TLI are less than one, the CFI is always greater than the TLI.
- In lavaan , you also obtain a p -value of close fit, that the RMSEA < 0.05. If you reject the model, it means your model is not a close fitting model.
The model chi-square is defined as either $NF_{ML}$ or $(N-1)(F_{ML})$ depending on the statistical package where $N$ is the sample size and $F_{ML}$ is the fit function from maximum likelihood, which is a statistical method used to estimate the parameters in your model.
$$F_{ML} = \log |\hat{\Sigma}(\theta)| + tr(S {\hat{\Sigma}}^{-1}(\theta))-\log|S|-(p+q)$$
where $|\cdot|$ is the determinant of the matrix, $tr$ indicates the trace or the sum of the diagonal elements, $\log$ is the natural log, $p$ is the number of exogenous variables, and $q$ is the number of endogenous variables. Then depending on the software,
$$N F_{ML} \sim \chi^2(df_{User})$$
Since $df > 0$ the model chi-square $\chi^2(df_{\mbox{User}})$ is a meaningful test only when you have an over-identified model (i.e., there are still degrees of freedom left over after accounting for all the free parameters in your model).
Comparing the Model Test User Model for Model 4A (over-identified) model to the saturated Model 4B, we see that the Test Statistic degrees of freedom is zero for Model 4B, whereas Model 4A has 1 degree of freedom indicating an over-identified model. The Test Statistic is 4.870 and there is an additional row with P-value (Chi-square) of 0.027 indicating that we reject the null hypothesis.
The larger the chi-square value the larger the difference between the sample implied covariance matrix $\Sigma{(\hat{\theta})}$ and the sample observed covariance matrix $S$, and the more likely you will reject your model. We can recreate the p-value which is essentially zero, using the density function of the chi-square with 1 degrees of freedom $\chi^2_{1}=3.84$. Since $p < 0.05$, using the model chi-square criteria alone we reject the null hypothesis that the model fits the data. It is well documented in CFA and SEM literature that the chi-square is often overly sensitive in model testing especially for large samples. David Kenny states that for models with 75 to 200 cases chi-square is a reasonable measure of fit, but for 400 cases or more it is nearly almost always significant.
True or False. The larger the model chi-square test statistic, the larger the residual covariance $S-\hat{\Sigma}(\theta)$.
Answer: True. Since the model chi-square is proportional to the discrepancy of $S$ and $\Sigma{(\hat{\theta})}$, the higher the chi-square the more positive the value of $S-\Sigma{(\hat{\theta})}$, defined as residual covariance.
Our sample of $N=500$ is considered somewhat large, hence our conclusion may be supplemented with other fit indices.
Model chi-square is sensitive to large sample sizes, but does that mean we stick with small samples? The answer is no, larger samples are always preferred. CFA and the general class of structural equation model are actually large sample techniques and much of the theory is based on the premise that your sample size is as large as possible. So how big of a sample do we need? Kline (2016) notes the $N:q$ rule, which states that the sample size should be determined by the number of $q$ parameters in your model, and the recommended ratio is $20:1$. This means that if you have 10 parameters, you should have n=200. A sample size less than 100 is almost always untenable according to Kline.
The baseline model can be thought of as the “worst-fitting” model and simply assumes that there is absolutely no covariance between variables. Suppose we modified Model 4A to become a baseline model, we would take out all paths and covariances; essentially estimating only the variances. Since there is are no regression paths, there are no endogenous variables in our model and we would only have $x$’s and $\phi$’s.
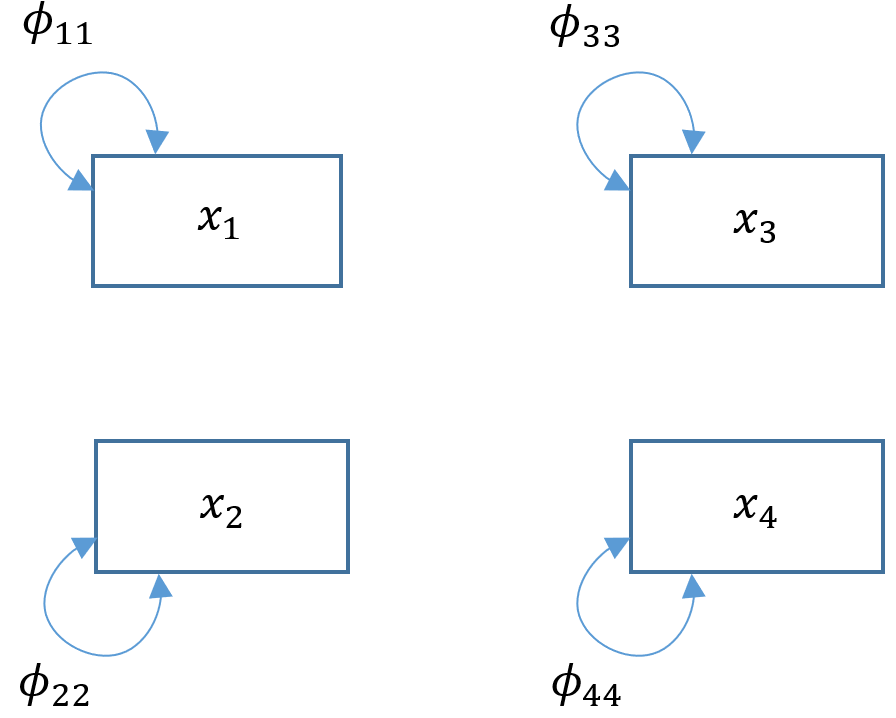
To confirm whether we have truly generated the baseline model, we compare our model to the Model Test Baseline Model in lavaan . We see that the User Model chi-square is 707.017 with 6 degrees of freedom, which matches the Baseline Model chi-square. We will see in the next section how baseline models are used in testing model fit.
Incremental versus absolute fit index
For over-identified models, there are many types of fit indexes available to the researcher. Historically, model chi-square was the only measure of fit but in practice the null hypothesis was often rejected due to the chi-square’s heightened sensitivity under large samples. To resolve this problem, approximate fit indexes that were not based on accepting or rejecting the null hypothesis were developed. Approximate fit indexes can be further classified into a) absolute and b) incremental or relative fit indexes. An incremental fit index (a.k.a. relative fit index) assesses the ratio of the deviation of the user model from the worst fitting model (a.k.a. the baseline model) against the deviation of the saturated model from the baseline model. Conceptually, if the deviation of the user model is the same as the deviation of the saturated model (a.k.a best fitting model), then the ratio should be 1. Alternatively, the more discrepant the two deviations, the closer the ratio is to 0 (see figure below). Examples of incremental fit indexes are the CFI and TLI.
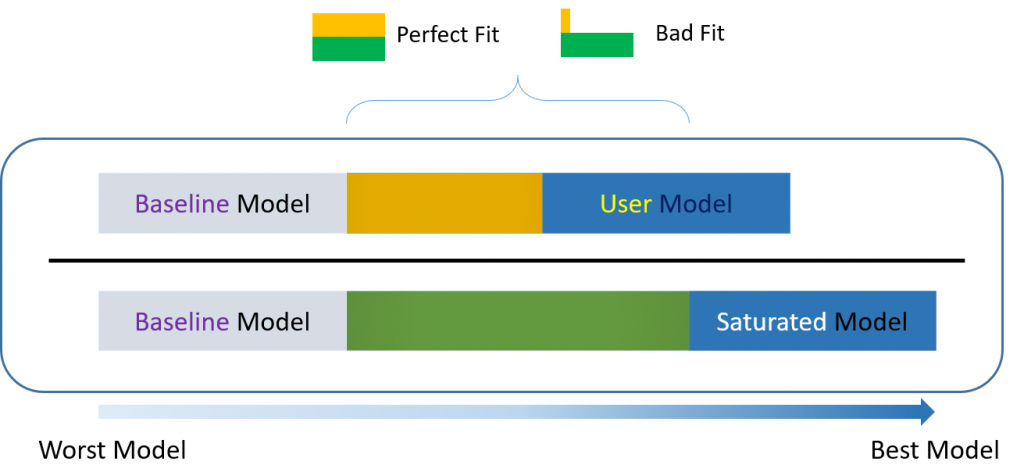
An absolute fit index on the other hand, does not compare the user model against a baseline model, but instead compares it to the observed data. An example of an absolute fit index is the RMSEA (see flowchart below).
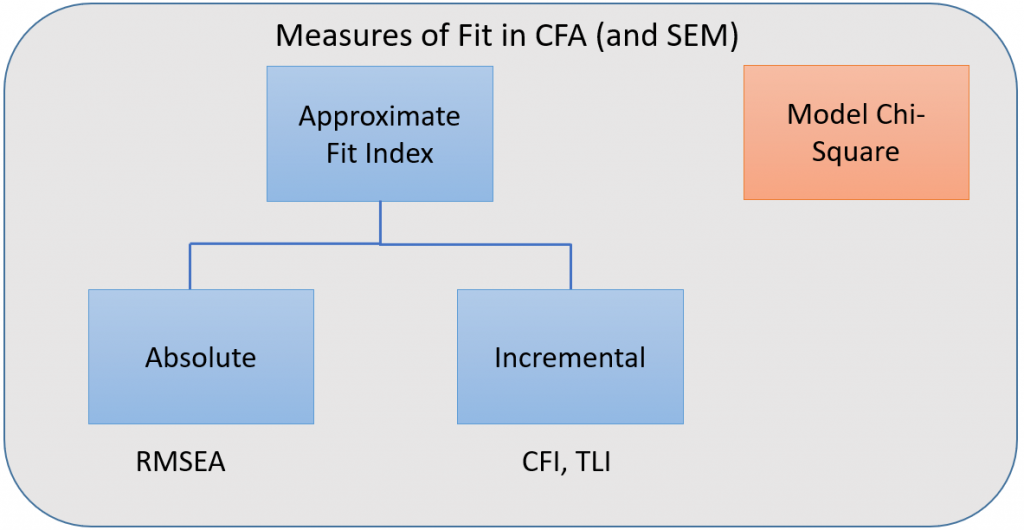
The CFI or comparative fit index is a popular fit index as a supplement to the model chi-square. Let $\delta=\chi^2 – df$ where $df$ is the degrees of freedom for that particular model. The closer $\delta$ is to zero, the more the model fits the data. The formula for the CFI is:
$$CFI= \frac{\delta(\mbox{Baseline}) – \delta(\mbox{User})}{\delta(\mbox{Baseline})} $$
To manually calculate the CFI, recall the selected output from Model 4A model:
Then $\chi^2(\mbox{Baseline}) = 674.748$ and $df({\mbox{Baseline}}) = 5$, and $\chi^2(\mbox{User}) = 4.87$ and $df(\mbox{User}) = 1$. So $\delta(\mbox{Baseline}) = 674.748 – 4.87 = 669.878$ and $\delta(\mbox{User} )= 4.87 – 1=3.87$. We can plug all of this into the following equation:
$$CFI=\frac{669.878-3.87}{669.878}=0.994$$
Verify that the calculations match lavaan output. If $\delta(\mbox{User})=0$, then it means that the user model is not misspecified, so the numerator becomes $\delta(\mbox{Baseline})$ and the ratio becomes 1. The closer the CFI is to 1, the better the fit of the model; with the maximum being 1. Some criteria claims 0.90 to 0.95 as a good cutoff for good fit [citation needed].
The Tucker Lewis Index is also an incremental fit index that is commonly outputted with the CFI in popular packages such as Mplus and in this case lavaan . The term used in the TLI is the relative chi-square (a.k.a. normed chi-square) defined as $\frac{\chi^2}{df}$. Compared to the model chi-square, relative chi-square is less sensitive to sample size. To understand relative chi-square, we need to know that the expected value or mean of a chi-square is its degrees of freedom (i.e., $E(\chi^2(df)) = df$). For example, given that the test statistic truly came from a chi-square distribution with 4 degrees of freedom, we would expect the average chi-square value across repeated samples would also be 4. Suppose the chi-square from our data actually came from a distribution with 10 degrees of freedom but our model says it came from a chi-square with 4 degrees of freedom. Over repeated sampling, the relative chi-square would be $10/4=2.5$. Thus, $\chi^2/df = 1$ indicates perfect fit, and some researchers say that a relative chi-square greater than 2 indicates poor fit (Byrne,1989), other researchers recommend using a ratio as low as 2 or as high as 5 to indicate a reasonable fit (Marsh and Hocevar, 1985).
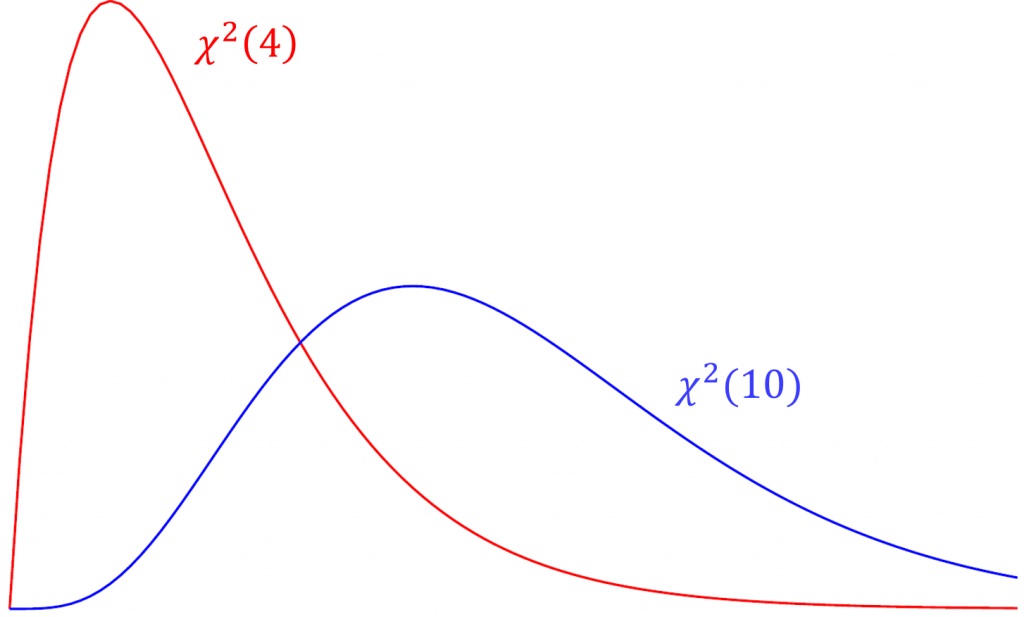
Suppose you ran a CFA with 20 degrees of freedom. What would be the acceptable range of chi-square values based on the criteria that the relative chi-square greater than 2 indicates poor fit?
The range of acceptable chi-square values ranges between 20 (indicating perfect fit) and 40, since 40/20 = 2.
The TLI is defined as
$$TLI= \frac{\chi^2(\mbox{Baseline})/df(\mbox{Baseline})-\chi^2(\mbox{User})/df(\mbox{User})}{\chi^2(\mbox{Baseline})/df(\mbox{Baseline})-1}$$
Note that the TLI can be greater than 1 but for practical purposes we round it to 1. Given the eight-item one factor model:
$$TLI= \frac{674.748/5-4.870/1}{674.748/5-1} =\frac{130.0796}{133.9496}=0.971.$$
We can confirm our answers for both the TLI and CFI which are reported together in lavaan
You can think of the TLI as the ratio of the deviation of the null (baseline) model from user model to the deviation of the baseline (or null) model to the perfect fit model $\chi^2/df = 1$. The more similar the deviation from the baseline model, the closer the ratio to one. A perfect fitting model which generate a TLI which equals 1. David Kenny states that if the CFI is less than one, then the CFI is always greater than the TLI. CFI pays a penalty of one for every parameter estimated. Because the TLI and CFI are highly correlated, only one of the two should be reported.
The root mean square error of approximation is an absolute measure of fit because it does not compare the discrepancy of the user model relative to a baseline model like the CFI or TLI. Instead, RMSEA defines $\delta$ as the non-centrality parameter which measures the degree of misspecification. Recall from the CFI that $\delta=\chi^2 – df$ where $df$ is the degrees of freedom for that particular model. The greater the $\delta$ the more misspecified the model.
$$ RMSEA = \sqrt{\frac{\delta}{df(N-1)}} $$
where $N$ is the total number of observations. The cutoff criteria as defined in Kline (2016, p.274-275)
- $\le 0.05$ ( close-fit )
- between $.05$ and $.08$ ( reasonable approximate fit , fails close-fit but also fails poor-fit)
- $>= 0.10$ ( poor-fit )
In Model 4A, $N=500$, $df(\mbox{User}) = 1$ and $\delta(\mbox{User} )= 4.87 – 1=3.87$ which we already known from calculating the CFI. Here $\delta$ is about 4 times the degrees of freedom.
$$ RMSEA = \sqrt{\frac{3.87}{1(499)}} = \sqrt{0.007755511}=0.088$$
Our RMSEA = 0.088 indicating reasonable approximate fit, as evidence by the large $\delta(\mbox{User} )$ relative to the degrees of freedom.
Given that the p-value of the model chi-square was less than 0.05, the CFI = 0.994 and the RMSEA = 0.088, and looking at the standardized loadings we report to the Principal Investigator that Model 4A is a reasonable model.
The Measurement Model
We have talked so far about how to model structural relationships between observed variables. A measurement model is essentially a multivariate regression model where the predictor is an exogenous or endogenous latent variable (a.k.a factor). The model is defined as
$$\mathbf{x= \tau_x + \Lambda_x \xi+ \delta}$$
- $\mathbf{x} =(x_1, \cdots, x_q)’$ vector of $x$-side indicators
- $\mathbf{\tau_x}$ vector of $q$ intercepts for $x$-side indicators
- $\mathbf{\xi}$ vector of $n$ latent exogenous variables
- $\mathbf{\delta}= ( \delta_1, \cdots, \delta_q)’$ vector of residuals for $x$-side indicators
- $\mathbf{\Lambda_x}$ matrix of loadings ($q \times n$) corresponding to the latent exogenous variables
- $\mathbf{\theta_{\delta}}$ variance or covariance of residuals for $x$-side indicators
The term $\tau_{x_{1}}$ means the intercept of the first item, and $\lambda^x_2$ is the loading of the second item with the factor and $\epsilon_{3}$ is the residual of the third item, after accounting for the only factor.
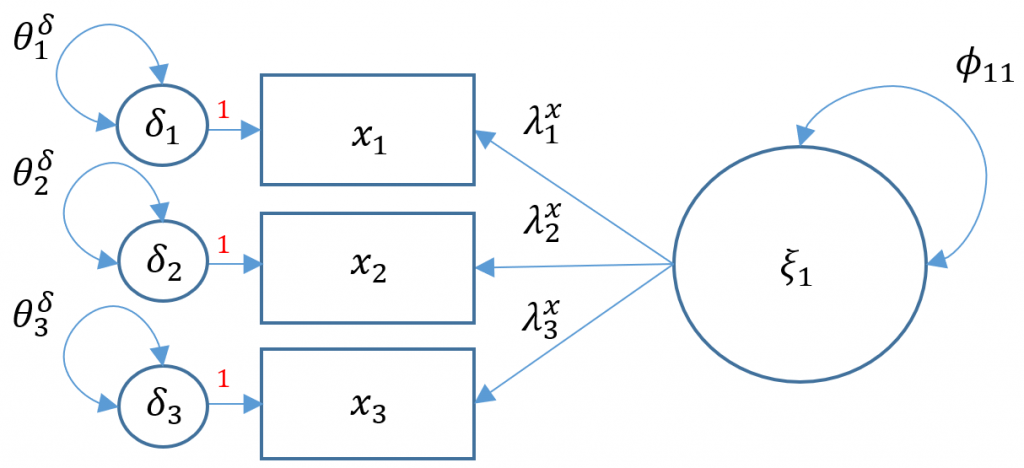
True or False. $\xi$ variables do not have residual covariances
Answer: True. $\xi$ are latent exogenous variables which do not have residuals hence no residual covariances
True or False. $x_i$ variables do not have residual covariances
Answer: False. $x_i$’s are manifest exogenous variables but in fact are explained by the latent exogenous variable, therefore it has a residual term and residual variance (unexplained variance), most notably $\theta_{\delta}$.
Identification of a three-item factor analysis
Identification of the one factor model with three items is necessary due to the fact that we have 7 parameters from the model-implied covariance matrix $\Sigma(\theta)$ (e.g., three factor loadings, three residual variances and one factor variance) but only $3(4)/2=6$ known values to work with. The extra parameter comes from the fact that we do not observe the factor but are estimating its variance. In order to identify a factor model with three or more items, there are two options known as the marker method and the variance standardization method. The
- marker method fixes the first loading of each factor to 1,
- variance standardization method fixes the variance of each factor to 1 but freely estimates all loadings.
In matrix notation, the marker method (Option 1) can be shown as
$$ \Sigma(\theta)= \phi_{11} \begin{pmatrix} 1 \\ \lambda^{x}_{2} \\ \lambda^{x}_{3} \end{pmatrix} \begin{pmatrix} 1 & \lambda^{x}_{2} & \lambda^{x}_{3} \\ \end{pmatrix} + \begin{pmatrix} \theta^{\delta}_{11} & 0 & 0 \\ 0 & \theta^{\delta}_{22} & 0 \\ 0 & 0 & \theta^{\delta}_{33} \\ \end{pmatrix} $$
In matrix notation, the variance standardization method (Option 2) looks like
$$ \Sigma(\theta)= (1) \begin{pmatrix} \lambda^{x}_{1} \\ \lambda^{x}_{2} \\ \lambda^{x}_{3} \end{pmatrix} \begin{pmatrix} \lambda^{x}_{1} & \lambda^{x}_{2} & \lambda^{x}_{3} \\ \end{pmatrix} + \begin{pmatrix} \theta^{\delta}_{11} & 0 & 0 \\ 0 & \theta^{\delta}_{22} & 0 \\ 0 & 0 & \theta^{\delta}_{33} \\ \end{pmatrix} $$
Notice in both models that the residual covariances stay freely estimated.
For the variance standardization method, go through the process of calculating the degrees of freedom. If we have six known values is this model just-identified, over-identified or under-identified?
Answer: We start with 10 unique parameters in the model-implied covariance matrix. Since we fix one factor variance, and 3 unique residual covariances, the number of free parameters is $10-(1+3)=6$. Since we have 6 known values, our degrees of freedom is $6-6=0$, which is defined to be saturated.
Let’s see how we can run a one-factor measurement in lavaan with Verbal IQ verbal , SES ses and Negative Parental Psychology ppsych as indicators of the factor (latent exogenous variable) Risk risk .
The first line is the model statement. Recall that =~ represents the indicator equation where the latent variable is on the left and the indicators (or observed variables) are to the right the symbol. Here we name our factor risk , which is indicated by verbal , ses and ppsych (note the names must match the variable names in the dataset). Here we explicitly model the intercepts of the indicators using verbal ~ 1 , ses ~ 1 and ppsych ~ 1 . Then store the model into object m5a for Model 5A. The second line is where we specify that we want to run the analysis using the sem function, which is actually a wrapper for the lavaan function. The model to be estimated is m5a and the dataset to be used is dat ; storing the output into object fit5a . Finally summary(fit5a, standardized=TRUE) requests a summary of the analysis, outputting the estimator used, the number of free parameters, the test statistic, estimated means, standardized loadings and standardized variances.
By default, lavaan chooses the marker method (Option 1) if nothing else is specified. To better interpret the factor loadings, often times you would request the standardized solutions. Notice two additional columns in the output, Std.lv and Std.all . For users of Mplus, Std.lv corresponds to STD and Std.all corresponds to STDYX. In the variance standardization method Std.lv , we only standardize by the predictor (the factor, X). Std.all not only standardizes by the variance of the latent variable ($\xi$) and also by the variance of outcome which in this case are the indicators $x$.
Try to fit the model using variance standardization method.
Answer: : Refer to Running a one-factor CFA in lavaan for more details on how perform variance standardization in lavaan .
Not all latent variables are exogenous. If in the case that the latent variable is endogenous we will rename the factor $\eta$.
Suppose again that we have three items except now they are labeled ($y_1,y_2,y_3$). For a latent endogenous variable, the structure of the measurement model remains the same except now the parameters are re-labeled as $y$-side variables. The path diagram for Model 5B is shown below (note, intercepts $\mathbf{\tau_y}$ are not shown but still implicitly modeled):
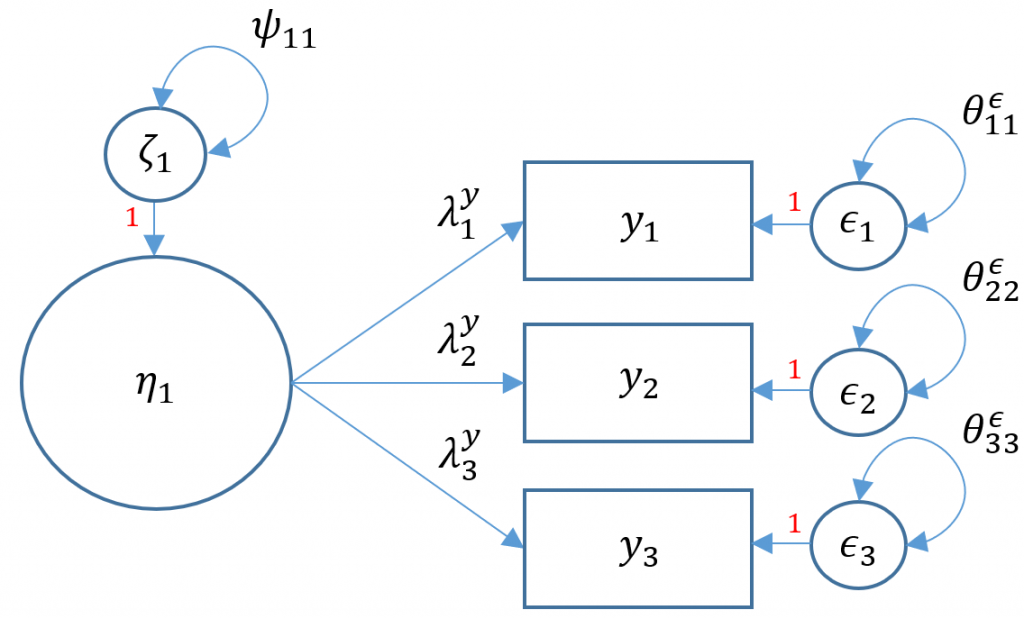
Note that the direction of the arrows face the right. In LISREL path diagram notation, exogenous latent variables have measurement arrows pointing to the left and endogenous latent variables have measurement arrows pointing to the right. Exogenous latent variables correspond to x -side models and endogenous latent variables to y -side models. Here the $y$-side measurement model is defined as
$$\mathbf{y= \tau_y + \Lambda_y \eta + \epsilon}$$
- $\mathbf{y} = (y_1, \cdots, y_p)’$ vector of $y$-side indicators
- $\mathbf{\tau_y}$ vector of $p$ intercepts for $y$-side indicators
- $\mathbf{\eta}$ vector of $m$ latent endogenous variables
- $\mathbf{\epsilon}= ( \epsilon_1, \cdots, \epsilon_p)’$ vector of residuals for $y$-side indicators
- $\mathbf{\Lambda_y}$ matrix of loadings ($m \times q$) corresponding to the latent endogenous variables
- $\mathbf{\theta_{\epsilon}}$ variance or covariance of residuals for $y$-side indicators
The main difference between Models 5A and 5B are simply that Model 5A is an exogenous latent factor analysis whereas Model 5B is an endogenous latent factor analysis, meaning that it is being predicted by another latent variable. Since we currently have no predictors of $\eta_1$, this is just a hypothetical model. In the section below on structural regression, we will see an endogenous latent variable modeled with real-world data. Exogenous latent measurement models are what are classified as x-side variables which point to the left in a path diagram and endogenous latent measurement models are known as y-side latent variables which point to the right in a path diagram.
Up to this point we have studied multivariate measurement models that define the relationship between indicators and latent variables, as well as multivariate regression and path models that define the causal relationship between observed endogenous and exogenous variables. In the next section, we will see how structural regression models allows us to model relationships between exogenous and endogenous latent variables.
True or False. In a LISREL path diagram, endogenous latent variables have right-pointing arrows to the indicators.
Answer: True. The indicators and latent endogenous variable is known as $y$-side variables.
The Structural Model
So far we have discussed all the individual components that make up the structural regression model. Recall that multivariate regression involves regression with simultaneous endogenous variables and path analysis allows for explanatory endogenous variables. Confirmatory factor analysis is a measurement model that links latent variables to indicators. Finally, structural regression unifies the measurement and structural models to allow for explanatory latent variables, whether endogenous or exogenous.
$$\mathbf{\eta = \alpha + B \eta + \Gamma \xi + \zeta}$$
Measurement variables
- $\mathbf{x} = (x_1, \cdots, x_q)’$ vector of $x$-side indicators
- $\mathbf{\tau_x}$ vector of $q$ intercept terms for $x$-side indicators
- $\mathbf{\tau_y}$ vector of $p$ intercept terms for $y$-side indicators
- $\mathbf{\Lambda_y}$ matrix of loadings ($p \times m$) corresponding to the latent endogenous variables
Structural variables
- $\mathbf{\alpha}$ a vector of $m$ intercepts
- $\Gamma$ a matrix of regression coefficients ($m \times n$) of latent exogenous to latent endogenous variables whose $i$-th row indicates the latent endogenous variable and $j$-th column indicates the latent exogenous variable
- $B$ a matrix of regression coefficients ($m \times m$) of latent endogenous to latent endogenous variables whose $i$-th row indicates the target endogenous variable and $j$-th column indicates the source endogenous variable.
- $\zeta= ( \zeta_1, \cdots, \zeta_m)’$ vector of residuals for the latent endogenous variable
- $\eta$ and $\xi$ are not observed
- $\epsilon$ and $\delta$ are errors of measurement for $y$ and $x$ respectively
- $\epsilon$ is uncorrelated with $\delta$
To specify the full structural regression model, it is more intuitive to start with the measurement model and then specify how the latent variables relate to each other (the structural model). In order for latent exogenous variables to explain latent endogenous variables, two separate measurement models must be established.
First let’s specify the latent exogenous measurement model with six items where the first three $x$-side indicators ($x_1, x_2, x_3$) are measured by $\xi_1$ and the latter three ($x_4,x_5,x_6$) are measured by $\xi_2$. The un-identified measurement model for two latent exogenous variables with three indicators each is:
$$ \begin{pmatrix} x_{1} \\ x_{2} \\ x_{3} \\ x_{4} \\ x_{5} \\ x_{6} \\ \end{pmatrix} = \begin{pmatrix} \tau_{x_{1}} \\ \tau_{x_{2}} \\ \tau_{x_{3}} \\ \tau_{x_{4}} \\ \tau_{x_{5}} \\ \tau_{x_{6}} \end{pmatrix} + \begin{pmatrix} \lambda^{x}_{11} & \lambda^{x}_{12} \\ \lambda^{x}_{21} & \lambda^{x}_{22} \\ \lambda^{x}_{31} & \lambda^{x}_{32} \\ \lambda^{x}_{41} & \lambda^{x}_{42} \\ \lambda^{x}_{51} & \lambda^{x}_{52} \\ \lambda^{x}_{61} & \lambda^{x}_{62} \end{pmatrix} \begin{pmatrix} \xi_{1} \\ \xi_{2} \end{pmatrix} + \begin{pmatrix} \delta_{1} \\ \delta_{2} \\ \delta_{3} \\ \delta_{4} \\ \delta_{5} \\ \delta_{6} \end{pmatrix} $$
Now that we’ve established the exogenous measurement model, let’s move to the endogenous measurement model. The three $y$-side indicators $y_1, y_2, y_3$ are measured by one factor $\eta_1$.
$$ \begin{pmatrix} y_{1} \\ y_{2} \\ y_{3} \end{pmatrix} = \begin{pmatrix} \tau_{y_{1}} \\ \tau_{y_{2}} \\ \tau_{y_{3}} \end{pmatrix} + \begin{pmatrix} \lambda^{y}_{11} \\ \lambda^{y}_{21} \\ \lambda^{y}_{31} \end{pmatrix} \begin{pmatrix} \eta_{1} \end{pmatrix} + \begin{pmatrix} \epsilon_{1}\\ \epsilon_{2} \\ \epsilon_{3} \end{pmatrix} $$
Now that we’ve established both $x$-side and $y$-side measurement models, we can specify the structural model.
Just as the structural model in multivariate regression and path analysis specifies relationships among observed variables, structural regression specifies the relationship between latent variables. In Model 6A, we have two latent exogenous variables ($\xi_1, \xi_2$) predicting one latent endogenous variable ($\eta_1$).
$$ \eta_{1} = \alpha_1 + \begin{pmatrix} \gamma_{11} & \gamma_{12} \end{pmatrix} \begin{pmatrix} \xi_1 \\ \xi_2 \end{pmatrix} + 0 \cdot \eta_1 + \zeta_{1} $$
Recall that the $\Gamma$ matrix specifies the relationship between exogenous and endogenous variables, so that $\gamma_{11}$ is the regression path of the first endogenous variable $\eta_1$ with the first exogenous variable $\xi_1$ and $\gamma_{12}$ is the regression path of $\eta_1$ with the second exogenous variable $\xi_2$. Since there are no endogenous variables predicting each other, $B=0$.
True or False. In Model 6A, $B=0$ here implies that there are no structural paths.
Answer: False. $B=0$ means that there are no endogenous variables predicting each other as in path analysis, but $\Gamma$ defines the structural paths between exogenous and endogenous variables.
True or False. $\eta = \eta_1$ because there is only endogenous variable, however $\Gamma$ is a $1 \times 2$ matrix because there are two exogenous variables.
Answer: True. The number of rows in $\eta$ tells you how many endogenous variables. Here there is only one outcome regardless the number of exogenous predictors.
True or False. You can simplify Model 6A model to the path analysis model by removing the two measurement models.
Answer: False (somewhat). Even if you remove the measurement models you still have the equation $\mathbf{\eta = \alpha + \Gamma \xi + B \eta + \zeta}$ which is defined only for latent endogenous variables. However, simply exchange $y$ for $\eta$ and $x$ for $\xi$ and you will obtain the path analysis model.
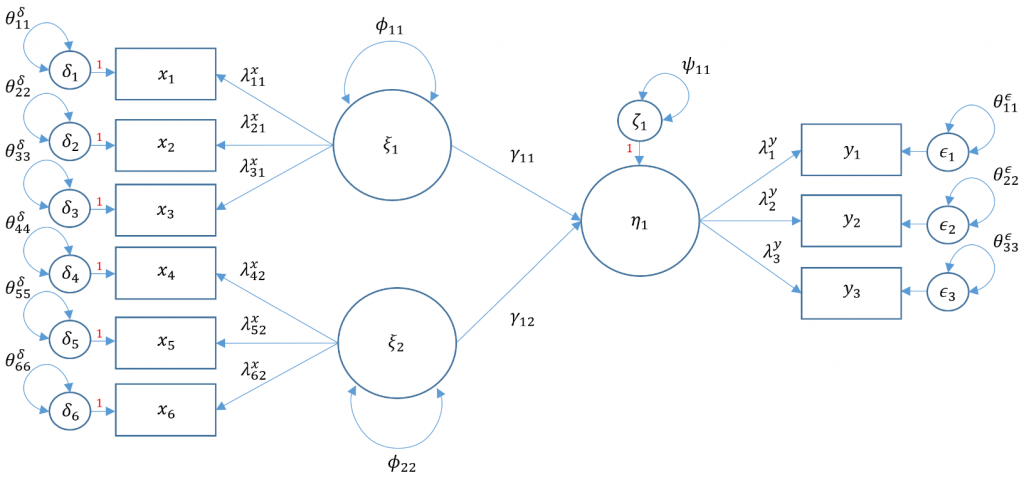
Suppose we want to consider a single exogenous latent variable $\xi_1$ predicting two endogenous latent variables $\eta_1, \eta_2$ and additionally that one of the endogenous variables predicts another. What additional matrix do you think we need? Since we have already established the measurement model in 6A we can re-use the specification, making note that the measurement model now has one $x$-side model and two $y$-side models. However, not only do we need $\Gamma$ to model the relationship of the exogenous variable to the two endogenous variables, we need the $B$ matrix to specify the relationship of the first endogenous variable to the other.
$$ \begin{pmatrix} \eta_{1} \\ \eta_{2} \end{pmatrix} = \begin{pmatrix} \alpha_1 \\ \alpha_2 \end{pmatrix} + \begin{pmatrix} \gamma_{11}\\ \gamma_{21} \end{pmatrix} \xi_1 + \begin{pmatrix} 0 & 0\\ \beta_{21} & 0 \end{pmatrix} \begin{pmatrix} \eta_1 \\ \eta_2 \end{pmatrix} + \begin{pmatrix} \zeta_{1}\\ \zeta_{2} \end{pmatrix} $$
Writing out the equations we get:
$$ \eta_{1} = \alpha_1 + \gamma_{11} \xi_1 + \zeta_{1} $$
$$ \eta_{2} = \alpha_2 + \gamma_{21} \xi_1 + \beta_{21} \eta_1 + \zeta_{2} $$
The first equation specifies that the first endogenous variable is being predicted only by the exogenous variable whereas the second equation specifies that the second endogenous variable is being predicted by both the exogenous variable and first endogenous variable.
True or False. The diagonal entries of $B$ are always zero.
Answer: True. You cannot have a variable predict itself so $\beta_{11}=\beta_{22}=0$.
True or False. $\beta_{21}$ means that the second endogenous variable is predicting the first endogenous variable.
True or False. $\gamma_{21}$ means that the second exogenous variable is predicting the first endogenous variable.
Answer: False. The first index specifies the endogenous variable, so $\gamma_{21}$ means the first exogenous variable predicts first endogenous variable (read it “backwards”). Since we only have one $\xi$ you can remove the second index and just say $\gamma_2$.
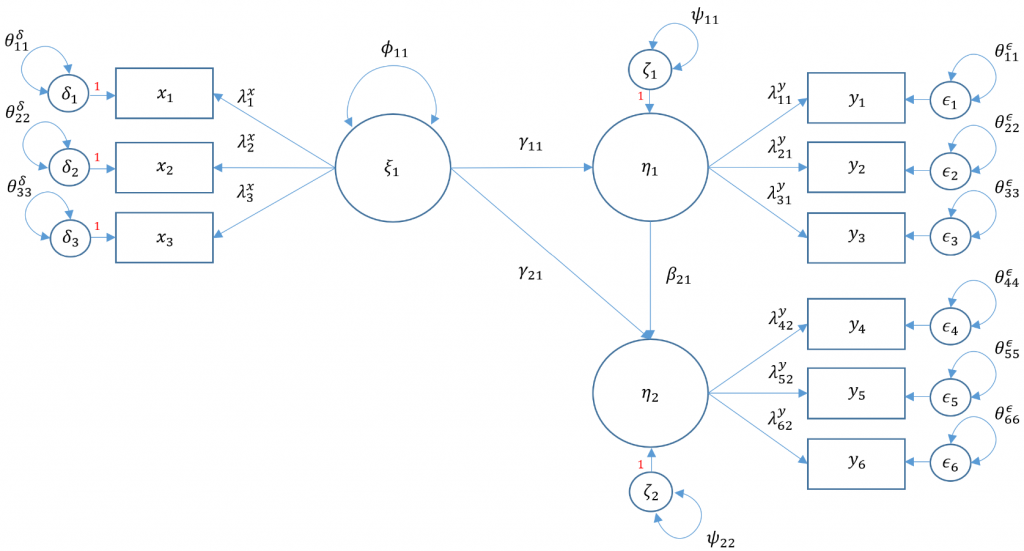
Since lavaan uses what are known as all $y$-side models, there is no need to different between exogenous and endogenous latent variables. As such, the measurement model in 6B is exactly the same as in Model 6A. The only modification then is the structural regression. We hypothesize that Risk negatively predicts Adjustment adjust ~ adjust and that Adjustment (an endogenous variable) and Risk (an exogenous variable) predict Achievement achieve ~ adjust + risk .
Structural regression with an observed endogenous variable (Model 6C)
Structural models relate latent to latent variables. Suppose you wanted to look at how Risk or Adjustment relate in particular to Reading scores rather than overall student achievement. In LISREL notation, the matrices $\Gamma$ or $B$ in the structural regression allow only for relationships among latent variables. However, lavaan and other software programs such as Mplus allow the user to easily specify the relationship between an observed and latent variable. The code is as easy as specifying read ~ adjust + risk where adjust and risk are latent variables.
The results suggest that Adjustment and Risk are positively associated with Reading scores, such that a one unit increase in adjust leads to a 0.285 increase in read and a one unit increase in risk leads to a 0.853 increase in read (adjusting for other variables in the model). In the following section, we will see how to circumvent the restriction that $\Gamma$ and $B$ are specified only between latent variables.
True or False. lavaan allows easy specification of paths between latent exogenous variables and observed endogenous variables.
True or False. In LISREL notation, the relationship between a latent variable and an observed variable can be defined in both measurement and structural models.
Answer: False. In LISREL notation, the relationship between a latent variable and an observed variable is only defined in a measurement model. We will see in the section below how lavaan is able to “bypass” this restriction.
Model 6C (Manual Specification)
The reason that lavaan makes it easy to specify the relationship between observed endogenous variables and latent exogenous variables in a structural regression is because it uses all $y$-side LISREL notation, which does not differentiate the $B$ and $\Gamma$ matrices. In traditional LISREL notation however, the $\Gamma$ and $B$ matrices contain the only allowable regression paths. As an instructional exercise, we will recreate Model 6C but still impose the restrictions on $B$. This workaround can be accomplished by setting read as the single indicator of the latent endogenous variable readf . For identification, constrain its loading to 1 and set the residual variance of the exogenous read to 0 (e.g., $\theta_{\epsilon}_{44}=0$). The path diagram can be visualized as:
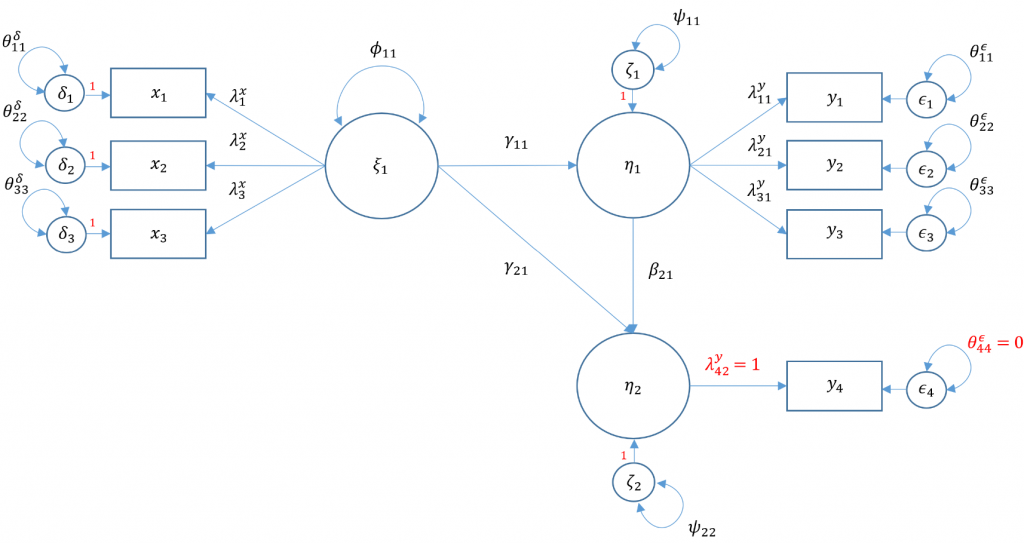
If you were to run this model in lavaan , it will give a warning like the one below.
This suggests that this single-indicator constraint may be incompatible with the default optimizer. To fix this error, re-run the analysis and change the default optimization method from NLMINB (Unconstrained and box-constrained optimization using PORT routines) to BFGS (Broyden–Fletcher–Goldfarb–Shanno algorithm) by adding the syntax optim.method=list("BFGS") . Note that using BFGS takes a whopping 4563 iterations but eventually converges.
The results we obtain are similar to the results from the output of m6c using NLMINB, given slight rounding errors due to the different optimization method used.
As we have seen, structural equation modeling is a broad framework that encompasses a vast array of linear models, namely linear regression, multivariate regression, path analysis, confirmatory factor analysis and structural regression. These models are parameterized rigorously under the LISREL (linear structural relations) framework developed by Karl Joreskög in 1969 and 1973. Understanding the matrix parameterizations is important not so much for practical implementation but to allow the data analyst to fully understand the nuances of each SEM model subtype. For example, a latent model must be identified by its corresponding observed indicators, a restriction that is not needed in path analysis models where all variables are observed. Additionally, a model that apparently predicts a latent variable to an observed endogenous variable is in fact a latent structural regression where the observed endogenous variable is forced to become a single indicator measurement model with constraints. These subtleties are not apparent to the causal analyst until he or she understands that $\Gamma$ and $B$ matrices in structural regression specify relationships only between latent variables. Although not a necessity for implementation, distinguishing between matrices such as $\Gamma$ and $B$ determine what type of model is considered. For example in a path analysis model, setting $B=0$ is equivalent to the multivariate regression where the only predictions are between observed exogenous and observed endogenous variables. Knowing that path analysis models do not contain $\eta$ or $\xi$ variables means understanding that path analysis is only appropriate for observed variables. Once the analyst is able to distinguish between these parameters, he or she will begin o understand the theoretical underpinnings of the structural equation model. However, distinguishing between $B$ and $\Gamma$ is not sufficient to understanding all of SEM, and we do not purport to instill mastery to the reader in one succinctly written website. Further pages may delve more deeply into estimation, as well as more complex topics such as multigroup SEM, latent growth models, and measurement invariance testing. There are also a tremendous number of literature and books on SEM that we hope the reader will take the time to read. At the very least, we hope you have found this introductory seminar to be useful, and we wish you best of luck on your research endeavors.
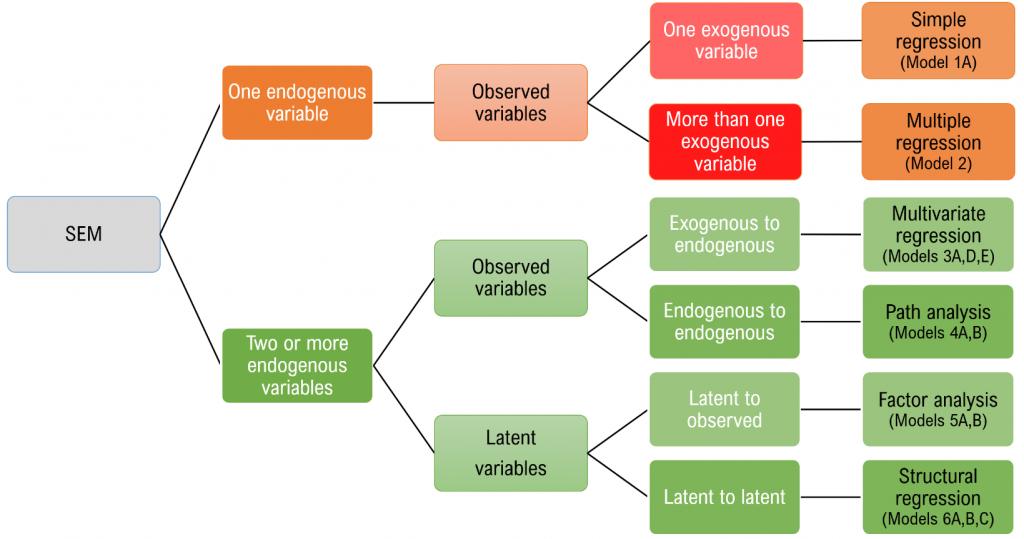
True or False. SEM encompasses a broad range of linear models and combines simultaneous linear equations with latent variable modeling.
Answer: True. Multivariate regression and path analysis are simultaneous equations of observed variables; factor analysis is a latent variable model, and structural regression combines the concepts of path analysis with factor analysis.
True or False. Multivariate regression means that there is always more than one exogenous predictor in my model.
Answer: False. Multivariate regression indicates more than one endogenous variable. You can certainly have only one exogenous predictor of multiple endogenous variables.
True or False. Structural regression models the regression paths only among latent variables.
Answer: True. Structural regression defines relationships between latent variables and path analysis defines relationships between observed variables.
Jöreskog, K. G., Olsson, U. H., & Wallentin, F. Y. (2016). Multivariate analysis with LISREL . Basel, Switzerland: Springer.
Kline, R. B. (2016). Principles and practice of structural equation modeling (4th ed.). Guilford publications.
For more information on:
- lavaan’s own tutorial http://lavaan.ugent.be/tutorial
- extracting objects from lavaan Inspect or extract information from a fitted lavaan object
Saturated versus baseline models
- What are the saturated and baseline models in sem?
- Google Forums
- Disentangling degrees of freedom
Fit indexes
- Research Gate Discussion about Chi-Square
- Assess whole SEM model–chi square and fit index
Your Name (required)
Your Email (must be a valid email for us to receive the report!)
Comment/Error Report (required)
How to cite this page
- © 2021 UC REGENTS
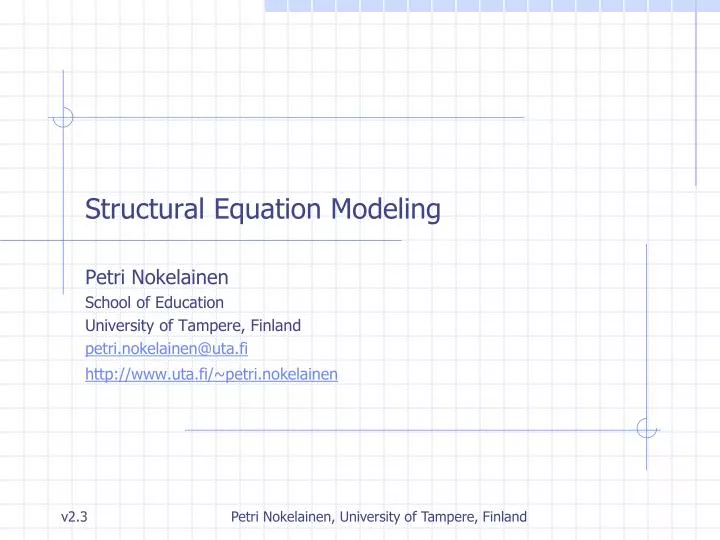
Structural Equation Modeling
Nov 09, 2011
1.52k likes | 1.96k Views
Structural Equation Modeling. Petri Nokelainen School of Education University of Tampere, Finland [email protected] http://www.uta.fi/~petri.nokelainen. Contents. Introduction Path Analysis Basic Concepts of Factor Analysis Model Constructing Model hypotheses Model specification
Share Presentation
- partial correlation coefficients
- systematic experiment
- model estimation
- dv iv petri nokelainen
- original measurement scale examination
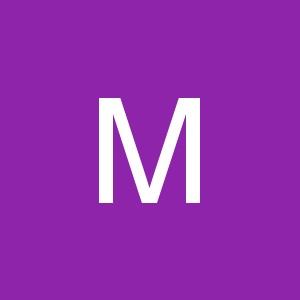
Presentation Transcript
Structural Equation Modeling Petri Nokelainen School of Education University of Tampere, Finland [email protected] http://www.uta.fi/~petri.nokelainen Petri Nokelainen, University of Tampere, Finland
Contents • Introduction • Path Analysis • Basic Concepts of Factor Analysis • Model Constructing • Model hypotheses • Model specification • Model identification • Model estimation • An Example of SEM: Commitment to Work and Organization • Conclusions • References Petri Nokelainen, University of Tampere, Finland
Introduction • Development of Western science is based on two great achievements: the invention of the formal logical system (in Euclidean geometry) by the Greek philosophers, and the possibility to find out causal relationships by systematic experiment (during the Renaissance). Albert Einstein (in Pearl, 2000) Petri Nokelainen, University of Tampere, Finland
Introduction • Structural equation modeling (SEM), as a concept, is a combination of statistical techniques such as exploratory factor analysis and multiple regression. • The purpose of SEM is to examine a set of relationships between one or more Independent Variables (IV) and one or more Dependent Variables (DV). Petri Nokelainen, University of Tampere, Finland
Introduction • Both IV’s and DV’s can be continuous or discrete. • Independent variables are usually considered either predictor or causal variables because they predict or cause the dependent variables (the response or outcome variables). Petri Nokelainen, University of Tampere, Finland
Introduction • Structural equation modeling is also known as ‘causal modeling’ or ‘analysis of covariance structures’. • Path analysis and confirmatory factor analysis (CFA) are special types of SEM. (Figure 1.) Petri Nokelainen, University of Tampere, Finland
Introduction • Genetics S. Wright (1921): “Prior knowledge of the causal relations is assumed as prerequisite … [in linear structural modeling]”. y =x + “In an ideal experiment where we control X to x and any other set Z of variables (not containing X or Y) to z, the value of Y is given by x + , where is not a function of the settings x and z.” (Pearl, 2000) Petri Nokelainen, University of Tampere, Finland
Introduction • According to JudeaPearl (2000), modern SEM is a far cry from the original causality modeling theme, mainly for the following two reasons: • Researchers have tried to build scientific ’credibility’ of SEM by isolating (or removing) references to causality. • Causal relationships do not have commonly accepted mathematical notation. Petri Nokelainen, University of Tampere, Finland
Two main components of SEM are presented in Figure 1. CFA operates with observed and latent variables, path analysis operates only with observed variables. Introduction Figure 1. Components of Structural Equation Modeling (Nokelainen, 1999.) Petri Nokelainen, University of Tampere, Finland
Path Analysis • Examines how nindependent(x, IV, Xi, ) variables are statistically related to a dependent (y, DV, Eta, ) variable. • Applies the techniques of regression analysis, aiming at more detailed resolution of the phenomena under investigation. • Allows • Causal interpretation of statistical dependencies • Examination of how data fits to a theoretical model Petri Nokelainen, University of Tampere, Finland
Path Analysis • Once the data is available, conduction of path analysis is straightforward: • Draw a path diagram according to the theory. • Conduct one or more regression analyses. • Compare the regression estimates (B) to the theoretical assumptions or (Beta) other studies. • If needed, modify the model by removing or adding connecting paths between the variables and redo stages 2 and 3. Petri Nokelainen, University of Tampere, Finland
Path Analysis • Data assumptions: • DV: • Continuous, normally distributed (univariate normality assumption) • IV: • Continuous (no dichotomy or categorical variables) • N: • About 30 observations for each IV Petri Nokelainen, University of Tampere, Finland
Path Analysis • Theoretical assumptions • Causality: • X1 and Y1 correlate. • X1 precedes Y1 chronologically. • X1 and Y1 are still related after controlling other dependencies. • Statistical assumptions • Model needs to be recursive. • It is OK to use ordinal data. • All variables are measured (and analyzed) without measurement error ( = 0). Petri Nokelainen, University of Tampere, Finland
Path Analysis • As stated earlier, path analysis assumes that the model is recursive. • Nature of causal dependency is unidirectional, like a ’one way road’ (arc with one head ). • If there is no a priori information available about the direction of causal dependency, it is assumed to be correlational (arc with two heads ). r Petri Nokelainen, University of Tampere, Finland
AGE EDUCATION TASK WILL Path Analysis r Petri Nokelainen, University of Tampere, Finland
AGE EDUCATION TASK WILL Path Analysis • Direct and indirect effect Petri Nokelainen, University of Tampere, Finland
Path Analysis • There are two types of observed variables: • Endogenous (y, DV, Eta ). • Exogenous (x, IV, Xi ). • For each endogenous (DV) variable, a regression analysis is performed. DV IV Petri Nokelainen, University of Tampere, Finland
Path Analysis x IV Xi EXOGENIOUS y DV Eta ENDOGENIOUS AGE EDUCATION TASK WILL Two regression analyses: 1) AGE + EDUCATION + WILL -> TASK 2) EDUCATION –> WILL Petri Nokelainen, University of Tampere, Finland
Path Analysis • Path coefficients are a product of one or more regression analyses. • They are indicators of statistical dependency between variables. DV1 pt,w IV3 Petri Nokelainen, University of Tampere, Finland
Path Analysis AGE Pt,a EDUCATION TASK Pt,e Pw,e Pt,w WILL Petri Nokelainen, University of Tampere, Finland
Path Analysis • Path coefficients are standardized (´Beta´) or unstandardized (´B´ or (´´) regression coefficients. • Strength of inter-variable dependencies are comparable to other studies when standardized values (z, where M = 0 and SD = 1) are used. • Unstandardized values allow the original measurement scale examination of inter-variable dependencies. Petri Nokelainen, University of Tampere, Finland
Path Analysis • Beta (B) AGE ,41 (,50) EDUCATION TASK ,12 (,13) ,23 (,31) ,31 (,39) WILL Petri Nokelainen, University of Tampere, Finland
Path Analysis • Path coefficient (pDV,IV) indicates the direct effect of IV to DV. • If the model contains only one IV and DV variable, the path coefficient equals to correlation coefficient. • In those models that have more than two variables (one IV and one DV), the path coefficients equal to partial correlation coefficients. • The other path coefficients are controlled while each individual path coefficient is calculated. Petri Nokelainen, University of Tampere, Finland
EDUCATION (a) ? ? DECAF COFFEE (g) SALARY (€) ? Path Analysis • No need to use LISREL or AMOS • Two separate regression analyses in SPSS (Analyze – Regression – Linear) Petri Nokelainen, University of Tampere, Finland
1. Data (N = 10) 2. First SPSS regression analysis (SALARY + EDUCATION -> DECAF_COFFEE) 3. Second SPSS regression analysis (EDUCATION -> SALARY) Petri Nokelainen, University of Tampere, Finland
EDUCATION (a) ,51 (33,22) ,84 ,67 (212,58) DECAF COFFEE (g) ,39 SALARY (€) ,52 (,11) Path Analysis Petri Nokelainen, University of Tampere, Finland
Path Analysis • Here is the same model in AMOS: Petri Nokelainen, University of Tampere, Finland
AMOS reports R square instead of more critical Adjusted R square. Path Analysis • And the results are naturally the same: • Standardized Petri Nokelainen, University of Tampere, Finland
Path Analysis • And the results are naturally the same: • Unstandardized Petri Nokelainen, University of Tampere, Finland
Basic Concepts of Factor Analysis • The fundamental idea underlying the factor analysis is that some but not all variables can be directly observed. • Those unobserved variables are referred to as either latent variables or factors. • Information about latent variables can be gained by observing their influence on observed variables. • Factor analysis examines covariation among a set of observed variables trying to generate a smaller number of latent variables. Petri Nokelainen, University of Tampere, Finland
Basic Concepts of Factor Analysis • Exploratory Factor Analysis • In exploratory factor analysis (EFA), observed variables are represented by squares and circles represent latent variables. • Causal effect of the latent variable on the observed variable is presented with straight line with arrowhead. Petri Nokelainen, University of Tampere, Finland
Basic Concepts of Factor Analysis • Exploratory Factor Analysis • The latent factors (ellipses) labeled with ’s (Xi) are called common factors and the ’s (delta) (usually in circles) are called errors in variables or residual variables. • Errors in variables have unique effects to one and only one observed variable - unlike the common factors that share their effects in common with more than one of the observed variables. Petri Nokelainen, University of Tampere, Finland
Basic Concepts of Factor Analysis Figure 2. Exploratory Factor Model (Nokelainen, 1999.) Petri Nokelainen, University of Tampere, Finland
Basic Concepts of Factor Analysis • Exploratory Factor Analysis • The EFA model in Figure 2 reflects the fact that researcher does not specify the structure of the relationships among the variables in the model. • When carrying out EFA, researcher must assume that • all common factors are correlated, • all observed variables are directly affected by all common factors, • errors in variables are uncorrelated with one another, • all observed variables are affected by a unique factor and • all ’s are uncorrelated with all ’s. (Long, 1983.) Petri Nokelainen, University of Tampere, Finland
Basic Concepts of Factor Analysis • Confirmatory Factor Analysis • One of the biggest problems in EFA is its inability to incorporate substantively meaningful constraints. • That is due to fact that algebraic mathematical solution to solve estimates is not trivial, instead one has to seek for other solutions. • That problem was partly solved by the development of the confirmatory factor model, which was based on an iterative algorithm (Jöreskog, 1969). Petri Nokelainen, University of Tampere, Finland
Basic Concepts of Factor Analysis • Confirmatory Factor Analysis • In confirmatory factor analysis (CFA), which is a special case of SEM, the correlations between the factors are an explicit part of the analysis because they are collected in a matrix of factor correlations. • With CFA, researcher is able to decide a priori whether the factors would correlate or not. (Tacq, 1997.) Petri Nokelainen, University of Tampere, Finland
Basic Concepts of Factor Analysis • Confirmatory Factor Analysis • Moreover, researcher is able to impose substantively motivated constraints, • which common factor pairs that are correlated, • which observed variables are affected by which common factors, • which observed variables are affected by a unique factor and • which pairs of unique factors are correlated. (Long, 1983.) Petri Nokelainen, University of Tampere, Finland
Basic Concepts of Factor Analysis Figure 3. Confirmatory Factor Model (Nokelainen, 1999.) Petri Nokelainen, University of Tampere, Finland
Model Constructing • One of the most well known covariance structure models is called LISREL (LInear Structural RELationships) or Jöreskog-Keesling-Wiley –model. • LISREL is also a name of the software (Jöreskog et al., 1979), which is later demonstrated in this presentation to analyze a latent variable model. • The other approach in this study field is Bentler-Weeks -model (Bentler et al., 1980) and EQS –software (Bentler, 1995). Petri Nokelainen, University of Tampere, Finland
Model Constructing • The latest software release attempting to implement SEM is graphical and intuitive AMOS (Arbuckle, 1997). • AMOS has since 2000 taken LISREL’s place as a module of a well-known statistical software package SPSS (Statistical Package for Social Sciences). • Also other high quality SEM programs exist, such as Mplus (Muthén & Muthén, 2000). • MPlus is targeted for professional users, it has only text input mode. Petri Nokelainen, University of Tampere, Finland
Model Constructing • In this presentation, I will use both the LISREL 8 –software and AMOS 5 for SEM analysis and PRELIS 2 –software (Jöreskog et al., 1985) for preliminary data analysis. • All the previously mentioned approaches to SEM use the same pattern for constructing the model: • model hypotheses, • model specification, • model identification and • model estimation. Petri Nokelainen, University of Tampere, Finland
1. Model Hypotheses • Next, we will perform a CFA model constructing process for a part of a “Commitment to Work and Organization” model. • This is quite technical approach but unavoidable in order to understand the underlying concepts and a way of statistical thinking. Petri Nokelainen, University of Tampere, Finland
1. Model Hypotheses • Next we study briefly basic concepts of factor analysis in order to understand the path which leads to structural equation modeling. • To demonstrate the process, we study the theoretical model of ‘growth-oriented atmosphere’ (Ruohotie, 1996, 1999) to analyze organizational commitment. • The data (N = 319), collected from Finnish polytechnic institute for higher education staff in 1998, contains six continuous summary variables (Table 1). By stating ’continuous’, we assume here that mean of n Likert scale items with frequency of more than 100 observations produce a summary item (component or factor) that behaves, according to central limit theorem, like a continuous variable with normal distribution. Petri Nokelainen, University of Tampere, Finland
1. Model Hypotheses Table 1. Variable Description S M U A P N P A O G R E T M I E V N E T F G U R N O C U T P I O N A L Petri Nokelainen, University of Tampere, Finland
1. Model Hypotheses • A sample of the data is presented in Table 2. Table 2. A Sample of the Raw Data Set Petri Nokelainen, University of Tampere, Finland
1. Model Hypotheses • The covariance matrix is presented in Table 3. Table 3. The Covariance Matrix Petri Nokelainen, University of Tampere, Finland
1. Model Hypotheses • What is covariance matrix? • Scatter, covariance, and correlation matrix form the basis of a multivariate method. • The correlation and the covariance matrix are also often used for a first inspection of relationships among the variables of a multivariate data set. • All of these matrices are calculated using the matrix multiplication (A · B). • The only difference between them is how the data is scaled before the matrix multiplication is executed: • scatter: no scaling • covariance: mean of each variable is subtracted before multiplication • correlation: each variable is standardized (mean subtracted, then divided by standard deviation) Petri Nokelainen, University of Tampere, Finland
- More by User
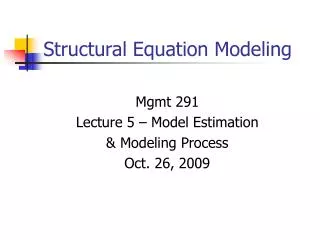
Structural Equation Modeling. Mgmt 291 Lecture 5 – Model Estimation & Modeling Process Oct. 26, 2009. About Estimation. When sample size big as infinite, est close to true value. 2 criteria 1) Unbiased ~ E(est) = true value (biased, inconsistent) 2) Efficient ~ Var(est) small
680 views • 29 slides
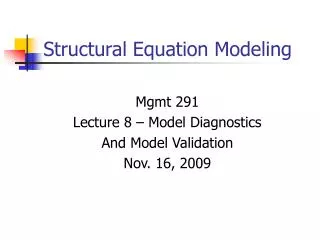
Structural Equation Modeling. Mgmt 291 Lecture 8 – Model Diagnostics And Model Validation Nov. 16, 2009. Computing Problem 1: “Not positive definite”. determinant of the matrix =< 0 makes Log Σ and Log S undefined computing work can not move forward.
583 views • 21 slides
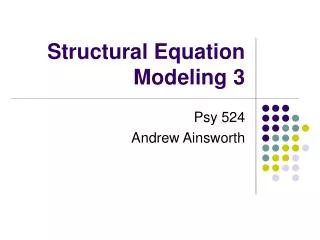
Structural Equation Modeling 3
Structural Equation Modeling 3. Psy 524 Andrew Ainsworth. Model Identification. Only identified models can be estimated in SEM A model is said to be identified if there is a unique solution for every estimate Y = 10 Y = a + b
556 views • 17 slides
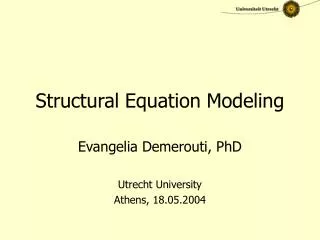
Structural Equation Modeling. Evangelia Demerouti, PhD Utrecht University Athens, 18.05.2004 . Structure. Introduction to SEM Examples Mediation Moderation Longitudinal data. Use of SEM. To test whether theoretical hypothesis about causal relationships fit to empirical data.
735 views • 51 slides
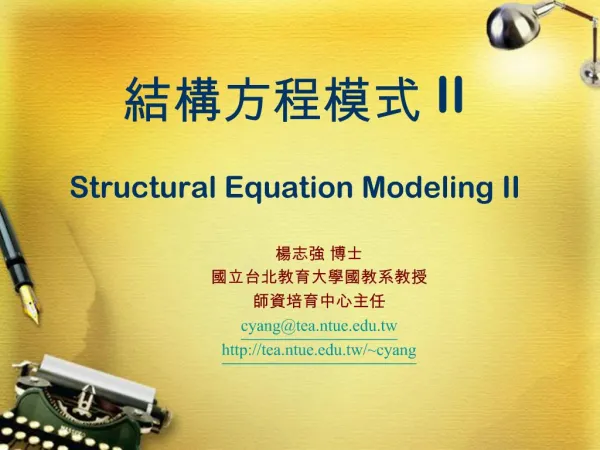
II Structural Equation Modeling II
??????? ?Exploratory Factor Analysis (EFA). ????????????. ???????????. ??????????????. ????????????. ?????. . ????. x1=1 l11f1 l12f2 l1qfq e1x2=2 l21f1 l22f2 l2qfq e2xp=p lp1f1 lp2f2 lpqfq epx?????;f?????l??????;e?????(???). . ????. ???????????????????????????
686 views • 50 slides
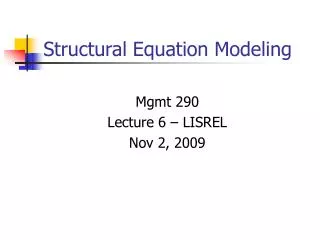
Structural Equation Modeling. Mgmt 290 Lecture 6 – LISREL Nov 2, 2009. LISREL Preparation: Diagram – Equations - Matrix. One Equation for Each Indicator in Measurement Model One Equation for Each Endogenous Variable in Matrix, Column affects Row.
930 views • 31 slides
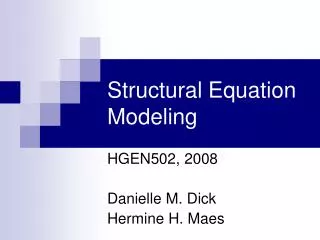
Structural Equation Modeling. HGEN502, 2008 Danielle M. Dick Hermine H. Maes. Role of model mediating between theory and data. Theory. Phenotype = Genotype+Environment (VP=VG+VE) Genetic factors (VG): Additive (VA) Dominance (VD) Environmental factors (VE): Common / Shared (VC)
370 views • 13 slides
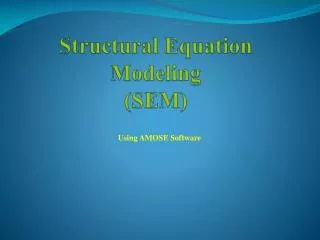
Structural Equation Modeling (SEM)
Structural Equation Modeling (SEM). Using AMOSE Software. What Is Structural Equation Modeling?.
566 views • 18 slides
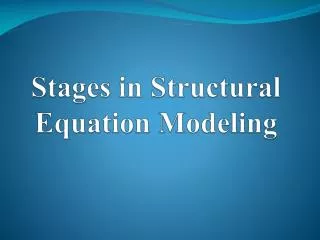
Stages in Structural Equation Modeling
Stages in Structural Equation Modeling. The Stages in Structural Equation Model. Stage 1) Assessing individual constructs Stage 2) Developing and assessing the measurement model validity Stage 3) Specifying the structural model Stage 4) Assessing structural model validity.
478 views • 24 slides
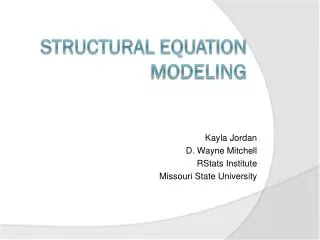
Kayla Jordan D. Wayne Mitchell RStats Institute Missouri State University. Structural Equation Modeling. What is SEM?. Statistical technique useful for testing theoretical models Theory-driven Confirmatory. Types of Variables. Manifest or Observed Variable. Error. Endogenous .
383 views • 16 slides
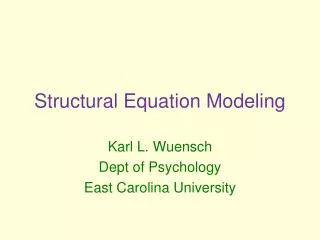
Structural Equation Modeling. Karl L. Wuensch Dept of Psychology East Carolina University. Nomenclature. AKA “causal modeling.” “analysis of covariance structure.” Two sets of variables Indicators – measured (observed, manifest) variables – diagramed within rectangles
597 views • 24 slides
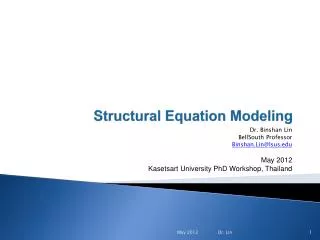
Structural Equation Modeling. Dr. Binshan Lin BellSouth Professor [email protected] May 2012 Kasetsart University PhD Workshop, Thailand. Instructor Profile.
712 views • 44 slides
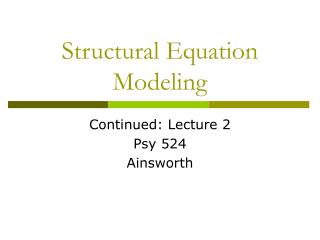
Structural Equation Modeling. Continued: Lecture 2 Psy 524 Ainsworth. Covariance Algebra. Underlying parameters in SEM Regression Coefficients Variances and Covariances A hypothesized model is used to estimate these parameters for the population (assuming the model is true)
568 views • 32 slides
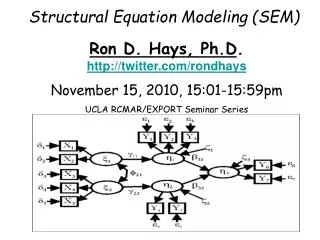
Ron D. Hays, Ph.D . http://twitter.com/rondhays November 15, 2010, 15:01-15:59pm UCLA RCMAR/EXPORT Seminar Series. Structural Equation Modeling (SEM). Acknowledgment of Support.
727 views • 21 slides
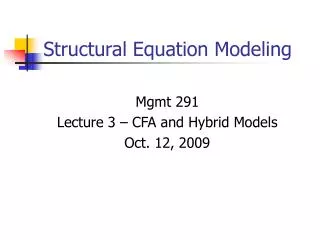
Structural Equation Modeling. Mgmt 291 Lecture 3 – CFA and Hybrid Models Oct. 12, 2009. Measurement is Everything. Nothing can be done with wrong or unreliable measurements. “Measurement is Everything”.
698 views • 40 slides
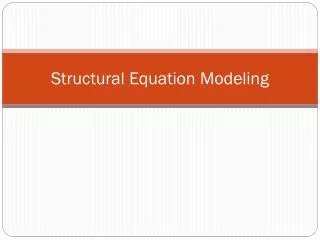
Structural Equation Modeling. What is SEM . Swiss Army Knife of Statistics Can replicate virtually any model from “canned” stats packages (some limitations of categorical variables) Can test process (B mediates the relationship between A and C) Can test competing models
513 views • 25 slides
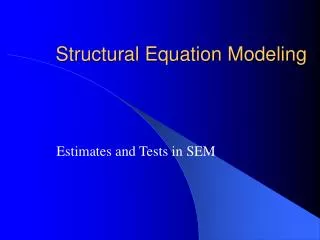
Structural Equation Modeling. Estimates and Tests in SEM. 資料來源 : 結構方程式 LISREL 的理論技術與應用 2003 邱皓政. 外衍觀測變數. 觀測變數. 內衍觀測變數. 外衍變數 exogenous variables (X). 潛在變數. 內衍潛在變數. 外衍潛在變數. 內衍變數 endogenous variables (Y). 外衍觀測變數. 觀測變數. 內衍觀測變數. Terminology.
434 views • 22 slides
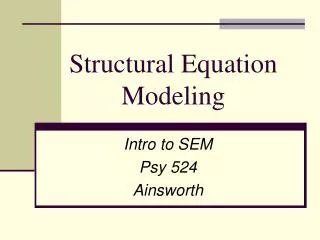
Structural Equation Modeling. Intro to SEM Psy 524 Ainsworth. AKA. SEM – Structural Equation Modeling CSA – Covariance Structure Analysis Causal Models Simultaneous Equations Path Analysis Confirmatory Factor Analysis. SEM in a nutshell. Combination of factor analysis and regression
536 views • 18 slides
- Search Menu
- Browse content in Arts and Humanities
- Browse content in Archaeology
- Anglo-Saxon and Medieval Archaeology
- Archaeological Methodology and Techniques
- Archaeology by Region
- Archaeology of Religion
- Archaeology of Trade and Exchange
- Biblical Archaeology
- Contemporary and Public Archaeology
- Environmental Archaeology
- Historical Archaeology
- History and Theory of Archaeology
- Industrial Archaeology
- Landscape Archaeology
- Mortuary Archaeology
- Prehistoric Archaeology
- Underwater Archaeology
- Urban Archaeology
- Zooarchaeology
- Browse content in Architecture
- Architectural Structure and Design
- History of Architecture
- Residential and Domestic Buildings
- Theory of Architecture
- Browse content in Art
- Art Subjects and Themes
- History of Art
- Industrial and Commercial Art
- Theory of Art
- Biographical Studies
- Byzantine Studies
- Browse content in Classical Studies
- Classical History
- Classical Philosophy
- Classical Mythology
- Classical Literature
- Classical Reception
- Classical Art and Architecture
- Classical Oratory and Rhetoric
- Greek and Roman Epigraphy
- Greek and Roman Law
- Greek and Roman Archaeology
- Greek and Roman Papyrology
- Late Antiquity
- Religion in the Ancient World
- Digital Humanities
- Browse content in History
- Colonialism and Imperialism
- Diplomatic History
- Environmental History
- Genealogy, Heraldry, Names, and Honours
- Genocide and Ethnic Cleansing
- Historical Geography
- History by Period
- History of Agriculture
- History of Education
- History of Emotions
- History of Gender and Sexuality
- Industrial History
- Intellectual History
- International History
- Labour History
- Legal and Constitutional History
- Local and Family History
- Maritime History
- Military History
- National Liberation and Post-Colonialism
- Oral History
- Political History
- Public History
- Regional and National History
- Revolutions and Rebellions
- Slavery and Abolition of Slavery
- Social and Cultural History
- Theory, Methods, and Historiography
- Urban History
- World History
- Browse content in Language Teaching and Learning
- Language Learning (Specific Skills)
- Language Teaching Theory and Methods
- Browse content in Linguistics
- Applied Linguistics
- Cognitive Linguistics
- Computational Linguistics
- Forensic Linguistics
- Grammar, Syntax and Morphology
- Historical and Diachronic Linguistics
- History of English
- Language Acquisition
- Language Variation
- Language Families
- Language Evolution
- Language Reference
- Lexicography
- Linguistic Theories
- Linguistic Typology
- Linguistic Anthropology
- Phonetics and Phonology
- Psycholinguistics
- Sociolinguistics
- Translation and Interpretation
- Writing Systems
- Browse content in Literature
- Bibliography
- Children's Literature Studies
- Literary Studies (Asian)
- Literary Studies (European)
- Literary Studies (Eco-criticism)
- Literary Studies (Modernism)
- Literary Studies (Romanticism)
- Literary Studies (American)
- Literary Studies - World
- Literary Studies (1500 to 1800)
- Literary Studies (19th Century)
- Literary Studies (20th Century onwards)
- Literary Studies (African American Literature)
- Literary Studies (British and Irish)
- Literary Studies (Early and Medieval)
- Literary Studies (Fiction, Novelists, and Prose Writers)
- Literary Studies (Gender Studies)
- Literary Studies (Graphic Novels)
- Literary Studies (History of the Book)
- Literary Studies (Plays and Playwrights)
- Literary Studies (Poetry and Poets)
- Literary Studies (Postcolonial Literature)
- Literary Studies (Queer Studies)
- Literary Studies (Science Fiction)
- Literary Studies (Travel Literature)
- Literary Studies (War Literature)
- Literary Studies (Women's Writing)
- Literary Theory and Cultural Studies
- Mythology and Folklore
- Shakespeare Studies and Criticism
- Browse content in Media Studies
- Browse content in Music
- Applied Music
- Dance and Music
- Ethics in Music
- Ethnomusicology
- Gender and Sexuality in Music
- Medicine and Music
- Music Cultures
- Music and Religion
- Music and Culture
- Music and Media
- Music Education and Pedagogy
- Music Theory and Analysis
- Musical Scores, Lyrics, and Libretti
- Musical Structures, Styles, and Techniques
- Musicology and Music History
- Performance Practice and Studies
- Race and Ethnicity in Music
- Sound Studies
- Browse content in Performing Arts
- Browse content in Philosophy
- Aesthetics and Philosophy of Art
- Epistemology
- Feminist Philosophy
- History of Western Philosophy
- Metaphysics
- Moral Philosophy
- Non-Western Philosophy
- Philosophy of Science
- Philosophy of Action
- Philosophy of Law
- Philosophy of Religion
- Philosophy of Language
- Philosophy of Mind
- Philosophy of Perception
- Philosophy of Mathematics and Logic
- Practical Ethics
- Social and Political Philosophy
- Browse content in Religion
- Biblical Studies
- Christianity
- East Asian Religions
- History of Religion
- Judaism and Jewish Studies
- Qumran Studies
- Religion and Education
- Religion and Health
- Religion and Politics
- Religion and Science
- Religion and Law
- Religion and Art, Literature, and Music
- Religious Studies
- Browse content in Society and Culture
- Cookery, Food, and Drink
- Cultural Studies
- Customs and Traditions
- Ethical Issues and Debates
- Hobbies, Games, Arts and Crafts
- Lifestyle, Home, and Garden
- Natural world, Country Life, and Pets
- Popular Beliefs and Controversial Knowledge
- Sports and Outdoor Recreation
- Technology and Society
- Travel and Holiday
- Visual Culture
- Browse content in Law
- Arbitration
- Browse content in Company and Commercial Law
- Commercial Law
- Company Law
- Browse content in Comparative Law
- Systems of Law
- Competition Law
- Browse content in Constitutional and Administrative Law
- Government Powers
- Judicial Review
- Local Government Law
- Military and Defence Law
- Parliamentary and Legislative Practice
- Construction Law
- Contract Law
- Browse content in Criminal Law
- Criminal Procedure
- Criminal Evidence Law
- Sentencing and Punishment
- Employment and Labour Law
- Environment and Energy Law
- Browse content in Financial Law
- Banking Law
- Insolvency Law
- History of Law
- Human Rights and Immigration
- Intellectual Property Law
- Browse content in International Law
- Private International Law and Conflict of Laws
- Public International Law
- IT and Communications Law
- Jurisprudence and Philosophy of Law
- Law and Politics
- Law and Society
- Browse content in Legal System and Practice
- Courts and Procedure
- Legal Skills and Practice
- Primary Sources of Law
- Regulation of Legal Profession
- Medical and Healthcare Law
- Browse content in Policing
- Criminal Investigation and Detection
- Police and Security Services
- Police Procedure and Law
- Police Regional Planning
- Browse content in Property Law
- Personal Property Law
- Study and Revision
- Terrorism and National Security Law
- Browse content in Trusts Law
- Wills and Probate or Succession
- Browse content in Medicine and Health
- Browse content in Allied Health Professions
- Arts Therapies
- Clinical Science
- Dietetics and Nutrition
- Occupational Therapy
- Operating Department Practice
- Physiotherapy
- Radiography
- Speech and Language Therapy
- Browse content in Anaesthetics
- General Anaesthesia
- Neuroanaesthesia
- Browse content in Clinical Medicine
- Acute Medicine
- Cardiovascular Medicine
- Clinical Genetics
- Clinical Pharmacology and Therapeutics
- Dermatology
- Endocrinology and Diabetes
- Gastroenterology
- Genito-urinary Medicine
- Geriatric Medicine
- Infectious Diseases
- Medical Oncology
- Medical Toxicology
- Pain Medicine
- Palliative Medicine
- Rehabilitation Medicine
- Respiratory Medicine and Pulmonology
- Rheumatology
- Sleep Medicine
- Sports and Exercise Medicine
- Clinical Neuroscience
- Community Medical Services
- Critical Care
- Emergency Medicine
- Forensic Medicine
- Haematology
- History of Medicine
- Browse content in Medical Dentistry
- Oral and Maxillofacial Surgery
- Paediatric Dentistry
- Restorative Dentistry and Orthodontics
- Surgical Dentistry
- Medical Ethics
- Browse content in Medical Skills
- Clinical Skills
- Communication Skills
- Nursing Skills
- Surgical Skills
- Medical Statistics and Methodology
- Browse content in Neurology
- Clinical Neurophysiology
- Neuropathology
- Nursing Studies
- Browse content in Obstetrics and Gynaecology
- Gynaecology
- Occupational Medicine
- Ophthalmology
- Otolaryngology (ENT)
- Browse content in Paediatrics
- Neonatology
- Browse content in Pathology
- Chemical Pathology
- Clinical Cytogenetics and Molecular Genetics
- Histopathology
- Medical Microbiology and Virology
- Patient Education and Information
- Browse content in Pharmacology
- Psychopharmacology
- Browse content in Popular Health
- Caring for Others
- Complementary and Alternative Medicine
- Self-help and Personal Development
- Browse content in Preclinical Medicine
- Cell Biology
- Molecular Biology and Genetics
- Reproduction, Growth and Development
- Primary Care
- Professional Development in Medicine
- Browse content in Psychiatry
- Addiction Medicine
- Child and Adolescent Psychiatry
- Forensic Psychiatry
- Learning Disabilities
- Old Age Psychiatry
- Psychotherapy
- Browse content in Public Health and Epidemiology
- Epidemiology
- Public Health
- Browse content in Radiology
- Clinical Radiology
- Interventional Radiology
- Nuclear Medicine
- Radiation Oncology
- Reproductive Medicine
- Browse content in Surgery
- Cardiothoracic Surgery
- Gastro-intestinal and Colorectal Surgery
- General Surgery
- Neurosurgery
- Paediatric Surgery
- Peri-operative Care
- Plastic and Reconstructive Surgery
- Surgical Oncology
- Transplant Surgery
- Trauma and Orthopaedic Surgery
- Vascular Surgery
- Browse content in Science and Mathematics
- Browse content in Biological Sciences
- Aquatic Biology
- Biochemistry
- Bioinformatics and Computational Biology
- Developmental Biology
- Ecology and Conservation
- Evolutionary Biology
- Genetics and Genomics
- Microbiology
- Molecular and Cell Biology
- Natural History
- Plant Sciences and Forestry
- Research Methods in Life Sciences
- Structural Biology
- Systems Biology
- Zoology and Animal Sciences
- Browse content in Chemistry
- Analytical Chemistry
- Computational Chemistry
- Crystallography
- Environmental Chemistry
- Industrial Chemistry
- Inorganic Chemistry
- Materials Chemistry
- Medicinal Chemistry
- Mineralogy and Gems
- Organic Chemistry
- Physical Chemistry
- Polymer Chemistry
- Study and Communication Skills in Chemistry
- Theoretical Chemistry
- Browse content in Computer Science
- Artificial Intelligence
- Computer Architecture and Logic Design
- Game Studies
- Human-Computer Interaction
- Mathematical Theory of Computation
- Programming Languages
- Software Engineering
- Systems Analysis and Design
- Virtual Reality
- Browse content in Computing
- Business Applications
- Computer Security
- Computer Games
- Computer Networking and Communications
- Digital Lifestyle
- Graphical and Digital Media Applications
- Operating Systems
- Browse content in Earth Sciences and Geography
- Atmospheric Sciences
- Environmental Geography
- Geology and the Lithosphere
- Maps and Map-making
- Meteorology and Climatology
- Oceanography and Hydrology
- Palaeontology
- Physical Geography and Topography
- Regional Geography
- Soil Science
- Urban Geography
- Browse content in Engineering and Technology
- Agriculture and Farming
- Biological Engineering
- Civil Engineering, Surveying, and Building
- Electronics and Communications Engineering
- Energy Technology
- Engineering (General)
- Environmental Science, Engineering, and Technology
- History of Engineering and Technology
- Mechanical Engineering and Materials
- Technology of Industrial Chemistry
- Transport Technology and Trades
- Browse content in Environmental Science
- Applied Ecology (Environmental Science)
- Conservation of the Environment (Environmental Science)
- Environmental Sustainability
- Environmentalist Thought and Ideology (Environmental Science)
- Management of Land and Natural Resources (Environmental Science)
- Natural Disasters (Environmental Science)
- Nuclear Issues (Environmental Science)
- Pollution and Threats to the Environment (Environmental Science)
- Social Impact of Environmental Issues (Environmental Science)
- History of Science and Technology
- Browse content in Materials Science
- Ceramics and Glasses
- Composite Materials
- Metals, Alloying, and Corrosion
- Nanotechnology
- Browse content in Mathematics
- Applied Mathematics
- Biomathematics and Statistics
- History of Mathematics
- Mathematical Education
- Mathematical Finance
- Mathematical Analysis
- Numerical and Computational Mathematics
- Probability and Statistics
- Pure Mathematics
- Browse content in Neuroscience
- Cognition and Behavioural Neuroscience
- Development of the Nervous System
- Disorders of the Nervous System
- History of Neuroscience
- Invertebrate Neurobiology
- Molecular and Cellular Systems
- Neuroendocrinology and Autonomic Nervous System
- Neuroscientific Techniques
- Sensory and Motor Systems
- Browse content in Physics
- Astronomy and Astrophysics
- Atomic, Molecular, and Optical Physics
- Biological and Medical Physics
- Classical Mechanics
- Computational Physics
- Condensed Matter Physics
- Electromagnetism, Optics, and Acoustics
- History of Physics
- Mathematical and Statistical Physics
- Measurement Science
- Nuclear Physics
- Particles and Fields
- Plasma Physics
- Quantum Physics
- Relativity and Gravitation
- Semiconductor and Mesoscopic Physics
- Browse content in Psychology
- Affective Sciences
- Clinical Psychology
- Cognitive Neuroscience
- Cognitive Psychology
- Criminal and Forensic Psychology
- Developmental Psychology
- Educational Psychology
- Evolutionary Psychology
- Health Psychology
- History and Systems in Psychology
- Music Psychology
- Neuropsychology
- Organizational Psychology
- Psychological Assessment and Testing
- Psychology of Human-Technology Interaction
- Psychology Professional Development and Training
- Research Methods in Psychology
- Social Psychology
- Browse content in Social Sciences
- Browse content in Anthropology
- Anthropology of Religion
- Human Evolution
- Medical Anthropology
- Physical Anthropology
- Regional Anthropology
- Social and Cultural Anthropology
- Theory and Practice of Anthropology
- Browse content in Business and Management
- Business Strategy
- Business History
- Business Ethics
- Business and Government
- Business and Technology
- Business and the Environment
- Comparative Management
- Corporate Governance
- Corporate Social Responsibility
- Entrepreneurship
- Health Management
- Human Resource Management
- Industrial and Employment Relations
- Industry Studies
- Information and Communication Technologies
- International Business
- Knowledge Management
- Management and Management Techniques
- Operations Management
- Organizational Theory and Behaviour
- Pensions and Pension Management
- Public and Nonprofit Management
- Strategic Management
- Supply Chain Management
- Browse content in Criminology and Criminal Justice
- Criminal Justice
- Criminology
- Forms of Crime
- International and Comparative Criminology
- Youth Violence and Juvenile Justice
- Development Studies
- Browse content in Economics
- Agricultural, Environmental, and Natural Resource Economics
- Asian Economics
- Behavioural Finance
- Behavioural Economics and Neuroeconomics
- Econometrics and Mathematical Economics
- Economic Systems
- Economic Methodology
- Economic History
- Economic Development and Growth
- Financial Markets
- Financial Institutions and Services
- General Economics and Teaching
- Health, Education, and Welfare
- History of Economic Thought
- International Economics
- Labour and Demographic Economics
- Law and Economics
- Macroeconomics and Monetary Economics
- Microeconomics
- Public Economics
- Urban, Rural, and Regional Economics
- Welfare Economics
- Browse content in Education
- Adult Education and Continuous Learning
- Care and Counselling of Students
- Early Childhood and Elementary Education
- Educational Equipment and Technology
- Educational Strategies and Policy
- Higher and Further Education
- Organization and Management of Education
- Philosophy and Theory of Education
- Schools Studies
- Secondary Education
- Teaching of a Specific Subject
- Teaching of Specific Groups and Special Educational Needs
- Teaching Skills and Techniques
- Browse content in Environment
- Applied Ecology (Social Science)
- Climate Change
- Conservation of the Environment (Social Science)
- Environmentalist Thought and Ideology (Social Science)
- Natural Disasters (Environment)
- Social Impact of Environmental Issues (Social Science)
- Browse content in Human Geography
- Cultural Geography
- Economic Geography
- Political Geography
- Browse content in Interdisciplinary Studies
- Communication Studies
- Museums, Libraries, and Information Sciences
- Browse content in Politics
- African Politics
- Asian Politics
- Chinese Politics
- Comparative Politics
- Conflict Politics
- Elections and Electoral Studies
- Environmental Politics
- European Union
- Foreign Policy
- Gender and Politics
- Human Rights and Politics
- Indian Politics
- International Relations
- International Organization (Politics)
- International Political Economy
- Irish Politics
- Latin American Politics
- Middle Eastern Politics
- Political Methodology
- Political Communication
- Political Philosophy
- Political Sociology
- Political Theory
- Political Behaviour
- Political Economy
- Political Institutions
- Politics and Law
- Public Administration
- Public Policy
- Quantitative Political Methodology
- Regional Political Studies
- Russian Politics
- Security Studies
- State and Local Government
- UK Politics
- US Politics
- Browse content in Regional and Area Studies
- African Studies
- Asian Studies
- East Asian Studies
- Japanese Studies
- Latin American Studies
- Middle Eastern Studies
- Native American Studies
- Scottish Studies
- Browse content in Research and Information
- Research Methods
- Browse content in Social Work
- Addictions and Substance Misuse
- Adoption and Fostering
- Care of the Elderly
- Child and Adolescent Social Work
- Couple and Family Social Work
- Developmental and Physical Disabilities Social Work
- Direct Practice and Clinical Social Work
- Emergency Services
- Human Behaviour and the Social Environment
- International and Global Issues in Social Work
- Mental and Behavioural Health
- Social Justice and Human Rights
- Social Policy and Advocacy
- Social Work and Crime and Justice
- Social Work Macro Practice
- Social Work Practice Settings
- Social Work Research and Evidence-based Practice
- Welfare and Benefit Systems
- Browse content in Sociology
- Childhood Studies
- Community Development
- Comparative and Historical Sociology
- Economic Sociology
- Gender and Sexuality
- Gerontology and Ageing
- Health, Illness, and Medicine
- Marriage and the Family
- Migration Studies
- Occupations, Professions, and Work
- Organizations
- Population and Demography
- Race and Ethnicity
- Social Theory
- Social Movements and Social Change
- Social Research and Statistics
- Social Stratification, Inequality, and Mobility
- Sociology of Religion
- Sociology of Education
- Sport and Leisure
- Urban and Rural Studies
- Browse content in Warfare and Defence
- Defence Strategy, Planning, and Research
- Land Forces and Warfare
- Military Administration
- Military Life and Institutions
- Naval Forces and Warfare
- Other Warfare and Defence Issues
- Peace Studies and Conflict Resolution
- Weapons and Equipment

- < Previous chapter
- Next chapter >

15 Structural Equation Models
John J. McArdle, Department of Psychology, University of Southern California, Los Angeles, CA
Kelly M. Kadlec, Department of Psychology, University of Southern California, Los Angeles, CA
- Published: 01 October 2013
- Cite Icon Cite
- Permissions Icon Permissions
The purpose of this chapter is to present an accessible overview of recent research on what are termed structural equation models (SEM). This presentation is intended for graduate level students in the behavioral sciences, possibly taking a SEM class, but formal algebra or calculus is not required. First, SEM is broadly defined, and the increasing use of this approach to data analysis is described. In general, SEM techniques are increasingly used in the behavioral and social sciences. Second, some technical features of SEM are presented to illustrate key benefits of SEM. Some classical issues are described that highlight issues SEM researchers usually find to be important, and the big appeal of SEM comes when if offers some hope to deal with these issues. Third, we consider the inclusion of common factors as latent variables in path models that can be incorporated into SEM. We claim that the inclusion of common factors is what really makes SEM different than other statistical approaches. Fourth, we describe how SEM calculation works, and this gives rise to various indices of goodness-of-fit. Many researchers herald these techniques, although this seems to be a leftover from prior statistical training. Fifth, we provide an illustration of contemporary data generation and computer programming (using CALIS, Mplus, and OpenMx). In the final section, we illustrate some options from our previous SEM work, answer specific questions about SEM practices, and include a discussion of issues for future SEM uses.
Introduction
“The effect of a concept-driven revolution is to explain old things in new ways. The effect of a tool-driven revolution is to discover new things that have to be explained .” (originally from Sir Frank Dyson, 1891; see Freeman Dyson, 1997 , Imagined Worlds, pp. 50–51).
We think it is fair to say that recent research involving structural equation models (SEM) constitutes both a concept-driven revolution and a tool-driven revolution. That is, principles of SEMs can be used both for developing concepts and these same principles can also be used as data analyses tools. What makes SEM unique is the emphasis on using unobserved or latent variables (LVs). This chapter will define these two separate SEM functions, the concepts and the tools, and then try to bring them back together with some examples.
Structural Equation Models Defined
The popular term structural equation models (SEM) can be used to represent many different kinds of multivariable ideas. Statistical analyses as seemingly diverse as analysis of variance (ANOVA), multiple regression, factor analysis, path analysis, multidimensional scaling, time series analysis, and so on, can be organized under the same SEM heading. This generalization is correct because in all such cases, we consider SEM a reflection of three key ideas in modern statistical data analysis: (1) The term “model” is used to represent a “theory” in terms of propositions about the relationships between variables. (2) The term “equation” means that the relationships between variables are expressed in a formal fashion using the strict rules of algebra. (3) The term “structural” is used to suggest that these algebraic equations form a specific restrictive pattern about real data that is consistent with the model, and hence the theory.
Any SEM representation of the model scores is used to create expectations about the basic summary statistics—means and covariances—of the observed data. This is important because these expectations can be compared to the data observations to form a test of goodness-of-fit—that is, expected means (μ) compared to observed means ( m ), and expected covariances (Σ) compared to observed covariances ( S ). This also highlights one of the big limitations of SEM—we only compare means and covariances, so any other potential model versus data differences (i.e., skewness) are assumed not to be critical. Of course, this is also true of many other data analysis techniques, such as multiple regression and ANOVA, principal components and factor analysis, and canonical correlation and regression, so SEM is a natural generalization of this classic work.
Given these statistical limits, many scientific theories can be represented as testable models. Most importantly, SEM gives researchers a way to represent theories as models using LVs that are not directly measured. This is easy to do and is a major benefit of SEM because many key constructs in the behavioral sciences simply cannot be directly observed and measured. Of course, hypothesis testing is not always possible because our theories can quickly require more elaborations than we can do with the available data. We are consoled only because we will know that our model, and hence our theory, is far beyond any evaluation by our available data.
If we create a SEM on an a priori basis, we can then determine how well the expected model fits the observed data by calculating a variety of a priori defined “goodness-of-fit” indices. Unfortunately, any truly confirmatory analysis requires a great deal of prior information. For example, to really test any model, we would probably need to have point hypotheses about all the group (i.e., fixed) parameters (i.e., the one-headed arrows). This is not the typical case, and we often settle for testing the pattern or structural hypotheses of the parameters—that is, is a specific correlation equal to the product of two parameters? Under these less rigid conditions, and if we assume all residuals are normally distributed, we can compare the model-based “likelihood-ratio” to a “chi-square” distribution and determine the probability of observing such an event—this model for these data—at random. This approach, of course, is virtually identical to calculating a p -value in an ANOVA or Regression model context (although a typical SEM often has more parameters), so all the assumptions and inference problems are similar as well. Although many people find SEM to have alleviated most statistical problems, it has not. Poor quality data will not miraculously provide robust results, just as cause-and-effect relationships cannot easily be formed from observational data. What SEM has done, and in a classical way, is allowed the representation of complex theories as complex models, and the basic expressions of models in terms of equations, so they can easily be used to examine ideas about the structure of a data set. Incidentally, we recognize that not all SEMs are based on a priori theory, and basically all we now ask is that SEM researchers try to tell us what they actually did ( see McArdle, 2010 ).
When we consider SEM in this fashion, we find SEM is all around us, and it has been here for a very long time. The broad ideas of SEM, as both concept and tool, probably started with the “common factor analysis” model of Spearman (1904 ; see Horn & McArdle, 1980 , 1992 , 2007 ), and this led to extensions such as the “path analysis” concepts of Wright (1918 , 1934 ) and the “variance components” ideas of Fisher (1918 , 1924 ; see Li, 1975 ). These SEM ideas seemed to be dormant in academic research for about 50 years and only recently revived by a combination of the efforts of quantitatively minded sociologists ( see Duncan, 1975 ; Goldberger & Duncan, 1973 ; Hauser& Goldberger, 1971 ) and psychologists (e.g., Werts & Linn, 1970 ). The early SEM seemed to be largely based on its conceptual and theoretical advantages rather than on the creation of analytic tools. But it also seems fair to say that these SEM concepts have enjoyed a revival in the last few decades, partially based on the generation of new SEM tools.
Confirmatory Factor Analysis and the Popular LISREL Movement
The current forms of SEM really took on a life of their own when they were placed into the context of what was termed a “confirmatory factor analysis” with “LVs ( see Joreskog, 1973 ; McDonald, 1985 ). This approach was probably best represented in the general framework termed the Linear Structural Equations Model (LISREL; Joreskog, 1973 ; Wiley, 1973 ; Joreskog & Sorbom, 1979 ). In the LISREL approach, the researchers were interested in creating a general approach to data analysis, but one that was far more general than the ANOVAbased general linear model (see Bock, 1975 ; Muller & Stewart, 2006 ). LISREL advocates seemed to suggest that we should always consider an analytic framework that included hypotheses about “unobserved” variables based on both means and covariances.
In simplest terms, the LISREL concept suggested that the econometric concept of multivariable regression could be merged with the psychometric concepts of common factors (for example, see Wiley, 1973 ). That is, in the LISREL approach we may have many X-variables (i.e., independent, inputs, predictors, exogenous, or right-hand side) and many Y-variables (i.e., dependent, outputs, endogenous, or left-hand side), and as usual, the X-variables can affect the Y-variables with specific coefficients. However, rather than simply calculating multiple regression coefficients for entire sets of predictors and outcomes, the LISREL model allowed researchers to pose hypotheses about the relationships between unobserved common factors with common factor scores; specifically, unobserved common factors of X-side (or left hand side) variables could be thought of as based on common factor scores (termed η, although not estimated), and Y-side (or left hand side) variables could also have common factor scores (termed ξ, although not estimated). The Y-side factors could then be regressed on the X-side factors, possibly with additional constraints such as some regression coefficients being zero. These equations for the scores could be represented in X-side matrices of factor loadings (Λ x ), unique covariances (©δ), and common factor covariances (ϕ), whereas the common factors of the Y-side variables could be represented with factor loadings (Λ y ), unique covariances (© ε ) regressions between the Y-side common factors and the X-side common factors (Γ), regressions within the Y-side common factors (B), and all with common factor residual covariances (Ψ 2 ).
This fairly complex LISREL concept allowed researchers to place their theories in terms of unobserved constructs and still provide tests of goodness-of-fit using observables. It seems to us that LISREL would not have gained such momentum if it were not associated with a working computer program— the SEM concept was matched with a SEM tool! In fact, the flexible computer programming of the first versions of LISREL ( see Joreskog & Sorbom, 1979 ; McDonald, 1985 ) allowed the unknown elements in these eight matrices of parameters to be (1) fixed at known values, (2) free to be estimated, or (3) estimated but equal to a different parameter. This new computer programming tool was a true innovation and came directly from the work of Joreskog (1969 ; see Lawley & Maxwell, 1971 ), where many new ideas for data analysis were emerging (e.g., Nesselroade & Baltes, 1984 ).
The SEM-LISREL approach allowed researchers both to think more clearly about what they were saying and, at the same time, fit models to data in ways that could not be done previously. This is viewed as a combination of concepts and tools. SEM-LISREL could be considered useful for its conceptual advances or because it added important tools (computer calculations) for behavioral scientists. For these kinds of reasons, it has been difficult to summarize all the advantages of SEM in one way (e.g., see Stapleton & Leite, 2005 ).
One thing that is clear is that the notion of confirmatory or a priori hypothesis-driven modeling represented powerful conceptual thinking. At the time LISREL was initiated, there was much work on the generic testing of hypotheses about specific multivariate patterns of group means, including repeated measures ( see Bock, 1975 ; O’Brien & Kaiser, 1985 ). However, these statistical tests of mean differences were carried out within a severely limited framework for hypotheses about covariances ( see Rao, 1965 ; Joreskog, 1973 ). Thus, the SEMLISREL program was a tool that permitted analyses well beyond the standard framework of estimation and tests of mean differences in the ANOVA-based general linear model.”
Because of the broad generality of the SEM-LISREL idea, and despite all the unfamiliar and occasionally odd choices of Greek terminology (i.e., Was there any real benefit in adding Greek letters for the unobserved and unestimated factors?), the SEM-LISREL concept and the related computer program became very popular because SEM-LISREL allowed seemingly unlimited ways to estimate and test hypotheses about both means and covariances. For these reasons, SEM-LISREL was studied and used in great depth ( see Joreskog & Sorbom, 1979 ; see Horn & McArdle, 1980 ; Meredith, 1993 ; Meredith & Horn, 2001 ) and is still popular today.
The Current Status of Structual Equation Model Research
The early SEM research seems to have been focused on the concepts, whereas more recent SEM research seems to be focused on the tools. But it is a bit difficult to know exactly who is using SEM for what purpose. One recent article by Stapleton and Leite (2005 ) attempted to summarize all current SEM syllabi in classroom usage, but the classic conceptual treatments were necessarily overlooked. There are also online blogs (SEM-NET) and Internet websites devoted to the SEM enterprise, but the same could be said about almost any faddish idea.
To consider this question of current SEM usage more seriously now, we conducted a Google Scholar search for scholarly articles containing the phrase “Structural Equation Model,” “Structural Equation Models,” or “Structural Equation Modeling” in their title (and done about 08/2010). We excluded (1) articles that had only been cited, rather than found online; (2) patents; and (3) legal opinions and journals. Separate searches were conducted for seven disciplines identified by Google Scholar: (1) Biology, Life Sciences, and Environmental Science; (2) Business, Administration, Finance, and Economics; (3) Chemistry and Materials Science; (4) Engineering, Computer Science, and Mathematics; (5) Medicine, Pharmacology, and Veterinary Science; (6) Physics, Astronomy, and Planetary Science; and (7) Social Sciences, Arts, and Humanities. Within each discipline, we searched by date starting with articles published between 1900 and 1999, followed by yearly searches between 2000 and 2010.
Our search produced a total of N = 1, 810 articles. Across disciplines, 22% of the articles on SEM were published during the twentieth century, whereas 78% were published during the first decade of the twenty-first century. A preponderance of the articles ( n = 856; 47%) were published in the social sciences/arts/humanities, followed by engineering/computer science/mathematics ( n + 483 27%), and then business/administration/finance/economics ( n = 378; 21%). In Figure 15.1 we have plotted the frequency of the term SEM in these articles over the past decade, and we can easily see that these three disciplines have also showed a steady increase of articles on SEM between 2000 and 2009. We did not distinguish the repeated authors, although we are certain there are many, and we did not take into account the likely increase in the number of overall publications. However, it is clear that almost half of scholarly articles on SEM are being produced by social scientists, and most all of them seem to be using SEM mainly as a new tool. But it seems that the SEM tools are now largely used in an appropriate fashion—to examine the utility of theoretical ideas about the effects of unobservable variables in terms of observable relationships.
Structural Equation Modeling As a Concept
A simple regression analysis (Fig. 15.2 ) does not really stimulate us to use SEM in any way, but some aspects of the formal basis of structural regression provide the needed motivation. In this case, the classic treatment of SEM by Goldberger (1973 ; in Goldberger & Duncan, 1973 ) is worth reconsidering. Goldberger initially suggested that the reason we do not use regression on all of our data analysis problems is that the simple linear model of regression is often incorrect and our results are biased. To be sure, it was well known that nonlinear relations may exist and additional predictor variables may be needed, but this was a larger problem. Indeed, this may surprise some readers who do not think of regression analysis as a concept, Goldberger starts with the idea that regression analysis is used to make a “statement about causes” ( see also Pearl, 2000 ). Goldberger seems to make the case that our standard data analyses should not be a search for the “highest” explained variance (i.e., maximum predictability) but the “correct” explained variance (i.e., maximum replicability). Of course, we can only begin to think this is true when our regression parameters remain the same from one analysis to another—Goldberger terms this principle “invariance” (p. 2). The search for invariance of parameters has now become a very basic principle in SEM.
A Chart of recent SEM publications.
A simple linear regression model with no intercept.
In general, this means any regression analysis can be incorrect for a number of reasons, leading to “biased” coefficients. Goldberger shows how SEM concepts and algebra can be used to consider the resulting biases, and his basic concepts will be demonstrated here using both algebra and path diagrams.
Using Path Analyses Diagrams
We often presume that one of the observed variables (X) is an input to the other (Y). For simplicity, it is typical to eliminate the intercept (β 0 ) and calculate a single linear regression coefficient (β) to describe an X → Y relationship. We write the linear regression expression
where the Greek letters are used to represent population parameters to be estimated, the intercept (β 0 ), and slope (β 1 ), and the residual scores ( e ) are not directly observed. We also assume
where the E is a symbol used for the expected values (rather than the usual summation symbol). This combined expression is considered the “structural equation model for the observed scores.”
One other useful feature—the path diagram— was originally a conceptual device. The relation of the algebraic equations and the path diagram is important, useful, and may explain some of the popularity of SEM among novices. In any path diagram used here, observed variables are drawn as squares, unobserved variables are drawn as circles, and a constant (needed when means or intercepts are used) is included as a triangle. Using this notation, a path diagram of the traditional model of simple linear regression is depicted in Figure 15.2 . This model has three variables: (1) an observed outcome (Y); (2) an observed predictor (X); and (3) an unobserved residual ( e ). The model also has three basic parameters: (1) aslope (β), (2) the variance of the predictor ( σ x 2 ) , , and (3) the variance of the residual ( ϕ e 2 ) .
Perhaps it is obvious that this path diagram is not a plot of the raw data but, rather, a topographical representation of some of the assumptions in the model of analysis—that is, the residual has no mean, no correlation with the predictor, and so on. The path diagram does not say anything about the distribution requirements for the predictor X (this can be most anything) or the residuals—that is, the unobserved residual scores ( e ) need to be “normally distributed” for the statistical tests to be exact. One might reasonably ask, “Do we need to know all this just to define a simple linear regression?” The answer to this question depends on what we are going to do next.
These path diagrams can be conceptually useful devices for understanding basic concepts or tools. These diagrams are conceptually useful because they require a potentially complex theory to be portrayed in a single display. These diagrams are also practically useful as a tool because they can be used to represent the input and output of any of the SEM computer programs. But one note of caution is important: The SEM path diagrams do not fully substitute for the SEM algebraic interpretations, so the SEM diagrams can often be misleading. A few examples are described later.
By assuming that the residuals are independent ( E [X, e ] = 0) of the predictors, we know (from calculus) that the best estimate of this coefficient can be found by multiplying the observed covariance of Y and X ( σ y x ) by the inverse of the variance of X ( σ xx − 1 ) . That is, if we write
Rather, we could simply write the key structural expectation of the XY covariance as
so, in simple regression, we can see exactly why the slope formula in Equation 2a works—the structural form expressed in Equation 2b when divided by the value of σ xx leaves us with β yx . Of course, this simple regression is one of the simplest models we will discuss, and not all SEM calculations are so easy.
Missing Predictor Biases in Regression
As a first alternative, let us consider the biases resulting from a missing predictor in a SEM. Let us assume the correct structural model is drawn here as Figure 15.3 . That is, the correct model is
This is very similar to a linear regression for the observed data (Y and X are measured), with the exception that we have included an unobserved variable ( z , drawn as a circle) that has an effect on the outcome (Y, with β yz ) and is allowed to be correlated with the other predictor (X, with σ yx ). This has a structure of observed covariances that is different than the standard linear regression model 2.
This implies that when we calculate the linear regression based on the expectation of the observables only (i.e., as β = σ yx σ xx − 1 ), we will calculate
The population model is a regression, but with a latent predictor.
the incorrect value because
That is, the typical regression estimate (β) will lead to an incorrect or biased estimate of the true regression coefficient (β) yx to the exact degree there is any non-zero effect of z on Y (β yz ) together with any non-zero correlation of z and Y (σ zx ). The missing z -variable can be any other variable, so this kind of bias is likely to occur with real data.
This kind of “missing predictor variable” bias can create changes of sign and is not strictly bounded. It is clear that one solution to this dilemma is to measure z and include it in the model together with X in a multiple regression. This is the reason why regression coefficients are altered when additional variables are included in the overall prediction model. This also means that when we find the parameter remains the same no matter what other variable is included, we assert parameter invariance and think we have found the structural parameter. The suggested solution, as usual, is to fit the correct model (Figure 15.3 ) directly to the observed data. Of course, this solution requires both the available data (measurements on X, Y, and Z) in addition to the regression tools.
Bias Resulting From Unreliability of Predictors
As a second alternative, let us consider the biases caused by the unreliability of a predictor in a SEM. Let us assume the correct model is one where the input variable that we measure (X) is decomposable into a true score ( x ∗ ) that produces the outcome (Y) and also an unreliable part ( u ) that does not. This is drawn in Figure 15.4 , and can be expressed as a structural model
If this is assumed to be the correct model, but we calculate the value using standard regression formulas (i.e., β = σ yx σ xx − 1 ), then we would be incorrect. That is, using standard regression we find
This means the effect of having unreliable predictors pushes the true value of the regression coefficient downward by the size of the unreliability (1 - [ ϕ ∗ 2 / σ x 2 ]) . It is useful to know that the estimated coefficient will always be smaller than the true coefficient, and this bias will not alter the sign of the coefficient, but this is actually not a desirable result. The suggested solution is to fit the correct model (Fig. 15.4 ) to the data, although this requires more data and more advanced programming (to be discussed).
The correct model is a regression with an unreliable predictor.
Bias Resulting From Unreliability of Outcomes
As another case, let us consider the biases resulting from the unreliability of an outcome in a SEM. Let us assume the correct model is one where the outcome variable that we measure (Y) is decomposable into a true score ( y ∗ ) that is produced by X and an unreliable part ( u ) that is not. This is drawn in Figure 15.5 and written as
where there are two residual terms representing the true noise terms ( e ∗ ) and the unreliability of the measurement ( u ). Indeed, unreliability of measures is clearly one of the big issues in SEM. If this is assumed to be the correct model, but we calculate
The correct model is a regression with an unreliable outcome.
the value using standard regression formulas (i.e., β = σ yx σ xx -1 ), then we find
It follows that data with unreliable outcomes does not alter the true value of the regression coefficient. But the size of the unreliability will affect the error variance (which will increase by the size of the unreliability ϕ e 2 + ψ u 2 ). Thus, the standardized coefficient and the explained variance will be lowered, and this is never a desirable result. The suggested solution is to fit the correct model (Fig. 15.5 ) directly to the data, again requiring more data and more programming.
Bias Resulting From Unreliability in Both Predictors and Outcomes
The biases resulting from the unreliability of both predictors and outcomes are an important part of SEM, so let us consider something not fully explored by Goldberger (1973 ). Let us assume the correct model is one in which the regression is a correct statement, but both measured outcome variables (Y) and measured predictor variables (X) have additional unobserved errors ( u and v ). This is drawn in Figure 15.6 as
If this is assumed to be the correct model, but we calculate the value using standard regression formulas (i.e., ft = Oyxo x - x ), then we find
Thus, if Model 6 is assumed to be the correct model, but we calculate the standard regression formulas, then we find the effect of having unreliable predictors and outcomes means both the true value of the regression coefficient will be biased downward by the size of the unreliability (1 - [ σ ∗ 2 / σ x 2 ]) , and the standardized coefficient and explained variance will be lowered. The suggested solution is to fit the correct model (Fig. 15.6 ) directly to the data, but this is not always possible. That is, to create a unique solution, we can use multiple indicators of the true variables ( y ∗ , x ∗ ).
Assume the correct model is a regression but both are unreliable.
Bias Resulting From True Feedback Loops
The final case considered by Goldberger was the most complex, and here he considered the biases resulting from the possible feedback of effects in a SEM. Here, he assumes the correct model can be represented by a set of recursive linear equations where the variables that we measure (X and Y) both presumably have an effect on one another. This is drawn in Figure 15.7 and written as
with two residual terms. If this is assumed to be the correct model, but we calculate the value using standard regression formulas (i.e., β = σ yx σ xx − 1 ), then we find
If this feedback (Fig. 15.7 ) is assumed to be the correct model, but we calculate the value using standard regression formulas, then we find the effect of
Assume the correct model is a feedback of “non-recursive” equations.
having a true feedback loop among the predictors means the true value of the regression coefficient will be biased in complex ways (i.e., the δ and K ). Thus, this general problem is not actually solved when we do a regression analysis and assume we calculate the true effect. The suggested solution is to fit the correct model (Fig. 15.7 ) directly to the data, but we now know this feedback model does not have any unique solution with just two or more measured variables ( see Hauser, Tsai, & Sewell, 1983 ).
Additional Issues When Considering Both Means and Covariances
Although we have not highlighted this issue here, the same general approach can be used to examine the intercept term (β 0 ) in regression, and this can be illustrated in the path diagram of Figure 15.8 . Once we estimate the slope, this leads to the estimates of other unknown parameters
To estimate the intercept parameter at the same time as the regression coefficient, we can include a constant term in the model (as a measured predictor, labeled 1, drawn here as a constant triangle). It follows that we need to include this constant into the model of expectations, but this is easily done by augmenting the covariance matrix with a mean vector or by estimating the model from the average crossproducts matrices ( after Rao, 1965 ). This approach will be described in more detail in the Technical Appendix. Most importantly, this general approach, using means and covariances together, forms the basis of many complex models being used today ( see McArdle, 1994 , 2007 , 2009 ). While the simple inclusion of means does not alter the prior biases, it does help us understand them better. That is, in all cases above, the intercept is biased as well as the slope. One solution to these problems is to represent the model in terms of the true parameters, often requiring more measurements to be included.
Including Common Factors/Latent Variables in Models
We think it is fair to say that a key reason researchers have moved toward SEM is because of the a priori inclusion of LVs representing common factors ( see Lawley & Maxwell, 1971 ; McDonald, 1985 ). That is, we include LVs because we are trying to estimate the unbiased parameters of the models just discussed (Figures 15.2 – 15.8 ) from the data. In this section, we will explore a few selected particular uses of SEM with LVs in path models. We try to point out how this can create an advantage for SEM over other forms of data analysis.
A simple regression model with an intercept.
The Structure of Common Factor Models
Figure 15.9 is a SEM path diagram representing a single common factor as a new LV. The common factor score (the circle termed F ) is not directly measured. But it is thought to have its own variability ( ϕ ? 2 ) and to produce the variation in each of the six observed variables ( Y(m )) through the common factor loadings (λ( m )). Each of the measured variables also has a unique variance (Ψ( m ) 2 ) that is assumed to be composed of variance that is both specific to that variable and variance that is based on errors of measurement. Using these terms, the model for the observed variables is thought to be
So, although we have many measured variables ( Y ( m )), we only have one unobserved common factor ( F ). This set of assumptions implies that the expectation we have for the covariance terms among measures includes only common factor variance, whereas the expected variance terms includes both common and specific variance. That is, if this model
A one-common factor “population” model.
is true, then each variance and covariance has a very simple structure describable as
The collective parameters of this model all include the factor variance term ( ϕ ? 2 ) , so an additional restriction will be needed to make the parameters “uniquely identified.” This is typically done by either restricting the unknown factor variance ( ϕ ? 2 = 1 ) or by restricting one of the factor loadings (λ(1) = 1). The specific choice of the identification “constraint” (also referred to as “setting the metric”) should not alter the estimation, fit, or interpretation of the result, but this is only guaranteed if the model is largely correct. If so, the unique property of the model is the ratio of the pairs of factor loadings (i.e., λ( j )/λ( k )).That is, after the identification constraint ( ϕ ? 2 = 1 ) , the new covariance expectations are now more restricted and can be written in the seemingly much simpler form of
As it turns out, this provides enough information for us to estimate the loadings and tell which measured variables are most closely aligned with the unobserved common factor and which are not—that is, the ratio of the loadings are used to provide a label for the unobserved common factor.
We can now examine whether the one-factor model fits the data by comparing the observed variances and covariance ( S ) to the model expected variances and covariance (Σ, from [9b]). If the model seems to fit, then there are many ways to restrict this model even further. For example, we could say that all the factor loadings are equal (as done in a Rasch-type model; see McDonald, 1999 ; McArdle, Grimm, Bowles, Hamagami, & Meredith, 2009 ). As a very general alternative, we could simply say there is no common factor at all (and all correlations are collectively zero), and this is a typical “baseline” model that we really must be able to say does not fit our data (i.e., if this model fits we are effectively finished). These are both testable alternatives to the one common factor model.
Alternatively, if this simple one common factor model does not seem to provide a good fit, then we can go the other way, and relax some of the model constraints. For example, we can posit the existence of a second common factor ( F 2 ), and one simple version of this model is drawn as Figure 15.10 . In this simple model, we posit that each common factor is related to a specific set of observed variables. In this case, each factor is indicated by three measured variables. Of course, because each common factor is unobserved, we need to identify the model by either fixing the common factor variances (e.g., ϕ 1 2 = 1 and ϕ 2 2 = 1 ) or by restricting a factor loading on each (e.g., λ(1) = 1 and λ(6) = 1). The only difference between this two-factor model and the one-factor model is the covariance among the common factors (φ 12 ). That is, if this value turns out to be the same as the variance terms (or the factor intercorrelation ρ 12 = 1), then this model reduces to become the previous one-factor model. This subtle difference could be important in the model fit, where there is now one degree of freedom difference between these models, testing the covariance hypothesis, and we can evaluate the gain in fit.
A simple two-common factor “population” model.
Unfortunately, these simple kinds of SEM are not the only ones that can be fitted. For example, we can start with this simple two-factor model and add two additional factor loadings, where factor 1 is indicated by variables 4 and 5, and two additional factor loadings, where factor 2 is indicated by variables 2 and 3. These additional parameters reduce the number of parameters we need to estimate, but the overall two-factor model is still identified, and unique estimates can be found for all parameters. It quickly becomes evident that these are not the only restrictions that can be allowed in the context of factor analysis. In this specific model with 6 variables and 2 common factors, at least 10 factor loadings can be placed in different locations. Because the overall two-factor model can be represented with different diagrams (i.e., “rotated” into a different position) without any change in fit, this means that we have possibly different parameter values and factorial interpretations.
In general, it is known that an alternative factor model cannot be judged by fit alone ( see McArdle & Cattell, 1994 ). Perhaps more importantly, this also illustrates that the highly restricted two-factor model (of Fig. 15.10 ) is a specific factorial hypothesis that can have a “unique solution” and “cannot be rotated” any further. This is the essential benefit of what is usually termed confirmatory factor analysis ( see Joreskog, 1970 ; Lawley & Maxwell, 1971 ; McDonald, 1985 ). Just as a clarifying note, in our view, if the factor loading values were all specified in advance, then this would really be considered a “confirmatory” approach to model evaluation. But to be sure, this somewhat rigid form of confirmatory analysis is hardly ever used.
The range of possibilities for factorial structure is so vast that it is rare to consider all possible alternatives. Instead we consider the goodness of fit of the models to be an indicator of whether a specific model does not fit the data, and we never explicitly know whether any specific model is the best one to fit. That is, using the classical arguments of Popper (1970 ), “we can reject models with data, but we can never know we have the best model for any set of data.” Another way to say this is that we can use the data to tell us which models are “false,” but we cannot use the data to tell us which models are “true.”
The careful reader will notice that we have also not mentioned the very big problem of the calculation of the unobserved common factor scores—this is because factor scores for individuals can be calculated in many alternative ways, so they are usually “indeterminant” (for elaboration, see McDonald, 1985 ). One simple way to understand this problem is to consider that a Pearson correlation coefficient (r yx ) can be uniquely calculated from observed data on two variables (Y and X), but if we simply know the size of the correlation (e.g., r yx = 0.6), then we cannot recreate the distribution of the observed data (on Y and X) that produced this correlation— there are an unlimited set of scores that could lead to this specific correlation. This is a considerable problem, because in SEM we do not usually obtain a plot of the unobserved common factor scores. It is typical for some to say the SEM evaluation “does not require factor score estimates” rather than they “cannot uniquely estimate factor scores.” This allows SEM users to appear to be correct, but also means that all the information about the factor scores must come from the model assumptions (i.e., normality of unique factors) rather than the empirical evidence from the score distribution.
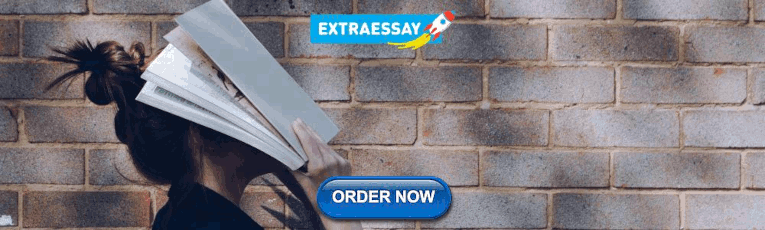
The Structure of Common Factors Within Latent Path Regression
We can now consider the addition of other variables into the LV model. If we include two measured input variables (X 1 and X 2 ) and consider the common factor score ( F ) to be the critical feature of all other outcomes (Y), then we can create a LV regression model with parameters (β(1) and β(2)) where the outcome has no error that could be attributed to its measurement. That is, the common factor model is used to separate the common variance from the unique variance, and the latter includes all errors of measurement. This scenario of a restricted LV path analysis is depicted in Figure 15.11 . This kind of model can be represented by two structural equations of the form
so the impacts of measured Xs on Ys are mediated through the common factor ( F ), and their impacts (β) are also estimated a function of the same factor loadings (λ( m )). Incidentally, because the factor scores, by definition, do not contain measurement error, the residuals ( d ) in the prediction of the factor scores do not contain measurement error and are often termed disturbances ( see Hsiao, 2003 ) to represent the effect of other unmeasured, but potentially reliable, sources of variation in the factor scores.
If the model of Equation 10a is true, then some key structural expectations of the variances and covariance are required to follow the specific pattern defined by
among many other covariance restrictions ( see Fig. 15.11 ). Thus, we hope to tell if this model
A “population” latent path model.
is grossly incorrect for our data. If this latent path model seems reasonable it can be used to test the accuracy of this pattern in real data here ( see Structural Equation Modeling As a Tool section), and it can also be used as a reasonable test of the available SEM computer software ( see the Computer Appendix section).
The Structure of Invariant Common Factors Over Time
One of the best features of SEM is that the basic ideas are broad and general and can be used to consider many different problems. For example, an alternative type of SEM naturally arises when we have common factors at both occasions, and this has some interesting additions. This is depicted in the path diagram of Figure 15.12 , and this is a model that can be used with multivariate longitudinal data ( see McArdle & Nesselroade, 1994 ; McArdle, 2005 ; McArdle, 2010 ). In these cases, we assume some of the same variables have been measured on both occasions. We term these M measured variables as Y( m )[1] and Y( m )[2]. Using a basic array of two-occasion data, we can consider two key SEM issues: (1) the “Invariance of the Common Factors” over occasions, and (2) the “Cross-Lagged Analysis” of the common factor scores.
The first consideration is fairly unique to SEM, but it is very useful. Because we have measured the same observed variables over time we might just assume we have measured the same unobserved factors over time. In principle, this should be an easy concept, especially because we have asserted we have measured the same persons on the same observed variables over two times. Indeed, this is one basic form of factorial equivalence, and it is almost always assumed using standard procedures such as MANOVA or Canonical Regression ( see Tabachinik & Fidell, 2007 ), and this would allow us to go directly to the Cross-Lagged Regression analysis, perhaps with some aggregated scores. But when we think about it a bit more, we come to realize that many things could change over time, including the people’s responses to the same stimuli. Thus, if we make the mistake of mislabeling the key factors at this initial level, then our mistakes are likely to become compounded in any subsequent analysis ( see McArdle, 2007 ). So SEM is useful because it can help us avoid a big mistake at this initial level. That is, SEM allows us to examine this interesting concept of Multiple Factors Invariance Over Time (MFIT) as a testable hypothesis. In fact, this a very basic test, because if the same people are not responding from the same latent sources at both occasions, this model should not fit very well. We do want to achieve this goal, so possibly we may need more than one common factor (as in Fig. 15.12 ) with a more complex pattern of loadings to achieve MFIT.
A theoretical model of cross-lagged regression applied to longitudinal common factor scores (from McArdle, 2010 ).
In SEM we first hypothesize a structural equation that could be applied at both occasions. Within this set of restrictions is the basic requirement of MFIT. That is, we can state
so the common factor ( F [ t ]) is assumed to have the same set of factor loadings within each time (λ( m )). Although the measured variable (Y( m )[ t ]) indicates the common factor score ( F [ t ]) in the same way at any time, the factor score itself can be changing. Here we only use a simple regression function of the prior t = 1 factor score. If this MFIT concept is true, then some key structural expectations might be
among many other covariance restrictions ( see Fig. 15.12 ). This latent path model is interesting because it is not necessarily true, and it can be tested using all observed variable expectations between time 1 and time 2. These expectations require the pattern of the correlations to be exactly proportional (via φ[2] 2 ); thus, all correlations can get higher or lower together, and because the loadings are required to be the same within each time, the relative ratios (λ( j )/λ( k )) and factorial interpretation must remain as well. This MFIT may not be easy to find on an empirical basis, but it is a desirable property to have because it implies we have found a set of common factors that provide a useful template for other changes. Of course, we may find more than one common factor (as in Fig. 15.12 ), possibly with some unusually complex combination of loadings ( see McArdle, 2007 ; McArdle & Cattell, 1994 ).
Once we assume the MFIT expressions, we can concentrate on the sequential changes in the factor scores. Assuming we have two factor scores ( G [ t ] and H [ t ]) within each time (as in Fig. 15.12 ), we can next consider a score model that comes from cross-lagged panel analysis ( see Joreskog & Sorbom, 1979 ; Hsiao, 2003 ) written here as
where we broadly assert that the “past leads to the future,” (i.e., scores at time 1 affect scores at time 2). Most importantly here, we suggest that the each common factor at time 1 has both a “lagged” effect (α g and α h ) on the same factor and a “crossed” effect (β g and β h ) on the other factor. Regressions are typically used in these SEM for simplicity, and there are two separate but possibly correlated disturbance terms ( d g and d h ). Besides working with factor scores that have no measurement error, we are also trying to understand their independent stability over time (1 − α) and their independent impact on the other (β g and β h ). This leads to some key structural expectations for the over-time variances and covariances, such as
among many other covariance restrictions. As it turns out, this initial model will fit just as well as the overall factor model, because all we have done is turn the covariances into regressions.
We can simply look at the values (and possibly the standard errors and significance) of the crossed coefficients to get some idea of the sequential impacts (i.e., does G → H or does H → G ) in light of the lagged features. But because crossed coefficients often represent critical issues of inference, we can use this general SEM approach in a more sophisticated fashion. To further specify and fit an alternative model where G [1] does not impact H [2] (i.e., β h = 0), we simply alter the expectations of Equation 12b . If this model still fits the data as well as the previous and less restricted model, then we conclude we have no evidence for an effect of G → H . To deal with the bigger questions, we can also fit alternative models where H [1] does not impact G [2] (i.e., β g = 0), or where neither factor affects the other (i.e., β g = 0, β h = 0), and possibly other models too.
This basic use of cross-lagged logic is one classical way to examine “causality in observational data” ( see Cochran & Cox, 1950 ; Duncan, 1975 ; Sobel, 1995 ; Pearl, 2000 ; Shrout, 2010 ), and although there are clear limits to our modeling (i.e., perhaps some other unobserved variables Z [ t ] cause both), at least we can test these basic ideas with variables that are assumed to have no measurement error. These kinds of SEMs are hopefully useful to create a basic understanding of the sequential inferences. Recent work on randomized experiments (see McArdle & Prindle, 2008 ; Prindle & McArdle, 2012 ) and other froms of mediation modeling is often being done in the absence of common factors, and this can be quite misleading (for good examples, see Cole & Maxwell, 2003 ).
The Structure of Common Factors for Multiple Repeated Measures
An important first set of models for repeated measures data have emerged from the “time-series” perspective ( see Anderson, 1957 ; Browne & Nessel-roade, 2005 ). These models essentially suggest, once again, that the “future is predicable from the past,”
An equal-time Markov model of longitudinal data used so the parameters are equal over unequal intervals of time.
and we should use this as a main feature of our analysis. The path diagram of Figure 15.13 describes some options for these kinds of time-series analyses. In this diagram everything in the past is used to produce (or predict) the future behaviors. This kind of model is termed fully recursive because it requires as many parameters to be calculated as there are observed correlations in the data so it is really not testing any major substantive idea. To be clear, in this popular formulation, we are not really able to test whether the past predicts the future, or even vice versa, but we can use this approach to calculate the predicted values.
The model in Figure 15.13 can be modified by adding some important constraints. For example, we can say that the only predictors needed for future scores are the ones in the immediate past. That is, the first affects the second, the second affects the third, and the third affects the fourth, and that is all that is needed to predict the future values. In general, we can eliminate three parameters from Figure 15.2 , and we can test this comparison of parameters as a formal hypothesis with three df . We emphasize that we are actually testing the importance of parameters that are not present rather than the ones that are present. By fitting this alternative model, we can examine whether these restrictions seem reasonable for our data.
This model is written where the future deviation at any time-point ([ t ]) was predicted from the deviation at the prior time-point ([ t − 1]) using a linear regression model with “fixed” group parameters (α[ t ]) and independent disturbances ( d [ t ]). One version of this model can be written as
so some of the expected variances and covariances are
The utility of time-series regression obviously comes when we add more variables and examine cross-lagged models, but this basic time-series structure resonates with some researchers more than others ( McArdle & Epstein, 1987 ; Walls & Schaffer, 2006 ).
We can add another important component to this basic model—a LV—typically drawn as a circle with an arrow pointing directly to the square. This kind of LV is introduced to indicate that the time-series process does not apply to the observed or “manifest variable” (MV) but, rather, to one key source of the variation in the MV—namely, the LV over time. Here we assume there is one LV at each time, that the part of the MV that is not attributable to the LV is “unique” (e.g., it may be partly “error of measurement”), and it has the same variation at all times. This is exactly like a common factor model, but here the common factor has only one indicator, so it is not broad in any sense, nor is it testable in a rigorous way. However, we should not underestimate the importance of this LV idea. One benefit of using LVs here is that it enables the estimation of the process in the presence of random error ( see Heise, 1975 ; Joreskog & Sorbom, 1979 ). In this formulation, the time series for the LV is “error-free,” so we expect it to have predictions that are absent of error, and thus higher in accuracy. The model may fit the data better using this LV approach, but we do not have, nor can we obtain, estimated scores for the LVs. What we can examine is the effect of measurement error on our understanding of the process.
There are some important conditions for this model to be useful. In interpretation of these timeseries models, we typically assume the time points are equally spaced, so any α coefficient could be substituted for another with the same specific timeinterval. We may need to use unobserved variables representing the time-points without data to deal with the “unbalanced” data over time ( see McArdle & Aber, 1990 ). But these are not typical constraints in this model. Another more typical restriction on time-series models is an assumption of “stationarity”—that the variances (and covariances) remain the same over all times because the system has reached a “steady state of equilibrium” ( see Walls & Schaffer, 2006 ). Equilibrium does not seem to be a reasonable assumption for many data sets, so this particular model is probably not a good idea. Finally, the means of the scores at each time are not considered so they do not count against us here. This may sound foolish at first, but there are many examples where these group level statistics are not needed. This problem does not create more misfit, but it can certainly be a problem when the change for the whole group is a key part of the substantive questions.
To ameliorate some of these problems, a seemingly completely different set of models was also used, and this is presented in Figure 15.14 . These are broadly termed latent curve models (LCMs), and they can be fitted by either approximate ANOVA methods or exact SEM methods (from Meredith & Tisak, 1990 ; see McArdle, 1986 , 1988 ; McArdle & Epstein, 1987 ). This LCM approach typically starts by assuming we have a trajectory equation for each occasion for each person formed as the sum of (1) unobserved or latent scores representing the individual’s initial level ( G {0}); (2) unobserved or latent scores representing the individual change over time or slope ( G {1}); and (3) unobserved and independent unique features of measurements ( u [ t ]). In this model, the arrows from the latent slopes to observed variables are a set of group coefficients or basis weights that define the timing or shape of the trajectory over time (e.g., α[ t ] = t − 1). Note how this carefully selected set of basis weights (as α[t] = [0, 1, 3, 5]) easily takes care of the unbalanced time delay between occasions.
In the LCM of Figure 15.14 , the initial level and slopes are often assumed to be random variables with “fixed” means (μ{0},μ{1}) but “random” variances (φ{0} 2 , φ{1} 2 ) and correlations (ρ{0, 1}). The standard deviations (φ{ j }) are drawn here to permit the direct representation of the correlations ( see McArdle & Hamagami, 1991 ). This LCM path diagram can also be interpreted as a two common factor model with means. The first latent factor score is an intercept or level score (labeled G {0}), and the second latent factor score is a slope or change score (labeled G {1}). The relationships between the latent levels G {0} and all observed scores Y[t] are fixed at a value of 1. In contrast, the relationships between the latent slopes G {1} and all observed scores Y[t] are assigned a value based on the time parameter α[ t ], which, depending on the application, may be fixed or estimated. For simplicity, the unique components ( u [ t ]) are defined as having constant unique deviations (Ψ) and are presumably uncorrelated with other components. This seems like a typical setup for such models.
In this longitudinal model, the change score ( G {1}) is assumed to be constant within an individual but it is not assumed to be the same between individuals. The LVs are written in italics ( G {0}, G {1}) because they are similar to the predicted scores in a standard regression equation. (That is, we do not use Greek notation because these scores do not need to be estimated). One new feature here is the use of a triangle to represent a measured variable that has no variation—that is, the unit constant. The key reason this new option is added to the path diagram is to allow us to make hypotheses about the group means. In LCM we want to evaluate hypotheses about the group means and covariances that require the same parameters of proportionality (i.e., the basis a[t] ) because this is an indirect way to evaluate the patterns of the score trajectories.
A path diagram for the latent curve model (LCM) with a linear “basis.”
Perhaps it is obvious, but this LCM is not the same as the prior time-series model of Equation 13 . This LCM structural equation model can be written as
so some of the expected means, variances, and covariances are
and the careful reader will see that this is a model for means as well as covariances, and these covariance assumptions are not identical to those of the timeseries model in Equation 13 .
Although a linear scaling of the basis is very popular ( see Singer & Willett, 2003 ), it is only one of many that could be used. For example, it is possible to add a lot of nonlinear complexity based on age or time to the simple growth curve models for the study of within-person changes. Wishart (1938 ) introduced a fundamental way to examine a nonlinear shape—the use of power polynomials to better fit the curvature apparent in growth data. The individual growth curve (consisting of t = 1, T occasions) is summarized with a small set of linear orthogonal polynomial coefficients based on a fixed power-series of time (α[ t ], ½α[ t ] 2 , 1/3 α[ t ] 3 ,… 1/p α[ t ] p ) describing the general nonlinear shape of the growth curve. A second-order (quadratic) polynomial growth model implies that the loadings of the second component are fixed to be a function of the first components (i.e., the derivative is linear with time).
The quadratic form of this basic model can be depicted as a path diagram as well (not included here). This can appear to be a bit complicated because it requires a third latent component with a basis that is related to the first one (i.e., ½α [ t ] 2 ), but all of this is done so the implied change is linear with time (i.e., we add acceleration). Additional variance and covariance terms can be used to account for individual differences in these new LVs. Typically we find that introducing some curvature allows the model to approximate the data points more closely. Of course, a model of growth data might require this form of a second-order (quadratic), third-order (cubic), or even higher-order polynomial model fitted to the data. The polynomial family kind of nonlinear models is very popular (e.g., Bryk & Raudenbush, 1992 ).
One reason researchers like the LCM logic is that it permits a rich variety of alternative extensions. For example, a different alternative to the linear growth model was brought to light by Meredith and Tisak (1990 )—the model proposed by Rao (1958 ) and Tucker (1958 ) in the form of summations of “latent curves.” These innovative techniques were important because they added the benefits of the SEM techniques – SEM made it possible to represent a wide range of alternative growth and change models ( McArdle, 1986 , 2009 ; McArdle & Epstein, 1987 ; McArdle & Anderson, 1990 ; McArdle & Hamagami, 1991 ). Our use of this latent curve concept can be accomplished with only a minor adjustment to the LCM of Figure 15.3a . We allow the curve basis to take on a form dictated by the empirical data. In this example we simply write a model for the same person at multiple occasions where the last two basis coefficients α[3] and α[4] are free to be estimated (the first two are still fixed at α[1] = 0 and α[2] = 1). The actual time of the measurement is known, but the basis parameters are allowed to be freely estimated so we can end up with different distances between time-points, an optimal shape for the whole curve. The estimated basis has been termed a “meta-meter” or “latent time” scale that can be plotted against the actual age curve for clearer interpretation ( Rao, 1958 ; Tucker, 1958 ; McArdle & Epstein, 1987 ). There are many ways to estimate these model parameters in a LCM framework, and all such options are not considered here.
The time series and LCMs are only two approaches to the analysis of within-person changes that, although representing major modeling approaches, barely scratch the surface of all the possibilities (e.g., see Walls & Schaffer, 2006 ; McArdle, 2009 ). These illustrations mainly show that any SEM, based on AR-type models or LCM-type models, is used to make a prediction about longitudinal trajectory. Thus, both kinds of models have their place in the array of possible analytic frameworks, and choices between models are not often so easy. Some of these choices are simply defined by the data.
For example, we might have a data set where the individuals are measured repeatedly at many more time-points (i.e., T = 100). In this sense, a collection of complete cases at a few time-points (T = 4) within a panel study design is fairly easy to consider, even the timing is unbalanced, and this has allowed us to go a bit further.
This LCM has become a very popular model, and it clearly highlights the differences between SEM and ANOVA. In SEM, the entire model is used to pattern the means and covariances ( see McArdle, 1988 ; McArdle & Epstein, 1987 ), whereas in ANOVA the means are fully patterned, and the covariances are either highly restricted (i.e., in repeated measures ANOVA we assume Compound Symmetry; see Muller & Stewart, 2006 ), or the covariances are allowed to be completely free (i.e., repeated measures MANOVA). If the univariate ANOVA assumptions are correct, then the tests of mean differences over time is the most powerful. But if these assumptions are not met, then the same questions about means differences are biased ( see Bock, 1975 ). The multivariate repeated measures model is always correct, but in general it will not be as powerful as the LCM. Thus, there are a lot of basic statistical reasons to use LCM rather than MANOVA.
The introduction of measured predictors of the LVs is a natural aspect of LV path models, and it is no different here. In fact, this reflects the best thinking in “multilevel” modeling ( Bryk & Raudenbush, 1992 ). Here, a set of the first level variables (Y[ t ]) are used to form the latent level and slopes ( G {0} and G {1}), and the second level variables (X) are used as predictors of the levels and slopes. There should be no doubt that multilevel thinking has allowed much progress in the simplicity of the required calculations, but there is also no doubt that this “new” approach does not automatically produce novel results ( see McArdle&Hamagami, 1996 ; Ferrer, Hamagami, & McArdle, 2004 ).
In this specific case, path diagrams of longitudinal data were originally used with autoregression models, and these models seemed to reflect actions or predictions moving forward in time (e.g., see Figure 15.13 ). However, it was very unclear whether any movement was actually taking place or if anyone was actually doing any traveling at all (not really). Subsequent work showed how these diagrams can be used in the context of growth and change with LVs (e.g., Fig. 15.14 ; McArdle, 1986 , 2009 ; McArdle & Epstein, 1987 ; McArdle & Anderson, 1990 ; McArdle & Woodcock, 1997 ).
Indeed, the usefulness of path diagrams as tools emerge as we move to the more complex examples.
Structual Equation Modeling As a Tool
When we want to calculate values for the parameters of a model, we may need specialized SEM software. There are many elegant treatments of SEM in the current literature (e.g., Loehlin, 2004 ; Kline, 2011 ), but a simple treatment is a worthwhile start. A sample of the current SEM programs are listed in Table 15.1 . As can be seen here, there are many other computer programs that carry out SEM, and many more are not listed or are under current development. But those listed will serve our purposes here. These are all computer programs that largely focus on SEM analyses, and it is useful to know what they are actually doing. The SEM programs listed here differ in several key options, including data entry, model construction, iterative schemas and options, model output, and general ease of use, so different but knowledgeable researchers will advocate one program over another (i.e., Mplus instead of CALIS).
Structural Equation Modeling As a General Data Analysis Technique
There is no doubt that these SEM computer programs listed above can be used to calculate a wide variety of regression-based parametric models, and some of these might be incorrect (as just seen). In fact, the SEM computer program can calculate almost any model, including square roots and exponents ( see McArdle & Boker, 1990 ). But these kinds of calculations do not represent the true benefits of SEM, or the real reason why so many researchers now use SEM programs.
In our own uses of SEM, we initially demonstrated that all available software for fitting a specific model with a specific data set (i.e., longitudinal growth models of the WISC) could be programmed to produce the same results (e.g., Ferrer, Hamagami, & McArdle, 2004 ). This led us to recognize an important general principle for SEM— All SEM computer programs produce the same result for the same model . To the degree this is true, it really should not matter which program is used as long as a correct expression of the model is our goal. Having said that, it is important to make sure that you have specified exactly the same model in each program—relying on computer program defaults may lead to different results for seemingly identical models. It is confusing why more researchers do not understand this basic
SEM principle, but it certainly seems they do not. Perhaps this is because the calculation of parameter estimates using SEM can be overly complicated. We will briefly describe some key SEM ideas here, and we will use a variety of SEM programs, such as SAS PROC Calis, Mplus, and OpenMx ( see the Technical Appendix). Some researchers have been surprised how many innovations have emerged first in SEM—dealing with multiple populations, incomplete data, and longitudinal data—and this made the original LISREL matrix concepts far less important than once thought ( see McArdle, 2005 ; Muthen & Muthen, 2002 ; for further reasons, see Kline, 2011 ).
As will be illustrated in the next section , any SEM program requires a tradeoff of SEM concepts for SEM tools, but the Mplus program offers a good example of easy input, correct calculations, and the need to work hard to carry out a real SEM analysis. Other programs we will use (CALIS and OpenMx) can produce the same estimates but are not as easy to use.
Creating Structural Equation Modeling Expectations
The way we create model expectations is not exactly clear to many researchers, but it is an essential technique. What we generally do is place values (or symbols) inside model matrices and then use matrix algebra to generate the expected values. This is required and automatically done by all of the computer programs, but not enough emphasis is placed on this calculation. Let us revisit an earlier thought—an SEM representation of the scores is used to create model expectations about the means (µ, for means m ) and covariances (Σ, for covariances S ) of the observed data. This is most important because it is these expectations that can be compared to the data observations to form a test of fit. The formal basis of LISREL notation was designed to produce a set of expected values (symbolized as E ) from the eight-matrix notation.
It is well-known now that a specific matrix algebra formulation such as SEM-LISREL is not the only way to create model expectations. Without any loss of enthusiasm for the basic premises of SEM, it is fair to say that there were other knowledgeable researchers who suggested that the LISREL concept and computer program was not actually the best way to deal with such problems, and McDonald (1980 ) was among the most vocal ( see McDonald, 1985 ; Loehlin, 2004 ). In McDonald’s alternative approach, the newly available computer programming he created allowed an unrestricted level of higher-order common factors, which he termed covariance structure analysis (COSAN). The resulting concept and computer program produced exactly the same values as LISREL, but it was not nearlyas popular as LISREL, which was unfortunate because COSAN was free software.
Our own interest in path diagrams also led to another innovation in the calculation schemes. Our own use of COSAN proved instrumental in asserting that the eight-matrix notation, and the entire LISREL concept, was not the only correct way to carry out SEM ( see Horn & McArdle, 1980 ). In fact, a practical comparison of COSAN and LISREL applications led directly to RAM theory ( McArdle & McDonald, 1984 ; McArdle, 2005 ) where only three model matrices were necessary to consider any model: (1) a filter matrix ( F ) of completely fixed ones and zeros designed to distinguish the manifest variables from the LVs in a model; (2) an arrow matrix ( A ) of potential one-headed arrows (regression coefficients and means) based on directional hypotheses; and (3) a sling matrix (Ω) of potential two-headed arrows based on nondirectional hypotheses.
Of course, the main point of RAM notation is that these three matrices also were based on a one-to-one identity with the path analysis graphics (for details, see McArdle, 2005 ; McArdle & Boker, 1990 ). In various demonstrations we showed how this three-matrix approach produced exactly the same values as the eight (or more)-matrix approach (e.g., McArdle & McDonald, 1984 ; McArdle, 2005 ). We also suggested that the existing SEM computer programs could be quite useful (e.g., LIS-REL, COSAN), but all available matrices in these programs were not needed because only these three parameter matrices were needed to produce all the correct model expectations (Σ). This simplified programming made exceedingly complex models relatively easy to consider (e.g., McArdle & Hamagami, 2003 ; Grimm & McArdle, 2005 ). Neverthless, any SEM only consists of variables that are either measured (squares) or not (circles) and relationships that are either directed (arrows) or not (slings). All other statements about the reasons why a specific approach should be used (i.e., combining econometrics with psychometrics) could still be useful, but they are certainly not essential. As with the earlier COSAN concept, it was no surprise that the RAM concept was not uniformly recognized by the community of scholars who had put so much time and energy into LISREL concepts, notation, and programming.
Statistical Indicators in Structural Equation Modeling
There are many statistical features that have been considered in SEM. A typical SEM has fewer parameters than observed covariances, so the model can be said to have degrees-of-freedom ( df ). Each df represents one way the model can be incorrect about the expectations. That is, the df represent ways the model does not need to look like the observed data, and the more of these df , the stronger the a priori nature of the model. Of course, not all df s represent the same parameter restrictions or model information, so we need to deal with this with one model at a time.
Any overall goodness-of-fit index typically requires some idea of the desired relation of the data observations ( m , S ) and the model expectations ([ μ , Σ ,). Some function of the distance between observations and expectations is chosen, and we can calculate individual “misfits.” If we want the model to reflect a good fit to the data, then we want the expectations to be close to the observations and, hence, small misfits. Of course, the term “close” can mean many things, so we need to define this in further detail. In simple regression, we often attempt to minimize the squared distance between the observations and the expectations— the least squares function (i.e., Σ[ O − E ) 2 ]). But there are other reasonable alternatives to consider. For example, we may wish to find the value that minimizes the squared distance of the observations and the expectations divided by the squared observations (i.e., Σ[ O − E ) 2 / O 2 ]). This is one expression of the principle of “generalized least squares” (GLS). Alternatively, we may wish to find the values that minimize the squared distance of the observations and the expectations divided by the squared expectations (i.e., Σ[ O − E ) 2 / E 2 ]). This is one simple expression of maximum likelihood estimation (MLE).
When we define a model on an a priori basis, the “probability of perfect fit” can be obtained by assuming the misfits are normally distributed, searching for GLS or MLE, and comparing the difference between the likelihood to a chi-square distribution (or random misfits). If the obtained misfits do not behave like random misfits, and the chi-square is relatively high for the associated df , then we conclude the model does not fit the data. If we are concerned that this index is asking for too much, which it usually is, then we may choose to estimate the “probability of close fit” using the root mean square error of approximation (e.g., ε a or RMSEA ; Browne & Cudeck, 1993 ). Often we do not define the model on an a priori basis, but we have used some key aspect of the data to help us choose a model, so we probably need to be careful to report this approach and we should not try to generate a probability statement at all ( see McArdle, 2010 ).
The estimate of any model parameter has some important features that follow the functional form. For example, if we estimate some models using the ordinary least squares (OLS), now termed unweighted least squares (ULS) function, we can say we have best linear unbiased estimates (BLUE; see Fox, 1997 ). If we estimate with a more complex function, such as GLS or MLE, then we can say we have GLS or MLE. In any case, the standard error of the parameter can also be calculated and used to create a confidence boundary for the parameter ( t = est / se ( est )), and this ratio is thought to be useful in model interpretation. In many cases, researchers ignore parameters that are not more than two standard errors away from zero, but they often do not say why they do this. It seems this is a rather crude evaluation of the individual virtue of a parameter in a model by assuming it is uncorrelated with other parameters, and this strategy that is probably based on the use of an arbitrary α < 0.05 criterion (i.e., because t > 1.96 and this is fairly close to t > 2 ).
Nevertheless, this overall principle was used in the simplification of otherwise complex models by Hauser, Tsai, and Sewell (1983 ) who unfortunately termed it “model trimming” and made it seem statistically defensible. Their tests actually represented a priori hypotheses so their particular application is not suspect. However, because key SEM parameters may be correlated with other parameters in the model, this “trimming” approach is in fact highly suspect. The only procedure that can be justified on a statistical basis is to set the key parameter to zero, allowing the other parameters to take the place of the key one, and see if the model fit is significantly altered (using / comparison logic). If there is a great loss of fit, then the key parameter is important. Although this approach can be much more tedious, there does not seem to be a simpler way to carry out this evaluation in a statistically meaningful fashion.
Structural Equation Modeling Estimation of Linear Multiple Regression
To illustrate these points in more detail, let us assume we have measured several persons ( N ) on two variables (Y and X) and we want to describe their functional relationship as X → Y. This expression is considered the “structural equation model for the observed scores.” For simplicity, we can eliminate the intercept (β 0 ) and calculate a single linear regression coefficient (β) to describe this X → Y relationship. By assuming that the residuals are independent of the predictors, we know (from calculus) that the best estimate of this coefficient has a specific numerical value ( β = σ yx σ xx -1 ) that is considered as the BLUE. So we use this prior and well-known result to calculate the regression coefficient, and we use this to define the other statistical features of the model ( see Fox, 1997 ).
Now that we have set up the structural model, we can examine several other ways to obtain reasonable values for the parameters of a SEM. In general, what we are trying to do is to find a set of parameters for the estimated relationships hypothesized that come as close to our observed relationships as possible. That is, we want to find those values that minimize some prespecified function of the observables, and we know this function could take on many different forms. So this definition of our goal can be accomplished in many ways, including by the direct result of the calculus (as above).
Now that we have created a functional goal, let us consider an iterative approach to model estimation. We can start by doing the following:
Create a Set of Starting Values —Make an educated guess for every parameter value. These can all be unities (ones) or they can be created in different ways based on previous findings from the substantive area of interest.
Calculate the Function at this Iteration —Take the values for every unknown model parameter and put these values into the positions of the matrix (in Table 15.A1 ). We can see we will create some differences between these guesses and the observations, and we can create a total function value for the overall model at this point.
Use Some Technique to Find Better Values —Here, there are several options to obtain an improved guess at the parameter values. If we are relying on standard SEM, then we would calculate the next best values by fundamental calculus—we estimate the first and second order derivatives of the parameters with respect to this function (and this can be complicated), and we then use the ratio of first and second derivatives as the most desired change in each parameter. Of course, if we use a Bayesian-type estimator (i.e., WINBUGS; see Lee, 2007 ; Ntzoufras, 2009 ) we might instead just simulate new scores for every LV and see how well these new values fit the data.
Put the New Values into the Calculation —After we obtain a new set of changes in the parameters, we can create new parameter values. We then plug these back into the matrix expressions, and we do the basic calculations (expression 2 and 3) all over again.
Terminate When We Cannot Do Better —We terminate the iterative sequence when we do not get a big enough change in the parameters from one iteration to the next. We term this as “convergence.”
There are a series of important proofs that show how this iterative approach can be used to estimate the best possible values for all model parameters (see Joreskog & Sorbom, 1979 ). This is true of multiple regression models for sure. Of most importance now is that this iterative approach can also be used when we do not know what values are optimal by straightforward algebra or calculus ( see Technical Appendix). This is often the case when we have more complicated SEMs, involving LVs, recursive relationships, or both. We will deal with some of these more complex models in a later section.
Considering Common Factors/Latent Variables in Models
As stated earlier, the merger of SEM concepts using the SEM tools is the basis of many real SEM applications. The SEM literature is filled with interesting forms of analysis, from the “Experimental” work of Blalock (1985a , 1985b) to the “Observational” examples in Rabe-Hesketh & Skrondal (2006 ; see Cochran & Cox, 1950 ). However, all such interesting applications include the concepts of LVs in path models, so we extend this merger by highlighting features of a few real data applications of our own.
Considering Benefits and Limitations of Including Common Factors
There are many ways we can use LVs or common factors in SEMs. But we must ask “Are we just fooling ourselves when we add unobservables to a model?” Some people seem to think so ( see McArdle, 1994 ), so it is important to reconsider what we are doing here. Let us list reasons why it is useful to consider LVs:
Unbiased —If LVs are used and not needed, then no bias should result. In this sense, LVs models are not dangerous.
Missing —If LVs are not used, but the data were actually created by a correlated predictor, then substantial bias can result.
Unreliable Inputs —If LVs are not used for input variables X , but are needed, then downward bias will result.
Unreliable Outcomes —If LVs are not used for output variables Y , but are needed, then there will be bias in standardized estimates.
Unreliable Inputs and Outcomes —If LVs are not used for X and Y , but are needed, then severe biases can result.
Because these cases are very likely in real data, it is wise to consider SEMs with LVs. At the same time, of course, there are also several problems that can emerge when using LVs:
Indeterminancy —In many models, there is no direct way to estimate a value for the LVs of an individual. This means the LVs cannot be plotted or explored in the usual way (i.e., for outliers, etc.), and new analyses need to be created.
Manifest Explanations —In many cases, there are alternative explanations for the data that are based only on MVs and are just as good (in terms of fit) as the first model with LVs.
Latent Explanations —In many cases, there are alternative explanations for the data that are based on other forms of LVs and are just as good (in terms of fit) as the first model of LVs. It is possible that researchers can fool themselves with LVs!
Communication —In many cases, the LV models are harder to explain in terms of basic theory and outcomes. Although these LV models may be correct, other may not understand.
Naming Fallacy —The wrong name may be attached an LV, partly because of low loadings or missing indicators, and this may persist and create more problems ( see Cliff, 1983).
Common Factors With Cross-Sectional Observations
Some research demonstrates the sequential nature of building a LV path model from crosssectional data gathered at a single occasion. In one of our analyses, McArdle and Prescott (1992 ) used data on N > 1, 600 adults from the national sample of data on the Wechsler Adult Intelligence Scale-Revised (WAIS-R; see Wechsler, 1981 ). Although there was much discussion of the merits of different models, the final model depicted in this article includes two common factors (termed Gf and Gc) and two key predictors (age and high school graduation status). Attempts were made here to consider many different kinds of models, including polynomial forms of age and nonlinear interactions with high school graduation. In this research, successive models with a sequence of restrictions suggested that that nonlinearity and interactions were not needed when the outcomes were the two most reliable common factors.
A related question is whether something can be gained by splitting persons into subgroups. Splitting data into groups is some evaluation of the evidence for the equality of the meaning of the LVs over groups. This introduction of multiple-group analysis, initially promoted by Lawley and Maxwell (1971 ) and Sörbom (1979 ), turns out to be a remarkable advance over classical ANOVA thinking. That is, nothing needs to be lost here, because there can be one overarching model for the whole data set, and parameters can be invariant over groups. This was demonstrated by Horn and McArdle (1992 ) using the same WAIS-R data to express these basic ideas. The data were split into four separate age groups, and a multiple-group analysis was conducted without path diagrams. What they tried to examine was the rationale for the equality or inequality of the basic factor structure over age groups.
Multiple-group concepts were put into path diagram form in McArdle etal. (2001 ), where data from the Hawaii High School study was used to examine multiple-group hypotheses using the CESD. In the models from the HHS study, persons who were ethnically different (Hawaiian vs. non-Hawaiian) were split into separate groups to see whether the factor patterns were the same across groups. They seemed to be, but others can check this out.
The multiple-group models were used in the theoretical analyses of McArdle and Cattell (1994 ) but go one step further. A multiple-group SEM was used to assert that if the model of factorial invariance did not hold over groups, we needed to relax some of the major simple structure restrictions on the factor pattern but not the invariance constraints. This was largely the opposite of the tradition at the time, where invariance constraints were added to simple structures. That is, when doing comparative factor analyses of real data, we may need many common factors (K) before we achieve factorial invariance, and we should not expect the resulting invariant factor pattern to also be simple. This research also showed how rotation of the final multifactor invariant pattern may be needed for substantive understanding.
This use of multiple-group factor invariance model leads to an interesting observation. If we can assume metric factorial invariance, then we do not need to measure every person on every variable to estimate SEMs. In this example, a one- versus two-factor model for the WAIS was considered the key issue, and a “fractional factorial design of measures” was chosen as a quick way to test the specific hypothesis. In this data collection design, presented in this paper, a first group of persons was chosen at random, and only four WAIS-R scales (of eight) were administered. Such a data collection, if it were actually undertaken, should take only about half of the usual testing time. A second randomly selected group was then administered another set of four WAIS-R scales. This process was repeated until eight groups with a different pattern of four measures each were obtained. The result from an eight-group SEM with factorial invariance constraints was compared for one versus two common factors, and the misfit suggested two factors were needed.
The power of the statistical tests for one versus two common factors was compared when there were between M = 1 and M = 8 variables measured in each required subgroup. The resulting power calculations, based on the Satorra and Saris (1985 ) method, were plotted for different sample sizes, and the results are surprising. For example, if we used the fractional factorial blocks design based on M = 4 variables in each group, and we wanted to achieve a power of 0.95, then we would only need about n = 80 persons in each of the eight subgroups. Of course, as the power requirements grow larger, so do the required samples sizes, and as the number of variables measured goes down, so does the resulting power. But, in general, to retain relatively high power for the test of one versus two common factors, it seems relatively few individuals are actually needed in each subgroup.
Common Factors With Longitudinal Observations
The case of repeated measures is an important one in modern data analysis, especially because of the importance placed on repeated measures in ANOVA ( see Bock, 1975 ). Our simplest model was presented here as Figure 15.12 , and this was used in McArdle and Nesselroade (1994 ). In that paper, we assumed the measurement of four different variables (W, X, Y, and Z) were repeated at two occasions. The data we used were four WISC Verbal subscales measured in the first grade and then again in the sixth grade on the same set of children ( N = 204).
Again the topic of metric factor invariance is raised, but now it can be applied to the same observations over time. The most obvious question, of course, is “Do the means change over time?” This informative question can be answered using a number of different ANOVA techniques ( see O’Brien & Kaiser, 1985 ). But the first SEM question raised is slightly different—“Is exactly the same common factor measured at each occasion?” The techniques of ANOVA typically assume the answer is yes, and we used these procedures here. However, this was not a testable assumption and it is not usually asked because, after all, we have measured the same people using the same instruments. So, although this may seem like an easy question to answer in the affirmative, it is actually not. That is, just because we can measure the same variables at each time does not mean the persons respond to the same features on the tests at both times. There are many real-life situations where we might think the people have changed in a qualitative way, and the factors have changed, so the comparison of means, even for the same observed variables, is really comparing latent apples to latent oranges.
This SEM question was initially answered by fitting a model to the invariance of the factor pattern over time in the WISC verbal scores. Once the best number of factors was determined within each timepoint, a model of metrically invariant loadings (same exact values) was fitted over time, and the loss of fit was examined. Now there is no doubt that we wanted to find invariant factors, so some loss of fit was deemed acceptable. Once the common factors were considered invariant, we could examine the mean changes in the common factor scores, and this became the focus of our analyses. We determined that this gave a different result than either the ANOVA or MANOVA, which created components that appeared to have maximum differences over time. In addition, we added several different features, including the possibility of specific factor covariances over time ( see Meredith & Horn, 2001 ). The correlation of the specific factors over time was also added to this analysis, but this set of parameters proved far less important.
As a byproduct of this two-time invariance analysis, we were also able to show that the factors of the sums over time was the same as the common factors of the changes over time. This was used to show several variations on the way means and covariances could be used, including the creation of a latent change score (LCS). In addition, this approach led to a more general expression and a test of the general assumption of metric factorial invariance in multilevel data ( see McArdle, 2007 ; McArdle, Fisher, & Kadlec, 2007 ).
In the next case of Figure 15.12 , we assumed that two factors were apparent at each of the two occasions ( see McArdle, 2010 ), and now we were interested in testing hypotheses about their dynamic nature over time using longitudinal panel data ( Blalock, 1985b ; Hsiao, 2003 ). That is, once the same factors are measured at both times, it is reasonable to consider their scores in a cross-lagged analysis. It is also reasonable to define latent factors that have particular patterns of change over time (i.e., G 1 produces H 2 , but not the other way around; see McArdle, 2007 ).
Common Factors With Multiple Longitudinal Observations
Next we assume we have a longitudinal sequence of repeated observations ( Y [t], where t > 2). In the past, these kinds of data would also typically be analyzed using the ANOVA models for repeated measures, perhaps adding polynomials ( see O’Brien & Kaiser, 1985 ). But the main SEM point here is that these GLM tests are mainly about the group differences in mean changes over repetitions and do not deal with hypotheses about covariances.
In the path diagram of Figure 15.14 , we considered an LCM (, after Meredith & Tisak, 1990 ; see McArdle, 1986 ). Inall cases, more than 200 children were measured at specific occasions (i.e., grades 1, 2, 4, and 6), and we used a LV approach to space the latent measurements out in an equal time lag. That is, when there was no observed data, we simply used a LV as a placeholder. That is, we assumed they had the scores but we never asked for them because of budgetary constraints (i.e., no funding for grades 3 and 5). This basic use of an LV as a placeholder turns out to be a very useful idea in SEMs.
The introduction of measured predictors of the LVs is a natural aspect of LV path models, and this reflects the best thinking in what is now termed hierarchical or multilevel modeling ( Bryk & Raudenbush, 1992 ). In one case ( McArdle, 2005 ), we fitted some cognitive data over many ages using a LCM, and we estimated the effect of educational attainment of the person, mother, and father on these latent scores. We found that the person’s education is mainly influenced by their mother, and this in turn influences the person’s slope in cognition over age. There are very few influences on the initial level, or from the father. Now, there should be no doubt that multilevel thinking has allowed much progress in the simplicity of the required calculations, but there is also no doubt that this “new” approach does not automatically produce novel results ( see McArdle & Hamagami, 1996 ; Ferrer et al., 2004 ; Ferrer & McArdle, 2010 ).
The final LCM is based on work done by McArdle and Woodcock (1997 ). Here we wanted to estimate a full LCM over many occasions, but a premium was placed on people’s time (largely because we wanted to administer up to 17 tests). So we used the basic logic of LVs as incomplete data to design a data collection plan. We measured each individual at a first occasion, and then at a second occasion that was spaced out in time by our data collection design. The basis of the LCM we fitted is drawn as a path diagram ( t = 0) or was not measured “circle in the square,” indicating the person may or may not have been measured, and we did this for up to four follow-up times ( t = 1 to 4). We applied this principle of a random “time-lag” to estimate and test developmental functions where the person was measured only twice but where we could estimate a multi-occasion LCM. We also found we could apply a second slope component that connected the two points in time, and this led to our independent estimate of a nontrivial practice function (with μ p and σ p 2 ). The same approach was applied to multiple indicators of multiple constructs where, by assuming factorial invariance, we could separate the true score changes from state dependent changes, and from test-specific practice effects ( see McArdle & Woodcock, 1997 ).
Much of the prior LV thinking was expressed in the recent overviews of McArdle (2009 ) and Ferrer and McArdle (2010 ). In one analysis of real data by McArdle and Hamagami (2006 ), the task was to examine longitudinal changes in both verbal skills and memory skills over age. The basic dynamic hypothesis (from the theory of J.L. Horn) was that memory losses would lead to verbal losses, but not the other way around. Figure 15.15 is a diagram of a univariate sequence of latent scores ( y [ t ]) where only six occasions of measurement (Y[t]) have been spread out over a 60-year period (from ages 5 to 65 years). One obvious complexity to this LCM was that the six occasions of measurement were spread out unequally over age, and one non-obvious complexity is that not everyone was measured at each of the six occasions. In addition, the full sample was relatively small ( N = 111), so only a minimal set of parameters could be accurately estimated. In this first model we proposed that there was a level of latent change scores (symbolized as Δ y [ t ] here) that occurred with regularity (Δ t = 5 years here) and were separated from the measurement uniquenesss ( u [ t ]). We then suggested that the source of the latent changes were two-part: (α) some of the variation in latent changes came from a constant latent slope ( G {1}), and (β) some of the variation in latent changes came from the immediately prior latent score ( y [ t − 1]). Very few parameters were considered here, resulting in an exponential shape for each function, but this LCS-LGM fit each univariate score at least as well as our previous latent basis LCMs.
A path diagram of a latent change score (LCS) model with incomplete data (from McArdle &Hamagami, 2006 ).
This result gave us some courage to fit a bivariate dynamic model where there are two sequences of latent changes, as depicted in Figure 15.16 . There is no doubt that this bivariate model requires more explanation and elaboration ( see McArdle, 2001 , 2009 ; Ferrer & McArdle, 2010 ), but it can be seen now as a model for the observed scores in terms of a combination of latent sources. The latent change in any variable is a three-part function: (α y ) some of the variation in latent changes came from a constant latent slope G {1}, (β y ) some of the variation in latent changes came from the immediately prior latent score ( y [ t − 1]), and (γ yx ) some of the variation in latent change came from the other variables prior latent score ( x [ t − 1]). We concluded that this effect was indeed in the predicted direction (M —> → V) and not the other way around.
The Future of Structural Equation Modeling
Up to this point, we have tried to set the stage in the need for SEM analysis, but we have been careful not to actually fit any SEMs to real data nor have we tried to explain why we need to make specific analytic choices. For interested readers, there are many good examples of SEM in the growing literature (e.g., Ferrer-Caja, Crawford, & Bryan, 2002 ; Widaman, 1985 ; King, King, & Foy, 1996 ; Kline, 2011 ). We can also say that a few novel SEM ideas were published in the context of a real problem in “Latent Variable Analysis of Age Trends in Tests of Cognitive Ability in the Health and Retirement Survey, 1992–2004” by McArdle, Fisher, & Kadlec (2007 ). As we quickly found, aspects of the available theory and available data led us to use very different SEMs than those that seem prominent in this literature.
A bivariate LCS model (from McArdle & Hamagami, 2006 )
There are so many contemporary statistical models that can be said to have a SEM form and can be fitted using SEM software, it is often best to say that SEM is an idea rather than a technique . In the terms used here, SEM is a concept rather than a tool. We know that one first necessary feature of SEM is of the requirement for clear model specification. When we write out a SEM—any SEM—we really need to know what we are thinking. If we do not have any thoughts, then we do not have any SEM either. This exerciseis very useful when creating simulations for demonstrations (as done here), for evaluation of software, or even for Monte Carlo simulation of complex mathematical-statistical issues. The SEM approach offers a level of precision not readily available to other approaches, and this is one reason it is so widely heralded. However, when it comes to actual data analysis, as shown here, the use of SEM does not always improve our understanding beyond that found by simpler and more standard methods ( see McArdle, 1994 ). So perhaps SEM is best thought of as a theoretical tool rather than a practical tool .
On the other hand, the SEM tools have become rather elegant. Perhaps it is now obvious that either CALIS or Mplus or OpenMx or any of the other programs in Table 15.1 can be used for SEM analyses, and it will not really matter which program we choose. As we have tried to show, the philosophy of CALIS and OpenMx, as with LISREL and others, is that the analyst must ask directly for the parameters of a specific SEM. This was a simple approach adopted by the original programmers of LISREL, and some of this rigidity is now changing. For example, in contrast to CALIS/LISREL and other programs, the working philosophy of Mplus/AMOS and other programs seems to be that the complexity of SEM should be simplified to the point where we do not have to know exactly what we are doing to make this SEM work. If we wanted to base all our analysis strictly around path diagrams, and avoid most algebra, then AMOS ( Wothke, 2000 ) can be a most useful tool. In some cases, we may worry that these elegant SEM tools are falling into the hands of people who do not know what they are doing— But then we should ask ourselves, who should be the judge here? The verdict is not yet out on many important issues about who will carry out the best data analysis and who will find the most result. These are intriguing questions.
So why do we use SEM at all? Well, it is not true that we use SEM because of the path diagrams—many traditional models can be represented using path diagrams, and we really do not need to use SEM tools to use SEM concepts. Also, some of the standard multivariate tools are now very easy to use. But there are three very good reasons why we use SEM for data analysis:
We use SEM because we have a priori ideas that we want to examine in real data, and some of these ideas are well beyond ANOVA and the so-called GLM framework. We would certainly like to know if we are wrong about these ideas, so we can appreciate much of the ongoing work on SEM estimators, statistical indices, and overall goodness-of-fit indices.
We use SEM because we want to consider the inclusion of unobserved variables in our models—that is, LV s . We often think about LVs in our theories, variables that are not directly measured or measurable, and we want to represent them in our models of these theories. In this sense, the inclusion of LV is for clarity, not for obscurity. It is also clear that the accurate representation of observed variable distributions may require more complex measurement models than the typical normality assumptions.
We use SEM because we would like to select the “true” or “correct” model, or at least an “adequate” model, for a set of data. We believe we can tell we have found an adequate model when we estimate parameters that do not differ with different samplings of person or variables or occasions—that is, the parameters are invariant . In the terms of linear regression, we do not always want the model with the highest explained variance for the current set of data, but we do want the model that is most likely to replicate over and over again.
Researchers who are interested in more details on SEM can consider the Technical Appendix that follows. Because these three goals listed above seem reasonable and continue to be part of most behavioral science research, SEM combined as both a concept and a tool is now very popular, and multivariate data analysis is likely to remain this way for a long time to come.
Technical Appendix: Algebraic Notes and Computer Programs for the SEMs Presented
1. reconsidering simple linear regression.
We often presume that one of the variables (X) is an input to the other (Y) and we repeat the common linear regression expression
Here, E is the symbol used for the expected values (rather than the usual greek summation symbol). This combined expression is considered the “structural equation model for the observed scores,” and a diagram of this simple model was presented earlier as Figure 15.8 .
The same general approach can be used to examine the intercept term (β 0 ). To estimate the intercept parameter at the same time as the regression coefficient, we need to include a constant term in the model (as a measured predictor, 1, drawn here as a constant triangle). It follows that we need to include this constant into the model of expectations, but this is easily done by augmenting the covariance matrix with a mean vector ( μ ) or by estimating the model from the average cross-products matrices ( Σ ; see Table 15.8; Rao, 1958 ). This approach using means and covariances together forms the basis of many complex models being used today ( see McArdle, 1994 , 2007 , 2009 ).
A simple way to create the required expected covariance matrix is to write out the SEM in terms of three RAM matrices. That is: (1) the unit elements of the F matrix means that the row variable (in the data) and column variable (of the model) should be assigned the same label; (2) the non-zero elements of the A matrix represents one-headed arrows where the row variable is input to the column variable; and (3) the non-zero elements of the Ω, matrix represents two-headed arrows where the row variable is connected to the column variable. These relations are used to form all model expectations ( μ and Σ ).
In using RAM notation, we first write the score vectors for all observed and unobserved variables using these definitions, which in this particular model can be listed as
Then we can write the three matrices of relationships among these variables as
This was not presented earlier, but this kind of specification in matrix form leads to the basic calculation of the resulting expected value matrix S??g???g measured ????????? g
?????g????????g????g????? and covariances (see McArdle, 2005 ). This is not always easy to understand from a matrix point of view, but it does allow us to use the path diagrams more effectively. From the resulting expressions we find that the model expected value of the covariance of Y and X is where Y and X intersect in this matrix (at column 1, row 2), and from our model we know this observed value (σ yx ) is supposedly composed of the regression weight (β) multiplied by the variance of X ( σ x 2 ) . Perhaps it is also easy to see that these matrices are simply descriptions of the path graphic display. All other models described below follow the same RAM matrix logic so they will not be presented in detail.
Of course, for simplicity, we can eliminate the intercept (β 0 ) and the constant (1) and just calculate a single linear regression coefficient (β 1 ) to describe this X → Y relationship. By assuming that the residuals are independent ( E [X, e ] = 0) of the predictors, we know (from calculus) that the best estimate of this coefficient can be found by multiplying the observed covariance of Y and X (σ yx ) by the inverse of the variance of X ( σ xx -1 ) , which in algebraic terms is the same as equations 2a and 2b . In simple regression, we can see exactly why this works by writing the structural expectations of the model in the matrix equation for the X. This matrix expression is considered the “structural equation model of the observed covariances.”
Furthermore, from this expression we can see that the model expected value of the covariance of Y and X, where Y and X intersect in this matrix (at column 1, row 2), and from our model we know this observed value (σ yx ) is supposedly composed of the regression weight (β) multiplied by the variance of X ( σ x 2 ) . Thus, if we simply divide by the variance of Y by the variance of X we will have the desired regression coefficient. If we assume the correct model is one of the ones presented in Figures 15.3 – 15.7 but we fit a standard regression model like Figure 15.2 we obtain the biases previously described.
2. An Example of Structural Equation Model Fitting
One good way to check the SEM computer programming is to create a fictictio us data set and then have the SEM program estimate the population values. This is accomplished here using a simple latent path model to illustrate the use of three different kinds of computer programs: (1) SEM in SAS-CALIS ( SAS Institute, 2006 ); (2) SEM in Mplus ( Muthen & Muthen, 2002 ); and (3) SEM in OpenMx ( Boker, 2011 ).
The three SEM programs were chosen for illustration because they are now widely used but seem to represent extremes of the philosophy of SEM techniques. That is, CALIS is one of the programs (like the early versions of LISREL and the current OpenMX) that requires the user to specify all model parameters to be fitted, so very few defaults are invoked, and there is extensive documentation of the techniques. The second program, Mplus (like AMOS or the current version of LISREL), requires the minimal amount of information about the model parameters, many defaults are invoked (including identification), and very little documentation of the techniques is available. The third program, OpenMx, is much like the first program in input style, but this program is completely free. The choice of which program to choose depends on the prior SEM experience of the user. Of course, any other SEM programs ( see Table 15.1 ) could be used instead. In addition to generating the data, each program allows a first set of analyses to be designed to see if we can recover SEM values using any statistical technique.
Let us assume a LV path model can be written for a set of six observed variables ( Y ( m ), m = 1 to 6) with two potentially correlated predictors (X(1) and X(2)). Here we assume a latent variable path model can be written for observed variables (Y) with two observed predictors (X). The general model might be written as
If we further assume the M λ(1,2,3) = 0.6,λ(4,5,6) = 0.4, andβ(1) = 0.2 β(2) = 0.2 then we have model thsn can be rewritten as
and this model appears back in Figure 15.10 .
This model can be placed in RAM notation by writing the score vectors for all observed and unobserved variables as
Then we can write the matrices of relationships as
Whereby using formula 1e above leads to the direct calculation of the structural model of covariances (later listed in Table 15.A1 ).
Let us next assume the values of A (1,2,3) = 0.6, A.(4, 5, 6) = 0.4, and/3(1)g = 0.0 and/S(2) = 0.8. In this factor with odel we have one six indicators, one measured variable (X(1)) that is unrelated to the common factor and one measured variable that does produce it (X(2)). The values chosen are relatively small (λ(1) = 0.6, λ(4) = 0.4, β(1) = 0.0, and β(2) = 0.8) and the additional variance terms, created so all residual variables in the population have zero means and unit variances, make the net effects even smaller. This places numerical values on the parameters of Figure 15.10 , and this is drawn in Figure 15.A1 , and it often called a multiple indicator multiple causes (MIMIC) model ( Hauser & Goldberger, 1971 ).
Details of the required computer program are presented in the Technical Appendix, and just a few details are presented here. Random score vectors that are consistent with this specific model may be created using many different computer programs (e.g., we present code for the SAS DATA step here using RANNOR functions in Table 15.A2 ). The output is generated by specific line of code, and it is a summary description of the simulated data in terms of means, standard deviations, and correlations, created from each program for N = 1,001 entities
A“population” latent path model useful for CALIS programming.
(another program option). Here, we have included the actual factor score created (F_score) as well as one of the many empirical estimates of this factor score (the F_aver).
Fitting the Simulated MIMIC Data With Standard Modeling Software
The three program outputs (Table 15.A3 – 15.A5 ) also show the results of scripts for running a few conventional analyses on these simulated data. For example, we might run a simple factor analysis of the six observed outcomes (Y; e.g., using SASFACTOR). The output of this analysis is presented in Table 15.A3 . Using the traditional Scree Plot and the Scree logic ( Cattell, 1978 ), we clearly find one dominant factor. Using the Maximum-Likelihood Factor extraction technique ( see Lawley & Maxwell, 1971 ), we obtain a test statistic that shows the zero-common factor model is a poor fit to the data (χ 2 = 860 on df = 15, p (perfect fit) < 0.0001), but the one-factor model is much better (χ 2 = 21 on df = 9, p (perfect fit) > 0.01), and the resulting difference in fit resulting from inclusion of 6 loadings was worthwhile ( d χ 2 = 839 on df = 6, p (no difference) < 0.0001). The ML estimated factor loadings Λ = [0.61, 0.60, 0.60, 0.46, 0.49, 0.47] are very close to the true values Λ = [0.6,0.6,0.6,0.4,0.4,0.4], and we would probably not be misled in any way. Of course, this is the correct model for the data so this seems appropriate. Obviously this standard approach to factor analysis seems very reasonable for these simple latent path scores, using either the Scree or ML approach.
After this, we might run a linear regression where one of the most reliable outcomes, the average of the Y ( m ), is regressed on the two input Xs. This results ( see Output Tables 15.A4 – 15.A5 ) in a significant overall F ( p < 0.0001), β(0) = 0.04 (ns), β(1) = 0.04 (ns), and β(2) = 0.39 ( p < 0.0001), with an R 2 = 0.26. This was obviously considered significant (i.e., accurately different than zero) because of the large sample size (N = 1001). In this case, the regression model is largely correct, but we know the true regression values should be β(0) = 0.0, β(1) = 0.0, and β(2) = λ(1) ∗ β3(2) = 0.6 ∗ 0.8 = 0.46, and the explained variance of the true factor scores is only R 2 = 0.8 2 /(0.8 2 + 1) = 0.19. Thus, the linear regression has done what it can to get the correct answer using the approximate solution because the average is presumed to account for all the unreliability – and it does not.
The next likely analysis is a canonical regression of the relationships between all Y(m ) variables and the two X input variables. The output (Table 15.A5 ) shows a significant canonical F = 29 on df b = 12 and df w = 1996, so p < 0.0001, and the canonical R 2 = 0.27, so this is close to the true R f 2 = 0 .19 , but it is somewhat inflated. However, the second canonical component is not significant ( p > 0.40) so only the first component would be considered in actual research. The canonical correlations between the six Y variables and the two X variable estimates the canonical weights as β = [−0.005,−1.000], correctly pointing to the second X variable as the source of this component. The canonical loadings on the Y side are estimated as Λ = [0.74, 0.71, 0.65, 0.52, 0.49, 0.63], rather than the true values of Λ = [0.6, 0.6,0.6, 0.4,0.4, 0.4], so these estimates are also slightly inflated. However, an this is impotant, the general canonical regression conclusion seems correct—there is at least one small but significant relationship between the X(2) and the Y(6) variables, and the first three outcomes (especially Y(1)) are more related to the relationship than the last three outcomes (the least of which is Y(5)).
Fitting the Simulated MIMIC Data With SEM-CALIS
These simulated data in SAS can be directly compared with the model expectations. Input (Table 15.A6 ) includes two SAS-CALIS script for a first SEM analyses we might carry out on the observed data. In the first script, LVP#0, we only consider the data from the now observed variables (Y(1)–Y(6) and X(1) and X(2)) and we use the RAM notation option of SAS-CALIS. In this option, the variables are assumed to be numbered by their entry in the observed data, so observed variables (1–8) are augmented with any LVs (numbers > 8). In this case, we have only described one new variable (number 9) and this is the common factor ( F ). Although it is possible to enter another LV for the disturbance term ( d , termed 10), for simplicity we do not need to do so here.
After doing this, each non-zero entry in the matrix is listed in terms of: (1) Matrix Number (or number of arrowheads), (2) Row Number (output variable), (3) Column Number (input variable), (4) Start Value (best guess), and (5) an optional label for the “free” parameters. This is done in order here for the first elements, which can be understood as “Matrix = 1, Row = 1, Column = 9, value = 0.6, fixed (resulting from no label),” indicating that LV 9 is input to manifest variable 1. The next five entries are similar, giving the specific fixed values of the factor loadings, and the seventh entry is understood as “Matrix = 1, Row = 9, Column = 7, Value = 0.0,” indicating that manifest variable 7 is input to LV 9 with a starting value = 0.0. The two-headed arrows of the model ( see Figure 15.10 ) are first entered as elements that can be understood as “Matrix = 2, Row = 1, Column = 1, value = 1, termed U12,” This is done to indicate that manifest variable 1 is connected to manifest variable 1 with a two-headed arrow termed “U12” that can be estimated from the data. The next five entries are similar, giving the starting values of the unique variances (Ψ{ m ) 2 ) at unity, but the seventh entry is understood as “Matrix = 2, Row = 9, Column = 9, Value = 1, Label = “Vd,” indicating that latent variable 9 has a variance term, and the last entry indicates LV 9 has a variance term. Figure 15.A1 can be termed a “compact diagram” because the unique factors have not been displayed. Any LVs that have fixed loadings of 1 and only produce one other outcome need not be put in the path diagram (e.g., McArdle, 2005 ), or in the program, so we only include their parameters. The “;” followed by the “RUN;” command is used to initiate the calculation.
The first output of the SAS-CALIS program gives a thorough description of the input model, input data, and iterative steps, but all this output is not included here. Instead, we simply jump to the results of goodness-of-fit listed in another section of the output (see Table 15.A7 ). It seems SAS-CALIS calculates and lists almost every statistical index ever invented (with the exception of some in Horn & McArdle, 1992 ). Without being hypercritical, we need to pick the indices of importance to our own work. In our SEM experience, the only indices that matter are: (1) the χ 2 = 38. (as usual, no decimal places are needed); (2) the dfs = 26; (3) the p (perfect) > 0.063; (4) the ε a = 0.021; and (5) the p (close fit) = 0.999, so this model fits the data very well. Incidentally, from the first two indices (and the N ), we can derive all other indices on the output.
The estimates from the model are presented in Table 15.A8 . Some of the other estimates are the unique variance terms ψ 2 = [0.67,0.64,0.60,0.86,0.94,0.90], and these are very close to the true values of ψ 2 = [0.64, 0.64,0.64,0.84,0.84, 0.84]. The other terms are related to the Xs, and the program seems to eliminate the X(1) score (it does not) because we have set β(1) = 0, while it keeps X(2) because it is related at β(2) = 0.8. The other two terms are the variance of the predictor (estimated σ x 2 = 1 .02 vs. true σ x 2 = 1 .00 ) and variance of the disturbance (estimated ϕ d 2 = 0 .89 vs. true ϕ d 2 = 0 .96 ). The standardized estimates (the estimates as if all variables have unit variance) are also listed, but these are less relevant here.
We can see that this Confirmatory LVP or MIMIC model fits these data very well indeed. This, of course, should be no surprise, because we generated the data in exactly this way. This result merely shows the SAS programming worked correctly. This confirmatory latent path model assumes we have the correct pattern hypothesis, as well as all oneheaded arrows. That is, we are not willing to let the algorithm optimize all parameter estimates. Incidentally, the reason we fixed the one-headed arrows in LVP #0 was that under some specific forms of sampling of persons, these parameters should be invariant ( see Meredith, 1964 , 1993 ; Meredith & Horn, 2001 ). On the other hand, the two-headed arrows would typically depend on the sample selection mechanism. In essence, the LVP #0 is exactly the kind of a priori model we hope to achieve, although this is certainly rare (i.e., more typically, only the structural pattern would count). The fact that the program recovered the correct answer for LVP #0 shows that the SAS-CALIS program actually works!
Next we try to fit this model to these data using other optional forms. In the other input of Table 15.A8 we use the same correct structural pattern, but now we try to estimate all but one of the true factor loadings (i.e., fixing λ(1) = 1) and the factor regression (done by supplying labels for all one-headed arrows). The output of this LVP #1 is the typical way models are fit to data. The output shows the fit is excellent (i.e., χ 2 =18., df s = 20, p (perfect) > 0.59, the ε a = 0.000, and p (close fit) = 1.000.) The estimated values in this output show how the ratio of the loadings is largely preserved by the estimates (λ 1 /λ 2 = 1/0.97 instead of 0.6/0.6, λ 1 /λ 4 = 1/0.69 instead of 0.6/0.4, and λ 4 /λ 5 = 0.69/0.67 instead of 0.4/0.4). This means SAS-CALIS worked again, even when we did not use a complete a priori specification of the loadings and regression in advance.
The next line of input scripts (LVP#2 and LVP#3 of Table 15.A9 ) are a bit different, because here we explicitly restrict the β(1) = 0 ( see line 8). This, of course, is the way we generated the data so this model should fit without failure, and it fits about the same as the prior model (χ 2 57. dfs =19, p (perfect) < 0.01, the ε a = 0.08, and p (close fit) = 0.36). In the next script we explicitly restrict the β(2) = 0 ( see line 8). This of course, is not the way we generated the data so this model should not fit so well anymore. The shows a χ 2 = 157., df s = 19, p (perfect) < 0.0001, the ε a = 0.18, and p (close fit) = 0.001. The same output also shows the other model parameters are affected but not by very much. As we know from many studies of statistical power (e.g., Satorra & Saris, 1985 ), the inclusion of small sized effects can only be found in large-scale data sets.
There are many other models that could be fit to these data, and we encourage the reader to try some of these. Another set of input scripts can be made which differ from the other SEMs in one important respect—here we include only variables Y(1), Y(2), Y(3), and X(2). That is, four potentially measured variables Y(4), Y(5), Y(6) and X(1) can been eliminated from the SAS-CALIS input. The question we can try to answer here is will the correct values emerge from the analysis even though all the variables are not used? The answer should be yes, because we have left just enough variables in the data to identify the model, but we are not sure how SAS-CALIS will like this selection of variables. As we find looking at the output (this can be tried by the reader), SAS-CALIS finds this to be a very reasonable model for the data (χ 2 = 1.0, dfs = 2, p (perfect) > 0.7, ε a = 0.02, and p (close fit) = 0.96). In addition, this shows the values for the parameters estimated are reasonable (λ 1 /λ 4 = 1.0/0.7 vs. 0.6/0.4) with the key β = 0.15 ( t > 5). These are not completely incorrect, but we must recognize that the LVP model has lost a lot of df s, because of seemingly missing observed scores, and the precision is lowered. This illustrates an important SEM message—if you want to pick up small effects and patterns in data, then you need large samples of variables and persons.
Fitting the Simulated MIMIC Data With SEM-Mplus
The same points made above will be illustrated using the Mplus computer program ( Muthen & Muthen, 2002 ). One way to write an Mplus script for the LVP model appears (Table 15.A10 ). The TITLE line and the DATA line have several options, and the previous data could been output from SAS simulation (of Input 1)to a text file (“sim_lvp1.dat”) with space delimiters and read back into Mplus. However, here we do not illustrate an option in the Mplus program that allows us to generate the data usingstatistical simulation—MONTECARLO. We could only ask for one replication (REP = 1) but we could ask for many more (i.e., REP = 101). In essence, the Mplus program allows the user to create repetitions of data based on one model and then fit the same or a different model to these data. The VARIABLE line allows names for all the variables in the file (and USEVAR is used to select the ones to consider in the model). Of course, we are not using many other useful features of Mplus (e.g., CATEGORICAL, MISSING). The ANALYSIS line is used to describe the kind of analysis required (TYPE = MEANSTRUCTURE). Once again, we are not using many other analytic features of Mplus (MISSING, CLUSTER, MIXTURE, etc.). The “MODEL POPULATION:” command allows us to create any population model we would like to examine. This allows us to add a LV to the model simply by creating a new name (F_score) that is not among the VARIABLES, and the BY statement (f_score BY Y1–Y3 0.6 Y4–Y6 * 0.6;) indicates that this is a common factor formed from the three Y( m ) variables but with different loadings. The second line asks for the addition of a regression of the “f_score ON X1 * 0.0 X2 * 0.8;” so this is the designation of the βs in the MIMIC model. The “MODEL:” command is a request to fit a specific model to the simulated data, and here we fit essentially the population model without fixed values (i.e., MODEL: f_score ON Y1–Y3; f_score ON X1 X2;), but this is not necessary. Any alternative could be attempted at this point. The OUPUT line allows many other options.
The first result from Mplus is simply a description of our data and main analysis problem. This output starts with a listing of the summary statistics calculated by Mplus from the raw data, and these are equivalent to the SAS results (in Table 15.A11 ). This result also gives an indication that the iterations have converged (so the model is identified), and the results are nearly equivalent to SAS-CALIS (χ 2 = 19, dfs = 19, p (perfect) > 0.8, the ε a = 0.000, and p (close fit) = 1.000). The estimated values in Table 15.A9 show how the ratio of the loadings is again largely preserved by the estimates (λ 1 /λ 2 = 1/.97 instead of 0.6/0.6), and here the Mplus program automatically created the required identification constraint (λ 1 = 1) and freely estimated the other parameters. The MLE, its standard error (S.E.), their ratio ( z -value), and a two-tailed p (non-zero) are all listed for every parameter. To get at some population values, Table 15.A9 may be useful, and here we see the standardized effect of the observed X on the unobserved F is β = 0.26 with R 2 = (1 − 0.932) = 0.068. Of course, this means Mplus worked even when we did not use an a priori specification of the loadings and regression in advance, and when we did not have to include all the indicators of the common factor.
Without any doubt, the Mplus program is easy to use, and many researchers find this to be the most critical feature ( see Maydeau-Olivares, 2000 ). In addition, Mplus now allows users to easily: (1) access advanced measurement techniques, such as dealing with measurement models such as ordinal, nominal, censored, and Poisson-based responses; (2) access advanced statistical techniques, such as sampling weights, multiple-group SEM, two-level SEM with a cluster structure, latent mixture SEMs of a general variety; (3) the direct inclusion of survival analyses with base-hazards and time-censoring; (4) the estimation of missing data using either maximum likelihood or multiple imputation; and (5) the use of Monte Carlo generation of simulated data uses the same input language. Among the most common complaints about Mplus are: (6) the default options do not always make the best sense (i.e., why correlate all LVs?); (7) there are few options for data input—that is, we can only use short names (up to 8 characters), we cannot read input data directly from standard statistical software, and we cannot read past a pre-specified number of columns; (8) user input errors are often hard to track down (i.e., it does not point to a line number in a script); and (9) as with many other SEM programs, Mplus does not allow Path Diagrams for input and/or output. All these problems can be overcome and they probably will be altered in future versions.
Fitting the Simulated MIMIC Data With R Code – OpenMx, lavaan, sem
The OpenMx program ( see Boker, 2011 ) is relatively new and seems largely untried, but it is also the only general SEM program that is entirely free to use ( see Table 15.1 ). This program has many different options, including the flexible use of matrix algebra and fitting functions. The example given here ( see Appendix Table 15.A12 ) is identical in every respect to the MIMIC model presented above, so it is not surprising that the SEM results are equivalent as well.
The first thing we will do is generate the data using the built in R “rnorm(1)” functions. Each line in the code generates a vector of scores, and these are placed together into a matrix of data (termed “MIMICData”). These data were defined to have the same features as the simulation previously described and we expect to find no differences – and we do not!
The second phase of the analysis is to try to fit a SEM to their data using the new “OpenMx” commands, and to do this we need to load the OpenMx library onto our machine (using “require(OpenMx)”). The “mxModel” command is used to accumulate the model components, and here we use another option (type = “RAM”) to invoke the use of RAM notation. From this point, we can then define each path coefficient in a SEM using the “mxPath” command/Each mxPath command line requires a simple statement of: (1) a “from” and “to” variable number or label; (2) the type of “arrowhead” proposed (1 or 2 headed); (3) whether the parameter is free of fixed (free = false); (4) the numerical value (a starting value or a fixed non-zero value); and (5) and optional label (useful if the parameter is equivalent to another). This “list of parameters” approach is the same as what has been used in PROC CALIS and is based on the list notation of McArdle (1988 , 2005 ; McArdle & Boker, 1990 ). Table 15.A12 Mplus Output for LVP #3 Open in new tab Table 15.A13 R Input for LVP Open in new tab
As stated before, this OpenMx program offers many other options, and some of the best have not been used here. But the fact that it can incorporate the RAM list means it will also work for SEMs of any complexity ( see McArdle, 2005 ). For this and other reasons, this program is worthwhile investigating further. The exact same sentiment applies to at least two other R code programs for SEM — the lavaan package (Rosell, 2012) and the sem package ( Fox, 2006 ). Both programs are free and easy to use, and these are worthy of further consideration.
Author Note
John J. McArdle, PhD, Senior Professor, Department of Psychology, University of Southern California.
Please send any written correspondence to SGM 711, 3620 S. McClintock Ave., University of Southern California, Los Angeles, CA 90089.
Funding for this chapter was provided by the National Institute on Aging (Grant # AG–07137–22).
Anderson, T.W. ( 1957 ). An Introduction to Multivariate Statistical Analysis . New York: Wiley.
Google Scholar
Google Preview
Blalock, H.M. (Ed., 1985 a). Causal Models in the Social Sciences , 2nd Ed. New York: Aldine.
Blalock, H.M. (Ed., 1985 b). Causal Models in Panel and Experimental Designs . New York: Aldine.
Bock, R. D. ( 1975 ). Multivariate statistical methods in behavioral research . New York: McGraw-Hill.
Boker, S.M. (2011). The OpenMx Project. See http://openmx.psyc.virginia.edu/about-openmx-project (as of November 2012).
Browne, M. W. & Cudeck, R. ( 1993 ). Alternative ways of assessing model fit. In: Bollen, K. A. & Long, J. S. (Eds.) Testing Structural Equation Models . (pp. 136–162). Beverly Hills, CA: Sage.
Browne, M. W. & Nesselroade, J. R. ( 2005 ). Representing psychological processes with dynamic factor models: Some promising uses and extensions of ARMA time series models. In A. Maydeau-Olivares & J. J. McArdle (Eds.), Advances in psychometrics: A festschrift to Roderick P. McDonald (pp. 415–451). Mahwah, NJ: Erlbaum.
Bryk, A. S. , & Raudenbush, S. W. ( 1992 ). Hierarchical linear models: Applications and data analysis methods . Newbury Park, CA: Sage.
Cattell, R.B. ( 1978 ). The Scientific Use of Factor Analysis in Behavioral and Life Sciences . New York: Plenum.
Cochran, W.G. & Cox, G.M. ( 1950 ). Experimental Design (2nd Ed.). New York: Wiley
Cole, D. A. , & Maxwell, S. E. ( 2003 ). Testing mediational models with longitudinal data: Questions and tips in the use of structural equation modeling. Journal of Abnormal Psychology, 112, 558–577.
Duncan, O.D. ( 1975 ). Introduction to Structural Equation Models . New York: Academic Press.
Dyson, F. ( 1997 ). Imagined Worlds . Cambridge, MA: Harvard University Press.
Ferrer, E. , Hamagami, F. , & McArdle, J.J. ( 2004 ). Modeling latent growth curves with incomplete data using different types of structural equation modeling and multilevel software. Structural Equation Modeling, 11 (3), 452–483.
Ferrer, E. & McArdle, J.J. ( 2010 ). Longitudinal Modeling of Developmental Changes in Psychological Research. Current Directions in Psychological Science, 19 (3), 149–154.
Ferrer-Caja, E. , Crawford, J.R. , & Bryan, J. ( 2002 ). A structural modeling examination of the executive decline hypothesis of cognitive aging through reanalysis. Aging, Neuropsychology, and Cognition, 9, 231–249.
Fisher, R.A. ( 1918 ). The correlation between relatives on the supposition of Mendelian inheritance. Transactions of the Royal Society of Edinburgh, 52, 399–433.
Fisher, R.A. ( 1924 ). The Conditions Under Which χ 2 Measures the Discrepancy Between Observation and Hypothesis. Journal of the Royal Statistical Society, 87, 442–450.
Fox, J. ( 1997 ). Applied Regression Analysis, Linear Models, and Related Methods . Thousand Oaks, CA: Sage.
Fox, J. ( 2006 ). Structural equation modeling with the sem packagein R. Structural Equation Modeling, 13 (3), 465–486.
Ghisletta, P. & McArdle, J.J. ( 2012 ). Latent Curve Models and Latent Change Score Models Estimated in R. Structural Equation Modeling, 19, 651–682.
Goldberger, A.S. ( 1973 ). Structural Equation Models: An Overview. In A.S. Goldberger & O.D. Duncan , (Eds.) Structural Equation Models in the Social Sciences . (pp. 1–18) New York: Seminar Press.
Goldberger, A.S. & Duncan, O.D. ( 1973 ). Structural Equation Models in the Social Sciences . New York: Seminar Press.
Grimm, K.J. & McArdle, J.J. ( 2005 ). A Note on the Computer Generation of Mean and Covariance Expectations in Latent Growth Curve Analysis. In F. Danserau & F.J. Yammarino (Ed.), Multi-level issues in strategy and Methods . (pp. 335–364). New York: Elsiver.
Hauser, R.M. & Goldberger, A.S. ( 1971 ). The treatment of unobservables in path analysis. In H.L. Costner (Ed.). Sociological Methodology . (pp. 81–117). San Francisco, CA: Jossey-Bass.
Hauser, R.M. , Tsai, S-L. , & Sewell, W.H. ( 1983 ). A model of stratification with response error in social and psychological variables. Sociology of Education, 56, 20–46.
Heise, D.R. ( 1975 ). Causal Analysis . New York: Wiley.
Horn, J.L. & McArdle, J.J. ( 1980 ). Perspectives on Mathematical and Statistical Model Building (MASMOB) in Research on Aging. In L. Poon (Ed.), Aging in the 1980’s: Psychological Issues . Washington, D. C.: American Psychological Association, 503 541.
Horn, J.L. & McArdle, J.J. ( 1992 ). A practical guide to measurement invariance in aging research. Experimental Aging Research, 18 (3), 117–144.
Horn, J. L. & McArdle, J.J. ( 2007 ). Understanding human intelligence since Spearman. In R. Cudeck & R. MacCallum , (Eds.). Factor Analysis at 100 years (pp. 205–247). Mahwah, NJ: Lawrence Erlbaum Associates.
Hsiao ( 2003 ). Analysis of panel data. Second edition. Cambridge, UK: Cambridge University Press.
Joreskog, K. & Sorbom, D. ( 1979 ). Advances in Factor Analysis and Structural Equation Models . Cambridge, MA; Abt Books.
Joreskog, K.G. ( 1969 ). A general approach to confirmatory maximum likelihood factor analysis. Psychometrika, 34, 183–202.
Joreskog, K.G. ( 1973 ). A general method for estimating a linear structural equation system. In A.S. Goldberger & O.D. Duncan , (Eds.) Structural Equation Models in the Social Sciences . (pp. 85–112). New York: Seminar Press.
King, D.W. , King, L.A. , Foy, D.W. ( 1996 ). Prewar factors in combat-related posttraumatic stress disorder: Structural equation modeling with a national sample of female and male Vietnam veterans. J. Consulting and Clinical Psychology, 64 (3), 520–531.
Kline, R.B. ( 2011 ). Principles and Practice of Structural Equation Modeling (3rd Ed). New York: Guilford Press.
Lawley, D.N. & Maxwell, A.E. ( 1971 ). Factor Analysis as a Statistical Method (2nd Ed.). London: Butterworths.
Lee, S-Y. ( 2007 ). Structural Equation Modeling: A Bayesian Approach . New York: Wiley.
Li, C.C. ( 1975 ). Path Analysis: A Primer . Pacific Grove, CA: Boxwood Press.
Loehlin, John C . ( 2004 ). Latent Variable Models: An Introduction to Factor, Path, and Structural Analysis (4th Ed.) Mahwah, NJ: Lawrence Erlbaum Associates.
Maydeau-Olivares, A. ( 2000 ). Review of Mplus. Multivariate Behavioral Research, 35 (4), 501–515.
McArdle, J.J. ( 1986 ). Latent variable growth within behavior genetic models. Behavior Genetics, 16 (1), 163–200.
McArdle, J.J. ( 1988 ). Dynamic but structural equation modeling of repeated measures data. In J.R. Nesselroade & R.B. Cattell (Eds.), The Handbook of Multivariate Experimental Psychology, Volume 2 . New York, Plenum Press, 561–614.
McArdle, J.J. ( 1994 ). Structural factor analysis experiments with incomplete data. Multivariate Behavioral Research, 29 (4), 409–454.
McArdle, J.J. ( 2001 ). A latent difference score approach to longitudinal dynamic structural analysis. In R. Cudeck , S. du Toit , & D. Sorbom (Eds.). Structural Equation Modeling: Present and future . (pp. 342–80). Lincolnwood, IL: Scientific Software International.
McArdle, J.J. ( 2005 ). The development of RAM rules for latent variable structural equation modeling. In A. Maydeau & J.J. McArdle , (Eds.). Contemporary Advances in Psychometrics . (pp. 225–273). Mahwah, NJ: Lawrence Erlbaum Associates.
McArdle, J.J. ( 2007 ). Five Steps in the Structural Factor Analysis of Longitudinal Data. In R. Cudeck & R. MacCallum , (Eds.). Factor Analysis at 100 years . (pp. 99–130). Mahwah, NJ: Lawrence Erlbaum Associates.
McArdle, J.J. ( 2009 ). Latent variable modeling of longitudinal data. Annual Review of Psychology, 60, 577–605.
McArdle, J.J. ( 2010 ). Some Ethical Issues in Factor Analysis. In A. Panter & S. Sterber (Eds.) Quantitative Methodology Viewed through an Ethical Lens . (pp. 313–339). Washington, DC: American Psychological Association Press.
McArdle, J.J. & Aber, M.S. ( 1990 ). Patterns of change within latent variable structural equation modeling. In A. von Eye (Eds.). New Statistical Methods in Developmental Research (pp. 151–224). New York: Academic Press.
McArdle, J.J. & Anderson, E. ( 1990 ). Latent variable growth models for research on aging. In J.E. Birren & K.W. Schaie (Eds.), The Handbook of the Psychology of Aging . (pp. 21–43). New York: Plenum Press.
McArdle, J.J. & Boker, S. M. ( 1990 ). RAMpath: A Computer Program for Automatic Path Diagrams . Hillsdale, NJ: Lawrence Erlbaum Publishers.
McArdle, J.J. & Cattell, R.B. ( 1994 ). Structural equation models of factorial invariance in parallel proportional profiles and oblique confactor problems. Multivariate Behavioral Research, 29 (1), 63–113.
McArdle, J.J. & Epstein, D.B. ( 1987 ). Latent growth curves within developmental structural equation models. Child Development, 58(1), 110–133.
McArdle, J.J. , Fisher, G.G. & Kadlec, K.M. ( 2007 ). Latent Variable Analysis of Age Trends in Tests of Cognitive Ability in the Health and Retirement Survey, 1992–2004. Psychology and Aging, 22(3), 525–545.
McArdle, J.J. , Grimm, K. , Hamagami, F. , Bowles, R. , & Meredith, W. ( 2009 ). Modeling Life-Span Growth Curves of Cognition Using Longitudinal Data With Multiple Samples and Changing Scales of Measurement. Psychological Methods, 14(2), 126–149.
McArdle, J.J. & Hamagami, F. ( 1991 ). Modeling incomplete longitudinal and cross-sectional data using latent growth structural models. In L. Collins & J.L. Horn (Eds.), Best Methods for the Analysis of Change (pp. 276 304). Washington, D.C.: APA Press.
McArdle, J.J. & Hamagami, F. ( 1996 ). Multilevel models from a multiple group structural equation perspective. In G. Marcoulides & R. Schumacker (Eds.), Advanced Structural Equation Modeling Techniques . (pp. 89–124). Hillsdale, NJ: Erlbaum.
McArdle, J.J. & Hamagami, F. ( 2003 ). Structural equation models for evaluating dynamic concepts within longitudinal twin analyses. Behavior Genetics, 33(2), 137–159.
McArdle, J.J. & Hamagami, F. ( 2006 ). Longitudinal tests of dynamic hypotheses on intellectual abilities measured over sixty years. In C. Bergeman & S.M. Boker (Eds.), Methodological Issues in Aging Research . (pp. 43–98). Mahwah: Erlbaum.
McArdle, J.J. , Johnson, R.C. , Hishinuma, E.S. , Myamoto, R.H. , & Andrade, N. ( 2001 ). Structural equation modeling of group differences in CES-D ratings of native Hawaiian and non-native Hawaiian high school students. Journal of Adolescent Research 16 (2), 108–149.
McArdle, J.J. & McDonald, R.P. ( 1984 ). Some algebraic properties of the Reticular Action Model for moment structures. British Journal of Mathematical and Statistical Psychology, 37, 234–251.
McArdle, J.J. & Nesselroade, J.R. ( 1994 ). Using multivariate data to structure developmental change. In S.H. Cohen & H.W. Reese (Eds.), Life Span Developmental Psychology: Methodological Innovations . (pp. 223–267). Hillsdale, NJ: Erlbaum.
McArdle, J.J. & Prescott, C.A. ( 1992 ). Age based construct validation using structural equation models. Experimental Aging Research, 18(3), 87–115.
McArdle, J.J. & Prindle, J.J. ( 2008 ). A latent change score analysis of a randomized clinical trial in reasoning training. Psychology and Aging, 23 (4), 702–719.
McArdle, J.J. & Woodcock, J.R. ( 1997 ). Expanding test rest designs to include developmental time lag components. Psychological Methods, 2 (4), 403–435.
McDonald, R.P. ( 1980 ). A simple comprehensive model for the analysis of covariance structures. British Journal of Mathematical and Statistical Psychology, 31, 161–183.
McDonald, R.P. ( 1985 ). Factor Analysis and Related Methods . Hillsdale, NJ: Erlbaum.
McDonald, R.P. ( 1999 ). Test Theory: A Unified Treatment . Mahwah, NJ: Erlbaum.
Meredith, W. ( 1964 ). Notes on factorial invariance. Psychometrika, 29, 177–185.
Meredith, W. ( 1993 ). Measurement invariance, factor analysis and factorial invariance. Psychometrika, 58, 525–543.
Meredith, W. , & Horn, J. ( 2001 ). The role of factorial invariance in modeling growth and change. In A. Sayer & L. Collins (Eds.), New methods for the analysis of change (pp. 203–240). Washington, DC: American Psychological Association.
Meredith, W. , & Tisak, J. ( 1990 ). Latent curve analysis. Psychometrika, 55, 107–22.
Muller, K.E. & Stewart, P.W. ( 2006 ). Linear Model Theory: Univariate, Multivariate, and Mixed Models . New York: Wiley.
Muthén, L.K. & Muthén, B.O. ( 2002 ). Mplus, the comprehensive modeling program for applied researchers user’s guide . Los Angeles, CA: Muthén & Muthén.
Nesselroade, J.R. & Baltes, P.B. ( 1984 ). From traditional factor analysis to structural-causal modeling in developmental research. In. V. Sarris & A. Parducci (Eds.). Perspectives in Psychological experimentation . (pp. 267–287). Hillsdale, NJ: Erlbaum
Ntzoufras, I. ( 2009 ). Bayesian Modeling Using WinBUGS . New York: Wiley.
O’Brien, R. G. & Kaiser, M.K. ( 1985 ). MANOVA Method for Analyzing Repeated Measures Designs: An Extensive Primer. Psychological Bulletin, 97 (2), 316–333.
Pearl, J. ( 2000 ). Causality: models, reasoning, and inference . Cambridge University Press.
Popper, K. ( 1970 ). Normal Science and its Dangers, In Lakatos, I. & Musgrave, A. (Eds.) Criticism and the Growth of Knowledge (pp. 51–58). Cambridge: Cambridge University Press.
Prindle, J. J. & McArdle, J.J. ( 2012 ). An Examination of Statistical Power in Multigroup Dynamic Structural Equation Models. Structural Equation Modeling, 19 (3) 351–372.
Rabe-Hesketh, S. & Skrondal, A. ( 2006 ). Multilevel and longitudinal modeling using Stata , 2nd Ed. College Station, Texas: Stats Press.
Rao, C. R. ( 1958 ). Some statistical methods for the comparison of growth curves. Biometrics, 14, 1–17.
Rao, C.R. ( 1965 ). Linear statistical inferences and its applications. New York: Wiley.
Roseell, Y. ( 2012 ). lavaan: an R package for structural equation modelling and more . Unpublished Manuscript, Department of Data analysis, Ghent University, Belguim.
SAS Institute (2006). PROC CALIS -> http://support.sas.com/documentation/cdl/en/statug/63347/HTML/default/viewer.htm#statug_calis_sect021.htm (as of November 2012).
Satorra, A. , & Saris, W. E. ( 1985 ). Power of the likelihood ratio test in covariance structure analysis. Psychometrika, 50, 83–90.
Shrout, P. ( 2010 ). Integrating causal analysis into psychopathol-ogy research. In Shrout, P.E. Keyes, K.M. & Ornstein, K. (Eds.). Causality and Psychopathology: Finding the Determinants of Disorders and Their Cures . (pp. 3–24). New York: Oxford University Press.
Singer, J.D. & Willett, J. B. ( 2003 ). Applied Longitudinal Data Analysis: Modeling Change and Event Occurrence . New York: Oxford University Press.
Sobel, M. ( 1995 ). Causal inference in the behavioral sciences. In Arminger, G. , Clogg, C.C. & Sobel, M.E. (Eds.). Handbook of Statistical Modeling for the Social and Behavioral Sciences . (pp. 1–38). New York: Plenum Press.
Spearman, C. ( 1904 ). “General intelligence” objectively determined and measured. American Journal of Psychology, 15, 201–293.
Stapleton, L.M. & Leite, W.L. ( 2005 ). Teacher’s Corner: A Review of Syllabi for a Sample of Structural Equation Modeling Courses. Structural Equation Modeling: A Multidisciplinary Journal, 12 (4), 642–664.
Sörbom, D. ( 1979 ). An alternative to the methodology for analysis of covariance. Psychometrika, 43, 381–396.
Tabachnick, B. G. , & Fidell, L. S. ( 2007 ). Using Multivariate Statistics , 5th ed. Boston: Allyn and Bacon.
Tucker, L.R ( 1958 ). Determination of parameters of a functional relation by factor analysis. Psychometrika, 23, 19–23.
Walls, T.A. & Schaffer, J.L. (Eds., 2006 ). Models for intensive longitudinal data . New York: Oxford University Press.
Werts, C.E. & Linn, R.L. ( 1970 ). Path analysis: Psychological examples. Psychological Bulletin, 74, 193–212.
Wechsler, D. ( 1981 ). WAIS-R: Wechsler Adult Intelligence Scale Revised . New York: Harcourt, Brace & Jovanovich.
Widaman, K. F. ( 1985 ). Hierarchically nested covariance structure models for multitrait- multimethod data. Applied Psychological Measurement, 9, 1–26.
Wiley, D. ( 1973 ). The identification problem for structural equation models with unmeasured variables. In A.S. Goldberger & O.D. Duncan , (Eds.) Structural Equation Models in the Social Sciences . (pp. 69–84). New York: Seminar Press.
Wishart, J. ( 1938 ). Growth rate determination in nutrition studies with the bacon pig, and their analyses. Biometrika, 30, 16–28.
Wright, S. ( 1918 ). On the Nature of Size Factors. Genetics 3, 367–374.
Wright, S. ( 1934 ). The Method of Path Coefficients. Annals of Mathematical Statistics 5, 161–215.
Wothke, W. ( 2000 ) Longitudinal and multi-group modeling with missing data. In T.D. Little , K.U. Schnabel , & J. Baumert (Eds.) Modeling longitudinal and multiple group data: Practical issues, applied approaches and specific examples . Mahwah, NJ: Lawrence Erlbaum Associates.
- About Oxford Academic
- Publish journals with us
- University press partners
- What we publish
- New features
- Open access
- Institutional account management
- Rights and permissions
- Get help with access
- Accessibility
- Advertising
- Media enquiries
- Oxford University Press
- Oxford Languages
- University of Oxford
Oxford University Press is a department of the University of Oxford. It furthers the University's objective of excellence in research, scholarship, and education by publishing worldwide
- Copyright © 2024 Oxford University Press
- Cookie settings
- Cookie policy
- Privacy policy
- Legal notice
This Feature Is Available To Subscribers Only
Sign In or Create an Account
This PDF is available to Subscribers Only
For full access to this pdf, sign in to an existing account, or purchase an annual subscription.
Academia.edu no longer supports Internet Explorer.
To browse Academia.edu and the wider internet faster and more securely, please take a few seconds to upgrade your browser .
Enter the email address you signed up with and we'll email you a reset link.
- We're Hiring!
- Help Center
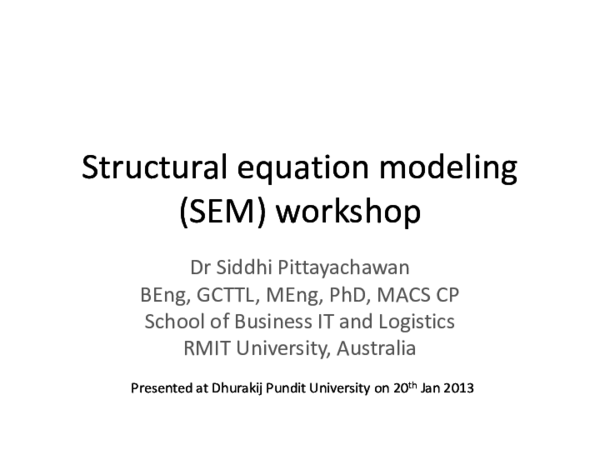
Structural equation modeling (SEM) workshop

Related Papers
Dr. P C Lai
This paper contributes to the existing literature by reviewing the research methodology and relevant literature with the focus on potential applications for the novelty technology of the single platform E-payment. The review includes subjects, populations, sample size requirement, data collection methods and measurement of variables, pilot study and statistical techniques for data analysis used in past researches. The review will shed some light and potential applications for future researchers, students and others to conceptualize operationalize and analyze the underlying research methodology in the development of their research for novelty technology.
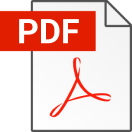
Educational Measurement: Issues and Practice
Dejan Dragan, Prof., PhD.
The paper addresses an introduction to the structural equation modeling (SEM), the insight into the methodology, and the importance of this statistical technique for practical applications. SEM is a very powerful statistical modeling tool, which incorporates the measurements models and the path models into the comprehensive covariance structure analysis framework. Herein, the exploratory analysis (EFA) and the confirmatory factor analysis (CFA) are usually employed as the intermediate stages of the modeling design. The main intent of this work is to inform the interesting readers about all the potentials, which can be conducted when use this technique. For encouraging the interested researchers, who are new in this field, the main steps and characteristics of SEM methodology are briefly presented. A short overview of applications based on this advanced statistical methodology is also provided, with emphasis on supply chain (SC) management applications, which study the impact of integration between individual players on the SC performance. An investigaton of Port Economics applications based on SEM is also inspected in this work.
David Gefen , Detmar Straub
Applications of Structural Equation Modeling in Social Sciences Research
JACKSON DECARVALHO
Structural equation modeling (SEM) is a comprehensive statistical modeling tool for analyzing multivariate data involving complex relationships between and among variables (Hoyle, 1995). SEM surpasses traditional regression models by including multiple independent and dependent variables to test associated hypothesizes about relationships among observed and latent variables. SEM explain why results occur while reducing misleading results by submitting all variables in the model to measurement error or uncontrolled variation of the measured variables. The purpose of this article is to provide basic knowledge of structural equation modeling methodology for testing relationships between indicator variables and latent constructs where SEM is the analysis technique of the research statistical design. It is noteworthy, SEM provides a way to test the specified set of relationships among observed and latent variables as a whole, and allow theory testing even when experiments are not possible. Consequently, these methodological approaches have become ubiquitous in the scientific research process of all disciplines.
Structural Equation Modeling
Anne Boomsma
Communications of the Association for Information Systems
Damon Campbell
Dr. Ramachandran Manickam
Structural equation modeling is many dimensions are a statistic is the technique of analysis, which is structural Used to analyze relationships. This technique includes factor analysis and multiple regression analysis and Is a combination of measured variables hidden constructions. Structural equations specify how the set of variables are interrelated based on linear equations, cause and effect (cause models) or paths through statistically (path analysis) sorted networks. Structural Equation Modeling (SEM) is a quantitative research technique that integrates standard methods. SEM is often used for research, rather than to explore or explain an event a research study is designed to verify the design. Structural Equation Modeling (SEM) is standard A quantity that integrates methods Is the research technique. Used show causal relationships between SEM variables. The relationships shown in the SEM refer to the researchers' hypotheses. In general, these relationships cannot be statis...
Wan Mohamad Asyraf Wan Afthanorhan
Academicians, researchers, as well as postgraduate students are developing theories concerning the relationships among certain hypothetical constructs. They are modeling their theorized relationships with the intention to test their theoretical model with the empirical data from the field. The example of a Theoretical Framework is given in Figure A. Figure A: The Schematic Diagram Showing the Theoretical Framework of a Study. The schematic diagram in Figure A is converted into Amos Graphic and analyzed using empirical data. In Amos Graphic, the rectangles represent the directly observed variables while the ellipses represent the unobserved variables or latent constructs. The schematic diagram of theoretical framework in Figure A is converted into Amos Graphic as shown in Figure B. The schematic diagram of the model for the study is developed based on debates in theory and literature. One needs to come out with a theoretical framework for the study.
ELANGOVAN N Christ (Deemed to be University)
The application of Structural Equation Modeling (SEM) in the business research is growing. The second-generation multivariate data analysis technique, SEM is easy to use and provides a high quality statistical analysis. Many visual SEM software programs help in a quick design of the theoretical model and to modify them graphically using simple drawing tools. Further it can estimate the model’s fit, make any modifications and arrive at a final valid model. It is essential to understand how to design the research process appropriate to the SEM analysis. This article describes the steps in SEM analysis, the conventions used in presenting a model, the elements of the SEM technique, the interpretation of the SEM results. The rule of thumb that evaluates the results and the other issues in SEM reporting are discussed.
Loading Preview
Sorry, preview is currently unavailable. You can download the paper by clicking the button above.
RELATED PAPERS
Journal of Medicinal Plants
Step In Colege
Dushyant Stepincollege
Allen Kardec Feitosa Oliveira
Radomir G . Zekavica
Annals of Public and Cooperative Economics
Michel Arcand
costituzionalismo.it
Gianluca Bascherini
José Henrique Barreto
Journal of Biological Sciences
Rodrigo Garrido
Jurnal Analis Kebijakan
bani adi darma
International Journal of Computer Information Systems and Industrial Management Applications
Ramanuj Lal
Rosalba Lucia Chávez Alcántara
Les mines et la métallurgie en Gaule et dans les provinces voisines, (dir.) R. Chevallier: 275-280. Paris: Errance
Maurizio Rossi
Importance of Visual communication in behavior change intentions
Anaekwe Chinaza
Horizontes Antropológicos
LUCIANO OLIVEIRA
European Journal of Gastroenterology & Hepatology
Journal of Chemical Information and Computer Sciences
Chaya Duraiswami
Boston毕业证文凭波士顿大学本科毕业证书 美国学历学位认证如何办理
Archives of Biological Sciences
romeo dobrin
Communications in agricultural and applied biological sciences
miriam Keikotlhaile
V. 16, n. 03
Revista Quaestio Iuris , Daniela Serra Castilhos
Philip Sisson
Journal of Neuroscience Methods
Jose-Manuel Alonso
Turkish Journal of Thoracic and Cardiovascular Surgery
Huseyin Melek
RELATED TOPICS
- We're Hiring!
- Help Center
- Find new research papers in:
- Health Sciences
- Earth Sciences
- Cognitive Science
- Mathematics
- Computer Science
- Academia ©2024
Thank you for visiting nature.com. You are using a browser version with limited support for CSS. To obtain the best experience, we recommend you use a more up to date browser (or turn off compatibility mode in Internet Explorer). In the meantime, to ensure continued support, we are displaying the site without styles and JavaScript.
- View all journals
- My Account Login
- Explore content
- About the journal
- Publish with us
- Sign up for alerts
- Open access
- Published: 08 May 2024
Structural basis and synergism of ATP and Na + activation in bacterial K + uptake system KtrAB
- Wesley Tien Chiang ORCID: orcid.org/0009-0008-0352-0315 1 na1 ,
- Yao-Kai Chang ORCID: orcid.org/0000-0003-0314-3165 2 na1 ,
- Wei-Han Hui 3 ,
- Shu-Wei Chang 3 , 4 ,
- Chen-Yi Liao 1 ,
- Yi-Chuan Chang 1 ,
- Chun-Jung Chen ORCID: orcid.org/0000-0002-5157-4288 5 ,
- Wei-Chen Wang ORCID: orcid.org/0009-0008-1682-9821 6 ,
- Chien-Chen Lai ORCID: orcid.org/0000-0002-7133-8266 6 , 7 ,
- Chun-Hsiung Wang 2 ,
- Siou-Ying Luo 2 ,
- Ya-Ping Huang 2 ,
- Shan-Ho Chou 1 ,
- Tzyy-Leng Horng 8 ,
- Ming-Hon Hou 9 ,
- Stephen P. Muench ORCID: orcid.org/0000-0001-6869-4414 10 ,
- Ren-Shiang Chen ORCID: orcid.org/0000-0001-6703-9864 11 ,
- Ming-Daw Tsai ORCID: orcid.org/0000-0003-1374-0414 2 , 12 &
- Nien-Jen Hu ORCID: orcid.org/0000-0002-0917-0677 1 , 13
Nature Communications volume 15 , Article number: 3850 ( 2024 ) Cite this article
2 Altmetric
Metrics details
- Cryoelectron microscopy
- Ion channels
- Membrane structure and assembly
The K + uptake system KtrAB is essential for bacterial survival in low K + environments. The activity of KtrAB is regulated by nucleotides and Na + . Previous studies proposed a putative gating mechanism of KtrB regulated by KtrA upon binding to ATP or ADP. However, how Na + activates KtrAB and the Na + binding site remain unknown. Here we present the cryo-EM structures of ATP- and ADP-bound KtrAB from Bacillus subtilis (BsKtrAB) both solved at 2.8 Å. A cryo-EM density at the intra-dimer interface of ATP-KtrA was identified as Na + , as supported by X-ray crystallography and ICP-MS. Thermostability assays and functional studies demonstrated that Na + binding stabilizes the ATP-bound BsKtrAB complex and enhances its K + flux activity. Comparing ATP- and ADP-BsKtrAB structures suggests that BsKtrB Arg417 and Phe91 serve as a channel gate. The synergism of ATP and Na + in activating BsKtrAB is likely applicable to Na + -activated K + channels in central nervous system.
Introduction
Bacteria can be found in a wide variety of habitats with fluctuating salinity, pH and temperature. Sophisticated machineries are therefore essential for bacteria to achieve an immediate physiological adjustment in order to survive in such variable environments 1 . Osmoadaptation is generally regulated by fine-tuning the concentrations of intracellular potassium ions and osmolytes in bacterial cells 2 , where TrkAH and KtrAB systems are responsible for the efficient uptake of K + ions in response to the osmoshock 3 , 4 . Both systems share a common protein quaternary structure: transmembrane subunits responsible for K + permeation, and regulatory subunits located in the cytosol, forming a ring-like structure for association with the transmembrane subunits. The regulatory subunits, TrkA and KtrA, which are referred to as regulator of K + conductance (RCK) proteins, can bind to signaling molecules in the cytosol and control the gating of the transmembrane pores, TrkH and KtrB, respectively. The KtrAB system is of particular interest due to its variety of binding ligands, such as NADH 5 , ATP and ADP 6 , 7 , c-di-AMP 8 , 9 , 10 , Ca 2+ and Mg 2+ 11 , and Na + 12 , 13 , 14 . However, the molecular mechanisms of these regulatory ligands toward KtrAB are not fully understood.
The KtrAB system is composed of the transmembrane KtrB dimer, each containing four structurally similar domains (D1~D4) (Fig. 1a and Supplementary Fig. 1 ) arranged around a four-fold pseudosymmetry axis normal to the cell membrane, and the regulatory KtrA octamer (tetramer of homodimer, Fig. 1a ) with a ring-like structure, known as the RCK gating ring. ATP binding to KtrA octamer activates K + flux activity of KtrB, whereas ADP binding inactivates it 6 , 7 , 15 . Crystal structures of ATP- and ADP-bound KtrA from Bacillus subtilis (BsKtrA) show a four-fold symmetric square-like ring and a two-fold symmetric diamond-like ring, respectively 7 . The crystal structure of BsKtrAB complex demonstrates that the square-like octameric ring of ATP-BsKtrA imposes a steric hindrance on the D1M2b helix of BsKtrB, forming a helical hairpin interacting with the BsKtrA octameric ring 7 . The cryo-EM structure of ADP-bound KtrAB from Vibrio alginolyticus (VaKtrAB) indicates that the D1M2b region is extended as a continuous helix penetrating into the diamond-like VaKtrA octameric ring 16 . The extended α-helix of D1M2b restricting the passage of K + was proposed to be a critical structural component in the gating of the VaKtrB pore 16 . Additionally, it was speculated that the intramembrane loop, a 15-amino-acid-long segment in the middle of the D3M2 helix protruding into the pore cavity under the selectivity filter, may also play a critical role in gating 17 , 18 . However, high-resolution structures of both ATP- and ADP-bound KtrAB complexes, preferably from a single bacterial species, are required for establishing the ligand-regulated gating mechanism at the atomic level.
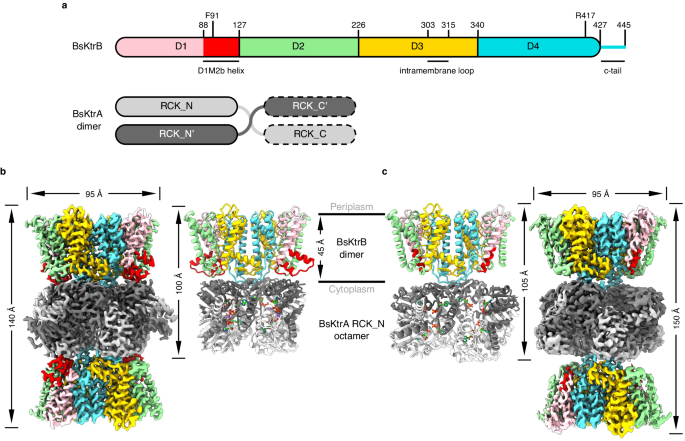
a The schematic representation of BsKtrB and BsKtrA. BsKtrB is colored by domains with the color scheme: D1 (pink), D2 (green), D3 (yellow) and D4 (cyan). The D1M2b helix in D1 domain of BsKtrB is highlighted in red. The intramembrane loop in D3 domain (303–315) is displayed. The essential residues (F91 and R417) involved in gating are indicated. Each subunit of a BsKtrA homodimer, colored in dark and light gray contains an RCK_N subdomain, a crossover helix and an RCK_C subdomain. The latter is shown with dashed line because the cryo-EM maps were not clearly resolved. b , c Cryo-EM density maps contoured with a threshold of 4.5 σ (showing the KtrB 2 A 8 B 2 arrangement). The structural models of ( b ) ATP-BsKtrAB (Structure II) and ( c ) ADP-BsKtrAB (structure III) are presented as cartoon and colored with the same color code as in ( a ). The partially built models of the BsKtrA RCK_C subdomains in ( b ) (Structure II) were not shown for clarity. The ATPs in ( b ) and ADPs in ( c ) are shown as lime sticks, and the Na + ions ( b ) are shown as purple spheres.
Furthermore, Na + ions have been suggested to play a role in the activation of the KtrAB complex 12 , 19 . In vivo observations implicated a sophisticated gating mechanism using Na + as the activator for K + influx, because high intracellular concentration of Na + disrupted many physiological functions 20 , and activation of K + uptake was a critical response to counteract the harmful effects caused by excessive Na + . The Na + -dependent activation of K + flux was also observed in Na + -activated K + (K Na ) channels, encoded by Slo2.1 and Slo2.2 , in the central nervous system 21 , 22 , 23 , which are essential to prevent the overload of depolarizing Na + . Dysfunction of K Na channels is associated with many neuropathological disorders 24 , 25 . K Na is a homotetrameric channel covalently linked to the RCK domain. The cryo-EM structures of chicken Slo2.2 at closed and open states have been reported 26 , 27 , and the Na + binding sites of human Slo2.2 have just been determined 28 . Interestingly, the Na + -dependent activation of K Na channels can be stimulated by binding of NAD + , presumably at the RCK domain 29 , implicating a synergy between Na + and nucleotides in activation of K + influx. However, little is known about the mechanism of activation from the structural perspective.
In this study, we uncover the Na + -binding site in ATP-bound BsKtrAB using cryo-EM and X-ray crystallography and demonstrate a synergistic activation mechanism of BsKtrAB involving both ATP and Na + . We elucidate the structural components responsible for K + gating by comparing the high-resolution cryo-EM structures of ATP- and ADP-bound BsKtrAB from a single bacterial species and proposed a comprehensive channel gating mechanism. These findings shed light on the K + uptake in response to Na + by coupling the ligand-induced conformational change of the RCK domain to the pore gating, and may provide a mechanistic framework for K Na channels.
Na + binding site in ATP-BsKtrAB and ATP-BsKtrA
As noted in earlier crystallographic studies, an electron density blob was observed in the intra-dimer interface of KtrA in the ATP-BsKtrAB complex, and Mg 2+ was assigned at this site 7 , 11 . We first tried to validate the assignment of Mg 2+ by solving the cryo-EM structures of ATP-BsKtrAB in the previously published buffer condition 2 with the addition of 2 mM Mg 2+ (Structure I) (Supplementary Fig. 2 ), and with the Mg 2+ removed by adding 2 mM EDTA and 1 mM EGTA (Structure II) (Fig. 1b ). The datasets were processed and refined to 2.5 Å and 2.8 Å, respectively (Supplementary Fig. 3 , 4 and Supplementary Table 1 ). We also solved the cryo-EM structure of ADP-BsKtrAB at the resolution 2.8 Å (Structure III) (Fig. 1c , Supplementary Fig. 5 and Supplementary Table 1 ). As previously observed, BsKtrAB complexes reveal a non-physiological quaternary structure of KtrB 2 A 8 B 2 assembly due to the identical interface for KtrB dimer binding on both sides of KtrA octameric ring 7 , 15 , 16 . The models were not completely built in BsKtrA RCK_C subdomain because the cryo-EM density maps were not clearly resolved in this region (Supplementary Table 2 ). Mg 2+ -added and Mg 2+ -free ATP-BsKtrAB cryo-EM structures (Structure I and Structure II) are virtually identical with an r.m.s.d. of 0.30 Å over 2104 C α atoms (Supplementary Fig. 6a ). Comparing our cryo-EM structures with previously published crystal structures, both ATP-BsKtrAB cryo-EM structures (Structure I and Structure II) are similar to the crystal structure of ATP-BsKtrAB 7 (PDB ID 4J7C ) (Supplementary Fig. 6b ), while ADP-BsKtrAB cryo-EM structure (Structure III) is structurally comparable to the low-resolution crystals structure of ADP-BsKtrA ΔC B 15 (PDB ID 5BUT ) (Supplementary Fig. 6c ).
ATP and ADP share the same binding site located at the intra-dimer interface of KtrA RCK_N subdomains 5 , 7 (Fig. 2a, b ), and the ligands can be unambiguously assigned in our cryo-EM structures (Fig. 2c, d ). A clear region of unassigned cryo-EM density between the two adjacent ATPs at the intra-dimer interface was observed in Structure I (Supplementary Fig. 7a ). Interestingly, the density at this site was still discernable in Structure II, despite the presence of chelators for divalent ions (Fig. 2c, e ). The density at the intra-dimer interface of BsKtrA (Structure II) appears to be right on the plane of the horizontal C2 symmetry axis parallel to the membrane, along the BsKtrA dimer interface (Supplementary Fig. 8a ). To further confirm the density, we reconstructed a map using the same dataset with a C2 symmetry axis oriented perpendicularly to the membrane bilayer at the BsKtrB dimer interface, and refined at a final resolution of 2.9 Å (Structure IIa) (Supplementary Fig. 8b–e and Supplementary Table 1 ). Additionally, a map without imposing symmetry (C1) was also reconstructed and refined to 3.0 Å (Structure IIb) (Supplementary Fig. 8f–i and Supplementary Table 1 ). The maps processed with the vertical C2 symmetry axis or C1 symmetry showed a visible density comparable to the original map with the horizontal C2 symmetry axis (Supplementary Fig. 9 ). Because the models (Structures II, IIa and IIb) revealed no obvious change in this region, we herein used Structure II for the following structural analysis and discussion due to the better resolution.
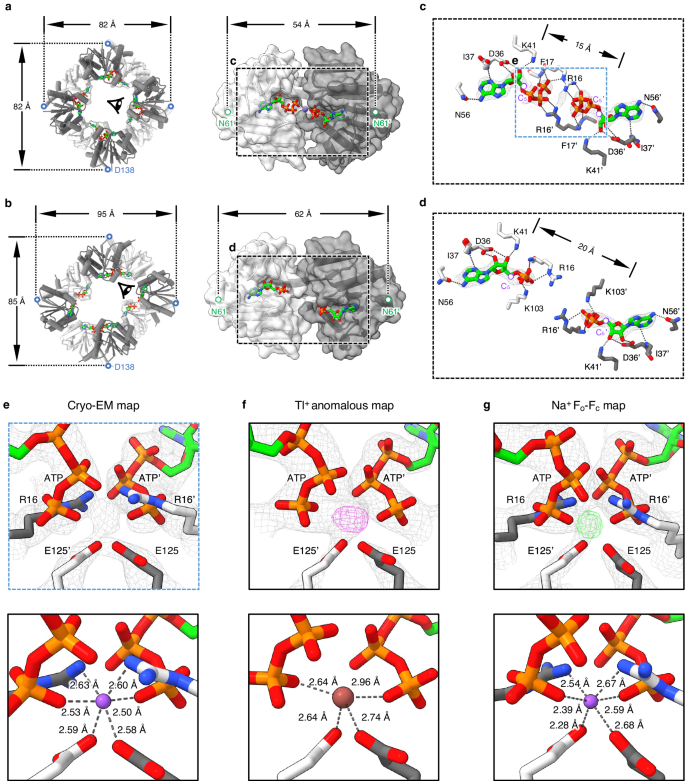
a , b Left panels, BsKtrA octameric rings in cylinder representation at ( a ) ATP- and ( b ) ADP-bound states from ATP-BsKtrAB (Structure II) and ADP-BsKtrAB (Structure III), respectively, with ATP and ADP shown in stick representation. The protomers of each BsKtrA dimer are colored in light and dark gray with semi-transparent surface and cartoon backbone representation. The RCK_C subdomains are omitted for clarity. Right panels, close-up views of ( a ) ATP- and ( b ) ADP-BsKtrA dimers from the perspective as indicated by the eye symbols shown in the left panels. The C α atoms of Asp138 and Asn61 are shown as blue and lime dots, respectively, with the double-head arrows indicating the distances between designated atoms. c , d Close-up views of the detailed ( c ) ATP- and ( d ) ADP-binding sites at the intra-dimer interface of BsKtrA magnified from the dashed boxes defined in ( a ) and ( b ), respectively. The cryo-EM densities of the nucleotides are contoured at 10 σ. The ribose C 5 atoms of the two bound ( c ) ATP or ( d ) ADP molecules are shown in purple dots with the respective distances indicated. e – g Upper panels, close-up views of the intra-dimer interface of ATP-BsKtrA from ( e ) the cryo-EM structure (Structure II), ( f ) the crystal structure of Tl + pretreated ATP-BsKtrA in the presence of 150 mM KCl, and ( g ) the crystal structure of Tl + pretreated ATP-BsKtrA in the presence of 150 mM Na + . The gray mesh represents the cryo-EM density map contoured at 7.0 σ in ( e ) and 2F o -F c electron density maps contoured at 2.3 σ in ( f ) and 2.0 σ in ( g ). The coordinating amino acid side chains and ATP γ-phosphates are shown in stick representation. The anomalous difference density map of Tl + (magenta mesh) in ( f ) is contoured at 5.0 σ, but the anomalous difference density cannot be observed in ( g ) even at the contoured level of 4.0 σ. The F o -F c omit map (green mesh) in ( g ) is contoured at 5.0 σ. Lower panels, coordination geometries of Na + (purple spheres) in ( e ) and ( g ) and Tl + (brown sphere) in ( f ) after structure refinement are depicted as dashed lines with distances indicated.
As previous studies have demonstrated that the activity of KtrAB is Na + -activated 12 , 19 , and the sample contained 70 mM Na + in the protein buffer, we suspected that Na + ions may bind at this site. Structural refinement of Na + at the site (Structure II) revealed an octahedral coordination geometry with the distances ranging from 2.50 Å to 2.63 Å (Fig. 2e ), which are within the range of Na + coordination distance of 2.13 Å to 2.76 Å 30 . The assigned Na + is coordinated by the carboxylate oxygens of Glu125 residues and the oxygens in the γ-phosphates of ATPs from both BsKtrA protomers in the intra-dimer interface. The side chains of Arg16 residues reveal an energetically unfavorable conformation pointing to Na + in the close vicinity. Nevertheless, the repulsive force would be compensated by the γ-phosphates of both ATPs and the carboxylate groups of both Glu125 residues. It is worth noting that the protein sample also contained 30 mM K + . Refinement of K + at the assigned site showed an identical coordination geometry with the same coordinating atoms as Na + with the distances ranging from 2.81 Å to 2.89 Å (Supplementary Fig. 7b ).
To scrutinize the unambiguity of the cation at the binding site, we co-crystallized BsKtrA with 2 mM thallium acetate in the presence of 150 mM K + and performed anomalous scattering experiments at its absorption edge (0.975 Å, 12,712 eV). Tl + provides a good mimic for K + due to a similar ionic radius 31 . However, anomalous scattering of Tl + was also utilized to identify Na + -binding sites in the glutamate transporter Glt Ph and the Na + /H + antiporter PaNhaP 32 , 33 . Surprisingly, the anomalous density peak representing Tl + is localized at the same site of the intra-dimer interface (Fig. 2f and Supplementary Table 3 ). We then performed a competition test by replacing K + in the BsKtrA protein buffer with 150 mM of Na + . The anomalous peak was diminished with a clear density peak at the site in the F o -F c omit map (Fig. 2g and Supplementary Table 3 ), supporting that Na + is the preferred ion to occupy the binding site at the intra-dimer interface of ATP-BsKtrA.
The ICP-MS analysis demonstrated that the Na + content of ATP-BsKtrA is higher than apo-BsKtrA, further indicating that Na + binding to BsKtrA is ATP-dependent (Supplementary Table 4 ). The molar ratio of specific ATP-associated Na + to BsKtrA is 0.48:1, approximately in agreement with the stoichiometric relationship of one Na + ion in an ATP-BsKtrA dimer. Furthermore, K + content was lower in either apo- or ATP-BsKtrA and no significant difference was shown between the two samples, suggesting that the site favors the binding of Na + .
Synergistic effects of Na + and ATP on BsKtrA
To investigate the possible functions of Na + binding to BsKtrA, we systematically characterized protein stability toward Na + and other cations. Based on the structural information, the cation could stabilize the ATP binding and thus the complex. We first investigated the stability of ATP-BsKtrA in the presence of Na + using urea-induced unfolding by monitoring the intrinsic tryptophan fluorescence 34 . BsKtrA was prepared in K + Buffer and Na + Buffer (containing 150 mM of either cation) with the titration against Na + and K + , respectively (see Methods for details). The midpoint urea unfolding concentration (C m ) of ATP-BsKtrA was found to be increased in a [Na]-dependent manner, in contrast to little or no effect with apo-BsKtrA and ADP-BsKtrA against Na + (Fig. 3a , Supplementary Fig. 10 ). It is noted that ATP-BsKtrA in K + Buffer revealed a C m at ~2 M (Fig. 3a , 0 mM Na + titration), but intriguingly, while prepared in Na + Buffer, ATP-BsKtrA showed a much higher C m at ~4.5 M (Fig. 3b , 0 mM K + titration) and titration against K + caused little effects on C m , implicating ATP-BsKtrA in the absence of Na + (K + Buffer) is more conformationally unstable, and, furthermore, K + cannot compete in the binding site of ATP-BsKtrA against Na + .
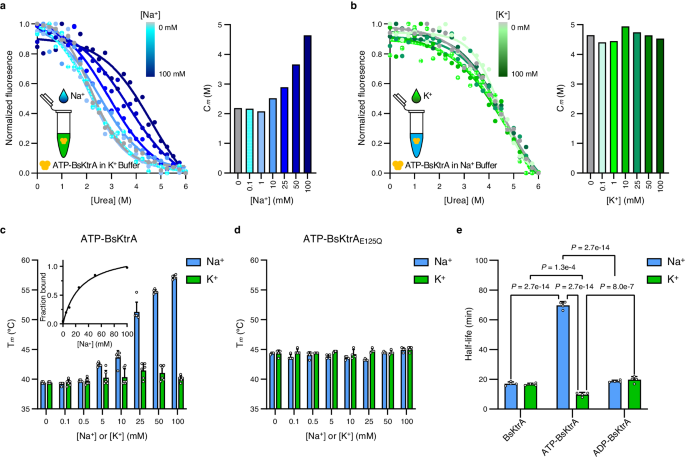
a , b Left panels: intrinsic tryptophan fluorescence-based urea unfolding assays of ATP-BsKtrA in ( a ) K + Buffer titrated against NaCl and in ( b ) Na + Buffer titrated against KCl, respectively. Right panels: the C m values plotted against [Na + ] and [K + ], respectively. The C m values were calculated by fitting the data to a sigmoidal 4PL model using GraphPad Prism. Refer to Supplementary Fig. 10a, b for original fluorescence data. c , d Differential scanning fluorimetry (DSF) assays demonstrating the T m of ( c ) ATP-BsKtrA and ( d ) ATP-BsKtrA E125Q titrated with NaCl (blue bars) and KCl (green bars). Data represent the mean ± s.d. with ( c ) n = 6 and ( d ) n = 4 independent experimental replicates. Inset c , the apparent dissociation constant ( K dapp ) of Na + binding with ATP-BsKtrA. The K dapp was analyzed by fitting the fraction bound derived from the DSF assays against the concentrations of NaCl to a one-site model using GraphPad Prism. Refer to Supplementary Fig. 11a, b for raw data. e Half-life constants of BsKtrA in the presence of Na + (blue bars) and K + (green bars). The half-life constants were determined by GraphPad Prism using exponential one-phase decay model. Data represent the mean ± s.d.; n = 4 independent experimental replicates. Statistical analyses were performed using two-way ANOVA. Refer to Supplementary Fig. 12 for raw data. Source data for a – e are provided as a Source Data file.
To individually characterize the effects of Na + and K + on the thermostability of ATP-bound BsKtrA, we prepared protein samples in Choline Buffer and performed differential scanning fluorimetry (DSF) 35 . The T m of ATP-BsKtrA showed a [Na + ]-dependent increase from 39 °C in the absence of Na + to 57 °C at 100 mM Na + . The apparent Na + -binding affinity ( K dapp ) was determined to be 27.5 ± 1.6 mM, while no thermostability effect was observed for K + ions on ATP-BsKtrA (Fig. 3c and Supplementary Fig. 11a ). Furthermore, both Na + and K + revealed no effect on apo-BsKtrA and ADP-BsKtrA (Supplementary Fig. 11c–f ). Substitution of BsKtrA Glu125, which is involved in the coordination with Na + (Fig. 2 ), with glutamine (E125Q) abolished the [Na + ]-dependent increase of T m while BsKtrA E125Q was pretreated with ATP (Fig. 3d and Supplementary Fig. 11b ), indicating the stabilizing effect of Na + on ATP-BsKtrA.
Half-life analysis of BsKtrA incubated at 40 °C also produced results in line with urea unfolding and thermostability assays: in the absence of Na + , ATP alone binding to BsKtrA diminished the protein stability (t 1/2 = 9.8 ± 1.4 min) compared to the t 1/2 of apo-BsKtrA (16.6 ± 0.8 min), but simultaneously addition of Na + and ATP synergistically increased the half-life of BsKtrA (t 1/2 = 70 ± 2.5 min) (Fig. 3e and Supplementary Fig. 12 ). Interestingly, BsKtrA to ATP binding affinities characterized using isothermal titration calorimetry (ITC) revealed comparable dissociation constants in the presence and absence of 200 mM Na + ( K d = 1.7 ± 0.3 μM and 5.5 ± 1.0 μM, respectively) (Supplementary Fig. 13 ), suggesting BsKtrA is capable of binding ATP even in the absence of Na + , although this particular ligand-binding state is actually unstable.
Altogether, these biophysical studies indicate that binding of ATP alone to BsKtrA is thermodynamically unstable, while Na + stabilizes BsKtrA in an ATP-dependent manner. The results are in great agreement with the structural finding that the two tethered ATP molecules at the intra-dimer interface of BsKtrA is energetically unfavorable; however, the electrostatic repulsion can be stabilized by Na + .
Ca 2+ binding to BsKtrA
The protein stability and ligand affinity assays described above provide solid evidence that Na + plays a critical role in stabilizing ATP-BsKtrA. These results in the roles of Na + are not necessarily in conflict with the roles of Ca 2+ and Mg 2+ reported previously 11 , which may also bind to the same Na + site or to different sites. To examine whether divalent cations could interact with BsKtrA in an ATP-dependent manner as previously implicated, we also characterized the thermostability of BsKtrA in the presence of Ca 2+ or Mg 2+ (Supplementary Fig. 14a–e ). Both Ca 2+ and Mg 2+ had no effect on ADP-BsKtrA and only a modest destabilizing effect on apo-BsKtrA (Supplementary Fig. 14a, b, d, e ). Interestingly, Ca 2+ increased the T m of ATP-BsKtrA in a concentration-dependent manner with an apparent Ca 2+ -binding affinity ( K dapp ) of 207 ± 29 μM (Supplementary Fig. 14d, f ), and the [Ca 2+ ]-dependent increase of T m was abolished in ATP-BsKtrA E125Q (Supplementary Fig. 14g, h ), but Mg 2+ caused little impact on the thermostability of ATP-BsKtrA (Supplementary Fig. 14c, e ). The ICP-MS analysis indicated that Ca 2+ content is higher in ATP-BsKtrA (2.529 ± 0.180 µg/ml) than in apo-BsKtrA (N.D.), further substantiating that the Na + binding site is also favorable for binding of Ca 2+ . However, Mg 2+ contents in both apo- or ATP-BsKtrA are at low level (0.113 ± 0.003 and 0.135 ± 0.004 µg/ml, respectively), and no Mg 2+ -dependent difference is observed between the two samples (Supplementary Table 4 ). Taken together, the results are in partial agreement with the recent study, suggesting that both Ca 2+ and Mg 2+ can bind to this site 11 . Notably, in that published results, Mg 2+ -induced activation of BsKtrAB was not exclusively ATP-dependent, and not even BsKtrA-dependent 11 . Further characterization is necessary to define the regulatory mechanism of divalent cations in KtrAB.
Na + facilitates ATP-BsKtrAB assembly and elevates the K + flux
The structural data and stability studies presented above suggest that Na + binding stabilizes the conformation ATP-BsKtrA, which could propagate to the BsKtrB to allow a stable quaternary structure of BsKtrAB with fully activated K + flux activity. To test this hypothesis, we first investigated the effects of Na + binding on BsKtrAB assembly using size exclusion chromatography (SEC). ATP-BsKtrA and BsKtrB mixture in the presence of Na + revealed a uniform species (Fig. 4a , black arrow), representing a stable assembly of ATP-BsKtrAB complex as demonstrated by the cryo-EM structure (Structure II) collected from the indicated fraction. Na + failed to produce a single species of ATP-BsKtrA E125Q B assembly (Fig. 4a ). In the absence of Na + , ATP-BsKtrA may still interact with BsKtrB as shown in Fig. 4a (ATP-BsKtrAB in K + Buffer with 0.03% DDM), but the mixture cannot form a monodisperse species. These results highlight the importance of Na + in ATP-BsKtrAB complex assembly: without the neutralizing effect of Na + at the middle of the two tethered ATP molecules in the BsKtrA dimer, the octameric ring at an energetically unstable state may reveal a stochastic conformation, deteriorating the proper assembly of ATP-BsKtrAB.
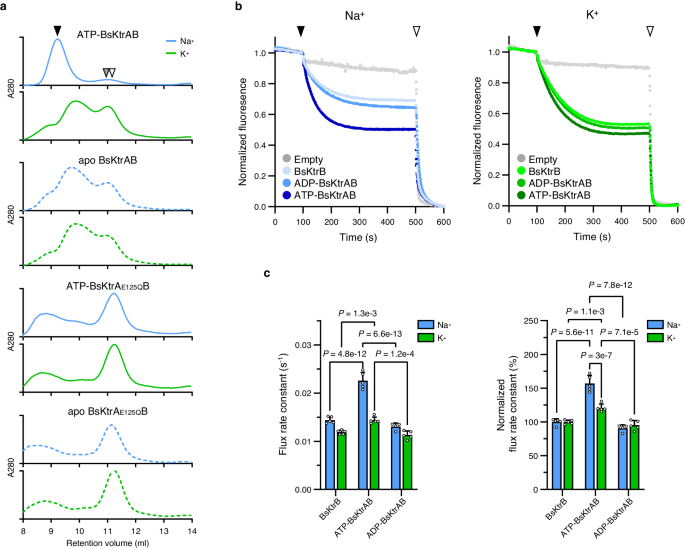
a The SEC profiles of BsKtrA (apo BsKtrA, ATP-BsKtrA, apo BsKtrA E125Q or ATP-BsKtrA E125Q ) mixed with BsKtrB in Na + Buffer or K + Buffer with 0.03% DDM. The retention volume for the BsKtrAB complex is benchmarked as the black arrow, while the retention volumes for BsKtrA octamer and BsKtrB dimer are benchmarked as the gray and white arrows, respectively. b Fluorescence-based K + flux assays using BsKtrB, ADP-BsKtrAB, and ATP-BsKtrAB in Swelling Na + Buffer (Na + , left panel) or Swelling K + Buffer (K + , right panel). The addition of H + ionophore CCCP and K + ionophore valinomycin are indicated as black and white arrows. K + flux rate constants were calculated by fitting the data (100–500 s) to a one-phase decay model using GraphPad Prism. c K + flux rate constants (left panel) and normalized flux rate constants (right panel) of BsKtrB, ATP-BsKtrAB and ADP-BsKtrAB in the presence of Na + (blue bars) and K + (green bars). The normalized K + flux rate constants were calculated using the respective K + flux rates of BsKtrB in the presence of either Na + or K + as 100%. Data represent mean ± s.d. with n = 4 (for ADP-BsKtrAB in Na + ) or n = 5 (for the others) independent experimental replicates. Statistical analyses were performed using two-way ANOVA. The schematic illustration of normalization and analysis are shown in Supplementary Fig. 15 . Source data for a – c are provided as a Source Data file.
Next, to correlate the protein assembly to the functionality, the liposomal K + flux assay was performed to validate the impact of Na + in the synergistic regulation of ATP-activation in the BsKtrAB complex. The K + flux activities of ADP-BsKtrAB showed inconsiderable difference in the presence of Na + or K + , suggesting no effect of either cation in the ADP-bound state. On the other hand, binding of ATP to BsKtrAB enhanced the K + flux rate by 20% in the absence of Na + (Swelling K + Buffer) and the activity was further elevated by 40% in the presence of Na + (Fig. 4b, c ). If Glu125, of which the side chain is involved in the coordination of Na + ions (Fig. 2 ), was replaced by glutamine, the Na + -dependent activation was no longer observed (Supplementary Fig. 16 ). Altogether, the results suggest that Na + binding at the BsKtrA intra-dimer interface coordinated by the γ-phosphates of ATPs and the carboxylate groups of Glu125 stabilizes the square-shaped ATP-BsKtrA octameric ring and maintains the pore-open conformation of BsKtrB (see below), leading to the activated state of BsKtrAB.
Comparison of ATP- and ADP-BsKtrAB
Having illustrated the detailed structure of ATP-BsKtrAB and established the structural basis of its activation by synergistic binding of ATP and Na + , we are in a strong position to examine the different structural effects of ATP and ADP as a way to further understand the gating mechanism of BsKtrAB. To minimize the uncertain effects that Mg 2+ may cause on the structure of BsKtrAB (Structure I), we hereafter used Mg 2+ -free ATP-BsKtrAB (Structure II) in comparison with ADP-BsKtrAB (Structure III) for the following structural analysis.
Our high-resolution structures of ATP- and ADP-BsKtrAB complexes with clear EM density of BsKtrB in the transmembrane region (Supplementary Fig. 17 ) allowed a reliable comparison between the two structures, showing distinct conformational rearrangements in both BsKtrA and BsKtrB. First, ADP binding to BsKtrA results in opening of the intra-dimer interface, as observed in the previous study 7 , making Na + binding at this site unfavorable (Fig. 2d ). In support, the biophysical data explains why the activating effects of Na + on BsKtrA are not significant at the ADP-bound state (Fig. 3e and Supplementary Fig. 11e ). The conformational change at the intra-dimer interface causes a dimer-to-dimer rotation, rendering the square- and diamond-shaped octameric rings. Second, in the ATP-BsKtrAB structure, the square-shaped ATP-BsKtrA octameric ring causes a steric hindrance for the D1M2b helix of BsKtrB, resulting in a helical hairpin conformation. The helical hairpin offers a contact interface with BsKtrB, which was previously denoted as the tip contact 7 (Supplementary Fig. 18a ). In the ADP-BsKtrAB structure, the long axis of the diamond-shaped ADP-BsKtrA octameric ring provides the space to accommodate the elongated D1M2b helices of the two BsKtrB protomers (Supplementary Fig. 18b ), although the density of the very C-terminal end of D1M2b helix (Gly103 to Gly124) is poor, likely due to high flexibility in this region. The conformational change is in general agreement with the cryo-EM structure of the ADP-VaKtrAB complex, where the two extended D1M2b helices penetrate into the octameric ring 16 . Albeit the overall conformational changes in ATP- and ADP-bound states are similar to the previous structural data, the high-resolution cryo-EM structures provide deeper insights into the gating mechanism.
BsKtrB Arg417 and Phe91 control the gate
Compared to ATP-BsKtrAB, the D4M2 of BsKtrB remains a discontinuous helix in ADP-BsKtrAB (Supplementary Fig. 19a ), unlike the extended and folded conformation observed in ADP-VaKtrAB 16 . The study of ADP-VaKtrAB proposed that the extended D4M2 helix along with a reorientation of the highly conserved arginine (Arg427) in the middle of D4M2 helix narrows the pore and closes the gate 16 . Interestingly, the equivalent residue Arg417 in BsKtrB is located below the selectivity filter with its side chain pointing to the pore, spatially close to the intramembrane loop and the kink in D1M2b helix 7 . To further examine the gating mechanism in greater detail, we investigated the detailed conformational changes in this region. Comparing the pore surfaces of BsKtrB in ATP- and ADP-bound states, it shows no obvious difference at the selectivity filter (Fig. 5a ). The pore radius analysis reveals the most constricted point near the intramembrane loop in the ADP-bound state (Fig. 5b ). Surprisingly, the intramembrane loop in both ATP- and ADP-BsKtrAB shows no pronounced open or closed conformational change (Fig. 5c, d and Supplementary Fig. 19a ). The intramembrane loop displaying no secondary structures is unexpectedly stable in both ATP- and ADP-bound BsKtrAB complex structures, as shown by the highly discernable cryo-EM density map (Supplementary Fig. 19b ). The loop contains a number of glycine residues (Gly304, Gly306 and Gly313), rendering the plasticity of torsion angle to afford the loop structure. A number of polar residues in the intramembrane loop, which are also well conserved in VaKtrB and other bacterial orthologues (Supplementary Fig. 20 ), exhibit hydrogen bonding interactions through the side chains with the neighboring residues in D2 domain (Fig. 5c, d ). The intramembrane loop is thus stabilized beneath the selectivity filter with a specific hydrogen bond network, and the aforementioned polar interactions remain nearly unchanged in ATP- and ADP-bound states (Fig. 5c, d ).
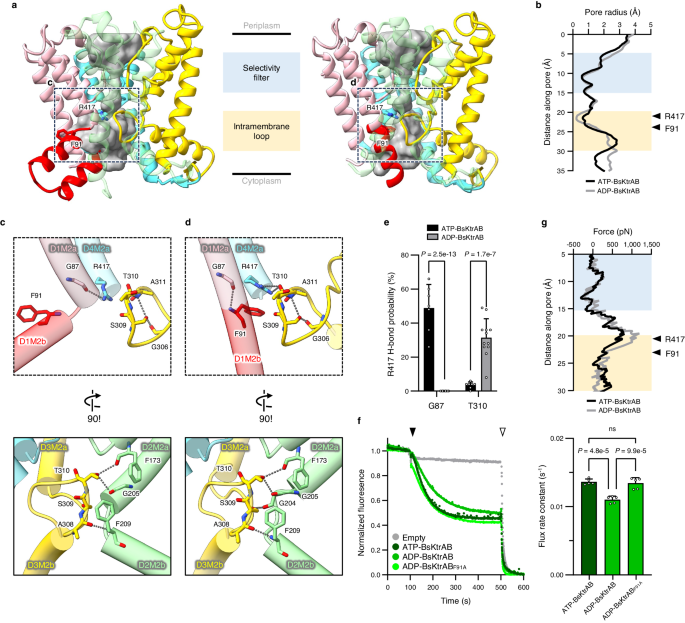
a Surface representation (gray) of BsKtrB pore in the ATP- (Structure II, left panel) and ADP-bound (Structure III, right panel) BsKtrAB complexes, calculated by HOLLOW. D1-D4 domains and D1M2b helix are highlighted with the same color code as in Fig. 1a . The D2 domain of BsKtrB is transparent for clarity. The side chains of Phe91 and Arg417 are shown in stick representation. b Pore radius as a function of the distance along the axis of BsKtrB pore, calculated by HOLE, is plotted with respect to the positions of selectivity filter and intramembrane loop shown in a . c , d A close-up view of the hydrogen bond interactions in the vicinity of the intramembrane loop and D1M2b helix of BsKtrB at ( c ) ATP- and ( d ) ADP-bound states as magnified from the dashed boxes defined in a . The side chains of the residues involved in the hydrogen bond interactions (gray dashed lines) are shown in stick representation. e The hydrogen bonding probabilities of Arg417-Gly87 and Arg417-Thr310 in the presence of ATP (black) and ADP (gray) are plotted on the basis of molecular dynamics simulation. Data represent the mean ± s.d. with n = 7 (ATP) and n = 12 (ADP) independent experimental replicates. Statistical analyses were performed using two-way ANOVA. f Left panel, fluorescence-based K + flux assays using wild-type BsKtrAB and KtrAB F91A in the presence of ATP or ADP. The addition of H + ionophore CCCP and valinomycin are indicated as black and white arrows. Right panel, plot of flux rate constants, which are calculated by fitting the data (100–500 s) to a one-phase decay model. Data represent the mean ± s.d. with n = 5 independent experimental replicates. Statistical analyses were performed by one-way ANOVA, and n.s. indicates no significance ( p ≥ 0.05). g The force required for K + to permeate cross the pore as a function of the distance along the pore analyzed using SMD simulation. Source data for ( b , e , g , f ) are provided as a Source Data file.
On the other hand, the side chain of BsKtrB Arg417, which was speculated to be a gating residue in the middle of D4M2 helix as mentioned above 17 , 36 , forms a hydrogen bond with the carbonyl oxygen of Thr310 in the intramembrane loop of ADP-BsKtrAB, providing a positively charged barrier that obstructs the passage of K + ions (Fig. 5d ). However, the polar interaction between Arg417 and Thr310 is interrupted in the ATP-BsKtrAB structure, where the side chain of Arg417 forms a hydrogen bond with the exposed carbonyl oxygen of Gly87 located at the kink of the discontinuous D1M2 helix (Fig. 5c ). In the ADP-BsKtrAB state, due to the fully extended and continuous helix conformation, Gly87 backbone oxygen is engaged in an intrahelical hydrogen bonding in D1M2 helix, hampering the polar interaction with Arg417. The hypothesis of the transition in hydrogen bonding was further supported by the results of MD simulation (Fig. 5e ). Notably, in ADP-BsKtrAB, the phenyl ring of Phe91 in D1M2b points toward the pore directly below the hydrogen bond between Arg417 and Thr310 (Fig. 5d ), serving as a hydrophobic gate. In ATP-BsKtrAB, the bulky side chain of Phe91 flips away from the pore due to the formation of a helical hairpin (Fig. 5c ). Sequence alignment indicates that Arg417 is highly conserved, and the equivalent residue of Phe91 in other bacterial orthologues is either phenylalanine or hydrophobic residues (Supplementary Fig. 20 ). Arg417 and Phe91 form the narrowest region in the pore at the ADP-bound state, and this region is widened at the ATP-bound state (Fig. 5b ), suggesting that these two residues play a pivotal role in the gating mechanism of BsKtrB in response to the ligand-induced conformation changes of BsKtrA octameric ring. Substituting the Phe91 with alanine enhanced the K + flux rate in the presence of ADP, showing a similar flux rate as ATP (Fig. 5f ); however, substitution of Arg417 resulted in protein aggregation, supporting its importance in protein stability. The experimental results were further substantiated by the SMD simulation, where the force profile indicates a free energy barrier at the region of Arg417 and Phe91 in the ADP-bound state (Fig. 5g ).
The crystallographic and electron microscopic data of BsKtrAB, together with the ligand binding characterization and activity analysis in this study provide comprehensive structural and functional evidence to deduce a detailed mechanism of the ligand-gated K + channel (Fig. 6 ). Furthermore, the structures of the ATP- and ADP-KtrAB complexes are both derived from B. subtilis , making the mechanistic analysis more reliable.
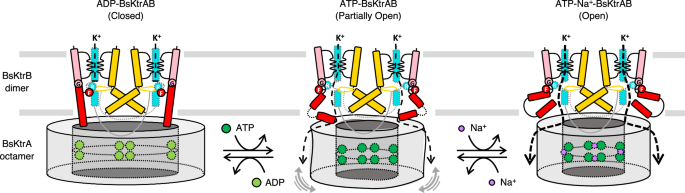
Left, ADP binding to BsKtrA induces a diamond-like octameric ring offers the space for BsKtrB D1M2 helix in an extended conformation, resulting in a hydrogen bond interaction of Arg417 (denoted as R) in the middle of D4M2 helix (cyan) with the intramembrane loop, which creates a positively charged barrier for K + passage. In this conformation, the hydrophobic side chain of Phe91 (denoted as F) faces to the permeation pathway, further blocking the aqueous flow in pore. Center, binding of ATP alone to BsKtrA causes instability to the octameric ring and difficulty in proper BsKtrAB assembly. The pore is partially open due to the transient interactions between BsKtrA and BsKtrB. Right, upon binding of both ATP and Na + to BsKtrA, the square-shaped octameric ring is thermodynamically stabilized, causing a steric hindrance for the D1M2 helix, and resulting in a helical hairpin (red). In this conformation, the side chain of Arg417 alternatively interacts with the backbone oxygen of Gly87 (denoted as G) in the discontinuous region of D1M2 helix, and the side chain of Phe91 swings away from the pore due to the conformational change of helical hairpin, by which the K + transport activity of BsKtrAB is activated. Figure adapted from Fig. 7 in Diskowski et al. 16 .
As known from previous structural studies 7 , 16 , ATP-binding to BsKtrA causes a square-shaped octameric ring, rendering a steric hindrance to BsKtrB D1M2b helix and thus inducing a helix hairpin conformation, which leads to an expansion in the pore. ADP-binding to BsKtrA induces a diamond-shaped ring, providing adequate space to allow D1M2b helix to be fully extended and thus restricting the pore. By analyzing the high-resolution cryo-EM structures in this study, we demonstrate that Arg417 and Phe91 serve as a gate blocking the K + flux in the closed state. The structural transition of D1M2b helix into hairpin alters the hydrogen bond interactions near the gate, resulting in the side chain conformational change of Arg417 and Phe91 in the open state. However, in ATP-bound state, BsKtrA octameric ring is intrinsically unstable because charge repulsion between the two tethered ATP molecules in BsKtrA dimer. Consequently, ATP-BsKtrA octameric ring may not be able to efficiently form a functionally active assembly of BsKtrAB complex. Na + compensates the negative charge and stabilizes ATP-BsKtrA thermodynamically, which in turn facilitates the interaction between ATP-BsKtrA and BsKtrB, and secures the complex assembly specifically at the functionally active state. Nevertheless, the synergism and gating mechanism proposed here are concluded on the basis of protein samples prepared in detergent micelles. Further structural studies using protein samples in lipid membranes are required to substantiate the mechanistic model.
The TrkHA complex, a member of SKT family consisting of the double-pore channel TrkH and the RCK tetrameric ring TrkA, displays similar signaling responses, gating elements and quaternary structures as those found in KtrAB 36 , 37 . In the TrkHA system, ATP binding results in a conformational change of TrkA, which triggers a movement of the intramembrane loop to the intracellular side, leading to an open channel conformation 38 . In the BsKtrAB complex, ATP binding does not cause a significant movement of the intramembrane loop to induce an open conformation. Another feature of BsKtrB differentiating itself from TrkH is its long C-terminal tail snaking into the cytoplasmic pore of the neighboring BsKtrB protomer. The carboxylate group of the very C-terminal residue (Gly445) interacts specifically with Lys315 in the intramembrane loop and the lining residues on the cytoplasmic pore, providing a platform for the interaction with the BsKtrA octameric ring, known as lateral contact 7 . The C-terminus of BsKtrB remains virtually unchanged in the ADP- and ATP-BsKtrAB structures, further fastening the conformation of the intramembrane loop. As a consequence, the open channel conformation of ATP-BsKtrAB is achieved mainly by the release of the constraints between D1M2b and the intramembrane loop.
As commented by Inga Hänelt and colleagues 2 , the high intracellular ATP/ADP ratio in normal cellular condition and the stronger ATP to KtrA binding affinity implicated that it is difficult for ADP to exert its inhibitory effect. Therefore, additional effectors might be involved in the regulatory process of ATP. It has been shown that in an euryhaline cyanobacterium Synechocystis , after being transferred into a saline medium, the intracellular [Na + ] rapidly increased within a few minutes in the early phase of osmoadaptation, and was subsequently replaced by K + ions, which lasted for hours to days 39 . As Na + ions have a deleterious effect on the function of bacterial cells, a surge of intracellular Na + concentration in hyperosmotic condition needs to be counter-balanced by enhancing the influx of K + . It was therefore postulated that Na + is involved in stimulating the uptake of K + . In this study, we demonstrated that Na + plays a critical role in the ATP-dependent activation of the BsKtrAB system for K + uptake by enhancing the stability of ATP-BsKtrA octameric ring and strengthening the BsKtrAB complex conformation at an open state. The thermostability assays and functional characterization indicated that binding ATP alone to BsKtrA is actually detrimental for maintaining an open pore state of BsKtrB. In contrast, ADP-BsKtrA is comparably more stable than ATP-BsKtrA if Na + is not bound. The millimolar range of Na + binding affinity for ATP-BsKtrA is consistent with the previously reported concentrations of Na + for KtrAB activation 12 , 13 , 40 . The relatively low affinity for Na + implies that the counteracting K + uptake is induced only when the intracellular [Na + ] is high.
Mg 2+ and Ca 2+ have been implicated in the Na + -binding site at the intradimer interface of ATP-BsKtrA 7 , 11 . Mg 2+ is a key cofactor in ATP hydrolysis. However, to the best of our knowledge, there is no previous study indicating that KtrA possesses the ATP hydrolysis activity, and our DSF studies clearly indicate that Mg 2+ cannot induce significant change in the thermostability of ATP-BsKtrA. Ca 2+ elevates the channel activity of MthK through directly binding to its RCK domain, as demonstrated by structural and electrophysiological analyses 41 , 42 . In this study, Ca 2+ seems to interact with BsKtrA, using a similar mechanism of Na + , to activate the channel activity of BsKtrB. However, the signaling pathway of Ca 2+ in bacterial cells remains to be elucidated 43 , and the physiological significance of Ca 2+ in KtrAB system requires a deeper investigation.
The Na + -dependent K + uptake systems are also present in animals 21 , 44 . K Na channels (Slo2.1 and Slo2.2) have received particular attention for their physiological importance in maintaining precise timing of action potential 45 and cell volume 46 . Sequence analysis and functional studies indicated that a consensus motif of NAD + -binding site similar to the Rossmann fold of BsKtrA is localized in the RCK2 domain of K Na 29 , and interestingly, K Na channels were activated by NAD + in a [Na + ]-dependent manner in a millimolar range 29 . In contrast, ATP seemed to inhibit the activity of Slo2.1 21 , but a recent study suggested that ATP has no effect on the activity of Slo2.1 47 . Further characterization is therefore necessary to define the regulatory role of ATP in K Na channels in the presence of Na + .
In summary, the high-resolution cryo-EM structures of BsKtrAB at the activated and inactivated states shed light on a mechanism of ligand-gated K + channels. The structural and functional studies illustrating the binding site of Na + of KtrA and its synergistic role in the ATP activation of KtrAB also provide an underlying framework to elucidate the mechanistic model of Na + -activated K Na channels in central nervous system neurons.
Protein expression and purification
The procedure for the purification of BsKtrA and BsKtrB was modified based on Vieira-Pires et al. 7 . Tag-less BsKtrA was overexpressed in E. coli BL21(DE3) in LB medium containing 100 μg/ml ampicillin at 20 °C for 16 h after 400 μM IPTG induction. Cell pellets were resuspended in Buffer A (50 mM Tris-HCl pH 8.0, 50 mM KCl, 5 mM DTT), and cell lysis was performed using a high-pressure cell disruptor (Constant Systems) at a pressure of 25 kpsi with Buffer A supplemented with Protease Inhibitor Cocktail (Roche), 1 µg/ml DNase (Sigma-Aldrich), and 1 mM MgCl 2 . The supernatant of the centrifuged lysate was loaded into an anion exchange column packed with Macro-Prep High Q Resin (Bio-Rad) and washed with Buffer B (50 mM Tris-HCl pH 8.0, 100 mM KCl, 5 mM DTT). The proteins in the lysate were fractionized using a KCl concentration gradient (from 100 mM to 600 mM) in Buffer B. The fractions containing BsKtrA were slowly loaded onto the N 6 -hexyl-ATP agarose (Jena Bioscience) column, which was then washed with Buffer C (50 mM Tris-HCl pH 8.0, 150 mM KCl, 5 mM DTT). BsKtrA was eluted with Buffer C containing 5 mM ATP or 5 mM ADP followed by a thorough dialysis (four times of 100-fold dialysis) in Buffer D (50 mM Tris-HCl pH 8.0, 150 mM KCl, 1 mM DTT, 1 mM EDTA) to remove bound ATP or ADP and divalent cations.
N-terminal His-tagged BsKtrB with the tobacco etch virus (TEV) protease cleavage site was overexpressed in E. coli C43 (DE3) in TB medium containing 100 μg/ml ampicillin at 25 °C for 16 h after 400 μM of IPTG induction. Cell pellets were resuspended and lysed in Buffer E (50 mM Tris-HCl pH 8.0, 120 mM NaCl, 30 mM KCl) supplemented with the protease inhibitor cocktail, 1 µg/ml DNase and 1 mM MgCl 2 . BsKtrB was extracted with 1 % DDM (n-dodecyl-β-D-maltoside, Carbosynth) in Buffer E at 4 °C for 2 h followed by ultracentrifugation at 150,000 g for 1 h. The supernatant was loaded onto a Ni-NTA (GE Healthcare) affinity chromatography column and washed with Buffer F (50 mM Tris-HCl pH 8.0, 120 mM NaCl, 30 mM KCl, 0.03% DDM). The protein was eluted with Buffer F supplemented with 250 mM imidazole. The fractions containing BsKtrB were dialyzed overnight at 4 °C in the presence of His-tagged TEV protease in Buffer F. The cleaved sample was loaded onto a Ni-NTA His-Trap column (GE Healthcare), and the flow-through containing BsKtrB was collected for further purification.
The purified BsKtrA and BsKtrB were subjected to size exclusion chromatography (SEC) using a Superdex 200 increase 10/300 (GE Healthcare) column for the final polish and buffer exchange. The SEC buffers for BsKtrA and BsKtrB are varied depending on the following experiments (see below). All purified protein samples were snap-frozen in liquid nitrogen and stored at −80 °C.
Cryo-EM sample preparation
For single-particle cryo-EM structure determination of BsKtrAB complexes, BsKtrA purified with K + Buffer (50 mM Tris-HCl pH 8.0, 150 mM KCl, 1 mM TCEP) and BsKtrB purified in Buffer F were mixed at the BsKtrA to BsKtrB molar ratio (2:1) with the addition of 1 mM ATP or ADP, respectively, and incubated for 2 h. The properly assembled ATP- or ADP-BsKtrAB complex samples were fractionized as monitored by SEC elution profiles using Superdex 200 increase 10/300 column with Cryo-EM Buffer (20 mM Tris-HCl pH 8.0, 70 mM NaCl, 30 mM KCl, 0.75 mM 6-cyclohexyl-1-hexyl-β-D-maltoside).
Cryo-EM grid preparation and data acquisition
For Mg 2+ -added ATP-BsKtrAB (Structure I) , 0.15 mg/ml BsKtrAB was mixed in the Cryo-EM Buffer containing 2 mM MgCl 2 and 1 mM ATP. For Mg 2+ -free ATP-BsKtrAB in the presence of EDTA/EGTA (Structure II), 4 mg/ml BsKtrAB was prepared in the Cryo-EM Buffer containing 1 mM ATP, 2 mM EDTA, and 1 mM EGTA. For ADP-BsKtrAB (Structure III), 0.24 mg/ml BsKtrAB was diluted in the Cryo-EM Buffer containing 100 μM ADP. All grids were prepared using the Vitrobot Mark IV (Thermo Scientific) at 4 °C and 100% humidity. 4 μl of protein samples were applied onto the freshly glow-discharged holey carbon films. Quantifoil R1.2/1.3 plus C2, graphene oxide coated UltrAuFoil R2/2, and Quantifoil R2/1 plus C2 were used for Mg 2+ -added ATP-BsKtrAB, Mg 2+ -free ATP-BsKtrAB, and ADP-BsKtrAB, respectively, with a 10 s wait time. The grids were then frozen in nitrogen-cooled liquid ethane after blotting for 3.5 s. All data were acquired on a 300 kV Titan Krios (Thermo Fisher) cryo-transmission electron microscopy equipped with a K3 Summit direct electron detector (Gatan) and GIF Quantum energy filter (Gatan) in super-resolution mode. The detailed parameters are summarized in Supplementary Table 1 .
Cryo-EM image processing
All raw movie stacks were motion-corrected and dose-weighted using MotionCor2 48 , which involved a two-fold binning factor, resulting in a pixel size of 0.83 Å per pixel. The motion-corrected movies were then transferred to CryoSPARC for the subsequent processes 49 , including CTF estimation, particle picking, 2D classification, 3D classification, and 3D map refinement as shown in Supplementary Figs. 3 – 5 . In brief, the micrographs with high resolution were selected for particle picking after CTF estimation. Initial particle picking employed Blob Picker were extracted with a 384-pixel box size for subsequent 2D classification. The classes with clear 2D views were adopted as the templates for the second round of particle picking. After three rounds of 2D classification, the remaining good particle stacks were selected for the 3D map ab-Initio reconstruction and classification.
For the Mg 2+ -added ATP-BsKtrAB (Structure I) dataset, 805,032 particles from 2D classification were subjected to ab-initio reconstruction and heterogeneous classification with C1 symmetry. 549,841 particles with KtrB 2 A 8 B 2 assembly from a single class were selected for homogeneous, non-uniform, and local refinement (using an entire protein complex mask) with C2 symmetry, resulting in a 2.48 Å Mg 2+ -added ATP-BsKtrAB map.
For the Mg 2+ -free ATP-BsKtrAB (Structure II) dataset, 755,437 particles from 2D classification were selected for two rounds of ab-initio reconstruction and heterogeneous classification with C1 symmetry. 527,427 particles with KtrB 2 A 8 B 2 assembly were then subjected to homogeneous and non-uniform refinement with C2 symmetry, resulting in a 2.82 Å Mg 2+ -free ATP-BsKtrAB map.
For the ADP-BsKtrAB (Structure III) dataset, 666,155 particles from 2D classification were used for ab-initio reconstruction and heterogeneous classification with C1 symmetry. 340,666 particles with KtrB 2 A 8 B 2 assembly were then subjected to the second round of 3D map generation and classification. 294,844 particles were selected for further homogeneous, non-uniform, and local refinement (using the entire protein mask) with C2 symmetry, resulting in a 2.83 Å ADP-BsKtrAB map. To improve the map quality, focused refinement with masking BsKtrA octameric ring region and BsKtrB dimer region were performed. For BsKtrB dimer, the symmetry was expanded and one KtrB dimer was masked during the refinement process.
Cryo-EM model building and refinement
For Mg 2+ -added ATP-BsKtrAB, the published crystal structure of ATP-BsKtrAB 7 (PDB code: 4J7C ) was used as an initial model and docked into the map using UCSF chimera. All residues from the fitted model were manually checked and refined using COOT 50 . DeepEMhancer sharpening map was applied to assist the model building of RCK_C domain 51 . The model building was then accomplished by several rounds of refinement by phenix.real_sapce_refine of the Phenix suite 52 and iteratively building using COOT. The final model of Mg 2+ -added ATP-BsKtrAB was further used as the initial model for the model building of Mg 2+ -free ATP-BsKtrAB. For ADP-BsKtrAB, the BsKtrA model from the published 5.97 Å ADP-BsKtrA ΔC B crystal structure 15 (PDB code: 5BUT ) and the BsKtrB model from the published 3.50 Å ATP-BsKtrAB crystal structure 7 (PDB code: 4J7C ) were used as the initial models and docked into the map by using phenix.dock_in_map . For the regions with low-resolution density maps, we employed focused-refined maps of the BsKtrA octamer and BsKtrB dimer to assist the model building process. The model building and refinement procedures were performed as described above. The statistics of the structure refinement were summarized in Supplementary Table 1 .
Full atomistic modeling
The full atomistic structures of ATP- and ADP-BsKtrAB complexes were constructed based on the cryo-EM resolved structures (Structures II and III). The missing residues Gly103 and Lys104 in the ATP-BsKtrAB were generated by homology modeling using SWISS-MODEL 53 . To obtain an initial structure of the missing part of ADP-BsKtrAB (Gly103 to Gly124), CCbuilder 54 was used to generate the D1M2b helix by using the amino acid sequences from Ile98 to Leu118. The initial model of the D1M2b helix in the ADP-BsKtrAB model was obtained by aligning the helix with cryo-EM resolved coordinates of the Ile98, Val99 and Met100. Homology modeling using SWISS-MODEL 53 was then applied to generate the molecular structures of other missing residues in ADP-BsKtrAB. After obtaining the full atomistic model of the ATP- and ADP-BsKtrAB complexes, we used the CHARMM-GUI server 55 to generate lipid bilayers and construct the protein/membrane complex. TIP3P water was used for all the simulations. The initial model box size is about 200x200x200 Å 3 and the KCl concentration is 0.15 mol/L. All the Molecular dynamics (MD) simulations are performed by Nanoscale molecular dynamics (NAMD) 56 with CHARMM force field. Visual molecular dynamics (VMD) 57 is used for the visualization and analysis of the simulation results.
To refine the molecular structures of the ATP- and ADP-BsKtrAB complexes, an energy minimization was performed firstly for the protein/membrane complex. After the energy minimization, an NPT ensemble simulation with a timestep of 1.0 fs is performed for 1 ns with unrelaxed lipid bilayers was implemented followed by 1 ns simulation with relaxed lipid bilayers. Then, the timestep was switched to 2.0 fs for additional 2 ns NPT ensemble simulation to ensure the protein/membrane system is stable. Finally, 45 ns NPT ensemble simulations with 2.0 fs timestep for both ATP- and ADP-BsKtrAB complexes were performed to investigate the structural stability (Supplementary Fig. 21 ) and differences.
Furthermore, steered molecular dynamics (SMD) simulations were performed by using final equilibrium structures of KtrB from MD simulations to study the molecular gating mechanism. The initial position of the POT is set at 10 Å above the selectivity filter. A spring was attached to the POT and the POT is pulled toward the selectivity filter. The harmonic spring was set as a constant of 7 kcal/mol/Å 2 , and the constant velocity was set at 0.01 Å/ps. In SMD simulations, we restricted the C α of the endpoint of the helices in the KtrB (Supplementary Fig. 22 ). The total SMD simulation time is 4 ns with a timestep of 2.0 fs. All of the results were calculated by three independent simulations for validation. For hydrogen bond analysis, the distance cutoff is 3.5 Angstrom and the Angle cutoff is 30 degrees. Three independent simulations were performed for the ATP- and ADP-bound models to calculate the hydrogen bond occupancy.
Protein crystallization
To obtain the Tl + -derivatized ATP-BsKtrA crystals, BsKtrA purified in K + Buffer was concentrated to 10 mg/ml and supplemented with 1 mM ATP dipotassium salt hydrate (KATP) and 10 mM TlOAc. The crystallization condition for the ATP-BsKtrB octamer was modified as previously described 7 . Crystals were grown at 20 °C using hanging-drop vapor diffusion by mixing 1 µl of protein with 1 µl of precipitant. For the sodium competition assay, BsKtrA was purified in Na + Buffer (50 mM Tris-HCl pH 8.0, 150 mM NaCl, 1 mM TCEP), followed by addition of 1 mM ATP disodium salt hydrate (NaATP) and 5 mM TlOAc before crystallization.
Data collection and structure determination
The X-ray diffraction data were collected using TPS 05A beamline at the National Synchrotron Radiation Research Center (NSRRC) in Taiwan. The diffraction data sets were indexed, integrated and scaled using the HKL-2000 package 58 . Molecular replacement was utilized to solve the structure by Phaser 59 with the published octameric ATP-BsKtrA coordinate 7 (PDB code: 4J90 ) as the search model. The structure was manually refined using COOT, and further structure refinement was performed with Phenix software suite 52 . Anomalous difference maps of Tl + were calculated using Phenix. The X-ray crystallographic data collection and refinement statistics are summarized in Supplementary Table 2 .
Isothermal titration calorimetry
ITC experiments were performed by using ITC200 calorimeter (MicroCal Inc.) at 25 °C with a 600 rpm stir speed. BsKtrA prepared in Na + Buffer or K + Buffer with a final concentration of 30 μM in a volume of 280 μl was titrated with 300 μM NaATP or 800 μM KATP, respectively. ITC measurements involved 20 injections of titrants with 2 μl for each injection. Each titration point was subtracted by the control experiments with ATP titration into either of the buffers. The experiments were performed in triplicates and all the titration points except for the first one were analyzed using the MicroCal ITC-Origin.
LC-MS/MS analysis
To obtain apo-BsKtrA, protein samples were dialyzed in K + Buffer in different folds of dialysis (100-, 10,000- and 1,000,000-fold) followed by further purification using Superdex 200 increase 10/300 (GE Healthcare) in K + Buffer. LC-MS/MS analysis was performed to examine the residual ATP bound to BsKtrA (Supplementary Fig. 23 ). 10 µg of BsKtrA from each preparation of dialysis folds was denatured at 95 °C for 5 min and the aggregated protein was removed by ultracentrifugation at 18,000 × g for 30 min. The supernatant was then collected, vacuum-dried, and resuspended in 15 µl of deionized water for triplicate LC-MS/MS analysis. The analysis was performed using an ACQUITY H-Class UPLC system coupled with an XEVO TQ-MS with an ESI ionization source (Waters, Milford). The 1 μl injection of samples were separated through a ZIC-cHILIC column (3 µm, 2.1 mm × 100 mm) at a flow rate of 0.25 ml/min at 35 °C. A binary gradient system consisting of mobile phases A and B, which were 0.1% formic acid (FA) in DI water and 0.1% FA in acetonitrile (J.T. Baker), respectively. The following gradient program was used: 0–2 min, 70% B; 2–2.5 min, 70%–5% B; 2.5–5 min, 5% B; 5–5.1 min, 5%–70% B; and 5.1–10 min, 70% B. The mass spectrometer was operated in negative ionization mode using multiple reaction monitoring (MRM) mode. The MRM transitions monitored were m/z 505 to m/z 158 for ATP, with the following parameters: cone voltage of 28 V, collision energy (CE) of 26 V, and a dwell time of 0.025 s. The following MS parameters were used: capillary voltage of 3.0 kV; desolvation temperature of 350 °C; desolvation gas flow of 600 L/h; collision gas flow of 0.25 ml/min. Both Q1 and Q3 quadrupoles were maintained at quantitative resolution. Peak data visualization and presentation were performed using MassLynx V4.1 software and GraphPad Prism.
ICP-MS analysis
ICP-MS was utilized to analyze the abundance of metal ions bound to ATP-BsKtrA. 5 mg/ml of either apo-BsKtrA or ATP-BsKtrA in Na + Buffer, K + Buffer, K + Buffer plus 10 mM CaCl 2 , or K + Buffer plus 10 mM MgCl 2 were prepared and incubated on ice for 1 h. Subsequently, buffer exchange in Choline Buffer (50 mM Tris-HCl pH 8.0, 150 mM choline chloride, 1 mM TCEP) was performed using a Superdex 200 increase 10/300 column (GE Healthcare). The collected protein fractions (300 μl) were treated with 5 ml of nitric acid. The digestion process was initiated using a microwave accelerated reaction system (CEM MARS 230/60) at 1600 W, with the temperature gradually increasing to 160 °C over 20 min, and held at that temperature for 40 min. The denatured protein samples were then diluted with deionized water to a final volume of 20 ml. The treated protein samples were analyzed with an ICP-MS system (NexION 300X, PerkinElmer), in the DRC mode (collision mode) at the Health Technology Center of Chung Shan Medical University.
Urea unfolding assay
The urea unfolding assay was performed using the intrinsic tryptophan fluorescence of BsKtrA as described previously 34 . Purified BsKtrA samples in K + Buffer or Na + Buffer were diluted to 1 µM using respective buffers, and titrated with increasing concentrations of K + or Na + , respectively, in the presence of 0.1 mM ATP or ADP, according to the experimental design, and then incubated for 1 h on ice. Protein samples were gently mixed with urea to reach different final urea concentrations and incubated at 25 °C for 30 min. The sample mixtures were transferred to a quartz cuvette (Starna scientific, Type 16.10/Q/10) for full-wavelength scanning of the fluorescence derived from the intrinsic tryptophan, using a spectrofluorophotometer (RF-6000, SHIMADZU) (Excitation: 295 nm, Emission: 310–380 nm). The tryptophan fluorescence emission intensities (330 nm) were normalized against the intensity of protein samples without urea treatment. The normalized fractions representing the percentages of folded BsKtrA were plotted against the concentration of urea. The unfolding concentration (C m ) of urea was analyzed using Sigmoidal 4PL model by GraphPad Prism.
Thermal stability assays
Thermal shifts of BsKtrA were monitored using differential scanning fluorimetry (DSF), as previously described 60 . Briefly, BsKtrA purified in Choline Buffer (50 mM Tris-HCl pH 8.0, 150 mM choline chloride, 1 mM TCEP) was diluted to 5 µM in the presence of 100 µM KATP or KADP with the titration of increasing concentrations of K + , Na + , Ca 2+ or Mg 2+ according to the experimental design. Sypro Orange 5000X (Sigma) was added to the mixtures to reach a final concentration of 5X, and the mixtures were placed in a 96-well PCR white plate (Bio-Rad). The assay was performed using a real-time PCR detection system (CFX Connect, Bio-Rad) with FRET channel scan mode. The temperature scan was performed from 25 °C to 85 °C with an increment of 0.3 °C per step with 12 s dwell time. The transition temperature ( T m ) was analyzed using CFX Manager (Bio-Rad), and then normalized with respect to the minimal (0%) and maximal (100%) change of T m detected. The normalized fractions were plotted against the concentrations of titrated cations, and the apparent dissociation constant ( K dapp ) was determined by one-site specific binding model using GraphPad Prism.
For protein unfolding half-life determination, the samples prepared as mentioned above were subjected to a real-time PCR thermocycler with a constant temperature at 40 °C. The fluorescence intensity was continuously measured for 175 min. The analysis of protein unfolding half-life was carried out as previously described 61 . The fraction of folded protein was calculated by the equation: 1-F i /F max , where F i is the fluorescence of each time point, and F max is the maximal fluorescence measured in that specific sample. The half-life constants were determined by GraphPad Prism using exponential one-phase decay model.
BsKtrAB complex assembly assay
Protein complex assembly was monitored using SEC. The elution volumes for BsKtrA octamer, BsKtrB dimer and BsKtrAB complex were benchmarked as demonstrated by Morais-Cabral and coworkers 7 . Individually purified BsKtrA (0.125 mg/ml) and BsKtrB (0.125 mg/ml) were mixed in a molar ratio of 2:1 in either K + Buffer or Na + Buffer, supplemented with 0.03% DDM in the presence of 50 µM KATP or NaATP, respectively, for 1 h incubation at 4 °C. The mixtures were subjected to Superdex 200 increase 10/300 column for SEC profile analysis.
Preparation of proteoliposomes
The preparation was modified based on the previous studies 11 . Briefly, E. coli polar lipids (Avanti) were dissolved in ether, followed by evaporation using an argon stream to remove any organic solvent. The lipids were then resuspended in Swelling Low K + Buffer (10 mM HEPES, 7 mM NMG pH 8.0, 0.2 mM EDTA, 150 mM KCl) to a final concentration of 10 mg/ml, followed by 3 rounds of freeze-thaw cycles in liquid nitrogen. Large unilamellar vesicles (LUVs) were prepared using an extruder (Avanti) with a membrane filter of 400 nm pore size. For proteoliposome reconstitution, the LUV solution was supplemented with 30 mM DM (n-Decyl-b-D-Maltoside, Anatrace), and properly assembled BsKtrAB complex was added to the LUV solution with a protein-to-lipid ratio of 1:100 (w:w) in the presence of 0.1 mM KATP or KADP for a gentle mixing at 25 °C for 1 h. The protein-lipid mixture was incubated twice with fresh SM-2 Biobeads (BioRad) at a bead-to-detergent ratio of 20:1 (w:w) at 25 °C for 1 h and then at 4 °C for overnight to remove residual detergents.
For the sodium-dependent K + flux assays, the salt concentration in Swelling Low K + Buffer for LUV preparation was increased to either 200 mM KCl (Swelling K + Buffer) or 150 mM KCl and 50 mM NaCl (Swelling Na + Buffer). Purified BsKtrB was reconstituted into the LUVs with a protein-to-lipid of 1:50 (w:w) as mentioned above, followed by incubation with BsKtrA with a molar ratio of 1:2 (KtrB:KtrA) at 4 °C for 1 h in the presence of 0.1 mM KATP or KADP. The proteoliposomes were then immediately used for K + flux assays.
Fluorescence-based K + flux assay
The fluorescence-based K + flux assay was carried out on the basis of previously published studies 11 . To establish the K + gradient, the proteoliposomes were diluted 100-fold in Flux Buffer (10 mM HEPES, 7 mM NMG pH 8.0, 0.2 mM EDTA, 150 mM sorbitol, 0.1 mM KATP or KADP). The samples were transferred to a quartz cuvette with a stirrer for fluorescence measurements at 25 °C using a spectrofluorometer (RF6000, Shimazu). The pH-sensitive dye ACMA (9-amino-6-chloro-2-methoxyacridine, Sigma) was then added to a final concentration of 500 nM and incubated for 5 min. The fluorescence was then monitored every 0.5 s (λ ex = 410 nm, λ em = 480 nm). The initial baseline was measured for 100 s, and the assay was initiated by adding 2 µM of H + ionophore CCCP (carbonyl cyanide m-chlorophenyl hydrazine, Sigma) and measured for 400 s. For the final baseline, 300 nM of the K + ionophore valinomycin (Sigma) was added and an additional 100 s of fluorescence intensity was recorded. For Na + -dependent flux assay, the sorbitol in Flux Buffer was increased to 200 mM, and the dilution of proteoliposomes into the Flux Buffer was increased to 200 folds. The normalization of fluorescence quenching curves and calculation of flux rate constants followed the previously described methods 11 . To normalize the fluorescence quenching curves, each dataset of individual experiment was normalized using the following equation: NF = (F − F val )/(F ini − F val ), where NF is the normalized fluorescence, F is the fluorescence of each time point, F ini is the last baseline point measured before CCCP addition, and F val is the lowest point measured after valinomycin addition The flux rate constants (100–500 s) were determined using the exponential one-phase decay model in GraphPad Prism. The schematic illustration of normalization and analysis are shown in Supplementary Fig. 15 .
Reporting summary
Further information on research design is available in the Nature Portfolio Reporting Summary linked to this article.
Data availability
The data that support this study are available from the corresponding authors upon request. The cryo-EM maps have been deposited in the Electron Microscopy Data Bank (EMDB) under accession codes EMD-36803 (Structure I); EMD-36804 (Structure II); EMD-38477 (Structure IIa); EMD-38478 (Structure IIb); EMD-36800 (Structure III); EMD-36801 (Structure III, focused refined on KtrA octamer); and EMD-36802 (Structure III, focused refined on KtrB dimer). The atomic coordinates have been deposited in the Protein Data Bank (PDB) under accession codes 8KIT (Structure I); 8K1U (Structure II); 8XMH (Structure IIa); 8XMI (Structure IIb); 8K1S (Structure III); 8K16 (Tl + -treated BsKtrA in K + Buffer); and 8K1K (Tl + -treated BsKtrA in Na + Buffer). Source data are provided with this paper.
Bremer, E. & Kramer, R. Responses of Microorganisms to Osmotic Stress. Annu. Rev. Microbiol . 73 , 313–334 (2019).
Stautz, J. et al. Molecular Mechanisms for Bacterial Potassium Homeostasis. J. Mol. Biol ., 33 , 166968 (2021).
Diskowski, M., Mikusevic, V., Stock, C. & Hänelt, I. Functional diversity of the superfamily of K + transporters to meet various requirements. Biol. Chem. 396 , 1003–1014 (2015).
Article CAS PubMed Google Scholar
Corratge-Faillie, C. et al. Potassium and sodium transport in non-animal cells: the Trk/Ktr/HKT transporter family. Cell. Mol. Life Sci. 67 , 2511–2532 (2010).
Albright, R. A., Ibar, J. L., Kim, C. U., Gruner, S. M. & Morais-Cabral, J. H. The RCK domain of the KtrAB K + transporter: multiple conformations of an octameric ring. Cell 126 , 1147–1159 (2006).
Kroning, N. et al. ATP binding to the KTN/RCK subunit KtrA from the K + -uptake system KtrAB of Vibrio alginolyticus: its role in the formation of the KtrAB complex and its requirement in vivo. J. Biol. Chem. 282 , 14018–14027 (2007).
Article PubMed Google Scholar
Vieira-Pires, R. S., Szollosi, A. & Morais-Cabral, J. H. The structure of the KtrAB potassium transporter. Nature 496 , 323–328 (2013).
Article ADS CAS PubMed Google Scholar
Corrigan, R. M. et al. Systematic identification of conserved bacterial c-di-AMP receptor proteins. Proc. Natl Acad. Sci. USA 110 , 9084–9089 (2013).
Article ADS CAS PubMed PubMed Central Google Scholar
Kim, H. et al. Structural Studies of Potassium Transport Protein KtrA Regulator of Conductance of K + (RCK) C domain in Complex with Cyclic Diadenosine Monophosphate (c-di-AMP). J. Biol. Chem . 290 , 16393–16402 (2015).
Bai, Y. et al. Cyclic di-AMP impairs potassium uptake mediated by a cyclic di-AMP binding protein in Streptococcus pneumoniae. J. Bacteriol. 196 , 614–623 (2014).
Article PubMed PubMed Central Google Scholar
Teixeira-Duarte, C. M., Fonseca, F. & Morais-Cabral, J. H. Activation of a nucleotide-dependent RCK domain requires binding of a cation cofactor to a conserved site. Elife 8 , e50661 (2019).
Article CAS PubMed PubMed Central Google Scholar
Tholema, N., Bakker, E. P., Suzuki, A. & Nakamura, T. Change to alanine of one out of four selectivity filter glycines in KtrB causes a two orders of magnitude decrease in the affinities for both K + and Na + of the Na + dependent K + uptake system KtrAB from Vibrio alginolyticus. FEBS Lett. 450 , 217–220 (1999).
Matsuda, N. et al. Na + -dependent K + uptake Ktr system from the cyanobacterium Synechocystis sp. PCC 6803 and its role in the early phases of cell adaptation to hyperosmotic shock. J. Biol. Chem. 279 , 54952–54962 (2004).
Zulkifli, L. et al. The KtrA and KtrE subunits are required for Na + -dependent K + uptake by KtrB across the plasma membrane in Synechocystis sp. strain PCC 6803. J. Bacteriol. 192 , 5063–5070 (2010).
Szollosi, A., Vieira-Pires, R. S., Teixeira-Duarte, C. M., Rocha, R. & Morais-Cabral, J. H. Dissecting the Molecular Mechanism of Nucleotide-Dependent Activation of the KtrAB K + Transporter. PLoS Biol. 14 , e1002356 (2016).
Diskowski, M. et al. Helical jackknives control the gates of the double-pore K + uptake system KtrAB. Elife 6 , e24303 (2017).
Hänelt, I. et al. Gain of function mutations in membrane region M2C2 of KtrB open a gate controlling K + transport by the KtrAB system from Vibrio alginolyticus. J. Biol. Chem. 285 , 10318–10327 (2010).
Hänelt, I. et al. Membrane region M2C2 in subunit KtrB of the K + uptake system KtrAB from Vibrio alginolyticus forms a flexible gate controlling K + flux: an electron paramagnetic resonance study. J. Biol. Chem. 285 , 28210–28219 (2010).
Tholema, N. et al. All four putative selectivity filter glycine residues in KtrB are essential for high affinity and selective K + uptake by the KtrAB system from Vibrio alginolyticus. J. Biol. Chem. 280 , 41146–41154 (2005).
Castle, A. M., Macnab, R. M. & Shulman, R. G. Measurement of intracellular sodium concentration and sodium transport in Escherichia coli by 23Na nuclear magnetic resonance. J. Biol. Chem. 261 , 3288–3294 (1986).
Bhattacharjee, A. et al. Slick (Slo2.1), a rapidly-gating sodium-activated potassium channel inhibited by ATP. J. Neurosci. 23 , 11681–11691 (2003).
Yuan, A. et al. The sodium-activated potassium channel is encoded by a member of the Slo gene family. Neuron 37 , 765–773 (2003).
Kaczmarek, L. K. Slack, Slick and Sodium-Activated Potassium Channels. ISRN Neurosci. 2013 , 354262 (2013).
Mao, X. et al. The Epilepsy of Infancy With Migrating Focal Seizures: Identification of de novo Mutations of the KCNT2 Gene That Exert Inhibitory Effects on the Corresponding Heteromeric K(Na)1.1/K(Na)1.2 Potassium Channel. Front. Cell. Neurosci. 14 , 1 (2020).
Ambrosino, P. et al. De novo gain-of-function variants in KCNT2 as a novel cause of developmental and epileptic encephalopathy. Ann. Neurol. 83 , 1198–1204 (2018).
Hite, R. K. et al. Cryo-electron microscopy structure of the Slo2.2 Na + -activated K + channel. Nature 527 , 198–203 (2015).
Hite, R. K. & MacKinnon, R. Structural Titration of Slo2.2, a Na + -Dependent K + Channel. Cell 168 , 390–399.e311 (2017).
Zhang, J. et al. Structural basis of human Slo2.2 channel gating and modulation. Cell Rep. 42 , 112858 (2023).
Tamsett, T. J., Picchione, K. E. & Bhattacharjee, A. NAD + activates KNa channels in dorsal root ganglion neurons. J. Neurosci. 29 , 5127–5134 (2009).
Harding, M. M. Metal-ligand geometry relevant to proteins and in proteins: sodium and potassium. Acta Crystallogr. Sect. D.-Biol. Crystallogr. 58 , 872–874 (2002).
Article ADS Google Scholar
Rossi, R. C. & Norby, J. G. Kinetics of K + -Stimulated Dephosphorylation and Simultaneous K + Occlusion by Na,K-Atpase, Studied with the K + Congener Tl + - the Possibility of Differences between the 1st Turnover and Steady-State. J. Biol. Chem. 268 , 12579–12590 (1993).
Boudker, O., Ryan, R. M., Yernool, D., Shimamoto, K. & Gouaux, E. Coupling substrate and ion binding to extracellular gate of a sodium-dependent aspartate transporter. Nature 445 , 387–393 (2007).
Wohlert, D., Kuhlbrandt, W. & Yildiz, O. Structure and substrate ion binding in the sodium/proton antiporter PaNhaP. Elife 3 , e03579 (2014).
Dong, J., Shi, N., Berke, I., Chen, L. & Jiang, Y. Structures of the MthK RCK domain and the effect of Ca 2+ on gating ring stability. J. Biol. Chem. 280 , 41716–41724 (2005).
Niesen, F. H., Berglund, H. & Vedadi, M. The use of differential scanning fluorimetry to detect ligand interactions that promote protein stability. Nat. Protoc. 2 , 2212–2221 (2007).
Cao, Y. et al. Crystal structure of a potassium ion transporter, TrkH. Nature 471 , 336–340 (2011).
Cao, Y. et al. Gating of the TrkH ion channel by its associated RCK protein TrkA. Nature 496 , 317–322 (2013).
Zhang, H. et al. TrkA undergoes a tetramer-to-dimer conversion to open TrkH which enables changes in membrane potential. Nat. Commun. 11 , 547 (2020).
Article ADS PubMed PubMed Central Google Scholar
Reed, R. H., Warr, S. R. C., Richardson, D. L., Moore, D. J. & Stewart, W. D. P. Multiphasic Osmotic Adjustment in a Euryhaline Cyanobacterium. FEMS Microbiol. Lett. 28 , 225–229 (1985).
Article CAS Google Scholar
Kawano, M., Abuki, R., Igarashi, K. & Kakinuma, Y. Evidence for Na + influx via the NtpJ protein of the KtrII K + uptake system in Enterococcus hirae. J. Bacteriol. 182 , 2507–2512 (2000).
Smith, F. J., Pau, V. P., Cingolani, G. & Rothberg, B. S. Structural basis of allosteric interactions among Ca 2+ -binding sites in a K + channel RCK domain. Nat. Commun. 4 , 2621 (2013).
Article ADS PubMed Google Scholar
Ye, S., Li, Y., Chen, L. & Jiang, Y. Crystal structures of a ligand-free MthK gating ring: insights into the ligand gating mechanism of K + channels. Cell 126 , 1161–1173 (2006).
King, M. M., Kayastha, B. B., Franklin, M. J. & Patrauchan, M. A. Calcium Regulation of Bacterial Virulence. Adv. Exp. Med. Biol. 1131 , 827–855 (2020).
Zhang, Z., Rosenhouse-Dantsker, A., Tang, Q. Y., Noskov, S. & Logothetis, D. E. The RCK2 domain uses a coordination site present in Kir channels to confer sodium sensitivity to Slo2.2 channels. J. Neurosci. 30 , 7554–7562 (2010).
Yang, B., Desai, R. & Kaczmarek, L. K. Slack and Slick K(Na) channels regulate the accuracy of timing of auditory neurons. J. Neurosci. 27 , 2617–2627 (2007).
Tejada, M. A., Hashem, N., Calloe, K. & Klaerke, D. A. Heteromeric Slick/Slack K + channels show graded sensitivity to cell volume changes. PloS one 12 , e0169914 (2017).
Garg, P. & Sanguinetti, M. C. Intracellular ATP does not inhibit Slo2.1 K + channels. Physiol. Rep . 2 e12118 (2014).
Zheng, S. Q. et al. MotionCor2: anisotropic correction of beam-induced motion for improved cryo-electron microscopy. Nat. Methods 14 , 331–332 (2017).
Punjani, A., Rubinstein, J. L., Fleet, D. J. & Brubaker, M. A. cryoSPARC: algorithms for rapid unsupervised cryo-EM structure determination. Nat. Methods 14 , 290–296 (2017).
Emsley, P., Lohkamp, B., Scott, W. G. & Cowtan, K. Features and development of Coot. Acta Crystallogr. D. Biol. Crystallogr. 66 , 486–501 (2010).
Sanchez-Garcia, R. et al. DeepEMhancer: a deep learning solution for cryo-EM volume post-processing. Commun. Biol. 4 , 874 (2021).
Liebschner, D. et al. Macromolecular structure determination using X-rays, neutrons and electrons: recent developments in Phenix. Acta Crystallogr. D. Struct. Biol. 75 , 861–877 (2019).
Waterhouse, A. et al. SWISS-MODEL: homology modelling of protein structures and complexes. Nucleic Acids Res 46 , W296–W303 (2018).
Wood, C. W. et al. CCBuilder: an interactive web-based tool for building, designing and assessing coiled-coil protein assemblies. Bioinformatics 30 , 3029–3035 (2014).
Jo, S., Kim, T., Iyer, V. G. & Im, W. CHARMM‐GUI: a web‐based graphical user interface for CHARMM. J. Comput. Chem. 29 , 1859–1865 (2008).
Phillips, J. C. et al. Scalable molecular dynamics on CPU and GPU architectures with NAMD. J. Chem. Phys. 153 , 044130 (2020).
Humphrey, W., Dalke, A. & Schulten, K. VMD: visual molecular dynamics. J. Mol. Graph. 14 , 33–38 (1996).
Otwinowski, Z. & Minor, W. Processing of X-ray diffraction data collected in oscillation mode. Meth. Enzymol. 276 , 307–326 (1997).
McCoy, A. J. et al. Phaser crystallographic software. J. Appl. Crystallogr. 40 , 658–674 (2007).
Boivin, S., Kozak, S. & Meijers, R. Optimization of protein purification and characterization using Thermofluor screens. Protein Expr. Puri. 91 , 192–206 (2013).
Sonoda, Y. et al. Benchmarking membrane protein detergent stability for improving throughput of high-resolution X-ray structures. Structure 19 , 17–25 (2011).
Download references
Acknowledgements
We thank Yuch-Cheng Jean and the staff of beamlines TLS 15A and TPS 05A, National Synchrotron Radiation Research Center (NSRRC) in Taiwan. The work research work was supported by research grants from National Science and Technology Council (NSTC110-2311-B-005 -005 -MY3, NSTC109-2311-B-005-005- and NSTC108-2311-B-005-003- to N.-J.H.). The cryo-EM work was supported by Academia Sinica, Taiwan Protein Project (AS-KPQ-105-TPP and AS-KPQ-109-TPP2 to M.-D.T.), and Taiwan Cryo-EM Consortium funded by National Council of Science and Technology (NSTC 112-2740-B-006-001). The cryo-EM experiments were performed at the Academia Sinica Cryo-EM Center (ASCEM) and the cryo-EM data were processed at the Academia Sinica Grid-computing Center (ASGC). ASCEM is supported by Academia Sinica (AS-CFII-108-110) and Taiwan Protein Project. ASGC is supported by Academia Sinica. We thank Dr. Shu-Chuan Jao in the Biophysics Core Facility, funded by Academia Sinica Core Facility and Innovative Instrument Project (AS-CFII-111-201), for providing technical assistance of ITC experiments. The authors thank Dr. Meng-Chiao Joseph Ho and Wen-Jin Winston Wu for useful discussions.
Author information
These authors contributed equally: Wesley Tien Chiang, Yao-Kai Chang
Authors and Affiliations
Graduate Institute of Biochemistry, National Chung Hsing University, Taichung, 402202, Taiwan
Wesley Tien Chiang, Chen-Yi Liao, Yi-Chuan Chang, Shan-Ho Chou & Nien-Jen Hu
Institute of Biological Chemistry, Academia Sinica, Taipei, 115201, Taiwan
Yao-Kai Chang, Chun-Hsiung Wang, Siou-Ying Luo, Ya-Ping Huang & Ming-Daw Tsai
Department of Civil Engineering, National Taiwan University, Taipei, 106319, Taiwan
Wei-Han Hui & Shu-Wei Chang
Department of Biomedical Engineering, National Taiwan University, Taipei, 10663, Taiwan
Shu-Wei Chang
Life Science Group, Scientific Research Division, National Synchrotron Radiation Research Center, Hsinchu, 30092, Taiwan
Chun-Jung Chen
Institute of Molecular Biology, National Chung Hsing University, Taichung, 402202, Taiwan
Wei-Chen Wang & Chien-Chen Lai
Graduate Institute of Chinese Medical Science, China Medical University, Taichung, 406040, Taiwan
Chien-Chen Lai
Department of Applied Mathematics, Feng Chia University, Taichung, 407102, Taiwan
Tzyy-Leng Horng
Institute of Genomics and Bioinformatics, National Chung Hsing University, Taichung, 402202, Taiwan
Ming-Hon Hou
School of Biomedical Sciences, Faculty of Biological Sciences and the Astbury Centre for Structural Molecular Biology, University of Leeds, Leeds, LS2 9JT, UK
Stephen P. Muench
Department of Life Science, Tunghai University, Taichung, 407224, Taiwan
Ren-Shiang Chen
Institute of Biochemical Sciences, National Taiwan University, Taipei, 106319, Taiwan
Ming-Daw Tsai
Ph.D Program in Translational Medicine, National Chung Hsing University, Taichung, 402202, Taiwan
Nien-Jen Hu
You can also search for this author in PubMed Google Scholar
Contributions
W.T.C., C.-Y.L., Y.-C.C., S.-Y.L. and Y.-K.C. conducted molecular cloning, point mutations, protein expression, purification, protein complex assembly, and biophysical studies. W.T.C. designed and performed K + flux assays. W.T.C., C.-Y.L., Y.-C.C. and C.-J.C. performed protein crystallization, X-ray diffraction experiments, anomalous scattering and crystal structure determination. Y.-K.C., C.-H.W., Y.-P.H. and S.P.M. carried out cryo-EM grid preparation, screening, data collection, processing and model building. W.-H.H., S.-W.C., T.-L.H. and R.-S.C. performed MD simulation and computational analysis. W.-C.W. and C.-C.L. carried out the LC MS/MS analysis. S.-H.C. and M.-H.H. assisted experimental designs and data analyses. W.T.C and Y.-K.C. prepared the figures. W.T.C., Y.-K.C., M.-D.T. and N.-J.H. wrote the manuscript. M.-D.T. and N.-J.H. conceived and supervised the research project.
Corresponding authors
Correspondence to Ming-Daw Tsai or Nien-Jen Hu .
Ethics declarations
Competing interests.
The authors declare no competing interests.
Peer review
Peer review information.
Nature Communications thanks Krishna Reddy and the other, anonymous, reviewer for their contribution to the peer review of this work. A peer review file is available.
Additional information
Publisher’s note Springer Nature remains neutral with regard to jurisdictional claims in published maps and institutional affiliations.
Supplementary information
Supplementary information, peer review file, description of additional supplementary files, supplementary data 1, supplementary data 2, supplementary data 3, supplementary data 4, supplementary data 5, reporting summary, source data, source data, rights and permissions.
Open Access This article is licensed under a Creative Commons Attribution 4.0 International License, which permits use, sharing, adaptation, distribution and reproduction in any medium or format, as long as you give appropriate credit to the original author(s) and the source, provide a link to the Creative Commons licence, and indicate if changes were made. The images or other third party material in this article are included in the article’s Creative Commons licence, unless indicated otherwise in a credit line to the material. If material is not included in the article’s Creative Commons licence and your intended use is not permitted by statutory regulation or exceeds the permitted use, you will need to obtain permission directly from the copyright holder. To view a copy of this licence, visit http://creativecommons.org/licenses/by/4.0/ .
Reprints and permissions
About this article
Cite this article.
Chiang, W.T., Chang, YK., Hui, WH. et al. Structural basis and synergism of ATP and Na + activation in bacterial K + uptake system KtrAB. Nat Commun 15 , 3850 (2024). https://doi.org/10.1038/s41467-024-48057-y
Download citation
Received : 14 September 2023
Accepted : 17 April 2024
Published : 08 May 2024
DOI : https://doi.org/10.1038/s41467-024-48057-y
Share this article
Anyone you share the following link with will be able to read this content:
Sorry, a shareable link is not currently available for this article.
Provided by the Springer Nature SharedIt content-sharing initiative
By submitting a comment you agree to abide by our Terms and Community Guidelines . If you find something abusive or that does not comply with our terms or guidelines please flag it as inappropriate.
Quick links
- Explore articles by subject
- Guide to authors
- Editorial policies
Sign up for the Nature Briefing newsletter — what matters in science, free to your inbox daily.

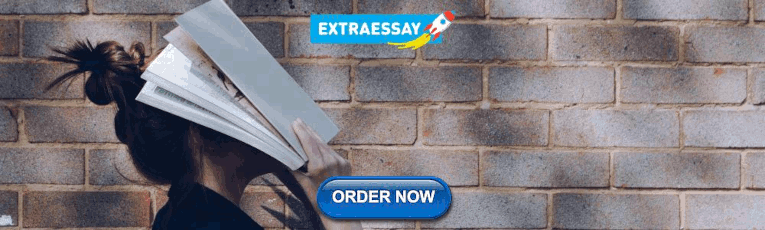
IMAGES
VIDEO
COMMENTS
Basics of Structural Equation Modeling. Feb 16, 2015 • Download as PPTX, PDF •. 35 likes • 23,152 views. S. smackinnon. These are some slides I use in my Multivariate Statistics course to teach psychology graduate student the basics of structural equation modeling using the lavaan package in R. Topics are at an introductory level, for ...
Learning Objectives. After reading this chapter, you should: 1. Understand the principles of structural equation modeling (SEM) 2. Describe the basic elements of a structural equation model. 3. Comprehend the basic concepts of partial least squares structural equation modeling (PLS-SEM) 4.
Kosuke Imai (Princeton) Structural Equation Modeling POL572 Spring 2016 16 / 39. Need for Sensitivity Analysis The sequential ignorability assumption is often too strong Need to assess the robustness of findings via sensitivity analysis Question: How large a departure from the key assumption must
Structural equation modeling (SEM) also known as latent variable modeling, latent variable path analysis, (means and) covariance (or moment) structure analysis, causal modeling, etc.; a technique for investigating relationships between latent (unobserved) variables or constructs that are measured by (multiple) manifest (observed) variables or ...
The scope of SEM is very well put by Stata's introduction to SEM: "Structural equation modeling is not just an estimation method for a particular model in the way that Stata's regress and probit commands are, or even in the way that stcox and mixed are. Structural equation modeling is a way of thinking, a way of writing, and a way of ...
Structural equation modeling is a multivariate data analysis method for analyzing complex relationships among constructs and indicators. To estimate structural equation models, researchers generally draw on two methods: covariance-based SEM (CB-SEM) and partial least squares SEM (PLS-SEM). Whereas CB-SEM is primarily used to confirm theories ...
Structural equation modeling (SEM) is a comprehensive statistical approach to testing hypotheses about relations among observed and latent variables (Hoyle, 1995). is a methodology for representing, estimating, and testing a theoretical network of (mostly) linear relations between variables (Rigdon, 1998).
Stata 12 has Structural equation modeling (SEM) using either graphical commands (like SIMPLIS) or command syntax in scalar algebra (like EQS), as well as GSEM (Generalized Structural Equation Models) and GLAMM (Generalized Linear Latent and Mixed Models). 3. R has John Fox's sem package and Yves Rosseel's lavann package.
The book describes a basic structural equation model followed by the presentation of several different types of structural equation models. Our approach in the text is both conceptual and ...
The goal of Structural Equation Modeling is to model the relations between measured and latent variables, or between multiple latent variables. If you want to use Structural Equation Modeling, you must try to identify the underlying concept that is important but not measurable. Secondly, Structural Equation Modeling applies mostly as a ...
Structural equation modeling (SEM) • is a comprehensive statistical approach to testing hypotheses about relations among observed and latent variables (Hoyle, 1995). • is a methodology for representing, estimating, and testing a theoretical network of (mostly) linear relations between variables (Rigdon, 1998). • tests hypothesized ...
This video describes best practices for how to present structural equation modeling results in a journal article. The video will discuss presenting the resu...
This paper is a tribute to researchers who have significantly contributed to improving and advancing structural equation modeling (SEM). It is, therefore, a brief overview of SEM and presents its beginnings, historical development, its usefulness in the social sciences and the statistical and philosophical (theoretical) controversies which have often appeared in the literature pertaining to SEM.
Technical Aspects Of Structural Equation Modeling. General model formulation for G groups. yig = vg + Λg ηig + Kg xig + εig, (26) ηig = αg + Bg ηig + Γg xig + ζig, (27) The covariance matrices. g Θ = V (ε ig) g and Ψ = V (ζ ig) are also allowed to vary across the G groups.
Purpose. This seminar will introduce basic concepts of structural equation modeling using lavaan in the R statistical programming language. Its emphasis is on identifying various manifestations of SEM models and interpreting the output rather than a thorough mathematical treatment or a comprehensive list of syntax options in lavaan.Since SEM is a broad topic, only the most fundamental topics ...
Evaluating structure - simultaneous equation models 4293 3.5. The role of nonexperimental data in structural modeling 4301 4. A framework for structural econometric models in IO 4303 4.1. The economic model 4304 ... Structural models 4365 8.1.3. Nonparametric identification and estimation 4368 8.2. Further issues 4374 8.3.
Structural equation modeling (SEM) is a research method that is widely used to verify complex phenomena in the field of nursing, related to various themes such as humans, health, and the environment. ... Table 5 provides an example of the presentation of the main results of the structural model.
Structural Equation Modelling. Jun 06, 2012. 890 likes | 1.87k Views. Structural Equation Modelling. Jouko Miettunen, PhD Department of Psychiatry University of Oulu e-mail: [email protected]. Topics of this presentation. Background Factor analyses Regression analyses Theory Modeling with AMOS References.
Introduction • Structural equation modeling (SEM), as a concept, is a combination of statistical techniques such as exploratory factor analysis and multiple regression. • The purpose of SEM is to examine a set of relationships between one or more Independent Variables (IV) and one or more Dependent Variables (DV).
The purpose of this chapter is to present an accessible overview of recent research on what are termed structural equation models (SEM). This presentation is intended for graduate level students in the behavioral sciences, possibly taking a SEM class, but formal algebra or calculus is not required.
2021 •. Dr. Ramachandran Manickam. Structural equation modeling is many dimensions are a statistic is the technique of analysis, which is structural Used to analyze relationships. This technique includes factor analysis and multiple regression analysis and Is a combination of measured variables hidden constructions.
Among the earliest publications of this sort is a brief invited article in Psychology and Aging "in response to confusion regarding ideal methods for reporting structural equation modeling " (Editor's Note in Raykov, Tomer, & Nesselroade, 1991, p. 499). The article offers a set of publication guidelines, ranging from the presentation of ...
The fraction of folded protein was calculated by the equation: 1-F i /F max, where F i is the fluorescence of each time point, and F max is the maximal fluorescence measured in that specific ...